- Research article
- Open access
- Published: 29 August 2023
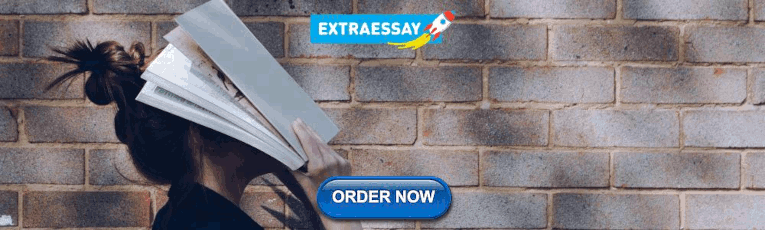
Associations of water, sanitation, and hygiene with typhoid fever in case–control studies: a systematic review and meta-analysis
- Chaelin Kim ORCID: orcid.org/0000-0001-7720-3349 1 ,
- Gerard R. Goucher 1 ,
- Birkneh Tilahun Tadesse ORCID: orcid.org/0000-0003-4005-8605 1 ,
- Woojoo Lee ORCID: orcid.org/0000-0001-7447-7045 2 ,
- Kaja Abbas ORCID: orcid.org/0000-0003-0563-1576 3 &
- Jong-Hoon Kim ORCID: orcid.org/0000-0002-9717-4044 1
BMC Infectious Diseases volume 23 , Article number: 562 ( 2023 ) Cite this article
2089 Accesses
2 Citations
7 Altmetric
Metrics details
Water, sanitation, and hygiene (WASH) play a pivotal role in controlling typhoid fever, as it is primarily transmitted through oral-fecal pathways. Given our constrained resources, staying current with the most recent research is crucial. This ensures we remain informed about practical insights regarding effective typhoid fever control strategies across various WASH components. We conducted a systematic review and meta-analysis of case-control studies to estimate the associations of water, sanitation, and hygiene exposures with typhoid fever.
We updated the previous review conducted by Brockett et al. We included new findings published between June 2018 and October 2022 in Web of Science, Embase, and PubMed. We used the Risk of Bias in Non-Randomized Studies of Interventions (ROBINS-I) tool for risk of bias (ROB) assessment. We classified WASH exposures according to the classification provided by the WHO/UNICEF Joint Monitoring Programme for Water Supply, Sanitation, and Hygiene (JMP) update in 2015. We conducted the meta-analyses by only including studies that did not have a critical ROB in both Bayesian and frequentist random-effects models.
We identified 8 new studies and analyzed 27 studies in total. Our analyses showed that while the general insights on the protective (or harmful) impact of improved (or unimproved) WASH remain the same, the pooled estimates of OR differed. Pooled estimates of limited hygiene (OR = 2.26, 95% CrI: 1.38 to 3.64), untreated water (OR = 1.96, 95% CrI: 1.28 to 3.27) and surface water (OR = 2.14, 95% CrI: 1.03 to 4.06) showed 3% increase, 18% decrease, and 16% increase, respectively, from the existing estimates. On the other hand, improved WASH reduced the odds of typhoid fever with pooled estimates for improved water source (OR = 0.54, 95% CrI: 0.31 to 1.08), basic hygiene (OR = 0.6, 95% CrI: 0.38 to 0.97) and treated water (OR = 0.54, 95% CrI: 0.36 to 0.8) showing 26% decrease, 15% increase, and 8% decrease, respectively, from the existing estimates.
Conclusions
The updated pooled estimates of ORs for the association of WASH with typhoid fever showed clear changes from the existing estimates. Our study affirms that relatively low-cost WASH strategies such as basic hygiene or water treatment can be an effective tool to provide protection against typhoid fever in addition to other resource-intensive ways to improve WASH.
Trial registration
PROSPERO 2021 CRD42021271881.
Peer Review reports
Typhoid fever, an infection caused by Salmonella enterica serovar Typhi ( S . Typhi), is a global public health problem. An estimated 11 to 20 million typhoid fever cases including 128,000 to 161,000 deaths occur each year [ 1 , 2 , 3 , 4 ] with the majority in low- and middle-income countries (LMICs) [ 5 , 6 ]. Although several effective treatment and prevention strategies are available [ 7 ], improving water, sanitation, and hygiene (WASH) is considered key to preventing typhoid fever considering that S . Typhi is transmitted via fecally contaminated water or food [ 8 ].
Understanding the relative strengths of the association between different components of WASH and typhoid fever may lead to more cost-effective strategies for implementing various WASH components that can provide the strongest protection against typhoid fever [ 9 ]. Designing such a strategy requires a detailed understanding of the strength of the association between different components of WASH and typhoid fever.
Population levels of access to improved WASH are monitored by the WHO/UNICEF Joint Monitoring Programme for Water Supply, Sanitation and Hygiene (JMP) in over 190 countries since 1990 [ 10 ]. The JMP WASH classification has three categories – drinking water, sanitation, and hygiene – and each category has service ladders indicating different levels of improvement. For instance, the drinking water category has five service ladders: safely managed, basic, limited, improved, unimproved, and surface water. JMP estimates on each of the different categories can be compared across each of the 190 countries that cover almost all of the LMICs.
Understanding the strength of the association between the levels of WASH and typhoid fever risk can create an opportunity to leverage the efforts of the JMP to better understand the risk of typhoid fever within and across countries. Although an association between typhoid fever and the levels of WASH practices is evident, the strength of this association tends to differ across studies. The systematic review and meta-analysis by Mogasale et al. [ 11 ] summarized the findings from case–control studies on the association between the levels of WASH and typhoid fever. This study focused only on the drinking water source and exposure categories of the included studies were not classified according to the JMP WASH categories. The systematic review and meta-analysis by Brockett et al. [ 12 ] included all three categories of WASH and categorized WASH exposures from case–control studies according to JMP WASH classification, but was applied in a broader level without using specific service ladders. Both studies included findings based on Widal-confirmed typhoid fever cases in addition to cases confirmed through blood culture, which may introduce bias because of the low specificity of the Widal test [ 13 ].
In this study, we aim to improve the estimates for the association between WASH exposures and typhoid fever by including new findings published since the previous review done by Brockett et al. [ 12 ], applying a rigorous risk of bias assessment, and clarifying the association between the JMP WASH categories and WASH exposures measured in case–control studies. Our study findings will be useful to infer actionable insights on the most effective ways to prevent the spread of typhoid fever and the ways to leverage the WHO/UNICEF JMP WASH data to explore the potential burden of typhoid fever.
Search strategy
We searched three databases – Web of Science, Embase, and PubMed – to find peer-reviewed articles in English. In each database, we searched using the following search terms: (“case control” OR “case–control”) AND “typhoid”. The search terms were consistent with the previous review done by Brokett et al. [ 12 ] except that we did not include “retrospective” to restrict our search to case–control studies. We restricted our search to articles published from June 2018 through Oct 2022 to identify articles that were published after the publication of Brockett et al. study [ 12 ], which included articles published between January 1990 and June 2018.
Inclusion and exclusion criteria
We developed inclusion and exclusion criteria based on the population, intervention, comparison, outcomes, and study design (PICOS) framework [ 14 ]. These predefined criteria were included in the protocol published in PROSPERO [ 15 ]. Eligible study populations encompassed populations of all ages, genders, and socioeconomic statuses living in low- and middle-income countries as defined by the World Bank [ 16 ]. Studies would be eligible for inclusion if they considered one of five WASH exposure categories, specifically: water source, water management, water treatment, sanitation, and hygiene. We excluded studies that were meant to evaluate vaccine efficacy in which the nature of interactions between WASH exposures and vaccination was not clear. Studies were considered eligible if they investigated association between typhoid fever and at least one WASH exposure using an odds ratio (OR).
WASH exposure categories
Studies varied in their WASH exposures, and we tried to systematically map the WASH exposures from included studies to the JMP WASH categories and service ladders (Table 1 ). The JMP provided service ladders for each of the three WASH categories: drinking water, sanitation, and hygiene. In addition to these three categories, we used two additional categories of water treatment and water management to delve into other important characteristics of water exposures. These two categories were also used in the previous review by Brockett et al . [ 12 ]. However, for hygiene, we aimed to utilize the JMP service ladder, which specifically focuses on handwashing practices by assessing the availability of handwashing facilities with soap and water at home. While we acknowledge the substantial role of food hygiene in typhoid infection, we did not include it in our study as we chose to follow the JMP's definition of hygiene [ 17 ].
We checked weather specific WASH exposures from included studies matched the JMP ladder definitions. If they matched one of these definitions, the exposure would be placed into the corresponding JMP ladder. For instance, basic in the JMP hygiene ladder was defined as “availability of a handwashing facility with soap and water at home”. Accordingly, we classified relevant exposures such as the use of soap for handwashing or soap available to wash hands under the basic hygiene category. We used the five WASH categories with 15 subcategories to synthesize the findings on the association between the WASH characteristics and typhoid fever.
Data extraction
We had three reviewers (CK, GG, JHK). Two reviewers assigned to each study determined the eligibility of articles in two separate phases. Any disagreements were resolved by discussion. Initially, titles and abstracts were screened to ensure that the studies used the case–control methodology, that the outcomes are typhoid cases, and that the context was in LMIC. Then, full manuscripts were read to ensure that articles met all of our PICOS criteria. Two reviewers (CK, GG) extracted data from the included studies, including author information, publication year, case/control definitions, WASH exposures, diagnostic methods, country, and effect size (odds ratio) for individual exposures. Google Sheets was used to manage the data.
Risk of bias assessment
We assessed the risk of bias of the included studies using the Cochrane Risk of Bias in Non-Randomized Studies of Interventions (ROBINS-I) tool [ 18 ] in seven domains: 1) confounding, 2) selection, 3) intervention classification, 4) intervention deviation, 5) missing data, 6) outcome measurement, and 7) selective reporting. Based on the assessment results in each domain, the studies were labeled as having a low, moderate, serious, or critical risk of bias. Two authors (CK, JHK) examined the risk of bias independently, and any discrepancies were resolved by discussion.
Statistical analysis
Data from studies that did not have critical risk of bias were used to generate the pooled estimates. Studies that did not use culture-confirmed cases were excluded in any data synthesis. The analyses were performed using the R statistical software (version 4.1.3). We developed a series of Bayesian random effects models using the brms package [ 19 ] to estimate the pooled ORs with 95% credible intervals (CrIs) for each exposure category with more than two studies. Random effects models were utilized as we assume that true effects may vary for each study depending on the contexts. Bayesian meta-analyses are particularly useful when the number of studies is small and enable us to use prior knowledge [ 20 ]. We assessed the possibility of publication bias through visual inspection of the funnel plots (Appendix B ). The repository for the data and software code of this study are publicly accessible at the GitHub repository [ 21 ].
Overview of included studies
The PRISMA flow diagram (Fig. 1 ) depicts the different phases of a systematic review. We identified 51, 44, and 50 articles from Web of Science, PubMed, and Embase, respectively. We obtained 101 unique articles after removing the duplicates. After reviewing the title and abstract, we excluded 89 non-eligible articles and reviewed the full-text copies of 12 studies. Following the full-text review, eight new studies were included in our review in addition to the 19 studies included in the previous review conducted by Brockett et al. [ 12 ], hence making a total of 27 studies included in our review. All extracted data from the included studies can be found in Appendix A . The newly identified studies are from the Democratic Republic of Congo, Fiji, India, Malawi, Pakistan and Uganda [ 22 , 23 , 24 , 25 , 26 , 27 , 28 , 29 ]. Among the 27 included studies, 18 studies (67%) used blood culture to define cases. The included studies showed variability in terms of the WASH exposures studied and the variables controlled when estimating the association between these WASH exposures and the odds of typhoid fever (Table 2 ). After removing the studies with potentially critical risk of bias, we included 18 studies for meta-analyses.
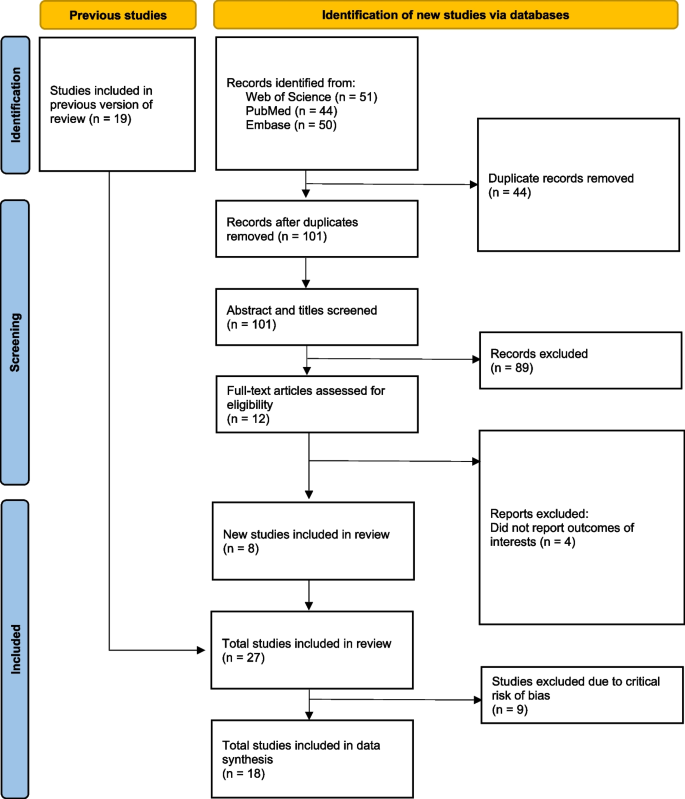
PRISMA flow diagram. The PRISMA (Preferred Reporting Items for Systematic Reviews and Meta-Analyses) flow diagram shows the number of articles at the different phases of identification, screening, and inclusion in the systematic review and meta-analysis
Except for six studies, which were categorized as having an overall moderate risk of bias, all other studies were classified as having an overall serious or critical risk of bias (Fig. 2 ). For the domain of confounding, 16 studies controlled for suspected confounding factors (i.e., age, sex, and socioeconomic characteristics) and were assessed as having a moderate risk of bias even though some level of confounding may still exist because of the inherent nature of the case–control study. For the domains of intervention classification, deviations from intended interventions, and the selection of the reported result, 23, 18, and 19 studies, respectively, were classified as having a moderate or low risk of bias. In addition, 13 studies were labeled as having a low risk of bias as they utilized a culture-confirmed typhoid fever diagnosis. However, 16 studies were rated as having a serious risk of bias as the case–control research design is prone to selection bias. Lastly, 13 studies did not provide adequate information to assess bias due to missing data. The figure on risk of bias assessment results broken down for each risk of bias criterion can be found in Appendix C .
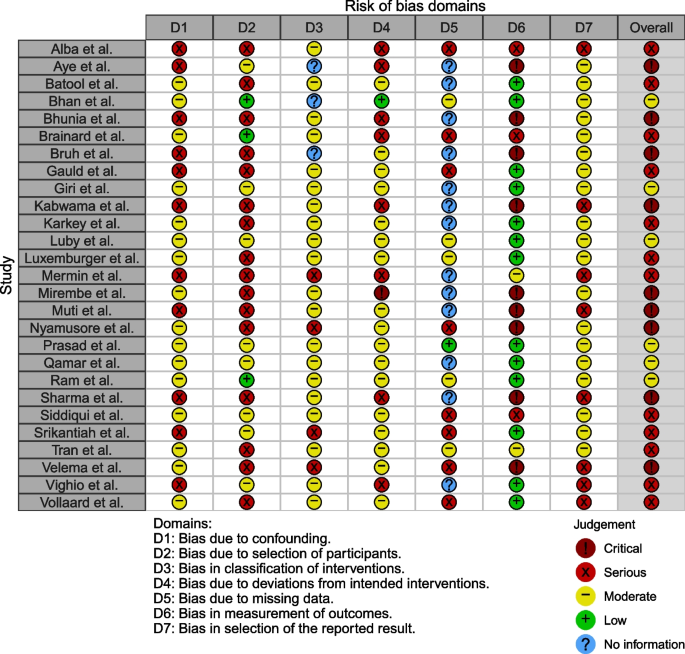
Risk of bias assessment using the Cochrane ROBINS-I tool. The studies included in the systematic review were assessed for risk of bias due to 1) confounding, 2) selection, 3) intervention classification, 4) intervention deviation, 5) missing data, 6) outcome measurement, and 7) selective reporting
Meta-analyses
We performed meta-analyses for the seven categories for which there were more than two studies. Overall, the potential risk factors doubled the odds of typhoid (OR = 1.91, 95% CrI: 1.38 to 2.79), while the potential protective factors reduced the odds by half (OR = 0.51, 95% CrI: 0.38 to 0.65) (Appendix E ).
Water source
JMP definition of improved water source includes piped water, protected dug wells, tube wells, protected springs, rainwater, and packaged water. While the improved water source can be further divided using the service ladders (i.e., safely managed, basic, or limited), we used only one category of improved water source because the number of studies is small and descriptions about the exposure were not detailed enough for further classification. Three studies reported data on the improved water source [ 41 , 44 , 47 ]. The pooled estimate of the ORs of improved water source was 0.54 (95% CrI: 0.31 to 1.08) with the between-study heterogeneity (τ) of 0.29.
Drinking water from an unimproved water source (i.e., unprotected dug well or spring) or directly from surface water are risk factors for typhoid fever. Five values fitted into the surface water group. Surface water sources increased the odds of typhoid by 2.14 (95% Crl: 1.03 to 4.06) with the between-study heterogeneity (τ) of 0.35 (Fig. 3 ).
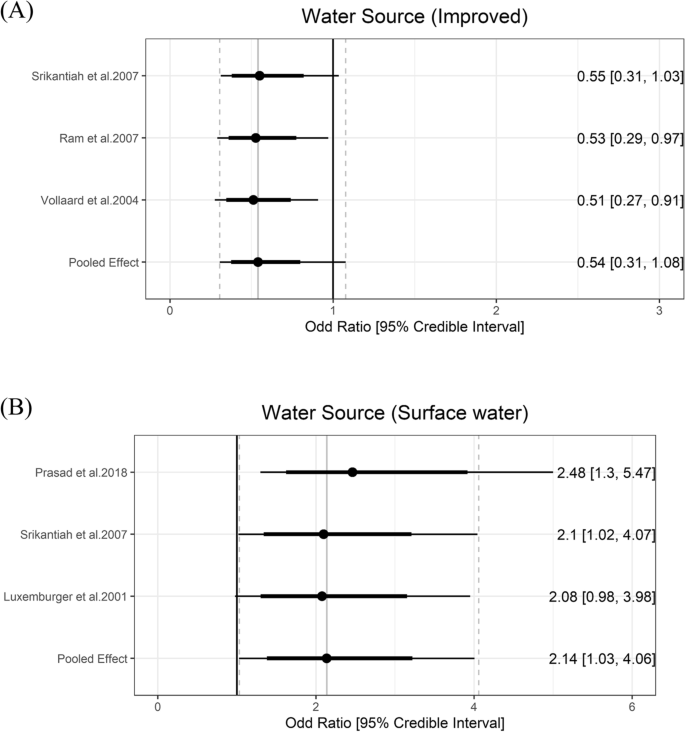
Association between water source and typhoid fever. The forest plot illustrates the association between water source and typhoid fever. Filled circles are posterior median values. Thick and thin black lines show 80% and 95% credible intervals, respectively
Water treatment
Household water treatment of any kind was included as a predicted protective factor due to prior evidence on decreasing typhoid fever burden [ 48 ]. Five studies reported information on water treatment and six exposures were classified as the water treatment group. The meta-analysis showed that any kind of household water treatment lowered the odds of typhoid by 0.54 (95% Crl = 0.36 to 0.8) with the between-study heterogeneity (τ) of 0.37. Using untreated water was a risk factor and increased the odds of typhoid fever by 1.96 (95% Crl = 1.28 to 3.27) with the between-study heterogeneity (τ) of 0.55 (Fig. 4 ).
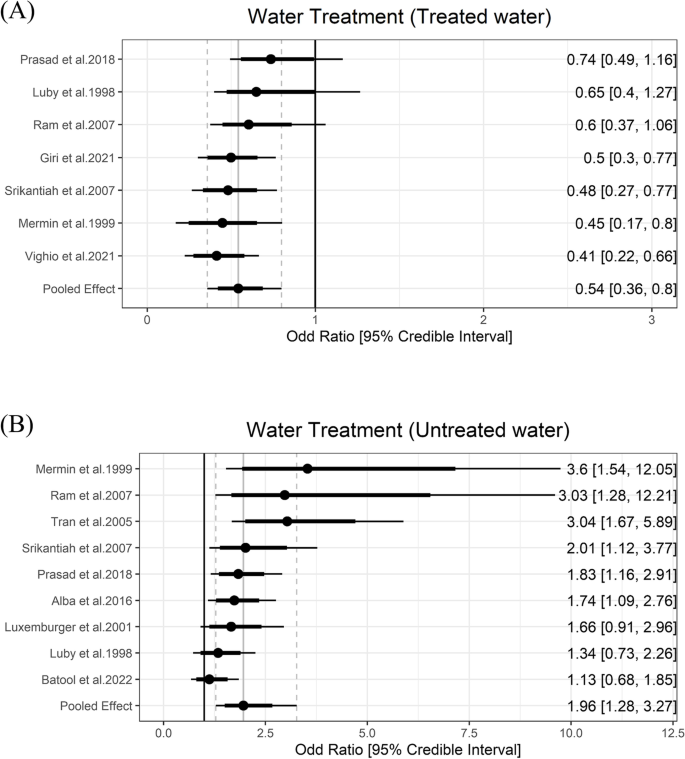
Association between water treatment and typhoid fever. The forest plot illustrates the association between water treatment and typhoid fever. Filled circles are posterior median values. Thick and thin black lines show 80% and 95% credible intervals, respectively
Water management
Safely managed water refers to water being stored in a narrow-mouthed, closed lid to prevent contamination [ 49 ], and is considered a protective factor against water-borne diseases. In order to expand the concept of safe water management and get a broader pool of data, we considered narrow-mouthed and/or closed lids in our exposure categories. Two studies measured the association between safely managed water and typhoid fever [ 35 , 44 ]. Using metal coverage of water storage and keeping water containers covered were associated with around 80% lower odds of having typhoid fever (odds ratio [OR]: 0.22, 95% confidence interval [95% CI]: 0.1 to 0.6; OR: 0.2, 95% CI: 0.04 to 1.1) [ 3 , 4 ]. Unsafe water management, such as the use of contaminated water storage, is a risk factor, and using dirty containers to store drinking water was associated with double the odds of having typhoid fever (aOR: 1.99, 95% CI: 0.6 to 6.65) [ 32 ]. Meta-analysis was not performed in the water management category due to less than three studies.
JMP defines improved sanitation facilities as those that prevent human contact with excreta. The categories of improved sanitation facilities can be further divided into safely managed, basic, and limited categories. No exposure categories from studies could be classified into these ladder rungs. Prasad et al. [ 24 ] measured that people who were using unimproved pit latrine had nearly 50 times greater odds of having typhoid than the controls (aOR: 49.47, 95% CI: 9.42 to 259.92). On the other hand, the pooled estimate of the ORs of open defecation was 1.21 (95% Crl = 0.64 to 3.41) with the between-study heterogeneity (τ) of 0.56 (Fig. 5 ).
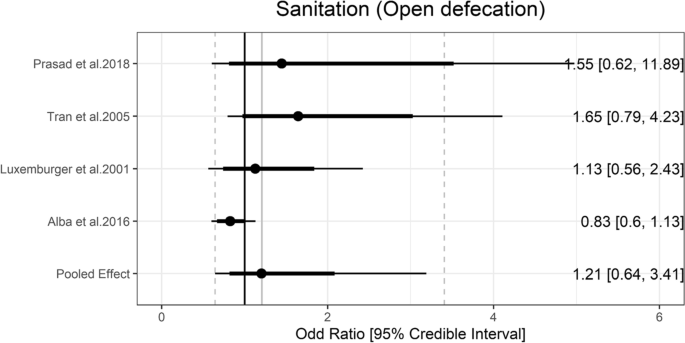
Association between sanitation and typhoid fever. The forest plot illustrates the association between sanitation and typhoid fever. Filled circles are posterior median values. Thick and thin black lines show 80% and 95% credible intervals, respectively
According to the JMP definitions, basic hygiene means that a handwashing facility with soap and water is available at home, and washing hands with soap is protective against diarrhea [ 48 ]. In meta-analysis, basic hygiene was associated with lower odds of typhoid (OR = 0.60, 95% Crl = 0.38 to 0.97) with the between-study heterogeneity (τ) of 0.24. Limited hygiene means that a handwashing facility is available at home without soap and/or water. Limited hygiene was associated higher odds of typhoid (OR = 2.26, 95% Crl = 1.38 to 3.64) with the between-study heterogeneity (τ) of 0.29 (Fig. 6 ).
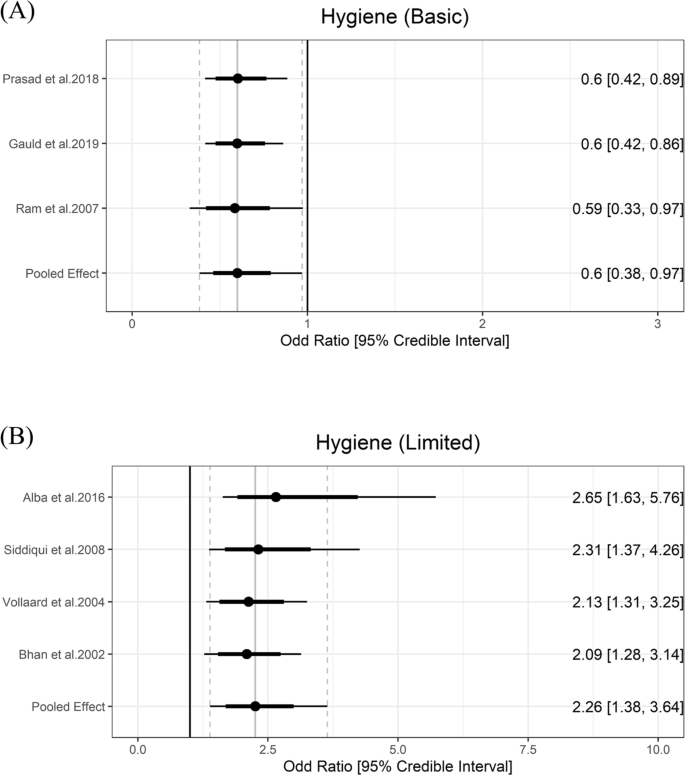
Association between hygiene and typhoid fever. The forest plot illustrates the association between hygiene and typhoid fever. Filled circles are posterior median values. Thick and thin black lines show 80% and 95% credible intervals, respectively
We conducted a systematic review and meta-analysis of case–control studies to infer the association between water, sanitation, and hygiene (WASH) and culture-confirmed typhoid fever. Our analyses updated the previous estimates of Brockett et al. [ 12 ] by adding the data published between June 2018 and Oct 2022 in addition to those included in the previous review and conducting a more comprehensive risk of bias assessment using the ROBINS-I tool. Our pooled estimates for ORs clearly varied from existing estimates while our study confirmed that improved WASH such as treated water and basic hygiene provided substantial protection against typhoid fever and limited hygiene, using untreated water and surface water increased the odds of typhoid fever.
Our meta-analyses of the newly compiled data yielded varied quantitative inferences regarding the association between WASH and typhoid fever compared to prior meta-analyses [ 12 ] (Appendix F ), particularly in terms of pooled estimates and confidence (and credible) intervals. In terms of protective factors, improved water sources and treated water demonstrated a greater reduction in the odds of typhoid fever than previously reported, while the confidence (and credible) intervals of the new analyses encompassed the estimates from the prior analyses. On the other hand, surface water and limited hygiene were found to increase the odds of typhoid fever to a larger extent and untreated water had a smaller effect on increasing the odds of typhoid fever compared to the prior analyses [ 12 ]. This discrepancy could be attributed to variations in the included studies for conducting meta-analyses.
The details of the methods differed between our study and the previous study by Brockett et al. [ 12 ], which led to a different data set and consequently different pooled estimates for ORs. Firstly, for the risk of bias assessment, the previous study used the adapted version of the Quality Assessment Tool for Quantitative Studies [ 50 ]. On the other hand, we used the ROBINS-I tool and removed studies classified having “critical” risk of bias, which resulted in a smaller number of studies in the meta-analysis. Compared with other risk of bias assessment tools, the ROBINS-I is more systematic and comprehensive and was specifically designed to address weaknesses in other tools [ 18 ]. Secondly, We adopted the Bayesian framework as our primary analysis because it could better characterize the uncertainty of the estimates, particularly when the number of studies is small [ 20 ], and the difference between these two approaches are most noticeable in the width of confidence or credible intervals. (Appendix F ). Thirdly, the previous review [ 12 ] included studies in which typhoid fever was confirmed through the Widal test or clinical signs as well as blood culture whereas we included only studies in which typhoid fever was confirmed through blood culture. Clinical symptoms of typhoid fever are not specific enough to differentiate from other enteric diseases [ 51 ]. Also, previous literature indicated that Widal test had low sensitivity and specificity (< 80%) and did not recommend using Widal test alone when diagnosing typhoid fever [ 13 ]. Fourthly, the previous study included more than one estimate from each sample whereas we only included only one estimate from each sample to avoid violating the assumption of independent findings (i.e., unit-of-analysis error) [ 52 ]. For instance, the previous review included two estimates from Alba et al. [ 30 ], sometimes treating water before drinking (i.e., sometimes vs. always) and never treating water before drinking (i.e., never vs. always), as inputs for meta-analysis of the untreated water category. We only included one of the two estimates as the two estimates came from the same sample, and we chose the “never vs. always” exposure as we believed it better reflected the risk of untreated water. Similarly, the previous review included both crude and adjusted estimates of the same exposure from the same sample. On the other hand, we included only adjusted estimates in the meta-analysis. Also, when there are multiple exposure estimates from the same study that can be classified into the same JMP WASH category (e.g., use of soap and soap near the toilet can be classified into the hygiene category), the previous review included them in the meta-analysis together. We included only one from each study that fits the JMP definition better (i.e., soap near the toilet in this case) in the analyses. Fifthly, we utilized more detailed WASH subcategories. For instance, although the exposures, ‘washing hands before meals regularly or after using the toilet’, was included in the lack of hygiene category in the previous review, we did not include in our JMP hygiene categories as washing hands does not imply washing hands with soap, which better reflects the JMP hygiene category [ 43 ].
Our study has limitations. First, case–control studies included in our meta-analyses varied not only in terms of study place and time, but also in how potential biases were controlled. Therefore, the variances observed in the data set may overrepresent the actual variance of the association between the WASH and typhoid fever. However, the heterogeneities of the OR estimates did not appear to be very high (Appendix F ). Second, there were discrepancies across studies in how the WASH exposure data was collected even if they were included in the same JMP WASH category. Only few studies collected data through the direct observation (e.g., observation of soap availability) [ 32 , 41 , 43 ], while the majority of other studies relied on self-reporting, which is prone to recall bias. Third, various WASH indicators may be related to the habits of an individual and thus correlated with one another. This implies that some of the included studies that do not control for other WASH factors can not differentiate the impacts of different WASH components. Some studies controlled for other WASH factors [ 22 , 23 , 24 , 25 , 26 , 30 , 31 , 32 , 33 , 36 , 37 , 38 , 40 , 44 ], but we did not conduct separate analyses of these due to the small number of estimates available. While the estimates do not seem to vary much between the studies that account for other WASH factors and those that do not, future studies need to pay attention to the multicollinearity among the WASH variables. Fourth, while we used our best judgment to categorize the WASH exposures in case–control studies according to JMP categories, actual WASH exposures included in the same JMP WASH category still varied. Lastly, we only included findings from case–control studies as we were updating the previous review of case–control studies and also the majority of the data are available in the form of case–control studies. Findings from randomized controlled trials [ 53 , 54 ] and cohort studies [ 55 ] are consistent with our analyses. For example, in the clinical trial conducted in Kolkata, India, living in a better WASH environment led to 57% (95% CI: 15—78) reduction in typhoid risk [ 53 ].
There is room for future research in this area. While we classified the effect measures (odds ratio estimates) for the WASH exposures on typhoid fever from each study using the updated WASH ladder metric, we had to resort to the old JMP metric of "improved/unimproved" when conducting meta-analyses because of the small number of studies to analyze. In particular, few or no existing studies examined the association between typhoid fever and WASH exposures that can be classified as unimproved water source, safely managed sanitation, basic sanitation, limited sanitation, or no hygiene facility. Future research should further investigate the association between WASH and typhoid fever in this area once more when OR estimates become available. Our findings, when combined with population-level JMP WASH trends, may be used to understand and forecast the population-level risk of typhoid fever, which can provide essential insights for decision-makers. Since the population levels of WASH have been monitored since 1990 in 191 countries, one can also analyse the longitudinal data to explore the country-level association and longitudinal trends between the levels of WASH and typhoid fever burden.
Our study findings will be useful to infer actionable insights on the most effective ways to control typhoid fever in LMICs. For instance, our findings reinforce the previous findings that, in addition to infrastructure improvements, behavioural changes such as washing hands with soap have a significant impact on the risk of contracting typhoid fever [ 9 ]. While major infrastructural improvements are crucial to reduce the burden of typhoid fever, they require resources that are difficult to commit to in LMICs. On the other hand, behaviour interventions may be feasible, affordable, and effective options to reduce disease risk in LMICs.
Availability of data and materials
All data and materials are publicly available in this published article and its GitHub repository.
Kirk MD, Pires SM, Black RE, Caipo M, Crump JA, Devleesschauwer B, et al. World Health Organization estimates of the global and regional disease burden of 22 foodborne bacterial, protozoal, and viral diseases, 2010: A data synthesis. PLoS Med. 2015;12(12):e1001921.
Kim J-H, Mogasale V, Im J, Ramani E, Marks F. Updated estimates of typhoid fever burden in sub-Saharan Africa. Lancet Glob Health. 2017;5(10): e969.
Article PubMed Google Scholar
GBD 2019 Demographics Collaborators. Global age-sex-specific fertility, mortality, healthy life expectancy (HALE), and population estimates in 204 countries and territories, 1950–2019: a comprehensive demographic analysis for the Global Burden of Disease Study 2019. Lancet. 2020;396(10258):1160–203.
Article Google Scholar
GBD 2019 Diseases and Injuries Collaborators. Global burden of 369 diseases and injuries in 204 countries and territories, 1990–2019: a systematic analysis for the Global Burden of Disease Study 2019. Lancet. 2020;396(10258):1204–22.
Mogasale V, Maskery B, Ochiai RL, Lee JS, Mogasale VV, Ramani E, et al. Burden of typhoid fever in low-income and middle-income countries: a systematic, literature-based update with risk-factor adjustment. Lancet Glob Health. 2014;2(10):e570–80.
GBD 2017 Typhoid and Paratyphoid Collaborators. The global burden of typhoid and paratyphoid fevers: a systematic analysis for the Global Burden of Disease Study 2017. Lancet Infect Dis. 2019;19(4):369–81.
Birger R, Antillón M, Bilcke J, Dolecek C, Dougan G, Pollard AJ, et al. Estimating the effect of vaccination on antimicrobial-resistant typhoid fever in 73 countries supported by Gavi: a mathematical modelling study. Lancet Infect Dis. 2022;22(5):679–91.
Article PubMed PubMed Central Google Scholar
Bhunia R, Hutin Y, Ramakrishnan R, Pal N, Sen T, Murhekar M. A typhoid fever outbreak in a slum of South Dumdum municipality, West Bengal, India, 2007: evidence for foodborne and waterborne transmission. BMC Public Health. 2009;9:115.
Im J, Islam MT, Ahmmed F, Kim DR, Islam Khan A, Zaman K, et al. Can existing improvements of water, sanitation, and hygiene (WASH) in urban slums reduce the burden of typhoid fever in these settings? Clin Infect Dis. 2021;72(11):e720–6.
World Health Organization, United Nations Children’s Fund (UNICEF). Progress on household drinking water, sanitation and hygiene 2000-2020: five years into the SDGs. Geneva: World Health Organization; 2021. Available from: https://apps.who.int/iris/handle/10665/345081 .
Mogasale VV, Ramani E, Mogasale V, Park JY, Wierzba TF. Estimating Typhoid Fever Risk Associated with Lack of Access to Safe Water: A Systematic Literature Review. J Environ Public Health. 2018;2018:9589208.
Brockett S, Wolfe MK, Hamot A, Appiah GD, Mintz ED, Lantagne D. Associations among Water, Sanitation, and Hygiene, and Food Exposures and Typhoid Fever in Case-Control Studies: A Systematic Review and Meta-Analysis. Am J Trop Med Hyg. 2020;103(3):1020–31.
Mengist HM, Tilahun K. Diagnostic value of Widal test in the diagnosis of typhoid fever: a systematic review. J Med Microbiol Diagn. 2017;6(01):1–4.
McKenzie JE, Brennan SE, Ryan RE, Thomson HJ, Johnston RV, Thomas J, et al. Defining the criteria for including studies and how they will be grouped for the synthesis. In: Higgins JPT, Thomas J, Chandler J, Cumpston M, Li T, Page MJ, et al., editors. Cochrane handbook for systematic reviews of interventions. Wiley; 2019. p. 33–65.
Chapter Google Scholar
Kim J-H, Goucher G. A Systematic Review and Meta-Analysis in Case-Control Studies of the Association between Water, Sanitation, and Hygiene Exposures and Cholera and Typhoid. PROSPERO 2021 CRD42021271881. https://www.crd.york.ac.uk/prospero/display_record.php?RecordID=271881 .
The World Bank. The World by Income and Region. [cited 2022 Dec 15]. https://datatopics.worldbank.org/world-development-indicators/the-world-by-income-and-region.html .
The JMP. Hygiene. [cited 2023 Jun 15]. https://washdata.org/monitoring/hygiene .
Sterne JAC, Hernán MA, Reeves BC, Savović J, Berkman ND, Viswanathan M, et al. ROBINS-I: a tool for assessing risk of bias in non-randomised studies of interventions. BMJ. 2016;355: i4919.
Bürkner PC. Advanced Bayesian multilevel modeling with the R package brms. arXiv preprint arXiv:1705.11123. 2017.
Sutton AJ, Abrams KR. Bayesian methods in meta-analysis and evidence synthesis. Stat Methods Med Res. 2001;10(4):277–303.
Article CAS PubMed Google Scholar
Kim C, Kim J-H. WASH_Typhoid. [cited 2022 Jul 14]. https://github.com/ckim0509/WASH_Typhoid .
Brainard J, D’hondt R, Ali E, Van den Bergh R, De Weggheleire A, Baudot Y, et al. Typhoid fever outbreak in the Democratic Republic of Congo: Case control and ecological study. PLoS Negl Trop Dis. 2018;12(10):e0006795.
Gauld JS, Olgemoeller F, Nkhata R, Li C, Chirambo A, Morse T, et al. Domestic River Water Use and Risk of Typhoid Fever: Results From a Case-control Study in Blantyre. Malawi Clin Infect Dis. 2020;70(7):1278–84.
PubMed Google Scholar
Prasad N, Jenkins AP, Naucukidi L, Rosa V, Sahu-Khan A, Kama M, et al. Epidemiology and risk factors for typhoid fever in Central Division, Fiji, 2014–2017: A case-control study. PLoS Negl Trop Dis. 2018;12(6): e0006571.
Qamar FN, Yousafzai MT, Khalid M, Kazi AM, Lohana H, Karim S, et al. Outbreak investigation of ceftriaxone-resistant Salmonella enterica serotype Typhi and its risk factors among the general population in Hyderabad, Pakistan: a matched case-control study. Lancet Infect Dis. 2018;18(12):1368–76.
Mirembe BB, Mazeri S, Callaby R, Nyakarahuka L, Kankya C, Muwonge A. Temporal, spatial and household dynamics of Typhoid fever in Kasese district, Uganda. PLoS One. 2019;14(4):e0214650.
Batool R, Qureshi S, Yousafzai MT, Kazi M, Ali M, Qamar FN. Risk factors associated with extensively drug-resistant typhoid in an outbreak setting of Lyari Town Karachi, Pakistan. Am J Trop Med. 2022;106(5):1379.
Giri S, Mohan VR, Srinivasan M, Kumar N, Kumar V, Dhanapal P, et al. Case-Control Study of Household and Environmental Transmission of Typhoid Fever in India. J Infect Dis. 2021;224(Supple 5):S584-92.
Vighio A, Syed MA, Hussain I, Zia SM, Fatima M, Masood N, et al. Risk Factors of Extensively Drug Resistant Typhoid Fever Among Children in Karachi: Case-Control Study. JMIR Public Health Surveill. 2021;7(5): e27276.
Alba S, Bakker MI, Hatta M, Scheelbeek PFD, Dwiyanti R, Usman R, et al. Risk factors of typhoid infection in the Indonesian archipelago. PLoS One. 2016;11(6):e0155286.
Aye TT, Siriarayapon P. Typhoid fever outbreak in Madaya Township, Mandalay Division, Myanmar, September 2000. J Med Assoc Thai. 2004;87(4):395–9.
Bhan MK, Bahl R, Sazawal S, Sinha A, Kumar R, Mahalanabis D, et al. Association between Helicobacter pylori infection and increased risk of typhoid fever. J Infect Dis. 2002;186(12):1857–60.
Bruh RH, Hadisaputro S, Budhi K, Sakundarno M, Widjanarko B. Host risk factors for typhoid fever in children (A case study of north sumatera province). Adv Sci Lett. 2017;23(4):3541–3.
Kabwama SN, Bulage L, Nsubuga F, Pande G, Oguttu DW, Mafigiri R, et al. A large and persistent outbreak of typhoid fever caused by consuming contaminated water and street-vended beverages: Kampala, Uganda, January - June 2015. BMC Public Health. 2017;17(1):23.
Karkey A, Thompson CN, Tran Vu Thieu N, Dongol S, Le Thi Phuong T, Voong Vinh P, et al. Differential epidemiology of Salmonella Typhi and Paratyphi A in Kathmandu, Nepal: a matched case control investigation in a highly endemic enteric fever setting. PLoS Negl Trop Dis. 2013;7(8):e2391.
Luby SP, Faizan MK, Fisher-Hoch SP, Syed A, Mintz ED, Bhutta ZA, et al. Risk factors for typhoid fever in an endemic setting, Karachi. Pakistan Epidemiol Infect. 1998;120(2):129–38.
Luxemburger C, Chau MC, Mai NL, Wain J, Tran TH, Simpson JA, et al. Risk factors for typhoid fever in the Mekong delta, southern Viet Nam: a case-control study. Trans R Soc Trop Med Hyg. 2001;95(1):19–23.
Mermin JH, Villar R, Carpenter J, Roberts L, Samaridden A, Gasanova L, et al. A massive epidemic of multidrug-resistant typhoid fever in Tajikistan associated with consumption of municipal water. J Infect Dis. 1999;179(6):1416–22.
Muti M, Gombe N, Tshimanga M, Takundwa L, Bangure D, Mungofa S, et al. Typhoid outbreak investigation in Dzivaresekwa, suburb of Harare City, Zimbabwe, 2011. Pan Afr Med J. 2014;18:309.
Nyamusore J, Nahimana MR, Ngoc CT, Olu O, Isiaka A, Ndahindwa V, et al. Risk factors for transmission of Salmonella Typhi in Mahama refugee camp, Rwanda: a matched case-control study. Pan Afr Med J. 2018;29:148.
Ram PK, Naheed A, Brooks WA, Hossain MA, Mintz ED, Breiman RF, et al. Risk factors for typhoid fever in a slum in Dhaka. Bangladesh Epidemiol Infect. 2007;135(3):458–65.
Sharma PK, Ramakrishnan R, Hutin Y, Manickam P, Gupte MD. Risk factors for typhoid in Darjeeling, West Bengal, India: evidence for practical action. Trop Med Int Health. 2009;14(6):696–702.
Siddiqui FJ, Haider SR, Bhutta ZA. Risk factors for typhoid fever in children in squatter settlements of Karachi: a nested case-control study. J Infect Public Health. 2008;1(2):113–20.
Srikantiah P, Vafokulov S, Luby SP, Ishmail T, Earhart K, Khodjaev N, et al. Epidemiology and risk factors for endemic typhoid fever in Uzbekistan. Trop Med Int Health. 2007;12(7):838–47.
Tran HH, Bjune G, Nguyen BM, Rottingen JA, Grais RF, Guerin PJ. Risk factors associated with typhoid fever in Son La province, northern Vietnam. Trans R Soc Trop Med Hyg. 2005;99(11):819–26.
Velema JP, van Wijnen G, Bult P, van Naerssen T, Jota S. Typhoid fever in Ujung Pandang, Indonesia–high-risk groups and high-risk behaviours. Trop Med Int Health. 1997;2(11):1088–94.
Vollaard AM, Ali S, van Asten HAGH, Widjaja S, Visser LG, Surjadi C, et al. Risk factors for typhoid and paratyphoid fever in Jakarta. Indonesia JAMA. 2004;291(21):2607–15.
CAS PubMed Google Scholar
Cairncross S, Hunt C, Boisson S, Bostoen K, Curtis V, Fung ICH, et al. Water, sanitation and hygiene for the prevention of diarrhoea. Int J Epidemiol. 2010;39 Suppl 1(Suppl 1):i193-205.
Mintz ED, Reiff FM, Tauxe RV. Safe water treatment and storage in the home. A practical new strategy to prevent waterborne disease. JAMA. 1995;273(12):948–53.
Effective Public Healthcare Panacea Project. Quality Assessment Tool for Quantitative Studies. [cited 2023 Jan 11]. Available from: https://www.ephpp.ca/quality-assessment-tool-for-quantitative-studies/ .
Crump JA, Sjölund-Karlsson M, Gordon MA, Parry CM. Epidemiology, clinical presentation, laboratory diagnosis, antimicrobial resistance, and antimicrobial management of invasive salmonella infections. Clin Microbiol Rev. 2015;28(4):901–37.
Article CAS PubMed PubMed Central Google Scholar
Higgins JP, Green S. How to include multiple groups from one study. Cochrane Handb Syst Rev Intervent. 2011;5:0.
Im J, Khanam F, Ahmmed F, Kim DR, Kang S, Tadesse BT, et al. Prevention of Typhoid Fever by Existing Improvements in Household Water, Sanitation, and Hygiene, and the Use of the Vi Polysaccharide Typhoid Vaccine in Poor Urban Slums: Results from a Cluster-Randomized Trial. Am J Trop Med Hyg. 2022;106(4):1149–55.
Tadesse BT, Khanam F, Ahmed F, Im J, Islam M, Kim DR, Kang SS, Liu X, Chowdhury F, Ahmed T, Aziz AB. Prevention of typhoid by Vi conjugate vaccine and achievable improvements in household WASH: evidence from a cluster-randomized trial in Dhaka, Bangladesh. Clin Infect Dis. 2022;75:1681–7.
Anand PK, Ramakrishnan R. Investigation of the outbreak of typhoid in a village of Thar Desert Rajasthan, India. Indian J Med Res. 2010;131(6):799–803.
Download references
Acknowledgements
We thank Justin Im (International Vaccine Institute) and John D. Clemens (International Vaccine Institute) for their review and feedback on this article.
This work was supported, in whole or in part, by Gavi, the Vaccine Alliance, Bowdoin College, and the Bill & Melinda Gates Foundation, via the Vaccine Impact Modelling Consortium (Grant Number OPP1157270 / INV-009125). The funders were not involved in the study design, data analysis, data interpretation, and writing of the manuscript. The authors alone are responsible for the views expressed in this article and they do not necessarily represent the decisions, policy, or views of their affiliated organisations.
Author information
Authors and affiliations.
International Vaccine Institute, Seoul, South Korea
Chaelin Kim, Gerard R. Goucher, Birkneh Tilahun Tadesse & Jong-Hoon Kim
Graduate School of Public Health, Seoul National University, Seoul, South Korea
London School of Hygiene & Tropical Medicine, London, UK
You can also search for this author in PubMed Google Scholar
Contributions
J-HK and CK conceptualised and designed the study. GG, CK, J-HK reviewed studies and extracted data from the included studies. CK and J-HK examined the risk of bias and conducted the meta-analyses using statistical software. CK, J-HK, and GG wrote the first draft. All authors (CK, GG, BTT, WL, KA, J-HK) contributed to interpretation of analysis and reviewing the manuscript for important intellectual content and have approved the final version.
Corresponding author
Correspondence to Jong-Hoon Kim .
Ethics declarations
Ethics approval and consent to participate.
Not applicable.
Consent for publication
Competing interests.
The authors declare that they have no competing interests.
Additional information
Publisher's note.
Springer Nature remains neutral with regard to jurisdictional claims in published maps and institutional affiliations.
Supplementary Information
Additional file 1., rights and permissions.
Open Access This article is licensed under a Creative Commons Attribution 4.0 International License, which permits use, sharing, adaptation, distribution and reproduction in any medium or format, as long as you give appropriate credit to the original author(s) and the source, provide a link to the Creative Commons licence, and indicate if changes were made. The images or other third party material in this article are included in the article's Creative Commons licence, unless indicated otherwise in a credit line to the material. If material is not included in the article's Creative Commons licence and your intended use is not permitted by statutory regulation or exceeds the permitted use, you will need to obtain permission directly from the copyright holder. To view a copy of this licence, visit http://creativecommons.org/licenses/by/4.0/ . The Creative Commons Public Domain Dedication waiver ( http://creativecommons.org/publicdomain/zero/1.0/ ) applies to the data made available in this article, unless otherwise stated in a credit line to the data.
Reprints and permissions
About this article
Cite this article.
Kim, C., Goucher, G.R., Tadesse, B.T. et al. Associations of water, sanitation, and hygiene with typhoid fever in case–control studies: a systematic review and meta-analysis. BMC Infect Dis 23 , 562 (2023). https://doi.org/10.1186/s12879-023-08452-0
Download citation
Received : 13 February 2023
Accepted : 11 July 2023
Published : 29 August 2023
DOI : https://doi.org/10.1186/s12879-023-08452-0
Share this article
Anyone you share the following link with will be able to read this content:
Sorry, a shareable link is not currently available for this article.
Provided by the Springer Nature SharedIt content-sharing initiative
- Typhoid fever; Water, sanitation, and hygiene (WASH)
- Case-control study
- Intervention strategy
- Bayesian meta-analysis
BMC Infectious Diseases
ISSN: 1471-2334
- Submission enquiries: [email protected]
- General enquiries: [email protected]
Thank you for visiting nature.com. You are using a browser version with limited support for CSS. To obtain the best experience, we recommend you use a more up to date browser (or turn off compatibility mode in Internet Explorer). In the meantime, to ensure continued support, we are displaying the site without styles and JavaScript.
- View all journals
- My Account Login
- Explore content
- About the journal
- Publish with us
- Sign up for alerts
- Open access
- Published: 11 December 2023
Numerical investigation of a typhoid disease model in fuzzy environment
- Fazal Dayan 1 ,
- Nauman Ahmed 2 , 3 , 4 ,
- Ali Hasan Ali 5 , 6 ,
- Muhammad Rafiq 3 , 7 &
- Ali Raza 3 , 4 , 8
Scientific Reports volume 13 , Article number: 21993 ( 2023 ) Cite this article
633 Accesses
Metrics details
- Applied mathematics
- Biological models
- Infectious diseases
Salmonella Typhi, a bacteria, is responsible for typhoid fever, a potentially dangerous infection. Typhoid fever affects a large number of people each year, estimated to be between 11 and 20 million, resulting in a high mortality rate of 128,000 to 161,000 deaths. This research investigates a robust numerical analytic strategy for typhoid fever that takes infection protection into consideration and incorporates fuzzy parameters. The use of fuzzy parameters acknowledges the variation in parameter values among individuals in the population, which leads to uncertainties. Because of their diverse histories, different age groups within this community may exhibit distinct customs, habits, and levels of resistance. Fuzzy theory appears as the most appropriate instrument for dealing with these uncertainty. With this in mind, a model of typhoid fever featuring fuzzy parameters is thoroughly examined. Two numerical techniques are developed within a fuzzy framework to address this model. We employ the non-standard finite difference (NSFD) scheme, which ensures the preservation of essential properties like dynamic consistency and positivity. Additionally, we conduct numerical simulations to illustrate the practical applicability of the developed technique. In contrast to many classical methods commonly found in the literature, the proposed approach exhibits unconditional convergence, solidifying its status as a dependable tool for investigating the dynamics of typhoid disease.
Similar content being viewed by others
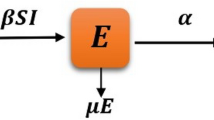
A reliable numerical investigation of an SEIR model of measles disease dynamics with fuzzy criteria
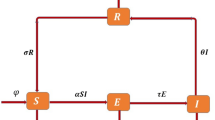
The stability analysis of a nonlinear mathematical model for typhoid fever disease
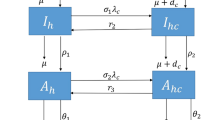
Computational study of a co-infection model of HIV/AIDS and hepatitis C virus models
Introduction.
Typhoid is a result of typhus, a condition with identical symptoms. This endemic illness is brought on by the extremely pathogenic bacteria Salmonella typhi. This bacterium was disseminated by polluted water and other carriers. Typhoid is characterized by a persistent fever, a very poor appetite, vomiting, a very bad headache, and exhaustion. The incubation period for typhoid is 7 to 14 days. The patient’s intestine, which is where the germ naturally dwells, serves as its home. There is an increase in the number of mononuclear phagocytic cells in the blood. The patient’s blood culture is a key factor in determining how to treat typhoid. Chloramphenicol is ingested if the strain is amoxicillin sensitive. The oral dose of ciprofloxacin or norfloxacin is used to eradicate the issue in the asymptomatic carrier. Due to multi-drug resistance bacteria, antibiotic treatment has grown more challenging globally. In many nations, eliminating the disease will only be possible with the provision of clean, safe, and sanitary living circumstances, wholesome food, and the aforementioned medical services. These actions may lessen or eradicate the condition, though it is difficult to reach this goal. Following the implementation of health education initiatives that alter behavior toward illness prevention and treatment, the public can be made more aware. Every year, typhoid affects millions of people throughout the world. Typhoid is currently treated with oral and injectable vaccines, however, these two are insufficient to eradicate the illness. The length of the sickness can be shortened if a drug-resistant strain is used to treat the infected person 1 , 2 .
The dynamics of infectious diseases have been studied and explained using a variety of mathematical models 3 . To investigate the spread of infection at various endemicity levels, Cvjetanovic et al. built a mathematical model for typhoid disease. The model was used to predict the likely impact of typhoid fever prevention strategies, such as mass vaccination campaigns and sanitation programs, on a chosen population in terms of illness prevention as well as in terms of relative costs and benefits 4 . In order to examine the dynamics of typhoid fever sickness while including infection resistance, Nthiiri et al. developed a mathematical model. Using the next-generation matrix technique, the model’s stable states are identified and the reproduction number is calculated. The model’s stability is analyzed to identify the factors that contribute to the disease’s spread within a specific community 5 . The dynamics of the typhoid fever model were investigated, as well as the existence and uniqueness of the solution, by Peter et al. For the model, stability analysis is also carried out 6 . Bakach et al. reviewed some mathematical models of typhoid 7 . Karunditu et al. formulated a mathematical model of typhoid fever incorporating unprotected humans. The local and global stability of equilibrium points is also studied 8 . A mathematical model for the transmission of typhoid was developed by Nyaberi and Musaili, and it examines the effects of treatment on the dynamics of the illness 9 . Birger et al. studied mathematical models of typhoid transmission by considering FQNS and multidrug resistance separately. The effect of vaccination was predicted on the basis of forecasts of vaccine coverage 10 . A mathematical model for typhoid fever spread in a population is formulated. The equilibrium points of the model and their stabilities are investigated 11 . By utilizing several optimal control strategies, Wameko et al. established a mathematical model to look into the dynamics of typhoid disease. Typhoid disinformation among the population is reduced when the three control techniques are quickly implemented, as demonstrated 12 .
Due to population-wide variations in susceptibility, exposure, infectivity, and recovery, the parameters employed in epidemic models are imprecise. If different age groups, population groups, and resistance patterns are taken into account, differences may result. To take into consideration these varying degrees of persons, more realistic models are required. Mishra et al. claim that due to the high level of uncertainty, epidemic systems, particularly those involving infectious diseases, require a new approach 13 . Fuzzy sets and fuzzy logic have been extensively utilized to tackle real-world problems across diverse domains, encompassing medicine, engineering, economics, and numerous other fields where human decision-making plays a pivotal role in assessment and logical reasoning 14 , 15 , 16 , 17 , 18 , just to mention a few. Moreover, scholars have harnessed this theoretical framework in epidemiology as well. Incorporating fuzzy theory and treating the transmission coefficient as a fuzzy set, Barros et al. suggested a SI model 19 . Fuzzy logic was used by Ortega et al. to predict issues with infectious disease epidemiology. A rabies model in dogs with incomplete vaccinations was discussed 20 .
Mondal et al. developed an SIS model for investigating the plague using the fuzzy set theory 21 . Das and Pal developed a SIR model and studied it mathematically and numerically 22 . Sadhukhan et al. conducted research on harvesting optimization in a food chain model in a fuzzy environment 23 . To capture the dynamics of coronavirus illness, Li et al. developed a fuzzy SEIR model supplemented by confidence index theory 24 . Abdy et al. presented an SIR model that incorporated fuzzy parameters to depict the dynamics of COVID-19 25 . Furthermore, Allehiany et al. explored a fuzzy SIR model employing Euler, RK-4, and NSFD methods 26 . The NSFD approach, first described by Micken 27 , has been used by a number of researchers for solving systems of differential equations 28 , 29 , 30 , to name a few. Adak and Jana investigated an SIS model involving treatment control with the utilization of fuzzy numbers 31 .
The existing mathematical models of typhoid are insufficient for the advancement of fuzzy numerical and mathematical procedures. We investigated a typhoid model with fuzzy parameters with this in mind. We can cope with the difficulties of uncertainty quantification in mathematical disease modeling by using fuzzy theory. As a result, the introduction of fuzzy parameters aids in our ability to comprehend the dynamics of typhoid transmission. Even the biological factors employed in mathematical models are not always constant because each community changes as the environment change. The majority of the issues linked to the rise in the earth’s average temperature are caused by global warming. The rate at which the virus spreads throughout the population is also impacted by temperature changes. Fuzzy mathematical models are more insightful than crisp models in this regard. The creation, use, and analysis of first order explicit numerical techniques in fuzzy non-standard finite difference situations are novel aspects of the current work.
The rest of this study is organized as follows: The formulation of the fuzzy model is discussed in the following section. Within the same section, we discuss equilibrium analysis, stability analysis, and the fuzzy basic reproduction number. Following that, in the numerical modeling section, we elaborate on the creation of the forward Euler scheme and NSFD schemes for the examined model. This section also includes an assessment of the NSFD scheme’s stability and consistency. The next part displays numerical simulations involving the developed techniques. Finally, in the final section, we summarize concluding observations and outline future research directions.
Typhoid fever model with fuzzy parameters
We examined the mathematical model previously discussed by Arif et al. 2
Here, \(T_1\) represents the proportion of humans who are protected, \(T_2\) represents the proportion of humans who are susceptible, \(T_3\) signifies the fraction of humans who are currently infected, and \(T_4\) indicates the fraction of humans receiving treatment. The rate at which humans receiving treatment transitions from the fraction of infected humans is denoted as \(\beta\) . The variable \(\alpha\) represents the rate at which individuals enroll in the protected human compartment against typhoid, while \((1-\alpha )\) denotes the rate of individuals who remain susceptible to the virus, \(\delta\) signifies the rate at which individuals experience a transient phase due to typhoid fever, and \(\theta\) reflects the per capita rate at which susceptible individuals contract typhoid fever infection. Lastly, \(\mu\) represents the natural rate of human death and birth. The depiction of the communication dynamics in the typhoid fever model is presented in Fig. 1 .
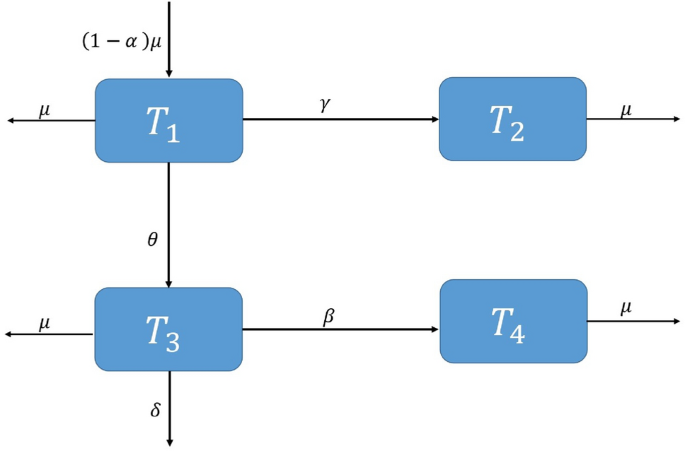
Flowchart of the model.
The fuzzy representation of the model mentioned above can be expressed as follows:
We assume that susceptible humans contract typhoid fever infection at a per capita rate denoted by \(\theta (\Omega )\) and the fraction of treated humans stemming from infected individuals, \(\beta (\Omega )\) , are represented as fuzzy numbers, contingent upon the individual’s virus load. The variable \(\theta (\Omega )\) can be defined as 19
The \(\theta (\Omega )\) reaches its peak when \(\Omega\) is at its maximum value, and it becomes insignificant when \(\Omega\) is at its minimum. \(\Omega _{min}\) represents the minimum virus load required for disease transmission, and disease transmission is at its highest when \(\Omega\) equals \(\Omega _M\) , reaching a value of 1. Similarly, \(\beta (\Omega )\) can be defined as 32
where \(\beta _0>0\) is the minimum treatment rate.
Equilibrium analysis
The analyzed model possesses a disease-free equilibrium point (DFE) and two endemic equilibrium points (EE).
If \(\Omega <\Omega _{min}\) and \(\beta (\Omega )>0\) then \(\theta (\Omega )=0\) and we obtain,
In this scenario, the human population remains free from typhoid, and this state is referred to as the DFE. From a biological perspective, typhoid disease is considered eradicated when the disease concentration within the population falls below the minimum threshold required for its sustained existence.
If \(\Omega _{min}\le \Omega \le \Omega _M\) and \(\beta (\Omega )>0\) then \(\theta (\Omega )=\frac{\Omega -\Omega _{min}}{\Omega _M-\Omega _{min}}\) and we obtain,
If \(\Omega _M<\Omega\) and \(\beta (\Omega )>0\) then \(\theta (\Omega )=1\) , and we obtain
The points \(T^*\) and \(T^{**}\) represent situations where the prevalence of typhoid disease exceeds the minimum threshold necessary for its propagation, resulting in the persistence of typhoid within the human population.
Stability analysis
To check the stability, let us assume the following system:
The Jacobian of the system ( 11 )–( 14 ) can be represented as:
The Jacobian at the DFE is
The local asymptotic stability of the steady-state is confirmed when the absolute eigenvalues of the Jacobian matrix mentioned earlier having negative real parts. Analyzing the Jacobian matrix, we find that the eigenvalues are as follows: \(\lambda _1= -(\gamma +\mu )\) , \(\lambda _2 = \lambda _4= - \mu\) , and \(\lambda _3 =- (\delta +\beta (\Omega )+\mu )\) . The fact that all of these eigenvalues having negative real parts validates the desired result.
Fuzzy basic reproductive number \(R_t^f\)
Using the next-generation matrix technique, we calculated the reproductive number, denoted as \(R_t\) .
Verma et al. 33 examined different scenarios by manipulating the parameters and, in each case, determined the reproduction number to assess whether the virus spread was effectively managed.
Now, \(R_t\) , being a function of the typhoid disease, can be analyzed as follows 34 :
If \(\Omega <\Omega _{min}\) and \(\beta (\Omega )>0\) then \(\theta (\Omega )=0\) and \(R_t(\Omega )=0\) .
If \(\Omega _{min}\le \Omega \le \Omega _M\) and \(\beta (\Omega )>0\) then \(\theta (\Omega )=\frac{\Omega -\Omega _{min}}{\Omega _M-\Omega _{min}}\) and \(R_t(\Omega )=\frac{\theta (\Omega )(\gamma +\mu -\alpha \mu )}{(\gamma +\mu )(\delta +\beta (\Omega )+\mu )}\) .
If \(\Omega _M<\Omega\) and \(\beta (\Omega )>0\) then \(\theta (\Omega )=1\) and \(R_t(\Omega )=\frac{\gamma +\mu -\alpha \mu }{(\gamma +\mu )(\delta +\beta (\Omega )+\mu )}\) . The disease-dependent function \(R_t(\Omega )\) correlates positively with the disease parameter \(\Omega\) , and its definition includes a fuzzy variable. As a result, the expected value of \(R_t(\Omega )\) is well defined, and its representation can be written as a triangular fuzzy number, as follows:
Now, \(R_t^f\) can be found as
and therefore,
Numerical modeling
Forward euler scheme.
The Forward Euler scheme is a well-known explicit first-order numerical approach for solving ordinary differential equations. It is computationally efficient and provides a rapid estimation of the behavior of solutions over time.
If \(\Omega <\Omega _{min}\) and \(\beta (\Omega )>0\) then \(\theta (\Omega )=0\) and
If \(\Omega _{min}\le \Omega \le \Omega _M\) and \(\beta (\Omega )>0\) then \(\theta (\Omega )=\frac{\Omega -\Omega _{min}}{\Omega _M-\Omega _{min}}\) and the Euler scheme becomes
If \(\Omega _M<\Omega\) and \(\beta (\Omega )>0\) then \(\theta (\Omega )=1\) and
Non-standard finite difference (NSFD) scheme
The NSFD scheme is a class of numerical methods for approximating solutions to differential equations. These approaches differ from traditional finite difference methods in their approach to discretizing the domain and approximating derivatives. It has the potential to improve accuracy in the solution of differential equations. The NSFD numerical model is formulated based on the NSFD theory introduced by Mickens 35 .
If \(\Omega <\Omega _{min}\) and \(\beta (\Omega )>0\) then \(\theta (\Omega )=0\) and the NSFD scheme becomes
If \(\Omega _{min}\le \Omega \le \Omega _M\) and \(\beta (\Omega )>0\) then \(\theta (\Omega )=\frac{\Omega -\Omega _{min}}{\Omega _M-\Omega _{min}}\) and the NSFD Scheme becomes
If \(\Omega _M<\Omega\) and \(\beta (\Omega )>0\) then \(\theta (\Omega )=1\) and the scheme becomes
Consistency analysis
To check the consistency of the proposed scheme, we start by taking Eq. ( 35 ), and we have
Taking into account the Taylor’s series expansion for \(T_1^{n+1}\) ,
Substituting the value of \(T_1^{n+1}\) in Eq. ( 51 ), we get
Taking \(h\rightarrow 0\) , we get
From Eq. ( 36 ), we have
Taking into account the Taylor’s series expansion for \(T_2^{n+1}\) ,
Substituting the value of \(T_2^{n+1}\) in Eq. ( 56 ), we get
From Eq. ( 37 ), we have
Similarly, taking into account the Taylor’s series expansion for \(T_3^{n+1}\) ,
Substituting the value of \(T_3^{n+1}\) in Eq. ( 61 ), we get
Simplifying Eq. ( 63 ) and taking \(h\rightarrow 0\) , we get
Similarly, we can get
by applying Taylor’s series on Eq. ( 38 ). Hence, we can conclude that our proposed scheme exhibits first-order consistency.
Stability of the NSFD scheme
To study the stability analysis, let us assume the following system:
The Jacobian matrix corresponding to the system ( 67 )–( 70 ) is
Jacobian at the DFE is
Eigenvalues of the above Jacobian matrix are \(\lambda _1=\frac{1}{1+h(\gamma +\mu )}<1\) , \(\lambda _2=\lambda _4=\frac{1}{1+h\mu }<1\) and \(\lambda _3=\frac{1}{1+h(\delta +\beta (\Omega )+\mu )}<1\) . Because all eigenvalues are less than one, this validates the desired outcome that the NSFD scheme is stable at the DFE 36 . NSFD schemes do not constitute a singular category of numerical methods. Numerous researchers have expanded upon the Mickens theory. For instance, Gurski 37 introduced a straightforward mathematical framework for NSFD schemes, specifically tailored for small systems of nonlinear differential equations. This approach leverages conventional techniques used in approximating differential equations, including the incorporation of artificial viscosity and the implementation of a predictor-corrector scheme. In their work, they examined both the NSFD scheme proposed by Mickens and the one developed by Erdogan and Ozis for first-order equations.
Numerical simulations
In this section, we present the simulation results of the performance of Euler method illustrated in Fig. 2 a–f, and the simulation results obtained using the NSFD method illustrated in Fig. 3 a–f.
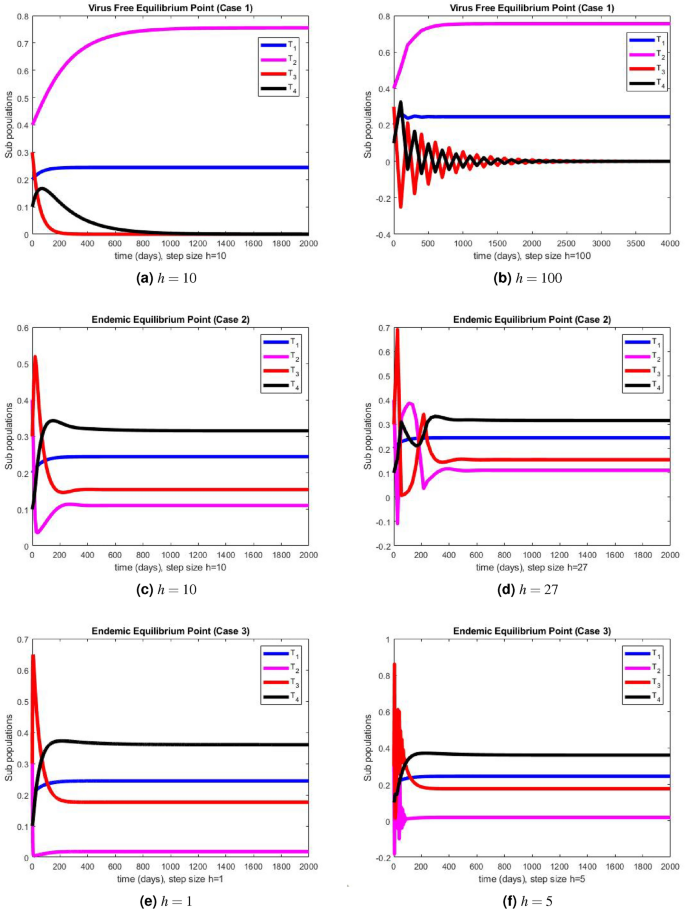
The portions of sub populations using Euler method at different step sizes.
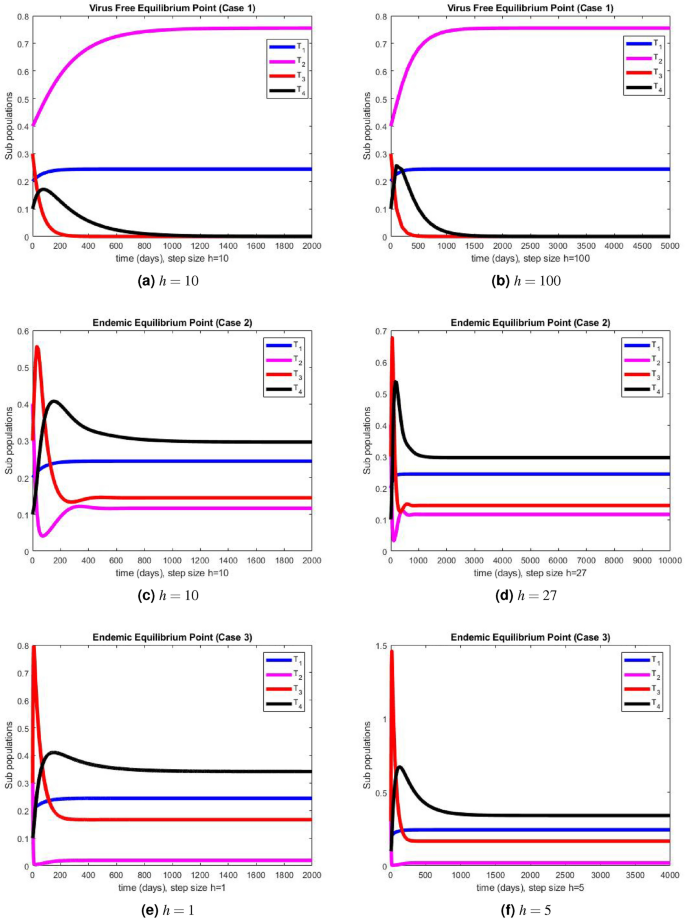
The portions of sub populations using NSFD method at different step sizes.
The graphical representations of the performance of Euler method are illustrated at various step sizes in Fig. 2 a–f. Initially, at a small step size \(h=1\) , the method exhibits stability, positivity, and convergence. However, as the step size is slightly increased to \(h=5\) , the method begins to oscillate and generates negative values in all three cases. In models like this, negative values are not meaningful since all compartments represent populations, and negativity is not feasible. Consequently, it can be deduced that this method is not a dependable tool for describing such models. In Fig. 3 a–f, we present the simulation results obtained using the NSFD method. This time, the method demonstrates stability, both at smaller and larger step sizes, and an increase in the step size does not adversely affect its convergence and positivity, which are crucial characteristics for modeling disease dynamics. The method consistently displays stable and convergent behavior across all three cases, underscoring its reliability for studying disease dynamics in such conditions. It is worth noting that many classical numerical schemes and their fuzzy counterparts tend to lose their convergence and positivity and struggle to maintain stability as the step size increases. In contrast, the proposed method proves to be more efficient and capable of addressing such issues. We provide the convergence analysis for proposed NSFD scheme analytically and then verify it numerically in numerical simulations section. It can be noted here that the proposed NSFD scheme gives unconditional convergence and remains consistent with the continuous dynamical system. Figure 3 a–f show the convergence of NSFD scheme to true equilibria, retaining all the essential features of continuous model unlike the Euler scheme which fails to do so (see Fig. 2 a–f).
Conclusions
In this study, we have considered a typhoid model with fuzzy parameters. We assumed that the infection does not transmit equally among the individual of the populations. Similarly, the treatment rate is also not the same for each individual. As a function of the virus concentrations, we treated the typhoid transmission rate \(\theta (\Omega )\) and the treatment rate \(\beta (\Omega )\) as fuzzy variables. In deterministic models, these parameters are fixed and independent of the viral load. As a result, it may be said that the fuzzy model is more adaptable and balanced than the crisp system. Fuzzy theory is used to address uncertainty quantification difficulties in mathematical disease modeling. We examined it for various virus loads because fuzzy variables are functions of virus load that depend on virus levels. In light of this, we addressed the studied model’s fuzzy equilibrium points while taking the population’s virus levels into account. We established that the disease-free equilibrium point is reached if the virus concentration is lower than the minimal concentration necessary for disease transmission in the population. When the population’s viral levels exceeded the bare minimum needed for disease transmission, we reached the endemic equilibrium points. For various viral concentrations, the basic reproduction rate is examined. We employed the expected value of a fuzzy number to ascertain the fuzzy basic reproduction number. Two numerical schemes are developed for the approximate solution of the studied model. The developed schemes are analyzed for different amounts of virus. The suggested numerical algorithms must maintain the positive nature of the solutions of such dynamic population models. The Euler method preserved this for only small values of the step sizes and generated negative values by increasing the step size. On the other hand, the NSFD preserved this for all large values of the step size too for different amounts of virus. Additionally, the convergence and consistency of the NSFD scheme are analyzed, demonstrating that the suggested approach is unconditionally convergent and consistent of order 1. The creation, application, and analysis of a non-standard finite difference technique for the numerical analysis of a typhoid illness model with fuzzy parameters are the main foci of the current work. Future developments may include fuzzy stochastic, fuzzy delayed, or fuzzy fractional dynamic senses. Age-structured fuzzy epidemic models could potentially be modeled using the NSFD modeling theory. This research focuses mostly on using triangular fuzzy numbers as membership functions. In the future, we may investigate the use of various fuzzy numbers as potential membership functions, such as trapezoidal, pentagonal, hexagonal, and so on.
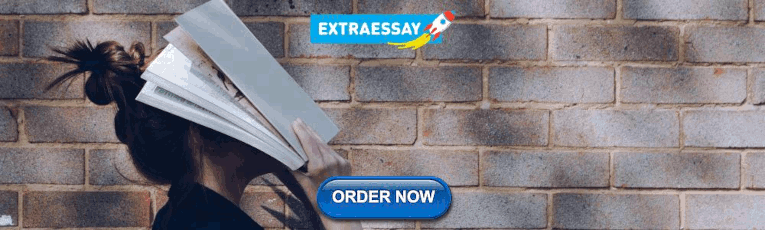
Data availability
The datasets analyzed during the current study are available from the corresponding author upon reasonable request.
Cai, L.-M. & Li, X.-Z. Global analysis of a vector-host epidemic model with nonlinear incidences. Appl. Math. Comput. 217 , 3531–3541 (2010).
Article MathSciNet MATH Google Scholar
Arif, M. S. et al. A reliable stochastic numerical analysis for typhoid fever incorporating with protection against infection. Comput. Mater. Continua 59 , 787–804 (2019).
Article Google Scholar
Alalhareth, F. K., Atta, U., Ali, A. H., Ahmad, A. & Alharbi, M. H. Analysis of leptospirosis transmission dynamics with environmental effects and bifurcation using fractional-order derivative. Alex. Eng. J. 80 , 372–382 (2023).
Cvjetanović, B., Grab, B. & Uemura, K. Epidemiological model of typhoid fever and its use in the planning and evaluation of antityphoid immunization and sanitation programmes. Bull. World Health Organ. 45 , 53–75 (1971).
PubMed PubMed Central Google Scholar
Nthiiri, J. K. et al. Mathematical modelling of typhoid fever disease incorporating protection against infection. Br. J. Math. Comput. Sci. 14 , 1–10 (2016).
Peter, O., Ibrahim, M., Akinduko, O. & Rabiu, M. Mathematical model for the control of typhoid fever. IOSR J. Math 13 , 60–66 (2017).
Google Scholar
Bakach, I., Just, M. R., Gambhir, M. & Fung, I.C.-H. Typhoid transmission: A historical perspective on mathematical model development. Trans. R. Soc. Trop. Med. Hyg. 109 , 679–689 (2015).
Article PubMed Google Scholar
Karunditu, J. W., Kimathi, G. & Osman, S. Mathematical modeling of typhoid fever disease incorporating unprotected humans in the spread dynamics. J. Adv. Math. Comput. Sci. 32 , 1–11 (2019).
Nyaberi, H. O. & Musaili, J. S. Mathematical modeling of the impact of treatment on the dynamics of typhoid. J. Egypt. Math. Soc. 29 , 15 (2021).
Birger, R. et al. Estimating the effect of vaccination on antimicrobial-resistant typhoid fever in 73 countries supported by Gavi: A mathematical modelling study. Lancet Infect. Dis. 22 , 679–691 (2022).
Article PubMed PubMed Central Google Scholar
Joshua, E. & ETUKUDO, U. Mathematical model of the spread of typhoid fever. World J. Appl. Sci. Technol. 3 , 10–12 (2011).
Wameko, M., Koya, P. & Wodajo, A. Mathematical model for transmission dynamics of typhoid fever with optimal control strategies. Int. J. Ind. Math. 12 , 283–296 (2020).
Mishra, B. K. & Prajapati, A. Spread of malicious objects in computer network: A fuzzy approach. Appl. Appl. Math. Int. J. 8 , 684–700 (2013).
MATH Google Scholar
Liao, T. W. A fuzzy multicriteria decision-making method for material selection. J. Manuf. Syst. 15 , 1–12 (1996).
Bates, J. H. & Young, M. P. Applying fuzzy logic to medical decision making in the intensive care unit. Am. J. Respir. Crit. Care Med. 167 , 948–952 (2003).
Fenton, N. & Wang, W. Risk and confidence analysis for fuzzy multicriteria decision making. Knowl.-Based Syst. 19 , 430–437 (2006).
Yeh, C.-H. & Chang, Y.-H. Modeling subjective evaluation for fuzzy group multicriteria decision making. Eur. J. Oper. Res. 194 , 464–473 (2009).
Article MATH Google Scholar
Saeed, M., Ali, U., Ali, J. & Dayan, F. Fuzzy soft relative method and its application in decision making problem: Fuzzy soft relative method and its application in decision making problem. Proc. Pak. Acad. Sci. A Phys. Comput. Sci. 57 , 21–30 (2020).
Barros, L. D., Leite, M. F. & Bassanezi, R. The SI epidemiological models with a fuzzy transmission parameter. Comput. Math. Appl. 45 , 1619–1628 (2003).
Ortega, N. R. S., Sallum, P. C. & Massad, E. Fuzzy dynamical systems in epidemic modelling. Kybernetes 29 , 201–218 (2000).
Mondal, P. K., Jana, S., Haldar, P. & Kar, T. K. Dynamical behavior of an epidemic model in a fuzzy transmission. Int. J. Uncertain. Fuzziness Knowl.-Based Syst. 23 , 651–665 (2015).
Das, A. & Pal, M. A mathematical study of an imprecise sir epidemic model with treatment control. J. Appl. Math. Comput. 56 , 477–500 (2018).
Sadhukhan, D., Sahoo, L., Mondal, B. & Maiti, M. Food chain model with optimal harvesting in fuzzy environment. J. Appl. Math. Comput. 34 , 1–18 (2010).
Li, C., Huang, J., Chen, Y.-H. & Zhao, H. A fuzzy susceptible-exposed-infected-recovered model based on the confidence index. Int. J. Fuzzy Syst. 23 , 907–917 (2021).
Abdy, M., Side, S., Annas, S., Nur, W. & Sanusi, W. An sir epidemic model for COVID-19 spread with fuzzy parameter: The case of Indonesia. Adv. Differ. Equ. 2021 , 1–17 (2021).
Article MathSciNet Google Scholar
Allehiany, F. et al. Bio-inspired numerical analysis of COVID-19 with fuzzy parameters. Comput. Mater. Continua 72 , 3213–3229 (2022).
Mickens, R. E. Dynamic consistency: A fundamental principle for constructing nonstandard finite difference schemes for differential equations. J. Differ. Equ. Appl. 11 , 645–653 (2005).
Cresson, J. & Pierret, F. Non standard finite difference scheme preserving dynamical properties. J. Comput. Appl. Math. 303 , 15–30 (2016).
Naveed, M. et al. Mathematical analysis of novel coronavirus (2019-ncov) delay pandemic model. Comput. Mater. Continua 64 , 1401–1414 (2020).
Shatanawi, W. et al. An effective numerical method for the solution of a stochastic coronavirus (2019-ncovid) pandemic model. Comput. Mater. Continua 66 , 1121–1137 (2021).
Adak, S. & Jana, S. Dynamical behavior of an epidemic model with fuzzy transmission and fuzzy treatment control. J. Appl. Math. Comput. 68 , 1929–1948 (2022).
Verma, R., Tiwari, S. & Upadhyay, R. K. Dynamical behaviors of fuzzy sir epidemic model. In Proceedings of the Conference of the European Society for Fuzzy Logic and Technology , 482–492 (Springer, 2017).
Verma, H., Mishra, V. N. & Mathur, P. Effectiveness of lock down to curtail the spread of corona virus: A mathematical model. ISA Trans. 124 , 124–134 (2022).
Mangongo, Y. T., Bukweli, J.-D.K. & Kampempe, J. D. B. Fuzzy global stability analysis of the dynamics of malaria with fuzzy transmission and recovery rates. Am. J. Oper. Res. 11 , 257–282 (2021).
Mickens, R. E. Numerical integration of population models satisfying conservation laws: NSFD methods. J. Biol. Dyn. 1 , 427–436 (2007).
Article MathSciNet PubMed MATH Google Scholar
Ratnam, K. V., Rao, P. R. S. & Shirisha, G. Stability preserving NSFD scheme for a cooperative and supportive network. Int. J. Dyn. Control 9 , 1576–1588 (2021).
Gurski, K. F. A simple construction of nonstandard finite-difference schemes for small nonlinear systems applied to sir models. Comput. Math. Appl. 66 , 2165–2177 (2013).
Download references
Author information
Authors and affiliations.
Department of Mathematics, School of Science, University of Management and Technology, Lahore, Pakistan
Fazal Dayan
Department of Mathematics and Statistics, The University of Lahore, Lahore, Pakistan
Nauman Ahmed
Department of Computer Science and Mathematics, Lebanese American University, Beirut, Lebanon
Nauman Ahmed, Muhammad Rafiq & Ali Raza
Department of Mathematics, Near East University, Mathematics Research Center, Near East Boulevard, 99138, Nicosia/Mersin 10, Turkey
Nauman Ahmed & Ali Raza
Department of Mathematics, College of Education for Pure Sciences, University of Basrah, Basrah, 61001, Iraq
Ali Hasan Ali
Institute of Mathematics, University of Debrecen, Pf. 400, Debrecen, 4002, Hungary
Department of Mathematics, Faculty of Science & Technology, University of Central Punjab, Lahore, Pakistan
Muhammad Rafiq
Department of Physical Sciences, The University of Chenab, Gujrat, Pakistan
You can also search for this author in PubMed Google Scholar
Contributions
Conceptualization, F.D. and N.A. Data curation, M.R. and A.R. Formal analysis, A.H.A. and A.R. Funding acquisition, N.A. and A.H.A. Investigation, N.A. and A.H.A. Methodology, F.D. and N.A. Project administration, N.A. and A.H.A. Resources, M.R. and A.R. Software, A.H.A. Supervision, N.A. Validation, M.R. and A.R. Writing—review and editing, F.D., N.A. and A.H.A. All authors have read and agreed to the final version of the manuscript.
Corresponding author
Correspondence to Ali Hasan Ali .
Ethics declarations
Competing interests.
The authors declare no competing interests.
Additional information
Publisher's note.
Springer Nature remains neutral with regard to jurisdictional claims in published maps and institutional affiliations.
Rights and permissions
Open Access This article is licensed under a Creative Commons Attribution 4.0 International License, which permits use, sharing, adaptation, distribution and reproduction in any medium or format, as long as you give appropriate credit to the original author(s) and the source, provide a link to the Creative Commons licence, and indicate if changes were made. The images or other third party material in this article are included in the article’s Creative Commons licence, unless indicated otherwise in a credit line to the material. If material is not included in the article’s Creative Commons licence and your intended use is not permitted by statutory regulation or exceeds the permitted use, you will need to obtain permission directly from the copyright holder. To view a copy of this licence, visit http://creativecommons.org/licenses/by/4.0/ .
Reprints and permissions
About this article
Cite this article.
Dayan, F., Ahmed, N., Ali, A.H. et al. Numerical investigation of a typhoid disease model in fuzzy environment. Sci Rep 13 , 21993 (2023). https://doi.org/10.1038/s41598-023-48405-w
Download citation
Received : 22 June 2023
Accepted : 26 November 2023
Published : 11 December 2023
DOI : https://doi.org/10.1038/s41598-023-48405-w
Share this article
Anyone you share the following link with will be able to read this content:
Sorry, a shareable link is not currently available for this article.
Provided by the Springer Nature SharedIt content-sharing initiative
By submitting a comment you agree to abide by our Terms and Community Guidelines . If you find something abusive or that does not comply with our terms or guidelines please flag it as inappropriate.
Quick links
- Explore articles by subject
- Guide to authors
- Editorial policies
Sign up for the Nature Briefing: AI and Robotics newsletter — what matters in AI and robotics research, free to your inbox weekly.

Loading metrics
Open Access
Peer-reviewed
Research Article
Mapping the incidence rate of typhoid fever in sub-Saharan Africa
Roles Conceptualization, Data curation, Funding acquisition, Investigation, Methodology, Project administration, Resources, Software, Supervision, Validation, Visualization, Writing – original draft
* E-mail: [email protected]
Affiliation International Vaccine Institute, Seoul, Republic of Korea

Roles Formal analysis, Methodology, Software, Validation, Visualization, Writing – original draft
Affiliation Department of Mathematics, Hanyang University, Seoul, Republic of Korea
Roles Data curation, Writing – review & editing
Roles Resources, Writing – review & editing
Affiliations International Vaccine Institute, Seoul, Republic of Korea, Graduate School of Public Health, Yonsei University, Seoul, Republic of Korea
Roles Investigation, Writing – review & editing
Current address: Department of Health Systems Governance and Financing, World Health Organization, Geneva, Switzerland
Roles Investigation, Resources, Writing – review & editing
Affiliations International Vaccine Institute, Seoul, Republic of Korea, Cambridge Institute of Therapeutic Immunology and Infectious Disease, University of Cambridge School of Clinical Medicine, Cambridge Biomedical Campus, Cambridge, United Kingdom, Madagascar Institute for Vaccine Research, University of Antananarivo, Antananarivo, Madagascar
Roles Validation, Writing – review & editing
Affiliation Malaria Atlas Project, Telethon Kids Institute, Perth, Australia
Affiliation School of Public Health, Kwame Nkrumah University of Science and Technology, Laing Building Complex J.W. Acheampong CI, Kumasi, Ghana
Affiliation Institut Supérieur des Sciences de la Population, Ouagadougou, Burkina Faso
Affiliation Armauer Hansen Research Institute, ALERT Compound Zenebework, Addis Ababa, Ethiopia
Affiliations Department of Microbiology, Institut National de Recherche Biomédicale, Kinshasa, Democratic Republic of Congo, Department of Medical Biology, Microbiology Service, University Teaching Hospital, Ave De L’hopital, Kinshasa, Democratic Republic of the Congo
Affiliation Centre for Tropical Medicine and Global Health, Nuffield Department of Medicine, University of Oxford, Oxford, United Kingdom
Affiliations Department of Epidemiology of Microbial Diseases, New Haven, Connecticut, United States of America, Yale Institute for Global Health, New Haven, Connecticut, United States of America
Affiliation Centre for International Health, Division of Health Sciences, University of Otago, Dunedin, New Zealand
Affiliations Institute for Health Metrics and Evaluation (IHME), University of Washington, Seattle, Washington, United States of America, Department of Health Metrics Sciences, University of Washington, Seattle, Washington, United States of America
- [ ... ],
Roles Funding acquisition, Investigation, Resources, Supervision, Validation, Writing – review & editing
Affiliations International Vaccine Institute, Seoul, Republic of Korea, Cambridge Institute of Therapeutic Immunology and Infectious Disease, University of Cambridge School of Clinical Medicine, Cambridge Biomedical Campus, Cambridge, United Kingdom, School of Public Health, Kwame Nkrumah University of Science and Technology, Laing Building Complex J.W. Acheampong CI, Kumasi, Ghana, Heidelberg Institute of Global Health, University of Heidelberg, Heidelberg, Germany
- [ view all ]
- [ view less ]
- Jong-Hoon Kim,
- Jungsoon Choi,
- Chaelin Kim,
- Gi Deok Pak,
- Prerana Parajulee,
- Andrea Haselbeck,
- Se Eun Park,
- Vittal Mogasale,
- Hyon Jin Jeon,
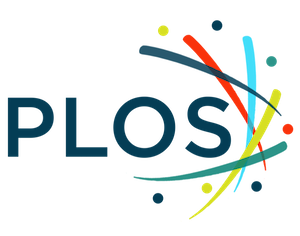
- Published: February 26, 2024
- https://doi.org/10.1371/journal.pntd.0011902
- Reader Comments
With more than 1.2 million illnesses and 29,000 deaths in sub-Saharan Africa in 2017, typhoid fever continues to be a major public health problem. Effective control of the disease would benefit from an understanding of the subnational geospatial distribution of the disease incidence.
We collated records of the incidence rate of typhoid fever confirmed by culture of blood in Africa from 2000 to 2022. We estimated the typhoid incidence rate for sub-Saharan Africa on 20 km × 20 km grids by exploring the association with geospatial covariates representing access to improved water and sanitation, health conditions of the population, and environmental conditions.
We identified six published articles and one pre-print representing incidence rate estimates in 22 sites in 2000–2022. Estimated incidence rates showed geospatial variation at sub-national, national, and regional levels. The incidence rate was high in Western and Eastern African subregions followed by Southern and Middle African subregions. By age, the incidence rate was highest among 5–14 yo followed by 2–4 yo, > 14 yo, and 0–1 yo. When aggregated across all age classes and grids that comprise each country, predicted incidence rates ranged from 43.7 (95% confidence interval: 0.6 to 591.2) in Zimbabwe to 2,957.8 (95% CI: 20.8 to 4,245.2) in South Sudan per 100,000 person-years. Sub-national heterogeneity was evident with the coefficient of variation at the 20 km × 20 km grid-level ranging from 0.7 to 3.3 and was generally lower in high-incidence countries and widely varying in low-incidence countries.
Our study provides estimates of 20 km × 20 km incidence rate of typhoid fever across sub-Saharan Africa based on data collected from 2000 through 2020. Increased understanding of the subnational geospatial variation of typhoid fever in Africa may inform more effective intervention programs by better targeting resources to heterogeneously disturbed disease risk.
Author summary
Typhoid fever remains a significant health challenge in low- and middle-income nations, especially in sub-Saharan Africa. A comprehensive understanding of the disease’s geospatial distribution is pivotal for its control—a gap previous studies overlooked. Addressing this, we undertook a study to chart the incidence of typhoid fever throughout sub-Saharan Africa. Using data from 2000 to 2022, we developed high-resolution maps with a granularity of 20 km by 20 km, detailing the spatial distribution of typhoid incidence. Our findings reveal pronounced disparities in typhoid incidence across different geospatial tiers: from local communities to entire nations and regions. Particularly, Western and Eastern Africa registered the highest incidences, with children aged 5–14 years being the most vulnerable. Distinctively, countries such as South Sudan reported alarmingly high figures, whereas Zimbabwe had notably fewer cases. Such insights are indispensable for health policymakers at local, national, and global levels. Pinpointing the areas hardest hit by typhoid allows for a more strategic allocation of resources and interventions. Armed with this data, we’re better positioned to fight typhoid effectively and safeguard lives in sub-Saharan Africa.
Citation: Kim J-H, Choi J, Kim C, Pak GD, Parajulee P, Haselbeck A, et al. (2024) Mapping the incidence rate of typhoid fever in sub-Saharan Africa. PLoS Negl Trop Dis 18(2): e0011902. https://doi.org/10.1371/journal.pntd.0011902
Editor: Qu Cheng, Huazhong University of Science and Technology Tongji Medical College, CHINA
Received: April 26, 2023; Accepted: January 4, 2024; Published: February 26, 2024
Copyright: © 2024 Kim et al. This is an open access article distributed under the terms of the Creative Commons Attribution License , which permits unrestricted use, distribution, and reproduction in any medium, provided the original author and source are credited.
Data Availability: The data and computer codes to generate the results are on Github ( https://github.com/kimfinale/TyphoidMapping ).
Funding: This work was supported, in whole or in part, by Gavi, the Vaccine Alliance, and the Bill & Melinda Gates Foundation, via the Vaccine Impact Modelling Consortium (Grant Number OPP1157270 / INV-009125) to J.-H.K and the Severe Typhoid Fever in Africa Program (Grant Number OPP1127988) and Typhoid Fever Surveillance in Africa Program (Grant Number OPPGH5231) to F.M. The funders had no role in study design, data collection and analysis, decision to publish, or preparation of the manuscript.
Competing interests: The authors have declared that no competing interests exist.
Introduction
Typhoid fever is a systemic infection caused by Salmonella enterica serovar Typhi ( S . Typhi) that is transmitted via water or food contaminated by human feces [ 1 ]. Typhoid fever often causes mild symptoms such as fever or weakness but untreated may progress to potentially fatal complications such as intestinal perforation [ 2 , 3 ]. Typhoid fever continues to be a major public health problem in low- and middle-income countries (LMICs) [ 4 – 8 ]. While being the cornerstone for control of typhoid fever, improving water, sanitation, and hygiene (WASH) and food safety is generally a resource-intensive and long-term goal. Other control strategies such as vaccination, that may protect unvaccinated people as well as vaccine recipients [ 9 ], and antimicrobials, that may reduce morbidity and mortality among those with typhoid fever while also reducing fecal carriage and onward transmission [ 1 ], may facilitate more immediate impact and near-term control. Limited resources for health mean that global and national policy makers need to identify efficient ways to implement these control strategies, which demands detailed understanding of the distribution of disease.
Typhoid fever is estimated to have caused 14 million cases in 2010 and 11 million cases in 2017 globally [ 6 ]. Earlier studies reported somewhat varying estimates: 21 million cases worldwide in 2000 [ 7 ]; 27 million (interquartile range: 18–36 million) cases in 2010 [ 10 ]; 12 million (95% confidence interval: 10–15 million) or slightly higher in 2010 [ 4 , 5 ]; and 17.8 million cases in 2015 but with much wider uncertainty intervals (95% credible interval: 6.9–48.4 million) [ 8 ].
Despite existing estimates, there are still knowledge gaps regarding the variation of typhoid fever incidence at the country or subnational levels. Understanding the subnational geospatial variation of the disease could lead to more efficient and effective intervention strategies by spatially targeting resources to areas at higher risk. Even for estimates that may agree at the global level, significant variation exists at the national and United Nations (UN) subregional levels [ 6 ]. Moreover, studies that estimate sub-national variation often rely on national-level covariates for modeling and prediction, potentially missing important local nuances [ 8 ]. In addition, existing studies do not account for observations during the recent typhoid surveillance in sub-Saharan Africa (SSA) and upating the estimates is necessary. Except for one prior study [ 8 ], the existing research has primarily examined the disease at the national level, neglecting sub-national variations. All estimates save two [ 5 , 6 ] rely on surveillance conducted in <20 sites before 2010, with only three sites from SSA including one from the 1980s.
We sought to address the limitations of existing studies and develop a more granular understanding of geospatial variation of typhoid fever incidence rates. In this study, we focused on SSA for which two multi-country surveillance studies, Typhoid Fever Surveillance in Africa Program (TSAP) conducted in 2012–2014, [ 11 ] and Severe Typhoid in Africa (SETA) conducted in 2016–2019 [ 12 , 13 ] provide recent data suggesting substatial burden of typhoid fever in the region. The issue of geospatial variation of typhoid fever is particualrly relevant to SSA because countries like the Democratic Republic of the Congo, Ghana, Malawi, and Zimbabwe are currently rolling out their typhoid vaccination programs [ 14 – 16 ]. We modeled age-stratified incidence rates by integrating data on the incidence rates and catchement area with recently synthesized geospatial covariates that represent factors that potentially influence the transmission of typhoid fever [ 17 – 20 ]. Using the predicted incidence rates on 20 km × 20 km grids of SSA, we characterized the geospatial variation of typhoid fever and potential implications.
We modeled the incidence rate of typhoid fever on 20 km × 20 km grids by exploring the association of observed incidence rates with geospatial covariates representing factors that may influence the transmission of S . Typhi, such as access to improved water and sanitation, health conditions of the population, or environmental conditions. We chose the resolution of 20 km × 20 km because this resolution allows us to explore the subnational heterogeneity with reasonable computational cost. The expected number of 20 km × 20 km grids per country varies from about 28 (e.g., Gambia) to about 5,862 (e.g., Democratic Republic of the Congo). A previous study explored the subnational heterogeneity of the burden of cholera in sub-Saharan Africa at a similar resolution [ 21 ]. We used multivariate regression models, assuming the typhoid case count per 100,000 person-years followed either a Poisson or negative binomial distribution. The negative binomial distribution was considered to address overdispersion.
Incidence rate data
The data on incidence rates were extracted as a part of a broader project investigating the occurrence of typhoid fever, regardless of whether the instances were documented through case reports, outbreak investigations, or longitudinal surveillance. The literatures search procedure has been detailed elsewhere [ 22 ] and only studies that reported incidence rates from longitudinal surveillance were included in this study ( Fig 1 ). In summary, we comprehensively searched through PubMed as well as preprint servers such as Social Science Research Network, bioRxiv, and medRxiv to identify relevant studies published between Jan 1, 2000 and Dec 31, 2022, using the search query "typhoid AND Africa.". Among 301 studies that reported occurrence of typhoid fever, five studies provided information on incidence rates of typhoid fever observed during longitudinal surveillance.
- PPT PowerPoint slide
- PNG larger image
- TIFF original image
https://doi.org/10.1371/journal.pntd.0011902.g001
Incidence rates for typhoid fever were available at 22 sites in 13 countries within SSA ( Fig 2 and Table A in S1 Information ) [ 11 , 23 – 29 ]. Estimates were based on hospital-based passive surveillance with the exception of one study that involved active surveillance of households [ 28 ]. All studies used multipliers in their calculation of incidence rates to account for healthcare seeking behavior and the recruitment proportion; some studies used an additional multiplier to account for blood culture sensitivity [ 24 , 25 , 27 ]. We standardized the observed incidence rates across different studies by using the estimates that accounted for healthcare seeking behavior and recruitment proportion during the surveillance period. We later applied a uniform multiplier of 1/0.6 to modeled incidence rates to account for blood culture sensitivity (estimated to be ~60%) [ 4 ]. The incidence rates were broken down by age: 0–1, 2–4 yo, 5–14 yo, and >14 yo. Three studies [ 24 , 26 , 27 ] were not included in the analysis as they did not report typhoid incidence for the relevant age classes. One of these studies presented an aggregated incidence across all age classes [ 24 ], while the remaining two studies reported incidence among children under the age of 5 years [ 26 ] and children under the 15 years of age [ 27 ], respectively. One study used an age category of 5–17 years instead of 5–14 years [ 28 ]; we included this study in the model without further adjustment assuming incidence rate observed among those aged 5–17 years would be the same as the one observed in those 5–14 years.
(A) Year and location of the surveillance. (B) Incidence rates per 100,000 person-years that do not account for the sensitivity of blood culture tests. Points and bars indicate mean and 95% confidence or credible intervals. Points with no bars represent zero incidence rates in which interval estimates were not reported. Shapefiles specific to the African continent are available for download from GADM at: https://gadm.org/license.html .
https://doi.org/10.1371/journal.pntd.0011902.g002
To model incidence rates using geospatial covariates, we linked observed incidence rates from each surveillance site to the geospatial covariates that fall on the catchment area of the relevant healthcare facilities. Catchment areas were based on unpublished catchment area information collected during the surveillance (Section A and Fig A in S1 Information ) [ 11 ] or the descriptions available in the studies [ 23 – 28 ].
Geospatial covariates
Variables that may influence the water-borne or food-borne transmission of S . Typhi and cover the entire continent of SSA with at least 20 km × 20 km resolution were explored for their predictive capacity for the incidence rate of typhoid fever ( Table 1 ). We explored access to safe drinking water and access to improved sanitation facilities [ 18 , 30 – 32 ] considering the water-borne or food-borne transmission of typhoid fever [ 1 ]. We included annual mean temperature [ 33 – 35 ], annual precipitation [ 34 ], elevation [ 36 ], and distance to water [ 37 ] because they may have the potential to influence the transmission of water-borne diseases and have been shown to be associated with an increased risk of typhoid fever. We also explored covariates that showed high predictive capacity in the previous modeling study [ 8 ], namely percent paved roads, percent of the population living in extreme poverty, prevalence of stunting, and prevalence of HIV [ 8 ]. Because the resolution of these covariates used in the previous modeling study [ 8 ] were low (i.e., national or first administrative unit level), we used high-resolution estimates that were recently synthesized: prevalence of stunting, wasting, and underweight under the age of five years [ 17 ] and the prevalence of HIV among adults aged 15–49 years [ 19 ]. We could not identify higher-resolution covariates for the two covariates—percent paved roads and people living in extreme poverty—and instead used a covariate indicating travel time to the nearest urban center [ 20 ] assuming all of these covariates partly capture infrastructure. We included human population density [ 38 ] as a covariate to reflect that the surveillance was conducted in sites with varying population density [ 39 , 40 ]. We created the population size for each 20 km × 20 km grids by aggregating the values from 1 km × 1 km grids using data from the WorldPop project [ 38 ].
https://doi.org/10.1371/journal.pntd.0011902.t001
These geospatial covariates were based on the estimates from previous analyses. For instance, the access to water and sanitation facilities are the mean estimates from the geostatistical modeling analyses that were based on Demographic and Health Survey (DHS), Multiple Indicator Cluster Surveys, and other household surveys and censuses [ 18 ]. Similarly, prevalence of stunting, wasting, and underweight under the age of five years [ 17 ], and the prevalence of HIV among adults aged 15–49 years [ 19 ] were the mean estimates of previous geostatistical modeling analyses of the data from DHS and other sources. Other covariates such as temperature and precipitation came from satellite observations [ 41 ]. Elevation data came from ‘elevatr’ package [ 36 ], which uses Terrain Tiles Public Dataset [ 42 ]. These covariates were at finer resolutions (e.g., 5 km × 5 km or 1 km × 1 km) and created 20 km × 20 km grids by aggregating smaller grids and setting the value of the grid as the mean of the comprising smaller grids. Geospatial distribution of the covariates appears in Figs B-Q in S1 Information .
We matched the year and location of data collection between observed incidence rates and geospatial covariates. For covariates whose values were available for the period of 2000 through 2017, we assumed the values after 2017 were the same as in 2017. Elevation and distance to water covariates were assumed to be time-invariant. To determine the location for the observed incidence rates, we identified grid cells that were covered by the catchment area during the surveillance (using ‘extract’ function of raster package in R). A grid cell was defined to be covered by an area if its center was inside the area. The average value of the covariates across the grids was taken as the representative value when multiple grids were covered by the area.
Predicting incidence rate
We employed a generalized linear modeling framework to explore the association between the outcome data and geospatial covariates. The outcome was quantified as case per 100,000 person-years for specific age groups (0–1 yo, 2–4 yo, 5–14 yo, and >14 yo) on 20 km × 20 km grids. Mathematical details about the models appear in Section B of S1 Information . For each model assuming a varying outcome distribution (Poisson or negative binomial), we conducted the following variable selection process. We removed covariates whose variance inflation factors (VIFs) were over ten, or that were highly correlated with other variables (Pearson’s r > 0.7) while having low correlation with the outcome (Pearson’s r < 0.1) to reduce multicollinearity [ 43 ]. We performed a forward stepwise variable selection method based on Akaike Information Criterion (AIC) [ 44 ] because AIC criteria can be straightforwardly applied to generalized models, including generalized linear models, non-linear models, and non-normal distributed data. A covariate was included in the model if its introduction led to a decrease in the AIC. Conversely, the covariate was not included in the model if its introduction didn’t lead to a decrease in AIC. The covariate with the highest positive or negative correlation with the outcome was tested first, followed by the covariate with the next highest correlation with the outcome, and so on. We further selected covariates based on their p -values, their impact on root mean-square error (RMSE) in the leave-one-out (LOO) cross-validation [ 45 ] (Section C in S1 Information ), and the age-distribution of the predicted incidence rates. We retained variables if their p -values were lower than 0.05, their addition reduced the RMSE in the LOO cross-validation, and they produced incidence rate that resembled the data in age distribution (i.e., incidence rates higher in 2–14 yo than in 0–1 yo and >14 yo). We chose the Poisson model for 0–1 yo and the negative binomial models for the other age classes. A list of covariates included in the final model and their associated parameter estimates appear in Table B in S1 Information .
To calculate the predicted number of cases at the grid level, we multiplied the predicted incidence rates with the population size for each 20 km × 20 km grid. Since the WorldPop data lacked age-specific population sizes, we estimated the age-specific population sizes by multiplying the population size at the grid level with the overall proportions of each age class at the country level, sourced from information in the UN World Population Prospectus [ 46 ]. To determine the predicted number of cases at the country level, we aggregated the grid-level cases across all the grids within the country of interest. Subsequently, we computed the country-level incidence rate by dividing the number of cases by the population size at the country level. To compare the predicted number of cases with estimates of previous studies, we assumed blood culture sensitivity at 60% as in the previous study [ 4 ] and used population size from 2017 [ 6 , 8 ]. We set the upper bound of the predicted incidence rates to 10,000 per 100,000 person-years if the predicted incidence rates were higher than 10,000 per 100,000 person-years.
Data sharing
All data and R code required to replicate the figures and tables presented in this paper are accessible in the GitHub repository [ 47 ]. Shapefiles specific to the African continent are available for download from GADM at: https://gadm.org/license.html . Information about the sources for all other publicly available data used in this study is documented in the respective sections where these data are referenced.
Model and predictor selection
The negative binomial regression model yielded the best predictive performance for age groups 2–4 yo, 5–14 yo, and >14 yo, while the Poisson model demonstrated superior performance for the 0–1 yo group (refer to Table C in S1 Information for RMSE values). These models with the best performance were then employed to predict the incidence rates of typhoid for the corresponding age group on 20 km × 20 km grids. Across all models, the covariates measuring the distance to water bodies and the proportion of people using surface water were included, and both demonstrated a positive association with the typhoid incidence rate, but to a varying extent (refer to Table 2 ). However, different covariates were additionally selected and therefore the final set of covariates varied by age group. For the 0–1 yo age group, population density and open defecation exhibited a positive association, whereas elevation, access to piped sanitation, and wasting showed a negative association with the incidence rate. For the 2–4 yo age group, only population density was additionally included in the model, and it had a positive association. For the 5–14 yo age group, only the proportion of population who have access to piped water was additionally included in the model, and it had a positive association.
Blank cells indicate the variables that were excluded during the variable selection process.
https://doi.org/10.1371/journal.pntd.0011902.t002
Comparison between observed and predicted incidence rates
Overall, 50 incidence rate estimates were available for 0–1 yo, 2–4 yo, 5–14 yo, and >14 yo age classes for 22 sites in 13 countries in sub-Saharan Africa ( Table A in S1 Information ). All studies reported incidence rates for all age classes except for one study [ 26 ] reporting incidence rates for those who were 4 years old or younger. Surveillance sites included both urban and rural sites and provided incidence rates observed over the period from 2006 through 2019. The surveillance duration for each site ranged from 13 months (East Wad Medani, Sudan for July 2012—July 2013) to >5 years (Asante Akim North, Ghana for March 2010—May 2012 and May 2016—May 2019).
A substantial fraction of the observed incidence rates was predicted by the model in the LOO cross-validation ( Fig 3 ). For the 0–1 yo, 2–4 yo, 5–14 yo, and >14 yo age groups, 13.6%, 50.0%, 53.8%, 62.5% of the observations were within the 95% confidence interval of the predictions, respectively.
Predicted against observed incidence rates per 100,000 person-years on log-log scale. Dots and bars represent the mean and 95% confidence intervals of predicted values.
https://doi.org/10.1371/journal.pntd.0011902.g003
Predicted incidence rate
Predicted incidence rates varied at the grid, subnational, country, and subregional levels. For instance, variations at the subnational level were evident in all age classes ( Fig 4A–4D ). Overall, 2–4 yo were predicted to have the highest incidence rates (417.5 [95% CI: 10.7 to 7291.5] per 100,000 person-years) followed by 5–14 yo (308.0 [95% CI: 33.7 to 6590.6]), and > 14 yo (46.2 [95% CI: 1.0 to 5661.3]); 0–1 yo were predicted to have the lowest incidence rates (21.0 [95% CI: 0.0 to 2840.7]) ( Fig R in S1 Information ).
Predicted incidence rates per 100,000 person-years for 0–1 yo (A), 2–4 yo (B), 5–14 yo (C), and >14 yo (D), summarized at subnational levels for 2017. Shapefiles specific to the African continent are available for download from GADM at: https://gadm.org/license.html .
https://doi.org/10.1371/journal.pntd.0011902.g004
Country-level variation of the predicted incidence is also evident in all age groups ( Fig S and Table D in S1 Information ). When aggregated across all age classes and grids that comprise each country, predicted incidence rates ranged from 43.7 (95% CI: 0.6 to 591.2) in Zimbabwe to 2,957.8 (95% CI: 20.8 to 4,245.2) in South Sudan per 100,000 person-years ( Fig 5 , Table D in S1 Information ). At the UN subregional level [ 48 ], Middle Africa had the highest incidence rate (1,017.1 [95% CI: 32.0 to 1,275.6] per 100,000 person-years), followed by Eastern Africa, Western Africa, and Southern Africa ( Table E in S1 Information ).
Country-level typhoid incidence rates per 100,000 person-years (A) and the coefficient of variation (CV) of 20 km × 20 km grid-level incidence rates (B). Mean country incidence rates varied from 43.7 to 2,957.8 and CV varies from 0.7 to 3.3. Shapefiles specific to the African continent are available for download from GADM at: https://gadm.org/license.html .
https://doi.org/10.1371/journal.pntd.0011902.g005
The expected annual number of cases in the continent adjusted for the 2017 population was 1,883,572 (95% CI: 127,474 to 6,535,604). By UN subregion, the number of typhoid fever cases was highest in Eastern Africa (4,782,220 [95% CI: 277,999 to 11,897,898] cases) followed by Western Africa (3,544,420 [95% CI: 1,904 to 9,874,415]), Middle Africa (2,515,459 [95% CI: 98,917 to 5,479,315]), and Southern Africa (145,130 [95% CI: 1,904 to 749,018]) ( Tables F and G in S1 Information ). Countries with high typhoid incidence (e.g., South Sudan) were associated with the lower coefficient of variation (CV) of 20 km × 20 km grid-level incidence rates whereas low-incidence countries were associated with a wide range of CVs from higher (e.g., Burkina Faso) and lower (e.g., Gambia) ( Fig 5 ) .
We estimated the age-specific incidence rates of typhoid fever for SSA on 20 km × 20 km grids using typhoid incidence rate data over the period of 2000 through 2022 including recently completed surveillance [ 13 ] and high-resolution geospatial covariates matched to the catchment area for the surveillance sites. Predicted incidence rates showed substantial geospatial variation at all levels: subnational, national and Africa subregional levels. To the best of our knowledge, this study represents the first exploration of the high-resolution distribution of typhoid incidence rates across the African continent, using the most current available data. While extracting actionable insights directly from the study results may pose challenges, achieving a more comprehensive understanding of the geospatial distribution of typhoid burden by age could lead to more effective and efficient targeted intervention programs [ 49 , 50 ].
While our model primarily focuses on predicting incidence rates per 100,000 people rather than providing explanations for the association between covariates and incidence rates, the selected covariates in the model appear to offer reasonable explanations. For instance, using surface water (all age groups), population density (0–1 yo and 2–4 yo age groups), and open defecation (0–1 yo) appear to show a positive association with typhoid incidence per capita. Conversely, covariates like access to piped sanitation and elevation indicate a negative association with the incidence rate, whereas a negative association between the prevalence of wasting and the incidence rate is not intuitively clear.
Existing estimates of the typhoid fever burden generally align on a global scale but exhibit considerable variation at the UN subregional level [ 6 ]. Our UN subregional-level estimates, overall, tend to surpass those of Mogasale et al .[ 4 ] and GBD estimates [ 6 ] while closely resembling the estimates presented by Antillón et al .[ 8 ]. Notably, there’s a substantial disparity for Eastern Africa, where our estimates are approximately twice as high as those reported by Antillón et al .[ 8 ]. ( Tables E and G in S1 Information ). However, due to the limited number of observations, both our estimates and those of by Antillón et al .[ 8 ] display significant uncertainty ranges, which considerably overlap and encompass the estimates of Mogasale et al . [ 4 ] whereas GBD estimates [ 6 ] persistently remain lower without overlapping with other estimates.
Intrepreting agreement or disagreement among estimates should be done with care, however, and needs to account for the context of the models and the data used in each study. For instance, estimates by Mogasale et al .[ 4 ] and Antillón et al .[ 8 ] did not account for the data from TSAP and SETA [ 11 , 12 ], which are a major source of data for incidence rate modeling in our study. Also, the methods used in the studies by Mogasale et al .[ 4 ] and Kim et al .[ 5 ] do not use any geospatial covariates to model observed incidence rates except for national-level estimates for the access to improved water source.
Incidence rate estimates from this study were characterized by large uncertainties. One of the main reasons for the wide confidence intervals is the small sample size. While we included all the datasets we found in the peer-reviewed literature including one pre-print as of January 2023, the number of observations was fewer than 30 for all age groups. The 95% confidence intervals of estimates from this study overlapped substantially with those of Antillón et al .[ 8 ] and encapsulate estimates of other studies ( Table I and Fig T in S1 Information ); however, the lower bounds of the confidence intervals tended to be lower than those of other studies with many of them close to zero, which might reflect the fact that the data set we used included zeros in 20% of the data.
Our study has limitations. First, while population-based surveillance provides reliable inputs for the model to predict the incidence of typhoid fever, these data were available from only 22 sites in 13 countries [ 11 , 13 , 23 – 28 ], which makes it challenging to infer incidence for all of SSA. While research collecting additional data and developing ways to use other information such as serosurveys [ 51 ] should continue, use of the most up-to-date incidence rate data and high-resolution geospatial covariates enable us to produce updated incidence rates at the subnational level. Second, like the estimates of previous studies for typhoid and other diseases [ 4 – 8 , 10 , 52 ], our estimates are predictions of annual incidence rates that are based on static and retrospective analyses. While these estimates can provide clues to the current incidence of disease, the burden of typhoid fever is seasonally and secularly variable, and effective policy making would require real-time data synthesis. Third, we set an upper limit for the incidence rates as 10,000 per 100,000 person-years, which is around 4 times higher than the highest of the observed incidence rates, while some of the model estimates at 20 km × 20 km grids go beyond the limit. This may introduce a bias that incidence rate estimates are lower for some areas. For 0–1 yo, 2–4 yo, 5–14 yo, and >14 yo, 0.2%, 5.6%, 6.2%, 5.1% of the total 60,535 valid grids had estimated values over 10,000 per 100,000 person-years. Fourth, while we used granular geospatial covariates to model the incidence rates, we had to rely on the nationally averaged age distribution to produce the age-specific predicted number of cases. Therefore, age-specific predicted number of cases may either overestimate or underestimate the true number of cases. Fifth, we modeled incidence rates of typhoid fever separately for each age group and this led to different sets of covariates for different age groups. Associations with the covariate in the models should not be interpreted as causal and many vary by age to the extent that certain covariates are not significant predictors of incidence in all age groups. Sixth, the predictive power of our model is limited for 0–1 yo, potentially because a large fraction of observations are zeroes. This warrants additional data and updates.
Our research offers the most detailed prediction to date of the typhoid fever incidence rate in Sub-Saharan Africa (SSA), utilizing the latest data on incidence rates and geospatial variables at a resolution of 20 km × 20 km. This study enhances understanding of the geographical variations in typhoid fever and provides valuable data that could inform targeted intervention strategies for typhoid control in the region.
Supporting information
S1 information. table a. longitudinal surveillance studies of typhoid fever incidence in africa..
Incidence rate data used for modeling come from four published articles and one preprint surveillance study that reported incidence rates measures in sub-Saharan Africa since 2000. Table B . Estimated coefficients of covariates in the proposed model, sub-Saharan Africa, 2017. Greyed cells indicate variables that were removed before modeling to reduce multicollinearity. Cells with the blue background indicate variables that were removed because the p-values were larger than or near to 0.05 and excluding them reduced the LOO cross-validation RMSE. Section A. Catchment area for the incidence rates Fig A. Catchment area represented as 20 km × 20 km grids. Shapefiles specific to the African continent are available for download from GADM at: https://gadm.org/license.html . Fig B . Percentage access to improved sanitation facilities based on Deshpande et al . [ 18 ]. The improved sanitation includes sewer or septic tanks and other improved sanitation facilities (improved latrines, ventilated improved latrines, composting toilets). The dataset is available at the IHME: https://cloud.ihme.washington.edu/s/bkH2X2tFQMejMxy . Fig C . Percentage access to sewer or septic sanitation facilities based on Deshpande et al . [ 18 ]. The dataset is available at the IHME: ttps://cloud.ihme.washington.edu/s/bkH2X2tFQMejMxy. Fig D . Percentage open defecation based on Deshpande et al . [ 18 ]. The dataset is available at the IHME: https://cloud.ihme.washington.edu/s/bkH2X2tFQMejMxy . Fig E . Access to improved drinking water based on Deshpande et al . [ 18 ]. The improved water indicates access to piped water according to the JMP definition and includes piped (piped on or off premises) and other improved (protected wells and springs, bottled water, rainwater collection, bought water) water. The dataset is available at the IHME: https://cloud.ihme.washington.edu/s/bkH2X2tFQMejMxy . Fig F . Access to piped drinking water based on Deshpande et al . [ 18 ]. The dataset is available at the IHME: https://cloud.ihme.washington.edu/s/bkH2X2tFQMejMxy . Fig G . Use of surface water based on Deshpande et al . [ 18 ]. The dataset is available at the IHME: https://cloud.ihme.washington.edu/s/bkH2X2tFQMejMxy . Fig H . Precipitation. The values indicate annual precipitation summed across daily precipitation data Climate Hazards group Infrared Precipitation with Stations (CHIRPS) data set [ 41 ]. The dataset is available at https://cds.climate.copernicus.eu/cdsapp#!/dataset/insitu-gridded-observations-global-and-regional?tab=form . Shapefiles specific to the African continent are available for download from GADM at: https://gadm.org/license.html . Fig I . Annual mean temperature for 2017. Monthly mean temperatures [ 41 ] were averaged. The dataset is available at https://cds.climate.copernicus.eu/cdsapp#!/dataset/insitu-gridded-observations-global-and-regional?tab=form . Shapefiles specific to the African continent are available for download from GADM at: https://gadm.org/license.html . Fig J . Prevalence of stunting among children under the age of 5 in Africa for 2017 based on the study by Kinyoki et al . [ 17 ]. The dataset is available at https://cloud.ihme.washington.edu/index.php/s/Q5CGeazb4iNsDQA . Shapefiles specific to the African continent are available for download from GADM at: https://gadm.org/license.html . Fig K . Prevalence of wasting among children under the age of 5 in Africa for 2017 based on the study by Kinyoki et al . [ 17 ]. The dataset is available at https://cloud.ihme.washington.edu/index.php/s/Q5CGeazb4iNsDQA . Shapefiles specific to the African continent are available for download from GADM at: https://gadm.org/license.html . Fig L . Prevalence of underweight among children under the age of 5 in Africa for 2017 based on the study by Kinyoki et al . [ 17 ]. The dataset is available at https://cloud.ihme.washington.edu/index.php/s/Q5CGeazb4iNsDQA . Shapefiles specific to the African continent are available for download from GADM at: https://gadm.org/license.html . Fig M. Prevalence of HIV infection among adults (15–49 years old) in sub-Saharan Africa for 2017 based on the study by Dwyer-Lindgren et al .[ 19 ]. The dataset is available at https://ghdx.healthdata.org/record/ihme-data/africa-hiv-prevalence-geospatial-estimates-2000-2017 . Shapefiles specific to the African continent are available for download from GADM at: https://gadm.org/license.html . Fig N . Travel time to cities (in minutes) in Africa for 2017 based on the study by Weiss et al . [ 20 ]. The dataset is available at https://data.malariaatlas.org/maps . Shapefiles specific to the African continent are available for download from GADM at: https://gadm.org/license.html . Fig O . Distance to water [ 37 ]. The value indicates the distance for each pixel to the nearest water cell (inland and sea) at 20 km × 20 km resolution. For the water cell the distance to water is 0 km. The dataset is available at https://data.ceda.ac.uk/neodc/globolakes/data/v1/limnology . Shapefiles specific to the African continent are available for download from GADM at: https://gadm.org/license.html . Fig P . Elevation [ 36 ]. Elevation data come from estimates by Mapzen that combine several digital elevation model (DEM) such as the Shuttle Radar Topography Mission (SRTM), the USGS National Elevation Dataset (NED), Global DEM (GDEM), and others. R package ‘elevatr’ serves as an API that enables an access to the elevation estimates by Mapzen hosted at Amazon Web Services Terrain Tiles. Shapefiles specific to the African continent are available for download from GADM at: https://gadm.org/license.html . Fig Q . Population count per pixel based on the WorldPop [ 38 ]. Mosaiced 1km resolution global dataset were aggregated to create 20 km resolution dataset. The dataset is available at https://hub.worldpop.org/geodata/listing?id=64 . Shapefiles specific to the African continent are available for download from GADM at: https://gadm.org/license.html . Section B . Multivariate regression for Poisson and negative binomial models. Section C. Model validation. Table C. The root mean squared error (RMSE) values of the proposed model (linear regression model) with the competing models (Poisson and Negative Binomial regression models). Fig R. Distribution of predicted incidence rates per 100,000 person years by age (A) and incidence rate ratio (B) with children aged 5–14 years as a reference group. Incidence rates were summarized at subnational levels. Red dots indicate observed incidence rates. Fig S. Predicted incidence rates per 100,000 person-years for 0–1 yo (A), 2–4 yo (B), 5–14 yo (C), and >14 yo (D) summarized at country level. Bold and thin lines inside Africa represent country borders and first-level administrative divisions, respectively. Shapefiles specific to the African continent are available for download from GADM at: https://gadm.org/license.html . Table D. Estimated incidence rates per 100,000 person years by country, sub-Saharan Africa, 2017. Table E. Estimated incidence rate of typhoid fever per 100,000 persons per year by Africa subregion, 2017. Table F. Estimated number of cases by country and age group, sub-Saharan Africa, 2017. Table G. Estimated number of cases by Africa subregion, 2017 (unit = thousands). Table H. Comparison of incidence rates per 100, 000 person-years by country. Fig T . Incidence rate estimates per 100,000 person-years by country. (A) shows existing incidence rate estimates at the country level. The upper bounds of some estimates by Antillón et al . and in the current study go over 4,000. (B) highlights the differences between the estimates by Antillón et al . and by this study that take a similar approach of using grid-level geospatial covariates.
https://doi.org/10.1371/journal.pntd.0011902.s001
Acknowledgments
We thank Justin Im (International Vaccine Institute) and John D. Clemens (International Vaccine Institute) for their review and feedback on this article. The authors alone are responsible for the views expressed in this article and they do not necessarily represent the decisions, policy, or views of their affiliated organizations.
- View Article
- PubMed/NCBI
- Google Scholar
- 14. Duff N. Liberia Becomes First Country in Africa to Introduce Typhoid Conjugate Vaccine. In: Take on Typhoid [Internet]. [cited 8 Aug 2022]. Available: https://www.coalitionagainsttyphoid.org/typhoid-news/liberia-becomes-first-country-in-africa-to-introduce-typhoid-conjugate-vaccine/
- 16. Africa’s first-ever mass typhoid fever vaccination campaign ends in Zimbabwe. In: Take on Typhoid [Internet]. [cited 8 Aug 2022]. Available: https://www.coalitionagainsttyphoid.org/typhoid-news/africas-first-ever-mass-typhoid-fever-vaccination-campaign-ends-in-zimbabwe/
- 29. Meiring JE, Shakya M, Khanam F, Voysey M, Phillips MT, Tonks S, et al. Burden of enteric fever at three urban sites in Africa and Asia: a multicentre population-based study. Lancet Glob Health. 2021;9: e1688–e1696. https://doi.org/10.1016/S2214-109X(21)00370-3 pmid:34798028
- 30. Brockett S, Wolfe MK, Hamot A, Appiah GD, Mintz ED, Lantagne D. Associations among Water, Sanitation, and Hygiene, and Food Exposures and Typhoid Fever in Case–Control Studies: A Systematic Review and Meta-Analysis. The American Journal of Tropical Medicine and Hygiene. 2020;103: 1020–1031. https://doi.org/10.4269/ajtmh.19-0479 pmid:32700668
- 31. Tadesse BT, Khanam F, Ahmed F, Im J, Islam MT, Kim DR, et al. Prevention of typhoid by Vi conjugate vaccine and achievable improvements in household WASH: Evidence from a cluster-randomized trial in Dhaka, Bangladesh. Clin Infect Dis. 2022; ciac289. https://doi.org/10.1093/cid/ciac289 pmid:35412603
- 32. Im J, Khanam F, Ahmmed F, Kim DR, Kang S, Tadesse BT, et al. Prevention of Typhoid Fever by Existing Improvements in Household Water, Sanitation, and Hygiene, and the Use of the Vi Polysaccharide Typhoid Vaccine in Poor Urban Slums: Results from a Cluster-Randomized Trial. Am J Trop Med Hyg. 2022;106: 1149–1155. https://doi.org/10.4269/ajtmh.21-1034 pmid:35385827
- 42. Terrain Tiles v1.1 Update · Mapzen. [cited 2 Aug 2023]. Available: https://www.mapzen.com/blog/terrain-tiles-v1.1/
- 47. Kim J-H. Mapping the incidence rate of typhoid fever in sub-Saharan Africa. Available: https://github.com/kimfinale/TyphoidMapping
- 48. United Nations Statistics Division–Standard Country and Area Codes Classifications. Available: https://unstats.un.org/unsd/methodology/m49/

An official website of the United States government
The .gov means it’s official. Federal government websites often end in .gov or .mil. Before sharing sensitive information, make sure you’re on a federal government site.
The site is secure. The https:// ensures that you are connecting to the official website and that any information you provide is encrypted and transmitted securely.
- Publications
- Account settings
Preview improvements coming to the PMC website in October 2024. Learn More or Try it out now .
- Advanced Search
- Journal List
- Res Rep Trop Med

The Burden of Typhoid Fever in Sub-Saharan Africa: A Perspective
Cara lynn kim.
1 International Vaccine Institute, Seoul, Republic of Korea
Ligia Maria Cruz Espinoza
Kirsten s vannice.
2 Enteric and Diarrheal Diseases, Bill & Melinda Gates Foundation, Seattle, WA, USA
Birkneh Tilahun Tadesse
3 Division of Clinical Pharmacology, Department of Laboratory Medicine, Karolinska Institutet, Karolinska University Hospital Huddinge, Stockholm, Sweden
4 Center for Innovative Drug Development and Therapeutic Trials for Africa, College of Health Sciences, Addis Ababa University, Addis Ababa, Ethiopia
Ellis Owusu-Dabo
5 School of Public Health, Kwame Nkrumah University of Science and Technology, Kumasi, Ghana
Raphaël Rakotozandrindrainy
6 University of Antananarivo, Antananarivo, Madagascar
Ilesh V Jani
7 Instituto Nacional de Saúde (INS), Maputo Province, Mozambique
Mekonnen Teferi
8 Armauer Hansen Research Institute, Addis Ababa, Ethiopia
Abdramane Bassiahi Soura
9 Institut Supérieur des Sciences de la Population, Université Joseph Ki-Zerbo de Ouagadougou, Ouagadougou, Burkina Faso
Octavie Lunguya
10 Department of Microbiology, Institut National de Recherche Biomédicale, Kinshasa, Democratic Republic of the Congo
11 Department of Medical Biology, University Teaching Hospital of Kinshasa, Kinshasa, Democratic Republic of the Congo
A Duncan Steele
Florian marks.
12 Cambridge Institute of Therapeutic Immunology and Infectious Disease, University of Cambridge School of Clinical Medicine, Cambridge Biomedical Campus, Cambridge, UK
13 Heidelberg Institute of Global Health, University of Heidelberg, Heidelberg, Germany
While typhoid fever has largely been eliminated in high-income regions which have developed modern water, sanitation, and hygiene facilities, it remains a significant public health burden resulting in morbidity and mortality among millions of individuals in resource-constrained settings. Prevention and control efforts are needed that integrate several high-impact interventions targeting facilities and infrastructure, including those addressing improvements in sanitation, access to safe water, and planned urbanization, together with parallel efforts directed at effective strategies for use of typhoid conjugate vaccines (TCV). The use of TCVs is a critical tool with the potential of having a rapid impact on typhoid fever disease burden; their introduction will also serve as an important strategy to combat evolving antimicrobial resistance to currently available typhoid fever treatments. Well-designed epidemiological surveillance studies play a critical role in establishing the need for, and monitoring the impact of, typhoid fever control and prevention strategies implemented by public health authorities. Here, we present a perspective based on a narrative review of the impact of typhoid fever on morbidity and mortality in sub-Saharan Africa and discuss ongoing surveillance activities and the role of vaccination in prevention and control efforts.
Introduction
Typhoid fever is a febrile illness caused by infection with the Gram-negative bacterium Salmonella enterica serovar Typhi ( S . Typhi). There are several Salmonella enterica species: the serovar Paratyphi causes paratyphoid fever; further, there are non-typhoidal serovars, the most prevalent being serovars Enteritidis, Typhimurium, and Dublin, which cause invasive non-typhoidal salmonellosis (iNTS). 1 , 2
Typhoid fever represents a significant public health burden, particularly in resource-constrained settings. 3 , 4 While typhoid fever has been largely eliminated in higher-income countries with modern sanitary facilities and safe drinking water, the disease persists as a significant public health issue in many low- and middle-income countries (LMICs) worldwide. 5 , 6 This public health issue is compounded by increasing antimicrobial resistance (AMR) and rampant economic loss due to the impact of this disease on productive members of the population. 7 The World Health Organization (WHO) estimates the global burden of typhoid fever at 11–20 million cases per year, resulting in ~140,000 deaths. 8
Typhoid conjugate vaccines (TCV) are highly effective and have been recommended for use in typhoid fever-endemic countries. 9 , 10 Vaccine introduction can address the enduring problem of pediatric typhoid fever as well as the evolving problem of multi-drug resistance (MDR) in affected regions.
Clinical Presentation and Diagnosis of Typhoid Fever
Typhoid fever is a systemic infection that presents with multisystem signs and symptoms albeit without pathognomonic clinical features. 4 Transmission usually occurs via the fecal-oral route, typically when an individual ingests contaminated food or water. 4 S . Typhi characteristically invades the gastrointestinal tract and progresses to systemic infection. 3 The risk of infection has previously been linked to factors such as exposure to contaminated water, inadequate waste management, poor hygiene conditions as well as inhabitation of urban slums. 11 Clinical diagnosis is challenging because of its nonspecific and diverse symptoms that include fever, diarrhea, and abdominal pain. 12
The gold standard for the diagnosis of typhoid fever is a bone-marrow culture. While this modality is highly sensitive, it is invasive and technically unfeasible in most settings. 13 Thus, this disease is typically diagnosed by the more practical method of blood culture despite its limited (40–60%) sensitivity and the challenges in establishing contamination-free blood culturing in LMIC settings. 14 , 15 While typhoid fever can usually be treated effectively with appropriate antibiotics if diagnosed accurately during the early stages of the disease, the limited resources available in most of the endemic regions often lead to delays in diagnosis and treatment, followed by empirical treatment only. 16 , 17 New diagnostic tools with improved accuracy, including antibody-based serologic assays used to evaluate samples collected through serosurveys, are currently under development and will hopefully be available for use in field settings. 18 , 19
Epidemiology of Typhoid Fever in Africa
S . Typhi has reportedly been cultured from patients in 42 of 57 African countries until 2018; however, this could result from limited diagnostic capacities and underreporting in the remaining 15 countries. 20 Several researchers have recently estimated the incidence of disease and mortality rates at levels that are similar, if not higher, than those identified in South/Southeast Asia. 20–22 Nevertheless, the results of one recent study show the high degree of heterogeneity in different settings and between countries. 20 In their 2017 review, Antillón et al 23 estimated the burden of typhoid fever in sub-Saharan Africa at 762 per 100,000 person-years. These findings highlight an incidence that is significantly higher than that identified in Southeast Asia, East Asia, and Oceania (estimated incidence of 108 per 100,000 person-years). This review applied a modeling approach which included factors such as population density, gross domestic product (GDP), Gini coefficients, access to improved sources of water, and sanitation as predictors of disease burden. Similarly, a 2019 comprehensive review by Marchello et al 24 estimated the African incidence of typhoid fever from previous results and presented an initial pooled estimate of 112.1/100,000 person-years, with a 95% confidence interval (CI) of 46.7–203.5. After application of adjustment factors, including access to health-care facilities (ie, eligible participants not seeking care), enrollment (ie, eligible participants without blood culture data), blood culture sensitivity, and the length of the surveillance period, the revised pooled estimate of the global burden of typhoid fever increased to 134.1/100,000 person-years (95% CI, 77.9–204.8). 24 The Institute for Health Metrics and Evaluation (IHME) Global Health Data Exchange provided similar estimates for 2019 at 114.8/100,000 person-years (95% CI, 73.5–171.0). 25
The incidence of typhoid fever has been reported higher among children, mainly those who are older than five years of age. A systematic analysis by Stanaway et al estimated 55.9% of cases in 2017 globally occurred among children younger than 15 years (95% CI, 50.3–61.6), and 12.6% among children younger than 5 years (95% CI, 8.7–17.7). 21 Another meta-analysis examining age-stratified disease occurrence reported a proportion of 0.24 (95% CI, 0.20–0.28) of pediatric enteric fever cases in Africa occurred in children under the age of five. 26 While these findings emphasize the substantial burden in pediatric populations, more comprehensive data on the burden of typhoid fever in younger age groups is needed.
It is not clear if the increased incidence reported for specific regions can be attributed to an actual increase in case numbers or whether it is the result of an increase in the number of studies undertaken with extended surveillance methods and more standardized diagnostic tools, in particular blood culture. Of note, few studies have compared incidence rates in urban versus rural areas in various LMICs. Those that have been undertaken have yielded mixed results, with some reporting higher and others documenting lower incidences in rural areas. 27–29 While higher incidences of typhoid fever in urban areas may be a direct result of higher population density, individuals residing in rural areas typically have more limited access to sanitation and sources of clean water. Similarly, fewer diagnostic tools of all sorts are available in rural areas compared to urban centers; this may lead to an inaccurately low calculated incidence of typhoid fever as many cases are likely to remain undiagnosed. Moreover, as there is usually a higher availability and accessibility of health-care services in urban areas, the higher incidences compared to rural areas could also be attributed to urban residents seeking health-care facilities more often when experiencing symptoms of infection. Nonetheless, given the extent of travel between urban and rural areas, the risk of exposure to typhoid fever remains ongoing in all locales.
The Typhoid Fever Surveillance in Africa Program (TSAP) that was conducted between the years 2010 and 2014 was setup in 13 sites in 10 Africa countries (Burkina Faso, Ghana, Guinea-Bissau, Senegal, Ethiopia, Sudan, Kenya, Tanzania, South Africa, and Madagascar) to determine age-stratified incidences of invasive Salmonella infections. Typhoid fever incidence estimates ranged from 0 in Sudan to 383/100,000 person-years in Burkina Faso. 30 Among age groups, the highest disease burden for typhoid fever was in the 5–8 years-of-age group. The TSAP study also highlighted variations in disease patterns between rural and urban sites in Ghana and reported age-adjusted incidence rates that were two times higher in the rural versus the urban site. 31 Factors contributing to this difference may include the poorer-quality sanitary and hygiene conditions in rural areas, compounded by less education and the availability of fewer typhoid interventions. However, other studies reported conflicting results, including much higher rates of typhoid fever in urban settlements. 28 Further research will be needed to clarify these results. The Severe Typhoid Program in Africa (SETA) was instituted as a follow-up to TSAP. In order to assess typhoid fever severity, the SETA provided continued surveillance of four of the original ten aforementioned higher-incidence countries (Burkina Faso, Ethiopia, Ghana, and Madagascar) and included two new sites in Nigeria and the Democratic Republic of the Congo (DRC). Results from this program will provide updated information on the severity, mortality, and economic burden of typhoid disease in these African countries. 32
Severity and Mortality of Typhoid Fever
Individuals infected with S . Typhi may develop complications by the second to fourth week of illness and could progress to death if left untreated. 4 , 33 Recent systematic reviews and meta-analyses focused on severity and mortality of typhoid fever in Africa identified an overall prevalence of complications at 37.7% 22 with an overall case fatality rate (CFR) of 3.82% (95% CI, 1.97–7.26%). 34 These results suggest that Africa has the highest prevalence of typhoid fever complications and mortality when compared to other WHO regions. For example, the CFR in the South-East Asian region has been estimated at 2.27% (95% CI, 0.91–5.55%). 22 , 24 , 34 , 35 Nevertheless, it is critical to recognize the considerable variations in the burden of disease both between and within these regions. 36–38
The most serious and well-characterized complication of typhoid fever is typhoid intestinal perforation (TIP). This condition is highly prevalent in Africa, with an overall incidence estimated at 7.6% of typhoid fever cases in Africa. 22 This rate is higher than that reported for the Americas (1.8%), Asia (0.7%), and Oceania (0.7%) combined. 22 TIP develops twice as frequently in males (66.3% of cases). 22 A review of this complication published in 2017 estimated the mortality associated with TIP at 5–80% in resource-poor countries. 39 The median CFR related to this complication estimated for the African region ranges from 19.5% (95% CI, 16.6–22.4%) 40 to 20.0% (13.7–28.0%). 22 These data can be compared to median CFRs estimated between 1.0% (0.0–8.4%) 22 and 10.7% (95% CI; 8.0–13.4%) 40 for the Asian region. The CFR associated with TIP among children under 18 years of age might be as high as 75%; 35 this rate is significantly higher than the overall estimated CFR for typhoid fever. There are data indicating that children under five are at a higher risk of experiencing severe illness and subsequently high mortality rates. 26 , 41 , 42 Similarly, Stanaway et al estimated highest mortality rates among young children; however, identifying a peak in the ages between 5 and 9 with 59.3% of global deaths occurring among children younger than 15 years of age. 21
Several factors may contribute to the high rates of severity and mortality associated with typhoid fever in Africa. Recent systematic reviews point to delays in diagnosis and/or treatment as factors that are associated with both increased severity and CFR. 22 , 43 The mean delay in the care provided for typhoid fever patients in Africa, defined as the duration of disease prior to hospitalization, has been estimated at 9.4 days. 22 Results from a recent study revealed that delay in care of an average 10 days was associated with three times greater odds of developing any typhoid fever complications (Odds Ratio [OR] 3.0; 95% CI, 2.14–4.17) and the more than two times greater odds of developing TIP (OR 2.5; 95% CI, 0.43–10.80). 43 A recent study focused on complications of typhoid fever specifically in Madagascar documented a substantial surge in intestinal perforations commencing at the time of onset of the Coronavirus-disease 2019 (COVID-19) pandemic, potentially as a result of delayed treatment due to general reluctance regarding visiting health-care facilities. 44 Other researchers have also identified severe typhoid fever disease among individuals who began treatment later in the course of the disease. 45–47 These data highlight the importance of early care and treatment for typhoid fever toward reducing morbidity and mortality. Furthermore, limited access to health services and specifically blood culture diagnostics in various settings in the African region preclude timely administration of appropriate antibiotic treatment based on pathogen susceptibility profile. This problem also contributes to poor outcomes secondary to treatment failure and the development of AMR. 22 , 35 , 47 While several researchers note that the increasing AMR of S . Typhi pathologic variants is a significant factor contributing to the severity of disease, 4 , 48 , 52 the relationship between the variants and severity of disease needs to be further investigated going forward.
While typhoid fever still significantly contributes to the morbidity and mortality inLMICs, socioeconomic impacts of this disease need also be considered. 49 Accurate estimations for the economic costs of typhoid fever are difficult to obtain given limited data availability and the use of heterogeneous research methods; one review cited an estimated cost per typhoid fever case at $171 in Tanzania while indicating the existence of a high variation between countries considering the estimated typhoid fever incidence in LMICs. 50 A primary evaluation of vaccination in LMIC settings found TCV to be cost-effective strategy in these settings. 51 Further studies for assessing cost of illness are also underway in the course of the SETA study.
Increasing Antibiotic Resistance
Early diagnosis and prompt initiation of appropriate treatment are considered to be the key factors that determine disease prognosis. 52 Typhoid fever responds well to treatment with antibiotics; however, the rise of MDR S . Typhi strains impairs successful treatment and may lead to a higher incidence of complications and disease severity. 36 , 53 , 54 This problem is exacerbated by the frequent inappropriate use of antibiotics that contribute to the rapid development of antibiotic resistance. 4 , 55 MDR strains, represented mainly by the H58 S . Typhi genotype, are resistant to first-line anti-typhoidal antibiotics that include chloramphenicol, ampicillin, and trimethoprim-sulfamethoxazole/co-trimoxazole. These MDR strains are also responsible for several outbreaks of typhoid fever across the African and Asian continents. 56 , 57 Zimbabwe, a location with a substantial endemic occurrence of typhoid fever, was one of the countries affected by repeated seasonal outbreaks with a high proportion of antimicrobial-resistant strains. Most culture-confirmed isolates (88%) from this region were resistant to two or more first-line anti-typhoid drugs with increasing trends observed between 2012 and 2017. 58 In response to these outbreaks, Zimbabwe carried out mass vaccination campaigns in 2019, aimed at children between the ages of 6 months and 15 years; this strategy was highly effective at reducing the incidence of typhoid fever in this age group. 59 MDR strains of S . Typhi are emerging at an increasing rate in other countries across the African continent. Although reported MDR rates vary greatly between countries, the overall rate in S . Typhi isolated from countries surveyed by the TSAP was estimated at 52%. 57 A study carried out in Bwamanda, DRC reported that 72.2% of S . Typhi isolates were co-resistant to ampicillin and trimethoprim-sulfamethoxazole, and an additional 33.3% were MDR. 37 Another study carried out in Nairobi, Kenya, revealed a high prevalence of H58-genotype in S . Typhi cases (97%, 96/99), with 76% of the strains being also MDR. 60
This increase in MDR strains of S . Typhi is particularly worrisome as this could have a profound impact on our ability to treat typhoid fever and thus result in a much higher CFR. To address this problem, current treatment strategies also include fluoroquinolones. However, recent findings have documented increases in transmission of extensively drug-resistant (XDR) S . Typhi strains, which are resistant to five classes of antibiotics: chloramphenicol, ampicillin, cotrimoxazole, fluoroquinolones, and third-generation cephalosporins. 61 Pakistan experienced its largest outbreak to date with XDR-typhoid in 2018 in Hyderabad, with an increased risk of infection for children younger than 15 years of age. 62 A surveillance study focused on the years 2016–2019 reported that 64% and 16% of the analyzed S . Typhi isolates from Pakistan were XDR and MDR, respectively. 63 In response, Pakistan became the first country to introduce TCVs which was administered to millions of children in 2019. 64 Recent studies have demonstrated that single-dose of the TCV was effective in children including against XDR-typhoid. 65 This result supports their future use, notably in countries with evolving and increasing evidence of AMR.
Typhoid Vaccines: Recent Developments
Typhoid vaccines constitute a major tool that can be used in the global effort to prevent typhoid fever. The WHO provides guidance for the programmatic use of typhoid vaccines to control typhoid fever including recommendations to include TCV into countries’ routine immunization programs as well as targeted catch-up vaccination. It also highlights the decision-making process for countries to decide on the best vaccination strategy. 10 Three typhoid vaccines are currently in use worldwide, including the newer generation TCV with the Vi-polysaccharide antigen, the unconjugated polysaccharide vaccine (Vi-PS), and a vaccine based on the administration of oral live attenuated Salmonella strain Ty21a. TCV is currently the preferred formulation because of its immunological properties, wider usage (can be administered to children ≥6 months of age), and longer-lasting protection after a single dose. The TCV formulation has overcome some of the challenges that previously hindered the success of both polysaccharide and live vaccines. 66 In this formulation, the S . Typhi Vi-polysaccharide is conjugated to a protein to elicit a stronger response from the immune system.
Several TCVs have been licensed nationally; 67 the Vi-TT and Vi-CRM 197 formulations are WHO-prequalified for use in children as young as 6 months and are thus the first typhoid vaccines to be approved for use in children under the age of 2 years ( Table 1 : Overview of WHO-Prequalified Typhoid Vaccines). This approval was particularly significant because of the high proportion of young children affected by typhoid fever. The WHO recommends the administration of TCVs in typhoid fever-endemic countries to children at 9 months of age or in the second year of life as a single dose. 9 , 10 Several additional vaccine candidates are currently in development; others have already received a national license. 68
Overview of WHO-Prequalified Typhoid Vaccines
Note: Adapted from World Health Organization. Comparison table of WHO prequalified typhoid conjugate vaccines (TCV); 2021. Available from: https://apps.who.int/iris/handle/10665/345367 . Licence: CC-BY-NC-SA 3.0 IGO. 69
As per currently available data, the two prequalified vaccines are expected to perform comparably. 69 The Typhoid Vaccine Acceleration Consortium (TyVAC) is conducting studies using the Vi-TT vaccine to evaluate efficacy (Malawi, Nepal), effectiveness (Bangladesh) and immunogenicity (Burkina Faso). 66 The results from a Phase 3 trial carried out in Malawi involving 28,130 participants demonstrated both safety and 80.7% efficacy (95% CI, 64.2–89.6%) of the Vi-TT vaccine in children from 9 months to 12 years of age. 70 Similar results have been reported from trials performed in Nepal and Bangladesh, with reports of 81.6% efficacy (95% CI, 58.8–91.8%) and 85.0% total vaccine protection (97.5% CI, 76.0–91.0%), respectively. 71 , 72 New data available from the two-year follow-up of the randomized-controlled trial in Nepal revealed a protective efficacy against blood-culture confirmed typhoid fever of 79.0% (95% CI, 61.9–88.5%). 73 These results were comparable to those from the interim analysis, which collectively indicate long-term vaccine protection and provide further support for its use in typhoid-endemic regions. Further, results also showed that the Vi-TT-TCV performed consistently well across different regions, populations and transmission settings with a robust safety profile.
The Typhoid Conjugate Vaccine Introduction in Africa program (THECA) has initiated a cluster-randomized trial in Ghana in 2021 and a mass vaccination campaign in the DRC in 2022 using the Vi-TT to generate information on its real-world effectiveness and impact. 74 Similar evaluations of the Vi-CRM 197 formulation are planned for Madagascar. It will be critical to assess vaccine efficacy and large-scale effectiveness particularly in settings with high disease burden and significant levels of AMR. However, the data currently available from Phase 3 trials and the first mass vaccinations permit optimism for the widespread application of Vi-TT, and potentially also other TCVs.
As emerging AMR remains a global concern for the treatment of typhoid fever as well as numerous other infectious diseases, vaccines are increasingly recognized as a potential solution. This point has received particular support from the findings of a study carried out in Pakistan that showed that Vi-TT was highly effective in combating an outbreak of XDR-typhoid fever (ie, 97% effectiveness against XDR- S . Typhi). 65 Vaccination campaigns carried out in endemic areas might limit not only the incidence but also the spread of typhoid fever, thereby reducing the need for antibiotics and the development of AMR.
Gavi, the Vaccine Alliance, pledged its support toward the introduction of TCV in designated countries starting in 2019. 75 Several countries have since applied for Gavi support with vaccine introductions planned for 2022. 76 Rollout of the TCV in sub-Saharan Africa has started and continued throughout the COVID-19 pandemic. In April 2021, Liberia became the first country in sub-Saharan Africa (and the second country globally after Pakistan) to introduce TCV in a program aimed at vaccinating more than one million children. This was followed by a similar program in Zimbabwe later that same year. 77 Malawi has recently received approval of their application to Gavi and plans to introduce TCV in the near future. 78
Data from ongoing and future trials will also help elucidate the optimal use of TCV, including efforts to assess the local burden of disease, design and implementation of national vaccination strategies throughout Africa, as well as duration of protection exhibited through a single dose of TCV. 76
While the development of typhoid fever vaccines has far progressed, the development of paratyphoid and iNTS vaccines are a decade behind. While there are currently no licensed vaccines available for either, there are several promising vaccine candidates of different compositions in the pipeline, with clinical trials ongoing. 77 , 79 , 80 Further investment and research efforts are needed to accelerate developments.
Conclusion and the Way Forward
Numerous studies carried out over the last decade that focused on typhoid fever specifically in Africa have improved our understanding of the epidemiology of the disease and the population at risk. Findings from these studies showed the higher incidence of disease as well as its increased severity and mortality in the region. They have provided insight into factors with the potential to contribute to these severe outcomes, including limited access to healthcare and delays in diagnosis and treatment. Ongoing multi-site surveillance programs that include more countries than any study performed previously are designed to collect a wide range of data with a systematic focus on disease severity, mortality, and complications, thus circumventing one of the major limitations of previous studies. The findings from these systematic investigations will provide a more accurate description of disease severity and mortality and will thus serve to guide vaccine introduction strategies in African countries. However, it may be important to expand surveillance to include more rural areas and to determine the feasibility of environmental surveillance and serological assays ( vide infra ) to achieve an improved understanding of the spatiotemporal distribution of the disease. Both methods are currently under evaluation as an addition to ongoing surveillance programs. If successful, such tools could become powerful and low-cost methodologies to support the incidence assessments of S . Typhi infection and the impact of vaccination strategies introduced in many endemic countries. 81 Additionally, as there is often a limited awareness and knowledge on infectious diseases in affected populations, another aspect to be considered is the education and community engagement in these respective populations, which could result in increased awareness and participation, as well as improved overall collaboration. 82 , 83
Future efforts might also focus on improving diagnostic strategies for use under field conditions and remote settings. Other efforts might be directed toward effective treatment options, to provide care as early as possible to achieve better outcomes. Simultaneously, preventive measures should be introduced, primarily vaccination with prequalified TCV-vaccines in highly affected regions. This would help provide sustained protection against typhoid fever in the short term. Widespread vaccination will also limit the spread of MDR and XDR strains. Finally, efforts to improve water, sanitation, and health infrastructure in Africa should be developed and accomplished as long-term goals towards the global UN Sustainable Development Goals.
The authors report no conflicts of interest in this work.
The Severe Typhoid Fever in Africa Program: Study Design and Methodology to Assess Disease Severity, Host Immunity, and Carriage Associated With Invasive Salmonellosis
Affiliations.
- 1 International Vaccine Institute, Seoul National University Research Park, Republic of Korea.
- 2 Oxford University Clinical Research Unit, Ho Chi Minh City, Vietnam.
- 3 Department of Microbiology and Infectious Disease, B. P. Koirala Institute of Health Sciences, Dharan, Nepal.
- 4 Institute of Medical Microbiology, University of Cologne, Germany.
- 5 Department of Medicine, Cambridge University, United Kingdom.
- 6 Global Health Institute, Emory University, Atlanta, Georgia.
- 7 School of Public Health, and, Kwame Nkrumah University of Science and Technology, Kumasi, Ghana.
- 8 Kumasi Centre for Collaborative Research in Tropical Medicine, Kwame Nkrumah University of Science and Technology, Kumasi, Ghana.
- 9 University of Antananarivo, Madagascar.
- 10 Institut Supérieur des Sciences de la Population, University of Ouagadougou, Ouagadougou, Burkina Faso.
- 11 Schiphra Hospital, Ouagadougou, Burkina Faso.
- 12 Armauer Hansen Research Institute, ALERT Campus, Addis Ababa, Ethiopia.
- 13 Faculty of Pharmacy, University of Ibadan.
- 14 Department of Medical Microbiology and Parasitology, College of Medicine, University of Ibadan.
- 15 Department of Medical Microbiology and Parasitology, University College Hospital.
- 16 Department of Community Medicine, College of Medicine, University of Ibadan.
- 17 Department of Community Medicine, University College Hospital, Ibadan, Nigeria.
- 18 Department of Microbiology and Immunology, KU Leuven.
- 19 Department of Clinical Sciences, Institute of Tropical Medicine, Antwerp, Belgium.
- 20 Institut National de Recherche Biomedicales, Kinshasa.
- 21 Service de Microbiologie, Cliniques Universitaires de Kinshasa, Democratic Republic of Congo.
- 22 Institute of Tropical Medicine, Eberhard-Karls University of Tübingen, Germany.
- 23 Duy Tan University, Da Nang, Vietnam.
- 24 Kilimanjaro Christian Medical Centre, Moshi, Tanzania.
- 25 Division of Infectious Diseases and International Health, Duke University Medical Center.
- 26 Duke Global Health Institute, Duke University, Durham, North Carolina.
- 27 Centre for International Health, University of Otago, Dunedin, New Zealand.
- 28 Jenner Institute, University of Oxford, United Kingdom.
- 29 Clinical Sciences, Liverpool School of Tropical Medicine.
- 30 Centre for Tropical Medicine, Nuffield Department of Medicine, University of Oxford, United Kingdom.
- 31 National Center for Emerging and Zoonotic Infectious Diseases, Centers for Disease Control and Prevention, Atlanta, Georgia.
- 32 icddr,b, Dhaka, Bangladesh.
- 33 Fielding School of Public Health, University of California, Los Angeles.
- PMID: 31665779
- PMCID: PMC6821161
- DOI: 10.1093/cid/ciz715
Background: Invasive salmonellosis is a common community-acquired bacteremia in persons residing in sub-Saharan Africa. However, there is a paucity of data on severe typhoid fever and its associated acute and chronic host immune response and carriage. The Severe Typhoid Fever in Africa (SETA) program, a multicountry surveillance study, aimed to address these research gaps and contribute to the control and prevention of invasive salmonellosis.
Methods: A prospective healthcare facility-based surveillance with active screening of enteric fever and clinically suspected severe typhoid fever with complications was performed using a standardized protocol across the study sites in Burkina Faso, the Democratic Republic of Congo (DRC), Ethiopia, Ghana, Madagascar, and Nigeria. Defined inclusion criteria were used for screening of eligible patients for enrollment into the study. Enrolled patients with confirmed invasive salmonellosis by blood culture or patients with clinically suspected severe typhoid fever with perforation were eligible for clinical follow-up. Asymptomatic neighborhood controls and immediate household contacts of each case were enrolled as a comparison group to assess the level of Salmonella-specific antibodies and shedding patterns. Healthcare utilization surveys were performed to permit adjustment of incidence estimations. Postmortem questionnaires were conducted in medically underserved areas to assess death attributed to invasive Salmonella infections in selected sites.
Results: Research data generated through SETA aimed to address scientific knowledge gaps concerning the severe typhoid fever and mortality, long-term host immune responses, and bacterial shedding and carriage associated with natural infection by invasive salmonellae.
Conclusions: SETA supports public health policy on typhoid immunization strategy in Africa.
Keywords: Severe typhoid fever; host immunity and carriage; invasive Salmonellosis; sub-Saharan Africa; surveillance protocol.
© The Author(s) 2019. Published by Oxford University Press for the Infectious Diseases Society of America.
Publication types
- Research Support, Non-U.S. Gov't
- Africa South of the Sahara / epidemiology
- Bacteremia / epidemiology
- Bacteremia / prevention & control
- Carrier State / epidemiology*
- Carrier State / microbiology
- Child, Preschool
- Community-Acquired Infections / epidemiology
- Community-Acquired Infections / microbiology
- Community-Acquired Infections / prevention & control
- Health Services Research / methods
- Health Services Research / organization & administration*
- Patient Acceptance of Health Care / statistics & numerical data*
- Prospective Studies
- Research Design
- Salmonella Infections / epidemiology*
- Salmonella Infections / immunology*
- Salmonella Infections / prevention & control
- Surveys and Questionnaires
- Typhoid Fever / epidemiology*
- Typhoid Fever / immunology
Grants and funding
- MR/P008852/1/MRC_/Medical Research Council/United Kingdom
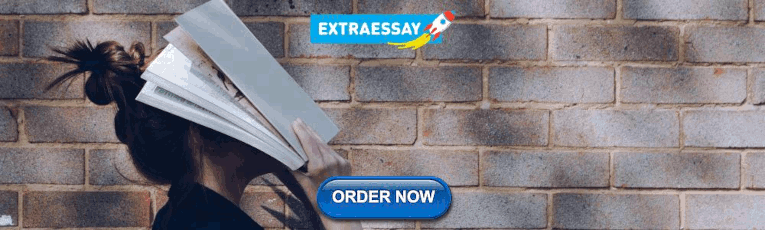
IMAGES
VIDEO
COMMENTS
Salmonella enterica subspecies enterica serovar Typhi (Salmonella Typhi) is the cause of typhoid fever. Typhoid fever is a systemic infection that is an important source of illness and death in low-resource areas [1, 2].Persons living in areas without access to improved sanitation facilities who are exposed to fecally contaminated water and food are at greatest risk for infection [].
Global estimates for the burden. of typhoid fever (defi ned as symptomatic infection with. S Typhi) are published regularly (26·9 million cases of. typhoid fever were reported in 2010) and ...
Abstract. Typhoid fever is a significant global health problem that impacts people living in areas without access to clean water and sanitation. However, collaborative international partnerships and new research have improved both knowledge of the burden in countries with endemic disease and the tools for improved surveillance, including ...
Despite notable progress, typhoid and paratyphoid fevers remain major causes of disability and death, with billions of people likely to be exposed to the pathogens. Although improvements in water and sanitation remain essential, increased vaccine use (including with typhoid conjugate vaccines that are effective in infants and young children and protective for longer periods) and improved data ...
Typhoid fever, an acute febrile illness caused by Salmonella enterica serovar Typhi (S.Typhi), is endemic in many low- and middle-income countries † ().In 2015, an estimated 11-21 million typhoid fever cases and 148,000-161,000 associated deaths occurred worldwide ().Effective prevention strategies include improved access to and use of infrastructure supporting safe water, sanitation ...
Most patients who present to hospitals with typhoid fever are children or young adults from 5 to 25 years of age. 1,52,53 However, community-based studies in areas of endemic disease indicate that ...
Typhoid fever, an infection caused by Salmonella enterica serovar Typhi (S.Typhi), is a global public health problem. An estimated 11 to 20 million typhoid fever cases including 128,000 to 161,000 deaths occur each year [1,2,3,4] with the majority in low- and middle-income countries (LMICs) [5, 6].Although several effective treatment and prevention strategies are available [], improving water ...
This research investigates a robust numerical analytic strategy for typhoid fever that takes infection protection into consideration and incorporates fuzzy parameters. The use of fuzzy parameters ...
Typhoid fever is one of the major causes of mortality and morbidity in overcrowded and unhygienic areas though comprehensive research and public health interventions have decreased the occurrence. The disease course ranges from early gastrointestinal distress to nonspecific systemic illness but ultimately may lead to multiple complications.
We conducted a literature review for typhoid outbreaks published since 1990. We found 47 publications describing 45,215 cases in outbreaks occurring in 25 countries from 1989 through 2018. Outbreak characteristics varied considerably by WHO region, with median outbreak size ranging from 12 to 1,101 cases, median duration from 23 to 140 days ...
Background: Contemporary incidence estimates of typhoid fever are needed to guide policy decisions and control measures and to improve future epidemiological studies. Methods: We systematically reviewed 3 databases (Ovid Medline, PubMed, and Scopus) without restriction on age, country, language, or time for studies reporting the incidence of blood culture-confirmed typhoid fever.
Typhoid fever is endemic in Laos, but there are limited epidemiological data. In a hospital-based study examining blood cultures at Mahosot hospital in the capital, Vientiane, between 2000 and 2018, there were a total of 913 culture-confirmed typhoid cases from just over 60 000 blood cultures (∼1.5% positivity). ... Research and Developments ...
Typhoid fever is a major public health problem in low-income and middle-income countries (LMICs) like Ethiopia where there are substandard hygiene and unsafe drinking water supplies and the quality of life is poor [1,2,3,4,5].Typhoid fever is a systemic infection caused by human-specific food and water-borne pathogens, such as Salmonella enterica subspecies, enterica serovar typhi (S. typhi ...
Author summary Modeling suggests that Oceania has surpassed Asia and sub-Saharan Africa as the region with the highest typhoid fever incidence. While Pacific Islands are often neglected due to small population sizes, there is an urgent need to understand the epidemiology of typhoid fever in the region. Fiji, an upper-middle income country in Oceania, has reported an increase in typhoid fever ...
overall prevalence of culture-confirmed typhoid fev5.0%(21/421).The prevalence of typhoid fever was sig-cantly associated with rural r(8.4%).As compared to the urban r,3.6e likely found to have culture-confirmed typhoid fever.The prevalence of typhoid fever was significantly associated with
Methods. the study carried out from March 1 st, 2017 to May 31 st, 2017 recruited patients who presented at the hospital with clinical signs and symptoms of typhoid fever and who had lab requests for stool culture requested by the resident physician.The prevalence of Salmonella typhi infections among the patients and the proportion of patients with adequate knowledge on the mode of ...
Author summary Typhoid fever remains a significant health challenge in low- and middle-income nations, especially in sub-Saharan Africa. A comprehensive understanding of the disease's geospatial distribution is pivotal for its control—a gap previous studies overlooked. Addressing this, we undertook a study to chart the incidence of typhoid fever throughout sub-Saharan Africa. Using data ...
This article is the introduction to a 12-paper supplement on global trends in typhoid fever. The Tackling Typhoid (T2) project was initiated in 2015 to synthesize the existing body of literature on typhoidal salmonellae and study national and regional typhoid fever trends. In addition to a global systematic review, eight case studies were undertaken to examine typhoid and paratyphoid fever ...
During the period of fever, up to 25% of patients show exanthem (rose spots), on the chest, abdomen and back. Complicated disease: Acute typhoid fever may be severe. Depending on the clinical setting and the quality of available medical care, up to 10% of typhoid patients may develop serious complications.
With data derived from meta-analyses of disease burdens in 73 countries eligible for support from Gavi, the Vaccine Alliance, Birger and colleagues have shown that introduction of routine immunisation of children from age 9 months with typhoid conjugate vaccines (TCVs), with a catch-up programme up to age 15 years, would avert 66·7 million cases and 826 000 deaths caused by typhoid fever over ...
The case fatality risk of typhoid fever was approximately 10%-30% in the preantimicrobial era [ 45 ]. With effective antimicrobials, the case fatality risk is usually <1%. Antimicrobial-resistant Salmonella Typhi is a major global health concern [ 46 ]. Clinical studies demonstrate the association between antimicrobial resistance and poor ...
The incidence of typhoid fever has been reported higher among children, mainly those who are older than five years of age. A systematic analysis by Stanaway et al estimated 55.9% of cases in 2017 globally occurred among children younger than 15 years (95% CI, 50.3-61.6), and 12.6% among children younger than 5 years (95% CI, 8.7-17.7). 21 ...
However, there is a paucity of data on severe typhoid fever and its associated acute and chronic host immune response and carriage. The Severe Typhoid Fever in Africa (SETA) program, a multicountry surveillance study, aimed to address these research gaps and contribute to the control and prevention of invasive salmonellosis.