- Privacy Policy

Home » Secondary Data – Types, Methods and Examples
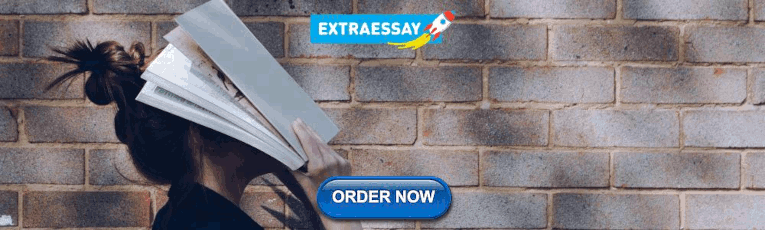
Secondary Data – Types, Methods and Examples
Table of Contents

Secondary Data
Definition:
Secondary data refers to information that has been collected, processed, and published by someone else, rather than the researcher gathering the data firsthand. This can include data from sources such as government publications, academic journals, market research reports, and other existing datasets.
Secondary Data Types
Types of secondary data are as follows:
- Published data: Published data refers to data that has been published in books, magazines, newspapers, and other print media. Examples include statistical reports, market research reports, and scholarly articles.
- Government data: Government data refers to data collected by government agencies and departments. This can include data on demographics, economic trends, crime rates, and health statistics.
- Commercial data: Commercial data is data collected by businesses for their own purposes. This can include sales data, customer feedback, and market research data.
- Academic data: Academic data refers to data collected by researchers for academic purposes. This can include data from experiments, surveys, and observational studies.
- Online data: Online data refers to data that is available on the internet. This can include social media posts, website analytics, and online customer reviews.
- Organizational data: Organizational data is data collected by businesses or organizations for their own purposes. This can include data on employee performance, financial records, and customer satisfaction.
- Historical data : Historical data refers to data that was collected in the past and is still available for research purposes. This can include census data, historical documents, and archival records.
- International data: International data refers to data collected from other countries for research purposes. This can include data on international trade, health statistics, and demographic trends.
- Public data : Public data refers to data that is available to the general public. This can include data from government agencies, non-profit organizations, and other sources.
- Private data: Private data refers to data that is not available to the general public. This can include confidential business data, personal medical records, and financial data.
- Big data: Big data refers to large, complex datasets that are difficult to manage and analyze using traditional data processing methods. This can include social media data, sensor data, and other types of data generated by digital devices.
Secondary Data Collection Methods
Secondary Data Collection Methods are as follows:
- Published sources: Researchers can gather secondary data from published sources such as books, journals, reports, and newspapers. These sources often provide comprehensive information on a variety of topics.
- Online sources: With the growth of the internet, researchers can now access a vast amount of secondary data online. This includes websites, databases, and online archives.
- Government sources : Government agencies often collect and publish a wide range of secondary data on topics such as demographics, crime rates, and health statistics. Researchers can obtain this data through government websites, publications, or data portals.
- Commercial sources: Businesses often collect and analyze data for marketing research or customer profiling. Researchers can obtain this data through commercial data providers or by purchasing market research reports.
- Academic sources: Researchers can also obtain secondary data from academic sources such as published research studies, academic journals, and dissertations.
- Personal contacts: Researchers can also obtain secondary data from personal contacts, such as experts in a particular field or individuals with specialized knowledge.
Secondary Data Formats
Secondary data can come in various formats depending on the source from which it is obtained. Here are some common formats of secondary data:
- Numeric Data: Numeric data is often in the form of statistics and numerical figures that have been compiled and reported by organizations such as government agencies, research institutions, and commercial enterprises. This can include data such as population figures, GDP, sales figures, and market share.
- Textual Data: Textual data is often in the form of written documents, such as reports, articles, and books. This can include qualitative data such as descriptions, opinions, and narratives.
- Audiovisual Data : Audiovisual data is often in the form of recordings, videos, and photographs. This can include data such as interviews, focus group discussions, and other types of qualitative data.
- Geospatial Data: Geospatial data is often in the form of maps, satellite images, and geographic information systems (GIS) data. This can include data such as demographic information, land use patterns, and transportation networks.
- Transactional Data : Transactional data is often in the form of digital records of financial and business transactions. This can include data such as purchase histories, customer behavior, and financial transactions.
- Social Media Data: Social media data is often in the form of user-generated content from social media platforms such as Facebook, Twitter, and Instagram. This can include data such as user demographics, content trends, and sentiment analysis.
Secondary Data Analysis Methods
Secondary data analysis involves the use of pre-existing data for research purposes. Here are some common methods of secondary data analysis:
- Descriptive Analysis: This method involves describing the characteristics of a dataset, such as the mean, standard deviation, and range of the data. Descriptive analysis can be used to summarize data and provide an overview of trends.
- Inferential Analysis: This method involves making inferences and drawing conclusions about a population based on a sample of data. Inferential analysis can be used to test hypotheses and determine the statistical significance of relationships between variables.
- Content Analysis: This method involves analyzing textual or visual data to identify patterns and themes. Content analysis can be used to study the content of documents, media coverage, and social media posts.
- Time-Series Analysis : This method involves analyzing data over time to identify trends and patterns. Time-series analysis can be used to study economic trends, climate change, and other phenomena that change over time.
- Spatial Analysis : This method involves analyzing data in relation to geographic location. Spatial analysis can be used to study patterns of disease spread, land use patterns, and the effects of environmental factors on health outcomes.
- Meta-Analysis: This method involves combining data from multiple studies to draw conclusions about a particular phenomenon. Meta-analysis can be used to synthesize the results of previous research and provide a more comprehensive understanding of a particular topic.
Secondary Data Gathering Guide
Here are some steps to follow when gathering secondary data:
- Define your research question: Start by defining your research question and identifying the specific information you need to answer it. This will help you identify the type of secondary data you need and where to find it.
- Identify relevant sources: Identify potential sources of secondary data, including published sources, online databases, government sources, and commercial data providers. Consider the reliability and validity of each source.
- Evaluate the quality of the data: Evaluate the quality and reliability of the data you plan to use. Consider the data collection methods, sample size, and potential biases. Make sure the data is relevant to your research question and is suitable for the type of analysis you plan to conduct.
- Collect the data: Collect the relevant data from the identified sources. Use a consistent method to record and organize the data to make analysis easier.
- Validate the data: Validate the data to ensure that it is accurate and reliable. Check for inconsistencies, missing data, and errors. Address any issues before analyzing the data.
- Analyze the data: Analyze the data using appropriate statistical and analytical methods. Use descriptive and inferential statistics to summarize and draw conclusions from the data.
- Interpret the results: Interpret the results of your analysis and draw conclusions based on the data. Make sure your conclusions are supported by the data and are relevant to your research question.
- Communicate the findings : Communicate your findings clearly and concisely. Use appropriate visual aids such as graphs and charts to help explain your results.
Examples of Secondary Data
Here are some examples of secondary data from different fields:
- Healthcare : Hospital records, medical journals, clinical trial data, and disease registries are examples of secondary data sources in healthcare. These sources can provide researchers with information on patient demographics, disease prevalence, and treatment outcomes.
- Marketing : Market research reports, customer surveys, and sales data are examples of secondary data sources in marketing. These sources can provide marketers with information on consumer preferences, market trends, and competitor activity.
- Education : Student test scores, graduation rates, and enrollment statistics are examples of secondary data sources in education. These sources can provide researchers with information on student achievement, teacher effectiveness, and educational disparities.
- Finance : Stock market data, financial statements, and credit reports are examples of secondary data sources in finance. These sources can provide investors with information on market trends, company performance, and creditworthiness.
- Social Science : Government statistics, census data, and survey data are examples of secondary data sources in social science. These sources can provide researchers with information on population demographics, social trends, and political attitudes.
- Environmental Science : Climate data, remote sensing data, and ecological monitoring data are examples of secondary data sources in environmental science. These sources can provide researchers with information on weather patterns, land use, and biodiversity.
Purpose of Secondary Data
The purpose of secondary data is to provide researchers with information that has already been collected by others for other purposes. Secondary data can be used to support research questions, test hypotheses, and answer research objectives. Some of the key purposes of secondary data are:
- To gain a better understanding of the research topic : Secondary data can be used to provide context and background information on a research topic. This can help researchers understand the historical and social context of their research and gain insights into relevant variables and relationships.
- To save time and resources: Collecting new primary data can be time-consuming and expensive. Using existing secondary data sources can save researchers time and resources by providing access to pre-existing data that has already been collected and organized.
- To provide comparative data : Secondary data can be used to compare and contrast findings across different studies or datasets. This can help researchers identify trends, patterns, and relationships that may not have been apparent from individual studies.
- To support triangulation: Triangulation is the process of using multiple sources of data to confirm or refute research findings. Secondary data can be used to support triangulation by providing additional sources of data to support or refute primary research findings.
- To supplement primary data : Secondary data can be used to supplement primary data by providing additional information or insights that were not captured by the primary research. This can help researchers gain a more complete understanding of the research topic and draw more robust conclusions.
When to use Secondary Data
Secondary data can be useful in a variety of research contexts, and there are several situations in which it may be appropriate to use secondary data. Some common situations in which secondary data may be used include:
- When primary data collection is not feasible : Collecting primary data can be time-consuming and expensive, and in some cases, it may not be feasible to collect primary data. In these situations, secondary data can provide valuable insights and information.
- When exploring a new research area : Secondary data can be a useful starting point for researchers who are exploring a new research area. Secondary data can provide context and background information on a research topic, and can help researchers identify key variables and relationships to explore further.
- When comparing and contrasting research findings: Secondary data can be used to compare and contrast findings across different studies or datasets. This can help researchers identify trends, patterns, and relationships that may not have been apparent from individual studies.
- When triangulating research findings: Triangulation is the process of using multiple sources of data to confirm or refute research findings. Secondary data can be used to support triangulation by providing additional sources of data to support or refute primary research findings.
- When validating research findings : Secondary data can be used to validate primary research findings by providing additional sources of data that support or refute the primary findings.
Characteristics of Secondary Data
Secondary data have several characteristics that distinguish them from primary data. Here are some of the key characteristics of secondary data:
- Non-reactive: Secondary data are non-reactive, meaning that they are not collected for the specific purpose of the research study. This means that the researcher has no control over the data collection process, and cannot influence how the data were collected.
- Time-saving: Secondary data are pre-existing, meaning that they have already been collected and organized by someone else. This can save the researcher time and resources, as they do not need to collect the data themselves.
- Wide-ranging : Secondary data sources can provide a wide range of information on a variety of topics. This can be useful for researchers who are exploring a new research area or seeking to compare and contrast research findings.
- Less expensive: Secondary data are generally less expensive than primary data, as they do not require the researcher to incur the costs associated with data collection.
- Potential for bias : Secondary data may be subject to biases that were present in the original data collection process. For example, data may have been collected using a biased sampling method or the data may be incomplete or inaccurate.
- Lack of control: The researcher has no control over the data collection process and cannot ensure that the data were collected using appropriate methods or measures.
- Requires careful evaluation : Secondary data sources must be evaluated carefully to ensure that they are appropriate for the research question and analysis. This includes assessing the quality, reliability, and validity of the data sources.
Advantages of Secondary Data
There are several advantages to using secondary data in research, including:
- Time-saving : Collecting primary data can be time-consuming and expensive. Secondary data can be accessed quickly and easily, which can save researchers time and resources.
- Cost-effective: Secondary data are generally less expensive than primary data, as they do not require the researcher to incur the costs associated with data collection.
- Large sample size : Secondary data sources often have larger sample sizes than primary data sources, which can increase the statistical power of the research.
- Access to historical data : Secondary data sources can provide access to historical data, which can be useful for researchers who are studying trends over time.
- No ethical concerns: Secondary data are already in existence, so there are no ethical concerns related to collecting data from human subjects.
- May be more objective : Secondary data may be more objective than primary data, as the data were not collected for the specific purpose of the research study.
Limitations of Secondary Data
While there are many advantages to using secondary data in research, there are also some limitations that should be considered. Some of the main limitations of secondary data include:
- Lack of control over data quality : Researchers do not have control over the data collection process, which means they cannot ensure the accuracy or completeness of the data.
- Limited availability: Secondary data may not be available for the specific research question or study design.
- Lack of information on sampling and data collection methods: Researchers may not have access to information on the sampling and data collection methods used to gather the secondary data. This can make it difficult to evaluate the quality of the data.
- Data may not be up-to-date: Secondary data may not be up-to-date or relevant to the current research question.
- Data may be incomplete or inaccurate : Secondary data may be incomplete or inaccurate due to missing or incorrect data points, data entry errors, or other factors.
- Biases in data collection: The data may have been collected using biased sampling or data collection methods, which can limit the validity of the data.
- Lack of control over variables: Researchers have limited control over the variables that were measured in the original data collection process, which can limit the ability to draw conclusions about causality.
About the author
Muhammad Hassan
Researcher, Academic Writer, Web developer
You may also like

Information in Research – Types and Examples

Qualitative Data – Types, Methods and Examples

Research Data – Types Methods and Examples

Quantitative Data – Types, Methods and Examples

Primary Data – Types, Methods and Examples
Root out friction in every digital experience, super-charge conversion rates, and optimize digital self-service
Uncover insights from any interaction, deliver AI-powered agent coaching, and reduce cost to serve
Increase revenue and loyalty with real-time insights and recommendations delivered to teams on the ground
Know how your people feel and empower managers to improve employee engagement, productivity, and retention
Take action in the moments that matter most along the employee journey and drive bottom line growth
Whatever they’re are saying, wherever they’re saying it, know exactly what’s going on with your people
Get faster, richer insights with qual and quant tools that make powerful market research available to everyone
Run concept tests, pricing studies, prototyping + more with fast, powerful studies designed by UX research experts
Track your brand performance 24/7 and act quickly to respond to opportunities and challenges in your market
Explore the platform powering Experience Management
- Free Account
- For Digital
- For Customer Care
- For Human Resources
- For Researchers
- Financial Services
- All Industries
Popular Use Cases
- Customer Experience
- Employee Experience
- Net Promoter Score
- Voice of Customer
- Customer Success Hub
- Product Documentation
- Training & Certification
- XM Institute
- Popular Resources
- Customer Stories
- Artificial Intelligence
Market Research
- Partnerships
- Marketplace
The annual gathering of the experience leaders at the world’s iconic brands building breakthrough business results, live in Salt Lake City.
- English/AU & NZ
- Español/Europa
- Español/América Latina
- Português Brasileiro
- REQUEST DEMO
- Experience Management
- Secondary Research
Try Qualtrics for free
Secondary research: definition, methods, & examples.
19 min read This ultimate guide to secondary research helps you understand changes in market trends, customers buying patterns and your competition using existing data sources.
In situations where you’re not involved in the data gathering process ( primary research ), you have to rely on existing information and data to arrive at specific research conclusions or outcomes. This approach is known as secondary research.
In this article, we’re going to explain what secondary research is, how it works, and share some examples of it in practice.
Free eBook: The ultimate guide to conducting market research
What is secondary research?
Secondary research, also known as desk research, is a research method that involves compiling existing data sourced from a variety of channels . This includes internal sources (e.g.in-house research) or, more commonly, external sources (such as government statistics, organizational bodies, and the internet).
Secondary research comes in several formats, such as published datasets, reports, and survey responses , and can also be sourced from websites, libraries, and museums.
The information is usually free — or available at a limited access cost — and gathered using surveys , telephone interviews, observation, face-to-face interviews, and more.
When using secondary research, researchers collect, verify, analyze and incorporate it to help them confirm research goals for the research period.
As well as the above, it can be used to review previous research into an area of interest. Researchers can look for patterns across data spanning several years and identify trends — or use it to verify early hypothesis statements and establish whether it’s worth continuing research into a prospective area.
How to conduct secondary research
There are five key steps to conducting secondary research effectively and efficiently:
1. Identify and define the research topic
First, understand what you will be researching and define the topic by thinking about the research questions you want to be answered.
Ask yourself: What is the point of conducting this research? Then, ask: What do we want to achieve?
This may indicate an exploratory reason (why something happened) or confirm a hypothesis. The answers may indicate ideas that need primary or secondary research (or a combination) to investigate them.
2. Find research and existing data sources
If secondary research is needed, think about where you might find the information. This helps you narrow down your secondary sources to those that help you answer your questions. What keywords do you need to use?
Which organizations are closely working on this topic already? Are there any competitors that you need to be aware of?
Create a list of the data sources, information, and people that could help you with your work.
3. Begin searching and collecting the existing data
Now that you have the list of data sources, start accessing the data and collect the information into an organized system. This may mean you start setting up research journal accounts or making telephone calls to book meetings with third-party research teams to verify the details around data results.
As you search and access information, remember to check the data’s date, the credibility of the source, the relevance of the material to your research topic, and the methodology used by the third-party researchers. Start small and as you gain results, investigate further in the areas that help your research’s aims.
4. Combine the data and compare the results
When you have your data in one place, you need to understand, filter, order, and combine it intelligently. Data may come in different formats where some data could be unusable, while other information may need to be deleted.
After this, you can start to look at different data sets to see what they tell you. You may find that you need to compare the same datasets over different periods for changes over time or compare different datasets to notice overlaps or trends. Ask yourself: What does this data mean to my research? Does it help or hinder my research?
5. Analyze your data and explore further
In this last stage of the process, look at the information you have and ask yourself if this answers your original questions for your research. Are there any gaps? Do you understand the information you’ve found? If you feel there is more to cover, repeat the steps and delve deeper into the topic so that you can get all the information you need.
If secondary research can’t provide these answers, consider supplementing your results with data gained from primary research. As you explore further, add to your knowledge and update your findings. This will help you present clear, credible information.
Primary vs secondary research
Unlike secondary research, primary research involves creating data first-hand by directly working with interviewees, target users, or a target market. Primary research focuses on the method for carrying out research, asking questions, and collecting data using approaches such as:
- Interviews (panel, face-to-face or over the phone)
- Questionnaires or surveys
- Focus groups
Using these methods, researchers can get in-depth, targeted responses to questions, making results more accurate and specific to their research goals. However, it does take time to do and administer.
Unlike primary research, secondary research uses existing data, which also includes published results from primary research. Researchers summarize the existing research and use the results to support their research goals.
Both primary and secondary research have their places. Primary research can support the findings found through secondary research (and fill knowledge gaps), while secondary research can be a starting point for further primary research. Because of this, these research methods are often combined for optimal research results that are accurate at both the micro and macro level.
Sources of Secondary Research
There are two types of secondary research sources: internal and external. Internal data refers to in-house data that can be gathered from the researcher’s organization. External data refers to data published outside of and not owned by the researcher’s organization.
Internal data
Internal data is a good first port of call for insights and knowledge, as you may already have relevant information stored in your systems. Because you own this information — and it won’t be available to other researchers — it can give you a competitive edge . Examples of internal data include:
- Database information on sales history and business goal conversions
- Information from website applications and mobile site data
- Customer-generated data on product and service efficiency and use
- Previous research results or supplemental research areas
- Previous campaign results
External data
External data is useful when you: 1) need information on a new topic, 2) want to fill in gaps in your knowledge, or 3) want data that breaks down a population or market for trend and pattern analysis. Examples of external data include:
- Government, non-government agencies, and trade body statistics
- Company reports and research
- Competitor research
- Public library collections
- Textbooks and research journals
- Media stories in newspapers
- Online journals and research sites
Three examples of secondary research methods in action
How and why might you conduct secondary research? Let’s look at a few examples:
1. Collecting factual information from the internet on a specific topic or market
There are plenty of sites that hold data for people to view and use in their research. For example, Google Scholar, ResearchGate, or Wiley Online Library all provide previous research on a particular topic. Researchers can create free accounts and use the search facilities to look into a topic by keyword, before following the instructions to download or export results for further analysis.
This can be useful for exploring a new market that your organization wants to consider entering. For instance, by viewing the U.S Census Bureau demographic data for that area, you can see what the demographics of your target audience are , and create compelling marketing campaigns accordingly.
2. Finding out the views of your target audience on a particular topic
If you’re interested in seeing the historical views on a particular topic, for example, attitudes to women’s rights in the US, you can turn to secondary sources.
Textbooks, news articles, reviews, and journal entries can all provide qualitative reports and interviews covering how people discussed women’s rights. There may be multimedia elements like video or documented posters of propaganda showing biased language usage.
By gathering this information, synthesizing it, and evaluating the language, who created it and when it was shared, you can create a timeline of how a topic was discussed over time.
3. When you want to know the latest thinking on a topic
Educational institutions, such as schools and colleges, create a lot of research-based reports on younger audiences or their academic specialisms. Dissertations from students also can be submitted to research journals, making these places useful places to see the latest insights from a new generation of academics.
Information can be requested — and sometimes academic institutions may want to collaborate and conduct research on your behalf. This can provide key primary data in areas that you want to research, as well as secondary data sources for your research.
Advantages of secondary research
There are several benefits of using secondary research, which we’ve outlined below:
- Easily and readily available data – There is an abundance of readily accessible data sources that have been pre-collected for use, in person at local libraries and online using the internet. This data is usually sorted by filters or can be exported into spreadsheet format, meaning that little technical expertise is needed to access and use the data.
- Faster research speeds – Since the data is already published and in the public arena, you don’t need to collect this information through primary research. This can make the research easier to do and faster, as you can get started with the data quickly.
- Low financial and time costs – Most secondary data sources can be accessed for free or at a small cost to the researcher, so the overall research costs are kept low. In addition, by saving on preliminary research, the time costs for the researcher are kept down as well.
- Secondary data can drive additional research actions – The insights gained can support future research activities (like conducting a follow-up survey or specifying future detailed research topics) or help add value to these activities.
- Secondary data can be useful pre-research insights – Secondary source data can provide pre-research insights and information on effects that can help resolve whether research should be conducted. It can also help highlight knowledge gaps, so subsequent research can consider this.
- Ability to scale up results – Secondary sources can include large datasets (like Census data results across several states) so research results can be scaled up quickly using large secondary data sources.
Disadvantages of secondary research
The disadvantages of secondary research are worth considering in advance of conducting research :
- Secondary research data can be out of date – Secondary sources can be updated regularly, but if you’re exploring the data between two updates, the data can be out of date. Researchers will need to consider whether the data available provides the right research coverage dates, so that insights are accurate and timely, or if the data needs to be updated. Also, fast-moving markets may find secondary data expires very quickly.
- Secondary research needs to be verified and interpreted – Where there’s a lot of data from one source, a researcher needs to review and analyze it. The data may need to be verified against other data sets or your hypotheses for accuracy and to ensure you’re using the right data for your research.
- The researcher has had no control over the secondary research – As the researcher has not been involved in the secondary research, invalid data can affect the results. It’s therefore vital that the methodology and controls are closely reviewed so that the data is collected in a systematic and error-free way.
- Secondary research data is not exclusive – As data sets are commonly available, there is no exclusivity and many researchers can use the same data. This can be problematic where researchers want to have exclusive rights over the research results and risk duplication of research in the future.
When do we conduct secondary research?
Now that you know the basics of secondary research, when do researchers normally conduct secondary research?
It’s often used at the beginning of research, when the researcher is trying to understand the current landscape . In addition, if the research area is new to the researcher, it can form crucial background context to help them understand what information exists already. This can plug knowledge gaps, supplement the researcher’s own learning or add to the research.
Secondary research can also be used in conjunction with primary research. Secondary research can become the formative research that helps pinpoint where further primary research is needed to find out specific information. It can also support or verify the findings from primary research.
You can use secondary research where high levels of control aren’t needed by the researcher, but a lot of knowledge on a topic is required from different angles.
Secondary research should not be used in place of primary research as both are very different and are used for various circumstances.
Questions to ask before conducting secondary research
Before you start your secondary research, ask yourself these questions:
- Is there similar internal data that we have created for a similar area in the past?
If your organization has past research, it’s best to review this work before starting a new project. The older work may provide you with the answers, and give you a starting dataset and context of how your organization approached the research before. However, be mindful that the work is probably out of date and view it with that note in mind. Read through and look for where this helps your research goals or where more work is needed.
- What am I trying to achieve with this research?
When you have clear goals, and understand what you need to achieve, you can look for the perfect type of secondary or primary research to support the aims. Different secondary research data will provide you with different information – for example, looking at news stories to tell you a breakdown of your market’s buying patterns won’t be as useful as internal or external data e-commerce and sales data sources.
- How credible will my research be?
If you are looking for credibility, you want to consider how accurate the research results will need to be, and if you can sacrifice credibility for speed by using secondary sources to get you started. Bear in mind which sources you choose — low-credibility data sites, like political party websites that are highly biased to favor their own party, would skew your results.
- What is the date of the secondary research?
When you’re looking to conduct research, you want the results to be as useful as possible , so using data that is 10 years old won’t be as accurate as using data that was created a year ago. Since a lot can change in a few years, note the date of your research and look for earlier data sets that can tell you a more recent picture of results. One caveat to this is using data collected over a long-term period for comparisons with earlier periods, which can tell you about the rate and direction of change.
- Can the data sources be verified? Does the information you have check out?
If you can’t verify the data by looking at the research methodology, speaking to the original team or cross-checking the facts with other research, it could be hard to be sure that the data is accurate. Think about whether you can use another source, or if it’s worth doing some supplementary primary research to replicate and verify results to help with this issue.
We created a front-to-back guide on conducting market research, The ultimate guide to conducting market research , so you can understand the research journey with confidence.
In it, you’ll learn more about:
- What effective market research looks like
- The use cases for market research
- The most important steps to conducting market research
- And how to take action on your research findings
Download the free guide for a clearer view on secondary research and other key research types for your business.
Related resources
Market intelligence 10 min read, marketing insights 11 min read, ethnographic research 11 min read, qualitative vs quantitative research 13 min read, qualitative research questions 11 min read, qualitative research design 12 min read, primary vs secondary research 14 min read, request demo.
Ready to learn more about Qualtrics?
What Is Secondary Data? A Complete Guide
What is secondary data, and why is it important? Find out in this post.
Within data analytics, there are many ways of categorizing data. A common distinction, for instance, is that between qualitative and quantitative data . In addition, you might also distinguish your data based on factors like sensitivity. For example, is it publicly available or is it highly confidential?
Probably the most fundamental distinction between different types of data is their source. Namely, are they primary, secondary, or third-party data? Each of these vital data sources supports the data analytics process in its own way. In this post, we’ll focus specifically on secondary data. We’ll look at its main characteristics, provide some examples, and highlight the main pros and cons of using secondary data in your analysis.
We’ll cover the following topics:
What is secondary data?
- What’s the difference between primary, secondary, and third-party data?
- What are some examples of secondary data?
- How to analyse secondary data
- Advantages of secondary data
- Disadvantages of secondary data
- Wrap-up and further reading
Ready to learn all about secondary data? Then let’s go.
1. What is secondary data?
Secondary data (also known as second-party data) refers to any dataset collected by any person other than the one using it.
Secondary data sources are extremely useful. They allow researchers and data analysts to build large, high-quality databases that help solve business problems. By expanding their datasets with secondary data, analysts can enhance the quality and accuracy of their insights. Most secondary data comes from external organizations. However, secondary data also refers to that collected within an organization and then repurposed.
Secondary data has various benefits and drawbacks, which we’ll explore in detail in section four. First, though, it’s essential to contextualize secondary data by understanding its relationship to two other sources of data: primary and third-party data. We’ll look at these next.
2. What’s the difference between primary, secondary, and third-party data?
To best understand secondary data, we need to know how it relates to the other main data sources: primary and third-party data.
What is primary data?
‘Primary data’ (also known as first-party data) are those directly collected or obtained by the organization or individual that intends to use them. Primary data are always collected for a specific purpose. This could be to inform a defined goal or objective or to address a particular business problem.
For example, a real estate organization might want to analyze current housing market trends. This might involve conducting interviews, collecting facts and figures through surveys and focus groups, or capturing data via electronic forms. Focusing only on the data required to complete the task at hand ensures that primary data remain highly relevant. They’re also well-structured and of high quality.
As explained, ‘secondary data’ describes those collected for a purpose other than the task at hand. Secondary data can come from within an organization but more commonly originate from an external source. If it helps to make the distinction, secondary data is essentially just another organization’s primary data.
Secondary data sources are so numerous that they’ve started playing an increasingly vital role in research and analytics. They are easier to source than primary data and can be repurposed to solve many different problems. While secondary data may be less relevant for a given task than primary data, they are generally still well-structured and highly reliable.
What is third-party data?
‘Third-party data’ (sometimes referred to as tertiary data) refers to data collected and aggregated from numerous discrete sources by third-party organizations. Because third-party data combine data from numerous sources and aren’t collected with a specific goal in mind, the quality can be lower.
Third-party data also tend to be largely unstructured. This means that they’re often beset by errors, duplicates, and so on, and require more processing to get them into a usable format. Nevertheless, used appropriately, third-party data are still a useful data analytics resource. You can learn more about structured vs unstructured data here .
OK, now that we’ve placed secondary data in context, let’s explore some common sources and types of secondary data.
3. What are some examples of secondary data?
External secondary data.
Before we get to examples of secondary data, we first need to understand the types of organizations that generally provide them. Frequent sources of secondary data include:
- Government departments
- Public sector organizations
- Industry associations
- Trade and industry bodies
- Educational institutions
- Private companies
- Market research providers
While all these organizations provide secondary data, government sources are perhaps the most freely accessible. They are legally obliged to keep records when registering people, providing services, and so on. This type of secondary data is known as administrative data. It’s especially useful for creating detailed segment profiles, where analysts hone in on a particular region, trend, market, or other demographic.
Types of secondary data vary. Popular examples of secondary data include:
- Tax records and social security data
- Census data (the U.S. Census Bureau is oft-referenced, as well as our favorite, the U.S. Bureau of Labor Statistics )
- Electoral statistics
- Health records
- Books, journals, or other print media
- Social media monitoring, internet searches, and other online data
- Sales figures or other reports from third-party companies
- Libraries and electronic filing systems
- App data, e.g. location data, GPS data, timestamp data, etc.
Internal secondary data
As mentioned, secondary data is not limited to that from a different organization. It can also come from within an organization itself.
Sources of internal secondary data might include:
- Sales reports
- Annual accounts
- Quarterly sales figures
- Customer relationship management systems
- Emails and metadata
- Website cookies
In the right context, we can define practically any type of data as secondary data. The key takeaway is that the term ‘secondary data’ doesn’t refer to any inherent quality of the data themselves, but to how they are used. Any data source (external or internal) used for a task other than that for which it was originally collected can be described as secondary data.
4. How to analyse secondary data
The process of analysing secondary data can be performed either quantitatively or qualitatively, depending on the kind of data the researcher is dealing with. The quantitative method of secondary data analysis is used on numerical data and is analyzed mathematically. The qualitative method uses words to provide in-depth information about data.
There are different stages of secondary data analysis, which involve events before, during, and after data collection. These stages include:
- Statement of purpose: Before collecting secondary data, you need to know your statement of purpose. This means you should have a clear awareness of the goal of the research work and how this data will help achieve it. This will guide you to collect the right data, then choosing the best data source and method of analysis.
- Research design: This is a plan on how the research activities will be carried out. It describes the kind of data to be collected, the sources of data collection, the method of data collection, tools used, and method of analysis. Once the purpose of the research has been identified, the researcher should design a research process that will guide the data analysis process.
- Developing the research questions: Once you’ve identified the research purpose, an analyst should also prepare research questions to help identify secondary data. For example, if a researcher is looking to learn more about why working adults are increasingly more interested in the “gig economy” as opposed to full-time work, they may ask, “What are the main factors that influence adults decisions to engage in freelance work?” or, “Does education level have an effect on how people engage in freelance work?
- Identifying secondary data: Using the research questions as a guide, researchers will then begin to identify relevant data from the sources provided. If the kind of data to be collected is qualitative, a researcher can filter out qualitative data—for example.
- Evaluating secondary data: Once relevant data has been identified and collates, it will be evaluated to ensure it fulfils the criteria of the research topic. Then, it is analyzed either using the quantitative or qualitative method, depending on the type of data it is.
You can learn more about secondary data analysis in this post .
5. Advantages of secondary data
Secondary data is suitable for any number of analytics activities. The only limitation is a dataset’s format, structure, and whether or not it relates to the topic or problem at hand.
When analyzing secondary data, the process has some minor differences, mainly in the preparation phase. Otherwise, it follows much the same path as any traditional data analytics project.
More broadly, though, what are the advantages and disadvantages of using secondary data? Let’s take a look.
Advantages of using secondary data
It’s an economic use of time and resources: Because secondary data have already been collected, cleaned, and stored, this saves analysts much of the hard work that comes from collecting these data firsthand. For instance, for qualitative data, the complex tasks of deciding on appropriate research questions or how best to record the answers have already been completed. Secondary data saves data analysts and data scientists from having to start from scratch.
It provides a unique, detailed picture of a population: Certain types of secondary data, especially government administrative data, can provide access to levels of detail that it would otherwise be extremely difficult (or impossible) for organizations to collect on their own. Data from public sources, for instance, can provide organizations and individuals with a far greater level of population detail than they could ever hope to gather in-house. You can also obtain data over larger intervals if you need it., e.g. stock market data which provides decades’-worth of information.
Secondary data can build useful relationships: Acquiring secondary data usually involves making connections with organizations and analysts in fields that share some common ground with your own. This opens the door to a cross-pollination of disciplinary knowledge. You never know what nuggets of information or additional data resources you might find by building these relationships.
Secondary data tend to be high-quality: Unlike some data sources, e.g. third-party data, secondary data tends to be in excellent shape. In general, secondary datasets have already been validated and therefore require minimal checking. Often, such as in the case of government data, datasets are also gathered and quality-assured by organizations with much more time and resources available. This further benefits the data quality , while benefiting smaller organizations that don’t have endless resources available.
It’s excellent for both data enrichment and informing primary data collection: Another benefit of secondary data is that they can be used to enhance and expand existing datasets. Secondary data can also inform primary data collection strategies. They can provide analysts or researchers with initial insights into the type of data they might want to collect themselves further down the line.
6. Disadvantages of secondary data
They aren’t always free: Sometimes, it’s unavoidable—you may have to pay for access to secondary data. However, while this can be a financial burden, in reality, the cost of purchasing a secondary dataset usually far outweighs the cost of having to plan for and collect the data firsthand.
The data isn’t always suited to the problem at hand: While secondary data may tick many boxes concerning its relevance to a business problem, this is not always true. For instance, secondary data collection might have been in a geographical location or time period ill-suited to your analysis. Because analysts were not present when the data were initially collected, this may also limit the insights they can extract.
The data may not be in the preferred format: Even when a dataset provides the necessary information, that doesn’t mean it’s appropriately stored. A basic example: numbers might be stored as categorical data rather than numerical data. Another issue is that there may be gaps in the data. Categories that are too vague may limit the information you can glean. For instance, a dataset of people’s hair color that is limited to ‘brown, blonde and other’ will tell you very little about people with auburn, black, white, or gray hair.
You can’t be sure how the data were collected: A structured, well-ordered secondary dataset may appear to be in good shape. However, it’s not always possible to know what issues might have occurred during data collection that will impact their quality. For instance, poor response rates will provide a limited view. While issues relating to data collection are sometimes made available alongside the datasets (e.g. for government data) this isn’t always the case. You should therefore treat secondary data with a reasonable degree of caution.
Being aware of these disadvantages is the first step towards mitigating them. While you should be aware of the risks associated with using secondary datasets, in general, the benefits far outweigh the drawbacks.
7. Wrap-up and further reading
In this post we’ve explored secondary data in detail. As we’ve seen, it’s not so different from other forms of data. What defines data as secondary data is how it is used rather than an inherent characteristic of the data themselves.
To learn more about data analytics, check out this free, five-day introductory data analytics short course . You can also check out these articles to learn more about the data analytics process:
- What is data cleaning and why is it important?
- What is data visualization? A complete introductory guide
- 10 Great places to find free datasets for your next project
- Login to Survey Tool Review Center
Secondary Research Advantages, Limitations, and Sources
Summary: secondary research should be a prerequisite to the collection of primary data, but it rarely provides all the answers you need. a thorough evaluation of the secondary data is needed to assess its relevance and accuracy..
5 minutes to read. By author Michaela Mora on January 25, 2022 Topics: Relevant Methods & Tips , Business Strategy , Market Research
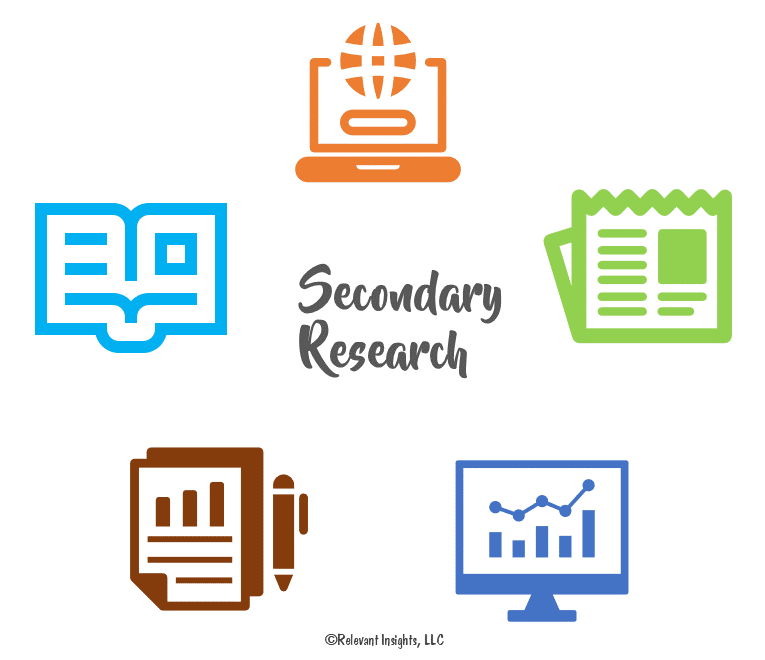
Secondary research is based on data already collected for purposes other than the specific problem you have. Secondary research is usually part of exploratory market research designs.
The connection between the specific purpose that originates the research is what differentiates secondary research from primary research. Primary research is designed to address specific problems. However, analysis of available secondary data should be a prerequisite to the collection of primary data.
Advantages of Secondary Research
Secondary data can be faster and cheaper to obtain, depending on the sources you use.
Secondary research can help to:
- Answer certain research questions and test some hypotheses.
- Formulate an appropriate research design (e.g., identify key variables).
- Interpret data from primary research as it can provide some insights into general trends in an industry or product category.
- Understand the competitive landscape.
Limitations of Secondary Research
The usefulness of secondary research tends to be limited often for two main reasons:
Lack of relevance
Secondary research rarely provides all the answers you need. The objectives and methodology used to collect the secondary data may not be appropriate for the problem at hand.
Given that it was designed to find answers to a different problem than yours, you will likely find gaps in answers to your problem. Furthermore, the data collection methods used may not provide the data type needed to support the business decisions you have to make (e.g., qualitative research methods are not appropriate for go/no-go decisions).
Lack of Accuracy
Secondary data may be incomplete and lack accuracy depending on;
- The research design (exploratory, descriptive, causal, primary vs. repackaged secondary data, the analytical plan, etc.)
- Sampling design and sources (target audiences, recruitment methods)
- Data collection method (qualitative and quantitative techniques)
- Analysis point of view (focus and omissions)
- Reporting stages (preliminary, final, peer-reviewed)
- Rate of change in the studied topic (slowly vs. rapidly evolving phenomenon, e.g., adoption of specific technologies).
- Lack of agreement between data sources.
Criteria for Evaluating Secondary Research Data
Before taking the information at face value, you should conduct a thorough evaluation of the secondary data you find using the following criteria:
- Purpose : Understanding why the data was collected and what questions it was trying to answer will tell us how relevant and useful it is since it may or may not be appropriate for your objectives.
- Methodology used to collect the data : Important to understand sources of bias.
- Accuracy of data: Sources of errors may include research design, sampling, data collection, analysis, and reporting.
- When the data was collected : Secondary data may not be current or updated frequently enough for the purpose that you need.
- Content of the data : Understanding the key variables, units of measurement, categories used and analyzed relationships may reveal how useful and relevant it is for your purposes.
- Source reputation : In the era of purposeful misinformation on the Internet, it is important to check the expertise, credibility, reputation, and trustworthiness of the data source.
Secondary Research Data Sources
Compared to primary research, the collection of secondary data can be faster and cheaper to obtain, depending on the sources you use.
Secondary data can come from internal or external sources.
Internal sources of secondary data include ready-to-use data or data that requires further processing available in internal management support systems your company may be using (e.g., invoices, sales transactions, Google Analytics for your website, etc.).
Prior primary qualitative and quantitative research conducted by the company are also common sources of secondary data. They often generate more questions and help formulate new primary research needed.
However, if there are no internal data collection systems yet or prior research, you probably won’t have much usable secondary data at your disposal.
External sources of secondary data include:
- Published materials
- External databases
- Syndicated services.
Published Materials
Published materials can be classified as:
- General business sources: Guides, directories, indexes, and statistical data.
- Government sources: Census data and other government publications.
External Databases
In many industries across a variety of topics, there are private and public databases that can bed accessed online or by downloading data for free, a fixed fee, or a subscription.
These databases can include bibliographic, numeric, full-text, directory, and special-purpose databases. Some public institutions make data collected through various methods, including surveys, available for others to analyze.
Syndicated Services
These services are offered by companies that collect and sell pools of data that have a commercial value and meet shared needs by a number of clients, even if the data is not collected for specific purposes those clients may have.
Syndicated services can be classified based on specific units of measurements (e.g., consumers, households, organizations, etc.).
The data collection methods for these data may include:
- Surveys (Psychographic and Lifestyle, advertising evaluations, general topics)
- Household panels (Purchase and media use)
- Electronic scanner services (volume tracking data, scanner panels, scanner panels with Cable TV)
- Audits (retailers, wholesalers)
- Direct inquiries to institutions
- Clipping services tracking PR for institutions
- Corporate reports
You can spend hours doing research on Google in search of external sources, but this is likely to yield limited insights. Books, articles journals, reports, blogs posts, and videos you may find online are usually analyses and summaries of data from a particular perspective. They may be useful and give you an indication of the type of data used, but they are not the actual data. Whenever possible, you should look at the actual raw data used to draw your own conclusion on its value for your research objectives. You should check professionally gathered secondary research.
Here are some external secondary data sources often used in market research that you may find useful as starting points in your research. Some are free, while others require payment.
- Pew Research Center : Reports about the issues, attitudes, and trends shaping the world. It conducts public opinion polling, demographic research, media content analysis, and other empirical social science research.
- Data.Census.gov : Data dissemination platform to access demographic and economic data from the U.S. Census Bureau.
- Data.gov : The US. government’s open data source with almost 200,00 datasets ranges in topics from health, agriculture, climate, ecosystems, public safety, finance, energy, manufacturing, education, and business.
- Google Scholar : A web search engine that indexes the full text or metadata of scholarly literature across an array of publishing formats and disciplines.
- Google Public Data Explorer : Makes large, public-interest datasets easy to explore, visualize and communicate.
- Google News Archive : Allows users to search historical newspapers and retrieve scanned images of their pages.
- Mckinsey & Company : Articles based on analyses of various industries.
- Statista : Business data platform with data across 170+ industries and 150+ countries.
- Claritas : Syndicated reports on various market segments.
- Mintel : Consumer reports combining exclusive consumer research with other market data and expert analysis.
- MarketResearch.com : Data aggregator with over 350 publishers covering every sector of the economy as well as emerging industries.
- Packaged Facts : Reports based on market research on consumer goods and services industries.
- Dun & Bradstreet : Company directory with business information.
Related Articles
- What Is Market Research?
- Step by Step Guide to the Market Research Process
- How to Leverage UX and Market Research To Understand Your Customers
- Why Your Business Needs Discovery Research
- Your Market Research Plan to Succeed As a Startup
- Top Reason Why Businesses Fail & What To Do About It
- What To Value In A Market Research Vendor
- Don’t Let The Budget Dictate Your Market Research Approach
- How To Use Research To Find High-Order Brand Benefits
- How To Prioritize What To Research
- Don’t Just Trust Your Gut — Do Research
- Understanding the Pros and Cons of Mixed-Mode Research
Subscribe to our newsletter to get notified about future articles
Subscribe and don’t miss anything!
Recent Articles
- How AI Can Further Remove Researchers in Search of Productivity and Lower Costs
- Re: Design/Growth Podcast – Researching User Experiences for Business Growth
- Why You Need Positioning Concept Testing in New Product Development
- Why Conjoint Analysis Is Best for Price Research
- The Rise of UX
- Making the Case Against the Van Westendorp Price Sensitivity Meter
- How to Future-Proof Experience Management and Your Business
- When Using Focus Groups Makes Sense
- How to Make Segmentation Research Actionable
- How To Integrate Market Research and UX Research for Desired Business Outcomes
Popular Articles
- Which Rating Scales Should I Use?
- What To Consider in Survey Design
- 6 Decisions To Make When Designing Product Concept Tests
- Write Winning Product Concepts To Get Accurate Results In Concept Tests
- How to Use Qualitative and Quantitative Research in Product Development
- The Opportunity of UX Research Webinar
- Myths & Misunderstandings About UX – MR Realities Podcast
- 12 Research Techniques to Solve Choice Overload
- Concept Testing for UX Researchers
- UX Research Geeks Podcast – Using Market Research for Better Context in UX
- A Researcher’s Path – Data Stories Leaders At Work Podcast
- How To Improve Racial and Gender Inclusion in Survey Design
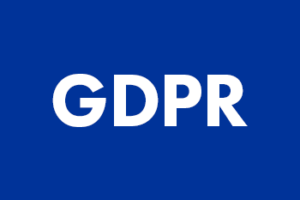
- Privacy Overview
- Strictly Necessary Cookies
This website uses cookies so that we can provide you with the best user experience possible. Cookie information is stored in your browser and performs functions such as recognising you when you return to our website and helping our team to understand which sections of the website you find most interesting and useful.
Strictly Necessary Cookie should be enabled at all times so that we can save your preferences for cookie settings.
If you disable this cookie, we will not be able to save your preferences. This means that every time you visit this website you will need to enable or disable cookies again.
- Open access
- Published: 30 May 2024
Healthcare use and costs in the last six months of life by level of care and cause of death
- Yvonne Anne Michel 1 , 2 ,
- Eline Aas 1 , 3 ,
- Liv Ariane Augestad 1 ,
- Emily Burger 1 , 4 ,
- Lisbeth Thoresen 5 &
- Gudrun Maria Waaler Bjørnelv ORCID: orcid.org/0000-0003-4997-5426 1 , 6
BMC Health Services Research volume 24 , Article number: 688 ( 2024 ) Cite this article
176 Accesses
Metrics details
Existing knowledge on healthcare use and costs in the last months of life is often limited to one patient group (i.e., cancer patients) and one level of healthcare (i.e., secondary care). Consequently, decision-makers lack knowledge in order to make informed decisions about the allocation of healthcare resources for all patients. Our aim is to elaborate the understanding of resource use and costs in the last six months of life by describing healthcare use and costs for all causes of death and by all levels of formal care.
Using five national registers, we gained access to patient-level data for all individuals who died in Norway between 2009 and 2013. We described healthcare use and costs for all levels of formal care—namely primary, secondary, and home- and community-based care —in the last six months of life, both in total and differentiated across three time periods (6-4 months, 3-2 months, and 1-month before death). Our analysis covers all causes of death categorized in ten ICD-10 categories.
During their last six months of life, individuals used an average of healthcare resources equivalent to €46,000, ranging from €32,000 (Injuries) to €64,000 (Diseases of the nervous system and sense organs). In terms of care level, 63% of healthcare resources were used in home- and community-based care (i.e., in-home nursing, practical assistance, or nursing home care), 35% in secondary care (mostly hospital care), and 2% in primary care (i.e., general practitioners). The amount and level of care varied by cause of death and by time to death. The proportion of home- and community-based care which individuals received during their last six months of life varied from 38% for cancer patients to 92% for individuals dying with mental diseases. The shorter the time to death, the more resources were needed: nearly 40% of all end-of-life healthcare costs were expended in the last month of life across all causes of death. The composition of care also differed depending on age. Individuals aged 80 years and older used more home- and community-based care (77%) than individuals dying at younger ages (40%) and less secondary care (old: 21% versus young: 57%).
Conclusions
Our analysis provides valuable evidence on how much healthcare individuals receive in their last six months of life and the associated costs, broken down by level of care and cause of death. Healthcare use and costs varied considerably by cause of death, but were generally higher the closer a person was to death. Our findings enable decision-makers to make more informed resource-allocation decisions and healthcare planners to better anticipate future healthcare needs.
Peer Review reports
Healthcare resources—such as trained staff, equipment, and beds in hospitals and nursing homes—are limited; therefore, decisions about how to use available healthcare resources are inevitable in publicly funded healthcare systems. Ideally, decision-makers base their resource-allocation decisions on valid, comprehensive evidence and societal preferences which indicate what is most important to the recipients of healthcare services. In reality, decision-makers have to make high-impact decisions under conditions of great uncertainty. As a result, scarce healthcare resources may be used inefficiently, due to significant knowledge gaps about which patient group needs which healthcare resources at which level of care.
The last months of life are known to be ‘resource intensive’ [ 1 , 2 , 3 ]. Existing knowledge on resource use during the last months of life is fragmented and incomprehensive, with studies focusing on single parameters of care and patient groups most commonly diagnosed with a specific type of cancer [ 4 , 5 , 6 , 7 , 8 , 9 ]. We identified two major knowledge gaps in the existing literature on resource use and costs in the last months of life.
For the first knowledge gap, extant research on resource use in the last months of life has focused predominantly on secondary healthcare services provided at hospitals; data on the use of primary healthcare (i.e., general practitioners (GPs), emergency primary healthcare) and home- and community-based care (i.e., care institutions, home nursing) is harder to find. Only if healthcare planners are provided with knowledge about healthcare use and costs at all levels of care can they fully optimise priorities when planning for future care needs.
We are aware of a limited number of studies which report on resource use and costs beyond secondary care. A systematic review summarised healthcare use in the last months of life in 3.7 million adult cancer patients [ 10 ]. Langton and colleagues found that secondary care received in hospitals was reported in most of the studies, while components of community care, was mentioned in 41% of the studies and physician visits as an indicator of primary care was mentioned in only 30% of the studies [ 10 ]. Nevertheless, none of the included studies provided data for all levels of formal care simultaneously. Tanuseputro’s population-based study looked into healthcare costs in the last 12 months of life in Ontario in 2010–2013 [ 11 ]. This study provided evidence on costs in the last year of life broken down by healthcare sector: total costs in the last year of life consisted of an average of 43% spent on inpatient care, while physician services, medications/devices, laboratories, and emergency rooms contributing to less than 20% of total costs; almost 16% was spent on long-term-care in institutions, and approximately 8% was spent on home care [ 11 ]. However, the study did not report resource use by cause of death. Finally, a recent registry-based study from 2022 investigated care pathways for patients with different cancer diagnoses in the last six months of life for all levels of formal care [ 12 ]. The authors found that, depending on their type of cancer, patients utilised 44–66% of resources in secondary care and 31–52% in home- and community-based care during their last six months of life [ 12 ]. To our knowledge, comparable estimates for all levels of formal care are not available for causes of death other than cancer.
For the second knowledge gap, knowledge on resource use and costs in the last months of life is only available for a limited number of causes of death, such as circulatory diseases [ 13 ], stroke [ 14 ], and respiratory diseases [ 15 ]. Still, most of the available evidence is on cancer patients’ use of secondary care in the last months of life [ 5 , 6 , 7 , 8 , 9 , 10 , 16 , 17 ]. Far less is known about resource use for individuals dying with mental diseases like dementia and Alzheimer’s disease, with existing studies focusing solely on costs [ 18 ]. Healthcare planners in publicly funded healthcare systems cannot afford inefficient allocation of scarce resources for a large and fast-growing patient group like dementia: the WHO expects that 75 million individuals will suffer from dementia in 2030, with the number rising to 132 million in 2050 [ 19 ]. Thus, ageing societies worldwide have an urgent need for evidence on resource use and costs for progressive mental diseases like dementia.
We aim to address these knowledge gaps by estimating healthcare use and costs in the six last months of life for all levels of formal care—primary, secondary, and home- and community-based care—for all causes of death, for two age groups, and for three time periods before death. In doing so, we aim to provide a more complete understanding of resource use and costs in the last six months of life. Our findings will support decision-makers in making more informed decisions regarding resource allocation and healthcare planners in better anticipating future healthcare needs.
In this study, we describe healthcare use at all levels of formal care (primary, secondary, and home- and community-based care) during the last six months of life of all individuals who died in Norway between 2009 and 2013. Using a healthcare perspective, we estimated the cost of healthcare during individuals’ last six months of life. To gather this information, we drew from five patient-level national registries.
Healthcare in Norway
Norway’s healthcare system is built on the principles of universal coverage and egalitarianism: healthcare is provided based on need for treatment, regardless of a person’s socioeconomic status, ethnicity, or area of residence. Healthcare is publicly funded, primarily through taxes, and membership in the public health insurance is mandatory [ 20 ]. Norwegian municipalities organise primary and home- and community-based care. In primary care, GPs play an important role and function as gatekeepers, referring patients to specialised healthcare when necessary. GPs provide primary care during office hours and emergency primary healthcare outside office hours [ 20 ]. The guiding principle for home- and community-based care is enabling patients to stay at home for as long as possible but to move to care facilities (i.e., nursing homes) when needed. Four state-owned Regional Health Authorities are responsible for organising specialised secondary care; inpatient care is provided at hospitals, while outpatient treatments are provided both at hospitals and by self-employed specialists in private practice [ 20 ].
National registries
We retrieved data from The Norwegian Causes of Death Register (CDR) [ 21 ], The Norwegian Patient Register (NPR) [ 22 ], Norwegian Control and Payment of Health Reimbursements Database (KUHR) [ 23 ], The Individual-based Statistics for Nursing and Care Services Register (IPLOS) [ 24 ], and Statistics Norway (SSB) [ 25 ].
Causes of death
Our study population contained all decedents in Norway in between 2009 and 2013, drawn from CDR. From this registry, we retrieved information on cause of death, coded as an individual’s underlying cause of death using ICD-10 codes [ 21 ]. Data on underlying cause of death was based on an individual’s death certificate, which was completed by a physician. For example, if a cancer patient died from pneumonia, the physician reported pneumonia as the immediate cause of death and cancer as the underlying cause of death. Only one underlying cause of death per person is recorded, identifying the diagnosis that most contributed to the individual’s death. In dialogue with the registries, we agreed on the following categories of underlying cause of death: Communicable diseases (ICD-10 codes A00–B99), Cancer (C00–C97), Endocrine, nutritional, and metabolic diseases (E00–E99), Mental and behavioural diseases (F00–99), Diseases of the nervous system and sense organs (G00–H95), Diseases of the circulatory system (I00–99), Diseases of the respiratory system (J00–99), Diseases of the digestive system (K00–93), Injuries (V01–Y89), and Other diseases (L, M, N, O, P, Q, R, S, T and U). In Table 1 , we list the five most common ICD-10 codes within each of the categories described above, providing the reader with an overview of which causes of death are represented in each category.
Healthcare use and costs
Primary care.
When a patient receives primary healthcare in Norway, the provider sends a claim to The Norwegian Health Economics Administration (HELFO) [ 26 ]. These claims, their associated costs, and information on patient co-payments are entered into KUHR. We used information on treatments provided by GPs, either at the GP’s office or as emergency primary healthcare outside normal office hours. We present primary healthcare use as number of visits. Costs of primary care were also retrieved from KUHR.
Secondary care
For each secondary care treatment provided at a hospital in Norway the patient’s diagnosis and the treatment provided are registered in NPR, including information on whether inpatient or outpatient treatment was provided. All patient-related activity in hospitals is grouped into approximately 900 diagnosis-related groups (DRGs), which reflect the treatment provided and its associated mean cost across several hospitals which provide the treatment [ 27 ]. DRG costs include direct costs associated with the treatment of the disease, cost of complications during the hospital stays, and overhead costs. Additionally, we retrieved laboratory and radiology costs and patients’ co-payments from KUHR. We used information on all hospital inpatient (including day and overnight treatments) and outpatient treatments, number of days in the hospital, and total costs during the last six months of life as estimated by DRGs.
- Home- and community-based care
All Norwegian municipalities must provide information to IPLOS [ 24 ]. We retrieved information on the number of days individuals spent in care institutions during their last six months of life. Additionally, we obtained information regarding whether individuals received home-based care in the form of practical or nursing assistance, which was measured in hours.
- Healthcare costs
We have used a healthcare perspective and show the estimated costs in 2013 euros (€) using the 2013 annual exchange rate. All costs were estimated at patient level.
To estimate the costs of primary care services, we used information on reimbursement claims and patient co-payments which are recorded in KUHR for each GP consultation and emergency primary care visit. Costs were estimated by dividing the sum of claims and patient co-payments by 0.3. This is in line with recommendations from the Norwegian Directorate of Health, who estimated that all claims and co-payments recorded in KUHR reflect approximately 30% of the total cost of primary care [ 28 ]. Other guidelines suggest using 0.5 [ 29 ], but a recent study found that this resulted in an underestimation of actual costs [ 30 ].
Secondary care costs were estimated by multiplying DRG weights by the yearly unit price of a DRG weight. The costs of radiology and laboratory services are recorded in KUHR. Similarly to other KUHR estimates, we summed costs of radiology and laboratory services as well as patient co-payments and dividing the total cost estimate by 0.3 [ 28 , 31 ]. We added these costs to the patient-level hospital costs.
To calculate costs of home- and community-based care, we multiplied days in care institutions by SSB’s official corrected gross operating expenses, published in KOSTRA (The Municipality- State- Reporting) [ 25 ]. To estimate the costs of practical and nursing assistance, we multiplied the number of hours of each type of care service that individuals received by the corresponding cost per hour, as estimated by Langeland and colleagues [ 31 ].
We estimated total healthcare costs by adding the costs in primary, secondary, and home- and community-based care. Variables of healthcare use and costs are detailed in Table 2 . To estimate country-specific costs, the readers can multiply their country-specific unit costs by the healthcare use estimates for all decedents as presented in Table 2 and decomposed for all causes of death and by age (younger and older than 80 years) in the detailed Supplementary Material 1 - 3 .
Place of living
Based on data from NPR [ 22 ] and IPLOS [ 24 ], we estimated how many days individuals spent at home, in care institutions—including short-term care and long-term care institutions (i.e., nursing homes, sheltered housing, other round-the-clock care, and sheltered housing with 24-hour care)—and in hospitals during their last six months of life. The number of days at home was estimated by subtracting days in hospitals and in care institutions from 186 days, which corresponds to six months. We allowed days in hospitals and in care institutions to overlap, since patients who receive treatment in hospitals often keep their place in their long-term care institution.
Statistical analysis
We used descriptive statistics to summarise the average healthcare use and costs during individuals’ last six months of life. We present both total healthcare use and costs by the following time periods: all six months before death (total), as well as 6 to 4 months, 3 to 2 months, and 1 month before death Footnote 1 . To enable comparison between time periods, we present healthcare use and costs as average resource use and costs per month for all time periods Footnote 2 . We present results for all decedents as well as stratified by cause of death. For all causes of death, we describe healthcare use and costs separately for those aged older than 80 years and for those younger than 80 years at the time of death. We provide supplementary materials with detailed cause-specific healthcare use and costs at all levels of formal care for the time periods 6 to 4 months, 3 to 2 months, and 1 month before death for all decedents (Supplementary Material 1 ), for those aged younger than 80 years (Supplementary Material 2 & 4 ) and for those aged 80 years or older (Supplementary Material 3 & 4 ). To estimate relevant healthcare use for other countries or contexts, our variables on resource use can be multiplied by country- or context-specific unit costs.
Between 2009 and 2013, a total of 207,299 individuals died in Norway, or approximately 41,000 individuals per year. The majority of those who died were older than 80 years at the time of death (Table 3 ). We list the categories of underlying cause of death in order of prevalence: Diseases of the circulatory system (31%), Cancer (26%), Diseases of the respiratory system (10%), Injuries (6%), Mental and behavioural diseases (5%), Diseases of the nervous system and sense organs (4%), Diseases of the digestive system (3%), Endocrine, nutritional, and metabolic diseases (2%), Communicable diseases (2%), and Other diseases (10%). Dementia was the most common underlying cause of death in both Mental and behavioural diseases (Unspecified dementia 77% + Vascular dementia 8%) and Diseases of the nervous system and sense organs (Alzheimer’s disease 45%) (Table 1 ). The most common causes of deaths in the other categories can be viewed in Table 1 .
All decedents
For the 207,299 decedents, the average healthcare costs per individual in the last six months of life was €46,166. The majority of healthcare resources were used in home- and community-based care (63%), followed by secondary care (35%) and primary care (2%). As death approached, healthcare use increased across all levels of care. On average, individuals used €17,801 in the last month of life, compared to €7,816 per month in the 3 to 2 months before death and €4,244 per month in the 6 to 4 months before death (Table 2 ). During their last six months of life, individuals spent most days at home (52%) and in care institutions (41%), and the fewest days in hospital (7%) (Table 2 ). The number of days individuals spent at home per month decreased as death approached (-6 days) (Table 2 ); correspondingly, the average number of days individuals spent in care institutions (+ 4 days) and at the hospital (+ 3 days) increased in the same time (Table 2 ).
On average, individuals received 2 inpatient and 3 outpatient treatments, visited their GP 9 times and had 3 emergency primary healthcare visits during their last six months of life (Table 2 ). They received 18 h of practical assistance and 56 h of nursing assistance during their last six months of life (Table 2 ). Similar to costs, healthcare use increased as death approached.
By cause of death
Average total healthcare costs in the last six months of life varied by cause of death, ranging from €32,276 (Injuries) to €64,123 (Diseases of the nervous system) (Fig. 1 ). Costs were lowest in primary care and highest in home- and community-based care for all causes of death except cancer, for which costs were highest in secondary care (Fig. 1 ). Individuals used different healthcare services depending on their cause of death. For example, individuals dying with endocrine/nutritional/metabolic diseases and individuals dying with cancer both used on average approximately €48,000 in the last six months of life; however, if total costs are decomposed by care level, it can be seen that cancer patients used more than twice as much in secondary care (€28,655) compared to individuals with endocrine/nutritional/metabolic diseases (€10,931), who in turn used twice as many resources in home- and community-based care (€36,262) compared to cancer patients (€18,454, Fig. 1 & Supplementary Material 1 ). Individuals dying with mental and nervous diseases, mostly dementia, received 86–92% of their care in the last six months of life outside secondary care, mostly in home- and community-based care. In contrast to individuals with dementia, individuals with digestive diseases or injuries used less resources in home- and community-based care, 38% and 58% respectively (Supplementary Material 1 ).
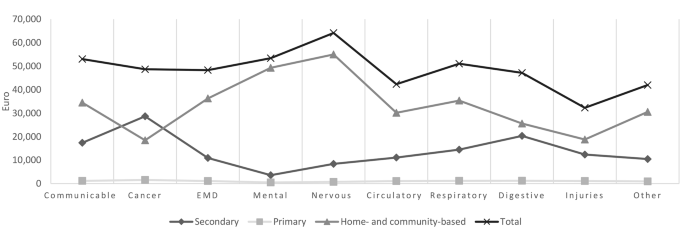
Total healthcare costs by level of care and cause of death
Place of living differed by cause of death. While individuals dying with communicable diseases, circulatory diseases, digestive diseases, injuries, or other diseases spent most days at home, individuals dying with mental and nervous diseases spent most days in care institutions. The number of days in hospital in the last six months of life varied considerably, from 3 days in hospital for patients with dementia to 24 days in hospital for cancer patients (Fig. 2 & Supplementary Material 1 ). Individuals with communicable diseases, respiratory diseases, and digestive diseases spent 12 to 15 days in hospital, while individuals with endocrine/nutritional/metabolic diseases, nervous diseases, circulatory diseases, and injuries spent 6 to 9 days in the hospital in the last six months of life (Fig. 2 & Supplementary Material 1 ).
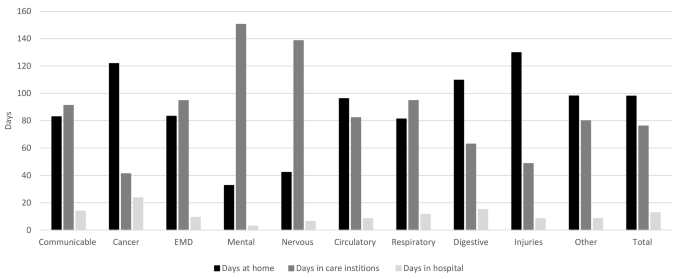
Place of living in the last six months of life by cause of death
Individuals dying with nervous diseases, including Parkinson’s and Alzheimer’s disease, used more practical (72 h) and nursing (110 h) assistance than those dying from other causes of death (Supplementary Material 1 ). The amount of nursing assistance received by individuals with injuries was the lowest, at 15 h, while cancer patients received the least practical assistance, at 10 h (Supplementary Material 1 ). On average, individuals with cancer received the highest number of inpatient, outpatient treatments and GP consultations, while individuals with mental and nervous diseases had the fewest (Supplementary Material 1 ).
Compared to the average cost in the last month of life (€17,800; Table 2 ), higher costs were observed for those dying with communicable, mental, nervous, endocrine/nutritional/metabolic, and respiratory diseases (Fig. 3 , Supplementary Material 1 ). In the last month of life, dying with nervous diseases was associated with the highest average costs (€29,000), while the lowest costs were observed for those dying with injuries (€11,000) (Fig. 3 , Supplementary Material 1 ). For individuals dying with all causes except cancer, home- and community-based care constituted approximately 80% of care in the last month of life. For individuals dying with mental and nervous diseases, 91–95% of care in the last month of life was provided through home- and community-based care (Fig. 3 , Supplementary Material 1 ). For detailed estimates of healthcare use and costs for all levels of care, for all causes of death and for all age groups, we refer to our comprehensive Supplementary Materials.
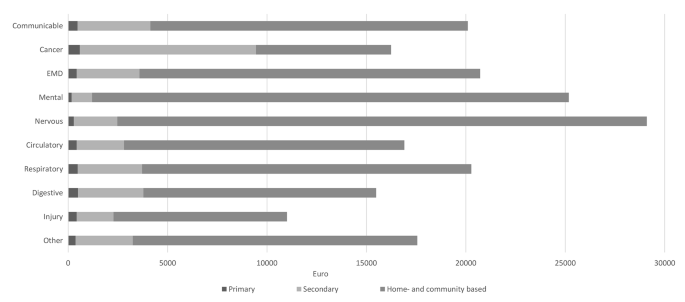
Healthcare costs in the last month of life by level of care and cause of death
The total healthcare cost during the last six months of life for individuals who died before the age of 80 years was €42,053, with these costs distributed as follows: 40% in home- and community-based care, 57% in secondary care, and 3% in primary care (Table 2 , Supplementary Material 2 ). For an individual who died at the age of 80 years or older, average total healthcare costs accumulated to €49,901, with 79% spent in home- and community-based care, 21% in secondary care, and 2% in primary care (Table 2 , Supplementary Material 3 ). Home- and community-based care was the dominant form of care for those aged 80 years and older, regardless of the cause of death (Table 2 , Supplementary Material 3 & 4 ). However, among those younger than 80 years, the level of care varied depending on the cause of death (Supplementary Material 2 & 4 ). For instance, for those aged 80 years or older, the proportion of overall expenses allocated to home- and community-based care ranged from 54% for individuals with cancer to 94% for individuals with mental and nervous diseases, mostly dementia (Supplementary Material 3 ). However, for those aged younger than 80 years at time of death, this proportion ranged from 25% (cancer) to 83% (mental and nervous diseases) (Supplementary Material 2 & 4 ). We provide comparable data for all causes of death by age (Supplementary Material 2 – 3 ), including a figure comparing age groups (Supplementary Material 4 ).
Healthcare use and costs differed by level of care, cause of death, age at death, and time to death. For all individuals who died in Norway between 2009 and 2013, the average total cost was €46,000 in the last six months of life. For all decedents, the majority of healthcare resources in the last six months of life were used at the level of home- and community-based care (63%, Fig. 1 ; Table 2 ). Whether most care was utilised in home- and community-based or secondary care differed by cause of death and by age (Supplementary Material 1 – 4 ). Those who died aged 80 years or older used most home- and community-based care across all causes of death (Supplementary Material 3 & 4 ). For those who died being younger than 80 years, the predominance of home- and community-based care was only true for individuals dying with mental and nervous diseases (Supplementary Material 2 & 4 ).
For all decedents, across all age groups, resource use increased, the shorter the time to death (Table 2 , Supplementary Material 1 ). On average, the last four weeks of life accounted for one third of all health care costs incurred in the last six months of life (Table 2 ). The costs associated with dying from injuries, circulatory diseases, and other diseases were lower than the average costs during the last six months of life, most likely due to sudden death (Supplementary Material 1 ). In contrast, individuals who died from mental and nervous diseases, communicable diseases, and respiratory diseases were more likely to have received care for a longer period of time before death, resulting in higher-than-average healthcare costs in the last months of life. Individuals dying with cancer, digestive diseases, and endocrine/nutritional/metabolic diseases had close to average costs during the last six months of life (Supplementary Material 1 & 4 ).
Our findings have important implications for decision-makers who are responsible for resource allocation in healthcare, as well as for healthcare planners who have to anticipate future healthcare needs. In the future, improved survival from some diseases will likely shift the causes of death of at the population level; for example, if improvements in cancer treatment prevent cancer-related deaths, more individuals will die from other diseases later in life rather than from cancer. Our analysis provides knowledge on resource use and costs associated with diseases beyond cancer which are common in older age, such as dementia. Dementia is currently the seventh-leading cause of death worldwide, and its prevalence is expected to double every 20 years [ 19 , 32 ]. Dementia is estimated to be one of the costliest diseases globally [ 33 ].
Kinge and colleagues estimated that dementia was the disease with the highest health spending, at 10.2% of total national health spending in Norway already in 2019 [ 30 ]. Evidence which facilitates assessment of the cost-effectiveness of new dementia drugs and which helps in planning the expected need for relevant healthcare is urgently needed around the world. We found that individuals with dementia used an above-average amount of healthcare resources in the last six months of life and that approximately 90% of these resources were used in home-and community-based care. These findings are in line with a 2023 Norwegian population-based registry study, which revealed that 78% of healthcare expenses related to dementia were spent on nursing homes [ 30 ]. Similarly, a systematic review summarized that individuals with dementia used more resources for professional home care and for nursing facilitates compared to individuals suffering from other diseases [ 18 ]. This type of cause-specific evidence can help healthcare planners prepare for future demands.
The validity of a decision-analytic model depends on the validity of the data used to populate the model. In the absence of cause-specific estimates on resource use and costs, modellers habitually use proxy parameters, which are available in the existing literature, or generic unit costs. Our study indicates that using proxy data from other disease types can be problematic: if cancer patients’ resource use is utilised to model resource use for dementia patients, this will systematically bias results—particularly the share of resource use taken up by home- and community-based care (38% for cancer patients vs. 92% for dementia patients) (Supplementary Material 1 - 4 ). Modellers should always strive to provide a complete picture of relevant disease pathways and to include the real-world economic burden of care at all levels for the entire lifespan [ 34 ]. Currently, due to gaps in knowledge regarding healthcare usage and costs, this is not feasible for all patient groups. Our findings enable the use of cause-specific estimates instead of proxy parameters, which has the potential to enhance estimates of resource use, models, and thus decisions allocating healthcare resources in various settings.
Previous studies on resource use and costs in the last months of life have often focused selectively on single causes of death and specific care variables, mainly secondary care variables. Methodological differences in samples, time frames, and healthcare settings make it difficult to compare parameters across studies. It is not possible to explain the variance in healthcare use and costs between previous studies and our findings based on the descriptive analyses we performed; nevertheless, it is helpful to put our findings into context. In the following, we focus solely on dementia, as it would be overwhelming to discuss findings for all causes of death.
The PAID 3.0, a Dutch tool initially created to incorporate future disease costs in economic evaluations, offers annual healthcare costs from the Netherlands, stratified by ICD-10 codes, age, and time to death [ 35 ]. This data is based on Dutch cost-of-illness data published in 2017 [ 36 ]. In the last year of life, the total average healthcare cost for individuals with mental and behavioural diseases (F00–99) was estimated with PAID to be €57,018 [ 35 ]. When we adjust our total cost estimate for mental diseases from 2013 to 2017, the two estimates are very similar (PAID: €57,018 vs. €58,736). The same is true for secondary care costs for individuals with mental diseases (PAID: €11,192 vs. €12,025), while for home- and community-based care, the Dutch estimate is higher than our findings (PAID: €45,826 vs. €39,891). The PAID data is based on the entire last year of life, while our findings summarize costs for the last six months of life; however, since the majority of healthcare costs occur when death approaches, we consider the comparison with PAID data to be valuable, despite the different time frames.
In a recently-published systematic review, Sontheimer and colleagues examined the costs of dementia from the time of diagnosis until death across different studies [ 18 ]. They found significant variation in total cost estimates, ranging from €1385 per person for 104 dementia patients in Argentina [ 37 ] to €48,655 per person for 541 dementia patients in residential care in Australia [ 38 ]. This wide range emphasises the importance of studies (like ours) which estimate healthcare costs in a common methodological framework. The reviewed studies support our finding that individuals with dementia receive most care through home- and community-based care: Patients with dementia had significantly higher costs for nursing facilities and professional home care for than patients without dementia. Interestingly, the total costs for inpatient and outpatient treatments were similar for patients with and without dementia. This finding supports our conclusion that the additional burden associated with dementia, compared to other causes of death, arises from demand in home and community-based care. This highlights the importance of reflecting healthcare use and costs from home and community-based care in decision analytic models.
Our findings might raise the question of whether our grouping of causes of death was detailed enough. For data anonymity reasons, the grouping of decedents into these categories of cause of death was predefined by the registries before the data were delivered to the researchers. We are nevertheless confident with the present grouping, since the categories of cause of death in this analysis cover the major causes of deaths and provide a wider range of causes of death than commonly seen in previous studies. An earlier study estimated healthcare use and costs for individuals dying with different types of cancer and showed that the specific cancer was less influential than other factors, such as individuals’ age and access to informal care [ 12 ]; whether this is true for subgroups for other causes of death could not be assessed with our dataset and thus remains largely unknown.
Generalisability
Some aspects regarding the generalisability of our findings must be discussed. First, our data come from 2009 to 2013; this time delay occurred because it took years to obtain access to comprehensive registry data. Since that period, several changes might have influenced individuals’ healthcare use in the last months of life. For instance, life-prolonging treatments might have increased survival, and patients who die today might differ from those who died in 2009–2013. Individuals dying today might be older, or they might die from different causes which can influence healthcare use. In addition, societal changes might have shifted individuals’ healthcare use. Importantly, Norway (along with other countries) is increasingly encouraging the shifting of treatment from secondary care to more local levels (i.e., the municipality); consequently, patients are meant to spend less time in hospitals, while stays in municipal care institutions are likely to increase. New analyses on updated data are needed in order to evaluate whether this has happened. To our knowledge, our estimates are currently the most comprehensive and updated with regard to resource use and costs for all decedents and for all causes of death.
Second, our findings can be generalised to settings which are similar to Norway, where healthcare is universally covered, out-of-pocket-payments are relatively low, and it is common to use formal care at the end of life. In healthcare settings with differences in incidence and severity of diseases, available healthcare resources, clinical practices, and relative price levels, our findings on healthcare use can still be informative [ 39 ]. To facilitate the adaptation of our results to other countries, we have reported our results for healthcare use and costs separately in the Supplementary Materials 1 – 4 . This enables readers to multiply our estimates on healthcare use with any other country-specific unit costs.
Third, we are aware that informal caregivers carry a considerable burden when individuals approach the end of their lives [ 40 , 41 ]. Cultural differences with regard to how much informal care families provide during this period will influence findings reporting the use of formal healthcare. In a study evaluating the number of individuals who died at home, Cohen and colleagues (2010) found that home death for persons dying with cancer varied from 12.8% in Norway to 22% in England, 23% in Wales, 28% in Belgium, 36% in Italy, and 45% in the Netherlands [ 42 ]. In 2022, 15% of all those who died from cancer in Norway died in private homes [ 21 ]. Place of death is likely connected to where individuals receive care; consequently, the amount of informal care and that of formal healthcare use might differ between these countries. In societies in which informal care is the dominant form of care in the last months of life, our findings can still be of interest, but they should be generalised with caution.
Finally, we consider it worth mentioning that it is challenging for physicians to identify the correct immediate cause of death. For this reason, we chose to use the underlying cause of death in our analysis. Still, using CDR as the source of cause of death has its limitations, primarily related to coding [ 43 ]: for example, there is a risk of different physicians coding multimorbid patients in different ways. We validated the underlying cause of death for all individuals with cancer by comparing the ICD-10 codes provided in CDR [ 21 ] with those in The Cancer Registry of Norway [ 44 ]. We found a reassuring overlap, which gives us confidence that CDR provided reliable information for all causes of death.
We report a comprehensive picture of the quantity of healthcare used during the last six months of life. At the same time, we acknowledge the relevance of assessing the quality of care. More research is needed to explore to what extent end-of-life care aligns with the preferences of patients and their next-of-kin. Unfortunately, our current dataset does not provide answers to these important questions, but we are optimistic that we can address them in future studies.
Using comprehensive, population-based registry data, we described healthcare use and costs in the last six months of life by level of care, for all decedents and stratified by ten major ICD-10 categories summarising all causes of death. Our research shows that healthcare use and costs in the last six months of life differ depending on cause of death: The total amount of healthcare varies, as does the level of care at which most resources were utilised (primary, secondary, or home- and community-based care). These findings enable decision-makers to make more informed decisions about recource allocation and healthcare planners to better anticipate future healthcare needs.
Data availability
Legal restrictions apply to the availability of the data underpinning the findings of this study, which were used under license for the current study. The data is not available upon request from the authors, and it cannot be made available to referees, editors, or readers upon request.
To preserve anonymity, we did not receive data for shorter time periods from the registries.
We have divided the numbers for the 3-month periods (e.g., 6-4 months before death) by 3 to obtain monthly estimates. For the entire analysis, we assumed no healthcare use for missing registrations.
Abbreviations
The Norwegian Causes of Death Register
Diagnosis-related group
International Classification of Diseases
General Practitioner
The Individual-based Statistics for Nursing and Care Services Register
The Municipality-State-Reporting
Norwegian Control and Payment of Health Reimbursements Database
The Norwegian Patient Register
World Health Organisation
Diernberger K, Luta X, Bowden J, Fallon M, Droney J, Lemmon E, Gray E, Marti J, Hall P. Healthcare use and costs in the last year of life: a national population data linkage study. BMJ Supportive Palliative Care. 2021. https://doi.org/10.1136/bmjspcare-2020-002708 . bmjspcare-2020-002708.
Article PubMed PubMed Central Google Scholar
Jo M, Lee Y, Kim T. Medical care costs at the end of life among older adults with cancer: a national health insurance data-based cohort study. BMC Palliat Care. 2023;22(1):76. https://doi.org/10.1186/s12904-023-01197-2 .
Chastek B, Harley C, Kallich J, Newcomer L, Paoli CJ, Teitelbaum AH. Health care costs for patients with cancer at the end of life. J Oncol Pract. 2012;8(6S):s75–80.
Article Google Scholar
Sun L, Legood R, dos-Santos-Silva I, Mathur Gaiha S, Sadique Z. Global treatment costs of breast cancer by stage: a systematic review. PLoS ONE. 2018;13(11):e0207993. https://journals.plos.org/plosone/article/file?id=10.1371/journal.pone.0207993&type=printable .
Bremner KE, Krahn MD, Warren JL, Hoch JS, Barrett MJ, Liu N, Barbera L, Yabroff KR. An international comparison of costs of end-of-life care for advanced lung cancer patients using health administrative data. Palliat Med. 2015;29(10):918–28.
Article PubMed Google Scholar
Dover LL, Dulaney CR, Williams CP, Fiveash JB, Jackson BE, Warren PP, Rocque GB. Hospice care, cancer-directed therapy, and Medicare expenditures among older patients dying with malignant brain tumors. Neurooncology. 2018;20(7):986–93.
Google Scholar
Kyeremanteng K, Ismail A, Wan C, Thavorn K, D’Egidio G. Outcomes and cost of patients with terminal cancer admitted to acute care in the final 2 weeks of life: a retrospective chart review. Am J Hospice Palliat Med. 2019;36(11):1020–5.
Reeve R, Srasuebkul P, Langton JM, Haas M, Viney R, Pearson SA. Health care use and costs at the end of life: a comparison of elderly Australian decedents with and without a cancer history. BMC Palliat care. 2018;17:1–10. & EOL-CC study authors
Shen C, Dasari A, Gu D, Chu Y, Zhou S, Xu Y, Shih YCT. Costs of Cancer Care for Elderly patients with neuroendocrine tumors. PharmacoEconomics. 2018;36:1005–13.
Langton JM, Blanch B, Drew AK, Haas M, Ingham JM, Pearson SA. Retrospective studies of end-of-life resource utilization and costs in cancer care using health administrative data: a systematic review. Palliat Med. 2014;28(10):1167–96.
Tanuseputro P, Wodchis WP, Fowler R, Walker P, Bai YQ, Bronskill SE, Manuel D. The health care cost of dying: a population-based retrospective cohort study of the last year of life in Ontario, Canada. PLoS ONE, 2015;10(3):e0121759.
Bjørnelv G, Hagen TP, Forma L, Aas E. Care pathways at end-of-life for cancer decedents: registry based analyses of the living situation, healthcare utilization and costs for all cancer decedents in Norway in 2009–2013 during their last 6 months of life. BMC Health Serv Res. 2022;22(1):1221.
Van Bulck L, Goossens E, Morin L, Luyckx K, Ombelet F, Willems R, Budts W, De Groote K, De Backer J, Annemans L, Moniotte S, de Hosson M, Marelli A, Moons P. Last year of life of adults with congenital heart diseases: causes of death and patterns of care. Eur Heart J. 2022;43(42):4483–92. https://doi.org/10.1093/eurheartj/ehac484 .
Levy SA, Pedowitz E, Stein LK, Dhamoon MS. Healthcare Utilization for Stroke Patients at the end of life: nationally Representative Data. J Stroke Cerebrovasc Dis. 2021;30(10):106008. https://doi.org/10.1016/j.jstrokecerebrovasdis.2021.106008 .
Faes K, De Frène V, Cohen J, Annemans L. Resource Use and Health Care costs of COPD patients at the end of life: a systematic review. J Pain Symptom Manag. 2016;52(4):588–99.
Bekelman JE, Halpern SD, Blankart CR, Bynum JP, Cohen J, Fowler R, Emanuel EJ. Comparison of site of death, health care utilization, and hospital expenditures for patients dying with cancer in 7 developed countries. JAMA. 2016;315(3):272–83.
Article CAS PubMed Google Scholar
Yabroff KR, Warren JL, Brown ML. Costs of cancer care in the USA: a descriptive review. Nat Clin Pract Oncol. 2007;4(11):643–56.
Sontheimer N, Konnopka A, König HH. The excess costs of dementia: a systematic review and Meta-analysis. J Alzheimers Dis. 2021;83(1):333–54. https://doi.org/10.3233/jad-210174 .
World Health Organisation. Global action plan on the public health response to dementia 2017–2025. Geneva: World Health Organization; 2017.
Ringard Å, Sagan A, Sperre Saunes I, Lindahl AK, World Health Organization. &. (2013). Norway: health system review.
The Norwegian Institute of Public Health. Dodsarsaksregisteret [The Norwegian Causes of Death Register]. Available from: https://www.fhi.no/hn/helseregistre-og-registre/dodsarsaksregisteret/ . Accessed 30 jan 2024.
The Norwegian Directorate of Health. Norsk Pasientregister [The Norwegian Patient Register]. Available from: https://helse direk torat et. no/norsk- pasie ntreg ister- npr. Accessed 30 jan 2024.
The Norwegian Directorate of Health. KUHR-databasen [The KUHR database]. Available from: https://www.helsedirektoratet.no/tema/statistikk-registre-og-rapporter/helsedata-og-helseregistre/kuhr . Accessed 30 jan 2024.
The Norwegian Directorate of Health. IPLOS-registeret [The IPLOS register]. Available from: Accessed 30 jan 2024. https://www.helsedirektoratet.no/tema/statistikk-registre-og-rapporter/helsedata-og-helseregistre/iplos-registeret
Statistics Norway. Kommune-Stat-Rapportering 2013 [The Municipality-State-Reporting]. Available from: Accessed 30 jan 2024. https://www.ssb.no/offentlig-sektor/kostra
The Norwegian Health Economics Administration HELFO. Available from: https://www.helfo.no/english/about-helfo . Accessed 30 jan 2024.
The Norwegian Directorate of Health. Innsatsstyrt finansiering 2016 [Activity based funding]. (2023). Available from: https://www.helsedirektoratet.no/tema/finansiering/innsatsstyrt-finansiering-og-drg-systemet/innsatsstyrt-finansiering-isf . Accessed 30 jan 2024.
The Norwegian Directorate of Health. Samfunnskostnader ved sykdom og ulykker Helsetap, helsetjenestekostnader og produksjonstap fordelt pa diagnoser og risikofaktorer [Societal costs of diseases and accidents. Health loss, healthcare services and production loss according to diagnoses and risk factors]. Available from: https://dokter.no/PDF-filer/Fastlegetariff_2013.pdf . (2013). Accessed 30 jan 2024.
The Norwegian Directorate of Health. (2012). Økonomisk evaluering av helsetiltak– en veileder [Economic evaluation of healthcare interventions– a guide]. Available from: https://www.helsedirektoratet.no/veiledere/okonomisk-evaluering-av-helsetiltak . Accessed 07 march 2024.
Kinge, J. M., Dieleman, J. L., Karlstad, Ø., Knudsen, A. K., Klitkou, S. T., Hay,S. I.,… Vollset, S. E. Disease-specific health spending by age, sex, and type of care in Norway: a national health registry study. BMC medicine, 2023;21(1):201.
Langeland E, Førland O, Aas E, Birkeland A, Folkestad B, Kjeken I. Modeler for hverdagsrehabilitering - en følgeevaluering i norske kommuner. Effekter for brukerne og gevinster for kommunene? [Models for everyday rehabilitation - a follow-up evaluation in Norwegian municipalities. Effects for the users and gains for the municipalities?] (2016). Available from: https://ntnuopen.ntnu.no/ntnu-xmlui/handle/11250/2389813 . Accessed 30 jan 2024.
Prince M, Wimo A, Guerchet M, Ali GC, Wu YT, Prina M. World Alzheimer report 2015. The global impact of dementia: an analysis of prevalence, incidence, cost and trends (Doctoral dissertation, Alzheimer’s disease international). 2015.
Launer LJ. Statistics on the burden of dementia: need for stronger data. Lancet Neurol. 2019;18:25–7.
McCaffrey N, Currow DC. Separated at birth? BMJ Supportive Palliative Care. 2015;5(1):2–3. https://doi.org/10.1136/bmjspcare-2015-000855 .
Kellerborg K, Perry-Duxbury M, de Vries L, van Baal P. Practical guidance for including future costs in economic evaluations in the Netherlands: introducing and applying PAID 3.0. Value Health. 2020;23(11):1453–61.
Rijksinstituut voor Volksgezondheid en Milieu (RIVM). [Dutch National Institute for Public Health and the Environment] https://www.volksgezondheidenzorg.info/ . Accessed 30 jan 2024.
Rojas G, Bartoloni L, Dillon C, Serrano CM, Iturry M, Allegri RF. Clinical and economic characteristics associated with direct costs of Alzheimer’s, frontotemporal and vascular dementia in Argentina. Int Psychogeriatr. 2011;23(4):554–61.
Gnanamanickam, E. S., Dyer, S. M., Milte, R., Harrison, S. L., Liu, E., Easton, T.,… Crotty, M. Direct health and residential care costs of people living with dementia in Australian residential aged care. International journal of geriatric psychiatry, 2018;33(7):859–866.
Sculpher, M. J., Pang, F. S., Manca, A., Drummond, M. F., Golder, S., Urdahl, H.,… Eastwood, A. (2004). Generalisability in economic evaluation studies in healthcare:a review and case studies.
Bauer JM, Sousa-Poza A. Impacts of informal caregiving on caregiver employment, health, and family. J Popul Ageing. 2015;8:113–45.
Bolin K, Lindgren B, Lundborg P. Informal and formal care among single-living elderly in Europe. Health Econ. 2008;17(3):393–409.
Cohen J, Houttekier D, Onwuteaka-Philipsen B, Miccinesi G, Addington-Hall J, Kaasa S, Deliens L. Which patients with cancer die at home? A study of six European countries using death certificate data. J Clin Oncol. 2010;28(13):2267–73.
Pedersen AG, Ellingsen CL. (2015). Data quality in the Causes of Death Registry. Journal of the Norwegian Medical Association Available from: https://tidsskriftet.no/en/2015/05/perspectives/data-quality-causes-death-registry . Accessed 30 jan 2024.
The Cancer Registry of Norway. Kreftregisteret [The Cancer Registry of Norway]. Available from: https://www.kreftregisteret.no/en/General/About-the-Cancer-Registry/ . Accessed 30 jan 2024.
Download references
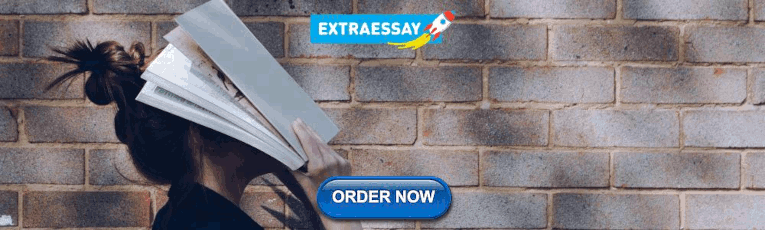
Acknowledgements
We would like to thank the Norwegian Cancer Society for funding this work as part of the SAFE project (research grant number: 208164). We thank Pauline Keller for her help in editing the final manuscript. We acknowledge two anonymous reviewers for their valuable feedback that helped us to improve this article.
The research was funded by the Norwegian Cancer Association, research grant number: 208164.
Open access funding provided by Norwegian University of Science and Technology
Author information
Authors and affiliations.
Department of Health Management and Health Economics, Institute of Health and Society, University of Oslo, Oslo, Norway
Yvonne Anne Michel, Eline Aas, Liv Ariane Augestad, Emily Burger & Gudrun Maria Waaler Bjørnelv
Faculty of Social Sciences, University of Applied Sciences Zittau/ Görlitz, Görlitz, Germany
Yvonne Anne Michel
Division for Health Services, Norwegian Institute of Public Health, Oslo, Norway
Center for Health Decision Science, Harvard T.H. Chan School of Public Health, Boston, MA, USA
Emily Burger
Department for Interdisciplinary Health Sciences, Institute of Health and Society, University of Oslo, Oslo, Norway
Lisbeth Thoresen
Department of Public Health and Nursing, Norwegian University of Science and Technology, Trondheim, Norway
Gudrun Maria Waaler Bjørnelv
You can also search for this author in PubMed Google Scholar
Contributions
All authors (YAM, EA, LAA, EB, LT, GWB) created a study plan. GWB and EA applied for ethical approval and collected the data. GWB conducted analyses in cooperation with YAM, and the results were continuously discussed with EA, LAA, EB, and LT. YAM drafted the manuscript, and EA, LAA, EB, LT, and GWB reviewed the manuscript throughout the process. All authors approved the final draft. We used a large language model, DeepL write ( www.deepl.com/write ), to improve the language of this article.
Corresponding author
Correspondence to Gudrun Maria Waaler Bjørnelv .
Ethics declarations
Ethics approval and consent to participate.
The Norwegian Ethics Committee and the Norwegian Data Protection Authority (ref no 2013/2090), in addition to all the registry owners, approved this study. Registry owners gave us administrative permission to access and use the data. The registry owners include the Norwegian Directorate of Health, the National Institute of Public Health, and Statistics Norway. The need for informed consent was waived by the Regional Committee for Medical Research Ethics South East Norway, since data was retrieved from national registries for the purpose of research, for which informed consent is not required. We confirm that all methods were carried out in accordance with relevant guidelines and regulations.
Consent for publication
Not applicable.
Competing interests
The authors declare no competing interests.
Additional information
Publisher’s note.
Springer Nature remains neutral with regard to jurisdictional claims in published maps and institutional affiliations.
Electronic supplementary material
Below is the link to the electronic supplementary material.
Supplementary Material 1: All decedents by all causes fo death
Supplementary material 2: decedents younger than 80 years by all causes of death, supplementary material 3: decedents older than 80 years by all causes of death, supplementary material 4: comparing healthcare costs by age at death, rights and permissions.
Open Access This article is licensed under a Creative Commons Attribution 4.0 International License, which permits use, sharing, adaptation, distribution and reproduction in any medium or format, as long as you give appropriate credit to the original author(s) and the source, provide a link to the Creative Commons licence, and indicate if changes were made. The images or other third party material in this article are included in the article’s Creative Commons licence, unless indicated otherwise in a credit line to the material. If material is not included in the article’s Creative Commons licence and your intended use is not permitted by statutory regulation or exceeds the permitted use, you will need to obtain permission directly from the copyright holder. To view a copy of this licence, visit http://creativecommons.org/licenses/by/4.0/ . The Creative Commons Public Domain Dedication waiver ( http://creativecommons.org/publicdomain/zero/1.0/ ) applies to the data made available in this article, unless otherwise stated in a credit line to the data.
Reprints and permissions
About this article
Cite this article.
Michel, Y.A., Aas, E., Augestad, L.A. et al. Healthcare use and costs in the last six months of life by level of care and cause of death. BMC Health Serv Res 24 , 688 (2024). https://doi.org/10.1186/s12913-024-10877-5
Download citation
Received : 29 March 2023
Accepted : 19 March 2024
Published : 30 May 2024
DOI : https://doi.org/10.1186/s12913-024-10877-5
Share this article
Anyone you share the following link with will be able to read this content:
Sorry, a shareable link is not currently available for this article.
Provided by the Springer Nature SharedIt content-sharing initiative
- End-of-life
- Healthcare use
- Cause of death
BMC Health Services Research
ISSN: 1472-6963
- General enquiries: [email protected]
Protecting against researcher bias in secondary data analysis: challenges and potential solutions
- Open access
- Published: 13 January 2022
- Volume 37 , pages 1–10, ( 2022 )
Cite this article
You have full access to this open access article
- Jessie R. Baldwin ORCID: orcid.org/0000-0002-5703-5058 1 , 2 ,
- Jean-Baptiste Pingault 1 , 2 ,
- Tabea Schoeler 1 ,
- Hannah M. Sallis 3 , 4 , 5 &
- Marcus R. Munafò 3 , 4 , 6
49k Accesses
33 Citations
181 Altmetric
Explore all metrics
Analysis of secondary data sources (such as cohort studies, survey data, and administrative records) has the potential to provide answers to science and society’s most pressing questions. However, researcher biases can lead to questionable research practices in secondary data analysis, which can distort the evidence base. While pre-registration can help to protect against researcher biases, it presents challenges for secondary data analysis. In this article, we describe these challenges and propose novel solutions and alternative approaches. Proposed solutions include approaches to (1) address bias linked to prior knowledge of the data, (2) enable pre-registration of non-hypothesis-driven research, (3) help ensure that pre-registered analyses will be appropriate for the data, and (4) address difficulties arising from reduced analytic flexibility in pre-registration. For each solution, we provide guidance on implementation for researchers and data guardians. The adoption of these practices can help to protect against researcher bias in secondary data analysis, to improve the robustness of research based on existing data.
Similar content being viewed by others
Reporting and Transparency in Big Data: The Nexus of Ethics and Methodology
Preregistration of Studies with Existing Data
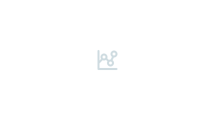
Secondary analysis of statutorily collected routine data
Avoid common mistakes on your manuscript.
Introduction
Secondary data analysis has the potential to provide answers to science and society’s most pressing questions. An abundance of secondary data exists—cohort studies, surveys, administrative data (e.g., health records, crime records, census data), financial data, and environmental data—that can be analysed by researchers in academia, industry, third-sector organisations, and the government. However, secondary data analysis is vulnerable to questionable research practices (QRPs) which can distort the evidence base. These QRPs include p-hacking (i.e., exploiting analytic flexibility to obtain statistically significant results), selective reporting of statistically significant, novel, or “clean” results, and hypothesising after the results are known (HARK-ing [i.e., presenting unexpected results as if they were predicted]; [ 1 ]. Indeed, findings obtained from secondary data analysis are not always replicable [ 2 , 3 ], reproducible [ 4 ], or robust to analytic choices [ 5 , 6 ]. Preventing QRPs in research based on secondary data is therefore critical for scientific and societal progress.
A primary cause of QRPs is common cognitive biases that affect the analysis, reporting, and interpretation of data [ 7 – 10 ]. For example, apophenia (the tendency to see patterns in random data) and confirmation bias (the tendency to focus on evidence that is consistent with one’s beliefs) can lead to particular analytical choices and selective reporting of “publishable” results [ 11 – 13 ]. In addition, hindsight bias (the tendency to view past events as predictable) can lead to HARK-ing, so that observed results appear more compelling.
The scope for these biases to distort research outputs from secondary data analysis is perhaps particularly acute, for two reasons. First, researchers now have increasing access to high-dimensional datasets that offer a multitude of ways to analyse the same data [ 6 ]. Such analytic flexibility can lead to different conclusions depending on the analytical choices made [ 5 , 14 , 15 ]. Second, current incentive structures in science reward researchers for publishing statistically significant, novel, and/or surprising findings [ 16 ]. This combination of opportunity and incentive may lead researchers—consciously or unconsciously—to run multiple analyses and only report the most “publishable” findings.
One way to help protect against the effects of researcher bias is to pre-register research plans [ 17 , 18 ]. This can be achieved by pre-specifying the rationale, hypotheses, methods, and analysis plans, and submitting these to either a third-party registry (e.g., the Open Science Framework [OSF]; https://osf.io/ ), or a journal in the form of a Registered Report [ 19 ]. Because research plans and hypotheses are specified before the results are known, pre-registration reduces the potential for cognitive biases to lead to p-hacking, selective reporting, and HARK-ing [ 20 ]. While pre-registration is not necessarily a panacea for preventing QRPs (Table 1 ), meta-scientific evidence has found that pre-registered studies and Registered Reports are more likely to report null results [ 21 – 23 ], smaller effect sizes [ 24 ], and be replicated [ 25 ]. Pre-registration is increasingly being adopted in epidemiological research [ 26 , 27 ], and is even required for access to data from certain cohorts (e.g., the Twins Early Development Study [ 28 ]). However, pre-registration (and other open science practices; Table 2 ) can pose particular challenges to researchers conducting secondary data analysis [ 29 ], motivating the need for alternative approaches and solutions. Here we describe such challenges, before proposing potential solutions to protect against researcher bias in secondary data analysis (summarised in Fig. 1 ).
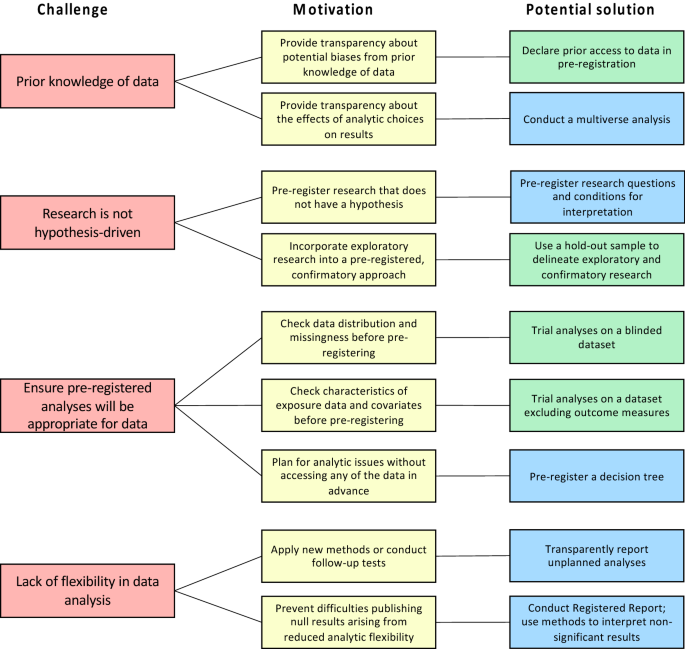
Challenges in pre-registering secondary data analysis and potential solutions (according to researcher motivations). Note : In the “Potential solution” column, blue boxes indicate solutions that are researcher-led; green boxes indicate solutions that should be facilitated by data guardians
Challenges of pre-registration for secondary data analysis
Prior knowledge of the data.
Researchers conducting secondary data analysis commonly analyse data from the same dataset multiple times throughout their careers. However, prior knowledge of the data increases risk of bias, as prior expectations about findings could motivate researchers to pursue certain analyses or questions. In the worst-case scenario, a researcher might perform multiple preliminary analyses, and only pursue those which lead to notable results (perhaps posting a pre-registration for these analyses, even though it is effectively post hoc). However, even if the researcher has not conducted specific analyses previously, they may be biased (either consciously or subconsciously) to pursue certain analyses after testing related questions with the same variables, or even by reading past studies on the dataset. As such, pre-registration cannot fully protect against researcher bias when researchers have previously accessed the data.
Research may not be hypothesis-driven
Pre-registration and Registered Reports are tailored towards hypothesis-driven, confirmatory research. For example, the OSF pre-registration template requires researchers to state “specific, concise, and testable hypotheses”, while Registered Reports do not permit purely exploratory research [ 30 ], although a new Exploratory Reports format now exists [ 31 ]. However, much research involving secondary data is not focused on hypothesis testing, but is exploratory, descriptive, or focused on estimation—in other words, examining the magnitude and robustness of an association as precisely as possible, rather than simply testing a point null. Furthermore, without a strong theoretical background, hypotheses will be arbitrary and could lead to unhelpful inferences [ 32 , 33 ], and so should be avoided in novel areas of research.
Pre-registered analyses are not appropriate for the data
With pre-registration, there is always a risk that the data will violate the assumptions of the pre-registered analyses [ 17 ]. For example, a researcher might pre-register a parametric test, only for the data to be non-normally distributed. However, in secondary data analysis, the extent to which the data shape the appropriate analysis can be considerable. First, longitudinal cohort studies are often subject to missing data and attrition. Approaches to deal with missing data (e.g., listwise deletion; multiple imputation) depend on the characteristics of missing data (e.g., the extent and patterns of missingness [ 34 ]), and so pre-specifying approaches to dealing with missingness may be difficult, or extremely complex. Second, certain analytical decisions depend on the nature of the observed data (e.g., the choice of covariates to include in a multiple regression might depend on the collinearity between the measures, or the degree of missingness of different measures that capture the same construct). Third, much secondary data (e.g., electronic health records and other administrative data) were never collected for research purposes, so can present several challenges that are impossible to predict in advance [ 35 ]. These issues can limit a researcher’s ability to pre-register a precise analytic plan prior to accessing secondary data.
Lack of flexibility in data analysis
Concerns have been raised that pre-registration limits flexibility in data analysis, including justifiable exploration [ 36 – 38 ]. For example, by requiring researchers to commit to a pre-registered analysis plan, pre-registration could prevent researchers from exploring novel questions (with a hypothesis-free approach), conducting follow-up analyses to investigate notable findings [ 39 ], or employing newly published methods with advantages over those pre-registered. While this concern is also likely to apply to primary data analysis, it is particularly relevant to certain fields involving secondary data analysis, such as genetic epidemiology, where new methods are rapidly being developed [ 40 ], and follow-up analyses are often required (e.g., in a genome-wide association study to further investigate the role of a genetic variant associated with a phenotype). However, this concern is perhaps over-stated – pre-registration does not preclude unplanned analyses; it simply makes it more transparent that these analyses are post hoc. Nevertheless, another understandable concern is that reduced analytic flexibility could lead to difficulties in publishing papers and accruing citations. For example, pre-registered studies are more likely to report null results [ 22 , 23 ], likely due to reduced analytic flexibility and selective reporting. While this is a positive outcome for research integrity, null results are less likely to be published [ 13 , 41 , 42 ] and cited [ 11 ], which could disadvantage researchers’ careers.
In this section, we describe potential solutions to address the challenges involved in pre-registering secondary data analysis, including approaches to (1) address bias linked to prior knowledge of the data, (2) enable pre-registration of non-hypothesis-driven research, (3) ensure that pre-planned analyses will be appropriate for the data, and (4) address potential difficulties arising from reduced analytic flexibility.
Challenge: Prior knowledge of the data
Declare prior access to data.
To increase transparency about potential biases arising from knowledge of the data, researchers could routinely report all prior data access in a pre-registration [ 29 ]. This would ideally include evidence from an independent gatekeeper (e.g., a data guardian of the study) stating whether data and relevant variables were accessed by each co-author. To facilitate this process, data guardians could set up a central “electronic checkout” system that records which researchers have accessed data, what data were accessed, and when [ 43 ]. The researcher or data guardian could then provide links to the checkout histories for all co-authors in the pre-registration, to verify their prior data access. If it is not feasible to provide such objective evidence, authors could self-certify their prior access to the dataset and where possible, relevant variables—preferably listing any publications and in-preparation studies based on the dataset [ 29 ]. Of course, self-certification relies on trust that researchers will accurately report prior data access, which could be challenging if the study involves a large number of authors, or authors who have been involved on many studies on the dataset. However, it is likely to be the most feasible option at present as many datasets do not have available electronic records of data access. For further guidance on self-certifying prior data access when pre-registering secondary data analysis studies on a third-party registry (e.g., the OSF), we recommend referring to the template by Van den Akker, Weston [ 29 ].
The extent to which prior access to data renders pre-registration invalid is debatable. On the one hand, even if data have been accessed previously, pre-registration is likely to reduce QRPs by encouraging researchers to commit to a pre-specified analytic strategy. On the other hand, pre-registration does not fully protect against researcher bias where data have already been accessed, and can lend added credibility to study claims, which may be unfounded. Reporting prior data access in a pre-registration is therefore important to make these potential biases transparent, so that readers and reviewers can judge the credibility of the findings accordingly. However, for a more rigorous solution which protects against researcher bias in the context of prior data access, researchers should consider adopting a multiverse approach.
Conduct a multiverse analysis
A multiverse analysis involves identifying all potential analytic choices that could justifiably be made to address a given research question (e.g., different ways to code a variable, combinations of covariates, and types of analytic model), implementing them all, and reporting the results [ 44 ]. Notably, this method differs from the traditional approach in which findings from only one analytic method are reported. It is conceptually similar to a sensitivity analysis, but it is far more comprehensive, as often hundreds or thousands of analytic choices are reported, rather than a handful. By showing the results from all defensible analytic approaches, multiverse analysis reduces scope for selective reporting and provides insight into the robustness of findings against analytical choices (for example, if there is a clear convergence of estimates, irrespective of most analytical choices). For causal questions in observational research, Directed Acyclic Graphs (DAGs) could be used to inform selection of covariates in multiverse approaches [ 45 ] (i.e., to ensure that confounders, rather than mediators or colliders, are controlled for).
Specification curve analysis [ 46 ] is a form of multiverse analysis that has been applied to examine the robustness of epidemiological findings to analytic choices [ 6 , 47 ]. Specification curve analysis involves three steps: (1) identifying all analytic choices – termed “specifications”, (2) displaying the results graphically with magnitude of effect size plotted against analytic choice, and (3) conducting joint inference across all results. When applied to the association between digital technology use and adolescent well-being [ 6 ], specification curve analysis showed that the (small, negative) association diminished after accounting for adequate control variables and recall bias – demonstrating the sensitivity of results to analytic choices.
Despite the benefits of the multiverse approach in addressing analytic flexibility, it is not without limitations. First, because each analytic choice is treated as equally valid, including less justifiable models could bias the results away from the truth. Second, the choice of specifications can be biased by prior knowledge (e.g., a researcher may choose to omit a covariate to obtain a particular result). Third, multiverse analysis may not entirely prevent selective reporting (e.g., if the full range of results are not reported), although pre-registering multiverse approaches (and specifying analytic choices) could mitigate this. Last, and perhaps most importantly, multiverse analysis is technically challenging (e.g., when there are hundreds or thousands of analytic choices) and can be impractical for complex analyses, very large datasets, or when computational resources are limited. However, this burden can be somewhat reduced by tutorials and packages which are being developed to standardise the procedure and reduce computational time [see 48 , 49 ].
Challenge: Research may not be hypothesis-driven
Pre-register research questions and conditions for interpreting findings.
Observational research arguably does not need to have a hypothesis to benefit from pre-registration. For studies that are descriptive or focused on estimation, we recommend pre-registering research questions, analysis plans, and criteria for interpretation. Analytic flexibility will be limited by pre-registering specific research questions and detailed analysis plans, while post hoc interpretation will be limited by pre-specifying criteria for interpretation [ 50 ]. The potential for HARK-ing will also be minimised because readers can compare the published study to the original pre-registration, where a-priori hypotheses were not specified.
Detailed guidance on how to pre-register research questions and analysis plans for secondary data is provided in Van den Akker’s [ 29 ] tutorial. To pre-specify conditions for interpretation, it is important to anticipate – as much as possible – all potential findings, and state how each would be interpreted. For example, suppose that a researcher aims to test a causal relationship between X and Y using a multivariate regression model with longitudinal data. Assuming that all potential confounders have been fully measured and controlled for (albeit a strong assumption) and statistical power is high, three broad sets of results and interpretations could be pre-specified. First, an association between X and Y that is similar in magnitude to the unadjusted association would be consistent with a causal relationship. Second, an association between X and Y that is attenuated after controlling for confounders would suggest that the relationship is partly causal and partly confounded. Third, a minimal, non-statistically significant adjusted association would suggest a lack of evidence for a causal effect of X on Y. Depending on the context of the study, criteria could also be provided on the threshold (or range of thresholds) at which the effect size would justify different interpretations [ 51 ], be considered practically meaningful, or the smallest effect size of interest for equivalence tests [ 52 ]. While researcher biases might still affect the pre-registered criteria for interpreting findings (e.g., toward over-interpreting a small effect size as meaningful), this bias will at least be transparent in the pre-registration.
Use a holdout sample to delineate exploratory and confirmatory research
Where researchers wish to integrate exploratory research into a pre-registered, confirmatory study, a holdout sample approach can be used [ 18 ]. Creating a holdout sample refers to the process of randomly splitting the dataset into two parts, often referred to as ‘training’ and ‘holdout’ datasets. To delineate exploratory and confirmatory research, researchers can first conduct exploratory data analysis on the training dataset (which should comprise a moderate fraction of the data, e.g., 35% [ 53 ]. Based on the results of the discovery process, researchers can pre-register hypotheses and analysis plans to formally test on the holdout dataset. This process has parallels with cross-validation in machine learning, in which the dataset is split and the model is developed on the training dataset, before being tested on the test dataset. The approach enables a flexible discovery process, before formally testing discoveries in a non-biased way.
When considering whether to use the holdout sample approach, three points should be noted. First, because the training dataset is not reusable, there will be a reduced sample size and loss of power relative to analysing the whole dataset. As such, the holdout sample approach will only be appropriate when the original dataset is large enough to provide sufficient power in the holdout dataset. Second, when the training dataset is used for exploration, subsequent confirmatory analyses on the holdout dataset may be overfitted (due to both datasets being drawn from the same sample), so replication in independent samples is recommended. Third, the holdout dataset should be created by an independent data manager or guardian, to ensure that the researcher does not have knowledge of the full dataset. However, it is straightforward to randomly split a dataset into a holdout and training sample and we provide example R code at: https://github.com/jr-baldwin/Researcher_Bias_Methods/blob/main/Holdout_script.md .
Challenge: Pre-registered analyses are not appropriate for the data
Use blinding to test proposed analyses.
One method to help ensure that pre-registered analyses will be appropriate for the data is to trial the analyses on a blinded dataset [ 54 ], before pre-registering. Data blinding involves obscuring the data values or labels prior to data analysis, so that the proposed analyses can be trialled on the data without observing the actual findings. Various types of blinding strategies exist [ 54 ], but one method that is appropriate for epidemiological data is “data scrambling” [ 55 ]. This involves randomly shuffling the data points so that any associations between variables are obscured, whilst the variable distributions (and amounts of missing data) remain the same. We provide a tutorial for how to implement this in R (see https://github.com/jr-baldwin/Researcher_Bias_Methods/blob/main/Data_scrambling_tutorial.md ). Ideally the data scrambling would be done by a data guardian who is independent of the research, to ensure that the main researcher does not access the data prior to pre-registering the analyses. Once the researcher is confident with the analyses, the study can be pre-registered, and the analyses conducted on the unscrambled dataset.
Blinded analysis offers several advantages for ensuring that pre-registered analyses are appropriate, with some limitations. First, blinded analysis allows researchers to directly check the distribution of variables and amounts of missingness, without having to make assumptions about the data that may not be met, or spend time planning contingencies for every possible scenario. Second, blinded analysis prevents researchers from gaining insight into the potential findings prior to pre-registration, because associations between variables are masked. However, because of this, blinded analysis does not enable researchers to check for collinearity, predictors of missing data, or other covariances that may be necessary for model specification. As such, blinded analysis will be most appropriate for researchers who wish to check the data distribution and amounts of missingness before pre-registering.
Trial analyses on a dataset excluding the outcome
Another method to help ensure that pre-registered analyses will be appropriate for the data is to trial analyses on a dataset excluding outcome data. For example, data managers could provide researchers with part of the dataset containing the exposure variable(s) plus any covariates and/or auxiliary variables. The researcher can then trial and refine the analyses ahead of pre-registering, without gaining insight into the main findings (which require the outcome data). This approach is used to mitigate bias in propensity score matching studies [ 26 , 56 ], as researchers use data on the exposure and covariates to create matched groups, prior to accessing any outcome data. Once the exposed and non-exposed groups have been matched effectively, researchers pre-register the protocol ahead of viewing the outcome data. Notably though, this approach could help researchers to identify and address other analytical challenges involving secondary data. For example, it could be used to check multivariable distributional characteristics, test for collinearity between multiple predictor variables, or identify predictors of missing data for multiple imputation.
This approach offers certain benefits for researchers keen to ensure that pre-registered analyses are appropriate for the observed data, with some limitations. Regarding benefits, researchers will be able to examine associations between variables (excluding the outcome), unlike the data scrambling approach described above. This would be helpful for checking certain assumptions (e.g., collinearity or characteristics of missing data such as whether it is missing at random). In addition, the approach is easy to implement, as the dataset can be initially created without the outcome variable, which can then be added after pre-registration, minimising burden on data guardians. Regarding limitations, it is possible that accessing variables in advance could provide some insight into the findings. For example, if a covariate is known to be highly correlated with the outcome, testing the association between the covariate and the exposure could give some indication of the relationship between the exposure and the outcome. To make this potential bias transparent, researchers should report the variables that they already accessed in the pre-registration. Another limitation is that researchers will not be able to identify analytical issues relating to the outcome data in advance of pre-registration. Therefore, this approach will be most appropriate where researchers wish to check various characteristics of the exposure variable(s) and covariates, rather than the outcome. However, a “mixed” approach could be applied in which outcome data is provided in scrambled format, to enable researchers to also assess distributional characteristics of the outcome. This would substantially reduce the number of potential challenges to be considered in pre-registered analytical pipelines.
Pre-register a decision tree
If it is not possible to access any of the data prior to pre-registering (e.g., to enable analyses to be trialled on a dataset that is blinded or missing outcome data), researchers could pre-register a decision tree. This defines the sequence of analyses and rules based on characteristics of the observed data [ 17 ]. For example, the decision tree could specify testing a normality assumption, and based on the results, whether to use a parametric or non-parametric test. Ideally, the decision tree should provide a contingency plan for each of the planned analyses, if assumptions are not fulfilled. Of course, it can be challenging and time consuming to anticipate every potential issue with the data and plan contingencies. However, investing time into pre-specifying a decision tree (or a set of contingency plans) could save time should issues arise during data analysis, and can reduce the likelihood of deviating from the pre-registration.
Challenge: Lack of flexibility in data analysis
Transparently report unplanned analyses.
Unplanned analyses (such as applying new methods or conducting follow-up tests to investigate an interesting or unexpected finding) are a natural and often important part of the scientific process. Despite common misconceptions, pre-registration does not permit such unplanned analyses from being included, as long as they are transparently reported as post-hoc. If there are methodological deviations, we recommend that researchers should (1) clearly state the reasons for using the new method, and (2) if possible, report results from both methods, to ideally show that the change in methods was not due to the results [ 57 ]. This information can either be provided in the manuscript or in an update to the original pre-registration (e.g., on the third-party registry such as the OSF), which can be useful when journal word limits are tight. Similarly, if researchers wish to include additional follow-up analyses to investigate an interesting or unexpected finding, this should be reported but labelled as “exploratory” or “post-hoc” in the manuscript.
Ensure a paper’s value does not depend on statistically significant results
Researchers may be concerned that reduced analytic flexibility from pre-registration could increase the likelihood of reporting null results [ 22 , 23 ], which are harder to publish [ 13 , 42 ]. To address this, we recommend taking steps to ensure that the value and success of a study does not depend on a significant p-value. First, methodologically strong research (e.g., with high statistical power, valid and reliable measures, robustness checks, and replication samples) will advance the field, whatever the findings. Second, methods can be applied to allow for the interpretation of statistically non-significant findings (e.g., Bayesian methods [ 58 ] or equivalence tests, which determine whether an observed effect is surprisingly small [ 52 , 59 , 60 ]. This means that the results will be informative whatever they show, in contrast to approaches relying solely on null hypothesis significance testing, where statistically non-significant findings cannot be interpreted as meaningful. Third, researchers can submit the proposed study as a Registered Report, where it will be evaluated before the results are available. This is arguably the strongest way to protect against publication bias, as in-principle study acceptance is granted without any knowledge of the results. In addition, Registered Reports can improve the methodology, as suggestions from expert reviewers can be incorporated into the pre-registered protocol.
Under a system that rewards novel and statistically significant findings, it is easy for subconscious human biases to lead to QRPs. However, researchers, along with data guardians, journals, funders, and institutions, have a responsibility to ensure that findings are reproducible and robust. While pre-registration can help to limit analytic flexibility and selective reporting, it involves several challenges for epidemiologists conducting secondary data analysis. The approaches described here aim to address these challenges (Fig. 1 ), to either improve the efficacy of pre-registration or provide an alternative approach to address analytic flexibility (e.g., a multiverse analysis). The responsibility in adopting these approaches should not only fall on researchers’ shoulders; data guardians also have an important role to play in recording and reporting access to data, providing blinded datasets and hold-out samples, and encouraging researchers to pre-register and adopt these solutions as part of their data request. Furthermore, wider stakeholders could incentivise these practices; for example, journals could provide a designated space for researchers to report deviations from the pre-registration, and funders could provide grants to establish best practice at the cohort level (e.g., data checkout systems, blinded datasets). Ease of adoption is key to ensure wide uptake, and we therefore encourage efforts to evaluate, simplify and improve these practices. Steps that could be taken to evaluate these practices are presented in Box 1.
More broadly, it is important to emphasise that researcher biases do not operate in isolation, but rather in the context of wider publication bias and a “publish or perish” culture. These incentive structures not only promote QRPs [ 61 ], but also discourage researchers from pre-registering and adopting other time-consuming reproducible methods. Therefore, in addition to targeting bias at the individual researcher level, wider initiatives from journals, funders, and institutions are required to address these institutional biases [ 7 ]. Systemic changes that reward rigorous and reproducible research will help researchers to provide unbiased answers to science and society’s most important questions.
Box 1. Evaluation of approaches
To evaluate, simplify and improve approaches to protect against researcher bias in secondary data analysis, the following steps could be taken.
Co-creation workshops to refine approaches
To obtain feedback on the approaches (including on any practical concerns or feasibility issues) co-creation workshops could be held with researchers, data managers, and wider stakeholders (e.g., journals, funders, and institutions).
Empirical research to evaluate efficacy of approaches
To evaluate the effectiveness of the approaches in preventing researcher bias and/or improving pre-registration, empirical research is needed. For example, to test the extent to which the multiverse analysis can reduce selective reporting, comparisons could be made between effect sizes from multiverse analyses versus effect sizes from meta-analyses (of non-pre-registered studies) addressing the same research question. If smaller effect sizes were found in multiverse analyses, it would suggest that the multiverse approach can reduce selective reporting. In addition, to test whether providing a blinded dataset or dataset missing outcome variables could help researchers develop an appropriate analytical protocol, researchers could be randomly assigned to receive such a dataset (or no dataset), prior to pre-registration. If researchers who received such a dataset had fewer eventual deviations from the pre-registered protocol (in the final study), it would suggest that this approach can help ensure that proposed analyses are appropriate for the data.
Pilot implementation of the measures
To assess the practical feasibility of the approaches, data managers could pilot measures for users of the dataset (e.g., required pre-registration for access to data, provision of datasets that are blinded or missing outcome variables). Feedback could then be collected from researchers and data managers via about the experience and ease of use.
Kerr NL. HARKing: Hypothesizing after the results are known. Pers Soc Psychol Rev. 1998;2(3):196–217.
CAS PubMed Google Scholar
Border R, Johnson EC, Evans LM, et al. No support for historical candidate gene or candidate gene-by-interaction hypotheses for major depression across multiple large samples. Am J Psychiatry. 2019;176(5):376–87.
PubMed PubMed Central Google Scholar
Duncan LE, Keller MC. A critical review of the first 10 years of candidate gene-by-environment interaction research in psychiatry. Am J Psychiatry. 2011;168(10):1041–9.
Seibold H, Czerny S, Decke S, et al. A computational reproducibility study of PLOS ONE articles featuring longitudinal data analyses. PLoS ONE. 2021;16(6):e0251194. https://doi.org/10.1371/journal.pone.0251194 .
Article CAS PubMed PubMed Central Google Scholar
Botvinik-Nezer R, Holzmeister F, Camerer CF, et al. Variability in the analysis of a single neuroimaging dataset by many teams. Nature. 2020;582:84–8.
CAS PubMed PubMed Central Google Scholar
Orben A, Przybylski AK. The association between adolescent well-being and digital technology use. Nat Hum Behav. 2019;3(2):173.
PubMed Google Scholar
Munafò MR, Nosek BA, Bishop DV, et al. A manifesto for reproducible science. Nat Hum Behav. 2017;1(1):0021.
Nuzzo R. How scientists fool themselves–and how they can stop. Nature News. 2015;526(7572):182.
CAS Google Scholar
Bishop DV. The psychology of experimental psychologists: Overcoming cognitive constraints to improve research: The 47th Sir Frederic Bartlett lecture. Q J Exp Psychol. 2020;73(1):1–19.
Google Scholar
Greenland S. Invited commentary: The need for cognitive science in methodology. Am J Epidemiol. 2017;186(6):639–45.
De Vries Y, Roest A, de Jonge P, Cuijpers P, Munafò M, Bastiaansen J. The cumulative effect of reporting and citation biases on the apparent efficacy of treatments: The case of depression. Psychol Med. 2018;48(15):2453–5.
Nickerson RS. Confirmation bias: A ubiquitous phenomenon in many guises. Rev Gen Psychol. 1998;2(2):175–220.
Franco A, Malhotra N, Simonovits G. Publication bias in the social sciences: Unlocking the file drawer. Science. 2014;345(6203):1502–5.
Silberzahn R, Uhlmann EL, Martin DP, et al. Many analysts, one data set: Making transparent how variations in analytic choices affect results. Adv Methods Pract Psychol Sci. 2018;1(3):337–56.
Simmons JP, Nelson LD, Simonsohn U. False-positive psychology: Undisclosed flexibility in data collection and analysis allows presenting anything as significant. Psychol Sci. 2011;22(11):1359–66.
Metcalfe J, Wheat, K., Munafo, M., Parry, J. Research integrity: A landscape study: UK Research and innovation 2020.
Nosek BA, Ebersole CR, DeHaven AC, Mellor DT. The preregistration revolution. Proc Natl Acad Sci. 2018;115(11):2600–6.
Wagenmakers E-J, Wetzels R, Borsboom D, van der Maas HL, Kievit RA. An agenda for purely confirmatory research. Perspect Psychol Sci. 2012;7(6):632–8.
Chambers CD. Registered reports: A new publishing initiative at Cortex. Cortex. 2013;49(3):609–10.
Nosek BA, Beck ED, Campbell L, et al. Preregistration is hard, and worthwhile. Trends Cogn Sci. 2019;23(10):815–8.
Kaplan RM, Irvin VL. Likelihood of null effects of large NHLBI clinical trials has increased over time. PLoS One. 2015;10(8):e0132382.
Allen C, Mehler DM. Open science challenges, benefits and tips in early career and beyond. PLoS Biol. 2019;17(5):e3000246.
Scheel AM, Schijen MR, Lakens D. An excess of positive results: Comparing the standard psychology literature with registered reports. Adv Methods Pract Psychol Sci. 2021;4(2):25152459211007468.
Schäfer T, Schwarz MA. The meaningfulness of effect sizes in psychological research: differences between sub-disciplines and the impact of potential biases. Front Psychol. 2019;10:813.
Protzko J, Krosnick J, Nelson LD, et al. High replicability of newly-discovered social-behavioral findings is achievable. PsyArXiv. 2020. doi: https://doi.org/10.31234/osf.io/n2a9x
Small DS, Firth D, Keele L, et al. Protocol for a study of the effect of surface mining in central appalachia on adverse birth outcomes. arXiv.org. 2020
Deshpande SK, Hasegawa RB, Weiss J, Small DS. Protocol for an observational study on the effects of playing football in adolescence on mental health in early adulthood. arXiv preprint 2018
Twins Early Development Study. TEDS Data Access Policy: 6. Pre-registration of analysis. https://www.teds.ac.uk/researchers/teds-data-access-policy#preregistration . Accessed 18 March 2021
Van den Akker O, Weston SJ, Campbell L, et al. Preregistration of secondary data analysis: a template and tutorial. PsyArXiv. 2019. doi: https://doi.org/10.31234/osf.io/hvfmr
Chambers C, Tzavella L. Registered reports: past, present and future. MetaArXiv. 2020. doi: https://doi.org/10.31222/osf.io/43298
McIntosh RD. Exploratory reports: A new article type for cortex. Cortex. 2017;96:A1–4.
Scheel AM, Tiokhin L, Isager PM, Lakens D. Why hypothesis testers should spend less time testing hypotheses. Perspect Psychol Sci. 2020;16(4):744–55.
Colhoun HM, McKeigue PM, Smith GD. Problems of reporting genetic associations with complex outcomes. Lancet. 2003;361(9360):865–72.
Hughes RA, Heron J, Sterne JAC, Tilling K. Accounting for missing data in statistical analyses: Multiple imputation is not always the answer. Int J Epidemiol. 2019;48(4):1294–304. https://doi.org/10.1093/ije/dyz032 .
Article PubMed PubMed Central Google Scholar
Goldstein BA. Five analytic challenges in working with electronic health records data to support clinical trials with some solutions. Clin Trials. 2020;17(4):370–6.
Goldin-Meadow S. Why preregistration makes me nervous. APS Observer. 2016;29(7).
Lash TL. Preregistration of study protocols is unlikely to improve the yield from our science, but other strategies might. Epidemiology. 2010;21(5):612–3. https://doi.org/10.1097/EDE.0b013e3181e9bba6 .
Article PubMed Google Scholar
Lawlor DA. Quality in epidemiological research: should we be submitting papers before we have the results and submitting more hypothesis-generating research? Int J Epidemiol. 2007;36(5):940–3.
Vandenbroucke JP. Preregistration of epidemiologic studies: An ill-founded mix of ideas. Epidemiology. 2010;21(5):619–20.
Pingault J-B, O’reilly PF, Schoeler T, Ploubidis GB, Rijsdijk F, Dudbridge F. Using genetic data to strengthen causal inference in observational research. Nat Rev Genet. 2018;19(9):566.
Fanelli D. Negative results are disappearing from most disciplines and countries. Scientometrics. 2012;90(3):891–904.
Greenwald AG. Consequences of prejudice against the null hypothesis. Psychol Bull. 1975;82(1):1.
Scott KM, Kline M. Enabling confirmatory secondary data analysis by logging data checkout. Adv Methods Pract Psychol Sci. 2019;2(1):45–54. https://doi.org/10.1177/2515245918815849 .
Article Google Scholar
Steegen S, Tuerlinckx F, Gelman A, Vanpaemel W. Increasing transparency through a multiverse analysis. Perspect Psychol Sci. 2016;11(5):702–12.
Del Giudice M, Gangestad SW. A traveler’s guide to the multiverse: Promises, pitfalls, and a framework for the evaluation of analytic decisions. Adv Methods Pract Psychol Sci. 2021;4(1):2515245920954925.
Simonsohn U, Simmons JP, Nelson LD. Specification curve: descriptive and inferential statistics on all reasonable specifications. SSRN. 2015. https://doi.org/10.2139/ssrn.2694998 .
Rohrer JM, Egloff B, Schmukle SC. Probing birth-order effects on narrow traits using specification-curve analysis. Psychol Sci. 2017;28(12):1821–32.
Masur P. How to do specification curve analyses in R: Introducing ‘specr’. 2020. https://philippmasur.de/2020/01/02/how-to-do-specification-curve-analyses-in-r-introducing-specr/ . Accessed 23rd July 2020.
Masur PK, Scharkow M. specr: Conducting and visualizing specification curve analyses: R package. (2020).
Kiyonaga A, Scimeca JM. Practical considerations for navigating registered reports. Trends Neurosci. 2019;42(9):568–72.
McPhetres J. What should a preregistration contain? PsyArXiv. (2020).
Lakens D. Equivalence tests: A practical primer for t tests, correlations, and meta-analyses. Soc Psychol Personal Sci. 2017;8(4):355–62.
Anderson ML, Magruder J. Split-sample strategies for avoiding false discoveries: National Bureau of Economic Research2017. Report No.: 0898-2937.
MacCoun R, Perlmutter S. Blind analysis: Hide results to seek the truth. Nature. 2015;526(7572):187–9.
MacCoun R, Perlmutter S. Blind analysis as a correction for confirmatory bias in physics and in psychology. Psychological science under scrutiny 2017. p. 295-322.
Rubin DB. The design versus the analysis of observational studies for causal effects: Parallels with the design of randomized trials. Stat Med. 2007;26(1):20–36.
Claesen A, Gomes SLBT, Tuerlinckx F, Vanpaemel W. Preregistration: Comparing dream to reality. 2019.
Schönbrodt FD, Wagenmakers E-J. Bayes factor design analysis: Planning for compelling evidence. Psychon Bull Rev. 2018;25(1):128–42.
Lakens D, Scheel AM, Isager PM. Equivalence testing for psychological research: A tutorial. Adv Methods Pract Psychol Sci. 2018;1(2):259–69.
Lakens D, McLatchie N, Isager PM, Scheel AM, Dienes Z. Improving inferences about null effects with Bayes factors and equivalence tests. J Gerontol Ser B. 2020;75(1):45–57.
Gopalakrishna G, ter Riet G, Vink G, Stoop I, Wicherts J, Bouter L. Prevalence of questionable research practices, research misconduct and their potential explanatory factors: a survey among academic researchers in The Netherlands. 2021.
Goldacre B, Drysdale, H., Powell-Smith, A., Dale, A., Milosevic, I., Slade, E., Hartley, H., Marston, C., Mahtani, K., Heneghan, C. The compare trials project. 2021. https://compare-trials.org . Accessed 23rd July 2020.
Mathieu S, Boutron I, Moher D, Altman DG, Ravaud P. Comparison of registered and published primary outcomes in randomized controlled trials. JAMA. 2009;302(9):977–84.
Rubin M. Does preregistration improve the credibility of research findings? arXiv preprint 2020.
Szollosi A, Kellen D, Navarro D, et al. Is preregistration worthwhile? Cell. 2019.
Quintana DS. A synthetic dataset primer for the biobehavioural sciences to promote reproducibility and hypothesis generation. Elife. 2020;9:e53275.
Weston SJ, Ritchie SJ, Rohrer JM, Przybylski AK. Recommendations for increasing the transparency of analysis of preexisting data sets. Adv Methods Pract Psychol Sci. 2019;2(3):214–27.
Thompson WH, Wright J, Bissett PG, Poldrack RA. Meta-research: dataset decay and the problem of sequential analyses on open datasets. Elife. 2020;9:e53498.
Download references
Acknowledgements
The authors are grateful to Professor George Davey for his helpful comments on this article.
J.R.B is funded by a Wellcome Trust Sir Henry Wellcome fellowship (grant 215917/Z/19/Z). J.B.P is a supported by the Medical Research Foundation 2018 Emerging Leaders 1 st Prize in Adolescent Mental Health (MRF-160–0002-ELP-PINGA). M.R.M and H.M.S work in a unit that receives funding from the University of Bristol and the UK Medical Research Council (MC_UU_00011/5, MC_UU_00011/7), and M.R.M is also supported by the National Institute for Health Research (NIHR) Biomedical Research Centre at the University Hospitals Bristol National Health Service Foundation Trust and the University of Bristol.
Author information
Authors and affiliations.
Department of Clinical, Educational and Health Psychology, Division of Psychology and Language Sciences, University College London, London, WC1H 0AP, UK
Jessie R. Baldwin, Jean-Baptiste Pingault & Tabea Schoeler
Social, Genetic and Developmental Psychiatry Centre, Institute of Psychiatry, Psychology and Neuroscience, King’s College London, London, UK
Jessie R. Baldwin & Jean-Baptiste Pingault
MRC Integrative Epidemiology Unit at the University of Bristol, Bristol Medical School, University of Bristol, Bristol, UK
Hannah M. Sallis & Marcus R. Munafò
School of Psychological Science, University of Bristol, Bristol, UK
Centre for Academic Mental Health, Population Health Sciences, University of Bristol, Bristol, UK
Hannah M. Sallis
NIHR Biomedical Research Centre, University Hospitals Bristol NHS Foundation Trust and University of Bristol, Bristol, UK
Marcus R. Munafò
You can also search for this author in PubMed Google Scholar
Contributions
JRB and MRM developed the idea for the article. The first draft of the manuscript was written by JRB, with support from MRM and all authors commented on previous versions of the manuscript. All authors read and approved the final manuscript.
Corresponding author
Correspondence to Jessie R. Baldwin .
Ethics declarations
Conflict of interest.
Author declares that they have no conflict of interest.
Additional information
Publisher's note.
Springer Nature remains neutral with regard to jurisdictional claims in published maps and institutional affiliations.
Rights and permissions
Open Access This article is licensed under a Creative Commons Attribution 4.0 International License, which permits use, sharing, adaptation, distribution and reproduction in any medium or format, as long as you give appropriate credit to the original author(s) and the source, provide a link to the Creative Commons licence, and indicate if changes were made. The images or other third party material in this article are included in the article's Creative Commons licence, unless indicated otherwise in a credit line to the material. If material is not included in the article's Creative Commons licence and your intended use is not permitted by statutory regulation or exceeds the permitted use, you will need to obtain permission directly from the copyright holder. To view a copy of this licence, visit http://creativecommons.org/licenses/by/4.0/ .
Reprints and permissions
About this article
Baldwin, J.R., Pingault, JB., Schoeler, T. et al. Protecting against researcher bias in secondary data analysis: challenges and potential solutions. Eur J Epidemiol 37 , 1–10 (2022). https://doi.org/10.1007/s10654-021-00839-0
Download citation
Received : 19 October 2021
Accepted : 28 December 2021
Published : 13 January 2022
Issue Date : January 2022
DOI : https://doi.org/10.1007/s10654-021-00839-0
Share this article
Anyone you share the following link with will be able to read this content:
Sorry, a shareable link is not currently available for this article.
Provided by the Springer Nature SharedIt content-sharing initiative
- Secondary data analysis
- Pre-registration
- Open science
- Researcher bias
Advertisement
- Find a journal
- Publish with us
- Track your research
- Open access
- Published: 05 June 2024
Single high-dose intravenous injection of Wharton’s jelly-derived mesenchymal stem cell exerts protective effects in a rat model of metabolic syndrome
- Alvin Man Lung Chan 1 , 5 ,
- Angela Min Hwei Ng 1 ,
- Mohd Heikal Mohd Yunus 2 ,
- Ruszymah Hj Idrus 1 ,
- Jia Xian Law 1 ,
- Muhammad Dain Yazid 1 ,
- Kok-Yong Chin 3 ,
- Mohd Rafizul Mohd Yusof 4 ,
- See Nguan Ng 5 ,
- Benson Koh 1 &
- Yogeswaran Lokanathan ORCID: orcid.org/0000-0002-9548-6490 1
Stem Cell Research & Therapy volume 15 , Article number: 160 ( 2024 ) Cite this article
Metrics details
Metabolic syndrome (MetS) is a significant epidemiological problem worldwide. It is a pre-morbid, chronic and low-grade inflammatory disorder that precedes many chronic diseases. Wharton’s jelly-derived mesenchymal stem cells (WJ-MSCs) could be used to treat MetS because they express high regenerative capacity, strong immunomodulatory properties and allogeneic biocompatibility. This study aims to investigate WJ-MSCs as a therapy against MetS in a rat model.
Twenty-four animals were fed with high-fat high-fructose (HFHF) diet ad libitum. After 16 weeks, the animals were randomised into treatment groups (n = 8/group) and received a single intravenous administration of vehicle, that is, 3 × 10 6 cells/kg or 10 × 10 6 cells/kg of WJ-MSCs. A healthy animal group (n = 6) fed with a normal diet received the same vehicle as the control (CTRL). All animals were periodically assessed (every 4 weeks) for physical measurements, serum biochemistry, glucose tolerance test, cardiovascular function test and whole-body composition. Post-euthanasia, organs were weighed and processed for histopathology. Serum was collected for C-reactive protein and inflammatory cytokine assay.
The results between HFHF-treated groups and healthy or HFHF-CTRL did not achieve statistical significance (α = 0.05). The effects of WJ-MSCs were masked by the manifestation of different disease subclusters and continuous supplementation of HFHF diet. Based on secondary analysis, WJ-MSCs had major implications in improving cardiopulmonary morbidities. The lungs, liver and heart show significantly better histopathology in the WJ-MSC-treated groups than in the untreated CTRL group. The cells produced a dose-dependent effect (high dose lasted until week 8) in preventing further metabolic decay in MetS animals.
Conclusions
The establishment of safety and therapeutic proof-of-concept encourages further studies by improving the current therapeutic model.
Metabolic syndrome (MetS) is a cluster of abnormal physiological conditions, often linked by genetic and environmental disruption. MetS is associated with many chronic diseases such as obesity, type 2 diabetes mellitus, cardiovascular diseases (CVDs), non-alcoholic fatty liver disease and even osteoporosis [ 1 , 2 , 3 , 4 , 5 ]. Operationally, MetS occurs when three of the five hallmarks, namely, increased body weight or abdominal circumference, hyperglycaemia (increased blood sugar levels), hypertension (high blood pressure), hypertriglyceridemia and dyslipidaemia (disproportionate cholesterol levels), are present [ 6 ]. Having these traits leads to increased mortality, morbidity and healthcare costs if left untreated [ 7 , 8 ]. Despite the availability of off-the-shelf pharmaceutics to manage conditions such as high cholesterol, hypertension or hyperglycaemia [ 9 , 10 , 11 , 12 ], they only offer temporary relief and do not address the root cause [ 13 , 14 ]. In response, major efforts have been taken in the field of regenerative medicine. Despite previous controversies, stem cell therapies have surged in the past decade. Thorough exploration and proper scientific communication have been delivered on its safety, which is a major hurdle for cell-based therapy [ 15 , 16 , 17 , 18 ].
This study aimed to establish the safety and efficacy of Wharton’s jelly-derived mesenchymal stem cell (WJ-MSC) for the treatment of MetS. Owing to potent MSCs, the previously discarded umbilical cord has been repurposed into a biological remedy [ 19 , 20 ]. Whilst bone marrow-derived MSCs (BM-MSCs) were thoroughly studied and said to be the gold standard for sourcing MSCs, the latest review has described WJ-MSCs to be equally competent, if not, superior [ 21 , 22 , 23 ]. MSCs from either source share a similar profile of high regenerative capacity and general immune privilege from allogeneic transplantation. As per the ISCT classification, the cells were also able to undergo trilineage differentiation (adipocyte, chondrocyte and osteoblast) when grown in a specific medium. Besides, MSCs participate in intercellular signalling via the secretion of active metabolites. These extracellular vesicles (canonically as ‘ exosomes ’) can stimulate regeneration in injured areas by modulating excess inflammation and supplementing growth factors [ 24 ]. An additional benefit of WJ-MSCs that remains debated is their homing and migratory properties [ 25 ]. The cells and their derivatives possess chemotactic proteins and/or receptors on their surface corresponding to the inflammatory signals or apoptotic bodies [ 26 , 27 ]. This feature enables migration into difficult-to-reach areas. Considering that MSCs and their paracrine effects influence multiple biological systems, they have the potential to alleviate the symptoms of MetS.
Following up on the safety study on WJ-MSCs, this paper aimed to establish the benefits of high or low doses of intravenously (IV)-administered WJ-MSCs versus no treatment for MetS in a similar animal model. The treatment was hypothesised to behave in a dose-dependent manner, where a higher dose could elicit a larger and prolonged effect. The results will be extrapolated and modelled into larger organisms for its intended human applications in the future.
Materials and methods
Culture of wj-mscs.
Umbilical cord was procured with consent from maternal volunteers undergoing elective Caesarean section at Universiti Kebangsaan Malaysia Medical Centre, Malaysia. The WJ-MSCs were pooled from three donors and qualified via characterisation, fibroblast-like cell morphology and attachment to plastic surfaces, expression of positive (≥ 95%; CD44, CD73, CD90 and CD105) and negative markers (≤ 3%; CD11b, CD19, CD34, CD45 and HLA-DR) by using a Human MSC Analysis Kit (BD Bioscience, USA) and trilineage differentiation using a manufacturer’s kit (Gibco, USA). The same source of cryopreserved cells was used in the safety study to ensure accurate representation of data for pre-clinical assessment of this cell therapy [ 28 ]. Briefly, the Passage 5 WJ-MSCs were thawed from cryo-storage and cultured into flasks the day before. After overnight culture, the attached cells were harvested, enumerated and prepared into syringes at 3 × 10 6 cells/kg [high-fat high-fructose (HFHF)-low dose (LD)] or 10 × 10 6 cells/kg body weight (BW, HFHF-high dose (HD)] for intravenous infusion.
Thirty male specific pathogen-free, 12-week-old Sprague Dawley rats were purchased from the Malaysia Institute of Pharmaceuticals & Nutraceuticals, National Institute of Biotechnology Malaysia (Penang, Malaysia). The animals were housed individually in closed ventilated cages via the Biobubble system (Allentown Inc., USA) located at the Centre for Tissue Engineering and Regenerative Medicine, Universiti Kebangsaan Malaysia. Additionally, the room was set at a temperature of 22 °C with a 12 h light-and-dark cycle. The rodents were given the standard lab chow (Altromin 1314; Lage, Germany) and autoclaved tap water ad libitum during the 2-week acclimatisation. The conduct and findings of the papers are reported in accordance to the ARRIVE Guidelines 2.0, ensuring rigorous and transparent reporting of our animal-based research.
Animal study plan
Induction of metabolic syndrome (from week − 16 to week 0).
After acclimatisation, the animals were randomly divided into two diet groups. The HFHF diet group (n = 24) was fed with high-fat pellet (Altromin C 1090–70; Lage, Germany) and 30% w/v of crystalline fructose (Aurora Industry Co. Ltd., China) dissolved in autoclaved tap water. The remaining animals (n = 6) were assigned to the normal diet (ND) group, which received standard lab chow (Gold Coin, Malaysia) and autoclaved tap water. Both diets were given ad libitum for 16 weeks. The success of MetS induction was determined by the presence of three out of the five MetS hallmarks; increased abdominal circumference, hypertension, hyperglycaemia, hypertriglyceridemia, and dyslipidaemia. Each of these physiological attributes was determined via measurable tests listed in Sect. 5.6.
Treatment of MetS with WJ-MSC (From Week 0 to Week 12)
After the animal model of MetS was established, the HFHF-diet animals were randomly assigned (n = 8 per group) to three treatment groups that received either 0.9% sodium chloride solution as blank or control (CTRL), 3 × 10 6 cells/kg BW as LD or 10 × 10 6 cells/kg BW as HD. The ND group received a blank solution. The treatment proceeded for 12 weeks as previously designed in the safety assessment study [ 28 ]. The animals were subjected to 12 weeks of periodic tests performed intermittently (every 4 weeks), which involved physical measurements, serum biochemistry, cardiovascular function test and whole-body composition analysis. Physical measurements and cardiovascular function tests were performed with minimal restraint. Blood collection via periorbital sinus and whole-body composition analysis required anaesthesia using 0.1 mL/kg BW of ketamine:xylazine (1:10) (K-X) cocktail (Troy Laboratories, Australia). Signs of morbidity and mortality were observed intermittently throughout the study. After 12 weeks, the animals were euthanised by chemical overdose via intraperitoneal (IP) administration of 2 mL pentobarbital (200 mg/mL, Vetoquinol, UK). Necropsy and histological staining of organs were performed thereafter.
Parameters for animal study
Signs of morbidity and mortality.
Physical observation of animals was performed intermittently, whenever possible. Their external and observable physiological states were adopted from ‘ Endpoint guidelines for animal use protocols ’ (2018), drafted by the University of Maryland (School of Medicine) and approved by the Institutional Animal Care and Use Committee (IACUC). Human endpoints were adopted and paraphrased from their protocol as described below:
Severe weight loss from anorexia and/or dehydration.
Dyspnoea (laboured breathing, hyperventilation and abdominal distension).
Prolonged hypothermia or hyperthermia (palpable temperature).
Stress and/or poor grooming (rough stained coat and porphyrin built around nose and eyes).
Lethargy, hunched posture and inability to rise or ambulate.
Poor reflex or irresponsiveness to external stimuli.
Tumour growth.
Physical measurement
The animals were lightly restrained using plastic decapicones without anaesthesia or sedatives. The BW, body length (nose to anus) and abdominal circumference (Abd. Circ.) were recorded. The body mass index (BMI) of the animals was calculated by the ratio of body weight to body length squared. Food intake was measured through the weight (g) of diet consumed per week. Water intake was calculated by volume (mL) consumed per week on the basis of the changed weight (g) of the bottled water. Physical measurements were taken weekly but presented in averages of study periods: weeks 0, 4, 8 and 12.
Blood serum analysis
Similarly, the animals were bled at weeks 0, 4, 8 and 12 via the tail vein after IP anaesthesia of the K-X cocktail. Blood samples were collected using clot-activator tubes (BD Biosciences, USA) and allowed to clot at room temperature for 15 min. Serum was collected via centrifugation at 3000 rcf for 10 min and subsequently stored in the freezer (− 20 °C) until needed. Serum biochemistry was conducted in the Haematology Laboratory located at the Veterinary Laboratory Service Unit, Universiti Putra Malaysia, Malaysia, to measure the concentration of aspartate aminotransferase (AST), alanine aminotransferase (ALT), creatinine (CREAT), cholesterol (CHOL), high-density lipoprotein (HDL), low-density lipoprotein (LDL), triglyceride (TGL) and serum glucose (GLUC).
Oral glucose tolerance test (OGTT)
Before the day of the experiment, the animals were fasted overnight with ad libitum access to autoclaved tap water only. On the following day, the fasting blood glucose (FBG) levels were measured using an ACCU-Check Performa glucometer (Roche Diagnostic, USA). After baseline measurement, the animals were given 20% dextrose solution (2 µL/g BW) via oral gavage. Afterwards, they were bled at intervals of 30, 60 and 120 min. In addition to weekly comparison of the different treatment groups, the area under the curve (AUC) was calculated and plotted as a summary of GLUC levels at different study periods.
Cardiovascular function test
Diastolic blood pressure (DBP), systolic blood pressure (SBP), mean arterial pressure (MAP), blood flow and volume and heart rate were measured using a non-invasive tail-cuff system (CODA, Kent Scientific Corporation, USA). The animals were restrained using plastic decapicones without anaesthesia and briefly warmed on a far-infrared warming platform (Kent Scientific Corporation, USA) before measurements were taken. TCODA software was programmed to measure five acclimatisation cycles and 10 experimental cycles, with each cycle spaced between 30 s to allow recovery of blood flow. Data were exported into Microsoft Excel 2019 MSO 64-bit (Microsoft Corp., USA) for further analysis.
Whole-body composition
The animals were anesthetised and laid in an anatomically prone position with their head, tail and limbs straightened outwards. The fat mass, lean mass, percentage of body fat, bone mineral content (BMC) and bone mineral density (BMD) were measured using Small Animal Analysis Software in a dual-energy X-ray absorptiometry machine (Hologic QDR-1000 System, Hologic Inc., USA). The short-term in-vivo coefficient of variation for whole-body BMD was 1.2% for this machine [ 29 ].
During the end of study at week 12, the animals were anaesthetised using the K-X cocktail and then euthanised via chemical overdose using 2 mL of pentobarbital sodium. When the animals were determined to be no longer conscious via toe-pinch response method, blood was drained from the animals’ carcass via cardiac puncture. Blood serum was collected and stored for supplementary experiments, excluding the final serum biochemistry test. The animals were dissected at the abdomen to reveal its subcutaneous adipose layer. Fat was collected via scalpel shaving. Then, the animals were dissected to reveal the thoracic and abdominal cavity. All the organs, including the heart, lung, liver, spleen, kidneys, pancreas and gut (large intestine); the bone (right femur); and the visceral fat were excised. Images were taken at all stages and during the harvesting of organs. The mass of the organs was recorded immediately using a weighing scale. The relative weight of the organs was calculated as below:
Histological staining of tissue sections
The harvested lung, liver, spleen and kidneys were preserved in a 4% paraformaldehyde solution before being embedded in paraffin. Sections were cut using a microtome (Leica Biosystems, Wetzlar, Germany), deparaffined with xylene and stained with haematoxylin and eosin (H&E). The stained section was observed under a light microscope by a blinded histopathologist to check for microanatomical conditions of the organs. The scoring was described as healthy or inflamed (minor, moderate or severe).
The serum concentration of C-reactive protein (CRP) was analysed using an ELISA kit purchased from Raybiotech (Georgia, USA). Serum dilutions and the assay procedure was performed in accordance with the manufacturer’s protocol.
Multiplex ELISA cytokine array
The Quantibody rat inflammation array was purchased from Raybiotech (Georgia, USA), and 96-well plates were designed to detect 10 inflammatory-associated cytokines: interferon-gamma (IFN-γ), interleukins (IL-10, IL-13, IL-1α, IL-1β, IL-2, IL-4 and IL-6), monocyte chemoattractant protein-1 (MCP-1) and tumour necrosis factor-alpha (TNF-α). Serum dilutions and the assay procedure were performed following the manufacturer’s protocol.
Statistical analysis
All sample sizes were determined by Mead’s resource equation. All statistical analysis was performed using GraphPad software (GraphPad Prism version 8.4.3, California, USA). The quantitative data or graphical results were presented as mean ± standard deviation (SD). Comparisons between the four treatment groups for physical measurement, serum biochemistry, cardiovascular function and whole-body composition were conducted through mixed-design ANOVA with Geisserg–Greenhouse correction. Time-fixed inter-group analysis was calculated via Holm Sidak’s post-hoc test ( P < 0.05). End-point comparisons, such as the relative weight of the organs, were performed using one-way ANOVA followed by Holm Sidak’s post-hoc test ( P < 0.05). Statistical significance was set at P < 0.05.
HFHF diet successfully induced MetS in animal model
After 16 weeks of the diet regime, the animals successfully achieved at least three of the five MetS hallmarks. The shared characteristics amongst all the treated animals (n = 24) were hypertension (increased SBP, DBP or MAP), hyperglycaemia (increased serum GLUC) and dyslipidaemia (reduced HDL cholesterol). All animals were screened individually and compared with the healthy CTRL animals (Appendix 1). Five animals achieved the minimum score of 3, whereas most animals achieved a higher score of 4 (n = 10) or 5 (n = 9). Furthermore, the animals showed signs of MetS-related stress, as shown in Table 1 . All HFHF animals had significant dyspnoea and secretion of porphyrin, indicating physical and metabolic stress. Some animals experienced severe weight loss, low food consumption, lethargy and poor response to stimuli.
WJ-MSC was unable to resolve MetS disorders in animal model
The results were consistent for the majority of the 26 observed parameters, proving that the animal model was successfully induced after 16 weeks. For the remainder of the study, the trend of results between the different doses of the WJ-MSC groups did not deviate from one another but remained significantly different ( P < 0.05) from the ND-CTRL. This finding was especially true for physical measurement, cardiovascular function tests and whole-body compositions, as shown in Fig. 1 .
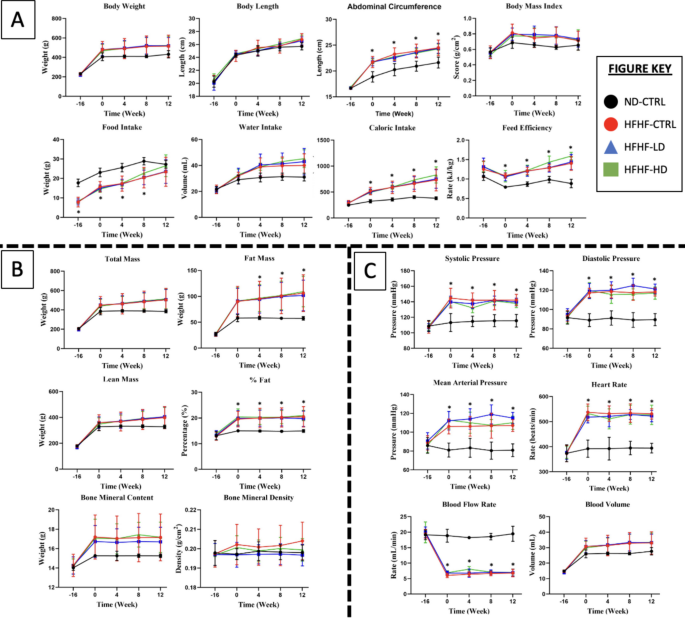
A Physical measurement, B whole-body composition and C cardiovascular function tests performed at weeks − 16, 0, 4, 8 and 12 for treatment (n = 8) and control (CTRL) animals (n = 6) in HFHF diet or ND diet groups. Symbols: (#) indicates that the HFHF group’s data are statistically significantly different ( P < 0.05) compared with those of the ND-CTRL group
In Fig. 2 , the serum biochemistry result showed no discernible pattern between receiving the diet or WJ-MSC treatment. The OGTT amongst the HFHF-diet groups did not differ, and no explicit changes were observed even after treatment with WJ-MSC or the vehicle solution. At week 0, the insulin response for the three HFHF diet groups to glucose bolus was delayed until 60 min compared with that for the ND-CTRL group, which reduced after 30 min. During week 4, the standard deviation values drastically varied for LD and HD WJ-MSC groups, suggesting that several animals benefited from receiving WJ-MSCs. By weeks 8 and 12, the insulin response improved in all HFHF diet groups because the glucose concentration reduced after 30 min. The calculated AUC presented a large standard deviation for all HFHF diet groups before treatment and later stabilised at week 8. By week 12, the deteriorated condition returned because the treated animals had statistically significant differences ( P < 0.05) with the ND-CTRL group.
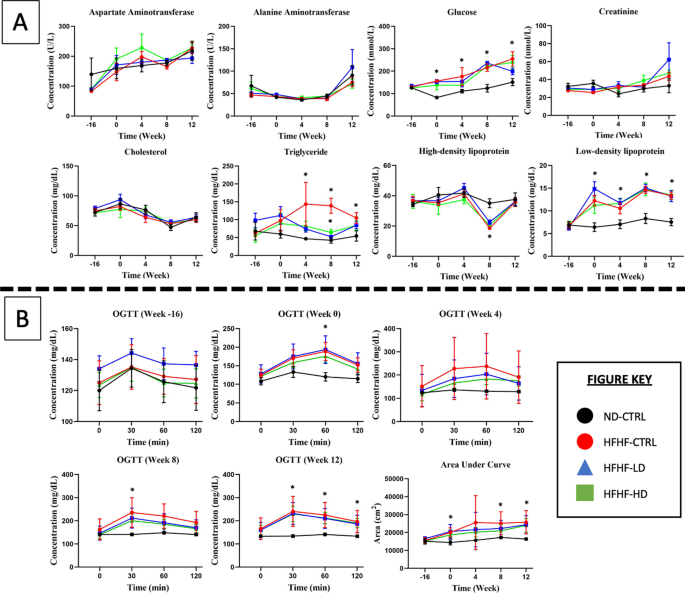
A Blood serum biochemistry and B oral glucose tolerance test performed at weeks − 16, 0, 4, 8 and 12 for the treatment (n = 8) and control (CTRL, n = 6) animals in HFHF diet or ND diet groups. Symbols: (#) indicates that the HFHF group’s data are statistically significantly different ( P < 0.05) compared with those of the ND-CTRL group
Subcluster analysis revealed the masked effects of WJ-MSC
As shown in Table 2 , comparison of the individual results of the treated animals (n = 6 – 8/group) with those of the HFHF-CTRL group (n = 8, Appendix 1) revealed the suspected masked effects of WJ-MSCs. Prior to these results, the largest MetS effect was increased hemodynamic indices (SBP, DBP and MAP). Subsequently, the number of animals in the HFHF-HD group that showed improvement in SBP, DBP and MAP results at week 4 were 6/8 ( P < 0.01), 3/8 ( P < 0.05) and 1/8 ( P < 0.05), respectively. At week 8, SBP and DBP were found to be reduced ( P < 0.05) in 1/8 and 3/8 of the animals only. By the end of week 12, SBP and DBP decreased ( P < 0.05) in 2/8 of the affected animals. The HFHF-LD group had a similar progression to the HFHF-HD group but only showed decreased ( P < 0.05) SBP and DBP at week 4 in 2/8 and 1/8 of MetS animals, respectively, and at week 8 in 1/8 and 3/8, respectively. Only SBP was corrected for 1/8 of the animals until week 12.
The categorised parameters for obesity, such as increased body weight and fat mass, were corrected in 1/6 of the affected animals for HFHF-LD and HFHF-HD groups during week 4 but did not remain thereafter. Diabetic-related parameters had notable improvements. The severe weight loss was resolved ( P < 0.05) in animals from the HFHF-LD (1/1) and HFHF-HD groups (1/2). Only the effects from a larger dose of WJ-MSCs prevented weight loss until week 8. Alternatively, the lean mass of the HFHF-HD group was higher ( P < 0.05) than that of the HFHF-CTRL group in weeks 4 and 8. Despite this finding, the primary indicator of diabetes is hyperglycaemia. According to the results, only 1/8 of the HFHF-HD animals had an improved glycaemic index ( P < 0.05) at week 8. Oddly, no prior recovery was seen for this during week 4. A similar result was found from OGTT (AUC), where 1/8 of the HFHF-LD animals recovered between weeks 4 and 12. Moreover, 1/8, 2/8 and 1/8 of the HFHF-HD animals overcame hyperglycaemia ( P < 0.05) during weeks 4, 8 and 12, respectively. Lastly, the BMC and BMD scores in the HFHF-HD group increased only in ½ and 1/4, respectively. Only the BMD score was sustained until week 8, extending the recovery of ½ of the affected animals.
Post-mortem analysis of histopathology showed the protective effect of WJ-MSCs
As shown in Fig. 3 , the necropsy revealed significant deposition of fat between the subcutaneous and muscular layers in the abdomen. However, the relative weight of the organs was statistically significant ( P < 0.05) for abdominal fat and liver only. Despite the hypertrophied heart, the relative weight was not statistically different as compensated by the increased body weight of the affected animals.
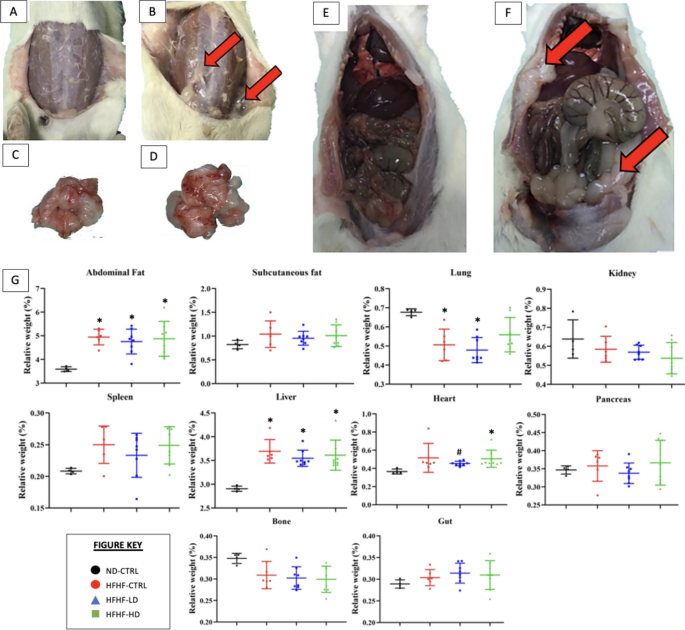
A – F necropsy and G relative weight of organs (n = 6–8) performed at the end of study (week 12). Images A, C and E represent the control (CTRL) healthy animals. Images B, D and F represent the typical MetS animals after induction of MetS via HFHF diet. Red arrows symbolise the deposition of fats on or around the organ. Symbols: (*) indicates that the HFHF group’s data are statistically significantly different ( P < 0.05) compared with those of the ND-CTRL group
In Fig. 4 , the liver presented clinically significant differences as a gradient from healthy to severe inflammation in the ND-CTRL, HFHF-HD, HFHF-LD and HFHF-CTRL groups. Signs of liver cirrhosis and fatty liver were identified by the degree of ‘ yellow ’ complexion from fatty deposits and the physically defined lobes as enlarged or round. The hearts of the HFHF animals were hypertrophied, as indicated by the enlarged and round ventricles. The lungs of HFHF-CTRL and HFHF-LD animals were pale, with mild inflammation, minor lesions and pulmonary blebs (white spotting). Conversely, the lungs of the HFHF-HD group had brighter red complexion with hints of minor inflammation (potential recovery) that were comparably similar to those of the healthy animals. All the lungs were unperforated, as determined by the ability to stay afloat in a saline-filled glass beaker (data not available). The kidneys and spleens of the HFHF groups appeared to be discoloured or pale compared with those of the ND-CTRL group. No differences were observed for the bone, gut and pancreas of the animals.
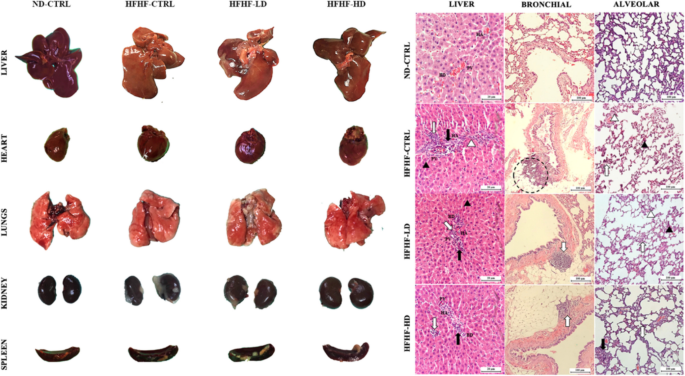
Physical observation of the harvested organs that include liver, heart, lungs, kidneys and spleen (chronological order). H&E-stained sections of the liver and lung (bronchial and alveolar) were captured at 10× and 40× magnifications with their respective scale bars (20 or 100 µm). The size and dimensions of the cropped images of the organs are not accurate representatives
The H&E images of the HFHF-CTRL group’s livers revealed major cellular hyperplasia in the surrounding parenchyma (hepatocyte cells) near the portal triads. The expanded cells constrict the interstitial spaces (sinusoidal spaces), leading to the loss or displacement of local Kupffer cells. The portal triad unit showed severe infiltration of leukocytes, necrosis and developed fibrous septum. Thus, the structures were disfigured, causing notable subunits, such as the bile duct, hepatic artery and portal vein, to be unidentifiable from severe vascular congestions. The HFHF-LD group showed similar but milder inflammation in the liver. Conversely, the HFHF-HD group preserved its microanatomical structures, with a minor leukocyte infiltration. However, the recovery may be transient or limited because a mass of leukocytes remained visible. The bronchial and alveolar sections of the lungs from the HFHF-CTRL group presented significant tissue necrosis and enhanced inflammatory status akin to the liver. A visible rupture of the bronchial wall can be observed, and it was likely a product of chronic accumulation of inflammatory damage. Meanwhile, diffused alveolar damage was manifested from the pronounced interstitial lymphocytic infiltrate into the alveolar septa. The same histopathology was seen in the lungs of the HFHF-LD group, but physical structures were more discernible. Lastly, the lungs of the HFHF-HD group were scored nearer to those of the ND-CTRL group than either the HFHF-CTRL or LD groups. No leukocyte invasion into the endothelial sections was found. However, the alveolar sacs were noticeably larger than in the other groups, which may signify the onset of respiratory stress from cardiovascular complications. No abnormalities were identified in the spleen or kidneys despite the paleness or discoloration mentioned above (Appendix 3).
Immunomodulatory benefits of WJ-MSCs followed subcluster and histopathology results
In addition to serum biochemistry, serum inflammation-associated proteins and cytokines were analysed to study the immunomodulatory properties of WJ-MSCs. The HFHF-CTRL group expressed the most significant changes at week 12 compared with those at week 0, which were seen through IL-1α (3.3-fold), IL-1β (0.63-fold), IL-4 (0.47-fold) and MCP-1 (1.9-fold). The HFHF-LD group showed briefly increased IL-4 (0.63-fold) and MCP-1 (1.5-fold) expression levels after 12 weeks. The HFHF-HD group results were significant for IL-1β (0.61-fold) only. Lastly, the ND-CTRL group had no significant changes for most parameters but showed decreased IL-1a (0.47-fold) and IL-2 (0.41-fold) expression levels compared with week 0. IL-1β, IL-2 and IL-4 had no identifiable trend in all groups. Throughout the study, the pro-inflammatory cytokines were highly expressed in the HFHF groups versus the ND group. No significant alterations were seen after the administration of WJ-MSCs.
The serum CRP produced the same inconsistencies with the serum biochemistry from Fig. 3 in “ Animals ” section. During week 0, all HFHF groups had significantly increased ( P < 0.05) CRP levels compared with the ND-CTRL group. By week 4, the animals infused with a higher dose of WJ-MSCs showed a major reduction ( P < 0.05, intra-group comparison). The groups that received LD or vehicle solution did not change for the rest of the study. At week 8, the reduced CRP levels of the HFHF-HD were not reproduced with the same magnitude as they had during weeks 0 − 4 but remained similar to the healthy animal group. By the end of the study, the CRP concentration increased, matching those of the other HFHF groups.
Based on the results presented above, the overall data showed that no significant changes occurred after treatment of WJ-MSC. An unexpected consequence was the manifestation of different subclusters of MetS. Different combinations of MetS hallmarks occurred from the diet, mirroring the diverse pathways of MetS to develop any of the metabolic-associated diseases mentioned before, such as the obesity subpopulation inferred by the increased body weight and lipid markers in this study (Appendix 1). A significant number of animals (n = 19) expectedly demonstrated an increase in body weight (highest at 600.6 g), whereas few (n = 5) experienced severe weight loss (lowest at 332.4 g) paired with visible signs of morbidity such as heavy perspiration, fatigue, accumulated porphyrin and decreased food and water intake. The underweight animals were below the weight range of the healthy untreated animals. This grouped data resulted in no statistically significant differences because the ND group (n = 6) and HFHF group (n = 24) had body weights of 410.65 ± 35.27 and 475 ± 66.07 g, respectively. Biased data between these subpopulations became increasingly distinct as the study progressed. The underweight animals co-expressed highest GLUC reading (11.0 mmol/L or 198 mg/dL), whereas the obesogenic animals had higher lipid-associated markers. Based on the symptoms presented, the smaller group were hypothesised to be pre-diabetic likely through acquired insulin resistance [ 30 , 31 , 32 , 33 , 34 , 35 ].
Previous studies have encountered different subclusters of MetS specifying their methodology and controlled conditions [ 36 , 37 , 38 ]. For example, Rozendaal et al. (2018) mapped the disease progression of MetS through in-vivo and in-silico models [ 39 ]. Although successful, the authors expressed concerns over their dynamic study model producing the unexpected outcome of two MetS phenotypes. In retrospect, this study’s animal model may have been selective towards obesity-focused MetS by excluding other subclusters. However, the fulfilment of the minimal criteria for MetS in other animals does not justify excluding potentially valuable data. Moreover, the inclusion of different metabolic diseases, obesity, diabetes, CVDs or MAFLD could benefit the scope of this study. However, the manifestation of polarising data in the same group was unprecedented. Besides the weight of the animals, several other parameters responded differently to HFHF diet, potentially negating the effects of infused WJ-MSCs. At least ≥ 10 of the 26 test parameters had mixed response (higher or lower) compared with the reference data from the healthy animals. For example, the BMC and BMD values may have been overestimated due to the obesity paradox. Increased body weight exerts a mechanical load that drives the increase in BMC and BMD in rats. However, adiposity contributes to increased chronic inflammation (e.g. SOD or MDA), which may cause bone deterioration [ 38 , 39 ]. The animals data were individually analysed versus the reference data from the untreated (HFHF-CTRL) group to circumvent this issue, corresponding to the same study period (Appendix 2). Based on the evidence presented in Sect. 2.2, WJ-MSCs were unable to treat MetS as a conventional means of a cure. In Figs. 1 and 2 , WJ-MSCs may have attenuated the effects of MetS. However, not all the animals responded similarly to the treatment.
In Sect. 2.3, WJ-MSCs were hypothesised to be responsible for delaying the metabolic derangement of MetS animals. The masked results of WJ-MSCs were obtained after each animal’s data (n = 8/group) were screened for 26 parameters during each study period (weeks 0, 4, 8 and 12; Table 2 ). WJ-MSCs positively affected the increased hemodynamic indices. This finding is consistent with many successful MSC applications for cardiopulmonary complications [ 42 , 43 , 44 , 45 , 46 ]. For example, Alencar et al. (2018) outlined the benefits of MSCs in improving the symptoms of pulmonary arterial hypertension [ 47 ]. The treatment enabled cardiac cell repolarisation, preventing further cell apoptosis and fibrosis. It also reduced muscle stiffness and the rigidity of vascular endothelial walls, restoring normal blood circulation. Whilst the mortality of diabetic animals was undetermined, major improvements were noted in the animal’s health (signs of morbidity) through returned weight and normal behaviour. The physical wellbeing of the animals did not deteriorate until weeks 4 and 8 for the LD and HD WJ-MSC groups, respectively, implying the importance of dose selection.
The necropsy and subsequent histological staining of organs were consistent with the subgroup analysis above. The liver, lungs, and heart had enhanced pathological outcome, as shown by the reduced inflammation and hypertrophy, following the administration of HD WJ-MSCs, similar to previous studies [ 48 , 49 , 50 ]. Given that the HFHF diet was given ad libitum until the end of the study, the effects of WJ-MSCs may have been reduced or negated. During MetS induction, only seven (29.1%) and five (20.1%) of the 24 HFHF diet-assigned animals had increased AST and ALT, respectively (Appendix 1). Even after WJ-MSC treatment, the levels remained unchanged. These findings were justified in previous literature that inferred that liver degeneration could be masked by ‘ normal ’ liver biomarkers [ 33 , 47 ]. In the present study, the authors speculated that the unchanged liver biomarkers were a byproduct of the continued HFHF diet. However, the histological images of the organs in the HFHF-HD group were less inflamed and structurally similar to those of the healthy animals and superior to those of the untreated and LD groups.
A major characteristic of MetS is persistent and damaging inflammation [ 51 , 52 ]. Hence, addressing the inflammation could supersede the relevancy of all other variables of MetS measured thus far. Based on Fig. 5 , the CRP levels at weeks 4 and 8 significantly reduced ( P < 0.05) compared with those at week 0 for the HFHF-HD group but not for the HFHF-LD nor HFHF-CTRL group. Oddly, the CRP of the HFHF-CTRL group drastically reduced, deviating from the expectations. Although CRP is known to increase inflammation, chronic developments, such as liver dysfunction or specific diseases (e.g. rheumatoid arthritis or lupus), could manifest normal-to-low serum levels [ 53 , 54 , 55 ]. Similar to serum biochemistry in Fig. 2 A, many inconsistencies within the inflammation array may be attributed to the limited samples and serum conditions. On the basis of the manufacturer’s instructions, a fold-change (cytokine concentration based on log–log regression standard curves) to stabilise the data with extreme variances was applied [ 56 ]. The inflammatory cytokine results between HFHF-LD and HFHF-HD had no significant changes after treatment, reaffirming the lack of WJ-MSC regenerative effect or overwhelming persistence of MetS inflammation.
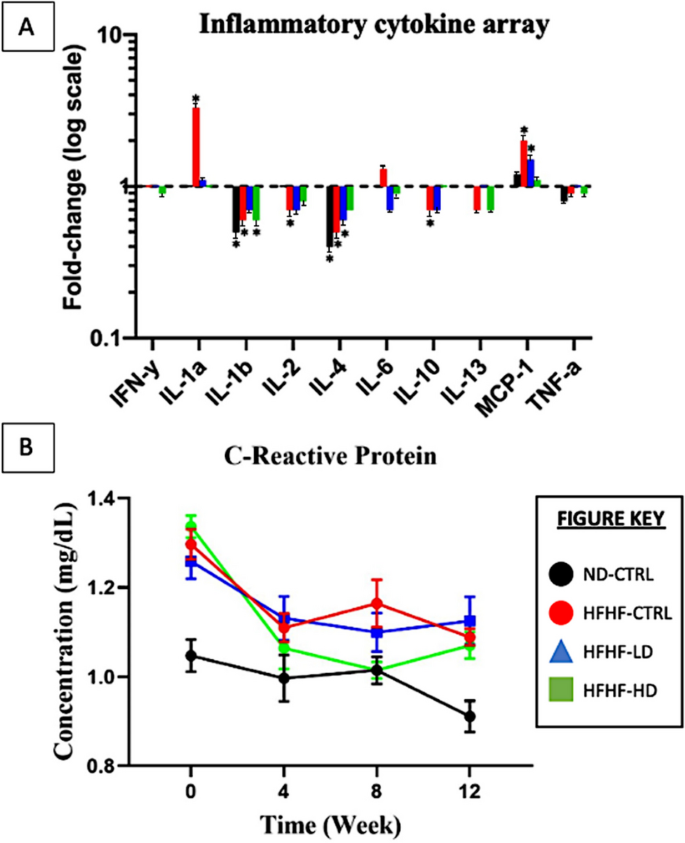
A Pro-inflammatory cytokines (IFN-γ, IL-1α, IL-1β, IL-2, IL-6, MCP-1 and TNF-α) and anti-inflammatory cytokines (IL-4, IL-10 and IL-13) expressed as fold-change (logarithmic scale) compared between the results (n = 4/group) from weeks 0 and 12. B C-reactive protein (n = 6) measured at weeks 0, 4, 8 and 12 for all animal groups. Symbol (*) indicates statistically significant change at ≥ 1.5-fold increase or ≥ 0.65-fold decrease
Concerningly, all serum-associated parameters performed through a standard statistical analysis were difficult to interpret. Although it is a major component of toxicological studies, the validity of serum analysis is often hindered by insufficient volume for replication, sample haemolysis, batch-to-batch variation, contamination and more [ 57 , 58 , 59 ]. Due to movement restrictions during the recent pandemic of 2021, the HFHF diet was forcibly extended from 12 to 16 weeks. This extension strengthened the characteristics of MetS and considerably eased the identification of subclusters [ 60 ]. However, whether the delayed treatment damaged the salvageability of the metabolic health in these animals was contemplated. The WJ-MSCs could have more explicit effects at sub-chronic periods of MetS as originally intended at 12 weeks. The continuous deterioration of MetS symptoms via HFHF diet supplementation has been a universal theme in this study, proving that the antagonists of poor dietary choices and sedentary lifestyles must be equally considered [ 38 ]. Nutritional studies have successfully demonstrated that dietary correction can reverse MetS symptoms [ 61 , 62 , 63 , 64 ]. The same was determined for physical exercises [ 65 , 66 , 67 , 68 ]. However, the removal of the HFHF diet or the introduction of any physical stimulus could conflict with the research objective of determining the potency of the cell therapy. Another potential modification is to implement multiple doses of the stem cells. Most studies have adopted multiple doses at a fixed period or intermittent intervals [ 67 , 69 , 70 ]. The reason for repeatedly introducing stem cells into patients is to supplement a continuous reparative effect. Based on a previous systematic review on the biodistribution of MSCs, the cells can survive for up to 72 h in-vivo, and the effects persisted for a maximum of 14 days [ 71 ]. This study’s method of systemic delivery may have also suffered from poor efficacy due to the accumulated loss of cells via lysis, impenetrability of the membrane, misroute or excretion [ 72 , 73 , 74 ]. However, the availability of systemic administration could benefit from difficult-to-reach areas [ 75 ].
In the context of cell passage, passages 1 − 4 are conventionally used to preserve MSC nascent qualities and prevent accumulation of senescent or genetic variation from long-term culture. However, previous evidence cited the safety and efficacy of cells from passages ≥ 5 and in similar Sprague Dawley models [ 76 , 77 , 78 ]. Wang et al. (2021) implied that the differences between passages 4 and 6 were not as significant as those amongst longer passages 8, 10 and 12, thus justifying the preferences of this study’s design [ 79 ]. Furthermore, the usage of cells from later passages for the experimental studies enabled the production of a sufficient number of cells to ensure the same batches of cells were used from the in-vitro characterisation phase to the safety and efficacy studies in the animal model study phase.
Another valid argument is the obstruction of transplanted human WJ-MSCs in the animal model. The authors foresaw that the effects of the cell therapy could be limited by unknown xenogeneic components [ 80 , 81 , 82 , 83 ]. An accurate allogeneic transplant (e.g. rodent-derived cells into animal model or human-derived cells into patients) may have produced different efficacy outcomes. However, the importance of testing medicinal products in pre-clinical models is majorly driven by safety [ 84 ]. Regardless of animal derivative studies, the succeeding phase (current study) for utilising human-derived cells must be tested using pre-clinical models before humans. Based on the previous and current studies, neither the healthy nor diseased animals responded adversely to the administered WJ-MSCs. The prospects for positive outcomes or recovery by stem cell therapy is promising.
This study has limitations. Firstly, one of the several issues that occurred was the insufficient serum volume from the blood, accompanied by minor-to-significant haemolysis. Due to the small size of the animals, blood was difficult to acquire, and therefore, the results were not fully representative of their metabolic health and inflammatory status. Secondly, the type of MetS animal models generated from diet-induced methods must be wholly considered early or prior into the study. The subcluster analysis was only a temporary solution. Thirdly, a single dose of MSC for 3 months could not elicit a significant reaction in the diseased animal model, much less in 2 weeks [ 71 ]. Lastly, the histological analyses, which had significant and relevant findings but were limited by the study’s resources, should have been further expanded. A suggestion for future studies is incorporating immunohistochemistry because it could provide valuable information on the treatment’s efficacy.
For future consideration, the authors proposed to include more than one dose at fixed intervals to explore the continuous effects of WJ-MSCs. A previous systematic review highlighted many recent studies that adopted multiple doses without any adverse outcomes [ 70 ]. Moreover, the heterogeneity of MetS is too diverse and complex to be compressed into a single study [ 38 ]. However, it may be compensated by adopting a larger sample size or additional inclusion and exclusion criteria to define the type of MetS studied [ 85 ]. Furthermore, recent efforts to study the functional, small extracellular vesicles from MSCs have proposed better clinical outcomes than cell therapy for future applications [ 24 ]. Consequently, MSCs may be better as support for other medications because they are well-positioned to stimulate regeneration and regulate inflammation simultaneously.
The WJ-MSCs were not able to correct the symptoms of MetS. However, further analysis showed that the continuous worsening state of the animals and subcluster profiles may have overwhelmed the effects of WJ-MSCs. Based on the new evidence presented, the WJ-MSCs exert a protective effect seen through delayed metabolic deterioration in vivo. The treatment effects were observed to be in a dose-dependent manner. The organs (lungs and liver) showed obvious preservation from receiving WJ-MSCs. The major MetS subclusters of cardiovascular impairments showed early improvements at week 4, which were sustained through week 8 through receiving a higher dose of the cells. Incidentally, CVD is the largest MetS comorbidity and the highest global cause of death by noncommunicable diseases. All the recovery made possible through WJ-MSC therapy was collectively reversed by week 12. Thus, this single infusion of WJ-MSCs was able to exert its regenerative and immunomodulatory effects for up to a maximum of 8 weeks. By securing the in-vivo safety in healthy and diseased animals, multiple doses of WJ-MSCs or co-therapy with pharmaceutical interventions, dietary changes, exercise regimes, and more are suggested for future exploration. Furthermore, the selection of specific MetS subclusters versus the whole MetS must be considered to validate any therapeutic efficacies in the future.
Availability of data and materials
Data will be made available upon reasonable request.
Abbreviations
Bone mineral content
Bone mineral density
C-reactive protein
Diastolic blood pressure
High-fat high-fructose
Mean arterial pressure
Monocyte chemoattractant protein-1
- Metabolic syndrome
Normal diet
Oral glucose tolerance test
Systolic blood pressure
Wharton’s jelly-derived mesenchymal stem cell
Moreira GC, Cipullo JP, Ciorlia LA, Cesarino CB, Vilela-Martin JF. Prevalence of metabolic syndrome: association with risk factors and cardiovascular complications in an urban population. PLoS ONE. 2014;9(9): e105056. https://doi.org/10.1371/journal.pone.0105056 .
Article CAS PubMed PubMed Central Google Scholar
Uzunlulu M, Telci Caklili O, Oguz A. Association between metabolic syndrome and cancer. Ann Nutr Metab. 2016;68(3):173–9. https://doi.org/10.1159/000443743 .
Article CAS PubMed Google Scholar
Tune JD, Goodwill AG, Sassoon DJ, Mather KJ. Cardiovascular consequences of metabolic syndrome. Transl Res. 2017;183:57–70. https://doi.org/10.1016/j.trsl.2017.01.001 .
Chin KY, Wong SK, Ekeuku SO, Pang KL. Relationship between metabolic syndrome and bone health - an evaluation of epidemiological studies and mechanisms involved. Diabetes Metab Syndr Obes. 2020;13(13):3667–90. https://doi.org/10.2147/DMSO.S275560 .
Article PubMed PubMed Central Google Scholar
Regufe VMG, Pinto CMCB, Perez PMVHC. Metabolic syndrome in type 2 diabetic patients: a review of current evidence. Porto Biomed J. 2020;5(6):e101. https://doi.org/10.1097/j.pbj.0000000000000101 .
Pammer LM, Lamina C, Schultheiss UT, Kotsis F, Kollerits B, Stockmann H, Lipovsek J, Meiselbach H, Busch M, Eckardt KU, Kronenberg F; GCKD Investigators (2021) Association of the metabolic syndrome with mortality and major adverse cardiac events: a large chronic kidney disease cohort. J Intern Med. 290(6):1219–1232. https://doi.org/10.1111/joim.13355 .
Alberti KG, Eckel RH, Grundy SM, Zimmet PZ, Cleeman JI, Donato KA, Fruchart JC, James WP, Loria CM, Smith SC Jr; International Diabetes Federation Task Force on Epidemiology and Prevention; Hational Heart, Lung, and Blood Institute; American Heart Association; World Heart Federation; International Atherosclerosis Society; International Association for the Study of Obesity (2009) Harmonizing the metabolic syndrome: a joint interim statement of the International Diabetes Federation Task Force on Epidemiology and Prevention; National Heart, Lung, and Blood Institute; American Heart Association; World Heart Federation; International Atherosclerosis Society; and International Association for the Study of Obesity. Circulation 120(16):1640–1645. https://doi.org/10.1161/CIRCULATIONAHA.109.192644 .
Tang X, Wu M, Wu S, Tian Y. Continuous metabolic syndrome severity score and the risk of CVD and all-cause mortality. Eur J Clin Invest. 2022;52(9): e13817. https://doi.org/10.1111/eci.13817 .
Article PubMed Google Scholar
Osadebe PO, Odoh EU, Uzor PF. Natural products as potential sources of antidiabetic drugs. JPRI. 2014;4(17):2075–9. https://doi.org/10.9734/BJPR/2014/8382 .
Article Google Scholar
Yanovski SZ, Yanovski JA. Long-term drug treatment for obesity: a systematic and clinical review. JAMA. 2014;311(1):74–86. https://doi.org/10.1001/jama.2013.281361 .
Cohen JB, Gadde KM. Weight Loss Medications in the Treatment of Obesity and Hypertension. Curr Hypertens Rep. 2019;21(2):16. https://doi.org/10.1007/s11906-019-0915-1 .
Yanovski SZ, Yanovski JA. Progress in pharmacotherapy for obesity. JAMA. 2021;326(2):129–30. https://doi.org/10.1001/jama.2021.9486 .
Müller TD, Clemmensen C, Finan B, DiMarchi RD, Tschöp MH. Anti-obesity therapy: from rainbow pills to polyagonists. Pharmacol Rev. 2018;70(4):712–46. https://doi.org/10.1124/pr.117.014803 .
Müller TD, Blüher M, Tschöp MH, DiMarchi RD. Anti-obesity drug discovery: advances and challenges. Nat Rev Drug Discov. 2022;21(3):201–23. https://doi.org/10.1038/s41573-021-00337-8 .
Kabat M, Bobkov I, Kumar S, Grumet M. Trends in mesenchymal stem cell clinical trials 2004–2018: Is efficacy optimal in a narrow dose range? Stem Cells Transl Med. 2020;9(1):17–27. https://doi.org/10.1002/sctm.19-0202 .
Planat-Benard V, Varin A, Casteilla L. MSCs and inflammatory cells crosstalk in regenerative medicine: concerted actions for optimized resolution driven by energy metabolism. Front Immunol. 2021;30(12): 626755. https://doi.org/10.3389/fimmu.2021.626755 .
Article CAS Google Scholar
Rodríguez-Fuentes DE, Fernández-Garza LE, Samia-Meza JA, Barrera-Barrera SA, Caplan AI, Barrera-Saldaña HA. Mesenchymal stem cells current clinical applications: a systematic review. Arch Med Res. 2021;52(1):93–101. https://doi.org/10.1016/j.arcmed.2020.08.006 .
Santra L, Gupta S, Singh AK, Sahu AR, Gandham RK, Naskar S, Maity SK, Ghosh J, Dhara SK. A comparative analysis of invasive and non-invasive method of bone marrow stromal cell isolation. AJAVA. 2015;10(10):549–55. https://doi.org/10.3923/ajava.2015.549.555 .
Weiss ML, Troyer DL. Stem cells in the umbilical cord. Stem Cell Rev. 2006;2(2):155–62. https://doi.org/10.1007/s12015-006-0022-y .
Vangsness CT Jr, Sternberg H, Harris L. Umbilical cord tissue offers the greatest number of harvestable mesenchymal stem cells for research and clinical application: a literature review of different harvest sites. Arthroscopy. 2015;31(9):1836–43. https://doi.org/10.1016/j.arthro.2015.03.014 .
Pittenger MF, Discher DE, Péault BM, Phinney DG, Hare JM, Caplan AI. Mesenchymal stem cell perspective: cell biology to clinical progress. NPJ Regen Med. 2019;2(4):22. https://doi.org/10.1038/s41536-019-0083-6 .
Musiał-Wysocka A, Kot M, Majka M. The pros and cons of mesenchymal stem cell-based therapies. Cell Transplant. 2019;28(7):801–12. https://doi.org/10.1177/0963689719837897 .
Bhat S, Viswanathan P, Chandanala S, Prasanna SJ, Seetharam RN. Expansion and characterization of bone marrow derived human mesenchymal stromal cells in serum-free conditions. Sci Rep. 2021;11(1):3403. https://doi.org/10.1038/s41598-021-83088-1 .
Muthu S, Bapat A, Jain R, Jeyaraman N, Jeyaraman M. Exosomal therapy-a new frontier in regenerative medicine. Stem Cell Investig. 2021;2(8):7. https://doi.org/10.21037/sci-2020-037 .
De Becker A, Riet IV. Homing and migration of mesenchymal stromal cells: How to improve the efficacy of cell therapy? World J Stem Cells. 2016;8(3):73–87. https://doi.org/10.4252/wjsc.v8.i3.73 .
Galleu A, Riffo-Vasquez Y, Trento C, Lomas C, Dolcetti L, Cheung TS, von Bonin M, Barbieri L, Halai K, Ward S, Weng L, Chakraverty R, Lombardi G, Watt FM, Orchard K, Marks DI, Apperley J, Bornhauser M, Walczak H, Bennett C, Dazzi F (2017) Apoptosis in mesenchymal stromal cells induces in vivo recipient-mediated immunomodulation. Science Transl Med 9(416):eaam7828. https://doi.org/10.1126/scitranslmed.aam7828
Cheung TS, Galleu A, von Bonin M, Bornhäuser M, Dazzi F. Apoptotic mesenchymal stromal cells induce prostaglandin E2 in monocytes: implications for the monitoring of mesenchymal stromal cell activity. Haematologica. 2019;104(10):e438–41. https://doi.org/10.3324/haematol.2018.214767 .
Chan AML, Ng AMH, Mohd Yunus MH, Hj Idrus RB, Law JX, Yazid MD, Chin KY, Shamsuddin SA, Mohd Yusof MR, Razali RA, Mat Afandi MA, Hassan MNF, Ng SN, Koh B, Lokanathan Y. Safety study of allogeneic mesenchymal stem cell therapy in animal model. Regen Ther. 2022;17(19):158–65. https://doi.org/10.1016/j.reth.2022.01.008 .
Alencar AKN, Pimentel-Coelho PM, Montes GC, da Silva MMC, Mendes LVP, Montagnoli TL, Silva AMS, Vasques JF, Rosado-de-Castro PH, Gutfilen B, Cunha VDMN, Fraga AGM, Silva PMRE, Martins MA, Ferreira TPT, Mendes-Otero R, Trachez MM, Sudo RT, Zapata-Sudo G. Human mesenchymal stem cell therapy reverses Su5416/hypoxia-induced pulmonary arterial hypertension in mice. Front Pharmacol. 2018;6(9):1395. https://doi.org/10.3389/fphar.2018.01395 .
Lominadze Z, Kallwitz ER. Misconception: you can’t have liver disease with normal liver chemistries. Clin Liver Dis (Hoboken). 2018;12(4):96–9. https://doi.org/10.1002/cld.742 .
Zafar M, Naqvi SN. Effects of STZ-induced diabetes on the relative weights of kidney, liver and pancreas in albino rats: a comparative study. J Morphol. 2010;28:135–42.
Google Scholar
Fujimaki S, Wakabayashi T, Takemasa T, Asashima M, Kuwabara T. Diabetes and stem cell function. Biomed Res Int. 2015;2015: 592915. https://doi.org/10.1155/2015/592915 .
Elksnis A, Martinell M, Eriksson O, Espes D. Heterogeneity of metabolic defects in type 2 diabetes and its relation to reactive oxygen species and alterations in beta-cell mass. Front Physiol. 2019;13(10):107. https://doi.org/10.3389/fphys.2019.00107 .
Zaki SM, Fattah SA, Hassan DS. The differential effects of high-fat and high- -fructose diets on the liver of male albino rat and the proposed underlying mechanisms. Folia Morphol (Warsz). 2019;78(1):124–36. https://doi.org/10.5603/FM.a2018.0063 .
Han L, Wang G, Zhou S, Situ C, He Z, Li Y, Qiu Y, Huang Y, Xu A, Ong MTY, Wang H, Zhang J, Wu Z. Muscle satellite cells are impaired in type 2 diabetic mice by elevated extracellular adenosine. Cell Rep. 2022;39(9): 110884. https://doi.org/10.1016/j.celrep.2022 .
Lee CM, Huxley RR, Woodward M, Zimmet P, Shaw J, Cho NH, Kim HR, Viali S, Tominaga M, Vistisen D, Borch-Johnsen K, Colagiuri S, DETECT-2 Collaboration (2008) The metabolic syndrome identifies a heterogeneous group of metabolic component combinations in the Asia-Pacific region. Diabetes Res Clin Pract 81(3):377–380. https://doi.org/10.1016/j.diabres.2008.05.011 .
Neeland IJ, Poirier P, Després JP. Cardiovascular and metabolic heterogeneity of obesity: clinical challenges and implications for management. Circulation. 2018;137(13):1391–406. https://doi.org/10.1161/CIRCULATIONAHA.117.029617 .
Chan AML, Ng AMH, Mohd Yunus MH, Idrus RBH, Law JX, Yazid MD, Chin KY, Shamsuddin SA, Lokanathan Y. Recent developments in rodent models of high-fructose diet-induced metabolic syndrome: a systematic review. Nutrients. 2021;13(8):2497. https://doi.org/10.3390/nu13082497 .
Rozendaal YJW, Wang Y, Paalvast Y, Tambyrajah LL, Li Z, Willems van Dijk K, Rensen PCN, Kuivenhoven JA, Groen AK, Hilbers PAJ, van Riel NAW (2018) In vivo and in silico dynamics of the development of Metabolic Syndrome. PLoS Comput Biol 14(6):e1006145. https://doi.org/10.1371/journal.pcbi.1006145 .
Fassio A, Idolazzi L, Rossini M, Gatti D, Adami G, Giollo A, Viapiana O (2018) The obesity paradox and osteoporosis. Eat Weight Disord 23(3):293–302. https://doi.org/10.1007/s40519-018-0505-2 . Epub 2018 Apr 11. Erratum in: Eat Weight Disord. 2018 May 2
Turcotte AF, O’Connor S, Morin SN, Gibbs JC, Willie BM, Jean S, Gagnon C. Association between obesity and risk of fracture, bone mineral density and bone quality in adults: a systematic review and meta-analysis. PLoS ONE. 2021;16(6): e0252487. https://doi.org/10.1371/journal.pone.0252487 .
Oliveira-Sales EB, Maquigussa E, Semedo P, Pereira LG, Ferreira VM, Câmara NO, Bergamaschi CT, Campos RR, Boim MA. Mesenchymal stem cells (MSC) prevented the progression of renovascular hypertension, improved renal function and architecture. PLoS ONE. 2013;8(11): e78464. https://doi.org/10.1371/journal.pone.0078464 .
Lee H, Lee JC, Kwon JH, Kim KC, Cho MS, Yang YS, Oh W, Choi SJ, Seo ES, Lee SJ, Wang TJ, Hong YM. The effect of umbilical cord blood derived mesenchymal stem cells in monocrotaline-induced pulmonary artery hypertension rats. J Kor Med Sci. 2015;30(5):576–85. https://doi.org/10.3346/jkms.2015.30.5.576 .
Lee H, Kim KC, Choi SJ, Hong YM. Optimal dose and timing of umbilical stem cells treatment in pulmonary arterial hypertensive rats. Yonsei Med J. 2017;58(3):570–80. https://doi.org/10.3349/ymj.2017.58.3.570 .
Van Linthout S, Hamdani N, Miteva K, Koschel A, Müller I, Pinzur L, Aberman Z, Pappritz K, Linke WA, Tschöpe C. Placenta-derived adherent stromal cells improve diabetes mellitus-associated left ventricular diastolic performance. Stem Cells Transl Med. 2017;6(12):2135–45. https://doi.org/10.1002/sctm.17-0130 .
Poomani MS, Mariappan I, Perumal R, Regurajan R, Muthan K, Subramanian V. Mesenchymal stem cell (MSCs) therapy for ischemic heart disease: a promising frontier. Glob Heart. 2022;17(1):19. https://doi.org/10.5334/gh.1098 .
Chin KY (2020) Calculating in-vivo short-term precision error of dual-energy X-ray absorptiometry in human and animal: a technical report. Med Health 15(1): 70–77. https://doi.org/10.17576/MH.2020.1501.06
Zhu H, Xiong Y, Xia Y, et al. (2017) Therapeutic Effects of Human Umbilical Cord-Derived Mesenchymal Stem Cells in Acute Lung Injury Mice. Sci Rep 7:39889. https://doi.org/10.1038/srep39889
Zhang GZ, Sun HC, Zheng LB, Guo JB, Zhang XL. In vivo hepatic differentiation potential of human umbilical cord-derived mesenchymal stem cells: therapeutic effect on liver fibrosis/cirrhosis. World J Gastroenterol. 2017;23(46):8152–68. https://doi.org/10.3748/wjg.v23.i46.8152 .
Park SJ, Kim RY, Park BW, et al. Dual stem cell therapy synergistically improves cardiac function and vascular regeneration following myocardial infarction. Nat Commun. 2019;10(1):3123. https://doi.org/10.1038/s41467-019-11091-2 .
Esposito K, Giugliano D. The metabolic syndrome and inflammation: association or causation? Nutr Metab Cardiovasc Dis. 2004;14(5):228–32. https://doi.org/10.1016/s0939-4753(04)80048-6 .
Lopez-Candales A, Hernández Burgos PM, Hernandez-Suarez DF, Harris D. Linking chronic inflammation with cardiovascular disease: from normal aging to the metabolic syndrome. J Nat Sci. 2017;3(4): e341.
PubMed PubMed Central Google Scholar
Pepys MB, Hirschfield GM. C-reactive protein: a critical update. J Clin Invest. 2003;111(12):1805–12. https://doi.org/10.1172/JCI18921.Erratum.In:JClinInvest.2003Jul;112(2):299 .
Suresh E. Diagnosis of early rheumatoid arthritis: what the non-specialist needs to know. J R Soc Med. 2004;97(9):421–4. https://doi.org/10.1177/014107680409700903 .
Enocsson H, Gullstrand B, Eloranta ML, Wetterö J, Leonard D, Rönnblom L, Bengtsson AA, Sjöwall C. C-reactive protein levels in systemic lupus erythematosus are modulated by the interferon gene signature and CRP gene polymorphism rs1205. Front Immunol. 2021;28(11): 622326. https://doi.org/10.3389/fimmu.2020.622326 .
Ambroise J, Bearzatto B, Robert A, Govaerts B, Macq B, Gala JL. Impact of the spotted microarray preprocessing method on fold-change compression and variance stability. BMC Bioinf. 2011;25(12):413. https://doi.org/10.1186/1471-2105-12-413 .
Weingand K, Brown G, Hall R, Davies D, Gossett K, Neptun D, Waner T, Matsuzawa T, Salemink P, Froelke W, Provost JP, Dal Negro G, Batchelor J, Nomura M, Groetsch H, Boink A, Kimball J, Woodman D, York M, Fabianson-Johnson E, Lupart M, Melloni E (1996) Harmonization of animal clinical pathology testing in toxicity and safety studies. The Joint Scientific Committee for International Harmonization of Clinical Pathology Testing. Fundam Appl Toxicol 29(2):198–201
Koseoglu M, Hur A, Atay A, Cuhadar S. Effects of hemolysis interferences on routine biochemistry parameters. Biochem Med (Zagreb). 2011;21(1):79–85. https://doi.org/10.11613/bm.2011.015 .
Ali D, Sacchetto E, Dumontet E, Le Carrer D, Orsonneau JL, Delaroche O, Bigot-Corbel E (2014) Interférence de l’hémolyse sur le dosage de vingt-deux paramètres biochimiques [Hemolysis influence on twenty-two biochemical parameters measurement]. Ann Biol Clin (Paris). 72(3):297–311. https://doi.org/10.1684/abc.2014.0952
Nunes-Souza V, César-Gomes CJ, Da Fonseca LJ, Guedes Gda S, Smaniotto S, Rabelo LA. Aging increases susceptibility to high fat diet-induced metabolic syndrome in C57BL/6 mice: improvement in glycemic and lipid profile after antioxidant therapy. Oxid Med Cell Longev. 2016;2016:1987960. https://doi.org/10.1155/2016/1987960 .
Hattori T, Murase T, Takatsu M, Nagasawa K, Matsuura N, Watanabe S, Murohara T, Nagata K. Dietary salt restriction improves cardiac and adipose tissue pathology independently of obesity in a rat model of metabolic syndrome. J Am Heart Assoc. 2014;3(6): e001312. https://doi.org/10.1161/JAHA.114.001312 .
Li N, Guenancia C, Rigal E, Hachet O, Chollet P, Desmoulins L, Leloup C, Rochette L, Vergely C. Short-term moderate diet restriction in adulthood can reverse oxidative, cardiovascular and metabolic alterations induced by postnatal overfeeding in mice. Sci Rep. 2016;28(6):30817. https://doi.org/10.1038/srep30817 .
Hyde PN, Sapper TN, Crabtree CD, LaFountain RA, Bowling ML, Buga A, Fell B, McSwiney FT, Dickerson RM, Miller VJ, Scandling D, Simonetti OP, Phinney SD, Kraemer WJ, King SA, Krauss RM, Volek JS. Dietary carbohydrate restriction improves metabolic syndrome independent of weight loss. JCI Insight. 2019;4(12): e128308. https://doi.org/10.1172/jci.insight.128308 .
Aouichat S, Chayah M, Bouguerra-Aouichat S, Agil A. Time-Restricted Feeding Improves Body Weight Gain, Lipid Profiles, and Atherogenic Indices in Cafeteria-Diet-Fed Rats: Role of Browning of Inguinal White Adipose Tissue. Nutrients. 2020;12(8):2185. https://doi.org/10.3390/nu12082185 .
Schmidt A, Bierwirth S, Weber S, Platen P, Schinköthe T, Bloch W. Short intensive exercise increases the migratory activity of mesenchymal stem cells. Br J Sports Med. 2009;43(3):195–8. https://doi.org/10.1136/bjsm.2007.043208 .
Mason C, Foster-Schubert KE, Imayama I, Kong A, Xiao L, Bain C, Campbell KL, Wang CY, Duggan CR, Ulrich CM, Alfano CM, Blackburn GL, McTiernan A. Dietary weight loss and exercise effects on insulin resistance in postmenopausal women. Am J Prev Med. 2011;41(4):366–75. https://doi.org/10.1016/j.amepre.2011.06.042 .
Zhang L, Li K, Liu X, Li D, Luo C, Fu B, Cui S, Zhu F, Zhao RC, Chen X. Repeated systemic administration of human adipose-derived stem cells attenuates overt diabetic nephropathy in rats. Stem Cells Dev. 2013;22(23):3074–86. https://doi.org/10.1089/scd.2013.0142 .
Bourzac C, Bensidhoum M, Pallu S, Portier H. Use of adult mesenchymal stromal cells in tissue repair: impact of physical exercise. Am J Physiol Cell Physiol. 2019;317(4):C642–54. https://doi.org/10.1152/ajpcell.00530.2018 .
Lee Y, Shin SH, Cho KA, Kim YH, Woo SY, Kim HS, Jung SC, Jo I, Jun HS, Park WJ, Park JW, Ryu KH. Administration of Tonsil-derived mesenchymal stem cells improves glucose tolerance in high fat diet-induced diabetic mice via insulin-like growth factor-binding protein 5-mediated endoplasmic reticulum stress modulation. Cells. 2019;8(4):368. https://doi.org/10.3390/cells8040368 .
Shamsuddin SA, Chan AML, Ng MH, Yazid MD, Law JX, Hj Idrus RB, Fauzi MB, Mohd Yunus MH, Lokanathan Y. Stem cells as a potential therapy in managing various disorders of metabolic syndrome: a systematic review. Am J Transl Res. 2021;13(11):12217–27.
CAS PubMed PubMed Central Google Scholar
Chan AML, Sampasivam Y, Lokanathan Y. Biodistribution of mesenchymal stem cells (MSCs) in animal models and implied role of exosomes following systemic delivery of MSCs: a systematic review. Am J Transl Res. 2022;14(4):2147–61.
Eggenhofer E, Luk F, Dahlke MH, Hoogduijn MJ. The life and fate of mesenchymal stem cells. Front Immunol. 2014;19(5):148. https://doi.org/10.3389/fimmu.2014.00148 .
Wang Y, Han ZB, Song YP, Han ZC. Safety of mesenchymal stem cells for clinical application. Stem Cells Int. 2012;2012: 652034. https://doi.org/10.1155/2012/652034 .
Choi D, Lee H, Kim H, Yang M, Heo J, Won Y, Jang S, Park JK, Son Y, Oh T, Lee E, Hong J. Cytoprotective self-assembled RGD peptide nanofilms for surface modification of viable mesenchymal stem cells. Chem Mater. 2017;29:2055–65. https://doi.org/10.1021/acs.chemmater.6b04096 .
Jin JF, Zhu LL, Chen M, Xu HM, Wang HF, Feng XQ, Zhu XP, Zhou Q. The optimal choice of medication administration route regarding intravenous, intramuscular, and subcutaneous injection. Patient Prefer Adherence. 2015;2(9):923–42. https://doi.org/10.2147/PPA.S87271 .
Yagi H, Soto-Gutierrez A, Kitagawa Y, Tilles AW, Tompkins RG, Yarmush ML. Bone marrow mesenchymal stromal cells attenuate organ injury induced by LPS and burn. Cell Transpl. 2010;19(6):823–30. https://doi.org/10.3727/096368910X508942 .
Lu G, Huang S, Chen Y, Ma K. Umbilical cord mesenchymal stem cell transplantation ameliorates burn-induced acute kidney injury in rats. Int J Low Extrem Wounds. 2013;12(3):205–11. https://doi.org/10.1177/1534734613502041 .
Li X, Liu L, Yang J, et al. Exosome derived from human umbilical cord mesenchymal stem cell mediates MiR-181c attenuating burn-induced excessive inflammation. EBioMedicine. 2016;8:72–82. https://doi.org/10.1016/j.ebiom.2016.04.030 .
Wang S, Wang Z, Su H, et al. Effects of long-term culture on the biological characteristics and RNA profiles of human bone-marrow-derived mesenchymal stem cells. Mol Ther Nucleic Acids. 2021;26:557–74. https://doi.org/10.1016/j.omtn.2021.08.013 .
Shanks N, Greek R, Greek J. Are animal models predictive for humans? Philos Ethics Humanit Med. 2009;15(4):2. https://doi.org/10.1186/1747-5341-4-2 .
Martić-Kehl MI, Schibli R, Schubiger PA. Can animal data predict human outcome? Problems and pitfalls of translational animal research. Eur J Nucl Med Mol Imaging. 2012;39(9):1492–6. https://doi.org/10.1007/s00259-012-2175-z .
Degeling C, Johnson J. Evaluating animal models: some taxonomic worries. J Med Philos. 2013;38(2):91–106. https://doi.org/10.1093/jmp/jht004 .
Pound P, Ritskes-Hoitinga M. Is it possible to overcome issues of external validity in preclinical animal research? Why most animal models are bound to fail. J Transl Med. 2018;16(1):304. https://doi.org/10.1186/s12967-018-1678-1 .
Leenaars CHC, Kouwenaar C, Stafleu FR, Bleich A, Ritskes-Hoitinga M, De Vries RBM, Meijboom FLB. Animal to human translation: a systematic scoping review of reported concordance rates. J Transl Med. 2019;17(1):223. https://doi.org/10.1186/s12967-019-1976-2 .
Ricci C, Baumgartner J, Malan L, Smuts CM. Determining sample size adequacy for animal model studies in nutrition research: limits and ethical challenges of ordinary power calculation procedures. Int J Food Sci Nutr. 2020;71(2):256–64. https://doi.org/10.1080/09637486.2019.1646714 .
Download references
Acknowledgements
The authors would like to acknowledge the veterinary officer, Dr. Mohd Hafidz Bin Mohd Izhar (Industrial Biotechnology Research Centre, Sirim Berhad) for his contribution to the animal study. Serum biochemistry was outsourced to Veterinary Laboratory Service Unit (VLSU) at Universiti Putra Malaysia, Malaysia.
This work was funded by Ming Medical Sdn. Bhd. (FF-2020–469) and Universiti Kebangsaan Malaysia (FF-2020–469/1 and DPK-2021–006).
Author information
Authors and affiliations.
Centre for Tissue Engineering and Regenerative Medicine, Faculty of Medicine, Universiti Kebangsaan Malaysia, 56000, Cheras, Kuala Lumpur, Malaysia
Alvin Man Lung Chan, Angela Min Hwei Ng, Ruszymah Hj Idrus, Jia Xian Law, Muhammad Dain Yazid, Benson Koh & Yogeswaran Lokanathan
Department of Physiology, Faculty of Medicine, Universiti Kebangsaan Malaysia, 56000, Kuala Lumpur, Malaysia
Mohd Heikal Mohd Yunus
Department of Pharmacology, Faculty of Medicine, Universiti Kebangsaan Malaysia, 56000, Kuala Lumpur, Malaysia
Kok-Yong Chin
Department of Parasitology and Medical Entomology, Faculty of Medicine, Universiti Kebangsaan Malaysia, 56000, Kuala Lumpur, Malaysia
Mohd Rafizul Mohd Yusof
Ming Medical Sdn Bhd, D3-3 (2nd Floor), Block D3 Dana 1 Commercial Centre, Jalan PJU 1a/46, 47301, Petaling Jaya, Selangor, Malaysia
Alvin Man Lung Chan & See Nguan Ng
You can also search for this author in PubMed Google Scholar
Contributions
A.M.H.N., M.H.M.Y., R.H.I., J.X.L., M.D.Y., K-Y.C., and Y.L.: concept and design; S.A.S., R.M.Y., B.K., and Y.L.: administrative support; Y.L.: provision of study material; S.N.N. and Y.L.: financial support; A.M.L.C. and R.M.Y.: collection of data; A.M.L.C, A.M.H.N., M.H.M.Y., and Y.L.: data analysis and interpretation, manuscript writing; ALL AUTHORS.: final approval of manuscript.
Corresponding author
Correspondence to Yogeswaran Lokanathan .
Ethics declarations
Ethics approval and consent to participate.
The animal study protocol was approved by the UKMREC (JEP-2020–790) and UKMAEC (TEC/FP/2020/YOGESWARAN/23-SEPT./1124-OCT.-2020-SEPT-2023) with adherence to Declaration of Helsinki, for project title “Safety and efficacy study of allogeneic mesenchymal stem cell therapy for treatment for Metabolic Syndrome in Sprague Dawley rat” from September 2020 to September 2023. Likewise, use of human WJ-MSC cells was acquired through approved Human Primary Cell Banking (UKM 1.5.3.5/244/FF-2015–376). The umbilical cord tissues were obtained with consent from maternal volunteers who were undergoing scheduled Caesarean sections.
Competing interests
All authors have declared no conflict of interest to report.
Additional information
Publisher's note.
Springer Nature remains neutral with regard to jurisdictional claims in published maps and institutional affiliations.
See Table 4 .
See Fig. 6 .
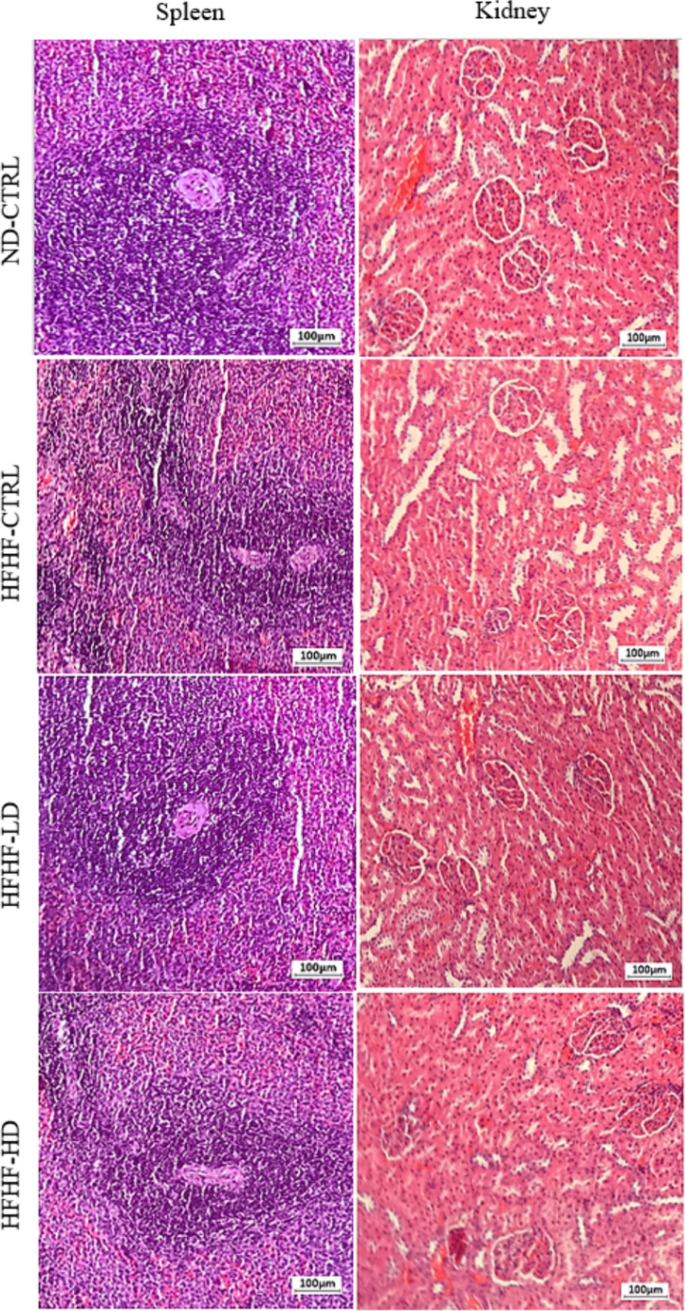
H&E images of kidney and spleen for all treatment groups (top to bottom rows): ND-CTRL, HFHF-CTRL, HFHF-LD and HFHF-HD. Images were captured at 10 × magnification with scale bar (100 µm)
Rights and permissions
Open Access This article is licensed under a Creative Commons Attribution 4.0 International License, which permits use, sharing, adaptation, distribution and reproduction in any medium or format, as long as you give appropriate credit to the original author(s) and the source, provide a link to the Creative Commons licence, and indicate if changes were made. The images or other third party material in this article are included in the article's Creative Commons licence, unless indicated otherwise in a credit line to the material. If material is not included in the article's Creative Commons licence and your intended use is not permitted by statutory regulation or exceeds the permitted use, you will need to obtain permission directly from the copyright holder. To view a copy of this licence, visit http://creativecommons.org/licenses/by/4.0/ . The Creative Commons Public Domain Dedication waiver ( http://creativecommons.org/publicdomain/zero/1.0/ ) applies to the data made available in this article, unless otherwise stated in a credit line to the data.
Reprints and permissions
About this article
Cite this article.
Chan, A.M.L., Ng, A.M.H., Yunus, M.H.M. et al. Single high-dose intravenous injection of Wharton’s jelly-derived mesenchymal stem cell exerts protective effects in a rat model of metabolic syndrome. Stem Cell Res Ther 15 , 160 (2024). https://doi.org/10.1186/s13287-024-03769-2
Download citation
Received : 02 March 2023
Accepted : 26 May 2024
Published : 05 June 2024
DOI : https://doi.org/10.1186/s13287-024-03769-2
Share this article
Anyone you share the following link with will be able to read this content:
Sorry, a shareable link is not currently available for this article.
Provided by the Springer Nature SharedIt content-sharing initiative
- Cell therapy
- Mesenchymal stem cell
- Syndrome X Wharton’s jelly
Stem Cell Research & Therapy
ISSN: 1757-6512
- Submission enquiries: Access here and click Contact Us
- General enquiries: [email protected]

An official website of the United States government
The .gov means it’s official. Federal government websites often end in .gov or .mil. Before sharing sensitive information, make sure you’re on a federal government site.
The site is secure. The https:// ensures that you are connecting to the official website and that any information you provide is encrypted and transmitted securely.
- Publications
- Account settings
Preview improvements coming to the PMC website in October 2024. Learn More or Try it out now .
- Advanced Search
- Journal List
- Iran J Public Health
- v.42(12); 2013 Dec
Secondary Data Analysis: Ethical Issues and Challenges
Research does not always involve collection of data from the participants. There is huge amount of data that is being collected through the routine management information system and other surveys or research activities. The existing data can be analyzed to generate new hypothesis or answer critical research questions. This saves lots of time, money and other resources. Also data from large sample surveys may be of higher quality and representative of the population. It avoids repetition of research & wastage of resources by detailed exploration of existing research data and also ensures that sensitive topics or hard to reach populations are not over researched ( 1 ). However, there are certain ethical issues pertaining to secondary data analysis which should be taken care of before handling such data.
Secondary data analysis
Secondary analysis refers to the use of existing research data to find answer to a question that was different from the original work ( 2 ). Secondary data can be large scale surveys or data collected as part of personal research. Although there is general agreement about sharing the results of large scale surveys, but little agreement exists about the second. While the fundamental ethical issues related to secondary use of research data remain the same, they have become more pressing with the advent of new technologies. Data sharing, compiling and storage have become much faster and easier. At the same time, there are fresh concerns about data confidentiality and security.
Issues in Secondary data analysis
Concerns about secondary use of data mostly revolve around potential harm to individual subjects and issue of return for consent. Secondary data vary in terms of the amount of identifying information in it. If the data has no identifying information or is completely devoid of such information or is appropriately coded so that the researcher does not have access to the codes, then it does not require a full review by the ethical board. The board just needs to confirm that the data is actually anonymous. However, if the data contains identifying information on participants or information that could be linked to identify participants, a complete review of the proposal will then be made by the board. The researcher will then have to explain why is it unavoidable to have identifying information to answer the research question and must also indicate how participants’ privacy and the confidentiality of the data will be protected. If the above said concerns are satisfactorily addressed, the researcher can then request for a waiver of consent.
If the data is freely available on the Internet, books or other public forum, permission for further use and analysis is implied. However, the ownership of the original data must be acknowledged. If the research is part of another research project and the data is not freely available, except to the original research team, explicit, written permission for the use of the data must be obtained from the research team and included in the application for ethical clearance.
However, there are certain other issues pertaining to the data that is procured for secondary analysis. The data obtained should be adequate, relevant but not excessive. In secondary data analysis, the original data was not collected to answer the present research question. Thus the data should be evaluated for certain criteria such as the methodology of data collection, accuracy, period of data collection, purpose for which it was collected and the content of the data. It shall be kept for no longer than is necessary for that purpose. It must be kept safe from unauthorized access, accidental loss or destruction. Data in the form of hardcopies should be kept in safe locked cabinets whereas softcopies should be kept as encrypted files in computers. It is the responsibility of the researcher conducting the secondary analysis to ensure that further analysis of the data conducted is appropriate. In some cases there is provision for analysis of secondary data in the original consent form with the condition that the secondary study is approved by the ethics review committee. According to the British Sociological Association’s Statement of Ethical Practice (2004) the researchers must inform participants regarding the use of data and obtain consent for the future use of the material as well. However it also says that consent is not a once-and-for-all event, but is subject to renegotiation over time ( 3 ). It appears that there are no guidelines about the specific conditions that require further consent.
Issues in Secondary analysis of Qualitative data
In qualitative research, the culture of data archiving is absent ( 4 ). Also, there is a concern that data archiving exposes subject’s personal views. However, the best practice is to plan anonymisation at the time of initial transcription. Use of pseudonyms or replacements can protect subject’s identity. A log of all replacements, aggregations or removals should be made and stored separately from the anonymised data files. But because of the circumstances, under which qualitative data is produced, their reinterpretation at some later date can be challenging and raises further ethical concerns.
There is a need for formulating specific guidelines regarding re-use of data, data protection and anonymisation and issues of consent in secondary data analysis.
Acknowledgements
The authors declare that there is no conflict of interest.
- Fielding NG, Fielding JL (2003). Resistance and adaptation to criminal identity: Using secondary analysis to evaluate classic studies of crime and deviance . Sociology , 34 ( 4 ): 671–689. [ Google Scholar ]
- Szabo V, Strang VR (1997). Secondary analysis of qualitative data . Advances in Nursing Science , 20 ( 2 ): 66–74. [ PubMed ] [ Google Scholar ]
- Statement of Ethical Practice for the British Sociological Association (2004). The British Sociological Association, Durham . Available at: http://www.york.ac.uk/media/abouttheuniversity/governanceandmanagement/governance/ethicscommittee/hssec/documents/BSA%20statement%20of%20ethical%20practice.pdf (Last accessed 24November2013)
- Archiving Qualitative Data: Prospects and Challenges of Data Preservation and Sharing among Australian Qualitative Researchers. Institute for Social Science Research, The University of Queensland, 2009 . Available at: http://www.assda.edu.au/forms/AQuAQualitativeArchiving_DiscussionPaper_FinalNov09.pdf (Last accessed 05September2013)
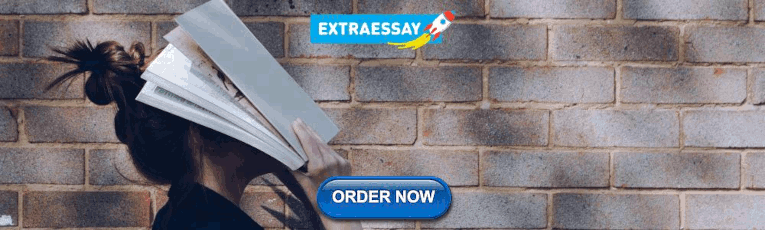
IMAGES
VIDEO
COMMENTS
Secondary analysis of data collected by another researcher for a different purpose, or SDA, is increasing in the medical and social sciences. This is not surprising, given the immense body of health care-related research performed worldwide and the potential beneficial clinical implications of the timely expansion of primary research (Johnston, 2014; Tripathy, 2013).
SDA involves investigations where data collected for a previous study is analyzed - either by the same researcher(s) or different researcher(s) - to explore new questions or use different analysis strategies that were not a part of the primary analysis (Szabo and Strang, 1997).For research involving quantitative data, SDA, and the process of sharing data for the purpose of SDA, has become ...
In simple terms, secondary data is every. dataset not obtained by the author, or "the analysis. of data gathered b y someone else" (Boslaugh, 2007:IX) to be more sp ecific. Secondary data may ...
Secondary analyses of large datasets provide a mechanism for researchers to address high impact questions that would otherwise be prohibitively expensive and time-consuming to study. This paper presents a guide to assist investigators interested in conducting secondary data analysis, including advice on the process of successful secondary data ...
Types of secondary data are as follows: Published data: Published data refers to data that has been published in books, magazines, newspapers, and other print media. Examples include statistical reports, market research reports, and scholarly articles. Government data: Government data refers to data collected by government agencies and departments.
Introduction. Secondary data analysis is a valuable research approach that can be used to advance knowledge across many disciplines through the use of quantitative, qualitative, or mixed methods data to answer new research questions (Polit & Beck, 2021).This research method dates to the 1960s and involves the utilization of existing or primary data, originally collected for a variety, diverse ...
Secondary research is a research method that uses data that was collected by someone else. In other words, whenever you conduct research using data that already exists, you are conducting secondary research. On the other hand, any type of research that you undertake yourself is called primary research. Example: Secondary research.
In addition to the challenges of secondary research as mentioned in subsection Secondary Data and Analysis, in current research realm of secondary analysis, there is a lack of rigor in the analysis and overall methodology (Ruggiano & Perry, 2019). This has the pitfall of possibly exaggerating the effects of researcher bias (Thorne, 1994, 1998 ...
However, researcher biases can lead to questionable research practices in secondary data analysis, which can distort the evidence base. While pre-registration can help to protect against researcher biases, it presents challenges for secondary data analysis. In this article, we describe these challenges and propose novel solutions and ...
increased, little is known about the current state of qualitative secondary data analysis or how researchers are conducting secondary data analysis with qualitative data. This critical interpretive synthesis examined research articles (n¼71) published between 2006 and 2016 that involved qualitative secondary data analysis and assessed the
This article—one in a series on clinical research by nurses—discusses the alignment of research goals with secondary data sources, explores sources of publicly available secondary data that might be of interest to nurse researchers, and outlines the costs and benefits of using secondary data. This article introduces the reader to secondary ...
the online version will vary from the pagination of the print book. 1. 2. Secondary data is usually defined in opposition to primary data. The latter is directly obtained. from first-hand sources ...
In the fourth seminar article by Edwards et al. [5], the authors review research using secondary data on nonprostate genitourinary malignancies. Again, a common theme here is the use of secondary data to inform the (many) questions where higher quality level 1 evidence does not exist. In many cases, such hypothesis-generating articles have led ...
This paper asserts that secondary data analysis is a viable method to utilize in the process of inquiry when a systematic procedure is followed and presents an illustrative research application ...
Secondary research, also known as desk research, is a research method that involves compiling existing data sourced from a variety of channels. This includes internal sources (e.g.in-house research) or, more commonly, external sources (such as government statistics, organizational bodies, and the internet).
The earliest reference to the use of secondary data analysis in the nursing literature can be found as far back as the 1980's, when Polit & Hungler (1983 ), in the second edition of their classic nursing research methods textbook, discussed this emerging approach to analysis. At that time, this method was rarely used by nursing researchers.
5. Advantages of secondary data. Secondary data is suitable for any number of analytics activities. The only limitation is a dataset's format, structure, and whether or not it relates to the topic or problem at hand. When analyzing secondary data, the process has some minor differences, mainly in the preparation phase.
Compared to primary research, the collection of secondary data can be faster and cheaper to obtain, depending on the sources you use. Secondary data can come from internal or external sources. Internal sources of secondary data include ready-to-use data or data that requires further processing available in internal management support systems ...
Our finding that secondary data-based research at the individual level remains scarce might similarly be the result of researchers' focus shifting from the research question to the data available. This can be highly problematic to the extent that it is a systematic shift. However, there is a range of novel sources of secondary data at the ...
A secondary source is a document or work where its author had an indirect part in a study or creation; an author is usually writing about or reporting the work or research done by someone else. Secondary sources can be used for additional or supporting information; they are not the direct product of research or the making of a creative work.
Secondary data analysis is one strategy to address this challenge. The use of existing data to test new hypotheses or answer new research questions has several advantages. It typically takes less time and resources, is low risk to participants, and allows access to large data sets and longitudinal data.
LLNL's HPC and data science capabilities play a significant role in international science research and innovation, and Lab researchers have won 10 R&D 100 Awards in the Software-Services category in the past decade. The latest issue of Science & Technology Review features several award-winning projects, including ZFP and CANDLE: (1) ZFP introduces a new method of compressing large data ...
Background Existing knowledge on healthcare use and costs in the last months of life is often limited to one patient group (i.e., cancer patients) and one level of healthcare (i.e., secondary care). Consequently, decision-makers lack knowledge in order to make informed decisions about the allocation of healthcare resources for all patients. Our aim is to elaborate the understanding of resource ...
Concerns about secondary data analysis when using qualitative data. The primary concerns about SDA with qualitative data surround rigor and ethics from a number of stakeholder perspectives, including research participants, funders, and the researchers themselves. Heaton (2004) suggests that a strength of secondary analysis of qualitative data ...
Analysis of secondary data sources (such as cohort studies, survey data, and administrative records) has the potential to provide answers to science and society's most pressing questions. However, researcher biases can lead to questionable research practices in secondary data analysis, which can distort the evidence base. While pre-registration can help to protect against researcher biases ...
Metabolic syndrome (MetS) is a significant epidemiological problem worldwide. It is a pre-morbid, chronic and low-grade inflammatory disorder that precedes many chronic diseases. Wharton's jelly-derived mesenchymal stem cells (WJ-MSCs) could be used to treat MetS because they express high regenerative capacity, strong immunomodulatory properties and allogeneic biocompatibility.
The sample size calculation was performed using G*power software version 3.1.9.2, which relied on data from the pilot study carried out as part of the current research project. The pilot revealed a large effect size of IOP between the studied groups (d = 0.4).
Secondary data analysis. Secondary analysis refers to the use of existing research data to find answer to a question that was different from the original work ( 2 ). Secondary data can be large scale surveys or data collected as part of personal research. Although there is general agreement about sharing the results of large scale surveys, but ...
Reports the retraction of "Predictors of depression among school adolescents in Northwest, Ethiopia, 2022: Institutional based cross-sectional" by Aklile Tsega Chekol, Mastewal Aschale Wale, Agmas Wassie Abate, Eyerusalem Abebe Beo, Eman Ali Said and Berhan Tsegaye Negash (BMC Psychiatry, 2023[Jun][14], Vol 23[1][429]). The Editors have retracted this article after concerns were raised.