
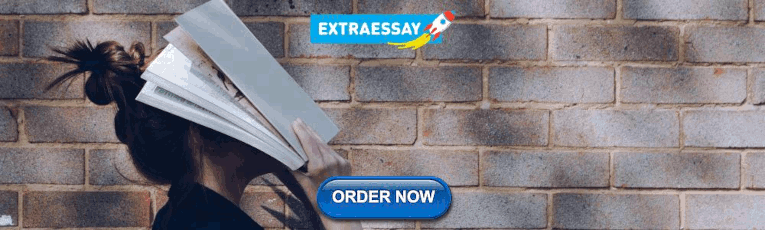
Paths to Preparedness
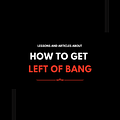
The Importance of Analysis Versus Assessment

There is a difference between an analysis and an assessment. I don’t know that I have always used these two words properly and, while it might sound minor or like just a semantic difference, the words do have specific and very different meanings.
According to the Merriam-Webster Dictionary, an “analysis” is defined as the careful study of something to learn about its parts, what they do and how they are related to each other. To analyze something is to separate a whole into its component parts, which allows a person to break something complex down into simpler and more basic elements. On the other hand, an assessment is defined as the act of making a judgment about something. To assess something, you are estimating the value or character of the object.
Behavioral analysis is used in a number of different contexts by different professionals in relation to threat recognition, child development, mental health concerns, employee development and countless other fields. The way that we use behavioral analysis is to explain how to use the uncontrollable and universal elements of nonverbal communication to facilitate on-the-ground decision-making and the proactive recognition of threats.
While the difference between an analysis and an assessment might seem superficial, distinguishing between the two helps to frame and understand what an organization requires in order to fill their security gaps. As you establish expectations for the time spent learning to read behavior, knowing the difference between behavioral analysis and behavioral assessments can help to provide some clarity about your new ability.
A Tactical Analysis
The Tactical Analysis ® program that we provide to our clients is an application of behavioral analysis to support and inform the decisions made by the members of our nation’s military, law enforcement officers and security professionals, tasked with protecting our country, our citizens and our freedoms. Being able to go through an analysis process to establish the baseline for people and the areas we visit is a crucial skill for the protectors who take our classes.
Tactical analysis is the way we break down people, situations and the environment into its component parts using the four pillars of observable behavior. These four pillars provide enough information to get a comprehensive understanding of a situation without slowing the decision-making process. The four pillars are:
Pillar #1: Individual people
Pillar #2: Groups of people
Pillar #3: How people relate to their environment
Pillar #4: The collective mood
By learning how to analyze behavior in this context, our nation’s protectors can read the human terrain, can identify which parts of their analysis need improvement and realize what information they need to improve their understanding of the situations they are in.
For the military, being able to conduct a tactical analysis of the areas being patrolled allows for a unit to quickly understand the dynamics of the location so they can identify the enemy targeting them more quickly and accurately. The process of collecting the information stemming from the four pillars allows for this group to become operationally effective much sooner in their deployment.
For police officers, being able to conduct a tactical analysis of an area allows a field-training officer to pass their experience on to a rookie officer to make them effective on the job sooner by providing a terminology for the behaviors being observed.
For private security professionals, being able to analyze situations and events using the four pillars means the advance work done by executive protection teams can be thorough and detailed.
Tactical Assessments
Tactical assessments are the fourteen different behavioral assessments that comprise the four pillars of observable behavior, but they are taught in relation to recognizing threats and identifying the members of criminal, insurgent and terrorist organizations. These are the assessments that our nation’s protectors need to make in order to do their job in the most effective way possible and to prevent violence from occurring. The fourteen assessments are:
Pillar #1: Individuals:
People displaying one of the four clusters of behavior:
Dominant, Submissive, Uncomfortable, or Comfortable.
Pillar #2: Groups of People:
The relationship between members of a group are:
Intimate, Personal, Acquaintances, Strangers
Pillar #3: Environment:
Places are either:
Habitual areas or anchor points
People are either:
Familiar or unfamiliar with their surroundings
Pillar #4: The Collective:
Places have either:
Positive atmospherics or negative atmospherics
By learning to make these different behavioral assessments and recognitions, our protectors develop the ability to use these assessments to support some essential decisions:
The four clusters of individual behavior are used to begin the process of identifying people who have violent intentions.
Assessments about group dynamics can be expanded to include how to identify the leaders of groups.
Assessments about the environment include the identification of patterns that reveal when people visit areas with violent intentions
Assessments about the collective mood are taught to identify when a shift in atmospherics reveals a change that a protector should take note of and investigate.
Tying It Back Together
By understanding the assessments you make and improving your ability to make them accurately and rapidly, a professional can more accurately analyze the situation they are in and determine if the existing conditions are in their best interest or if they need to be changed. By developing your ability to use the terminology established in the tactical assessments, veteran operators with a wealth of experience and intuitive understanding of events can analyze them more clearly and articulate how they perceived a situation using the component parts. This helps to develop a novice’s ability to make intelligent decisions through mental preparation and development, but most importantly, provides the structure to go through an analysis process in the same way, each and every time. Developing the habit of conducting a tactical analysis, using the tactical assessments, is what will help our nation’s protectors accelerate through the decision-making process and take action more quickly than the criminal can.

Ready for more?
Analyze vs. Assess
What's the difference.
Analyze and assess are two terms commonly used in research, evaluation, and decision-making processes. While they share similarities, they have distinct differences. Analyze refers to the process of breaking down a complex problem or situation into its constituent parts to gain a deeper understanding. It involves examining the details, patterns, and relationships within the data or information. On the other hand, assess focuses on evaluating or judging the quality, value, or significance of something. It involves making judgments or drawing conclusions based on the analysis conducted. In summary, analyze is about understanding the components, while assess is about evaluating the overall worth or impact.
Further Detail
Comparisons may contain inaccurate information about people, places, or facts. Please report any issues.
- Skip to primary navigation
- Skip to main content
- Skip to footer

Student Affairs Planning, Assessment & Research
Division of Student Affairs
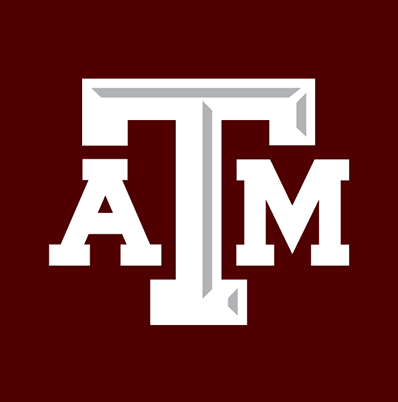
Header Right
- Search website...
Assessment vs. Research: What’s the Difference?
November 1, 2018 by Darby
You may have heard the terms “assessment” and “research” used interchangeably. Are they really the same thing? Does it matter? (And that doesn’t even include throwing “evaluation” into the mix!) There have even been recent debates among professionals about it. (<a href=”http://www.presence.io/blog/assessment-and-research-are-different-things-and-thats-okay/”>http://www.presence.io/blog/assessment-and-research-are-different-things-and-thats-okay/</a>, <a href=”https://www.insidehighered.com/views/2016/11/21/how-assessment-falls-significantly-short-valid-research-essay”>https://www.insidehighered.com/views/2016/11/21/how-assessment-falls-significantly-short-valid-research-essay</a>, <a href=”https://onlinelibrary.wiley.com/doi/abs/10.1002/abc.21273″>https://onlinelibrary.wiley.com/doi/abs/10.1002/abc.21273</a> )
In my opinion, assessment and research have a lot in common. They are about collecting data to learn something, they use similar data collection methodologies (qualitative and quantitative), they require knowledge and practice to be effective, and they are important to student affairs and higher education. There are expectations of good practice in both areas.
On the other hand, there are some key differences. The purpose of research is to create generalizable knowledge, that is, to be able to make credible statements about groups of people beyond one campus. It might be about first year college students, new professionals in student affairs, or college graduates in STEM fields. Research may also be used to develop new theories or test hypotheses. Assessment is typically confined to one program, one campus, or one group. In that case, the purpose is to collect information for improvement to that particular area of interest. Assessment rarely would set up an experimental design to test a hypothesis. The results are not meant to apply to a broader area, but they are key to decision making. Assessment can provide reasonably accurate information to the people who need it, in a complex, changing environment.
The timing of research and assessment may differ. Research may have more flexibility in the time it takes for data collection because it may not be tied to one particular program, service, or experience that will change. Alternatively, assessment may be time bound, because the information is being collected about a particular program or service, so changes can be implemented. It may be an event that occurs on an annual basis, information is needed for a budget request, or data needs to be provided for an annual report.
The expectations of response rate may also be different. Of course, everyone wants a high response rate that reflects the population of interest. Realistically, though, that may not happen. In research, there may be more effort and resources to recruit respondents over a longer time or use already collected large data sets. There may be effort to determine if late responders were similar to early responders or if more recruitment needs to happen. In assessment, partially because of the time-bound nature, and the over-assessment of college students, staff may have to settle for the response rate they get and decide if the results are credible.
The audience may also differ. Ideally, all professionals should be keeping up with the literature in their field based on sound research. Research results are published in journals for other researchers to see and use. More narrow, though, assessment provides (hopefully) useful information to decision makers and practitioners about their particular area. In the big picture, assessment results can inform research questions and vice versa.
Research typically requires Institutional Review Board (IRB) approval, before collecting data from “human subjects.” That board wants to ensure that people are not harmed and appropriate processes are followed. Because of the narrow focus, and usually low risk nature, assessment is typically excused from the IRB process.
All in all, both assessment and research belong in student affairs and higher education. They are important to individual campuses and departments. They just may look a little different in the structure and use. Practitioners need to access both to be the best they can be.
Copyright 2024 • Student Affairs Planning, Assessment & Research | Division of Student Affairs • All Rights Reserved. • Hosted by Division of Student Affairs Department of IT
Explainer: how and why is research assessed?
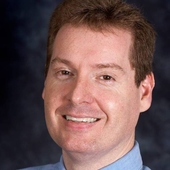
Professor, University of Newcastle
Disclosure statement
Derek R. Smith does not work for, consult, own shares in or receive funding from any company or organisation that would benefit from this article, and has disclosed no relevant affiliations beyond their academic appointment.
University of Newcastle provides funding as a member of The Conversation AU.
View all partners
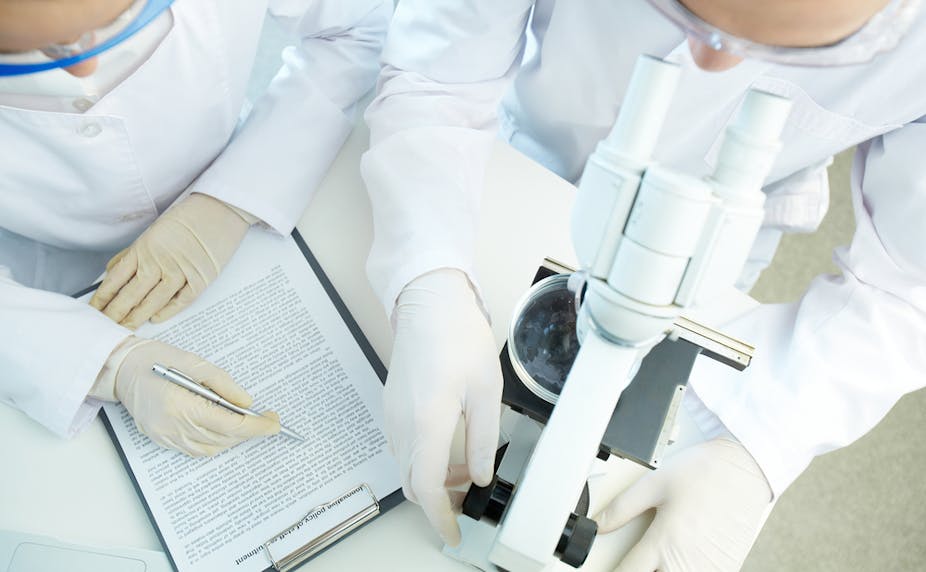
Governments and taxpayers deserve to know that their money is being spent on something worthwhile to society . Individuals and groups who are making the greatest contribution to science and to the community deserve to be recognised . For these reasons, all research has to be assessed.
Judging the importance of research is often done by looking at the number of citations a piece of research receives after it has been published.
Let’s say Researcher A figures out something important (such as how to cure a disease). He or she then publishes this information in a scientific journal, which Researcher B reads. Researcher B then does their own experiments and writes up the results in a scientific journal, which refers to the original work of Researcher A. Researcher B has now cited Researcher A.
Thousands of experiments are conducted around the world each year, but not all of the results are useful. In fact, a lot of scientific research that governments pay for is often ignored after it’s published. For example, of the 38 million scientific articles published between 1900 and 2005, half were not cited at all .
To ensure the research they are paying for is of use, governments need a way to decide which researchers and topics they should continue to support. Any system should be fair and, ideally, all researchers should be scored using the same measure.
This is why the field of bibliometrics has become so important in recent years. Bibliometric analysis helps governments to number and rank researchers, making them easier to compare.
Let’s say the disease that Researcher A studies is pretty common, such as cancer, which means that many people are looking at ways to cure it. In the mix now there would be Researchers C, D and E, all publishing their own work on cancer. Governments take notice if, for example, ten people cite the work of Researcher A and only two cite the work of Researcher C.
If everyone in the world who works in the same field as Researcher A gets their research cited on average (say) twice each time they publish, then the international citation benchmark for that topic (in bibliometrics) would be two. The work of Researcher A, with his or her citation rate of ten (five times higher than the world average), is now going to get noticed.
Excellence for Research in Australia
Bibliometric analysis and citation benchmarks form a key part of how research is assessed in Australia. The Excellence for Research in Australia ( ERA ) process evaluates the quality of research being undertaken at Australian universities against national and international benchmarks. It is administered by the Australian Research Council ( ARC ) and helps the government decide what research is important and what should continue to receive support.
Although these are not the only components assessed in the ERA process, bibliometric data and citation analysis are still a big part of the performance scores that universities and institutions receive.
Many other countries apply formal research assessment systems to universities and have done so for many years. The United Kingdom, for example, operated a process known as the Research Assessment Exercise between 1986 and 2001. This was superseded by the Research Excellence Framework in 2014.
A bibliometrics-based performance model has also been employed in Norway since 2002. This model was first used to influence budget allocations in 2006, based on scientific publications from the previous year.
Although many articles don’t end up getting cited, this doesn’t always mean the research itself didn’t matter. Take, for example, the polio vaccine developed by Albert Sabin last century, which saves over 300,000 lives around the world each year.
Sabin and others published the main findings in 1960 in what has now become one of the most important scientific articles of all time. By the late 1980s, however, Sabin’s article had not even been cited 100 times .
On the other hand, we have Oliver Lowry, who in 1951 published an article describing a new method for measuring the amount of protein in solutions,. This has become the most highly cited article of all time (over 300,000 citations and counting). Even Lowry was surprised by its “success”, pointing out that he wasn’t really a genius and that this study was by no means his best work.
The history of research assessment
While some may regard the assessment of research as a modern phenomenon inspired by a new generation of faceless bean-counters, the concept has been around for centuries.
Sir Francis Galton , a celebrated geneticist and statistician, was probably the first well-known person to examine the performance of individual scientists, publishing a landmark book, English Men of Science , in the 1870s.
Galton’s work evidently inspired others, with an American book, American Men of Science , appearing in the early 1900s.
Productivity rates for scientists and academics (precursors to today’s performance benchmarks and KPIs) have also existed in one form or another for many years. One of the first performance “benchmarks” appeared in a 1940s book, The Academic Man , which described the output of American academics.
This book is probably most famous for coining the phrase “publish or perish” - the belief that an academic’s fate is doomed if they don’t get their research published. It’s a fate that bibliometric analysis and other citation benchmarks now reinforce.
- Research assessment

Data Manager

Research Support Officer

Director, Social Policy

Head, School of Psychology

Senior Research Fellow - Women's Health Services
- Popular Tools
Customer Relationship Management
- Web Development & Design
- Video Communication
User Experience
- Hosting Tools
- Automation Tools
- Marketing & Analytics
- Fintech and Banking
- Streaming Services
No products in the cart.
Analysis vs. Assessment: Sequencing and Importance in Decision-Making
04 January 2024 - Business Solutions
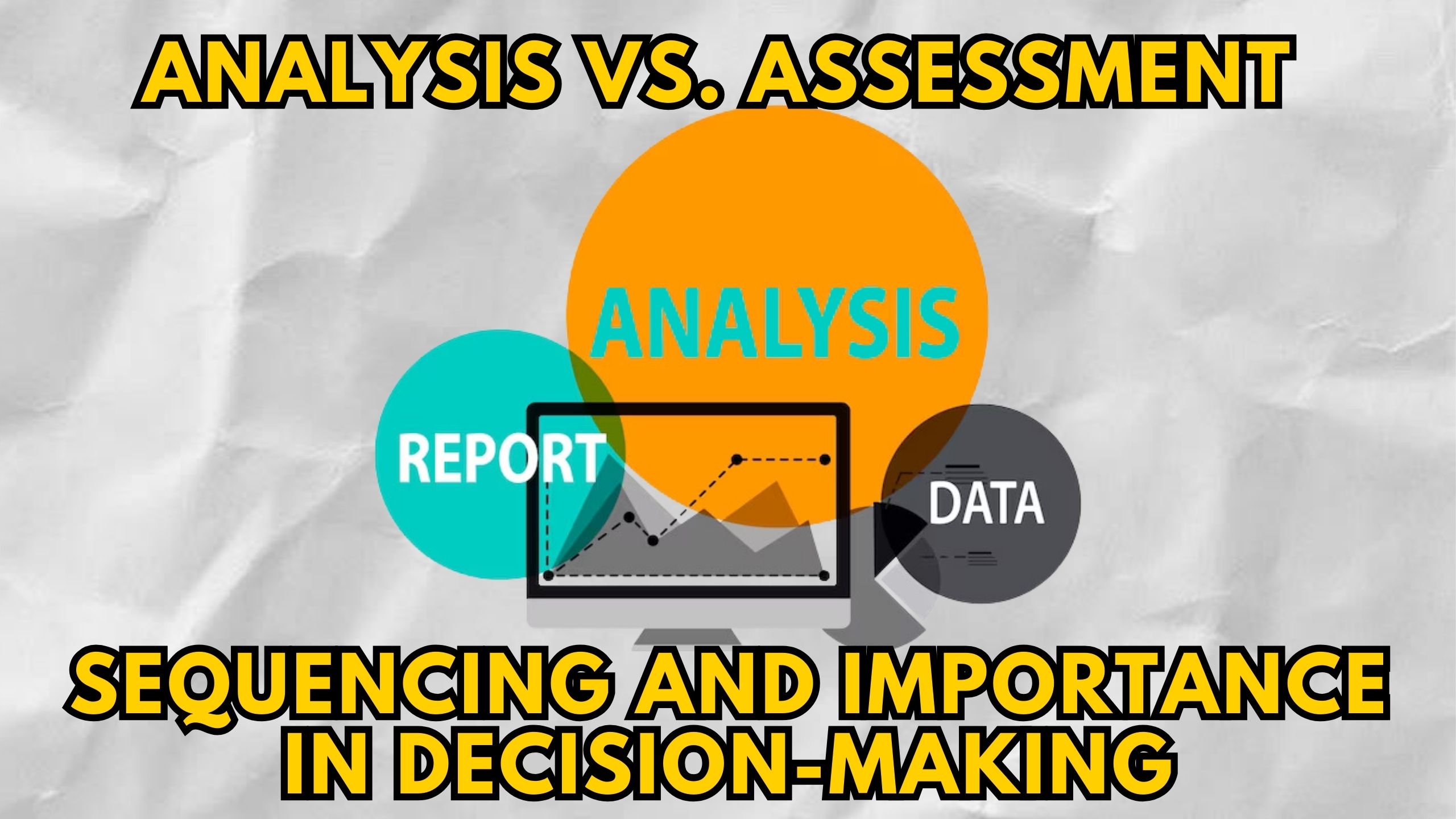
Share Insight
Share the comparison insight with others
In the realm of decision-making, understanding the nuances between analysis and assessment is crucial. This blog delves into the sequencing and importance of these processes, exploring their distinct roles in informed decision-making. We’ll also introduce five relevant SaaS products designed to streamline these critical aspects.
The Dynamics of Analysis and Assessment
Unveiling the process of analysis.
Analysis involves breaking down complex information into simpler components, facilitating a detailed understanding. In decision-making, this often comes as the initial step, providing a foundation for subsequent assessments.
Navigating the Terrain of Assessment
Assessment, on the other hand, focuses on evaluating and gauging the significance of analyzed information. It’s a holistic process that considers various factors to make informed judgments or decisions.
Sequencing Dilemma: Which Comes First?
The age-old question persists: which comes first, analysis or assessment? While both are interlinked, establishing a sequence depends on the context. Effective decision-making often sees analysis paving the way for assessments, ensuring a comprehensive understanding before evaluations.
Relevant SaaS Products Transforming Decision-Making
Empowering analysis with intuitive data visualization, Tableau transforms raw data into actionable insights. Its relevance lies in providing a clear visual narrative for in-depth analysis.
2. Qualtrics
As a versatile assessment tool, Qualtrics enables businesses to gather valuable feedback and insights. It’s a vital component in the decision-making process, ensuring a robust understanding of stakeholder perspectives.
3. Google Analytics
A cornerstone in analyzing online data, Google Analytics offers profound insights into user behavior. Understanding user interactions is pivotal for strategic assessments in digital landscapes.
Streamlining communication analysis, Crisp provides real-time data on customer interactions. This tool is relevant for assessing customer engagement and tailoring communication strategies.
5. Zoho Analytics
Bridging the gap between analysis and assessment, Zoho Analytics offers a comprehensive platform. It allows businesses to analyze data intricately and assess performance across various domains.
In the intricate dance of decision-making, analysis and assessment perform unique yet intertwined roles. Recognizing their sequencing and importance is paramount for navigating the complexities of choices. Now armed with this knowledge, embark on a journey of informed decision-making.
Revolutionize your decision-making prowess with Subscribed.fyi :
Unlock Secret Deals and Save Big: Exclusive deals on 100+ SaaS tools await. Your savings of $100,000+ per year are just a click away.
Manage All Subscriptions in One Place: Effortlessly track, monitor, and control your subscriptions for enhanced decision-making.
Compare and Evaluate: Make informed decisions by comparing SaaS tools side by side.
Join Subscribed.fyi for free and revolutionize your SaaS stack. Sign up today to unlock a world of possibilities in decision-making.
Relevant Links:
- Google Analytics
- Zoho Analytics
Other articles
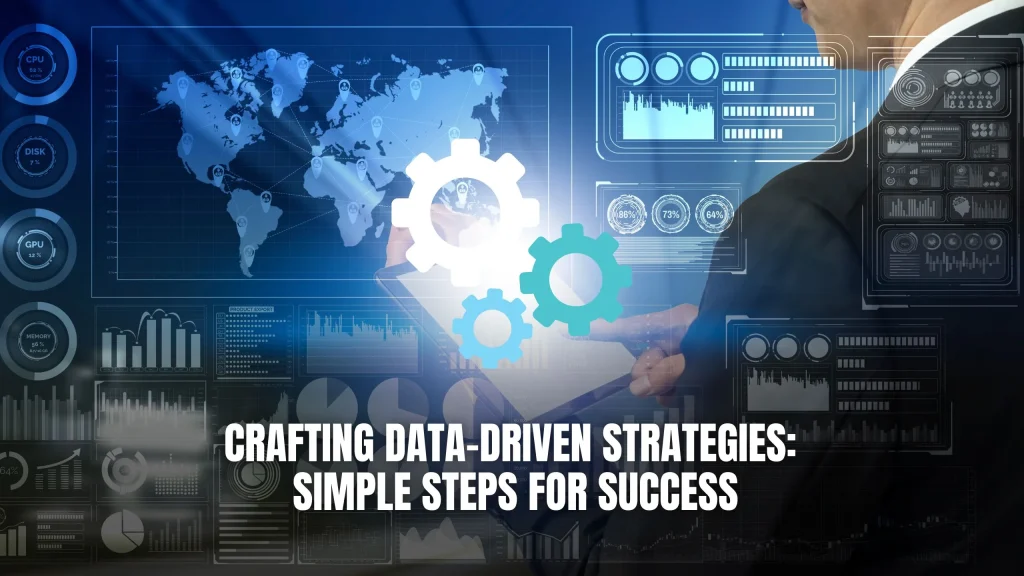
Analytics and Data Tools
Crafting Data-Driven Strategies: Simple Steps for Success
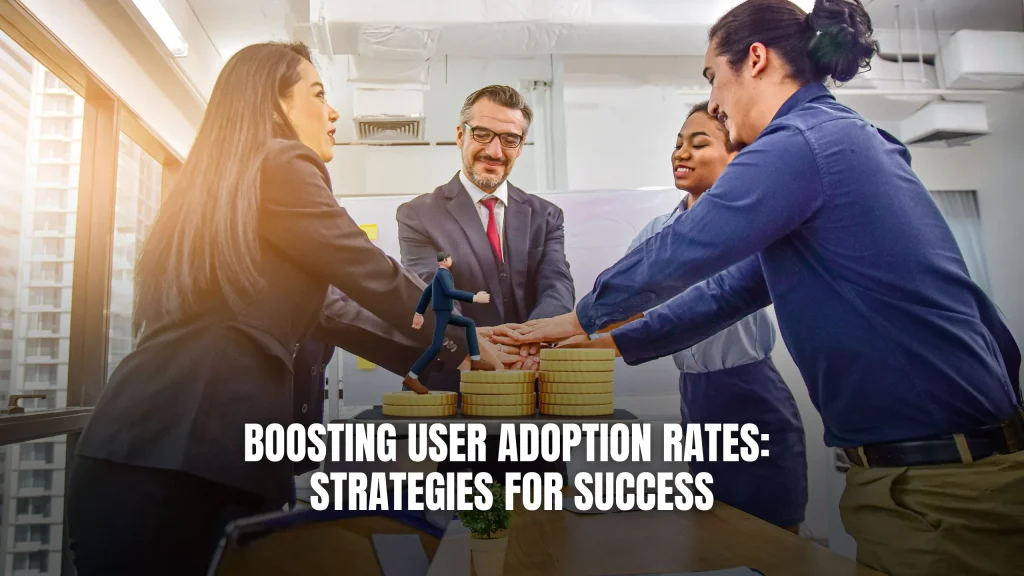
Boosting User Adoption Rates: Strategies for Success
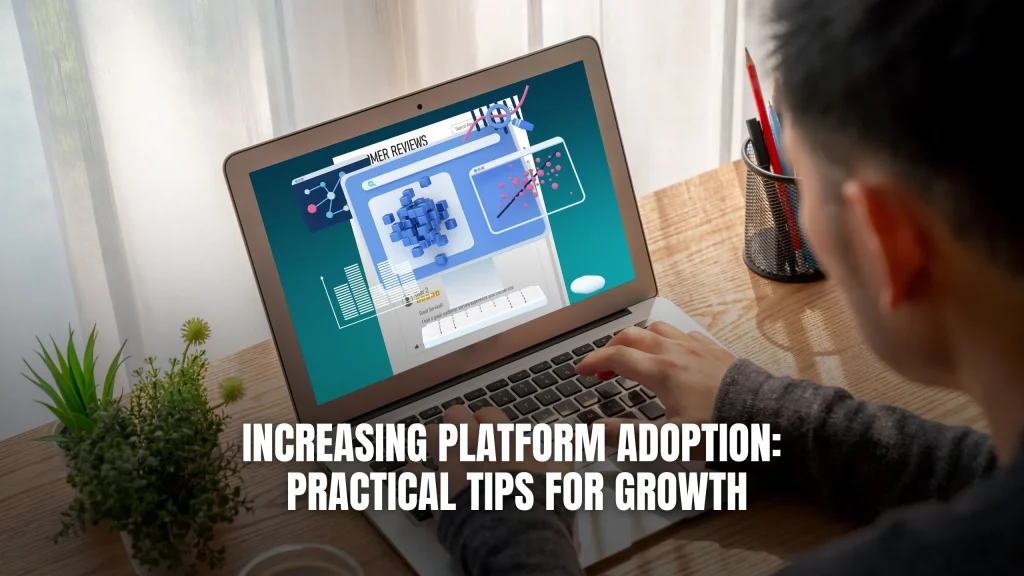
Increasing Platform Adoption: Practical Tips for Growth
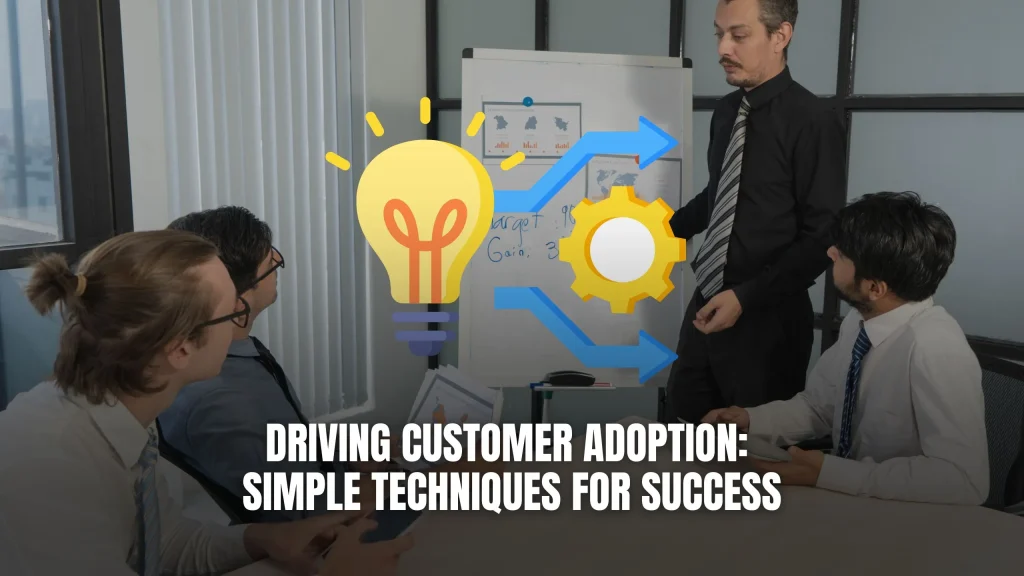
Driving Customer Adoption: Simple Techniques for Success
- Search Menu
- Sign in through your institution
- Advance articles
- Author Guidelines
- Submission Site
- Open Access
- Why Publish?
- About Research Evaluation
- Editorial Board
- Advertising and Corporate Services
- Journals Career Network
- Self-Archiving Policy
- Dispatch Dates
- Journals on Oxford Academic
- Books on Oxford Academic
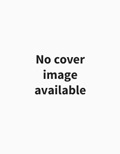
Article Contents
1. introduction, what is meant by impact, 2. why evaluate research impact, 3. evaluating research impact, 4. impact and the ref, 5. the challenges of impact evaluation, 6. developing systems and taxonomies for capturing impact, 7. indicators, evidence, and impact within systems, 8. conclusions and recommendations.
- < Previous
Assessment, evaluations, and definitions of research impact: A review
- Article contents
- Figures & tables
- Supplementary Data
Teresa Penfield, Matthew J. Baker, Rosa Scoble, Michael C. Wykes, Assessment, evaluations, and definitions of research impact: A review, Research Evaluation , Volume 23, Issue 1, January 2014, Pages 21–32, https://doi.org/10.1093/reseval/rvt021
- Permissions Icon Permissions
This article aims to explore what is understood by the term ‘research impact’ and to provide a comprehensive assimilation of available literature and information, drawing on global experiences to understand the potential for methods and frameworks of impact assessment being implemented for UK impact assessment. We take a more focused look at the impact component of the UK Research Excellence Framework taking place in 2014 and some of the challenges to evaluating impact and the role that systems might play in the future for capturing the links between research and impact and the requirements we have for these systems.
When considering the impact that is generated as a result of research, a number of authors and government recommendations have advised that a clear definition of impact is required ( Duryea, Hochman, and Parfitt 2007 ; Grant et al. 2009 ; Russell Group 2009 ). From the outset, we note that the understanding of the term impact differs between users and audiences. There is a distinction between ‘academic impact’ understood as the intellectual contribution to one’s field of study within academia and ‘external socio-economic impact’ beyond academia. In the UK, evaluation of academic and broader socio-economic impact takes place separately. ‘Impact’ has become the term of choice in the UK for research influence beyond academia. This distinction is not so clear in impact assessments outside of the UK, where academic outputs and socio-economic impacts are often viewed as one, to give an overall assessment of value and change created through research.
an effect on, change or benefit to the economy, society, culture, public policy or services, health, the environment or quality of life, beyond academia
Impact is assessed alongside research outputs and environment to provide an evaluation of research taking place within an institution. As such research outputs, for example, knowledge generated and publications, can be translated into outcomes, for example, new products and services, and impacts or added value ( Duryea et al. 2007 ). Although some might find the distinction somewhat marginal or even confusing, this differentiation between outputs, outcomes, and impacts is important, and has been highlighted, not only for the impacts derived from university research ( Kelly and McNicol 2011 ) but also for work done in the charitable sector ( Ebrahim and Rangan, 2010 ; Berg and Månsson 2011 ; Kelly and McNicoll 2011 ). The Social Return on Investment (SROI) guide ( The SROI Network 2012 ) suggests that ‘The language varies “impact”, “returns”, “benefits”, “value” but the questions around what sort of difference and how much of a difference we are making are the same’. It is perhaps assumed here that a positive or beneficial effect will be considered as an impact but what about changes that are perceived to be negative? Wooding et al. (2007) adapted the terminology of the Payback Framework, developed for the health and biomedical sciences from ‘benefit’ to ‘impact’ when modifying the framework for the social sciences, arguing that the positive or negative nature of a change was subjective and can also change with time, as has commonly been highlighted with the drug thalidomide, which was introduced in the 1950s to help with, among other things, morning sickness but due to teratogenic effects, which resulted in birth defects, was withdrawn in the early 1960s. Thalidomide has since been found to have beneficial effects in the treatment of certain types of cancer. Clearly the impact of thalidomide would have been viewed very differently in the 1950s compared with the 1960s or today.
In viewing impact evaluations it is important to consider not only who has evaluated the work but the purpose of the evaluation to determine the limits and relevance of an assessment exercise. In this article, we draw on a broad range of examples with a focus on methods of evaluation for research impact within Higher Education Institutions (HEIs). As part of this review, we aim to explore the following questions:
What are the reasons behind trying to understand and evaluate research impact?
What are the methodologies and frameworks that have been employed globally to assess research impact and how do these compare?
What are the challenges associated with understanding and evaluating research impact?
What indicators, evidence, and impacts need to be captured within developing systems
What are the reasons behind trying to understand and evaluate research impact? Throughout history, the activities of a university have been to provide both education and research, but the fundamental purpose of a university was perhaps described in the writings of mathematician and philosopher Alfred North Whitehead (1929) .
‘The justification for a university is that it preserves the connection between knowledge and the zest of life, by uniting the young and the old in the imaginative consideration of learning. The university imparts information, but it imparts it imaginatively. At least, this is the function which it should perform for society. A university which fails in this respect has no reason for existence. This atmosphere of excitement, arising from imaginative consideration transforms knowledge.’
In undertaking excellent research, we anticipate that great things will come and as such one of the fundamental reasons for undertaking research is that we will generate and transform knowledge that will benefit society as a whole.
One might consider that by funding excellent research, impacts (including those that are unforeseen) will follow, and traditionally, assessment of university research focused on academic quality and productivity. Aspects of impact, such as value of Intellectual Property, are currently recorded by universities in the UK through their Higher Education Business and Community Interaction Survey return to Higher Education Statistics Agency; however, as with other public and charitable sector organizations, showcasing impact is an important part of attracting and retaining donors and support ( Kelly and McNicoll 2011 ).
The reasoning behind the move towards assessing research impact is undoubtedly complex, involving both political and socio-economic factors, but, nevertheless, we can differentiate between four primary purposes.
HEIs overview. To enable research organizations including HEIs to monitor and manage their performance and understand and disseminate the contribution that they are making to local, national, and international communities.
Accountability. To demonstrate to government, stakeholders, and the wider public the value of research. There has been a drive from the UK government through Higher Education Funding Council for England (HEFCE) and the Research Councils ( HM Treasury 2004 ) to account for the spending of public money by demonstrating the value of research to tax payers, voters, and the public in terms of socio-economic benefits ( European Science Foundation 2009 ), in effect, justifying this expenditure ( Davies Nutley, and Walter 2005 ; Hanney and González-Block 2011 ).
Inform funding. To understand the socio-economic value of research and subsequently inform funding decisions. By evaluating the contribution that research makes to society and the economy, future funding can be allocated where it is perceived to bring about the desired impact. As Donovan (2011) comments, ‘Impact is a strong weapon for making an evidence based case to governments for enhanced research support’.
Understand. To understand the method and routes by which research leads to impacts to maximize on the findings that come out of research and develop better ways of delivering impact.
The growing trend for accountability within the university system is not limited to research and is mirrored in assessments of teaching quality, which now feed into evaluation of universities to ensure fee-paying students’ satisfaction. In demonstrating research impact, we can provide accountability upwards to funders and downwards to users on a project and strategic basis ( Kelly and McNicoll 2011 ). Organizations may be interested in reviewing and assessing research impact for one or more of the aforementioned purposes and this will influence the way in which evaluation is approached.
It is important to emphasize that ‘Not everyone within the higher education sector itself is convinced that evaluation of higher education activity is a worthwhile task’ ( Kelly and McNicoll 2011 ). The University and College Union ( University and College Union 2011 ) organized a petition calling on the UK funding councils to withdraw the inclusion of impact assessment from the REF proposals once plans for the new assessment of university research were released. This petition was signed by 17,570 academics (52,409 academics were returned to the 2008 Research Assessment Exercise), including Nobel laureates and Fellows of the Royal Society ( University and College Union 2011 ). Impact assessments raise concerns over the steer of research towards disciplines and topics in which impact is more easily evidenced and that provide economic impacts that could subsequently lead to a devaluation of ‘blue skies’ research. Johnston ( Johnston 1995 ) notes that by developing relationships between researchers and industry, new research strategies can be developed. This raises the questions of whether UK business and industry should not invest in the research that will deliver them impacts and who will fund basic research if not the government? Donovan (2011) asserts that there should be no disincentive for conducting basic research. By asking academics to consider the impact of the research they undertake and by reviewing and funding them accordingly, the result may be to compromise research by steering it away from the imaginative and creative quest for knowledge. Professor James Ladyman, at the University of Bristol, a vocal adversary of awarding funding based on the assessment of research impact, has been quoted as saying that ‘…inclusion of impact in the REF will create “selection pressure,” promoting academic research that has “more direct economic impact” or which is easier to explain to the public’ ( Corbyn 2009 ).
Despite the concerns raised, the broader socio-economic impacts of research will be included and count for 20% of the overall research assessment, as part of the REF in 2014. From an international perspective, this represents a step change in the comprehensive nature to which impact will be assessed within universities and research institutes, incorporating impact from across all research disciplines. Understanding what impact looks like across the various strands of research and the variety of indicators and proxies used to evidence impact will be important to developing a meaningful assessment.
What are the methodologies and frameworks that have been employed globally to evaluate research impact and how do these compare? The traditional form of evaluation of university research in the UK was based on measuring academic impact and quality through a process of peer review ( Grant 2006 ). Evidence of academic impact may be derived through various bibliometric methods, one example of which is the H index, which has incorporated factors such as the number of publications and citations. These metrics may be used in the UK to understand the benefits of research within academia and are often incorporated into the broader perspective of impact seen internationally, for example, within the Excellence in Research for Australia and using Star Metrics in the USA, in which quantitative measures are used to assess impact, for example, publications, citation, and research income. These ‘traditional’ bibliometric techniques can be regarded as giving only a partial picture of full impact ( Bornmann and Marx 2013 ) with no link to causality. Standard approaches actively used in programme evaluation such as surveys, case studies, bibliometrics, econometrics and statistical analyses, content analysis, and expert judgment are each considered by some (Vonortas and Link, 2012) to have shortcomings when used to measure impacts.
Incorporating assessment of the wider socio-economic impact began using metrics-based indicators such as Intellectual Property registered and commercial income generated ( Australian Research Council 2008 ). In the UK, more sophisticated assessments of impact incorporating wider socio-economic benefits were first investigated within the fields of Biomedical and Health Sciences ( Grant 2006 ), an area of research that wanted to be able to justify the significant investment it received. Frameworks for assessing impact have been designed and are employed at an organizational level addressing the specific requirements of the organization and stakeholders. As a result, numerous and widely varying models and frameworks for assessing impact exist. Here we outline a few of the most notable models that demonstrate the contrast in approaches available.
The Payback Framework is possibly the most widely used and adapted model for impact assessment ( Wooding et al. 2007 ; Nason et al. 2008 ), developed during the mid-1990s by Buxton and Hanney, working at Brunel University. It incorporates both academic outputs and wider societal benefits ( Donovan and Hanney 2011 ) to assess outcomes of health sciences research. The Payback Framework systematically links research with the associated benefits ( Scoble et al. 2010 ; Hanney and González-Block 2011 ) and can be thought of in two parts: a model that allows the research and subsequent dissemination process to be broken into specific components within which the benefits of research can be studied, and second, a multi-dimensional classification scheme into which the various outputs, outcomes, and impacts can be placed ( Hanney and Gonzalez Block 2011 ). The Payback Framework has been adopted internationally, largely within the health sector, by organizations such as the Canadian Institute of Health Research, the Dutch Public Health Authority, the Australian National Health and Medical Research Council, and the Welfare Bureau in Hong Kong ( Bernstein et al. 2006 ; Nason et al. 2008 ; CAHS 2009; Spaapen et al. n.d. ). The Payback Framework enables health and medical research and impact to be linked and the process by which impact occurs to be traced. For more extensive reviews of the Payback Framework, see Davies et al. (2005) , Wooding et al. (2007) , Nason et al. (2008) , and Hanney and González-Block (2011) .
A very different approach known as Social Impact Assessment Methods for research and funding instruments through the study of Productive Interactions (SIAMPI) was developed from the Dutch project Evaluating Research in Context and has a central theme of capturing ‘productive interactions’ between researchers and stakeholders by analysing the networks that evolve during research programmes ( Spaapen and Drooge, 2011 ; Spaapen et al. n.d. ). SIAMPI is based on the widely held assumption that interactions between researchers and stakeholder are an important pre-requisite to achieving impact ( Donovan 2011 ; Hughes and Martin 2012 ; Spaapen et al. n.d. ). This framework is intended to be used as a learning tool to develop a better understanding of how research interactions lead to social impact rather than as an assessment tool for judging, showcasing, or even linking impact to a specific piece of research. SIAMPI has been used within the Netherlands Institute for health Services Research ( SIAMPI n.d. ). ‘Productive interactions’, which can perhaps be viewed as instances of knowledge exchange, are widely valued and supported internationally as mechanisms for enabling impact and are often supported financially for example by Canada’s Social Sciences and Humanities Research Council, which aims to support knowledge exchange (financially) with a view to enabling long-term impact. In the UK, UK Department for Business, Innovation, and Skills provided funding of £150 million for knowledge exchange in 2011–12 to ‘help universities and colleges support the economic recovery and growth, and contribute to wider society’ ( Department for Business, Innovation and Skills 2012 ). While valuing and supporting knowledge exchange is important, SIAMPI perhaps takes this a step further in enabling these exchange events to be captured and analysed. One of the advantages of this method is that less input is required compared with capturing the full route from research to impact. A comprehensive assessment of impact itself is not undertaken with SIAMPI, which make it a less-suitable method where showcasing the benefits of research is desirable or where this justification of funding based on impact is required.
The first attempt globally to comprehensively capture the socio-economic impact of research across all disciplines was undertaken for the Australian Research Quality Framework (RQF), using a case study approach. The RQF was developed to demonstrate and justify public expenditure on research, and as part of this framework, a pilot assessment was undertaken by the Australian Technology Network. Researchers were asked to evidence the economic, societal, environmental, and cultural impact of their research within broad categories, which were then verified by an expert panel ( Duryea et al. 2007 ) who concluded that the researchers and case studies could provide enough qualitative and quantitative evidence for reviewers to assess the impact arising from their research ( Duryea et al. 2007 ). To evaluate impact, case studies were interrogated and verifiable indicators assessed to determine whether research had led to reciprocal engagement, adoption of research findings, or public value. The RQF pioneered the case study approach to assessing research impact; however, with a change in government in 2007, this framework was never implemented in Australia, although it has since been taken up and adapted for the UK REF.
In developing the UK REF, HEFCE commissioned a report, in 2009, from RAND to review international practice for assessing research impact and provide recommendations to inform the development of the REF. RAND selected four frameworks to represent the international arena ( Grant et al. 2009 ). One of these, the RQF, they identified as providing a ‘promising basis for developing an impact approach for the REF’ using the case study approach. HEFCE developed an initial methodology that was then tested through a pilot exercise. The case study approach, recommended by the RQF, was combined with ‘significance’ and ‘reach’ as criteria for assessment. The criteria for assessment were also supported by a model developed by Brunel for ‘measurement’ of impact that used similar measures defined as depth and spread. In the Brunel model, depth refers to the degree to which the research has influenced or caused change, whereas spread refers to the extent to which the change has occurred and influenced end users. Evaluation of impact in terms of reach and significance allows all disciplines of research and types of impact to be assessed side-by-side ( Scoble et al. 2010 ).
The range and diversity of frameworks developed reflect the variation in purpose of evaluation including the stakeholders for whom the assessment takes place, along with the type of impact and evidence anticipated. The most appropriate type of evaluation will vary according to the stakeholder whom we are wishing to inform. Studies ( Buxton, Hanney and Jones 2004 ) into the economic gains from biomedical and health sciences determined that different methodologies provide different ways of considering economic benefits. A discussion on the benefits and drawbacks of a range of evaluation tools (bibliometrics, economic rate of return, peer review, case study, logic modelling, and benchmarking) can be found in the article by Grant (2006) .
Evaluation of impact is becoming increasingly important, both within the UK and internationally, and research and development into impact evaluation continues, for example, researchers at Brunel have developed the concept of depth and spread further into the Brunel Impact Device for Evaluation, which also assesses the degree of separation between research and impact ( Scoble et al. working paper ).
Although based on the RQF, the REF did not adopt all of the suggestions held within, for example, the option of allowing research groups to opt out of impact assessment should the nature or stage of research deem it unsuitable ( Donovan 2008 ). In 2009–10, the REF team conducted a pilot study for the REF involving 29 institutions, submitting case studies to one of five units of assessment (in clinical medicine, physics, earth systems and environmental sciences, social work and social policy, and English language and literature) ( REF2014 2010 ). These case studies were reviewed by expert panels and, as with the RQF, they found that it was possible to assess impact and develop ‘impact profiles’ using the case study approach ( REF2014 2010 ).
From 2014, research within UK universities and institutions will be assessed through the REF; this will replace the Research Assessment Exercise, which has been used to assess UK research since the 1980s. Differences between these two assessments include the removal of indicators of esteem and the addition of assessment of socio-economic research impact. The REF will therefore assess three aspects of research:
Environment
Research impact is assessed in two formats, first, through an impact template that describes the approach to enabling impact within a unit of assessment, and second, using impact case studies that describe the impact taking place following excellent research within a unit of assessment ( REF2014 2011a ). HEFCE indicated that impact should merit a 25% weighting within the REF ( REF2014 2011b ); however, this has been reduced for the 2014 REF to 20%, perhaps as a result of feedback and lobbying, for example, from the Russell Group and Million + group of Universities who called for impact to count for 15% ( Russell Group 2009 ; Jump 2011 ) and following guidance from the expert panels undertaking the pilot exercise who suggested that during the 2014 REF, impact assessment would be in a developmental phase and that a lower weighting for impact would be appropriate with the expectation that this would be increased in subsequent assessments ( REF2014 2010 ).
The quality and reliability of impact indicators will vary according to the impact we are trying to describe and link to research. In the UK, evidence and research impacts will be assessed for the REF within research disciplines. Although it can be envisaged that the range of impacts derived from research of different disciplines are likely to vary, one might question whether it makes sense to compare impacts within disciplines when the range of impact can vary enormously, for example, from business development to cultural changes or saving lives? An alternative approach was suggested for the RQF in Australia, where it was proposed that types of impact be compared rather than impact from specific disciplines.
Providing advice and guidance within specific disciplines is undoubtedly helpful. It can be seen from the panel guidance produced by HEFCE to illustrate impacts and evidence that it is expected that impact and evidence will vary according to discipline ( REF2014 2012 ). Why should this be the case? Two areas of research impact health and biomedical sciences and the social sciences have received particular attention in the literature by comparison with, for example, the arts. Reviews and guidance on developing and evidencing impact in particular disciplines include the London School of Economics (LSE) Public Policy Group’s impact handbook (LSE n.d.), a review of the social and economic impacts arising from the arts produced by Reeve ( Reeves 2002 ), and a review by Kuruvilla et al. (2006) on the impact arising from health research. Perhaps it is time for a generic guide based on types of impact rather than research discipline?
What are the challenges associated with understanding and evaluating research impact? In endeavouring to assess or evaluate impact, a number of difficulties emerge and these may be specific to certain types of impact. Given that the type of impact we might expect varies according to research discipline, impact-specific challenges present us with the problem that an evaluation mechanism may not fairly compare impact between research disciplines.
5.1 Time lag
The time lag between research and impact varies enormously. For example, the development of a spin out can take place in a very short period, whereas it took around 30 years from the discovery of DNA before technology was developed to enable DNA fingerprinting. In development of the RQF, The Allen Consulting Group (2005) highlighted that defining a time lag between research and impact was difficult. In the UK, the Russell Group Universities responded to the REF consultation by recommending that no time lag be put on the delivery of impact from a piece of research citing examples such as the development of cardiovascular disease treatments, which take between 10 and 25 years from research to impact ( Russell Group 2009 ). To be considered for inclusion within the REF, impact must be underpinned by research that took place between 1 January 1993 and 31 December 2013, with impact occurring during an assessment window from 1 January 2008 to 31 July 2013. However, there has been recognition that this time window may be insufficient in some instances, with architecture being granted an additional 5-year period ( REF2014 2012 ); why only architecture has been granted this dispensation is not clear, when similar cases could be made for medicine, physics, or even English literature. Recommendations from the REF pilot were that the panel should be able to extend the time frame where appropriate; this, however, poses difficult decisions when submitting a case study to the REF as to what the view of the panel will be and whether if deemed inappropriate this will render the case study ‘unclassified’.
5.2 The developmental nature of impact
Impact is not static, it will develop and change over time, and this development may be an increase or decrease in the current degree of impact. Impact can be temporary or long-lasting. The point at which assessment takes place will therefore influence the degree and significance of that impact. For example, following the discovery of a new potential drug, preclinical work is required, followed by Phase 1, 2, and 3 trials, and then regulatory approval is granted before the drug is used to deliver potential health benefits. Clearly there is the possibility that the potential new drug will fail at any one of these phases but each phase can be classed as an interim impact of the original discovery work on route to the delivery of health benefits, but the time at which an impact assessment takes place will influence the degree of impact that has taken place. If impact is short-lived and has come and gone within an assessment period, how will it be viewed and considered? Again the objective and perspective of the individuals and organizations assessing impact will be key to understanding how temporal and dissipated impact will be valued in comparison with longer-term impact.
5.3 Attribution
Impact is derived not only from targeted research but from serendipitous findings, good fortune, and complex networks interacting and translating knowledge and research. The exploitation of research to provide impact occurs through a complex variety of processes, individuals, and organizations, and therefore, attributing the contribution made by a specific individual, piece of research, funding, strategy, or organization to an impact is not straight forward. Husbands-Fealing suggests that to assist identification of causality for impact assessment, it is useful to develop a theoretical framework to map the actors, activities, linkages, outputs, and impacts within the system under evaluation, which shows how later phases result from earlier ones. Such a framework should be not linear but recursive, including elements from contextual environments that influence and/or interact with various aspects of the system. Impact is often the culmination of work within spanning research communities ( Duryea et al. 2007 ). Concerns over how to attribute impacts have been raised many times ( The Allen Consulting Group 2005 ; Duryea et al. 2007 ; Grant et al. 2009 ), and differentiating between the various major and minor contributions that lead to impact is a significant challenge.
Figure 1 , replicated from Hughes and Martin (2012) , illustrates how the ease with which impact can be attributed decreases with time, whereas the impact, or effect of complementary assets, increases, highlighting the problem that it may take a considerable amount of time for the full impact of a piece of research to develop but because of this time and the increase in complexity of the networks involved in translating the research and interim impacts, it is more difficult to attribute and link back to a contributing piece of research.

Time, attribution, impact. Replicated from ( Hughes and Martin 2012 ).
This presents particular difficulties in research disciplines conducting basic research, such as pure mathematics, where the impact of research is unlikely to be foreseen. Research findings will be taken up in other branches of research and developed further before socio-economic impact occurs, by which point, attribution becomes a huge challenge. If this research is to be assessed alongside more applied research, it is important that we are able to at least determine the contribution of basic research. It has been acknowledged that outstanding leaps forward in knowledge and understanding come from immersing in a background of intellectual thinking that ‘one is able to see further by standing on the shoulders of giants’.
5.4 Knowledge creep
It is acknowledged that one of the outcomes of developing new knowledge through research can be ‘knowledge creep’ where new data or information becomes accepted and gets absorbed over time. This is particularly recognized in the development of new government policy where findings can influence policy debate and policy change, without recognition of the contributing research ( Davies et al. 2005 ; Wooding et al. 2007 ). This is recognized as being particularly problematic within the social sciences where informing policy is a likely impact of research. In putting together evidence for the REF, impact can be attributed to a specific piece of research if it made a ‘distinctive contribution’ ( REF2014 2011a ). The difficulty then is how to determine what the contribution has been in the absence of adequate evidence and how we ensure that research that results in impacts that cannot be evidenced is valued and supported.
5.5 Gathering evidence
Gathering evidence of the links between research and impact is not only a challenge where that evidence is lacking. The introduction of impact assessments with the requirement to collate evidence retrospectively poses difficulties because evidence, measurements, and baselines have, in many cases, not been collected and may no longer be available. While looking forward, we will be able to reduce this problem in the future, identifying, capturing, and storing the evidence in such a way that it can be used in the decades to come is a difficulty that we will need to tackle.
Collating the evidence and indicators of impact is a significant task that is being undertaken within universities and institutions globally. Decker et al. (2007) surveyed researchers in the US top research institutions during 2005; the survey of more than 6000 researchers found that, on average, more than 40% of their time was spent doing administrative tasks. It is desirable that the assignation of administrative tasks to researchers is limited, and therefore, to assist the tracking and collating of impact data, systems are being developed involving numerous projects and developments internationally, including Star Metrics in the USA, the ERC (European Research Council) Research Information System, and Lattes in Brazil ( Lane 2010 ; Mugabushaka and Papazoglou 2012 ).
Ideally, systems within universities internationally would be able to share data allowing direct comparisons, accurate storage of information developed in collaborations, and transfer of comparable data as researchers move between institutions. To achieve compatible systems, a shared language is required. CERIF (Common European Research Information Format) was developed for this purpose, first released in 1991; a number of projects and systems across Europe such as the ERC Research Information System ( Mugabushaka and Papazoglou 2012 ) are being developed as CERIF-compatible.
In the UK, there have been several Jisc-funded projects in recent years to develop systems capable of storing research information, for example, MICE (Measuring Impacts Under CERIF), UK Research Information Shared Service, and Integrated Research Input and Output System, all based on the CERIF standard. To allow comparisons between institutions, identifying a comprehensive taxonomy of impact, and the evidence for it, that can be used universally is seen to be very valuable. However, the Achilles heel of any such attempt, as critics suggest, is the creation of a system that rewards what it can measure and codify, with the knock-on effect of directing research projects to deliver within the measures and categories that reward.
Attempts have been made to categorize impact evidence and data, for example, the aim of the MICE Project was to develop a set of impact indicators to enable impact to be fed into a based system. Indicators were identified from documents produced for the REF, by Research Councils UK, in unpublished draft case studies undertaken at King’s College London or outlined in relevant publications (MICE Project n.d.). A taxonomy of impact categories was then produced onto which impact could be mapped. What emerged on testing the MICE taxonomy ( Cooke and Nadim 2011 ), by mapping impacts from case studies, was that detailed categorization of impact was found to be too prescriptive. Every piece of research results in a unique tapestry of impact and despite the MICE taxonomy having more than 100 indicators, it was found that these did not suffice. It is perhaps worth noting that the expert panels, who assessed the pilot exercise for the REF, commented that the evidence provided by research institutes to demonstrate impact were ‘a unique collection’. Where quantitative data were available, for example, audience numbers or book sales, these numbers rarely reflected the degree of impact, as no context or baseline was available. Cooke and Nadim (2011) also noted that using a linear-style taxonomy did not reflect the complex networks of impacts that are generally found. The Goldsmith report ( Cooke and Nadim 2011 ) recommended making indicators ‘value free’, enabling the value or quality to be established in an impact descriptor that could be assessed by expert panels. The Goldsmith report concluded that general categories of evidence would be more useful such that indicators could encompass dissemination and circulation, re-use and influence, collaboration and boundary work, and innovation and invention.
While defining the terminology used to understand impact and indicators will enable comparable data to be stored and shared between organizations, we would recommend that any categorization of impacts be flexible such that impacts arising from non-standard routes can be placed. It is worth considering the degree to which indicators are defined and provide broader definitions with greater flexibility.
It is possible to incorporate both metrics and narratives within systems, for example, within the Research Outcomes System and Researchfish, currently used by several of the UK research councils to allow impacts to be recorded; although recording narratives has the advantage of allowing some context to be documented, it may make the evidence less flexible for use by different stakeholder groups (which include government, funding bodies, research assessment agencies, research providers, and user communities) for whom the purpose of analysis may vary ( Davies et al. 2005 ). Any tool for impact evaluation needs to be flexible, such that it enables access to impact data for a variety of purposes (Scoble et al. n.d.). Systems need to be able to capture links between and evidence of the full pathway from research to impact, including knowledge exchange, outputs, outcomes, and interim impacts, to allow the route to impact to be traced. This database of evidence needs to establish both where impact can be directly attributed to a piece of research as well as various contributions to impact made during the pathway.
Baselines and controls need to be captured alongside change to demonstrate the degree of impact. In many instances, controls are not feasible as we cannot look at what impact would have occurred if a piece of research had not taken place; however, indications of the picture before and after impact are valuable and worth collecting for impact that can be predicted.
It is now possible to use data-mining tools to extract specific data from narratives or unstructured data ( Mugabushaka and Papazoglou 2012 ). This is being done for collation of academic impact and outputs, for example, Research Portfolio Online Reporting Tools, which uses PubMed and text mining to cluster research projects, and STAR Metrics in the US, which uses administrative records and research outputs and is also being implemented by the ERC using data in the public domain ( Mugabushaka and Papazoglou 2012 ). These techniques have the potential to provide a transformation in data capture and impact assessment ( Jones and Grant 2013 ). It is acknowledged in the article by Mugabushaka and Papazoglou (2012) that it will take years to fully incorporate the impacts of ERC funding. For systems to be able to capture a full range of systems, definitions and categories of impact need to be determined that can be incorporated into system development. To adequately capture interactions taking place between researchers, institutions, and stakeholders, the introduction of tools to enable this would be very valuable. If knowledge exchange events could be captured, for example, electronically as they occur or automatically if flagged from an electronic calendar or a diary, then far more of these events could be recorded with relative ease. Capturing knowledge exchange events would greatly assist the linking of research with impact.
The transition to routine capture of impact data not only requires the development of tools and systems to help with implementation but also a cultural change to develop practices, currently undertaken by a few to be incorporated as standard behaviour among researchers and universities.
What indicators, evidence, and impacts need to be captured within developing systems? There is a great deal of interest in collating terms for impact and indicators of impact. Consortia for Advancing Standards in Research Administration Information, for example, has put together a data dictionary with the aim of setting the standards for terminology used to describe impact and indicators that can be incorporated into systems internationally and seems to be building a certain momentum in this area. A variety of types of indicators can be captured within systems; however, it is important that these are universally understood. Here we address types of evidence that need to be captured to enable an overview of impact to be developed. In the majority of cases, a number of types of evidence will be required to provide an overview of impact.
7.1 Metrics
Metrics have commonly been used as a measure of impact, for example, in terms of profit made, number of jobs provided, number of trained personnel recruited, number of visitors to an exhibition, number of items purchased, and so on. Metrics in themselves cannot convey the full impact; however, they are often viewed as powerful and unequivocal forms of evidence. If metrics are available as impact evidence, they should, where possible, also capture any baseline or control data. Any information on the context of the data will be valuable to understanding the degree to which impact has taken place.
Perhaps, SROI indicates the desire to be able to demonstrate the monetary value of investment and impact by some organizations. SROI aims to provide a valuation of the broader social, environmental, and economic impacts, providing a metric that can be used for demonstration of worth. This is a metric that has been used within the charitable sector ( Berg and Månsson 2011 ) and also features as evidence in the REF guidance for panel D ( REF2014 2012 ). More details on SROI can be found in ‘A guide to Social Return on Investment’ produced by The SROI Network (2012) .
Although metrics can provide evidence of quantitative changes or impacts from our research, they are unable to adequately provide evidence of the qualitative impacts that take place and hence are not suitable for all of the impact we will encounter. The main risks associated with the use of standardized metrics are that
The full impact will not be realized, as we focus on easily quantifiable indicators
We will focus attention towards generating results that enable boxes to be ticked rather than delivering real value for money and innovative research.
They risk being monetized or converted into a lowest common denominator in an attempt to compare the cost of a new theatre against that of a hospital.
7.2 Narratives
Narratives can be used to describe impact; the use of narratives enables a story to be told and the impact to be placed in context and can make good use of qualitative information. They are often written with a reader from a particular stakeholder group in mind and will present a view of impact from a particular perspective. The risk of relying on narratives to assess impact is that they often lack the evidence required to judge whether the research and impact are linked appropriately. Where narratives are used in conjunction with metrics, a complete picture of impact can be developed, again from a particular perspective but with the evidence available to corroborate the claims made. Table 1 summarizes some of the advantages and disadvantages of the case study approach.
The advantages and disadvantages of the case study approach
By allowing impact to be placed in context, we answer the ‘so what?’ question that can result from quantitative data analyses, but is there a risk that the full picture may not be presented to demonstrate impact in a positive light? Case studies are ideal for showcasing impact, but should they be used to critically evaluate impact?
7.3 Surveys and testimonies
One way in which change of opinion and user perceptions can be evidenced is by gathering of stakeholder and user testimonies or undertaking surveys. This might describe support for and development of research with end users, public engagement and evidence of knowledge exchange, or a demonstration of change in public opinion as a result of research. Collecting this type of evidence is time-consuming, and again, it can be difficult to gather the required evidence retrospectively when, for example, the appropriate user group might have dispersed.
The ability to record and log these type of data is important for enabling the path from research to impact to be established and the development of systems that can capture this would be very valuable.
7.4 Citations (outside of academia) and documentation
Citations (outside of academia) and documentation can be used as evidence to demonstrate the use research findings in developing new ideas and products for example. This might include the citation of a piece of research in policy documents or reference to a piece of research being cited within the media. A collation of several indicators of impact may be enough to convince that an impact has taken place. Even where we can evidence changes and benefits linked to our research, understanding the causal relationship may be difficult. Media coverage is a useful means of disseminating our research and ideas and may be considered alongside other evidence as contributing to or an indicator of impact.
The fast-moving developments in the field of altmetrics (or alternative metrics) are providing a richer understanding of how research is being used, viewed, and moved. The transfer of information electronically can be traced and reviewed to provide data on where and to whom research findings are going.
The understanding of the term impact varies considerably and as such the objectives of an impact assessment need to be thoroughly understood before evidence is collated.
While aspects of impact can be adequately interpreted using metrics, narratives, and other evidence, the mixed-method case study approach is an excellent means of pulling all available information, data, and evidence together, allowing a comprehensive summary of the impact within context. While the case study is a useful way of showcasing impact, its limitations must be understood if we are to use this for evaluation purposes. The case study does present evidence from a particular perspective and may need to be adapted for use with different stakeholders. It is time-intensive to both assimilate and review case studies and we therefore need to ensure that the resources required for this type of evaluation are justified by the knowledge gained. The ability to write a persuasive well-evidenced case study may influence the assessment of impact. Over the past year, there have been a number of new posts created within universities, such as writing impact case studies, and a number of companies are now offering this as a contract service. A key concern here is that we could find that universities which can afford to employ either consultants or impact ‘administrators’ will generate the best case studies.
The development of tools and systems for assisting with impact evaluation would be very valuable. We suggest that developing systems that focus on recording impact information alone will not provide all that is required to link research to ensuing events and impacts, systems require the capacity to capture any interactions between researchers, the institution, and external stakeholders and link these with research findings and outputs or interim impacts to provide a network of data. In designing systems and tools for collating data related to impact, it is important to consider who will populate the database and ensure that the time and capability required for capture of information is considered. Capturing data, interactions, and indicators as they emerge increases the chance of capturing all relevant information and tools to enable researchers to capture much of this would be valuable. However, it must be remembered that in the case of the UK REF, impact is only considered that is based on research that has taken place within the institution submitting the case study. It is therefore in an institution’s interest to have a process by which all the necessary information is captured to enable a story to be developed in the absence of a researcher who may have left the employment of the institution. Figure 2 demonstrates the information that systems will need to capture and link.
Research findings including outputs (e.g., presentations and publications)
Communications and interactions with stakeholders and the wider public (emails, visits, workshops, media publicity, etc)
Feedback from stakeholders and communication summaries (e.g., testimonials and altmetrics)
Research developments (based on stakeholder input and discussions)
Outcomes (e.g., commercial and cultural, citations)
Impacts (changes, e.g., behavioural and economic)

Overview of the types of information that systems need to capture and link.
Attempting to evaluate impact to justify expenditure, showcase our work, and inform future funding decisions will only prove to be a valuable use of time and resources if we can take measures to ensure that assessment attempts will not ultimately have a negative influence on the impact of our research. There are areas of basic research where the impacts are so far removed from the research or are impractical to demonstrate; in these cases, it might be prudent to accept the limitations of impact assessment, and provide the potential for exclusion in appropriate circumstances.
This work was supported by Jisc [DIINN10].
Google Scholar
Google Preview
Email alerts
Citing articles via.
- Recommend to your Library
Affiliations
- Online ISSN 1471-5449
- Print ISSN 0958-2029
- Copyright © 2024 Oxford University Press
- About Oxford Academic
- Publish journals with us
- University press partners
- What we publish
- New features
- Open access
- Institutional account management
- Rights and permissions
- Get help with access
- Accessibility
- Advertising
- Media enquiries
- Oxford University Press
- Oxford Languages
- University of Oxford
Oxford University Press is a department of the University of Oxford. It furthers the University's objective of excellence in research, scholarship, and education by publishing worldwide
- Copyright © 2024 Oxford University Press
- Cookie settings
- Cookie policy
- Privacy policy
- Legal notice
This Feature Is Available To Subscribers Only
Sign In or Create an Account
This PDF is available to Subscribers Only
For full access to this pdf, sign in to an existing account, or purchase an annual subscription.
ASSESSMENT, MEASUREMENT, EVALUATION & RESEARCH Science: A way of knowing
Citation: Huitt, W. (2004). Science: A way of knowing. Educational Psychology Interactive . Valdosta, GA: Valdosta State University. http:// www.edpsycinteractive.org/topics intro/sciknow.html
Return to: | EdPsyc Interactive: Courses | Home Page |
Having a true or correct view of the universe, how it works, and how we as human beings are influenced by our nature and our surroundings are important goals for educators. In general, there are four ways or methods by which truth about phenomena can be ascertained. First, we can know something is true because we trust the source of the information. For example, we may read a textbook or review a research study. We can also use references such as religious literature (e.g., the Talmud, the Bible, the Koran, etc.) In both cases, the information has been revealed to us and we trust the source of the information. Second, we may know something is true through intuition or personal inspiration. We may feel strongly that we have been "guided" to truth through an insight that is unique and personal. A third way of knowing is through personal experience. This is often a powerful approach to many people. A fourth way of knowing is through reason or thinking logically and critically about the first three.
Each of these ways of knowing is potentially flawed. We may read something from an otherwise credible source who has made a mistake relative to a particular issue. We may also have an inspiration that upon further investigation proves to be incorrect. The possibility of error through personal experience is well known by way of optical illusions. And obviously, reason is capable of error since so many scientists have different explanations for the same set of data and teachers of religion have different explanations of the same inspired text.
Kerlinger (1973) summarizing the writings of the philosopher Charles Pierce (as cited in Buchler, 1955 and Cohen & Nagel, 1934), provides a slightly different view of the four methods by which we determine truth. The first is the method of tenacity whereby truth is what is known to the individual or group. It simply is true. The second is the method of authority in which truth is established through a trusted source such as God, tradition, or public sanction. The third is the a prori method or the method of intuition. The fourth method is the scientific method which attempts to define a process for defining truth that produces results verifiable by others and is self-correcting. Kerlinger's definition of scientific research is that it is a "systematic, controlled, empirical, and critical investigation of hypothetical propositions about the presumed relations among natural phenomena" (p. 11).
Science, in terms of the ways of knowing discussed by Kerlinger (1973), might be considered a special case of the combination of experience and reason. While inspiration or intuition often plays an important role in scientific discovery, it must be subjected to experience that can be publicly verified and reason before it is accepted. The same holds true of revealed information; it is expected that we replicate or test out someone else's experience or ideas as reported in scientific or nonscientific literature or religious scripture.
My conceptualization of this topic is to focus on the sources of knowledge we might use. For example, we might use personal experience as known through the senses, unconscious knowledge or insight, or the methods and criteria associated with religion, philosophy or science. Each of these sources can be discussed in terms of such dimensions as the primary focus of the approach, the foundation upon which knowledge is based, the methods for acquiring knowledge and so forth. The following table presents an overview of this conceptualization.
- A common foundation for all approaches is the independent investigation and search for truth
- Faith--unquestioning belief; certitude; complete trust, confidence or reliance; conscious knowledge; generally has emotional connotation; often based on unprovable assumptions
If one accepts that a human being is an entirely physical or material phenomena (i.e., no aspect of the human being is spiritual or nonmaterial (see Hunt, 1994), then the primary use of the scientific approach to discover how human beings are structured, how they function, and how they change over time within and among different contexts or ecologies seems to be warranted. However, if that basic proposition or assumption is not accepted, rather if it is accepted that the human being is, in essence, a spiritual being (de Chardin, 1980), then the exclusive use of the scientific method (or for that matter personal experience or material philosophy) to discern truth about human beings and human behavior is probably not warranted.
The issue of the best source of "truth" is a major controversy in our society today. However, since educational and developmental psychology are considered disciplines within the science of psychology, we will use the scientific method as the basis for discerning truth in this course. This is not meant to invalidate other ways of knowing in terms of making a significant contribution to our study of human beings. Rather it is to remind us that, while other ways of knowing can make important contributions to our understanding of human behavior, the power of scientific knowledge and methods is to show us how our perceptions of knowledge arrived at through these other ways are sometimes incorrect. Conflicts among understandings derived from separate ways of knowing point to the need for, or perhaps highlight, possible new understandings that can resolve the conflict. After all, truth is truth in whichever form we observe it. Ultimately, if a concept, principle, or theory is true, it will be validated by each and every way of knowing. That is, when all four or five ways of knowing (using one of the classification systems presented above) produce similar results, our confidence that we have discovered "truth" is greatly increased. We must keep this point in mind as we explore the findings of science presented in this course.
Based on this analysis of different ways of knowing I have begun to construct a statement of my viewpoint or philosophy of human nature . I hope you can use it as a starting point to develop your own.
Science is one of those ways; scientists have established a set of rules and methodology by which truth is verified (Kuhn, 1962). The process of science generally follows a paradigm that defines the rules and describes procedures, instrumentation and methods of interpretation of data (Wilber, 1998). The results of science are formulated into a hierarchy of increasing complexity of knowledge : facts, concepts, principles, theories, and laws. When engaged in the process of science, scientists formulate hypotheses or educated guesses about the relationships between or among different facets of knowledge.
Assessment, measurement, research, and evaluation are part of the processes of science and issues related to each topic often overlap. Assessment refers to the collection of data to describe or better understand an issue. This can involve qualitative data (words and/or pictures) or quantitative data (numbers). Measurement is the term used for quantitative data as it is "the process of quantifying observations [or descriptions] about a quality or attribute of a thing or person" (Thondike and Hagen, 1986, p. 5). The process of measurement involves three steps:
- identifying and defining the quality or attribute that is to be measured;
- determining a set of operations by which the attribute may be made manifest and perceivable; and
- establishing a set of procedures or definitions for translating observations into quantitative statements of degree or amount. (p. 9)
Assessment and/or measurement are done with respect to variables (phenomena that can take on more than one value or level). For example, the variable "gender" has the values or levels of male and female and data could be collected relative to this variable. Data, both qualitative and quantitative, are generally collected through one or more of the following methods:
- Paper/pencil--Collection of data through self-reports, interviews, questionnaires, tests or other instruments
- Systematic observation--Researcher looks for specific actions or activities, but is not involved in the actions being observed
- Participant observation--Researcher is actively involved in the process being described and writes up observations at a later time
- Clinical--Data are collected by specialists in the process of treatment
Research refers to the use of data for the purpose of describing, predicting, and controlling as a means toward better understanding the phenomena under consideration, and evaluation refers to the comparison of data to a standard for the purpose of judging worth or quality. Three types of research studies are normally performed: descriptive, correlational, and experimental. The issues of research validity are discussed from a general perspective by Campbell and Stanley (1966).
Collecting data (assessment), quantifying that data (measurement), making judgments (evaluation), and developing understanding about the data (research) always raise issues of reliability and validity. The issue of reliability is essentially the same for all aspects of assessment, research, and evaluation. Reliability attempts to answer our concerns about the consistency of the information collected (i.e., can we depend on the data or findings?), while validity focuses on accuracy or truth. The relationship between reliability and validity can be confusing because measurements (e.g., tests) and research can be reliable without being valid, but they cannot be valid unless they are reliable. This simply means that for a test or study to be valid it must consistently (reliability) do what it purports to do (validity). For a measurement (e.g., a test score) to be judged reliable it should produce a consistent score; for the research study to be considered reliable each time it is replicated it too should produce similar results.
Dictionary definitions of terms used in measurement often give one only part of the picture. For example, validity is given as the adverb of valid which means "strong." Unfortunately, this type of definition is not specific enough when the term is used in certain contexts such as research or evaluation. Additionally, education and psychology use validity in multiple ways, each having several components. For example, research findings may be reliable (consistent across studies), but not valid (accurate or true statements about relationships among "variables"), but findings may not be valid if they are not reliable. At a minimum, for an instrument to be reliable a consistent set of data must be produced each time it is used; for a research study to be reliable it should produce consistent results each time it is performed.
References:
- Buchler, J. (Ed.). (1955). Philosophical writings of Peirce (Chapter 2). New York: Dover.
- Campbell, D. T., & Stanley, J. C. (1966). Experimental and quasi-experimental designs for research . Chicago: Rand McNally.
- Cohen, M., & Nagel, E. (1934). An introduction to logic and scientific method . New York: Harcourt.
- deChardin, P. T. (1980). The phenomena of man . New York: HarperCollins.
- Hunt, M. (1994, Fall). The "Soul": Modern psychological interpretations. Free Inquiry , 22-25.
- Kerlinger, F. (1973). Foundations of behavioral research . New York: Holt, Reinhart & Winston.
- Kuhn, T. (1962). The structure of scientific revolutions . Chicago: University of Chicago Press.
- Thorndike, R., & Hagen, E. (1986). Measurement and evaluation in psychology and education (4th ed.). New York: Wiley.
- Wilber, K. (1998). The marriage of sense and soul: Integrating science and religion. New York: Random House.
| Internet Resources | Electronic Files |
All materials on this website [http://www.edpsycinteractive.org] are, unless otherwise stated, the property of William G. Huitt. Copyright and other intellectual property laws protect these materials. Reproduction or retransmission of the materials, in whole or in part, in any manner, without the prior written consent of the copyright holder, is a violation of copyright law.
We’re reviewing our resources this spring (May-August 2024). We will do our best to minimize disruption, but you might notice changes over the next few months as we correct errors & delete redundant resources.
Critical Analysis and Evaluation
Many assignments ask you to critique and evaluate a source. Sources might include journal articles, books, websites, government documents, portfolios, podcasts, or presentations.
When you critique, you offer both negative and positive analysis of the content, writing, and structure of a source.
When you evaluate , you assess how successful a source is at presenting information, measured against a standard or certain criteria.
Elements of a critical analysis:
opinion + evidence from the article + justification
Your opinion is your thoughtful reaction to the piece.
Evidence from the article offers some proof to back up your opinion.
The justification is an explanation of how you arrived at your opinion or why you think it’s true.
How do you critique and evaluate?
When critiquing and evaluating someone else’s writing/research, your purpose is to reach an informed opinion about a source. In order to do that, try these three steps:
- How do you feel?
- What surprised you?
- What left you confused?
- What pleased or annoyed you?
- What was interesting?
- What is the purpose of this text?
- Who is the intended audience?
- What kind of bias is there?
- What was missing?
- See our resource on analysis and synthesis ( Move From Research to Writing: How to Think ) for other examples of questions to ask.
- sophisticated
- interesting
- undocumented
- disorganized
- superficial
- unconventional
- inappropriate interpretation of evidence
- unsound or discredited methodology
- traditional
- unsubstantiated
- unsupported
- well-researched
- easy to understand
- Opinion : This article’s assessment of the power balance in cities is confusing.
- Evidence: It first says that the power to shape policy is evenly distributed among citizens, local government, and business (Rajal, 232).
- Justification : but then it goes on to focus almost exclusively on business. Next, in a much shorter section, it combines the idea of citizens and local government into a single point of evidence. This leaves the reader with the impression that the citizens have no voice at all. It is not helpful in trying to determine the role of the common voter in shaping public policy.
Sample criteria for critical analysis
Sometimes the assignment will specify what criteria to use when critiquing and evaluating a source. If not, consider the following prompts to approach your analysis. Choose the questions that are most suitable for your source.
- What do you think about the quality of the research? Is it significant?
- Did the author answer the question they set out to? Did the author prove their thesis?
- Did you find contradictions to other things you know?
- What new insight or connections did the author make?
- How does this piece fit within the context of your course, or the larger body of research in the field?
- The structure of an article or book is often dictated by standards of the discipline or a theoretical model. Did the piece meet those standards?
- Did the piece meet the needs of the intended audience?
- Was the material presented in an organized and logical fashion?
- Is the argument cohesive and convincing? Is the reasoning sound? Is there enough evidence?
- Is it easy to read? Is it clear and easy to understand, even if the concepts are sophisticated?
How to conduct a meta-analysis in eight steps: a practical guide
- Open access
- Published: 30 November 2021
- Volume 72 , pages 1–19, ( 2022 )
Cite this article
You have full access to this open access article
- Christopher Hansen 1 ,
- Holger Steinmetz 2 &
- Jörn Block 3 , 4 , 5
153k Accesses
47 Citations
158 Altmetric
Explore all metrics
Avoid common mistakes on your manuscript.
1 Introduction
“Scientists have known for centuries that a single study will not resolve a major issue. Indeed, a small sample study will not even resolve a minor issue. Thus, the foundation of science is the cumulation of knowledge from the results of many studies.” (Hunter et al. 1982 , p. 10)
Meta-analysis is a central method for knowledge accumulation in many scientific fields (Aguinis et al. 2011c ; Kepes et al. 2013 ). Similar to a narrative review, it serves as a synopsis of a research question or field. However, going beyond a narrative summary of key findings, a meta-analysis adds value in providing a quantitative assessment of the relationship between two target variables or the effectiveness of an intervention (Gurevitch et al. 2018 ). Also, it can be used to test competing theoretical assumptions against each other or to identify important moderators where the results of different primary studies differ from each other (Aguinis et al. 2011b ; Bergh et al. 2016 ). Rooted in the synthesis of the effectiveness of medical and psychological interventions in the 1970s (Glass 2015 ; Gurevitch et al. 2018 ), meta-analysis is nowadays also an established method in management research and related fields.
The increasing importance of meta-analysis in management research has resulted in the publication of guidelines in recent years that discuss the merits and best practices in various fields, such as general management (Bergh et al. 2016 ; Combs et al. 2019 ; Gonzalez-Mulé and Aguinis 2018 ), international business (Steel et al. 2021 ), economics and finance (Geyer-Klingeberg et al. 2020 ; Havranek et al. 2020 ), marketing (Eisend 2017 ; Grewal et al. 2018 ), and organizational studies (DeSimone et al. 2020 ; Rudolph et al. 2020 ). These articles discuss existing and trending methods and propose solutions for often experienced problems. This editorial briefly summarizes the insights of these papers; provides a workflow of the essential steps in conducting a meta-analysis; suggests state-of-the art methodological procedures; and points to other articles for in-depth investigation. Thus, this article has two goals: (1) based on the findings of previous editorials and methodological articles, it defines methodological recommendations for meta-analyses submitted to Management Review Quarterly (MRQ); and (2) it serves as a practical guide for researchers who have little experience with meta-analysis as a method but plan to conduct one in the future.
2 Eight steps in conducting a meta-analysis
2.1 step 1: defining the research question.
The first step in conducting a meta-analysis, as with any other empirical study, is the definition of the research question. Most importantly, the research question determines the realm of constructs to be considered or the type of interventions whose effects shall be analyzed. When defining the research question, two hurdles might develop. First, when defining an adequate study scope, researchers must consider that the number of publications has grown exponentially in many fields of research in recent decades (Fortunato et al. 2018 ). On the one hand, a larger number of studies increases the potentially relevant literature basis and enables researchers to conduct meta-analyses. Conversely, scanning a large amount of studies that could be potentially relevant for the meta-analysis results in a perhaps unmanageable workload. Thus, Steel et al. ( 2021 ) highlight the importance of balancing manageability and relevance when defining the research question. Second, similar to the number of primary studies also the number of meta-analyses in management research has grown strongly in recent years (Geyer-Klingeberg et al. 2020 ; Rauch 2020 ; Schwab 2015 ). Therefore, it is likely that one or several meta-analyses for many topics of high scholarly interest already exist. However, this should not deter researchers from investigating their research questions. One possibility is to consider moderators or mediators of a relationship that have previously been ignored. For example, a meta-analysis about startup performance could investigate the impact of different ways to measure the performance construct (e.g., growth vs. profitability vs. survival time) or certain characteristics of the founders as moderators. Another possibility is to replicate previous meta-analyses and test whether their findings can be confirmed with an updated sample of primary studies or newly developed methods. Frequent replications and updates of meta-analyses are important contributions to cumulative science and are increasingly called for by the research community (Anderson & Kichkha 2017 ; Steel et al. 2021 ). Consistent with its focus on replication studies (Block and Kuckertz 2018 ), MRQ therefore also invites authors to submit replication meta-analyses.
2.2 Step 2: literature search
2.2.1 search strategies.
Similar to conducting a literature review, the search process of a meta-analysis should be systematic, reproducible, and transparent, resulting in a sample that includes all relevant studies (Fisch and Block 2018 ; Gusenbauer and Haddaway 2020 ). There are several identification strategies for relevant primary studies when compiling meta-analytical datasets (Harari et al. 2020 ). First, previous meta-analyses on the same or a related topic may provide lists of included studies that offer a good starting point to identify and become familiar with the relevant literature. This practice is also applicable to topic-related literature reviews, which often summarize the central findings of the reviewed articles in systematic tables. Both article types likely include the most prominent studies of a research field. The most common and important search strategy, however, is a keyword search in electronic databases (Harari et al. 2020 ). This strategy will probably yield the largest number of relevant studies, particularly so-called ‘grey literature’, which may not be considered by literature reviews. Gusenbauer and Haddaway ( 2020 ) provide a detailed overview of 34 scientific databases, of which 18 are multidisciplinary or have a focus on management sciences, along with their suitability for literature synthesis. To prevent biased results due to the scope or journal coverage of one database, researchers should use at least two different databases (DeSimone et al. 2020 ; Martín-Martín et al. 2021 ; Mongeon & Paul-Hus 2016 ). However, a database search can easily lead to an overload of potentially relevant studies. For example, key term searches in Google Scholar for “entrepreneurial intention” and “firm diversification” resulted in more than 660,000 and 810,000 hits, respectively. Footnote 1 Therefore, a precise research question and precise search terms using Boolean operators are advisable (Gusenbauer and Haddaway 2020 ). Addressing the challenge of identifying relevant articles in the growing number of database publications, (semi)automated approaches using text mining and machine learning (Bosco et al. 2017 ; O’Mara-Eves et al. 2015 ; Ouzzani et al. 2016 ; Thomas et al. 2017 ) can also be promising and time-saving search tools in the future. Also, some electronic databases offer the possibility to track forward citations of influential studies and thereby identify further relevant articles. Finally, collecting unpublished or undetected studies through conferences, personal contact with (leading) scholars, or listservs can be strategies to increase the study sample size (Grewal et al. 2018 ; Harari et al. 2020 ; Pigott and Polanin 2020 ).
2.2.2 Study inclusion criteria and sample composition
Next, researchers must decide which studies to include in the meta-analysis. Some guidelines for literature reviews recommend limiting the sample to studies published in renowned academic journals to ensure the quality of findings (e.g., Kraus et al. 2020 ). For meta-analysis, however, Steel et al. ( 2021 ) advocate for the inclusion of all available studies, including grey literature, to prevent selection biases based on availability, cost, familiarity, and language (Rothstein et al. 2005 ), or the “Matthew effect”, which denotes the phenomenon that highly cited articles are found faster than less cited articles (Merton 1968 ). Harrison et al. ( 2017 ) find that the effects of published studies in management are inflated on average by 30% compared to unpublished studies. This so-called publication bias or “file drawer problem” (Rosenthal 1979 ) results from the preference of academia to publish more statistically significant and less statistically insignificant study results. Owen and Li ( 2020 ) showed that publication bias is particularly severe when variables of interest are used as key variables rather than control variables. To consider the true effect size of a target variable or relationship, the inclusion of all types of research outputs is therefore recommended (Polanin et al. 2016 ). Different test procedures to identify publication bias are discussed subsequently in Step 7.
In addition to the decision of whether to include certain study types (i.e., published vs. unpublished studies), there can be other reasons to exclude studies that are identified in the search process. These reasons can be manifold and are primarily related to the specific research question and methodological peculiarities. For example, studies identified by keyword search might not qualify thematically after all, may use unsuitable variable measurements, or may not report usable effect sizes. Furthermore, there might be multiple studies by the same authors using similar datasets. If they do not differ sufficiently in terms of their sample characteristics or variables used, only one of these studies should be included to prevent bias from duplicates (Wood 2008 ; see this article for a detection heuristic).
In general, the screening process should be conducted stepwise, beginning with a removal of duplicate citations from different databases, followed by abstract screening to exclude clearly unsuitable studies and a final full-text screening of the remaining articles (Pigott and Polanin 2020 ). A graphical tool to systematically document the sample selection process is the PRISMA flow diagram (Moher et al. 2009 ). Page et al. ( 2021 ) recently presented an updated version of the PRISMA statement, including an extended item checklist and flow diagram to report the study process and findings.
2.3 Step 3: choice of the effect size measure
2.3.1 types of effect sizes.
The two most common meta-analytical effect size measures in management studies are (z-transformed) correlation coefficients and standardized mean differences (Aguinis et al. 2011a ; Geyskens et al. 2009 ). However, meta-analyses in management science and related fields may not be limited to those two effect size measures but rather depend on the subfield of investigation (Borenstein 2009 ; Stanley and Doucouliagos 2012 ). In economics and finance, researchers are more interested in the examination of elasticities and marginal effects extracted from regression models than in pure bivariate correlations (Stanley and Doucouliagos 2012 ). Regression coefficients can also be converted to partial correlation coefficients based on their t-statistics to make regression results comparable across studies (Stanley and Doucouliagos 2012 ). Although some meta-analyses in management research have combined bivariate and partial correlations in their study samples, Aloe ( 2015 ) and Combs et al. ( 2019 ) advise researchers not to use this practice. Most importantly, they argue that the effect size strength of partial correlations depends on the other variables included in the regression model and is therefore incomparable to bivariate correlations (Schmidt and Hunter 2015 ), resulting in a possible bias of the meta-analytic results (Roth et al. 2018 ). We endorse this opinion. If at all, we recommend separate analyses for each measure. In addition to these measures, survival rates, risk ratios or odds ratios, which are common measures in medical research (Borenstein 2009 ), can be suitable effect sizes for specific management research questions, such as understanding the determinants of the survival of startup companies. To summarize, the choice of a suitable effect size is often taken away from the researcher because it is typically dependent on the investigated research question as well as the conventions of the specific research field (Cheung and Vijayakumar 2016 ).
2.3.2 Conversion of effect sizes to a common measure
After having defined the primary effect size measure for the meta-analysis, it might become necessary in the later coding process to convert study findings that are reported in effect sizes that are different from the chosen primary effect size. For example, a study might report only descriptive statistics for two study groups but no correlation coefficient, which is used as the primary effect size measure in the meta-analysis. Different effect size measures can be harmonized using conversion formulae, which are provided by standard method books such as Borenstein et al. ( 2009 ) or Lipsey and Wilson ( 2001 ). There also exist online effect size calculators for meta-analysis. Footnote 2
2.4 Step 4: choice of the analytical method used
Choosing which meta-analytical method to use is directly connected to the research question of the meta-analysis. Research questions in meta-analyses can address a relationship between constructs or an effect of an intervention in a general manner, or they can focus on moderating or mediating effects. There are four meta-analytical methods that are primarily used in contemporary management research (Combs et al. 2019 ; Geyer-Klingeberg et al. 2020 ), which allow the investigation of these different types of research questions: traditional univariate meta-analysis, meta-regression, meta-analytic structural equation modeling, and qualitative meta-analysis (Hoon 2013 ). While the first three are quantitative, the latter summarizes qualitative findings. Table 1 summarizes the key characteristics of the three quantitative methods.
2.4.1 Univariate meta-analysis
In its traditional form, a meta-analysis reports a weighted mean effect size for the relationship or intervention of investigation and provides information on the magnitude of variance among primary studies (Aguinis et al. 2011c ; Borenstein et al. 2009 ). Accordingly, it serves as a quantitative synthesis of a research field (Borenstein et al. 2009 ; Geyskens et al. 2009 ). Prominent traditional approaches have been developed, for example, by Hedges and Olkin ( 1985 ) or Hunter and Schmidt ( 1990 , 2004 ). However, going beyond its simple summary function, the traditional approach has limitations in explaining the observed variance among findings (Gonzalez-Mulé and Aguinis 2018 ). To identify moderators (or boundary conditions) of the relationship of interest, meta-analysts can create subgroups and investigate differences between those groups (Borenstein and Higgins 2013 ; Hunter and Schmidt 2004 ). Potential moderators can be study characteristics (e.g., whether a study is published vs. unpublished), sample characteristics (e.g., study country, industry focus, or type of survey/experiment participants), or measurement artifacts (e.g., different types of variable measurements). The univariate approach is thus suitable to identify the overall direction of a relationship and can serve as a good starting point for additional analyses. However, due to its limitations in examining boundary conditions and developing theory, the univariate approach on its own is currently oftentimes viewed as not sufficient (Rauch 2020 ; Shaw and Ertug 2017 ).
2.4.2 Meta-regression analysis
Meta-regression analysis (Hedges and Olkin 1985 ; Lipsey and Wilson 2001 ; Stanley and Jarrell 1989 ) aims to investigate the heterogeneity among observed effect sizes by testing multiple potential moderators simultaneously. In meta-regression, the coded effect size is used as the dependent variable and is regressed on a list of moderator variables. These moderator variables can be categorical variables as described previously in the traditional univariate approach or (semi)continuous variables such as country scores that are merged with the meta-analytical data. Thus, meta-regression analysis overcomes the disadvantages of the traditional approach, which only allows us to investigate moderators singularly using dichotomized subgroups (Combs et al. 2019 ; Gonzalez-Mulé and Aguinis 2018 ). These possibilities allow a more fine-grained analysis of research questions that are related to moderating effects. However, Schmidt ( 2017 ) critically notes that the number of effect sizes in the meta-analytical sample must be sufficiently large to produce reliable results when investigating multiple moderators simultaneously in a meta-regression. For further reading, Tipton et al. ( 2019 ) outline the technical, conceptual, and practical developments of meta-regression over the last decades. Gonzalez-Mulé and Aguinis ( 2018 ) provide an overview of methodological choices and develop evidence-based best practices for future meta-analyses in management using meta-regression.
2.4.3 Meta-analytic structural equation modeling (MASEM)
MASEM is a combination of meta-analysis and structural equation modeling and allows to simultaneously investigate the relationships among several constructs in a path model. Researchers can use MASEM to test several competing theoretical models against each other or to identify mediation mechanisms in a chain of relationships (Bergh et al. 2016 ). This method is typically performed in two steps (Cheung and Chan 2005 ): In Step 1, a pooled correlation matrix is derived, which includes the meta-analytical mean effect sizes for all variable combinations; Step 2 then uses this matrix to fit the path model. While MASEM was based primarily on traditional univariate meta-analysis to derive the pooled correlation matrix in its early years (Viswesvaran and Ones 1995 ), more advanced methods, such as the GLS approach (Becker 1992 , 1995 ) or the TSSEM approach (Cheung and Chan 2005 ), have been subsequently developed. Cheung ( 2015a ) and Jak ( 2015 ) provide an overview of these approaches in their books with exemplary code. For datasets with more complex data structures, Wilson et al. ( 2016 ) also developed a multilevel approach that is related to the TSSEM approach in the second step. Bergh et al. ( 2016 ) discuss nine decision points and develop best practices for MASEM studies.
2.4.4 Qualitative meta-analysis
While the approaches explained above focus on quantitative outcomes of empirical studies, qualitative meta-analysis aims to synthesize qualitative findings from case studies (Hoon 2013 ; Rauch et al. 2014 ). The distinctive feature of qualitative case studies is their potential to provide in-depth information about specific contextual factors or to shed light on reasons for certain phenomena that cannot usually be investigated by quantitative studies (Rauch 2020 ; Rauch et al. 2014 ). In a qualitative meta-analysis, the identified case studies are systematically coded in a meta-synthesis protocol, which is then used to identify influential variables or patterns and to derive a meta-causal network (Hoon 2013 ). Thus, the insights of contextualized and typically nongeneralizable single studies are aggregated to a larger, more generalizable picture (Habersang et al. 2019 ). Although still the exception, this method can thus provide important contributions for academics in terms of theory development (Combs et al., 2019 ; Hoon 2013 ) and for practitioners in terms of evidence-based management or entrepreneurship (Rauch et al. 2014 ). Levitt ( 2018 ) provides a guide and discusses conceptual issues for conducting qualitative meta-analysis in psychology, which is also useful for management researchers.
2.5 Step 5: choice of software
Software solutions to perform meta-analyses range from built-in functions or additional packages of statistical software to software purely focused on meta-analyses and from commercial to open-source solutions. However, in addition to personal preferences, the choice of the most suitable software depends on the complexity of the methods used and the dataset itself (Cheung and Vijayakumar 2016 ). Meta-analysts therefore must carefully check if their preferred software is capable of performing the intended analysis.
Among commercial software providers, Stata (from version 16 on) offers built-in functions to perform various meta-analytical analyses or to produce various plots (Palmer and Sterne 2016 ). For SPSS and SAS, there exist several macros for meta-analyses provided by scholars, such as David B. Wilson or Andy P. Field and Raphael Gillet (Field and Gillett 2010 ). Footnote 3 Footnote 4 For researchers using the open-source software R (R Core Team 2021 ), Polanin et al. ( 2017 ) provide an overview of 63 meta-analysis packages and their functionalities. For new users, they recommend the package metafor (Viechtbauer 2010 ), which includes most necessary functions and for which the author Wolfgang Viechtbauer provides tutorials on his project website. Footnote 5 Footnote 6 In addition to packages and macros for statistical software, templates for Microsoft Excel have also been developed to conduct simple meta-analyses, such as Meta-Essentials by Suurmond et al. ( 2017 ). Footnote 7 Finally, programs purely dedicated to meta-analysis also exist, such as Comprehensive Meta-Analysis (Borenstein et al. 2013 ) or RevMan by The Cochrane Collaboration ( 2020 ).
2.6 Step 6: coding of effect sizes
2.6.1 coding sheet.
The first step in the coding process is the design of the coding sheet. A universal template does not exist because the design of the coding sheet depends on the methods used, the respective software, and the complexity of the research design. For univariate meta-analysis or meta-regression, data are typically coded in wide format. In its simplest form, when investigating a correlational relationship between two variables using the univariate approach, the coding sheet would contain a column for the study name or identifier, the effect size coded from the primary study, and the study sample size. However, such simple relationships are unlikely in management research because the included studies are typically not identical but differ in several respects. With more complex data structures or moderator variables being investigated, additional columns are added to the coding sheet to reflect the data characteristics. These variables can be coded as dummy, factor, or (semi)continuous variables and later used to perform a subgroup analysis or meta regression. For MASEM, the required data input format can deviate depending on the method used (e.g., TSSEM requires a list of correlation matrices as data input). For qualitative meta-analysis, the coding scheme typically summarizes the key qualitative findings and important contextual and conceptual information (see Hoon ( 2013 ) for a coding scheme for qualitative meta-analysis). Figure 1 shows an exemplary coding scheme for a quantitative meta-analysis on the correlational relationship between top-management team diversity and profitability. In addition to effect and sample sizes, information about the study country, firm type, and variable operationalizations are coded. The list could be extended by further study and sample characteristics.

Exemplary coding sheet for a meta-analysis on the relationship (correlation) between top-management team diversity and profitability
2.6.2 Inclusion of moderator or control variables
It is generally important to consider the intended research model and relevant nontarget variables before coding a meta-analytic dataset. For example, study characteristics can be important moderators or function as control variables in a meta-regression model. Similarly, control variables may be relevant in a MASEM approach to reduce confounding bias. Coding additional variables or constructs subsequently can be arduous if the sample of primary studies is large. However, the decision to include respective moderator or control variables, as in any empirical analysis, should always be based on strong (theoretical) rationales about how these variables can impact the investigated effect (Bernerth and Aguinis 2016 ; Bernerth et al. 2018 ; Thompson and Higgins 2002 ). While substantive moderators refer to theoretical constructs that act as buffers or enhancers of a supposed causal process, methodological moderators are features of the respective research designs that denote the methodological context of the observations and are important to control for systematic statistical particularities (Rudolph et al. 2020 ). Havranek et al. ( 2020 ) provide a list of recommended variables to code as potential moderators. While researchers may have clear expectations about the effects for some of these moderators, the concerns for other moderators may be tentative, and moderator analysis may be approached in a rather exploratory fashion. Thus, we argue that researchers should make full use of the meta-analytical design to obtain insights about potential context dependence that a primary study cannot achieve.
2.6.3 Treatment of multiple effect sizes in a study
A long-debated issue in conducting meta-analyses is whether to use only one or all available effect sizes for the same construct within a single primary study. For meta-analyses in management research, this question is fundamental because many empirical studies, particularly those relying on company databases, use multiple variables for the same construct to perform sensitivity analyses, resulting in multiple relevant effect sizes. In this case, researchers can either (randomly) select a single value, calculate a study average, or use the complete set of effect sizes (Bijmolt and Pieters 2001 ; López-López et al. 2018 ). Multiple effect sizes from the same study enrich the meta-analytic dataset and allow us to investigate the heterogeneity of the relationship of interest, such as different variable operationalizations (López-López et al. 2018 ; Moeyaert et al. 2017 ). However, including more than one effect size from the same study violates the independency assumption of observations (Cheung 2019 ; López-López et al. 2018 ), which can lead to biased results and erroneous conclusions (Gooty et al. 2021 ). We follow the recommendation of current best practice guides to take advantage of using all available effect size observations but to carefully consider interdependencies using appropriate methods such as multilevel models, panel regression models, or robust variance estimation (Cheung 2019 ; Geyer-Klingeberg et al. 2020 ; Gooty et al. 2021 ; López-López et al. 2018 ; Moeyaert et al. 2017 ).
2.7 Step 7: analysis
2.7.1 outlier analysis and tests for publication bias.
Before conducting the primary analysis, some preliminary sensitivity analyses might be necessary, which should ensure the robustness of the meta-analytical findings (Rudolph et al. 2020 ). First, influential outlier observations could potentially bias the observed results, particularly if the number of total effect sizes is small. Several statistical methods can be used to identify outliers in meta-analytical datasets (Aguinis et al. 2013 ; Viechtbauer and Cheung 2010 ). However, there is a debate about whether to keep or omit these observations. Anyhow, relevant studies should be closely inspected to infer an explanation about their deviating results. As in any other primary study, outliers can be a valid representation, albeit representing a different population, measure, construct, design or procedure. Thus, inferences about outliers can provide the basis to infer potential moderators (Aguinis et al. 2013 ; Steel et al. 2021 ). On the other hand, outliers can indicate invalid research, for instance, when unrealistically strong correlations are due to construct overlap (i.e., lack of a clear demarcation between independent and dependent variables), invalid measures, or simply typing errors when coding effect sizes. An advisable step is therefore to compare the results both with and without outliers and base the decision on whether to exclude outlier observations with careful consideration (Geyskens et al. 2009 ; Grewal et al. 2018 ; Kepes et al. 2013 ). However, instead of simply focusing on the size of the outlier, its leverage should be considered. Thus, Viechtbauer and Cheung ( 2010 ) propose considering a combination of standardized deviation and a study’s leverage.
Second, as mentioned in the context of a literature search, potential publication bias may be an issue. Publication bias can be examined in multiple ways (Rothstein et al. 2005 ). First, the funnel plot is a simple graphical tool that can provide an overview of the effect size distribution and help to detect publication bias (Stanley and Doucouliagos 2010 ). A funnel plot can also support in identifying potential outliers. As mentioned above, a graphical display of deviation (e.g., studentized residuals) and leverage (Cook’s distance) can help detect the presence of outliers and evaluate their influence (Viechtbauer and Cheung 2010 ). Moreover, several statistical procedures can be used to test for publication bias (Harrison et al. 2017 ; Kepes et al. 2012 ), including subgroup comparisons between published and unpublished studies, Begg and Mazumdar’s ( 1994 ) rank correlation test, cumulative meta-analysis (Borenstein et al. 2009 ), the trim and fill method (Duval and Tweedie 2000a , b ), Egger et al.’s ( 1997 ) regression test, failsafe N (Rosenthal 1979 ), or selection models (Hedges and Vevea 2005 ; Vevea and Woods 2005 ). In examining potential publication bias, Kepes et al. ( 2012 ) and Harrison et al. ( 2017 ) both recommend not relying only on a single test but rather using multiple conceptionally different test procedures (i.e., the so-called “triangulation approach”).
2.7.2 Model choice
After controlling and correcting for the potential presence of impactful outliers or publication bias, the next step in meta-analysis is the primary analysis, where meta-analysts must decide between two different types of models that are based on different assumptions: fixed-effects and random-effects (Borenstein et al. 2010 ). Fixed-effects models assume that all observations share a common mean effect size, which means that differences are only due to sampling error, while random-effects models assume heterogeneity and allow for a variation of the true effect sizes across studies (Borenstein et al. 2010 ; Cheung and Vijayakumar 2016 ; Hunter and Schmidt 2004 ). Both models are explained in detail in standard textbooks (e.g., Borenstein et al. 2009 ; Hunter and Schmidt 2004 ; Lipsey and Wilson 2001 ).
In general, the presence of heterogeneity is likely in management meta-analyses because most studies do not have identical empirical settings, which can yield different effect size strengths or directions for the same investigated phenomenon. For example, the identified studies have been conducted in different countries with different institutional settings, or the type of study participants varies (e.g., students vs. employees, blue-collar vs. white-collar workers, or manufacturing vs. service firms). Thus, the vast majority of meta-analyses in management research and related fields use random-effects models (Aguinis et al. 2011a ). In a meta-regression, the random-effects model turns into a so-called mixed-effects model because moderator variables are added as fixed effects to explain the impact of observed study characteristics on effect size variations (Raudenbush 2009 ).
2.8 Step 8: reporting results
2.8.1 reporting in the article.
The final step in performing a meta-analysis is reporting its results. Most importantly, all steps and methodological decisions should be comprehensible to the reader. DeSimone et al. ( 2020 ) provide an extensive checklist for journal reviewers of meta-analytical studies. This checklist can also be used by authors when performing their analyses and reporting their results to ensure that all important aspects have been addressed. Alternative checklists are provided, for example, by Appelbaum et al. ( 2018 ) or Page et al. ( 2021 ). Similarly, Levitt et al. ( 2018 ) provide a detailed guide for qualitative meta-analysis reporting standards.
For quantitative meta-analyses, tables reporting results should include all important information and test statistics, including mean effect sizes; standard errors and confidence intervals; the number of observations and study samples included; and heterogeneity measures. If the meta-analytic sample is rather small, a forest plot provides a good overview of the different findings and their accuracy. However, this figure will be less feasible for meta-analyses with several hundred effect sizes included. Also, results displayed in the tables and figures must be explained verbally in the results and discussion sections. Most importantly, authors must answer the primary research question, i.e., whether there is a positive, negative, or no relationship between the variables of interest, or whether the examined intervention has a certain effect. These results should be interpreted with regard to their magnitude (or significance), both economically and statistically. However, when discussing meta-analytical results, authors must describe the complexity of the results, including the identified heterogeneity and important moderators, future research directions, and theoretical relevance (DeSimone et al. 2019 ). In particular, the discussion of identified heterogeneity and underlying moderator effects is critical; not including this information can lead to false conclusions among readers, who interpret the reported mean effect size as universal for all included primary studies and ignore the variability of findings when citing the meta-analytic results in their research (Aytug et al. 2012 ; DeSimone et al. 2019 ).
2.8.2 Open-science practices
Another increasingly important topic is the public provision of meta-analytical datasets and statistical codes via open-source repositories. Open-science practices allow for results validation and for the use of coded data in subsequent meta-analyses ( Polanin et al. 2020 ), contributing to the development of cumulative science. Steel et al. ( 2021 ) refer to open science meta-analyses as a step towards “living systematic reviews” (Elliott et al. 2017 ) with continuous updates in real time. MRQ supports this development and encourages authors to make their datasets publicly available. Moreau and Gamble ( 2020 ), for example, provide various templates and video tutorials to conduct open science meta-analyses. There exist several open science repositories, such as the Open Science Foundation (OSF; for a tutorial, see Soderberg 2018 ), to preregister and make documents publicly available. Furthermore, several initiatives in the social sciences have been established to develop dynamic meta-analyses, such as metaBUS (Bosco et al. 2015 , 2017 ), MetaLab (Bergmann et al. 2018 ), or PsychOpen CAMA (Burgard et al. 2021 ).
3 Conclusion
This editorial provides a comprehensive overview of the essential steps in conducting and reporting a meta-analysis with references to more in-depth methodological articles. It also serves as a guide for meta-analyses submitted to MRQ and other management journals. MRQ welcomes all types of meta-analyses from all subfields and disciplines of management research.
Gusenbauer and Haddaway ( 2020 ), however, point out that Google Scholar is not appropriate as a primary search engine due to a lack of reproducibility of search results.
One effect size calculator by David B. Wilson is accessible via: https://www.campbellcollaboration.org/escalc/html/EffectSizeCalculator-Home.php .
The macros of David B. Wilson can be downloaded from: http://mason.gmu.edu/~dwilsonb/ .
The macros of Field and Gillet ( 2010 ) can be downloaded from: https://www.discoveringstatistics.com/repository/fieldgillett/how_to_do_a_meta_analysis.html .
The tutorials can be found via: https://www.metafor-project.org/doku.php .
Metafor does currently not provide functions to conduct MASEM. For MASEM, users can, for instance, use the package metaSEM (Cheung 2015b ).
The workbooks can be downloaded from: https://www.erim.eur.nl/research-support/meta-essentials/ .
Aguinis H, Dalton DR, Bosco FA, Pierce CA, Dalton CM (2011a) Meta-analytic choices and judgment calls: Implications for theory building and testing, obtained effect sizes, and scholarly impact. J Manag 37(1):5–38
Google Scholar
Aguinis H, Gottfredson RK, Joo H (2013) Best-practice recommendations for defining, identifying, and handling outliers. Organ Res Methods 16(2):270–301
Article Google Scholar
Aguinis H, Gottfredson RK, Wright TA (2011b) Best-practice recommendations for estimating interaction effects using meta-analysis. J Organ Behav 32(8):1033–1043
Aguinis H, Pierce CA, Bosco FA, Dalton DR, Dalton CM (2011c) Debunking myths and urban legends about meta-analysis. Organ Res Methods 14(2):306–331
Aloe AM (2015) Inaccuracy of regression results in replacing bivariate correlations. Res Synth Methods 6(1):21–27
Anderson RG, Kichkha A (2017) Replication, meta-analysis, and research synthesis in economics. Am Econ Rev 107(5):56–59
Appelbaum M, Cooper H, Kline RB, Mayo-Wilson E, Nezu AM, Rao SM (2018) Journal article reporting standards for quantitative research in psychology: the APA publications and communications BOARD task force report. Am Psychol 73(1):3–25
Aytug ZG, Rothstein HR, Zhou W, Kern MC (2012) Revealed or concealed? Transparency of procedures, decisions, and judgment calls in meta-analyses. Organ Res Methods 15(1):103–133
Begg CB, Mazumdar M (1994) Operating characteristics of a rank correlation test for publication bias. Biometrics 50(4):1088–1101. https://doi.org/10.2307/2533446
Bergh DD, Aguinis H, Heavey C, Ketchen DJ, Boyd BK, Su P, Lau CLL, Joo H (2016) Using meta-analytic structural equation modeling to advance strategic management research: Guidelines and an empirical illustration via the strategic leadership-performance relationship. Strateg Manag J 37(3):477–497
Becker BJ (1992) Using results from replicated studies to estimate linear models. J Educ Stat 17(4):341–362
Becker BJ (1995) Corrections to “Using results from replicated studies to estimate linear models.” J Edu Behav Stat 20(1):100–102
Bergmann C, Tsuji S, Piccinini PE, Lewis ML, Braginsky M, Frank MC, Cristia A (2018) Promoting replicability in developmental research through meta-analyses: Insights from language acquisition research. Child Dev 89(6):1996–2009
Bernerth JB, Aguinis H (2016) A critical review and best-practice recommendations for control variable usage. Pers Psychol 69(1):229–283
Bernerth JB, Cole MS, Taylor EC, Walker HJ (2018) Control variables in leadership research: A qualitative and quantitative review. J Manag 44(1):131–160
Bijmolt TH, Pieters RG (2001) Meta-analysis in marketing when studies contain multiple measurements. Mark Lett 12(2):157–169
Block J, Kuckertz A (2018) Seven principles of effective replication studies: Strengthening the evidence base of management research. Manag Rev Quart 68:355–359
Borenstein M (2009) Effect sizes for continuous data. In: Cooper H, Hedges LV, Valentine JC (eds) The handbook of research synthesis and meta-analysis. Russell Sage Foundation, pp 221–235
Borenstein M, Hedges LV, Higgins JPT, Rothstein HR (2009) Introduction to meta-analysis. John Wiley, Chichester
Book Google Scholar
Borenstein M, Hedges LV, Higgins JPT, Rothstein HR (2010) A basic introduction to fixed-effect and random-effects models for meta-analysis. Res Synth Methods 1(2):97–111
Borenstein M, Hedges L, Higgins J, Rothstein H (2013) Comprehensive meta-analysis (version 3). Biostat, Englewood, NJ
Borenstein M, Higgins JP (2013) Meta-analysis and subgroups. Prev Sci 14(2):134–143
Bosco FA, Steel P, Oswald FL, Uggerslev K, Field JG (2015) Cloud-based meta-analysis to bridge science and practice: Welcome to metaBUS. Person Assess Decis 1(1):3–17
Bosco FA, Uggerslev KL, Steel P (2017) MetaBUS as a vehicle for facilitating meta-analysis. Hum Resour Manag Rev 27(1):237–254
Burgard T, Bošnjak M, Studtrucker R (2021) Community-augmented meta-analyses (CAMAs) in psychology: potentials and current systems. Zeitschrift Für Psychologie 229(1):15–23
Cheung MWL (2015a) Meta-analysis: A structural equation modeling approach. John Wiley & Sons, Chichester
Cheung MWL (2015b) metaSEM: An R package for meta-analysis using structural equation modeling. Front Psychol 5:1521
Cheung MWL (2019) A guide to conducting a meta-analysis with non-independent effect sizes. Neuropsychol Rev 29(4):387–396
Cheung MWL, Chan W (2005) Meta-analytic structural equation modeling: a two-stage approach. Psychol Methods 10(1):40–64
Cheung MWL, Vijayakumar R (2016) A guide to conducting a meta-analysis. Neuropsychol Rev 26(2):121–128
Combs JG, Crook TR, Rauch A (2019) Meta-analytic research in management: contemporary approaches unresolved controversies and rising standards. J Manag Stud 56(1):1–18. https://doi.org/10.1111/joms.12427
DeSimone JA, Köhler T, Schoen JL (2019) If it were only that easy: the use of meta-analytic research by organizational scholars. Organ Res Methods 22(4):867–891. https://doi.org/10.1177/1094428118756743
DeSimone JA, Brannick MT, O’Boyle EH, Ryu JW (2020) Recommendations for reviewing meta-analyses in organizational research. Organ Res Methods 56:455–463
Duval S, Tweedie R (2000a) Trim and fill: a simple funnel-plot–based method of testing and adjusting for publication bias in meta-analysis. Biometrics 56(2):455–463
Duval S, Tweedie R (2000b) A nonparametric “trim and fill” method of accounting for publication bias in meta-analysis. J Am Stat Assoc 95(449):89–98
Egger M, Smith GD, Schneider M, Minder C (1997) Bias in meta-analysis detected by a simple, graphical test. BMJ 315(7109):629–634
Eisend M (2017) Meta-Analysis in advertising research. J Advert 46(1):21–35
Elliott JH, Synnot A, Turner T, Simmons M, Akl EA, McDonald S, Salanti G, Meerpohl J, MacLehose H, Hilton J, Tovey D, Shemilt I, Thomas J (2017) Living systematic review: 1. Introduction—the why, what, when, and how. J Clin Epidemiol 91:2330. https://doi.org/10.1016/j.jclinepi.2017.08.010
Field AP, Gillett R (2010) How to do a meta-analysis. Br J Math Stat Psychol 63(3):665–694
Fisch C, Block J (2018) Six tips for your (systematic) literature review in business and management research. Manag Rev Quart 68:103–106
Fortunato S, Bergstrom CT, Börner K, Evans JA, Helbing D, Milojević S, Petersen AM, Radicchi F, Sinatra R, Uzzi B, Vespignani A (2018) Science of science. Science 359(6379). https://doi.org/10.1126/science.aao0185
Geyer-Klingeberg J, Hang M, Rathgeber A (2020) Meta-analysis in finance research: Opportunities, challenges, and contemporary applications. Int Rev Finan Anal 71:101524
Geyskens I, Krishnan R, Steenkamp JBE, Cunha PV (2009) A review and evaluation of meta-analysis practices in management research. J Manag 35(2):393–419
Glass GV (2015) Meta-analysis at middle age: a personal history. Res Synth Methods 6(3):221–231
Gonzalez-Mulé E, Aguinis H (2018) Advancing theory by assessing boundary conditions with metaregression: a critical review and best-practice recommendations. J Manag 44(6):2246–2273
Gooty J, Banks GC, Loignon AC, Tonidandel S, Williams CE (2021) Meta-analyses as a multi-level model. Organ Res Methods 24(2):389–411. https://doi.org/10.1177/1094428119857471
Grewal D, Puccinelli N, Monroe KB (2018) Meta-analysis: integrating accumulated knowledge. J Acad Mark Sci 46(1):9–30
Gurevitch J, Koricheva J, Nakagawa S, Stewart G (2018) Meta-analysis and the science of research synthesis. Nature 555(7695):175–182
Gusenbauer M, Haddaway NR (2020) Which academic search systems are suitable for systematic reviews or meta-analyses? Evaluating retrieval qualities of Google Scholar, PubMed, and 26 other resources. Res Synth Methods 11(2):181–217
Habersang S, Küberling-Jost J, Reihlen M, Seckler C (2019) A process perspective on organizational failure: a qualitative meta-analysis. J Manage Stud 56(1):19–56
Harari MB, Parola HR, Hartwell CJ, Riegelman A (2020) Literature searches in systematic reviews and meta-analyses: A review, evaluation, and recommendations. J Vocat Behav 118:103377
Harrison JS, Banks GC, Pollack JM, O’Boyle EH, Short J (2017) Publication bias in strategic management research. J Manag 43(2):400–425
Havránek T, Stanley TD, Doucouliagos H, Bom P, Geyer-Klingeberg J, Iwasaki I, Reed WR, Rost K, Van Aert RCM (2020) Reporting guidelines for meta-analysis in economics. J Econ Surveys 34(3):469–475
Hedges LV, Olkin I (1985) Statistical methods for meta-analysis. Academic Press, Orlando
Hedges LV, Vevea JL (2005) Selection methods approaches. In: Rothstein HR, Sutton A, Borenstein M (eds) Publication bias in meta-analysis: prevention, assessment, and adjustments. Wiley, Chichester, pp 145–174
Hoon C (2013) Meta-synthesis of qualitative case studies: an approach to theory building. Organ Res Methods 16(4):522–556
Hunter JE, Schmidt FL (1990) Methods of meta-analysis: correcting error and bias in research findings. Sage, Newbury Park
Hunter JE, Schmidt FL (2004) Methods of meta-analysis: correcting error and bias in research findings, 2nd edn. Sage, Thousand Oaks
Hunter JE, Schmidt FL, Jackson GB (1982) Meta-analysis: cumulating research findings across studies. Sage Publications, Beverly Hills
Jak S (2015) Meta-analytic structural equation modelling. Springer, New York, NY
Kepes S, Banks GC, McDaniel M, Whetzel DL (2012) Publication bias in the organizational sciences. Organ Res Methods 15(4):624–662
Kepes S, McDaniel MA, Brannick MT, Banks GC (2013) Meta-analytic reviews in the organizational sciences: Two meta-analytic schools on the way to MARS (the Meta-Analytic Reporting Standards). J Bus Psychol 28(2):123–143
Kraus S, Breier M, Dasí-Rodríguez S (2020) The art of crafting a systematic literature review in entrepreneurship research. Int Entrepreneur Manag J 16(3):1023–1042
Levitt HM (2018) How to conduct a qualitative meta-analysis: tailoring methods to enhance methodological integrity. Psychother Res 28(3):367–378
Levitt HM, Bamberg M, Creswell JW, Frost DM, Josselson R, Suárez-Orozco C (2018) Journal article reporting standards for qualitative primary, qualitative meta-analytic, and mixed methods research in psychology: the APA publications and communications board task force report. Am Psychol 73(1):26
Lipsey MW, Wilson DB (2001) Practical meta-analysis. Sage Publications, Inc.
López-López JA, Page MJ, Lipsey MW, Higgins JP (2018) Dealing with effect size multiplicity in systematic reviews and meta-analyses. Res Synth Methods 9(3):336–351
Martín-Martín A, Thelwall M, Orduna-Malea E, López-Cózar ED (2021) Google Scholar, Microsoft Academic, Scopus, Dimensions, Web of Science, and OpenCitations’ COCI: a multidisciplinary comparison of coverage via citations. Scientometrics 126(1):871–906
Merton RK (1968) The Matthew effect in science: the reward and communication systems of science are considered. Science 159(3810):56–63
Moeyaert M, Ugille M, Natasha Beretvas S, Ferron J, Bunuan R, Van den Noortgate W (2017) Methods for dealing with multiple outcomes in meta-analysis: a comparison between averaging effect sizes, robust variance estimation and multilevel meta-analysis. Int J Soc Res Methodol 20(6):559–572
Moher D, Liberati A, Tetzlaff J, Altman DG, Prisma Group (2009) Preferred reporting items for systematic reviews and meta-analyses: the PRISMA statement. PLoS medicine. 6(7):e1000097
Mongeon P, Paul-Hus A (2016) The journal coverage of Web of Science and Scopus: a comparative analysis. Scientometrics 106(1):213–228
Moreau D, Gamble B (2020) Conducting a meta-analysis in the age of open science: Tools, tips, and practical recommendations. Psychol Methods. https://doi.org/10.1037/met0000351
O’Mara-Eves A, Thomas J, McNaught J, Miwa M, Ananiadou S (2015) Using text mining for study identification in systematic reviews: a systematic review of current approaches. Syst Rev 4(1):1–22
Ouzzani M, Hammady H, Fedorowicz Z, Elmagarmid A (2016) Rayyan—a web and mobile app for systematic reviews. Syst Rev 5(1):1–10
Owen E, Li Q (2021) The conditional nature of publication bias: a meta-regression analysis. Polit Sci Res Methods 9(4):867–877
Page MJ, McKenzie JE, Bossuyt PM, Boutron I, Hoffmann TC, Mulrow CD, Shamseer L, Tetzlaff JM, Akl EA, Brennan SE, Chou R, Glanville J, Grimshaw JM, Hróbjartsson A, Lalu MM, Li T, Loder EW, Mayo-Wilson E,McDonald S,McGuinness LA, Stewart LA, Thomas J, Tricco AC, Welch VA, Whiting P, Moher D (2021) The PRISMA 2020 statement: an updated guideline for reporting systematic reviews. BMJ 372. https://doi.org/10.1136/bmj.n71
Palmer TM, Sterne JAC (eds) (2016) Meta-analysis in stata: an updated collection from the stata journal, 2nd edn. Stata Press, College Station, TX
Pigott TD, Polanin JR (2020) Methodological guidance paper: High-quality meta-analysis in a systematic review. Rev Educ Res 90(1):24–46
Polanin JR, Tanner-Smith EE, Hennessy EA (2016) Estimating the difference between published and unpublished effect sizes: a meta-review. Rev Educ Res 86(1):207–236
Polanin JR, Hennessy EA, Tanner-Smith EE (2017) A review of meta-analysis packages in R. J Edu Behav Stat 42(2):206–242
Polanin JR, Hennessy EA, Tsuji S (2020) Transparency and reproducibility of meta-analyses in psychology: a meta-review. Perspect Psychol Sci 15(4):1026–1041. https://doi.org/10.1177/17456916209064
R Core Team (2021). R: A language and environment for statistical computing . R Foundation for Statistical Computing, Vienna, Austria. URL https://www.R-project.org/ .
Rauch A (2020) Opportunities and threats in reviewing entrepreneurship theory and practice. Entrep Theory Pract 44(5):847–860
Rauch A, van Doorn R, Hulsink W (2014) A qualitative approach to evidence–based entrepreneurship: theoretical considerations and an example involving business clusters. Entrep Theory Pract 38(2):333–368
Raudenbush SW (2009) Analyzing effect sizes: Random-effects models. In: Cooper H, Hedges LV, Valentine JC (eds) The handbook of research synthesis and meta-analysis, 2nd edn. Russell Sage Foundation, New York, NY, pp 295–315
Rosenthal R (1979) The file drawer problem and tolerance for null results. Psychol Bull 86(3):638
Rothstein HR, Sutton AJ, Borenstein M (2005) Publication bias in meta-analysis: prevention, assessment and adjustments. Wiley, Chichester
Roth PL, Le H, Oh I-S, Van Iddekinge CH, Bobko P (2018) Using beta coefficients to impute missing correlations in meta-analysis research: Reasons for caution. J Appl Psychol 103(6):644–658. https://doi.org/10.1037/apl0000293
Rudolph CW, Chang CK, Rauvola RS, Zacher H (2020) Meta-analysis in vocational behavior: a systematic review and recommendations for best practices. J Vocat Behav 118:103397
Schmidt FL (2017) Statistical and measurement pitfalls in the use of meta-regression in meta-analysis. Career Dev Int 22(5):469–476
Schmidt FL, Hunter JE (2015) Methods of meta-analysis: correcting error and bias in research findings. Sage, Thousand Oaks
Schwab A (2015) Why all researchers should report effect sizes and their confidence intervals: Paving the way for meta–analysis and evidence–based management practices. Entrepreneurship Theory Pract 39(4):719–725. https://doi.org/10.1111/etap.12158
Shaw JD, Ertug G (2017) The suitability of simulations and meta-analyses for submissions to Academy of Management Journal. Acad Manag J 60(6):2045–2049
Soderberg CK (2018) Using OSF to share data: A step-by-step guide. Adv Methods Pract Psychol Sci 1(1):115–120
Stanley TD, Doucouliagos H (2010) Picture this: a simple graph that reveals much ado about research. J Econ Surveys 24(1):170–191
Stanley TD, Doucouliagos H (2012) Meta-regression analysis in economics and business. Routledge, London
Stanley TD, Jarrell SB (1989) Meta-regression analysis: a quantitative method of literature surveys. J Econ Surveys 3:54–67
Steel P, Beugelsdijk S, Aguinis H (2021) The anatomy of an award-winning meta-analysis: Recommendations for authors, reviewers, and readers of meta-analytic reviews. J Int Bus Stud 52(1):23–44
Suurmond R, van Rhee H, Hak T (2017) Introduction, comparison, and validation of Meta-Essentials: a free and simple tool for meta-analysis. Res Synth Methods 8(4):537–553
The Cochrane Collaboration (2020). Review Manager (RevMan) [Computer program] (Version 5.4).
Thomas J, Noel-Storr A, Marshall I, Wallace B, McDonald S, Mavergames C, Glasziou P, Shemilt I, Synnot A, Turner T, Elliot J (2017) Living systematic reviews: 2. Combining human and machine effort. J Clin Epidemiol 91:31–37
Thompson SG, Higgins JP (2002) How should meta-regression analyses be undertaken and interpreted? Stat Med 21(11):1559–1573
Tipton E, Pustejovsky JE, Ahmadi H (2019) A history of meta-regression: technical, conceptual, and practical developments between 1974 and 2018. Res Synth Methods 10(2):161–179
Vevea JL, Woods CM (2005) Publication bias in research synthesis: Sensitivity analysis using a priori weight functions. Psychol Methods 10(4):428–443
Viechtbauer W (2010) Conducting meta-analyses in R with the metafor package. J Stat Softw 36(3):1–48
Viechtbauer W, Cheung MWL (2010) Outlier and influence diagnostics for meta-analysis. Res Synth Methods 1(2):112–125
Viswesvaran C, Ones DS (1995) Theory testing: combining psychometric meta-analysis and structural equations modeling. Pers Psychol 48(4):865–885
Wilson SJ, Polanin JR, Lipsey MW (2016) Fitting meta-analytic structural equation models with complex datasets. Res Synth Methods 7(2):121–139. https://doi.org/10.1002/jrsm.1199
Wood JA (2008) Methodology for dealing with duplicate study effects in a meta-analysis. Organ Res Methods 11(1):79–95
Download references
Open Access funding enabled and organized by Projekt DEAL. No funding was received to assist with the preparation of this manuscript.
Author information
Authors and affiliations.
University of Luxembourg, Luxembourg, Luxembourg
Christopher Hansen
Leibniz Institute for Psychology (ZPID), Trier, Germany
Holger Steinmetz
Trier University, Trier, Germany
Erasmus University Rotterdam, Rotterdam, The Netherlands
Wittener Institut Für Familienunternehmen, Universität Witten/Herdecke, Witten, Germany
You can also search for this author in PubMed Google Scholar
Corresponding author
Correspondence to Jörn Block .
Ethics declarations
Conflict of interest.
The authors have no relevant financial or non-financial interests to disclose.
Additional information
Publisher's note.
Springer Nature remains neutral with regard to jurisdictional claims in published maps and institutional affiliations.
See Table 1 .
Rights and permissions
Open Access This article is licensed under a Creative Commons Attribution 4.0 International License, which permits use, sharing, adaptation, distribution and reproduction in any medium or format, as long as you give appropriate credit to the original author(s) and the source, provide a link to the Creative Commons licence, and indicate if changes were made. The images or other third party material in this article are included in the article's Creative Commons licence, unless indicated otherwise in a credit line to the material. If material is not included in the article's Creative Commons licence and your intended use is not permitted by statutory regulation or exceeds the permitted use, you will need to obtain permission directly from the copyright holder. To view a copy of this licence, visit http://creativecommons.org/licenses/by/4.0/ .
Reprints and permissions
About this article
Hansen, C., Steinmetz, H. & Block, J. How to conduct a meta-analysis in eight steps: a practical guide. Manag Rev Q 72 , 1–19 (2022). https://doi.org/10.1007/s11301-021-00247-4
Download citation
Published : 30 November 2021
Issue Date : February 2022
DOI : https://doi.org/10.1007/s11301-021-00247-4
Share this article
Anyone you share the following link with will be able to read this content:
Sorry, a shareable link is not currently available for this article.
Provided by the Springer Nature SharedIt content-sharing initiative
- Find a journal
- Publish with us
- Track your research

An official website of the United States government
The .gov means it’s official. Federal government websites often end in .gov or .mil. Before sharing sensitive information, make sure you’re on a federal government site.
The site is secure. The https:// ensures that you are connecting to the official website and that any information you provide is encrypted and transmitted securely.
- Publications
- Account settings
Preview improvements coming to the PMC website in October 2024. Learn More or Try it out now .
- Advanced Search
- Journal List
- Korean J Anesthesiol
- v.71(2); 2018 Apr
Introduction to systematic review and meta-analysis
1 Department of Anesthesiology and Pain Medicine, Inje University Seoul Paik Hospital, Seoul, Korea
2 Department of Anesthesiology and Pain Medicine, Chung-Ang University College of Medicine, Seoul, Korea
Systematic reviews and meta-analyses present results by combining and analyzing data from different studies conducted on similar research topics. In recent years, systematic reviews and meta-analyses have been actively performed in various fields including anesthesiology. These research methods are powerful tools that can overcome the difficulties in performing large-scale randomized controlled trials. However, the inclusion of studies with any biases or improperly assessed quality of evidence in systematic reviews and meta-analyses could yield misleading results. Therefore, various guidelines have been suggested for conducting systematic reviews and meta-analyses to help standardize them and improve their quality. Nonetheless, accepting the conclusions of many studies without understanding the meta-analysis can be dangerous. Therefore, this article provides an easy introduction to clinicians on performing and understanding meta-analyses.
Introduction
A systematic review collects all possible studies related to a given topic and design, and reviews and analyzes their results [ 1 ]. During the systematic review process, the quality of studies is evaluated, and a statistical meta-analysis of the study results is conducted on the basis of their quality. A meta-analysis is a valid, objective, and scientific method of analyzing and combining different results. Usually, in order to obtain more reliable results, a meta-analysis is mainly conducted on randomized controlled trials (RCTs), which have a high level of evidence [ 2 ] ( Fig. 1 ). Since 1999, various papers have presented guidelines for reporting meta-analyses of RCTs. Following the Quality of Reporting of Meta-analyses (QUORUM) statement [ 3 ], and the appearance of registers such as Cochrane Library’s Methodology Register, a large number of systematic literature reviews have been registered. In 2009, the Preferred Reporting Items for Systematic Reviews and Meta-Analyses (PRISMA) statement [ 4 ] was published, and it greatly helped standardize and improve the quality of systematic reviews and meta-analyses [ 5 ].

Levels of evidence.
In anesthesiology, the importance of systematic reviews and meta-analyses has been highlighted, and they provide diagnostic and therapeutic value to various areas, including not only perioperative management but also intensive care and outpatient anesthesia [6–13]. Systematic reviews and meta-analyses include various topics, such as comparing various treatments of postoperative nausea and vomiting [ 14 , 15 ], comparing general anesthesia and regional anesthesia [ 16 – 18 ], comparing airway maintenance devices [ 8 , 19 ], comparing various methods of postoperative pain control (e.g., patient-controlled analgesia pumps, nerve block, or analgesics) [ 20 – 23 ], comparing the precision of various monitoring instruments [ 7 ], and meta-analysis of dose-response in various drugs [ 12 ].
Thus, literature reviews and meta-analyses are being conducted in diverse medical fields, and the aim of highlighting their importance is to help better extract accurate, good quality data from the flood of data being produced. However, a lack of understanding about systematic reviews and meta-analyses can lead to incorrect outcomes being derived from the review and analysis processes. If readers indiscriminately accept the results of the many meta-analyses that are published, incorrect data may be obtained. Therefore, in this review, we aim to describe the contents and methods used in systematic reviews and meta-analyses in a way that is easy to understand for future authors and readers of systematic review and meta-analysis.
Study Planning
It is easy to confuse systematic reviews and meta-analyses. A systematic review is an objective, reproducible method to find answers to a certain research question, by collecting all available studies related to that question and reviewing and analyzing their results. A meta-analysis differs from a systematic review in that it uses statistical methods on estimates from two or more different studies to form a pooled estimate [ 1 ]. Following a systematic review, if it is not possible to form a pooled estimate, it can be published as is without progressing to a meta-analysis; however, if it is possible to form a pooled estimate from the extracted data, a meta-analysis can be attempted. Systematic reviews and meta-analyses usually proceed according to the flowchart presented in Fig. 2 . We explain each of the stages below.

Flowchart illustrating a systematic review.
Formulating research questions
A systematic review attempts to gather all available empirical research by using clearly defined, systematic methods to obtain answers to a specific question. A meta-analysis is the statistical process of analyzing and combining results from several similar studies. Here, the definition of the word “similar” is not made clear, but when selecting a topic for the meta-analysis, it is essential to ensure that the different studies present data that can be combined. If the studies contain data on the same topic that can be combined, a meta-analysis can even be performed using data from only two studies. However, study selection via a systematic review is a precondition for performing a meta-analysis, and it is important to clearly define the Population, Intervention, Comparison, Outcomes (PICO) parameters that are central to evidence-based research. In addition, selection of the research topic is based on logical evidence, and it is important to select a topic that is familiar to readers without clearly confirmed the evidence [ 24 ].
Protocols and registration
In systematic reviews, prior registration of a detailed research plan is very important. In order to make the research process transparent, primary/secondary outcomes and methods are set in advance, and in the event of changes to the method, other researchers and readers are informed when, how, and why. Many studies are registered with an organization like PROSPERO ( http://www.crd.york.ac.uk/PROSPERO/ ), and the registration number is recorded when reporting the study, in order to share the protocol at the time of planning.
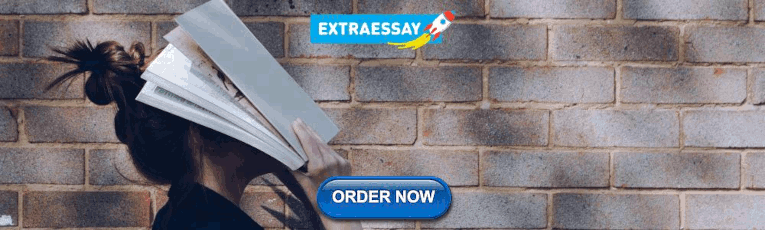
Defining inclusion and exclusion criteria
Information is included on the study design, patient characteristics, publication status (published or unpublished), language used, and research period. If there is a discrepancy between the number of patients included in the study and the number of patients included in the analysis, this needs to be clearly explained while describing the patient characteristics, to avoid confusing the reader.
Literature search and study selection
In order to secure proper basis for evidence-based research, it is essential to perform a broad search that includes as many studies as possible that meet the inclusion and exclusion criteria. Typically, the three bibliographic databases Medline, Embase, and Cochrane Central Register of Controlled Trials (CENTRAL) are used. In domestic studies, the Korean databases KoreaMed, KMBASE, and RISS4U may be included. Effort is required to identify not only published studies but also abstracts, ongoing studies, and studies awaiting publication. Among the studies retrieved in the search, the researchers remove duplicate studies, select studies that meet the inclusion/exclusion criteria based on the abstracts, and then make the final selection of studies based on their full text. In order to maintain transparency and objectivity throughout this process, study selection is conducted independently by at least two investigators. When there is a inconsistency in opinions, intervention is required via debate or by a third reviewer. The methods for this process also need to be planned in advance. It is essential to ensure the reproducibility of the literature selection process [ 25 ].
Quality of evidence
However, well planned the systematic review or meta-analysis is, if the quality of evidence in the studies is low, the quality of the meta-analysis decreases and incorrect results can be obtained [ 26 ]. Even when using randomized studies with a high quality of evidence, evaluating the quality of evidence precisely helps determine the strength of recommendations in the meta-analysis. One method of evaluating the quality of evidence in non-randomized studies is the Newcastle-Ottawa Scale, provided by the Ottawa Hospital Research Institute 1) . However, we are mostly focusing on meta-analyses that use randomized studies.
If the Grading of Recommendations, Assessment, Development and Evaluations (GRADE) system ( http://www.gradeworkinggroup.org/ ) is used, the quality of evidence is evaluated on the basis of the study limitations, inaccuracies, incompleteness of outcome data, indirectness of evidence, and risk of publication bias, and this is used to determine the strength of recommendations [ 27 ]. As shown in Table 1 , the study limitations are evaluated using the “risk of bias” method proposed by Cochrane 2) . This method classifies bias in randomized studies as “low,” “high,” or “unclear” on the basis of the presence or absence of six processes (random sequence generation, allocation concealment, blinding participants or investigators, incomplete outcome data, selective reporting, and other biases) [ 28 ].
The Cochrane Collaboration’s Tool for Assessing the Risk of Bias [ 28 ]
Data extraction
Two different investigators extract data based on the objectives and form of the study; thereafter, the extracted data are reviewed. Since the size and format of each variable are different, the size and format of the outcomes are also different, and slight changes may be required when combining the data [ 29 ]. If there are differences in the size and format of the outcome variables that cause difficulties combining the data, such as the use of different evaluation instruments or different evaluation timepoints, the analysis may be limited to a systematic review. The investigators resolve differences of opinion by debate, and if they fail to reach a consensus, a third-reviewer is consulted.
Data Analysis
The aim of a meta-analysis is to derive a conclusion with increased power and accuracy than what could not be able to achieve in individual studies. Therefore, before analysis, it is crucial to evaluate the direction of effect, size of effect, homogeneity of effects among studies, and strength of evidence [ 30 ]. Thereafter, the data are reviewed qualitatively and quantitatively. If it is determined that the different research outcomes cannot be combined, all the results and characteristics of the individual studies are displayed in a table or in a descriptive form; this is referred to as a qualitative review. A meta-analysis is a quantitative review, in which the clinical effectiveness is evaluated by calculating the weighted pooled estimate for the interventions in at least two separate studies.
The pooled estimate is the outcome of the meta-analysis, and is typically explained using a forest plot ( Figs. 3 and and4). 4 ). The black squares in the forest plot are the odds ratios (ORs) and 95% confidence intervals in each study. The area of the squares represents the weight reflected in the meta-analysis. The black diamond represents the OR and 95% confidence interval calculated across all the included studies. The bold vertical line represents a lack of therapeutic effect (OR = 1); if the confidence interval includes OR = 1, it means no significant difference was found between the treatment and control groups.

Forest plot analyzed by two different models using the same data. (A) Fixed-effect model. (B) Random-effect model. The figure depicts individual trials as filled squares with the relative sample size and the solid line as the 95% confidence interval of the difference. The diamond shape indicates the pooled estimate and uncertainty for the combined effect. The vertical line indicates the treatment group shows no effect (OR = 1). Moreover, if the confidence interval includes 1, then the result shows no evidence of difference between the treatment and control groups.

Forest plot representing homogeneous data.
Dichotomous variables and continuous variables
In data analysis, outcome variables can be considered broadly in terms of dichotomous variables and continuous variables. When combining data from continuous variables, the mean difference (MD) and standardized mean difference (SMD) are used ( Table 2 ).
Summary of Meta-analysis Methods Available in RevMan [ 28 ]
The MD is the absolute difference in mean values between the groups, and the SMD is the mean difference between groups divided by the standard deviation. When results are presented in the same units, the MD can be used, but when results are presented in different units, the SMD should be used. When the MD is used, the combined units must be shown. A value of “0” for the MD or SMD indicates that the effects of the new treatment method and the existing treatment method are the same. A value lower than “0” means the new treatment method is less effective than the existing method, and a value greater than “0” means the new treatment is more effective than the existing method.
When combining data for dichotomous variables, the OR, risk ratio (RR), or risk difference (RD) can be used. The RR and RD can be used for RCTs, quasi-experimental studies, or cohort studies, and the OR can be used for other case-control studies or cross-sectional studies. However, because the OR is difficult to interpret, using the RR and RD, if possible, is recommended. If the outcome variable is a dichotomous variable, it can be presented as the number needed to treat (NNT), which is the minimum number of patients who need to be treated in the intervention group, compared to the control group, for a given event to occur in at least one patient. Based on Table 3 , in an RCT, if x is the probability of the event occurring in the control group and y is the probability of the event occurring in the intervention group, then x = c/(c + d), y = a/(a + b), and the absolute risk reduction (ARR) = x − y. NNT can be obtained as the reciprocal, 1/ARR.
Calculation of the Number Needed to Treat in the Dichotomous table
Fixed-effect models and random-effect models
In order to analyze effect size, two types of models can be used: a fixed-effect model or a random-effect model. A fixed-effect model assumes that the effect of treatment is the same, and that variation between results in different studies is due to random error. Thus, a fixed-effect model can be used when the studies are considered to have the same design and methodology, or when the variability in results within a study is small, and the variance is thought to be due to random error. Three common methods are used for weighted estimation in a fixed-effect model: 1) inverse variance-weighted estimation 3) , 2) Mantel-Haenszel estimation 4) , and 3) Peto estimation 5) .
A random-effect model assumes heterogeneity between the studies being combined, and these models are used when the studies are assumed different, even if a heterogeneity test does not show a significant result. Unlike a fixed-effect model, a random-effect model assumes that the size of the effect of treatment differs among studies. Thus, differences in variation among studies are thought to be due to not only random error but also between-study variability in results. Therefore, weight does not decrease greatly for studies with a small number of patients. Among methods for weighted estimation in a random-effect model, the DerSimonian and Laird method 6) is mostly used for dichotomous variables, as the simplest method, while inverse variance-weighted estimation is used for continuous variables, as with fixed-effect models. These four methods are all used in Review Manager software (The Cochrane Collaboration, UK), and are described in a study by Deeks et al. [ 31 ] ( Table 2 ). However, when the number of studies included in the analysis is less than 10, the Hartung-Knapp-Sidik-Jonkman method 7) can better reduce the risk of type 1 error than does the DerSimonian and Laird method [ 32 ].
Fig. 3 shows the results of analyzing outcome data using a fixed-effect model (A) and a random-effect model (B). As shown in Fig. 3 , while the results from large studies are weighted more heavily in the fixed-effect model, studies are given relatively similar weights irrespective of study size in the random-effect model. Although identical data were being analyzed, as shown in Fig. 3 , the significant result in the fixed-effect model was no longer significant in the random-effect model. One representative example of the small study effect in a random-effect model is the meta-analysis by Li et al. [ 33 ]. In a large-scale study, intravenous injection of magnesium was unrelated to acute myocardial infarction, but in the random-effect model, which included numerous small studies, the small study effect resulted in an association being found between intravenous injection of magnesium and myocardial infarction. This small study effect can be controlled for by using a sensitivity analysis, which is performed to examine the contribution of each of the included studies to the final meta-analysis result. In particular, when heterogeneity is suspected in the study methods or results, by changing certain data or analytical methods, this method makes it possible to verify whether the changes affect the robustness of the results, and to examine the causes of such effects [ 34 ].
Heterogeneity
Homogeneity test is a method whether the degree of heterogeneity is greater than would be expected to occur naturally when the effect size calculated from several studies is higher than the sampling error. This makes it possible to test whether the effect size calculated from several studies is the same. Three types of homogeneity tests can be used: 1) forest plot, 2) Cochrane’s Q test (chi-squared), and 3) Higgins I 2 statistics. In the forest plot, as shown in Fig. 4 , greater overlap between the confidence intervals indicates greater homogeneity. For the Q statistic, when the P value of the chi-squared test, calculated from the forest plot in Fig. 4 , is less than 0.1, it is considered to show statistical heterogeneity and a random-effect can be used. Finally, I 2 can be used [ 35 ].
I 2 , calculated as shown above, returns a value between 0 and 100%. A value less than 25% is considered to show strong homogeneity, a value of 50% is average, and a value greater than 75% indicates strong heterogeneity.
Even when the data cannot be shown to be homogeneous, a fixed-effect model can be used, ignoring the heterogeneity, and all the study results can be presented individually, without combining them. However, in many cases, a random-effect model is applied, as described above, and a subgroup analysis or meta-regression analysis is performed to explain the heterogeneity. In a subgroup analysis, the data are divided into subgroups that are expected to be homogeneous, and these subgroups are analyzed. This needs to be planned in the predetermined protocol before starting the meta-analysis. A meta-regression analysis is similar to a normal regression analysis, except that the heterogeneity between studies is modeled. This process involves performing a regression analysis of the pooled estimate for covariance at the study level, and so it is usually not considered when the number of studies is less than 10. Here, univariate and multivariate regression analyses can both be considered.
Publication bias
Publication bias is the most common type of reporting bias in meta-analyses. This refers to the distortion of meta-analysis outcomes due to the higher likelihood of publication of statistically significant studies rather than non-significant studies. In order to test the presence or absence of publication bias, first, a funnel plot can be used ( Fig. 5 ). Studies are plotted on a scatter plot with effect size on the x-axis and precision or total sample size on the y-axis. If the points form an upside-down funnel shape, with a broad base that narrows towards the top of the plot, this indicates the absence of a publication bias ( Fig. 5A ) [ 29 , 36 ]. On the other hand, if the plot shows an asymmetric shape, with no points on one side of the graph, then publication bias can be suspected ( Fig. 5B ). Second, to test publication bias statistically, Begg and Mazumdar’s rank correlation test 8) [ 37 ] or Egger’s test 9) [ 29 ] can be used. If publication bias is detected, the trim-and-fill method 10) can be used to correct the bias [ 38 ]. Fig. 6 displays results that show publication bias in Egger’s test, which has then been corrected using the trim-and-fill method using Comprehensive Meta-Analysis software (Biostat, USA).

Funnel plot showing the effect size on the x-axis and sample size on the y-axis as a scatter plot. (A) Funnel plot without publication bias. The individual plots are broader at the bottom and narrower at the top. (B) Funnel plot with publication bias. The individual plots are located asymmetrically.

Funnel plot adjusted using the trim-and-fill method. White circles: comparisons included. Black circles: inputted comparisons using the trim-and-fill method. White diamond: pooled observed log risk ratio. Black diamond: pooled inputted log risk ratio.
Result Presentation
When reporting the results of a systematic review or meta-analysis, the analytical content and methods should be described in detail. First, a flowchart is displayed with the literature search and selection process according to the inclusion/exclusion criteria. Second, a table is shown with the characteristics of the included studies. A table should also be included with information related to the quality of evidence, such as GRADE ( Table 4 ). Third, the results of data analysis are shown in a forest plot and funnel plot. Fourth, if the results use dichotomous data, the NNT values can be reported, as described above.
The GRADE Evidence Quality for Each Outcome
N: number of studies, ROB: risk of bias, PON: postoperative nausea, POV: postoperative vomiting, PONV: postoperative nausea and vomiting, CI: confidence interval, RR: risk ratio, AR: absolute risk.
When Review Manager software (The Cochrane Collaboration, UK) is used for the analysis, two types of P values are given. The first is the P value from the z-test, which tests the null hypothesis that the intervention has no effect. The second P value is from the chi-squared test, which tests the null hypothesis for a lack of heterogeneity. The statistical result for the intervention effect, which is generally considered the most important result in meta-analyses, is the z-test P value.
A common mistake when reporting results is, given a z-test P value greater than 0.05, to say there was “no statistical significance” or “no difference.” When evaluating statistical significance in a meta-analysis, a P value lower than 0.05 can be explained as “a significant difference in the effects of the two treatment methods.” However, the P value may appear non-significant whether or not there is a difference between the two treatment methods. In such a situation, it is better to announce “there was no strong evidence for an effect,” and to present the P value and confidence intervals. Another common mistake is to think that a smaller P value is indicative of a more significant effect. In meta-analyses of large-scale studies, the P value is more greatly affected by the number of studies and patients included, rather than by the significance of the results; therefore, care should be taken when interpreting the results of a meta-analysis.
When performing a systematic literature review or meta-analysis, if the quality of studies is not properly evaluated or if proper methodology is not strictly applied, the results can be biased and the outcomes can be incorrect. However, when systematic reviews and meta-analyses are properly implemented, they can yield powerful results that could usually only be achieved using large-scale RCTs, which are difficult to perform in individual studies. As our understanding of evidence-based medicine increases and its importance is better appreciated, the number of systematic reviews and meta-analyses will keep increasing. However, indiscriminate acceptance of the results of all these meta-analyses can be dangerous, and hence, we recommend that their results be received critically on the basis of a more accurate understanding.
1) http://www.ohri.ca .
2) http://methods.cochrane.org/bias/assessing-risk-bias-included-studies .
3) The inverse variance-weighted estimation method is useful if the number of studies is small with large sample sizes.
4) The Mantel-Haenszel estimation method is useful if the number of studies is large with small sample sizes.
5) The Peto estimation method is useful if the event rate is low or one of the two groups shows zero incidence.
6) The most popular and simplest statistical method used in Review Manager and Comprehensive Meta-analysis software.
7) Alternative random-effect model meta-analysis that has more adequate error rates than does the common DerSimonian and Laird method, especially when the number of studies is small. However, even with the Hartung-Knapp-Sidik-Jonkman method, when there are less than five studies with very unequal sizes, extra caution is needed.
8) The Begg and Mazumdar rank correlation test uses the correlation between the ranks of effect sizes and the ranks of their variances [ 37 ].
9) The degree of funnel plot asymmetry as measured by the intercept from the regression of standard normal deviates against precision [ 29 ].
10) If there are more small studies on one side, we expect the suppression of studies on the other side. Trimming yields the adjusted effect size and reduces the variance of the effects by adding the original studies back into the analysis as a mirror image of each study.
- Introduction
- Article Information
Data are demonstrated among the derivation (National Inpatient Sample, 2019; Panel A) and validation (National Inpatient Sample, 2020; Panel B) populations including hospitalizations for major diagnostic or therapeutic operating room procedures. Line graphs with observed (black) and projected (blue) mortality connecting survey-weighted, population-based point estimates (dots) and associated 95% CIs (error bars) across the integerized RAI-ICD.
eTable 1. Data Elements and Specific Variables from National Inpatient Sample
eTable 2. Adaptation of Cancer Categorizations Into Clinically Comparable ICD-10-CM Codes and Corresponding Remission Codes
eTable 3. Finalized Adaptation of the Risk Analysis Index Parameters Into Clinically Comparable ICD-10 Codes
eTable 4. Final RAI-ICD Thresholds and Measures of Diagnostic Accuracy for Inpatient Mortality as Calibrated in the Validation National Inpatient Sample Population (2020)
eFigure 1. Survey-Weighted and Estimated Populations in National Inpatient Sample (NIS)
eTable 5. Data Missingness in Operative National Impatient Populations Among Adult Hospitalizations
eTable 6. Logistic Regression for In-Hospital Mortality Weighting Risk Analysis Index Parameters in the National Inpatient Sample Derivation Population (2019)
eFigure 2. Decision Curve Analysis for RAI-ICD for Alterative Cancer Parameter Definitions
eTable 7. Model Performance Based on ICD-10-CM Codes for Each Cancer Categorization in National Inpatient Sample Derivation Population (2019)
eTable 8. Baseline Characteristics of Sensitivity Cohorts Including Operative and Non-Operative Hospitalizations
eFigure 3. Observed and Predicted Mortality by the Integerized RAI-ICD Score for Sensitivity Analysis
eTable 9. Spearman Correlation Between Various Frailty Tools
eFigure 4. Distribution of Frailty Categorization Among UPMC Hospitalizations with an Available Outpatient Risk Analysis Index – Clinical (RAI-C) Score
eReferences.
Data Sharing Statement
See More About
Sign up for emails based on your interests, select your interests.
Customize your JAMA Network experience by selecting one or more topics from the list below.
- Academic Medicine
- Acid Base, Electrolytes, Fluids
- Allergy and Clinical Immunology
- American Indian or Alaska Natives
- Anesthesiology
- Anticoagulation
- Art and Images in Psychiatry
- Artificial Intelligence
- Assisted Reproduction
- Bleeding and Transfusion
- Caring for the Critically Ill Patient
- Challenges in Clinical Electrocardiography
- Climate and Health
- Climate Change
- Clinical Challenge
- Clinical Decision Support
- Clinical Implications of Basic Neuroscience
- Clinical Pharmacy and Pharmacology
- Complementary and Alternative Medicine
- Consensus Statements
- Coronavirus (COVID-19)
- Critical Care Medicine
- Cultural Competency
- Dental Medicine
- Dermatology
- Diabetes and Endocrinology
- Diagnostic Test Interpretation
- Drug Development
- Electronic Health Records
- Emergency Medicine
- End of Life, Hospice, Palliative Care
- Environmental Health
- Equity, Diversity, and Inclusion
- Facial Plastic Surgery
- Gastroenterology and Hepatology
- Genetics and Genomics
- Genomics and Precision Health
- Global Health
- Guide to Statistics and Methods
- Hair Disorders
- Health Care Delivery Models
- Health Care Economics, Insurance, Payment
- Health Care Quality
- Health Care Reform
- Health Care Safety
- Health Care Workforce
- Health Disparities
- Health Inequities
- Health Policy
- Health Systems Science
- History of Medicine
- Hypertension
- Images in Neurology
- Implementation Science
- Infectious Diseases
- Innovations in Health Care Delivery
- JAMA Infographic
- Law and Medicine
- Leading Change
- Less is More
- LGBTQIA Medicine
- Lifestyle Behaviors
- Medical Coding
- Medical Devices and Equipment
- Medical Education
- Medical Education and Training
- Medical Journals and Publishing
- Mobile Health and Telemedicine
- Narrative Medicine
- Neuroscience and Psychiatry
- Notable Notes
- Nutrition, Obesity, Exercise
- Obstetrics and Gynecology
- Occupational Health
- Ophthalmology
- Orthopedics
- Otolaryngology
- Pain Medicine
- Palliative Care
- Pathology and Laboratory Medicine
- Patient Care
- Patient Information
- Performance Improvement
- Performance Measures
- Perioperative Care and Consultation
- Pharmacoeconomics
- Pharmacoepidemiology
- Pharmacogenetics
- Pharmacy and Clinical Pharmacology
- Physical Medicine and Rehabilitation
- Physical Therapy
- Physician Leadership
- Population Health
- Primary Care
- Professional Well-being
- Professionalism
- Psychiatry and Behavioral Health
- Public Health
- Pulmonary Medicine
- Regulatory Agencies
- Reproductive Health
- Research, Methods, Statistics
- Resuscitation
- Rheumatology
- Risk Management
- Scientific Discovery and the Future of Medicine
- Shared Decision Making and Communication
- Sleep Medicine
- Sports Medicine
- Stem Cell Transplantation
- Substance Use and Addiction Medicine
- Surgical Innovation
- Surgical Pearls
- Teachable Moment
- Technology and Finance
- The Art of JAMA
- The Arts and Medicine
- The Rational Clinical Examination
- Tobacco and e-Cigarettes
- Translational Medicine
- Trauma and Injury
- Treatment Adherence
- Ultrasonography
- Users' Guide to the Medical Literature
- Vaccination
- Venous Thromboembolism
- Veterans Health
- Women's Health
- Workflow and Process
- Wound Care, Infection, Healing
Get the latest research based on your areas of interest.
Others also liked.
- Download PDF
- X Facebook More LinkedIn
Dicpinigaitis AJ , Khamzina Y , Hall DE, et al. Adaptation of the Risk Analysis Index for Frailty Assessment Using Diagnostic Codes. JAMA Netw Open. 2024;7(5):e2413166. doi:10.1001/jamanetworkopen.2024.13166
Manage citations:
© 2024
- Permissions
Adaptation of the Risk Analysis Index for Frailty Assessment Using Diagnostic Codes
- 1 Department of Neurology, New York Presbyterian–Weill Cornell Medical Center, New York, New York
- 2 Bowers Neurosurgical Frailty and Outcomes Data Science Lab, Albuquerque, New Mexico
- 3 Department of Surgery, University of Pittsburgh, Pittsburgh, Pennsylvania
- 4 Department of Surgery, Veterans Affairs Pittsburgh Healthcare System, Pittsburgh, Pennsylvania
- 5 Center for Health Equity Research and Promotion, Veterans Affairs Pittsburgh Healthcare System, Pittsburgh, Pennsylvania
- 6 Wolff Center, UPMC, Pittsburgh, Pennsylvania
- 7 Clinical Research, Investigation, and Systems Modeling of Acute Illness (CRISMA) Center, Pittsburgh, Pennsylvania
- 8 Department of Critical Care Medicine, University of Pittsburgh, Pittsburgh, Pennsylvania
- 9 Division of Vascular Surgery, University of Pittsburgh, Pittsburgh, Pennsylvania
Question Can the Risk Analysis Index (RAI), a validated frailty assessment, be adapted to and validated in inpatient administrative data ubiquitously available in retrospective datasets and electronic health records?
Findings In this cohort study that included data from more than 9.5 million hospitalized adults, when the RAI parameters were adapted to the International Statistical Classification of Diseases, Tenth Revision, Clinical Modification (RAI-ICD), increasing RAI-ICD scores were associated with an increase in hospital length of stay, hospital charges, and in-hospital mortality.
Meaning These findings suggest that the rigorously adapted, derived, and validated RAI-ICD extends the quantification of frailty to administrative inpatient hospitalization data.
Importance Frailty is associated with adverse outcomes after even minor physiologic stressors. The validated Risk Analysis Index (RAI) quantifies frailty; however, existing methods limit application to in-person interview (clinical RAI) and quality improvement datasets (administrative RAI).
Objective To expand the utility of the RAI utility to available International Classification of Diseases, Tenth Revision, Clinical Modification ( ICD-10-CM ) administrative data, using the National Inpatient Sample (NIS).
Design, Setting, and Participants RAI parameters were systematically adapted to ICD-10-CM codes (RAI-ICD) and were derived (NIS 2019) and validated (NIS 2020) . The primary analysis included survey-weighed discharge data among adults undergoing major surgical procedures. Additional external validation occurred by including all operative and nonoperative hospitalizations in the NIS (2020) and in a multihospital health care system (UPMC, 2021-2022). Data analysis was conducted from January to May 2023.
Exposures RAI parameters and in-hospital mortality.
Main Outcomes and Measures The association of RAI parameters with in-hospital mortality was calculated and weighted using logistic regression, generating an integerized RAI-ICD score. After initial validation, thresholds defining categories of frailty were selected by a full complement of test statistics. Rates of elective admission, length of stay, hospital charges, and in-hospital mortality were compared across frailty categories. C statistics estimated model discrimination.
Results RAI-ICD parameters were weighted in the 9 548 206 patients who were hospitalized (mean [SE] age, 55.4 (0.1) years; 3 742 330 male [weighted percentage, 39.2%] and 5 804 431 female [weighted percentage, 60.8%]), modeling in-hospital mortality (2.1%; 95% CI, 2.1%-2.2%) with excellent derivation discrimination (C statistic, 0.810; 95% CI, 0.808-0.813). The 11 RAI-ICD parameters were adapted to 323 ICD-10-CM codes. The operative validation population of 8 113 950 patients (mean [SE] age, 54.4 (0.1) years; 3 148 273 male [weighted percentage, 38.8%] and 4 965 737 female [weighted percentage, 61.2%]; in-hospital mortality, 2.5% [95% CI, 2.4%-2.5%]) mirrored the derivation population. In validation, the weighted and integerized RAI-ICD yielded good to excellent discrimination in the NIS operative sample (C statistic, 0.784; 95% CI, 0.782-0.786), NIS operative and nonoperative sample (C statistic, 0.778; 95% CI, 0.777-0.779), and the UPMC operative and nonoperative sample (C statistic, 0.860; 95% CI, 0.857-0.862). Thresholds defining robust (RAI-ICD <27), normal (RAI-ICD, 27-35), frail (RAI-ICD, 36-45), and very frail (RAI-ICD >45) strata of frailty maximized precision (F1 = 0.33) and sensitivity and specificity (Matthews correlation coefficient = 0.26). Adverse outcomes increased with increasing frailty.
Conclusion and Relevance In this cohort study of hospitalized adults, the RAI-ICD was rigorously adapted, derived, and validated. These findings suggest that the RAI-ICD can extend the quantification of frailty to inpatient adult ICD-10-CM –coded patient care datasets.
Frailty is a syndrome defined by vulnerability to stressors, resulting in adverse outcomes accounting for an increased risk of health care utilization, loss of functional independence, and mortality. 1 - 7 Multiple validated instruments quantify frailty using varying statistical and conceptual models, each applicable to discrete and disparate data sources. 2 , 8 Applying different frailty models leads to inconsistent results and variable conclusions with an inability to equitably compare findings across studies, limiting overall generalizability. Therefore, a reliable conceptual framework and measure of frailty applicable to numerous clinical settings and datasets is needed.
The Risk Analysis Index (RAI) represents a robust metric based on the deficit accumulation frailty model, reliably projecting short-term and long-term mortality in both surgical and nonsurgical adult populations. 9 Initially developed and prospectively validated as a 14-item questionnaire, the RAI is the only frailty assessment proven feasible for real-time, point-of-care frailty assessment of predominantly robust populations. When used to inform preoperative decision-making, the clinical RAI (RAI-C) is associated with the pragmatic improvement of postoperative outcomes, including reduced mortality. 10 , 11 The prospective screening RAI-C is complemented by the administrative RAI (RAI-A), adapted for retrospective use of available frailty-associated variables exclusively applicable to surgical quality datasets. 12 - 14 To date, the RAI has not been applied to, or optimized for use with, other administrative data based on the globally available International Classification of Diseases, Tenth Revision, Clinical Modification ( ICD-10-CM ) codes. We aim to map the conceptual framework of the validated RAI parameters to data ubiquitously available in inpatient administrative data, including ICD-10-CM codes, thereby allowing frailty investigation to substantially expand beyond in-person evaluation and surgical quality datasets to a broader assessment of surgical and nonsurgical diagnoses.
This cohort study followed the Standards for Reporting of Diagnostic Accuracy ( STARD ) reporting guideline 15 and Strengthening the Reporting of Observational Studies in Epidemiology ( STROBE ) reporting guideline. In 4 steps, we adapted, derived, stratified, and validated the RAI using ICD-10-CM codes (RAI-ICD) in the National Inpatient Sample (NIS). Secondary external validation occurred using data abstracted from 14 community and academic hospitals in an integrated health care system (UPMC). The secondary analysis of both datasets was reviewed by the University of Pittsburgh Human Research Protection Office with usage exempt from human participants review (NIS) or with a waiver of informed consent (UPMC) because the study used deidentified data in accordance with the Common Rule. All data were presented in accordance with the NIS, Healthcare Cost and Utilization Project (HCUP), and Agency for Healthcare Research and Quality use agreements. 16
The primary analysis was completed using the NIS (2019-2020). The NIS, developed and maintained by HCUP, includes unweighted data for approximately 7 000 000 annual hospitalizations in the US regardless of expected payer, reflecting a 20% stratified sample of HCUP-participating, nonfederal, acute-care hospitals. 16 Data elements include patient demographic and hospital characteristics; 40 ICD-10-CM and 20 International Statistical Classification of Diseases, Tenth Revision, Procedure Coding System (ICD-10-PCS) codes; and discharge disposition. Race and ethnicity are categorized as 1 data element. Race and ethnicity were categorized as Asian, Black, White, other (defined as Hispanic, Native American, or any other race or ethnicity not otherwise specified), and missing. Race and ethnicity were included to to denote inclusivity and understand the population of patients included. ICD-10-PCS defines major diagnostic or therapeutic operating room procedures. 17 In-hospital mortality was defined by discharge disposition or an assigned ICD-10-CM code initiating transition to comfort care (Z51.5). Missingness of reported data were quantified (eTable 1 in Supplement 1 ).
The inpatient UPMC administrative and electronic health record (EHR) data were abstracted from the Clinical Data Warehouse including hospitalization demographics, with an uncapped number of potential ICD-10-CM codes. 18 The inpatient data were supplemented with outpatient RAI-C scores computed at surgical clinics within 90 days of hospitalization. 10
The widely validated RAI is composed of 11 parameters and 2 statistical interactions. 12 Age and sex were adapted to NIS variables. All pertinent ICD-10-CM codes were explored by 2 independent reviewers (A.D and Y.K.) for unintentional weight loss, poor appetite, congestive heart failure, shortness of breath, kidney failure, cancer, functional status (ie, level of independency), cognitive decline, and institutional living status at hospital admission. 19 , 20 Malignant neoplasm codes (C00-C96) for cancer parameter definition were reviewed with additional scrutiny and categorized into severe, moderate, or mild based upon 5-year survival rates 21 - 24 and were exclusive to active cancer diagnoses (eMethods, eTable 2, and eTable 3 in Supplement 1 ). The institutional living status parameter was omitted due to the absence of an applicable NIS variable or suitable ICD-10-CM code. All initial reviewer discrepancies were discussed among 3 additional reviewers (D.H., K.R., and C.B.) yielding consensus. For each hospitalization, the presence of more than 1 of the ICD-10-CM codes within each RAI parameter was interpreted as present.
All analyses were completed using Stata statistical software version 17 (StataCorp) and Prism version 9 (GraphPad) with code available . 25 Data analysis occurred from January to May 2023.
The RAI-ICD was NIS derived (2019) and validated (2020) using discharge data among inpatient hospitalizations for adults (≥18 years) undergoing major diagnostic or therapeutic operating room procedures. All NIS data analyses were survey weighted. Demographics, RAI-ICD parameters, and outcomes were presented as mean with standard error (SE) or proportion with 95% CI. Logistic regression using a robust sandwich estimator generated β coefficients among hospitalization data without missingness. Model selection among cancer categories was informed by discrimination and calibration estimated with C statistics and additional testing (eMethods in Supplement 1 ).
Logistic regression derived the RAI-ICD including 10 RAI parameters with 2 statistical interactions (age × cancer and functional status × cognitive decline) projecting in-hospital mortality. Mirroring the initial RAI-A derivation, the effect sizes (β coefficients) weighted each RAI parameter, generating an integerized RAI-ICD scoring system (range, 0-81 with a higher score indicating increased frailty). 9 , 12
The integerized RAI-ICD scoring system was then validated (NIS 2020) among hospitalizations with major diagnostic or therapeutic operating room procedures. For each integer value of RAI-ICD, we calculated the observed and projected mortality, cumulative proportion of frailty, sensitivity, specificity, positive predictive value, negative predictive value, F1 score, 26 and Matthews correlation coefficient (MCC). 27 Projected mortality was computed with postprojection margins adjusting for the integerized RAI-ICD. The single best performing cancer categorization model was selected using model performance and testing parameters in combination with decision curve analysis. The RAI-ICD was then stratified into 4 categories of increasing mortality risk: robust, normal, frail, and very frail in accordance with methods previously established for RAI calibration (eTable 4 in Supplement 1 ). 12 , 13 Secondary outcomes were compared across frailty categories and included elective admission, hospital length of stay, and hospital charges.
Sensitivity analyses evaluating the external validity and result robustness included replicating the analysis in 2 alternative cohorts and testing an alternative mortality definition. The alternative cohorts included hospitalizations both with and without major diagnostic or therapeutic operating room procedures in the NIS (2020) and UPMC (2021-2022). The alterative in-hospital mortality definition included only the discharge disposition. Finally, we assessed convergent validity by Spearman rank correlation coefficients comparing the RAI-ICD with the RAI-C in the UPMC data as well as 2 alternative frailty indices in the NIS 2020: Hospital Frailty Risk Score (HFRS) 28 and US Department of Veterans Affairs Frailty Index (VA-FI-10) 29 modified to exclude Current Procedural Terminology ( CPT ) codes unavailable in the NIS. 30
The estimated derivation population comprised 9 548 206 survey-weighted hospitalizations of patients (mean [SE] age, 55.4 [0.1] years; 3 742 330 males [weighted percentage, 39.8%; 95% CI, 38.8%-39.6%] and 5 804 431 females [weighted percentage, 60.8%; 95% CI, 60.4%-61.2%]; 1 136 237 Black individuals [weighted percentage, 11.9%; 95% CI, 11.3%-12.5%]; 6 445 039 White individuals [weighted percentage, 67.5%; 95% CI, 66.4-68.5]; and 1 413 134 individuals with another race or ethnicity [weighted percentage, 14.8%; 95% CI, 14.0%-15.7%]) ( Table 1 and eFigure 1 in Supplement 1 ). It mirrored the primary survey-weighted validation population of 8 113 950 patients (mean [SE] age, 54.4 [0.1] years; 3 148 213 males [weighted percentage, 38.8%; 95% CI, 38.3%-39.3%] and 4 965 737 females [weighted percentage, 61.2%; 95% CI, 60.7%-61.7%]; 989 902 Black individuals [weighted percentage, 12.2%; 95% CI, 11.6%-12.8%]; 5 363 321 White individuals [weighted percentage, 66.1%; 95% CI, 65.1%-67.2%]; 1 265 776 individuals with another race or ethnicity [weighted percentage, 15.6%; 95% CI, 14.7%-16.4%]). Overall observed in-hospital mortality was 2.1% (95% CI, 2.1%-2.2%) for the derivation population and 2.5% (95% CI, 2.4%-2.5%) for the for the validation populations. Data missingness was less than 5% (eTable 5 in Supplement 1 ).
Of the approximately 68 000 available ICD-10-CM codes, 323 were selected for the final RAI-ICD (eTable 3 in Supplement 1 ). Together with age and sex, each adapted ICD-10-CM parameter definition and 2 interaction terms were weighted (eTable 6 in Supplement 1 ). The severe cancer categorization performed optimally (eResults, eFigure 2, and eTable 7 in Supplement 1 ) and constituted the final RAI-ICD cancer parameter definition, generating excellent derivation discrimination (C statistic, 0.810; 95% CI, 0.808-0.813). After weighting and integerizing the RAI-ICD ( Table 2 ), the mean (SE) integerized RAI-ICD was 20.7 (0.1) for the derivation population and 20.3 (0.1) for the validation population. Observed mortality mirrored projected mortality, rising with increasing RAI-ICD score ( Figure ). The integerized RAI-ICD achieved good discrimination for in-hospital mortality ( Table 3 ).
Observed and projected mortality, the cumulative proportion of the population, and performance statistics at each RAI-ICD integer are reported in eTable 4 in Supplement 1 . Following methods established previously for the RAI-A and RAI-C, 13 the RAI-ICD integer of 36 defined the threshold for frailty because projected mortality at this level (4.98%; 95% CI, 4.86%-5.09%) was approximately double that of the mortality observed within the validation population (2.48%; 95% CI, 2.42%-2.55%). This threshold also correlates with maximal F1 score (0.33) and MCC (0.26), representing an optimal balance between sensitivity and specificity, accuracy, and precision. Very frail scores (RAI-ICD >45) corresponded to mortality at least 4 times the overall observed mortality, whereas robust scores (RAI-ICD <27) reflected mortality below the overall observed mortality. Normal scores (RAI-ICD, 27-35) corresponded to observed mortalities between robust and frail (RAI-ICD, 36-45) (eTable 4 in Supplement 1 ). In-hospital mortality, emergent admissions, hospital length of stay, and total charges increased with increasing RAI-ICD frailty categorization ( Table 4 ).
In sensitivity analysis, the alterative definition of mortality occurred among 126 724 NIS hospitalizations (1.6%), yielding good discrimination (C statistic, 0.741; 95% CI, 0.738-0.743) ( Table 3 ). Hospitalizations with and without major diagnostic or therapeutic operating room procedures resulted in good discrimination for the 27 668 666 individuals in the NIS operative and nonoperative sample (C statistic, 0.778; 95% CI, 0.777-0.779) and the NIS operative sample (C statistic, 0.784; 95% CI, 0.782-0.786) and excellent discrimination for the 1 316 544 individuals in the UPMC sample (C statistic, 0.860; 95% CI, 0.857-0.862) ( Table 3 and eTable 8 in Supplement 1 ). Outcome differentiation across the RAI-ICD integers and frailty categories mirrored that of the primary analysis (eFigure 3 in Supplement 1 ).
Exploration of convergent validity between the RAI-ICD, RAI-C, VA-FI-10, and HFRS demonstrated similar discrimination (C statistic for RAI-C, 0.814 [95% CI, 0.809-0.819]; C statistic for HFRS, 0.868 [95% CI, 0.867-0.870]; and C statistic for VA-FI-10, 0.758 [95% CI, 0.756-0.760]). The RAI-ICD and the VA-FI-10 were strongly correlated (ρ, 0.701; 95% CI, 0.700-0.702), but the HFRS and RAI-ICD (ρ, 0.560; 95%CI, 0.559-0.561) and HFRS and VA-FI-10 (ρ, 0.669; 95% CI, 0.669-0.670) were moderately correlated (eTable 9 and eFigure 4 in Supplement 1 ).
In this cohort study, we successfully adapted, derived, stratified, and validated the RAI frailty score using ICD-10-CM codes in a population estimate including millions of contemporary US hospitalizations. The RAI-ICD was systematically developed using a broad range of ICD-10-CM codes that were carefully adapted to previously validated parameters defining frailty. 12 In both the NIS population and a large multihospital system cohort, the RAI-ICD achieved excellent discrimination for in-hospital mortality with increasing frailty and was associated with increasing hospital resource utilization. The performance of the RAI-ICD was comparable with previously validated RAI iterations (C statistic range, 0.77-0.86). 9 , 12 , 13 These data support and further strengthen the RAI as a robust and versatile frailty assessment tool among operative and nonoperative patient populations that may now be applied to any dataset including ICD-10 codes.
Frailty affects millions of older adults worldwide, generating not only an increased risk of adverse patient outcomes but also contributing to ever-growing health care expenditures. For example, while representing nearly 4% of the US Medicare population, frail individuals were responsible for nearly one-half of the overall potentially preventable high cost spendings. 5 In the NIS, which includes all payers, the RAI-ICD again demonstrates frailty was associated with both increasing in-hospital mortality and resource utilization.
Prospectively applied, validated, and survey-based assessment tools identifying frail patients have demonstrated that targeted interventions (eg, nutritional, social, and physical support) may improve outcomes. 8 However, consensus on a precise definition of frailty remains elusive, 31 and apart from the Comprehensive Geriatric Assessment requiring 60 to 90 minutes of face-to-face time with a geriatrician specialist, no single benchmark frailty assessment has emerged. The resulting proliferation of frailty tools and ongoing disagreement about the underlying conceptual models led a recent National Institute of Health consensus panel 32 to recommend using existing measures whenever possible, moving beyond conceptual disagreements, and focusing on clinical practice. We agree and offer the RAI-ICD as an extension of the existing RAI-A and RAI-C, applying a uniform conceptual model to a wide variety of clinical contexts. The RAI-ICD is applicable to many publicly available and EHR datasets for cross-disciplinary, retrospective investigation. Furthermore, the findings from such investigations can be immediately implemented at the bedside using the prospective, widely validated RAI-C survey with proven feasibility in as little as 30 seconds. 11
The RAI-ICD is not the only claims-based frailty tool utilizing ICD-10-CM codes, and should be considered in context with alternatives such as the HFRS 28 and the claims-based frailty indices (CFI) by Kim et al (CFI-Kim) 33 and Segal et al (CFI-Segal), 34 along with the Orkaby et al 19 CFI for use among US military veterans (VA-FI-10). Each of these frailty indices demonstrates similar model discrimination even as they tend to identify frailty among differing sets of patients with only modest intersection. 12 , 35 As such, the choice of any given tool depends less on model performance and more on the tool’s conceptual validity, interpretability, and feasibility.
Each of the aforementioned ICD-10-CM –based frailty assessments were informed by existing conceptual models of frailty, meeting a baseline threshold of face validity. Frailty-related ranges of administrative diagnostic and procedural codes were selected based on expert opinion alone (HFRS) or mapping to either the Rockwood cumulative deficit (RAI-ICD, CFI-Kim, and VA-FI-10) or frailty phenotype (CFI-Segal) conceptual models. 28 , 36 - 38 However, the initial, broad, clinician-guided selection of codes for the HFRS, CFI-Kim, and CFI-Segal tools were then tapered down and finalized using black-box, machine-learning techniques. Because the Kim, Segal and Orkaby CFIs include approximately 27 000, 2600, and 7000 ICD-10 codes, respectively, a data-driven approach is necessary. On close inspection, some included codes are unrelated to frailty and thus conceptually inappropriate. For example, the HRFS relies heavily on acute diagnoses (eg, acute kidney failure or hypotension); the CFI-Kim includes infectious diseases (eg, pneumonia and influenza), lacerations, and paternity tests 33 ; and both the CFI-Segal and VA-FI include comorbidities (eg, hypertension and hyperlipidemia) highly prevalent in robust populations and not particular to frailty. As such, the face validity of these tools can be challenged, and these inconsistencies may explain their poor performance in specific disease states and among those that are critically ill. 39 , 40 Furthermore, the excellent discrimination of the HRFS for in-hospital mortality in the NIS may, in fact, highlight the tool’s selection of highly morbid, acute conditions without necessarily demonstrating specificity for frailty. For example, a 25-year-old professional athlete who slips on their stairs at home and falls again upon getting up with a resulting concussion and small traumatic intracranial hemorrhage would obtain an HFRS score consistent with frailty despite being an elite athlete (eMethods in Supplement 1 ). Finally, although the originating authors of the HFRS caution it is validated only for patients older than 75 years and is not intended for individualized clinical patient decision-making, 41 , 42 growing literature applies it for precisely these purposes. 43 - 46
Across all ICD-10-CM –based frailty tools, one potential advantage is the capacity to automate and embed frailty calculations within an EHR. Operationalizing is not trivial 47 - 49 ; however, automated frailty assessment could facilitate efficient, prospective, population-level assessments, 28 including EHR-embedded pragmatic trials promising for both containment of ever-rising trial costs 50 - 54 and improving outcomes in efficient, self-learning health care systems. 55 , 56 Notably, such automaticity is possible only for patients with previously documented diagnoses. Therefore, an appropriate frailty designation will both change fluidly with care and may misclassify a robust status among the most isolated and, therefore, vulnerable patients without prior exposure to the health care system for diagnoses. Therefore, automated code-based assessments must be supplemented by alterative, rapid, and feasible bedside assessment. The prospectively generated RAI-C is one such assessment, 11 successfully embedded in Epic, 57 Cerner, 58 and the VA Computerized Patient Record System 59 EHRs.
The applicability of each ICD-10-CM –based tool has limits. First, the HFRS (Hospital Episode Statistics database, >75 years), ICF-Kim (Cardiovascular Health Study, >65 years), 33 ICF-Segal (Medicare beneficiaries), 34 and VA-FI-10 (Veterans Health Association, >75 years) 19 were all derived and validated in datasets including exclusively older cohorts, limiting their application. While age and frailty often correlate, they are independent. Elderly patients can be robust, and young patients can be frail. Second, the data elements required to compute the index delineate its applicability. CFI-Kim ( CPT and Healthcare Common Procedure Coding System), CFI-Segal (history of prior admission), VA-FI-10 ( CPT ) all include data elements beyond demographic data and ICD-10 codes. Simultaneously leveraging multiple code catalogs (ie, ICD-10 or CPT ) may theoretically increase model performance, 60 , 61 but at a cost; not all datasets contain the code catalogs required to compute these indices, again limiting their application. Furthermore, the theoretical performance improvement of including codes across catalogs has not yet demonstrated clear benefit. For example, the NIS does not contain CPT codes, forcing their omission from VA-FI-10 scoring here. And yet, the VA-FI-10 generated good discrimination and convergent validity with the RAI-ICD, suggesting the increased complexity of numerous coding catalogs may not be required. Finally, CFI-Segal has not yet been translated from International Classification of Diseases, Ninth Revision, Clinical Modification (ICD-9-CM) to ICD-10-CM , highlighting the need to continuously adapt and recalibrate tools.
Common to all ICD-9-CM and ICD-10-CM based frailty assessments are 2 additional limitations. First, the number of data elements available within datasets varies. For example, the maximal number of ICD-9-CM and ICD-10-CM codes recorded for each hospitalization is 20 for the Hospital Episode Statistics database, 40 for the NIS, and unlimited for the UPMC EHR. This heterogeneity may alter model performance across datasets, introducing bias. Second, ICD-9-CM and ICD-10-CM coding assignments are notorious sources of inaccuracy with omission of granular details and unwarranted variability across clinicians, coders, hospital-systems, regions, and time. As a result, highly utilized, accurate codes in one setting or era may be omitted or misused elsewhere. Although these problems reduced over time, 62 - 64 they are especially prevalent for ICD-10-CM Z-codes. They allow for inclusion of previously omitted frailty concepts including functional capacity. Specifically, they represent factors associated with health status that have contact with and dependence on the health care system, which conceptually match to frailty such as problems related to life-management difficulty. 28 , 65 Although payers and clinicians alike promoted Z-code use, they remain both vastly underutilized and their application varies by patient factors (eg, gender and race), hospitals (eg, location and teaching status), and geography. 65
This study has limitations specific to the RAI-ICD. First, the RAI-ICD was optimized and validated exclusively from retrospectively obtained, in-hospital data in which the characteristics of the validation cohort mirrored the derivation cohort, potentially limiting generalizability. Second, although we tested the RAI-ICD in multiple datasets, further evaluation of generalizability is required. Third, the complete ICD-10-CM code review was completed by 2 authors (A.D. and Y.K.) with discrepancies discussed among three additional authors (D.H., K.R., and C.B.) with an iterative focus on maximizing specificity; however, interrater reliability was not recorded. Fourth, in optimizing test statistics, the RAI-ICD was highly specific but less sensitive, minimizing the misclassification of nonfrail patients as frail; however, the RAI-ICD may warrant further context specific calibration (eg, screening) if greater sensitivity is required.
In this cohort study, when the RAI parameters were adapted to the ICD-10-CM , increasing RAI-ICD scores were associated with an increase in hospital length of stay, hospital charges, and in-hospital mortality. With over 60 frailty indices available, each conceptually unique and applicable to disparate datasets, the main benefit of the RAI-ICD is that it extends the quantification of frailty to datasets with access to administrative data including ICD-10-CM codes using a unified conceptual framework validated in both prospective and retrospective applications.
Accepted for Publication: March 23, 2024.
Published: May 24, 2024. doi:10.1001/jamanetworkopen.2024.13166
Open Access: This is an open access article distributed under the terms of the CC-BY License . © 2024 Dicpinigaitis AJ et al. JAMA Network Open .
Corresponding Author: Katherine M. Reitz, MD, MSc, Division of Vascular Surgery, University of Pittsburgh, E362.4 South Tower PUH, Pittsburgh, PA 15213 ( [email protected] ).
Author Contributions: Drs Reitz and Khamzina had full access to all of the data in the study and take responsibility for the integrity of the data and the accuracy of the data analysis. Drs Dicpinigaitis and Khamzina are cofirst authors. Drs Reitz and Bowers are cosenior authors.
Concept and design: Dicpinigaitis, Khamzina, Hall, Seymour, Schmidt, Reitz, Bowers.
Acquisition, analysis, or interpretation of data: Dicpinigaitis, Khamzina, Hall, Nassereldine, Kennedy, Seymour, Reitz, Bowers.
Drafting of the manuscript: Dicpinigaitis, Khamzina, Hall, Reitz.
Critical review of the manuscript for important intellectual content: All authors.
Statistical analysis: Dicpinigaitis, Khamzina, Kennedy, Reitz.
Administrative, technical, or material support: Nassereldine, Seymour, Schmidt, Reitz.
Supervision: Hall, Schmidt, Reitz, Bowers.
Conflict of Interest Disclosures: Dr Hall reported receiving grants from the Veterans Affairs Office of Research and Development and honoraria from FutureAssure outside the submitted work. Dr Kennedy reported receiving grants from the National Institutes of Health outside the submitted work. Dr Seymour reported receiving personal fees from Octapharma, Inotrem, and Beckman Coulter outside the submitted work. No other disclosures were reported.
Disclaimer: Dr Seymor is associate editor of JAMA but was not involved in any of the decisions regarding review of the manuscript or its acceptance.
Funding/Support: This study was supported in part by the National Institute of Health (L30 AG064730 to Dr Reitz).
Role of the Funder/Sponsor: The funder had no role in the design and conduct of the study; collection, management, analysis, and interpretation of the data; preparation, review, or approval of the manuscript; and decision to submit the manuscript for publication.
Data Sharing Statement: See Supplement 2 .
Additional Contributions: The authors thank the staff at the Biostatistics and Data Management Core at the Clinical Research, Investigation, and Systems Modeling of Acute Illness (CRISMA) Center in the Department of Critical Care Medicine at the University of Pittsburgh for curating and managing the University of Pittsburgh Medical Center data. The authors also thank the Healthcare Cost and Utilization Project Nationwide databases data partners: Alaska, Arizona, Arkansas, California, Colorado, Connecticut, Delaware, District of Columbia, Florida, Georgia, Hawaii, Illinois, Indiana, Iowa, Kansas, Kentucky, Louisiana, Maine, Maryland, Massachusetts, Michigan, Minnesota, Mississippi, Missouri, Montana, Nebraska, Nevada, New Hampshire, New Jersey, New Mexico, New York, North Carolina, North Dakota, Ohio, Oklahoma, Oregon, Pennsylvania, Rhode Island, South Carolina, South Dakota, Tennessee, Texas, Utah, Vermont, Virginia, Washington, West Virginia, Wisconsin, and Wyoming. Finally, the authors thank Kavelin Rumalla, MD (Bowers Neurosurgical Frailty and Outcomes Data Science Lab), for his assistance with data analysis; Dr Rumalla did not receive compensation for their contributions.
- Register for email alerts with links to free full-text articles
- Access PDFs of free articles
- Manage your interests
- Save searches and receive search alerts

An official website of the United States government
Here’s how you know
Official websites use .gov A .gov website belongs to an official government organization in the United States.
Secure .gov websites use HTTPS A lock ( Lock A locked padlock ) or https:// means you’ve safely connected to the .gov website. Share sensitive information only on official, secure websites.
JavaScript appears to be disabled on this computer. Please click here to see any active alerts .
Celebrating EPA Researcher
EPA environmental epidemiologist Shannon Griffin received the 2023 Arthur S. Flemming Award, which honors outstanding federal employees, for creatively advancing laboratory-based biomarker techniques.
Read more here
Healthy & Resilient Communities Research Webinar
Join us at our June 11th webinar from 3:00 -4:00 p.m. ET to hear from an EPA researcher about geospatial model development and applications in cumulative impacts research.
Register here
Science is the foundation
EPA is one of the world’s leading environmental and human health research organizations.
The Office of Research and Development is EPA's scientific research arm. On this page you can access our products, tools, and events, and learn about grant and job opportunities.
Research Topics
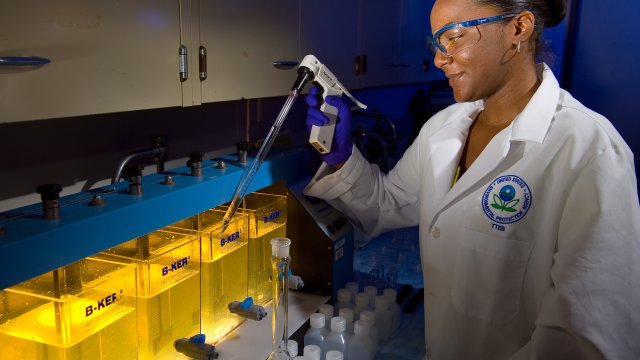
EPA does research on many different topics, including air, water, climate, and more.
Publications
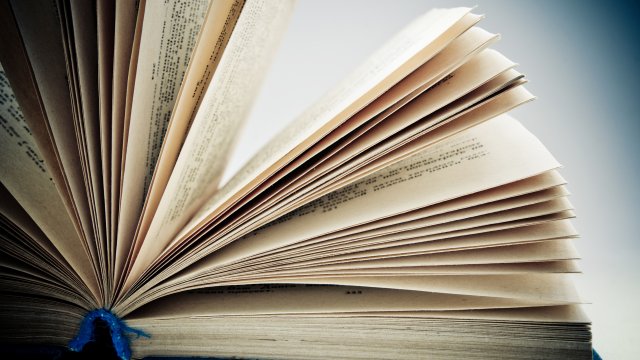
Read the latest scientific publications, reports, articles, and more.
Funding & Career Opportunities
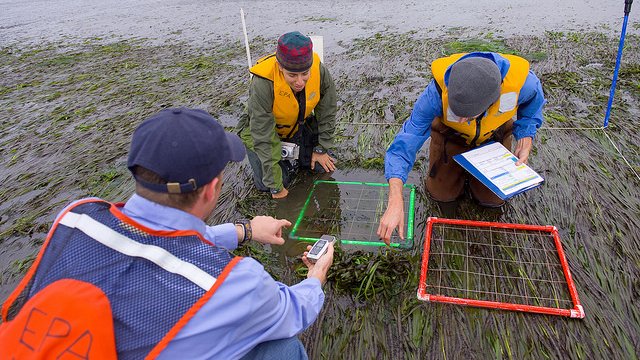
Find research funding opportunities, small business grants, jobs, and ways to license EPA’s technology.
Upcoming Events
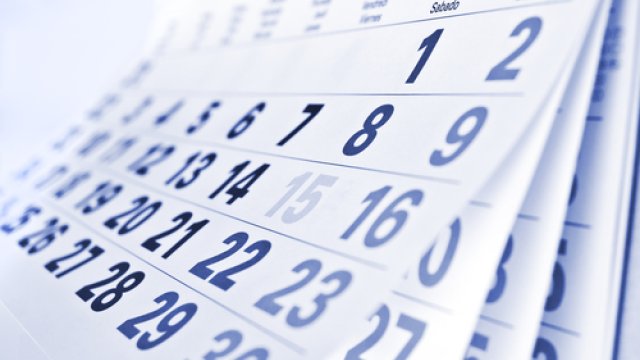
See what webinars and events EPA researchers are hosting, presenting at, or attending.
Stay Connected
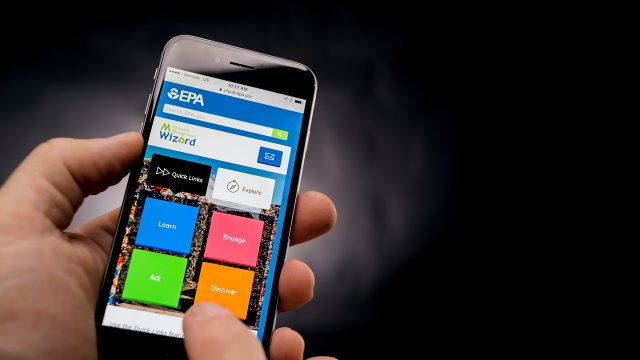
Keep up to date with the latest Science Matters newsletter, social media posts, and press releases.
About Our Research
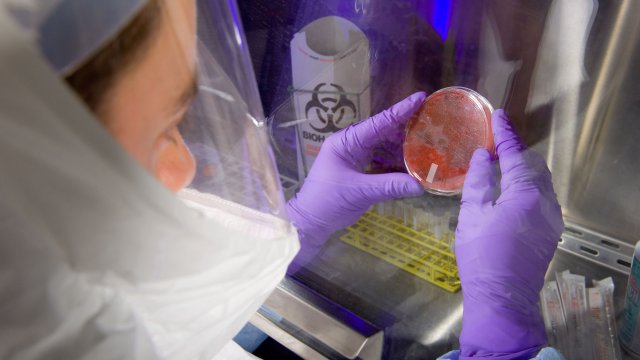
Learn about EPA’s research organization, research facilities, and the research planning process.
Research Tools
EPA Science Models and Research Tools (SMaRT) Search is a searchable inventory of freely available models, tools, and databases from EPA's Office of Research and Development (ORD).
In Your Community
See how EPA's Office of Research and Development (ORD) engages and collaborates with states , including tools and other resources available to states.
Thank you for visiting nature.com. You are using a browser version with limited support for CSS. To obtain the best experience, we recommend you use a more up to date browser (or turn off compatibility mode in Internet Explorer). In the meantime, to ensure continued support, we are displaying the site without styles and JavaScript.
- View all journals
- My Account Login
- Explore content
- About the journal
- Publish with us
- Sign up for alerts
- Open access
- Published: 17 October 2023
The impact of founder personalities on startup success
- Paul X. McCarthy 1 , 2 ,
- Xian Gong 3 ,
- Fabian Braesemann 4 , 5 ,
- Fabian Stephany 4 , 5 ,
- Marian-Andrei Rizoiu 3 &
- Margaret L. Kern 6
Scientific Reports volume 13 , Article number: 17200 ( 2023 ) Cite this article
60k Accesses
2 Citations
305 Altmetric
Metrics details
- Human behaviour
- Information technology
An Author Correction to this article was published on 07 May 2024
This article has been updated
Startup companies solve many of today’s most challenging problems, such as the decarbonisation of the economy or the development of novel life-saving vaccines. Startups are a vital source of innovation, yet the most innovative are also the least likely to survive. The probability of success of startups has been shown to relate to several firm-level factors such as industry, location and the economy of the day. Still, attention has increasingly considered internal factors relating to the firm’s founding team, including their previous experiences and failures, their centrality in a global network of other founders and investors, as well as the team’s size. The effects of founders’ personalities on the success of new ventures are, however, mainly unknown. Here, we show that founder personality traits are a significant feature of a firm’s ultimate success. We draw upon detailed data about the success of a large-scale global sample of startups (n = 21,187). We find that the Big Five personality traits of startup founders across 30 dimensions significantly differ from that of the population at large. Key personality facets that distinguish successful entrepreneurs include a preference for variety, novelty and starting new things (openness to adventure), like being the centre of attention (lower levels of modesty) and being exuberant (higher activity levels). We do not find one ’Founder-type’ personality; instead, six different personality types appear. Our results also demonstrate the benefits of larger, personality-diverse teams in startups, which show an increased likelihood of success. The findings emphasise the role of the diversity of personality types as a novel dimension of team diversity that influences performance and success.
Similar content being viewed by others
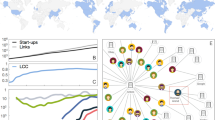
Predicting success in the worldwide start-up network
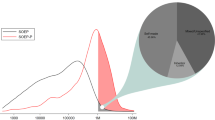
The personality traits of self-made and inherited millionaires
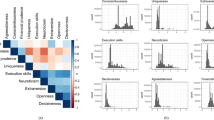
The nexus of top executives’ attributes, firm strategies, and outcomes: Large firms versus SMEs
Introduction.
The success of startups is vital to economic growth and renewal, with a small number of young, high-growth firms creating a disproportionately large share of all new jobs 1 , 2 . Startups create jobs and drive economic growth, and they are also an essential vehicle for solving some of society’s most pressing challenges.
As a poignant example, six centuries ago, the German city of Mainz was abuzz as the birthplace of the world’s first moveable-type press created by Johannes Gutenberg. However, in the early part of this century, it faced several economic challenges, including rising unemployment and a significant and growing municipal debt. Then in 2008, two Turkish immigrants formed the company BioNTech in Mainz with another university research colleague. Together they pioneered new mRNA-based technologies. In 2020, BioNTech partnered with US pharmaceutical giant Pfizer to create one of only a handful of vaccines worldwide for Covid-19, saving an estimated six million lives 3 . The economic benefit to Europe and, in particular, the German city where the vaccine was developed has been significant, with windfall tax receipts to the government clearing Mainz’s €1.3bn debt and enabling tax rates to be reduced, attracting other businesses to the region as well as inspiring a whole new generation of startups 4 .
While stories such as the success of BioNTech are often retold and remembered, their success is the exception rather than the rule. The overwhelming majority of startups ultimately fail. One study of 775 startups in Canada that successfully attracted external investment found only 35% were still operating seven years later 5 .
But what determines the success of these ‘lucky few’? When assessing the success factors of startups, especially in the early-stage unproven phase, venture capitalists and other investors offer valuable insights. Three different schools of thought characterise their perspectives: first, supply-side or product investors : those who prioritise investing in firms they consider to have novel and superior products and services, investing in companies with intellectual property such as patents and trademarks. Secondly, demand-side or market-based investors : those who prioritise investing in areas of highest market interest, such as in hot areas of technology like quantum computing or recurrent or emerging large-scale social and economic challenges such as the decarbonisation of the economy. Thirdly, talent investors : those who prioritise the foundation team above the startup’s initial products or what industry or problem it is looking to address.
Investors who adopt the third perspective and prioritise talent often recognise that a good team can overcome many challenges in the lead-up to product-market fit. And while the initial products of a startup may or may not work a successful and well-functioning team has the potential to pivot to new markets and new products, even if the initial ones prove untenable. Not surprisingly, an industry ‘autopsy’ into 101 tech startup failures found 23% were due to not having the right team—the number three cause of failure ahead of running out of cash or not having a product that meets the market need 6 .
Accordingly, early entrepreneurship research was focused on the personality of founders, but the focus shifted away in the mid-1980s onwards towards more environmental factors such as venture capital financing 7 , 8 , 9 , networks 10 , location 11 and due to a range of issues and challenges identified with the early entrepreneurship personality research 12 , 13 . At the turn of the 21st century, some scholars began exploring ways to combine context and personality and reconcile entrepreneurs’ individual traits with features of their environment. In her influential work ’The Sociology of Entrepreneurship’, Patricia H. Thornton 14 discusses two perspectives on entrepreneurship: the supply-side perspective (personality theory) and the demand-side perspective (environmental approach). The supply-side perspective focuses on the individual traits of entrepreneurs. In contrast, the demand-side perspective focuses on the context in which entrepreneurship occurs, with factors such as finance, industry and geography each playing their part. In the past two decades, there has been a revival of interest and research that explores how entrepreneurs’ personality relates to the success of their ventures. This new and growing body of research includes several reviews and meta-studies, which show that personality traits play an important role in both career success and entrepreneurship 15 , 16 , 17 , 18 , 19 , that there is heterogeneity in definitions and samples used in research on entrepreneurship 16 , 18 , and that founder personality plays an important role in overall startup outcomes 17 , 19 .
Motivated by the pivotal role of the personality of founders on startup success outlined in these recent contributions, we investigate two main research questions:
Which personality features characterise founders?
Do their personalities, particularly the diversity of personality types in founder teams, play a role in startup success?
We aim to understand whether certain founder personalities and their combinations relate to startup success, defined as whether their company has been acquired, acquired another company or listed on a public stock exchange. For the quantitative analysis, we draw on a previously published methodology 20 , which matches people to their ‘ideal’ jobs based on social media-inferred personality traits.
We find that personality traits matter for startup success. In addition to firm-level factors of location, industry and company age, we show that founders’ specific Big Five personality traits, such as adventurousness and openness, are significantly more widespread among successful startups. As we find that companies with multi-founder teams are more likely to succeed, we cluster founders in six different and distinct personality groups to underline the relevance of the complementarity in personality traits among founder teams. Startups with diverse and specific combinations of founder types (e. g., an adventurous ‘Leader’, a conscientious ‘Accomplisher’, and an extroverted ‘Developer’) have significantly higher odds of success.
We organise the rest of this paper as follows. In the Section " Results ", we introduce the data used and the methods applied to relate founders’ psychological traits with their startups’ success. We introduce the natural language processing method to derive individual and team personality characteristics and the clustering technique to identify personality groups. Then, we present the result for multi-variate regression analysis that allows us to relate firm success with external and personality features. Subsequently, the Section " Discussion " mentions limitations and opportunities for future research in this domain. In the Section " Methods ", we describe the data, the variables in use, and the clustering in greater detail. Robustness checks and additional analyses can be found in the Supplementary Information.
Our analysis relies on two datasets. We infer individual personality facets via a previously published methodology 20 from Twitter user profiles. Here, we restrict our analysis to founders with a Crunchbase profile. Crunchbase is the world’s largest directory on startups. It provides information about more than one million companies, primarily focused on funding and investors. A company’s public Crunchbase profile can be considered a digital business card of an early-stage venture. As such, the founding teams tend to provide information about themselves, including their educational background or a link to their Twitter account.
We infer the personality profiles of the founding teams of early-stage ventures from their publicly available Twitter profiles, using the methodology described by Kern et al. 20 . Then, we correlate this information to data from Crunchbase to determine whether particular combinations of personality traits correspond to the success of early-stage ventures. The final dataset used in the success prediction model contains n = 21,187 startup companies (for more details on the data see the Methods section and SI section A.5 ).
Revisions of Crunchbase as a data source for investigations on a firm and industry level confirm the platform to be a useful and valuable source of data for startups research, as comparisons with other sources at micro-level, e.g., VentureXpert or PwC, also suggest that the platform’s coverage is very comprehensive, especially for start-ups located in the United States 21 . Moreover, aggregate statistics on funding rounds by country and year are quite similar to those produced with other established sources, going to validate the use of Crunchbase as a reliable source in terms of coverage of funded ventures. For instance, Crunchbase covers about the same number of investment rounds in the analogous sectors as collected by the National Venture Capital Association 22 . However, we acknowledge that the data source might suffer from registration latency (a certain delay between the foundation of the company and its actual registration on Crunchbase) and success bias in company status (the likeliness that failed companies decide to delete their profile from the database).
The definition of startup success
The success of startups is uncertain, dependent on many factors and can be measured in various ways. Due to the likelihood of failure in startups, some large-scale studies have looked at which features predict startup survival rates 23 , and others focus on fundraising from external investors at various stages 24 . Success for startups can be measured in multiple ways, such as the amount of external investment attracted, the number of new products shipped or the annual growth in revenue. But sometimes external investments are misguided, revenue growth can be short-lived, and new products may fail to find traction.
Success in a startup is typically staged and can appear in different forms and times. For example, a startup may be seen to be successful when it finds a clear solution to a widely recognised problem, such as developing a successful vaccine. On the other hand, it could be achieving some measure of commercial success, such as rapidly accelerating sales or becoming profitable or at least cash positive. Or it could be reaching an exit for foundation investors via a trade sale, acquisition or listing of its shares for sale on a public stock exchange via an Initial Public Offering (IPO).
For our study, we focused on the startup’s extrinsic success rather than the founders’ intrinsic success per se, as its more visible, objective and measurable. A frequently considered measure of success is the attraction of external investment by venture capitalists 25 . However, this is not in and of itself a good measure of clear, incontrovertible success, particularly for early-stage ventures. This is because it reflects investors’ expectations of a startup’s success potential rather than actual business success. Similarly, we considered other measures like revenue growth 26 , liquidity events 27 , 28 , 29 , profitability 30 and social impact 31 , all of which have benefits as they capture incremental success, but each also comes with operational measurement challenges.
Therefore, we apply the success definition initially introduced by Bonaventura et al. 32 , namely that a startup is acquired, acquires another company or has an initial public offering (IPO). We consider any of these major capital liquidation events as a clear threshold signal that the company has matured from an early-stage venture to becoming or is on its way to becoming a mature company with clear and often significant business growth prospects. Together these three major liquidity events capture the primary forms of exit for external investors (an acquisition or trade sale and an IPO). For companies with a longer autonomous growth runway, acquiring another company marks a similar milestone of scale, maturity and capability.
Using multifactor analysis and a binary classification prediction model of startup success, we looked at many variables together and their relative influence on the probability of the success of startups. We looked at seven categories of factors through three lenses of firm-level factors: (1) location, (2) industry, (3) age of the startup; founder-level factors: (4) number of founders, (5) gender of founders, (6) personality characteristics of founders and; lastly team-level factors: (7) founder-team personality combinations. The model performance and relative impacts on the probability of startup success of each of these categories of founders are illustrated in more detail in section A.6 of the Supplementary Information (in particular Extended Data Fig. 19 and Extended Data Fig. 20 ). In total, we considered over three hundred variables (n = 323) and their relative significant associations with success.
The personality of founders
Besides product-market, industry, and firm-level factors (see SI section A.1 ), research suggests that the personalities of founders play a crucial role in startup success 19 . Therefore, we examine the personality characteristics of individual startup founders and teams of founders in relationship to their firm’s success by applying the success definition used by Bonaventura et al. 32 .
Employing established methods 33 , 34 , 35 , we inferred the personality traits across 30 dimensions (Big Five facets) of a large global sample of startup founders. The startup founders cohort was created from a subset of founders from the global startup industry directory Crunchbase, who are also active on the social media platform Twitter.
To measure the personality of the founders, we used the Big Five, a popular model of personality which includes five core traits: Openness to Experience, Conscientiousness, Extraversion, Agreeableness, and Emotional stability. Each of these traits can be further broken down into thirty distinct facets. Studies have found that the Big Five predict meaningful life outcomes, such as physical and mental health, longevity, social relationships, health-related behaviours, antisocial behaviour, and social contribution, at levels on par with intelligence and socioeconomic status 36 Using machine learning to infer personality traits by analysing the use of language and activity on social media has been shown to be more accurate than predictions of coworkers, friends and family and similar in accuracy to the judgement of spouses 37 . Further, as other research has shown, we assume that personality traits remain stable in adulthood even through significant life events 38 , 39 , 40 . Personality traits have been shown to emerge continuously from those already evident in adolescence 41 and are not significantly influenced by external life events such as becoming divorced or unemployed 42 . This suggests that the direction of any measurable effect goes from founder personalities to startup success and not vice versa.
As a first investigation to what extent personality traits might relate to entrepreneurship, we use the personality characteristics of individuals to predict whether they were an entrepreneur or an employee. We trained and tested a machine-learning random forest classifier to distinguish and classify entrepreneurs from employees and vice-versa using inferred personality vectors alone. As a result, we found we could correctly predict entrepreneurs with 77% accuracy and employees with 88% accuracy (Fig. 1 A). Thus, based on personality information alone, we correctly predict all unseen new samples with 82.5% accuracy (See SI section A.2 for more details on this analysis, the classification modelling and prediction accuracy).
We explored in greater detail which personality features are most prominent among entrepreneurs. We found that the subdomain or facet of Adventurousness within the Big Five Domain of Openness was significant and had the largest effect size. The facet of Modesty within the Big Five Domain of Agreeableness and Activity Level within the Big Five Domain of Extraversion was the subsequent most considerable effect (Fig. 1 B). Adventurousness in the Big Five framework is defined as the preference for variety, novelty and starting new things—which are consistent with the role of a startup founder whose role, especially in the early life of the company, is to explore things that do not scale easily 43 and is about developing and testing new products, services and business models with the market.
Once we derived and tested the Big Five personality features for each entrepreneur in our data set, we examined whether there is evidence indicating that startup founders naturally cluster according to their personality features using a Hopkins test (see Extended Data Figure 6 ). We discovered clear clustering tendencies in the data compared with other renowned reference data sets known to have clusters. Then, once we established the founder data clusters, we used agglomerative hierarchical clustering. This ‘bottom-up’ clustering technique initially treats each observation as an individual cluster. Then it merges them to create a hierarchy of possible cluster schemes with differing numbers of groups (See Extended Data Fig. 7 ). And lastly, we identified the optimum number of clusters based on the outcome of four different clustering performance measurements: Davies-Bouldin Index, Silhouette coefficients, Calinski-Harabas Index and Dunn Index (see Extended Data Figure 8 ). We find that the optimum number of clusters of startup founders based on their personality features is six (labelled #0 through to #5), as shown in Fig. 1 C.
To better understand the context of different founder types, we positioned each of the six types of founders within an occupation-personality matrix established from previous research 44 . This research showed that ‘each job has its own personality’ using a substantial sample of employees across various jobs. Utilising the methodology employed in this study, we assigned labels to the cluster names #0 to #5, which correspond to the identified occupation tribes that best describe the personality facets represented by the clusters (see Extended Data Fig. 9 for an overview of these tribes, as identified by McCarthy et al. 44 ).
Utilising this approach, we identify three ’purebred’ clusters: #0, #2 and #5, whose members are dominated by a single tribe (larger than 60% of all individuals in each cluster are characterised by one tribe). Thus, these clusters represent and share personality attributes of these previously identified occupation-personality tribes 44 , which have the following known distinctive personality attributes (see also Table 1 ):
Accomplishers (#0) —Organised & outgoing. confident, down-to-earth, content, accommodating, mild-tempered & self-assured.
Leaders (#2) —Adventurous, persistent, dispassionate, assertive, self-controlled, calm under pressure, philosophical, excitement-seeking & confident.
Fighters (#5) —Spontaneous and impulsive, tough, sceptical, and uncompromising.
We labelled these clusters with the tribe names, acknowledging that labels are somewhat arbitrary, based on our best interpretation of the data (See SI section A.3 for more details).
For the remaining three clusters #1, #3 and #4, we can see they are ‘hybrids’, meaning that the founders within them come from a mix of different tribes, with no one tribe representing more than 50% of the members of that cluster. However, the tribes with the largest share were noted as #1 Experts/Engineers, #3 Fighters, and #4 Operators.
To label these three hybrid clusters, we examined the closest occupations to the median personality features of each cluster. We selected a name that reflected the common themes of these occupations, namely:
Experts/Engineers (#1) as the closest roles included Materials Engineers and Chemical Engineers. This is consistent with this cluster’s personality footprint, which is highest in openness in the facets of imagination and intellect.
Developers (#3) as the closest roles include Application Developers and related technology roles such as Business Systems Analysts and Product Managers.
Operators (#4) as the closest roles include service, maintenance and operations functions, including Bicycle Mechanic, Mechanic and Service Manager. This is also consistent with one of the key personality traits of high conscientiousness in the facet of orderliness and high agreeableness in the facet of humility for founders in this cluster.
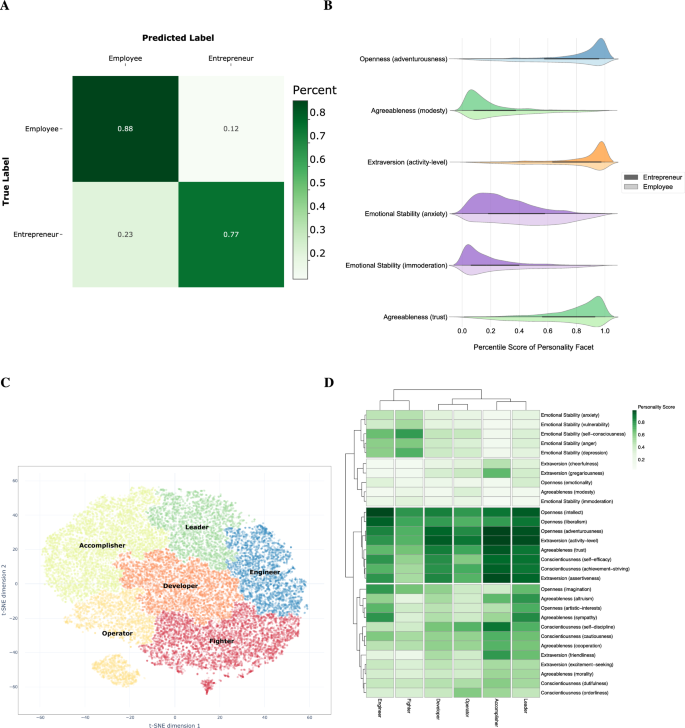
Founder-Level Factors of Startup Success. ( A ), Successful entrepreneurs differ from successful employees. They can be accurately distinguished using a classifier with personality information alone. ( B ), Successful entrepreneurs have different Big Five facet distributions, especially on adventurousness, modesty and activity level. ( C ), Founders come in six different types: Fighters, Operators, Accomplishers, Leaders, Engineers and Developers (FOALED) ( D ), Each founder Personality-Type has its distinct facet.
Together, these six different types of startup founders (Fig. 1 C) represent a framework we call the FOALED model of founder types—an acronym of Fighters, Operators, Accomplishers, Leaders, Engineers and D evelopers.
Each founder’s personality type has its distinct facet footprint (for more details, see Extended Data Figure 10 in SI section A.3 ). Also, we observe a central core of correlated features that are high for all types of entrepreneurs, including intellect, adventurousness and activity level (Fig. 1 D).To test the robustness of the clustering of the personality facets, we compare the mean scores of the individual facets per cluster with a 20-fold resampling of the data and find that the clusters are, overall, largely robust against resampling (see Extended Data Figure 11 in SI section A.3 for more details).
We also find that the clusters accord with the distribution of founders’ roles in their startups. For example, Accomplishers are often Chief Executive Officers, Chief Financial Officers, or Chief Operating Officers, while Fighters tend to be Chief Technical Officers, Chief Product Officers, or Chief Commercial Officers (see Extended Data Fig. 12 in SI section A.4 for more details).
The ensemble theory of success
While founders’ individual personality traits, such as Adventurousness or Openness, show to be related to their firms’ success, we also hypothesise that the combination, or ensemble, of personality characteristics of a founding team impacts the chances of success. The logic behind this reasoning is complementarity, which is proposed by contemporary research on the functional roles of founder teams. Examples of these clear functional roles have evolved in established industries such as film and television, construction, and advertising 45 . When we subsequently explored the combinations of personality types among founders and their relationship to the probability of startup success, adjusted for a range of other factors in a multi-factorial analysis, we found significantly increased chances of success for mixed foundation teams:
Initially, we find that firms with multiple founders are more likely to succeed, as illustrated in Fig. 2 A, which shows firms with three or more founders are more than twice as likely to succeed than solo-founded startups. This finding is consistent with investors’ advice to founders and previous studies 46 . We also noted that some personality types of founders increase the probability of success more than others, as shown in SI section A.6 (Extended Data Figures 16 and 17 ). Also, we note that gender differences play out in the distribution of personality facets: successful female founders and successful male founders show facet scores that are more similar to each other than are non-successful female founders to non-successful male founders (see Extended Data Figure 18 ).
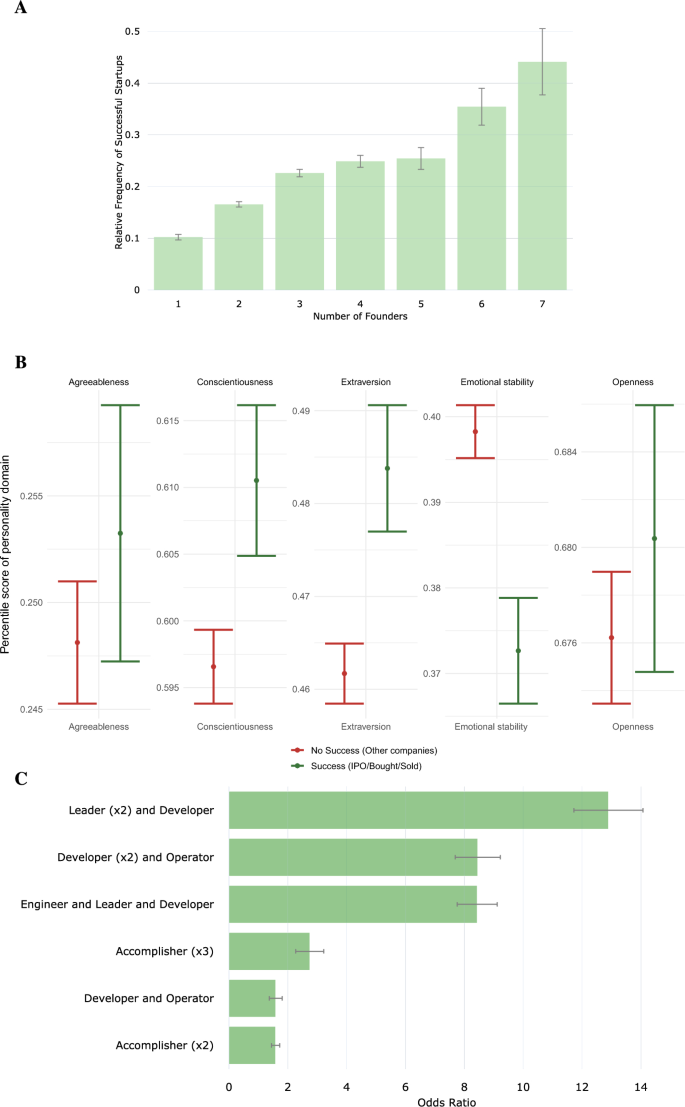
The Ensemble Theory of Team-Level Factors of Startup Success. ( A ) Having a larger founder team elevates the chances of success. This can be due to multiple reasons, e.g., a more extensive network or knowledge base but also personality diversity. ( B ) We show that joint personality combinations of founders are significantly related to higher chances of success. This is because it takes more than one founder to cover all beneficial personality traits that ‘breed’ success. ( C ) In our multifactor model, we show that firms with diverse and specific combinations of types of founders have significantly higher odds of success.
Access to more extensive networks and capital could explain the benefits of having more founders. Still, as we find here, it also offers a greater diversity of combined personalities, naturally providing a broader range of maximum traits. So, for example, one founder may be more open and adventurous, and another could be highly agreeable and trustworthy, thus, potentially complementing each other’s particular strengths associated with startup success.
The benefits of larger and more personality-diverse foundation teams can be seen in the apparent differences between successful and unsuccessful firms based on their combined Big Five personality team footprints, as illustrated in Fig. 2 B. Here, maximum values for each Big Five trait of a startup’s co-founders are mapped; stratified by successful and non-successful companies. Founder teams of successful startups tend to score higher on Openness, Conscientiousness, Extraversion, and Agreeableness.
When examining the combinations of founders with different personality types, we find that some ensembles of personalities were significantly correlated with greater chances of startup success—while controlling for other variables in the model—as shown in Fig. 2 C (for more details on the modelling, the predictive performance and the coefficient estimates of the final model, see Extended Data Figures 19 , 20 , and 21 in SI section A.6 ).
Three combinations of trio-founder companies were more than twice as likely to succeed than other combinations, namely teams with (1) a Leader and two Developers , (2) an Operator and two Developers , and (3) an Expert/Engineer , Leader and Developer . To illustrate the potential mechanisms on how personality traits might influence the success of startups, we provide some examples of well-known, successful startup founders and their characteristic personality traits in Extended Data Figure 22 .
Startups are one of the key mechanisms for brilliant ideas to become solutions to some of the world’s most challenging economic and social problems. Examples include the Google search algorithm, disability technology startup Fingerwork’s touchscreen technology that became the basis of the Apple iPhone, or the Biontech mRNA technology that powered Pfizer’s COVID-19 vaccine.
We have shown that founders’ personalities and the combination of personalities in the founding team of a startup have a material and significant impact on its likelihood of success. We have also shown that successful startup founders’ personality traits are significantly different from those of successful employees—so much so that a simple predictor can be trained to distinguish between employees and entrepreneurs with more than 80% accuracy using personality trait data alone.
Just as occupation-personality maps derived from data can provide career guidance tools, so too can data on successful entrepreneurs’ personality traits help people decide whether becoming a founder may be a good choice for them.
We have learnt through this research that there is not one type of ideal ’entrepreneurial’ personality but six different types. Many successful startups have multiple co-founders with a combination of these different personality types.
To a large extent, founding a startup is a team sport; therefore, diversity and complementarity of personalities matter in the foundation team. It has an outsized impact on the company’s likelihood of success. While all startups are high risk, the risk becomes lower with more founders, particularly if they have distinct personality traits.
Our work demonstrates the benefits of personality diversity among the founding team of startups. Greater awareness of this novel form of diversity may help create more resilient startups capable of more significant innovation and impact.
The data-driven research approach presented here comes with certain methodological limitations. The principal data sources of this study—Crunchbase and Twitter—are extensive and comprehensive, but there are characterised by some known and likely sample biases.
Crunchbase is the principal public chronicle of venture capital funding. So, there is some likely sample bias toward: (1) Startup companies that are funded externally: self-funded or bootstrapped companies are less likely to be represented in Crunchbase; (2) technology companies, as that is Crunchbase’s roots; (3) multi-founder companies; (4) male founders: while the representation of female founders is now double that of the mid-2000s, women still represent less than 25% of the sample; (5) companies that succeed: companies that fail, especially those that fail early, are likely to be less represented in the data.
Samples were also limited to those founders who are active on Twitter, which adds additional selection biases. For example, Twitter users typically are younger, more educated and have a higher median income 47 . Another limitation of our approach is the potentially biased presentation of a person’s digital identity on social media, which is the basis for identifying personality traits. For example, recent research suggests that the language and emotional tone used by entrepreneurs in social media can be affected by events such as business failure 48 , which might complicate the personality trait inference.
In addition to sampling biases within the data, there are also significant historical biases in startup culture. For many aspects of the entrepreneurship ecosystem, women, for example, are at a disadvantage 49 . Male-founded companies have historically dominated most startup ecosystems worldwide, representing the majority of founders and the overwhelming majority of venture capital investors. As a result, startups with women have historically attracted significantly fewer funds 50 , in part due to the male bias among venture investors, although this is now changing, albeit slowly 51 .
The research presented here provides quantitative evidence for the relevance of personality types and the diversity of personalities in startups. At the same time, it brings up other questions on how personality traits are related to other factors associated with success, such as:
Will the recent growing focus on promoting and investing in female founders change the nature, composition and dynamics of startups and their personalities leading to a more diverse personality landscape in startups?
Will the growth of startups outside of the United States change what success looks like to investors and hence the role of different personality traits and their association to diverse success metrics?
Many of today’s most renowned entrepreneurs are either Baby Boomers (such as Gates, Branson, Bloomberg) or Generation Xers (such as Benioff, Cannon-Brookes, Musk). However, as we can see, personality is both a predictor and driver of success in entrepreneurship. Will generation-wide differences in personality and outlook affect startups and their success?
Moreover, the findings shown here have natural extensions and applications beyond startups, such as for new projects within large established companies. While not technically startups, many large enterprises and industries such as construction, engineering and the film industry rely on forming new project-based, cross-functional teams that are often new ventures and share many characteristics of startups.
There is also potential for extending this research in other settings in government, NGOs, and within the research community. In scientific research, for example, team diversity in terms of age, ethnicity and gender has been shown to be predictive of impact, and personality diversity may be another critical dimension 52 .
Another extension of the study could investigate the development of the language used by startup founders on social media over time. Such an extension could investigate whether the language (and inferred psychological characteristics) change as the entrepreneurs’ ventures go through major business events such as foundation, funding, or exit.
Overall, this study demonstrates, first, that startup founders have significantly different personalities than employees. Secondly, besides firm-level factors, which are known to influence firm success, we show that a range of founder-level factors, notably the character traits of its founders, significantly impact a startup’s likelihood of success. Lastly, we looked at team-level factors. We discovered in a multifactor analysis that personality-diverse teams have the most considerable impact on the probability of a startup’s success, underlining the importance of personality diversity as a relevant factor of team performance and success.
Data sources
Entrepreneurs dataset.
Data about the founders of startups were collected from Crunchbase (Table 2 ), an open reference platform for business information about private and public companies, primarily early-stage startups. It is one of the largest and most comprehensive data sets of its kind and has been used in over 100 peer-reviewed research articles about economic and managerial research.
Crunchbase contains data on over two million companies - mainly startup companies and the companies who partner with them, acquire them and invest in them, as well as profiles on well over one million individuals active in the entrepreneurial ecosystem worldwide from over 200 countries and spans. Crunchbase started in the technology startup space, and it now covers all sectors, specifically focusing on entrepreneurship, investment and high-growth companies.
While Crunchbase contains data on over one million individuals in the entrepreneurial ecosystem, some are not entrepreneurs or startup founders but play other roles, such as investors, lawyers or executives at companies that acquire startups. To create a subset of only entrepreneurs, we selected a subset of 32,732 who self-identify as founders and co-founders (by job title) and who are also publicly active on the social media platform Twitter. We also removed those who also are venture capitalists to distinguish between investors and founders.
We selected founders active on Twitter to be able to use natural language processing to infer their Big Five personality features using an open-vocabulary approach shown to be accurate in the previous research by analysing users’ unstructured text, such as Twitter posts in our case. For this project, as with previous research 20 , we employed a commercial service, IBM Watson Personality Insight, to infer personality facets. This service provides raw scores and percentile scores of Big Five Domains (Openness, Conscientiousness, Extraversion, Agreeableness and Emotional Stability) and the corresponding 30 subdomains or facets. In addition, the public content of Twitter posts was collected, and there are 32,732 profiles that each had enough Twitter posts (more than 150 words) to get relatively accurate personality scores (less than 12.7% Average Mean Absolute Error).
The entrepreneurs’ dataset is analysed in combination with other data about the companies they founded to explore questions about the nature and patterns of personality traits of entrepreneurs and the relationships between these patterns and company success.
For the multifactor analysis, we further filtered the data in several preparatory steps for the success prediction modelling (for more details, see SI section A.5 ). In particular, we removed data points with missing values (Extended Data Fig. 13 ) and kept only companies in the data that were founded from 1990 onward to ensure consistency with previous research 32 (see Extended Data Fig. 14 ). After cleaning, filtering and pre-processing the data, we ended up with data from 25,214 founders who founded 21,187 startup companies to be used in the multifactor analysis. Of those, 3442 startups in the data were successful, 2362 in the first seven years after they were founded (see Extended Data Figure 15 for more details).
Entrepreneurs and employees dataset
To investigate whether startup founders show personality traits that are similar or different from the population at large (i. e. the entrepreneurs vs employees sub-analysis shown in Fig. 1 A and B), we filtered the entrepreneurs’ data further: we reduced the sample to those founders of companies, which attracted more than US$100k in investment to create a reference set of successful entrepreneurs (n \(=\) 4400).
To create a control group of employees who are not also entrepreneurs or very unlikely to be of have been entrepreneurs, we leveraged the fact that while some occupational titles like CEO, CTO and Public Speaker are commonly shared by founders and co-founders, some others such as Cashier , Zoologist and Detective very rarely co-occur seem to be founders or co-founders. To illustrate, many company founders also adopt regular occupation titles such as CEO or CTO. Many founders will be Founder and CEO or Co-founder and CTO. While founders are often CEOs or CTOs, the reverse is not necessarily true, as many CEOs are professional executives that were not involved in the establishment or ownership of the firm.
Using data from LinkedIn, we created an Entrepreneurial Occupation Index (EOI) based on the ratio of entrepreneurs for each of the 624 occupations used in a previous study of occupation-personality fit 44 . It was calculated based on the percentage of all people working in the occupation from LinkedIn compared to those who shared the title Founder or Co-founder (See SI section A.2 for more details). A reference set of employees (n=6685) was then selected across the 112 different occupations with the lowest propensity for entrepreneurship (less than 0.5% EOI) from a large corpus of Twitter users with known occupations, which is also drawn from the previous occupational-personality fit study 44 .
These two data sets were used to test whether it may be possible to distinguish successful entrepreneurs from successful employees based on the different patterns of personality traits alone.
Hierarchical clustering
We applied several clustering techniques and tests to the personality vectors of the entrepreneurs’ data set to determine if there are natural clusters and, if so, how many are the optimum number.
Firstly, to determine if there is a natural typology to founder personalities, we applied the Hopkins statistic—a statistical test we used to answer whether the entrepreneurs’ dataset contains inherent clusters. It measures the clustering tendency based on the ratio of the sum of distances of real points within a sample of the entrepreneurs’ dataset to their nearest neighbours and the sum of distances of randomly selected artificial points from a simulated uniform distribution to their nearest neighbours in the real entrepreneurs’ dataset. The ratio measures the difference between the entrepreneurs’ data distribution and the simulated uniform distribution, which tests the randomness of the data. The range of Hopkins statistics is from 0 to 1. The scores are close to 0, 0.5 and 1, respectively, indicating whether the dataset is uniformly distributed, randomly distributed or highly clustered.
To cluster the founders by personality facets, we used Agglomerative Hierarchical Clustering (AHC)—a bottom-up approach that treats an individual data point as a singleton cluster and then iteratively merges pairs of clusters until all data points are included in the single big collection. Ward’s linkage method is used to choose the pair of groups for minimising the increase in the within-cluster variance after combining. AHC was widely applied to clustering analysis since a tree hierarchy output is more informative and interpretable than K-means. Dendrograms were used to visualise the hierarchy to provide the perspective of the optimal number of clusters. The heights of the dendrogram represent the distance between groups, with lower heights representing more similar groups of observations. A horizontal line through the dendrogram was drawn to distinguish the number of significantly different clusters with higher heights. However, as it is not possible to determine the optimum number of clusters from the dendrogram, we applied other clustering performance metrics to analyse the optimal number of groups.
A range of Clustering performance metrics were used to help determine the optimal number of clusters in the dataset after an apparent clustering tendency was confirmed. The following metrics were implemented to evaluate the differences between within-cluster and between-cluster distances comprehensively: Dunn Index, Calinski-Harabasz Index, Davies-Bouldin Index and Silhouette Index. The Dunn Index measures the ratio of the minimum inter-cluster separation and the maximum intra-cluster diameter. At the same time, the Calinski-Harabasz Index improves the measurement of the Dunn Index by calculating the ratio of the average sum of squared dispersion of inter-cluster and intra-cluster. The Davies-Bouldin Index simplifies the process by treating each cluster individually. It compares the sum of the average distance among intra-cluster data points to the cluster centre of two separate groups with the distance between their centre points. Finally, the Silhouette Index is the overall average of the silhouette coefficients for each sample. The coefficient measures the similarity of the data point to its cluster compared with the other groups. Higher scores of the Dunn, Calinski-Harabasz and Silhouette Index and a lower score of the Davies-Bouldin Index indicate better clustering configuration.
Classification modelling
Classification algorithms.
To obtain a comprehensive and robust conclusion in the analysis predicting whether a given set of personality traits corresponds to an entrepreneur or an employee, we explored the following classifiers: Naïve Bayes, Elastic Net regularisation, Support Vector Machine, Random Forest, Gradient Boosting and Stacked Ensemble. The Naïve Bayes classifier is a probabilistic algorithm based on Bayes’ theorem with assumptions of independent features and equiprobable classes. Compared with other more complex classifiers, it saves computing time for large datasets and performs better if the assumptions hold. However, in the real world, those assumptions are generally violated. Elastic Net regularisation combines the penalties of Lasso and Ridge to regularise the Logistic classifier. It eliminates the limitation of multicollinearity in the Lasso method and improves the limitation of feature selection in the Ridge method. Even though Elastic Net is as simple as the Naïve Bayes classifier, it is more time-consuming. The Support Vector Machine (SVM) aims to find the ideal line or hyperplane to separate successful entrepreneurs and employees in this study. The dividing line can be non-linear based on a non-linear kernel, such as the Radial Basis Function Kernel. Therefore, it performs well on high-dimensional data while the ’right’ kernel selection needs to be tuned. Random Forest (RF) and Gradient Boosting Trees (GBT) are ensembles of decision trees. All trees are trained independently and simultaneously in RF, while a new tree is trained each time and corrected by previously trained trees in GBT. RF is a more robust and straightforward model since it does not have many hyperparameters to tune. GBT optimises the objective function and learns a more accurate model since there is a successive learning and correction process. Stacked Ensemble combines all existing classifiers through a Logistic Regression. Better than bagging with only variance reduction and boosting with only bias reduction, the ensemble leverages the benefit of model diversity with both lower variance and bias. All the above classification algorithms distinguish successful entrepreneurs and employees based on the personality matrix.
Evaluation metrics
A range of evaluation metrics comprehensively explains the performance of a classification prediction. The most straightforward metric is accuracy, which measures the overall portion of correct predictions. It will mislead the performance of an imbalanced dataset. The F1 score is better than accuracy by combining precision and recall and considering the False Negatives and False Positives. Specificity measures the proportion of detecting the true negative rate that correctly identifies employees, while Positive Predictive Value (PPV) calculates the probability of accurately predicting successful entrepreneurs. Area Under the Receiver Operating Characteristic Curve (AUROC) determines the capability of the algorithm to distinguish between successful entrepreneurs and employees. A higher value means the classifier performs better on separating the classes.
Feature importance
To further understand and interpret the classifier, it is critical to identify variables with significant predictive power on the target. Feature importance of tree-based models measures Gini importance scores for all predictors, which evaluate the overall impact of the model after cutting off the specific feature. The measurements consider all interactions among features. However, it does not provide insights into the directions of impacts since the importance only indicates the ability to distinguish different classes.
Statistical analysis
T-test, Cohen’s D and two-sample Kolmogorov-Smirnov test are introduced to explore how the mean values and distributions of personality facets between entrepreneurs and employees differ. The T-test is applied to determine whether the mean of personality facets of two group samples are significantly different from one another or not. The facets with significant differences detected by the hypothesis testing are critical to separate the two groups. Cohen’s d is to measure the effect size of the results of the previous t-test, which is the ratio of the mean difference to the pooled standard deviation. A larger Cohen’s d score indicates that the mean difference is greater than the variability of the whole sample. Moreover, it is interesting to check whether the two groups’ personality facets’ probability distributions are from the same distribution through the two-sample Kolmogorov-Smirnov test. There is no assumption about the distributions, but the test is sensitive to deviations near the centre rather than the tail.
Privacy and ethics
The focus of this research is to provide high-level insights about groups of startups, founders and types of founder teams rather than on specific individuals or companies. While we used unit record data from the publicly available data of company profiles from Crunchbase , we removed all identifiers from the underlying data on individual companies and founders and generated aggregate results, which formed the basis for our analysis and conclusions.
Data availability
A dataset which includes only aggregated statistics about the success of startups and the factors that influence is released as part of this research. Underlying data for all figures and the code to reproduce them are available on GitHub: https://github.com/Braesemann/FounderPersonalities . Please contact Fabian Braesemann ( [email protected] ) in case you have any further questions.
Change history
07 may 2024.
A Correction to this paper has been published: https://doi.org/10.1038/s41598-024-61082-7
Henrekson, M. & Johansson, D. Gazelles as job creators: A survey and interpretation of the evidence. Small Bus. Econ. 35 , 227–244 (2010).
Article Google Scholar
Davila, A., Foster, G., He, X. & Shimizu, C. The rise and fall of startups: Creation and destruction of revenue and jobs by young companies. Aust. J. Manag. 40 , 6–35 (2015).
Which vaccine saved the most lives in 2021?: Covid-19. The Economist (Online) (2022). noteName - AstraZeneca; Pfizer Inc; BioNTech SE; Copyright - Copyright The Economist Newspaper NA, Inc. Jul 14, 2022; Last updated - 2022-11-29.
Oltermann, P. Pfizer/biontech tax windfall brings mainz an early christmas present (2021). noteName - Pfizer Inc; BioNTech SE; Copyright - Copyright Guardian News & Media Limited Dec 27, 2021; Last updated - 2021-12-28.
Grant, K. A., Croteau, M. & Aziz, O. The survival rate of startups funded by angel investors. I-INC WHITE PAPER SER.: MAR 2019 , 1–21 (2019).
Google Scholar
Top 20 reasons start-ups fail - cb insights version (2019). noteCopyright - Copyright Newstex Oct 21, 2019; Last updated - 2022-10-25.
Hochberg, Y. V., Ljungqvist, A. & Lu, Y. Whom you know matters: Venture capital networks and investment performance. J. Financ. 62 , 251–301 (2007).
Fracassi, C., Garmaise, M. J., Kogan, S. & Natividad, G. Business microloans for us subprime borrowers. J. Financ. Quantitative Ana. 51 , 55–83 (2016).
Davila, A., Foster, G. & Gupta, M. Venture capital financing and the growth of startup firms. J. Bus. Ventur. 18 , 689–708 (2003).
Nann, S. et al. Comparing the structure of virtual entrepreneur networks with business effectiveness. Proc. Soc. Behav. Sci. 2 , 6483–6496 (2010).
Guzman, J. & Stern, S. Where is silicon valley?. Science 347 , 606–609 (2015).
Article ADS CAS PubMed Google Scholar
Aldrich, H. E. & Wiedenmayer, G. From traits to rates: An ecological perspective on organizational foundings. 61–97 (2019).
Gartner, W. B. Who is an entrepreneur? is the wrong question. Am. J. Small Bus. 12 , 11–32 (1988).
Thornton, P. H. The sociology of entrepreneurship. Ann. Rev. Sociol. 25 , 19–46 (1999).
Eikelboom, M. E., Gelderman, C. & Semeijn, J. Sustainable innovation in public procurement: The decisive role of the individual. J. Public Procure. 18 , 190–201 (2018).
Kerr, S. P. et al. Personality traits of entrepreneurs: A review of recent literature. Found. Trends Entrep. 14 , 279–356 (2018).
Hamilton, B. H., Papageorge, N. W. & Pande, N. The right stuff? Personality and entrepreneurship. Quant. Econ. 10 , 643–691 (2019).
Salmony, F. U. & Kanbach, D. K. Personality trait differences across types of entrepreneurs: A systematic literature review. RMS 16 , 713–749 (2022).
Freiberg, B. & Matz, S. C. Founder personality and entrepreneurial outcomes: A large-scale field study of technology startups. Proc. Natl. Acad. Sci. 120 , e2215829120 (2023).
Article CAS PubMed PubMed Central Google Scholar
Kern, M. L., McCarthy, P. X., Chakrabarty, D. & Rizoiu, M.-A. Social media-predicted personality traits and values can help match people to their ideal jobs. Proc. Natl. Acad. Sci. 116 , 26459–26464 (2019).
Article ADS CAS PubMed PubMed Central Google Scholar
Dalle, J.-M., Den Besten, M. & Menon, C. Using crunchbase for economic and managerial research. (2017).
Block, J. & Sandner, P. What is the effect of the financial crisis on venture capital financing? Empirical evidence from us internet start-ups. Ventur. Cap. 11 , 295–309 (2009).
Antretter, T., Blohm, I. & Grichnik, D. Predicting startup survival from digital traces: Towards a procedure for early stage investors (2018).
Dworak, D. Analysis of founder background as a predictor for start-up success in achieving successive fundraising rounds. (2022).
Hsu, D. H. Venture capitalists and cooperative start-up commercialization strategy. Manage. Sci. 52 , 204–219 (2006).
Blank, S. Why the lean start-up changes everything (2018).
Kaplan, S. N. & Lerner, J. It ain’t broke: The past, present, and future of venture capital. J. Appl. Corp. Financ. 22 , 36–47 (2010).
Hallen, B. L. & Eisenhardt, K. M. Catalyzing strategies and efficient tie formation: How entrepreneurial firms obtain investment ties. Acad. Manag. J. 55 , 35–70 (2012).
Gompers, P. A. & Lerner, J. The Venture Capital Cycle (MIT Press, 2004).
Shane, S. & Venkataraman, S. The promise of entrepreneurship as a field of research. Acad. Manag. Rev. 25 , 217–226 (2000).
Zahra, S. A. & Wright, M. Understanding the social role of entrepreneurship. J. Manage. Stud. 53 , 610–629 (2016).
Bonaventura, M. et al. Predicting success in the worldwide start-up network. Sci. Rep. 10 , 1–6 (2020).
Schwartz, H. A. et al. Personality, gender, and age in the language of social media: The open-vocabulary approach. PLoS ONE 8 , e73791 (2013).
Plank, B. & Hovy, D. Personality traits on twitter-or-how to get 1,500 personality tests in a week. In Proceedings of the 6th workshop on computational approaches to subjectivity, sentiment and social media analysis , pp 92–98 (2015).
Arnoux, P.-H. et al. 25 tweets to know you: A new model to predict personality with social media. In booktitleEleventh international AAAI conference on web and social media (2017).
Roberts, B. W., Kuncel, N. R., Shiner, R., Caspi, A. & Goldberg, L. R. The power of personality: The comparative validity of personality traits, socioeconomic status, and cognitive ability for predicting important life outcomes. Perspect. Psychol. Sci. 2 , 313–345 (2007).
Article PubMed PubMed Central Google Scholar
Youyou, W., Kosinski, M. & Stillwell, D. Computer-based personality judgments are more accurate than those made by humans. Proc. Natl. Acad. Sci. 112 , 1036–1040 (2015).
Soldz, S. & Vaillant, G. E. The big five personality traits and the life course: A 45-year longitudinal study. J. Res. Pers. 33 , 208–232 (1999).
Damian, R. I., Spengler, M., Sutu, A. & Roberts, B. W. Sixteen going on sixty-six: A longitudinal study of personality stability and change across 50 years. J. Pers. Soc. Psychol. 117 , 674 (2019).
Article PubMed Google Scholar
Rantanen, J., Metsäpelto, R.-L., Feldt, T., Pulkkinen, L. & Kokko, K. Long-term stability in the big five personality traits in adulthood. Scand. J. Psychol. 48 , 511–518 (2007).
Roberts, B. W., Caspi, A. & Moffitt, T. E. The kids are alright: Growth and stability in personality development from adolescence to adulthood. J. Pers. Soc. Psychol. 81 , 670 (2001).
Article CAS PubMed Google Scholar
Cobb-Clark, D. A. & Schurer, S. The stability of big-five personality traits. Econ. Lett. 115 , 11–15 (2012).
Graham, P. Do Things that Don’t Scale (Paul Graham, 2013).
McCarthy, P. X., Kern, M. L., Gong, X., Parker, M. & Rizoiu, M.-A. Occupation-personality fit is associated with higher employee engagement and happiness. (2022).
Pratt, A. C. Advertising and creativity, a governance approach: A case study of creative agencies in London. Environ. Plan A 38 , 1883–1899 (2006).
Klotz, A. C., Hmieleski, K. M., Bradley, B. H. & Busenitz, L. W. New venture teams: A review of the literature and roadmap for future research. J. Manag. 40 , 226–255 (2014).
Duggan, M., Ellison, N. B., Lampe, C., Lenhart, A. & Madden, M. Demographics of key social networking platforms. Pew Res. Center 9 (2015).
Fisch, C. & Block, J. H. How does entrepreneurial failure change an entrepreneur’s digital identity? Evidence from twitter data. J. Bus. Ventur. 36 , 106015 (2021).
Brush, C., Edelman, L. F., Manolova, T. & Welter, F. A gendered look at entrepreneurship ecosystems. Small Bus. Econ. 53 , 393–408 (2019).
Kanze, D., Huang, L., Conley, M. A. & Higgins, E. T. We ask men to win and women not to lose: Closing the gender gap in startup funding. Acad. Manag. J. 61 , 586–614 (2018).
Fan, J. S. Startup biases. UC Davis Law Review (2022).
AlShebli, B. K., Rahwan, T. & Woon, W. L. The preeminence of ethnic diversity in scientific collaboration. Nat. Commun. 9 , 1–10 (2018).
Article CAS Google Scholar
Żbikowski, K. & Antosiuk, P. A machine learning, bias-free approach for predicting business success using crunchbase data. Inf. Process. Manag. 58 , 102555 (2021).
Corea, F., Bertinetti, G. & Cervellati, E. M. Hacking the venture industry: An early-stage startups investment framework for data-driven investors. Mach. Learn. Appl. 5 , 100062 (2021).
Chapman, G. & Hottenrott, H. Founder personality and start-up subsidies. Founder Personality and Start-up Subsidies (2021).
Antoncic, B., Bratkovicregar, T., Singh, G. & DeNoble, A. F. The big five personality-entrepreneurship relationship: Evidence from slovenia. J. Small Bus. Manage. 53 , 819–841 (2015).
Download references
Acknowledgements
We thank Gary Brewer from BuiltWith ; Leni Mayo from Influx , Rachel Slattery from TeamSlatts and Daniel Petre from AirTree Ventures for their ongoing generosity and insights about startups, founders and venture investments. We also thank Tim Li from Crunchbase for advice and liaison regarding data on startups and Richard Slatter for advice and referrals in Twitter .
Author information
Authors and affiliations.
The Data Science Institute, University of Technology Sydney, Sydney, NSW, Australia
Paul X. McCarthy
School of Computer Science and Engineering, UNSW Sydney, Sydney, NSW, Australia
Faculty of Engineering and Information Technology, University of Technology Sydney, Sydney, Australia
Xian Gong & Marian-Andrei Rizoiu
Oxford Internet Institute, University of Oxford, Oxford, UK
Fabian Braesemann & Fabian Stephany
DWG Datenwissenschaftliche Gesellschaft Berlin, Berlin, Germany
Melbourne Graduate School of Education, The University of Melbourne, Parkville, VIC, Australia
Margaret L. Kern
You can also search for this author in PubMed Google Scholar
Contributions
All authors designed research; All authors analysed data and undertook investigation; F.B. and F.S. led multi-factor analysis; P.M., X.G. and M.A.R. led the founder/employee prediction; M.L.K. led personality insights; X.G. collected and tabulated the data; X.G., F.B., and F.S. created figures; X.G. created final art, and all authors wrote the paper.
Corresponding author
Correspondence to Fabian Braesemann .
Ethics declarations
Competing interests.
The authors declare no competing interests.
Additional information
Publisher's note.
Springer Nature remains neutral with regard to jurisdictional claims in published maps and institutional affiliations.
The original online version of this Article was revised: The Data Availability section in the original version of this Article was incomplete, the link to the GitHub repository was omitted. Full information regarding the corrections made can be found in the correction for this Article.
Supplementary Information
Supplementary information., rights and permissions.
Open Access This article is licensed under a Creative Commons Attribution 4.0 International License, which permits use, sharing, adaptation, distribution and reproduction in any medium or format, as long as you give appropriate credit to the original author(s) and the source, provide a link to the Creative Commons licence, and indicate if changes were made. The images or other third party material in this article are included in the article's Creative Commons licence, unless indicated otherwise in a credit line to the material. If material is not included in the article's Creative Commons licence and your intended use is not permitted by statutory regulation or exceeds the permitted use, you will need to obtain permission directly from the copyright holder. To view a copy of this licence, visit http://creativecommons.org/licenses/by/4.0/ .
Reprints and permissions
About this article
Cite this article.
McCarthy, P.X., Gong, X., Braesemann, F. et al. The impact of founder personalities on startup success. Sci Rep 13 , 17200 (2023). https://doi.org/10.1038/s41598-023-41980-y
Download citation
Received : 15 February 2023
Accepted : 04 September 2023
Published : 17 October 2023
DOI : https://doi.org/10.1038/s41598-023-41980-y
Share this article
Anyone you share the following link with will be able to read this content:
Sorry, a shareable link is not currently available for this article.
Provided by the Springer Nature SharedIt content-sharing initiative
By submitting a comment you agree to abide by our Terms and Community Guidelines . If you find something abusive or that does not comply with our terms or guidelines please flag it as inappropriate.
Quick links
- Explore articles by subject
- Guide to authors
- Editorial policies
Sign up for the Nature Briefing: AI and Robotics newsletter — what matters in AI and robotics research, free to your inbox weekly.

This paper is in the following e-collection/theme issue:
Published on 31.5.2024 in Vol 26 (2024)
Comparison of the Working Alliance in Blended Cognitive Behavioral Therapy and Treatment as Usual for Depression in Europe: Secondary Data Analysis of the E-COMPARED Randomized Controlled Trial
Authors of this article:

Original Paper
- Asmae Doukani 1 , MSc ;
- Matteo Quartagno 2 , PhD ;
- Francesco Sera 3 , PhD ;
- Caroline Free 1 , PhD ;
- Ritsuko Kakuma 1 , PhD ;
- Heleen Riper 4 , PhD ;
- Annet Kleiboer 5, 6 , PhD ;
- Arlinda Cerga-Pashoja 1 , PhD ;
- Anneke van Schaik 4, 7 , PhD ;
- Cristina Botella 8, 9 , PhD ;
- Thomas Berger 10 , PhD ;
- Karine Chevreul 11, 12 , PhD ;
- Maria Matynia 13 , PhD ;
- Tobias Krieger 10 , DPhil ;
- Jean-Baptiste Hazo 11, 12 , PhD ;
- Stasja Draisma 14 , PhD ;
- Ingrid Titzler 15 , PhD ;
- Naira Topooco 16 , PhD ;
- Kim Mathiasen 17, 18 , PhD ;
- Kristofer Vernmark 16 , PhD ;
- Antoine Urech 10, 19 , PhD ;
- Anna Maj 13 , PhD ;
- Gerhard Andersson 20, 21, 22 , PhD ;
- Matthias Berking 23 , MD, PhD ;
- Rosa María Baños 9, 24 , PhD ;
- Ricardo Araya 25 , PhD
1 Department of Population Health, London School of Hygiene & Tropical Medicine, London, United Kingdom
2 Medical Research Council Clinical Trials Unit, University College London, London, United Kingdom
3 Department of Statistics, Computer Science and Applications “G. Parenti”, University of Florence, Florance, Italy
4 Department of Psychiatry, Amsterdam University Medial Centre, Vrije Universiteit Amsterdam, Amsterdam, Netherlands
5 Department Clinical, Neuro, and Developmental Psychology, Vrije Universiteit Amsterdam, Amsterdam, Netherlands
6 Amsterdam Public Health Institute, Amsterdam, Netherlands
7 Academic Department for Depressive Disorders, Dutch Mental Health Care, Amsterdam, Netherlands
8 Department of Basic Psychology, Clinical and Psychobiology, Universitat Jaume I, Castellón de la Plana, Spain
9 Centro de Investigación Biomédica en Red Fisiopatología Obesidad y Nutrición, Instituto Carlos III, Madrid, Spain
10 Department of Clinical Psychology and Psychotherapy, University of Bern, Bern, Switzerland
11 Unité de Recherche Clinique in Health Economics, Assistance Publique–Hôpitaux de Paris, Paris, France
12 Health Economics Research Unit, Inserm, University of Paris, Paris, France
13 Faculty of Psychology, SWPS University, Warsaw, Poland
14 Department on Aging, Netherlands Institute of Mental Health and Addiction (Trimbos Institute), Utrecht, Netherlands
15 Department of Clinical Psychology and Psychotherapy, Friedrich-Alexander-Universität Erlangen-Nürnberg, Erlangen, Germany
16 Department of Behavioural Sciences and Learning, Linköping University, Linköping, Sweden
17 Department of Clinical Medicine, Faculty of Health Sciences, University of Southern Denmark, Odense, Denmark
18 Centre for Digital Psychiatry, Mental Health Services of Southern Denmark, Odense, Denmark
19 Department of Neurology, Inselspital Bern, Bern University Hospital, Bern, Switzerland
20 Department of Behavioral Sciences and Learning, Linköping University, Linköping, Sweden
21 Department of Clinical Neuroscience, Karolinska Institute, Stockholm, Sweden
22 Department of Biomedical and Clinical Sciences, Linköping University, Linköping, Sweden
23 Department of Clinical Psychology and Psychotherapy, Friedrich-Alexander-University Erlangen-Nürnberg, Erlangen, Germany
24 Department of Personality, Evaluation and Psychological Treatments, Universidad de Valencia, Valencia, Spain
25 Department of Health Service and Population Research, Institute of Psychiatry, Psychology & Neuroscience, King’s College London, London, United Kingdom
Corresponding Author:
Asmae Doukani, MSc
Department of Population Health
London School of Hygiene & Tropical Medicine
Keppel Street
London, WC1E 7HT
United Kingdom
Phone: 44 020 7636 8636 ext 2463
Email: [email protected]
Background: Increasing interest has centered on the psychotherapeutic working alliance as a means of understanding clinical change in digital mental health interventions in recent years. However, little is understood about how and to what extent a digital mental health program can have an impact on the working alliance and clinical outcomes in a blended (therapist plus digital program) cognitive behavioral therapy (bCBT) intervention for depression.
Objective: This study aimed to test the difference in working alliance scores between bCBT and treatment as usual (TAU), examine the association between working alliance and depression severity scores in both arms, and test for an interaction between system usability and working alliance with regard to the association between working alliance and depression scores in bCBT at 3-month assessments.
Methods: We conducted a secondary data analysis of the E-COMPARED (European Comparative Effectiveness Research on Blended Depression Treatment versus Treatment-as-usual) trial, which compared bCBT with TAU across 9 European countries. Data were collected in primary care and specialized services between April 2015 and December 2017. Eligible participants aged 18 years or older and diagnosed with major depressive disorder were randomized to either bCBT (n=476) or TAU (n=467). bCBT consisted of 6-20 sessions of bCBT (involving face-to-face sessions with a therapist and an internet-based program). TAU consisted of usual care for depression. The main outcomes were scores of the working alliance (Working Alliance Inventory-Short Revised–Client [WAI-SR-C]) and depressive symptoms (Patient Health Questionnaire-9 [PHQ-9]) at 3 months after randomization. Other variables included system usability scores (System Usability Scale-Client [SUS-C]) at 3 months and baseline demographic information. Data from baseline and 3-month assessments were analyzed using linear regression models that adjusted for a set of baseline variables.
Results: Of the 945 included participants, 644 (68.2%) were female, and the mean age was 38.96 years (IQR 38). bCBT was associated with higher composite WAI-SR-C scores compared to TAU ( B =5.67, 95% CI 4.48-6.86). There was an inverse association between WAI-SR-C and PHQ-9 in bCBT ( B =−0.12, 95% CI −0.17 to −0.06) and TAU ( B =−0.06, 95% CI −0.11 to −0.02), in which as WAI-SR-C scores increased, PHQ-9 scores decreased. Finally, there was a significant interaction between SUS-C and WAI-SR-C with regard to an inverse association between higher WAI-SR-C scores and lower PHQ-9 scores in bCBT ( b =−0.030, 95% CI −0.05 to −0.01; P =.005).
Conclusions: To our knowledge, this is the first study to show that bCBT may enhance the client working alliance when compared to evidence-based routine care for depression that services reported offering. The working alliance in bCBT was also associated with clinical improvements that appear to be enhanced by good program usability. Our findings add further weight to the view that the addition of internet-delivered CBT to face-to-face CBT may positively augment experiences of the working alliance.
Trial Registration: ClinicalTrials.gov NCT02542891, https://clinicaltrials.gov/study/NCT02542891; German Clinical Trials Register DRKS00006866, https://drks.de/search/en/trial/DRKS00006866; Netherlands Trials Register NTR4962, https://www.onderzoekmetmensen.nl/en/trial/25452; ClinicalTrials.Gov NCT02389660, https://clinicaltrials.gov/study/NCT02389660; ClinicalTrials.gov NCT02361684, https://clinicaltrials.gov/study/NCT02361684; ClinicalTrials.gov NCT02449447, https://clinicaltrials.gov/study/NCT02449447; ClinicalTrials.gov NCT02410616, https://clinicaltrials.gov/study/NCT02410616; ISRCTN Registry ISRCTN12388725, https://www.isrctn.com/ISRCTN12388725?q=ISRCTN12388725&filters=&sort=&offset=1&totalResults=1&page=1&pageSize=10; ClinicalTrials.gov NCT02796573, https://classic.clinicaltrials.gov/ct2/show/NCT02796573
International Registered Report Identifier (IRRID): RR2-10.1186/s13063-016-1511-1
Introduction
Depression is one of the most significant contributors to the global disease burden, affecting an estimated 264 million people globally [ 1 , 2 ]. Depression accounts for 7.2% of the overall disease burden in Europe, costing an estimated €113,405 billion (US $123,038 billion) per year. However, 45% of people with major depression will go untreated [ 3 ]. High costs and suboptimal access to mental health care are among the many reasons to foster digital mental health interventions (DMHIs), which promise greater quality of care and lower costs of delivery [ 4 , 5 ].
Evidence concerning the effectiveness of DMHIs has increased substantially over the past decade. Growing evidence indicates that internet-delivered cognitive behavioral therapy (iCBT) might be just as effective as face-to-face cognitive behavioral therapy (CBT) for a range of mental health conditions, particularly depression [ 6 - 13 ]. iCBT is delivered with varying degrees of support ranging from a stand-alone self-administered digital program to a blended treatment with the active involvement of a therapist through regular face-to-face meetings. Blended psychotherapies provide higher levels of therapist support compared to guided approaches that provide minimal or some guidance from a mental health practitioner [ 4 ]. Blended delivery has gained interest, with emerging evidence suggesting that such interventions can lead to improved adherence and treatment outcomes [ 14 ].
As interest in DMHIs increases, considerable attention has centered around the concept of the client-therapist alliance, of which there are many variations (therapeutic, working, helping, etc). While different therapeutic approaches have historically failed to agree on a definition of the alliance, Edward Bordin [ 15 - 17 ] proposed a pan-theoretical tripartite conceptualization called the working alliance that is characterized by 3 key dimensions, including the emotional “bond” between the client and the therapist, the agreement on the therapeutic “goals,” and the “task” needed to advance the client’s goals toward clinical improvement. This concept is particularly important because it has consistently predicted positive treatment outcomes for a range of psychological approaches, including CBT for depression [ 18 - 20 ].
The client-therapist alliance was identified as a key research priority for research policy and funding in digital technologies in mental health care, in a large consensus study involving people with lived experiences of mental health problems and service use, their carers, and mental health practitioners [ 21 ]. The integration of digital technologies in psychotherapy has led to changes in the way the alliance is conceptualized and assessed [ 19 ], with variability depending on the type of DMHI (digital program [ 22 ], avatar [ 23 ], or mobile app [ 24 ]).
The literature investigating the client-therapist alliance has largely focused on addressing 2 key questions. The first question is “Do alliance scores predict changes in clinical outcomes?” [ 21 , 25 - 29 ], and the second question, which has been focused on to a lesser extent, is “Does the alliance vary depending on how psychotherapy is delivered?” Systematic reviews that have addressed these questions specifically in relation to interventions that are guided, adopt CBT [ 21 ], or target the treatment of depression [ 27 ] found that the working alliance can be established in guided DMHIs at a comparable level to face-to-face therapy [ 21 ]; however, the literature on the outcome-alliance relationship is mixed [ 21 , 26 , 27 ].
To this end, only 3 studies have examined the working alliance in blended CBT (bCBT). The first was an uncontrolled study in Sweden, which offered 4 face-to-face and 10 iCBT sessions to a total of 73 participants in primary care services and which was part of the E-COMPARED (European Comparative Effectiveness Research on Blended Depression Treatment versus Treatment-as-usual) study [ 30 ]. The findings showed that the alliance was rated highly by both clients and therapists. However, only therapist alliance ratings were associated with client score changes in depression, while client ratings were not.
The second study was conducted in the Netherlands and recruited 102 participants from specialist care services. Participants were either randomized to bCBT (n=47), which consisted of a 20-week intervention (10 face-to-face and 10 online sessions), or a control condition (n=45), which consisted of 15-20 face-to-face CBT sessions [ 31 ]. Similar to the findings from the study conducted in Sweden [ 30 ], the working alliance was rated highly by both clients and therapists, and no differences were observed between scores. Client ratings of the working alliance were associated with lower depression scores over time in face-to-face CBT but not in bCBT. Therapist working alliance ratings were not significantly associated with depression scores over time in both treatment conditions [ 31 ].
The third and most recent study was conducted in Denmark. The study recruited a total of 76 participants who were either randomized to bCBT (n=38), which consisted of 6 face-to-face sessions that were alternated with 6-8 online modules of an internet-based program, or a control condition (n=38), which consisted of 12 face-to-face CBT sessions [ 32 ]. The findings showed a significant difference in client and therapist working alliance scores, in which clients rated their working alliance higher than therapists. However, only the therapist ratings across conditions were significantly associated with outcomes in depression. Working alliance ratings across face-to-face CBT and bCBT were comparable. Working alliance ratings in both face-to-face CBT and bCBT did not significantly predict treatment outcomes. It is not clear why an in-group effect was found for therapists across the pooled data and not within treatment conditions [ 32 ]. These findings might indicate that the study was not powered enough to detect an association for client ratings in each treatment condition.
While research has mainly focused on measuring the alliance between the client and therapist, emerging qualitative research suggests that DMHIs may offer additional relational alliance benefits [ 29 , 33 , 34 ]. An example comes from a qualitative study that examined the working alliance demands in a bCBT intervention for people with mild-to-moderate depression in the United Kingdom, as part of the E-COMPARED trial [ 35 ]. Qualitative data indicated a potential fourth dimension called “usability heuristics,” which appeared to uniquely promote the working alliance in bCBT. Usability heuristics defines the digital program’s role in promoting active engagement, self-discovery, and autonomous problem-solving, with higher levels expected to enhance the quality of the working alliance. Features that enable “usability heuristics” include digital technologies that increase access and immediacy to the therapeutic task (availability), appropriately respond to the client’s input (interactivity), are easy to use, have esthetic appeal, and promote self-directed therapy [ 36 ]. Findings regarding usability heuristics and the respective subfeatures were also reported in another qualitative study that tested this framework in a Spanish sample of participants who experienced self-guided or low-intensity supported iCBT [ 37 ]. It is therefore possible that experiences of digital program features may influence the way that the working alliance is experienced in blended formats of CBT [ 36 ].
Aims and Objectives
To our knowledge, we report the largest investigation of the working alliance in bCBT for depression, using pooled data from 9 country sites involved in a pragmatic noninferiority randomized controlled trial investigating the effectiveness of bCBT for depression when compared with treatment as usual (TAU) [ 35 ]. Further to this, our study will explore if system usability, a newly conceptualized feature of the working alliance, in bCBT interacts with the working alliance and treatment outcome association [ 36 ]. Our primary objectives are to test the difference in working alliance scores between bCBT and TAU (objective 1), and determine if working alliance scores are associated with depression scores (objective 2). Our secondary objective is to test for an interaction between system usability and the working alliance with regard to an association between the working alliance and depression scores in bCBT (objective 3).
Study Design and Settings
We conducted a nonprespecified secondary analysis of data collected in the E-COMPARED study, a large European 2-arm, noninferiority randomized controlled trial investigating the effectiveness of bCBT compared with TAU across 9 European countries (France: ClinicalTrials.gov NCT02542891, September 4, 2015; Germany: German Clinical Trials Register DRKS00006866, December 2, 2014; The Netherlands: Netherlands Trials Register NTR4962, January 5, 2015; Poland: ClinicalTrials.gov NCT02389660, February 18, 2015; Spain: ClinicalTrials.gov NCT02361684, January 8, 2015; Sweden: ClinicalTrials.gov NCT02449447, March 30, 2015; Switzerland: ClinicalTrials.gov NCT02410616, April 2, 2015; United Kingdom: ISRCTN Registry ISRCTN12388725, March 20, 2015; Denmark: ClinicalTrials.gov NCT02796573, June 1, 2016) [ 35 , 38 ]. Data were collected between April 2015 and December 2017. Clients seeking treatment for depression were recruited, assessed, and treated across routine primary care in Germany, Poland, Spain, Sweden, and the United Kingdom, and specialized mental health services in France, the Netherlands, Switzerland, and Denmark [ 35 ]. Following the start of recruitment, an additional satellite site was added in Denmark to boost recruitment [ 38 ]. The E-COMPARED trial was funded by the European Commission FP7-Health-2013-Innovation-1 program (grant agreement number: 603098).
Participants
Recruitment procedures differed in each country, but all sites screened new clients seeking help for depression, who scored 5 or higher on the Patient Health Questionnaire-9 (PHQ-9) [ 39 ]. The study was explained to potential participants either face-to-face or over a telephone call. Clients who agreed to take part in the study were invited to an initial appointment to assess eligibility. The inclusion criteria applied at all sites were as follows: age ≥18 years and meeting the diagnostic criteria for major depressive disorder as confirmed by the MINI International Neuropsychiatric Interview (M.I.N.I) version 5.0 [ 40 ]. The exclusion criteria were as follows: high risk of suicide and psychiatric comorbidity (ie, substance dependence, bipolar affective disorder, psychotic illness, or obsessive compulsive disorder) assessed during the M.I.N.I. interview; receiving psychological treatment for depression in primary or specialized mental health care at the point of recruitment; inability to comprehend the spoken and written language of the country site; lacking access to a computer or a fast internet connection (ie, broadband or comparable); and lacking a smartphone or being unwilling to carry a smartphone if one was provided by the research team [ 35 ].
After baseline assessments, participants were randomized to 1 of 2 treatment arms (bCBT or TAU) using block randomization, with stratification by country [ 35 ]. All participants provided written informed consent before taking part in the trial [ 35 ].
Ethical Considerations
The trial was conducted in accordance with the Declaration of Helsinki and was approved by all local ethics committees. Ethics approval to conduct a secondary analysis was obtained from the London School of Hygiene and Tropical Medicine Research Ethics Committee on October 7, 2019 (ethics reference number: 17852). For further information on the trial, including local ethics approvals and the randomization process, see the trial protocol [ 35 ].
Interventions: bCBT and TAU
bCBT for depression consisted of integrating a digital program (iCBT plus mobile app) with face-to-face CBT in a single treatment protocol [ 35 , 41 ]. iCBT programs included 4 mandatory core modules of CBT (ie, psychoeducation, behavioral activation, cognitive restructuring, and relapse prevention) plus optional modules (eg, physical exercise and problem solving) typically completed at home, while face-to-face CBT was delivered in the clinic [ 35 ]. Clients worked through treatment modules, completed exercises, and monitored their symptoms on the digital program, while face-to-face sessions were used by the therapist to set up modules, monitor client progress, and address client-specific needs. Sequencing and time spent on each module were flexibly applied; however, the 4 mandatory modules on the digital program had to be completed. Data on treatment and dosage were not collected for TAU in the trial. See Table 1 for a breakdown of recruitment, bCBT format and dosage, and treatments offered in TAU across all country sites [ 30 , 35 , 42 ]. It was not possible to blind therapists to treatment allocation; however, assessors were blinded [ 35 ].
a bCBT: blended cognitive behavioral therapy.
b TAU: treatment as usual.
c Sequencing of face-to-face and online sessions can include more than one session per week for either component.
d CBT: cognitive behavioral therapy.
e GP: general practitioner.
f IAPT: improving access to psychological therapy.
g NHS: National Health Service.
h Denmark was added as a satellite recruitment site [ 38 ] after the commencement of the project.
Based on the registered data, 194 therapists delivered trial interventions. In Germany, therapists only delivered bCBT in the treatment arm, whereas therapists from the remaining 8 country sites delivered interventions across both treatment arms. The risk of contamination was not perceived as a concern, as CBT was also offered in TAU, with the focus of the trial on investigating the blending of an internet-based intervention with face-to-face CBT when compared to routine care. Data on therapist ratings of the working alliance will be published in a separate paper to enable comprehensive reporting and discussion of the findings.
Diagnostic Assessment
In the E-COMPARED study [ 35 ], a diagnosis of major depression according to the Diagnostic and Statistical Manual of Mental Disorders IV (DSM-IV) was established at baseline using the M.I.N.I [ 40 ], a structured diagnostic interview that has been translated into 65 languages and is used for both clinical and research practice. The interview compares well with the Structured Clinical Interview for DSM-IV disorders [ 43 ] and the Composite International Diagnostic Interview [ 40 , 43 ]. The M.I.N.I. was also used to assess the following comorbid disorders that were part of the exclusion criteria: substance dependence, bipolar affective disorder, psychotic illness, and obsessive-compulsive disorder. The M.I.N.I was administered face-to-face or via telephone at baseline and 12-month follow-up assessments. Telephone administration of diagnostic interviews has shown good validity and reliability [ 44 , 45 ].
Primary Measures
The study outcomes were the working alliance and depression severity, which were measured using the Working Alliance Inventory-Short Revised–Client (WAI-SR-C) [ 46 ] and the PHQ-9 [ 39 ], respectively. The WAI-SR-C scale is based on the theory of working alliance containing 3-item subscales assessing bond, task, and goals by Bordin [ 15 , 16 ]. The 12 items are rated on a 5-point scale from 1 (seldom) to 5 (always), with total scores ranging between 12 and 60. Higher scores on the scale indicate better working alliance. The WAI-SR-C scale has demonstrated good reliability (internal consistency) for all 3 factors, including the bond, task, and goals subscales (Cronbach α=0.92, 0.92, and 0.89, respectively) [ 47 ]. The scale has been shown to be correlated with other therapeutic alliance scales such as the California Therapeutic Alliance Rating System [ 19 , 48 ] and the Helping Alliance Questionnaire-II [ 19 , 49 ]. The WAI-SR-C scale was only administered at 3-month assessments. Data for the WAI-SR-C scale were not collected in the TAU arm of the Swedish country site.
The PHQ-9 [ 39 ] was used to assess depression as the trial’s primary clinical outcome. The PHQ-9 is a 9-item scale that can be used to screen and diagnose people for depressive disorders. Each of the 9 items is scored on a 4-point scale from 0 (not at all) to 3 (nearly every day). The total score ranges between 0 and 27, with higher scores indicating greater symptom severity. Depression severity can be grouped into the following: mild (score 0-5), moderate (6-10), moderately severe (11-15), and severe (≥16). The PHQ-9 has been shown to have good psychometric properties [ 39 ] and has demonstrated its utility as a valid diagnostic tool [ 50 ]. The PHQ-9 was administered at the baseline and 3-, 6-, and 12-month assessments; however, this study only used baseline and 3-month assessment data as the study was interested in investigating depression scores that generally corresponded to before and after treatment.
Other Measures
System Usability Scale-Client (SUS-C) [ 51 , 52 ] was used to assess the usability of the digital programs. The SUS-C is a 10-item self-reported questionnaire. Items are measured on a 5-point scale ranging from 1 (strongly disagree) to 5 (strongly agree). The total SUS-C score ranges between 10 and 50 to produce a global score. Higher scores indicate better system usability. The total sum score has been found to be a valid and interpretable measure to assess the usability of internet-based interventions by professionals in mental health care settings [ 53 ]. The SUS has shown high internal reliability (eg, coefficient Ω=0.91) and good concurrent validity and sensitivity [ 52 , 53 ]. The SUS-C was administered at the 3-month follow-up assessment.
Demographic data on the participant’s gender, age, educational attainment, marital status, and country site were collected at baseline. Baseline variables entered as covariates in the regression models included age, gender (male, female, and other), marital status (single, divorced, widowed, living together, and married), and educational level (low, middle, and high, corresponding to secondary school education or equivalent [low], college or equivalent [middle], and university degree or higher [high]).
Baseline data were completed online, face-to-face, via telephone, or a combination of these approaches. The 3-month follow-up assessments were largely completed online, with the exception of the PHQ-9 that was collected via telephone to maximize data collection of the trial’s primary outcome. Data that were directly collected by researchers (ie, either in person or via telephone) were double entered to increase the accuracy of the data entry process.
Statistical Analysis
The study used an intention-to-treat (ITT) population for the data analysis [ 54 ]. While the ITT approach is standard for RCTs, some methodologists advise that a per-protocol population is more suitable for pragmatic noninferiority trials owing to concerns that a “flawed trial” is likely to incorrectly demonstrate noninferiority (eg, a trial that loses the ability to distinguish any true differences between treatment groups that are present). However, contrary to the primary analysis in the E-COMPARED trial, noninferiority tests were not performed in our analyses. A decision was made to use a pure ITT population in order to maintain the original treatment group composition achieved after the random allocation of trial participants, therefore minimizing the confounding between the treatment groups and providing unbiased estimates of the treatment effects on the working alliance [ 54 ].
Data of the E-COMPARED trial were downloaded from a data repository. All analyses employed an ITT population. All models were adjusted for baseline PHQ-9 scores, age, gender, marital status, educational attainment, and country site. Analyses were performed on SPSS (version 26 or above) [ 55 ], STATA (version 16 or above) [ 56 ], and PROCESS Macro plug-in for SPSS (version 3.5 or above) [ 57 ]. Reported P values are 2-tailed, with significance levels at P ≤.05.
Treatment Assignment as a Predictor for WAI-SR-C Scores at 3-Month Assessments
In order to test if treatment assignment predicted WAI-SR-C scores at 3-month assessments (objective 1), a fixed effects linear regression model [ 58 ] was fitted separately for WAI-SR-C composite and subscale scores (goals, task, and bond). Four models were fitted altogether.
Association Between PHQ-9 Scores and WAI-SR-C Scores at 3-Month Assessments
To determine if WAI-SR-C scores were associated with PHQ-9 scores at 3-month assessments (objective 2), a fixed effects linear regression model was fitted to investigate this association separately for the bCBT and TAU arms in order to understand the alliance-outcome association within different treatment conditions in the trial. The model was also fitted separately for WAI-SR-C composite and subscale scores. Eight models were fitted altogether.
Testing the Interaction Between WAI-SR-C and SUS-C Scores With Regard to the Relationship Between WAI-SR-C and PHQ-9 Scores
To test the interaction between 3-month SUS-C and 3-month WAI-SR-C scores in a model examining the relationship between 3-month WAI-SR-C and 3-month PHQ-9 scores, a multiple regression model was fitted separately for WAI-SR-C composite and subscale scores in order to estimate the size of the interaction. Four models were fitted altogether.
Missing Data
Multiple imputation was used to handle high levels of missing data, under the missing at random (MAR) assumption. In particular, 36.6% (345/943) of data were missing for the PHQ-9, 20.7% (195/943) were missing for the WAI-SR-C, and 27.9% (133/476) were missing for the SUS-C at 3-month assessments. We imputed data sets using the chained equation approach [ 59 ]. Tabulations of missing data across treatment conditions and country sites are presented in Tables S1-S3 in Multimedia Appendix 1 . Chi-square results of differences in missing and complete data between E-COMPARED country sites are presented in Tables S4 and S5 in Multimedia Appendix 1 . In the imputation model, we included all variables that were part of the analyses, including observations from the PHQ-9 at baseline and demographic variables. To account for the interaction term in the regression model, data were imputed using the just another variable (JAV) approach [ 60 ]. Multiple imputation was performed separately for bCBT and TAU to allow for condition-specific variables to be considered. For example, the SUS-C variable was only entered in the bCBT arm, as those in the TAU arm did not use a digital program.
Post hoc Analysis
Post hoc sensitivity analyses were conducted to examine if the multiple imputation approach that was used to handle missing data would lead to different conclusions when compared to a complete case analysis. Under the MAR assumption, consistent findings between the primary analysis and sensitivity analysis can strengthen the reliability of the findings [ 61 - 64 ], at least in situations where both the primary and sensitivity analyses are expected to be valid under similar assumptions (eg, multiple imputation and complete case analysis under the MAR assumption in the outcome variable only).
Owing to the heterogeneity of interventions offered in the TAU arm within the current pragmatic trial, a subgroup analysis was conducted to explore the magnitude of treatment effects on the working alliance when using a subset of the sample, which compared bCBT with face-to-face CBT offered in the TAU arm in Denmark, France, Poland, Switzerland, and the United Kingdom country sites of the E-COMPARED trial [ 35 ]. The subanalysis replicated the main analysis in just 5 country sites. This enabled the working alliance in bCBT to be directly compared with a defined comparator. Results between the primary analysis and the subanalysis were compared to understand if results vary when there are multiple interventions in TAU and when there is a defined comparator (ie, face-to-face CBT) [ 65 - 67 ].
Clinical and Demographic Characteristics
Table 2 summarizes the baseline characteristics. Among the 943 participants who consented and were randomized in the trial (bCBT=476; TAU=467) (See Figure S1 in Multimedia Appendix 1 for the trial’s profile), most were female (644/943, 68.3%), were middle-aged, and had a university degree or higher (447/943, 47.4). The PHQ-9 scores (median 15, IQR 7) reflected depression of moderate severity. PHQ-9 scores at 3 months will be reported in the main trial paper, which is being prepared. The median WAI-SR-C score was 47.42 (IQR 6) in the bCBT arm and 42 (IQR 8) in the TAU arm. The median SUS-C score was 42 (IQR 9) in the bCBT arm. See Table 3 for the median (IQR) values of the WAI-SR-C and SUS-C scores across treatment groups, and see Tables S6-S8 in Multimedia Appendix 1 for the median (IQR) values of the WAI-SR-C and SUS-C scores by country site.
c Data collected were in respect to what would be considered low, middle, and high levels of education in each setting. Data were missing for 1 of 943 (0.2%) individuals in the bCBT arm.
d Self-reported country of birth can be found in Table S9 in Multimedia Appendix 1 .
e PHQ-9: Patient Health Questionnaire-9.
f PHQ-9 severity cutoff points are as follows: 5-9, mild depression; 1-14, moderate depression; 15-19, moderately severe depression; and ≥20, severe depression [ 39 ].
c WAI-SR-C: Working Alliance Inventory-Short Revised–Client.
d SUS-C: System Usability Scale-Client.
e N/A: not applicable.
Treatment Assignment as a Predictor for WAI-SR-C Scores
Treatment assignment significantly predicted WAI-SR-C composite, goals, task, and bond scores (See Table 4 for model summaries). Being allocated to bCBT predicted higher WAI-SR-C composite and subscale scores at 3-month assessments when compared to TAU.
a WAI-SR-C: Working Alliance Inventory-Short Revised–Client.
b Separate models were generated for WAI-SR-C composite and subscale scores (ie, goals, task, and bond).
c Unstandardized beta.
Across both treatment arms, WAI-SR-C composite scores and goals and task subscale scores were significantly associated with PHQ-9 scores, in which lower PHQ-9 scores were associated with higher WAI-SR-C composite scores and goals and task subscale scores. WAI-SR-C bond scores were not significantly associated with PHQ-9 scores in both treatment arms (see Table 5 for model summaries).
c bCBT: blended cognitive behavioral therapy.
d TAU: treatment as usual.
e Unstandardized beta.
There was a significant interaction between WAI-SR-C and SUS-C scores with regard to the association between WAI-SR-C composite scores and PHQ-9 scores at 3 months ( b =−0.008, 95% CI −0.01 to −0.00; P =.03). Similar findings were noted for the goals ( b =−0.021, 95% CI −0.04 to −0.00; P =.03) and task ( b =−0.028, 95% CI −0.05 to −0.01; P =.003) subscales but not for the bond subscale ( b =−0.010, 95% CI −0.03 to 0.01; P =.30). Figure 1 shows the presence of an inverse association between composite WAI-SR-C (for composite, and the goals and task subscales but not the bond subscale) and PHQ-9 scores among those with high SUS-C scores.

Sensitivity and Subgroup Results
The sensitivity analysis with the complete case data set and subgroup analysis of 5 country sites that only offered face-to-face CBT in the TAU arm produced results that were comparable to those reported in the main paper. However, the interaction between SUS-C (and all subscales) and WAI-SR-C scores with regard to the association between WAI-SR-C and PHQ-9 scores was not significant in terms of sensitivity. Other differences are summarized in Results S2 in Multimedia Appendix 1 , while the full results of the sensitivity and subgroup analyses can be found in Results S3 and S4 in Multimedia Appendix 1 .
Principal Findings
This study investigated the client-rated working alliance in a bCBT intervention for depression when compared to TAU [ 35 ]. Overall, our study found that treatment allocation (bCBT versus TAU) was a significant predictor of working alliance scores, in which ratings of the working alliance (composite scale and goals, task, and bond subscales) were higher in bCBT than in TAU. The working alliance was significantly associated with treatment outcomes. Across both bCBT and TAU groups, as working alliance scores increased, PHQ-9 scores decreased for composite, goals, and task scores but not for bond scores. Finally, there was a significant interaction between average and above-average system usability and higher working alliance (composite scale and goals and task subscales, but not bond subscale) scores when examining the relationship between the working alliance and PHQ-9 scores at 3-month assessments.
To our knowledge, our study is the first to report that working alliance composite scores and all subscale scores were higher in bCBT than in TAU. A post hoc analysis using data from country sites that only offered face-to-face CBT in the TAU arm found that the working alliance was significantly higher in the bCBT arm compared to face-to-face CBT. These findings indicate that a blended approach may offer additional alliance-building benefits when compared to face-to-face CBT and other types of usual care for depression offered in TAU such as talking therapies and psychopharmacological interventions. A possible explanation for our findings is that the digital elements of the intervention may enable better definition and coverage of the goals and the task than what might be possible in face-to-face sessions alone [ 68 ]. A study exploring program usage across 4 country sites of the E-COMPARED study found that clients received an average of 10 messages from their therapists online [ 69 ]. Features of the digital program that enabled the client to receive contact from the therapist away from the clinic may therefore play a role in increasing the availability of the therapist and enhancing opportunities to further strengthen the working alliance [ 69 ].
Further support for our findings comes from a qualitative study that examined the working alliance in bCBT in the United Kingdom country site of the E-COMPARED trial [ 36 ], which found that participants preferred bCBT compared to face-to-face CBT alone. The “immediacy” of access to the therapeutic task was reported to enhance engagement with the intervention and provide a higher sense of control and independence. The digital program was also described as a “secure base” that allowed participants to progressively explore self-directed treatment [ 36 ]. Similarly, a qualitative study from the German country site of the E-COMPARED trial found that bCBT was perceived to strengthen patient self-management and autonomy in relation to place and location [ 70 ].
Our study appears to be the first to identify a significant association between lower depression scores and higher working alliance composite scores and goals and task subscale scores but not bond subscale scores. In alignment with our findings, a narrative review of the working alliance in online therapy found that most guided iCBT studies included in the review reported significant associations between outcomes and the task and goals subscale scores but not the bond subscale scores [ 26 ]. A possible explanation could be that the bond is experienced differently in bCBT compared to traditional formats of CBT [ 26 ]. Bordin’s [ 15 , 16 ] conceptualization of the working alliance suggests that while the pan-theoretical theory allows for the basic measurement of the goals, task, and bond to produce beneficial therapeutic change, the ideal alliance profile is likely to be different across therapeutic approaches and interventions [ 15 , 16 , 18 ]. The findings may therefore indicate that the working alliance profile might differ in b-CBT. However, further research is needed to investigate this.
Finally, our finding that average and higher system usability ratings may strengthen the working alliance (especially the task subscale) may point to the digital programs’ influence on how the working alliance is experienced. This is not surprising given that CBT activities (eg, content and exercises) were primarily completed in the iCBT program and may indicate its relevance in the building of the working alliance and in supporting the task within a capacity that is potentially parallel to the bond. These findings partially test and support a conceptual framework of the working alliance that incorporates features that are derived from the digital program within a blended setting called “digital heuristics” (the promotion of active engagement and autonomous problem solving) in which “ease of use” and “interactivity” were identified as key features for optimizing “active engagement” with the task in the iCBT program [ 36 ]. These qualitative findings were mirrored in another study that tested the abovementioned framework, in which digital heuristics emerged as a fourth dimension when examining the working alliance in self-guided and low-intensity supported iCBT for depression [ 37 ]. High and low iCBT program functionalities were also identified by therapists as facilitators and barriers, in building the working alliance in bCBT in the German and UK country sites of the E-COMPARED trial [ 36 , 70 - 72 ]. Although our findings remain preliminary and do not show a causal effect, further investigation concerning the effect of the digital program on the working alliance may be a fruitful direction for future research.
Collectively, our findings suggest that blending face-to-face CBT with an iCBT program may enhance the working alliance and treatment outcomes for depression. These findings hold important implications for clinical practice, especially following the COVID-19 pandemic that resulted in major shifts from in-person care to blended health care provision. The findings of this study suggest that a blended approach may enhance rather than worsen mental health care. Our study’s findings regarding the interaction between system usability and the working alliance in terms of treatment outcomes represent a preliminary step to quantitively understand the influence of the digital program and its role in how the working alliance is experienced. While further research is required to explore digital taxonomies that contribute toward fostering the working alliance in bCBT, our findings build on previous qualitative research [ 29 , 34 , 36 , 68 ] to explore a conceptualization of the working alliance that goes beyond the client and the therapist in order to consider the role of the digital program. The impact of the digital program on the working alliance may support the case of employing digital navigators who can help clients to use the intervention and troubleshoot technology and program usability issues, and remove the added burden of managing program-related problems that would otherwise fall on the therapist [ 70 , 72 , 73 ].
We propose 4 directions for future research. First, future research is required to build a comprehensive understanding of what, how, and when digital features (eg, usage, interface, interactivity, and accessibility) influence the working alliance [ 36 ]. Second, psychometric scales measuring the working alliance in bCBT should be adapted or developed to conceptually reflect a construct that also incorporates the client-program working alliance [ 42 ]. Third, the working alliance should be investigated early in the intervention and across multiple stages of treatment [ 74 ]. Fourth, future research should investigate if our results can be replicated across different DMHIs and treatment dosages.
Limitations
Several study limitations should be noted. First, working alliance data were collected at a single point that corresponded with 3-month assessments. While this is common in clinical trials [ 25 , 58 ], the measurement of the alliance is recommended early in treatment within the first 5 sessions and at different points across treatment [ 74 - 77 ]. However, the number of face-to-face sessions varied between the 9 country sites (eg, 5 to 10 sessions), which would have posed significant challenges for the systematic data collection required in a clinical trial [ 54 ]. Second, the study engaged in multiple comparisons, which may have increased the risk of type 1 error (a positive result may be due to chance). However, given the exploratory nature of this analysis and the fact that different outcomes are likely to be highly correlated, a multiple adjustment comparison was not deemed necessary [ 78 ]. Third, the results of the analysis are valid under the MAR assumption, which we believe to be plausible because the effect of country sites appears to influence the missingness of the main outcome variables, stemming from country-specific data collection procedures and experiences. This is supported by chi-square analyses that indicate significantly higher rates of missing data for the PHQ-9 and WAI-SR-C across some countries compared to others. Nevertheless, it should be noted that this paper cannot rule out that data are missing not at random. Future research can explore this further using a sensitivity analysis. Fourth, the heterogeneity of interventions offered in the TAU group limits the study from conclusively tying causation to a specific comparator intervention. However, it should be noted that interventions offered by services in TAU were regarded as evidence-based, largely consisting of CBT and psychopharmacological interventions [ 35 ]. This may reduce the limitations associated with the multiple treatments offered in TAU [ 66 , 79 ] and adhering to the pragmatic trial’s ancillary objective to not impose specific constraints on clients and clinicians concerning data collection [ 79 ]. However, additional steps were also taken to address this limitation by conducting a subanalysis with a subset of trial country sites that only offered face-to-face CBT in TAU. The findings showed comparable results to those of the main analysis, highlighting that the addition of iCBT to face-to-face CBT may improve the quality of the working alliance. Fifth, another potential limitation is related to the variation in how bCBT was delivered across the trial’s country sites, concerning the number of sessions and the types of iCBT programs delivered, across different country sites. However, it should be noted that the study was focused on investigating the noninferiority of blending CBT given that there is a sufficient level of evidence concerning key treatment components, such as the CBT approach, and different delivery formats, including in-person and internet-based delivery of CBT for depression [ 80 , 81 ]. Although the number of treatment sessions varied between settings, to our knowledge, there is no evidence to suggest that the number of sessions of CBT effect the client-therapist alliance as the alliance is typically developed early in treatment and within the first 5 sessions [ 74 - 77 ]. Moreover, another study exploring the usage of different components of bCBT and treatment engagement when compared to intended use in the E-COMPARED study concluded that personalized blended care was more suitable compared to attempting to achieve a standardized optimal blend [ 69 ]. Variations in the number of treatment sessions described may enable a pragmatic understanding of the working alliance in bCBT interventions in real-world clinical settings [ 66 ].
Conclusions
To our knowledge, this is the first study to show that bCBT may enhance the working alliance when compared to routine care for depression and when compared to face-to-face CBT. The working alliance in bCBT was also associated with clinical improvements in depression, which appear to be enhanced by good program usability. Collectively, our findings appear to add further weight to the view that the addition of iCBT to face-to-face CBT may positively augment experiences of the working alliance.
Authors' Contributions
AD had full access to all of the data and takes full responsibility for the integrity of the data and the accuracy of the data analysis. AD, MQ, FS, RA, and CF contributed to the design concept. AD drafted the manuscript. AD, RA, MQ, FS, CF, RK, HR, AK, ACP, AvS, CB, TB, KC, MM, TK, JBH, SD, IT, NT, KM, KV, AU, GA, MB, and RMB critically revised the manuscript for important intellectual content. AD, MQ, RA, HR, FS, AK, RK, CF, ACP, and SD contributed to data acquisition, analysis, and interpretation. AD contributed to statistical analysis. HR, SD, AK, MB, and ACP provided administrative, technical, or material support. AD, RA, MQ, FS, RK, and CF supervised the study.
Conflicts of Interest
None declared.
Supplementary methods and results that include information on: Participants' country of birth, information on missing data, medians and IQR for working alliance, participant characteristics, depression and system usability scores, trial profile, and results of the sensitivity analysis and subgroup analysis.
CONSORT-eHEALTH checklist (V.1.6.1).
- GBD 2017 DiseaseInjury IncidencePrevalence Collaborators. Global, regional, and national incidence, prevalence, and years lived with disability for 354 diseases and injuries for 195 countries and territories, 1990-2017: a systematic analysis for the Global Burden of Disease Study 2017. Lancet. Nov 10, 2018;392(10159):1789-1858. [ FREE Full text ] [ CrossRef ] [ Medline ]
- Fact Sheet: Suicide. World Health Organization. URL: https://www.who.int/news-room/fact-sheets/detail/suicide [accessed 2024-03-24]
- Kohn R, Saxena S, Levav I, Saraceno B. The treatment gap in mental health care. Bull World Health Organ. Nov 2004;82(11):858-866. [ FREE Full text ] [ Medline ]
- Fairburn CG, Patel V. The impact of digital technology on psychological treatments and their dissemination. Behav Res Ther. Jan 2017;88:19-25. [ FREE Full text ] [ CrossRef ] [ Medline ]
- Torous J, Jän Myrick K, Rauseo-Ricupero N, Firth J. Digital Mental Health and COVID-19: Using Technology Today to Accelerate the Curve on Access and Quality Tomorrow. JMIR Ment Health. Mar 26, 2020;7(3):e18848. [ FREE Full text ] [ CrossRef ] [ Medline ]
- Kaltenthaler E, Parry G, Beverley C. Computerized Cognitive Behaviour Therapy: A Systematic Review. Behav. Cogn. Psychother. Feb 18, 2004;32(1):31-55. [ CrossRef ]
- Ruwaard J, Lange A, Schrieken B, Emmelkamp P. Efficacy and effectiveness of online cognitive behavioral treatment: a decade of interapy research. Stud Health Technol Inform. 2011;167:9-14. [ Medline ]
- Foroushani P, Schneider J, Assareh N. Meta-review of the effectiveness of computerised CBT in treating depression. BMC Psychiatry. Aug 12, 2011;11(1):131. [ FREE Full text ] [ CrossRef ] [ Medline ]
- Ivarsson D, Blom M, Hesser H, Carlbring P, Enderby P, Nordberg R, et al. Guided internet-delivered cognitive behavior therapy for post-traumatic stress disorder: A randomized controlled trial. Internet Interventions. Mar 2014;1(1):33-40. [ FREE Full text ] [ CrossRef ]
- Cuijpers P, Donker T, Johansson R, Mohr DC, van Straten A, Andersson G. Self-guided psychological treatment for depressive symptoms: a meta-analysis. PLoS One. Jun 21, 2011;6(6):e21274. [ FREE Full text ] [ CrossRef ] [ Medline ]
- Karyotaki E, Ebert DD, Donkin L, Riper H, Twisk J, Burger S, et al. Do guided internet-based interventions result in clinically relevant changes for patients with depression? An individual participant data meta-analysis. Clin Psychol Rev. Jul 2018;63:80-92. [ CrossRef ] [ Medline ]
- Andrews G, Basu A, Cuijpers P, Craske M, McEvoy P, English C, et al. Computer therapy for the anxiety and depression disorders is effective, acceptable and practical health care: An updated meta-analysis. J Anxiety Disord. Apr 2018;55:70-78. [ FREE Full text ] [ CrossRef ] [ Medline ]
- Josephine K, Josefine L, Philipp D, David E, Harald B. Internet- and mobile-based depression interventions for people with diagnosed depression: A systematic review and meta-analysis. J Affect Disord. Dec 01, 2017;223:28-40. [ CrossRef ] [ Medline ]
- Erbe D, Eichert H, Riper H, Ebert D. Blending Face-to-Face and Internet-Based Interventions for the Treatment of Mental Disorders in Adults: Systematic Review. J Med Internet Res. Sep 15, 2017;19(9):e306. [ FREE Full text ] [ CrossRef ] [ Medline ]
- Bordin ES. The generalizability of the psychoanalytic concept of the working alliance. Psychotherapy: Theory, Research & Practice. 1979;16(3):252-260. [ CrossRef ]
- Bordin ES. Theory and research on the therapeutic working alliance: New directions. In: Horvath AO, Greenberg LS, editors. The working alliance: Theory, research, and practice. New York, NY. John Wiley & Sons; 1994:13-37.
- Raue P, Goldfried M. The therapeutic alliance in cognitive-behavior therapy. In: Horvath AO, Greenberg LS, editors. The working alliance: Theory, research, and practice. New York, NY. John Wiley & Sons; 1994:131-152.
- Lambert MJ. Psychotherapy outcome research: Implications for integrative and eclectical therapists. In: Norcross JC, Goldfried MR, editors. Handbook of psychotherapy integration. New York, NY. Basic Books; 1992:94-129.
- Norcross JC, Lambert MJ. Psychotherapy relationships that work II. Psychotherapy (Chic). Mar 2011;48(1):4-8. [ CrossRef ] [ Medline ]
- Cameron S, Rodgers J, Dagnan D. The relationship between the therapeutic alliance and clinical outcomes in cognitive behaviour therapy for adults with depression: A meta-analytic review. Clin Psychol Psychother. May 2018;25(3):446-456. [ CrossRef ] [ Medline ]
- Pihlaja S, Stenberg J, Joutsenniemi K, Mehik H, Ritola V, Joffe G. Therapeutic alliance in guided internet therapy programs for depression and anxiety disorders - A systematic review. Internet Interv. Mar 2018;11:1-10. [ FREE Full text ] [ CrossRef ] [ Medline ]
- Gómez Penedo J, Berger T, Grosse Holtforth M, Krieger T, Schröder J, Hohagen F, et al. The Working Alliance Inventory for guided Internet interventions (WAI-I). J Clin Psychol. Jun 2020;76(6):973-986. [ CrossRef ] [ Medline ]
- Heim E, Rötger A, Lorenz N, Maercker A. Working alliance with an avatar: How far can we go with internet interventions? Internet Interv. Mar 1, 2018;11:41-46. [ FREE Full text ] [ CrossRef ] [ Medline ]
- Henson P, Wisniewski H, Hollis C, Keshavan M, Torous J. Digital mental health apps and the therapeutic alliance: initial review. BJPsych Open. Jan 2019;5(1):e15. [ FREE Full text ] [ CrossRef ] [ Medline ]
- Sucala M, Schnur JB, Constantino MJ, Miller SJ, Brackman EH, Montgomery GH. The therapeutic relationship in e-therapy for mental health: a systematic review. J Med Internet Res. Aug 02, 2012;14(4):e110. [ FREE Full text ] [ CrossRef ] [ Medline ]
- Berger T. The therapeutic alliance in internet interventions: A narrative review and suggestions for future research. Psychother Res. Sep 2017;27(5):511-524. [ CrossRef ] [ Medline ]
- Wehmann E, Köhnen M, Härter M, Liebherz S. Therapeutic Alliance in Technology-Based Interventions for the Treatment of Depression: Systematic Review. J Med Internet Res. Jun 11, 2020;22(6):e17195. [ FREE Full text ] [ CrossRef ] [ Medline ]
- Hayati R, Bastani P, Kabir M, Kavosi Z, Sobhani G. Scoping literature review on the basic health benefit package and its determinant criteria. Global Health. Mar 02, 2018;14(1):26. [ FREE Full text ] [ CrossRef ] [ Medline ]
- Tremain H, McEnery C, Fletcher K, Murray G. The Therapeutic Alliance in Digital Mental Health Interventions for Serious Mental Illnesses: Narrative Review. JMIR Ment Health. Aug 07, 2020;7(8):e17204. [ FREE Full text ] [ CrossRef ] [ Medline ]
- Vernmark K, Hesser H, Topooco N, Berger T, Riper H, Luuk L, et al. Working alliance as a predictor of change in depression during blended cognitive behaviour therapy. Cogn Behav Ther. Jul 2019;48(4):285-299. [ FREE Full text ] [ CrossRef ] [ Medline ]
- Kooistra L, Ruwaard J, Wiersma J, van Oppen P, Riper H. Working Alliance in Blended Versus Face-to-Face Cognitive Behavioral Treatment for Patients with Depression in Specialized Mental Health Care. J Clin Med. Jan 27, 2020;9(2):347. [ FREE Full text ] [ CrossRef ] [ Medline ]
- Askjer S, Mathiasen K. The working alliance in blended versus face-to-face cognitive therapy for depression: A secondary analysis of a randomized controlled trial. Internet Interv. Sep 2021;25:100404. [ FREE Full text ] [ CrossRef ] [ Medline ]
- Barazzone N, Cavanagh K, Richards D. Computerized cognitive behavioural therapy and the therapeutic alliance: a qualitative enquiry. Br J Clin Psychol. Nov 2012;51(4):396-417. [ CrossRef ] [ Medline ]
- Clarke J, Proudfoot J, Whitton A, Birch M, Boyd M, Parker G, et al. Therapeutic Alliance With a Fully Automated Mobile Phone and Web-Based Intervention: Secondary Analysis of a Randomized Controlled Trial. JMIR Ment Health. Feb 25, 2016;3(1):e10. [ FREE Full text ] [ CrossRef ] [ Medline ]
- Kleiboer A, Smit J, Bosmans J, Ruwaard J, Andersson G, Topooco N, et al. European COMPARative Effectiveness research on blended Depression treatment versus treatment-as-usual (E-COMPARED): study protocol for a randomized controlled, non-inferiority trial in eight European countries. Trials. Aug 03, 2016;17(1):387. [ FREE Full text ] [ CrossRef ] [ Medline ]
- Doukani A, Free C, Michelson D, Araya R, Montero-Marin J, Smith S, et al. Towards a conceptual framework of the working alliance in a blended low-intensity cognitive behavioural therapy intervention for depression in primary mental health care: a qualitative study. BMJ Open. Sep 23, 2020;10(9):e036299. [ FREE Full text ] [ CrossRef ] [ Medline ]
- Barceló-Soler A, García-Campayo J, Araya R, Doukani A, Gili M, García-Palacios A, et al. Working alliance in low-intensity internet-based cognitive behavioral therapy for depression in primary care in Spain: A qualitative study. Front Psychol. 2023;14:1024966. [ FREE Full text ] [ CrossRef ] [ Medline ]
- Mathiasen K, Andersen TE, Riper H, Kleiboer AAM, Roessler KK. Blended CBT versus face-to-face CBT: a randomised non-inferiority trial. BMC Psychiatry. Dec 05, 2016;16(1):432. [ FREE Full text ] [ CrossRef ] [ Medline ]
- Kroenke K, Spitzer RL, Williams JBW. The PHQ-9: validity of a brief depression severity measure. J Gen Intern Med. Sep 2001;16(9):606-613. [ FREE Full text ] [ CrossRef ] [ Medline ]
- Lecrubier Y, Sheehan D, Weiller E, Amorim P, Bonora I, Sheehan KH, et al. The Mini International Neuropsychiatric Interview (MINI). A short diagnostic structured interview: reliability and validity according to the CIDI. Eur. psychiatr. Apr 16, 2020;12(5):224-231. [ CrossRef ]
- van der Vaart R, Witting M, Riper H, Kooistra L, Bohlmeijer E, van Gemert-Pijnen L. Blending online therapy into regular face-to-face therapy for depression: content, ratio and preconditions according to patients and therapists using a Delphi study. BMC Psychiatry. Dec 14, 2014;14:355. [ FREE Full text ] [ CrossRef ] [ Medline ]
- Herrero R, Vara M, Miragall M, Botella C, García-Palacios A, Riper H, et al. Working Alliance Inventory for Online Interventions-Short Form (WAI-TECH-SF): The Role of the Therapeutic Alliance between Patient and Online Program in Therapeutic Outcomes. Int J Environ Res Public Health. Aug 25, 2020;17(17):6169. [ FREE Full text ] [ CrossRef ] [ Medline ]
- Sheehan D, Lecrubier Y, Harnett Sheehan K, Janavs J, Weiller E, Keskiner A, et al. The validity of the Mini International Neuropsychiatric Interview (MINI) according to the SCID-P and its reliability. European Psychiatry. 1997;12(5):232-241. [ FREE Full text ] [ CrossRef ]
- Rohde P, Lewinsohn PM, Seeley JR. Comparability of telephone and face-to-face interviews in assessing axis I and II disorders. Am J Psychiatry. Nov 1997;154(11):1593-1598. [ CrossRef ] [ Medline ]
- Ruskin PE, Reed S, Kumar R, Kling MA, Siegel E, Rosen M, et al. Reliability and acceptability of psychiatric diagnosis via telecommunication and audiovisual technology. Psychiatr Serv. Aug 1998;49(8):1086-1088. [ CrossRef ] [ Medline ]
- Horvath AO, Greenberg LS. Development and validation of the Working Alliance Inventory. Journal of Counseling Psychology. 1989;36(2):223-233. [ CrossRef ]
- Cahill J, Barkham M, Hardy G, Gilbody S, Richards D, Bower P, et al. A review and critical appraisal of measures of therapist-patient interactions in mental health settings. Health Technol Assess. Jun 2008;12(24):iii, ix-iii, 47. [ FREE Full text ] [ CrossRef ] [ Medline ]
- Fenton L, Cecero J, Nich C, Frankforter T, Carroll K. Perspective is everything: the predictive validity of six working alliance instruments. J Psychother Pract Res. 2001;10(4):262-268. [ FREE Full text ] [ Medline ]
- Luborsky L, Barber J, Siqueland L, Johnson S, Najavits L, Frank A, et al. The Revised Helping Alliance Questionnaire (HAq-II) : Psychometric Properties. J Psychother Pract Res. 1996;5(3):260-271. [ FREE Full text ] [ Medline ]
- Wittkampf KA, Naeije L, Schene AH, Huyser J, van Weert HC. Diagnostic accuracy of the mood module of the Patient Health Questionnaire: a systematic review. Gen Hosp Psychiatry. Sep 2007;29(5):388-395. [ CrossRef ] [ Medline ]
- Brooke J. SUS: A 'Quick and Dirty' Usability Scale. In: Jordan PW, Thomas B, McClelland IL, Weerdmeester B, editors. Usability Evaluation In Industry. London, UK. CRC Press; 1996.
- Bangor A, Kortum PT, Miller JT. An Empirical Evaluation of the System Usability Scale. International Journal of Human-Computer Interaction. Jul 30, 2008;24(6):574-594. [ CrossRef ]
- Mol M, van Schaik A, Dozeman E, Ruwaard J, Vis C, Ebert D, et al. Dimensionality of the system usability scale among professionals using internet-based interventions for depression: a confirmatory factor analysis. BMC Psychiatry. May 12, 2020;20(1):218. [ FREE Full text ] [ CrossRef ] [ Medline ]
- Ranganathan P, Pramesh C, Aggarwal R. Common pitfalls in statistical analysis: Intention-to-treat versus per-protocol analysis. Perspect Clin Res. 2016;7(3):144-146. [ FREE Full text ] [ CrossRef ] [ Medline ]
- IBM SPSS Statistics 26. IBM Corp. URL: https://www.ibm.com/support/pages/ibm-spss-statistics-26-documentation [accessed 2024-03-24]
- Stata Statistical Software: Release 16. Stata Corp. URL: https://www.scirp.org/reference/referencespapers?referenceid=2757660 [accessed 2024-03-24]
- Hayes AF. Introduction to Mediation, Moderation, and Conditional Process Analysis (Second Edition): A Regression-Based Approach. New York, NY. Guilford Press; 2017.
- Kirkwood BR, Stern JAC. Essential Medical Statistics, 2nd Edition. Hoboken, NJ. Wiley; 2003.
- Carpenter JR, Kenward MG. Multiple Imputation and its Application. New York, NY. John Wiley & Sons; 2013.
- von Hippel PT. 8. How to Impute Interactions, Squares, and other Transformed Variables. Sociological Methodology. Aug 01, 2009;39(1):265-291. [ FREE Full text ] [ CrossRef ]
- de Souza R, Eisen R, Perera S, Bantoto B, Bawor M, Dennis B, et al. Best (but oft-forgotten) practices: sensitivity analyses in randomized controlled trials. Am J Clin Nutr. Jan 2016;103(1):5-17. [ FREE Full text ] [ CrossRef ] [ Medline ]
- Thabane L, Mbuagbaw L, Zhang S, Samaan Z, Marcucci M, Ye C, et al. A tutorial on sensitivity analyses in clinical trials: the what, why, when and how. BMC Med Res Methodol. Jul 16, 2013;13:92. [ FREE Full text ] [ CrossRef ] [ Medline ]
- Parpia S, Morris T, Phillips M, Wykoff C, Steel D, Thabane L, et al. Retina Evidence Trials InterNational Alliance (R.E.T.I.N.A.) Study Group. Sensitivity analysis in clinical trials: three criteria for a valid sensitivity analysis. Eye (Lond). Nov 2022;36(11):2073-2074. [ FREE Full text ] [ CrossRef ] [ Medline ]
- Morris TP, Kahan BC, White IR. Choosing sensitivity analyses for randomised trials: principles. BMC Med Res Methodol. Jan 24, 2014;14(1):11. [ FREE Full text ] [ CrossRef ] [ Medline ]
- Burke J, Sussman J, Kent D, Hayward R. Three simple rules to ensure reasonably credible subgroup analyses. BMJ. Nov 04, 2015;351:h5651. [ FREE Full text ] [ CrossRef ] [ Medline ]
- Dawson L, Zarin D, Emanuel E, Friedman L, Chaudhari B, Goodman S. Considering usual medical care in clinical trial design. PLoS Med. Sep 2009;6(9):e1000111. [ FREE Full text ] [ CrossRef ] [ Medline ]
- Farrokhyar F, Skorzewski P, Phillips M, Garg S, Sarraf D, Thabane L, et al. Retina Evidence Trials InterNational Alliance (R.E.T.I.N.A.) Study Group. When to believe a subgroup analysis: revisiting the 11 criteria. Eye (Lond). Nov 2022;36(11):2075-2077. [ FREE Full text ] [ CrossRef ] [ Medline ]
- Doukani A, Free C, Araya R, Michelson D, Cerga-Pashoja A, Kakuma BR. Practitioners' experience of the working alliance in a blended cognitive-behavioural therapy intervention for depression: qualitative study of barriers and facilitators. BJPsych Open. Jul 25, 2022;8(4):e142. [ FREE Full text ] [ CrossRef ] [ Medline ]
- Kemmeren LL, van Schaik A, Smit JH, Ruwaard J, Rocha A, Henriques M, et al. Unraveling the Black Box: Exploring Usage Patterns of a Blended Treatment for Depression in a Multicenter Study. JMIR Ment Health. Jul 25, 2019;6(7):e12707. [ FREE Full text ] [ CrossRef ] [ Medline ]
- Titzler I, Saruhanjan K, Berking M, Riper H, Ebert D. Barriers and facilitators for the implementation of blended psychotherapy for depression: A qualitative pilot study of therapists' perspective. Internet Interv. Jun 2018;12:150-164. [ FREE Full text ] [ CrossRef ] [ Medline ]
- Titzler I, Berking M, Schlicker S, Riper H, Ebert D. Barriers and Facilitators for Referrals of Primary Care Patients to Blended Internet-Based Psychotherapy for Depression: Mixed Methods Study of General Practitioners' Views. JMIR Ment Health. Aug 18, 2020;7(8):e18642. [ FREE Full text ] [ CrossRef ] [ Medline ]
- Cerga-Pashoja A, Doukani A, Gega L, Walke J, Araya R. Added value or added burden? A qualitative investigation of blending internet self-help with face-to-face cognitive behaviour therapy for depression. Psychother Res. Nov 05, 2020;30(8):998-1010. [ CrossRef ] [ Medline ]
- Wisniewski H, Torous J. Digital navigators to implement smartphone and digital tools in care. Acta Psychiatr Scand. Apr 2020;141(4):350-355. [ FREE Full text ] [ CrossRef ] [ Medline ]
- Crits-Christoph P, Gibbons MBC, Hamilton J, Ring-Kurtz S, Gallop R. The dependability of alliance assessments: the alliance-outcome correlation is larger than you might think. J Consult Clin Psychol. Jun 2011;79(3):267-278. [ FREE Full text ] [ CrossRef ] [ Medline ]
- Piper W, Azim H, Joyce A, McCallum M. Transference interpretations, therapeutic alliance, and outcome in short-term individual psychotherapy. Arch Gen Psychiatry. Oct 1991;48(10):946-953. [ FREE Full text ] [ CrossRef ] [ Medline ]
- Eames V, Roth A. Patient attachment orientation and the early working alliance-a study of patient and therapist reports of alliance quality and ruptures. Psychother Res. Dec 23, 2000;10(4):421-434. [ CrossRef ] [ Medline ]
- Norcross JC, Wampold BE. Evidence-based therapy relationships: research conclusions and clinical practices. Psychotherapy (Chic). Mar 2011;48(1):98-102. [ CrossRef ] [ Medline ]
- Rothman K. No Adjustments Are Needed for Multiple Comparisons. Epidemiology. 1990;1(1):43-46. [ FREE Full text ] [ CrossRef ]
- Giraudeau B, Caille A, Eldridge S, Weijer C, Zwarenstein M, Taljaard M. Heterogeneity in pragmatic randomised trials: sources and management. BMC Med. Oct 28, 2022;20(1):372. [ FREE Full text ] [ CrossRef ] [ Medline ]
- Karyotaki E, Efthimiou O, Miguel C, Bermpohl F, Furukawa T, Cuijpers P, Individual Patient Data Meta-Analyses for Depression (IPDMA-DE) Collaboration, et al. Internet-Based Cognitive Behavioral Therapy for Depression: A Systematic Review and Individual Patient Data Network Meta-analysis. JAMA Psychiatry. Apr 01, 2021;78(4):361-371. [ FREE Full text ] [ CrossRef ] [ Medline ]
- Kambeitz-Ilankovic L, Rzayeva U, Völkel L, Wenzel J, Weiske J, Jessen F, et al. A systematic review of digital and face-to-face cognitive behavioral therapy for depression. NPJ Digit Med. Sep 15, 2022;5(1):144. [ FREE Full text ] [ CrossRef ] [ Medline ]
Abbreviations
Edited by Y Hong; submitted 29.03.23; peer-reviewed by A AL-Asadi, A González-Robles; comments to author 26.01.24; revised version received 09.02.24; accepted 11.02.24; published 31.05.24.
©Asmae Doukani, Matteo Quartagno, Francesco Sera, Caroline Free, Ritsuko Kakuma, Heleen Riper, Annet Kleiboer, Arlinda Cerga-Pashoja, Anneke van Schaik, Cristina Botella, Thomas Berger, Karine Chevreul, Maria Matynia, Tobias Krieger, Jean-Baptiste Hazo, Stasja Draisma, Ingrid Titzler, Naira Topooco, Kim Mathiasen, Kristofer Vernmark, Antoine Urech, Anna Maj, Gerhard Andersson, Matthias Berking, Rosa María Baños, Ricardo Araya. Originally published in the Journal of Medical Internet Research (https://www.jmir.org), 31.05.2024.
This is an open-access article distributed under the terms of the Creative Commons Attribution License (https://creativecommons.org/licenses/by/4.0/), which permits unrestricted use, distribution, and reproduction in any medium, provided the original work, first published in the Journal of Medical Internet Research, is properly cited. The complete bibliographic information, a link to the original publication on https://www.jmir.org/, as well as this copyright and license information must be included.
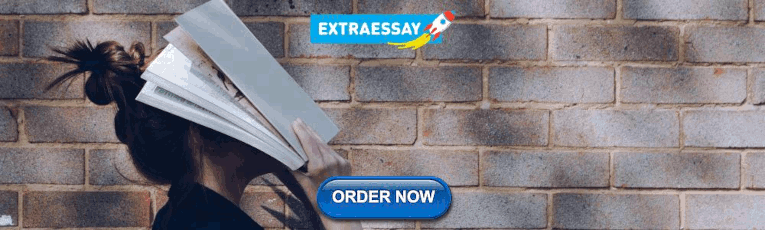
IMAGES
VIDEO
COMMENTS
Assessments follow the analysis process, usually focused more on observations and one-on-one meetings with employees or groups, where they can gain direct feedback and insight on their performance. Typically, analysis requires more thought as managers attempt to quantify performance while assessment focuses on establishing the final outcome.
It involves gathering information, measuring performance, and making judgments. While analysis is more focused on understanding, assessment is more concerned with evaluating and making informed decisions. Both processes are crucial in decision-making and problem-solving, but they serve different purposes in the evaluation and research process ...
Mistake #2: Failing To Recognize The Scope Of Each Term. Another common mistake is overlooking the differing scopes of "assess" and "analysis.". While "assess" typically involves a broader evaluation or estimation, "analysis" focuses on a more detailed and systematic examination.
"Assessment is a multi-stage, multi-dimensional process - a vehicle - for bringing clarity and balance to an individual activity or set of activities." Assessment, Evaluation, and Research - Similarities with Distinctions Upcraft and Schuh (2001) distinguish between assessment and research, noting that research guides theory
Introduction. Statistical analysis is necessary for any research project seeking to make quantitative conclusions. The following is a primer for research-based statistical analysis. It is intended to be a high-level overview of appropriate statistical testing, while not diving too deep into any specific methodology.
There is a difference between an analysis and an assessment. I don't know that I have always used these two words properly and, while it might sound minor or like just a semantic difference, the words do have specific and very different meanings. According to the Merriam-Webster Dictionary, an "analysis" is defined as the careful study of something to learn about its parts, what they do ...
Analyze and assess are two terms commonly used in research, evaluation, and decision-making processes. While they share similarities, they have distinct differences. ... or significance of something. It involves making judgments or drawing conclusions based on the analysis conducted. In summary, analyze is about understanding the components ...
Assessment can provide reasonably accurate information to the people who need it, in a complex, changing environment. The timing of research and assessment may differ. Research may have more flexibility in the time it takes for data collection because it may not be tied to one particular program, service, or experience that will change ...
Bibliometric analysis helps governments to number and rank researchers, making them easier to compare. ... Many other countries apply formal research assessment systems to universities and have ...
And trying to adhere to the standards of research may get in the way of doing effective assessment. Get full access to this article View all access and purchase options for this article.
The Dynamics of Analysis and Assessment Unveiling the Process of Analysis. Analysis involves breaking down complex information into simpler components, facilitating a detailed understanding. In decision-making, this often comes as the initial step, providing a foundation for subsequent assessments. Navigating the Terrain of Assessment
1. Introduction, what is meant by impact? When considering the impact that is generated as a result of research, a number of authors and government recommendations have advised that a clear definition of impact is required (Duryea, Hochman, and Parfitt 2007; Grant et al. 2009; Russell Group 2009).From the outset, we note that the understanding of the term impact differs between users and ...
Assessment, measurement, research, and evaluation are part of the processes of science and issues related to each topic often overlap. Assessment refers to the collection of data to describe or better understand an issue. This can involve qualitative data (words and/or pictures) or quantitative data (numbers).
Goals. The goals of experimental research and program assessment differ significantly. While research focuses on the creation of new knowledge, testing an experimental hypothesis, or documenting new knowledge, assessment and evaluation focus on program accountability, program management, or decision-making and budgeting.
See our resource on analysis and synthesis (Move From Research to Writing: How to Think) for other examples of questions to ask. Form an assessment. The questions you asked in the last step should lead you to form an assessment. Here are some assessment/opinion words that might help you build your critique and evaluation: illogical; helpful
On the basis of an initial review of a larger set of decision-making techniques, we have selected three analytical approaches as most applicable to the needs for prospective and retrospective assessment as defined by BSR: Bibliometric analysis of the results of research and the connections among research efforts, reputational studies (such as ...
Thematic analysis is a research method used to identify and interpret patterns or themes in a data set; it often leads to new insights and understanding (Boyatzis, 1998; Elliott, 2018; Thomas, 2006).However, it is critical that researchers avoid letting their own preconceptions interfere with the identification of key themes (Morse & Mitcham, 2002; Patton, 2015).
2.1 Step 1: defining the research question. The first step in conducting a meta-analysis, as with any other empirical study, is the definition of the research question. Most importantly, the research question determines the realm of constructs to be considered or the type of interventions whose effects shall be analyzed.
It is easy to confuse systematic reviews and meta-analyses. A systematic review is an objective, reproducible method to find answers to a certain research question, by collecting all available studies related to that question and reviewing and analyzing their results. A meta-analysis differs from a systematic review in that it uses statistical ...
In this discussion, assessment is compared and contrasted with research to explain how these forms of inquiry differ though methodology is similar. (Author) Descriptors: Evaluation , Higher Education , Measurement Techniques , Qualitative Research , Research and Development , Research Methodology , Statistical Analysis , Theory Practice ...
13.1 Introduction. This chapter explores the concept of risk-benefit analysis in health research regulation, as well as ethical and practical questions raised by identifying, quantifying, and weighing risks and benefits. It argues that the pursuit of objectivity in risk-benefit analysis is ultimately futile, as the very concepts of risk and ...
When collecting and analyzing data, quantitative research deals with numbers and statistics, while qualitative research deals with words and meanings. Both are important for gaining different kinds of knowledge. Quantitative research. Quantitative research is expressed in numbers and graphs. It is used to test or confirm theories and assumptions.
The purpose of assessment is formative, i.e. to increase quality whereas. evaluation is all about judging quality, therefore the purpose is summative. 5. Assessment is concerned with process ...
The Risk Analysis Index (RAI) represents a robust metric based on the deficit accumulation frailty model, reliably projecting short-term and long-term mortality in both surgical and nonsurgical adult populations. 9 Initially developed and prospectively validated as a 14-item questionnaire, the RAI is the only frailty assessment proven feasible ...
In Proceedings of the 2023 Symposium on Eye Tracking Research and Applications. 1-7. Google Scholar Digital Library; Kerstin Gidlöf, Annika Wallin, Richard Dewhurst, and Kenneth Holmqvist. 2013. Using eye tracking to trace a cognitive process: Gaze behaviour during decision making in a natural environment. Journal of eye movement research 6 ...
Science is the foundation. EPA is one of the world's leading environmental and human health research organizations. The Office of Research and Development is EPA's scientific research arm. On this page you can access our products, tools, and events, and learn about grant and job opportunities.
The research aims to determine differences in attention allocation between high- and low-ability participants during programming problem-solving. Utilizing the TS-AOI method, findings show that high-ability participants maintain more focused attention and demonstrate shifts in attention from the early to the late phases of tasks, unlike their ...
This study outlines the assessment of the cyber-physical system SPERTA, which was designed to evaluate the real-time performance of Taekwondo athletes. The system conducts performance analyses focusing on speed, acceleration, strength, and identifying and quantifying the athlete's movements. The research involved administering an online questionnaire to athletes and coaches to evaluate the ...
This new and growing body of research includes several reviews and meta-studies, which show that personality traits play an important role in both career success and entrepreneurship 15,16,17,18 ...
Methods: We conducted a secondary data analysis of the E-COMPARED (European Comparative Effectiveness Research on Blended Depression Treatment versus Treatment-as-usual) trial, which compared bCBT with TAU across 9 European countries. Data were collected in primary care and specialized services between April 2015 and December 2017.