Qualitative case study data analysis: an example from practice
Affiliation.
- 1 School of Nursing and Midwifery, National University of Ireland, Galway, Republic of Ireland.
- PMID: 25976531
- DOI: 10.7748/nr.22.5.8.e1307
Aim: To illustrate an approach to data analysis in qualitative case study methodology.
Background: There is often little detail in case study research about how data were analysed. However, it is important that comprehensive analysis procedures are used because there are often large sets of data from multiple sources of evidence. Furthermore, the ability to describe in detail how the analysis was conducted ensures rigour in reporting qualitative research.
Data sources: The research example used is a multiple case study that explored the role of the clinical skills laboratory in preparing students for the real world of practice. Data analysis was conducted using a framework guided by the four stages of analysis outlined by Morse ( 1994 ): comprehending, synthesising, theorising and recontextualising. The specific strategies for analysis in these stages centred on the work of Miles and Huberman ( 1994 ), which has been successfully used in case study research. The data were managed using NVivo software.
Review methods: Literature examining qualitative data analysis was reviewed and strategies illustrated by the case study example provided. Discussion Each stage of the analysis framework is described with illustration from the research example for the purpose of highlighting the benefits of a systematic approach to handling large data sets from multiple sources.
Conclusion: By providing an example of how each stage of the analysis was conducted, it is hoped that researchers will be able to consider the benefits of such an approach to their own case study analysis.
Implications for research/practice: This paper illustrates specific strategies that can be employed when conducting data analysis in case study research and other qualitative research designs.
Keywords: Case study data analysis; case study research methodology; clinical skills research; qualitative case study methodology; qualitative data analysis; qualitative research.
- Case-Control Studies*
- Data Interpretation, Statistical*
- Nursing Research / methods*
- Qualitative Research*
- Research Design
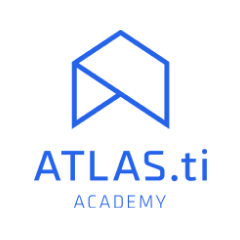
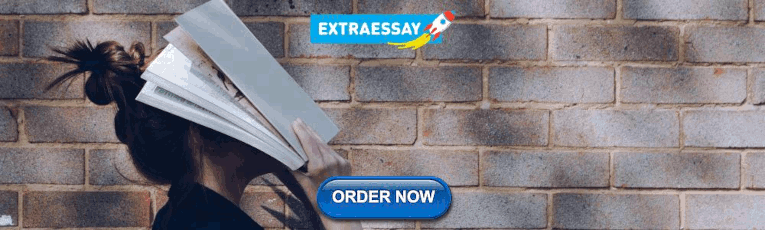
The Ultimate Guide to Qualitative Research - Part 1: The Basics
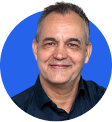
- Introduction and overview
- What is qualitative research?
- What is qualitative data?
- Examples of qualitative data
- Qualitative vs. quantitative research
- Mixed methods
- Qualitative research preparation
- Theoretical perspective
- Theoretical framework
- Literature reviews
Research question
- Conceptual framework
- Conceptual vs. theoretical framework
Data collection
- Qualitative research methods
- Focus groups
- Observational research
What is a case study?
Applications for case study research, what is a good case study, process of case study design, benefits and limitations of case studies.
- Ethnographical research
- Ethical considerations
- Confidentiality and privacy
- Power dynamics
- Reflexivity
Case studies
Case studies are essential to qualitative research , offering a lens through which researchers can investigate complex phenomena within their real-life contexts. This chapter explores the concept, purpose, applications, examples, and types of case studies and provides guidance on how to conduct case study research effectively.
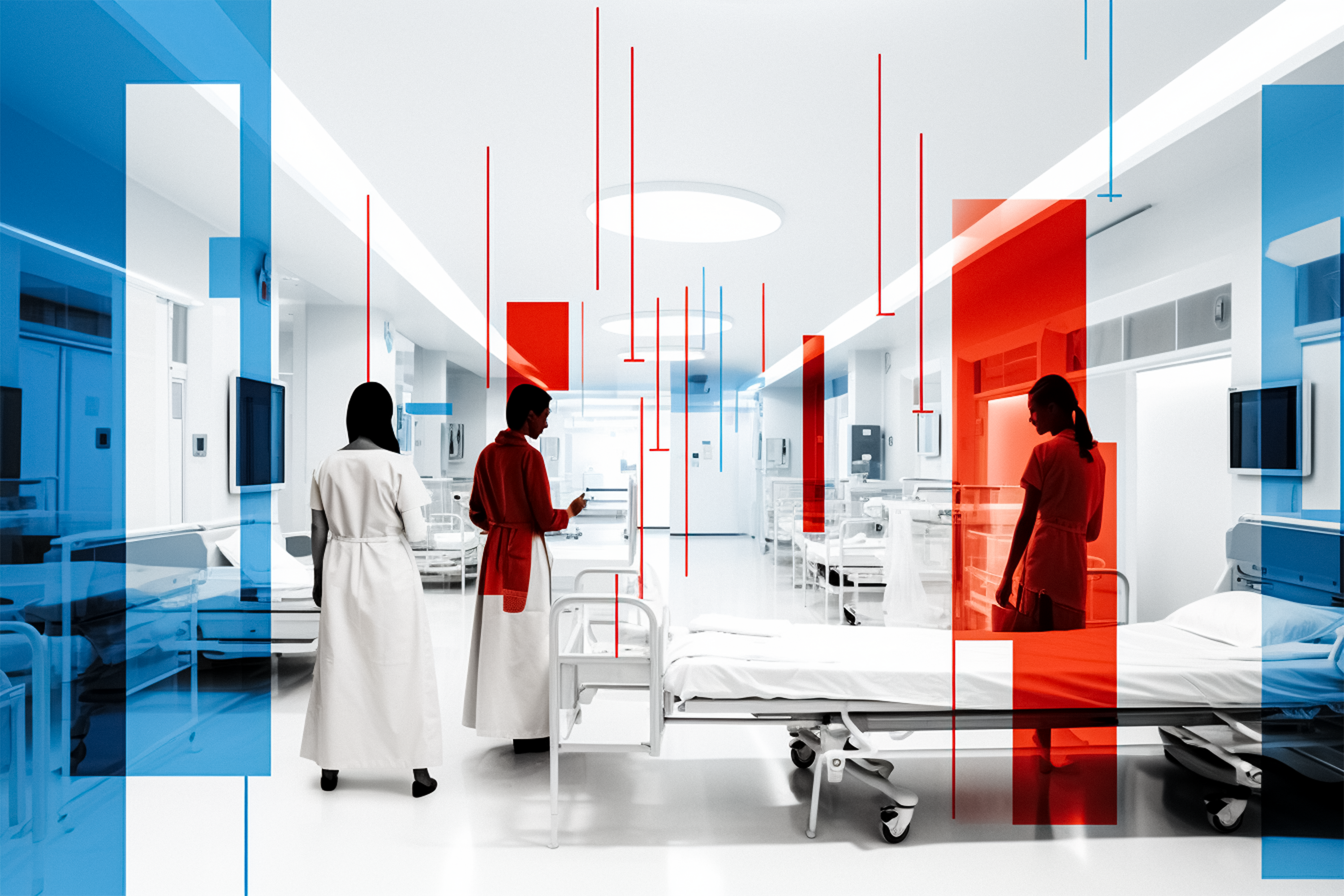
Whereas quantitative methods look at phenomena at scale, case study research looks at a concept or phenomenon in considerable detail. While analyzing a single case can help understand one perspective regarding the object of research inquiry, analyzing multiple cases can help obtain a more holistic sense of the topic or issue. Let's provide a basic definition of a case study, then explore its characteristics and role in the qualitative research process.
Definition of a case study
A case study in qualitative research is a strategy of inquiry that involves an in-depth investigation of a phenomenon within its real-world context. It provides researchers with the opportunity to acquire an in-depth understanding of intricate details that might not be as apparent or accessible through other methods of research. The specific case or cases being studied can be a single person, group, or organization – demarcating what constitutes a relevant case worth studying depends on the researcher and their research question .
Among qualitative research methods , a case study relies on multiple sources of evidence, such as documents, artifacts, interviews , or observations , to present a complete and nuanced understanding of the phenomenon under investigation. The objective is to illuminate the readers' understanding of the phenomenon beyond its abstract statistical or theoretical explanations.
Characteristics of case studies
Case studies typically possess a number of distinct characteristics that set them apart from other research methods. These characteristics include a focus on holistic description and explanation, flexibility in the design and data collection methods, reliance on multiple sources of evidence, and emphasis on the context in which the phenomenon occurs.
Furthermore, case studies can often involve a longitudinal examination of the case, meaning they study the case over a period of time. These characteristics allow case studies to yield comprehensive, in-depth, and richly contextualized insights about the phenomenon of interest.
The role of case studies in research
Case studies hold a unique position in the broader landscape of research methods aimed at theory development. They are instrumental when the primary research interest is to gain an intensive, detailed understanding of a phenomenon in its real-life context.
In addition, case studies can serve different purposes within research - they can be used for exploratory, descriptive, or explanatory purposes, depending on the research question and objectives. This flexibility and depth make case studies a valuable tool in the toolkit of qualitative researchers.
Remember, a well-conducted case study can offer a rich, insightful contribution to both academic and practical knowledge through theory development or theory verification, thus enhancing our understanding of complex phenomena in their real-world contexts.
What is the purpose of a case study?
Case study research aims for a more comprehensive understanding of phenomena, requiring various research methods to gather information for qualitative analysis . Ultimately, a case study can allow the researcher to gain insight into a particular object of inquiry and develop a theoretical framework relevant to the research inquiry.
Why use case studies in qualitative research?
Using case studies as a research strategy depends mainly on the nature of the research question and the researcher's access to the data.
Conducting case study research provides a level of detail and contextual richness that other research methods might not offer. They are beneficial when there's a need to understand complex social phenomena within their natural contexts.
The explanatory, exploratory, and descriptive roles of case studies
Case studies can take on various roles depending on the research objectives. They can be exploratory when the research aims to discover new phenomena or define new research questions; they are descriptive when the objective is to depict a phenomenon within its context in a detailed manner; and they can be explanatory if the goal is to understand specific relationships within the studied context. Thus, the versatility of case studies allows researchers to approach their topic from different angles, offering multiple ways to uncover and interpret the data .
The impact of case studies on knowledge development
Case studies play a significant role in knowledge development across various disciplines. Analysis of cases provides an avenue for researchers to explore phenomena within their context based on the collected data.
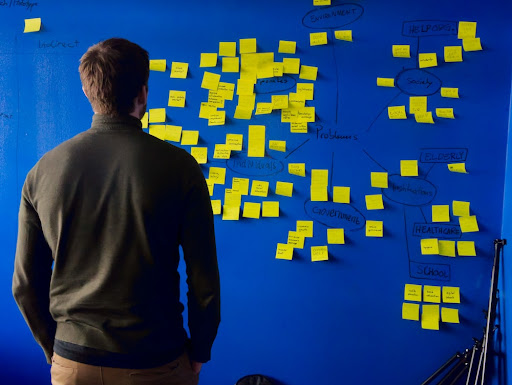
This can result in the production of rich, practical insights that can be instrumental in both theory-building and practice. Case studies allow researchers to delve into the intricacies and complexities of real-life situations, uncovering insights that might otherwise remain hidden.
Types of case studies
In qualitative research , a case study is not a one-size-fits-all approach. Depending on the nature of the research question and the specific objectives of the study, researchers might choose to use different types of case studies. These types differ in their focus, methodology, and the level of detail they provide about the phenomenon under investigation.
Understanding these types is crucial for selecting the most appropriate approach for your research project and effectively achieving your research goals. Let's briefly look at the main types of case studies.
Exploratory case studies
Exploratory case studies are typically conducted to develop a theory or framework around an understudied phenomenon. They can also serve as a precursor to a larger-scale research project. Exploratory case studies are useful when a researcher wants to identify the key issues or questions which can spur more extensive study or be used to develop propositions for further research. These case studies are characterized by flexibility, allowing researchers to explore various aspects of a phenomenon as they emerge, which can also form the foundation for subsequent studies.
Descriptive case studies
Descriptive case studies aim to provide a complete and accurate representation of a phenomenon or event within its context. These case studies are often based on an established theoretical framework, which guides how data is collected and analyzed. The researcher is concerned with describing the phenomenon in detail, as it occurs naturally, without trying to influence or manipulate it.
Explanatory case studies
Explanatory case studies are focused on explanation - they seek to clarify how or why certain phenomena occur. Often used in complex, real-life situations, they can be particularly valuable in clarifying causal relationships among concepts and understanding the interplay between different factors within a specific context.
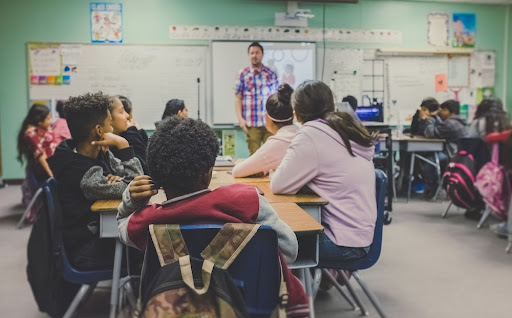
Intrinsic, instrumental, and collective case studies
These three categories of case studies focus on the nature and purpose of the study. An intrinsic case study is conducted when a researcher has an inherent interest in the case itself. Instrumental case studies are employed when the case is used to provide insight into a particular issue or phenomenon. A collective case study, on the other hand, involves studying multiple cases simultaneously to investigate some general phenomena.
Each type of case study serves a different purpose and has its own strengths and challenges. The selection of the type should be guided by the research question and objectives, as well as the context and constraints of the research.
The flexibility, depth, and contextual richness offered by case studies make this approach an excellent research method for various fields of study. They enable researchers to investigate real-world phenomena within their specific contexts, capturing nuances that other research methods might miss. Across numerous fields, case studies provide valuable insights into complex issues.
Critical information systems research
Case studies provide a detailed understanding of the role and impact of information systems in different contexts. They offer a platform to explore how information systems are designed, implemented, and used and how they interact with various social, economic, and political factors. Case studies in this field often focus on examining the intricate relationship between technology, organizational processes, and user behavior, helping to uncover insights that can inform better system design and implementation.
Health research
Health research is another field where case studies are highly valuable. They offer a way to explore patient experiences, healthcare delivery processes, and the impact of various interventions in a real-world context.
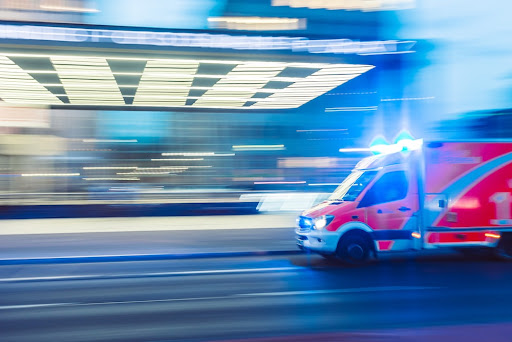
Case studies can provide a deep understanding of a patient's journey, giving insights into the intricacies of disease progression, treatment effects, and the psychosocial aspects of health and illness.
Asthma research studies
Specifically within medical research, studies on asthma often employ case studies to explore the individual and environmental factors that influence asthma development, management, and outcomes. A case study can provide rich, detailed data about individual patients' experiences, from the triggers and symptoms they experience to the effectiveness of various management strategies. This can be crucial for developing patient-centered asthma care approaches.
Other fields
Apart from the fields mentioned, case studies are also extensively used in business and management research, education research, and political sciences, among many others. They provide an opportunity to delve into the intricacies of real-world situations, allowing for a comprehensive understanding of various phenomena.
Case studies, with their depth and contextual focus, offer unique insights across these varied fields. They allow researchers to illuminate the complexities of real-life situations, contributing to both theory and practice.
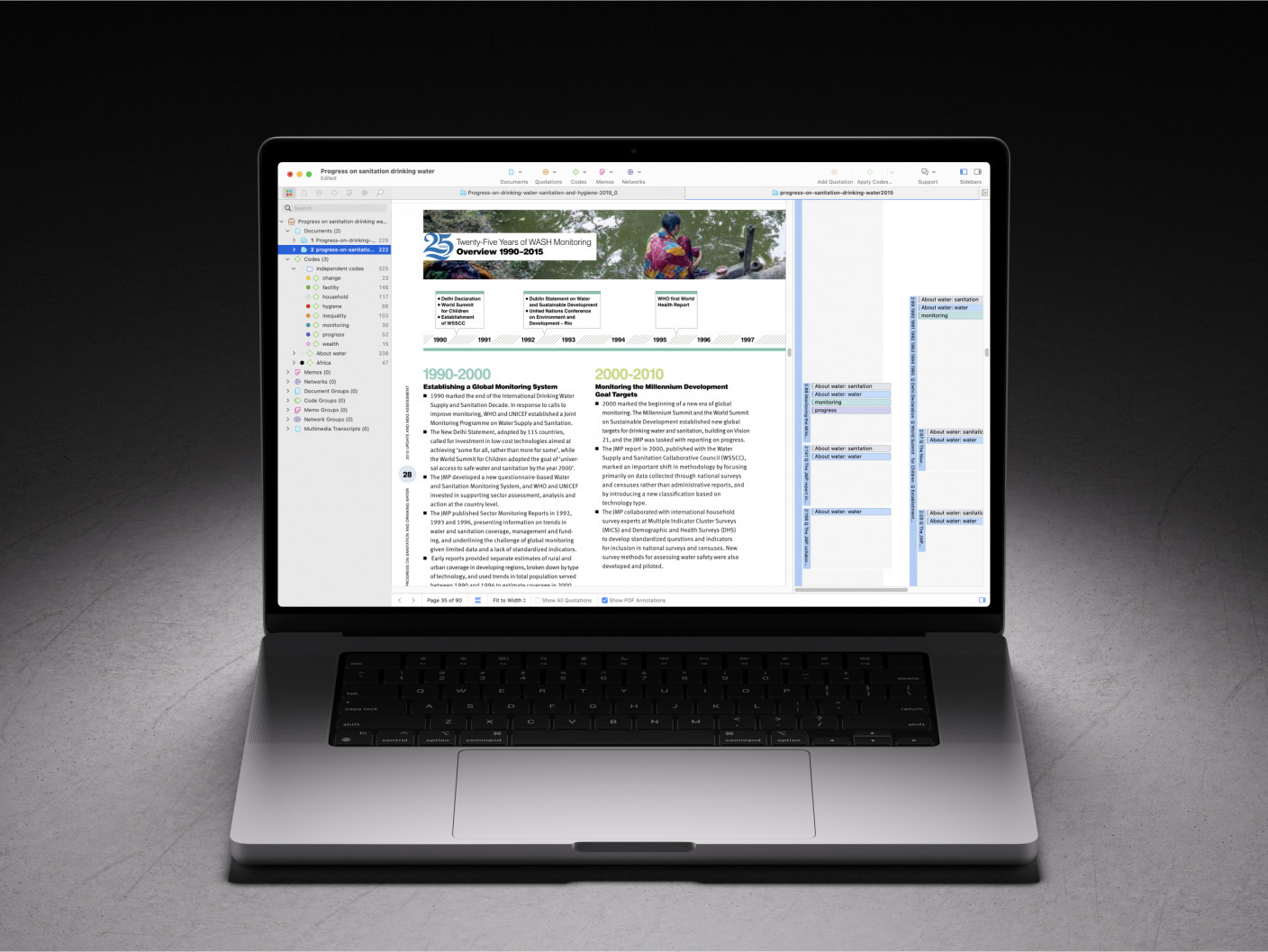
Whatever field you're in, ATLAS.ti puts your data to work for you
Download a free trial of ATLAS.ti to turn your data into insights.
Understanding the key elements of case study design is crucial for conducting rigorous and impactful case study research. A well-structured design guides the researcher through the process, ensuring that the study is methodologically sound and its findings are reliable and valid. The main elements of case study design include the research question , propositions, units of analysis, and the logic linking the data to the propositions.
The research question is the foundation of any research study. A good research question guides the direction of the study and informs the selection of the case, the methods of collecting data, and the analysis techniques. A well-formulated research question in case study research is typically clear, focused, and complex enough to merit further detailed examination of the relevant case(s).
Propositions
Propositions, though not necessary in every case study, provide a direction by stating what we might expect to find in the data collected. They guide how data is collected and analyzed by helping researchers focus on specific aspects of the case. They are particularly important in explanatory case studies, which seek to understand the relationships among concepts within the studied phenomenon.
Units of analysis
The unit of analysis refers to the case, or the main entity or entities that are being analyzed in the study. In case study research, the unit of analysis can be an individual, a group, an organization, a decision, an event, or even a time period. It's crucial to clearly define the unit of analysis, as it shapes the qualitative data analysis process by allowing the researcher to analyze a particular case and synthesize analysis across multiple case studies to draw conclusions.
Argumentation
This refers to the inferential model that allows researchers to draw conclusions from the data. The researcher needs to ensure that there is a clear link between the data, the propositions (if any), and the conclusions drawn. This argumentation is what enables the researcher to make valid and credible inferences about the phenomenon under study.
Understanding and carefully considering these elements in the design phase of a case study can significantly enhance the quality of the research. It can help ensure that the study is methodologically sound and its findings contribute meaningful insights about the case.
Ready to jumpstart your research with ATLAS.ti?
Conceptualize your research project with our intuitive data analysis interface. Download a free trial today.
Conducting a case study involves several steps, from defining the research question and selecting the case to collecting and analyzing data . This section outlines these key stages, providing a practical guide on how to conduct case study research.
Defining the research question
The first step in case study research is defining a clear, focused research question. This question should guide the entire research process, from case selection to analysis. It's crucial to ensure that the research question is suitable for a case study approach. Typically, such questions are exploratory or descriptive in nature and focus on understanding a phenomenon within its real-life context.
Selecting and defining the case
The selection of the case should be based on the research question and the objectives of the study. It involves choosing a unique example or a set of examples that provide rich, in-depth data about the phenomenon under investigation. After selecting the case, it's crucial to define it clearly, setting the boundaries of the case, including the time period and the specific context.
Previous research can help guide the case study design. When considering a case study, an example of a case could be taken from previous case study research and used to define cases in a new research inquiry. Considering recently published examples can help understand how to select and define cases effectively.
Developing a detailed case study protocol
A case study protocol outlines the procedures and general rules to be followed during the case study. This includes the data collection methods to be used, the sources of data, and the procedures for analysis. Having a detailed case study protocol ensures consistency and reliability in the study.
The protocol should also consider how to work with the people involved in the research context to grant the research team access to collecting data. As mentioned in previous sections of this guide, establishing rapport is an essential component of qualitative research as it shapes the overall potential for collecting and analyzing data.
Collecting data
Gathering data in case study research often involves multiple sources of evidence, including documents, archival records, interviews, observations, and physical artifacts. This allows for a comprehensive understanding of the case. The process for gathering data should be systematic and carefully documented to ensure the reliability and validity of the study.
Analyzing and interpreting data
The next step is analyzing the data. This involves organizing the data , categorizing it into themes or patterns , and interpreting these patterns to answer the research question. The analysis might also involve comparing the findings with prior research or theoretical propositions.
Writing the case study report
The final step is writing the case study report . This should provide a detailed description of the case, the data, the analysis process, and the findings. The report should be clear, organized, and carefully written to ensure that the reader can understand the case and the conclusions drawn from it.
Each of these steps is crucial in ensuring that the case study research is rigorous, reliable, and provides valuable insights about the case.
The type, depth, and quality of data in your study can significantly influence the validity and utility of the study. In case study research, data is usually collected from multiple sources to provide a comprehensive and nuanced understanding of the case. This section will outline the various methods of collecting data used in case study research and discuss considerations for ensuring the quality of the data.
Interviews are a common method of gathering data in case study research. They can provide rich, in-depth data about the perspectives, experiences, and interpretations of the individuals involved in the case. Interviews can be structured , semi-structured , or unstructured , depending on the research question and the degree of flexibility needed.
Observations
Observations involve the researcher observing the case in its natural setting, providing first-hand information about the case and its context. Observations can provide data that might not be revealed in interviews or documents, such as non-verbal cues or contextual information.
Documents and artifacts
Documents and archival records provide a valuable source of data in case study research. They can include reports, letters, memos, meeting minutes, email correspondence, and various public and private documents related to the case.
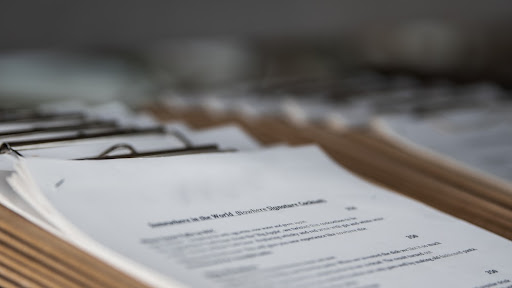
These records can provide historical context, corroborate evidence from other sources, and offer insights into the case that might not be apparent from interviews or observations.
Physical artifacts refer to any physical evidence related to the case, such as tools, products, or physical environments. These artifacts can provide tangible insights into the case, complementing the data gathered from other sources.
Ensuring the quality of data collection
Determining the quality of data in case study research requires careful planning and execution. It's crucial to ensure that the data is reliable, accurate, and relevant to the research question. This involves selecting appropriate methods of collecting data, properly training interviewers or observers, and systematically recording and storing the data. It also includes considering ethical issues related to collecting and handling data, such as obtaining informed consent and ensuring the privacy and confidentiality of the participants.
Data analysis
Analyzing case study research involves making sense of the rich, detailed data to answer the research question. This process can be challenging due to the volume and complexity of case study data. However, a systematic and rigorous approach to analysis can ensure that the findings are credible and meaningful. This section outlines the main steps and considerations in analyzing data in case study research.
Organizing the data
The first step in the analysis is organizing the data. This involves sorting the data into manageable sections, often according to the data source or the theme. This step can also involve transcribing interviews, digitizing physical artifacts, or organizing observational data.
Categorizing and coding the data
Once the data is organized, the next step is to categorize or code the data. This involves identifying common themes, patterns, or concepts in the data and assigning codes to relevant data segments. Coding can be done manually or with the help of software tools, and in either case, qualitative analysis software can greatly facilitate the entire coding process. Coding helps to reduce the data to a set of themes or categories that can be more easily analyzed.
Identifying patterns and themes
After coding the data, the researcher looks for patterns or themes in the coded data. This involves comparing and contrasting the codes and looking for relationships or patterns among them. The identified patterns and themes should help answer the research question.
Interpreting the data
Once patterns and themes have been identified, the next step is to interpret these findings. This involves explaining what the patterns or themes mean in the context of the research question and the case. This interpretation should be grounded in the data, but it can also involve drawing on theoretical concepts or prior research.
Verification of the data
The last step in the analysis is verification. This involves checking the accuracy and consistency of the analysis process and confirming that the findings are supported by the data. This can involve re-checking the original data, checking the consistency of codes, or seeking feedback from research participants or peers.
Like any research method , case study research has its strengths and limitations. Researchers must be aware of these, as they can influence the design, conduct, and interpretation of the study.
Understanding the strengths and limitations of case study research can also guide researchers in deciding whether this approach is suitable for their research question . This section outlines some of the key strengths and limitations of case study research.
Benefits include the following:
- Rich, detailed data: One of the main strengths of case study research is that it can generate rich, detailed data about the case. This can provide a deep understanding of the case and its context, which can be valuable in exploring complex phenomena.
- Flexibility: Case study research is flexible in terms of design , data collection , and analysis . A sufficient degree of flexibility allows the researcher to adapt the study according to the case and the emerging findings.
- Real-world context: Case study research involves studying the case in its real-world context, which can provide valuable insights into the interplay between the case and its context.
- Multiple sources of evidence: Case study research often involves collecting data from multiple sources , which can enhance the robustness and validity of the findings.
On the other hand, researchers should consider the following limitations:
- Generalizability: A common criticism of case study research is that its findings might not be generalizable to other cases due to the specificity and uniqueness of each case.
- Time and resource intensive: Case study research can be time and resource intensive due to the depth of the investigation and the amount of collected data.
- Complexity of analysis: The rich, detailed data generated in case study research can make analyzing the data challenging.
- Subjectivity: Given the nature of case study research, there may be a higher degree of subjectivity in interpreting the data , so researchers need to reflect on this and transparently convey to audiences how the research was conducted.
Being aware of these strengths and limitations can help researchers design and conduct case study research effectively and interpret and report the findings appropriately.
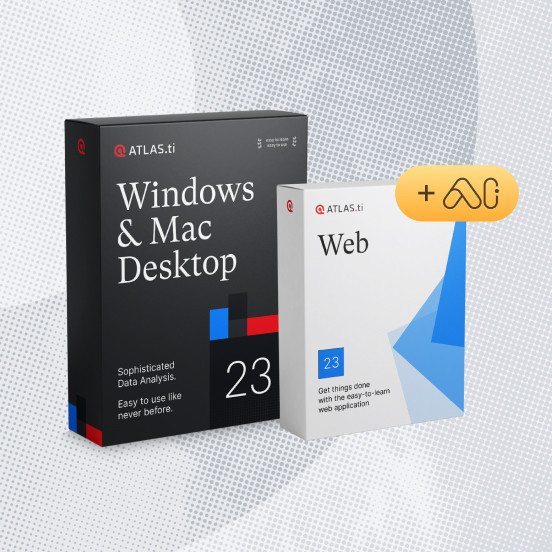
Ready to analyze your data with ATLAS.ti?
See how our intuitive software can draw key insights from your data with a free trial today.
- AI & NLP
- Churn & Loyalty
- Customer Experience
- Customer Journeys
- Customer Metrics
- Feedback Analysis
- Product Experience
- Product Updates
- Sentiment Analysis
- Surveys & Feedback Collection
- Try Thematic
Welcome to the community
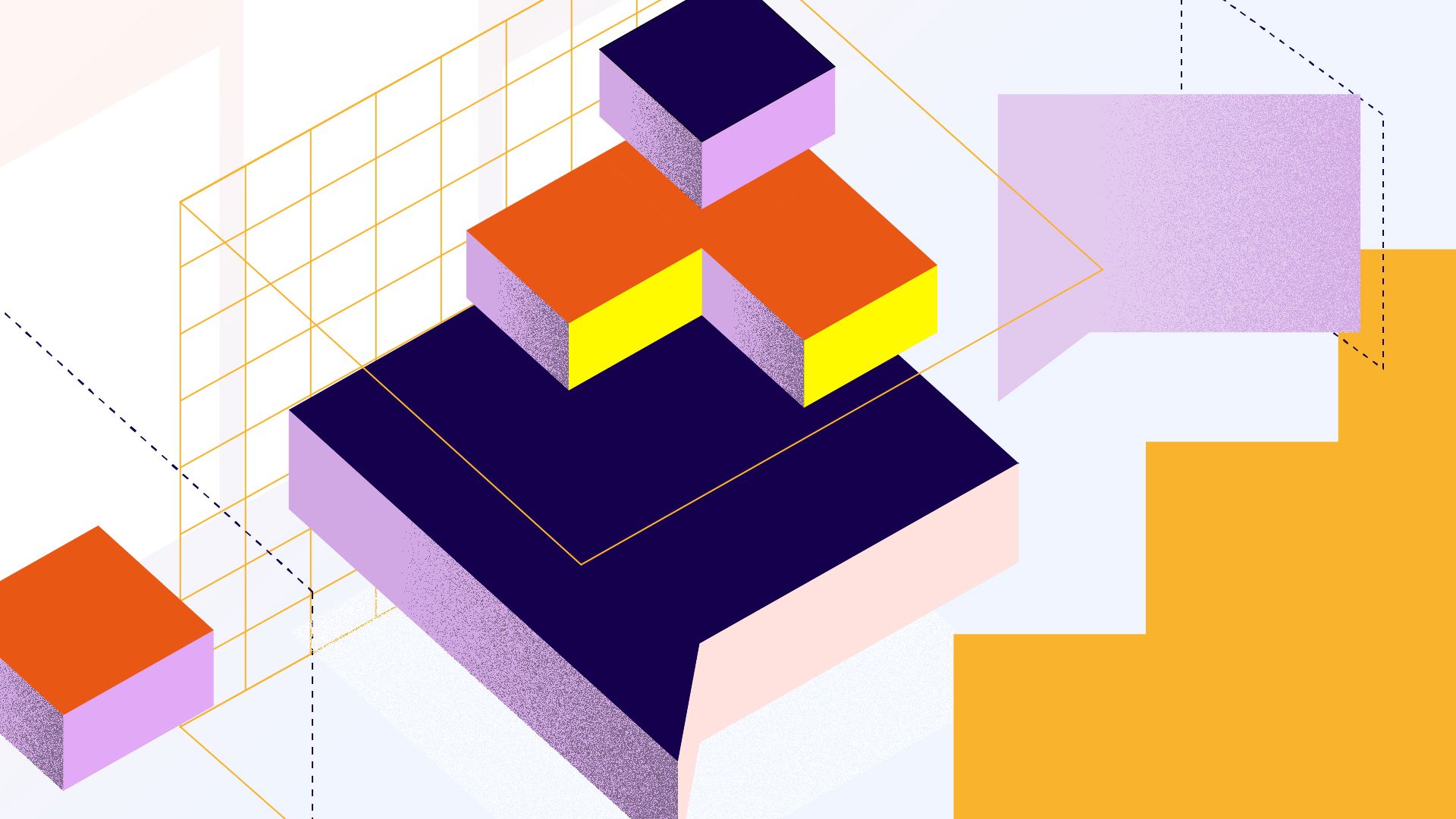
Qualitative Data Analysis: Step-by-Step Guide (Manual vs. Automatic)
When we conduct qualitative methods of research, need to explain changes in metrics or understand people's opinions, we always turn to qualitative data. Qualitative data is typically generated through:
- Interview transcripts
- Surveys with open-ended questions
- Contact center transcripts
- Texts and documents
- Audio and video recordings
- Observational notes
Compared to quantitative data, which captures structured information, qualitative data is unstructured and has more depth. It can answer our questions, can help formulate hypotheses and build understanding.
It's important to understand the differences between quantitative data & qualitative data . But unfortunately, analyzing qualitative data is difficult. While tools like Excel, Tableau and PowerBI crunch and visualize quantitative data with ease, there are a limited number of mainstream tools for analyzing qualitative data . The majority of qualitative data analysis still happens manually.
That said, there are two new trends that are changing this. First, there are advances in natural language processing (NLP) which is focused on understanding human language. Second, there is an explosion of user-friendly software designed for both researchers and businesses. Both help automate the qualitative data analysis process.
In this post we want to teach you how to conduct a successful qualitative data analysis. There are two primary qualitative data analysis methods; manual & automatic. We will teach you how to conduct the analysis manually, and also, automatically using software solutions powered by NLP. We’ll guide you through the steps to conduct a manual analysis, and look at what is involved and the role technology can play in automating this process.
More businesses are switching to fully-automated analysis of qualitative customer data because it is cheaper, faster, and just as accurate. Primarily, businesses purchase subscriptions to feedback analytics platforms so that they can understand customer pain points and sentiment.
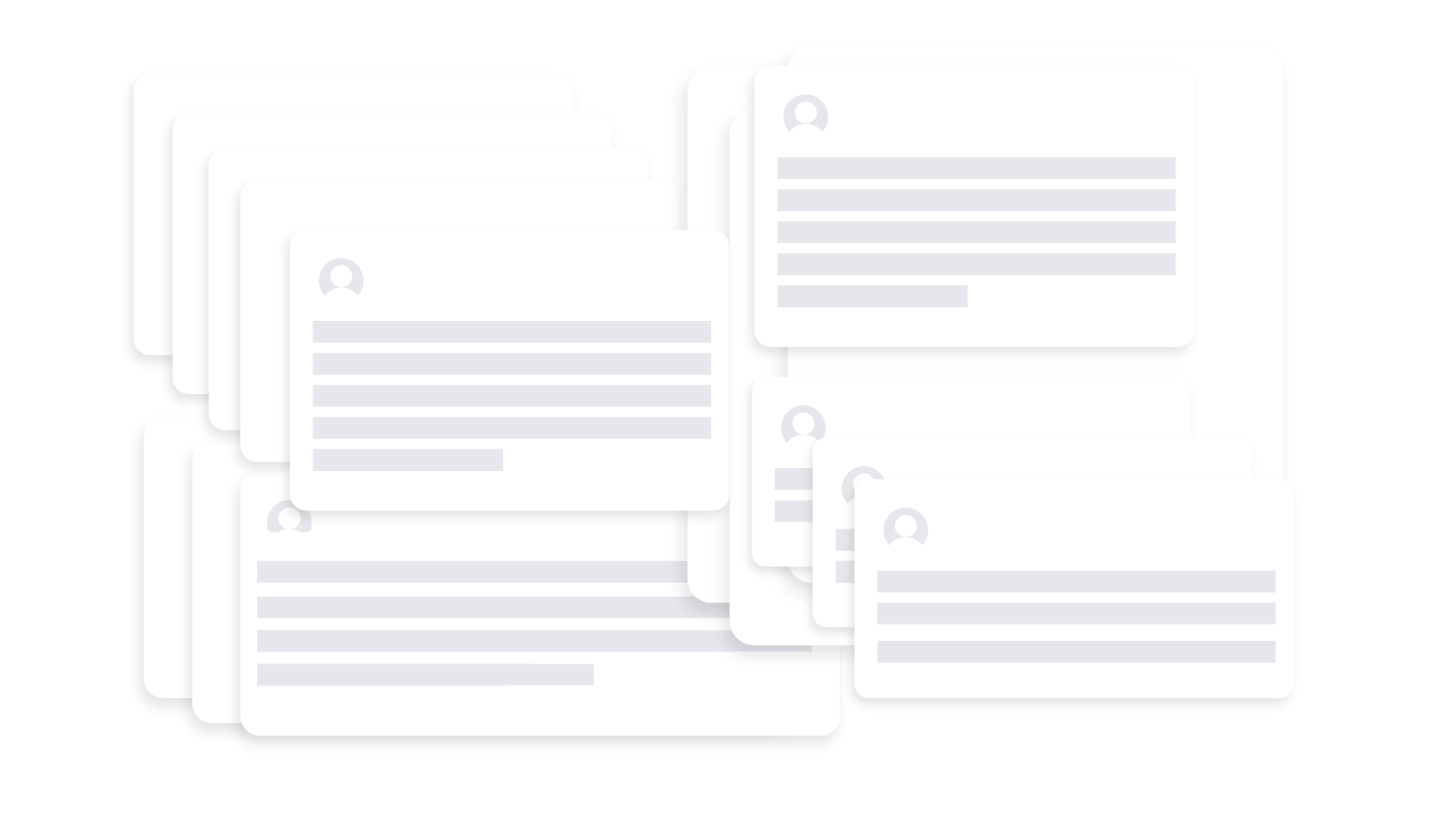
We’ll take you through 5 steps to conduct a successful qualitative data analysis. Within each step we will highlight the key difference between the manual, and automated approach of qualitative researchers. Here's an overview of the steps:
The 5 steps to doing qualitative data analysis
- Gathering and collecting your qualitative data
- Organizing and connecting into your qualitative data
- Coding your qualitative data
- Analyzing the qualitative data for insights
- Reporting on the insights derived from your analysis
What is Qualitative Data Analysis?
Qualitative data analysis is a process of gathering, structuring and interpreting qualitative data to understand what it represents.
Qualitative data is non-numerical and unstructured. Qualitative data generally refers to text, such as open-ended responses to survey questions or user interviews, but also includes audio, photos and video.
Businesses often perform qualitative data analysis on customer feedback. And within this context, qualitative data generally refers to verbatim text data collected from sources such as reviews, complaints, chat messages, support centre interactions, customer interviews, case notes or social media comments.
How is qualitative data analysis different from quantitative data analysis?
Understanding the differences between quantitative & qualitative data is important. When it comes to analyzing data, Qualitative Data Analysis serves a very different role to Quantitative Data Analysis. But what sets them apart?
Qualitative Data Analysis dives into the stories hidden in non-numerical data such as interviews, open-ended survey answers, or notes from observations. It uncovers the ‘whys’ and ‘hows’ giving a deep understanding of people’s experiences and emotions.
Quantitative Data Analysis on the other hand deals with numerical data, using statistics to measure differences, identify preferred options, and pinpoint root causes of issues. It steps back to address questions like "how many" or "what percentage" to offer broad insights we can apply to larger groups.
In short, Qualitative Data Analysis is like a microscope, helping us understand specific detail. Quantitative Data Analysis is like the telescope, giving us a broader perspective. Both are important, working together to decode data for different objectives.
Qualitative Data Analysis methods
Once all the data has been captured, there are a variety of analysis techniques available and the choice is determined by your specific research objectives and the kind of data you’ve gathered. Common qualitative data analysis methods include:
Content Analysis
This is a popular approach to qualitative data analysis. Other qualitative analysis techniques may fit within the broad scope of content analysis. Thematic analysis is a part of the content analysis. Content analysis is used to identify the patterns that emerge from text, by grouping content into words, concepts, and themes. Content analysis is useful to quantify the relationship between all of the grouped content. The Columbia School of Public Health has a detailed breakdown of content analysis .
Narrative Analysis
Narrative analysis focuses on the stories people tell and the language they use to make sense of them. It is particularly useful in qualitative research methods where customer stories are used to get a deep understanding of customers’ perspectives on a specific issue. A narrative analysis might enable us to summarize the outcomes of a focused case study.
Discourse Analysis
Discourse analysis is used to get a thorough understanding of the political, cultural and power dynamics that exist in specific situations. The focus of discourse analysis here is on the way people express themselves in different social contexts. Discourse analysis is commonly used by brand strategists who hope to understand why a group of people feel the way they do about a brand or product.
Thematic Analysis
Thematic analysis is used to deduce the meaning behind the words people use. This is accomplished by discovering repeating themes in text. These meaningful themes reveal key insights into data and can be quantified, particularly when paired with sentiment analysis . Often, the outcome of thematic analysis is a code frame that captures themes in terms of codes, also called categories. So the process of thematic analysis is also referred to as “coding”. A common use-case for thematic analysis in companies is analysis of customer feedback.
Grounded Theory
Grounded theory is a useful approach when little is known about a subject. Grounded theory starts by formulating a theory around a single data case. This means that the theory is “grounded”. Grounded theory analysis is based on actual data, and not entirely speculative. Then additional cases can be examined to see if they are relevant and can add to the original grounded theory.
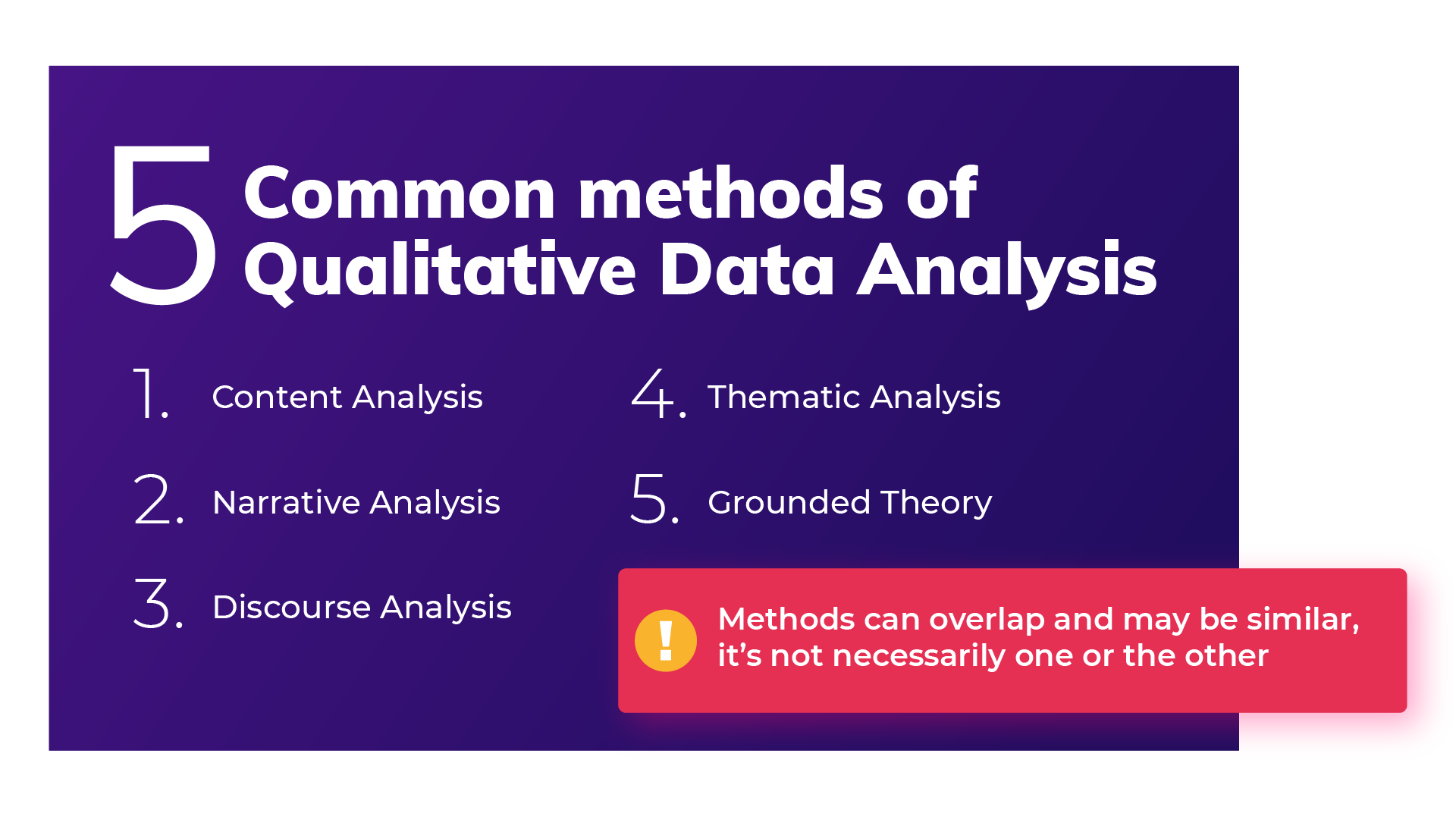
Challenges of Qualitative Data Analysis
While Qualitative Data Analysis offers rich insights, it comes with its challenges. Each unique QDA method has its unique hurdles. Let’s take a look at the challenges researchers and analysts might face, depending on the chosen method.
- Time and Effort (Narrative Analysis): Narrative analysis, which focuses on personal stories, demands patience. Sifting through lengthy narratives to find meaningful insights can be time-consuming, requires dedicated effort.
- Being Objective (Grounded Theory): Grounded theory, building theories from data, faces the challenges of personal biases. Staying objective while interpreting data is crucial, ensuring conclusions are rooted in the data itself.
- Complexity (Thematic Analysis): Thematic analysis involves identifying themes within data, a process that can be intricate. Categorizing and understanding themes can be complex, especially when each piece of data varies in context and structure. Thematic Analysis software can simplify this process.
- Generalizing Findings (Narrative Analysis): Narrative analysis, dealing with individual stories, makes drawing broad challenging. Extending findings from a single narrative to a broader context requires careful consideration.
- Managing Data (Thematic Analysis): Thematic analysis involves organizing and managing vast amounts of unstructured data, like interview transcripts. Managing this can be a hefty task, requiring effective data management strategies.
- Skill Level (Grounded Theory): Grounded theory demands specific skills to build theories from the ground up. Finding or training analysts with these skills poses a challenge, requiring investment in building expertise.
Benefits of qualitative data analysis
Qualitative Data Analysis (QDA) is like a versatile toolkit, offering a tailored approach to understanding your data. The benefits it offers are as diverse as the methods. Let’s explore why choosing the right method matters.
- Tailored Methods for Specific Needs: QDA isn't one-size-fits-all. Depending on your research objectives and the type of data at hand, different methods offer unique benefits. If you want emotive customer stories, narrative analysis paints a strong picture. When you want to explain a score, thematic analysis reveals insightful patterns
- Flexibility with Thematic Analysis: thematic analysis is like a chameleon in the toolkit of QDA. It adapts well to different types of data and research objectives, making it a top choice for any qualitative analysis.
- Deeper Understanding, Better Products: QDA helps you dive into people's thoughts and feelings. This deep understanding helps you build products and services that truly matches what people want, ensuring satisfied customers
- Finding the Unexpected: Qualitative data often reveals surprises that we miss in quantitative data. QDA offers us new ideas and perspectives, for insights we might otherwise miss.
- Building Effective Strategies: Insights from QDA are like strategic guides. They help businesses in crafting plans that match people’s desires.
- Creating Genuine Connections: Understanding people’s experiences lets businesses connect on a real level. This genuine connection helps build trust and loyalty, priceless for any business.
How to do Qualitative Data Analysis: 5 steps
Now we are going to show how you can do your own qualitative data analysis. We will guide you through this process step by step. As mentioned earlier, you will learn how to do qualitative data analysis manually , and also automatically using modern qualitative data and thematic analysis software.
To get best value from the analysis process and research process, it’s important to be super clear about the nature and scope of the question that’s being researched. This will help you select the research collection channels that are most likely to help you answer your question.
Depending on if you are a business looking to understand customer sentiment, or an academic surveying a school, your approach to qualitative data analysis will be unique.
Once you’re clear, there’s a sequence to follow. And, though there are differences in the manual and automatic approaches, the process steps are mostly the same.
The use case for our step-by-step guide is a company looking to collect data (customer feedback data), and analyze the customer feedback - in order to improve customer experience. By analyzing the customer feedback the company derives insights about their business and their customers. You can follow these same steps regardless of the nature of your research. Let’s get started.
Step 1: Gather your qualitative data and conduct research (Conduct qualitative research)
The first step of qualitative research is to do data collection. Put simply, data collection is gathering all of your data for analysis. A common situation is when qualitative data is spread across various sources.
Classic methods of gathering qualitative data
Most companies use traditional methods for gathering qualitative data: conducting interviews with research participants, running surveys, and running focus groups. This data is typically stored in documents, CRMs, databases and knowledge bases. It’s important to examine which data is available and needs to be included in your research project, based on its scope.
Using your existing qualitative feedback
As it becomes easier for customers to engage across a range of different channels, companies are gathering increasingly large amounts of both solicited and unsolicited qualitative feedback.
Most organizations have now invested in Voice of Customer programs , support ticketing systems, chatbot and support conversations, emails and even customer Slack chats.
These new channels provide companies with new ways of getting feedback, and also allow the collection of unstructured feedback data at scale.
The great thing about this data is that it contains a wealth of valubale insights and that it’s already there! When you have a new question about user behavior or your customers, you don’t need to create a new research study or set up a focus group. You can find most answers in the data you already have.
Typically, this data is stored in third-party solutions or a central database, but there are ways to export it or connect to a feedback analysis solution through integrations or an API.
Utilize untapped qualitative data channels
There are many online qualitative data sources you may not have considered. For example, you can find useful qualitative data in social media channels like Twitter or Facebook. Online forums, review sites, and online communities such as Discourse or Reddit also contain valuable data about your customers, or research questions.
If you are considering performing a qualitative benchmark analysis against competitors - the internet is your best friend, and review analysis is a great place to start. Gathering feedback in competitor reviews on sites like Trustpilot, G2, Capterra, Better Business Bureau or on app stores is a great way to perform a competitor benchmark analysis.
Customer feedback analysis software often has integrations into social media and review sites, or you could use a solution like DataMiner to scrape the reviews.
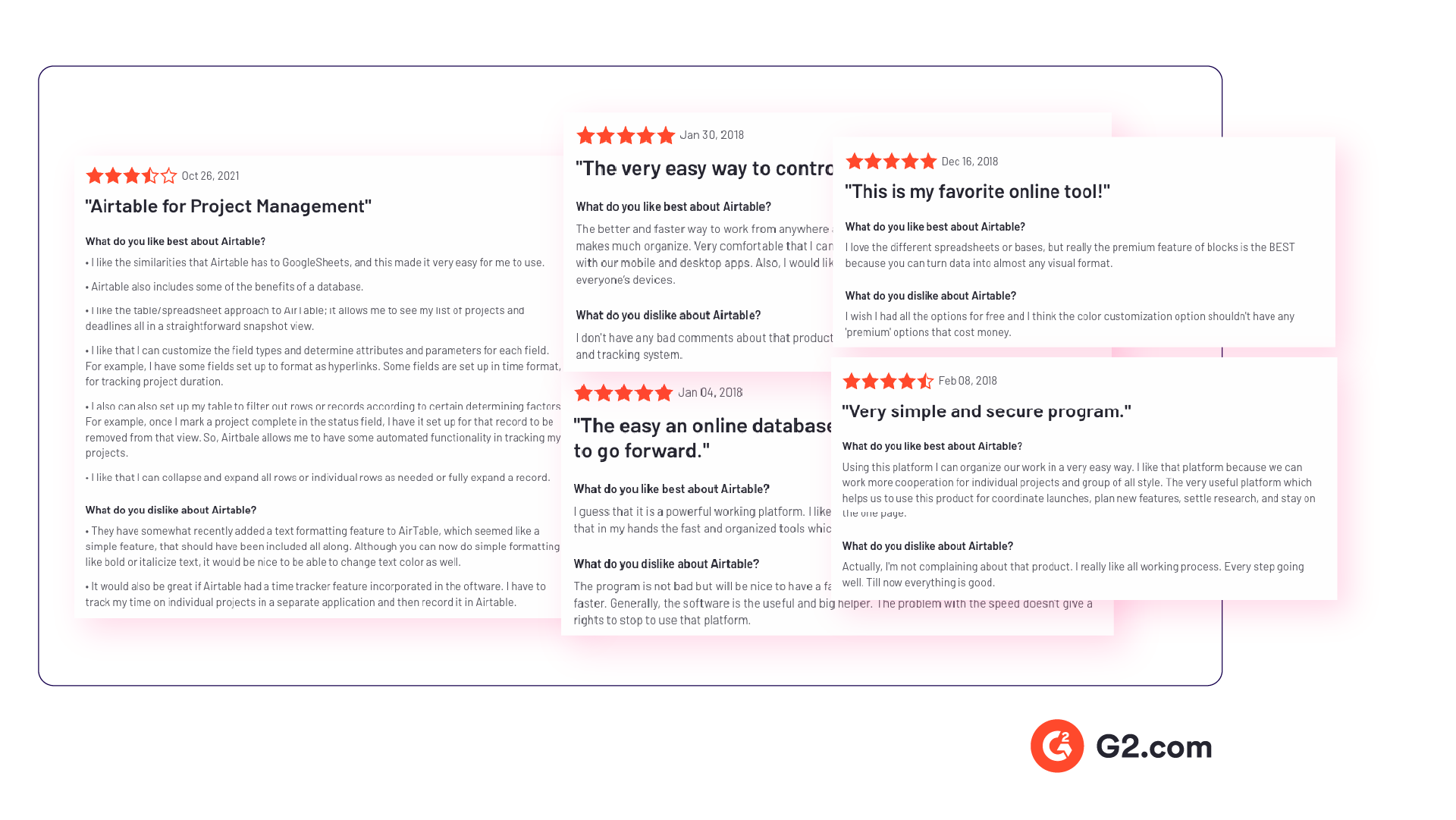
Step 2: Connect & organize all your qualitative data
Now you all have this qualitative data but there’s a problem, the data is unstructured. Before feedback can be analyzed and assigned any value, it needs to be organized in a single place. Why is this important? Consistency!
If all data is easily accessible in one place and analyzed in a consistent manner, you will have an easier time summarizing and making decisions based on this data.
The manual approach to organizing your data
The classic method of structuring qualitative data is to plot all the raw data you’ve gathered into a spreadsheet.
Typically, research and support teams would share large Excel sheets and different business units would make sense of the qualitative feedback data on their own. Each team collects and organizes the data in a way that best suits them, which means the feedback tends to be kept in separate silos.
An alternative and a more robust solution is to store feedback in a central database, like Snowflake or Amazon Redshift .
Keep in mind that when you organize your data in this way, you are often preparing it to be imported into another software. If you go the route of a database, you would need to use an API to push the feedback into a third-party software.
Computer-assisted qualitative data analysis software (CAQDAS)
Traditionally within the manual analysis approach (but not always), qualitative data is imported into CAQDAS software for coding.
In the early 2000s, CAQDAS software was popularised by developers such as ATLAS.ti, NVivo and MAXQDA and eagerly adopted by researchers to assist with the organizing and coding of data.
The benefits of using computer-assisted qualitative data analysis software:
- Assists in the organizing of your data
- Opens you up to exploring different interpretations of your data analysis
- Allows you to share your dataset easier and allows group collaboration (allows for secondary analysis)
However you still need to code the data, uncover the themes and do the analysis yourself. Therefore it is still a manual approach.
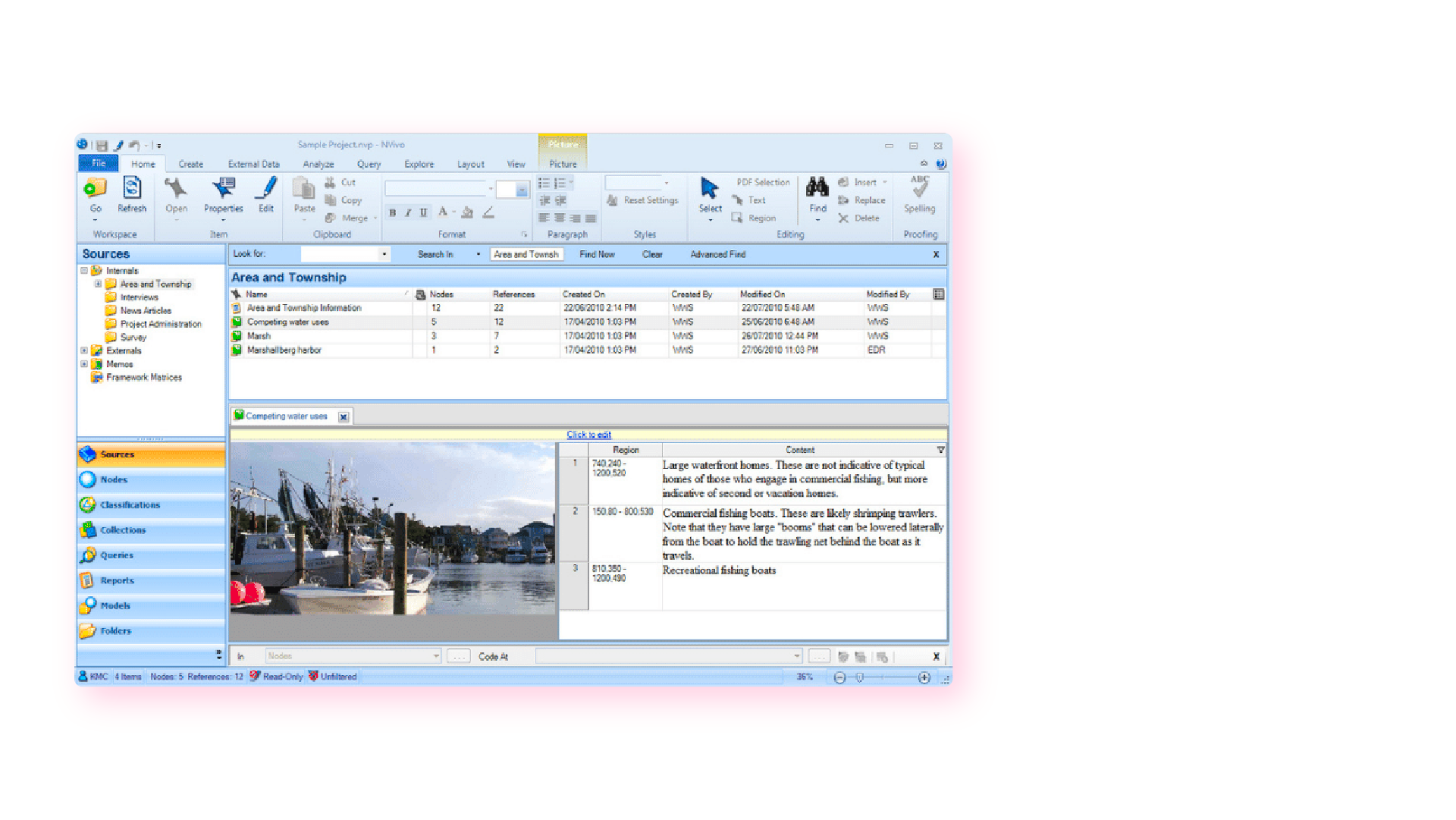
Organizing your qualitative data in a feedback repository
Another solution to organizing your qualitative data is to upload it into a feedback repository where it can be unified with your other data , and easily searchable and taggable. There are a number of software solutions that act as a central repository for your qualitative research data. Here are a couple solutions that you could investigate:
- Dovetail: Dovetail is a research repository with a focus on video and audio transcriptions. You can tag your transcriptions within the platform for theme analysis. You can also upload your other qualitative data such as research reports, survey responses, support conversations, and customer interviews. Dovetail acts as a single, searchable repository. And makes it easier to collaborate with other people around your qualitative research.
- EnjoyHQ: EnjoyHQ is another research repository with similar functionality to Dovetail. It boasts a more sophisticated search engine, but it has a higher starting subscription cost.
Organizing your qualitative data in a feedback analytics platform
If you have a lot of qualitative customer or employee feedback, from the likes of customer surveys or employee surveys, you will benefit from a feedback analytics platform. A feedback analytics platform is a software that automates the process of both sentiment analysis and thematic analysis . Companies use the integrations offered by these platforms to directly tap into their qualitative data sources (review sites, social media, survey responses, etc.). The data collected is then organized and analyzed consistently within the platform.
If you have data prepared in a spreadsheet, it can also be imported into feedback analytics platforms.
Once all this rich data has been organized within the feedback analytics platform, it is ready to be coded and themed, within the same platform. Thematic is a feedback analytics platform that offers one of the largest libraries of integrations with qualitative data sources.
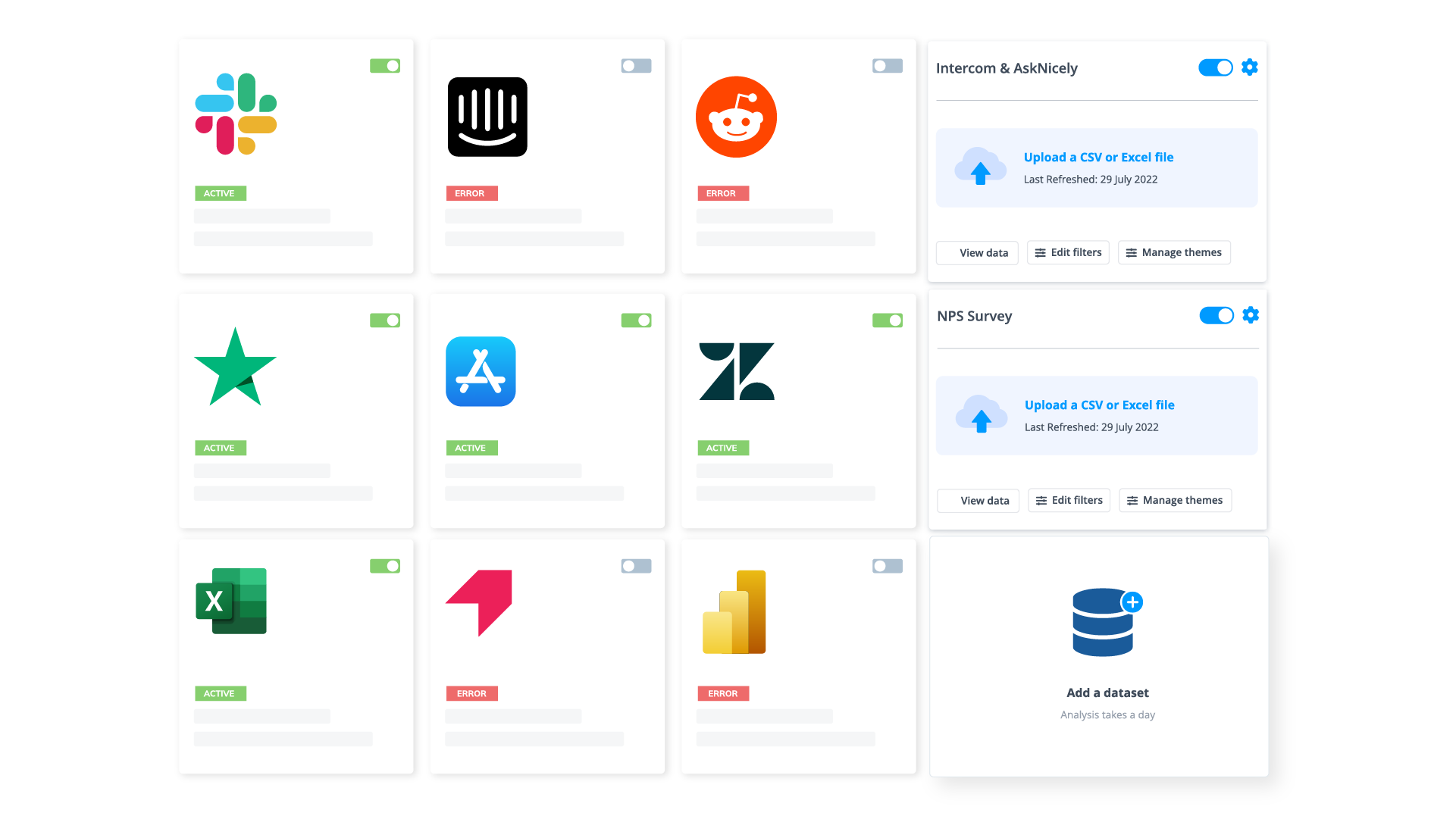
Step 3: Coding your qualitative data
Your feedback data is now organized in one place. Either within your spreadsheet, CAQDAS, feedback repository or within your feedback analytics platform. The next step is to code your feedback data so we can extract meaningful insights in the next step.
Coding is the process of labelling and organizing your data in such a way that you can then identify themes in the data, and the relationships between these themes.
To simplify the coding process, you will take small samples of your customer feedback data, come up with a set of codes, or categories capturing themes, and label each piece of feedback, systematically, for patterns and meaning. Then you will take a larger sample of data, revising and refining the codes for greater accuracy and consistency as you go.
If you choose to use a feedback analytics platform, much of this process will be automated and accomplished for you.
The terms to describe different categories of meaning (‘theme’, ‘code’, ‘tag’, ‘category’ etc) can be confusing as they are often used interchangeably. For clarity, this article will use the term ‘code’.
To code means to identify key words or phrases and assign them to a category of meaning. “I really hate the customer service of this computer software company” would be coded as “poor customer service”.
How to manually code your qualitative data
- Decide whether you will use deductive or inductive coding. Deductive coding is when you create a list of predefined codes, and then assign them to the qualitative data. Inductive coding is the opposite of this, you create codes based on the data itself. Codes arise directly from the data and you label them as you go. You need to weigh up the pros and cons of each coding method and select the most appropriate.
- Read through the feedback data to get a broad sense of what it reveals. Now it’s time to start assigning your first set of codes to statements and sections of text.
- Keep repeating step 2, adding new codes and revising the code description as often as necessary. Once it has all been coded, go through everything again, to be sure there are no inconsistencies and that nothing has been overlooked.
- Create a code frame to group your codes. The coding frame is the organizational structure of all your codes. And there are two commonly used types of coding frames, flat, or hierarchical. A hierarchical code frame will make it easier for you to derive insights from your analysis.
- Based on the number of times a particular code occurs, you can now see the common themes in your feedback data. This is insightful! If ‘bad customer service’ is a common code, it’s time to take action.
We have a detailed guide dedicated to manually coding your qualitative data .
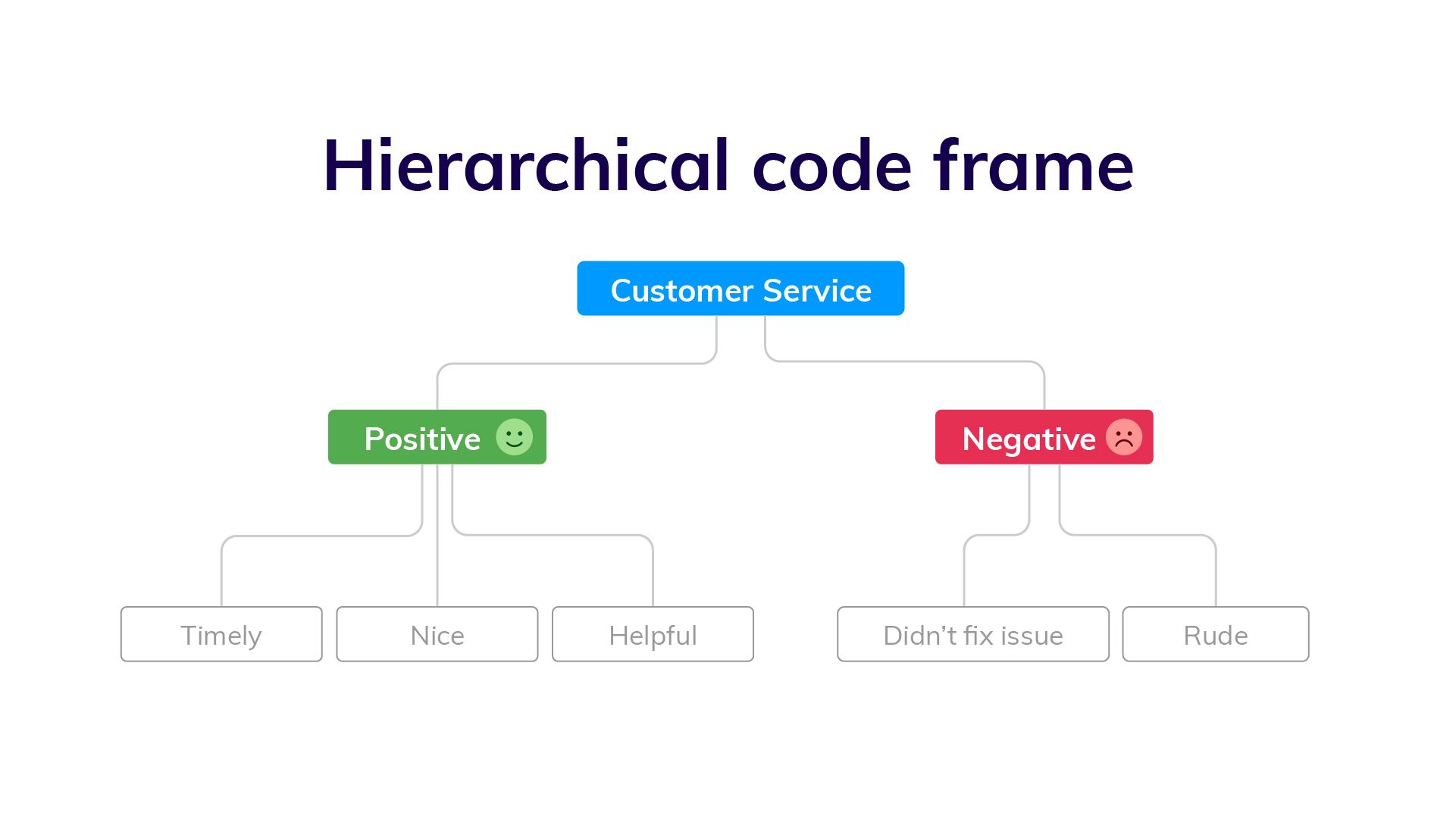
Using software to speed up manual coding of qualitative data
An Excel spreadsheet is still a popular method for coding. But various software solutions can help speed up this process. Here are some examples.
- CAQDAS / NVivo - CAQDAS software has built-in functionality that allows you to code text within their software. You may find the interface the software offers easier for managing codes than a spreadsheet.
- Dovetail/EnjoyHQ - You can tag transcripts and other textual data within these solutions. As they are also repositories you may find it simpler to keep the coding in one platform.
- IBM SPSS - SPSS is a statistical analysis software that may make coding easier than in a spreadsheet.
- Ascribe - Ascribe’s ‘Coder’ is a coding management system. Its user interface will make it easier for you to manage your codes.
Automating the qualitative coding process using thematic analysis software
In solutions which speed up the manual coding process, you still have to come up with valid codes and often apply codes manually to pieces of feedback. But there are also solutions that automate both the discovery and the application of codes.
Advances in machine learning have now made it possible to read, code and structure qualitative data automatically. This type of automated coding is offered by thematic analysis software .
Automation makes it far simpler and faster to code the feedback and group it into themes. By incorporating natural language processing (NLP) into the software, the AI looks across sentences and phrases to identify common themes meaningful statements. Some automated solutions detect repeating patterns and assign codes to them, others make you train the AI by providing examples. You could say that the AI learns the meaning of the feedback on its own.
Thematic automates the coding of qualitative feedback regardless of source. There’s no need to set up themes or categories in advance. Simply upload your data and wait a few minutes. You can also manually edit the codes to further refine their accuracy. Experiments conducted indicate that Thematic’s automated coding is just as accurate as manual coding .
Paired with sentiment analysis and advanced text analytics - these automated solutions become powerful for deriving quality business or research insights.
You could also build your own , if you have the resources!
The key benefits of using an automated coding solution
Automated analysis can often be set up fast and there’s the potential to uncover things that would never have been revealed if you had given the software a prescribed list of themes to look for.
Because the model applies a consistent rule to the data, it captures phrases or statements that a human eye might have missed.
Complete and consistent analysis of customer feedback enables more meaningful findings. Leading us into step 4.
Step 4: Analyze your data: Find meaningful insights
Now we are going to analyze our data to find insights. This is where we start to answer our research questions. Keep in mind that step 4 and step 5 (tell the story) have some overlap . This is because creating visualizations is both part of analysis process and reporting.
The task of uncovering insights is to scour through the codes that emerge from the data and draw meaningful correlations from them. It is also about making sure each insight is distinct and has enough data to support it.
Part of the analysis is to establish how much each code relates to different demographics and customer profiles, and identify whether there’s any relationship between these data points.
Manually create sub-codes to improve the quality of insights
If your code frame only has one level, you may find that your codes are too broad to be able to extract meaningful insights. This is where it is valuable to create sub-codes to your primary codes. This process is sometimes referred to as meta coding.
Note: If you take an inductive coding approach, you can create sub-codes as you are reading through your feedback data and coding it.
While time-consuming, this exercise will improve the quality of your analysis. Here is an example of what sub-codes could look like.
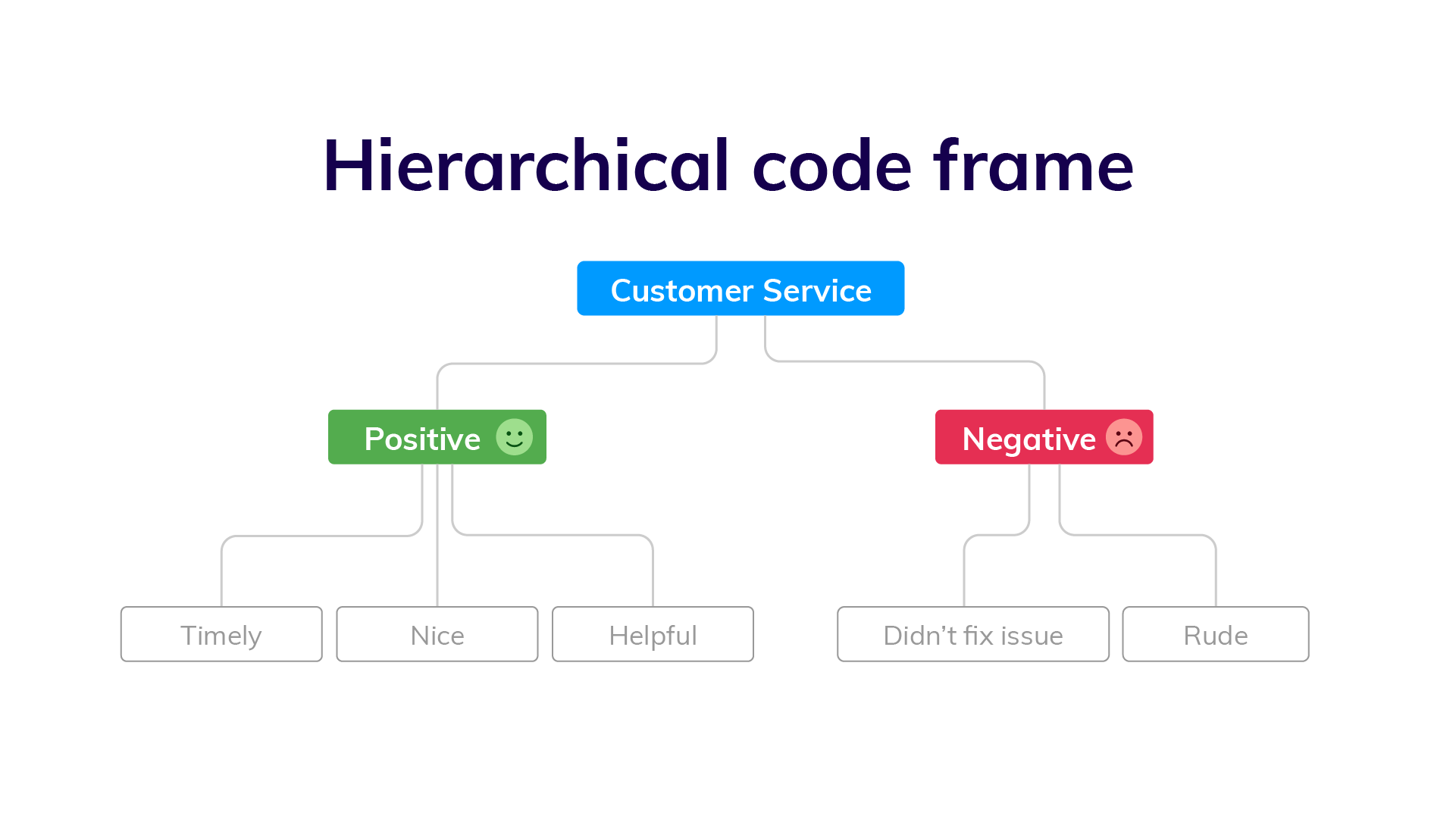
You need to carefully read your qualitative data to create quality sub-codes. But as you can see, the depth of analysis is greatly improved. By calculating the frequency of these sub-codes you can get insight into which customer service problems you can immediately address.
Correlate the frequency of codes to customer segments
Many businesses use customer segmentation . And you may have your own respondent segments that you can apply to your qualitative analysis. Segmentation is the practise of dividing customers or research respondents into subgroups.
Segments can be based on:
- Demographic
- And any other data type that you care to segment by
It is particularly useful to see the occurrence of codes within your segments. If one of your customer segments is considered unimportant to your business, but they are the cause of nearly all customer service complaints, it may be in your best interest to focus attention elsewhere. This is a useful insight!
Manually visualizing coded qualitative data
There are formulas you can use to visualize key insights in your data. The formulas we will suggest are imperative if you are measuring a score alongside your feedback.
If you are collecting a metric alongside your qualitative data this is a key visualization. Impact answers the question: “What’s the impact of a code on my overall score?”. Using Net Promoter Score (NPS) as an example, first you need to:
- Calculate overall NPS
- Calculate NPS in the subset of responses that do not contain that theme
- Subtract B from A
Then you can use this simple formula to calculate code impact on NPS .
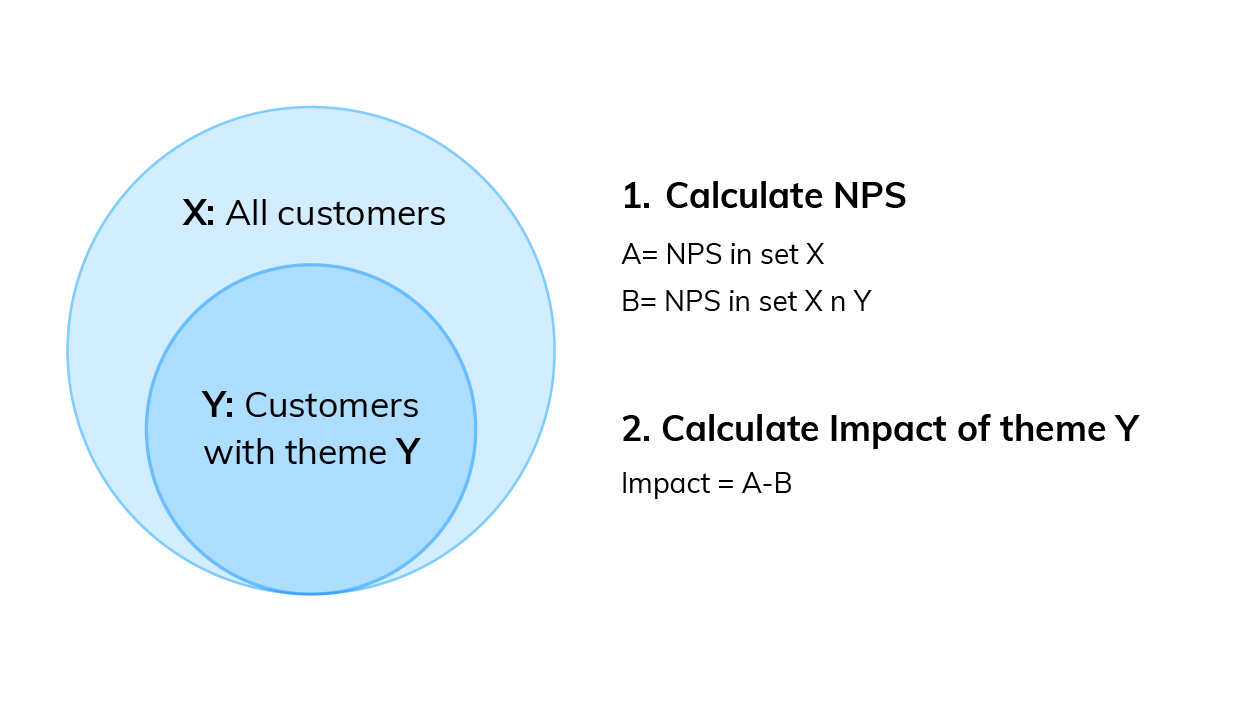
You can then visualize this data using a bar chart.
You can download our CX toolkit - it includes a template to recreate this.
Trends over time
This analysis can help you answer questions like: “Which codes are linked to decreases or increases in my score over time?”
We need to compare two sequences of numbers: NPS over time and code frequency over time . Using Excel, calculate the correlation between the two sequences, which can be either positive (the more codes the higher the NPS, see picture below), or negative (the more codes the lower the NPS).
Now you need to plot code frequency against the absolute value of code correlation with NPS. Here is the formula:
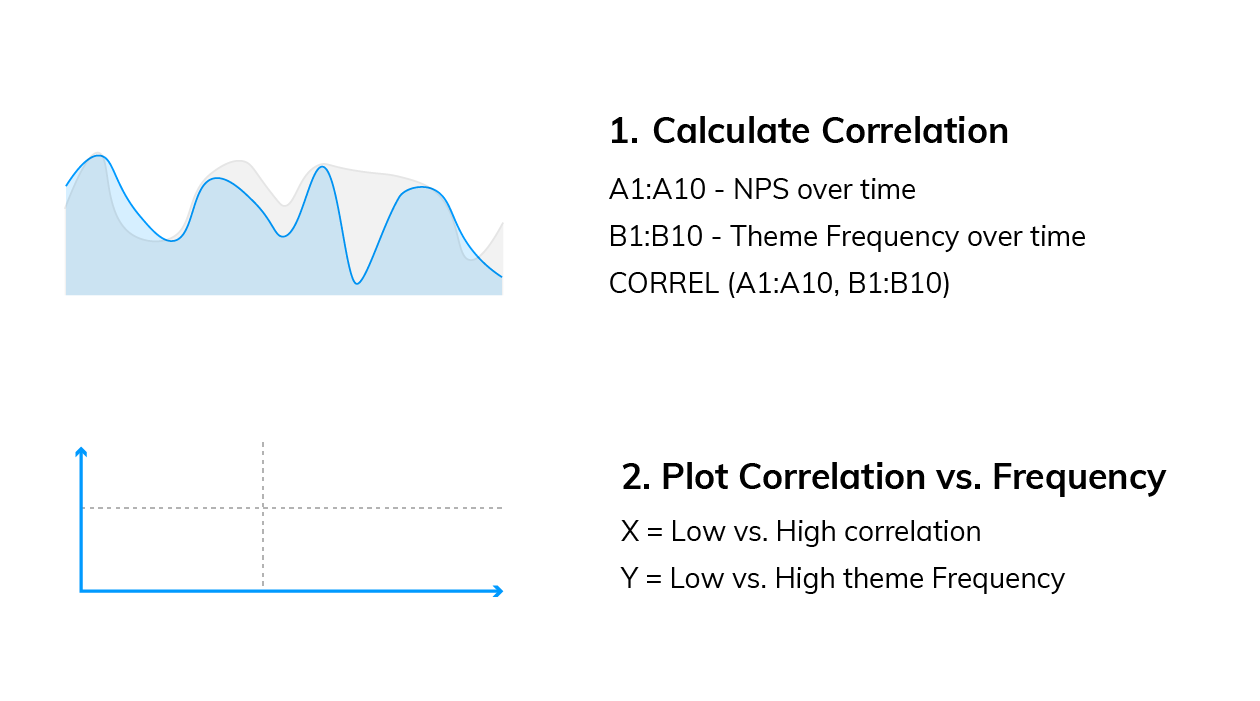
The visualization could look like this:
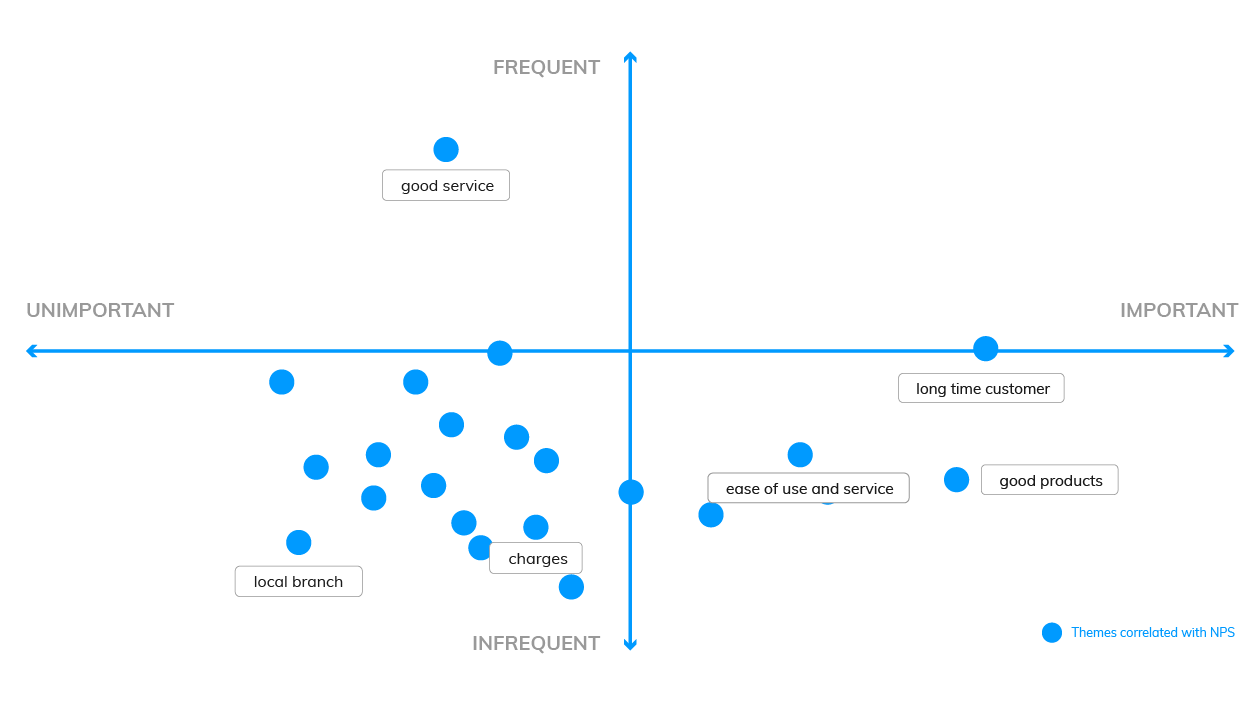
These are two examples, but there are more. For a third manual formula, and to learn why word clouds are not an insightful form of analysis, read our visualizations article .
Using a text analytics solution to automate analysis
Automated text analytics solutions enable codes and sub-codes to be pulled out of the data automatically. This makes it far faster and easier to identify what’s driving negative or positive results. And to pick up emerging trends and find all manner of rich insights in the data.
Another benefit of AI-driven text analytics software is its built-in capability for sentiment analysis, which provides the emotive context behind your feedback and other qualitative textual data therein.
Thematic provides text analytics that goes further by allowing users to apply their expertise on business context to edit or augment the AI-generated outputs.
Since the move away from manual research is generally about reducing the human element, adding human input to the technology might sound counter-intuitive. However, this is mostly to make sure important business nuances in the feedback aren’t missed during coding. The result is a higher accuracy of analysis. This is sometimes referred to as augmented intelligence .
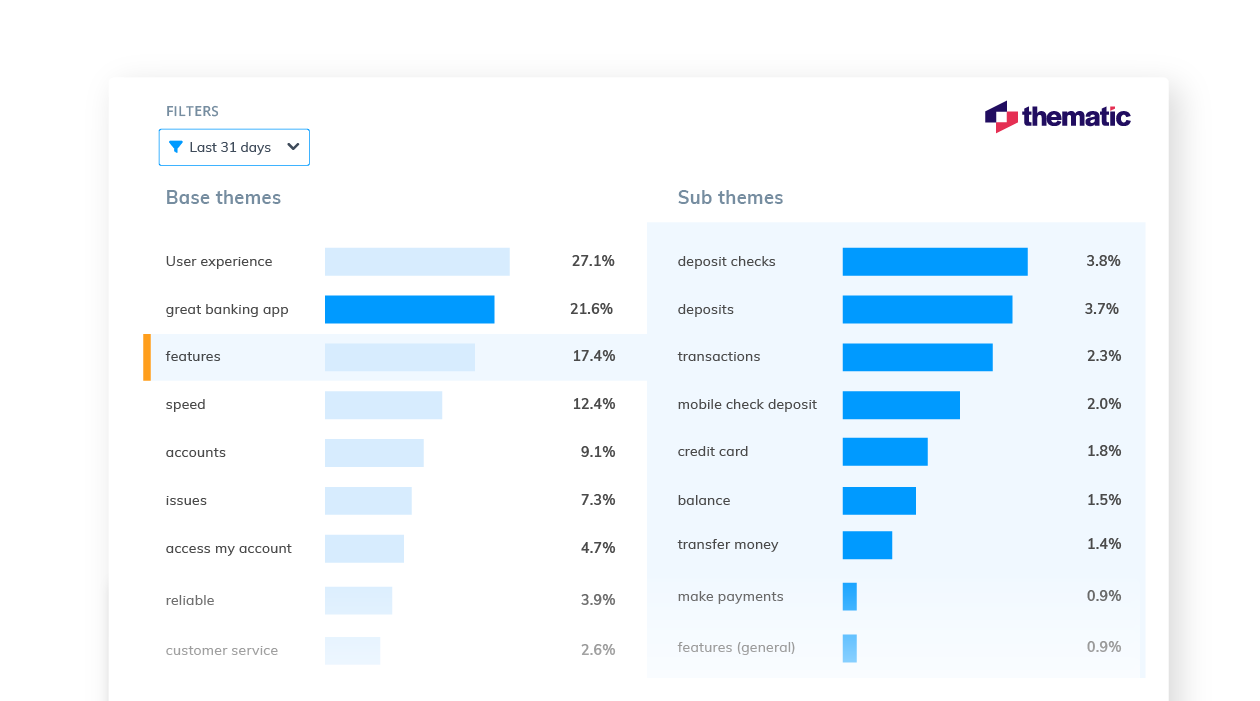
Step 5: Report on your data: Tell the story
The last step of analyzing your qualitative data is to report on it, to tell the story. At this point, the codes are fully developed and the focus is on communicating the narrative to the audience.
A coherent outline of the qualitative research, the findings and the insights is vital for stakeholders to discuss and debate before they can devise a meaningful course of action.
Creating graphs and reporting in Powerpoint
Typically, qualitative researchers take the tried and tested approach of distilling their report into a series of charts, tables and other visuals which are woven into a narrative for presentation in Powerpoint.
Using visualization software for reporting
With data transformation and APIs, the analyzed data can be shared with data visualisation software, such as Power BI or Tableau , Google Studio or Looker. Power BI and Tableau are among the most preferred options.
Visualizing your insights inside a feedback analytics platform
Feedback analytics platforms, like Thematic, incorporate visualisation tools that intuitively turn key data and insights into graphs. This removes the time consuming work of constructing charts to visually identify patterns and creates more time to focus on building a compelling narrative that highlights the insights, in bite-size chunks, for executive teams to review.
Using a feedback analytics platform with visualization tools means you don’t have to use a separate product for visualizations. You can export graphs into Powerpoints straight from the platforms.
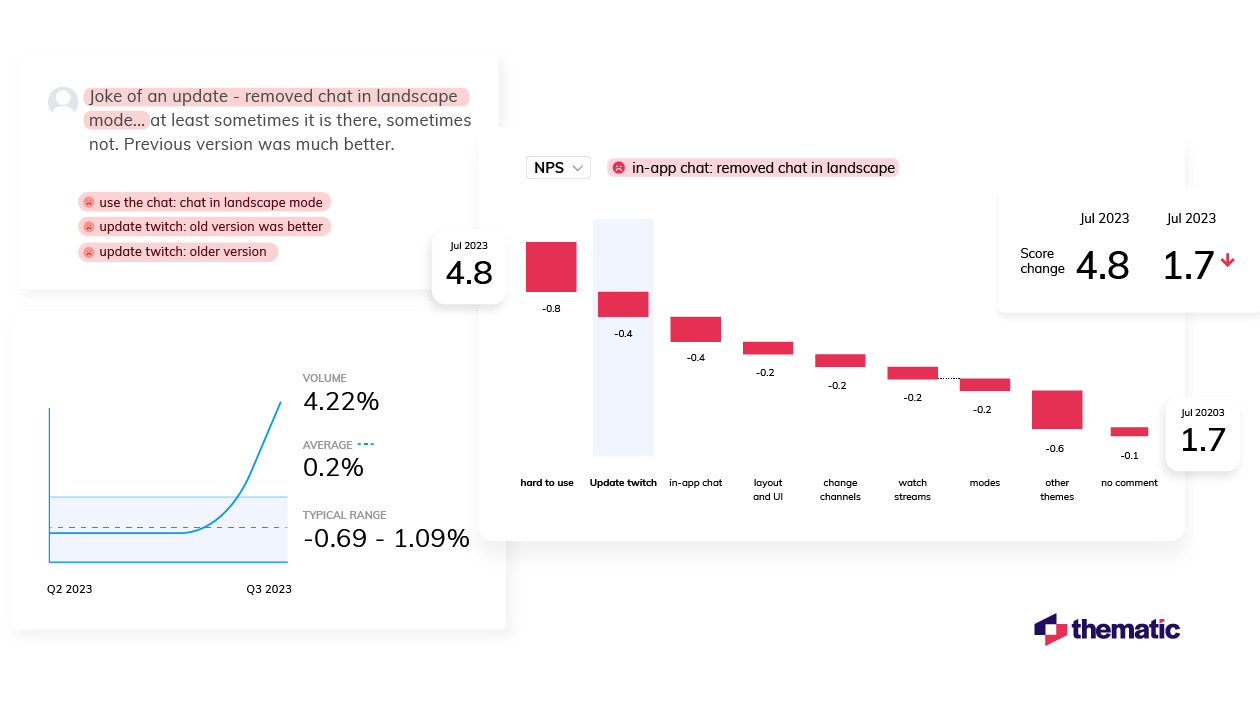
Conclusion - Manual or Automated?
There are those who remain deeply invested in the manual approach - because it’s familiar, because they’re reluctant to spend money and time learning new software, or because they’ve been burned by the overpromises of AI.
For projects that involve small datasets, manual analysis makes sense. For example, if the objective is simply to quantify a simple question like “Do customers prefer X concepts to Y?”. If the findings are being extracted from a small set of focus groups and interviews, sometimes it’s easier to just read them
However, as new generations come into the workplace, it’s technology-driven solutions that feel more comfortable and practical. And the merits are undeniable. Especially if the objective is to go deeper and understand the ‘why’ behind customers’ preference for X or Y. And even more especially if time and money are considerations.
The ability to collect a free flow of qualitative feedback data at the same time as the metric means AI can cost-effectively scan, crunch, score and analyze a ton of feedback from one system in one go. And time-intensive processes like focus groups, or coding, that used to take weeks, can now be completed in a matter of hours or days.
But aside from the ever-present business case to speed things up and keep costs down, there are also powerful research imperatives for automated analysis of qualitative data: namely, accuracy and consistency.
Finding insights hidden in feedback requires consistency, especially in coding. Not to mention catching all the ‘unknown unknowns’ that can skew research findings and steering clear of cognitive bias.
Some say without manual data analysis researchers won’t get an accurate “feel” for the insights. However, the larger data sets are, the harder it is to sort through the feedback and organize feedback that has been pulled from different places. And, the more difficult it is to stay on course, the greater the risk of drawing incorrect, or incomplete, conclusions grows.
Though the process steps for qualitative data analysis have remained pretty much unchanged since psychologist Paul Felix Lazarsfeld paved the path a hundred years ago, the impact digital technology has had on types of qualitative feedback data and the approach to the analysis are profound.
If you want to try an automated feedback analysis solution on your own qualitative data, you can get started with Thematic .
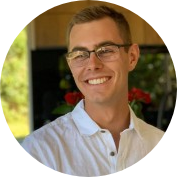
Community & Marketing
Tyler manages our community of CX, insights & analytics professionals. Tyler's goal is to help unite insights professionals around common challenges.
We make it easy to discover the customer and product issues that matter.
Unlock the value of feedback at scale, in one platform. Try it for free now!
- Questions to ask your Feedback Analytics vendor
- How to end customer churn for good
- Scalable analysis of NPS verbatims
- 5 Text analytics approaches
- How to calculate the ROI of CX
Our experts will show you how Thematic works, how to discover pain points and track the ROI of decisions. To access your free trial, book a personal demo today.
Recent posts
When two major storms wreaked havoc on Auckland and Watercare’s infrastructurem the utility went through a CX crisis. With a massive influx of calls to their support center, Thematic helped them get inisghts from this data to forge a new approach to restore services and satisfaction levels.
Become a qualitative theming pro! Creating a perfect code frame is hard, but thematic analysis software makes the process much easier.
Qualtrics is one of the most well-known and powerful Customer Feedback Management platforms. But even so, it has limitations. We recently hosted a live panel where data analysts from two well-known brands shared their experiences with Qualtrics, and how they extended this platform’s capabilities. Below, we’ll share the
- Privacy Policy

Home » Case Study – Methods, Examples and Guide
Case Study – Methods, Examples and Guide
Table of Contents

A case study is a research method that involves an in-depth examination and analysis of a particular phenomenon or case, such as an individual, organization, community, event, or situation.
It is a qualitative research approach that aims to provide a detailed and comprehensive understanding of the case being studied. Case studies typically involve multiple sources of data, including interviews, observations, documents, and artifacts, which are analyzed using various techniques, such as content analysis, thematic analysis, and grounded theory. The findings of a case study are often used to develop theories, inform policy or practice, or generate new research questions.
Types of Case Study
Types and Methods of Case Study are as follows:
Single-Case Study
A single-case study is an in-depth analysis of a single case. This type of case study is useful when the researcher wants to understand a specific phenomenon in detail.
For Example , A researcher might conduct a single-case study on a particular individual to understand their experiences with a particular health condition or a specific organization to explore their management practices. The researcher collects data from multiple sources, such as interviews, observations, and documents, and uses various techniques to analyze the data, such as content analysis or thematic analysis. The findings of a single-case study are often used to generate new research questions, develop theories, or inform policy or practice.
Multiple-Case Study
A multiple-case study involves the analysis of several cases that are similar in nature. This type of case study is useful when the researcher wants to identify similarities and differences between the cases.
For Example, a researcher might conduct a multiple-case study on several companies to explore the factors that contribute to their success or failure. The researcher collects data from each case, compares and contrasts the findings, and uses various techniques to analyze the data, such as comparative analysis or pattern-matching. The findings of a multiple-case study can be used to develop theories, inform policy or practice, or generate new research questions.
Exploratory Case Study
An exploratory case study is used to explore a new or understudied phenomenon. This type of case study is useful when the researcher wants to generate hypotheses or theories about the phenomenon.
For Example, a researcher might conduct an exploratory case study on a new technology to understand its potential impact on society. The researcher collects data from multiple sources, such as interviews, observations, and documents, and uses various techniques to analyze the data, such as grounded theory or content analysis. The findings of an exploratory case study can be used to generate new research questions, develop theories, or inform policy or practice.
Descriptive Case Study
A descriptive case study is used to describe a particular phenomenon in detail. This type of case study is useful when the researcher wants to provide a comprehensive account of the phenomenon.
For Example, a researcher might conduct a descriptive case study on a particular community to understand its social and economic characteristics. The researcher collects data from multiple sources, such as interviews, observations, and documents, and uses various techniques to analyze the data, such as content analysis or thematic analysis. The findings of a descriptive case study can be used to inform policy or practice or generate new research questions.
Instrumental Case Study
An instrumental case study is used to understand a particular phenomenon that is instrumental in achieving a particular goal. This type of case study is useful when the researcher wants to understand the role of the phenomenon in achieving the goal.
For Example, a researcher might conduct an instrumental case study on a particular policy to understand its impact on achieving a particular goal, such as reducing poverty. The researcher collects data from multiple sources, such as interviews, observations, and documents, and uses various techniques to analyze the data, such as content analysis or thematic analysis. The findings of an instrumental case study can be used to inform policy or practice or generate new research questions.
Case Study Data Collection Methods
Here are some common data collection methods for case studies:
Interviews involve asking questions to individuals who have knowledge or experience relevant to the case study. Interviews can be structured (where the same questions are asked to all participants) or unstructured (where the interviewer follows up on the responses with further questions). Interviews can be conducted in person, over the phone, or through video conferencing.
Observations
Observations involve watching and recording the behavior and activities of individuals or groups relevant to the case study. Observations can be participant (where the researcher actively participates in the activities) or non-participant (where the researcher observes from a distance). Observations can be recorded using notes, audio or video recordings, or photographs.
Documents can be used as a source of information for case studies. Documents can include reports, memos, emails, letters, and other written materials related to the case study. Documents can be collected from the case study participants or from public sources.
Surveys involve asking a set of questions to a sample of individuals relevant to the case study. Surveys can be administered in person, over the phone, through mail or email, or online. Surveys can be used to gather information on attitudes, opinions, or behaviors related to the case study.
Artifacts are physical objects relevant to the case study. Artifacts can include tools, equipment, products, or other objects that provide insights into the case study phenomenon.
How to conduct Case Study Research
Conducting a case study research involves several steps that need to be followed to ensure the quality and rigor of the study. Here are the steps to conduct case study research:
- Define the research questions: The first step in conducting a case study research is to define the research questions. The research questions should be specific, measurable, and relevant to the case study phenomenon under investigation.
- Select the case: The next step is to select the case or cases to be studied. The case should be relevant to the research questions and should provide rich and diverse data that can be used to answer the research questions.
- Collect data: Data can be collected using various methods, such as interviews, observations, documents, surveys, and artifacts. The data collection method should be selected based on the research questions and the nature of the case study phenomenon.
- Analyze the data: The data collected from the case study should be analyzed using various techniques, such as content analysis, thematic analysis, or grounded theory. The analysis should be guided by the research questions and should aim to provide insights and conclusions relevant to the research questions.
- Draw conclusions: The conclusions drawn from the case study should be based on the data analysis and should be relevant to the research questions. The conclusions should be supported by evidence and should be clearly stated.
- Validate the findings: The findings of the case study should be validated by reviewing the data and the analysis with participants or other experts in the field. This helps to ensure the validity and reliability of the findings.
- Write the report: The final step is to write the report of the case study research. The report should provide a clear description of the case study phenomenon, the research questions, the data collection methods, the data analysis, the findings, and the conclusions. The report should be written in a clear and concise manner and should follow the guidelines for academic writing.
Examples of Case Study
Here are some examples of case study research:
- The Hawthorne Studies : Conducted between 1924 and 1932, the Hawthorne Studies were a series of case studies conducted by Elton Mayo and his colleagues to examine the impact of work environment on employee productivity. The studies were conducted at the Hawthorne Works plant of the Western Electric Company in Chicago and included interviews, observations, and experiments.
- The Stanford Prison Experiment: Conducted in 1971, the Stanford Prison Experiment was a case study conducted by Philip Zimbardo to examine the psychological effects of power and authority. The study involved simulating a prison environment and assigning participants to the role of guards or prisoners. The study was controversial due to the ethical issues it raised.
- The Challenger Disaster: The Challenger Disaster was a case study conducted to examine the causes of the Space Shuttle Challenger explosion in 1986. The study included interviews, observations, and analysis of data to identify the technical, organizational, and cultural factors that contributed to the disaster.
- The Enron Scandal: The Enron Scandal was a case study conducted to examine the causes of the Enron Corporation’s bankruptcy in 2001. The study included interviews, analysis of financial data, and review of documents to identify the accounting practices, corporate culture, and ethical issues that led to the company’s downfall.
- The Fukushima Nuclear Disaster : The Fukushima Nuclear Disaster was a case study conducted to examine the causes of the nuclear accident that occurred at the Fukushima Daiichi Nuclear Power Plant in Japan in 2011. The study included interviews, analysis of data, and review of documents to identify the technical, organizational, and cultural factors that contributed to the disaster.
Application of Case Study
Case studies have a wide range of applications across various fields and industries. Here are some examples:
Business and Management
Case studies are widely used in business and management to examine real-life situations and develop problem-solving skills. Case studies can help students and professionals to develop a deep understanding of business concepts, theories, and best practices.
Case studies are used in healthcare to examine patient care, treatment options, and outcomes. Case studies can help healthcare professionals to develop critical thinking skills, diagnose complex medical conditions, and develop effective treatment plans.
Case studies are used in education to examine teaching and learning practices. Case studies can help educators to develop effective teaching strategies, evaluate student progress, and identify areas for improvement.
Social Sciences
Case studies are widely used in social sciences to examine human behavior, social phenomena, and cultural practices. Case studies can help researchers to develop theories, test hypotheses, and gain insights into complex social issues.
Law and Ethics
Case studies are used in law and ethics to examine legal and ethical dilemmas. Case studies can help lawyers, policymakers, and ethical professionals to develop critical thinking skills, analyze complex cases, and make informed decisions.
Purpose of Case Study
The purpose of a case study is to provide a detailed analysis of a specific phenomenon, issue, or problem in its real-life context. A case study is a qualitative research method that involves the in-depth exploration and analysis of a particular case, which can be an individual, group, organization, event, or community.
The primary purpose of a case study is to generate a comprehensive and nuanced understanding of the case, including its history, context, and dynamics. Case studies can help researchers to identify and examine the underlying factors, processes, and mechanisms that contribute to the case and its outcomes. This can help to develop a more accurate and detailed understanding of the case, which can inform future research, practice, or policy.
Case studies can also serve other purposes, including:
- Illustrating a theory or concept: Case studies can be used to illustrate and explain theoretical concepts and frameworks, providing concrete examples of how they can be applied in real-life situations.
- Developing hypotheses: Case studies can help to generate hypotheses about the causal relationships between different factors and outcomes, which can be tested through further research.
- Providing insight into complex issues: Case studies can provide insights into complex and multifaceted issues, which may be difficult to understand through other research methods.
- Informing practice or policy: Case studies can be used to inform practice or policy by identifying best practices, lessons learned, or areas for improvement.
Advantages of Case Study Research
There are several advantages of case study research, including:
- In-depth exploration: Case study research allows for a detailed exploration and analysis of a specific phenomenon, issue, or problem in its real-life context. This can provide a comprehensive understanding of the case and its dynamics, which may not be possible through other research methods.
- Rich data: Case study research can generate rich and detailed data, including qualitative data such as interviews, observations, and documents. This can provide a nuanced understanding of the case and its complexity.
- Holistic perspective: Case study research allows for a holistic perspective of the case, taking into account the various factors, processes, and mechanisms that contribute to the case and its outcomes. This can help to develop a more accurate and comprehensive understanding of the case.
- Theory development: Case study research can help to develop and refine theories and concepts by providing empirical evidence and concrete examples of how they can be applied in real-life situations.
- Practical application: Case study research can inform practice or policy by identifying best practices, lessons learned, or areas for improvement.
- Contextualization: Case study research takes into account the specific context in which the case is situated, which can help to understand how the case is influenced by the social, cultural, and historical factors of its environment.
Limitations of Case Study Research
There are several limitations of case study research, including:
- Limited generalizability : Case studies are typically focused on a single case or a small number of cases, which limits the generalizability of the findings. The unique characteristics of the case may not be applicable to other contexts or populations, which may limit the external validity of the research.
- Biased sampling: Case studies may rely on purposive or convenience sampling, which can introduce bias into the sample selection process. This may limit the representativeness of the sample and the generalizability of the findings.
- Subjectivity: Case studies rely on the interpretation of the researcher, which can introduce subjectivity into the analysis. The researcher’s own biases, assumptions, and perspectives may influence the findings, which may limit the objectivity of the research.
- Limited control: Case studies are typically conducted in naturalistic settings, which limits the control that the researcher has over the environment and the variables being studied. This may limit the ability to establish causal relationships between variables.
- Time-consuming: Case studies can be time-consuming to conduct, as they typically involve a detailed exploration and analysis of a specific case. This may limit the feasibility of conducting multiple case studies or conducting case studies in a timely manner.
- Resource-intensive: Case studies may require significant resources, including time, funding, and expertise. This may limit the ability of researchers to conduct case studies in resource-constrained settings.
About the author
Muhammad Hassan
Researcher, Academic Writer, Web developer
You may also like

One-to-One Interview – Methods and Guide

Exploratory Research – Types, Methods and...

Research Methods – Types, Examples and Guide

Ethnographic Research -Types, Methods and Guide

Quantitative Research – Methods, Types and...

Qualitative Research Methods

Research Writing and Analysis
- NVivo Group and Study Sessions
- SPSS This link opens in a new window
- Statistical Analysis Group sessions
- Using Qualtrics
- Dissertation and Data Analysis Group Sessions
- Defense Schedule - Commons Calendar This link opens in a new window
- Research Process Flow Chart
- Research Alignment Chapter 1 This link opens in a new window
- Step 1: Seek Out Evidence
- Step 2: Explain
- Step 3: The Big Picture
- Step 4: Own It
- Step 5: Illustrate
- Annotated Bibliography
- Literature Review This link opens in a new window
- Systematic Reviews & Meta-Analyses
- How to Synthesize and Analyze
- Synthesis and Analysis Practice
- Synthesis and Analysis Group Sessions
- Problem Statement
- Purpose Statement
- Conceptual Framework
- Theoretical Framework
- Locating Theoretical and Conceptual Frameworks This link opens in a new window
- Quantitative Research Questions
- Qualitative Research Questions
- Trustworthiness of Qualitative Data
- Analysis and Coding Example- Qualitative Data
- Thematic Data Analysis in Qualitative Design
- Dissertation to Journal Article This link opens in a new window
- International Journal of Online Graduate Education (IJOGE) This link opens in a new window
- Journal of Research in Innovative Teaching & Learning (JRIT&L) This link opens in a new window
Writing a Case Study

What is a case study?

A Case study is:
- An in-depth research design that primarily uses a qualitative methodology but sometimes includes quantitative methodology.
- Used to examine an identifiable problem confirmed through research.
- Used to investigate an individual, group of people, organization, or event.
- Used to mostly answer "how" and "why" questions.
What are the different types of case studies?

Note: These are the primary case studies. As you continue to research and learn
about case studies you will begin to find a robust list of different types.
Who are your case study participants?

What is triangulation ?
Validity and credibility are an essential part of the case study. Therefore, the researcher should include triangulation to ensure trustworthiness while accurately reflecting what the researcher seeks to investigate.

How to write a Case Study?
When developing a case study, there are different ways you could present the information, but remember to include the five parts for your case study.

Was this resource helpful?
- << Previous: Thematic Data Analysis in Qualitative Design
- Next: Journal Article Reporting Standards (JARS) >>
- Last Updated: May 29, 2024 8:05 AM
- URL: https://resources.nu.edu/researchtools

Case Study Research in Software Engineering: Guidelines and Examples by Per Runeson, Martin Höst, Austen Rainer, Björn Regnell
Get full access to Case Study Research in Software Engineering: Guidelines and Examples and 60K+ other titles, with a free 10-day trial of O'Reilly.
There are also live events, courses curated by job role, and more.
DATA ANALYSIS AND INTERPRETATION
5.1 introduction.
Once data has been collected the focus shifts to analysis of data. It can be said that in this phase, data is used to understand what actually has happened in the studied case, and where the researcher understands the details of the case and seeks patterns in the data. This means that there inevitably is some analysis going on also in the data collection phase where the data is studied, and for example when data from an interview is transcribed. The understandings in the earlier phases are of course also valid and important, but this chapter is more focusing on the separate phase that starts after the data has been collected.
Data analysis is conducted differently for quantitative and qualitative data. Sections 5.2 – 5.5 describe how to analyze qualitative data and how to assess the validity of this type of analysis. In Section 5.6 , a short introduction to quantitative analysis methods is given. Since quantitative analysis is covered extensively in textbooks on statistical analysis, and case study research to a large extent relies on qualitative data, this section is kept short.
5.2 ANALYSIS OF DATA IN FLEXIBLE RESEARCH
5.2.1 introduction.
As case study research is a flexible research method, qualitative data analysis methods are commonly used [176]. The basic objective of the analysis is, as in any other analysis, to derive conclusions from the data, keeping a clear chain of evidence. The chain of evidence means that a reader ...
Get Case Study Research in Software Engineering: Guidelines and Examples now with the O’Reilly learning platform.
O’Reilly members experience books, live events, courses curated by job role, and more from O’Reilly and nearly 200 top publishers.
Don’t leave empty-handed
Get Mark Richards’s Software Architecture Patterns ebook to better understand how to design components—and how they should interact.
It’s yours, free.
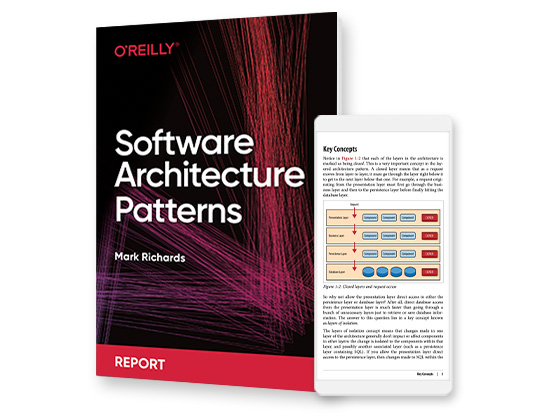
Check it out now on O’Reilly
Dive in for free with a 10-day trial of the O’Reilly learning platform—then explore all the other resources our members count on to build skills and solve problems every day.
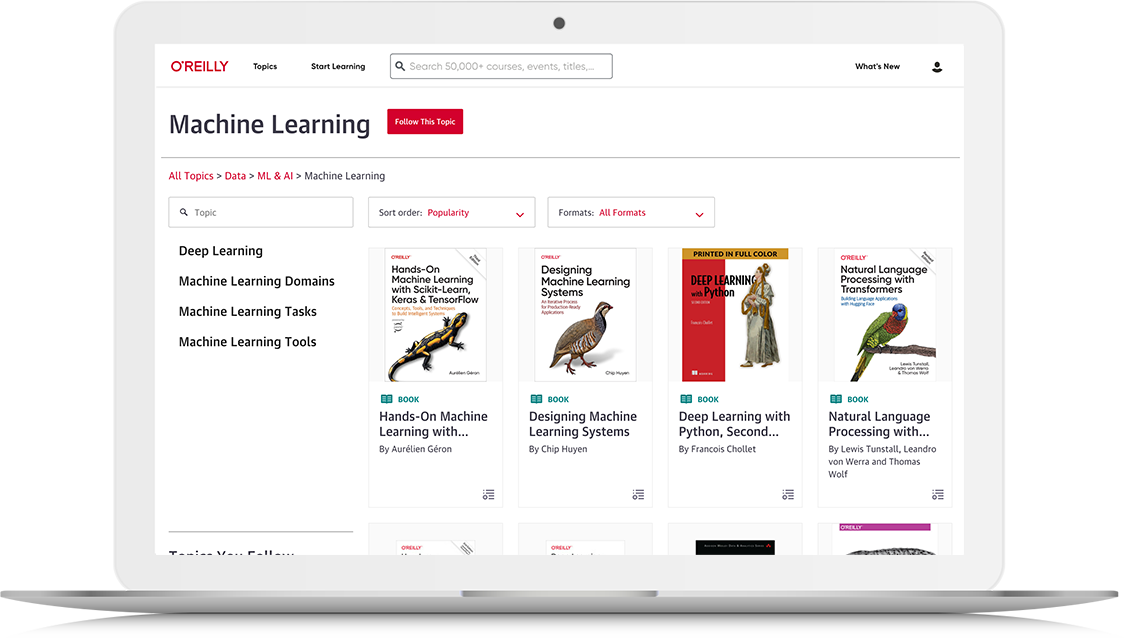
- Article Writing Affordable Article Writing Services
- Blog Writing Blogs that optimise your visibility
- Product Description Website that optimise your visibility
- Website Writing Website that optimise your visibility
- Proofreading Website that optimise your visibility
- Translation Website that optimise your visibility
- Agriculture Affordable Article Writing Services
- Health & Beauty Blogs that optimise your visibility
- Automotive Website that optimise your visibility
- Sports & fitness Website that optimise your visibility
- Real Estate Website that optimise your visibility
- Entertainment Website that optimise your visibility
- Blogs Affordable Article Writing Services
- Samples Blogs that optimise your visibility
- Case Study Website that optimise your visibility
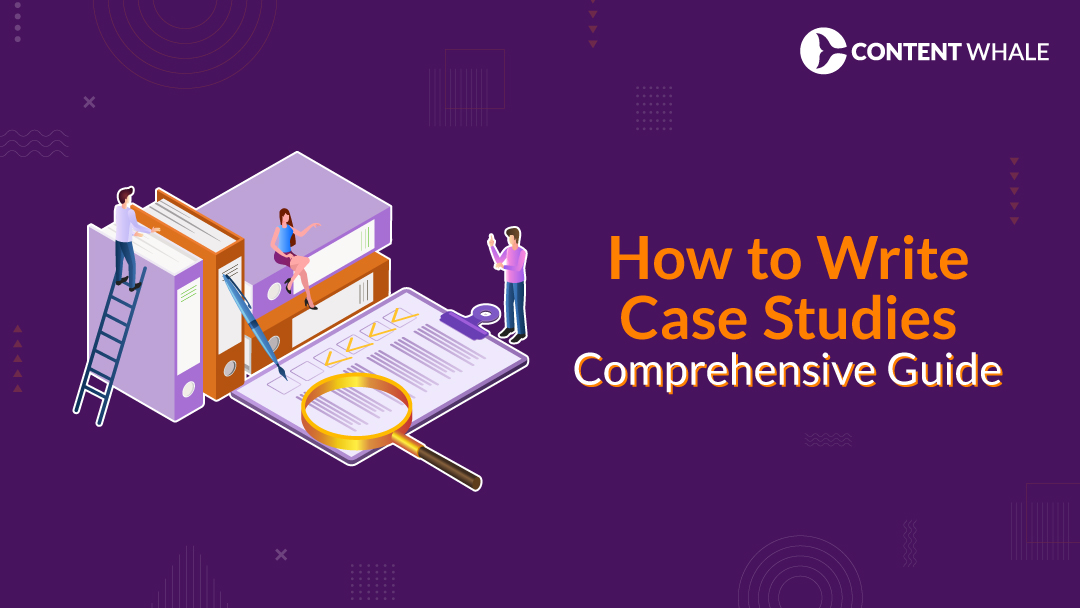
“How to Write Case Studies: A Comprehensive Guide”
Case studies are essential for marketing and research, offering in-depth insights into successes and problem-solving methods. This blog explains how to write case studies, including steps for creating them, tips for analysis, and case study examples. You'll also find case study templates to simplify the process. Effective case studies establish credibility, enhance marketing efforts, and provide valuable insights for future projects.
Case studies are detailed examinations of subjects like businesses, organizations, or individuals. They are used to highlight successes and problem-solving methods. They are crucial in marketing, education, and research to provide concrete examples and insights.
This blog will explain how to write case studies and their importance. We will cover different applications of case studies and a step-by-step process to create them. You’ll find tips for conducting case study analysis, along with case study examples and case study templates.
Effective case studies are vital. They showcase success stories and problem-solving skills, establishing credibility. This guide will teach you how to create a case study that engages your audience and enhances your marketing and research efforts.
What are Case Studies?
1. Definition and Purpose of a Case Study
Case studies are in-depth explorations of specific subjects to understand dynamics and outcomes. They provide detailed insights that can be generalized to broader contexts.
2. Different Types of Case Studies
- Exploratory: Investigates an area with limited information.
- Explanatory: Explains reasons behind a phenomenon.
- Descriptive: Provides a detailed account of the subject.
- Intrinsic : Focuses on a unique subject.
- Instrumental: Uses the case to understand a broader issue.
3. Benefits of Using Case Studies
Case studies offer many benefits. They provide real-world examples to illustrate theories or concepts. Businesses can demonstrate the effectiveness of their products or services. Researchers gain detailed insights into specific phenomena. Educators use them to teach through practical examples. Learning how to write case studies can enhance your marketing and research efforts.
Understanding how to create a case study involves recognizing these benefits. Case study examples show practical applications. Using case study templates can simplify the process.
5 Steps to Write a Case Study
1. Identifying the Subject or Case
Choose a subject that aligns with your objectives and offers valuable insights. Ensure the subject has a clear narrative and relevance to your audience. The subject should illustrate key points and provide substantial learning opportunities. Common subjects include successful projects, client stories, or significant business challenges.
2. Conducting Thorough Research and Data Collection
Gather comprehensive data from multiple sources. Conduct interviews with key stakeholders, such as clients, team members, or industry experts. Use surveys to collect quantitative data. Review documents, reports, and any relevant records. Ensure the information is accurate, relevant, and up-to-date. This thorough research forms the foundation for how to write case studies that are credible and informative.
3. Structuring the Case Study
Organize your case study into these sections:
- Introduction: Introduce the subject and its significance. Provide an overview of what will be covered.
- Background: Provide context and background information. Describe the subject’s history, environment, and any relevant details.
- Case Presentation: Detail the case, including the problem or challenge faced. Discuss the actions taken to address the issue.
- Analysis: Analyze the data and discuss the findings. Highlight key insights, patterns, and outcomes.
- Conclusion: Summarize the outcomes and key takeaways. Reflect on the broader implications and lessons learned.
4. Writing a Compelling Introduction
The introduction should grab the reader’s attention. Start with a hook, such as an interesting fact, quote, or question. Provide a brief overview of the subject and its importance. Explain why this case is relevant and worth studying. An engaging introduction sets the stage for how to create a case study that keeps readers interested.
5. Providing Background Information and Context
Give readers the necessary background to understand the case. Include details about the subject’s history, environment, and any relevant circumstances. Explain the context in which the case exists, such as the industry, market conditions, or organizational culture. Providing a solid foundation helps readers grasp the significance of the case and enhances the credibility of your study.
Understanding how to write a case study involves meticulous research and a clear structure. Utilizing case study examples and templates can guide you through the process, ensuring you present your findings effectively. These steps are essential for writing informative, engaging, and impactful case studies.
How to Write Case Study Analysis
1. Analyzing the Data Collected
Examine the data to identify patterns, trends, and key findings. Use qualitative and quantitative methods to ensure a comprehensive analysis. Validate the data’s accuracy and relevance to the subject. Look for correlations and causations that can provide deeper insights.
2. Identifying Key Issues and Problems
Pinpoint the main issues or challenges faced by the subject. Determine the root causes of these problems. Use tools like SWOT analysis (Strengths, Weaknesses, Opportunities, Threats) to get a clear picture. Prioritize the issues based on their impact and urgency.
3. Discussing Possible Solutions and Their Implementation
Explore various solutions that address the identified issues. Compare the potential effectiveness of each solution. Discuss the steps taken to implement the chosen solutions. Highlight the decision-making process and the rationale behind it. Include any obstacles faced during implementation and how they were overcome.
4. Evaluating the Results and Outcomes
Assess the outcomes of the implemented solutions. Use metrics and KPIs (Key Performance Indicators) to measure success. Compare the results with the initial objectives and expectations. Discuss any deviations and their reasons. Provide evidence to support your evaluation, such as before-and-after data or testimonials.
5. Providing Insights and Lessons Learned
Reflect on the insights gained from the case study. Discuss what worked well and what didn’t. Highlight lessons that can be applied to similar situations. Provide actionable recommendations for future projects. This section should offer valuable takeaways for the readers, helping them understand how to create a case study that is insightful and practical.
Mastering how to write case studies involves understanding each part of the analysis. Use case study examples to see how these elements are applied. Case study templates can help you structure your work. Knowing how to make a case study analysis will make your findings clear and actionable.
Case Study Examples and Templates
1. Showcasing Successful Case Studies
Georgia tech athletics increase season ticket sales by 80%.
Georgia Tech Athletics aimed to enhance their season ticket sales and engagement with fans. Their initial strategy involved multiple outbound phone calls without targeting. They partnered with Salesloft to improve their sales process with a more structured inbound approach. This allowed sales reps to target communications effectively. As a result, Georgia Tech saw an 80% increase in season ticket sales, with improved employee engagement and fan relationships.
WeightWatchers Revamps Enterprise Sales Process with HubSpot
WeightWatchers sought to improve their sales efficiency. Their previous system lacked automation, requiring extensive manual effort. By adopting HubSpot’s CRM, WeightWatchers streamlined their sales process. The automation capabilities of HubSpot allowed them to manage customer interactions more effectively. This transition significantly enhanced their operational efficiency and sales performance.
2. Breakdown of What Makes These Examples Effective
These case study examples are effective due to their clear structure and compelling storytelling. They:
- Identify the problem: Each case study begins by outlining the challenges faced by the client.
- Detail the solution: They explain the specific solutions implemented to address these challenges.
- Showcase the results: Quantifiable results and improvements are highlighted, demonstrating the effectiveness of the solutions.
- Use visuals and quotes: Incorporating images, charts, and client testimonials enhances engagement and credibility.
3. Providing Case Study Templates
To assist in creating your own case studies, here are some recommended case study templates:
1. General Case Study Template
- Suitable for various industries and applications.
- Includes sections for background, problem, solution, and results.
- Helps provide a structured narrative for any case study.
2. Data-Driven Case Study Template
- Focuses on presenting metrics and data.
- Ideal for showcasing quantitative achievements.
- Structured to highlight significant performance improvements and achievements.
3. Product-Specific Case Study Template
- Emphasizes customer experiences and satisfaction with a specific product.
- Highlights benefits and features of the product rather than the process.
4. Tips for Customizing Templates to Fit Your Needs
When using case study templates, tailor them to match the specific context of your study. Consider the following tips:
- Adapt the language and tone: Ensure it aligns with your brand voice and audience.
- Include relevant visuals: Add charts, graphs, and images to support your narrative.
- Personalize the content: Use specific details about the subject to make the case study unique and relatable.
Utilizing these examples and templates will guide you in how to write case studies effectively. They provide a clear framework for how to create a case study that is engaging and informative. Learning how to make a case study becomes more manageable with these resources and examples.
Tips for Creating Compelling Case Studies
1. Using Storytelling Techniques to Engage Readers
Incorporate storytelling techniques to make your case study engaging. A compelling narrative holds the reader’s attention.
2. Including Quotes and Testimonials from Participants
Add quotes and testimonials to add credibility. Participant feedback enhances the authenticity of your study.
3. Visual Aids: Charts, Graphs, and Images to Support Your Case
Use charts, graphs, and images to illustrate key points. Visual aids help in better understanding and retention.
4. Ensuring Clarity and Conciseness in Writing
Write clearly and concisely to maintain reader interest. Avoid jargon and ensure your writing is easy to follow.
5. Highlighting the Impact and Benefits
Emphasize the positive outcomes and benefits. Show how the subject has improved or achieved success.
Understanding how to write case studies involves using effective storytelling and visuals. Case study examples show how to engage readers, and case study templates help organize your content. Learning how to make a case study ensures that it is clear and impactful.
Benefits of Using Case Studies
1. Establishing Authority and Credibility
How to write case studies can effectively establish your authority. Showcasing success stories builds credibility in your field.
2. Demonstrating Practical Applications of Your Product or Service
Case study examples demonstrate how your product or service solves real-world problems. This practical evidence is convincing for potential clients.
3. Enhancing Marketing and Sales Efforts
Use case studies to support your marketing and sales strategies. They highlight your successes and attract new customers.
4. Providing Valuable Insights for Future Projects
Case studies offer insights that can guide future projects. Learning how to create a case study helps in applying these lessons effectively.
5. Engaging and Educating Your Audience
Case studies are engaging and educational. They provide detailed examples and valuable lessons. Using case study templates can make this process easier and more effective. Understanding how to make a case study ensures you can communicate these benefits clearly.
Writing effective case studies involves thorough research, clear structure, and engaging content. By following these steps, you’ll learn how to write case studies that showcase your success stories and problem-solving skills. Use the case study examples and case study templates provided to get started. Well-crafted case studies are valuable tools for marketing, research, and education. Start learning how to make a case study today and share your success stories with the world.
What is the purpose of a case study?
A case study provides detailed insights into a subject, illustrating successes and solutions. It helps in understanding complex issues.
How do I choose a subject for my case study?
Select a subject that aligns with your objectives and offers valuable insights. Ensure it has a clear narrative.
What are the key components of a case study analysis?
A case study analysis includes data collection, identifying key issues, discussing solutions, evaluating outcomes, and providing insights.
Where can I find case study templates?
You can find downloadable case study templates online. They simplify the process of creating a case study.
How can case studies benefit my business?
Case studies establish credibility, demonstrate practical applications, enhance marketing efforts, and provide insights for future projects. Learning how to create a case study can significantly benefit your business.
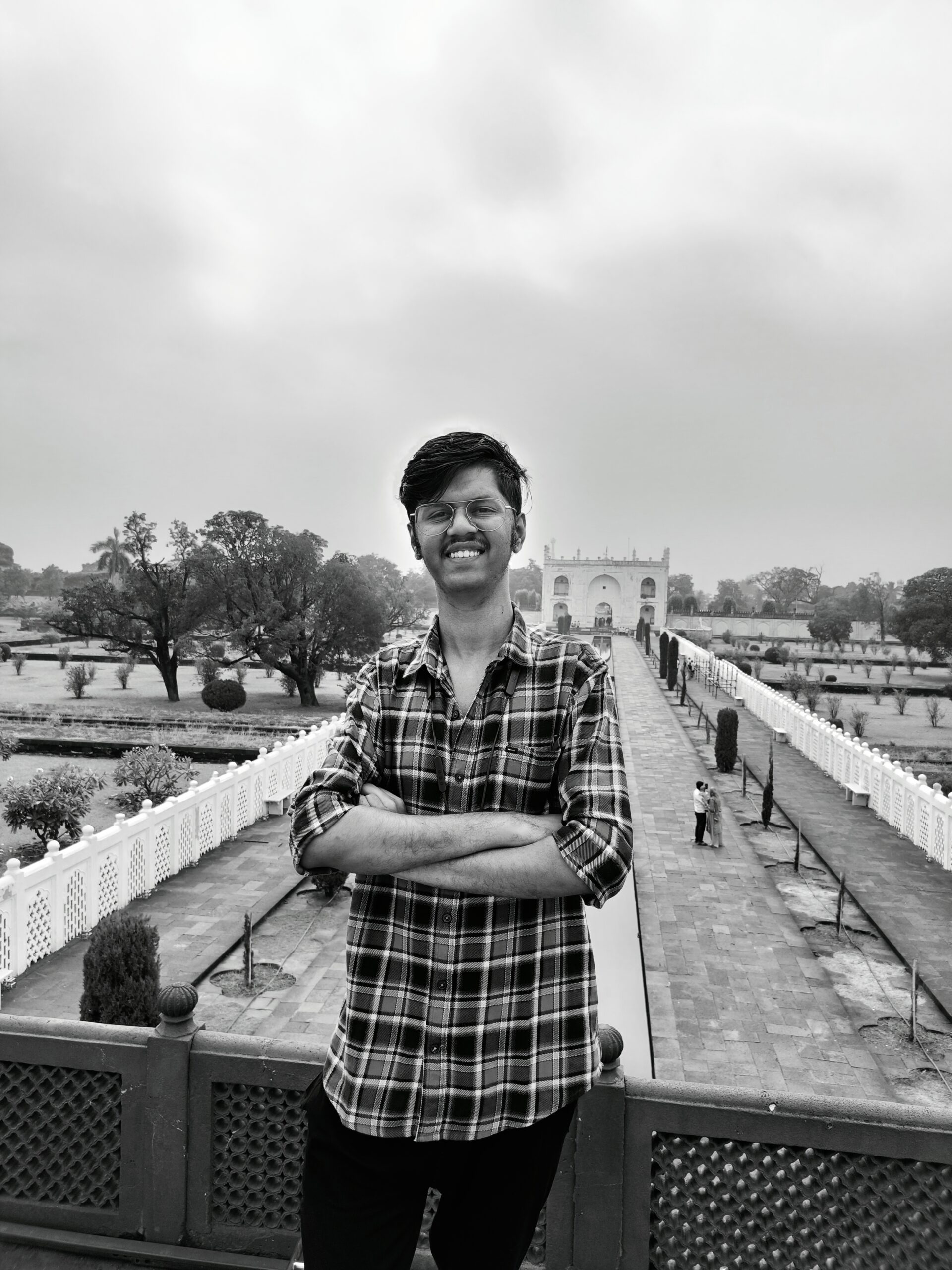
I am currently pursuing my Masters in Communication and Journalism from University of Mumbai. I am the author of four self published books. I am interested inv writing for films and TV. I run a blog where I write about film reviews.
More details for blogs
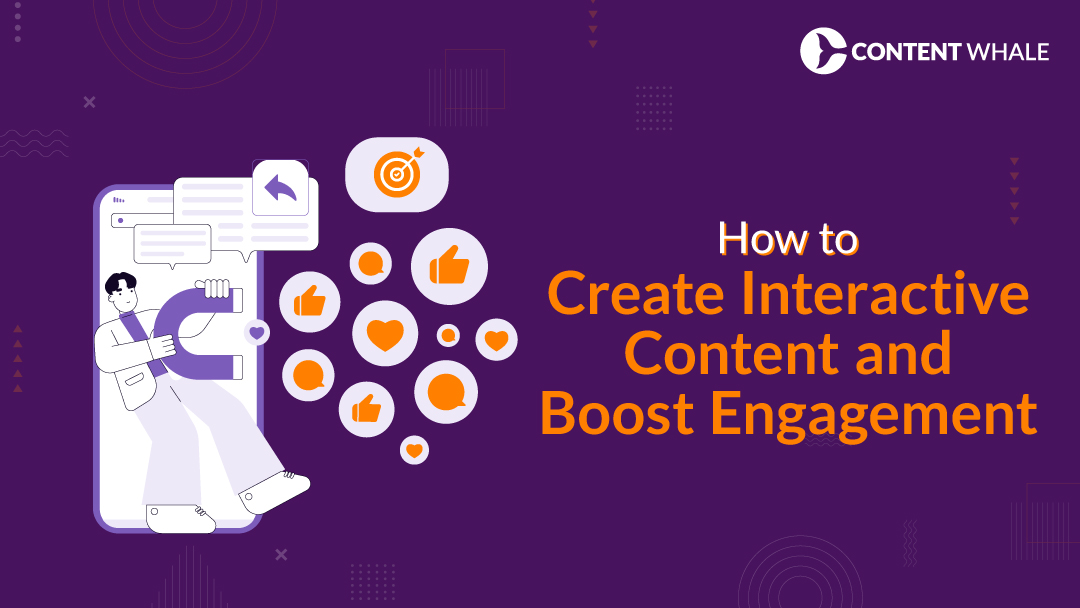
How to Create Interactive Content and Boost Engagement
Learn how to create interactive content to engage your audience. Discover tools, strategies, and benefits of using interactive elements.
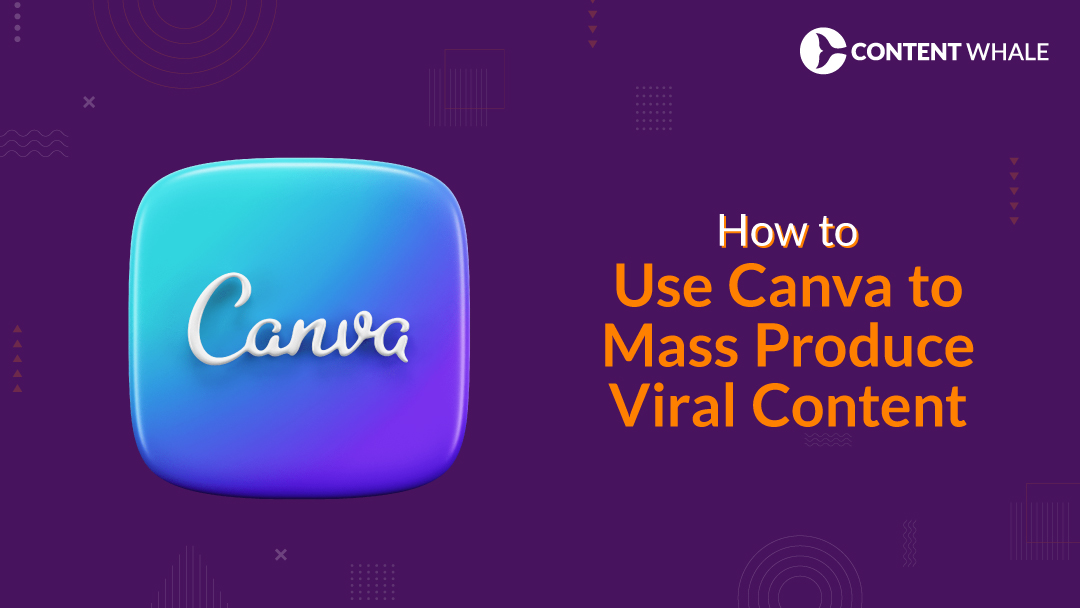
How to Use Canva to Mass Produce Viral Content
Discover how to use Canva to mass produce viral content with these expert tips and strategies. Boost your content creation game today!
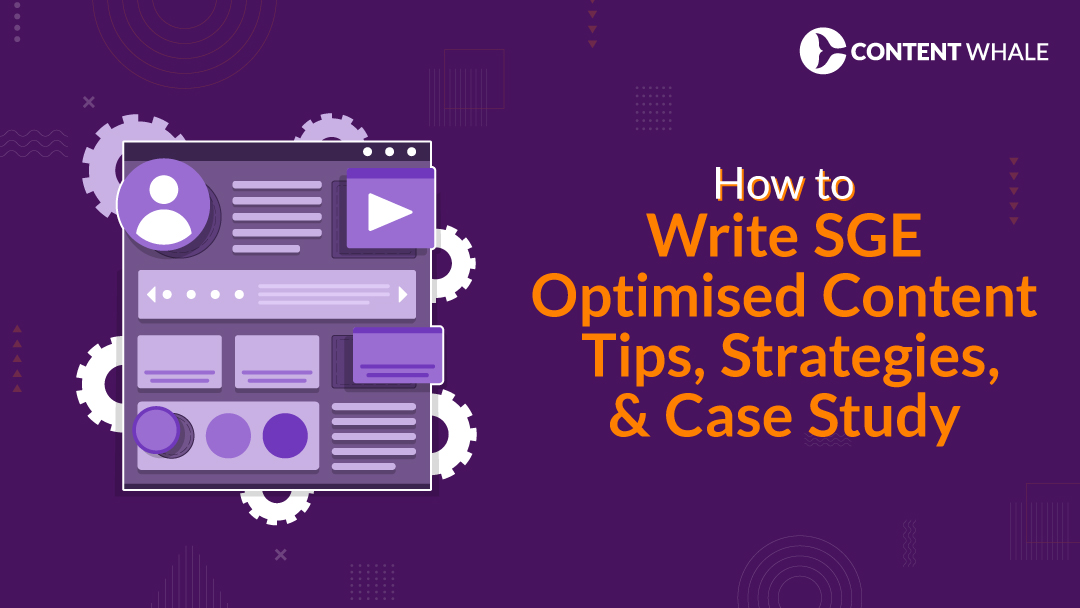
SGE Ranking Strategies: How to Get Rankings on SGEs
Learn how to create answers that rank for SGE with our comprehensive guide on SGE ranking strategies. Powerful tips and examples!
Need assistance with something
Speak with our expert right away to receive free service-related advice.
Sales CRM Terms
What is Case Study Analysis? (Explained With Examples)
Oct 11, 2023
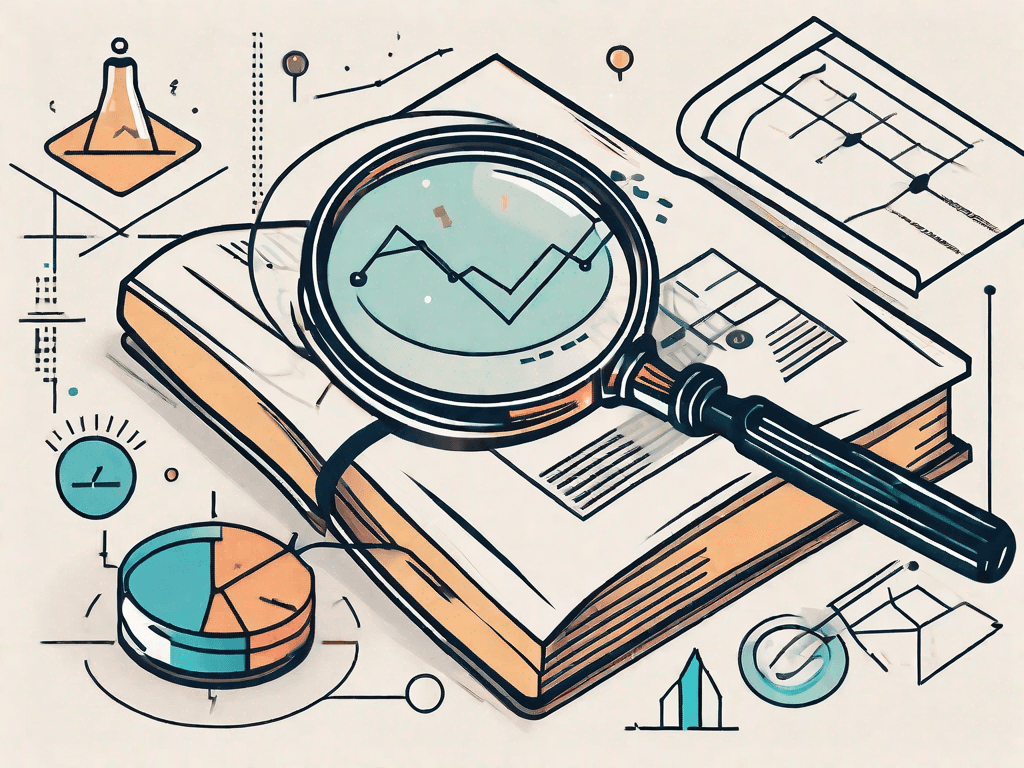
Case Study Analysis is a widely used research method that examines in-depth information about a particular individual, group, organization, or event. It is a comprehensive investigative approach that aims to understand the intricacies and complexities of the subject under study. Through the analysis of real-life scenarios and inquiry into various data sources, Case Study Analysis provides valuable insights and knowledge that can be used to inform decision-making and problem-solving strategies.
1°) What is Case Study Analysis?
Case Study Analysis is a research methodology that involves the systematic investigation of a specific case or cases to gain a deep understanding of the subject matter. This analysis encompasses collecting and analyzing various types of data, including qualitative and quantitative information. By examining multiple aspects of the case, such as its context, background, influences, and outcomes, researchers can draw meaningful conclusions and provide valuable insights for various fields of study.
When conducting a Case Study Analysis, researchers typically begin by selecting a case or multiple cases that are relevant to their research question or area of interest. This can involve choosing a specific organization, individual, event, or phenomenon to study. Once the case is selected, researchers gather relevant data through various methods, such as interviews, observations, document analysis, and artifact examination.
The data collected during a Case Study Analysis is then carefully analyzed and interpreted. Researchers use different analytical frameworks and techniques to make sense of the information and identify patterns, themes, and relationships within the data. This process involves coding and categorizing the data, conducting comparative analysis, and drawing conclusions based on the findings.
One of the key strengths of Case Study Analysis is its ability to provide a rich and detailed understanding of a specific case. This method allows researchers to delve deep into the complexities and nuances of the subject matter, uncovering insights that may not be captured through other research methods. By examining the case in its natural context, researchers can gain a holistic perspective and explore the various factors and variables that contribute to the case.
1.1 - Definition of Case Study Analysis
Case Study Analysis can be defined as an in-depth examination and exploration of a particular case or cases to unravel relevant details and complexities associated with the subject being studied. It involves a comprehensive and detailed analysis of various factors and variables that contribute to the case, aiming to answer research questions and uncover insights that can be applied in real-world scenarios.
When conducting a Case Study Analysis, researchers employ a range of research methods and techniques to collect and analyze data. These methods can include interviews, surveys, observations, document analysis, and experiments, among others. By using multiple sources of data, researchers can triangulate their findings and ensure the validity and reliability of their analysis.
Furthermore, Case Study Analysis often involves the use of theoretical frameworks and models to guide the research process. These frameworks provide a structured approach to analyzing the case and help researchers make sense of the data collected. By applying relevant theories and concepts, researchers can gain a deeper understanding of the underlying factors and dynamics at play in the case.
1.2 - Advantages of Case Study Analysis
Case Study Analysis offers numerous advantages that make it a popular research method across different disciplines. One significant advantage is its ability to provide rich and detailed information about a specific case, allowing researchers to gain a holistic understanding of the subject matter. Additionally, Case Study Analysis enables researchers to explore complex issues and phenomena in their natural context, capturing the intricacies and nuances that may not be captured through other research methods.
Moreover, Case Study Analysis allows researchers to investigate rare or unique cases that may not be easily replicated or studied through experimental methods. This method is particularly useful when studying phenomena that are complex, multifaceted, or involve multiple variables. By examining real-world cases, researchers can gain insights that can be applied to similar situations or inform future research and practice.
Furthermore, this research method allows for the analysis of multiple sources of data, such as interviews, observations, documents, and artifacts, which can contribute to a comprehensive and well-rounded examination of the case. Case Study Analysis also facilitates the exploration and identification of patterns, trends, and relationships within the data, generating valuable insights and knowledge for future reference and application.
1.3 - Disadvantages of Case Study Analysis
While Case Study Analysis offers various advantages, it also comes with certain limitations and challenges. One major limitation is the potential for researcher bias, as the interpretation of data and findings can be influenced by preconceived notions and personal perspectives. Researchers must be aware of their own biases and take steps to minimize their impact on the analysis.
Additionally, Case Study Analysis may suffer from limited generalizability, as it focuses on specific cases and contexts, which might not be applicable or representative of broader populations or situations. The findings of a case study may not be easily generalized to other settings or individuals, and caution should be exercised when applying the results to different contexts.
Moreover, Case Study Analysis can require significant time and resources due to its in-depth nature and the need for meticulous data collection and analysis. This can pose challenges for researchers working with limited budgets or tight deadlines. However, the thoroughness and depth of the analysis often outweigh the resource constraints, as the insights gained from a well-conducted case study can be highly valuable.
Finally, ethical considerations also play a crucial role in Case Study Analysis, as researchers must ensure the protection of participant confidentiality and privacy. Researchers must obtain informed consent from participants and take measures to safeguard their identities and personal information. Ethical guidelines and protocols should be followed to ensure the rights and well-being of the individuals involved in the case study.
2°) Examples of Case Study Analysis
Real-world examples of Case Study Analysis demonstrate the method's practical application and showcase its usefulness across various fields. The following examples provide insights into different scenarios where Case Study Analysis has been employed successfully.
2.1 - Example in a Startup Context
In a startup context, a Case Study Analysis might explore the factors that contributed to the success of a particular startup company. It would involve examining the organization's background, strategies, market conditions, and key decision-making processes. This analysis could reveal valuable lessons and insights for aspiring entrepreneurs and those interested in understanding the intricacies of startup success.
2.2 - Example in a Consulting Context
In the consulting industry, Case Study Analysis is often utilized to understand and develop solutions for complex business problems. For instance, a consulting firm might conduct a Case Study Analysis on a company facing challenges in its supply chain management. This analysis would involve identifying the underlying issues, evaluating different options, and proposing recommendations based on the findings. This approach enables consultants to apply their expertise and provide practical solutions to their clients.
2.3 - Example in a Digital Marketing Agency Context
Within a digital marketing agency, Case Study Analysis can be used to examine successful marketing campaigns. By analyzing various factors such as target audience, message effectiveness, channel selection, and campaign metrics, this analysis can provide valuable insights into the strategies and tactics that contribute to successful marketing initiatives. Digital marketers can then apply these insights to optimize future campaigns and drive better results for their clients.
2.4 - Example with Analogies
Case Study Analysis can also be utilized with analogies to investigate specific scenarios and draw parallels to similar situations. For instance, a Case Study Analysis could explore the response of different countries to natural disasters and draw analogies to inform disaster management strategies in other regions. These analogies can help policymakers and researchers develop more effective approaches to mitigate the impact of disasters and protect vulnerable populations.
In conclusion, Case Study Analysis is a powerful research method that provides a comprehensive understanding of a particular individual, group, organization, or event. By analyzing real-life cases and exploring various data sources, researchers can unravel complexities, generate valuable insights, and inform decision-making processes. With its advantages and limitations, Case Study Analysis offers a unique approach to gaining in-depth knowledge and practical application across numerous fields.
About the author

Arnaud Belinga

Close deals x2 faster with
Breakcold sales crm.
SEE PRICING
*No credit card required
Related Articles
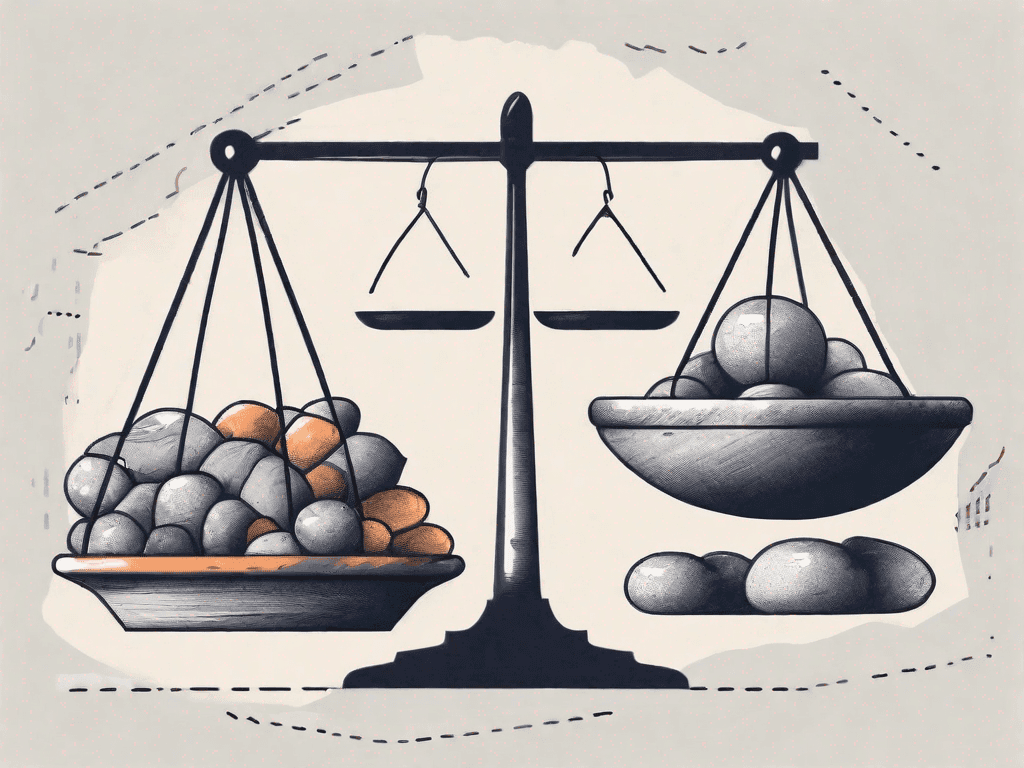
What is the 80-20 rule? (Explained With Examples)

What is the ABCD Sales Method? (Explained With Examples)
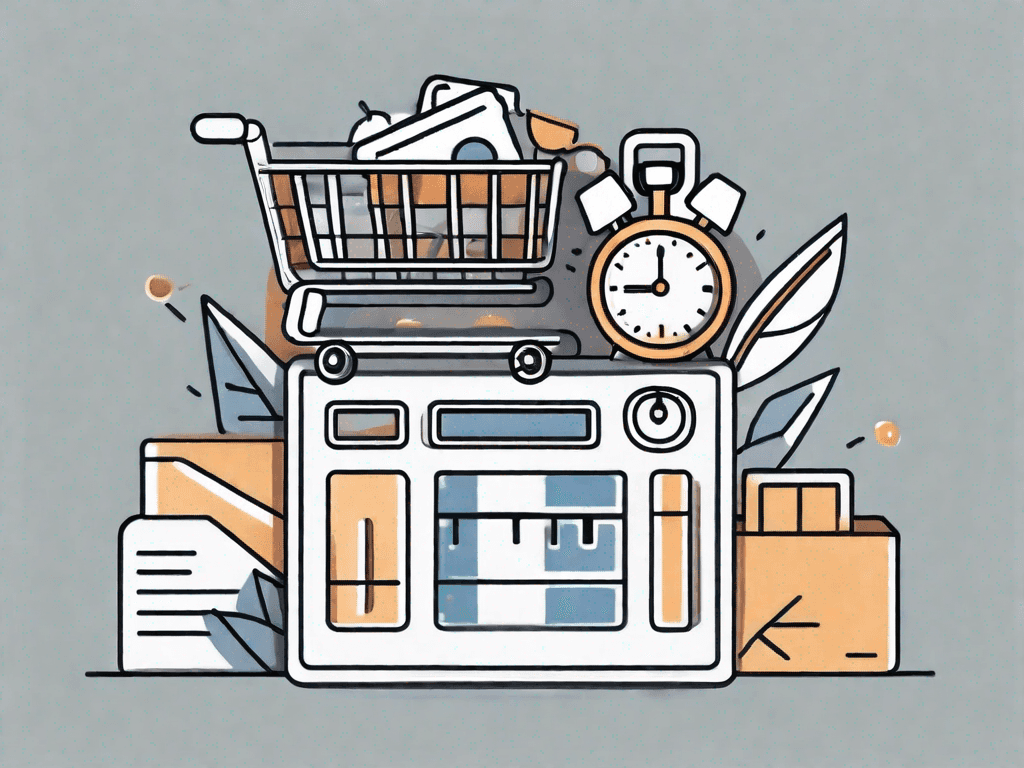
What is an Accelerated Sales Cycle? (Explained With Examples)

What is Account-Based Marketing (ABM)? (Explained With Examples)

What is an Account Manager? (Explained With Examples)
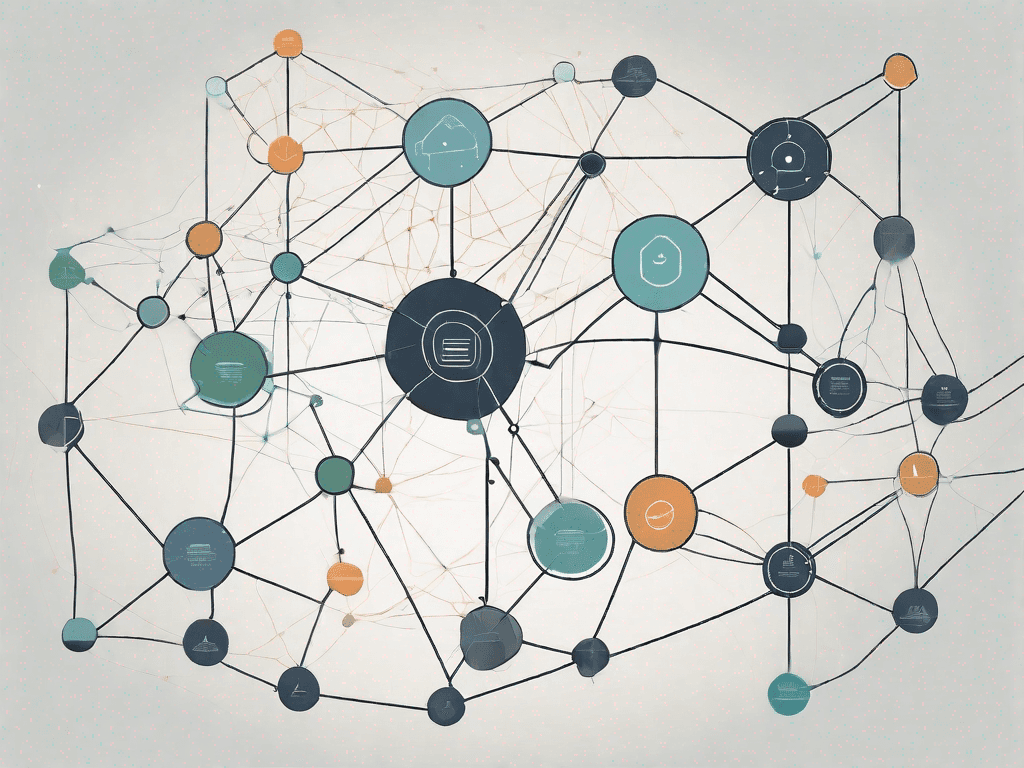
What is Account Mapping? (Explained With Examples)

What is Account-Based Selling? (Explained With Examples)
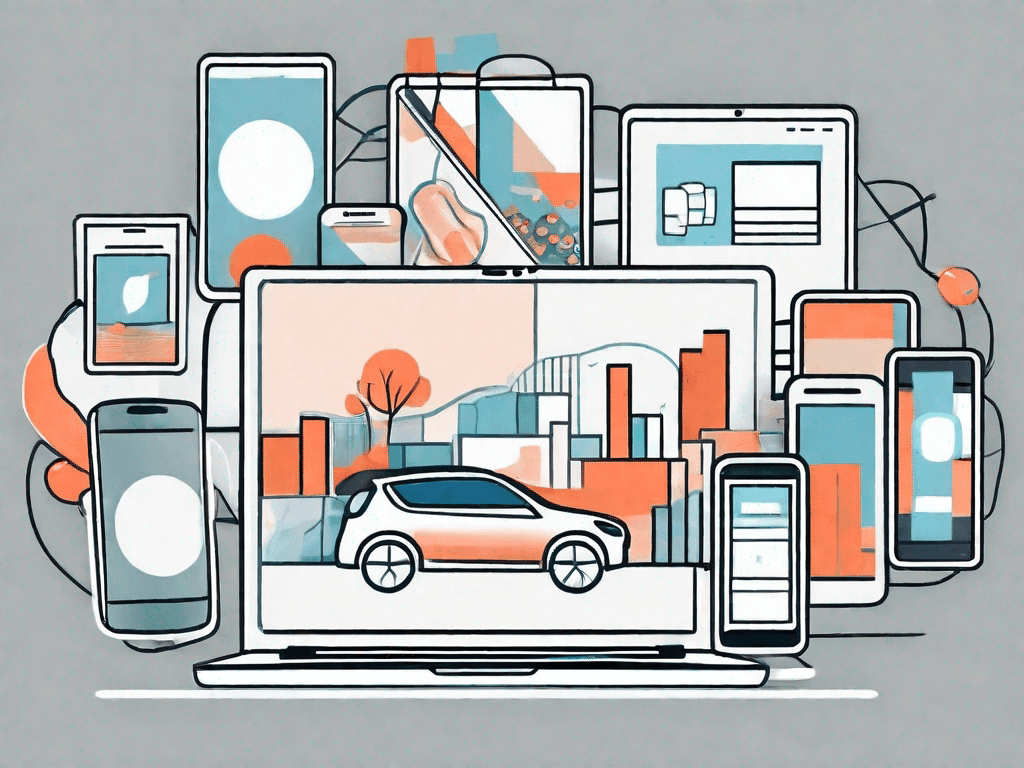
What is Ad Targeting? (Explained With Examples)

What is the Addressable Market? (Explained With Examples)

What is the Adoption Curve? (Explained With Examples)
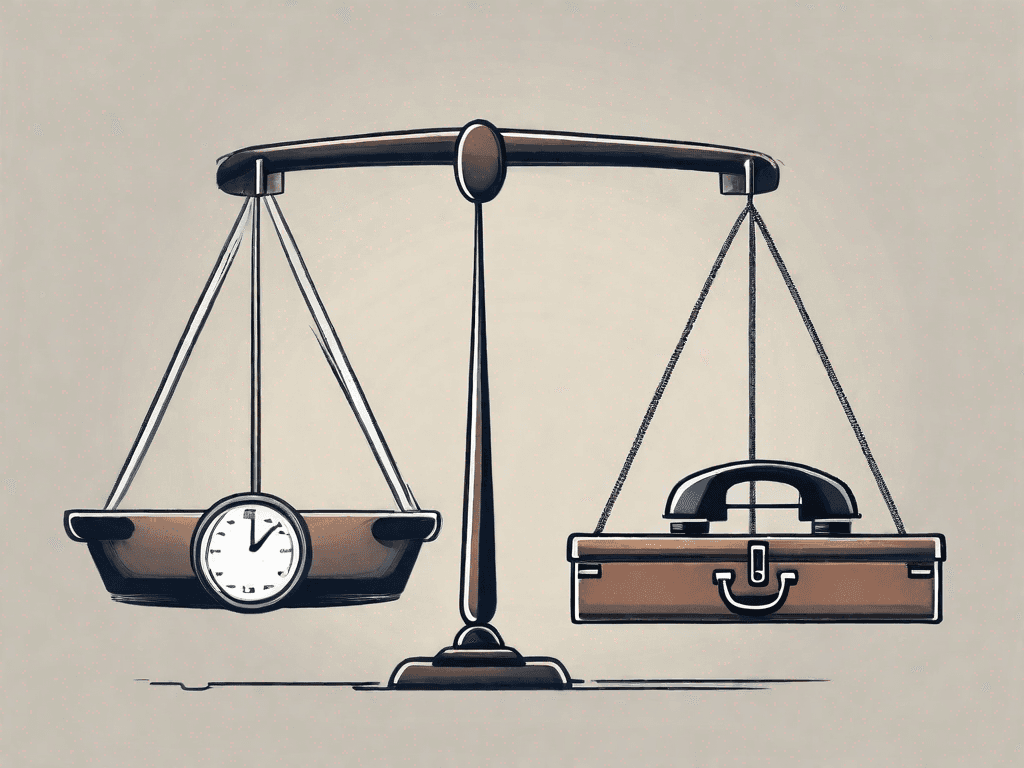
What is an AE (Account Executive)? (Explained With Examples)

What is Affiliate Marketing? (Explained With Examples)

What is AI in Sales? (Explained With Examples)
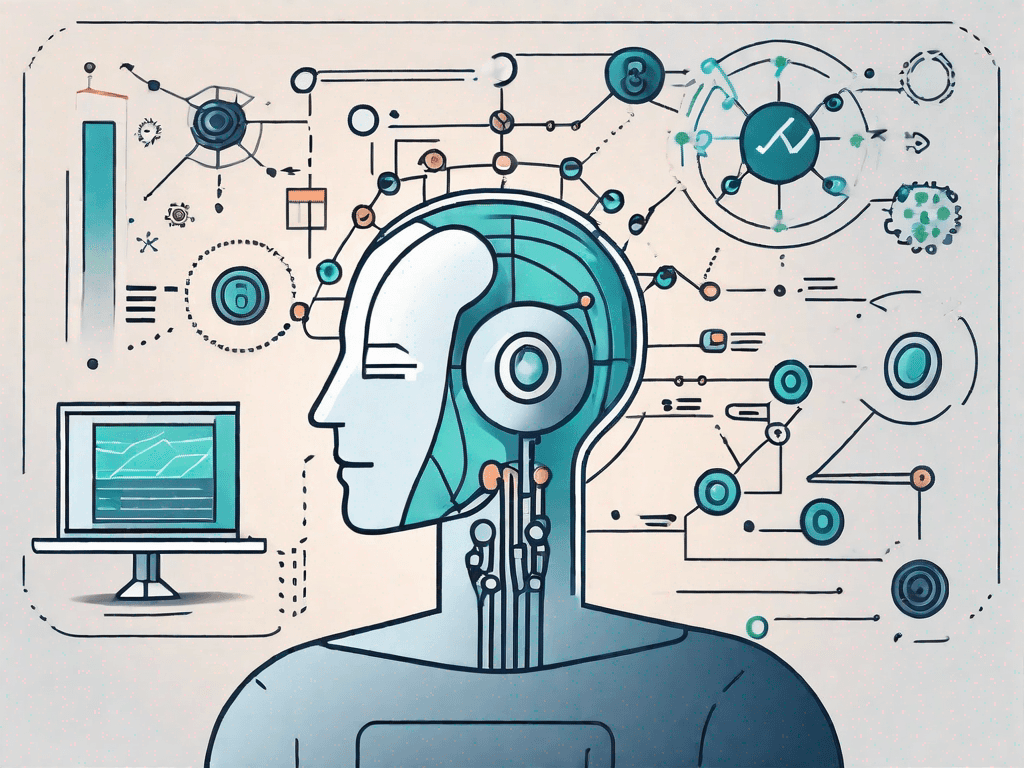
What is an AI-Powered CRM? (Explained With Examples)
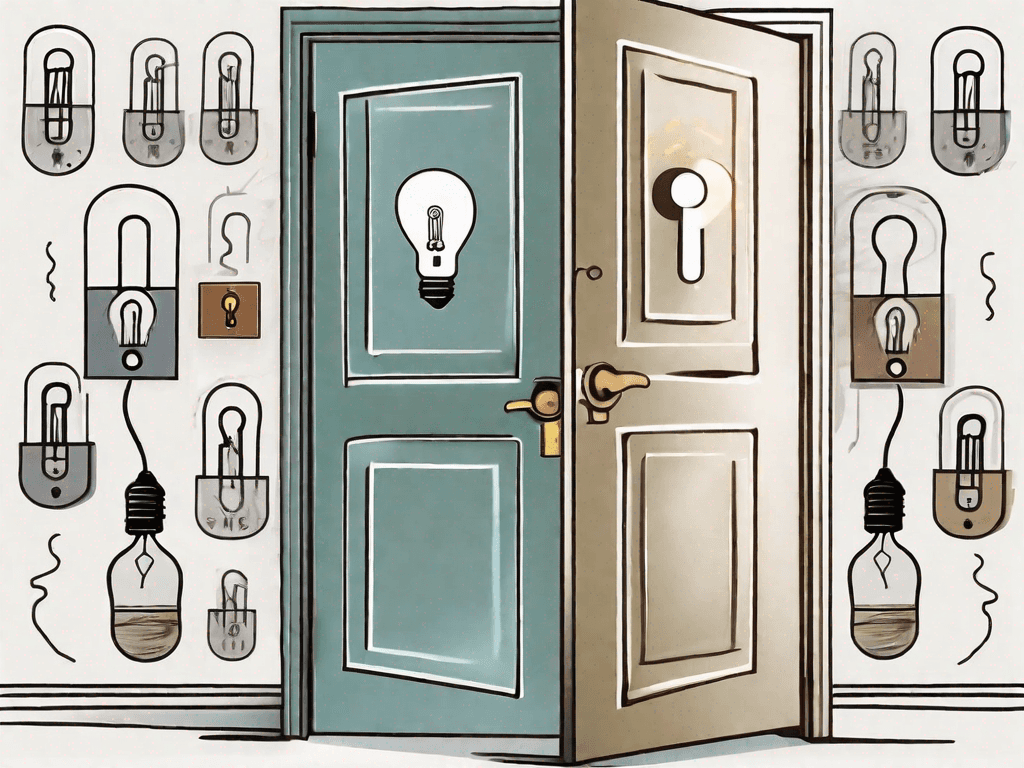
What is an Alternative Close? (Explained With Examples)

What is the Annual Contract Value? (ACV - Explained With Examples)

What are Appointments Set? (Explained With Examples)

What is an Assumptive Close? (Explained With Examples)

What is Automated Outreach? (Explained With Examples)

What is Average Revenue Per Account (ARPA)? (Explained With Examples)

What is B2B (Business-to-Business)? (Explained With Examples)

What is B2G (Business-to-Government)? (Explained With Examples)

What is B2P (Business-to-Partner)? (Explained With Examples)

What is BANT (Budget, Authority, Need, Timing)? (Explained With Examples)

What is Behavioral Economics in Sales? (Explained With Examples)

What is Benchmark Data? (Explained With Examples)

What is Benefit Selling? (Explained With Examples)
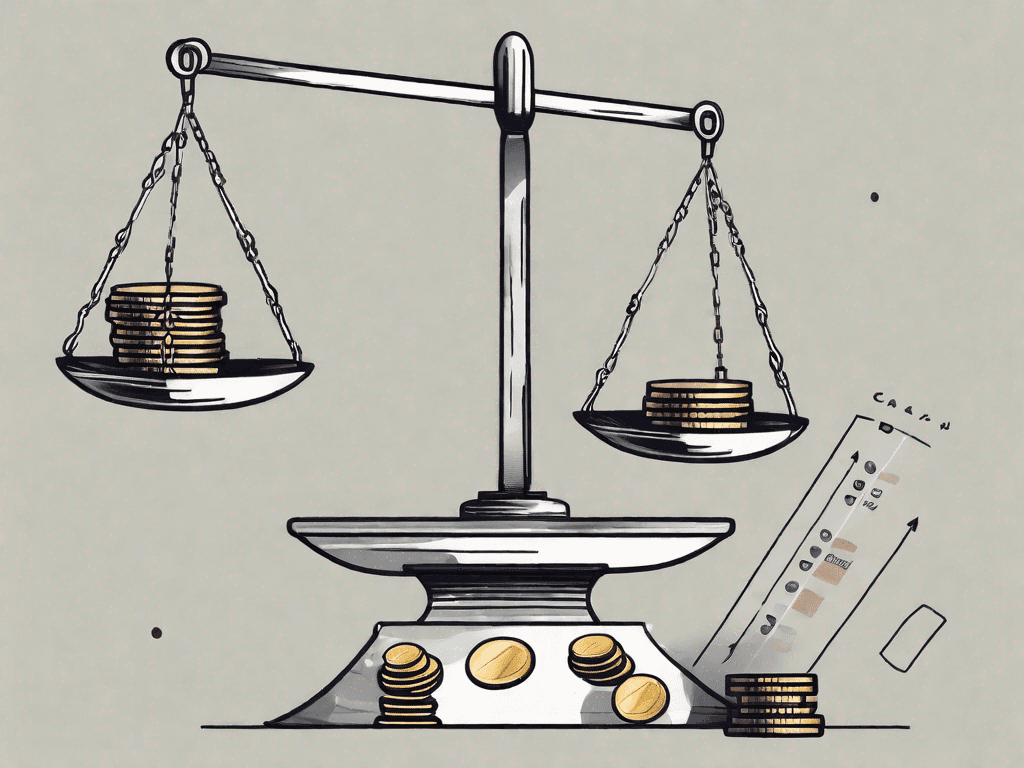
What are Benefit Statements? (Explained With Examples)

What is Beyond the Obvious? (Explained With Examples)

What is a Bootstrapped Startup? (Explained With Examples)

What is the Bottom of the Funnel (BOFU)? (Explained With Examples)

What is Bounce Rate? (Explained With Examples)

What is Brand Awareness? (Explained With Examples)

What is the Break-Even Point? (Explained With Examples)

What is a Breakup Email? (Explained With Examples)

What is Business Development? (Explained With Examples)

What are Business Insights? (Explained With Examples)

What is Business Process Automation? (Explained With Examples)

What is a Buyer Persona? (Explained With Examples)

What is the Buyer's Journey? (Explained With Examples)

What is the Buying Cycle? (Explained With Examples)

What is a Buying Signal? (Explained With Examples)
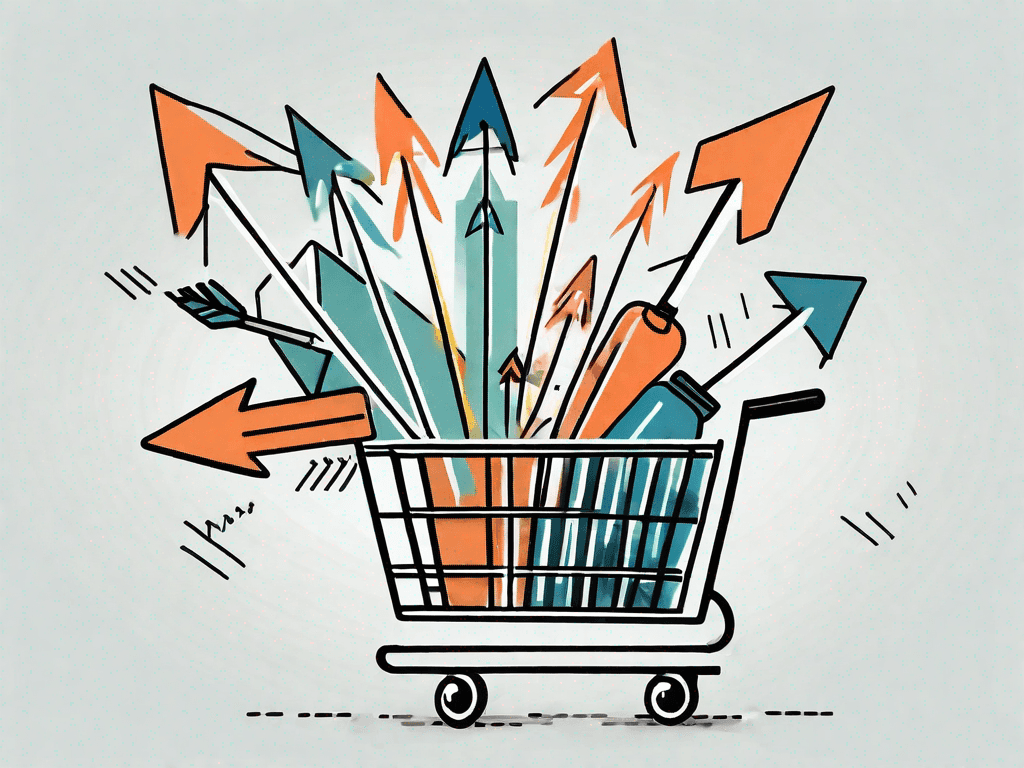
What is a Buying Team? (Explained With Examples)

What is a C-Level Executive? (Explained With Examples)
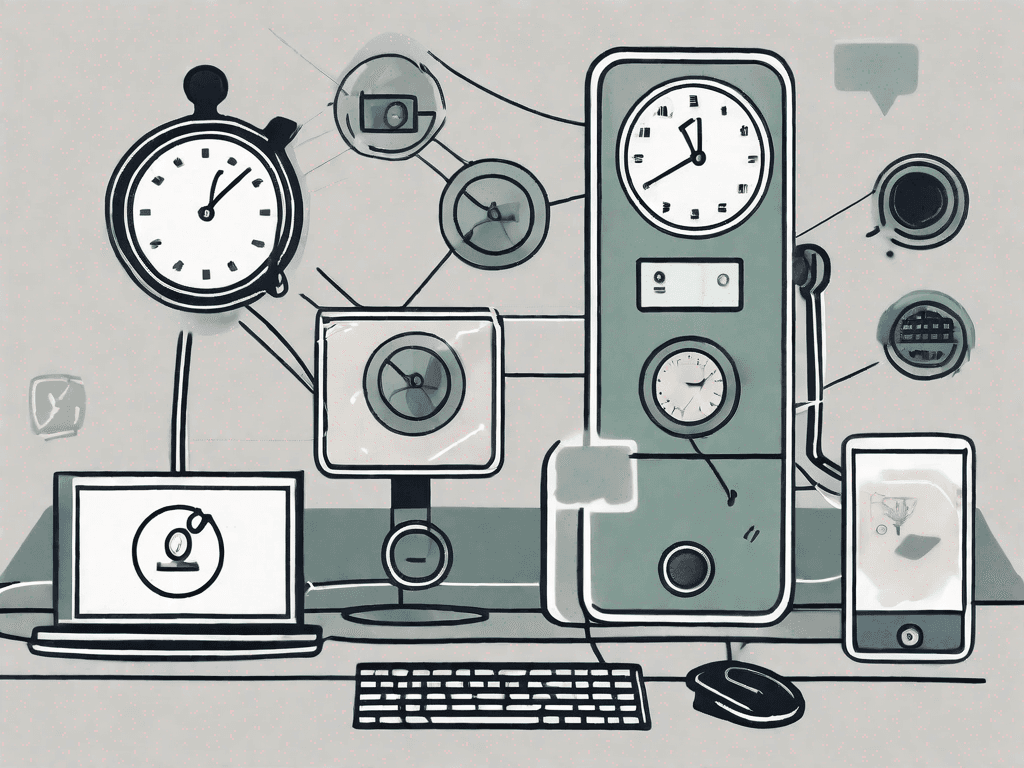
What is Call Logging? (Explained With Examples)

What is Call Recording? (Explained With Examples)
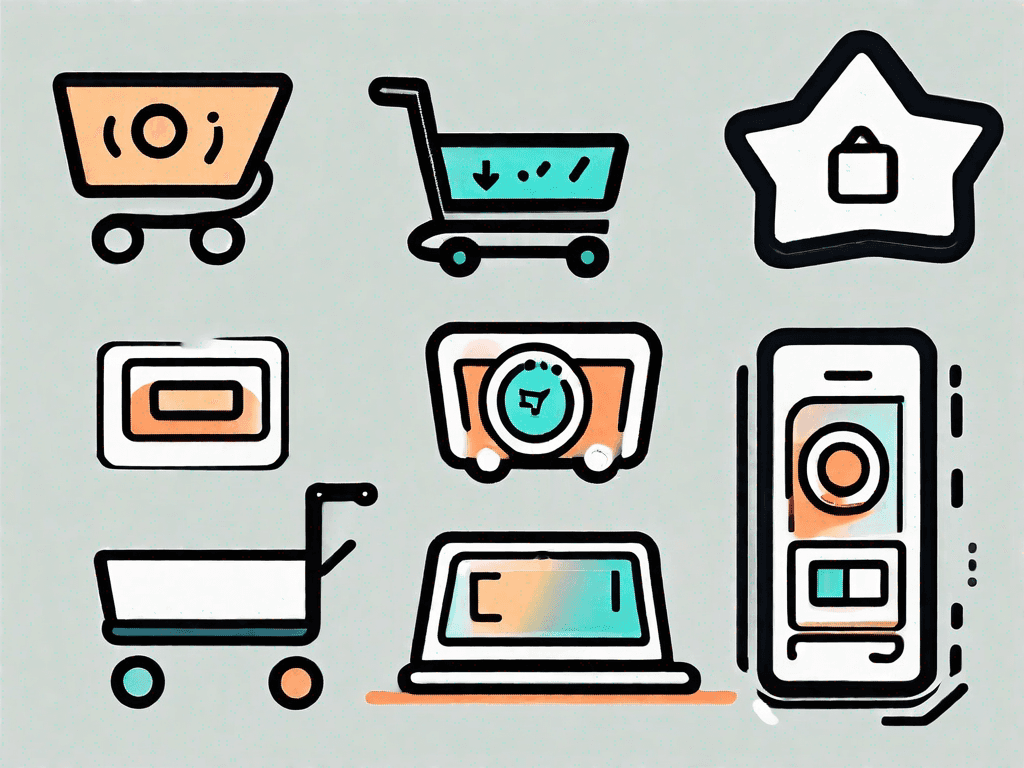
What is a Call-to-Action (CTA)? (Explained With Examples)

What is Challenger Sales? (Explained With Examples)
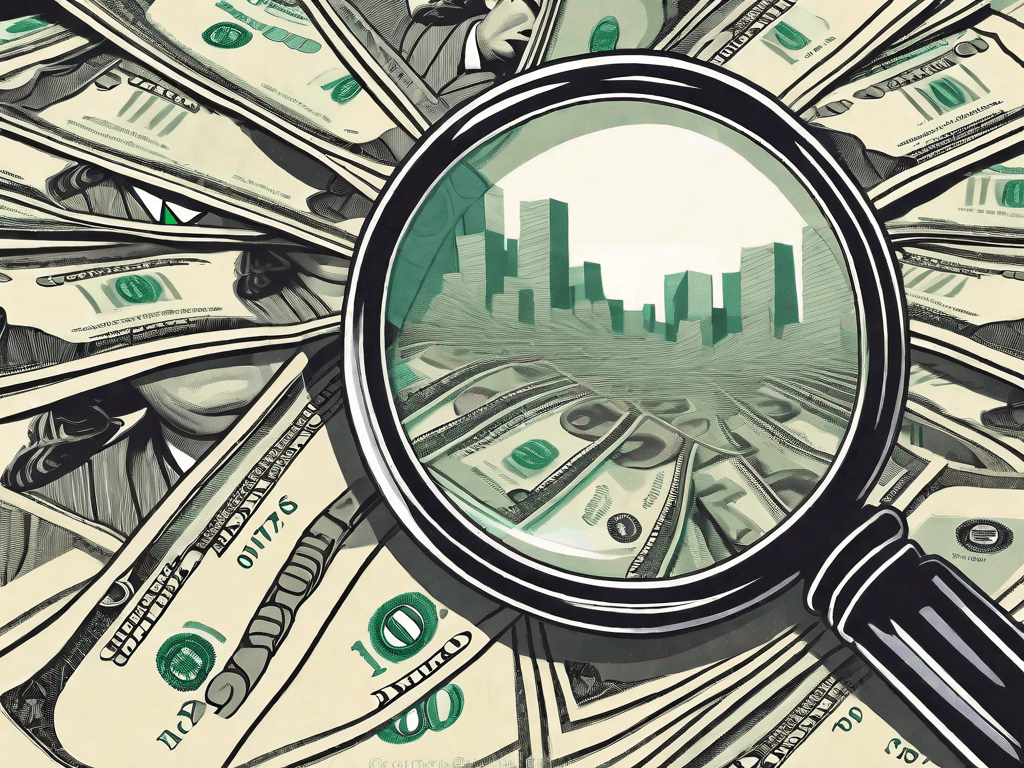
What is Chasing Lost Deals? (Explained With Examples)
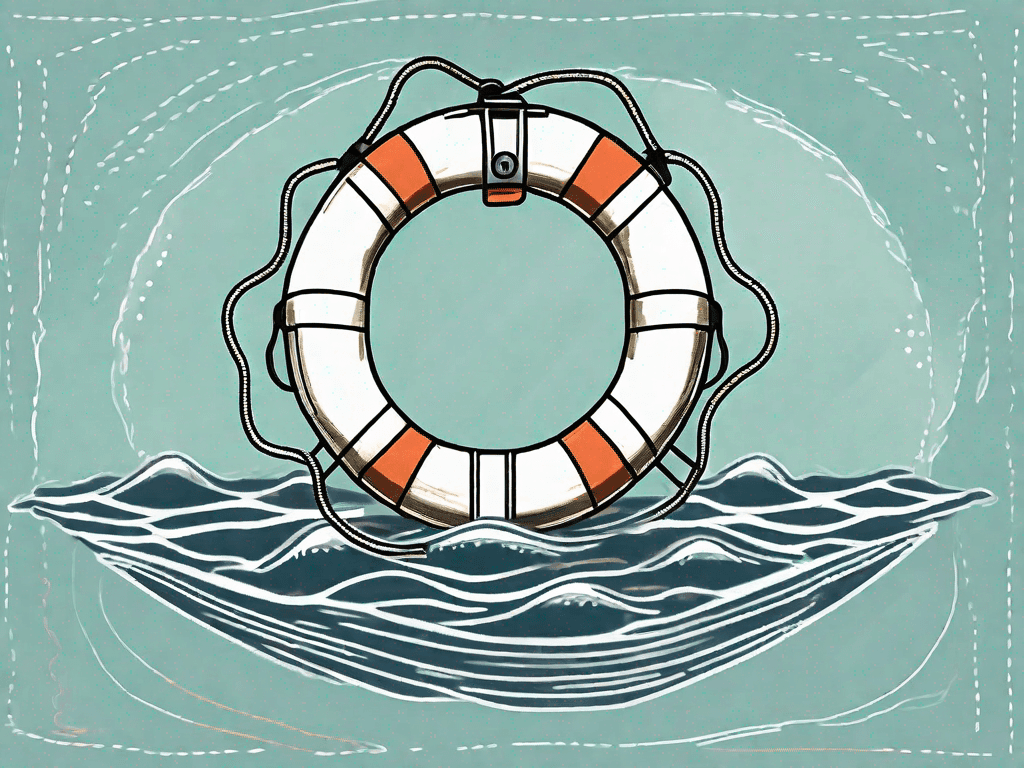
What is Churn Prevention? (Explained With Examples)

What is Churn Rate? (Explained With Examples)
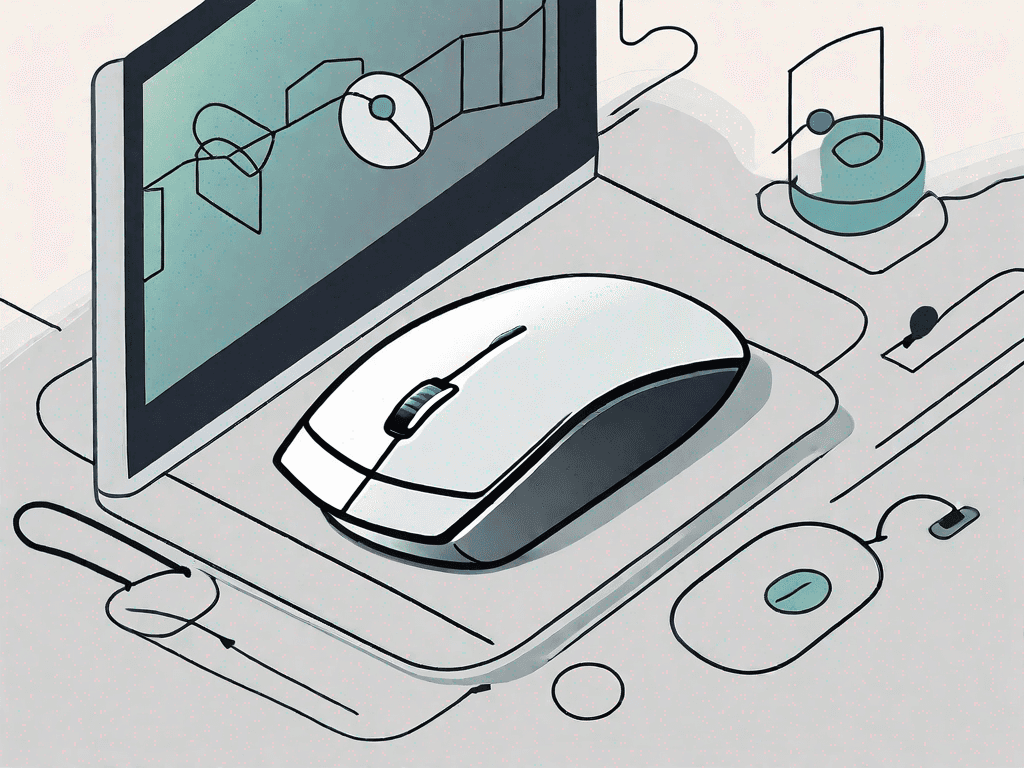
What is Click-Through Rate (CTR)? (Explained With Examples)

What is Client Acquisition? (Explained With Examples)
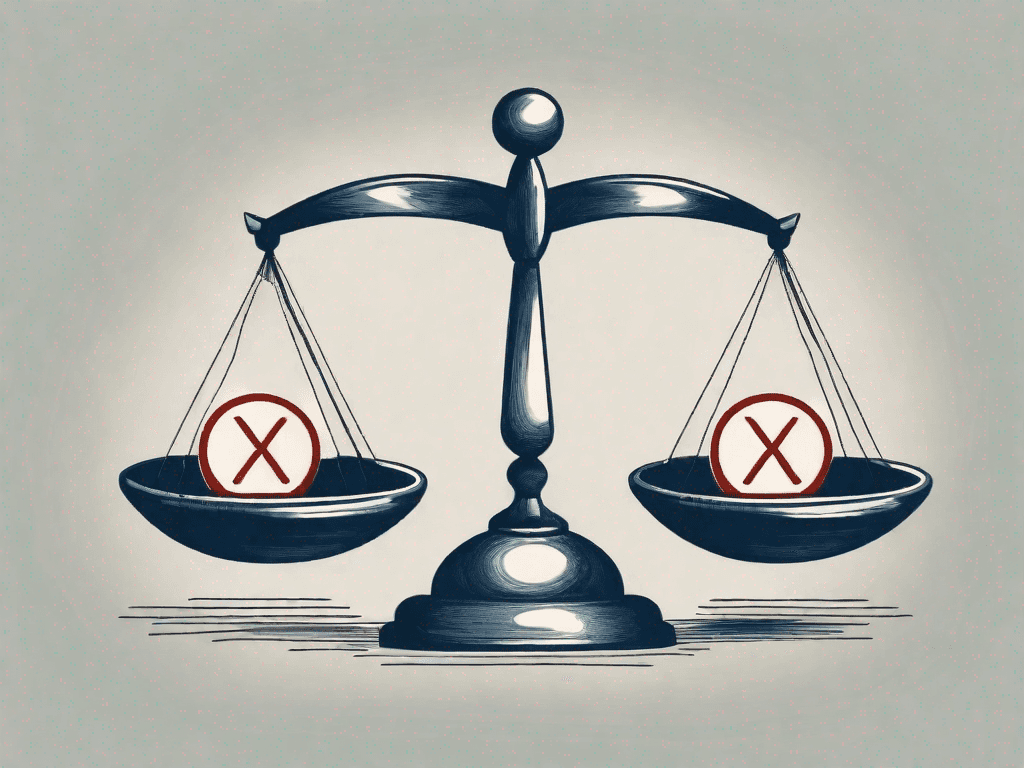
What is the Closing Ratio? (Explained With Examples)
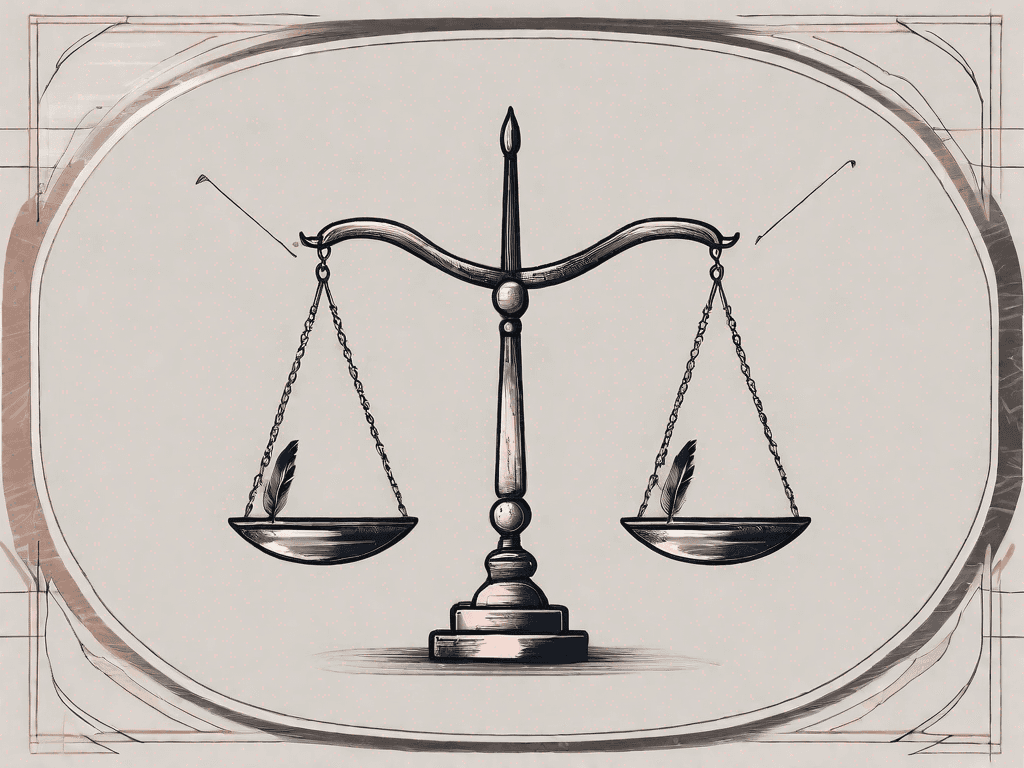
What is the Ben Franklin Close? (Explained With Examples)
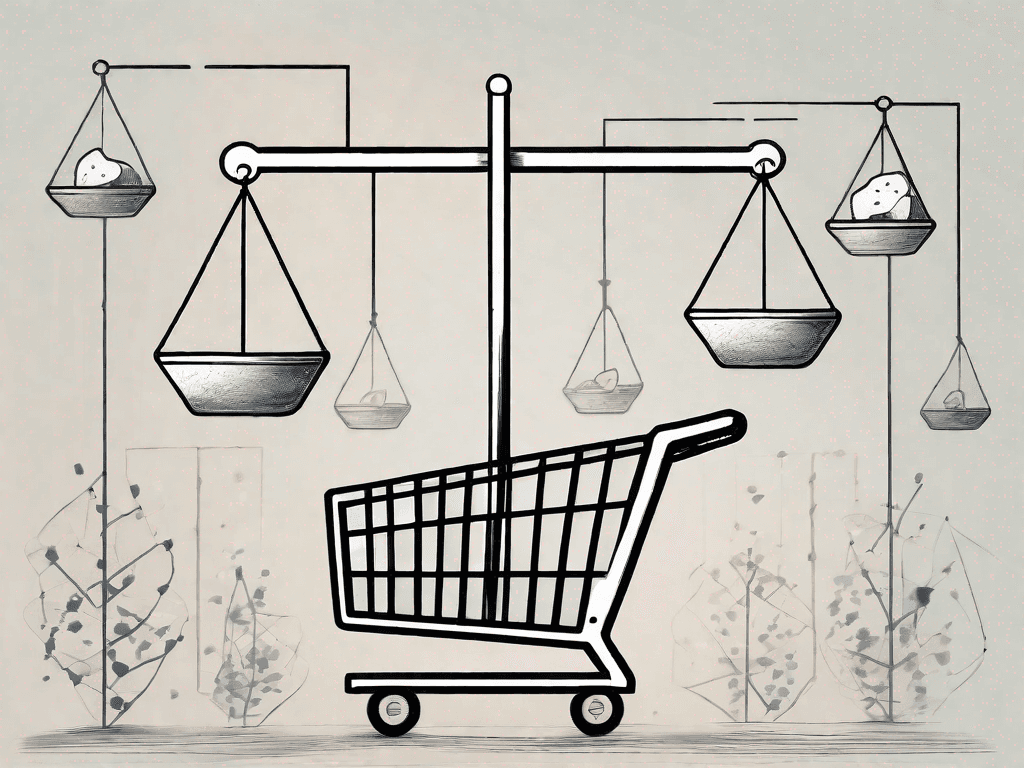
What is Cognitive Bias in Sales? (Explained With Examples)

What is Cognitive Dissonance in Sales? (Explained With Examples)

What is Cold Calling? (Explained With Examples)

What is Cold Outreach? (Explained With Examples)

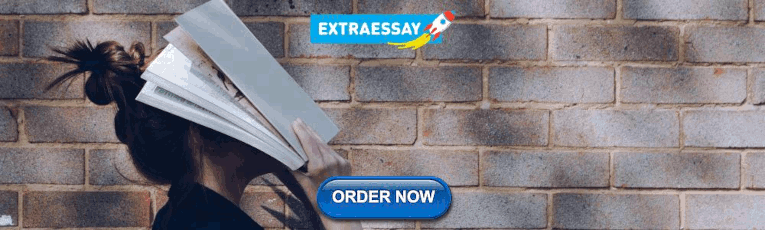
What is a Competitive Advantage? (Explained With Examples)

What is a Competitive Analysis? (Explained With Examples)

What is Competitive Positioning? (Explained With Examples)

What is Conceptual Selling? (Explained With Examples)

What is Consultative Closing? (Explained With Examples)

What is Consultative Negotiation? (Explained With Examples)

What is Consultative Prospecting? (Explained With Examples)
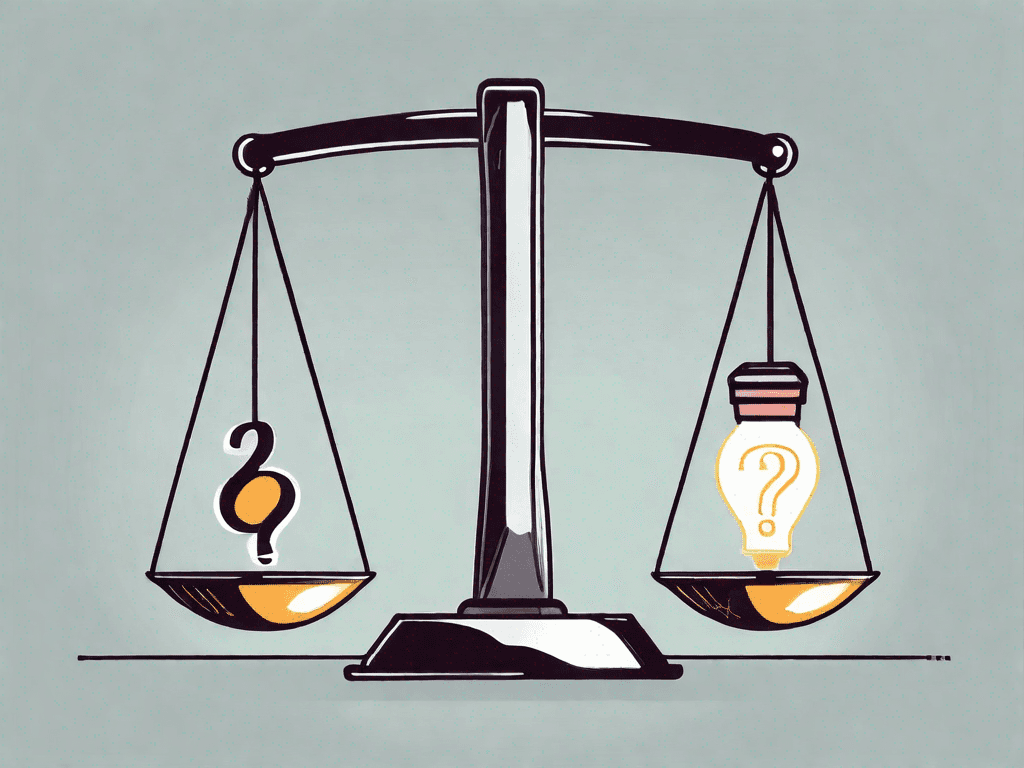
What is Consultative Selling? (Explained With Examples)

What is Content Marketing? (Explained With Examples)

What is Content Syndication? (Explained With Examples)

What is a Conversion Funnel? (Explained With Examples)

What is Conversion Optimization? (Explained With Examples)

What is a Conversion Path? (Explained With Examples)

What is Conversion Rate? (Explained With Examples)

What is Cost-Per-Click (CPC)? (Explained With Examples)

What is a CRM (Customer Relationship Management)? (Explained With Examples)

What is Cross-Cultural Selling? (Explained With Examples)
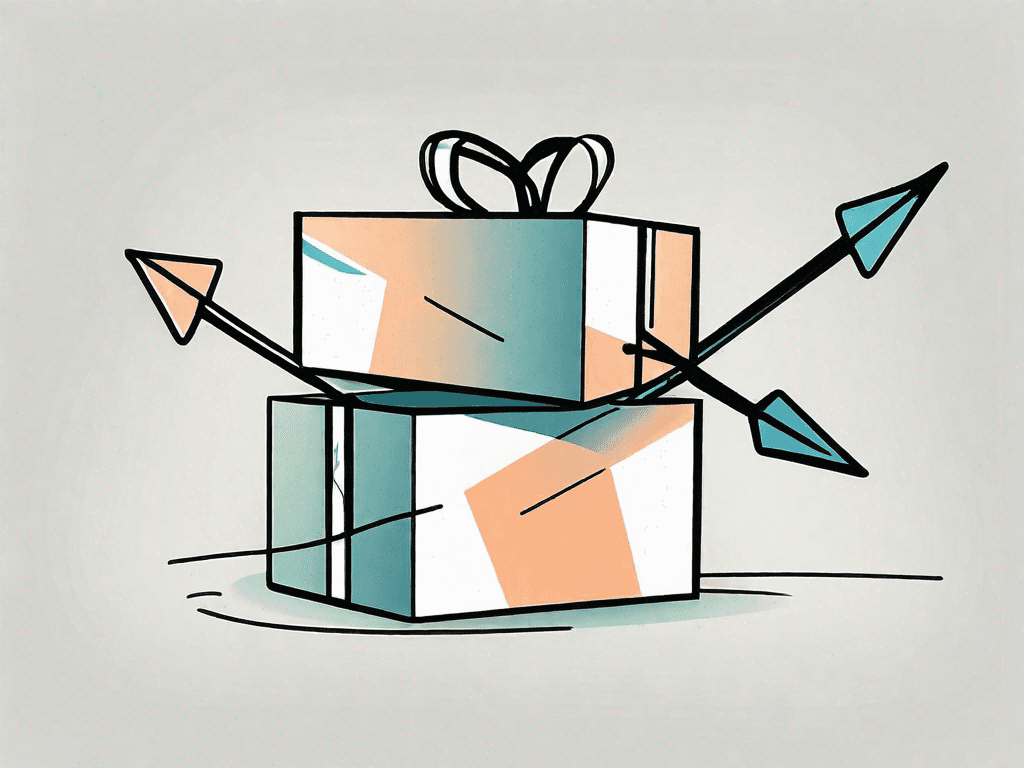
What is a Cross-Sell Ratio? (Explained With Examples)

What is Cross-Selling? (Explained With Examples)

What is Customer Acquisition Cost (CAC)? (Explained With Examples)

What is Customer-Centric Marketing? (Explained With Examples)

What is Customer-Centric Selling? (Explained With Examples)

What is Customer Journey Mapping? (Explained With Examples)

What is the Customer Journey? (Explained With Examples)
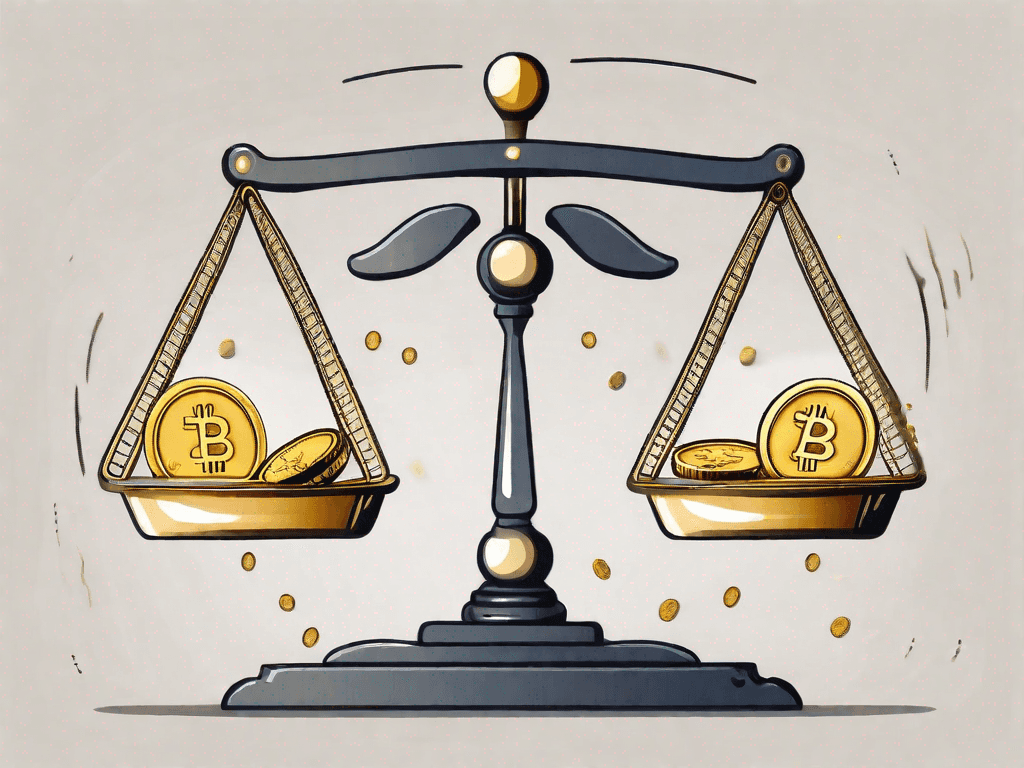
What is the Customer Lifetime Value (CLV)? (Explained With Examples)
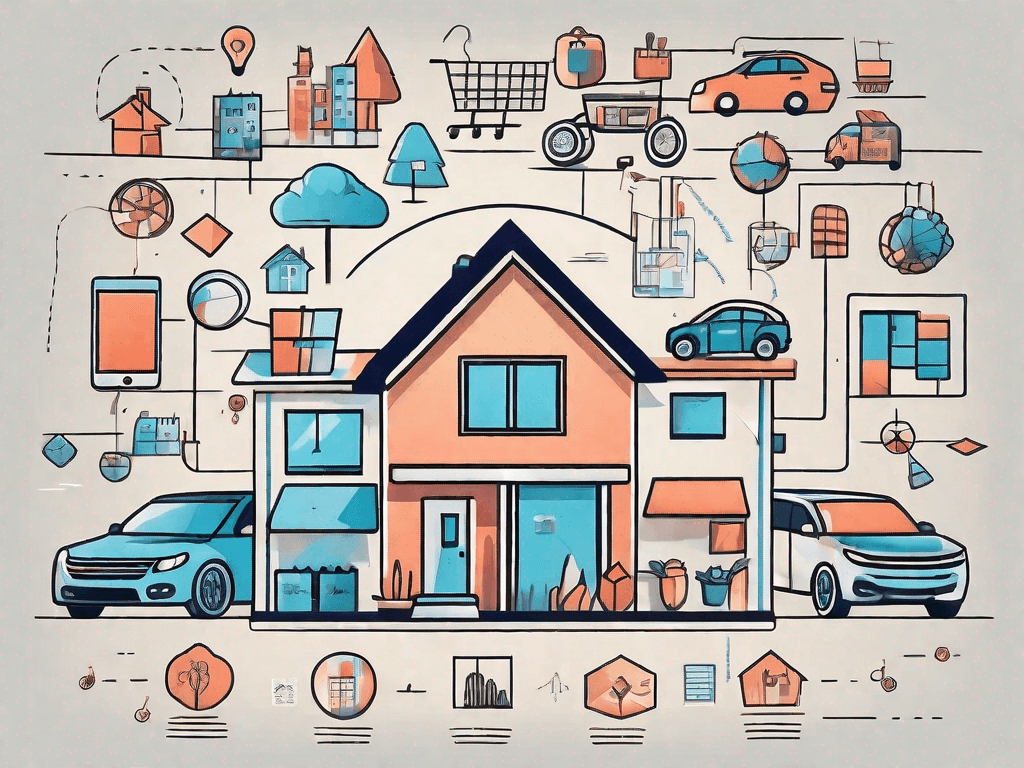
What is Customer Profiling? (Explained With Examples)

What is Customer Retention? (Explained With Examples)

What is Dark Social? (Explained With Examples)
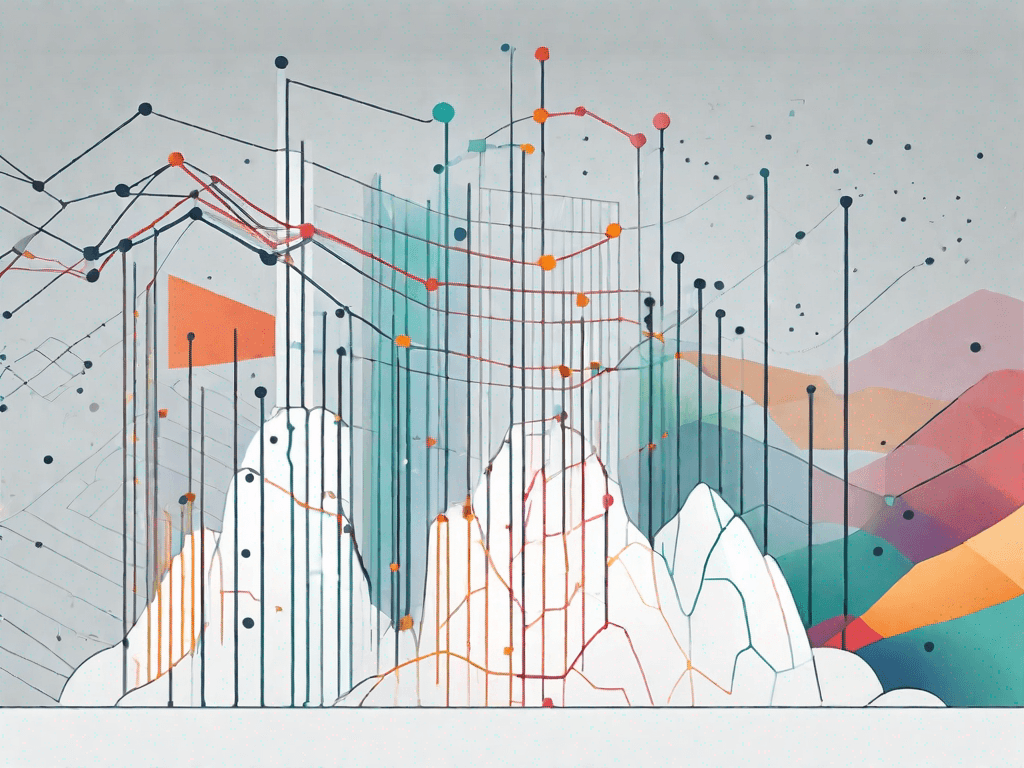
What is Data Enrichment? (Explained With Examples)

What is Data Segmentation? (Explained With Examples)
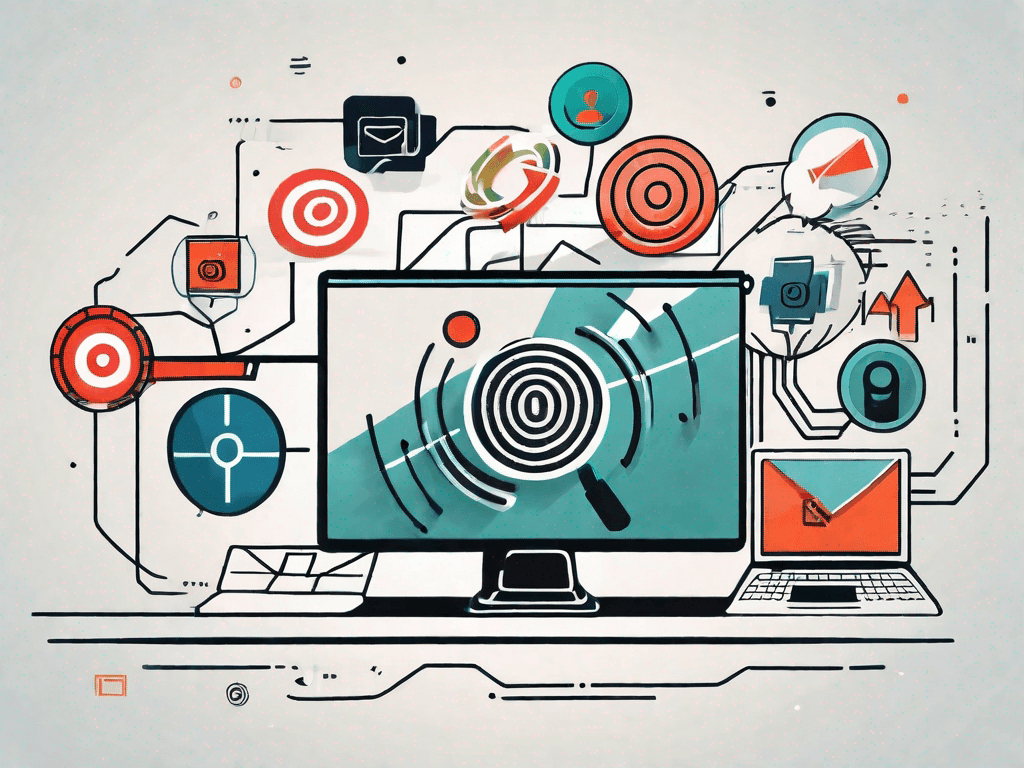
What is Database Marketing? (Explained With Examples)

What are Decision Criteria? (Explained With Examples)
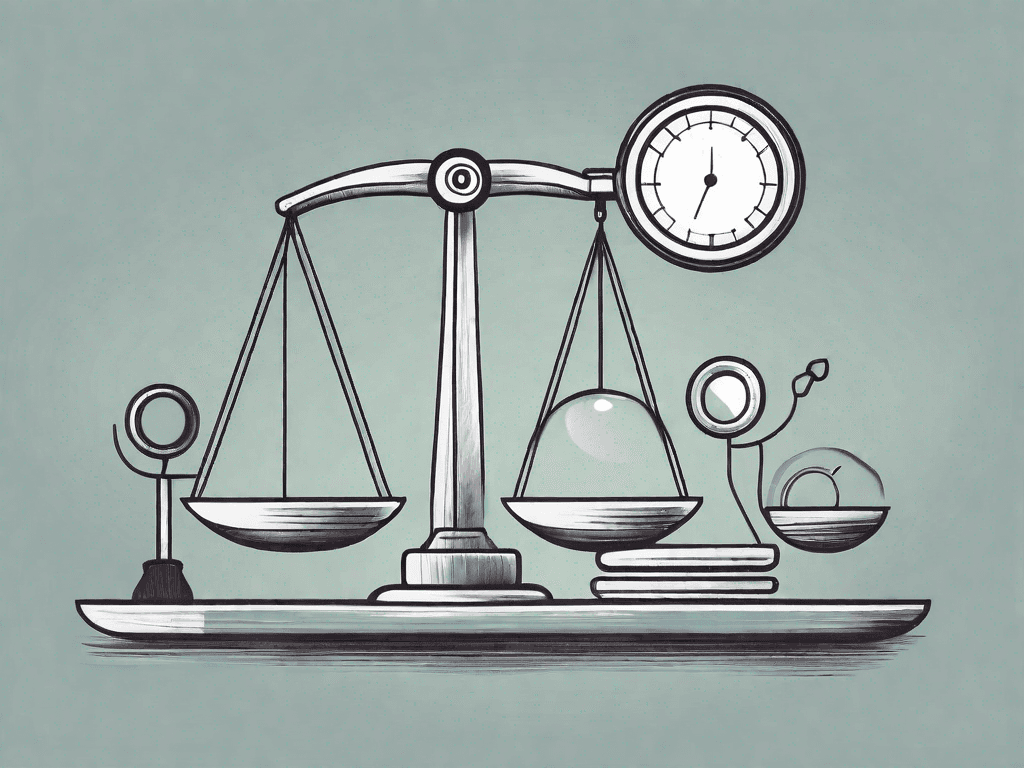
What is a Decision Maker? (Explained With Examples)

What is a Decision-Making Unit (DMU)? (Explained With Examples)

What is Demand Generation? (Explained With Examples)
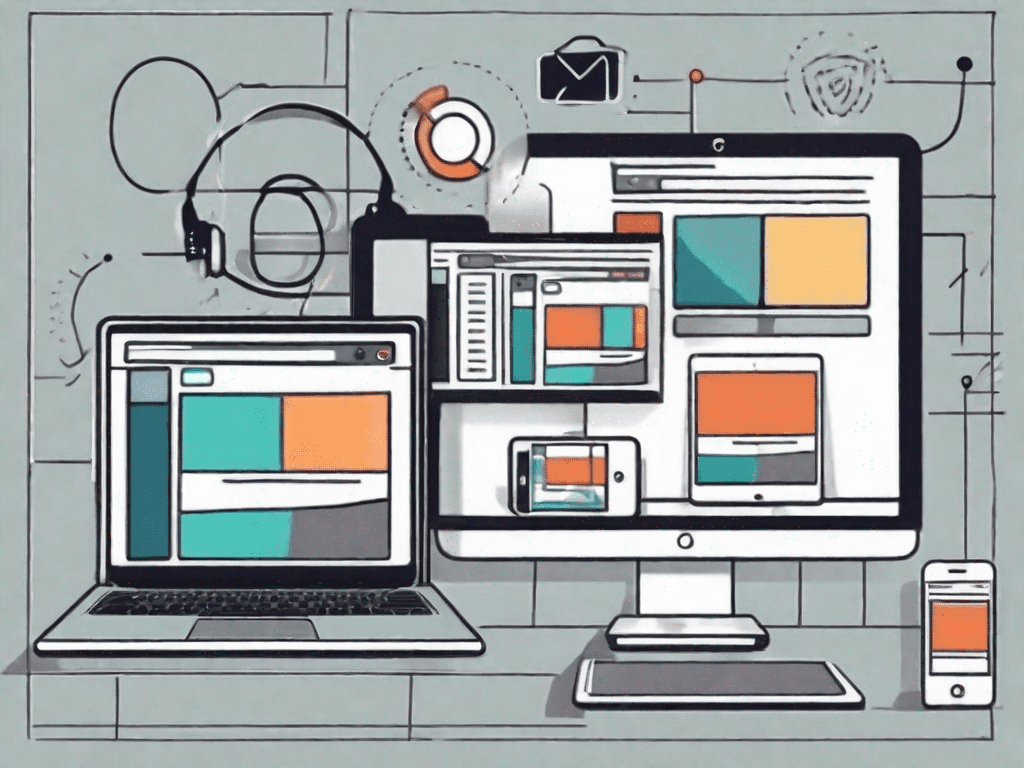
What is Digital Marketing? (Explained With Examples)

What is Direct Marketing? (Explained With Examples)
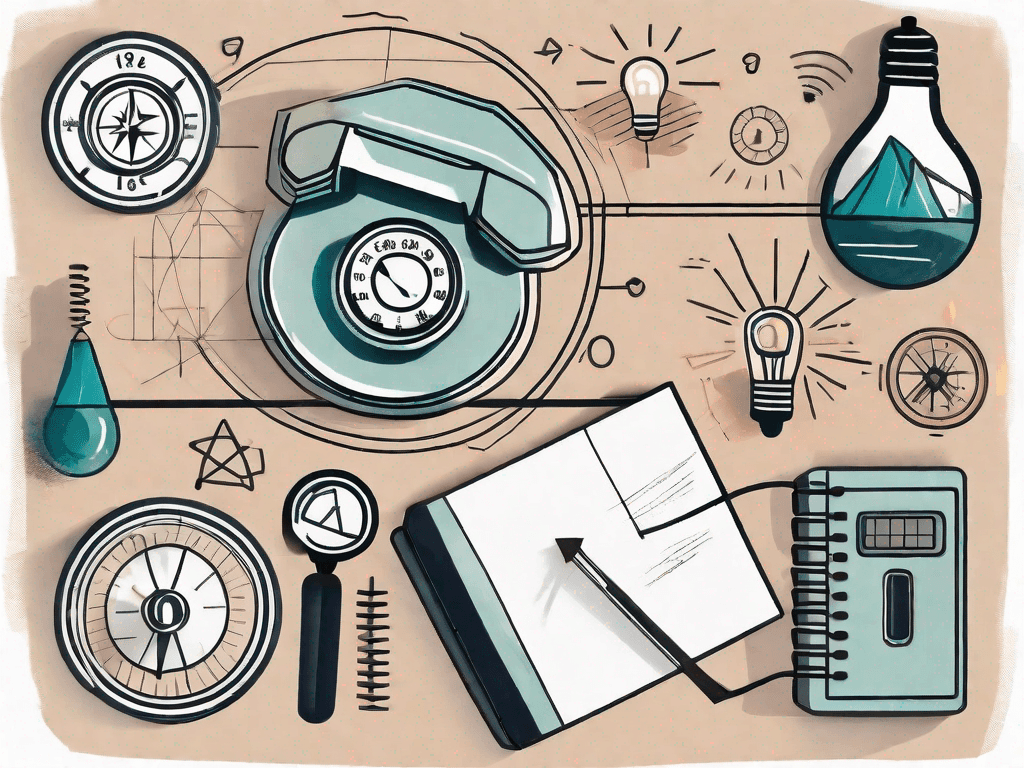
What is a Discovery Call? (Explained With Examples)
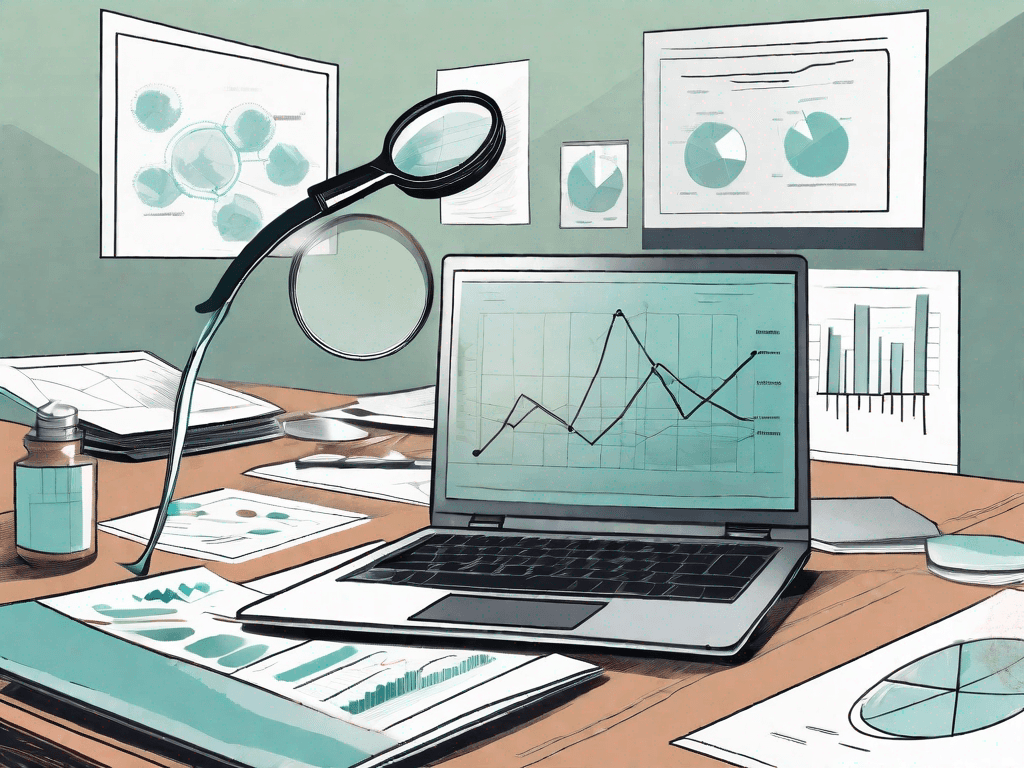
What is a Discovery Meeting? (Explained With Examples)
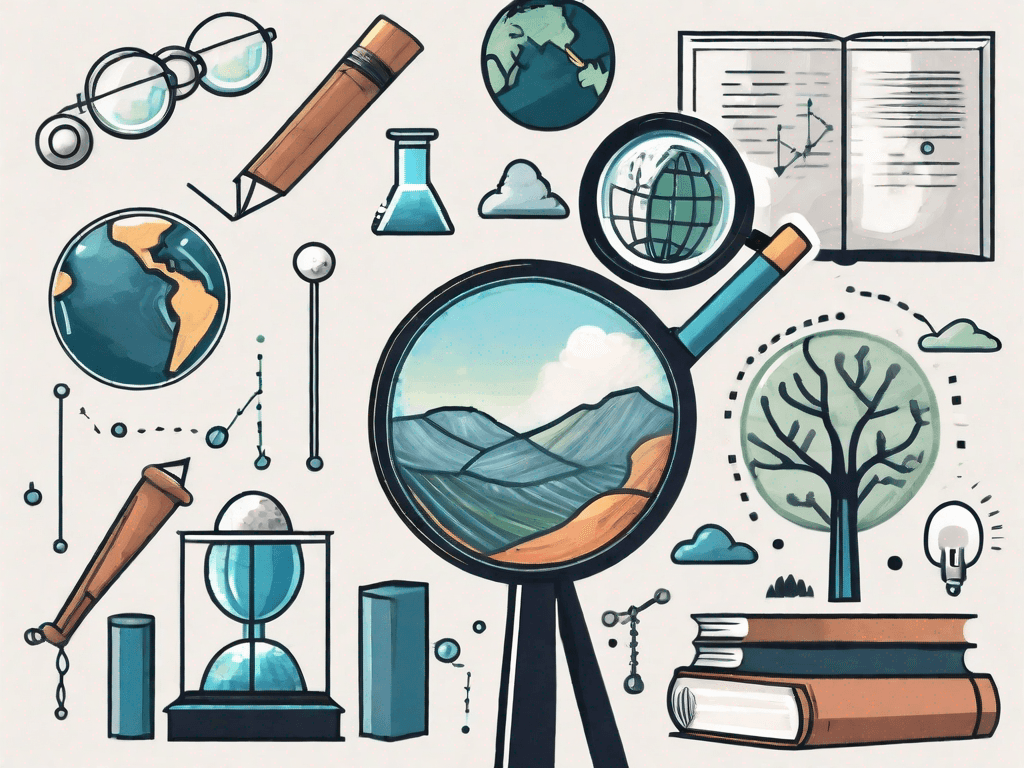
What are Discovery Questions? (Explained With Examples)

What is Door-to-Door Sales? (Explained With Examples)

What is a Drip Campaign? (Explained With Examples)

What is Dunning? (Explained With Examples)

What is an Early Adopter? (Explained With Examples)

What is Elevator Pitch? (Explained With Examples)

What is Email Hygiene? (Explained With Examples)
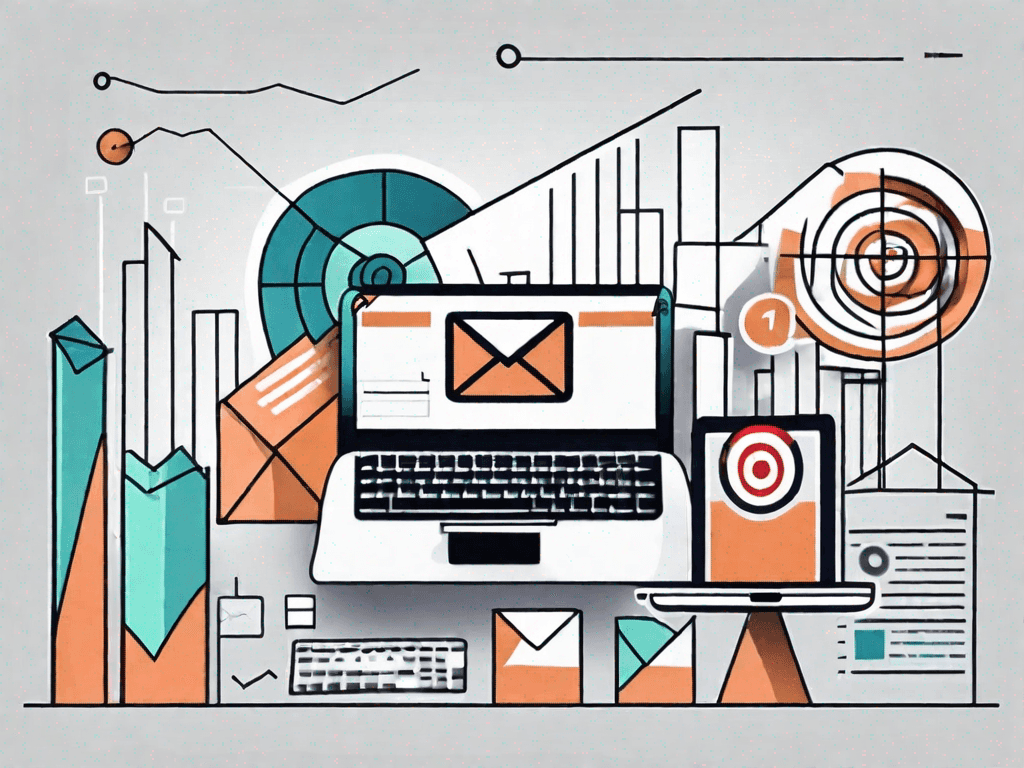
What is Email Marketing? (Explained With Examples)

What is Emotional Intelligence Selling? (Explained With Examples)
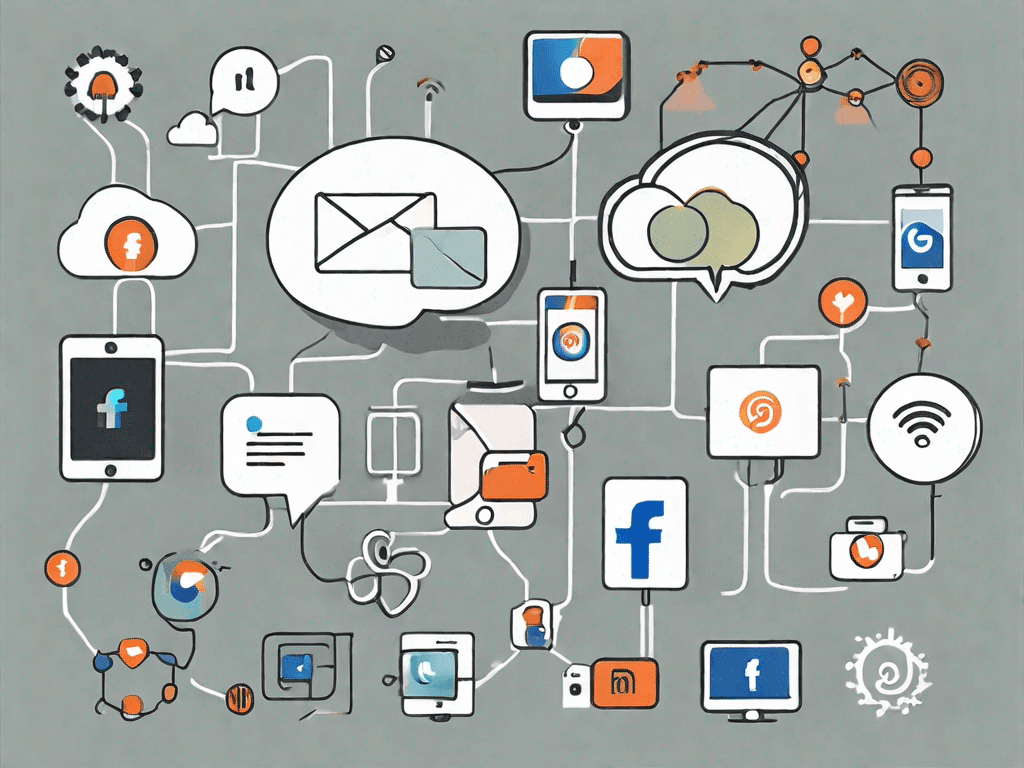
What is Engagement Marketing? (Explained With Examples)

What is Engagement Rate? (Explained With Examples)

What is Engagement Strategy? (Explained With Examples)

What is Feature-Benefit Selling? (Explained With Examples)

What is Field Sales? (Explained With Examples)

What is a Follow-Up? (Explained With Examples)

What is Forecast Accuracy? (Explained With Examples)

What is a Funnel? (Explained With Examples)
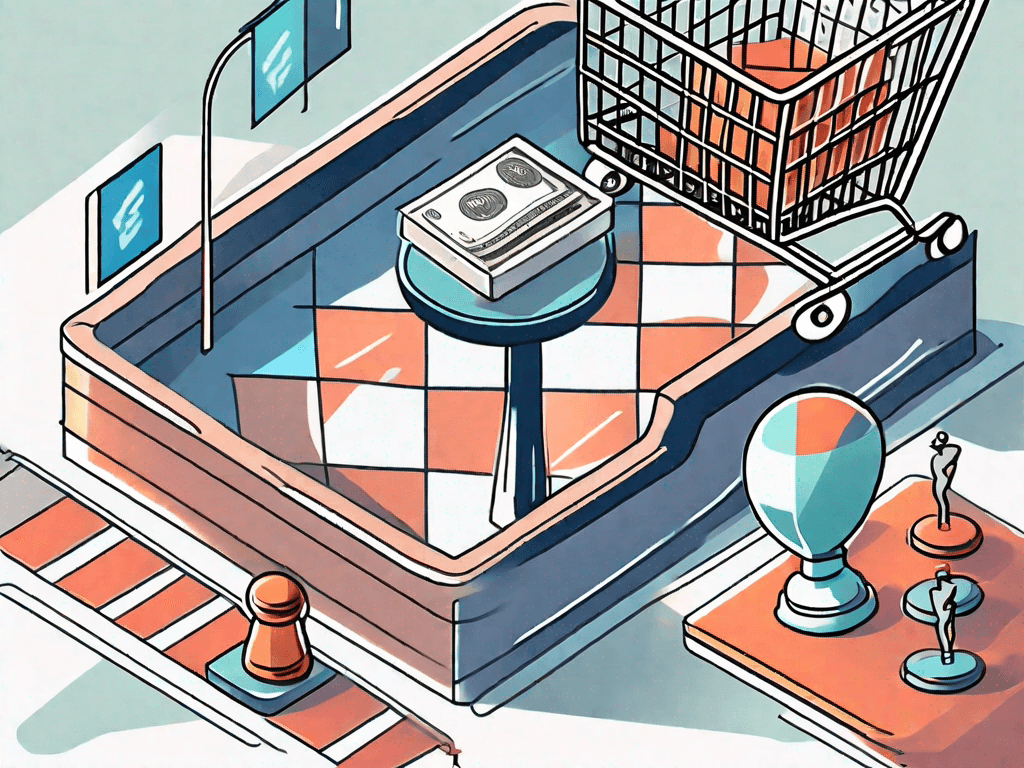
What is Gamification in Sales? (Explained With Examples)

What is Gatekeeper Strategy? (Explained With Examples)

What is Gatekeeper? (Explained With Examples)

What is a Go-to Market Strategy? (Explained With Examples)

What is Growth Hacking? (Explained With Examples)
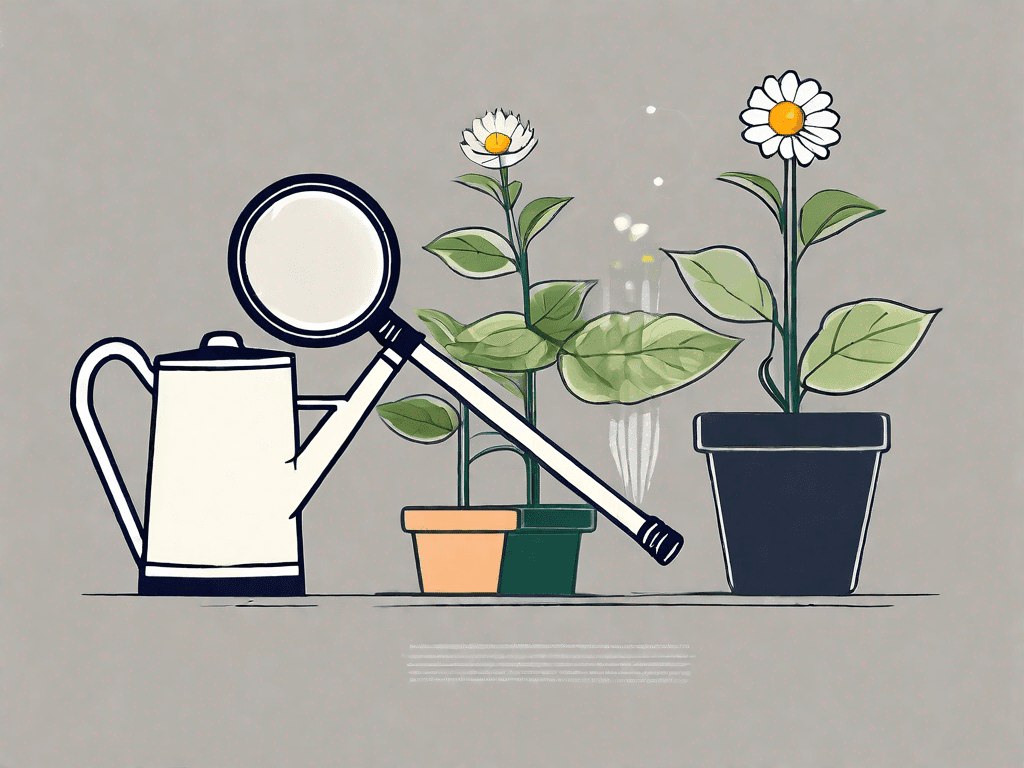
What is Growth Marketing? (Explained With Examples)

What is Guerrilla Marketing? (Explained With Examples)

What is High-Ticket Sales? (Explained With Examples)

What is Holistic Selling? (Explained With Examples)

What is Ideal Customer Profile (ICP)? (Explained With Examples)

What is Inbound Lead Generation? (Explained With Examples)

What is an Inbound Lead? (Explained With Examples)
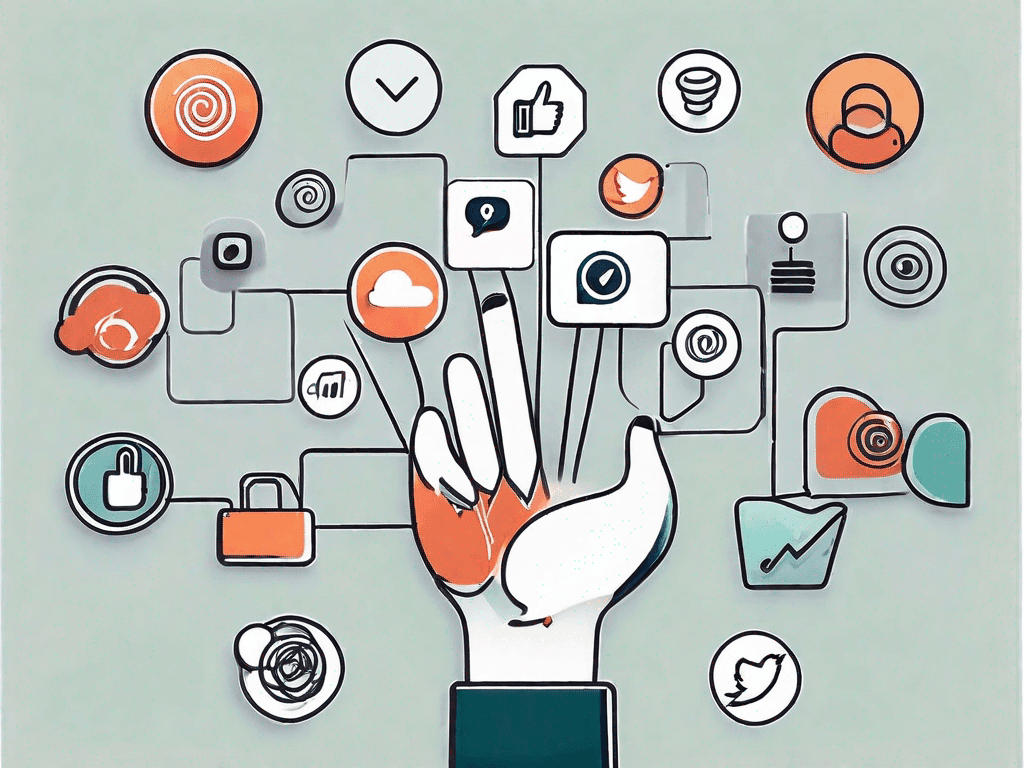
What is Inbound Marketing? (Explained With Examples)
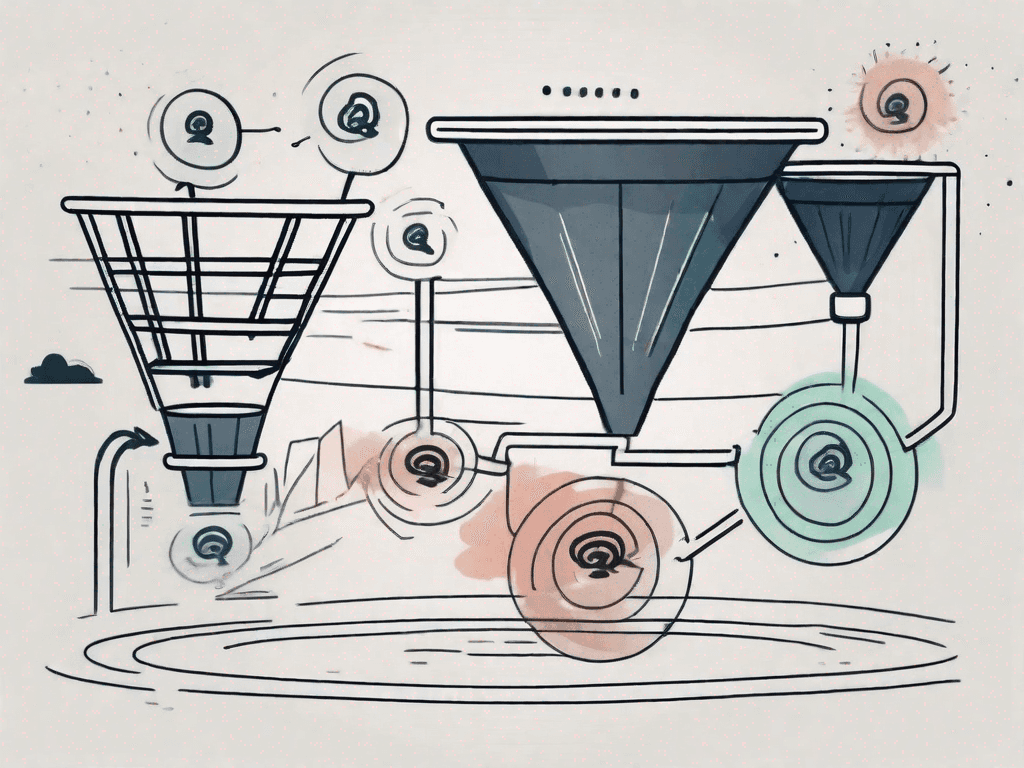
What is Inbound Sales? (Explained With Examples)

What is Influencer Marketing? (Explained With Examples)

What is Inside Sales Representative? (Explained With Examples)

What is Inside Sales? (Explained With Examples)

What is Insight Selling? (Explained With Examples)

What is a Key Account? (Explained With Examples)

What is a Key Performance Indicator (KPI)? (Explained With Examples)

What is a Landing Page? (Explained With Examples)
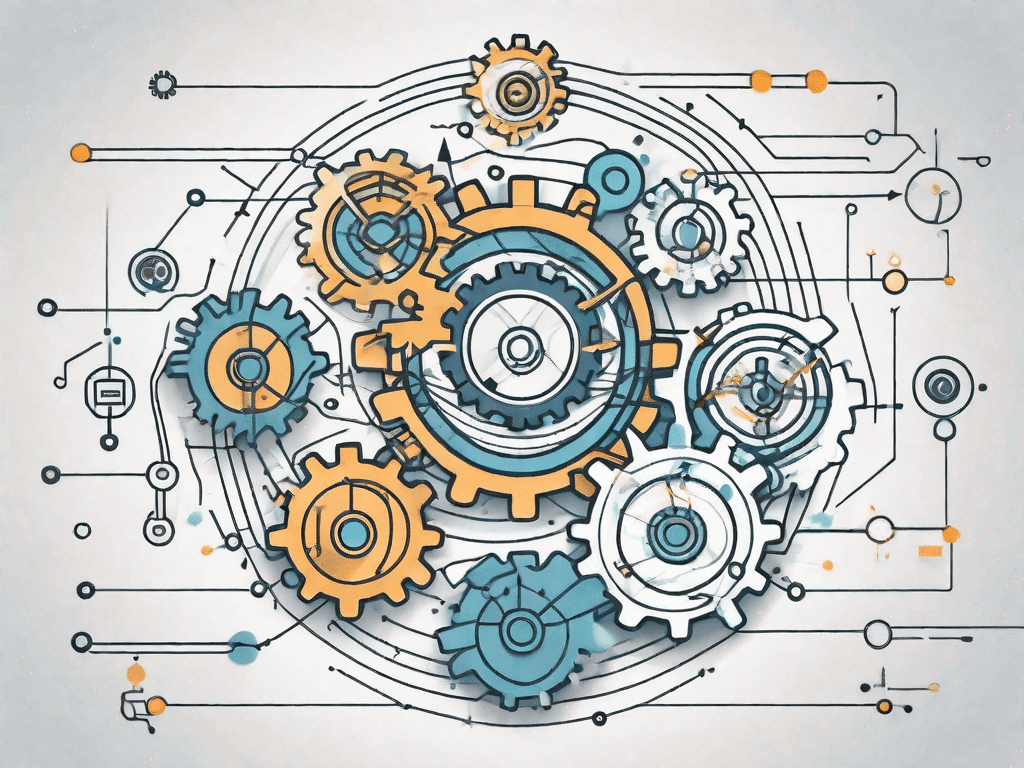
What is Lead Database? (Explained With Examples)

What is a Lead Enrichment? (Explained With Examples)

What is Lead Generation? (Explained With Examples)

What is Lead Nurturing? (Explained With Examples)

What is Lead Qualification? (Explained With Examples)
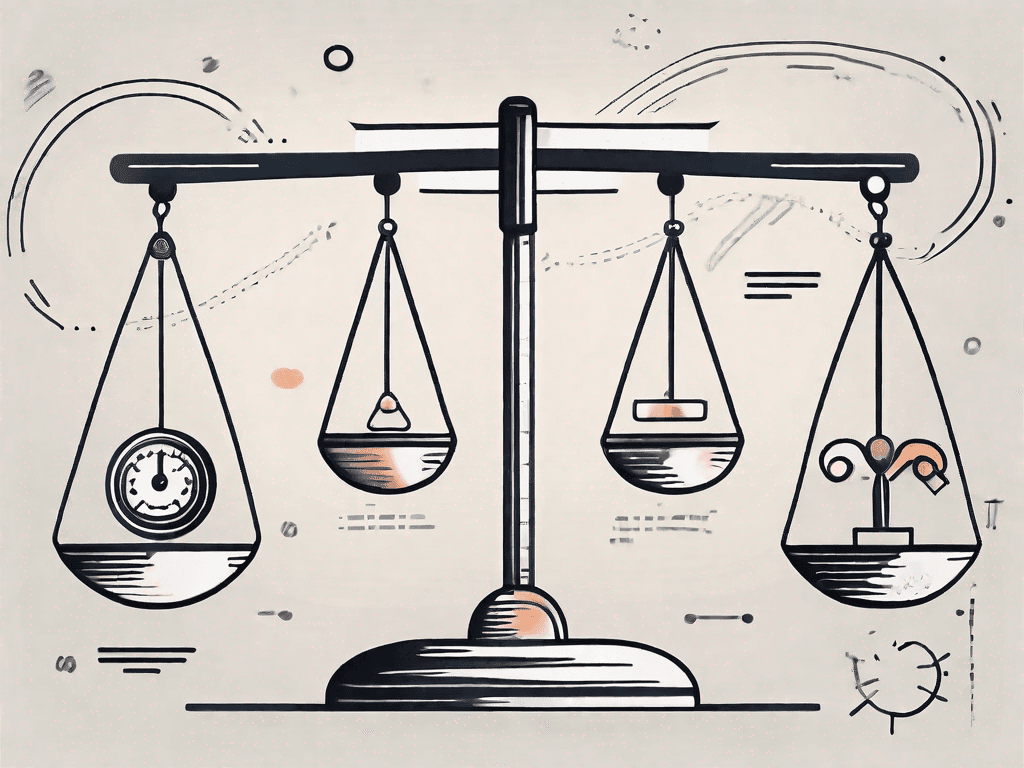
What is Lead Scoring? (Explained With Examples)
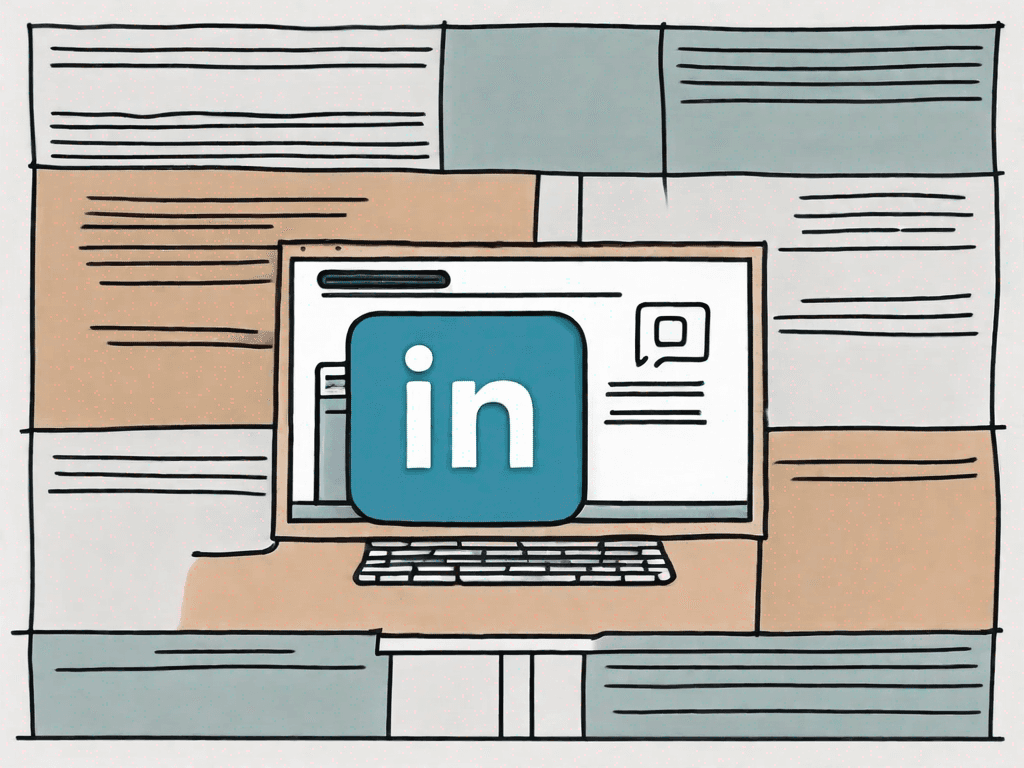
What are LinkedIn InMails? (Explained With Examples)

What is LinkedIn Sales Navigator? (Explained With Examples)

What is Lost Opportunity? (Explained With Examples)

What is Market Positioning? (Explained With Examples)

What is Market Research? (Explained With Examples)
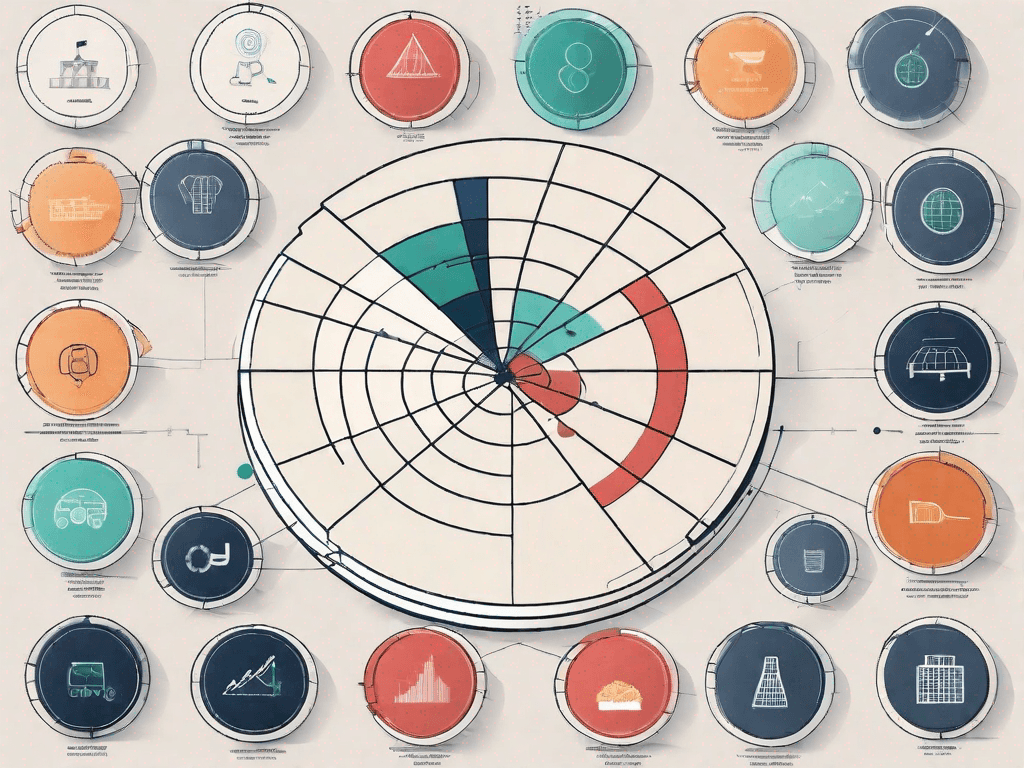
What is Market Segmentation? (Explained With Examples)

What is MEDDIC? (Explained With Examples)

What is Middle Of The Funnel (MOFU)? (Explained With Examples)

What is Motivational Selling? (Explained With Examples)

What is a MQL (Marketing Qualified Lead)? (Explained With Examples)

What is MRR Growth? (Explained With Examples)
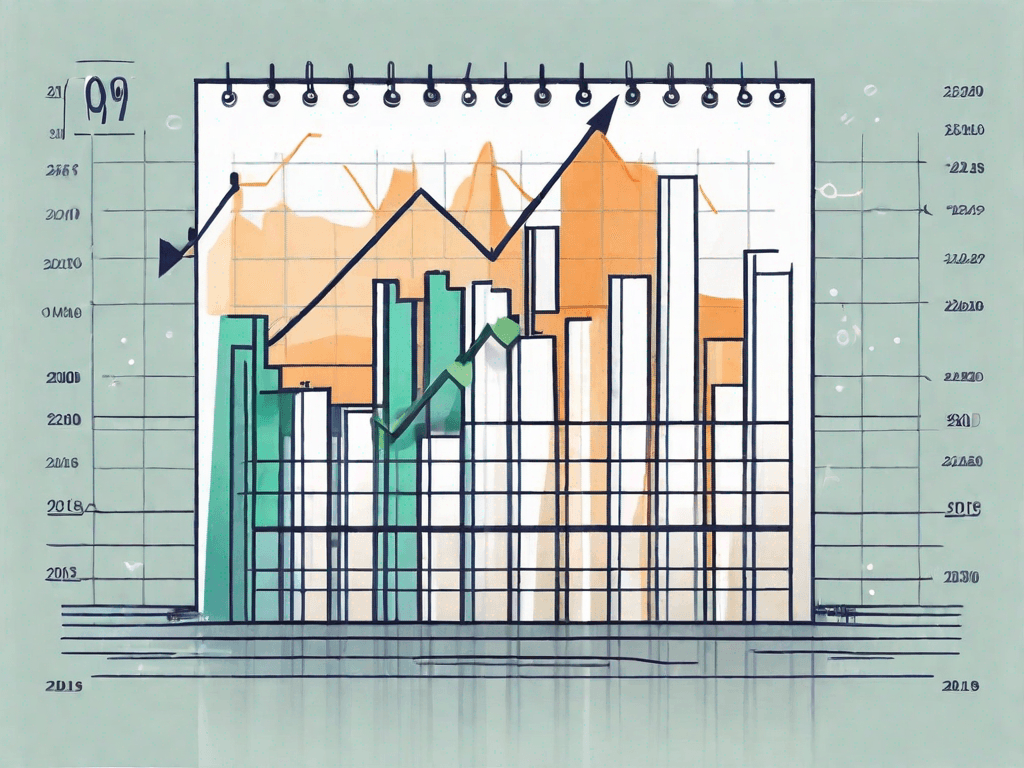
What is MRR (Monthly Recurring Revenue)? (Explained With Examples)
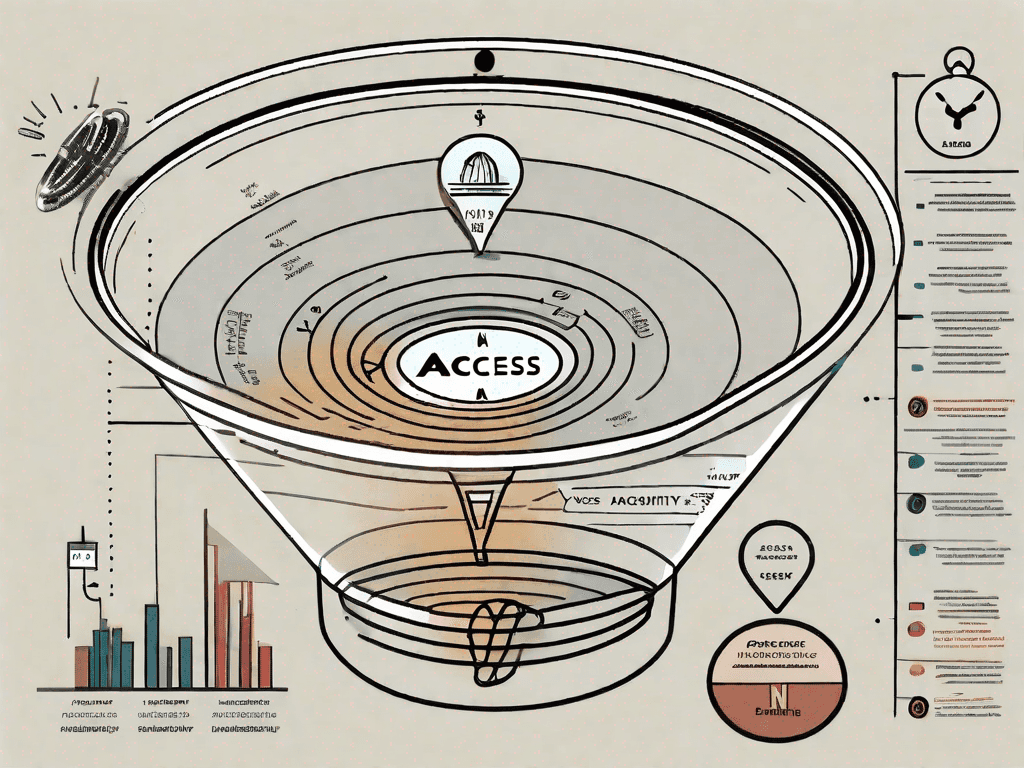
What is N.E.A.T. Selling? (Explained With Examples)

What is Neil Rackham's Sales Tactics? (Explained With Examples)
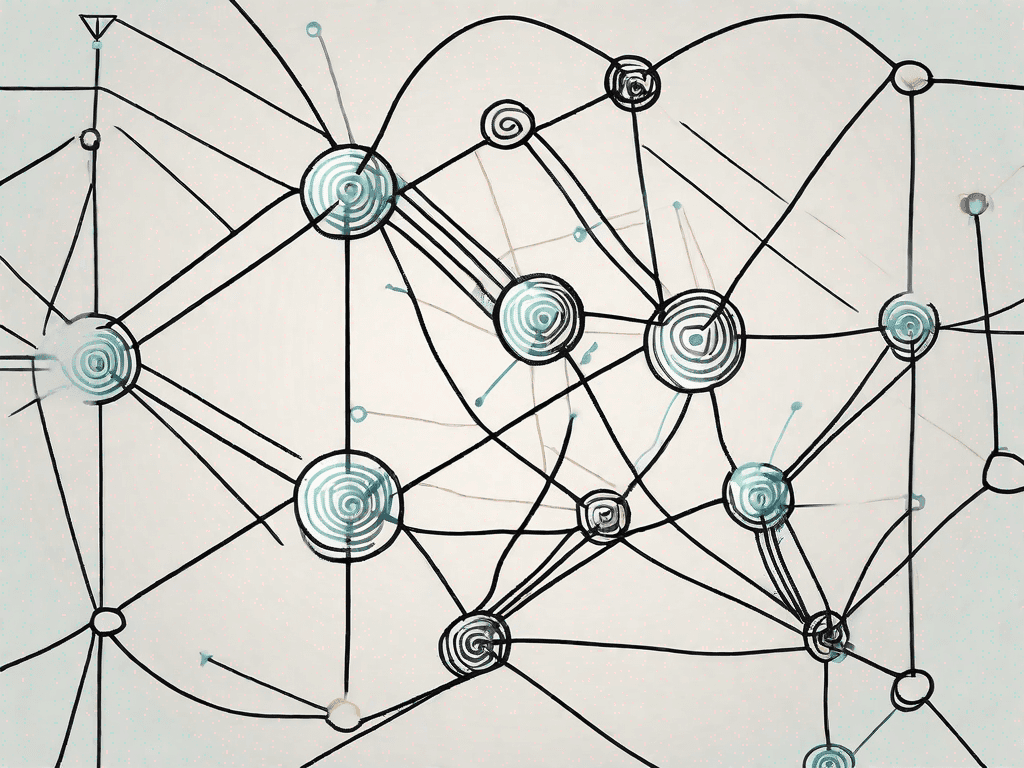
What is Networking? (Explained With Examples)

What is NLP Sales Techniques? (Explained With Examples)

What is the Net Promotion Score? (NPS - Explained With Examples)
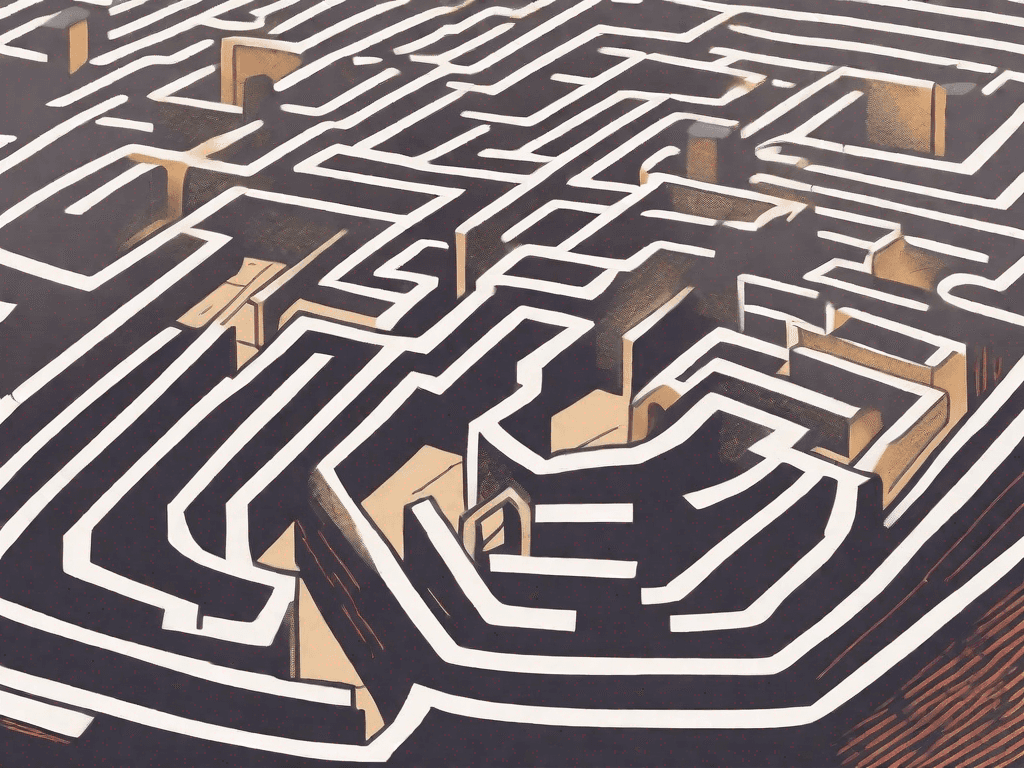
What is Objection Handling Framework? (Explained With Examples)

What is On-Hold Messaging? (Explained With Examples)

What is Onboarding in Sales? (Explained With Examples)

What is Online Advertising? (Explained With Examples)

What is Outbound Sales? (Explained With Examples)

What is Pain Points Analysis? (Explained With Examples)

What is Permission Marketing? (Explained With Examples)

What is Personality-Based Selling? (Explained With Examples)
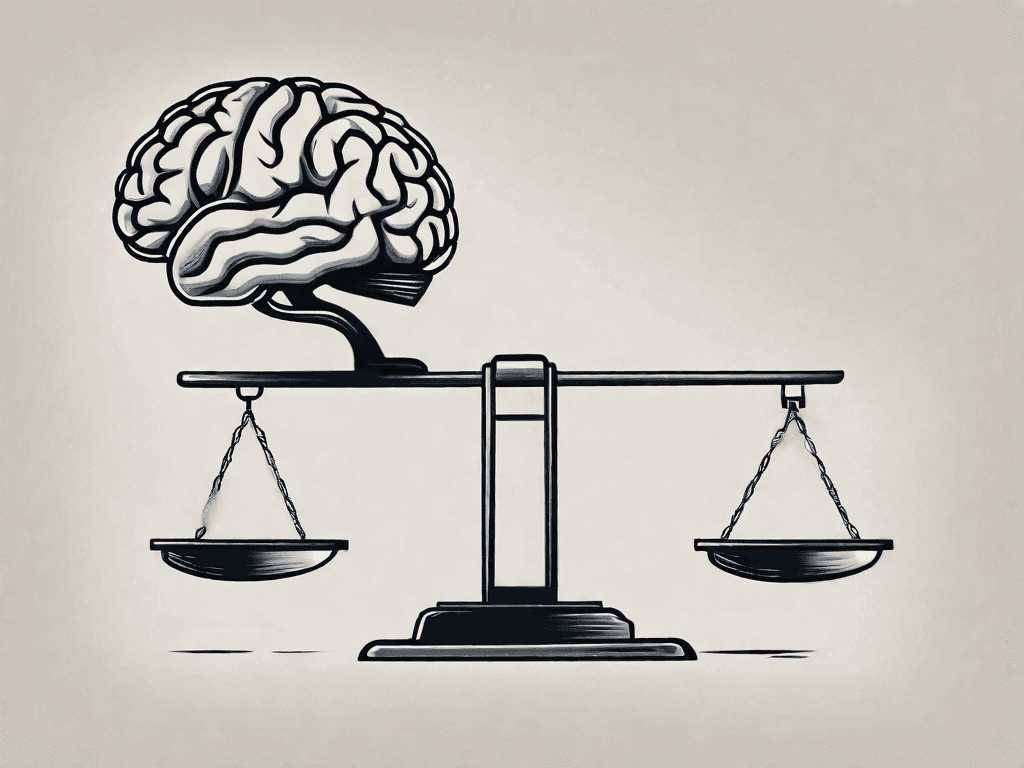
What is Persuasion Selling? (Explained With Examples)
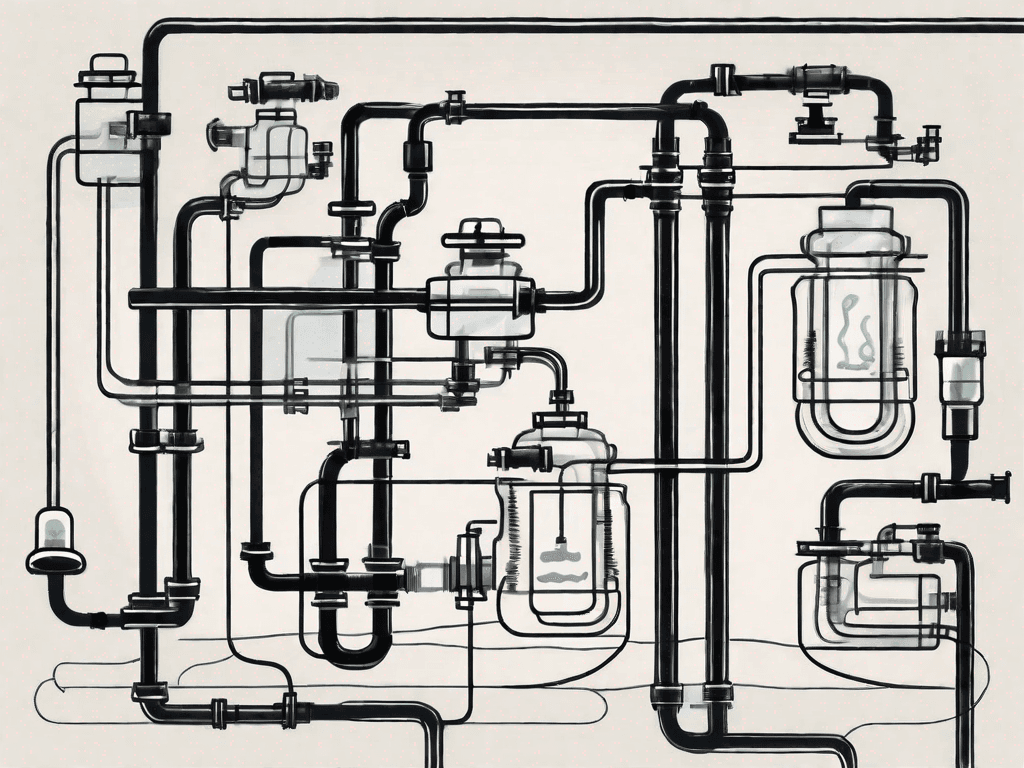
What is Pipeline Management? (Explained With Examples)

What is Pipeline Velocity? (Explained With Examples)

What is Predictive Lead Scoring? (Explained With Examples)

What is Price Negotiation? (Explained With Examples)
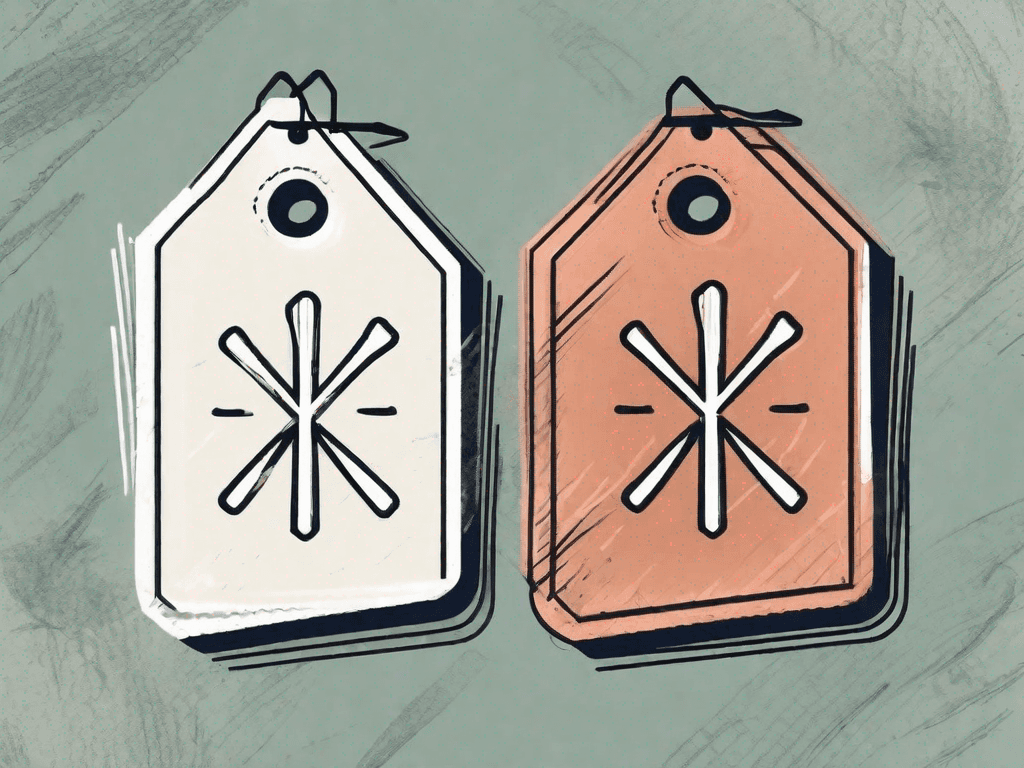
What is Price Objection? (Explained With Examples)

What is Price Sensitivity? (Explained With Examples)

What is Problem-Solution Selling? (Explained With Examples)
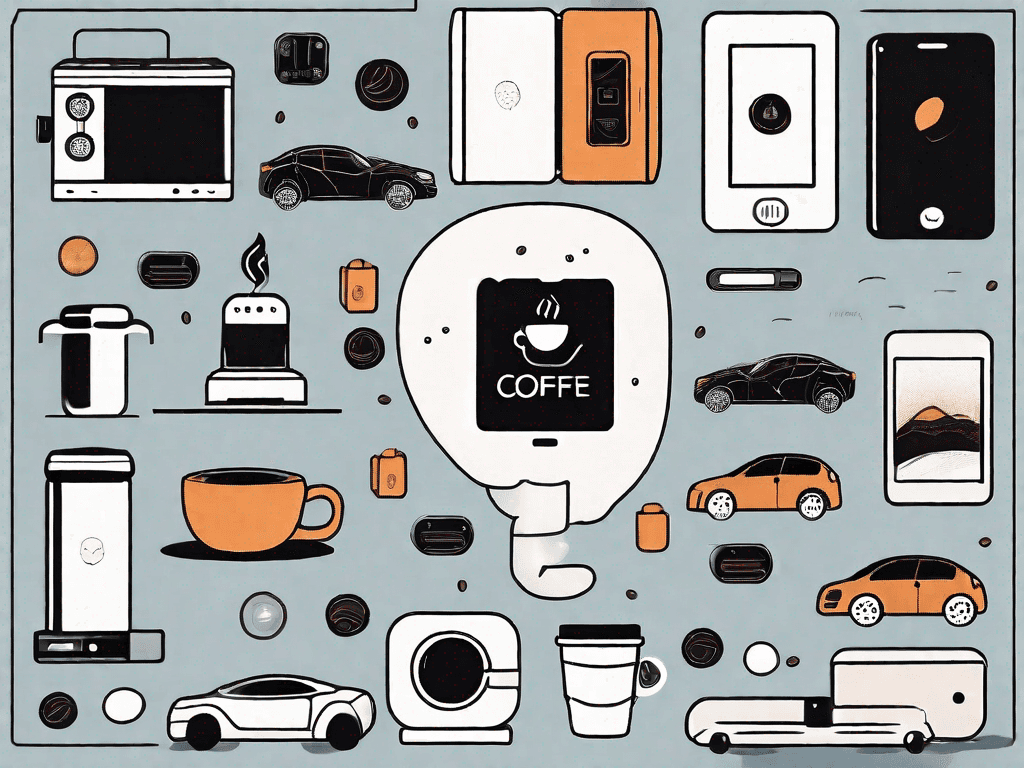
What is Product Knowledge? (Explained With Examples)

What is Product-Led-Growth? (Explained With Examples)
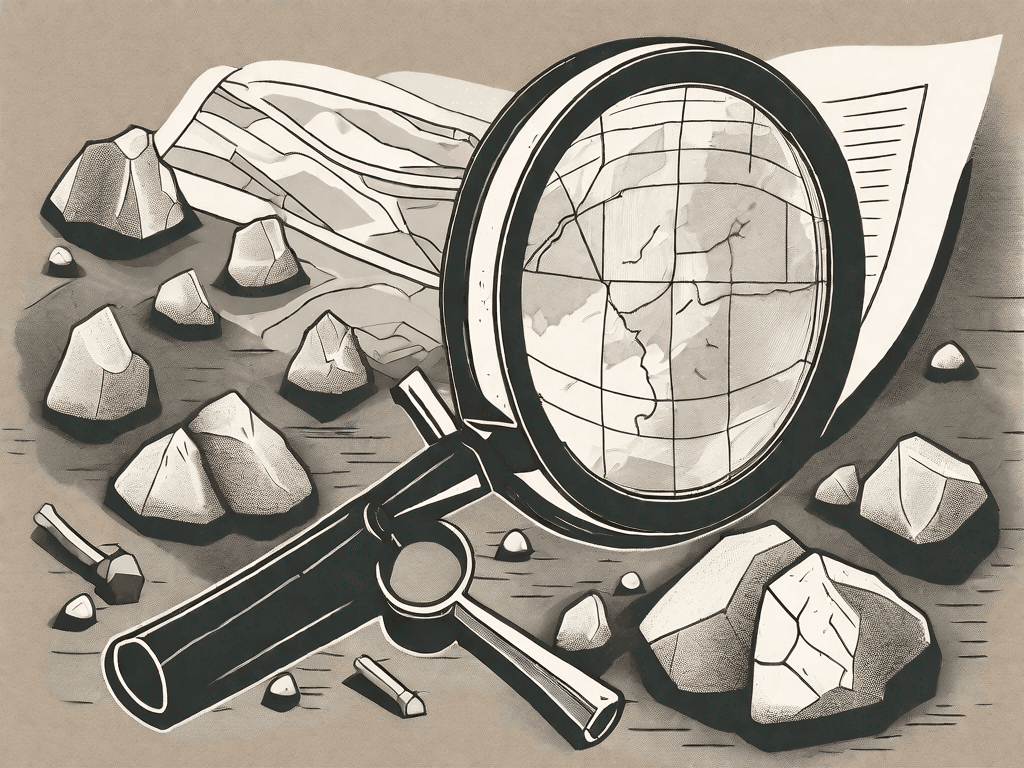
What is Prospecting? (Explained With Examples)

What is a Qualified Lead? (Explained With Examples)

What is Question-Based Selling? (Explained With Examples)
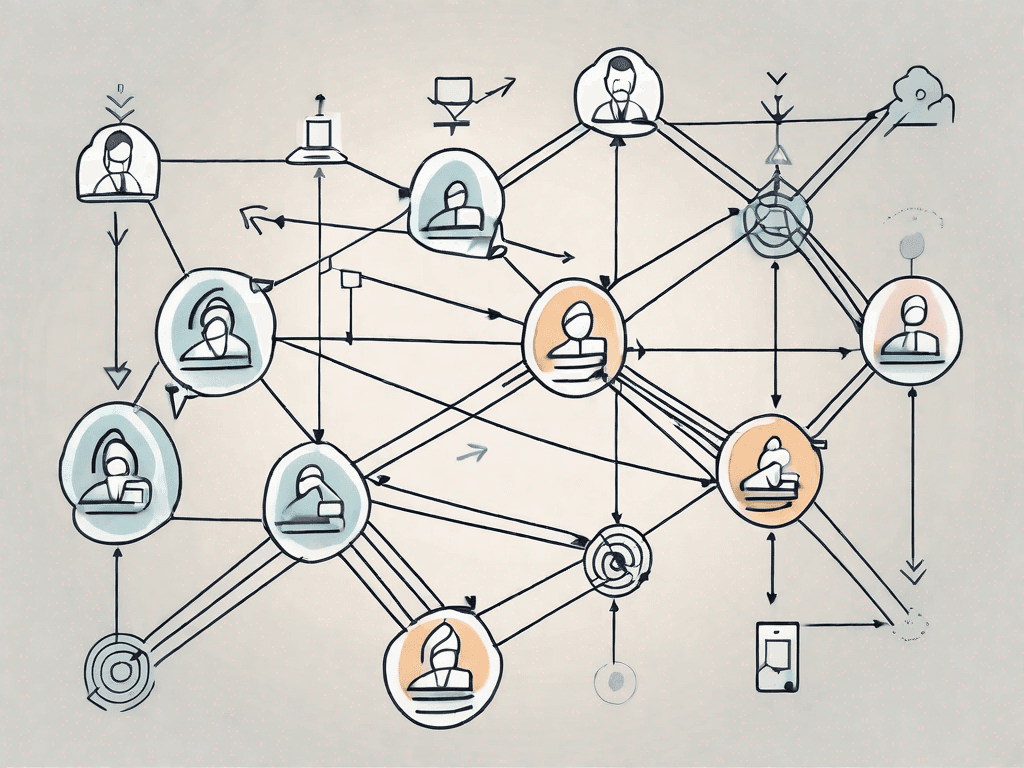
What is Referral Marketing? (Explained With Examples)

What is Relationship Building? (Explained With Examples)
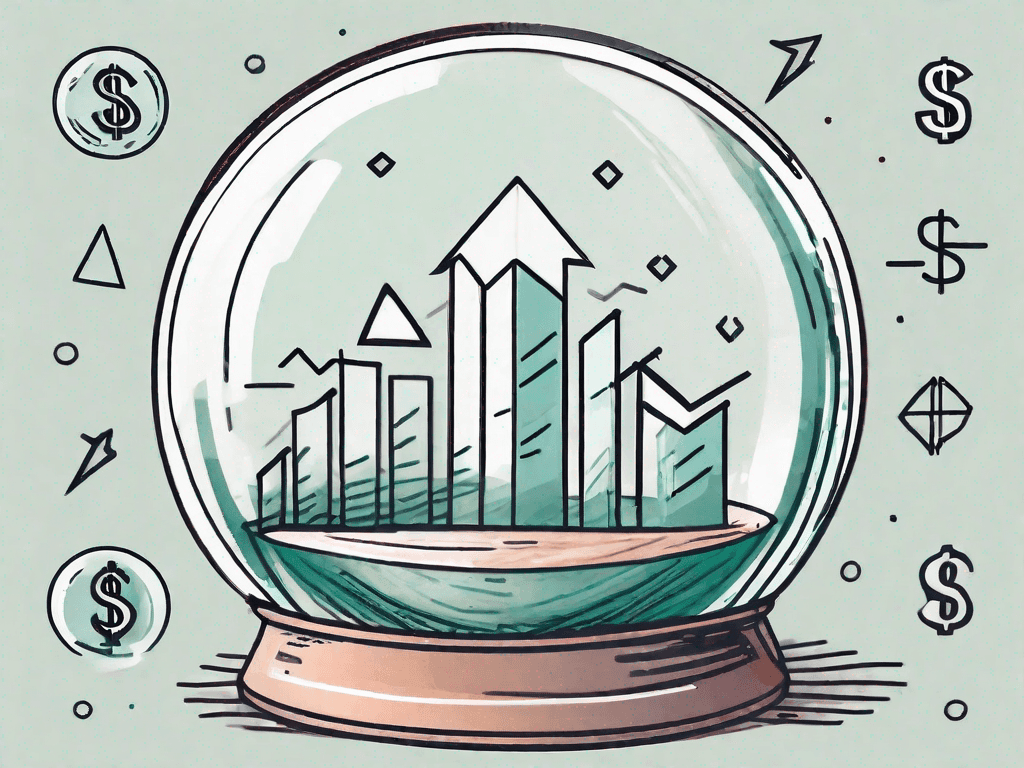
What is Revenue Forecast? (Explained With Examples)

What is a ROI? (Explained With Examples)

What is Sales Automation? (Explained With Examples)

What is a Sales Bonus Plan? (Explained With Examples)

What is a Sales Champion? (Explained With Examples)

What is a Sales Collateral? (Explained With Examples)

What is a Sales Commission Structure Plan? (Explained With Examples)

What is a Sales CRM? (Explained With Examples)

What is a Sales Cycle? (Explained With Examples)
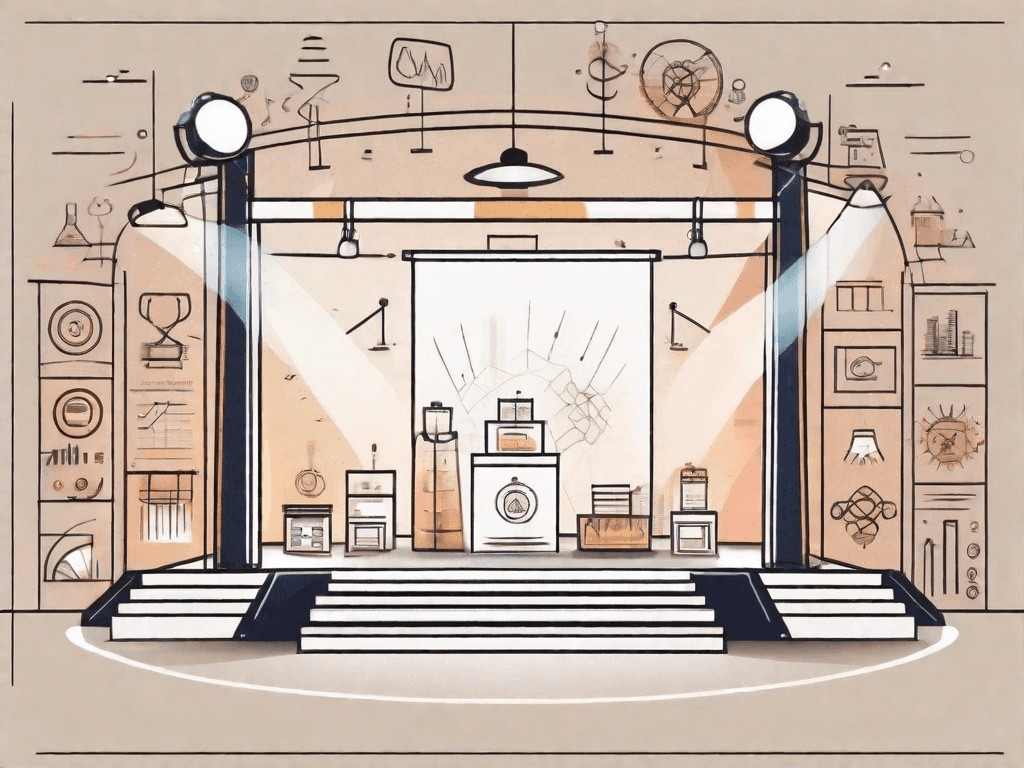
What is a Sales Demo? (Explained With Examples)
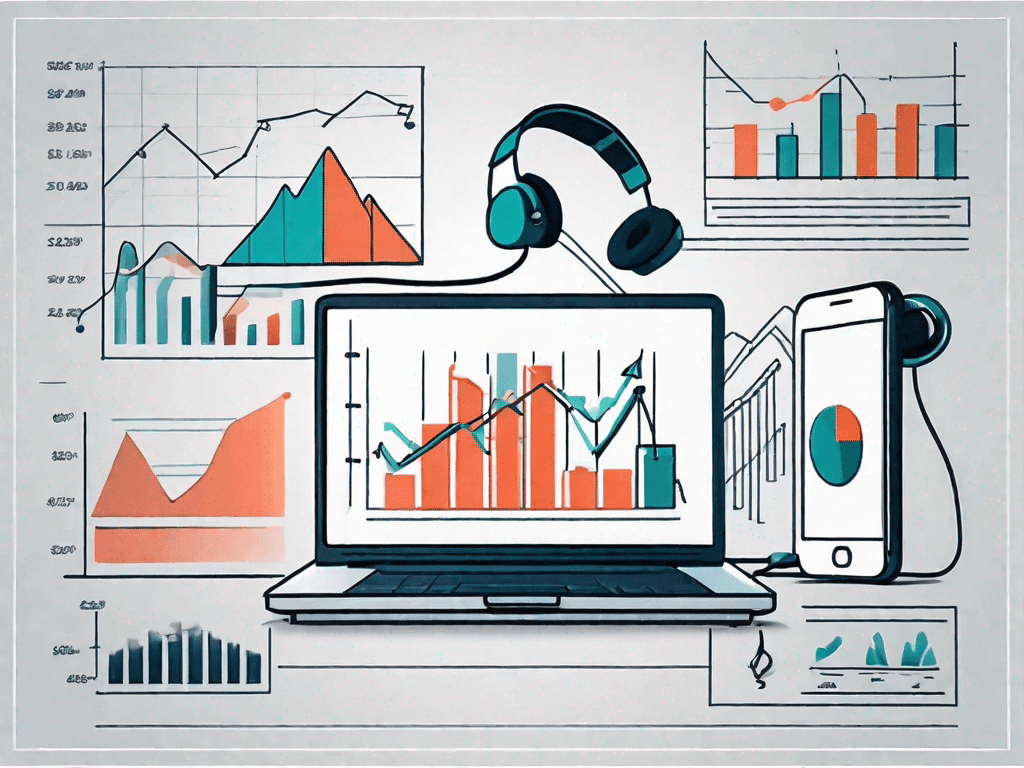
What is Sales Enablement? (Explained With Examples)

What is a Sales Flywheel? (Explained With Examples)

What is a Sales Funnel? (Explained With Examples)
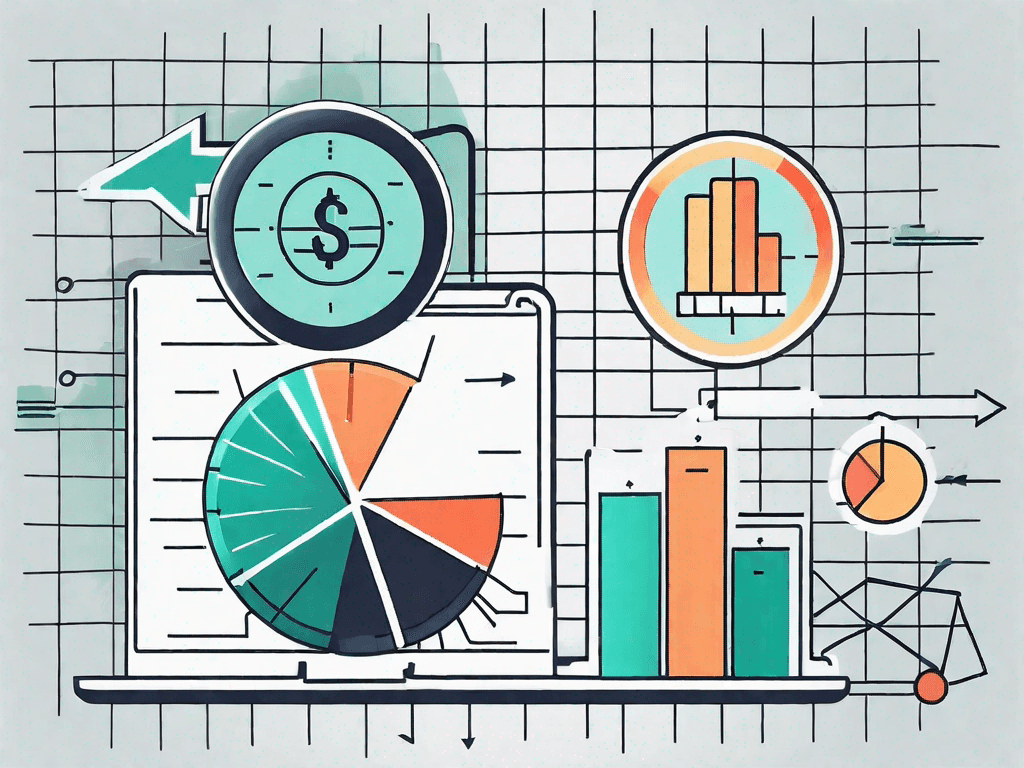
What are Sales KPIs? (Explained With Examples)

What is a Sales Meetup? (Explained With Examples)

What is a Sales Pipeline? (Explained With Examples)

What is a Sales Pitch? (Explained With Examples)

What is a Sales Playbook? (Explained With Examples)
Try breakcold now, are you ready to accelerate your sales pipeline.
Join over +1000 agencies, startups & consultants closing deals with Breakcold Sales CRM
Get Started for free
Sales CRM Features
Sales CRM Software
Sales Pipeline
Sales Lead Tracking
CRM with social media integrations
Social Selling Software
Contact Management
CRM Unified Email LinkedIn Inbox
Breakcold works for many industries
CRM for Agencies
CRM for Startups
CRM for Consultants
CRM for Small Business
CRM for LinkedIn
CRM for Coaches
Sales CRM & Sales Pipeline Tutorials
The 8 Sales Pipeline Stages
The Best CRMs for Agencies
The Best CRMs for Consultants
The Best LinkedIn CRMs
How to close deals in 2024, not in 2010
CRM automation: from 0 to PRO in 5 minutes
LinkedIn Inbox Management
LinkedIn Account-Based Marketing (2024 Tutorial with video)
Tools & more
Sales Pipeline Templates
Alternatives
Integrations
CRM integration with LinkedIn
© 2024 Breakcold
Privacy Policy
Terms of Service
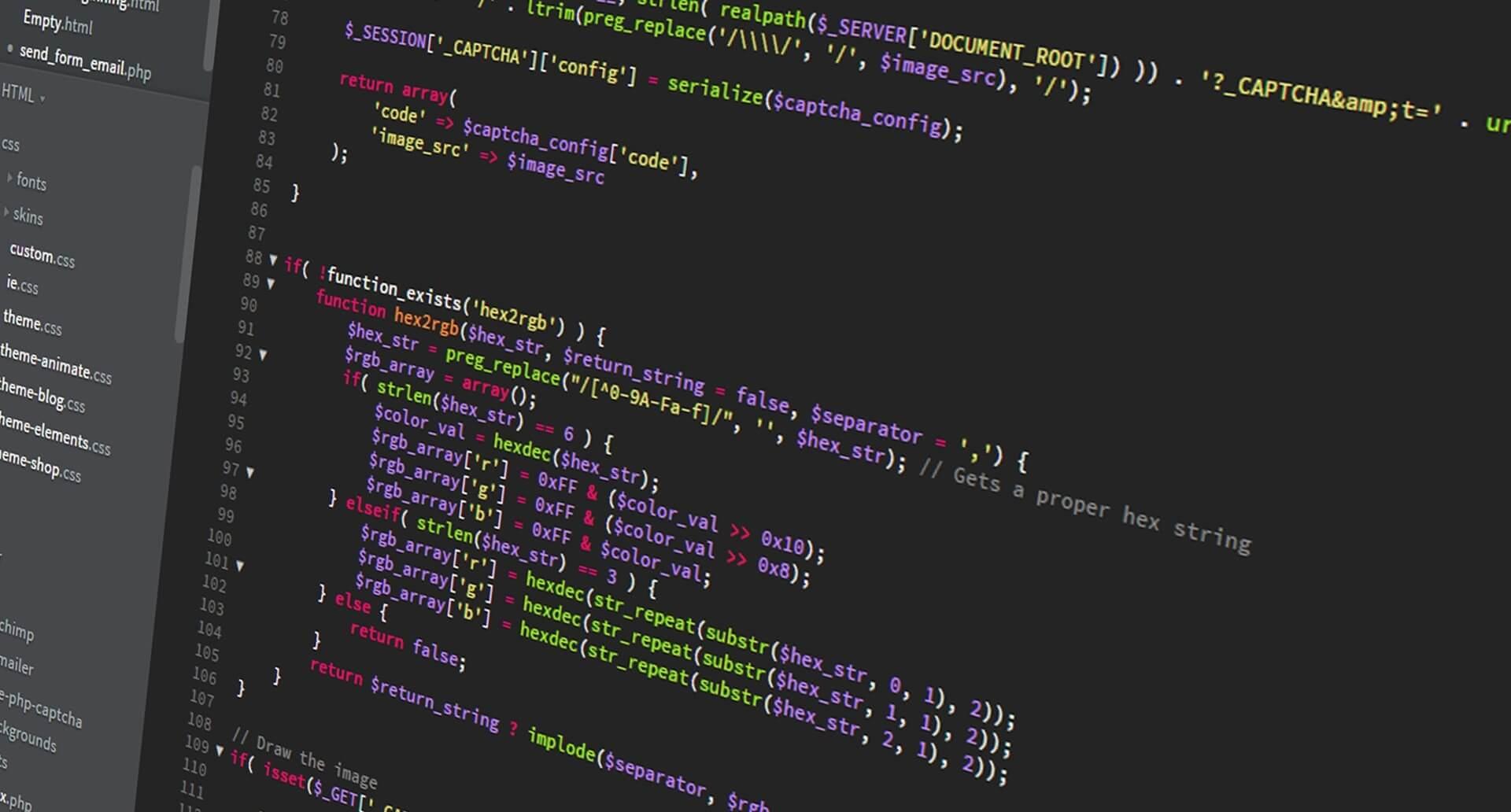
Data Analytics Case Study: Complete Guide in 2024
What are data analytics case study interviews.
When you’re trying to land a data analyst job, the last thing to stand in your way is the data analytics case study interview.
One reason they’re so challenging is that case studies don’t typically have a right or wrong answer.
Instead, case study interviews require you to come up with a hypothesis for an analytics question and then produce data to support or validate your hypothesis. In other words, it’s not just about your technical skills; you’re also being tested on creative problem-solving and your ability to communicate with stakeholders.
This article provides an overview of how to answer data analytics case study interview questions. You can find an in-depth course in the data analytics learning path .
How to Solve Data Analytics Case Questions
Check out our video below on How to solve a Data Analytics case study problem:
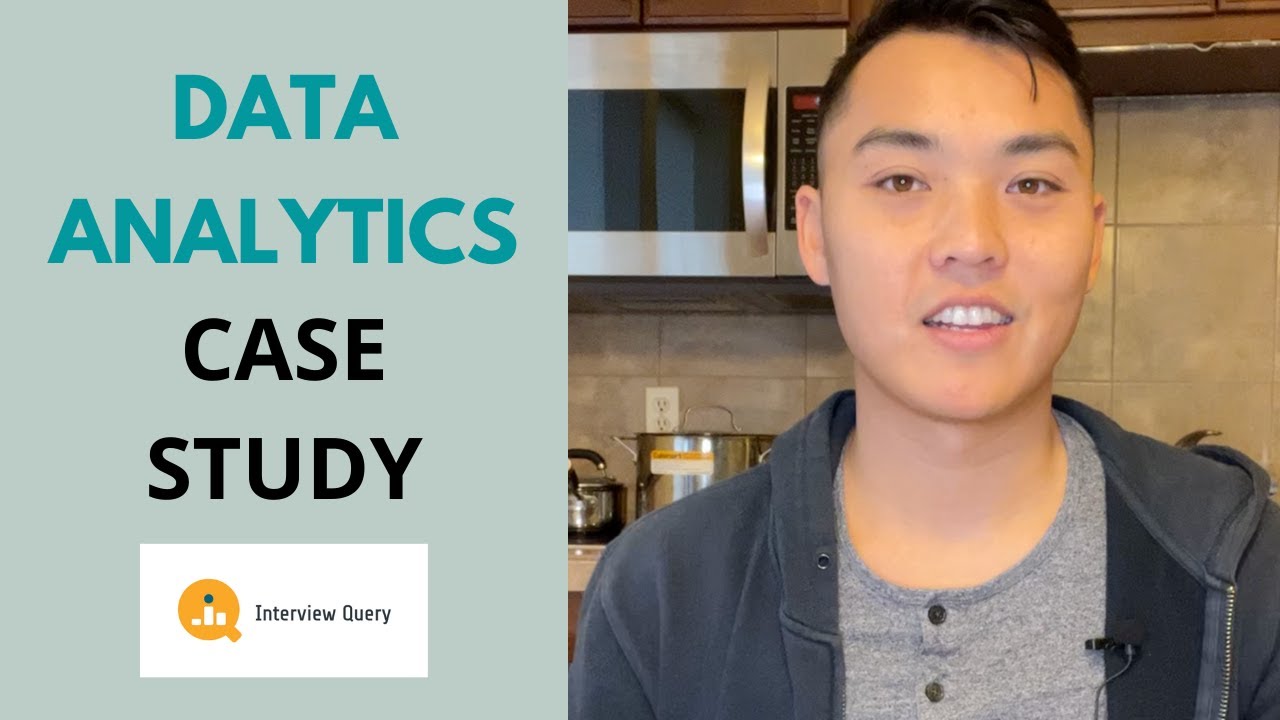
With data analyst case questions, you will need to answer two key questions:
- What metrics should I propose?
- How do I write a SQL query to get the metrics I need?
In short, to ace a data analytics case interview, you not only need to brush up on case questions, but you also should be adept at writing all types of SQL queries and have strong data sense.
These questions are especially challenging to answer if you don’t have a framework or know how to answer them. To help you prepare, we created this step-by-step guide to answering data analytics case questions.
We show you how to use a framework to answer case questions, provide example analytics questions, and help you understand the difference between analytics case studies and product metrics case studies .
Data Analytics Cases vs Product Metrics Questions
Product case questions sometimes get lumped in with data analytics cases.
Ultimately, the type of case question you are asked will depend on the role. For example, product analysts will likely face more product-oriented questions.
Product metrics cases tend to focus on a hypothetical situation. You might be asked to:
Investigate Metrics - One of the most common types will ask you to investigate a metric, usually one that’s going up or down. For example, “Why are Facebook friend requests falling by 10 percent?”
Measure Product/Feature Success - A lot of analytics cases revolve around the measurement of product success and feature changes. For example, “We want to add X feature to product Y. What metrics would you track to make sure that’s a good idea?”
With product data cases, the key difference is that you may or may not be required to write the SQL query to find the metric.
Instead, these interviews are more theoretical and are designed to assess your product sense and ability to think about analytics problems from a product perspective. Product metrics questions may also show up in the data analyst interview , but likely only for product data analyst roles.
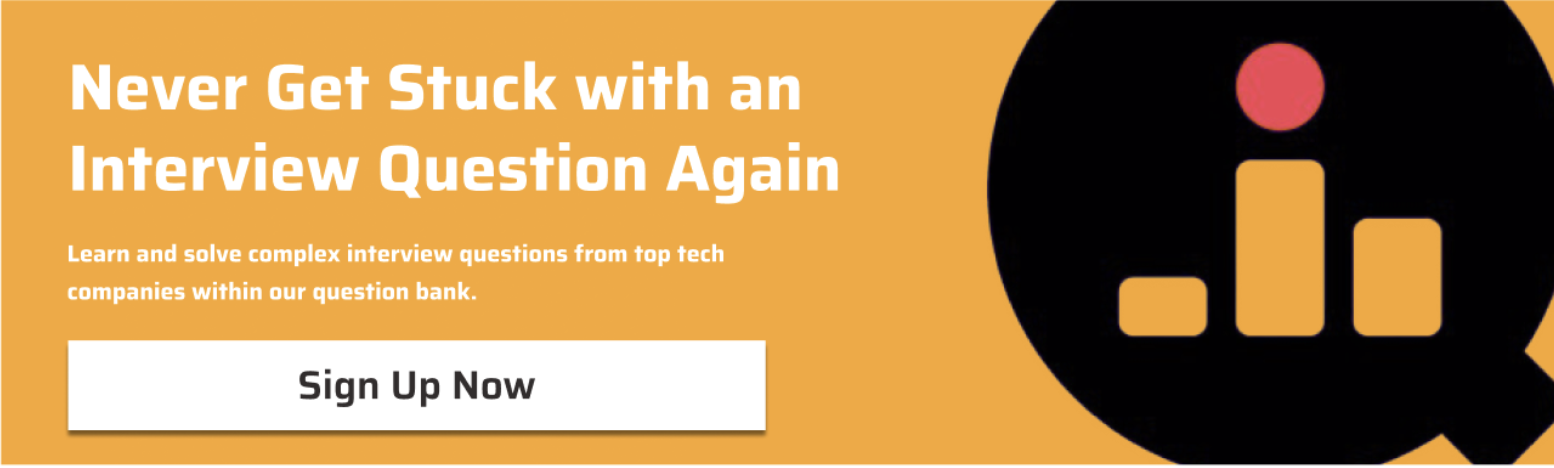
TRY CHECKING: Marketing Analytics Case Study Guide
Data Analytics Case Study Question: Sample Solution
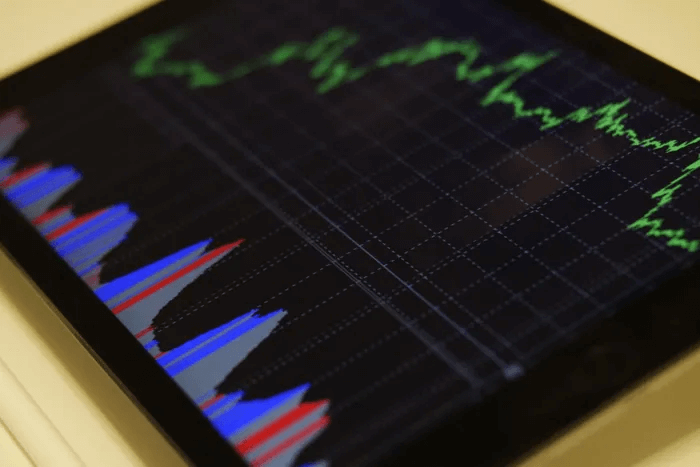
Let’s start with an example data analytics case question :
You’re given a table that represents search results from searches on Facebook. The query column is the search term, the position column represents each position the search result came in, and the rating column represents the human rating from 1 to 5, where 5 is high relevance, and 1 is low relevance.
Each row in the search_events table represents a single search, with the has_clicked column representing if a user clicked on a result or not. We have a hypothesis that the CTR is dependent on the search result rating.
Write a query to return data to support or disprove this hypothesis.
search_results table:
search_events table
Step 1: With Data Analytics Case Studies, Start by Making Assumptions
Hint: Start by making assumptions and thinking out loud. With this question, focus on coming up with a metric to support the hypothesis. If the question is unclear or if you think you need more information, be sure to ask.
Answer. The hypothesis is that CTR is dependent on search result rating. Therefore, we want to focus on the CTR metric, and we can assume:
- If CTR is high when search result ratings are high, and CTR is low when the search result ratings are low, then the hypothesis is correct.
- If CTR is low when the search ratings are high, or there is no proven correlation between the two, then our hypothesis is not proven.
Step 2: Provide a Solution for the Case Question
Hint: Walk the interviewer through your reasoning. Talking about the decisions you make and why you’re making them shows off your problem-solving approach.
Answer. One way we can investigate the hypothesis is to look at the results split into different search rating buckets. For example, if we measure the CTR for results rated at 1, then those rated at 2, and so on, we can identify if an increase in rating is correlated with an increase in CTR.
First, I’d write a query to get the number of results for each query in each bucket. We want to look at the distribution of results that are less than a rating threshold, which will help us see the relationship between search rating and CTR.
This CTE aggregates the number of results that are less than a certain rating threshold. Later, we can use this to see the percentage that are in each bucket. If we re-join to the search_events table, we can calculate the CTR by then grouping by each bucket.
Step 3: Use Analysis to Backup Your Solution
Hint: Be prepared to justify your solution. Interviewers will follow up with questions about your reasoning, and ask why you make certain assumptions.
Answer. By using the CASE WHEN statement, I calculated each ratings bucket by checking to see if all the search results were less than 1, 2, or 3 by subtracting the total from the number within the bucket and seeing if it equates to 0.
I did that to get away from averages in our bucketing system. Outliers would make it more difficult to measure the effect of bad ratings. For example, if a query had a 1 rating and another had a 5 rating, that would equate to an average of 3. Whereas in my solution, a query with all of the results under 1, 2, or 3 lets us know that it actually has bad ratings.
Product Data Case Question: Sample Solution
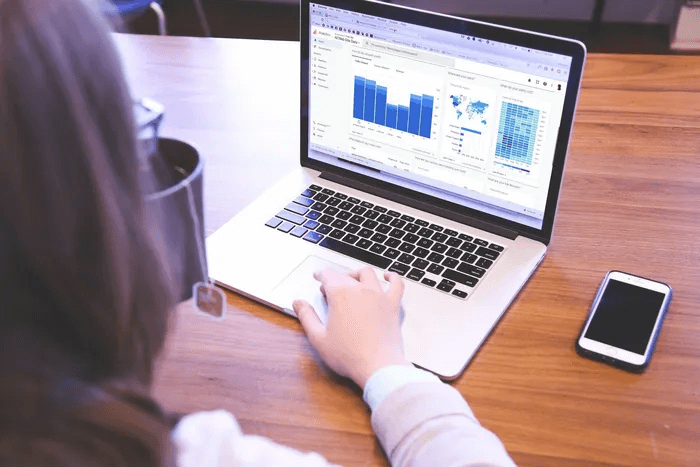
In product metrics interviews, you’ll likely be asked about analytics, but the discussion will be more theoretical. You’ll propose a solution to a problem, and supply the metrics you’ll use to investigate or solve it. You may or may not be required to write a SQL query to get those metrics.
We’ll start with an example product metrics case study question :
Let’s say you work for a social media company that has just done a launch in a new city. Looking at weekly metrics, you see a slow decrease in the average number of comments per user from January to March in this city.
The company has been consistently growing new users in the city from January to March.
What are some reasons why the average number of comments per user would be decreasing and what metrics would you look into?
Step 1: Ask Clarifying Questions Specific to the Case
Hint: This question is very vague. It’s all hypothetical, so we don’t know very much about users, what the product is, and how people might be interacting. Be sure you ask questions upfront about the product.
Answer: Before I jump into an answer, I’d like to ask a few questions:
- Who uses this social network? How do they interact with each other?
- Has there been any performance issues that might be causing the problem?
- What are the goals of this particular launch?
- Has there been any changes to the comment features in recent weeks?
For the sake of this example, let’s say we learn that it’s a social network similar to Facebook with a young audience, and the goals of the launch are to grow the user base. Also, there have been no performance issues and the commenting feature hasn’t been changed since launch.
Step 2: Use the Case Question to Make Assumptions
Hint: Look for clues in the question. For example, this case gives you a metric, “average number of comments per user.” Consider if the clue might be helpful in your solution. But be careful, sometimes questions are designed to throw you off track.
Answer: From the question, we can hypothesize a little bit. For example, we know that user count is increasing linearly. That means two things:
- The decreasing comments issue isn’t a result of a declining user base.
- The cause isn’t loss of platform.
We can also model out the data to help us get a better picture of the average number of comments per user metric:
- January: 10000 users, 30000 comments, 3 comments/user
- February: 20000 users, 50000 comments, 2.5 comments/user
- March: 30000 users, 60000 comments, 2 comments/user
One thing to note: Although this is an interesting metric, I’m not sure if it will help us solve this question. For one, average comments per user doesn’t account for churn. We might assume that during the three-month period users are churning off the platform. Let’s say the churn rate is 25% in January, 20% in February and 15% in March.
Step 3: Make a Hypothesis About the Data
Hint: Don’t worry too much about making a correct hypothesis. Instead, interviewers want to get a sense of your product initiation and that you’re on the right track. Also, be prepared to measure your hypothesis.
Answer. I would say that average comments per user isn’t a great metric to use, because it doesn’t reveal insights into what’s really causing this issue.
That’s because it doesn’t account for active users, which are the users who are actually commenting. A better metric to investigate would be retained users and monthly active users.
What I suspect is causing the issue is that active users are commenting frequently and are responsible for the increase in comments month-to-month. New users, on the other hand, aren’t as engaged and aren’t commenting as often.
Step 4: Provide Metrics and Data Analysis
Hint: Within your solution, include key metrics that you’d like to investigate that will help you measure success.
Answer: I’d say there are a few ways we could investigate the cause of this problem, but the one I’d be most interested in would be the engagement of monthly active users.
If the growth in comments is coming from active users, that would help us understand how we’re doing at retaining users. Plus, it will also show if new users are less engaged and commenting less frequently.
One way that we could dig into this would be to segment users by their onboarding date, which would help us to visualize engagement and see how engaged some of our longest-retained users are.
If engagement of new users is the issue, that will give us some options in terms of strategies for addressing the problem. For example, we could test new onboarding or commenting features designed to generate engagement.
Step 5: Propose a Solution for the Case Question
Hint: In the majority of cases, your initial assumptions might be incorrect, or the interviewer might throw you a curveball. Be prepared to make new hypotheses or discuss the pitfalls of your analysis.
Answer. If the cause wasn’t due to a lack of engagement among new users, then I’d want to investigate active users. One potential cause would be active users commenting less. In that case, we’d know that our earliest users were churning out, and that engagement among new users was potentially growing.
Again, I think we’d want to focus on user engagement since the onboarding date. That would help us understand if we were seeing higher levels of churn among active users, and we could start to identify some solutions there.
Tip: Use a Framework to Solve Data Analytics Case Questions
Analytics case questions can be challenging, but they’re much more challenging if you don’t use a framework. Without a framework, it’s easier to get lost in your answer, to get stuck, and really lose the confidence of your interviewer. Find helpful frameworks for data analytics questions in our data analytics learning path and our product metrics learning path .
Once you have the framework down, what’s the best way to practice? Mock interviews with our coaches are very effective, as you’ll get feedback and helpful tips as you answer. You can also learn a lot by practicing P2P mock interviews with other Interview Query students. No data analytics background? Check out how to become a data analyst without a degree .
Finally, if you’re looking for sample data analytics case questions and other types of interview questions, see our guide on the top data analyst interview questions .
Currently taking bookings for July >>
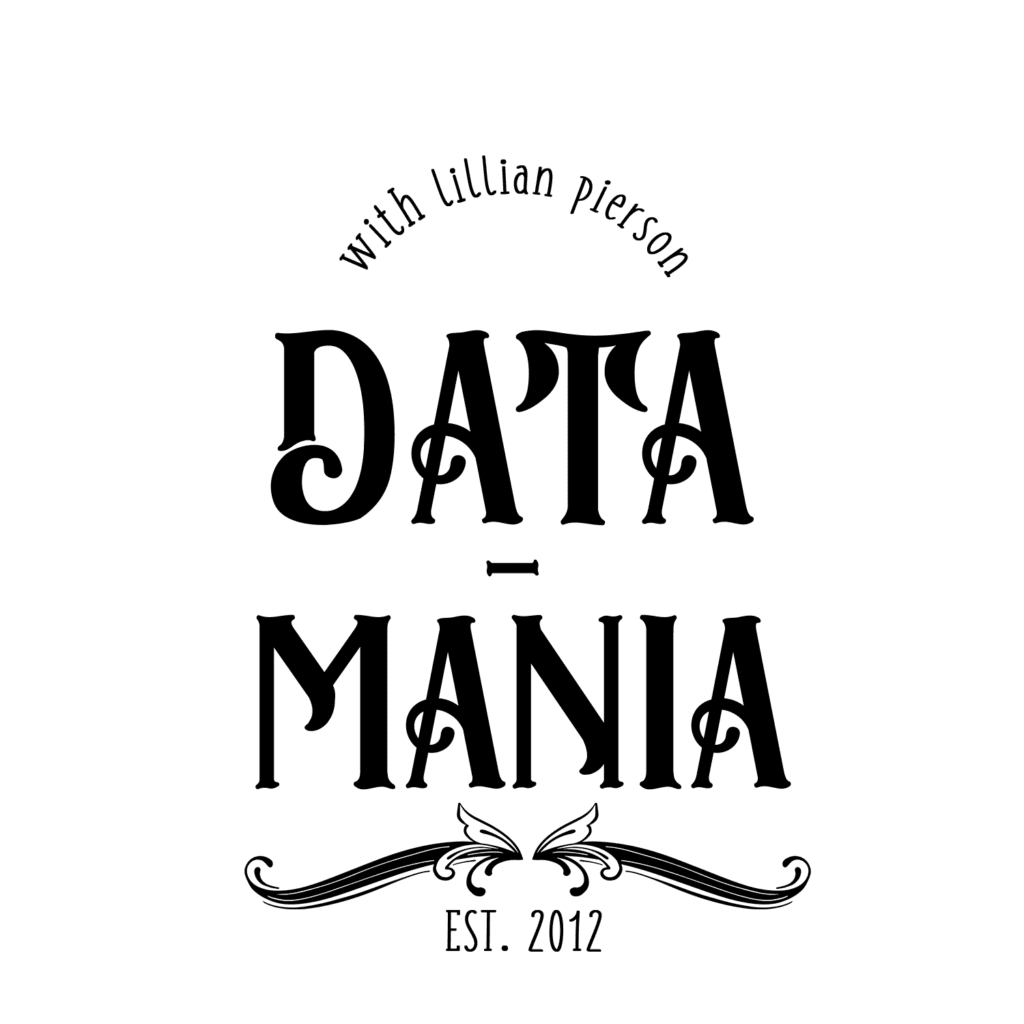
Data Analysis Case Study: Learn From Humana’s Automated Data Analysis Project
Lillian Pierson, P.E.
Playback speed:
Got data? Great! Looking for that perfect data analysis case study to help you get started using it? You’re in the right place.
If you’ve ever struggled to decide what to do next with your data projects, to actually find meaning in the data, or even to decide what kind of data to collect, then KEEP READING…
Deep down, you know what needs to happen. You need to initiate and execute a data strategy that really moves the needle for your organization. One that produces seriously awesome business results.
But how you’re in the right place to find out..
As a data strategist who has worked with 10 percent of Fortune 100 companies, today I’m sharing with you a case study that demonstrates just how real businesses are making real wins with data analysis.
In the post below, we’ll look at:
- A shining data success story;
- What went on ‘under-the-hood’ to support that successful data project; and
- The exact data technologies used by the vendor, to take this project from pure strategy to pure success
If you prefer to watch this information rather than read it, it’s captured in the video below:
Here’s the url too: https://youtu.be/xMwZObIqvLQ
3 Action Items You Need To Take
To actually use the data analysis case study you’re about to get – you need to take 3 main steps. Those are:
- Reflect upon your organization as it is today (I left you some prompts below – to help you get started)
- Review winning data case collections (starting with the one I’m sharing here) and identify 5 that seem the most promising for your organization given it’s current set-up
- Assess your organization AND those 5 winning case collections. Based on that assessment, select the “QUICK WIN” data use case that offers your organization the most bang for it’s buck
Step 1: Reflect Upon Your Organization
Whenever you evaluate data case collections to decide if they’re a good fit for your organization, the first thing you need to do is organize your thoughts with respect to your organization as it is today.
Before moving into the data analysis case study, STOP and ANSWER THE FOLLOWING QUESTIONS – just to remind yourself:
- What is the business vision for our organization?
- What industries do we primarily support?
- What data technologies do we already have up and running, that we could use to generate even more value?
- What team members do we have to support a new data project? And what are their data skillsets like?
- What type of data are we mostly looking to generate value from? Structured? Semi-Structured? Un-structured? Real-time data? Huge data sets? What are our data resources like?
Jot down some notes while you’re here. Then keep them in mind as you read on to find out how one company, Humana, used its data to achieve a 28 percent increase in customer satisfaction. Also include its 63 percent increase in employee engagement! (That’s such a seriously impressive outcome, right?!)
Step 2: Review Data Case Studies
Here we are, already at step 2. It’s time for you to start reviewing data analysis case studies (starting with the one I’m sharing below). I dentify 5 that seem the most promising for your organization given its current set-up.
Humana’s Automated Data Analysis Case Study
The key thing to note here is that the approach to creating a successful data program varies from industry to industry .
Let’s start with one to demonstrate the kind of value you can glean from these kinds of success stories.
Humana has provided health insurance to Americans for over 50 years. It is a service company focused on fulfilling the needs of its customers. A great deal of Humana’s success as a company rides on customer satisfaction, and the frontline of that battle for customers’ hearts and minds is Humana’s customer service center.
Call centers are hard to get right. A lot of emotions can arise during a customer service call, especially one relating to health and health insurance. Sometimes people are frustrated. At times, they’re upset. Also, there are times the customer service representative becomes aggravated, and the overall tone and progression of the phone call goes downhill. This is of course very bad for customer satisfaction.
Humana wanted to use artificial intelligence to improve customer satisfaction (and thus, customer retention rates & profits per customer).
Humana wanted to find a way to use artificial intelligence to monitor their phone calls and help their agents do a better job connecting with their customers in order to improve customer satisfaction (and thus, customer retention rates & profits per customer ).
In light of their business need, Humana worked with a company called Cogito, which specializes in voice analytics technology.
Cogito offers a piece of AI technology called Cogito Dialogue. It’s been trained to identify certain conversational cues as a way of helping call center representatives and supervisors stay actively engaged in a call with a customer.
The AI listens to cues like the customer’s voice pitch.
If it’s rising, or if the call representative and the customer talk over each other, then the dialogue tool will send out electronic alerts to the agent during the call.
Humana fed the dialogue tool customer service data from 10,000 calls and allowed it to analyze cues such as keywords, interruptions, and pauses, and these cues were then linked with specific outcomes. For example, if the representative is receiving a particular type of cues, they are likely to get a specific customer satisfaction result.
The Outcome
Customers were happier, and customer service representatives were more engaged..
This automated solution for data analysis has now been deployed in 200 Humana call centers and the company plans to roll it out to 100 percent of its centers in the future.
The initiative was so successful, Humana has been able to focus on next steps in its data program. The company now plans to begin predicting the type of calls that are likely to go unresolved, so they can send those calls over to management before they become frustrating to the customer and customer service representative alike.
What does this mean for you and your business?
Well, if you’re looking for new ways to generate value by improving the quantity and quality of the decision support that you’re providing to your customer service personnel, then this may be a perfect example of how you can do so.
Humana’s Business Use Cases
Humana’s data analysis case study includes two key business use cases:
- Analyzing customer sentiment; and
- Suggesting actions to customer service representatives.
Analyzing Customer Sentiment
First things first, before you go ahead and collect data, you need to ask yourself who and what is involved in making things happen within the business.
In the case of Humana, the actors were:
- The health insurance system itself
- The customer, and
- The customer service representative
As you can see in the use case diagram above, the relational aspect is pretty simple. You have a customer service representative and a customer. They are both producing audio data, and that audio data is being fed into the system.
Humana focused on collecting the key data points, shown in the image below, from their customer service operations.
By collecting data about speech style, pitch, silence, stress in customers’ voices, length of call, speed of customers’ speech, intonation, articulation, silence, and representatives’ manner of speaking, Humana was able to analyze customer sentiment and introduce techniques for improved customer satisfaction.
Having strategically defined these data points, the Cogito technology was able to generate reports about customer sentiment during the calls.
Suggesting actions to customer service representatives.
The second use case for the Humana data program follows on from the data gathered in the first case.
In Humana’s case, Cogito generated a host of call analyses and reports about key call issues.
In the second business use case, Cogito was able to suggest actions to customer service representatives, in real-time , to make use of incoming data and help improve customer satisfaction on the spot.
The technology Humana used provided suggestions via text message to the customer service representative, offering the following types of feedback:
- The tone of voice is too tense
- The speed of speaking is high
- The customer representative and customer are speaking at the same time
These alerts allowed the Humana customer service representatives to alter their approach immediately , improving the quality of the interaction and, subsequently, the customer satisfaction.
The preconditions for success in this use case were:
- The call-related data must be collected and stored
- The AI models must be in place to generate analysis on the data points that are recorded during the calls
Evidence of success can subsequently be found in a system that offers real-time suggestions for courses of action that the customer service representative can take to improve customer satisfaction.
Thanks to this data-intensive business use case, Humana was able to increase customer satisfaction, improve customer retention rates, and drive profits per customer.
The Technology That Supports This Data Analysis Case Study
I promised to dip into the tech side of things. This is especially for those of you who are interested in the ins and outs of how projects like this one are actually rolled out.
Here’s a little rundown of the main technologies we discovered when we investigated how Cogito runs in support of its clients like Humana.
- For cloud data management Cogito uses AWS, specifically the Athena product
- For on-premise big data management, the company used Apache HDFS – the distributed file system for storing big data
- They utilize MapReduce, for processing their data
- And Cogito also has traditional systems and relational database management systems such as PostgreSQL
- In terms of analytics and data visualization tools, Cogito makes use of Tableau
- And for its machine learning technology, these use cases required people with knowledge in Python, R, and SQL, as well as deep learning (Cogito uses the PyTorch library and the TensorFlow library)
These data science skill sets support the effective computing, deep learning , and natural language processing applications employed by Humana for this use case.
If you’re looking to hire people to help with your own data initiative, then people with those skills listed above, and with experience in these specific technologies, would be a huge help.
Step 3: S elect The “Quick Win” Data Use Case
Still there? Great!
It’s time to close the loop.
Remember those notes you took before you reviewed the study? I want you to STOP here and assess. Does this Humana case study seem applicable and promising as a solution, given your organization’s current set-up…
YES ▶ Excellent!
Earmark it and continue exploring other winning data use cases until you’ve identified 5 that seem like great fits for your businesses needs. Evaluate those against your organization’s needs, and select the very best fit to be your “quick win” data use case. Develop your data strategy around that.
NO , Lillian – It’s not applicable. ▶ No problem.
Discard the information and continue exploring the winning data use cases we’ve categorized for you according to business function and industry. Save time by dialing down into the business function you know your business really needs help with now. Identify 5 winning data use cases that seem like great fits for your businesses needs. Evaluate those against your organization’s needs, and select the very best fit to be your “quick win” data use case. Develop your data strategy around that data use case.
More resources to get ahead...
Get income-generating ideas for data professionals, are you tired of relying on one employer for your income are you dreaming of a side hustle that won’t put you at risk of getting fired or sued well, my friend, you’re in luck..
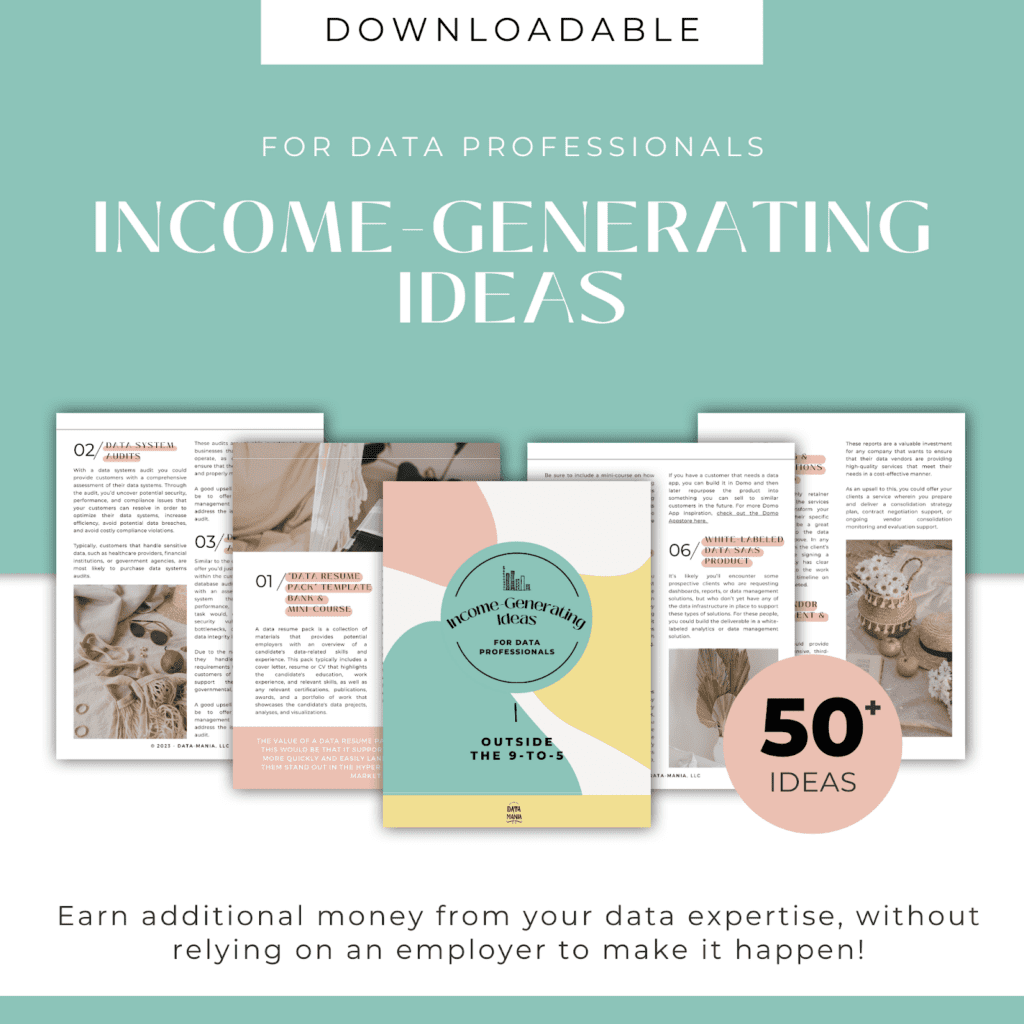
This 48-page listing is here to rescue you from the drudgery of corporate slavery and set you on the path to start earning more money from your existing data expertise. Spend just 1 hour with this pdf and I can guarantee you’ll be bursting at the seams with practical, proven & profitable ideas for new income-streams you can create from your existing expertise. Learn more here!
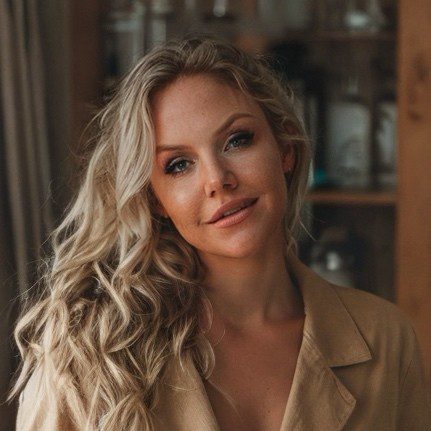
Apply To Work Together
Get featured, join the convergence newsletter.
Our newsletter is exclusively written for operators in the data & AI industry. Hi, I'm Lillian Pierson, Data-Mania's founder. We welcome you to our little corner of the internet. Data-Mania offers fractional CMO and marketing consulting services to deep tech B2B businesses. The Convergence community is sponsored by Data-Mania, as a tribute to the data community from which we sprung. You are welcome anytime.
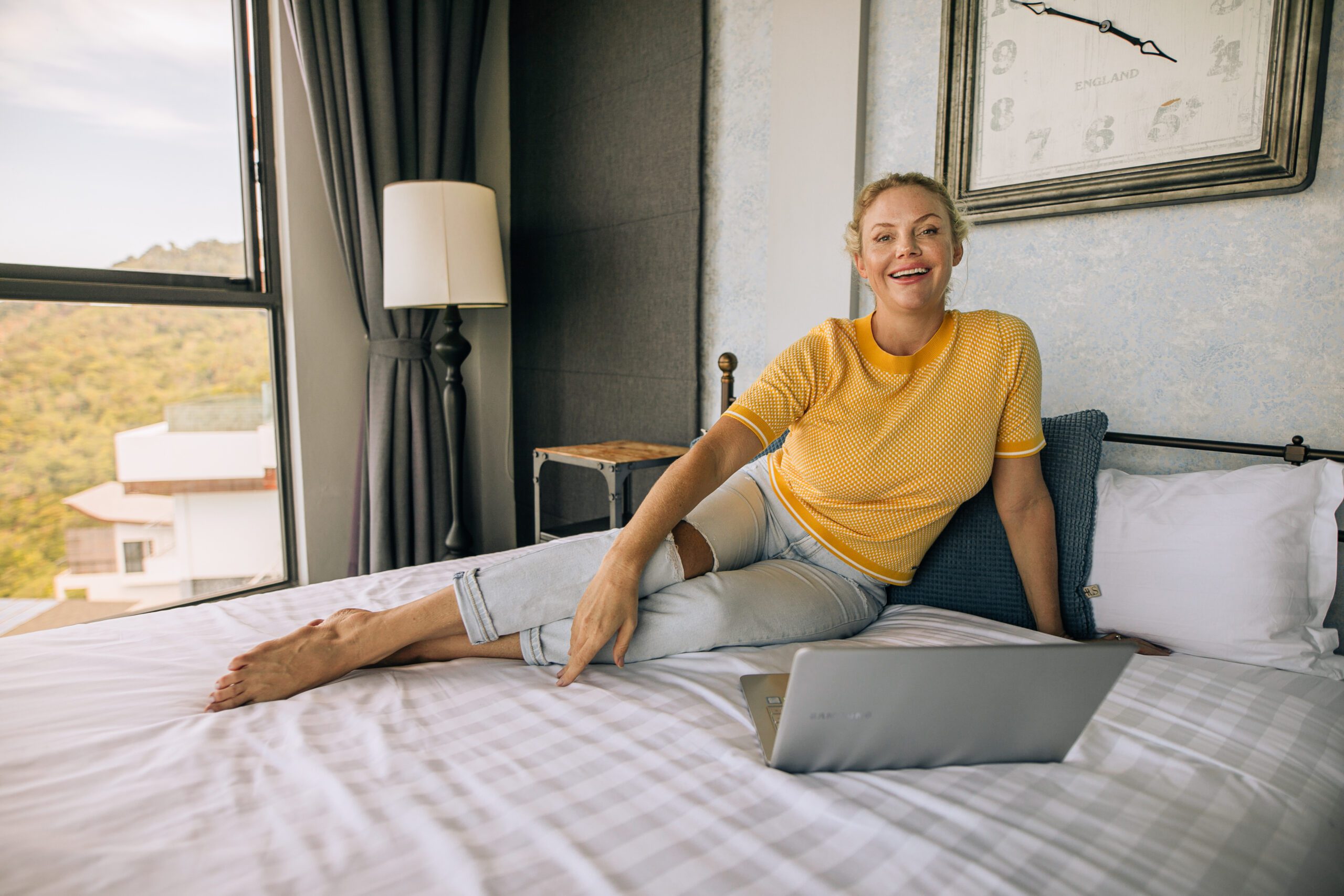
Get more actionable advice by joining The Convergence Newsletter for free below.
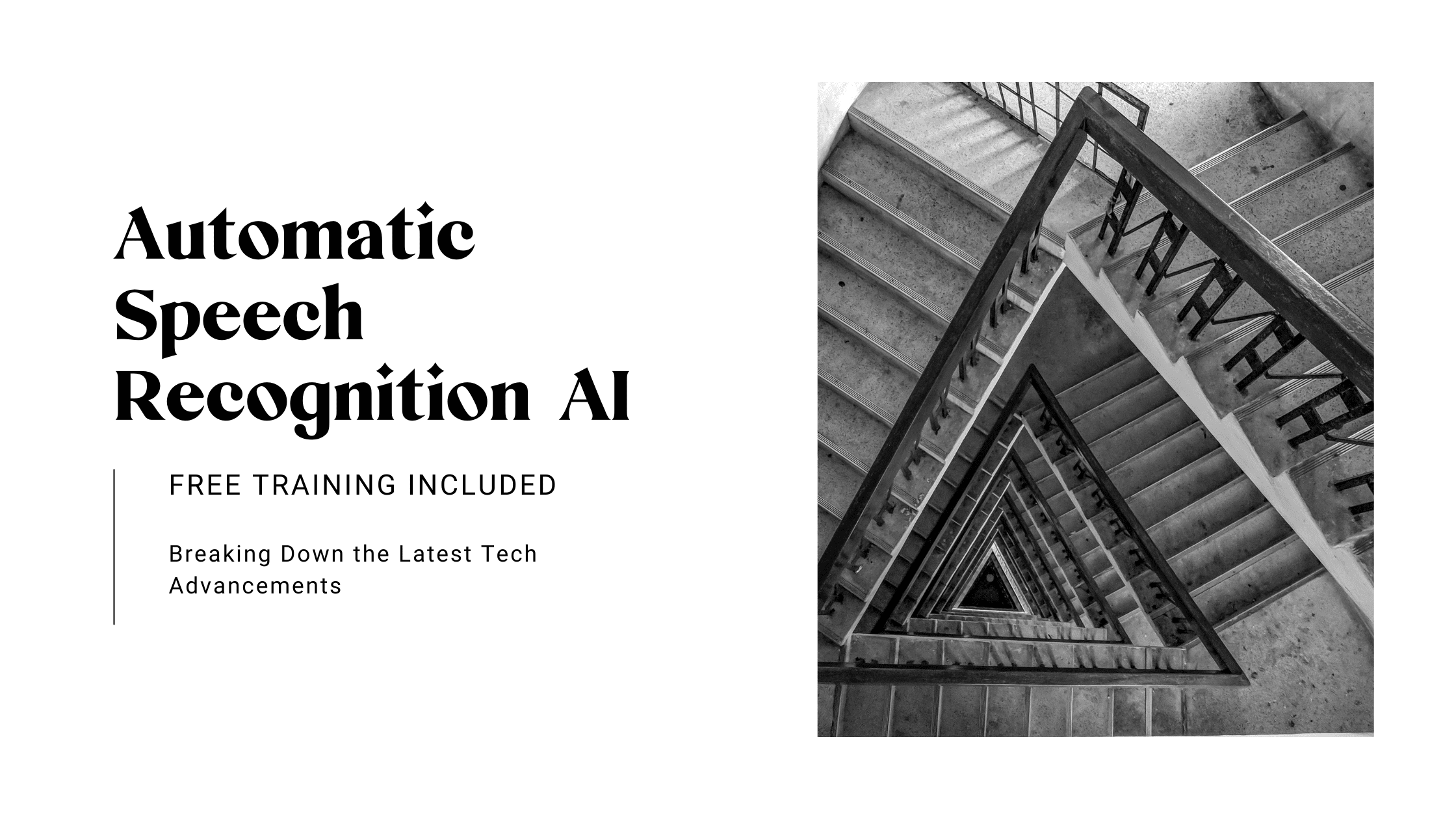
Automatic Speech Recognition AI: Breaking Down the Latest Tech Advancements [Free Training Included]
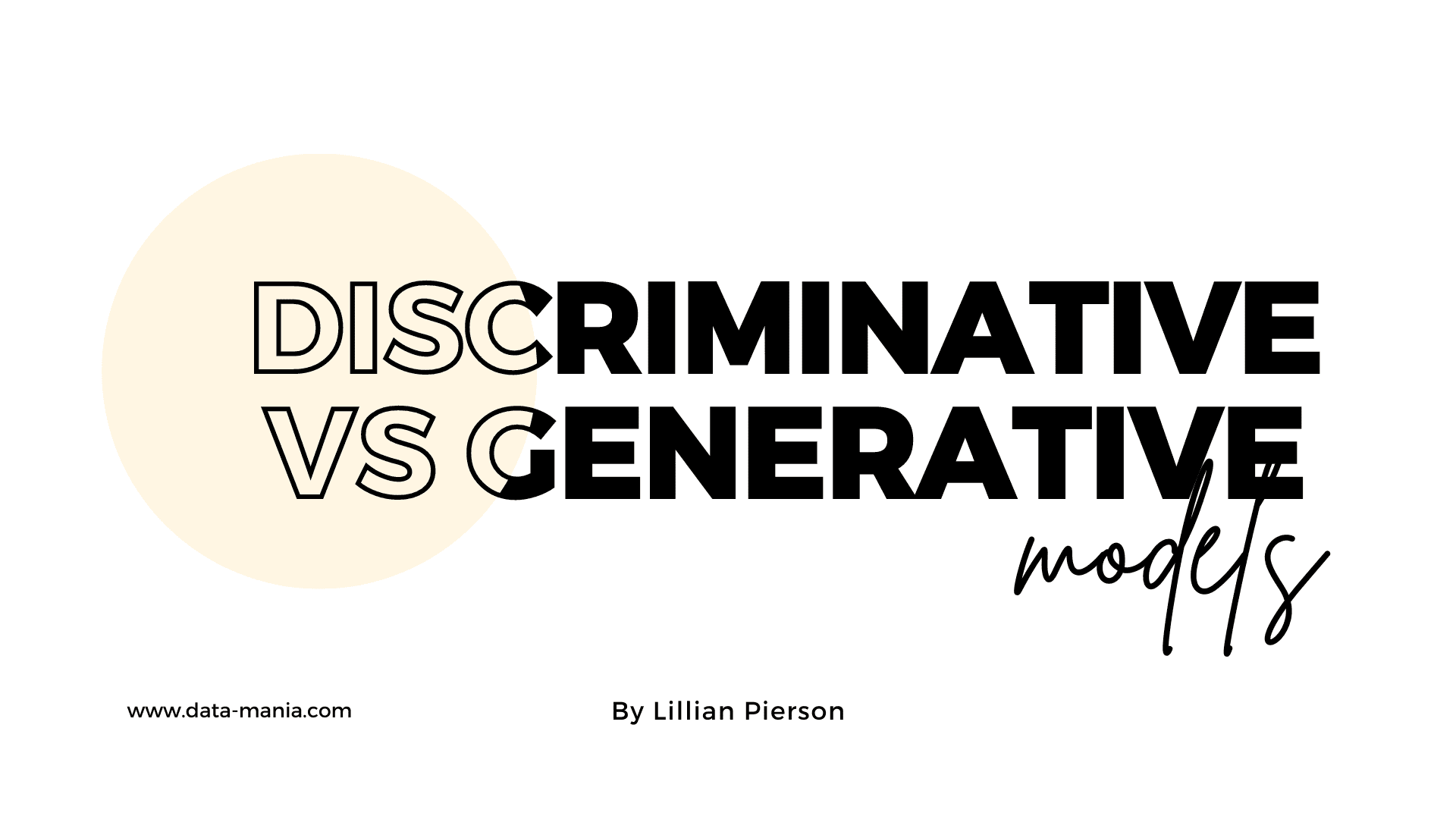
Choosing Between Discriminative vs Generative Models
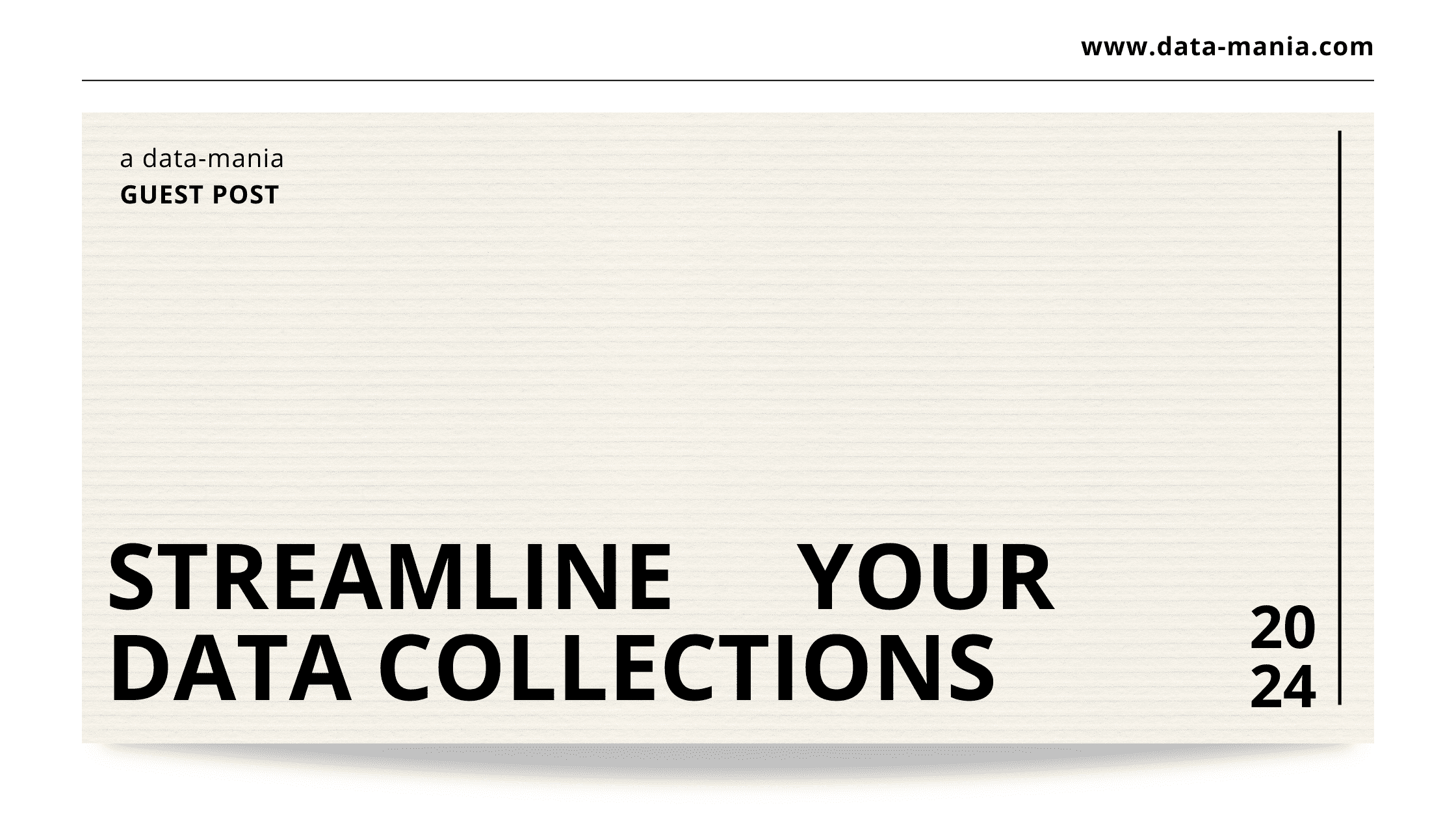
5 Ways AI Helps Streamline Data Collection
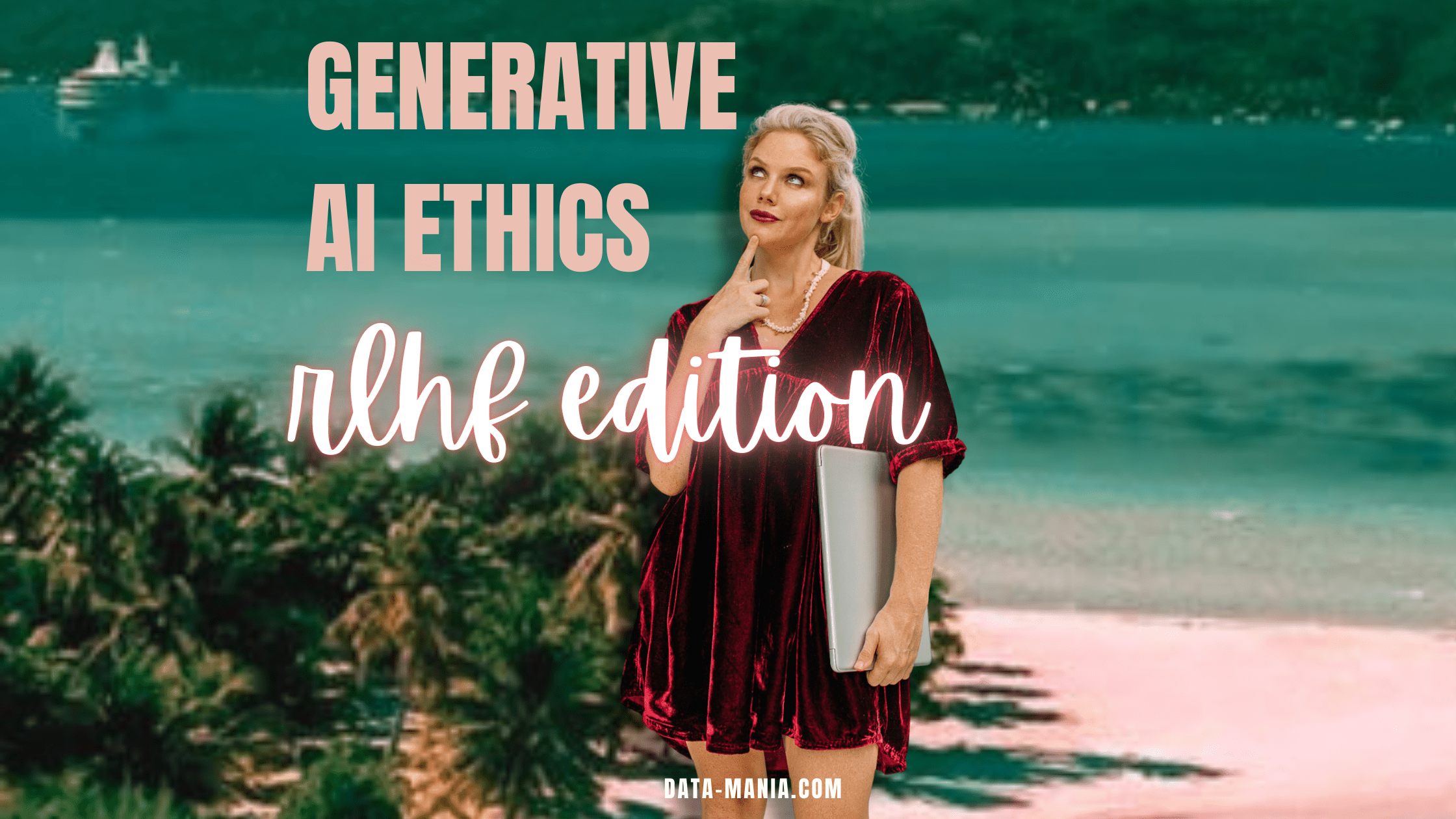
Ugly Generative AI Ethics Concerns: RLHF Edition
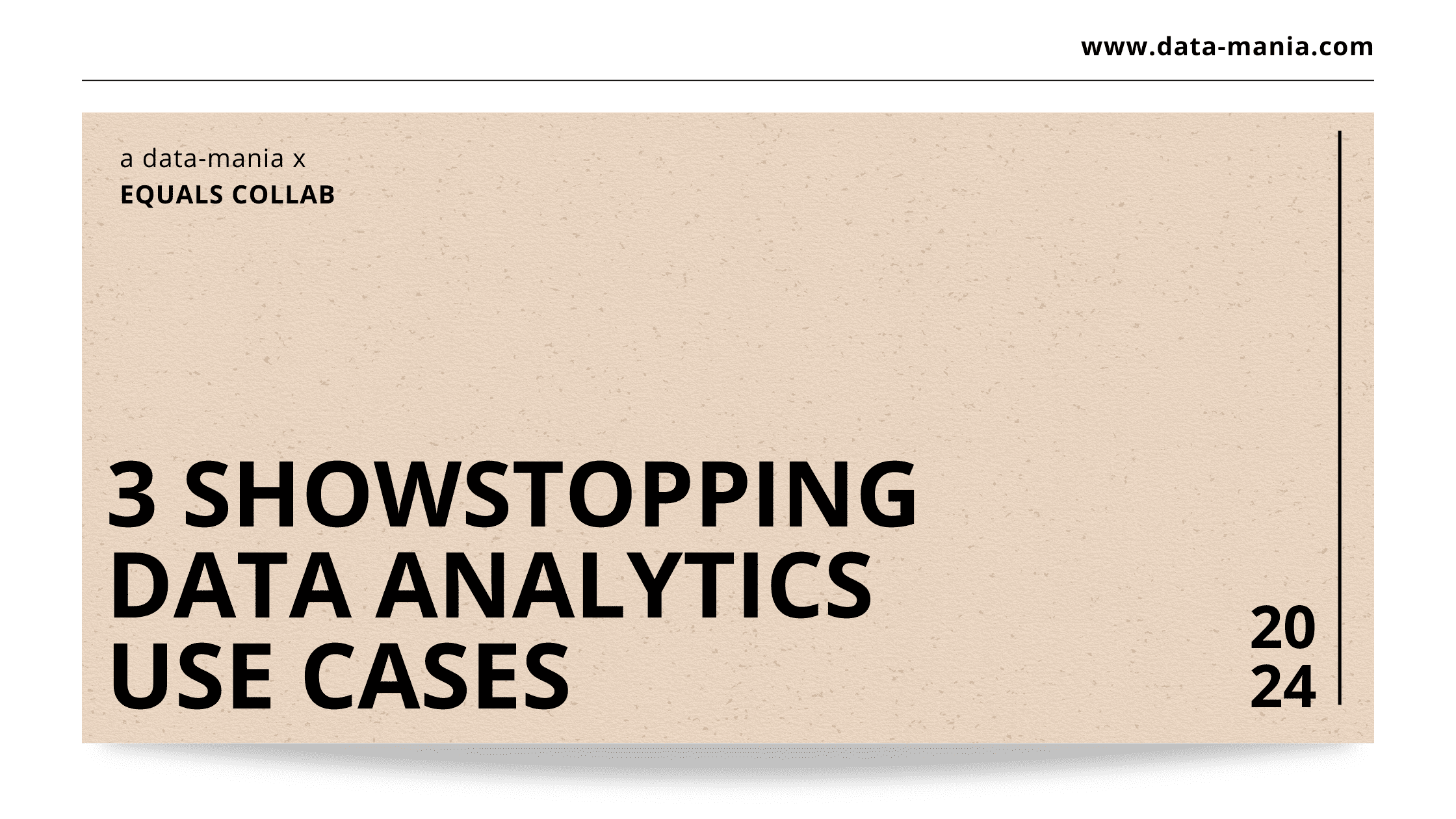
3 Showstopping Data Analytics Use Cases To Uplevel Your Startup Profit-Margins
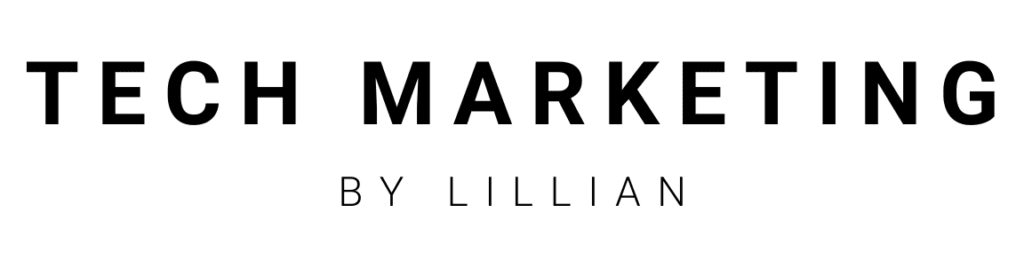
Fractional CMO for deep tech B2B businesses. Specializing in go-to-market strategy, SaaS product growth, and consulting revenue growth. American expat serving clients worldwide since 2012.
Get connected, © data-mania, 2012 - 2024+, all rights reserved - terms & conditions - privacy policy | products protected by copyscape, privacy overview.
Get The Newsletter
- Open access
- Published: 30 May 2024
Exploring the tradeoff between data privacy and utility with a clinical data analysis use case
- Eunyoung Im 1 , 2 ,
- Hyeoneui Kim 1 , 2 , 3 ,
- Hyungbok Lee 1 , 5 ,
- Xiaoqian Jiang 4 &
- Ju Han Kim 5 , 6
BMC Medical Informatics and Decision Making volume 24 , Article number: 147 ( 2024 ) Cite this article
141 Accesses
Metrics details
Securing adequate data privacy is critical for the productive utilization of data. De-identification, involving masking or replacing specific values in a dataset, could damage the dataset’s utility. However, finding a reasonable balance between data privacy and utility is not straightforward. Nonetheless, few studies investigated how data de-identification efforts affect data analysis results. This study aimed to demonstrate the effect of different de-identification methods on a dataset’s utility with a clinical analytic use case and assess the feasibility of finding a workable tradeoff between data privacy and utility.
Predictive modeling of emergency department length of stay was used as a data analysis use case. A logistic regression model was developed with 1155 patient cases extracted from a clinical data warehouse of an academic medical center located in Seoul, South Korea. Nineteen de-identified datasets were generated based on various de-identification configurations using ARX, an open-source software for anonymizing sensitive personal data. The variable distributions and prediction results were compared between the de-identified datasets and the original dataset. We examined the association between data privacy and utility to determine whether it is feasible to identify a viable tradeoff between the two.
All 19 de-identification scenarios significantly decreased re-identification risk. Nevertheless, the de-identification processes resulted in record suppression and complete masking of variables used as predictors, thereby compromising dataset utility. A significant correlation was observed only between the re-identification reduction rates and the ARX utility scores.
Conclusions
As the importance of health data analysis increases, so does the need for effective privacy protection methods. While existing guidelines provide a basis for de-identifying datasets, achieving a balance between high privacy and utility is a complex task that requires understanding the data’s intended use and involving input from data users. This approach could help find a suitable compromise between data privacy and utility.
Peer Review reports
Clinical data gathered through Electronic Health Records (EHR) is an invaluable asset for producing meaningful insights into patient care and healthcare service management. However, as this data includes sensitive personal information, there is a heightened risk of financial or social damage to individuals if their health data is improperly disclosed [ 1 , 2 ]. To address these concerns, many countries have implemented stringent regulations to safeguard patient privacy while still enabling the efficient use of data for health advancements [ 3 ]. In the United States, for example, the Health Insurance Portability and Accountability Act (HIPAA) sets forth provisions for data protection and usage [ 4 ]. Similarly, the General Data Protection Regulation (GDPR) offers a comprehensive data privacy framework within the European Union [ 5 ]. Additionally, South Korea’s Personal Information Protection Act delineates the guidelines for secure and permissible data handling [ 6 ].
The growing imperative for data privacy has spurred significant progress in privacy-preserving technologies. Differential Privacy (DP) safeguards data by integrating controlled random noise, thus ensuring individual data points remain confidential while aggregate analysis remains accurate [ 7 ]. In the biomedical field, DP is extensively employed in data query systems; the noise integrated into query responses helps protect sensitive inquiries pertaining to uncommon cases [ 8 , 9 ]. Current research in DP focuses on solving complex problems such as determining optimal privacy budgets and noise levels to balance confidentiality with data utility [ 8 , 10 , 11 ].
Homomorphic Encryption (HE) represents a breakthrough in cryptography for preserving privacy, enabling computations on encrypted data without altering the original values [ 12 ]. Recent research has validated the practicality of performing data analysis using HE [ 13 , 14 , 15 ]. Nonetheless, HE has not become mainstream in healthcare applications, primarily due to its substantial computational demands, intricate implementation, and the limited range of analytics that can be performed on data in its encrypted form [ 12 , 16 ].
Blockchain technology, recognized for its immutable, decentralized, and transparent nature [ 17 ], is gaining attention as an innovative approach for data privacy [ 18 , 19 , 20 ]. Despite this interest, the real-world application of blockchain is contingent upon enhancements in its capacity to process substantial data volumes, simplification of its implementation, and resolution of related regulatory challenges [ 21 , 22 , 23 , 24 ].
When preparing datasets with personal health information for secondary analysis, the prevailing practice is to mitigate the risk of re-identification of the subjects in the dataset by employing stringent de-identification procedures [ 25 , 26 ]. This involves the removal of direct identifiers that can uniquely pinpoint individual subjects within the dataset and altering quasi-identifiers, which alone do not identify subjects but could do so when merged with other data sources. Furthermore, the process considers sensitive information that, despite not directly identifying subjects, could have detrimental effects if disclosed, ensuring such data is also considered during the de-identification process.
The leading method for data de-identification employs strategies like K-anonymity, L-diversity, and T-closeness to modify data. K-anonymity safeguards against linkage attacks by ensuring that there are at least K identical records for any set of quasi-identifiers within a dataset, making it impossible to distinguish one individual from K-1 others [ 27 ]. In line with this, South Korea’s data publishing guidelines recommend adhering to a minimum of ‘K = 3’ for K-anonymity [ 28 , 29 ]. Additionally, L-diversity mandates a sensitive variable must have at least L distinct values, thereby offering protection against homogeneity attacks [ 30 ]. T-closeness, on the other hand, ensures that the distribution of a sensitive variable within any subset of the dataset closely approximates the distribution of that variable of the entire dataset, adhering to a specified threshold [ 31 ]. T-closeness prevents the likelihood that knowledge of the variable’s distribution could be exploited to reveal an individual’s identity [ 31 ]. The process of de-identification, which often involves masking or altering certain data values, can result in information loss and potentially reduce the utility of the dataset [ 32 ].
Determining the optimal threshold between data privacy and utility remains a complex challenge. Several studies have investigated how various de-identification strategies, specifically K-anonymity, L-diversity, and T-closeness, influence data utility. This is typically assessed by comparing the analytical results of de-identified datasets with those derived from the original dataset. Some researchers advocate that the privacy enhancements are overshadowed by a substantial reduction in data utility [ 33 , 34 ], while others argue that such utility loss might not be as severe as some studies imply [ 35 ]. However, these studies evaluated each de-identification technique in isolation, often resorting to simplified models that fail to fully capture the complexities of real-world data use, and led to mixed conclusions [ 34 , 35 ].
Moreover, the insights offered by such research into the tangible effects of data de-identification on actual data analysis tasks are somewhat restricted. This is because the analyses were either performed using overly simplistic examples [ 28 , 34 ] or on public datasets that have already undergone some form of de-identification [ 35 , 36 ], or focusing on theoretical aspects [ 37 ]. Therefore, there is a need for more intricate research that closely mirrors the complexities of real-life data analytics tasks and considers the multifaceted nature of data utility and privacy in actual applications.
This study explores the effects of different de-identification strategies on clinical datasets prepared for secondary analysis, with a focus on their implications for practical data analysis tasks. The aims of this study are twofold: firstly, to assess the effects of de-identification on both the dataset’s integrity and the outcomes of data analyses; and secondly, to ascertain if discernible trends emerge from the application of various de-identification techniques that could guide the establishment of a feasible balance between data privacy and data utility.
Data analysis use case
This study explores the impact of various de-identification techniques on datasets and their subsequent analysis results using a data analytic use case. The analytic use case involved predicting the Length of Stay (LOS) of high-acuity patients transferred to the emergency department (ED) of an academic medical center located in Seoul, South Korea. LOS in the ED serves as a crucial quality metric for ED services [ 38 , 39 , 40 ]. In Korea, an ED LOS under six hours is considered optimal [ 41 ]. Nonetheless, the overcrowding issues prevalent in tertiary hospital EDs elevate the risk of prolonged ED stays for patients transferred from other facilities for specialized care [ 42 , 43 ]. Understanding the factors affecting the ED LOS of transferred high-acuity patients is essential to providing timely care. The authors, HK and HL, previously developed a model to predict ED LOS using logistic regression, Random Forest, and Naïve Bayes techniques [ 44 ]. Building on insights from this earlier research, the current use case was crafted to develop a logistic regression model to predict ED LOS based on variables including the patient’s sex, age, medical conditions, the type and location of the transferring hospital, and the treatment outcomes.
The prediction model for ED LOS was developed using data from 1,155 patients who were transferred to the study site’s ED between January 2019 and December 2019. Patient demographics, clinical details, and transfer-related information were extracted from the study site’s Clinical Data Warehouse (CDW). The variables collected for this study are listed in Table 1 .
De-identification of the datasets
Developing de-identification scenarios.
Identifiers such as patient names and medical record numbers were removed. Quasi-identifiers play a critical role in de-identification as they form the foundation for assessing the adequacy of de-identification efforts and undergo most data transformations. To select the variables to test as quasi-identifiers, we first examined the extent to which each variable could uniquely link to individual subjects within the dataset, potentially identifying them. Table 2 displays the percentage of subjects in the dataset uniquely linked to either a single variable or a combination of variables. For instance, the sending hospital and primary diagnosis were uniquely linked to 27.71% and 17.75% of the subjects, respectively, and their combination linked up to 94% of the subjects. Consequently, information regarding the sending hospital and the primary diagnosis , coded using the International Classification of Disease (ICD) [ 45 ], were utilized as quasi-identifiers, along with sex and age , which are commonly considered quasi-identifiers in various de-identification efforts [ 4 , 46 ]. Treatment outcomes were identified as sensitive information. We developed 19 de-identification scenarios by varying the quasi-identifiers and sensitive information, and applying diverse configurations of privacy-preserving techniques such as K-anonymity, L-diversity, and T-closeness to each scenario.
Data transformation for de-identification
De-identification was performed using ARX, a publicly accessible and well-validated data anonymization tool that supports various de-identification methods [ 47 , 48 , 49 ]. We employed generalization and micro-aggregation techniques to modify the quasi-identifiers, both aimed at reducing the risk of re-identification by transforming original data into more general values. Generalization involves building a hierarchy for the given values by specifying minimum and maximum generalization levels. Generalization involves creating a hierarchy of values by specifying minimum and maximum levels, which can be adjusted based on criteria such as the number of digits masked in zip codes, size of intervals for age , condensation of 5-point Likert scores to 3-point scales, and generalization of full dates to broader time units such as week, month, or year [ 50 ]. Micro-aggregation, on the other hand, assigns representative values for alphanumeric data, such as using the mode for sex and the mean for age [ 50 ].
In our de-identification process, quasi-identifiers such as the sending hospital and primary diagnosis were transformed using generalization, while sex was modified through micro-aggregation. Age was subjected to both generalization and micro-aggregation. The generalization hierarchy for age included three levels with intervals of 5, 10, and 30 years respectively. For micro-aggregation, mean age values were used. The primary diagnosis was generalized into two levels based on higher-level ICD codes. For instance, a primary diagnosis with the ICD code I20.0, representing unstable angina , was generalized to I20 (i.e., angina pectoris ) at level 1, and further to I20-I25 (i.e., ischemic heart diseases ) at level 2. Generalization of the sending hospital also included two levels, where a specific facility such as “Hanmaeum Clinic in Jongno-gu, Seoul city” was generalized to the county level as “facility in Jongno-gu” at level 1 and then to the city level as “facility in Seoul” at level 2. For sex , micro-aggregation was employed, setting the mode as the representative value.
K-anonymity, L-diversity, and T-closeness were employed concurrently with specific parameters set for each: K and L were both set at 3, and T was set at 0.5. K-anonymity was specifically applied to quasi-identifiers to ensure that each individual is indistinguishable from at least two others. L-diversity and T-closeness, on the other hand, were applied to the variable designated as sensitive, ensuring that sensitive information is both sufficiently diverse and closely aligned with the overall distribution of the dataset. Table 3 details these 19 de-identification scenarios.
Data transformation was carried out in ARX according to the de-identification scenarios outlined in Table 3 . ARX provides options to adjust additional transformation parameters: the suppression limit , which sets the maximum proportion of records that can be omitted from the original dataset; approximation , which prioritizes solutions with shorter execution times; and precomputation , which determines the threshold for the fraction of unique data values in the dataset [ 50 ]. For this study, we utilized the default settings in ARX, where the suppression limit was set to 100%, and both approximation and precomputation features were disabled.
During execution, ARX evaluated various combinations of generalization and micro-aggregation levels to meet the requirements for K-anonymity, L-diversity, and T-closeness, ultimately recommending an optimal solution based on the balance between minimizing re-identification risk and preserving data utility. Figure 1 displays a screenshot of the data transformation solutions for the scenario where age , primary diagnosis , and sending hospital were designated as quasi-identifiers. Ultimately, we produced 19 versions of de-identified datasets, each based on the transformation solution that ARX identified as optimal.
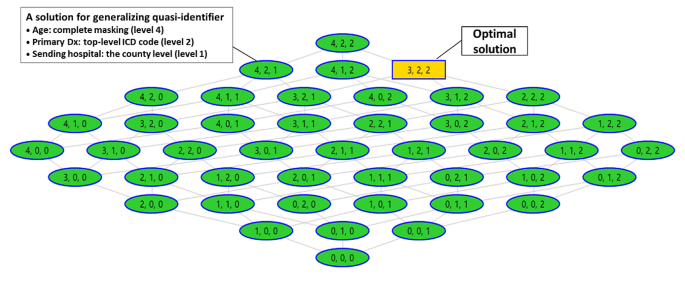
The data transformation solutions suggested by ARX
Examination of the de-identified datasets
We reviewed the reduction in re-identification risk and the data utility scores that ARX estimated for the 19 de-identified datasets. To assess the similarity between each de-identified dataset and the original dataset, we employed Earth Mover’s Distance (EMD) [ 51 ]. Additionally, we calculated the dataset retention ratio. This metric is derived by dividing the number of data points in the transformed dataset by the number of data points in the original dataset. EMD and dataset retention ratio quantitatively evaluate the dissimilarity between the original dataset and the de-identified datasets, offering insights into how much the data has been altered through de-identification.
Testing the effects of de-identification on ED LOS prediction
Variable creation for predictive modeling.
To construct a logistic regression model for predicting ED LOS, we defined outcome and predictor variables. ED LOS, the outcome variable, was dichotomized into two categories: 6 h or less, and more than 6 h. We identified 13 predictors, including patient sex, age, medical conditions, treatment outcome, and the sending hospital type. Age , sending hospital location , and treatment outcome were dichotomized. Five dummy variables were created from primary diagnosis to represent high priority disease , neoplastic disease , circulatory disease , respiratory disease , and injury-related visits . The sending hospital type was derived from the sending hospital information . These variables, detailed in Table 4 , were consistently defined across all 19 de-identified datasets as well as the original dataset to facilitate comparative analyses.
Data analysis
After defining the outcome and predictor variables for logistic regression, we examined their distributions across the 19 de-identified datasets and the original dataset. To assess the differences in variable distributions, we utilized the proportion test [ 52 ]. Subsequently, logistic regression analysis was conducted using both the de-identified and original dataset. The predictive performance of these models was evaluated using the Area Under the Curve (AUC) of the Receiver Operating Characteristic (ROC) curve. We compared the AUC scores (AUROC) of the logistic regression models derived from the 19 de-identified datasets to that from the original dataset, employing the DeLong test [ 53 ]. Additionally, we analyzed the differences in the odds ratios of the predictors and their statistical significance to assess any impact the de-identification process might have had on the predictive capability of the models. All analyses were performed using R (version 4.0.4) [ 54 ].
Data transformation configurations applied for the de-identification of the datasets
Table 5 displays the optimal configurations for data transformation used in the 19 de-identified datasets. Variables subjected to generalization or micro-aggregation were designated as quasi-identifiers. Sensitive information is identified as ‘SI’ within the table. It is important to note that empty cells signify that the corresponding variable was treated as non-sensitive information in the specific dataset.
The de-identified datasets
Table 6 displays the re-identification reduction rates, ARX utility scores, EMD scores, and dataset retention ratios for the 19 transformed datasets. Additionally, the table presents the number of records retained post-transformation and the number of predictor variables generated. The ARX utility score reflects the extent of information loss, with a higher score indicating lower utility. It is important to note that the baseline re-identification risk varied among the datasets due to differences in the configuration of quasi-identifiers.
Overall, all 19 de-identification scenarios significantly reduced re-identification risk. However, the data transformation processes involved in de-identification led to record suppression and complete masking of variables used as predictors, thereby compromising dataset utility. Notably, except for three datasets (13, 15, 16), which used only sex and age as quasi-identifiers, there was a loss of one or more predictor variables. Datasets 13, 15, and 16 demonstrated the highest retention ratios and the lowest ARX utility and EMD scores, indicating minimal information loss and the highest similarity to the original dataset, thus reflecting superior dataset utility. They also exhibited the lowest baseline and post-transformation re-identification risks.
Datasets 7 and 8 underwent a transformation under the most complex de-identification scenarios, employing three quasi-identifiers and applying both L-diversity and T-closeness to two sensitive variables. Although these datasets achieved complete re-identification risk reduction, the extensive data transformation allowed only seven predictor variables to be generated. The de-identification scenarios 1 and 3, 2 and 4, and 13, 15, and 16 shared identical configurations of quasi-identifiers but varied in the L-diversity and T-closeness conditions applied to sensitive information, resulting in identical de-identified datasets (see Table 3 ).
Table 7 details the differences in variable distribution between each transformed dataset and the original dataset. As expected, variables designated as quasi-identifiers underwent the most transformation, leading to significant changes. Variables derived from these quasi-identifiers, such as sending hospital type, circulatory disease , and high priority disease , also exhibited notable distributional changes.
The prediction results
Logistic regression models were developed using both the original dataset and 19 de-identified datasets. The complete masking of variables classified as quasi-identifiers in some de-identified datasets resulted in differences in the number and types of predictors available for constructing the logistic regression models. Additionally, the number of records included in the regression analysis varied due to record suppression associated with the de-identification process. Figure 2 illustrates the ROC curves and the AUC values for all 20 datasets. The AUC values ranged from 0.695 to 0.787. The models generated from datasets 7 and 8, which only retained seven predictors due to extensive data masking, exhibited a statistically significant difference in AUC when compared to the original dataset, with a p-value of 0.002. For the models derived from the other datasets, no significant differences in AUC values were observed.
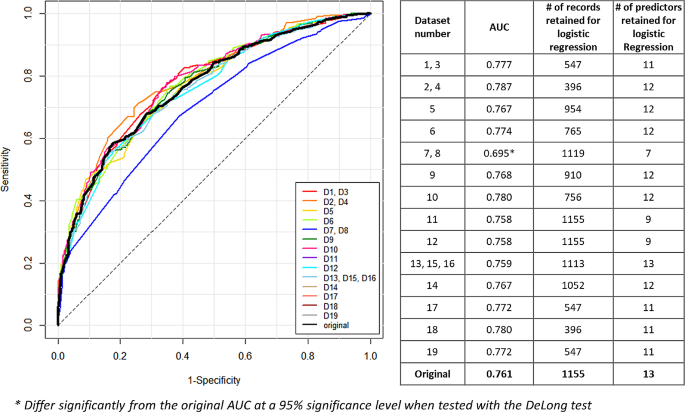
The number of records and predictors included in each model and the model performance
Figure 3 displays the Odds Ratios (OR) for predictors from selected datasets. Datasets 13, 15, and 16 were chosen because they retained all 13 predictor variables (Fig. 3 (a)). Dataset 9 was selected for having the next highest number of predictors ( N = 12) and for utilizing three quasi-identifiers: the sending hospital , which is identified as the most revealing variable in Table 2 , along with sex and age , which are commonly used as quasi-identifiers (Fig. 3 (b)). Dataset 19 was also included because it was configured using only the sending hospital and primary diagnosis as quasi-identifiers (Fig. 3 (c)). The ORs for all 19 datasets are detailed in Additional file 1: Figure S1 .
As depicted in Fig. 3 (a), the original dataset and de-identified datasets 13, 15, and 16 showed comparable prediction outcomes, with sex being the only predictor that displayed an OR notably different from the original dataset; however, it was not statistically significant in either model. Figure 3 (b) indicates that the ORs of the 12 predictors in dataset 9 were similar to those in the original dataset, although the OR for injury-related visits became insignificant. In contrast, dataset 19, which excluded two predictors, showed more pronounced differences in the ORs of the 11 remaining predictors (Fig. 3 (c)). Additionally, neoplastic disease and respiratory disease , significant predictors in the original dataset, became insignificant in dataset 9, while injury-related visits , previously insignificant, became significant (Fig. 3 (c)).
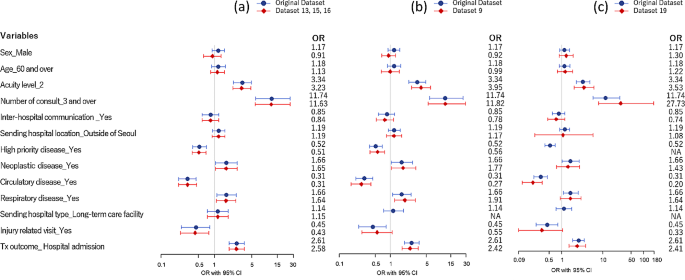
The Odds-Ratios of the predictors from the original dataset and the selected de-identified datasets
Data utility vs. data privacy
Figure 4 presents the correlations between re-identification risk reduction rates, ARX utility scores, EMD, and dataset retention ratios. There is a significant correlation between the re-identification reduction rate and the ARX utility score, indicating that greater reductions in re-identification risk are typically accompanied by larger losses of information. Conversely, the re-identification reduction rate exhibits a slight negative correlation with both EMD and dataset retention ratio; however, these correlations are not statistically significant.
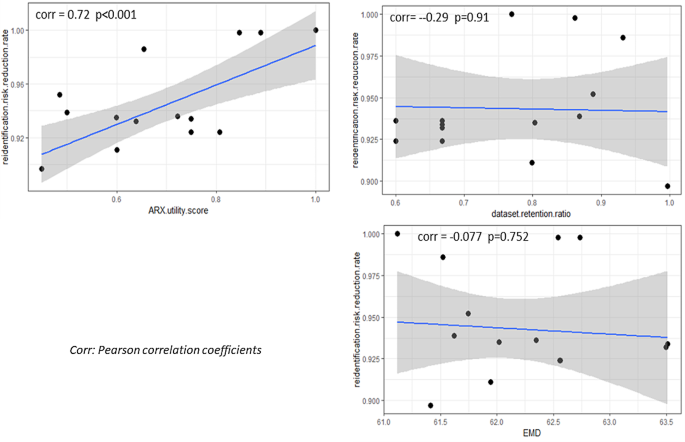
The correlations between re-identification risk reduction and features of the de-identified datasets
This study tested various de-identification strategies on a clinical dataset, adjusting the number and types of quasi-identifiers and sensitive information, and configuring K-anonymity, L-diversity, and T-closeness in diverse ways. It aimed to address gaps left by earlier studies that utilized simplistic data use cases and de-identification configurations [ 28 , 34 , 35 ].
The results indicated that de-identification led to the suppression of records and variables, precluding the replication of analyses performed on the original dataset. Consequently, logistic regression models for predicting ED LOS yielded differing conclusions based on the de-identification approach, as illustrated in Fig. 3 . This highlights the need for the evolution of privacy technologies that maintain data integrity. Additionally, it cautions data users about potential biases introduced when working with de-identified datasets.
The study found optimal data utility when only sex and age were classified as quasi-identifiers, maintaining all variables and losing only six records. This configuration also significantly reduced the baseline re-identification risk, albeit sex and age by themselves did not strongly individualize records. However, this configuration did not account for the additional re-identification risk posed by the sending hospital and primary diagnosis , both of which were considered the most identifying variables in the dataset (Table 2 ). To eliminate any alterations to sex and age —key variables for clinical research—we examined the impact of designating only the sending hospital and primary diagnosis as quasi-identifiers (dataset 19). This strategy greatly reduced the chance of re-identification but at a considerable cost to data utility, resulting in the loss of over half the dataset and two predictor variables: the sending hospital type and high priority disease .
Seeking a compromise, datasets 5–12 incorporated sex , age , and either sending hospital or primary diagnosis as quasi-identifiers. In this series, datasets 7 and 8 achieved zero re-identification risk post-de-identification but sacrificed nearly half of the predictor variables. Datasets 11 and 12, while managing to retain all records, were considered less favorable due to the loss of four predictor variables. Datasets 5 and 6 struck a more acceptable balance, offering substantial re-identification risk reduction, retaining over 78% of records, and sacrificing only one predictor variable. Although dataset 5 had marginally better scores for risk reduction and data utility, dataset 6 was preferred because it retained information on high priority disease , a key predictor of ED LOS.
In this study, three different data utility metrics were examined, but only the ARX utility score exhibited a statistically significant correlation with the re-identification risk reduction rate. The EMD and dataset retention ratio both showed minor negative correlations with re-identification risk reduction; however, these were not statistically significant. This could suggest that the structural aspects of a dataset may not alone be adequate for assessing its utility, although further studies with a broader array of datasets would be required to substantiate this preliminary indication.
The scope of this research was limited to a single use case, analyzing data obtained from one hospital. Moreover, the range of de-identification scenarios tested did not encompass the full spectrum of complex configurations that could be employed. Despite these constraints, the research offers valuable insights into the nuanced interplay between data de-identification processes and data utility. It contributes to the ongoing conversation about how to approach data privacy in a way that still enables effective data usage.
As health data analysis grows more critical, so does the imperative to devise effective methods for ensuring data privacy. While established guidelines [ 47 ] offer a foundation for the de-identification of datasets, crafting a dataset that maintains a high level of privacy without unduly compromising its utility remains a nuanced challenge. It demands a thorough grasp of the data’s intended application. Incorporating input from data users during the de-identification process and considering the variety of potential data use cases could prove beneficial in finding a workable tradeoff between data privacy and utility.
Data availability
The clinical dataset used in this study is not made available due to the sensitive nature of clinical data. However, de-identified analytic datasets are available upon reasonable request from the corresponding author and with permission of Seoul National University Hospital.
Abbreviations
Area Under the receiver operating characteristic (ROC) Curve
Differential Privacy
Emergency Department
Earth Mover’s Distance
Homomorphic Encryption
International Classification of Disease
Length Of Stay
Receiver Operating Characteristic
Price WN, Cohen IG. Privacy in the age of medical big data. Nat Med. 2019;25(1):37–43.
Article CAS PubMed PubMed Central Google Scholar
Gostin LO, Halabi SF, Wilson K. Health data and privacy in the digital era. JAMA. 2018;320(3):233–4.
Article PubMed Google Scholar
Data Protection and Privacy Legislation Worldwide | UNCTAD. https://unctad.org/page/data-protection-and-privacy-legislation-worldwide . Accessed 6 Oct 2022.
Health and Human Services. Guidance Regarding Methods for De-identification of Protected Health Information in Accordance with the Health Insurance Portability and Accountability Act (HIPAA) Privacy Rule. https://www.hhs.gov/hipaa/for-professionals/privacy/special-topics/de-identification/index.html#coveredentities (2022). Accessed 28 Mar 2024.
General Data Protection Regulation (GDPR). Article 32 GDPR( https://gdprhub.eu/index.php?title=Article_32_GDPR (2023). Accessed 4 Apr 2024.
Personal Information Protection Commission. Pseudonymization Guidelines. Korea;2024.
Thapa C, Camtepe S. Precision health data: requirements, challenges and existing techniques for data security and privacy. Comput Biol Med. 2021;129:104130.
Cho H, Simmons S, Kim R, Berger B. Privacy-preserving biomedical database queries with optimal privacy-utility trade-offs. Cell Syst. 2020;10(5):408–16. e9.
Article CAS PubMed Google Scholar
Deldar F, Abadi M. Differentially private count queries over personalized-location trajectory databases. Data Brief. 2018;20:1510–4.
Article PubMed PubMed Central Google Scholar
Venkatesaramani R, Wan Z, Malin BA, Vorobeychik Y. Enabling tradeoffs in privacy and utility in genomic data beacons and summary statistics. Genome Res. 2023;33(7):1113–23.
PubMed PubMed Central Google Scholar
Xiong L, Post A, Jiang X, Ohno-Mochado L. New Methods to Protect Privacy When Using Patient Health Data to Compare Treatments. 2021.
Scheibner J, Raisaro JL, Troncoso-Pastoriza JR, Ienca M, Fellay J, Vayena E, et al. Revolutionizing medical data sharing using advanced privacy-enhancing technologies: technical, legal, and ethical synthesis. J Med Internet Res. 2021;23(2):e25120.
Bataa M, Song S, Park K, Kim M, Cheon JH, Kim S. Finding highly similar regions of genomic sequences through homomorphic encryption. J Comput Biol. 2024;31(3):197–212.
Kim D, Son Y, Kim D, Kim A, Hong S, Cheon JH. Privacy-preserving approximate GWAS computation based on homomorphic encryption. BMC Med Genom. 2020;13:1–12.
Article Google Scholar
Rovida L, Leporati A. Encrypted image classification with low memory footprint using fully homomorphic encryption. Cryptology ePrint Archive; 2024.
Acar A, Aksu H, Uluagac AS, Conti M. A survey on homomorphic encryption schemes: theory and implementation. ACM Comput Surv (Csur). 2018;51(4):1–35.
Kuo T-T, Kim H-E, Ohno-Machado L. Blockchain distributed ledger technologies for biomedical and health care applications. J Am Med Inform Assoc. 2017;24(6):1211–20.
Zhang F, Zhang Y, Ji S, Han Z. Secure and decentralized Federated Learning Framework with Non-IID Data based on Blockchain. Heliyon. 2024.
Wu C, Tang YM, Kuo WT, Yip HT, Chau KY. Healthcare 5.0: a secure and distributed network for system informatics in medical surgery. Int J Med Informatics. 2024:105415.
Ali A, Al-Rimy BAS, Tin TT, Altamimi SN, Qasem SN, Saeed F. Empowering Precision Medicine: Unlocking Revolutionary insights through Blockchain-enabled Federated Learning and Electronic Medical Records. Sensors. 2023;23(17):7476.
Chukwu E, Garg L. A systematic review of blockchain in healthcare: frameworks, prototypes, and implementations. Ieee Access. 2020;8:21196–214.
Fan C, Ghaemi S, Khazaei H, Musilek P. Performance evaluation of blockchain systems: a systematic survey. IEEE Access. 2020;8:126927–50.
Thantilage RD, Le-Khac N-A, Kechadi M-T. Healthcare data security and privacy in Data Warehouse architectures. Inf Med Unlocked. 2023:101270.
Tandon A, Dhir A, Islam AN, Mäntymäki M. Blockchain in healthcare: a systematic literature review, synthesizing framework and future research agenda. Comput Ind. 2020;122:103290.
Ahmed T, Aziz MMA, Mohammed N. De-identification of electronic health record using neural network. Sci Rep. 2020;10(1):18600.
Ahmed T, Aziz MMA, Mohammed N, Jiang X, editors. Privacy preserving neural networks for electronic health records de-identification. Proceedings of the 12th ACM Conference on Bioinformatics, Computational Biology, and Health Informatics; 2021.
Sweeney L. Achieving k-anonymity privacy protection using generalization and suppression. Int J Uncertain Fuzziness Knowledge-Based Syst. 2002;10(05):571–88.
Jeon S, Seo J, Kim S, Lee J, Kim J-H, Sohn JW, et al. Proposal and assessment of a de-identification strategy to enhance anonymity of the observational medical outcomes partnership common data model (OMOP-CDM) in a public cloud-computing environment: anonymization of medical data using privacy models. J Med Internet Res. 2020;22(11):e19597.
Personal Information Protection Commission. uidelines for Personal Information De-identification Measures. 2016.
Machanavajjhala A, Kifer D, Gehrke J, Venkitasubramaniam M. l-diversity: privacy beyond k-anonymity. Acm Trans Knowl Discovery data (tkdd). 2007;1(1):3–es.
Li N, Li T, Venkatasubramanian S, editors. t-closeness: Privacy beyond k-anonymity and l-diversity. 2007 IEEE 23rd international conference on data engineering; 2006: IEEE.
Tomashchuk O, Van Landuyt D, Pletea D, Wuyts K, Joosen W, editors. A data utility-driven benchmark for de-identification methods. Trust, Privacy and Security in Digital Business: 16th International Conference, TrustBus 2019, Linz, Austria, August 26–29, 2019, Proceedings 16; 2019: Springer.
Brickell J, Shmatikov V, editors. The cost of privacy: destruction of data-mining utility in anonymized data publishing. Proceedings of the 14th ACM SIGKDD international conference on Knowledge discovery and data mining; 2008.
Wu L, He H, Zaïane OR, editors. Utility of privacy preservation for health data publishing. Proceedings of the 26th IEEE International Symposium on Computer-Based Medical Systems; 2013: IEEE.
Li T, Li N, editors. On the tradeoff between privacy and utility in data publishing. Proceedings of the 15th ACM SIGKDD international conference on Knowledge discovery and data mining; 2009.
Karagiannis S, Ntantogian C, Magkos E, Tsohou A, Ribeiro LL. Mastering data privacy: leveraging K-anonymity for robust health data sharing. Int J Inf Secur. 2024:1–13.
Zamani A, Oechtering TJ, Skoglund M. On the privacy-utility trade-off with and without direct access to the private data. IEEE Trans Inf Theory. 2023.
Baek S-M, Seo D-W, Kim Y-J, Jeong J, Kang H, Han KS, et al. Analysis of emergency department length of stay in patient with severe illness code. J Korean Soc Emerg Med. 2020;31(5):518–25.
Google Scholar
Laam LA, Wary AA, Strony RS, Fitzpatrick MH, Kraus CK. Quantifying the impact of patient boarding on emergency department length of stay: all admitted patients are negatively affected by boarding. J Am Coll Emerg Physicians Open. 2021;2(2):e12401.
Otto R, Blaschke S, Schirrmeister W, Drynda S, Walcher F, Greiner F. Length of stay as quality indicator in emergency departments: analysis of determinants in the German Emergency Department Data Registry (AKTIN registry). Intern Emerg Med. 2022;17(4):1199–209.
National Emergency Medical Center: Statistical yearbook of National Emergency Department Information System. https://www.e-gen.or.kr/nemc/statistics_annual_report.do?%20brdclscd=02 (2022). Accessed 7 Oct 2022.
Chang Y-H, Shih H-M, Chen C-Y, Chen W-K, Huang F-W, Muo C-H. Association of sudden in-hospital cardiac arrest with emergency department crowding. Resuscitation. 2019;138:106–9.
Kim J-s, Bae H-J, Sohn CH, Cho S-E, Hwang J, Kim WY, et al. Maximum emergency department overcrowding is correlated with occurrence of unexpected cardiac arrest. Crit Care. 2020;24:1–8.
Lee H, Lee S, Kim H. Factors affecting the length of stay in the emergency department for critically ill patients transferred to regional emergency medical center. Nurs Open. 2023;10(5):3220–31.
World Health Organization(WHO). International Statistical Classification of Diseases and Related Health Problems(ICD). https://www.who.int/standards/classifications/classification-of-diseases/1 (2019). Accessed 11 Oct, 2022.
Eicher J, Kuhn KA, Prasser F. An experimental comparison of quality models for health data de-identification. MEDINFO 2017: Precision Healthcare through Informatics: IOS; 2017. p. 704–8.
Jakob CE, Kohlmayer F, Meurers T, Vehreschild JJ, Prasser F. Design and evaluation of a data anonymization pipeline to promote Open Science on COVID-19. Sci data. 2020;7(1):435.
Meurers T, Bild R, Do K-M, Prasser F. A scalable software solution for anonymizing high-dimensional biomedical data. GigaScience. 2021;10(10):giab068.
Prasser F, Kohlmayer F, Lautenschläger R, Kuhn KA, editors. Arx-a comprehensive tool for anonymizing biomedical data. AMIA Annual Symposium Proceedings; 2014: American Medical Informatics Association.
ARX Configuration. n.d. https://arx.deidentifier.org/anonymization-tool/configuration/ . Accessed 4 Apr 2024.
Pele O, Werman M, editors. Fast and robust earth mover’s distances. 2009 IEEE 12th international conference on computer vision; 2009: IEEE.
Gart JJ. The comparison of proportions: a review of significance tests, confidence intervals and adjustments for stratification. Revue de l’Institut International de Statistique; 1971. pp. 148–69.
DeLong ER, DeLong DM, Clarke-Pearson DL. Comparing the areas under two or more correlated receiver operating characteristic curves: a nonparametric approach. Biometrics. 1988:837–45.
R Core Team. R: a language and environment for statistical. Version 4.0.4. Vienna. Austria: R Foundation for Statistical Computing; 2021.
Download references
Acknowledgements
EI received a scholarship from the BK21 education program (Center for World-leading Human-care Nurse Leaders for the Future).
This study was supported in part by a research grant from the Korean Healthcare Bigdata showcase Project by the Korea Disease Control and Prevention Agency in the Republic of Korea (no.4800-4848-501). The funding body played no role in the design of the study and collection, analysis, interpretation of data, and writing the manuscript.
Author information
Authors and affiliations.
College of Nursing, Seoul National University, Seoul, South Korea
Eunyoung Im, Hyeoneui Kim & Hyungbok Lee
Center for World-leading Human-care Nurse Leaders for the Future by Brain Korea 21 (BK 21) four project, College of Nursing, Seoul National University, Seoul, South Korea
Eunyoung Im & Hyeoneui Kim
The Research Institute of Nursing Science, Seoul National University, Seoul, South Korea
Hyeoneui Kim
School of Biomedical Informatics, UTHealth, Houston, TX, USA
Xiaoqian Jiang
Seoul National University Hospital, Seoul, South Korea
Hyungbok Lee & Ju Han Kim
College of Medicine, Seoul National University, Seoul, South Korea
You can also search for this author in PubMed Google Scholar
Contributions
EI conducted data de-identification and data analysis. HK conceived the initial project idea and interpreted the results. EI and HK designed the study and wrote the manuscript. HL prepared the clinical data and analyzed the utility of the de-identified dataset. XJ and JK interpreted the analysis results and provided critical insights into the data de-identification approaches. All authors read and approved the final manuscript.
Corresponding author
Correspondence to Hyeoneui Kim .
Ethics declarations
Ethics approval and consent to participate.
This study utilized retrospective EHR data and was approved by the Institutional Review Board of the Seoul National University Hospital Biomedical Research Institute (IRB approval No: H-2009-156-1159). In accordance with Article 16 of the Korean Bioethics Law, informed consent was waived by the IRB. All experiments were performed in accordance with relevant guidelines and regulations.
Consent for publication
Not applicable.
Competing interests
The authors declare no competing interests.
Additional information
Publisher’s note.
Springer Nature remains neutral with regard to jurisdictional claims in published maps and institutional affiliations.
Electronic supplementary material
Below is the link to the electronic supplementary material.
Supplementary Material 1
Rights and permissions.
Open Access This article is licensed under a Creative Commons Attribution 4.0 International License, which permits use, sharing, adaptation, distribution and reproduction in any medium or format, as long as you give appropriate credit to the original author(s) and the source, provide a link to the Creative Commons licence, and indicate if changes were made. The images or other third party material in this article are included in the article’s Creative Commons licence, unless indicated otherwise in a credit line to the material. If material is not included in the article’s Creative Commons licence and your intended use is not permitted by statutory regulation or exceeds the permitted use, you will need to obtain permission directly from the copyright holder. To view a copy of this licence, visit http://creativecommons.org/licenses/by/4.0/ . The Creative Commons Public Domain Dedication waiver ( http://creativecommons.org/publicdomain/zero/1.0/ ) applies to the data made available in this article, unless otherwise stated in a credit line to the data.
Reprints and permissions
About this article
Cite this article.
Im, E., Kim, H., Lee, H. et al. Exploring the tradeoff between data privacy and utility with a clinical data analysis use case. BMC Med Inform Decis Mak 24 , 147 (2024). https://doi.org/10.1186/s12911-024-02545-9
Download citation
Received : 01 June 2023
Accepted : 21 May 2024
Published : 30 May 2024
DOI : https://doi.org/10.1186/s12911-024-02545-9
Share this article
Anyone you share the following link with will be able to read this content:
Sorry, a shareable link is not currently available for this article.
Provided by the Springer Nature SharedIt content-sharing initiative
- Data privacy
- Data utility
- Data de-identification
- Clinical data analysis
BMC Medical Informatics and Decision Making
ISSN: 1472-6947
- General enquiries: [email protected]
Changing urban land types and its locational impact on groundwater resources: a case study on Megacity Kolkata
- Published: 06 June 2024
Cite this article
- Suddhasil Bose ORCID: orcid.org/0000-0003-4836-7779 1 ,
- Asis Mazumdar 1 &
- Snehamanju Basu 2
Groundwater exploitation poses significant challenges in urban areas, as the depletion of groundwater levels (GWL) and degradation of groundwater quality (GWQ) are pervasive issues worldwide. This article aims to examine the locational influence of significant urban land types on groundwater resources, with Kolkata selected as the study area due to its pronounced GWL depletion and deteriorated GWQ. By utilizing remote sensing technology, this study individually identifies different urban land categories, such as built-up areas, green spaces, and surface water bodies, at intervals of a decade. The combined impact of these urban land types is then analysed and clustered into three distinct segments. To assess the spatial variation of GWQ, data on groundwater resources from the study area are collected, and the water quality index (WQI) is generated. Additionally, spatial autocorrelation (SA) analysis is employed to comprehend the spatial distribution of groundwater resources. Correlation coefficients are calculated to establish the relationship between different urban land types and groundwater resources. Subsequently, geographically weighted regression (GWR) is implemented to observe and identify local variations in GWL and GWQ concerning built-up areas, green spaces, and surface water bodies. The results obtained from this modelling approach demonstrate that the expansion of built-up areas positively contributes to groundwater degradation, while the presence of greater green spaces and surface water bodies in urban areas helps mitigate groundwater deterioration. The accuracy of the findings is evaluated, yielding satisfactory results with a quasi R 2 value exceeding 0.80 for groundwater resources in both years separately and by mapping local R 2 values. This study successfully identifies the impact of different urban land types on groundwater resources at a local level, thereby revealing spatial variations among the variables. The findings offer valuable insights for fostering better sustainable development at the local scale within cities.
This is a preview of subscription content, log in via an institution to check access.
Access this article
Price includes VAT (Russian Federation)
Instant access to the full article PDF.
Rent this article via DeepDyve
Institutional subscriptions
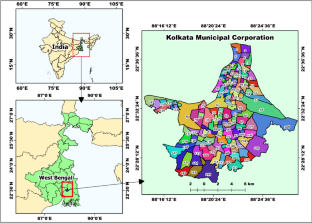
Data availability
Availability of data can be available upon request.
Abed, A. O., & Mohsin, M. K. (2022). Using the NDVI and NDBI indicators to study the urban expansion of the city of mahaweel for the period from 2003 to 2020 using geographic information systems. Journal of Optoelectronics Laser, 41 (6), 924–932. https://doi.org/10.5772/intechopen.97350
Article Google Scholar
Almadrones-Reyes, K. J., & Dagamac, N. H. A. (2022). Land-use/land cover change and land surface temperature in Metropolitan Manila, Philippines using landsat imagery. GeoJournal . https://doi.org/10.1007/s10708-022-10701-9
Al-Sefry, S. A., & Şen, Z. (2006). Groundwater rise problem and risk evaluation in major cities of arid lands—Jedddah Case in Kingdom of Saudi Arabia. Water Resources Management, 20 (1), 91–108. https://doi.org/10.1007/s11269-006-4636-2
An, X., Jin, W., Long, X., Chen, S., Qi, S., & Zhang, M. (2022). Spatial and temporal evolution of carbon stocks in Dongting Lake wetlands based on remote sensing data. Geocarto International, 8 , 1–20. https://doi.org/10.1080/10106049.2022.2093412
Aragaw, T. T., & Gnanachandrasamy, G. (2021). Evaluation of groundwater quality for drinking and irrigation purposes using GIS-based water quality index in urban area of Abaya-Chemo sub-basin of Great Rift Valley, Ethiopia. Applied Water Science, 11 (9), 1–20. https://doi.org/10.1007/s13201-021-01482-6
Article CAS Google Scholar
Ball, R. O., & Church, R. L. (1980). Water quality indexing and scoring. Journal of the Environmental Engineering Division, 106 (4), 757–771. https://doi.org/10.1061/JEEGAV.0001067
Boateng, T. K., Opoku, F., Acquaah, S. O., & Akoto, O. (2016). Groundwater quality assessment using statistical approach and water quality index in Ejisu-Juaben Municipality, Ghana. Environmental Earth Sciences, 75 (6), 1–14. https://doi.org/10.1007/s12665-015-5105-0
Bose, S, Mazumdar, A., & Basu, S. (2020). Review on present situation of groundwater scenario on Kolkata Municipal Area. In IOP conference series: Earth and environmental science (Vol. 505, p. 12022). https://doi.org/10.1088/1755-1315/505/1/012022
Bose, S., Mazumdar, A., & Basu, S. (2023). Evolution of groundwater quality assessment on urban area—A bibliometric analysis. Groundwater for Sustainable Development, 20 , 100894. https://doi.org/10.1016/j.gsd.2022.100894
Bose, S., Mazumdar, A., & Halder, S. (2024). Groundwater sustainability in the face of urban expansion: A case study on Kolkata’s ongoing challenge. Groundwater for Sustainable Development, 25 , 101162. https://doi.org/10.1016/j.gsd.2024.101162
Brown, R. M., McClelland, N. I., Deininger, R. A., & Tozer, R. G. (1970). A water quality index-do we dare. Water and Sewage Works, 117 , 10.
Google Scholar
Brunsdon, C., Fotheringham, S., & Charlton, M. (1998). Geographically weighted regression. Journal of the Royal Statistical Society: Series D (The Statistician), 47 (3), 431–443.
Brunsdont, C., Fotheringham, S., & Charlton, M. (1998). Geographically weighted regression—Modelling spatial non-stationarity. Journal of the Royal Statistical Society: Series D Statistics, 47 (3), 431–443. https://doi.org/10.1111/1467-9884.00145
Dinius, S. H. (1987). Design of an index of water quality. Journal of the American Water Resources Association, 23 (5), 833–843. https://doi.org/10.1111/j.1752-1688.1987.tb02959.x
Dutta, K., Basu, D., & Agrawal, S. (2021). Synergetic interaction between spatial land cover dynamics and expanding urban heat islands. Environmental Monitoring and Assessment, 193 (4), 184. https://doi.org/10.1007/s10661-021-08969-4
Elmahdy, S., Mohamed, M., & Ali, T. (2020). Land use/land cover changes impact on groundwater level and quality in the northern part of the United Arab Emirates. Remote Sensing, 12 (11), 1715. https://doi.org/10.3390/RS12111715
Fatemi, M., & Narangifard, M. (2019). Monitoring LULC changes and its impact on the LST and NDVI in District 1 of Shiraz City. Arabian Journal of Geosciences, 12 (4), 1–12. https://doi.org/10.1007/s12517-019-4259-6
Gascon, M., Cirach, M., Martinez, D., Dadvand, P., Valentin, A., Plasència, A., & Nieuwenhuijsen, M. J. (2016). Normalized difference vegetation index (NDVI) as a marker of surrounding greenness in epidemiological studies: The case of Barcelona city. Urban Forestry & Urban Greening, 19 , 88–94. https://doi.org/10.1016/j.ufug.2016.07.001
Gesels, J., Dollé, F., Leclercq, J., Jurado, A., & Brouyère, S. (2021). Groundwater quality changes in peri-urban areas of the Walloon region of Belgium. Journal of Contaminant Hydrology, 240 , 103780. https://doi.org/10.1016/j.jconhyd.2021.103780
Getis, A. (2007). Reflections on spatial autocorrelation. Regional Science and Urban Economics, 37 (4), 491–496. https://doi.org/10.1016/j.regsciurbeco.2007.04.005
Ghosh, S., Kumar, D., & Kumari, R. (2022). Assessing spatiotemporal dynamics of land surface temperature and satellite-derived indices for new town development and suburbanization planning. Urban Governance . https://doi.org/10.1016/j.ugj.2022.05.001
He, S., & Wu, J. (2019). Relationships of groundwater quality and associated health risks with land use/land cover patterns: A case study in a loess area, Northwest China. Human and Ecological Risk Assessment: an International Journal, 25 (1–2), 354–373. https://doi.org/10.1080/10807039.2019.1570463
Horton, R. K. (1965). An index number system for rating water quality. Journal of Water Pollution Control Federation, 37 (3), 300–306.
Hussain, S., Mubeen, M., Ahmad, A., Majeed, H., Qaisrani, S. A., Hammad, H. M., & Nasim, W. (2022). Assessment of land use/land cover changes and its effect on land surface temperature using remote sensing techniques in Southern Punjab, Pakistan. Environmental Science and Pollution Research . https://doi.org/10.1007/s11356-022-21650-8
John, B., Das, S., & Das, R. (2022). Natural groundwater level fluctuations of Kolkata City based on seasonal field data and population growth using geo-spatial application and characterised statistical techniques. Environment, Development and Sustainability . https://doi.org/10.1007/s10668-022-02313-7
Khan, A., Atta-Ur-rahman, S., & Ali, M. (2019). Impact of built environment on groundwater depletion in Peshawar, Pakistan. Journal of Himalayan Earth Sciences, 52 (1), 86–105.
Khan, M. R., Koneshloo, M., Knappett, P. S. K., Ahmed, K. M., Bostick, B. C., Mailloux, B. J., et al. (2016). Megacity pumping and preferential flow threaten groundwater quality. Nature Communications, 7 (1), 12833. https://doi.org/10.1038/ncomms12833
Krishna Kumar, S., Logeshkumaran, A., Magesh, N. S., Godson, P. S., & Chandrasekar, N. (2015). Hydro-geochemistry and application of water quality index (WQI) for groundwater quality assessment, Anna Nagar, part of Chennai City, Tamil Nadu, India. Applied Water Science, 5 (4), 335–343. https://doi.org/10.1007/s13201-014-0196-4
Krogulec, E., Małecki, J. J., Porowska, D., & Wojdalska, A. (2020). Assessment of causes and effects of groundwater level change in an urban area (Warsaw, Poland). Water, 12 (11), 3107. https://doi.org/10.3390/w12113107
Li, W., Zhang, W., Li, Z., Wang, Y., Chen, H., Gao, H., et al. (2022). A new method for surface water extraction using multi-temporal Landsat 8 images based on maximum entropy model. European Journal of Remote Sensing, 55 (1), 303–312. https://doi.org/10.1080/22797254.2022.2062054
Liaqat, M. U., Mohamed, M. M., Chowdhury, R., Elmahdy, S. I., Khan, Q., & Ansari, R. (2021). Impact of land use/land cover changes on groundwater resources in Al Ain region of the United Arab Emirates using remote sensing and GIS techniques. Groundwater for Sustainable Development, 14 , 100587. https://doi.org/10.1016/j.gsd.2021.100587
Lopez-Gunn, E., Llamas, M. R., Garrido, A., & Sanz, D. (2011). Groundwater management. In Treatise on water science (Vol. 1, pp. 97–127). Elsevier. https://doi.org/10.1016/B978-0-444-53199-5.00010-5
Lv, G., Zheng, S., & Hu, W. (2022). Exploring the relationship between the built environment and block vitality based on multi-source big data: An analysis in Shenzhen, China. Geomatics, Natural Hazards and Risk, 13 (1), 1593–1613. https://doi.org/10.1080/19475705.2022.2091484
Mansour, K., Aziz, M. A., Hashim, S., & Effat, H. (2022). Impact of anthropogenic activities on urban heat islands in major cities of El-Minya Governorate, Egypt. The Egyptian Journal of Remote Sensing and Space Science . https://doi.org/10.1016/j.ejrs.2022.03.014
Mishra, B., Sandifer, J., & Gyawali, B. R. (2019). Urban heat island in kathmandu, nepal: Evaluating relationship between ndvi and lst from 2000 to 2018. International Journal of Environment, 8 (1), 17–29. https://doi.org/10.3126/ije.v8i1.22546
Montgomery, D. C., Peck, E. A., & Vining, G. G. (2021). Introduction to linear regression analysis . John Wiley & Sons.
Mukherjee, F. (2022). Environmental impacts of urban Sprawl in Surat, Gujarat: An examination using Landsat data. Journal of the Indian Society of Remote Sensing . https://doi.org/10.1007/s12524-022-01509-8
Mulyadi, A., Dede, M., & Widiawaty, M. A. (2020). Spatial interaction of groundwater and surface topographic using geographically weighted regression in built-up area. In IOP conference series: Earth and environmental science (Vol. 477, p. 012023). IOP Publishing. https://doi.org/10.1088/1755-1315/477/1/012023
Okotto, L., Okotto-Okotto, J., Price, H., Pedley, S., & Wright, J. (2015). Socio-economic aspects of domestic groundwater consumption, vending and use in Kisumu, Kenya. Applied Geography, 58 , 189–197. https://doi.org/10.1016/j.apgeog.2015.02.009
Patra, S., Sahoo, S., Mishra, P., & Mahapatra, S. C. (2018). Impacts of urbanization on land use /cover changes and its probable implications on local climate and groundwater level. Journal of Urban Management, 7 (2), 70–84. https://doi.org/10.1016/j.jum.2018.04.006
Pius, A., Jerome, C., & Sharma, N. (2011). Evaluation of groundwater quality in and around Peenya industrial area of Bangalore, South India using GIS techniques. Environmental Monitoring and Assessment, 184 (7), 4067–4077. https://doi.org/10.1007/S10661-011-2244-Y
Prasad, S., & Singh, R. B. (2022). Urban Heat Island (UHI) assessment using the satellite data: A case study of Varanasi City, India. In Smart cities for sustainable development (pp. 287–299). Springer. https://doi.org/10.1007/978-981-16-7410-5_17
Ray, B., & Shaw, R. (2016). Water stress in the megacity of Kolkata, India, and its implications for urban resilience. In Urban disasters and resilience in Asia (pp. 317–336). Elsevier. https://doi.org/10.1016/B978-0-12-802169-9.00020-3
Siddik, M. S., Tulip, S. S., Rahman, A., Islam, M. N., Haghighi, A. T., & Mustafa, S. M. T. (2022). The impact of land use and land cover change on groundwater recharge in northwestern Bangladesh. Journal of Environmental Management, 315 , 115130. https://doi.org/10.1016/j.jenvman.2022.115130
Sinaga, K. P., & Yang, M. S. (2020). Unsupervised K-means clustering algorithm. IEEE Access, 8 , 80716–80727. https://doi.org/10.1109/ACCESS.2020.2988796
Tavares, A. O., Pato, R. L., & Magalhães, M. C. (2012). Spatial and temporal land use change and occupation over the last half century in a peri-urban area. Applied Geography, 34 (2), 432–444. https://doi.org/10.1016/j.apgeog.2012.01.009
Vaddiraju, S. C., & Reshma, T. (2022). Urbanization implications on hydro-meteorological parameters of Saroor Nagar Watershed of Telangana. Environmental Challenges . https://doi.org/10.1016/j.envc.2022.100562
Vázquez-Suñé, E., Sánchez-Vila, X., & Carrera, J. (2005). Introductory review of specific factors influencing urban groundwater, an emerging branch of hydrogeology, with reference to Barcelona, Spain. Hydrogeology Journal, 13 (3), 522–533. https://doi.org/10.1007/s10040-004-0360-2
Verma, P., Singh, P., & Srivastava, S. K. (2020). Impact of land use change dynamics on sustainability of groundwater resources using earth observation data. Environment, Development and Sustainability, 22 (6), 5185–5198. https://doi.org/10.1007/s10668-019-00420-6
Wakode, H. B., Baier, K., Jha, R., & Azzam, R. (2018). Impact of urbanization on groundwater recharge and urban water balance for the city of Hyderabad, India. International Soil and Water Conservation Research, 6 (1), 51–62. https://doi.org/10.1016/j.iswcr.2017.10.003
Xiao, S., Fügener, T., Wende, W., Yan, W., Chen, H., Syrbe, R., & Xue, B. (2022). The dynamics of vegetation and implications for ecosystem services in the context of urbanisation: An example from Huangyan-Taizhou, China. Ecological Engineering, 179 , 106614. https://doi.org/10.1016/j.ecoleng.2022.106614
Yadav, V., & Bhagat, R. B. (2015). Spatial dynamics of population in Kolkata urban agglomeration. In Urban development challenges, risks and resilience in Asian mega cities (pp. 157–173). Springer. https://doi.org/10.1007/978-4-431-55043-3_9
Yang, W., Deng, M., Tang, J., & Luo, L. (2022). Geographically weighted regression with the integration of machine learning for spatial prediction. Journal of Geographical Systems . https://doi.org/10.1007/s10109-022-00387-5
Yang, X., & Chen, L. (2017). Evaluation of automated urban surface water extraction from Sentinel-2A imagery using different water indices. Journal of Applied Remote Sensing, 11 (2), 026016. https://doi.org/10.1117/1.jrs.11.026016
Zhu, K., Bayer, P., Grathwohl, P., & Blum, P. (2015). Groundwater temperature evolution in the subsurface urban heat island of Cologne, Germany. Hydrological Processes, 29 (6), 965–978. https://doi.org/10.1002/hyp.10209
Download references
This research received no specific grant from any funding agency in the public, commercial, or not-for-profit sectors.
Author information
Authors and affiliations.
School of Water Resources Engineering, Jadavpur University, Kolkata, West Bengal, 700032, India
Suddhasil Bose & Asis Mazumdar
Department of Geography, Lady Brabourne College, Kolkata, West Bengal, 700017, India
Snehamanju Basu
You can also search for this author in PubMed Google Scholar
Corresponding author
Correspondence to Suddhasil Bose .
Ethics declarations
Conflict of interest.
The authors declare that they have no competing interests.
Additional information
Publisher's note.
Springer Nature remains neutral with regard to jurisdictional claims in published maps and institutional affiliations.
Rights and permissions
Springer Nature or its licensor (e.g. a society or other partner) holds exclusive rights to this article under a publishing agreement with the author(s) or other rightsholder(s); author self-archiving of the accepted manuscript version of this article is solely governed by the terms of such publishing agreement and applicable law.
Reprints and permissions
About this article
Bose, S., Mazumdar, A. & Basu, S. Changing urban land types and its locational impact on groundwater resources: a case study on Megacity Kolkata. Environ Dev Sustain (2024). https://doi.org/10.1007/s10668-024-05095-2
Download citation
Received : 17 July 2022
Accepted : 27 May 2024
Published : 06 June 2024
DOI : https://doi.org/10.1007/s10668-024-05095-2
Share this article
Anyone you share the following link with will be able to read this content:
Sorry, a shareable link is not currently available for this article.
Provided by the Springer Nature SharedIt content-sharing initiative
- Urban lands
- Groundwater resources
- Locational impact
- Water quality index
- Spatial autocorrelation
- Geographical weightage regression
- Find a journal
- Publish with us
- Track your research

An official website of the United States government
Here's how you know
Official websites use .gov A .gov website belongs to an official government organization in the United States.
Secure .gov websites use HTTPS A lock ( Lock Locked padlock ) or https:// means you’ve safely connected to the .gov website. Share sensitive information only on official, secure websites.

FHFA Statistics Measuring Price Effects from Disasters Using Public Data: A Case Study of Hurricane Ian
What happens to home values after a disaster? In a recent working paper , we address this question with a combination of readily available public data sources that track insurance claims and house prices. Focusing on a specific storm, Hurricane Ian, we use leading statistical estimation approaches, difference-in-differences (DiD) and synthetic control methods (SCM), to provide some insights. A key finding is that data limitations create problems for identifying precise price effects. This blogpost discusses the data, methodological choices, and basic findings.
As a preview, we find positive price effects for homes affected by the hurricane. However, our results should not be interpreted as causal, but more correlational. Several factors, including COVID-19 and measurement error, could have confounding influences which make it difficult to measure the effects of Ian’s damage on home prices. Still, the exercise is useful because it demonstrates how one might use public information and why it is useful to continue improving such data releases to support policy analyses.
Defining Damages from Hurricane Ian to Estimate Price Effects
Hurricane Ian struck southwest Florida on September 23, 2022, damaging homes across the state. According to the National Hurricane Center Tropical Cyclone Report, Hurricane Ian “was responsible for over 150 direct and indirect deaths and over $112 billion in damage, making it the costliest hurricane in Florida’s history and the third costliest in the United States history”. 1 Figure 1 shows that path of Ian through Florida. Real estate listings are shown with yellow dots (larger circles indicating counties with more properties for sale) and darker blue shading indicates places that had more claims filed for damages. The figure shows a large concentration of insurance claims in southwest Florida, which also has a large number of real estate listings. We focus on Lee County, which has the darkest shading and is located on the Gulf Coast side of the state where the storm first landed.
Figure 1: Hurricane Ian’s path through Florida in 2022
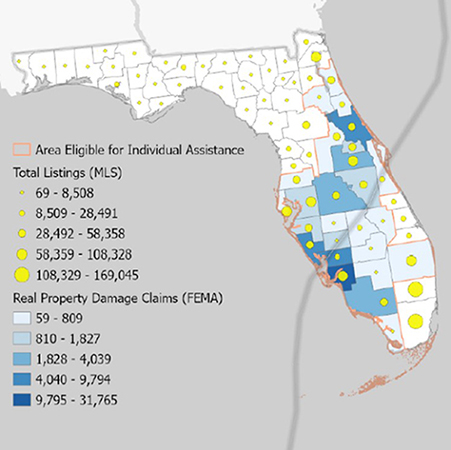
We estimate price effects by combining publicly available damages data with real estate listings data for southwest Florida between March 2015 and June 2023. The Federal Emergency Management Agency (FEMA) determines real property damage to properties for claims with limited or no insurance as part of the Individuals and Households Program (IHP). This information is combined with real estate market activity from Multiple Listing Service (MLS) data sourced by CoreLogic.
The key challenge we face is that many damage-related reports rely on publicly available disaster data, which for privacy reasons typically only include coarse descriptions of location. As a result, individual properties must be assigned damages from an aggregate level (e.g., county or ZIP code), thus introducing measurement error. This is a problem because previous research has shown mismeasured “treatment” variables like damages to a home can create problems for estimating causal price effects. 2
After merging IHP and MLS data, treated areas are classified at the county or ZIP code levels and with each home in an area assigned the same treatment status. This occurs regardless of whether the home was damaged. We consider multiple ways to assign treatment at the county and ZIP code levels. While the full set of aggregate treatment definitions are shown in the paper, this blogpost only presents a preferred specification at the county level (with damages aggregated for all homes therein) where treatment indicates county damages exceed the median for other counties.
Preliminary Evidence
Real estate market measures are illustrated in two panels within Figure 2. While not shown here, the trends are similar between Lee County (the focus area) and the entire state of Florida. The left panel presents median list (solid navy line) and close prices (solid maroon line) for each month during our sample. Both price trends are noisy and lack a sharp change when the storm hit, which is denoted by the dashed vertical black line. The right panel shows a reduction in the number of new listings, suggesting that prices could have been propped up by a decrease in home supply. Alternatively, homes that sold after the storm could be disproportionately better quality and command a premium. We are agnostic about the correct channel at work here: more careful analysis is needed to identify the mechanism(s) operating to affect prices. Finally, a potential COVID-19 effect starts after 2020 with prices increasing more quickly in the left panel and greater listing volatility in the right panel.
The lack of a clear and sustained impact on home prices suggests a more rigorous empirical analysis is needed for prices. We emphasize that we do not claim to establish a causal relationship nor that a price adjustment mechanism is identified correctly. We simply aim to estimate any aggregate price effect while using public data and documenting the challenges even when using leading statistical approaches.
Figure 2: Real estate market activity in Lee County, Florida
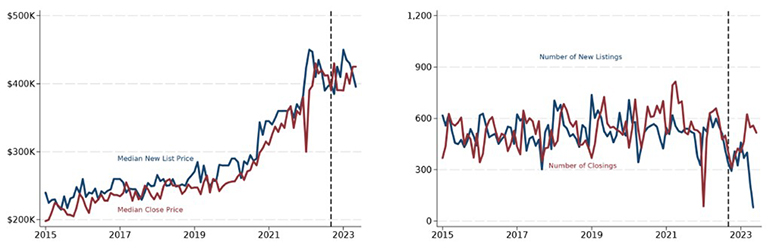
Difference-in-Differences Method for Price Effects
The most common approach used in the disaster literature for estimating price effects is a difference-in-differences (DiD) or event study approach. 3 In generalized terms, this means comparing price trends in affected (“treated”) areas with price trends in unaffected (“control”) areas.
Figure 3: Dynamic DiD for Lee County where Hurricane Ian made landfall
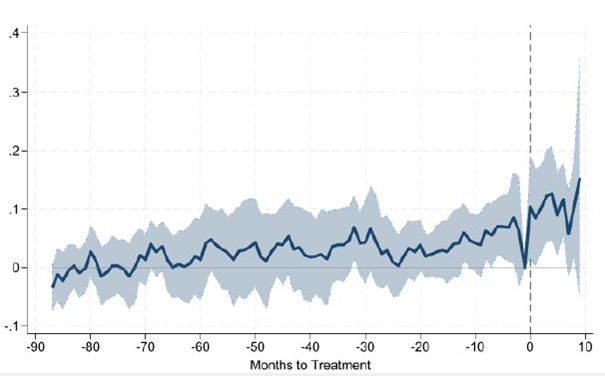
Figure 3 conveys the DiD results for a dynamic treatment (“event study”). The horizontal axis represents time relative to Ian, with the vertical dashed line indicating Ian’s landfall. The vertical axis shows an estimated parameter that is the difference in price trends between affected and unaffected areas at each time, with the shaded area representing the 95% confidence interval of the parameter. Higher prices are correlated with damages post-Ian. Where is this shown? By the price trend increasing above the zero line after the dashed vertical line.
However, the key assumption underlying DiD is the parallel trends assumption, which says that absent Hurricane Ian the areas affected and not affected would have had similar price trends. To test whether this assumption holds, researchers commonly conduct “pre-trends” tests, checking whether before Ian affected and not affected areas had similar price trends. In Figure 3, this corresponds to the difference in price trends being close to zero prior to the dashed line. As the figure shows, there is evidence that parallel trends does appear to be violated about 20 to 25 months prior to Ian’s arrival. What happened at that time? That was the early period of COVID. This is problematic statistically because the homes that saw prices increase during the early stages of the pandemic were the same homes that were damaged by Ian, making identification of the true Ian price effects challenging.
Synthetic Control Method for Price Effects
Another approach one can take is to construct a suitable control using the synthetic control method (SCM) at the aggregated level. The SCM determines an optimal weighted average of untreated counties (i.e. its synthetic control) to match the pre-Ian price trends of Lee County. Figure 4 below illustrates Lee County and its synthetic control. The figure suggests a time-varying price premium of at least five percent that peaks in the low double digits a few months after Ian, similar to the DiD finding. However, despite the apparent good fit in the figure with the blue and red lines appearing to be on top of each other until the dashed vertical line, more sensitive goodness of fit tests indicate these estimates lack statistical significance.
Figure 4: Synthetic control for Lee County
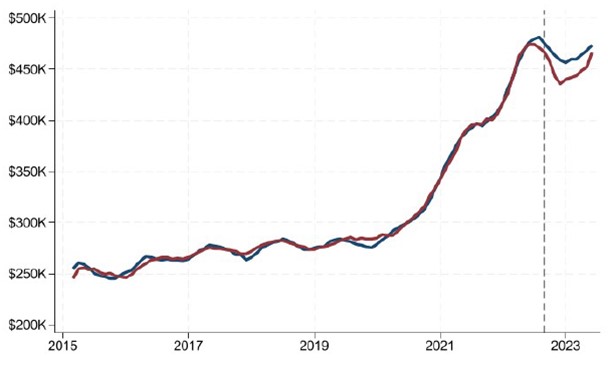
In summary, using publicly available data it may be difficult to estimate price effects even when employing leading empirical techniques. While we record some evidence for positive price effects for Ian, it is possible that declines across many markets but less so in the target county. Either way, these results should be viewed with some skepticism because of an inability to establishing actual property-level damages when using public data. Given the local nature of damages, when one is unable to precisely identify which units are treated or not, finding a suitable control group is extremely difficult. For those interested in working with such data, we offer several suggestions in the paper and encourage you to read further.
1 See https://www.nhc.noaa.gov/data/tcr/AL092022_Ian.pdf .
2 For recent academic research on this type of problem in a difference-in-differences setting, see Denteh Kedagni (2022) and Negi Negi (2022), for example. The problem is the mismeasurement of the treatment variable, often called a “misclassification” problem.
3 See another one of FHFA’s working papers, for a recent review of the applied disaster literature: Justin Contat, Carrie Hopkins, Luis Mejia, Matthew Suandi. 2024. “When Climate Meets Real Estate: A Survey of the Literature." Real Estate Economics. https://doi.org/10.1111/1540-6229.12489 .
By:Justin Contat
Senior Economist
Division of Research and Statistics
By:Will Doerner
Supervisory Economist
By:Robert Renner
Senior Geographer
By:Malcolm Rogers
Tagged: FHFA Stats Blog; Source: FHFA; Natural Disasters; Natural Disaster Price Effects; hurricane; real estate valuation
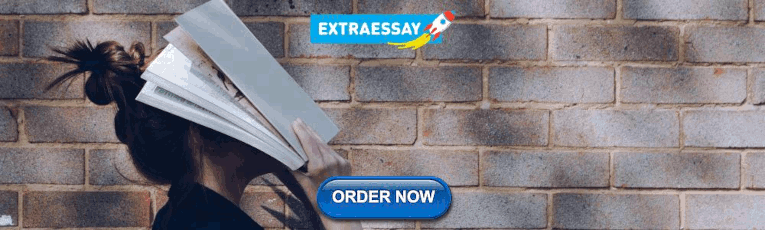
IMAGES
VIDEO
COMMENTS
internal validity and external validity in doing case studies (see Chapter 2). EXERCISE 5.2 Creating a General Analytic Strategy Assume that you have begun analyzing your case study data but still do not have an overall analytic strategy. Instead of staying stalled at this analytic step, move to the next step and speculate how you might ...
Data sources: The research example used is a multiple case study that explored the role of the clinical skills laboratory in preparing students for the real world of practice. Data analysis was conducted using a framework guided by the four stages of analysis outlined by Morse ( 1994 ): comprehending, synthesising, theorising and recontextualising.
Data analysis. Analyzing case study research involves making sense of the rich, detailed data to answer the research question. This process can be challenging due to the volume and complexity of case study data. However, a systematic and rigorous approach to analysis can ensure that the findings are credible and meaningful.
Step 1: Gather your qualitative data and conduct research (Conduct qualitative research) The first step of qualitative research is to do data collection. Put simply, data collection is gathering all of your data for analysis. A common situation is when qualitative data is spread across various sources.
Revised on November 20, 2023. A case study is a detailed study of a specific subject, such as a person, group, place, event, organization, or phenomenon. Case studies are commonly used in social, educational, clinical, and business research. A case study research design usually involves qualitative methods, but quantitative methods are ...
The data collection method should be selected based on the research questions and the nature of the case study phenomenon. Analyze the data: The data collected from the case study should be analyzed using various techniques, such as content analysis, thematic analysis, or grounded theory. The analysis should be guided by the research questions ...
A Case study is: An in-depth research design that primarily uses a qualitative methodology but sometimes includes quantitative methodology. Used to examine an identifiable problem confirmed through research. Used to investigate an individual, group of people, organization, or event. Used to mostly answer "how" and "why" questions.
As case study research is a flexible research method, qualitative data analysis methods are commonly used [176]. The basic objective of the analysis is, as in any other analysis, to derive conclusions from the data, keeping a clear chain of evidence.
How to Write Case Study Analysis 1. Analyzing the Data Collected. Examine the data to identify patterns, trends, and key findings. Use qualitative and quantitative methods to ensure a comprehensive analysis. Validate the data's accuracy and relevance to the subject. Look for correlations and causations that can provide deeper insights.
The data collected during a Case Study Analysis is then carefully analyzed and interpreted. Researchers use different analytical frameworks and techniques to make sense of the information and identify patterns, themes, and relationships within the data. This process involves coding and categorizing the data, conducting comparative analysis, and ...
Qualitative analysis involves analyzing non-numerical data from sources like interviews, observations, documents, and images in a case study. It helps explore context, meaning, and patterns to ...
Through conducting a case study among 12 Chinese college students and qualitatively analyzing the reflections and interview data collected over a semester, the present research, adopting the ...
propose an approach to the analysis of case study data by logically linking the data to a series of propositions and then interpreting the subsequent information. Like the Yin (1994) strategy, the Miles and Huberman (1994) process of analysis of case study data, although quite detailed, may still be insufficient to guide the novice researcher.
How to Analyze a Case Study Adapted from Ellet, W. (2007). The case study handbook. Boston, MA: Harvard Business School. A business case simulates a real situation and has three characteristics: 1. a significant issue, 2. enough information to reach a reasonable conclusion, 3. no stated conclusion. A case may include 1. irrelevant information 2.
Identify the key problems and issues in the case study. Formulate and include a thesis statement, summarizing the outcome of your analysis in 1-2 sentences. Background. Set the scene: background information, relevant facts, and the most important issues. Demonstrate that you have researched the problems in this case study. Evaluation of the Case
1. Solving a Data Science case study means analyzing and solving a problem statement intensively. Solving case studies will help you show unique and amazing data science use cases in your ...
Step 1: With Data Analytics Case Studies, Start by Making Assumptions. Hint: Start by making assumptions and thinking out loud. With this question, focus on coming up with a metric to support the hypothesis. If the question is unclear or if you think you need more information, be sure to ask.
Humana's Automated Data Analysis Case Study. The key thing to note here is that the approach to creating a successful data program varies from industry to industry. Let's start with one to demonstrate the kind of value you can glean from these kinds of success stories. Humana has provided health insurance to Americans for over 50 years.
7 Top Data Science Case Studies . Here are 7 top case studies that show how companies and organizations have approached common challenges with some seriously inventive data science solutions: Geosciences. Data science is a powerful tool that can help us to understand better and predict geoscience phenomena.
Data analysis use case. This study explores the impact of various de-identification techniques on datasets and their subsequent analysis results using a data analytic use case. The analytic use case involved predicting the Length of Stay (LOS) of high-acuity patients transferred to the emergency department (ED) of an academic medical center ...
Groundwater exploitation poses significant challenges in urban areas, as the depletion of groundwater levels (GWL) and degradation of groundwater quality (GWQ) are pervasive issues worldwide. This article aims to examine the locational influence of significant urban land types on groundwater resources, with Kolkata selected as the study area due to its pronounced GWL depletion and deteriorated ...
COVID-19 Risk Analysis Based on Population Migration Big Data: A Case Study of Wuhan. Pages 940-946. Previous Chapter Next Chapter. ABSTRACT. Population movement between regions is one of the main ways for the spread of COVID-19. The Chinese government has adopted unprecedented population movement controls to restrain the spread of COVID-19.
We utilized 2006-2015 claims data of the Taiwan National Health Insurance Program as a data source. A case-control study was conducted, involving individuals 20 years or older with and without colon polyps. ... However, more studies combining the biomarker analysis and molecular pathways are needed to prompt a comprehensive exploration of ...
As the medium of geological information, groundwater provides an indirect method to solve the secondary disasters of mining activities. Identifying the groundwater regime of overburden aquifers induced by the mining disturbance is significant in mining safety and geological environment protection. This study proposes the novel data-driven algorithm based on the combination of machine learning ...
What happens to home values after a disaster? In a recent working paper, we address this question with a combination of readily available public data sources that track insurance claims and house prices. Focusing on a specific storm, Hurricane Ian, we use leading statistical estimation approaches, difference-in-differences (DiD) and synthetic control methods (SCM), to provide some insights. A ...