
An official website of the United States government
The .gov means it’s official. Federal government websites often end in .gov or .mil. Before sharing sensitive information, make sure you’re on a federal government site.
The site is secure. The https:// ensures that you are connecting to the official website and that any information you provide is encrypted and transmitted securely.
- Publications
- Account settings
Preview improvements coming to the PMC website in October 2024. Learn More or Try it out now .
- Advanced Search
- Journal List
- Int J Environ Res Public Health

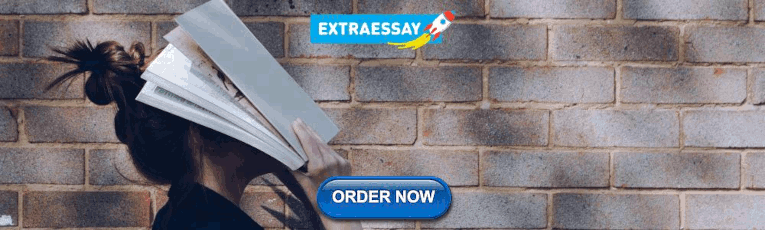
Machine Learning and Criminal Justice: A Systematic Review of Advanced Methodology for Recidivism Risk Prediction
Guido vittorio travaini.
1 School of Medicine, Vita-Salute San Raffaele University, 20132 Milan, Italy
Federico Pacchioni
Silvia bellumore, marta bosia.
2 Department of Clinical Neurosciences, IRCCS San Raffaele Scientific Institute, 20132 Milan, Italy
Francesco De Micco
3 Bioethics and Humanities Research Unit, Campus Bio-Medico University of Rome, 00128 Rome, Italy
4 Department of Clinical Affairs, Campus Bio-Medico University Hospital Foundation, 00128 Rome, Italy
Associated Data
Not applicable.
Recent evolution in the field of data science has revealed the potential utility of machine learning (ML) applied to criminal justice. Hence, the literature focused on finding better techniques to predict criminal recidivism risk is rapidly flourishing. However, it is difficult to make a state of the art for the application of ML in recidivism prediction. In this systematic review, out of 79 studies from Scopus and PubMed online databases we selected, 12 studies that guarantee the replicability of the models across different datasets and their applicability to recidivism prediction. The different datasets and ML techniques used in each of the 12 studies have been compared using the two selected metrics. This study shows how each method applied achieves good performance, with an average score of 0.81 for ACC and 0.74 for AUC. This systematic review highlights key points that could allow criminal justice professionals to routinely exploit predictions of recidivism risk based on ML techniques. These include the presence of performance metrics, the use of transparent algorithms or explainable artificial intelligence (XAI) techniques, as well as the high quality of input data.
1. Introduction
Recidivism rates have a major impact on public safety and increase the cost of incarceration. An estimate of economic and social costs of recidivism has been provided by one study conducted in England and Wales on a 12-month follow-up, indicating GBP 18.1 billion for the 2016 criminal cohort [ 1 ]. This incidence is also influenced by the high rate of criminal recidivism, stated as high as 50% in many international jurisdictions [ 2 ]. In addition to the social costs, it is also important to mention the devastating consequences that recidivism causes for victims, communities and offenders as well as their own families, which are usually not even mentioned among the injured parties. So, it is very important to try to reduce the high rate of criminal recidivism and reduce these effects.
A discipline that in recent years has provided an important contribution by attempting to provide for criminal recidivism is data science. Indeed, data science is the application of quantitative and qualitative methods to solve relevant problems and predict outcomes [ 3 , 4 , 5 ]. In particular, using the potential of risk assessment tools and machine learning (ML) algorithms in predicting the risk of criminal recidivism in order to reduce its spread has been outlined in literature since the 1920s [ 6 ]. Over the years, the methodologies improved becoming more reliable due to the development of various datasets and ML models able to support judicial decisions on probation, length of sentence or application of better rehabilitation strategies. ML models can indeed be applied in various criminal justice areas. At the group level, they can be profitably used to monitor or predict the effects of criminal justice policies on reducing recidivism. At the individual level, they can be applied to support judicial decisions, assessing specific risk of recidivism.
This review aims to deepen these debated topics. Indeed, despite the extensive development of these approaches and a promising assessment of the reliability of ML models to support criminal justice [ 7 ], they are still underutilized in judicial practice [ 8 ]. This stems from the controversial opinions debated in the scientific and jurisprudential literature [ 9 , 10 , 11 , 12 ]. The foremost reason is the fear of delegating decisions entirely to an algorithm, as reported by Rees “we would be entitled to feel uneasy, even if presented with compelling evidence that, on average, the machines make better decisions than the humans” [ 13 ]. Despite the perplexities, it is hard to imagine our future ignoring the potentials of ML model’s application [ 14 ]. Indeed, the ability of ML systems to predict complex outputs with high certainty has led them to become increasingly pervasive and has received growing attention in recent years to optimize public policy and criminal justice [ 15 , 16 , 17 , 18 ]. Thus, researchers have sought to improve prediction scales by looking for strong indicators that predict different types of recidivism thus improving the accuracy of the ML model. However, it is also important to focus on previously overlooked aspects and interactions, such as standardizing data collection and applying data pre-processing, two process that can increase the performances of ML models. The different variables considered for the prediction of recidivism and the constantly evolving ML techniques have raised the need for clarity in the vast literature that has rapidly followed over the years. Leveraging the potential of a review written according to the Preferred Reporting Items for Systematic Reviews and Meta-Analyses (PRISMA) Statement [ 19 ], this study aims for the first time to delineate the state of the art in recidivism prediction techniques with a critical analysis of their potentialities and limitations.
2. Materials and Methods
2.1. eligibility criteria.
The methods for systematic review have been structured according to the PRISMA Statement. No language, publication date, or publication status restrictions were imposed. The features of included studies are the following: (1) the aim of the study is to predict recidivism; (2) the study has explicit accounting of data collection methods; and (3) the study has proper description of the methodologies, including the applied machine learning methods.
In contrast, the authors did not include studies in which one or more of the following criteria were satisfied: (1) the main purpose of the study is to reduce the bias of the ML model (e.g., race-based bias); (2) the study has the aim to predict the psychiatric characteristics in the reoffender (e.g., mental illness); and (3) the study lacks the accuracy (ACC) or the Area Under the Curve (AUC) metrics necessary to evaluate machine learning models.
2.2. Information Sources and Search Strategy
Studies have been selected from two online databases: Scopus and Pubmed. We used the following equation searching by title, abstract and keywords for: ((“crim*”) OR (“offen*”) OR (“violat*”)) AND ((“recidiv*”) OR (“relapse”)) AND ((“machine learning”) OR (“artificial intelligence”) OR (“deep learning”)) resulting in a total of 79 bibliographic records. References listed in the included papers have also been examined to identify studies meeting our inclusion and exclusion criteria. The search was last performed in January 2022. We first screened titles and excluded those clearly not meeting the eligibility criteria. Then abstracts were examined, and lastly, full texts were read, eventually leading to the inclusion or exclusion of the papers according to the above described criteria. The screening of the literature was performed in blind by two investigators (S.B. and F.P.) In the case of disagreement, a third reviewer (M.B.) assessed the paper to achieve a consensus.
2.3. Assessment of Risk of Bias
The ROBIS tool was used to assess the risk of bias of the included systematic reviews [ 20 ]. The ROBIS tool is a method for assessing bias in systematic reviews that consists of three phases: (1) assessing relevance (optional); (2) identifying concerns with the review process; and (3) judging risk of bias in the review. Phase two involves assessing the review across four domains: (1) study eligibility criteria; (2) identification and selection of studies; (3) data collection and study appraisal; and (4) synthesis and findings. The third phase summarizes the concerns identified during phase two. The first two phases were performed independently by two authors (SB, FP) and resulting discrepancies were addressed by a third author (GT or MB).
3.1. Study Selection
A total of 16 duplicates were identified and removed from the 79 preliminary results. In addition, 33 articles were removed by looking at the title and abstract only since they did not match the criteria. The remaining papers were selected or excluded after a comprehensive analysis of the full text. Among those papers, 18 were excluded since they did not satisfy eligibility criteria for the following reasons:
- The purpose of fourteen papers was to reduce model bias;
- One paper assessed only the psychiatric characteristic of repeat offenders;
- Three papers did not clearly describe the methodology.
A total of 12 studies were finally selected. Figure 1 summarizes the results of the study selection, represented as a PRISMA flow diagram obtained following the guidelines published by Page and colleagues [ 19 ].

Flowchart showing the process of inclusion of publications.
3.2. Study Characteristics
To present the results of the studies, we divided them into three sections. The first one analyses dataset and ML techniques applied within the study. We first focused on the characteristics of datasets. Then we checked whether in the studies the authors have used ML techniques such as data pre-processing or cross validation (CV). The first one is a technique to transforming raw data into an understandable format for the ML models. The CV, instead, is a technique to estimate if the ML models are able to correctly predict data not yet observed. This first section is important because different datasets and different ML techniques can greatly influence the final performance of ML models. In the second section, we analysed the type of recidivism that each study aimed to predict. Then we selected the ML model that in the study obtained the best performance. Finally, in the third section, by dividing the studies into four categories based on their aim, we compared the performance of each ML model based on specific metrics.
3.3. Characteristics of Dataset and ML Techniques
The main features of the considered studies are listed in Table 1 .
Dataset combined and ML techniques applied.
CV: cross validation; ANOVA: analysis of variance.
The datasets are different in each study. Two of them used only data from the correctional institution or the justice system. The first one was based on 3061 youth charged with a sexual offense in Florida who have been monitored over two years after the initial charge to determine sexual recidivism [ 26 ]. The aim of Ozkan and colleagues was to examine whether ML models could provide a better prediction than classical statistical tools. Thus, the authors took advantage of the statistical models using several predictor variables, including historical risk assessment data and a rich set of developmental factors for all youth reported for delinquency by the Florida Department of Juvenile Justice (FDJJ). The second study published by Butsara [ 21 ] used a dataset from a central correctional institution for drug addicts and a central women’s correctional institution in Thailand. The sample consists in 300 male and 298 female inmates. The authors proposed a method to find the crucial factors for predicting recidivism in drug distribution and investigated the power of ML in predicting recidivism.
Five papers refer to risk assessment tools created specifically to predict recidivism across the country. Among them Karimi-Haghighi and Castillo used RisCanvi, a risk assessment protocol for violence prevention introduced in the Catalan prison during 2009 in which professionals conducted interviews resulting in the creation of a risk score through some risk elements [ 25 ]. The elements are included in five risk areas of prisoners: criminal/penitentiary, family/social, clinical and attitudinal/personal factors. The dataset used includes 2634 cases. The Duwe and Kim study considers 27,772 offenders released from Minnesota prisons between 2003 and 2006 [ 22 ]. The authors used a dataset from the Minnesota Screening Tool Assessing Recidivism Risk (MnSTARR), which assesses the risk of five different types of recidivism, taking advantage of the Minnesota Sex Offender Screening Tool-3 (MnSOST-3), used to analyse sexual recidivism risk for Minnesota sex offenders. Tollenaar and colleagues in two different studies used the StatRec scale with static information from the Dutch Offender Index (DOI) [ 31 , 32 ]. In both studies, the recidivism prediction is divided into three categories: general, criminal and violent recidivism. The dataset is based on offenders over the age of 12 found guilty during a criminal case that ended in 2005. In the more recent one, the authors also included public access data from the North Carolina prison in the dataset to investigate the generalizability of the results. These data feature all individuals released from July 1977 to June 1978 and from July 1979 to June 1980. Both cohorts were tested with ML models, but for this review we consider only the 1977–1978 data excluding the Tollenaar’s 2019 study [ 32 ] because the 1980 cohort showed a worse calibration probability. The dynamic elements of the Finnish Risk and Needs Assessment Form (RITA) were used by Salo and colleagues [ 27 ] to predict general and violent recidivism. The sample included 746 men sentenced to a new term of imprisonment. All individuals must have the full RITA, which considers 52 items such as aggression, alcohol problems, drug use, work problems, coping with economic problems and resistance to change.
Another study that combined statistical features with other specific risk assessment tools is by Tolan and collaborators [ 30 ]. The authors compared different combinations of datasets and ML models in terms of AUC and then showed the results with Structured Assessment of Violence Risk in Youth (SAVRY) features in which the ML model outperforms. The SAVRY is a violent risk assessment tool including 24 risk factors and 6 predictive factors. These risk factors are divided into historical, individual and social/contextual categories. The data analysed in the study were extracted from the Catalonia juvenile justice system and included 853 juvenile offenders between the ages of 12 and 17 who finished a sentence in 2010 and were subjected to a SAVRY analysis.
Only one study used the Historical, Clinical and Risk Management–20 (HCR-20) with 16 other clinical and non-clinical risk assessment factors in order to determine the likelihood of recidivism among first-time offenders (FTOs) [ 28 ]. Data were collected from various prisons in the Indian state of Jharkhand. The study was conducted on 204 male inmates aged between 18 and 30, most of them below the poverty line.
Finally, it is important to mention the paper of Haarsma and his working group [ 24 ] in which the authors used the NeuroCognitive Risk Assessment (NCRA), a neurocognitive test-based risk assessment software able to measure key criminogenic factors related to recidivism. In this study, 730 participants in the Harris County Department of Community Supervision and Corrections self-administered the NCRA. The individual’s recidivism risk score by NCRA combined with a set of demographic features was quantified using a ML model.
After compiling the dataset, each study used different ML techniques to analyse and improve the reading of the data and results. Four studies used pre-processing to improve the datasets. Two of them preferred a feature selection technique [ 24 , 26 ]. One study used a generic data standardization and then applied feature selection [ 21 ]. The last one used ANOVA to identify relevant attributes for the current dataset [ 28 ].
Another relevant aspect to mention is the author’s choice to use a ML technique called cross validation (CV) in order to estimate the ability of ML models to generalize to data not yet observed. Among the studies included in the review, nine used CV.
3.4. Aim of the Studies and ML Model Applied
For a better comparison of the studies, it is possible to perform a sorting by the type of recurrence they aim to predict. The sorting leads to four categories: general [ 22 , 23 , 24 , 28 , 31 , 32 ], sexual [ 31 , 32 ], violent [ 22 , 25 , 31 ] and all other recidivism. The last category includes studies that considered a specific type of crime [ 21 ] or referred only to males [ 27 ] or youth [ 26 , 29 , 30 ].
The previously observed datasets ( Table 1 ) were used to train ML models to generate recidivism predictions. There are different types of models that you can select based on the available data and type of target you want to predict. So, all the studies compared different models to obtain the best results in predicting recidivism. The metrics used to compare the models were accuracy (ACC) and area under curve (AUC). The ACC is used to measure how often the algorithm correctly classifies a data point and represents the ratio of correctly classified observation to the total number of the predictions. The AUC measures the ability of the ML models to distinguish recidivism from non-recidivism. Both metrics provide a result in the range [0,1]. When the score is near 0, it means that ML models have the worst ability to predict, a score close to 0.5 means that the models randomly predict the probability of recidivism. On the other hand, a score close to 1 means that ML models have a perfect ability to distinguish recidivism from non-recidivism.
In this review, for each study, we considered only the ML models that performed better, according to the author’s observation ( Table 2 ). The most used ML model is the logistic regression [ 21 , 30 , 31 , 32 ] and two of its variants, the LogitBoost [ 22 ] and the generalized linear models with ridge and lasso regularization (Glmnet) [ 24 ]. The second most popular model is the random forest [ 23 , 26 , 27 , 33 ]. The other ML models to mention are multi-layer perceptron (MLP) [ 25 ], linear discriminant analysis (LDA) [ 31 ] and penalized LDA [ 32 ].
Purpose of datasets, ML models and their evaluation.
ACC: accuracy; AUC: area under the curve; Thailand: data by central correctional institution for drug addicts and central women correctional institution in Thailand; MnSTARR+: Minnesota Screening Tool Assessing Recidivism Risk + Minnesota Sex Offender Screening Tool-3; LS/CMI: Level of Service/Case Management Inventory; NCRA+: NeuroCognitive Risk.
3.5. Results of Syntheses
Table 3 , Table 4 , Table 5 and Table 6 describe the results for the analysis of ACC and AUC in general, sexual, violent and all the other recidivism in which all ML models perform well. The ACC scores are in the range [0.65, 0.96], and the AUC scores are in the range [0.69, 0.78]. The highest scores have been highlighted in Table 3 , Table 4 , Table 5 and Table 6 . Table 3 shows the results obtained from the different studies predicting generic recidivism. The ensemble model trained with an HCR-20+ dataset leads to improved performance and seems to be the most effective method in terms of the ACC [ 28 ]. Considering the AUC, the logistic regression yields the highest score with the use of the MnSTARR+ and StatRec datasets [ 22 , 31 ].
Evaluation sexual recidivism.
AUC: area under curve; ACC: accuracy; StatRec: static recidivism risk (Static Recidiverisico); DOI: Dutch Offender’s Index. The highest scores are highlighted in bold.
Evaluation violent recidivism.
AUC: area under curve; ACC: accuracy; RisCanvi: risk assessment protocol for violence prevention introduced in the Catalan prison; StatRec: static recidivism risk (Static Recidiverisico); DOI: Dutch Offender’s Index. The highest scores are highlighted in bold.
Evaluation all the other recidivism.
AUC: area under curve; ACC: accuracy; Thailand: Thailand: data by central correctional institution for drug addicts and central women correctional institution in Thailand; FDJJ: Florida Department of Juvenile Justice; RITA+: Finnish Risk and Needs Assessment Form + static predictors; YLS/CMI: Youth Level of Service/Case Management Inventory 2.0; SAVRY+: Structured Assessment of Violence Risk in Youth + static features. The highest scores are highlighted in bold.
Evaluation general recidivism.
AUC: area under curve; ACC: accuracy; MnSTARR+: Minnesota Screening Tool Assessing Recidivism Risk + Minnesota Sex Offender Screening Tool-3; LS/CMI: Level of Service/Case Management Inventory; NCRA+: NeuroCognitive Risk Assessment + demographic feature set; HCR-20+: Historical, Clinical and Risk Management–20 + clinical and non-clinical risk assessment factors; StatRec: static recidivism risk (Static Recidiverisico); DOI: Dutch Offender’s Index. The highest scores are highlighted in bold.
In Table 3 , Table 4 and Table 5 , the comparison between the StatRec and DOI datasets shows that they produce the same results except for general recidivism with a difference of 0.05 [ 31 , 32 ]. However, this result was not surprising since both studies used data from the Dutch Offender’s Index.
In contrast, for violent recidivism ( Table 5 ), the multi-layer perceptron (MLP) trained with the RisCanvi dataset performed better than the other techniques [ 26 ].
Table 6 shows the results of studies that include all other recidivism. These studies are difficult to compare because each one has a different sample type, such as juvenile offenders or males or aims to predict a specific recidivism (e.g., recidivism in drug distribution). However, we observed that the results obtained reflect an overall effectiveness of prediction models. The Thailand dataset has a particular relevance with an ACC of 0.90 obtained with logistic regression [ 21 ]. In term of AUC, a model obtained a significant score of 0.78 using the RITA+ dataset trained with random forest [ 27 ].
3.6. Factors Involved in Predicting Recidivism
In some of the studies considered in this review, the authors described the variables that contributed most to the final evaluation. Some considerations about these results are discussed in Section 4 . Below we report the variables most implicated in the results of each model (when provided by the authors).
Four top factors are identified: royal pardons or suspension, first offending age, encouragement of family members and frequency of substance abuse [ 21 ]. Among the items in the LS/CMI they identify: Items A18 (charge laid, probation breached, parole suspended during prior community supervision), A 14 (three or more present offenses), A 423 (could make a better use of time) and A 735 (current drug problem) [ 23 ]. A total of 13 tests are selected within NCRA, in particular: balloon analog risk task (time collected), point-subtraction aggression paradigm (grow, punish ratio), reading the mind through the eyes (correct, time median), emotional stroop (test time, black time, icon color time, Pos Neg time) and Tower of London (aborted, dup moves, illegal moves first move frac) [ 24 ]. The stronger predictors in this model (sexual recidivism) are prior felony sex offense referrals, number of prior misdemeanor sexual misconduct referrals and number of prior felony offenses [ 26 ]. Among the dynamic factors extracted from RITA’s items, the most important seem to be problem’s managing one’s economy for general recidivism and aggressiveness for violent recidivism [ 27 ]. The most significant variables affecting the model accuracy is the total YLS score followed by difficulty in controlling behavior, age at first arrest, history of running away and family circumstances [ 29 ]. The most important features for the logistic regression model include almost all static features (sex, ethnicity, age at main crime, crime in 10 years, etc.,) and only one SAVRY feature (evaluation of the expert). For the MLP model, all static features are more important than SAVRY features [ 30 ]. For general recidivism (logistic regression model), the most powerful predictors are age, conviction density, specific offense types (property offence and public order offence), the number of previous offences and home country. Considering violent recidivism (logistic regression model), the largest effects can be seen in the number of previous convictions, the most severe offence type (property crime with violence), offence type present in the index case (property crime without violence, public order, other offence) and country of origin. Lastly, for sexual recidivism (linear discriminant analysis), three main coefficients are identified: previous sexual offences and country of origin have the greater positive affect on the probability of sexual recidivism, while the number of previous public prosecutor’s disposals has the largest negative effect [ 31 ].
3.7. Reporting Biases
In this systematic review, the datasets and ML models included have been compared simultaneously. However, for a critical evaluation of the results of ML models, it is also necessary to focus on the type of dataset used to train the model.
In each study, the method of data collection is different. In some papers, the data come from the countries’ institutions, while in others they come from the checklists of risk assessment tools or neurocognitive tests. Consequently, datasets have different characteristics such as sample size, mean age, type of crime and years of recidivism follow-up. These variables can significantly modify the assessment of the ML model [ 34 ].
Another relevant aspect to mention is that not all the papers declare the use of data pre-processing or are clearly explicit about the process used. As mentioned above, we applied the ROBIS assessment to highlight any possible systematic distortion. Table 7 and Figure 2 summarize the risk of bias. In detail, in Table 7 , for each study the relative risk of bias in each of the four domains is assigned on a three-level scale indicating “low” or “high” risk of bias or “unclear” risk when no information is available to judge a particular item. Figure 2 depicts the relative risk of bias (as in table N, from “high” to “low” and “unclear” risk) among all the included studies for each domain assessed as well as the “overall risk”. Of the considered studies, nine were evaluated as low risk overall, one as high risk, and two studies resulted in an unclear risk. The main concerns relate to the inhomogeneity of the samples and the poor description of data pre-processing and analysis which makes it harder to compare different ML techniques.

Graphical display for ROBIS results.
Tabular presentation for ROBIS results.
☺ = low risk; ☹ = high risk and ? = unclear risk.
4. Discussion
The current state of data science reveals that ML algorithms may be very efficient. Accordingly, the results of the papers considered in this review show that each ML model has a good performance. Taking into consideration the ACC, the mid-score is 0.81. In contrast, considering the AUC the mid-score is 0.74. Considering that the range of ACC and the AUC are from 0 (no predictability) to 1 (perfect predictability), both mid-scores show a good predictability of the models. Some studies use a risk assessment for a specific type of recidivism, crime or population, while others measure the risk of general recidivism. However, comparing the two types of studies, no significant differences emerge. The only difference observed in this review is an increase in ACC of 0.03 for the specific type of recidivism. Thus, there is no evidence that the use of a more specific risk assessment could significantly improve the ability to predict criminal recidivism.
This review compares different ML methods, models and datasets used to predict recidivism. Analysing the available models, the most common technique used in these papers to predict recidivism is logistic regression, while more complex algorithms are less common. In terms of performance, a simpler prediction model such as logistic regression and more complex ones such as the random forest show similar predictive validity and performance. What emerges from the results is that it does not seem essential to focus on model complexity to improve the predictability of criminal recidivism.
However, literature analysed in this systematic review pinpointed some limitations. First of all, the performance of each model does not depend only on the ML model or the dataset. The method of data collection and data pre-processing are also important. Both are aspects that can significantly affect model performance [ 34 , 35 ]. We observed that in the literature, it is uncommon to focus on data pre-processing techniques, which makes it difficult to compare different studies. In addition, all ML models used in the literature return a binary result, and for many of them, depending on the model and its description, it is difficult to understand which variables most influenced the final assessment. As a matter of fact, since the use of risk assessments has become more widespread and successful, this issue also emerged in recent studies in which the possibility of racial bias has been highlighted [ 36 ]. Age estimation-related biases, which in the forensic field are of significant relevance, should also be considered [ 37 , 38 , 39 ].
In Section 3.6 , we reported the variables that have the greatest impact on the assessment of the risk of recidivism for each model. In this regard, it is good to make some considerations. As specified by the authors themselves, the most important variables for the final evaluation should be assessed limited only to the ML model used and to the specific dataset of that ML model. Hence, the results may not generalize to more specific subsamples or different subpopulations [ 31 ]. Moreover, for the final evaluation, it is not always possible to consider the single variables separately since the ML algorithms take into account the way in which the individual variables are combined [ 23 ]. Lastly, the complexity of the models that use interactions and nonlinear effects makes it challenging to explain individual-level predictions, and the difficulty grows along with the complexity of the model [ 22 ].
Using the ML method for risk assessment in criminal justice to predict recidivism has increased in recent years [ 14 , 23 ]. However, it is still a controversial topic due to the large amount of research on algorithmic fairness [ 40 ]. The purpose of this review is to analyse the state of the art of the techniques applied to predict criminal recidivism. Clearly, we did not aim to show the perfect prediction of the ML method nor to claim that it is possible to rely solely on the ML model to predict recidivism. Conversely, we highlight the strengths and limitations of data science applied to the humanities.
First, we would like to point out that it would be important to pay more attention to the dataset and data processing. Taking a few steps back and focusing on these aspects could improve model performance and reduce possible bias [ 35 ].
Moreover, in order to facilitate comparison, it would be useful to learn to compare models by having the same evaluation metrics available. Considering metrics available in the analysed papers, we observed a good overall performance of the models. This allows us to emphasize the concrete support that these tools can bring to human judgment, which is also not free of bias [ 41 ]. The binary result is a limitation for this approach, as well as algorithmic unfairness [ 17 ].
The latest machine learning models are like ‘black boxes’ because they have such a complex design that users cannot understand how an AI system converts data into decisions [ 42 ]. The lack of accessibility of the models and algorithms used in judicial decisions could undermine the principles of transparency, impartiality and fairness and lead to the development of discrimination between individuals or groups of individuals [ 43 ]. It would be useful to develop transparent algorithms or use explainable AI. The explainable AI consists in AI systems that can explain their rationale to a human user and characterize their strengths and weaknesses [ 44 ]. With these techniques, we could know how much each variable affected the outcome, helping to form knowledgeable opinions usable from criminal justice professionals to motivate their decision [ 45 , 46 ]. In this regard, it would be useful to use a human-in-the-loop approach that leverages the strengths of collaboration between humans and machines to produce the best results, reinforcing the importance of the synergistic work [ 47 , 48 ].
5. Conclusions
The implementation of quantitative and qualitative methods to predict criminal recidivism could be a useful tool in the field of criminal justice [ 3 , 4 , 5 ] However, although research in this area is steadily increasing [ 7 ], its use in judicial practice is still limited [ 8 ] due to the controversial views [ 9 , 10 , 11 , 12 ]. This systematic review shows the state of the art regarding the application of ML techniques to the risk of reoffending and highlights key points useful for criminal justice professionals to exploit these new technologies. Each method applied achieves good performance, with an average score of 0.81 for ACC and 0.74 for AUC. However, the application of artificial intelligence in this field is still a controversial topic due to the significant critical issues [ 37 ]. To overcome these critical issues, it will be imperative to face and overcome a new challenge, that of making algorithms transparent and accessible so that the application of these new technologies contributes to decisions based on the principles of transparency, impartiality and fairness. In this regard, the integration of methods from the natural and social sciences according to a systemic orientation would allow the correlation between e-tech data and the human interpretation of the same [ 49 ], keeping the human operator at the head of the human–computer system in accordance with the integrated cognitive system [ 50 ].
The use of artificial intelligence in judicial proceedings and the resulting decision-making processes will be a field of wide reflection among scientists, jurists and bioethicists. This systematic review is a thorough synthesis of the best available evidence, but it is also a contribution in a field that presents many ethical, deontological and legal critical issues in the state of the art.
Funding Statement
This research received no external funding.
Author Contributions
Conceptualization, G.V.T. and F.P.; methodology, S.B.; software, S.B.; validation, G.V.T. and F.D.M.; formal analysis, F.P., S.B. and M.B.; investigation, F.P.; resources, S.B.; data curation, F.P.; writing—original draft preparation, G.V.T. and F.P.; writing—review and editing, F.D.M.; visualization, M.B.; supervision, G.V.T. and F.D.M.; project administration, G.V.T. All authors have read and agreed to the published version of the manuscript.
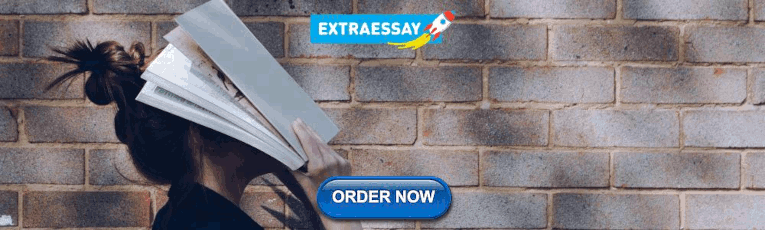
Institutional Review Board Statement
Informed consent statement, data availability statement, conflicts of interest.
The authors declare no conflict of interest.
Publisher’s Note: MDPI stays neutral with regard to jurisdictional claims in published maps and institutional affiliations.
Survey on Crime Analysis, Forecasting, and Prediction Using Machine Learning
- Conference paper
- First Online: 23 July 2023
- Cite this conference paper
- Iqbal Singh Saini 13 &
- Navneet Kaur 13
Part of the book series: Lecture Notes in Networks and Systems ((LNNS,volume 703))
Included in the following conference series:
- International Conference On Innovative Computing And Communication
232 Accesses
The duty of ensuring people’s safety and preserving peace is of the utmost importance, and crime is the biggest barrier. An excellent technology that can significantly contribute to reducing and eradicating crime is artificial intelligence and machine learning. This study offers a review of the past five years' worth of research on crime. The survey mainly focuses on the algorithms utilized by the researchers in their work for crime prediction and the performance metrics used to validate the outcomes of the predictive model. Additionally, the goal, outcomes, strengths, shortcomings, and potential application of the research are all described. This paper will guide the young researchers to proceed with the most appropriate algorithms while performing the research in crime analysis field.
This is a preview of subscription content, log in via an institution to check access.
Access this chapter
- Available as PDF
- Read on any device
- Instant download
- Own it forever
- Available as EPUB and PDF
- Compact, lightweight edition
- Dispatched in 3 to 5 business days
- Free shipping worldwide - see info
Tax calculation will be finalised at checkout
Purchases are for personal use only
Institutional subscriptions
Feng M, Zheng J, Han Y, Ren J, Liu Q (2018) Big data analytics and mining for crime data analysis, visualization and prediction. In: International conference on brain inspired cognitive systems. Springer, Cham, pp 605–614
Google Scholar
Kitchen P, Williams A (2010) Quality of life and perceptions of crime in Saskatoon, Canada. Soc Indicators Res 95(1):33–61, Springer
Hanson RF, Sawyer GK, Begle AM, Hubel GS (2010) The impact of crime victimization on quality of life. J Traumatic Stress: Official Publ Int Soc Traumatic Stress Stud 23(2):189–197
Aos S, Phipps P, Barnoski R, Lieb R (2001) The comparative costs and benefits of programs to reduce crime. Version 4.0
Kiran P, Mounika B, Naveen P, Tejaswini N, Karthik B (2022) Crime data analysis using machine learning. South Asian J Eng Technol 12(3):61–68
Article Google Scholar
Kim S, Joshi P, Kalsi PS, Taheri P (2018) Crime analysis through machine learning. In: 2018 IEEE 9th annual information technology, electronics and mobile communication conference (IEMCON), IEEE, pp 415–420
Bandekar SR, Vijayalakshmi C (2020) Design and analysis of machine learning algorithms for the reduction of crime rates in India. Proc Comput Sci 172:122–127
Ingilevich V, Ivanov S (2018) Crime rate prediction in the urban environment using social factors. Proc Comput Sci 136:472–478
Das P, Das AK (2019) Application of classification techniques for prediction and analysis of crime in India. In: Computational intelligence in data mining. Springer, Singapore, pp 191–201
ToppiReddy HK, Reddy BS, Mahajan G (2018) Crime prediction and monitoring framework based on spatial analysis. Proc Comput Sci 132:696–705
Hajela G, Chawla M, Rasool A (2020) A clustering based hotspot identification approach for crime prediction. Proc Comput Sci 167:1462–1470
Wang J, Jun H, Shen S, Zhuang J, Ni S (2020) Crime risk analysis through big data algorithm with urban metrics. Physica A 545:123627
Letourneau S, Ell N, Cheung P, McCaskill J, El-Hajj M (2018) The effects of neighbourhood characteristics on crime incidence. In: 2018 IEEE 8th annual computing and communication workshop and conference (CCWC). IEEE, pp 720–726
Lal S, Tiwari L, Ranjan R, Verma A, Sardana N, Mourya R (2020) Analysis and classification of crime tweets. Procedia computer science 167:1911–1919
Dakalbab F, Talib MA, Waraga OA, Nassif AB, Abbas S, Nasir Q (2022) Artificial intelligence and crime prediction: a systematic literature review. Soc Sci Human Open 6(1):100342
David H, Suruliandi A (2017) Survey on crime analysis and prediction using data mining techniques. ICTACT J Soft Comput 7(3)
Nath SV (2006) Crime pattern detection using data mining. In: 2006 IEEE/WIC/ACM international conference on web intelligence and intelligent agent technology workshops. IEEE, 41–44
Rish I (2001) An empirical study of the Naive Bayes classifier. In: IJCAI 2001 workshop on empirical methods in artificial intelligence, vol 3(22), pp 41–46
Zhang S, Li X, Zong M, Zhu X, Cheng D (2017) Learning k for knn classification. ACM Trans Intell Syst Technol (TIST) 8(3):1–19
Xu M, Watanachaturaporn P, Varshney PK, Arora MK (2005) Decision tree regression for soft classification of remote sensing data. Remote Sens Environ 97(3):322–336
Segal MR (2004) Machine learning benchmarks and random forest regression
Montgomery DC, Peck EA, Vining GG (2021) Introduction to linear regression analysis. Wiley
Kleinbaum DG, Dietz K, Gail M, Klein M, Klein M (2002) Logistic regression. New York, Springer
Chen T, He T, Benesty M, Khotilovich V, Tang Y, Cho H, Chen K (2015) Xgboost: extreme gradient boosting. R Package Version 0.4–2 1(4):1–4.
Likas A, Vlassis N, Verbeek JJ (2003) The global k-means clustering algorithm. Pattern Recogn 36(2):451–461
Ranstam J, Cook JA (2018) LASSO regression. J Br Surg 105(10):1348–1348
Geurts P, Ernst D, Wehenkel L (2006) Extremely randomized trees. Mach Learn 63(1):3–42
Article MATH Google Scholar
Schapire RE (2013) Explaining adaboost. In: Empirical inference. Springer, Berlin, Heidelberg, 37–52
Naser MZ, Alavi A (2020) Insights into performance fitness and error metrics for machine learning. arXiv preprint arXiv:2006.00887
Download references
Author information
Authors and affiliations.
Department of Computer Science and Engineering, Chandigarh University, Mohali, India
Iqbal Singh Saini & Navneet Kaur
You can also search for this author in PubMed Google Scholar
Corresponding author
Correspondence to Iqbal Singh Saini .
Editor information
Editors and affiliations.
IT Department, Faculty of Computers and Information, Cairo University, Giza, Egypt
Aboul Ella Hassanien
Tijuana Institute of Technology, Tijuana, Mexico
Oscar Castillo
Department of Computer Science, Shaheed Sukhdev College of Business Studies, University of Delhi, New Delhi, India
Sameer Anand
Ajay Jaiswal
Rights and permissions
Reprints and permissions
Copyright information
© 2023 The Author(s), under exclusive license to Springer Nature Singapore Pte Ltd.
About this paper
Cite this paper.
Saini, I.S., Kaur, N. (2023). Survey on Crime Analysis, Forecasting, and Prediction Using Machine Learning. In: Hassanien, A.E., Castillo, O., Anand, S., Jaiswal, A. (eds) International Conference on Innovative Computing and Communications. ICICC 2023. Lecture Notes in Networks and Systems, vol 703. Springer, Singapore. https://doi.org/10.1007/978-981-99-3315-0_22
Download citation
DOI : https://doi.org/10.1007/978-981-99-3315-0_22
Published : 23 July 2023
Publisher Name : Springer, Singapore
Print ISBN : 978-981-99-3314-3
Online ISBN : 978-981-99-3315-0
eBook Packages : Engineering Engineering (R0)
Share this paper
Anyone you share the following link with will be able to read this content:
Sorry, a shareable link is not currently available for this article.
Provided by the Springer Nature SharedIt content-sharing initiative
- Publish with us
Policies and ethics
- Find a journal
- Track your research
Academia.edu no longer supports Internet Explorer.
To browse Academia.edu and the wider internet faster and more securely, please take a few seconds to upgrade your browser .
Enter the email address you signed up with and we'll email you a reset link.
- We're Hiring!
- Help Center
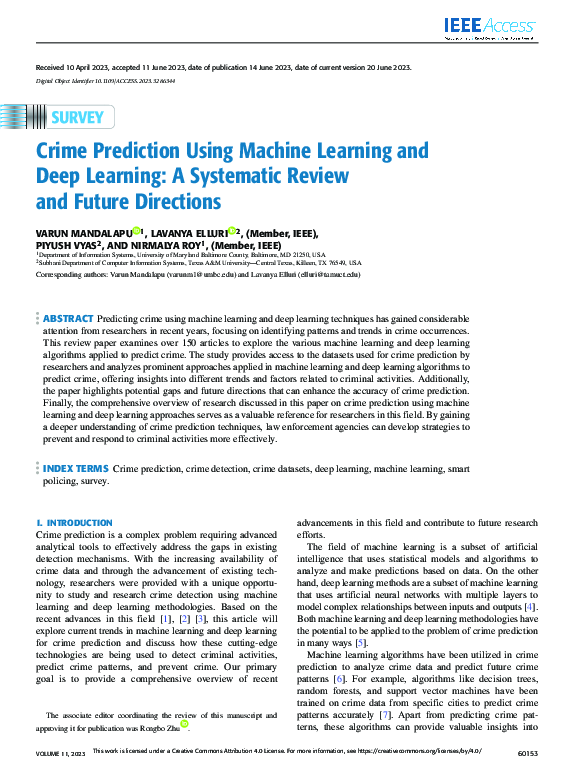
Crime Prediction Using Machine Learning and Deep Learning: A Systematic Review and Future Directions

IEEE Access
Related Papers
Forecasting
Theodoros Semertzidis
In this paper, a detailed study on crime classification and prediction using deep learning architectures is presented. We examine the effectiveness of deep learning algorithms in this domain and provide recommendations for designing and training deep learning systems for predicting crime areas, using open data from police reports. Having time-series of crime types per location as training data, a comparative study of 10 state-of-the-art methods against 3 different deep learning configurations is conducted. In our experiments with 5 publicly available datasets, we demonstrate that the deep learning-based methods consistently outperform the existing best-performing methods. Moreover, we evaluate the effectiveness of different parameters in the deep learning architectures and give insights for configuring them to achieve improved performance in crime classification and finally crime prediction.
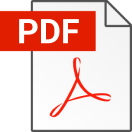
INTERNATIONAL JOURNAL OF ADVANCE RESEARCH, IDEAS AND INNOVATIONS IN TECHNOLOGY
Ijariit Journal , Sheikh Mohammad Younis
Due to the escalation in the rate of crimes, there is a requirement of the system that will detect and predict crimes at the dynamic time. The objective of this survey is to learn Data Mining techniques that will go on to help in detecting and predicting crimes using association rule mining, decision trees and naive Bayes, k-means clustering and Machine learning techniques such as deep neural network and artificial neural network. Noticeable findings from this survey were that when the dataset instances have a large number of missing values pre-processing becomes an important task and crime does not occur uniformly across urban landscapes but concentrates in specific areas. Hence, predicting crime hotspots is a very crucial task and also applying post-processing will help in decreasing the rate of crimes.
Bulletin of Electrical Engineering and Informatics
beei iaes , Mostafa Azizi
In recent years, the use of artificial intelligence (AI) for image and videobased crime detection has gained significant attention from law enforcement agencies and security experts. Indeed, deep learning (DL) models can learn complex patterns from data and help law enforcement agencies save time and resources by automatically identifying and tracking potential criminals. This contributes to make deep investigations and better steer their targets' searches. Among others, handheld firearms and bladed weapons are the most frequent objects encountered at crime scenes. In this paper, we propose a DL-based surveillance system that can detect the presence of tracked objects, such as handheld firearms and bladed weapons, as well as may proceed to alert authorities regarding eventual threats before an incident occurs. After making a comparison of different DL-based object detection techniques, such as you only look once (YOLO), single shot multibox detector (SSD), or faster region-based convolutional neural networks (R-CNN), YOLO achieves the optimal balance of mean average precision (mAP) and inference speed for real-time prediction. Thus, we retain YOLOv5 for the implementation of our solution.
Ceza Hukuku ve Kriminoloji Dergisi-Journal of Penal Law and Criminology
Emre Cihan Ateş
Along with the rapid change of information technologies and the widespread use of the internet over time, data stacks with ample diversity and quite large volumes has emerged. The use of data mining is increasing day by day due to the huge part it plays in the acquisition of information by making necessary analyses especially within a large amount of data. Obtaining accurate information is a key factor affecting decision-making processes. Crime data is included among the application areas of data mining, being one of the data stacks which is rapidly growing with each passing day. Crime events constitute unwanted behaviour in every society. For this reason, it is important to extract meaningful information from crime data. This article aims to provide an overview of the use of data mining and machine learning in crime data and to give a new perspective on the decision-making processes by presenting examples of the use of data mining for a crime. For this purpose, some examples of data mining and machine learning in crime and security areas are presented by giving a conceptual framework in the subject of big data, data mining, machine learning, and deep learning along with task types, processes, and methods.
Paolo Contardo
Deep learning is rapidly growing, obtaining groundbreaking results in speech recognition, image processing, pattern recognition, and many other application domains. Following the success of deep learning, many automatic data analysis techniques are becoming common also in law enforcement agencies. To this end, we present a survey about the potential impact of deep learning on three application domains, peculiar to law enforcement agencies. Specifically, we analyze the findings about deep learning for Face Recognition, Fingerprint Recognition, and Violence Detection. In fact, combining 1) data from the routine procedure of collecting a subject frontal and profile pictures and her/his fingerprints, 2) the pervasiveness of surveillance cameras, and 3) the capability of learning from a huge amount of data, might support the next steps in crime prevention.
Eur. Chem. Bull. 2023
Taranpreet Ruprah , Hemang Shrivastav
Crime Scene detection predict the chances of happening the crime without any involvement of human intervention is always the crucial task in the field of artificial intelligence. In this paper crime forecasting based on the weapon detection and tracking with the person can help investigator to understand the sequence of action took place during the crime. The images are manually annotated, which is a process where an expert goes through each images and mark the position and class of object within the image. Object detection and classification algorithms provides the necessary ground to verify data for the algorithms. The models like SSD, YOLO and Faster RCNN are used for weapons detection and mediapipe library is used to generate the human body datapoints and calculate the relation between weapons with the human. The maximum accuracy of Faster RCNN with mediapipe library is 93%.
Crime prediction with machine learning
Duncan Mulongo
This study aggregates, summarizes, and evaluates the spatial-temporal crime prediction and detection techniques through conducting a Systematic Literature Review (SLR). A systematic Literature review identifies, selects and critically appraises research in order to provide a solution to a well formulated problem. (A, 2016)) . [The systematic review follows a clearly defined protocol or a plan where criteria is clearly stated before the review is conducted]. It was possible to find a comprehensive study on crime hotspot detection and prediction while conducting the SLR. However, there was an attempt to critically analyze the existing literature reviews while also studying the challenges facing the current crime prediction and detection systems. A thorough study was done in determining the type of operations and undertakings carried out by major security bodies in Kenya which included; The Kenya police Service (KPS), Directorate of Criminal Investigation (DCI), National Intelligence service (NIS) and National Crime Research Centre (NCRC). A keen evaluation on the techniques used by the NCRC was performed to obtain vital information on crime and how the body manipulate data to gain useful insights on crime. It was found out clearly that NCRC perform crime research and study across the country after every two years. The claim is that, one year is used for collection of data from across the country based on public opinion on crime while the other year is used for analysis of crime data. With this type of technique the current data being used may not be up to date or even sufficient and accurate enough for accurate prediction of crime. Furthermore, the whole process of crime data analysis is not a real time process and thus it is rendered infective. A study on other different sources pertaining the studies on crime hotspot detection and prediction was also performed. These included the use of machine learning and data analysis; more specifically on the use of Classification & Clustering Algorithms like the KK-means, Decision trees, Time series Analysis and the Bayes theorem in predicting crime incidences. Hereby, it is presented in great details how the fore mentioned techniques can be used in predicting crime proactively.
Machine Learning and Applications: An International Journal
Ana Laura Lira Cortes
This work presents research based on evidence with neural networks for the development of predictive crime models, finding the data sets used are focused on historical crime data, crime classification, types of theft at different scales of space and time, counting crime and conflict points in urban areas. Among some results, 81% precision is observed in the prediction of the Neural Network algorithm and ranges in the prediction of crime occurrence at a space-time point between 75% and 90% using LSTM (Long-ShortSpace-Time). It is also observed in this review, that in the field of justice, systems based on intelligent technologies have been incorporated, to carry out activities such as legal advice, prediction and decisionmaking, national and international cooperation in the fight against crime, police and intelligence services, control systems with facial recognition, search and processing of legal information, predictive surveillance, the definition of criminal models under the crit...
IJCSMC Journal
It is critical to recognise crime patterns in order to be better prepared to respond to criminal behavior. We study crime data of states in india that was scrapped publicly available websites kaggle for our project. The goal is to estimate which type of crime is most likely to occur at a given time and location. The use of AI and machine learning to identify crime using sound or video is now in use, has been demonstrated to function, and is likely to grow. The use of AI/ML to forecast crimes or a person’s chance of committing a crime has potential, but it is still a work in progress. The most difficult task will most likely be “proving” to legislators that it works. It’s tough to establish the negative when a system is meant to prevent something from happening. A positive feedback loop would certainly benefit companies who are directly involved in providing governments with AI capabilities to monitor areas or predict crime. Improvements in crime prevention technology will almost certainly lead to an increase in overall spending on this technology. We also try to make our categorization work more relevant by grouping many classes together into larger groups. Finally, we present and discuss our findings using several classifiers, as well as future research directions.
IRJET Journal
RELATED PAPERS
ANÁLISIS TÉCNICO: SISTEMAS AUTOMÁTICOS DE TRADING
Revista Cubana de Alimentación y Nutrición
Jesus Barreto Penie
Noida City Center Call Girls
Theoretical and Applied Climatology
Toxicological Sciences
Kenneth Koeplinger
IMISCOE research series
Parvati Raghuram
2006 IEEE International Conference on Communications
Ting-Kai Huang
International Journal of Distributed Sensor Networks
zahoor alam khan
Ciencia Latina Revista Científica Multidisciplinar
JOSE HUAMANI ARONE
UCLA文凭证书 加州大学洛杉矶分校文凭证书 klhjkgh
Revista Panamericana de Salud Pública
Ignacio Cabrera-Samith
Xavier Ribes
Renewable and Sustainable Energy Reviews
Thomas Trabold
Annals of the New York Academy of Sciences
Philip Avner
Marges, Els: revista de llengua i literatura
Carles Batlle i Jordà
Journal für Hypertonie - Austrian Journal of Hypertension
Reinhold Glehr
Investigaciones de Historia Económica
mukulumpangi mpagi
The American Journal of Clinical Nutrition
JESSICA KATHERINE CAMARGO ALVAREZ
Journal of Bodywork and Movement Therapies
Journal of Applied Business Research (JABR)
Xavier Garza
- We're Hiring!
- Help Center
- Find new research papers in:
- Health Sciences
- Earth Sciences
- Cognitive Science
- Mathematics
- Computer Science
- Academia ©2024
Artificial intelligence & crime prediction: A systematic literature review
- University of Sharjah
Research output : Contribution to journal › Review article › peer-review
The security of a community is its topmost priority; hence, governments must take proper actions to reduce the crime rate. Consequently, the application of artificial intelligence (AI) in crime prediction is a significant and well-researched area. This study investigates AI strategies in crime prediction. We conduct a systematic literature review (SLR). Our review evaluates the models from numerous points of view, including the crime analysis type, crimes studied, prediction technique, performance metrics and evaluations, strengths and weaknesses of the proposed method, and limitations and future directions. We review 120 research papers published between 2008 and 2021 that cover AI approaches for crime prediction. We provide 34 crime categories researched by researchers and 23 distinct crime analysis methodologies after analyzing the selected research articles. On the other hand, we identify 64 different machine learning (ML) techniques for crime prediction. In addition, we observe that the most applied approach in crime prediction is the supervised learning approach. Furthermore, we discuss the evaluation and performance metrics, as well as the tools utilized in building the models and their strengths and weaknesses. Crime prediction AI techniques are a promising field of study, and there are several ML models that researchers have applied. Consequently, based upon this review, we provide advice and guidance for researchers working in this area of study.
Access to Document
- 10.1016/j.ssaho.2022.100342
Other files and links
- Link to publication in Scopus
Fingerprint
- Artificial Intelligence Computer Science 100%
- Systematic Review Social Sciences 100%
- Machine Learning Economics, Econometrics and Finance 100%
- Performance Metric Computer Science 40%
- Crime Analysis Social Sciences 40%
- Performance Evaluation Computer Science 20%
- Research Paper Computer Science 20%
- Machine Learning Technique Computer Science 20%
T1 - Artificial intelligence & crime prediction
T2 - A systematic literature review
AU - Dakalbab, Fatima
AU - Abu Talib, Manar
AU - Abu Waraga, Omnia
AU - Bou Nassif, Ali
AU - Abbas, Sohail
AU - Nasir, Qassim
N1 - Publisher Copyright: © 2022 The Authors
PY - 2022/1
Y1 - 2022/1
N2 - The security of a community is its topmost priority; hence, governments must take proper actions to reduce the crime rate. Consequently, the application of artificial intelligence (AI) in crime prediction is a significant and well-researched area. This study investigates AI strategies in crime prediction. We conduct a systematic literature review (SLR). Our review evaluates the models from numerous points of view, including the crime analysis type, crimes studied, prediction technique, performance metrics and evaluations, strengths and weaknesses of the proposed method, and limitations and future directions. We review 120 research papers published between 2008 and 2021 that cover AI approaches for crime prediction. We provide 34 crime categories researched by researchers and 23 distinct crime analysis methodologies after analyzing the selected research articles. On the other hand, we identify 64 different machine learning (ML) techniques for crime prediction. In addition, we observe that the most applied approach in crime prediction is the supervised learning approach. Furthermore, we discuss the evaluation and performance metrics, as well as the tools utilized in building the models and their strengths and weaknesses. Crime prediction AI techniques are a promising field of study, and there are several ML models that researchers have applied. Consequently, based upon this review, we provide advice and guidance for researchers working in this area of study.
AB - The security of a community is its topmost priority; hence, governments must take proper actions to reduce the crime rate. Consequently, the application of artificial intelligence (AI) in crime prediction is a significant and well-researched area. This study investigates AI strategies in crime prediction. We conduct a systematic literature review (SLR). Our review evaluates the models from numerous points of view, including the crime analysis type, crimes studied, prediction technique, performance metrics and evaluations, strengths and weaknesses of the proposed method, and limitations and future directions. We review 120 research papers published between 2008 and 2021 that cover AI approaches for crime prediction. We provide 34 crime categories researched by researchers and 23 distinct crime analysis methodologies after analyzing the selected research articles. On the other hand, we identify 64 different machine learning (ML) techniques for crime prediction. In addition, we observe that the most applied approach in crime prediction is the supervised learning approach. Furthermore, we discuss the evaluation and performance metrics, as well as the tools utilized in building the models and their strengths and weaknesses. Crime prediction AI techniques are a promising field of study, and there are several ML models that researchers have applied. Consequently, based upon this review, we provide advice and guidance for researchers working in this area of study.
UR - http://www.scopus.com/inward/record.url?scp=85147277863&partnerID=8YFLogxK
U2 - 10.1016/j.ssaho.2022.100342
DO - 10.1016/j.ssaho.2022.100342
M3 - Review article
AN - SCOPUS:85147277863
SN - 2590-2911
JO - Social Sciences and Humanities Open
JF - Social Sciences and Humanities Open
M1 - 100342
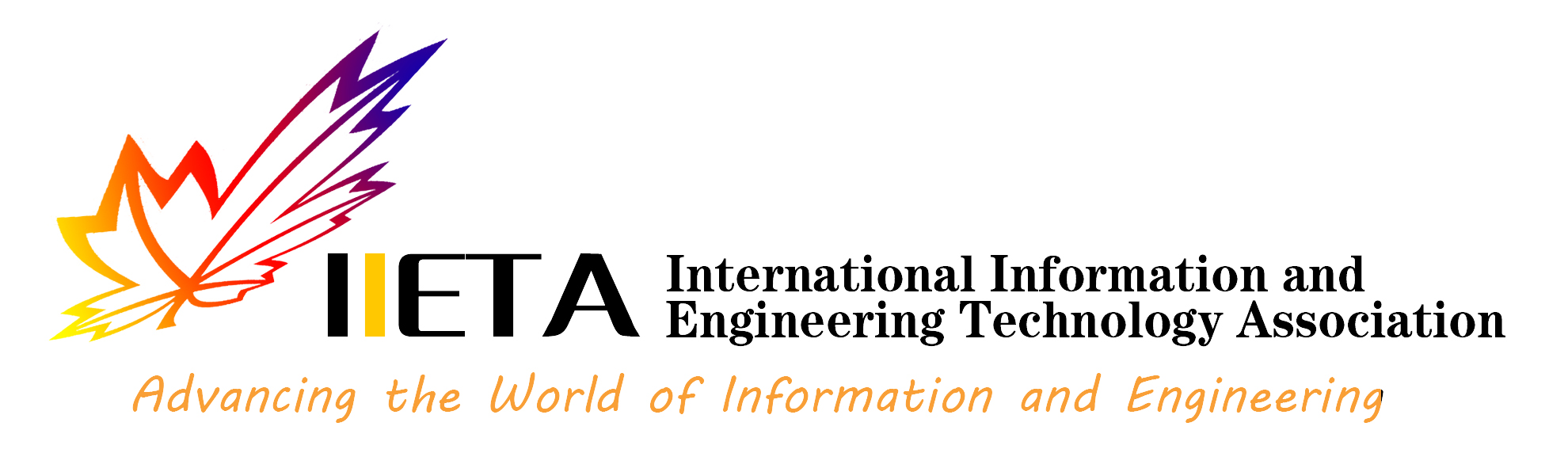
Search form
Systematic literature review of crime prediction and data mining.
Adesola Falade * | Ambrose Azeta | Aderonke Oni | Isaac Odun-ayo
OPEN ACCESS
Crime is a social menace that impacts negatively on social economic development of a nation. Crime has been in existence from time immemorial and violent crime is the main enemy of the society. One of the primary responsibilities of any government is security of life and properties which translates to reduction of crime rate and provisioning of adequate security to its citizenry. To this end, government must wake up to its responsibilities by reducing crime rate and provide adequate security to its citizenry through effective, efficient and proactive policing. Any research in this direction that can help in analyzing and predicting the future occurrence of violent crime by using crime dataset is laudable. Predicting future occurrence of crime from crime dataset is well reported in literature, therefore it has become imperative to come up with an overview of the present state of the art on crime prediction and control.
The systematic review present in this study focuses on crime prediction and data mining as well as the techniques employed in the past studies. The existing work is classified and grouped into different categories and are presented by using visualization approach. It is found that more studies adopted supervised learning approaches to crime prediction and control compared to other methods. The challenges encountered were also reported. Crime prediction has become hot research area in recent time because of its intending benefits to socio-economic development of a nation.
FIRs-first information report, CCTV-closed circuit television, IB-Intelligence Bureau, NCB-Narcotics Control Bureau, SVM-support vector machine, DNN-deep neural network, ML-machine learning, NoSQL-no structured query language
Crime can be described as a cankerworm that negatively impact on social and economic development of any nation. In an area where crime is endemic, no development can take place [1]. Individual and nation are retrogressively affected by this monster called crime. However, it is the responsibility of any government to provide a secured environment for its citizenry. Several researches have gone into crime prediction, prevention and control in the past, all in an effort to facilitate the implementation of an efficient and effective predictive policing. Police and other law enforcement agency are the custodian of past crime records and they maintained the crime database [2-4]. Data obtained from this source should be analyzed to gain useful insights and knowledge to assist in decision making process. Numerous studies in the past have reported a number of techniques for analyzing crime data among which are statistical and machine learning techniques [3]. Therefore crime data analytics can provide information about crime statistics and report for a region or country [4]. Thus this will be useful to law enforcement agencies for aiding in proactive decision making in order to provide adequate security and safety for their citizenry [5]. Crime prediction and data mining information may be of different types and can be seen as categorized in Figure 1.
The main motivation of this study is to explore the various crime prediction and data mining techniques and challenges reported in literature as well as to know which state of the arts are relevant. The analysis in the study will offer knowledge to other researchers in knowing which categories of crime prediction and data mining measures and techniques as well as challenges that have been covered in the previous research studies and also help to identify gaps. The objectives of the study are to systematically review crime prediction and data mining approaches, issues and challenges faced in the existing studies. This review will help researchers gain state of the arts methods of crime prediction and data mining and more so help highlighting research gaps.
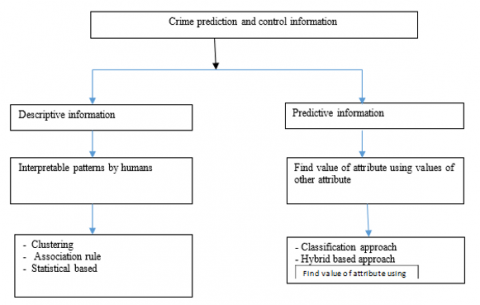
Figure 1. Crime prediction and data mining information categories
The rest of the paper is organized as follows: Research methodology used for the study is described in Section two, while classification of crime prediction and crime data analyses is presented in Section three using the following criteria: (1) Techniques used. (2) Technologies used and (3) various challenges addressed. Sources of crime dataset and types of crime is discussed in section four. Result of the review is discussed in section five, while conclusion and future directions is presented in section six.
The methodology employed in this study is composed of three steps. The first step involves getting related research work in crime prediction and data mining. The second step involves establishing a classification scheme illustrated in Section three. Presentation of the summary of findings and report of detailed literature review is the third step. The following research questions will be investigated:
RQ1: What are the different techniques used in crime prediction and crime data mining? To provide answer to this question, bar chart in section three explain and illustrate frequency of publications corresponding to different techniques in this area.
RQ2: What are the various techniques and measures used in crime prediction and data mining? To be able to answer this question, Table four in Section three described the techniques used in crime prediction and data mining.
RQ3: What are the various challenges involved in crime prediction and data mining. In providing answer to this question, pie chart in section three describes the various challenges and issues faced.
3.1 Screening & search strategy
In choosing relevant articles for this study, five major databases were selected. For this purpose, these major databases of electronic journals were searched. The following are the digital libraries considered:
•IEEE Xplore (http://ieeexplore.ieee.org),
•ACM Digital Library (www.acm.org/dl),
•Science Direct (http://www.sciencedirect.com),
•Springer (http://www.springerlink.com),
•Wiley Inter science (http://www.interscience.wiley.org).
Study Selection: Journal published research papers, conference proceedings and workshops which are thought to be worthy of consideration are used for the study. Keyword based search is used to pick the most relevant work. The keywords used are: violent crime prediction and data mining. Those that are not directly related to crime prediction and data mining were discarded. Omission of any research paper was based on the following criteria:
• Research paper that have not been published, non-English related papers, text-books, Master and Doctoral dissertations, and papers that are not peer-reviewed.
• Crime prediction and data mining researches published between 2004 and 2018 which are current and recent were considered. The search strategies and the number of outcomes obtained is presented in Table 1. Some of the studies which are not relevant are excluded from the returned studies. The abstract of articles returned during search which could not be estimated based on titles were considered. After reading such papers, if the abstract is not evident, the paper is considered irrelevant and then excluded. it.
Table 1. Search selection criteria used
3.2 Classification scheme establishment
The chosen selection process used for this study resulted in 225 papers altogether, eliminating papers repeated from five different digital libraries on 30th November 2018, 12.30 PM. The papers were carefully classified and accessed and were further classified in accordance with the criteria formulated in section 2 of this paper.
3.3 Papers distribution and summary of researches in crime prediction
The results obtained after classification proffer important guidelines for future research direction on crime prediction and data mining. The number of related literature to crime prediction and data mining has increased enormously. Papers from 2004 to 2018 were reviewed. These papers and their distribution over the years are presented in Table 2. Also presented in Table 6 is the summary of the researches in crime prediction and data mining according to the contribution of the past studies.
Table 2. Distribution of papers over the years
Different The following criteria are used to classify the research papers:
(1) Techniques used, (2) Technologies used and (3) Gaps and issues addressed.
4.1 Classifications based on the techniques used
The information on Table 3 shows the crime prediction’s various techniques applied on the basis of papers reviewed. The various corresponding techniques used along with frequency of publications in crime prediction and data mining are illustrated using a bar chart in Figure 2.
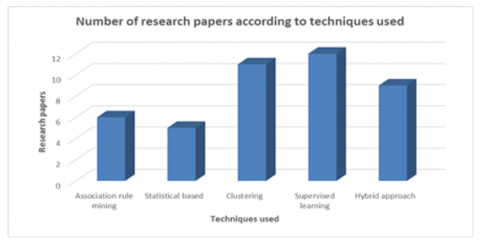
Figure 2. Number of research papers according to techniques used
Table 3. Various crime prediction and mining techniques used
4.2 Classifications based on the challenges addressed
The gaps found and addressed in the papers reviewed were applied as the third classification criteria. Difficulty in data collection, data preprocessing, data storage, performance and integration are mostly discussed [6-8]. Other issues addressed involved dealing with data that does not have specified structure, selection of appropriate methods, selection of appropriate technologies and collection of data from reliable sources [9-11]. The various gaps addressed by various research papers are presented using a pie-chart in Figure 3.
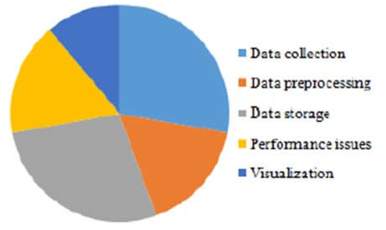
Figure 3. Challenges addressed by the research papers
4.2.1 Data collection and integration
Input process is critical in the crime prediction and control process [9, 11]. Data pertaining to crime is collected from various heterogeneous sources such as criminal records, social media, news, and police reports (FIRs) etc. Collecting such a huge volume of data is highly challenging, according to [12].
4.2.2 Data preprocessing
The data about crime collected are usually in various formats such as text, images, graph, audio, relational data, unstructured datand semi-structured data [13]. According to [14], the process of transforming these various data in different formats to the understandable and desired format is a major challenge in crime prediction.
4.2.3 Data storage issues
Data associated with crime is characterized by huge amount of data. Crime prediction requires a large amount of storage and this necessitate a new data management technique located on distributed processing systems [15]. New database technology NoSQL such as Apache Hadoop is becoming a new standard for crime prediction and data analytics [16].
4.2.4 Performance related issues
Issues bordering on performance are usually concerned with processing time, reliability and precision [17-19]. Technologies and techniques used affect the processing time. In resolving this problem, there is need to evolve algorithms and technologies to increase the precision and accuracy.
4.2.5 Issues on visualization
Visualizing the results from crime prediction and crime data analysis is a major challenge [11]. Knowledge representation using bar-charts, graphs, and pie-chart is often referred to as visualization [20]. It is in most cases difficult to lay hands on a user-friendly visualization for the massive data [20].
Discovering a successful investigation and the right insights, it becomes very important to recognize the available data sources of crime and the various types of crime [21].
5.1 Crime data sources and analysis
Report from the Police and other law enforcement agencies were discovered. Crime report from Police FIRs (First Information Report) archives historical crime data that contain important information about crime, complainants and the suspects.
First Information Report (FIRs) made by the police from the victim are normally written by police staff on paper and usually in an unstructured format. This is one of the reliable ways of crime data collection [22].
5.2 Investigation files
The police use previous investigation files of the suspect to identify a previously convicted suspect, these files are usually in text, photo, credit card statements, CCTV video files, phone call, bank account, e-mail send & receive records, forensic reports, witness & victim statements and lawyer statements [23, 24].
5.3 Intelligence reports
Data and information about criminals are usually maintained by intelligent agencies. National Intelligence Agencies (NIA) of Nigeria, Intelligence Bureau (IB), and Narcotics Control Bureau (NCB) are some of these agencies [25, 26].
5.4 Intelligence findings
Intelligence findings that are open source based are extracted from the web, search engines, and social media platforms such as (Facebook, Twitter, and LinkedIn). This information is highly in an unstructured format [27, 28].
5.5 Police records
Criminal suspects and their records are usually maintained by police officers for future references and use. These records are kept in relational data format or text format [29].
5.6 Type of crimes
Different crime categories are presented in Table 5, which are classified by various law-enforcement agencies. Different types of crime, such as arm robbery and kidnapping concern police at various divisions and levels of government [27, 30]. Other types of crime such as cybercrimes and terrorism are usually investigated by local, national and international agencies [31].
This systematic review brings together the evidence for the collection of data used for crime prediction and control in Nigeria. A total of 42 studies were included in the review. Out of this total, six (6) used Association rule mining for crime pattern analysis from crime data. The limitation of this approach is processing time and visualization were not considered. Five (5) other studies applied statistical based techniques such as Principal Component Analysis, Spatial Statistics Analysis for crime prediction. The result obtained were inefficient and inaccurate as it takes time to make crime prediction. Eleven (11) out of the reviewed studies applied Clustering approach to crime prediction where it produces unreliable prediction result with noisy data and when the number of clusters are small. Thirteen (13) concentrated on supervised learning techniques where some of the authors use Frequent pattern mining, emotion mining, Support Vector Machine, Decision Tree and Neural Network techniques on crime data. Some of these techniques have some inherent deficiencies that really affected the output and performance of the model developed. For example, decision tree is prone to over-fitting when dataset is huge and also deep neural network will produce inaccurate results when dataset is small. Lastly, only seven (7) researchers adopted hybrid approach for crime prediction and data mining and only five out these actually focused on spatio-temporal crime prediction. Some of the authors that applied hybrid approaches to crime prediction and data mining problem, use association rule mining and Genetic Algorithms, while some of authors applied IF-IDF and Bayesian Network while some also applied K-means and Deep Neural Network. But some noticeable gap in all of these is that there is an appreciable reduction in the overall performance of the system when dataset become huge. Some studies used demographic and social media data for their crime prediction while in some other studies, historical crime data was used for prediction the occurrence of crime. The summary of the review result is presented in Table 4.
Table 4. Technologies used in crime prediction and data mining
This systematic literature review is put together from 42 publications. The summary of the researches done in crime prediction and data mining is presented in the Table 5. It all shows that the techniques such as statistical methods, clustering, association rule mining, classification and hybrid approaches can be applied for crime prediction and data mining problem. It is worthy of note that Figure 4 depicted highest number of research papers adopted supervised learning approach with 31% of the total studies to crime prediction, and this is followed by Clustering with 26%, Hybrid approach with 22%, Association rule mining with 14% and the least which is statistical based approach with 7% respectively. Various studies affirmed that application of crime prediction and data mining techniques is majorly dependent on situation available and objectives involved.
Table 5. Research summary of crime prediction and data mining
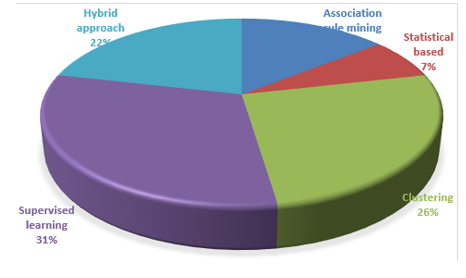
Figure 4. Summary of findings
Crime prediction and data mining has become hot research areas in recent time because of its attendance benefits to socio-economic development of a nation. Outcome of this research will definitely benefit and help potential researchers to have good understanding of the available and the state of the arts techniques for crime prediction and control. The analytics of crime prediction and data mining can be used to give statistical reporting of crime in a particular area or region. This will ultimately benefit the society by making law enforcement agency and the government understand the various factors and causes of crime. Hence agencies of government can take a better and proactive decision towards improving the safety of the citizens.
This is to acknowledge the financial support of Center for ICT/ICE Research, known as Covenant University Centre for Research, Innovation and Discovery (CUCRID), Ota, Nigeria.
[1] Gok, M., Celik, A., Ozal, Y., Ozgul, F. (2012). Mining hate crimes to figure out reasons behind. IEEE/ACM International Conference on Advances in Social Networks Analysis and Mining, Istanbul, Turkey, pp. 887-889. https://doi.org/10.1109/ASONAM.2012.159 [2] Chung, W., Xu, J.J., Wang, G., Qin, Y., Chau, M., Chen, H. (2004). Crime data mining: A general framework and some examples. Computer, IEEE, 37(4): 50-56. https://doi.org/10.1109/MC.2004.1297301 [3] Chen, Y.F., Huang, H., Ma, L.H. (2010). AK-modes: A weighted clustering algorithm for finding similar case subsets. 2010 IEEE International Conference on Intelligent Systems and Knowledge Engineering, Hangzhou, China, pp. 218-223. https://doi.org/10.1109/ISKE.2010.5680876 [4] Atzenbeck, C., Celik, A., Erdem, Z., Ozgul, F. (2011). Incorporating data sources and methodologies for crime data mining. Proceedings of 2011 IEEE International Conference on Intelligence and Security Informatics, pp. 176-180. https://doi.org/10.1109/ISI.2011.5983995 [5] Adesola, F., Misra, S., Omoregbe, N., Damasevicius, R., Maskeliunas, R. (2019). An IOT-Based Architecture for Crime Management in Nigeria. In: R. K. Shukla et al. (eds) Springer Nature Singapore Pte Ltd. [6] Li, C.T., Jeng, S.K., Huang, Y.Y. (2015). Mining location-based social networks for criminal activity prediction. Wireless and Optical Communication Conference (WOCC), IEEE, pp. 185-189. https://doi.org/10.1109/WOCC.2015.7346202 [7] Ahishakiye, E., Opiyo, E. D. Taremwa, D., Niyonzima, I. (2017). Crime prediction using decision tree (J48) classification algorithm. International Journal of Computer and Information Technology, 6(3). [8] Wang, B., Zhang, D.H., Brantingham, P.J., Bertozzi, A.L. (2017). Deep learning for real time crime forecasting. eprint arXiv:1707.03340, NOLTA, 2017arXiv170703340W. [9] Gifford, C.M., Buczak, A.L. (2010). Fuzzy association rule mining for community crime pattern discovery. ISI-KDD, Washington D.C, U.S.A, ACM, pp. 1-10. https://doi.org/10.1145/1938606.1938608 [10] Ester, M., Glasser, U., Brantingham, P.L., Tayebi, M.A. (2014). CRIMETRACER: Activity space based crime location prediction. IEEE/ACM International Conference on Advances in Social Networks Analysis and Mining (ASONAM 2014), Beijing, China, pp. 472-480. https://doi.org/10.1109/ASONAM.2014.6921628 [11] Chang, K.C., Hsing, T.P., Chou, S., Chen, P.S. (2006). Mining criminal databases to finding investigation clues-by example of stolen automobiles database. Intelligence and Security Informatics, pp. 91-102. https://doi.org/10.1007/11734628_12 [12] Elzinga, P., Viaene, S., Van Hulle, M.M., Dedene, G., Poelmans, J. (2009). Gaining insight in domestic violence with emergent self-organizing maps. Expert Systems with Applications, 36(9): 11864-11874. https://doi.org/10.1016/j.eswa.2009.04.027 [13] Lee, I., Phillips, P. (2011). Crime analysis through spatial areal aggregated density patterns. Geoinformatica, 15(1): 49-74. https://doi.org/10.1007/s10707-010-0116-1 [14] Bagula, A., Berman, S., Isafiadea, O. (2015). A revised frequent pattern model for crime situation recognition based on floor-ceil quartile function. Procedia Computer Science, 55: 251-260. https://doi.org/10.1016/j.procs.2015.07.042 [15] Corcoran, J., Higgs, G., Brunsdon, C. (2007). Visualising space and time in crime patterns: A comparison of methods. Computers, Environment and Urban Systems, 31(1): 52-75. https://doi.org/10.1016/j.compenvurbsys.2005.07.009 [16] Oh, G.S., Paek, S.Y., Park, H.H. (2012). Measuring the crime displacement and diffusion of benefit effects of open-street CCTV in South Korea. International Journal of Law, Crime and Justice, 40(3): 179-191. https://doi.org/10.1016/j.ijlcj.2012.03.003 [17] Javideh, M., Ebrahimi, M.R., Keyvanpour, M.R. (2011). Detecting and investigating crime by means of data mining: A general crime matching framework. Procedia Computer Science, 3: 872-880. https://doi.org/10.1016/j.procs.2010.12.143 [18] Leroy, G., Ku, C.H. (2014). A decision support system: Automated crime report analysis and classification for e-government. Government Information Quarterly, 31(4): 534-544. https://doi.org/10.1016/j.giq.2014.08.003 [19] Bhatnagar, V., Jain, A. (2016). Crime data analysis using pig with Hadoop. Procedia Computer Science, 78: 571- 578. https://doi.org/10.1016/j.procs.2016.02.104 [20] Li, X.G., Juhola, M. (2014). Country crime analysis using the self-organizing map with special regard to demographic factors. Springer, AI & Soc, 29(1): 53-68. https://doi.org/10.1007/s00146-013-0441-7 [21] Erdem, Z., Bowerman, C., Bondy, B., Ozgul, F. (2010). Combined detection model for criminal network detection. PAISI, Springer, pp. 1-14. https://doi.org/10.1007/978-3-642-13601-6_1 [22] Yan, Q.B., Zhang, L., Peng, L.Z., Han, H.B., Chen, Z.X. (2015). Using map-based interactive interface for understanding and characterizing crime data in cities. ICSI-CCI, Springer, pp. 479-490. https://doi.org/10.1007/978-3-319-20469-7_51 [23] Gupta, M., Gupta, M.P., Chandra, B. (2007). Adaptive query interface for mining crime data. DNIS, Springer, pp. 285-296. [24] Lee, I., Phillips, P. (2012). Mining co-distribution patterns for large crime datasets. Expert Systems with Applications, 39(14): 11556-11563. https://doi.org/10.1016/j.eswa.2012.03.071 [25] Jain, A., Arora, S., Agarwal, S., Gupta, T., Tyagi, N., Tayal, D.K. (2015). Crime detection and criminal identification in India using data mining techniques. Springer, AI & Soc, 30(1): 117-127. https://doi.org/10.1007/s00146-014-0539-6 [26] Evans, M., Kang, J., Mohan, P., Shekhar, S. (2011). Identifying patterns inspatial information: A survey of methods. Wiley Online Library, 1(3): 193-214. https://doi.org/10.1002/widm.25 [27] Huang, X., Silva, E., Ghodsi, M., Hassani, H. (2016). A review of data mining applications in crime. Statistical Analysis and Data Mining: The ASA Data Science Journal, 9(3): 139-154. https://doi.org/10.1002/sam.11312 [28] Ewart, B., Oatley, G. (2011). Data mining and crime analysis. Wiley Online Library, 1(2): 147-153. https://doi.org/10.1002/widm.6 [29] Quick, M., Chan, P.W., Law, J. (2014). Analyzing hotspots of crime using a Bayesian spatiotemporal modeling approach: A case study of violent crime in the greater Toronto area. Geographical Analysis, 47(1): 1-19. https://doi.org/10.1111/gean.12047 [30] Leroy, G., Ku, J. (2011). A crime reports analysis system to identify related crimes. Journal of the American Society for Information Science and Technology, 62(8): 1533-1547. http://doi.org/10.1002/asi.21552 [31] Xu, J.J., Chen, H.C., Chau, M., Schroeder, J. (2007). Automated criminal link analysis based on domain knowledge. Journal of the American Society for Information Science and Technology, 58(6): 842-855. http://doi.org/10.1002/asi.20552 [32] Packer, M.W., Thomason, M.B., Wesley, J.C., Hansen, P.J., Conklin, J.H., Brown, D.E., Lyons, A.M. (2006). Uniform crime report "super clean" data cleaning tool. Proceedings of the 2006 Systems and Information Engineering Design Symposium, Charlottesville, VA, USA, pp. 14-18. http://doi.org/10.1109/SIEDS.2006.278706 [33] Lee, I., Phillips, P. (2009). Criminal cross correlation mining and visualization. PAISI, Springer, pp. 2-13. https://doi.org/10.1007/978-3-642-01393-5_2 [34] Kang, H.W., Kang H.B. (2017). Prediction of crime occurrence from multi-modal data using deep learning. PLoS ONE, 12(4): e0176244. https://doi.org/10.1371/journal.pone.0176244 [35] Yang, D.Q., Heaney, T., Tonon, A., Cudre-Mauroux, P. (2017). CrimeTelescope: Crime hotspot prediction based on urban and social media data fusion. World Wide Web, 21(5): 1323-1347. https://doi.org/10.1007/s11280-017-0515-4 [36] Abdul, A., Jakaria, R., Imran, R. (2017). Using data mining technique to analyze crime of Bangladesh. IJCSN-International Journal of Computer Science and Network, 6(4): 489-494. [37] Gulumbe, S.U., Dikko, H.G., Bello, Y. (2014). Analysis of crime data using principal component analysis: A case study of Katsina State. CBN Journal of Applied Statistics, 3(2): 39-49. [38] Srisuk, S., Thongtae, P. (2008). An analysis of data mining applications in crime domain. 2008 IEEE 8th International Conference on Computer and Information Technology Workshops, Sydney, QLD, Australia, pp. 122-126. https://doi.org/10.1109/CIT.2008.Workshops.80 [39] Usha, D., Rameshkumar, K. (2014). Application of frequent pattern mining and association rule mining on crime pattern mining. Journal of Advances in Computer Science and Technology, 3(4): 264-275. [40] Waduge, N., Ranathunga, L. (2017). Machine learning approaches for detecting crime patterns. https://www.reseachgate.net/publication/319465093, accessed on 16 April 2019. [41] Devan, M.S., Gangadharan. S., Sathyadevan, S. (2014). Crime analysis and prediction using data mining. in 2014 First International Conference on Networks & Soft Computing, Guntur, India, pp. 406-412. https://doi.org/10.1109/CNSC.2014.6906719 [42] Jabar, E.K., Hashem, S.H., Hessian, E.M. (2013). Propose data mining AR-GA model to advance crime analysis. IOSR Journal of Computer Engineering (IOSR-JCE), 14(5): 38-45. https://doi.org/10.9790/0661-1453845 [43] Jeon, J., Jeong, S. (2016). Designing a crime-prevention system by converging big data and IoT. Journal of Internet Computing and Services (JICS), 17(3): 115-128. https://doi.org/10.7472/jksii.2016.17.3.115 [44] Byun, J.Y., Nasridinov, A., Park, Y.H. (2014). Internet of things for smart crime detection. Contemporary Engineering Sciences, 7(15): 749-754. http://dx.doi.org/10.12988/ces.2014.4685 [45] Moona, T., Heoa, S., Leeb, S. (2014). Ubiquitous crime prevention system (UCPS) for a safer city. Procedia Environmental Sciences, 22: 288-301. https://doi.org/10.1016/j.proenv.2014.11.028 [46] Reddy, P., Rajesh, D. (2016). A data analytics applied for crime identification over IOT. International Journal of Science and Research (IJSR), 5(2): 243-246. [47] Ristea, A., Leitner, M. (2018). Integration of social media in spatial crime analysis and prediction models for events. AGILE 2018 – Lund, June 12-15. [48] Bao, F., Lu, C.T., Chen, I.R., Shah, S. Crowdsafe: crowd sourcing of crime incidents and safe. in ACM SIGSPATIAL GIS’11, Chicago, IL, USA, ACM, pp. 521-524. https://doi.org/10.1145/2093973.2094064
Phone: + 1 825 436 9306
Email: [email protected]
Subscription
Language support
Please sign up to receive notifications on new issues and newsletters from IIETA
Select Journal/Journals:
Copyright © 2024 IIETA. All Rights Reserved.
Citation Analysis
- Google Scholar
- Scholar Metrics
- Web of Science
Quick Links
- Editorial Boards
- Author Guidelines
- Online Submission
- Peer Review Process
- Publication Fee
- Abstracting and Indexing
- Publication Ethics
- Visitor Statistics
- Registration for IJ-AI's Professional Reviewers
- For Readers
- For Authors
- For Librarians
- Announcements
A systematic literature review of machine learning methods in predicting court decisions
- There are currently no refbacks.

IAES International Journal of Artificial Intelligence (IJ-AI) ISSN/e-ISSN 2089-4872/2252-8938 This journal is published by the Institute of Advanced Engineering and Science (IAES) in collaboration with Intelektual Pustaka Media Utama (IPMU) .
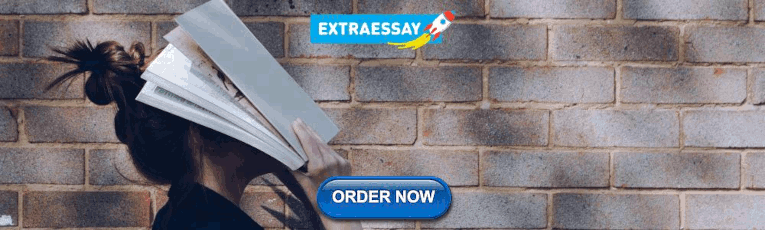
IMAGES
VIDEO
COMMENTS
Consequently, the application of artificial intelligence (AI) in crime prediction is a significant and well-researched area. This study investigates AI strategies in crime prediction. We conduct a systematic literature review (SLR). Our review evaluates the models from numerous points of view, including the crime analysis type, crimes studied ...
We conduct a Systematic Literature Review (SLR). Our review evaluates the models from numerous points of view; the crime analysis type, the crimes studied types, the prediction technique, the performance metrics and evaluations, the strength and weakness of the proposed method, and the limitation and future direction.
This study investigates AI strategies in crime prediction. We conduct a systematic literature review (SLR). Our review evaluates the models from numerous points of view, including the crime ...
DOI: 10.2139/ssrn.4039691 Corpus ID: 247098701; Artificial Intelligence & Crime Prediction: A Systematic Literature Review @article{Dakalbab2022ArtificialI, title={Artificial Intelligence \& Crime Prediction: A Systematic Literature Review}, author={Fatima Mohamad Dakalbab and Manar Abu Talib and Omnia Elmutasim and Ali Bou Nassif and Sohail Abbas and Qassim Nasir}, journal={SSRN Electronic ...
Using a Systematic Literature Review (SLR) methodology, we aim to collect and synthesize the required knowledge regarding machine learning-based crime prediction and help both law enforcement authorities and scientists to mitigate and prevent future crime occurrences. We focus primarily on 68 selected machine learning papers that predict crime.
Keywords: machine learning, recidivism, crime prediction, artificial intelligence, explainable artificial intelligence. 1. Introduction ... However, literature analysed in this systematic review pinpointed some limitations. First of all, the performance of each model does not depend only on the ML model or the dataset. The method of data ...
Using a Systematic Literature Review (SLR) methodology, we aim to collect and synthesize the required knowledge regarding machine learning-based crime prediction and help both law enforcement authorities and scientists to mitigate and prevent future crime occurrences. We focus primarily on 68 selected machine learning papers that predict crime.
Abstract. The duty of ensuring people's safety and preserving peace is of the utmost importance, and crime is the biggest barrier. An excellent technology that can significantly contribute to reducing and eradicating crime is artificial intelligence and machine learning. This study offers a review of the past five years' worth of research on ...
In the next five years, it is anticipated that more than 2.5 million devices will be fully online. This paper focuses on artificial intelligence (AI), crime prediction and crime prevention. A ...
This study aggregates, summarizes, and evaluates the spatial-temporal crime prediction and detection techniques through conducting a Systematic Literature Review (SLR). A systematic Literature review identifies, selects and critically appraises research in order to provide a solution to a well formulated problem. (A, 2016)) .
Smart policing refers to the use of advanced technologies such as artificial intelligence to enhance policing activities in terms of crime prevention or crime reduction. Artificial intelligence tools, including machine learning and natural language processing, have widespread applications across various fields, such as healthcare, business, and law enforcement. By means of these technologies ...
Artificial Intelligence & Crime Prediction: A Systematic Literature Review. F Dakalbab, MA Talib, OA Waraga, AB Nassif, S Abbas, Q Nasir ... Artificial intelligence techniques in financial trading: A systematic literature review. F Dakalbab, M Abu Talib, Q Nasir, T Saroufil. Journal of King Saud University - Computer and Information Sciences 36 ...
Using a Systematic Literature Review (SLR) methodology, we aim to collect and synthesize the required knowledge regarding machine learning-based crime prediction and help both law enforcement ...
We conduct a systematic literature review (SLR). Our review evaluates the models from numerous points of view, including the crime analysis type, crimes studied, prediction technique, performance metrics and evaluations, strengths and weaknesses of the proposed method, and limitations and future directions.
Artificial intelligence & crime prediction: A systematic literature review. Fatima Dakalbab, Manar Abu Talib, Omnia Abu Waraga, Ali Bou Nassif, Sohail Abbas and Qassim Nasir. Journal: Social Sciences & Humanities Open, 2022, Volume 6, Number 1, Page 100342. DOI: 10.1016/j.ssaho.2022.100342. Read Online
The systematic review present in this study focuses on crime prediction and data mining as well as the techniques employed in the past studies. The existing work is classified and grouped into different categories and are presented by using visualization approach. It is found that more studies adopted supervised learning approaches to crime ...
The systematic literature review (SLR) is the gold standard in providing research a firm evidence foundation to support decision-making. ... The growth of Artificial Intelligence (AI) is changing the way that research is conducted across a variety of fields and disciplines ... Predictive text and handwriting recognition, web search engines, and ...
Prediction is possible in various cases, such as predicting the outcome of construction litigation, crime-related cases, parental rights, worker types, divorces, and tax law. The machine learning methods can function as support decision tools in the legal system with artificial intelligence's advancement.
The aim of this thesis was to conduct a broad systematic review of available predictions of BIM development and directions for future BIM research to present the current and future challenges facing the AECOO industry. BIM is the basis for arriving at the idea of the digital twin, which in turn brings the construction industry closer to the concept of the circular economy. BIM enters into ...
BACKGROUND: Artificial intelligence (AI) uses computer systems to simulate cognitive capacities to accomplish goals like problem-solving and decision-making. Machine learning (ML), a branch of AI, makes algorithms find connections between preset variables, thereby producing prediction models. ML can aid shoulder surgeons in determining which patients may be susceptible to worse outcomes and ...
We blended narrative, integrative, and systematic literature review and propose future steps for more balanced NBS development. The findings of this work suggest that AI presents an opportunity for a more balanced NBS design through its applications in climate change prediction, water management, and project visualisation.