To read this content please select one of the options below:
Please note you do not have access to teaching notes, accounting, accountability, social media and big data: revolution or hype.
Accounting, Auditing & Accountability Journal
ISSN : 0951-3574
Article publication date: 15 May 2017
The purpose of this paper is to outline an agenda for researching the relationship between technology-enabled networks – such as social media and big data – and the accounting function. In doing so, it links the contents of an unfolding area research with the papers published in this special issue of Accounting, Auditing and Accountability Journal .
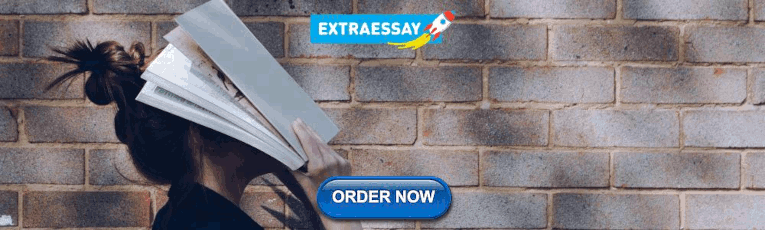
Design/methodology/approach
The paper surveys the existing literature, which is still in its infancy, and proposes ways in which to frame early and future research. The intention is not to offer a comprehensive review, but to stimulate and conversation.
The authors review several existing studies exploring technology-enabled networks and highlight some of the key aspects featuring social media and big data, before offering a classification of existing research efforts, as well as opportunities for future research. Three areas of investigation are identified: new performance indicators based on social media and big data; governance of social media and big data information resources; and, finally, social media and big data’s alteration of information and decision-making processes.
Originality/value
The authors are currently experiencing a technological revolution that will fundamentally change the way in which organisations, as well as individuals, operate. It is claimed that many knowledge-based jobs are being automated, as well as others transformed with, for example, data scientists ready to replace even the most qualified accountants. But, of course, similar claims have been made before and therefore, as academics, the authors are called upon to explore the impact of these technology-enabled networks further. This paper contributes by starting a debate and speculating on the possible research agendas ahead.
- Social media
- Management control
Acknowledgements
The authors wish to acknowledge the helpful comments of the reviewers. These have greatly improved the quality of the manuscript and the arguments contained within. In addition, the authors would like to thank Gloria Parker and Rainbow Shum for their expert help in liaising with Emerald and working through Scholar One. The authors are also appreciative of the effort and support of the authors who submitted papers to the special issue and reviewers who devoted their time and effort to the refereeing process. Finally, the authors are immensely grateful for Professor James Guthrie for his practical support intellectual encouragement, and wise counsel as the authors brought this special issue to fruition.
This paper forms part of the Accounting, Accountability, Social Media and Big Data: Revolution or Hype? Special issue.
Arnaboldi, M. , Busco, C. and Cuganesan, S. (2017), "Accounting, accountability, social media and big data: revolution or hype?", Accounting, Auditing & Accountability Journal , Vol. 30 No. 4, pp. 762-776. https://doi.org/10.1108/AAAJ-03-2017-2880
Emerald Publishing Limited
Copyright © 2017, Emerald Publishing Limited
Related articles
We’re listening — tell us what you think, something didn’t work….
Report bugs here
All feedback is valuable
Please share your general feedback
Join us on our journey
Platform update page.
Visit emeraldpublishing.com/platformupdate to discover the latest news and updates
Questions & More Information
Answers to the most commonly asked questions here
- - Google Chrome
Intended for healthcare professionals
- Access provided by Google Indexer
- My email alerts
- BMA member login
- Username * Password * Forgot your log in details? Need to activate BMA Member Log In Log in via OpenAthens Log in via your institution

Search form
- Advanced search
- Search responses
- Search blogs
- News & Views
- What’s holding up the...
What’s holding up the big data revolution in healthcare?
- Related content
- Peer review
- Kiret Dhindsa , postdoctoral fellow 1 ,
- Mohit Bhandari , professor 2 ,
- Ranil R Sonnadara , associate professor 2
- 1 Vector Institute for Artificial Intelligence, Toronto, Ontario, Canada
- 2 Department of Surgery, McMaster University, Hamilton, Ontario, Canada
- Correspondence to: K Dhindsa dhindsj{at}mcmaster.ca
Poor data quality, incompatible datasets, inadequate expertise, and hype
Big data refers to datasets that are too large or complex to analyse with traditional methods. 1 Instead we rely on machine learning—self updating algorithms that build predictive models by finding patterns in data. 2 In recent years, a so called “big data revolution” in healthcare has been promised 3 4 5 so often that researchers are now asking why this supposed inevitability has not happened. 6 Although some technical barriers have been correctly identified, 7 there is a deeper issue: many of the data are of poor quality and in the form of small, incompatible datasets.
Current practices around collection, curation, and sharing of data make it difficult to apply machine learning to healthcare on a large scale. We need to develop, evaluate, and adopt modern health data standards that guarantee data quality, ensure that datasets from different institutions are compatible for pooling, and allow timely access to datasets by researchers and others. These prerequisites for machine learning have not yet been met.
Part of the problem is that the hype surrounding machine learning obscures the reality that it is just a tool for data science with its own requirements and limitations. The hype also fails to acknowledge that all healthcare tools must work within a wide range of human constraints, from the molecular to the social and political. Each of these will limit what can be achieved: even big technical advances may have only modest effects when integrated into the complex framework of clinical practice and healthcare delivery.
Although machine learning is the state of the art in predictive big data analytics, it is still susceptible to poor data quality, 8 9 sometimes in uniquely problematic ways. 2 Machine learning, including its more recent incarnation deep learning, 10 performs tasks involving pattern recognition (generally a combination of classification, regression, dimensionality reduction, and clustering 11 ). The ability to detect even the most subtle patterns in raw data is a double edged sword: machine learning algorithms, like humans, can easily be misdirected by spurious and irrelevant patterns. 12
For example, medical imaging datasets are often riddled with annotations—made directly on the images—that carry information about specific diagnostic features found by clinicians. This is disastrous in the machine learning context, where an algorithm trained using datasets that include annotated images will seem to perform extremely well on standard tests but fail to work in a real world scenario where similar annotations are not available. Since these algorithms find patterns regardless of how meaningful those patterns are to humans, the rule “garbage in, garbage out” may apply even more than usual. 13
Even if we had good data, would we have enough? Healthcare data are currently distributed across multiple institutions, collected using different procedures, and formatted in different ways. Machine learning algorithms recognise patterns by exploiting sources of variance in large datasets. But inconsistencies across institutions mean that combining datasets to achieve the required size easily introduces an insurmountable degree of non-predictive variability. This makes it all too easy for a machine learning algorithm to miss the truly important patterns and latch onto the more dominating patterns introduced by institutional differences.
Holistic solution
A holistic solution to problems of data quality and quantity would include adoption of consistent health data standards across institutions, complete with new data sharing policies that ensure ongoing protection of patient privacy. If healthcare leaders see an opportunity to advance patient care with big data and machine learning, they must take the initiative to establish new data policies in consultation with clinicians, data scientists, patients, and the public.
Improved data management is clearly necessary if machine learning algorithms are to generate models that can transition successfully from the laboratory to clinical practice. How should we go about it? Effective data management requires specialist training in data science and information technology, and detailed knowledge of the nuances associated with data types, applications, and domains, including how they relate to machine learning. This points to a growing role for data management specialists and knowledge engineers who can pool and curate datasets; such experts may become as essential to modern healthcare as imaging technicians are now. 14 Clinicians will also need training as collectors of health data and users of machine learning tools. 15
To truly realise the potential of big data in healthcare we need to bring together up-to-date data management practices, specialists who can maximise the usability and quality of health data, and a new policy framework that recognises the need for data sharing. Until then, the big data revolution (or at least a realistic version of it) remains on hold.
Competing interests: We have read and understood BMJ policy on declaration of interests and declare the following: RRS reports board membership for Compute Ontario, SHARCNET, and SOSCIP—all non-profit advanced research computing organisations. MB reports personal fees from Stryker, Sanofi, Ferring, and Pendopharm and grants from Acumed, DJO, and Sanofi outside the submitted work.
Provenance and peer review: Not commissioned; externally peer reviewed.
- De Mauro A ,
- Michalski RS ,
- Carbonell JG ,
- Mitchell TM
- Fernandes L ,
- O’Connor M ,
- Raghupathi W ,
- Raghupathi V
- Murdoch TB ,
- ↵ Cortes C, Jackel LD, Chiang WP. Limits on learning machine accuracy imposed by data quality. In: Advances in neural information processing systems. MIT Press, 1995:239-46.
- Najafabadi MM ,
- Villanustre F ,
- Khoshgoftaar TM ,
- Muharemagic E
- ↵ Papernot N, McDaniel P, Jha S, Fredrikson M, Celik ZB, Swami A. The limitations of deep learning in adversarial settings. In: Security and Privacy (EuroS&P), 2016 IEEE European Symposium on IEEE. 2016:372-87.
- Jones-Farmer LA
- Furlong LI ,
- Albanell J ,
MURAL - Maynooth University Research Archive Library
- Browse by Year
- Browse by Academic Unit
- Browse by Author
- Repository Policies
- Cite from MURAL
- MURAL Libguide
Big Data – Hype or Revolution?
Kitchin, Rob (2016) Big Data – Hype or Revolution? In: The SAGE Handbook of Social Media Research Methods. SAGE Publications, pp. 27-39. ISBN 9781473916326
Share your research

Abstract included in text.
Actions (login required)
Repository staff only(login required).
Downloads per month over past year
View more statistics
Origin of downloads

More From Forbes
The next big data leap: how ai is reshaping data and analytics roles.

- Share to Facebook
- Share to Twitter
- Share to Linkedin
Avinash Tripathi is an analytics evangelist, thought leader and keynote speaker with over 20 years of experience in higher education.
As we approach the halfway point in 2024, we can look back and see that the past eighteen months have brought a massive shift in the field of data and analytics, especially due to AI.
The substantial investments in AI startups and the global data analytics market are indicative of what’s to come; venture capitalists injected $42.5 billion into AI startups last year, according to CB Insights, cited by the Financial Times .
This trend is corroborated by survey 2023 Statista research that shows that data and analytics as a focus area for business investments. In fact, the global data analytics market is expected to witness expansion with projections showing it skyrocketing from $61.44 billion in 2023 to $581.34 billion in 2033.
These substantial investments are driving an upsurge in job opportunities within the data field, and data-related roles are set to evolve. The outlook appears incredibly promising for careers in data analytics and data science spurred by the escalating volume of data and the critical necessity to derive insights from it.
Several data-related fields are projected for growth compared to the overall job market trends. According to the U.S. Bureau of Labor Statistics, for example, there is a projected 35% increase in data scientist positions between 2022 and 2032. The need for professionals exceeds the workforce, and this pattern seems unlikely to change.
iOS 17 5 Apple Issues Update Now Warning To All iPhone Users
Northern lights could show up yet again tonight: here’s an updated aurora borealis forecast, biden vs trump 2024 election polls trump leads biden by 1 point latest survey shows, the human edge in the age of ai: why analysts will thrive, not be replaced.
The future lies at the dynamic intersection of data analytics and AI. While AI will undoubtedly transform how we analyze data, there's no replacing the human strengths of interpretation, strategic thinking and insightful analysis.
Here are some key trends shaping data science roles in the coming years.
Beyond Data Puppets: Analysts As Navigators, Not Processors
Analysts will remain crucial in navigating businesses through the ever-growing sea of data. Their role will evolve from data processors to data storytellers , uncovering valuable insights and driving data-informed decisions. The key will be to avoid being overwhelmed by information and AI, focusing instead on critical thinking and communication.
The Rise Of AI Collaboration: New Roles And A Human-AI Team
The growth of AI and machine learning (ML) will present opportunities to automate tasks like cleaning large datasets, data pipeline management, provisioning, model training and basic analysis. This frees analysts and engineers to concentrate on higher-value activities like problem formulation, feature selection and model interpretation.
A rise in new job positions designed to facilitate this partnership is likely, including AI trainers, specialists in AI operations and roles like AI ethicists and data privacy experts who understand well the compliance that's important with data regulations.
Specialization Is Key: In-Demand Skills For The Future
While specific tasks get automated, the demand for analysts with specialized skills could soar. As data becomes integral to all roles. There could be an increasing desire to use data strategically for industry-specific problem-solving.
Analysts with deep expertise in areas like healthcare, education or finance may be crucial for exploring complex problems.
Highlighting The Importance Of Communication And Collaboration
As data-related careers evolve, success in data-related fields will require more than just technical prowess. Effective communication and collaboration will be central to navigating the rise of artificial intelligence (AI) across organizations, including leadership.
Algorithms—often complex "black boxes"—can be biased, favoring their training data. Interpersonal and cross-functional collaboration can help to mitigate these biases, uncovering new possibilities. Storytelling, cultural sensitivity, virtual presence and inclusive communication for potentially dispersed teams will become more and more valued.
Ethical And Responsible Data Champions
As laws around data privacy evolve and concerns about bias in AI systems increase, professionals in analytics will participate in advocating for proper data handling.
The increasing emphasis on data security, innovation in product development and governance of AI will create opportunities for roles such as AI governance specialists and data security officers. As the focus on data protection and regulations compliance grows, all analysts will need to be knowledgeable about data security and applicable laws.
Continuous Learning: A Key To Success
The fields of data science and advanced analytics undergo constant change, with new technologies emerging regularly.
To succeed in this setting, professionals engaged in data must foster a growth-oriented mindset, emphasizing ongoing learning. This involves consistently refining skills, staying abreast of emerging technologies and adapting to the evolving industry terrain.
Specialization is valuable, especially when it's built upon a strong foundation. While advanced courses in cloud computing, data mining, big data processing and specific programming languages like R, Python or Java can provide a competitive edge, a robust educational foundation will enable you to specialize more effectively.
This foundation is in core mathematics, statistics, data science and business analytics. It provides the framework for understanding complex algorithms and how to interpret their outputs effectively. In the next 10 years, data analysts who can seamlessly combine a strong foundation with expertise in AI/ML, excellent communication skills and industry-specific knowledge will likely be at the forefront of data-driven advancements.
The Future Of Data Is Human: Communication, Collaboration And Continuous Learning
Pivoting from the "Great Resignation" of last year, a study from the University of Phoenix Career Institute® found that we have reached "a moment of talent stagnation —both for employers and workers." (Disclosure: I am the vice president of analytics at the University of Phoenix.) While workers are optimistic about their careers, they feel undervalued and stuck.
Companies struggle to find talent externally, but a disconnect exists—workers say they lack growth opportunities within their companies, contradicting employer claims of abundant internal advancement. The solution? Invest in your existing workforce—it's cheaper and more effective than external recruitment in the long run.
The key for professionals in data-related fields will be to develop skills in working with AI tools and harnessing their capabilities to augment their expertise. This future of work demands a focus on upskilling and complementing human capabilities with AI, fostering a partnership that drives innovation and growth in the data-driven economy.
Forbes Technology Council is an invitation-only community for world-class CIOs, CTOs and technology executives. Do I qualify?
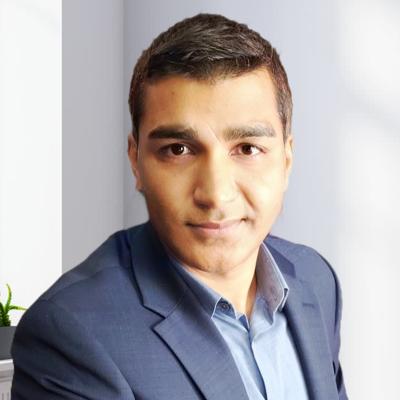
- Editorial Standards
- Reprints & Permissions
Accounting, accountability, social media and big data: Revolution or hype?
- Centre for Sustainability and Responsible Management
Research output : Contribution to journal › Article › peer-review
Access to Document
- 10.1108/AAAJ-03-2017-2880
- Accounting, accountability, social media and big data: Revolution or hype? Accepted author manuscript, 496 KB Licence: CC BY-NC
- https://doi.org/10.1108/AAAJ-03-2017-2880
T1 - Accounting, accountability, social media and big data: Revolution or hype?
AU - Arnaboldi, Michela
AU - Busco, Cristiano
AU - Cuganesan, Suresh
PY - 2017/5/15
Y1 - 2017/5/15
N2 - Purpose – This paper outlines an agenda for researching the relationship between technology enabled networks – such as social media and big data - and the accounting function. In doing so, it links the contents of an unfolding area research with the papers published in this Accounting, Auditing and Accountability Journal special issue.Design/methodology – The paper surveys the existing literature, which is still in its infancy, and proposes ways in which to frame early and future research. The intention is not to offer a comprehensive review, but to stimulate and conversation.Findings – We review several existing studies exploring technology enabled networks and highlight some of the key aspects featuring social media and big data, before offering a classification of existing research efforts, as well as opportunities for future research. Three areas of investigation are identified: new performance indicators based on social media and big data;governance of social media and big data information resources; and, finally, social media and big data’s alteration of information and decision-making processes.Originality/value – We are currently experiencing a technological revolution that will fundamentally change the way in which organisations, as well as individuals, operate. It is claimed that many knowledge-based jobs are being automated, as well as others transformed with, for example, data scientists ready to replace even the most qualified accountants. But, of course, similar claims have been made before and therefore, as academics, we are called upon to explore the impact of these technology enabled networks further. This paper contributes by starting a debate and speculating on the possible research agendas ahead. © 2017, Emerald Group Publishing . The attached document (embargoed until 15/05/2019) is an author produced version of a paper published in Accounting, Auditing & Accountability Journal, uploaded in accordance with the publisher’s self- archiving policy. The final published version (version of record) is available online at https://doi.org/10.1108/AAAJ-03-2017-2880. Some minor differences between this version and the final published version may remain. We suggest you refer to the final published version should you wish to cite from it.
AB - Purpose – This paper outlines an agenda for researching the relationship between technology enabled networks – such as social media and big data - and the accounting function. In doing so, it links the contents of an unfolding area research with the papers published in this Accounting, Auditing and Accountability Journal special issue.Design/methodology – The paper surveys the existing literature, which is still in its infancy, and proposes ways in which to frame early and future research. The intention is not to offer a comprehensive review, but to stimulate and conversation.Findings – We review several existing studies exploring technology enabled networks and highlight some of the key aspects featuring social media and big data, before offering a classification of existing research efforts, as well as opportunities for future research. Three areas of investigation are identified: new performance indicators based on social media and big data;governance of social media and big data information resources; and, finally, social media and big data’s alteration of information and decision-making processes.Originality/value – We are currently experiencing a technological revolution that will fundamentally change the way in which organisations, as well as individuals, operate. It is claimed that many knowledge-based jobs are being automated, as well as others transformed with, for example, data scientists ready to replace even the most qualified accountants. But, of course, similar claims have been made before and therefore, as academics, we are called upon to explore the impact of these technology enabled networks further. This paper contributes by starting a debate and speculating on the possible research agendas ahead. © 2017, Emerald Group Publishing . The attached document (embargoed until 15/05/2019) is an author produced version of a paper published in Accounting, Auditing & Accountability Journal, uploaded in accordance with the publisher’s self- archiving policy. The final published version (version of record) is available online at https://doi.org/10.1108/AAAJ-03-2017-2880. Some minor differences between this version and the final published version may remain. We suggest you refer to the final published version should you wish to cite from it.
U2 - 10.1108/AAAJ-03-2017-2880
DO - 10.1108/AAAJ-03-2017-2880
M3 - Article
SN - 0951-3574
JO - Accounting, Auditing & Accountability Journal
JF - Accounting, Auditing & Accountability Journal
- OII >
- Research >
- publications >
Big Data: A Revolution That Will Transform How We Live, Work and Think
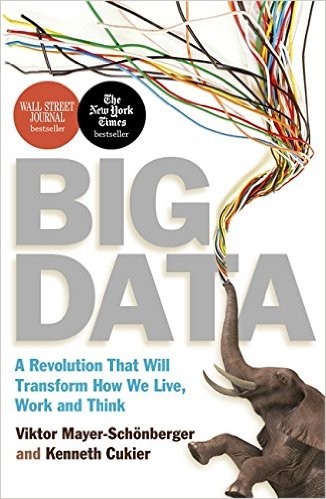
We have always used information to make decisions, but there is a radical change taking place. Society is going from a constant shortage of data to a surfeit of information – and this upends everything.
For centuries, we have only collected and crunched a sliver of information because of the cost and complexity of processing larger amounts. We relied on data of the cleanest, highest quality possible, since we only tapped a little of it. And we tried to uncover the reasons behind how the world worked, to generalise. Yet all this was actually a function of a small-data world, when we never had enough information. Change that, and a lot of other things need to change as well.
Think of a car engine. Breakdowns rarely ever happen all at once. Instead, one hears strange noises or the driving “feels funny” a few days in advance. Many vehicles are fitted with sensors that can measure the heat and vibration from the engine. By capturing it in data, one can know what a healthy engine’s “data signature” looks like, as well as how it changes prior to a breakdown. That way, one can identify when a part is about to fail before it actually breaks. The car can alert the driver to visit a service station to get it repaired, as if it is clairvoyant. But we needed lots of data, needed to accept messy data, and had to give up knowing why the engine was about to break for the practical knowledge that it was, without a cause.
That’s big data. It ushers in three big shifts: more, messy and correlations (the book’s chapters 2, 3 and 4). First, more. We can finally harness a vast quantity of information, and in some cases, we can analyse all the data about a phenomenon. This lets us drill down into the details we could never see before. Second, messy. When we harness more data, we can shed our preference for data that’s only of the best calibre, and let in some imperfections. The benefits of using more data outweighs cleaner but less data. Third, correlations. Instead of trying to uncover causality, the reasons behind things, it is often sufficient to simply uncover practical answers. So if some combinations of aspirin and orange juice puts a deadly disease into remission, it is less important to know what the biological mechanism is than to just drink the potion. For many things, with big data it is faster, cheaper and good enough to learn “what,” not “why.”
A reason that we can do these things is that we have so much more data, and one reason for that is because we are taking more aspects of society and rendering it into a data form (discussed in chapter 5). With so much data around, and the ability to process it, big data is the bedrock of new companies.
The value of data is in its secondary uses, not simply in the primary purpose for which it was initially collected, which is the way we tended to value it in the past (noted in chapter 6). Hence, a big delivery company can reuse data on who sends packages to whom to make economic forecasts. A travel site crunches billions of old flight-price records from airlines, to predict whether a given airfare is a good one, or if the price is likely to increase or decrease. These extraordinary data services require three things: the data, the skills, and a big data mindset (examined in chapter 7). Today, the skills are lacking, few have the mindset even though the data seems abundant. But over time, the skills and creativity will become commonplace — and the most prized part will be the data itself.
Big data also has a dark side (chapter 8). Privacy is harder to protect because the traditional legal and technical mechanisms don’t work well with big data. And a new problem emerges: propensity — penalizing people based on what they are predicted to do, not what the have done. At the same time, there will be an increasing need to stay vigilant so that we don’t fall victim to the “dictatorship of data,” the idea that we shut off our reasoned judgment and endow in the data-driven decisions more than they deserve.
Solutions to these thorny problems (raised in chapter 9) include a fundamental rethink of privacy law and the technology to protect personal information. Also, a new class of professional called the “algorithmist” that will do for the big data age what accountants and auditors did for an era 100 years ago, when the cornucopia of information swamping society was in the form of financial data.
What role is left for humanity? For intuition, experience and acting in defiance of what the data suggests? Big data is set to change not only how we interact with the world, but ourselves.
What people are saying
“This brilliant book cuts through the hype surrounding big data. A must-read for anyone in business, information technology, public policy…. And anyone else who is just plain curious about the future.” – John Seely Brown, Former director of Xerox PARC
“Just as water is wet in a way that individual water molecules aren’t, big data can reveal information in a way that individual bits of data can’t. The authors show us the surprising ways that enormous, complex, and messy collections of data can be used to predict everything from shopping patterns to flu outbreaks.” – Clay Shirky, author of Cognitive Surplus and Here Comes Everybody
“An optimistic and practical look at the Big Data revolution — just the thing to get your head around the big changes already underway and the bigger changes to come.” – Cory Doctorow, boingboing.com
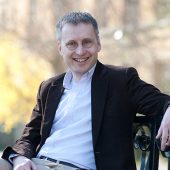
Professor Viktor Mayer-Schönberger
Professor of Internet Governance and Regulation
Viktor Mayer-Schönberger is the OII's Professor of Internet Governance and Regulation. His research focuses on the role of information in a networked economy.
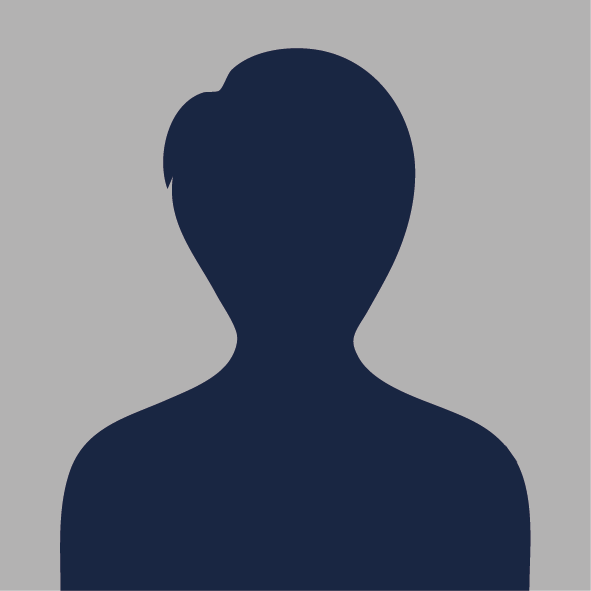
Kenneth Neil Cukier
Press coverage.
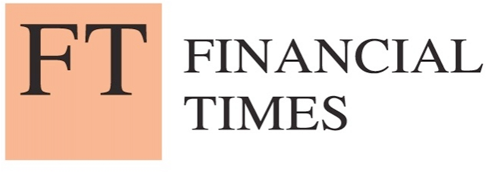
Business Book of the Year 2013
Financial Times, 18 September 2013
Big Data: A Revolution That Will Transform How We Live, Work, and Think, the bestselling book by Viktor Mayer-Schönberger and Kenneth Cukier has been shortlisted for the Financial Times/Goldman Sachs Business Book of the Year.
Sign in » warwick.ac.uk
- Acceptable use policy
Browse Econ Literature
- Working papers
- Software components
- Book chapters
- JEL classification
More features
- Subscribe to new research
RePEc Biblio
Author registration.
- Economics Virtual Seminar Calendar NEW!
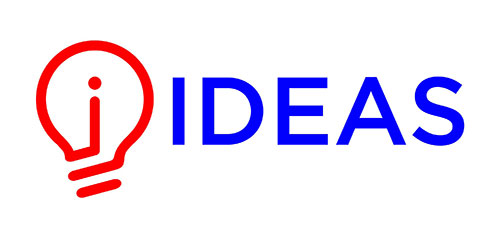
Accounting, accountability, social media and big data: revolution or hype?
- Author & abstract
- 33 Citations
- Related works & more
Corrections
More services and features.
Follow serials, authors, keywords & more
Public profiles for Economics researchers
Various research rankings in Economics
RePEc Genealogy
Who was a student of whom, using RePEc
Curated articles & papers on economics topics
Upload your paper to be listed on RePEc and IDEAS
New papers by email
Subscribe to new additions to RePEc
EconAcademics
Blog aggregator for economics research
Cases of plagiarism in Economics
About RePEc
Initiative for open bibliographies in Economics
News about RePEc
Questions about IDEAS and RePEc
RePEc volunteers
Participating archives
Publishers indexing in RePEc
Privacy statement
Found an error or omission?
Opportunities to help RePEc
Get papers listed
Have your research listed on RePEc
Open a RePEc archive
Have your institution's/publisher's output listed on RePEc
Get RePEc data
Use data assembled by RePEc
Advertisement
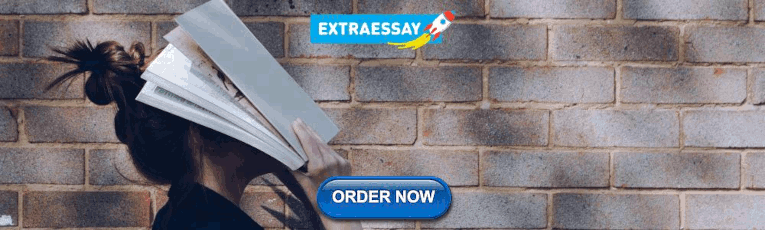
Awaiting the Second Big Data Revolution: From Digital Noise to Value Creation
- Open access
- Published: 18 February 2015
- Volume 15 , pages 35–47, ( 2015 )
Cite this article
You have full access to this open access article
- Mark Huberty 1
“Big data”—the collection of vast quantities of data about individual behavior via online, mobile, and other data-driven services—has been heralded as the agent of a third industrial revolution—one with raw materials measured in bits, rather than tons of steel or barrels of oil. Yet the industrial revolution transformed not just how firms made things, but the fundamental approach to value creation in industrial economies. To date, big data has not achieved this distinction. Instead, today’s successful big data business models largely use data to scale old modes of value creation, rather than invent new ones altogether. Moreover, today’s big data cannot deliver the promised revolution. In this way, today’s big data landscape resembles the early phases of the first industrial revolution, rather than the culmination of the second a century later. Realizing the second big data revolution will require fundamentally different kinds of data, different innovations, and different business models than those seen to date. That fact has profound consequences for the kinds of investments and innovations firms must seek, and the economic, political, and social consequences that those innovations portend.
Similar content being viewed by others
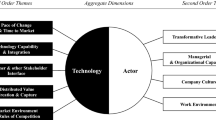
Digital transformation: a review, synthesis and opportunities for future research
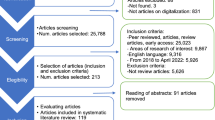
The role of digitalization in business and management: a systematic literature review
Trends and Future Perspective Challenges in Big Data
Avoid common mistakes on your manuscript.
1 Introduction
We believe that we live in an era of “big data”. Firms today accumulate, often nearly by accident, vast quantities of data about their customers, suppliers, and the world at large. Technology firms like Google or Facebook have led the pack in finding uses for such data, but its imprint is visible throughout the economy. The expanding sources and uses of data suggest to many the dawn of a new industrial revolution. Those who cheer lead for this revolution proclaim that these changes, over time, will bring about the same scope of change to economic and social prosperity in the 21st century, that rail, steam, or steel did in the 19th.
Yet this “big data” revolution has so far fallen short of its promise. Precious few firms transmutate data into novel products. Instead, most rely on data to operate, at unprecedented scale, business models with long pedigree in the media and retail sectors. Big data, despite protests to the contrary, is thus an incremental change—and its revolution one of degree, not kind.
The reasons for these shortcomings point to the challenges we face in realizing the promise of the big data revolution. Today’s advances in search, e-commerce, and social media relied on the creative application of marginal improvements in computational processing power and data storage. In contrast, tomorrow’s hopes for transforming real-world outcomes in areas like health care, education, energy, and other complex phenomena pose scientific and engineering challenges of an entirely different scale.
2 The Implausibility of Big Data
Our present enthusiasm for big data stems from the confusion of data and knowledge. Firms today can gather more data, at lower cost, about a wider variety of subjects, than ever before. Big data’s advocates claim that this data will become the raw material of a new industrial revolution. As with its 19th century predecessor, this revolution will alter how we govern, work, play, and live. But unlike the 19th century, we are told, the raw materials driving this revolution are so cheap and abundant that the horizon is bounded only by the supply of smart people capable of molding these materials into the next generation of innovations (Manyika et al. 2011 ).
This utopia of data is badly flawed. Those who promote it rely on a series of dubious assumptions about the origins and uses of data, none of which hold up to serious scrutiny. In aggregate, these assumptions all fail to address whether the data we have actually provides the raw materials needed for a data-driven Industrial Revolution we need. Taken together, these failures point out the limits of a revolution built on the raw materials that today seem so abundant.
Four of these assumptions merit special attention: First, N = all , or the claim that our data allow a clear and unbiased study of humanity; second, that today = tomorrow , or the claim that understanding online behavior today implies that we will still understand it tomorrow; third, offline = online , the claim that understanding online behavior offers a window into economic and social phenomena in the physical world; and fourth, that complex patterns of social behavior, once understood, will remain stable enough to become the basis of new data-driven, predictive products and services in sectors well beyond social and media markets. Each of these has its issues. Taken together, those issues limit the future of a revolution that relies, as today’s does, on the “digital exhaust” of social networks, e-commerce, and other online services. The true revolution must lie elsewhere.
2.1 N = All
Gathering data via traditional methods has always been difficult. Small samples were unreliable; large samples were expensive; samples might not be representative, despite researchers’ best efforts; tracking the same sample over many years required organizations and budgets that few organizations outside governments could justify. None of this, moreover, was very scalable: researchers needed a new sample for every question, or had to divine in advance a battery of questions and hope that this proved adequate. No wonder social research proceeded so slowly.
Mayer-Schönberger and Cukier ( 2013 ) argue that big data will eliminate these problems. Instead of having to rely on samples, online data, they claim, allows us to measure the universe of online behavior, where N (the number of people in the sample) is basically all (the entire population of people we care about). Hence we no longer need worry, they claim, about the problems that have plagued researchers in the past. When N = all , large samples are cheap and representative, new data on individuals arrives constantly, monitoring data over time poses no added difficulty, and cheap storage permits us to ask new questions of the same data again and again. With this new database of what people are saying or buying, where they go and when, how their social networks change and evolve, and myriad other factors, the prior restrictions borne of the cost and complexity of sampling will melt away.
But N ≠ all . Most of the data that dazzles those infatuated by “big data”—Mayer-Schönberger and Cukier included—comes from what McKinsey & Company termed “digital exhaust” (Manyika et al. 2011 ): the web server logs, e-commerce purchasing histories, social media relations, and other data thrown off by systems in the course of serving web pages, online shopping, or person-to-person communication. The N covered by that data concerns only those who use these services—not society at large. In practice, this distinction turns out to matter quite a lot. The demographics of any given online service usually differ dramatically from the population at large, whether we measure by age, gender, race, education, and myriad other factors.
Hence the uses of that data are limited. It’s very relevant for understanding web search behavior, purchasing, or how people behave on social media. But the N here is skewed in ways both known and unknown—perhaps younger than average, or more tech-savvy, or wealthier than the general population. The fact that we have enormous quantities of data about these people may not prove very useful to understanding society writ large.
2.2 Today = Tomorrow
But let’s say that we truly believe this assumption—that everyone is (or soon will be) online. Surely the proliferation of smart phones and other devices is bringing that world closer, at least in the developed world. This brings up the second assumption—that we know where to go find all these people. Several years ago, MySpace was the leading social media website, a treasure trove of new data on social relations. Today, it’s the punchline to a joke. The rate of change in online commerce, social media, search, and other services undermines any claim that we can actually know that our N = all sample that works today will work tomorrow. Instead, we only know about new developments—and the data and populations they cover—well after they have already become big. Hence our N = all sample is persistently biased in favor of the old. Moreover, we have no way of systematically checking how biased the sample is, without resorting to traditional survey methods and polling—the very methods that big data is supposed to render obsolete.
2.3 Online Behavior = Offline Behavior
But let’s again assume that problem away. Let’s assume that we have all the data, about all the people, for all the online behavior, gathered from the digital exhaust of all the relevant products and services out there. Perhaps, in this context, we can make progress understanding human behavior online. But that is not the revolution that big data has promised. Most of the “big data” hype has ambitions beyond improving web search, online shopping, socializing, or other online activity. Instead, big data should help cure disease, detect epidemics, monitor physical infrastructure, and aid first responders in emergencies.
To satisfy these goals, we need a new assumption: that what people do online mirrors what they do offline. Otherwise, all the digital exhaust in the world won’t describe the actual problems we care about.
There’s little reason to think that offline life faithfully mirrors online behavior. Research has consistently shown that individuals’ online identities vary widely from their offline selves. In some cases, that means people are more cautious about revealing their true selves. Danah Boyd’s work (Boyd and Marwick 2011 ) has shown that teenagers cultivate online identities very different from their offline selves—whether for creative, privacy, or other reasons. In others, it may mean that people are more vitriolic, or take more extreme positions. Online political discussions—another favorite subject of big data enthusiasts—suffer from levels of vitriol and partisanship far beyond anything seen offline (Conover et al. 2011 ). Of course, online and offline identity aren’t entirely separate. That would invite suggestions of schizophrenia among internet users. But the problem remains—we don’t know what part of a person is faithfully represented online, and what part is not.
Furthermore, even where online behavior may echo offline preferences or beliefs, that echo is often very weak. In statistical terms, our ability to distinguish “significant” from “insignificant” results improves with the sample size—but statistical significance is not actual significance. Knowing, say, that a history of purchasing some basket of products is associated with an increased risk of being a criminal may be helpful. But if that association is weak—say a one-hundredth of a percent increase—it’s practical import is effectively zero. Big data may permit us to find these associations, but it does not promise that they will be useful.
2.4 Behavior of All (Today) = Behavior of All (Tomorrow)
OK, but you say, surely we can determine how these distortions work, and incorporate them into our models? After all, doesn’t statistics have a long history of trying to gain insight from messy, biased, or otherwise incomplete data?
Perhaps we could build such a map, one that allows us to connect the observed behaviors of a skewed and selective online population to offline developments writ large. This suffices only if we care primarily about describing the past. But much of the promise of big data comes from predicting the future—where and when people will get sick in an epidemic, which bridges might need the most attention next month, whether today’s disgruntled high school student will become tomorrow’s mass shooter.
Satisfying these predictive goals requires yet another assumption. It is not enough to have all the data, about all the people, and a map that connects that data to real-world behaviors and outcomes. We also have to assume that the map we have today will still describe the world we want to predict tomorrow.
Two obvious and unknowable sources of change stand in our way. First, people change. Online behavior is a culmination of culture, language, social norms and other factors that shape both people and how they express their identity. These factors are in constant flux. The controversies and issues of yesterday are not those of tomorrow; the language we used to discuss anger, love, hatred, or envy change. The pathologies that afflict humanity may endure, but the ways we express them do not.
Second, technological systems change. The data we observe in the “digital exhaust” of the internet is created by individuals acting in the context of systems with rules of their own. Those rules are set, intentionally or not, by the designers and programmers that decide what we can and cannot do with them. And those rules are in constant flux. What we can and cannot buy, who we can and cannot contact on Facebook, what photos we can or cannot see on Flickr vary, often unpredictably. Facebook alone is rumored to run up to a thousand different variants on its site at one time. Hence even if culture never changed, our map from online to offline behavior would still decay as the rules of online systems continued to evolve.
An anonymous reviewer pointed out, correctly, that social researchers have always faced this problem. This is certainly true but many of the features of social systems—political and cultural institutions, demography, and other factors—change on a much longer timeframe than today’s data-driven internet services. For instance, US Congressional elections operate very differently now compared with a century ago; but change little between any two elections. Contrast that with the pace of change for major social media services, for which 2 years may be a lifetime.
A recent controversy illustrates this problem to a T. Facebook recently published a study (Kramer et al. 2014 ) in which they selectively manipulated the news feeds of a randomized sample of users, to determine whether they could manipulate users’ emotional states. The revelation of this study prompted fury on the part of users, who found this sort of manipulation unpalatable. Whether they should, of course, given that Facebook routinely runs experiments on its site to determine how best to satisfy (i.e., make happier) its users, is an interesting question. But the broader point remains—someone watching the emotional state of Facebook users might have concluded that overall happiness was on the rise, perhaps consequence of the improving American economy. But in fact this increase was entirely spurious, driven by Facebook’s successful experiment at manipulating its users.
Compounding this problem, we cannot know, in advance, which of the social and technological changes we do know about will matter to our map. That only becomes apparent in the aftermath, as real-world outcomes diverge from predictions cast using the exhaust of online systems.
Lest this come off as statistical nihilism, consider the differences in two papers that both purport to use big data to project the outcome of US elections. DiGrazia et al. ( 2013 ) claim that merely counting the tweets that reference a Congressional candidate—with no adjustments for demography, or spam, or even name confusion—can forecast whether that candidate will win his or her election. This is a purely “digital exhaust” approach. They speculate—but cannot know—whether this approach works because (to paraphrase their words) “one tweet equals one vote”, or “all attention on Twitter is better”. Moreover, it turns out that the predictive performance of this simple model provides no utility. As Huberty ( 2013 ) shows, their estimates perform no better than an approach that simply guesses that the incumbent party would win—a simple and powerful predictor of success in American elections. Big data provided little value.
Contrast this with Wang et al. ( 2014 ). They use the Xbox gaming platform as a polling instrument, which they hope might help compensate for the rising non-response rates that have plagued traditional telephone polls. As with Twitter, N ≠ all : the Xbox user community is younger, more male, less politically involved. But the paper nevertheless succeeds in generating accurate estimates of general electoral sentiment. The key difference lies in their use of demographic data to re-weight respondents’ electoral sentiments to look like the electorate at large. The Xbox data were no less skewed than Twitter data; but the process of data collection provided the means to compensate. The black box of Twitter’s digital exhaust, lacking this data, did not. The difference? DiGrazia et al. ( 2013 ) sought to reuse data created for one purpose in order to do something entirely different; Wang et al. ( 2014 ) set out to gather data explicitly tailored to their purpose alone.
2.5 The Implausibility of Big Data 1.0
Taken together, the assumptions that we have to make to fulfill the promise of today’s big data hype appear wildly implausible. To recap, we must assume that:
everyone we care about is online;
we know where to find them today, and tomorrow;
they represent themselves online consistent with how they behave offline, and;
they will continue to represent themselves online—in behavior, language, and other factors—in the same way, for long periods of time.
Nothing in the history of the internet suggests that even one of these statements holds true. Everyone was not online in the past; and likely will not be online in the future. The constant, often wrenching changes in the speed, diversity, and capacity of online services means those who are online move around constantly. They do not, as we’ve seen, behave in ways necessarily consistent with their offline selves. And the choices they make about how to behave online evolve in unpredictable ways, shaped by a complex and usually opaque amalgam of social norms and algorithmic influences.
But if each of these statements fall down, then how have companies like Amazon, Facebook, or Google built such successful business models? The answer lies in two parts. First, most of what these companies do is self-referential: they use data about how people search, shop, or socialize online to improve and expand services targeted at searching, shopping, or socializing. Google, by definition, has an N = all sample of Google users’ online search behavior. Amazon knows the shopping behaviors of Amazon users. Of course, these populations are subject to change their behaviors, their self-representation, or their expectations at any point. But at least Google or Amazon can plausibly claim to have a valid sample of the primary populations they care about.
Second, the consequences of failure are, on the margins, very low. Google relies heavily on predictive models of user behavior to sell the advertising that accounts for most of its revenue. But the consequences of errors in that model are low—Google suffers little from serving the wrong ad on the margins. Of course, persistent and critical errors of understanding will undermine products and lead to lost customers. But there’s usually plenty of time to correct course before that happens. So long as Google does better than its competitors at targeting advertising, it will continue to win the competitive fight for advertising dollars.
But if we move even a little beyond these low-risk, self-referential systems, the usefulness of the data that underpin them quickly erodes. Google Flu provides a valuable lesson in this regard. In 2008, Google announced a new collaboration with the Centers for Disease Control (CDC) to track and report rates of influenza infection. Historically, the CDC had monitored US flu infection patterns through a network of doctors that tracked and reported “influenza-like illness” in their clinics and hospitals. But doctors’ reports took up to 2 weeks to reach the CDC—a long time in a world confronting SARS or avian flu. Developing countries with weaker public health capabilities faced even greater challenges. Google hypothesized that, when individuals or their family members got the flu, they went looking on the internet—via Google, of course—for medical advice. In a highly cited paper, Ginsberg et al. ( 2008 ) showed that they could predict region-specific influenza infection rates in the United States using Google search frequency data. Here was the true promise of big data—that we capitalize on virtual data to better understand, and react to, the physical world around us.
The subsequent history of Google Flu illustrates the shortcomings of the first big data revolution. While Google Flu has performed well in many seasons, it has failed twice, both times in the kind of abnormal flu season during which accurate data are most valuable. The patterns of and reasons for failure speak to the limits of prediction. In 2009, Google Flu under-predicted flu rates during the H1N1 pandemic. Post-hoc analysis suggested that the different viral characteristics of H1N1 compared with garden-variety strains of influenza likely meant that individuals didn’t know they had a flu strain, and thus didn’t go looking for flu-related information (Cook et al. 2011 ). Conversely, in 2012, Google Flu over-predicted influenza infections. Google has yet to discuss why, but speculation has centered on the intensive media coverage of an early-onset flu season, which may have sparked interest in the flu among healthy individuals (Butler 2013 ).
The problems experienced by Google Flu provide a particularly acute warning of the risks inherent in trying to predict what will happen in the real world based on the exhaust of the digital one. Google Flu relied on a map—a mathematical relationship between online behavior and real-world infection. Google built that map on historic patterns of flu infection and search behavior. It assumed that such patterns would continue to hold in the future. But there was nothing fundamental about those patterns. Either a change in the physical world (a new virus) or the virtual one (media coverage) were enough to render the map inaccurate. The CDC’s old reporting networks out-performed big data when it mattered most.
3 A Revolution Constrained: Data, Potential, and Value Creation
Despite ostensibly free raw materials, mass-manufacturing insight from digital exhaust has thus proven far more difficult than big data’s advocates would let on. It’s thus unsurprising that this revolution has had similarly underwhelming effects on business models. Amazon, Facebook, and Google are enormously successful businesses, underpinned by technologies operating at unprecedented scale. But they still rely on centuries-old business models for most of their revenue. Google and Amazon differ in degree, but not kind, from a newspaper or a large department store when it comes to making money. This is a weak showing from a revolution that was supposed to change the 21st century in the way that steam, steel, or rail changed the 19th. Big data has so far made it easier to sell things, target ads, or stalk long-lost friends or lovers. But it hasn’t yet fundamentally reworked patterns of economic life, generated entirely new occupations, or radically altered relationships with the physical world. Instead, it remains oddly self-referential: we generate massive amounts of data in the process of online buying, viewing, or socializing; but find that data truly useful only for improving online sales and search.
Understanding how we might get from here to there requires a better understanding of how and why data—big or small—might create value in a world of better algorithms and cheap compute capacity. Close examination shows that firms have largely used big data to improve on existing business models, rather than adopt new ones; and that those improvements have relied on data to describe and predict activity in worlds largely of their own making. Where firms have ventured beyond these self-constructed virtual worlds, the data have proven far less useful, and products built atop data far more prone to failure.
3.1 Refining Data into Value
The Google Flu example suggests the limits to big data as a source of mass-manufactured insight about the real world. But Google itself, and its fellow big-data success stories, also illustrate the shortcomings of big data as a source of fundamentally new forms of value creation. Most headline big data business models have used their enhanced capacity to describe, predict, or infer in order to implement—albeit at impressive scale and complexity—centuries-old business models. Those models create value not from the direct exchange between consumer and producer, but via a web of transactions several orders removed from the creation of the data itself. Categorizing today’s big data business models based on just how far they separate data generation from value creation quickly illustrates how isolated the monetary value of firms’ data is from their primary customers. Having promised a first-order world, big data has delivered a third-order reality.
Realizing the promise of the big data revolution will require a different approach. The same problems that greeted flu prediction have plagued other attempts to build big data applications that forecast the real world. Engineering solutions to these problems that draw on the potential of cheap computation and powerful algorithms will require not different methods, but different raw materials. The data those materials require must originate from a first-order approach to studying and understanding the worlds we want to improve. Such approaches will require very different models of firm organization than those exploited by Google and its competitors in the first big data revolution.
3.1.1 Third-Order Value Creation: The Newspaper Model
Most headline big data business models do not make much money directly from their customers. Instead, they rely on third parties—mostly advertisers—to generate profits from data. The actual creation and processing of data is only useful insofar as it’s of use to those third parties. In doing so, these models have merely implemented, at impressive scale and complexity, the very old business model used by the newspapers they have largely replaced.
If we reach back into the dim past when newspapers were viable businesses (rather than hobbies of the civic-minded wealthy), we will remember that their business model had three major components:
gather, filter, and analyze news;
attract readers by providing that news at far below cost, and;
profit by selling access to those readers to advertisers.
The market for access matured along with the newspapers that provided it. Both newspapers and advertisers realized that people who read the business pages differed from those who read the front page, or the style section. Front-page ads were more visible to readers than those buried on page A6. Newspapers soon started pricing access to their readers accordingly. Bankers paid one price to advertise in the business section, clothing designers another for the style pages. This segmentation of the ad market evolved as the ad buyers and sellers learned more about whose eyeballs were worth how much, when, and where.
Newspapers were thus third-order models. The news services they provided were valuable in their own right. But readers didn’t pay for them. Instead, news was a means of generating attention and data, which was only valuable when sold to third parties in the form of ad space. Data didn’t directly contribute to improving the headline product—news—except insofar as it generated revenue that could be plowed back into news gathering. The existence of a tabloid press of dubious quality but healthy revenues proved the weakness of the link between good journalism and profit.
From a value creation perspective, Google, Yahoo, and other ad-driven big data businesses are nothing more than newspapers at scale. They too provide useful services (then news, now email or search) to users at rates far below cost. They too profit by selling access to those users to third-party advertisers. They too accumulate and use data to carve up the ad market. The scale of data they have available, of course, dwarfs that of their newsprint ancestors. This data, combined with cheap computation and powerful statistics, has enabled operational efficiency, scale, and effectiveness far beyond what newspapers could ever have managed. But the business model itself—the actual means by which these firms earn revenues—is identical.
Finally, that value model does not emerge, fully-formed, from the data itself. The data alone are no more valuable than the unrefined iron ore or crude oil of past industrial revolutions. Rather, the data were mere inputs to a production process that depended on human insight—that what people looked for on the internet might be a good proxy for their consumer interests.
3.1.2 Second-Order Value Creation: The Retail Model
Big-box retail ranks as the other substantial success for big data. Large retailers like Amazon, Wal-Mart, or Target have harvested very fine-grained data about customer preferences to make increasingly accurate predictions of what individual customers wish to buy, in what quantities and combinations, at what times of the year, at what price. These predictions are occasionally shocking in their accuracy—as with Target’s implicit identification of a pregnant teenager well before her father knew it himself, based solely on subtle changes in her purchasing habits.
From this data, these retailers can, and have, built a detailed understanding of retail markets: what products are complements or substitutes for each other; exactly how much more people are willing to pay for brand names versus generics; how size, packaging, and placement in stores and on shelves matters to sales volumes.
Insights built on such data have prompted two significant changes in retail markets. First, they have made large retailers highly effective at optimizing supply chains, identifying retail trends in their infancy, and managing logistical difficulties to minimize the impact on sales and lost competitiveness. This has multiplied their effectiveness versus smaller retailers, who lack such capabilities and are correspondingly less able to compete on price.
But it has also changed, fundamentally, the relationship of these retailers to their suppliers. Big box retailers have increasingly become monopsony buyers of some goods—books for Amazon, music for iTunes. But they are also now monopoly sellers of information back to their suppliers. Amazon, Target and Wal-Mart have a much better understanding of their suppliers’ customers than the customers themselves. They also understand these suppliers’ competitors far better. Hence their aggregation of information has given them substantial power over suppliers. This has had profound consequences for the suppliers. Wal-Mart famously squeezes suppliers on cost—either across the board, or by pitting suppliers against one another based on detailed information of their comparative cost efficiencies and customer demand.
Hence big data has shifted the power structure of the retail sector and its manufacturing supply chains. The scope and scale of the data owned by Amazon or Wal-Mart about who purchases what, when, and in what combinations often means that they understand the market for a product far better than the manufacturer. Big data, in this case, comes from big business—a firm that markets to the world also owns data about the world’s wants, needs, and peculiarities. Even as they are monopsony buyers of many goods (think e-books for Amazon), they are correspondingly monopoly sellers of data. And that has made them into huge market powers on two dimensions, enabling them to squeeze suppliers to the absolute minimum price, packaging, size, and other product features that are most advantageous to them—and perhaps to their customers.
But big data has not changed the fundamental means of value creation in the retail sector. Whatever its distributional consequences, the basic retail transaction—of individuals buying goods from retail intermediaries, remains unchanged from earlier eras. The same economies of scale and opportunities for cross-marketing that made Montgomery Ward a retail powerhouse in the 19th century act on Amazon and Wal-Mart in the 21st. Big data may have exacerbated trends already present in the retail sector; but the basics of how that sector creates value for customers and generates profits for investors are by no means new. Retailers have yet to build truly new products or services that rely on data itself—instead, that data is an input into a longstanding process of optimization of supply chain relations, marketing, and product placement in service of a very old value model: the final close of sale between a customer and the retailer.
3.1.3 First-order Value Creation: The Opportunity
Second- and third-order models find value in data several steps removed from the actual transaction that generates the data. However, as the Google Flu example illustrated, that data may have far less value when separated from its virtual context. Thus while these businesses enjoy effectively free raw materials, the potential uses of those materials are in fact quite limited. Digital exhaust from web browsing, shopping, or socializing has proven enormously useful in the self-referential task of improving future web browsing, shopping, and socializing. But that success has not translated success at tasks far removed from the virtual world that generated this exhaust. Digital exhaust may be plentiful and convenient to collect, but it offers limited support for understanding or responding to real-world problems.
First-order models, in contrast, escape the Flu trap by building atop purpose-specific data, conceived and collected with the intent of solving specific problems. In doing so, they capitalize on the cheap storage, powerful algorithms, and inexpensive computing power that made the first wave of big data firms possible. But they do so in pursuit of a rather different class of problems.
First order products remain in their infancy. But some nascent examples suggest what might be possible. IBM’s Watson famously used its natural language and pattern recognition abilities to win the Jeopardy! game show. Doing so constituted a major technical feat: the ability to understand unstructured, potentially obfuscated Jeopardy! game show answers, and respond with properly-structured questions based on information gleaned from vast databases of unstructured information on history, popular culture, art, science, or almost any other domain.
The question now is whether IBM can adapt this technology to other problems. Its first attempts at improving medical diagnosis appear promising. By learning from disease and health data gathered from millions of patients, initial tests suggest that Watson can improve the quality, accuracy, and efficacy of medical diagnosis and service to future patients (Steadman 2013 ). Watson closes the data value loop: patient data is made valuable because it improves patient services, not because it helps with insurance underwriting or product manufacturing or logistics or some other third-party activity.
Premise Corporation provides another example. Premise has built a mobile-phone based data gathering network to measure macroeconomic aggregates like inflation and food scarcity. This network allows them to monitor economic change at a very detailed level, in regions of the world where official statistics are unavailable or unreliable. This sensor network is the foundation of the products and services that Premise sells to financial services firms, development agencies, and other clients. As compared with the attenuated link between data and value in second- or third-order businesses, Premise’s business model links the design of the data generation process directly to the value of its final products.
Optimum Energy (OE) provides a final example. OE monitors and aggregates data on building energy use—principally data centers—across building types, environments, and locations. That data enables it to build models for building energy use and efficiency optimization. Those models, by learning building behaviors across many different kinds of inputs and buildings, can perform better than single-building models with limited scope. Most importantly, OE creates value for clients by using this data to optimize energy efficiency and reduce energy costs.
These first-order business models all rely on data specifically obtained for their products. This reliance on purpose-specific data contrasts with third-order models that rely on the “digital exhaust” of conventional big data wisdom. To use the newspaper example, third-order models assume—but can’t specifically verify—that those who read the style section are interested in purchasing new fashions. Google’s success stemmed from closing this information gap a bit—showing that people who viewed web pages on fashion were likely to click on fashion ads. But again, the data that supports this is data generated by processes unrelated to actual purchasing—activities like web surfing and search or email exchange. And so the gap remains. Google appears to realize this, and has launched Consumer Surveys as an attempt to bridge that gap. In brief, it offers people the chance to skip ads in favor of providing brand feedback.
3.2 The Unrealized Promise of Unreasonable Data
We should remember the root of the claim about big data. That claim was perhaps best summarized by Halevy et al. ( 2009 ) in what they termed “the unreasonable effectiveness of data”—that, when seeking to improve the performance of predictive systems, more data appeared to yield better returns on effort than better algorithms. Most appear to have taken that to mean that data—and particularly more data—are unreasonably effective everywhere—and that, by extension, even noisy or skewed data could suffice to answer hard questions if we could simply get enough of it. But that misstates the authors’ claims. They did not claim that more data was always better. Rather, they argued that, for specific kinds of applications, history suggested that gathering more data paid better dividends than inventing better algorithms.
Where data are sparse or the phenomenon under measurement noisy, more data allow a more complete picture of what we are interested in. Machine translation provides a very pertinent example: human speech and writing varies enormously within one language, let alone two. Faced with the choice between better algorithms for understanding human language, and more data to quantify the variance in language, more data appears to work better. But for other applications, the “bigness” of data may not matter at all. If I want to know who will win an election, polling a thousand people might be enough. Relying on the aggregated voices of a nation’s Twitter users, in contrast, will probably fail (Gayo-Avello et al. 2011 ; Gayo-Avello 2012 ; Huberty 2013 ). Not only are we not, as section 2 discussed, in the N = all world that infatuated Mayer-Schönberger and Cukier ( 2013 ); but for most problems we likely don’t care to be. Having the right data—and consequently identifying the right question to ask beforehand—is far more important than having a lot of data of limited relevance to the answers we seek.
4 Consequences
Big data therefore falls short of the proclamation that it represents the biggest change in technological and economic possibility since the industrial revolution. That revolution, in the span of a century or so, fundamentally transformed almost every facet of human life. Someone born in 1860, who lived to be 70 years old, grew up in a world of horses for travel, candles for light, salting and canning for food preservation, and telegraphs for communication. The world of their passing had cars and airplanes, electric light and refrigerators, and telephones, radio, and motion pictures. Having ranked big data with the industrial revolution, we find ourselves wondering why our present progress seems so paltry in comparison.
But much of what we associate with the industrial revolution—the advances in automobile transport, chemistry, communication, and medicine—came much later. The businesses that produced them were fundamentally different from the small collections of tinkerers and craftsmen that built the first power looms. Instead, these firms invested in huge industrial research and development operations to discover and then commercialize new scientific discoveries. These changes were expensive, complicated, and slow—so slow that John Stuart Mill despaired, as late as 1871, of human progress. But in time, they produced a world inconceivable to even the industrial enthusiasts of the 1840s.
In today’s revolution, we have our looms, but we envision the possibility of a Model T. Today, we can see glimmers of that possibility in IBM’s Watson, Google’s self-driving car, or Nest’s thermostats that learn the climate preferences of a home’s occupants. These and other technologies are deeply embedded in, and reliant on, data generated from and around real-world phenomena. None rely on “digital exhaust”. They do not create value by parsing customer data or optimizing ad click-through rates (though presumably they could). They are not the product of a relatively few, straightforward (if ultimately quite useful) insights. Instead, IBM, Google, and Nest have dedicated substantial resources to studying natural language processing, large-scale machine learning, knowledge extraction, and other problems. The resulting products represent an industrial synthesis of a series of complex innovations, linking machine intelligence, real-time sensing, and industrial design. These products are thus much closer to what big data’s proponents have promised—but their methods are a world away from the easy hype about mass-manufactured insights from the free raw material of digital exhaust.
5 Towards the Second Big Data Revolution
We’re stuck in the first industrial revolution. We have the power looms and the water mills, but wonder, given all the hype, at the absence of the Model Ts and telephones of our dreams. The answer is a hard one. The big gains from big data will require a transformation of organizational, technological, and economic operations on par with that of the second industrial revolution. Then, as now, firms had to invest heavily in industrial research and development to build the foundations of entirely new forms of value creation. Those foundations permitted entirely new business models, in contrast to the marginal changes of the first industrial revolution. And the raw materials of the first revolution proved only tangentially useful to the innovations of the second.
These differences portend a revolution of greater consequence and complexity. Firms will likely be larger. Innovation will rely less on small entrepreneurs, who lack the funds and scale for systems-level innovation. Where entrepreneurs do remain, they will play far more niche roles. As Rao ( 2012 ) has argued, startups will increasingly become outsourced R&D, whose innovations are acquired to become features of existing products rather than standalone products themselves. The success of systems-level innovation will threaten a range of current jobs—white collar and service sector as well as blue collar and manufacturing—as expanding algorithmic capacity widens the scope of digitizeable tasks. But unlike past revolutions, that expanding capacity also begs the question of where this revolution will find new forms of employment insulated from these technological forces; and if it does not, how we manage the social instability that will surely follow. With luck, we will resist the temptation to use those same algorithmic tools for social control. But human history on that point is not encouraging.
Regardless, we should resist the temptation to assume that a world of ubiquitous data means a world of cheap, abundant, and relevant raw materials for a new epoch of economic prosperity. The most abundant of those materials today turn out to have limited uses outside the narrow products and services that generate them. Overcoming that hurdle requires more than just smarter statisticians, better algorithms, or faster computation. Instead, it will require new business models capable of nurturing both new sources of data and new technologies into truly new products and services.
Boyd D, Marwick AE (2011) Social privacy in networked publics: teens’ attitudes, practices, and strategies. In: A decade in internet time: symposium on the dynamics of the internet and society. pp 1–29
Butler D (2013) When Google got flu wrong. Nature 494(7436):155
Article Google Scholar
Conover MD, Ratkiewicz J, Francisco M, Goncalves B, Flammini A, Menczer F (2011) Political polarization on Twitter. In: Proceedings of the Fifth International AAAI Conference on Weblogs and Social Media
Cook S, Conrad C, Fowlkes AL, Mohebbi MH (2011) Assessing Google Flu trends performance in the United States during the 2009 influenza virus a (H1N1) pandemic. PLoS One 6(8):1–8
DiGrazia J, McKelvey K, Bollen J, Rojas F (2013) More tweets, more votes: social media as a quantitative indicator of political behavior. PLoS ONE 8(11):1–5
Gayo-Avello D (2012) I wanted to predict elections with Twitter and all I got was this lousy paper: a balanced survey on election prediction using twitter data. arXiv preprint arXiv:1204.6441
Gayo-Avello D, Metaxas PT, Mustafaraj E (2011) Limits of electoral predictions using Twitter. In: Proceedings of the International Conference on Weblogs and Social Media (ICWSM) 21:2011
Ginsberg J, Mohebbi MH, Patel RS, Brammer L, Smolinski MS, Brilliant L (2008) Detecting influenza epidemics using search engine query data. Nature 457(7232):1012–1014
Halevy A, Norvig P, Pereira F (2009) The unreasonable effectiveness of data. Intell Syst IEEE 24(2):8–12
Huberty M (2013) Multi-cycle forecasting of congressional elections with social media. In: Proceedings of the 2nd Workshop on Politics, Elections, and Data (PLEAD), pp 23–30
Kramer A, Guillory J, Hancock J (2014) Experimental evidence of massive-scale emotional contagion through social networks. Proc Natl Acad Sci 111(24):8788–8790
Manyika J, Chui M, Brown B, Bughin J, Dobbs R, Roxburgh C, Byers AH (2011) Big data: the next frontier for innovation, competition, and productivity. McKinsey Global Institute Report
Mayer-Schönberger V, Cukier K (2013) Big data: a revolution that will transform how we live, work, and think. Eamon Dolan/Houghton Mifflin Harcourt
Rao V (2012) Entrepreneurs are the new labor. Forbes. http://www.forbes.com/sites/venkateshrao/2012/09/03/entrepreneurs-are-the-new-labor-part-i/ . Accessed 3 Sept 2014
Steadman I (2013) IBM’s Watson is better at diagnosing cancer than human doctors. Wired UK, February 11th
Wang W, Rothschild D, Goel S, Gelman A (2014) Forecasting elections with non-representative polls. Int J Forecast Forthcoming
Download references
Acknowledgments
This research is a part of the ongoing collaboration of BRIE, the Berkeley Roundtable on the International Economy at the University of California at Berkeley, and ETLA, The Research Institute of the Finnish Economy. This paper has benefited from extended discussions with Cathryn Carson, Drew Conway, Chris Diehl, Stu Feldman, David Gutelius, Jonathan Murray, Joseph Reisinger, Sean Taylor, Georg Zachmann, and John Zysman. All errors committed, and opinions expressed, remain solely my own.
Author information
Authors and affiliations.
Berkeley Roundtable on the International Economy, Berkeley, CA, USA
Mark Huberty
You can also search for this author in PubMed Google Scholar
Corresponding author
Correspondence to Mark Huberty .
Rights and permissions
Open Access This article is distributed under the terms of the Creative Commons Attribution License which permits any use, distribution, and reproduction in any medium, provided the original author(s) and the source are credited.
Reprints and permissions
About this article
Huberty, M. Awaiting the Second Big Data Revolution: From Digital Noise to Value Creation. J Ind Compet Trade 15 , 35–47 (2015). https://doi.org/10.1007/s10842-014-0190-4
Download citation
Received : 14 April 2014
Revised : 13 September 2014
Accepted : 03 December 2014
Published : 18 February 2015
Issue Date : March 2015
DOI : https://doi.org/10.1007/s10842-014-0190-4
Share this article
Anyone you share the following link with will be able to read this content:
Sorry, a shareable link is not currently available for this article.
Provided by the Springer Nature SharedIt content-sharing initiative
- Digitalization
- Value creation
- Business models
- Technological change
JEL Classification
- Find a journal
- Publish with us
- Track your research
IRIS - Institutional Research Information System
Purpose: The purpose of this paper is to outline an agenda for researching the relationship between technology-enabled networks – such as social media and big data – and the accounting function. In doing so, it links the contents of an unfolding area research with the papers published in this special issue of Accounting, Auditing and Accountability Journal. Design/methodology/approach: The paper surveys the existing literature, which is still in its infancy, and proposes ways in which to frame early and future research. The intention is not to offer a comprehensive review, but to stimulate and conversation. Findings: The authors review several existing studies exploring technology-enabled networks and highlight some of the key aspects featuring social media and big data, before offering a classification of existing research efforts, as well as opportunities for future research. Three areas of investigation are identified: new performance indicators based on social media and big data; governance of social media and big data information resources; and, finally, social media and big data’s alteration of information and decision-making processes. Originality/value: The authors are currently experiencing a technological revolution that will fundamentally change the way in which organisations, as well as individuals, operate. It is claimed that many knowledge-based jobs are being automated, as well as others transformed with, for example, data scientists ready to replace even the most qualified accountants. But, of course, similar claims have been made before and therefore, as academics, the authors are called upon to explore the impact of these technology-enabled networks further. This paper contributes by starting a debate and speculating on the possible research agendas ahead.
Accounting, accountability, social media and big data: revolution or hype? / Arnaboldi, Michela; Busco, Cristiano; Cuganesan, Suresh. - In: ACCOUNTING, AUDITING & ACCOUNTABILITY JOURNAL. - ISSN 1368-0668. - 30:4(2017), pp. 762-776. [10.1108/AAAJ-03-2017-2880]
Accounting, accountability, social media and big data: revolution or hype?
Arnaboldi, michela; busco, cristiano ; cuganesan, suresh, scheda breve scheda completa scheda completa (dc), pubblicazioni consigliate.
I documenti in IRIS sono protetti da copyright e tutti i diritti sono riservati, salvo diversa indicazione.
Informazioni

social impact
Conferma cancellazione.
Sei sicuro che questo prodotto debba essere cancellato?
simulazione ASN
- Data, AI, & Machine Learning
- Managing Technology
- Social Responsibility
- Workplace, Teams, & Culture
- AI & Machine Learning
- Diversity & Inclusion
- Big ideas Research Projects
Artificial Intelligence and Business Strategy
- Responsible AI
- Future of the Workforce
- Future of Leadership
- All Research Projects
- AI in Action
- Most Popular
- The Truth Behind the Nursing Crisis
- Work/23: The Big Shift
- Coaching for the Future-Forward Leader
- Measuring Culture
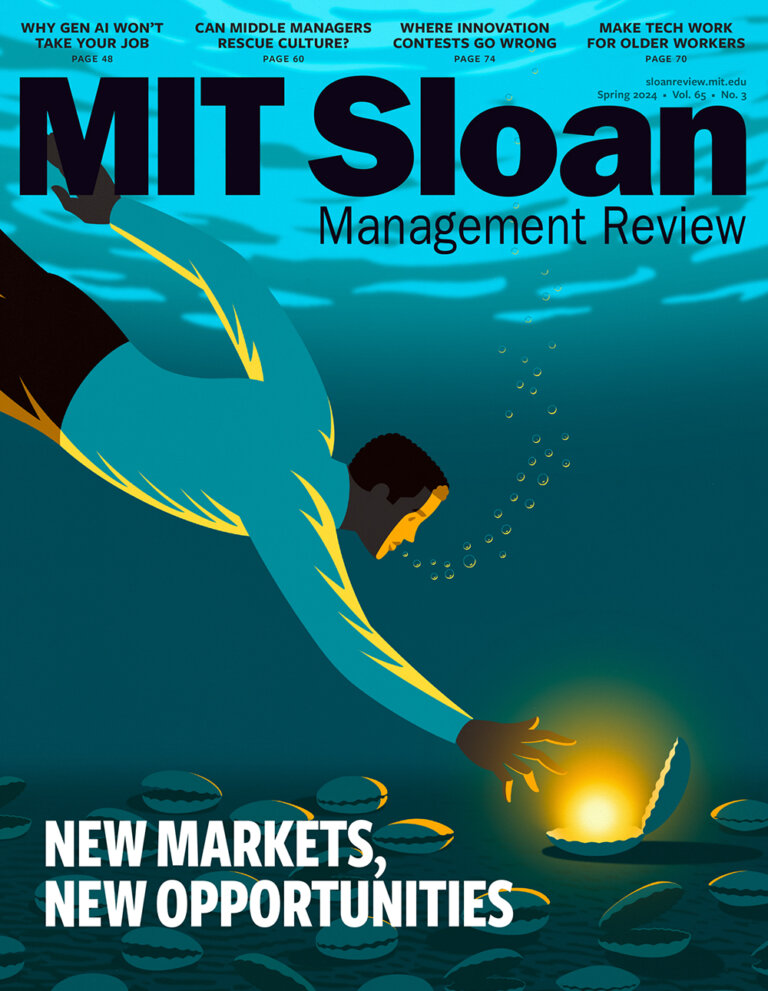
The spring 2024 issue’s special report looks at how to take advantage of market opportunities in the digital space, and provides advice on building culture and friendships at work; maximizing the benefits of LLMs, corporate venture capital initiatives, and innovation contests; and scaling automation and digital health platform.
- Past Issues
- Upcoming Events
- Video Archive
- Me, Myself, and AI
- Three Big Points
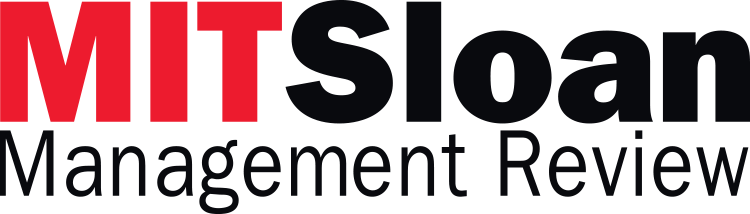
Me, Myself, and AI Episode 905
Ai hype and skepticism: economist paul romer.
The Nobel Prize-winning economist shares his views on artificial intelligence and innovation.

- Data, AI, & Machine Learning
- AI & Machine Learning
In collaboration with
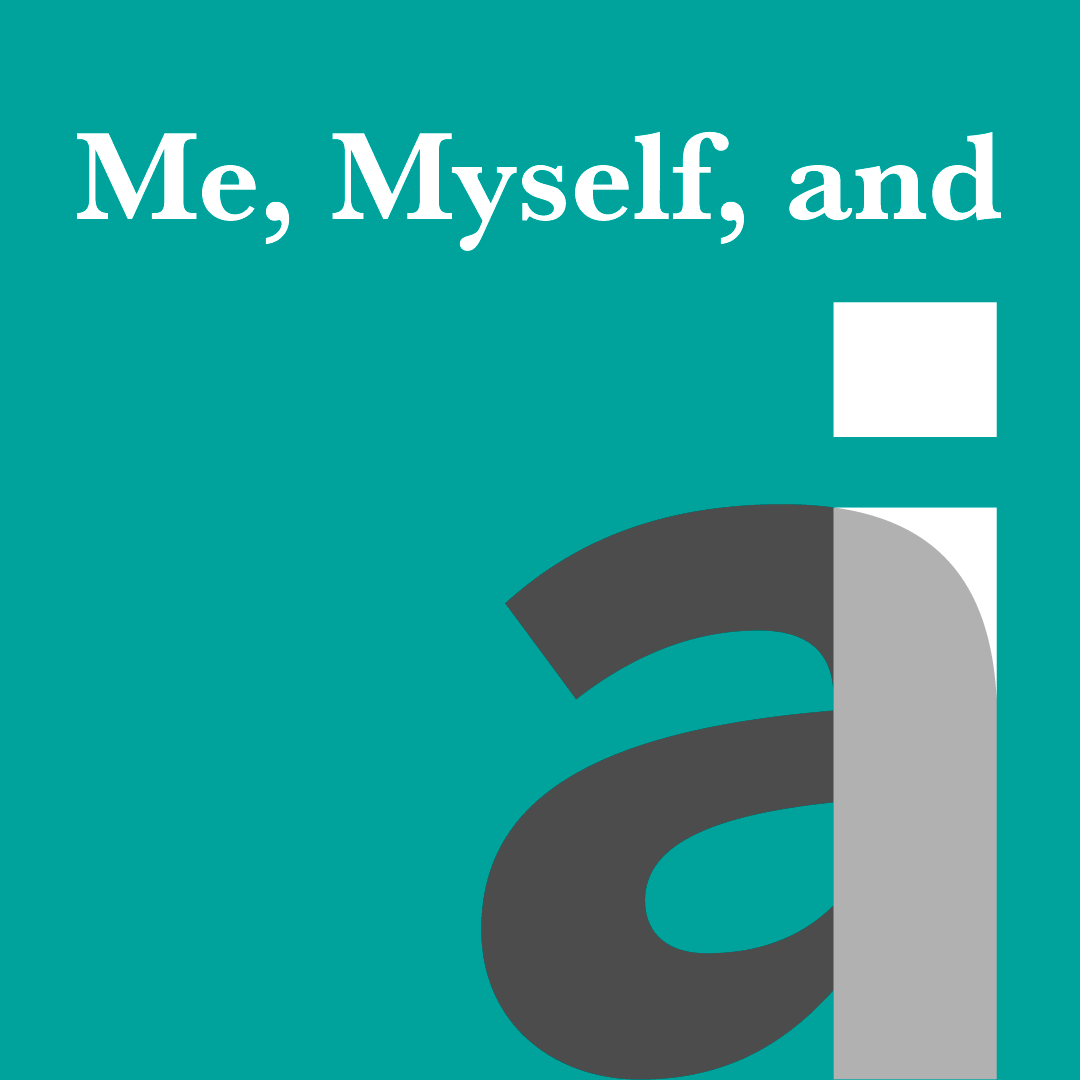
Get updates by email
Please enter a valid email address
Thank you for signing up
Privacy Policy
Paul Romer once considered himself the most optimistic economist. He rightfully predicted that technology would blow up as an economic driver coming out of the inflation of the 1970s but acknowledges he did not foresee the inequality that technology advances would lead to.
On this episode of the Me, Myself, and AI podcast, Paul shares his views on AI advances and their implications for society. Rather than pave the way for full automation, he is a proponent of keeping humans in the loop and believes that, rather than slowing down technology, it can be pointed in a direction for more meaningful and beneficial use, citing education as an area ripe to benefit from AI.
Subscribe to Me, Myself, and AI on Apple Podcasts or Spotify .
Shervin Khodabandeh: What does a Nobel Prize-winning economist think the future of AI holds? Find out on today’s episode.
Paul Romer: I’m economist Paul Romer, and you are listening to Me, Myself, and AI .
Sam Ransbotham: Welcome to Me, Myself, and AI , a podcast on artificial intelligence in business. Each episode, we introduce you to someone innovating with AI. I’m Sam Ransbotham, professor of analytics at Boston College. I’m also the AI and business strategy guest editor at MIT Sloan Management Review .
Shervin Khodabandeh: And I’m Shervin Khodabandeh, senior partner with BCG and one of the leaders of our AI business. Together, MIT SMR and BCG have been researching and publishing on AI since 2017, interviewing hundreds of practitioners and surveying thousands of companies on what it takes to build and to deploy and scale AI capabilities, and really transform the way organizations operate.
Sam Ransbotham: Hi, everyone. Today, Shervin and I have a fun treat for you. We’re joined by Paul Romer, winner of the 2018 Nobel Prize in economics. He’s a professor at Boston College and directs its new Center for the Economics of Ideas. Paul has a fascinating background, including time as the chief economist at the World Bank, founder of multiple companies, and avid contributor to public policy discussion everywhere. He’s really at the forefront of thinking about how the economics of ideas differs from the traditional economics of scarce physical objects. Beyond that, he is an interesting and nice person, so we’re thrilled to have him with us today. Thanks for taking the time to talk with us.
Paul Romer: Well, I’m glad to be here. It’s always fun to talk.
Sam Ransbotham: All right, let’s start off with the elephant in the room. There is a whole lot of talk about artificial intelligence right now. If we think about the spectrum of technologies and how they affect society, we might have the wheel and fire and electricity and the steam engine on one side and maybe transistors over there. And on the other hand, we have something like Segways and Clippy. Where are we in this spectrum with artificial intelligence, do you think?
Paul Romer: Let me start by repeating something I said in class yesterday, because my students are very excited about AI. I’m a little more skeptical. I think there’s a lot that will be possible because of AI, but I think people are buying a little bit too much of the hype and they’re losing perspective. I told them that I don’t think AI is actually the big revolution that we’re living through right now. The real revolution, I think, is stuff we’re seeing coming out of the war in Ukraine. The nature of that revolution is that sensors and devices are giving people the ability to do things that they could never do before.
The problem with the way most people are framing AI is they’re thinking about autonomous vehicles where you’re taking the human out of the loop; the technology is the replacement for the human. That is not working that well. The autonomous vehicles were supposed to be the killer application of AI, and it’s turning out to be a bust. There’s a reason Apple just canceled its car project.
But if you go back to using technology to enhance what people can do — you have an interface that lets a human interact with data from a lot of sensors and software that then translates what the human does in the interface into things that are happening out in the world — incredible things are going to come out of that. But this kind of just turn it over to the machine and let go, I think that’s going to turn out to be very disappointing.
Part of the way I tried to explain to the students how big this revolution is: If you look at an organization like the Marines, the Marines recognized a while ago [that] the tank is just history. The weapons like the tank are not going to be important going forward. And they’re thinking about things like electric motorcycles and portable missiles. The Air Force just canceled a big project that they had where they were building helicopters. They said the same thing; the helicopter is not going to work in this new world of sensors and devices.
So very big things are happening. I still think that this idea of just train the machine, use unsupervised learning, and it just gets really smart and does things for us [is not] where the action is going to be.
Shervin Khodabandeh: I agree with you, Paul, that today you can’t hand it over to the machine and expect it to take over. And I do believe that we humans have this amazing ability to make everything completely black and white. It’s either AI or nothing, or human or nothing. Totally aligned with you there. You also made the point that there is a real revolution going on, which is the proliferation of information and data and just our ability in instrumentation … and measurements. Where I might disagree with you is when Sam was making a parallel to other technologies [like] the wheel and the electric motor. All those things were disruptions and changed the way of working. They changed how humans worked in a factory. The electric motor changed how people worked, whether [they were] all aligned with one main shaft or in departments or compartments. What I would say is AI has begun to do that. And the reason I believe that is if I look at things that were uniquely human, [our] cognitive abilities — whether it’s vision or it’s logic or creating images and things like that — we’re seeing evidence that AI is doing those.
We’re also seeing evidence that AI is doing them now much faster than it used to do before. So whereas it maybe took 10 years for computer vision to surpass human ability, it took [AI] maybe one year or two. Of course, it built on decades of research, but since its introduction, it maybe took a couple of years for AI to be able to summarize books and text or create images and content. So I guess where I would debate what you’re saying is, don’t you think that if you play forward this evolution, maybe another three or five or 10 years, there will be a world where AI will do much more of the things that humans had a monopoly on?
Paul Romer: Yeah. So to recap — and it’s good to disagree. For academics, this is how we make our living. You disagree with people, and it’s the conversation [that] converges toward some notion of truth. So I’m arguing the way it’s always been and, in effect, the way it will always be, is that you keep the human in the loop and the tools make the human more powerful. Whereas you’re saying no, we’re starting to see demands where you don’t even need the human in the loop — the machines take over.
Shervin Khodabandeh: No, actually, I would agree with you that we always need a human in the loop. But I also would say that we need a lot less of a human in the loop, therefore that human could do a lot more other things, whatever those things might be. But I actually agree with you that you cannot have AI completely replace humans. On that, I agree with you.
Paul Romer: But I also mean this: Not for ethical reasons or to kind of supervise but just to get the job done, I think you’re going to need the human in the loop. And so I guess, let me try and restate the distinction: You’re thinking there are things that, say, people could just offload. We let the machine do it, and the human can build on that. There are some places where we’re close to that. I think one where I’ve actually used it myself is there’s a Whisper AI that will give you a text transcript of an audio recording. Maybe you produce text from this podcast. The AI, the machine, is pretty good at that. There are still mistakes. I still have fixed up those transcripts, but they’re pretty close to being things you could just turn loose and not participate.
But they still make mistakes. And so I still have to do a few fixes there. And I think that’s still going to be a lot of the experience. And I think that what we’ve seen repeatedly is that very rapid progress early on makes people think, “Oh, we’re going to very soon get to point X.”
We fail to anticipate how quickly the diminishing returns or the extra effort kicks in. Autonomous vehicles made rapid progress initially. Now they’re finding that the few rare cases that come up, that are very difficult to anticipate, are very hard to solve. So I think we’ve got to be careful about this extrapolation from rapid progress. And we’ve got to look and say, “Where are the cases where we’ve actually gotten all the way to the point where we’ve just taken the human out of the loop?” And if it’s hard to get there, in most cases, we’re going to make systematic mistakes if we’re betting based on the high rate of initial progress.
Shervin Khodabandeh: I totally agree with you. And I think that there might be even no-fly zones altogether in areas where this expectation that AI is 100% removing humans is a false expectation.
Paul Romer: But let me drill in on this. Do you think that autonomous vehicles are going to work? Is there money to be made investing in autonomous vehicles?
Shervin Khodabandeh: I don’t know the full economics of it, right? But from a capability perspective, I would say that there is a future, maybe it’s in 10 years, maybe it’s in five. It’s not in one or two, but not in 50. I think there’s a future where there will be autonomous vehicles that, in aggregate, would make far fewer errors and fatalities than human drivers do.
Sam Ransbotham: Shervin, that’s an important point. Our benchmark isn’t perfect humans. We had 43,000 deaths last year in the U.S. from human drivers. One way to think about this would be to separate out the problem. For example, think about the difference between long-haul trucking and driving around narrow Boston streets. It’s much easier for me to see that we’d make progress on long-haul trucking first. We talk about these problems in big clumps, but we just don’t have to.
Paul Romer: I think I’m still a lot more skeptical than you about the autonomous vehicles. I think we’ll actually create some new domains where semiautonomous or fully autonomous vehicles can operate, like tractors. You know, John Deere is pursuing this.
But I think it’s revealing that Apple has just decided there’s no money to be made in this line of investment after investing a bunch of money. I also think it’s important to remember that what matters here is the social acceptability of these technologies. It isn’t just whether somebody can argue in statistical terms that this is safer than what we usually see with people. If you look at airplanes, you could argue that we’re making airplane travel way too safe, because it’s much more dangerous to ride on a bus or take a train, but [it’s] very clear the public does not want aircraft travel to be more dangerous. So we have to just accept that even if, on average, the autonomous vehicles are, say, safer than the people, voters are not going to accept machines that kill people.
Shervin Khodabandeh: Or the same could be said in medicine, right? I mean, there are examples of AI in radiology where it is performing better than radiologists. I would still like a real radiologist and a real doctor.
Sam Ransbotham: But, Shervin, you want the doctor, because you live in Los Angeles, where you’ve got access to great medical facilities there and so do we in Boston. I think if we had a conversation with some different people, we might have a different perspective.
Shervin Khodabandeh: Yeah, that’s a good point, too. When I think about most of what humans do, when I think about the rest of everything that people do in their regular jobs — which is sort through documents, summarize things, search knowledge — more and more of those things can be done with AI, arguably better.
Paul Romer: I think my answer is, let’s take it one step at a time. Let’s start with the things where I think we can make some adjustments, trying manufacturing, but watching that alone may not be enough. Then I think there are tools we could use. You could, for example, use the tax system that subsidizes employment of, say, manufacturing workers, so that the trade-off for any one firm might be, “OK, well, I can get subsidized workers, or I can just pay the full freight.” Or you could tax higher-income workers to subsidize the lower-income workers, or you could tax the machines or tax the transistors but use the revenues to then, say, subsidize the employment of the workers.
Shervin Khodabandeh: Do you think one of these tools could be or should be in [the] regulation of AI? And, look, I’m hardly the person to advocate something against innovation. Right? It’s like my own passion and my own career. And so I don’t mean it in a way of completely stifling innovation, but do you think that there is a time and place for governments to play a much heavier, a stronger sort of role in no-fly zones with AI, things that you can and cannot do? I mean, [this is] not that different [than] what we did with some of the genetic sciences, right? There are certain things that we don’t allow people to do, even though we can do it.
Paul Romer: Let me get at what I think is part of your question and even sharpen it. This idea of allowing the globalization of ideas and sharing of ideas, I was saying we’re still committed to the discovery of new ideas and to innovation. Other people are saying, “Well, we not only want to limit how you turn those ideas into physical products. We may even want to steer innovation in a particular direction, like steer it away from some things and steer it toward other things, but then we might even get to a point where we say, ‘No, we just want to slow the innovation down. We want to slow down progress because it’s just too hard to cope with the stresses that will cause.’”
I haven’t gotten to that point yet where I say, as a voter, I’m ready to slow things down. But I can see the possibility of coming to that conclusion. If you think the choice is we’re going to lose democracy and rule of law or we’re going to slow down technology, I wouldn’t have much trouble making that choice. I don’t think we’re there. At least that’s not the first thing we should try. But I think we should think carefully about what our priorities are. And I don’t think technology for its own sake is the be-all and end-all.
Sam Ransbotham: I like the idea of pausing. It’s always appealing. I have teenage kids, Shervin has teenage kids. I think the idea of pausing and enjoying that period longer, that seems great, but I don’t know how realistic that is. You know, my background was working with the United Nations and the weapons inspectors in the weapons of mass destruction phase. We’ve done a very good job of limiting that. We haven’t had any big explosions since 1945.
But that had physical goods to it. It had a physical thing that you could control. And the same thing is true with, for example, food safety. We have inspectors. We have regulations, supply chain. We have things we can regulate. Do we have the same tools that we had in prior iterations of technology, like biotech?
Paul Romer: I was thinking the same thing before Sam spoke, which is that even if I agreed [that] things have gotten so serious [that] I’m ready to try and slow down discovery, you have to ask, Is it even feasible for us to slow down? And that’s going to be a real constraint.
Sam Ransbotham: Some of your ideas here about jobs seem like they depended on the idea that there’s still going to be this Zeno’s paradox of approaching full automation. And as long as that exists, we’ll still have a human role. So that’s a pretty appealing argument as a human. I like that idea.
Paul Romer: Yeah. But if I go to this older idea of the race between education and technology, I think one of the things we have to keep in mind is that we could slow down the technology, but we could also speed up education. And we should think really hard about what do we do to keep raising skill levels. Traditionally, we did that through things like extending the period of life that somebody spends in school. That’s getting harder and harder to do. We might get better at getting more productivity out of the years that people spend in school. But a lot of people put work into that, and we haven’t made a lot of progress there either. The point that I got focused on at the World Bank was that a huge amount of skill acquisition actually comes on the job.
If you look at a society, a typical society, it may produce about as much skill through learning on the job as it produces in its school system. The average amount of skill we think is produced per year in school is higher than the amount for, say, one person for a year in school, or one person for a year in work. You don’t learn as much when you work, but there are a lot more people who are working. So you can end up producing as much human capital on the job. And I think we should be paying much more attention to the potential for jobs to actually enhance skill. This is actually one of my biggest concerns about some of the applications of AI right now.
Have you had Sal Khan from Khan Academy on your podcast?
Sam Ransbotham: No.
Paul Romer: I haven’t spoken to him ever, but I know he’s an optimist about how AI might actually be able to improve the rate of learning.
Sam Ransbotham: We did have [Zan Gilani from] Duolingo . I think Duolingo was a great example of exactly that.
Paul Romer: They’re another one. And they’ve kind of delivered, I think, on that. So those are the people who are optimistic about AI, who I frankly put some stock in what they’re saying. If we took that seriously and said that the only way this will be socially acceptable is [if] we do a better job of educating, we’d better put a lot of resources into figuring out how to use AI to improve education and to measure so we know that we’re really improving. And if we did that, we might come out OK.
Sam Ransbotham: We have to be careful because we’re university professors here, but the idea of this micro learning is a big deal. We’ve somehow gotten wrapped in this idea that the 15-week semester is the magic unit of time, and three hours per week is magic. And there’s nothing that says that’s the case.
Paul Romer: My wife is a big user of Duolingo, and she keeps learning another new language. It seems to work for her, but I’d love to have some more evidence about how Duolingo or how Khan Academy can work. Right now, the big successes in those areas seem to be in enhancing opportunities for people who are already pretty good at learning languages or learning skills, so what would really be great is to see how we use AI to help the bottom half of the class in school. But maybe that’s the optimistic message to come out of this. It’s a little bit like what I was saying — instead of trying to slow down technology, let’s point it in a direction.
I’m really impressed with what the military is doing in trying to understand what you can do with these technologies and do something to meet their mission. If we were as serious about using AI in improving education as the military is — part of what I like about the military is they know they can’t survive on hype. It’s really got to deliver or they’re going to fail. But if we were that serious about using AI and developing it to help us in education, then we might actually end up with better technology and benefits that are more widely shared.
Shervin Khodabandeh: And now the question is, How can we use the capability and direct it in that way? And I think what I’m taking away from this conversation is it’s just not going to happen necessarily automatically. Because automatically what would happen is probably what you said, which is more efficiency, think more widgets per hour, less skills. That’s probably [taken] for granted.
Paul Romer: And more and more inequality.
Shervin Khodabandeh: Yep.
Paul Romer: It’s interesting, as I listened to you, I used to say that I was the most optimistic economist I knew. Back in the 1980s, as we were coming out of the inflation of the ’70s and limits to growth, and just this doomster kind of mindset, I was saying, “No, look, technology can go like gangbusters,” and I was kind of right about that. What I didn’t anticipate, though, is that it could also — if we didn’t take the right supporting measures — lead to a lot of inequality. I’m really disturbed by the degree to which U.S. society has both benefited from very rapid technology but has opened up these big, growing inequality gaps . And it’s very hard to get at this with just the straight income inequality data, but look at life expectancy. People who are just high school educated are not increasing their life expectancy the way people who are college educated have; they’re even suffering real decreases in life expectancy. Life is really not turning out as well for them. I really feel like the failure was not [about having] enough technological development, but we didn’t share the gains widely enough. And we’ve got to work hard at figuring out how to do a better job with that, going forward.
Shervin Khodabandeh: Yeah. Well said.
Sam Ransbotham: One of the [ways] we close this show is by asking five questions. Our first question is usually, What’s the biggest opportunity with AI? I think you’ve just mentioned learning, and I think we’ll take that as your first answer.
Paul Romer: OK.
Sam Ransbotham: But what’s the biggest misconception about AI people have?
Paul Romer: The idea that AI can write software. The idea that it can write code. I think that’s just claiming way too much.
Sam Ransbotham: What’s the first career you wanted?
Paul Romer: I wanted to be a physicist. I switched from physics as an undergrad to economics because I thought physics would be too hard. And it was also a time when the U.S. was closing down the space program, and there weren’t as many jobs in physics and so forth. But for most of my life, I wanted to be a software developer, and now I’ve had a chance to play at that for five years. Maybe I could try and be a physicist in another five years.
Sam Ransbotham: When are we using too much AI? When do we need less AI?
Paul Romer: You and I have talked about this, but I think we’ve got to make documentation easier for students to access, particularly the Python documentation, because if it were easier or a little bit more responsive, I think they’d spend more time checking out the documentation and they’d learn a lot from that. Just asking one-off queries, like, “How do I do this? How do I do that?” I don’t think they’re seeing the big picture. We’re doing too much of ChatGPT-type questions about Python and not enough students reading good documentation.
Sam Ransbotham: Just the backstory. Paul and I spend a lot of time arguing about Python and how to help people get sharper on Python. What’s one thing you wish AI could do that it can’t?
Paul Romer: I wish I could manage my time better. I think a lot of what we learn is not so much facts we retain, but we learn to keep going even when things are going badly. [Say we hit] a wall and we’re stuck. How do you keep going on things? I wish AI could help me, almost like a coach. I wish I had an AI coach that helped me know, because it’s a very subtle decision. Sometimes you’ve got to just drop it. It’s not going to work. Give up on that path, go do something else.
Shervin Khodabandeh: I was going to ask, Would you listen to it?
Paul Romer: If I controlled it, if I could control it, yeah, maybe.
Sam Ransbotham: We didn’t even get into that — the whole thing about the domination by the tech giants here. Paul, it’s been great. I know we’re running short on time. It’s been great talking to you. Thanks for taking the time to talk with us today.
Paul Romer: Well, maybe we should make this an annual thing. Have me back again in a year and we can say, “OK, let’s update which direction have things gone? Have they gone the way we thought? Or what was new?”
Shervin Khodabandeh: We’d love that. At the rate it’s going, maybe it should be semiannual.
Thanks for joining us today. Next time, Sam and I meet Mario Rodriguez at GitHub. Talk to you then.
Allison Ryder: Thanks for listening to Me, Myself, and AI . We believe, like you, that the conversation about AI implementation doesn’t start and stop with this podcast. That’s why we’ve created a group on LinkedIn specifically for listeners like you. It’s called AI for Leaders, and if you join us, you can chat with show creators and hosts, ask your own questions, share your insights, and gain access to valuable resources about AI implementation from MIT SMR and BCG. You can access it by visiting mitsmr.com/AIforLeaders . We’ll put that link in the show notes, and we hope to see you there.
About the Hosts
Sam Ransbotham ( @ransbotham ) is a professor in the information systems department at the Carroll School of Management at Boston College, as well as guest editor for MIT Sloan Management Review ’s Artificial Intelligence and Business Strategy Big Ideas initiative. Shervin Khodabandeh is a senior partner and managing director at BCG and the coleader of BCG GAMMA (BCG’s AI practice) in North America. He can be contacted at [email protected] .
Me, Myself, and AI is a collaborative podcast from MIT Sloan Management Review and Boston Consulting Group and is hosted by Sam Ransbotham and Shervin Khodabandeh. Our engineer is David Lishansky, and the coordinating producers are Allison Ryder and Sophie Rüdinger.
More Like This
Add a comment cancel reply.
You must sign in to post a comment. First time here? Sign up for a free account : Comment on articles and get access to many more articles.
Subscribe to Me, Myself, and AI
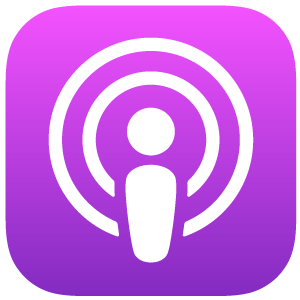

- SUGGESTED TOPICS
- The Magazine
- Newsletters
- Managing Yourself
- Managing Teams
- Work-life Balance
- The Big Idea
- Data & Visuals
- Reading Lists
- Case Selections
- HBR Learning
- Topic Feeds
- Account Settings
- Email Preferences
Data Readiness for the AI Revolution
Pulse Survey Sponsored By Microsoft and Profisee

Download the report
Businesses are bracing themselves for an era of supercharged innovation and productivity sparked by artificial intelligence (AI) and the arrival of generative AI (gen AI). But as companies take bold steps into the world of AI—or imagine what this future could look like for them—many are finding they do not have the solid and reliable data foundation needed to harness this technology in a safe and value-enhancing way.
OpenAI may have finally cracked the code on voice interactions with AI
- This post originally appeared in the Insider Today newsletter.
- You can sign up for Business Insider's daily newsletter here .

Hello! Miss Teen USA is turning into the pageant no one seems to want to win. After the initial winner resigned her position, this year's first runner-up declined to take over as queen . Here's a timeline of all the drama .
In today's big story, everyone's talking — literally — about the big update for OpenAI's ChatGPT that could make your job a lot easier .
What's on deck:
- Markets: The leader of the meme-stock revolution reemerged.
- Tech: Apple needs a W in the form of a big, new product .
- Business: Walmart's CEO has some career advice, and a big piece of it is living in the moment .
But first, let's chat.
If this was forwarded to you, sign up here.
The big story
Putting the chat in chatgpt.
First came HAL. Then came Her. Now there is GPT-4o.
Hollywood has a history of humanlike AI talking to people. But their real-world counterparts never seemed to live up to the hype, sounding too robotic or taking too long to respond.
OpenAI may have finally cracked the code on voice interactions with AI, though. The company unveiled an upgrade for ChatGPT that includes an impressively humanlike voice feature made possible by its new flagship model, GPT-4o .
During a live presentation, OpenAI's latest product helped with breathing exercises, walked a user through a math equation, and served as a translator. OpenAI says GPT-4o's average response to audio inputs is 320 milliseconds, which is on par with a typical human-to-human conversation.
The internet was impressed, to say the least. Sharing your screen with ChatGPT while talking through problems could be a game changer for coding and data-focused work , writes Business Insider's Alex Bitter.
One developer I texted after the event raved about the potential and was excited to test it out.
Typing out class or API-specific names into questions for ChatGPT while coding was tedious, they said. The demo made it seem as if they could talk to ChatGPT like a coworker.
"They've essentially made text input almost be the barbaric form of communicating with ChatGPT (or any AI) now," the developer said.
OpenAI's big reveal shows the shockwaves the startup keeps sending through the tech world.
This time around, it's likely Apple that is feeling the heat , writes BI's Katie Notopoulos.
Before Monday's event, a report detailed how ChatGPT prompted Apple executives to realize the digital assistant Siri needed a major upgrade . Those updates, which are expected to come during Apple's Worldwide Developers Conference in June, will now undoubtedly be judged against GPT-4o.
But it's not just Apple that's been put on its back foot.
For months there has been heavy speculation that OpenAI is developing a web search product to compete with Google Search . OpenAI is even reportedly trying to snag Googlers from its search team.
In the meantime, Google I/O, the company's biggest developer event, kicks off today, and AI will surely be at the forefront.
Even those deeply invested in OpenAI's success are getting competitive with the red-hot startup.
Microsoft, which has poured $10 billion into OpenAI, is reportedly building its own AI model in an effort to show the tech giant doesn't plan to just ride OpenAI's coattails during the AI wars.
3 things in markets
- The dollar can't be dethroned. That's according to Morgan Stanley strategists, who outlined three reasons the greenback will maintain its status as the world's dominant currency on a podcast last week. "Bottom line, King dollar doesn't really have any challengers," Michael Zezas, the bank's head of US public policy research, said.
- Roaring Kitty's back, and meme stocks are loving it. Keith Gill, a key figure in the GameStop short squeeze of 2021, posted on X for the first time in nearly three years . Naturally, r/wallstreetbets took it in stride, and by that, I mean shares of GameStop surged as much as 118% .
- A top economist predicts the US's debt-fueled growth will catch up to it in 2025. Apollo's Torsten Slok said the economy will be alright for a few more quarters, but next year will be a different story . Consumers and businesses are carrying too much debt, and a hard landing is coming, he said.
3 things in tech
- Apple desperately needs its Next Big Thing. Under Tim Cook's tenure, there have been no earth-shattering product launches — which is exactly what Apple needs right now . With iPhone sales slowing and revenue falling, it's time for the company to reclaim its innovative edge.
- Mayhem at Tesla continues. The company rescinded job offers for incoming full-time employees . The move is part of broader cuts at Tesla, which have spanned the past several weeks and aim to eliminate over 10% of its workforce.
- Happy birthday, Zuck. Meta CEO Mark Zuckerberg turns 40 today . Over the past 10 years, the world's fourth-richest man's wealth has surged — and he's used his ballooning personal fortune to build up a $200 million real estate portfolio and buy himself a megayacht.
3 things in business
- Melinda French Gates steps down. In a statement on X, French Gates announced she'd resigned as co-chair of the Bill & Melinda Gates Foundation . She'll have "an additional $12.5 billion to commit to my work on behalf of women and families," she said, thanks to an arrangement with her ex-husband, Bill Gates.
- Tips for success, according to Walmart's CEO. Speaking at the University of Arkansas' commencement ceremony, CEO Doug McMillon shared three pieces of advice : Be present, choose a career that doesn't feel like work, and assume positive intent from others.
- It's not all plain sailing for child-free adults. DINKs (dual-income couples with no children) are known for having disposable income and spending their paychecks on major investments, luxury vacations, and early retirement. But a growing number of child-free adults are living paycheck to paycheck because it's harder for them to access tax credits and government assistance.
What's happening today
- Today's earnings: Alibaba, Home Depot, Sony, and other companies are reporting .
- Disney is hosting its upfront presentation, unveiling its 2024-25 program lineups.
- Google's Input/Output annual developer conference is today.
- Google's new Pixel 8a smartphone is available to buy.
- Cannes Film Festival kicks off today.
The Insider Today team: Dan DeFrancesco , deputy editor and anchor, in New York. Jordan Parker Erb , editor, in New York. Hallam Bullock , senior editor, in London. George Glover , reporter, in London.
Axel Springer, Business Insider's parent company, has a global deal to allow OpenAI to train its models on its media brands' reporting.
Watch: What is ChatGPT, and should we be afraid of AI chatbots?
- Main content
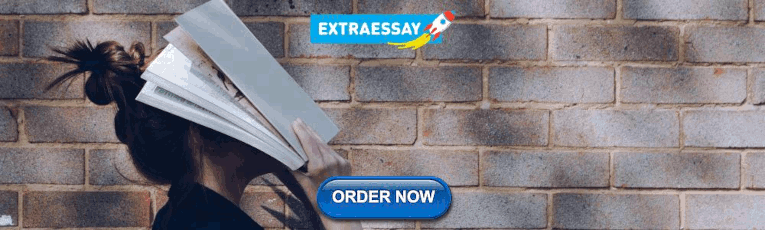
IMAGES
VIDEO
COMMENTS
Module Outline. Big data is said to be transforming science and social science. In this module, you will critically engage with this claim and explore the ways in which the rapid rise of big data impacts on research processes and practices in a growing range of disciplinary areas and fields of study. In particular, the module considers the ...
Big Data - Hype or Revolution? Rob Kitchin IntroductIon The etymology of 'big data' can be traced to the mid-1990s, first used to refer to the han-dling and analysis of massive datasets (Diebold, 2012). Laney (2001) refined the definition to refer to data characterized by the now standard 3Vs, with big data being:
You will also examine how we might we use big data research both as a way to resist and/or shape global transformations, how big data might impact on the future of social science, and what challenges lie ahead for social science research given the impact of big data.
Module Description. Big data is said to be transforming science and social science. In this module, you will critically engage with this claim and explore the ways in which the rapid rise of big data impacts on research processes and practices in a growing range of disciplinary areas and fields of study. In particular, the module considers the ...
Semantic Scholar extracted view of "Big Data - Hype or Revolution?" by Rob Kitchin. Skip to search form Skip to main content Skip to account menu. Semantic Scholar's Logo. Search 216,881,724 papers from all fields of science. Search. Sign In Create Free Account. DOI: 10.4135/9781473983847.N3;
This research is justified by the need to identify governance structures that promote the expansion of ways of processing accounting data and that address the changes brought about by the current ...
The intention is not to offer a comprehensive review, but to stimulate and conversation.,The authors review several existing studies exploring technology-enabled networks and highlight some of the key aspects featuring social media and big data, before offering a classification of existing research efforts, as well as opportunities for future ...
Poor data quality, incompatible datasets, inadequate expertise, and hype Big data refers to datasets that are too large or complex to analyse with traditional methods.1 Instead we rely on machine learning—self updating algorithms that build predictive models by finding patterns in data.2 In recent years, a so called "big data revolution" in healthcare has been promised345 so often that ...
Big Data reached the peak of its hype cycle in 2014, and then outgrew its own position - in 2015, Forrester dropped Big Data off of its annual hype cycle for emerging technologies report, their lead analyst, Betsy Burton, explaining that "Big Data has become a part of many hype cycles."1 Illustrating this fact,
The Data Revolution is a must read for anyone interested in why data have become so important in the contemporary era. Thoroughly updated, including ten new chapters, the book provides an accessible and comprehensive: introduction to thinking conceptually about the nature of data and the field of critical data studies overview of big data, open ...
Kitchin, Rob (2016) Big Data - Hype or Revolution? In: The SAGE Handbook of Social Media Research Methods. SAGE Publications, pp. 27-39. ISBN 9781473916326
The differences between big data and analytics are a matter of volume, velocity, and variety: More data now cross the internet every second than were stored in the entire internet 20 years ago.
In fact, the global data analytics market is expected to witness expansion with projections showing it skyrocketing from $61.44 billion in 2023 to $581.34 billion in 2033. These substantial ...
Three areas of investigation are identified: new performance indicators based on social media and big data;governance of social media and big data information resources; and, finally, social media and big data's alteration of information and decision-making processes.Originality/value - We are currently experiencing a technological ...
Downloadable (with restrictions)! Purpose - The purpose of this paper is to outline an agenda for researching the relationship between technology-enabled networks - such as social media and big data - and the accounting function. In doing so, it links the contents of an unfolding area research with the papers published in this special issue ofAccounting, Auditing and Accountability Journal.
Publication date: October 2013. Publisher: John Murray. ISBN: 9781848547926. Society is going from a constant shortage of data to a surfeit of information - and this upends everything. In this groundbreaking and fascinating book, two of the world's most-respected data experts reveal the reality of a big data world.
"Carefully distinguishing between big data and open data, and exploring various data infrastructures, Kitchin vividly illustrates how the data landscape is rapidly changing and calls for a revolution in how we think about data." - Evelyn Ruppert, Goldsmiths, University of London "Deconstructs the hype around the 'data revolution' to carefully guide us through the histories and the futures ...
Accounting, accountability, social media and big data: revolution or hype? Michela Arnaboldi, Cristiano Busco and Suresh Cuganesan. Accounting, Auditing & Accountability Journal, 2017, vol. 30, issue 4, 762-776 . Abstract: Purpose - The purpose of this paper is to outline an agenda for researching the relationship between technology-enabled networks - such as social media and big data ...
Content and teaching | Assessment | Availability. Module content and teaching Principal aims • To introduce students to some of the rising issues and challenges at the forefront of big data research; • To explore the extent to which big data problematise core methodological issues in research; • To apply general issues involved in doing research with big data to more specific thematic ...
Downloadable (with restrictions)! Author(s): Michela Arnaboldi & Cristiano Busco & Suresh Cuganesan. 2017 Abstract: Purpose - The purpose of this paper is to outline an agenda for researching the relationship between technology-enabled networks - such as social media and big data - and the accounting function. In doing so, it links the contents of an unfolding area research with the papers ...
"Big data"—the collection of vast quantities of data about individual behavior via online, mobile, and other data-driven services—has been heralded as the agent of a third industrial revolution—one with raw materials measured in bits, rather than tons of steel or barrels of oil. Yet the industrial revolution transformed not just how firms made things, but the fundamental approach to ...
An agenda for researching the relationship between technology-enabled networks - such as social media and big data - and the accounting function is outlined and a classification of existing research efforts, as well as opportunities for future research are offered. Purpose - The purpose of this paper is to outline an agenda for researching the relationship between technology-enabled ...
A first step toward an inclusive big data research agenda for IS is offered by focusing on the interplay between big data's characteristics, the information value chain encompassing people-process-technology, and the three dominant IS research traditions (behavioral, design, and economics of IS). Expand
research. 2. Big Data and Implications for Accounting . A large attention by practitioner and academics on has emerged from the Big Data diffusion of social media application, and the related possibility to observe and crawl data from public interfaces. However, the term Big Data does not refer solely to Social Media Data but
Accounting, accountability, social media and big data: revolution or hype? BUSCO, CRISTIANO; 2017 Abstract Purpose: The purpose of this paper is to outline an agenda for researching the relationship between technology-enabled networks - such as social media and big data - and the accounting function.
A recipient of the 2018 Nobel Prize in economics, Romer is a research associate at the National Bureau of Economic Research, a fellow of the American Academy of Arts and Sciences, and a nonresident scholar at the Macdonald-Laurier Institute. He previously served as the chief economist at the World Bank and was the founding director of New York ...
Data Readiness for the AI Revolution. May 01, 2024. Download the report. Businesses are bracing themselves for an era of supercharged innovation and productivity sparked by artificial intelligence ...
The company unveiled an upgrade for ChatGPT that includes an impressively humanlike voice feature made possible by its new flagship model, GPT-4o. During a live presentation, OpenAI's latest ...