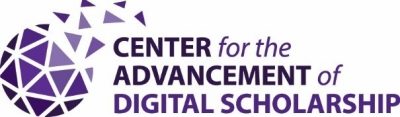
Want to create or adapt books like this? Learn more about how Pressbooks supports open publishing practices.
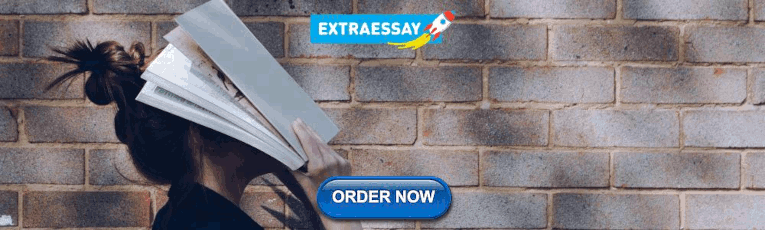
6 Analyzing Data from Your Classroom
ESSENTIAL QUESTIONS
- What are the best ways to organize and analyze your data?
- What methods of data analysis will be most effective for your study?
- What claims can you make after analyzing your data?
- How do your claims contribute to the knowledge base?
You’ve determined the methods for data collection and then collected that data for your action research project. It is now time to conduct the analysis of your data, which precedes drawing conclusions and sharing your findings. During your action research project, you have been informally analyzing your data and now you can formally analyze to develop findings and reflect on their implications for practice. This will also provide an opportunity to identify unanswered questions and possible new directions. As an action researcher, you will create a coherent and reliable story from all the data collected. This is a key part of the professional development or professional learning aspect of action research. As an action researcher, you are looking to create meaning from your practice by utilizing rich descriptions and narratives, and you are developing expertise by examining situations closely and analyzing them.
Beginning the Meaning Making Process
Before you begin your data analysis, you should revisit the intended goals of the project. Equally, you should think about your research question and reacquaint yourself with your literature review to clearly envision what you have been investigating and why. The goal of data analysis is to identify themes and patterns to provide robust evidence for any claims you are able to make from your findings. You will need to look at the data you have collected from several sources and relate these to your original, expected, outcomes. Of course, you will also be mindful of unexpected outcomes which may be of significance to your study too. Your conclusions should relate to the original intended objectives of the study. Again, your literature review will also help with the analysis, and it will provide distinctions in terms of what we know and don’t know as a field of study. Your findings should either confirm previous literature or provide new knowledge in relation to previous literature.
During this stage of the project, it is also important for you to reflect on the research process itself. Did your project go as planned? What would you do differently? What were the biggest challenges? For those interested in your study, they will be interested in knowing about your challenges, as well as your successes.
Organizing your Data
You will want to make a note of everything you possibly can when you collect data. As you organize your data, take a look at the notes or personal journal you have kept during your data collection period. Your notes may reveal that you have initiated important data analysis in the time and space of collecting the data. This sort of analysis could be personal notes on the themes which related to your original research aims and questions. You may have even made determinations about whether to gather additional data. More importantly, you may have noted some unanticipated themes or ideas that emerged during data collection. I think it is valuable to put these things to the front of your mind as you organize your data and begin the analysis stage.
Analysis and Presentation of your Data
I would like to address some general issues related to analyzing and presenting your data. Here are links to specific examples of data analysis in four action research articles, in the journal Networks: An Online Journal for Teacher Research. These are not necessarily ideal examples, but they provide a variety to spark thinking about your own study and discussion among your classmates.
Using quantitative data
You may have collected some quantitative data to provide demographic, contextual, or academic background for your study. Quantitative data helps support, supplement, and complement the qualitative data you have collected. While it is likely that you have not collected a massive amount of quantitative data, any amount will support a stronger argument. You should be able to analyze and represent the quantitative data using tables or charts. Computer software, such as Microsoft Excel, is suitable for this purpose, and can even handle basic correlations between multiple sets of data (e.g., gender and test scores). If an action research project involves several sites and the data are extensive, you may consider using a statistical package, such as IBM’s SPSS. Your quantitative data can be presented in charts and graphs developed by these programs. Including charts and graphs is worthwhile for two reasons. First, a visual representation is often easier for many readers to understand when digesting data-based information. Second, visual representations break up continuous narratives which can useful when conveying a considerable amount of numerical data and the subsequent correlations. Visual representations can also be an effective way to present qualitative data, or to at least give the reader a glimpse or preview of the data as a whole representation before reading the narrative.
Using qualitative data
Since your action research project was probably located within your professional context and focused on your practice, you likely explored attitudes, behaviors, and feelings that required collecting qualitative data. Most of this data will be in the form of descriptive text or short answer text, which you will need to analyze and interpret. For qualitative data, analysis of the text will require you to develop an analytical framework to use as the basis of analysis. This framework can also be subjective, so being clear and upfront about your framework is important for validity and reliability. If your data collection resulted in a large amount of descriptive text, it may seem overwhelming to analyze. This is quite normal for a qualitative study and having a lot of data means that you will have plenty to work from. If you have a considerable amount of descriptive data, you could use computer software (as outlined later in this chapter) which is relatively simple to use.
Transcription of your data is something that is often overlooked, or avoided, for several reasons. For some types of data collection (e.g., interviews, focus groups, discussions, etc.) it is useful to have the recording transcribed so that you can analyze the text more easily than listening to the recording. Transcribing is often avoided because of time constraints (or because the researcher cannot afford to have someone transcribe for them). However, if you are going to analyze the data, you should not think of it as purely transcription – it is your first opportunity to engage with the data. This will facilitate a more efficient process of analysis as it will more than likely be the second time you have engaged with the data. If you have previewed or already experienced the data, to save time you might transcribe only the parts that are pertinent to the study or your interest.
Analyzing qualitative data
For better, or worse, there is no universally correct way to analyze qualitative data; however, it is important to be systematic in your method of analysis. As I mentioned earlier, your data analysis probably started initially during your data collection. The questions you asked, the frameworks that you used, and the types of documents you collected would have provided some themes and categories that naturally developed as part of this process. I have suggested to new researchers a step-by-step approach to help them get started:
- Organize your data. Begin by listing the different sets of data you have collected, show how they are related, and how they will support each other (triangulation).
- Read the content. You need to read the data, probably several times, to develop a sense of what the data are indicating. All your data – observation notes, field diaries, policy documents and so on – need to be looked at. Common words and themes should start to emerge.
- Highlight relevant sections and aspects of the data.
- Develop categories to sort evidence. As you examine the data you will need to use actual evidence (numbers, actual quotes, artifacts, etc.) from your data to support your claims. You want these pieces of evidence to be the most vivid or clear representation for the categories you develop. For example, if you interviewed fifteen students and twelve of the interview transcripts provided evidence that the students’ understanding had grown due to your instructional intervention, you would want to note that twelve of fifteen students interviewed demonstrated growth in understanding, and possibly provide a quote or sample of how this was demonstrated from one of those twelve students. This sort of evidence enhances the trustworthiness of your findings.
- Code your data. Codes will develop from the categories you use to sort the evidence you find in the range of data. Codes also help you when you do a second or third analysis of the data as it guides your examination of the data. (Coding is discussed later in this chapter.)
- Review and narrow the codes. You may begin with a lot of initial codes, but you will want to narrow these to the most significant, well-evidenced, or best triangulated data. Most likely, these narrow codes will become the significant themes to report on your study.
- Interpret your findings. Once you have narrowed your codes, and have evidence in place to support those codes, it is time to interpret the data and develop meaning within the context of your study, and field. This is where your literature review will be useful again.
- Validate the findings. Validation, in addition to this process (see figure 6.1 below), can take many forms. In previous chapters, I had discussed using critical friends to confirm the validity of your interpretations.
- Create report and plan dissemination .
A framework for qualitative data analysis and interpretation
If you are feeling a bit overwhelmed by the amount of qualitative data you collected, you may find Creswell’s (2009) framework to analyze and interpret qualitative data useful (See figure 6.1).

Figure 6.1 Qualitative Data Analysis, interpreted from Creswell (Creswell, 2009, p. 185)
Similarly to above, Creswell also proposes a step-by-step approach to provide practitioners with a guide to undertake action research. I have summarized this in the following section.
Step 1. Organize the data for analysis. You will need to transcribe interviews, scan material, type up your notes, and sort or arrange the different types of data.
Step 2. Read all the data thoroughly. Get a general sense of the data and reflect on their overall meaning. You may have received an initial impression from the data collection, but make notes in the margins or spaces and record any other initial thoughts at this stage.
Step 3. Begin detailed coding and analysis. Coding organizes the material into meaningful chunks of text. When coding, think about:
- code based on previous literature and common sense;
- code what is surprising and unanticipated;
- code for the unusual which may be of conceptual interest to readers.
You may want to hand-code the data, use highlighting colors, or cut and paste text segments onto cards. You may also use a computer software package to help to code, organize and sort the information (e.g., NVivo)
Step 4. Codes should be representative of the categories, topic, setting, or people that are part of the analysis. Creswell suggests generating 5-7 categories. These will be supported with quotations and specific evidence form the data and may represent headings in your report.
Step 5. Decide how you will represent the codes, themes, and descriptions in the narrative. The narrative will summarize the findings from the analysis. This could be a discussion that outlines the project chronologically, a detailed discussion of several themes (including sub-themes, specific illustrations, multiple perspectives from individuals, and quotations), or a discussion with interconnecting themes. Visuals, graphs, figures, or tables are also useful to support the discussion.
Step 6. This final step involves making an interpretation or deriving meaning from the data. Meaning might come from, but is not limited to, lessons learned from the data. Meaning can also be derived when comparing findings to the literature or theories from the literature review.
Positionality and qualitative data
When analyzing qualitative data, the issue of your own positionality will need to be addressed. Positionality was mentioned in a previous chapter; however, addressing your positionality involves how your own social identity and experiences may impact your interpretation of the data. For example, an educator-researcher may have complex identities that they need to be aware of when they are analyzing the data. As a privileged white male with a terminal degree of education, I have to realize I may not fully relate to the experiences of many of my students, and this is important if I am analyzing the attitudes and beliefs of my students. I need to keep that under consideration throughout the research process, but especially as a I deriving meaning from the perspectives of my students. Therefore, positionality is very important for an educator-researcher who is planning and implementing action in a classroom, while they are also a teacher. It is also important to consider the possible impact of being an educator-researcher and acknowledge the possible influence this may have on the interpretations they make and any bias which may influence the research process. Qualitative research is interpretive research with the researcher typically involved in a sustained and intensive experience with the participants, which opens up a range of potential ethical and personal issues into the qualitative research process.
Many action research reports include a section on positionality, in which the researchers write a narrative describing their positionality and keep that visible as they analyze data. Below are some questions regarding what would constitute a positionality statement:
Positionality Statements
- Who am I? (Including demographics, epistemologies and philosophies, journey in education, etc.)
- What do I believe about teaching/learning?
- What do I believe about this topic?
- What are my expectations of this study?
- What are my connections or dis-connections with the participants?
- What are my experiences with the context of this study?
Analysis with computer software
It is now common for data to be analyzed using computer software. However, as Mertler (2008) notes, it is a misconception to think that the software will do the analysis, as data analysis still requires the use of inductive logic, and therefore, advanced technologies cannot take the place of the human brain. Computer software primarily helps researchers organize and store data. Software such as NVivo can also provide very efficient systems for coding a lot data, as well as many different types of data, including social media and video. Software like NVivo can be expensive for an educator-researcher. There are several free and/or cheaper applications and software that provide many of the same features.
Coding your data is such an important part of the analysis process, I want to devote a bit more discussion to the process. Simply put, coding entails identifying the main themes and patterns within your data. Coding is meant to help you conceptualize and condense your data into meaningful and manageable chunks from which to make conclusions. Coding data can take many shapes and forms. Regardless of how you choose to code your data, it is important to keep your research goals and research questions in the forefront of your mind. After immersing yourself in your data sources, it is possible to feel somewhat overcome by thinking and possibilities sparked by the data. This feeling may be caused by one of two issues you will have to deal with in your analysis. First, it is possible you may think that nearly everything you have collected is relevant and significant to the study, which could lead to some stress in how to determine what to focus on, or what is most significant. Coding should help reduce this stress by bringing patterns and themes to the forefront to help you prioritize some aspects of the data, and make it feel much more manageable. Once you begin to realize you are coding only those things which are relevant, you will ease the stress and begin to enjoy the analysis and coding process. Second, coding can be taxing work because of the constant processing, categorizing, and depth of thinking. Like it is suggested when revising writing, take regular breaks to maintain your full concentration, and in the case of research, to also review your coding criteria.
Using Evidence and Generating Knowledge
The main purpose of gathering data, through a research process, is to provide evidence. In order to provide evidence, you need to analyze the data you have collected. Again, it is important to remember the starting place of your inquiry, and what you are looking for in the study. You began with goals at the start of your research and mapped out your data collection strategically, now you have the data which will provide evidence for articulating your claims and developing pedagogical theories.
Regardless of the type of data you have collected, quantitative data or qualitative data or a combination of the two, ultimately the significance and impact of your research will depend on the quality of data you have collected, the interpretations you make, and your reflections and conclusions. Therefore, the significance of your study will depend upon the quality of the data you have collected and depth of your data analysis.
While you are engaged in data analysis, it might be useful to highlight the data that could be used as evidence to support your claims when you share your research. In the past, I have color coded different types of evidence.
So, what do we mean when we say provide evidence? When researchers provide evidence, they are providing pieces of data that support their claims about what their study did or did not demonstrate. In the next chapter, we will discuss how to share or report your findings. When you share or report you can think of it as an argument that you are making about your findings and subsequent claims. The data is used as evidence to support your claims and strengthen your argument. It is important to remember that to develop valid claims to knowledge, you will need to support your claims with evidence using relevant parts of your data. Therefore, evidence may take the form of survey results, quotes or extracts from interview transcripts, selections from your classroom observation notes, artifacts, photographs, and examples of students’ work.
Generating Knowledge
The purpose of research is to generate new knowledge. As an educator and researcher, the knowledge you produce will be based on your practice. Once you have findings and claims, this will most likely affect your practice. You will articulate knowledge that is generated from how your research has affected your practice and contemplate what significance it may have for other practitioners. This process amounts to you building personal theories about what you have done and demonstrated in your study. Therefore, your theories will emerge from your practice and this will contribute new knowledge to the existing knowledge base. Your data will provide illustrative examples of what happened in your classroom and you will cite relevant evidence. When you develop knowledge from your study, the claims you make and the theories you formulate are original, as you have employed your own critical thinking skills and informed judgement. Your critical thinking and informed judgement are demonstrated in the evidence you provide to validate your claims to knowledge.
Creating Trustworthy Claims to Knowledge
Your research findings and claims to knowledge are much more impactful if demonstrated as trustworthy. Action research is often conducted in collaborative teams, involving communities of educator researchers. Collaborative teams have built in opportunities to increase trustworthiness of studies. Having multiple people make interpretations of the same data creates trustworthiness through common understandings, making the findings more representative. The trustworthiness of research is also based on readers or consumers of your research accept your claims to knowledge. It is scary for many to think they need to validate claims to knowledge, and readers or consumers will critically evaluate their claims. As mentioned in previous chapters, trustworthiness can be accomplished methodologically, and when you report or share your findings you need to simply articulate your methods for trustworthiness. For example:
Achieving Trustworthiness
- Articulate your procedures clearly;
- Explain how you conducted your research thoroughly;
- Describe the robustness of your data collection methods;
- Make clear how triangulation was achieved.
Expect to be challenged on any aspect of your research claims. This is where validation meetings with different groups of people are useful, to have them consider their research processes and findings from different perspectives both during their research and at the conclusion of the project. When you report or share your research, you should include details of your validation meetings and any developments that resulted from these meetings.
Critical Friends can also be useful in regard to trustworthiness. When you establish the project recruit, Critical Friends. Explain that their role is to evaluate all aspects of the research by challenging your assumptions and considering ways to reduce subjectivity and ethical issues. Critical Friends can be helpful in thinking about all aspects of your research, even the implications, usefulness, and replicability of your research. You can utilize Critical Friends either individually or as a group to provide formative feedback at different points of the research.
Data Analysis Checklist
- What is your positionality in regard to the data? How will it affect your analysis?
- How will you organize and prepare your raw data for analysis?
- Read and engage with all of your data.
- Code. What themes or categories are emerging across the data?
- What descriptions will you use to define and characterize your codes?
- Are the codes and/or themes interrelated? Are there sub-codes?
- How will you represent codes in the final report?
- What theories can you use to interpret the codes?
- What do your themes, codes, and descriptions mean in relation to your research question(s)?
- Would a Critical Friend or colleague’s review of your analysis add to the trustworthiness of the study?
Action Research Copyright © by J. Spencer Clark; Suzanne Porath; Julie Thiele; and Morgan Jobe is licensed under a Creative Commons Attribution-NonCommercial 4.0 International License , except where otherwise noted.
Share This Book
Action Research Tutorials-CCAR
Action research tutorials, tutorial 8: analyzing data - evidence, tutorial 8: resources, a. understanding your data.
Action Research is not a single research project; rather it is an ongoing iterative approach that takes place across cycles of innovation and reflection. It is a way of learning from and through systematic inquiry into one's practice. Central to this process is the collection and analysis of data. The image below (Rie1, 2014) uses color to represent the growing knowledge of the action researcher. After a number of cycles, there is a reporting out or sharing function. In the last tutorial, we discussed what data artifacts you could collect. Now we are going to talk about the analysis of these data artifacts. This is often the step that practitioners find most difficult because they have not been trained to analyze data.
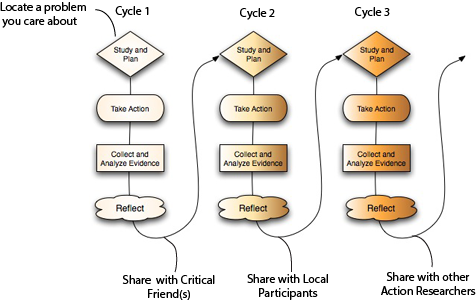
B. Organizing your data -- What is your storyline?
You will need to report your "findings" that you discover from exploring your data. To develop findings, you have to engage in some serious "looking" to find meaning from what you collected. Often new action researchers will simply describe a survey and then paste the questions with the group responses in their reports. But this is not helping the reader to make sense of what you learned. You haven't processed the data to learn from it. It is helpful to begin with your research questions or your near and medium outcomes on your logic model. What does the data you collected tell you that will help answer your research question? How does the data help you to understand if you see evidence of the outcomes that you predicted in your logic model? To answer these questions, take the responses to your survey and group them around what you are trying to learn. You need to find the storyline that you will be sharing with your audience. AN EXAMPLE-- Katherine Korte Flips her Government Course For example, consider an educator teaching a government class who flipped her classroom as her "action" and, for data collection, asked her students to provide some feedback on their learning in a survey. RESEARCH QUESTION: If I flipped my classroom using video to send lessons home and ibook technology, how will this affect the quality of knowledge building dialogues in the classroom? She was interested in what students learned, how they learned, and changes in their attitudes and engagement in learning. Therefore, she might take her ten survey questions and organize them under these three topics with one question not fitting this process.
(1) knowledge ....(three survey questions) (2) process ....(four survey questions) (3) attitudes, ....(two survey questions)
Now she would compute responses to these groups of questions and think about other data sources besides the survey -- what tests or quizzes did students take that would help answer the first question about knowledge? How might field notes shed light on what students were learning? What artifacts were produced that could be examined? For the second topic, there were student responses that addressed their work in discussion circles, and what they learned from each other and some of the problems from group work. How did students' comments match her notes? Were there more or fewer problems that students needed help with? Then she might organize the analysis into three sections:
Changes in Student Knowledge ....analysis of three survey questions ....classroom quizzes, ....end of term assessment, ....ibook notetaking, ....performances Changes in the Way Students worked ....analysis of four survey questions ....field notes on how well the groups worked ....what problems they had, ....teacher assessment of how prepared they were for the classroom discussions, ....speed at which they accomplished their tasks Student Reactions to the flipped Classroom ....analysis of two survey questions ....students blogs ....observations about the comfort of students contributing ideas or making presentations
This organization helps her to find a storyline that she will tell about the data.
Validity of Measures
You can see that now that multiple sources of data are being examined to see what they say about the outcomes that the educator cares about. The analysis should be an honest hunt to figure out what can be learned from what happened. You are looking for valid measures that will answer your question. For example, if a teacher listed grades as evidence of student engagement, one might challenge this measure as not a valid measure of engagement because a student could have high grades and not be engaged and another student could be engaged and yet have a low grade. This is why researchers consider the validity of measures.
Participatory Data Collection
Empowering the group to participate in data collection and analysis helps everyone to have a sense of ownership over the outcomes and the deep understanding of change. These guides and articles might help you think about who should be involved in the data collection/analysis work in your action research. Even if you collect and analyze data within a group, you still have your own reflections on what this process means to you as an action researcher.
Guijt, I.,(2014). Participatory Approaches: This guide, written by Irene Guijt for UNICEF , looks at the use of participatory approaches in impact evaluation. While not exactly the same as action research it is very close and this guide will be useful if you are involving stakeholders in your process of data collection and analysis
Increasing Participation in Evaluating – Bruner Foundation Guide - This guide discusses how Organization Staff, Evaluators and Funders are involved in Participatory Evaluation.
Campilan, D. (2000). Participatory Evaluation of Participatory Research. Forum on Evaluation of International Cooperation Projects: Centering on Development of Human Resources in the Field of Agriculture. Nagoya, Japan, International Potato Center. http://ir.nul.nagoya-u.ac.jp/jspui/bitstream/2237/8890/1/39-56.pdf
Chambers, R. (2009) Making the Poor Count: Using Participatory Options for Impact Evaluation in Chambers, R., Karlan, D., Ravallion, M. and Rogers, P. (Eds) Designing impact evaluations: different perspectives . New Delhi, India, International Initiative for Impact Evaluation. http://www.3ieimpact.org/admin/pdfs_papers/50.pdf
Guijt, I. and J. Gaventa (1998). Participatory Monitoring and Evaluation: Learning from Change. IDS Policy Briefing. Brighton, UK, University of Sussex- In participatory monitoring and evaluation, stakeholders work together to decide how progress should be measured and how to understand the outcomes of change.
Zukoski, A. and M. Luluquisen (2002). "Participatory Evaluation: What is it? Why do it? What are the challenges?" Policy & Practice(5). http://depts.washington.edu/ccph/pdf_files/Evaluation.pdf
C. Exploring your data -- What is your story?
Coding your Data
The Center for Evaluation Research at the University of Calif, Davis has a good and brief guide on coding as well as other tools for data analysis that will be helpful to read before you begin your coding process. If you are doing coding of data-- for example coding what students write in their blogs, it will be helpful to develop a codebook where you list the codes and examples to help you make decisions. This serves to create consistency or reliability in your coding. Good research practice involves creating a codebook for any qualitative analysis you do. This can be very simple or more complex depending on the nature of your data and you scale of analysis you plan to do. This is similar to create a rubric to assess student work. If you are writing an action research dissertation, the use of a codebook is highly recommended. If you want to see how researchers use codebooks and coding schemes , you will find a number of them have been collected by Kimberly Neuendorf as an accompaniment to her Content Analysis Guidebook. I enjoyed exploring the codebook for the study of female roles in James Bond 007 movies . In this video, Kevin Meethan also describes the process of going from an interview to codes-- if you go to the youtube version, I think you can find a link to the texts that are given in the examples, but you can just listen to the examples and a get sense for the coding process.
Reliability In any coding, an important question to ask is: how reliable is the coding process? In other words, if this teacher told us what theme or content to look for and we coded the data would it be the same as her coding? To achieve reliability, researchers often have two people code data and compare. If they agree on 85% of the cases, then we say the coding is reliable. If you cannot find another person to compare your coding, you can check your coding with yourself at different times. Code a few cases and then wait a day and recode. Do they match? The closer the match the more reliable your coding. Teachers often engage in a very similar process to grade papers in a consistent or reliable way. They create a rubric and then use that rubric to assess the student work. The rubric is their codebook and the access is the coding process. Content Analysis Let's stay with the teacher who flipped her classroom and had students keep notes in their iBooks as they watched the videos at home. This teacher wants to analyze this textual data. How should she approach this? Depending on which category the action researcher chooses, there are lots of ways to do a qualitative analysis of data. Some are more time consuming than others and a large amount of data might be more than a teacher can examine. Our teacher might want to create a sample to analyze. She could take a random set of three entries for each student, or all students' last blog before the test, or she might decide to examine all of the blogs of six students. These six could be randomly selected from all of the students or from groups of students. For example, the teacher might select two students at random from those that received an A, B or C grade in the course, or in a previous course, or one student from each discussion group. A content analysis helps us to know what was contained in these blogs. Suppose there are some central ideas or concepts and our teacher wants to see how often, if at all, they appear in student writing. To access this information, she might use a single blog, or response to a question as the "unit of analysis" and then mark yes or no for a number of ideas that she has decided on. She would take each entry and code it, say 1 or 0, for each of the concepts. This would help her see how frequently individuals and the class as a whole explored these concepts. A different approach to content analysis would involve coding all of the topics that were covered in each entry. Then she could examine the topics that students selected as important. This approach would help her to understand what topics students judged to be important. Theme Analysis Theme analysis is similar to content analysis but a bit more difficult. Here, you are not looking for concepts but for themes. Some process themes might be positive or negative attitudes about the different forms of technology used in the classroom. As in content analysis, these could be set up by the teacher or they could emerge from the data as when the action researcher discovers themes through repeated reading, grouping them, and constructing a final set of themes to use. Developing a Critical Eye Action research is not about proving how successful you were at some new attempt. It is about learning from your efforts. It is not so much about whether it worked or not, but a more thoughtful examination of how it worked, or for whom it worked, and why things turned out as they did. So be ready to hear that your plans did not work as you expected. Try not to be defensive. Instead, see this as a time to learn from your inquiry. What worked and why? What did not work and why? it is important to examine evidence that contradicts or opposes your research question. You might find the " What Else Test " developed by Jess Dart will be helpful as you develop your critical eye.
Advice for Data Analysis in Social Services
The Institute for Research and Innovation in Social Services (IRISS) is a charitable company with a mission to:…promote positive outcomes for the people who use Scotland’s social services by enhancing the capacity and capability of the social services workforce to access and make use of knowledge and research for service innovation and improvement. They have a number of resources can help think about action research starting with this animated video:
Outcomes ToolboxA toolbox of resources relevant to an outcomes-focused approach in social services Developed by IRISS in partnership with Coalition of Care and Support Providers in Scotland (CCPS), the Outcomes Toolbox brings together a range of resources and knowledge relevant to an outcomes-focused approach in the social services. Understanding and Measuring Outcomes The role of Qualitative Data Emma Miller and Ellen Daly
D. Displaying your Data -- How will you Tell a Compelling Research Story?
Think about what your data has told you and tell others what you learned. Use chart, graphs or tables to help people quickly see what you have found. With all of the choices you will find yourself thinking which type of chart should I use. While there are suggested guidelines about these choices, you might find it works just as well to try displaying the data in a number of different ways and look at it and see what you learn. Then test it with someone who does not know the data. What do they see from your chart? Consider the signal to noise ratio. The signal is your data and the noise is graphical elements around the data you might not need. You want to encourage the eye to quickly zero in on what is relevant. This video shows some examples might help you think about how to represent your data without unnecessary distortion. While design can be subjective (and while I don't agree with all of the comments) the suggestion to minimize "chart junk" is important. Try to find ways to clearly communicate your data in an honest and pleasing image.
Presentation and Visualization of Data A site that might be helpful for understanding some basic concepts in statistics and how to display data can be found at http://www.shodor.org/interactivate/lessons/HistogramsBarGraph/ This site is arranged to support for high school math teachers but there some interactives that might help you think about data displays. (And it is a fun way to play with math and statistic concepts). Many eyes ( http://www.many-eyes.com ) is a free tool for creating a visualization of data sets. for examples see... http://www-958.ibm.com/software/data/cognos/manyeyes/visualizations?sort=rating
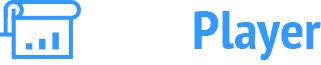
- My presentations
Auth with social network:
Download presentation
We think you have liked this presentation. If you wish to download it, please recommend it to your friends in any social system. Share buttons are a little bit lower. Thank you!
Presentation is loading. Please wait.
DATA ANALYSIS WRITING CHAPTER FOUR. Chapter Four, Data Analysis, is the section of the thesis for the action research study that provides the reader with.
Published by Laura Lindsey Modified over 7 years ago
Similar presentations
Presentation on theme: "DATA ANALYSIS WRITING CHAPTER FOUR. Chapter Four, Data Analysis, is the section of the thesis for the action research study that provides the reader with."— Presentation transcript:
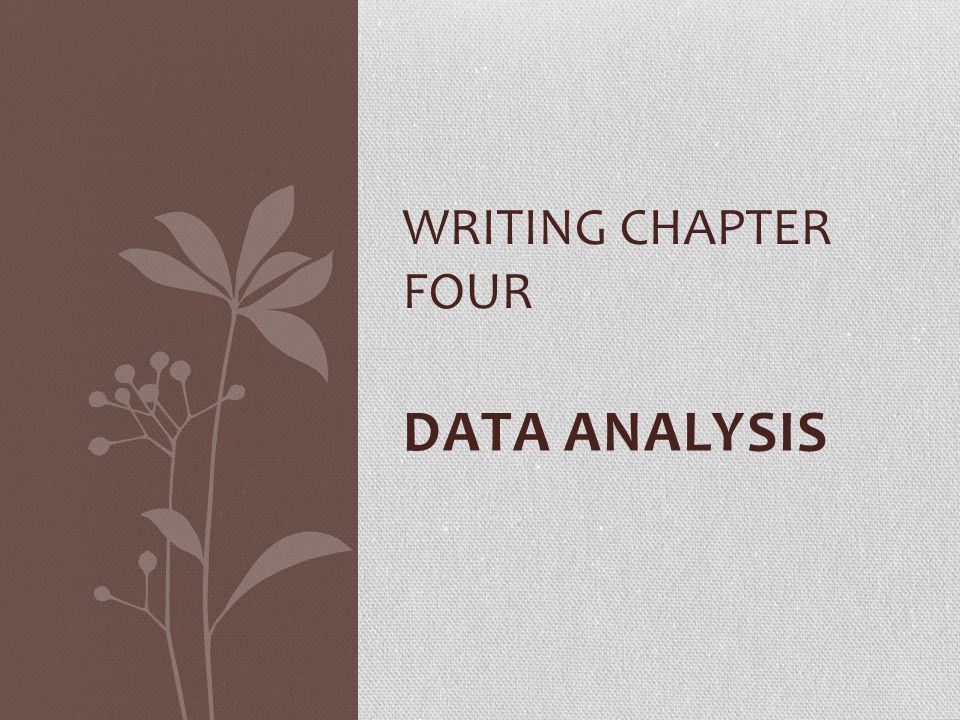
Bullying, Academic Performance, and High School Dropout in Adolescence Victoria Hirsch 1, Meredith Henry, Sylvie Mrug 1 and Michael Windle 2 1 University.
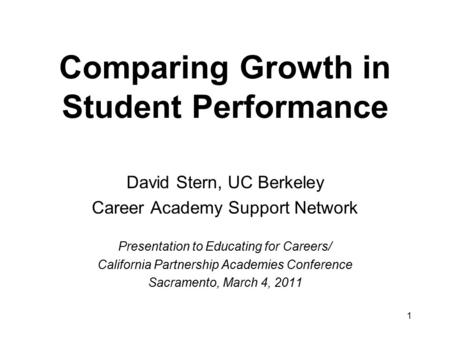
Comparing Growth in Student Performance David Stern, UC Berkeley Career Academy Support Network Presentation to Educating for Careers/ California Partnership.
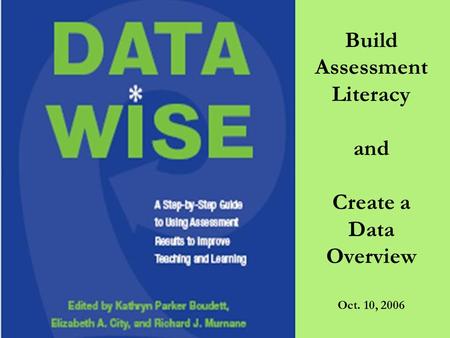
Build Assessment Literacy and Create a Data Overview Oct. 10, 2006.
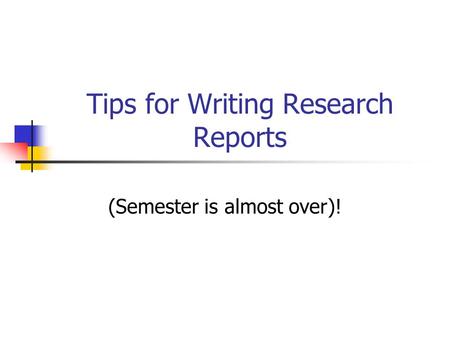
Tips for Writing Research Reports (Semester is almost over)!
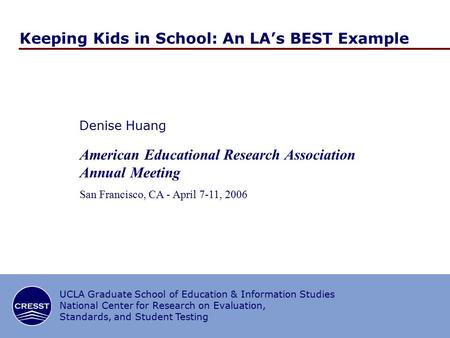
UCLA Graduate School of Education & Information Studies National Center for Research on Evaluation, Standards, and Student Testing Keeping Kids in School:
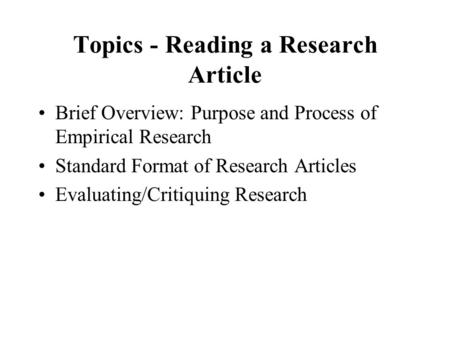
Topics - Reading a Research Article Brief Overview: Purpose and Process of Empirical Research Standard Format of Research Articles Evaluating/Critiquing.
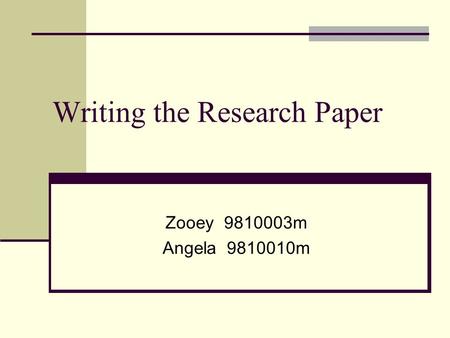
Writing the Research Paper
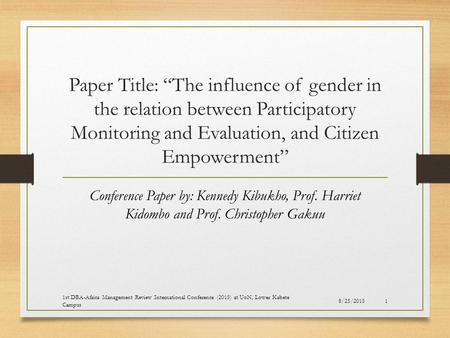
Paper Title: “The influence of gender in the relation between Participatory Monitoring and Evaluation, and Citizen Empowerment” Conference Paper by: Kennedy.
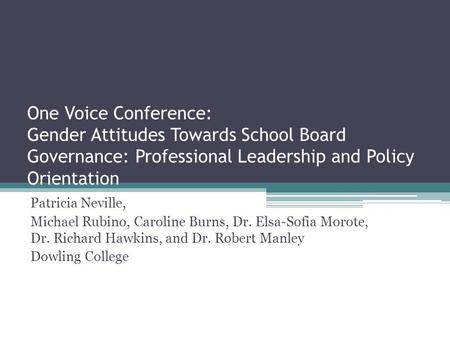
One Voice Conference: Gender Attitudes Towards School Board Governance: Professional Leadership and Policy Orientation Patricia Neville, Michael Rubino,
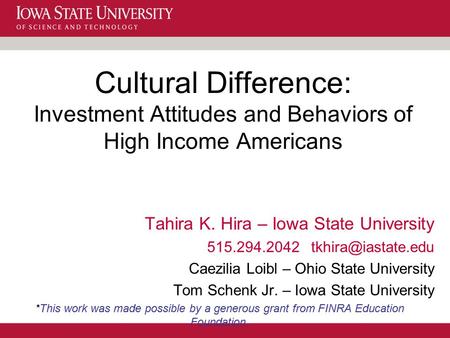
Cultural Difference: Investment Attitudes and Behaviors of High Income Americans Tahira K. Hira – Iowa State University
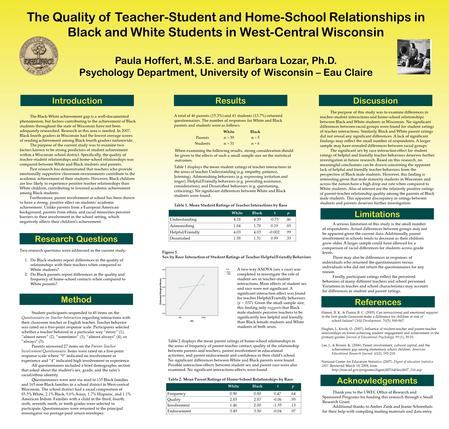
The Quality of Teacher-Student and Home-School Relationships in Black and White Students in West-Central Wisconsin Paula Hoffert, M.S.E. and Barbara Lozar,
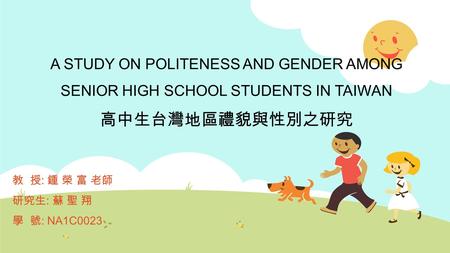
A STUDY ON POLITENESS AND GENDER AMONG SENIOR HIGH SCHOOL STUDENTS IN TAIWAN 高中生台灣地區禮貌與性別之研究 教 授 : 鍾 榮 富 老師 研究生 : 蘇 聖 翔 學 號 : NA1C0023.
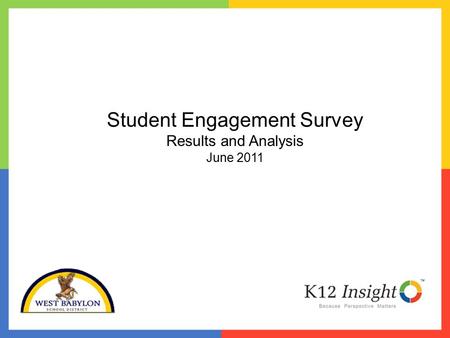
Student Engagement Survey Results and Analysis June 2011.
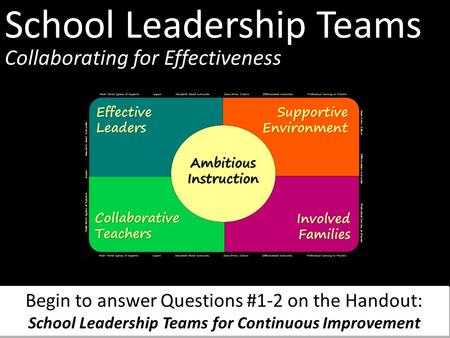
School Leadership Teams Collaborating for Effectiveness Begin to answer Questions #1-2 on the Handout: School Leadership Teams for Continuous Improvement.
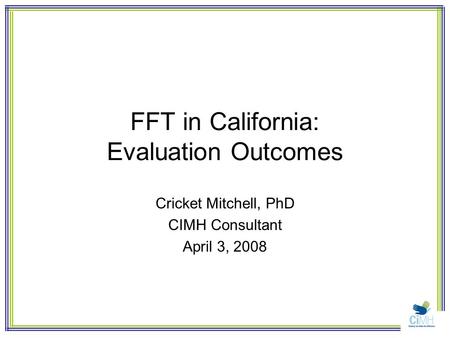
FFT in California: Evaluation Outcomes Cricket Mitchell, PhD CIMH Consultant April 3, 2008.
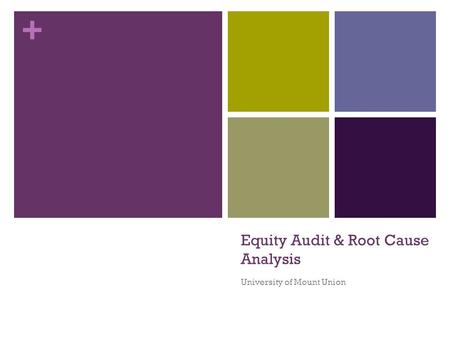
+ Equity Audit & Root Cause Analysis University of Mount Union.
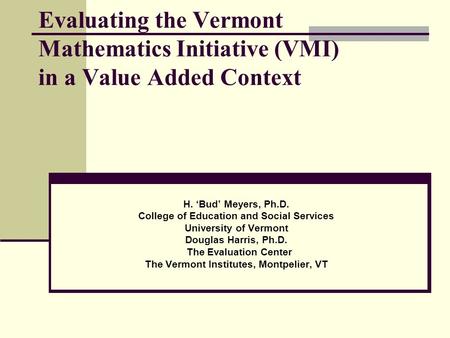
Evaluating the Vermont Mathematics Initiative (VMI) in a Value Added Context H. ‘Bud’ Meyers, Ph.D. College of Education and Social Services University.
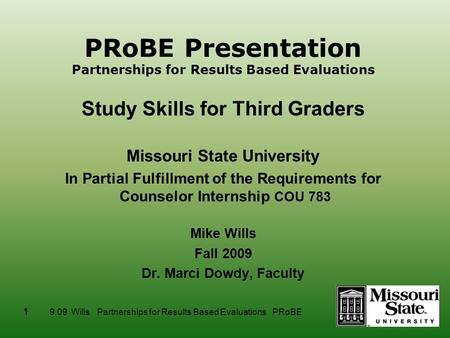
Wills Partnerships for Results Based Evaluations PRoBE PRoBE Presentation Partnerships for Results Based Evaluations Study Skills for Third Graders.
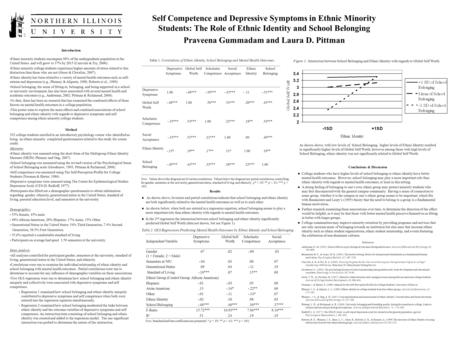
Self Competence and Depressive Symptoms in Ethnic Minority Students: The Role of Ethnic Identity and School Belonging Praveena Gummadam and Laura D. Pittman.
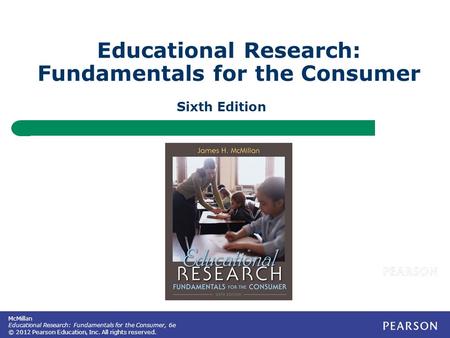
McMillan Educational Research: Fundamentals for the Consumer, 6e © 2012 Pearson Education, Inc. All rights reserved. Educational Research: Fundamentals.
About project
© 2024 SlidePlayer.com Inc. All rights reserved.
Academia.edu no longer supports Internet Explorer.
To browse Academia.edu and the wider internet faster and more securely, please take a few seconds to upgrade your browser .
Enter the email address you signed up with and we'll email you a reset link.
- We're Hiring!
- Help Center
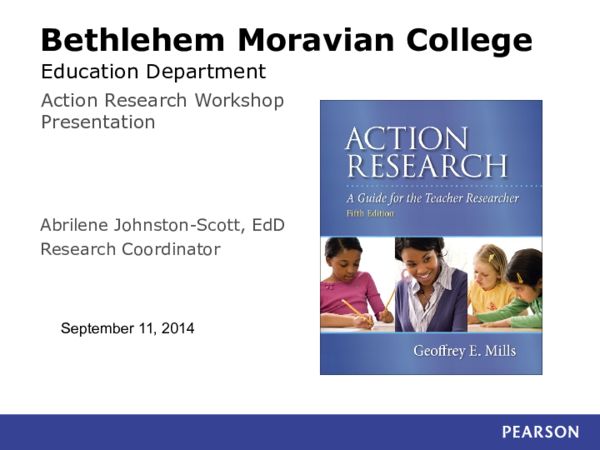
Action Research Process Presentation

Related Papers
Andrew Johnson
Action research can be defined as a systematic observation of one’s own teaching practice. It is a way to link theories and research directly to classroom practice. It also empowers teachers to make the changes that are best for their own teaching situations. In this sense, action research is an effective and economical way to attend to the professional development of teachers. This article describes the basic elements of action research.
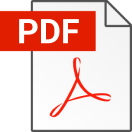
Sir CP Dr. Benedict David, KCR, Ph.D, DHC, CA
Irene Lacia
Action research is a type of research related to one’s professional practice. In the field of education, it can be defined as the process of studying a school, classroom, or teaching-learning situation with the purpose of understanding and improving the quality of actions or instruction. In this sense, it is the ultimate form of teacher reflection. Described in this chapter expert are the basic elements and the steps of action research.
Allan Feldman
Alberta Journal of Educational Research
Ali Kemal Tekin
Although action research has become one of the major methods employed in educational research, there has always been a scarcity of solid informational textbooks and journal articles outlining and giving in-depth information about this trendy research methodology. However, it is a well-accepted research methodology and has become one of the most critical research approaches that can be used by educators in order to improve their knowledge and practices (Tekin & Kotaman, 2013). Therefore, it is considered a method of inquiry especially suitable for practicing teachers. Today's teachers are not only expected to teach students but also to act in many areas related to education. They are burdened with many responsibilities such as administrative tasks, advocacy for children, and fundraising. Beyond these duties, teachers have been increasingly seen as researchers as they are expected to constantly improve their teaching practices for the benefit of children's education. Therefore, action research provides them with a methodology they can use while they are working on their regular duties as it is a practical approach conducted with a focus on the teaching and learning process. They can blend research and practice since the researchers draw their findings and conclusions predominantly on their own experiences and observations from their practices. As the author states, "A main focus of action research is the improvement of classroom practice" (p. 23). Therefore, this research experience can contribute to teachers' own and other teachers' practices when they design and revise curriculum. It can also help them make the whole educational process more meaningful for themselves, students, and the school community. The author also identifies clear pathways that have the potential to improve teachers' practices, such as systematic reflections about how teachers would improve their practices with action research methodology. Any research approach excluding the related practitioners' perspectives and contributions should not be seen as a complete process since it is the practitioners who are expected to apply the implications and suggestions drawn by the studies. Action research methodology provides these opportunities and fills a huge gap that needs attention in educational research. To benefit from this experiential methodology, teachers should understand the action research approach. Action research books currently on the market contain too much theoretical information and have a philosophical background, which makes it hard for a practicing teacher to understand. They also provide too few meaningful examples. Most books about action research also suffer from a lack of good quality organization, which can make curious future
International Journal of Scientific and Management Research
Honelly Mae Cascolan
The overall goal of this study was to assess the efficacy and impact of action research to secondary education in Pangasinan Division II mother high schools. Specifically, it looked into the experiences and challenges faced by the teachers in conducting action research. Later on, the researchers devised a strategy or a plan of action to help the teachers in doing action research and address the problems they have encountered. A combination of quantitative and qualitative methods of research was used and it utilized 50 secondary teachers from the 21 mother high schools in Pangasinan Division II as respondents. These were the findings: (1) Action research, according to the teachers, has a positive impact in the teaching-learning process (2) It was also helpful in teacher’s professional development, particularly in teacher’s promotion (3) It has also a positive impact in curriculum creation or development. (4) For the teacher-respondents, doing action research is challenging due to fin...
Action research can be defined as the process of studying a real school or classroom situation to understand and improve the quality of actions or instruction. It is a systematic and orderly way for teachers to observe their practice or to explore a problem and a possible course of action. This chapter provides an overview of this powerful teaching tool.
Vivienne Marie Baumfield
Universal Journal of Educational Research
Darin Jan Tindowen
This study was conducted to describe the conceptions and difficulties of Junior High School teachers in the conduct of action research. A combination of quantitative and qualitative types of research was utilized in the study involving 60 teachers in the Junior High School department. The results reveal that action research is a valuable tool for teachers to improve teaching and learning process, to increase pedagogical and instructional knowledge, and to positively impact students' learning. Furthermore, teachers have difficulties in the conduct of action research especially literature search, presentation and publication of results, and data collection. Finally, four major themes emerged as the major issues and challenges of teachers in the conduct of action research, which are additional workload and burden in the part of the teacher, writing anxiety, lack of time, and inadequate knowledge in the conduct of action research.
RELATED PAPERS
Confabulario. Suplemento cultural de alta difusión cultural del periódico El Universal. Monografia especial. 14/08/2021. México: El Universal, Compañía Periodística Nacional, S.A. de C.V.
Rossend Rovira Morgado
Shoaib Mughal
International Journal of Research in Medical Sciences
tariq masood
Literatura de Chinelo
Marcos Ramos
International Journal of Electronics Signals and Systems
Ashwini Holla
Jutta Winsemann
Saudi Journal of Biological Sciences
Nilam Wulandari
Produsen Cold Storage Tuban
Prosedur C O L D S T O R A G E Tuban
arXiv (Cornell University)
Deepak Pachpatte
Cancer Genetics and Cytogenetics
Michele Shuster
Alejandro Pages
在线办理concordia学位证书 康考迪亚大学毕业证学历学位证书学费发票原版一模一样
Juan Cruellas
ANZ Journal of Surgery
Shaun Whitmore
Applied Optics
gérard gouesbet
Journal of Nanoscience and Nanotechnology
Ngọc Thành Lê
Acta Limnologica Brasiliensia
Rayana Picolotto
Springer eBooks
Rainer Doemer
Cancer Research
Louise Lutze-Mann
Japan Geoscience Union
Naoshi Hirata
Journal of Clinical Neuroscience
- We're Hiring!
- Help Center
- Find new research papers in:
- Health Sciences
- Earth Sciences
- Cognitive Science
- Mathematics
- Computer Science
- Academia ©2024
- Skip to main content
- Skip to primary sidebar
- Skip to footer
- QuestionPro

- Solutions Industries Gaming Automotive Sports and events Education Government Travel & Hospitality Financial Services Healthcare Cannabis Technology Use Case NPS+ Communities Audience Contactless surveys Mobile LivePolls Member Experience GDPR Positive People Science 360 Feedback Surveys
- Resources Blog eBooks Survey Templates Case Studies Training Help center

Home Market Research
Data Analysis in Research: Types & Methods
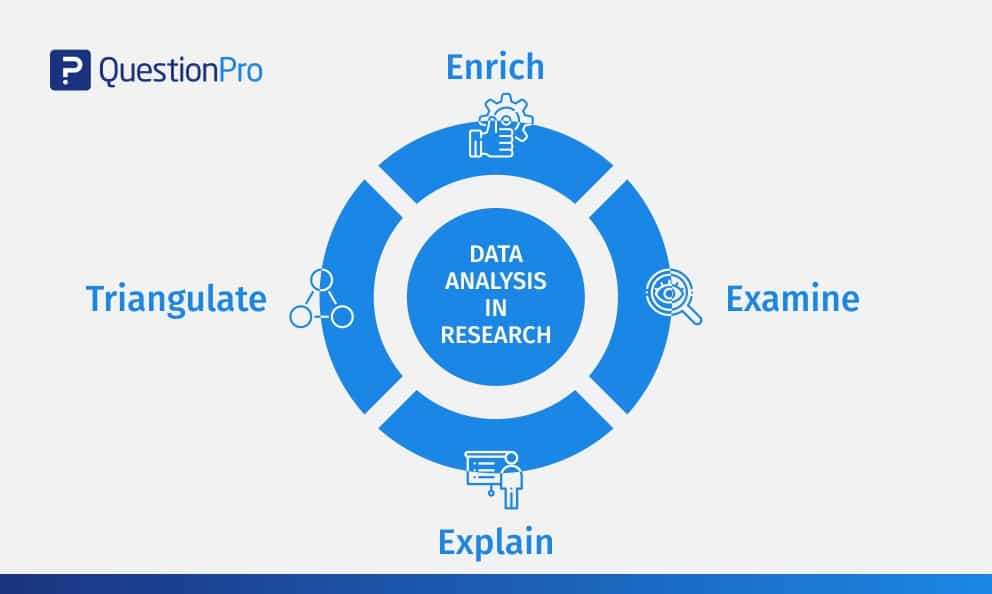
Content Index
Why analyze data in research?
Types of data in research, finding patterns in the qualitative data, methods used for data analysis in qualitative research, preparing data for analysis, methods used for data analysis in quantitative research, considerations in research data analysis, what is data analysis in research.
Definition of research in data analysis: According to LeCompte and Schensul, research data analysis is a process used by researchers to reduce data to a story and interpret it to derive insights. The data analysis process helps reduce a large chunk of data into smaller fragments, which makes sense.
Three essential things occur during the data analysis process — the first is data organization . Summarization and categorization together contribute to becoming the second known method used for data reduction. It helps find patterns and themes in the data for easy identification and linking. The third and last way is data analysis – researchers do it in both top-down and bottom-up fashion.
LEARN ABOUT: Research Process Steps
On the other hand, Marshall and Rossman describe data analysis as a messy, ambiguous, and time-consuming but creative and fascinating process through which a mass of collected data is brought to order, structure and meaning.
We can say that “the data analysis and data interpretation is a process representing the application of deductive and inductive logic to the research and data analysis.”
Researchers rely heavily on data as they have a story to tell or research problems to solve. It starts with a question, and data is nothing but an answer to that question. But, what if there is no question to ask? Well! It is possible to explore data even without a problem – we call it ‘Data Mining’, which often reveals some interesting patterns within the data that are worth exploring.
Irrelevant to the type of data researchers explore, their mission and audiences’ vision guide them to find the patterns to shape the story they want to tell. One of the essential things expected from researchers while analyzing data is to stay open and remain unbiased toward unexpected patterns, expressions, and results. Remember, sometimes, data analysis tells the most unforeseen yet exciting stories that were not expected when initiating data analysis. Therefore, rely on the data you have at hand and enjoy the journey of exploratory research.
Create a Free Account
Every kind of data has a rare quality of describing things after assigning a specific value to it. For analysis, you need to organize these values, processed and presented in a given context, to make it useful. Data can be in different forms; here are the primary data types.
- Qualitative data: When the data presented has words and descriptions, then we call it qualitative data . Although you can observe this data, it is subjective and harder to analyze data in research, especially for comparison. Example: Quality data represents everything describing taste, experience, texture, or an opinion that is considered quality data. This type of data is usually collected through focus groups, personal qualitative interviews , qualitative observation or using open-ended questions in surveys.
- Quantitative data: Any data expressed in numbers of numerical figures are called quantitative data . This type of data can be distinguished into categories, grouped, measured, calculated, or ranked. Example: questions such as age, rank, cost, length, weight, scores, etc. everything comes under this type of data. You can present such data in graphical format, charts, or apply statistical analysis methods to this data. The (Outcomes Measurement Systems) OMS questionnaires in surveys are a significant source of collecting numeric data.
- Categorical data: It is data presented in groups. However, an item included in the categorical data cannot belong to more than one group. Example: A person responding to a survey by telling his living style, marital status, smoking habit, or drinking habit comes under the categorical data. A chi-square test is a standard method used to analyze this data.
Learn More : Examples of Qualitative Data in Education
Data analysis in qualitative research
Data analysis and qualitative data research work a little differently from the numerical data as the quality data is made up of words, descriptions, images, objects, and sometimes symbols. Getting insight from such complicated information is a complicated process. Hence it is typically used for exploratory research and data analysis .
Although there are several ways to find patterns in the textual information, a word-based method is the most relied and widely used global technique for research and data analysis. Notably, the data analysis process in qualitative research is manual. Here the researchers usually read the available data and find repetitive or commonly used words.
For example, while studying data collected from African countries to understand the most pressing issues people face, researchers might find “food” and “hunger” are the most commonly used words and will highlight them for further analysis.
LEARN ABOUT: Level of Analysis
The keyword context is another widely used word-based technique. In this method, the researcher tries to understand the concept by analyzing the context in which the participants use a particular keyword.
For example , researchers conducting research and data analysis for studying the concept of ‘diabetes’ amongst respondents might analyze the context of when and how the respondent has used or referred to the word ‘diabetes.’
The scrutiny-based technique is also one of the highly recommended text analysis methods used to identify a quality data pattern. Compare and contrast is the widely used method under this technique to differentiate how a specific text is similar or different from each other.
For example: To find out the “importance of resident doctor in a company,” the collected data is divided into people who think it is necessary to hire a resident doctor and those who think it is unnecessary. Compare and contrast is the best method that can be used to analyze the polls having single-answer questions types .
Metaphors can be used to reduce the data pile and find patterns in it so that it becomes easier to connect data with theory.
Variable Partitioning is another technique used to split variables so that researchers can find more coherent descriptions and explanations from the enormous data.
LEARN ABOUT: Qualitative Research Questions and Questionnaires
There are several techniques to analyze the data in qualitative research, but here are some commonly used methods,
- Content Analysis: It is widely accepted and the most frequently employed technique for data analysis in research methodology. It can be used to analyze the documented information from text, images, and sometimes from the physical items. It depends on the research questions to predict when and where to use this method.
- Narrative Analysis: This method is used to analyze content gathered from various sources such as personal interviews, field observation, and surveys . The majority of times, stories, or opinions shared by people are focused on finding answers to the research questions.
- Discourse Analysis: Similar to narrative analysis, discourse analysis is used to analyze the interactions with people. Nevertheless, this particular method considers the social context under which or within which the communication between the researcher and respondent takes place. In addition to that, discourse analysis also focuses on the lifestyle and day-to-day environment while deriving any conclusion.
- Grounded Theory: When you want to explain why a particular phenomenon happened, then using grounded theory for analyzing quality data is the best resort. Grounded theory is applied to study data about the host of similar cases occurring in different settings. When researchers are using this method, they might alter explanations or produce new ones until they arrive at some conclusion.
LEARN ABOUT: 12 Best Tools for Researchers
Data analysis in quantitative research
The first stage in research and data analysis is to make it for the analysis so that the nominal data can be converted into something meaningful. Data preparation consists of the below phases.
Phase I: Data Validation
Data validation is done to understand if the collected data sample is per the pre-set standards, or it is a biased data sample again divided into four different stages
- Fraud: To ensure an actual human being records each response to the survey or the questionnaire
- Screening: To make sure each participant or respondent is selected or chosen in compliance with the research criteria
- Procedure: To ensure ethical standards were maintained while collecting the data sample
- Completeness: To ensure that the respondent has answered all the questions in an online survey. Else, the interviewer had asked all the questions devised in the questionnaire.
Phase II: Data Editing
More often, an extensive research data sample comes loaded with errors. Respondents sometimes fill in some fields incorrectly or sometimes skip them accidentally. Data editing is a process wherein the researchers have to confirm that the provided data is free of such errors. They need to conduct necessary checks and outlier checks to edit the raw edit and make it ready for analysis.
Phase III: Data Coding
Out of all three, this is the most critical phase of data preparation associated with grouping and assigning values to the survey responses . If a survey is completed with a 1000 sample size, the researcher will create an age bracket to distinguish the respondents based on their age. Thus, it becomes easier to analyze small data buckets rather than deal with the massive data pile.
LEARN ABOUT: Steps in Qualitative Research
After the data is prepared for analysis, researchers are open to using different research and data analysis methods to derive meaningful insights. For sure, statistical analysis plans are the most favored to analyze numerical data. In statistical analysis, distinguishing between categorical data and numerical data is essential, as categorical data involves distinct categories or labels, while numerical data consists of measurable quantities. The method is again classified into two groups. First, ‘Descriptive Statistics’ used to describe data. Second, ‘Inferential statistics’ that helps in comparing the data .
Descriptive statistics
This method is used to describe the basic features of versatile types of data in research. It presents the data in such a meaningful way that pattern in the data starts making sense. Nevertheless, the descriptive analysis does not go beyond making conclusions. The conclusions are again based on the hypothesis researchers have formulated so far. Here are a few major types of descriptive analysis methods.
Measures of Frequency
- Count, Percent, Frequency
- It is used to denote home often a particular event occurs.
- Researchers use it when they want to showcase how often a response is given.
Measures of Central Tendency
- Mean, Median, Mode
- The method is widely used to demonstrate distribution by various points.
- Researchers use this method when they want to showcase the most commonly or averagely indicated response.
Measures of Dispersion or Variation
- Range, Variance, Standard deviation
- Here the field equals high/low points.
- Variance standard deviation = difference between the observed score and mean
- It is used to identify the spread of scores by stating intervals.
- Researchers use this method to showcase data spread out. It helps them identify the depth until which the data is spread out that it directly affects the mean.
Measures of Position
- Percentile ranks, Quartile ranks
- It relies on standardized scores helping researchers to identify the relationship between different scores.
- It is often used when researchers want to compare scores with the average count.
For quantitative research use of descriptive analysis often give absolute numbers, but the in-depth analysis is never sufficient to demonstrate the rationale behind those numbers. Nevertheless, it is necessary to think of the best method for research and data analysis suiting your survey questionnaire and what story researchers want to tell. For example, the mean is the best way to demonstrate the students’ average scores in schools. It is better to rely on the descriptive statistics when the researchers intend to keep the research or outcome limited to the provided sample without generalizing it. For example, when you want to compare average voting done in two different cities, differential statistics are enough.
Descriptive analysis is also called a ‘univariate analysis’ since it is commonly used to analyze a single variable.
Inferential statistics
Inferential statistics are used to make predictions about a larger population after research and data analysis of the representing population’s collected sample. For example, you can ask some odd 100 audiences at a movie theater if they like the movie they are watching. Researchers then use inferential statistics on the collected sample to reason that about 80-90% of people like the movie.
Here are two significant areas of inferential statistics.
- Estimating parameters: It takes statistics from the sample research data and demonstrates something about the population parameter.
- Hypothesis test: I t’s about sampling research data to answer the survey research questions. For example, researchers might be interested to understand if the new shade of lipstick recently launched is good or not, or if the multivitamin capsules help children to perform better at games.
These are sophisticated analysis methods used to showcase the relationship between different variables instead of describing a single variable. It is often used when researchers want something beyond absolute numbers to understand the relationship between variables.
Here are some of the commonly used methods for data analysis in research.
- Correlation: When researchers are not conducting experimental research or quasi-experimental research wherein the researchers are interested to understand the relationship between two or more variables, they opt for correlational research methods.
- Cross-tabulation: Also called contingency tables, cross-tabulation is used to analyze the relationship between multiple variables. Suppose provided data has age and gender categories presented in rows and columns. A two-dimensional cross-tabulation helps for seamless data analysis and research by showing the number of males and females in each age category.
- Regression analysis: For understanding the strong relationship between two variables, researchers do not look beyond the primary and commonly used regression analysis method, which is also a type of predictive analysis used. In this method, you have an essential factor called the dependent variable. You also have multiple independent variables in regression analysis. You undertake efforts to find out the impact of independent variables on the dependent variable. The values of both independent and dependent variables are assumed as being ascertained in an error-free random manner.
- Frequency tables: The statistical procedure is used for testing the degree to which two or more vary or differ in an experiment. A considerable degree of variation means research findings were significant. In many contexts, ANOVA testing and variance analysis are similar.
- Analysis of variance: The statistical procedure is used for testing the degree to which two or more vary or differ in an experiment. A considerable degree of variation means research findings were significant. In many contexts, ANOVA testing and variance analysis are similar.
- Researchers must have the necessary research skills to analyze and manipulation the data , Getting trained to demonstrate a high standard of research practice. Ideally, researchers must possess more than a basic understanding of the rationale of selecting one statistical method over the other to obtain better data insights.
- Usually, research and data analytics projects differ by scientific discipline; therefore, getting statistical advice at the beginning of analysis helps design a survey questionnaire, select data collection methods , and choose samples.
LEARN ABOUT: Best Data Collection Tools
- The primary aim of data research and analysis is to derive ultimate insights that are unbiased. Any mistake in or keeping a biased mind to collect data, selecting an analysis method, or choosing audience sample il to draw a biased inference.
- Irrelevant to the sophistication used in research data and analysis is enough to rectify the poorly defined objective outcome measurements. It does not matter if the design is at fault or intentions are not clear, but lack of clarity might mislead readers, so avoid the practice.
- The motive behind data analysis in research is to present accurate and reliable data. As far as possible, avoid statistical errors, and find a way to deal with everyday challenges like outliers, missing data, data altering, data mining , or developing graphical representation.
LEARN MORE: Descriptive Research vs Correlational Research The sheer amount of data generated daily is frightening. Especially when data analysis has taken center stage. in 2018. In last year, the total data supply amounted to 2.8 trillion gigabytes. Hence, it is clear that the enterprises willing to survive in the hypercompetitive world must possess an excellent capability to analyze complex research data, derive actionable insights, and adapt to the new market needs.
LEARN ABOUT: Average Order Value
QuestionPro is an online survey platform that empowers organizations in data analysis and research and provides them a medium to collect data by creating appealing surveys.
MORE LIKE THIS
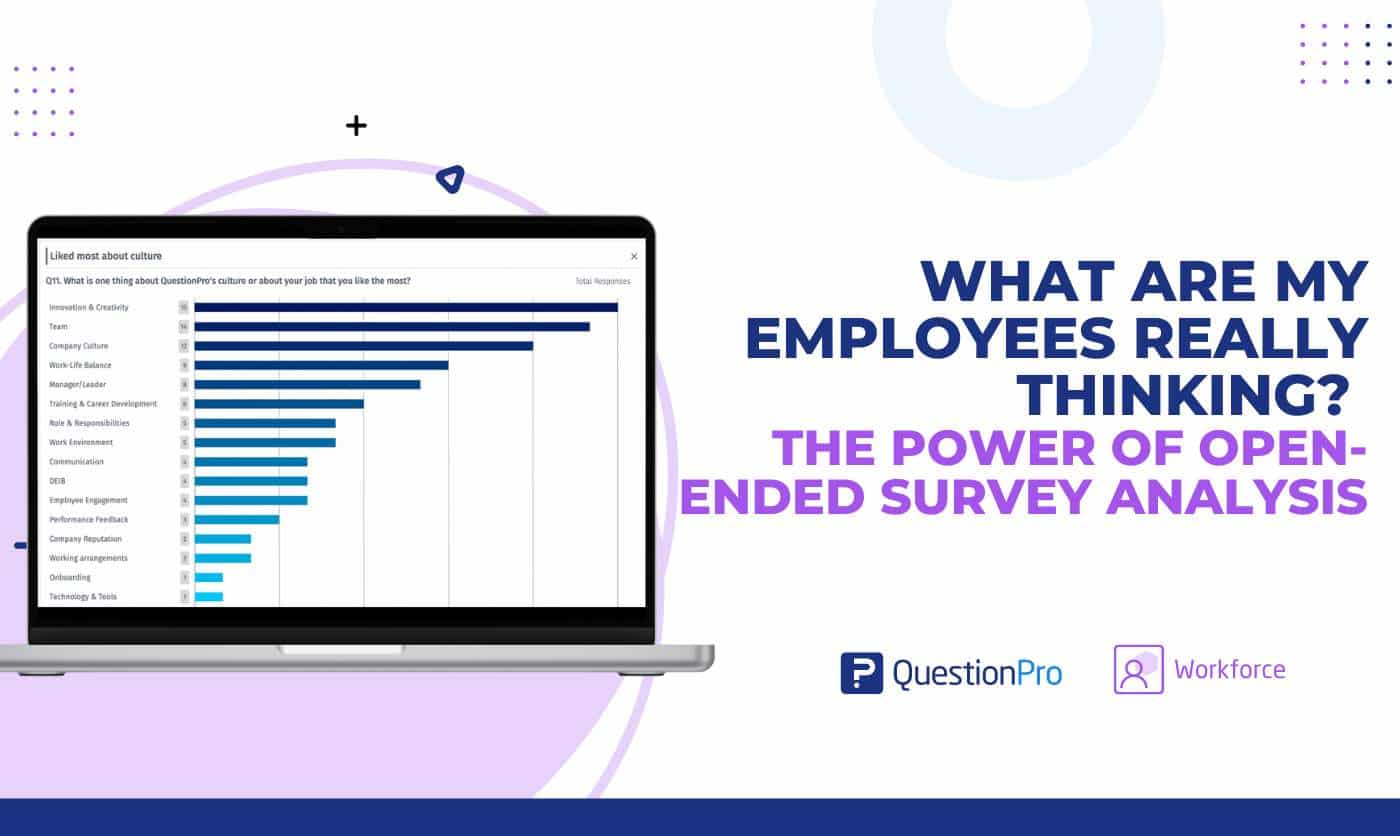
What Are My Employees Really Thinking? The Power of Open-ended Survey Analysis
May 24, 2024
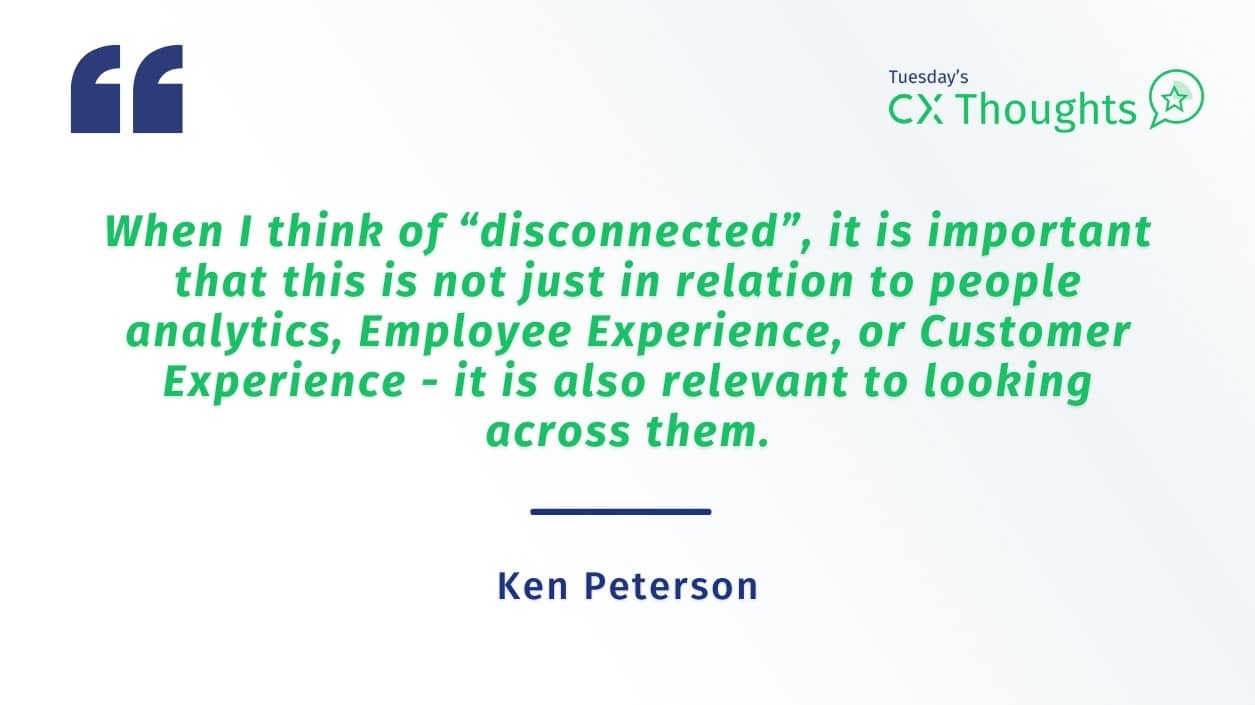
I Am Disconnected – Tuesday CX Thoughts
May 21, 2024
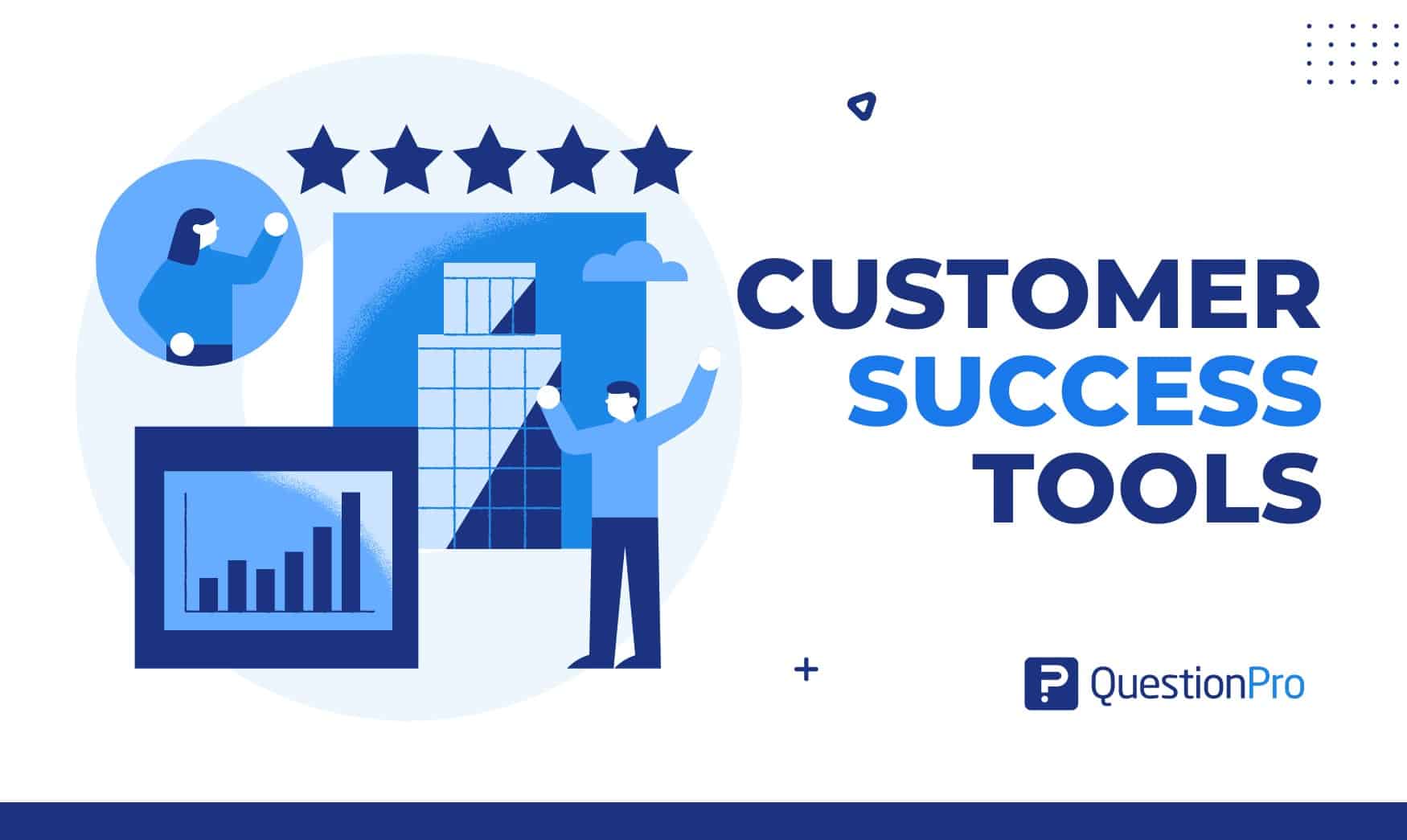
20 Best Customer Success Tools of 2024
May 20, 2024
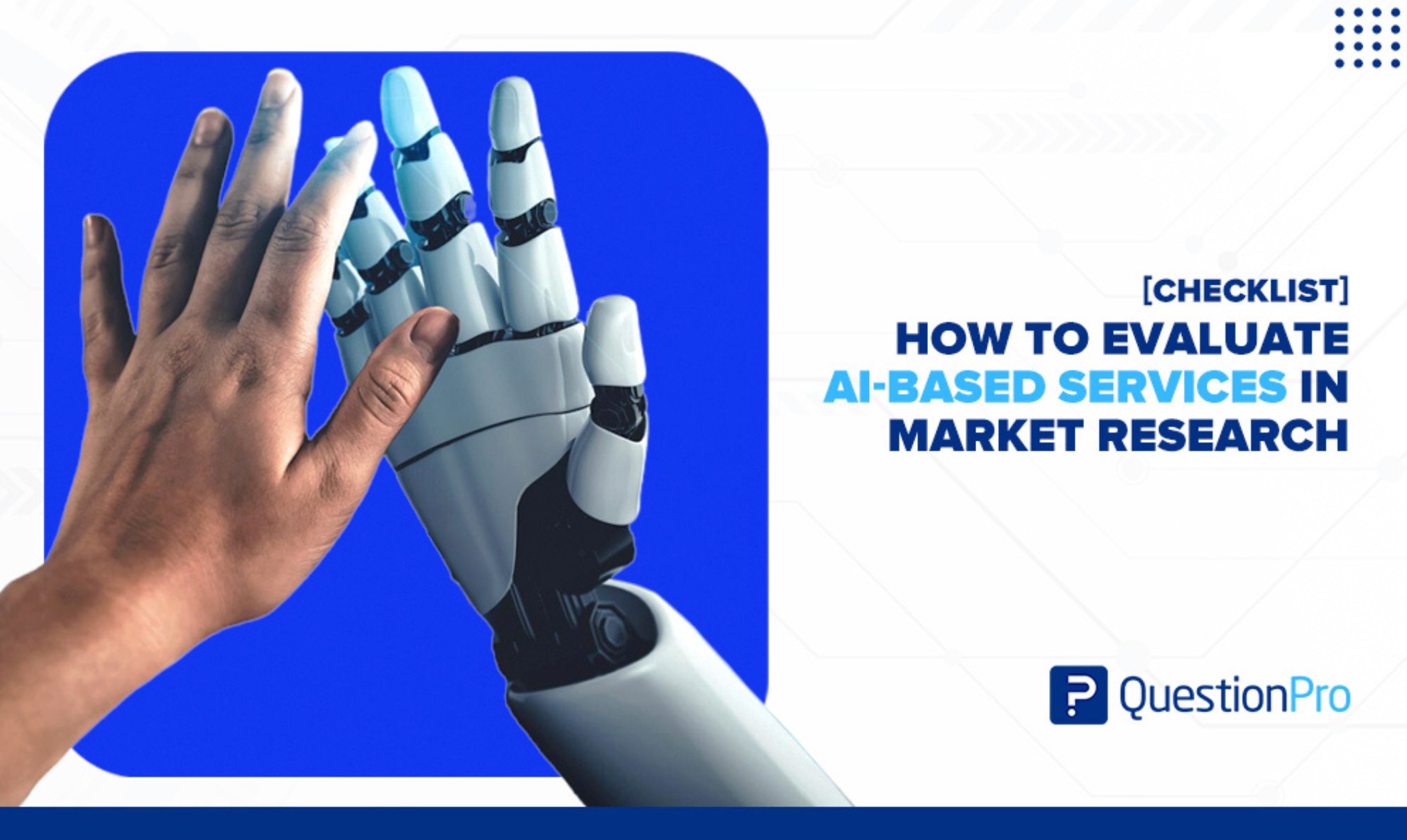
AI-Based Services Buying Guide for Market Research (based on ESOMAR’s 20 Questions)
Other categories.
- Academic Research
- Artificial Intelligence
- Assessments
- Brand Awareness
- Case Studies
- Communities
- Consumer Insights
- Customer effort score
- Customer Engagement
- Customer Experience
- Customer Loyalty
- Customer Research
- Customer Satisfaction
- Employee Benefits
- Employee Engagement
- Employee Retention
- Friday Five
- General Data Protection Regulation
- Insights Hub
- Life@QuestionPro
- Market Research
- Mobile diaries
- Mobile Surveys
- New Features
- Online Communities
- Question Types
- Questionnaire
- QuestionPro Products
- Release Notes
- Research Tools and Apps
- Revenue at Risk
- Survey Templates
- Training Tips
- Uncategorized
- Video Learning Series
- What’s Coming Up
- Workforce Intelligence

Skills for Learning : Research Skills
Data analysis is an ongoing process that should occur throughout your research project. Suitable data-analysis methods must be selected when you write your research proposal. The nature of your data (i.e. quantitative or qualitative) will be influenced by your research design and purpose. The data will also influence the analysis methods selected.
We run interactive workshops to help you develop skills related to doing research, such as data analysis, writing literature reviews and preparing for dissertations. Find out more on the Skills for Learning Workshops page.
We have online academic skills modules within MyBeckett for all levels of university study. These modules will help your academic development and support your success at LBU. You can work through the modules at your own pace, revisiting them as required. Find out more from our FAQ What academic skills modules are available?
Quantitative data analysis
Broadly speaking, 'statistics' refers to methods, tools and techniques used to collect, organise and interpret data. The goal of statistics is to gain understanding from data. Therefore, you need to know how to:
- Produce data – for example, by handing out a questionnaire or doing an experiment.
- Organise, summarise, present and analyse data.
- Draw valid conclusions from findings.
There are a number of statistical methods you can use to analyse data. Choosing an appropriate statistical method should follow naturally, however, from your research design. Therefore, you should think about data analysis at the early stages of your study design. You may need to consult a statistician for help with this.
Tips for working with statistical data
- Plan so that the data you get has a good chance of successfully tackling the research problem. This will involve reading literature on your subject, as well as on what makes a good study.
- To reach useful conclusions, you need to reduce uncertainties or 'noise'. Thus, you will need a sufficiently large data sample. A large sample will improve precision. However, this must be balanced against the 'costs' (time and money) of collection.
- Consider the logistics. Will there be problems in obtaining sufficient high-quality data? Think about accuracy, trustworthiness and completeness.
- Statistics are based on random samples. Consider whether your sample will be suited to this sort of analysis. Might there be biases to think about?
- How will you deal with missing values (any data that is not recorded for some reason)? These can result from gaps in a record or whole records being missed out.
- When analysing data, start by looking at each variable separately. Conduct initial/exploratory data analysis using graphical displays. Do this before looking at variables in conjunction or anything more complicated. This process can help locate errors in the data and also gives you a 'feel' for the data.
- Look out for patterns of 'missingness'. They are likely to alert you if there’s a problem. If the 'missingness' is not random, then it will have an impact on the results.
- Be vigilant and think through what you are doing at all times. Think critically. Statistics are not just mathematical tricks that a computer sorts out. Rather, analysing statistical data is a process that the human mind must interpret!
Top tips! Try inventing or generating the sort of data you might get and see if you can analyse it. Make sure that your process works before gathering actual data. Think what the output of an analytic procedure will look like before doing it for real.
(Note: it is actually difficult to generate realistic data. There are fraud-detection methods in place to identify data that has been fabricated. So, remember to get rid of your practice data before analysing the real stuff!)
Statistical software packages
Software packages can be used to analyse and present data. The most widely used ones are SPSS and NVivo.
SPSS is a statistical-analysis and data-management package for quantitative data analysis. Click on ‘ How do I install SPSS? ’ to learn how to download SPSS to your personal device. SPSS can perform a wide variety of statistical procedures. Some examples are:
- Data management (i.e. creating subsets of data or transforming data).
- Summarising, describing or presenting data (i.e. mean, median and frequency).
- Looking at the distribution of data (i.e. standard deviation).
- Comparing groups for significant differences using parametric (i.e. t-test) and non-parametric (i.e. Chi-square) tests.
- Identifying significant relationships between variables (i.e. correlation).
NVivo can be used for qualitative data analysis. It is suitable for use with a wide range of methodologies. Click on ‘ How do I access NVivo ’ to learn how to download NVivo to your personal device. NVivo supports grounded theory, survey data, case studies, focus groups, phenomenology, field research and action research.
- Process data such as interview transcripts, literature or media extracts, and historical documents.
- Code data on screen and explore all coding and documents interactively.
- Rearrange, restructure, extend and edit text, coding and coding relationships.
- Search imported text for words, phrases or patterns, and automatically code the results.
Qualitative data analysis
Miles and Huberman (1994) point out that there are diverse approaches to qualitative research and analysis. They suggest, however, that it is possible to identify 'a fairly classic set of analytic moves arranged in sequence'. This involves:
- Affixing codes to a set of field notes drawn from observation or interviews.
- Noting reflections or other remarks in the margins.
- Sorting/sifting through these materials to identify: a) similar phrases, relationships between variables, patterns and themes and b) distinct differences between subgroups and common sequences.
- Isolating these patterns/processes and commonalties/differences. Then, taking them out to the field in the next wave of data collection.
- Highlighting generalisations and relating them to your original research themes.
- Taking the generalisations and analysing them in relation to theoretical perspectives.
(Miles and Huberman, 1994.)
Patterns and generalisations are usually arrived at through a process of analytic induction (see above points 5 and 6). Qualitative analysis rarely involves statistical analysis of relationships between variables. Qualitative analysis aims to gain in-depth understanding of concepts, opinions or experiences.
Presenting information
There are a number of different ways of presenting and communicating information. The particular format you use is dependent upon the type of data generated from the methods you have employed.
Here are some appropriate ways of presenting information for different types of data:
Bar charts: These may be useful for comparing relative sizes. However, they tend to use a large amount of ink to display a relatively small amount of information. Consider a simple line chart as an alternative.
Pie charts: These have the benefit of indicating that the data must add up to 100%. However, they make it difficult for viewers to distinguish relative sizes, especially if two slices have a difference of less than 10%.
Other examples of presenting data in graphical form include line charts and scatter plots .
Qualitative data is more likely to be presented in text form. For example, using quotations from interviews or field diaries.
- Plan ahead, thinking carefully about how you will analyse and present your data.
- Think through possible restrictions to resources you may encounter and plan accordingly.
- Find out about the different IT packages available for analysing your data and select the most appropriate.
- If necessary, allow time to attend an introductory course on a particular computer package. You can book SPSS and NVivo workshops via MyHub .
- Code your data appropriately, assigning conceptual or numerical codes as suitable.
- Organise your data so it can be analysed and presented easily.
- Choose the most suitable way of presenting your information, according to the type of data collected. This will allow your information to be understood and interpreted better.
Primary, secondary and tertiary sources
Information sources are sometimes categorised as primary, secondary or tertiary sources depending on whether or not they are ‘original’ materials or data. For some research projects, you may need to use primary sources as well as secondary or tertiary sources. However the distinction between primary and secondary sources is not always clear and depends on the context. For example, a newspaper article might usually be categorised as a secondary source. But it could also be regarded as a primary source if it were an article giving a first-hand account of a historical event written close to the time it occurred.
- Primary sources
- Secondary sources
- Tertiary sources
- Grey literature
Primary sources are original sources of information that provide first-hand accounts of what is being experienced or researched. They enable you to get as close to the actual event or research as possible. They are useful for getting the most contemporary information about a topic.
Examples include diary entries, newspaper articles, census data, journal articles with original reports of research, letters, email or other correspondence, original manuscripts and archives, interviews, research data and reports, statistics, autobiographies, exhibitions, films, and artists' writings.
Some information will be available on an Open Access basis, freely accessible online. However, many academic sources are paywalled, and you may need to login as a Leeds Beckett student to access them. Where Leeds Beckett does not have access to a source, you can use our Request It! Service .
Secondary sources interpret, evaluate or analyse primary sources. They're useful for providing background information on a topic, or for looking back at an event from a current perspective. The majority of your literature searching will probably be done to find secondary sources on your topic.
Examples include journal articles which review or interpret original findings, popular magazine articles commenting on more serious research, textbooks and biographies.
The term tertiary sources isn't used a great deal. There's overlap between what might be considered a secondary source and a tertiary source. One definition is that a tertiary source brings together secondary sources.
Examples include almanacs, fact books, bibliographies, dictionaries and encyclopaedias, directories, indexes and abstracts. They can be useful for introductory information or an overview of a topic in the early stages of research.
Depending on your subject of study, grey literature may be another source you need to use. Grey literature includes technical or research reports, theses and dissertations, conference papers, government documents, white papers, and so on.
Artificial intelligence tools
Before using any generative artificial intelligence or paraphrasing tools in your assessments, you should check if this is permitted on your course.
If their use is permitted on your course, you must acknowledge any use of generative artificial intelligence tools such as ChatGPT or paraphrasing tools (e.g., Grammarly, Quillbot, etc.), even if you have only used them to generate ideas for your assessments or for proofreading.
- Academic Integrity Module in MyBeckett
- Assignment Calculator
- Building on Feedback
- Disability Advice
- Essay X-ray tool
- International Students' Academic Introduction
- Manchester Academic Phrasebank
- Quote, Unquote
- Skills and Subject Suppor t
- Turnitin Grammar Checker
{{You can add more boxes below for links specific to this page [this note will not appear on user pages] }}
- Research Methods Checklist
- Sampling Checklist
Skills for Learning FAQs

0113 812 1000
- University Disclaimer
- Accessibility
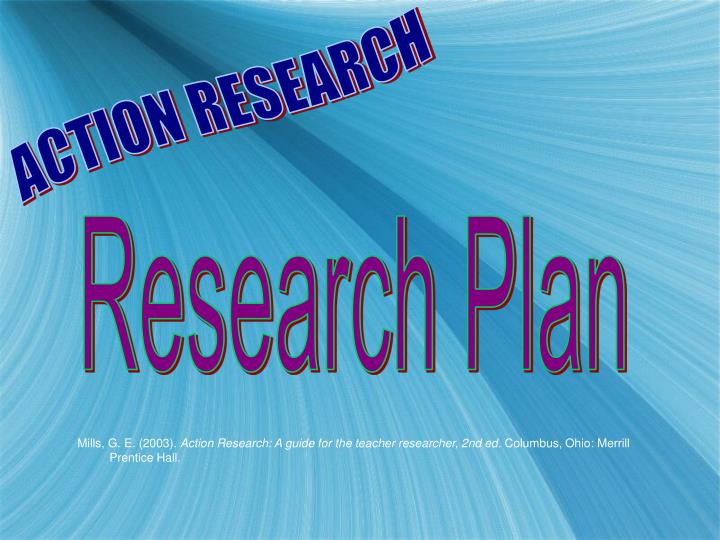
ACTION RESEARCH
Mar 27, 2019
9.46k likes | 20.54k Views
ACTION RESEARCH. Research Plan. Mills, G. E. (2003). Action Research: A guide for the teacher researcher, 2nd ed. Columbus, Ohio: Merrill Prentice Hall.
Share Presentation
- research question
- roles responsibilities
- dialectic action research plan
- action research group
- data collection data analysis

Presentation Transcript
ACTION RESEARCH Research Plan Mills, G. E. (2003). Action Research: A guide for the teacher researcher, 2nd ed. Columbus, Ohio: Merrill Prentice Hall.
… is systematic inquiry done by teachers (or other individuals in the teaching/learning environment) to gather information about- and subsequently improve- how their particular schools operate, how they teach, and how well their students learn. ACTION RESEARCH
Chapter 1: Understanding Action ResearchAction Research Teaching Focus Area Objectives Implement lesson Reflect on whether students achieved objectives Data Collection Data Analysis & Interpretation Reflect on Lesson Reflect on how today’s lesson affects tomorrow’s lesson Action Planning
The Dialectic Action Research Plan Mills, G. E. (2003). Action Research: A guide for the teacher researcher, 2nd ed. Columbus, Ohio: Merrill Prentice Hall. Identify an Area of Focus Develop an Action Plan Collect Data Analyze and Interpret Data
To Do: Select an appropriate area of focus. Do reconnaissance. Review related literature. Write and research plan to guide your work. Steps to Planning an Action Research Project
1. Criteria for Selecting a General Idea/Area of Focus: Involve teaching and learning and should focus on your own practice Be something within your locus of control Be something you feel passionate about Be something you would like to change or improve Take a statement/observation and turn it into a Research Question. It should…
Steps to create a good Area of Focus • Identify a problem or situation you would like to change or improve. Example: My ESL students don’t like to read in English. • Turn that problem into a question.* Example: What reading strategies can a ESL teacher use to motivate her students to enjoy reading in English? * This question will guide yourreconnaissance and eventually your development of final research questions.
3. Review of Related Literature 2. Reconnaissance • Explore your own understanding of your Area of Focus. • Write a Reflective Memo • Search for others’ theories and research on your topic. • Write a Theoretical Framework of theories that support your study. • Write a Literature Review of research studies that pertain to your study.
4. Create a Research Plan: A research plan summarizes your action research thoughts in a plan that will guide you through your classroom research work.
Steps for writing a goodResearch Plan Write (re-write) an area-of-focus statement Define variables Develop research questions Describe the intervention or innovations Describe the membership of the action research group Describe negotiations that need to be undertaken Develop a timeline Develop a statement of resources Develop data collection ideas Develop a triangulation matrix
Step 1: Area of Focus Statement * In the following samples of Area of Focus Statements, try to identify… The structure and key words The variables An Area of Focus Statement … • Defines the purpose of your study • Identifies the variables that you will study
Samples: Area of Focus Statements The purpose of this study is to describe the effects of an integrated problem-solving mathematics curriculum on student transfer of problem-solving skills and the retention of basic math facts and functions. What do these have in common? 2. The purpose of this study is to describe the impact of bringing audience members into an interactive relationship with teen theater productions on participants’ abilities to identify issues and incorporate solutions to similar problems in their own lives. 3. The purpose of this study is to describe the effects of student-led conferences on parent and student satisfaction with the conferencing process.
Area of Focus Statement “The purpose of this study is to…” Key words … describe the effects of… on… … describe the impact of… on…
A Variable… Is a characteristic of your study that is subject to change. Step 2: Define the Variables In the following samples of Area of Focus Statements, try to… Identify the variables Notice how specifically they are written
What are the variables? The purpose of this study is to describe the effects of an integrated problem-solving mathematics curriculum on student transfer of problem-solving skills and the retention of basic math facts and functions. Samples: Area of Focus Statements/Variables 2. The purpose of this study is to describe the impact of bringing audience members into an interactive relationship with teen theater productions on participants’ abilities to identify issues and incorporate solutions to similar problems in their own lives. 3. The purpose of this study is to describe the effects of student-led conferences on parent and student satisfaction with the conferencing process.
Define the Variables Write definitions of what you will really focus on in the study. • Make definitions clear and specific to your study. Think…. • What does the variable LOOK like? • How will I know it when I see it?
TITLE:__________________________ AUTHOR: __________________ • Educational Problem: It is not known if the software program students use in the lab is actually effective in improving the pronunciation skills of basic level ESL students. • Research Question: Arethe CALL program Tell Me More effective in improving the pronunciation skills of Basic ESL students? • Area-of-focus statement: The purpose of this study is…to find out the effectiveness of the program Tell Me More in improving the pronunciation skills of basic level ESL students. • 2. The problem is… …turned into a question and …turned into an Area of Focus statement. Variables are determined and defined according to the study.
Step 3: Develop Research Questions * In the following sample of a Research Plan try to identify… How variable definitions are turned into specific research questions How the research questions will guide the research and gather the answers needed. Research Questions are… • Specific questions that guide and focus your research.
Variables turn into research questions… • 3. Research Questions: • How does the CALL program Tell Me More help improve the pronunciation skills of basic level ESL students? • How do the students feel about the improvement (or not) of their pronunciation? • Does the students’ pronunciation improve after using the CALL software Tell Me More? • 4. Intervention or innovation: I will… observe and keep a record of the students’ performance to see if their pronunciation improves after using the software. I will survey and hold informal talks with the students to find out how they feel about using the software and if they feel that their pronunciation improves after using it. This information will help the institute and lab choose appropriate pronunciation software and shed light onto how to make the best use of the software. …which then determine the intervention or innovation that will occur.
Develop Research Questions What are your specific questions? What are you really going to focus on? • Help focus the data collection plan • Help validate that you have a workable way to proceed with your investigation
Step 4: Intervention/Innovation “I will…” … implement… … include… … incorporate… An Intervention/Innovation is… • What you will do*, change, or introduce in your study to improve the educational problem mentioned. * Note: It is also possible to do a descriptive research project whose intervention is in the action plan for the future.
Step 5: Membership The members of your study are… • Anyone who plays a part in your research. • Yourself • Students • Administrator • Parents
Describe the Membership of the Action Research Group Who will you be working with? Why are they important? What will be each person’s roles/responsibilities?
5. Membership • 6. Negotiations • I will need to ask the permission of the CCA to do observations in the Computer lab. • I will need to ask the students to take surveys about their practice with the software in the lab. Clarifying the members of the study… …leads to determining negotiations that must be made in order to get the information you need.
Step 6: Negotiations Negotiations are... • Conversations and/or permission that needs take place before you can gather your data… • Permission to gather information, hold interviews, do observations, audiotape, videotape, use workspace, etc. • Confirmation of cooperation from others in any aspect of the study
Describe Negotiations that Need to be Taken What negotiations do you have to undertake with others to implement your action research plan? How will you get access to the site? Do you need permission? From whom? How will you get it?
Step 7: Timeline A timeline... • Guides and organizes your research • Keeps you on track
Develop a Timeline Who will be doing what and when?* *Hint: Work backwards in time! Phase 1 (July-Nov): Identity area focus, review literature develop research questions, reconnaissance. Phase 2 (date-date)… Phase 3 (date-date)… (July-Nov) (date) (date) Phase 1 Phase 2 Phase 3
Step 8: Resources Resources are... • Materials you will need in order to perform your research study. • All researchers need a journal and pencil! • Technology- video camera, tape recorder, computer, etc. (Don’t forget batteries!) • Graphic organizers, class documents, etc.
Step 9: Data Collection Ideas • Data that… • Answers your research questions • Provides evidence for your reflections on what you are researching • Can be supported by other sources of data (triangulation of data)
Develop Data Collection Ideas What kind of data will provide you the evidence you need? From what source will you get it? Naturally occurring data: test scores, attendance records, writing samples, portfolios, anecdotal records,... Other types of data: interviews, surveys, questionnaires, videotapes, audiotapes, maps, photos, observations,…
7. Timeline • 8. Statement of Resources: • Notebook/pencil for fieldnotes & journal • Survey • Questions for informal conversations • 9. Data Collection Ideas: All this work requires… …a timeline for completion… (Hint: count backwards) …a list of what you’ll need… …and ideas on how to best get the information you need to answer your research questions.
3. Research Questions: • How does the CALL program Tell Me More help improve the pronunciation skills of basic level ESL students? • How do the students feel about the improvement (or not) of their pronunciation? • Does the students’ pronunciation improve after using the CALL software Tell Me More? Research questions should be directly related to… • 9. Data Collection Ideas: …the data collection ideas …and the data sources from which data are gathered
Step 10: Develop Triangulation Matrix * In the following samples of a Triangulation Matrix, try to identify… The variables which became research questions The data sources that will answer those questions A Triangulation Matrix… • Shows various data sources that will be used to answer each research question. • Assures that your findings will be supported by multiple sources
Sample 1: Triangulation Matrix Methods of Data Collection
Sample 2: Triangulation Matrix Methods of Data Collection
PUT THEINTO ACTION PLAN ACTION !
Don’t stop there… Write an Action Research Paper! Why? • The process of writing requires the writer to clarify meaning. • The act of putting information on paper for your peers and teachers necessitates honesty, accuracy, clarity, and thought. • Research, once written, can be shared with a wider audience.
- More by User
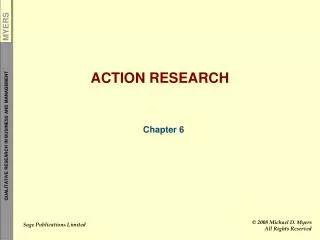
ACTION RESEARCH. Chapter 6. Introduction. Action research is a research method that aims to solve current practical problems while expanding scientific knowledge It is oriented towards collaboration and change involving researchers and subjects. Definition.
651 views • 7 slides
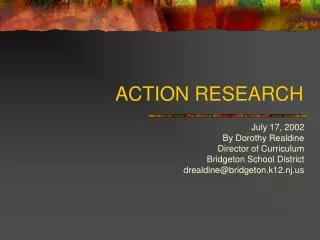
ACTION RESEARCH. July 17, 2002 By Dorothy Realdine Director of Curriculum Bridgeton School District [email protected] . What is Action Research?. A form of self-reflective inquiry by practitioners into their own educational practices and situations.
718 views • 10 slides
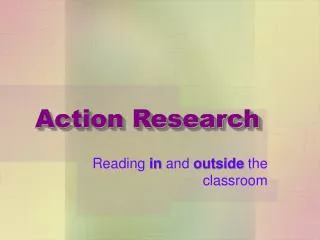
Action Research
Action Research. Reading in and outside the classroom. Guidelines. Our team Research rationale Theoretical background Classroom context Description of action research Conclusions. Our team. Núcleo de Estágio de Português e Inglês da Escola Secundária Carolina Michaëlis Ana Silva
1.04k views • 45 slides
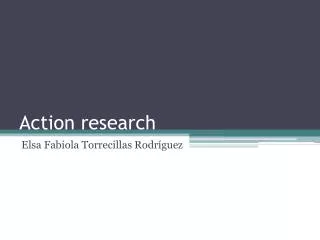
Action research
Action research. Elsa Fabiola Torrecillas Rodríguez. What is action research ?. Action research is a kind of research that teachers carry out in order to improve or solve a problem they have in their classroom environment .
1.63k views • 12 slides
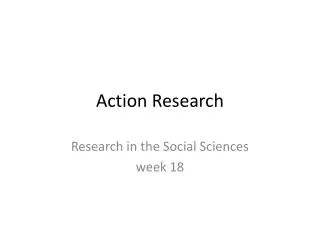
Action Research. Research in the Social Sciences week 18. Outline. What is action research? Participatory action research Insider action research External action research Why action research? Stages in Action Research Ethics Activity. Key Resources. Journal: Action Research
797 views • 15 slides
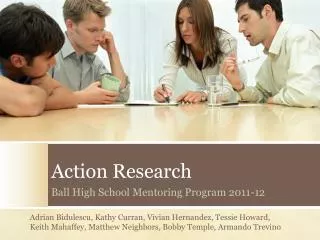
Action Research. Ball High School Mentoring Program 2011-12. Adrian Bidulescu, Kathy Curran, Vivian Hernandez, Tessie Howard, Keith Mahaffey, Matthew Neighbors, Bobby Temple, Armando Trevino. Research Question. Does academic mentoring of African American males affect their attendance?.
260 views • 10 slides
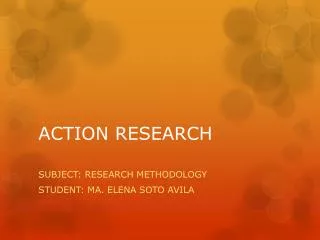
ACTION RESEARCH. SUBJECT: RESEARCH METHODOLOGY STUDENT: MA. ELENA SOTO AVILA. WHAT IS ACTION RESEARCH?.
398 views • 7 slides
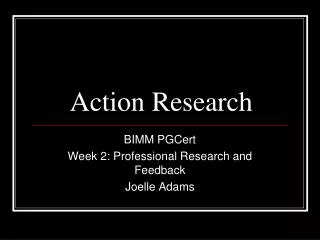
Action Research. BIMM PGCert Week 2: Professional Research and Feedback Joelle Adams. Catching Up. How was your teaching this week? Did you have any challenges? How did/might you overcome them? Does anyone else have any suggestions?. Following Up. Presentations
621 views • 20 slides
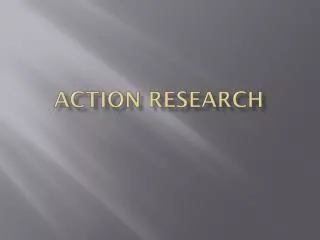
Action Research. Goals. Rationale Action Research Cycle Process Role of Teachers Accomplished Teaching. Rationale.
655 views • 19 slides
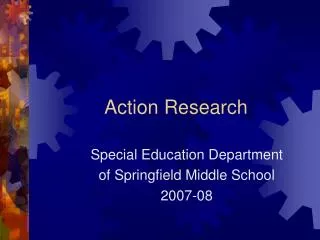
Action Research. Special Education Department of Springfield Middle School 2007-08. The Question?. Will applying content-area support have a greater impact on student achievement than grade level support?. The Rationale:.
351 views • 13 slides
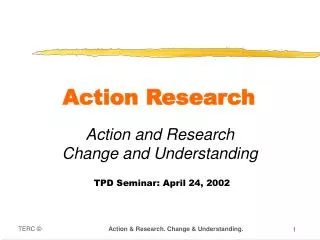
Action Research. Action and Research Change and Understanding. TPD Seminar: April 24, 2002. Professional Practice: Focus 1. Think-Pair-Share (TPS) For each partner think about and discuss: One element of your TPD practice that you have tried to improve
542 views • 10 slides
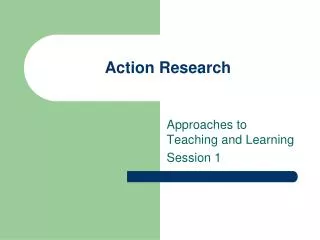
Action Research. Approaches to Teaching and Learning Session 1. What is action research?. Carried out by teacher(s). In his/her own class. With his/her own students. To improve some aspect of learning/teaching. Action Research. What is Action Research?
744 views • 13 slides
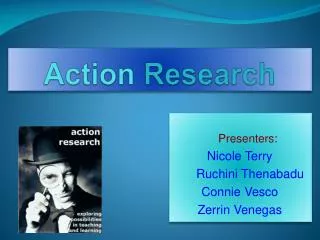
Action Research. Presenters: Nicole Terry Ruchini Thenabadu Connie Vesco Zerrin Venegas. “The secret of success in the teaching profession is to continually grow and learn. Action research is a way to continue to grow and learn by making use of your own experiences.”
613 views • 22 slides
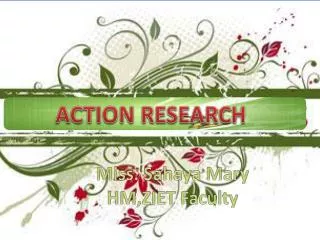
ACTION RESEARCH. Miss. Sahaya Mary HM,ZIET Faculty.
963 views • 22 slides
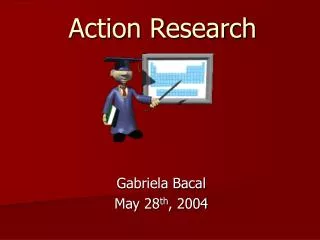
Action Research. Gabriela Bacal May 28 th , 2004. Exploring Additional Classes. Nanotechnology http://nanotech.nanopolis.net/ Chemistry http://www.prenhall.com/divisions/esm/chem_central/chemcentral/ Atomic and Nuclear Physics http://www.colorado.edu/physics/2000/index.pl?Type=TOC.
451 views • 18 slides
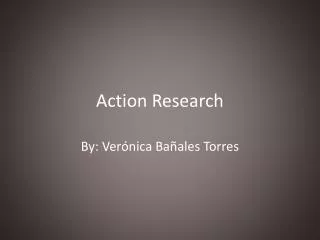
Action Research. By : Verónica Bañales Torres. What is Action Research (AR)?. Action research is a type of research related of the idea of ‘ reflective practice ’.
529 views • 10 slides
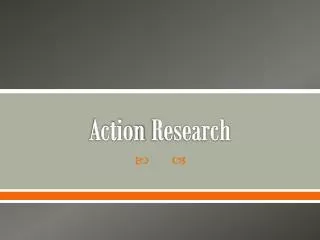
Action Research. What is action research ?. Action research is a study/investigation applied to a classroom setting with the objective of working issues which changes/alters the learning environment, and further on find a solutions to them.
745 views • 8 slides
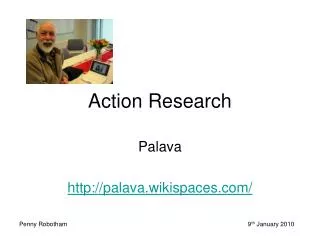
Action Research. Palava http://palava.wikispaces.com/. Some of our interests. Effective use of exercise books Revision strategies and intervention Modelling Cognitive dissonance. Youtube and You in the classroom. A little light relief …. Your task …. Give it a go. The ScienceVideoBank.
514 views • 27 slides
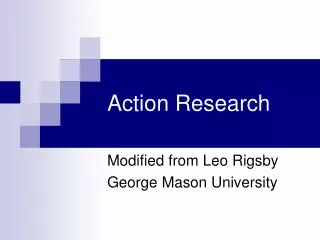
Action Research. Modified from Leo Rigsby George Mason University. How is Action Research Defined?. Action Research is a three step spiral process of (1) planning which involves reconnaissance; (2) taking actions; and (3) fact-finding about the results of the action (Kurt Lewin, 1947).
626 views • 12 slides
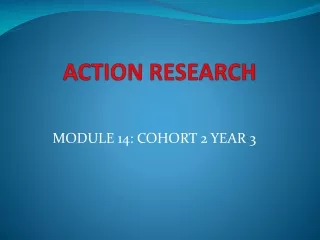
ACTION RESEARCH. MODULE 14: COHORT 2 YEAR 3. PLANNING. Staff:. German Hernandez (International Professor) [email protected] Milton Top (Local Professor) [email protected] Prewien Mohan (Teaching Assistant) [email protected] Julian Mac Lean (Teaching Assistant)
576 views • 12 slides
Home PowerPoint Templates Data Analysis
Data Analysis PowerPoint Templates & Presentation Slides
Download 100% editable data analysis PowerPoint templates and backgrounds for presentations in Microsoft PowerPoint.
Featured Templates
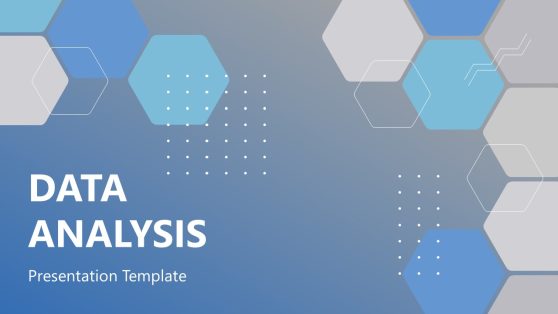
Data Analysis PowerPoint Template
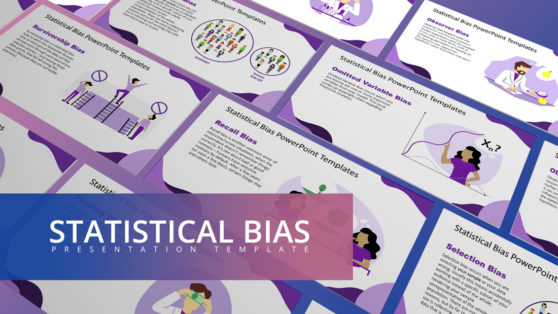
Statistical Bias PowerPoint Templates
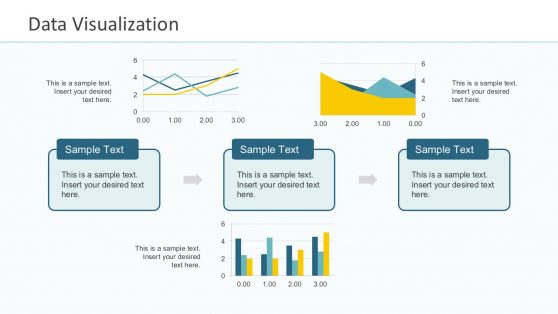
Data Visualization PowerPoint Template
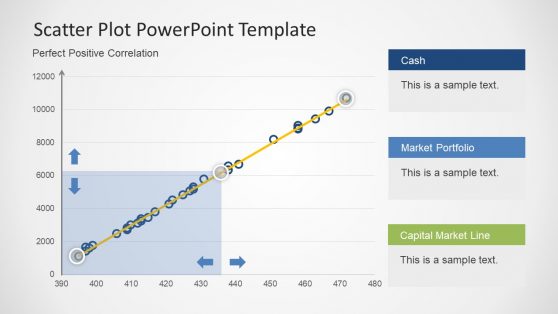
Scatter Plots Correlations PowerPoint Templates
Latest templates.
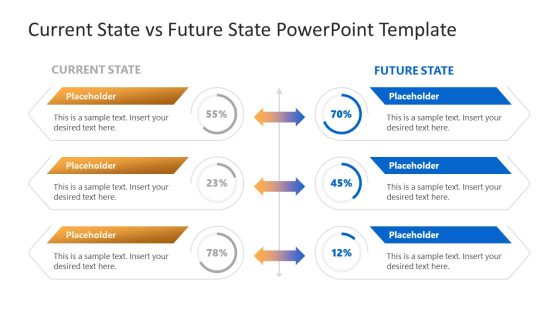
Current State vs Future State PowerPoint Template
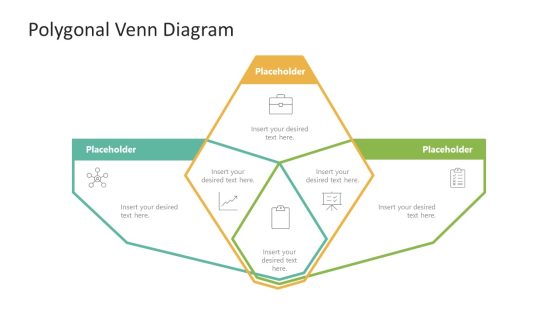
Polygonal Venn Diagram
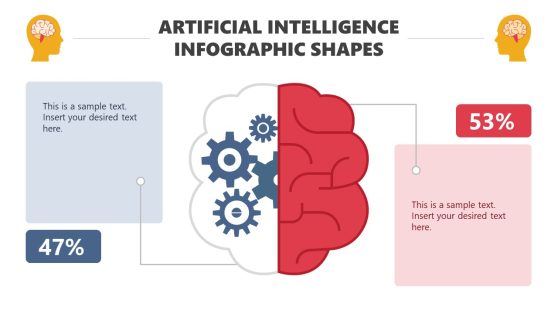
Artificial Intelligence Infographic Shapes for PowerPoint
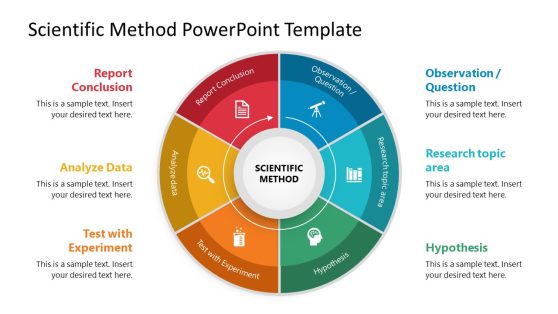
Scientific Method Diagram PowerPoint Template
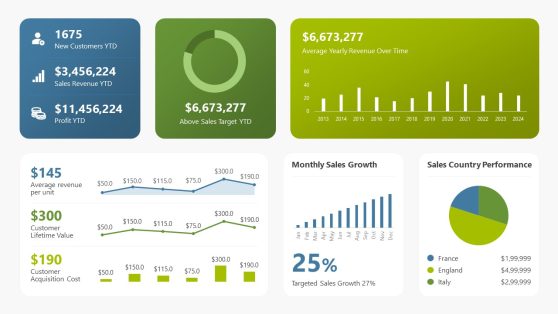
Sales Performance Dashboard PowerPoint Template
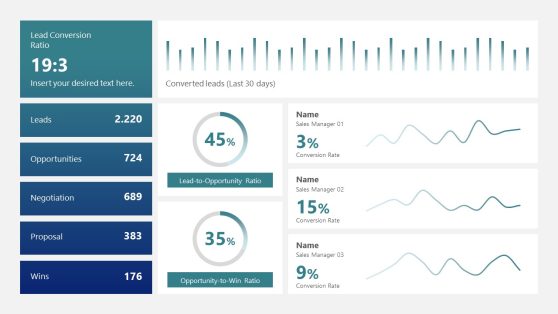
Sales Dashboard Template for PowerPoint
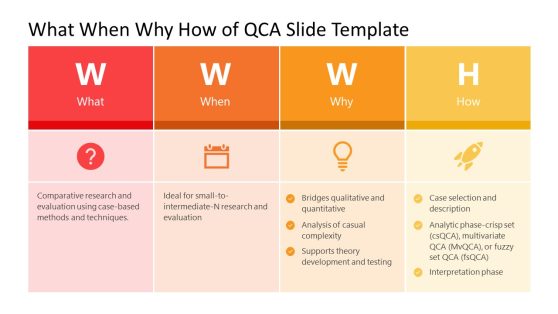
What When Why How of QCA Template for PowerPoint
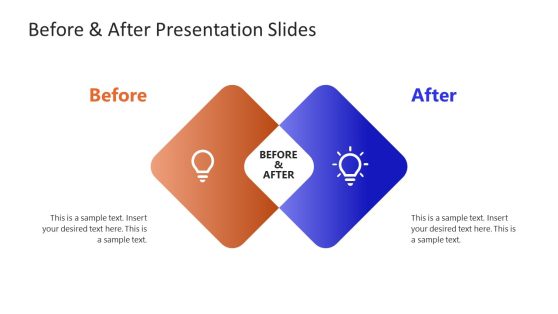
Before & After Presentation Slides
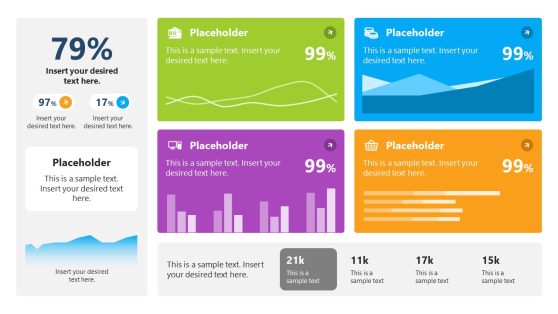
Executive Dashboard PowerPoint Template
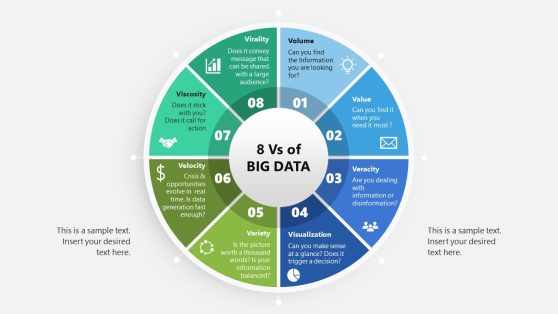
8Vs of Big Data PowerPoint Template
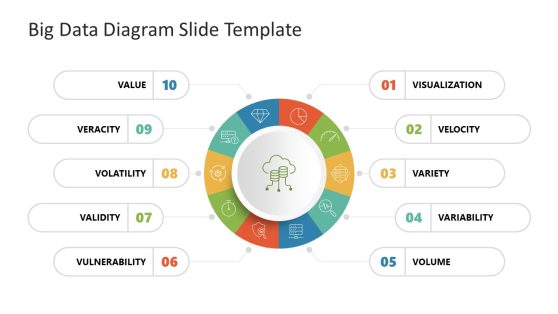
Big Data Diagram PowerPoint Template
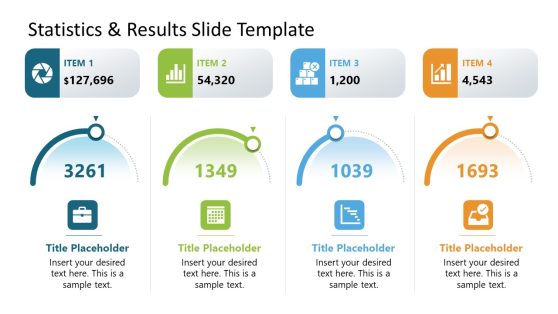
Statistics & Results PowerPoint Template
Data Analysis PowerPoint presentation templates are pre-designed slides that can be used for presenting results, insights, and conclusions derived from the analysis of various kinds of data. They often contain a variety of slide layouts, diagrams, charts, and other graphic elements that can effectively communicate complex data in a visually engaging and digestible manner.
Our editable data analysis presentation slides can help to prepare impeccable business reports and data analysis presentations with the help of editable & high-quality data analysis slide templates compatible with PowerPoint & Google Slides presentations.
Possible use cases, applications and presentation ideas for data analysis slide templates:
- Business Intelligence: A company might use data analysis templates to present results from its business intelligence efforts. This could include data about sales trends, customer demographics, and operational efficiency.
- Academic Research: Researchers can use data analysis presentation templates to present their research findings in conferences or seminars. They can showcase data about a variety of subjects, from social sciences to natural sciences.
- Marketing Campaign Analysis: Marketing professionals might use data analysis PowerPoint templates to present the results of a marketing campaign, analyzing data like audience engagement, conversion rates, and return on investment.
- SEO Strategy: A data analysis can also be used in a SEO-oriented presentation. This can help digital marketing teams, businesses, and SEO agencies to plan, implement, and report their SEO strategies effectively. The use of tools such as Google’s BigQuery can also demonstrate the ability to handle and analyze big data, which is increasingly important in today’s data-driven marketing landscape.
- Financial Analysis: Financial analysts could use slide templates on data analysis to present financial data such as revenue trends, cost analysis, budgeting, and forecasting.
- Healthcare Data Analysis: In the healthcare sector, data analysis templates can be used to present data on patient demographics, treatment effectiveness, and disease prevalence, for example.
- Consulting: Consultants and consulting firms often need to present data-driven insights to their clients. A data analysis PowerPoint template or presentation template for Google Slides would be suitable for this.
- Government & Public Policy: Government officials or policy analysts may use data analysis presentation templates to present data on social issues, economic trends, or the impact of certain policies.
These data analysis infographics and charts can help to prepare compelling data analysis presentation designs with charts and visually appealing graphics.
Download Unlimited Content
Our annual unlimited plan let you download unlimited content from slidemodel. save hours of manual work and use awesome slide designs in your next presentation..
Case Study Research Method in Psychology
Saul Mcleod, PhD
Editor-in-Chief for Simply Psychology
BSc (Hons) Psychology, MRes, PhD, University of Manchester
Saul Mcleod, PhD., is a qualified psychology teacher with over 18 years of experience in further and higher education. He has been published in peer-reviewed journals, including the Journal of Clinical Psychology.
Learn about our Editorial Process
Olivia Guy-Evans, MSc
Associate Editor for Simply Psychology
BSc (Hons) Psychology, MSc Psychology of Education
Olivia Guy-Evans is a writer and associate editor for Simply Psychology. She has previously worked in healthcare and educational sectors.
On This Page:
Case studies are in-depth investigations of a person, group, event, or community. Typically, data is gathered from various sources using several methods (e.g., observations & interviews).
The case study research method originated in clinical medicine (the case history, i.e., the patient’s personal history). In psychology, case studies are often confined to the study of a particular individual.
The information is mainly biographical and relates to events in the individual’s past (i.e., retrospective), as well as to significant events that are currently occurring in his or her everyday life.
The case study is not a research method, but researchers select methods of data collection and analysis that will generate material suitable for case studies.
Freud (1909a, 1909b) conducted very detailed investigations into the private lives of his patients in an attempt to both understand and help them overcome their illnesses.
This makes it clear that the case study is a method that should only be used by a psychologist, therapist, or psychiatrist, i.e., someone with a professional qualification.
There is an ethical issue of competence. Only someone qualified to diagnose and treat a person can conduct a formal case study relating to atypical (i.e., abnormal) behavior or atypical development.
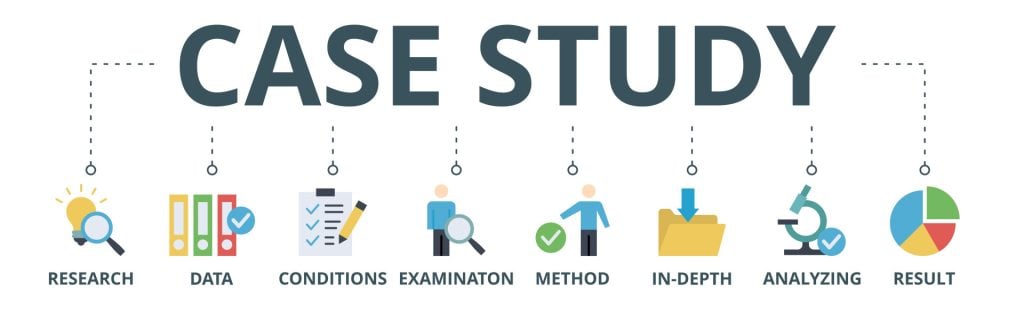
Famous Case Studies
- Anna O – One of the most famous case studies, documenting psychoanalyst Josef Breuer’s treatment of “Anna O” (real name Bertha Pappenheim) for hysteria in the late 1800s using early psychoanalytic theory.
- Little Hans – A child psychoanalysis case study published by Sigmund Freud in 1909 analyzing his five-year-old patient Herbert Graf’s house phobia as related to the Oedipus complex.
- Bruce/Brenda – Gender identity case of the boy (Bruce) whose botched circumcision led psychologist John Money to advise gender reassignment and raise him as a girl (Brenda) in the 1960s.
- Genie Wiley – Linguistics/psychological development case of the victim of extreme isolation abuse who was studied in 1970s California for effects of early language deprivation on acquiring speech later in life.
- Phineas Gage – One of the most famous neuropsychology case studies analyzes personality changes in railroad worker Phineas Gage after an 1848 brain injury involving a tamping iron piercing his skull.
Clinical Case Studies
- Studying the effectiveness of psychotherapy approaches with an individual patient
- Assessing and treating mental illnesses like depression, anxiety disorders, PTSD
- Neuropsychological cases investigating brain injuries or disorders
Child Psychology Case Studies
- Studying psychological development from birth through adolescence
- Cases of learning disabilities, autism spectrum disorders, ADHD
- Effects of trauma, abuse, deprivation on development
Types of Case Studies
- Explanatory case studies : Used to explore causation in order to find underlying principles. Helpful for doing qualitative analysis to explain presumed causal links.
- Exploratory case studies : Used to explore situations where an intervention being evaluated has no clear set of outcomes. It helps define questions and hypotheses for future research.
- Descriptive case studies : Describe an intervention or phenomenon and the real-life context in which it occurred. It is helpful for illustrating certain topics within an evaluation.
- Multiple-case studies : Used to explore differences between cases and replicate findings across cases. Helpful for comparing and contrasting specific cases.
- Intrinsic : Used to gain a better understanding of a particular case. Helpful for capturing the complexity of a single case.
- Collective : Used to explore a general phenomenon using multiple case studies. Helpful for jointly studying a group of cases in order to inquire into the phenomenon.
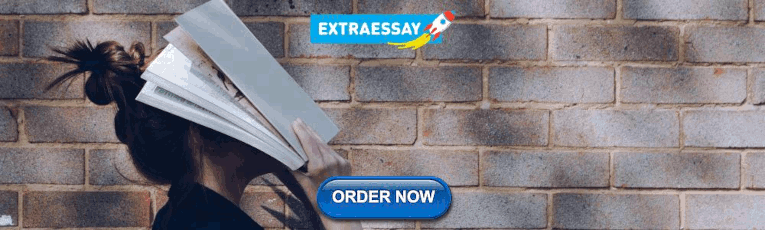
Where Do You Find Data for a Case Study?
There are several places to find data for a case study. The key is to gather data from multiple sources to get a complete picture of the case and corroborate facts or findings through triangulation of evidence. Most of this information is likely qualitative (i.e., verbal description rather than measurement), but the psychologist might also collect numerical data.
1. Primary sources
- Interviews – Interviewing key people related to the case to get their perspectives and insights. The interview is an extremely effective procedure for obtaining information about an individual, and it may be used to collect comments from the person’s friends, parents, employer, workmates, and others who have a good knowledge of the person, as well as to obtain facts from the person him or herself.
- Observations – Observing behaviors, interactions, processes, etc., related to the case as they unfold in real-time.
- Documents & Records – Reviewing private documents, diaries, public records, correspondence, meeting minutes, etc., relevant to the case.
2. Secondary sources
- News/Media – News coverage of events related to the case study.
- Academic articles – Journal articles, dissertations etc. that discuss the case.
- Government reports – Official data and records related to the case context.
- Books/films – Books, documentaries or films discussing the case.
3. Archival records
Searching historical archives, museum collections and databases to find relevant documents, visual/audio records related to the case history and context.
Public archives like newspapers, organizational records, photographic collections could all include potentially relevant pieces of information to shed light on attitudes, cultural perspectives, common practices and historical contexts related to psychology.
4. Organizational records
Organizational records offer the advantage of often having large datasets collected over time that can reveal or confirm psychological insights.
Of course, privacy and ethical concerns regarding confidential data must be navigated carefully.
However, with proper protocols, organizational records can provide invaluable context and empirical depth to qualitative case studies exploring the intersection of psychology and organizations.
- Organizational/industrial psychology research : Organizational records like employee surveys, turnover/retention data, policies, incident reports etc. may provide insight into topics like job satisfaction, workplace culture and dynamics, leadership issues, employee behaviors etc.
- Clinical psychology : Therapists/hospitals may grant access to anonymized medical records to study aspects like assessments, diagnoses, treatment plans etc. This could shed light on clinical practices.
- School psychology : Studies could utilize anonymized student records like test scores, grades, disciplinary issues, and counseling referrals to study child development, learning barriers, effectiveness of support programs, and more.
How do I Write a Case Study in Psychology?
Follow specified case study guidelines provided by a journal or your psychology tutor. General components of clinical case studies include: background, symptoms, assessments, diagnosis, treatment, and outcomes. Interpreting the information means the researcher decides what to include or leave out. A good case study should always clarify which information is the factual description and which is an inference or the researcher’s opinion.
1. Introduction
- Provide background on the case context and why it is of interest, presenting background information like demographics, relevant history, and presenting problem.
- Compare briefly to similar published cases if applicable. Clearly state the focus/importance of the case.
2. Case Presentation
- Describe the presenting problem in detail, including symptoms, duration,and impact on daily life.
- Include client demographics like age and gender, information about social relationships, and mental health history.
- Describe all physical, emotional, and/or sensory symptoms reported by the client.
- Use patient quotes to describe the initial complaint verbatim. Follow with full-sentence summaries of relevant history details gathered, including key components that led to a working diagnosis.
- Summarize clinical exam results, namely orthopedic/neurological tests, imaging, lab tests, etc. Note actual results rather than subjective conclusions. Provide images if clearly reproducible/anonymized.
- Clearly state the working diagnosis or clinical impression before transitioning to management.
3. Management and Outcome
- Indicate the total duration of care and number of treatments given over what timeframe. Use specific names/descriptions for any therapies/interventions applied.
- Present the results of the intervention,including any quantitative or qualitative data collected.
- For outcomes, utilize visual analog scales for pain, medication usage logs, etc., if possible. Include patient self-reports of improvement/worsening of symptoms. Note the reason for discharge/end of care.
4. Discussion
- Analyze the case, exploring contributing factors, limitations of the study, and connections to existing research.
- Analyze the effectiveness of the intervention,considering factors like participant adherence, limitations of the study, and potential alternative explanations for the results.
- Identify any questions raised in the case analysis and relate insights to established theories and current research if applicable. Avoid definitive claims about physiological explanations.
- Offer clinical implications, and suggest future research directions.
5. Additional Items
- Thank specific assistants for writing support only. No patient acknowledgments.
- References should directly support any key claims or quotes included.
- Use tables/figures/images only if substantially informative. Include permissions and legends/explanatory notes.
- Provides detailed (rich qualitative) information.
- Provides insight for further research.
- Permitting investigation of otherwise impractical (or unethical) situations.
Case studies allow a researcher to investigate a topic in far more detail than might be possible if they were trying to deal with a large number of research participants (nomothetic approach) with the aim of ‘averaging’.
Because of their in-depth, multi-sided approach, case studies often shed light on aspects of human thinking and behavior that would be unethical or impractical to study in other ways.
Research that only looks into the measurable aspects of human behavior is not likely to give us insights into the subjective dimension of experience, which is important to psychoanalytic and humanistic psychologists.
Case studies are often used in exploratory research. They can help us generate new ideas (that might be tested by other methods). They are an important way of illustrating theories and can help show how different aspects of a person’s life are related to each other.
The method is, therefore, important for psychologists who adopt a holistic point of view (i.e., humanistic psychologists ).
Limitations
- Lacking scientific rigor and providing little basis for generalization of results to the wider population.
- Researchers’ own subjective feelings may influence the case study (researcher bias).
- Difficult to replicate.
- Time-consuming and expensive.
- The volume of data, together with the time restrictions in place, impacted the depth of analysis that was possible within the available resources.
Because a case study deals with only one person/event/group, we can never be sure if the case study investigated is representative of the wider body of “similar” instances. This means the conclusions drawn from a particular case may not be transferable to other settings.
Because case studies are based on the analysis of qualitative (i.e., descriptive) data , a lot depends on the psychologist’s interpretation of the information she has acquired.
This means that there is a lot of scope for Anna O , and it could be that the subjective opinions of the psychologist intrude in the assessment of what the data means.
For example, Freud has been criticized for producing case studies in which the information was sometimes distorted to fit particular behavioral theories (e.g., Little Hans ).
This is also true of Money’s interpretation of the Bruce/Brenda case study (Diamond, 1997) when he ignored evidence that went against his theory.
Breuer, J., & Freud, S. (1895). Studies on hysteria . Standard Edition 2: London.
Curtiss, S. (1981). Genie: The case of a modern wild child .
Diamond, M., & Sigmundson, K. (1997). Sex Reassignment at Birth: Long-term Review and Clinical Implications. Archives of Pediatrics & Adolescent Medicine , 151(3), 298-304
Freud, S. (1909a). Analysis of a phobia of a five year old boy. In The Pelican Freud Library (1977), Vol 8, Case Histories 1, pages 169-306
Freud, S. (1909b). Bemerkungen über einen Fall von Zwangsneurose (Der “Rattenmann”). Jb. psychoanal. psychopathol. Forsch ., I, p. 357-421; GW, VII, p. 379-463; Notes upon a case of obsessional neurosis, SE , 10: 151-318.
Harlow J. M. (1848). Passage of an iron rod through the head. Boston Medical and Surgical Journal, 39 , 389–393.
Harlow, J. M. (1868). Recovery from the Passage of an Iron Bar through the Head . Publications of the Massachusetts Medical Society. 2 (3), 327-347.
Money, J., & Ehrhardt, A. A. (1972). Man & Woman, Boy & Girl : The Differentiation and Dimorphism of Gender Identity from Conception to Maturity. Baltimore, Maryland: Johns Hopkins University Press.
Money, J., & Tucker, P. (1975). Sexual signatures: On being a man or a woman.
Further Information
- Case Study Approach
- Case Study Method
- Enhancing the Quality of Case Studies in Health Services Research
- “We do things together” A case study of “couplehood” in dementia
- Using mixed methods for evaluating an integrative approach to cancer care: a case study

Related Articles
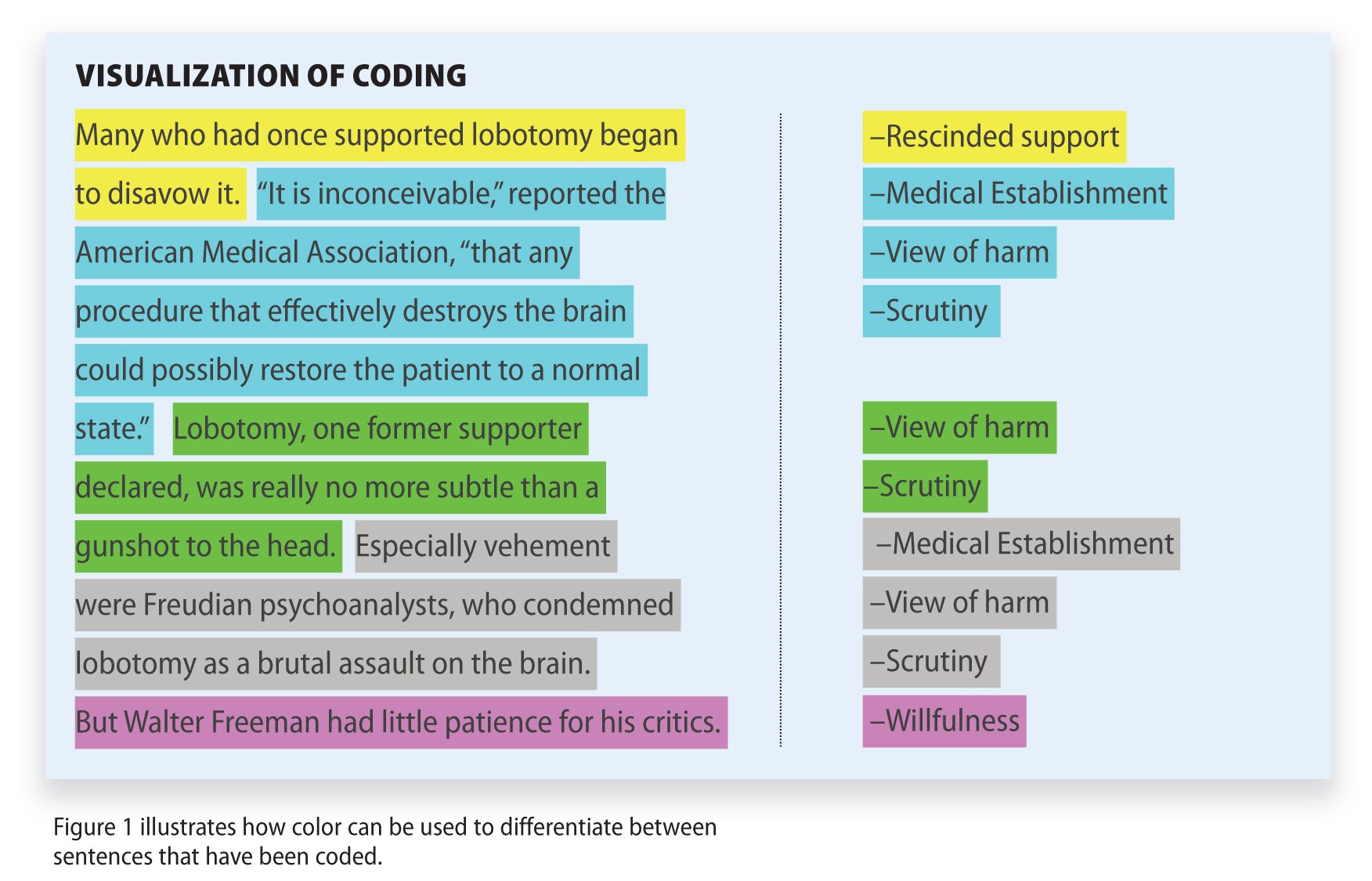
Research Methodology
Qualitative Data Coding
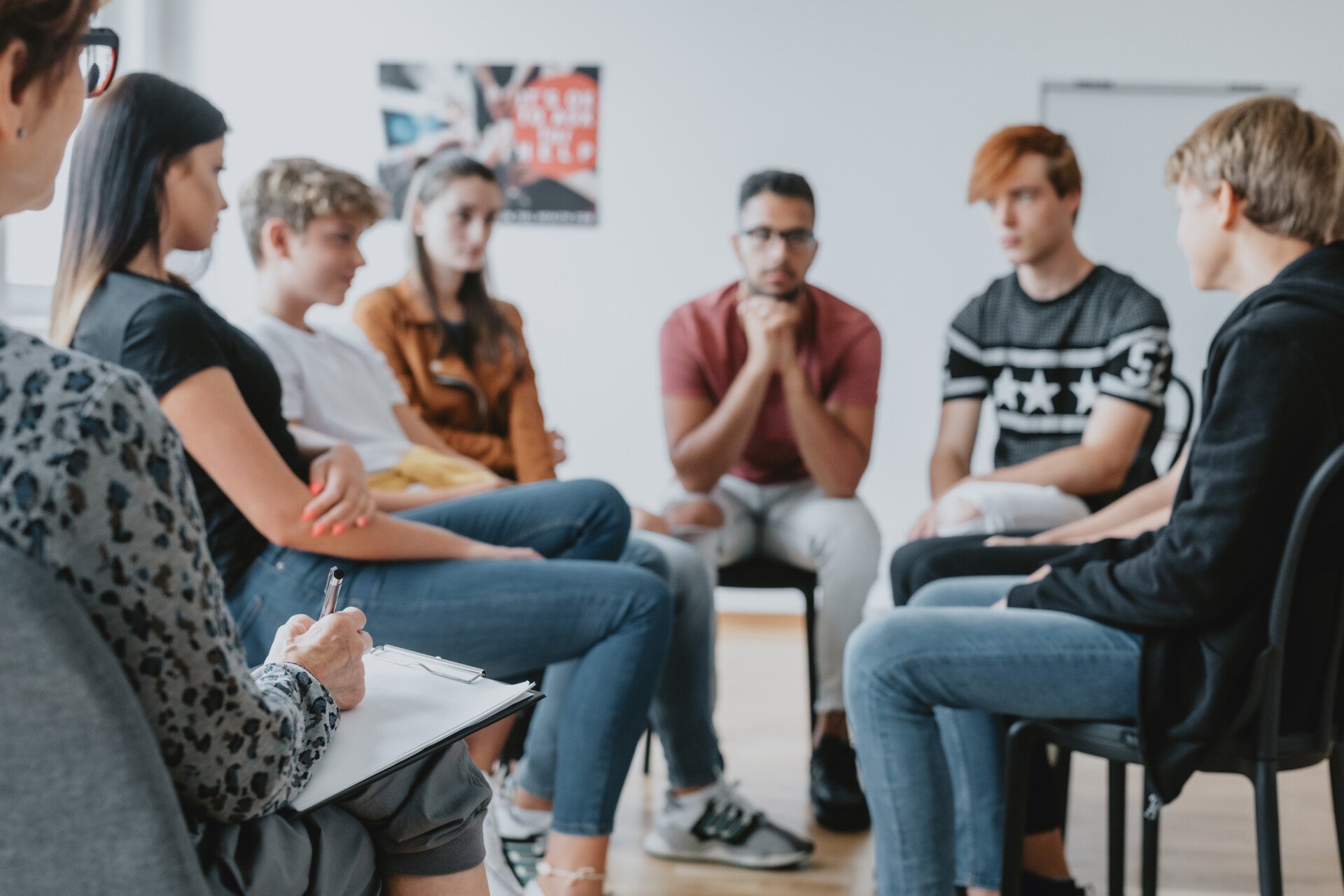
What Is a Focus Group?
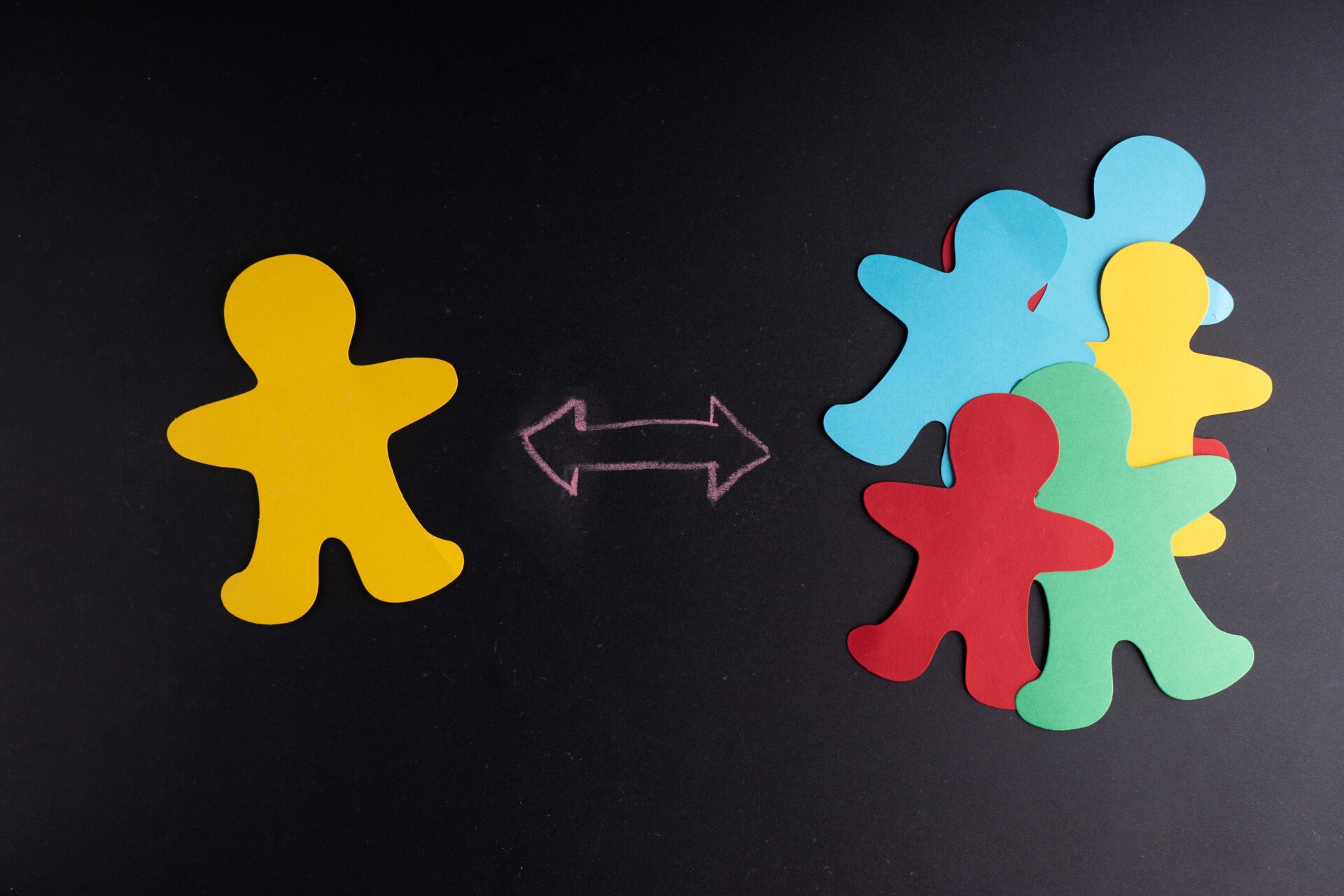
Cross-Cultural Research Methodology In Psychology
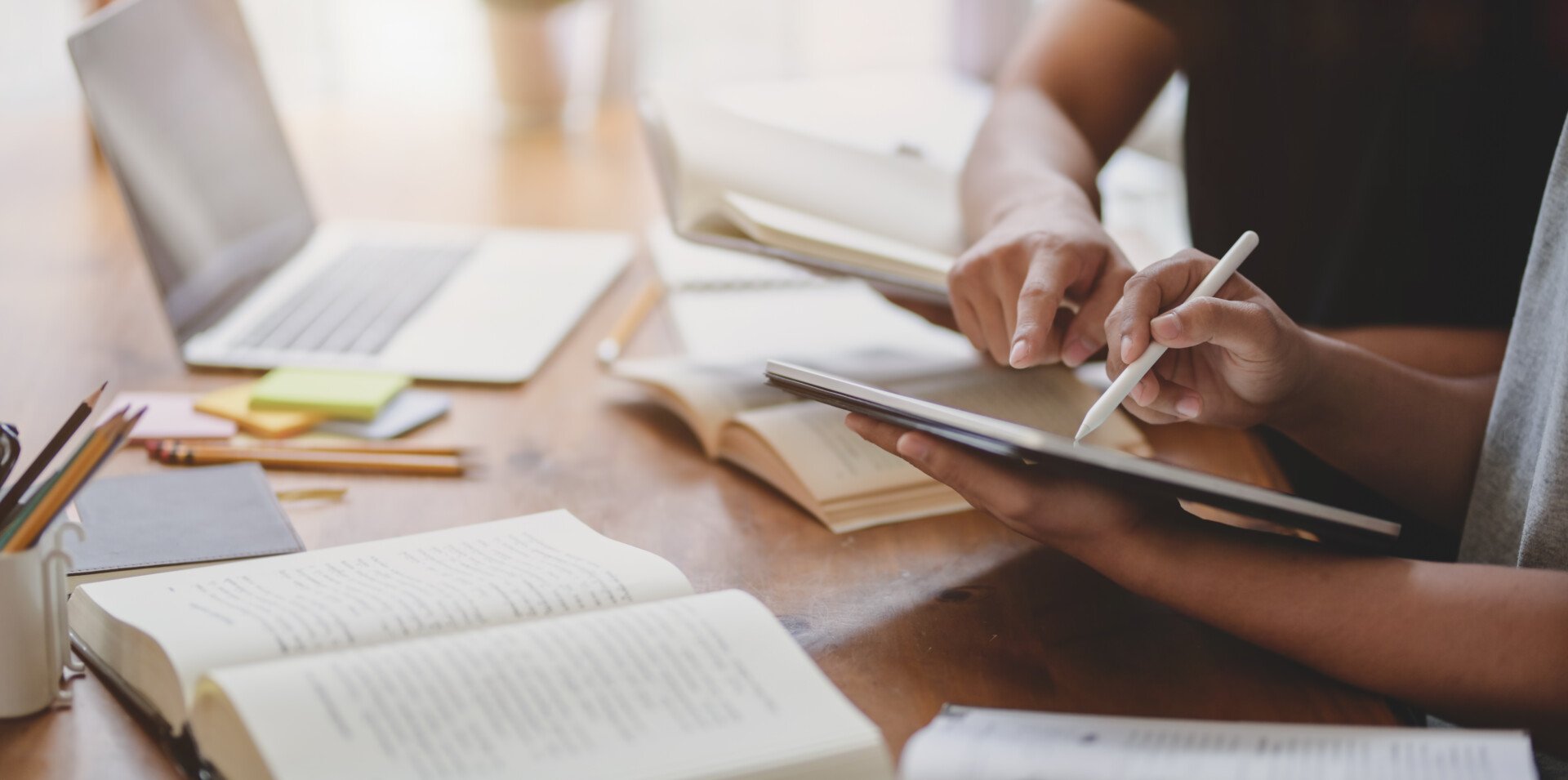
What Is Internal Validity In Research?
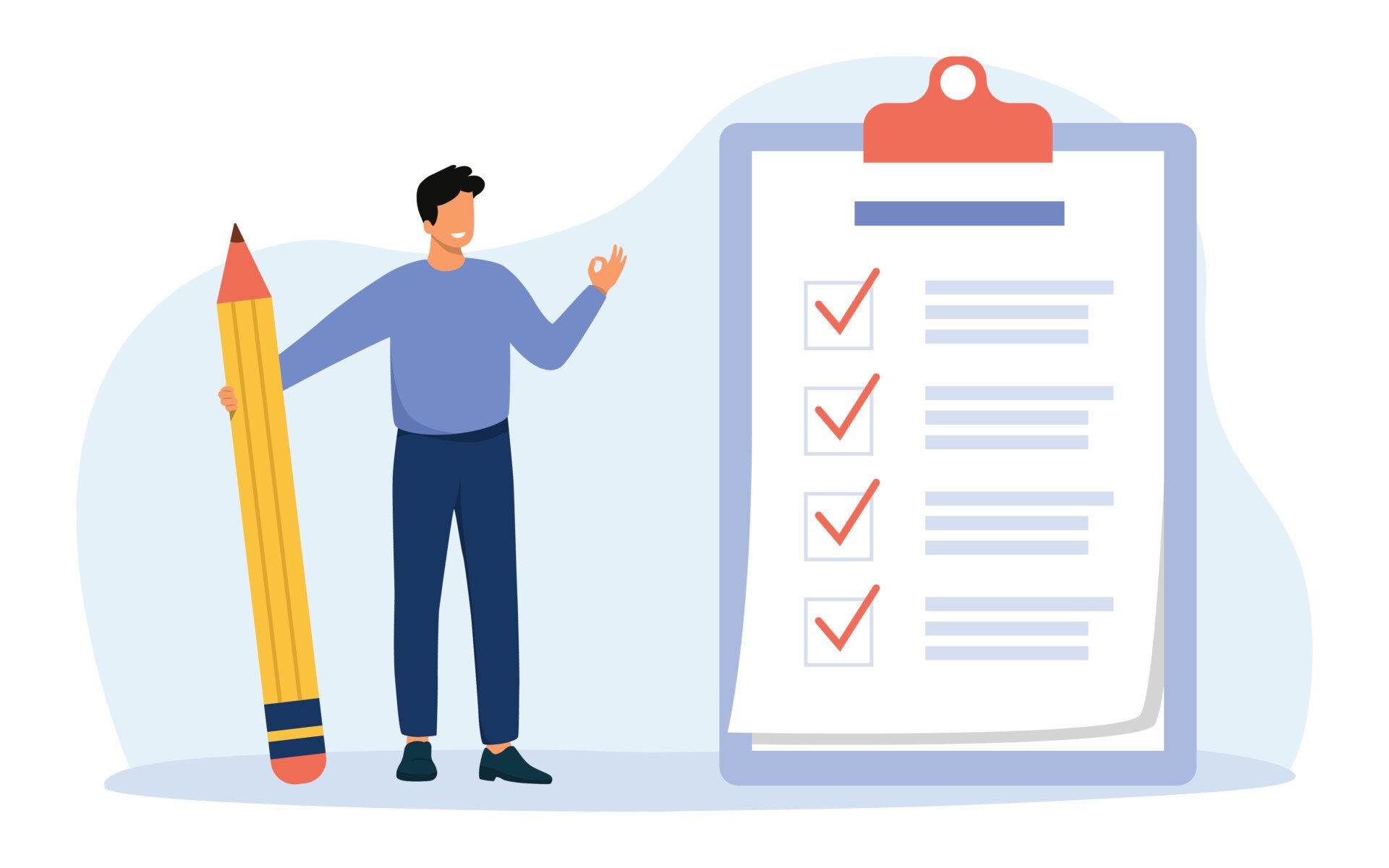
Research Methodology , Statistics
What Is Face Validity In Research? Importance & How To Measure
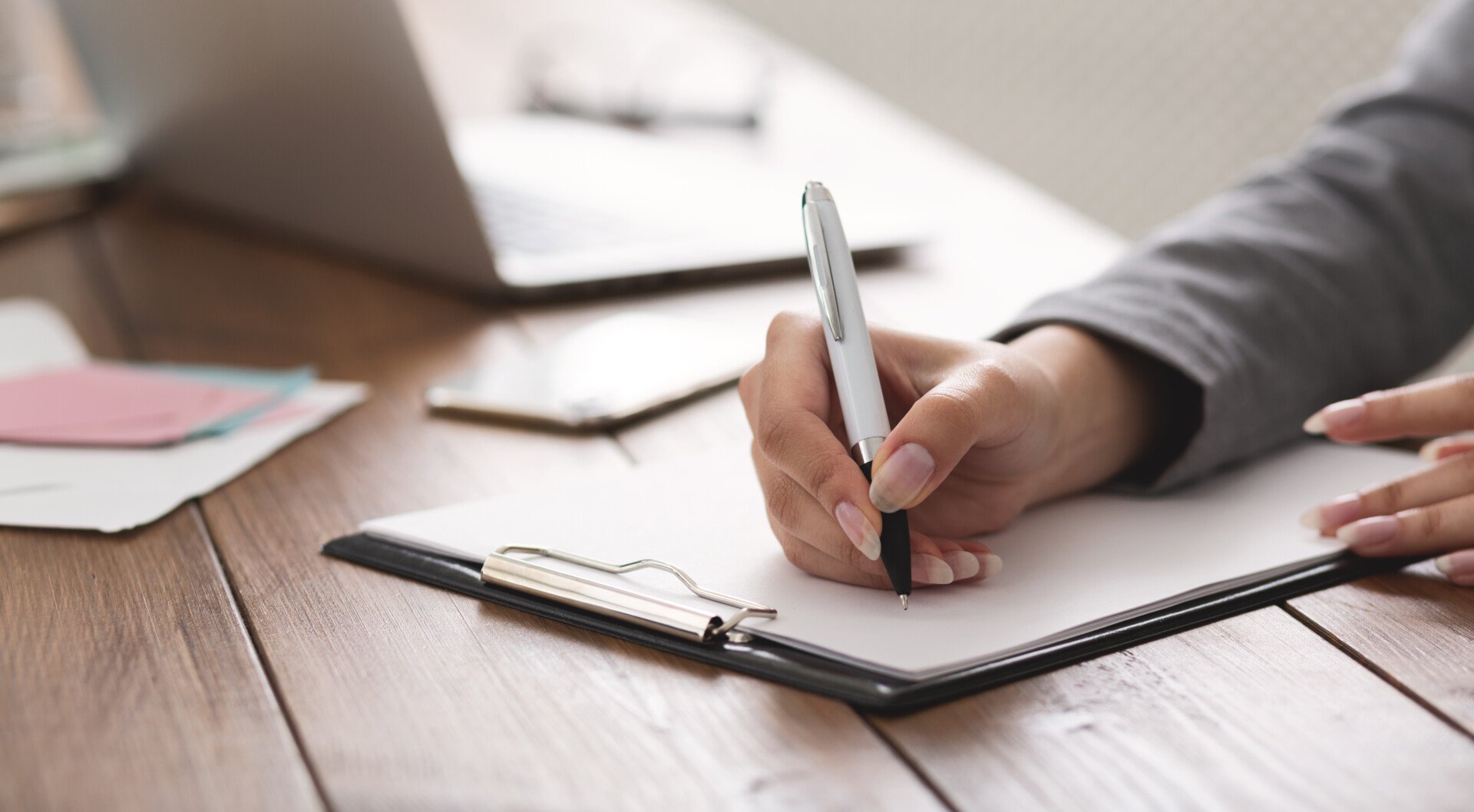
Criterion Validity: Definition & Examples
The independent source for health policy research, polling, and news.
Abortion in the United States Dashboard
On june 24, 2022, the supreme court overturned roe v. wade, eliminating the federal constitutional standard that had protected the right to abortion. without any federal standard regarding abortion access, states will set their own policies to ban or protect abortion. the abortion in the united states dashboard is an ongoing research project tracking state abortion policies and litigation following the overturning of roe v. wade. be sure to click on the buttons or scroll down to see all the content. it will be updated as new information is available., new releases, medication abortion, related health, dobbs-era abortion bans and restrictions: early insights about implications for pregnancy loss.
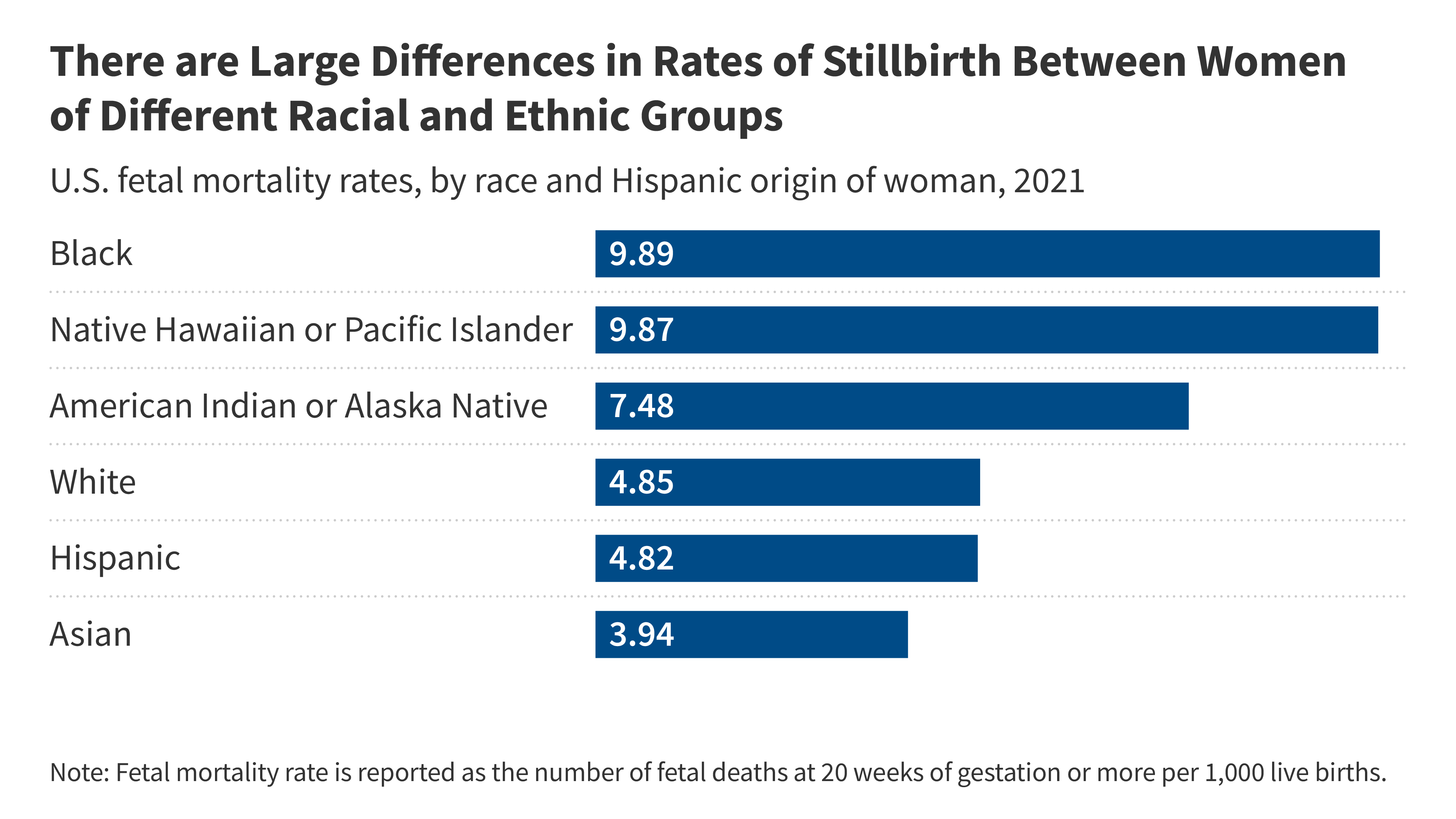
This brief examines pregnancy loss management in the Dobbs era and explores how limiting or banning abortion may have negative consequences on people experiencing miscarriage or stillbirth.
Policy Tracker: Exceptions to State Abortion Bans and Early Gestational Limits
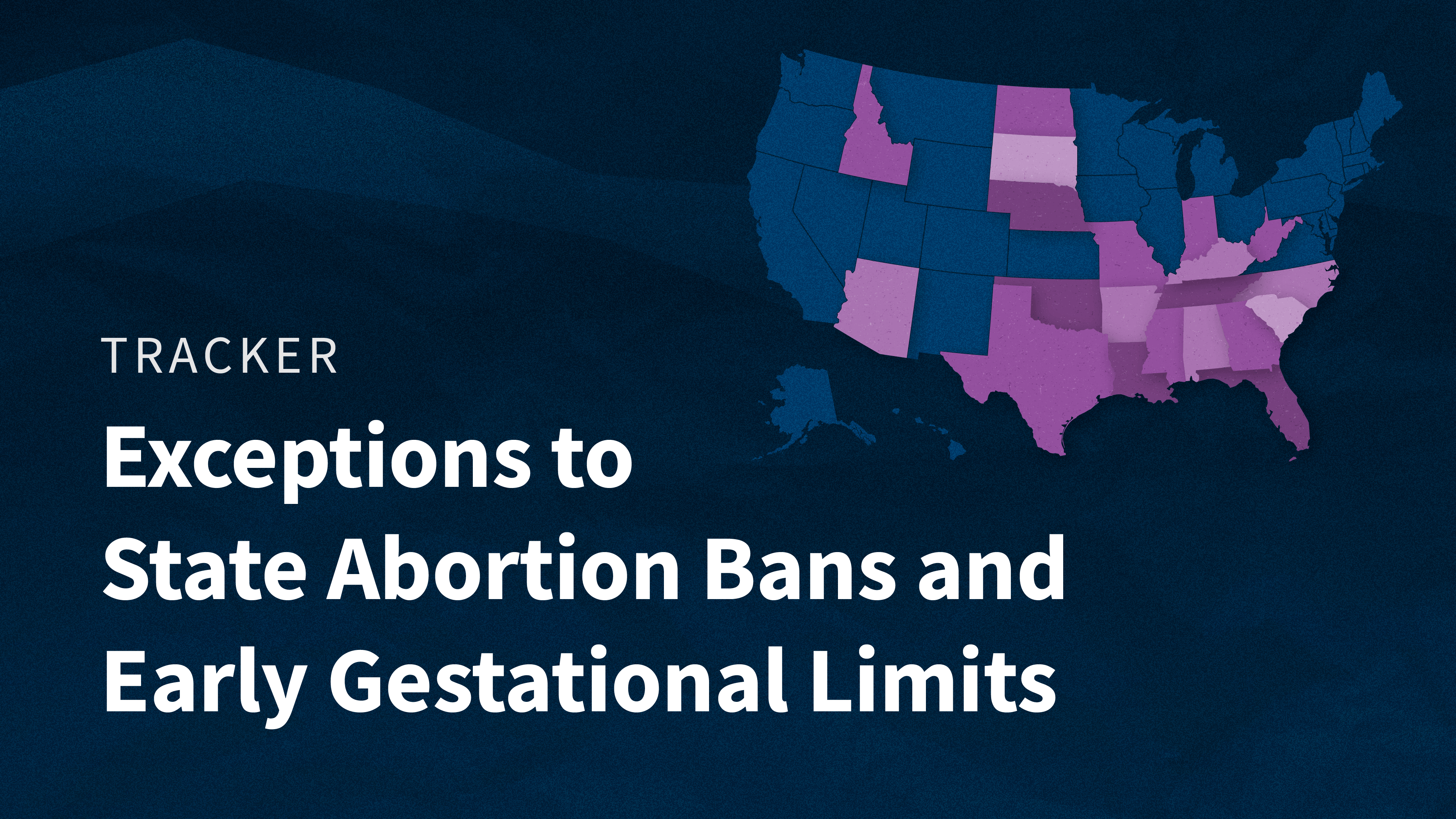
Abortion is currently banned in 14 states and 6 states have early gestational limits between 6 weeks and 15 weeks in effect. Nearly all of these bans include exceptions, which generally fall into four categories: to prevent the death of the pregnant person, when there is risk to the health of the pregnant person, when the pregnancy is the result of rape or incest, and when there is a lethal fetal anomaly.
Ballot Tracker: Status of Abortion-Related State Constitutional Amendment Measures for the 2024 Election
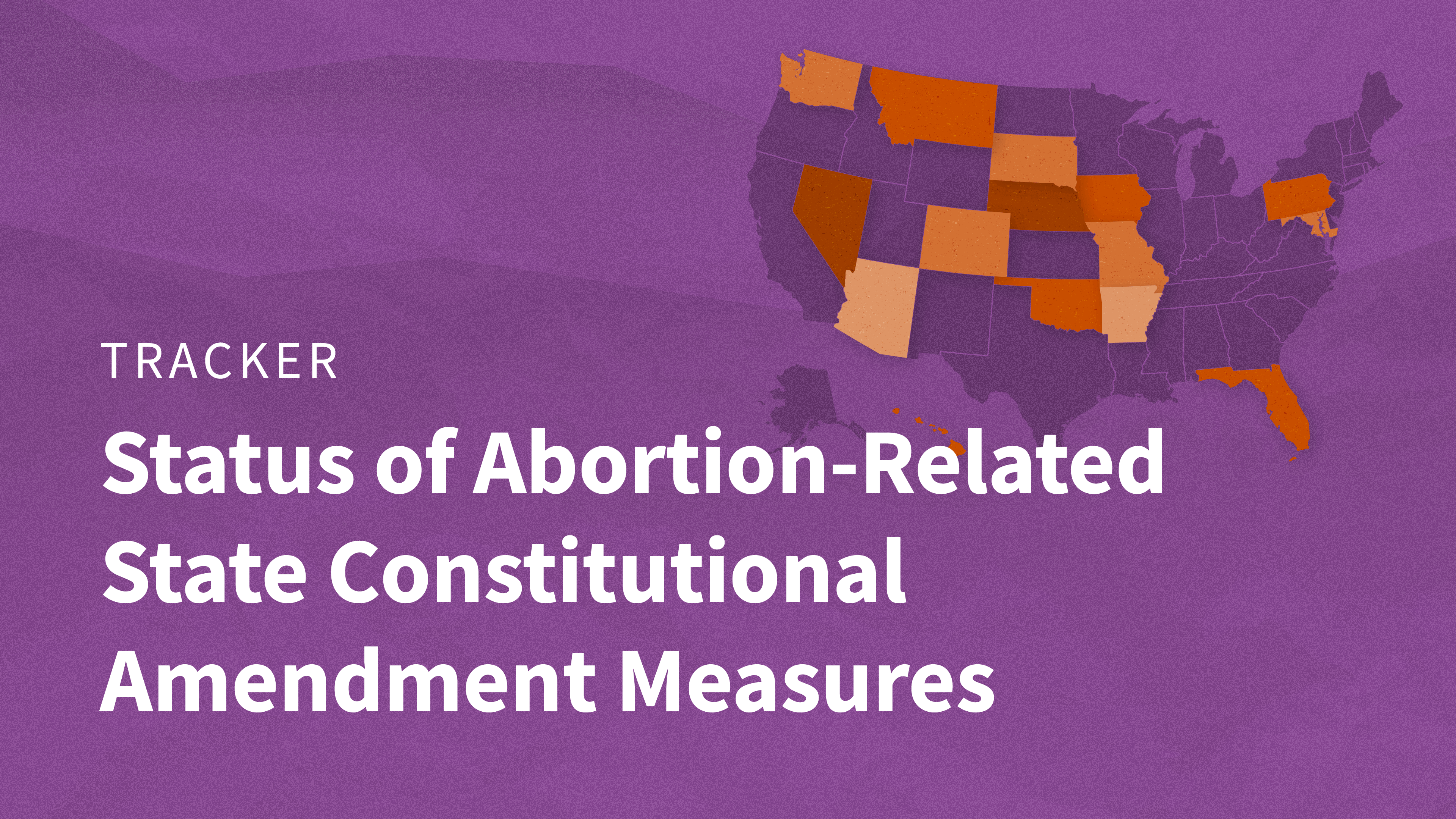
Since the Supreme Court’s Dobbs decision overturning Roe v. Wade , voters in 6 states have weighed in on constitutional amendments regarding abortion, and the side favoring access to abortion prevailed in every state. In 2024, up to 15 states may have abortion measures on their ballot seeking to either affirm that the state constitution protects the right to abortion or that nothing in the constitution confers such a right.
What are the Implications of the Dobbs Ruling for Racial Disparities?
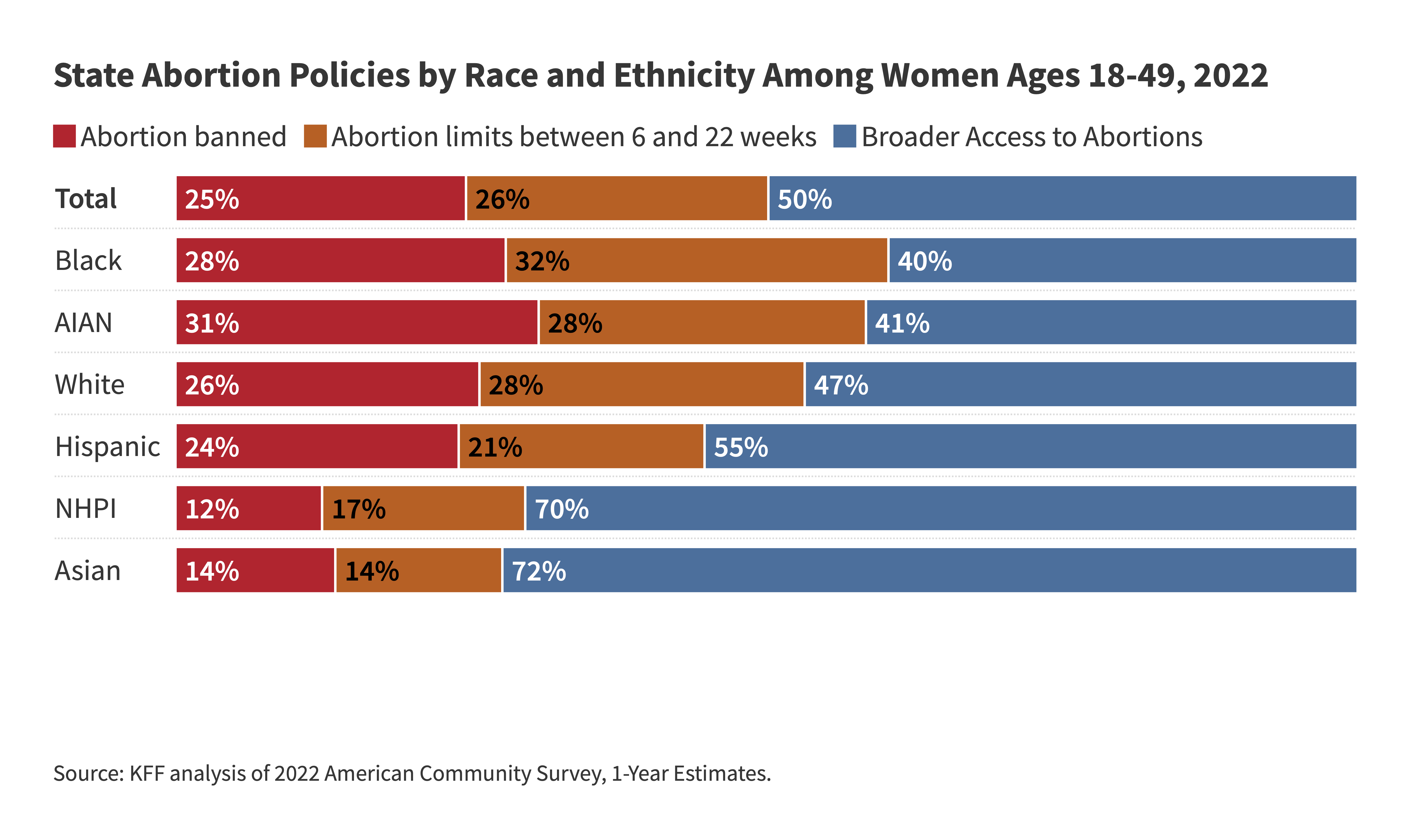
This analysis examines the implications of the Dobbs decision and state restrictions on abortion coverage for racial disparities in access to care and health outcomes.
81% of Abortions Occur Before 10 Weeks
Over four in ten (45%) abortions occur by six weeks of gestation, 36% are between seven and nine weeks, and 13% at 10-13 weeks. Just 7% of abortions occur after the first trimester.
Out-of-Pocket Abortion Costs
In 2021, the median costs for people paying out of pocket in the first trimester were $568 for a medication abortion and $625 for a procedural abortion. The Federal Reserve estimates that nationally about one-third of people do not have $400 on hand for unexpected expenses. For low-income people, who are more likely to need abortion care, these costs are often unaffordable.
Abortion Back at SCOTUS: Can States Ban Emergency Abortion Care for Pregnant Patients?
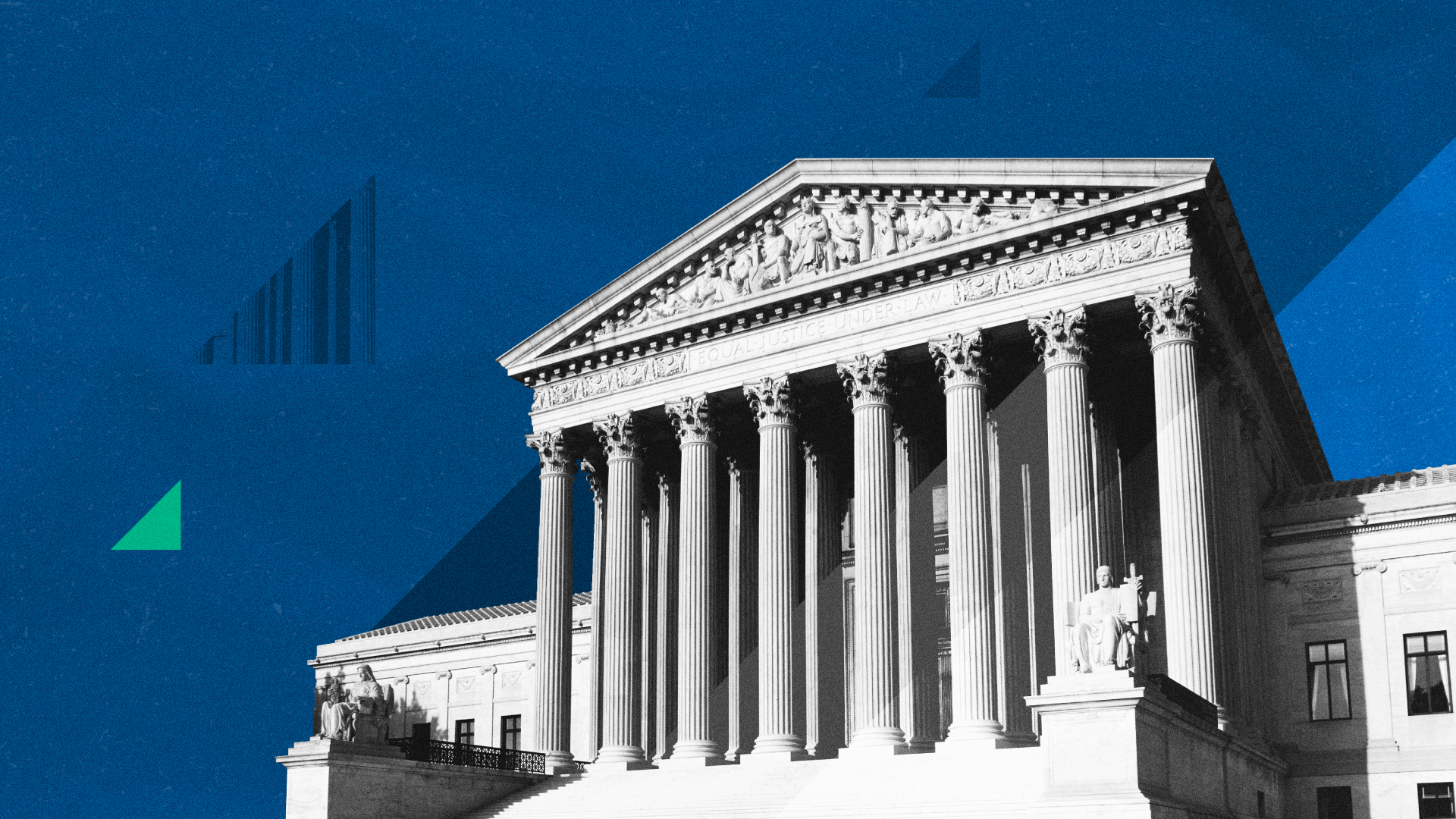
On April 24, 2024, the Supreme Court will hear the second case this term involving access to abortion: Idaho v. United States. At stake in this case is whether the Emergency Medical Treatment and Active Labor Act, a federal law requiring hospitals to provide stabilizing treatment to patients who present to their emergency rooms, preempts state abortion laws and requires hospitals that accept Medicare to provide abortion care when it is necessary to stabilize a patient’s condition, even when this abortion care violates state law.
What’s at Stake for Access to Medication Abortion and the FDA in the Supreme Court Case FDA v. the Alliance for Hippocratic Medicine?
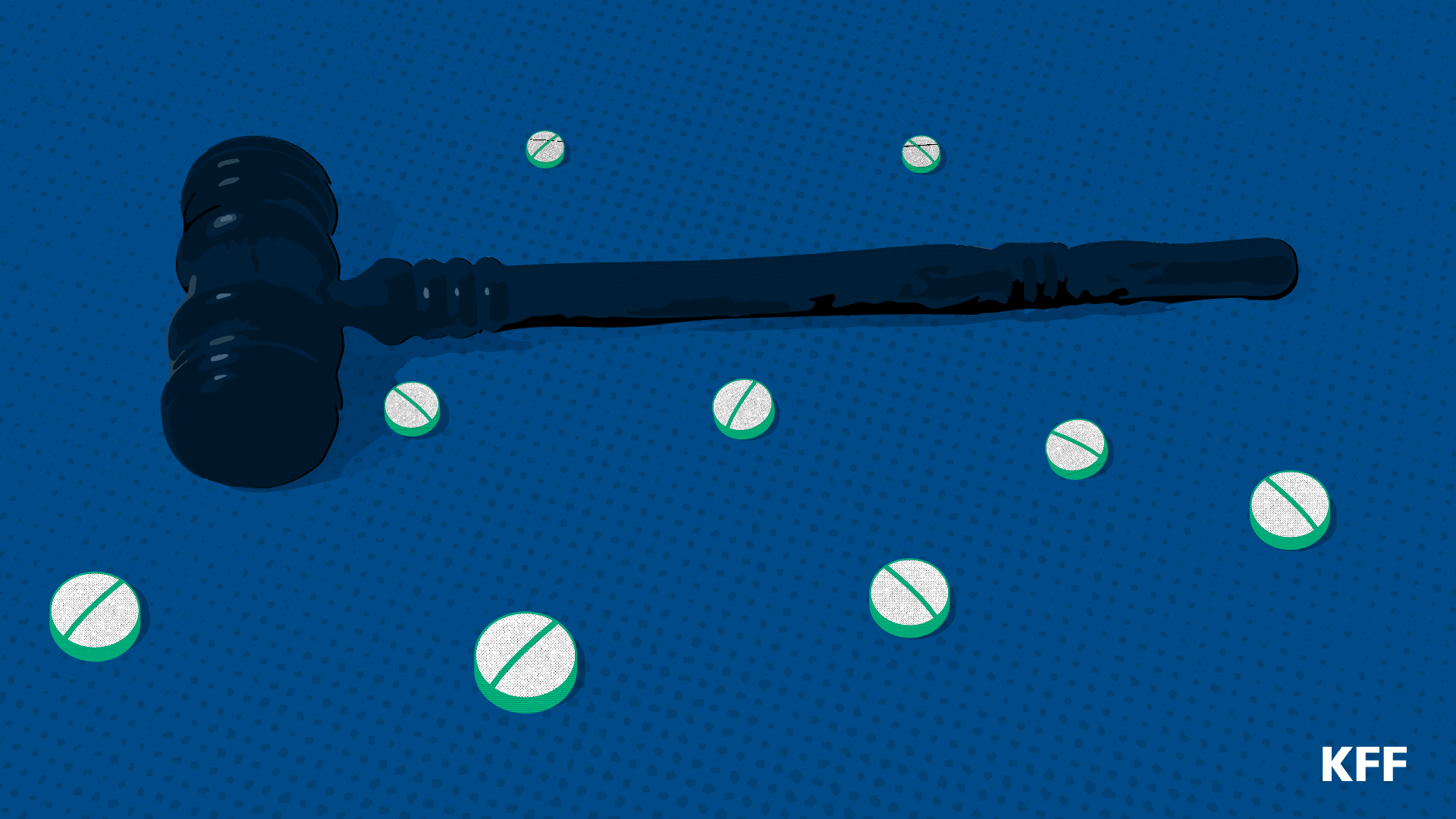
The Supreme Court will be hearing oral arguments for the case FDA v. Alliance for Hippocratic Medicine. This brief explains the issues at stake before the court and their implications for the drug regulatory process and medication abortion access throughout the country.
The Comstock Act: Implications for Abortion Care Nationwide
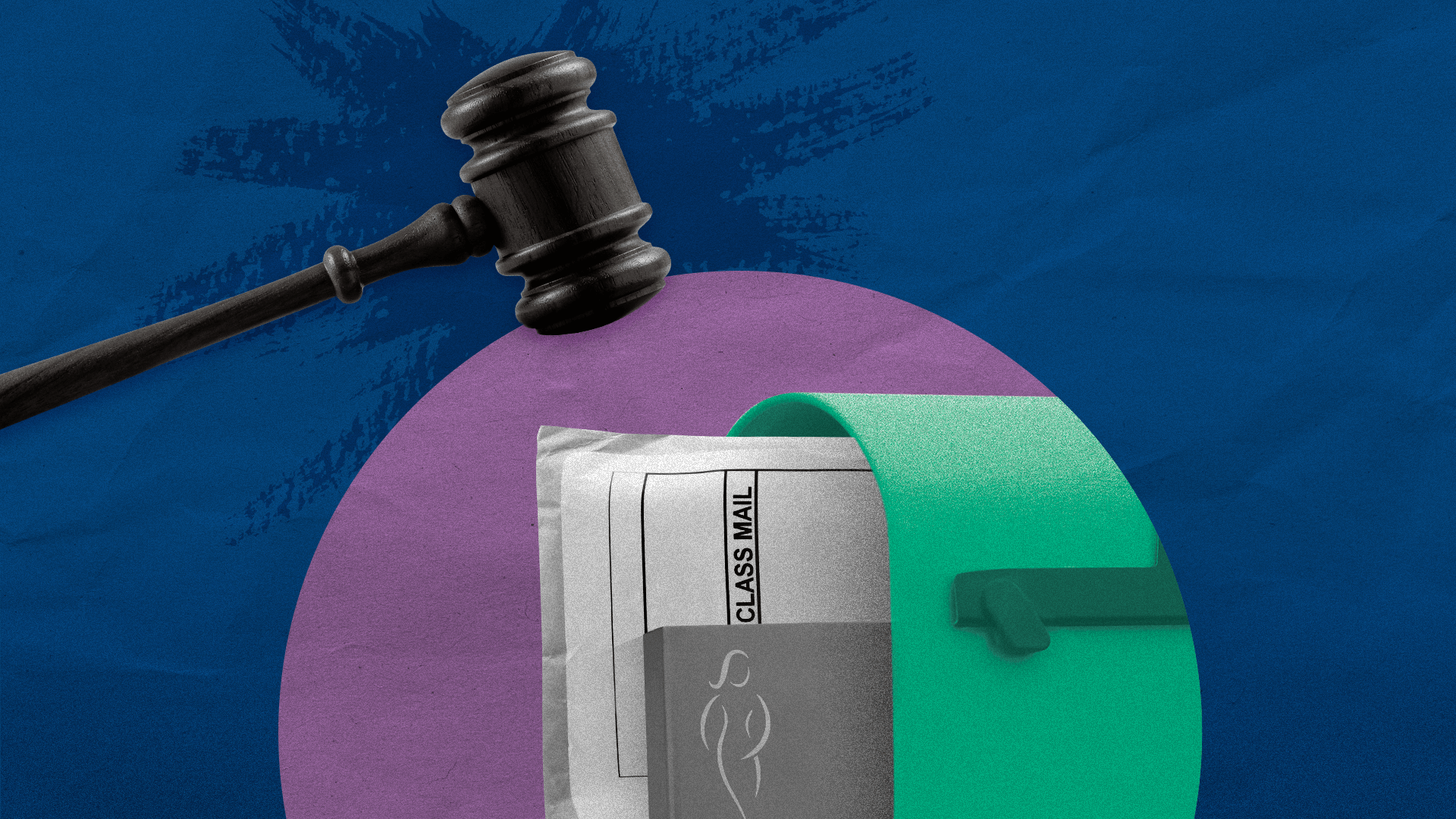
This brief provides background on the Comstock Act, reviews how it has been interpreted by the Biden Administration’s DOJ, and considers how it could be enforced by an administration that is hostile toward abortion to severely restrict the distribution of drugs and supplies used for abortion, with implications for abortion access in all states across the country.
State and Federal Reproductive Rights and Abortion Litigation Tracker
This State and Federal Reproductive Rights Litigation tracker aggregates information about ongoing litigation regarding abortion bans and restrictions, FDA approval of Mifepristone (an abortion pill) and other federal regulations.
Addressing Abortion Access through State Ballot Initiatives
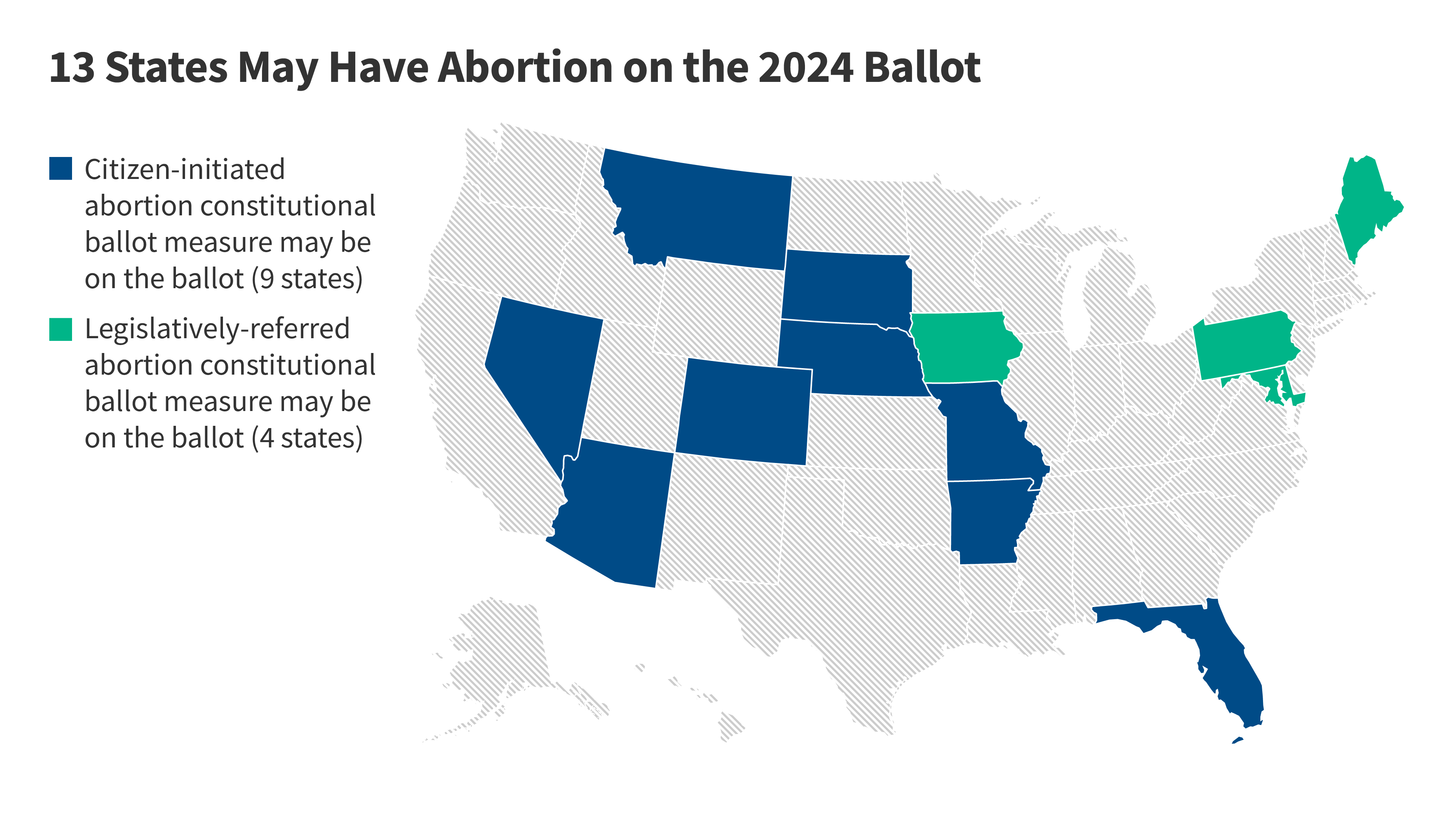
This issue brief explains why constitutional amendment ballot measures have become so popular with advocates on both sides of the abortion issue, reviews the current initiatives that are in progress and may appear in on state ballots the next general election, and outlines the processes states have available to them to use to place initiatives on the ballot.
Who Decides When a Patient Qualifies for an Abortion Ban Exception? Doctors vs. the Courts
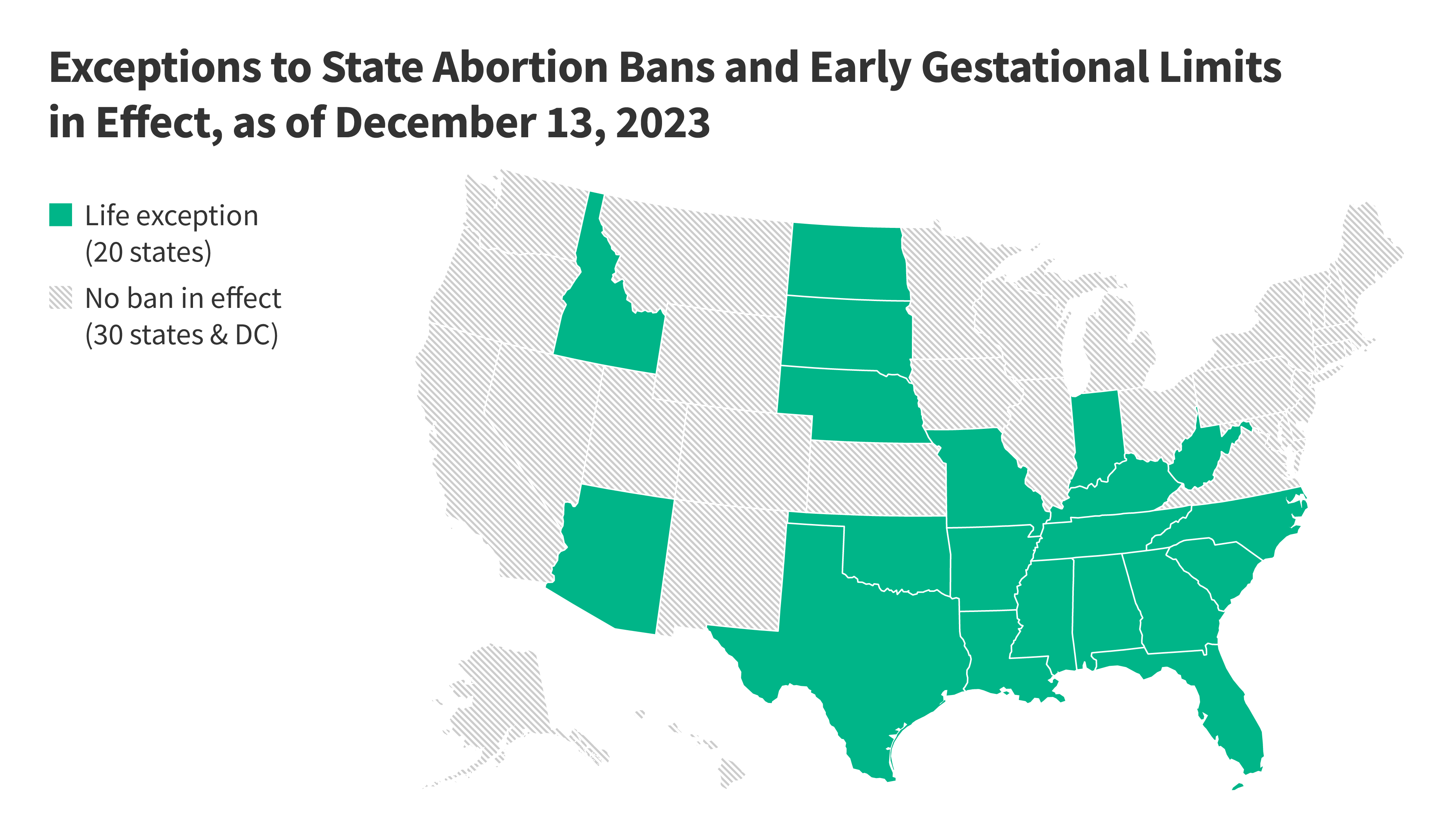
While all eyes were on Texas and the recent case of Kate Cox, a woman seeking a court order allowing her abortion under an exception to the Texas abortion ban, the conflict could have played out in many states. The risk to doctors is so high that many doctors are hesitant to provide life-saving abortion care unless the threat to life is imminent.
Abortions Later in Pregnancy in a Post-Dobbs Era
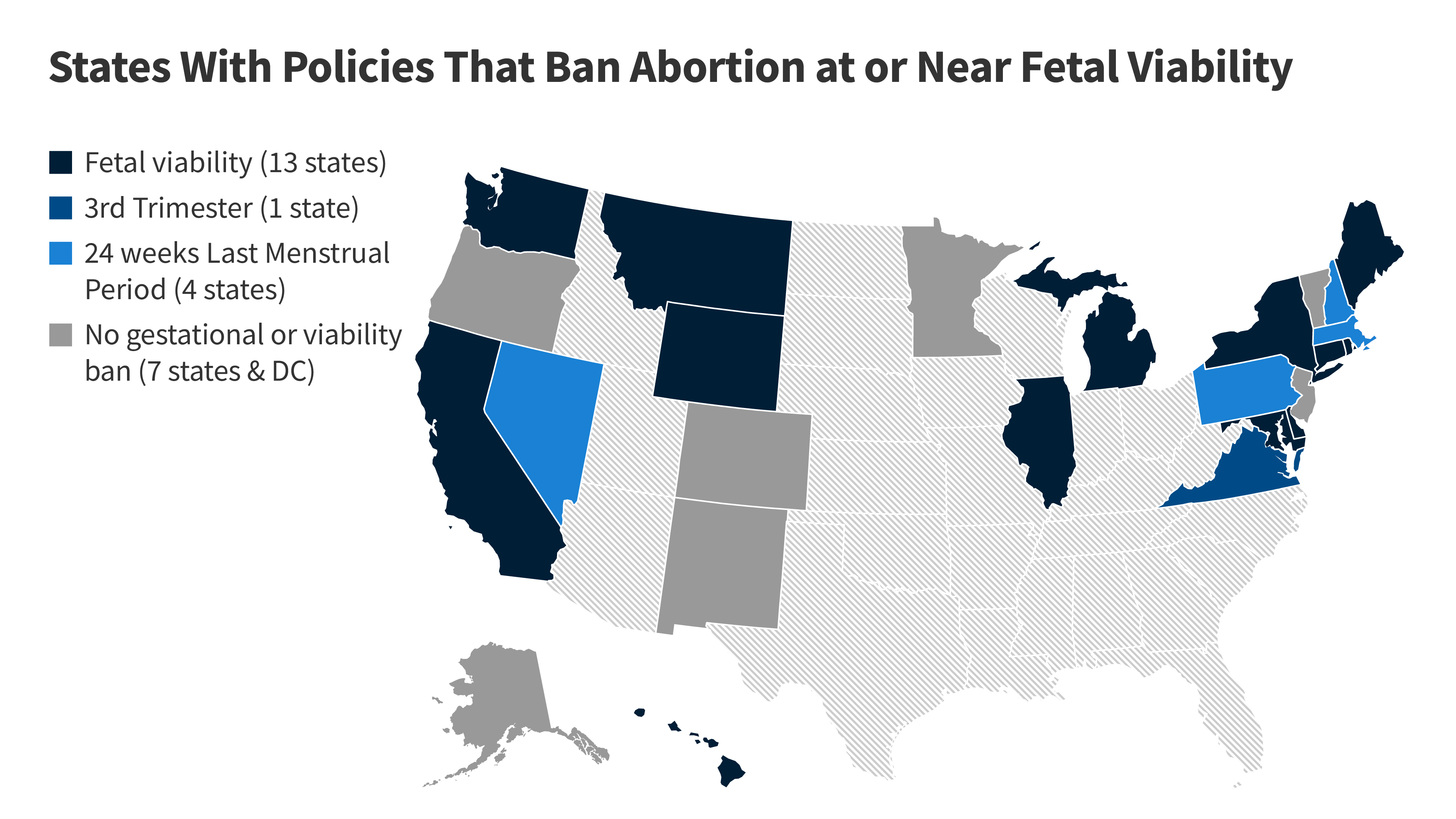
This brief explains why individuals may seek abortions later in pregnancy, how often these procedures occur, and the various laws which regulate access to abortions later in pregnancy across the country.
As part of efforts to limit abortion access, some states have taken action to block the use of telehealth for abortion. Among the 36 states & DC that have not banned abortion, twelve states have at least one restriction that requires at least one trip to the clinic, and effectively ban telehealth for medication abortion
The Availability and Use of Medication Abortion
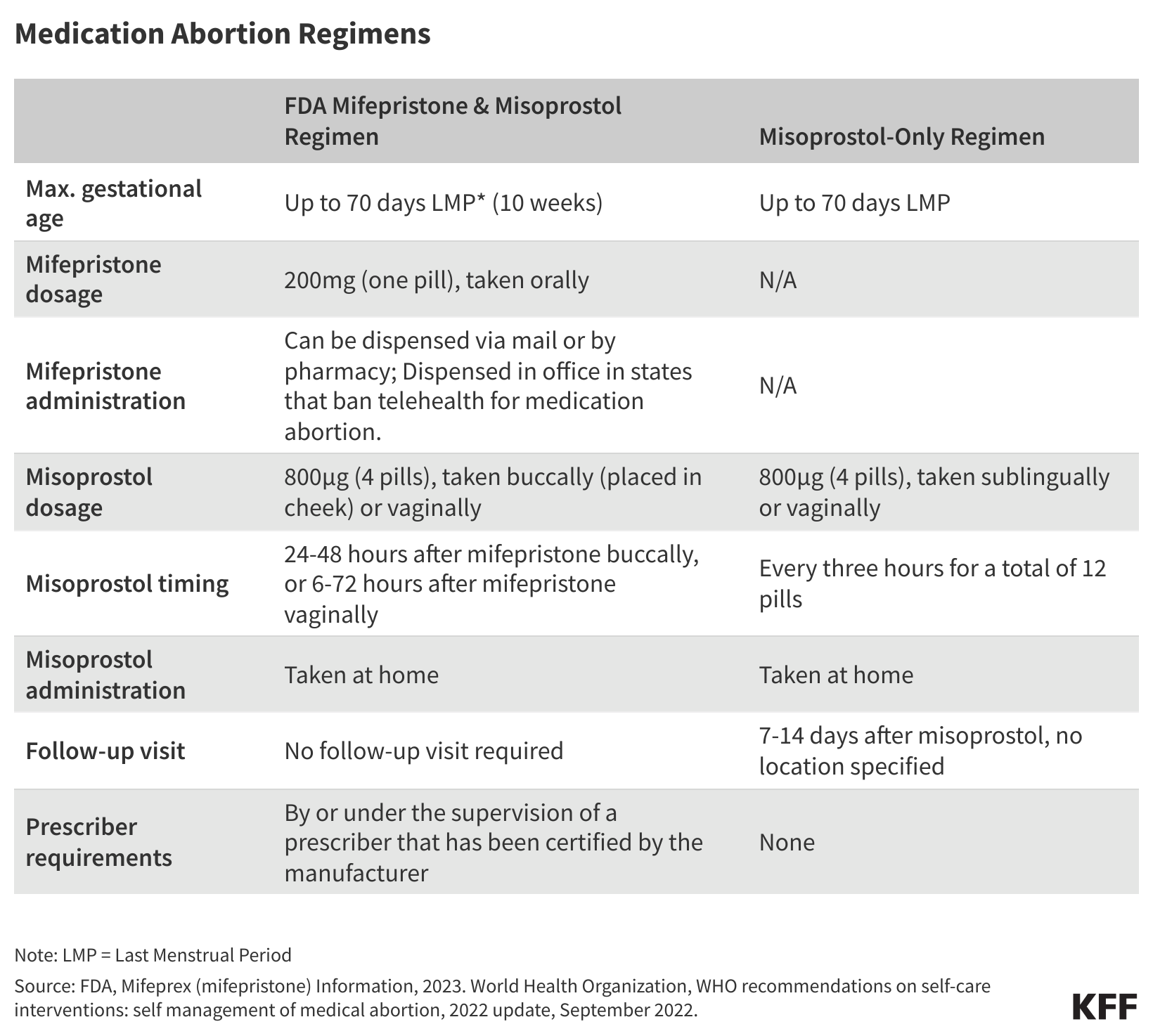
This factsheet provides an overview of medication abortion, with a focus on federal and state regulations pertaining to its provision and coverage, and the role of the drug in self-managed abortions.
Current Abortion Coverage Restrictions
Several states have enacted private plan restrictions and have also banned abortion coverage from ACA Marketplace plans. Currently, there are 10 states that restrict abortion coverage in private plans and 25 that ban coverage in any Marketplace plans.
Abortion Decision Renews Questions About Employer Access to Health Information
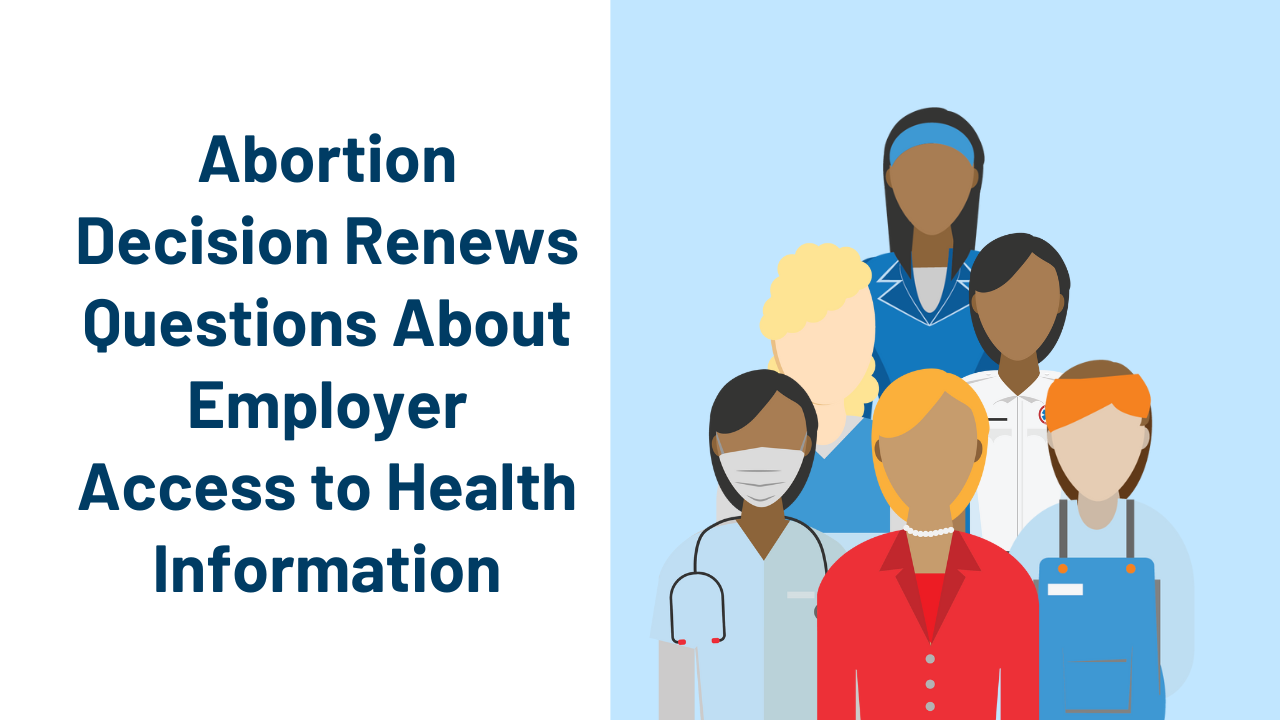
This Policy Watch takes a look at employers ability to access abortion information when their health plan covers abortion services. With some states criminalizing entities who assist in abortions, employers and providers face legal jeopardy and existing privacy laws such as HIPAA (the Health Insurance Portability and Accountability Act) may be limited in their privacy protections.
Variability in Payment Rates for Abortion Services Under Medicaid
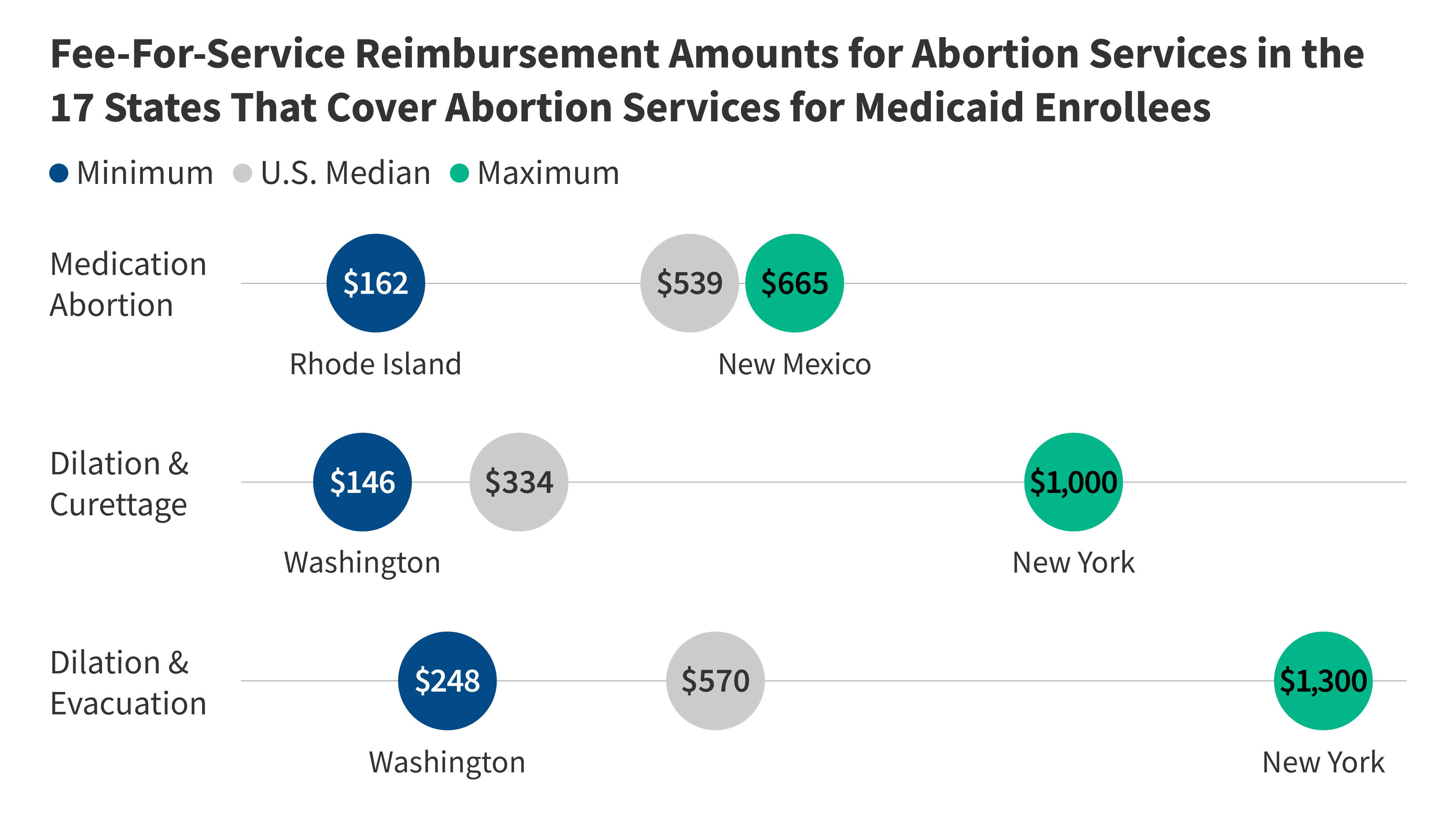
This brief looks at Medicaid reimbursement rates for abortion services across states, including D&C and D&E procedures, and medication abortion. There is tremendous variability in how much states reimburse for abortion services.
The Hyde Amendment and Coverage for Abortion Services Under Medicaid in the Post-Roe Era
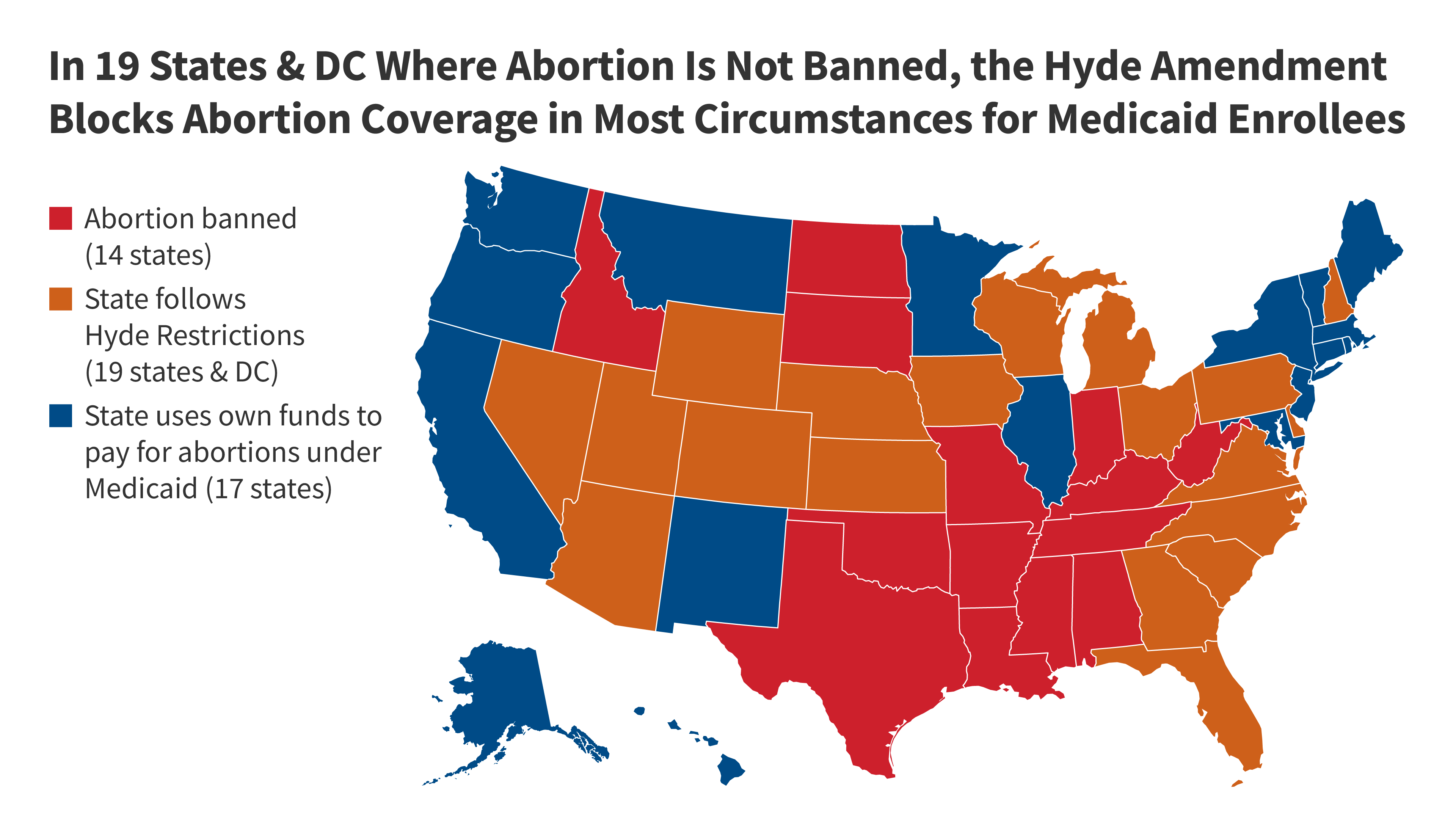
This brief details the federal programs that are affected by the Hyde Amendment and laws and regulations that have a similar goal, provides estimates on the share of women insured by Medicaid affected by the law, reviews the impact of the law on their access to abortion services, and discusses the potential effect if the law were to be repealed.
Employer Assistance with Abortion Related Travel Costs
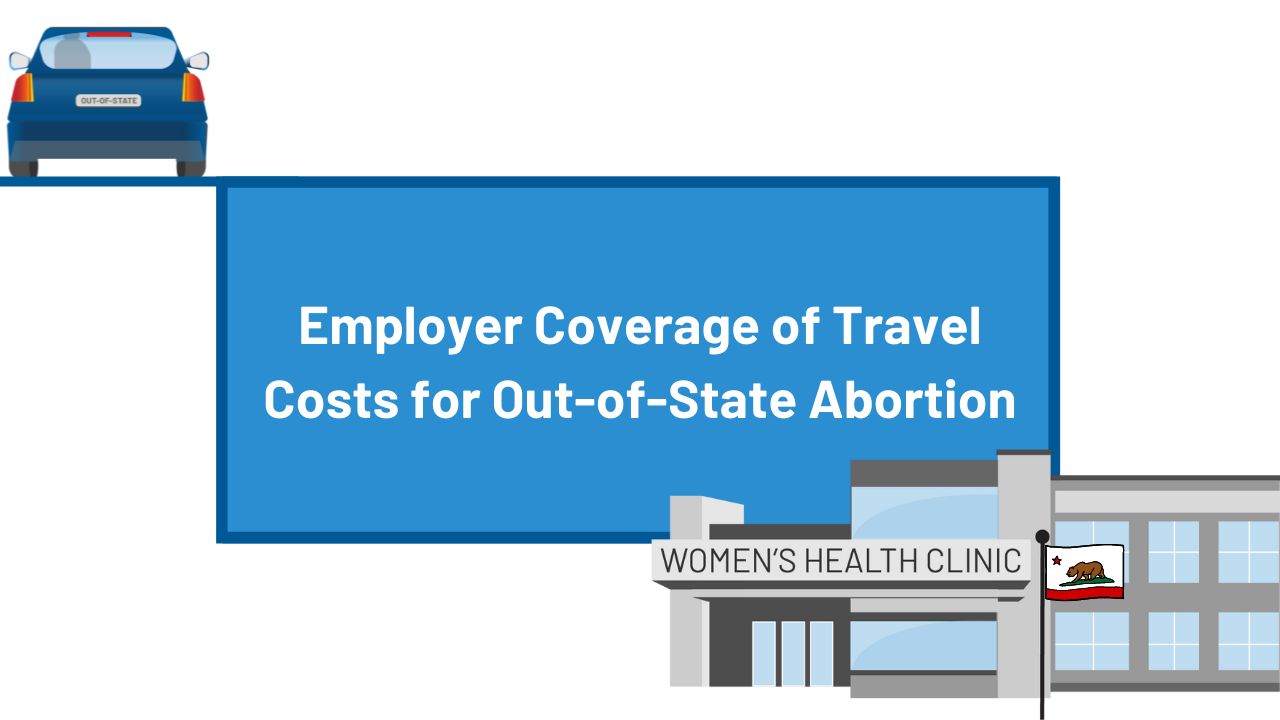
This Policy Watch gives an overview of employers offering to cover travel expenses for workers who need to go out of state for an abortion in the context of increasing restrictions on abortion around the country. We discuss who is offering these benefits, the implications for workers, and some of the legal and political concerns for employers.
Coverage of Abortion in Large Employer-Sponsored Plans in 2023
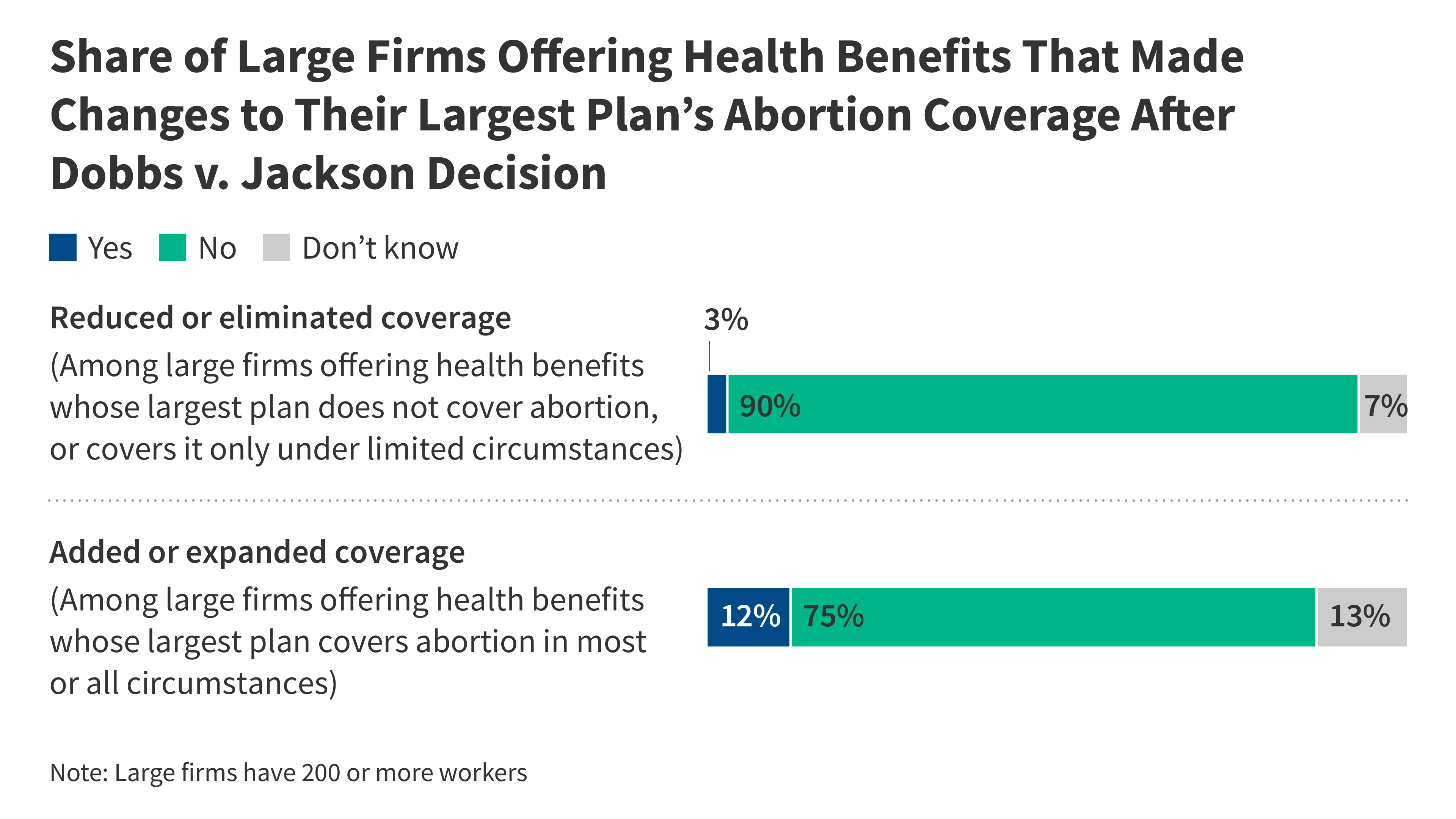
This brief presents findings from the 2023 KFF Employer Health Benefits Survey on coverage of abortion services in large employer-sponsored health plans, changes employers made to abortion coverage since the 2022 Supreme Court ruling, and employers’ provision of financial assistance for travel out of state to obtain an abortion.
KFF Health Tracking Poll March 2024: Abortion in the 2024 Election and Beyond
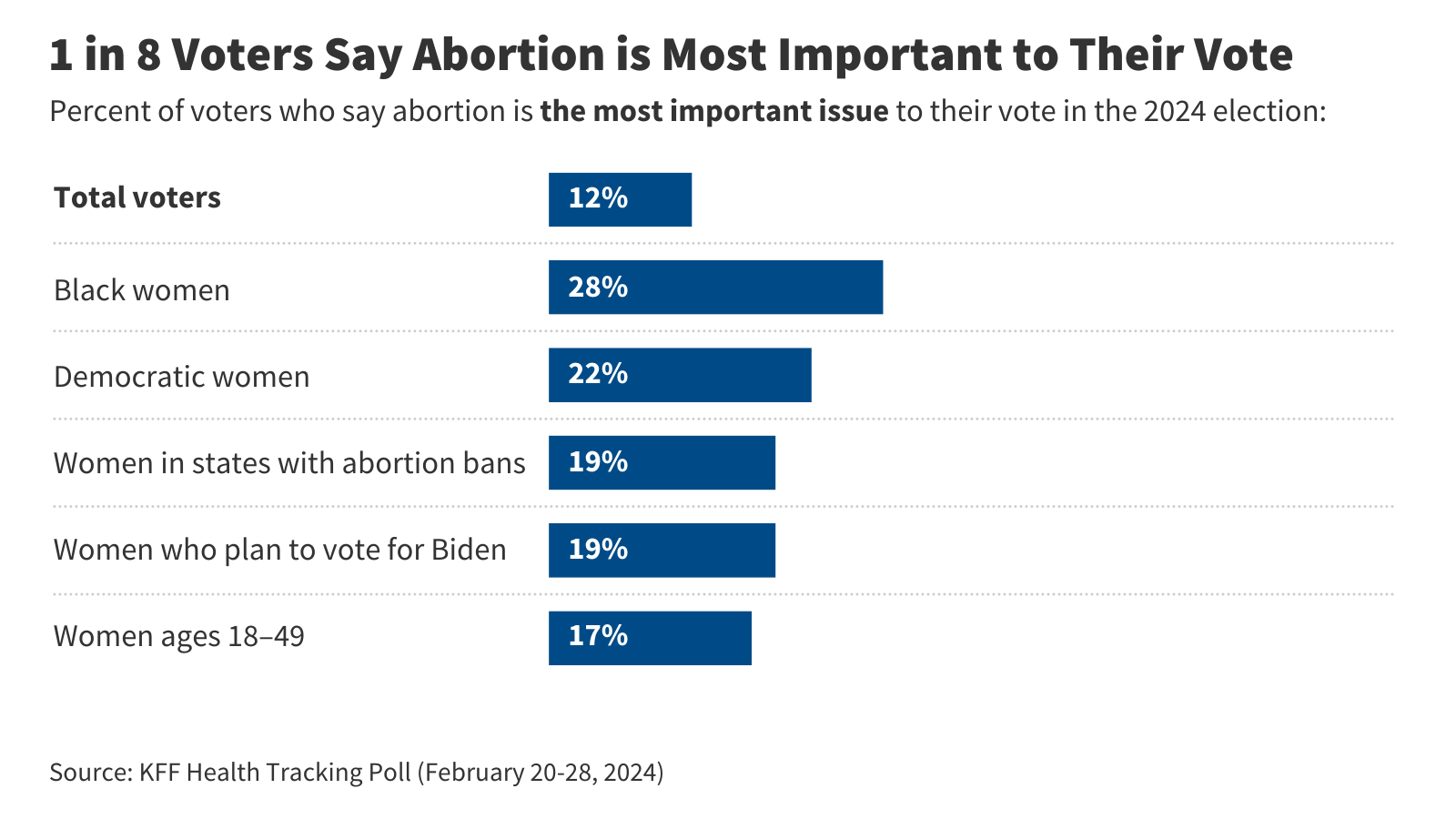
This poll finds 1 in 8 voters say abortion is the most important issue to their vote. They are younger, lean Democratic, and generally want abortion to be legal in all or most cases. The poll also gauges the public's views on abortion-related policies, including a national 16-week abortion ban and allowing abortion for pregnancy-related emergencies.
Women’s Views of Abortion Access and Policies in the Dobbs Era: Insights From the KFF Health Tracking Poll
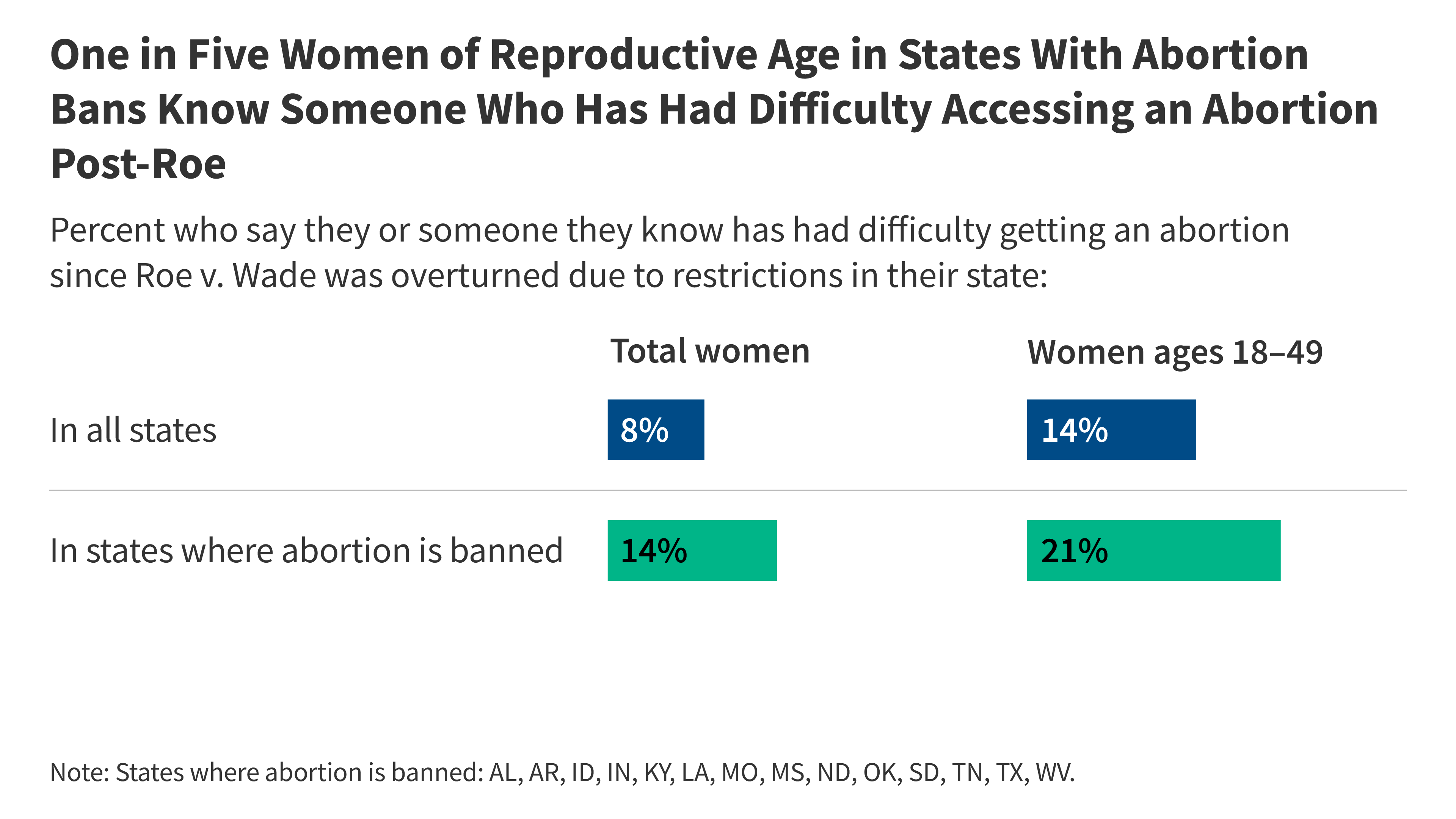
Our latest poll finds one in five women of reproductive age in states with abortion bans say either they or someone they personally know has had difficulty obtaining an abortion. Majorities of women across states—including in those with abortion bans—think abortion should be legal in all or most cases and support a range of policies that protect abortion access.
Pregnancy-Related Mortality (per 100,000 births) by Race and Ethnicity, 2017-2019
Native Hawaiian or Pacific Islander, American Indian or Alaskan Native and Black people are more likely to die while pregnant or within a year of the end of pregnancy compared to White people
State Abortion Policies by Race and Ethnicity Among Women Ages 18-49, 2022
Six in ten of Black (60%) and AIAN (59%) women ages 18-49 live in states with abortion bans or restrictions. Just over half (53%) of White women ages 18-49 live in states with bans or restrictions, while less than half of Hispanic (45%) and about three in ten Asian (28%) and NHPI (29%) women ages 18-49 live in these states
A National Survey of OBGYNs’ Experiences After Dobbs
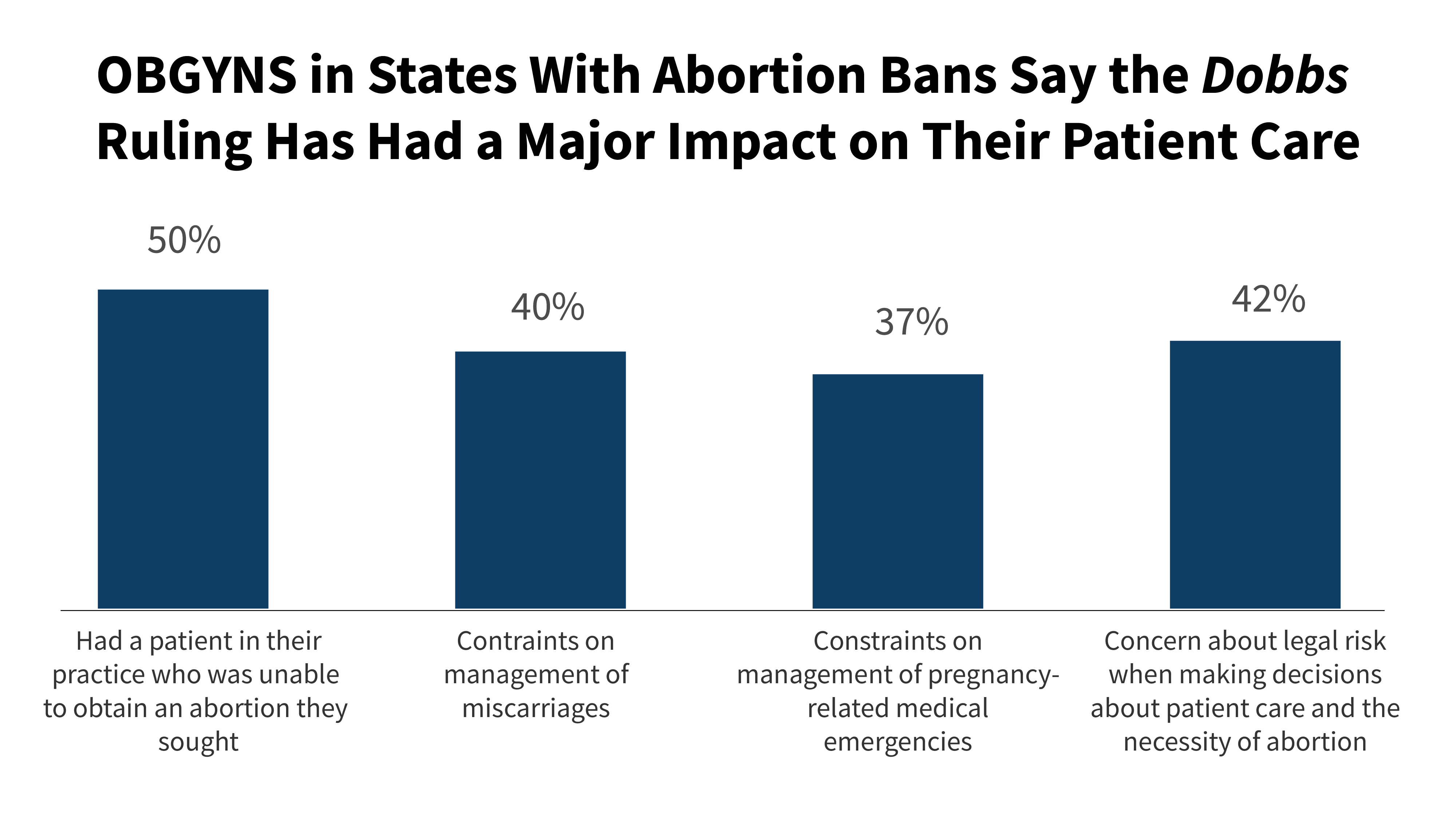
This report, based on a nationally representative survey of office-based OBGYNs practicing in the United States, examines the provision of sexual and reproductive health care provided by OBGYNs before and after the Dobbs decision, comparing the experiences of OBGYNs practicing in states where abortion is fully banned, states with gestational restrictions, and states where abortion remains available under most circumstances.
Abortion Statistics by State
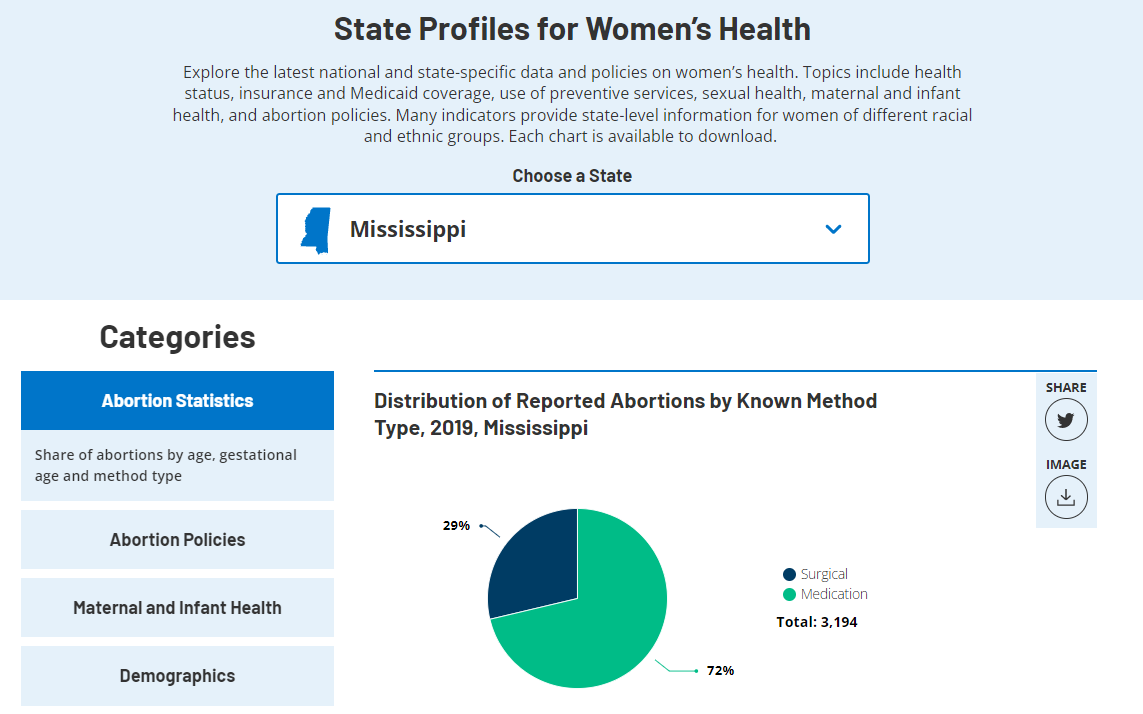
Share of abortions by age, gestational age and method type.
Abortion Policies by State
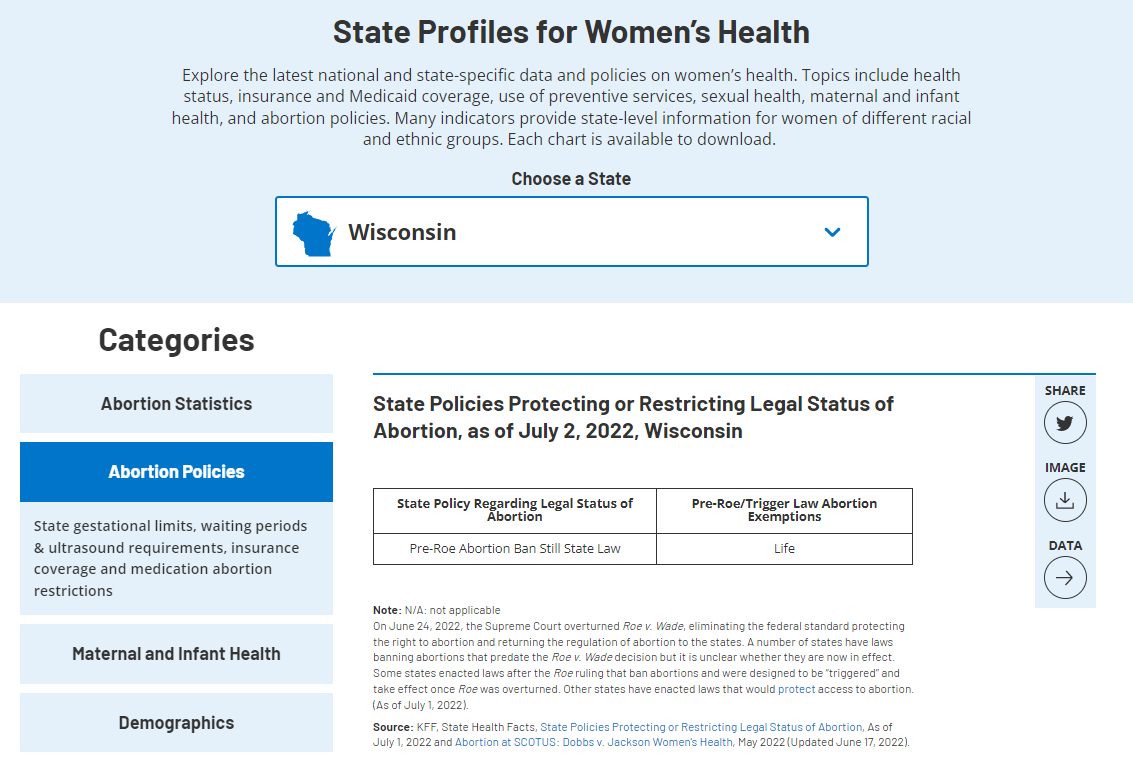
State gestational limits, waiting periods & ultrasound requirements, insurance coverage, and medication abortion restrictions.
Latest Resources From KFF

The Right to Contraception: State and Federal Actions, Misinformation, and the Courts
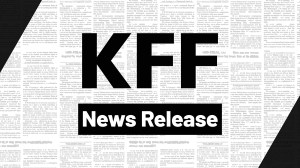
KFF Examines New Rule Giving LGBTQ+ People More Protections Against Discrimination in Health Care
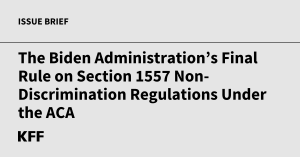
The Biden Administration’s Final Rule on Section 1557 Non-Discrimination Regulations Under the ACA
Abortion gestational limits and exceptions.
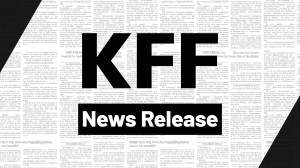
KFF Examines Implications of Dobbs-era Abortion Bans and Restrictions for Miscarriage or Stillbirth
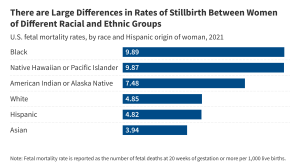

A .gov website belongs to an official government organization in the United States.
A lock ( ) or https:// means you've safely connected to the .gov website. Share sensitive information only on official, secure websites.
- About Adverse Childhood Experiences
- Risk and Protective Factors
- Program: Essentials for Childhood: Preventing Adverse Childhood Experiences through Data to Action
- Adverse childhood experiences can have long-term impacts on health, opportunity and well-being.
- Adverse childhood experiences are common and some groups experience them more than others.
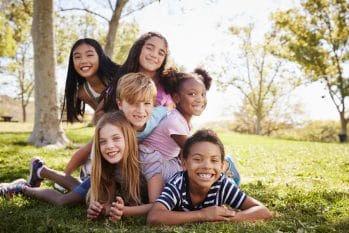
What are adverse childhood experiences?
Adverse childhood experiences, or ACEs, are potentially traumatic events that occur in childhood (0-17 years). Examples include: 1
- Experiencing violence, abuse, or neglect.
- Witnessing violence in the home or community.
- Having a family member attempt or die by suicide.
Also included are aspects of the child’s environment that can undermine their sense of safety, stability, and bonding. Examples can include growing up in a household with: 1
- Substance use problems.
- Mental health problems.
- Instability due to parental separation.
- Instability due to household members being in jail or prison.
The examples above are not a complete list of adverse experiences. Many other traumatic experiences could impact health and well-being. This can include not having enough food to eat, experiencing homelessness or unstable housing, or experiencing discrimination. 2 3 4 5 6
Quick facts and stats
ACEs are common. About 64% of adults in the United States reported they had experienced at least one type of ACE before age 18. Nearly one in six (17.3%) adults reported they had experienced four or more types of ACEs. 7
Preventing ACEs could potentially reduce many health conditions. Estimates show up to 1.9 million heart disease cases and 21 million depression cases potentially could have been avoided by preventing ACEs. 1
Some people are at greater risk of experiencing one or more ACEs than others. While all children are at risk of ACEs, numerous studies show inequities in such experiences. These inequalities are linked to the historical, social, and economic environments in which some families live. 5 6 ACEs were highest among females, non-Hispanic American Indian or Alaska Native adults, and adults who are unemployed or unable to work. 7
ACEs are costly. ACEs-related health consequences cost an estimated economic burden of $748 billion annually in Bermuda, Canada, and the United States. 8
ACEs can have lasting effects on health and well-being in childhood and life opportunities well into adulthood. 9 Life opportunities include things like education and job potential. These experiences can increase the risks of injury, sexually transmitted infections, and involvement in sex trafficking. They can also increase risks for maternal and child health problems including teen pregnancy, pregnancy complications, and fetal death. Also included are a range of chronic diseases and leading causes of death, such as cancer, diabetes, heart disease, and suicide. 1 10 11 12 13 14 15 16 17
ACEs and associated social determinants of health, such as living in under-resourced or racially segregated neighborhoods, can cause toxic stress. Toxic stress, or extended or prolonged stress, from ACEs can negatively affect children’s brain development, immune systems, and stress-response systems. These changes can affect children’s attention, decision-making, and learning. 18
Children growing up with toxic stress may have difficulty forming healthy and stable relationships. They may also have unstable work histories as adults and struggle with finances, jobs, and depression throughout life. 18 These effects can also be passed on to their own children. 19 20 21 Some children may face further exposure to toxic stress from historical and ongoing traumas. These historical and ongoing traumas refer to experiences of racial discrimination or the impacts of poverty resulting from limited educational and economic opportunities. 1 6
Adverse childhood experiences can be prevented. Certain factors may increase or decrease the risk of experiencing adverse childhood experiences.
Preventing adverse childhood experiences requires understanding and addressing the factors that put people at risk for or protect them from violence.
Creating safe, stable, nurturing relationships and environments for all children can prevent ACEs and help all children reach their full potential. We all have a role to play.
- Merrick MT, Ford DC, Ports KA, et al. Vital Signs: Estimated Proportion of Adult Health Problems Attributable to Adverse Childhood Experiences and Implications for Prevention — 25 States, 2015–2017. MMWR Morb Mortal Wkly Rep 2019;68:999-1005. DOI: http://dx.doi.org/10.15585/mmwr.mm6844e1 .
- Cain KS, Meyer SC, Cummer E, Patel KK, Casacchia NJ, Montez K, Palakshappa D, Brown CL. Association of Food Insecurity with Mental Health Outcomes in Parents and Children. Science Direct. 2022; 22:7; 1105-1114. DOI: https://doi.org/10.1016/j.acap.2022.04.010 .
- Smith-Grant J, Kilmer G, Brener N, Robin L, Underwood M. Risk Behaviors and Experiences Among Youth Experiencing Homelessness—Youth Risk Behavior Survey, 23 U.S. States and 11 Local School Districts. Journal of Community Health. 2022; 47: 324-333.
- Experiencing discrimination: Early Childhood Adversity, Toxic Stress, and the Impacts of Racism on the Foundations of Health | Annual Review of Public Health https://doi.org/10.1146/annurev-publhealth-090419-101940 .
- Sedlak A, Mettenburg J, Basena M, et al. Fourth national incidence study of child abuse and neglect (NIS-4): Report to Congress. Executive Summary. Washington, DC: U.S. Department of Health an Human Services, Administration for Children and Families.; 2010.
- Font S, Maguire-Jack K. Pathways from childhood abuse and other adversities to adult health risks: The role of adult socioeconomic conditions. Child Abuse Negl. 2016;51:390-399.
- Swedo EA, Aslam MV, Dahlberg LL, et al. Prevalence of Adverse Childhood Experiences Among U.S. Adults — Behavioral Risk Factor Surveillance System, 2011–2020. MMWR Morb Mortal Wkly Rep 2023;72:707–715. DOI: http://dx.doi.org/10.15585/mmwr.mm7226a2 .
- Bellis, MA, et al. Life Course Health Consequences and Associated Annual Costs of Adverse Childhood Experiences Across Europe and North America: A Systematic Review and Meta-Analysis. Lancet Public Health 2019.
- Adverse Childhood Experiences During the COVID-19 Pandemic and Associations with Poor Mental Health and Suicidal Behaviors Among High School Students — Adolescent Behaviors and Experiences Survey, United States, January–June 2021 | MMWR
- Hillis SD, Anda RF, Dube SR, Felitti VJ, Marchbanks PA, Marks JS. The association between adverse childhood experiences and adolescent pregnancy, long-term psychosocial consequences, and fetal death. Pediatrics. 2004 Feb;113(2):320-7.
- Miller ES, Fleming O, Ekpe EE, Grobman WA, Heard-Garris N. Association Between Adverse Childhood Experiences and Adverse Pregnancy Outcomes. Obstetrics & Gynecology . 2021;138(5):770-776. https://doi.org/10.1097/AOG.0000000000004570 .
- Sulaiman S, Premji SS, Tavangar F, et al. Total Adverse Childhood Experiences and Preterm Birth: A Systematic Review. Matern Child Health J . 2021;25(10):1581-1594. https://doi.org/10.1007/s10995-021-03176-6 .
- Ciciolla L, Shreffler KM, Tiemeyer S. Maternal Childhood Adversity as a Risk for Perinatal Complications and NICU Hospitalization. Journal of Pediatric Psychology . 2021;46(7):801-813. https://doi.org/10.1093/jpepsy/jsab027 .
- Mersky JP, Lee CP. Adverse childhood experiences and poor birth outcomes in a diverse, low-income sample. BMC pregnancy and childbirth. 2019;19(1). https://doi.org/10.1186/s12884-019-2560-8 .
- Reid JA, Baglivio MT, Piquero AR, Greenwald MA, Epps N. No youth left behind to human trafficking: Exploring profiles of risk. American journal of orthopsychiatry. 2019;89(6):704.
- Diamond-Welch B, Kosloski AE. Adverse childhood experiences and propensity to participate in the commercialized sex market. Child Abuse & Neglect. 2020 Jun 1;104:104468.
- Shonkoff, J. P., Garner, A. S., Committee on Psychosocial Aspects of Child and Family Health, Committee on Early Childhood, Adoption, and Dependent Care, & Section on Developmental and Behavioral Pediatrics (2012). The lifelong effects of early childhood adversity and toxic stress. Pediatrics, 129(1), e232–e246. https://doi.org/10.1542/peds.2011-2663
- Narayan AJ, Kalstabakken AW, Labella MH, Nerenberg LS, Monn AR, Masten AS. Intergenerational continuity of adverse childhood experiences in homeless families: unpacking exposure to maltreatment versus family dysfunction. Am J Orthopsych. 2017;87(1):3. https://doi.org/10.1037/ort0000133 .
- Schofield TJ, Donnellan MB, Merrick MT, Ports KA, Klevens J, Leeb R. Intergenerational continuity in adverse childhood experiences and rural community environments. Am J Public Health. 2018;108(9):1148-1152. https://doi.org/10.2105/AJPH.2018.304598 .
- Schofield TJ, Lee RD, Merrick MT. Safe, stable, nurturing relationships as a moderator of intergenerational continuity of child maltreatment: a meta-analysis. J Adolesc Health. 2013;53(4 Suppl):S32-38. https://doi.org/10.1016/j.jadohealth.2013.05.004 .
Adverse Childhood Experiences (ACEs)
ACEs can have a tremendous impact on lifelong health and opportunity. CDC works to understand ACEs and prevent them.
McKinsey Global Private Markets Review 2024: Private markets in a slower era
At a glance, macroeconomic challenges continued.

McKinsey Global Private Markets Review 2024: Private markets: A slower era
If 2022 was a tale of two halves, with robust fundraising and deal activity in the first six months followed by a slowdown in the second half, then 2023 might be considered a tale of one whole. Macroeconomic headwinds persisted throughout the year, with rising financing costs, and an uncertain growth outlook taking a toll on private markets. Full-year fundraising continued to decline from 2021’s lofty peak, weighed down by the “denominator effect” that persisted in part due to a less active deal market. Managers largely held onto assets to avoid selling in a lower-multiple environment, fueling an activity-dampening cycle in which distribution-starved limited partners (LPs) reined in new commitments.
About the authors
This article is a summary of a larger report, available as a PDF, that is a collaborative effort by Fredrik Dahlqvist , Alastair Green , Paul Maia, Alexandra Nee , David Quigley , Aditya Sanghvi , Connor Mangan, John Spivey, Rahel Schneider, and Brian Vickery , representing views from McKinsey’s Private Equity & Principal Investors Practice.
Performance in most private asset classes remained below historical averages for a second consecutive year. Decade-long tailwinds from low and falling interest rates and consistently expanding multiples seem to be things of the past. As private market managers look to boost performance in this new era of investing, a deeper focus on revenue growth and margin expansion will be needed now more than ever.

Perspectives on a slower era in private markets
Global fundraising contracted.
Fundraising fell 22 percent across private market asset classes globally to just over $1 trillion, as of year-end reported data—the lowest total since 2017. Fundraising in North America, a rare bright spot in 2022, declined in line with global totals, while in Europe, fundraising proved most resilient, falling just 3 percent. In Asia, fundraising fell precipitously and now sits 72 percent below the region’s 2018 peak.
Despite difficult fundraising conditions, headwinds did not affect all strategies or managers equally. Private equity (PE) buyout strategies posted their best fundraising year ever, and larger managers and vehicles also fared well, continuing the prior year’s trend toward greater fundraising concentration.
The numerator effect persisted
Despite a marked recovery in the denominator—the 1,000 largest US retirement funds grew 7 percent in the year ending September 2023, after falling 14 percent the prior year, for example 1 “U.S. retirement plans recover half of 2022 losses amid no-show recession,” Pensions and Investments , February 12, 2024. —many LPs remain overexposed to private markets relative to their target allocations. LPs started 2023 overweight: according to analysis from CEM Benchmarking, average allocations across PE, infrastructure, and real estate were at or above target allocations as of the beginning of the year. And the numerator grew throughout the year, as a lack of exits and rebounding valuations drove net asset values (NAVs) higher. While not all LPs strictly follow asset allocation targets, our analysis in partnership with global private markets firm StepStone Group suggests that an overallocation of just one percentage point can reduce planned commitments by as much as 10 to 12 percent per year for five years or more.
Despite these headwinds, recent surveys indicate that LPs remain broadly committed to private markets. In fact, the majority plan to maintain or increase allocations over the medium to long term.
Investors fled to known names and larger funds
Fundraising concentration reached its highest level in over a decade, as investors continued to shift new commitments in favor of the largest fund managers. The 25 most successful fundraisers collected 41 percent of aggregate commitments to closed-end funds (with the top five managers accounting for nearly half that total). Closed-end fundraising totals may understate the extent of concentration in the industry overall, as the largest managers also tend to be more successful in raising non-institutional capital.
While the largest funds grew even larger—the largest vehicles on record were raised in buyout, real estate, infrastructure, and private debt in 2023—smaller and newer funds struggled. Fewer than 1,700 funds of less than $1 billion were closed during the year, half as many as closed in 2022 and the fewest of any year since 2012. New manager formation also fell to the lowest level since 2012, with just 651 new firms launched in 2023.
Whether recent fundraising concentration and a spate of M&A activity signals the beginning of oft-rumored consolidation in the private markets remains uncertain, as a similar pattern developed in each of the last two fundraising downturns before giving way to renewed entrepreneurialism among general partners (GPs) and commitment diversification among LPs. Compared with how things played out in the last two downturns, perhaps this movie really is different, or perhaps we’re watching a trilogy reusing a familiar plotline.
Dry powder inventory spiked (again)
Private markets assets under management totaled $13.1 trillion as of June 30, 2023, and have grown nearly 20 percent per annum since 2018. Dry powder reserves—the amount of capital committed but not yet deployed—increased to $3.7 trillion, marking the ninth consecutive year of growth. Dry powder inventory—the amount of capital available to GPs expressed as a multiple of annual deployment—increased for the second consecutive year in PE, as new commitments continued to outpace deal activity. Inventory sat at 1.6 years in 2023, up markedly from the 0.9 years recorded at the end of 2021 but still within the historical range. NAV grew as well, largely driven by the reluctance of managers to exit positions and crystallize returns in a depressed multiple environment.
Private equity strategies diverged
Buyout and venture capital, the two largest PE sub-asset classes, charted wildly different courses over the past 18 months. Buyout notched its highest fundraising year ever in 2023, and its performance improved, with funds posting a (still paltry) 5 percent net internal rate of return through September 30. And although buyout deal volumes declined by 19 percent, 2023 was still the third-most-active year on record. In contrast, venture capital (VC) fundraising declined by nearly 60 percent, equaling its lowest total since 2015, and deal volume fell by 36 percent to the lowest level since 2019. VC funds returned –3 percent through September, posting negative returns for seven consecutive quarters. VC was the fastest-growing—as well as the highest-performing—PE strategy by a significant margin from 2010 to 2022, but investors appear to be reevaluating their approach in the current environment.
Private equity entry multiples contracted
PE buyout entry multiples declined by roughly one turn from 11.9 to 11.0 times EBITDA, slightly outpacing the decline in public market multiples (down from 12.1 to 11.3 times EBITDA), through the first nine months of 2023. For nearly a decade leading up to 2022, managers consistently sold assets into a higher-multiple environment than that in which they had bought those assets, providing a substantial performance tailwind for the industry. Nowhere has this been truer than in technology. After experiencing more than eight turns of multiple expansion from 2009 to 2021 (the most of any sector), technology multiples have declined by nearly three turns in the past two years, 50 percent more than in any other sector. Overall, roughly two-thirds of the total return for buyout deals that were entered in 2010 or later and exited in 2021 or before can be attributed to market multiple expansion and leverage. Now, with falling multiples and higher financing costs, revenue growth and margin expansion are taking center stage for GPs.
Real estate receded
Demand uncertainty, slowing rent growth, and elevated financing costs drove cap rates higher and made price discovery challenging, all of which weighed on deal volume, fundraising, and investment performance. Global closed-end fundraising declined 34 percent year over year, and funds returned −4 percent in the first nine months of the year, losing money for the first time since the 2007–08 global financial crisis. Capital shifted away from core and core-plus strategies as investors sought liquidity via redemptions in open-end vehicles, from which net outflows reached their highest level in at least two decades. Opportunistic strategies benefited from this shift, with investors focusing on capital appreciation over income generation in a market where alternative sources of yield have grown more attractive. Rising interest rates widened bid–ask spreads and impaired deal volume across food groups, including in what were formerly hot sectors: multifamily and industrial.
Private debt pays dividends
Debt again proved to be the most resilient private asset class against a turbulent market backdrop. Fundraising declined just 13 percent, largely driven by lower commitments to direct lending strategies, for which a slower PE deal environment has made capital deployment challenging. The asset class also posted the highest returns among all private asset classes through September 30. Many private debt securities are tied to floating rates, which enhance returns in a rising-rate environment. Thus far, managers appear to have successfully navigated the rising incidence of default and distress exhibited across the broader leveraged-lending market. Although direct lending deal volume declined from 2022, private lenders financed an all-time high 59 percent of leveraged buyout transactions last year and are now expanding into additional strategies to drive the next era of growth.
Infrastructure took a detour
After several years of robust growth and strong performance, infrastructure and natural resources fundraising declined by 53 percent to the lowest total since 2013. Supply-side timing is partially to blame: five of the seven largest infrastructure managers closed a flagship vehicle in 2021 or 2022, and none of those five held a final close last year. As in real estate, investors shied away from core and core-plus investments in a higher-yield environment. Yet there are reasons to believe infrastructure’s growth will bounce back. Limited partners (LPs) surveyed by McKinsey remain bullish on their deployment to the asset class, and at least a dozen vehicles targeting more than $10 billion were actively fundraising as of the end of 2023. Multiple recent acquisitions of large infrastructure GPs by global multi-asset-class managers also indicate marketwide conviction in the asset class’s potential.
Private markets still have work to do on diversity
Private markets firms are slowly improving their representation of females (up two percentage points over the prior year) and ethnic and racial minorities (up one percentage point). On some diversity metrics, including entry-level representation of women, private markets now compare favorably with corporate America. Yet broad-based parity remains elusive and too slow in the making. Ethnic, racial, and gender imbalances are particularly stark across more influential investing roles and senior positions. In fact, McKinsey’s research reveals that at the current pace, it would take several decades for private markets firms to reach gender parity at senior levels. Increasing representation across all levels will require managers to take fresh approaches to hiring, retention, and promotion.
Artificial intelligence generating excitement
The transformative potential of generative AI was perhaps 2023’s hottest topic (beyond Taylor Swift). Private markets players are excited about the potential for the technology to optimize their approach to thesis generation, deal sourcing, investment due diligence, and portfolio performance, among other areas. While the technology is still nascent and few GPs can boast scaled implementations, pilot programs are already in flight across the industry, particularly within portfolio companies. Adoption seems nearly certain to accelerate throughout 2024.
Private markets in a slower era
If private markets investors entered 2023 hoping for a return to the heady days of 2021, they likely left the year disappointed. Many of the headwinds that emerged in the latter half of 2022 persisted throughout the year, pressuring fundraising, dealmaking, and performance. Inflation moderated somewhat over the course of the year but remained stubbornly elevated by recent historical standards. Interest rates started high and rose higher, increasing the cost of financing. A reinvigorated public equity market recovered most of 2022’s losses but did little to resolve the valuation uncertainty private market investors have faced for the past 18 months.
Within private markets, the denominator effect remained in play, despite the public market recovery, as the numerator continued to expand. An activity-dampening cycle emerged: higher cost of capital and lower multiples limited the ability or willingness of general partners (GPs) to exit positions; fewer exits, coupled with continuing capital calls, pushed LP allocations higher, thereby limiting their ability or willingness to make new commitments. These conditions weighed on managers’ ability to fundraise. Based on data reported as of year-end 2023, private markets fundraising fell 22 percent from the prior year to just over $1 trillion, the largest such drop since 2009 (Exhibit 1).
The impact of the fundraising environment was not felt equally among GPs. Continuing a trend that emerged in 2022, and consistent with prior downturns in fundraising, LPs favored larger vehicles and the scaled GPs that typically manage them. Smaller and newer managers struggled, and the number of sub–$1 billion vehicles and new firm launches each declined to its lowest level in more than a decade.
Despite the decline in fundraising, private markets assets under management (AUM) continued to grow, increasing 12 percent to $13.1 trillion as of June 30, 2023. 2023 fundraising was still the sixth-highest annual haul on record, pushing dry powder higher, while the slowdown in deal making limited distributions.
Investment performance across private market asset classes fell short of historical averages. Private equity (PE) got back in the black but generated the lowest annual performance in the past 15 years, excluding 2022. Closed-end real estate produced negative returns for the first time since 2009, as capitalization (cap) rates expanded across sectors and rent growth dissipated in formerly hot sectors, including multifamily and industrial. The performance of infrastructure funds was less than half of its long-term average and even further below the double-digit returns generated in 2021 and 2022. Private debt was the standout performer (if there was one), outperforming all other private asset classes and illustrating the asset class’s countercyclical appeal.
Private equity down but not out
Higher financing costs, lower multiples, and an uncertain macroeconomic environment created a challenging backdrop for private equity managers in 2023. Fundraising declined for the second year in a row, falling 15 percent to $649 billion, as LPs grappled with the denominator effect and a slowdown in distributions. Managers were on the fundraising trail longer to raise this capital: funds that closed in 2023 were open for a record-high average of 20.1 months, notably longer than 18.7 months in 2022 and 14.1 months in 2018. VC and growth equity strategies led the decline, dropping to their lowest level of cumulative capital raised since 2015. Fundraising in Asia fell for the fourth year of the last five, with the greatest decline in China.
Despite the difficult fundraising context, a subset of strategies and managers prevailed. Buyout managers collectively had their best fundraising year on record, raising more than $400 billion. Fundraising in Europe surged by more than 50 percent, resulting in the region’s biggest haul ever. The largest managers raised an outsized share of the total for a second consecutive year, making 2023 the most concentrated fundraising year of the last decade (Exhibit 2).
Despite the drop in aggregate fundraising, PE assets under management increased 8 percent to $8.2 trillion. Only a small part of this growth was performance driven: PE funds produced a net IRR of just 2.5 percent through September 30, 2023. Buyouts and growth equity generated positive returns, while VC lost money. PE performance, dating back to the beginning of 2022, remains negative, highlighting the difficulty of generating attractive investment returns in a higher interest rate and lower multiple environment. As PE managers devise value creation strategies to improve performance, their focus includes ensuring operating efficiency and profitability of their portfolio companies.
Deal activity volume and count fell sharply, by 21 percent and 24 percent, respectively, which continued the slower pace set in the second half of 2022. Sponsors largely opted to hold assets longer rather than lock in underwhelming returns. While higher financing costs and valuation mismatches weighed on overall deal activity, certain types of M&A gained share. Add-on deals, for example, accounted for a record 46 percent of total buyout deal volume last year.
Real estate recedes
For real estate, 2023 was a year of transition, characterized by a litany of new and familiar challenges. Pandemic-driven demand issues continued, while elevated financing costs, expanding cap rates, and valuation uncertainty weighed on commercial real estate deal volumes, fundraising, and investment performance.
Managers faced one of the toughest fundraising environments in many years. Global closed-end fundraising declined 34 percent to $125 billion. While fundraising challenges were widespread, they were not ubiquitous across strategies. Dollars continued to shift to large, multi-asset class platforms, with the top five managers accounting for 37 percent of aggregate closed-end real estate fundraising. In April, the largest real estate fund ever raised closed on a record $30 billion.
Capital shifted away from core and core-plus strategies as investors sought liquidity through redemptions in open-end vehicles and reduced gross contributions to the lowest level since 2009. Opportunistic strategies benefited from this shift, as investors turned their attention toward capital appreciation over income generation in a market where alternative sources of yield have grown more attractive.
In the United States, for instance, open-end funds, as represented by the National Council of Real Estate Investment Fiduciaries Fund Index—Open-End Equity (NFI-OE), recorded $13 billion in net outflows in 2023, reversing the trend of positive net inflows throughout the 2010s. The negative flows mainly reflected $9 billion in core outflows, with core-plus funds accounting for the remaining outflows, which reversed a 20-year run of net inflows.
As a result, the NAV in US open-end funds fell roughly 16 percent year over year. Meanwhile, global assets under management in closed-end funds reached a new peak of $1.7 trillion as of June 2023, growing 14 percent between June 2022 and June 2023.
Real estate underperformed historical averages in 2023, as previously high-performing multifamily and industrial sectors joined office in producing negative returns caused by slowing demand growth and cap rate expansion. Closed-end funds generated a pooled net IRR of −3.5 percent in the first nine months of 2023, losing money for the first time since the global financial crisis. The lone bright spot among major sectors was hospitality, which—thanks to a rush of postpandemic travel—returned 10.3 percent in 2023. 2 Based on NCREIFs NPI index. Hotels represent 1 percent of total properties in the index. As a whole, the average pooled lifetime net IRRs for closed-end real estate funds from 2011–20 vintages remained around historical levels (9.8 percent).
Global deal volume declined 47 percent in 2023 to reach a ten-year low of $650 billion, driven by widening bid–ask spreads amid valuation uncertainty and higher costs of financing (Exhibit 3). 3 CBRE, Real Capital Analytics Deal flow in the office sector remained depressed, partly as a result of continued uncertainty in the demand for space in a hybrid working world.
During a turbulent year for private markets, private debt was a relative bright spot, topping private markets asset classes in terms of fundraising growth, AUM growth, and performance.
Fundraising for private debt declined just 13 percent year over year, nearly ten percentage points less than the private markets overall. Despite the decline in fundraising, AUM surged 27 percent to $1.7 trillion. And private debt posted the highest investment returns of any private asset class through the first three quarters of 2023.
Private debt’s risk/return characteristics are well suited to the current environment. With interest rates at their highest in more than a decade, current yields in the asset class have grown more attractive on both an absolute and relative basis, particularly if higher rates sustain and put downward pressure on equity returns (Exhibit 4). The built-in security derived from debt’s privileged position in the capital structure, moreover, appeals to investors that are wary of market volatility and valuation uncertainty.
Direct lending continued to be the largest strategy in 2023, with fundraising for the mostly-senior-debt strategy accounting for almost half of the asset class’s total haul (despite declining from the previous year). Separately, mezzanine debt fundraising hit a new high, thanks to the closings of three of the largest funds ever raised in the strategy.
Over the longer term, growth in private debt has largely been driven by institutional investors rotating out of traditional fixed income in favor of private alternatives. Despite this growth in commitments, LPs remain underweight in this asset class relative to their targets. In fact, the allocation gap has only grown wider in recent years, a sharp contrast to other private asset classes, for which LPs’ current allocations exceed their targets on average. According to data from CEM Benchmarking, the private debt allocation gap now stands at 1.4 percent, which means that, in aggregate, investors must commit hundreds of billions in net new capital to the asset class just to reach current targets.
Private debt was not completely immune to the macroeconomic conditions last year, however. Fundraising declined for the second consecutive year and now sits 23 percent below 2021’s peak. Furthermore, though private lenders took share in 2023 from other capital sources, overall deal volumes also declined for the second year in a row. The drop was largely driven by a less active PE deal environment: private debt is predominantly used to finance PE-backed companies, though managers are increasingly diversifying their origination capabilities to include a broad new range of companies and asset types.
Infrastructure and natural resources take a detour
For infrastructure and natural resources fundraising, 2023 was an exceptionally challenging year. Aggregate capital raised declined 53 percent year over year to $82 billion, the lowest annual total since 2013. The size of the drop is particularly surprising in light of infrastructure’s recent momentum. The asset class had set fundraising records in four of the previous five years, and infrastructure is often considered an attractive investment in uncertain markets.
While there is little doubt that the broader fundraising headwinds discussed elsewhere in this report affected infrastructure and natural resources fundraising last year, dynamics specific to the asset class were at play as well. One issue was supply-side timing: nine of the ten largest infrastructure GPs did not close a flagship fund in 2023. Second was the migration of investor dollars away from core and core-plus investments, which have historically accounted for the bulk of infrastructure fundraising, in a higher rate environment.
The asset class had some notable bright spots last year. Fundraising for higher-returning opportunistic strategies more than doubled the prior year’s total (Exhibit 5). AUM grew 18 percent, reaching a new high of $1.5 trillion. Infrastructure funds returned a net IRR of 3.4 percent in 2023; this was below historical averages but still the second-best return among private asset classes. And as was the case in other asset classes, investors concentrated commitments in larger funds and managers in 2023, including in the largest infrastructure fund ever raised.
The outlook for the asset class, moreover, remains positive. Funds targeting a record amount of capital were in the market at year-end, providing a robust foundation for fundraising in 2024 and 2025. A recent spate of infrastructure GP acquisitions signal multi-asset managers’ long-term conviction in the asset class, despite short-term headwinds. Global megatrends like decarbonization and digitization, as well as revolutions in energy and mobility, have spurred new infrastructure investment opportunities around the world, particularly for value-oriented investors that are willing to take on more risk.
Private markets make measured progress in DEI
Diversity, equity, and inclusion (DEI) has become an important part of the fundraising, talent, and investing landscape for private market participants. Encouragingly, incremental progress has been made in recent years, including more diverse talent being brought to entry-level positions, investing roles, and investment committees. The scope of DEI metrics provided to institutional investors during fundraising has also increased in recent years: more than half of PE firms now provide data across investing teams, portfolio company boards, and portfolio company management (versus investment team data only). 4 “ The state of diversity in global private markets: 2023 ,” McKinsey, August 22, 2023.
In 2023, McKinsey surveyed 66 global private markets firms that collectively employ more than 60,000 people for the second annual State of diversity in global private markets report. 5 “ The state of diversity in global private markets: 2023 ,” McKinsey, August 22, 2023. The research offers insight into the representation of women and ethnic and racial minorities in private investing as of year-end 2022. In this chapter, we discuss where the numbers stand and how firms can bring a more diverse set of perspectives to the table.
The statistics indicate signs of modest advancement. Overall representation of women in private markets increased two percentage points to 35 percent, and ethnic and racial minorities increased one percentage point to 30 percent (Exhibit 6). Entry-level positions have nearly reached gender parity, with female representation at 48 percent. The share of women holding C-suite roles globally increased 3 percentage points, while the share of people from ethnic and racial minorities in investment committees increased 9 percentage points. There is growing evidence that external hiring is gradually helping close the diversity gap, especially at senior levels. For example, 33 percent of external hires at the managing director level were ethnic or racial minorities, higher than their existing representation level (19 percent).
Yet, the scope of the challenge remains substantial. Women and minorities continue to be underrepresented in senior positions and investing roles. They also experience uneven rates of progress due to lower promotion and higher attrition rates, particularly at smaller firms. Firms are also navigating an increasingly polarized workplace today, with additional scrutiny and a growing number of lawsuits against corporate diversity and inclusion programs, particularly in the US, which threatens to impact the industry’s pace of progress.
Fredrik Dahlqvist is a senior partner in McKinsey’s Stockholm office; Alastair Green is a senior partner in the Washington, DC, office, where Paul Maia and Alexandra Nee are partners; David Quigley is a senior partner in the New York office, where Connor Mangan is an associate partner and Aditya Sanghvi is a senior partner; Rahel Schneider is an associate partner in the Bay Area office; John Spivey is a partner in the Charlotte office; and Brian Vickery is a partner in the Boston office.
The authors wish to thank Jonathan Christy, Louis Dufau, Vaibhav Gujral, Graham Healy-Day, Laura Johnson, Ryan Luby, Tripp Norton, Alastair Rami, Henri Torbey, and Alex Wolkomir for their contributions
The authors would also like to thank CEM Benchmarking and the StepStone Group for their partnership in this year's report.
This article was edited by Arshiya Khullar, an editor in the Gurugram office.
Explore a career with us
Related articles.

CEO alpha: A new approach to generating private equity outperformance
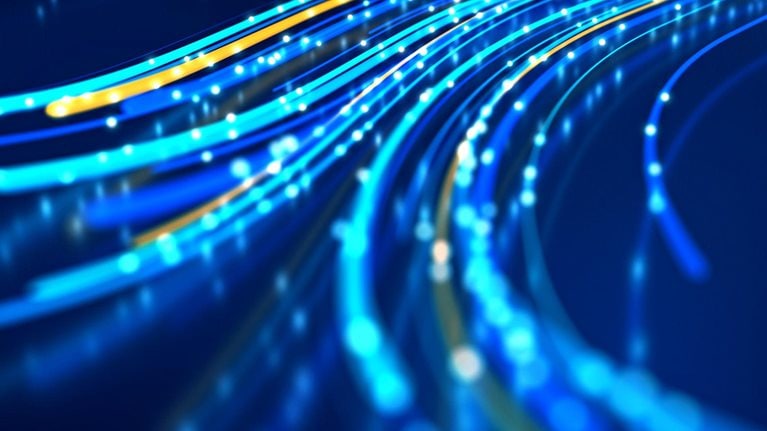
Private equity turns to resiliency strategies for software investments

The state of diversity in global private markets: 2022
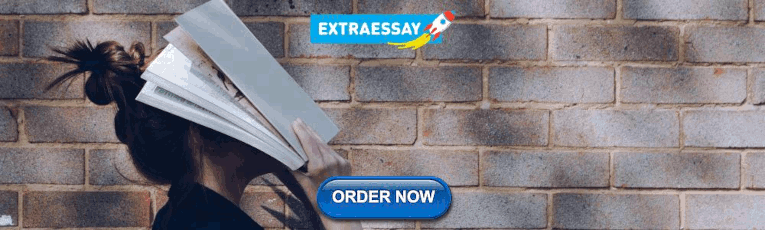
IMAGES
VIDEO
COMMENTS
This critical component of the action research process is called data analysis and interpretation, and it needs to be carefully thought out. 4. 1. Data Analysis Data analysis is an attempt by the teacher researcher to summarize collected data in a dependable and accurate manner. The type of data you collect will determine the data analysis ...
The 'observing' stage of action research involves collecting data, for example via surveys, focus groups, interviews, observations, reflective journal writing, and/or assessments. For language ...
Analysis and Presentation of your Data. I would like to address some general issues related to analyzing and presenting your data. Here are links to specific examples of data analysis in four action research articles, in the journal Networks: An Online Journal for Teacher Research.
Action Research is not a single research project; rather it is an ongoing iterative approach that takes place across cycles of innovation and reflection. It is a way of learning from and through systematic inquiry into one's practice. Central to this process is the collection and analysis of data. The image below (Rie1, 2014) uses color to ...
ENC 706: Action Research 4: Data Analysis & Reporting in Action Research PowerPoint 5.2: Inferential & Predictive Statistics (Chapters 21-28) graduating college seniors. In this case, the t test is based on repeated measures, where the same group of students is tested on two different occasions. In both of these examples, notice
5-2 Steps in Conducting Action Research (6 of 7) Analyzing the Data. Data analysis should not wait until the end of the data collection period . Action research takes time. Examine the data for relevant themes or patterns. Present the results for others to see and analyze, using tables, diagrams, and graphs
In PowerPoint 5.2, we looked at several inferential statistics, including t tests and ANOVA tests and one nonparametric test: the Chi-square. In PowerPoint 5.3, we will look at statistics that can be used to clarify and predict relationships. This PowerPoint focuses on the last five chapters of the Making Sense of Statistics text, chapters 29 ...
ENC 706: Action Research 4: Data Analysis & Reporting in Action Research PowerPoint 4: Confirming & Communicating Data Analysis As you explore these tactics, you will probably notice that you have already used some of them intuitively, simply because it made sense to do so. Now, you can attach an official name to key steps of your data analysis ...
Off course, you can always COPY AND PASTE your XL data into POWERPOINT for a presentation. The next two slides are some sample XL graphs that were moved into POWERPOINT for reference. ... Action Research Workshop Data Analysis GI Group April 2011. Bendik Bygstad Institute of Informatics, UiO Norwegian School of IT. Data analysis in the research ...
Action Research Data Analysis Tutorial - Free download as Powerpoint Presentation (.ppt), PDF File (.pdf), Text File (.txt) or view presentation slides online. Action Research Data Analysis Tutorial
Action research is a research method that aims to simultaneously investigate and solve an issue. In other words, as its name suggests, action research conducts research and takes action at the same time. It was first coined as a term in 1944 by MIT professor Kurt Lewin.A highly interactive method, action research is often used in the social ...
2 Chapter Four, Data Analysis, is the section of the thesis for the action research study that provides the reader with a summary of all data in response to each research question. This power point presentation will help you organize the chapter to make the data summaries, descriptions, analyses, and interpretations clearer to the reader.
Action research can be defined as a systematic observation of one's own teaching practice. It is a way to link theories and research directly to classroom practice. It also empowers teachers to make the changes that are best for their own teaching situations. In this sense, action research is an effective and economical way to attend to the ...
Action Research - Download as a PDF or view online for free ... This PowerPoint presentation provides an in-depth analysis of "The Ball Poem" by John Berryman. It covers the poem's themes, literary devices, and the author's background, offering a comprehensive understanding of the text. ... Collection and organization of data 3. Interpretation ...
The Role of Analysis • Data analysis is an attempt by the teacher researcher to summarize the data that have been collected in a dependable, accurate, reliable, and correct manner. • It is the presentation of the findings in a manner that has an air of undeniability. The Role of Interpretation • Data interpretation is an attempt by the ...
Definition of research in data analysis: According to LeCompte and Schensul, research data analysis is a process used by researchers to reduce data to a story and interpret it to derive insights. The data analysis process helps reduce a large chunk of data into smaller fragments, which makes sense. Three essential things occur during the data ...
Overview. Data analysis is an ongoing process that should occur throughout your research project. Suitable data-analysis methods must be selected when you write your research proposal. The nature of your data (i.e. quantitative or qualitative) will be influenced by your research design and purpose. The data will also influence the analysis ...
Analysis and Discussion of Results in an Action Research - Free download as Powerpoint Presentation (.ppt / .pptx), PDF File (.pdf), Text File (.txt) or view presentation slides online. This document outlines an action research study that examined the effectiveness of differentiated instruction in teaching English to 4th grade students. It discusses the problem statement, research questions ...
Step 1: Define Your Data Hierarchy. While presenting data on the budget allocation, start by outlining the hierarchical structure. The sequence will be like the overall budget at the top, followed by departments, projects within each department, and finally, individual cost categories for each project. Example:
ACTION RESEARCH. The Dialectic Action Research Plan Mills, G. E. (2003). Action Research: A guide for the teacher researcher, 2nd ed. Columbus, Ohio: Merrill Prentice Hall. Identify an Area of Focus Develop an Action Plan Collect Data Analyze and Interpret Data. To Do: Select an appropriate area of focus.
These 20 free PowerPoint and Google Slides templates for data presentations will help you cut down your preparation time significantly. You'll be able to focus on what matters most - ensuring the integrity of your data and its analysis. We'll take care of the design end for you!
Statistics & Results PowerPoint Template. Data Analysis PowerPoint presentation templates are pre-designed slides that can be used for presenting results, insights, and conclusions derived from the analysis of various kinds of data. They often contain a variety of slide layouts, diagrams, charts, and other graphic elements that can effectively ...
Case studies are in-depth investigations of a person, group, event, or community. Typically, data is gathered from various sources using several methods (e.g., observations & interviews). The case study research method originated in clinical medicine (the case history, i.e., the patient's personal history). In psychology, case studies are ...
Daniel E. Dawes is a health care and public health leader, health policy expert, educator, researcher, and author. Dawes is the Founding Dean of the School of Global Health at Meharry Medical College. Dawes also holds positions on the White House COVID-19 Health Equity Task Force, the CDC's Advisory Committee to the Director, and the NIH's National Advisory Council for Nursing Research.
The Abortion in the U.S. Dashboard is an ongoing research project tracking state abortion policies and litigation following the overturning of Roe v. Wade.
Toxic stress, or extended or prolonged stress, from ACEs can negatively affect children's brain development, immune systems, and stress-response systems. These changes can affect children's attention, decision-making, and learning. 18. Children growing up with toxic stress may have difficulty forming healthy and stable relationships.
Background Suicidal thoughts and behaviours (STB) are a common reason for presentation to emergency departments and general hospitals. A meta‐analysis of the strength of clinical risk factors for subsequent suicide might aid understanding of suicidal behaviour and help suicide prevention. Methods We conducted a meta‐analysis of cohort and controlled studies on clinical risk factors and ...
Custom copilot is pre-populated with information from the file/folder selection. The copilot has a default folder name, branding, description, sources you've selected, and other fields already. You can keep these fields and parameters as-is, or easily update them. Customize the identity with a name change. Customize the grounding knowledge.
Fundraising fell 22 percent across private market asset classes globally to just over $1 trillion, as of year-end reported data—the lowest total since 2017. Fundraising in North America, a rare bright spot in 2022, declined in line with global totals, while in Europe, fundraising proved most resilient, falling just 3 percent.