How to Generate and Validate Product Hypotheses

Every product owner knows that it takes effort to build something that'll cater to user needs. You'll have to make many tough calls if you wish to grow the company and evolve the product so it delivers more value. But how do you decide what to change in the product, your marketing strategy, or the overall direction to succeed? And how do you make a product that truly resonates with your target audience?
There are many unknowns in business, so many fundamental decisions start from a simple "what if?". But they can't be based on guesses, as you need some proof to fill in the blanks reasonably.
Because there's no universal recipe for successfully building a product, teams collect data, do research, study the dynamics, and generate hypotheses according to the given facts. They then take corresponding actions to find out whether they were right or wrong, make conclusions, and most likely restart the process again.
On this page, we thoroughly inspect product hypotheses. We'll go over what they are, how to create hypothesis statements and validate them, and what goes after this step.
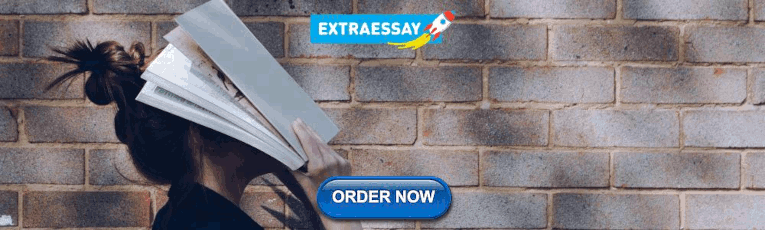
What Is a Hypothesis in Product Management?
A hypothesis in product development and product management is a statement or assumption about the product, planned feature, market, or customer (e.g., their needs, behavior, or expectations) that you can put to the test, evaluate, and base your further decisions on . This may, for instance, regard the upcoming product changes as well as the impact they can result in.
A hypothesis implies that there is limited knowledge. Hence, the teams need to undergo testing activities to validate their ideas and confirm whether they are true or false.
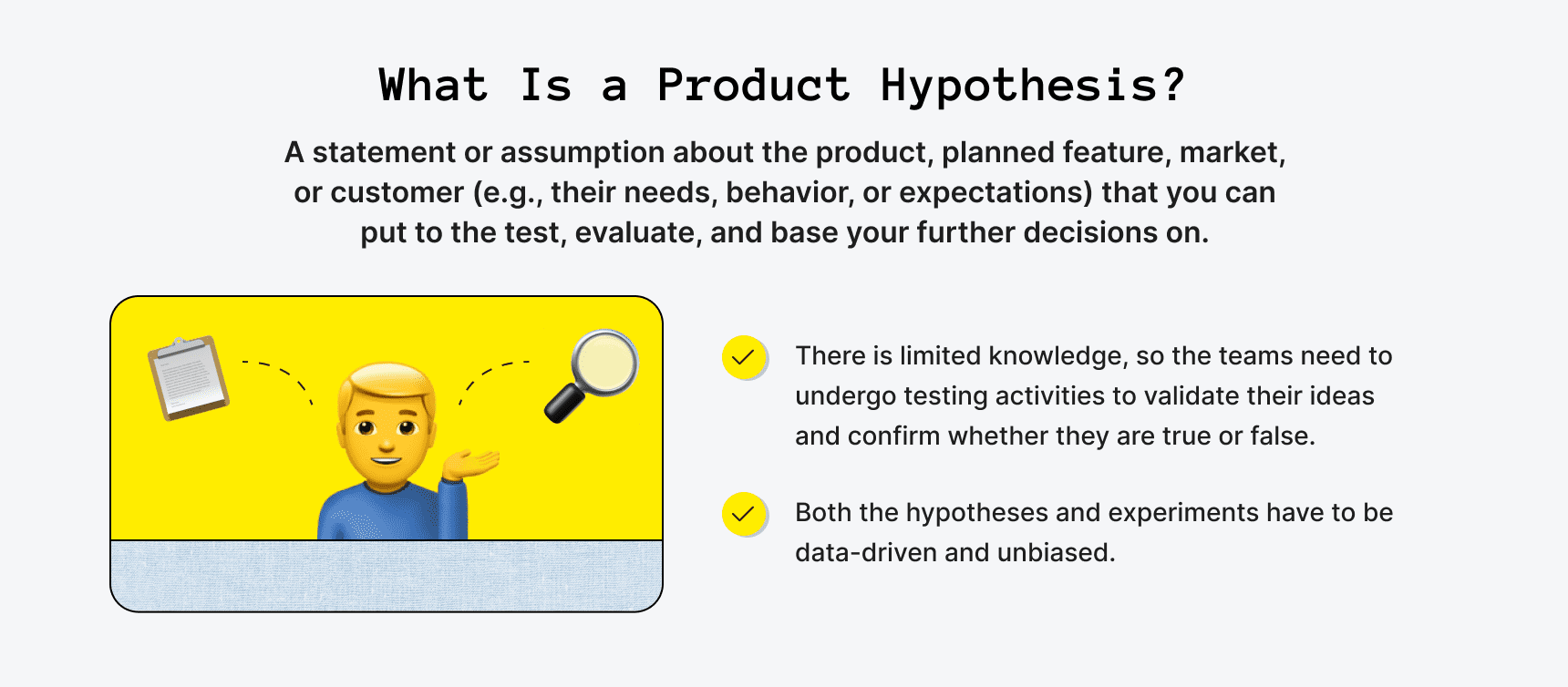
Hypotheses guide the product development process and may point at important findings to help build a better product that'll serve user needs. In essence, teams create hypothesis statements in an attempt to improve the offering, boost engagement, increase revenue, find product-market fit quicker, or for other business-related reasons.
It's sort of like an experiment with trial and error, yet, it is data-driven and should be unbiased . This means that teams don't make assumptions out of the blue. Instead, they turn to the collected data, conducted market research , and factual information, which helps avoid completely missing the mark. The obtained results are then carefully analyzed and may influence decision-making.
Such experiments backed by data and analysis are an integral aspect of successful product development and allow startups or businesses to dodge costly startup mistakes .
When do teams create hypothesis statements and validate them? To some extent, hypothesis testing is an ongoing process to work on constantly. It may occur during various product development life cycle stages, from early phases like initiation to late ones like scaling.
In any event, the key here is learning how to generate hypothesis statements and validate them effectively. We'll go over this in more detail later on.
Idea vs. Hypothesis Compared
You might be wondering whether ideas and hypotheses are the same thing. Well, there are a few distinctions.
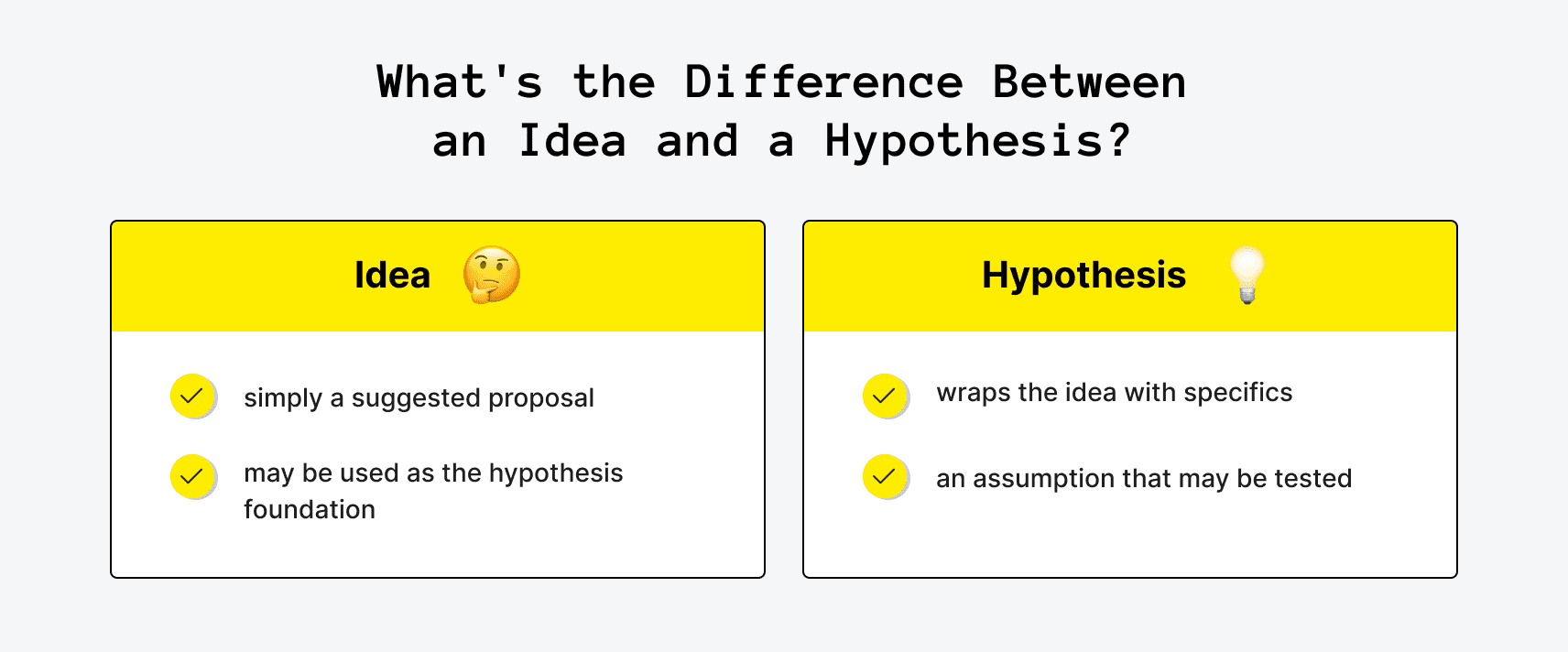
An idea is simply a suggested proposal. Say, a teammate comes up with something you can bring to life during a brainstorming session or pitches in a suggestion like "How about we shorten the checkout process?". You can jot down such ideas and then consider working on them if they'll truly make a difference and improve the product, strategy, or result in other business benefits. Ideas may thus be used as the hypothesis foundation when you decide to prove a concept.
A hypothesis is the next step, when an idea gets wrapped with specifics to become an assumption that may be tested. As such, you can refine the idea by adding details to it. The previously mentioned idea can be worded into a product hypothesis statement like: "The cart abandonment rate is high, and many users flee at checkout. But if we shorten the checkout process by cutting down the number of steps to only two and get rid of four excessive fields, we'll simplify the user journey, boost satisfaction, and may get up to 15% more completed orders".
A hypothesis is something you can test in an attempt to reach a certain goal. Testing isn't obligatory in this scenario, of course, but the idea may be tested if you weigh the pros and cons and decide that the required effort is worth a try. We'll explain how to create hypothesis statements next.
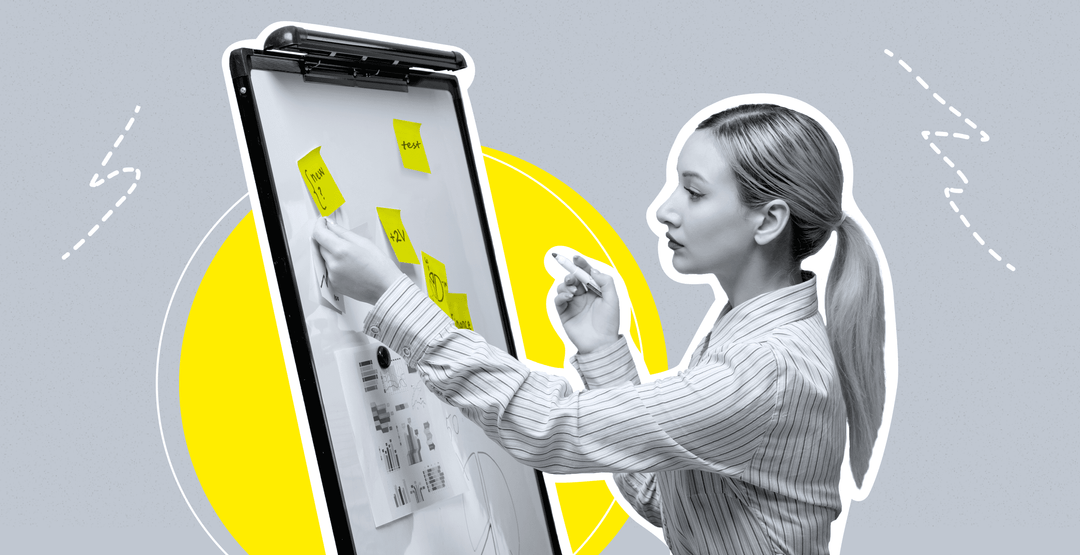
How to Generate a Hypothesis for a Product
The last thing those developing a product want is to invest time and effort into something that won't bring any visible results, fall short of customer expectations, or won't live up to their needs. Therefore, to increase the chances of achieving a successful outcome and product-led growth , teams may need to revisit their product development approach by optimizing one of the starting points of the process: learning to make reasonable product hypotheses.
If the entire procedure is structured, this may assist you during such stages as the discovery phase and raise the odds of reaching your product goals and setting your business up for success. Yet, what's the entire process like?
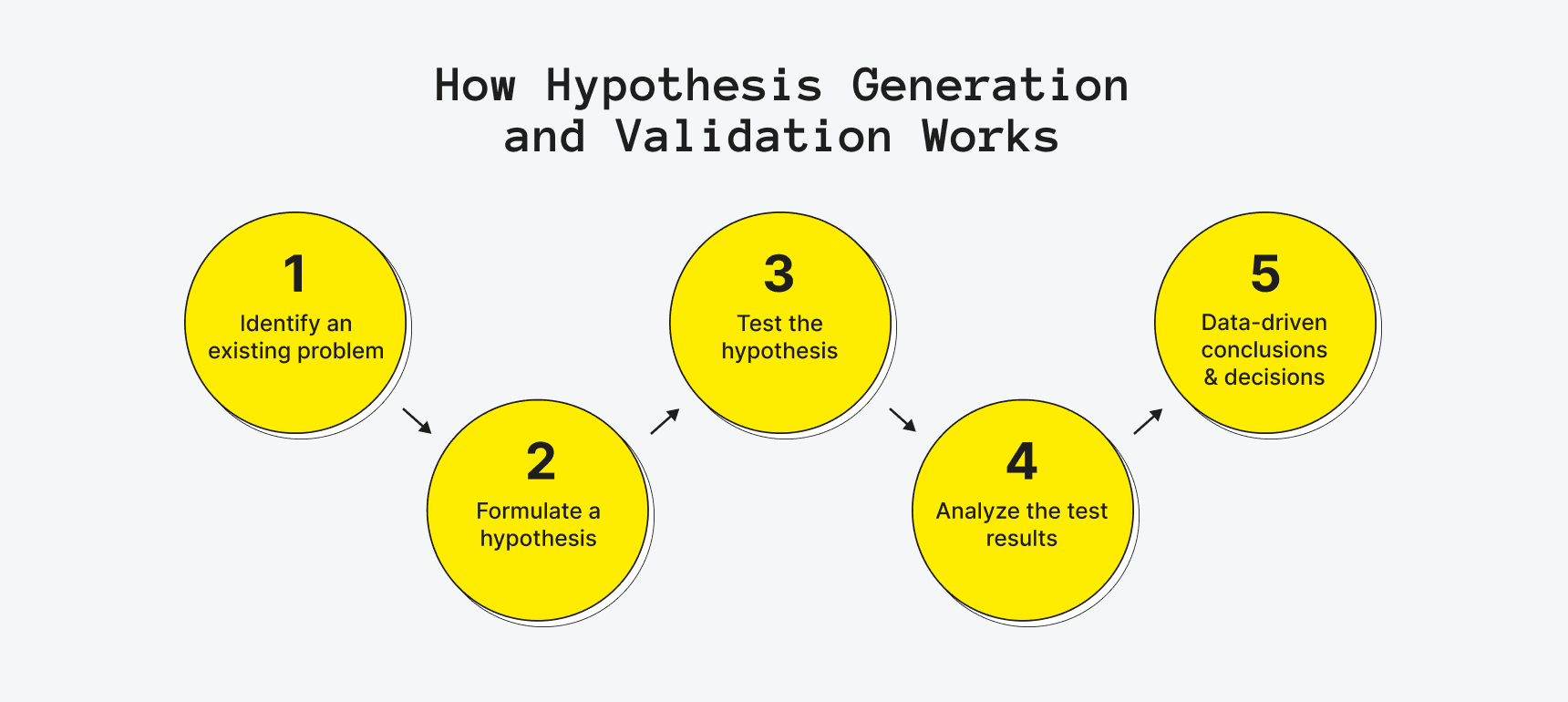
- It all starts with identifying an existing problem . Is there a product area that's experiencing a downfall, a visible trend, or a market gap? Are users often complaining about something in their feedback? Or is there something you're willing to change (say, if you aim to get more profit, increase engagement, optimize a process, expand to a new market, or reach your OKRs and KPIs faster)?
- Teams then need to work on formulating a hypothesis . They put the statement into concise and short wording that describes what is expected to achieve. Importantly, it has to be relevant, actionable, backed by data, and without generalizations.
- Next, they have to test the hypothesis by running experiments to validate it (for instance, via A/B or multivariate testing, prototyping, feedback collection, or other ways).
- Then, the obtained results of the test must be analyzed . Did one element or page version outperform the other? Depending on what you're testing, you can look into various merits or product performance metrics (such as the click rate, bounce rate, or the number of sign-ups) to assess whether your prediction was correct.
- Finally, the teams can make conclusions that could lead to data-driven decisions. For example, they can make corresponding changes or roll back a step.
How Else Can You Generate Product Hypotheses?
Such processes imply sharing ideas when a problem is spotted by digging deep into facts and studying the possible risks, goals, benefits, and outcomes. You may apply various MVP tools like (FigJam, Notion, or Miro) that were designed to simplify brainstorming sessions, systemize pitched suggestions, and keep everyone organized without losing any ideas.
Predictive product analysis can also be integrated into this process, leveraging data and insights to anticipate market trends and consumer preferences, thus enhancing decision-making and product development strategies. This approach fosters a more proactive and informed approach to innovation, ensuring products are not only relevant but also resonate with the target audience, ultimately increasing their chances of success in the market.
Besides, you can settle on one of the many frameworks that facilitate decision-making processes , ideation phases, or feature prioritization . Such frameworks are best applicable if you need to test your assumptions and structure the validation process. These are a few common ones if you're looking toward a systematic approach:
- Business Model Canvas (used to establish the foundation of the business model and helps find answers to vitals like your value proposition, finding the right customer segment, or the ways to make revenue);
- Lean Startup framework (the lean startup framework uses a diagram-like format for capturing major processes and can be handy for testing various hypotheses like how much value a product brings or assumptions on personas, the problem, growth, etc.);
- Design Thinking Process (is all about interactive learning and involves getting an in-depth understanding of the customer needs and pain points, which can be formulated into hypotheses followed by simple prototypes and tests).
Need a hand with product development?
Upsilon's team of pros is ready to share our expertise in building tech products.

How to Make a Hypothesis Statement for a Product
Once you've indicated the addressable problem or opportunity and broken down the issue in focus, you need to work on formulating the hypotheses and associated tasks. By the way, it works the same way if you want to prove that something will be false (a.k.a null hypothesis).
If you're unsure how to write a hypothesis statement, let's explore the essential steps that'll set you on the right track.
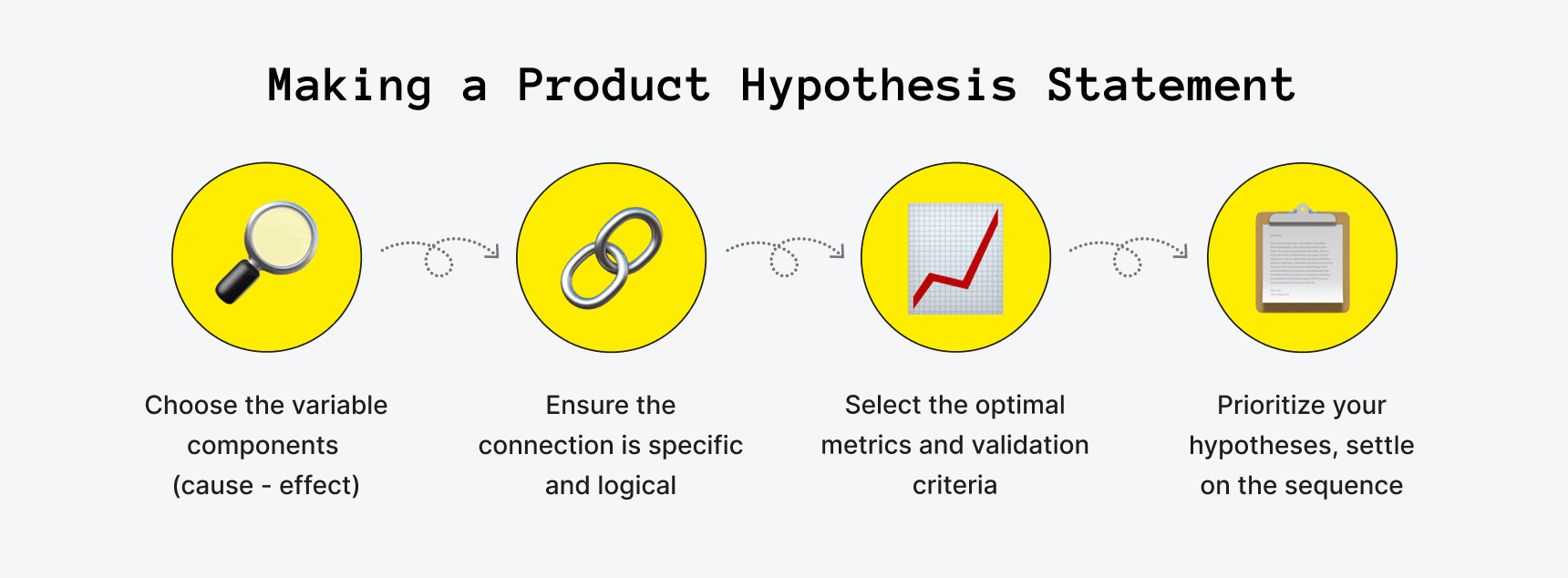
Step 1: Allocate the Variable Components
Product hypotheses are generally different for each case, so begin by pinpointing the major variables, i.e., the cause and effect . You'll need to outline what you think is supposed to happen if a change or action gets implemented.
Put simply, the "cause" is what you're planning to change, and the "effect" is what will indicate whether the change is bringing in the expected results. Falling back on the example we brought up earlier, the ineffective checkout process can be the cause, while the increased percentage of completed orders is the metric that'll show the effect.
Make sure to also note such vital points as:
- what the problem and solution are;
- what are the benefits or the expected impact/successful outcome;
- which user group is affected;
- what are the risks;
- what kind of experiments can help test the hypothesis;
- what can measure whether you were right or wrong.
Step 2: Ensure the Connection Is Specific and Logical
Mind that generic connections that lack specifics will get you nowhere. So if you're thinking about how to word a hypothesis statement, make sure that the cause and effect include clear reasons and a logical dependency .
Think about what can be the precise and link showing why A affects B. In our checkout example, it could be: fewer steps in the checkout and the removed excessive fields will speed up the process, help avoid confusion, irritate users less, and lead to more completed orders. That's much more explicit than just stating the fact that the checkout needs to be changed to get more completed orders.
Step 3: Decide on the Data You'll Collect
Certainly, multiple things can be used to measure the effect. Therefore, you need to choose the optimal metrics and validation criteria that'll best envision if you're moving in the right direction.
If you need a tip on how to create hypothesis statements that won't result in a waste of time, try to avoid vagueness and be as specific as you can when selecting what can best measure and assess the results of your hypothesis test. The criteria must be measurable and tied to the hypotheses . This can be a realistic percentage or number (say, you expect a 15% increase in completed orders or 2x fewer cart abandonment cases during the checkout phase).
Once again, if you're not realistic, then you might end up misinterpreting the results. Remember that sometimes an increase that's even as little as 2% can make a huge difference, so why make 50% the merit if it's not achievable in the first place?
Step 4: Settle on the Sequence
It's quite common that you'll end up with multiple product hypotheses. Some are more important than others, of course, and some will require more effort and input.
Therefore, just as with the features on your product development roadmap , prioritize your hypotheses according to their impact and importance. Then, group and order them, especially if the results of some hypotheses influence others on your list.
Product Hypothesis Examples
To demonstrate how to formulate your assumptions clearly, here are several more apart from the example of a hypothesis statement given above:
- Adding a wishlist feature to the cart with the possibility to send a gift hint to friends via email will increase the likelihood of making a sale and bring in additional sign-ups.
- Placing a limited-time promo code banner stripe on the home page will increase the number of sales in March.
- Moving up the call to action element on the landing page and changing the button text will increase the click-through rate twice.
- By highlighting a new way to use the product, we'll target a niche customer segment (i.e., single parents under 30) and acquire 5% more leads.

How to Validate Hypothesis Statements: The Process Explained
There are multiple options when it comes to validating hypothesis statements. To get appropriate results, you have to come up with the right experiment that'll help you test the hypothesis. You'll need a control group or people who represent your target audience segments or groups to participate (otherwise, your results might not be accurate).
What can serve as the experiment you may run? Experiments may take tons of different forms, and you'll need to choose the one that clicks best with your hypothesis goals (and your available resources, of course). The same goes for how long you'll have to carry out the test (say, a time period of two months or as little as two weeks). Here are several to get you started.

Feedback and User Testing
Talking to users, potential customers, or members of your own online startup community can be another way to test your hypotheses. You may use surveys, questionnaires, or opt for more extensive interviews to validate hypothesis statements and find out what people think. This assumption validation approach involves your existing or potential users and might require some additional time, but can bring you many insights.
Conduct A/B or Multivariate Tests
One of the experiments you may develop involves making more than one version of an element or page to see which option resonates with the users more. As such, you can have a call to action block with different wording or play around with the colors, imagery, visuals, and other things.
To run such split experiments, you can apply tools like VWO that allows to easily construct alternative designs and split what your users see (e.g., one half of the users will see version one, while the other half will see version two). You can track various metrics and apply heatmaps, click maps, and screen recordings to learn more about user response and behavior. Mind, though, that the key to such tests is to get as many users as you can give the tests time. Don't jump to conclusions too soon or if very few people participated in your experiment.
Build Prototypes and Fake Doors
Demos and clickable prototypes can be a great way to save time and money on costly feature or product development. A prototype also allows you to refine the design. However, they can also serve as experiments for validating hypotheses, collecting data, and getting feedback.
For instance, if you have a new feature in mind and want to ensure there is interest, you can utilize such MVP types as fake doors . Make a short demo recording of the feature and place it on your landing page to track interest or test how many people sign up.
Usability Testing
Similarly, you can run experiments to observe how users interact with the feature, page, product, etc. Usually, such experiments are held on prototype testing platforms with a focus group representing your target visitors. By showing a prototype or early version of the design to users, you can view how people use the solution, where they face problems, or what they don't understand. This may be very helpful if you have hypotheses regarding redesigns and user experience improvements before you move on from prototype to MVP development.
You can even take it a few steps further and build a barebone feature version that people can really interact with, yet you'll be the one behind the curtain to make it happen. There were many MVP examples when companies applied Wizard of Oz or concierge MVPs to validate their hypotheses.
Or you can actually develop some functionality but release it for only a limited number of people to see. This is referred to as a feature flag , which can show really specific results but is effort-intensive.
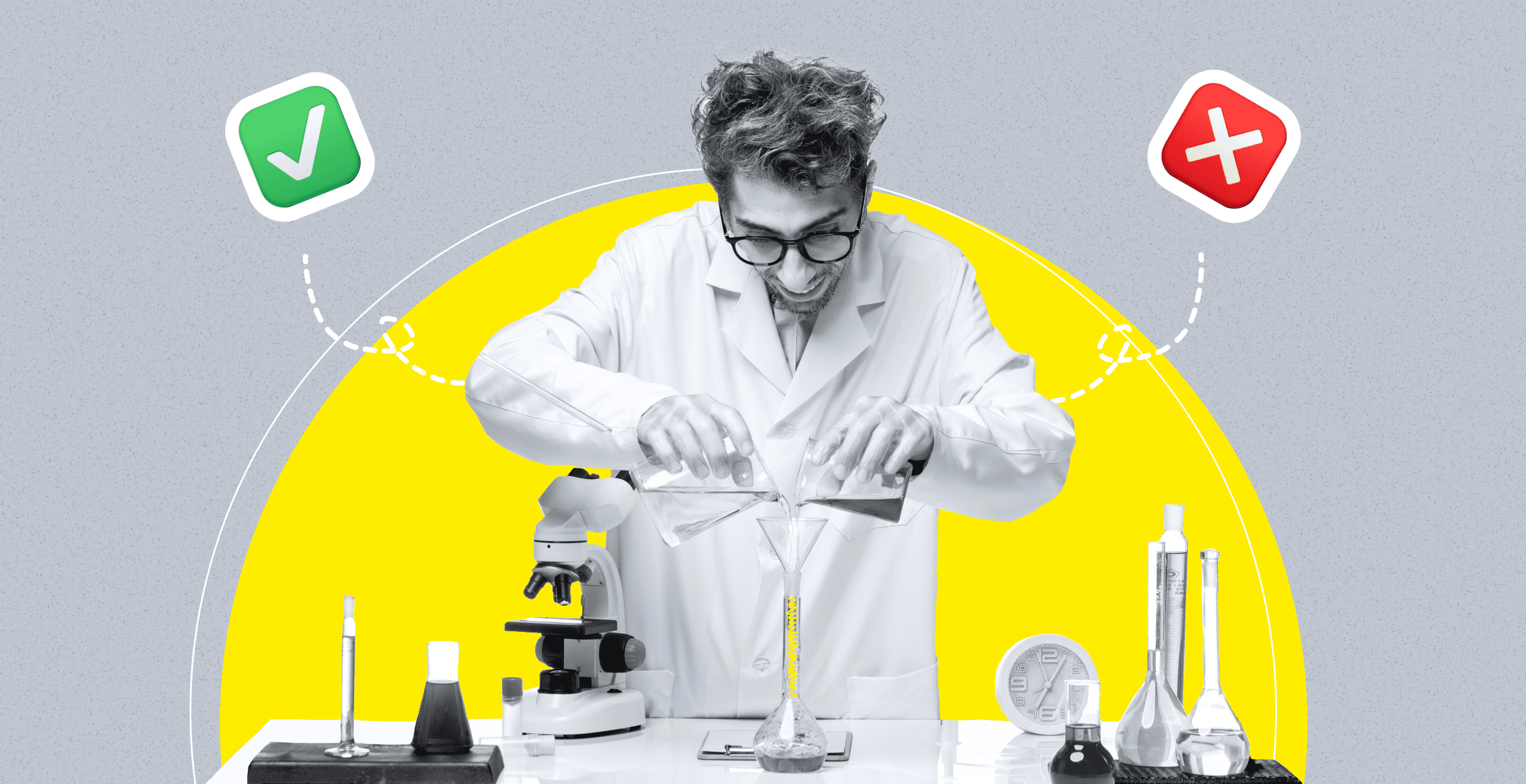
What Comes After Hypothesis Validation?
Analysis is what you move on to once you've run the experiment. This is the time to review the collected data, metrics, and feedback to validate (or invalidate) the hypothesis.
You have to evaluate the experiment's results to determine whether your product hypotheses were valid or not. For example, if you were testing two versions of an element design, color scheme, or copy, look into which one performed best.
It is crucial to be certain that you have enough data to draw conclusions, though, and that it's accurate and unbiased . Because if you don't, this may be a sign that your experiment needs to be run for some additional time, be altered, or held once again. You won't want to make a solid decision based on uncertain or misleading results, right?
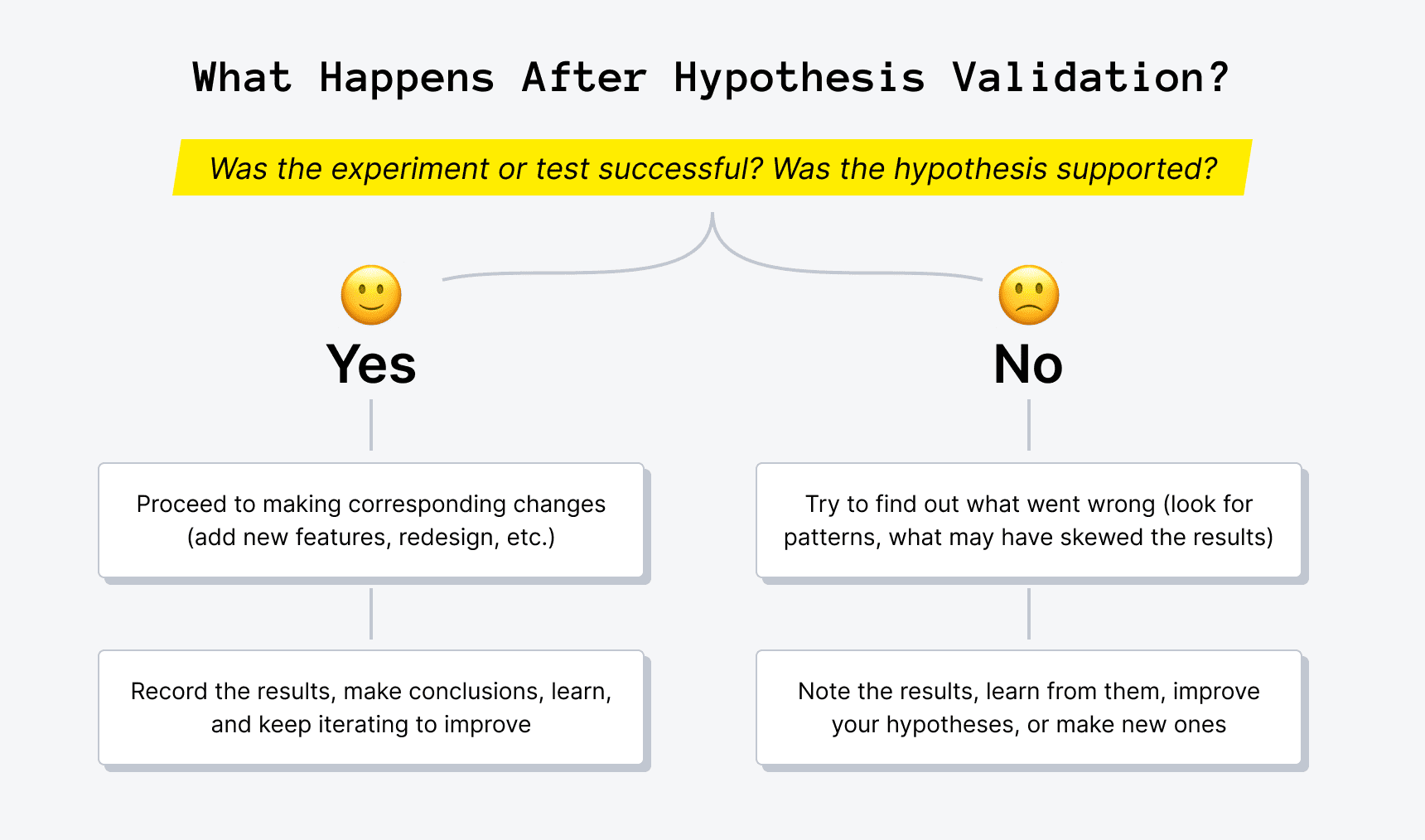
- If the hypothesis was supported , proceed to making corresponding changes (such as implementing a new feature, changing the design, rephrasing your copy, etc.). Remember that your aim was to learn and iterate to improve.
- If your hypothesis was proven false , think of it as a valuable learning experience. The main goal is to learn from the results and be able to adjust your processes accordingly. Dig deep to find out what went wrong, look for patterns and things that may have skewed the results. But if all signs show that you were wrong with your hypothesis, accept this outcome as a fact, and move on. This can help you make conclusions on how to better formulate your product hypotheses next time. Don't be too judgemental, though, as a failed experiment might only mean that you need to improve the current hypothesis, revise it, or create a new one based on the results of this experiment, and run the process once more.
On another note, make sure to record your hypotheses and experiment results . Some companies use CRMs to jot down the key findings, while others use something as simple as Google Docs. Either way, this can be your single source of truth that can help you avoid running the same experiments or allow you to compare results over time.
Have doubts about how to bring your product to life?
Upsilon's team of pros can help you build a product most optimally.
Final Thoughts on Product Hypotheses
The hypothesis-driven approach in product development is a great way to avoid uncalled-for risks and pricey mistakes. You can back up your assumptions with facts, observe your target audience's reactions, and be more certain that this move will deliver value.
However, this only makes sense if the validation of hypothesis statements is backed by relevant data that'll allow you to determine whether the hypothesis is valid or not. By doing so, you can be certain that you're developing and testing hypotheses to accelerate your product management and avoiding decisions based on guesswork.
Certainly, a failed experiment may bring you just as much knowledge and findings as one that succeeds. Teams have to learn from their mistakes, boost their hypothesis generation and testing knowledge, and make improvements according to the results of their experiments. This is an ongoing process, of course, as no product can grow if it isn't iterated and improved.
If you're only planning to or are currently building a product, Upsilon can lend you a helping hand. Our team has years of experience providing product development services for growth-stage startups and building MVPs for early-stage businesses , so you can use our expertise and knowledge to dodge many mistakes. Don't be shy to contact us to discuss your needs!

How to Conduct a Product Experiment: Tips, Tools, and Process

How to Build an AI App: The Ultimate Guide
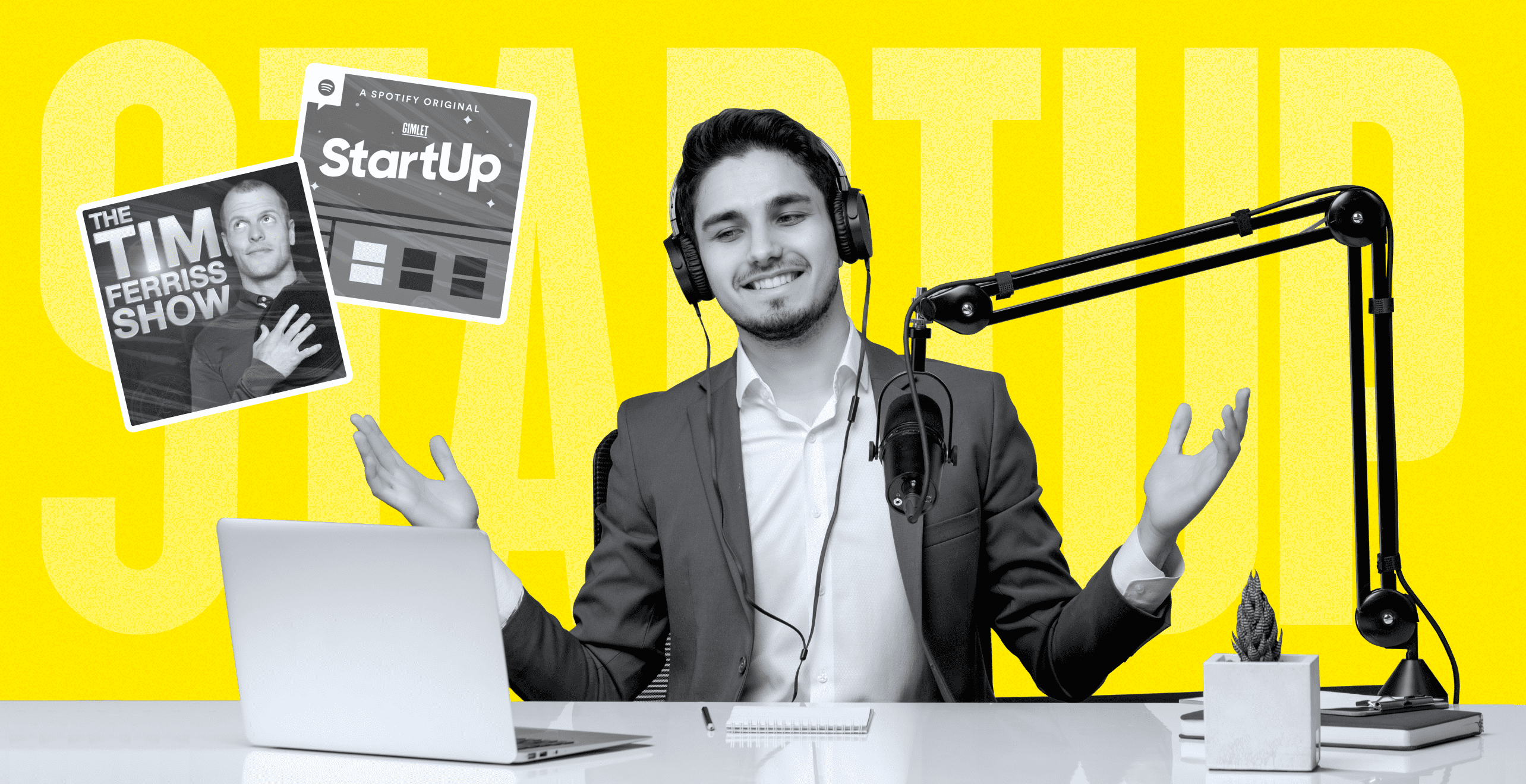
Best Startup Podcasts to Grow and Inspire Your Business
Never miss an update.

- Hypothesis Testing: Definition, Uses, Limitations + Examples

Hypothesis testing is as old as the scientific method and is at the heart of the research process.
Research exists to validate or disprove assumptions about various phenomena. The process of validation involves testing and it is in this context that we will explore hypothesis testing.
What is a Hypothesis?
A hypothesis is a calculated prediction or assumption about a population parameter based on limited evidence. The whole idea behind hypothesis formulation is testing—this means the researcher subjects his or her calculated assumption to a series of evaluations to know whether they are true or false.
Typically, every research starts with a hypothesis—the investigator makes a claim and experiments to prove that this claim is true or false . For instance, if you predict that students who drink milk before class perform better than those who don’t, then this becomes a hypothesis that can be confirmed or refuted using an experiment.
Read: What is Empirical Research Study? [Examples & Method]
What are the Types of Hypotheses?
1. simple hypothesis.
Also known as a basic hypothesis, a simple hypothesis suggests that an independent variable is responsible for a corresponding dependent variable. In other words, an occurrence of the independent variable inevitably leads to an occurrence of the dependent variable.
Typically, simple hypotheses are considered as generally true, and they establish a causal relationship between two variables.
Examples of Simple Hypothesis
- Drinking soda and other sugary drinks can cause obesity.
- Smoking cigarettes daily leads to lung cancer.
2. Complex Hypothesis
A complex hypothesis is also known as a modal. It accounts for the causal relationship between two independent variables and the resulting dependent variables. This means that the combination of the independent variables leads to the occurrence of the dependent variables .
Examples of Complex Hypotheses
- Adults who do not smoke and drink are less likely to develop liver-related conditions.
- Global warming causes icebergs to melt which in turn causes major changes in weather patterns.
3. Null Hypothesis
As the name suggests, a null hypothesis is formed when a researcher suspects that there’s no relationship between the variables in an observation. In this case, the purpose of the research is to approve or disapprove this assumption.
Examples of Null Hypothesis
- This is no significant change in a student’s performance if they drink coffee or tea before classes.
- There’s no significant change in the growth of a plant if one uses distilled water only or vitamin-rich water.
Read: Research Report: Definition, Types + [Writing Guide]
4. Alternative Hypothesis
To disapprove a null hypothesis, the researcher has to come up with an opposite assumption—this assumption is known as the alternative hypothesis. This means if the null hypothesis says that A is false, the alternative hypothesis assumes that A is true.
An alternative hypothesis can be directional or non-directional depending on the direction of the difference. A directional alternative hypothesis specifies the direction of the tested relationship, stating that one variable is predicted to be larger or smaller than the null value while a non-directional hypothesis only validates the existence of a difference without stating its direction.
Examples of Alternative Hypotheses
- Starting your day with a cup of tea instead of a cup of coffee can make you more alert in the morning.
- The growth of a plant improves significantly when it receives distilled water instead of vitamin-rich water.
5. Logical Hypothesis
Logical hypotheses are some of the most common types of calculated assumptions in systematic investigations. It is an attempt to use your reasoning to connect different pieces in research and build a theory using little evidence. In this case, the researcher uses any data available to him, to form a plausible assumption that can be tested.
Examples of Logical Hypothesis
- Waking up early helps you to have a more productive day.
- Beings from Mars would not be able to breathe the air in the atmosphere of the Earth.
6. Empirical Hypothesis
After forming a logical hypothesis, the next step is to create an empirical or working hypothesis. At this stage, your logical hypothesis undergoes systematic testing to prove or disprove the assumption. An empirical hypothesis is subject to several variables that can trigger changes and lead to specific outcomes.
Examples of Empirical Testing
- People who eat more fish run faster than people who eat meat.
- Women taking vitamin E grow hair faster than those taking vitamin K.
7. Statistical Hypothesis
When forming a statistical hypothesis, the researcher examines the portion of a population of interest and makes a calculated assumption based on the data from this sample. A statistical hypothesis is most common with systematic investigations involving a large target audience. Here, it’s impossible to collect responses from every member of the population so you have to depend on data from your sample and extrapolate the results to the wider population.
Examples of Statistical Hypothesis
- 45% of students in Louisiana have middle-income parents.
- 80% of the UK’s population gets a divorce because of irreconcilable differences.
What is Hypothesis Testing?
Hypothesis testing is an assessment method that allows researchers to determine the plausibility of a hypothesis. It involves testing an assumption about a specific population parameter to know whether it’s true or false. These population parameters include variance, standard deviation, and median.
Typically, hypothesis testing starts with developing a null hypothesis and then performing several tests that support or reject the null hypothesis. The researcher uses test statistics to compare the association or relationship between two or more variables.
Explore: Research Bias: Definition, Types + Examples
Researchers also use hypothesis testing to calculate the coefficient of variation and determine if the regression relationship and the correlation coefficient are statistically significant.
How Hypothesis Testing Works
The basis of hypothesis testing is to examine and analyze the null hypothesis and alternative hypothesis to know which one is the most plausible assumption. Since both assumptions are mutually exclusive, only one can be true. In other words, the occurrence of a null hypothesis destroys the chances of the alternative coming to life, and vice-versa.
Interesting: 21 Chrome Extensions for Academic Researchers in 2021
What Are The Stages of Hypothesis Testing?
To successfully confirm or refute an assumption, the researcher goes through five (5) stages of hypothesis testing;
- Determine the null hypothesis
- Specify the alternative hypothesis
- Set the significance level
- Calculate the test statistics and corresponding P-value
- Draw your conclusion
- Determine the Null Hypothesis
Like we mentioned earlier, hypothesis testing starts with creating a null hypothesis which stands as an assumption that a certain statement is false or implausible. For example, the null hypothesis (H0) could suggest that different subgroups in the research population react to a variable in the same way.
- Specify the Alternative Hypothesis
Once you know the variables for the null hypothesis, the next step is to determine the alternative hypothesis. The alternative hypothesis counters the null assumption by suggesting the statement or assertion is true. Depending on the purpose of your research, the alternative hypothesis can be one-sided or two-sided.
Using the example we established earlier, the alternative hypothesis may argue that the different sub-groups react differently to the same variable based on several internal and external factors.
- Set the Significance Level
Many researchers create a 5% allowance for accepting the value of an alternative hypothesis, even if the value is untrue. This means that there is a 0.05 chance that one would go with the value of the alternative hypothesis, despite the truth of the null hypothesis.
Something to note here is that the smaller the significance level, the greater the burden of proof needed to reject the null hypothesis and support the alternative hypothesis.
Explore: What is Data Interpretation? + [Types, Method & Tools]
- Calculate the Test Statistics and Corresponding P-Value
Test statistics in hypothesis testing allow you to compare different groups between variables while the p-value accounts for the probability of obtaining sample statistics if your null hypothesis is true. In this case, your test statistics can be the mean, median and similar parameters.
If your p-value is 0.65, for example, then it means that the variable in your hypothesis will happen 65 in100 times by pure chance. Use this formula to determine the p-value for your data:
- Draw Your Conclusions
After conducting a series of tests, you should be able to agree or refute the hypothesis based on feedback and insights from your sample data.
Applications of Hypothesis Testing in Research
Hypothesis testing isn’t only confined to numbers and calculations; it also has several real-life applications in business, manufacturing, advertising, and medicine.
In a factory or other manufacturing plants, hypothesis testing is an important part of quality and production control before the final products are approved and sent out to the consumer.
During ideation and strategy development, C-level executives use hypothesis testing to evaluate their theories and assumptions before any form of implementation. For example, they could leverage hypothesis testing to determine whether or not some new advertising campaign, marketing technique, etc. causes increased sales.
In addition, hypothesis testing is used during clinical trials to prove the efficacy of a drug or new medical method before its approval for widespread human usage.
What is an Example of Hypothesis Testing?
An employer claims that her workers are of above-average intelligence. She takes a random sample of 20 of them and gets the following results:
Mean IQ Scores: 110
Standard Deviation: 15
Mean Population IQ: 100
Step 1: Using the value of the mean population IQ, we establish the null hypothesis as 100.
Step 2: State that the alternative hypothesis is greater than 100.
Step 3: State the alpha level as 0.05 or 5%
Step 4: Find the rejection region area (given by your alpha level above) from the z-table. An area of .05 is equal to a z-score of 1.645.
Step 5: Calculate the test statistics using this formula
Z = (110–100) ÷ (15÷√20)
10 ÷ 3.35 = 2.99
If the value of the test statistics is higher than the value of the rejection region, then you should reject the null hypothesis. If it is less, then you cannot reject the null.
In this case, 2.99 > 1.645 so we reject the null.
Importance/Benefits of Hypothesis Testing
The most significant benefit of hypothesis testing is it allows you to evaluate the strength of your claim or assumption before implementing it in your data set. Also, hypothesis testing is the only valid method to prove that something “is or is not”. Other benefits include:
- Hypothesis testing provides a reliable framework for making any data decisions for your population of interest.
- It helps the researcher to successfully extrapolate data from the sample to the larger population.
- Hypothesis testing allows the researcher to determine whether the data from the sample is statistically significant.
- Hypothesis testing is one of the most important processes for measuring the validity and reliability of outcomes in any systematic investigation.
- It helps to provide links to the underlying theory and specific research questions.
Criticism and Limitations of Hypothesis Testing
Several limitations of hypothesis testing can affect the quality of data you get from this process. Some of these limitations include:
- The interpretation of a p-value for observation depends on the stopping rule and definition of multiple comparisons. This makes it difficult to calculate since the stopping rule is subject to numerous interpretations, plus “multiple comparisons” are unavoidably ambiguous.
- Conceptual issues often arise in hypothesis testing, especially if the researcher merges Fisher and Neyman-Pearson’s methods which are conceptually distinct.
- In an attempt to focus on the statistical significance of the data, the researcher might ignore the estimation and confirmation by repeated experiments.
- Hypothesis testing can trigger publication bias, especially when it requires statistical significance as a criterion for publication.
- When used to detect whether a difference exists between groups, hypothesis testing can trigger absurd assumptions that affect the reliability of your observation.
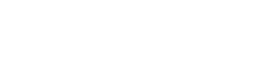
Connect to Formplus, Get Started Now - It's Free!
- alternative hypothesis
- alternative vs null hypothesis
- complex hypothesis
- empirical hypothesis
- hypothesis testing
- logical hypothesis
- simple hypothesis
- statistical hypothesis
- busayo.longe
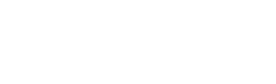
You may also like:
Alternative vs Null Hypothesis: Pros, Cons, Uses & Examples
We are going to discuss alternative hypotheses and null hypotheses in this post and how they work in research.

Type I vs Type II Errors: Causes, Examples & Prevention
This article will discuss the two different types of errors in hypothesis testing and how you can prevent them from occurring in your research
What is Pure or Basic Research? + [Examples & Method]
Simple guide on pure or basic research, its methods, characteristics, advantages, and examples in science, medicine, education and psychology
Internal Validity in Research: Definition, Threats, Examples
In this article, we will discuss the concept of internal validity, some clear examples, its importance, and how to test it.
Formplus - For Seamless Data Collection
Collect data the right way with a versatile data collection tool. try formplus and transform your work productivity today..
- Business Essentials
- Leadership & Management
- Credential of Leadership, Impact, and Management in Business (CLIMB)
- Entrepreneurship & Innovation
- Digital Transformation
- Finance & Accounting
- Business in Society
- For Organizations
- Support Portal
- Media Coverage
- Founding Donors
- Leadership Team
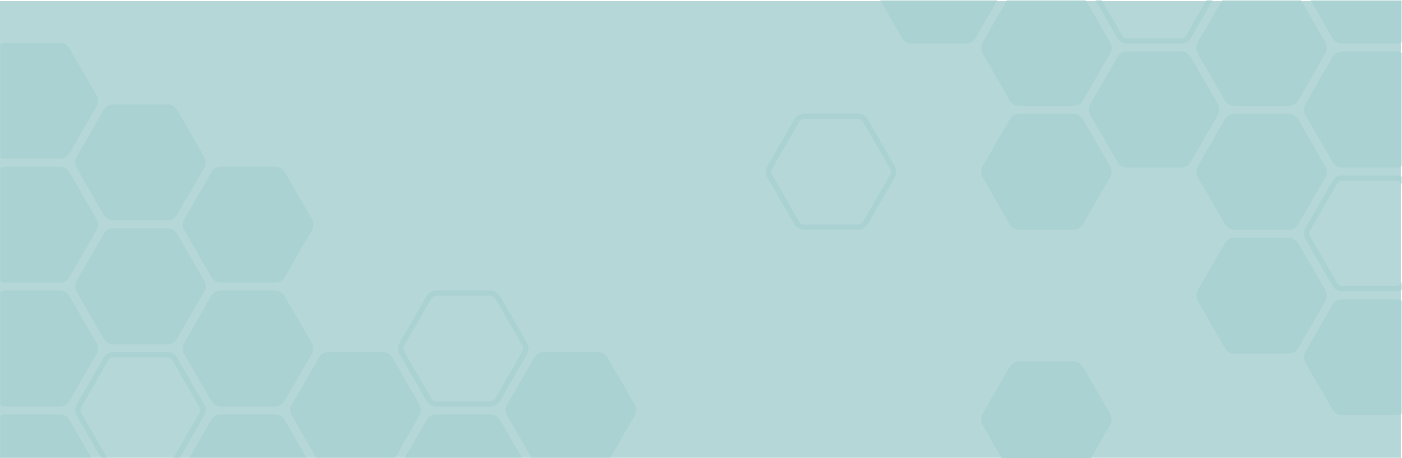
- Harvard Business School →
- HBS Online →
- Business Insights →
Business Insights
Harvard Business School Online's Business Insights Blog provides the career insights you need to achieve your goals and gain confidence in your business skills.
- Career Development
- Communication
- Decision-Making
- Earning Your MBA
- Negotiation
- News & Events
- Productivity
- Staff Spotlight
- Student Profiles
- Work-Life Balance
- AI Essentials for Business
- Alternative Investments
- Business Analytics
- Business Strategy
- Business and Climate Change
- Design Thinking and Innovation
- Digital Marketing Strategy
- Disruptive Strategy
- Economics for Managers
- Entrepreneurship Essentials
- Financial Accounting
- Global Business
- Launching Tech Ventures
- Leadership Principles
- Leadership, Ethics, and Corporate Accountability
- Leading Change and Organizational Renewal
- Leading with Finance
- Management Essentials
- Negotiation Mastery
- Organizational Leadership
- Power and Influence for Positive Impact
- Strategy Execution
- Sustainable Business Strategy
- Sustainable Investing
- Winning with Digital Platforms
A Beginner’s Guide to Hypothesis Testing in Business
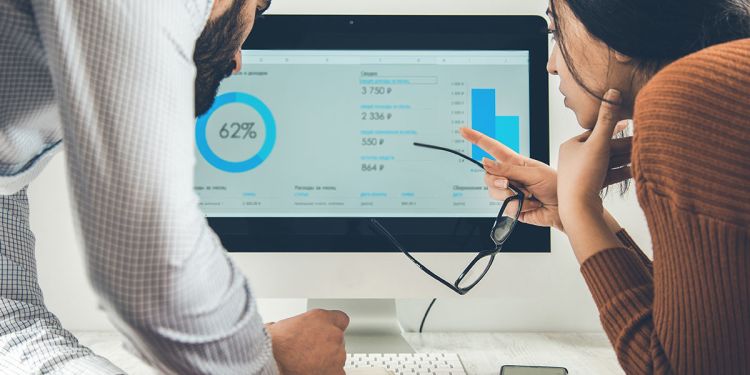
- 30 Mar 2021
Becoming a more data-driven decision-maker can bring several benefits to your organization, enabling you to identify new opportunities to pursue and threats to abate. Rather than allowing subjective thinking to guide your business strategy, backing your decisions with data can empower your company to become more innovative and, ultimately, profitable.
If you’re new to data-driven decision-making, you might be wondering how data translates into business strategy. The answer lies in generating a hypothesis and verifying or rejecting it based on what various forms of data tell you.
Below is a look at hypothesis testing and the role it plays in helping businesses become more data-driven.
Access your free e-book today.
What Is Hypothesis Testing?
To understand what hypothesis testing is, it’s important first to understand what a hypothesis is.
A hypothesis or hypothesis statement seeks to explain why something has happened, or what might happen, under certain conditions. It can also be used to understand how different variables relate to each other. Hypotheses are often written as if-then statements; for example, “If this happens, then this will happen.”
Hypothesis testing , then, is a statistical means of testing an assumption stated in a hypothesis. While the specific methodology leveraged depends on the nature of the hypothesis and data available, hypothesis testing typically uses sample data to extrapolate insights about a larger population.
Hypothesis Testing in Business
When it comes to data-driven decision-making, there’s a certain amount of risk that can mislead a professional. This could be due to flawed thinking or observations, incomplete or inaccurate data , or the presence of unknown variables. The danger in this is that, if major strategic decisions are made based on flawed insights, it can lead to wasted resources, missed opportunities, and catastrophic outcomes.
The real value of hypothesis testing in business is that it allows professionals to test their theories and assumptions before putting them into action. This essentially allows an organization to verify its analysis is correct before committing resources to implement a broader strategy.
As one example, consider a company that wishes to launch a new marketing campaign to revitalize sales during a slow period. Doing so could be an incredibly expensive endeavor, depending on the campaign’s size and complexity. The company, therefore, may wish to test the campaign on a smaller scale to understand how it will perform.
In this example, the hypothesis that’s being tested would fall along the lines of: “If the company launches a new marketing campaign, then it will translate into an increase in sales.” It may even be possible to quantify how much of a lift in sales the company expects to see from the effort. Pending the results of the pilot campaign, the business would then know whether it makes sense to roll it out more broadly.
Related: 9 Fundamental Data Science Skills for Business Professionals
Key Considerations for Hypothesis Testing
1. alternative hypothesis and null hypothesis.
In hypothesis testing, the hypothesis that’s being tested is known as the alternative hypothesis . Often, it’s expressed as a correlation or statistical relationship between variables. The null hypothesis , on the other hand, is a statement that’s meant to show there’s no statistical relationship between the variables being tested. It’s typically the exact opposite of whatever is stated in the alternative hypothesis.
For example, consider a company’s leadership team that historically and reliably sees $12 million in monthly revenue. They want to understand if reducing the price of their services will attract more customers and, in turn, increase revenue.
In this case, the alternative hypothesis may take the form of a statement such as: “If we reduce the price of our flagship service by five percent, then we’ll see an increase in sales and realize revenues greater than $12 million in the next month.”
The null hypothesis, on the other hand, would indicate that revenues wouldn’t increase from the base of $12 million, or might even decrease.
Check out the video below about the difference between an alternative and a null hypothesis, and subscribe to our YouTube channel for more explainer content.
2. Significance Level and P-Value
Statistically speaking, if you were to run the same scenario 100 times, you’d likely receive somewhat different results each time. If you were to plot these results in a distribution plot, you’d see the most likely outcome is at the tallest point in the graph, with less likely outcomes falling to the right and left of that point.

With this in mind, imagine you’ve completed your hypothesis test and have your results, which indicate there may be a correlation between the variables you were testing. To understand your results' significance, you’ll need to identify a p-value for the test, which helps note how confident you are in the test results.
In statistics, the p-value depicts the probability that, assuming the null hypothesis is correct, you might still observe results that are at least as extreme as the results of your hypothesis test. The smaller the p-value, the more likely the alternative hypothesis is correct, and the greater the significance of your results.
3. One-Sided vs. Two-Sided Testing
When it’s time to test your hypothesis, it’s important to leverage the correct testing method. The two most common hypothesis testing methods are one-sided and two-sided tests , or one-tailed and two-tailed tests, respectively.
Typically, you’d leverage a one-sided test when you have a strong conviction about the direction of change you expect to see due to your hypothesis test. You’d leverage a two-sided test when you’re less confident in the direction of change.

4. Sampling
To perform hypothesis testing in the first place, you need to collect a sample of data to be analyzed. Depending on the question you’re seeking to answer or investigate, you might collect samples through surveys, observational studies, or experiments.
A survey involves asking a series of questions to a random population sample and recording self-reported responses.
Observational studies involve a researcher observing a sample population and collecting data as it occurs naturally, without intervention.
Finally, an experiment involves dividing a sample into multiple groups, one of which acts as the control group. For each non-control group, the variable being studied is manipulated to determine how the data collected differs from that of the control group.

Learn How to Perform Hypothesis Testing
Hypothesis testing is a complex process involving different moving pieces that can allow an organization to effectively leverage its data and inform strategic decisions.
If you’re interested in better understanding hypothesis testing and the role it can play within your organization, one option is to complete a course that focuses on the process. Doing so can lay the statistical and analytical foundation you need to succeed.
Do you want to learn more about hypothesis testing? Explore Business Analytics —one of our online business essentials courses —and download our Beginner’s Guide to Data & Analytics .

About the Author
- Product Management
How to Generate and Validate Product Hypotheses
What is a product hypothesis.
A hypothesis is a testable statement that predicts the relationship between two or more variables. In product development, we generate hypotheses to validate assumptions about customer behavior, market needs, or the potential impact of product changes. These experimental efforts help us refine the user experience and get closer to finding a product-market fit.
Product hypotheses are a key element of data-driven product development and decision-making. Testing them enables us to solve problems more efficiently and remove our own biases from the solutions we put forward.
Here’s an example: ‘If we improve the page load speed on our website (variable 1), then we will increase the number of signups by 15% (variable 2).’ So if we improve the page load speed, and the number of signups increases, then our hypothesis has been proven. If the number did not increase significantly (or not at all), then our hypothesis has been disproven.
In general, product managers are constantly creating and testing hypotheses. But in the context of new product development , hypothesis generation/testing occurs during the validation stage, right after idea screening .
Now before we go any further, let’s get one thing straight: What’s the difference between an idea and a hypothesis?
Idea vs hypothesis
Innovation expert Michael Schrage makes this distinction between hypotheses and ideas – unlike an idea, a hypothesis comes with built-in accountability. “But what’s the accountability for a good idea?” Schrage asks. “The fact that a lot of people think it’s a good idea? That’s a popularity contest.” So, not only should a hypothesis be tested, but by its very nature, it can be tested.
At Railsware, we’ve built our product development services on the careful selection, prioritization, and validation of ideas. Here’s how we distinguish between ideas and hypotheses:
Idea: A creative suggestion about how we might exploit a gap in the market, add value to an existing product, or bring attention to our product. Crucially, an idea is just a thought. It can form the basis of a hypothesis but it is not necessarily expected to be proven or disproven.
- We should get an interview with the CEO of our company published on TechCrunch.
- Why don’t we redesign our website?
- The Coupler.io team should create video tutorials on how to export data from different apps, and publish them on YouTube.
- Why not add a new ‘email templates’ feature to our Mailtrap product?
Hypothesis: A way of framing an idea or assumption so that it is testable, specific, and aligns with our wider product/team/organizational goals.
Examples:
- If we add a new ‘email templates’ feature to Mailtrap, we’ll see an increase in active usage of our email-sending API.
- Creating relevant video tutorials and uploading them to YouTube will lead to an increase in Coupler.io signups.
- If we publish an interview with our CEO on TechCrunch, 500 people will visit our website and 10 of them will install our product.
Now, it’s worth mentioning that not all hypotheses require testing . Sometimes, the process of creating hypotheses is just an exercise in critical thinking. And the simple act of analyzing your statement tells whether you should run an experiment or not. Remember: testing isn’t mandatory, but your hypotheses should always be inherently testable.
Let’s consider the TechCrunch article example again. In that hypothesis, we expect 500 readers to visit our product website, and a 2% conversion rate of those unique visitors to product users i.e. 10 people. But is that marginal increase worth all the effort? Conducting an interview with our CEO, creating the content, and collaborating with the TechCrunch content team – all of these tasks take time (and money) to execute. And by formulating that hypothesis, we can clearly see that in this case, the drawbacks (efforts) outweigh the benefits. So, no need to test it.
In a similar vein, a hypothesis statement can be a tool to prioritize your activities based on impact. We typically use the following criteria:
- The quality of impact
- The size of the impact
- The probability of impact
This lets us organize our efforts according to their potential outcomes – not the coolness of the idea, its popularity among the team, etc.
Now that we’ve established what a product hypothesis is, let’s discuss how to create one.
Start with a problem statement
Before you jump into product hypothesis generation, we highly recommend formulating a problem statement. This is a short, concise description of the issue you are trying to solve. It helps teams stay on track as they formalize the hypothesis and design the product experiments. It can also be shared with stakeholders to ensure that everyone is on the same page.
The statement can be worded however you like, as long as it’s actionable, specific, and based on data-driven insights or research. It should clearly outline the problem or opportunity you want to address.
Here’s an example: Our bounce rate is high (more than 90%) and we are struggling to convert website visitors into actual users. How might we improve site performance to boost our conversion rate?
How to generate product hypotheses
Now let’s explore some common, everyday scenarios that lead to product hypothesis generation. For our teams here at Railsware, it’s when:
- There’s a problem with an unclear root cause e.g. a sudden drop in one part of the onboarding funnel. We identify these issues by checking our product metrics or reviewing customer complaints.
- We are running ideation sessions on how to reach our goals (increase MRR, increase the number of users invited to an account, etc.)
- We are exploring growth opportunities e.g. changing a pricing plan, making product improvements , breaking into a new market.
- We receive customer feedback. For example, some users have complained about difficulties setting up a workspace within the product. So, we build a hypothesis on how to help them with the setup.
BRIDGES framework for ideation
When we are tackling a complex problem or looking for ways to grow the product, our teams use BRIDGeS – a robust decision-making and ideation framework. BRIDGeS makes our product discovery sessions more efficient. It lets us dive deep into the context of our problem so that we can develop targeted solutions worthy of testing.
Between 2-8 stakeholders take part in a BRIDGeS session. The ideation sessions are usually led by a product manager and can include other subject matter experts such as developers, designers, data analysts, or marketing specialists. You can use a virtual whiteboard such as Figjam or Miro (see our Figma template ) to record each colored note.
In the first half of a BRIDGeS session, participants examine the Benefits, Risks, Issues, and Goals of their subject in the ‘Problem Space.’ A subject is anything that is being described or dealt with; for instance, Coupler.io’s growth opportunities. Benefits are the value that a future solution can bring, Risks are potential issues they might face, Issues are their existing problems, and Goals are what the subject hopes to gain from the future solution. Each descriptor should have a designated color.
After we have broken down the problem using each of these descriptors, we move into the Solution Space. This is where we develop solution variations based on all of the benefits/risks/issues identified in the Problem Space (see the Uber case study for an in-depth example).
In the Solution Space, we start prioritizing those solutions and deciding which ones are worthy of further exploration outside of the framework – via product hypothesis formulation and testing, for example. At the very least, after the session, we will have a list of epics and nested tasks ready to add to our product roadmap.
How to write a product hypothesis statement
Across organizations, product hypothesis statements might vary in their subject, tone, and precise wording. But some elements never change. As we mentioned earlier, a hypothesis statement must always have two or more variables and a connecting factor.
1. Identify variables
Since these components form the bulk of a hypothesis statement, let’s start with a brief definition.
First of all, variables in a hypothesis statement can be split into two camps: dependent and independent. Without getting too theoretical, we can describe the independent variable as the cause, and the dependent variable as the effect . So in the Mailtrap example we mentioned earlier, the ‘add email templates feature’ is the cause i.e. the element we want to manipulate. Meanwhile, ‘increased usage of email sending API’ is the effect i.e the element we will observe.
Independent variables can be any change you plan to make to your product. For example, tweaking some landing page copy, adding a chatbot to the homepage, or enhancing the search bar filter functionality.
Dependent variables are usually metrics. Here are a few that we often test in product development:
- Number of sign-ups
- Number of purchases
- Activation rate (activation signals differ from product to product)
- Number of specific plans purchased
- Feature usage (API activation, for example)
- Number of active users
Bear in mind that your concept or desired change can be measured with different metrics. Make sure that your variables are well-defined, and be deliberate in how you measure your concepts so that there’s no room for misinterpretation or ambiguity.
For example, in the hypothesis ‘Users drop off because they find it hard to set up a project’ variables are poorly defined. Phrases like ‘drop off’ and ‘hard to set up’ are too vague. A much better way of saying it would be: If project automation rules are pre-defined (email sequence to responsible, scheduled tickets creation), we’ll see a decrease in churn. In this example, it’s clear which dependent variable has been chosen and why.
And remember, when product managers focus on delighting users and building something of value, it’s easier to market and monetize it. That’s why at Railsware, our product hypotheses often focus on how to increase the usage of a feature or product. If users love our product(s) and know how to leverage its benefits, we can spend less time worrying about how to improve conversion rates or actively grow our revenue, and more time enhancing the user experience and nurturing our audience.
2. Make the connection
The relationship between variables should be clear and logical. If it’s not, then it doesn’t matter how well-chosen your variables are – your test results won’t be reliable.
To demonstrate this point, let’s explore a previous example again: page load speed and signups.
Through prior research, you might already know that conversion rates are 3x higher for sites that load in 1 second compared to sites that take 5 seconds to load. Since there appears to be a strong connection between load speed and signups in general, you might want to see if this is also true for your product.
Here are some common pitfalls to avoid when defining the relationship between two or more variables:
Relationship is weak. Let’s say you hypothesize that an increase in website traffic will lead to an increase in sign-ups. This is a weak connection since website visitors aren’t necessarily motivated to use your product; there are more steps involved. A better example is ‘If we change the CTA on the pricing page, then the number of signups will increase.’ This connection is much stronger and more direct.
Relationship is far-fetched. This often happens when one of the variables is founded on a vanity metric. For example, increasing the number of social media subscribers will lead to an increase in sign-ups. However, there’s no particular reason why a social media follower would be interested in using your product. Oftentimes, it’s simply your social media content that appeals to them (and your audience isn’t interested in a product).
Variables are co-dependent. Variables should always be isolated from one another. Let’s say we removed the option “Register with Google” from our app. In this case, we can expect fewer users with Google workspace accounts to register. Obviously, it’s because there’s a direct dependency between variables (no registration with Google→no users with Google workspace accounts).
3. Set validation criteria
First, build some confirmation criteria into your statement . Think in terms of percentages (e.g. increase/decrease by 5%) and choose a relevant product metric to track e.g. activation rate if your hypothesis relates to onboarding. Consider that you don’t always have to hit the bullseye for your hypothesis to be considered valid. Perhaps a 3% increase is just as acceptable as a 5% one. And it still proves that a connection between your variables exists.
Secondly, you should also make sure that your hypothesis statement is realistic . Let’s say you have a hypothesis that ‘If we show users a banner with our new feature, then feature usage will increase by 10%.’ A few questions to ask yourself are: Is 10% a reasonable increase, based on your current feature usage data? Do you have the resources to create the tests (experimenting with multiple variations, distributing on different channels: in-app, emails, blog posts)?
Null hypothesis and alternative hypothesis
In statistical research, there are two ways of stating a hypothesis: null or alternative. But this scientific method has its place in hypothesis-driven development too…
Alternative hypothesis: A statement that you intend to prove as being true by running an experiment and analyzing the results. Hint: it’s the same as the other hypothesis examples we’ve described so far.
Example: If we change the landing page copy, then the number of signups will increase.
Null hypothesis: A statement you want to disprove by running an experiment and analyzing the results. It predicts that your new feature or change to the user experience will not have the desired effect.
Example: The number of signups will not increase if we make a change to the landing page copy.
What’s the point? Well, let’s consider the phrase ‘innocent until proven guilty’ as a version of a null hypothesis. We don’t assume that there is any relationship between the ‘defendant’ and the ‘crime’ until we have proof. So, we run a test, gather data, and analyze our findings — which gives us enough proof to reject the null hypothesis and validate the alternative. All of this helps us to have more confidence in our results.
Now that you have generated your hypotheses, and created statements, it’s time to prepare your list for testing.
Prioritizing hypotheses for testing
Not all hypotheses are created equal. Some will be essential to your immediate goal of growing the product e.g. adding a new data destination for Coupler.io. Others will be based on nice-to-haves or small fixes e.g. updating graphics on the website homepage.
Prioritization helps us focus on the most impactful solutions as we are building a product roadmap or narrowing down the backlog . To determine which hypotheses are the most critical, we use the MoSCoW framework. It allows us to assign a level of urgency and importance to each product hypothesis so we can filter the best 3-5 for testing.
MoSCoW is an acronym for Must-have, Should-have, Could-have, and Won’t-have. Here’s a breakdown:
- Must-have – hypotheses that must be tested, because they are strongly linked to our immediate project goals.
- Should-have – hypotheses that are closely related to our immediate project goals, but aren’t the top priority.
- Could-have – hypotheses of nice-to-haves that can wait until later for testing.
- Won’t-have – low-priority hypotheses that we may or may not test later on when we have more time.
How to test product hypotheses
Once you have selected a hypothesis, it’s time to test it. This will involve running one or more product experiments in order to check the validity of your claim.
The tricky part is deciding what type of experiment to run, and how many. Ultimately, this all depends on the subject of your hypothesis – whether it’s a simple copy change or a whole new feature. For instance, it’s not necessary to create a clickable prototype for a landing page redesign. In that case, a user-wide update would do.
On that note, here are some of the approaches we take to hypothesis testing at Railsware:
A/B testing
A/B or split testing involves creating two or more different versions of a webpage/feature/functionality and collecting information about how users respond to them.
Let’s say you wanted to validate a hypothesis about the placement of a search bar on your application homepage. You could design an A/B test that shows two different versions of that search bar’s placement to your users (who have been split equally into two camps: a control group and a variant group). Then, you would choose the best option based on user data. A/B tests are suitable for testing responses to user experience changes, especially if you have more than one solution to test.
Prototyping
When it comes to testing a new product design, prototyping is the method of choice for many Lean startups and organizations. It’s a cost-effective way of collecting feedback from users, fast, and it’s possible to create prototypes of individual features too. You may take this approach to hypothesis testing if you are working on rolling out a significant new change e.g adding a brand-new feature, redesigning some aspect of the user flow, etc. To control costs at this point in the new product development process , choose the right tools — think Figma for clickable walkthroughs or no-code platforms like Bubble.
Deliveroo feature prototype example
Let’s look at how feature prototyping worked for the food delivery app, Deliveroo, when their product team wanted to ‘explore personalized recommendations, better filtering and improved search’ in 2018. To begin, they created a prototype of the customer discovery feature using web design application, Framer.
One of the most important aspects of this feature prototype was that it contained live data — real restaurants, real locations. For test users, this made the hypothetical feature feel more authentic. They were seeing listings and recommendations for real restaurants in their area, which helped immerse them in the user experience, and generate more honest and specific feedback. Deliveroo was then able to implement this feedback in subsequent iterations.
Asking your users
Interviewing customers is an excellent way to validate product hypotheses. It’s a form of qualitative testing that, in our experience, produces better insights than user surveys or general user research. Sessions are typically run by product managers and involve asking in-depth interview questions to one customer at a time. They can be conducted in person or online (through a virtual call center , for instance) and last anywhere between 30 minutes to 1 hour.
Although CustDev interviews may require more effort to execute than other tests (the process of finding participants, devising questions, organizing interviews, and honing interview skills can be time-consuming), it’s still a highly rewarding approach. You can quickly validate assumptions by asking customers about their pain points, concerns, habits, processes they follow, and analyzing how your solution fits into all of that.
Wizard of Oz
The Wizard of Oz approach is suitable for gauging user interest in new features or functionalities. It’s done by creating a prototype of a fake or future feature and monitoring how your customers or test users interact with it.
For example, you might have a hypothesis that your number of active users will increase by 15% if you introduce a new feature. So, you design a new bare-bones page or simple button that invites users to access it. But when they click on the button, a pop-up appears with a message such as ‘coming soon.’
By measuring the frequency of those clicks, you could learn a lot about the demand for this new feature/functionality. However, while these tests can deliver fast results, they carry the risk of backfiring. Some customers may find fake features misleading, making them less likely to engage with your product in the future.
User-wide updates
One of the speediest ways to test your hypothesis is by rolling out an update for all users. It can take less time and effort to set up than other tests (depending on how big of an update it is). But due to the risk involved, you should stick to only performing these kinds of tests on small-scale hypotheses. Our teams only take this approach when we are almost certain that our hypothesis is valid.
For example, we once had an assumption that the name of one of Mailtrap ’s entities was the root cause of a low activation rate. Being an active Mailtrap customer meant that you were regularly sending test emails to a place called ‘Demo Inbox.’ We hypothesized that the name was confusing (the word ‘demo’ implied it was not the main inbox) and this was preventing new users from engaging with their accounts. So, we updated the page, changed the name to ‘My Inbox’ and added some ‘to-do’ steps for new users. We saw an increase in our activation rate almost immediately, validating our hypothesis.
Feature flags
Creating feature flags involves only releasing a new feature to a particular subset or small percentage of users. These features come with a built-in kill switch; a piece of code that can be executed or skipped, depending on who’s interacting with your product.
Since you are only showing this new feature to a selected group, feature flags are an especially low-risk method of testing your product hypothesis (compared to Wizard of Oz, for example, where you have much less control). However, they are also a little bit more complex to execute than the others — you will need to have an actual coded product for starters, as well as some technical knowledge, in order to add the modifiers ( only when… ) to your new coded feature.
Let’s revisit the landing page copy example again, this time in the context of testing.
So, for the hypothesis ‘If we change the landing page copy, then the number of signups will increase,’ there are several options for experimentation. We could share the copy with a small sample of our users, or even release a user-wide update. But A/B testing is probably the best fit for this task. Depending on our budget and goal, we could test several different pieces of copy, such as:
- The current landing page copy
- Copy that we paid a marketing agency 10 grand for
- Generic copy we wrote ourselves, or removing most of the original copy – just to see how making even a small change might affect our numbers.
Remember, every hypothesis test must have a reasonable endpoint. The exact length of the test will depend on the type of feature/functionality you are testing, the size of your user base, and how much data you need to gather. Just make sure that the experiment running time matches the hypothesis scope. For instance, there is no need to spend 8 weeks experimenting with a piece of landing page copy. That timeline is more appropriate for say, a Wizard of Oz feature.
Recording hypotheses statements and test results
Finally, it’s time to talk about where you will write down and keep track of your hypotheses. Creating a single source of truth will enable you to track all aspects of hypothesis generation and testing with ease.
At Railsware, our product managers create a document for each individual hypothesis, using tools such as Coda or Google Sheets. In that document, we record the hypothesis statement, as well as our plans, process, results, screenshots, product metrics, and assumptions.
We share this document with our team and stakeholders, to ensure transparency and invite feedback. It’s also a resource we can refer back to when we are discussing a new hypothesis — a place where we can quickly access information relating to a previous test.
Understanding test results and taking action
The other half of validating product hypotheses involves evaluating data and drawing reasonable conclusions based on what you find. We do so by analyzing our chosen product metric(s) and deciding whether there is enough data available to make a solid decision. If not, we may extend the test’s duration or run another one. Otherwise, we move forward. An experimental feature becomes a real feature, a chatbot gets implemented on the customer support page, and so on.
Something to keep in mind: the integrity of your data is tied to how well the test was executed, so here are a few points to consider when you are testing and analyzing results:
Gather and analyze data carefully. Ensure that your data is clean and up-to-date when running quantitative tests and tracking responses via analytics dashboards. If you are doing customer interviews, make sure to record the meetings (with consent) so that your notes will be as accurate as possible.
Conduct the right amount of product experiments. It can take more than one test to determine whether your hypothesis is valid or invalid. However, don’t waste too much time experimenting in the hopes of getting the result you want. Know when to accept the evidence and move on.
Choose the right audience segment. Don’t cast your net too wide. Be specific about who you want to collect data from prior to running the test. Otherwise, your test results will be misleading and you won’t learn anything new.
Watch out for bias. Avoid confirmation bias at all costs. Don’t make the mistake of including irrelevant data just because it bolsters your results. For example, if you are gathering data about how users are interacting with your product Monday-Friday, don’t include weekend data just because doing so would alter the data and ‘validate’ your hypothesis.
- Not all failed hypotheses should be treated as losses. Even if you didn’t get the outcome you were hoping for, you may still have improved your product. Let’s say you implemented SSO authentication for premium users, but unfortunately, your free users didn’t end up switching to premium plans. In this case, you still added value to the product by streamlining the login process for paying users.
- Yes, taking a hypothesis-driven approach to product development is important. But remember, you don’t have to test everything . Use common sense first. For example, if your website copy is confusing and doesn’t portray the value of the product, then you should still strive to replace it with better copy – regardless of how this affects your numbers in the short term.
Wrapping Up
The process of generating and validating product hypotheses is actually pretty straightforward once you’ve got the hang of it. All you need is a valid question or problem, a testable statement, and a method of validation. Sure, hypothesis-driven development requires more of a time commitment than just ‘giving it a go.’ But ultimately, it will help you tune the product to the wants and needs of your customers.
If you share our data-driven approach to product development and engineering, check out our services page to learn more about how we work with our clients!
Advisory boards aren’t only for executives. Join the LogRocket Content Advisory Board today →
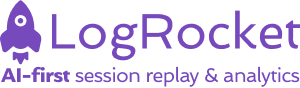
- Product Management
- Solve User-Reported Issues
- Find Issues Faster
- Optimize Conversion and Adoption
How to write an effective hypothesis
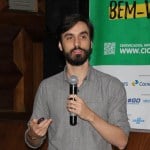
Hypothesis validation is the bread and butter of product discovery. Understanding what should be prioritized and why is the most important task of a product manager. It doesn’t matter how well you validate your findings if you’re trying to answer the wrong question.
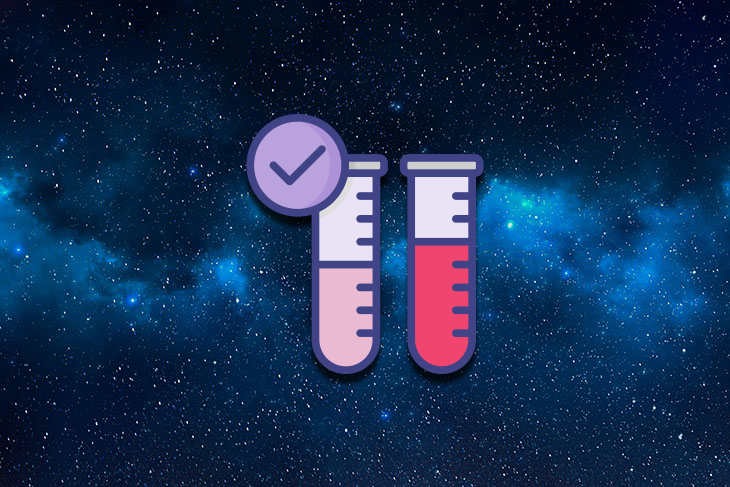
A question is as good as the answer it can provide. If your hypothesis is well written, but you can’t read its conclusion, it’s a bad hypothesis. Alternatively, if your hypothesis has embedded bias and answers itself, it’s also not going to help you.
There are several different tools available to build hypotheses, and it would be exhaustive to list them all. Apart from being superficial, focusing on the frameworks alone shifts the attention away from the hypothesis itself.
In this article, you will learn what a hypothesis is, the fundamental aspects of a good hypothesis, and what you should expect to get out of one.
The 4 product risks
Mitigating the four product risks is the reason why product managers exist in the first place and it’s where good hypothesis crafting starts.
The four product risks are assessments of everything that could go wrong with your delivery. Our natural thought process is to focus on the happy path at the expense of unknown traps. The risks are a constant reminder that knowing why something won’t work is probably more important than knowing why something might work.
These are the fundamental questions that should fuel your hypothesis creation:
Is it viable for the business?
Is it relevant for the user, can we build it, is it ethical to deliver.
Is this hypothesis the best one to validate now? Is this the most cost-effective initiative we can take? Will this answer help us achieve our goals? How much money can we make from it?
Has the user manifested interest in this solution? Will they be able to use it? Does it solve our users’ challenges? Is it aesthetically pleasing? Is it vital for the user, or just a luxury?
Do we have the resources and know-how to deliver it? Can we scale this solution? How much will it cost? Will it depreciate fast? Is it the best cost-effective solution? Will it deliver on what the user needs?
Is this solution safe both for the user and for the business? Is it inclusive enough? Is there a risk of public opinion whiplash? Is our solution enabling wrongdoers? Are we jeopardizing some to privilege others?
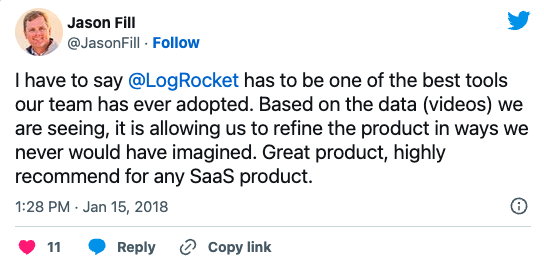
Over 200k developers and product managers use LogRocket to create better digital experiences

There is an infinite amount of questions that can surface from these risks, and most of those will be context dependent. Your industry, company, marketplace, team composition, and even the type of product you handle will impose different questions, but the risks remain the same.
How to decide whether your hypothesis is worthy of validation
Assuming you came up with a hefty batch of risks to validate, you must now address them. To address a risk, you could do one of three things: collect concrete evidence that you can mitigate that risk, infer possible ways you can mitigate a risk and, finally, deep dive into that risk because you’re not sure about its repercussions.
This three way road can be illustrated by a CSD matrix :
Certainties
Suppositions.
Everything you’re sure can help you to mitigate whatever risk. An example would be, on the risk “how to build it,” assessing if your engineering team is capable of integrating with a certain API. If your team has made it a thousand times in the past, it’s not something worth validating. You can assume it is true and mark this particular risk as solved.
To put it simply, a supposition is something that you think you know, but you’re not sure. This is the most fertile ground to explore hypotheses, since this is the precise type of answer that needs validation. The most common usage of supposition is addressing the “is it relevant for the user” risk. You presume that clients will enjoy a new feature, but before you talk to them, you can’t say you are sure.
Doubts are different from suppositions because they have no answer whatsoever. A doubt is an open question about a risk which you have no clue on how to solve. A product manager that tries to mitigate the “is it ethical to deliver” risk from an industry that they have absolute no familiarity with is poised to generate a lot of doubts, but no suppositions or certainties. Doubts are not good hypothesis sources, since you have no idea on how to validate it.
A hypothesis worth validating comes from a place of uncertainty, not confidence or doubt. If you are sure about a risk mitigation, coming up with a hypothesis to validate it is just a waste of time and resources. Alternatively, trying to come up with a risk assessment for a problem you are clueless about will probably generate hypotheses disconnected with the problem itself.
That said, it’s important to make it clear that suppositions are different from hypotheses. A supposition is merely a mental exercise, creativity executed. A hypothesis is a measurable, cartesian instrument to transform suppositions into certainties, therefore making sure you can mitigate a risk.
How to craft a hypothesis
A good hypothesis comes from a supposed solution to a specific product risk. That alone is good enough to build half of a good hypothesis, but you also need to have measurable confidence.
More great articles from LogRocket:
- How to implement issue management to improve your product
- 8 ways to reduce cycle time and build a better product
- What is a PERT chart and how to make one
- Discover how to use behavioral analytics to create a great product experience
- Explore six tried and true product management frameworks you should know
- Advisory boards aren’t just for executives. Join LogRocket’s Content Advisory Board. You’ll help inform the type of content we create and get access to exclusive meetups, social accreditation, and swag.
You’ll rarely transform a supposition into a certainty without an objective. Returning to the API example we gave when talking about certainties, you know the “can we build it” risk doesn’t need validation because your team has made tens of API integrations before. The “tens” is the quantifiable, measurable indication that gives you the confidence to be sure about mitigating a risk.
What you need from your hypothesis is exactly this quantifiable evidence, the number or hard fact able to give you enough confidence to treat your supposition as a certainty. To achieve that goal, you must come up with a target when creating the hypothesis. A hypothesis without a target can’t be validated, and therefore it’s useless.
Imagine you’re the product manager for an ecommerce app. Your users are predominantly mobile users, and your objective is to increase sales conversions. After some research, you came across the one click check-out experience, made famous by Amazon, but broadly used by ecommerces everywhere.
You know you can build it, but it’s a huge endeavor for your team. You best make sure your bet on one click check-out will work out, otherwise you’ll waste a lot of time and resources on something that won’t be able to influence the sales conversion KPI.
You identify your first risk then: is it valuable to the business?
Literature is abundant on the topic, so you are almost sure that it will bear results, but you’re not sure enough. You only can suppose that implementing the one click functionality will increase sales conversion.
During case study and data exploration, you have reasons to believe that a 30 percent increase of sales conversion is a reasonable target to be achieved. To make sure one click check-out is valuable to the business then, you would have a hypothesis such as this:
We believe that if we implement a one-click checkout on our ecommerce, we can grow our sales conversion by 30 percent
This hypothesis can be played with in all sorts of ways. If you’re trying to improve user-experience, for example, you could make it look something like this:
We believe that if we implement a one-click checkout on our ecommerce, we can reduce the time to conversion by 10 percent
You can also validate different solutions having the same criteria, building an opportunity tree to explore a multitude of hypothesis to find the better one:
We believe that if we implement a user review section on the listing page, we can grow our sales conversion by 30 percent
Sometimes you’re clueless about impact, or maybe any win is a good enough win. In that case, your criteria of validation can be a fact rather than a metric:
We believe that if we implement a one-click checkout on our ecommerce, we can reduce the time to conversion
As long as you are sure of the risk you’re mitigating, the supposition you want to transform into a certainty, and the criteria you’ll use to make that decision, you don’t need to worry so much about “right” or “wrong” when it comes to hypothesis formatting.
That’s why I avoided following up frameworks on this article. You can apply a neat hypothesis design to your product thinking, but if you’re not sure why you’re doing it, you’ll extract nothing out of it.
What comes after a good hypothesis?
The final piece of this puzzle comes after the hypothesis crafting. A hypothesis is only as good as the validation it provides, and that means you have to test it.
If we were to test the first hypothesis we crafted, “we believe that if we implement a one-click checkout on our ecommerce, we can grow our sales conversion by 30 percent,” you could come up with a testing roadmap to build up evidence that would eventually confirm or deny your hypothesis. Some examples of tests are:
A/B testing — Launch a quick and dirty one-click checkout MVP for a controlled group of users and compare their sales conversion rates against a control group. This will provide direct evidence on the effect of the feature on sales conversions
Customer support feedback — Track any inquiries or complaints related to the checkout process. You can use organic user complaints as an indirect measure of latent demand for one-click checkout feature
User survey — Ask why carts were abandoned for a cohort of shoppers that left the checkout step close to completion. Their reasons might indicate the possible success of your hypothesis
Effective hypothesis crafting is at the center of product management. It’s the link between dealing with risks and coming up with solutions that are both viable and valuable. However, it’s important to recognize that the formulation of a hypothesis is just the first step.
The real value of a hypothesis is made possible by rigorous testing. It’s through systematic validation that product managers can transform suppositions into certainties, ensuring the right product decisions are made. Without validation, even the most well-thought-out hypothesis remains unverified.
Featured image source: IconScout
LogRocket generates product insights that lead to meaningful action
Get your teams on the same page — try LogRocket today.
Share this:
- Click to share on Twitter (Opens in new window)
- Click to share on Reddit (Opens in new window)
- Click to share on LinkedIn (Opens in new window)
- Click to share on Facebook (Opens in new window)
- #product strategy
- #project management
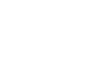
Stop guessing about your digital experience with LogRocket
Recent posts:.
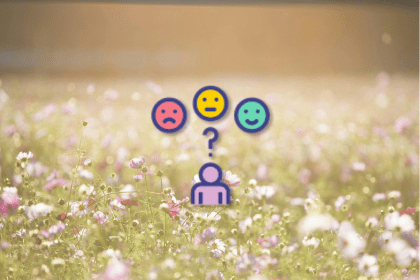
Techniques for running customer behavior analysis
Customer behavior analysis (CBA) is the study of how individual customers, groups, or segments act when interacting with your product.
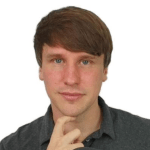
How to use — but not abuse — frameworks
While frameworks have clear benefits, it’s important to understand how and when to use them, as they are often overused or used in the wrong context or setting.
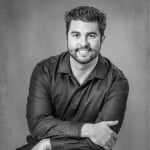
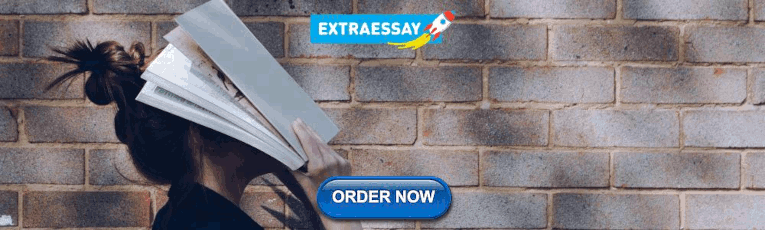
Key lessons from failed products
All the metrics, data, and analysis you made will make a difference, but success isn’t always directly proportional to the effort you put in.
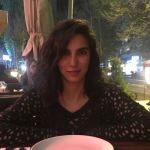
Leader Spotlight: Aligning to a ‘healthy days’ North Star, with Nupur Srivastava
Nupur Srivastava, COO of Included Health, talks about using the number of days a member considers themselves healthy as a North Star.
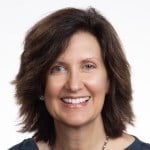
Leave a Reply Cancel reply
- Prompt Library
- DS/AI Trends
- Stats Tools
- Interview Questions
- Generative AI
- Machine Learning
- Deep Learning
Hypothesis Testing Steps & Examples
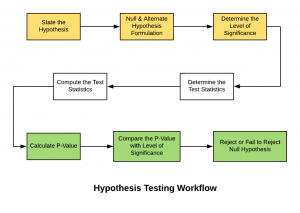
Table of Contents
What is a Hypothesis testing?
As per the definition from Oxford languages, a hypothesis is a supposition or proposed explanation made on the basis of limited evidence as a starting point for further investigation. As per the Dictionary page on Hypothesis , Hypothesis means a proposition or set of propositions, set forth as an explanation for the occurrence of some specified group of phenomena, either asserted merely as a provisional conjecture to guide investigation (working hypothesis) or accepted as highly probable in the light of established facts.
The hypothesis can be defined as the claim that can either be related to the truth about something that exists in the world, or, truth about something that’s needs to be established a fresh . In simple words, another word for the hypothesis is the “claim” . Until the claim is proven to be true, it is called the hypothesis. Once the claim is proved, it becomes the new truth or new knowledge about the thing. For example , let’s say that a claim is made that students studying for more than 6 hours a day gets more than 90% of marks in their examination. Now, this is just a claim or a hypothesis and not the truth in the real world. However, in order for the claim to become the truth for widespread adoption, it needs to be proved using pieces of evidence, e.g., data. In order to reject this claim or otherwise, one needs to do some empirical analysis by gathering data samples and evaluating the claim. The process of gathering data and evaluating the claims or hypotheses with the goal to reject or otherwise (failing to reject) can be called as hypothesis testing . Note the wordings – “failing to reject”. It means that we don’t have enough evidence to reject the claim. Thus, until the time that new evidence comes up, the claim can be considered the truth. There are different techniques to test the hypothesis in order to reach the conclusion of whether the hypothesis can be used to represent the truth of the world.
One must note that the hypothesis testing never constitutes a proof that the hypothesis is absolute truth based on the observations. It only provides added support to consider the hypothesis as truth until the time that new evidences can against the hypotheses can be gathered. We can never be 100% sure about truth related to those hypotheses based on the hypothesis testing.
Simply speaking, hypothesis testing is a framework that can be used to assert whether the claim or the hypothesis made about a real-world/real-life event can be seen as the truth or otherwise based on the given data (evidences).
Hypothesis Testing Examples
Before we get ahead and start understanding more details about hypothesis and hypothesis testing steps, lets take a look at some real-world examples of how to think about hypothesis and hypothesis testing when dealing with real-world problems :
- Customers are churning because they ain’t getting response to their complaints or issues
- Customers are churning because there are other competitive services in the market which are providing these services at lower cost.
- Customers are churning because there are other competitive services which are providing more services at the same cost.
- It is claimed that a 500 gm sugar packet for a particular brand, say XYZA, contains sugar of less than 500 gm, say around 480gm. Can this claim be taken as truth? How do we know that this claim is true? This is a hypothesis until proved.
- A group of doctors claims that quitting smoking increases lifespan. Can this claim be taken as new truth? The hypothesis is that quitting smoking results in an increase in lifespan.
- It is claimed that brisk walking for half an hour every day reverses diabetes. In order to accept this in your lifestyle, you may need evidence that supports this claim or hypothesis.
- It is claimed that doing Pranayama yoga for 30 minutes a day can help in easing stress by 50%. This can be termed as hypothesis and would require testing / validation for it to be established as a truth and recommended for widespread adoption.
- One common real-life example of hypothesis testing is election polling. In order to predict the outcome of an election, pollsters take a sample of the population and ask them who they plan to vote for. They then use hypothesis testing to assess whether their sample is representative of the population as a whole. If the results of the hypothesis test are significant, it means that the sample is representative and that the poll can be used to predict the outcome of the election. However, if the results are not significant, it means that the sample is not representative and that the poll should not be used to make predictions.
- Machine learning models make predictions based on the input data. Each of the machine learning model representing a function approximation can be taken as a hypothesis. All different models constitute what is called as hypothesis space .
- As part of a linear regression machine learning model , it is claimed that there is a relationship between the response variables and predictor variables? Can this hypothesis or claim be taken as truth? Let’s say, the hypothesis is that the housing price depends upon the average income of people already staying in the locality. How true is this hypothesis or claim? The relationship between response variable and each of the predictor variables can be evaluated using T-test and T-statistics .
- For linear regression model , one of the hypothesis is that there is no relationship between the response variable and any of the predictor variables. Thus, if b1, b2, b3 are three parameters, all of them is equal to 0. b1 = b2 = b3 = 0. This is where one performs F-test and use F-statistics to test this hypothesis.
You may note different hypotheses which are listed above. The next step would be validate some of these hypotheses. This is where data scientists will come into picture. One or more data scientists may be asked to work on different hypotheses. This would result in these data scientists looking for appropriate data related to the hypothesis they are working. This section will be detailed out in near future.
State the Hypothesis to begin Hypothesis Testing
The first step to hypothesis testing is defining or stating a hypothesis. Before the hypothesis can be tested, we need to formulate the hypothesis in terms of mathematical expressions. There are two important aspects to pay attention to, prior to the formulation of the hypothesis. The following represents different types of hypothesis that could be put to hypothesis testing:
- Claim made against the well-established fact : The case in which a fact is well-established, or accepted as truth or “knowledge” and a new claim is made about this well-established fact. For example , when you buy a packet of 500 gm of sugar, you assume that the packet does contain at the minimum 500 gm of sugar and not any less, based on the label of 500 gm on the packet. In this case, the fact is given or assumed to be the truth. A new claim can be made that the 500 gm sugar contains sugar weighing less than 500 gm. This claim needs to be tested before it is accepted as truth. Such cases could be considered for hypothesis testing if this is claimed that the assumption or the default state of being is not true. The claim to be established as new truth can be stated as “alternate hypothesis”. The opposite state can be stated as “null hypothesis”. Here the claim that the 500 gm packet consists of sugar less than 500 grams would be stated as alternate hypothesis. The opposite state which is the sugar packet consists 500 gm is null hypothesis.
- Claim to establish the new truth : The case in which there is some claim made about the reality that exists in the world (fact). For example , the fact that the housing price depends upon the average income of people already staying in the locality can be considered as a claim and not assumed to be true. Another example could be the claim that running 5 miles a day would result in a reduction of 10 kg of weight within a month. There could be varied such claims which when required to be proved as true have to go through hypothesis testing. The claim to be established as new truth can be stated as “alternate hypothesis”. The opposite state can be stated as “null hypothesis”. Running 5 miles a day would result in reduction of 10 kg within a month would be stated as alternate hypothesis.
Based on the above considerations, the following hypothesis can be stated for doing hypothesis testing.
- The packet of 500 gm of sugar contains sugar of weight less than 500 gm. (Claim made against the established fact). This is a new knowledge which requires hypothesis testing to get established and acted upon.
- The housing price depends upon the average income of the people staying in the locality. This is a new knowledge which requires hypothesis testing to get established and acted upon.
- Running 5 miles a day results in a reduction of 10 kg of weight within a month. This is a new knowledge which requires hypothesis testing to get established for widespread adoption.
Formulate Null & Alternate Hypothesis as Next Step
Once the hypothesis is defined or stated, the next step is to formulate the null and alternate hypothesis in order to begin hypothesis testing as described above.
What is a null hypothesis?
In the case where the given statement is a well-established fact or default state of being in the real world, one can call it a null hypothesis (in the simpler word, nothing new). Well-established facts don’t need any hypothesis testing and hence can be called the null hypothesis. In cases, when there are any new claims made which is not well established in the real world, the null hypothesis can be thought of as the default state or opposite state of that claim. For example , in the previous section, the claim or hypothesis is made that the students studying for more than 6 hours a day gets more than 90% of marks in their examination. The null hypothesis, in this case, will be that the claim is not true or real. The null hypothesis can be stated that there is no relationship or association between the students reading more than 6 hours a day and they getting 90% of the marks. Any occurrence is only a chance occurrence. Another example of hypothesis is when somebody is alleged that they have performed a crime.
Null hypothesis is denoted by letter H with 0, e.g., [latex]H_0[/latex]
What is an alternate hypothesis?
When the given statement is a claim (unexpected event in the real world) and not yet proven, one can call/formulate it as an alternate hypothesis and accordingly define a null hypothesis which is the opposite state of the hypothesis. The alternate hypothesis is a new knowledge or truth that needs to be established. In simple words, the hypothesis or claim that needs to be tested against reality in the real world can be termed the alternate hypothesis. In order to reach a conclusion that the claim (alternate hypothesis) can be considered the new knowledge or truth (based on the available evidence), it would be important to reject the null hypothesis. It should be noted that null and alternate hypotheses are mutually exclusive and at the same time asymmetric. In the example given in the previous section, the claim that the students studying for more than 6 hours get more than 90% of marks can be termed as the alternate hypothesis.
Alternate hypothesis is denoted with H subscript a, e.g., [latex]H_a[/latex]
Once the hypothesis is formulated as null([latex]H_0[/latex]) and alternate hypothesis ([latex]H_a[/latex]), there are two possible outcomes that can happen from hypothesis testing. These outcomes are the following:
- Reject the null hypothesis : There is enough evidence based on which one can reject the null hypothesis. Let’s understand this with the help of an example provided earlier in this section. The null hypothesis is that there is no relationship between the students studying more than 6 hours a day and getting more than 90% marks. In a sample of 30 students studying more than 6 hours a day, it was found that they scored 91% marks. Given that the null hypothesis is true, this kind of hypothesis testing result will be highly unlikely. This kind of result can’t happen by chance. That would mean that the claim can be taken as the new truth or new knowledge in the real world. One can go and take further samples of 30 students to perform some more testing to validate the hypothesis. If similar results show up with other tests, it can be said with very high confidence that there is enough evidence to reject the null hypothesis that there is no relationship between the students studying more than 6 hours a day and getting more than 90% marks. In such cases, one can go to accept the claim as new truth that the students studying more than 6 hours a day get more than 90% marks. The hypothesis can be considered the new truth until the time that new tests provide evidence against this claim.
- Fail to reject the null hypothesis : There is not enough evidence-based on which one can reject the null hypothesis (well-established fact or reality). Thus, one would fail to reject the null hypothesis. In a sample of 30 students studying more than 6 hours a day, the students were found to score 75%. Given that the null hypothesis is true, this kind of result is fairly likely or expected. With the given sample, one can’t reject the null hypothesis that there is no relationship between the students studying more than 6 hours a day and getting more than 90% marks.
Examples of formulating the null and alternate hypothesis
The following are some examples of the null and alternate hypothesis.
Hypothesis Testing Steps
Here is the diagram which represents the workflow of Hypothesis Testing.
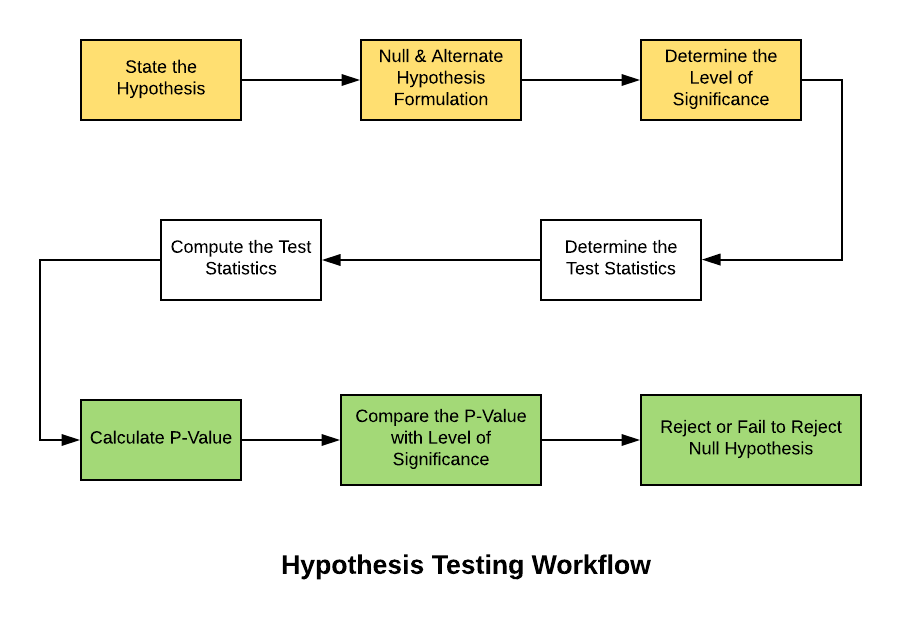
Figure 1. Hypothesis Testing Steps
Based on the above, the following are some of the steps to be taken when doing hypothesis testing:
- State the hypothesis : First and foremost, the hypothesis needs to be stated. The hypothesis could either be the statement that is assumed to be true or the claim which is made to be true.
- Formulate the hypothesis : This step requires one to identify the Null and Alternate hypotheses or in simple words, formulate the hypothesis. Take an example of the canned sauce weighing 500 gm as the Null Hypothesis.
- Set the criteria for a decision : Identify test statistics that could be used to assess the Null Hypothesis. The test statistics with the above example would be the average weight of the sugar packet, and t-statistics would be used to determine the P-value. For different kinds of problems, different kinds of statistics including Z-statistics, T-statistics, F-statistics, etc can be used.
- Identify the level of significance (alpha) : Before starting the hypothesis testing, one would be required to set the significance level (also called as alpha ) which represents the value for which a P-value less than or equal to alpha is considered statistically significant. Typical values of alpha are 0.1, 0.05, and 0.01. In case the P-value is evaluated as statistically significant, the null hypothesis is rejected. In case, the P-value is more than the alpha value, the null hypothesis is failed to be rejected.
- Compute the test statistics : Next step is to calculate the test statistics (z-test, t-test, f-test, etc) to determine the P-value. If the sample size is more than 30, it is recommended to use z-statistics. Otherwise, t-statistics could be used. In the current example where 20 packets of canned sauce is selected for hypothesis testing, t-statistics will be calculated for the mean value of 505 gm (sample mean). The t-statistics would then be calculated as the difference of 505 gm (sample mean) and the population means (500 gm) divided by the sample standard deviation divided by the square root of sample size (20).
- Calculate the P-value of the test statistics : Once the test statistics have been calculated, find the P-value using either of t-table or a z-table. P-value is the probability of obtaining a test statistic (t-score or z-score) equal to or more extreme than the result obtained from the sample data, given that the null hypothesis H0 is true.
- Compare P-value with the level of significance : The significance level is set as the allowable range within which if the value appears, one will be failed to reject the Null Hypothesis. This region is also called as Non-rejection region . The value of alpha is compared with the p-value. If the p-value is less than the significance level, the test is statistically significant and hence, the null hypothesis will be rejected.
P-Value: Key to Statistical Hypothesis Testing
Once you formulate the hypotheses, there is the need to test those hypotheses. Meaning, say that the null hypothesis is stated as the statement that housing price does not depend upon the average income of people staying in the locality, it would be required to be tested by taking samples of housing prices and, based on the test results, this Null hypothesis could either be rejected or failed to be rejected . In hypothesis testing, the following two are the outcomes:
- Reject the Null hypothesis
- Fail to Reject the Null hypothesis
Take the above example of the sugar packet weighing 500 gm. The Null hypothesis is set as the statement that the sugar packet weighs 500 gm. After taking a sample of 20 sugar packets and testing/taking its weight, it was found that the average weight of the sugar packets came to 495 gm. The test statistics (t-statistics) were calculated for this sample and the P-value was determined. Let’s say the P-value was found to be 15%. Assuming that the level of significance is selected to be 5%, the test statistic is not statistically significant (P-value > 5%) and thus, the null hypothesis fails to get rejected. Thus, one could safely conclude that the sugar packet does weigh 500 gm. However, if the average weight of canned sauce would have found to be 465 gm, this is way beyond/away from the mean value of 500 gm and one could have ended up rejecting the Null Hypothesis based on the P-value .
Hypothesis Testing for Problem Analysis & Solution Implementation
Hypothesis testing can be applied in both problem analysis and solution implementation. The following represents method on how you can apply hypothesis testing technique for both problem and solution space:
- Problem Analysis : Hypothesis testing is a systematic way to validate assumptions or educated guesses during problem analysis. It allows for a structured investigation into the nature of a problem and its potential root causes. In this process, a null hypothesis and an alternative hypothesis are usually defined. The null hypothesis generally asserts that no significant change or effect exists, while the alternative hypothesis posits the opposite. Through controlled experiments, data collection, or statistical analysis, these hypotheses are then tested to determine their validity. For example, if a software company notices a sudden increase in user churn rate, they might hypothesize that the recent update to their application is the root cause. The null hypothesis could be that the update has no effect on churn rate, while the alternative hypothesis would assert that the update significantly impacts the churn rate. By analyzing user behavior and feedback before and after the update, and perhaps running A/B tests where one user group has the update and another doesn’t, the company can test these hypotheses. If the alternative hypothesis is confirmed, the company can then focus on identifying specific issues in the update that may be causing the increased churn, thereby moving closer to a solution.
- Solution Implementation : Hypothesis testing can also be a valuable tool during the solution implementation phase, serving as a method to evaluate the effectiveness of proposed remedies. By setting up a specific hypothesis about the expected outcome of a solution, organizations can create targeted metrics and KPIs to measure success. For example, if a retail business is facing low customer retention rates, they might implement a loyalty program as a solution. The hypothesis could be that introducing a loyalty program will increase customer retention by at least 15% within six months. The null hypothesis would state that the loyalty program has no significant effect on retention rates. To test this, the company can compare retention metrics from before and after the program’s implementation, possibly even setting up control groups for more robust analysis. By applying statistical tests to this data, the company can determine whether their hypothesis is confirmed or refuted, thereby gauging the effectiveness of their solution and making data-driven decisions for future actions.
- Tests of Significance
- Hypothesis testing for the Mean
- z-statistics vs t-statistics (Khan Academy)
Hypothesis testing quiz
The claim that needs to be established is set as ____________, the outcome of hypothesis testing is _________.
Please select 2 correct answers
P-value is defined as the probability of obtaining the result as extreme given the null hypothesis is true
There is a claim that doing pranayama yoga results in reversing diabetes. which of the following is true about null hypothesis.
In this post, you learned about hypothesis testing and related nuances such as the null and alternate hypothesis formulation techniques, ways to go about doing hypothesis testing etc. In data science, one of the reasons why one needs to understand the concepts of hypothesis testing is the need to verify the relationship between the dependent (response) and independent (predictor) variables. One would, thus, need to understand the related concepts such as hypothesis formulation into null and alternate hypothesis, level of significance, test statistics calculation, P-value, etc. Given that the relationship between dependent and independent variables is a sort of hypothesis or claim , the null hypothesis could be set as the scenario where there is no relationship between dependent and independent variables.
Recent Posts
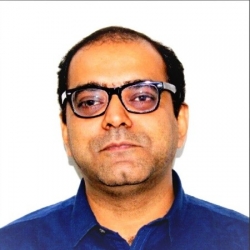
- Python Pickle Security Issues / Risk - May 31, 2024
- Pricing Analytics in Banking: Strategies, Examples - May 15, 2024
- How to Learn Effectively: A Holistic Approach - May 13, 2024
Ajitesh Kumar
Leave a reply cancel reply.
Your email address will not be published. Required fields are marked *
- Search for:
- Excellence Awaits: IITs, NITs & IIITs Journey
ChatGPT Prompts (250+)
- Generate Design Ideas for App
- Expand Feature Set of App
- Create a User Journey Map for App
- Generate Visual Design Ideas for App
- Generate a List of Competitors for App
- Python Pickle Security Issues / Risk
- Pricing Analytics in Banking: Strategies, Examples
- How to Learn Effectively: A Holistic Approach
- How to Choose Right Statistical Tests: Examples
- Data Lakehouses Fundamentals & Examples
Data Science / AI Trends
- • Prepend any arxiv.org link with talk2 to load the paper into a responsive chat application
- • Custom LLM and AI Agents (RAG) On Structured + Unstructured Data - AI Brain For Your Organization
- • Guides, papers, lecture, notebooks and resources for prompt engineering
- • Common tricks to make LLMs efficient and stable
- • Machine learning in finance
Free Online Tools
- Create Scatter Plots Online for your Excel Data
- Histogram / Frequency Distribution Creation Tool
- Online Pie Chart Maker Tool
- Z-test vs T-test Decision Tool
- Independent samples t-test calculator
Recent Comments
I found it very helpful. However the differences are not too understandable for me
Very Nice Explaination. Thankyiu very much,
in your case E respresent Member or Oraganization which include on e or more peers?
Such a informative post. Keep it up
Thank you....for your support. you given a good solution for me.
Hypothesis validation
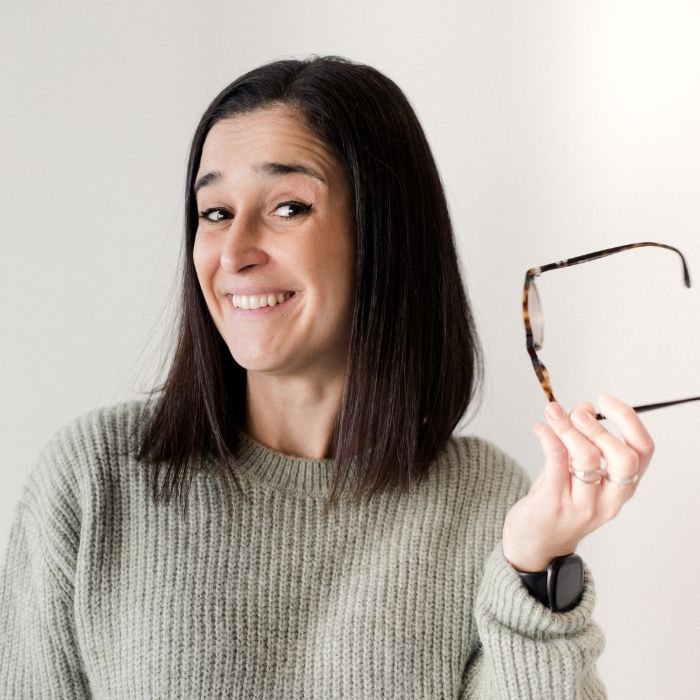
- May 24, 2022
- Product Ownership
- user stories
Share This Post
Table of Contents
Hi everyone!!
This article is based on the scenario presented in our article ‘ User Story Splitting ‘. If you didn’t have the chance yet to take a look at it, we encourage you to do it before continue with this article’s reading. We just want to be sure that you are not missing context but, just as a quick recap…
- …we are working in the product department of a company called ‘Frame Store’. We sell frames …
- … what we used to get from our business people is a big idea, inspirational ( sometimes not 100% realistic and rational! ) of a very nice and cool feature …
And… these are the assumptions we would like to validate:
- If the users are able to customize their menu, they will be able to find the options easily, improving performance and satisfaction.
- If the users are able to search menu options, they will be able to find the options easily, improving performance and satisfaction.
Validating these hypotheses we are seeking to reduce the risk of failure and to be sure we are giving real value to the user.
Let’s jump to the next sections where you will discover in more details how you can reduce the risks, this is ‘as simple as’ follow a hypothesis-driven validation or, what is the same, work on the hypothesis (with empiric data) before to take any decision and move forward.
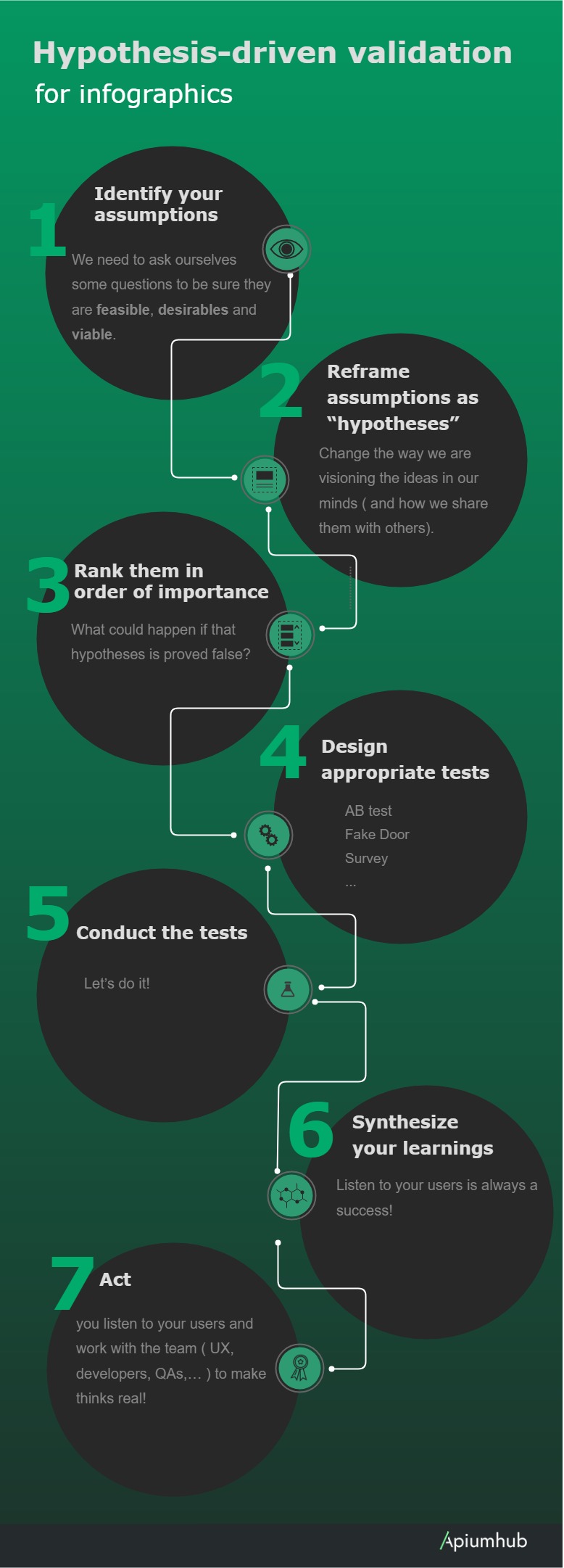
Identify your assumptions
For our example, we have the following assumptions:
We need to ask ourselves some questions to be sure they are feasible , desirables and viable .
Some examples of these questions could be…
- Are we facing some technological challenges that could make these hypotheses not realistic?
- Are we trying to solve a real pain point for our users?
- Does make sense for our company to improve that part of the process right now?
With this 3 questions we can have a very high level idea if our hypotheses is feasible, desirable and viable. We could iterate more in each of them, trying to go more deep on any of these characteristics. This is just a good point to start.
Reframe assumptions as “hypotheses”
This step could seems unnecessary but it’s really required to change the way we are visioning the ideas in our minds ( and how we share them with others).
Assumptions are something we take from granted, it is what it is. On the other hand, hypotheses are like a tentative assumption. We are implicitly saying that we have not proof of the certainty of the assumption.
- We believe that , if the users are able to customize their menu, they will be able to find them easily, improving performance and satisfaction.
- We believe that , if the users are able to search menu options, they will be able to find them easily, improving performance and satisfaction.
A good exercise in here is also the create ‘null hypotheses’, like:
- We believe that, if the users are able to customize their menu, they will be able to find the options in the same way than before, not improving performance and satisfaction.
- We believe that, if the users are able to search menu options, they will be able to find the options in the same way than before, not improving performance and satisfaction.
These are the ones we want to prove false.
Rank them in order of importance
One good advice in order to prioritize hypothesis is to evaluate what could happen if the hypothesis is proven false. In another words, what’s the Cost of Delay ( a concept some of you already know from Safe methodology).
What would be the impact for our app, or even higher level, for our company!
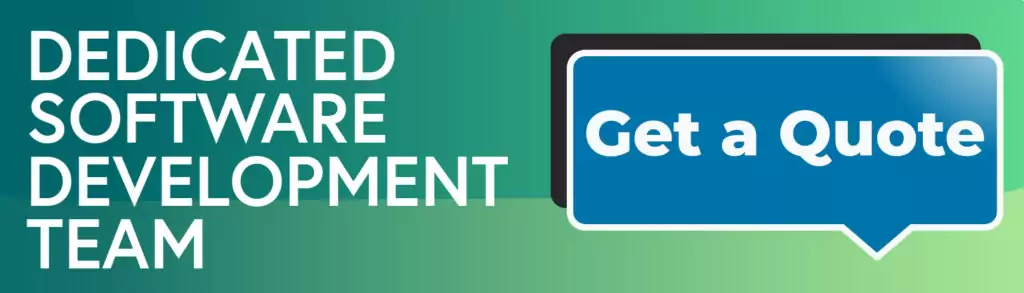
Design appropriate tests
As a good advice I would say ‘ test first what has more risk ‘. This could be apply to anything, do not start with the easy part just to realize that the more complex one is impossible to achieve. Let’s discard it ASAP.

There are multiple ways to perform a test on the hypothesis (Qualitative and Quantitative) … we will go through few of them:
Implement a minimum valuable product with the hypotheses we need to validate. We don’t need to have the full functionality implemented, just the most important features.
If we want to validate our hypothesis ‘ We believe that, if the users are able to search menu options, they will be able to find them easily, improving performance and satisfaction. ‘, let’s implement a search field but that’s everything, nothing else, nothing fancy.
Let’s release it to half of our users and see how it goes. Do not spend a lot of time in something that maybe nobody want. Once we proof that our users likes it and is giving value, we will work to improve it!
This will require even less implementation than the AB testing of the feature BUT could generate more frustration to our users. To validate our hypothesis we will…
- Add the search field to our landing page
- Let the users write the information they need to search
- Do not perform any search, the portal could prompt a message like ‘Sorry, this feature is not still available, we are working on it!’.
- Send to our analytics system how many people is actually using the feature.
As I said before, I believe that this kind of communication need to be handle appropriately to reduce frustration from the users ( some companies uses messages like ‘sorry, this feature is not available in your country yet’… a kind of white lie but could be less harming ).
This validation process could be executed inside an AB test, just replacing the MVP implementation with this Fate Door.
Let’s ask to our users and see what’s the outcome. What is important in here is to not ask direct questions where we are driving the users to the answer we expect is the correct one.
During all this years, I heard like a hundred times ‘remember the yellow Walkman!’ … how can I forget about it!! That example teach us what we don’t have to ask but, the real value is on ‘what do we have to ask?’. It took me few days to come with just few ‘none interfering’ questions!
As advice, try to formulate questions following :
- Do you believe that …
- How do you feel when…
Try to avoid yes/no answers. I would always prefer to go with a scale from ‘less likely’ to ‘very likely’ or similar.
Take in consideration that our product needs to be designed and implemented considering hypotheses validation since day 1. We can not delay the integration of an AB test framework to the last one of our implementations, this needs to be prioritize high!
Conduct the tests
You can user tools like Firebase to perform your AB. Let’s place in there the segmentation of your users you want to move to the test and wait until the data you receive is significative enough ( some tools like Firebase tells it itself).
How long will take? Depends on the traffic you have on that part of the portal/app. Changes on the landing page will give you significative data sooner that changes on some detailed page, like users profiles or any other with less traffic.
About surveys, pick well your candidates to have a realistic representation of your users. Imagine that more than 75% of our users in the Frame Store Portal are people older than 50 years. Our candidates need to represents this distribution so, do not pick a lot of 20-30 years old candidates!
Synthesize your learnings
We need to be openminded at this stage of the hypothesis validation. Maybe, the hypothesis is proved false. This should never be consider a mistake! this is a success ! We where able to save time and money for the company stopping of pursuing something that has no value.
A lot of companies failed because they were unable to validate hypotheses but not just that. I saw cases where, after designed and conducted the appropriate test, they failed reading the results. How could that be possible?? that is just because people is too committed to the assumption that they do not accept that it could be proved false. People sold the idea to upper management before prove it right and now,… ‘ Wow… I can not go back and tell them that what I thought was not true, that I was wrong! ’. This is a big mistake, the biggest one we can commit at this point. We spend time and money to validate an hypothesis and now, we will spend time implementing it even if it not useful for anybody!. Crazy stuff…
Be openminded, change your thought to ‘ Wow… luckily we proved that our assumption where false before move forward with it. Now we know more about our users needs! we can formulate new assumptions! ’. Nobody failed!
Implementation with the certainty of giving value to your users is the best feeling ever! you listen to them and work with the team ( UX, developers, QAs,… ) to make thinks real!
Is there a small chance of failure ( will be almost impossible to reduce the risk to 0! ) but nothing if you compare it with the changes of failure when you put your team to work implementing an assumption that you didn’t validate!
Conclusions
Hope this article gives you some clues to improve your hypothesis validation process. Our advice…validate, validate and … iterate!
We wish you all the best on this long but very fulfilling process!
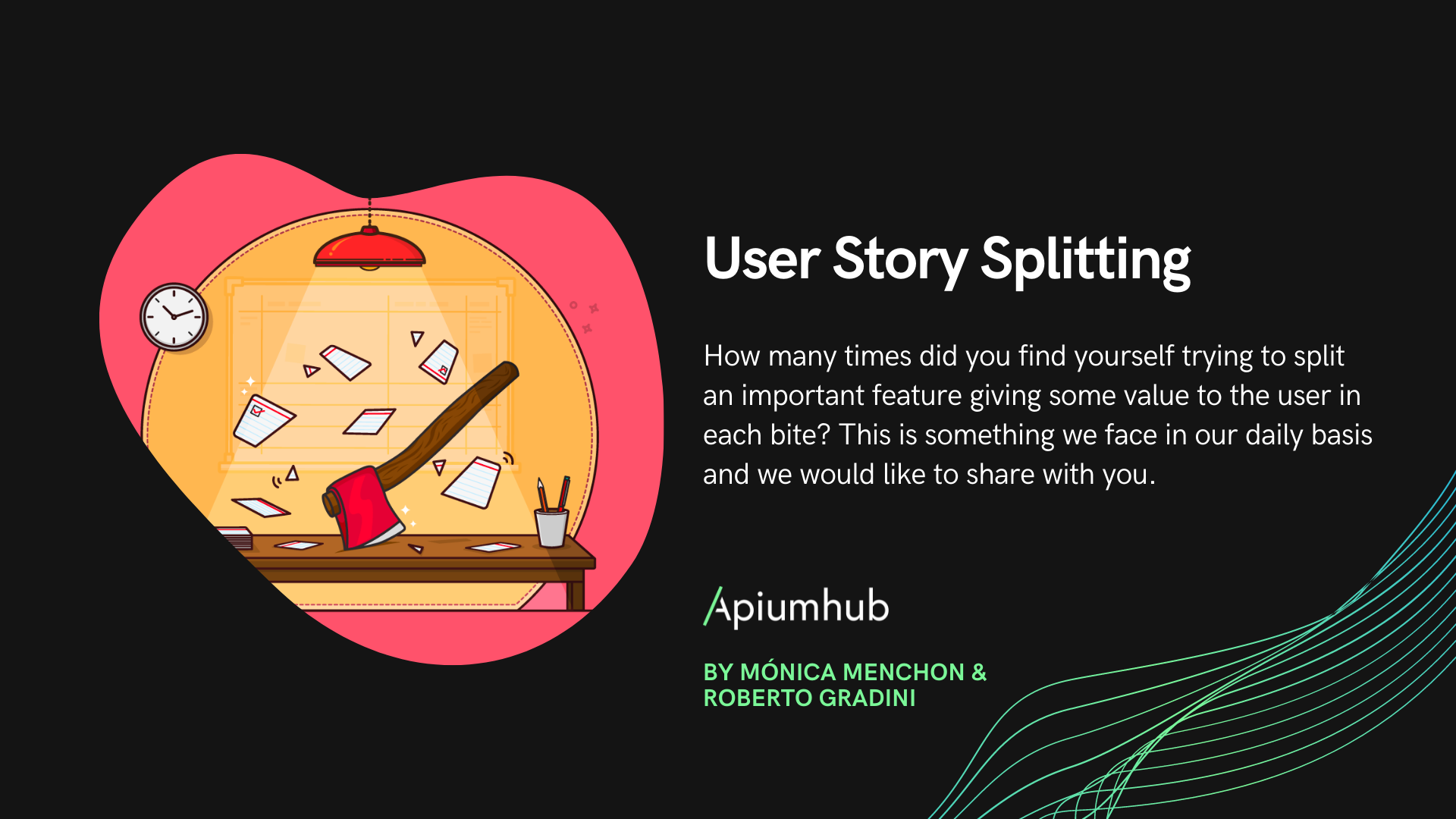
Leave a Reply Cancel Reply
Your email address will not be published. Required fields are marked *
You may use these HTML tags and attributes: <a href="" title=""> <abbr title=""> <acronym title=""> <b> <blockquote cite=""> <cite> <code> <del datetime=""> <em> <i> <q cite=""> <s> <strike> <strong>
Save my name, email, and website in this browser for the next time I comment.
Subscribe To Our Newsletter
Get updates from our latest tech findings
About Apiumhub
Apiumhub brings together a community of software developers & architects to help you transform your idea into a powerful and scalable product. Our Tech Hub specialises in Software Architecture , Web Development & Mobile App Development . Here we share with you industry tips & best practices, based on our experience.
Estimate Your Project
- Agile web and app development
- Data Science
- Offshoring and outsourcing
- Software architecture
- Software Architecture Sonar
- Software Quality Assurance Category
- Technology industry trends
- User Experience Design
Popular posts
- Custom Software Development Services & Modern Practices
- 5 Major Software Architecture Patterns
- Software Development Service Providers You Can Trust
- Software Outsourcing: Interesting Statistics, Predictions, Facts & Key Players
- Tech Of The Future: Technology Predictions For Our World In 2050
Get our Book : Software Architecture Metrics
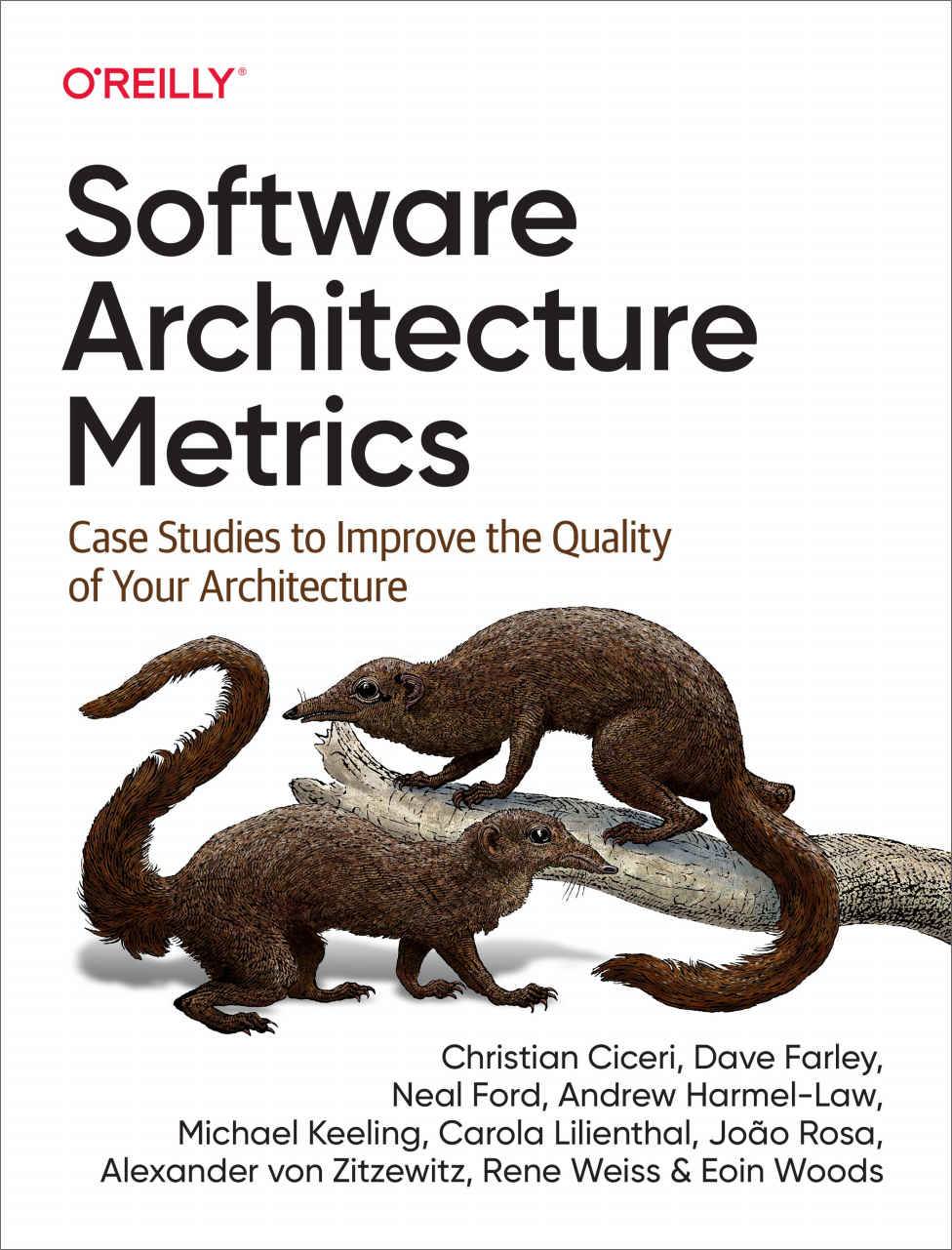
Have a challenging project?
We Can Work On It Together
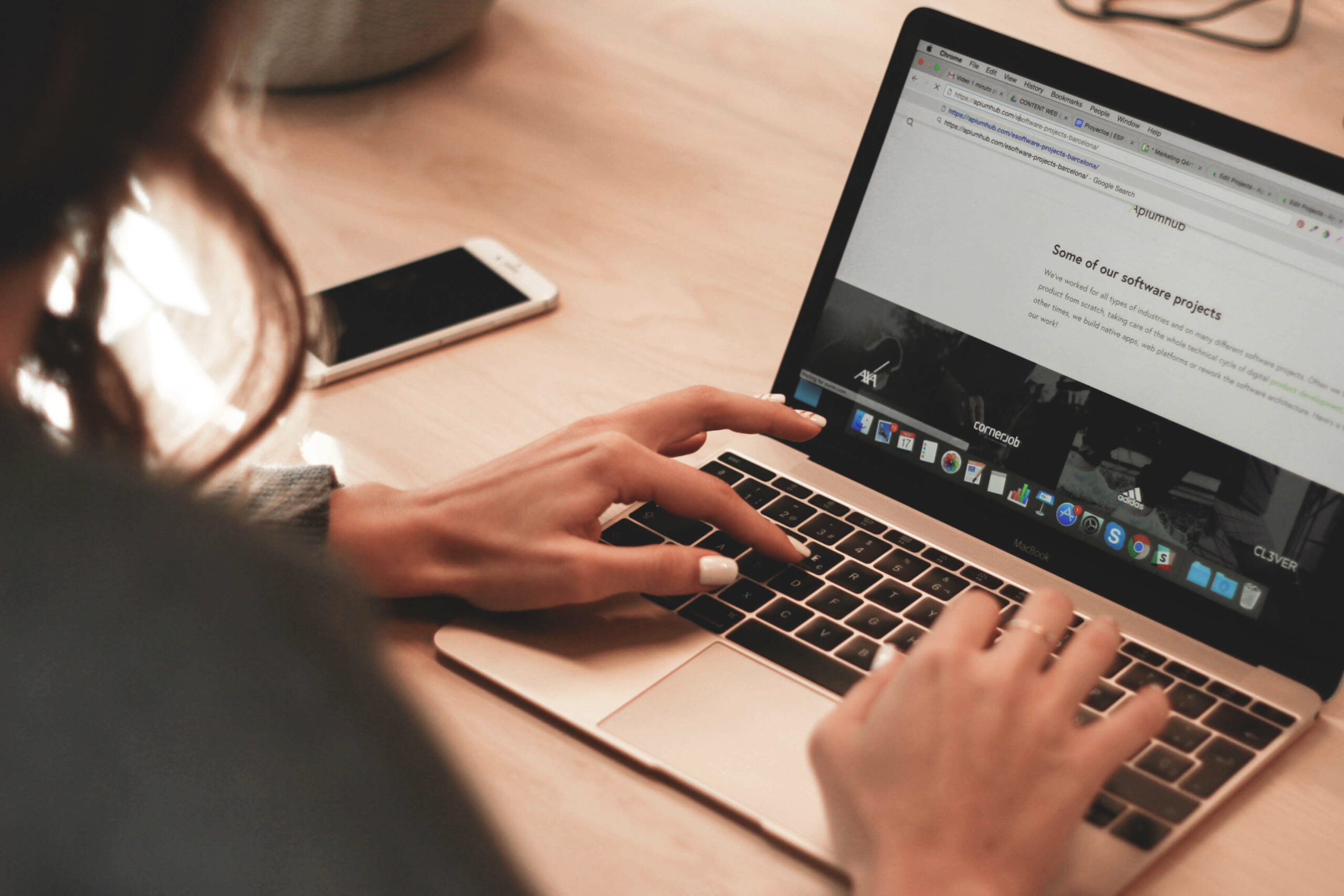
- (+34) 934 815 085
- [email protected]
- Consell de Cent, 333, 7o, 08007 Barcelona
OUR SERVICES
- Dedicated Team
- Team Extension
- Software Architecture
- Web Development
- Mobile App Development
- Agile Product Manager
- QA Automation
LATEST BLOG NEWS
React 19: Comprehensive Guide to the Latest Features
Artificial Intelligence Conferences 2024
Artificial Intelligence 101: What It Is, Its Evolution and Potential Jobs
Exploring Quarkus vs Spring Boot
USEFUL LINKS
- Free Discovery Session
- Green Software
- Become a Contributor
- Privacy Policy
- Whistleblower Channel
Receive the latest tech industry news and events

- Python for Machine Learning
- Machine Learning with R
- Machine Learning Algorithms
- Math for Machine Learning
- Machine Learning Interview Questions
- ML Projects
- Deep Learning
- Computer vision
- Data Science
- Artificial Intelligence
Hypothesis in Machine Learning
- Demystifying Machine Learning
- Bayes Theorem in Machine learning
- What is Machine Learning?
- Best IDEs For Machine Learning
- Learn Machine Learning in 45 Days
- Interpolation in Machine Learning
- How does Machine Learning Works?
- Machine Learning for Healthcare
- Applications of Machine Learning
- Machine Learning - Learning VS Designing
- Continual Learning in Machine Learning
- Meta-Learning in Machine Learning
- P-value in Machine Learning
- Why Machine Learning is The Future?
- How Does NASA Use Machine Learning?
- Few-shot learning in Machine Learning
- Machine Learning Jobs in Hyderabad
The concept of a hypothesis is fundamental in Machine Learning and data science endeavours. In the realm of machine learning, a hypothesis serves as an initial assumption made by data scientists and ML professionals when attempting to address a problem. Machine learning involves conducting experiments based on past experiences, and these hypotheses are crucial in formulating potential solutions.
It’s important to note that in machine learning discussions, the terms “hypothesis” and “model” are sometimes used interchangeably. However, a hypothesis represents an assumption, while a model is a mathematical representation employed to test that hypothesis. This section on “Hypothesis in Machine Learning” explores key aspects related to hypotheses in machine learning and their significance.
Table of Content
How does a Hypothesis work?
Hypothesis space and representation in machine learning, hypothesis in statistics, faqs on hypothesis in machine learning.
A hypothesis in machine learning is the model’s presumption regarding the connection between the input features and the result. It is an illustration of the mapping function that the algorithm is attempting to discover using the training set. To minimize the discrepancy between the expected and actual outputs, the learning process involves modifying the weights that parameterize the hypothesis. The objective is to optimize the model’s parameters to achieve the best predictive performance on new, unseen data, and a cost function is used to assess the hypothesis’ accuracy.
In most supervised machine learning algorithms, our main goal is to find a possible hypothesis from the hypothesis space that could map out the inputs to the proper outputs. The following figure shows the common method to find out the possible hypothesis from the Hypothesis space:
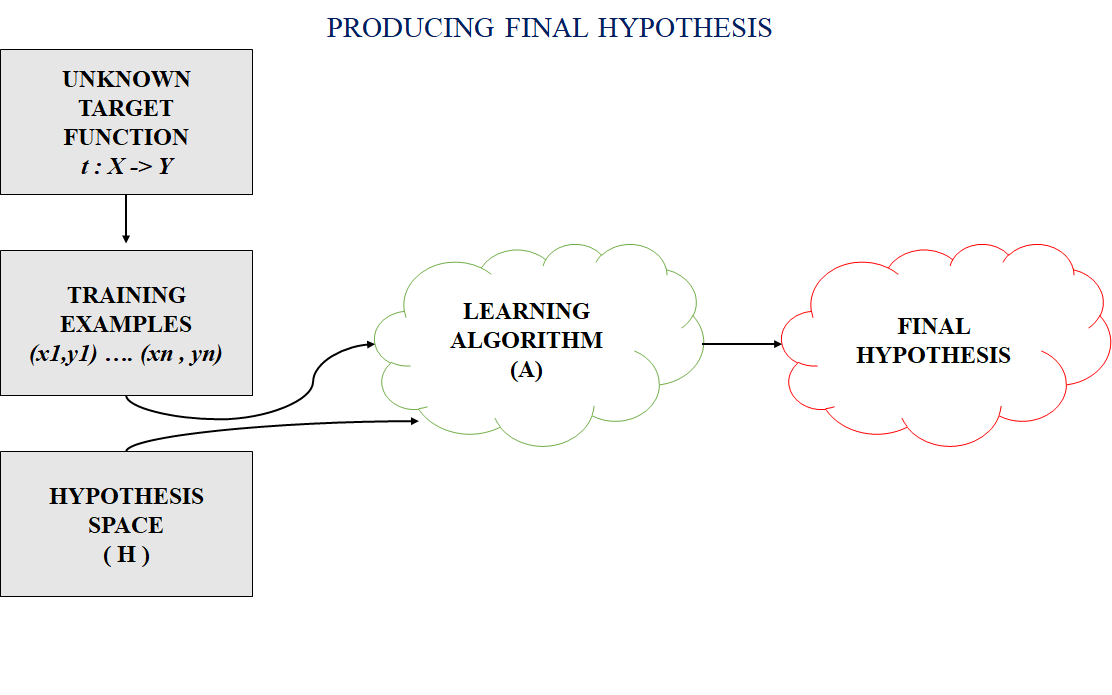
Hypothesis Space (H)
Hypothesis space is the set of all the possible legal hypothesis. This is the set from which the machine learning algorithm would determine the best possible (only one) which would best describe the target function or the outputs.
Hypothesis (h)
A hypothesis is a function that best describes the target in supervised machine learning. The hypothesis that an algorithm would come up depends upon the data and also depends upon the restrictions and bias that we have imposed on the data.
The Hypothesis can be calculated as:
[Tex]y = mx + b [/Tex]
- m = slope of the lines
- b = intercept
To better understand the Hypothesis Space and Hypothesis consider the following coordinate that shows the distribution of some data:
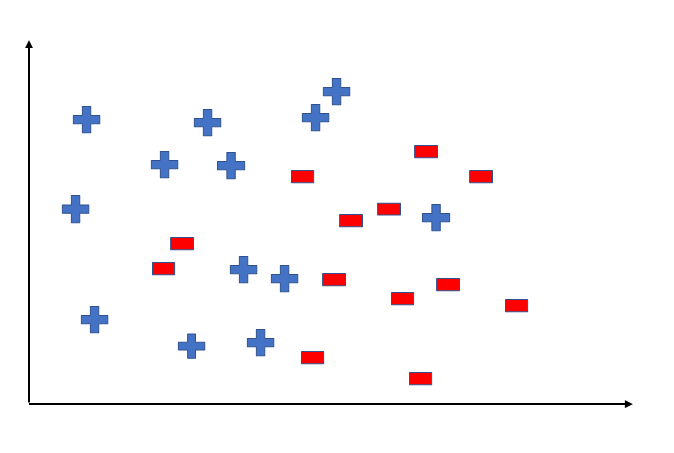
Say suppose we have test data for which we have to determine the outputs or results. The test data is as shown below:
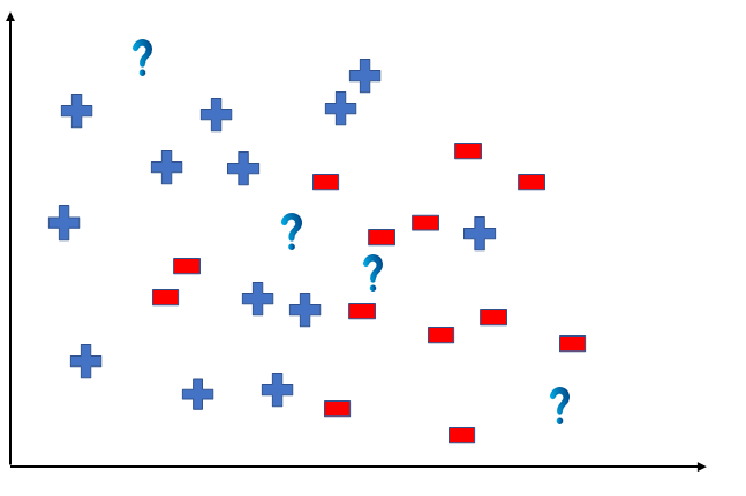
We can predict the outcomes by dividing the coordinate as shown below:
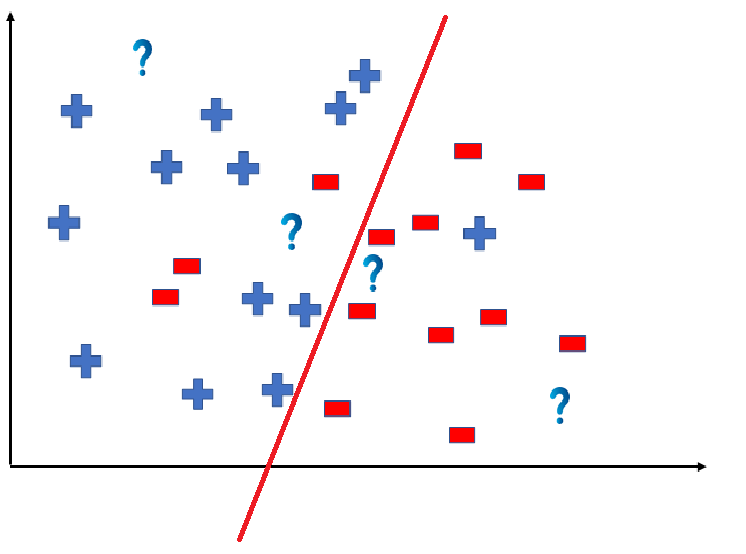
So the test data would yield the following result:
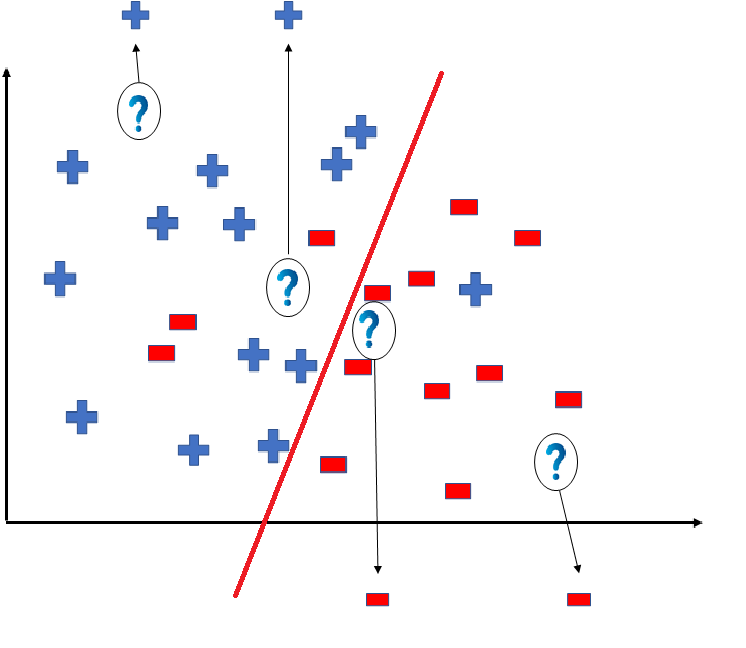
But note here that we could have divided the coordinate plane as:
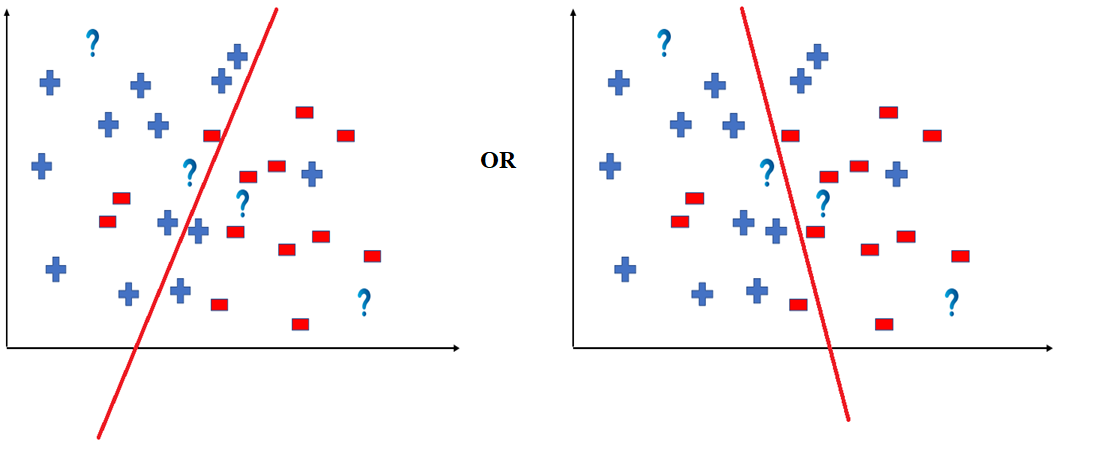
The way in which the coordinate would be divided depends on the data, algorithm and constraints.
- All these legal possible ways in which we can divide the coordinate plane to predict the outcome of the test data composes of the Hypothesis Space.
- Each individual possible way is known as the hypothesis.
Hence, in this example the hypothesis space would be like:
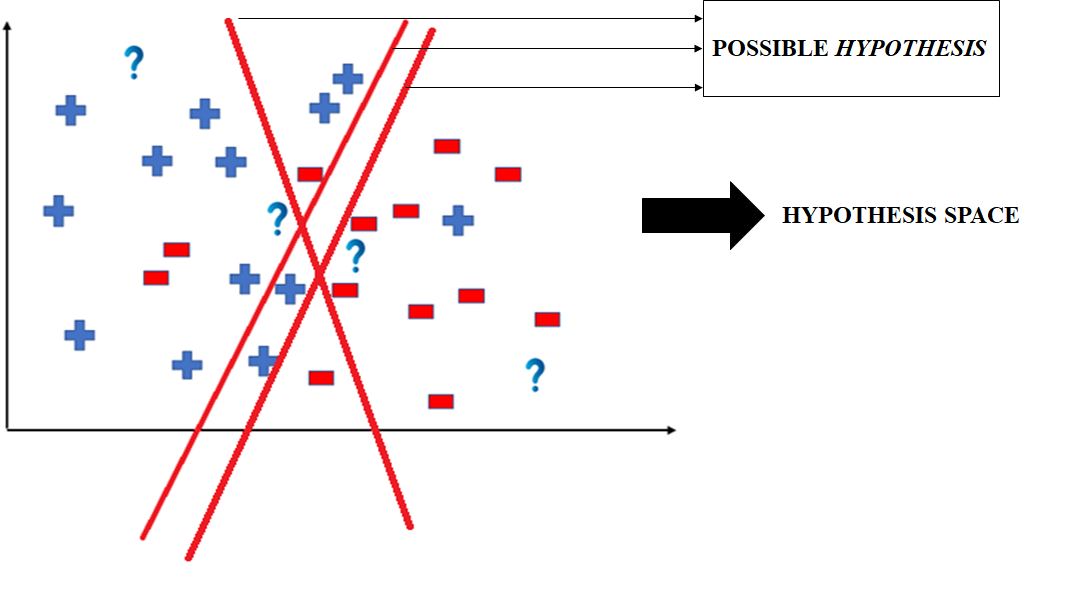
The hypothesis space comprises all possible legal hypotheses that a machine learning algorithm can consider. Hypotheses are formulated based on various algorithms and techniques, including linear regression, decision trees, and neural networks. These hypotheses capture the mapping function transforming input data into predictions.
Hypothesis Formulation and Representation in Machine Learning
Hypotheses in machine learning are formulated based on various algorithms and techniques, each with its representation. For example:
- Linear Regression : [Tex] h(X) = \theta_0 + \theta_1 X_1 + \theta_2 X_2 + … + \theta_n X_n[/Tex]
- Decision Trees : [Tex]h(X) = \text{Tree}(X)[/Tex]
- Neural Networks : [Tex]h(X) = \text{NN}(X)[/Tex]
In the case of complex models like neural networks, the hypothesis may involve multiple layers of interconnected nodes, each performing a specific computation.
Hypothesis Evaluation:
The process of machine learning involves not only formulating hypotheses but also evaluating their performance. This evaluation is typically done using a loss function or an evaluation metric that quantifies the disparity between predicted outputs and ground truth labels. Common evaluation metrics include mean squared error (MSE), accuracy, precision, recall, F1-score, and others. By comparing the predictions of the hypothesis with the actual outcomes on a validation or test dataset, one can assess the effectiveness of the model.
Hypothesis Testing and Generalization:
Once a hypothesis is formulated and evaluated, the next step is to test its generalization capabilities. Generalization refers to the ability of a model to make accurate predictions on unseen data. A hypothesis that performs well on the training dataset but fails to generalize to new instances is said to suffer from overfitting. Conversely, a hypothesis that generalizes well to unseen data is deemed robust and reliable.
The process of hypothesis formulation, evaluation, testing, and generalization is often iterative in nature. It involves refining the hypothesis based on insights gained from model performance, feature importance, and domain knowledge. Techniques such as hyperparameter tuning, feature engineering, and model selection play a crucial role in this iterative refinement process.
In statistics , a hypothesis refers to a statement or assumption about a population parameter. It is a proposition or educated guess that helps guide statistical analyses. There are two types of hypotheses: the null hypothesis (H0) and the alternative hypothesis (H1 or Ha).
- Null Hypothesis(H 0 ): This hypothesis suggests that there is no significant difference or effect, and any observed results are due to chance. It often represents the status quo or a baseline assumption.
- Aternative Hypothesis(H 1 or H a ): This hypothesis contradicts the null hypothesis, proposing that there is a significant difference or effect in the population. It is what researchers aim to support with evidence.
Q. How does the training process use the hypothesis?
The learning algorithm uses the hypothesis as a guide to minimise the discrepancy between expected and actual outputs by adjusting its parameters during training.
Q. How is the hypothesis’s accuracy assessed?
Usually, a cost function that calculates the difference between expected and actual values is used to assess accuracy. Optimising the model to reduce this expense is the aim.
Q. What is Hypothesis testing?
Hypothesis testing is a statistical method for determining whether or not a hypothesis is correct. The hypothesis can be about two variables in a dataset, about an association between two groups, or about a situation.
Q. What distinguishes the null hypothesis from the alternative hypothesis in machine learning experiments?
The null hypothesis (H0) assumes no significant effect, while the alternative hypothesis (H1 or Ha) contradicts H0, suggesting a meaningful impact. Statistical testing is employed to decide between these hypotheses.
Please Login to comment...
Similar reads.
- Machine Learning
Improve your Coding Skills with Practice
What kind of Experience do you want to share?

Blog » Value Hypothesis & Growth Hypothesis: lean startup validation
Value Hypothesis & Growth Hypothesis: lean startup validation
Posted on September 16, 2021 |
You’ve come up with a fantastic idea for a startup and you need to discuss the hypothesis and its value? But you’re not sure if it’s a viable one or not. What do you do next? It’s essential to get your ideas right before you start developing them. 95% of new products fail in their first year of launch. Or to put it another way, only one in twenty product ideas succeed. In this article, we’ll be taking a look at why it’s so important to validate your startup idea before you start spending a lot of time and money developing it. And that’s where the Lean Startup Validation process gets into, alongside the growth hypothesis and value hypothesis. We’ll also be looking at the questions that you need to ask.
Table of contents
The lean startup validation methodology, the benefits of validating your startup idea, the value hypothesis, the growth hypothesis, recommendations and questions for creating and running a good hypothesis, in conclusion – take the time to validate your product.

What does it mean to validate a lean startup?
Validating your lean startup idea may sound like a complicated process, but it’s a lot simpler than you may think. It may be the case that you were already planning on carrying out some of the work.
Essentially, validating your startup when you check your idea to see if it solves a problem that your prospective customers have. You can do this by creating hypotheses and then carrying out research to see if these hypotheses are true or false.
The best startups have always been about finding a gap in the market and offering a product or service that solves the problem. For example, take Airbnb . Before Airbnb launched, people only had the option of staying in hotels. Airbnb opened up the hospitality industry, offering cheaper accommodation to people who could not afford to stay inexpensive hotels.

“Don’t be in a rush to get big. Be in a rush to have a great product” – Eric Ries
Validation is a crucial part of the lean startup methodology, which was devised by entrepreneur Eric Ries. The lean startup methodology is all about optimizing the amount of time that is needed to ensure a product or service is viable.
Lean Startup Validation is a critical part of the lean startup process as it helps make sure that an idea will be successful before time is spent developing the final product.
As an example of a failed idea where more validation could have helped, take Google Glass . It sounded like a good idea on paper, but the technology failed spectacularly. Customer research would have shown that $1,500 was too much money, that people were worried about health and safety, and most importantly… there was no apparent benefit to the product.
Find out more about lean startup methodology on our blog
How to create a mobile app using lean startup methodology
The key benefit of validating your lean startup idea is to make sure that the idea you have is a viable one before you start using resources to build and promote it.
There are other less obvious benefits too:
- It can help you fine-tune your idea. So, it may be the case that you wanted your idea to go in a particular direction, but user research shows that pivoting may be the best thing to do
- It can help you get funding. Investors may be more likely to invest in your startup idea if you have evidence that your idea is a viable one
The value hypothesis and the growth hypothesis – are two ways to validate your idea
“To grow a successful business, validate your idea with customers” – Chad Boyda
In Eric Rie’s book ‘ The Lean Startup’ , he identifies two different types of hypotheses that entrepreneurs can use to validate their startup idea – the growth hypothesis and the value hypothesis.
Let’s look at the two different ideas, how they compare, and how you can use them to see if your startup idea could work.

The value hypothesis tests whether your product or service provides customers with enough value and most importantly, whether they are prepared to pay for this value.
For example, let’s say that you want to develop a mobile app to help dog owners find people to help walk their dogs while they are at work. Before you start spending serious time and money developing the app, you’ll want to see if it is something of interest to your target audience.
Your value hypothesis could say, “we believe that 60% of dog owners aged between 30 and 40 would be willing to pay upwards of €10 a month for this service.”
You then find dog owners in this age range and ask them the question. You’re pleased to see that 75% say that they would be willing to pay this amount! Your hypothesis has worked! This means that you should focus your app and your advertising on this target audience.
If the data comes back and says your prospective target audience isn’t willing to pay, then it means you have to rethink and reframe your app before running another hypothesis. For example, you may want to focus on another demographic, or look at reducing the price of the subscription.
Shoe retailer Zappos used a value hypothesis when starting out. Founder Nick Swinmurn went to local shoe stores, taking photos of the shoes and posting them on the Zappos website. Then, if customers bought the shoes, he’d buy them from the store and send them out to them. This allowed him to see if there was interest in his website, without having to spend lots of money on stock.

The growth hypothesis tests how your customers will find your product or service and shows how your potential product could grow over the years.
Let’s go back to the dog-walking app we talked about earlier. You think that 80% of app downloads will come from word-of-mouth recommendations.
You create a minimal viable product ( MVP for short ) – this is a basic version of your app that may not contain all of the features just yet. So, you then upload it to the app stores and wait for people to start downloading it. When you have a baseline of customers, you send them an email asking them how they heard of your app.
When the feedback comes back, it shows that only 30% of downloads have come from word-of-mouth recommendations. This means that your growth hypothesis has not been successful in this scenario.
Does this mean that your idea is a bad one? Not necessarily. It just means that you may have to look at other ways of promoting your app. If you are relying on word-of-mouth recommendations to advertise it, then it could potentially fail.
Dropbox used growth hypotheses to its advantage when creating its software. The file-storage company constantly tweaked its website, running A/B tests to see which features and changes were most popular with customers, using them in the final product.

Like any good science experiment, there are things that you need to bear in mind when running your hypotheses. Here are our recommendations:
- You may be wondering which type of hypothesis you should carry out first – a growth hypothesis or a value hypothesis. Eric Ries recommends carrying out a value hypothesis first, as it makes sense to see if there is interest before seeing how many people are interested. However, the precise order may depend on the type of product or service you want to sell;
- You will probably need to run multiple hypotheses to validate your product or service. If you do this, be sure to only test one hypothesis at a time. If you end up testing multiple ones in one go, you may not be sure which hypothesis has had which result;
- Test your most critical assumption first – this is one that you are most worried about, and could affect your idea the most. It may be that solving this issue makes your product or service a viable one;
- Specific – is your hypothesis simple? If it’s jumbled or confusing, you’re not going to get the best results from it. If you’re struggling to put together a clear hypothesis, it’s probably a sign to go back to the drawing board.
- Measurable – can your hypothesis be measured? You’ll want to get tangible results so you can check if the changes you have made have worked.
- Achievable – is your hypothesis attainable? If not, you may want to break it down into smaller goals.
- Relevant – will your hypothesis prove the validity of your product or service?
- Timely – can your hypothesis be measured in a set amount of time? You don’t want a goal that will take years to monitor and measure!
- Be as critical as possible. If you have created an idea, it is only natural that you want it to succeed. However, being objective rather than subjective will help your startup most in the long term;
- When you are carrying out customer research, use as vast a pool of people as time and money will allow. This will result in more accurate data. The great news is that you can use social media and other networking sites to reach out to potential customers and ask them their opinions;
- When carrying out customer research, be sure to ask the questions that matter. Bear in mind that liking your product or service isn’t the same as buying it. If a customer is enthusiastic about your idea, be sure to ask follow-on questions about why they like it, or if they would be willing to spend money on it. Otherwise, your data may end up being useless;
- While it is essential to have as many relevant hypotheses as possible, be careful not to have too many. While it may sound like a good idea to try out lots of different ideas, it can actually be counter-productive. As Eric Ries said:
“Don’t bog new teams down with too much information about falsifiable hypotheses. Because if we load our teams up with too much theory, they can easily get stuck in analysis paralysis. I’ve worked with teams that have come up with hundreds of leap-of-faith assumptions. They listed so many assumptions that were so detailed and complicated that they couldn’t decide what to do next. They were paralyzed by the just sheer quantity of the list.”

“We must learn what customers really want, not what they say they want or what we think they should want.” – Eric Ries
According to CB Insights , the number one reason why startups fail is that there is no demand for the product. Many entrepreneurs have gone ahead and launched a product that they think people want, only to find that there is no market at all.
Lean Startup Validation is essential in helping your business idea to succeed. While it may seem like extra work, the additional work you do in the beginning will be of a critical advantage later down the line.
Still not 100% convinced? Take HubSpot . Before HubSpot launched its sales and marketing services, it started off as a blog. Co-founders Dharmesh Shah and Brian Halligan used this blog to validate their ideas and see what their visitors wanted. This helped them confirm that their concept was on the right lines and meant they could launch a product that people actually wanted to use.
Validating a startup idea before development is crucial because it ensures that the idea is viable and addresses a real problem that customers have. With a high failure rate of new products, validation helps avoid wasting time and resources on ideas that might not succeed.
The value hypothesis tests whether customers find enough value in a product or service to pay for it. The growth hypothesis examines how customers will discover and adopt the product over time. Both hypotheses are essential for validating the viability of a startup idea.
Eric Ries recommends starting with a value hypothesis before a growth hypothesis. Validating whether the idea provides value is crucial before considering how to promote and grow it.
When creating and running a hypothesis, consider the following: 1. Focus on testing one hypothesis at a time. 2. Test your most critical assumptions first. 3. Ensure your hypothesis follows SMART goals (Specific, Measurable, Achievable, Relevant, Timely). 4. Use a wide pool of potential customers for accurate data. 5. Ask relevant and probing questions during customer research. 6. Avoid overwhelming your team with excessive hypotheses.
Validating your product idea before development helps you avoid the top reason for startup failure—lack of demand for the product. By confirming that there is a market need and interest in your idea, you increase the chances of building a successful product.
Lean Startup Validation helps entrepreneurs avoid the mistake of launching a product that doesn’t address a genuine need. By gathering evidence and feedback early, you can make informed decisions about pivoting or refining your idea before investing significant time and resources.
Certainly. Suppose you’re developing a mobile app for dog owners to find dog-walking services. Your value hypothesis could be: “We believe that 60% of dog owners aged between 30 and 40 would be willing to pay upwards of €10 a month for this service.” You then validate this hypothesis by surveying dog owners in that age range and analyzing their responses.
The growth hypothesis examines how customers will discover and adopt your product. If, for example, you expect 80% of app downloads to come from word-of-mouth recommendations, but feedback shows only 30% are from this source, you may need to reevaluate your promotion strategy.
Yes, Lean Startup Validation can be applied to startups across various industries. Whether you’re offering a product or service, the process of testing hypotheses and gathering evidence applies universally to ensure the viability of your idea.
To gather accurate data, focus on reaching a diverse pool of potential customers through various channels, including social media and networking sites. Ask relevant questions about their preferences, willingness to pay, and potential pain points related to your idea
Being critical and objective during validation helps you avoid confirmation bias and wishful thinking. Objectivity allows you to assess whether your idea truly addresses a problem and resonates with customers, ensuring that your startup’s foundation is built on solid evidence.
Launching Startups that get Success Stories
Contact us:
Quick links
© 2016 - 2024 URLAUNCHED LTD. All Rights Reserved
Identification and validation of gestational diabetes subgroups by data-driven cluster analysis
- Open access
- Published: 27 May 2024
Cite this article
You have full access to this open access article
- Benedetta Salvatori ORCID: orcid.org/0000-0002-4878-8699 1 ,
- Silke Wegener 2 ,
- Grammata Kotzaeridi 3 ,
- Annika Herding 3 ,
- Florian Eppel 3 ,
- Iris Dressler-Steinbach ORCID: orcid.org/0000-0002-9448-475X 2 ,
- Wolfgang Henrich ORCID: orcid.org/0000-0002-0759-832X 2 ,
- Agnese Piersanti ORCID: orcid.org/0000-0002-1921-838X 4 ,
- Micaela Morettini ORCID: orcid.org/0000-0002-8327-8379 4 ,
- Andrea Tura ORCID: orcid.org/0000-0003-3466-5900 1 &
- Christian S. Göbl ORCID: orcid.org/0000-0002-3922-7443 3 , 5
858 Accesses
4 Altmetric
Explore all metrics
Aims/hypothesis
Gestational diabetes mellitus (GDM) is a heterogeneous condition. Given such variability among patients, the ability to recognise distinct GDM subgroups using routine clinical variables may guide more personalised treatments. Our main aim was to identify distinct GDM subtypes through cluster analysis using routine clinical variables, and analyse treatment needs and pregnancy outcomes across these subgroups.
In this cohort study, we analysed datasets from a total of 2682 women with GDM treated at two central European hospitals (1865 participants from Charité University Hospital in Berlin and 817 participants from the Medical University of Vienna), collected between 2015 and 2022. We evaluated various clustering models, including k -means, k -medoids and agglomerative hierarchical clustering. Internal validation techniques were used to guide best model selection, while external validation on independent test sets was used to assess model generalisability. Clinical outcomes such as specific treatment needs and maternal and fetal complications were analysed across the identified clusters.
Our optimal model identified three clusters from routinely available variables, i.e. maternal age, pre-pregnancy BMI (BMIPG) and glucose levels at fasting and 60 and 120 min after the diagnostic OGTT (OGTT0, OGTT60 and OGTT120, respectively). Cluster 1 was characterised by the highest OGTT values and obesity prevalence. Cluster 2 displayed intermediate BMIPG and elevated OGTT0, while cluster 3 consisted mainly of participants with normal BMIPG and high values for OGTT60 and OGTT120. Treatment modalities and clinical outcomes varied among clusters. In particular, cluster 1 participants showed a much higher need for glucose-lowering medications (39.6% of participants, compared with 12.9% and 10.0% in clusters 2 and 3, respectively, p <0.0001). Cluster 1 participants were also at higher risk of delivering large-for-gestational-age infants. Differences in the type of insulin-based treatment between cluster 2 and cluster 3 were observed in the external validation cohort.
Conclusions/interpretation
Our findings confirm the heterogeneity of GDM. The identification of subgroups (clusters) has the potential to help clinicians define more tailored treatment approaches for improved maternal and neonatal outcomes.
Graphical Abstract
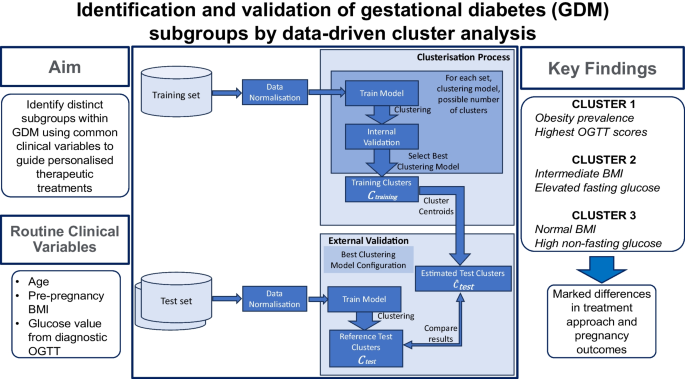
Similar content being viewed by others
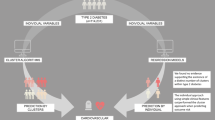
Comparison between data-driven clusters and models based on clinical features to predict outcomes in type 2 diabetes: nationwide observational study
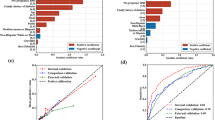
Early prediction of gestational diabetes mellitus using maternal demographic and clinical risk factors
Prediction models for the risk of gestational diabetes: a systematic review.
Avoid common mistakes on your manuscript.
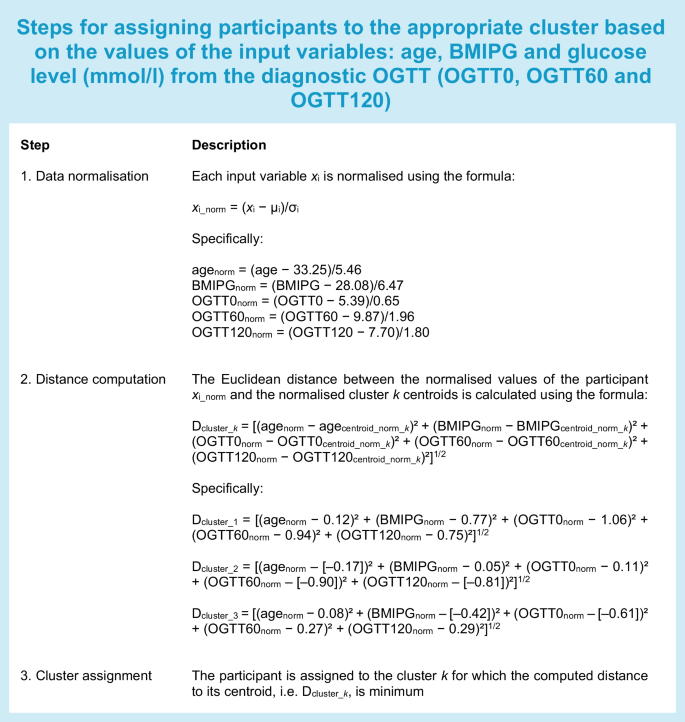
Introduction
Diabetes mellitus is a heterogeneous disease. Thus, there is currently growing interest in exploiting analytical techniques, particularly unsupervised machine learning, to identify subtypes of patients within those with diabetes that could be the basis for defining more targeted therapeutic strategies [ 1 , 2 ]. A well-known example of such approach is the study by Ahlqvist et al, which introduced a classification of adult onset diabetes based on cluster analysis [ 3 ]. Studies with a similar approach have been performed subsequently [ 4 , 5 ].
Gestational diabetes mellitus (GDM) is similarly characterised by considerable ‘phenotypic heterogeneity’ [ 6 ], which was recently identified as an important research gap by the National Institute of Diabetes and Digestive and Kidney Diseases Workshop [ 7 ]. As such, women with GDM may differ in terms of pregnancy outcomes and required treatment strategy. Some women achieve good glycaemic control through diet and lifestyle modification, whereas other women require long-acting insulin (to improve fasting glucose) or short-acting insulin (to achieve acceptable postprandial glucose), and some women will need both short- and long-acting insulin, possibly with further treatment in addition (e.g. metformin). However, to our knowledge, no previous studies have identified GDM subgroups through cluster analysis using only variables commonly measured in the clinical routine of GDM treatment. Therefore, this study aimed to identify clusters of GDM using basic clinical variables and investigate whether the identified clusters are associated with specific treatment needs/modalities or clinical outcomes, including the occurrence of pregnancy complications.
Participants and experimental procedures
A prospectively compiled dataset was analysed that comprised all singleton pregnancies with GDM diagnosis attending the Pregnancy Outpatient Department at Charité University Hospital (Berlin, Germany) between 2015 and 2022. Another cohort was used for external validation, and comprised women attending the Pregnancy Outpatient Department at the Medical University of Vienna (Vienna, Austria) in the same years. In both cohorts, women with pre-gestational diabetes or with multiple pregnancies were excluded. If any woman had multiple deliveries during the study period, we focused on the first pregnancy. Hence, the final sample consisted of 1865 and 817 women for Berlin and Vienna, respectively. A flow chart with detailed information for included and excluded patients is provided in the electronic supplemental material (ESM) Fig. 1 . A list of all variables in Berlin and Vienna datasets is provided in ESM Table 1 . Due to the nature of the present study, detailed information about ethnicity and socioeconomic status of the included patients was not available. GDM diagnosis was established by performing a 75 g OGTT in the late second or early third trimester (i.e. between 24 and 28 weeks of gestation), with fasting, 60 and 120 min glucose concentrations equal to or exceeding 5.1, 10 and 8.5 mmol/l (or 92, 180 and 153 mg/dl), respectively [ 8 , 9 ]. In participants at high risk of GDM or those with elevated fasting glucose concentrations at early pregnancy, the presence of GDM was verified by early OGTT testing before 24 weeks, according to local guidelines [ 10 ]. All participants with GDM received lifestyle advice and medical nutrition therapy, and guidance on capillary blood glucose measurement. Glucose‐lowering medication was initiated if the fasting or 1 h postprandial capillary blood glucose exceeded 5.3 or 7.8 mmol/l (95 or 140 mg/dl), with intermediate (or long-acting) insulin being prescribed for elevated fasting glucose and short-acting insulin being prescribed for postprandial hyperglycaemia [ 11 ]. The therapeutic approaches and treatment goals in Berlin and Vienna were comparable, as the same guidelines and recommendations are valid in these countries [ 10 ]. Metformin was used in some participants, especially insulin-resistant women, in addition to insulin and/or lifestyle modification. A more detailed description about indications for and use of glucose-lowering medication is provided in ESM Methods . The study was approved by the local ethics committees (Berlin: EA2/097/23, Vienna: 1542/2019), and performed in accordance with the Declaration of Helsinki. Further details are reported in ESM Methods .
Identification and validation of clusters
Definition of training and test datasets, input and outcome variables.
The pre-processing workflow for deriving training and test sets is described in ESM Methods . The Berlin dataset was randomly partitioned into a training set (70%) and a test set (30%). The Vienna dataset was used as an independent test set for validation of identified clustering models [ 3 ]. Different sets of input variables were considered to identify potential clusters. The variable sets were selected with the aim of identifying meaningful clusters while ensuring applicability in routine GDM clinical practice. One set comprised age, pre-pregnancy BMI (BMIPG), and the glucose values from the OGTT (fasting, 60 and 120 min: OGTT0, OGTT60 and OGTT120, respectively). Another set included the same variables excluding age. Further sets included age, BMIPG and either mean OGTT glucose or simply fasting glucose. Outcome variables were defined in relation to clinical outcomes of interest (see ESM Table 1 ). Table 1 reports summary statistics for the training set, Berlin test set and Vienna test set.
Clustering models implementation and validation
Various clustering algorithms were tested: k -means using Euclidean distances, and k -medoids and agglomerative hierarchical clustering [ 12 ] using both Euclidean and Manhattan distances. A hierarchical clustering algorithm was implemented with three agglomeration methodologies: complete, average and ward.D2 linkages [ 12 ]. We also explored the use of two density-based clustering methods: DBSCAN (density-based spatial clustering of applications with noise) [ 12 ] and HDBSCAN (hierarchical density-based spatial clustering) [ 13 ].
To determine the possible number of clusters for each algorithm and set of input variables, several methods were employed: the Gap statistic method [ 12 ], silhouette maximisation [ 12 ] and the function NbClust from the ‘NbClust’ R package [ 14 ], whereby various indices are calculated and the number of clusters proposed by the majority of indices is selected. Lastly, the elbow method [ 12 ] was applied for further heuristic assessment of the potential number of clusters through visualisation of cluster compactness.
We then applied a range of internal validation techniques on the training set to select valid clustering solutions and identify the optimal one. First, we ensured the solution stability (indicated by a Jaccard index above 0.75) and its compactness (indicated by a non-negative mean silhouette) for each cluster [ 15 , 16 ]. The significance of each input variable was evaluated as discussed below [ 16 ]. Moreover, a twofold cross-validation was implemented on two random subsets from the training set [ 16 ]. These ‘partial models’ were then compared with the ‘original model’ (i.e. derived from the entire training set) across three key aspects: input similarity, result similarity and outcome significance consistency. With regard to result similarity, several metrics were employed, including the adjusted Rand index and classification metrics such as sensitivity, specificity and the F1 score (see ESM Methods for details) [ 17 ].
Following identification and selection of the optimal model, its clustering results underwent an external validation phase on the two test sets to assess generalisability. For a given clustering algorithm and input variables, we defined the training cluster C training (clustering results from the training set) and the estimated test cluster \(\widehat{C}_{\mathrm{test}}\) (clustering results for the test set using the model determined from the training set). In \(\widehat{C}_{\mathrm{test}}\) , each participant was assigned to the cluster whose centroid was nearest. We also defined the reference test cluster C test , representing the clustering results on the test set obtained by applying the same clustering algorithm directly to the test set itself. Comparison between C training and \(\widehat{C}_{\mathrm{test}}\) allowed assessment of possible differences in each cluster between the training set and the test sets. Finally, comparison between \(\widehat{C}_{\mathrm{test}}\) and C test allowed validation of the clustering results on the test sets, evaluating the consistency of cluster assignments [ 18 ] (see ESM Methods and ESM Table 2 ). All procedures were performed in R, version 4.2.2 ( https://cran.rstudio.com/ ). ESM Fig. 2 shows the implemented clustering analysis procedure.
Statistical analysis
Each input variable was evaluated in terms of significant differences across clusters, this being one of the criteria for acceptance of a potential clustering solution [ 16 ]. The normality of variable distribution was checked via a Shapiro–Wilk test and a graphical test of normal distribution. ANOVA followed by Fisher’s protected least significant difference post hoc test was used for normally distributed variables to achieve 95% coverage probability, while the Kruskal–Wallis and Dunn post hoc tests [ 19 ] were used for non-normally distributed variables [ 19 ]. The p values in Tables 2 , 3 and 4 were interpreted in an explorative manner.
Similar methodology was applied to compare the continuous outcome variables. For binary outcomes, χ 2 or Fisher’s exact tests and logistic regression analysis were performed [ 19 ]. Logistic regression results further underwent ANOVA (likelihood ratio test), and, if significant, subsequent pairwise comparisons. These analyses on the outcome variables were performed separately on the training and test sets, and on partial datasets derived from twofold cross-validation. Statistical analysis was performed using R, version 4.2.2. For all tests, the two-sided significance level was set to 0.05. All p values were interpreted in an explorative manner, with the aim of generating new hypotheses. Therefore, no further adjustment for multiplicity was performed in this study, unless otherwise indicated in the text.
Selection of the optimal clustering model configuration
The selected clustering model employed the k -means algorithm and identified k =3 clusters from age, BMIPG, OGTT0, OGTT60 and OGTT120 as input variables. This configuration was selected based on the performance of the tested models against our implemented internal validation criteria. Specifically, both k -medoids and all hierarchical clustering models failed to reach the required threshold of 0.75 for the Jaccard index for each cluster, one of the internal validation criteria that was implemented to deem a proposed solution as acceptable. The reduced stability of these solutions was also confirmed by the unsatisfactory metrics achieved in twofold cross-validation, such as the adjusted Rand index. Use of DBSCAN and HDBSCAN was not pursued further as they yielded unsatisfactory results (see ESM Results for details).
Of note, when using k -means, the Gap statistic method suggested k =1 for the clustering model, while the silhouette method and the majority of the NbClust indices proposed k =2. However, upon further analysis, the k =2 clustering solution was not found to meet our internal validation criteria, which require that all variables within a proposed clustering solution must be significantly different across the identified clusters ( p <0.05). Specifically, age did not exhibit significant differences across the two clusters, leading us to discard this partitioning. Thus, k =3, the second option identified by NbClust (with modest differentiation compared with the first option), was considered the best choice.
Main characteristics of identified clusters
The cluster centroids are listed in ESM Table 3 . Figure 1 shows the cluster plot, with the proportion of participants assigned to each cluster (Fig. 1 a–c) and the variable distribution within each cluster (Fig. 1 d–f).
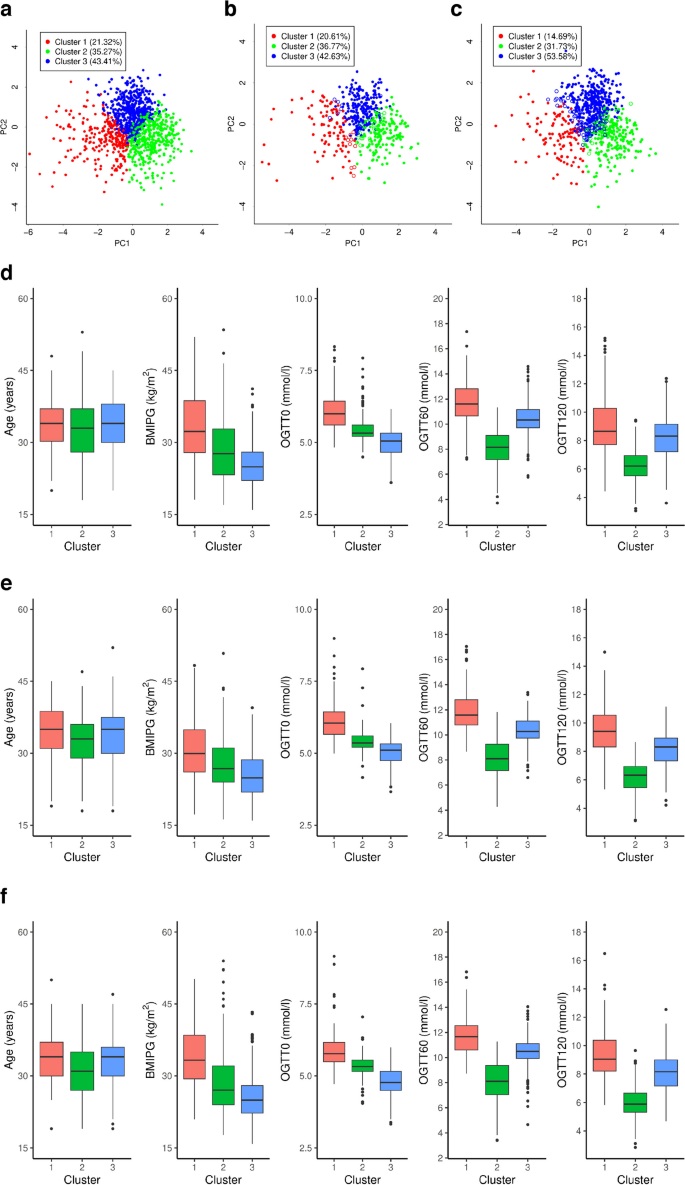
( a – c ) Representation of identified clusters on the principal components space (first two principal components, PC1 and PC2) for the training set ( a ), and of estimated clusters for the Berlin test set ( b ) and Vienna test set ( c ). Each point corresponds to a participant, and different colours represent the assigned cluster; for test sets, filled circles indicate participants correctly assigned to a cluster, whereas empty circles indicate participants assigned to a different cluster than that in the related reference clusters. The percentage of participants assigned to each cluster is also reported. ( d – f ) Distribution of input variables in the identified clusters for the training set ( d ), Berlin test set ( e ) and Vienna test set ( f )
Cluster 1, which included 246 out of 1154 participants (21.3%), exhibited the highest values across all variables, with the majority of participants being obese before pregnancy and having elevated glucose levels throughout the OGTT. Cluster 2 included 407 participants (35.3%) with lower median age and intermediate BMI, but elevated fasting glucose often exceeding the GDM threshold. Cluster 3 included 501 participants (43.4%) whose age was similar to that of cluster 1, with typically normal BMI and elevated post-load glucose levels (OGTT60 and OGTT120).
Internal validation results
The three clusters identified showed a high Jaccard index (0.88, 0.86 and 0.86 for clusters 1, 2 and 3, respectively) and non-negative silhouette values. These clusters were validated through implementation of twofold cross-validation, showing robustness and reproducibility for cluster characteristics. Differences in input variables were found in almost all pairs of clusters, confirming the importance of the input variables. Further details of the internal validation results are reported in ESM Results , and goodness-of-fit statistics are reported in ESM Table 4 .
External validation results
Assigning the participants in the test sets to clusters.
Assignment to the clusters was performed for each participant in each of the two test sets as described below (see text box).
Comparison between training set and test sets
The distribution of participants among clusters in the Berlin test set (Fig. 1 b) closely mirrored that of the training set (application of a χ 2 test on the contingency table of cluster assignment proportions led to a p value of 0.84). Furthermore, no differences were found in the values of variables between these sets, except for BMIPG and OGTT120 in cluster 1. The Vienna test set (the external validation cohort) showed some differences in cluster proportions (Fig. 1 c) compared with the training set, but, despite this, showed interesting results in terms of differences among clusters for some outcome variables, as discussed below.
Further information on distribution of variables across clusters is shown in Fig. 1 e,f. Other details of external validation results are reported in ESM Results .
Comparison between estimated and reference clusters in test sets ( \(\bf\widehat{C}_{\mathrm{test}}\) and C test )
On the test sets, k -means clustering with k =3 was reiterated as previously illustrated in the text above, providing the C test clusters that served as reference for comparison with the estimated clusters ( \(\widehat{C}_{\mathrm{test}}\) ). In the Berlin test set, the Jaccard indices for comparison between \(\widehat{C}_{\mathrm{test}}\) and C test were 0.87, 0.78 and 0.77, for clusters 1, 2 and 3, respectively, while the Vienna test set (the external cohort) showed indices of 0.80, 0.85 and 0.88, respectively. Figure 1 b,c show the cluster assignments for both sets. Overall, the adjusted Rand indices were 0.81 and 0.74 for the Berlin and Vienna test sets, with total accuracies of 93.3% and 90.5%, respectively. Specific goodness-of-fit metrics are reported in ESM Table 5 .
Comparison of clinical outcomes across clusters
Clinical outcomes were first analysed on the training set (Table 2 ). The need for glucose-lowering medications was higher in cluster 1 (39.6%) compared with clusters 2 (12.9%) and 3 (10.0%) ( p <0.0001). Likewise, birthweight >4000 g and being large for gestational age (LGA) proportions were also higher in cluster 1 (19.7% and 30.5% for birthweight >4000 g and LGA proportion, respectively) compared with cluster 2 (12.1% and 22.4%, respectively) and cluster 3 (13.1% and 22.0%, respectively) ( p <0.05). Finally, the low and in-range base excess were significantly different between cluster 1 and cluster 3. The significant differences identified in the training set were further explored in the two partial models obtained through twofold cross-validation (see ESM Tables 6 and 7 ).
The difference in drug prescription rates between cluster 1 vs clusters 2 and 3 was confirmed in the Berlin test set ( p <0.0001) (Table 3 ). The increased drug prescription rates in cluster 1 ( p <0.0001), the higher proportion of babies who were LGA, the higher values for birthweight percentiles, and the difference in low and in-range base excess in cluster 1 vs cluster 3 were confirmed in the Vienna test set (Table 4 ).
Some outcomes were available for the external validation cohort (Vienna test set) but not for the Berlin sets. Interestingly, all pharmacological treatment outcomes, except use of metformin only, showed differences across clusters (Table 4 ). Cluster 1 had higher insulin use (68.1%) than in clusters 2 and 3 (32.9% and 29.0%, respectively; p <0.0001). Use of rapid-acting insulin was higher in cluster 1 (34.5%) than in clusters 2 and 3 (6.6% and 14.7%, respectively; p <0.0001), and lower in cluster 2 vs cluster 3 ( p <0.0001). Use of neutral protamine Hagedorn (NPH) insulin was again higher in cluster 1 (54.9%) than in clusters 2 and 3 (32.1% and 24.7%, respectively; p <0.0001), and higher in cluster 2 vs cluster 3 ( p <0.05). Use of long-acting insulin was also higher in cluster 1 (10.0%) than in clusters 2 and 3 (0.4% and 1.0%, respectively; p <0.002).
The OR for the training set, the partial models from twofold cross-validation, and the two test sets were calculated as described in ESM Results and are reported in ESM Tables 8 – 12 .
Assigning a new patient to a cluster
Having defined the clustering model, a new patient may be assigned to the appropriate cluster using the same procedure used to assign the patients in the test sets. The steps for patient assignment are summarised in the text box.
This study aimed to assess clusters of GDM through an unsupervised machine learning technique known as data-driven clustering, using easily accessible clinical variables. We identified three clusters, one of which, cluster 1, exhibited a higher risk for the need for glucose-lowering medications, indicating its potential for targeted intervention strategies. This cluster, characterised by a higher BMI and hyperglycaemia at fasting as well as after oral glucose load, may represent a GDM subgroup with severe glucometabolic impairment and a higher need for pharmacological treatment (required by approximately 40% of patients). Moreover, the identified clusters showed differences in treatment modalities, even between clusters 2 and 3. Basal insulin was typically prescribed to participants in cluster 2, while rapid-acting insulin was more often prescribed in cluster 3. Our finding concerning differences in the need for glucose-lowering medications was extremely robust, as comparable differences between the clusters were observed in both test sets and the validation cohort. Furthermore, our analysis revealed additional differences among the three clusters for various outcome variables associated with pregnancy disorders, maternal delivery and fetal complications, although with lower degree of evidence (in some cases, mostly seen in the external validation cohort). In particular, infants of participants in cluster 3 had lower birthweight percentiles and a lower risk for being LGA, suggesting more favourable pregnancy outcomes. These observations underline the possible clinical importance of our study. On the other hand, it should be acknowledged that the differences in neonatal outcomes among clusters, such as the LGA prevalence, were not totally consistent among all participants in the Berlin and Vienna datasets, which was unexpected given that all participants were adequately treated by the same guidelines and recommendations [ 10 ]. Consequently, we consider the identified cluster differences in terms of the need for glucose-lowering medications as a more robust and relevant result, suggesting a more severe phenotype of the disease.
One may question the advantages of identifying clusters rather than developing predictors of the clinical outcomes of interest. In fact, developing a predictor is typically easier than identifying clusters because of the lower risk of methodological flaws. However, predictors usually focus on one outcome (such as pharmacological treatment risk), whereas clustering defines subgroups aiming to identify meaningful phenotypes of the disease, which may comprise several physiological/pathophysiological and clinical characteristics. Thus, the cluster definition may be progressively improved by specification of further characteristics. An example of this is seen in the present study, in which specific information about the type of insulin prescription was available in the external validation cohort (Vienna), and we identified differences among clusters for this important clinical aspect. Conversely, this is difficult to assess with a predictor-based approach. With the predictor-based approach, if an investigator focuses on a new outcome (such as predicting the risk for an event or condition that was not addressed before), it is likely that a completely new predictor must be developed, with no relation with the previous predictors. With the cluster-based approach, future studies may instead add characteristics (including risk for events/conditions) that were not addressed originally. Thus, it is possible to rely on the previously identified clusters without the need to perform the necessary methodological steps for new cluster definition (including collection of sufficiently large datasets). As a fact, compared with predictors, cluster-based approaches have the potential to provide a more holistic view of the disease under investigation [ 20 ]. It is also worth noting that, when we investigated the performance of logistic regression analysis (a typical predictor-based approach) using the same datasets (the training set and the Berlin and Vienna test sets) and the same input variables (age, BMI and OGTT glucose levels, either separately or together) for prediction of pharmacological treatment requirements, we obtained unsatisfactory results (details not shown). This suggests that, even for prediction of a single clinical outcome of interest, the prediction-based approach may not be adequate or superior to the cluster-based approach.
Our approach has the advantage of being simple and easily applicable in clinical practice, as the defined GDM clusters relied on only five input variables that are consistently available for patients with GDM (age, BMI and three OGTT glucose levels). Based on this approach, every clinician in charge of patients with GDM will be able to easily categorise each patient into a cluster. This has several clinical implications. For patients assigned to cluster 1, the clinician will gain awareness of the elevated risk of need for glucose-lowering medications and especially high insulin requirements, thus providing an indication of possible aggressive titration needs. On the other hand, patients in cluster 1, evaluated by the clinician as requiring intensive lifestyle intervention, may be trained with specific educational programmes to potentially avoid pharmacological treatment. Likewise, for patients in cluster 2 rather than cluster 3, and vice versa, the clinician will be aware that specific treatment modalities may be preferable, as patients in cluster 3 required a treatment approach based on fast-acting insulin formulations, while intermediate-acting insulin formulations were more often required for patients in cluster 2. Future prospective studies may clarify whether specific interventions (e.g. medical nutrition therapy vs pharmacotherapy) are more or less effective in a specific cluster. On the other hand, further studies to generate different cluster definitions may be also pertinent. In our approach, we aimed to use a minimum number of input variables to ensure wide clinical applicability, but future studies may define clusters based on more input variables, with more restricted clinical applicability but probably an improved ability to predict specific clinical outcomes.
Clinicians may wonder whether the assignment of their patients with GDM to the clusters identified in our study can be deemed reliable. Our methodological procedure was careful, with several alternatives tested to obtain the most accurate results. Furthermore, we validated our findings thoroughly, and, most importantly, using two independent datasets, one of which originated in a different clinic (Vienna) to the training set (Berlin). It is worth noting that, on average, the values of the five input variables exploited for clustering were typically different between the Vienna and Berlin datasets. This may be the common situation for clinicians applying our methodology to assign clusters for their patients. Despite this, the validation results were satisfactory. Thus, it is reasonable to expect correct assignment of new patients to our identified clusters, at least for women in a European setting.
Comparing our findings to prior studies is challenging, as, to our knowledge, our study is the first to identify GDM clusters based on routine clinical variables. In a previous study, we found that fasting hyperglycaemia, either isolated or in combination with post-load hyperglycaemia, was associated with a more frequent need for glucose-lowering medications [ 21 ]. In another study, we observed different treatment modalities in participants with GDM and higher BMI, demonstrating increased requirement of insulin [ 22 ]. We also applied a supervised learning technique to build a predictor of pharmacological requirements on a subset of the Vienna cohort analysed in the present study, in which the relevant prediction variables were glucose levels at fasting and at 60 min after the OGTT, plus age [ 23 ]. These previous findings are essentially consistent with those of the present study. Other investigators developed similar approaches [ 24 , 25 , 26 , 27 , 28 ], again with findings that are typically consistent with ours. However, none of those previous studies used unsupervised learning such as cluster analysis.
The necessary number of observations in relation to the number of input variables to ensure accurate results in cluster analysis has previously been reported to be 70 observations per input variable [ 29 ]. This value is higher than that required for supervised machine learning [ 30 , 31 ], suggesting more challenging requirements for unsupervised vs supervised approaches. As the number of participants per input variable was much higher in our study (230 participants per variable in the training set), and we observed consistent results in training and test datasets (both of which respected the necessary observation/input ratio), we believe that our sample size was certainly adequate. One limitation may be that, among all investigated outcomes, only some showed differences among clusters. On the other hand, we considered only five variables for cluster definition: given that such limited data were exploited for GDM cluster definition, it is plausible that the identified clusters may not yield all the clinical information of possible interest. In fact, the extent of the clinical information provided by our cluster definition aligns reasonably with the limited data required.
In summary, our study identifies novel GDM subgroups through unsupervised machine learning using routine clinical variables. The subgroups derived by cluster analysis showed remarkable differences in terms of glucose-lowering medication needs and treatment modalities (e.g. rapid-acting vs intermediate- or long-acting insulin), which is of major clinical relevance. In general, therefore, our methodology holds promises for guiding personalised treatment strategies and enhancing our understanding of GDM heterogeneity.
Abbreviations
Pre-pregnancy BMI
Density-based spatial clustering of applications with noise
- Gestational diabetes mellitus
Hierarchical density-based spatial clustering
Large for gestational age
Glucose level from the diagnostic OGTT at fasting
Glucose level from the diagnostic OGTT at 60 min
Glucose level from the diagnostic OGTT at 120 min
Small for gestational age
Tanabe H, Masuzaki H, Shimabukuro M (2021) Novel strategies for glycaemic control and preventing diabetic complications applying the clustering-based classification of adult-onset diabetes mellitus: a perspective. Diabetes Res Clin Pract 180:109067. https://doi.org/10.1016/j.diabres.2021.109067
Article CAS PubMed Google Scholar
Sarría-Santamera A, Orazumbekova B, Maulenkul T, Gaipov A, Atageldiyeva K (2020) The identification of diabetes mellitus subtypes applying cluster analysis techniques: a systematic review. Int J Environ Res Public Health 17(24):9523. https://doi.org/10.3390/ijerph17249523
Article CAS PubMed PubMed Central Google Scholar
Ahlqvist E, Storm P, Käräjämäki A et al (2018) Novel subgroups of adult-onset diabetes and their association with outcomes: a data-driven cluster analysis of six variables. Lancet Diabetes Endocrinol 6(5):361–369. https://doi.org/10.1016/S2213-8587(18)30051-2
Article PubMed Google Scholar
Li X, van Giessen A, Altunkaya J et al (2023) Potential value of identifying type 2 diabetes subgroups for guiding intensive treatment: a comparison of novel data-driven clustering with risk-driven subgroups. Diabetes Care 46(7):1395–1403. https://doi.org/10.2337/dc22-2170
Lugner M, Gudbjörnsdottir S, Sattar N et al (2021) Comparison between data-driven clusters and models based on clinical features to predict outcomes in type 2 diabetes: nationwide observational study. Diabetologia 64(9):1973–1981. https://doi.org/10.1007/s00125-021-05485-5
Powe CE, Hivert M-F, Udler MS (2020) Defining heterogeneity among women with gestational diabetes mellitus. Diabetes 69(10):2064–2074. https://doi.org/10.2337/dbi20-0004
Wexler DJ, Powe CE, Barbour LA et al (2018) Research gaps in gestational diabetes mellitus: executive summary of a National Institute of Diabetes and Digestive and Kidney Diseases Workshop. Obstet Gynecol 132(2):496–505. https://doi.org/10.1097/AOG.0000000000002726
Article PubMed PubMed Central Google Scholar
International Association of Diabetes and Pregnancy Study Groups Consensus Panel (2010) International Association of Diabetes and Pregnancy Study Groups recommendations on the diagnosis and classification of hyperglycemia in pregnancy. Diabetes Care 33(3):676–682. https://doi.org/10.2337/dc09-1848
Article CAS PubMed Central Google Scholar
World Health Organization (2013) Diagnostic criteria and classification of hyperglycaemia first detected in pregnancy. World Health Organization, Geneva, Switzerland
Google Scholar
Schäfer-Graf U, Gembruch U, Kainer F et al (2018) Gestational diabetes mellitus (GDM) – diagnosis, treatment and follow-up. Guideline of the DDG and DGGG (S3 Level, AWMF Registry Number 057/008, February 2018). Geburtshilfe Frauenheilkd 78(12):1219–1231. https://doi.org/10.1055/a-0659-2596
ElSayed NA, Aleppo G, Aroda VR et al (2022) 15. Management of diabetes in pregnancy: standards of care in diabetes – 2023. Diabetes Care 46(Suppl 1):S254–S266. https://doi.org/10.2337/dc23-S015
Article PubMed Central Google Scholar
Kassambara A (2017) Practical guide to cluster analysis in R: unsupervised machine learning, 1st edn. STHDA, United States
McInnes L, Healy J, Astels S (2017) hdbscan: hierarchical density-based clustering. J Open Source Softw 2(11):205. https://doi.org/10.21105/joss.00205
Article Google Scholar
Charrad M, Ghazzali N, Boiteau V, Niknafs A (2014) NbClust: an R package for determining the relevant number of clusters in a data set. J Stat Softw 61:1–36
Hennig C (2007) Cluster-wise assessment of cluster stability. Comput Stat Data Anal 52(1):258–271. https://doi.org/10.1016/j.csda.2006.11.025
Tkaczynski A (2017) Segmentation using two-step cluster analysis. In: Dietrich T, Rundle-Thiele S, Kubacki K (eds) Segmentation in social marketing. Springer, Singapore, pp 109–125. https://doi.org/10.1007/978-981-10-1835-0_8
Hubert L, Arabie P (1985) Comparing partitions. J Classif 2:193–218. https://doi.org/10.1007/BF01908075
Ullmann T, Hennig C, Boulesteix A-L (2022) Validation of cluster analysis results on validation data: a systematic framework. WIREs: Data Mining Knowl Discov 12(3):e1444. https://doi.org/10.1002/widm.1444
Field A, Miles J, Field Z (2012) Discovering statistics using R. Sage, London
Ahlqvist E, Tuomi T, Groop L (2019) Clusters provide a better holistic view of type 2 diabetes than simple clinical features. Lancet Diabetes Endocrinol 7(9):668–669. https://doi.org/10.1016/S2213-8587(19)30257-8
Kotzaeridi G, Blätter J, Eppel D et al (2021) Characteristics of gestational diabetes subtypes classified by oral glucose tolerance test values. Eur J Clin Invest 51(9):e13628. https://doi.org/10.1111/eci.13628
Linder T, Eder A, Monod C et al (2022) Impact of prepregnancy overweight and obesity on treatment modality and pregnancy outcome in women with gestational diabetes mellitus. Front Endocrinol 13:799625. https://doi.org/10.3389/fendo.2022.799625
Yerlikaya G, Falcone V, Stopp T et al (2018) To predict the requirement of pharmacotherapy by OGTT glucose levels in women with GDM classified by the IADPSG criteria. J Diabetes Res 2018:3243754. https://doi.org/10.1155/2018/3243754
Alunni ML, Roeder HA, Moore TR, Ramos GA (2015) First trimester gestational diabetes screening – change in incidence and pharmacotherapy need. Diabetes Res Clin Pract 109(1):135–140. https://doi.org/10.1016/j.diabres.2015.04.027
Brzozowska MM, Puvanendran A, Bliuc D, Zuschmann A, Piotrowicz AK, O’Sullivan A (2023) Predictors for pharmacological therapy and perinatal outcomes with metformin treatment in women with gestational diabetes. Front Endocrinol 14:1119134. https://doi.org/10.3389/fendo.2023.1119134
Meshel S, Schejter E, Harel T et al (2016) Can we predict the need for pharmacological treatment according to demographic and clinical characteristics in gestational diabetes? J Matern Fetal Neonatal Med 29(13):2062–2066. https://doi.org/10.3109/14767058.2015.1077225
Martine-Edith G, Johnson W, Hunsicker E, Hamer M, Petherick ES (2021) Associations between maternal characteristics and pharmaceutical treatment of gestational diabetes: an analysis of the UK Born in Bradford (BiB) cohort study. BMJ Open 11(11):e053753. https://doi.org/10.1136/bmjopen-2021-053753
Rodrigo N, Randall D, Al-Hial FA, Pak KLM, Kim AJ, Glastras SJ (2023) Fasting glucose level on the oral glucose tolerance test is associated with the need for pharmacotherapy in gestational diabetes mellitus. Nutrients 15(5):1226. https://doi.org/10.3390/nu15051226
Dolnicar S, Grün B, Leisch F, Schmidt K (2014) Required sample sizes for data-driven market segmentation analyses in tourism. J Travel Res 53(3):296–306. https://doi.org/10.1177/0047287513496475
Salvatori B, Linder T, Eppel D et al (2022) TyGIS: improved triglyceride–glucose index for the assessment of insulin sensitivity during pregnancy. Cardiovasc Diabetol 21(1):215. https://doi.org/10.1186/s12933-022-01649-8
Morgan BL, Voorhis CRWV (2007) Understanding power and rules of thumb for determining sample sizes. Tutor Quant Methods Psychol 3(2):43–50. https://doi.org/10.20982/tqmp.03.2.p043
Download references
Author information
Authors and affiliations.
CNR Institute of Neuroscience, Padua, Italy
Benedetta Salvatori & Andrea Tura
Department of Obstetrics, Charité -Universitätsmedizin Berlin, Corporate Member of Freie Universität Berlin, Humboldt-Universität zu Berlin and Berlin Institute of Health, Berlin, Germany
Silke Wegener, Iris Dressler-Steinbach & Wolfgang Henrich
Department of Obstetrics and Gynaecology, Medical University of Vienna, Vienna, Austria
Grammata Kotzaeridi, Annika Herding, Florian Eppel & Christian S. Göbl
Department of Information Engineering, Università Politecnica delle Marche, Ancona, Italy
Agnese Piersanti & Micaela Morettini
Department of Obstetrics and Gynaecology, Division of Obstetrics, Medical University of Graz, Graz, Austria
Christian S. Göbl
You can also search for this author in PubMed Google Scholar
Corresponding authors
Correspondence to Andrea Tura or Christian S. Göbl .
Ethics declarations
Acknowledgements.
We thank all the health personnel involved in the study. BS is currently supported by an Orio Carlini Scholarship (Call 2022) from Consortium GARR, Rome, Italy.
Data availability
The datasets analysed in the current study are available from the corresponding author upon reasonable request. The request must include an appropriate protocol and analysis plan, and institutional approvals must be in place before transfer of any information.
Open access funding provided by Medical University of Vienna. This research received no specific grant from any funding agency in the public, commercial or not-for-profit sectors.
Authors’ relationships and activities
The authors declare that there are no relationships or activities that might bias, or be perceived to bias, their work.
Contribution statement
CSG conceptualised the study. BS, AP, MM, AT and CSG developed the methodology. CSG, SW, GK, AH, FE, ID-S and WH curated the data. BS, SW, CSG and AT prepared the original draft. GK, AH, FE, ID-S, WH, AP and MM reviewed and edited the manuscript. All authors were involved in data interpretation and critically revising the report, and reviewed and approved the report for submission. BS, SW, CSG and AT had full access to all the data in the study, take responsibility for the integrity of the data and the accuracy of the data analysis, and are the guarantors of the study.
Additional information
Publisher's note.
Springer Nature remains neutral with regard to jurisdictional claims in published maps and institutional affiliations.
Benedetta Salvatori and Silke Wegener are joint first authors.
Andrea Tura and Christian S. Göbl are joint senior authors.
Supplementary Information
Below is the link to the electronic supplementary material.
ESM (PDF 1217 KB)
Rights and permissions.
Open Access This article is licensed under a Creative Commons Attribution 4.0 International License, which permits use, sharing, adaptation, distribution and reproduction in any medium or format, as long as you give appropriate credit to the original author(s) and the source, provide a link to the Creative Commons licence, and indicate if changes were made. The images or other third party material in this article are included in the article's Creative Commons licence, unless indicated otherwise in a credit line to the material. If material is not included in the article's Creative Commons licence and your intended use is not permitted by statutory regulation or exceeds the permitted use, you will need to obtain permission directly from the copyright holder. To view a copy of this licence, visit http://creativecommons.org/licenses/by/4.0/ .
Reprints and permissions
About this article
Salvatori, B., Wegener, S., Kotzaeridi, G. et al. Identification and validation of gestational diabetes subgroups by data-driven cluster analysis. Diabetologia (2024). https://doi.org/10.1007/s00125-024-06184-7
Download citation
Received : 20 March 2024
Accepted : 19 April 2024
Published : 27 May 2024
DOI : https://doi.org/10.1007/s00125-024-06184-7
Share this article
Anyone you share the following link with will be able to read this content:
Sorry, a shareable link is not currently available for this article.
Provided by the Springer Nature SharedIt content-sharing initiative
- Cluster analysis
- Data-driven clustering
- Oral glucose tolerance test
- Pregnancy outcomes
- Treatment stratification
- Unsupervised machine learning
- Find a journal
- Publish with us
- Track your research
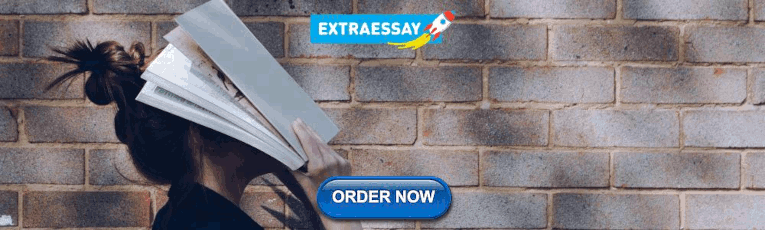
IMAGES
VIDEO
COMMENTS
Product Hypothesis Examples. To demonstrate how to formulate your assumptions clearly, here are several more apart from the example of a hypothesis statement given above: ... However, this only makes sense if the validation of hypothesis statements is backed by relevant data that'll allow you to determine whether the hypothesis is valid or not ...
There are 5 main steps in hypothesis testing: State your research hypothesis as a null hypothesis and alternate hypothesis (H o) and (H a or H 1 ). Collect data in a way designed to test the hypothesis. Perform an appropriate statistical test. Decide whether to reject or fail to reject your null hypothesis. Present the findings in your results ...
By introducing hypothesis-driven validation into your process, ... Here are some sample test types: Quantitative: Surveys (these can be as informal as a Google Form or Twitter poll), data analysis ...
Mean Population IQ: 100. Step 1: Using the value of the mean population IQ, we establish the null hypothesis as 100. Step 2: State that the alternative hypothesis is greater than 100. Step 3: State the alpha level as 0.05 or 5%. Step 4: Find the rejection region area (given by your alpha level above) from the z-table.
A hypothesis or hypothesis statement seeks to explain why something has happened, or what might happen, under certain conditions. It can also be used to understand how different variables relate to each other. Hypotheses are often written as if-then statements; for example, "If this happens, then this will happen.".
Step 1. Define the Objective. One of the main foundations for the development of a product hypothesis is the problem statement, which involves a brief and clear description of the objective to be addressed. This objective description - specific, flexible, and data-driven - serves as a guide for the design of your hypothesis experiments.
Set validation criteria. First, build some confirmation criteria into your statement. Think in terms of percentages (e.g. increase/decrease by 5%) and choose a relevant product metric to track e.g. activation rate if your hypothesis relates to onboarding.
Hypothesis Statements. A hypothesis is a statement made with limited knowledge about a given situation that requires validation to be confirmed as true or false to such a degree where the team can ...
A/B Testing. One of the most common use cases to achieve hypothesis validation is randomized A/B testing, in which a change or feature is released at random to one-half of users (A) and withheld from the other half (B). Returning to the hypothesis of bigger product images improving conversion on Amazon, one-half of users will be shown the ...
It tests the null hypothesis that the population variances are equal (called homogeneity of variance or homoscedasticity). Suppose the resulting p-value of Levene's test is less than the significance level (typically 0.05).In that case, the obtained differences in sample variances are unlikely to have occurred based on random sampling from a population with equal variances.
Hypothesis validation is the bread and butter of product discovery. Understanding what should be prioritized and why is the most important task of a product manager. ... 30 percent," you could come up with a testing roadmap to build up evidence that would eventually confirm or deny your hypothesis. Some examples of tests are:
It is claimed that doing Pranayama yoga for 30 minutes a day can help in easing stress by 50%. This can be termed as hypothesis and would require testing / validation for it to be established as a truth and recommended for widespread adoption. One common real-life example of hypothesis testing is election polling.
How do you formulate a digital product hypothesis? How do you validate it? Can a negative hypothesis validation bring positive results? Find the answers in this article!
Step 3 - learn. In this step you analyze the data and gain insights. You systematically connect the evidence and data from experiments back to the initial hypotheses, how you tested them, and what you learned. This is where you identify if your initial hypotheses were right, wrong, or still unclear. You might learn that you have to reshape your ...
For our example, we have the following assumptions: If the users are able to customize their menu, ... Hope this article gives you some clues to improve your hypothesis validation process. Our advice…validate, validate and … iterate! We wish you all the best on this long but very fulfilling process! More to Explore.
Construct Validity | Definition, Types, & Examples. Published on February 17, 2022 by Pritha Bhandari.Revised on June 22, 2023. Construct validity is about how well a test measures the concept it was designed to evaluate. It's crucial to establishing the overall validity of a method.. Assessing construct validity is especially important when you're researching something that can't be ...
An outcome can be, for example, the onset of a disease. Criterion validity consists of two subtypes depending on the time at which the two measures (the criterion and your test) are obtained: Concurrent validity is a validation strategy where the the scores of a test and the criterion are obtained at the same time.
A good alternative hypothesis example is "Attending physiotherapy sessions improves athletes' on-field performance." or "Water evaporates at 100°C. ... Also referred to as the working hypothesis, an empirical hypothesis claims a theory's validation via experiments and observation. This way, the statement appears justifiable and different ...
What is Hypothesis Validation. Hypothesis validation is different. In this case, you already have an idea of what is wrong, and you have an idea of how you might possibly fix it. You now have to go out and do some research to figure out if your assumptions and decisions were correct. For our fictional shoe-buying product, hypothesis validation ...
Hypothesis testing is a statistical method that is used to make a statistical decision using experimental data. Hypothesis testing is basically an assumption that we make about a population parameter. It evaluates two mutually exclusive statements about a population to determine which statement is best supported by the sample data.
A hypothesis is a function that best describes the target in supervised machine learning. The hypothesis that an algorithm would come up depends upon the data and also depends upon the restrictions and bias that we have imposed on the data. The Hypothesis can be calculated as: y = mx + b y =mx+b. Where, y = range. m = slope of the lines.
Developing a hypothesis (with example) Step 1. Ask a question. Writing a hypothesis begins with a research question that you want to answer. The question should be focused, specific, and researchable within the constraints of your project. Example: Research question.
The value hypothesis and the growth hypothesis - are two ways to validate your idea. "To grow a successful business, validate your idea with customers" - Chad Boyda. In Eric Rie's book ' The Lean Startup', he identifies two different types of hypotheses that entrepreneurs can use to validate their startup idea - the growth ...
It was determined that discrepancies resulted from clerical errors (49.09%), samples from transgender participants (3.64%) and stem cell or bone marrow transplant patients (7.27%) along with undetermined sample mix-ups (40%) for which sample swaps occurred prior to arrival at genome centers, however the exact cause of the events at the sampling ...
Aims/hypothesis Gestational diabetes mellitus (GDM) is a heterogeneous condition. Given such variability among patients, the ability to recognise distinct GDM subgroups using routine clinical variables may guide more personalised treatments. Our main aim was to identify distinct GDM subtypes through cluster analysis using routine clinical variables, and analyse treatment needs and pregnancy ...
Model selection was implemented using leave-one-out cross validation. Results reported here were based on the winning models (electronic supplementary material, table S4). Pairwise comparisons among species were implemented using the method of estimated marginal means via the emmeans package [ 36 ].