Please sign in
You need to log in to use the bookmarking feature.

- Baker Library
- Special Collections
- Fast Answers
- All Services
- Plan Your Visit
- Working Knowledge
- Academic Programs
- Faculty & Research
- Harvard Business Review
- Initiatives
- Map / Directions
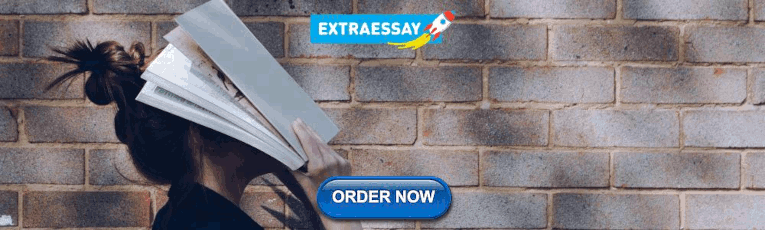
PMR Journals (Portfolio Management Research)
Full-text issues (current and archival) of quarterly publications covering research on global investment and finance.
About this Database
Publications include The Journal of Alternative Investments , The Journal of Derivatives , The Journal of Fixed Income , The Journal of Investing , The Journal of Investment Compliance , The Journal of Portfolio Management , The Journal of Private Equity , The Journal of Structured Finance , The Journal of Trading , and The Journal of Wealth Management .
Formerly known as IMR and Institutional Investor Journals.
Go To Database
Access method
Authorized Baker Visitors HarvardKey
Related Resources
Financial Markets Hedge Funds
McKinsey Global Private Markets Review 2024: Private markets in a slower era
At a glance, macroeconomic challenges continued.

McKinsey Global Private Markets Review 2024: Private markets: A slower era
If 2022 was a tale of two halves, with robust fundraising and deal activity in the first six months followed by a slowdown in the second half, then 2023 might be considered a tale of one whole. Macroeconomic headwinds persisted throughout the year, with rising financing costs, and an uncertain growth outlook taking a toll on private markets. Full-year fundraising continued to decline from 2021’s lofty peak, weighed down by the “denominator effect” that persisted in part due to a less active deal market. Managers largely held onto assets to avoid selling in a lower-multiple environment, fueling an activity-dampening cycle in which distribution-starved limited partners (LPs) reined in new commitments.
About the authors
This article is a summary of a larger report, available as a PDF, that is a collaborative effort by Fredrik Dahlqvist , Alastair Green , Paul Maia, Alexandra Nee , David Quigley , Aditya Sanghvi , Connor Mangan, John Spivey, Rahel Schneider, and Brian Vickery , representing views from McKinsey’s Private Equity & Principal Investors Practice.
Performance in most private asset classes remained below historical averages for a second consecutive year. Decade-long tailwinds from low and falling interest rates and consistently expanding multiples seem to be things of the past. As private market managers look to boost performance in this new era of investing, a deeper focus on revenue growth and margin expansion will be needed now more than ever.

Perspectives on a slower era in private markets
Global fundraising contracted.
Fundraising fell 22 percent across private market asset classes globally to just over $1 trillion, as of year-end reported data—the lowest total since 2017. Fundraising in North America, a rare bright spot in 2022, declined in line with global totals, while in Europe, fundraising proved most resilient, falling just 3 percent. In Asia, fundraising fell precipitously and now sits 72 percent below the region’s 2018 peak.
Despite difficult fundraising conditions, headwinds did not affect all strategies or managers equally. Private equity (PE) buyout strategies posted their best fundraising year ever, and larger managers and vehicles also fared well, continuing the prior year’s trend toward greater fundraising concentration.
The numerator effect persisted
Despite a marked recovery in the denominator—the 1,000 largest US retirement funds grew 7 percent in the year ending September 2023, after falling 14 percent the prior year, for example 1 “U.S. retirement plans recover half of 2022 losses amid no-show recession,” Pensions and Investments , February 12, 2024. —many LPs remain overexposed to private markets relative to their target allocations. LPs started 2023 overweight: according to analysis from CEM Benchmarking, average allocations across PE, infrastructure, and real estate were at or above target allocations as of the beginning of the year. And the numerator grew throughout the year, as a lack of exits and rebounding valuations drove net asset values (NAVs) higher. While not all LPs strictly follow asset allocation targets, our analysis in partnership with global private markets firm StepStone Group suggests that an overallocation of just one percentage point can reduce planned commitments by as much as 10 to 12 percent per year for five years or more.
Despite these headwinds, recent surveys indicate that LPs remain broadly committed to private markets. In fact, the majority plan to maintain or increase allocations over the medium to long term.
Investors fled to known names and larger funds
Fundraising concentration reached its highest level in over a decade, as investors continued to shift new commitments in favor of the largest fund managers. The 25 most successful fundraisers collected 41 percent of aggregate commitments to closed-end funds (with the top five managers accounting for nearly half that total). Closed-end fundraising totals may understate the extent of concentration in the industry overall, as the largest managers also tend to be more successful in raising non-institutional capital.
While the largest funds grew even larger—the largest vehicles on record were raised in buyout, real estate, infrastructure, and private debt in 2023—smaller and newer funds struggled. Fewer than 1,700 funds of less than $1 billion were closed during the year, half as many as closed in 2022 and the fewest of any year since 2012. New manager formation also fell to the lowest level since 2012, with just 651 new firms launched in 2023.
Whether recent fundraising concentration and a spate of M&A activity signals the beginning of oft-rumored consolidation in the private markets remains uncertain, as a similar pattern developed in each of the last two fundraising downturns before giving way to renewed entrepreneurialism among general partners (GPs) and commitment diversification among LPs. Compared with how things played out in the last two downturns, perhaps this movie really is different, or perhaps we’re watching a trilogy reusing a familiar plotline.
Dry powder inventory spiked (again)
Private markets assets under management totaled $13.1 trillion as of June 30, 2023, and have grown nearly 20 percent per annum since 2018. Dry powder reserves—the amount of capital committed but not yet deployed—increased to $3.7 trillion, marking the ninth consecutive year of growth. Dry powder inventory—the amount of capital available to GPs expressed as a multiple of annual deployment—increased for the second consecutive year in PE, as new commitments continued to outpace deal activity. Inventory sat at 1.6 years in 2023, up markedly from the 0.9 years recorded at the end of 2021 but still within the historical range. NAV grew as well, largely driven by the reluctance of managers to exit positions and crystallize returns in a depressed multiple environment.
Private equity strategies diverged
Buyout and venture capital, the two largest PE sub-asset classes, charted wildly different courses over the past 18 months. Buyout notched its highest fundraising year ever in 2023, and its performance improved, with funds posting a (still paltry) 5 percent net internal rate of return through September 30. And although buyout deal volumes declined by 19 percent, 2023 was still the third-most-active year on record. In contrast, venture capital (VC) fundraising declined by nearly 60 percent, equaling its lowest total since 2015, and deal volume fell by 36 percent to the lowest level since 2019. VC funds returned –3 percent through September, posting negative returns for seven consecutive quarters. VC was the fastest-growing—as well as the highest-performing—PE strategy by a significant margin from 2010 to 2022, but investors appear to be reevaluating their approach in the current environment.
Private equity entry multiples contracted
PE buyout entry multiples declined by roughly one turn from 11.9 to 11.0 times EBITDA, slightly outpacing the decline in public market multiples (down from 12.1 to 11.3 times EBITDA), through the first nine months of 2023. For nearly a decade leading up to 2022, managers consistently sold assets into a higher-multiple environment than that in which they had bought those assets, providing a substantial performance tailwind for the industry. Nowhere has this been truer than in technology. After experiencing more than eight turns of multiple expansion from 2009 to 2021 (the most of any sector), technology multiples have declined by nearly three turns in the past two years, 50 percent more than in any other sector. Overall, roughly two-thirds of the total return for buyout deals that were entered in 2010 or later and exited in 2021 or before can be attributed to market multiple expansion and leverage. Now, with falling multiples and higher financing costs, revenue growth and margin expansion are taking center stage for GPs.
Real estate receded
Demand uncertainty, slowing rent growth, and elevated financing costs drove cap rates higher and made price discovery challenging, all of which weighed on deal volume, fundraising, and investment performance. Global closed-end fundraising declined 34 percent year over year, and funds returned −4 percent in the first nine months of the year, losing money for the first time since the 2007–08 global financial crisis. Capital shifted away from core and core-plus strategies as investors sought liquidity via redemptions in open-end vehicles, from which net outflows reached their highest level in at least two decades. Opportunistic strategies benefited from this shift, with investors focusing on capital appreciation over income generation in a market where alternative sources of yield have grown more attractive. Rising interest rates widened bid–ask spreads and impaired deal volume across food groups, including in what were formerly hot sectors: multifamily and industrial.
Private debt pays dividends
Debt again proved to be the most resilient private asset class against a turbulent market backdrop. Fundraising declined just 13 percent, largely driven by lower commitments to direct lending strategies, for which a slower PE deal environment has made capital deployment challenging. The asset class also posted the highest returns among all private asset classes through September 30. Many private debt securities are tied to floating rates, which enhance returns in a rising-rate environment. Thus far, managers appear to have successfully navigated the rising incidence of default and distress exhibited across the broader leveraged-lending market. Although direct lending deal volume declined from 2022, private lenders financed an all-time high 59 percent of leveraged buyout transactions last year and are now expanding into additional strategies to drive the next era of growth.
Infrastructure took a detour
After several years of robust growth and strong performance, infrastructure and natural resources fundraising declined by 53 percent to the lowest total since 2013. Supply-side timing is partially to blame: five of the seven largest infrastructure managers closed a flagship vehicle in 2021 or 2022, and none of those five held a final close last year. As in real estate, investors shied away from core and core-plus investments in a higher-yield environment. Yet there are reasons to believe infrastructure’s growth will bounce back. Limited partners (LPs) surveyed by McKinsey remain bullish on their deployment to the asset class, and at least a dozen vehicles targeting more than $10 billion were actively fundraising as of the end of 2023. Multiple recent acquisitions of large infrastructure GPs by global multi-asset-class managers also indicate marketwide conviction in the asset class’s potential.
Private markets still have work to do on diversity
Private markets firms are slowly improving their representation of females (up two percentage points over the prior year) and ethnic and racial minorities (up one percentage point). On some diversity metrics, including entry-level representation of women, private markets now compare favorably with corporate America. Yet broad-based parity remains elusive and too slow in the making. Ethnic, racial, and gender imbalances are particularly stark across more influential investing roles and senior positions. In fact, McKinsey’s research reveals that at the current pace, it would take several decades for private markets firms to reach gender parity at senior levels. Increasing representation across all levels will require managers to take fresh approaches to hiring, retention, and promotion.
Artificial intelligence generating excitement
The transformative potential of generative AI was perhaps 2023’s hottest topic (beyond Taylor Swift). Private markets players are excited about the potential for the technology to optimize their approach to thesis generation, deal sourcing, investment due diligence, and portfolio performance, among other areas. While the technology is still nascent and few GPs can boast scaled implementations, pilot programs are already in flight across the industry, particularly within portfolio companies. Adoption seems nearly certain to accelerate throughout 2024.
Private markets in a slower era
If private markets investors entered 2023 hoping for a return to the heady days of 2021, they likely left the year disappointed. Many of the headwinds that emerged in the latter half of 2022 persisted throughout the year, pressuring fundraising, dealmaking, and performance. Inflation moderated somewhat over the course of the year but remained stubbornly elevated by recent historical standards. Interest rates started high and rose higher, increasing the cost of financing. A reinvigorated public equity market recovered most of 2022’s losses but did little to resolve the valuation uncertainty private market investors have faced for the past 18 months.
Within private markets, the denominator effect remained in play, despite the public market recovery, as the numerator continued to expand. An activity-dampening cycle emerged: higher cost of capital and lower multiples limited the ability or willingness of general partners (GPs) to exit positions; fewer exits, coupled with continuing capital calls, pushed LP allocations higher, thereby limiting their ability or willingness to make new commitments. These conditions weighed on managers’ ability to fundraise. Based on data reported as of year-end 2023, private markets fundraising fell 22 percent from the prior year to just over $1 trillion, the largest such drop since 2009 (Exhibit 1).
The impact of the fundraising environment was not felt equally among GPs. Continuing a trend that emerged in 2022, and consistent with prior downturns in fundraising, LPs favored larger vehicles and the scaled GPs that typically manage them. Smaller and newer managers struggled, and the number of sub–$1 billion vehicles and new firm launches each declined to its lowest level in more than a decade.
Despite the decline in fundraising, private markets assets under management (AUM) continued to grow, increasing 12 percent to $13.1 trillion as of June 30, 2023. 2023 fundraising was still the sixth-highest annual haul on record, pushing dry powder higher, while the slowdown in deal making limited distributions.
Investment performance across private market asset classes fell short of historical averages. Private equity (PE) got back in the black but generated the lowest annual performance in the past 15 years, excluding 2022. Closed-end real estate produced negative returns for the first time since 2009, as capitalization (cap) rates expanded across sectors and rent growth dissipated in formerly hot sectors, including multifamily and industrial. The performance of infrastructure funds was less than half of its long-term average and even further below the double-digit returns generated in 2021 and 2022. Private debt was the standout performer (if there was one), outperforming all other private asset classes and illustrating the asset class’s countercyclical appeal.
Private equity down but not out
Higher financing costs, lower multiples, and an uncertain macroeconomic environment created a challenging backdrop for private equity managers in 2023. Fundraising declined for the second year in a row, falling 15 percent to $649 billion, as LPs grappled with the denominator effect and a slowdown in distributions. Managers were on the fundraising trail longer to raise this capital: funds that closed in 2023 were open for a record-high average of 20.1 months, notably longer than 18.7 months in 2022 and 14.1 months in 2018. VC and growth equity strategies led the decline, dropping to their lowest level of cumulative capital raised since 2015. Fundraising in Asia fell for the fourth year of the last five, with the greatest decline in China.
Despite the difficult fundraising context, a subset of strategies and managers prevailed. Buyout managers collectively had their best fundraising year on record, raising more than $400 billion. Fundraising in Europe surged by more than 50 percent, resulting in the region’s biggest haul ever. The largest managers raised an outsized share of the total for a second consecutive year, making 2023 the most concentrated fundraising year of the last decade (Exhibit 2).
Despite the drop in aggregate fundraising, PE assets under management increased 8 percent to $8.2 trillion. Only a small part of this growth was performance driven: PE funds produced a net IRR of just 2.5 percent through September 30, 2023. Buyouts and growth equity generated positive returns, while VC lost money. PE performance, dating back to the beginning of 2022, remains negative, highlighting the difficulty of generating attractive investment returns in a higher interest rate and lower multiple environment. As PE managers devise value creation strategies to improve performance, their focus includes ensuring operating efficiency and profitability of their portfolio companies.
Deal activity volume and count fell sharply, by 21 percent and 24 percent, respectively, which continued the slower pace set in the second half of 2022. Sponsors largely opted to hold assets longer rather than lock in underwhelming returns. While higher financing costs and valuation mismatches weighed on overall deal activity, certain types of M&A gained share. Add-on deals, for example, accounted for a record 46 percent of total buyout deal volume last year.
Real estate recedes
For real estate, 2023 was a year of transition, characterized by a litany of new and familiar challenges. Pandemic-driven demand issues continued, while elevated financing costs, expanding cap rates, and valuation uncertainty weighed on commercial real estate deal volumes, fundraising, and investment performance.
Managers faced one of the toughest fundraising environments in many years. Global closed-end fundraising declined 34 percent to $125 billion. While fundraising challenges were widespread, they were not ubiquitous across strategies. Dollars continued to shift to large, multi-asset class platforms, with the top five managers accounting for 37 percent of aggregate closed-end real estate fundraising. In April, the largest real estate fund ever raised closed on a record $30 billion.
Capital shifted away from core and core-plus strategies as investors sought liquidity through redemptions in open-end vehicles and reduced gross contributions to the lowest level since 2009. Opportunistic strategies benefited from this shift, as investors turned their attention toward capital appreciation over income generation in a market where alternative sources of yield have grown more attractive.
In the United States, for instance, open-end funds, as represented by the National Council of Real Estate Investment Fiduciaries Fund Index—Open-End Equity (NFI-OE), recorded $13 billion in net outflows in 2023, reversing the trend of positive net inflows throughout the 2010s. The negative flows mainly reflected $9 billion in core outflows, with core-plus funds accounting for the remaining outflows, which reversed a 20-year run of net inflows.
As a result, the NAV in US open-end funds fell roughly 16 percent year over year. Meanwhile, global assets under management in closed-end funds reached a new peak of $1.7 trillion as of June 2023, growing 14 percent between June 2022 and June 2023.
Real estate underperformed historical averages in 2023, as previously high-performing multifamily and industrial sectors joined office in producing negative returns caused by slowing demand growth and cap rate expansion. Closed-end funds generated a pooled net IRR of −3.5 percent in the first nine months of 2023, losing money for the first time since the global financial crisis. The lone bright spot among major sectors was hospitality, which—thanks to a rush of postpandemic travel—returned 10.3 percent in 2023. 2 Based on NCREIFs NPI index. Hotels represent 1 percent of total properties in the index. As a whole, the average pooled lifetime net IRRs for closed-end real estate funds from 2011–20 vintages remained around historical levels (9.8 percent).
Global deal volume declined 47 percent in 2023 to reach a ten-year low of $650 billion, driven by widening bid–ask spreads amid valuation uncertainty and higher costs of financing (Exhibit 3). 3 CBRE, Real Capital Analytics Deal flow in the office sector remained depressed, partly as a result of continued uncertainty in the demand for space in a hybrid working world.
During a turbulent year for private markets, private debt was a relative bright spot, topping private markets asset classes in terms of fundraising growth, AUM growth, and performance.
Fundraising for private debt declined just 13 percent year over year, nearly ten percentage points less than the private markets overall. Despite the decline in fundraising, AUM surged 27 percent to $1.7 trillion. And private debt posted the highest investment returns of any private asset class through the first three quarters of 2023.
Private debt’s risk/return characteristics are well suited to the current environment. With interest rates at their highest in more than a decade, current yields in the asset class have grown more attractive on both an absolute and relative basis, particularly if higher rates sustain and put downward pressure on equity returns (Exhibit 4). The built-in security derived from debt’s privileged position in the capital structure, moreover, appeals to investors that are wary of market volatility and valuation uncertainty.
Direct lending continued to be the largest strategy in 2023, with fundraising for the mostly-senior-debt strategy accounting for almost half of the asset class’s total haul (despite declining from the previous year). Separately, mezzanine debt fundraising hit a new high, thanks to the closings of three of the largest funds ever raised in the strategy.
Over the longer term, growth in private debt has largely been driven by institutional investors rotating out of traditional fixed income in favor of private alternatives. Despite this growth in commitments, LPs remain underweight in this asset class relative to their targets. In fact, the allocation gap has only grown wider in recent years, a sharp contrast to other private asset classes, for which LPs’ current allocations exceed their targets on average. According to data from CEM Benchmarking, the private debt allocation gap now stands at 1.4 percent, which means that, in aggregate, investors must commit hundreds of billions in net new capital to the asset class just to reach current targets.
Private debt was not completely immune to the macroeconomic conditions last year, however. Fundraising declined for the second consecutive year and now sits 23 percent below 2021’s peak. Furthermore, though private lenders took share in 2023 from other capital sources, overall deal volumes also declined for the second year in a row. The drop was largely driven by a less active PE deal environment: private debt is predominantly used to finance PE-backed companies, though managers are increasingly diversifying their origination capabilities to include a broad new range of companies and asset types.
Infrastructure and natural resources take a detour
For infrastructure and natural resources fundraising, 2023 was an exceptionally challenging year. Aggregate capital raised declined 53 percent year over year to $82 billion, the lowest annual total since 2013. The size of the drop is particularly surprising in light of infrastructure’s recent momentum. The asset class had set fundraising records in four of the previous five years, and infrastructure is often considered an attractive investment in uncertain markets.
While there is little doubt that the broader fundraising headwinds discussed elsewhere in this report affected infrastructure and natural resources fundraising last year, dynamics specific to the asset class were at play as well. One issue was supply-side timing: nine of the ten largest infrastructure GPs did not close a flagship fund in 2023. Second was the migration of investor dollars away from core and core-plus investments, which have historically accounted for the bulk of infrastructure fundraising, in a higher rate environment.
The asset class had some notable bright spots last year. Fundraising for higher-returning opportunistic strategies more than doubled the prior year’s total (Exhibit 5). AUM grew 18 percent, reaching a new high of $1.5 trillion. Infrastructure funds returned a net IRR of 3.4 percent in 2023; this was below historical averages but still the second-best return among private asset classes. And as was the case in other asset classes, investors concentrated commitments in larger funds and managers in 2023, including in the largest infrastructure fund ever raised.
The outlook for the asset class, moreover, remains positive. Funds targeting a record amount of capital were in the market at year-end, providing a robust foundation for fundraising in 2024 and 2025. A recent spate of infrastructure GP acquisitions signal multi-asset managers’ long-term conviction in the asset class, despite short-term headwinds. Global megatrends like decarbonization and digitization, as well as revolutions in energy and mobility, have spurred new infrastructure investment opportunities around the world, particularly for value-oriented investors that are willing to take on more risk.
Private markets make measured progress in DEI
Diversity, equity, and inclusion (DEI) has become an important part of the fundraising, talent, and investing landscape for private market participants. Encouragingly, incremental progress has been made in recent years, including more diverse talent being brought to entry-level positions, investing roles, and investment committees. The scope of DEI metrics provided to institutional investors during fundraising has also increased in recent years: more than half of PE firms now provide data across investing teams, portfolio company boards, and portfolio company management (versus investment team data only). 4 “ The state of diversity in global private markets: 2023 ,” McKinsey, August 22, 2023.
In 2023, McKinsey surveyed 66 global private markets firms that collectively employ more than 60,000 people for the second annual State of diversity in global private markets report. 5 “ The state of diversity in global private markets: 2023 ,” McKinsey, August 22, 2023. The research offers insight into the representation of women and ethnic and racial minorities in private investing as of year-end 2022. In this chapter, we discuss where the numbers stand and how firms can bring a more diverse set of perspectives to the table.
The statistics indicate signs of modest advancement. Overall representation of women in private markets increased two percentage points to 35 percent, and ethnic and racial minorities increased one percentage point to 30 percent (Exhibit 6). Entry-level positions have nearly reached gender parity, with female representation at 48 percent. The share of women holding C-suite roles globally increased 3 percentage points, while the share of people from ethnic and racial minorities in investment committees increased 9 percentage points. There is growing evidence that external hiring is gradually helping close the diversity gap, especially at senior levels. For example, 33 percent of external hires at the managing director level were ethnic or racial minorities, higher than their existing representation level (19 percent).
Yet, the scope of the challenge remains substantial. Women and minorities continue to be underrepresented in senior positions and investing roles. They also experience uneven rates of progress due to lower promotion and higher attrition rates, particularly at smaller firms. Firms are also navigating an increasingly polarized workplace today, with additional scrutiny and a growing number of lawsuits against corporate diversity and inclusion programs, particularly in the US, which threatens to impact the industry’s pace of progress.
Fredrik Dahlqvist is a senior partner in McKinsey’s Stockholm office; Alastair Green is a senior partner in the Washington, DC, office, where Paul Maia and Alexandra Nee are partners; David Quigley is a senior partner in the New York office, where Connor Mangan is an associate partner and Aditya Sanghvi is a senior partner; Rahel Schneider is an associate partner in the Bay Area office; John Spivey is a partner in the Charlotte office; and Brian Vickery is a partner in the Boston office.
The authors wish to thank Jonathan Christy, Louis Dufau, Vaibhav Gujral, Graham Healy-Day, Laura Johnson, Ryan Luby, Tripp Norton, Alastair Rami, Henri Torbey, and Alex Wolkomir for their contributions
The authors would also like to thank CEM Benchmarking and the StepStone Group for their partnership in this year's report.
This article was edited by Arshiya Khullar, an editor in the Gurugram office.
Explore a career with us
Related articles.

CEO alpha: A new approach to generating private equity outperformance
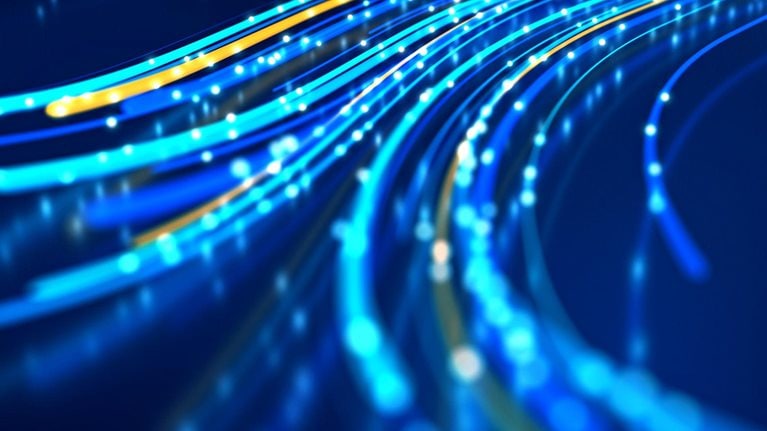
Private equity turns to resiliency strategies for software investments

The state of diversity in global private markets: 2022
Stock prediction and mutual fund portfolio management using curve fitting techniques
- Original Research
- Published: 02 January 2021
- Volume 12 , pages 9521–9534, ( 2021 )
Cite this article
- Giridhar Maji 1 ,
- Debomita Mondal 2 ,
- Nilanjan Dey 3 ,
- Narayan C. Debnath 4 &
- Soumya Sen ORCID: orcid.org/0000-0002-9178-6410 2
7690 Accesses
12 Citations
Explore all metrics
Investment in the share market helps generate more profit than the other financial instruments but has the threat of market risk that might lead to a high loss. This risk factor refrains many potential investors from investing in the share market directly. Instead, they invest in different mutual funds that are being managed by experienced portfolio managers. To avoid the risk factors and increase the gain, they put the accumulated capital in multiple stocks. They need to perform many calculations and predictions to overcome the uncertainties and unpredictability and need to ensure higher gains to the investors of that mutual fund. In this research work initially, a data mining based approach employs a curve fitting/regression technique to forecast the individual stock price. Based on the above analysis, we propose a framework to diversify the investment of the capital fund. This method employs buy and hold strategy using both statistical features and basic domain knowledge of the share market. The proposed framework distributes the capital first, by distributing sector-wise, and then for each sector, investing company-wise, as a diversified approach among different stocks for higher return but maintaining lower risks. Experimental results show that the proposed framework performs well and generates a good yield compared to some benchmark and ranked mutual funds in the Indian stock market.
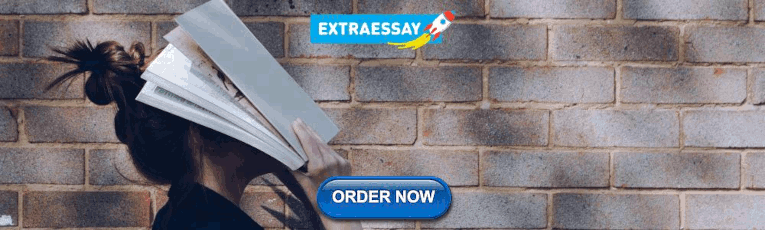
Similar content being viewed by others
A Predictive System for Efficient Portfolio Management: An Application of ANN and Technical Indicators
Stock market prediction and portfolio selection models: a survey.
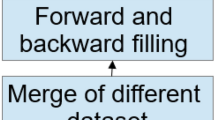
Portfolio optimization in stocks using mean–variance optimization and the efficient frontier
Avoid common mistakes on your manuscript.
1 Introduction
A company’s future growth and hence return to the shareholders depends on a lot of internal and external factors. A mutual fund (MF) manager collects funds from many different clients and then invests in many different stocks, bonds, and many other money market instruments to earn a good return. The size of the MF industry in India is around INR 26.07 trillion as of June 30, 2020, and the corpus size has increased up to 4 times in the last 10 years (Association of Mutual Funds in India, 2020). It implies a whopping 400% growth in just over 10 years that indicates people’s interest to invest in MFs. This sustained trend shows that general people (retail investors) are investing heavily in different MFs. One of the reasons for interest in an MF is that most investors have the appetite for higher returns but do not want to take the risk to invest in the share market directly. They feel it safer to invest in the market indirectly via some trusted MFs. Another reason for interest in MFs is the falling interest rate on the bank’s fixed deposits. Again, due to the high inflation, the effective yield from fixed deposit becomes negligible. Hence general people look into the different investment alternatives that will give reasonable returns with minimum possible uncertainties. Generally, the investment in individual stock may earn a good profit, but it also risks an unbounded loss. Therefore, individual investors often avoid investing in the share market directly. Instead, they prefer MFs because these are managed by expert professionals (portfolio managers) and invest in multiple stocks belonging to different sectors to mitigate the risk factors.
There are many different types of mutual funds in the market with varied stated objectives and investment time-horizons. The fixed income MFs (aka debt funds) generally invest in secured instruments that guarantee capital protection but yield less return. Again, the equity-based MFs are the high-risk, high-return group where most of the capital goes into buying shares of companies listed in the stock exchanges. The hybrid and balanced MFs are medium risk funds where total corpus gets invested into risk-free, secured bonds, and high-risk equity stocks.
There are many other varieties of MFs such as (1) a gold fund that mainly invests in gold, (2) the gilt funds that earn from investing in fixed interest government securities, (3) the Exchange Traded Funds (ETF) (Agapova 2011 ), where a proportion of different stocks are combined and listed in the stock market for trading like a single stock, (4) Equity-linked savings scheme (ELSS) with a minimum lock-in period of 3 years, during which investors can not redeem. It also provides certain tax benefits.
In this research work, we consider only equity stocks though other money market instruments may very well be incorporated. Here we take different company stocks from varied business domains. This research work is generic for analysis on all the share markets and mutual funds across the world. We have chosen the Indian share market for the experiments and analysis purposes. The SENSEX is the indexing measure of the Bombay Stock Exchange (BSE) and comprises thirty well-established and well-performing companies (BSE 2019a). The NIFTY 50 is a well-known index representing the National Stock Exchange (NSE) and is Indiaś benchmark stock market index for the Indian equity market. It covers around twenty-two sectors of the Indian economy and offers investment manager exposure to the Indian market in one portfolio. Some of the companies in NIFTY from different sectors are ACC Ltd. (Cement), Asian Paints (Paints), Axis Bank (Private Bank), BHEL (Electric Equipment), Bharti Airtel (Telecommunication), Cipla (Pharmaceuticals), Coal India (Mining and Metals), ITC (Cigarette Hotels), Infosys (IT and Software), L&T, ONGC (Oil and Gas), SBI (public bank), TCS (IT and Software), Tata Steel (Steel), Zee entertainment (Entertainment), etc. (NSE 2019). Most of the above stocks also belong to SENSEX. We understand that the SENSEX covers almost all the industrial sectors, and therefore tracking its performance will give the pulse of the whole stock market.
Historical stock price movement data, as well as many other time-series data related to the internal as well as external factors associated to a particular stock or a group of similar stocks (like banking stocks, Metal stocks, automobile stocks, IT stocks, etc.), can be analytically processed by different data mining tools (Liao et al. 2012 ; Hajizadeh et al. 2010 ; Zhang and Zhou 2004 ) to predict the future movement of the stock market. However, there is no guaranty that the result of the analysis will be accurate. As many external factors are involved, many well-performing stocks may suddenly become loss-making. One of the most convincing examples is the spreading of Coronavirus Disease (COVID-19) (Fong et al. 2020 ) across the world. Due to lockdown in many parts of the world, economic activities reduced drastically, resulting in a rapid fall of share prices across the globe. COVID-19 was an unplanned event and affected almost all business sectors. However, some pharmaceutical companies, gloves makers, sanitizer makers, etc. have benefited due to the sudden increase in demand for their products. It implies that it is safe to invest in a different sector to reduce the risk of investment. Even if a company from a well-performing business sector may go through some financial loss, bankruptcy, or scams that can lead to a sharp fall in the share price. It suggests investing in multiple companies belong to the same business domain. It becomes difficult for many individual investors to keep track of the several companies belonging to the different sectors and maintain a diversified portfolio. Henceforth to stay invested in the stock market with a diversified portfolio, a mutual fund is the best option for many retail investors. It gives different AMCs (Asset Management Companies) opportunities to introduce new mutual funds to the market with varying risk factors, return expectations, etc. The competition is increasing between different AMCs to attract more investors. The role of the portfolio manager is getting challenging day-by-day. In this research work, we focus on developing a framework for the portfolio manager to maintain a diversified portfolio with less risk and high return probability. The main contributions of the paper are as follows.
At first, we do a business level analysis on the individual stock to perform a data mining-based approach by applying curve fittings (regression) to find the accuracy of the prediction curve.
In the next level, based on these predictions, a framework is derived for the portfolio managers to invest in diversified stocks to increase the return on investment.
The rest of the paper is organized as follows. Related works are discussed in Sect. 2 , followed by the proposed framework and methodology employed for mutual fund portfolio management in Sect. 4 . Experimental results with BSE stock market data are presented in Sect. 5 . Finally, the study is concluded in Sect. 6 with the future direction of work.
2 Related work
A large number of studies have been conducted on stock exchange index movement forecast and individual stock price prediction using data mining and statistical analysis (Chikhi and Diebolt 2010 ). Stock market movement and the impact of different intrinsic and external factors such as gold price, crude oil price, political stability, dollar exchange rate, bank interest rate, etc. on the stock market are discussed in Mondal et al. ( 2018 ). A review of the stock market is presented in Rusu and Rusu ( 2003 ), Atsalakis and Valavanis ( 2009 ) and Preethi and Santhi ( 2012 ). Authors have identified three different categories of analysis: fundamental analysis, technical analysis, and efficient market theory.
The fundamental analysis performed on the basis of macroeconomic factors such as prevailing interest rate, inflation data, industry/sector a company belongs to, profit/loss of the company, the amount of dividend paid, brand value, crude oil price (Zhu et al. 2014 ), gold price (Weng et al. 2020 ), foreign capital inflow etc. It employs statistical models and uses financial algorithms to the financial statements and other periodical market information to estimate the share price of the company.
The underlying assumption of the technical analysis is that stock price variation itself incorporates the effects of all economic, political (Narang 2015 ), financial, and external factors. Technical analysis studies the short-term changes in stock price with the expectation that historical stock prices behavior will continue in the near future. Accurate and clean historical data such as days closing price, the dividend paid, bonus share issued, split ratio, buyback of shares, etc., are abundant from stock exchanges. Hence, it becomes promising to employ data mining techniques to predict the future trends of stock prices for investors on these datasets.
Important properties of the efficient market theory say (1) market prices are known to all stakeholders as soon as they change, (2) all related market information is freely available to all participants, (3) share prices reflect their fundamental values, and (4) stock prices follow a random walk.
Many neuro-fuzzy (Asadi 2019 ; Chandar 2019 ) models were proposed and different techniques like Artificial Neural Network with backpropagation (Mingyue et al. 2016 ; Singh and Mohanty 2018 ), Recurrent Neural Network (RNN) (Zhao et al. 2020 ; Ghosh et al. 2019 ), evolutionary techniques like genetic algorithms (Weng et al. 2020 ; Ding et al. 2020 ), the firefly algorithm combined with the support vector regression (Zhang et al. 2019 ), the linear regression (Altay and Satman 2005 ; Ji et al. 2020 ) etc. were applied to predict stock prices. Zhang and Zhou ( 2004 ) reviewed data mining in the context of financial application from both technical and application perspectives. Then they compared different data mining techniques to solve financial problems and discuss important data mining issues involved in specific financial applications. Most of the above models other than regression are complex in design, use many parameters which are difficult to select, model settings such as the number of hidden layers, initial values of variables, use of optimizers, etc. They require a very long training phase, and the problem of over-fitting or under-fitting exists too.
Chandar ( 2019 ) utilized discrete wavelet transform (DWT) to decompose time series data and combined it with an adaptive fuzzy inference system to forecast the closing price of a stock. Data visualization (Moere and Offenhuber 2009 ) is important to represent the movement of the stocks and to understand the performance using the visual tools. This aspect enables the ambient computing (Curran 2011 ) by integrating cognitive processing with the visualization for better human interaction and understanding of the system.
Gottschlich and Hinz ( 2014 ) proposed a decision support system design that enables investors to include the crowd’s recommendations in their investment decisions and use it to manage a portfolio. Here a serious concern is the presence of noise in the data. Common investors (here the crowd) have much less in-depth knowledge regarding the intricacies of the stock market and stock price movement based on sporadic rumors or speculation does not yield stable growth or returns. Hence it may provide higher gains for a very short period but may not be a beneficial tool for a stable mutual fund portfolio management.
Qian and Rasheed ( 2007 ) employed the Hurst exponent to select a time window when prediction probability would be higher and then used different machine learning techniques to be trained with data from that time period. They used different heuristics to generate the parameters for classification and tested on the Dow Jones Industrial Average index to achieve an average accuracy of 65%. In the context of the present study, it would not be wise to adapt as an MF portfolio that runs throughout the year, and it can not be open to investment for a selected time window. Also, the complexity of the above method will be rigorous when used for a bucket of different stocks.
Park et al. ( 2019 ) used stock indices movement data of eight countries and used them to predict S&P index movement. They have considered a total of 10 years day’s closing index values and calculated their movement as upward and downward to use different binary classifiers such as support vector machine (SVM), k-nearest neighbor (KNN), probabilistic neural network (PNN) etc. Ananthi and Vijayakumar ( 2020 ) have recently applied KNN based regression model on different market indicator like MACD , RSI , Bollinger bands , and candle stick patterns to generate buy/sell signals. The main limitation here is the forecasting horizon as the above work can only predict for a few days in advance, but we observe that regression based tools yield comparable prediction without large computational burden.
Maji et al. ( 2017 ) considered lagged correlation among the upward and downward movement of a sectoral index with some day-lag and applied association rule mining to predict future movement with a forecasting horizon the same as the day-lag. Authors have used Association Rule Mining (ARM) to find the correlation between different stock prices and generate association rules between different stock prices. Baralis et al. ( 2017 ) employed frequent itemset mining to supporting buy-and-hold investors in technical analyses by automatically identifying promising sets of high-yield yet diversified stocks to buy. Both of the above works forecast for a very limited time window, a few days in the future, and most useful for short term traders.
A stock market portfolio recommender system has been proposed in (Paranjape-Voditel and Deshpande 2013 ). Sankar et al. ( 2015 ) proposed a trust-based stock recommender system utilizing social network analysis techniques. They have utilized the stock holding and buying/selling information from other trusted mutual funds to recommend stocks. This approach is entirely dependent on the collective wisdom of other fund managers. Some authors propose recommender systems based on users’ contextual short term information and sentiment analysis (Richthammer and Pernul 2018 ; Gottschlich and Hinz 2014 ). All of the stock recommender systems are again useful to individual regular traders for short term gains.
Majority of the research works discussed above focused on the share market directly by forecasting the future price or movement direction. In almost all the cases (except Maji et al. 2017 ; Paranjape-Voditel and Deshpande 2013 ), many financial indicators (short-term, long-term, macro-economic, and micro-economic parameters) have been used to predict the future price.
Our framework is based on the assumption that historical stock prices incorporate the impact of all such parameters. Hence, we simplify our framework by only considering historical stock prices as a proxy to all such financial parameters. A point to note is that investors who invest in the share market look into the closing price of different periods to check the growth or decay for that period. The closing price of the stock is the standard representation of the stocks for historical analysis.
We observe that financial time series regression tools are the most widely used and simple to compute. Many recent studies utilized regression techniques in stock price forecasting. To keep the computations simple and easy, without compromising on forecasting accuracy, we have employed regression tools in the initial prediction phase of our proposed framework of MF portfolio management.
3 Problem statement and contribution
Formation of a mutual fund portfolio to avoid the risk of investment on individual/few stocks by investing in multiple stocks belonging to different business sectors. It is difficult to manage and analyze many stocks at a time. Henceforth a framework is required to work with multiple stocks from various business sectors to increase the profit over time but reducing the risk factors.
Contributions
Analysis is performed only on the closing stock price but it includes the effect of various parameters such as dividend, bonus, split, etc. This simplifies the overhead of analysing with multiple parameters and avoids the use of complex tools.
Diversified portfolio management by analyzing multiple stocks (company-wise) from different business sectors (sector-wise).
Analysis and comparison of the different business sectors performance wise.
A systematic portfolio management framework to increase the return over a longer period but minimizing the risks.
Flexible to support the analysis on individual stock, group of stocks, business domains and that for any time period.
4 Proposed framework to forecast and allocate fund across sectors
This section derives a framework for portfolio managers to invest for many different time horizons in stocks to maximize investors’ gain. Here, the analysis is done on multiple sectors to ensure diversified portfolio management to reduce investors’ risk.
As already discussed that there are various perspectives of investors while investing their funds to gain more profit. Share markets are highly sensitive, and there are numerous factors directly or indirectly associated with it that control the market sentiment (Gottschlich and Hinz 2014 ). A group of investors foresees an appreciation in stock valuation so, they buy stocks at the current market price and expect to sell them in future and book profit. Again, at the same time, another group of investors assumes that stock prices will fall in the future so, they sell at the current market price, and in the future, they may buy again at dips. In reality, there exists simultaneously many sellers and buyers in the market due to the different perception of future stock valuation. In the future, any one group of investors will benefit, and others may have to incur a loss. Many different statistical techniques and time-series analyses have been used with mixed success. We aim to use well-tested regression techniques as a starting tool to screen individual stocks along with domain knowledge in bucketing company stocks into industrial sectors. Therefore, the objective is to propose an analytical approach to predict the share price of different companies and invest total capital across various diversified sectors to earn better profit percentages and mitigate the overall risk. Moreover, we aim to maximize the profit over time as the investors prefer to get a higher return for long term investments.
4.1 Analysis of different statistical method and finding a suitable one
Statistics being the body of methods meant for the study of numerical data, the first step in any statistical inquiry must be a collection of relevant numerical data. Once data are collected, a knowledge discovery may analyze the behavior of the data. However, as the different data are different, a suitable statistical method is to be identified for data processing belongs to a particular application.
In this present work, one of the primary challenges is finding a preferable method that would help us forecast/predict the companies’ share value. However, in reality, it is impossible to predict the share value of the companies accurately, as several issues control the movement of the share market. Many parameters may not be directly related to the up/down of the share market, but indirectly related to the share market by affecting parameters responsible for changing the share value directly. Even some new cases/issues (e.g., COVID-19, terrorist attacks, etc.) may evolve in the future that is unknown at the time of analysis. The proposed prediction model will be based on historical prices of shares as it reflects the effects of all events and parameters that determine the stock prices.
There are several statistical methods such as standard deviation , linear regression , non-linear regression, correlation , time series analysis , etc. that are useful for prediction. Standard deviation is the simplest one. The result produced by the standard deviation is used for basic calculation. It gives a high error rate for complex data. Therefore, for more satisfactory precision, it is not a suitable method.
In correlation analysis, the concern is the mutual relationship between the two variables. It uses a measure of the interdependence of the two variables, known as the correlation coefficient.
Because of the close connection between the correlation coefficient and linear regression, it is clear that the former can serve as a satisfactory measure of the strength of the relationship between the two variables only when that relationship is of the linear type. Hence a low value of the correlation coefficient does not rule out the possibility that the variables are related in some other manner. However, as the problem domain is the capital market, it might be possible that the correlation coefficient is small in the measure. Therefore, while dealing with real financial time series data, it is not possible to guarantee that the correlation coefficient remains greater than a particular value all the time. For this limitation, correlation is not considered in the present work as a tool of prediction.
In the share market, the stock prices of companies change over time, and it does not maintain any specific pattern. Different companies follow different patterns of change. Not only this, but a particular company may also show varying patterns over time. Therefore, it is necessary to understand the pattern of change in the share price. Once the values of the shares plotted against time, different types of curves get generated. In mathematical analysis, several forms of curves are identified over time. Among these numerous forms, we intend to look for the one that best fits the given pattern.
There are several forms of curves used in mathematical analysis. Out of those commonly used are listed below in Table 1 . Now the best-fitted form of curve is identified by comparing the actual values with the values generated by solving the regression equation associated with each type of curve. In this problem domain, the independent variable is the time ( x ) and the dependent variable is the closing stock price ( y ).
This comparison helps to calculate the error value for each curve defined above. Now the curve for which the minimum error value is obtained is identified as the best-fitted curve to represent the share movement of that particular company.
Regression analysis is very much useful, as it helps us in the prediction, and forming the curves which help to compute the error values. In regression analysis, one of the two variables (say x ) is the independent ’ variable and the other ( y ) as the dependent ’ variable, and the objective is to be investigate the dependence of the variable y on the independent variable x . The major problem in the case of linear regression is to express the relationship between y and x through a mathematical function such as a linear equation and, then only it is possible to use the resulting equation to predict y in terms of x .
Non-linear regression is another variation of regression techniques. But in the case of non-linear regression, there is no hard and first rule to establish the relationship between two variables in a linear pattern like linear regression. The relationship between the two variables in non-linear regression is non-linear.
4.2 Methodology
One of the main objectives is to predict the share price of an individual company. Actual share prices plotted in a 1-month interval up to the current year. The present-day stock price of a company is estimated using regression on the historical price data (say last P monthly closing price). The regression method is applied to solve the different types of curve-fitting models and compare those values with the current one (actual market price). Next, the difference between the estimated price and the actual price is computed. The percentage of difference gives the error rate for each type of curve. The minimum squared error percentage value would be chosen and, therefore, the corresponding curve would be identified as the best-fitted curve, and the corresponding share value is considered for further processing. The best fit curve then predicts the stock price for the next time period for the company. The overall flow of the methodology is depicted in Fig. 1 .
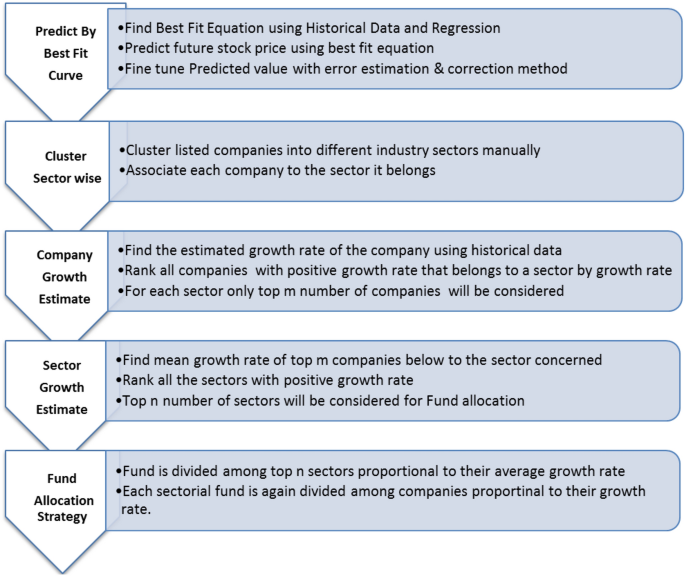
Overview of different phases of the proposed investment strategy
In order to get better prediction value refinement is done on the predicted value generated so far (using the best-fit regression line) by removing some error to some extent, by implementing an error estimation technique discussed below.
In the case of the share market, the impact of the share price of recent years is more influential than the previous years. However, a long-time analysis is also required to understand the trend of the particular stock. With these issues as critical consideration, It uses data for a longer time with a higher impact on recent prices in the proposed formulation. It puts more weight in recent years and gradually decrease the weight for previous years. Then after calculating an error measure, It adjusts on the error value with the predicted value for better estimation.
It gives a set of predicted values of different companies, and that is the input data set for further activities. Therefore, it is needed to allocate the fund into the market such that the net return is comparatively higher. Investing total funds into a single company would not always maximize the returns as a company showing a higher growth-rate might not continue the same in the future. The same is also true for the companies having a low-profit percentage at present, might show better return in the future due to different factors such as the launching of new products, new investment from the investors, acquisition, etc. Therefore, the capital should be invested across many companies (diversified investment) that belong to diverse industry sectors to maintain better returns while reducing the risk. The proposed strategy is to diversify into many sectors for better portfolio management.
After predicting the share values, the companies are clustered sector-wise for diversified fund allocation. These different sectors with a different rate of growth are identified. Therefore, we propose a mathematical approach to allocate funds sector-wise.
Before the allocation of funds, it needs to calculate the growth rate of all individual companies belongs to a sector. The calculation is on the growth rate between the two same time period for all the companies and to give more focus on the recent growth rate as compared to the older period. Some weighting factors are set for the previous periods that would get multiplied with their corresponding growth rate where their sum up gives the overall growth of that company. Similarly, we need to calculate the growth rate of all the companies within a sector. Then, the mean of the growth rate of all the companies except those with negative growth would reflect the overall growth rate of a particular industry sector. Likewise, the net growth rate of all the sectors is calculated. The philosophy here is to allocate a bigger chunk of the fund to the high growth sectors and less to the moderately growing sectors. The same logic is applied while selecting the candidate companies within an industry sector.
4.3 Algorithm for diversified fund allocation across Companies as well as sector-wise to maximize the net return
In the proposed methodology, the prediction of the current share value is done based on the data from the previous p months. The month-wise weight ( \(Y_i\) ) is used for calculating the impact of growth/fall of the price several times in the proposed algorithm for consecutive years to compute the error values and in case of the computing growth rate of an individual company in subsequent years. It is calculated for the i th month using Eq. ( 1 ):
Different industry sectors are identified by consulting financial news sources and NSE and BSE web portals. Companies that belong to these sectors are also available from the same sources. Historical stock prices of those listed companies are also available from NSE and BSE portal (BSE 2019b).
[PREDICTION OF STOCK PRICE OF SELECTED COMPANIES]
A: [IDENTIFYING BEST FITTED CURVE AND DATA SET GENERATION]
i: Collect the historical stock prices of a company for the last p months. This constitutes the initial dataset for the model.
ii: Solve different curve-fitting models by a regression method to predict the stock price for different time periods between p and 0 months (present). Therefore, generating some predicted share values of that company across the different time period.
iii: Calculate the percentage deviation of the forecasted values from the actual values.
iv: Choose the curve as best fitted for which the rate of deviation ( Root Mean Square Error (RMSE)) is lowest and R-squared value is maximum then put the predicted values of the corresponding curve in our data set.
v: Predict the share value (for historical data for which actual prices are also known) after a certain period (when we would need to withdraw our fund may be after 3, 6, 12 months in future) by solving the best-fitted curve using regression. Insert the predicted share value into the dataset.
vi: Repeat the steps from 1.A.i to 1.A.v for every company to generate our data set.
B. [FINE-TUNING OF PREDICTED STOCK PRICE BY ERROR ESTIMATION]
i: Calculate the deviation of predicted share value from the actual value at each considered time period
ii: Suppose the deviations at P different time periods are, \(\ldots ,d_p\) . Where \(d_k\) is the deviation of share value corresponding to its actual share value k period (months) earlier.
iii: Calculate the Company Net Error ( CNE ) by the following formula:
where \(CNE_j\) is the Company Net Error of the \(j\) th company (where \(j=1 \ldots m\) ). Y values for each time period are calculated following Eq. ( 1 ).
iv: Add the \(CNE_j\) to the corresponding predicted share value of the company to generate FPV ( Final Predicted Value ) and insert FPV into our data set.
v: Repeat step 1.B.i to 1.B.iv until FPV ( Final Predicted Value ) of all the companies get calculated.
[BUSINESS DOMAIN IDENTIFICATION]
Group all listed companies into different industry sectors or domains based on the business areas of the company with the help of domain experts.
[SELECTION OF SECTORS AND COMPANIES BELONG TO THAT SECTOR FOR INVESTMENT]
A: [COMPANY-WISE WITHIN A SECTOR]
i: Pick up a company from a particular sector.
ii: Find the percentages of the growth rate of the company for different time periods with respect to the month immediately earlier. Calculate this into present day from the initial time period (say p months ago). Suppose the growth rate between i th previous month and \((i-1)\) th previous month is \(Gr_i\) , where \(i=1\) to P , considering current month as 0th month. Therefore, \(Gr_i\) is the growth rate of \((i-1)\) th time period w.r.t its immediate earlier month i.e. i th month. To maximize the impact of current growth over the growth of older year, we would develop a mathematical formula stated below. Suppose the growth rates of a company are \(Gr_1, Gr_2, \ldots , Gr_p\) respectively from present to P years earlier.
iii: Calculate the Company Net Growth Rate (CNGR) by the following formula:
Where \(CNGR_j\) is the Company Net Growth Rate of the \(j^{th}\) company (where j=1 to m). \(Y_i\) is calculated following Eq. ( 1 ).
iv: Repeat step 3.A.i to 3.A.iii until Company Net Growth Rate ( CNGR ) of all the companies of that particular sector gets calculated.
v: Consider only the companies having positive growth rate to invest our fund and discard all the companies having a negative growth rate for that time period.
B: [SECTORWISE]
Calculate the net growth rate of a particular sector by finding the mean value of the growth rate of all the companies belong to that sector.
Repeat step 3.A and 3.B for each sector.
4: [ALLOCATION OF FUND]
In the case of fund allocation, the motive is to allocate more funds in such sectors and companies having better growth rate over the sectors and the companies having a lower rate of growth to enlarge overall profit. Say overall fund is F .
A. [SECTORWISE]
i. Find out the Sector Multiplying Factor ( SMF ) by the following formula:
Where \(G_i\) is the growth rate of sectors \(S_i\) , n is the number of sectors selected for investment.
ii: Determine the sector-wise fund to be invested by the mathematical formula given below:
Where \(SA_i\) denotes sector-wise percentage allocation. Thus sector-wise allocation is given by
iii: Repeat step 4.A.i to step 4.A.ii for all the selected sector.
B. [COMPANYWISE] Repeat for each sector \(S_i\) where \(i=1\) to n Let each sector \(S_i\) consists of m number of companies \(C_1\) to \(C_m\) with growth percentages of \(G_1\) to \(G_m\) respectively.
i: Find out the Company Multiplying Factor ( CMF ) by the following formula:
Where \(G_j\) is the growth rate of a sector containing m number of companies \(C_j\) (where \(j=1 \ldots m\) ) respectively.
ii: Determine the company wise fund to be invested by the mathematical formula given below: \( CA_k = g_k * CMF \) for sector \(C_i\) . (where \(k = 1 \ldots m\) ) Where \(CA_k\) denotes company wise allocation percentage wise Thus company wise allocation is given by
iii: End of Repeat Step 4B
5 Experimental results
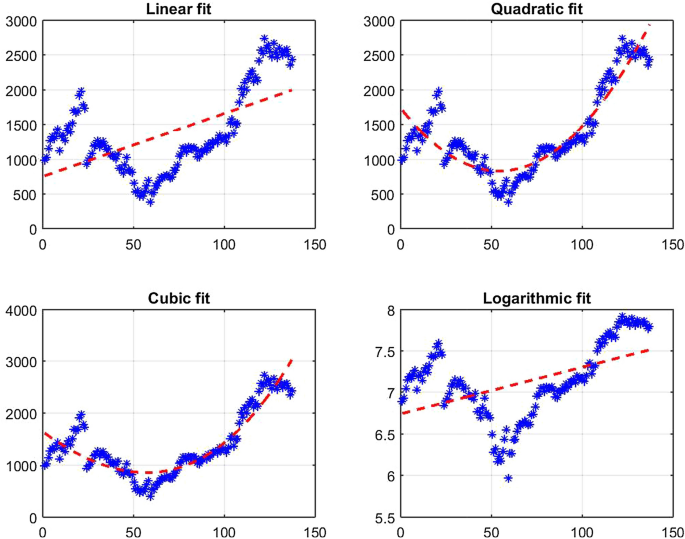
Different curves fitting with monthly closing stock prices of TCS
In this experiment, 137 months of (Aug 2004–Dec 2015) closing stock prices of 20 different companies from BSE along with their dividend paid, bonus share issued, share split, etc. (These parameters are used for the mutual fund profit calculation not for share price forecasting) are used as the training set. Data from January 2016 to Dec 2016 has been used for validation, evaluation, and comparison of the proposed portfolio with other CRISIL (CRISIL 2020; Annapoorna and Gupta 2013 ) ranked mutual funds and benchmark indexes. For the experiments, we first perform regression on different company’s historical closing prices and select the best fitting curve for further processing. As an example, we have shown different curves fitted with the stock prices of TCS in Fig. 2 . The trend line gives the curve equation and corresponding coefficient values. We choose the curve with minimum RMS error for each of the fitted curves. Table 2 shows the sample calculations of the best curve fitting of TCS with different parameter values like coefficients of fitted curve, RMSE, and R-squared value. We compute the same with the other 19 companies and the best fit curve for each of these companies along with the different parameters are shown in Table 3 . Next, we allocate the fund into multiple industrial sectors based on the cumulative performance of the stocks from each such sectors as presented in Table 4 . We have considered a total capital of Rs. 1000,000 for investment by the portfolio manager to evaluate the return on investment at different time periods. Finally, Table 5 shows the fund allocated to each company from each sector depending on their ranking based on expected earning.
The main motive is to allocate more funds to the companies with a higher growth rate than those with a comparatively lower growth rate within a particular sector to earn a better profit. Therefore, the percentage of total funds allocated, along with the amount of funds allocated to an individual company within a particular sector (say, IT) shown in Table 4 . For example, the fund allocated to the IT sector is Rs. 1,10,000/-. Therefore, this amount of funds further allocated across various companies within the IT sector shown in the first four rows of Table 5 .
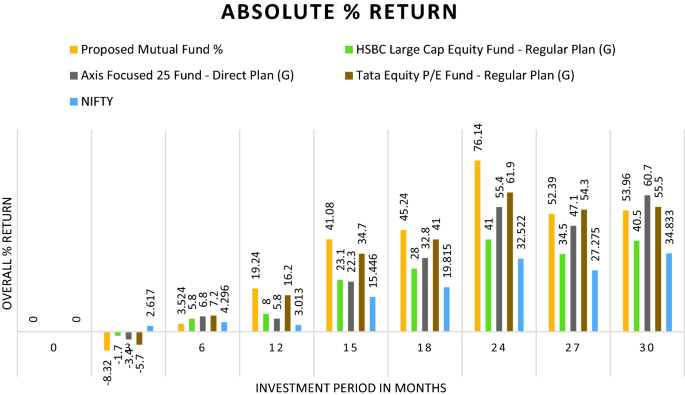
Absolute overall gain/return (%) on investment over the period of 30 months for proposed mutual fund and three other well-established funds. (HSBC large cap equity fund regular plan (G) is ranked-1 MF by (CRISIL 2020) and Axis focused 25 fund direct plan is ranked-1 in diversified equity MFs in last 3 years)
Let us assume that funds allocated to different companies as per the framework, and stocks brought in December 2015, and the funds remain invested for the next 30 months. For simplicity, let us also assume that no more fund inflow or redemption took place in-between. Next, we calculated the market value of the stocks bought at the end of every successive quarter. The dividend amounts, given by the companies from time to time, also added to arrive at the final value of the invested capital. Next, the absolute percentage return on investment is deduced for all subsequent quarters. Detailed calculation sheet considering the actual stock prices from December 2015 to June 2018 placed in the Supplementary Material. We compared the gains with some well-established funds’ performance during the same period. HSBC Large Cap Equity Fund—Regular Plan (G) is ranked first by CRISIL (2020) for the period, and also, Axis Focused 25 Fund—Direct Plan (G) placed at rank-1 in diversified equity MFs in the last 3 years. Table 6 presents the comparative results between our proposed methodology and the top-performing MFs. Figure 3 represents the comparative performance graphically. We observe from the bar chart that proposed MF generates quite a good income in terms of capital appreciation as well as return on investment. We have followed a buy-and-hold strategy during the evaluation of the investment framework. It means that during the whole period of 30 months, no stocks have been sold out partially on peaks, and no new stocks are brought on dips. The above ensures that our framework does not depend much on the market timing of the fund managers. Our return could have been more if we would have re-invested the dividend amounts in the same stock, but in this study, we have kept the dividend amount aside and never invested for simplicity. If we carefully observe the results in Fig. 3 , we see that during the first 3 months almost all the MFs including the proposed scheme have negative returns and after 6 months all go to green and increase subsequently. The period is from January 2016 to June 2016 and certain global events caused those bearish patterns such as economic slowdown of China (Hu et al. 2018 ), the Brexit issue (Mora-Cantallops et al. 2019 ) and volatile crude oil price.
6 Conclusion
This paper proposes a novel methodology to build up a mutual fund portfolio to increase the profit over time and avoid risks. The people with the goal of the investment generally look for higher returns over time. The proposed methodology helps build a robust portfolio over time by analyzing the historical data based on regression analysis. Experimental results show that it performs as good as India’s top-performing mutual funds or even beat them over a long period. The majority of the research works in this domain are focused on predicting the individual stock price. Here we extend and reframe the problem by identifying the different business sectors and then compute the price of every stock selected for these sectors and finally group these stocks in such a cumulative manner so that the risk of investment is reduced but the percentage of return increases. The proposed method can be used by portfolio managers to benchmark mutual funds’ performances for a considerably long period. Though the experiment performed on the Indian share market data, it is also applicable to different share markets across the globe. This framework has the advantage of being less dependent on market-timing of buy/sell by expert fund managers. We have excluded the options of buying on dips and selling on ups to enhance profit margin. Instead, we have focused on making it robust against market volatility by using a buy-and-hold strategy. Dividends received are also not re-invested in the framework for simplicity. We have shown that it is possible to gain a decent return with much less market timing dependence and complex financial indicator analysis. The high net worth individuals (HNI) could also leverage the proposed framework for their portfolio management. In our future study, strategies other than buy-and-hold could be explored by adding complexity to the framework. Every quarter buy/sell strategy could be incorporated based on the forecasted change in the upcoming months. The dividend amount could also be used to hedge the market risk by investing in gold/government securities/bonds, etc. We can study the curve’s changing pattern for varying periods for a specific domain/company for better prediction.
Agapova A (2011) Conventional mutual index funds versus exchange-traded funds. J Financ Mark 14(2):323–343
Article Google Scholar
Altay E, Satman MH (2005) Stock market forecasting: artificial neural network and linear regression comparison in an emerging market. J Financ Manag Anal 18(2):18
Google Scholar
Ananthi M, Vijayakumar K (2020) Stock market analysis using candlestick regression and market trend prediction (CKRM). J Ambient Intell Humaniz Comput. https://doi.org/10.1007/s12652-020-01892-5
Annapoorna M, Gupta PK (2013) A comparative analysis of returns of mutual fund schemes ranked 1 by CRISIL. Tactful Manag Res J 2(1):1–6
Asadi S (2019) Evolutionary fuzzification of ripper for regression: case study of stock prediction. Neurocomputing 331:121–137
Association of Mutual Funds in India (2020) Industry trends June 2020. https://www.amfiindia.com/Themes/Theme1/downloads/home/Industry-Trends.pdf . Accessed 19 July 2020
Atsalakis GS, Valavanis KP (2009) Surveying stock market forecasting techniques-Part II: soft computing methods. Expert Syst Appl 36(3):5932–5941
Baralis E, Cagliero L, Garza P (2017) Planning stock portfolios by means of weighted frequent itemsets. Expert Syst Appl 86:1–17
BSE (2019a) Bombay stock exchange, India. http://www.bseindia.com . Accessed 30 Nov 2019
BSE (2019b) BSE India stock monthly closing price dataset. http://www.bseindia.com/markets/equity/EQReports/StockPrcHistori.aspx?scripcode=512289&flag=sp&Submit=G . Accessed 30 Sept 2019
Chandar SK (2019) Fusion model of wavelet transform and adaptive neuro fuzzy inference system for stock market prediction. J Ambient Intell Humaniz Comput 1–9
Chikhi M, Diebolt C (2010) Nonparametric analysis of financial time series by the kernel methodology. Qual Quantity 44(5):865–880
CRISIL (2020) Mutual fund ranking. https://www.crisil.com/en/home/what-we-do/financial-products/mf-ranking.html . Accessed 19 July 2020
Curran K (2011) Ubiquitous developments in ambient computing and intelligence: human-centered applications. Artif Intell 2021:229
Ding S, Cui T, Xiong X et al (2020) Forecasting stock market return with nonlinearity: a genetic programming approach. J Ambient Intell Human Comput 11:4927–4939. https://doi.org/10.1007/s12652-020-01762-0
Fong SJ, Dey N, Chaki J (2020) Artificial intelligence for coronavirus outbreak. Springer, New York. https://doi.org/10.1007/978-981-15-5936-5
Book Google Scholar
Ghosh A, Bose S, Maji G, Debnath N, Sen S (2019) Stock price prediction using LSTM on Indian share market. In: Proceedings of 32nd international conference on, vol 63, pp 101–110
Gottschlich J, Hinz O (2014) A decision support system for stock investment recommendations using collective wisdom. Decis Support Syst 59:52–62
Hajizadeh E, Ardakani HD, Shahrabi J (2010) Application of data mining techniques in stock markets: a survey. J Econ Int Financ 2(7):109–118
Hu S, Liu M, Fong S, Song W, Dey N, Wong R (2018) Forecasting China future MNP by deep learning in behavior engineering and applications. Springer, New York, pp 169–210
Ji M, Zhang K, Wu Q (2020) Introducing a hybrid model SAE-BP for regression analysis of soil temperature with hyperspectral data. Int J Ambient Comput Intell (IJACI) 11(3):66–79
Liao SH, Chu PH, Hsiao PY (2012) Data mining techniques and applications—a decade review from 2000 to 2011. Expert Syst Appl 39(12):11303–11311
Maji G, Sen S, Sarkar A (2017) Share market sectoral indices movement forecast with lagged correlation and association rule mining. In: IFIP International conference on computer information systems and industrial management, Springer, pp 327–340
Mingyue Q, Cheng L, Yu S (2016) Application of the artificial neural network in predicting the direction of stock market index. In: 2016 10th International conference on complex, intelligent, and software intensive systems (CISIS), IEEE, pp 219–223
Moere AV, Offenhuber D (2009) Beyond ambient display: a contextual taxonomy of alternative information display. Int J Ambient Comput Intell (IJACI) 1(2):39–46
Mondal D, Maji G, Goto T, Debnath NC, Sen S (2018) A data warehouse based modelling technique for stock market analysis. Int J Eng Technol 7(313):165–170
Mora-Cantallops M, Sánchez-Alonso S, Visvizi A (2019) The influence of external political events on social networks: the case of the Brexit twitter network. J Ambient Intell Human Comput. https://doi.org/10.1007/s12652-019-01273-7
Narang V (2015) Impact of politics on sensex. Int J Econ Bus Rev 3(1):165–168
NSE (2019) National stock exchange, India. http://www.nseindia.com . Accessed 30 Nov 2019
Paranjape-Voditel P, Deshpande U (2013) A stock market portfolio recommender system based on association rule mining. Appl Soft Comput 13(2):1055–1063
Park M, Lee ML, Lee J et al (2019) Predicting stock market indices using classification tools. Asian Econ Financ Rev 9(2):243–256
Preethi G, Santhi B (2012) Stock market forecasting techniques: a survey. J Theor Appl Inf Technol 46(1):24
Qian B, Rasheed K (2007) Stock market prediction with multiple classifiers. Appl Intell 26(1):25–33
Richthammer C, Pernul G (2020) Situation awareness for recommender systems. Electron Commerce Res 20:783–806. https://doi.org/10.1007/s10660-018-9321-z
Rusu V, Rusu C (2003) Forecasting methods and stock market analysis. Creative Math 12:103–110
Sankar CP, Vidyaraj R, Kumar KS (2015) Trust based stock recommendation system—a social network analysis approach. Proced Comput Sci 46:299–305
Singh N, Mohanty S (2018) Short term price forecasting using adaptive generalized neuron model. Int J Ambient Comput Intell (IJACI) 9(3):44–56
Weng F, Chen Y, Wang Z, Hou M, Luo J, Tian Z (2020) Gold price forecasting research based on an improved online extreme learning machine algorithm. J Ambient Intell Human Comput 1–11
Zhang D, Zhou L (2004) Discovering golden nuggets: data mining in financial application. IEEE Trans Syst Man Cybern Part C (Appl Rev) 34(4):513–522
Zhang J, Teng YF, Chen W (2019) Support vector regression with modified firefly algorithm for stock price forecasting. Appl Intell 49(5):1658–1674
Zhao J, Zeng D, Liang S, Kang H, Liu Q (2020) Prediction model for stock price trend based on recurrent neural network. J Ambient Intell Human Comput. https://doi.org/10.1007/s12652-020-02057-0
Zhu HM, Li R, Li S (2014) Modelling dynamic dependence between crude oil prices and Asia-Pacific stock market returns. Int Rev Econ Financ 29:208–223
Download references
Author information
Authors and affiliations.
Department of Electrical Engineering, Asansol Polytechnic, Asansol, 713302, India
Giridhar Maji
A.K. Choudhury School of Information Technology, University of Calcutta, Salt Lake, 700106, India
Debomita Mondal & Soumya Sen
Department of Computer Science and Engineering, JIS University, Kolkata, India
Nilanjan Dey
School of Computing and Information Technology, Eastern International University, Thu Dau Mot, Vietnam
Narayan C. Debnath
You can also search for this author in PubMed Google Scholar
Corresponding author
Correspondence to Soumya Sen .
Additional information
Publisher's note.
Springer Nature remains neutral with regard to jurisdictional claims in published maps and institutional affiliations.
Rights and permissions
Reprints and permissions
About this article
Maji, G., Mondal, D., Dey, N. et al. Stock prediction and mutual fund portfolio management using curve fitting techniques. J Ambient Intell Human Comput 12 , 9521–9534 (2021). https://doi.org/10.1007/s12652-020-02693-6
Download citation
Received : 26 April 2020
Accepted : 10 November 2020
Published : 02 January 2021
Issue Date : October 2021
DOI : https://doi.org/10.1007/s12652-020-02693-6
Share this article
Anyone you share the following link with will be able to read this content:
Sorry, a shareable link is not currently available for this article.
Provided by the Springer Nature SharedIt content-sharing initiative
- Stock market analysis
- Sector-wise stock selection
- Stock price prediction
- Curve fitting
- Mutual fund portfolio management
- Find a journal
- Publish with us
- Track your research
- Skip to main content
- Skip to search
- Skip to footer
Products and Services
Contact cisco.
To get global contact information, please make your selections in the drop-down menus.
Country/region and language
Get in touch
Please reach out to sales for general inquiries or to chat with a live agent.
Sales inquiries
1 800 553 6387 , press 1
Order and billing
1 800 553 6387 , press 2-1
Monday to Friday 8 a.m. to 5 p.m. Eastern Time Chat is available to you 24/7.
Find technical support for products and licensing, access to support case manager, and chat with support assistant. Technical support is available 24/7.
Enterprise and service providers
1 800 553 2447 (U.S. and Canada)
Small business
1 866 606 1866 (U.S. and Canada)
Training and certifications
1 800 553 6387 , press 4
Explore support
Explore certification support
Cisco partners
Become a partner, locate a partner, get updates, and partner support.
Explore Cisco partners
Get partner support
Find a Cisco office
Find offices around the world.
Locate offices
Corporate headquarters
300 East Tasman Drive San Jose, CA 95134
Legal mailing address
Cisco Systems, Inc. 170 West Tasman Drive San Jose, California 95134

Complete the form below or log in and the form will autofill. One of our sales specialists will call you within 15 minutes or on a date or time you request. Specialists are available Monday through Friday, 8 a.m. to 5 p.m. Eastern Time. We are currently experiencing delays in response times. If you require an immediate sales response – please call us 1 800-553-6387. Otherwise, a sales advisor will call you as soon as possible. * Required
Want to use a different email? Sign out * Required
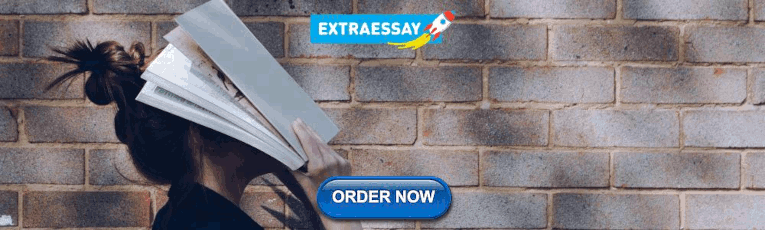
IMAGES
VIDEO
COMMENTS
ABOUT THE JOURNAL OF PORTFOLIO MANAGEMENT. The Journal of Portfolio Management (JPM) is a definitive source of thought-leading analyses and practical techniques that many institutional investors turn to for insight on the financial markets.The JPM offers cutting-edge research on all major topics in investments, including asset allocation, performance measurement, market trends, portfolio ...
Sustainability integration in project portfolio management helps shape strategic, organizational, and project-based contexts. The authors conducted a structured literature review from 2000 to 2021 and developed a novel integrative framework presenting a holistic view highlighting three substantive research themes: sustainability mindset, sustainability assessment, and sustainability ...
Advancing project and portfolio management research: Applying strategic management theories. International Journal of Project Management, 30(5), 525-538. Crossref. Google Scholar. Killen C. P., Kjaer C. (2012). Understanding project interdependencies: The role of visual representation, culture and process. ... Call for papers, Internatio nal ...
The Journal of Portfolio Management Editor: Dr. Frank J. Fabozzi 858 Tower View Circle New Hope, PA 18938 Email: [email protected] . Journal of Portfolio Management (JPM) Content Guidelines. The aim and objective identified by most journals is to publish papers that will make an important contribution to the finance literature.
Quickly implement key research with our weekly Practical Applications—short reports that bridge the gap between theory and practice. We select our most technical published research and distill it down to the central concepts and practical takeaways. Ideal for highlighting the most actionable findings, they are simply presented and easy to ...
This study provides a systematic overview of portfolio management approaches and research advances of the past decades, and it explores the root causes of innovation portfolio management challenges. ... The six groups emerged through an iterative process of reviewing the papers among the three authors, applying a few distinct criteria: the ...
Explore the latest full-text research PDFs, articles, conference papers, preprints and more on PORTFOLIO MANAGEMENT. Find methods information, sources, references or conduct a literature review on ...
The paper concludes with hypotheses for future investigation. ... These hypotheses can advance a public administration-oriented portfolio management research agenda into portfolio management that could illuminate concepts of scholarly interest as well as assist practitioners. There are many more discrete research questions that could also ...
Showcasing the revolutionary developments in financial theory and practice . In 1974, Paul Samuelson laid down a challenge in The Journal of Portfolio Management, and John C. Bogle answered the call by creating the world's first index mutual fund.More trailblazing ideas have evolved on the pages of our 13 titles, including the Sharpe Ratio, the Black-Scholes Model, and the concept of goals ...
Financial Markets and Portfolio Management (FMPM), addresses all areas of finance, including financial markets, portfolio theory and wealth management, asset pricing, corporate finance, corporate governance, alternative investments, risk management, and regulation.. Official publication of the Swiss Financial Analysts Association. Serves as a bridge between innovative research and its ...
Portfolio managements in financial markets involve risk management strategies and opportunistic responses to individual trading behaviours. Optimal portfolios constructed aim to have a minimal risk with highest accompanying investment returns, regardless of market conditions. This paper focuses on providing an alternative view in maximising portfolio returns using Reinforcement Learning (RL ...
Diversification is one of the major components of investment decision-making under risk or uncertainty. However, paradoxically, as the 2007-2009 financial crisis revealed, the concept remains misunderstood. Our goal in writing this paper is to correct this issue by reviewing the concept in portfolio theory. The core of our review focuses on the following diversification principles: law of ...
This section provides a conceptual background to digital service innovation and portfolio management. More specifically, Sect. 2.1 provides historical context to the development of PM in research and differentiates innovation PM to other streams of PM research. Section 2.2 defines digital services and discusses how they distinguish from physical products and non-digital services.
Full-text issues (current and archival) of quarterly publications covering research on global investment and finance. Publications include The Journal of Alternative Investments, The Journal of Derivatives, The Journal of Fixed Income, The Journal of Investing, The Journal of Investment Compliance, The Journal of Portfolio Management, The Journal of Private Equity, The Journal of Structured ...
This paper primarily employs the. literature review method ology and comparative analysis methodology. Firstly, it collects, summarizes, and analyzes multiple papers on portfolio management models ...
The annual awards, co-founded and generously funded by Bruce Jacobs and Ken Levy of Jacobs Levy Equity Management, consist of a $5,000 prize for the Best Article and $2,500 prizes for each of three Outstanding Articles. Cast your vote for the best papers that appeared in The Journal of Portfolio Management in 2021, including all the special issues.
Abstract. The task of creating an investment portfolio by a financial institution is considered. Funds for. creating a portfolio are taken from two sources: enterprise's equity funds and borrowed ...
It requires a systematicapproach, professional Expertise and scientific knowledge. Portfolio management is the exclusive way by which an investor can get maximum Returns, while it minimizes the risk at the same time. So,objectives of portfolio management can be Stated as: -. Minimization of risk. Capital growth.
The main goal of this research is to examine the role that AI plays in the financial markets, with an emphasis on how it affects trading, portfolio management, and price prediction. Today artificial intelligence (AI) has become an indispensable assistance for human traders. AI systems provide human traders with numerous advantages, such as the capability to conduct genuine high-frequency ...
Portfolio management means selecting the right investment policy for the individuals in terms of minimize risk and maximum return. The term portfolio refers to any collection of financial assets such as stocks, bonds and cash. It is generally accepted that a portfolio is designed according to the investors risk, time frame and investment objective.
Bhalla, V. K. (2011). Investment Management Security Analysis and Portfolio Management (17th Revised ed.). New Delhi: S.Chand & Company Ltd. PDF | It presentation of lecture. It consist various ...
Portfolio optimization has always been a challenging proposition in finance and management. Portfolio optimization facilitates in selection of portfolios in a volatile market situation. In this paper, different classical, statistical and intelligent approaches employed for portfolio optimization and management are reviewed. A brief study is performed to understand why portfolio is important ...
maturity issues such as money market funds, commercial papers and shares that are widely traded. c.) Safety: An, serious constraint to be considered by the investor is the safety of the principal ... Professional portfolio management, backed by research is now being adopted by mutual funds, investment consultants, individual investors and big ...
The scope of DEI metrics provided to institutional investors during fundraising has also increased in recent years: more than half of PE firms now provide data across investing teams, portfolio company boards, and portfolio company management (versus investment team data only). 4 "The state of diversity in global private markets: 2023 ...
The authors then discuss how to estimate the alpha and beta of private investment funds and move onto a second set of topics concerned with portfolio construction and risk management. They examine the distorting effects that serially correlated return streams can have on risk analysis and asset allocation decisions and finally conclude with a ...
The role of the portfolio manager is getting challenging day-by-day. In this research work, we focus on developing a framework for the portfolio manager to maintain a diversified portfolio with less risk and high return probability. The main contributions of the paper are as follows.
Get in touch. Please reach out to sales for general inquiries or to chat with a live agent. Sales inquiries. 1 800 553 6387, press 1. Order and billing. 1 800 553 6387 , press 2-1. Monday to Friday