A Review on Quality of Service and SERVQUAL Model
- Conference paper
- First Online: 10 July 2020
- Cite this conference paper
- Zhengyu Shi 10 &
- Huifang Shang 10
Part of the book series: Lecture Notes in Computer Science ((LNISA,volume 12204))
Included in the following conference series:
- International Conference on Human-Computer Interaction
5457 Accesses
6 Citations
In field of service design, the research and application of service quality plays an important role in the development and competition of enterprises by establishing brand image and generating market effect. Therefore, experts in management and marketing have studied it and found that the quality of service in the industry has a great impact on consumer satisfaction, consumer experience and brand loyalty. Based on the research and development of the concept of service quality, PZB, a famous American marketing expert team, established SERVQUAL (SQ) model through the test of retail cases, and constantly revised and improved it, which was applied to multiple service industries. Through literature review, this paper analyzes the application of SERVQUAL model in China and abroad, mainly involving retail industry, medical service industry, e-commerce industry, tourism service industry and other service fields. The study found that SERVQUAL model plays a guiding role in evaluating the management of emerging enterprises, consumers’ preference for services, and resource allocation of service industries in developing countries. In addition, this paper compares the application of SERVQUAL (SQ) model and its derivative SERVPERF (SP) model in the service field, and finds that SP model is mainly a result-oriented quality of service study, while SERVQUAL model is mainly a result-oriented quality of service study based on process dynamic change. In the multi-field studies, it is found that SERVQUAL model, as a common basic model, combines the Fuzzy theory, Functional quality deployment and Kano model to comprehensively evaluate the service quality in the application field and provide decision support for enterprise development. Finally, this article discusses and summarizes the study of service quality, revises and improves the research model, and looks forward and proposes future service quality studies to provide more market and social value to service industry.
This is a preview of subscription content, log in via an institution to check access.
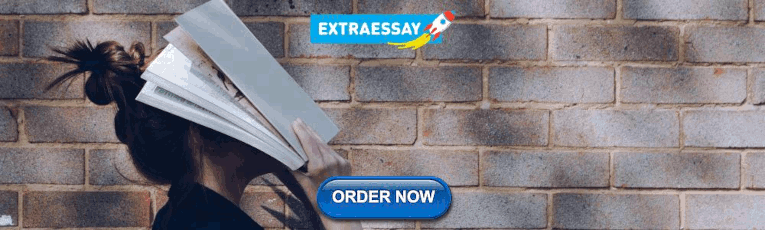
Access this chapter
- Available as PDF
- Read on any device
- Instant download
- Own it forever
- Available as EPUB and PDF
- Compact, lightweight edition
- Dispatched in 3 to 5 business days
- Free shipping worldwide - see info
Tax calculation will be finalised at checkout
Purchases are for personal use only
Institutional subscriptions
Gronroos, C.: Marketing in Service Companies. Liber, Malmo (1983)
Google Scholar
Lehtinen, J.R., Lehtinen, U.: Service quality: a study of quality dimensions. Unpublished working paper, Service Management Institute, Helsinki (1982)
Lewis, R.C., Booms, B.H.: The marketing aspects of service quality in emerging perspectives on service marketing. In: Berry, L., Shostack, G., Upah, G. (ed.) American Marketing, Chicago, pp. 99–107 (1983)
Parasuraman, A., Zeithaml, V.A., Berry, L.L.: SERVQUAL: a multiple-item scale for measuring consumer perceptions of service quality. J. Retail. 64 (1), 12–40 (1988). https://doi.org/10.1016/0737-6782(88)90045-8
Article Google Scholar
Leblanc, G., Nguyen, N.: Customers’ perceptions of service quality in financial institutions. Int. J. Bank Mark. 6 (4), 7–18 (1988). https://doi.org/10.1108/eb010834
Hedvall, M.-B., Paltschik, M.: An investigation in, and generation of, service quality concepts. In: Avlonitis, G.J., et al. (eds.) Marketing Thought and Practice in the 1990s, European Marketing Academy, Athens, pp. 473–83 (1989)
Liu, W., Liu, G.: Quality Management, p. 220. Yanshi Press, Beijing (2004)
Parasuraman, A., Zeithaml, V.A., Berry, L.L.: A conceptual model of service quality and its implications for future research. J. Mark. 49 (4), 41–50 (1985). https://doi.org/10.1177/002224298504900403
Parasuraman, A., Berry, L.L., Zeithaml, V.A.: Perceived service quality as a customer-based performance measure: an empirical examination of organizational barriers using an extended service quality model. 30 (3), 335–364 (1991). https://doi.org/10.1002/hrm.3930300304
Parasuraman, A., Berry, L.L., Zeithaml, V.A.: Refinement and reassessment of the SERVQUAL scale. J. Retail. 67 (8), 1463–1467 (1991). https://doi.org/10.1021/nl0492436
Carman, J.M.: Consumer perceptions of service quality: an assessment of the SERVQUAL dimensions. J. Retail. 66 (2), 33–55 (1990). https://doi.org/10.1016/0737-6782(90)90032-A
Taylor, S.A., Cronin, J.J.: Modelling patient satisfaction and service quality. J. Health Care Mark. 14 (1), 34–44 (1994)
Wang, Y.L., Luor, T., Luarn, P., Lu, H.S.: Contribution and trend to quality research–a literature review of SERVQUAL model from 1998 to 2013. Informatica Economica 19 (1), 34–45 (2015). https://doi.org/10.12948/issn14531305/19.1.2015.03
Baker, D.A., Crompton, J.L.: Quality, satisfaction and behavioral intentions. Ann. Tour. Res. 27 (3), 785–804 (2000). https://doi.org/10.1016/S0160-7383(99)00108-5
Dabholkar, P.A., Shepherd, C.D., Thorpe, D.I.: A comprehensive framework for service quality: an investigation of critical conceptual and measurement issues through a longitudinal study. J. Retail. 76 (2), 139 (2000). https://doi.org/10.1016/S0022-4359(00)00029-4
Devaraj, S., Ming, F., Kohli, R.: Antecedents of B2C channel satisfaction and preference: validating e-commerce metrics. Inf. Syst. Res. 13 (3), 316–333 (2002). https://doi.org/10.1287/isre.13.3.316.77
Chhabra, N.: Measurement of consumer’s perception of service quality in organized retail using SERVQUAL instrument. Manage. Dyn. 13 (1), 70–82 (2013)
MathSciNet Google Scholar
Mobarakeh, S.K., Ghahnavieh, F.R.: A survey on the performance of Siahat Gasht tour and travel agency from the viewpoint of customers using SERVQUAL model. Int. J. Sci. Manage. Dev. 3 (6), 394–402 (2015)
Palese, B., Usai, A.: The relative importance of service quality dimensions in e-commerce experiences. Int. J. Inf. Manage. 40 , 132–140 (2018). https://doi.org/10.1016/j.ijinfomgt.2018.02.001
Khare, A., Parveen, C., Rai, R.: Retailer behavior as determinant of service quality in Indian retailing. J. Retail Leisure Prop. 9 (4), 303–317 (2010). https://doi.org/10.1057/rlp.2010.14
Vassiliadis, C.A., Fotiadis, A.K., Tavlaridou, E.: The effect of creating new secondary health services on patients’ perceptions: a Kano service quality analysis approach. Total Qual. Manage. Bus. Excell. 25 (7–8), 897–907 (2014). https://doi.org/10.1080/14783363.2014.904564
Bansal, A., Gaur, G., Chauhan, V.: Analysis of service quality provided by goibibo.com in tourism industry. Glob. J. Enterpr. Inf. Syst. 8 (2), 40–47 (2016)
Chakravarty, A.: Evaluation of service quality of hospital outpatient department services. Med. J. Armed Forces India 67 (3), 224 (2011). https://doi.org/10.1016/s0377-1237(11)60045-2
Meesala, A., Paul, J.: Service quality, consumer satisfaction and loyalty in hospitals: thinking for the future. J. Retail. Consum. Serv. (2016). https://doi.org/10.1016/j.jretconser.2016.10.011
Hong, Z., Su, Q., Huo, J.: Study on the research of service quality management. Manage. Rev. 24 (7), 154–165 (2012). https://doi.org/10.14120/j.cnki.cn11-5057/f.2012.07.016
Cui, L., Chen, S.: Research on service quality evaluation and improvement countermeasures of commercial Banks in China – based on improved SERVQUAL model. Res. Dev. 149 (04), 92–95 (2010). https://doi.org/10.13483/j.cnki.kfyj.2010.04.039
Zhu, M., Miao, S., Zhuo, J.: An empirical study on chinese express industry with SERVQUAL. Sci. Technol. Manage Res. 31 (08), 45–52 (2011)
Zuo, W., Zhu, W.: Research on service quality management of online car-hailing based on SERVQUAL in sharing economy: case study of Didichuxing and Uber. J. Manage. Case Stud. 11 (4), 349–367 (2018)
Cronin Jr., J.J., Taylor, S.A.: Measuring service quality: a reexamination and extension. J. Mark. 56 (3), 55–68 (1992). https://doi.org/10.1177/002224299205600304
Boulding, W., Kalra, A., Staelin, R., Zeithaml, V.A.: A dynamic process model of service quality: from expectations to behavioral intentions. J. Mark. Res. (JMR) 30 (1), 7–27 (1993). https://doi.org/10.1177/002224379303000102
Hartline, M.D., Ferrell, O.C.: The management of customer-contact service employees: an empirical investigation. J. Mark. 60 (4), 52–70 (1996). https://doi.org/10.1177/002224299606000406
Marshall, K.P., Smith, J.R.: SERVPERF utility for predicting neighborhood shopping behavior. J. Nonprofit Public Sect. Mark. 7 (4), 45 (2000). https://doi.org/10.1300/J054v07n04_05
Hossain, M.J., Islam, M.A., Saadi, M.S.: Evaluating users’ experience of service performance using SERVPERF scale: a case study of some private university libraries in Bangladesh. Ann. Libr. Inform. Stud. 60 (4), 249–259 (2013)
Le Tan, P., Fitzgerald, G.: Applying the SERVPERF scale to evaluate quality of care in two public hospitals at Khanh Hoa Province, Vietnam. Asia Pac. J. Health Manage. 9 (2), 66–76 (2014)
Mahmoud, A.B., Khalifa, B.: A confirmatory factor analysis for SERVPERF instrument based on a sample of students from syrian universities. Educ. Train. 57 (3), 343–359 (2015)
Parasuraman, A., Zeithaml, V.A., Berry, L.L.: Reassessment of expectations as a comparison standard in measuring service quality: implications for further research. J. Mark. 58 (1), 111–124 (1994). https://doi.org/10.1177/002224299405800109
Zadeh, L.A.: Fuzzy sets. Inf. Control 8 (3), 338–353 (1965)
Hu, H.-Y., Lee, Y.-C., Yen, T.-M.: Service quality gaps analysis based on Fuzzy linguistic SERVQUAL with a case study in hospital out-patient services. TQM J. 22 (5), 499–515 (2010). https://doi.org/10.1108/17542731011072847
Lin, C.J., Wu, W.W.: A causal analytical method for group decision-making under fuzzy environment. Expert Syst. Appl. 34 (1), 205–213 (2008). https://doi.org/10.1016/j.eswa.2006.08.012
Article MathSciNet Google Scholar
Wu, W.Y., Hsiao, S.W., Kuo, H.P.: Fuzzy set theory based decision model for determining market position and developing strategy for hospital service quality. Total Qual. Manage. Bus. Excell. 15 (4), 439–456 (2004). https://doi.org/10.1080/1478336042000183587
Aydin, O., Pakdil, F.: Fuzzy SERVQUAL analysis in airline services. Organizacija 41 (3), 108–115 (2008)
Braendle, U., Sepasi, S., Rahdari, A.H.: Fuzzy evaluation of service quality in the banking sector: a decision support system. Fuzzy Econ. Rev. 19 (2), 47–79 (2014)
Mazur, G.: QFD for service industries. In Proceedings of the Fifth Symposium on Quality Function (1993)
Lampa, S., Mazur, G.: Bagel sales double at host marriott: Using quality function deployment. Japan Business Consultants (1996)
Dube, L., Johnson, M.D., Renaghan, L.M.: Adapting the QFD approach to extended service transactions. Prod. Oper. Manage. 8 (3), 301–317 (1999). https://doi.org/10.1111/j.1937-5956.1999.tb00310.x
Yildirim, K.E., Yildirim, A., Ozcan, S.: Integrated usage of the SERVQUAL and quality function deployment techniques in the assessment of public service quality: the case of Ardahan Municipality. Bus. Econ. Res. J. 10 (4), 885–901 (2019)
Cho, I.J., Kim, Y.J., Kwak, C.: Application of SERVQUAL and fuzzy quality function deployment to service improvement in service centres of electronics companies. Total Qual. Manage. Bus. Excell. 27 (3/4), 368–381 (2016). https://doi.org/10.1080/14783363.2014.997111
Kano, N., et al.: Attractive quality and must-be quality. J. Jpn. Soc. Qual. Control 41 (2), 39–48 (1984)
Vassiliadis, C.A., Fotiadis, A.K., Tavlaridou, E.: The effect of creating new secondary health services on patients’ perceptions: a Kano service quality analysis approach. Total Qual. Manage. Bus. Excell. 25 (7/8), 897–907 (2014). https://doi.org/10.1080/14783363.2014.904564
Chiang, T.-Y., Perng, Y.-H.: A new model to improve service quality in the property management industry. Int. J. Strateg. Prop. Manag. 22 (5), 436–446 (2018)
Download references
Author information
Authors and affiliations.
School of Art Design and Media, East China University of Science and Technology, Shanghai, 200237, China
Zhengyu Shi & Huifang Shang
You can also search for this author in PubMed Google Scholar
Corresponding author
Correspondence to Huifang Shang .
Editor information
Editors and affiliations.
Missouri University of Science and Technology, Rolla, MO, USA
Fiona Fui-Hoon Nah
Rights and permissions
Reprints and permissions
Copyright information
© 2020 Springer Nature Switzerland AG
About this paper
Cite this paper.
Shi, Z., Shang, H. (2020). A Review on Quality of Service and SERVQUAL Model. In: Nah, FH., Siau, K. (eds) HCI in Business, Government and Organizations. HCII 2020. Lecture Notes in Computer Science(), vol 12204. Springer, Cham. https://doi.org/10.1007/978-3-030-50341-3_15
Download citation
DOI : https://doi.org/10.1007/978-3-030-50341-3_15
Published : 10 July 2020
Publisher Name : Springer, Cham
Print ISBN : 978-3-030-50340-6
Online ISBN : 978-3-030-50341-3
eBook Packages : Computer Science Computer Science (R0)
Share this paper
Anyone you share the following link with will be able to read this content:
Sorry, a shareable link is not currently available for this article.
Provided by the Springer Nature SharedIt content-sharing initiative
- Publish with us
Policies and ethics
- Find a journal
- Track your research
Service quality in the healthcare sector: a systematic review and meta-analysis
LBS Journal of Management & Research
ISSN : 0972-8031
Article publication date: 16 January 2023
Issue publication date: 4 September 2023
The purpose of this study is to summarize the available pool of literature on service quality to identify different dimensions of service quality in the healthcare industry and understand how it is measured. The study attempts to explore the research gaps in the literature about different service quality dimensions and patient satisfaction.
Design/methodology/approach
A systematic literature review process was followed to achieve the objectives of the study. Various inclusion and exclusion criteria were used to select relevant research articles from 2000–2020 for the study, and a total of 100 research articles were selected.
The study identified 41 different dimensions of healthcare service quality measurement and classified these dimensions into four categories, namely servicescape, personnel, hospital administration and patients. It can be concluded that SERVQUAL is the most widely used service quality measurement tool.
Originality/value
The study identified that a majority of the researchers deduced a positive relationship between SERVQUAL dimensions and the quality of healthcare services. The findings of study will assist hospital executives in formulating effective strategies to ensure that patients receive superior quality healthcare services.
- Healthcare sector
- Service quality
- Systematic review
Darzi, M.A. , Islam, S.B. , Khursheed, S.O. and Bhat, S.A. (2023), "Service quality in the healthcare sector: a systematic review and meta-analysis", LBS Journal of Management & Research , Vol. 21 No. 1, pp. 13-29. https://doi.org/10.1108/LBSJMR-06-2022-0025
Emerald Publishing Limited
Copyright © 2022, Mushtaq Ahmad Darzi, Sheikh Basharul Islam, Syed Owais Khursheed and Suhail Ahmad Bhat
Published in LBS Journal of Management & Research . Published by Emerald Publishing Limited. This article is published under the Creative Commons Attribution (CC BY 4.0) licence. Anyone may reproduce, distribute, translate and create derivative works of this article (for both commercial and no commercial purposes), subject to full attribution to the original publication and authors. The full terms of this licence may be seen at http://creativecommons.org/licences/by/4.0/legalcode
Introduction
The quality of healthcare services has long been a subject of concern for both private and public healthcare service providers across the globe. According to Senic and Marinkovic (2013) , integrity and competitiveness of a nation's healthcare structure are gauged by the quality of healthcare services rendered. Indian National Health Policy 2017 envisions that everyone should have access to high-quality healthcare without facing financial suffering ( MoHFW, 2017 ). Adherence to quality standards and improved quality design results in a better-perceived value, which leads to better prices, better income and greater profitability ( Zeithaml, 2000 ). Customers of the healthcare industry in developing countries are becoming more and more aware of their right to quality healthcare. Consequently, delivering high-quality service by healthcare service providers is gaining momentum ( Abuosi & Atinga, 2013 ). According to Yee, Yeung, and Cheng (2010) , healthcare service providers need to provide high-quality services to sustain the trustworthiness of patients. Demand for superior service quality is growing due to an increase in the per capita income of customers and increased aspirations of the customer ( Singh & Prasher, 2019 ). Also, as a result of competition from private healthcare service providers, public care providers are facing pressing demand for delivering high-quality services ( Zarei, Arab, Froushani, Rashidian, & Ghazi-Tabatabaei, 2012 ).
Mosadeghrad (2014, p. 78) defined healthcare quality as “ consistently delighting the patient by providing efficacious, effective and efficient healthcare services according to the latest clinical guidelines and standards, which meet the patient ' s needs and satisfies providers ”. Ovretveit (2009, p. 4) defines quality care as the “ p rovision of care that exceeds patient expectations and achieves the highest possible clinical outcomes with the resources available ”. Parasuraman, Zeithaml and Berry (1985) described service quality as the gap between a customer's expectations of service and the customer's perception of service after the service is rendered. When perception exceeds expectations, the customer will be satisfied ( Kalaja, Myshketa, & Scalera, 2016 ). Several studies have confirmed that customer expectations of service are much higher than the customer perception of services rendered by both public and private sector institutions ( Andaleeb, Siddiqui, & Khandakar, 2007 ; Zarei et al. , 2012 ; Manulik, Rosińczuk, & Karniej, 2016 ). A firm provides quality service when its services at least meet or exceed the expectations of the customer ( Owusu-Frimpong, Nwankwo, & Dason, 2010 ). Service quality evaluation varies from the service provider's and service receiver's point of view. Service delivery professionals evaluate service based on delivery and design aspects, while receivers of service evaluate it based on their overall perception after consuming the service ( Brown & Swartz, 1989 ). Traditionally healthcare quality was judged based on some objective criteria such as mortality rate, morbidity rate, infant mortality rate, etc. However, as time passes, the structure of the industry changed, and the role of patients in deciding quality has been given more and more consideration ( Dagger, Sweeney, & Johnson, 2007 ). To survive in the modern competitive markets, it has become of utmost importance for service providers to understand the needs and expectations of customers. They must deliver what the customer is expected instead of what they feel is important for a customer to maintain the business demand ( Singh & Prasher, 2019 ). Kotler and Keller (2006) suggest that in the consumer-oriented healthcare market where healthcare delivery is commodified and patient-led, the patient should be the judge of service quality. Hence, to provide better quality services, healthcare service providers need to identify the main dimensions of service quality in healthcare and focus on those dimensions rated more important by the patients ( Singh & Prasher, 2019 ).
Studies on healthcare service quality have been conducted in a variety of settings worldwide, namely Albania ( Kalaja et al. , 2016 ), Australia ( Copnell et al. , 2009 ; Dagger et al. , 2007 ; Levesque & Sutherland, 2020 ), Bangladesh ( Andaleeb et al. , 2007 ), China ( Li et al. , 2015 ; Wu, Li, & Li, 2016 ), Denmark ( Engelbrecht, 2005 ; Groene, Skau, & Frølich, 2008 ), Ghana ( Abuosi & Atinga, 2013 ; Agyapong, Afi, & Kwateng, 2018 ), India ( Chahal, 2008 ; Aagja & Garg, 2010 ; Chahal & Kumari, 2010 ; Gupta & Rokade, 2016 ; Singh & Prasher, 2019 ; Upadhyai, Jain, Roy, & Pant, 2019 ; Jog et al. , 2020 ), Iran ( Goshtasebi et al. , 2009 ; Mohammadkarim, Jamil, Pejman, Seyyed, & Mostafa, 2011 ; Mosadeghrad, 2014 ), Malaysia ( Ahmad & Sungip, 2008 ; Hasan, Ilias, Rahman, & Razak, 2009 ), Pakistan ( Irfan & Ijaz, 2011 ; Shabbir, Malik, & Malik, 2016 ; Fatima, Malik, & Shabbir, 2018 ; Dhahri, Iqbal, & Khan, 2020 ), Turkey ( Beyan & Baykal, 2012 ) and USA ( Lee, 2003 ; Hegji & Self, 2009 ; Mustafa, Yang, Mortezavi, Vadamalai, & Ramsey, 2020 ; Thompson, Shen, & Lee, 2020 ). The purpose of this paper is to investigate and summarize the available literature on healthcare service quality to understand what constitutes healthcare service quality and its principal dimensions and also to highlight the prominent research gaps that will provide direction for future research.
Methodology
The study followed a systematic review process to obtain research articles relevant to the research problem understudy. The systematic review process is a structured way of identifying, evaluating and interpreting the available literature related to any particular area ( Kamboj & Rahman, 2015 ). A systematic literature review is a two-step process. First, defining the criteria for inclusion of articles and second, identifying databases and research studies ( McLean & Antony, 2014 ).
Inclusion criteria
Papers published during 2000–2020 were considered for the study. This was done by applying a custom range filter. The reason for selecting the above mention time frame is the most recent two decades were selected for article search.
Research articles related to healthcare service quality were included in the review process. The criterion was adopted in line with the primary objective of the review process.
Empirical and review articles published in peer-reviewed journals were considered.
Only papers in the English language were included.
Database and article selection
The literature search was conducted in the autumn of 2021. The databases selected for the literature search included Emerald, Elsevier, Sage, Taylor and Francis and Google Scholar. Filters such as custom range and sort by relevance were applied to restrict the search results to keywords. The systematic review process is presented in Figure 1 . In stage 1 of the review process, the literature was searched using the keywords such as healthcare, healthcare services, service quality and SERVQUAL. The search obtained 209 research articles. The research papers were selected based on relevance to the topic understudy and the popularity of the articles. Researchers such as Beaulieu (2015) argued that the popularity of journal articles with above 10 citations are considered in top 24% of the highest cited articles, and articles that receive 100 citations are considered among 1.8% of the most popular articles across the globe, which makes the current study a worth addition to the existing body of literature. In stage 2, the screening of articles was then conducted first based on title and abstract and then based on inclusion criteria. Screening of articles based on the title and abstract resulted in the exclusion of 63 research articles, and 146 articles were moved to the next level of screening.
Then articles were screened by applying inclusion criteria to exclude articles that do not fulfill the above-stated criteria ( Kamboj & Rahman, 2015 ). This screening obtained 100 research articles that were finally considered for review, and the rest of the articles (46) were excluded from the study. Finally, in stage 3 of the review process, the study provides a summary (publication trend, journal-wise distribution, methodology that includes sampling method and data analysis tools used and key findings) of the 100 articles included in the review.
Common characteristics of reviewed articles
Classification of articles by research type and hospital setting
Table 1 displays the classification of research articles based on research type and hospital setting. The research type describes the nature of the research and yields that a maximum number of articles were quantitative studies (62 articles) followed by qualitative studies (15 articles) and only 07 studies that were both qualitative and quantitative. A few review articles (14 articles) were also considered during the process. The results of the review substantiate that there is a need of conducting qualitative research that can provide an in-depth understanding of how various service quality dimensions affect the perceived quality of care among patients and the treatment satisfaction level. Qualitative studies can also provide insights into the priorities of patients while receiving medical services.
The classification based on hospital setting yields more than 77 articles that have purposively chosen a specific hospital setting and the rest have collected data from respondents in general. Out of 77 articles, 49% of research studies were conducted in a public hospital setting, and 25% were conducted in a private hospital setting. Around 26% of research were conducted in both public and private hospital settings. The direct comparison of healthcare services and perceived service quality among patients was observed as the main motivator in choosing both hospital settings ( Ovretveit, 2000 ; Mostafa, 2005 ; Taner & Antony, 2006 ; Andaleeb et al. , 2007 ; Owusu-Frimpong et al. , 2010 ; Manulik et al. , 2016 ; Dhahri et al. , 2020 ).
Data analysis tool
Figure 2 presents the frequency of various data analysis tools used by researchers to obtain meaningful results. The examination of articles selected for review revealed that 15 different data analysis techniques have been utilized in the past two decades. Descriptive statistics (29 articles) including mean and standard deviation has been the most frequently applied technique in healthcare service quality research followed by t -test (18 articles). It was also found that both techniques have been applied in combination because service quality can be obtained by ascertaining the difference between service perception and service expectation of patients using the SERVQUAL model ( Ahmad & Sungip, 2008 ; Irfan & Ijaz, 2011 ; Zarei, Daneshkohan, Khabiri, & Arab, 2015 ; Torabipour, Sayaf, Salehi, & Ghasemzadeh, 2016 ). Other major techniques preferred by researchers include correlation (17 articles), regression (17 articles), systematic literature review (12 articles) and ANOVA (11 articles). However, only 20 articles in total have applied structural equation modeling (SEM), MANOVA, content analysis, chi-square test, Shapiro–Wilk test, Mann–Whitney U-test, Kruskal–Wallis tests and Wilcoxon test, making them among the least preferred techniques in healthcare service quality research.
Sampling method
Articles selected for review depict that both nonprobability and probability sampling have been applied to study healthcare service quality and patient satisfaction. The articles have adopted 08 different sampling methods in addition to the complete enumeration (Census), which was employed for 03 articles. From nonprobability sampling techniques, convenience sampling (18 articles) is the most widely used sampling technique, and simple random sampling (19 articles) is the most frequently applied sampling method from the probability sampling group. Cluster sampling was found to be the least applied sampling technique among probability sampling methods because most of the studies were focused on specific regions with a limited geographical area. Targeting a smaller geographical area or specific site increases the feasibility of reaching out to sampling units because of the limited population spread. Therefore, when further segregation based on the geographical area seems impossible, the applicability of cluster sampling becomes impractical ( Cameron & Miller, 2015 ).
Findings and discussion
The systematic review of 100 articles has fetched several important findings in terms of measures of healthcare service quality and the theories applied in examining healthcare service quality.
Measures of healthcare service quality
Healthcare service quality, because of its intangible character and subjective nature, is difficult to define and measure. The comprehensive study of research articles about healthcare service quality illustrated that service quality in healthcare is examined by using different measures primarily related to servicescape, personnel, hospital administration and patients. The study has identified 41 distinctive measures of healthcare service quality ( Table 2 ). The factors commonly used to measure the quality of servicescape are identified as physical environmental quality, diagnostic aspect of care, resources and capacity, tangibility, financial and physical access to care and access ( Herstein & Gamliel, 2006 ; Ahmad & Sungip, 2008 ; Sharma & Narang, 2011 ; Simou, Pliatsika, Koutsogeorgou, & Roumeliotou, 2014 ; Marzban, Najafi, Etedal, Moradi, & Rajaee, 2015 ). Among the mentioned dimensions of servicescape, utilization has been less studied in the past. Future researchers can explore these areas because often in healthcare centers, the infrastructure capacity is overutilized or underutilized, which hinders the delivery of healthcare services. The determinants mostly employed to determine the quality of human resources (personnel) include healthcare personnel conduct, efficacy, efficiency, empathy, interaction quality, physician and staff performance, provider competency/performance, reliability, responsiveness, timeliness and trustworthiness ( Chahal & Kumari, 2012 ; Manulik et al. , 2016 ; Singh & Prasher, 2019 ). Some of the fewer studied factors under personnel characteristics include quality of patient-staff communication, outcome quality, professional quality, provider motivation and satisfaction encounters. These factors can influence the service quality of healthcare centers but are less researched in the past. The factors concerning quality aspects of hospital management/administration include admission, assurance, healthcare delivery system, infection rate, standard operating procedures, leadership and management and medical service ( Ovretveit, 2000 ; Herstein & Gamliel, 2006 ; Taner & Antony, 2006 ; Aagja & Garg, 2010 ; Irfan & Ijaz, 2011 ; Gupta & Rokade, 2016 ; Torabipour et al. , 2016 ). Among the determinants of hospital administration availability of doctors and paramedical staff, discharge mechanism of patients, documentation procedure in the hospital, social responsibility consciousness among the staff, management quality and drug availability in the hospital are some of the key factors that influence the service encounters between staff and patients. These determinants are less studied in the literature. Future researchers can build their research on these less studied variables. Lastly, the factors affecting service quality in terms of patient characteristics include patient satisfaction, the average length of stay, patient cooperation, patient quality/illness and patient socio-demographic variables ( Ovretveit, 2000 ; Mosadeghrad, 2014 ; Gupta & Rokade, 2016 ). It was observed that most of the service quality determinants identified can be summarized under the major 05 SERVQUAL determinants.
Theories applied to healthcare service quality
The list of popular theories that have been applied to examine healthcare service quality across the globe is presented in Figure 3 . A total of 11 different theories were identified during the review process. Less than 50% of papers identified for review have adopted one or the other service quality measurement framework and around 70% (32 research articles) among them have applied the SERVQUAL framework by Parasuraman, Zeithaml, and Berry (1988) . This makes SERVQUAL the mostly widely applied service quality framework. The other theories that have been utilized in the recent decade to examine the service quality of healthcare system include total quality management, fuzzy analytical hierarchy process, service performance model and health monitoring indicators system: health map ( Chahal & Kumari, 2012 ; Ramez, 2012 ; Zarei et al. , 2015 ; Amole, Oyatoye, & Adebiyi, 2015 ; Singh & Prasher, 2019 ; Zaid, Arqawi, Mwais, Al Shobaki, & Abu-Naser, 2020 ). The elements used to measure the perceived service quality of hospitals under different theories other than the SERVQUAL model can largely be classified under five SERVQUAL dimensions. However, outcome quality, process quality, administrative/management quality, utilization, technical quality and trustworthiness are identified as additional new dimensions being used to examine the service quality of hospitals ( Ovretveit, 2000 ; Chahal & Kumari, 2010 ; Simou et al. , 2014 ; Singh & Prasher, 2019 ; Zaid et al. , 2020 ).
Limitations and future research directions
The current study has some shortcomings which open up opportunities for future research. The present study followed a systematic review process to obtain research articles from different databases, like Emerald, Elsevier, Sage, Taylor and Francis and Google Scholar. Several inclusion criteria were applied, and only those full-text articles that are available in the English language were selected for the review. Therefore, there is the possibility of excluding some articles that are not available in these databases or are available in some other languages. Further, most of the studies selected for review were from developed nations. There is a lot of difference between the healthcare system of developed and developing nations. Thus, the findings of the present study cannot be generalized to developing nations without additional validation ( Kamboj & Rahman, 2015 ). Therefore, there is a need of carrying out empirical research in developing nations in this area.
The review of available literature has revealed that there are a large number of measurement tools available for the assessment of service quality in healthcare. However, the majority of these measurement instruments developed by the researchers assess quality from patients' perspectives and do not take into consideration service providers' perspectives. The technical aspect of service quality cannot be assessed by patients alone ( Upadhyai et al. , 2019 ). For a better understanding of service quality evaluation and satisfaction of service encounters, both service providers' and receivers' perspectives should be taken into consideration ( Brown & Swartz, 1989 ). Therefore, future researchers need to explore the knowledge gap (gap 1) of the SERVQUAL gap given proposed by Parasuraman et al. (1985) .
Practical implications
The study has attempted to identify and describe all dimensions and measurement tools relevant to healthcare service quality in light of the available literature. The study provides a thorough description of a vast number of investigations and reflects their outcomes. This research could help understand the diverse conceptualizations of service quality in healthcare compared to other types of services. The study also identified various gaps in the available literature that could be answered by future research.
The results of this study will help hospital executives in understanding the various constituents of quality and their impact on patient satisfaction. This will help hospital managers in formulating strategies that will improve patient satisfaction and ultimately improving the overall performance of hospitals. The study also highlighted the factors in which patients weigh more, thereby helping hospital managers to set priorities and help in proper resource utilization.
The current study presents an in-depth review of the literature concerning service quality and patient satisfaction in the healthcare industry. Service quality is a subjective measure and hence tends to vary from place to place and from patient to patient based on preference. The study has identified different measures that have been utilized to date to examine service quality or quality gaps in various hospital settings. Most of the studies selected for review have employed SERVQUAL dimensions of quality as service quality parameters. Service quality in the majority of the studies was established based on a difference between perceived and expected scores of service quality determinants, and the t -test was identified as the widely used statistical measure for testing its significance. In addition to this, various measures to determine patient satisfaction were identified and classified based on extra 3Ps of services marketing, namely physical evidence, people and process. The maximum number and most weighted factors affecting patient satisfaction are related to human resources actively engaged in providing medical services. It was observed that SERVQUAL determinants are popularly being used as a tool to determine the level of satisfaction among patients. All SERVQUAL determinants were found to have a significant positive relationship with patient satisfaction. Finally, 11 popular theories were identified among which SERVQUAL is widely applied.
Systematic literature review process
Theories applied in healthcare service quality
Aagja , J. P. , & Garg , R. ( 2010 ). Measuring perceived service quality for public hospitals (PubHosQual) in the Indian context . International Journal of Pharmaceutical and Healthcare Marketing , 4 ( 1 ), 60 – 83 .
Abu-Kharmeh , S. S. ( 2012 ). Evaluating the quality of health care services in the Hashemite Kingdom of Jordan . International Journal of Business and Management , 7 ( 4 ), 195 – 205 .
Abuosi , A. A. , & Atinga , R. A. ( 2013 ). Service quality in healthcare institutions: Establishing the gaps for policy action . International Journal of Health Care Quality Assurance , 26 ( 5 ), 481 – 492 .
Adebayo , E. T. , Adesina , B. A. , Ahaji , L. E. , & Hussein , N. A. ( 2014 ). Patient assessment of the quality of dental care services in a Nigerian hospital . Journal of Hospital Administration , 3 ( 6 ), 20 – 28 .
Aghamolaei , T. , Eftekhaari , T. E. , Rafati , S. , Kahnouji , K. , Ahangari , S. , Shahrzad , M. E. , & Hoseini , S. H. ( 2014 ). Service quality assessment of a referral hospital in southern Iran with SERVQUAL technique: Patients' perspective . BMC Health Services Research , 14 ( 1 ), 1 – 5 .
Agyapong , A. , Afi , J. D. , & Kwateng , K. O. ( 2018 ). Examining the effect of perceived service quality of health care delivery in Ghana on behavioural intentions of patients: The mediating role of customer satisfaction . International Journal of Healthcare Management , 11 ( 4 ), 276 – 288 .
Ahmad , A. , & Sungip , Z. ( 2008 ). An assessment on service quality in Malaysia insurance industry . Communications of the IBIMA , 1 , 13 – 26 . Available from: https://www.airitilibrary.com/Publication/alDetailedMesh?docid=19437765-200802-201406040030-201406040030-13-26
Ahmed , R. , & Samreen , H. ( 2011 ). Assessing the Service quality of some selected hospitals in Karachi based on the SERVQUAL model . Pakistan Business Review , 32 ( 5 ), 266 – 314 .
Al Fraihi , K. J. , Famco , D. , & Latif , S. A. ( 2016 ). Evaluation of outpatient service quality in Eastern Saudi Arabia: Patient's expectations and perceptions . Saudi Medical Journal , 37 ( 4 ), 420 – 428 .
Amole , B. B. , Oyatoye , E. O. , & Adebiyi , S. O. ( 2015 ). Prioritization of service quality influences on patients satisfaction using analytic hierarchy process: The Nigeria experience . Economics and Applied Informatics , 3 , 25 – 35 . Available from: https://www.ceeol.com/search/article-detail?id=530660
Andaleeb , S. S. , Siddiqui , N. , & Khandakar , S. ( 2007 ). Patient satisfaction with health services in Bangladesh . Health Policy and Planning , 22 ( 4 ), 263 – 273 .
Andrews , M. A. , Areekal , B. , Rajesh , K. R. , Krishnan , J. , Suryakala , R. , Krishnan , B. , & Santhosh , P. V. ( 2020 ). First confirmed case of COVID-19 infection in India: A case report . The Indian Journal of Medical Research , 151 ( 5 ), 490 – 492 .
Bahadori , M. , Raadabadi , M. , Jamebozorgi , M. H. , Salesi , M. , & Ravangard , R. ( 2014 ). Measuring the quality of provided services for patients with chronic kidney disease . Nephro-urology Monthly , 6 ( 5 ), e21810 .
Beaulieu , L. ( 2015 ). How many citations are actually a lot of citations? . Available from: https://lucbeaulieu.com/2015/11/19/how-many-citations-are-actually-a-lot-of-citations/ (accessed on: 11 December 2021) .
Beyan , O. D. , & Baykal , N. ( 2012 ). A knowledge-based search tool for performance measures in health care systems . Journal of Medical Systems , 36 ( 1 ), 201 – 221 .
Boshoff , C. , & Gray , B. ( 2004 ). The relationship between service quality, customer satisfaction and buying intentions in the private hospital industry . South African Journal of Business Management , 35 ( 4 ), 27 – 37 .
Brady , M. K. , & Cronin , J. J. ( 2001 ). Some new thoughts on conceptualizing perceived service quality: A hierarchical approach . Journal of Marketing , 65 , 34 – 49 .
Brown , S. W. , & Swartz , T. A. ( 1989 ). A gap analysis of professional service quality . The Journal of Marketing , 53 ( 2 ), 92 – 98 .
Cameron , A. C. , & Miller , D. L. ( 2015 ). A practitioner’s guide to cluster-robust inference . Journal of Human Resources , 50 ( 2 ), 317 – 372 .
Carini , E. , Gabutti , I. , Frisicale , E. M. , Di Pilla , A. , Pezzullo , A. M. , de Waure , C. , & Specchia , M. L. ( 2020 ). Assessing hospital performance indicators. What dimensions? Evidence from an umbrella review . BMC Health Services Research , 20 ( 1 ), 1 – 13 .
Chahal , H. ( 2008 ). Predicting patient loyalty and service quality relationship: A case study of civil hospital, Ahmedabad, India . Vision , 12 ( 4 ), 45 – 55 .
Chahal , H. , & Kumari , N. ( 2010 ). Development of multidimensional scale for healthcare service quality (HCSQ) in Indian context . Journal of Indian Business Research , 2 ( 4 ), 230 – 255 .
Chahal , H. , & Kumari , N. ( 2012 ). Service quality and performance in the public health-care sector . Health Marketing Quarterly , 29 ( 3 ), 181 – 205 .
Chahal , H. , & Mehta , S. ( 2013 ). Modeling patient satisfaction construct in the Indian health care context . International Journal of Pharmaceutical and Healthcare Marketing , 7 ( 1 ), 75 – 92 .
Chakravarty , A. ( 2011 ). Evaluation of service quality of hospital outpatient department services . Medical Journal Armed Forces India , 67 ( 3 ), 221 – 224 .
Chaudhury , N. , Hammer , J. , Knemer , M. , Muralidharan , K. , & Rogers , F. H. ( 2006 ). Missing in action: Teacher and health worker absence in developing countries . Journal of Economic Perspectives , 20 ( 1 ), 91 – 116 .
Choi , K.-S. , Lee , H. , Kim , C. , & Lee , S. ( 2005 ). The service quality dimensions and patient satisfaction relationships in South Korea: Across gender, age and type of service . Journal of Service Marketing , 19 ( 3 ), 140 – 149 .
Conly , J. , Seto , W. H. , Pittet , D. , Holmes , A. , Chu , M. , & Hunter , P. R. ( 2020 ). Use of medical face masks versus particulate respirators as a component of personal protective equipment for health care workers in the context of the COVID-19 pandemic . Antimicrobial Resistance and Infection Control , 9 ( 1 ), 1 – 7 .
Copnell , B. , Hagger , V. , Wilson , S. G. , Evans , S. M. , Sprivulis , P. C. , & Cameron , P. A. ( 2009 ). Measuring the quality of hospital care: An inventory of indicators . Internal Medicine Journal , 39 ( 6 ), 352 – 360 .
Dagger , T. S. , Sweeney , J. C. , & Johnson , L. W. ( 2007 ). A hierarchical model of health service quality: Scale development and investigation of an integrated model . Journal of Service Research , 10 ( 2 ), 123 – 142 .
De los Santos , J. A. A. , & Labrague , L. J. ( 2021 ). The impact of fear of COVID-19 on job stress, and turnover intentions of frontline nurses in the community: A cross-sectional study in the Philippines . Traumatology , 27 ( 1 ), 52 – 59 .
Dhahri , A. A. , Iqbal , M. R. , & Khan , A. F. A. ( 2020 ). A cross-sectional survey on availability of facilities to healthcare workers in Pakistan during the COVID-19 pandemic . Annals of Medicine and Surgery , 59 , 127 – 130 .
D'Souza , S. C. , & Sequeira , A. H. ( 2011 ). Application of MBNQA for service quality management and performance in healthcare organizations . International Journal of Engineering, Science and Technology , 3 ( 7 ), 73 – 88 .
Engelbrecht , S. ( 2005 ). Motivation and burnout in human service work: The case of midwifery in Denmark: Roskilde university, faculty of psychology . Philosophy and Science Studies .
Fatima , T. , Malik , S. A. , & Shabbir , A. ( 2018 ). Hospital healthcare service quality, patient satisfaction and loyalty: An investigation in context of private healthcare systems . International Journal of Quality and Reliability Management , 35 ( 6 ), 1195 – 1214 .
Gandjour , A. , Kleinschmit , F. , Littmann , V. , & Lauterbach , K. W. ( 2002 ). An evidence-based evaluation of quality and efficiency indicators . Quality Management in Healthcare , 10 ( 4 ), 41 – 52 .
Goshtasebi , A. , Vahdaninia , M. , Gorgipour , R. , Samanpour , A. , Maftoon , F. , Farzadi , F. , & Ahmadi , F. ( 2009 ). Assessing hospital performance by the pabon Lasso model . Iranian Journal of Public Health , 38 ( 2 ), 119 – 124 .
Groene , O. , Skau , J. K. , & Frølich , A. ( 2008 ). An international review of projects on hospital performance assessment . International Journal for Quality in Health Care , 20 ( 3 ), 162 – 171 .
Gupta , K. S. , & Rokade , V. ( 2016 ). Importance of quality in health care sector: A review . Journal of Health Management , 18 ( 1 ), 84 – 94 .
Gustafsson , A. , Johnson , M. D. , & Roos , I. ( 2005 ). The effects of customer satisfaction, relationship commitment dimensions, and triggers on customer retention . Journal of Marketing , 69 ( 4 ), 210 – 218 .
Haque , A. , Sarwar , A. A. I.-M. , Yasmin , F. , & Nuruzzaman , A. A. ( 2012 ). The impact of customer perceived service quality on customer satisfaction for private health centre in Malaysia: A structural equation modeling approach . Information Management and Business Review , 4 ( 5 ), 257 – 267 .
Hasan , H. F. A. , Ilias , A. , Rahman , R. A. , & Razak , M. Z. A. ( 2009 ). Service quality and student satisfaction: A case study at private higher education institutions . International Business Research , 1 ( 3 ), 163 – 175 .
Hegji , C. E. , & Self , D. R. ( 2009 ). The impact of hospital quality on profits, volume, and length of stay . Health Marketing Quarterly , 26 , 209 – 223 .
Herstein , R. , & Gamliel , E. ( 2006 ). The role of private branding in improving service quality . Managing Service Quality , 16 ( 4 ), 306 – 319 .
Herzlinger , R. E. ( 2003 ). Consumer-driven health care: Implications for providers, payers, and policy-makers . Healthplan , 44 ( 6 ), 26 – 29 .
Irfan , S. M. , & Ijaz , A. ( 2011 ). Comparison of service quality between private and public hospitals: Empirical evidences from Pakistan . Journal of Quality and Technology Management , 7 ( 1 ), 1 – 22 .
Israr , M. , Awan , N. , Jan , D. , Ahmad , N. , & Ahmad , S. ( 2016 ). Patients' perception, views and satisfaction with community health center services at mardan district of Khyber Pakhtunkhwa . American Journal of Public Health Research , 4 ( 3 ), 79 – 87 .
Iyengar , S. , & Dholakia , R. H. ( 2012 ). Access of the rural poor to primary healthcare in India . Review of Market Integration , 4 ( 1 ), 71 – 109 .
Jog , S. , Kelkar , D. , Bhat , M. , Patwardhan , S. , Godavarthy , P. , Dhundi , U. , & Bhavsar , R. ( 2020 ). Preparedness of acute care facility and a hospital for COVID-19 pandemic: What we did! . Indian Journal of Critical Care Medicine: Peer-Reviewed, Official Publication of Indian Society of Critical Care Medicine , 24 ( 6 ), 385 .
Kalaja , R. , Myshketa , R. , & Scalera , F. ( 2016 ). Service quality assessment in health care sector: The case of Durres public hospital . Procedia-Social and Behavioral Sciences , 235 , 557 – 565 .
Kamboj , S. , & Rahman , Z. ( 2015 ). Marketing capabilities and firm performance: Literature review and future research agenda . International Journal of Productivity and Performance Management , 64 ( 8 ), 1041 – 1067 .
Karydis , A. , Komboli‐Kodovazeniti , M. , Hatzigeorgiou , D. , & Panis , V. ( 2001 ). Expectations and perceptions of Greek patients regarding the quality of dental health care . International Journal for Quality in Health Care , 13 ( 5 ), 409 – 416 .
Kazemi , N. , Ehsani , P. , Abdi , F. , & Bighami , M. ( 2013 ). Measuring hospital service quality and its influence on patient satisfaction: An empirical study using structural equation modelling . Management Science Letters , 3 ( 7 ), 2125 – 2136 .
Kotler , P. , & Keller , K. ( 2006 ). Marketing management ( 12th ed. ). London : Pearson Education .
Lee , F. ( 2003 ). To build loyalty, hospitals need to exceed customer's expectation . Marketing Health Service , ( Summer ), 33 – 37 .
Levesque , J. F. , & Sutherland , K. ( 2017 ). What role does performance information play in securing improvement in healthcare? A conceptual framework for levers of change . BMJ Open , 7 ( 8 ), 1 – 9 , e014825 .
Levesque , J. F. , & Sutherland , K. ( 2020 ). Combining patient, clinical and system perspectives in assessing performance in healthcare: An integrated measurement framework . BMC Health Services Research , 20 ( 1 ), 1 – 14 .
Li , M. , Lowrie , D. B. , Huang , C. Y. , Lu , X. C. , Zhu , Y. C. , Wu , X. H. … Lu , H. Z. ( 2015 ). Evaluating patients' perception of service quality at hospitals in nine Chinese cities by use of the ServQual scale . Asian Pacific Journal of Tropical Biomedicine , 5 ( 6 ), 497 – 504 .
Lim , J. , Lim , K. , Heinrichs , J. , Al-Aali , K. , Aamir , A. , & Qureshi , M. ( 2018 ). The role of hospital service quality in developing the satisfaction of the patients and hospital performance . Management Science Letters , 8 ( 12 ), 1353 – 1362 .
Liu , J. W. , Lu , S. N. , Chen , S. S. , Yang , K. D. , Lin , M. C. , Wu , C. C. … Chen , C. L. ( 2006 ). Epidemiologic study and containment of a nosocomial outbreak of severe acute respiratory syndrome in a medical center in Kaohsiung, Taiwan . Infection Control and Hospital Epidemiology , 27 ( 5 ), 466 – 472 .
Loiacono , E. T. , Watson , R. T. , & Goodhue , D. L. ( 2007 ). WebQual: An instrument for consumer evaluation of web sites . International Journal of Electronic Commerce , 11 ( 3 ), 51 – 87 .
Mahajan , N. N. , Pednekar , R. , Patil , S. R. , Subramanyam , A. A. , Rathi , S. , Malik , S. … Srivastava , S.A. ( 2020 ). Preparedness, administrative challenges for establishing obstetric services, and experience of delivering over 400 women at a tertiary care COVID‐19 hospital in India . International Journal of Gynecology and Obstetrics , 151 ( 2 ), 188 – 196 .
Manulik , S. , Rosińczuk , J. , & Karniej , P. ( 2016 ). Evaluation of health care service quality in Poland with the use of SERVQUAL method at the specialist ambulatory health care center . Patient Preference and Adherence , 10 , 1435 – 1442 .
Marzban , S. , Najafi , M. , Etedal , M. G. , Moradi , S. , & Rajaee , R. ( 2015 ). The evaluation of outpatient quality services in physiotherapy in the teaching health centers of Shahid Beheshti University based on SERVQUAL tools . European Journal of Biology and Medical Science Research , 3 ( 3 ), 46 – 53 .
McLean , R. , & Antony , J. ( 2014 ). Why continuous improvement initiatives fail in manufacturing environments? A systematic review of the evidence . International Journal of Productivity and Performance Management , 63 ( 3 ), 370 – 376 .
Ministry of Health and Family Welfare (MoHFW) ( 2017 ). National health policy 2017 . Available from: https://main.mohfw.gov.in/documents/policy ( accessed 15 January 2022 ).
Mohammadkarim , B. , Jamil , S. , Pejman , H. , Seyyed , M. H. , & Mostafa , N. ( 2011 ). Combining multiple indicators to assess hospital performance in Iran using the Pabon Lasso Model . The Australasian Medical Journal , 4 ( 4 ), 175 – 179 .
Mosadeghrad , A. M. ( 2014 ). Factors influencing healthcare service quality . International Journal of Health Policy and Management , 3 ( 2 ), 77 – 89 .
Mostafa , M. M. ( 2005 ). An empirical study of patient's expectations and satisfactions in Egyptian hospitals . International Journal of Health Care Quality Assurance , 18 ( 7 ), 516 – 532 .
Murti , A. , Deshpande , A. , & Srivastava , N. ( 2013 ). Service quality, customer (patient) satisfaction and behavioural intention in health care services: Exploring the Indian perspective . Journal of Health Management , 15 ( 1 ), 29 – 44 .
Mustafa , S. S. , Yang , L. , Mortezavi , M. , Vadamalai , K. , & Ramsey , A. ( 2020 ). Patient satisfaction with telemedicine encounters in an allergy and immunology practice during the coronavirus disease 2019 pandemic . Annals of Allergy, Asthma and Immunology , 125 ( 4 ), 478 – 479 .
Nasiripour , A. A. , Kazemi , M. A. A. , & Izadi , A. ( 2012 ). Designing a hospital performance assessment model based on balanced scorecard . HealthMED , 6 ( 9 ), 2983 – 2989 .
Ovretveit , J. ( 2000 ). Total quality management in European healthcare . International Journal of Health Care Quality Assurance , 13 ( 2 ), 74 – 80 .
Ovretveit , J. ( 2009 ). Does improving quality save money? . In A Review of Evidence of Which Improvements to Quality Reduce Costs to Health Service Providers , London : The Health Foundation .
Owusu-Frimpong , N. , Nwankwo , S. , & Dason , B. ( 2010 ). Measuring service quality and patient satisfaction with access to public and private healthcare delivery . International Journal of Public Sector Management , 23 ( 3 ), 203 – 220 .
Pandey , N. , Kaushal , V. , Puri , G. D. , Taneja , S. , Biswal , M. , Mahajan , P. , … Agarwal , R. ( 2020 ). Transforming a general hospital to an infectious disease hospital for COVID-19 over 2 weeks . Frontiers in Public Health , 8 , 1 – 8 .
Parasuraman , A. , Zeithaml , V. A. , & Berry , L. L. ( 1985 ). A conceptual model of service quality and its implications for future study . Journal of Marketing , 49 ( 10 ), 41 – 50 .
Parasuraman , A. , Zeithaml , V. A. , & Berry , L. ( 1988 ). Servqual: A multiple-item scale for measuring consumer perceptions of service quality . Journal of Retailing , 64 ( 1 ), 12 – 40 .
Prajoko , Y. W. , & Supit , T. ( 2020 ). Cancer patient satisfaction and perception of chemotherapy services during COVID-19 pandemic in central Java, Indonesia . Asian Pacific Journal of Cancer Care , 5 ( 1 ), 43 – 50 .
Rameshan , P. , & Singh , S. ( 2004 ). Quality of service of primary health centres: Insights from a field study . Vikalpa , 29 ( 3 ), 71 – 82 .
Ramez , W. S. ( 2012 ). Patients' perception of health care quality, satisfaction and behavioral intention: An empirical study in Bahrain . International Journal of Business and Social Science , 3 ( 18 ), 131 – 141 .
Robb , C. E. , de Jager , C. A. , Ahmadi-Abhari , S. , Giannakopoulou , P. , Udeh-Momoh , C. , McKeand , J. , & Middleton , L. ( 2020 ). Associations of social isolation with anxiety and depression during the early COVID-19 pandemic: A survey of older adults in London, UK . Frontiers in Psychiatry , 11 , 1 – 12 .
Saleh , S. , Alameddine , M. , Mourad , Y. , & Natafgi , N. ( 2015 ). Quality of care in primary health care setting in the eastern mediterranean region: A systematic review of the literature . International Journal for Quality in Health Care , 27 ( 2 ), 79 – 88 .
Senic , V. , & Marinkovic , V. ( 2013 ). Patient care, satisfaction and service quality in health care . International Journal of Consumer Studies , 37 ( 3 ), 312 – 319 .
Shabbir , A. , Malik , S. A. , & Malik , S. A. ( 2016 ). Measuring patients' healthcare service quality perceptions, satisfaction, and loyalty in public and private sector hospitals in Pakistan . International Journal of Quality and Reliability Management , 33 ( 5 ), 538 – 557 .
Sharma , J. K. , & Narang , R. ( 2011 ). Quality of healthcare services in rural India: The user perspective . Vikalpa , 36 ( 1 ), 51 – 60 .
Sharma , A. , Prinja , S. , & Aggarwal , A. K. ( 2019 ). Comprehensive measurement of health system performance at district level in India: Generation of a composite index . The International Journal of Health Planning and Management , 34 ( 4 ), e1783 – e1799 .
Simou , E. , Pliatsika , P. , Koutsogeorgou , E. , & Roumeliotou , A. ( 2014 ). Developing a national framework of quality indicators for public hospitals . The International Journal of Health Planning and Management , 29 ( 3 ), e187 – e206 .
Singh , A. , & Prasher , A. ( 2019 ). Measuring healthcare service quality from patients' perspective: Using fuzzy AHP application . Total Quality Management and Business Excellence , 30 ( 3-4 ), 284 – 300 .
Sohail , M. S. ( 2003 ). Service quality in hospitals: More favourable than you might think . Managing Service Quality , 13 ( 3 ), 197 – 206 .
Taner , T. , & Antony , J. ( 2006 ). Comparing public and private hospital care service quality in Turkey . Leadership in Health Services , 19 ( 2 ), 1 – 10 .
Taqi , M. , Bidhuri , S. , Sarkar , S. , Ahmad , W. S. , & Wangchok , P. ( 2017 ). Rural healthcare infrastructural disparities in India: A critical analysis of availability and accessibility . Journal of Multidisciplinary Research in Healthcare , 3 ( 2 ), 125 – 149 .
Tashobya , C. K. , da Silveira , V. C. , Ssengooba , F. , Nabyonga-Orem , J. , Macq , J. , & Criel , B. ( 2014 ). Health systems performance assessment in low-income countries: Learning from international experiences . Globalization and Health , 10 ( 1 ), 1 – 19 .
Taylor , G. ( 2012 ). Readability of OHS documents–A comparison of surface characteristics of OHS text between some languages . Safety Science , 50 ( 7 ), 1627 – 1635 .
Thompson , C. C. , Shen , L. , & Lee , L. S. ( 2020 ). COVID-19 in endoscopy: Time to do more? Gastrointestinal Endoscopy , 92 ( 2 ), 435 – 439 .
Torabipour , A. , Sayaf , R. , Salehi , R. , & Ghasemzadeh , R. ( 2016 ). Analyzing the quality Gapsin the services of rehabilitation centers using the SERVQUAL technique in Ahvaz, Iran . Jundishapur Journal of Health Sciences , 8 ( 1 ), 25 – 30 .
Tucker , J. L. III. , & Adams , S. R. ( 2001 ). Incorporating patient's assessment of satisfaction and quality: An integrative model of patient's evaluations of their care . Managing Service Quality , 11 ( 4 ), 272 – 286 .
Upadhyai , R. , Jain , A. K. , Roy , H. , & Pant , V. ( 2019 ). A review of healthcare service quality dimensions and their measurement . Journal of Health Management , 21 ( 1 ), 102 – 127 .
Wiesniewski , M. , & Wiesniewski , H. ( 2005 ). Measuring service in a hospital colposcopy clinic . International Journal of Health Care Quality Assurance , 18 ( 3 ), 217 – 228 .
Wu , H. Y. , Lin , Y. K. , & Chang , C. H. ( 2011 ). Performance evaluation of extension education centres in universities based on the balanced scorecard . Evaluation and Program Planning , 34 ( 1 ), 37 – 50 .
Wu , H. C. , Li , T. , & Li , M. Y. ( 2016 ). A study of behavioral intentions, patient satisfaction, perceived value, patient trust and experiential quality for medical tourists . Journal of Quality Assurance in Hospitality and Tourism , 17 ( 2 ), 114 – 150 .
Wu , B. , Xiao , H. , Dong , X. , Wang , M. , & Xue , L. ( 2012 ). Tourism knowledge domains: A keyword analysis . Asia Pacific Journal of Tourism Research , 17 ( 4 ), 355 – 380 .
Yee , R. W. , Yeung , A. C. , & Cheng , T. E. ( 2010 ). An empirical study of employee loyalty, service quality and firm performance in the service industry . International Journal of Production Economics , 124 ( 1 ), 109 – 120 .
Yeşilada , F. , & Direktör , E. ( 2010 ). Health care service quality: A comparison of public and private hospitals . African Journal of Business Management , 4 ( 6 ), 962 – 971 .
Zaid , A. A. , Arqawi , S. M. , Mwais , R. M. A. , Al Shobaki , M. J. , & Abu-Naser , S. S. ( 2020 ). The impact of total quality management and perceived service quality on patient satisfaction and behavior intention in Palestinian healthcare organizations . Technology Reports of Kansai University , 62 ( 03 ), 221 – 232 .
Zarei , A. , Arab , M. , Froushani , A. R. , Rashidian , A. , & Ghazi-Tabatabaei , S. M. ( 2012 ). Service quality of private hospitals: The Iranian Patients' perspective . BMC Health Services Research , 12 ( 31 ), 66 – 76 .
Zarei , E. , Daneshkohan , A. , Khabiri , R. , & Arab , M. ( 2015 ). The effect of hospital service quality on patient's trust . Iranian Red Crescent Medical Journal , 17 ( 1 ), 1 – 5 .
Zeithaml , V. A. ( 2000 ). Service quality, profitability, and the economic worth of customers: what we know and what we need to learn . Journal of the Academy of Marketing Science , 28 ( 1 ), 67 – 85 .
Zineldin , M. ( 2006 ). The quality of health care and patient satisfaction: An exploratory investigation of the 5Qs model at some Egyptian and Jordanian medical clinics . International Journal of Health Care Quality Assurance , 19 , 60 – 92 .
Corresponding author
About the authors.
Related articles
We’re listening — tell us what you think, something didn’t work….
Report bugs here
All feedback is valuable
Please share your general feedback
Join us on our journey
Platform update page.
Visit emeraldpublishing.com/platformupdate to discover the latest news and updates
Questions & More Information
Answers to the most commonly asked questions here

- SUGGESTED TOPICS
- The Magazine
- Newsletters
- Managing Yourself
- Managing Teams
- Work-life Balance
- The Big Idea
- Data & Visuals
- Reading Lists
- Case Selections
- HBR Learning
- Topic Feeds
- Account Settings
- Email Preferences
Transformations That Work
- Michael Mankins
- Patrick Litre

More than a third of large organizations have some type of transformation program underway at any given time, and many launch one major change initiative after another. Though they kick off with a lot of fanfare, most of these efforts fail to deliver. Only 12% produce lasting results, and that figure hasn’t budged in the past two decades, despite everything we’ve learned over the years about how to lead change.
Clearly, businesses need a new model for transformation. In this article the authors present one based on research with dozens of leading companies that have defied the odds, such as Ford, Dell, Amgen, T-Mobile, Adobe, and Virgin Australia. The successful programs, the authors found, employed six critical practices: treating transformation as a continuous process; building it into the company’s operating rhythm; explicitly managing organizational energy; using aspirations, not benchmarks, to set goals; driving change from the middle of the organization out; and tapping significant external capital to fund the effort from the start.
Lessons from companies that are defying the odds
Idea in Brief
The problem.
Although companies frequently engage in transformation initiatives, few are actually transformative. Research indicates that only 12% of major change programs produce lasting results.
Why It Happens
Leaders are increasingly content with incremental improvements. As a result, they experience fewer outright failures but equally fewer real transformations.
The Solution
To deliver, change programs must treat transformation as a continuous process, build it into the company’s operating rhythm, explicitly manage organizational energy, state aspirations rather than set targets, drive change from the middle out, and be funded by serious capital investments.
Nearly every major corporation has embarked on some sort of transformation in recent years. By our estimates, at any given time more than a third of large organizations have a transformation program underway. When asked, roughly 50% of CEOs we’ve interviewed report that their company has undertaken two or more major change efforts within the past five years, with nearly 20% reporting three or more.
- Michael Mankins is a leader in Bain’s Organization and Strategy practices and is a partner based in Austin, Texas. He is a coauthor of Time, Talent, Energy: Overcome Organizational Drag and Unleash Your Team’s Productive Power (Harvard Business Review Press, 2017).
- PL Patrick Litre leads Bain’s Global Transformation and Change practice and is a partner based in Atlanta.
Partner Center
ORIGINAL RESEARCH article
Predictive analysis of groundwater balance and assessment of safe yield using a probabilistic groundwater model for the dead sea basin.
- 1 CSIRO Environment Dutton Park, Brisbane, Australia
- 2 School of Engineering and Built Environment, Griffith Sciences, Griffith University, Nathan, Queensland, Australia
- 3 Cities Research Institute, Griffith University, Nathan, Queensland, Australia
- 4 Australian Rivers Institute, Griffith University, Nathan, Queensland, Australia
- 5 CSIRO Environment, Adelaide, Australia
The final, formatted version of the article will be published soon.
Select one of your emails
You have multiple emails registered with Frontiers:
Notify me on publication
Please enter your email address:
If you already have an account, please login
You don't have a Frontiers account ? You can register here
Groundwater in the Middle East and North Africa region is a critical component of the water supply budget due to a (semi-)arid climate and hence limited surface water resources. Despite the significance, factors affecting the groundwater balance and overall sustainability of the resource are often poorly understood. This often includes recharge and discharge characteristics, groundwater extraction and impacts of climate change. The present study investigates the groundwater balance in the Dead Sea Basin aquifer in Jordan using a groundwater flow model developed using the MODFLOW. The study aimed to simulate groundwater balance components and their effect on estimation of the aquifer's safe yield, and to also undertake a preliminary analysis of the impact of climate change on groundwater levels in the aquifer. Model calibration and predictive analysis was undertaken using a probabilistic modelling workflow. Spatially heterogeneous groundwater recharge for the historical period was estimated as a function of rainfall by simultaneously calibrating the recharge and aquifer hydraulic property parameters. The model indicated that annual average recharge constituted 5.1% of the precipitation over a simulation period of 6 years. The effect of groundwater recharge and discharge components were evaluated in the context of estimation of safe yield of the aquifer. The average annual safe yield is estimated as ~8.0mm corresponding to the 80% of the calibrated recharge value. Simulated groundwater levels matched well with the declining trends in observed water levels which are indicative of unsustainable use. Long-term simulation of groundwater levels indicated that current conditions would result in large drawdown in groundwater levels by the end of the century. Simulation of climate change scenarios using projected estimates of rainfall and evaporation indicates that climate change scenarios would further exacerbate groundwater levels by relatively small amounts.
Keywords: Dead Sea basin, Groundwater, Climate Change, Safe yield, Pest
Received: 02 Feb 2024; Accepted: 30 Apr 2024.
Copyright: © 2024 Al Atawneh, J., Cartwright, Bertone and Doble. This is an open-access article distributed under the terms of the Creative Commons Attribution License (CC BY) . The use, distribution or reproduction in other forums is permitted, provided the original author(s) or licensor are credited and that the original publication in this journal is cited, in accordance with accepted academic practice. No use, distribution or reproduction is permitted which does not comply with these terms.
* Correspondence: Dima Al Atawneh, CSIRO Environment Dutton Park, Brisbane, Australia Sreekanth J., CSIRO Environment Dutton Park, Brisbane, Australia
Disclaimer: All claims expressed in this article are solely those of the authors and do not necessarily represent those of their affiliated organizations, or those of the publisher, the editors and the reviewers. Any product that may be evaluated in this article or claim that may be made by its manufacturer is not guaranteed or endorsed by the publisher.
Numbers, Facts and Trends Shaping Your World
Read our research on:
Full Topic List
Regions & Countries
- Publications
- Our Methods
- Short Reads
- Tools & Resources
Read Our Research On:
Discrimination Experiences Shape Most Asian Americans’ Lives
3. asian americans and the ‘model minority’ stereotype, table of contents.
- Key findings from the survey
- Most Asian Americans have been treated as foreigners in some way, no matter where they were born
- Most Asian Americans have been subjected to ‘model minority’ stereotypes, but many haven’t heard of the term
- Experiences with other daily and race-based discrimination incidents
- In their own words: Key findings from qualitative research on Asian Americans and discrimination experiences
- Discrimination in interpersonal encounters with strangers
- Racial discrimination at security checkpoints
- Encounters with police because of race or ethnicity
- Racial discrimination in the workplace
- Quality of service in restaurants and stores
- Discrimination in neighborhoods
- Experiences with name mispronunciation
- Discrimination experiences of being treated as foreigners
- In their own words: How Asian Americans would react if their friend was told to ‘go back to their home country’
- Awareness of the term ‘model minority’
- Views of the term ‘model minority’
- How knowledge of Asian American history impacts awareness and views of the ‘model minority’ label
- Most Asian Americans have experienced ‘model minority’ stereotypes
- In their own words: Asian Americans’ experiences with the ‘model minority’ stereotype
- Asian adults who personally know an Asian person who has been threatened or attacked since COVID-19
- In their own words: Asian Americans’ experiences with discrimination during the COVID-19 pandemic
- Experiences with talking about racial discrimination while growing up
- Is enough attention being paid to anti-Asian racism in the U.S.?
- Acknowledgments
- Sample design
- Data collection
- Weighting and variance estimation
- Methodology: 2021 focus groups of Asian Americans
- Appendix: Supplemental tables
In the survey, we asked Asian Americans about their views and experiences with another stereotype: Asians in the U.S. being a “model minority.” Asian adults were asked about their awareness of the label “model minority,” their views on whether the term is a good or bad thing, and their experiences with being treated in ways that reflect the stereotype.
What is the ‘model minority’ stereotype?
Amid the Civil Rights Movement in the 1960s, another narrative about Asian Americans became widespread: being characterized as a “model” minority. In 1966, two articles were published in The New York Times Magazine and U.S. News and World Report that portrayed Japanese and Chinese Americans as examples of successful minorities. Additionally, in 1987 Time magazine published a cover story on “those Asian American whiz kids.” The model minority stereotype has characterized the nation’s Asian population as high-achieving economically and educationally, which has been attributed to Asians being hardworking and deferential to parental and authority figures, among other factors. The stereotype generalizes Asians in the U.S. as intelligent, well-off, and able to excel in fields such as math and science. Additionally, the model minority myth positions Asian Americans in comparison with other non-White groups such as Black and Hispanic Americans.
For many Asians living in the United States, these characterizations do not align with their lived experiences or reflect their diverse socioeconomic backgrounds . Among Asian origin groups in the U.S., there are wide differences in economic and social experiences. Additionally, academic research has investigated how the pressures of the model minority stereotype can impact Asian Americans’ mental health and academic performance . Critics of the myth have also pointed to its impact on other racial and ethnic groups, especially Black Americans. Some argue that the myth has been used to minimize racial discrimination and justify policies that overlook the historical circumstances and impacts of colonialism, slavery and segregation on other non-White racial and ethnic groups.
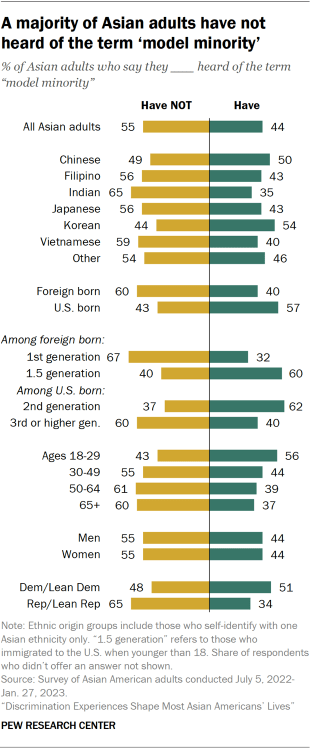
More than half of Asian adults (55%) say they have not heard of the term “model minority.” Just under half (44%) say they have heard of the term.
There are some differences in awareness of the term across demographic groups:
- Ethnic origin: About half of Korean and Chinese adults say they have heard of the term, while only about one-third of Indian adults say the same.
- Nativity: 57% of U.S.-born Asian adults have heard the term “model minority,” compared with 40% of immigrants.
- Immigrant generation: Among immigrants, 60% of those who came to the U.S. as children (“1.5 generation” in this report) say they have heard of the term “model minority,” compared with 32% of those who came to the U.S. as adults (first generation). And among U.S.-born Asian Americans, those who are second generation are more likely than those who are third or higher generation to say the same (62% vs. 40%).
- Age: 56% of Asian adults under 30 say they have heard of the term, compared with fewer than half among older Asian adults.
- Party: 51% of Asian adults who identify with or lean to the Democratic Party say they’ve heard the term, compared with 34% of those who identify with or lean to the Republican Party.
Awareness of the term ‘model minority’ varies across education and income
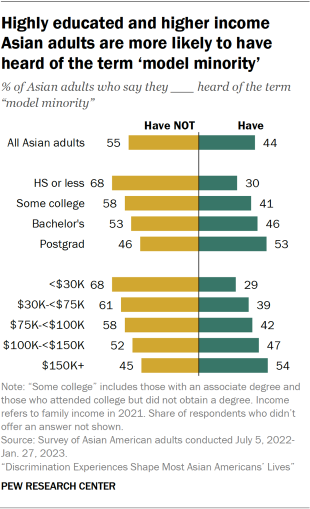
Asian adults with higher levels of formal education and higher family income are more likely to say they have heard of the term “model minority”:
- 53% of Asian adults with a postgraduate degree say they have heard the term, compared with smaller shares of those with a bachelor’s degree or less.
- 54% of Asian adults who make $150,000 or more say they have heard the term, higher than the shares among those with lower incomes. Among Asian Americans who make less than $30,000, only 29% say they have heard of the term “model minority.”
Notably, awareness of the term is higher among those born in the U.S. than immigrants across all levels of education and income.
Among Asian adults who have heard of the term “model minority,” about four-in-ten say using it to describe Asians in the U.S. is a bad thing. Another 28% say using it is neither good nor bad, 17% say using it is a good thing, and 12% say they are not sure.
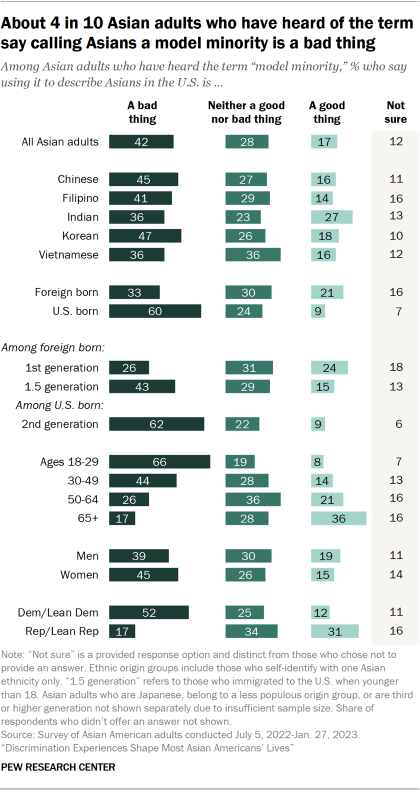
These views vary by ethnic origin, nativity, age and party. Among those who have heard of the term:
- Ethnic origin: Among Indian adults, the gap between those who say the term “model minority” is a bad thing and those who say it is a good thing (36% vs. 27%) is smaller than among other ethnic origin groups.
- Nativity: 60% of U.S.-born Asian adults say describing Asians as a model minority is a bad thing, while 9% say it is a good thing. Meanwhile, immigrants’ views of the model minority stereotype are more split (33% vs. 21%, respectively).
- Immigrant generation: Among immigrants, 43% of 1.5-generation Asian adults say using the term “model minority” is a bad thing, compared with 26% of first-generation Asian adults.
- Age: Asian adults under 30 are far more likely to say the model minority label is a bad thing than a good thing (66% vs. 8%). Meanwhile, Asian adults 65 and older are more likely to say describing Asian Americans as a model minority is a good thing (36%) than a bad thing (17%).
- Party: 52% of Asian Democrats say describing Asians as a model minority is a bad thing, about three times the share of Asian Republicans who say the same (17%).
Among those who know the term “model minority,” views of whether using it to describe Asians in the U.S. is a good or bad thing does not vary significantly across education levels. By income, Asian adults who make less than $30,000 are somewhat less likely to say it is a bad thing than those with higher incomes. 18
Views of the ‘model minority’ label are linked to perceptions of the American dream
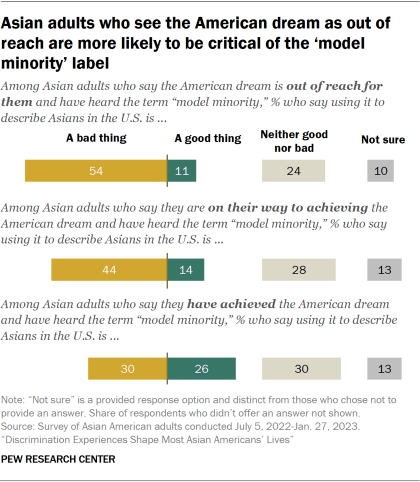
In the survey, we asked Asian Americans if they believe they have achieved the American dream, are on their way to achieving it, or if they believe the American dream is out of their reach. Among those who have heard of the term “model minority”:
- 54% of Asian adults who believe the American dream is out of their reach say describing Asian Americans as a model minority is a bad thing. This is higher than the shares among those who believe they are on their way to achieving (44%) or believe they have already achieved the American dream (30%).
- Meanwhile, 26% of Asian adults who believe they have achieved the American dream say the model minority label is a good thing. In comparison, 14% of those who believe they are on their way to achieving the American dream and 11% of those who believe that the American dream is out of their reach say the same.
In this survey, we asked Asian Americans how informed they are about the history of Asians in the U.S.
Whether Asian adults have heard of the model minority label is linked to their knowledge of Asian American history:
- 62% of Asian adults who are extremely or very informed of U.S. Asian history have heard of the term “model minority.”
- Smaller shares of those who are somewhat informed (44%) or a little or not at all informed (29%) about U.S. Asian history say they are aware of the term.
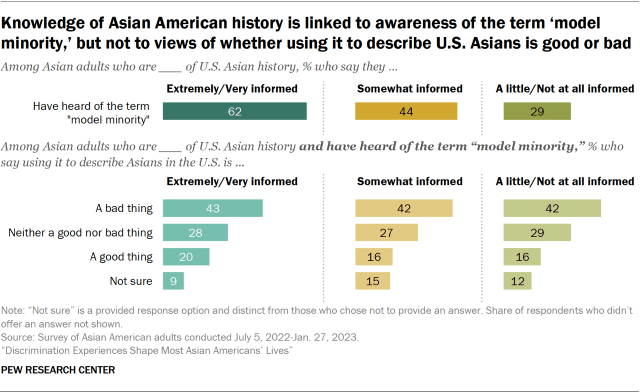
However, among those who have heard of the “model minority” label, views on whether using it to describe Asian Americans is good or bad are similar regardless of how informed they are on Asian American history. About four-in-ten across knowledge levels say describing Asian Americans as a model minority is a bad thing.
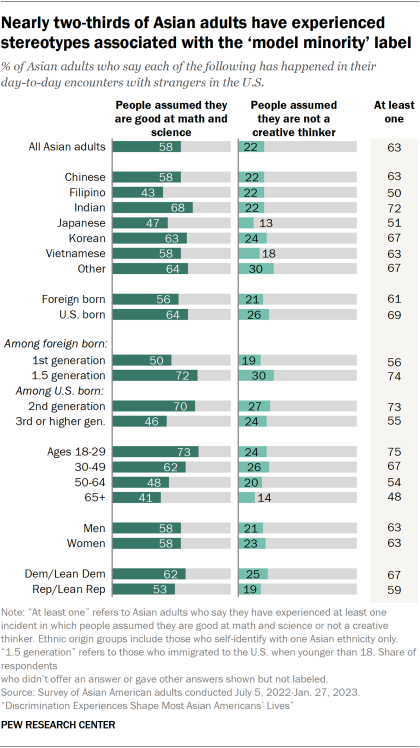
The model minority stereotype often paints Asian Americans as intellectually and financially successful, deferential to authority, and competent but robotic or unemotional , especially in comparison with other racial and ethnic groups. Additionally, some stereotypes associated with the model minority characterize Asian Americans as successful in fields such as math and science, as well as lacking in creativity.
Nearly two-thirds of Asian adults (63%) say that in their day-to-day encounters with strangers, they have at least one experience in which someone assumed they are good at math and science or not a creative thinker.
Broadly, Asian adults are far more likely to say someone has assumed they are good at math and science (58%) than not a creative thinker (22%).
Across these experiences, there are some differences by demographic groups:
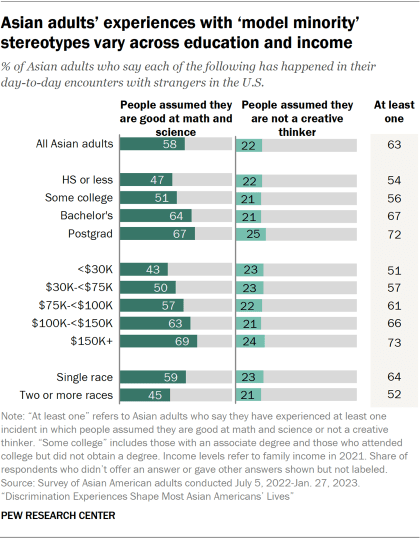
- Ethnic origin: 68% of Indian adults say strangers have assumed they are good at math and science, a higher share than among most other origin groups. Meanwhile, about half or fewer of Japanese (47%) and Filipino (43%) adults say people have made this assumption about them.
- Immigrant generation: About seven-in-ten Asian adults who are 1.5 generation and second generation each say people have assumed they are good at math and science, compared with 50% among the first generation and 46% among third or higher generations.
- Education: About two-thirds of Asian adults with a postgraduate degree or a bachelor’s degree say strangers have assumed they are good at math and science, compared with roughly half of those with some college experience or less. Similar shares regardless of education say people have assumed they are not a creative thinker.
- Income: 69% of those who make $150,000 or more say strangers have assumed they are good at math and science, compared with 43% of those who make less than $30,000.
- Race: 59% of single-race Asian adults (those who identify as Asian and no other race) say someone assumed they are good at math and science, compared with 45% of Asian adults who identify with two or more races (those who identify as Asian and at least one other race).
In our 2021 focus groups of Asian Americans, participants talked about their views of and experiences with the “model minority” stereotype.
Many U.S.-born Asian participants shared how it has been harmful , with some discussing the social pressures associated with it. Others spoke about how the stereotype portrays Asians as monolithic and compares them with other racial and ethnic groups.
“You have to be polished. There’s no room for failure. There’s no room for imperfections. You have to be well-spoken, well-educated, have the right opinions, be good-looking, be tall. [You] have to have a family structure. There’s no room for any sort of freedom in identity except for the mold that you’ve been painted as – as a model citizen.”
–U.S.-born man of Pakistani origin in early 30s
“As an Asian person, I feel like there’s a stereotype that Asian students are high achievers academically. They’re good at math and science. … I was a pretty mediocre student, and math and science were actually my weakest subjects, so I feel like it’s either way you lose. Teachers expect you to fit a certain stereotype and if you’re not, then you’re a disappointment, but at the same time, even if you are good at math and science, that just means that you’re fitting a stereotype. It’s [actually] your own achievement, but your teachers might think ‘Oh, it’s because they’re Asian,’ and that diminishes your achievement.”
–U.S.-born woman of Korean origin in late 20s
“The model minority myth … mak[es] us as Asians [and] South Asians monoliths. … I’ve had people go, ‘Oh, so your dad’s a doctor? Is he a lawyer? Do you have money? Do you have this? Do you have that? Are you [in] an arranged marriage?’ And just the kind of image that portrays and gives us. But the expectations put on us as being high performing and everyone assumes you’re going to be smart. … I am a black sheep in many ways, not only within my family, but within Asian [and] South Asian culture, being [in my profession], someone who’s not a doctor, who hasn’t gone the professional, traditional, educational route. So, it’s very harmful, that too, for those communities within the Asian diaspora who have come to the United States. … [M]any of them come from impoverished and underrepresented communities and the expectations put on them to produce or the types of jobs and menial labor they have to take on as a result is really a very poisonous mythos to have out there.”
–U.S.-born woman of Indian origin in early 40s
“One of the reasons the model minority fallacy works so well as an argument against affirmative action [for Indians is] they are a newer immigrant group that has come here and … [t]here’s a lot of education [in India]. People have opportunity there that then they can come [to America] and continue with those connections. Whereas Blacks and Hispanics have had generations of oppression, so they don’t have anything to build off of. So when you bucket everybody – Black, Hispanics and Asians – into one group, then you can make those arguments of, ‘Oh, [Asians] are the model minority, they can do it.’”
Some participants talked about having mixed feelings about being called the “model minority” and how they felt like it put them in a kind of “middle ground.”
“I feel like Asians are kind of known as the model minority. That kind of puts us in an interesting position where I feel like we’re supposed to excel and succeed in the media, or we’re seen in the media as exceeding in all these things as smart. All of us are not by any means. Yeah, I feel like we’re in this weird middle ground.”
–U.S.-born man of Chinese origin in early 20s
“A lot of people believe that Japanese are the most humble and honest people, even among other Asians. I feel like I need to live up to that. I have to try hard when people say things like that. Of course, it is good, but it’s a lot of work sometimes. As Japanese, and for my family, I try hard.”
–Immigrant man of Japanese origin in mid-40s (translated from Japanese)
Others had more positive impressions of the model minority label, saying it made them proud to be Asian and have others see them that way:
“Whenever I apply for any job, in the drop-down there is an option to choose the ethnicity, and I write Asian American proudly because everyone knows us Asians as hardworking, they recognize us as loyal and hardworking.”
–Immigrant woman of Nepalese origin in mid-40s (translated from Nepali)
“I think any model is a good thing. I mean the cognitive, the word ‘model,’ when you model after somebody it’s a positive meaning to it. So personally for me I have no issues with being called the model minority because it only tells me that I’m doing something right.”
–U.S.-born man of Hmong origin in early 40s
- Some of these groups had relatively small sample sizes. For shares of Asian adults who have heard of the term “model minority” and say using the term to describe the U.S. Asian population is a good or bad thing, by education and income, refer to the Appendix . ↩
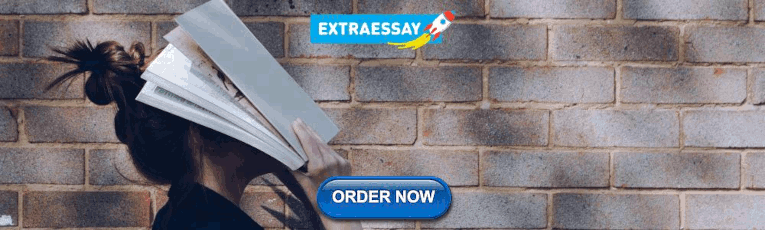
Sign up for our weekly newsletter
Fresh data delivery Saturday mornings
Sign up for The Briefing
Weekly updates on the world of news & information
- Asian Americans
- Discrimination & Prejudice
- Immigration Issues
- Race Relations
- Racial Bias & Discrimination
Key facts about Asian Americans living in poverty
Methodology: 2023 focus groups of asian americans, 1 in 10: redefining the asian american dream (short film), the hardships and dreams of asian americans living in poverty, key facts about asian american eligible voters in 2024, most popular, report materials.
1615 L St. NW, Suite 800 Washington, DC 20036 USA (+1) 202-419-4300 | Main (+1) 202-857-8562 | Fax (+1) 202-419-4372 | Media Inquiries
Research Topics
- Age & Generations
- Coronavirus (COVID-19)
- Economy & Work
- Family & Relationships
- Gender & LGBTQ
- Immigration & Migration
- International Affairs
- Internet & Technology
- Methodological Research
- News Habits & Media
- Non-U.S. Governments
- Other Topics
- Politics & Policy
- Race & Ethnicity
- Email Newsletters
ABOUT PEW RESEARCH CENTER Pew Research Center is a nonpartisan fact tank that informs the public about the issues, attitudes and trends shaping the world. It conducts public opinion polling, demographic research, media content analysis and other empirical social science research. Pew Research Center does not take policy positions. It is a subsidiary of The Pew Charitable Trusts .
Copyright 2024 Pew Research Center
Terms & Conditions
Privacy Policy
Cookie Settings
Reprints, Permissions & Use Policy
- Mobile Site
- Staff Directory
- Advertise with Ars
Filter by topic
- Biz & IT
- Gaming & Culture
Front page layout
Inside the Apple core —
Apple releases eight small ai language models aimed at on-device use, openelm mirrors efforts by microsoft to make useful small ai language models that run locally..
Benj Edwards - Apr 25, 2024 8:55 pm UTC
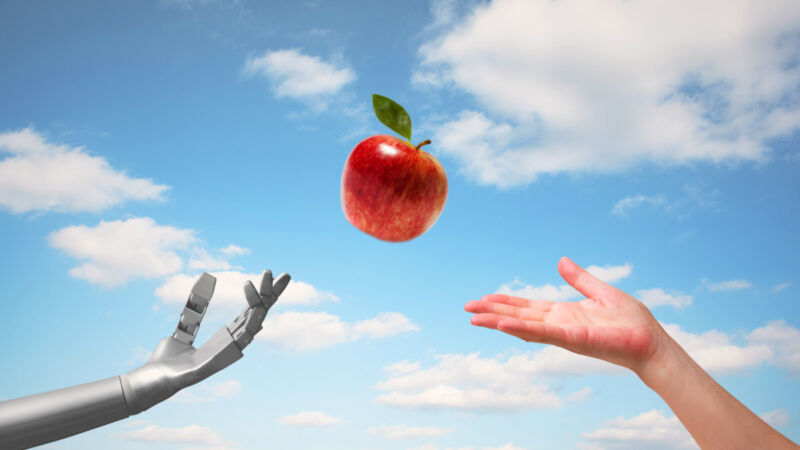
In the world of AI, what might be called "small language models" have been growing in popularity recently because they can be run on a local device instead of requiring data center-grade computers in the cloud. On Wednesday, Apple introduced a set of tiny source-available AI language models called OpenELM that are small enough to run directly on a smartphone. They're mostly proof-of-concept research models for now, but they could form the basis of future on-device AI offerings from Apple.
Further Reading
Apple's new AI models, collectively named OpenELM for "Open-source Efficient Language Models," are currently available on the Hugging Face under an Apple Sample Code License . Since there are some restrictions in the license, it may not fit the commonly accepted definition of "open source," but the source code for OpenELM is available.
On Tuesday, we covered Microsoft's Phi-3 models , which aim to achieve something similar: a useful level of language understanding and processing performance in small AI models that can run locally. Phi-3-mini features 3.8 billion parameters, but some of Apple's OpenELM models are much smaller, ranging from 270 million to 3 billion parameters in eight distinct models.
In comparison, the largest model yet released in Meta's Llama 3 family includes 70 billion parameters (with a 400 billion version on the way), and OpenAI's GPT-3 from 2020 shipped with 175 billion parameters. Parameter count serves as a rough measure of AI model capability and complexity, but recent research has focused on making smaller AI language models as capable as larger ones were a few years ago.
The eight OpenELM models come in two flavors: four as "pretrained" (basically a raw, next-token version of the model) and four as instruction-tuned (fine-tuned for instruction following, which is more ideal for developing AI assistants and chatbots):
- OpenELM-270M
- OpenELM-450M
- OpenELM-1_1B
- OpenELM-270M-Instruct
- OpenELM-450M-Instruct
- OpenELM-1_1B-Instruct
- OpenELM-3B-Instruct
OpenELM features a 2048-token maximum context window. The models were trained on the publicly available datasets RefinedWeb , a version of PILE with duplications removed, a subset of RedPajama , and a subset of Dolma v1.6 , which Apple says totals around 1.8 trillion tokens of data. Tokens are fragmented representations of data used by AI language models for processing.
Apple says its approach with OpenELM includes a "layer-wise scaling strategy" that reportedly allocates parameters more efficiently across each layer, saving not only computational resources but also improving the model's performance while being trained on fewer tokens. According to Apple's released white paper , this strategy has enabled OpenELM to achieve a 2.36 percent improvement in accuracy over Allen AI's OLMo 1B (another small language model) while requiring half as many pre-training tokens.
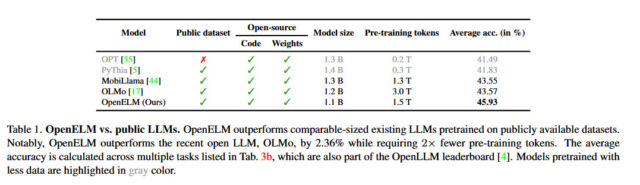
Apple also released the code for CoreNet , a library it used to train OpenELM—and it also included reproducible training recipes that allow the weights (neural network files) to be replicated, which is unusual for a major tech company so far. As Apple says in its OpenELM paper abstract, transparency is a key goal for the company: "The reproducibility and transparency of large language models are crucial for advancing open research, ensuring the trustworthiness of results, and enabling investigations into data and model biases, as well as potential risks."
By releasing the source code, model weights, and training materials, Apple says it aims to "empower and enrich the open research community." However, it also cautions that since the models were trained on publicly sourced datasets, "there exists the possibility of these models producing outputs that are inaccurate, harmful, biased, or objectionable in response to user prompts."
While Apple has not yet integrated this new wave of AI language model capabilities into its consumer devices, the upcoming iOS 18 update (expected to be revealed in June at WWDC) is rumored to include new AI features that utilize on-device processing to ensure user privacy—though the company may potentially hire Google or OpenAI to handle more complex, off-device AI processing to give Siri a long-overdue boost.
reader comments
Channel ars technica.
This paper is in the following e-collection/theme issue:
Published on 1.5.2024 in Vol 26 (2024)
Machine Learning for Predicting Risk and Prognosis of Acute Kidney Disease in Critically Ill Elderly Patients During Hospitalization: Internet-Based and Interpretable Model Study
Authors of this article:

Original Paper
- Mingxia Li 1, 2 * , PhD ;
- Shuzhe Han 3 * , MM ;
- Fang Liang 4 , MD ;
- Chenghuan Hu 1 , MD ;
- Buyao Zhang 1 , MM ;
- Qinlan Hou 1 , MM ;
- Shuangping Zhao 1, 5, 6 , MD
1 Department of Critical Care Medicine, Xiangya Hospital of Central South University, Changsha, China
2 Department of Critical Care Medicine, ZhuJiang Hospital of Southern Medical University, Guangzhou, China
3 Department of Obstetrics and Gynecology, 967th Hospital of the Joint Logistics Support Force of the Chinese People's Liberation Army, Dalian, China
4 Department of Hematology and Critical Care Medicine, The Third Xiangya Hospital, Central South University, Changsha, China
5 National Clinical Research Center for Geriatric Disorders, Changsha, China
6 Hunan Provincial Clinical Research Center of Intensive Care Medicine, Changsha, China
*these authors contributed equally
Corresponding Author:
Shuangping Zhao, MD
Department of Critical Care Medicine
Xiangya Hospital of Central South University
No 87, Xiangya Road, Kaifu District
Changsha, 410008
Phone: 86 1397495302
Email: [email protected]
Background: Acute kidney disease (AKD) affects more than half of critically ill elderly patients with acute kidney injury (AKI), which leads to worse short-term outcomes.
Objective: We aimed to establish 2 machine learning models to predict the risk and prognosis of AKD in the elderly and to deploy the models as online apps.
Methods: Data on elderly patients with AKI (n=3542) and AKD (n=2661) from the Medical Information Mart for Intensive Care IV (MIMIC-IV) database were used to develop 2 models for predicting the AKD risk and in-hospital mortality, respectively. Data collected from Xiangya Hospital of Central South University were for external validation. A bootstrap method was used for internal validation to obtain relatively stable results. We extracted the indicators within 24 hours of the first diagnosis of AKI and the fluctuation range of some indicators, namely delta (day 3 after AKI minus day 1), as features. Six machine learning algorithms were used for modeling; the area under the receiver operating characteristic curve (AUROC), decision curve analysis, and calibration curve for evaluating; Shapley additive explanation (SHAP) analysis for visually interpreting; and the Heroku platform for deploying the best-performing models as web-based apps.
Results: For the model of predicting the risk of AKD in elderly patients with AKI during hospitalization, the Light Gradient Boosting Machine (LightGBM) showed the best overall performance in the training (AUROC=0.844, 95% CI 0.831-0.857), internal validation (AUROC=0.853, 95% CI 0.841-0.865), and external (AUROC=0.755, 95% CI 0.699–0.811) cohorts. In addition, LightGBM performed well for the AKD prognostic prediction in the training (AUROC=0.861, 95% CI 0.843-0.878), internal validation (AUROC=0.868, 95% CI 0.851-0.885), and external (AUROC=0.746, 95% CI 0.673-0.820) cohorts. The models deployed as online prediction apps allowed users to predict and provide feedback to submit new data for model iteration. In the importance ranking and correlation visualization of the model’s top 10 influencing factors conducted based on the SHAP value, partial dependence plots revealed the optimal cutoff of some interventionable indicators. The top 5 factors predicting the risk of AKD were creatinine on day 3, sepsis, delta blood urea nitrogen (BUN), diastolic blood pressure (DBP), and heart rate, while the top 5 factors determining in-hospital mortality were age, BUN on day 1, vasopressor use, BUN on day 3, and partial pressure of carbon dioxide (PaCO 2 ).
Conclusions: We developed and validated 2 online apps for predicting the risk of AKD and its prognostic mortality in elderly patients, respectively. The top 10 factors that influenced the AKD risk and mortality during hospitalization were identified and explained visually, which might provide useful applications for intelligent management and suggestions for future prospective research.
Introduction
Acute kidney injury (AKI), as a common complex heterogeneous syndrome in critically ill patients, is associated with an increased risk of death and adverse renal events [ 1 - 3 ]. AKI is more common in elderly patients in the intensive care unit (ICU), with sustained impaired renal function associated with a poor prognosis for survival [ 4 , 5 ]. Therefore, in this study, we focused on AKI in the elderly with renal function impairment for more than 7 days during hospitalization, that is, acute kidney disease (AKD). In 2012, Kidney Disease: Improving Global Outcomes (KDIGO) first proposed the term “AKD,” but at this time, it was viewed as a period of kidney pathology following AKI, not as an independent definition [ 6 ]. In a comparative study on the epidemiology of AKD, patients with AKI who developed AKD had a higher risk of chronic kidney disease (CKD) and end-stage renal disease (ESRD), suggesting the potential clinical research value for AKD as a novel term [ 7 ]. In 2017, the Acute Disease Quality Initiative (ADQI) 16 Workgroup presented an expert consensus on AKD, defining AKD as AKI with KDIGO stage 1 or higher within 7-90 days of the first diagnosis of AKI [ 8 ]. There is a distinct disease course between AKI, AKD, and CKD. AKD highlights the key intervenable period in the transformation process from AKI to CKD and lays the foundation for the construction of management strategies for renal function recovery.
Recently, a multicenter study indicated that more than half of the hospitalized patients with AKI developed AKD, which increased the risk of long-term mortality [ 9 ]. Nevertheless, an epidemiological study by Andonovic et al [ 10 ] found that patients with AKD in the ICU have a higher short-term risk of death but no statistically significant difference in long-term survival. Moreover, Chen et al [ 11 ] reported that patients with AKD are more likely to require long-term dialysis. Considering the high incidence and mortality rate of AKD, researchers have conducted exploratory early warning studies on AKD. Current predictive research on AKD has focused primarily on patients with AKI during hospitalization, sepsis-related AKI, coronary heart disease, and renal cell carcinoma postoperatively [ 9 , 12 - 14 ]. However, it should be noted that elderly patients with diminished renal function have received insufficient attention, and little is known about the AKD risk and prognostic mortality in the elderly. Further, some studies have explored the use of artificial intelligence (AI) algorithms to predict the onset and progression of diseases, but only a few have developed user-friendly online prediction apps for clinical practice. Zhou et al [ 15 ] established an online calculator using Extreme Gradient Boosting (XGBoost) to predict AKI in patients with sepsis-associated acute respiratory distress syndrome. Regrettably, this risk calculator has not been validated externally to determine generalization capacity. Thus, it is imperative to use big data and AI technology to conduct research on the diagnosis and prognosis prediction of AKD in the elderly, and transform the AI models into internet-based apps to assist clinicians in timely intervention to maximize the improvement in renal function and survival outcomes.
Therefore, this study intended to develop 2 machine learning prediction models: one was a model for predicting the risk of AKD in critically ill patients during hospitalization to address problems regarding renal function recovery and early detection of AKD; the other was for predicting the in-hospital mortality in AKD to deal with the adverse outcomes of AKD. In addition, Shapley additive explanation (SHAP) analysis was used to rank the importance and visualize the correlation of the features affecting the occurrence and outcome of AKD [ 16 ]. Importantly, we deployed the models with the most comprehensive performance as web-based prediction apps to facilitate doctors' decision-making.
Ethical Considerations
This study was conducted strictly in accordance with the Guidelines for Developing and Reporting Machine Learning Predictive Models in Biomedical Research [ 17 ]. The MIMIC-IV database was approved by the Ethics Review Boards of the MIT and the Beth Israel Deaconess Medical Center. This study obtained access and download permission from the MIMIC-IV database (no. 41817305) and passed the retrospective ethics review of the Medical Ethics Committee of Xiangya Hospital Central South University (no. 202105200). Due to the deprivacy of the data for this retrospective study, it was exempted from patients’ informed consent.
Study Design
A retrospective cohort study was conducted using electronic health records (EHRs) from the Medical Information Mart for Intensive Care IV (MIMIC-IV) data and patients’ data from Xiangya Hospital Central South University (Hunan, China) [ 18 ]. In June 2022, the Massachusetts Institute of Technology (MIT) released the revised version of MIMIC-IV 2.0, which contained in-hospital diagnosis and treatment records, as well as in- and out-of-hospital death information for about 40,000 patients in ICUs, and achieved data privacy by deleting patient identification numbers and drifting data through time. The MIMIC-IV cohorts were used to develop machine learning predictive models. Further, the Department of Critical Care Medicine at Xiangya Hospital Central South University is the National Key Clinical Specialty. Approximately 2500 critically ill patients are admitted to the department each year for the treatment of various diseases. Patients were enrolled in the Department of Critical Care Medicine from 2017 to 2021 for external validation of models.
Data on AKI in the Elderly
Inclusion and exclusion criteria.
Data on AKI in elderly patients in the ICU were collected for the construction and external verification of an early warning model for the risk of AKD. According to the Chinese Healthy Elderly Standard issued by the National Health Commission of China, those 60 years old and above were defined as the elderly. The inclusion criteria were (1) age≥60 years, (2) ICU stay of at least 48 hours, (3) EHRs of patients admitted to the ICU for the first time, and (4) patients with AKI who met KDIGO criteria. The exclusion criteria were (1) patients with ESRD and (2) missing data on the diagnosis of AKI. Figure 1 shows the data extraction process in detail.

Outcome Definition
The occurrence of AKD during hospitalization was considered the outcome of the risk prediction study. AKI was diagnosed and staged in accordance with the AKI guidelines issued by the KDIGO in 2012 [ 6 ]. According to the expert consensus of the ADQI-16 Workgroup in 2017, AKD was defined as the presence of at least stage 1 AKI within 7-90 days after the initial diagnosis of AKI [ 8 ]. In this study, patients with AKI who met this definition during hospitalization were regarded as having AKD ( Multimedia Appendix 1 ).
Data Extraction
Navicat Premium (version 15.0.13) was used for MIMIC-IV database management and PostgreSQL (version 9.6; PostgreSQL Global Development Group) for variable extraction. Patients with AKI were identified based on their serum creatinine and urine output levels. Patients with AKI stage higher than or equal to 1 between 7 days following AKI and discharge were considered to have AKD during their hospitalization. Finally, 33 variables were determined and extracted from the Xiangya Hospital data set and the MIMIC-IV database, including age, gender, and the AKI stage as basic characteristics; sepsis, hypertension, diabetes, chronic kidney disease (CKD), chronic pulmonary disease (CPD), and chronic liver disease (CLD) as comorbidities; mechanical ventilation (MV), renal replacement therapy (RRT), and vasopressors as interventions; heart rate, respiratory rate, systolic blood pressure (SBP), diastolic blood pressure (DBP) as vital signs; and white blood cell (WBC) count, red blood cell (RBC) count, hemoglobin, hematocrit, potassium, calcium, anion gap, partial pressure of oxygen (PaO 2 ), partial pressure of carbon dioxide (PaCO 2 ), pH, glucose, blood urea nitrogen (BUN), and serum creatinine as laboratory tests. These examination indicators were measured on day 1 of AKI diagnosis. We also obtained BUN and serum creatinine levels on day 3 following AKI diagnosis, as well as corresponding delta BUN and delta creatinine values on day 3 minus day 1.
Data on AKD in the Elderly
Following the aforementioned study on AKI in the elderly, further extracted the data concerning critically ill patients with AKD to construct and verify a model that predicted poor prognostic mortality during hospitalization. The inclusion criteria of elderly patients with AKD were as follows: (1) age≥60 years, (2) length of stay in the ICU for more than 48 hours, (3) patients admitted to the ICU for the first time, and (4) patients with AKD who met the ADQI consensus of 2017. The exclusion criteria were (1) patients who had ESRD and (2) missing data related to AKD diagnosis. A detailed description of the data extraction can be seen in Figure 1 .
Outcome Definition and Data Extraction
In-hospital death was the outcome of the prognostic prediction study of AKD in the elderly.
This study on prognostic mortality prediction and the aforementioned study on risk prediction of AKD in the elderly were similar in terms of the content and timing for extracting 33 variables.
Construction and Validation of Models
Several supervised learning algorithms were selected to solve classification prediction problems in this study: logistic regression model (LRM), XGBoost, Light Gradient Boosting Machine (LightGBM), multilayer perceptron (MLP), random forest (RF), and the K-nearest neighbor (KNN) algorithm. Two models were developed using the MIMIC-IV cohort: one for predicting AKD occurrence among the elderly and the other for predicting the prognostic mortality in AKD. To prevent overfitting and improve generalization, a 10-fold cross-validation method was applied to assess the models, and the final models were constructed based on repeated iterations. Multimedia Appendix 2 shows the optimal hyperparameters of the AKD risk and AKD mortality models. Through the GridSearchCV module, we conducted a grid search that traversed all parameter values and returned the parameter combination that provided the best overall performance. The models constructed from the MIMIC-IV database were internally validated by bootstrap resampling with replacement to evaluate performance. The established training model was externally validated with the Xiangya Hospital cohort.
Evaluation and Deployment of Models
The classification prediction effect of the models was evaluated using the area under the receiver operating characteristic curve (AUROC), sensitivity, specificity, positive predictive value (PPV), and negative predictive value (NPV) under the optimal cutoff value. Moreover, a calibration curve was developed to determine the models’ predictive accuracy, and clinical decision curve analysis (DCA) was performed to assess their clinical utility. To enhance the interpretability of machine learning black-box models, we performed SHAP analysis by visualizing each feature’s marginal contribution to the models’ prediction in importance-ranking plots and showing how each feature impacts the outcome in partial dependence plots. Lastly, we selected machine learning algorithm models with the best comprehensive performance from the training and validation cohorts and deployed them to the online server for the convenience of clinical workers or patients. The web-based apps were managed by Heroku.
Statistical Analysis
Data were analyzed using Python (version 3.9.7) and R (version 4.2.0; R Foundation for Statistical Computing). Variables with a missing ratio higher than 35% were deleted, and the mice package (version 3.14.0) in R was used to fill in the missing values using the multiple imputation method. In data preprocessing, the Z-score method was used to scale the continuous variables with the StandardScaler function. Categorical variables were represented as numbers (percentages) and compared between groups using the chi-square test. Depending on whether the continuous variables were normally distributed, the mean (SD) or median (IQR) was expressed and compared using the 2-tailed t test or the Mann-Whitney U test. By analyzing the Youden index, the optimal cutoff value of the receiver operating characteristic (ROC) curve was calculated, as well as the sensitivity, specificity, PPV, and NPV of the models. Statistical significance was set at P <.05.
Model 1: Predicting the risk of AKD in the Elderly
Baseline characteristics.
In the study on the risk prediction of AKD in critically ill elderly patients during hospitalization, a total of 3542 elderly patients with AKI from the MIMIC-IV database and 280 from Xiangya Hospital were retrospectively included after screening by the inclusion and exclusion criteria as the training and external validation cohorts, respectively. AKD incidence was 75.1% (2661/3542) in the training cohort and 66.4% (186/280) in the external validation cohort. A comparison of baseline characteristics and stratification of the 2 cohorts according to the presence or absence of AKD is shown in Multimedia Appendix 3 . In the MIMIC-IV cohort, patients with AKD had a higher proportion of comorbidities (sepsis, diabetes, CKD, and CPD) and a lower proportion of hypertension ( P <.05); a higher proportion of interventions (MV, RRT, and vasopressor use; P <.05); a higher heart rate and lower SBP and DBP in terms of vital signs ( P <.05); and higher potassium, anion gap, BUN on day 1 (following AKI diagnosis), serum creatinine on day 1, BUN on day 3 (following AKI diagnosis), and serum creatinine on day 3 and lower PaO 2 in terms of laboratory tests ( P <.05). Furthermore, more patients with AKD in the Xiangya Hospital cohort were males and had stage 3 AKI ( P <.05). The features of the Xiangya Hospital cohort with a similar trend to the MIMIC-IV cohort were as follows: diabetes, CKD, RRT, vasopressor use, potassium, anion gap, PaO 2 , BUN on day 1, serum creatinine on day 1, BUN on day 3, and serum creatinine on day 3, while hypertension and SBP had opposite trends and statistical results ( P <.05).
Model Comparison
We included all the variables shown in Multimedia Appendix 3 in the model construction since these indicators are common and easily obtainable in clinical practice. Table 1 shows the performance comparison of the 6 machine learning models for predicting AKD risk in the training, internal validation, and external cohorts. In the training cohort, the algorithm with the greatest performance was LightGBM, with AUROC=0.844 (95% CI 0.831-0.857), sensitivity=0.788 (95% CI 0.759-0.814), specificity=0.761 (95% CI 0.745-0.777), PPV=0.522 (95% CI 0.495-0.549), and NPV=0.915 (95% CI 0.903-0.927). In the external validation cohort, the best-predicting model was the LRM, which had AUROC=0.763 (95% CI 0.707-0.818), sensitivity=0.830 (95% CI 0.738-0.899), specificity=0.586 (95% CI 0.512-0.658), PPV=0.503 (95% CI 0.422-0.584), and NPV=0.872 (95% CI 0.800-0.925). LightGBM also demonstrated the ability to distinguish patients at a higher risk of AKD during hospitalization in the validation cohort: AUROC=0.853 (95% CI 0.841-0.865), sensitivity=0.817 (95% CI 0.791-0.842), specificity=0.759 (95% CI 0.742-0.775), PPV=0.534 (95% CI 0.507-0.560), and NPV=0.925 (95% CI 0.913-0.936) in the internal validation cohort and AUROC=0.755 (95% CI 0.699-0.811), sensitivity=0.851 (95% CI 0.763-0.916), specificity=0.597 (95% CI 0.523-0.668), PPV=0.516 (95% CI 0.435-0.597), and NPV=0.888 (95% CI 0.819-0.937) in the external cohort. Figures 2 A and 2B provide the ROC curves of the prediction models in the training and external cohorts, among which LightGBM showed the best overall performance. Multimedia Appendix 4 exhibits the ROC curves for the internal validation cohort. We selected the 3 algorithms (LightGBM, RF, XGBoost) with better performance in the validation cohort to conduct DCA; Figure 2 C shows that when the threshold probability of AKD reached 60%, the net benefit ratio of taking intervention measures was 0.5, showing good clinical applicability of LightGBM. Further, the calibration curves for the 3 algorithms are presented in Figure 2 D displaying the relative consistency between predictions and actual values. However, according to Figure 2 D, when the threshold probability was low, the prediction probability of the model was high with overfitting, which was consistent with Figure 2 C: when the threshold probability was low, the net benefit of the model hardly increased.

a AKD: acute kidney disease.
b AUROC: area under the receiver operating characteristic curve.
c PPV: positive predictive value.
d NPV: negative predictive value.
e LRM: logistic regression model.
f XGBoost: Extreme Gradient Boosting.
g LightGBM: Light Gradient Boosting Machine.
h MLP: multilayer perceptron.
i RF: random forest.
j KNN: K-nearest neighbor.
Model Interpretability
To better explain the clinical significance of certain features, this study quantified the features’ importance as SHAP values. As shown in Figure 3 A, variables were given a ranking based on their contribution to the risk prediction of AKD, with creatinine on day 3, sepsis, delta BUN, DBP, heart rate, delta creatinine, creatinine on day 1, respiratory rate, pH, and diabetes as the top 10 predictors of developing AKD during hospitalization in the elderly. Figure 3 B shows a detailed relationship between each feature and AKD risk, indicating that the positively related features were as follows: creatinine on day 3, sepsis, delta BUN, heart rate, delta creatinine, creatinine on day 1, pH, and diabetes (the higher the value of these features or the presence of complications, the higher the probability of developing AKD in elderly patients with AKI). Further, the protective effect was associated with a higher DBP. However, the relationship between respiratory rate and AKD during hospitalization was not clearly demonstrated. Furthermore, partial dependence plots were drawn in Figure 4 for the first 4 continuous variables in Figure 3 A. The partial dependence plots visually displayed the global relationship between feature and risk distribution. According to Figure 4 A, the change curve between creatinine on day 3 (abscissa) and AKD risk (ordinate) indicated a cutoff value of 110 for this feature, meaning that when the creatinine level on day 3 exceeded 110 umol/L, the risk of AKD during hospitalization also increased. Similarly, Figures 4 B, 4C, and 4D demonstrate that 0 was the cutoff for delta BUN (positive correlation), 80 mmHg for the DBP (negative correlation), and 110 beats/minute for the heart rate (positive correlation). Thus, targeted feature management may assist in reducing the risk of AKD in elderly patients with AKI during hospitalization based on the cutoff values in partial dependency plots.

Model Application
We deployed the LightGBM algorithm as an online app because the LightGBM AKD risk model had a relatively high AUROC in the training, internal validation, and external cohorts. After the 10-fold cross-validation grid search, the LightGBM hyperparameters were finally tuned as follows: “num_leaves”: 10, “max_depth”: 5, “max_bin”: 135, “min_data_in_leaf”: 11, “feature_fraction”: 1.0, “bagging_fraction”: 1.0, “bagging_freq”: 45, “lambda_l1”: 0.0, “lambda_l2”: 0.001, “min_split_gain”: 0.4. Further, a web-based app for predicting AKD risk in the elderly was designed, which could be accessed online at any time by medical staff or patients ( Multimedia Appendix 5 ) [ 19 ]. For an elderly patient with AKI being diagnosed for the first time in the ICU, physicians collected and input all variables’ values correctly in Multimedia Appendix 5 and then clicked the Predict button to obtain the predicted result (AKD or non-AKD) during hospitalization. Moreover, users could enter variables’ values and the author’s email address and click the Feedback button, enabling new data to be sent to the author to facilitate model iteration. When the result showed that the patient was at high risk of AKD, early intervention could be implemented based on the partial dependence plots in Figure 4 and interventionable indicators might be controlled as close to the cutoff value as possible to prevent the progression of AKI and reduce the risk of AKD.

Model 2 : Predicting Prognostic Mortality in Elderly Patients With AKD
In this study on predicting the prognostic mortality in elderly patients with AKD, a total of 2661 elderly patients with AKD from the MIMIC-IV database (training cohort) and 186 from Xiangya Hospital (external validation cohort) were screened out and enrolled. The in-hospital mortality of elderly patients with AKD was 29.6% (788/2661) in the training cohort and 41.3% (77/186) in the external validation cohort. Multimedia Appendix 6 provides the differences in baseline characteristics between the 2 cohorts stratified by in-hospital death. In the MIMIC-IV cohort, compared with survivors, patients who died in the hospital were more likely to be older ( P <.05), with a higher proportion of comorbidities (sepsis, CKD, and CLDl P <.05); a higher proportion of interventions (RRT and vasopressor use; P <.05); a higher heart rate and lower SBP in terms of vital signs ( P <.05); and higher WBC count, potassium, anion gap, glucose, BUN on day 1, creatinine on day 1, BUN on day 3, creatinine on day 3, delta BUN, and delta creatinine and lower RBC count, hemoglobin, hematocrit, and PaCO 2 in terms of laboratory tests ( P <.05). Additionally, in the Xiangya Hospital cohort, sepsis, RRT, vasopressor use, heart rate, anion gap, and BUN on day 1 had similar statistical trends to those in the MIMIC-IV cohort ( P <.05).
The performance of the in-hospital death prediction model for AKD in elderly patients in the training and external cohorts is presented in Table 2 . In the training cohort, the best-performing algorithm was XGBoost, with AUROC=0.870 (95% CI 0.853-0.886), sensitivity=0.772 (95% CI 0.752-0.791), specificity=0.793 (95% CI 0.763-0.821), PPV=0.594 (95% CI 0.564-0.624), and NPV=0.899 (95% CI 0.883-0.913). In the external validation cohort, the LRM provided the best prediction, with AUROC=0.772 (95% CI 0.701-0.843), sensitivity=0.706 (95% CI 0.612-0.790), specificity=0.740 (95% CI 0.628-0.834), PPV=0.640 (95% CI 0.532-0.739), and NPV=0.794 (95% CI 0.700-0.869). However, comprehensively comparing the prediction performance of the training cohort and the generalization of the validation cohort, the LightGBM algorithm showed good overall performance, as demonstrated by an AUROC of 0.861 (95% CI 0.843-0.878) in the training cohort, 0.868 (95% CI 0.851-0.885) in the internal validation cohort, and 0.746 (95% CI 0.673-0.820) in the external cohort, in accordance with the ROC curves in Figure 5 A, Multimedia Appendix 7 , and Figure 5 B. Figure 5 C indicates that in DCA, when the probability of death during hospitalization reached 10%, the net benefit of intervention measures was 0.2, suggesting good efficacy of LightGBM. Moreover, the calibration curve in Figure 5 D shows that the predicted curve of the model surrounded the actual probability line, indicating relative accuracy.

Using SHAP values, we performed a visual analysis of a model for predicting AKD prognostic mortality of the elderly. Figure 6 A shows the top 10 predictors of in-hospital death in patients with AKD, as follows: age, BUN on day 1, vasopressor use, BUN on day 3, PaCO 2 , RRT, delta creatinine, RBC count, respiratory rate, and creatinine on day 1. Figure 6 B indicates a more detailed representation of the positive and negative relationships between features and outcomes. The risk of death due to AKD during hospitalization was positively associated with the following features: older age, higher BUN on day 1, use of vasopressors, higher BUN on day 3, higher PaCO 2 , use of RRT, higher delta creatinine, and creatinine on day 1. The RBC count and respiratory rate were higher in hospitalized survivors among elderly patients with AKD. For the first 4 continuous variables in Figure 6 A, partial dependence plots were drawn ( Figure 7 ). According to Figure 7 A, the probability of in-hospital death increased from 0 when the patient reached 75 years of age. Similarly, Figures 7 B, 7C, and 7D show that the cutoff values of BUN on day 1, BUN on day 3 and PaCO 2 affecting the risk of death were 15 mmol/L, 10 mmol/L, and 45 mmHg, respectively, which might contribute to guiding patients’ management and reducing the in-hospital risk of death for those with AKD.

We chose the LightGBM algorithm, which exhibited good AUROC values in the training and validation cohorts, to deploy the prognostic mortality function in the online version of the AKD model. The optimal combination of hyperparameters for the LightGBM prognostic model was as follows: “num_leaves”: 10, “max_depth”: 4, “max_bin”: 35, “min_data_in_leaf”: 100, “feature_fraction”: 1.0, “bagging_fraction”: 0.7, “bagging_freq”: 5, “lambda_l1”: 0.0, “lambda_l2”: 0.1, “min_split_gain”: 0.0. The web-based app to predict in-hospital death in elderly patients with AKD could be accessed online ( Multimedia Appendix 8 ) [ 20 ]. When elderly patients were diagnosed with AKD for the first time, we entered all the indicators correctly on the web page and clicked the Predict button to predict the prognosis (death or survival) of elderly patients with AKD during hospitalization. Additionally, if users found a prediction error, they could enter the variable’s value and their own email address and then click the Feedback button, enabling the corresponding data to be automatically sent to the author’s email address. Using this feedback function could facilitate the collection of new data for model iterations. At the same time, according to the cutoff value shown in the partial dependence plots in Figure 7 , targeted interventions were performed on patients at risk of death due to AKD, with the potential to improve the survival of patients with AKD.

Principal Findings
Predicting the risk of akd in the elderly.
As part of this study, we focused on model construction and feature analysis for AKD risk during hospitalization, and LightGBM was selected as the best algorithm for online deployment (training cohort AUROC=0.844, 95% CI 0.831-0.857; validation cohort AUROC=0.755, 95% CI 0.699-0.811). To the best of our knowledge, our study was the first to analyze the risk characteristics of AKD in critically ill elderly patients during hospitalization and to develop an easy-to-use online AKD risk identification app.
In addition to basic information, comorbidities, vital signs, and laboratory indicators on day 1 of AKI diagnosis, some indicators on day 3 and their fluctuations were also selected as features, including creatinine on day 3, BUN on day 3, delta BUN, and delta creatinine. A previous study found that the maximum creatinine level is reached on day 3 within 1-5 days after cardiac surgery in elderly patients [ 21 ]. Treiber et al [ 22 ] demonstrated that in neonatal patients with perinatal hypoxia, the AUROC of serum creatinine on day 3 after birth as a single predictor for AKI is 0.660, indicating a certain predictive value. Similar to these studies, our study revealed that serum creatinine on day 3 is higher than that on day 1 of AKD diagnosis in the elderly, ranking first in the feature of the AKD risk prediction model. Thus, serum creatinine on day 3 might be considered a focused experimental indicator for clinical research on patients with AKD in the ICU.
Delta BUN is commonly used to evaluate changes in renal function; however, the definition of the specific delta BUN varies. According to a study on patients with acute heart failure, delta BUN refers to the difference between the day before and after the administration of loop diuretics, but there was no statistical difference between the treatment and control groups ( P >.05) [ 23 ]. Moreover, delta BUN was defined as the difference between 1 year after transplantation and at transplantation to evaluate renal function in a retrospective study conducted by Ewald et al [ 24 ]. In our study, we found that delta BUN (day 3 – day 1 after AKI diagnosis) is significantly positively correlated with AKD in elderly patients, with higher BUN on day 3 than on day 1 (delta BUN>0). Wu et al [ 25 ] also observed a gradual increase in BUN after AKI, in which BUN peaked at day 3 following cisplatin-induced AKI. Additionally, we determined that delta creatinine and creatinine on day 1 are associated with an increased risk of AKD during hospitalization. In a prospective study on adult patients after cardiac surgery, researchers defined delta creatinine as baseline – first postoperative creatinine and concluded that delta creatinine combined with biomarkers has a good predictive effect on mortality[ 24 ]. Furthermore, Garner et al [ 26 ] defined delta creatinine to be higher than 26 μmol/L within 30 days of admission, enabling 98% of hospitalized patients with AKI to be identified.
At present, many studies have examined the factors associated with sepsis-related AKI, such as age, CKD, diabetes, infective endocarditis, and intra-abdominal infections [ 27 - 30 ]. However, there are relatively few studies conducted on sepsis and AKD. According to a single-center retrospective study, 46.9% of patients with sepsis developed AKD; in other words, sepsis is a critical factor contributing to the development of AKD in patients with AKI [ 31 ]. As a result of this study, it was also found that sepsis has a significant influence on renal function recovery of elderly patients with AKI.
Renal dysfunction is primarily caused by insufficient renal perfusion, indicating that improving the patient’s hemodynamics to increase perfusion pressure might be an effective strategy for reversing kidney damage [ 32 ]. Previously, it was demonstrated that the DBP might be a valuable target for hemodynamic therapy in AKI by affecting renal perfusion [ 33 ]. Additionally, we found that the DBP is a major factor in the occurrence of AKD in elderly patients with AKI and that the risk of AKD gradually increases as the DBP decreases from 80 mmHg. However, a study of patients with severe coronary artery disease found that the risk of AKD is higher when the DBP is less than 50 mmHg [ 34 ]. This difference in the cutoff of the DBP for predicting AKD might reflect varying patient populations. As an indicator of overall health, the heart rate is affected by many factors, including pain stimulation, temperature, blood volume, and inflammatory responses. In a randomized controlled trial of β-receptor blockers in heart failure, maintaining a heart rate of 60 beats/minute was found to be beneficial to patient outcomes [ 35 ]. Additionally, a heart rate higher than 100 beats/minute might be a predictor of sepsis in patients not on advanced life support [ 36 ]. Our study also revealed that AKD is more likely to develop when the heart rate exceeds 110 beats/minute.
There is evidence that an abnormal respiratory rate could interfere with the baroreceptor reflex and cardiovascular variability [ 37 ]. We also found that an excessively high or low respiratory rate might adversely affect renal function recovery and lead to AKD in elderly patients with AKI. Metabolic acidosis is a common and life-threatening homeostatic disorder in the ICU, especially in patients with sepsis [ 38 ]. Furthermore, acidosis-related hemodynamic changes and decreased pH also contribute to the risk of AKI [ 39 ]. However, there has been relatively little attention given to metabolic alkalosis resulting from mass gastric fluid loss, a compensatory response to respiratory acidosis, or excess diuresis in critically ill patients. In a retrospective study of patients with septic shock, metabolic alkalosis was a significant predictor of the length of stay [ 40 ]. Likewise, we found that elevated pH is also a predictor of patients with AKD in the ICU, suggesting persistent renal impairment. Diabetes as a chronic disease is preventable and controllable. Currently, some studies have indicated that AKI is more common among patients with diabetes and that diabetes might increase AKI risk [ 41 ]. According to a national study of hospitalization trends in AKI in the United States between 2000 and 2014, the incidence of AKI among patients with diabetes was significantly higher than among patients without diabetes [ 42 ]. Our study also demonstrated that diabetes contributes to the development of AKD in elderly patients with AKI in the ICU.
Predicting Prognostic Mortality in Elderly Patients With AKD
After analyzing and predicting the risk of AKD during hospitalization for elderly patients with AKI in the ICU, a further machine learning prediction study was conducted on the hospital prognostic mortality of patients with AKD. Finally, the LightGBM algorithm was selected and deployed as a user-friendly web app, which performed well in both the training (AUROC=0.861, 95% CI 0.843-0.878) and external validation (AUROC=0.746, 95% CI 0.673-0.820) cohorts. In our opinion, this study was the first to construct and validate online machine learning models for continuously predicting the AKD risk and prognostic mortality in elderly patients.
Notably, we found that among the top 10 significant variables for predicting the occurrence of AKD in patients with AKI and predicting the prognostic mortality in AKD, delta creatinine, creatinine on day 1, and respiratory frequency all had good predictive values. In the prediction of hospital death in the elderly with AKD, creatinine on day 1 following renal injury was proportional to the likelihood of death. Some studies have shown that serum creatinine and mortality risk are significantly correlated. Thongprayoon et al [ 43 ] concluded that the serum creatinine level is a reliable predictor of mortality in critically ill patients. According to a retrospective study by Pooja et al [ 44 ], hepatorenal syndrome–related death is independently affected by high serum creatinine levels. Further, our study observed a positive correlation between delta creatinine and hospital mortality in elderly patients with AKD, which also appeared relevant to the risk of developing AKD in elderly patients with AKI. In patients with liver cirrhosis, researchers have found that creatinine variability (ie, delta creatinine) can serve as an effective indicator for predicting mortality [ 45 ]. Bradypnea is often seen in patients with central respiratory failure, sleep apnea syndrome, and high intracranial pressure. There is evidence to suggest that slow breathing can lead to death in patients with many diseases, including traumatic brain injury and stroke [ 46 , 47 ]. Additionally, Gooneratne et al [ 48 ] revealed that slow breathing with drowsiness results in increased mortality in the elderly. In this study, we also found that slow breathing is highly predictive of mortality in elderly patients with AKD.
In previous studies, age has been found to be a critical factor in the development of AKI [ 49 ]. Further, a retrospective study of Chinese multicenter patients with AKI revealed that age is an independent predictor of AKD progression in the logistic regression model but is not considered a risk factor for death [ 9 ]. As found in our study, age ranked first among the factors influencing in-hospital death in elderly patients with AKD, with patients aged over 75 years showing higher mortality and those 60-75 years old not appearing to present a significant risk of death. Patients in the ICU with septic shock or cardiogenic shock usually require vasopressors, such as norepinephrine, epinephrine, and dopamine. Plurad et al [ 50 ] found that early administration of vasopressors in the ICU is independently associated with the risk of death regardless of the volume status at admission. In our study, vasopressor use was also a key predictor of mortality in elderly patients with AKD. Research has indicating that BUN is closely related to mortality risk in those with critical illness, acute pancreatitis, and heart failure [ 51 - 53 ]. In our study, we found that BUN on day 1 and day 3 after AKI diagnosis contributes to the risk of death due to AKD. Importantly, the cutoff values for BUN on day 1 and day 3 to predict in-hospital death were determined to be 15 and 20 mmol/L, respectively. However, Wernly et al [ 54 ] calculated 9.7 mmol/L as the optimal cutoff for BUN using Youden’s index to predict patients’ mortality in the ICU. Among patients with acute myocardial infarction complicated by cardiogenic shock, Zhu et al [ 55 ] observed that patients with BUN levels higher than 8.95 mmol/L on admission have an adverse short-term outcome [ 55 ].
As the main indicator of respiratory health and acid-base homeostasis, PaCO 2 levels higher than 45 mmHg often indicate the presence of hypercapnia. In a prospective observational study, patients with hypercapnia experienced higher in-hospital and long-term mortality [ 56 ]. Additionally, we observed that elderly patients with AKD who had PaCO 2 levels higher than 45 mmHg are more likely to die during hospitalization. RRT has been widely used as an effective intervention in patients with AKI, acute severe pancreatitis, and poisoning. Our study suggested that elderly patients with AKD requiring RRT might be at higher risk of in-hospital death, similar to the fact that patients with AKI in need for RRT usually have poorer survival and less renal function recovery, although RRT could delay or even stop this adverse process [ 57 ]. Based on our findings, AKD is associated with decreased RBC counts, which are observed in aplastic anemia, iron deficiency anemia, and massive bleeding. Recently, the RBC distribution width (RDW) has been widely regarded as a predictor of prognosis, especially in patients with coronary heart disease, AKI, and CKD [ 58 - 60 ]. Nevertheless, because of the high missing proportion of the RDW, we did not include it in our constructed model.
We focused on elderly individuals with AKD for the first time, identified features affecting AKD risk and prognostic mortality, and developed 2 web-based prediction apps. After the users input the apps’ URLs on the mobile phone or computer and manually entered the variables’ values, they could click the Predict button to obtain the predictions or the Feedback button to send us new data. Although our online apps are easy to use, the calibration tool deployed by Sun et al [ 61 ] is more convenient and can be automated for use at different hospitals without manual data preparation, which could serve as a reference for further iterative development. Of note, data sets from Xiangya Hospital (China) were used for external validation, with good performance. However, the performance of the AKI prediction model in different sites has shown significant degradation [ 61 ], which might be due to the following measures we took to minimize performance degradation caused by a data shift. First, we adopted relatively strict inclusion and exclusion criteria to reduce the heterogeneity of enrolled patients. In addition, AKI and AKD were defined based on laboratory measurements, which prevented errors in medical record text recognition. Third, the units of the variables in the MIMIC-IV and Xiangya Hospital cohorts were unified. Finally, we added the Feedback button to 2 online forecasting apps to gather new training data through user feedback, that is, coping with data shifts by adhering to the fundamental principle of increasing training data.
Limitations
However, there are still some limitations. First, since detailed information about patients after discharge was lacking, the emphasis was placed on AKD diagnosis and prognosis during hospitalization. Second, the prediction models were based on machine learning classification algorithms, which could only identify a high or a low risk of AKD and patients’ survival but could not display detailed risk values. Finally, although the 2 prediction models constructed in this study were externally validated and demonstrated good generalization abilities, additional variables, such as biomarkers, were needed to ensure better performance, as well as prospective experiments to further evaluate the online apps.
In conclusion, 2 online apps with machine learning algorithms were successfully constructed and deployed for predicting the AKD risk and prognostic mortality in elderly patients. SHAP can intuitively explain the rankings of importance, threshold values for partial features, and positive or negative correlations between features and outcomes, thereby aiding medical staff in early identification and targeted management to promote renal function recovery and patient survival to a certain extent.
Acknowledgments
The author thanks all the contributors of the MIMIC-IV database and all the enrolled patients, in appreciation of their contribution to the research of medical big data. This study was supported by the National Key R&D Program of China (no. 2020YFC2005000), the Natural Science Foundation of the Hunan Province of China (no. 2020JJ4929), and the Research Project of the Hunan Provincial Health Commission of China (no. 202217015418).
Data Availability
The data sets used and analyzed during this study are available from the corresponding author upon reasonable request.
Authors' Contributions
ML and SH contributed equally to this work. SZ and CH were responsible for study design; BZ and QH for data extraction; ML and FL for data analysis; ML and SH for model construction and application; and ML and SZ for manuscript preparation. All authors have reviewed and approved the final manuscript.
Conflicts of Interest
None declared.
The timeline plot of AKI and AKD during hospitalization. AKD: acute kidney disease; AKI: acute kidney injury.
The optimal hyperparameters.
The characteristics of elderly patients with AKI. AKI: acute kidney injury.
The ROC curves in the internal validation of AKD risk prediction models.
The internet-based app of the LightGBM model for predicting the AKD risk. AKD: acute kidney disease; LightGBM: Light Gradient Boosting Machine.
The characteristics of elderly patients with AKD. AKD: acute kidney disease.
The ROC curves in the internal validation of prognostic mortality prediction models.
The internet-based app of the LightGBM model for predicting AKD mortality. AKD: acute kidney disease; LightGBM: Light Gradient Boosting Machine.
- Ronco C, Bellomo R, Kellum JA. Acute kidney injury. Lancet. Nov 23, 2019;394(10212):1949-1964. [ CrossRef ] [ Medline ]
- Hoste EAJ, Bagshaw SM, Bellomo R, Cely CM, Colman R, Cruz DN, et al. Epidemiology of acute kidney injury in critically ill patients: the multinational AKI-EPI study. Intensive Care Med. Aug 11, 2015;41(8):1411-1423. [ CrossRef ] [ Medline ]
- Meyer D, Mohan A, Subev E, Sarav M, Sturgill D. Acute kidney injury incidence in hospitalized patients and implications for nutrition support. Nutr Clin Pract. Dec 03, 2020;35(6):987-1000. [ CrossRef ] [ Medline ]
- Kane-Gill SL, Sileanu FE, Murugan R, Trietley GS, Handler SM, Kellum JA. Risk factors for acute kidney injury in older adults with critical illness: a retrospective cohort study. Am J Kidney Dis. Jun 2015;65(6):860-869. [ CrossRef ] [ Medline ]
- Li Q, Zhao M, Wang X. The impact of transient and persistent acute kidney injury on short-term outcomes in very elderly patients. Clin Interv Aging. 2017;12:1013-1020. [ FREE Full text ] [ CrossRef ] [ Medline ]
- Lameire N, Kellum JA, KDIGO AKI Guideline Work Group. Contrast-induced acute kidney injury and renal support for acute kidney injury: a KDIGO summary (part 2). Crit Care. Feb 04, 2013;17(1):205. [ CrossRef ] [ Medline ]
- See EJ, Polkinghorne KR, Toussaint ND, Bailey M, Johnson DW, Bellomo R. Epidemiology and outcomes of acute kidney diseases: a comparative analysis. Am J Nephrol. Apr 27, 2021;52(4):342-350. [ CrossRef ] [ Medline ]
- Chawla LS, Bellomo R, Bihorac A, Goldstein SL, Siew ED, Bagshaw SM, et al. Acute Disease Quality Initiative Workgroup 16. Acute kidney disease and renal recovery: consensus report of the Acute Disease Quality Initiative (ADQI) 16 Workgroup. Nat Rev Nephrol. Apr 27, 2017;13(4):241-257. [ CrossRef ] [ Medline ]
- Xiao Y, Cheng W, Wu X, Yan P, Feng L, Zhang N, et al. Novel risk models to predict acute kidney disease and its outcomes in a Chinese hospitalized population with acute kidney injury. Sci Rep. Sep 24, 2020;10(1):15636. [ CrossRef ] [ Medline ]
- Andonovic M, Traynor JP, Shaw M, Sim MA, Mark PB, Puxty KA. Short- and long-term outcomes of intensive care patients with acute kidney disease. EClinicalMedicine. Feb 2022;44:101291. [ CrossRef ] [ Medline ]
- Chen Y, Wu M, Mao C, Yeh Y, Chen T, Liao C, et al. Severe acute kidney disease is associated with worse kidney outcome among acute kidney injury patients. Sci Rep. Apr 20, 2022;12(1):6492. [ CrossRef ] [ Medline ]
- He J, Lin J, Duan M. Application of machine learning to predict acute kidney disease in patients with sepsis associated acute kidney injury. Front Med (Lausanne). Dec 10, 2021;8:792974. [ CrossRef ] [ Medline ]
- Chen Y, Jenq C, Hsu C, Yu Y, Chang C, Fan P, et al. Acute kidney disease and acute kidney injury biomarkers in coronary care unit patients. BMC Nephrol. Jun 01, 2020;21(1):207. [ CrossRef ] [ Medline ]
- Hu X, Liu D, Qiao Y, Zheng X, Duan J, Pan S, et al. Development and Validation of a Nomogram Model to Predict Acute Kidney Disease After Nephrectomy in Patients with Renal Cell Carcinoma. Cancer Manag Res. 2020;12:11783-11791. [ FREE Full text ] [ CrossRef ] [ Medline ]
- Zhou Y, Feng J, Mei S, Zhong H, Tang R, Xing S, et al. MACHINE LEARNING MODELS FOR PREDICTING ACUTE KIDNEY INJURY IN PATIENTS WITH SEPSIS-ASSOCIATED ACUTE RESPIRATORY DISTRESS SYNDROME. Shock. Mar 01, 2023;59(3):352-359. [ CrossRef ] [ Medline ]
- Van den Broeck G, Lykov A, Schleich M, Suciu D. On the tractability of SHAP explanations. J Artif Intell Res. Jun 23, 2022;74:851-886. [ CrossRef ]
- Luo W, Phung D, Tran T, Gupta S, Rana S, Karmakar C, et al. Guidelines for developing and reporting machine learning predictive models in biomedical research: a multidisciplinary view. J Med Internet Res. Dec 16, 2016;18(12):e323. [ CrossRef ] [ Medline ]
- Johnson A, Bulgarelli L, Pollard T, Horng S, Celi L, Mark R. MIMIC-IV (version 2). PhysioNet. Jun 12, 2022. URL: https://physionet.org/content/mimiciv/2.0/ [accessed 2024-04-23]
- AKD prediction in elderly AKD patients. Heroku. URL: https://predict---akd-f59a631788c2.herokuapp.com/ [accessed 2024-04-23]
- Mortality prediction in elderly AKD patients. Heroku. URL: https://predict---death-260031eeda1c.herokuapp.com/ [accessed 2024-04-23]
- Ristikankare A, Pöyhiä R, Kuitunen A, Skrifvars M, Hämmäinen P, Salmenperä M, et al. Serum cystatin C in elderly cardiac surgery patients. Ann Thorac Surg. Mar 2010;89(3):689-694. [ CrossRef ] [ Medline ]
- Treiber M, Gorenjak M, Pecovnik Balon B. Serum cystatin-C as a marker of acute kidney injury in the newborn after perinatal hypoxia/asphyxia. Ther Apher Dial. Feb 03, 2014;18(1):57-67. [ CrossRef ] [ Medline ]
- Yamamoto T, Miura S, Shirai K, Urata H. Renoprotective benefit of tolvaptan in acute decompensated heart failure patients with loop diuretic-resistant status. J Clin Med Res. Jan 2019;11(1):49-55. [ CrossRef ] [ Medline ]
- Ewald C, Swanson BJ, Vargas L, Grant WJ, Mercer DF, Langnas AN, et al. Including colon in intestinal transplantation: a focus on post-transplant renal function - a retrospective study. Transpl Int. Feb 10, 2020;33(2):142-148. [ CrossRef ] [ Medline ]
- Wu C, Liu L, Xu W, Jian J, Zhang N, Wang X, et al. [Correlation analysis of inflammatory response and Klotho expression in renal tissue of mice with acute renal injury induced by cisplatin]. Xi Bao Yu Fen Zi Mian Yi Xue Za Zhi. Aug 2019;35(8):702-706. [ Medline ]
- Garner AE, Lewington AJP, Barth JH. Detection of patients with acute kidney injury by the clinical laboratory using rises in serum creatinine: comparison of proposed definitions and a laboratory delta check. Ann Clin Biochem. Jan 30, 2012;49(Pt 1):59-62. [ CrossRef ] [ Medline ]
- Bagshaw SM, Lapinsky S, Dial S, Arabi Y, Dodek P, Wood G, et al. Cooperative Antimicrobial Therapy of Septic Shock (CATSS) Database Research Group. Acute kidney injury in septic shock: clinical outcomes and impact of duration of hypotension prior to initiation of antimicrobial therapy. Intensive Care Med. May 9, 2009;35(5):871-881. [ CrossRef ] [ Medline ]
- Bagshaw S, Uchino S, Bellomo R, Morimatsu H, Morgera S, Schetz M, et al. BeginningEnding Supportive Therapy for the Kidney (BEST Kidney) Investigators. Septic acute kidney injury in critically ill patients: clinical characteristics and outcomes. Clin J Am Soc Nephrol. May 2007;2(3):431-439. [ CrossRef ] [ Medline ]
- Hoste E, Lameire N, Vanholder R, Benoit D, Decruyenaere J, Colardyn F. Acute renal failure in patients with sepsis in a surgical ICU: predictive factors, incidence, comorbidity, and outcome. J Am Soc Nephrol. Apr 2003;14(4):1022-1030. [ CrossRef ] [ Medline ]
- Fan Z, Jiang J, Xiao C, Chen Y, Xia Q, Wang J, et al. Construction and validation of prognostic models in critically Ill patients with sepsis-associated acute kidney injury: interpretable machine learning approach. J Transl Med. Jun 22, 2023;21(1):406. [ CrossRef ] [ Medline ]
- Flannery AH, Li X, Delozier NL, Toto RD, Moe OW, Yee J, et al. Sepsis-associated acute kidney disease and long-term kidney outcomes. Kidney Med. Jul 2021;3(4):507-514.e1. [ CrossRef ] [ Medline ]
- Gattinoni L, Brazzi L, Pelosi P, Latini R, Tognoni G, Pesenti A, et al. A trial of goal-oriented hemodynamic therapy in critically ill patients. SvO2 Collaborative Group. N Engl J Med. Oct 19, 1995;333(16):1025-1032. [ CrossRef ] [ Medline ]
- Legrand M, Dupuis C, Simon C, Gayat E, Mateo J, Lukaszewicz A, et al. Association between systemic hemodynamics and septic acute kidney injury in critically ill patients: a retrospective observational study. Crit Care. Nov 29, 2013;17(6):R278. [ CrossRef ] [ Medline ]
- Gong K, Xie X. An interpretable ensemble model of acute kidney disease risk prediction for patients in coronary care units. In: AI and Analytics for Smart Cities and Service Systems: Proceedings of the 2021 INFORMS International Conference on Service Science. Switzerland. Springer; 2021;76-90.
- Kotecha D, Flather MD, Altman DG, Holmes J, Rosano G, Wikstrand J, Hjalmarson, et al. Beta-Blockers in Heart Failure Collaborative Group. Heart rate and rhythm and the benefit of beta-blockers in patients with heart failure. J Am Coll Cardiol. Jun 20, 2017;69(24):2885-2896. [ CrossRef ] [ Medline ]
- Wahab A, Smith RJ, Lal A, Flurin L, Malinchoc M, Dong Y, et al. CHARACTERISTICS AND PREDICTORS OF PATIENTS WITH SEPSIS WHO ARE CANDIDATES FOR MINIMALLY INVASIVE APPROACH OUTSIDE OF INTENSIVE CARE UNIT. Shock. May 01, 2023;59(5):702-707. [ FREE Full text ] [ CrossRef ] [ Medline ]
- Radaelli A, Mancia G, Balestri G, Bonfanti D, Castiglioni P. Respiratory patterns and baroreflex function in heart failure. Sci Rep. Feb 08, 2023;13(1):2220. [ CrossRef ] [ Medline ]
- Kellum JA, Bellomo R, Kramer DJ, Pinsky MR. Etiology of metabolic acidosis during saline resuscitation in endotoxemia. Shock. May 1998;9(5):364-368. [ CrossRef ] [ Medline ]
- Husain-Syed F, Slutsky AS, Ronco C. Lung-Kidney Cross-Talk in the Critically Ill Patient. Am J Respir Crit Care Med. Aug 15, 2016;194(4):402-414. [ CrossRef ] [ Medline ]
- Kreü S, Jazrawi A, Miller J, Baigi A, Chew M. Alkalosis in critically ill patients with severe sepsis and septic shock. PLoS One. Jan 3, 2017;12(1):e0168563. [ CrossRef ] [ Medline ]
- Advani A. Acute kidney injury: a bona fide complication of diabetes. Diabetes. Nov 2020;69(11):2229-2237. [ CrossRef ] [ Medline ]
- Pavkov ME, Harding JL, Burrows NR. Trends in hospitalizations for acute kidney injury—United States, 2000–2014. MMWR Morb Mortal Wkly Rep. Mar 16, 2018;67(10):289-293. [ CrossRef ] [ Medline ]
- Thongprayoon C, Cheungpasitporn W, Kashani K. Serum creatinine level, a surrogate of muscle mass, predicts mortality in critically ill patients. J Thorac Dis. May 2016;8(5):E305-E311. [ CrossRef ] [ Medline ]
- Pooja B, Rajpurohit S, Nagaraju SP, Musunuri B, Bhat G, Shetty S. Clinical profile and outcome of hepatorenal syndrome in tertiary care hospital based on new ICA criteria: a retrospective study. J Clin Exp Hepatol. 2022;12:S41. [ CrossRef ]
- Cullaro G, Hsu C, Lai JC. Variability in serum creatinine is associated with waitlist and post-liver transplant mortality in patients with cirrhosis. Hepatology. Oct 15, 2022;76(4):1069-1078. [ CrossRef ] [ Medline ]
- Saadat S, Akbari H, Khorramirouz R, Mofid R, Rahimi-Movaghar V. Determinants of mortality in patients with traumatic brain injury. Ulus Travma Acil Cerrahi Derg. May 2012;18(3):219-224. [ FREE Full text ] [ Medline ]
- Bayir A, Ak A, Kara H, Sahin TK. Serum and cerebrospinal fluid magnesium levels, Glasgow Coma Scores, and in-hospital mortality in patients with acute stroke. Biol Trace Elem Res. Jul 23, 2009;130(1):7-12. [ CrossRef ] [ Medline ]
- Gooneratne N, Richards K, Joffe M, Lam R, Pack F, Staley B, et al. Sleep disordered breathing with excessive daytime sleepiness is a risk factor for mortality in older adults. Sleep. Apr 01, 2011;34(4):435-442. [ CrossRef ] [ Medline ]
- Shen J, Chu Y, Wang C, Yan S. Risk factors for acute kidney injury after major abdominal surgery in the elderly aged 75 years and above. BMC Nephrol. Jun 23, 2022;23(1):224. [ CrossRef ] [ Medline ]
- Plurad D, Talving P, Lam L, Inaba K, Green D, Demetriades D. Early vasopressor use in critical injury is associated with mortality independent from volume status. J Trauma. Sep 2011;71(3):565-70; discussion 570. [ CrossRef ] [ Medline ]
- Arihan O, Wernly B, Lichtenauer M, Franz M, Kabisch B, Muessig J, et al. Blood urea nitrogen (BUN) is independently associated with mortality in critically ill patients admitted to ICU. PLoS One. Jan 25, 2018;13(1):e0191697. [ CrossRef ] [ Medline ]
- Wu BU, Johannes RS, Sun X, Conwell DL, Banks PA. Early changes in blood urea nitrogen predict mortality in acute pancreatitis. Gastroenterology. Jul 2009;137(1):129-135. [ CrossRef ] [ Medline ]
- Cauthen CA, Lipinski MJ, Abbate A, Appleton D, Nusca A, Varma A, et al. Relation of blood urea nitrogen to long-term mortality in patients with heart failure. Am J Cardiol. Jun 01, 2008;101(11):1643-1647. [ CrossRef ] [ Medline ]
- Wernly B, Lichtenauer M, Vellinga NA, Boerma EC, Ince C, Kelm M, et al. Blood urea nitrogen (BUN) independently predicts mortality in critically ill patients admitted to ICU: A multicenter study. Clin Hemorheol Microcirc. 2018;69(1-2):123-131. [ CrossRef ] [ Medline ]
- Zhu Y, Sasmita BR, Hu X, Xue Y, Gan H, Xiang Z, et al. Blood urea nitrogen for short-term prognosis in patients with cardiogenic shock complicating acute myocardial infarction. Int J Clin Pract. Mar 15, 2022;2022:9396088. [ CrossRef ] [ Medline ]
- Vonderbank S, Gibis N, Schulz A, Boyko M, Erbuth A, Gürleyen H, et al. Hypercapnia at Hospital Admission as a Predictor of Mortality. Open Access Emerg Med. 2020;12:173-180. [ FREE Full text ] [ CrossRef ] [ Medline ]
- Cerdá J, Liu K, Cruz D, Jaber B, Koyner J, Heung M, et al. AKI Advisory Group of the American Society of Nephrology. Promoting Kidney Function Recovery in Patients with AKI Requiring RRT. Clin J Am Soc Nephrol. Oct 07, 2015;10(10):1859-1867. [ FREE Full text ] [ CrossRef ] [ Medline ]
- Oh HJ, Park JT, Kim J, Yoo DE, Kim SJ, Han SH, et al. Red blood cell distribution width is an independent predictor of mortality in acute kidney injury patients treated with continuous renal replacement therapy. Nephrol Dial Transplant. Feb 28, 2012;27(2):589-594. [ CrossRef ] [ Medline ]
- Hu Y, Liu H, Fu S, Wan J, Li X. Red blood cell distribution width is an independent predictor of AKI and mortality in patients in the coronary care unit. Kidney Blood Press Res. Dec 8, 2017;42(6):1193-1204. [ CrossRef ] [ Medline ]
- Zhang T, Li J, Lin Y, Yang H, Cao S. Association between red blood cell distribution width and all-cause mortality in chronic kidney disease patients: a systematic review and meta-analysis. Arch Med Res. May 2017;48(4):378-385. [ CrossRef ] [ Medline ]
- Sun H, Depraetere K, Meesseman L, Cabanillas Silva P, Szymanowsky R, Fliegenschmidt J, et al. Machine learning-based prediction models for different clinical risks in different hospitals: evaluation of live performance. J Med Internet Res. Jun 07, 2022;24(6):e34295. [ CrossRef ] [ Medline ]
Abbreviations
Edited by T de Azevedo Cardoso; submitted 28.07.23; peer-reviewed by H Sun, F Zhang; comments to author 05.12.23; revised version received 23.01.24; accepted 17.04.24; published 01.05.24.
©Mingxia Li, Shuzhe Han, Fang Liang, Chenghuan Hu, Buyao Zhang, Qinlan Hou, Shuangping Zhao. Originally published in the Journal of Medical Internet Research (https://www.jmir.org), 01.05.2024.
This is an open-access article distributed under the terms of the Creative Commons Attribution License (https://creativecommons.org/licenses/by/4.0/), which permits unrestricted use, distribution, and reproduction in any medium, provided the original work, first published in the Journal of Medical Internet Research, is properly cited. The complete bibliographic information, a link to the original publication on https://www.jmir.org/, as well as this copyright and license information must be included.
- Category: AI
Tiny but mighty: The Phi-3 small language models with big potential
- Sally Beatty
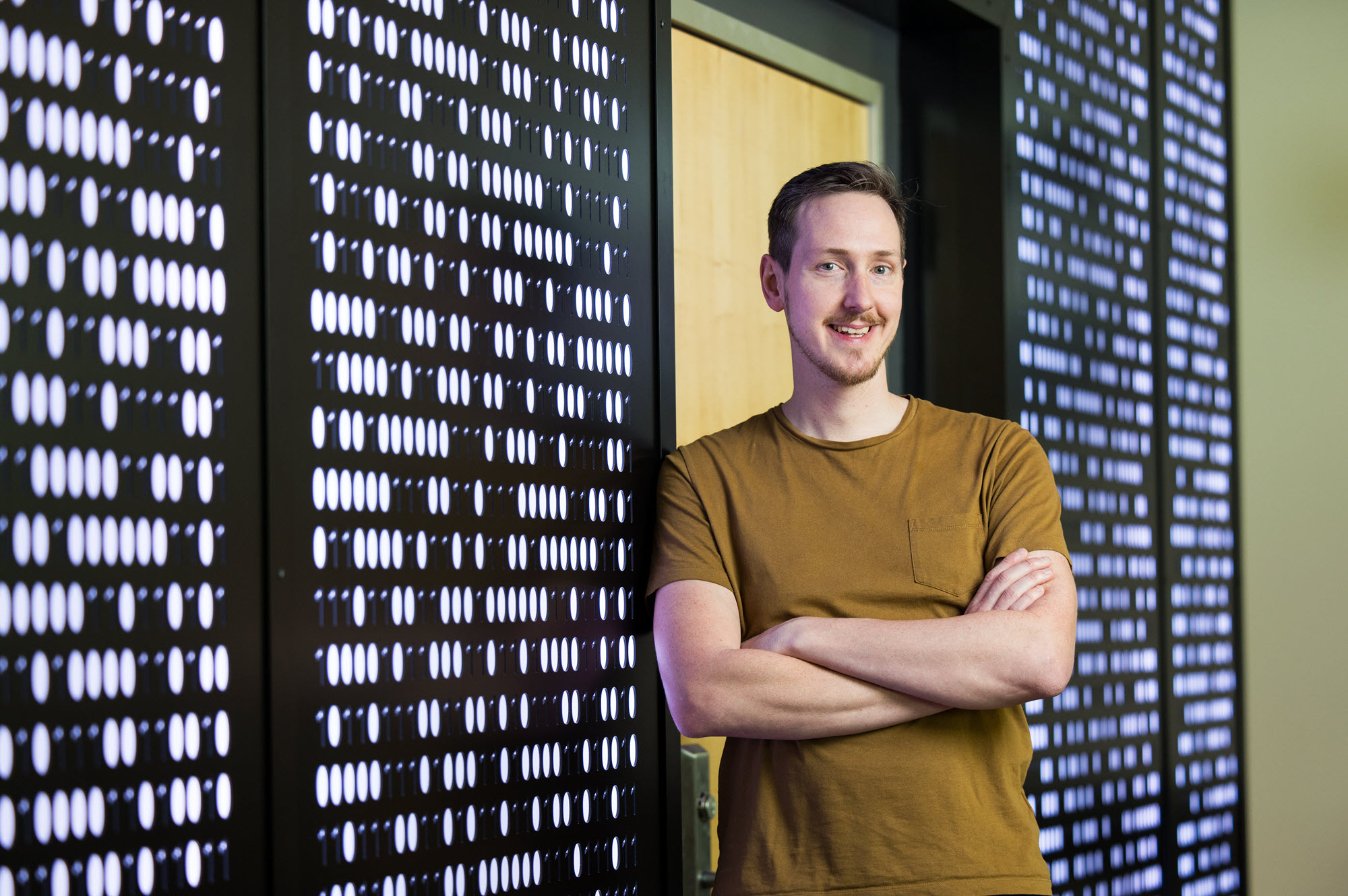
Sometimes the best way to solve a complex problem is to take a page from a children’s book. That’s the lesson Microsoft researchers learned by figuring out how to pack more punch into a much smaller package.
Last year, after spending his workday thinking through potential solutions to machine learning riddles, Microsoft’s Ronen Eldan was reading bedtime stories to his daughter when he thought to himself, “how did she learn this word? How does she know how to connect these words?”
That led the Microsoft Research machine learning expert to wonder how much an AI model could learn using only words a 4-year-old could understand – and ultimately to an innovative training approach that’s produced a new class of more capable small language models that promises to make AI more accessible to more people.
Large language models (LLMs) have created exciting new opportunities to be more productive and creative using AI. But their size means they can require significant computing resources to operate.
While those models will still be the gold standard for solving many types of complex tasks, Microsoft has been developing a series of small language models (SLMs) that offer many of the same capabilities found in LLMs but are smaller in size and are trained on smaller amounts of data.
The company announced today the Phi-3 family of open models , the most capable and cost-effective small language models available. Phi-3 models outperform models of the same size and next size up across a variety of benchmarks that evaluate language, coding and math capabilities, thanks to training innovations developed by Microsoft researchers.
Microsoft is now making the first in that family of more powerful small language models publicly available: Phi-3-mini , measuring 3.8 billion parameters, which performs better than models twice its size, the company said.
Starting today, it will be available in the Microsoft Azure AI Model Catalog and on Hugging Face , a platform for machine learning models, as well as Ollama , a lightweight framework for running models on a local machine. It will also be available as an NVIDIA NIM microservice with a standard API interface that can be deployed anywhere.
Microsoft also announced additional models to the Phi-3 family are coming soon to offer more choice across quality and cost. Phi-3-small (7 billion parameters) and Phi-3-medium (14 billion parameters) will be available in the Azure AI Model Catalog and other model gardens shortly.
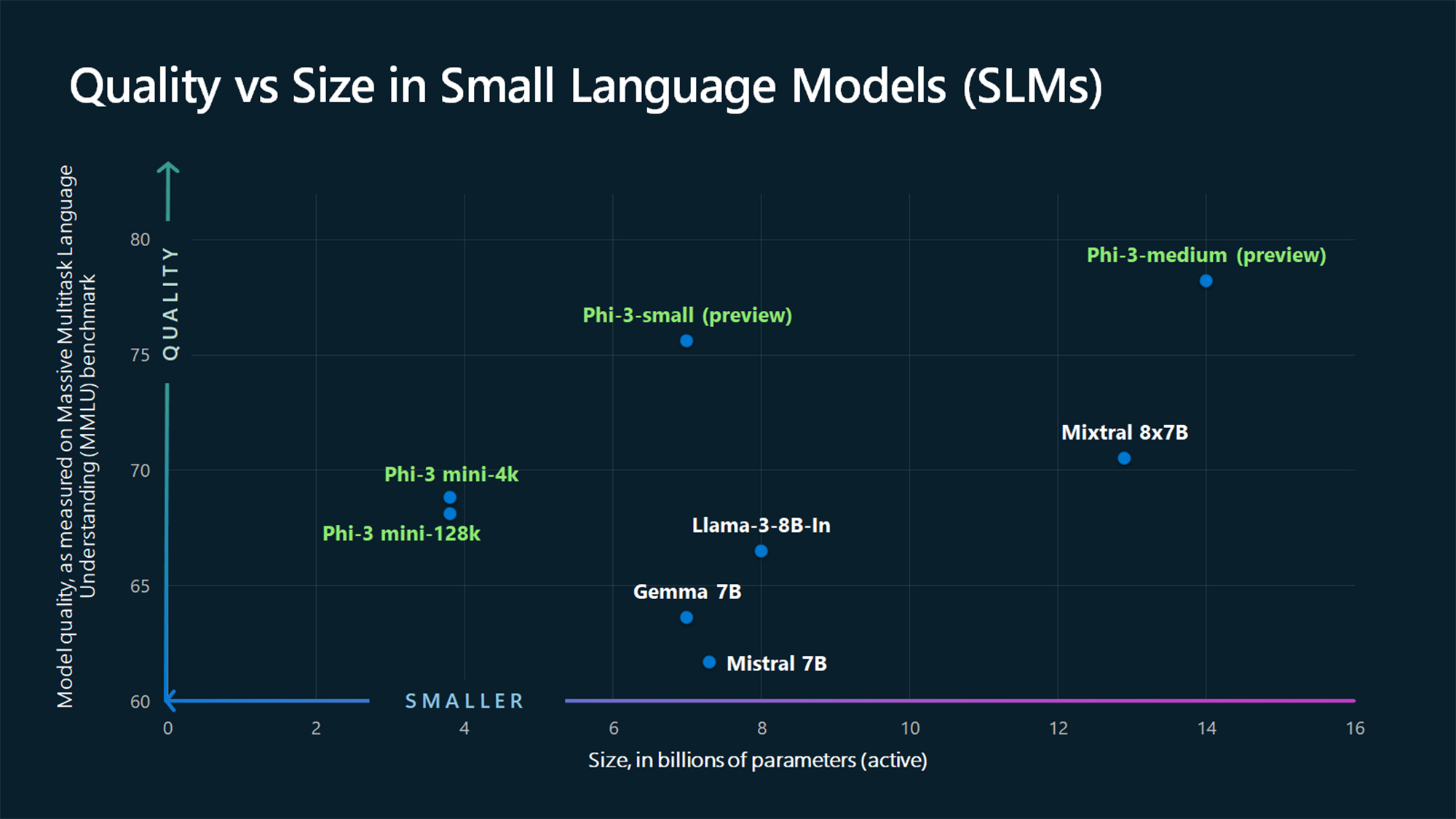
Small language models are designed to perform well for simpler tasks, are more accessible and easier to use for organizations with limited resources and they can be more easily fine-tuned to meet specific needs.
“What we’re going to start to see is not a shift from large to small, but a shift from a singular category of models to a portfolio of models where customers get the ability to make a decision on what is the best model for their scenario,” said Sonali Yadav, principal product manager for Generative AI at Microsoft.
“Some customers may only need small models, some will need big models and many are going to want to combine both in a variety of ways,” said Luis Vargas, vice president of AI at Microsoft.
Choosing the right language model depends on an organization’s specific needs, the complexity of the task and available resources. Small language models are well suited for organizations looking to build applications that can run locally on a device (as opposed to the cloud) and where a task doesn’t require extensive reasoning or a quick response is needed.
Large language models are more suited for applications that need orchestration of complex tasks involving advanced reasoning, data analysis and understanding of context.
Small language models also offer potential solutions for regulated industries and sectors that encounter situations where they need high quality results but want to keep data on their own premises, said Yadav.
Vargas and Yadav are particularly excited about the opportunities to place more capable SLMs on smartphones and other mobile devices that operate “at the edge,” not connected to the cloud. (Think of car computers, PCs without Wi-Fi, traffic systems, smart sensors on a factory floor, remote cameras or devices that monitor environmental compliance.) By keeping data within the device, users can “minimize latency and maximize privacy,” said Vargas.
Latency refers to the delay that can occur when LLMs communicate with the cloud to retrieve information used to generate answers to users prompts. In some instances, high-quality answers are worth waiting for while in other scenarios speed is more important to user satisfaction.
Because SLMs can work offline, more people will be able to put AI to work in ways that haven’t previously been possible, Vargas said.
For instance, SLMs could also be put to use in rural areas that lack cell service. Consider a farmer inspecting crops who finds signs of disease on a leaf or branch. Using a SLM with visual capability, the farmer could take a picture of the crop at issue and get immediate recommendations on how to treat pests or disease.
“If you are in a part of the world that doesn’t have a good network,” said Vargas, “you are still going to be able to have AI experiences on your device.”
The role of high-quality data
Just as the name implies, compared to LLMs, SLMs are tiny, at least by AI standards. Phi-3-mini has “only” 3.8 billion parameters – a unit of measure that refers to the algorithmic knobs on a model that help determine its output. By contrast, the biggest large language models are many orders of magnitude larger.
The huge advances in generative AI ushered in by large language models were largely thought to be enabled by their sheer size. But the Microsoft team was able to develop small language models that can deliver outsized results in a tiny package. This breakthrough was enabled by a highly selective approach to training data – which is where children’s books come into play.
To date, the standard way to train large language models has been to use massive amounts of data from the internet. This was thought to be the only way to meet this type of model’s huge appetite for content, which it needs to “learn” to understand the nuances of language and generate intelligent answers to user prompts. But Microsoft researchers had a different idea.
“Instead of training on just raw web data, why don’t you look for data which is of extremely high quality?” asked Sebastien Bubeck, Microsoft vice president of generative AI research who has led the company’s efforts to develop more capable small language models. But where to focus?
Inspired by Eldan’s nightly reading ritual with his daughter, Microsoft researchers decided to create a discrete dataset starting with 3,000 words – including a roughly equal number of nouns, verbs and adjectives. Then they asked a large language model to create a children’s story using one noun, one verb and one adjective from the list – a prompt they repeated millions of times over several days, generating millions of tiny children’s stories.
They dubbed the resulting dataset “TinyStories” and used it to train very small language models of around 10 million parameters. To their surprise, when prompted to create its own stories, the small language model trained on TinyStories generated fluent narratives with perfect grammar.
Next, they took their experiment up a grade, so to speak. This time a bigger group of researchers used carefully selected publicly-available data that was filtered based on educational value and content quality to train Phi-1. After collecting publicly available information into an initial dataset, they used a prompting and seeding formula inspired by the one used for TinyStories, but took it one step further and made it more sophisticated, so that it would capture a wider scope of data. To ensure high quality, they repeatedly filtered the resulting content before feeding it back into a LLM for further synthesizing. In this way, over several weeks, they built up a corpus of data large enough to train a more capable SLM.
“A lot of care goes into producing these synthetic data,” Bubeck said, referring to data generated by AI, “looking over it, making sure it makes sense, filtering it out. We don’t take everything that we produce.” They dubbed this dataset “CodeTextbook.”
The researchers further enhanced the dataset by approaching data selection like a teacher breaking down difficult concepts for a student. “Because it’s reading from textbook-like material, from quality documents that explain things very, very well,” said Bubeck, “you make the task of the language model to read and understand this material much easier.”
Distinguishing between high- and low-quality information isn’t difficult for a human, but sorting through more than a terabyte of data that Microsoft researchers determined they would need to train their SLM would be impossible without help from a LLM.
“The power of the current generation of large language models is really an enabler that we didn’t have before in terms of synthetic data generation,” said Ece Kamar, a Microsoft vice president who leads the Microsoft Research AI Frontiers Lab, where the new training approach was developed.
Starting with carefully selected data helps reduce the likelihood of models returning unwanted or inappropriate responses, but it’s not sufficient to guard against all potential safety challenges. As with all generative AI model releases, Microsoft’s product and responsible AI teams used a multi-layered approach to manage and mitigate risks in developing Phi-3 models.
For instance, after initial training they provided additional examples and feedback on how the models should ideally respond, which builds in an additional safety layer and helps the model generate high-quality results. Each model also undergoes assessment, testing and manual red-teaming, in which experts identify and address potential vulnerabilities.
Finally, developers using the Phi-3 model family can also take advantage of a suite of tools available in Azure AI to help them build safer and more trustworthy applications.
Choosing the right-size language model for the right task
But even small language models trained on high quality data have limitations. They are not designed for in-depth knowledge retrieval, where large language models excel due to their greater capacity and training using much larger data sets.
LLMs are better than SLMs at complex reasoning over large amounts of information due to their size and processing power. That’s a function that could be relevant for drug discovery, for example, by helping to pore through vast stores of scientific papers, analyze complex patterns and understand interactions between genes, proteins or chemicals.
“Anything that involves things like planning where you have a task, and the task is complicated enough that you need to figure out how to partition that task into a set of sub tasks, and sometimes sub-sub tasks, and then execute through all of those to come with a final answer … are really going to be in the domain of large models for a while,” said Vargas.
Based on ongoing conversations with customers, Vargas and Yadav expect to see some companies “offloading” some tasks to small models if the task is not too complex.
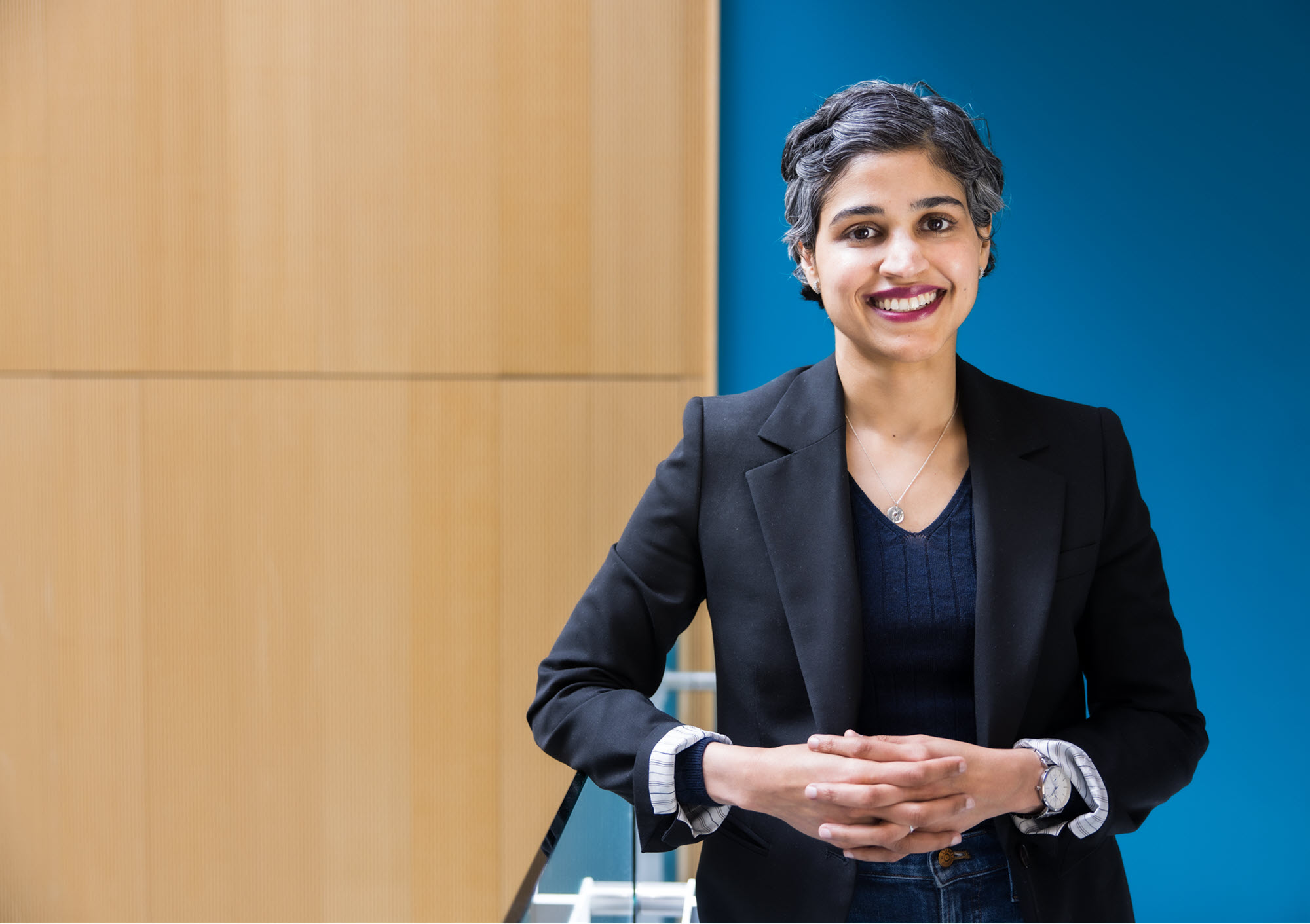
For instance, a business could use Phi-3 to summarize the main points of a long document or extract relevant insights and industry trends from market research reports. Another organization might use Phi-3 to generate copy, helping create content for marketing or sales teams such as product descriptions or social media posts. Or, a company might use Phi-3 to power a support chatbot to answer customers’ basic questions about their plan, or service upgrades.
Internally, Microsoft is already using suites of models, where large language models play the role of router, to direct certain queries that require less computing power to small language models, while tackling other more complex requests itself.
“The claim here is not that SLMs are going to substitute or replace large language models,” said Kamar. Instead, SLMs “are uniquely positioned for computation on the edge, computation on the device, computations where you don’t need to go to the cloud to get things done. That’s why it is important for us to understand the strengths and weaknesses of this model portfolio.”
And size carries important advantages. There’s still a gap between small language models and the level of intelligence that you can get from the big models on the cloud, said Bubeck. “And maybe there will always be a gap because you know – the big models are going to keep making progress.”
Related links:
- Read more: Introducing Phi-3, redefining what’s possible with SLMs
- Learn more: Azure AI
- Read more: Phi-3 Technical Report: A Highly Capable Language Model Locally on Your Phone
Top image: Sebastien Bubeck, Microsoft vice president of Generative AI research who has led the company’s efforts to develop more capable small language models. (Photo by Dan DeLong for Microsoft)
- Work & Careers
- Life & Arts
OpenAI’s model all but matches doctors in assessing eye problems

- OpenAI’s model all but matches doctors in assessing eye problems on x (opens in a new window)
- OpenAI’s model all but matches doctors in assessing eye problems on facebook (opens in a new window)
- OpenAI’s model all but matches doctors in assessing eye problems on linkedin (opens in a new window)
- OpenAI’s model all but matches doctors in assessing eye problems on whatsapp (opens in a new window)
Michael Peel in London
Simply sign up to the Artificial intelligence myFT Digest -- delivered directly to your inbox.
OpenAI’s latest artificial intelligence model has almost matched expert doctors in analysing eye conditions, according to research that highlights the technology’s potential in medicine.
The Microsoft-backed start-up’s GPT-4 model surpassed or achieved the same scores as all but the top-scoring specialist medics in assessing ocular problems and suggesting treatments, according to a paper published on Wednesday.
Ophthalmology has been a big focus of efforts to put AI to clinical use and fix obstacles to take-up, such as the tendency of models to “hallucinate” by creating fictitious data.
“What this work shows is that the knowledge and reasoning ability of these large language models in an eye health context is now almost indistinguishable from experts,” said Arun Thirunavukarasu, the lead author of a paper on the findings published in PLOS Digital Health journal. “We are seeing the ability to answer quite complicated questions,” he added.
The research used 87 different patient scenarios to test the performance of GPT-4 against non-specialist junior doctors and both trainee and expert eye medics. The model outperformed the juniors and achieved similar results to many of the specialists, the paper said.
The study is notable because it compares the AI model’s abilities with those of practising doctors rather than with examination results, the researchers said. It also deploys the broad powers of generative AI, rather than narrower capabilities tested in some previous AI medical studies such as diagnosing cancer risks from patient scans.
The model performed equally well on questions that demanded first-order recall and those requiring higher-order reasoning, such as the ability to interpolate, interpret and process information.
“We are now training in a much more open-ended way and we are discovering almost abilities in these models that they weren’t explicitly trained for,” said Thirunavukarasu, who carried out the research while studying at the University of Cambridge’s school of clinical medicine.
The model could be refined further by training it on an expanded data set including management algorithms, deidentified patient notes and textbooks, said Thirunavukarasu, who is now based at Oxford university.
He added that this would demand a “tricky balance” between expanding the number and nature of sources, while ensuring the information remained of good quality. Potential clinical uses could be in the triage of patients or where access to specialist healthcare professionals was limited.
Interest in deploying AI in a clinical setting has soared with evidence of its contribution to diagnostics, such as flagging early-stage breast cancers that may be missed by doctors. At the same time, researchers are grappling with how to manage serious risks, given the damage that false diagnoses can cause to patients.
The latest study was “exciting” and its idea of using AI to benchmark experts’ performance “super-interesting”, said Pearse Keane, professor of artificial medical intelligence at University College London.
Keane, who is also affiliated with Moorfields Eye Hospital in London, agreed that more work was needed before introducing the techniques in a clinical context.
Keane cited an example from his own research last year in which he asked a large language model about macular degeneration in the eye, only for it to give “made-up” references in its reply.
“We just have to balance our excitement about this technology and the potential massive benefits . . . with caution and scepticism,” he said.
Promoted Content
Follow the topics in this article.
- US & Canadian companies Add to myFT
- Health Add to myFT
- Technology sector Add to myFT
- Healthcare Add to myFT
- Medical science Add to myFT
International Edition
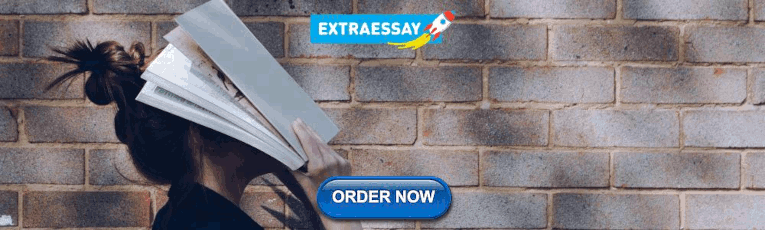
IMAGES
VIDEO
COMMENTS
"A Conceptual Model of Service Quality and Its Implications for Future Research" , used with the permission of the publisher of the original article. The SERVQUAL model, which is a research tool, determines the relative impact of five dimensions, namely, tangibility, reliability, responsibility, confidence, and empathy, on customer ...
This definition was the basis for the creation of the SERVQUAL model. In the original form of the model from 1985, the study consisted of an analysis of ten dimensions of service quality: (1 ...
Research article. First published online February 1, 2017. ... (1997) implemented the SERVQUAL model to measure service quality using the same questionnaire to a group of faculty and students and suggested that different stakeholders have different perspectives regarding the quality of the business school.
Trend of Service Quality Research in the last decade (2005-2015) ... The SERVQUAL model is a service quality model designed by Parasuraman et al. in 1985 [5] and has been used in several studies ...
This study attempts to examine the impact of service quality on customer loyalty and customer satisfaction using the SERVQUAL model for four main Islamic banks in the Sultanate of Oman. This is a quantitative nature of a study, which involved a structured, self-administered questionnaire based on a convenience sampling method gathering data ...
Furthermore, it is widely accepted for its conceptualization and assessment of service quality. In a literature review of the SERVQUAL model from 1998 to 2013 by Wang and his colleagues, the SERVQUAL model was found to be a hot research topic of academic researchers and a significant contributor to service quality research .
The results of other authors show that the service quality element of personal interactions and relationships is one of the most important components, affecting the patients' perception of service quality [29,30,31]. In addition, their research shows that human factors have a greater impact on patients' perceptions of quality than non-human ...
This paper presents a comprehensive framework for identifying and prioritizing the critical factors that affect service quality and customer satisfaction. It integrates the SERVQUAL model, which ...
RESEARCH includes research articles that focus on the ... The foundation for the SERVQUAL scale is the gap model proposed by Parasuraman, Zeithaml and Berry (1985, 1988). With roots in disconfirmation paradigm,1 ... service quality as being a gap between customer's ex-
The assessment of service quality has received increasing attention over the past few decades. The SERVQUAL model is an instrument that is commonly used to quantify the quality of a service. It divides "service quality" into five dimensions: tangibles, responsiveness, reliability, assurance, and empathy.
Keywords: Service quality · SERVQUAL model · Quality evaluation 1 Introduction In the early 1970s, as the economic recovery in western countries gradually emerged, ... method of engineering system, the structure of the service quality model is optimized, and the research and development of service quality are discussed and prospected.
Parasuraman et al. considered that service quality was subjectively perceived by customers, and it was the gap between customer expectations and service performance.They chose retail banking, credit card, securities brokerage, and product repair and maintenance as investigated service industries, conducted several focus groups and in-depth interviews as research method, and built a ...
In Chinese literature, Zhisheng Hong et al. (2012) published "Study on the Research of Service Quality Management" , which was cited for 224 times, mainly introduced the research field of service quality and the application of SERVQUAL model, as well as the prospect of future dynamic changes of service quality and service management in the ...
The SERVQUAL model has been validated in diverse service industries such as healthcare, banking, hospitality, and education (Bekhet, Al-Alak, & El-Refae, 2014; Sann, Lai, Liaw, & Chen, 2023; Shekarchizadeh, Rasli, & Hon-Tat, 2011). The model assesses the perceived service quality of students in higher education institutions (Saliba & Zoran ...
Quality gap model, on the basis of Parasuraman A. et al. "A Conceptual Model of Service Quality and Its Implications for Future Research" [4], used with the permission of the publisher of the original article. The SERVQUAL model, which is a research tool, determines the relative impact of
The increased competitive academic environment pushes higher institutions to improve their service quality for meeting the market demands. It is thus necessary to assess the factors that satisfy students and make them loyal to the university. This study has focused on assessing service quality, using the SERVQUAL Model to measure students' satisfaction with private universities in Bangladesh ...
The second half of the 20th century saw the development of a new trend in the management of medical services across Europe. Those shifts were associated with the transformation of various spheres of human life, both on professional and private levels. The service market then turned back to "quality", already known in antiquity. According to Aristotle, "quality" is one of the basic ...
Attending to student perceptions of program/service quality (SERVQUAL) is a means to identify areas that have the greatest return on investment. ... The paper aims to discuss these issues.,This study explores how a program has applied the SERVQUAL model and survey to identify areas for growth.,The survey of 57 students in a cohort-based ...
A Review and Critique of Research Using Servqual: A review and critique of research using SERVQUAL. Lisa J. Morrison Coulthard View all authors and affiliations. ... Andersson T. D. (1992) Another model of service quality: a model of causes and effects of service quality tested on a case within the restaurant industry. In Kunst P., & Lemmick J ...
Various inclusion and exclusion criteria were used to select relevant research articles from 2000-2020 for the study, and a total of 100 research articles were selected.,The study identified 41 different dimensions of healthcare service quality measurement and classified these dimensions into four categories, namely servicescape, personnel ...
Clearly, businesses need a new model for transformation. In this article the authors present one based on research with dozens of leading companies that have defied the odds, such as Ford, Dell ...
The measurement of service quality had an important role in managing service provided, diagnosing the problem, and assessing service performance. ... Baldwin and Sohal in their study showed that SERVQUAL was a good model in the field of assessing the quality of dental services in terms of validity and ... Articles from Dental Research Journal ...
Scenario studies examine alternative future socioeconomic development pathways and their impacts on direct drivers of biodiversity loss such as land-use and climate, often using integrated assessment models ().Consequences of these scenarios for biodiversity and ecosystem services can be assessed using biodiversity and ecosystem function and services models (6, 7).
Printed in the United States of America. Service Quality: A Case Study Using. SERVQUAL Model. Nor Atiqah Aima Roslan, Norasmiha Mohd Nor, Eta W ahab. Faculty of Technology Management and Business ...
This article is part of the Research Topic Computational Advances in Water Resources Modelling and Optimisation View all 4 articles. ... The model indicated that annual average recharge constituted 5.1% of the precipitation over a simulation period of 6 years. The effect of groundwater recharge and discharge components were evaluated in the ...
Party: 52% of Asian Democrats say describing Asians as a model minority is a bad thing, about three times the share of Asian Republicans who say the same (17%). Among those who know the term "model minority," views of whether using it to describe Asians in the U.S. is a good or bad thing does not vary significantly across education levels.
By releasing the source code, model weights, and training materials, Apple says it aims to "empower and enrich the open research community." However, it also cautions that since the models were ...
Background: Acute kidney disease (AKD) affects more than half of critically ill elderly patients with acute kidney injury (AKI), which leads to worse short-term outcomes. Objective: We aimed to establish 2 machine learning models to predict the risk and prognosis of AKD in the elderly and to deploy the models as online apps. Methods: Data on elderly patients with AKI (n=3542) and AKD (n=2661 ...
Phi-3-small (7 billion parameters) and Phi-3-medium (14 billion parameters) will be available in the Azure AI Model Catalog and other model gardens shortly. Graphic illustrating how the quality of new Phi-3 models, as measured by performance on the Massive Multitask Language Understanding (MMLU) benchmark, compares to other models of similar size.
Keane cited an example from his own research last year in which he asked a large language model about macular degeneration in the eye, only for it to give "made-up" references in its reply.