The Geography of Transport Systems
The spatial organization of transportation and mobility
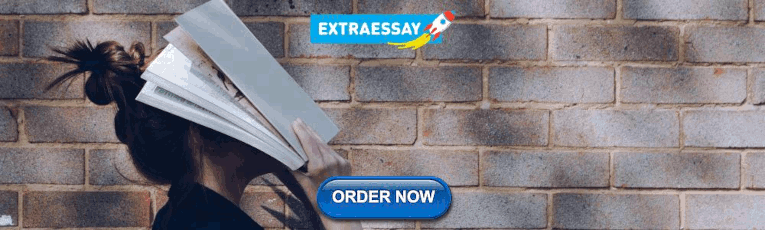
5.1 – Transportation Modes, Modal Competition and Modal Shift
Author: dr. jean-paul rodrigue.
Transport modes are the means supporting the mobility of passengers and freight. They are mobile transport assets and fall into three basic types; land (road, rail, pipelines), water (shipping), and air.
1. A Diversity of Modes
Transport modes are designed to carry passengers or freight , but most modes combine both . For instance, an automobile has the capacity to carry some freight, while a passenger plane has a bellyhold that is used for luggage and cargo. Each mode is characterized by technical, operational, and commercial characteristics defining its market opportunities and economies of scale . Technical characteristics relate to attributes such as speed, capacity, and motive technology, while operational characteristics involve the context in which modes operate, including speed limits, safety conditions, or operating hours. The demand for transport and the ownership of modes are dominant commercial characteristics, as transportation modes are used to support economic activities and generate income.

a. Road transportation
Road infrastructures are large consumers of space with the lowest level of physical constraints among transportation modes. However, physiographical constraints are significant in road construction, with substantial additional costs to overcome features such as rivers or rugged terrain. While historically, road transportation was developed to support non-motorized forms of transportation (walking, domestic animals, and cycling at the end of the 19th century), it is motorization that has shaped most of its development since the beginning of the 20th century, particularly with the setting of national highway systems.
Road transportation has average operational flexibility as vehicles can serve several purposes but can rarely operate outside roads. Road transport systems have low barriers of entry, but high maintenance costs, both for the vehicles and infrastructures, which are related to low life spans of less than 10 years for a vehicle. They are mainly linked to light industries and freight distribution, where rapid freight movements in small loads are the norm. With containerization, road transportation has become a crucial link in freight distribution between ports and commercial hinterlands.
b. Rail transportation and pipelines
Railways are composed of a traced path for a right of way on which wheeled vehicles are bound. Rail transportation also includes monorails and maglev, which are more recent developments of guided rail technology. They have an average level of physical constraints, and a low gradient is required, particularly for freight. Heavy industries are traditionally linked with rail transport systems, although containerization has improved the flexibility of rail transportation through its connectivity with road and maritime modes. Rail is the land transportation mode offering the highest capacity, with a 23,000 tons fully loaded coal unit train being the heaviest load ever carried. Gauges , however, vary around the world, often challenging the integration of rail systems.
Pipeline routes are practically unlimited as they can be laid on land or underwater. They aim to move liquids such as petroleum products over long distances cost-effectively. The longest gas pipeline links Alberta to Sarnia (Canada), which is 2,911 km in length. The longest oil pipeline is the Transiberian, extending over 9,344 km from the Russian Arctic oilfields in eastern Siberia to Western Europe. Physical constraints are low and include the landscape and pergelisol in arctic environments. Pipeline construction costs vary according to the diameter and increase proportionally with the distance and viscosity of fluids (from low-viscosity gas to high-viscosity oil). The Trans Alaskan pipeline, which is 1,300 km long, was built under challenging conditions and had to be above ground for most of its path. Pipeline terminals are essential since they correspond to refineries and harbors.

c. Maritime transportation
With physical properties such as buoyancy and limited friction, maritime transportation is the most effective mode of moving large quantities of cargo over long distances. Main maritime routes are composed of oceans, coasts, seas, lakes, rivers, and channels. However, due to the location of economic activities, maritime circulation takes place in specific parts of the maritime space, particularly over the North Atlantic and the North Pacific. The construction of channels, locks, and dredging are attempting to facilitate maritime circulation by reducing its discontinuity, but such endeavors are highly expensive. Comprehensive inland waterway systems include Western Europe, the Volga / Don system, the St. Lawrence / Great Lakes system, the Mississippi and its tributaries, the Amazon, the Panama / Paraguay, and the interior of China.
Maritime transportation has high terminal costs since port infrastructures are among the most expensive to build, maintain, and operate. These high costs also relate to maritime shipping, where the construction, operation, and maintenance of ships are capital-intensive. More than any other mode, maritime transportation is linked to heavy industries, such as steel and petrochemical facilities adjacent to port sites. Yet, with containerization, maritime shipping has become the linchpin of globalization, allowing the trading of a wide range of goods and commodities.
d. Air transportation
The core advantage of air transportation is speed and flexibility in network configuration. Air routes are practically unlimited but denser over the North Atlantic, inside North America and Europe, and over the North Pacific. Even if planes can have a long range, the majority of services link city pairs less than 2 hours apart. Air transport constraints are multidimensional and include the site (a commercial plane needs about 3,300 meters of runway for landing and take-off), the climate, fog, and wind currents. Air activities are linked to the tertiary and quaternary sectors, notably finance and tourism, which lean on the long-distance mobility of people. More recently, air transportation has accommodated growing quantities of high-value freight and is playing an increasing role in global logistics.
e. Intermodal transportation
Intermodalism concerns a variety of modes used in combination so that the respective advantages of each mode are advantaged. Although intermodal transportation applies to passenger movements, such as using the different, interconnected modes of a public transit system, it is over freight transportation that the most significant impacts of intermodalism have been observed. Containerization has been a powerful vector of intermodal integration, enabling maritime and land transportation systems to interconnect.
f. Telecommunications
Telecommunication systems are paradoxical in terms of if they can be considered as a transport mode since telecommunications often do not have an apparent physicality. This physicality is real since they are structured as high-capacity networks with low constraints, which may include the physiography and oceanic masses crossed by fiber optic cables . They provide for the instantaneous movement of information (speed of light). Because of their limited coverage, wave transmissions often require substations, such as for cellular phone and data networks where WiFi connections are of even more limited range. Satellites are often using a geostationary orbit, which is getting crowded.
High network costs and low distribution costs characterize many telecommunication networks, which are linked to the tertiary and quaternary sectors (stock markets, business-to-business information networks, etc.). Telecommunications can provide a substitution for personal mobility in some economic sectors, but the major impact is e-commerce, which has opened a range of commercial opportunities. More recently,

2. Modal Competition
Each transportation mode has key operational and commercial advantages and properties . However, contemporary demand is influenced by integrated transportation systems that require flexibility in the respective use of each mode. As a result, modal competition exists to various degrees and takes several dimensions. Modes can compete or complement one another in cost , speed, accessibility, frequency, safety, comfort, etc. Three main conditions ensure that some modes complement one another:
- Different geographical markets . If different markets are involved, modes will enable continuity within the transport system, particularly if different scales are concerned, such as between national and international transportation. This requires an interconnection, commonly known as a gateway, where transferring from one mode to another is possible. Intermodal transportation has been particularly relevant to improving the complementarity and connectivity of different geographical markets.
- Different transport markets . The nature of what is being transported, such as passengers or freight, often indicates a level of complementarity. Even if the same market area is serviced, it may not be equally accessible, depending on the mode used. Thus, in some markets, rail and road transportation can be complementary as one may focus on passengers and the other on freight.
- Different levels of service . For a similar market and accessibility, two modes offering different service levels will tend to complement one another with niche services. The most prevailing complementarity concerns cost versus time.
Thus, there is modal competition when there is an overlap in geography, transport markets, and level of service. Cost is one of the most important considerations in modal choice. Because each mode has its price/performance profile , competition between the modes depends primarily upon the distance traveled, the quantities shipped, and their value. While maritime transport might offer the lowest variable costs, road transport tends to be most competitive over short distances and for small bundles of goods. A critical factor is the terminal cost structure for each mode, where the costs (and delays) of loading and unloading a unit impose fixed costs incurred independent of the distance traveled.

With increasing income levels, the propensity for people to travel rises . At the same time, international trade in manufactured goods and parts has increased. These trends in travel demand act differently upon modes. Those that offer faster and more reliable services gain over modes that might provide a lower cost but slower alternative. For passenger services, rail is challenged by road transport competition over short distances and aircraft for longer trips. Freight, rail, and shipping have been impacted by competition from road and air modes. While shipping, pipelines, and rail still perform well for bulk shipments, competition has seen road and air modes capture an important market share of the high revenue-generating goods over the last decades. Road transportation continues to dominate the passenger and freight transportation markets.
Although intermodal transportation has opened many opportunities for complementarity between modes, transport operators are now competing over many modes in the transport chain. A growing paradigm thus involves supply chain competition with the modal competition component occurring over three dimensions :
- Modal usage . A competition that involves the comparative advantage of using a specific or a combination of modes. Distance remains one of the primary determinants of modal utilization for passenger transportation. However, for a similar distance, costs, speed, and comfort can be significant factors behind the choice of a mode.
- Infrastructure usage . Competition resulting from the presence of freight and passenger traffic on the same itineraries linking the same nodes. Therefore, each level of capacity a mode uses is at the expense of the other mode.
- Market area . Competition between transport terminals for using new locations (terminal relocation or expansion) or capturing new markets (hinterland).

It is generally advocated that modal equality (or modal neutrality ) should be part of public policy, where each mode would compete based on its inherent characteristics and merits. Since different transport modes are under different jurisdictions and funding mechanisms, modal equality is conceptually impossible, as some modes will always be more advantageous than others. Modal competition is influenced by public policy, particularly over the funding of infrastructure and regulation issues. The public sector usually provides roads, while many other transport infrastructures are financed by their operators. This is the case for rail, air, and maritime transportation. For instance, in the United States, the Federal Government would finance 80% of the costs of a highway project, leaving the state government to supply the remaining 20%. This share is 50% for public transit, while the Federal Government will not provide any funding for passenger rail. Under such circumstances, public policy shapes modal preferences.
3. Modal Shift
The technological evolution in the transport industry aims at adapting transport infrastructures to growing needs and requirements. When a transport mode becomes more advantageous than another over the same route or market, a modal shift is likely to take place.
A modal shift involves the growth in the demand of a transport mode at the expense of another, although a modal shift can involve an absolute growth in both concerned modes.
The comparative advantages behind a modal shift can be in terms of costs, convenience, speed, or reliability. For passengers, this involved a transition in modal preferences as incomes increased, such as from collective (public transit) to individual modes (motorbikes, automobiles) of transportation. For freight , this has implied a shift to faster and more flexible modes when possible and cost-effective, namely trucking and air freight. A modal shift can further be nuanced by time shift , for which the same mode takes place at another time period, likely when there is less congestion. During congestion, it is thus likely that a time shift will be preferred to a modal shift , particularly if the time shift is relatively marginal (e.g. a few hours). An individual may delay travel at a later time while a freight delivery can be rescheduled.
There are important geographical variations in modal competition . The availability of transport infrastructures and networks varies enormously, with corridors subject to the highest modal competition level. Corridors have many different modes that, in combination, provide a range of transport services that ensure an efficient commercial environment. Thus, in contrast to the European Union, China, and Japan, rail freight transport occupies a more significant market share in North America, but passenger rail has a negligible share. However, there are limited services in many parts of the world, and some critical modes, such as rail, may be absent altogether. This limits the choices for passengers and shippers and acts to limit accessibility. Passengers and freight are forced to use the only available modes that may not be the most effective to support their mobility. Areas with limited modal choices tend to be among the least developed. On the other hand, advanced economies possess a wide range of modes that can provide services to meet the needs of society and the economy.
All modes are affected by energy price volatility , particularly petroleum , from the individual car owner to the corporation operating a fleet of hundreds of aircraft or ships. Different pricing mechanisms are used, namely direct rate adjustments, as in the case of shipping, or indirect adjustments, as in the case of airlines, with the reliance on fuel surcharges when energy prices are increasing. In the context of higher energy prices and environmental concerns, and higher input costs for transportation, the following can be expected:
- Higher transport costs increase the friction of distance and constrain mobility. As a major consumer of petroleum, the transport industry must increase rates. Across-the-board increases cause people to rethink their movement patterns and companies to adjust their supply chains.
- Because energy costs impact modes differently, a modal shift can be anticipated. Road and air transport are more energy-intensive than the other modes, so energy price increases are likely to impact them more severely than other modes. This could lead to a shift towards water and rail transport in particular.
- Higher fuel prices incite a greater fuel economy across modes. This can be achieved by reducing speed, through engine and fuel innovations, or electrification.

If a modal shift results in higher costs and less efficiency, then this shift is not sustainable. Modal shift strategies often supported by public policy goals have initial difficulties competing with existing transport alternatives. Evidence underlines that subsidies are usually required to support initial attempts at modal shifts, but if the shift needs to be constantly subsidized, it may not be a viable option.
4. Passengers versus Freight
There is a complementarity between passenger and freight transport systems . With some exceptions, such as buses and pipelines, most transport modes have been developed to handle freight and passenger traffic, but measuring performance is completely different for freight. In some cases, both are carried in the same vehicle, as in air transport, where about 80% of the freight is transported in the cargo holds of passenger aircraft. In others, different types of vehicles have been developed for freight and passenger traffic, but they share the same infrastructure, such as rail and road traffic. In shipping, passengers and freight shared the same vessels and often the same terminals. Since the 1950s, specialization has occurred, and the two are now entirely distinct, except for ferries and some RORO services.

Sharing freight and passenger modes is not without difficulties , and indeed, some of the major problems confronting transportation occur when the two compete for the use of scarce transport infrastructure. For example, trucks in urban areas are seen as a nuisance and cause congestion by passenger transport users. Daytime deliveries and double-parked trucks are perceived as a particular nuisance. The poor performance of some modes, such as rail, is seen as the outcome of freight and passengers having to share routes. There is growing interest in using transit system segments to move freight, particularly in central areas. This raises the question as to what extent and under which circumstances freight and passengers are compatible. The main advantages of joint operations are:
- High capital costs can be justified and amortized with a diverse revenue stream.
- Operating and maintenance costs can be spread over a broader base.
- The same modes or traction sources can be used for freight and passengers , particularly for rail.
The main disadvantages of joint operations are:
- Unmatching demand locations . The origins and destinations of freight flows are usually quite distinct from passenger traffic.
- Frequency of demand . Passengers usually need high-frequency services, while freight, except air cargo, tends to be less critical.
- Timing of service . Demand for passenger services has specific peaks during the day. For freight, it tends to be more evenly spread throughout the day. Several freight operations prefer night services since they ensure that shipments arrive at their destination in the morning.
- Traffic balance . On a daily basis, passenger flows tend to be in equilibrium, irrespective of the distance involved (e.g. commuting or air transportation). For freight, market imbalances produce empty flows that require repositioning assets such as trucks or containers.
- Reliability . Although freight traffic increasingly demands quality service, delays (diversion from posted schedules) are unacceptable for passengers.
- Sharing routes . Favors passenger traffic, with passenger trains often given priority or trucks excluded from specific areas at certain times of the day.
- Different operational speeds . Passengers demand faster service but specific cargo, such as parcels, face similar requirements.
- Security screening measures . Require different procedures for passengers and freight.
Consequently, the ongoing separation of passengers and freight on specific gateways and corridors likely involves a growing divergence of flows, modes, and terminals.
5. A Growing Divergence
Passengers and freight are increasingly divergent activities as they reflect different transportation markets. Passenger and freight transport are being unbundled in several modes and across many regions.
a. Shipping
It has already been mentioned that passenger services have become separated from freight operations in the maritime sector. Ferry services are the exception, where ro-ro ships on high-frequency services adapt to the needs of both passenger and freight market segments. These ferry ships can transport cars, buses, and trucks carrying freight with the respective proportions determined by the demand. Deep-sea passenger travel is dominated by cruise shipping, which has no freight-handling capabilities and cargo ships rarely have an interest or the ability to transport passengers.
Most rail systems improved passenger and freight services, maintaining both segments sharing the same infrastructures . Proportions have a strong geographical variation, with much of the developing world having passenger rail as the dominant mode for inter-city transport, particularly in India and China. In Europe, national rail systems have also prioritized passenger service to expand regional mobility. Significant investments have improved the comfort of trains and passenger rail stations, but most notable has been upgrading track and equipment to achieve higher operational speeds. Rail freight transport has tended to lose out because of the emphasis on passengers since such systems were optimized for passenger flows. Because of their lower operational speeds, freight trains are frequently excluded from day-time slots, when passenger trains are most in demand. Overnight journeys may not meet the needs of cargo owners. This incompatibility is a factor in the loss of freight business by most rail systems still trying to operate freight and passenger operations.
The separation between freight and passenger rail business in North America is the most extensive. Private railway companies could not compete against the automobile and airline industry for passenger traffic and withdrew from the passenger business in the 1970s. They were left to operate a freight-only system, which has generally been successful, especially with the introduction of intermodality. Public agencies have taken over the passenger business, AMTRAK in the US, and VIA Rail in Canada. The major problem is that they have to lease trackage from the freight railways; thus, slower freight trains are prioritized.
Freight and passenger vehicles still share the roads. The growth of freight traffic is increasing road congestion, and in many cities, concerns are being raised about the presence of trucks. There are already restrictions on truck dimensions and weights in certain parts of cities, and there are growing pressures to limit truck access to non-daylight hours. For example, certain highways exclude truck traffic, which is likely to become a growing trend; the need to separate trucks from passenger vehicle traffic. This separation can be spatial (separate roads or lanes) or temporal (evening deliveries).
d. Air transport
Air transport is the mode where freight and passengers are the most integrated. First, they share the same terminal facilities, although there is a specialization with some airports focusing on freight activity. Yet, even here, a divergence is being noted. The growth of all-freight airlines and freight-only planes operated by some major carriers, such as Singapore Airlines, is a trend. The interests of the shippers, including the timing of the shipments and the destinations, are sometimes better served than in passenger aircraft. The divergence between passengers and freight is also accentuated by the growing importance of charter and low-cost carriers. Their interest in freight is minimal, especially when their business is oriented toward tourism, as tourist destinations tend to be lean freight-generating locations.
Related Topics
- 5.2 – Road Transportation
- 5.3 – Rail Transportation and Pipelines
- 5.4 – Maritime Transportation
- 5.5 – Air Transport
- 5.6 – Intermodal Transportation and Containerization
- 3.3 – Transport Costs
- 3.4 – The Provision and Demand of Transport Services
Bibliography
- BTS [Bureau of Transportation Statistics] (2006) America on the Go: Long Distance Transportation Patterns: Mode Choice.
- Donovan, A. (2000) “Intermodal Transportation in Historical Perspective”, Transportation Law Journal, Vol. 27, No. 3, pp 317-344.
- ITF (2022) Mode Choice in Freight Transport, ITF Research Reports, OECD Publishing, Paris.
- Sultana, S. and J. Weber (eds) (2017) Minicars, Maglevs, and Mopeds: Modern Modes of Transportation around the World, Santa Barbara, CA: ABC-CLIO.
Oslo – Promoting Active Transport Modes
- Clean and energy-efficient vehicles
- Mobility management
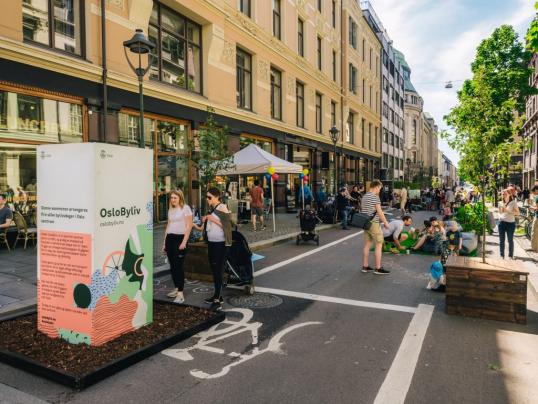
First published on 5 February 2021.
As with many cities in recent decades, the Norwegian capital, Oslo, was increasingly giving up urban space to cars. Since the 1960s, massive road building schemes were undertaken to accommodate the explosive growth in the ownership and use of private cars. However, the ever-increasing growth of the capital over the past decades, its projected continued growth, and mounting worries over poor air quality and climate emissions, forced new thinking on planning and catering for mobility to protect the environment and improve the quality of the city for its inhabitants.
The course that Oslo has taken to change the hierarchy of urban activities and modes of mobility, including prioritising people over cars, is unlike that taken in other cities. With the range and scope of its measures, which have at times been bold, Oslo provides a unique and interesting example of an integrated approach to city development. Oslo’s Car-free ‘Livability Programme’ is a crucial factor in the strategy to create a greener, livelier and more inclusive city.
Oslo’s population is one of the fastest growing in Europe, with an increase of 30% since 2000. While this has spurred economic activity, it has placed increasing pressure on the environment and air quality. With a current population of 680,000 and another 30% increase in population expected by 2040, the city intends to implement measures that will manage the impact of this growth on the environment and climate.
In 2015, a new city administration came into office and set an ambitious agenda to halve greenhouse gas emissions by 2020, become fossil fuel free by 2030 and to make Oslo an even better place to live. At that time, transport accounted for some 60% of the city’s carbon dioxide (CO2) emissions with almost 40% coming from private cars. A key part of the strategy to achieve the new objectives was to reduce car traffic by 20% by 2019 and by 33% by 2030 (compared with 2015 levels). Continuing to facilitate mobility whilst achieving these objectives would require significantly increasing the share of public transport, cycling and walking.
In 2014, the ‘Public Space Public Life’ (PSPL) survey was commissioned by the city. It concluded that, despite the city’s compact size, much could be improved in the connectivity and accessibility of urban spaces (especially for walking and cycling) and in relation to the quality of space. In response to these challenges, Oslo launched the Car-free ‘Livability Programme’ in 2016. Its purpose was to create a greener, livelier and more inclusive city by reducing traffic and space for private cars in the city centre during the 4-year mandate of the City Council (2015-2019).
The initial intention of the Car-free ‘Livability Programme’ was to impose vehicle access restrictions. These would prohibit most vehicles from driving through the part of the city centre confined within Ring 1, the innermost of Oslo’s three motorway ring roads which has an area of approximately 1.7 km2. With just under 1,100 residents (in 2015), it is not a major residential area. However, it is a centre of activity and hosts around 90,000 jobs (2015), facilitating the central railway station, Oslo’s main commercial streets, shopping malls and major nightlife districts.
Although car ownership (around 15%) and the share of cars commuting to the centre (around 10%) are low, the proposed car-free zone in the centre faced significant opposition, especially from businesses. Therefore, the city decided to change its approach to a more gradual one, which aimed at having the least possible number of vehicles in the centre instead of having a car-free zone.
The Car-free ‘Livability Programme’ was implemented in three phases. In phase one, on-street parking spaces were removed within the central Ring 1 and so were some parking spaces in surrounding areas that were deemed to be ‘in conflict with bike development’. Car parks in and around the central zone were left in place, although many other on-street parking spaces around the central zone were freed up for alternative uses.
In a limited number of pilot areas, including Dronnings gate and Fridtjof Nansens square, measures were introduced to encourage pedestrian-friendly areas prioritising culture and city life. People in Oslo were invited to provide their input – these included representatives of leisure clubs, cultural groups, businesses, restaurants, senior residents, the young, and people living, visiting and working in the centre. They could share views and launch ideas during a range of meetings, presentations and online via the municipality website. A variety of measures – large and small, temporary and permanent – were implemented. These included creating terraces and playgrounds, placing more street furniture (such as benches and trees in planters), using art in urban spaces, organising events and improving amenities (such as water taps and public toilets).
Phase two of the Car-free ‘Livability Programme’, implemented in 2018, was the stage introducing the most changes. It was also the year Oslo commenced the implementation of its ‘Action Plan for increased City Life 2018-2027’, which was commissioned by the City Council as a follow-up to the PSPL survey.
To improve city life, it was recommended that the City Council commence work to improve connections to and through the city centre (area within Ring 1) to increase interaction and synergies between target points in the city, and to highlight and encourage the use of hidden urban spaces. These recommendations were included in the Car-free ‘Livability Programme’.
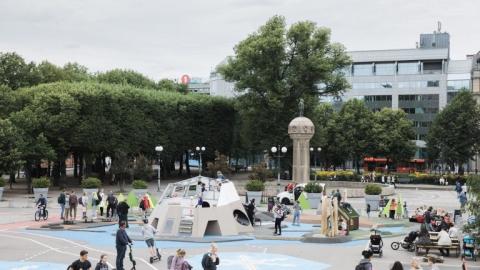
Traffic routes through the city centre were altered and several streets were closed to vehicles, although some exceptions were made for goods deliveries and parking for the disabled. In addition, the pedestrian network was extended, new bike lanes were created and the number of on-street parking spaces was further reduced. The City Council also continued to cooperate with local stakeholders to develop and implement an annual calendar of activities and events.
The activities, events and works to implement the Car-free ‘Livability Programme’ continued in 2019 (Phase 3 and the final year of the programme). The total number of parking spaces that were removed was almost 800, while the first major physical street renovations were completed in Dronnings gate and Øvre Slottsgate. The City Council also evaluated the entire Car-free ‘Livability Programme’ in 2019. Even though it moved away from its initial idea of creating a car-free city centre, the City Council indicated that it would continue to assess whether access restrictions were necessary to reduce traffic.
Oslo has decided to continue the gradual approach adopted in the Car-free ‘Livability Programme’. Since its adoption, the Action Plan for increased City Life 2018-2027 has provided the strategic direction for implementing the Car-free ‘Livability Programme’. Further investments after 2019 have been linked to the implementation of the Action Plan and to the Area Regulation of Streets and Urban Spaces in central Oslo. The latter is the city’s zoning plan, which was adopted in 2019. This is the long-term plan to further improve accessibility for cyclists and pedestrians, and provide descriptions of the measures that will be implemented to facilitate car-free city life.
To assess the results and learn from the experience of the Car-free ‘Livability Programme’, evaluations were conducted during each year of the programme, comparing the results with the ‘baseline situation’ at the start of the programme. It was concluded that the Car-free ‘Livability Programme’ had a clear local effect and, even if some traffic had moved to alternative routes, the measures were likely to have contributed to an overall traffic reduction in Oslo by making driving and parking in the city centre more difficult.
Traffic data collected as part of the evaluations showed that within the city centre, car traffic reduced by 11% in the period 2016 to 2018, and by 19% between 2018 and 2019. Surveys also revealed that the measures have not impacted on origin-destination patterns within the city centre, although drivers are making fewer trips by car, while the occupancy rate has increased from 1.41 to 1.85 passengers per vehicle.
It has been difficult to establish the effect of individual measures. Furthermore, in addition to the measures implemented through the Car-free ‘Livability Programme’, several others have been implemented within and outside the project area, which may have also affected traffic in the area. These include introducing new ‘ toll rings’ , time- and environment-differentiated tariffs on the ‘toll rings’, and construction work in the city centre. Although it is likely that these measures have also had an impact on traffic during the evaluation period, it is assumed that the effect has been synergetic in reducing the number of cars in the centre.
Annual random surveys of the number of pedestrians in various streets and the number of people spending time in different urban spaces show an overall increase (14% and 43% respectively) over the period of the Car-free ‘Livability Programme’. At the same time, overall figures from the travel habits survey for Oslo’s city centre show that the share of pedestrians is stable, while the share of public transport and bicycles has increased slightly, and the share of vehicles has decreased. Since the pedestrian counts were conducted during short periods and do not cover the same areas as the travel habits survey, it is uncertain whether the increase in pedestrians in the surveyed streets is a trend or random variation.
According to polls, more than 50% of the inhabitants of Oslo are now open to a city centre with fewer vehicles, and this share has been increasing over the years. After a period with trials, testing and construction, it is only now that people are able to see and experience the results. The majority of people are also positive about the future prospects of a car-free city centre and how it can attract more people to the area.
The most criticism relating to the objective of a car-free city centre has probably come from people in the business community. Their key concerns have been over potential problems with their supply logistics and, particularly, a fear of losing customers. Research by the City Council has shown that, although there has been a decline in retail turnover, this decline was due to broader trends within the economy, and turnover in the city centre was at the same or even higher levels than in competing areas outside the car-free zone.
Challenges, opportunities and transferability
The Car-free ‘Livability Programme’ has generated interest and curiosity from all corners, especially since Oslo was awarded the European Green Capital Award 2019 . Oslo has shown that it is possible to quickly and effectively reduce the number of cars in a city centre, and to prioritise pedestrians, cyclists and public transport users while making the city greener.
Still, Oslo’s efforts to improve city life have not only provoked enthusiasm, but also resistance. Some of this resistance is attributable to a sense that the changes are happening too quickly and without sufficient information or public engagement. The focus in the early stages of the Car-free ‘Livability Programme’ on removing car parking spaces and reducing car access triggered resistance. While the perceived downside of the plans (such as reduced car access for visitors and residents, and problems in logistics) became quickly apparent, the upside (a liveable city) received less attention. In addition, members of the business community in particular felt that their contribution to city life (such as trade, jobs and shopping) was taken too much for granted, while their concerns received insufficient attention.
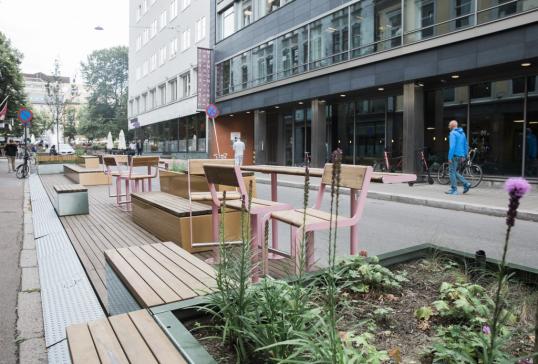
Oslo has responded by adopting a gradual approach to continue with the transformation of the urban centre where cars will become subordinate to pedestrians, cyclists and public transport. Reducing car use is not an objective in itself, but merely a means to help achieve a greener and cleaner city. In this way, measures to reduce car use have become part of a wider strategy to improve urban life.
Even more careful consideration is being given to ensuring that reducing car use in the city works for the benefit of everyone. This means ensuring that the city remains accessible to everyone. Oslo has shown that by investing in public transport and cycling infrastructure, these modes can provide a viable alternative to private car use, especially in densely populated areas.
Without doubt, Oslo provides an inspiring example of how urban space can be shared between users. Oslo is actively working to improve urban logistics. Many of the reclaimed parking spaces have been made available for goods deliveries. In addition, the number of disabled parking spaces in the city centre has increased and Oslo continues to cooperate with interest groups for the elderly and people with disabilities to further improve street design and facilities. Finally, the Car free 'Livability Programme' has been an arena for innovation and learning.
Through experimental and exploratory urban space projects, Oslo has highlighted the city’s potential and set interesting examples for others. Continuous monitoring and evaluation has provided Oslo with useful information on the immediate effects. As experience builds and new initiatives are implemented to promote walking, cycling and public transport, the City of Oslo will continue to be a city to watch in the future.
More information:
- Oslo’s Car free Livability Programme (Norwegian)
- Oslo’s Car free Livability Programme (English)
- European Green Capital Award
Share this page
An Open Access Journal
- Original Paper
- Open access
- Published: 12 July 2022
Assessing robustness in multimodal transportation systems: a case study in Lisbon
- Joao Tiago Aparicio 1 ,
- Elisabete Arsenio ORCID: orcid.org/0000-0002-8520-8665 2 &
- Rui Henriques 3
European Transport Research Review volume 14 , Article number: 28 ( 2022 ) Cite this article
5287 Accesses
12 Citations
1 Altmetric
Metrics details
Introduction
Worldwide public transport systems are exposed to disruptions caused by malfunctions, accidents, maintenance, reduced fleet, and disasters, compromising mobility. Transport networks’ multimodal planning and management can be explored to increase their robustness against these events. In this context, this research paper proposes and empirically compares methods to assess the robustness of a multimodal transport network, looking at aspects regarding the single-mode and multimodal network topology.
Materials and Methods
We hypothesize that the appropriate multilayered and traffic sensitive modeling of a multimodal transport network can help characterize robustness and further unravel vulnerabilities related to the integration of different transport modes. Using metric-based targeting, we evaluate how the network decreases performance when simulating failures on stations and pathways using different scenarios. The following six extraction strategies for nodes and edges were used in the simulation: Random removal; Initial Degree removal; Initial Betweenness removal; Recalculate Degree removal; Recalculate Betweenness removal; and Multimodal Hubs removal. Lisbon’s public transport is used as a case study and is modeled as a multiplex network integrating eight different modes of transport. Proposing a novel normalized version of assessing the impact of failures, we were able to compare side by side the robustness of each modality layer, regardless of their size. Lastly, we simulate cascading events such as the breakdown of an entire transportation line.
Conclusions
Using different ways to induce failures in the network, we observe that to leave all nodes completely disconnected, we would need to remove about half the network nodes, highlighting the robustness of the Lisbon public transport network. Comparing different failure scenarios, methods that rely on recalculating network metrics yield a higher impact on the network robustness assessment. The impact of different events is quantified, showing that failures in stations are generally more dangerous than in pathways and offering views on the consequences of deactivating particular network modules. Overall, the results of this study allow decision-makers to gain further understanding of the topological vulnerabilities of a transportation network.
1 Introduction
Around 55% of the world's population lives in urban areas [ 37 ]. However, such areas represent only a minor percentage of the total surface area. Therefore, daily commuting patterns and mobility services’ consumption impact factors such as the usage of natural resources and impact time in transportation, pollution, and quality of life, among others, yield extreme importance in sustainable development goals [ 3 , 4 , 6 ]). In this context, numerous studies attempt to optimize transportation systems planning and usage in urban scenarios [ 10 , 28 , 30 ].
Among the studied solutions, the importance of a multimodal transportation system arises due to the high demand for some traffic corridors. According to [ 21 ], an improved articulation of multimodality aspects within a transport system is significantly associated with lower carbon emissions. This assertion leads us to believe that successfully coordinating the interfaces in this kind of network is a viable solution for promoting carbon neutrality in the long run. However, according to [ 15 ], the study of multimodal transportation systems “has historically been underrepresented in travel surveying efforts. This lack of consideration has implications for widely accepted statistics for nonmotorized travel behavior (walking, bicycling, among others). It affects researchers and professionals in travel modeling, urban planning, public health, and urban design”. An integrated view of how specific failures affect the overall multimodal system is also relevant. Since it is relatively common to have planned or unplanned disruptions (i.e., construction works, technical failures, attacks, and natural disasters), causing delays and possibly inhibiting the usage of a pathway or station [ 11 ]. This observation raises the need to assess how robust these transportation systems are. Therefore, our main research objective is to assess the robustness of multimodal transportation systems in an urban scenario. In the context of this study, this assessment is applied at a topological level. Particularly we would like to understand how the different types of percolation tests have different results depending on network topology, the main topological fragilities regrading mono and multimodal public transport, and the differences between them and a metric to access the topological robustness of the network, that allows for direct comparison regardless of the network size and shape.
Making stops along our path from origin to our destination is often required, and the more stops we make, the more time we spend on transportation. So naturally, how nodes and edges failures affect the Average Path Length (APL) is an important research question that we are interested in answering. This is the case since the effectiveness of a path between two stations can be assessed by the number of stops in a trip (the APL). Besides the effects on the APL, understanding how many nodes (or stations) have to fail to fragment the network into isolated components is a requirement to understand further the impact of a disaster on a station or stop (in creating isolated components). In addition, we also aim to infer the effect of removing edges (pathways) versus nodes (stations) since this helps us infer if incidents have a higher impact on pathways or stations. To empirically test the simulation-based robustness testing, we aim to understand if metrics should be recalculated after each extraction and the measurement of the impact of cascading failures. Such as what happens if a station failure causes chain reactions on other stations located along the same line or in the nearby stations or stops from other modes.
The current study contributes to increasing the knowledge of the robustness assessment of multimodal transportation systems. Hence, we do a comprehensive review of modeling multimodal transportation systems and quantitatively assess their robustness. Another contribution of this study is the possibility of supporting public transportation policies focusing on actionable priorities for a more resilient system design. For instance, where should the focus of actionability be in case of an accident on different stations or pathways. By understanding which elements are more important for network integration.
For this research, the city of Lisbon is used as an example to improve the integration of the current multimodal transport network. This analysis is fundamental in the context of the Lisbon Metropolitan Area (LMA), where the average occupancy rate for individual private transport (cars) is 1.60 passengers per vehicle. This is also where the daily traffic inflow in the county of Lisbon is also the cumulative result of commuting traffic inflows between Lisbon and the eighteen municipalities that integrate the LMA [ 24 ]. Several road traffic corridors are flooded daily with single occupancy vehicles that could use another public transport alternative. Despite stable establishments and integrating the operators’ systems with a single card, challenges to the public transport network's integrated operation and multimodal planning still persist within LMA.
Additionally, as noted previously, in a general sense, it is essential to note the impact of natural hazards on disrupting a working transportation system as well. Previous literature [ 32 ] denotes the natural hazard risk in different zones of the LMA (see Fig. 1 ). This map shows us the spatial distribution of risk for earthquakes, flooding, and landslides in the county of Lisbon. This stresses the importance of understanding various risk impacts on the network's structure. Along with the general risk from natural incidents, other incidents through the city have a higher incidence rate, and these usually have a more localized effect [ 19 ]. Such incidents may be caused by faults in energy and water suppliers, traffic accidents, and fires that disable stations and pathways. [ 19 ] rendered a map of incidents from a diverse standpoint (see Fig. 2 ). This shows us that a study on the impact of network robustness at a local level is relevant.
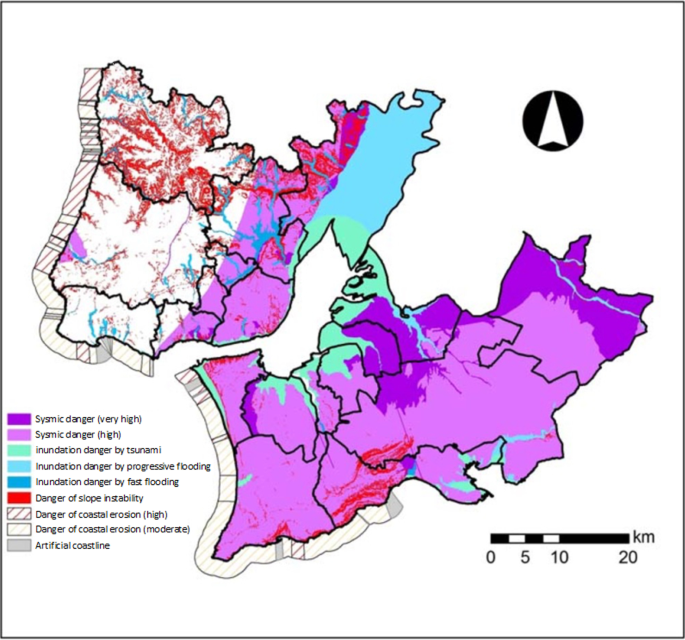
Lisbon Metropolitan Area, natural hazard map, extracted and translated from [ 32 ]
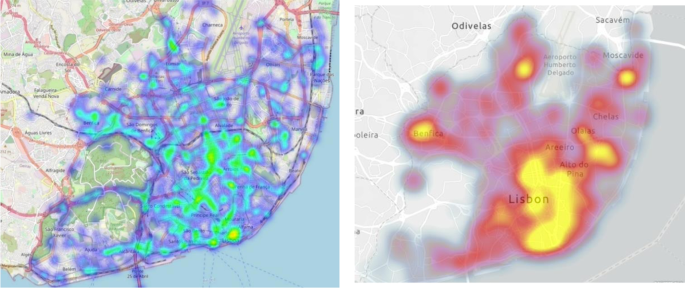
Distribution of incidents in the city of Lisbon from [ 19 ]. On the right is the occurrence of incidents and on the left, their impact on pathways, i.e., the number of roads affected
Since the Lisbon public transportation network is susceptible to these failures that can affect the daily commute of its users, a way to guarantee and improve the robustness of this critical component of our public services is crucial. To tackle this problem, we aim to understand how robust the Lisbon public transport network is. This assessment starts by quantitatively measuring robustness [ 40 ]. Since the Lisbon public transportation network is also complex, examining how different stations and stops may have similar properties, bridging different lines (routes as linear road/rail/other mode infrastructures) and transportation modes is essential. Topological attributes and concepts of network resilience, besides simulation, will play a role in this research study. We ask ourselves which nodes (e.g., stations) are central and the role of degree-degree correlations. This is, if central stations connect to other central stations in this particular topology, this may cause a higher fragility [ 41 ]. Also, looking at how different extraction strategies (or simulations) for quantifying robustness affect the connectedness of the network will shed some light on which method has the highest impact on breaking the critical pathways that keep the transportation system usable throughout the city.
The above concepts will be applied to Lisbon’s multimodal transport network in the ILU (Integrative Learning from Urban data and the situational context for city mobility optimization) project. This project joins research institutes, the Lisbon municipality, and public transportation operators to promote more efficient and sustainable mobility.
2 Literature review
Robustness can be understood as the quality of the capability of a system to work under disruptions [ 23 ]. Robustness has been alternatively defined as an optimization problem [ 26 ]. A robust solution can be seen as having the best performance under high-stress conditions. To further understand this property of the system perturbation, the literature review shows that experiments are a dominant approach, which can be done in silico (using computer simulations) [ 29 ]. In the context of a multimodal transportation system, we can inherently see the redundancy since the transportation modes usually provide alternative routes for the same places.
Previous studies indicate that the first step in measuring the robustness of a transportation system is to model the transportation network. Accordingly, the modeling of multimodal transportation systems recurring to multilayer networks has been previously proposed to analyze urban transportation systems [ 2 ]. This allowed the analysis of superlayer interdependence. Multilayer networks are also used to model air transportation networks. This can, for instance, be used to study how the degree–degree correlations of a network affect the spreading of an epidemic [ 5 ]. Different types of multimodal transport networks have been modeled in several empirical studies, as shown in Table 1 [ 39 ]. Note that air travel can be modeled as a heterogeneous mode of transport, as different air travel companies constitute different layers of a network [ 20 ].
Different authors have modeled and assessed diverse types of networks. [ 8 ] addressed the evolution along time using historical data. [ 36 ] developed a method for choosing different routes in shipment according to demand. [ 16 ] studied connectedness in India using community membership and betweenness centrality. [ 38 ] applied a weighted multiplex to air traffic to then make a network analysis with metrics such as average degree and assortativity.
[ 18 ] modeled an air traffic network in layers based on flight frequency and concluded that it is less redundant in the Chinese network than the worldwide network. [ 5 ] modeled an air traffic network and assessed assortativity and rich-club effect (nodes with high degrees connect to nodes with high degrees) in international flights. [ 2 ] modeled the Madrid urban transport network, the measured overlap between different modes and transfers, and the time from one station to another. [ 17 ] studied how the network's topology affected the formation of communities and how betweenness centrality and closeness centrality affect growth. [ 27 ] create a methodology to assess the spatial accessibility of public transportation networks using a case study in Shanghai, China.
To answer the need for measuring the resilience of networks, [ 25 ] noted in their robustness and resilience review that a network should be considered resilient if it sustains a high number of node failures before it becomes disconnected. In that sense, performing node percolation tests is suggested to measure resilience. Measuring this property in transport networks has been the focus of several studies. [ 35 ] have evaluated system-wide robustness by finding critical isolating links in road networks. They reduced the link capacity and measured the travel time to measure robustness. This allowed for a dynamic perspective as well. Later on, [ 42 ] conducted a similar study but induced the vulnerabilities by blocking lanes instead, same as deleting an edge, which ranked the links by how critical they were building upon the much earlier work that did just that [ 33 ].
These studies are related to the earlier studies on reliability by [ 13 ], where they measured the reliability of networks given the demand change based on route choice models. This study extended capacity reliability to network equilibrium models as well as accounts for route-choice actions of drivers. [ 1 ] measured the reliability using network capacity and travel time by changing demand and inducing link degradation and non-persistent congestion. These studies show how different demand levels affect capacity and reliability in an analytical way. It is important to note that reliability is fundamentally different from resilience. Reliability is usually a measure of susceptibility to the interruption of service. Resilience, on the other hand, measures the ability to recover from such interruptions in service [ 14 ]. Robustness, on the other hand, can be seen as a dimension of resilience and as a cause for reliability.
Studies of network resilience have also been done in the context of multimodal transport. [ 31 ] recently showed that the same idea of percolation could be used in multiplex networks. This notion opens this test as a way to measure the robustness of multimodal transport networks accurately.
Another view on resilience measurement on multimodal transport networks is given by [ 7 ], who postulates that a whirlpool network structure on a multimodal transport network leads to a cyclic steady state, unlike a tree structure, under the flow simulations proposed. A whirlpool state is one that points towards another, and the following states recursively point to the following until it completes a circle. Then the measurement of robustness is given by the ratio of states in a whirlpool structure and the total number of states in the multimodal transport network.
Besides a topological resilience test, dynamic measurement of such property has also been studied in multimodal urban transport. [ 34 ] studied resilience to extreme weather events (EWE) in several European cities by measuring the percentage of change in working segments during the EWE. Then claimed this could be used for prediction tasks by using past rail, air, and road usage data. However, this method lacked actionable information because prevention is only attainable if we know where to act. Later on, [ 12 ] defined a framework to assess robustness. This helped the description of robustness assessment as an economic problem. This kind of assessment is very relevant for this study because it provides a baseline for understanding the impact of different disruption scenarios in specific links, quantifying their criticality. This is a type of assessment that provides specific enough data to be actionable in the real world, showing that the number of critical links distribution follows a left-skewed behavior (Fig. 3 ). This is relevant since we can see that most links are not that critical, and others are significant to keep the network connected. By identifying such links and providing redundancy, priority policies, and other measures, the robustness of the network can actually increase.
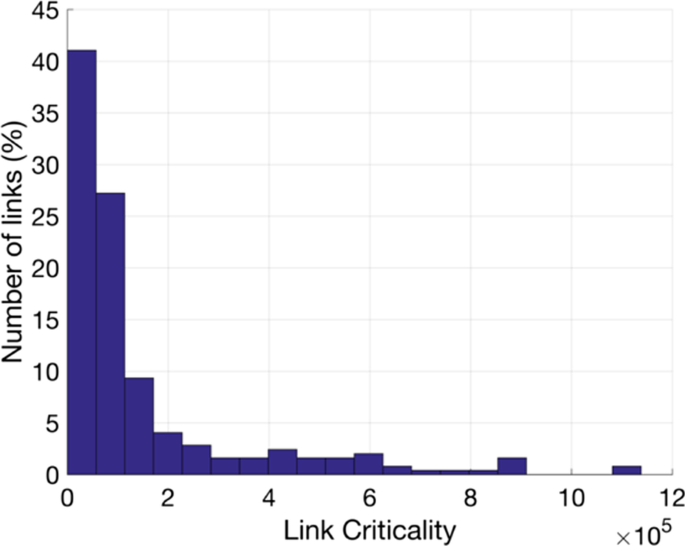
[ 12 ] link criticality study results
3 Methods and modelling
3.1 methods.
To answer the questions stated in the introduction regarding the topological robustness, we use different strategies. As we have seen, there are several approaches to assessing robustness. One of them is removing nodes or edges from a network to measure the impact of a simulated station failure [ 31 ]. The simplest way to do this is by injecting random failures in the network, i.e., randomly removing nodes from the network. It is expected that this simple strategy may not maximize the damage to the network, but it can be a reasonably realistic approach since the Lisbon public transport network may have station failures due to unpredictable and unexpected events.
Other more precise strategies were defined by [ 22 ], and we followed their strategy. We also simulated the failure of nodes and edges to be able to compare, which yields the highest impact, as the optimization stress handling can be part of the definition of robustness, as seen previously. These more precise node extractions (simulated station failures) utilize attributes from the network, such as degree and centrality. To test a network with more precision, an effective strategy should be to extract the most critical node. This notion of importance can vary depending on the attribute to which we give attention.
We could select nodes in descending order of degree. This extraction is called Initial Degree removal—ID removal—because we base the extraction on only the initial calculation of the degrees, and we do not update it after removing nodes.
Another critical attribute is the centrality of a node. There are a few types of centralities, but the betweenness centrality is crucial since it represents the nodes that have edges that unify communities/groups inside the network. These nodes are the ones with the highest criticality for network connectivity since they connect the communities of the network. This extraction is called Initial Betweenness removal—IB removal—like ID removal, it only calculates the initial betweenness centrality of each node at the beginning and does not update it.
Both extractions only calculate the attributes, degree distribution, and betweenness centrality at the beginning of the algorithm. When we remove nodes from the network, it changes the degree of the neighbors of the removed node, and it modifies the betweenness centrality of all the nodes. Two new strategies appear called Recalculate Degree removal—RD removal—and Recalculate Betweenness removal—RB removal. These strategies are identical to the ID and IB ones, except they update/recalculate the degree and the betweenness centrality after each node/edge removal. The RD and RB algorithms are considerably more time and computation expensive for each interaction, but we wanted to study if they are more efficient at disconnecting the network. Efficiency, in this case, is a way to simulate the highest stress levels.
ID and RD removal are local strategies because when a node is removed, it only changes the degree of the neighbors of that node. Thus, only these local nodes need to be updated. On the other hand, IB and RB removal are global strategies since by removing a node, we need to recalculate the betweenness centrality globally, i.e., of all nodes.
Every calculation of the betweenness centrality of the network costs around 5 min, and since we must apply thousands of recalculations (one per node removal), this would be very time-consuming and less sustainable. Because of that, we decided only to recalculate the betweenness centrality when batches of 5% of the nodes were removed. Being N the number of Nodes and E the number of Edges , the ID algorithm is \({\text{O}}\left( {{\text{N}} + {\text{E}}} \right)\) , the RD is \({\text{O}}\left( {{\text{N}}\left( {{\text{N}} + {\text{E}}} \right)} \right)\) , and the IB is \({\text{O}}\left( {{\text{NE}}} \right){ }\) (using a faster algorithm for betweenness centrality created by [ 9 ]), and the RB is \({\text{O}}\left( {N^{2} {\text{E}}} \right)\) .
3.2 Modeling
Most worldwide public transportation networks are multimodal. Therefore, these are modeled as multilayers. Moreover, we also wanted to test if removing nodes that connect layers of the graph is critical, i.e., if it disconnects the network faster. We call these nodes multimodal hubs, and this extraction strategy is Multimodal Hubs removal. For example, two multimodal hubs are Alameda , which connects Metro and Carris, and Gare do Oriente , which connects Carris, Metro, and CP. It is worth noting that the Multimodal Hubs removal strategy only makes sense when evaluating the multilayer network. When evaluating each modality, this strategy is invalid because there are no multimodal hubs.
Removing nodes from this network may correspond to a stop being deactivated or being in construction, while removing edges may correspond to cutting roads or accidents preventing any vehicle from passing through. Edges can also fail. All our node extraction strategies can be applied to edges with a few modifications. For example, how can we get the degree of an edge? The edge degree depends on the nodes that are connected by it. We used the following four different methods to calculate edge degrees:
\(k_{e} = k_{v} \cdot k_{w}\)
\(k_{e} = k_{v} + k_{w}\)
\(k_{e} = {\text{min}}(k_{v} , k_{w} )\)
\(k_{e} = {\text{max}}(k_{v} , k_{w} )\)
where ke is the edge degree and kv and kw are the degree of the nodes that are connected by the edge e. Since our network is directed, we also assume that v is the source and w is the destination node of the edge. However, there are other ways to calculate the degree of an edge, such as ke = kv or ke = kw.
In accordance with the study of [ 22 ], where they concluded that method A. ke = kv ∗ kw was the best fit for the majority of the network types that they studied. Method A showed the best correlation of the four methods between the edge degree and the edge betweenness centrality. It is expected that the node with a higher degree should also have a higher betweenness centrality. To calculate the betweenness centrality of an edge, we also used the [ 9 ] \({\text{O}}\left( {\text{ NE}} \right)\) algorithm.
We can expect that the extractions based on centrality measures may be more harmful than the random failures. Concerning the nodes vs. edges, we believe that there could be a slight difference between these extractions, but this is hard to predict. Degree-based strategies probably will split the network into many subgraphs of vertices with low degrees. Betweenness centrality strategies can create clusters that are highly connected since they tend to destroy the bridges that connect communities first.
We understand that not only single nodes fail but also more catastrophic events can happen in the network, bringing the notion of cascading effect. For the cascading effects, we described and implemented two extraction strategies.
The cascading effect simulating a Line Failure removes an entire transportation line in the network. This could happen with an underground implosion that made the whole line stop. We simulate this by removing all the nodes that have the attribute of that line. Some nodes may belong to more than one line, even from different modalities. For example, Gare do Oriente stop is a multimodal hub that belongs to Metro, Carris, and CP (railway company).
The cascading effect simulating a Neighbours Failure removes neighbors from several layers of a failed node. One example of this in the Lisbon public transportation network could be a recursive failing of transports in the same spot. When a station fails, the neighbor stations could experience overflows because of relocated traffic, and this could potentially cause them to fail as well. To simulate this type of cascading effect, we collect several layers of the node’s neighbors, and then remove all these nodes at the same time. An example of this could be a flood that affected all transport modes in a particular spot of the city to the point of not being usable. In conclusion, we used the six following extraction strategies for nodes and edges:
Random removal
Initial Degree removal (ID)
Initial Betweenness removal (IB)
Recalculate Degree removal (RD)
Recalculate Betweenness removal (RB)
Multimodal Hubs removal
We also used the two following extraction strategies for cascading effects:
Line Failure
Neighbours’ Failure
3.3 Assessment metrics
To assess the impact of each strategy, we explore the impact of each removal based on different metrics through the simulations. To understand how connectedness is affected, we measure the average path length (also known as average geodesic distance), average degree, number of isolated components, and the size of the strongly connected component [ 5 , 16 , 38 ]. To understand how the average path length is impacted by each removal, we only average out the shortest paths that still exist in the network. This approach allows us not to need to calculate the inverse path length, \(l^{ - 1}\) . As the study of this network is applied to a multimodal transportation network, we also measure the impact of the described extractions on the use of multimodality.
By implementing the different extraction strategies discussed previously. For each iteration, we compute the largest SCC and its size. This size decreases with the removal, and if a critical station is removed, the size decreases even faster. Ergo the strategy which has a faster decrease has a more significant impact on the network. This means that networks that exhibit a steeper decrease sooner, as the percentage of iteration steps, are less robust. In the same fashion, a high-performance extraction strategy is one in which the SCC descends faster.
To compare different removal strategies, we propose a novel metric, the discrete normalized Area Under Curve (AUC), to compare the resilience of the different layers and the multilayer network. The normalized AUC ⊂ [0,100] allows us to compare the values of networks of different sizes and shapes. This is calculated using the following formula:
where \({\upchi }\) is the number of steps of the simulation, \({\uptau }_{i}\) , is the size of the largest strongly connected component at timestep \(i\) , and \(V\) is the number of nodes of the network. This measurement allows us to compare the different resilience side by side. It is important to note that this metric might have a higher variability (for both inflation and deflation) in smaller networks, given the granularity.
4 Results and discussion
4.1 data extraction and pre-processing.
Firstly, we identified the criteria used for the inference of the multiplex network, followed by an analysis of the robustness of the topology. To transform route planning from GTFS data files of each mode into a network, we joined the routes and the stops of each one of the transportation modalities: Carris, CP, Fertagus, Metro, Rodlisboa, Sulfertagus, Transtejo, and TST (bus, railway, riverway, subway, tram companies’). This merge contains information regarding the id, geographical position, sequence within the line, and name of the station. This allows us to verify which stops are connected to one another.
Given the structure of the data, we created a directed graph (digraph) for each transportation modality (bus, railway, riverway, subway, tram), forming a layer for each. We resorted to digraphs for each layer because transport does not always flow in both directions within the same path. This allows us to create each layer of our multilayer network. We apply a multilayer representation hence the edges of different layers have different types that represent different realities. Modeling such characteristics is not possible with a single layer (or monolayer) network.
After the representation of each layer, we must account for possible multimodal interactions, i.e., the possibility to shift to different transportation modes within a trip. These links are of extreme importance because they allow us to assess the connectivity of the transportation system as a whole. To accurately understand where these edges could be located, we created a script that extracted the Lisbon city map and calculated the walking distance between every two stations, and combined it with a standard coordinate distance calculation to get faster calculations (Fig. 4 ). After getting this result, we selected the pairs of stations from different modes and linked them based on distance. The intermodal interfaces are about 0,0040% of the distances calculated. We connected the eight modes of transportation in the city of Lisbon using the described method.
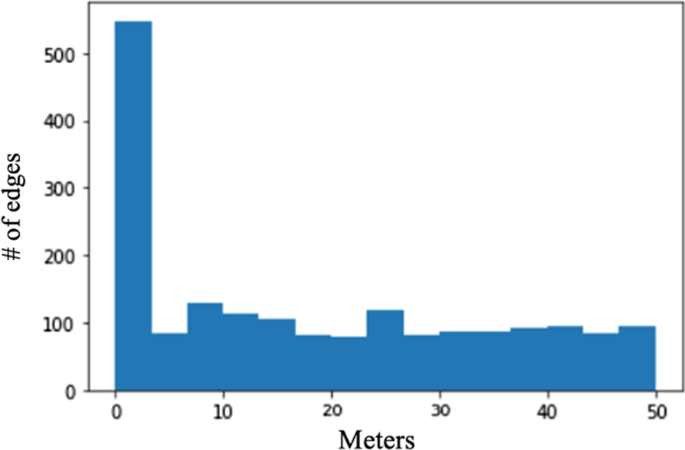
The distribution of distances associated with the 1878 intermodal connections has a very high cardinality of distances close to 0 m. This represents the stations/stops that simultaneously link layers
By programming a 3-dimensional method for visualizing such a network, using the geographical information available, we were able to see its structure (Fig. 5 ). This network is composed of 8 layers, 7972 nodes, and 11,892 edges. The complete network required the computation of 46 399 492 distances to join all the layers with the multimodal connections.
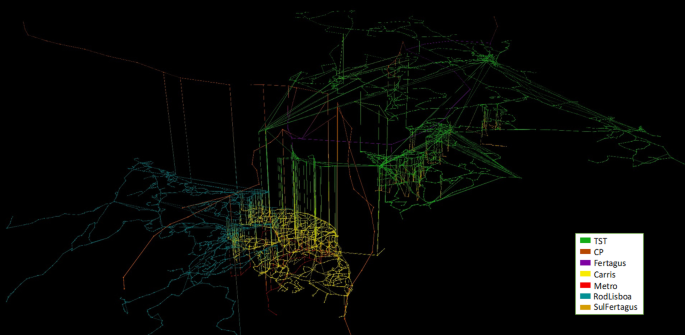
Multilayer Lisbon transport network topology on a 3D representation with all the layers
4.2 Network analysis
The number of nodes and edges per layer is disclosed in Table 2 . It is clear that the distribution of stations is not equitable across layers. Additionally to the edges in each layer, 1502 edges represent multimodal changes. The average in and out-degree are approximately the same at 1.4917, which means that the majority of the edges are reciprocally directed.
As we are studying a directed network, it is essential to assess the strongly connected components (SCCs) since it measures the numbers that connect with each other regarding the direction of each pathway. These are graph partitions, where the nodes are connected through a path. It is quite normal to have many single-node SCCs in unidirectional lines. This is precisely the case in this network. We observe 417 SCCs in the whole network as some layers, such as METRO and CP, have only bidirectional relationships between nodes. An example that would cause a higher number of SCCs would be, for instance, a set of isolating lines from the rest of the network by eliminating a critical station. Lines that are naturally isolated within the present topology happen in the TST Sesimbra line and the RODLISBOA Vila nova line. The remaining small SCCs in the multilayer network are single stations where the flow is unidirectional. The biggest strongly connected component has 7512 nodes which are about 94% of the total nodes of the network. The number of SCC’s per each layer are: CARRIS: 67, CP: 3, FERTAGUS: 1, METRO: 1, RODLISBOA: 263, SOFLUSA: 1, SULFERTAGUS: 6, TRANSTEJO: 3, TST: 177. The fact that the number of SCCs of the individual layers summed is higher than the SCCs in the multilayer network means that the multimodal edges are significantly well placed on improving network connectivity.
4.3 How many stations are connected with each station?
In this multilayered network, we can examine the kind of degree distribution we have, shown in Fig. 6 . We can see a high tendency of having both the in-degree and out-degree equal to one (part of a line) and two to four (stations that have intersections of lines of one or more modes). We can also see some nodes with an out-degree of 0. These are start and end stations, respectively, that have no reciprocal edges in the opposite direction. Stations with in-degree and out-degree equal to one can be caused by a significant amount of stations that flow only in one direction, or career end stops have different identifiers depending on orientation. This fact is also explaining the high number of low distances in the figure above. The in-degree is always very close to the out-degree. Since we are looking at a transportation network, this tells us that the majority of the connections between stations flow both ways, forming what is known as a chain (or a line) , even though there are apparent exceptions (stations that connect with many stations) as we can see on the tail of the plot with a log scale.
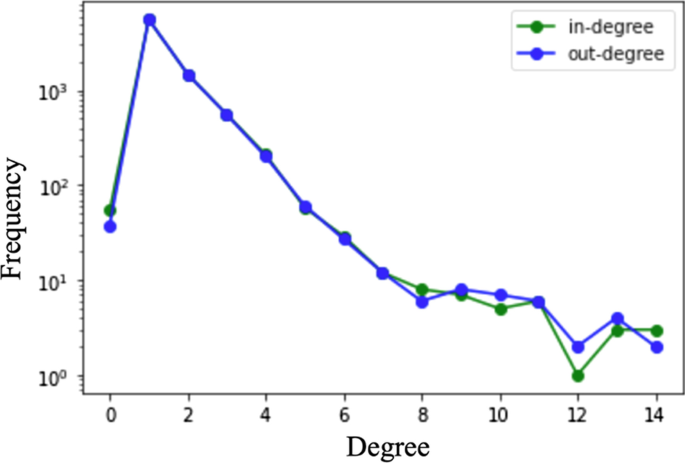
Degree distribution of the multimodal transport network
4.4 How many stops do we have on average between each station?
To answer this question, we look at the average path length in the number of stops between two stations (not the length of the path itself). For the largest mutually connected component, the average path length (APL) is about 34.6878. This means that, on average, to get from a station/stop to any other on the network, we have to go through about 35 stations/stops before reaching the destination (this number includes multimodal travels as well). Per layer, this value is usually smaller (METRO: 7.7176, CP: 10.2072, FERTAGUS: 5.0, CARRIS: 25.8734, RODLISBOA: 36.9525, SULFERTAGUS: 34.7543, TRANSTEJO: 1.5, TST: 43.3558). However, each layer covers a smaller area than the composition of all the layers. In the case of TST, the APL is higher than in the composition of all the layers. This means that multimodality can be helpful in avoiding many stops.
4.5 Which stations connect travelers from different parts of the city?
In the case of transportation networks, it is interesting to measure the betweenness centrality to understand which nodes connect different communities of stations, i.e., sets of interconnected stations within a region. In the multilayer network, we identify some stations that have a very high centrality (See Fig. 7 ). These are mainly from TST. This may be a sign that TST is a kind of bridging layer in some zones. Some examples of such bridging stations are Lisboa Gare do Oriente (0,2529), Setúbal Ciprestes (0,1639), Lisboa Alcântra (0,1592) at TST. METRO also has three stations with exceptionally high betweenness centrality, including Campo Grande (0,1813), Oriente (0,1504), and Cidade Unversitária (0,1480). Rod Lisboa also has a station with a very high betweenness centrality, Lisboa Campo Grande (0,1879). With this simple analysis, we can see that both Lisboa Campo Grande and Lisboa Oriente are multimodality hubs that bridge across different layers.
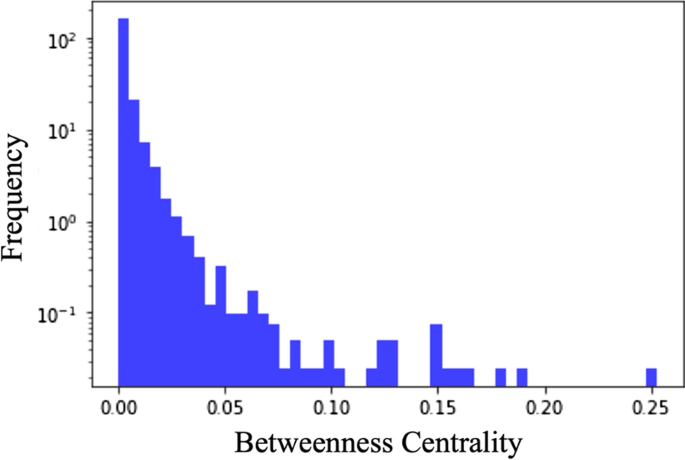
Betweenness centrality distribution: In the graph, we can clearly see a few nodes with very high centrality. These are the nodes that represent the stations mentioned above
The left skew on the betweenness centrality may indicate the same type of distribution on node criticality since the betweenness centrality on the node measures the number of shortest paths that include that node. So these results are similar to the ones found in the literature [ 12 ].
4.6 Are the central stations directly connected with one another?
To understand the role of degree-degree correlations, we look at degree assortativity to understand if central stations are connected to one another. This measures the similarity of connected nodes concerning their degree. [ 5 ] noted that in multilayer networks, degree-degree correlations should be measured system-wide. In that same study, they generalize this concept for these types of networks and apply it to an airport transportation multilayer network. There they also note that the rich-club effect is, in fact, present in such networks, masked due to the high number of peripheral nodes that connect the hubs. However, intralayer, the networks tend to be disassortative as they focus on one specific region.
We observed a similar behavior from Arruda et al. regarding the assortativity, even with the differences, in reality, being represented (air travel versus urban transportation). We calculated the assortativity for the multilayer network and for each layer, observing the same results. In Fig. 8 , we see the same pattern described by [ 5 ]. We can observe a much higher assortativity in the multilayer network than in any of the single layers. This is reasonably simple to understand since the assortativity is influenced by the high number of multimodal hubs that connect to one another. Analogous to the properties found in past case studies, we may find a kind of a rich-club effect that may not be there because of the many peripheral nodes. This means that between the central stations within the layer, there are less central stations connected to more central stations. Since this is not the focus of this work, it will be left for future studies.
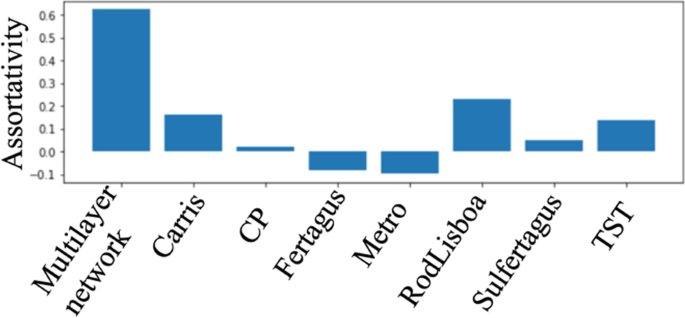
Assortativity distribution of each layer and the multilayer network
4.7 Impact of different extraction strategies on network connectedness
In this section, we attempt to answer this question by analyzing the behavior of the size of the largest strongly connected component—SCC—over time for the duration of the simulation. Observing Table 3 , the most resilient layer is FERTAGUS, and the least resilient layer is RODLISBOA.
Table 3 shows that the RD and RB strategies usually yield the best results across all layers. In other words, this is more often than not the best strategy to measure robustness. Nevertheless, the RB strategy tends to have a faster descent among the different layers, as we can see in Fig. 9 . Hence, the failure of intermodal hubs that have a high degree has devastating effects on the multimodal topology. Moreover, the IB and Random strategies seem to have the least impact on the size of the largest SCC. This is expected, and it is a good baseline to understand that the targeting techniques work to measure robustness. There seems to be no strong reason why the ID strategy has a better result than the RD. However, this is the case in SULFERTAGUS, and it should be investigated further. However, the degree is clearly not the targeting metric that yields the most effective stress strategy, so this may be due to the random removal of stations with the same degree and lower betweenness. We postulate that this happens because there may be reasonably large components that have nodes with a high degree; however, removing nodes from these components does not affect the size of the largest component. So, this phenomenon probably has more to do with the metric we are using than the strategy itself. So this means that single-mode networks are more robust targeting in high degree stations. In Fig. 9 , we observe that the RD strategy yields a faster decrease. This checks out with the Normalised AUC with the lowest value. In the third graph, we observe that we still need many more edge removals to get the same result of the size of the largest SCC as in node strategies.
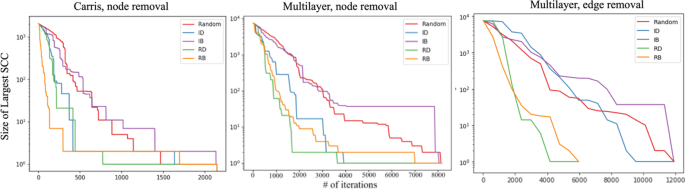
Evolution of SCC size along node extraction strategies for a single layer, CARRIS (left), all layers (middle), and edge removal in multilayer network (right)
4.8 Impact of node and edge targeting on average path length
To understand the evolution of path length when targeting stations and pathways, we calculated the APL only for existing paths along with the network. So, if there was no path using the transportation system between two points, this was not accounted for. It is important to note that this strategy may not be the best to measure the robustness of on-road transportation since alternative paths may be available on roads that are not on the usual route. So, we expect the APL to reduce along each time step quickly. We ran the result for each layer and the whole network as well.
Regarding the results for station and pathway targeting of the CARRIS network in Figure 11 , the RD is the best strategy. This means that removing the stops with the highest degree has the highest impact on the length of stations one can reach. Note that it may change with alternative routes and redundancy techniques. The remaining strategies have about the same efficiency, with RB being slightly ahead towards the end of the simulation. This result is not unanimous, as we can see in Fig. 10 . As we see, RB can either be the best or the worst strategy, depending on the network. This can be due to nodes with high betweenness centrality being part of paths that do not have alternatives. RB providing an efficient strategy indicates lower network robustness. This is the case because it is a topological fragility to have most of the shortest paths within a network going through a single station. In the multimodal network with all the layers (Fig. 11 ), we see that RB is a strategy that promotes a fast decrease in the APL. This indicates lower network robustness. In the multimodal network, we see that many shortest paths go through the intermodal hubs, allowing parts of the city that have a single mode to be connected with the rest of the city. We can see that the APL decreases much faster in the RB than in the RD for the multilayer, unlike in the CARRIS network. We can also see a slight increase at the beginning of the simulation (only for other extraction strategies). This is due to the removal of less critical stations. The number of removals needed to get the same APL is much higher for edge strategies, meaning node removal is more effective for decreasing APL.
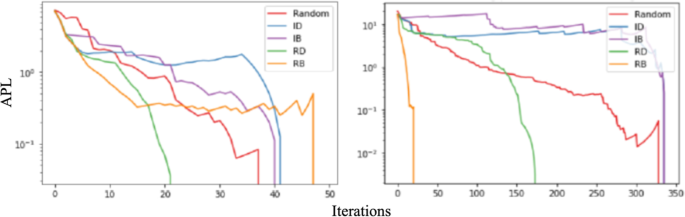
Evolution of APL along node extraction strategies for METRO (left) and SULFERTAGUS (right)
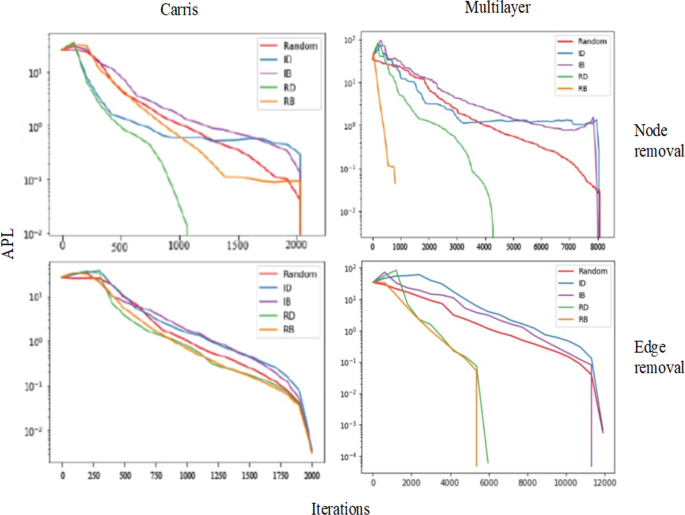
Evolution of APL along node (above) and edge (bellow) extraction strategies for the CARRIS and the Multilayer network
4.9 How many nodes or edges do we have to delete to fragment the network into isolated components?
An isolated component is when a node loses all its edges. Since our graph is directed, when we talk about all the edges, we are mentioning both the in and out edges. To be able to answer this question, we used the extraction strategies proposed in the methods and evaluated the evolution of the network by showing the distribution of the isolated components. To accurately understand the extraction strategies, we ran them across every layer in isolation and then in the complete network, except for multimodal hub removal on isolated layers because there are no multimodal hubs in isolated layers. Figure 12 shows the evolution of isolated components for each strategy in the CARRIS layer. In these graphs, we clearly see that RD had the best results. This is the only one that stopped before the end of the simulation because there are only isolated components when it stops. We obtained about the same results in every layer and for multilayer network is also very similar.
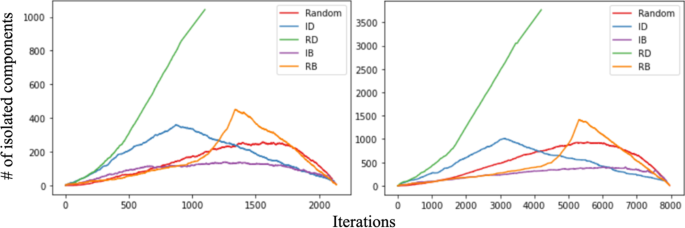
Evolution of the Isolated components (IC) in the CARRIS layer (left) and multimodality network (right) for node removal
We also find that all extraction strategies, except RD, increase and then decrease the number of isolated components (Fig. 12 ). This means that after we reach the maximum number of ICs, we are only removing isolated nodes. This means that the lower the maximum and the later it is reached, the less effective at measuring the robustness of the isolation of different parts of the city the strategy is. Halfway through RB, we start to see rapid growth. This is because after removing the main bridges from communities, there still are redundancy paths. Once these paths start to be removed, it is simpler to disconnect each community individually. It should be noted that it is likely that a node with a high degree also has a high betweenness centrality since multimodal hubs are often points that connect communities. However, this is not the case since less-connected stations may be essential in connecting different parts of the city. The IB extraction is the worst because we are dividing the problem infinitely. This is virtually dividing the path from A to B into two recursively. In summation, the only effective strategy to measure the vulnerability of disconnecting the network from different parts of the city is RD.
So, to answer the highlighted question in this section, we look at the number of iterations when RD ends the simulation. This is the number of nodes that have been removed when all the remaining nodes are isolated. The higher the percentage of nodes that need to be removed from the total of nodes in this network, the more robust the network is, see Fig. 13 . We can see that the robustness across all networks using this metric is about the same.
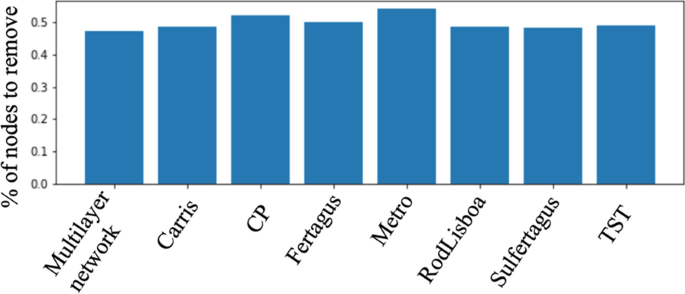
Minimum percentage of nodes that need to be removed to separate the network into only isolated components, using targeted extractions. The lower this value, the less resilient this network is
To understand the multimodal hub removal, we recurred to another graph due to scale and purpose. As the graph reaches a pique and then decreases, we can see that we do not need to remove all the multimodal nodes to get isolated layers. As we can see, there is a slight variation when some nodes are removed. This means that removing specific nodes has more impact on the interlayer connections (Fig. 14 ). We can see that node removal is much better than edge removal, which makes sense since in edges, we only separate in at most 8 ICs (single layers). This is not directly comparable with other strategies, as it only encompasses the removal of about 10% of the nodes. However, from what we can assess, in the beginning, the growth in the number of isolated components follows approximately a linear function, which is slower than RD. However, we can safely conclude that the redundancy of intermodal hubs is the best way to ensure connectedness in this multimodal network since not all layers are redundant in all areas of the city. Redundancy in all areas of the city would be much more costly and may even cause other issues like consuming space of the already established routes.
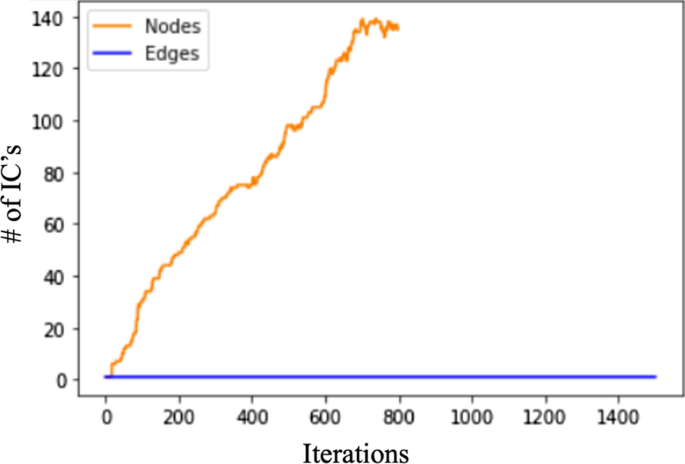
Evolution of the Isolated components using the Multimodal Hubs removal strategy
On the other hand, there are edge extractions. In this case, it is expected that we have to remove all the edges to have all nodes isolated; Fig. 15 shows precisely that. The behavior of extraction per layer and in the multilayer network is identical, as can be seen in both graphs in Fig. 15 . Contrary to node targeting, the worst strategy is RD, and the best strategy is IB. The RD is always the worst strategy. This is the case because we are removing edges from nodes with high degrees. We can conclude that the betweenness centrality is an adequate metric for edge targeting and degree is better for node targeting. This means that removing single pathways that are in many shortest paths has a higher impact than removing single pathways that lead to high degree stations. However, deactivation or failure of high degree stations has the highest impact. High degree stations are the ones that are usually more central geographically and have a more critical role in connecting different modes of transport.
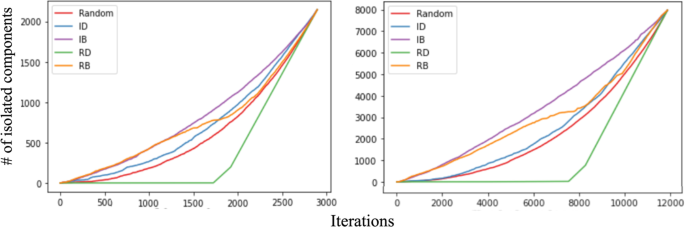
Evolution of Isolated components along edge extraction strategies for CARRIS (left) and for the multilayer network (right)
4.9.1 Impact of removing nodes versus edges
We found that node extractions are more efficient than extracting the edges, as we need more removals to get the same increase of ICs and decrease of APL and decrease of SCC. This means that removing the high degree stations has a much higher impact than removing every single pathway that leads to each important station individually. As previous Figures indicate, it takes much more iterations to disconnect a network by extracting the edges. This happens for various reasons. Expectedly, there are more edges than nodes, so more iterations are needed to create the same impact. Another important reason is that when we remove a node, we also remove all the edges of that specific node, and the contrary is not the case. This happens because an incident in a pathway to a station may not impact the station, and an incident on a station may more probably impact the pathways through it. This makes the node removal more damaging to the network.
On the SCC and the APL evaluation, we also see that edges’ extractions are very similar to each other and also less efficient at disconnecting the network than nodes removal. We can conclude that the network has various redundant paths. Hence, by removing edges, we can go to the same destination by going through more stations.
It is also important to note that in the IC evaluation, the ID and RD removal extractions on the edges are not as efficient as in the nodes and have a slow impact. With a slow impact, we mean that we need to remove more edges to create the same damage as the node removal. This happens because, when we base the extraction on the edge degree, we are extracting edges of hub nodes (nodes with high degrees). This means that we are removing edges from nodes with lots of edges and so it does not show the effect at first.
4.9.2 Should we recalculate the degree and betweenness after each extraction?
Often, the random strategy is better than the strategies which do not recalculate the new metrics of the new graph (IB and ID). On the other hand, RB and RD removal strategies can be very effective (Table 3 ) yet not so computationally efficient. They take much more computational power to compute metrics, especially the RB. As can be seen in previous sections, recalculating degree after each node removal has proven to be beneficial, but recalculating betweenness centrality has yielded relatively stable results for ICs and wildly variable results in APL evolution. Intuitively this could probably be explained by the lack of redundant paths in some networks, which is a fair way to assess its robustness. Both RB and RD strategies have the same similar results across layers and are the best ones to reduce the SSC.
For the edge removing strategies, in the case of the goal being generating more isolated components, recalculating the betweenness centrality yields the worst results. These results are curious as they are quite different from the ones proposed by [ 22 ]:1), “ suggesting that the network structure changes as important vertices or edges are removed .”
Despite the RD and RB strategy overall having better results for node extractions, in massive graphs seems unfeasible to calculate the betweenness centrality per each iteration. Another possible strategy is, instead of calculating per each iteration, i.e., each node removal, to calculate this metric per each percentage of removals. In this way, we are avoiding the complexity with the trade-off of slightly disrupting the result. This is a suboptimal solution; however, it is shown to be viable to the extent of the empirical results.
4.9.3 What is the impact of cascading failures?
The cascading failures are a complex topic, and we have only scratched the surface. On cascading failures, we used two removal strategies: entirely deleting a transportation line (e.g., route) and removing the neighbors of a given node (e.g., station). For the first removal, we simulated the crash of each line separately, that is, one line at a time, and measured the number of ICs, then repeated the process for each line after resetting the network to its initial condition. We were able to get the maximum number of 6 separated components from a single line by doing this assessment. This line/route connects Pontinha and Campo Grande, operated by RodLisboa (bus road operator). It is mainly a commuting route that connects passengers living in Pontinha (county within LMA) with Lisbon city, mainly for home-to-work purposes.
In our second simulation, we applied the failure removal approach of a neighbor. Line Failure was used as a model for the simulation. We estimated the neighbors of each node, eliminated them, and gathered the metric of the isolated component. We chose three layers of a node’s neighbors since the average number was closer to the average number of each line when comparing the two. Odivelas was discovered to be the key point in this method, with a maximum number of isolated components of 10.
We can see that the nodes that connect Lisbon city with other counties within LMA are critical to the organization of the city’s public transportation system. Nonetheless, this Neighbour Failure technique has much more devastating effects than the Line Failure strategy, with nearly double the network damage (6 vs. 10), but this is based on a small sample. These results tell us that the impact of a localized failure that deactivates that spot in several layers has a higher impact than a line failure in this topology. However, this result may vary depending on the region since localized failures in remote areas have a lower relative impact.
5 Conclusions and future works
In this work, we propose a measurement method of the topological robustness of a multimodal network by using different network analysis metrics and by performing node and edge percolation with specific criteria. Then we proceed to apply these measurements to the Lisbon Metropolitan Area Multimodal network, to further understand applicability. We modeled and analyzed the structure of Lisbon’s public transport network to measure its robustness according to a multimodal stance. Results allow us to understand the geographic information inherent to the multiplex network by programming a 3D plotting method. After calculating and interpreting some of the network metrics, results indicate that multimodal coordination can help diminish the number of stops in a pathway. After identifying stations that work as bridges between different layers and communities, we then analyzed different extraction strategies based on previous work. This is an important step to guide the assessment of classic robustness metrics in the context of multimodal transport networks. The target metrics include the size of the largest SCC (strongly connected components), APL (average path length), and ICs (isolated components), as well as a proposed normalized AUC (area under the curve). Hence, we observed that the selection of metrics should be constrained to the goal and type of removal (edge vs. node).
Results show that the strategies that depend on recalculating metrics are generally more effective, with the exception of a particular case of edge removal using betweenness centrality to maximize ICs, which counter-intuitively had better results for the IB (initial betweenness) strategy. Even though we were able to postulate on this phenomenon, further research needs to be done to understand it. We also showed that the resilience tests needed to remove about half the nodes of the network to leave all the remaining nodes wholly disconnected. This is a phenomenon that happens in all layers and the multilayer network as well, suggesting that betweenness targeting is the best way to measure robustness across the different strategies. We also verified higher assortativity phenomena in multilayered networks, in contrast to single layers, highlighting the importance of intermodal hub redundancy.
Based on the robustness tests, we were able to conclude that the most effective method for targeting nodes is RD (recalculate degree). However, in some cases, RB (recalculate betweenness) yielded better results for multilayer APL decadence (both for nodes and edges strategies), although it showed higher variability. This means that the number of pathways to a station is less important than how many shortest paths go through that station in a multimodal scenario when completely disconnecting the network. For decreasing the size of the largest SCC, RD yielded better results for the multilayer network. However, for most of its individual layers, the best strategy was actually RB. This means that to divide a multimodal network into disconnected regions, high degree station failures have a higher impact than high betweenness station failures. However, to yield the same result in a single-mode network, betweenness is a more relevant metric, highlighting the impact of the network topology as the vulnerabilities linked to a multimodal network considerably differ from a single-layer network.
With the normalized AUC, we were able to compare, side by side, the robustness of each transport operator-specific network, regardless of its size. Carris, RodLisboa, SulFertagus, and TST show inferior robustness to the remaining networks. The two most robust networks were Fertagus and Transtejo. Nevertheless, this result may be due to inflation on smaller sizes, pinpointing the need for non-uniform scaling factors for the AUC normalization. The multilayer network was more resilient than one-half of the layers but less than the other. We also performed cascading failures in the network to understand some of the expected impacts. Still, since it is a vast and exciting topic, we focused on two major strategies. We showed that neighbor failure is more effective than line failure in this particular network, even though they are moderately similar in the structure of the present network. The impact yield from different cascading events can be the subject of a future study. For example, instead of simulating random line crashes, using simulated failures on more than one line at a time and using a specific measure would be more effective in determining which lines are more critical, like what we have done with the degree and betweenness centrality targeting. The second elimination could be investigated further by experimenting with more layers of neighbors. The archetype of these might be a combination of these two strategies, attempting to fail lines that are close to other lines using information from each node’s neighbors. We also identified that further research is needed on the effect of the rich club on peripheral nodes on robustness and cascading effects in the context of this network. Last but not least, we recognize the importance of the analysis of the capacity, ridership and bottlenecks. Nevetheless, topological views also yield interesting results. More specifically, the gathered results in this study suggest that robustness can be objectively measured using network metrics and percolation simulations. The impact of such simulations can be compared regardless of the network size or structure in any multimodal transportation scenario. Moreover, research findings point out that we can use the targeting techniques to understand network recoverability (resilience stance) by focusing on stations with hub characteristics (higher centrality) or high betweenness. This study allows practitioners and urban transportation policymakers to tackle the impact of negative disruption in multimodal transportation networks.
Availability of data and materials
The data are available for collaborations in the context of the ILU project under NDA agreements with CML, CARRIS and METRO.
Al-Deek, H., & Emam, E. B. (2006). New methodology for estimating reliability in transportation networks with degraded link capacities. Journal of Intelligent Transportation Systems, 10 (3), 117–129.
Article Google Scholar
Aleta, A., Meloni, S., & Moreno, Y. (2017). A multilayer perspective for the analysis of urban transportation systems. Scientific Reports, 7 , 44359.
Aparicio, J. T., Arsenio, E., & Henriques, R. (2021). Understanding the Impacts of the COVID-19 pandemic on public transportation travel patterns in the city of Lisbon. Sustainability, 13 (15), 8342.
Aparicio, J. T., Trinca, M., Castro, D., Henriques, R. (2021b). “Vehicle Smart Grid Allocation Using Multi-Agent Systems Sensitive to Irrational Behavior and Unstable Power Supply.” Pp. 1–6 in ( 2021b) 16th Iberian Conference on Information Systems and Technologies (CISTI) .
de Arruda, G., Ferraz, E. C., Moreno, Y., & Rodrigues, F. A. (2016). On degree-degree correlations in multilayer networks. Physica D: Nonlinear Phenomena, 323 , 5–11.
Article MathSciNet Google Scholar
Bernardo, M. R., Neto, M. D. C., de Lisboa, N., Aparicio, M., de Lisboa. (2019). “Smart Mobility: A Multimodal Services Study in the Metropolitan Area of Lisbon.” Smart Mobility 20.
Bocewicz, G. (2014). “Robustness of multimodal transportation networks.” Eksploatacja i Niezawodność 16.
Bogart, D. (2009). Inter-modal network externalities and transport development: evidence from roads, canals, and ports during the english industrial revolution. Networks and Spatial Economics, 9 (3), 309–338.
Brandes, U. (2001). A faster algorithm for betweenness centrality. Journal of Mathematical Sociology, 25 (2), 163–177.
Campbell, A. M., & Van Woensel, T. (2019). Special issue on recent advances in urban transport and logistics through optimization and analytics. Transportation Science, 53 (1), 1–5. https://doi.org/10.1287/trsc.2018.0882
Cats, O., & Jenelius, E. (2018). Beyond a complete failure: The impact of partial capacity degradation on public transport network vulnerability. Transportmetrica B: Transport Dynamics, 6 (2), 77–96.
Google Scholar
Cats, O., Koppenol, G.-J., & Warnier, M. (2017). Robustness assessment of link capacity reduction for complex networks: Application for public transport systems. Reliability Engineering & System Safety, 167 , 544–553.
Chen, A., Yang, H., Lo, H. K., & Tang, W. H. (2002). Capacity reliability of a road network: An assessment methodology and numerical results. Transportation Research Part B: Methodological, 36 (3), 225–252.
Clark-Ginsberg, A. (2016). “What’s the Difference between Reliability and Resilience.” Department of Homeland Security. March .
Clifton, K., & Muhs, C. D. (2012). Capturing and representing multimodal trips in travel surveys: review of the practice. Transportation Research Record, 2285 (1), 74–83.
Derudder, B., Liu, X., Kunaka, C., & Roberts, M. (2014). The connectivity of south asian cities in infrastructure networks. Journal of Maps, 10 (1), 47–52.
Ding, R., Ujang N., bin Hamid, H., Abd Manan, M. S, He, Y, Li, R., Wu, J. (2018). “Detecting the urban traffic network structure dynamics through the growth and analysis of multi-layer networks.” Physica A: Statistical Mechanics and Its Applications 503:800–817
Du, W.-B., Zhou, X.-L., Lordan, O., Wang, Z., Zhao, C., & Zhu, Y.-B. (2016). Analysis of the chinese airline network as multi-layer networks. Transportation Research Part E: Logistics and Transportation Review, 89 , 108–116.
Elvas, L. B., Mataloto, B. M., Martins, A. L., & Ferreira, J. C. (2021). Disaster management in smart cities. Smart Cities, 4 (2), 819–839.
Fernandes, N., Moro, S., Costa, C. J., & Aparício, M. (2020). Factors influencing charter flight departure delay. Research in Transportation Business & Management, 34 , 100413. https://doi.org/10.1016/j.rtbm.2019.100413
Heinen, E., & Mattioli, G. (2019). Multimodality and CO2 emissions: A relationship moderated by distance. Transportation Research Part D: Transport and Environment, 75 , 179–196.
Holme, P., Kim, B. J., Yoon, C. N., & Han, S. K. (2002). Attack vulnerability of complex networks. Physical Review E, 65 (5), 056109.
Immers, B., Yperman, I., Stada J., Bleukx, A. (2002). “Reliability and Robustness of Transportation Networks.” Problem Survey and Examples .
INE, I. P. (2018). Mobilidade e Funcionalidade Do Território Nas Áreas Metropolitanas Do Porto e de Lisboa 2017 . Instituto Nacional de Estatística, I. P. Av.António José de Almeida,1000–043 Lisboa, Portugal: Instituto Nacional de Estatística, I. P.
Klau, G. W., & Weiskircher, R. (2005). Robustness and resilience. In U. Brandes & T. Erlebach (Eds.), Network analysis (pp. 417–437). Berlin: Springer. https://doi.org/10.1007/978-3-540-31955-9_15
Chapter Google Scholar
Kouvelis, P., Gang, Y. (2013). Robust Discrete Optimization and Its Applications . Vol. 14. Springer Science & Business Media.
Liu, R., Chen, Y., Jianping, Wu., Tingbao, Xu., Gao, L., & Zhao, X. (2018). Mapping spatial accessibility of public transportation network in an urban area–a case study of shanghai Hongqiao transportation hub. Transportation Research Part D: Transport and Environment, 59 , 478–495.
Lu, H., Wang, J., & Zhang, P. (2004). Urban transport structure optimization based on energy consumption. Journal-Tsinghua University, 44 (3), 383–386.
Masel, J., & Siegal, M. L. (2009). Robustness: Mechanisms and consequences. Trends in Genetics, 25 (9), 395–403.
Migliore, M., & Catalano, M. (2007). “Urban public transport optimization by bus ways: a neural network-based methodology”. Urban transport xiii–urban transport and the environment in the 21st century. Book Series: WIT Transactions on the Built Environment, 96 , 347–356.
Montes-Orozco, E., Mora-Gutiérrez, R. A., Obregón-Quintana, B., de-los-Cobos-Silva, SG., Rincón-García, EA., Lara-Velázquez, P., Gutiérrez-Andrade, MA. (2020). “Inverse Percolation to Quantify Robustness in Multiplex Networks.” Complexity 2020.
Ramos, C., Zêzere, J. L., & Reis, E. (2010). “Avaliação Da Susceptibilidade Aos Perigos Naturais Da Região de Lisboa e Vale Do Tejo”. Prospectiva e Planeamento . Ordenamento Territorial e Sustentabilidade, 17 , 57–73.
Scott, D. M., Novak, D. C., Aultman-Hall, L., & Guo, F. (2006). Network robustness index: A new method for identifying critical links and evaluating the performance of transportation networks. Journal of Transport Geography, 14 (3), 215–227.
Stamos, I., Mitsakis, E., Salanova, J. M., & Aifadopoulou, G. (2015). Impact assessment of extreme weather events on transport networks: A Data-driven approach. Transportation Research Part D: Transport and Environment, 34 , 168–178.
Sullivan, J. L., Novak, D. C., Aultman-Hall, L., & Scott, D. M. (2010). Identifying critical road segments and measuring system-wide robustness in transportation networks with isolating links: A link-based capacity-reduction approach. Transportation Research Part A: Policy and Practice, 44 (5), 323–336.
Tavasszy, L., Minderhoud, M., Perrin, J.-F., & Notteboom, T. (2011). A Strategic network choice model for global container flows: Specification, estimation and application. Journal of Transport Geography, 19 (6), 1163–1172.
UN. (2018). “World Urbanization Prospects.”
Varga, I. (2016). Weighted multiplex network of air transportation. The European Physical Journal B, 89 (6), 139.
Zhang, H., Cui, H., Wang, W., & Song, W. (2020). Properties of Chinese railway network: Multilayer structures based on timetable data. Physica A: Statistical Mechanics and Its Applications, 560 , 125184.
Zhang, X., Miller-Hooks, E., & Denny, K. (2015). Assessing the role of network topology in transportation network resilience. Journal of Transport Geography, 46 , 35–45.
Zhou, Di., Eugene Stanley, H., D’Agostino, G., & Scala, A. (2012). Assortativity decreases the robustness of interdependent networks. Physical Review E, 86 (6), 066103.
Zhou, Y., & Wang, J. (2017). Critical link analysis for urban transportation systems. IEEE Transactions on Intelligent Transportation Systems, 19 (2), 402–415.
Download references
Acknowledgements
We thank Daniel Castro and Miguel Trinca for their contributions regarding the code and insightful discussions along with Professor Francisco C. Santos for the proposed challenge. We also thank CARRIS, METRO and Câmara Municipal de Lisboa (Gabinete de Mobilidade and Centro de Operações Integrado) for the provision of data, support, and valuable feedback. This work was further supported by national funds through Fundação para a Ciência e Tecnologia (FCT) under project ILU (DSAIPA/DS/0111/2018) and INESC-ID pluriannual (UIDB/50021/2020).
This work is further supported by national funds through Fundação para a Ciência e Tecnologia under project ILU—Integrative Learning from Urban Data and Situational Context for City Mobility Optimization (DSAIPA/DS/0111/2018) and INESC-ID pluriannual (UIDB/50021/2020).
Author information
Authors and affiliations.
LNEC I.P. and INESC-ID, Instituto, Superior Técnico, University of Lisbon, Lisbon, Portugal
Joao Tiago Aparicio
LNEC I.P. Department of Transport, Lisbon, Portugal
Elisabete Arsenio
INESC-ID, Instituto Superior Técnico, University of Lisbon, Lisbon, Portugal
Rui Henriques
You can also search for this author in PubMed Google Scholar
Contributions
All authors (JTA, EA, RH) contributed to the research study. JTA conducted the simulation and data analysis, and prepared the first version of the manuscript. EA and RH carried out the research supervision and validation of the use case. RH was responsible for the project administration. All authors (JTA, EA, RH) approved the final manuscript.
Corresponding author
Correspondence to Elisabete Arsenio .
Ethics declarations
Competing interests.
The authors declare that they have no competing interest.
Additional information
Publisher's note.
Springer Nature remains neutral with regard to jurisdictional claims in published maps and institutional affiliations.
Rights and permissions
Open Access This article is licensed under a Creative Commons Attribution 4.0 International License, which permits use, sharing, adaptation, distribution and reproduction in any medium or format, as long as you give appropriate credit to the original author(s) and the source, provide a link to the Creative Commons licence, and indicate if changes were made. The images or other third party material in this article are included in the article's Creative Commons licence, unless indicated otherwise in a credit line to the material. If material is not included in the article's Creative Commons licence and your intended use is not permitted by statutory regulation or exceeds the permitted use, you will need to obtain permission directly from the copyright holder. To view a copy of this licence, visit http://creativecommons.org/licenses/by/4.0/ .
Reprints and permissions
About this article
Cite this article.
Aparicio, J.T., Arsenio, E. & Henriques, R. Assessing robustness in multimodal transportation systems: a case study in Lisbon. Eur. Transp. Res. Rev. 14 , 28 (2022). https://doi.org/10.1186/s12544-022-00552-3
Download citation
Received : 22 February 2022
Accepted : 14 June 2022
Published : 12 July 2022
DOI : https://doi.org/10.1186/s12544-022-00552-3
Share this article
Anyone you share the following link with will be able to read this content:
Sorry, a shareable link is not currently available for this article.
Provided by the Springer Nature SharedIt content-sharing initiative
- Multimodality
- Transport resilience
- Multiplex networks
- Robustness measurement
- City by city
- Current issues
- General election 2024
- Metro mayors
- Levelling up
- Productivity

Transport Case Studies
How different cities are improving their transport connections using our case study library
Case study library
Why improve transport?
Effective transport is vital for the economy. Good transport connections have direct benefits to people, businesses, the environment, and the economy overall. They can support innovation, help people access jobs, shape greener and healthier places, and attract new firms.
Having more control over transport can help cities make the most of their infrastructure by reducing uncertainty and short timescale of funding, improving the bus system, and ensuring integration of transport, economic development and infrastructure.
Use the table below to find out more about how and what cities in the UK and abroad have done to improve transport. These case studies highlight what the cities did and their self-reported outcomes.
To find out more about what types of transport what types of policy interventions have an impact on local economic growth from across OECD countries, as well as evidence-based policy design guides, take a look at the What Works Centre pages on Transport.
Case studies
click the case study title to find out more
More Case Studies on Housing and Transport
Delivering change: making transport work for cities.
Zach Wilcox and Nada Nohrová
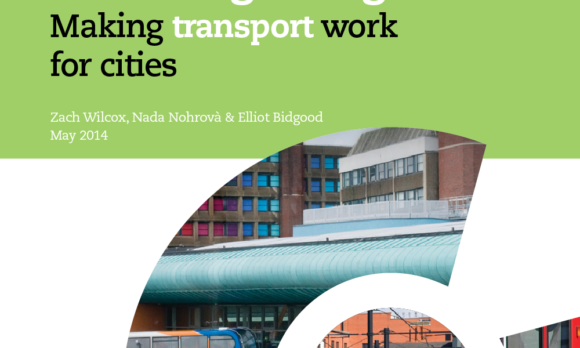
Delivering change: Putting city centres at the heart of the local economy
Louise McGough and Elli Thomas

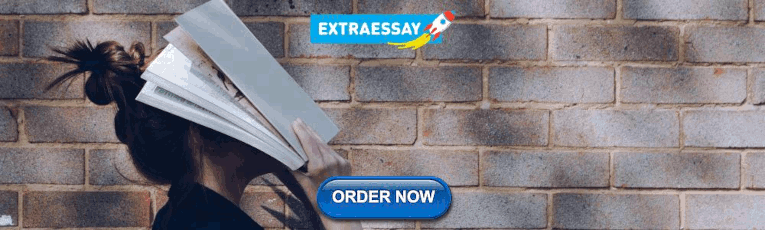
Open Data or Closed Doors?
Maire Williams

Funding and financing inclusive growth in cities
Naomi Clayton, Simon Jeffrey and Anthony Breach

How can UK cities clean up the air we breathe?
Adeline Bailly

Policy Officer
James Evans
Series: City Talks
Paul Swinney
Austin Kilroy
Combined authorities
Agent-based decision-support model for bus route redesign in networks of small cities and towns: case study of Agder, Norway
- Case Study and Application
- Open access
- Published: 03 June 2024
Cite this article
You have full access to this open access article
- Sinziana I. Rasca ORCID: orcid.org/0000-0002-2925-5176 1 na1 ,
- Bin Hu 2 na1 ,
- Benjamin Biesinger 2 &
- Matthias Prandtstetter 2
Small cities and towns often struggle to provide high-quality public transport services to daily commuters. This is reflected in the modal split, where the share of car users dominates. Such a problem requires a modern solution, where transport planners can verify the impact of potential transport network improvements on the travel behavior of the residents before the changes are actually deployed. This study aims to demonstrate the usefulness of employing an agent-based simulation tool in the decision process for redesigning an express service regional bus route connecting a network of small cities and towns. The model was initially developed as a Mobility as a Service simulation solution for suburban areas of European metropolises. The model is adapted and applied to a case study for the region of Agder, Norway, simulating the impact of nine different scenarios on the patronage of a specific bus route. The simulation model proposes to upgrade the classic agent structure to a persona profile designed specifically for the case study. The main objective of this research is to identify the scenario that maximizes patronage while minimizing total route travel time and additional costs. The results suggest that the proposed model can be successfully adapted from suburban metropolitan areas to the realities of the considered case study, and potentially other similar regions. Specifically, out of the nine proposed scenarios, the model identified four promising ones. One of the four scenarios also fits the cost constraints imposed by the transport provider. The model provides a solid approach for analyzing complex transport systems that are practically impossible to consider in detail if the analysis is done without computer support. Thus, the results can be used as a decision support system for public transport planning and operations in networks of small cities and towns.
Avoid common mistakes on your manuscript.
1 Introduction
The increase in accessibility to motorized transport for the wider public has shifted the commuting patterns of humans, increasing their daily travel ranges. Regional public transportation (PT) systems represent a crucial element in ensuring access to a more diverse job market for the population living outside walking or biking distance from their jobs, and also in reducing the environmental footprint of daily commuting at a regional level. Nevertheless, if the PT offer cannot compete with the advantages of car commuting, the cost of running the PT system becomes economically unviable, which increases car dependency. As a result, there is a clear need to maximize the return on investment for PT by attracting more paying customers towards this sustainable transport mode.
Transport network planning has become increasingly complex in recent decades. Consequently, PT providers are exploring new avenues for verifying the feasibility of proposed solutions for routing and optimization problems. In recent years, transport planners have used computer-based simulation models to evaluate route option alternatives and the impact of schedule changes on ridership and occupancy with increasing success. Several studies have addressed such approaches (Manser et al. 2020 ; Narayan et al. 2017 ; Cats et al. 2014 ). Nevertheless, there have been few such applications employed by PT providers working in geographical areas where small cities and towns represent the predominating urban forms. This is generally due to the lack of resources, both human and financial, that PT providers in such areas are confronted with, but also because such research generally focuses on large cities and metropolises such as Melbourne, Australia (Liyanage and Dia 2020 ) and Zurich, Switzerland (Manser et al. 2020 ).
The use of agent-based simulation programs, such as PTV’s Visum (PTVGroup 2021 ) or MATSim (MATSim 2021 ), is even more of a rarity in small-size public transport authorities (PTAs), due to the extra layer of travel data and effort necessary for defining the activity of the agents. Therefore, most of the PT planning work is still carried out in a classic way, without employing simulation models. The increased volume and complexity of the available data, combined with the necessity to better respond to customers’ needs, topped by a growing competition with private vehicles, is now exceeding the capacity of human analysis. To achieve better results, there is a clear need to employ computer simulations in public transport improvement decision-making processes, even in the case of smaller PTAs.
The present research aims to bridge the gap between using classic PT network design processes and employing an agent-based simulation model for solving PT planning-related problems. This approach is applied to a specific case study, simulating the viability of different scenarios for modifying a current express service (ES) bus route, as defined by Vuchic ( 2007 ), connecting a network of small cities and towns. The study demonstrates the usefulness of employing an agent-based simulation tool in the decision process for redesigning the route. The simulation model also proposes to upgrade the classic agent structure with qualitative data input from a set of persona profiles designed specifically for the case study.
The model was initially developed as a Mobility as a Service (MaaS) simulation solution for suburban areas of European metropolises in the frame of the OptiMaaS project. Footnote 1 MaaS is a relatively new concept, developed in the past decade, and its definition is still under development. According to Arias-Molinares and García-Palomares ( 2020 , p. 254), the core elements of MaaS are: “a unique single platform (app or website), real-time information on all available modes in the city (public and private), multimodal transportation (intermodal journey planners), technological integration to plan, book and pay for mobility needs, and personalized bundled mobility packages according to user’s particular requirements”. The only aspect of MaaS considered in the present case study is the multimodality, highlighted by Tønnesen et al. ( 2021 ) as an under-investigated aspect in Norwegian transport planning from the perspective of access, egress, and transfer. The model is adapted to the region of Agder with its current realities. The main objective of the study is to develop a decision-support system for PT planning, based on the initial OptiMaaS model. The decision-support system would be targeted at public transport providers and planners and would help identify the scenario that maximizes PT patronage while minimizing total route travel time for the users and additional costs for the operators.
The remainder of this paper is organized as follows: Sect. 2 presents the current state of the art with regards to agent-based modeling and simulation for PT and also the use of persona profiles in PT research; Sect. 3 gives an overview of the methodology applied in the present study; Sect. 4 presents the selected case study; Sect. 5 gives an overview of the persona profiles generation and allocation; Sect. 6 describes the simulated scenarios; Sect. 7 introduces the achieved results; Sect. 8 presents the discussion while Sect. 9 provides the gained conclusions based on the results.
2 State of the art
2.1 current approaches for route planning and optimization.
Route and tour planning are fundamental problems in transport optimization and have a long history. Finding the shortest route or path from A to B on a given road network can be solved in polynomial time (Dijkstra 1959 ). Even if the basic shortest path finding algorithm is simple, there have still been advances in recent years. The most relevant developments are in the following topics:
Performance and scalability, such as computing the optimal route in (fractions of) milliseconds on a continental scale (Botea 2011 ; Bast et al. 2006 ).
Multiobjective and non-linear criteria in shortest path algorithms (Berger et al. 2010 ; Aifadopoulou et al. 2007 ).
Dynamic algorithms, that take into account real-time traffic conditions (Schultes and Sanders 2007 ; Delling and Nannicini 2012 ).
Multimodality, i.e., consideration of multiple travel modes for the trip (Aifadopoulou et al. 2007 ; Delling et al. 2013 ).
A more detailed overview of path planning algorithms is given in a survey paper by Bast et al. ( 2016 ). The most relevant variant for this article is multimodality. The simulation model presented in the current research employs an intermodal route planner, where multiple transport modes can be used on the same trip (Prandtstetter et al. 2020 ).
2.2 Agent-based modeling and simulation in the field of transport
A vast gap exists between purely mathematical approaches and solutions that include user behavior aspects in the simulation process for PT planning and optimization (Venturini et al. 2019 ; Schafer 2012 ). One possible explanation for the existence of this gap could be the difficulty of standardizing the generation of user profiles relevant for different regions around the globe, combined with the need for complex data sets for running such simulation models.
The introduction of user behavior into simulation models created the agent-based simulation approach. Classic simulation models overlook the element of randomness, an element that is present in the decision-making process of users regarding their daily travel behavior and activity scheduling (Kaddoura et al. 2015 ). This element is introduced in the agent-based modeling and simulation (ABMS), which is “a relatively new approach to modelling systems composed of autonomous, interacting agents” (Macal and North 2010 , p. 151).
In the case of mobility behavior, the attributes and behaviors of the agents, as well as the relationships and methods of interaction, are generated using quantitative data sets, such as travel surveys or mobile tracking GPS data (Chen et al. 2016 ). So far, no references were found where ABMS agents were designed using a blend of quantitative (e.g., travel surveys) and qualitative data (e.g., interviews).
Two important applications for ABMS in the field of transport research are the prediction of travel demand (Balmer et al. 2008 ) and travel behavior (Leng and Corman 2020 ; Čertickỳ et al. 2015 ). Using ABMS offers PTAs the opportunity to verify the outcome of implementing different scenarios (routing, frequency, capacity, etc.) before the actual deployment of a project. This approach, which makes ABMS a central element of decision support systems, already exists in fields such as disaster relief distribution (Fikar et al. 2016 ; Othman et al. 2017 ) and e-commerce architecture (Šperka and Slaninová 2012 ). Therefore, ABMS can also be employed both as central elements of decision support systems for PT planners and decision makers and also as exploratory tools for researchers and transport developers.
2.3 User profiles in transport planning
The need to forecast travel demand is a core element in the design of any PT system. For that purpose, human travel behavior analysis developed as a research field in its own right. In most cases, the user segmentation approach employed in travel behavior analyses is quantitative (Haustein and Hunecke 2013 ), with different variables identified in quantitative data sets being scrutinized (Chng et al. 2016 ; Ha et al. 2020 ; Ma and Ye 2019 ). Nevertheless, such data lacks an important feature: the inclusion of user attitudes and needs as factors in the travel mode choice decision-making process of the users (Markvica et al. 2017 ; Le Loo et al. 2015 ).
A new type of user behavior analysis and profiling, entitled “personas”, has been introduced in transport research relatively recently (Hörold et al. 2013 ; Oliveira et al. 2017 ), being initially developed for the field of software design (Brickey et al. 2011 ). Personas are a generalization of potential behavior types, which do not claim completeness (Grudin and Pruitt 2002 ) but can provide help in progressing from abstract goals towards more tangible assumptions (Pfeifer et al. 2019 ). Some popular uses of personas in mobility behavior analyses include research on development and acceptance of future mobility concepts (Kong et al. 2018 ) and scenario thinking for urban mobility developments (Vallet et al. 2020 ).
There has been criticism on the lack of solid empirical grounding in relation to the use of personas as core elements of user-centric approaches (Salminen et al. 2020 ; Chapman and Milham 2006 ; Miaskiewicz and Kozar 2011 ). Therefore, methodologies for creating more “reality grounded” personas have been further developed (Salminen et al. 2020 , 2022 ). Gonzalez de Heredia et al. ( 2018 ) proposed three methods for creating holistic and detailed personas: based on new survey data, combining data from multiple existing surveys, and iterating between qualitative data from interviews linked with quantitative data from surveys.
The persona profiles used in the present research were developed using the third method proposed by Gonzalez de Heredia et al. ( 2018 ), a similar data sourcing approach as the one employed by Hörold et al. ( 2013 ). For that purpose, both quantitative (travel surveys) and qualitative (interviews and expert group input) data were collected and analyzed for the development of the persona profiles.
2.4 Present study
The present study proposes a comprehensive approach for simulating transport networks. The approach moves beyond classical mathematical optimization models, introducing specifically designed user profiles as part of an agent-based computer simulation-optimization framework. These similarities and differences are summarized in Table 1 . The results are meant to help PTAs, urban planners and other decision makers verify the potential impact of public transport route and network modifications. They are also meant to facilitate decision making with regard to public transport planning and operation.
Shim et al. ( 2002 , p. 111) presented the three core components of classic decision support systems: “(i) sophisticated database management capabilities [...], (ii) powerful modelling functions [...], and (iii) powerful, yet simple user interface designs that enable interactive queries, reporting, and graphing functions”. Our proposed approach is in line with the first two core components proposed by Shim et al. and is meant to be a stepping stone for the development of a decision support system targeted at PTAs providing transport services in networks of small cities and towns.
The primary contributions of this study are:
Showcasing the adaptation potential of an existing transport simulation model, initially designed for metropolitan urban areas, to a regional setting with the purpose of evaluating potential express service PT solutions.
Bridging the gap between persona use in mobility research and agent-based simulations.
Proposing a novel agent design and data collection procedure based on survey data to facilitate the introduction of user behavior and user needs in the transport simulation model and the modal choice decision-making process.
Applying the adapted model to the specific case of a small cities and towns network.
Highlighting the potential impact of using transport simulation models in supporting decision making for public transport planning.
3 Methodology
The general data flow and processing consists of three parts, presented in the following: Subsect. 3.1 describes the data processing; Subsect. 3.2 the simulation-optimization framework; and Subsect. 3.3 the simulation/evaluation module. Figure 1 illustrates the overall simulation-optimization framework.
3.1 Data processing
Several types of input data are needed, as can be seen in the flowchart presented in Fig. 1 . At the very beginning, the mobility demand generator (see Subsect. 3.2.1 ) pre-processes raw data from travel surveys, public transport usage data, map data, and other meta-data about the considered region. The output from the generator is a fixed demand in the form of a list of trips a number of agents need to perform within a certain time period (for example, from Origin A to Destination B at Time C as part of a normal working day routine). Additionally, information about the user types, in the form of persona profiles (for example, age group, car ownership, children in care), is fed into this model so that it can later be used to simulate the travel behavior.
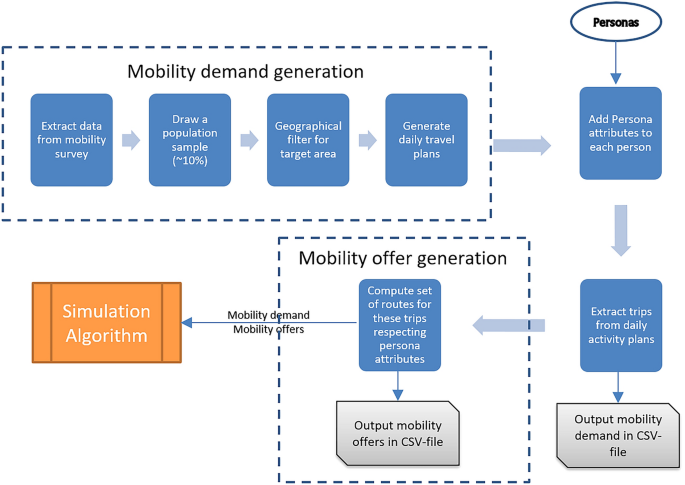
Flowchart for gathering and processing input data for the simulation algorithm
Based on the resulting mobility demand, which combines the needs and preferences of the users with the existing transport infrastructure, initial mobility offers are generated. Mobility offers are represented by the most common transport options for each trip (such as public transport, car, bike, or walking route). They are computed by using an intermodal routing engine (Prandtstetter et al. 2020 ), because the model should allow intermodal routes (for example, when a person uses a bike and changes to a bus for his commuting trip). The routing engine requires data about the underlying map (street network) and the public transport schedules, which are commonly available in General Transit Feed Specification (GTFS) format. Both the mobility demand and the initial mobility offers are computed only once in the data pre-processing step and are fed into the simulation algorithm.
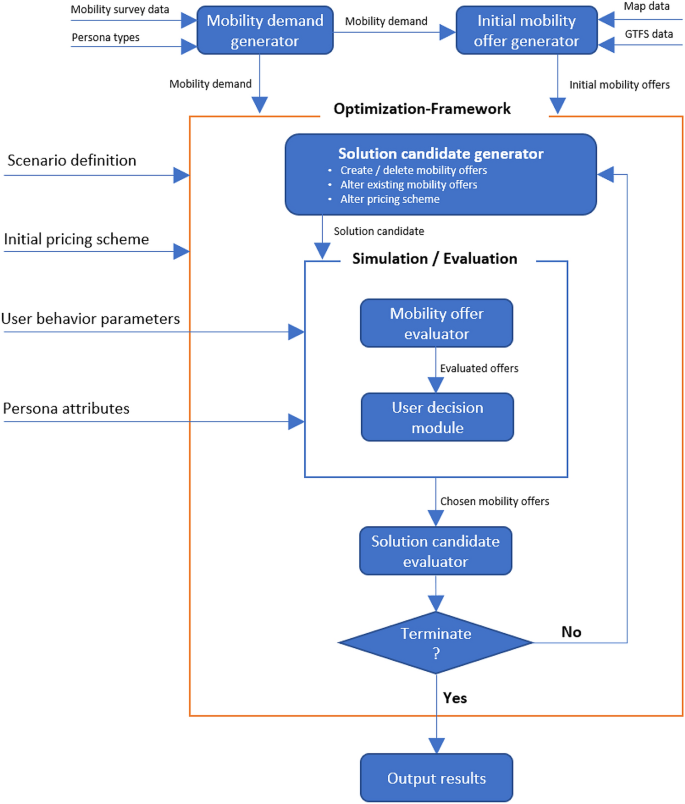
Overview of the proposed simulation-optimization framework architecture and employed data sets
3.2 Simulation-optimization framework
In this framework, a specific scenario is considered, such as an increased frequency for the bus schedule. Based on the scenario specification, the search space of the optimization algorithm is defined. In the bus schedule example, this could consist of adding three extra buses within a certain time period, and the goal is to search for the best updated schedule. Together with the mobility demand, the initial mobility offers, and an initial pricing scheme for these offers, it forms the input data for optimization.
New solution candidates are generated by either adding/deleting mobility offers (e.g., adding a new bus line), altering existing mobility offers (e.g., reducing the walking time to the nearest public transport stop), or altering the pricing scheme of the mobility offers. These changes to the transport system are evaluated by simulating the user behavior in response to the changes. For this, a numerical value, or a so-called utility value (see Sect. 3.3.1) is assigned to each mobility offer based on a choice model, which takes user behavior parameters and persona attribute values into account. To model the user decisions, for each mobility demand the mobility offer is chosen with a likelihood proportional to the utility rate. In the end, all chosen mobility offers are subsequently passed to the evaluation solution evaluator as described above. A sketch of this framework is shown in Fig. 2 . The following sections provide a more detailed description of each component.
3.2.1 Mobility demand generator
The mobility demand generator generates a set of mobility demands based on the steps in Fig. 1 . This is later used to represent the mobility patterns of the analyzed user groups in the given context. Each mobility demand consists of a user ID, a corresponding persona ID, an origin location, a destination location, and a trip start time. The sequences included in the mobility demand generator are:
Extract data from a mobility survey : The extraction of mobility demand data is the key for the whole model. The start and end location of the trip, trip start time, and user data must be provided. This is usually based on available travel journals or (national, regional, or local) travel surveys. Hörl and Balać ( 2020 ) described an example of such a methodology.
Draw a population sample : The model requires a minimum sample size in order to provide robust results. According to Llorca and Moeckel ( 2019 ), a sample size of at least five percent is necessary to correctly represent the behavior of the population. Other researchers have recommended larger sample sizes (Kagho et al. 2022 ; Ben-Dor et al. 2021 ). Depending on the sample size of the mobility survey (previous point), the extracted data can be completed by other input data sources (if the sample size is too small) or reduced (if the sample size is too large). The survey sample employed in the present research (1800 trips) represents approximately three percent of the whole population aged 20–66 (according to data provided by Agder Fylkeskommune) living in the coastal municipalities served by Line 100. If we only consider the employee group, our survey sample size is around five percent.
Geographical filter for a target area : If a nationwide travel survey is used, the input data can be filtered so that it represents the considered area. A basic approach is to only consider trips starting or ending within the area. Additionally, customized personas are used to reflect differences in the overall mobility behavior of several representative user groups of the considered area. This information is often not contained in national travel surveys but can be inserted in a separate step either in an elaborate way when specifically considered in the survey design (e.g., Rasca et al. 2023 ), or in a simpler way by considering demographic data of the region of interest.
Generate daily travel plans : The goal of this step is to align trips with respect to the semantics of the traveling person. Trips should not be considered independently, but as a sequence (activity chains). This not only influences the mode choice (that is, which modes are preferred for which trip purpose) but also adds constraints (for example, beginning a trip on a day with a private car should end with returning the car to the home location).
3.2.2 Initial mobility offer generator
The goal of the initial mobility offer generator is to generate a set of mobility offers for each mobility demand generated in the previous step. Each mobility offer consists of a list of route segments, in which the overall route is partitioned. Each partition represents a continuous trip using the same mode. A mode switch (or a transfer between two different public transport lines) occurs between two consecutive route segments of the same offer. The information about the transport mode (and specific lines for public transport), the travel time (calculated by the intermodal routing engine), the length, and the quality of the partition are stored for further use. Note that this module only creates the initially available offers; that is, the offers of the baseline scenario without any additional options induced by measures.
The users’ choice of these routes is often based not only on the travel time but includes other factors, such as the number of transfers for public transport; therefore, many different reasonable routes are computed. There are several approaches described in the literature on how to find a set of alternative routes from a given source node to a given destination node, see, e.g., Bader et al. ( 2011 ), Delling and Wagner ( 2009 ), and Bast et al. ( 2013 ). The present model uses a via-node approach, in which the fastest route from the source to the destination node is computed while visiting a node out of a list of via nodes in between. The list of via nodes is given by nodes on a perpendicular line of a direct line from the source to the destination. An intermodal routing engine then computes routes using single or multiple modes.
3.2.3 Solution generation and optimization
The simulation-optimization framework is able to optimize the solutions with respect to implementing mobility stations, finding optimal locations, etc. The algorithms within the optimization module are based on generating an initial candidate solution and applying metaheuristic methods (Glover and Kochenberger 2006 ) such as variable neighborhood search. Since optimization is not used for strategic planning of the transport system in our particular study, we will not go into the details of this component.
3.3 Simulation/evaluation module
In the simulation component, the user choices of the routes are simulated. When an optimization part is used, this represents the evaluation component of the optimization algorithm. If no optimization is used, this is the main component of the overall framework. The goal of this part is to simulate the user’s choice of a mobility offer for each of their mobility demands. This is achieved by parametrized utility functions assigning a utility value to each mobility offer, a value that is based on the properties of the routes and the personal preferences of the users (extracted from the persona profiles).
The first step in this method is to sort the mobility demands by their departure times in ascending order. Then a user choice is simulated for each mobility demand following the ascending order. However, in some cases, previously chosen offers by the same or a different agent might influence the choice possibilities of the current mobility demand, depending on the realities of the simulated case. For example, in Agder, car and bike sharing were not available as transport options at the time that the present research was conducted. Therefore, if a private car or bike is used for a trip by an agent in the simulation, it will be used for the return trip as well. To ensure this is correctly represented in the model, before a choice is made, a filter is employed to remove infeasible mobility offers/trip chains. Our assumption for the case of Agder is that the private cars/bikes are always available at the home location of the corresponding agent at the start of the day and have to be returned to their home locations by the end of the day (for example, after the last mobility demand of the agent). For each mobility offer, the following conditions are checked:
If the offer uses a limited resource, check its availability.
If the first chosen offer of the same agent is not a private car/bike, all further car/bike choices are infeasible until the agent returns to the home location.
If the previously chosen offer of the same agent was a car/bike and the current location will not be visited again, set all other modes as infeasible. This ensures that the car/bike always returns to its home location.
This reality might differ depending on the case selected. For example, in the OptiMaaS model, car and bike sharing were included as transport options, meaning that the agents could have return trip chains with diverse transport modes.
In the next sections, we will show how the user choice is simulated given that all remaining offers are feasible.
3.3.1 Mobility offer evaluation
A key component in the simulation is the evaluation of mobility offers. Each offer is evaluated using a set of evaluation functions taking the offer properties, the user behavior model, and the persona attributes into account. The evaluation approach is based on utility functions which are often used in the literature for estimating a personal utility of a choice (Modesti and Sciomachen 1998 ). In the present study, the approach is based on the utility functions of the route choices (Balac et al. 2019 ; Becker et al. 2020 ) which used the following functions for the different modes of transport:
The parameters \(\alpha\) and \(\beta\) reflect the user attitudes towards the different modes and the associated importance of the attributes (e.g., travel time, distance) of the routes. These values are often estimated by applying a multinomial logistic regression model to the results of a stated preference travel behavior survey (Frei et al. 2017 ). Since performing such a survey is out of the scope of this project, in a first step we used the \(\alpha\) and \(\beta\) values from a previous Norwegian study (Raustøl 2013 ) in combination with the most recent Norwegian official values of travel time (Flügel et al. 2020 ). As the set of values identified in Raustøl ( 2013 ) was incomplete (see Table 2 ), the missing \(\beta\) values were estimated starting from the latest Norwegian values of travel time (VOT) and the proportion of \(\beta\) values available in Frei et al. ( 2017 ). The \(\beta\) values for travel time are measured in minutes, while the \(\beta\) value for travel cost is measured in Norwegian Kroner (NOK). The VOT formula employed, sourced from Athira et al. ( 2016 ), is:
In a second step, we applied the automatic parameter tuning package irace (López-Ibáñez et al. 2016 ) to calibrate the two missing \(\alpha\) parameters towards the area under investigation. With a known mobility modal split for the target area, calculated from the results of the survey (Car 66.03%, Bike 18.25%, Walking 9.19%, and Public Transport 6.53%), the goal was to obtain a parametrized user behavior model that approximates these values. After running irace for 20 000 iterations, the results of the calibrated model reflected the modal split resulting from the survey. Therefore, the \(\alpha\) parameters could be used. Studies similar to ours use the values computed for Zurich, Switzerland, which were originally presented in Frei et al. ( 2017 ) and used in Balac et al. ( 2019 ) and Becker et al. ( 2020 ).
In addition to the \(\alpha\) and \(\beta\) parameters, the mode preferences of the agents were considered by utilizing their preferred transport mode in the utility functions. This was achieved by assigning a numerical value on a scale of one (poor option) to five (very good option) for each persona and for each transport mode based on the outputs from the persona profile design (Rasca 2022 ). This assigned preference value can modify the utility rate with a range between 10 percent and 50 percent (corresponding to 1–5). For example, the utility rate for walking for persona \(p_1\) is computed as follows:
where \(p^w_1\) is the preference value for walking of persona \(p_1\) . Note that, in our approach, the trip options are generally multimodal, which means that when PT is chosen, the first leg and the last leg are performed by walking and their (dis)utility is added to the trip utility.
3.3.2 Look-ahead approach for mobility offer evaluation
Considering the choices of the mobility demands of the users independently generates a bias towards the most attractive mode for the first mobility demand. As the choice of a car or bicycle as a transport mode for the first trip often determines the remaining choices for the other mobility demands of the day, a “looking ahead” mobility offer evaluator was developed to reduce this bias. This was achieved by changing the utility computation of each offer through also considering the offers for the future mobility demands of the same person. Specifically, for a given mobility demand, each offer is temporarily fixed and all the remaining choices of the agent are simulated given that the fixed offer is chosen. As a result, the new utility of the offer is the sum of the utility of the offer and all the chosen future offers (determined by simulation). This total value is then used instead of only the utility value of the actual offer for the user decision module.
3.3.3 User decision module
After evaluating the mobility offer, the user decision module simulates the decision of the user about the chosen offer based on the utility values. Like many others before (Hörl et al. 2018 ; Grether et al. 2009 ; Jánošíková et al. 2014 ), the present approach also relies on a multinomial choice model for the probabilistic decision-making, which is as follows. Let \(O_d\) be the set of mobility offers of mobility demand \(d \in D\) , and \(\hbox {S}_{{di}}\) the utility of mobility offer i with \(i \in O_d\) . Then, the probability that offer i is chosen out of the set \(O_d\) is given by:
where \(\mu\) is a scale parameter to set the importance of the utility value. The choice for each user and demand is then simulated by taking a sample of the resulting probability distribution, which gives the set of chosen mobility demands.
3.3.4 Limitations
The limitations of the current research need to be addressed before presenting the results. We will highlight two types of limitations: data-related, and methodology-connected.
Concerning the data-related limitations, note that the survey over-represented individuals with higher education. This issue has been previously encountered in electronically-conducted surveys in Norway (Roche-Cerasi et al. 2013 ). Furthermore, the distribution of the survey respondents in situ was not entirely consistent with the real population distribution. For example, very few respondents living in Fevik were registered, despite Fevik being an important component of Grimstad municipality. Furthermore, no detailed travel journals were collected.
As the data collected did not allow for the calculation of a dedicated VOT, the most recent pre-determined VOTs for Norway were used (Flügel et al. 2020 ), as well as pre-determined \(\beta\) parameters, where available. One limitation regarding the sourced \(\beta\) parameters is that they were calculated for a specific sample of cyclists. Nevertheless, when calculating the VOT based on the formula sourced from Athira et al. ( 2016 ), we found the results achieved with the \(\beta\) parameters sourced from Raustøl ( 2013 ) to be very close (on average a deviation of approximately six percent) to the VOTs used as reference.
In terms of methodological limitations, only one express-service bus route’s redesign was considered in the present case study. Nevertheless, the model can be employed for more complex endeavours. For the sake of simplicity, the model, which is regarded as a pilot study, did not include an occupancy feature or a crowding penalty and crowding disutility.
4 Case study
4.1 the region of agder, norway.
Agder is the southernmost region of Norway, covering a territory of 16,434 \(\hbox {km}^{2}\) and hosting a population of approximately 300,000 inhabitants. Roughly 80 percent of the population is concentrated in the coastal area. The municipality of Kristiansand, with a population of approximately 112,000 inhabitants, is the largest urban area and also the capital of the region. The predominant urban forms in Agder are small cities and towns (SCTs). Regional bus routes connect Kristiansand with the other main urban settlements in the region, the most popular route being bus Line 100 connecting Kristiansand to Arendal via Lillesand and Grimstad (Fig. 3 ).
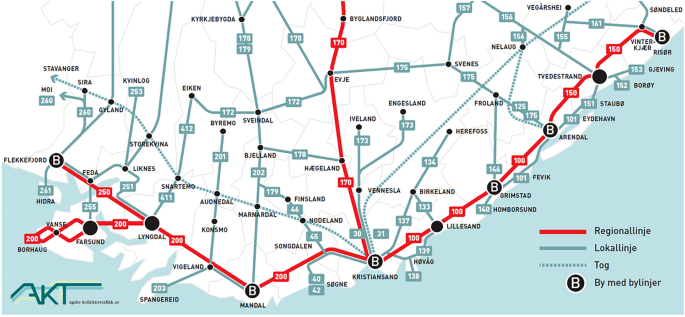
Map of the regional bus network in Agder (courtesy of Agder Kollektivtrafikk AS)
The public transport provision outside the boundaries of Kristiansand is limited in terms of frequency and density of the PT network. This, combined with a low-density of habitation, increasing urban sprawl, high car dependency and easy access to free parking generates a modal split that strongly favors the car (approximately 70 percent) to the disadvantage of public transport (approximately 5 percent). The mode share of PT is situated below the national average of Norway, which was 10.9 percent in 2019 (Statista 2020 ).
As a signatory of the Paris Agreement, Norway has introduced new sustainability targets for the public transport sector, challenging all regions to increase their share of PT usage. Agder Kollektivtrafikk A.S. Footnote 2 (AKT), which is the PTA in Agder, is looking for strategies that would help attract new customers and increase the sustainability of the mobility sector in this region.
4.1.1 Bus Line 100
The regional “Line 100” bus route connects Kristiansand to Arendal (see Appendix A ) and consists of four main routes, each with its own variations in terms of end stops and route lengths (see Table 3 ). The line is the most popular in the region, connecting the largest municipalities in Agder (Kristiansand and Arendal) and the two campuses of the University of Agder, located in Kristiansand and Grimstad. The route also includes the largest Norwegian shopping area, Sørlandssenteret, and the most visited Norwegian tourist attraction: the Dyreparken zoo and amusement park.
4.1.2 Redesign of the express service for Line 100
Line 100 has 62-seat single articulated buses equipped with seat belts, onboarding through the front door and alighting through the middle door, pre-paid tickets with on-board validation at the front door, maximum driving speed of 90 km/h on highway, stations part of the sidewalk or curb environment, with modest passenger shelters, and middle-door lifts for passengers with disabilities. The stops and stations are designed to allow in-line, double berthing. Larger stations allow for bike transfer (bike parking facilities available in the stations’ vicinity) and a limited number of stations provide park-and-ride facilities. In all scenarios considered in the present research, the conditions stated above are assumed.
There are several transfer options between Line 100 and other important regional routes (see Fig. 3 ), such as Line 150 (Arendal to Tvedestrand), Line 133 (Lillesand to Birkeland), or Line 35 (Kristiansand to Kjevik airport).
Bus services are subcontracted by the PTA in Agder. The costs associated with 1 km of service on the L100 in January 2021 when the study was performed were 40 NOK (Norwegian Kroner), which gives an approximate cost of 221 592 NOK for a typical workday schedule for the entire Line 100.
An analysis of the survey data collected in 2019 in the Agder region revealed that the minimum desired frequency for buses in Agder is 20 min (Rasca and Saeed 2022 ). When the present study was conducted, the regular frequency of Line 100 was 30 min between 5:00 and 16:30, with exceptions in the rush hours when the ES routes (100D and 100E) are running. Currently, the schedule of the ES routes is not optimized for providing a higher frequency with regular intervals during rush hours. The multiple variations in the routes for the same line (see Table 3 and Appendix A ) can make it difficult for new potential customers to understand the schedule.
AKT has been exploring options to reorganize the two ES route options (100D and 100E) into one unified route (100 Express or 100E). For that purpose, they performed a consultation of the current users of the existing two express lines (100D and 100E). The results of the consultation revealed that the customers wish for more direct connections between the municipalities, faster transit times and schedules better fitting to their needs. Therefore, the transport planning team at AKT proposed a revision of the ES route options of Line 100 by merging the 100D and 100E route variations into a new 100E.
The new route would have direct, non-stop buses connecting Kristiansand to Lillesand, Grimstad and Arendal, running in parallel in the morning rush hours towards Kristiansand and in the afternoon rush hours outwards from it. For the opposite direction at the rush hour times, the buses would run between Kristiansand and Arendal passing through the regular 100C route in Grimstad. The proposed schedule can be seen in Appendix A .
4.1.3 Requirements from the Public Transport Authority in Agder
The model architecture and solutions evaluation for the case of Agder were designed in accordance with the requirements of AKT, the PTA in the region. These requirements formed a set of key performance indicators (KPIs) used in the solution candidate evaluator step. The five main requirements, targeted at the employees’ user group, were:
KPI.1—minimize end-to-end transit time for the ES route of Line 100.
KPI.2—increase ES line patronage compared to current values. Patronage estimations were based on the results from RS 2019.
KPI.3—increase rush hour frequency on Line 100.
KPI.4—minimize waiting time for users.
KPI.5—identify best solutions (increased patronage and frequency) for a daily cost increase on the ES line of maximum 10 percent.
KPI.6—maintain the purpose of the ES line as an inter-city commuting service.
4.1.4 Data collection
The present research employed both quantitative and qualitative data. This section presents the data collection methods and sources.
Quantitative data collection
The data sets used for the model initialization cover the regional transport infrastructure (roads, speed limits) and the public transport network, with a focus on Line 100 (location and name of PT stations, routes, timetables and trips of the individual vehicles with their respective sequences of stations and arrival/departure times). The data for Line 100 are loaded from GTFS files provided by AKT, the PTA in Agder.
Demographic data for the population of Agder (age, education, family size), and car ownership data for the region were provided by the Agder Regional Council. The demographic and car ownership data were used for the persona distribution in the frame of the simulation.
Collecting travel behavior survey data is considered time-consuming and expensive, so researchers recommend the use of existing travel survey data where available (Heyken Soares et al. 2019 ). In the case of Agder, the latest national travel survey data available at the time the present study was conducted, were from 2011. The current PTA in Agder was formed in 2015 through the merger of two previous PTAs, with consequent changes in the operations and PT offer. Therefore, we could not consider the 2011 data as reflective of the current daily commuting realities. As a result, a dedicated Regional Travel Survey (RS 2019) was performed between June and September 2019 in Agder as part of the OPTCORA Footnote 3 project. The survey data were used for two purposes: to construct the persona profiles and as input data for the simulation model (agent routes, schedules and movement). The RS 2019 survey structure and the collection method for the N = 1861 responses are described in detail by Rasca et al. ( 2023 ).
The RS 2019 survey data used in the simulation model for the trip generation were: postal code of home location, GPS coordinates of employment location, time of travel, and travel mode. The last component was used to determine the modal split used in the calibration phase. For the persona profile creation, the following data were used: demographic profile, car ownership, parking availability, commuting habits, public transport accessibility and satisfaction, and personal drivers for increasing PT use.
The collected qualitative data was a critical element in structuring the persona profiles. The process, presented in detail in the research of Rasca et al. ( 2023 ), employed: (a) a panel of local and international experts in the field of public transport who supported the development and validation of the persona profiles; and (b) a series of 32 semi-structured interviews performed with employees of organizations located in the proximity of Line 100 that took part in the survey. No incentives were offered for participating in the interviews.
5 Persona profiles generation and allocation
The process of generating the 20 persona profiles for Agder is described in detail in Rasca et al. ( 2023 ). The core steps of the process are presented in Fig. 4 , showing how both qualitative (semi-structured interviews, expert panel) and quantitative (travel survey and latent class cluster analysis (LCCA)) methods were employed. Once the persona profiles were created (see Appendix B for overview of persona profiles), a preference for different transport modes used for the daily commute was calculated. The method for this calculation is presented in Rasca ( 2022 ).
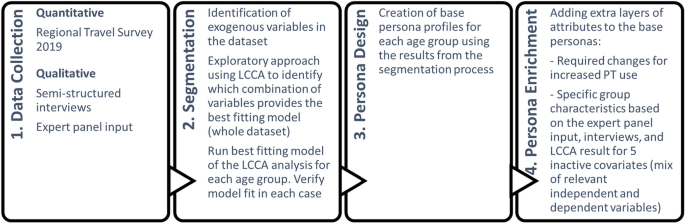
Steps of the persona design process (Rasca et al. 2023 )
The 20 persona profiles (see Appendix B ) were distributed in the analyzed area, striving to represent as closely as possible the target population group (adults aged 18–66). The process included (1) the segmentation of the adult population in each municipality of Agder in groups of similar age and education level as the personas designed; (2) separating each group in representative proportions for each of the corresponding four base persona profiles, as defined by Rasca et al. ( 2023 ); (3) randomly allocating personas to the trips starting in the postal codes of the studied area, respecting the distribution achieved in the previous two steps.
6 Scenario description
The application of the simulation-optimization framework is based on inputting predefined scenarios into the model, once the model has been calibrated, and comparing the results to the initially assessed situation.
The nine scenarios (see Table 4 ) were generated by a group of experts composed of transport planners employed by the PTA in Agder, transport researchers from the University of Agder and the AIT Austrian Institute of Technology. The scenarios were based on: data gathered from users of PT in Agder based on the regular customer surveys conducted by AKT, data gathered from non PT-users (RS 2019 and interviews), and the professional experience of the experts themselves. The scenarios aimed to include both improvement requests from the inhabitants of Agder (users and non-users of PT) and employees of the companies that participated in the RS 2019 (for example, increased frequency, shorter travel time, better timetable), as well as requests formulated by the PTA (increase of patronage, minimization of operating costs).
The approach to generating the nine scenarios took into account two main perspectives: the routing aspect and the frequency of service provision. The routing analysis included aspects such as: catchment areas for existing bus stops and proposed ones, occupancy profile of the line based on onboarding and alighting on each station, density of the inhabited area on the bus route, and density of the available services (employment, education, health, leisure) on the bus route.
Table 4 presents an overview of all scenarios proposed for simulation. The base case, or Scenario zero (S0), represents the current status of the network achieved by calibrating the model. Scenario 1 (S1) represents the solution proposed by AKT with the introduction of the new 100E, while the remaining scenarios are those proposed by the expert group. Scenarios 2–6 (S2–S6) simulate different route alternatives with similar schedules and were intended to verify the best route option in relation to transit time, costs and increase in usage. Scenarios 7–9 (S7–S9) simulate the introduction of a 20-minute minimum frequency covering different lengths of rush hours for the best route option identified in the previous step.
The request of the PTA to keep the cost increase limited to 10 percent meant that the possible frequency variations were limited to be almost non-existent. Therefore, the decision was to simulate two scenarios (S7 and S8) with moderate schedule changes, and one scenario (S9) with large schedule changes, with the goal of showcasing the PTA the potential patronage increase brought by improving the service frequency.
It should be noted that adding an off-highway stop to the ES route, in order to service a new location (such as Lillesand), brings an additional transit time of at least three minutes to the end-to-end route.
The proposed scenarios were separated into two categories: the first six scenarios (S1–S6) provided route variations, while the last three provided frequency changes using the best route solution identified in the previous step. The following two subsections present the results achieved when comparing simulation outputs for scenarios in each of the two categories.
7.1 Selection of the fast transit route option
For the identification of the best performing fast transit route scenario, the following KPIs were employed: KPI.1 (minimize transit time), KPI.2 (increase patronage), KPI.4 (minimize waiting time, both at the first stop of the journey and in transit stations for users traveling with multiple buses to the destination), and KPI.6 (maintain focus on intercity trips). The data sets presented in Tables 5 and 6 match the results achieved with each of the four KPIs. In the case of KPI.6, both the information sets regarding the average travel distance and the share of long trips were found to contribute to the information regarding intercity trips. Therefore, KPI.6 is repeated in the headings of the tables.
The selection of the best-performing route for 100X occurred in a three-step process, using the four KPIs as reference for the identification of best-performing solutions: (1) simulate the route with a minimum number of stops based on prioritized stops identified by the transport planners (S1–S2); (2) choose the best-performing solution from the previous step and simulate three route options that contained a fast transit stop in Lillesand (S3–S5); (3) choose the best-performing solution from the previous step and add a stop in Sørlandssenter shopping area (S6). Once all three steps were performed, the results were analyzed and the route with the best potential outcomes according to the KPIs could be identified.
Table 5 summarizes the simulation results for the base-case scenario and scenarios S1–S5. The four KPIs analyzed are presented in comparison, with statistically significant results for KPI.2, representing the patronage variation, marked in bold.
An increase in waiting time is a clear negative aspect and can be observed in all scenarios. Note that the schedule of the connecting feeder lines and connecting routes was not altered to fit to the newly proposed route schedules, so waiting times may be negatively affected by this aspect. In terms of the average travel time (KPI.1), it is not possible to clearly determine its variation as having a positive or negative impact. This is because an increase in average travel distance may also trigger an increase in average travel time per trip. Therefore, the ES patronage variation (KPI.2) and share of long trips in all bus trips (KPI.6) were used to determine the best-performing scenario in this phase.
We observe that S2 clearly outperforms S1 in terms of positive patronage variation. The two scenarios (S1 and S2) show similar average waiting times, average travel times per trip, and shares of long trips in all bus trips. The only notable difference between the two scenarios is in the average travel distance, which is slightly higher in the case of S1. Considering these results, the conclusion of the first step of the process is that the proposed 100X (S2) route will be used for further analysis in step two.
The introduction of an ES stop in Lillesand (S3–S5) brings a clear increase in patronage, but also increases the average travel time and length of the trips compared to S2. The best performing scenario that includes a stop in Lillesand is S4, with a 105.3% patronage increase (considerably higher than S3 and S5), similar waiting times to S5 but better than S3, and a slightly higher average travel time and average travel distance compared to S5. Overall, it can be concluded that S4 is the scenario that should be used for further simulations in the third step.
It has to be noted that S4 and S5 are using theoretical ES stops for Lillesand, as the physical bus stop infrastructure for these cases does not exist yet. Therefore, the best-performing S4 would need additional infrastructure investments for an ES stop at Møglestu in Lillesand before it can be deployed.
The third step of the process simulates the addition of a stop in Sørlandssenter to the route S6. The results for S6 show that the patronage suffers losses compared to S4, and does not show statistically significant changes in terms of waiting time compared to S4. A clear decrease in the average travel distance is visible, as well as a reduction in the share of long trips. Therefore, the addition of a stop in Sørlandssenter is not considered beneficial, as the increase in overall route length (and therefore cost) is not compensated by a ridership increase.
Given that S4 requires an infrastructure investment for building a new bus stop, and that S4 surpasses the 10% cost increase even before altering the ES route’s frequency, the PTA opted to choose the route solution that did not include Lillesand. Therefore, the selected route was the one proposed in S2. In the following scenario simulations, targeted at testing the impact of different frequencies on the best-performing route, S2 will be used as the ES route option.
7.2 Selection of the best frequency option
In this section, we present the results achieved when altering the schedule and frequency of the ES line (scenarios S7 to S9). The results are summarized in Table 6 .
In the case of Scenario 7, we observe that a moderate price increase (20.95 percent, see Table 3), produces a large impact on the ES patronage (89.5 percent). At the same time, the impact of a larger price increase (74.88 percent) which is simulated in the case of S8 further improves the patronage compared to S7 by only 31.6 percent. All other KPI results tend to be similar between S7 and S8.
In the data from RS2019, 33 percent of the respondents stated that their work schedule usually starts between 07:00 and 08:00, 29.3 percent between 08:00 and 09:00, the rest either having flexible work hours (with a start most commonly between 07:00 and 09:00 in Norway) or working in shifts. Therefore, the fact that an increased frequency relevant to the morning and afternoon rush hours has the biggest impact on the ridership and is consistent with the user profiles in Agder and their travel habits.
The results analysis for S9 suggests that a 10-min frequency in the rush hours for the same duration as S7 has the potential to bring an additional 63.1% increase in patronage compared to S7 and brings more than two and a half times more customers to the ES lines than the base-case scenario S0, at a 127.29% cost increase. This highlights the importance of having frequencies that allow users to “forget the timetable”.
The introduction of regular time intervals and increased frequencies, combined with a unique ES route proved to have a strong positive effect on the patronage. Nevertheless, once the timetable and routing were regularized for the ES option, the price increase was higher than anticipated. Therefore, none of the proposed schedules falls within the 10% cost increase limit required by the PTA.
8 Discussion
To better understand the outcomes of the study and for an easier comparison between the impact of different scenarios on the patronage of the ES option of Line 100, a simplified decision tree has been constructed (Fig. 5 ) based on the results presented in the previous section.
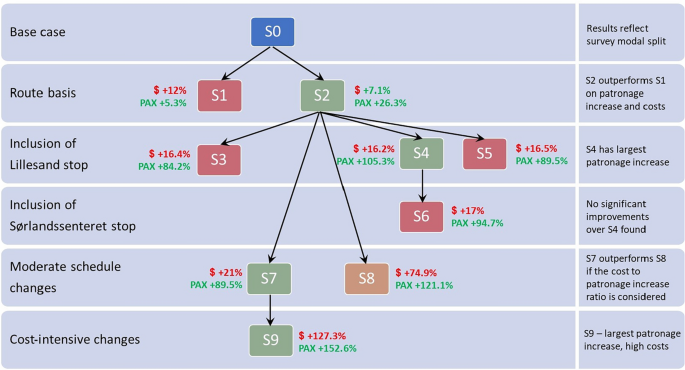
Decision tree based on simulation results for costs and ridership for the nine scenarios
The analysis of the route options for the ES of Line 100 (S1–S6) suggests that it is necessary to prioritize higher density urban areas outside Kristiansand (Lillesand, Grimstad, Arendal) that are not serviced by metropolitan transport lines, as Sørlandssenteret is. At the same time, the positioning of the ES stops needs to be carefully considered. Low-density locations, which make it necessary for users to employ another means of transport to reach the bus stop (as is the case in S3), have a negative impact on patronage. It is also important to consider the connection with other local and regional feeder lines, the simulation for S4 where the location of the bus stop also serves Line 133 showing a clear increase in patronage. This confirms the findings of Hansson et al. ( 2022 ) and Tønnesen et al. ( 2021 ), who stressed the need to ensure a high level of accessibility to bus stops, both from the perspective of service quality as well as multimodal access, such as car and bicycle parking possibilities. The routing simulations used an identical schedule for S0 and for S2–S6, indicating that a redesign of the ES route is crucial in increasing patronage for the user group.
The imposed financial limit of a maximum 10% cost increase left very little room for possible measures in relation to frequency improvements. Financial limitations are some of the most common issues for PTAs serving low-density areas, whose services often depend on national and regional subsidies. Employing a simulation model like the one proposed in the present study may support PTAs in motivating the need for increased funding for service improvements with data-based predictions, which have a better chance of success in accessing extra funding.
The schedule and frequency alterations present considerable opportunities. When analyzing the results for S7, we observe that the simple regularization of the schedule to a predictable frequency of maximum 20-minute intervals for two hours during the morning and two rush hours in the afternoon brings an additional 63.2 percent in patronage compared to S2. Extending the same frequency interval to a duration of six hours of ES service per day (three in the morning and three in the afternoon) further increases the patronage by 31.6 percent in the case of S8.
An increase in frequency to 10-min intervals (S9) for 4 h a day brings an additional 126.3% increase in patronage compared to S2. Consequently, if major financial investments are to be made in order to attract more car commuters towards PT, the results of our research suggest that the first focus area should be the increase of frequency during rush hours and not necessarily the extension of the ES service during lower demand periods of the day.
When analyzing the ES average travel distance in general and average travel distance per long trip in particular, we observe that the introduction of more intermediate stops (S3–S6) has a clear negative effect on both (KPI.6). This shows that more trips with a shorter distance are happening, possibly connecting intermediate stops directly. Such an effect can be seen as both negative and positive, depending on the purpose set by the PTA. If the total end-to-end travel time (KPI.1) of scenarios S2–S6 is considered in relation to KPI.6, an increase in travel time for the same share of long trips is observed for S6. That goes against the requirement of the PTA to reduce the travel time of the passengers.
An interesting aspect of the results is the average waiting time for all scenarios simulated, which increases for almost all newly proposed scenarios with values up to 63.2 percent. The only scenario that comes close to the waiting time of the base-case scenario is S9. This peculiar behavior can be explained by the fact that the schedule of the feeder lines and connecting routes which is now designed to meet the timetables of S0, has not been adapted to the proposed scenarios. Therefore, waiting times for S1–S9 should be compared to each other, rather than with S0.
The ES patronage increased considerably (more than 70 percent) in seven out of the nine proposed scenarios, irrespective of end-to-end route travel time. While this percentage increase seems very high, the head count is in fact a reasonable number (the initial value was of 19 passengers, and the simulated values reached 35–42 passengers) when one considers the initial low share of PT users in the modal split. However, we cannot expect this change to take effect immediately, since it takes time for people to learn about the changes and to adapt their commuting behavior. The indicated increase should be seen as a medium to long-term target after the system settles.
The route simulations also showed that direct rush hour connections between the serviced municipalities, which ignore intermediate stops and consequently decrease the frequency possibilities due to budgetary constraints (as in the case of S1), may not have a strong positive impact on the patronage compared to today’s figures. Therefore, it is recommended that a single ES route is put in place, preferably with regular service intervals that allow for predictability for the customer’s route planning.
The results of the present research confirm the potential of ABMS models to support the development and optimization of future mobility services, such as MaaS, taking into account both the realities of multi-modal journeys and the behavior of simulated agents (Cisterna et al. 2021 ; Djavadian and Chow 2017 ). The achieved results underline the importance of being able to verify proposed routing and frequency solutions before deployment. Even though S1 responded to the stated preferences of current ES users, the simulation results for it did not show any added value to the patronage, despite cost increases. Therefore, investing resources into calibrating a simulation model like the one proposed in the present study and using it to verify the potential impact of diverse PT network redesign initiatives can provide PTAs with support in decision-making processes. This falls in line with the first two core components of decision support systems defined by Shim et al. ( 2002 ) and sets the foundation for developing the model into a decision support tool for transport planners and PTAs.
The challenges of using the proposed methodology in other case studies around the world also need to be discussed. The current approach demonstrates the adaptability of such a model to two different urban typologies: suburban areas of European metropolises and networks of small cities and towns. Nevertheless, the lack of a user interface makes it challenging to employ the model for a wider audience. Furthermore, an extensive amount of data is necessary to calibrate the model, which can be difficult to collect and organize in a correct format.
9 Conclusions and further research
The results of the present study suggest that the proposed model can be successfully adapted from simulating transport behavior in complex suburban metropolitan areas to the less complex realities of coastal regions hosting networks of small cities and towns serviced by ES bus-based PT. The model proved useful in assessing the viability of different routing and frequency scenarios for the same ES bus route. The model provides a solid approach for analyzing complex transport systems, adding the extra layer of user profiles and preferences to the traditional statistical data set. Thus, the results can be used as decision support systems for transport and urban planners, PTAs, or even national authorities when route improvements are considered.
When discussing frequency improvements versus an extension of service time span for the ES routes, the results suggest that frequency increases during critical time windows have better results in terms of patronage increase than extending the ES service.
The results also show clear differences when adding stops in low-density habitation areas versus higher density areas. This underlines the importance of focusing on the accessibility level of PT stops on ES routes, as the station-to-station transit time is not a sufficient incentive for choosing a specific mode of transport.
The present research combines methods that were previously employed for the simulation of travel habits, using multi-modal transport means, in European metropolitan suburban areas with a fresh approach to integrating tailor-made user profiles (or personas) in agent-based simulations. The proposed approach shows a high potential for increasing the accuracy of travel behavior simulations for specific regions.
The proposed method’s applicability, tested in this case on an ES bus route, is not limited to bus-based services or to the long-distance commuting of employees. Future applications may include a whole range of other transport services (rail-based, car or bike sharing, etc.), depending on the purpose and local realities of the specific studies.
Several other points of interest have also been identified as presenting potential for further research. Firstly, we have observed two major improvement points for the model analysis and output: (1) an occupancy feature to identify the need for supplementing capacity at different service times; (2) a crowding penalty should be introduced in the user decision model to better simulate the user decision-making process. Regarding the usability of the model, a user-friendly interface would be necessary to increase the chances that the staff of the PTAs and other actors in the transport sector would employ the model.
For more realistic results, the model should be applied to a wider user database, covering more diverse user groups (highschool and university students, pensioners, unemployed individuals, etc.). This, combined with a more detailed travel journal for the agents, would provide a more detailed picture of the agents’ travel mode decisions and the interactions between the agents themselves. At the same time, further studies that determine the regional VOTs, passenger’s cost (sum of access, waiting, riding, and egress time values), and crowding disutility would be necessary for further development of the model in representing user behavior and decision making.
Some questions about the implementation and validation remain unanswered until the results of an analysis performed with the use of the model are applied. The results themselves can not be validated until they are tested in real life, and the question of “How long will it take for the users to adapt to the new schedule?” is also part of the validation process.
Data Availability
The datasets generated during and/or analyzed during the current study are available from the corresponding author upon reasonable request.
https://www.optimaas.eu/ .
https://www.akt.no/ .
https://www.uia.no/en/news/computer-simulation-shows-how-bus-transport-can-be-improved . Acessed: 2021-09-22.
Aifadopoulou G, Ziliaskopoulos A, Chrisohoou E (2007) Multiobjective optimum path algorithm for passenger pretrip planning in multimodal transportation networks. Transp Res Rec 2032(1):26–34
Article Google Scholar
Arias-Molinares D, García-Palomares JC (2020) The Ws of MaaS: understanding mobility as a service from a literature review. IATSS Res 44(3):253–263
Athira I, Muneera C, Krishnamurthy K, Anjaneyulu M (2016) Estimation of value of travel time for work trips. Transport Res Procedia 17:116–123
Bader R, Dees J, Geisberger R, Sanders P (2011) Alternative route graphs in road networks. In: International Conference on Theory and Practice of Algorithms in (Computer) Systems. Springer, pp 21–32
Balac M, Becker H, Ciari F, Axhausen KW (2019) Modeling competing free-floating carsharing operators—a case study for Zurich, Switzerland. Transp Res Part C: Emerg Technol 98:101–117
Balmer M, Meister K, Rieser M, Nagel K, Axhausen KW (2008) Agent-based simulation of travel demand: structure and computational performance of MATSim-T. Arbeitsberichte Verkehrs- und Raumplanung 504:1–38
Google Scholar
Bast H, Funke S, Matijevic D (2006) Transit ultrafast shortest-path queries with linear-time preprocessing. In: 9th DIMACS Implementation Challenge [1]
Bast H, Brodesser M, Storandt S (2013) Result diversity for multi-modal route planning. In: 13th Workshop on Algorithmic Approaches for Transportation Modelling, Optimization, and Systems
Bast H, Delling D, Goldberg A, Müller-Hannemann M, Pajor T, Sanders P, Wagner D, Werneck RF (2016) Route planning in transportation networks, algorithm engineering. Springer, Berlin, pp 19–80
Becker H, Balac M, Ciari F, Axhausen KW (2020) Assessing the welfare impacts of Shared Mobility and Mobility as a Service (MaaS). Transport Res Part A: Policy Pract 131:228–243
Ben-Dor G, Ben-Elia E, Benenson I (2021) Population downscaling in multi-agent transportation simulations: a review and case study. Simul Model Pract Theory 108:102233
Berger A, Grimmer M, Müller-Hannemann M (2010) Fully dynamic speed-up techniques for multi-criteria shortest path searches in time-dependent networks. In: International Symposium on Experimental Algorithms. Springer, pp 35–46
Botea A (2011) Ultra-fast optimal pathfinding without runtime search. In: Proceedings of the AAAI Conference on Artificial Intelligence and Interactive Digital Entertainment, vol 6
Brickey J, Walczak S, Burgess T (2011) Comparing semi-automated clustering methods for persona development. IEEE Trans Softw Eng 38(3):537–546
Cats O, Rufi FM, Koutsopoulos HN (2014) Optimizing the number and location of time point stops. Public Transport 6(3):215–235
Čertickỳ M, Drchal J, Cuchỳ M, Jakob M (2015) Fully agent-based simulation model of multimodal mobility in European cities. In: 2015 International Conference on Models and Technologies for Intelligent Transportation Systems (MT-ITS). IEEE, pp 229–236
Chapman CN, Milham RP (2006) The personas’ new clothes: methodological and practical arguments against a popular method. In Proceedings of the human factors and ergonomics society annual meeting, vol 50. SAGE Publications Sage CA: Los Angeles, CA, pp 634–636
Chen C, Ma J, Susilo Y, Liu Y, Wang M (2016) The promises of big data and small data for travel behavior (aka human mobility) analysis. Transport Res Part C: Emerg Technol 68:285–299
Chng S, White M, Abraham C, Skippon S (2016) Commuting and wellbeing in London: the roles of commute mode and local public transport connectivity. Prev Med 88:182–188. https://doi.org/10.1016/j.ypmed.2016.04.014
Cisterna C, Giorgione G, Viti F (2021) Explorative analysis of potential MaaS customers: an agent-based scenario. Procedia Comput Sci 184:629–634
Delling D, Nannicini G (2012) Core routing on dynamic time-dependent road networks. INFORMS J Comput 24(2):187–201
Delling D, Wagner D (2009) Pareto paths with SHARC. In: International Symposium on Experimental Algorithms. Springer, pp 125–136
Delling D, Dibbelt J, Pajor T, Wagner D, Werneck RF (2013) Computing multimodal journeys in practice. In: International Symposium on Experimental Algorithms. Springer, pp 260–271
Dijkstra EW (1959) A note on two problems in connection with graphs. Numeriche Mathematik 1:269–271
Djavadian S, Chow JY (2017) An agent-based day-to-day adjustment process for modeling ‘Mobility as a Service’ with a two-sided flexible transport market. Transport Res Part B: Methodol 104:36–57
Fikar C, Gronalt M, Hirsch P (2016) A decision support system for coordinated disaster relief distribution. Expert Syst Appl 57:104–116
Flügel S, Askill Halse H, Hulleberg N, Jordbakke GN, Veisten K, Sundfør HB, Kouwenhoven M (2020) Value of travel time and related factors—Technical report, the Norwegian valuation study 2018-2020. Technical Report 1762/2020, Institute of Transport Economics (TØI)
Frei C, Hyland M, Mahmassani HS (2017) Flexing service schedules: assessing the potential for demand-adaptive hybrid transit via a stated preference approach. Transport Res Part C: Emerg Technol 76:71–89
Glover FW, Kochenberger GA (2006) Handbook of metaheuristics, vol 57. Springer Science & Business Media, Berlin
Gonzalez de Heredia A, Goodman-Deane J, Waller S, Clarkson PJ, Justel D, Iriarte I, Hernández J et al. (2018) Personas for policy-making and healthcare design. In: DS 92: Proceedings of the DESIGN 2018 15th International Design Conference, pp 2645–2656
Graser A, Widhalm P, Dragaschnig M (2020) Extracting patterns from large movement datasets. GI Forum 2020(8):153–163
Grether D, Kickhöfer B, Nagel K (2009) Policy evaluation in multi-agent transport simulations considering income-dependent user preferences. In: Proceedings of the 12th Conference of the International Association for Travel Behaviour Research (IATBR), Jaipur, India, pp 09–13
Grudin J, Pruitt J (2002) Personas, participatory design and product development: an infrastructure for engagement. Proc PDC 2:144–152
Ha J, Lee S, Ko J (2020) Unraveling the impact of travel time, cost, and transit burdens on commute mode choice for different income and age groups. Transport Res Part A: Policy Pract 141:147–166
Hansson J, Pettersson-Löfstedt F, Svensson H, Wretstrand A (2022) Effects of rural bus stops on travel time and reliability. Public Transport 14(3):683–704
Haustein S, Hunecke M (2013) Identifying target groups for environmentally sustainable transport: assessment of different segmentation approaches. Curr Opin Environ Sustain 5(2):197–204
Heyken Soares P, Mumford CL, Amponsah K, Mao Y (2019) An adaptive scaled network for public transport route optimisation. Public Transport 11(2):379–412
Hörl S, Balać M (2020) Reproducible scenarios for agent-based transport simulation: a case study for Paris and île-de-France
Hörl S, Balac M, Axhausen KW (2018) A first look at bridging discrete choice modeling and agent-based microsimulation in MATSim. Procedia Comput Sci 130:900–907
Hörold S, Mayas C, Krömker H (2013) User-oriented information systems in public transport. Contemp Ergon Hum Factors 2013:160–167
Jánošíková L, Slavík J, Koháni M (2014) Estimation of a route choice model for urban public transport using smart card data. Transp Plan Technol 37(7):638–648
Kaddoura I, Kickhöfer B, Neumann A, Tirachini A (2015) Agent-based optimisation of public transport supply and pricing: impacts of activity scheduling decisions and simulation randomness. Transportation 42(6):1039–1061
Kagho GO, Meli J, Walser D, Balac M (2022) Effects of population sampling on agent-based transport simulation of on-demand services. Procedia Comput Sci 201:305–312
Kong P, Cornet H, Frenkler F (2018) Personas and emotional design for public service robots: a case study with autonomous vehicles in public transportation. In: 2018 international conference on cyberworlds (cw). IEEE, pp 284–287
Le Loo LY, Corcoran J, Mateo-Babiano D, Zahnow R (2015) Transport mode choice in South East Asia: investigating the relationship between transport users’ perception and travel behaviour in Johor Bahru, Malaysia. J Transp Geogr 46:99–111
Leng N, Corman F (2020) How the issue time of information affects passengers in public transport disruptions: an agent-based simulation approach. Procedia Comput Sci 170:382–389
Liyanage S, Dia H (2020) An agent-based simulation approach for evaluating the performance of on-demand bus services. Sustainability 12(10):4117
Llorca C, Moeckel R (2019) Effects of scaling down the population for agent-based traffic simulations. Procedia Comput Sci 151:782–787
López-Ibáñez M, Dubois-Lacoste J, Cáceres LP, Birattari M, Stützle T (2016) The irace package: iterated racing for automatic algorithm configuration. Oper Res Perspect 3:43–58
Ma L, Ye R (2019) Does daily commuting behavior matter to employee productivity? J Transp Geogr 76:130–141
Macal C, North M (2010) Tutorial on agent-based modelling and simulation. J Simul 4(3):151–162
Manser P, Becker H, Hörl S, Axhausen KW (2020) Designing a large-scale public transport network using agent-based microsimulation. Transport Res Part A: Policy Pract 137:1–15
Markvica K, Millonig A, Rudloff C (2017) Introducing additional low emission mobility offers in a well connected area: Challenges and opportunities. In: REAL CORP 2017–PANTA RHEI–A World in Constant Motion. Proceedings of 22nd International Conference on Urban Planning, Regional Development and Information Society, pp 309–318
MATSim (2021) https://www.matsim.org/ . Accessed: 29 Sep 2021
Miaskiewicz T, Kozar KA (2011) Personas and user-centered design: How can personas benefit product design processes? Des Stud 32(5):417–430
Modesti P, Sciomachen A (1998) A utility measure for finding multiobjective shortest paths in urban multimodal transportation networks. Eur J Oper Res 111(3):495–508
Narayan J, Cats O, van Oort N, Hoogendoorn S (2017) Performance assessment of fixed and flexible public transport in a multi agent simulation framework. Transport Res Procedia 27:109–116
Nitsche P, Widhalm P, Breuss S, Brändle N, Maurer P (2014) Supporting large-scale travel surveys with smartphones—a practical approach. Transport Res Part C: Emerg Technol 43:212–221
Oliveira L, Bradley C, Birrell S, Tinworth N, Davies A, Cain R (2017) Using passenger personas to design technological innovation for the rail industry. In: First International Conference on Intelligent Transport Systems. Springer, pp 67–75
Othman SB, Zgaya H, Dotoli M, Hammadi S (2017) An agent-based decision support system for resources’ scheduling in emergency supply chains. Control Eng Pract 59:27–43
Pfeifer M, Lindinger C, Naveau N (2019) Berufe der Zukunft in einer automatisierten Mobilitätsumgebung. Technical report, KFV Sicher Leben
Prandtstetter M, Seragiotto C, Straub M, Magoutas B, Bothos E, Bradesko L (2020) Providing intermodal route alternatives. In: Proceedings of 7th Transport Research Arena TRA 2018
PTVGroup (2021) Ptv visum. https://www.ptvgroup.com/en/solutions/products/ptv-visum/ . Accessed: 29 Sep 2021
Rasca S (2022) Numerical estimation of travel mode preferences for agents in agent-based simulations. In: 2022 Smart City Symposium Prague (SCSP). IEEE, pp 1–6
Rasca S, Saeed N (2022) Exploring the factors influencing the use of public transport by commuters living in networks of small cities and towns. Travel Behav Soc 28:249–263
Rasca SI, Markvica K, Biesinger B (2023) Persona design methodology for work-commute travel behaviour using latent class cluster analysis. Multimodal Transport 2(4):100095
Raustøl J (2013) Value of travel time savings—estimates on Norwegian cyclists. Master’s thesis, Norges teknisk-naturvitenskapelige universitet, Fakultet for samfunnsvitenskap og teknologiledelse, Institutt for samfunnsokonomi
Roche-Cerasi I, Rundmo T, Sigurdson JF, Moe D (2013) Transport mode preferences, risk perception and worry in a Norwegian urban population. Accident Anal Prev 50:698–704
Salminen J, Guan K, Jung SG, Chowdhury SA, Jansen BJ (2020) A literature review of quantitative persona creation. In: Proceedings of the 2020 CHI Conference on Human Factors in Computing Systems, pp 1–14
Salminen J, Wenyun Guan K, Jung SG, Jansen B (2022) Use cases for design personas: a systematic review and new frontiers. In: Proceedings of the 2022 CHI Conference on Human Factors in Computing Systems, pp 1–21
Schafer A (2012) Introducing behavioral change in transportation into energy/economy/environment models. Technical Report 6234, World Bank Policy Research Working Paper
Schultes D, Sanders P (2007) Dynamic highway-node routing. In: International Workshop on Experimental and Efficient Algorithms. Springer, pp 66–79
Shim JP, Warkentin M, Courtney JF, Power DJ, Sharda R, Carlsson C (2002) Past, present, and future of decision support technology. Decis Support Syst 33(2):111–126
Šperka R, Slaninová K (2012) The usability of agent-based simulation in decision support system of e-commerce architecture. IJ Inform Eng Electron Bus 4:10–17
Statista (2020) Distribution of passenger-kilometers travelled by land in Norway in 2018*, by mode of transport. https://www.statista.com/statistics/449477/norway-modal-split-of-passenger-transport-on-land/ . Accessed: 10 Dec 2020
Tønnesen A, Knapskog M, Uteng TP, Øksenholt KV (2021) The integration of active travel and public transport in Norwegian policy packages: a study on ‘access, egress and transfer’ and their positioning in two multilevel contractual agreements. Res Transport Bus Manag 40:100546
Vallet F, Puchinger J, Millonig A, Lamé G, Nicolaï I (2020) Tangible futures: combining scenario thinking and personas—a pilot study on urban mobility. Futures 117:102513
Venturini G, Tattini J, Mulholland E, Gallachóir BÓ (2019) Improvements in the representation of behavior in integrated energy and transport models. Int J Sustain Transp 13(4):294–313
Vuchic VR (2007) Urban transit systems and technology. Wiley, Hoboken
Book Google Scholar
Download references
Acknowledgements
The current study was developed as part of the OPTCORA project financed in partnership by the Regionale Forskningsfond Agder, Agder Kollektivtrafikk A.S. and the University of Agder (grant number 309046). Special thanks are extended to: the members of the AKT team, namely Thomas Ruud Jensen, Kenneth Risan, Ståle Sætre, and Roald Morvik; the AIT Austrian Institute of Technology and especially to Karin Markvica for support in the creation of the persona profiles; Agder Fylkeskommune and Gunnar Ogwyn Lindaas for the support throughout the data collection and analysis; Bynett Sør and William Fagerheim and to the municipalities and companies in Agder who supported the data collection. Thanks are also extended to the supervision team supporting the PhD studies of Sinziana Rasca, namely Helge Liltved, Naima Saeed, Jonas Høgli Major, and Mikaela Vasstrøm.
Open access funding provided by University of Agder.
Author information
Sinziana I. Rasca and Bin Hu have contributed equally to this work.
Authors and Affiliations
Department of Engineering Sciences, University of Agder, 9 Jon Lilletunsvei, Grimstad, 4879, Norway
Sinziana I. Rasca
Center for Energy, AIT Austrian Institute of Technology GmbH, Giefinggasse 4, Vienna, 1210, Austria
Bin Hu, Benjamin Biesinger & Matthias Prandtstetter
You can also search for this author in PubMed Google Scholar
Corresponding author
Correspondence to Sinziana I. Rasca .
Additional information
Publisher's note.
Springer Nature remains neutral with regard to jurisdictional claims in published maps and institutional affiliations.
Appendix A: Overview of the current schedule for Line 100 and the proposed schedule for express service 100E
See Fig. 6 and 7 .
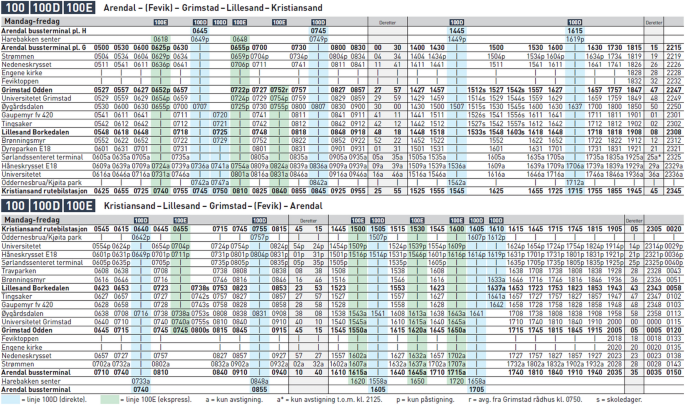
Current schedule for Line100. Scenario 0
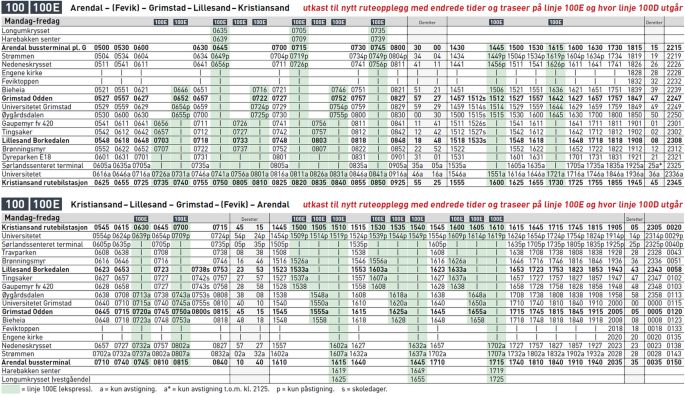
Proposed schedule by AKT for new 100E express service route variation of Line100. Scenario 1
Appendix B: Overview of persona profiles
See Fig. 8 .
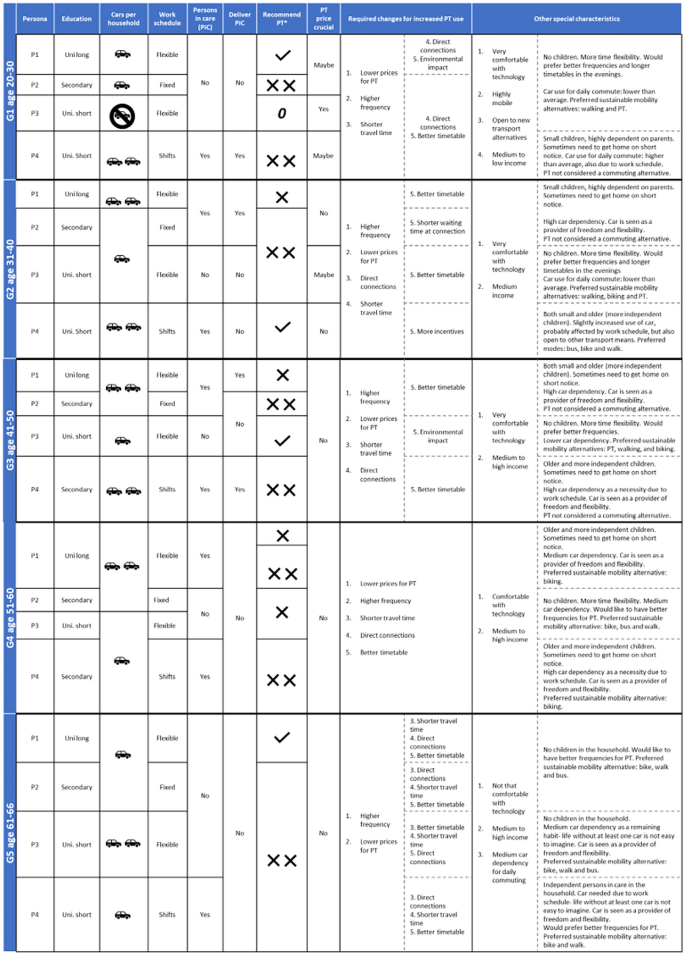
Overview of the persona profiles used in the study (Rasca et al. 2023 )
Rights and permissions
Open Access This article is licensed under a Creative Commons Attribution 4.0 International License, which permits use, sharing, adaptation, distribution and reproduction in any medium or format, as long as you give appropriate credit to the original author(s) and the source, provide a link to the Creative Commons licence, and indicate if changes were made. The images or other third party material in this article are included in the article's Creative Commons licence, unless indicated otherwise in a credit line to the material. If material is not included in the article's Creative Commons licence and your intended use is not permitted by statutory regulation or exceeds the permitted use, you will need to obtain permission directly from the copyright holder. To view a copy of this licence, visit http://creativecommons.org/licenses/by/4.0/ .
Reprints and permissions
About this article
Rasca, S.I., Hu, B., Biesinger, B. et al. Agent-based decision-support model for bus route redesign in networks of small cities and towns: case study of Agder, Norway. Public Transp (2024). https://doi.org/10.1007/s12469-024-00358-7
Download citation
Accepted : 04 March 2024
Published : 03 June 2024
DOI : https://doi.org/10.1007/s12469-024-00358-7
Share this article
Anyone you share the following link with will be able to read this content:
Sorry, a shareable link is not currently available for this article.
Provided by the Springer Nature SharedIt content-sharing initiative
- Public transport
- Agent-based simulation
- Decision support system
- Small cities and towns
- Find a journal
- Publish with us
- Track your research
- Skip to content
- Skip to search
- Skip to footer
Support & Downloads
- Worldwide - English
- Arabic - عربي
- Brazil - Português
- Canada - Français
- China - 简体中文
- China - 繁體中文 (臺灣)
- Germany - Deutsch
- Italy - Italiano
- Japan - 日本語
- Korea - 한국어
- Latin America - Español
- Netherlands - Nederlands">Netherlands - Nederlands
- Helpful Links
- Licensing Support
- Technology Support
- Support for Cisco Acquisitions
- Support Tools
- Cisco Community

To open or view a case, you need a service contract
Get instant updates on your TAC Case and more

Contact TAC by Phone
800-553-2447 US/Canada
866-606-1866 US/Canada
- Returns Portal
Products by Category
- Unified Communications
- Networking Software (IOS & NX-OS)
- Collaboration Endpoints and Phones
Status Tools
The Cisco Security portal provides actionable intelligence for security threats and vulnerabilities in Cisco products and services and third-party products.
Get to know any significant issues, other than security vulnerability-related issues, that directly involve Cisco products and typically require an upgrade, workaround, or other customer action.
Check the current status of services and components for Cisco's cloud-based Webex, Security and IoT offerings.
The Cisco Support Assistant (formerly TAC Connect Bot) provides a self-service experience for common case inquiries and basic transactions without waiting in a queue.
Suite of tools to assist you in the day to day operations of your Collaboration infrastructure.
The Cisco CLI Analyzer (formerly ASA CLI Analyzer) is a smart SSH client with internal TAC tools and knowledge integrated. It is designed to help troubleshoot and check the overall health of your Cisco supported software.
My Notifications allows an user to subscribe and receive notifications for Cisco Security Advisories, End of Life Announcements, Field Notices, and Software & Bug updates for specific Cisco products and technologies.
More Support
- Partner Support
- Small Business Product Support
- Business Critical Services
- Customer Experience
- DevNet Developer Support
- Cisco Trust Portal
Cisco Communities
Generate and manage PAK-based and other device licenses, including demo licenses.
Track and manage Smart Software Licenses.
Generate and manage licenses from Enterprise Agreements.
Solve common licensing issues on your own.
Software and Downloads
Find software bugs based on product, release and keyword.
View Cisco suggestions for supported products.
Use the Cisco Software Checker to search for Cisco Security Advisories that apply to specific Cisco IOS, IOS XE, NX-OS and NX-OS in ACI Mode software releases.
Get the latest updates, patches and releases of Cisco Software.
Search form
Thales has completed the sale of its ground transportation systems business.
Share this article
With around 9,000 employees, Ground Transportation Systems is a global leader in Rail Signaling and Train Control Systems, Telecommunications and Supervision systems and Fare collection solutions.
Through this divestment, Thales reinforces its strategic focus on 3 long-term high technology growth markets: Aeronautics & Space, Defense & Security, and Cybersecurity & Digital Identity.
The impact of the sale will be accounted for in Q2 24.
Note : In accordance with standard IFRS5, the financial data for the “Ground Transportation Systems” operating segment for 2022 and 2023 had been classified under “discontinued operations” following entry into exclusive negotiations with Hitachi Rail with a view to disposing of this business.
For information from Hitachi Rail, please contact:
Adam Love at [email protected] or on +1 437 234 4024
Ed Brown at [email protected] or on +44 7850 071 287
For press information about Hitachi Rail, please go here .
- Thales has completed the sale of its Ground Transportation Systems business - Press release - 31 May 2024.pdf

PT Len and Thales Sign JV Agreement to Strengthen Indonesian Defence

Thales’ FlytEDGE – the first cloud-based IFE in the world Winner of Crystal Cabin Award

Thales’ FlytEDGE digitally remasters the inflight entertainment experience
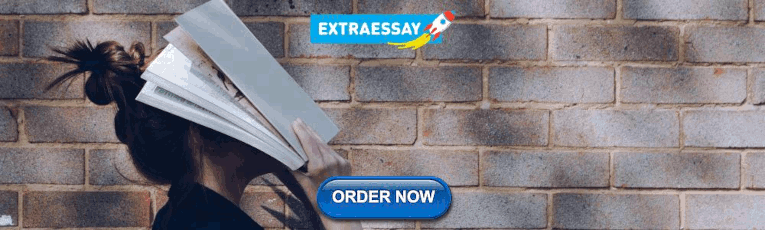
IMAGES
VIDEO
COMMENTS
Transport modes are the means supporting the mobility of passengers and freight. They are mobile transport assets and fall into three basic types; land (road, rail, pipelines), water (shipping), and air. 1. A Diversity of Modes. Transport modes are designed to carry passengers or freight, but most modes combine both.
This study aims to understand the reasons behind people's modal preferences in commuting trips, which contribute to transportation-related problems and to develop and prioritize policies that increase the use of sustainable modes. For this reason, in this study, a comparative case study was conducted to evaluate the factors that lead to ...
Exploring Preferences for Transportation Modes in an Urban Air Mobility Environment: Munich Case Study. Mengying Fu [email protected], Raoul Rothfeld, and Constantinos Antoniou View all authors and affiliations. ... This preliminary research intends to gain insight into the travel behavior impacts of autonomous transportation modes, especially ...
influencing the use of a certain transport mode. Most studies focus on binary options [2-6] by analyzing two transport modes (e.g., car and public transport) or motorized or non-motorized mode categories. Other studies investigated the mode choice through multinomial models (i.e., with more than two options for the dependent variable) [7-11].
for regional mobility: a case study. Nicholas Beresford Taylor MA (Oxon), PhD, CEng, FIET, FCIHT. Independent Researcher, Member of Transport Planning Society affiliated. to ICE, Little Sandhurst ...
Limits to mobility are imposed by geography and the speed, access, energy and ergonomic constraints of travel modes, which cannot be manipulated arbitrarily by design or technology. Achieving 'sustainable, efficient and sufficient' regional mobility needs thinking in terms of a 'nexus' between transport, human settlement and activity, and geography. A travel mode's characteristics and ...
Factors and Pairwise Comparisons: A Case Study Saman Hassanzadeh Amin, Ning Yan and Danielle Morris Additional information is available at the end of the chapter ... transportation mode consists of multiple modes of transportation (e.g., water and truck) being used. Analyzing and selecting the right transportation mode is a challenging task because
The proportion of students who live in their own house or share a house with friends is 19.7%. Although commuting to campus by walking reduces travel time, the average travel time for commuting is about 56 min. Shuttle busses (49.0%) were the most commonly used mode of transportation.
2.1 Transport Mode Detection. Transport Mode Detection (TMD) is a classification problem whose goal is to detect the transport mode of a human subject, e.g., walking, cycling, using any sensor.With inertial sensors, multiple methods coexist: some use handcrafted features with traditional learning methods (see [1, 6, 9], for instance), while others use directly use Deep Neural networks ...
Urban transport infrastructure is under increasing pressure from rising travel demand in many cities worldwide. It is no longer sustainable or even economically viable to cope with increased demand by continually adding capacity to transport networks. Instead, travel demand must be managed by encouraging passengers to adapt their travel behaviour. This approach necessitates a significantly ...
Oslo - Promoting Active Transport Modes. First published on 5 February 2021. As with many cities in recent decades, the Norwegian capital, Oslo, was increasingly giving up urban space to cars. Since the 1960s, massive road building schemes were undertaken to accommodate the explosive growth in the ownership and use of private cars.
Lisbon's public transport is used as a case study and is modeled as a multiplex network integrating eight different modes of transport. Proposing a novel normalized version of assessing the impact of failures, we were able to compare side by side the robustness of each modality layer, regardless of their size.
The European Council (2001, p.15- 16) defined a sustainable transport system as one that: (1) allows the basic access and. development needs of individuals, companies and societies to be met ...
Transport mode selection, load assignment, and transport operations are taken into account, which increases the complexity of the problem. Because of that, most researchers have focused on single-mode transport systems. Road transport is the dominant mode in Turkey. Road traffic crashes and deaths cause loses of 10,000 lives every year in ...
ECONOMIC AND SOCIAL COMMISSION FOR ASIA AND THE PACIFIC. CHAPTER III. CASE STUDY METHODOLOGY. A number of models have been developed to aid transport decision makers in choosing the most effective transport mode or combination of modes that not only minimize costs and risk, but also satisfy various on-time service requirements within the ...
In this study, we divide modes of transport into two nests - (i) public transport and (ii) individual. transport. Public transport includes two alternatives - (i/a) bus and (i/b) train, individual ...
Case study of development of the Tokyo metropolitan area and its corresponding transportation models A metropolitan area, sometimes referred to as a metropolitan region, metro area, or simply metro, is a region consisting of a densely populated urban core and its less populated surrounding territories, sharing industry, infrastructure, and ...
This study's goal was to show where the focus of the previous literature has been and to emphasize where the focus should be for future publications. From a cohort of 358 case studies, the authors categorized each case study into twenty categories based on transportation modes and eleven categories based on the authors' primary areas of ...
It also tries to establish a better understanding of which industrial sectors are using which mode of logistics services and why. According to the analysis, the high value and especially high price/kg ratio of products, short life cycles and worldwide markets are typical reasons to use rapid modes of transport.
Case study Policy aim Location Country Lead organisation(s) 1. Using existing powers over bus stops, timetables and routes : Improve bus quality and services : Nottingham : UK : Nottingham City Council : 2. Integrating the planning of bus services with other modes of transport in the region : Improve bus quality and services : Helsinki : Finland
The model is adapted and applied to a case study for the region of Agder, Norway, simulating the impact of nine different scenarios on the patronage of a specific bus route. ... need to maximize the return on investment for PT by attracting more paying customers towards this sustainable transport mode. Transport network planning has become ...
Emissions depend more on fleet's structure (age, traction), than the mode itself. New strict emission limits make road transport the least burdensome transport mode. Electrification of railway transport is required to make railways the greenest mode. ... Environmental burden of different transport modes - Real case study in Slovakia
The Incentivizing Active and Shared Travel Pilot Program studied how people choose to get around. It looked at travel behavior and the tradeoffs people consider when choosing to drive alone, or travel by a method that is more environmentally friendly. Plan Bay Area 2050 is the region's long-range plan for reducing greenhouse gas (GHG) emissions.
The case study showed that although rail transport is presented as an environmentally friendly transport mode in terms of emissions production, this is not generally the case. The vehicle fleet's structure has the greatest impact on selected ecological impacts of individual transport modes, from the viewpoint of its age and the type of traction ...
In rural areas with low population density, demand-responsive transport (DRT) is considered a promising alternative to conventional public transport (PT). With a fleet of smaller vehicles, DRT provides a much more flexible and convenient service. This characteristic makes DRT also a potential mode of transport to serve students in rural areas. If DRT vehicles are used to serve students, then ...
Urban air mobility (UAM) enabled by electric vertical take-off and landing (eVTOL) aircraft presents an innovative transportation system for mega-cities. An analysis of the techno-economic feasibility of eVTOL air taxis can provide insights into the development and potential impacts of this emerging mobility solution. This study examines eVTOL configurations and proposes a conceptual model for ...
Check the current status of services and components for Cisco's cloud-based Webex, Security and IoT offerings. Cisco Support Assistant. The Cisco Support Assistant (formerly TAC Connect Bot) provides a self-service experience for common case inquiries and basic transactions without waiting in a queue.
Ed Brown at [email protected] or on +44 7850 071 287. For press information about Hitachi Rail, please go here. Thales (Euronext Paris: HO) announces the completion on 31 May 2024 of the sale of its Ground Transportation Systems Global Business Unit for an enterprise value of € 1,660 million to Hitachi Rail.
Informal public transport services, as the term suggests, refer to public transport services that are provided differently as compared to the typical government-provided bus- and rail-based transport in cities. Existing mostly in the form of shared services, informal transport modes use vehicles with seating capacity ranging from 3 to 20 people.