
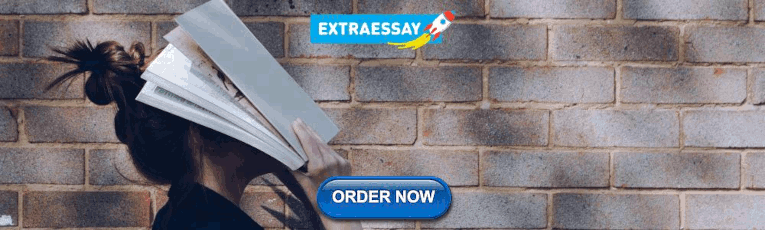
Research Variables 101
Independent variables, dependent variables, control variables and more
By: Derek Jansen (MBA) | Expert Reviewed By: Kerryn Warren (PhD) | January 2023
If you’re new to the world of research, especially scientific research, you’re bound to run into the concept of variables , sooner or later. If you’re feeling a little confused, don’t worry – you’re not the only one! Independent variables, dependent variables, confounding variables – it’s a lot of jargon. In this post, we’ll unpack the terminology surrounding research variables using straightforward language and loads of examples .
Overview: Variables In Research
What (exactly) is a variable.
The simplest way to understand a variable is as any characteristic or attribute that can experience change or vary over time or context – hence the name “variable”. For example, the dosage of a particular medicine could be classified as a variable, as the amount can vary (i.e., a higher dose or a lower dose). Similarly, gender, age or ethnicity could be considered demographic variables, because each person varies in these respects.
Within research, especially scientific research, variables form the foundation of studies, as researchers are often interested in how one variable impacts another, and the relationships between different variables. For example:
- How someone’s age impacts their sleep quality
- How different teaching methods impact learning outcomes
- How diet impacts weight (gain or loss)
As you can see, variables are often used to explain relationships between different elements and phenomena. In scientific studies, especially experimental studies, the objective is often to understand the causal relationships between variables. In other words, the role of cause and effect between variables. This is achieved by manipulating certain variables while controlling others – and then observing the outcome. But, we’ll get into that a little later…
The “Big 3” Variables
Variables can be a little intimidating for new researchers because there are a wide variety of variables, and oftentimes, there are multiple labels for the same thing. To lay a firm foundation, we’ll first look at the three main types of variables, namely:
- Independent variables (IV)
- Dependant variables (DV)
- Control variables
What is an independent variable?
Simply put, the independent variable is the “ cause ” in the relationship between two (or more) variables. In other words, when the independent variable changes, it has an impact on another variable.
For example:
- Increasing the dosage of a medication (Variable A) could result in better (or worse) health outcomes for a patient (Variable B)
- Changing a teaching method (Variable A) could impact the test scores that students earn in a standardised test (Variable B)
- Varying one’s diet (Variable A) could result in weight loss or gain (Variable B).
It’s useful to know that independent variables can go by a few different names, including, explanatory variables (because they explain an event or outcome) and predictor variables (because they predict the value of another variable). Terminology aside though, the most important takeaway is that independent variables are assumed to be the “cause” in any cause-effect relationship. As you can imagine, these types of variables are of major interest to researchers, as many studies seek to understand the causal factors behind a phenomenon.
Need a helping hand?
What is a dependent variable?
While the independent variable is the “ cause ”, the dependent variable is the “ effect ” – or rather, the affected variable . In other words, the dependent variable is the variable that is assumed to change as a result of a change in the independent variable.
Keeping with the previous example, let’s look at some dependent variables in action:
- Health outcomes (DV) could be impacted by dosage changes of a medication (IV)
- Students’ scores (DV) could be impacted by teaching methods (IV)
- Weight gain or loss (DV) could be impacted by diet (IV)
In scientific studies, researchers will typically pay very close attention to the dependent variable (or variables), carefully measuring any changes in response to hypothesised independent variables. This can be tricky in practice, as it’s not always easy to reliably measure specific phenomena or outcomes – or to be certain that the actual cause of the change is in fact the independent variable.
As the adage goes, correlation is not causation . In other words, just because two variables have a relationship doesn’t mean that it’s a causal relationship – they may just happen to vary together. For example, you could find a correlation between the number of people who own a certain brand of car and the number of people who have a certain type of job. Just because the number of people who own that brand of car and the number of people who have that type of job is correlated, it doesn’t mean that owning that brand of car causes someone to have that type of job or vice versa. The correlation could, for example, be caused by another factor such as income level or age group, which would affect both car ownership and job type.
To confidently establish a causal relationship between an independent variable and a dependent variable (i.e., X causes Y), you’ll typically need an experimental design , where you have complete control over the environmen t and the variables of interest. But even so, this doesn’t always translate into the “real world”. Simply put, what happens in the lab sometimes stays in the lab!
As an alternative to pure experimental research, correlational or “ quasi-experimental ” research (where the researcher cannot manipulate or change variables) can be done on a much larger scale more easily, allowing one to understand specific relationships in the real world. These types of studies also assume some causality between independent and dependent variables, but it’s not always clear. So, if you go this route, you need to be cautious in terms of how you describe the impact and causality between variables and be sure to acknowledge any limitations in your own research.
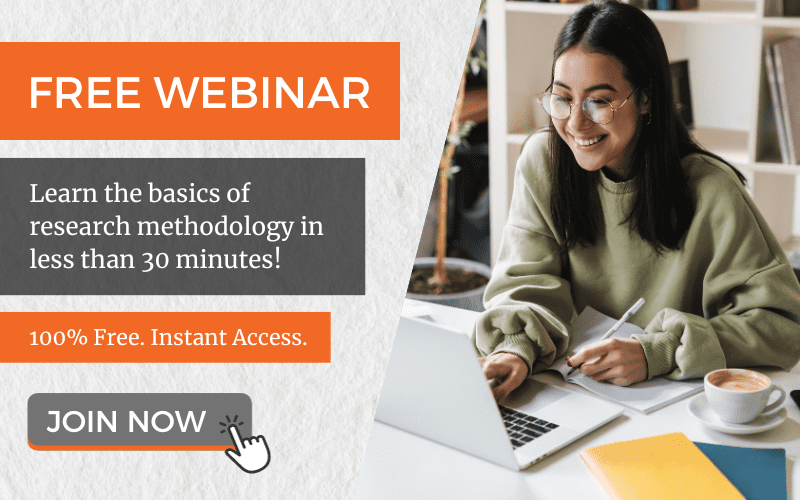
What is a control variable?
In an experimental design, a control variable (or controlled variable) is a variable that is intentionally held constant to ensure it doesn’t have an influence on any other variables. As a result, this variable remains unchanged throughout the course of the study. In other words, it’s a variable that’s not allowed to vary – tough life 🙂
As we mentioned earlier, one of the major challenges in identifying and measuring causal relationships is that it’s difficult to isolate the impact of variables other than the independent variable. Simply put, there’s always a risk that there are factors beyond the ones you’re specifically looking at that might be impacting the results of your study. So, to minimise the risk of this, researchers will attempt (as best possible) to hold other variables constant . These factors are then considered control variables.
Some examples of variables that you may need to control include:
- Temperature
- Time of day
- Noise or distractions
Which specific variables need to be controlled for will vary tremendously depending on the research project at hand, so there’s no generic list of control variables to consult. As a researcher, you’ll need to think carefully about all the factors that could vary within your research context and then consider how you’ll go about controlling them. A good starting point is to look at previous studies similar to yours and pay close attention to which variables they controlled for.
Of course, you won’t always be able to control every possible variable, and so, in many cases, you’ll just have to acknowledge their potential impact and account for them in the conclusions you draw. Every study has its limitations , so don’t get fixated or discouraged by troublesome variables. Nevertheless, always think carefully about the factors beyond what you’re focusing on – don’t make assumptions!
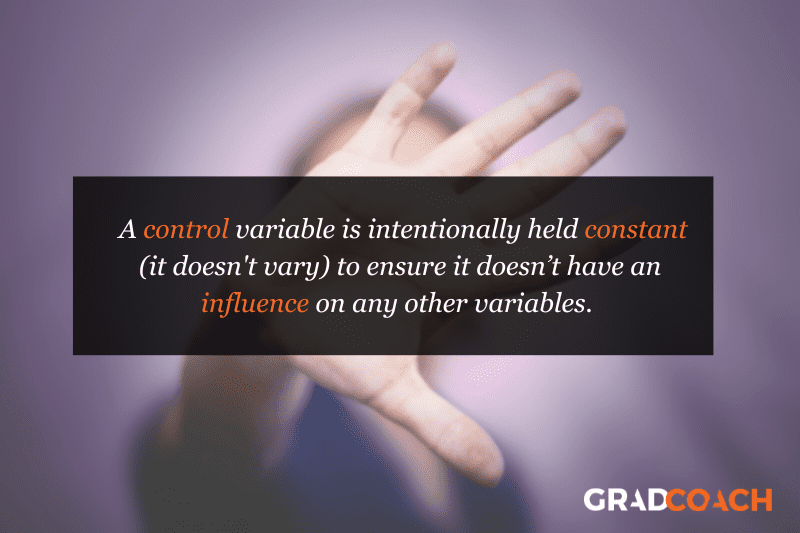
Other types of variables
As we mentioned, independent, dependent and control variables are the most common variables you’ll come across in your research, but they’re certainly not the only ones you need to be aware of. Next, we’ll look at a few “secondary” variables that you need to keep in mind as you design your research.
- Moderating variables
- Mediating variables
- Confounding variables
- Latent variables
Let’s jump into it…
What is a moderating variable?
A moderating variable is a variable that influences the strength or direction of the relationship between an independent variable and a dependent variable. In other words, moderating variables affect how much (or how little) the IV affects the DV, or whether the IV has a positive or negative relationship with the DV (i.e., moves in the same or opposite direction).
For example, in a study about the effects of sleep deprivation on academic performance, gender could be used as a moderating variable to see if there are any differences in how men and women respond to a lack of sleep. In such a case, one may find that gender has an influence on how much students’ scores suffer when they’re deprived of sleep.
It’s important to note that while moderators can have an influence on outcomes , they don’t necessarily cause them ; rather they modify or “moderate” existing relationships between other variables. This means that it’s possible for two different groups with similar characteristics, but different levels of moderation, to experience very different results from the same experiment or study design.
What is a mediating variable?
Mediating variables are often used to explain the relationship between the independent and dependent variable (s). For example, if you were researching the effects of age on job satisfaction, then education level could be considered a mediating variable, as it may explain why older people have higher job satisfaction than younger people – they may have more experience or better qualifications, which lead to greater job satisfaction.
Mediating variables also help researchers understand how different factors interact with each other to influence outcomes. For instance, if you wanted to study the effect of stress on academic performance, then coping strategies might act as a mediating factor by influencing both stress levels and academic performance simultaneously. For example, students who use effective coping strategies might be less stressed but also perform better academically due to their improved mental state.
In addition, mediating variables can provide insight into causal relationships between two variables by helping researchers determine whether changes in one factor directly cause changes in another – or whether there is an indirect relationship between them mediated by some third factor(s). For instance, if you wanted to investigate the impact of parental involvement on student achievement, you would need to consider family dynamics as a potential mediator, since it could influence both parental involvement and student achievement simultaneously.
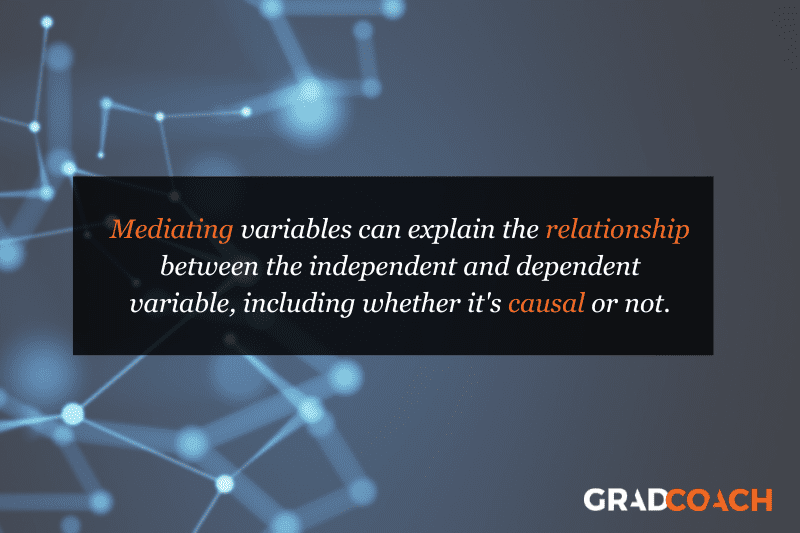
What is a confounding variable?
A confounding variable (also known as a third variable or lurking variable ) is an extraneous factor that can influence the relationship between two variables being studied. Specifically, for a variable to be considered a confounding variable, it needs to meet two criteria:
- It must be correlated with the independent variable (this can be causal or not)
- It must have a causal impact on the dependent variable (i.e., influence the DV)
Some common examples of confounding variables include demographic factors such as gender, ethnicity, socioeconomic status, age, education level, and health status. In addition to these, there are also environmental factors to consider. For example, air pollution could confound the impact of the variables of interest in a study investigating health outcomes.
Naturally, it’s important to identify as many confounding variables as possible when conducting your research, as they can heavily distort the results and lead you to draw incorrect conclusions . So, always think carefully about what factors may have a confounding effect on your variables of interest and try to manage these as best you can.
What is a latent variable?
Latent variables are unobservable factors that can influence the behaviour of individuals and explain certain outcomes within a study. They’re also known as hidden or underlying variables , and what makes them rather tricky is that they can’t be directly observed or measured . Instead, latent variables must be inferred from other observable data points such as responses to surveys or experiments.
For example, in a study of mental health, the variable “resilience” could be considered a latent variable. It can’t be directly measured , but it can be inferred from measures of mental health symptoms, stress, and coping mechanisms. The same applies to a lot of concepts we encounter every day – for example:
- Emotional intelligence
- Quality of life
- Business confidence
- Ease of use
One way in which we overcome the challenge of measuring the immeasurable is latent variable models (LVMs). An LVM is a type of statistical model that describes a relationship between observed variables and one or more unobserved (latent) variables. These models allow researchers to uncover patterns in their data which may not have been visible before, thanks to their complexity and interrelatedness with other variables. Those patterns can then inform hypotheses about cause-and-effect relationships among those same variables which were previously unknown prior to running the LVM. Powerful stuff, we say!
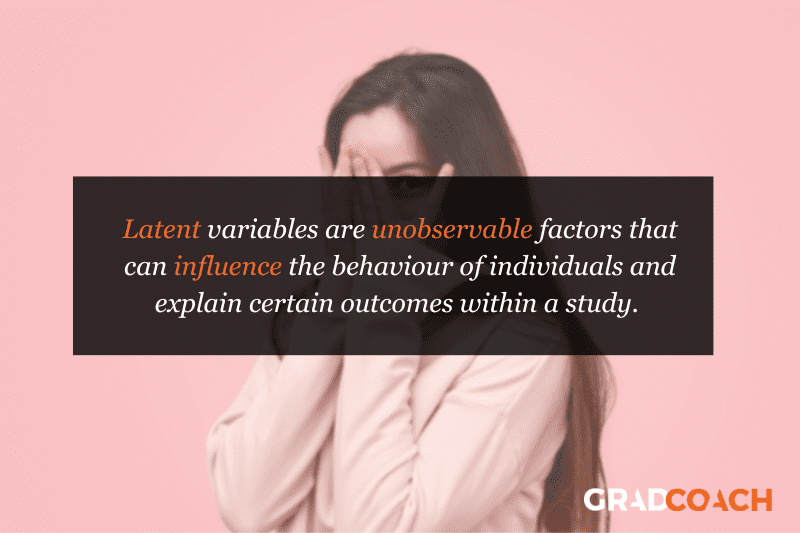
Let’s recap
In the world of scientific research, there’s no shortage of variable types, some of which have multiple names and some of which overlap with each other. In this post, we’ve covered some of the popular ones, but remember that this is not an exhaustive list .
To recap, we’ve explored:
- Independent variables (the “cause”)
- Dependent variables (the “effect”)
- Control variables (the variable that’s not allowed to vary)
If you’re still feeling a bit lost and need a helping hand with your research project, check out our 1-on-1 coaching service , where we guide you through each step of the research journey. Also, be sure to check out our free dissertation writing course and our collection of free, fully-editable chapter templates .
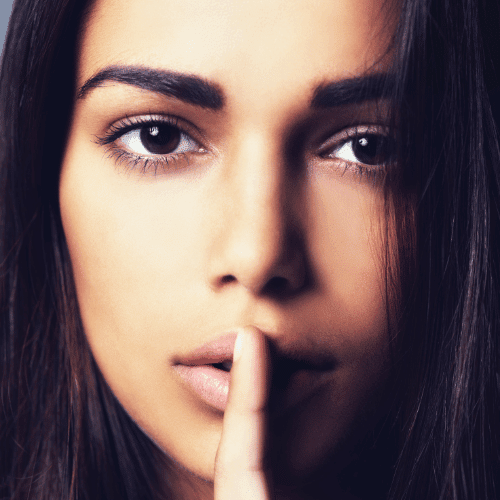
Psst... there’s more!
This post was based on one of our popular Research Bootcamps . If you're working on a research project, you'll definitely want to check this out ...
You Might Also Like:
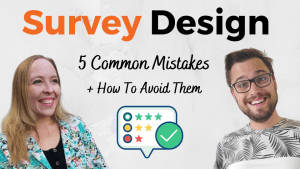
Very informative, concise and helpful. Thank you
Helping information.Thanks
practical and well-demonstrated
Very helpful and insightful
Submit a Comment Cancel reply
Your email address will not be published. Required fields are marked *
Save my name, email, and website in this browser for the next time I comment.
- Print Friendly
- USC Libraries
- Research Guides
Organizing Your Social Sciences Research Paper
- Independent and Dependent Variables
- Purpose of Guide
- Design Flaws to Avoid
- Glossary of Research Terms
- Reading Research Effectively
- Narrowing a Topic Idea
- Broadening a Topic Idea
- Extending the Timeliness of a Topic Idea
- Academic Writing Style
- Applying Critical Thinking
- Choosing a Title
- Making an Outline
- Paragraph Development
- Research Process Video Series
- Executive Summary
- The C.A.R.S. Model
- Background Information
- The Research Problem/Question
- Theoretical Framework
- Citation Tracking
- Content Alert Services
- Evaluating Sources
- Primary Sources
- Secondary Sources
- Tiertiary Sources
- Scholarly vs. Popular Publications
- Qualitative Methods
- Quantitative Methods
- Insiderness
- Using Non-Textual Elements
- Limitations of the Study
- Common Grammar Mistakes
- Writing Concisely
- Avoiding Plagiarism
- Footnotes or Endnotes?
- Further Readings
- Generative AI and Writing
- USC Libraries Tutorials and Other Guides
- Bibliography
Definitions
Dependent Variable The variable that depends on other factors that are measured. These variables are expected to change as a result of an experimental manipulation of the independent variable or variables. It is the presumed effect.
Independent Variable The variable that is stable and unaffected by the other variables you are trying to measure. It refers to the condition of an experiment that is systematically manipulated by the investigator. It is the presumed cause.
Cramer, Duncan and Dennis Howitt. The SAGE Dictionary of Statistics . London: SAGE, 2004; Penslar, Robin Levin and Joan P. Porter. Institutional Review Board Guidebook: Introduction . Washington, DC: United States Department of Health and Human Services, 2010; "What are Dependent and Independent Variables?" Graphic Tutorial.
Identifying Dependent and Independent Variables
Don't feel bad if you are confused about what is the dependent variable and what is the independent variable in social and behavioral sciences research . However, it's important that you learn the difference because framing a study using these variables is a common approach to organizing the elements of a social sciences research study in order to discover relevant and meaningful results. Specifically, it is important for these two reasons:
- You need to understand and be able to evaluate their application in other people's research.
- You need to apply them correctly in your own research.
A variable in research simply refers to a person, place, thing, or phenomenon that you are trying to measure in some way. The best way to understand the difference between a dependent and independent variable is that the meaning of each is implied by what the words tell us about the variable you are using. You can do this with a simple exercise from the website, Graphic Tutorial. Take the sentence, "The [independent variable] causes a change in [dependent variable] and it is not possible that [dependent variable] could cause a change in [independent variable]." Insert the names of variables you are using in the sentence in the way that makes the most sense. This will help you identify each type of variable. If you're still not sure, consult with your professor before you begin to write.
Fan, Shihe. "Independent Variable." In Encyclopedia of Research Design. Neil J. Salkind, editor. (Thousand Oaks, CA: SAGE, 2010), pp. 592-594; "What are Dependent and Independent Variables?" Graphic Tutorial; Salkind, Neil J. "Dependent Variable." In Encyclopedia of Research Design , Neil J. Salkind, editor. (Thousand Oaks, CA: SAGE, 2010), pp. 348-349;
Structure and Writing Style
The process of examining a research problem in the social and behavioral sciences is often framed around methods of analysis that compare, contrast, correlate, average, or integrate relationships between or among variables . Techniques include associations, sampling, random selection, and blind selection. Designation of the dependent and independent variable involves unpacking the research problem in a way that identifies a general cause and effect and classifying these variables as either independent or dependent.
The variables should be outlined in the introduction of your paper and explained in more detail in the methods section . There are no rules about the structure and style for writing about independent or dependent variables but, as with any academic writing, clarity and being succinct is most important.
After you have described the research problem and its significance in relation to prior research, explain why you have chosen to examine the problem using a method of analysis that investigates the relationships between or among independent and dependent variables . State what it is about the research problem that lends itself to this type of analysis. For example, if you are investigating the relationship between corporate environmental sustainability efforts [the independent variable] and dependent variables associated with measuring employee satisfaction at work using a survey instrument, you would first identify each variable and then provide background information about the variables. What is meant by "environmental sustainability"? Are you looking at a particular company [e.g., General Motors] or are you investigating an industry [e.g., the meat packing industry]? Why is employee satisfaction in the workplace important? How does a company make their employees aware of sustainability efforts and why would a company even care that its employees know about these efforts?
Identify each variable for the reader and define each . In the introduction, this information can be presented in a paragraph or two when you describe how you are going to study the research problem. In the methods section, you build on the literature review of prior studies about the research problem to describe in detail background about each variable, breaking each down for measurement and analysis. For example, what activities do you examine that reflect a company's commitment to environmental sustainability? Levels of employee satisfaction can be measured by a survey that asks about things like volunteerism or a desire to stay at the company for a long time.
The structure and writing style of describing the variables and their application to analyzing the research problem should be stated and unpacked in such a way that the reader obtains a clear understanding of the relationships between the variables and why they are important. This is also important so that the study can be replicated in the future using the same variables but applied in a different way.
Fan, Shihe. "Independent Variable." In Encyclopedia of Research Design. Neil J. Salkind, editor. (Thousand Oaks, CA: SAGE, 2010), pp. 592-594; "What are Dependent and Independent Variables?" Graphic Tutorial; “Case Example for Independent and Dependent Variables.” ORI Curriculum Examples. U.S. Department of Health and Human Services, Office of Research Integrity; Salkind, Neil J. "Dependent Variable." In Encyclopedia of Research Design , Neil J. Salkind, editor. (Thousand Oaks, CA: SAGE, 2010), pp. 348-349; “Independent Variables and Dependent Variables.” Karl L. Wuensch, Department of Psychology, East Carolina University [posted email exchange]; “Variables.” Elements of Research. Dr. Camille Nebeker, San Diego State University.
- << Previous: Design Flaws to Avoid
- Next: Glossary of Research Terms >>
- Last Updated: May 30, 2024 9:38 AM
- URL: https://libguides.usc.edu/writingguide
Independent and Dependent Variables
Saul Mcleod, PhD
Editor-in-Chief for Simply Psychology
BSc (Hons) Psychology, MRes, PhD, University of Manchester
Saul Mcleod, PhD., is a qualified psychology teacher with over 18 years of experience in further and higher education. He has been published in peer-reviewed journals, including the Journal of Clinical Psychology.
Learn about our Editorial Process
Olivia Guy-Evans, MSc
Associate Editor for Simply Psychology
BSc (Hons) Psychology, MSc Psychology of Education
Olivia Guy-Evans is a writer and associate editor for Simply Psychology. She has previously worked in healthcare and educational sectors.
On This Page:
In research, a variable is any characteristic, number, or quantity that can be measured or counted in experimental investigations . One is called the dependent variable, and the other is the independent variable.
In research, the independent variable is manipulated to observe its effect, while the dependent variable is the measured outcome. Essentially, the independent variable is the presumed cause, and the dependent variable is the observed effect.
Variables provide the foundation for examining relationships, drawing conclusions, and making predictions in research studies.

Independent Variable
In psychology, the independent variable is the variable the experimenter manipulates or changes and is assumed to directly affect the dependent variable.
It’s considered the cause or factor that drives change, allowing psychologists to observe how it influences behavior, emotions, or other dependent variables in an experimental setting. Essentially, it’s the presumed cause in cause-and-effect relationships being studied.
For example, allocating participants to drug or placebo conditions (independent variable) to measure any changes in the intensity of their anxiety (dependent variable).
In a well-designed experimental study , the independent variable is the only important difference between the experimental (e.g., treatment) and control (e.g., placebo) groups.
By changing the independent variable and holding other factors constant, psychologists aim to determine if it causes a change in another variable, called the dependent variable.
For example, in a study investigating the effects of sleep on memory, the amount of sleep (e.g., 4 hours, 8 hours, 12 hours) would be the independent variable, as the researcher might manipulate or categorize it to see its impact on memory recall, which would be the dependent variable.
Dependent Variable
In psychology, the dependent variable is the variable being tested and measured in an experiment and is “dependent” on the independent variable.
In psychology, a dependent variable represents the outcome or results and can change based on the manipulations of the independent variable. Essentially, it’s the presumed effect in a cause-and-effect relationship being studied.
An example of a dependent variable is depression symptoms, which depend on the independent variable (type of therapy).
In an experiment, the researcher looks for the possible effect on the dependent variable that might be caused by changing the independent variable.
For instance, in a study examining the effects of a new study technique on exam performance, the technique would be the independent variable (as it is being introduced or manipulated), while the exam scores would be the dependent variable (as they represent the outcome of interest that’s being measured).
Examples in Research Studies
For example, we might change the type of information (e.g., organized or random) given to participants to see how this might affect the amount of information remembered.
In this example, the type of information is the independent variable (because it changes), and the amount of information remembered is the dependent variable (because this is being measured).
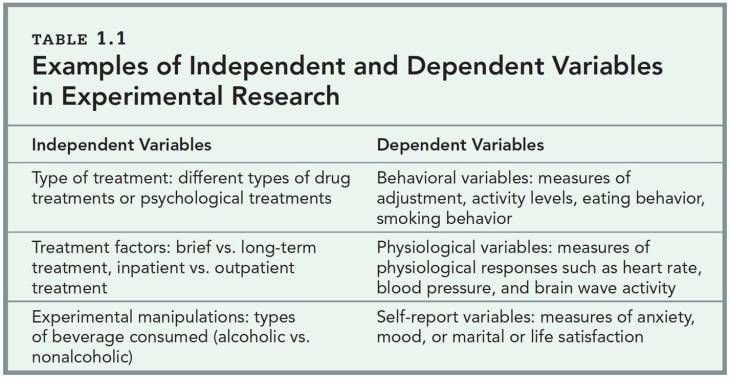
For the following hypotheses, name the IV and the DV.
1. Lack of sleep significantly affects learning in 10-year-old boys.
IV……………………………………………………
DV…………………………………………………..
2. Social class has a significant effect on IQ scores.
DV……………………………………………….…
3. Stressful experiences significantly increase the likelihood of headaches.
4. Time of day has a significant effect on alertness.
Operationalizing Variables
To ensure cause and effect are established, it is important that we identify exactly how the independent and dependent variables will be measured; this is known as operationalizing the variables.
Operational variables (or operationalizing definitions) refer to how you will define and measure a specific variable as it is used in your study. This enables another psychologist to replicate your research and is essential in establishing reliability (achieving consistency in the results).
For example, if we are concerned with the effect of media violence on aggression, then we need to be very clear about what we mean by the different terms. In this case, we must state what we mean by the terms “media violence” and “aggression” as we will study them.
Therefore, you could state that “media violence” is operationally defined (in your experiment) as ‘exposure to a 15-minute film showing scenes of physical assault’; “aggression” is operationally defined as ‘levels of electrical shocks administered to a second ‘participant’ in another room.
In another example, the hypothesis “Young participants will have significantly better memories than older participants” is not operationalized. How do we define “young,” “old,” or “memory”? “Participants aged between 16 – 30 will recall significantly more nouns from a list of twenty than participants aged between 55 – 70” is operationalized.
The key point here is that we have clarified what we mean by the terms as they were studied and measured in our experiment.
If we didn’t do this, it would be very difficult (if not impossible) to compare the findings of different studies to the same behavior.
Operationalization has the advantage of generally providing a clear and objective definition of even complex variables. It also makes it easier for other researchers to replicate a study and check for reliability .
For the following hypotheses, name the IV and the DV and operationalize both variables.
1. Women are more attracted to men without earrings than men with earrings.
I.V._____________________________________________________________
D.V. ____________________________________________________________
Operational definitions:
I.V. ____________________________________________________________
2. People learn more when they study in a quiet versus noisy place.
I.V. _________________________________________________________
D.V. ___________________________________________________________
3. People who exercise regularly sleep better at night.
Can there be more than one independent or dependent variable in a study?
Yes, it is possible to have more than one independent or dependent variable in a study.
In some studies, researchers may want to explore how multiple factors affect the outcome, so they include more than one independent variable.
Similarly, they may measure multiple things to see how they are influenced, resulting in multiple dependent variables. This allows for a more comprehensive understanding of the topic being studied.
What are some ethical considerations related to independent and dependent variables?
Ethical considerations related to independent and dependent variables involve treating participants fairly and protecting their rights.
Researchers must ensure that participants provide informed consent and that their privacy and confidentiality are respected. Additionally, it is important to avoid manipulating independent variables in ways that could cause harm or discomfort to participants.
Researchers should also consider the potential impact of their study on vulnerable populations and ensure that their methods are unbiased and free from discrimination.
Ethical guidelines help ensure that research is conducted responsibly and with respect for the well-being of the participants involved.
Can qualitative data have independent and dependent variables?
Yes, both quantitative and qualitative data can have independent and dependent variables.
In quantitative research, independent variables are usually measured numerically and manipulated to understand their impact on the dependent variable. In qualitative research, independent variables can be qualitative in nature, such as individual experiences, cultural factors, or social contexts, influencing the phenomenon of interest.
The dependent variable, in both cases, is what is being observed or studied to see how it changes in response to the independent variable.
So, regardless of the type of data, researchers analyze the relationship between independent and dependent variables to gain insights into their research questions.
Can the same variable be independent in one study and dependent in another?
Yes, the same variable can be independent in one study and dependent in another.
The classification of a variable as independent or dependent depends on how it is used within a specific study. In one study, a variable might be manipulated or controlled to see its effect on another variable, making it independent.
However, in a different study, that same variable might be the one being measured or observed to understand its relationship with another variable, making it dependent.
The role of a variable as independent or dependent can vary depending on the research question and study design.

Related Articles
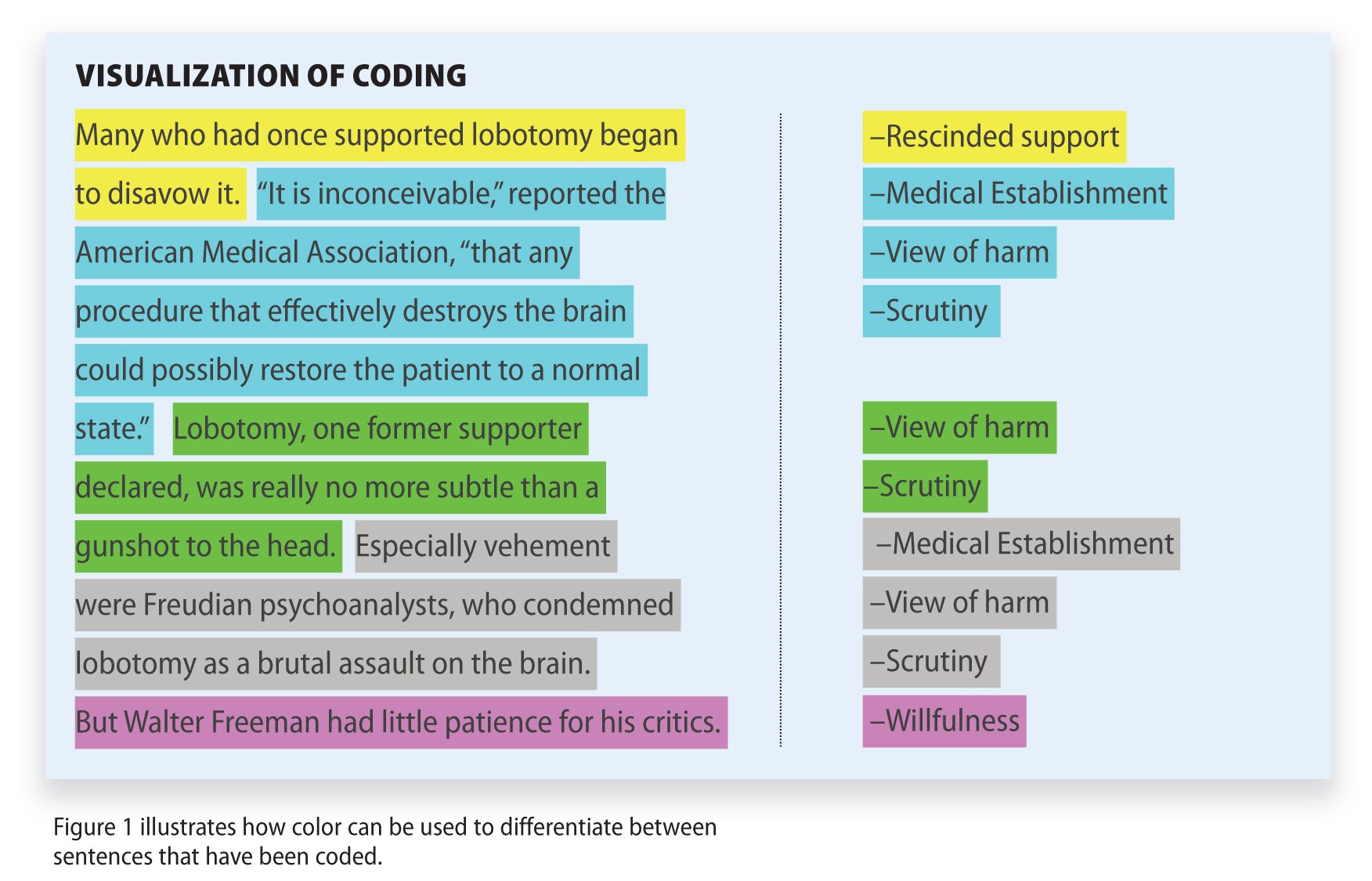
Research Methodology
Qualitative Data Coding
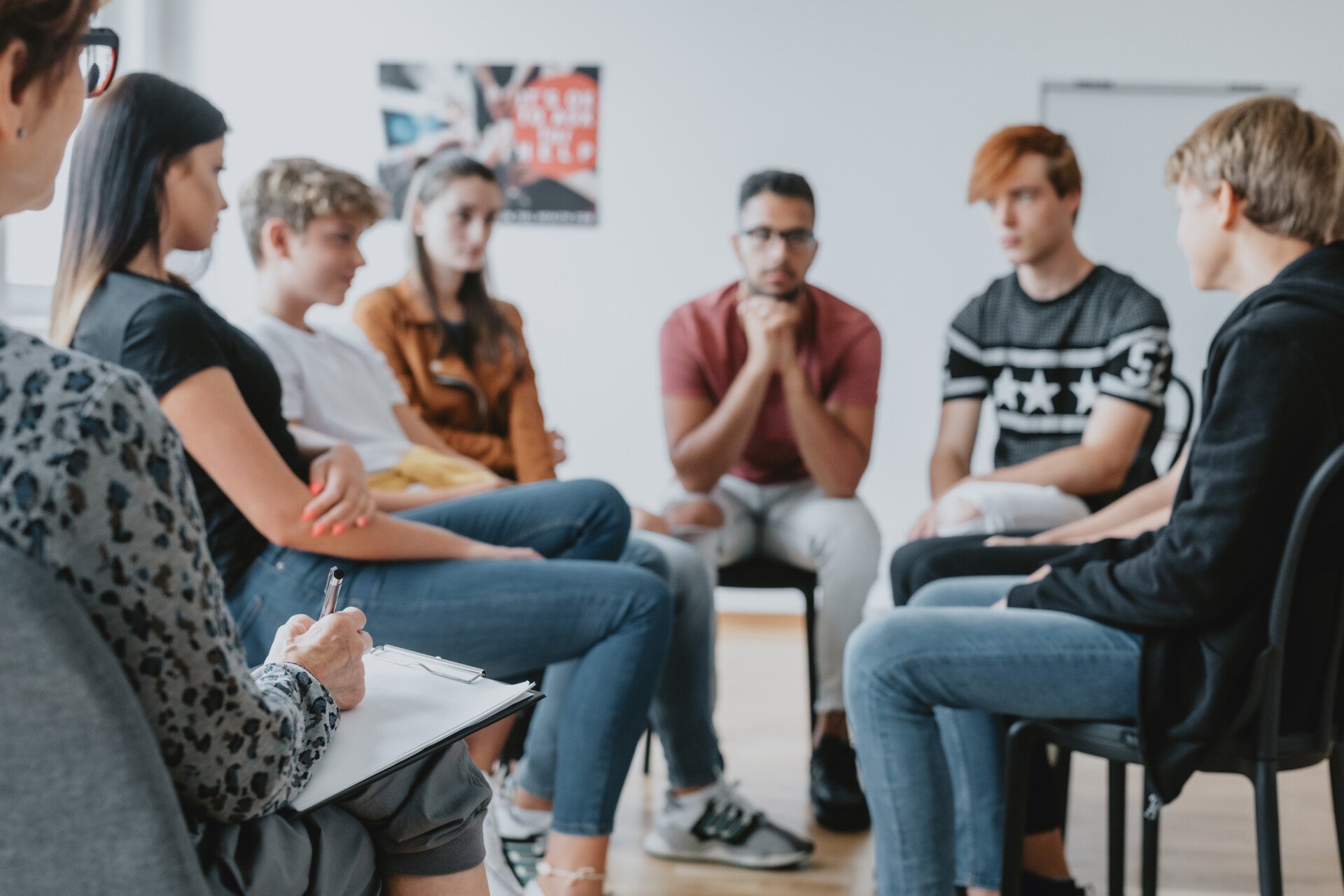
What Is a Focus Group?
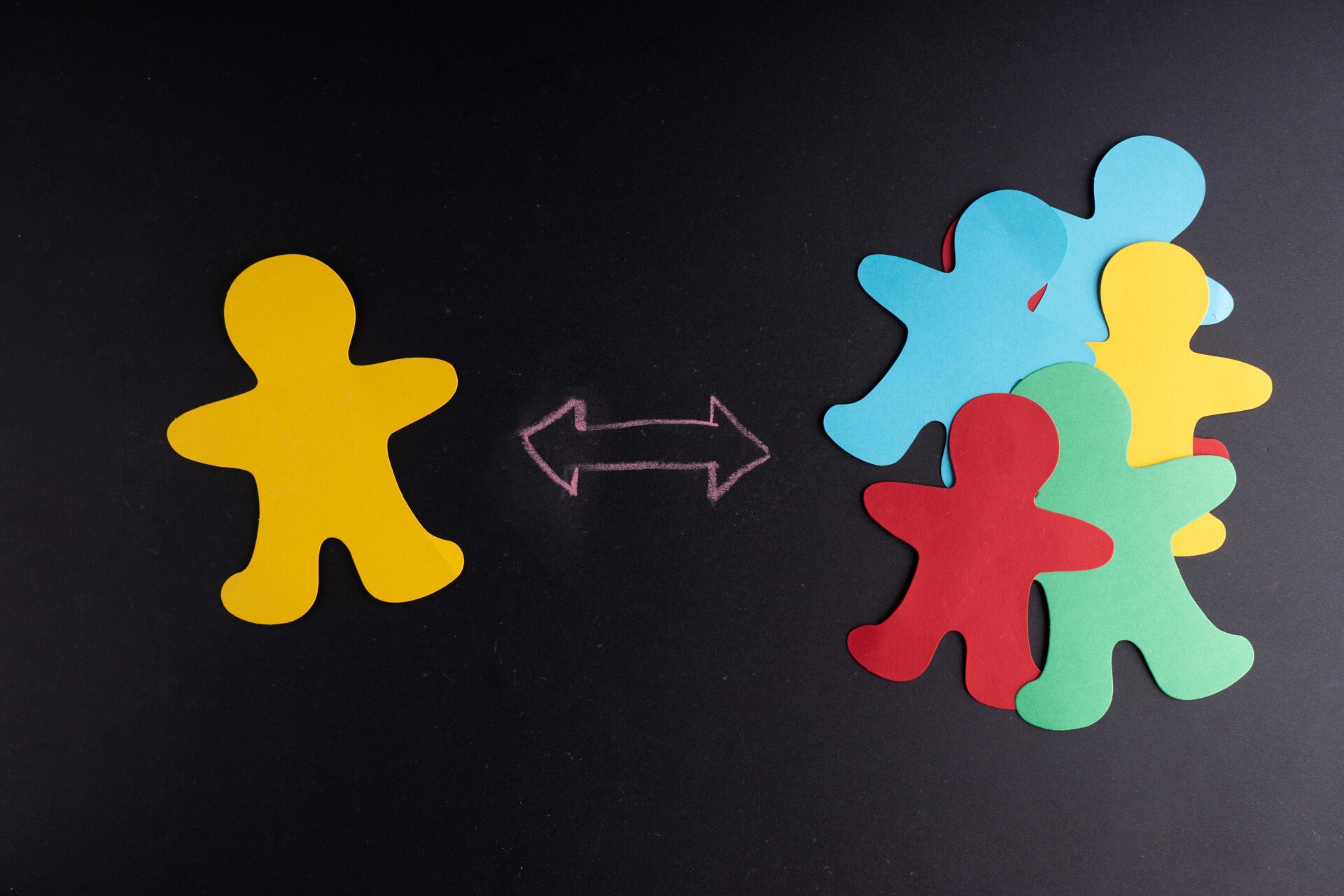
Cross-Cultural Research Methodology In Psychology
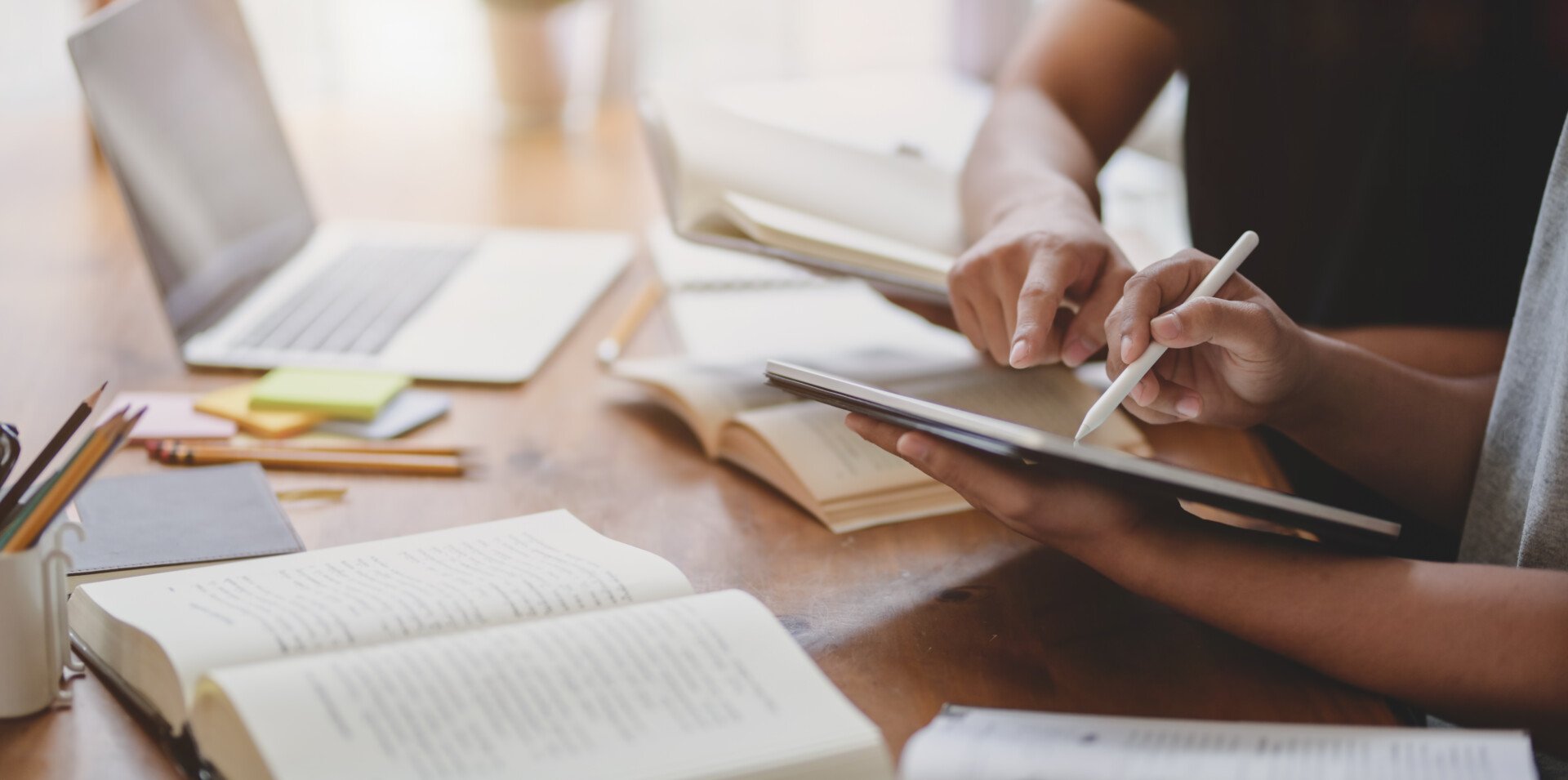
What Is Internal Validity In Research?
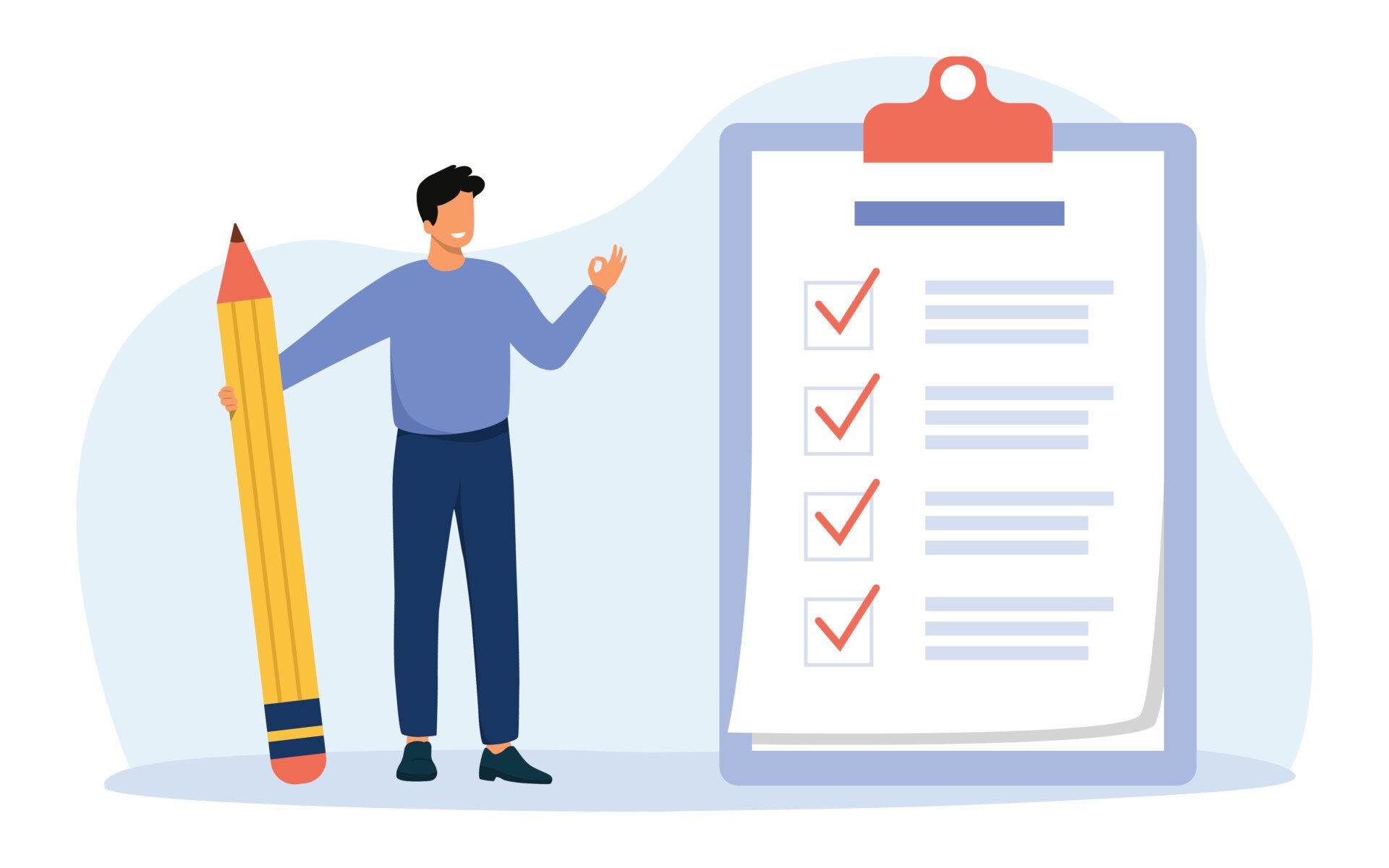
Research Methodology , Statistics
What Is Face Validity In Research? Importance & How To Measure
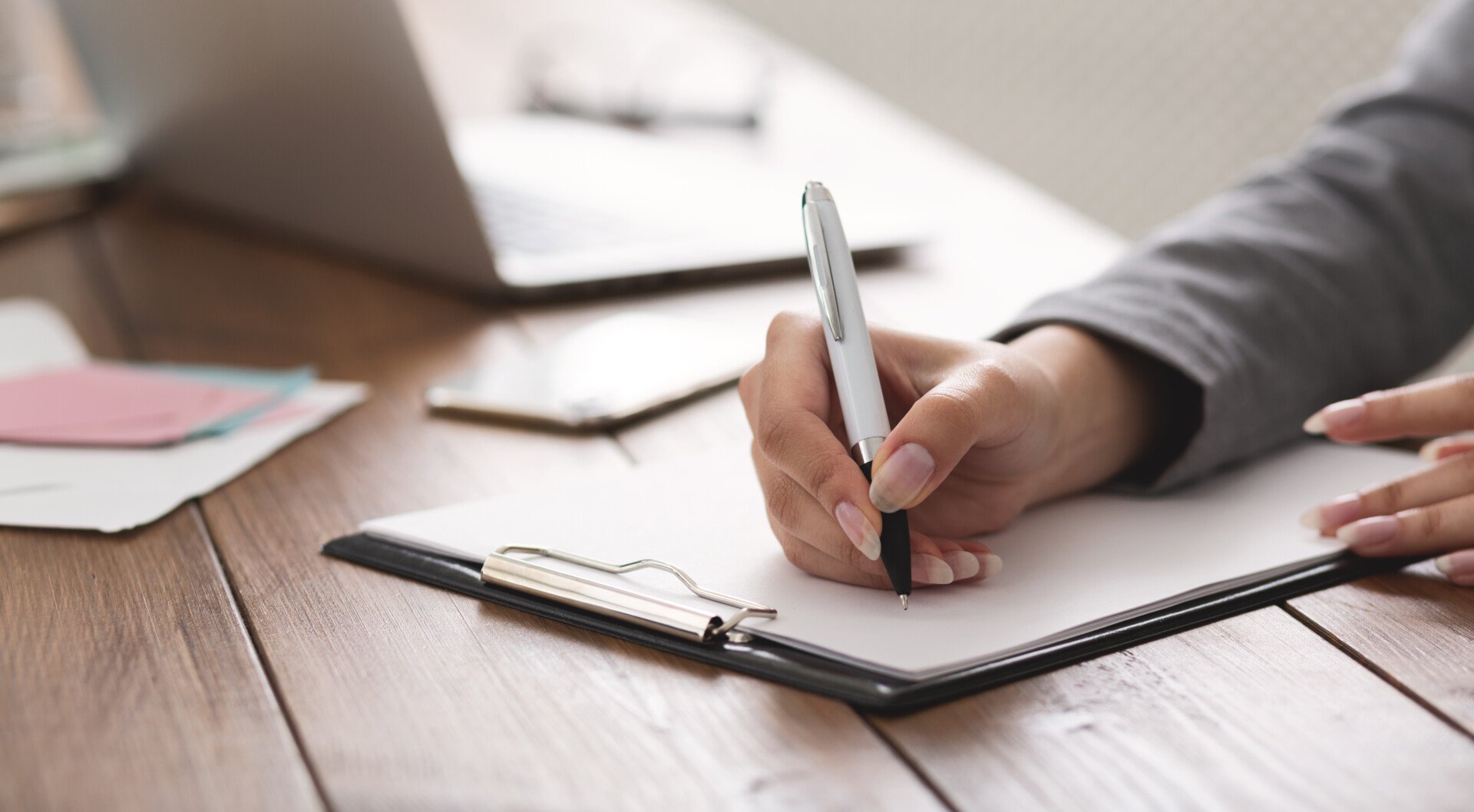
Criterion Validity: Definition & Examples

An official website of the United States government
The .gov means it’s official. Federal government websites often end in .gov or .mil. Before sharing sensitive information, make sure you’re on a federal government site.
The site is secure. The https:// ensures that you are connecting to the official website and that any information you provide is encrypted and transmitted securely.
- Publications
- Account settings
Preview improvements coming to the PMC website in October 2024. Learn More or Try it out now .
- Advanced Search
- Journal List
- Indian J Psychol Med
- v.43(2); 2021 Mar
A Student’s Guide to the Classification and Operationalization of Variables in the Conceptualization and Design of a Clinical Study: Part 1
Chittaranjan andrade.
1 Dept. of Clinical Psychopharmacology and Neurotoxicology, National Institute of Mental Health and Neurosciences, Bengaluru, Karnataka, India.
Students without prior research experience may not know how to conceptualize and design a study. This article explains how an understanding of the classification and operationalization of variables is the key to the process. Variables describe aspects of the sample that is under study; they are so called because they vary in value from subject to subject in the sample. Variables may be independent or dependent. Independent variables influence the value of other variables; dependent variables are influenced in value by other variables. A hypothesis states an expected relationship between variables. A significant relationship between an independent and dependent variable does not prove cause and effect; the relationship may partly or wholly be explained by one or more confounding variables. Variables need to be operationalized; that is, defined in a way that permits their accurate measurement. These and other concepts are explained with the help of clinically relevant examples.
Key Message:
This article explains the following concepts: Independent variables, dependent variables, confounding variables, operationalization of variables, and construction of hypotheses.
In any body of research, the subject of study requires to be described and understood. For example, if we wish to study predictors of response to antidepressant drugs (ADs) in patients with major depressive disorder (MDD), we might select patient age, sex, age at onset of MDD, number of previous episodes of depression, duration of current depressive episode, presence of psychotic symptoms, past history of response to ADs, and other patient and illness characteristics as potential predictors. These characteristics or descriptors are called variables. Whether or not the patient responds to AD treatment is also a variable. A solid understanding of variables is the cornerstone in the conceptualization and preparation of a research protocol, and in the framing of study hypotheses. This subject is presented in two parts. This article, Part 1, explains what independent and dependent variables are, how an understanding of these is important in framing hypotheses, and what operationalization of a variable entails.
Variables are defined as characteristics of the sample that are examined, measured, described, and interpreted. Variables are so called because they vary in value from subject to subject in the study. As an example, if we wish to examine the relationship between age and height in a sample of children, age and height are the variables of interest; their values vary from child to child. In the earlier example, patients vary in age, sex, duration of current depressive episode, and response to ADs. Variables are classified as dependent and independent variables and are usually analyzed as categorical or continuous variables.
Independent and Dependent Variables
Independent variables are defined as those the values of which influence other variables. For example, age, sex, current smoking, LDL cholesterol level, and blood pressure are independent variables because their values (e.g., greater age, positive for current smoking, and higher LDL cholesterol level) influence the risk of myocardial infarction. Dependent variables are defined as those the values of which are influenced by other variables. For example, the risk of myocardial infarction is a dependent variable the value of which is influenced by variables such as age, sex, current smoking, LDL cholesterol level, and blood pressure. The risk is higher in older persons, in men, in current smokers, and so on.
There may be a cause–effect relationship between independent and dependent variables. For example, consider a clinical trial with treatment (iron supplement vs placebo) as the independent variable and hemoglobin level as the dependent variable. In children with anemia, an iron supplement will raise the hemoglobin level to a greater extent than will placebo; this is a cause–effect relationship because iron is necessary for the synthesis of hemoglobin. However, consider the variables teeth and weight . An alien from outer space who has no knowledge of human physiology may study human children below the age of 5 years and find that, as the number of teeth increases, weight increases. Should the alien conclude that there is a cause–effect relationship here, and that growing teeth causes weight gain? No, because a third variable, age, is a confounding variable 1 – 3 that is responsible for both increase in the number of teeth and increase in weight. In general, therefore, it is more proper to state that independent variables are associated with variations in the values of the dependent variables rather than state that independent variables cause variations in the values of the dependent variables. For causality to be asserted, other criteria must be fulfilled; this is out of the scope of the present article, and interested readers may refer to Schunemann et al. 4
As a side note, here, whether a particular variable is independent or dependent will depend on the question that is being asked. For example, in a study of factors influencing patient satisfaction with outpatient department (OPD) services, patient satisfaction is the dependent variable. But, in a study of factors influencing OPD attendance at a hospital, OPD attendance is the dependent variable, and patient satisfaction is merely one of many possible independent variables that can influence OPD attendance.
Importance of Variables in Stating the Research Objectives
Students must have a clear idea about what they want to study in order to conceptualize and frame a research protocol. The first matters that they need to address are “What are my research questions?” and “What are my hypotheses?” Both questions can be answered only after choosing the dependent variables and then the independent variables for study.
In the case of a student who is interested in studying predictors of AD outcomes in patients with MDD, treatment response is the dependent variable and patient and clinical characteristics are possible independent variables. So, the selection of dependent and independent variables helps defines the objectives of the study:
- To determine whether sociodemographic variables, such as age and sex, predict the outcome of an episode of depression in MDD patients who are treated with an AD.
- To determine whether clinical variables, such as age at onset of depression, number of previous depressive episodes, duration of current depressive episode, and the presence of soft neurological signs, predict the outcome of an episode of depression in MDD patients who are treated with an AD.
Note that in a formal research protocol, the student will need to state all the independent variables and not merely list examples. The student may also choose to include additional independent variables, such as baseline biochemical, psychophysiological, and neuroradiological measures.
Importance of Variables in Framing Hypotheses
A hypothesis is a clear statement of what the researcher expects to find in the study. As an example, a researcher may hypothesize that longer duration of current depression is associated with poorer response to ADs. In this hypothesis, the duration of the current episode of depression is the independent variable and treatment response is the dependent variable. It should be obvious, now, that a hypothesis can also be defined as the statement of an expected relationship between an independent and a dependent variable . Or, expressed visually, (independent variable) (arrow) (dependent variable) = hypothesis.
It would be a waste of time and energy to do a study to examine only one question: whether duration of current depression predicts treatment response. So, it is usual for research protocols to include many independent variables and many dependent variables in the generation of many hypotheses, as shown in Table 1 . Pairing each variable in the “independent variable” column with each variable in the “dependent variable” column would result in the generation of these hypotheses. Table 2 shows how this is done for age. Sets of hypotheses can likewise be constructed for the remaining independent and dependent variables in Table 1 . Importantly, the student must select one of these hypotheses as the primary hypothesis; the remaining hypotheses, no matter how many they are, would be secondary hypotheses. It is necessary to have only one hypothesis as the primary hypothesis in order to calculate the sample size necessary for an adequately powered study and to reduce the risk of false positive findings in the analysis. 5 In rare situations, two hypotheses may be considered equally important and may be stated as coprimary hypotheses.
Independent Variables and Dependent Variables in a Study on Sociodemographic and Clinical Prediction of Response of Major Depressive Disorder to Antidepressant Drug Treatment
Combinations of Age with Dependent Variables in the Generation of Hypotheses
Operationalization of Variables
In Table 1 , suicidality is listed as an independent variable and severity of depression, as a dependent variable. These variables need to be operationalized; that is, stated in a way that explains how they will be measured. Table 3 presents three ways in which suicidality can be measured and four ways in which (reduction in) the severity of depression can be measured. Now, each way of measurement in the “independent variable” column can be paired with a way of measurement in the “dependent variable” column, making a total of 12 possible hypotheses. In like manner, the many variables listed in Table 1 can each be operationalized in several different ways, resulting in the generation of a very large number of hypotheses. As already stated, the student must select only one hypothesis as the primary hypothesis.
Possible Ways of Operationalization of Suicidality and Depression
HAM-D: Hamilton Depression Rating Scale, MADRS: Montgomery–Asberg Depression Rating Scale.
Much thought should be given to the operationalization of variables because variables that are carelessly operationalized will be poorly measured; the data collected will then be of poor quality, and the study will yield unreliable results. For example, socioeconomic status may be operationalized as lower, middle, or upper class, depending on the patient’s monthly income, on the total monthly income of the family, or using a validated socioeconomic status assessment scale that takes into consideration income, education, occupation, and place of residence. The student must choose the method that would best suit the needs of the study, and the method that has the greatest scientific acceptability. However, it is also permissible to operationalize the same variable in many different ways and to include all these different operationalizations in the study, as shown in Table 3 . This is because conceptualizing variables in different ways can help understand the subject of the study in different ways.
Operationalization of variables requires a consideration of the reliability and validity of the method of operationalization; discussions on reliability and validity are out of the scope of this article. Operationalization of variables also requires specification of the scale of measurement: nominal, ordinal, interval, or ratio; this is also out of the scope of the present article. Finally, operationalization of variables can also specify details of the measurement procedure. As an example, in a study on the use of metformin to reduce olanzapine-associated weight gain, we may state that we will obtain the weight of the patient but fail to explain how we will do it. Better would be to state that the same weighing scale will be used. Still better would be to state that we will use a weighing instrument that works on the principle of moving weights on a levered arm, and that the same instrument will be used for all patients. And best would be to add that we will weigh patients, dressed in standard hospital gowns, after they have voided their bladder but before they have eaten breakfast. When the way in which a variable will be measured is defined, measurement of that variable becomes more objective and uniform
Concluding Notes
The next article, Part 2, will address what categorical and continuous variables are, why continuous variables should not be converted into categorical variables and when this rule can be broken, and what confounding variables are.
Declaration of Conflicting Interests: The author declared no potential conflicts of interest with respect to the research, authorship, and/or publication of this article.
Funding: The author received no financial support for the research, authorship, and/or publication of this article.
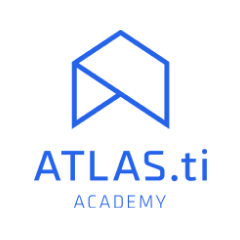
Dependent vs. Independent Variables in Research
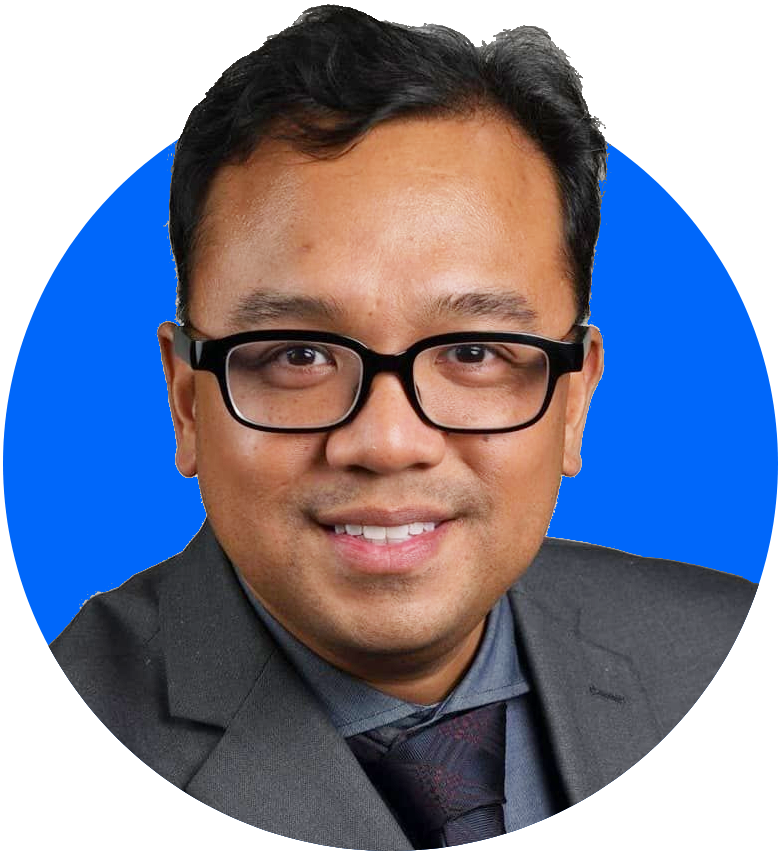
Introduction
Independent and dependent variables in research, can qualitative data have independent and dependent variables.
Experiments rely on capturing the relationship between independent and dependent variables to understand causal patterns. Researchers can observe what happens when they change a condition in their experiment or if there is any effect at all.
It's important to understand the difference between the independent variable and dependent variable. We'll look at the notion of independent and dependent variables in this article. If you are conducting experimental research, defining the variables in your study is essential for realizing rigorous research .
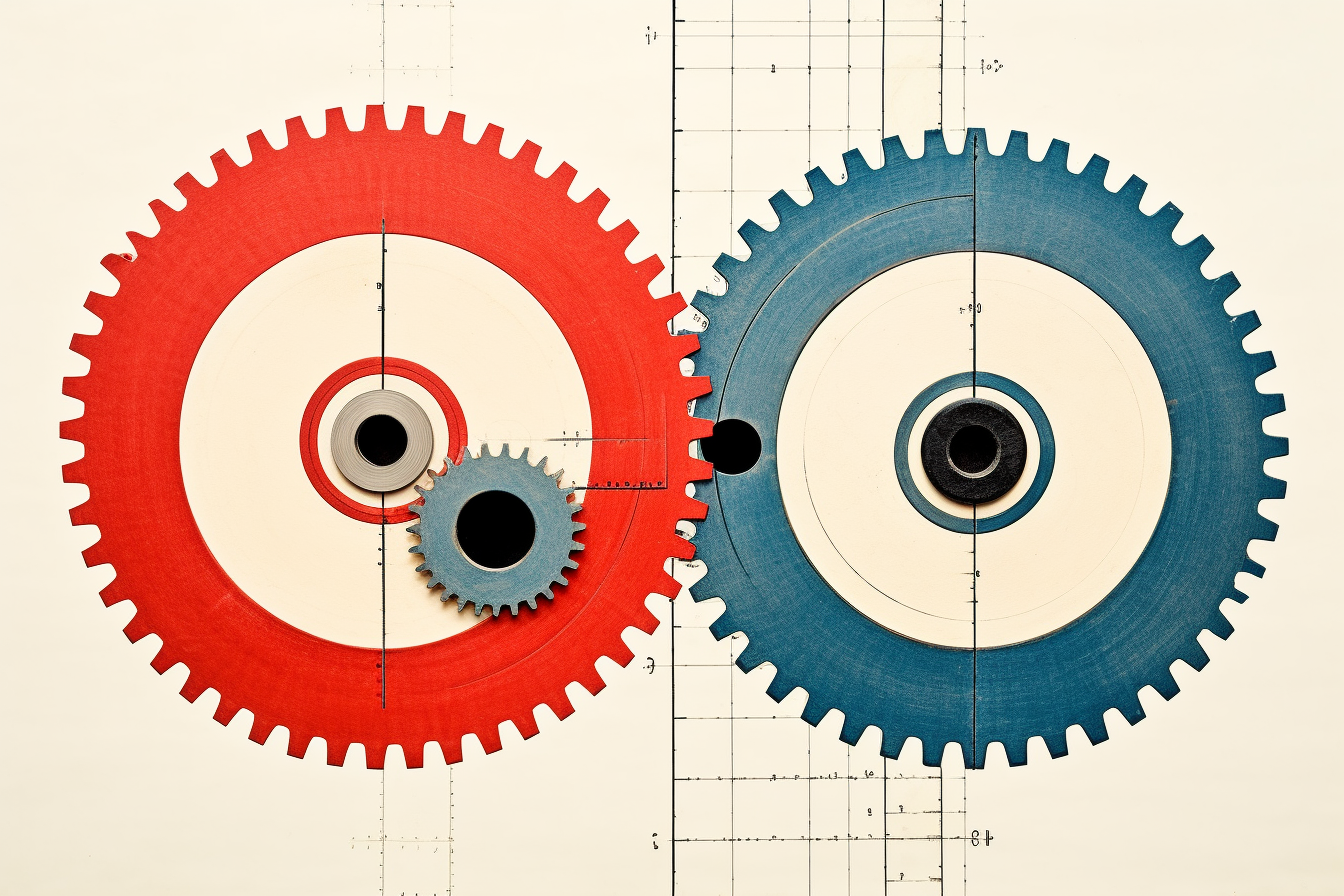
In experimental research, a variable refers to the phenomenon, person, or thing that is being measured and observed by the researcher. A researcher conducts a study to see how one variable affects another and make assertions about the relationship between different variables.
A typical research question in an experimental study addresses a hypothesized relationship between the independent variable manipulated by the researcher and the dependent variable that is the outcome of interest presumably influenced by the researcher's manipulation.
Take a simple experiment on plants as an example. Suppose you have a control group of plants on one side of a garden and an experimental group of plants on the other side. All things such as sunlight, water, and fertilizer being equal, both plants should be expected to grow at the same rate.
Now imagine that the plants in the experimental group are given a new plant fertilizer under the assumption that they will grow faster. Then you will need to measure the difference in growth between the two groups in your study.
In this case, the independent variable is the type of fertilizer used on your plants while the dependent variable is the rate of growth among your plants. If there is a significant difference in growth between the two groups, then your study provides support to suggest that the fertilizer causes higher rates of plant growth.
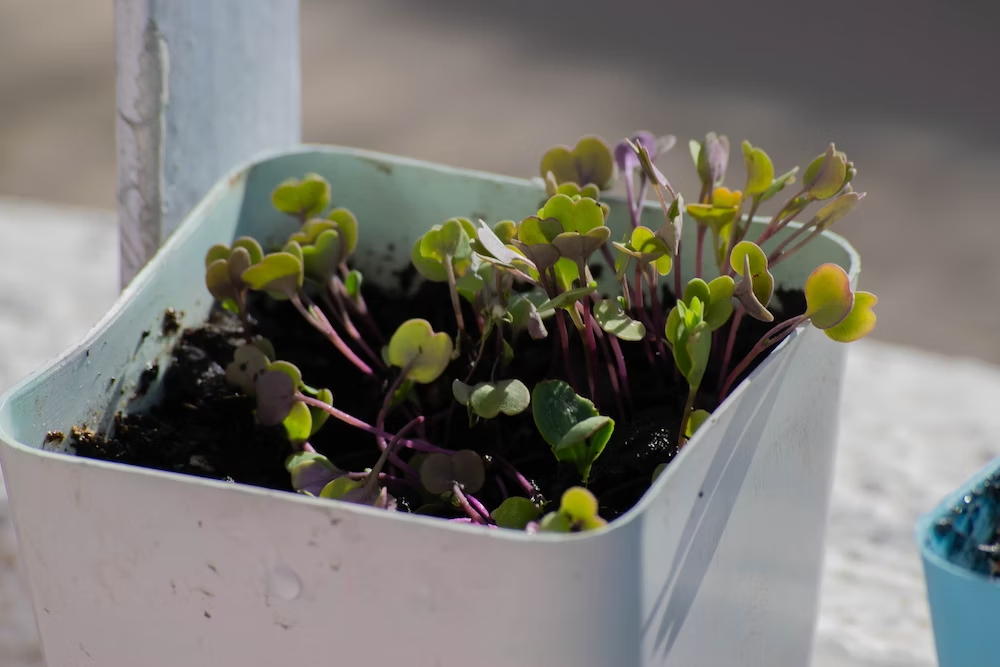
What is the key difference between independent and dependent variables?
The independent variable is the element in your study that you intentionally change, which is why it can also be referred to as the manipulated variable.
You manipulate this variable to see how it might affect the other variables you observe, all other factors being equal. This means that you can observe the cause and effect relationships between one independent variable and one or multiple dependent variables.
Independent variables are directly manipulated by the researcher, while dependent variables are not. They are "dependent" because they are affected by the independent variable in the experiment. Researchers can thus study how manipulating the independent variable leads to changes in the main outcome of interest being measured as the dependent variable.
Note that while you can have multiple dependent variables, it is challenging to establish research rigor for multiple independent variables. If you are making so many changes in an experiment, how do you know which change is responsible for the outcome produced by the study? Studying more than one independent variable would require running an experiment for each independent variable to isolate its effects on the dependent variable.
This being said, it is certainly possible to employ a study design that involves multiple independent and dependent variables, as is the case with what is called a factorial experiment. For example, a psychological study examining the effects of sleep and stress levels on work productivity and social interaction would have two independent variables and two dependent variables, respectively.
Such a study would be complex and require careful planning to establish the necessary research rigor , however. If possible, consider narrowing your research to the examination of one independent variable to make it more manageable and easier to understand.
Independent variable examples
Let's consider an experiment in the social studies. Suppose you want to determine the effectiveness of a new textbook compared to current textbooks in a particular school.
The new textbook is supposed to be better, but how can you prove it? Besides all the selling points that the textbook publisher makes, how do you know if the new textbook is any good? A rigorous study examining the effects of the textbook on classroom outcomes is in order.
The textbook given to students makes up the independent variable in your experimental study. The shift from the existing textbooks to the new one represents the manipulation of the independent variable in this study.
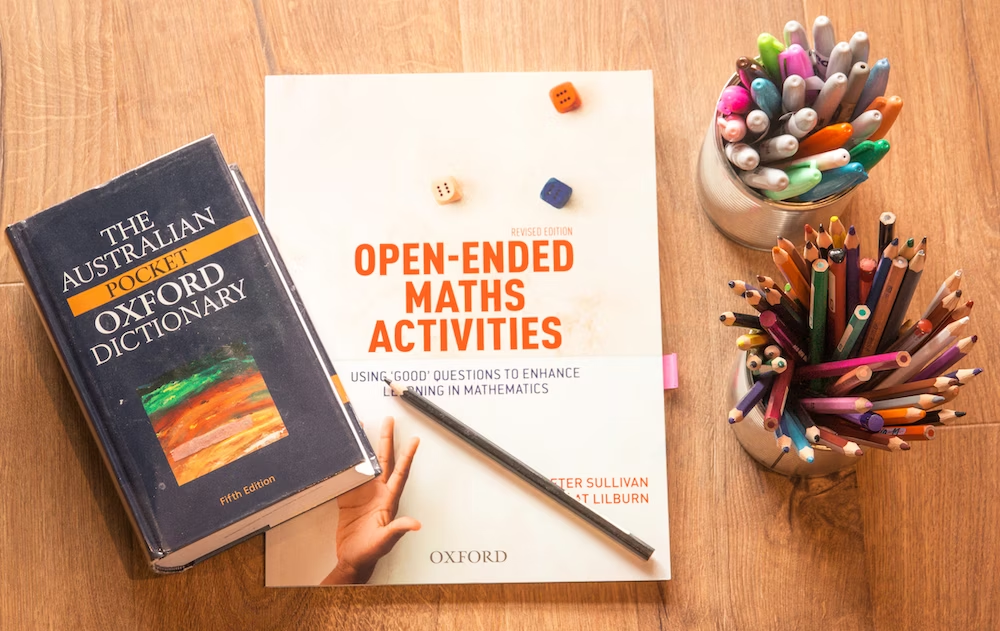
Dependent variable examples
In any experiment, the dependent variable is observed to measure how it is affected by changes to the independent variable. Outcomes such as test scores and other performance metrics can make up the data for the dependent variable.
Now that we are changing the textbook in the experiment above, we should examine if there are any effects.
To do this, we will need two classrooms of students. As best as possible, the two sets of students should be of similar proficiency (or at least of similar backgrounds) and placed within similar conditions for teaching and learning (e.g., physical space, lesson planning).
The control group in our study will be one set of students using the existing textbook. By examining their performance, we can establish a baseline. The performance of the experimental group, which is the set of students using the new textbook, can then be compared with the baseline performance.
As a result, the change in the test scores make up the data for our dependent variable. We cannot directly affect how well students perform on the test, but we can conclude from our experiment whether the use of the new textbook might impact students' performance.
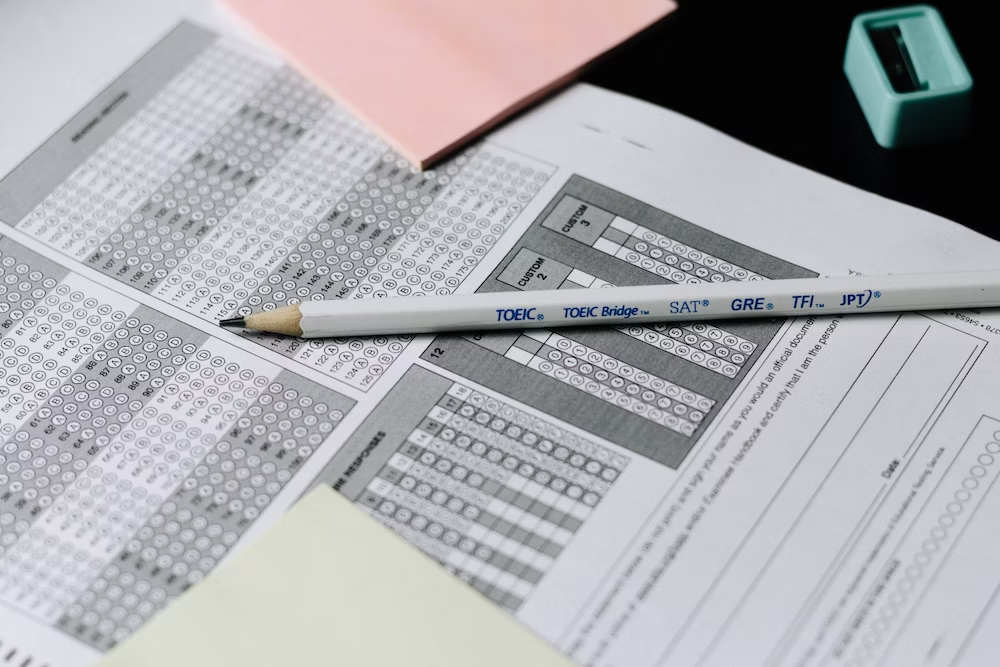
Turn data into valuable insights with ATLAS.ti
Rely on our powerful data analysis interface for your research, starting with a free trial.
How do you know if a variable is independent or dependent?
We can typically think of an independent variable as something a researcher can directly change. In the above example, we can change the textbook used by the teacher in class. If we're talking about plants, we can change the fertilizer.
Conversely, the dependent variable is something that we do not directly influence or manipulate. Strictly speaking, we cannot directly manipulate a student's performance on a test or the rate of growth of a plant, not without other factors such as new teaching methods or new fertilizer, respectively.
Understanding the distinction between a dependent variable and an independent variable is key to experimental research. Ultimately, the distinction can be reduced to which element in a study has been directly influenced by the researcher.
Other variables
Given the potential complexities encountered in research, there is essential terminology for other variables in any experimental study. You might employ this terminology or encounter them while reading other research.
A control variable is any factor that the researcher tries to keep constant as the independent variable changes. In the plant experiment described earlier in this article, the sunlight and water are each a controlled variable while the type of fertilizer used is the manipulated variable across control and experimental groups.
To ensure research rigor, the researcher needs to keep these control variables constant to dispel any concerns that differences in growth rate were being driven by sunlight or water, as opposed to the fertilizer being used.
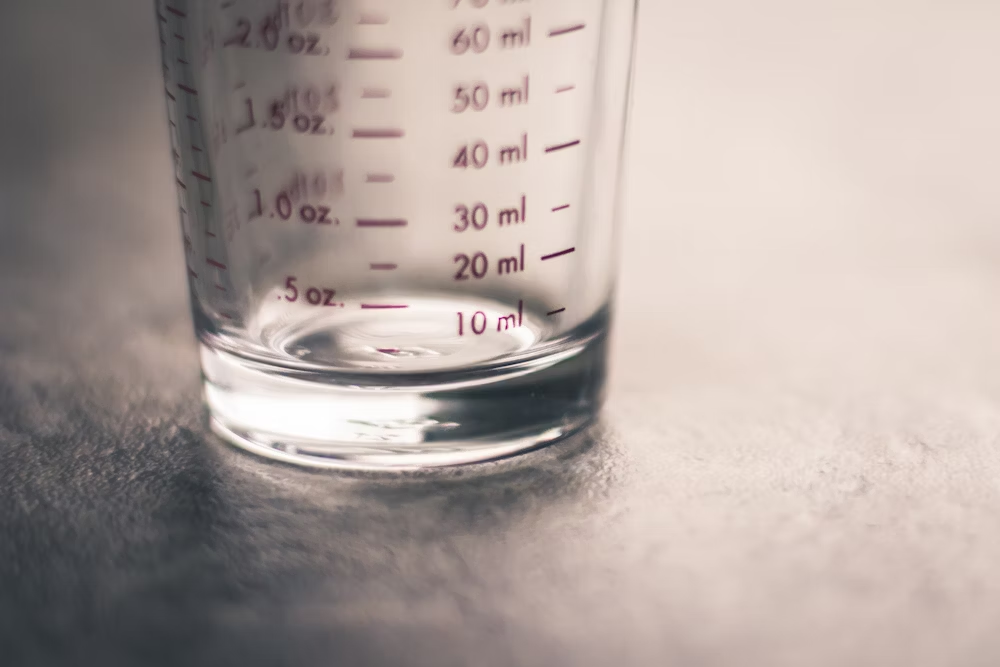
Extraneous variables refer to any unwanted influence on the dependent variable that may confound the analysis of the study. For example, if bugs or animals ate the plants in your fertilizer study, this was greatly impact the rates of plant growth. This is why it would be important to control the environment and protect it from such threats.
Finally, independent variables can go by different names such as subject variables or predictor variables. Dependent variables can also be referred to as the responding variable or outcome variable. Whatever the language, they all serve the same role of influencing the dependent variable in an experiment.
The use of the word " variables " is typically associated with quantitative and confirmatory research. Naturalistic qualitative research typically does not employ experimental designs or establish causality. Qualitative research often draws on observations , interviews , focus groups , and other forms of data collection that are allow researchers to study the naturally occurring "messiness" of the social world, rather than controlling all variables to isolate a cause-and-effect relationship.
In limited circumstances, the idea of experimental variables can apply to participant observations in ethnography , where the researcher should be mindful of their influence on the environment they are observing.
However, the experimental paradigm is best left to quantitative studies and confirmatory research questions. Qualitative researchers in the social sciences are oftentimes more interested in observing and describing socially-constructed phenomena rather than testing hypotheses .
Nonetheless, the notion of independent and dependent variables does hold important lessons for qualitative researchers. Even if they don't employ variables in their study design, qualitative researchers often observe how one thing affects another. A theoretical or conceptual framework can then suggest potential cause-and-effect relationships in their study.
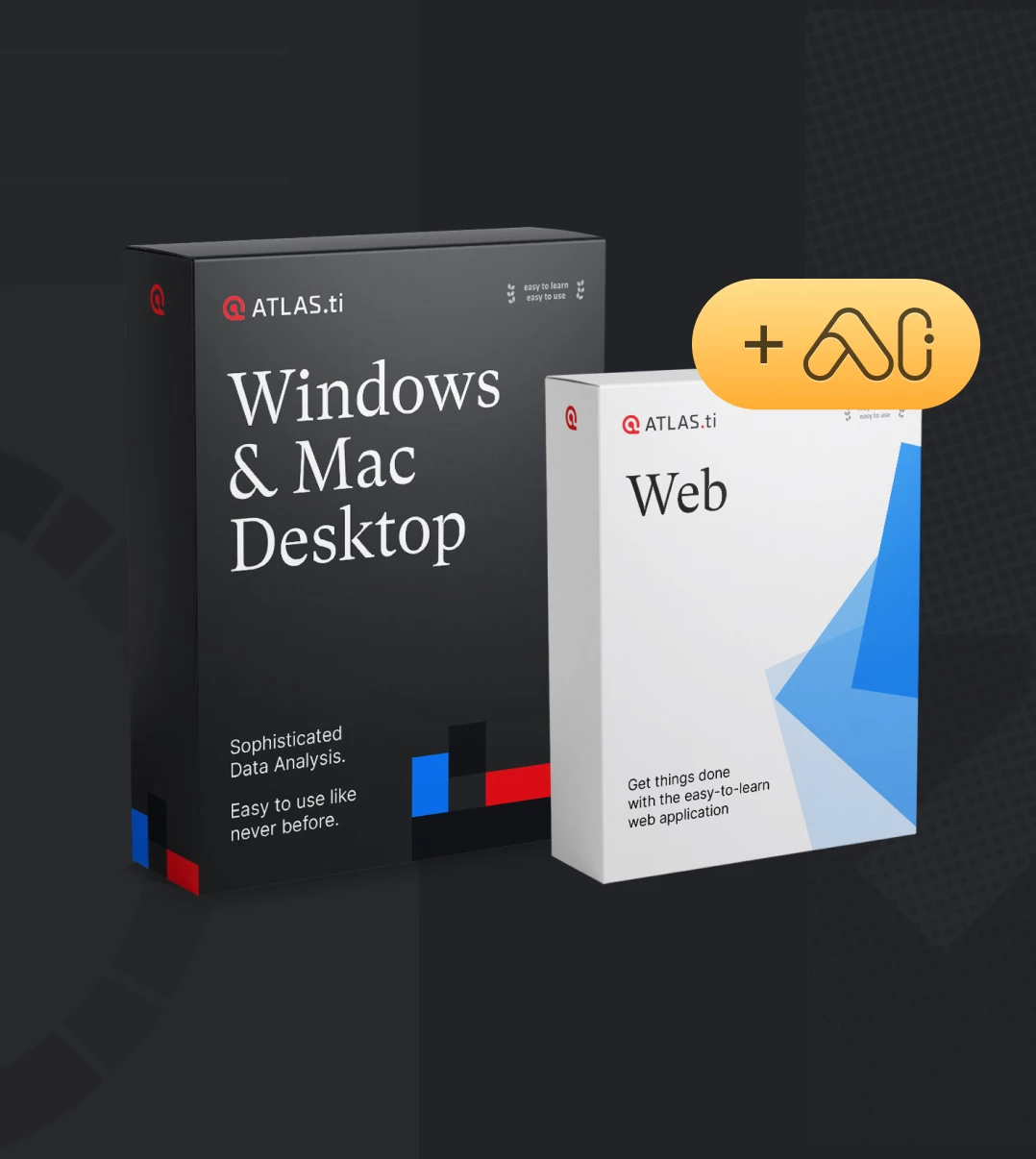
With ATLAS.ti, insightful data analysis is at your fingertips
Download a free trial of ATLAS.ti to see how you can make the most of your data.
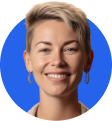
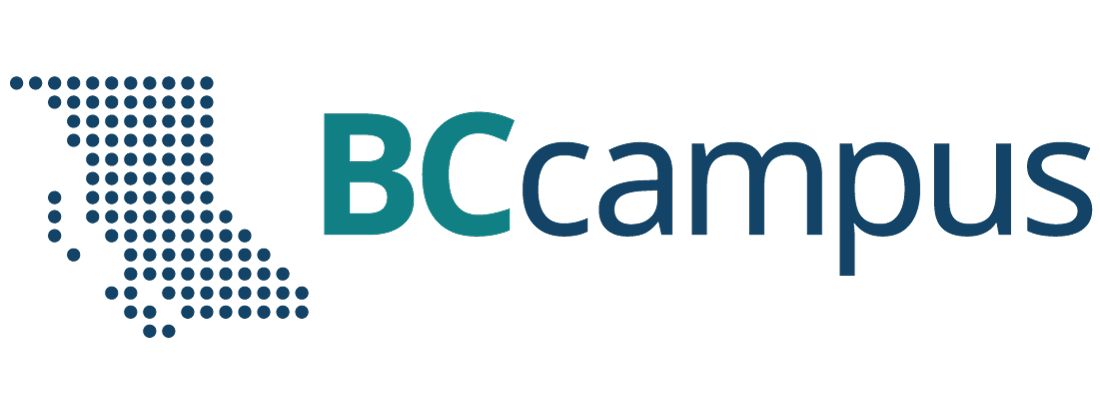
Want to create or adapt books like this? Learn more about how Pressbooks supports open publishing practices.
Measurement and Units of Analysis
25 Independent and Dependent Variables
When one variable causes another variable, we have what researchers call independent and dependent variables. In the example where gender was found to be causally linked to cell phone addiction, gender would be the independent variable and cell phone addiction would be the dependent variable. An independent variable is one that causes another. A dependent variable is one that is caused by the other. Dependent variables depend on independent variables. If you are struggling to figure out which is the dependent and which is the independent variable, there is a little trick, as follows:
Ask yourself the following question: Is X dependent upon Y. Now substitute words for X and Y. For example, is the level of success in an online class dependent upon the time spent online? Success in an online class is the dependent variable, because it is dependent upon something. In this case, we are asking if the level of success in an online class is dependent upon the time spent online. Time spent online is the independent variable. Table 4.2 provides you with an opportunity to practice identifying the dependent and the independent variable
- Dependent variable = success in online class; Independent variable = gender
- Dependent variable = prevalence of PTSD in BC; independent variable = level of funding for early intervention
- Dependent variable = reporting of high school bullying; independent variable = anti-bullying programs in high schools
- Dependent variable = survival rate of female heart attack victims; independent variable = hospital emergency room procedures
Extraneous variables (from Adjei, n.d.)
While it is very common to hear the terms independent and dependent variable, extraneous variables are less common, which is surprising because an extraneous variable can destroy the integrity of a research study that claims to show a cause and effect relationship. An extraneous variable is a variable that may compete with the independent variable in explaining the outcome. Remember this, if you are ever interested in identifying cause and effect relationships you must always determine whether there are any extraneous variables you need to worry about. If an extraneous variable really is the reason for an outcome (rather than the IV) then we sometimes like to call it a confounding variable because it has confused or confounded the relationship we are interested in (see example below).
Suppose we want to determine the effectiveness of new course curriculum for an online research methods class. We want to test how effective the new course curriculum is on student learning, compared to the old course curriculum. We are unable to use random assignment to equate our groups. Instead, we ask one of the college´s most experienced online teachers to use the new online curriculum with one class of online students and the old curriculum with the other class of online students. Imagine that the students taking the new curriculum course (the experimental group) got higher grades than the control group (the old curriculum). Do you see any problems with claiming that the reason for the difference between the two groups is because of the new curriculum? The problem is that there are alternative explanations.
First, perhaps the difference is because the group of students in the new curriculum course were more experienced students, both in terms of age and where they were in their studies (more third year students than first year students). Perhaps the old curriculum class had a higher percentage of students for whom English is not their first language and they struggled with some of the material because of language barriers, which had nothing to do with then old curriculum. In other words, we have a problem, in that there could be alternative explanations for our findings. These alternative explanations are called extraneous variables and they can occur when we do not have random assignation. Indeed, it is very possible that the difference we saw between the two groups was due to other variables (i.e. experience level of students, English language proficiency), rather than the IV (new versus old curriculum).
It is important to note that researchers can and should attempt to control for extraneous variables, as much as possible. This can be done in two ways. The first is by employing standardized procedures . This means that the researcher attempts to ensure that all aspects of the experiment are the same, with the exception of the independent variable. For example, the researchers would use the same method for recruiting participants and they would conduct the experiment in the same setting. They would ensure that they give the same explanation to the participants at the beginning of the study and any feedback at the end of the study in exactly the same way. Any rewards for participation would be offered for all participants in the same manner. They could also ensure that the experiment occurs on the same day of the week (or month), or at the same time of day, and that the lab is kept at a constant temperature, a constant level of brightness, and a constant level of noise (Explore Psychology, 2019).
The second way that a researcher in an experiment can control for extraneous variables is to employ random assignation to reduce the likelihood that characteristics specific to some of the participants have influenced the independent variable. Random assignment means that every person chosen for an experiment has an equal chance of being assigned to either the test group of the control group (Explore Psychology, 2019). Chapter 6 provides more detail on random assignment, and explains the difference between a test group and a control group.
Text Attributions
- “Extraneous variables” by J.K. Adjei – CHECK LICENCE.
An Introduction to Research Methods in Sociology Copyright © 2019 by Valerie A. Sheppard is licensed under a Creative Commons Attribution-NonCommercial-ShareAlike 4.0 International License , except where otherwise noted.
Share This Book

- Science Notes Posts
- Contact Science Notes
- Todd Helmenstine Biography
- Anne Helmenstine Biography
- Free Printable Periodic Tables (PDF and PNG)
- Periodic Table Wallpapers
- Interactive Periodic Table
- Periodic Table Posters
- How to Grow Crystals
- Chemistry Projects
- Fire and Flames Projects
- Holiday Science
- Chemistry Problems With Answers
- Physics Problems
- Unit Conversion Example Problems
- Chemistry Worksheets
- Biology Worksheets
- Periodic Table Worksheets
- Physical Science Worksheets
- Science Lab Worksheets
- My Amazon Books
Independent and Dependent Variables Examples

The independent and dependent variables are key to any scientific experiment, but how do you tell them apart? Here are the definitions of independent and dependent variables, examples of each type, and tips for telling them apart and graphing them.
Independent Variable
The independent variable is the factor the researcher changes or controls in an experiment. It is called independent because it does not depend on any other variable. The independent variable may be called the “controlled variable” because it is the one that is changed or controlled. This is different from the “ control variable ,” which is variable that is held constant so it won’t influence the outcome of the experiment.
Dependent Variable
The dependent variable is the factor that changes in response to the independent variable. It is the variable that you measure in an experiment. The dependent variable may be called the “responding variable.”
Examples of Independent and Dependent Variables
Here are several examples of independent and dependent variables in experiments:
- In a study to determine whether how long a student sleeps affects test scores, the independent variable is the length of time spent sleeping while the dependent variable is the test score.
- You want to know which brand of fertilizer is best for your plants. The brand of fertilizer is the independent variable. The health of the plants (height, amount and size of flowers and fruit, color) is the dependent variable.
- You want to compare brands of paper towels, to see which holds the most liquid. The independent variable is the brand of paper towel. The dependent variable is the volume of liquid absorbed by the paper towel.
- You suspect the amount of television a person watches is related to their age. Age is the independent variable. How many minutes or hours of television a person watches is the dependent variable.
- You think rising sea temperatures might affect the amount of algae in the water. The water temperature is the independent variable. The mass of algae is the dependent variable.
- In an experiment to determine how far people can see into the infrared part of the spectrum, the wavelength of light is the independent variable and whether the light is observed is the dependent variable.
- If you want to know whether caffeine affects your appetite, the presence/absence or amount of caffeine is the independent variable. Appetite is the dependent variable.
- You want to know which brand of microwave popcorn pops the best. The brand of popcorn is the independent variable. The number of popped kernels is the dependent variable. Of course, you could also measure the number of unpopped kernels instead.
- You want to determine whether a chemical is essential for rat nutrition, so you design an experiment. The presence/absence of the chemical is the independent variable. The health of the rat (whether it lives and reproduces) is the dependent variable. A follow-up experiment might determine how much of the chemical is needed. Here, the amount of chemical is the independent variable and the rat health is the dependent variable.
How to Tell the Independent and Dependent Variable Apart
If you’re having trouble identifying the independent and dependent variable, here are a few ways to tell them apart. First, remember the dependent variable depends on the independent variable. It helps to write out the variables as an if-then or cause-and-effect sentence that shows the independent variable causes an effect on the dependent variable. If you mix up the variables, the sentence won’t make sense. Example : The amount of eat (independent variable) affects how much you weigh (dependent variable).
This makes sense, but if you write the sentence the other way, you can tell it’s incorrect: Example : How much you weigh affects how much you eat. (Well, it could make sense, but you can see it’s an entirely different experiment.) If-then statements also work: Example : If you change the color of light (independent variable), then it affects plant growth (dependent variable). Switching the variables makes no sense: Example : If plant growth rate changes, then it affects the color of light. Sometimes you don’t control either variable, like when you gather data to see if there is a relationship between two factors. This can make identifying the variables a bit trickier, but establishing a logical cause and effect relationship helps: Example : If you increase age (independent variable), then average salary increases (dependent variable). If you switch them, the statement doesn’t make sense: Example : If you increase salary, then age increases.
How to Graph Independent and Dependent Variables
Plot or graph independent and dependent variables using the standard method. The independent variable is the x-axis, while the dependent variable is the y-axis. Remember the acronym DRY MIX to keep the variables straight: D = Dependent variable R = Responding variable/ Y = Graph on the y-axis or vertical axis M = Manipulated variable I = Independent variable X = Graph on the x-axis or horizontal axis
- Babbie, Earl R. (2009). The Practice of Social Research (12th ed.) Wadsworth Publishing. ISBN 0-495-59841-0.
- di Francia, G. Toraldo (1981). The Investigation of the Physical World . Cambridge University Press. ISBN 978-0-521-29925-1.
- Gauch, Hugh G. Jr. (2003). Scientific Method in Practice . Cambridge University Press. ISBN 978-0-521-01708-4.
- Popper, Karl R. (2003). Conjectures and Refutations: The Growth of Scientific Knowledge . Routledge. ISBN 0-415-28594-1.
Related Posts

Statistics Made Easy
Independent vs. Dependent Variables: What’s the Difference?
In an experiment, there are two main variables:
The independent variable: the variable that an experimenter changes or controls so that they can observe the effects on the dependent variable.
The dependent variable: the variable being measured in an experiment that is “dependent” on the independent variable.

In an experiment, an experimenter is interested in seeing how the dependent variable changes as a result of the independent being changed or manipulated in some way.
Example of an Independent and Dependent Variable
For example, a researcher might change the amount of water they provide to a certain plant to observe how it affects the growth rate of the plant.
In this example, the amount of water given to the plant is controlled by the researcher and, thus, is the independent variable . The growth rate is the dependent variable because it is directly dependent on the amount of water that the plant receives and it’s the variable we’re interested in measuring.

How to Remember the Difference Between Independent and Dependent Variables
An easy way to remember the difference between independent and dependent variables is to insert the two variables into the following sentence in such a way that it makes sense:
Changing (independent variable) affects the value of (dependent variable) .
For example, it would make sense to say:
Changing the amount of water affects the value of the plant growth rate .
This is how we know that amount of water is the independent variable and plant growth rate is the dependent variable.
If we tried reversing the positions of these two variables, the sentence wouldn’t make sense:
Changing the plant growth rate affects the value of the amount of water .
Thus, we know that we must have the independent and dependent variables switched around.
More Examples
Here are a few more examples of independent and dependent variables.
A marketer changes the amount of money they spend on advertisements to see how it affects total sales.
Independent variable: amount spent on advertisements
Dependent variable: total sales
A doctor changes the dose of a particular medicine to see how it affects the blood pressure of a patient.
Independent variable: dosage level of medicine
Dependent variable: blood pressure
A researcher changes the version of a study guide given to students to see how it affects exam scores.
Independent variable: the version of the study guide
Dependent variable: exam scores
Independent vs. Dependent Variables on a Graph
When we create a graph, the independent variable will go on the x-axis and the dependent variable will go on the y-axis.
For example, suppose a researcher provides different amounts of water for 20 different plants and measures the growth rate of each plant. The following scatterplot shows the amount of water and the growth rate for each plant:
The independent variable (amount of water) is shown on the x-axis while the dependent variable (growth rate) is shown on the y-axis:

Featured Posts

Hey there. My name is Zach Bobbitt. I have a Masters of Science degree in Applied Statistics and I’ve worked on machine learning algorithms for professional businesses in both healthcare and retail. I’m passionate about statistics, machine learning, and data visualization and I created Statology to be a resource for both students and teachers alike. My goal with this site is to help you learn statistics through using simple terms, plenty of real-world examples, and helpful illustrations.
Leave a Reply Cancel reply
Your email address will not be published. Required fields are marked *
Join the Statology Community
Sign up to receive Statology's exclusive study resource: 100 practice problems with step-by-step solutions. Plus, get our latest insights, tutorials, and data analysis tips straight to your inbox!
By subscribing you accept Statology's Privacy Policy.
- Bipolar Disorder
- Therapy Center
- When To See a Therapist
- Types of Therapy
- Best Online Therapy
- Best Couples Therapy
- Best Family Therapy
- Managing Stress
- Sleep and Dreaming
- Understanding Emotions
- Self-Improvement
- Healthy Relationships
- Student Resources
- Personality Types
- Guided Meditations
- Verywell Mind Insights
- 2024 Verywell Mind 25
- Mental Health in the Classroom
- Editorial Process
- Meet Our Review Board
- Crisis Support
Types of Variables in Psychology Research
Examples of Independent and Dependent Variables
Kendra Cherry, MS, is a psychosocial rehabilitation specialist, psychology educator, and author of the "Everything Psychology Book."
:max_bytes(150000):strip_icc():format(webp)/IMG_9791-89504ab694d54b66bbd72cb84ffb860e.jpg)
James Lacy, MLS, is a fact-checker and researcher.
:max_bytes(150000):strip_icc():format(webp)/James-Lacy-1000-73de2239670146618c03f8b77f02f84e.jpg)
Dependent and Independent Variables
- Intervening Variables
- Extraneous Variables
- Controlled Variables
- Confounding Variables
- Operationalizing Variables
Frequently Asked Questions
Variables in psychology are things that can be changed or altered, such as a characteristic or value. Variables are generally used in psychology experiments to determine if changes to one thing result in changes to another.
Variables in psychology play a critical role in the research process. By systematically changing some variables in an experiment and measuring what happens as a result, researchers are able to learn more about cause-and-effect relationships.
The two main types of variables in psychology are the independent variable and the dependent variable. Both variables are important in the process of collecting data about psychological phenomena.
This article discusses different types of variables that are used in psychology research. It also covers how to operationalize these variables when conducting experiments.
Students often report problems with identifying the independent and dependent variables in an experiment. While this task can become more difficult as the complexity of an experiment increases, in a psychology experiment:
- The independent variable is the variable that is manipulated by the experimenter. An example of an independent variable in psychology: In an experiment on the impact of sleep deprivation on test performance, sleep deprivation would be the independent variable. The experimenters would have some of the study participants be sleep-deprived while others would be fully rested.
- The dependent variable is the variable that is measured by the experimenter. In the previous example, the scores on the test performance measure would be the dependent variable.
So how do you differentiate between the independent and dependent variables? Start by asking yourself what the experimenter is manipulating. The things that change, either naturally or through direct manipulation from the experimenter, are generally the independent variables. What is being measured? The dependent variable is the one that the experimenter is measuring.
Intervening Variables in Psychology
Intervening variables, also sometimes called intermediate or mediator variables, are factors that play a role in the relationship between two other variables. In the previous example, sleep problems in university students are often influenced by factors such as stress. As a result, stress might be an intervening variable that plays a role in how much sleep people get, which may then influence how well they perform on exams.
Extraneous Variables in Psychology
Independent and dependent variables are not the only variables present in many experiments. In some cases, extraneous variables may also play a role. This type of variable is one that may have an impact on the relationship between the independent and dependent variables.
For example, in our previous example of an experiment on the effects of sleep deprivation on test performance, other factors such as age, gender, and academic background may have an impact on the results. In such cases, the experimenter will note the values of these extraneous variables so any impact can be controlled for.
There are two basic types of extraneous variables:
- Participant variables : These extraneous variables are related to the individual characteristics of each study participant that may impact how they respond. These factors can include background differences, mood, anxiety, intelligence, awareness, and other characteristics that are unique to each person.
- Situational variables : These extraneous variables are related to things in the environment that may impact how each participant responds. For example, if a participant is taking a test in a chilly room, the temperature would be considered an extraneous variable. Some participants may not be affected by the cold, but others might be distracted or annoyed by the temperature of the room.
Other extraneous variables include the following:
- Demand characteristics : Clues in the environment that suggest how a participant should behave
- Experimenter effects : When a researcher unintentionally suggests clues for how a participant should behave
Controlled Variables in Psychology
In many cases, extraneous variables are controlled for by the experimenter. A controlled variable is one that is held constant throughout an experiment.
In the case of participant variables, the experiment might select participants that are the same in background and temperament to ensure that these factors don't interfere with the results. Holding these variables constant is important for an experiment because it allows researchers to be sure that all other variables remain the same across all conditions.
Using controlled variables means that when changes occur, the researchers can be sure that these changes are due to the manipulation of the independent variable and not caused by changes in other variables.
It is important to also note that a controlled variable is not the same thing as a control group . The control group in a study is the group of participants who do not receive the treatment or change in the independent variable.
All other variables between the control group and experimental group are held constant (i.e., they are controlled). The dependent variable being measured is then compared between the control group and experimental group to see what changes occurred because of the treatment.
Confounding Variables in Psychology
If a variable cannot be controlled for, it becomes what is known as a confounding variabl e. This type of variable can have an impact on the dependent variable, which can make it difficult to determine if the results are due to the influence of the independent variable, the confounding variable, or an interaction of the two.
Operationalizing Variables in Psychology
An operational definition describes how the variables are measured and defined in the study. Before conducting a psychology experiment , it is essential to create firm operational definitions for both the independent variable and dependent variables.
For example, in our imaginary experiment on the effects of sleep deprivation on test performance, we would need to create very specific operational definitions for our two variables. If our hypothesis is "Students who are sleep deprived will score significantly lower on a test," then we would have a few different concepts to define:
- Students : First, what do we mean by "students?" In our example, let’s define students as participants enrolled in an introductory university-level psychology course.
- Sleep deprivation : Next, we need to operationally define the "sleep deprivation" variable. In our example, let’s say that sleep deprivation refers to those participants who have had less than five hours of sleep the night before the test.
- Test variable : Finally, we need to create an operational definition for the test variable. For this example, the test variable will be defined as a student’s score on a chapter exam in the introductory psychology course.
Once all the variables are operationalized, we're ready to conduct the experiment.
Variables play an important part in psychology research. Manipulating an independent variable and measuring the dependent variable allows researchers to determine if there is a cause-and-effect relationship between them.
A Word From Verywell
Understanding the different types of variables used in psychology research is important if you want to conduct your own psychology experiments. It is also helpful for people who want to better understand what the results of psychology research really mean and become more informed consumers of psychology information .
Independent and dependent variables are used in experimental research. Unlike some other types of research (such as correlational studies ), experiments allow researchers to evaluate cause-and-effect relationships between two variables.
Researchers can use statistical analyses to determine the strength of a relationship between two variables in an experiment. Two of the most common ways to do this are to calculate a p-value or a correlation. The p-value indicates if the results are statistically significant while the correlation can indicate the strength of the relationship.
In an experiment on how sugar affects short-term memory, sugar intake would be the independent variable and scores on a short-term memory task would be the independent variable.
In an experiment looking at how caffeine intake affects test anxiety, the amount of caffeine consumed before a test would be the independent variable and scores on a test anxiety assessment would be the dependent variable.
Just as with other types of research, the independent variable in a cognitive psychology study would be the variable that the researchers manipulate. The specific independent variable would vary depending on the specific study, but it might be focused on some aspect of thinking, memory, attention, language, or decision-making.
American Psychological Association. Operational definition . APA Dictionary of Psychology.
American Psychological Association. Mediator . APA Dictionary of Psychology.
Altun I, Cınar N, Dede C. The contributing factors to poor sleep experiences in according to the university students: A cross-sectional study . J Res Med Sci . 2012;17(6):557-561. PMID:23626634
Skelly AC, Dettori JR, Brodt ED. Assessing bias: The importance of considering confounding . Evid Based Spine Care J . 2012;3(1):9-12. doi:10.1055/s-0031-1298595
- Evans, AN & Rooney, BJ. Methods in Psychological Research. Thousand Oaks, CA: SAGE Publications; 2014.
- Kantowitz, BH, Roediger, HL, & Elmes, DG. Experimental Psychology. Stamfort, CT: Cengage Learning; 2015.
By Kendra Cherry, MSEd Kendra Cherry, MS, is a psychosocial rehabilitation specialist, psychology educator, and author of the "Everything Psychology Book."
Have a language expert improve your writing
Run a free plagiarism check in 10 minutes, automatically generate references for free.
- Knowledge Base
- Methodology
- Independent vs Dependent Variables | Definition & Examples
Independent vs Dependent Variables | Definition & Examples
Published on 4 May 2022 by Pritha Bhandari . Revised on 17 October 2022.
In research, variables are any characteristics that can take on different values, such as height, age, temperature, or test scores.
Researchers often manipulate or measure independent and dependent variables in studies to test cause-and-effect relationships.
- The independent variable is the cause. Its value is independent of other variables in your study.
- The dependent variable is the effect. Its value depends on changes in the independent variable.
Your independent variable is the temperature of the room. You vary the room temperature by making it cooler for half the participants, and warmer for the other half.
Table of contents
What is an independent variable, types of independent variables, what is a dependent variable, identifying independent vs dependent variables, independent and dependent variables in research, visualising independent and dependent variables, frequently asked questions about independent and dependent variables.
An independent variable is the variable you manipulate or vary in an experimental study to explore its effects. It’s called ‘independent’ because it’s not influenced by any other variables in the study.
Independent variables are also called:
- Explanatory variables (they explain an event or outcome)
- Predictor variables (they can be used to predict the value of a dependent variable)
- Right-hand-side variables (they appear on the right-hand side of a regression equation).
These terms are especially used in statistics , where you estimate the extent to which an independent variable change can explain or predict changes in the dependent variable.
Prevent plagiarism, run a free check.
There are two main types of independent variables.
- Experimental independent variables can be directly manipulated by researchers.
- Subject variables cannot be manipulated by researchers, but they can be used to group research subjects categorically.
Experimental variables
In experiments, you manipulate independent variables directly to see how they affect your dependent variable. The independent variable is usually applied at different levels to see how the outcomes differ.
You can apply just two levels in order to find out if an independent variable has an effect at all.
You can also apply multiple levels to find out how the independent variable affects the dependent variable.
You have three independent variable levels, and each group gets a different level of treatment.
You randomly assign your patients to one of the three groups:
- A low-dose experimental group
- A high-dose experimental group
- A placebo group
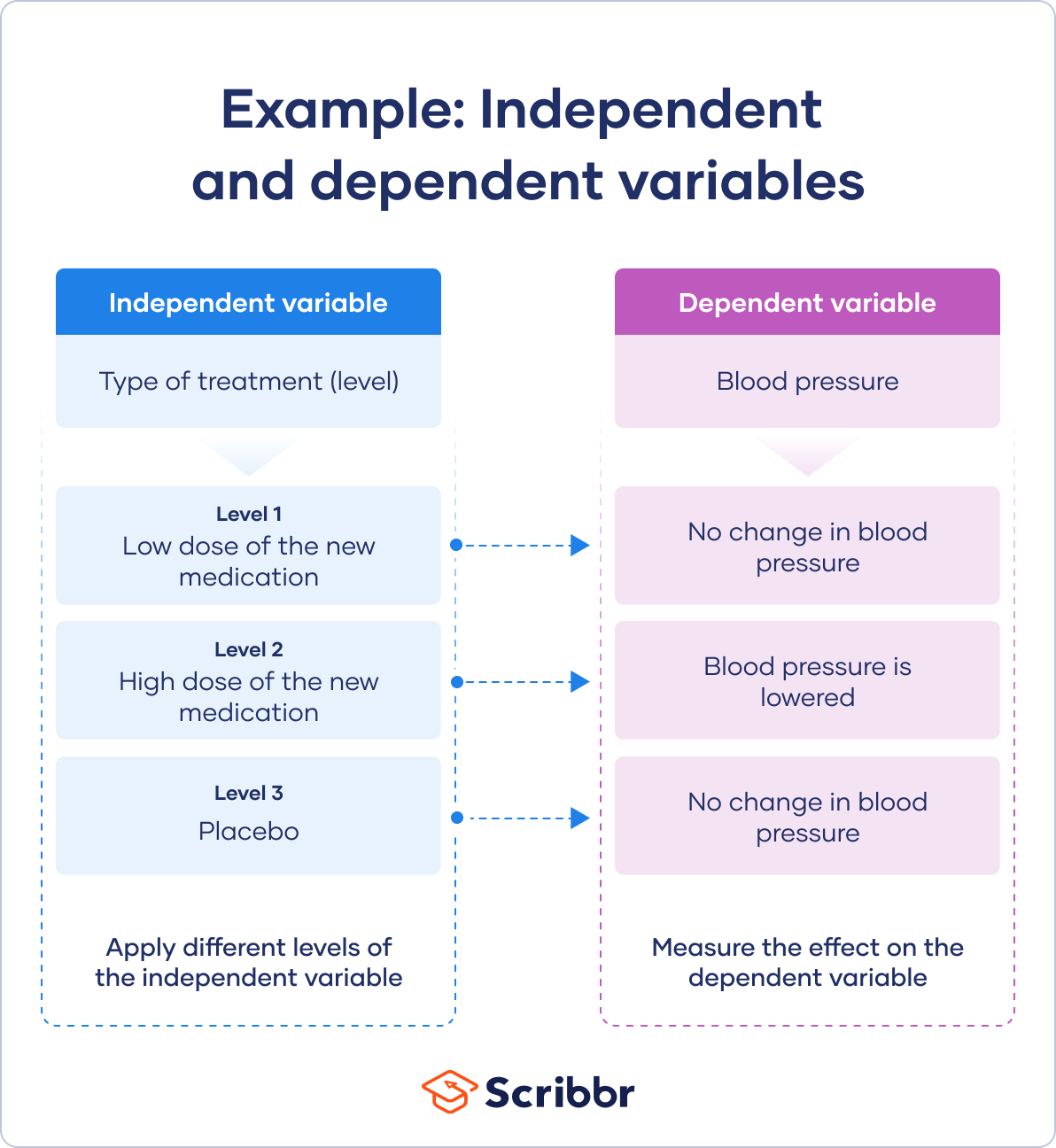
A true experiment requires you to randomly assign different levels of an independent variable to your participants.
Random assignment helps you control participant characteristics, so that they don’t affect your experimental results. This helps you to have confidence that your dependent variable results come solely from the independent variable manipulation.
Subject variables
Subject variables are characteristics that vary across participants, and they can’t be manipulated by researchers. For example, gender identity, ethnicity, race, income, and education are all important subject variables that social researchers treat as independent variables.
It’s not possible to randomly assign these to participants, since these are characteristics of already existing groups. Instead, you can create a research design where you compare the outcomes of groups of participants with characteristics. This is a quasi-experimental design because there’s no random assignment.
Your independent variable is a subject variable, namely the gender identity of the participants. You have three groups: men, women, and other.
Your dependent variable is the brain activity response to hearing infant cries. You record brain activity with fMRI scans when participants hear infant cries without their awareness.
A dependent variable is the variable that changes as a result of the independent variable manipulation. It’s the outcome you’re interested in measuring, and it ‘depends’ on your independent variable.
In statistics , dependent variables are also called:
- Response variables (they respond to a change in another variable)
- Outcome variables (they represent the outcome you want to measure)
- Left-hand-side variables (they appear on the left-hand side of a regression equation)
The dependent variable is what you record after you’ve manipulated the independent variable. You use this measurement data to check whether and to what extent your independent variable influences the dependent variable by conducting statistical analyses.
Based on your findings, you can estimate the degree to which your independent variable variation drives changes in your dependent variable. You can also predict how much your dependent variable will change as a result of variation in the independent variable.
Distinguishing between independent and dependent variables can be tricky when designing a complex study or reading an academic paper.
A dependent variable from one study can be the independent variable in another study, so it’s important to pay attention to research design.
Here are some tips for identifying each variable type.
Recognising independent variables
Use this list of questions to check whether you’re dealing with an independent variable:
- Is the variable manipulated, controlled, or used as a subject grouping method by the researcher?
- Does this variable come before the other variable in time?
- Is the researcher trying to understand whether or how this variable affects another variable?
Recognising dependent variables
Check whether you’re dealing with a dependent variable:
- Is this variable measured as an outcome of the study?
- Is this variable dependent on another variable in the study?
- Does this variable get measured only after other variables are altered?
Independent and dependent variables are generally used in experimental and quasi-experimental research.
Here are some examples of research questions and corresponding independent and dependent variables.
For experimental data, you analyse your results by generating descriptive statistics and visualising your findings. Then, you select an appropriate statistical test to test your hypothesis .
The type of test is determined by:
- Your variable types
- Level of measurement
- Number of independent variable levels
You’ll often use t tests or ANOVAs to analyse your data and answer your research questions.
In quantitative research , it’s good practice to use charts or graphs to visualise the results of studies. Generally, the independent variable goes on the x -axis (horizontal) and the dependent variable on the y -axis (vertical).
The type of visualisation you use depends on the variable types in your research questions:
- A bar chart is ideal when you have a categorical independent variable.
- A scatterplot or line graph is best when your independent and dependent variables are both quantitative.
To inspect your data, you place your independent variable of treatment level on the x -axis and the dependent variable of blood pressure on the y -axis.
You plot bars for each treatment group before and after the treatment to show the difference in blood pressure.
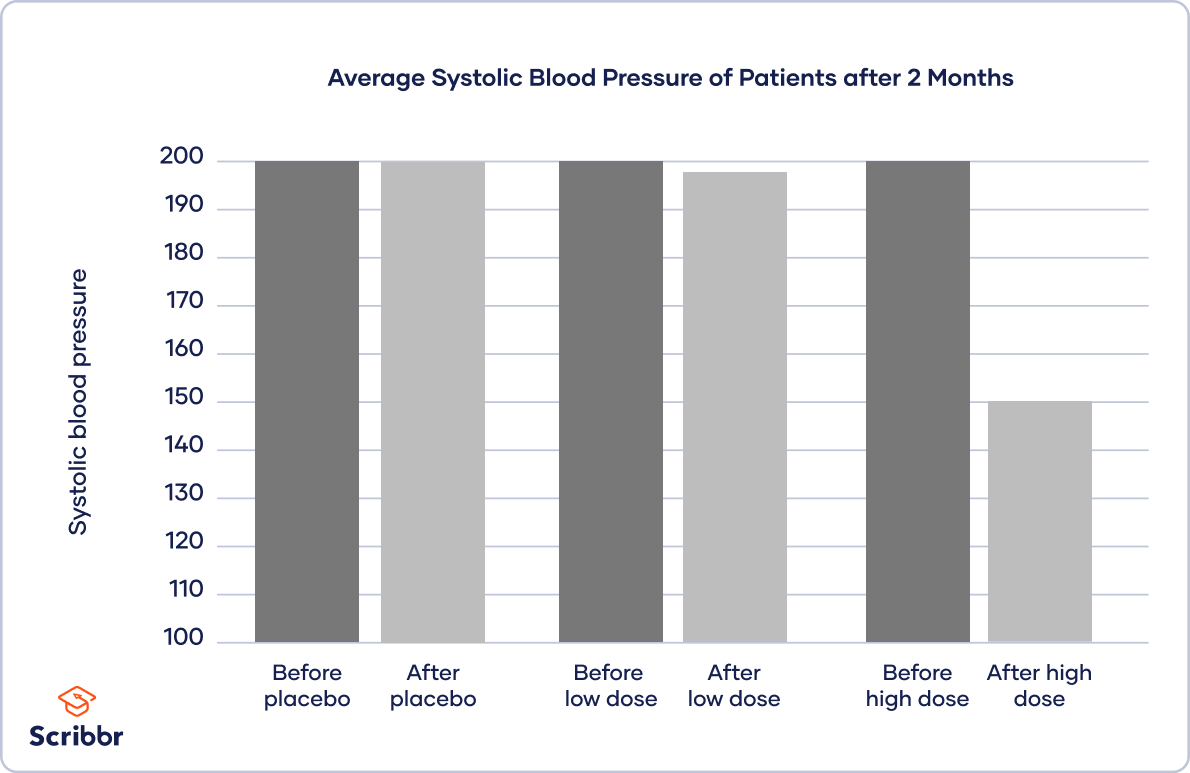
An independent variable is the variable you manipulate, control, or vary in an experimental study to explore its effects. It’s called ‘independent’ because it’s not influenced by any other variables in the study.
- Right-hand-side variables (they appear on the right-hand side of a regression equation)
A dependent variable is what changes as a result of the independent variable manipulation in experiments . It’s what you’re interested in measuring, and it ‘depends’ on your independent variable.
In statistics, dependent variables are also called:
Determining cause and effect is one of the most important parts of scientific research. It’s essential to know which is the cause – the independent variable – and which is the effect – the dependent variable.
You want to find out how blood sugar levels are affected by drinking diet cola and regular cola, so you conduct an experiment .
- The type of cola – diet or regular – is the independent variable .
- The level of blood sugar that you measure is the dependent variable – it changes depending on the type of cola.
Yes, but including more than one of either type requires multiple research questions .
For example, if you are interested in the effect of a diet on health, you can use multiple measures of health: blood sugar, blood pressure, weight, pulse, and many more. Each of these is its own dependent variable with its own research question.
You could also choose to look at the effect of exercise levels as well as diet, or even the additional effect of the two combined. Each of these is a separate independent variable .
To ensure the internal validity of an experiment , you should only change one independent variable at a time.
No. The value of a dependent variable depends on an independent variable, so a variable cannot be both independent and dependent at the same time. It must be either the cause or the effect, not both.
Cite this Scribbr article
If you want to cite this source, you can copy and paste the citation or click the ‘Cite this Scribbr article’ button to automatically add the citation to our free Reference Generator.
Bhandari, P. (2022, October 17). Independent vs Dependent Variables | Definition & Examples. Scribbr. Retrieved 31 May 2024, from https://www.scribbr.co.uk/research-methods/independent-vs-dependent-variables/
Is this article helpful?
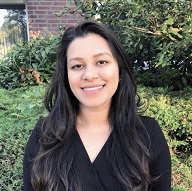
Pritha Bhandari
Other students also liked, a quick guide to experimental design | 5 steps & examples, quasi-experimental design | definition, types & examples, types of variables in research | definitions & examples.
Difference Between Independent and Dependent Variables
Independent vs. Dependent Variables
- Chemical Laws
- Periodic Table
- Projects & Experiments
- Scientific Method
- Biochemistry
- Physical Chemistry
- Medical Chemistry
- Chemistry In Everyday Life
- Famous Chemists
- Activities for Kids
- Abbreviations & Acronyms
- Weather & Climate
The two main variables in a scientific experiment are the independent and dependent variables. An independent variable is changed or controlled in a scientific experiment to test the effects on another variable. This variable being tested and measured is called the dependent variable.
As its name suggests, the dependent variable is "dependent" on the independent variable. As the experimenter changes the independent variable, the effect on the dependent variable is observed and recorded.
Key Takeaways
- There can be many variables in an experiment, but the two key variables that are always present are the independent and dependent variables.
- The independent variable is the one the researcher intentionally changes or controls.
- The dependent variable is the factor that the research measures. It changes in response to the independent variable; in other words, it depends on it.
- Examples of Independent and Dependent Variables
Let's say a scientist wants to see if the brightness of light has any effect on a moth's attraction to the light. The brightness of the light is controlled by the scientist. This would be the independent variable . How the moth reacts to the different light levels (such as its distance to the light source) would be the dependent variable .
As another example, say you want to know whether eating breakfast affects student test scores. The factor under the experimenter's control is the presence or absence of breakfast, so you know it is the independent variable. The experiment measures test scores of students who ate breakfast versus those who did not. Theoretically, the test results depend on breakfast, so the test results are the dependent variable. Note that test scores are the dependent variable even if it turns out there is no relationship between scores and breakfast.
For another experiment, a scientist wants to determine whether one drug is more effective than another at controlling high blood pressure. The independent variable is the drug, while the patient's blood pressure is the dependent variable. In some ways, this experiment resembles the one with breakfast and test scores. However, when comparing two different treatments, such as drug A and drug B, it's usual to add another variable, called the control variable. The control variable , which in this case is a placebo that contains the same inactive ingredients as the drugs, makes it possible to tell whether either drug actually affects blood pressure.
How to Tell Independent and Dependent Variables Apart
The independent and dependent variables in an experiment may be viewed in terms of cause and effect. If the independent variable is changed, then an effect is seen, or measured, in the dependent variable. Remember, the values of both variables may change in an experiment and are recorded. The difference is that the value of the independent variable is controlled by the experimenter, while the value of the dependent variable only changes in response to the independent variable.
Remembering Variables and How to Plot Them
When results are plotted in graphs, the convention is to use the independent variable as the x-axis and the dependent variable as the y-axis. The DRY MIX acronym can help keep the variables straight:
D is the dependent variable R is the responding variable Y is the axis on which the dependent or responding variable is graphed (the vertical axis)
M is the manipulated variable or the one that is changed in an experiment I is the independent variable X is the axis on which the independent or manipulated variable is graphed (the horizontal axis)
- Null Hypothesis Examples
- What Is a Dependent Variable?
- Independent Variable Definition and Examples
- Popular Math Terms and Definitions
- Dependent Variable Definition and Examples
- Scientific Variable
- Scientific Method Vocabulary Terms
- DRY MIX Experiment Variables Acronym
- The Difference Between Control Group and Experimental Group
- What Is a Variable in Science?
- What Is an Experiment? Definition and Design
- The Role of a Controlled Variable in an Experiment
- The Differences Between Explanatory and Response Variables
- Six Steps of the Scientific Method
- Understanding Experimental Groups

Advertisement
The Independent Variable vs. Dependent Variable in Research
- Share Content on Facebook
- Share Content on LinkedIn
- Share Content on Flipboard
- Share Content on Reddit
- Share Content via Email
In any scientific research, there are typically two variables of interest: independent variables and dependent variables. In forming the backbone of scientific experiments , they help scientists understand relationships, predict outcomes and, in general, make sense of the factors that they're investigating.
Understanding the independent variable vs. dependent variable is so fundamental to scientific research that you need to have a good handle on both if you want to design your own research study or interpret others' findings.
To grasp the distinction between the two, let's delve into their definitions and roles.
What Is an Independent Variable?
What is a dependent variable, research study example, predictor variables vs. outcome variables, other variables, the relationship between independent and dependent variables.
The independent variable, often denoted as X, is the variable that is manipulated or controlled by the researcher intentionally. It's the factor that researchers believe may have a causal effect on the dependent variable.
In simpler terms, the independent variable is the variable you change or vary in an experiment so you can observe its impact on the dependent variable.
The dependent variable, often represented as Y, is the variable that is observed and measured to determine the outcome of the experiment.
In other words, the dependent variable is the variable that is affected by the changes in the independent variable. The values of the dependent variable always depend on the independent variable.
Let's consider an example to illustrate these concepts. Imagine you're conducting a research study aiming to investigate the effect of studying techniques on test scores among students.
In this scenario, the independent variable manipulated would be the studying technique, which you could vary by employing different methods, such as spaced repetition, summarization or practice testing.
The dependent variable, in this case, would be the test scores of the students. As the researcher following the scientific method , you would manipulate the independent variable (the studying technique) and then measure its impact on the dependent variable (the test scores).
You can also categorize variables as predictor variables or outcome variables. Sometimes a researcher will refer to the independent variable as the predictor variable since they use it to predict or explain changes in the dependent variable, which is also known as the outcome variable.
When conducting an experiment or study, it's crucial to acknowledge the presence of other variables, or extraneous variables, which may influence the outcome of the experiment but are not the focus of study.
These variables can potentially confound the results if they aren't controlled. In the example from above, other variables might include the students' prior knowledge, level of motivation, time spent studying and preferred learning style.
As a researcher, it would be your goal to control these extraneous variables to ensure you can attribute any observed differences in the dependent variable to changes in the independent variable. In practice, however, it's not always possible to control every variable.
The distinction between independent and dependent variables is essential for designing and conducting research studies and experiments effectively.
By manipulating the independent variable and measuring its impact on the dependent variable while controlling for other factors, researchers can gain insights into the factors that influence outcomes in their respective fields.
Whether investigating the effects of a new drug on blood pressure or studying the relationship between socioeconomic factors and academic performance, understanding the role of independent and dependent variables is essential for advancing knowledge and making informed decisions.
Correlation vs. Causation
Understanding the relationship between independent and dependent variables is essential for making sense of research findings. Depending on the nature of this relationship, researchers may identify correlations or infer causation between the variables.
Correlation implies that changes in one variable are associated with changes in another variable, while causation suggests that changes in the independent variable directly cause changes in the dependent variable.
Control and Intervention
In experimental research, the researcher has control over the independent variable, allowing them to manipulate it to observe its effects on the dependent variable. This controlled manipulation distinguishes experiments from other types of research designs.
For example, in observational studies, researchers merely observe variables without intervention, meaning they don't control or manipulate any variables.
Context and Analysis
Whether it's intentional or unintentional, independent, dependent and other variables can vary in different contexts, and their effects may differ based on various factors, such as age, characteristics of the participants, environmental influences and so on.
Researchers employ statistical analysis techniques to measure and analyze the relationships between these variables, helping them to draw meaningful conclusions from their data.
We created this article in conjunction with AI technology, then made sure it was fact-checked and edited by a HowStuffWorks editor.
Please copy/paste the following text to properly cite this HowStuffWorks.com article:
- What is New
- Download Your Software
- Behavioral Research
- Software for Consumer Research
- Software for Human Factors R&D
- Request Live Demo
- Contact Sales
Sensor Hardware
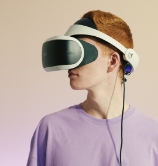
We carry a range of biosensors from the top hardware producers. All compatible with iMotions
iMotions for Higher Education
Imotions for business.
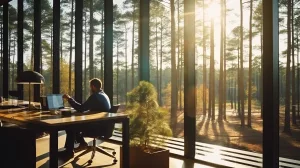
How Does Environmental Ergonomics Affect Behavior?
Ergonomics Testing
Morten Pedersen
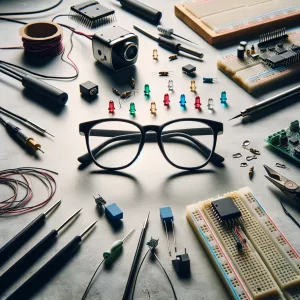
Can You Build Your Own Eye Tracking Glasses?
News & events.
- iMotions Lab
- iMotions Online
- Eye Tracking
- Eye Tracking Screen Based
- Eye Tracking VR
- Eye Tracking Glasses
- Eye Tracking Webcam
- FEA (Facial Expression Analysis)
- Voice Analysis
- EDA/GSR (Electrodermal Activity)
- EEG (Electroencephalography)
- ECG (Electrocardiography)
- EMG (Electromyography)
- Respiration
- iMotions Lab: New features
- iMotions Lab: Developers
- EEG sensors
- Sensory and Perceptual
- Consumer Inights
- Human Factors R&D
- Work Environments, Training and Safety
- Customer Stories
- Published Research Papers
- Document Library
- Customer Support Program
- Help Center
- Release Notes
- Contact Support
- Partnerships
- Mission Statement
- Ownership and Structure
- Executive Management
- Job Opportunities
Publications
- Newsletter Sign Up
Roles of Independent and Dependent Variables in Research
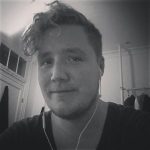
Explore the essential roles of independent and dependent variables in research. This guide delves into their definitions, significance in experiments, and their critical relationship. Learn how these variables are the foundation of research design, influencing hypothesis testing, theory development, and statistical analysis, empowering researchers to understand and predict outcomes of research studies.
Table of Contents
Introduction.
At the very base of scientific inquiry and research design , variables act as the fundamental steps, guiding the rhythm and direction of research. This is particularly true in human behavior research, where the quest to understand the complexities of human actions and reactions hinges on the meticulous manipulation and observation of these variables. At the heart of this endeavor lie two different types of variables, namely: independent and dependent variables, whose roles and interplay are critical in scientific discovery.
Understanding the distinction between independent and dependent variables is not merely an academic exercise; it is essential for anyone venturing into the field of research. This article aims to demystify these concepts, offering clarity on their definitions, roles, and the nuances of their relationship in the study of human behavior, and in science generally. We will cover hypothesis testing and theory development, illuminating how these variables serve as the cornerstone of experimental design and statistical analysis.
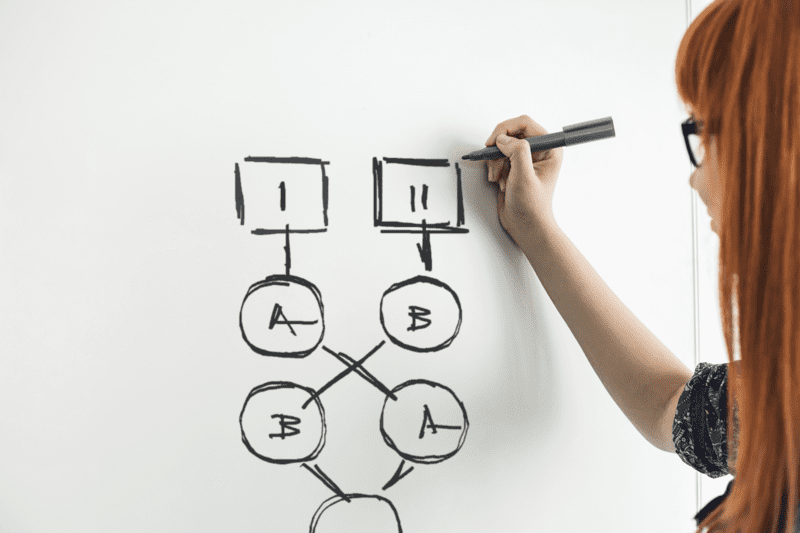
The significance of grasping the difference between independent and dependent variables extends beyond the confines of academia. It empowers researchers to design robust studies, enables critical evaluation of research findings, and fosters an appreciation for the complexity of human behavior research. As we delve into this exploration, our objective is clear: to equip readers with a deep understanding of these fundamental concepts, enhancing their ability to contribute to the ever-evolving field of human behavior research.
Chapter 1: The Role of Independent Variables in Human Behavior Research
In the realm of human behavior research, independent variables are the keystones around which studies are designed and hypotheses are tested. Independent variables are the factors or conditions that researchers manipulate or observe to examine their effects on dependent variables, which typically reflect aspects of human behavior or psychological phenomena. Understanding the role of independent variables is crucial for designing robust research methodologies, ensuring the reliability and validity of findings.
Defining Independent Variables
Independent variables are those variables that are changed or controlled in a scientific experiment to test the effects on dependent variables. In studies focusing on human behavior, these can range from psychological interventions (e.g., cognitive-behavioral therapy), environmental adjustments (e.g., noise levels, lighting, smells, etc), to societal factors (e.g., social media use). For example, in an experiment investigating the impact of sleep on cognitive performance, the amount of sleep participants receive is the independent variable.
Selection and Manipulation
Selecting an independent variable requires careful consideration of the research question and the theoretical framework guiding the study. Researchers must ensure that their chosen variable can be effectively, and consistently manipulated or measured and is ethically and practically feasible, particularly when dealing with human subjects.
Manipulating an independent variable involves creating different conditions (e.g., treatment vs. control groups) to observe how changes in the variable affect outcomes. For instance, researchers studying the effect of educational interventions on learning outcomes might vary the type of instructional material (digital vs. traditional) to assess differences in student performance.
Challenges in Human Behavior Research
Manipulating independent variables in human behavior research presents unique challenges. Ethical considerations are paramount, as interventions must not harm participants. For example, studies involving vulnerable populations or sensitive topics require rigorous ethical oversight to ensure that the manipulation of independent variables does not result in adverse effects.
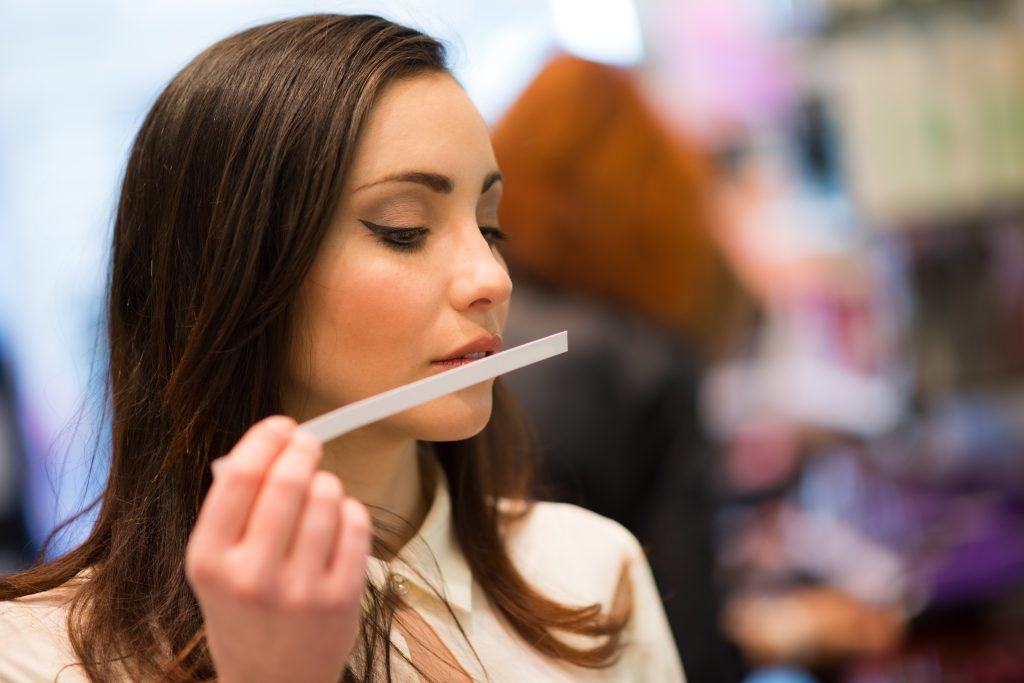
Practical limitations also come into play, such as controlling for extraneous variables that could influence the outcomes. In the aforementioned example of sleep and cognitive performance, factors like caffeine consumption or stress levels could confound the results. Researchers employ various methodological strategies, such as random assignment and controlled environments, to mitigate these influences.
Chapter 2: Dependent Variables: Measuring Human Behavior
The dependent variable in human behavior research acts as a mirror, reflecting the outcomes or effects resulting from variations in the independent variable. It is the aspect of human experience or behavior that researchers aim to understand, predict, or change through their studies. This section explores how dependent variables are measured, the significance of their accurate measurement, and the inherent challenges in capturing the complexities of human behavior.
Defining Dependent Variables
Dependent variables are the responses or outcomes that researchers measure in an experiment, expecting them to vary as a direct result of changes in the independent variable. In the context of human behavior research, dependent variables could include measures of emotional well-being, cognitive performance, social interactions, or any other aspect of human behavior influenced by the experimental manipulation. For instance, in a study examining the effect of exercise on stress levels, stress level would be the dependent variable, measured through various psychological assessments or physiological markers.
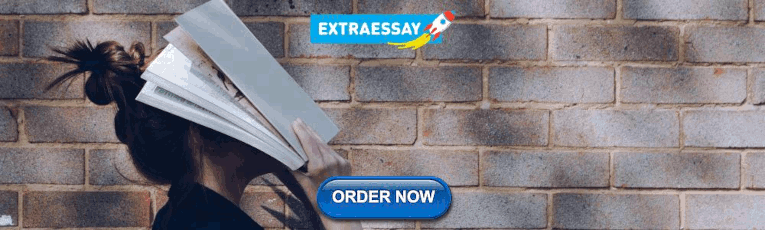
Measurement Methods and Tools
Measuring dependent variables in human behavior research involves a diverse array of methodologies, ranging from self-reported questionnaires and interviews to physiological measurements and behavioral observations. The choice of measurement tool depends on the nature of the dependent variable and the objectives of the study.
- Self-reported Measures: Often used for assessing psychological states or subjective experiences, such as anxiety, satisfaction, or mood. These measures rely on participants’ introspection and honesty, posing challenges in terms of accuracy and bias.
- Behavioral Observations: Involve the direct observation and recording of participants’ behavior in natural or controlled settings. This method is used for behaviors that can be externally observed and quantified, such as social interactions or task performance.
- Physiological Measurements: Include the use of technology to measure physical responses that indicate psychological states, such as heart rate, cortisol levels, or brain activity. These measures can provide objective data about the physiological aspects of human behavior.
Reliability and Validity
The reliability and validity of the measurement of dependent variables are critical to the integrity of human behavior research.
- Reliability refers to the consistency of a measure; a reliable tool yields similar results under consistent conditions.
- Validity pertains to the accuracy of the measure; a valid tool accurately reflects the concept it aims to measure.
Ensuring reliability and validity often involves the use of established measurement instruments with proven track records, pilot testing new instruments, and applying rigorous statistical analyses to evaluate measurement properties.
Challenges in Measuring Human Behavior
Measuring human behavior presents challenges due to its complexity and the influence of multiple, often interrelated, variables. Researchers must contend with issues such as participant bias, environmental influences, and the subjective nature of many psychological constructs. Additionally, the dynamic nature of human behavior means that it can change over time, necessitating careful consideration of when and how measurements are taken.
Section 3: Relationship between Independent and Dependent Variables
Understanding the relationship between independent and dependent variables is at the core of research in human behavior. This relationship is what researchers aim to elucidate, whether they seek to explain, predict, or influence human actions and psychological states. This section explores the nature of this relationship, the means by which it is analyzed, and common misconceptions that may arise.
The Nature of the Relationship
The relationship between independent and dependent variables can manifest in various forms—direct, indirect, linear, nonlinear, and may be moderated or mediated by other variables. At its most basic, this relationship is often conceptualized as cause and effect: the independent variable (the cause) influences the dependent variable (the effect). For instance, increased physical activity (independent variable) may lead to decreased stress levels (dependent variable).
Analyzing the Relationship
Statistical analyses play a pivotal role in examining the relationship between independent and dependent variables. Techniques vary depending on the nature of the variables and the research design, ranging from simple correlation and regression analyses for quantifying the strength and form of relationships, to complex multivariate analyses for exploring relationships among multiple variables simultaneously.
- Correlation Analysis : Used to determine the degree to which two variables are related. However, it’s crucial to note that correlation does not imply causation.
- Regression Analysis : Goes a step further by not only assessing the strength of the relationship but also predicting the value of the dependent variable based on the independent variable.
- Experimental Design : Provides a more robust framework for inferring causality, where manipulation of the independent variable and control of confounding factors allow researchers to directly observe the impact on the dependent variable.
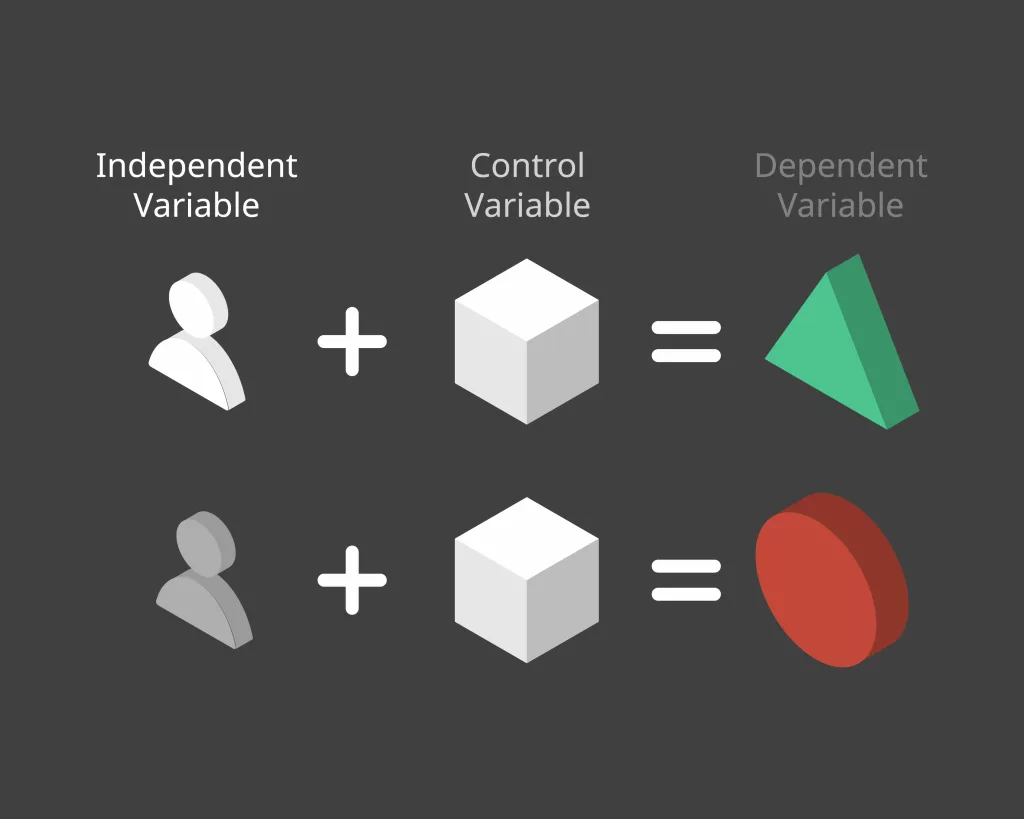
Causality vs. Correlation
A fundamental consideration in human behavior research is the distinction between causality and correlation. Causality implies that changes in the independent variable cause changes in the dependent variable. Correlation, on the other hand, indicates that two variables are related but does not establish a cause-effect relationship. Confounding variables may influence both, creating the appearance of a direct relationship where none exists. Understanding this distinction is crucial for accurate interpretation of research findings.
Common Misinterpretations
The complexity of human behavior and the myriad factors that influence it often lead to challenges in interpreting the relationship between independent and dependent variables. Researchers must be wary of:
- Overestimating the strength of causal relationships based on correlational data.
- Ignoring potential confounding variables that may influence the observed relationship.
- Assuming the directionality of the relationship without adequate evidence.
This exploration highlights the importance of understanding independent and dependent variables in human behavior research. Independent variables act as the initiating factors in experiments, influencing the observed behaviors, while dependent variables reflect the results of these influences, providing insights into human emotions and actions.
Ethical and practical challenges arise, especially in experiments involving human participants, necessitating careful consideration to respect participants’ well-being. The measurement of these variables is critical for testing theories and validating hypotheses, with their relationship offering potential insights into causality and correlation within human behavior.
Rigorous statistical analysis and cautious interpretation of findings are essential to avoid misconceptions. Overall, the study of these variables is fundamental to advancing human behavior research, guiding researchers towards deeper understanding and potential interventions to improve the human condition.
Free 44-page Experimental Design Guide
For Beginners and Intermediates
- Introduction to experimental methods
- Respondent management with groups and populations
- How to set up stimulus selection and arrangement
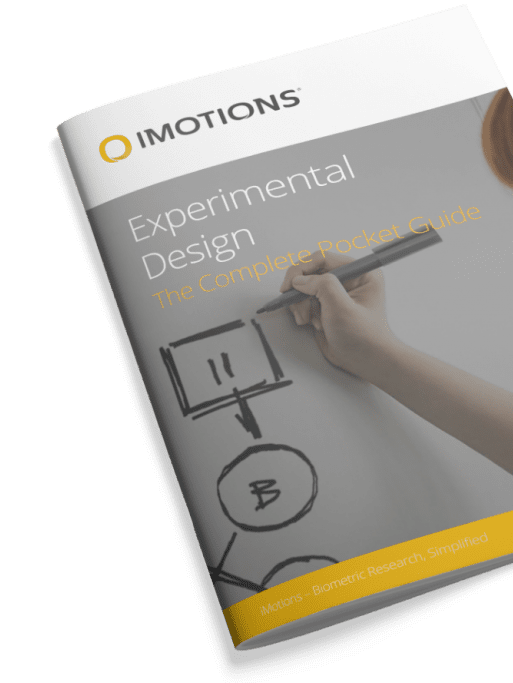
Last edited
About the author
See what is next in human behavior research
Follow our newsletter to get the latest insights and events send to your inbox.
Related Posts
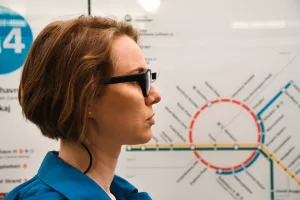
Exploring Mobile Eye Trackers: How Eye Tracking Glasses Work and Their Applications
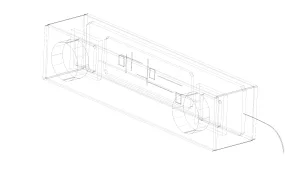
Understanding Screen-Based Eye Trackers: How They Work and Their Applications
Product guides.
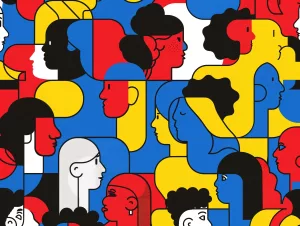
What is Social Behavior?
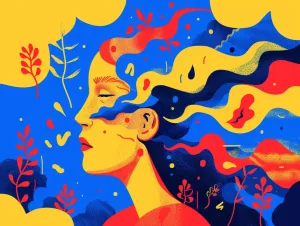
What is Covert Behavior?
You might also like these.
Human Factors and UX
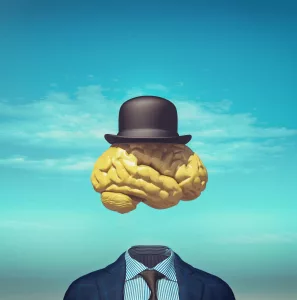
Managing and Measuring Cognitive Performance
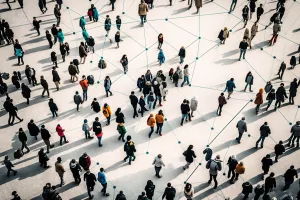
The World Runs on Behavioral Insights
Consumer Insights
Case Stories
Explore Blog Categories
Best Practice
Collaboration, product news, research fundamentals, research insights, 🍪 use of cookies.
We are committed to protecting your privacy and only use cookies to improve the user experience.
Chose which third-party services that you will allow to drop cookies. You can always change your cookie settings via the Cookie Settings link in the footer of the website. For more information read our Privacy Policy.
- gtag This tag is from Google and is used to associate user actions with Google Ad campaigns to measure their effectiveness. Enabling this will load the gtag and allow for the website to share information with Google. This service is essential and can not be disabled.
- Livechat Livechat provides you with direct access to the experts in our office. The service tracks visitors to the website but does not store any information unless consent is given. This service is essential and can not be disabled.
- Pardot Collects information such as the IP address, browser type, and referring URL. This information is used to create reports on website traffic and track the effectiveness of marketing campaigns.
- Third-party iFrames Allows you to see thirdparty iFrames.
Organizing Your Social Sciences Research Paper: Independent and Dependent Variables
- Purpose of Guide
- Writing a Research Proposal
- Design Flaws to Avoid
- Independent and Dependent Variables
- Narrowing a Topic Idea
- Broadening a Topic Idea
- The Research Problem/Question
- Academic Writing Style
- Choosing a Title
- Making an Outline
- Paragraph Development
- The C.A.R.S. Model
- Background Information
- Theoretical Framework
- Citation Tracking
- Evaluating Sources
- Reading Research Effectively
- Primary Sources
- Secondary Sources
- What Is Scholarly vs. Popular?
- Is it Peer-Reviewed?
- Qualitative Methods
- Quantitative Methods
- Common Grammar Mistakes
- Writing Concisely
- Avoiding Plagiarism [linked guide]
- Annotated Bibliography
- Grading Someone Else's Paper
Definitions
Dependent Variable The variable that depends on other factors that are measured. These variables are expected to change as a result of an experimental manipulation of the independent variable or variables. It is the presumed effect.
Independent Variable The variable that is stable and unaffected by the other variables you are trying to measure. It refers to the condition of an experiment that is systematically manipulated by the investigator. It is the presumed cause.
Cramer, Duncan and Dennis Howitt. The SAGE Dictionary of Statistics . London: SAGE, 2004; Penslar, Robin Levin and Joan P. Porter. Institutional Review Board Guidebook: Introduction . Washington, DC: United States Department of Health and Human Services, 2010; "What are Dependent and Independent Variables?" Graphic Tutorial .
Identifying Dependent and Indepent Variables
Don't feel bad if you are confused about what is the dependent variable and what is the independent variable in social and behavioral sciences research . However, it's important that you learn the difference because framing a study using these variables is a common approach to organizing the elements of a social sciences research study in order to discover relevant and meaningful results. Specifically, it is important for these two reasons:
- You need to understand and be able to evaluate their application in other people's research.
- You need to apply them correctly in your own research.
A variable in research simply refers to a person, place, thing, or phenomenon that you are trying to measure in some way. The best way to understand the difference between a dependent and independent variable is that the meaning of each is implied by what the words tell us about the variable you are using. You can do this with a simple exercise from the website, Graphic Tutorial. Take the sentence, "The [independent variable] causes a change in [dependent variable] and it is not possible that [dependent variable] could cause a change in [independent variable]." Insert the names of variables you are using in the sentence in the way that makes the most sense. This will help you identify each type of variable. If you're still not sure, consult with your professor before you begin to write.
Fan, Shihe. "Independent Variable." In Encyclopedia of Research Design. Neil J. Salkind, editor. (Thousand Oaks, CA: SAGE, 2010), pp. 592-594; "What are Dependent and Independent Variables?" Graphic Tutorial ; Salkind, Neil J. "Dependent Variable." In Encyclopedia of Research Design , Neil J. Salkind, editor. (Thousand Oaks, CA: SAGE, 2010), pp. 348-349;
Structure and Writing Style
The process of examining a research problem in the social and behavioral sciences is often framed around methods of analysis that compare, contrast, correlate, average, or integrate relationships between or among variables . Techniques include associations, sampling, random selection, and blind selection. Designation of the dependent and independent variable involves unpacking the research problem in a way that identifies a general cause and effect and classifying these variables as either independent or dependent.
The variables should be outlined in the introduction of your paper and explained in more detail in the methods section . There are no rules about the structure and style for writing about independent or dependent variables but, as with any academic writing, clarity and being succinct is most important.
After you have described the research problem and its significance in relation to prior research, explain why you have chosen to examine the problem using a method of analysis that investigates the relationships between or among independent and dependent variables . State what it is about the research problem that lends itself to this type of analysis. For example, if you are investigating the relationship between corporate environmental sustainability efforts [the independent variable] and dependent variables associated with measuring employee satisfaction at work using a survey instrument, you would first identify each variable and then provide background information about the variables. What is meant by "environmental sustainability"? Are you looking at a particular company [e.g., General Motors] or are you investigating an industry [e.g., the meat packing industry]? Why is employee satisfaction in the workplace important? How does a company make their employees aware of sustainability efforts and why would a company even care that its employees know about these efforts?
Identify each variable for the reader and define each . In the introduction, this information can be presented in a paragraph or two when you describe how you are going to study the research problem. In the methods section, you build on the literature review of prior studies about the research problem to describe in detail background about each variable, breaking each down for measurement and analysis. For example, what activities do you examine that reflect a company's commitment to environmental sustainability? Levels of employee satisfaction can be measured by a survey that asks about things like volunteerism or a desire to stay at the company for a long time.
The structure and writing style of describing the variables and their application to analyzing the research problem should be stated and unpacked in such a way that the reader obtains a clear understanding of the relationships between the variables and why they are important. This is also important so that the study can be replicated in the future using the same variables but applied in a different way.
Fan, Shihe. "Independent Variable." In Encyclopedia of Research Design. Neil J. Salkind, editor. (Thousand Oaks, CA: SAGE, 2010), pp. 592-594; "What are Dependent and Independent Variables?" Graphic Tutorial ; “ Case Example for Independent and Dependent Variables .” ORI Curriculum Examples. U.S. Department of Health and Human Services, Office of Research Integrity; Salkind, Neil J. "Dependent Variable." In Encyclopedia of Research Design , Neil J. Salkind, editor. (Thousand Oaks, CA: SAGE, 2010), pp. 348-349; “ Independent Variables and Dependent Variables .” Karl L. Wuensch, Department of Psychology, East Carolina University [posted email exchange]; “ Variables .” Elements of Research. Dr. Camille Nebeker, San Diego State University.
- << Previous: Design Flaws to Avoid
- Next: Choosing a Research Problem >>
- Last Updated: Sep 8, 2023 12:19 PM
- URL: https://guides.library.txstate.edu/socialscienceresearch
- Privacy Policy

Home » Independent Variable – Definition, Types and Examples
Independent Variable – Definition, Types and Examples
Table of Contents

Independent Variable
Definition:
Independent variable is a variable that is manipulated or changed by the researcher to observe its effect on the dependent variable. It is also known as the predictor variable or explanatory variable
The independent variable is the presumed cause in an experiment or study, while the dependent variable is the presumed effect or outcome. The relationship between the independent variable and the dependent variable is often analyzed using statistical methods to determine the strength and direction of the relationship.
Types of Independent Variables
Types of Independent Variables are as follows:
Categorical Independent Variables
These variables are categorical or nominal in nature and represent a group or category. Examples of categorical independent variables include gender, ethnicity, marital status, and educational level.
Continuous Independent Variables
These variables are continuous in nature and can take any value on a continuous scale. Examples of continuous independent variables include age, height, weight, temperature, and blood pressure.
Discrete Independent Variables
These variables are discrete in nature and can only take on specific values. Examples of discrete independent variables include the number of siblings, the number of children in a family, and the number of pets owned.
Binary Independent Variables
These variables are dichotomous or binary in nature, meaning they can take on only two values. Examples of binary independent variables include yes or no questions, such as whether a participant is a smoker or non-smoker.
Controlled Independent Variables
These variables are manipulated or controlled by the researcher to observe their effect on the dependent variable. Examples of controlled independent variables include the type of treatment or therapy given, the dosage of a medication, or the amount of exposure to a stimulus.
Independent Variable and dependent variable Analysis Methods
Following analysis methods that can be used to examine the relationship between an independent variable and a dependent variable:
Correlation Analysis
This method is used to determine the strength and direction of the relationship between two continuous variables. Correlation coefficients such as Pearson’s r or Spearman’s rho are used to quantify the strength and direction of the relationship.
ANOVA (Analysis of Variance)
This method is used to compare the means of two or more groups for a continuous dependent variable. ANOVA can be used to test the effect of a categorical independent variable on a continuous dependent variable.
Regression Analysis
This method is used to examine the relationship between a dependent variable and one or more independent variables. Linear regression is a common type of regression analysis that can be used to predict the value of the dependent variable based on the value of one or more independent variables.
Chi-square Test
This method is used to test the association between two categorical variables. It can be used to examine the relationship between a categorical independent variable and a categorical dependent variable.
This method is used to compare the means of two groups for a continuous dependent variable. It can be used to test the effect of a binary independent variable on a continuous dependent variable.
Measuring Scales of Independent Variable
There are four commonly used Measuring Scales of Independent Variables:
- Nominal Scale : This scale is used for variables that can be categorized but have no inherent order or numerical value. Examples of nominal variables include gender, race, and occupation.
- Ordinal Scale : This scale is used for variables that can be categorized and have a natural order but no specific numerical value. Examples of ordinal variables include levels of education (e.g., high school, bachelor’s degree, master’s degree), socioeconomic status (e.g., low, middle, high), and Likert scales (e.g., strongly disagree, disagree, neutral, agree, strongly agree).
- I nterval Scale : This scale is used for variables that have a numerical value and a consistent unit of measurement but no true zero point. Examples of interval variables include temperature in Celsius or Fahrenheit, IQ scores, and time of day.
- Ratio Scale: This scale is used for variables that have a numerical value, a consistent unit of measurement, and a true zero point. Examples of ratio variables include height, weight, and income.
Independent Variable Examples
Here are some examples of independent variables:
- In a study examining the effects of a new medication on blood pressure, the independent variable would be the medication itself.
- In a study comparing the academic performance of male and female students, the independent variable would be gender.
- In a study investigating the effects of different types of exercise on weight loss, the independent variable would be the type of exercise performed.
- In a study examining the relationship between age and income, the independent variable would be age.
- In a study investigating the effects of different types of music on mood, the independent variable would be the type of music played.
- In a study examining the effects of different teaching strategies on student test scores, the independent variable would be the teaching strategy used.
- In a study investigating the effects of caffeine on reaction time, the independent variable would be the amount of caffeine consumed.
- In a study comparing the effects of two different fertilizers on plant growth, the independent variable would be the type of fertilizer used.
Independent variable vs Dependent variable
Applications of independent variable.
Applications of Independent Variable in different fields are as follows:
- Scientific experiments : Independent variables are commonly used in scientific experiments to study the cause-and-effect relationships between different variables. By controlling and manipulating the independent variable, scientists can observe how changes in that variable affect the dependent variable.
- Market research: Independent variables are also used in market research to study consumer behavior. For example, researchers may manipulate the price of a product (independent variable) to see how it affects consumer demand (dependent variable).
- Psychology: In psychology, independent variables are often used to study the effects of different treatments or therapies on mental health conditions. For example, researchers may manipulate the type of therapy (independent variable) to see how it affects a patient’s symptoms (dependent variable).
- Education: Independent variables are used in educational research to study the effects of different teaching methods or interventions on student learning outcomes. For example, researchers may manipulate the teaching method (independent variable) to see how it affects student performance on a test (dependent variable).
Purpose of Independent Variable
The purpose of an independent variable is to manipulate or control it in order to observe its effect on the dependent variable. In other words, the independent variable is the variable that is being tested or studied to see if it has an effect on the dependent variable.
The independent variable is often manipulated by the researcher in order to create different experimental conditions. By varying the independent variable, the researcher can observe how the dependent variable changes in response. For example, in a study of the effects of caffeine on memory, the independent variable would be the amount of caffeine consumed, while the dependent variable would be memory performance.
The main purpose of the independent variable is to determine causality. By manipulating the independent variable and observing its effect on the dependent variable, researchers can determine whether there is a causal relationship between the two variables. This is important for understanding how different variables affect each other and for making predictions about how changes in one variable will affect other variables.
When to use Independent Variable
Here are some situations when an independent variable may be used:
- When studying cause-and-effect relationships: Independent variables are often used in studies that aim to establish causal relationships between variables. By manipulating the independent variable and observing the effect on the dependent variable, researchers can determine whether there is a cause-and-effect relationship between the two variables.
- When comparing groups or conditions: Independent variables can also be used to compare groups or conditions. For example, a researcher might manipulate an independent variable (such as a treatment or intervention) and observe the effect on a dependent variable (such as a symptom or behavior) in two different groups of participants (such as a treatment group and a control group).
- When testing hypotheses: Independent variables are used to test hypotheses about how different variables are related. By manipulating the independent variable and observing the effect on the dependent variable, researchers can test whether their hypotheses are supported or not.
Characteristics of Independent Variable
Here are some of the characteristics of independent variables:
- Manipulation: The independent variable is manipulated by the researcher in order to create different experimental conditions. The researcher changes the level or value of the independent variable to observe how it affects the dependent variable.
- Control : The independent variable is controlled by the researcher to ensure that it is the only variable that is changing in the experiment. By controlling other variables that might affect the dependent variable, the researcher can isolate the effect of the independent variable on the dependent variable.
- Categorical or continuous: Independent variables can be either categorical or continuous. Categorical independent variables have distinct categories or levels that are not ordered (e.g., gender, ethnicity), while continuous independent variables are measured on a scale (e.g., age, temperature).
- Treatment : In some experiments, the independent variable represents a treatment or intervention that is being tested. For example, a researcher might manipulate the independent variable by giving participants a new medication or therapy.
- Random assignment : In order to control for extraneous variables and ensure that the independent variable is the only variable that is changing, participants are often randomly assigned to different levels of the independent variable. This helps to ensure that any differences between the groups are not due to pre-existing differences between the participants.
Advantages of Independent Variable
Independent variables have several advantages, including:
- Control : Independent variables allow researchers to control the variables being studied, which helps to establish cause-and-effect relationships. By manipulating the independent variable, researchers can see how changes in that variable affect the dependent variable.
- Replication : Manipulating independent variables allows researchers to replicate studies to confirm or refute previous findings. By controlling the independent variable, researchers can ensure that any differences in the dependent variable are due to the manipulation of the independent variable, rather than other factors.
- Predictive Powe r: Independent variables can be used to predict future outcomes. By examining how changes in the independent variable affect the dependent variable, researchers can make predictions about how the dependent variable will respond in the future.
- Precision : Independent variables can help to increase the precision of a study by allowing researchers to control for extraneous variables that might otherwise confound the results. This can lead to more accurate and reliable findings.
- Generalizability : Independent variables can help to increase the generalizability of a study by allowing researchers to manipulate variables in a way that reflects real-world conditions. This can help to ensure that findings are applicable to a wider range of situations and contexts.
Disadvantages of Independent Variable
Independent variables also have several disadvantages, including:
- Artificiality : In some cases, manipulating the independent variable in a study may create an artificial environment that does not reflect real-world conditions. This can limit the generalizability of the findings.
- Ethical concerns: Manipulating independent variables in some studies may raise ethical concerns, such as when human participants are subjected to potentially harmful or uncomfortable conditions.
- Limitations in measuring variables: Some variables may be difficult or impossible to manipulate in a study. For example, it may be difficult to manipulate someone’s age or gender, which can limit the researcher’s ability to study the effects of these variables.
- Complexity : Some variables may be very complex, making it difficult to determine which variables are independent and which are dependent. This can make it challenging to design a study that effectively examines the relationship between variables.
- Extraneous variables : Even when researchers manipulate the independent variable, other variables may still affect the results. These extraneous variables can confound the results, making it difficult to draw clear conclusions about the relationship between the independent and dependent variables.
About the author
Muhammad Hassan
Researcher, Academic Writer, Web developer
You may also like

Control Variable – Definition, Types and Examples

Moderating Variable – Definition, Analysis...

Qualitative Variable – Types and Examples

Variables in Research – Definition, Types and...

Categorical Variable – Definition, Types and...

Ratio Variable – Definition, Purpose and Examples
Log in using your username and password
- Search More Search for this keyword Advanced search
- Latest content
- Current issue
- BMJ Journals More You are viewing from: Google Indexer
You are here
- Online First
- Factors associated with disease flare following SARS-CoV-2 vaccination in people with inflammatory rheumatic and musculoskeletal diseases: results from the physician-reported EULAR Coronavirus Vaccine (COVAX) Registry
- Article Text
- Article info
- Citation Tools
- Rapid Responses
- Article metrics

- http://orcid.org/0000-0002-9394-1103 Bayram Farisogullari 1 , 2 ,
- http://orcid.org/0000-0002-8611-162X Saskia Lawson-Tovey 3 , 4 ,
- http://orcid.org/0000-0001-8242-9262 Kimme L Hyrich 4 , 5 ,
- http://orcid.org/0000-0002-4528-310X Laure Gossec 6 ,
- http://orcid.org/0000-0002-4401-2551 Loreto Carmona 7 ,
- http://orcid.org/0000-0002-6233-022X Anja Strangfeld 8 ,
- http://orcid.org/0000-0003-0059-2141 Elsa F Mateus 9 , 10 ,
- http://orcid.org/0000-0001-6487-3634 Martin Schäfer 8 ,
- Ana Rodrigues 11 , 12 , 13 ,
- http://orcid.org/0000-0001-7432-847X Eric Hachulla 14 ,
- http://orcid.org/0000-0001-8177-702X Jose A Gomez-Puerta 15 , 16 ,
- Marta Mosca 17 ,
- http://orcid.org/0000-0002-7156-2356 Patrick Durez 18 ,
- http://orcid.org/0000-0001-5454-903X Ludovic Trefond 19 ,
- Tiphaine Goulenok 20 ,
- Martina Cornalba 21 , 22 ,
- Emoke Stenova 23 ,
- Inita Bulina 24 ,
- Eva Strakova 25 ,
- Julija Zepa 26 ,
- http://orcid.org/0000-0002-7719-1736 Nicolas Roux 27 ,
- Olivier Brocq 28 ,
- Eric Veillard 29 ,
- http://orcid.org/0000-0002-7719-1736 Bernd Raffeiner 30 ,
- http://orcid.org/0000-0001-7518-1131 Gerd R Burmester 31 ,
- http://orcid.org/0000-0002-4244-5417 Xavier Mariette 32 ,
- http://orcid.org/0000-0002-8411-7972 Pedro M Machado 1 , 33 , 34 , 35
- EULAR COVAX Registry
- 1 Department of Neuromuscular Diseases, UCL Queen Square Institute of Neurology, University College London , London , UK
- 2 Division of Rheumatology, Department of Internal Medicine, Faculty of Medicine, Hacettepe University , Ankara , Türkiye
- 3 Centre for Genetics and Genomics Versus Arthritis, Centre for Musculoskeletal Research, University of Manchester , Manchester , UK
- 4 National Institute of Health Research Manchester Biomedical Research Centre, Manchester University NHS Foundation Trust, Manchester Academic Health Science Centre , Manchester , UK
- 5 Centre for Epidemiology Versus Arthritis, The University of Manchester, Manchester Academic Health Science Centre , Manchester , UK
- 6 Sorbonne Université, INSERM, Institut Pierre Louis d'Epidémiologie et de Santé Publique, Paris France; Pitié-Salpêtrière hospital, AP-HP, Rheumatology Department , Paris , France
- 7 Instituto de Salud Musculoesquelética , Madrid , Spain
- 8 German Rheumatism Research Center (DRFZ Berlin), Epidemiology Unit, and Charité University Medicine , Berlin , Germany
- 9 Portuguese League Against Rheumatic Diseases (LPCDR) , Lisbon , Portugal
- 10 European Alliance of Associations for Rheumatology (EULAR) Standing Committee of People with Arthritis/Rheumatism in Europe (PARE) , Kilchberg , Switzerland
- 11 Reuma.pt, Sociedade Portuguesa de Reumatologia , Lisbon , Portugal
- 12 EpiDoC unit, CEDOC, Nova Medical School , Lisbon , Portugal
- 13 Rheumatology Unit, Hospital dos Lusíadas , Lisbon , Portugal
- 14 Service de Médecine Interne et Immunologie Clinique, Centre de référence des maladies autoimmunes systémiques rares du Nord et Nord-Ouest de France (CeRAINO), Univ. Lille, Inserm, CHU Lille, U1286 - INFINITE - Institute for Translational Research in Inflammation, F-59000 , Lille , France
- 15 Rheumatology Department, Hospital Clinic , Barcelona , Spain
- 16 University of Barcelona , Barcelona , Spain
- 17 University of Pisa and Azienda Ospedaliero Universitaria Pisana , Pisa , Italy
- 18 Cliniques Universitaires Saint-Luc – Université catholique de Louvain (UCLouvain) – Institut de Recherche Expérimentale et Clinique (IREC), Rheumatology , Brussels , Belgium
- 19 Université Clermont Auvergne, CHU Clermont-Ferrand, Service de Médecine Interne, Centre de Référence pour les Maladies auto immunes et auto inflammatoires Systémiques Rares d'Auvergne, Hôpital Gabriel Montpied, Inserm U1071, INRA USC2018, M2iSH, 63000 , Clermont-Ferrand , France
- 20 Service de Médecine Interne, Hôpital Bichat-Claude Bernard, Assistance Publique Hôpitaux de Paris, Université de Paris , Paris , France
- 21 University of Milan, Department of Clinical Sciences and Community Health , Milan , Italy
- 22 Dipartimento di Reumatologia e Scienze Mediche, ASST Gaetano Pini - CTO, Clinical and Pediatric Rheumatology Unit , Milan , Italy
- 23 1st Department of Internal Medicine, Faculty of Medicine, University Hospital, Comenius University, Mickiewiczova 13 , 82101 Bratislava , Slovakia
- 24 Pauls Stradins Clinical University Hospital, Riga, Latvia; Riga Stradins University, Riga, Latvia, University of Latvia , Riga , Latvia
- 25 Department of Internal Medicine, Faculty Hospital Prešov , Prešov , Slovakia
- 26 Pauls Stradins Clinical University Hospital, Riga, Latvia; Riga Stradins University , Riga , Latvia
- 27 Service de Rhumatologie, Hôpital Robert Schuman , Metz , France
- 28 Rheumatology Department, Princess Grace Hospital , Monaco
- 29 Cabinet de Rhumatologie des "Marines de Chasles" , Saint Malo , France
- 30 Department of Rheumatology, Central Hospital of Bolzano , Bolzano , Italy
- 31 Department of Rheumatology and Clinical Immunology, Charité - Universitätsmedizin Berlin , Berlin , Germany
- 32 Department of Rheumatology, Université Paris-Saclay, Assistance Publique-Hôpitaux de Paris, Hôpital Bicêtre, INSERM UMR1184 , Le Kremlin Bicêtre , France
- 33 Centre for Rheumatology, UCL Division of Medicine, University College London , London , UK
- 34 National Institute for Health Research University College London Hospitals Biomedical Research Centre, University College London Hospitals NHS Foundation Trust , London , UK
- 35 Department of Rheumatology, Northwick Park Hospital, London North West University Healthcare NHS Trust , London , UK
- Correspondence to Professor Pedro M Machado, Department of Neuromuscular Diseases, University College London, London, UK; p.machado{at}ucl.ac.uk
Objectives To investigate the frequency and factors associated with disease flare following vaccination against SARS-CoV-2 in people with inflammatory/autoimmune rheumatic and musculoskeletal diseases (I-RMDs).
Methods Data from the European Alliance of Associations for Rheumatology Coronavirus Vaccine physician-reported registry were used. Factors associated with flare in patients with I-RMDs were investigated using multivariable logistic regression adjusted for demographic and clinical factors.
Results The study included 7336 patients with I-RMD, with 272 of 7336 (3.7%) experiencing flares and 121 of 7336 (1.6%) experiencing flares requiring starting a new medication or increasing the dosage of an existing medication. Factors independently associated with increased odds of flare were: female sex (OR=1.40, 95% CI=1.05 to 1.87), active disease at the time of vaccination (low disease activity (LDA), OR=1.45, 95% CI=1.08 to 1.94; moderate/high disease activity (M/HDA), OR=1.37, 95% CI=0.97 to 1.95; vs remission), and cessation/reduction of antirheumatic medication before or after vaccination (OR=4.76, 95% CI=3.44 to 6.58); factors associated with decreased odds of flare were: higher age (OR=0.90, 95% CI=0.83 to 0.98), non-Pfizer/AstraZeneca/Moderna vaccines (OR=0.10, 95% CI=0.01 to 0.74; vs Pfizer), and exposure to methotrexate (OR=0.57, 95% CI=0.37 to 0.90), tumour necrosis factor inhibitors (OR=0.55, 95% CI=0.36 to 0.85) or rituximab (OR=0.27, 95% CI=0.11 to 0.66), versus no antirheumatic treatment. In a multivariable model using new medication or dosage increase due to flare as the dependent variable, only the following independent associations were observed: active disease (LDA, OR=1.47, 95% CI=0.94 to 2.29; M/HDA, OR=3.08, 95% CI=1.91 to 4.97; vs remission), cessation/reduction of antirheumatic medication before or after vaccination (OR=2.24, 95% CI=1.33 to 3.78), and exposure to methotrexate (OR=0.48, 95% CI=0.26 to 0.89) or rituximab (OR=0.10, 95% CI=0.01 to 0.77), versus no antirheumatic treatment.
Conclusion I-RMD flares following SARS-CoV-2 vaccination were uncommon. Factors associated with flares were identified, namely higher disease activity and cessation/reduction of antirheumatic medications before or after vaccination.
- Antirheumatic Agents
- Autoimmune Diseases
- Epidemiology
- Vaccination
Data availability statement
Data are available upon reasonable request. Applications to access the data will be considered by the European Alliance of Associations for Rheumatology (EULAR) on a case-by-case basis, and data will be shared if the request is considered reasonable, of scientific interest, and legally and ethically possible.
This is an open access article distributed in accordance with the Creative Commons Attribution Non Commercial (CC BY-NC 4.0) license, which permits others to distribute, remix, adapt, build upon this work non-commercially, and license their derivative works on different terms, provided the original work is properly cited, appropriate credit is given, any changes made indicated, and the use is non-commercial. See: http://creativecommons.org/licenses/by-nc/4.0/ .
https://doi.org/10.1136/ard-2024-225869
Statistics from Altmetric.com
Request permissions.
If you wish to reuse any or all of this article please use the link below which will take you to the Copyright Clearance Center’s RightsLink service. You will be able to get a quick price and instant permission to reuse the content in many different ways.
WHAT IS ALREADY KNOWN ON THIS TOPIC
Post-SARS-CoV-2 vaccination disease flares are not frequent in people with inflammatory/autoimmune rheumatic and musculoskeletal diseases (I-RMDs).
Previous studies have been smaller and mainly analysed patient-reported flares, with flare rates typically higher compared with physician-reported flares, often failing to distinguish I-RMD flares from short-term vaccine reactogenicity.
WHAT THIS STUDY ADDS
In this large international physician-reported registry, I-RMD flares and flares requiring medication following vaccination against SARS-CoV-2 were infrequent (3.7 and 1.6%, respectively).
Higher disease activity at the time of SARS-CoV-2 vaccination and cessation/reduction of antirheumatic medications before or after vaccination were associated with an increased probability of flare, while exposure to certain medications such as methotrexate and rituximab was associated with a decreased probability of flare.
HOW THIS STUDY MIGHT AFFECT RESEARCH, PRACTICE OR POLICY
These data will aid patients, clinicians and other healthcare professionals making shared informed decisions regarding I-RMD management in the context of vaccination.
These findings will help to inform vaccination strategies in patients with I-RMDs, specifically decisions regarding stopping or reducing antirheumatic medications around the time of vaccination.
Introduction
The COVID-19 pandemic caused unprecedented pressure on healthcare systems and resulted in a dramatic loss of human life worldwide. 1 2 The development of highly effective vaccines against SARS-CoV-2 changed the course of the pandemic, with many lives saved by immunisation against SARS-CoV-2, including the lives of patients with inflammatory/autoimmune rheumatic and musculoskeletal diseases (I-RMDs). 3–10
Using early data from the international observational European Alliance of Associations for Rheumatology (EULAR) Coronavirus Vaccine (COVAX) physician-reported registry, we previously reported that COVID-19 vaccines were well tolerated by patients with I-RMD with infrequent reports of I-RMD flare (4.4%, 1.5% requiring medication changes) and very infrequent reports of serious adverse events (AEs) (0.4%). 8 Other studies have mainly analysed patient-reported flares, with flare rates typically being higher compared with physician-reported flares, and often failing to distinguish I-RMD flares from short-term vaccine reactogenicity. 11–23
The possibility of I-RMD flares has raised concerns, not only because there might be specific demographic or clinical features associated with increased risk of post-vaccination flare, but also because some organisations have recommended conventional and targeted synthetic disease-modifying antirheumatic drugs (cs/tsDMARDs) to be withheld ‘for 1–2 weeks (as disease activity allows) after each COVID-19 vaccine dose’, a strategy that could contribute to increased frequency of post-vaccination I-RMD flares. 24
Our aim was to investigate the frequency and factors associated with I-RMD flare following vaccination against SARS-CoV-2 in people with I-RMD.
Data collection
The EULAR COVAX physician-reported registry ( https://www.eular.org/eular-covax-registry ) 8 was an observational registry of patients with a pre-existing I-RMD or non-I-RMD who received one or more doses of any vaccine against SARS-CoV-2. The registry was launched in February 2021 and closed in October 2022. Data were recorded voluntarily by rheumatologists or other members of the clinical rheumatology team directly into an online data entry system (using REDCap, 25 26 which is a secure web application for building and managing online surveys and databases) or transferred from a national registry (for Portugal). Cases included in this study had a pre-existing I-RMD diagnosis and received one or two doses of the same COVID-19 vaccine. Patients receiving a combination of vaccines were excluded. Patients receiving more than two doses of the same vaccine were also excluded because disease activity data were only collected at baseline (ie, at the time of first dose of primary vaccination schedule).
Data collected included patients’ age (years), sex, country of residence, primary I-RMD diagnosis, COVID-19 vaccine received, number of doses and dates, physician global assessment of disease activity at the time of first dose of primary vaccination schedule (categorised as remission, low, moderate or high disease activity), exposure to immunomodulatory/immunosuppressive treatments at the time of vaccination, cessation/reduction of antirheumatic medication before or after vaccination, development of post-vaccination I-RMD flares and their characteristics, and prescription of new antirheumatic medication or dosage increase due to flare.
Providers were asked to report as many cases as possible of patients with RMDs vaccinated against SARS-CoV-2, with or without flares. Cases could be collected in outpatient, day care or inpatient settings, with the number of reported cases per session varying depending on feasibility. When reporting only a subset of patients from, for example, a full clinic list, providers were asked to select cases randomly, to avoid selection bias. Furthermore, the time from vaccination to the reporting of the case/outcome/flare was allowed to vary between individuals. Providers were also asked to distinguish between I-RMD flares and AEs, namely AEs within 7 days from vaccination (reactogenicity) and AEs of special interest.
Diagnostic groups
Diagnostic groups were defined based on the physician-reported primary I-RMD diagnosis: (1) inflammatory joint diseases (IJD), (2) connective tissue diseases (CTDs), (3) vasculitis and (4) other I-RMDs. Rheumatic diseases included in each category are listed in table 1 .
- View inline
Patients’ demographics and clinical characteristics
Antirheumatic medications
Exposure to the following immunomodulatory/immunosuppressive treatments at the time of COVID-19 vaccination was gathered: (1) csDMARDs: antimalarials (hydroxychloroquine and chloroquine), leflunomide, methotrexate and sulfasalazine; (2) biological DMARDs (bDMARDs): abatacept, belimumab, rituximab, interleukin (IL)-1 inhibitors (anakinra, canakinumab and rilonacept), IL-6 inhibitors (tocilizumab, sarilumab), IL-12/23 inhibitors (ustekinumab), IL-23 inhibitors (guselkumab, risankizumab and tildrakizumab), IL-17 inhibitors (secukinumab, ixekizumab and brodalumab) and tumour necrosis factor (TNF) inhibitors (adalimumab, certolizumab, etanercept, golimumab, infliximab and TNF inhibitor biosimilars); (3) tsDMARDs: apremilast and Janus kinase (JAK) inhibitors (tofacitinib, baricitinib and upadacitinib); (4) immunosuppressants: glucocorticoids, azathioprine/6-mercaptopurine, cyclophosphamide, ciclosporin, mycophenolate mofetil and tacrolimus; (5) other drugs, including intravenous immunoglobulin and antifibrotics.
I-RMD flare characteristics and definition
Flare was defined by physician report (signs and symptoms interpreted by the local physician as being suggestive of post-vaccination I-RMD flare). The following detailed information about flares was collected: (1) type of flare (including fever, weight loss, increase in fatigue, increase in dryness, enlarged lymph nodes, arthralgia, arthritis flare, cutaneous, pulmonary, renal, neurological, muscular, cardiac, gastrointestinal or haematological flare, or other types of flare); (2) severity of flare (mild, moderate, severe without hospitalisation and severe with hospitalisation); (3) information about changes in antirheumatic medication (including dosage increase or new medication) due to the flare; and (4) period of time between vaccination and the flare.
Statistical analyses
Data were reported descriptively for the entire cohort of patients with I-RMDs and for each diagnostic group. Factors associated with COVID-19 vaccination-related disease flares were estimated using univariable and multivariable logistic regression analyses and reported as OR and 95% CI. Two separate multivariable models were built, one with ‘I-RMD flare’ as dependent variable and one with ‘new antirheumatic medication or dosage increase due to flare’ as dependent variable.
Covariates included in the models were the following: age (per decade of life), sex, rheumatic disease diagnostic group (IJD (reference), CTD, vasculitis and other inflammatory rheumatic diseases), disease activity (remission (reference), low disease activity, and moderate or high disease activity), vaccine type (Pfizer/BioNTech (reference), Moderna, AstraZeneca and other vaccines), rheumatic disease treatment and cessation/reduction of antirheumatic medications at the time of vaccination.
For patients being treated with more than one of the medications of interest (except glucocorticoids), we created a medication hierarchy based on clinical expertise to categorise patients, as previously reported. 10 This process creates disjoint categories, allowing a clear reference group for interpretation of the regression models and avoiding collinearities. The following hierarchy of treatment allocation was used: immunosuppressants (azathioprine/6-mercaptopurine, cyclophosphamide, ciclosporin, mycophenolate mofetil and tacrolimus)>methotrexate>leflunomide>sulfasalazine>antimalarials. Patients receiving b/tsDMARDs were considered solely in the b/tsDMARD group. Glucocorticoids were analysed separately in the model.
Missing values for vaccine type and disease activity were derived by multiple imputation using full conditional specification (age, sex, flare, country of residence, disease group, disease activity, vaccine type and exposure to glucocorticoid treatment were included in the imputation model). Results of the logistic regression analyses for 100 imputed datasets were pooled by Rubin’s rules. Secondary models (sensitivity analysis) were performed without data imputation.
General characteristics
Of 10 569 patients reported to the registry, 7336 patients with I-RMDs were included in this study. Reasons for exclusion are presented in figure 1 . The majority were female (68%) and mean age was 58.3 (SD 15.5) years. Most patients had IJD (71%), followed by CTD (18%), vasculitis (9.4%) and other I-RMDs (1.7%). Three-quarters of the diagnoses consisted of rheumatoid arthritis (RA; 36.1%), axial spondyloarthritis (axSpA; 16.1%), psoriatic arthritis (PsA; 12.2%), systemic lupus erythematosus (SLE; 7.4%) and polymyalgia rheumatica (PMR; 3.7%) ( table 1 ).
- Download figure
- Open in new tab
- Download powerpoint
Study flow chart. Some patients were excluded for more than one reason. bDMARD, biological disease-modifying antirheumatic drug; I-RMDs, inflammatory rheumatic and musculoskeletal diseases; NI-RMD, non-inflammatory rheumatic and musculoskeletal disease; tsDMARD, targeted synthetic disease-modifying antirheumatic drug.
While the majority of patients were in remission (39.7%) or had low disease activity (27.4%), 17.8% of all cases had missing data on disease activity, and the remaining patients (15.1%) had moderate or high disease activity. The most frequently used medication groups were csDMARDs (58.2%), ts/bDMARDs (49%) and immunosuppressants (35.2%), respectively. The rate of not using antirheumatic medications was 7% ( table 2 ). The most common individual medications were methotrexate (34.1%), glucocorticoids (30.3%) and TNF inhibitors (30.3%). In terms of disease subgroups, the use of csDMARDs and b/tsDMARDs was highest in IJD (63.2% and 63%, respectively), while the use of immunosuppressants was highest in vasculitis (72.2%).
Inflammatory/autoimmune rheumatic and musculoskeletal disease activity and medications
In terms of vaccination, 17.7% of the included cases received one dose, while 82.3% received two doses ( table 3 ). Among those who received two doses, the majority received the Pfizer/BioNTech vaccine (74.1%), followed by the AstraZeneca (13.2%) and Moderna vaccines (9.4%). Similarly, among patients who received only one dose, the Pfizer/BioNTech, AstraZeneca and Moderna vaccines were also the most commonly administered, accounting for 42.8%, 9.2% and 30.8% of cases, respectively.
SARS-CoV-2 vaccination and flares in patients with inflammatory/autoimmune rheumatic and musculoskeletal diseases
Demographic and clinical characteristics of patients with post-vaccination I-RMD flare
Disease flares were reported in 272 (3.7%) of the 7336 patients. Of these patients, 206 (4%) were in the IJD group, 38 (2.9%) in the CTD group, 22 (3.2%) in the vasculitis group and 6 (4.9%) in the other I-RMD group. Mean age of patients with post-vaccination flare was 56.2 (SD 14.3) years and most patients were female (204 of 272, 75%). Most patients had low disease activity (37.9%) before vaccination, followed by remission (36%), moderate (18.8) and high disease activity (1.5%). The most recent vaccine dose before flare was the first in 38.6% and the second in 36% of patients, although these data were missing in 25% of cases. Mean time between flare and the most recent vaccine dose was 7.2 days (SD 8.2). Mean time between vaccination and case reporting was 115 days (SD 98). Most flares were mild (34.2%) or moderate (45.6%), although severe flares with or without hospitalisation occurred in 4.4% and 9.2% of the flare cases, respectively. Flare of arthritis (49.6%), polyarthralgia (37.9%) and increase in fatigue (12.1%) were the most common types of flare. In addition, 44.5% of patients with a flare (1.6% of all patients) started on a new medication or increased the dosage of an existing medication because of the flare ( table 4 and online supplemental table 1 ). TNF inhibitors (30.5), methotrexate (30.1%) and glucocorticoids (24.6%) were the most commonly used drugs, when a flare was observed ( online supplemental table 2 ).
Supplemental material
Demographic and clinical characteristics of patients with post-vaccination flare
Factors associated with I-RMD flare
In univariable analyses, the following variables were found to be associated with I-RMD flare: age, female sex, low disease activity (vs remission), other vaccine types (vs Pfizer/AstraZeneca/Moderna vaccines) and cessation/reduction of antirheumatic medications at the time of vaccination. Furthermore, moderate/high disease activity (vs remission), exposure to methotrexate, exposure to rituximab and cessation/reduction of antirheumatic medications at the time of vaccination were associated with new medication or dosage increase due to flare ( online supplemental table 3 ).
Two separate multivariable models were built, one with ‘I-RMD flare’ as dependent variable and one with ‘new medication or dosage increase due to flare’ as dependent variable ( table 5 ).
Multivariable logistic regression analysis of factors associated with flare, using either ‘I-RMD flare‘ or ‘new antirheumatic medication or dosage increase due to flare’ as the dependent variable
Independent factors associated with I-RMD flare were: higher age (per decade of life: OR=0.90, 95% CI=0.83 to 0.98), female sex (OR=1.40, 95% CI=1.05 to 1.87), active disease (low disease activity, OR=1.45, 95% CI=1.08 to 1.94; moderate/high disease activity, OR=1.37, 95% CI=0.97 to 1.95; vs remission), non-Pfizer/AstraZeneca/Moderna vaccines (OR=0.10, 95% CI=0.01 to 0.74; vs Pfizer), cessation/reduction of antirheumatic medication before or after vaccination (OR=4.76, 95% CI=3.44 to 6.58), and exposure to methotrexate (OR=0.57, 95% CI=0.37 to 0.90), TNF inhibitors (OR=0.55, 95% CI=0.36 to 0.85) and rituximab (OR=0.27, 95% CI=0.11 to 0.66), compared with no antirheumatic treatment.
In the multivariable model with new medication or dosage increase due to flare as the dependent variable, the only independent associations observed were: active disease (low disease activity, OR=1.47, 95% CI=0.94 to 2.29; moderate/high disease activity, OR=3.08, 95% CI=1.91 to 4.97; vs remission), cessation/reduction of antirheumatic medication before or after vaccination (OR=2.24, 95% CI=1.33 to 3.78), and exposure to methotrexate (OR=0.48, 95% CI=0.26 to 0.89) and rituximab (OR=0.10, 95% CI=0.01 to 0.77), compared with no antirheumatic treatment.
Secondary models (sensitivity analysis) performed without data imputation yield very similar results to the primary analysis ( online supplemental table 4 ).
The EULAR COVAX physician-reported registry represents the largest international case series of individuals with I-RMDs who received vaccination against SARS-CoV-2. Within this study, flares were reported in 3.7% of patients with I-RMD, while flares requiring starting a new medication or increasing the dosage of an existing medication were reported in 1.6% of the patients. Higher disease activity and cessation/reduction of antirheumatic medications before or after vaccination were associated with an increased probability of flare, while exposure to certain medications such as methotrexate and rituximab was associated with a decreased probability of flare (both for flares in general as well as for flares requiring starting a new antirheumatic medication or change the dose of a current medication). Flares were also more likely to occur in younger patients and females, and less likely to occur with non-Pfizer/AstraZeneca/Moderna vaccines; however, these associations were only observed for flares in general, but not for flares requiring starting a new antirheumatic medication or change the dose of a current medication.
The reported incidences of flare and need to start a new antirheumatic medication or to increase the dosage of an existing medication are in line with the initial results of the EULAR COVAX Registry, where we reported incidences of 4.4% and 1.5%, respectively. 8 Other studies have reported similar or higher incidences, up to 26.7%, depending on the definition of flare (including patient or physician reported), study methodology and study population investigated. 11–23 The highest incidence of flare (26.7%) was reported in a small group of 191 individuals with autoimmune rheumatic diseases who reported minimal clinically important worsening in the 10-item PROMIS physical function form. 17 Importantly, the reported incidences of flare and need to start a new antirheumatic medication or to increase the dosage of an existing medication are not beyond the expected underlying risk of flare based on the natural history of I-RMDs. 27 28
Post-vaccination flares in individuals with I-RMDs may occur with any vaccination, possibly due to non-specific adjuvant effects or molecular mimicry triggering immune activation, leading to dysregulation of both adaptive and innate immune responses. This dysregulation may involve disordered nucleic acid metabolism and abnormal interferon-induced gene signatures via the TLR-7/9 pathways, contributing to robust early innate immune responses. 29 Our findings confirm that while flares in I-RMDs following SARS-CoV-2 vaccination are possible, they are infrequently observed.
In our study, younger age and female sex were found to be more likely associated with flare occurrence. Several studies have explored the association between demographic factors and post-SARS-CoV-2-vaccination flare. For example, in a patient-reported study assessing risk factors for flare requiring treatment after COVID-19 vaccination, female sex emerged as a significant predictor, while age did not show a significant association with flare. 20 Other studies have reported the association of age and sex with SARS-CoV-2 vaccination-related flare as non-significant factors. 12 15 17 These discrepancies should be interpreted taking into account differences in mean age, sex distribution, disease types, and other study-related methods and characteristics.
o specific disease group was identified as associated with flare. Likewise, another study 15 found that specific I-RMD subgroups were not a risk factor for flare. However, a patient-reported study suggested that certain diseases, such as SLE, PsA and PMR, were associated with higher odds of flare, while idiopathic inflammatory myopathies were associated with lower odds of flare compared with RA. 20 Data regarding which rheumatic diseases or disease groups are more prone to flare are limited.
The relationship between vaccine type and flare has been depicted differently across various studies. In our study, we showed that vaccination with other vaccines (excluding Pfizer, AstraZeneca and Moderna) was less likely to be associated with flare. This observation should be interpreted with caution, given the low number of non-Pfizer/AstraZeneca/Moderna vaccines administered and the potential reporting bias. Some studies reported higher flare incidence with AstraZeneca 20 and Moderna, 17 while others did not report a relationship between vaccine type and flare. 15 30
Importantly, we found that higher disease activity and cessation/reduction of antirheumatic medications before or after vaccination were associated with an increased probability of flare, both for flares in general as well as for flares requiring starting a new antirheumatic medication or change the dose of a current medication. This finding highlights the importance of I-RMD control when considering vaccination schedules. There is limited knowledge on post-vaccination disease flare in patients with active disease. The potential mechanism underlying post-vaccination flare in such patients may result from persistent immune pathway dysregulation, even during periods of low disease activity, further exacerbated by vaccination. While EULAR recommendations suggest that vaccination should preferably be administered during quiescent disease states, 31 in the context of a severe pandemic like COVID-19, vaccination is crucial irrespective of disease activity state.
The knowledge regarding the use, reduction or discontinuation of antirheumatic drugs in the context of SARS-CoV-2 vaccination is still scarce. Both EULAR and the American College of Rheumatology (ACR) provided recommendations on these drugs. Unlike the ACR, which recommends withholding certain drugs, including methotrexate, JAK inhibitors, abatacept, mycophenolate mofetil and rituximab, in patients with controlled disease, 24 EULAR does not recommend temporarily reducing or holding any of these drugs except for rituximab. 6 32 A recent randomised trial investigated the effects of a 2-week discontinuation of methotrexate in patients with RA following administration of an inactivated SARS-CoV-2 vaccine. The study found that discontinuing methotrexate improved the anti-SARS-CoV-2 IgG response; however, methotrexate discontinuation was also associated with an increase in disease flares after vaccination compared with patients who continued methotrexate. 33 Similarly, in an open-label, multicentre, randomised-controlled superiority trial involving individuals with immune-mediated inflammatory diseases, including RA, psoriasis with or without arthritis, axSpA, atopic dermatitis, PMR and SLE, a 2-week interruption of methotrexate therapy resulted in increased antibody responses against the receptor-binding domain of the SARS-CoV-2 spike protein, although with a short-term significant increase in the risk of disease flare after COVID-19 booster vaccination compared with continued methotrexate therapy. 34 The clinical significance of interruption of methotrexate after vaccination and improved humoral response is yet to be determined (ie, the clinical impact of this strategy in terms of protection against SARS-CoV-2 infection or prevention of worse COVID-19 outcomes is still unknown), and a shared decision process is needed to weigh the potential benefit of enhancing the humoral response against SARS-CoV-2 against the potential risk of I-RMD exacerbation.
Finally, in our study, patients exposed to methotrexate, TNF inhibitors (overall flare rate only) and rituximab had a lower incidence of flares in our study. In another study, 12 receiving csDMARDs or biological therapy was associated with a lower incidence of flares, while in another study, use of mycophenolate mofetil and glucocorticoids was also linked to lower flare incidences. 17 However, a cross-sectional study of patients with SLE found no significant association between flare and SLE medications. 14 It is known that some immunosuppressive or immunomodulatory drugs reduce seroconversion rates, rendering the vaccine less immunogenic and potentially impacting flare rates. 4
This study has limitations. First, there is potential selection bias since the COVAX Registry relies on voluntary case reporting; however, this could in principle have led to over-reporting of flares and AEs; therefore, the low rate of flares consistent with other publications is reassuring. Second, as the data are collected from multiple centres across Europe, they represent subjective evaluations across different settings that could influence results, particularly regarding disease activity and flare assessment. Third, time between vaccination and case reporting is also variable, limiting data interpretation and not allowing us to draw any conclusions regarding the long-term safety profile of vaccines against SARS-CoV-2. Fourth, for some signs/symptoms, it can be difficult to determine if the event should be considered an I-RMD flare or simply a transient reactogenicity effect of the vaccine (eg, polyarthralgia); in our study, this decision was left to the reporting physician, which can be considered a study limitation. Importantly, no causal conclusions regarding vaccination and the development of flares can firmly be drawn from this dataset. The strengths of the current study lie in its clinician-reported nature, the large number of patients, provision of multicentre and international real-life data, and detailed examination of clinical and demographic factors associated with flare across various rheumatic diseases.
In conclusion, our findings suggest that disease flare following SARS-CoV-2 vaccination in individuals with immune-mediated rheumatic diseases (I-RMDs) is uncommon. Higher disease activity and cessation/reduction of antirheumatic medications before or after vaccination were associated with an increased probability of flare, while exposure to certain medications such as methotrexate and rituximab was associated with a decreased probability of flare. These findings will assist patients, clinicians and other healthcare professionals in making informed decisions regarding the management of I-RMDs in the context of SARS-CoV-2 vaccination and contribute to the development of the most appropriate vaccination strategies for patients with I-RMDs.
Ethics statements
Patient consent for publication.
Not applicable.
Ethics approval
Given the registry collects anonymous non-interventional data, the UK Health Research Authority (HRA) does not class the registry as a research study (in line with the HRA decision tool) and patient consent is not required.
Acknowledgments
We wish to thank all healthcare providers who entered data into the registry
- Callaway E ,
- Cyranoski D ,
- Mallapaty S , et al
- Cucinotta D ,
- Kepten E , et al
- Kroon FPB ,
- Alunno A , et al
- Landewé RB ,
- Machado PM ,
- Kroon F , et al
- Landewé RBM ,
- Sadarangani M ,
- Marchant A ,
- Kollmann TR
- Lawson-Tovey S ,
- Strangfeld A , et al
- Strangfeld A ,
- Schäfer M ,
- Gianfrancesco MA , et al
- Barbhaiya M ,
- Levine JM ,
- Bykerk VP , et al
- Connolly CM ,
- Boyarsky BJ , et al
- Wang Y , et al
- Dubois M , et al
- Fragoulis GE ,
- Bournia V-K ,
- Mavrea E , et al
- Agrawat H ,
- Day J , et al
- Yeung WWY , et al
- Mohanasundaram K ,
- Santhanam S ,
- Natarajan R , et al
- Wilkerson J , et al
- Sattui SE ,
- Kennedy K , et al
- Cardelli C ,
- Depascale R , et al
- Zavala-Flores E ,
- Salcedo-Matienzo J ,
- Quiroz-Alva A , et al
- Curtis JR ,
- Johnson SR ,
- Anthony DD , et al
- Harris PA ,
- Minor BL , et al
- Thielke R , et al
- Bykerk VP ,
- Shadick N ,
- Frits M , et al
- Markusse IM ,
- Gerards AH , et al
- De Marco G ,
- Mahajna H , et al
- Ionescu GD , et al
- Rondaan C ,
- Heijstek MW , et al
- Araujo CSR ,
- Medeiros-Ribeiro AC ,
- Saad CGS , et al
- Abhishek A ,
- Boyton RJ ,
- Peckham N , et al
Supplementary materials
Supplementary data.
This web only file has been produced by the BMJ Publishing Group from an electronic file supplied by the author(s) and has not been edited for content.
- Data supplement 1
XM and PMM are joint senior authors.
Handling editor Josef S Smolen
X @saskiaamber, @LGossec, @carmona_loreto, @initabulina, @pedrommcmachado
Presented at The work reported in this manuscript has previously been presented at ACR Convergence 2022: Farisoğulları B, et al . Factors Associated with Disease Flare Following SARS-CoV-2 Vaccination in People with Inflammatory Rheumatic and Musculoskeletal Diseases–Results from the Physician-Reported EULAR Coronavirus Vaccine (COVAX) Registry [abstract]. Arthritis Rheumatol. 2022; 74 (suppl 9). https://acrabstracts.org/abstract/factors-associated-with-disease-flare-following-sars-cov-2-vaccination-in-people-with-inflammatory-rheumatic-and-musculoskeletal-diseases-results-from-the-physician-reported-eular-coronaviru/ . Accessed 14 May 2024.
Collaborators In addition to the authors listed above, the following colleagues also contributed to the EULAR COVAX Registry by submitting at least 10 cases each: Viviane Queyrel, Julien Henry, Raphaele Seror, Eric Toussirot, Emoke Stenova, Azeddine Dellal, Vanda Mlynarikova, Romain Forestier, François Lamer, Hélène Maillard, Amélie Leurs, Thierry Zenone, Daniel Wendling, Amélie Florent, Theodoros Dimitroulas, Simona Rednic, Bernard Combe, Yves Piette, Jozef Odnoga, Giovanna Cuomo, Ioannis Raftakis, Jean-Camille Meric, Sylvain Lanot, Marion Mirabel, Mikhail Protopopov, Katalin Törõcsik, John Brockbank, Marion Jacob, Pascal Coquerelle, Christophe Richez, Elisabeth Gervais, Séverine Verlinden, Antoine Froissart, Fabienne Roux, Marion Couderc, Renaud Desbarbieux, Alojzija Hocevar, Pierre-Yves Jeandel, Sophie Rivière, Luciana Popa, Fabienne Coury, Inita Bulina, Jean-Jacques Dubost, Lionel Spielmann, Marie-Hélène Guyot, Nicolas Deseyne, Isabelle Amigues, Dagmar Mičeková, Loraine Gauzere, Gaëlle Viadere, Natalia de la Torre-Rubio, Victor Strotz.
Contributors BF and PMM had access to the study data, developed the figures and tables, wrote the first draft of the manuscript, and vouch for the data and analyses. PMM directed the work, had final responsibility for the decision to submit for publication and is responsible for the overall content as guarantor. All authors contributed to data collection, interpretation of the data and intellectual content during the drafting and revision of the work and approved the final version to be published.
Funding Financial support from the European Alliance of Associations for Rheumatology (EULAR).
Competing interests BF does not report conflicts of interest. SL-T does not report conflicts of interest. KLH reports non-personal speaker's fees from AbbVie and grant income from BMS and Pfizer, all unrelated to this manuscript; and her institute was supported by EULAR for COVAX database management. LG reports research grants from Amgen, Galapagos, Lilly, Pfizer and Sandoz; and consulting fees from AbbVie, Amgen, BMS, Celltrion, Galapagos, Gilead, GSK, Janssen, Lilly, Novartis, Pfizer, Samsung Bioepis, Sanofi-Aventis and UCB, all unrelated to this manuscript. LC has not received fees or personal grants from any laboratory, but her institute works by contract for laboratories among other institutions, such as Galapagos, Pfizer, Lilly, MSD, Novartis, Roche, Sanofi Aventis, BMS and Sandoz; she also reports safety board participation, not paid personally. AS reports personal fees from lectures for AbbVie, Galapagos, Lilly, Pfizer and Takeda. EFM does not report conflicts of interest. MS does not report conflicts of interest. AR does not report conflicts of interest. EH does not report conflicts of interest. JAG-P does not report conflicts of interest. MM reports having received payment or honoraria for lectures, presentations, speakers bureaus, manuscript writing or educational events from AstraZeneca, all unrelated to this manuscript. PD reports speaker's fees from AbbVie, Lilly, Galapagos, Janssen and Biogen, all unrelated to this manuscript. LT does not report conflicts of interest. TG does not report conflicts of interest. MC does not report conflicts of interest. ES reports receiving grants from AbbVie; payment or honoraria for lectures, presentations, speakers bureaus, manuscript writing or educational events from Novartis, AbbVie, Pfizer, Eli Lilly, UCB, Sobi, Roche, Sanofi, Boehringer-Ingelheim, Viatris, Sandoz, Mylan, MSD, Amgen and Janssen-Johnson & Johnson; support for attending meetings and/or travel from AbbVie and Janssen-Johnson & Johnson; participation in a data safety monitoring board or advisory board from AbbVie, Eli Lilly, UCB, Sobi, Boehringer-Ingelheim and Janssen-Johnson & Johnson; and is a committee member of Slovak Society of Rheumatology, all unrelated to this manuscript. IB reports receiving speaker's fees from AbbVie, Pfizer, Boehringer Ingelheim and Janssen; and has received support for attending meetings and/or travel from AbbVie, all unrelated to this manuscript. ES does not report conflicts of interest. JZ reports consulting fees from AbbVie, Janssen, Novartis and Boehringer-Ingelheim; speaker's fees from AbbVie, Janssen, Novartis, Boehringer Ingelheim Latvia and UAB Viasana; receiving support for attending meetings and/or travel from AbbVie, UAB Viasana and Johnson & Johnson; and is a board member of Latvian Society of Adult Rheumatology; all unrelated to this manuscript. NR has nothing to disclose. OB has nothing to disclose. EV has nothing to disclose. BR has nothing to disclose. GRB has nothing to disclose. XM reports personal consultant fees from BMS, Galapagos, GSK, Novartis and Pfizer; and having received support for attending meetings and/or travel from Novartis, all unrelated to this manuscript. PMM has received consulting/speaker’s fees from AbbVie, BMS, Celgene, Eli Lilly, Galapagos, Janssen, MSD, Novartis, Orphazyme, Pfizer, Roche and UCB, all unrelated to this manuscript.
Patient and public involvement Patients and/or the public were involved in the design, or conduct, or reporting, or dissemination plans of this research. Refer to the Methods section for further details.
Provenance and peer review Not commissioned; externally peer reviewed.
Supplemental material This content has been supplied by the author(s). It has not been vetted by BMJ Publishing Group Limited (BMJ) and may not have been peer-reviewed. Any opinions or recommendations discussed are solely those of the author(s) and are not endorsed by BMJ. BMJ disclaims all liability and responsibility arising from any reliance placed on the content. Where the content includes any translated material, BMJ does not warrant the accuracy and reliability of the translations (including but not limited to local regulations, clinical guidelines, terminology, drug names and drug dosages), and is not responsible for any error and/or omissions arising from translation and adaptation or otherwise.
Read the full text or download the PDF:
- Open access
- Published: 18 May 2024
Independent relationship between sleep apnea-specific hypoxic burden and glucolipid metabolism disorder: a cross-sectional study
- Chenyang Li 1 , 2 na1 ,
- Yu Peng 1 , 2 na1 ,
- Xiaoyue Zhu 1 , 2 na1 ,
- Yupu Liu 1 , 2 ,
- Jianyin Zou 1 , 2 ,
- Huaming Zhu 1 , 2 ,
- Xinyi Li 1 , 2 ,
- Hongliang Yi 1 , 2 ,
- Jian Guan 1 , 2 ,
- Xu Zhang 3 ,
- Huajun Xu 1 , 2 &
- Shankai Yin 1 , 2
Respiratory Research volume 25 , Article number: 214 ( 2024 ) Cite this article
300 Accesses
Metrics details
Obstructive sleep apnea (OSA) is associated with abnormal glucose and lipid metabolism. However, whether there is an independent association between Sleep Apnea-Specific Hypoxic Burden (SASHB) and glycolipid metabolism disorders in patients with OSA is unknown.
We enrolled 2,173 participants with suspected OSA from January 2019 to July 2023 in this study. Polysomnographic variables, biochemical indicators, and physical measurements were collected from each participant. Multiple linear regression analyses were used to evaluate independent associations between SASHB, AHI, CT90 and glucose as well as lipid profile. Furthermore, logistic regressions were used to determine the odds ratios (ORs) for abnormal glucose and lipid metabolism across various SASHB, AHI, CT90 quartiles.
The SASHB was independently associated with fasting blood glucose (FBG) (β = 0.058, P = 0.016), fasting insulin (FIN) (β = 0.073, P < 0.001), homeostasis model assessment of insulin resistance (HOMA-IR) (β = 0.058, P = 0.011), total cholesterol (TC) (β = 0.100, P < 0.001), total triglycerides (TG) (β = 0.063, P = 0.011), low-density lipoprotein cholesterol (LDL-C) (β = 0.075, P = 0.003), apolipoprotein A-I (apoA-I) (β = 0.051, P = 0.049), apolipoprotein B (apoB) (β = 0.136, P < 0.001), apolipoprotein E (apoE) (β = 0.088, P < 0.001) after adjustments for confounding factors. Furthermore, the ORs for hyperinsulinemia across the higher SASHB quartiles were 1.527, 1.545, and 2.024 respectively, compared with the lowest quartile ( P < 0.001 for a linear trend); the ORs for hyper-total cholesterolemia across the higher SASHB quartiles were 1.762, 1.998, and 2.708, compared with the lowest quartile ( P < 0.001 for a linear trend) and the ORs for hyper-LDL cholesterolemia across the higher SASHB quartiles were 1.663, 1.695, and 2.316, compared with the lowest quartile ( P < 0.001 for a linear trend). Notably, the ORs for hyper-triglyceridemia{1.471, 1.773, 2.099} and abnormal HOMA-IR{1.510, 1.492, 1.937} maintained a consistent trend across the SASHB quartiles.
Conclusions
We found SASHB was independently associated with hyperinsulinemia, abnormal HOMA-IR, hyper-total cholesterolemia, hyper-triglyceridemia and hyper-LDL cholesterolemia in Chinese Han population. Further prospective studies are needed to confirm that SASHB can be used as a predictor of abnormal glycolipid metabolism disorders in patients with OSA.
Trial registration
ChiCTR1900025714 { http://www.chictr.org.cn/ }; Prospectively registered on 6 September 2019; China.
Introduction
Obstructive sleep apnea (OSA) is a highly prevalent sleep disordered breathing, with estimated prevalence rates of 17% in females and 34% in males within the general population, and increasingly with age and obesity [ 1 , 2 ]. OSA is characterized by repeated intermittent hypoxia (IH), frequent arousals, and daytime drowsiness. A significant association between OSA and the risk of metabolic syndrome was recently established and attracted considerable attention [ 3 , 4 ]. Dyslipidemia and abnormal glucose metabolism emerge as two primary components of metabolic disorders, and both of them are commonly intertwined with clinical outcomes associated with OSA [ 5 , 6 ]. Previous studies have confirmed that OSA is associated with dyslipidemia, abnormal glucose metabolism and such an association is mainly attributable to nocturnal IH and sleep fragmentation (SF) [ 7 , 8 , 9 , 10 ]. Previous rodent studies have suggested that IH can impair pancreatic islet beta-cell function, subsequently increasing fasting glucose levels and generating insulin resistance [ 11 , 12 ], thereby further exacerbating the development of diabetes [ 13 , 14 ].
In our previous study [ 15 ], we found Sleep Apnea-Specific Hypoxic Burden (SASHB), a new pulse oximetry (SpO 2 )-related index and defined as the sum of the areas under the baseline SpO 2 curves corresponding to respiratory events, has shown promise in identifying people at risk of OSA in Chinese Han population. In addition to quantifying the frequency of respiratory events, SASHB captures the depth and duration of hypoxemia associated with OSA. Series of studies have shown that higher SASHB in OSA were associated with higher risks of cardiovascular mortality, major cardiovascular event rates [ 16 ], blood pressure [ 17 ], stroke [ 18 ], heart failure [ 19 ], and chronic kidney disease in the clinical setting [ 20 ]. However, whether SASHB was independently associated with abnormal glucose and lipid metabolism remains unknown.
In order to clearly address such an association, we performed such comprehensive cross-sectional study. Furthermore, we calculated adjusted odds ratios (ORs) for different abnormal glucose and lipid metabolism categories among OSA patients, stratified by varying levels of SASHB.
Subjects and methods
Study design and population.
We enrolled 2,914 subjects with suspected OSA who underwent overnight polysomnography (PSG) in the sleep laboratory of Shanghai Jiao Tong University of Medicine Affiliated Sixth People’s Hospital from January 2019 to July 2023. The exclusion criteria were as follows: (1) history of OSA treatment; (2) age < 18 years; (3) severe systemic disease such as heart, liver, lung, and renal failure; (4) other non-OSA sleep disorders; (5) severe psychiatric disorders or malignancy; (6) administration of glucose-lowering or lipid-lowering medications; and (7) missing clinical PSG data. Ultimately, a total of 2,173 subjects met the inclusion criteria for this study. We learned about their general health status including habits such as smoking, alcohol consumption, and medication use through a comprehensive questionnaire. The recruitment flow chart is shown in Fig. 1 . This study was conducted in accordance with the Helsinki Declaration and was approved by the Ethics Review Committee of the Sixth People’s Hospital which is affiliated with the Medical College of Shanghai Jiaotong University (approval no. 2019-KY-050 [K]); the study was registered in the China Clinical Trials Registry (serial number ChiCTR1900025714). All participants gave written informed consent.
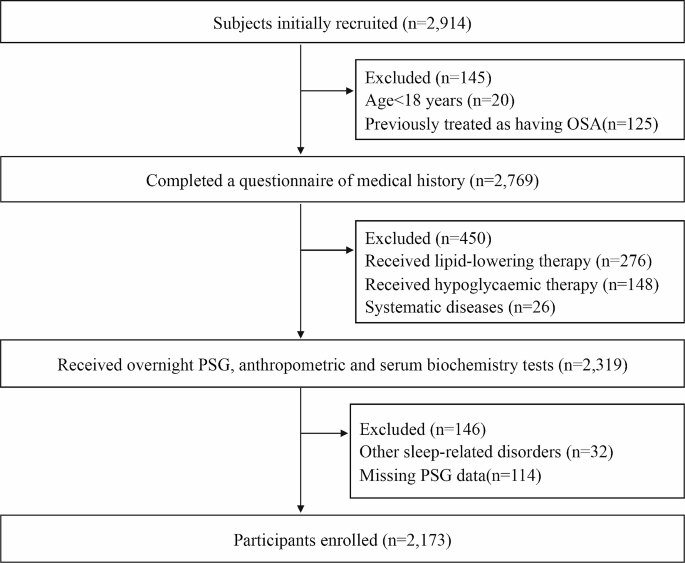
Screening flow chat of participants
Polysomnography and definitions
To obtain precise and objective sleep parameters, sleep was monitored in the sleep laboratory by overnight PSG (Alice-5, Alice-6; Respironics, Pittsburgh, Pennsylvania, USA). Bilateral electroencephalogram (EEG) channels (C3-M2 and C4-M1), bilateral electrooculogram (EOG), chin electromyogram (EMG), lowest oxygen saturation, chest and abdominal wall motion, airflow, and body position were recorded for all study participants. The scoring of respiratory events, oxygen desaturations adhered to the guidelines established by the American Academy of Sleep Medicine (AASM) in 2017 [ 21 ]. Scoring of micro-arousals followed the Rechtschaffen and Kales (R&K) rule [ 22 ]. A micro-arousal event was defined as an abrupt shift in EEG frequency, encompassing alpha, theta and/or frequencies > 16 Hz (excluding spindles) that lasted at least 3s, with at least 10s of stable sleep preceding the observed change. Additionally, scoring of arousal during rapid eye movement (REM) requires a concurrent increase in submental EMG lasting at least 1s. We operationally defined the microarousal index (MAI) as the tally of abrupt EEG frequency shifts, each lasting at least 3 s, per hour of recorded sleep. Moreover, the apnea hypopnea index (AHI) was quantified as the number of apnea and hypopnea events occurring per hour during the sleep period.
Biochemical indicators
For each study participant, a fasting blood sample was collected from the antecubital vein on the morning following the PSG evaluation. Fasting blood glucose (FBG) and fasting insulin (FIN), and serum lipid profiles, which included total cholesterol (TC), total triglycerides (TG), high-density lipoprotein cholesterol (HDL-C), and low-density lipoprotein cholesterol (LDL-C), apolipoprotein A-I (apoA-I), apolipoprotein B (apoB), apolipoprotein E (apoE) were measured for each participant. FBG levels were quantified using the H-7600 autoanalyzer (Hitachi, Tokyo, Japan), while FIN levels were determined through immunoradiological assays. Calibration of the analyzer and quality control operations were routinely carried out. The homeostasis model assessment of insulin resistance (HOMA-IR) was calculated to quantify insulin resistance using FIN and FBG as follows: HOMA-IR = FIN (uIU/mL)×FBG (mmol/L)/22.5 [ 23 ]. We defined FBG of 6.1 mmol/L or greater as hyperglycemia, FIN of 12.2 uIU/mL or greater as hyperinsulinemia, and HOMA-IR of 2.5 or greater as insulin resistance [ 23 , 24 , 25 ].
Serum lipid profiles were assessed in the hospital laboratory utilizing standard procedures. According to the US National Cholesterol Education Program Adult Treatment Panel III (NCEPIII) [ 26 ] and the Joint Committee for Developing Chinese Guidelines on the Prevention and Treatment of Dyslipidemia in Adults (JCDCG) [ 27 ], dyslipidemia in terms of TC, LDL-c, HDL-c and TG, were defined as TC levels > 5.17mmol/L, LDL-c levels≥3.37mmol/L, HDL-c levels < 1.03 mmol/L, and TG levels ≥ 1.7 mmol/L, separately.
Anthropometric measurements
All participants were instructed to wear light clothing and removed shoes. Height, weight, neck circumference (NC), waist circumference (WC), and hip circumference (HC) were measured with a meter ruler and weighing scale, respectively, following established procedures. Body Mass Index (BMI) was calculated as weight (kg)/height 2 (m 2 ); the neck height ratio (NHR) = NC/height and the waist-hip ratio (WHR) = WC/HC were also calculated. Daytime blood pressure (BP) was measured after at least 5 min of rest in a seated position employing a mercury sphygmomanometer, following the American Society of Hypertension Guidelines, and the mean of three measurements was recorded for each participant. Hypertension was defined as a systolic BP ≥ 140mmHg, a diastolic BP ≥ 90mmHg, or current use of antihypertensive medication [ 28 ].
The SASHB calculation flow
The SASHB was determined by assessing the respiratory event-associated area under the desaturation curve commencing from a pre-event baseline. Our SASHB calculations are based on those of Dr. Azarbarzin’s research team, but the methods are not identical, differing principally in the definition of the pre-event SpO 2 baseline level [ 15 ]. For each apnea or hypopnea event, the Azarbarzin team defined the pre-event baseline saturation as the maximum SpO 2 during the 100 s prior to the end of the event; in our study, the maximum value at the start point of the SpO 2 trend evident in the search window at the time of an apnea or hypopnea event served as the SpO 2 baseline level. The search window used to detect respiratory events is shown in Fig. 2 .
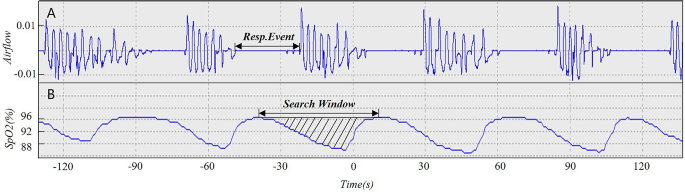
Calculation of SASHB for individual respiratory events corresponding to specific search window
The specific SASHB calculation process was: First, take the maximum value of the starting point of SpO 2 trend in the search window for each respiratory event as the SpO 2 baseline level; Second, calculate \({S}_{i}\) , thus the area of the similar triangle for which the SpO 2 level serves as the horizontal baseline in each search window, and the downward and upward trends in the SpO 2 , the other sides of the triangle; Third, S= \({\sum }_{i=1}^{n}{S}_{i}\) , which is the sum of all respiratory events in the similar triangle of the specific search window, where i is the number of apnea or hypopnea events during the night; Fourth, the total area is divided by the total night recording time to yield the SASHB in units of %min/h; Fifth, a SASHB of 40% min/h corresponds to a 4% reduction in SpO 2 below baseline for 10 min during every hour of sleep or a 5% reduction below baseline for 8 min every hour.
The calculation of SASHB relies on computer-based analysis rather than manual operation. In this study, the calculation of the SASHB index is rooted in laboratory test data, encompassing nasal airflow and blood oxygen saturation trend maps. We developed the operation program of SASHB by using MATLAB (MathWorks, R2018a, USA), and this software can realize the batch processing of the original SpO 2 data. A description of the quality control criteria for the SpO 2 trend graph (recording duration and artifact management) and details of the original calculation codes for SASHB were provided in the supplementary material.
Statistical analysis
Data are presented as mean values ± standard deviation (SD) for continuous variables and percentages for categorical variables. Descriptive statistics were computed across the quartiles of SASHB. Inter-group differences in descriptive statistics were examined using analysis of variance (ANOVA) for continuous variables and chi-square tests for categorical variables. A polynomial linear trend test was used to evaluate linear trends across SASHB quartiles for continuous variables, and a linear-by-linear association test was applied for dichotomous variables. The treatment of missing data in the dataset is done using the maximum likelihood estimate method, where missing values are estimated from the marginal distribution of the observations.
Stepwise multiple regression analyses were executed separately to explore the independent associations of SASHB, AHI, CT90 with glucose metabolism indicators (FBG, FIN, HOMA-IR) and lipid profiles including TC, LDL-c, HDL-c, TG, apoA-I, apoB and apoE. Binary logistic regression analyses were employed to determine risk factors for hyperglycemia, hyperinsulinemia, abnormal HOMA-IR, hyper-total cholesterolemia, hyper-LDL cholesterolemia, hypo-HDL cholesterolemia, and hypertriglyceridemia. Linear trends were assessed by examining the median SASHB value for each quartile and conducting the overall F-test for that value. Additionally, odds ratios (ORs) and 95% confidence intervals (CIs) were also computed. Importantly, the statistical analysis was preceded using collinearity diagnostics to eliminate potential multicollinearity among variables. The two steps of the collinearity analyses were: (1) a preliminary analysis using a Spearman correlation and (2) collinearity diagnostics to determine the selected covariates in the multivariate linear regression analyses. For detail, please see Supplementary (Tables S28 - S30 ). Following the collinearity diagnosis, Model 1 was adjusted for age, and BMI as continuous variables, as well as sex as categorical variables. Model 2 included the following covariates: age, BMI as a continuous variable; and sex, hypertension, smoking status, and alcohol consumption as categorical variables. Furthermore, Model 3 further added the MAI to Model 2. Of note, SASHB and CT90 were examined as both a classified variable according to its quartiles as well as a continuous one. These yielded similar conclusions, and we presented only the results employing the continuous variables in linear regression analyses and the quartile variables in logistic regression analyses for simplicity. All statistical analyses employed SPSS ver. 26.0 (SPSS Inc., Chicago, IL, USA). Statistical significance was defined as a bilateral p -value < 0.05.
Baseline characteristics and univariate analysis
In total, 2,173 patients with suspected OSA were enrolled in this study. Of these, 1634 were male and 539 were female. Participants were categorized by SASHB quartiles (≤20.84, 20.84–77.11, 77.11–214.53, and > 214.53). Demographic characteristics (age, height, BMI, NC, WC, HC, NHR, WHR) and sleep indices (AHI, MAI) differed significantly across SASHB quartiles (all P for trend < 0.001; Table 1 ); Continuous variables such as FBG, FIN, HOMA-IR, TC, TG, HDL-C, LDL-C, apoB and apoE were also differed across the SASHB quartiles (all P for trend < 0.001; Table 1 ). Specifically, a positive dose-response relationship was observed between SASHB and FBG, FIN, HOMA-IR, TC, LDL-c, and TG levels, while conversely, a negative dose-response relationship was observed between SASHB and HDL-c levels (Fig. 3 ). Furthermore, the prevalence rates of Hyperglycemia, Hyperinsulinemia, insulin resistance, Hyper-total cholesterolemia, Hypo-HDL cholesterolemia, Hyper-LDL cholesterolemia, and Hyper-triglyceridemia increased with the SASHB quartile from 14.90 to 33.70%, 24.50–50.20%, 30.60–60.30%, 17.90–36.40%, 42.20–57.70%, 15.80–30.20%, and 26.10–55.00%, respectively (linear trends, p < 0.001) (Table 1 ).
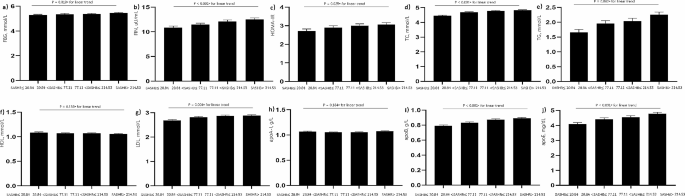
Adjusted mean values of the glucose and lipid levels in model 1. (a) FBG - SASHB; (b) FIN - SASHB; (c) HOMA-IR - SASHB; (d) TC - SASHB; (e) TG - SASHB; (f) HDL - SASHB; (g) LDL - SASHB; (h) apoA-I - SASHB; (i) apoB - SASHB; and (j) apoE - SASHB. Abbreviations : The data were adjusted for age, body mass index (BMI), and sex. FBG: Fasting blood glucose; FIN: Fasting insulin; HOMA-IR: Homeostasis model assessment of insulin resistance; TC: Total cholesterol; TG: Total triglycerides; HDL-C: High-density lipoprotein cholesterol; LDL-C: Low-density lipoprotein cholesterol; apoA-I: apolipoprotein A-I; apoB: apolipoprotein B; apoE: apolipoprotein E; SASHB: Sleep Apnea-Specific Hypoxic Burden
Relationship between SASHB and glucose metabolism
After adjusting for age, gender, BMI, MAP, smoking status, alcohol consumption and MAI, the SASHB was found to be independently associated with FBG (β = 0.058, P = 0.017), FIN (β = 0.073, P < 0.001), and the HOMA-IR (β = 0.058, P = 0.005) (Table S1 , Model 3, Figs. 4 and 5 ).
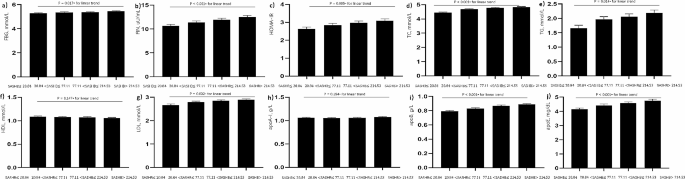
Adjusted mean values of the glucose and lipid levels in model 2. (a) FBG - SASHB; (b) FIN - SASHB; (c) HOMA-IR - SASHB; (d) TC - SASHB; (e) TG - SASHB; (f) HDL - SASHB; (g) LDL - SASHB; (h) apoA-I - SASHB; (i) apoB - SASHB; and (j) apoE - SASHB. Abbreviations : The data were adjusted for age, body mass index (BMI), sex, smoking status, mean artery pressure, and alcohol consumption. FBG: Fasting blood glucose; FIN: Fasting insulin; HOMA-IR: Homeostasis model assessment of insulin resistance; TC: Total cholesterol; TG: Total triglycerides; HDL-C: High-density lipoprotein cholesterol; LDL-C: Low-density lipoprotein cholesterol; apoA-I: apolipoprotein A-I; apoB: apolipoprotein B; apoE: apolipoprotein E; SASHB: Sleep Apnea-Specific Hypoxic Burden
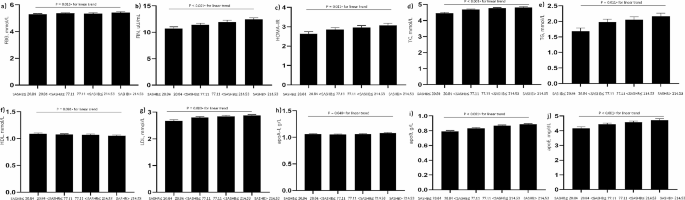
Adjusted mean values of the glucose and lipid levels in model 3. (a) FBG - SASHB; (b) FIN - SASHB; (c) HOMA-IR - SASHB; (d) TC - SASHB; (e) TG - SASHB; (f) HDL - SASHB; (g) LDL - SASHB; (h) apoA-I - SASHB; (i) apoB - SASHB; and (j) apoE - SASHB. Abbreviations : The data were adjusted for age, body mass index (BMI), sex, smoking status, mean artery pressure, alcohol consumption and microarousal index. FBG: Fasting blood glucose; FIN: Fasting insulin; HOMA-IR: Homeostasis model assessment of insulin resistance; TC: Total cholesterol; TG: Total triglycerides; HDL-C: High-density lipoprotein cholesterol; LDL-C: Low-density lipoprotein cholesterol; apoA-I: apolipoprotein A-I; apoB: apolipoprotein B; apoE: apolipoprotein E; SASHB: Sleep Apnea-Specific Hypoxic Burden
As shown in Table S7 , after adjusting for age, gender, and BMI in Model 1, as well as accounting for the MAP, smoking status, and alcohol consumption in Model 2, logistic regression models were employed to assess the association between the SASHB and abnormal glucose metabolism (i.e., hyperglycemia, hyperinsulinemia, and an abnormal HOMA-IR). Upon the incorporation of the MAI into Model 2, the ORs (95% CI) for hyperinsulinemia and abnormal HOMA-IR remained significant in the linear trend test, with ORs (95% CI) for SASHB quartiles being 1 (reference), hyperinsulinemia{1.527 (1.077, 2.166), 1.545 (1.083, 2.204), and 2.024 (1.400, 2.926), respectively ( p < 0.001 for a linear trend)}; abnormal HOMA-IR {1.510 (1.085, 2.104), 1.492(1.064, 2.092), 1.937(1.356, 2.767), respectively ( p = 0.001 for a linear trend)}.
Relationship between SASHB and lipid profile
Upon adjusting for age, gender, BMI, MAP, smoking status, alcohol consumption and MAI, the SASHB was found to be independently associated with TC (β = 0.100, P < 0.001), TG (β = 0.063, P = 0.011), LDL-C (β = 0.075, P = 0.003), apoB (β = 0.136, P < 0.001) and apoE (β = 0.088, P < 0.001) (Table S4 , Model 3, Figs. 4 and 5 ).
Logistic regression analyses revealed positive associations between all abnormal lipid metabolism ORs (95% CI) and increasing SASHB quartiles. These results persisted even after the MAI was incorporated into the model, with ORs (95% CI) of 1 (reference), 1.762 (1.243, 2.499), 1.998 (1.399, 2.856), and 2.708 (1.871, 3.919) ( P < 0.001 for a linear trend) for Hyper-total cholesterolemia; ORs (95% CI) of 1 (reference), 1.663 (1.156, 2.392), 1.695 (1.164, 2.467), and 2.316 (1.574, 3.407) ( p < 0.001 for a linear trend) for Hyper-LDL cholesterolemia; and ORs (95% CI) of 1 (reference), 1.471 (1.078, 2.007), 1.773 (1.293, 2.433), and 2.099 (1.505, 2.928) ( p < 0.001 for a linear trend), respectively, for Hyper-triglyceridemia across SASHB quartiles (Table S7 , Model 3).
Relationship between AHI, CT90 and glycolipid metabolism
Logistic regression models were employed to assess the association between the AHI and abnormal glucose and lipid metabolism. Within Model 3, significant positive linear trends were observed for abnormal glucose and lipid metabolism (Hyperinsulinemia, HOMA-IR ≥ 2.5, Hyper-total cholesterolemia, Hyper-LDL cholesterolemia, Hyper-triglyceridemia) ORs and 95% CIs with increasing AHI quartiles (all P for trend≤0.001). Specific ORs (95% CI) are shown in the supplemental file Table S8 . Similarly, we analyzed CT90, Logistic regression models were employed to assess the association between the CT90 and abnormal glucose and lipid metabolism (Table S9 ). The results of the stepwise multiple linear regression (AHI, CT90) of glucose metabolism index in models 1, 2 and 3 are presented in the supplemental file (Table S2 , 3 , 5 , 6 ).
Association of AHI, CT90 and SASHB with glycolipid metabolism in male and female concentration
In the male subset, Logistic regression models were employed to assess the association between the SASHB and abnormal glucose and lipid metabolism. Within Model 3, significant positive linear trends were observed for abnormal glycolipid metabolism (hyperinsulinemia, abnormal HOMA-IR, Hyper-total cholesterolemia, Hyper-LDL cholesterolemia) ORs and 95% CIs with increasing SASHB quartiles (Table S16 ). The results of the AHI and CT90 are shown in supplemental file Tables 17 and 18 .
In the female subset, Logistic regression models were employed to assess the association between the SASHB and abnormal glucose and lipid metabolism. Within Model 3, significant positive linear trends were observed for abnormal glycolipid metabolism (hyperinsulinemia, Hyperinsulinemia, Hyper-triglyceridemia) ORs and 95% CIs with increasing SASHB quartiles (Table S25 ). The results of the AHI and CT90 are shown in supplemental file Tables 26 and 27 .
In the men’s and female’s subset, the results of the stepwise multiple linear regression (SASHB, AHI, CT90) of glucose metabolism index in models 1, 2 and 3 are presented in the supplemental file (Table S2 , 3, 5, 6).
The present study demonstrated independent associations between SASHB and abnormal glucose as well as lipid metabolism with substantial sample, objective PSG data, and rigorous multivariate adjustments. Our findings indicate a positive linear trend for the risk of hyperinsulinemia and abnormal HOMA-IR across SASHB quartiles in abnormal glucose metabolism after adjusting for multiple variables; In terms of abnormal lipid metabolism, we observed a positive linear trend for risk of Hyper-total cholesterolemia, Hyper-LDL cholesterolemia and Hyper-triglyceridemia across SASHB quartiles after adjusting for multiple variables.
Because SpO 2 is readily available from laboratory and home sleep studies, it makes sense to include the depth and duration of IH among the metrics that have predictive value for abnormalities in glycolipid metabolism. SASHB is a candidate metric designed to predict the likelihood of the occurrence of abnormalities in glycolipid metabolism by capturing the frequency of respiratory events, and the depth and duration of the hypoxia associated with them. Azarbarzin et al. [ 29 ] first explored the association between SASHB and cardiovascular mortality, this study suggested that SASHB can be considered as early warning, diagnosis and prevention of cardiovascular diseases in OSA patients. Blanchard et al. examined the relationship between SASHB and incidence of new cerebrovascular events, they found that SASHB presented a higher prognostic value of cerebrovascular events when compared with other sleep variables (HR = 1.28); they also noted that SASHB may be a robust risk factor for stroke stratification in OSA [ 30 ].
Previous studies have confirmed that SASHB is one of the important early warning indicators of risk for cardiovascular morbidity and mortality, and given that abnormalities in glucose and lipid metabolism are established risk factors for cardiovascular morbidity and mortality [ 31 ], there has been an ongoing effort to find potential factors associated with glucose and lipid homeostasis. The impact of SASHB, an important early warning indicator for assessing IH in patients with OSA, on glucose metabolism and lipid metabolism remains unknown. Our findings support an independent correlation between SASHB and a range of metabolic abnormalities, including hyperinsulinemia, abnormal HOMA-IR, Hyper-cholesterolemia, Hyper -LDL cholesterolemia and Hyper-triglyceridemia. IH stimulation has been found to result in reduced insulin sensitivity and impaired glucose tolerance, with potential mechanisms of influence including activation of the sympathetic and hypothalamic-pituitary-adrenal systems, with the release of catecholamines that reduce insulin receptor sensitivity and decrease tissue insulin-mediated glucose uptake, while stimulating gluconeogenesis [ 32 ]. A previous study also found that SF could induce abnormal glucose metabolism through increased activity of the hypothalamic–pituitary–adrenal axis, resulting in higher circulating cortisol concentrations [ 33 ]. In rodent studies, SF was also associated with the development of glucose intolerance and insulin resistance [ 34 , 35 ]. Sleep fragmentation is a stressor that causes the elevation of hormones such as adrenocorticotropic hormone and cortisol. These hormones play a role in lipolysis, which might affect lipid levels [ 36 , 37 ] A previous study [ 38 ] reported an association between sleep fragmentation and dyslipidemia in a population of approximately 700 OSA patients. However, the included subjects in that studies were non-consecutive, which could have induced selection bias. Studies performed in animal models showed that repeated arousal from sleep could cause impaired lipid levels [ 39 , 40 ].
Rodent studies demonstrated that sleep disruption and IH could lead to insulin resistance [ 12 , 35 ]. Many clinical studies exploring the relationship between OSA and glucose metabolism were performed, with inconsistent results. Some studies suggested a link between OSA and abnormal glucose metabolism prior to the manifestation of diabetes [ 41 , 42 ], and further demonstrated that two pathophysiological processes of OSA (SF and IH) could increase circulating glucose by decreasing insulin sensitivity and reducing glucose effectiveness [ 13 , 43 , 44 , 45 ]. A cross-sectional study recruiting 1,834 patients with suspected OSA demonstrated that SF was independently associated with hyperinsulinemia, whereas IH was associated with hyperglycemia, hyperinsulinemia, and abnormal HOMA-IR abnormalities [ 46 ]. An epidemiologic study of 2,686 patients with suspected OSA suggests that sleep fragmentation is strongly associated with high LDL cholesterolemia in patients with OSA. It is warranted to investigate the causal relationship between sleep fragmentation and dyslipidemia, as well as the underlying mechanisms of this association further in prospective cohort studies [ 47 ].
A case-control study [ 48 ] observed inflammatory markers (IL-6, IL-8, IL-17, IL-18, MIF, Hs CRP, TNF- \(\alpha\) , PAI-1 and leptin) were significantly associated with OSA as compared to those without OSAs. Fang Y et al. revealed the association between autoantibodies against inflammatory factors and OSA, and the combination of auto antibodies against CRP, IL-6, IL-8 and TNF- \(\alpha\) may function as novel biomarker for monitoring the presence of OSA [ 49 ]. It has also been found that IH can stimulate the expression of NF-ĸB [ 50 ], leading to an increase in the expression of downstream inflammatory mediators, such as tumor necrosis factor- \({\alpha }\) and interleukin-8, which results in damage to pancreatic islet cells and decreases insulin sensitivity in the liver, muscle, and adipose tissues, ultimately leading to disturbances in glucose metabolism in patients with OSA. It has also been suggested [ 51 ] that the increase in FFA levels may be mediated by hypoxia-induced up-regulation of adipose triglyceride lipase activators such as protein kinase A. OSA, in addition to affecting circulating levels of TC and FFA, also regulates lipid function through oxidative stress. oxidative stress to regulate lipid function. We speculate that the depth and duration of hypoxia may be a possible factor in the excitation of sympathetic nerve activity, expression of inflammatory mediators, and oxidative stress, although further prospective studies are needed to elucidate this potential relationship.
Combining the duration and depth of respiratory events and their associated desaturation may provide useful information for more precise identification and management of patients with OSA (precision medicine). For example, studies have shown that longer [ 52 , 53 ] and deeper [ 54 ] apneas and hypoventilation elicit a greater cardiovascular response than shorter and milder apneas and hypoventilation. The autonomous relationship between SASHB and abnormalities of glucose and lipid metabolism observed in this study highlights the need for improvement with a focus on IH when developing novel treatment strategies for OSA patients with comorbid abnormalities of glucose metabolism. For example, when considering oxygen therapy for OSA, the frequency, depth and duration of oxygen were all important. This integrated approach has the potential to improve the prognosis and outcome of OSA patients with comorbid glucose metabolism abnormalities.
The highlight of this study is that all OSA indices were collected by laboratory-based PSG monitoring rather than surrogate measures (e.g., witnessed apnea or portable PSG). Additionally, we meticulously excluded individuals undergoing treatment with hypoglycemic and lipid-lowering medications. Finally, the substantial sample size and adjustment for confounding factors enhance the accuracy and credibility of our findings. Despite these merits, our study carries several limitations that merit discussion in the interpretation of our results. First, the present report is limited by the fact that it was based on clinical samples and observational research, and could not provide the causative evidence. Second, diet and physical activity are two important factors that influence glucose and lipid metabolism. Although only residents in east China with roughly analogous lifestyles were enrolled, and we strictly excluded patients who had been treated with hypoglycemic and lipid-lowering drugs, not controlling these two confounding factors is a potential limitation of our study. Third, the non-community-based prospective design of our study is a limitation worth noting. Fourth, these data are valid only for the examined population. Fifth, Morphological changes (android obesity) also play a major role in explaining both the higher hypoxic burden and the dysmetabolisms. However, we did not have the classic morphological co-variates of the metabolic syndrome been correctly entered into the model. Sixth, currently the calculation of the SASHB is based on the identification of desaturating events from ventilatory traces and qualified respiratory events. Other oximetric techniques propose to do without the detection of respiratory events and are perhaps more interesting.
In conclusion, our study revealed a positive linear trend for risk of hyperinsulinemia and HOMA-IR across SASHB quartiles; Similarly, concerning abnormal lipid metabolism, we observed a positive linear trend for risk of Hyper-total cholesterolemia, Hyper-LDL cholesterolemia and Hyper-triglyceridemia across SASHB quartiles. These findings underscore the imperative need to delve deeper into the causal connection between hypoxic burden and abnormal glucose and lipid metabolism. Future research endeavors should focus on elucidating the underlying mechanisms of such an association through prospective cohort studies.
Data availability
No datasets were generated or analysed during the current study.
Abbreviations
Obstructive sleep apnea
- Sleep apnea-specific hypoxic burden
Fasting blood glucose
Fasting insulin
Total cholesterol
Total triglycerides
High-density lipoprotein cholesterol
Low-density lipoprotein cholesterol
Apolipoprotein A-I
Apolipoprotein B
Apolipoprotein E
Intermittent hypoxia
Polysomnography
Electrooculogram
Electromyogram
American Academy of Sleep Medicine
Rapid eye movement
Microarousal index
Apnea hypopnea index
Homeostasis model assessment of insulin resistance
Neck circumference, WC, waist circumference
Hip circumference
Blood pressure
Body mass index
Mean arterial pressure
Gottlieb DJ, Punjabi NM. Diagnosis and management of obstructive sleep apnea: a review. JAMA. 2020;323:1389–400.
Article PubMed Google Scholar
Yeghiazarians Y, Jneid H, Tietjens JR, Redline S, Brown DL, El-Sherif N, et al. Obstructive Sleep Apnea and Cardiovascular Disease: A Scientific Statement from the American Heart Association. Circulation. 2021;144:e56–67.
Article CAS PubMed Google Scholar
Xu H, Liu F, Li Z, et al. Genome-Wide Association Study of Obstructive Sleep Apnea and Objective Sleep-related traits identifies novel risk loci in Han Chinese individuals. Am J Respir Crit Care Med. 2022;206(12):1534–45.
Xia Y, You K, Xiong Y. Interaction effects between characteristics of obstructive sleep apnea and obesity on dyslipidemia. Auris. Nasus Larynx. 2022;49(3):437–44.
Article Google Scholar
Huang T, Sands SA, Stampfer MJ, Tworoger SS, Hu FB, Redline S. Insulin resistance, hyperglycemia, and risk of developing obstructive sleep apnea in men and women in the United States. Annals Am Thorac Soc. 2022;19(10):1740–9.
Gündüz C, Basoglu OK, Hedner J et al. Obstructive sleep apnoea independently predicts lipid levels: data from the European Sleep Apnea Database. Respirology. 2018;23(12):1180–9
André S, Andreozzi F, Van Overstraeten C, et al. Cardiometabolic comorbidities in obstructive sleep apnea patients are related to disease severity, nocturnal hypoxemia, and decreased sleep quality. Respir Res. 2020;21(1):35.
Article PubMed PubMed Central Google Scholar
Martínez-Cerón E, Casitas R, Galera R, et al. Contribution of sleep characteristics to the association between obstructive sleep apnea and dyslipidemia. Sleep Med. 2021;84:63–72.
Meszaros M, Bikov A. Obstructive sleep apnoea and lipid metabolism: the Summary of evidence and future perspectives in the pathophysiology of OSA-Associated Dyslipidaemia. Biomedicines. 2022;10(11):2754.
Article CAS PubMed PubMed Central Google Scholar
Zou J, Xia Y, Xu H, Fu Y, Qian Y, Li X, et al. Independent relationships between cardinal features of obstructive sleep apnea and glycometabolism: a cross-sectional study. Metab Clin Exp. 2018;85:340–7.
Polak J, Shimoda LA, Drager LF, et al. Intermittent hypoxia impairs glucose homeostasis in C57BL6/J mice: partial improvement with cessation of the exposure. Sleep. 2013;36(10):1483–B1490.
Iiyori N, Alonso LC, Li J, et al. Intermittent hypoxia causes insulin resistance in lean mice independent of autonomic activity. Am J Respir Crit Care Med. 2007;175(8):851–7.
Tanno S, Tanigawa T, Saito I, et al. Sleep-related intermittent hypoxemia and glucose intolerance: a community-based study. Sleep Med. 2014;15(10):1212–8.
Muraki I, Tanigawa T, Yamagishi K, et al. Nocturnal intermittent hypoxia and the development of type 2 diabetes: the circulatory risk in communities Study (CIRCS). Diabetologia. 2010;53(3):481–8.
Li C, Gao Y, Huang W, et al. The use of the sleep apnea-specific hypoxic burden to predict obstructive sleep apnea hypopnea syndrome: evidence from a large cross-sectional study. Sleep Med. 2023;111:94–100.
Trzepizur W, Blanchard M, Ganem T, Balusson F, Feuilloy M, Girault JM, et al. Sleep apnea-specific hypoxic Burden, Symptom subtypes, and risk of Cardiovascular events and all-cause mortality. Am J Respir Crit Care Med. 2022;205:108–17.
Caples SM, Anderson WM, Calero K, Howell M, Hashmi SD. Use of polysomnography and home sleep apnea tests for the longitudinal management of obstructive sleep apnea in adults: an American Academy of Sleep Medicine clinical guidance statement. J Clin Sleep Med. 2021;17:1287–93.
Liu X, Lam DC, Chan KPF, Chan HY, Ip MS, Lau KK. Prevalence and determinants of Sleep Apnea in patients with stroke: a Meta-analysis. J Stroke Cerebrovasc Dis. 2021;30:106129.
Mediano O, Cano-Pumarega I, Sánchez-de-la-Torre M, Alonso-Álvarez ML, Troncoso MF, García-Río F, et al. Upcoming scenarios for the Comprehensive Management of Obstructive Sleep Apnea: an overview of the Spanish Sleep Network. Arch Bronconeumol. 2020;56:35–41.
Lin CH, Lurie RC, Lyons OD. Sleep apnea and chronic kidney disease: a state-of-the-art review. Chest. 2020;157(3):673–85.
Cao W, Luo J, Xiao Y. A review of current tools used for evaluating the severity of obstructive sleep apnea. Nat Sci Sleep. 2020;12:1023–31.
Moser D, Anderer P, Gruber G, et al. Sleep classification according to AASM and Rechtschaffen & Kales: effects on sleep scoring parameters. Sleep. 2009;32(2):139–49.
Mund C, Kellellu CK, Rattan R, Mahapatra S, Lamare AA, Jena S. Study of serum apelin and Insulin Resistance in type 2 diabetes Mellitus patients with or without obesity. Cureus J Med Sci. 2023;15(8):e43401.
Google Scholar
Park YM, Choi MK, Lee SS, et al. Dietary inflammatory potential and risk of mortality in metabolically healthy and unhealthy phenotypes among overweight and obese adults. Clin Nutr. 2019;38(2):682–8.
Yang CD, Pan WQ, Feng S, et al. Insulin resistance is Associated With Heart failure with recovered ejection fraction in patients without diabetes. J Am Heart Association. 2022;11(19):e026184.
Hirode G, Wong RJ. Trends in the prevalence of metabolic syndrome in the United States, 2011–2016. JAMA. 2020;323(24):2526–8.
Chinese guidelines on prevention and treatment of dyslipidemia in adults. Zhonghua Xin xue guan bing za zhi. 2007;35:390–419.
Mancusi C, de Simone G, Asteggiano R, Richter D, Williams B, Ferrini M. Survey on arterial hypertension management: a report from the ESC Council for Cardiology Practice and the ESC Council on Hypertension. Eur Heart J Open. 2021;1(2):oeab013.
Azarbarzin A, Sands SA, Stone KL, Taranto-Montemurro L, Messineo L, Terrill PI, et al. The hypoxic burden of sleep apnoea predicts cardiovascular disease-related mortality: the osteoporotic fractures in men study and the Sleep Heart Health Study. Eur Heart J. 2019;40:1149–57.
Blanchard M, Gervès-Pinquié C, Feuilloy M, Le Vaillant M, Trzepizur W, Meslier N et al. Hypoxic burden and heart rate variability predict stroke incidence in sleep apnoea. Eur Respir J. 2021;57.
Mehrabani-Zeinabad K, Haghighatdoost F, Mohammadifard N, et al. Impact of diabesity phenotype on cardiovascular diseases, major cardiovascular events and all-cause mortality. Sci Rep. 2023;13(1):11266.
Dungan K M, Braithwaite S S, Preiser JC. Stress hyperglycaemia[J]. Lancet. 2009;373(9677):1798–807.
Ekstedt M, Akerstedt T, Söderström M. Microarousals during sleep are associated with increased levels of lipids, cortisol, and blood pressure. Psychosom Med. 2004;66(6):925–31.
Baud MO, Magistretti PJ, Petit JM. Sustained sleep fragmentation affects brain temperature, food intake and glucose tolerance in mice. J Sleep Res. 2013;22(1):3–12.
Poroyko VA, Carreras A, Khalyfa A, et al. Chronic sleep disruption alters gut microbiota, induces systemic and adipose tissue inflammation and insulin resistance in mice. Sci Rep. 2016;6:35405.
Brindley DN, McCann BS, Niaura R, Stoney CM, Suarez EC. Stress and lipoprotein metabolism: modulators and mechanisms. Metabolism. 1993;42(9 Suppl 1):3–15.
Späth-Schwalbe E, Gofferje M, Kern W, Born J, Fehm HL. Sleep disruption alters nocturnal ACTH and cortisol secretory patterns. Biol Psychiatry. 1991;29(6):575–84.
Trzepizur W, Le Vaillant M, Meslier N, et al. Independent association between nocturnal intermittent hypoxemia and metabolic dyslipidemia. Chest. 2013;143(6):1584–9.
Perry JC, D’Almeida V, Souza FG, Schoorlemmer GH, Colombari E, Tufik S. Consequences of subchronic and chronic exposure to intermittent hypoxia and sleep deprivation on cardiovascular risk factors in rats. Respir Physiol Neurobiol. 2007;156(3):250–8.
Andersen ML, Martins PJ, D’Almeida V, Santos RF, Bignotto M, Tufik S. Effects of paradoxical sleep deprivation on blood parameters associated with cardiovascular risk in aged rats. Exp Gerontol. 2004;39(5):817–24.
Lindberg E, Theorell-Haglöw J, Svensson M, Gislason T, Berne C, Janson C. Sleep apnea and glucose metabolism: a long-term follow-up in a community-based sample. Chest. 2012;142(4):935–42.
Meslier N, Gagnadoux F, Giraud P, et al. Impaired glucose-insulin metabolism in males with obstructive sleep apnoea syndrome. Eur Respir J. 2003;22(1):156–60.
Eckel RH, Depner CM, Perreault L, et al. Morning circadian misalignment during short sleep duration impacts insulin sensitivity. Curr Biol. 2015;25(22):3004–10.
Stamatakis KA, Punjabi NM. Effects of sleep fragmentation on glucose metabolism in normal subjects. Chest. 2010;137(1):95–101.
Newhouse LP, Joyner MJ, Curry TB, et al. Three hours of intermittent hypoxia increases circulating glucose levels in healthy adults. Physiol Rep. 2017;5(1):e13106.
Zou J, Xia Y, Xu H, et al. Independent relationships between cardinal features of obstructive sleep apnea and glycometabolism: a cross-sectional study. Metabolism. 2018;85:340–7.
Qian Y, Yi H, Zou J, et al. Independent Association between Sleep Fragmentation and Dyslipidemia in patients with obstructive sleep apnea. Sci Rep. 2016;6:26089.
Bhatt SP, Guleria R, Kabra SK. Metabolic alterations and systemic inflammation in overweight/obese children with obstructive sleep apnea. PLoS ONE. 2021;16(6):e0252353.
Fang Y, Su J, Zhang B, et al. Autoantibodies of inflammatory cytokines as serum biomarkers in OSA patients. Clin Chim Acta. 2023;547:117399.
RYAN S, TAYLOR C T, MCNICHOLAS W T. Predictors of elevated nuclear factor-kappab-dependent genes in obstructive sleep apnea syndrome[J]. Am J Respir Crit Care Med. 2006;174(7):824–30.
XIONG YL, QU Z, CHEN N, et al. The local corticotropinreleasing hormone receptor 2 signalling pathway partly mediates hypoxia-induced increases in lipolysis via the cAMP-protein kinase a signalling pathway in white adipose tissue[J]. Mol Cell Endocrinol. 2014;392(1/2):106–14.
Butler MP, Emch JT, Rueschman M, Sands SA, Shea SA, Wellman A, et al. Apnea-hypopnea event Duration predicts Mortality in men and women in the Sleep Heart Health Study. Am J Respir Crit Care Med. 2019;199:903–12.
Hietakoste S, Korkalainen H, Kainulainen S, Sillanmäki S, Nikkonen S, Myllymaa S, et al. Longer apneas and hypopneas are associated with greater ultra-short-term HRV in obstructive sleep apnea. Sci Rep. 2020;10:21556.
Azarbarzin A, Ostrowski M, Moussavi Z, Hanly P, Younes M. Contribution of arousal from sleep to postevent tachycardia in patients with obstructive sleep apnea. Sleep. 2013;36:881–9.
Download references
Acknowledgements
The authors acknowledge all the participants and survey staffs for their participation.
This study was funded by the Ministry of Science and Technology of the People’s Republic of China (STI2030-Major Projects 2021ZD0201900); Interdisciplinary Program of Shanghai Jiao Tong University (YG2023LC11); Shanghai Three Year Action Plan for Traditional Chinese Medicine ZY(2021–2023)-0205-04.
Author information
Chenyang Li, Yu Peng and Xiaoyue Zhu contributed equally to this work.
Authors and Affiliations
Department of Otolaryngology-Head and Neck Surgery & Center of Sleep Medicine, Shanghai Jiao Tong University School of Medicine Affiliated Sixth People’s Hospital, Shanghai, China
Chenyang Li, Yu Peng, Xiaoyue Zhu, Yupu Liu, Jianyin Zou, Huaming Zhu, Xinyi Li, Hongliang Yi, Jian Guan, Huajun Xu & Shankai Yin
Shanghai Key Laboratory of Sleep Disordered Breathing, Shanghai, China
School of Biomedical Engineering, Shenzhen University Medical School, Shenzhen, China
You can also search for this author in PubMed Google Scholar
Contributions
The authors take responsibility and vouch for the accuracy and completeness of the data and analyses. Prof. SY, JG and HX had full access to all of the data in the study and took responsibility for the integrity of the data and the accuracy of the data analysis. Study design: CL, YP, XZ and SY; Data collection: YL, JZ and HZ; Statistical analysis: XL, XZ, HY; Manuscript draft: CL, YP, JG and HX. The authors have seen and approved the manuscript.
Corresponding authors
Correspondence to Jian Guan , Xu Zhang or Huajun Xu .
Ethics declarations
Ethics approval and consent to participate.
The study was conducted according to the World Medical Association Declaration of Helsinki in 1975, as revised in 1983, and was approved by the Ethic Committee of Shanghai Jiao Tong University Affiliated Sixth People’s Hospital (Trial registration number: ChiCTR1900025714). All subjects provided their informed written consent.
Consent for publication
Not applicable.
Competing interests
The authors declare no competing interests.
Additional information
Publisher’s note.
Springer Nature remains neutral with regard to jurisdictional claims in published maps and institutional affiliations.
Electronic supplementary material
Below is the link to the electronic supplementary material.
Supplementary Material 1
Rights and permissions.
Open Access This article is licensed under a Creative Commons Attribution 4.0 International License, which permits use, sharing, adaptation, distribution and reproduction in any medium or format, as long as you give appropriate credit to the original author(s) and the source, provide a link to the Creative Commons licence, and indicate if changes were made. The images or other third party material in this article are included in the article’s Creative Commons licence, unless indicated otherwise in a credit line to the material. If material is not included in the article’s Creative Commons licence and your intended use is not permitted by statutory regulation or exceeds the permitted use, you will need to obtain permission directly from the copyright holder. To view a copy of this licence, visit http://creativecommons.org/licenses/by/4.0/ . The Creative Commons Public Domain Dedication waiver ( http://creativecommons.org/publicdomain/zero/1.0/ ) applies to the data made available in this article, unless otherwise stated in a credit line to the data.
Reprints and permissions
About this article
Cite this article.
Li, C., Peng, Y., Zhu, X. et al. Independent relationship between sleep apnea-specific hypoxic burden and glucolipid metabolism disorder: a cross-sectional study. Respir Res 25 , 214 (2024). https://doi.org/10.1186/s12931-024-02846-7
Download citation
Received : 08 December 2023
Accepted : 12 May 2024
Published : 18 May 2024
DOI : https://doi.org/10.1186/s12931-024-02846-7
Share this article
Anyone you share the following link with will be able to read this content:
Sorry, a shareable link is not currently available for this article.
Provided by the Springer Nature SharedIt content-sharing initiative
- Obstructive sleep apnea hypopnea syndrome
- Abnormal glucose and lipid metabolism.
Respiratory Research
ISSN: 1465-993X
- General enquiries: [email protected]

Pengaruh Green Accounting Dan Kinerja Lingkungan Terhadap Kinerja Keuangan Perusahaan Sub Sektor Tambang Batubara
- Defa Putri Maharani Program Studi Akuntansi, Fakultas Ekonomi Universitas Gunadarma
- Dyah Palupi Universitas Gunadarma
- Dassaad Dassaad Program Studi Akuntansi, Fakultas Ekonomi Universitas Gunadarma
- Budi Wahyudi Program Studi Akuntansi, Fakultas Ekonomi Universitas Gunadarma
- Riyanti Riyanti Program Studi Akuntansi, Fakultas Ekonomi Universitas Gunadarma
This research aims to determine and analyze the influence of Green Accounting on financial performance, the influence of environmental performance on financial performance, and the influence of Green Accounting and environmental performance on financial performance in coal mining subsector companies listed on the Indonesia Stock Exchange (BEI) for the 2018-2022 period. This research uses 35 samples of coal mining subsector companies listed on the Indonesia Stock Exchange (BEI). The sampling technique uses the purposive sampling method. Purposive sampling is a sampling method taken based on criteria determined by the researcher. The variable in this research is financial performance which uses profitability ratios as measured by ROA and ROE as dependent variables and Green Accounting and Environmental Performance as independent variables. This research uses multiple linear analysis methods and uses SPSS version 25 software as a tool for testing multiple linear regression and descriptive statistical tests. The results of this research show that Green Accounting and financial performance calculated using ROA have an influence while using ROE calculations have no effect. Environmental performance does not have a significant effect on financial performance calculated using ROA, while that calculated using ROE has a significant effect. Green Accounting and environmental performance on financial performance simultaneously independent variables have an influence on the dependent variable.
Keywords : Green Accounting, Environmental Performance, Financial Performance (ROA) and (ROE)

- Klik disini untuk file PDF
Copyright (c) 2024 JURNAL MANEKSI

This work is licensed under a Creative Commons Attribution-NonCommercial 4.0 International License .

Decision Of The Director General Of Strengthening Research And Development Ministry Of Research, Technology, And Higher Education Republic Of Indonesia:

Journal Statistics
Information
- For Readers
- For Authors
- For Librarians
Editorial Office POLITEKNIK NEGERI AMBON PUSAT PENELITIAN DAN PENGABDIAN MASYARAKAT JL. Ir. M. Putuhena, Wailela-Rumahtiga, Ambon Maluku, Indonesia Kode Pos: 97234 Contact: 081343016488

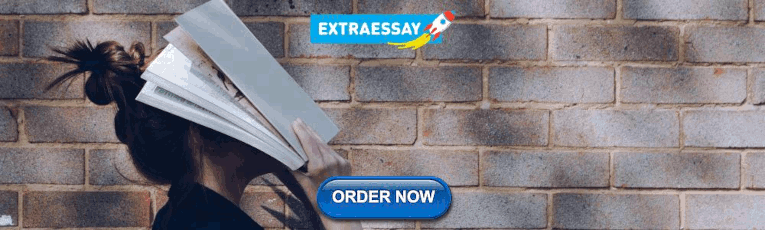
IMAGES
VIDEO
COMMENTS
The independent variable is the cause. Its value is independent of other variables in your study. The dependent variable is the effect. Its value depends on changes in the independent variable. Example: Independent and dependent variables. You design a study to test whether changes in room temperature have an effect on math test scores.
While the independent variable is the " cause ", the dependent variable is the " effect " - or rather, the affected variable. In other words, the dependent variable is the variable that is assumed to change as a result of a change in the independent variable. Keeping with the previous example, let's look at some dependent variables ...
A variable in research simply refers to a person, place, thing, or phenomenon that you are trying to measure in some way. ... Designation of the dependent and independent variable involves unpacking the research problem in a way that identifies a general cause and effect and classifying these variables as either independent or dependent.
In research, a variable is any characteristic, number, or quantity that can be measured or counted in experimental investigations. One is called the dependent variable, and the other is the independent variable. In research, the independent variable is manipulated to observe its effect, while the dependent variable is the measured outcome.
Pairing each variable in the "independent variable" column with each variable in the "dependent variable" column would result in the generation of these hypotheses. Table 2 shows how this is done for age. Sets of hypotheses can likewise be constructed for the remaining independent and dependent variables in Table 1. Importantly, the ...
Independent variables and dependent variables are the two fundamental types of variables in statistical modeling and experimental designs. ... That process involves in-depth research and many subject-area, theoretical, and statistical considerations. At its most basic level, you'll want to include the predictors you are specifically assessing ...
Variables are an important concept in experimental and hypothesis-testing research, so understanding independent/dependent variables is key to understanding research design. In this article, we will talk about what separates a dependent variable from an independent variable and how the concept applies to research.
Extraneous variables (from Adjei, n.d.) While it is very common to hear the terms independent and dependent variable, extraneous variables are less common, which is surprising because an extraneous variable can destroy the integrity of a research study that claims to show a cause and effect relationship. An extraneous variable is a variable that may compete with the independent variable in ...
Plot or graph independent and dependent variables using the standard method. The independent variable is the x-axis, while the dependent variable is the y-axis. ... The Practice of Social Research (12th ed.) Wadsworth Publishing. ISBN -495-59841-. di Francia, G. Toraldo (1981). The Investigation of the Physical World. Cambridge University Press.
Independent and Dependent Variables, Explained With Examples. Written by MasterClass. Last updated: Mar 21, 2022 • 4 min read. In experiments that test cause and effect, two types of variables come into play. One is an independent variable and the other is a dependent variable, and together they play an integral role in research design.
The independent variable is the cause and the dependent variable is the effect, that is, independent variables influence dependent variables. In research, a dependent variable is the outcome of interest of the study and the independent variable is the factor that may influence the outcome. Let's explain this with an independent and dependent ...
Independent vs. Dependent Variables on a Graph. When we create a graph, the independent variable will go on the x-axis and the dependent variable will go on the y-axis. For example, suppose a researcher provides different amounts of water for 20 different plants and measures the growth rate of each plant. The following scatterplot shows the ...
A variable is considered dependent if it depends on an independent variable. Dependent variables are studied under the supposition or demand that ... Situational variables are features of the environment in which the study or research was conducted, which have a bearing on the outcome of the experiment in a negative way. Included are the air ...
Visually depicting independent and dependent variables . Depending on your research topic, you can portray dependent and independent variables using the following methods: 1. Scatterplot . This format is used where both, the independent and dependent variables are continuous.
The two main types of variables in psychology are the independent variable and the dependent variable. Both variables are important in the process of collecting data about psychological phenomena. This article discusses different types of variables that are used in psychology research. It also covers how to operationalize these variables when ...
The independent variable is the cause. Its value is independent of other variables in your study. The dependent variable is the effect. Its value depends on changes in the independent variable. Example: Independent and dependent variables. You design a study to test whether changes in room temperature have an effect on maths test scores.
The independent variable is the drug, while the patient's blood pressure is the dependent variable. In some ways, this experiment resembles the one with breakfast and test scores. However, when comparing two different treatments, such as drug A and drug B, it's usual to add another variable, called the control variable.
The independent variable, often denoted as X, is the variable that is manipulated or controlled by the researcher intentionally. It's the factor that researchers believe may have a causal effect on the dependent variable. In simpler terms, the independent variable is the variable you change or vary in an experiment so you can observe its impact ...
The relationship between independent and dependent variables can manifest in various forms—direct, indirect, linear, nonlinear, and may be moderated or mediated by other variables. At its most basic, this relationship is often conceptualized as cause and effect: the independent variable (the cause) influences the dependent variable (the effect).
Compare the independent variable and dependent variable in research. See other types of variables in research, including confounding and extraneous variables. Updated: 11/21/2023
Designation of the dependent and independent variable involves unpacking the research problem in a way that identifies a general cause and effect and classifying these variables as either independent or dependent. The variables should be outlined in the introduction of your paper and explained in more detail in the methods section. There are no ...
Learn about the most popular types of variables in research, including dependent, independent and control variables - as well as mediating, moderating and co...
Definition: Independent variable is a variable that is manipulated or changed by the researcher to observe its effect on the dependent variable. It is also known as the predictor variable or explanatory variable. The independent variable is the presumed cause in an experiment or study, while the dependent variable is the presumed effect or outcome.
S, n.d). So, the dependent variable is the one we want to understand or predict, while the independent variable is used to help us understand or predict the dependent variable. The goal is to determine how much the changes in the independent variables are associated with changes in the dependent variable. (Beers. B, 2023).
Dependent variable. To measure the dependent variable, a proxy is used to reflect radical innovation performance (Garriga et al., 2013; Laursen & Salter, 2006; Monteiro et al., 2017). The questionnaire asks firms to report the fraction of the firm's turnover from products and services that are new to the world (i.e., innovations that the firm ...
Clinical and Experimental Dental Research is an open access ... and periapical status according to the Periapical Index. The main outcome of the study, AP, was used as the dependent variable and all analyses were performed at root level. ... Logistic regression analysis of associations between independent variables and the dependent variable AP ...
8 German Rheumatism Research Center (DRFZ Berlin), Epidemiology Unit, ... In the multivariable model with new medication or dosage increase due to flare as the dependent variable, the only independent associations observed were: active disease (low disease activity, OR=1.47, 95% CI=0.94 to 2.29; moderate/high disease activity, OR=3.08, 95% CI=1 ...
Objectives Obstructive sleep apnea (OSA) is associated with abnormal glucose and lipid metabolism. However, whether there is an independent association between Sleep Apnea-Specific Hypoxic Burden (SASHB) and glycolipid metabolism disorders in patients with OSA is unknown. Methods We enrolled 2,173 participants with suspected OSA from January 2019 to July 2023 in this study. Polysomnographic ...
The variable in this research is financial performance which uses profitability ratios as measured by ROA and ROE as dependent variables and Green Accounting and Environmental Performance as independent variables. This research uses multiple linear analysis methods and uses SPSS version 25 software as a tool for testing multiple linear ...