- Increase Font Size
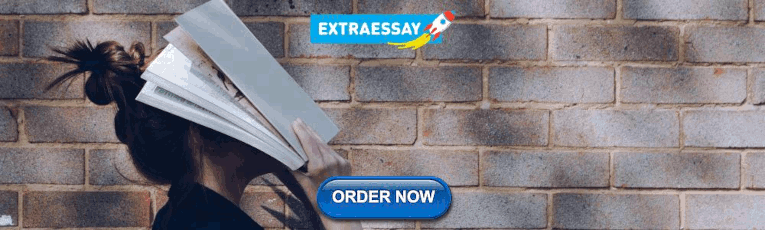
46 Presentation of data II – Graphical representation
Pa . Raajeswari
Graphical representation is the visual display of data using plots and charts. It is used in many academic and professional disciplines but most widely so in the fields of mathematics, medicine and sciences. Graphical representation helps to quantify, sort and present data in a method that is understandable to a large variety of audiences. A graph is the representation of data by using graphical symbols such as lines, bars, pie slices, dots etc. A graph does represent a numerical data in the form of a qualitative structure and provides important information.
Statistical surveys and experiments provides valuable information about numerical scores. For better understanding and making conclusions and interpretations, the data should be managed and organized in a systematic form.
Graphs also enable in studying both time series and frequency distribution as they give clear account and precise picture of problem. Above all graphs are also easy to understand and eye catching and can create a storing impact on memory.
General Principles of Graphic Representation:
There are some algebraic principles which apply to all types of graphic representation of data. In a graph there are two lines called coordinate axes. One is vertical known as Y axis and the other is horizontal called X axis. These two lines are perpendicular to each other. Where these two lines intersect each other is called ‘0’ or the Origin. On the X axis the distances right to the origin have positive value and distances left to the origin have negative value. On the Y axis distances above the origin have a positive value and below the origin have a negative value.
TYPES OF GRAPHICAL REPRESENTATON:
The various types of graphical representations of the data are
- Circle Graph
- Histogram and Frequency Polygon
1. Dot Plots
The dot plot is one of the most simplest ways of graphical representation of the statistical data. As the name itself suggests, a dot plot uses the dots. It is a graphic display which usually compares frequency within different categories. The dot plot is composed of dots that are to be plotted on a graph paper.
In the dot plot, every dot denotes a specific number of observations belonging to a data set. One dot usually represents one observation. These dots are to be marked in the form of a column for each category. In this way, the height of each column shows the corresponding frequency of some category. The dot plots are quite useful when there are small amount of data is given within the small number of categories.
2. Bar Graph
A bar graph is a very frequently used graph in statistics as well as in media. A bar graph is a type of graph which contains rectangles or rectangular bars. The lengths of these bars should be proportional to the numerical values represented by them. In bar graph, the bars may be plotted either horizontally or vertically. But a vertical bar graph (also known as column bar graph) is used more than a horizontal one.
A vertical bar graph is shown below:
Number of students went to different countries for study:
The rectangular bars are separated by some distance in order to distinguish them from one another. The bar graph shows comparison among the given categories.
Mostly, horizontal axis of the graph represents specific categories and vertical axis shows the discrete numerical values.
3.Line Graph
A line graph is a kind of graph which represents data in a way that a series of points are to be connected by segments of straight lines. In a line graph, the data points are plotted on a graph and they are joined together with straight line.
A sample line graph is illustrated in the following diagram:
The line graphs are used in the science, statistics and media. Line graphs are very easy to create. These are quite popular in comparison with other graphs since they visualize characteristics revealing data trends very clearly. A line graph gives a clear visual comparison between two variables which are represented on X-axis and Y-axis.
4.Circle Graph
A circle graph is also known as a pie graph or pie chart. It is called so since it is similar to slice of a “pie”. A pie graph is defined as a graph which contains a circle which is divided into sectors. These sectors illustrate the numerical proportion of the data.
A pie chart are shown in the following diagram:
The arc lengths of the sectors, in pie chart, are proportional to the numerical value they represent.Circle graphs are quite commonly seen in mass media as well as in business world.
5. Histogram and Frequency Polygon
The histograms and frequency polygons are very common graphs in statistics. A histogram is defined as a graphical representation of the mutually exclusive events. A histogram is quite similar to the bar graph. Both are made up of rectangular bars. The difference is that there is no gap between any two bars in the histogram. The histogram is used to represent the continuous data.
A histogram may look like the following graph:
The frequency polygon is a type of graphical representation which gives us better understanding of the shape of given distribution. Frequency polygons serve almost the similar purpose as histograms do. But the frequency polygon is quite helpful for the purpose of comparing two or more sets of data. The frequency polygons are said to be the extension of the histogram. When the midpoints of tops of the rectangular bars are joined together, the frequency polygon is made.
Few examples of graphical representation of statistical data are given below:
Example 1: Draw a dot plot for the following data.
Solution: The pie graph of the above data is:
Methods to Represent a Frequency Distribution:
Generally four methods are used to represent a frequency distribution graphically. These are Histogram, Smoothed frequency graph and Ogive or Cumulative frequency graph and pie diagram.
1. Histogram:
Histogram is a non-cumulative frequency graph, it is drawn on a natural scale in which the representative frequencies of the different class of values are represented through vertical rectangles drawn closed to each other. Measure of central tendency, mode can be easily determined with the help of this graph.
How to draw a Histogram:
Represent the class intervals of the variables along the X axis and their frequencies along the Y-axis on natural scale.
Start X axis with the lower limit of the lowest class interval. When the lower limit happens to be a distant score from the origin give a break in the X-axis n to indicate that the vertical axis has been moved in for convenience.
Now draw rectangular bars in parallel to Y axis above each of the class intervals with class units as base: The areas of rectangles must be proportional to the frequencies of the corresponding classes.
In this graph we shall take class intervals in the X axis and frequencies in the Y axis. Before plotting the graph we have to convert the class into their exact limits.
Advantages of histogram:
1. It is easy to draw and simple to understand.
2. It helps us to understand the distribution easily and quickly.
3. It is more precise than the polygene.
Limitations of histogram:
1. It is not possible to plot more than one distribution on same axes as histogram.
2. Comparison of more than one frequency distribution on the same axes is not possible.
3. It is not possible to make it smooth.
Uses of histogram:
1.Represents the data in graphic form.
2.Provides the knowledge of how the scores in the group are distributed. Whether the scores are piled up at the lower or higher end of the distribution or are evenly and regularly distributed throughout the scale.
3.Frequency Polygon. The frequency polygon is a frequency graph which is drawn by joining the coordinating points of the mid-values of the class intervals and their corresponding fre-quencies.
How to draw a frequency polygon:
Draw a horizontal line at the bottom of graph paper named ‘OX’ axis. Mark off the exact limits of the class intervals along this axis. It is better to start with i. of lowest value. When the lowest score in the distribution is a large number we cannot show it graphically if we start with the origin. Therefore put a break in the X axis to indicate that the vertical axis has been moved in for convenience. Two additional points may be added to the two extreme ends.
Draw a vertical line through the extreme end of the horizontal axis known as OY axis. Along this line mark off the units to represent the frequencies of the class intervals. The scale should be chosen in such a way that it will make the largest frequency (height) of the polygon approximately 75 percent of the width of the figure.
Plot the points at a height proportional to the frequencies directly above the point on the horizontal axis representing the mid-point of each class interval.
After plotting all the points on the graph join these points by a series of short straight lines to form the frequency polygon. In order to complete the figure two additional intervals at the high end and low end of the distribution should be included. The frequency of these two intervals will be zero.
Illustration: No. 7.3:
Draw a frequency polygon from the following data:
Advantages of frequency polygon:
2. It is possible to plot two distributions at a time on same axes.
3. Comparison of two distributions can be made through frequency polygon.
4. It is possible to make it smooth.
Limitations of frequency polygon:
1. It is less precise.
2. It is not accurate in terms of area the frequency upon each interval.
Uses of frequency polygon:
1. When two or more distributions are to be compared the frequency polygon is used.
2. It represents the data in graphic form.
3. It provides knowledge of how the scores in one or more group are distributed. Whether the scores are piled up at the lower or higher end of the distribution or are evenly and regularly distributed throughout the scale.
2. Smoothed Frequency Polygon:
When the sample is very small and the frequency distribution is irregular the polygon is very jig-jag. In order to wipe out the irregularities and “also get a better notion of how the figure might look if the data were more numerous, the frequency polygon may be smoothed.”
In this process to adjust the frequencies we take a series of ‘moving’ or ‘running’ averages. To get an adjusted or smoothed frequency we add the frequency of a class interval with the two adjacent intervals, just below and above the class interval. Then the sum is divided by 3. When these adjusted frequencies are plotted against the class intervals on a graph we get a smoothed frequency polygon.
Illustration 7.4:
Draw a smoothed frequency polygon, of the data given in the illustration No. 7.3:
Here we have to first convert the class intervals into their exact limits. Then we have to determine the adjusted or smoothed frequencies.
3. Ogive or Cumulative Frequency Polygon:
Ogive is a cumulative frequency graphs drawn on natural scale to determine the values of certain factors like median, Quartile, Percentile etc. In these graphs the exact limits of the class intervals are shown along the X-axis and the cumulative frequencies are shown along the Y-axis. Below are given the steps to draw an ogive.
Get the cumulative frequency by adding the frequencies cumulatively, from the lower end (to get a less than ogive) or from the upper end (to get a more than ogive).
Mark off the class intervals in the X-axis.
Represent the cumulative frequencies along the Y-axis beginning with zero at the base.
Put dots at each of the coordinating points of the upper limit and the corresponding frequencies.
Join all the dots with a line drawing smoothly. This will result in curve called ogive.
Illustration No. 7.5:
Draw an ogive from the data given below:
To plot this graph first we have to convert, the class intervals into their exact limits. Then we have to calculate the cumulative frequencies of the distribution.
Uses of Ogive:
1. Ogive is useful to determine the number of students below and above a particular score.
2. When the median as a measure of central tendency is wanted.
3. When the quartiles, deciles and percentiles are wanted.
4. By plotting the scores of two groups on a same scale we can compare both the groups.
4. The Pie Diagram:
Figure given below shows the distribution of elementary pupils by their academic achievement in a school. Of the total, 60% are high achievers, 25% middle achievers and 15% low achievers. The construction of this pie diagram is quite simple. There are 360 degree in the circle. Hence, 60% of 360′ or 216° are counted off as shown in the diagram; this sector represents the proportion of high achievers students.
Ninety degrees counted off for the middle achiever students (25%) and 54 degrees for low achiever students (15%). The pie-diagram is useful when one wishes to picture proportions of the total in a striking way. Numbers of degrees may be measured off “by eye” or more accurately with a protractor.
Uses of Pie diagram:
1. Pie diagram is useful when one wants to picture proportions of the total in a striking way.
2. When a population is stratified and each strata is to be presented as a percentage at that time pie diagram is used.
PURPOSE OF GRAPHICAL REPRESENTATION:
The purpose of graphical presentation of data is to provide a quick and easy-to-read picture of information that clearly shows what otherwise takes a great deal of explanation. The impact of graphical data is typically more pointed and memorable than paragraphs of written information
For example, a person making a presentation regarding sales in various states across the country establishes the point of the presentation to the audience more quickly by using a color-coded map rather than merely stating the sales figures for each state. Observers quickly determine which states are ahead and which are behind in sales, and they know where emphasis needs to be placed. Alternatively, when making a presentation on sales by age groups using a pie chart that divides the pie into various ages, the audience quickly sees the results of sales by age. This means that the audience is more likely to retain that information than if the presenter simply reads the results aloud or puts it into writing.
GENERAL RULES DISPLAYING DATA
- Simpler is Better
- Graphs, Tables and charts can be used together
- Use clear Description, title and labels
- Provide a narrative Description of the highlights
- Don’t compare variables with different scales of magnitude.
- A Diagram must be attractive, well proportioned,neat and pleasing to the eyes.
- They should be geometrically Accurate
- Size of the diagram should be proportional to paper should not be too big or too small
- Different colors should be used to classify data’s.
ADVANTAGES:
- Acceptability: graphical report is acceptable to the busy persons because it easily highlights about the theme of the report. This helps to avoid wastage of time.
- Comparative Analysis : Information can be compared in terms of graphical representation. Â Such comparative analysis helps for quick understanding and attention.
- Less cost : Information if descriptive involves huge time to present properly. It involves more money to print the information but graphical presentation can be made in short but catchy view to make the report understandable. It obviously involves less cost.
- Decision Making: Business executives can view the graphs at a glance and can make decision very quickly which is hardly possible through descriptive report.
- Logical Ideas: If tables, design and graphs are used to represent information then a logical sequence is created to clear the idea of the audience.
- Helpful for less literate Audience: Less literate or illiterate people can understand graphical representation easily because it does not involve going through line by line of any descriptive report.
- Less Effort and Time: To present any table, design, image or graphs require less effort and time. Furthermore, such presentation makes quick understanding of the information.
- Less Error and Mistakes: Qualitative or informative or descriptive reports involve errors or mistakes. As graphical representations are exhibited through numerical figures, tables or graphs, it usually involves less error and mistake.
- A complete Idea: Such representation creates clear and complete idea in the mind of audience. Reading hundred pages may not give any scope to make decision. But an instant view or looking at a glance obviously makes an impression in  the mind of audience regarding the topic or subject.
- Use in the Notice Board: Such representation can be hanged in the notice board to quickly raise the attention of employees in any organization.
DISADVANTAGES:
Graphical representation of reports is not free from limitations. The following are the problems of graphical representation of data or reports:
- Costly : Graphical representation pf reports are costly because it involves images, colors and paints. Combination of material with human efforts makes the graphical presentation expensive.
- More time : Normal report involves less time to represent but graphical representation involves more time as it requires graphs and figures which are dependent to more time.
- Errors and Mistakes : Since graphical representations are complex, there is- each and every chance of errors and mistake. This causes problems for better understanding to general people.
- Lack of Secrecy: Graphical representation makes full presentation of information which may hamper the objective to keep something secret.
- Problems to select the suitable method: Information can be presented through various graphical methods and ways. Which should be the suitable method is very hard to select.
- Problem of Understanding: All may not be able to get the meaning of graphical representation because it involves various technical matters which are complex to general people.
Last of all it can be said that graphical representation does not provide proper information to general people.
CONCLUSION:
Graphical representation makes the datamore possible to easily draw; visual impression of data. Graphical representation of data enhances the understandings of the observer. It makes comparisons easy. This kind of method creates an imprint on mind for a long period of time. Well in this chapter we have discussed about the definition ,types ,advantages and disadvantages in detail with relevant examples which will have an impact in the power of understanding. I request you all to go through the various types of graphs commonly used in research studies in with reference to home science research studies to explore new ideas in the field of research.
- http://shodhganga.inflibnet.ac.in/bitstream/10603/143688/2/file%202%20chapter%201 %20data%20representation%20techniques.pdf
- http://www.mas.ncl.ac.uk/~ndah6/teaching/MAS1403/notes_chapter2.pdf https://www.ncbi.nlm.nih.gov/pmc/articles/PMC5453888/
- http://cec.nic.in/wpresources/module/Anthropology/PaperIX/9/content/downloads/file1. pdf
- https://www.kluniversity.in/arp/uploads/2096.pdf
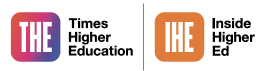
How to develop a graphical framework to chart your research
Graphic representations or frameworks can be powerful tools to explain research processes and outcomes. David Waller explains how researchers can develop effective visual models to chart their work

David Waller
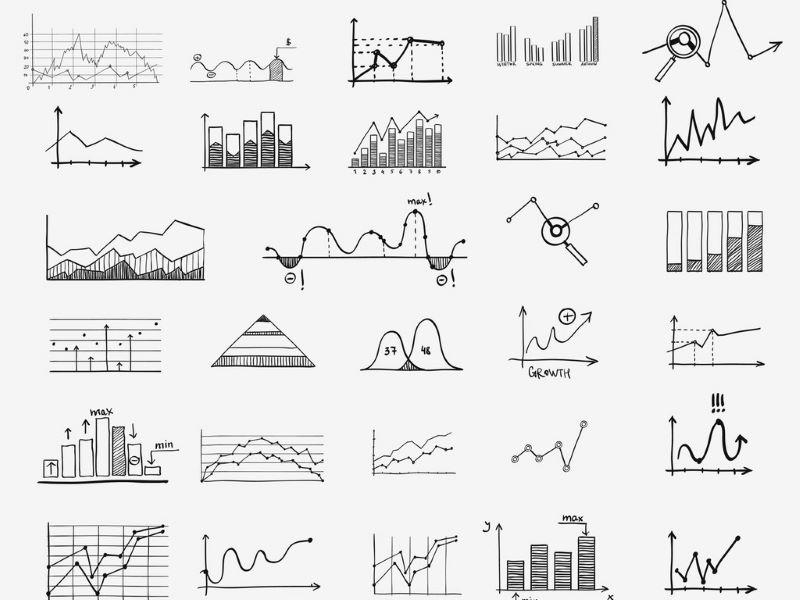
You may also like
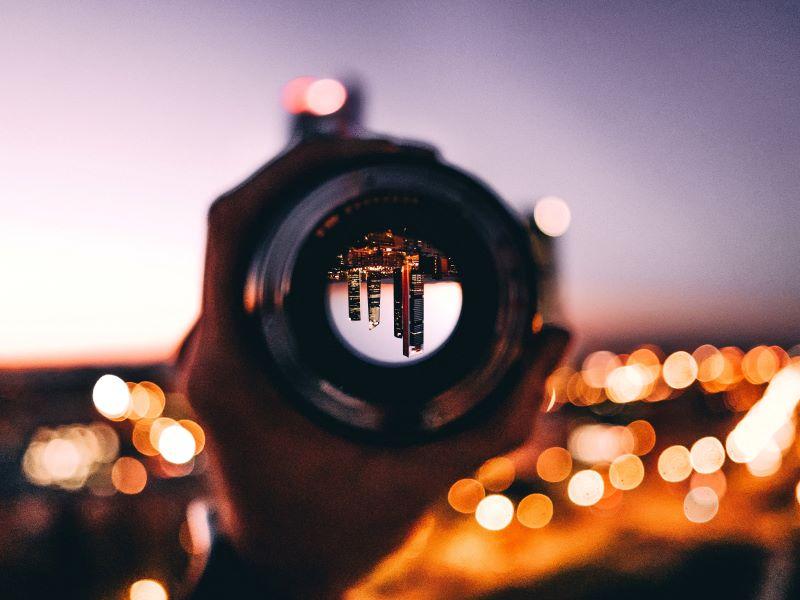
Popular resources
.css-1txxx8u{overflow:hidden;max-height:81px;text-indent:0px;} Students using generative AI to write essays isn't a crisis
How students’ genai skills affect assignment instructions, turn individual wins into team achievements in group work, access and equity: two crucial aspects of applied learning, emotions and learning: what role do emotions play in how and why students learn.
While undertaking a study, researchers can uncover insights, connections and findings that are extremely valuable to anyone likely to read their eventual paper. Thus, it is important for the researcher to clearly present and explain the ideas and potential relationships. One important way of presenting findings and relationships is by developing a graphical conceptual framework.
A graphical conceptual framework is a visual model that assists readers by illustrating how concepts, constructs, themes or processes work. It is an image designed to help the viewer understand how various factors interrelate and affect outcomes, such as a chart, graph or map.
These are commonly used in research to show outcomes but also to create, develop, test, support and criticise various ideas and models. The use of a conceptual framework can vary depending on whether it is being used for qualitative or quantitative research.
- Using literature reviews to strengthen research: tips for PhDs and supervisors
- Get your research out there: 7 strategies for high-impact science communication
- Understanding peer review: what it is, how it works and why it is important
There are many forms that a graphical conceptual framework can take, which can depend on the topic, the type of research or findings, and what can best present the story.
Below are examples of frameworks based on qualitative and quantitative research.
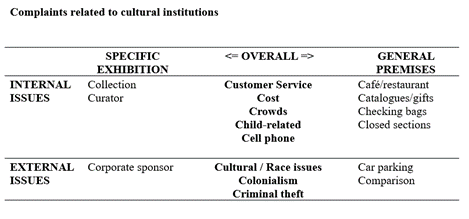
As shown by the table below, in qualitative research the conceptual framework is developed at the end of the study to illustrate the factors or issues presented in the qualitative data. It is designed to assist in theory building and the visual understanding of the exploratory findings. It can also be used to develop a framework in preparation for testing the proposition using quantitative research.
In quantitative research a conceptual framework can be used to synthesise the literature and theoretical concepts at the beginning of the study to present a model that will be tested in the statistical analysis of the research.
It is important to understand that the role of a conceptual framework differs depending on the type of research that is being undertaken.
So how should you go about creating a conceptual framework? After undertaking some studies where I have developed conceptual frameworks, here is a simple model based on “Six Rs”: Review, Reflect, Relationships, Reflect, Review, and Repeat.
Process for developing conceptual frameworks:
Review: literature/themes/theory.
Reflect: what are the main concepts/issues?
Relationships: what are their relationships?
Reflect: does the diagram represent it sufficiently?
Review: check it with theory, colleagues, stakeholders, etc.
Repeat: review and revise it to see if something better occurs.
This is not an easy process. It is important to begin by reviewing what has been presented in previous studies in the literature or in practice. This provides a solid background to the proposed model as it can show how it relates to accepted theoretical concepts or practical examples, and helps make sure that it is grounded in logical sense.
It can start with pen and paper, but after reviewing you should reflect to consider if the proposed framework takes into account the main concepts and issues, and the potential relationships that have been presented on the topic in previous works.
It may take a few versions before you are happy with the final framework, so it is worth continuing to reflect on the model and review its worth by reassessing it to determine if the model is consistent with the literature and theories. It can also be useful to discuss the idea with colleagues or to present preliminary ideas at a conference or workshop – be open to changes.
Even after you come up with a potential model it is good to repeat the process to review the framework and be prepared to revise it as this can help in refining the model. Over time you may develop a number of models with each one superseding the previous one.
A concern is that some students hold on to the framework they first thought of and worry that developing or changing it will be seen as a weakness in their research. However, a revised and refined model can be an important factor in justifying the value of the research.
Plenty of possibilities and theoretical topics could be considered to enhance the model. Whether it ultimately supports the theoretical constructs of the research will be dependent on what occurs when it is tested. As social psychologist, Kurt Lewin, famously said “ There's nothing so practical as good theory ”.
The final result after doing your reviewing and reflecting should be a clear graphical presentation that will help the reader understand what the research is about as well as where it is heading.
It doesn’t need to be complex. A simple diagram or table can clarify the nature of a process and help in its analysis, which can be important for the researcher when communicating to their audience. As the saying goes: “ A picture is worth 1000 words ”. The same goes for a good conceptual framework, when explaining a research process or findings.
David Waller is an associate professor at the University of Technology Sydney .
If you found this interesting and want advice and insight from academics and university staff delivered direct to your inbox each week, sign up for the THE Campus newsletter .
Students using generative AI to write essays isn't a crisis
Eleven ways to support international students, indigenising teaching through traditional knowledge, seven exercises to use in your gender studies classes, rather than restrict the use of ai, embrace the challenge, how hard can it be testing ai detection tools.
Register for free
and unlock a host of features on the THE site
- Research article
- Open access
- Published: 27 January 2011
A multidisciplinary systematic review of the use of diagrams as a means of collecting data from research subjects: application, benefits and recommendations
- Muriah J Umoquit 1 ,
- Peggy Tso 1 , 2 ,
- Helen ED Burchett 3 &
- Mark J Dobrow 1 , 2
BMC Medical Research Methodology volume 11 , Article number: 11 ( 2011 ) Cite this article
18k Accesses
35 Citations
4 Altmetric
Metrics details
In research, diagrams are most commonly used in the analysis of data and visual presentation of results. However there has been a substantial growth in the use of diagrams in earlier stages of the research process to collect data. Despite this growth, guidance on this technique is often isolated within disciplines.
A multidisciplinary systematic review was performed, which included 13 traditional healthcare and non-health-focused indexes, non-indexed searches and contacting experts in the field. English-language articles that used diagrams as a data collection tool and reflected on the process were included in the review, with no restriction on publication date.
The search identified 2690 documents, of which 80 were included in the final analysis. The choice to use diagrams for data collection is often determined by requirements of the research topic, such as the need to understand research subjects' knowledge or cognitive structure, to overcome cultural and linguistic differences, or to understand highly complex subject matter. How diagrams were used for data collection varied by the degrees of instruction for, and freedom in, diagram creation, the number of diagrams created or edited and the use of diagrams in conjunction with other data collection methods. Depending on how data collection is structured, a variety of options for qualitative and quantitative analysis are available to the researcher. The review identified a number of benefits to using diagrams in data collection, including the ease with which the method can be adapted to complement other data collection methods and its ability to focus discussion. However it is clear that the benefits and challenges of diagramming depend on the nature of its application and the type of diagrams used.
Discussion/Conclusion
The results of this multidisciplinary systematic review examine the application of diagrams in data collection and the methods for analyzing the unique datasets elicited. Three recommendations are presented. Firstly, the diagrammatic approach should be chosen based on the type of data needed. Secondly, appropriate instructions will depend on the approach chosen. And thirdly, the final results should present examples of original or recreated diagrams. This review also highlighted the need for a standardized terminology of the method and a supporting theoretical framework.
Peer Review reports
Diagrams are graphic representations used to explain the relationships and connections between the parts it illustrates. There are many subcategories of the broader term 'diagram', which are distinguished by the elements they incorporate or their overall topic. Two dominant subcategories include 'concept maps' and 'mind maps'[ 1 ]. Diagrams are typically brought into the research process in later stages of data analysis or when summarizing and presenting final results. It is commonplace to see a diagram illustrating how concepts or themes relate to each other or to explain how the research data relates to an underlying theory. These diagrams can be developed through the researchers' inductive reasoning of the data collected or may be assisted by computer software[ 2 ].
The use of diagrams in earlier stages of the research process (i.e. to collect data) is a relatively new method and is not a common data collection approach at present. However, their use is developing in multiple disciplines, including healthcare research. Diagrams have been used to collect data from research subjects by asking them to either draw a diagram themselves or modify a prototypic diagram supplied by the researcher. The use of diagrams in data collection has been viewed favorably in helping to gather rich data on healthcare topics. These research topics are widely varied and include collecting information to improve patient safety with medication[ 3 ], understanding neighborhood characteristics related to mental well-being[ 4 ], mapping out healthcare networks[ 5 ], evaluating patient educational programs[ 6 , 7 ], understanding how different populations view microbial illnesses[ 8 ], diagramming as part of nursing education that is evidence-based[ 9 ] and involves critical thinking[ 10 , 11 ], to engage youth in healthcare consultations[ 12 ], and to gain insights on physician professional growth[ 13 ] and their accountability relationships[ 14 ].
Despite the increasing use of diagrams in data collection, there lacks a strong "supportive structure" (pg. 343) for researchers choosing this method[ 15 ]. The use of diagrams in data collection has developed independently in multiple disciplines under a number of different names, making knowledge transfer regarding this technique difficult. For example, little has been published on process mapping outside of the organizational literature until fairly recently[ 5 , 16 , 17 ]. This has limited the exchange of best practices between disciplines. Researchers are often starting from scratch when designing their diagramming data collection approaches and their analysis of the unique data collected[ 15 ].
By conducting a multidisciplinary systematic review, as defined in the PRISMA statement[ 18 ], we hope to consolidate lessons learned and offer recommendations for researchers in healthcare and other disciplines about how diagrams may be incorporated into their data collection process. The questions that guided our search for relevant studies were:
(1) What drives the selection of a diagramming approach for data collection?
(2) What are the different approaches to diagramming for data collection?
(3) What are the different approaches to analyzing data collected with diagramming?
(4) What are the benefits and challenges of using diagramming for data collection?
Diagramming techniques used for data collection in the research process is a challenging area to review, given the variable terminology across, and even within, fields. A preliminary survey of the literature helped identify some key terminology used in different disciplines (e.g. "graphic elicitation" or "participatory diagramming"). The terms used in the titles and abstracts of the preliminary articles identified, as well as the keywords used to index them in databases, formed the basis of our multidisciplinary search strategy. We combined these specific terms with general 'diagram' terms and with general 'data collection' and/or 'analysis' terms.
In December 2009, we electronically searched 13 indexed sources, including traditional health care related indexes and non-health focused indexes (EMBASE; HealthSTAR; Medline; Cumulative Index to Nursing and Allied Health Literature; GEOBASE; InfoTrac Environmental Issues & Policy eCollection; ProQUEST Dissertations; Design and Applied Arts Index; Education Resources Information Center; International Bibliography of the Social Sciences; PsychINFO; Public Affairs Information Services; and Social Science Citation Index). To ensure that all appropriate references were identified and to limit publication bias, non-indexed sources were also searched via general search tools (i.e. Google Scholar and Google Books) to uncover any additional publications. To supplement the search, 35 experts, identified by our searches, were contacted and asked to identify additional relevant articles and grey literature.
Reference Manager 11 was used to support the review. Following the removal of duplicates, articles were screened based on their title and abstract. The full-text was then screened for articles not excluded based on their title/abstract. Articles were excluded if they were not written in English, did not use diagramming techniques in the data collection process (i.e. research subjects did not create or edit diagrams) or were not evaluative or reflective about the data collection process and/or analysis of data collected from diagramming methods. No publication date or publication type restrictions were imposed; research studies, theoretical articles, method articles and opinion pieces were included if they met the above criteria.
The screening was undertaken by two authors (MJU, PT). Double screening was done at regular intervals to ensure inter-rater reliability. Further, the two researchers met weekly during the screening and data extraction phases to discuss the nuances of the articles and to resolve differences by deliberation until consensus was reached.
A total of 2690 references were identified, after the removal of duplicates. Given our search had no publication date restrictions and included dissertations, full-text articles were sometimes difficult to retrieve. Authors were contacted when the article could not be found online or through the University of Toronto's library system. While 4 articles were retrieved in this manner, 27 full-text articles still could not be found and were ultimately excluded. In total, 233 full-text articles were screened and a total of 80 articles were included in the study's review. Figure 1 presents a flow diagram of our search and screening. Data was extracted on the general characteristics of the articles and the four objectives detailed earlier (see Table 1 ).
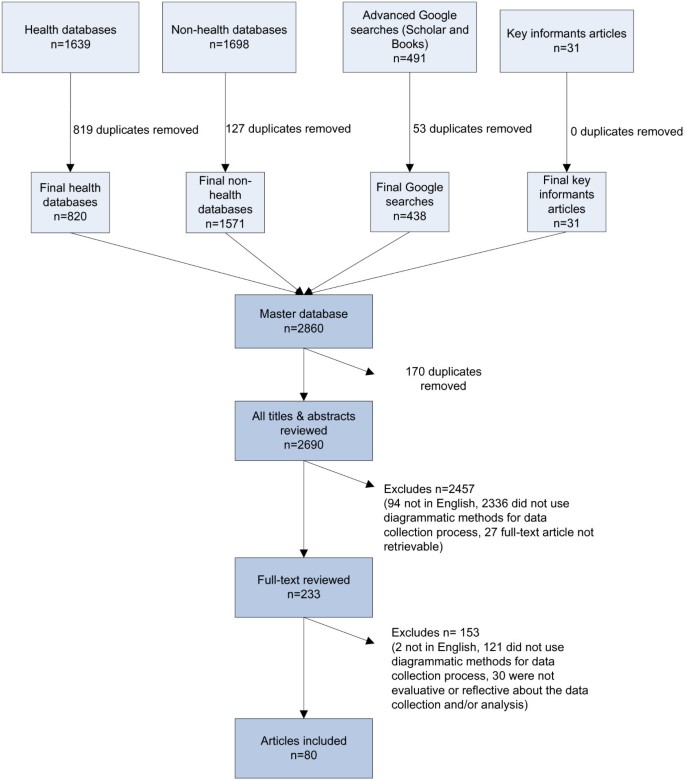
Flow of articles through the systematic review .
General characteristics
Of the 80 articles included in our review, 53 were published studies[ 1 , 4 – 15 , 19 – 58 ], 19 were dissertations[ 59 – 77 ], 2 were books[ 78 , 79 ] and 6 represented grey literature[ 3 , 80 – 84 ], including unpublished working papers submitted by key experts and reports available on the internet. These articles were published between 1986 and 2010, with the majority published after 2000 and a substantial increase after 2006. This suggests that interest in these techniques has been increasing in recent years.
The most common discipline, determined by the lead author's affiliation and/or publication title, was from the education field. Other disciplines included healthcare, engineering, environmental science, geography, industrial design, psychology, and social science. The majority of articles clearly specified the study sample size, which averaged 36 research subjects, with a range of 2 to 243. Diagramming methods were used with a wide variety of research subjects, including students (elementary to graduate school), farmers, nurses, physicians, engineers, administrators and graphic designers.
What drives the selection of a diagramming approach for data collection?
The majority of articles specified at least one explicit reason why a form of diagramming was selected for data collection. These reasons fall into two broad categories: requirements or challenges of the research topic and the unique dataset that results from using diagrams.
The specific research topic examined was the most common reason for researchers choosing a diagramming technique for data collection. For some research topics, past studies have validated diagramming data collection techniques as a useful way to collect data. For example, research has established the usefulness of diagrams in collecting data about research subjects' knowledge or cognitive structures[ 19 – 22 , 59 , 80 ]. Diagrams in data collection have also been validated as a means of measuring changes over time[ 6 , 10 , 20 , 22 – 24 , 60 , 85 ] and differences between participant groups[ 20 , 25 , 61 , 81 ]. Diagramming methods were also sought out when research topics were not conducive to the more common qualitative data collection methods, such as interviews alone. These reasons include a research topic that deals with a population with linguistic, cultural, social or intuitional barriers the researcher wants to overcome[ 12 , 14 , 15 , 26 – 29 , 79 ] or with highly complex subject matter[ 12 , 14 , 25 , 30 – 32 , 85 ]. Examples of highly complex subject matters include the abstract nature of the research topic of 'pedagogical constructs'[ 25 ] and the multifaceted and diverse nature of 'clinical accountability relationships'[ 14 ].
Secondly, researchers sought out diagramming data collection methods because of the benefits previous studies found regarding the quality and uniqueness of the collected dataset. When research subjects drew diagrams without prompts, previous studies concluded that it minimized the influence of the researcher on the participant and their responses[ 1 , 33 – 35 , 61 , 62 , 81 , 82 ]. Studies have also found that diagramming is a reflective tool for the research subjects[ 28 , 29 , 36 , 63 ]. Since diagrams can represent both concrete and theoretical notions[ 37 ], diagramming offers a more holistic coverage of the topic[ 29 , 38 , 61 ], with more uncensored and unique data gathered[ 1 , 24 , 28 , 35 , 39 , 40 , 58 ] than more traditional qualitative data collection methods.
What are the different approaches to diagramming for data collection?
A range of applications were identified, which varied widely based on the degree of instruction, degree of freedom in diagram creation, the number of diagrams created or edited and the use of diagram in conjunction with other data collection methods.
Half of the studies did not report the details of the instructions provided to the research subjects, except for describing the basic request to create a diagram. One study explicitly observed that specific instructions are needed to ensure the research participants create a diagram and not another form of written material[ 32 ], such as a drawing or table. Simple or short instructions were often given to research subjects when the diagram sought by the researchers did not have to conform to a rigid structure, such as life-cycle[ 13 ] and professional practice diagrams[ 32 ].
When the researcher sought highly structured diagrams, the degree of instruction provided to the research subjects ranged from the preferred method of giving specific and detailed instructions on what elements should be included (e.g. hierarchies, arrows) to showing an example of the type of diagram the researcher would like the participant to create. For example, in comparison to other diagramming techniques, concept maps have a fairly rigid definition and a very specific set of elements that the end diagram should contain. Such diagrams may require more detailed instructions. One study had associate nursing degree students draw their own concept maps after a 20-minute introductory tutorial, presentation of a sample diagram, discussion and question period, and instructions listing all elements to be included (e.g. arrangement of items, hierarchal order, linking concepts with arrow, labeling propositions, identifying cross-links/relationships)[ 9 ]. In some instances, research subjects were given the opportunity to practice the diagramming method and receive corrective feedback prior to data collection[ 19 , 23 , 41 , 64 ].
It was most common for research subjects to create an original diagram on their own, in groups or a combination of both. Alternatively, some studies had the participant edit either a designated diagram provided by the researcher[ 3 , 14 , 15 , 42 ] or a researcher-created diagram generated by the researcher during the interview with participant input[ 4 , 5 , 7 , 43 ]. A few studies also chose the middle ground between original and prepared diagrams. For example, some provided a central concept or word to create the diagram around[ 14 , 44 ], or included some words or shapes to fill in on a prepared diagram[ 45 ] and others gave research subjects a list of words to use in the creation of their diagram[ 65 , 80 ].
Half of the studies used diagrams at multiple times within the data collection process as a means of comparison. A subset used a pre-/post- (or time series) approach to data collection, allowing researchers to track changes before, after, and sometimes during an intervention. This was found primarily within the discipline of education. For example, Rios asked a sample of teachers to create concept maps at multiple time intervals in order to identify their conceptual models and examine the impact of student interactions on the teachers' subject matter structure or vice versa[ 66 ].
Some researchers explicitly expressed the idea that diagrams alone would not capture complete perspectives from the research subjects[ 57 , 77 ], suggesting that diagrams should be used in combination with other data collection methods. The majority of the studies did use diagramming data collection techniques in addition to other methods. In some cases the additional data collection methods were used explicitly in conjunction with the diagramming techniques, such as creating the diagram within interviews or discussion of the diagrams in later focus groups or interviews[ 8 , 23 , 39 , 59 , 60 , 67 ].
What are the different approaches to analyzing data collected with diagramming?
The majority of the articles reported details about the analysis of diagram data. The use of only quantitative or qualitative analysis, or a mixture of both types of analysis was fairly equally distributed among the articles. Within each of these three categories of analysis, there were a variety of different techniques used that are briefly outlined below.
The majority of studies comparing diagrams across time or across research subjects chose either quantitative techniques only or a mix of qualitative and quantitative techniques for their analysis. Quantitative analysis techniques included counting (e.g. number of concepts identified, number of links between concepts, number of examples given, levels in hierarchy) and scoring. The two most common scoring methods were structural scoring and relational scoring[ 22 ]. Structural scoring refers to when weights are assigned to hierarchical structures, links between concepts and other elements. For example, a link between two concepts may be 10 points, an example 1 point and invalid examples and cross links 0 points[ 40 ]. Relational scoring reflects the quality or importance of each concept or link as determined by the researcher, by comparison to a similar diagram created by an expert or by other research subjects[ 36 , 38 , 60 , 62 , 68 ]. Some studies using quantitative analysis showed diagrams to illustrate how the final counting and scoring of a diagram was completed in the presentation of final study results[ 35 , 40 , 41 , 46 – 49 , 54 , 62 ]. For example, Kesby had focus groups in Zimbabwe create scored diagrams with local materials of rocks, string and bottle caps[ 54 ]. These diagrams were photographed and also reproduced on computer for legibility. Including an original diagram in the presentation of results also helps to orient the reader to the type of diagram that was used.
Studies which had diagrams completed or edited in the presence of the researcher, included additional data collection methods, and/or studies using less structured diagrams to collect data were likely to use either qualitative techniques only or a mix of qualitative and quantitative techniques for their analysis. For example, Haidet et al. gave medical school research subjects less structure and encouraged creativity in their diagramming exercise within interviews[ 13 ]. The diagram was used as a prompt to stimulate discussion, which was then analyzed through the interview transcripts. The diagram itself was then displayed in the final results to visually summarize the verbal exchange. Qualitative analysis included thematic and content analysis of the diagrams and additional data sources, such as transcripts, to identify prominent topics, themes and patterns in the diagrams[ 13 , 25 , 26 , 34 , 63 , 66 , 69 – 71 ].
In some studies that used mixed-methods, the collected diagrams were the primary source of data and guided the analysis of additional data sources, for example, by providing the core themes for transcript analysis. In other cases the additional data sources played the dominant role in analysis and the diagrams were used almost as verification or visual representation to illustrate conclusions[ 72 ].
What are the benefits and challenges of using diagramming for data collection?
Some of the benefits to using diagrams for data collection have already been discussed in the section on why researchers chose diagramming data collection approaches. In addition to these, diagramming approaches that were seen as complementary to other data collection approaches were commonly used in interviews and focus groups[ 60 , 73 ]. They were found to help focus discussions on particular themes[ 32 ] and enabled research subjects to more easily reflect on a topic or their beliefs by helping them to express thoughts in a more structured and organized manner[ 50 , 51 ]. The use of diagrams was also seen to increase recall[ 52 ] and self-reflectiveness[ 53 , 54 ]. In 1992, Powell found that interviews which made use of diagramming approaches were more introspective and tended to be more theoretical and philosophical than those that did not use diagramming methods[ 25 ].
Over half of the articles discussed at least one challenge of using a diagramming method for data collection. Interestingly, these challenges were often contradicted by other articles. All studies completed their data collection with diagrams, with some reporting that the diagramming allowed research subjects to overcome challenges of verbal communication[ 12 , 14 , 15 , 26 – 29 , 75 , 79 ]. However, many studies found that at least some of the research subjects expressed difficulty or discomfort with the diagramming task[ 1 , 9 , 10 , 14 , 28 , 35 , 55 , 56 , 58 , 62 , 74 , 76 , 83 ]. Some identified the ease and speed of data collection as benefits of using diagramming approaches[ 24 , 31 , 54 ], while others saw it as being time-intensive, particularly for analysis[ 6 , 11 , 26 , 62 ]. Related to the visual organization and structure of knowledge that the diagrams presented, an advantage to using diagramming approaches for data collection is their ability to obtain unique and unsolicited data[ 7 , 14 , 15 , 26 , 29 , 33 , 42 , 43 , 58 ]. In contrast, there were also concerns regarding the data it did not collect, such as non-verbal communication, that require the discretion and experience of researchers to identify and interpret[ 15 , 24 , 84 ]. These contradictions illustrate that the benefits and challenges to using diagramming approaches for data collection depend on the application and type of diagram used in each research study.
This systematic review represents the first overview of diagrams being used as a data collection approach in multiple disciplines. In 2006, Nesbit & Adesope[ 86 ] conducted a widely cited meta-analysis looking at peer-reviewed articles focusing on learning with concept and knowledge maps and found that the interest in using diagrams appeared to be on the rise[ 86 ]. While our systematic review concurs that interest is growing, it differs from their meta-analysis in two ways. Firstly our definition of a diagram is much broader, encompassing a variety of diagrams that extend beyond concept and knowledge maps. As well as the structured diagrams that Nesbit & Adescope focused on, our review also includes less structured diagrams, such as the life-cycle and professional practice discussed in our results section. Secondly, our focus is solely on diagrams being used as a data collection approach, whereas their meta-analysis included diagrams used as analysis techniques as well.
Given our broad definition of a diagram, we have reviewed approaches for collecting data through a wide spectrum of diagrams, from highly structured concept maps to less defined diagrams. We have provided an overview of the instruction options for research subjects, the creation and analysis of diagramming as a data collection approach, as well as highlighted some of the benefits and challenges. While there is variation regarding the guidance in instructions and approaches to the construction of different diagrams, use of diagrams as a data collection tool as a whole is clearly increasing in healthcare and in other disciplines. This systematic review is the first step in consolidating this information to assist in the refinement of this approach. For those considering using diagramming as a data collection approach, we offer three recommendations. Firstly, the diagrammatic approach should be chosen based on the type of data needed to answer the research question(s). Secondly, based on the diagrammatic approach chosen, it is important to select the appropriate instructions needed. Finally, presentation of final results should include examples of the original or recreated diagrams.
Choice of Diagramming Approach
The most important considerations for choosing the diagramming approach is the type of data needed to answer the research question (e.g., examining change over time, exploring people's experiences or views) and the type of analysis preferred by the researcher. For example, highly structured diagrams allowed for valid quantitative analysis, such as counting and ranking, which could be compared across research subjects. It should be noted that there is some controversy whether items should even be counted and that both an over- or under-reliance may be dangerous to the final research conclusions[ 28 ]. In comparison, other approaches that used a less structured diagrams relied heavily on qualitative analysis.
Instruction and Creation
The appropriateness of different approaches to instructions to guide diagram creation is an important consideration in ensuring the validity of data. It is clear from our review that the initial instructions given to research subjects varied in structure but had a great impact on the resulting diagrams and their potential for different analysis techniques. If researchers require highly structured diagrams it may be useful to give research subjects more detailed instructions and the opportunity for practice and feedback[ 30 , 64 ].
Presentation of results
The last recommendation is that studies using diagramming data collection approaches should include visual presentations of the findings in their results sections. The use of diagrams often results in the collection of unique and unsolicited data through a visual component, which can then be displayed along with the final analysis. Just as diagrams can provide data not easily obtained through verbal data collection techniques, visual presentation of the collected diagrams may provide insights not as easily grasped through verbal communication of study results. While providing a scanned image of the original diagram can be difficult at times, it is also possible to present a photograph of the original diagram or a computer-generated recreation of the diagram. This is especially important given the variation in terminology between disciplines, as it relays to the reader the type and structure of diagrams created or used.
In addition to the recommendations for researchers considering the use of diagramming data collection approaches, this multidisciplinary review also identifies areas where future research is needed. This review required a substantial amount of preliminary work to understand the terminology used to describe diagrams and this data collection approach across different disciplines and fields. The intent was to devise a sensitive search, so as to cast a wide net in order to capture articles in a range of disciplines where the terminology is not standardized. Cole et al. illustrate this issue by identifying over a dozen different terms that are used to describe concept maps[ 56 ]. Thus far, development of terminology has focused on the end result, i.e. what type of diagram is created based on the elements it contains, rather than focusing on the actual data collection approach itself. This has created different data collection approaches that use diagrams separately, isolating research done across disciplines and even within disciplines. Therefore, it is our recommendation that efforts are directed towards standardizing the terminology for this data collection method. This would allow researchers to maintain the work they have done regarding specific types of diagrams, whilst providing an umbrella term to help with the sharing of best practices. Future research should also be directed at identification of the underpinning theory of the method as the review demonstrated a gap in this area[ 15 , 32 , 35 ]. Such a theory may help to further inform researchers regarding the appropriate use and applications for diagramming data collection approaches.
A limitation of our review is that the database search strategy did not capture general articles on visual data collection methods, which may include specific information on diagramming data collection approaches. However the non-indexed searches and articles identified by experts did pick up some of these articles. While efforts were made to contact authors to retrieve articles from our search, twenty-seven full-text articles were irretrievable. Ten of these were dissertations and five were books. This may have contributed to an incomplete representation of what the literature has to offer about diagramming data collection approaches.
There has been a growing interest in the use of diagrams for data collection in the research process over recent years, as shown by the increase in publications and the wide range of approaches developed for diagramming data collection and diagram data analysis. As noted earlier, diagrams have been used to collect rich data on a variety of healthcare topics and it is expected that the use of this method will continue to grow. The results of this multidisciplinary systematic review provide an overview of the application of diagrams in research data collection and the methods for analyzing the unique datasets elicited. Recommendations are presented to assist researchers considering the use of diagrams in their data collection process. This review also highlighted the need for a standardized terminology of the method and a supporting theoretical framework.
Wheeldon J, Faubert J: Framing experience: concept maps, mind maps, and data collection in qualitative research. International Journal of Qualitative Methods. 2009, 8: 68-83.
Google Scholar
Forbes MA: Hope in the older adult with chronic illness: A comparison of two research methods in theory building. Advances in Nursing Science. 1999, 22: 74-87.
Article CAS PubMed Google Scholar
Jafri T, Lyons MN, Clarkson PJ: The supply of medicines in the NHS. 2008, Improving Patient Safety: Cambridge, UK
O'Campo P, Salmon C, Burke J: Neighbourhoods and mental well-being: what are the pathways?. Health and Place. 2009, 15: 56-68.
Article PubMed Google Scholar
Pluto DM, Hirshorn BA: Process mapping as a tool for home health network analysis. Home Health Care Services Quarterly. 2003, 22: 1-16. 10.1300/J027v22n02_01.
Franca S, d'Ivernois JF, Marchand C, Haenni C, Ybarra J, Golay A: Evaluation of nutritional education using concept mapping. Patient Education and Counseling. 2004, 52: 183-192. 10.1016/S0738-3991(03)00037-5.
Marchand C, d'Ivernois JF, Assal JP, Slama G, Hivon R: An analysis, using concept mapping, of diabetic patients' knowledge, before and after patient education. Medical Teacher. 2002, 24: 90-99. 10.1080/01421590120091087.
Jones MG, Rua MJ: Conceptual representations of flu and microbial illness held by students, teachers, and medical professionals. Social Science and Mathematics. 2008, 108: 263-278.
Article Google Scholar
Abel WM, Freeze M: Evaluation of concept mapping in an associate degree nursing program. Journal of Nursing Education. 2006, 45: 356-364.
PubMed Google Scholar
Daley BJ, Shaw CR, Balistrieri T, Glasenapp K, Piacentine L: Concept maps: a strategy to teach and evaluate critical thinking. Journal of Nursing Education. 1999, 38: 42-47.
CAS PubMed Google Scholar
Hicks-Moore SL, Pastirik PJ: Evaluating critical thinking in clinical concept maps: A pilot study. International Journal of Nursing Education Scholarship. 2006, 3: 1-15. 10.2202/1548-923X.1314.
Coad J: Using art-based techniques in engaging children and young people in health care consultations and/or research... including commentary by Bishop V and Picard C. Journal of Research in Nursing. 2007, 12: 487-497. 10.1177/1744987107081250.
Haidet P, Hatem DS, Fecile ML, Stein HF, Haley H-L, Kimmel B, et al: The role of relationships in the professional formation of physicians: Case report and illustration of an elicitation technique. Patient Education and Counseling. 2008, 72: 382-387. 10.1016/j.pec.2008.05.016.
Umoquit MJ, Dobrow MJ, Lemieux-Charles L, Ritvo PG, Urbach DR, Wodchis WP: The efficiency and effectiveness of utilizing diagrams in interviews: An assessment of participatory diagramming and graphic elicitation. BMC Medical Research Methodology. 2008, 8-
Crilly N, Blackwell A, Clarkson P: Graphic elicitation: using research diagrams as interview stimuli. Qualitative Research. 2006, 6: 341-366. 10.1177/1468794106065007.
Jun GT, Ward JR, Morris ZS, Clarkson PJ: Health care process modelling: which method when?. International Journal for Quality in Health Care. 2009, 21: 214-224. 10.1093/intqhc/mzp016.
Jun GT, Ward JR, Clarkson PJ: Systems modelling approaches to the design of safe healthcare delivery:ease of use and usefulness percived by healthcare works. Ergonomics. 2010, 53: 829-847. 10.1080/00140139.2010.489653.
Liberati A, Altman DG, Tetzlaff J, Mulrow C, Gotzsche PC, Ioannidis JP, et al: The PRISMA statement for reporting systematic reviews and meta-analyses of studies that evaluate health care interventions: explanation and elaboration. PLoS Medicine. 2009, 6: e100100-10.1371/journal.pmed.1000100.
Zhang L, Wang Y, Dong B, Zhou Z: The comparison study of Chinese and American secondary school students' knowledge structure--An experimental research based on concept map assessment technique. Frontiers of Education in China. 2009, 4: 286-297. 10.1007/s11516-009-0015-0.
Hay DB: Using concept maps to measure deep, surface and non-learning outcomes. Studies in Higher Education. 2007, 32: 39-57. 10.1080/03075070601099432.
Lavigne NC, Salkind SJ, Yan J: Exploring college students' mental representations of inferential statistics. The Journal of Mathematical Behavior. 2008, 27: 11-32. 10.1016/j.jmathb.2007.10.003.
West DC, Park JK, Pomeroy JR, Sandoval J: Concept mapping assessment in medical education: A comparison of two scoring systems. Medical Education. 2002, 36: 820-826. 10.1046/j.1365-2923.2002.01292.x.
Carter G, Jones MG, Rua M: Effects of partner's ability on the achievement and conceptual organization of high-achieving fifth-grade students. Science Education. 2002, 87: 94-111. 10.1002/sce.10031.
Hay DB, Wells H, Kinchin IM: Quantitative and qualitative measures of student learning at university level. Higher Education. 2008, 56: 221-240. 10.1007/s10734-007-9099-8.
Powell RR: The influence of prior experiences on pedagogical constructs of traditional and nontraditional preservice teachers. Teaching and Teacher Education. 1992, 8: 225-238. 10.1016/0742-051X(92)90022-U.
Kesby M: Participatory diagramming as a means to improve communication about sex in rural Zimbabwe: a pilot study. Social Science & Medicine. 2000, 50: 1723-1741.
Article CAS Google Scholar
Van der Riet M: Diagramming as mediational means: Vygotskian theory and participatory research. South African Journal of Psychology. 2008, 38: 455-465.
Wheeldon J: Is a picture worth a thousand words? Using mind maps to facilitate participant recall in qualitative research. Qualitative Report.
Bagnoli A: Beyond the standard interview: The use of graphic elicitation and arts-based methods. Qualitative Research. 2009, 9: 547-571. 10.1177/1468794109343625.
Lim SE, Chan Cheng PW, Lam MS, Ngan SF: Developing reflective and thinking skills by means of semantic mapping strategies in kindergarten teacher education. Early Child Development and Care. 2003, 173 (1): 55-72. 10.1080/0300443022000022422.
Ozesmi U, Ozesmi SL: Ecological models based on people's knowledge: A multi-step fuzzy cognitive mapping approach. Ecological Modeling. 2004, 176: 43-64. 10.1016/j.ecolmodel.2003.10.027.
Varga-Atkins T, O'Brien M: From drawings to diagrams: Maintaining researcher control during graphic elicitation in qualitative interviews. International Journal of Research & Methods in Education. 2009, 32: 53-67.
Williams CG: Using concept maps to assess conceptual knowledge of function. Journal for Research in Mathematics Education. 1998, 29: 414-421. 10.2307/749858.
Strean WB, Holt NL: Coaches', athletes', and parents' perceptions of fun in youth sports: assumptions about learning and implications for practice. AVANTE. 2000, 6: 83-98.
Wheeldon J: Mapping mixed methods research: Methods, measures, and meaning. Journal of Mixed Methods Research. 2010, 48: 87-102. 10.1177/1558689809358755.
Rye JA, Rubba PA: An exploration of the concept map as an interview tool to facilitate the externalization of students' understandings about global atmospheric change. Journal of Research in Science Teaching. 1998, 35: 521-546. 10.1002/(SICI)1098-2736(199805)35:5<521::AID-TEA4>3.0.CO;2-R.
Lourdel N, Gondran N, Laforest V, Debray B, Brodhag C: Sustainable development cognitive map: A new method of evaluating student understanding. International Journal of Sustainability in Higher Education. 2007, 8: 170-182. 10.1108/14676370710726634.
Koul R, Clariana RB, Salehi R: Comparing several human and computer-based methods for scoring concept maps and essays. Journal of Educational Computing Research. 2005, 32: 227-239. 10.2190/5X9Y-0ETN-213U-8FV7.
Shymansky JA, Yore LD, Treagust DF, Thiele RB, Harrison A, Waldrip BG, et al: Examining the construction process: A study of changes in level 10 students' understanding of classical mechanics. Journal of Research in Science Teaching. 1997, 34: 571-593. 10.1002/(SICI)1098-2736(199708)34:6<571::AID-TEA3>3.0.CO;2-K.
West DC, Pomeroy JR, Park JK, Gerstenberger EA, Sandoval J: Critical thinking in graduate medical education: A role for concept mapping assessment?. Journal of the American Medical Association. 2000, 284: 1105-1110. 10.1001/jama.284.9.1105.
Lian MWS: An investigation into high-achiever and low-achiever knowledge organisation and knowledge processing in concept mapping: A case study. Research in Science Education. 1998, 28: 337-352. 10.1007/BF02461567.
Crilly N, Clarkson P, Blackwell A: Using research diagrams for member validation in qualitative research. Diagrams. 2006, 4045: 258-262.
Cox P, Niewoehner J, Pidgeon N, Gerrard S, Fischhoff B, Riley D: The use of mental models in chemical risk protection: Developing a generic workplace methodology. Risk Analysis. 2003, 23: 311-324. 10.1111/1539-6924.00311.
Koury K, Hollingsead C, Fitzgerald G, Miller K, Mitchem K, Tsai HH, et al: Case-based instruction in different delivery contexts: The impact of time in cases. Journal of Interactive Learning Research. 2009, 20: 445-467.
Gill PE, Persson M: On using concept-maps to study school-children's understanding of leisure-time. Leisure Studies. 2008, 27: 213-220. 10.1080/02614360802048795.
Wheeldon J: To guide or provoke? Maps, pedagogy, and the value(s) of teaching criminal justice ethics. Criminal Justice Education.
Mouratiadou I, Moran D: Mapping public participation in the Water Framework Directive: A case study of the Pinios River Basin, Greece. Ecological Economics. 2007, 62: 66-76. 10.1016/j.ecolecon.2007.01.009.
Kimber K, Pillay H, Richards C: Technoliteracy and learning: An analysis of the quality of knowledge in electronic representations of understanding. Computers & Education. 2007, 48: 59-79.
Artiles AJ, Barreto RM, Pena L, McClafferty K: Pathways to teacher learning in multicultural contexts - A longitudinal case study of two novice bilingual teachers in urban schools. Remedial and Special Education. 1998, 19: 70-90. 10.1177/074193259801900203.
Farrell TSC: Critical reflection in a TESL Course: Mapping conceptual change. ELT Journal. 2008, 63: 221-229. 10.1093/elt/ccn058.
Lourdel N, Gondran N, Laforest V, Brodhag C: Introduction of sustainable development in engineers' curricula: Problematic and evaluation methods. International Journal of Sustainability in Higher Education. 2005, 6: 254-264. 10.1108/14676370510607223.
Naykki P, Jarvela S: How pictorial knowledge representations mediate collaborative knowledge construction in groups. Journal of Research on Technology in Education. 2008, 40: 359-
Conceicao SC, Taylor LD: Using a constructivist approach with online concept maps: relationship between theory and nursing education. Nursing Education Perspectives. 2007, 28: 268-275.
Kesby M: Participatory diagramming: Deploying qualitative methods through an action research epistemology. Area. 2000, 32: 423-435. 10.1111/j.1475-4762.2000.tb00158.x.
Ahmed R, Ali NA: Performance appraisal decision in Malaysian public service. International Journal of Public Sector Management. 17: 48-64. 10.1108/09513550410515565. 4 A.D
Cole C, Lin Y, Leide J, Large A, Beheshti J: A classification of mental models of undergraduates seeking information for a course essay in history and psychology: preliminary investigations into aligning their mental models with online thesauri. Journal of the American Society for Information Science & Technology. 2007, 58: 2092-2104.
Rowe AL, Cooke NJ: Measuring mental models: Choosing the right tools for the job. Human Resources Development Quarterly. 1995, 6: 243-255. 10.1002/hrdq.3920060303.
Wheeldon J: Learning from Latvia: Adoption, adaptation, and evidence based justice reform. Journal of Baltic Studies.
Garegae KGM: . Teachers, beliefs about mathematics, its teaching and learning and the communication of these beliefs to students: A case study in Botswana. Ph.D. 2002, Canada: The University of Manitoba
McWhirter LJ: Conceptual development and retention within the learning cycle. Ph.D. 1998, United States -- Oklahoma: The University of Oklahoma
Williams CG: . Using concepts maps to determine differences in the concept image of function held by students in reform and traditional calculus classes. Ph.D. 1994, United States -- California: University of California
Puukari S: . Video programmes as learning tools: Teaching the gas laws and behaviour of gases in Finnish and Canadian senior high schools. Ed.D. 2003, Finland: Jyvaskylan Yliopisto
Mathews S: An ethnographic examination of perspective consciousness and intercultural competence among social studies student-teachers in Kenya, East Africa. Ph.D. 2008, United States -- Indiana: Indiana University
Skidmore L: Concept mapping to promote meaningful learning at the community college level. 2008, United States -- Minnesota: Walden University, Ed.D
Yin Y, Vanides J, Ruiz-Primo MA, Ayala CC, Shavelson R: A Comparison of Two Construct-a-Concept-Map Science Assessments: Created Linking Phrases and Selected Linking Phrases. 2004, The Regents of the University of California, 1-28. unknown CSE Report 624
Rios JM: Studies of contrast: Relationships between subject matter structures and teaching. 1995, United States -- Wisconsin: The University of Wisconsin, Ph.D
Feldsine JE: The construction of concept maps facilitates the learning of general college chemistry: A case study. 1987, United States -- New York: Cornell University, Ph.D
Butler KA: Scaffolding software: How does it influence student conceptual understanding and motivation?. 2004, United States -- Illinois: Southern Illinois University at Carbondale, Ph.D
Alexander LM: Variations of professional identity over time: A study of physician assistants. 2003, United States -- District of Columbia: The George Washington University, Ed.D
Cantu DA: A naturalistic investigation of the relationship between secondary social studies teachers' beliefs and practice. 1997, United States -- Illinois: Southern Illinois University at Carbondale, Ph.D
Cozza B: Concept mapping through logs and metacognitive reflection during third graders' scientific problem-solving. 1996, United States -- New York: Fordham University, Ph.D
Ballard BG: Preservice teachers' beliefs about classroom management before and after student teaching. 2002, United States -- Nevada: University of Nevada, Las Vegas, Ed.D
Lowe M: Continuing medical education for physicians: Key factors contributing to learning and change. 2007, United States -- Connecticut: University of Connecticut, Ph.D
Yue H: Concept maps as assessment tools in mathematics: Comparison with clinical interviews. 2008, United States -- Texas: The University of Texas at El Paso, MAT
White E: Institutional effectiveness: The integration of program review, strategic planning and budgeting processes in two California community colleges. 2007, Andrews University, Berrien Springs, MI
Grunow JEM: Using concept maps in a professional development program to assess and enhance teachers' understanding of rational number. 1998, United States -- Wisconsin: The University of Wisconsin, Ph.D
Rowe AL: Mental models of physical systems: Examining the relationship between knowing and doing. 1995, Rice University
Kane M, Trochim WMK: Concept mapping for planning and evaluation. 2007, Thousand Oaks, CA, US: Sage Publications, Inc
Chapter Google Scholar
Kesby M: Participatory diagramming and the ethical and practical challenges of helping Africans themselves to move HIV work 'beyond epidemiology'. HIV/AIDS in Africa: Beyond Epidemiology. Edited by: Kalipendi E, Craddock S, Oppong J, Ghosh J. 2004, Blackwell, 217-228.
Schreiber DA, Abegg GL: Scoring Student-Generated Concept Maps in Introductory College Chemistry. 1991, The National Association for Research in Science Teaching, 1-19. Annual meeting
Williams CG: Concept Maps as Research Tools in Mathematics. 18 April, 1995. 1995, 1-65.
Varga-Atkins T, O'Brien M: Graphic elicitation as a research technique: reflecting on drawings and diagrams as complementary methods. 2008, 1-22. Ref Type: Report
Emmel N: Participatory Mapping: An innovative sociological method. Toolkit #3. 2008, ESRC National Centre for Research Methods, 1-8. Ref Type: Report
Beyerbach BA: Concept Mapping in Assessing Prospective Teachers' Concept Development. n/a, 1-16. 1986, US Department of Education, Ref Type: Report
Kane M, Trochim WMK: Concept Mapping for Planning and Evaluation. 2007, Thousand Oaks, CA, US: Sage Publications, Inc
Nesbit JC, Adescope O: Learning with concept and knowledge maps: A meta-analysis. Review of Education Research. 2006, 76: 413-448. 10.3102/00346543076003413.
Pre-publication history
The pre-publication history for this paper can be accessed here: http://www.biomedcentral.com/1471-2288/11/11/prepub
Download references
Acknowledgements
This study was supported by funding from a grant from the Canadian Institutes of Health Research.
Author information
Authors and affiliations.
Cancer Services & Policy Research Unit, Cancer Care Ontario, (620 University Ave), Toronto, (M5G 2L7), Canada
Muriah J Umoquit, Peggy Tso & Mark J Dobrow
Department of Health Policy, Management and Evaluation, University of Toronto, (155 College Street), Toronto, (M5T 3M6), Canada
Peggy Tso & Mark J Dobrow
Department of Global Health and Development, Faculty of Public Health and Policy, London School of Hygiene and Tropical Medicine, (15-17 Tavistock Place), London, (WC1H 9SH), UK
Helen ED Burchett
You can also search for this author in PubMed Google Scholar
Corresponding author
Correspondence to Mark J Dobrow .
Additional information
Competing interests.
The authors declare that they have no competing interests.
Authors' contributions
MJU, MJD, HEDB participated in the conception and design of the study. Data collection, abstraction and analysis was carried out by MJU and PT. MJU and PT prepared the original draft of the manuscript, and all authors reviewed and critically revised the original and subsequent manuscript drafts and approved the final manuscript.
Authors’ original submitted files for images
Below are the links to the authors’ original submitted files for images.
Authors’ original file for figure 1
Rights and permissions.
Open Access This article is published under license to BioMed Central Ltd. This is an Open Access article is distributed under the terms of the Creative Commons Attribution License ( https://creativecommons.org/licenses/by/2.0 ), which permits unrestricted use, distribution, and reproduction in any medium, provided the original work is properly cited.
Reprints and permissions
About this article
Cite this article.
Umoquit, M.J., Tso, P., Burchett, H.E. et al. A multidisciplinary systematic review of the use of diagrams as a means of collecting data from research subjects: application, benefits and recommendations. BMC Med Res Methodol 11 , 11 (2011). https://doi.org/10.1186/1471-2288-11-11
Download citation
Received : 31 August 2010
Accepted : 27 January 2011
Published : 27 January 2011
DOI : https://doi.org/10.1186/1471-2288-11-11
Share this article
Anyone you share the following link with will be able to read this content:
Sorry, a shareable link is not currently available for this article.
Provided by the Springer Nature SharedIt content-sharing initiative
- Research Subject
- Data Collection Method
- Data Collection Process
- Structure Diagram
- Data Collection Approach
BMC Medical Research Methodology
ISSN: 1471-2288
- General enquiries: [email protected]

An official website of the United States government
The .gov means it’s official. Federal government websites often end in .gov or .mil. Before sharing sensitive information, make sure you’re on a federal government site.
The site is secure. The https:// ensures that you are connecting to the official website and that any information you provide is encrypted and transmitted securely.
- Publications
- Account settings
Preview improvements coming to the PMC website in October 2024. Learn More or Try it out now .
- Advanced Search
- Journal List
- BMC Med Res Methodol

A multidisciplinary systematic review of the use of diagrams as a means of collecting data from research subjects: application, benefits and recommendations
Muriah j umoquit.
1 Cancer Services & Policy Research Unit, Cancer Care Ontario, (620 University Ave), Toronto, (M5G 2L7), Canada
2 Department of Health Policy, Management and Evaluation, University of Toronto, (155 College Street), Toronto, (M5T 3M6), Canada
Helen ED Burchett
3 Department of Global Health and Development, Faculty of Public Health and Policy, London School of Hygiene and Tropical Medicine, (15-17 Tavistock Place), London, (WC1H 9SH), UK
Mark J Dobrow
In research, diagrams are most commonly used in the analysis of data and visual presentation of results. However there has been a substantial growth in the use of diagrams in earlier stages of the research process to collect data. Despite this growth, guidance on this technique is often isolated within disciplines.
A multidisciplinary systematic review was performed, which included 13 traditional healthcare and non-health-focused indexes, non-indexed searches and contacting experts in the field. English-language articles that used diagrams as a data collection tool and reflected on the process were included in the review, with no restriction on publication date.
The search identified 2690 documents, of which 80 were included in the final analysis. The choice to use diagrams for data collection is often determined by requirements of the research topic, such as the need to understand research subjects' knowledge or cognitive structure, to overcome cultural and linguistic differences, or to understand highly complex subject matter. How diagrams were used for data collection varied by the degrees of instruction for, and freedom in, diagram creation, the number of diagrams created or edited and the use of diagrams in conjunction with other data collection methods. Depending on how data collection is structured, a variety of options for qualitative and quantitative analysis are available to the researcher. The review identified a number of benefits to using diagrams in data collection, including the ease with which the method can be adapted to complement other data collection methods and its ability to focus discussion. However it is clear that the benefits and challenges of diagramming depend on the nature of its application and the type of diagrams used.
Discussion/Conclusion
The results of this multidisciplinary systematic review examine the application of diagrams in data collection and the methods for analyzing the unique datasets elicited. Three recommendations are presented. Firstly, the diagrammatic approach should be chosen based on the type of data needed. Secondly, appropriate instructions will depend on the approach chosen. And thirdly, the final results should present examples of original or recreated diagrams. This review also highlighted the need for a standardized terminology of the method and a supporting theoretical framework.
Diagrams are graphic representations used to explain the relationships and connections between the parts it illustrates. There are many subcategories of the broader term 'diagram', which are distinguished by the elements they incorporate or their overall topic. Two dominant subcategories include 'concept maps' and 'mind maps'[ 1 ]. Diagrams are typically brought into the research process in later stages of data analysis or when summarizing and presenting final results. It is commonplace to see a diagram illustrating how concepts or themes relate to each other or to explain how the research data relates to an underlying theory. These diagrams can be developed through the researchers' inductive reasoning of the data collected or may be assisted by computer software[ 2 ].
The use of diagrams in earlier stages of the research process (i.e. to collect data) is a relatively new method and is not a common data collection approach at present. However, their use is developing in multiple disciplines, including healthcare research. Diagrams have been used to collect data from research subjects by asking them to either draw a diagram themselves or modify a prototypic diagram supplied by the researcher. The use of diagrams in data collection has been viewed favorably in helping to gather rich data on healthcare topics. These research topics are widely varied and include collecting information to improve patient safety with medication[ 3 ], understanding neighborhood characteristics related to mental well-being[ 4 ], mapping out healthcare networks[ 5 ], evaluating patient educational programs[ 6 , 7 ], understanding how different populations view microbial illnesses[ 8 ], diagramming as part of nursing education that is evidence-based[ 9 ] and involves critical thinking[ 10 , 11 ], to engage youth in healthcare consultations[ 12 ], and to gain insights on physician professional growth[ 13 ] and their accountability relationships[ 14 ].
Despite the increasing use of diagrams in data collection, there lacks a strong "supportive structure" (pg. 343) for researchers choosing this method[ 15 ]. The use of diagrams in data collection has developed independently in multiple disciplines under a number of different names, making knowledge transfer regarding this technique difficult. For example, little has been published on process mapping outside of the organizational literature until fairly recently[ 5 , 16 , 17 ]. This has limited the exchange of best practices between disciplines. Researchers are often starting from scratch when designing their diagramming data collection approaches and their analysis of the unique data collected[ 15 ].
By conducting a multidisciplinary systematic review, as defined in the PRISMA statement[ 18 ], we hope to consolidate lessons learned and offer recommendations for researchers in healthcare and other disciplines about how diagrams may be incorporated into their data collection process. The questions that guided our search for relevant studies were:
(1) What drives the selection of a diagramming approach for data collection?
(2) What are the different approaches to diagramming for data collection?
(3) What are the different approaches to analyzing data collected with diagramming?
(4) What are the benefits and challenges of using diagramming for data collection?
Diagramming techniques used for data collection in the research process is a challenging area to review, given the variable terminology across, and even within, fields. A preliminary survey of the literature helped identify some key terminology used in different disciplines (e.g. "graphic elicitation" or "participatory diagramming"). The terms used in the titles and abstracts of the preliminary articles identified, as well as the keywords used to index them in databases, formed the basis of our multidisciplinary search strategy. We combined these specific terms with general 'diagram' terms and with general 'data collection' and/or 'analysis' terms.
In December 2009, we electronically searched 13 indexed sources, including traditional health care related indexes and non-health focused indexes (EMBASE; HealthSTAR; Medline; Cumulative Index to Nursing and Allied Health Literature; GEOBASE; InfoTrac Environmental Issues & Policy eCollection; ProQUEST Dissertations; Design and Applied Arts Index; Education Resources Information Center; International Bibliography of the Social Sciences; PsychINFO; Public Affairs Information Services; and Social Science Citation Index). To ensure that all appropriate references were identified and to limit publication bias, non-indexed sources were also searched via general search tools (i.e. Google Scholar and Google Books) to uncover any additional publications. To supplement the search, 35 experts, identified by our searches, were contacted and asked to identify additional relevant articles and grey literature.
Reference Manager 11 was used to support the review. Following the removal of duplicates, articles were screened based on their title and abstract. The full-text was then screened for articles not excluded based on their title/abstract. Articles were excluded if they were not written in English, did not use diagramming techniques in the data collection process (i.e. research subjects did not create or edit diagrams) or were not evaluative or reflective about the data collection process and/or analysis of data collected from diagramming methods. No publication date or publication type restrictions were imposed; research studies, theoretical articles, method articles and opinion pieces were included if they met the above criteria.
The screening was undertaken by two authors (MJU, PT). Double screening was done at regular intervals to ensure inter-rater reliability. Further, the two researchers met weekly during the screening and data extraction phases to discuss the nuances of the articles and to resolve differences by deliberation until consensus was reached.
A total of 2690 references were identified, after the removal of duplicates. Given our search had no publication date restrictions and included dissertations, full-text articles were sometimes difficult to retrieve. Authors were contacted when the article could not be found online or through the University of Toronto's library system. While 4 articles were retrieved in this manner, 27 full-text articles still could not be found and were ultimately excluded. In total, 233 full-text articles were screened and a total of 80 articles were included in the study's review. Figure Figure1 1 presents a flow diagram of our search and screening. Data was extracted on the general characteristics of the articles and the four objectives detailed earlier (see Table Table1 1 ).

Flow of articles through the systematic review .
Characteristics of reviewed studies using diagramming data collection approaches
General characteristics
Of the 80 articles included in our review, 53 were published studies[ 1 , 4 - 15 , 19 - 58 ], 19 were dissertations[ 59 - 77 ], 2 were books[ 78 , 79 ] and 6 represented grey literature[ 3 , 80 - 84 ], including unpublished working papers submitted by key experts and reports available on the internet. These articles were published between 1986 and 2010, with the majority published after 2000 and a substantial increase after 2006. This suggests that interest in these techniques has been increasing in recent years.
The most common discipline, determined by the lead author's affiliation and/or publication title, was from the education field. Other disciplines included healthcare, engineering, environmental science, geography, industrial design, psychology, and social science. The majority of articles clearly specified the study sample size, which averaged 36 research subjects, with a range of 2 to 243. Diagramming methods were used with a wide variety of research subjects, including students (elementary to graduate school), farmers, nurses, physicians, engineers, administrators and graphic designers.
What drives the selection of a diagramming approach for data collection?
The majority of articles specified at least one explicit reason why a form of diagramming was selected for data collection. These reasons fall into two broad categories: requirements or challenges of the research topic and the unique dataset that results from using diagrams.
The specific research topic examined was the most common reason for researchers choosing a diagramming technique for data collection. For some research topics, past studies have validated diagramming data collection techniques as a useful way to collect data. For example, research has established the usefulness of diagrams in collecting data about research subjects' knowledge or cognitive structures[ 19 - 22 , 59 , 80 ]. Diagrams in data collection have also been validated as a means of measuring changes over time[ 6 , 10 , 20 , 22 - 24 , 60 , 85 ] and differences between participant groups[ 20 , 25 , 61 , 81 ]. Diagramming methods were also sought out when research topics were not conducive to the more common qualitative data collection methods, such as interviews alone. These reasons include a research topic that deals with a population with linguistic, cultural, social or intuitional barriers the researcher wants to overcome[ 12 , 14 , 15 , 26 - 29 , 79 ] or with highly complex subject matter[ 12 , 14 , 25 , 30 - 32 , 85 ]. Examples of highly complex subject matters include the abstract nature of the research topic of 'pedagogical constructs'[ 25 ] and the multifaceted and diverse nature of 'clinical accountability relationships'[ 14 ].
Secondly, researchers sought out diagramming data collection methods because of the benefits previous studies found regarding the quality and uniqueness of the collected dataset. When research subjects drew diagrams without prompts, previous studies concluded that it minimized the influence of the researcher on the participant and their responses[ 1 , 33 - 35 , 61 , 62 , 81 , 82 ]. Studies have also found that diagramming is a reflective tool for the research subjects[ 28 , 29 , 36 , 63 ]. Since diagrams can represent both concrete and theoretical notions[ 37 ], diagramming offers a more holistic coverage of the topic[ 29 , 38 , 61 ], with more uncensored and unique data gathered[ 1 , 24 , 28 , 35 , 39 , 40 , 58 ] than more traditional qualitative data collection methods.
What are the different approaches to diagramming for data collection?
A range of applications were identified, which varied widely based on the degree of instruction, degree of freedom in diagram creation, the number of diagrams created or edited and the use of diagram in conjunction with other data collection methods.
Half of the studies did not report the details of the instructions provided to the research subjects, except for describing the basic request to create a diagram. One study explicitly observed that specific instructions are needed to ensure the research participants create a diagram and not another form of written material[ 32 ], such as a drawing or table. Simple or short instructions were often given to research subjects when the diagram sought by the researchers did not have to conform to a rigid structure, such as life-cycle[ 13 ] and professional practice diagrams[ 32 ].
When the researcher sought highly structured diagrams, the degree of instruction provided to the research subjects ranged from the preferred method of giving specific and detailed instructions on what elements should be included (e.g. hierarchies, arrows) to showing an example of the type of diagram the researcher would like the participant to create. For example, in comparison to other diagramming techniques, concept maps have a fairly rigid definition and a very specific set of elements that the end diagram should contain. Such diagrams may require more detailed instructions. One study had associate nursing degree students draw their own concept maps after a 20-minute introductory tutorial, presentation of a sample diagram, discussion and question period, and instructions listing all elements to be included (e.g. arrangement of items, hierarchal order, linking concepts with arrow, labeling propositions, identifying cross-links/relationships)[ 9 ]. In some instances, research subjects were given the opportunity to practice the diagramming method and receive corrective feedback prior to data collection[ 19 , 23 , 41 , 64 ].
It was most common for research subjects to create an original diagram on their own, in groups or a combination of both. Alternatively, some studies had the participant edit either a designated diagram provided by the researcher[ 3 , 14 , 15 , 42 ] or a researcher-created diagram generated by the researcher during the interview with participant input[ 4 , 5 , 7 , 43 ]. A few studies also chose the middle ground between original and prepared diagrams. For example, some provided a central concept or word to create the diagram around[ 14 , 44 ], or included some words or shapes to fill in on a prepared diagram[ 45 ] and others gave research subjects a list of words to use in the creation of their diagram[ 65 , 80 ].
Half of the studies used diagrams at multiple times within the data collection process as a means of comparison. A subset used a pre-/post- (or time series) approach to data collection, allowing researchers to track changes before, after, and sometimes during an intervention. This was found primarily within the discipline of education. For example, Rios asked a sample of teachers to create concept maps at multiple time intervals in order to identify their conceptual models and examine the impact of student interactions on the teachers' subject matter structure or vice versa[ 66 ].
Some researchers explicitly expressed the idea that diagrams alone would not capture complete perspectives from the research subjects[ 57 , 77 ], suggesting that diagrams should be used in combination with other data collection methods. The majority of the studies did use diagramming data collection techniques in addition to other methods. In some cases the additional data collection methods were used explicitly in conjunction with the diagramming techniques, such as creating the diagram within interviews or discussion of the diagrams in later focus groups or interviews[ 8 , 23 , 39 , 59 , 60 , 67 ].
What are the different approaches to analyzing data collected with diagramming?
The majority of the articles reported details about the analysis of diagram data. The use of only quantitative or qualitative analysis, or a mixture of both types of analysis was fairly equally distributed among the articles. Within each of these three categories of analysis, there were a variety of different techniques used that are briefly outlined below.
The majority of studies comparing diagrams across time or across research subjects chose either quantitative techniques only or a mix of qualitative and quantitative techniques for their analysis. Quantitative analysis techniques included counting (e.g. number of concepts identified, number of links between concepts, number of examples given, levels in hierarchy) and scoring. The two most common scoring methods were structural scoring and relational scoring[ 22 ]. Structural scoring refers to when weights are assigned to hierarchical structures, links between concepts and other elements. For example, a link between two concepts may be 10 points, an example 1 point and invalid examples and cross links 0 points[ 40 ]. Relational scoring reflects the quality or importance of each concept or link as determined by the researcher, by comparison to a similar diagram created by an expert or by other research subjects[ 36 , 38 , 60 , 62 , 68 ]. Some studies using quantitative analysis showed diagrams to illustrate how the final counting and scoring of a diagram was completed in the presentation of final study results[ 35 , 40 , 41 , 46 - 49 , 54 , 62 ]. For example, Kesby had focus groups in Zimbabwe create scored diagrams with local materials of rocks, string and bottle caps[ 54 ]. These diagrams were photographed and also reproduced on computer for legibility. Including an original diagram in the presentation of results also helps to orient the reader to the type of diagram that was used.
Studies which had diagrams completed or edited in the presence of the researcher, included additional data collection methods, and/or studies using less structured diagrams to collect data were likely to use either qualitative techniques only or a mix of qualitative and quantitative techniques for their analysis. For example, Haidet et al. gave medical school research subjects less structure and encouraged creativity in their diagramming exercise within interviews[ 13 ]. The diagram was used as a prompt to stimulate discussion, which was then analyzed through the interview transcripts. The diagram itself was then displayed in the final results to visually summarize the verbal exchange. Qualitative analysis included thematic and content analysis of the diagrams and additional data sources, such as transcripts, to identify prominent topics, themes and patterns in the diagrams[ 13 , 25 , 26 , 34 , 63 , 66 , 69 - 71 ].
In some studies that used mixed-methods, the collected diagrams were the primary source of data and guided the analysis of additional data sources, for example, by providing the core themes for transcript analysis. In other cases the additional data sources played the dominant role in analysis and the diagrams were used almost as verification or visual representation to illustrate conclusions[ 72 ].
What are the benefits and challenges of using diagramming for data collection?
Some of the benefits to using diagrams for data collection have already been discussed in the section on why researchers chose diagramming data collection approaches. In addition to these, diagramming approaches that were seen as complementary to other data collection approaches were commonly used in interviews and focus groups[ 60 , 73 ]. They were found to help focus discussions on particular themes[ 32 ] and enabled research subjects to more easily reflect on a topic or their beliefs by helping them to express thoughts in a more structured and organized manner[ 50 , 51 ]. The use of diagrams was also seen to increase recall[ 52 ] and self-reflectiveness[ 53 , 54 ]. In 1992, Powell found that interviews which made use of diagramming approaches were more introspective and tended to be more theoretical and philosophical than those that did not use diagramming methods[ 25 ].
Over half of the articles discussed at least one challenge of using a diagramming method for data collection. Interestingly, these challenges were often contradicted by other articles. All studies completed their data collection with diagrams, with some reporting that the diagramming allowed research subjects to overcome challenges of verbal communication[ 12 , 14 , 15 , 26 - 29 , 75 , 79 ]. However, many studies found that at least some of the research subjects expressed difficulty or discomfort with the diagramming task[ 1 , 9 , 10 , 14 , 28 , 35 , 55 , 56 , 58 , 62 , 74 , 76 , 83 ]. Some identified the ease and speed of data collection as benefits of using diagramming approaches[ 24 , 31 , 54 ], while others saw it as being time-intensive, particularly for analysis[ 6 , 11 , 26 , 62 ]. Related to the visual organization and structure of knowledge that the diagrams presented, an advantage to using diagramming approaches for data collection is their ability to obtain unique and unsolicited data[ 7 , 14 , 15 , 26 , 29 , 33 , 42 , 43 , 58 ]. In contrast, there were also concerns regarding the data it did not collect, such as non-verbal communication, that require the discretion and experience of researchers to identify and interpret[ 15 , 24 , 84 ]. These contradictions illustrate that the benefits and challenges to using diagramming approaches for data collection depend on the application and type of diagram used in each research study.
This systematic review represents the first overview of diagrams being used as a data collection approach in multiple disciplines. In 2006, Nesbit & Adesope[ 86 ] conducted a widely cited meta-analysis looking at peer-reviewed articles focusing on learning with concept and knowledge maps and found that the interest in using diagrams appeared to be on the rise[ 86 ]. While our systematic review concurs that interest is growing, it differs from their meta-analysis in two ways. Firstly our definition of a diagram is much broader, encompassing a variety of diagrams that extend beyond concept and knowledge maps. As well as the structured diagrams that Nesbit & Adescope focused on, our review also includes less structured diagrams, such as the life-cycle and professional practice discussed in our results section. Secondly, our focus is solely on diagrams being used as a data collection approach, whereas their meta-analysis included diagrams used as analysis techniques as well.
Given our broad definition of a diagram, we have reviewed approaches for collecting data through a wide spectrum of diagrams, from highly structured concept maps to less defined diagrams. We have provided an overview of the instruction options for research subjects, the creation and analysis of diagramming as a data collection approach, as well as highlighted some of the benefits and challenges. While there is variation regarding the guidance in instructions and approaches to the construction of different diagrams, use of diagrams as a data collection tool as a whole is clearly increasing in healthcare and in other disciplines. This systematic review is the first step in consolidating this information to assist in the refinement of this approach. For those considering using diagramming as a data collection approach, we offer three recommendations. Firstly, the diagrammatic approach should be chosen based on the type of data needed to answer the research question(s). Secondly, based on the diagrammatic approach chosen, it is important to select the appropriate instructions needed. Finally, presentation of final results should include examples of the original or recreated diagrams.
Choice of Diagramming Approach
The most important considerations for choosing the diagramming approach is the type of data needed to answer the research question (e.g., examining change over time, exploring people's experiences or views) and the type of analysis preferred by the researcher. For example, highly structured diagrams allowed for valid quantitative analysis, such as counting and ranking, which could be compared across research subjects. It should be noted that there is some controversy whether items should even be counted and that both an over- or under-reliance may be dangerous to the final research conclusions[ 28 ]. In comparison, other approaches that used a less structured diagrams relied heavily on qualitative analysis.
Instruction and Creation
The appropriateness of different approaches to instructions to guide diagram creation is an important consideration in ensuring the validity of data. It is clear from our review that the initial instructions given to research subjects varied in structure but had a great impact on the resulting diagrams and their potential for different analysis techniques. If researchers require highly structured diagrams it may be useful to give research subjects more detailed instructions and the opportunity for practice and feedback[ 30 , 64 ].
Presentation of results
The last recommendation is that studies using diagramming data collection approaches should include visual presentations of the findings in their results sections. The use of diagrams often results in the collection of unique and unsolicited data through a visual component, which can then be displayed along with the final analysis. Just as diagrams can provide data not easily obtained through verbal data collection techniques, visual presentation of the collected diagrams may provide insights not as easily grasped through verbal communication of study results. While providing a scanned image of the original diagram can be difficult at times, it is also possible to present a photograph of the original diagram or a computer-generated recreation of the diagram. This is especially important given the variation in terminology between disciplines, as it relays to the reader the type and structure of diagrams created or used.
In addition to the recommendations for researchers considering the use of diagramming data collection approaches, this multidisciplinary review also identifies areas where future research is needed. This review required a substantial amount of preliminary work to understand the terminology used to describe diagrams and this data collection approach across different disciplines and fields. The intent was to devise a sensitive search, so as to cast a wide net in order to capture articles in a range of disciplines where the terminology is not standardized. Cole et al. illustrate this issue by identifying over a dozen different terms that are used to describe concept maps[ 56 ]. Thus far, development of terminology has focused on the end result, i.e. what type of diagram is created based on the elements it contains, rather than focusing on the actual data collection approach itself. This has created different data collection approaches that use diagrams separately, isolating research done across disciplines and even within disciplines. Therefore, it is our recommendation that efforts are directed towards standardizing the terminology for this data collection method. This would allow researchers to maintain the work they have done regarding specific types of diagrams, whilst providing an umbrella term to help with the sharing of best practices. Future research should also be directed at identification of the underpinning theory of the method as the review demonstrated a gap in this area[ 15 , 32 , 35 ]. Such a theory may help to further inform researchers regarding the appropriate use and applications for diagramming data collection approaches.
A limitation of our review is that the database search strategy did not capture general articles on visual data collection methods, which may include specific information on diagramming data collection approaches. However the non-indexed searches and articles identified by experts did pick up some of these articles. While efforts were made to contact authors to retrieve articles from our search, twenty-seven full-text articles were irretrievable. Ten of these were dissertations and five were books. This may have contributed to an incomplete representation of what the literature has to offer about diagramming data collection approaches.
There has been a growing interest in the use of diagrams for data collection in the research process over recent years, as shown by the increase in publications and the wide range of approaches developed for diagramming data collection and diagram data analysis. As noted earlier, diagrams have been used to collect rich data on a variety of healthcare topics and it is expected that the use of this method will continue to grow. The results of this multidisciplinary systematic review provide an overview of the application of diagrams in research data collection and the methods for analyzing the unique datasets elicited. Recommendations are presented to assist researchers considering the use of diagrams in their data collection process. This review also highlighted the need for a standardized terminology of the method and a supporting theoretical framework.
Competing interests
The authors declare that they have no competing interests.
Authors' contributions
MJU, MJD, HEDB participated in the conception and design of the study. Data collection, abstraction and analysis was carried out by MJU and PT. MJU and PT prepared the original draft of the manuscript, and all authors reviewed and critically revised the original and subsequent manuscript drafts and approved the final manuscript.
Pre-publication history
The pre-publication history for this paper can be accessed here:
http://www.biomedcentral.com/1471-2288/11/11/prepub
Acknowledgements
This study was supported by funding from a grant from the Canadian Institutes of Health Research.
- Wheeldon J, Faubert J. Framing experience: concept maps, mind maps, and data collection in qualitative research. International Journal of Qualitative Methods. 2009; 8 :68–83. [ Google Scholar ]
- Forbes MA. Hope in the older adult with chronic illness: A comparison of two research methods in theory building. Advances in Nursing Science. 1999; 22 :74–87. [ PubMed ] [ Google Scholar ]
- Jafri T, Lyons MN, Clarkson PJ. The supply of medicines in the NHS. Improving Patient Safety: Cambridge, UK; 2008. [ Google Scholar ]
- O'Campo P, Salmon C, Burke J. Neighbourhoods and mental well-being: what are the pathways? Health and Place. 2009; 15 :56–68. [ PubMed ] [ Google Scholar ]
- Pluto DM, Hirshorn BA. Process mapping as a tool for home health network analysis. Home Health Care Services Quarterly. 2003; 22 :1–16. doi: 10.1300/J027v22n02_01. [ PubMed ] [ CrossRef ] [ Google Scholar ]
- Franca S, d'Ivernois JF, Marchand C, Haenni C, Ybarra J, Golay A. Evaluation of nutritional education using concept mapping. Patient Education and Counseling. 2004; 52 :183–192. doi: 10.1016/S0738-3991(03)00037-5. [ PubMed ] [ CrossRef ] [ Google Scholar ]
- Marchand C, d'Ivernois JF, Assal JP, Slama G, Hivon R. An analysis, using concept mapping, of diabetic patients' knowledge, before and after patient education. Medical Teacher. 2002; 24 :90–99. doi: 10.1080/01421590120091087. [ PubMed ] [ CrossRef ] [ Google Scholar ]
- Jones MG, Rua MJ. Conceptual representations of flu and microbial illness held by students, teachers, and medical professionals. Social Science and Mathematics. 2008; 108 :263–278. [ Google Scholar ]
- Abel WM, Freeze M. Evaluation of concept mapping in an associate degree nursing program. Journal of Nursing Education. 2006; 45 :356–364. [ PubMed ] [ Google Scholar ]
- Daley BJ, Shaw CR, Balistrieri T, Glasenapp K, Piacentine L. Concept maps: a strategy to teach and evaluate critical thinking. Journal of Nursing Education. 1999; 38 :42–47. [ PubMed ] [ Google Scholar ]
- Hicks-Moore SL, Pastirik PJ. Evaluating critical thinking in clinical concept maps: A pilot study. International Journal of Nursing Education Scholarship. 2006; 3 :1–15. doi: 10.2202/1548-923X.1314. [ PubMed ] [ CrossRef ] [ Google Scholar ]
- Coad J. Using art-based techniques in engaging children and young people in health care consultations and/or research... including commentary by Bishop V and Picard C. Journal of Research in Nursing. 2007; 12 :487–497. doi: 10.1177/1744987107081250. [ CrossRef ] [ Google Scholar ]
- Haidet P, Hatem DS, Fecile ML, Stein HF, Haley H-L, Kimmel B. et al. The role of relationships in the professional formation of physicians: Case report and illustration of an elicitation technique. Patient Education and Counseling. 2008; 72 :382–387. doi: 10.1016/j.pec.2008.05.016. [ PubMed ] [ CrossRef ] [ Google Scholar ]
- Umoquit MJ, Dobrow MJ, Lemieux-Charles L, Ritvo PG, Urbach DR, Wodchis WP. The efficiency and effectiveness of utilizing diagrams in interviews: An assessment of participatory diagramming and graphic elicitation. BMC Medical Research Methodology. 2008. p. 8. [ PMC free article ] [ PubMed ]
- Crilly N, Blackwell A, Clarkson P. Graphic elicitation: using research diagrams as interview stimuli. Qualitative Research. 2006; 6 :341–366. doi: 10.1177/1468794106065007. [ CrossRef ] [ Google Scholar ]
- Jun GT, Ward JR, Morris ZS, Clarkson PJ. Health care process modelling: which method when? International Journal for Quality in Health Care. 2009; 21 :214–224. doi: 10.1093/intqhc/mzp016. [ PubMed ] [ CrossRef ] [ Google Scholar ]
- Jun GT, Ward JR, Clarkson PJ. Systems modelling approaches to the design of safe healthcare delivery:ease of use and usefulness percived by healthcare works. Ergonomics. 2010; 53 :829–847. doi: 10.1080/00140139.2010.489653. [ PubMed ] [ CrossRef ] [ Google Scholar ]
- Liberati A, Altman DG, Tetzlaff J, Mulrow C, Gotzsche PC, Ioannidis JP. et al. The PRISMA statement for reporting systematic reviews and meta-analyses of studies that evaluate health care interventions: explanation and elaboration. PLoS Medicine. 2009; 6 :e100100. doi: 10.1371/journal.pmed.1000100. [ PMC free article ] [ PubMed ] [ CrossRef ] [ Google Scholar ]
- Zhang L, Wang Y, Dong B, Zhou Z. The comparison study of Chinese and American secondary school students' knowledge structure--An experimental research based on concept map assessment technique. Frontiers of Education in China. 2009; 4 :286–297. doi: 10.1007/s11516-009-0015-0. [ CrossRef ] [ Google Scholar ]
- Hay DB. Using concept maps to measure deep, surface and non-learning outcomes. Studies in Higher Education. 2007; 32 :39–57. doi: 10.1080/03075070601099432. [ CrossRef ] [ Google Scholar ]
- Lavigne NC, Salkind SJ, Yan J. Exploring college students' mental representations of inferential statistics. The Journal of Mathematical Behavior. 2008; 27 :11–32. doi: 10.1016/j.jmathb.2007.10.003. [ CrossRef ] [ Google Scholar ]
- West DC, Park JK, Pomeroy JR, Sandoval J. Concept mapping assessment in medical education: A comparison of two scoring systems. Medical Education. 2002; 36 :820–826. doi: 10.1046/j.1365-2923.2002.01292.x. [ PubMed ] [ CrossRef ] [ Google Scholar ]
- Carter G, Jones MG, Rua M. Effects of partner's ability on the achievement and conceptual organization of high-achieving fifth-grade students. Science Education. 2002; 87 :94–111. doi: 10.1002/sce.10031. [ CrossRef ] [ Google Scholar ]
- Hay DB, Wells H, Kinchin IM. Quantitative and qualitative measures of student learning at university level. Higher Education. 2008; 56 :221–240. doi: 10.1007/s10734-007-9099-8. [ CrossRef ] [ Google Scholar ]
- Powell RR. The influence of prior experiences on pedagogical constructs of traditional and nontraditional preservice teachers. Teaching and Teacher Education. 1992; 8 :225–238. doi: 10.1016/0742-051X(92)90022-U. [ CrossRef ] [ Google Scholar ]
- Kesby M. Participatory diagramming as a means to improve communication about sex in rural Zimbabwe: a pilot study. Social Science & Medicine. 2000; 50 :1723–1741. [ PubMed ] [ Google Scholar ]
- Van der Riet M. Diagramming as mediational means: Vygotskian theory and participatory research. South African Journal of Psychology. 2008; 38 :455–465. [ Google Scholar ]
- Wheeldon J. Is a picture worth a thousand words? Using mind maps to facilitate participant recall in qualitative research. Qualitative Report. in press .
- Bagnoli A. Beyond the standard interview: The use of graphic elicitation and arts-based methods. Qualitative Research. 2009; 9 :547–571. doi: 10.1177/1468794109343625. [ CrossRef ] [ Google Scholar ]
- Lim SE, Chan PW Cheng, Lam MS, Ngan SF. Developing reflective and thinking skills by means of semantic mapping strategies in kindergarten teacher education. Early Child Development and Care. 2003; 173 (1):55–72. doi: 10.1080/0300443022000022422. [ CrossRef ] [ Google Scholar ]
- Ozesmi U, Ozesmi SL. Ecological models based on people's knowledge: A multi-step fuzzy cognitive mapping approach. Ecological Modeling. 2004; 176 :43–64. doi: 10.1016/j.ecolmodel.2003.10.027. [ CrossRef ] [ Google Scholar ]
- Varga-Atkins T, O'Brien M. From drawings to diagrams: Maintaining researcher control during graphic elicitation in qualitative interviews. International Journal of Research & Methods in Education. 2009; 32 :53–67. [ Google Scholar ]
- Williams CG. Using concept maps to assess conceptual knowledge of function. Journal for Research in Mathematics Education. 1998; 29 :414–421. doi: 10.2307/749858. [ CrossRef ] [ Google Scholar ]
- Strean WB, Holt NL. Coaches', athletes', and parents' perceptions of fun in youth sports: assumptions about learning and implications for practice. AVANTE. 2000; 6 :83–98. [ Google Scholar ]
- Wheeldon J. Mapping mixed methods research: Methods, measures, and meaning. Journal of Mixed Methods Research. 2010; 48 :87–102. doi: 10.1177/1558689809358755. [ CrossRef ] [ Google Scholar ]
- Rye JA, Rubba PA. An exploration of the concept map as an interview tool to facilitate the externalization of students' understandings about global atmospheric change. Journal of Research in Science Teaching. 1998; 35 :521–546. doi: 10.1002/(SICI)1098-2736(199805)35:5<521::AID-TEA4>3.0.CO;2-R. [ CrossRef ] [ Google Scholar ]
- Lourdel N, Gondran N, Laforest V, Debray B, Brodhag C. Sustainable development cognitive map: A new method of evaluating student understanding. International Journal of Sustainability in Higher Education. 2007; 8 :170–182. doi: 10.1108/14676370710726634. [ CrossRef ] [ Google Scholar ]
- Koul R, Clariana RB, Salehi R. Comparing several human and computer-based methods for scoring concept maps and essays. Journal of Educational Computing Research. 2005; 32 :227–239. doi: 10.2190/5X9Y-0ETN-213U-8FV7. [ CrossRef ] [ Google Scholar ]
- Shymansky JA, Yore LD, Treagust DF, Thiele RB, Harrison A, Waldrip BG. et al. Examining the construction process: A study of changes in level 10 students' understanding of classical mechanics. Journal of Research in Science Teaching. 1997; 34 :571–593. doi: 10.1002/(SICI)1098-2736(199708)34:6<571::AID-TEA3>3.0.CO;2-K. [ CrossRef ] [ Google Scholar ]
- West DC, Pomeroy JR, Park JK, Gerstenberger EA, Sandoval J. Critical thinking in graduate medical education: A role for concept mapping assessment? Journal of the American Medical Association. 2000; 284 :1105–1110. doi: 10.1001/jama.284.9.1105. [ PubMed ] [ CrossRef ] [ Google Scholar ]
- Lian MWS. An investigation into high-achiever and low-achiever knowledge organisation and knowledge processing in concept mapping: A case study. Research in Science Education. 1998; 28 :337–352. doi: 10.1007/BF02461567. [ CrossRef ] [ Google Scholar ]
- Crilly N, Clarkson P, Blackwell A. Using research diagrams for member validation in qualitative research. Diagrams. 2006; 4045 :258–262. [ Google Scholar ]
- Cox P, Niewoehner J, Pidgeon N, Gerrard S, Fischhoff B, Riley D. The use of mental models in chemical risk protection: Developing a generic workplace methodology. Risk Analysis. 2003; 23 :311–324. doi: 10.1111/1539-6924.00311. [ PubMed ] [ CrossRef ] [ Google Scholar ]
- Koury K, Hollingsead C, Fitzgerald G, Miller K, Mitchem K, Tsai HH. et al. Case-based instruction in different delivery contexts: The impact of time in cases. Journal of Interactive Learning Research. 2009; 20 :445–467. [ Google Scholar ]
- Gill PE, Persson M. On using concept-maps to study school-children's understanding of leisure-time. Leisure Studies. 2008; 27 :213–220. doi: 10.1080/02614360802048795. [ CrossRef ] [ Google Scholar ]
- Wheeldon J. To guide or provoke? Maps, pedagogy, and the value(s) of teaching criminal justice ethics. Criminal Justice Education. in press .
- Mouratiadou I, Moran D. Mapping public participation in the Water Framework Directive: A case study of the Pinios River Basin, Greece. Ecological Economics. 2007; 62 :66–76. doi: 10.1016/j.ecolecon.2007.01.009. [ CrossRef ] [ Google Scholar ]
- Kimber K, Pillay H, Richards C. Technoliteracy and learning: An analysis of the quality of knowledge in electronic representations of understanding. Computers & Education. 2007; 48 :59–79. [ Google Scholar ]
- Artiles AJ, Barreto RM, Pena L, McClafferty K. Pathways to teacher learning in multicultural contexts - A longitudinal case study of two novice bilingual teachers in urban schools. Remedial and Special Education. 1998; 19 :70–90. doi: 10.1177/074193259801900203. [ CrossRef ] [ Google Scholar ]
- Farrell TSC. Critical reflection in a TESL Course: Mapping conceptual change. ELT Journal. 2008; 63 :221–229. doi: 10.1093/elt/ccn058. [ CrossRef ] [ Google Scholar ]
- Lourdel N, Gondran N, Laforest V, Brodhag C. Introduction of sustainable development in engineers' curricula: Problematic and evaluation methods. International Journal of Sustainability in Higher Education. 2005; 6 :254–264. doi: 10.1108/14676370510607223. [ CrossRef ] [ Google Scholar ]
- Naykki P, Jarvela S. How pictorial knowledge representations mediate collaborative knowledge construction in groups. Journal of Research on Technology in Education. 2008; 40 :359. [ Google Scholar ]
- Conceicao SC, Taylor LD. Using a constructivist approach with online concept maps: relationship between theory and nursing education. Nursing Education Perspectives. 2007; 28 :268–275. [ PubMed ] [ Google Scholar ]
- Kesby M. Participatory diagramming: Deploying qualitative methods through an action research epistemology. Area. 2000; 32 :423–435. doi: 10.1111/j.1475-4762.2000.tb00158.x. [ CrossRef ] [ Google Scholar ]
- Ahmed R, Ali NA. Performance appraisal decision in Malaysian public service. International Journal of Public Sector Management. pp. 48–64. 4 A.D. [ CrossRef ]
- Cole C, Lin Y, Leide J, Large A, Beheshti J. A classification of mental models of undergraduates seeking information for a course essay in history and psychology: preliminary investigations into aligning their mental models with online thesauri. Journal of the American Society for Information Science & Technology. 2007; 58 :2092–2104. [ Google Scholar ]
- Rowe AL, Cooke NJ. Measuring mental models: Choosing the right tools for the job. Human Resources Development Quarterly. 1995; 6 :243–255. doi: 10.1002/hrdq.3920060303. [ CrossRef ] [ Google Scholar ]
- Wheeldon J. Learning from Latvia: Adoption, adaptation, and evidence based justice reform. Journal of Baltic Studies. in press .
- Garegae KGM. Teachers, beliefs about mathematics, its teaching and learning and the communication of these beliefs to students: A case study in Botswana. Ph.D. Canada: The University of Manitoba; 2002. [ Google Scholar ]
- McWhirter LJ. Conceptual development and retention within the learning cycle. Ph.D. United States -- Oklahoma: The University of Oklahoma; 1998. [ Google Scholar ]
- Williams CG. Using concepts maps to determine differences in the concept image of function held by students in reform and traditional calculus classes. Ph.D. United States -- California: University of California; 1994. [ Google Scholar ]
- Puukari S. Video programmes as learning tools: Teaching the gas laws and behaviour of gases in Finnish and Canadian senior high schools. Ed.D. Finland: Jyvaskylan Yliopisto; 2003. [ Google Scholar ]
- Mathews S. An ethnographic examination of perspective consciousness and intercultural competence among social studies student-teachers in Kenya, East Africa. Ph.D. United States -- Indiana: Indiana University; 2008. [ Google Scholar ]
- Skidmore L. Concept mapping to promote meaningful learning at the community college level. United States -- Minnesota: Walden University; 2008. Ed.D. [ Google Scholar ]
- Yin Y, Vanides J, Ruiz-Primo MA, Ayala CC, Shavelson R. A Comparison of Two Construct-a-Concept-Map Science Assessments: Created Linking Phrases and Selected Linking Phrases. The Regents of the University of California; 2004. pp. 1–28. unknown CSE Report 624. [ Google Scholar ]
- Rios JM. Studies of contrast: Relationships between subject matter structures and teaching. United States -- Wisconsin: The University of Wisconsin; 1995. Ph.D. [ Google Scholar ]
- Feldsine JE. The construction of concept maps facilitates the learning of general college chemistry: A case study. United States -- New York: Cornell University; 1987. Ph.D. [ Google Scholar ]
- Butler KA. Scaffolding software: How does it influence student conceptual understanding and motivation? United States -- Illinois: Southern Illinois University at Carbondale; 2004. Ph.D. [ Google Scholar ]
- Alexander LM. Variations of professional identity over time: A study of physician assistants. United States -- District of Columbia: The George Washington University; 2003. Ed.D. [ Google Scholar ]
- Cantu DA. A naturalistic investigation of the relationship between secondary social studies teachers' beliefs and practice. United States -- Illinois: Southern Illinois University at Carbondale; 1997. Ph.D. [ Google Scholar ]
- Cozza B. Concept mapping through logs and metacognitive reflection during third graders' scientific problem-solving. United States -- New York: Fordham University; 1996. Ph.D. [ Google Scholar ]
- Ballard BG. Preservice teachers' beliefs about classroom management before and after student teaching. United States -- Nevada: University of Nevada, Las Vegas; 2002. Ed.D. [ Google Scholar ]
- Lowe M. Continuing medical education for physicians: Key factors contributing to learning and change. United States -- Connecticut: University of Connecticut; 2007. Ph.D. [ Google Scholar ]
- Yue H. Concept maps as assessment tools in mathematics: Comparison with clinical interviews. United States -- Texas: The University of Texas at El Paso; 2008. MAT. [ Google Scholar ]
- White E. Institutional effectiveness: The integration of program review, strategic planning and budgeting processes in two California community colleges. Andrews University, Berrien Springs, MI; 2007. [ Google Scholar ]
- Grunow JEM. Using concept maps in a professional development program to assess and enhance teachers' understanding of rational number. United States -- Wisconsin: The University of Wisconsin; 1998. Ph.D. [ Google Scholar ]
- Rowe AL. Mental models of physical systems: Examining the relationship between knowing and doing. Rice University; 1995. [ Google Scholar ]
- Kane M, Trochim WMK. Concept mapping for planning and evaluation. Thousand Oaks, CA, US: Sage Publications, Inc; 2007. [ Google Scholar ]
- Kesby M. In: HIV/AIDS in Africa: Beyond Epidemiology. Kalipendi E, Craddock S, Oppong J, Ghosh J, editor. Blackwell; 2004. Participatory diagramming and the ethical and practical challenges of helping Africans themselves to move HIV work 'beyond epidemiology'; pp. 217–228. [ Google Scholar ]
- Schreiber DA, Abegg GL. Scoring Student-Generated Concept Maps in Introductory College Chemistry. The National Association for Research in Science Teaching; 1991. pp. 1–19. Annual meeting. [ Google Scholar ]
- Williams CG. Concept Maps as Research Tools in Mathematics. 18 April, 1995. 1995. pp. 1–65.
- Varga-Atkins T, O'Brien M. Graphic elicitation as a research technique: reflecting on drawings and diagrams as complementary methods. 2008. pp. 1–22. Ref Type: Report.
- Emmel N. Participatory Mapping: An innovative sociological method. Toolkit #3. ESRC National Centre for Research Methods; 2008. pp. 1–8. Ref Type: Report. [ Google Scholar ]
- Beyerbach BA. Concept Mapping in Assessing Prospective Teachers' Concept Development. n/a, 1-16. US Department of Education; 1986. Ref Type: Report. [ Google Scholar ]
- Kane M, Trochim WMK. Concept Mapping for Planning and Evaluation. Thousand Oaks, CA, US: Sage Publications, Inc; 2007. [ Google Scholar ]
- Nesbit JC, Adescope O. Learning with concept and knowledge maps: A meta-analysis. Review of Education Research. 2006; 76 :413–448. doi: 10.3102/00346543076003413. [ CrossRef ] [ Google Scholar ]
Diagramming Research Designs
Cite this chapter.
- Herman J. Adèr
700 Accesses
In the social sciences different graphical methods have been developed to represent research designs. The chapter starts with a review of some of these methods: figures often turn out to be ambiguous, in particular where the interpretation of the arrows between nodes is concerned. Furthermore, rendering the placement in time of the various operations stays mostly implicit, while indication of strategy is not depicted graphically. A functional notation is sketched which allows the drawing of well-defined diagrams using a graphical node-and-arrow language. An alternative to the representation of an experimental design is given first and, at the end, a diagram of an actual survey study. Finally, the consequences for various fields are discussed. This kind of diagramming could be beneficially used in methodological consultation and education. Modelling meta data and meta analysis are other potential application fields.
This is a preview of subscription content, log in via an institution to check access.
Access this chapter
- Available as PDF
- Read on any device
- Instant download
- Own it forever
- Compact, lightweight edition
- Dispatched in 3 to 5 business days
- Free shipping worldwide - see info
Tax calculation will be finalised at checkout
Purchases are for personal use only
Institutional subscriptions
Unable to display preview. Download preview PDF.
Adèr, H.J. (1995). Methodological knowledge: Notation and implementation in expert systems. Phd thesis, University of Amsterdam
Google Scholar
Eveline Bleiker, E., Van der Ploeg, H.M., Adèr, H.J., Van Daal, W.A.J. and Hendriks, J.H.C.L. (1995). Personality traits of women with breast cancer: Before and after diagnosis. Psychological Reports 76:1139–1146.
Article Google Scholar
Cook, T.D. and Campbell, D.T. (1979). Quasi-experimentation: Design and analysis issues for field settings. Chicago: RandMcNally.
Dube, R. and Weiss, S.M. (1989). A state transition model for building rule-based systems. International Journal of Expert Systems 2(3/4):291–329.
Genesereth, M.R. and Nillson, N.J. (1987). Logical foundations of artificial intelligence. Los Altos, CA: Morgan Kaufmann.
Judd, C.M., Smith, E.R. and Kidder, L.H. (1991). Research methods in social relations. (6th edn). New York: Holt, Rinehart & Winston.
Stenning, K. and Inder, R. (1995). Applying semantic concepts to analyzing media and modalities. In J. Glasgow, N.H. Narayanan, and B. Chandrasekaran (Eds), Diagrammatic reasoning: Cognitive and computational perspectives, Ch. 10. Menlo Park, CA: AAAI Press /MIT Press,
Sewel Wright, S. (1934). The method of path coefficients. Annals of Mathematical Statistics 5:161–215.
Download references
You can also search for this author in PubMed Google Scholar
Editor information
Editors and affiliations.
Department of Computer and Information Sciences, Fordham University, 441 East Fordham Road, Bronx, NY, 10458, USA
Michael Anderson MSc, PhD
School of Computer Science and Software Engineering, Monash University, PO Box 26, Clayton Campus, Victoria, 3800, Australia
Bernd Meyer Dr rer nat
Department of Computer Science, University of York, Heslington, York, YO10 5DD, UK
Patrick Olivier MA, MSc, PhD
Rights and permissions
Reprints and permissions
Copyright information
© 2002 Springer-Verlag London
About this chapter
Adèr, H.J. (2002). Diagramming Research Designs. In: Anderson, M., Meyer, B., Olivier, P. (eds) Diagrammatic Representation and Reasoning. Springer, London. https://doi.org/10.1007/978-1-4471-0109-3_27
Download citation
DOI : https://doi.org/10.1007/978-1-4471-0109-3_27
Publisher Name : Springer, London
Print ISBN : 978-1-85233-242-6
Online ISBN : 978-1-4471-0109-3
eBook Packages : Springer Book Archive
Share this chapter
Anyone you share the following link with will be able to read this content:
Sorry, a shareable link is not currently available for this article.
Provided by the Springer Nature SharedIt content-sharing initiative
- Publish with us
Policies and ethics
- Find a journal
- Track your research
- Privacy Policy

Home » Graphical Methods – Types, Examples and Guide
Graphical Methods – Types, Examples and Guide
Table of Contents

Graphical Methods
Definition:
Graphical methods refer to techniques used to visually represent data, relationships, or processes using charts, graphs, diagrams, or other graphical formats. These methods are widely used in various fields such as science, engineering, business, and social sciences, among others, to analyze, interpret and communicate complex information in a concise and understandable way.
Types of Graphical Methods
Here are some of the most common types of graphical methods for data analysis and visual presentation:
Line Graphs
These are commonly used to show trends over time, such as the stock prices of a particular company or the temperature over a certain period. They consist of a series of data points connected by a line that shows the trend of the data over time. Line graphs are useful for identifying patterns in data, such as seasonal changes or long-term trends.
These are commonly used to compare values of different categories, such as sales figures for different products or the number of students in different grade levels. Bar charts use bars that are either horizontal or vertical and represent the data values. They are useful for comparing data visually and identifying differences between categories.
These are used to show how a whole is divided into parts, such as the percentage of students in a school who are enrolled in different programs. Pie charts use a circle that is divided into sectors, with each sector representing a portion of the whole. They are useful for showing proportions and identifying which parts of a whole are larger or smaller.
Scatter Plots
These are used to visualize the relationship between two variables, such as the correlation between a person’s height and weight. Scatter plots consist of a series of data points that are plotted on a graph and connected by a line or curve. They are useful for identifying trends and relationships between variables.
These are used to show the distribution of data across a two-dimensional plane, such as a map of a city showing the density of population in different areas. Heat maps use color-coded cells to represent different levels of data, with darker colors indicating higher values. They are useful for identifying areas of high or low density and for highlighting patterns in data.
These are used to show the distribution of data in a single variable, such as the distribution of ages of a group of people. Histograms use bars that represent the frequency of each data value, with taller bars indicating a higher frequency. They are useful for identifying the shape of a distribution and for identifying outliers or unusual data values.
Network Diagrams
These are used to show the relationships between different entities or nodes, such as the relationships between people in a social network. Network diagrams consist of nodes that are connected by lines that represent the relationship. They are useful for identifying patterns in complex data and for understanding the structure of a network.
Box plots, also known as box-and-whisker plots, are a type of graphical method used to show the distribution of data in a single variable. They consist of a box with whiskers extending from the top and bottom of the box. The box represents the middle 50% of the data, with the median value indicated by a line inside the box. The whiskers represent the range of the data, with any data points outside the whiskers indicated as outliers. Box plots are useful for identifying the spread and shape of a distribution and for identifying outliers or unusual data values.
Applications of Graphical Methods
Graphical methods have a wide range of applications in various fields, including:
- Business : Graphical methods are commonly used in business to analyze sales data, financial data, and other types of data. They are useful for identifying trends, patterns, and outliers, as well as for presenting data in a clear and concise manner to stakeholders.
- Science and engineering: Graphical methods are used extensively in scientific and engineering fields to analyze data and to present research findings. They are useful for visualizing complex data sets and for identifying relationships between variables.
- Social sciences: Graphical methods are used in social sciences to analyze and present data related to human behavior, such as demographics, survey results, and statistical analyses. They are useful for identifying trends and patterns in large data sets and for communicating findings to a broader audience.
- Education : Graphical methods are used in education to present information to students and to help them understand complex concepts. They are useful for visualizing data and for presenting information in a way that is easy to understand.
- Healthcare : Graphical methods are used in healthcare to analyze patient data, to track disease outbreaks, and to present medical information to patients. They are useful for identifying patterns and trends in patient data and for communicating medical information in a clear and concise manner.
- Sports : Graphical methods are used in sports to analyze and present data related to player performance, team statistics, and game outcomes. They are useful for identifying trends and patterns in player and team data and for communicating this information to coaches, players, and fans.
Examples of Graphical Methods
Here are some examples of real-time applications of graphical methods:
- Stock Market: Line graphs, candlestick charts, and bar charts are widely used in real-time trading systems to display stock prices and trends over time. Traders use these charts to analyze historical data and make informed decisions about buying and selling stocks in real-time.
- Weather Forecasting : Heat maps and radar maps are commonly used in weather forecasting to display current weather conditions and to predict future weather patterns. These maps are useful for tracking the movement of storms, identifying areas of high and low pressure, and predicting the likelihood of severe weather events.
- Social Media Analytics: Scatter plots and network diagrams are commonly used in social media analytics to track the spread of information across social networks. Analysts use these graphs to identify patterns in user behavior, to track the popularity of specific topics or hashtags, and to monitor the influence of key opinion leaders.
- Traffic Analysis: Heat maps and network diagrams are used in traffic analysis to visualize traffic flow patterns and to identify areas of congestion or accidents. These graphs are useful for predicting traffic patterns, optimizing traffic flow, and improving transportation infrastructure.
- Medical Diagnostics: Box plots and histograms are commonly used in medical diagnostics to display the distribution of patient data, such as blood pressure, heart rate, or blood sugar levels. These graphs are useful for identifying patterns in patient data, diagnosing medical conditions, and monitoring the effectiveness of treatments in real-time.
- Cybersecurity: Heat maps and network diagrams are used in cybersecurity to visualize network traffic patterns and to identify potential security threats. These graphs are useful for identifying anomalies in network traffic, detecting and mitigating cyber attacks, and improving network security protocols.
How to use Graphical Methods
Here are some general steps to follow when using graphical methods to analyze and present data:
- Identify the research question: Before creating any graphs, it’s important to identify the research question or hypothesis you want to explore. This will help you select the appropriate type of graph and ensure that the data you collect is relevant to your research question.
- Collect and organize the data: Collect the data you need to answer your research question and organize it in a way that makes it easy to work with. This may involve sorting, filtering, or cleaning the data to ensure that it is accurate and relevant.
- Select the appropriate graph : There are many different types of graphs available, each with its own strengths and weaknesses. Select the appropriate graph based on the type of data you have and the research question you are exploring. For example, a scatterplot may be appropriate for exploring the relationship between two continuous variables, while a bar chart may be appropriate for comparing categorical data.
- Create the graph: Once you have selected the appropriate graph, create it using software or a tool that allows you to customize the graph based on your needs. Be sure to include appropriate labels and titles, and ensure that the graph is clearly legible.
- Analyze the graph: Once you have created the graph, analyze it to identify patterns, trends, and relationships in the data. Look for outliers or other anomalies that may require further investigation.
- Draw conclusions: Based on your analysis of the graph, draw conclusions about the research question you are exploring. Use the graph to support your conclusions and to communicate your findings to others.
- Iterate and refine: Finally, refine your graph or create additional graphs as needed to further explore your research question. Iteratively refining and revising your graphs can help to ensure that you are accurately representing the data and that you are drawing the appropriate conclusions.
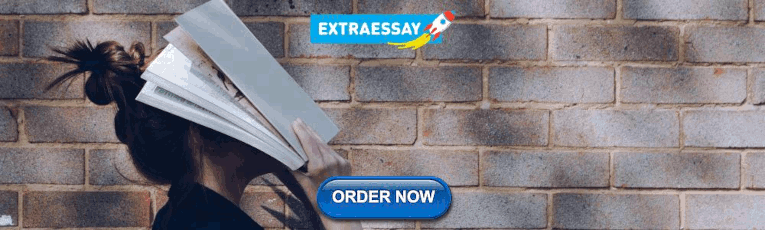
When to use Graphical Methods
Graphical methods can be used in a variety of situations to help analyze, interpret, and communicate data. Here are some general guidelines on when to use graphical methods:
- To identify patterns and trends: Graphical methods are useful for identifying patterns and trends in data, which may be difficult to see in raw data tables or spreadsheets. Graphs can reveal trends that may not be immediately apparent in the data, making it easier to draw conclusions and make predictions.
- To compare data: Graphs can be used to compare data from different sources or over different time periods. Graphical comparisons can make it easier to identify differences or similarities in the data, which can be useful for making decisions and taking action.
- To summarize data : Graphs can be used to summarize large amounts of data in a single visual display. This can be particularly useful when presenting data to a broad audience, as it can help to simplify complex data sets and make them more accessible.
- To communicate data: Graphs can be used to communicate data and findings to a variety of audiences, including stakeholders, colleagues, and the general public. Graphs can be particularly useful in situations where data needs to be presented quickly and in a way that is easy to understand.
- To identify outliers: Graphical methods are useful for identifying outliers or anomalies in the data. Outliers can be indicative of errors or unusual events, and may warrant further investigation.
Purpose of Graphical Methods
The purpose of graphical methods is to help people analyze, interpret, and communicate data in a way that is both accurate and understandable. Graphical methods provide visual representations of data that can be easier to interpret than tables of numbers or raw data sets. Graphical methods help to reveal patterns and trends that may not be immediately apparent in the data, making it easier to draw conclusions and make predictions. They can also help to identify outliers or unusual data points that may warrant further investigation.
In addition to helping people analyze and interpret data, graphical methods also serve an important communication function. Graphs can be used to present data to a wide range of audiences, including stakeholders, colleagues, and the general public. Graphs can help to simplify complex data sets, making them more accessible and easier to understand. By presenting data in a clear and concise way, graphical methods can help people make informed decisions and take action based on the data.
Overall, the purpose of graphical methods is to provide a powerful tool for analyzing, interpreting, and communicating data. Graphical methods help people to better understand the data they are working with, to identify patterns and trends, and to make informed decisions based on the data.
Characteristics of Graphical Methods
Here are some characteristics of graphical methods:
- Visual Representation: Graphical methods provide a visual representation of data, which can be easier to interpret than tables of numbers or raw data sets. Graphs can help to reveal patterns and trends that may not be immediately apparent in the data.
- Simplicity : Graphical methods simplify complex data sets, making them more accessible and easier to understand. By presenting data in a clear and concise way, graphical methods can help people make informed decisions and take action based on the data.
- Comparability : Graphical methods can be used to compare data from different sources or over different time periods. This can help to identify differences or similarities in the data, which can be useful for making decisions and taking action.
- Flexibility : Graphical methods can be adapted to different types of data, including continuous, categorical, and ordinal data. Different types of graphs can be used to display different types of data, depending on the characteristics of the data and the research question.
- Accuracy : Graphical methods should accurately represent the data being analyzed. Graphs should be properly scaled and labeled to avoid distorting the data or misleading viewers.
- Clarity : Graphical methods should be clear and easy to read. Graphs should be designed with the viewer in mind, using appropriate colors, labels, and titles to ensure that the message of the graph is conveyed effectively.
Advantages of Graphical Methods
Graphical methods offer several advantages for analyzing and presenting data, including:
- Clear visualization: Graphical methods provide a clear and intuitive visual representation of data that can help people understand complex relationships, trends, and patterns in the data. This can be particularly useful when dealing with large and complex data sets.
- Efficient communication: Graphical methods can help to communicate complex data sets in an efficient and accessible way. Visual representations can be easier to understand than numerical data alone, and can help to convey key messages quickly.
- Effective comparison: Graphical methods allow for easy comparison between different data sets, making it easier to identify trends, patterns, and differences. This can help in making decisions, identifying areas for improvement, or developing new insights.
- Improved decision-making: Graphical methods can help to inform decision-making by presenting data in a clear and easy-to-understand format. They can also help to identify key areas of focus, enabling individuals or teams to make more informed decisions.
- Increased engagement: Graphical methods can help to engage audiences by presenting data in an engaging and interactive way. This can be particularly useful in presentations or reports, where visual representations can help to maintain audience attention and interest.
- Better understanding: Graphical methods can help individuals to better understand the data they are working with, by providing a clear and intuitive visual representation of the data. This can lead to improved insights and decision-making, as well as better understanding of the implications of the data.
Limitations of Graphical Methods
Here are a few limitations to consider:
- Misleading representation: Graphical methods can potentially misrepresent data if they are not designed properly. For example, inappropriate scaling or labeling of the axes or the use of certain types of graphs can create a distorted view of the data.
- Limited scope: Graphical methods can only display a limited amount of data, which can make it difficult to capture the full complexity of a data set. Additionally, some types of data may be difficult to represent visually.
- Time-consuming : Creating graphs can be a time-consuming process, particularly if multiple graphs need to be created and analyzed. This can be a limitation in situations where time is limited or resources are scarce.
- Technical skills: Some graphical methods require technical skills to create and interpret. For example, certain types of graphs may require knowledge of specialized software or programming languages.
- Interpretation : Interpreting graphs can be subjective, and the same graph can be interpreted in different ways by different people. This can lead to confusion or disagreements when using graphs to communicate data.
- Accessibility : Some graphical methods may not be accessible to all audiences, particularly those with visual impairments. Additionally, some types of graphs may not be accessible to those with limited literacy or numeracy skills.
About the author
Muhammad Hassan
Researcher, Academic Writer, Web developer
You may also like

Cluster Analysis – Types, Methods and Examples

Discriminant Analysis – Methods, Types and...

MANOVA (Multivariate Analysis of Variance) –...

Documentary Analysis – Methods, Applications and...

ANOVA (Analysis of variance) – Formulas, Types...

Substantive Framework – Types, Methods and...
Graphical Representation of Data
Graphical representation of data is an attractive method of showcasing numerical data that help in analyzing and representing quantitative data visually. A graph is a kind of a chart where data are plotted as variables across the coordinate. It became easy to analyze the extent of change of one variable based on the change of other variables. Graphical representation of data is done through different mediums such as lines, plots, diagrams, etc. Let us learn more about this interesting concept of graphical representation of data, the different types, and solve a few examples.
Definition of Graphical Representation of Data
A graphical representation is a visual representation of data statistics-based results using graphs, plots, and charts. This kind of representation is more effective in understanding and comparing data than seen in a tabular form. Graphical representation helps to qualify, sort, and present data in a method that is simple to understand for a larger audience. Graphs enable in studying the cause and effect relationship between two variables through both time series and frequency distribution. The data that is obtained from different surveying is infused into a graphical representation by the use of some symbols, such as lines on a line graph, bars on a bar chart, or slices of a pie chart. This visual representation helps in clarity, comparison, and understanding of numerical data.
Representation of Data
The word data is from the Latin word Datum, which means something given. The numerical figures collected through a survey are called data and can be represented in two forms - tabular form and visual form through graphs. Once the data is collected through constant observations, it is arranged, summarized, and classified to finally represented in the form of a graph. There are two kinds of data - quantitative and qualitative. Quantitative data is more structured, continuous, and discrete with statistical data whereas qualitative is unstructured where the data cannot be analyzed.
Principles of Graphical Representation of Data
The principles of graphical representation are algebraic. In a graph, there are two lines known as Axis or Coordinate axis. These are the X-axis and Y-axis. The horizontal axis is the X-axis and the vertical axis is the Y-axis. They are perpendicular to each other and intersect at O or point of Origin. On the right side of the Origin, the Xaxis has a positive value and on the left side, it has a negative value. In the same way, the upper side of the Origin Y-axis has a positive value where the down one is with a negative value. When -axis and y-axis intersect each other at the origin it divides the plane into four parts which are called Quadrant I, Quadrant II, Quadrant III, Quadrant IV. This form of representation is seen in a frequency distribution that is represented in four methods, namely Histogram, Smoothed frequency graph, Pie diagram or Pie chart, Cumulative or ogive frequency graph, and Frequency Polygon.
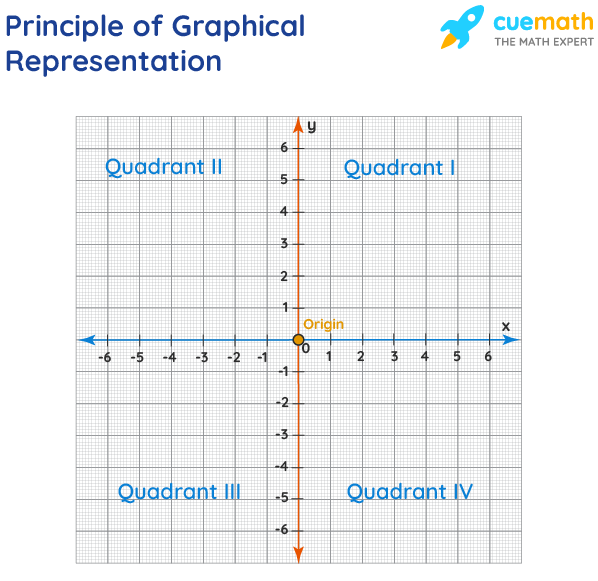
Advantages and Disadvantages of Graphical Representation of Data
Listed below are some advantages and disadvantages of using a graphical representation of data:
- It improves the way of analyzing and learning as the graphical representation makes the data easy to understand.
- It can be used in almost all fields from mathematics to physics to psychology and so on.
- It is easy to understand for its visual impacts.
- It shows the whole and huge data in an instance.
- It is mainly used in statistics to determine the mean, median, and mode for different data
The main disadvantage of graphical representation of data is that it takes a lot of effort as well as resources to find the most appropriate data and then represent it graphically.
Rules of Graphical Representation of Data
While presenting data graphically, there are certain rules that need to be followed. They are listed below:
- Suitable Title: The title of the graph should be appropriate that indicate the subject of the presentation.
- Measurement Unit: The measurement unit in the graph should be mentioned.
- Proper Scale: A proper scale needs to be chosen to represent the data accurately.
- Index: For better understanding, index the appropriate colors, shades, lines, designs in the graphs.
- Data Sources: Data should be included wherever it is necessary at the bottom of the graph.
- Simple: The construction of a graph should be easily understood.
- Neat: The graph should be visually neat in terms of size and font to read the data accurately.
Uses of Graphical Representation of Data
The main use of a graphical representation of data is understanding and identifying the trends and patterns of the data. It helps in analyzing large quantities, comparing two or more data, making predictions, and building a firm decision. The visual display of data also helps in avoiding confusion and overlapping of any information. Graphs like line graphs and bar graphs, display two or more data clearly for easy comparison. This is important in communicating our findings to others and our understanding and analysis of the data.
Types of Graphical Representation of Data
Data is represented in different types of graphs such as plots, pies, diagrams, etc. They are as follows,
Related Topics
Listed below are a few interesting topics that are related to the graphical representation of data, take a look.
- x and y graph
- Frequency Polygon
- Cumulative Frequency
Examples on Graphical Representation of Data
Example 1 : A pie chart is divided into 3 parts with the angles measuring as 2x, 8x, and 10x respectively. Find the value of x in degrees.
We know, the sum of all angles in a pie chart would give 360º as result. ⇒ 2x + 8x + 10x = 360º ⇒ 20 x = 360º ⇒ x = 360º/20 ⇒ x = 18º Therefore, the value of x is 18º.
Example 2: Ben is trying to read the plot given below. His teacher has given him stem and leaf plot worksheets. Can you help him answer the questions? i) What is the mode of the plot? ii) What is the mean of the plot? iii) Find the range.
Solution: i) Mode is the number that appears often in the data. Leaf 4 occurs twice on the plot against stem 5.
Hence, mode = 54
ii) The sum of all data values is 12 + 14 + 21 + 25 + 28 + 32 + 34 + 36 + 50 + 53 + 54 + 54 + 62 + 65 + 67 + 83 + 88 + 89 + 91 = 958
To find the mean, we have to divide the sum by the total number of values.
Mean = Sum of all data values ÷ 19 = 958 ÷ 19 = 50.42
iii) Range = the highest value - the lowest value = 91 - 12 = 79
go to slide go to slide
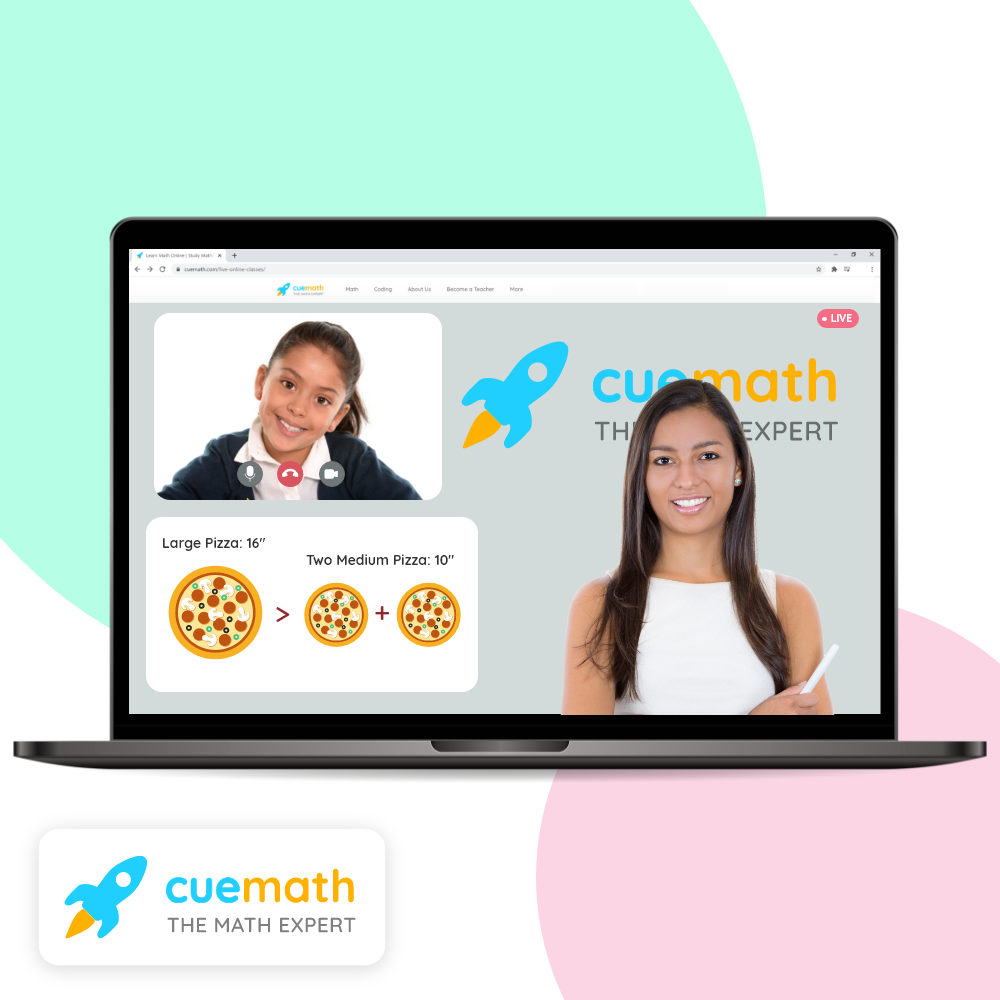
Book a Free Trial Class
Practice Questions on Graphical Representation of Data
Faqs on graphical representation of data, what is graphical representation.
Graphical representation is a form of visually displaying data through various methods like graphs, diagrams, charts, and plots. It helps in sorting, visualizing, and presenting data in a clear manner through different types of graphs. Statistics mainly use graphical representation to show data.
What are the Different Types of Graphical Representation?
The different types of graphical representation of data are:
- Stem and leaf plot
- Scatter diagrams
- Frequency Distribution
Is the Graphical Representation of Numerical Data?
Yes, these graphical representations are numerical data that has been accumulated through various surveys and observations. The method of presenting these numerical data is called a chart. There are different kinds of charts such as a pie chart, bar graph, line graph, etc, that help in clearly showcasing the data.
What is the Use of Graphical Representation of Data?
Graphical representation of data is useful in clarifying, interpreting, and analyzing data plotting points and drawing line segments , surfaces, and other geometric forms or symbols.
What are the Ways to Represent Data?
Tables, charts, and graphs are all ways of representing data, and they can be used for two broad purposes. The first is to support the collection, organization, and analysis of data as part of the process of a scientific study.
What is the Objective of Graphical Representation of Data?
The main objective of representing data graphically is to display information visually that helps in understanding the information efficiently, clearly, and accurately. This is important to communicate the findings as well as analyze the data.
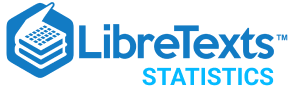
- school Campus Bookshelves
- menu_book Bookshelves
- perm_media Learning Objects
- login Login
- how_to_reg Request Instructor Account
- hub Instructor Commons
Margin Size
- Download Page (PDF)
- Download Full Book (PDF)
- Periodic Table
- Physics Constants
- Scientific Calculator
- Reference & Cite
- Tools expand_more
- Readability
selected template will load here
This action is not available.
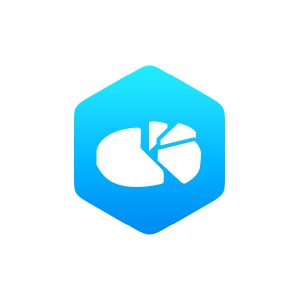
2: Graphical Representations of Data
- Last updated
- Save as PDF
- Page ID 22222
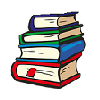
\( \newcommand{\vecs}[1]{\overset { \scriptstyle \rightharpoonup} {\mathbf{#1}} } \)
\( \newcommand{\vecd}[1]{\overset{-\!-\!\rightharpoonup}{\vphantom{a}\smash {#1}}} \)
\( \newcommand{\id}{\mathrm{id}}\) \( \newcommand{\Span}{\mathrm{span}}\)
( \newcommand{\kernel}{\mathrm{null}\,}\) \( \newcommand{\range}{\mathrm{range}\,}\)
\( \newcommand{\RealPart}{\mathrm{Re}}\) \( \newcommand{\ImaginaryPart}{\mathrm{Im}}\)
\( \newcommand{\Argument}{\mathrm{Arg}}\) \( \newcommand{\norm}[1]{\| #1 \|}\)
\( \newcommand{\inner}[2]{\langle #1, #2 \rangle}\)
\( \newcommand{\Span}{\mathrm{span}}\)
\( \newcommand{\id}{\mathrm{id}}\)
\( \newcommand{\kernel}{\mathrm{null}\,}\)
\( \newcommand{\range}{\mathrm{range}\,}\)
\( \newcommand{\RealPart}{\mathrm{Re}}\)
\( \newcommand{\ImaginaryPart}{\mathrm{Im}}\)
\( \newcommand{\Argument}{\mathrm{Arg}}\)
\( \newcommand{\norm}[1]{\| #1 \|}\)
\( \newcommand{\Span}{\mathrm{span}}\) \( \newcommand{\AA}{\unicode[.8,0]{x212B}}\)
\( \newcommand{\vectorA}[1]{\vec{#1}} % arrow\)
\( \newcommand{\vectorAt}[1]{\vec{\text{#1}}} % arrow\)
\( \newcommand{\vectorB}[1]{\overset { \scriptstyle \rightharpoonup} {\mathbf{#1}} } \)
\( \newcommand{\vectorC}[1]{\textbf{#1}} \)
\( \newcommand{\vectorD}[1]{\overrightarrow{#1}} \)
\( \newcommand{\vectorDt}[1]{\overrightarrow{\text{#1}}} \)
\( \newcommand{\vectE}[1]{\overset{-\!-\!\rightharpoonup}{\vphantom{a}\smash{\mathbf {#1}}}} \)
In this chapter, you will study numerical and graphical ways to describe and display your data. This area of statistics is called "Descriptive Statistics." You will learn how to calculate, and even more importantly, how to interpret these measurements and graphs.
- 2.1: Introduction In this chapter, you will study numerical and graphical ways to describe and display your data. This area of statistics is called "Descriptive Statistics." You will learn how to calculate, and even more importantly, how to interpret these measurements and graphs. In this chapter, we will briefly look at stem-and-leaf plots, line graphs, and bar graphs, as well as frequency polygons, and time series graphs. Our emphasis will be on histograms and box plots.
- 2.2: Stem-and-Leaf Graphs (Stemplots), Line Graphs, and Bar Graphs A stem-and-leaf plot is a way to plot data and look at the distribution, where all data values within a class are visible. The advantage in a stem-and-leaf plot is that all values are listed, unlike a histogram, which gives classes of data values. A line graph is often used to represent a set of data values in which a quantity varies with time. These graphs are useful for finding trends. A bar graph is a chart that uses either horizontal or vertical bars to show comparisons among categories.
- 2.3: Histograms, Frequency Polygons, and Time Series Graphs A histogram is a graphic version of a frequency distribution. The graph consists of bars of equal width drawn adjacent to each other. The horizontal scale represents classes of quantitative data values and the vertical scale represents frequencies. The heights of the bars correspond to frequency values. Histograms are typically used for large, continuous, quantitative data sets. A frequency polygon can also be used when graphing large data sets with data points that repeat.
- 2.4: Using Excel to Create Graphs Using technology to create graphs will make the graphs faster to create, more precise, and give the ability to use larger amounts of data. This section focuses on using Excel to create graphs.
- 2.5: Graphs that Deceive It's common to see graphs displayed in a misleading manner in social media and other instances. This could be done purposefully to make a point, or it could be accidental. Either way, it's important to recognize these instances to ensure you are not misled.
- 2.E: Graphical Representations of Data (Exercises) These are homework exercises to accompany the Textmap created for "Introductory Statistics" by OpenStax.
Contributors and Attributions
Barbara Illowsky and Susan Dean (De Anza College) with many other contributing authors. Content produced by OpenStax College is licensed under a Creative Commons Attribution License 4.0 license. Download for free at http://cnx.org/contents/[email protected] .
- Accountancy
- Business Studies
- Organisational Behaviour
- Human Resource Management
- Entrepreneurship
- CBSE Class 11 Statistics for Economics Notes
Chapter 1: Concept of Economics and Significance of Statistics in Economics
- Statistics for Economics | Functions, Importance, and Limitations
Chapter 2: Collection of Data
- Data Collection & Its Methods
- Sources of Data Collection | Primary and Secondary Sources
- Direct Personal Investigation: Meaning, Suitability, Merits, Demerits and Precautions
- Indirect Oral Investigation : Suitability, Merits, Demerits and Precautions
- Difference between Direct Personal Investigation and Indirect Oral Investigation
- Information from Local Source or Correspondents: Meaning, Suitability, Merits, and Demerits
- Questionnaires and Schedules Method of Data Collection
- Difference between Questionnaire and Schedule
- Qualities of a Good Questionnaire and types of Questions
- What are the Published Sources of Collecting Secondary Data?
- What Precautions should be taken before using Secondary Data?
- Two Important Sources of Secondary Data: Census of India and Reports & Publications of NSSO
- What is National Sample Survey Organisation (NSSO)?
- What is Census Method of Collecting Data?
- Sample Method of Collection of Data
- Methods of Sampling
- Father of Indian Census
- What makes a Sampling Data Reliable?
- Difference between Census Method and Sampling Method of Collecting Data
- What are Statistical Errors?
Chapter 3: Organisation of Data
- Organization of Data
- Objectives and Characteristics of Classification of Data
- Classification of Data in Statistics | Meaning and Basis of Classification of Data
- Concept of Variable and Raw Data
- Types of Statistical Series
- Difference between Frequency Array and Frequency Distribution
- Types of Frequency Distribution
Chapter 4: Presentation of Data: Textual and Tabular
- Textual Presentation of Data: Meaning, Suitability, and Drawbacks
- Tabular Presentation of Data: Meaning, Objectives, Features and Merits
- Different Types of Tables
- Classification and Tabulation of Data
Chapter 5: Diagrammatic Presentation of Data
- Diagrammatic Presentation of Data: Meaning , Features, Guidelines, Advantages and Disadvantages
- Types of Diagrams
- Bar Graph | Meaning, Types, and Examples
- Pie Diagrams | Meaning, Example and Steps to Construct
- Histogram | Meaning, Example, Types and Steps to Draw
- Frequency Polygon | Meaning, Steps to Draw and Examples
- Ogive (Cumulative Frequency Curve) and its Types
- What is Arithmetic Line-Graph or Time-Series Graph?
Diagrammatic and Graphic Presentation of Data
Chapter 6: measures of central tendency: arithmetic mean.
- Measures of Central Tendency in Statistics
- Arithmetic Mean: Meaning, Example, Types, Merits, and Demerits
- What is Simple Arithmetic Mean?
- Calculation of Mean in Individual Series | Formula of Mean
- Calculation of Mean in Discrete Series | Formula of Mean
- Calculation of Mean in Continuous Series | Formula of Mean
- Calculation of Arithmetic Mean in Special Cases
- Weighted Arithmetic Mean
Chapter 7: Measures of Central Tendency: Median and Mode
- Median(Measures of Central Tendency): Meaning, Formula, Merits, Demerits, and Examples
- Calculation of Median for Different Types of Statistical Series
- Calculation of Median in Individual Series | Formula of Median
- Calculation of Median in Discrete Series | Formula of Median
- Calculation of Median in Continuous Series | Formula of Median
- Graphical determination of Median
- Mode: Meaning, Formula, Merits, Demerits, and Examples
- Calculation of Mode in Individual Series | Formula of Mode
- Calculation of Mode in Discrete Series | Formula of Mode
- Grouping Method of Calculating Mode in Discrete Series | Formula of Mode
- Calculation of Mode in Continuous Series | Formula of Mode
- Calculation of Mode in Special Cases
- Calculation of Mode by Graphical Method
- Mean, Median and Mode| Comparison, Relationship and Calculation
Chapter 8: Measures of Dispersion
- Measures of Dispersion | Meaning, Absolute and Relative Measures of Dispersion
- Range | Meaning, Coefficient of Range, Merits and Demerits, Calculation of Range
- Calculation of Range and Coefficient of Range
- Interquartile Range and Quartile Deviation
- Partition Value | Quartiles, Deciles and Percentiles
- Quartile Deviation and Coefficient of Quartile Deviation: Meaning, Formula, Calculation, and Examples
- Quartile Deviation in Discrete Series | Formula, Calculation and Examples
- Quartile Deviation in Continuous Series | Formula, Calculation and Examples
- Mean Deviation: Coefficient of Mean Deviation, Merits, and Demerits
- Calculation of Mean Deviation for different types of Statistical Series
- Mean Deviation from Mean | Individual, Discrete, and Continuous Series
- Mean Deviation from Median | Individual, Discrete, and Continuous Series
- Standard Deviation: Meaning, Coefficient of Standard Deviation, Merits, and Demerits
- Standard Deviation in Individual Series
- Methods of Calculating Standard Deviation in Discrete Series
- Methods of calculation of Standard Deviation in frequency distribution series
- Combined Standard Deviation: Meaning, Formula, and Example
- How to calculate Variance?
- Coefficient of Variation: Meaning, Formula and Examples
- Lorenz Curveb : Meaning, Construction, and Application
Chapter 9: Correlation
- Correlation: Meaning, Significance, Types and Degree of Correlation
- Methods of measurements of Correlation
- Calculation of Correlation with Scattered Diagram
- Spearman's Rank Correlation Coefficient
- Karl Pearson's Coefficient of Correlation
- Karl Pearson's Coefficient of Correlation | Methods and Examples
Chapter 10: Index Number
- Index Number | Meaning, Characteristics, Uses and Limitations
- Methods of Construction of Index Number
- Unweighted or Simple Index Numbers: Meaning and Methods
- Methods of calculating Weighted Index Numbers
- Fisher's Index Number as an Ideal Method
- Fisher's Method of calculating Weighted Index Number
- Paasche's Method of calculating Weighted Index Number
- Laspeyre's Method of calculating Weighted Index Number
- Laspeyre's, Paasche's, and Fisher's Methods of Calculating Index Number
- Consumer Price Index (CPI) or Cost of Living Index Number: Construction of Consumer Price Index|Difficulties and Uses of Consumer Price Index
- Methods of Constructing Consumer Price Index (CPI)
- Wholesale Price Index (WPI) | Meaning, Uses, Merits, and Demerits
- Index Number of Industrial Production : Characteristics, Construction & Example
- Inflation and Index Number
Important Formulas in Statistics for Economics
- Important Formulas in Statistics for Economics | Class 11
Diagrammatic and graphic presentation of data means visual representation of the data. It shows a comparison between two or more sets of data and helps in the presentation of highly complex data in its simplest form. Diagrams and graphs are clear and easy to read and understand. In the diagrammatic presentation of data, bar charts, rectangles, sub-divided rectangles, pie charts, or circle diagrams are used. In the graphic presentation of data, graphs like histograms, frequency polygon, frequency curves, cumulative frequency polygon, and graphs of time series are used.
General Rules for Construction of Diagrammatic and Graphic Presentations:
1. Chronic Number: Each outline or chart should have a chronic number. It is important to recognize one from the other.
2. Title: A title should be given to each outline or chart. From the title, one can understand what the graph or diagram is. The title ought to be brief and simple. It is normally positioned at the top.
3. Legitimate size and scale: An outline or chart ought to be of ordinary size and drawn with an appropriate scale. The scale in a chart indicates the size of the unit.
4. Neatness: Outlines should be pretty much as straightforward as could be expected. Further, they should be very perfect and clean. They ought to likewise be dropped to check out.
5. File: Each outline or chart should be joined by a record. This outlines various sorts of lines, shades or tones utilized in the graph.
6. Commentary: Commentaries might be given at the lower part of an outline. It explains specific focuses in the chart.
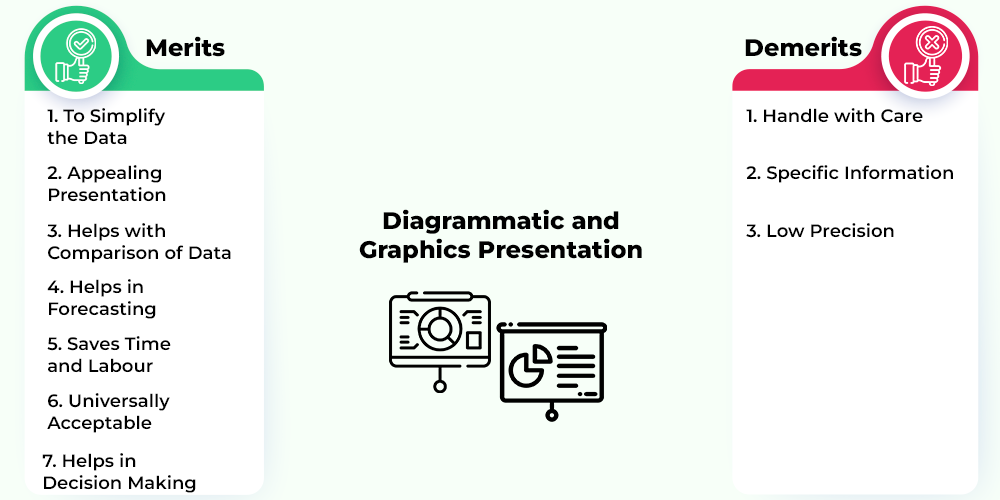
Merits of Diagrammatic and Graphics Presentation:
The fundamental benefits or merits of a diagrammatic and graphical representation of data are as follows:
1. To simplify the data: Outlines and charts present information in a simple manner that can be perceived by anyone without any problem. Huge volume of data can be easily presented using graphs and diagrams.
2. Appealing presentation: Outlines and charts present complex information and data in an understandable and engaging manner and leave a great visual effect. In this way, the diagrammatic and graphical representation of information effectively draws the attention of users.
3. Helps with comparison of data: With the help of outlines and charts, comparison and examination data between various arrangements of information is possible.
4. Helps in forecasting: The diagrammatic and graphical representation of information has past patterns, which helps in forecasting and making various policies for the future.
5. Saves time and labour: Charts and graphs make the complex data into a simple form, which can be easily understood by anyone without having prior knowledge of the data. It gives ready to use information, and the user can use it accordingly. In this way, it saves a lot of time and labour.
6. Universally acceptable: Graphs and diagrams are used in every field and can be easily understood by anyone. Hence they are universally acceptable.
7. Helps in decision making: Diagrams and graphs give the real data about the past patterns, trends, outcomes, etc., which helps in future preparation.
Demerits of Diagrammatic and Graphics Presentation:
The demerits of diagrammatic and graphics presentation of data are as follows:
1. Handle with care: Drawing, surmising and understanding from graphs and diagrams needs proper insight and care. A person with little knowledge of statistics cannot analyze or use the data properly.
2. Specific information: Graphs and diagrams do not depict true or precise information. They are generally founded on approximations. The information provided is limited and specific.
3. Low precision: Graphs and diagrams can give misleading results, as they are mostly based on approximation of data. Personal judgement is used to study or analyze the data, which can make the information biased. Also, data can easily be manipulated.
Please Login to comment...
Similar reads.
- Statistics for Economics
Improve your Coding Skills with Practice
What kind of Experience do you want to share?
- Math Article
Graphical Representation
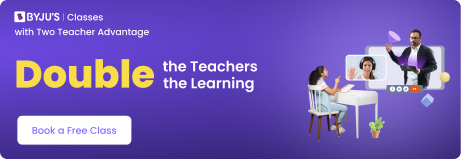
Graphical Representation is a way of analysing numerical data. It exhibits the relation between data, ideas, information and concepts in a diagram. It is easy to understand and it is one of the most important learning strategies. It always depends on the type of information in a particular domain. There are different types of graphical representation. Some of them are as follows:
- Line Graphs – Line graph or the linear graph is used to display the continuous data and it is useful for predicting future events over time.
- Bar Graphs – Bar Graph is used to display the category of data and it compares the data using solid bars to represent the quantities.
- Histograms – The graph that uses bars to represent the frequency of numerical data that are organised into intervals. Since all the intervals are equal and continuous, all the bars have the same width.
- Line Plot – It shows the frequency of data on a given number line. ‘ x ‘ is placed above a number line each time when that data occurs again.
- Frequency Table – The table shows the number of pieces of data that falls within the given interval.
- Circle Graph – Also known as the pie chart that shows the relationships of the parts of the whole. The circle is considered with 100% and the categories occupied is represented with that specific percentage like 15%, 56%, etc.
- Stem and Leaf Plot – In the stem and leaf plot, the data are organised from least value to the greatest value. The digits of the least place values from the leaves and the next place value digit forms the stems.
- Box and Whisker Plot – The plot diagram summarises the data by dividing into four parts. Box and whisker show the range (spread) and the middle ( median) of the data.
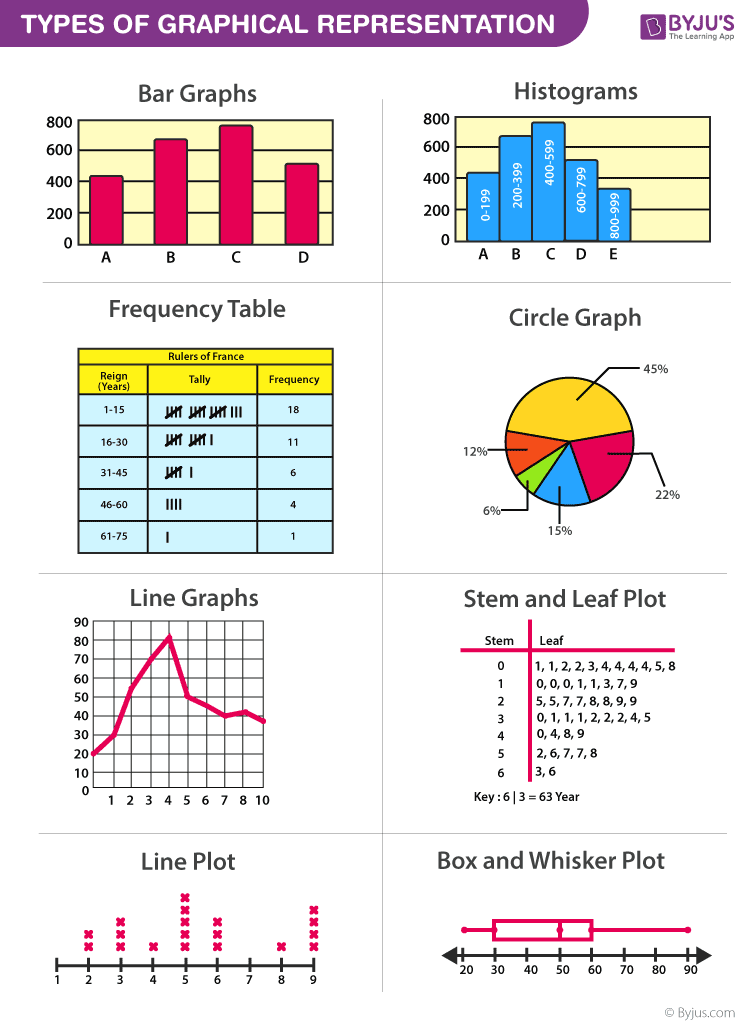
General Rules for Graphical Representation of Data
There are certain rules to effectively present the information in the graphical representation. They are:
- Suitable Title: Make sure that the appropriate title is given to the graph which indicates the subject of the presentation.
- Measurement Unit: Mention the measurement unit in the graph.
- Proper Scale: To represent the data in an accurate manner, choose a proper scale.
- Index: Index the appropriate colours, shades, lines, design in the graphs for better understanding.
- Data Sources: Include the source of information wherever it is necessary at the bottom of the graph.
- Keep it Simple: Construct a graph in an easy way that everyone can understand.
- Neat: Choose the correct size, fonts, colours etc in such a way that the graph should be a visual aid for the presentation of information.
Graphical Representation in Maths
In Mathematics, a graph is defined as a chart with statistical data, which are represented in the form of curves or lines drawn across the coordinate point plotted on its surface. It helps to study the relationship between two variables where it helps to measure the change in the variable amount with respect to another variable within a given interval of time. It helps to study the series distribution and frequency distribution for a given problem. There are two types of graphs to visually depict the information. They are:
- Time Series Graphs – Example: Line Graph
- Frequency Distribution Graphs – Example: Frequency Polygon Graph
Principles of Graphical Representation
Algebraic principles are applied to all types of graphical representation of data. In graphs, it is represented using two lines called coordinate axes. The horizontal axis is denoted as the x-axis and the vertical axis is denoted as the y-axis. The point at which two lines intersect is called an origin ‘O’. Consider x-axis, the distance from the origin to the right side will take a positive value and the distance from the origin to the left side will take a negative value. Similarly, for the y-axis, the points above the origin will take a positive value, and the points below the origin will a negative value.
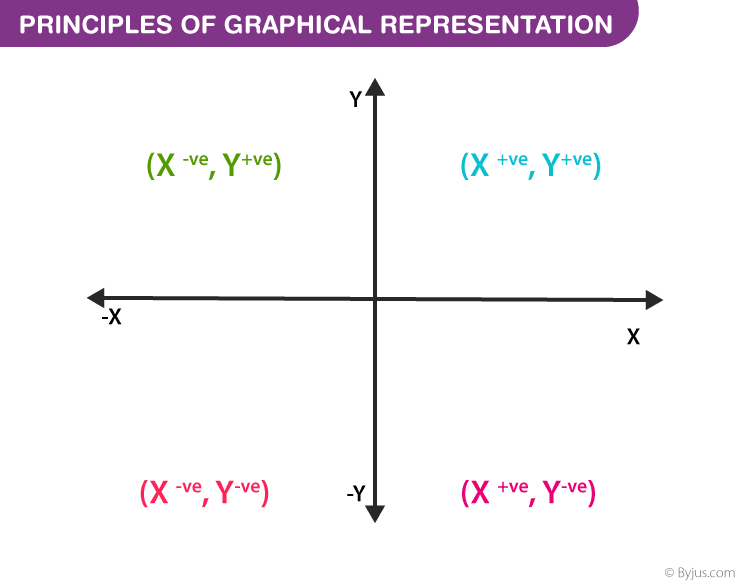
Generally, the frequency distribution is represented in four methods, namely
- Smoothed frequency graph
- Pie diagram
- Cumulative or ogive frequency graph
- Frequency Polygon
Merits of Using Graphs
Some of the merits of using graphs are as follows:
- The graph is easily understood by everyone without any prior knowledge.
- It saves time
- It allows us to relate and compare the data for different time periods
- It is used in statistics to determine the mean, median and mode for different data, as well as in the interpolation and the extrapolation of data.
Example for Frequency polygonGraph
Here are the steps to follow to find the frequency distribution of a frequency polygon and it is represented in a graphical way.
- Obtain the frequency distribution and find the midpoints of each class interval.
- Represent the midpoints along x-axis and frequencies along the y-axis.
- Plot the points corresponding to the frequency at each midpoint.
- Join these points, using lines in order.
- To complete the polygon, join the point at each end immediately to the lower or higher class marks on the x-axis.
Draw the frequency polygon for the following data
Mark the class interval along x-axis and frequencies along the y-axis.
Let assume that class interval 0-10 with frequency zero and 90-100 with frequency zero.
Now calculate the midpoint of the class interval.
Using the midpoint and the frequency value from the above table, plot the points A (5, 0), B (15, 4), C (25, 6), D (35, 8), E (45, 10), F (55, 12), G (65, 14), H (75, 7), I (85, 5) and J (95, 0).
To obtain the frequency polygon ABCDEFGHIJ, draw the line segments AB, BC, CD, DE, EF, FG, GH, HI, IJ, and connect all the points.
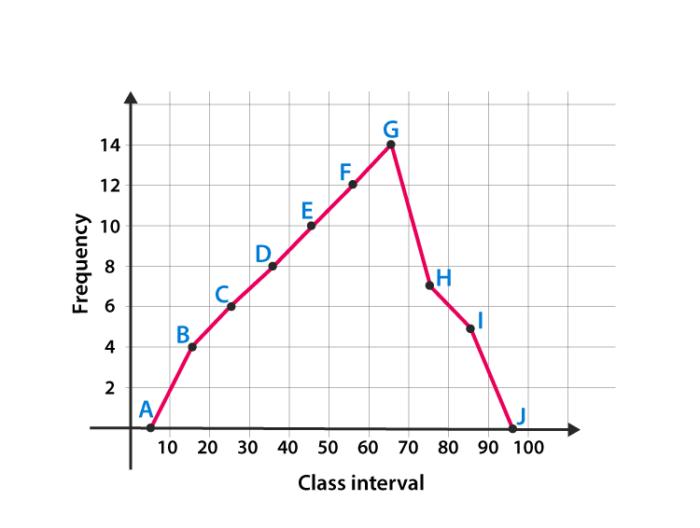
Frequently Asked Questions
What are the different types of graphical representation.
Some of the various types of graphical representation include:
- Line Graphs
- Frequency Table
- Circle Graph, etc.
Read More: Types of Graphs
What are the Advantages of Graphical Method?
Some of the advantages of graphical representation are:
- It makes data more easily understandable.
- It saves time.
- It makes the comparison of data more efficient.
Leave a Comment Cancel reply
Your Mobile number and Email id will not be published. Required fields are marked *
Request OTP on Voice Call
Post My Comment

Very useful for understand the basic concepts in simple and easy way. Its very useful to all students whether they are school students or college sudents
Thanks very much for the information

Register with BYJU'S & Download Free PDFs
Register with byju's & watch live videos.
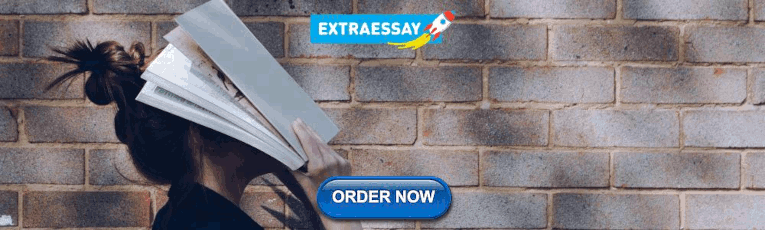
COMMENTS
Graphical representation is the visual display of data using plots and charts. It is used in many academic and professional disciplines but most widely so in the fields of mathematics, medicine and sciences. Graphical representation helps to quantify, sort and present data in a method that is understandable to a large variety of audiences.
How to develop a graphical framework to chart your research. Graphic representations or frameworks can be powerful tools to explain research processes and outcomes. David Waller explains how researchers can develop effective visual models to chart their work. Outreach and communication. Writing tips.
Background In research, diagrams are most commonly used in the analysis of data and visual presentation of results. However there has been a substantial growth in the use of diagrams in earlier stages of the research process to collect data. Despite this growth, guidance on this technique is often isolated within disciplines. Methods A multidisciplinary systematic review was performed, which ...
Data display in a graphic format is a way of portraying information succinctly and efficiently, illustrating details provided in longer textual information. Visual displays provide a multidimensional space to organize data and show connections between different pieces of relevant data (Dey, 1993). A main goal of any diagram is to provide ready
Graph, plot, and chart often refer to the display of data, data ... this may still represent a challenge to some. However, figure making is a method like anything else, and in order to do it, new methodologies may need to be learned. ... geometries are representations of the data in different forms, and often there may be more than one geometry ...
Discussion/Conclusion. The results of this multidisciplinary systematic review examine the application of diagrams in data collection and the methods for analyzing the unique datasets elicited. Three recommendations are presented. Firstly, the diagrammatic approach should be chosen based on the type of data needed.
A graph is a pictorial representation of data. In fact, it is a mathematical picture which presents the data in a visual mode. ... Bar diagram is a graphical representation of data in the form of rectangular bars shown vertically or horizontally ... J.P. (2019). Graphical Presentation of Data. In: Statistics and Research Methods in Psychology ...
1. Diagrams are meant only to give a pictorial representation to the quantitative data with a view to make them comprehensive. 2. Diagrams do not approve or disapprove a particular fact. 3. Diagrams are not suitable for further analysis of data which could only be possible from tables with numeric values. 4.
An alternative to the representation of an experimental design is given first and, at the end, a diagram of an actual survey study. Finally, the consequences for various fields are discussed. This kind of diagramming could be beneficially used in methodological consultation and education. Modelling meta data and meta analysis are other ...
Here, the interval width is too large, resulting in only two intervals for our data. With such few intervals it is difficult to identify any patterns in the data. We can get a better idea about what is going on if we choose a smaller interval width - say 5. Doing so gives the following stem and leaf plot: 2 4.
In qualitative research, graphic representation tools are more commonly used for data analysis and reporting than for the elicitation of data from participants (Umoquit, Tso, Burchett, & Dobrow, 2011; Wheeldon, 2010), although their use for data collection does appear to be on the rise (Umoquit et al., 2011). Graphic elicitation is used when ...
Grounded theorists believe that creating visual representations of the emerging theories is an intrinsic and essential step in theory building (Clarke, 2005; Charmaz, 2006; Strauss, 1987; Strauss & Corbin, 1998).This qualitative tradition of inquiry strongly encourages the use of diagrams and figures to synthesize major theoretical concepts and their connections.
Here are some examples of real-time applications of graphical methods: Stock Market: Line graphs, candlestick charts, and bar charts are widely used in real-time trading systems to display stock prices and trends over time. Traders use these charts to analyze historical data and make informed decisions about buying and selling stocks in real-time.
Graphical representation is a form of visually displaying data through various methods like graphs, diagrams, charts, and plots. It helps in sorting, visualizing, and presenting data in a clear manner through different types of graphs. Statistics mainly use graphical representation to show data.
A histogram is a graphic version of a frequency distribution. The graph consists of bars of equal width drawn adjacent to each other. The horizontal scale represents classes of quantitative data values and the vertical scale represents frequencies. The heights of the bars correspond to frequency values. Histograms are typically used for large ...
Diagrammatic and Graphic Presentation 29 6) They have become an integral part of research: In fact, now a days it is difficult to find any research work without visual support. The reason is that this is the most convincing and appealing way of presenting the data. You can find diagrammatic and graphic presentation of data in journals, magazines,
Part 6 (Graphic representation of data) of Chapter 3 (Research methods): Research methodology textbook on assessing the impact of urban built form on micro-temperature change - Chapter preview
13. Sub-divided Bar Diagram • In a sub-divided bar diagram, the bar is sub-divided into various parts in proportion to the values given in the data and the whole bar represent the total. • Such diagrams are also called Component Bar diagrams. • The sub divisions are distinguished by different colors or crossings or dotting.
The fundamental benefits or merits of a diagrammatic and graphical representation of data are as follows: 1. To simplify the data: Outlines and charts present information in a simple manner that can be perceived by anyone without any problem. Huge volume of data can be easily presented using graphs and diagrams. 2.
Graphical Representation is a way of analysing numerical data. It exhibits the relation between data, ideas, information and concepts in a diagram. It is easy to understand and it is one of the most important learning strategies. It always depends on the type of information in a particular domain. There are different types of graphical ...
The diagrammatic representation of data is a method used in the analysis and exploration of information with the help of diagrams. It refers to different methods that convert numbers into graphic forms, such as bar graphs, circle charts, and histograms. This also includes the use of color, layout, and shape to encode data.