- Search Menu
- Sign in through your institution
- Advanced Articles
- Editor's Choice
- Author Guidelines
- Publish with us
- Submission Site
- Open Access
- Self-Archiving Policy
- About Clean Energy
- About the National Institute of Clean and Low-Carbon Energy
- Editorial Board
- Instructions for Reviewers
- Advertising & Corporate Services
- Journals Career Network
- Journals on Oxford Academic
- Books on Oxford Academic

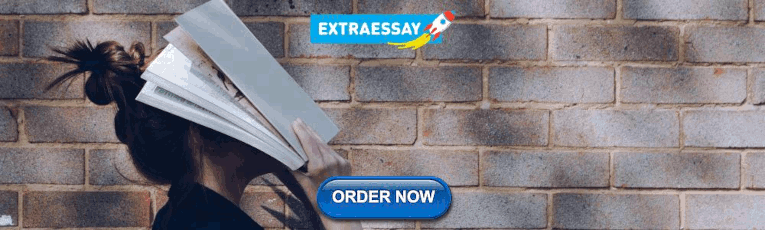
Article Contents
Introduction, 1 smart-home definition, 2 smart-home infrastructures, 3 smart-home energy-management scheme, 4 technical challenges of smart homes, 5 conclusion, conflict of interest.
- < Previous
Smart homes: potentials and challenges
- Article contents
- Figures & tables
- Supplementary Data
Rasha El-Azab, Smart homes: potentials and challenges, Clean Energy , Volume 5, Issue 2, June 2021, Pages 302–315, https://doi.org/10.1093/ce/zkab010
- Permissions Icon Permissions
Decentralized distributed clean-energy sources have become an essential need for smart grids to reduce the harmful effects of conventional power plants. Smart homes with a suitable sizing process and proper energy-management schemes can share in reducing the whole grid demand and even sell clean energy to the utility. Smart homes have been introduced recently as an alternative solution to classical power-system problems, such as the emissions of thermal plants and blackout hazards due to bulk plants/transmission outages. The appliances, sources and energy storage of smart homes should be coordinated with the requirements of homeowners via a suitable energy-management scheme. Energy-management systems are the main key to optimizing both home sources and the operation of loads to maximize home-economic benefits while keeping a comfortable lifestyle. The intermittent uncertain nature of smart homes may badly affect the whole grid performance. The prospective high penetration of smart homes on a smart power grid will introduce new, unusual scenarios in both generation and loading. In this paper, the main features and requirements of smart homes are defined. This review aims also to address recent proposed smart-home energy-management schemes. Moreover, smart-grid challenges with a high penetration of smart-home power are discussed.

Smart homes provide comfortable, fully controlled and secure lifestyles to their occupants. Moreover, smart homes can save energy and money with the possibility of profiting from selling clean renewable energy to the grid. On the other hand, the probable decrease in total domestic-energy loads encourages many governments to support promising smart-home technologies. Some countries have already put out many rules, laws and subsidy programmes to encourage the integration of smart homes, such as encouraging the optimization of the heating system, supporting building energy storage and/or deploying smart meters. For instance, the European Standard EN 15232 [ 1 ] and the Energy Performance of Building Directive 2010/31/EU [ 2 ], which is in line with Directive 2009/72/EC as well as the Energy Road Map 2050 [ 3 ], encourage the integration of smart-home technologies to decrease power demand in residential areas.
To control the environment, a smart home is automated by controlling some appliances, such as those used for lighting and heating, based on different climatic conditions. Now, recent control schemes adapt many functions besides classical switching ones. They can monitor the internal environment and the activities of the home occupants. They also can independently take pre-programmed actions and operate devices in set predefined patterns, independently or according to the user’s requirements. Besides the ease of life, smart homes confirm efficient usage of electricity, lowering peak load, reducing energy bills and minimizing greenhouse-gas emissions [ 4 , 5 ].
Smart homes can be studied from many points of view. The communication systems [ 6 ], social impacts [ 7 ], thermal characteristics [ 8 ], technologies and trends of smart homes [ 9 ] are reviewed individually. Moreover, the monitoring and modelling of smart-home appliances via smart meters are reviewed for accurate load forecasting, as in [ 10 , 11 ]. Recently, power-grid authorities have modified residential electrical tariffs to encourage proper demand-side management by homeowners. Different from previous reviews, this paper introduces smart homes from the electrical/economic point of view. It also discusses smart-home energy-management systems (SHEMS) in two different modes, offline load scheduling and real-time management. The prospective impacts of unusual smart-home power profiles on future smart grids are also summarized.
After this introductory section, Section 1 describes the different definitions of smart homes within the last two decades. Smart-home communication schemes and other infrastructures of smart homes are discussed in Section 2. Section 3 discusses in more detail the existing functions of SHEMS, their pre-proposed optimization techniques and related technical/economical objective functions. The impacts of smart homes on modern grids are also discussed in Section 4. Finally, in Section 5, the main conclusions and contributions of the paper are highlighted.
The term ‘smart home’ has been commonly used for about two decades to describe houses with controlled energy schemes. This automation scheme confirms easier lifestyles for homeowners than normal un-automated homes, especially for elderly or disabled persons. Recently, the concept of ‘smart home’ has a wider description to include many applications of technologies in one place.
Sowah et al. [ 12 ] define smart homes as: ‘Houses that provide their occupants a comfortable, secure, and energy efficient environment with minimum possible costs regardless their occupants.’ The Smart Homes Association defines a smart home as: ‘The integration of technology and services through home networking for a better quality of living’ [ 13 ].
Makhadmeh et al. define them as: ‘Incorporated residential houses with smart technology to improve the comfort level of users (residents) by enhancing safety and healthcare and optimizing power consumption. Users can control and monitor smart-home appliances remotely through the home energy-management system (HEMS), which provides a remote monitoring system that uses telecommunication technology’ [ 14 ].
Smart homes can be defined as: any residential buildings using different communication schemes and optimization algorithms to predict, analyse, optimize and control its energy-consumption patterns according to preset users’ preferences to maximize home-economic benefits while preserving predefined conditions of a comfortable lifestyle.
Distributed clean energy generated by smart homes provides many benefits for prospective smart grids. Consequently, the effects of smart homes on future power grids should be extensively studied. In the near future, smart homes will play a major role as a power supplier in modern grids, not only as a power consumer.
The general infrastructure of smart homes consists of control centres, resources of electricity, smart meters and communication tools, as shown in Fig. 1 . Each component of the smart-home model will be discussed in the following subsections.

Infrastructure of SHEMS source
2.1 The control centre
The control centre provides home users with proper units to monitor and control different home appliances [ 15 ]. All real-time data are collected by SHEMS to optimize the demand/generation coordination and verify the predefined objectives. The main functions of the control centre can be summarized as follows [ 15 ]:
(i) collecting data from different meters, homeowners’ commands and grid utility via a proper communication system;
(ii) providing proper monitoring and analysing of home-energy consumption for homeowners;
(iii) coordinating between different appliances and resources to satisfy the optimal solution for predefined objectives.
2.2 Smart meter
The smart meter receives a demand-response signal from power utilities as an input to the SHEMS system [ 16 , 17 ]. Recently, advanced smart-metering infrastructures can monitor many home features such as electrical consumption, gas, water and heating [ 18 ].
2.3 Appliances
Smart-home loads can be divided according to their operating nature into two categories: schedulable and non-schedulable loads. Non-schedulable loads are operated occasionally according to the homeowner’s desires without any predictable operating patterns, such as printers, televisions and hairdryers, whereas schedulable loads have a predictable operating pattern that can be shifted or controlled via SHEMS, such as washing machines and air conditioners [ 19 ].
According to [ 19 ], controllable devices are also classified into interruptible and non-interruptible load according to the effect of supply interruption on their tasks. Electric vehicles (EVs) can be considered as an exceptional load [ 20 , 21 ]. EVs have two operating modes: charging and discharging. Therefore, EVs are interruptible schedulable loads during the charging mode. Moreover, EV battery energy can also be discharged to supply power to the grid during critical events, which is known as vehicle-to-grid [ 22 ]. By SHEMS, EVs can participate in supplying loads during high-priced power periods. In low-priced power periods, EVs restore their energy from the grid [ 23 , 24 ].
2.4 Resources of electricity
Solar and wind plants are the most mature renewable-energy sources in modern grids. Nowadays, many buildings have installed photovoltaic (PV) modules, thermal solar heaters or micro wind turbines. For smart homes, various functions can be supplied by solar energy besides generating electricity, such as a solar water heater (SWH), solar dryer and solar cooler [ 25 ]. Moreover, PV plants are cheap with low requirements of maintenance [ 26 ], whereas hot water produced by SWHs can be used in many home functions, such as washing and cooking, which increases the home-energy efficiency [ 27 ].
Energy storage may be considered as the cornerstone for any SHEMS. SHEMS are usually installed with energy-storage systems (ESSs) to manage their stored energy according to predefined objectives. Many energy-storage technologies are available in the power markets. Batteries and fuel cells are the most compatible energy-storage types of smart-home applications [ 28 ]. A fuel-cell structure is very similar to a battery. During the charging process, hydrogen fuel cells use electricity to produce hydrogen. Hydrogen feeds the fuel cell to create electricity during the discharging process. Fuel cells have relatively low efficiency compared to batteries. Fuel cells provide extra clean storage environments with the capability of storing extra hydrogen tanks. That perfectly matches isolated homes in remote areas [ 29 ].
Although wind energy is more economical for large-scale plants, it has a very limited market for micro wind turbines in homes. Typically, micro wind turbines require at least a wind speed of 2.7 m/s to generate minimum power, 25 m/s for rated power and 40 m/s for continuous generated power [ 30 ]. A micro wind turbine is relatively expensive, intermittent and needs special maintenance requirements and constraints compared to a solar plant [ 31 ].
Recently, biomass energy has been a promising renewable resource alternative for smart homes. Many pieces of research have recommended biomass energy for different types of buildings [ 32 ]. Heating is the main function of biomass in smart homes, as discussed in [ 33 , 34 ]. In addition, a biomass-fuelled generation system is examined for many buildings [ 35 , 36 ].
2.5 Communication schemes
Recently, communication systems are installed as built-in modules in smart homes. Both home users and grid operators will be able to monitor and control several home appliances in the near future to satisfy the optimum home-energy profile while preserving a comfortable lifestyle. Therefore, both wired and wireless communication schemes are utilized, which is known as a home area network (HAN), to cover remote-control signals as home occupants’ ones. Fig. 1 shows an example of a HAN that consists of Wi-Fi and cloud computing networks for both indoor and outdoor data exchange, respectively [ 37 , 38 ].
Energy-management systems for homes require three main components: the computational embedded controllers, the local-area network communication middleware and the transmission control protocol/internet protocol (TCP/IP) communication for wide-area integration with the utility company using wide-area network communication [ 37 ].
According to home characteristics, many wired communication schemes can be selected, such as power-line communication (PLC), inter-integrated circuit (I2C) and serial peripheral interface or wireless technologies such as Zigbee, Wi-Fi, radio-frequency identification (RFID) and the Internet of Things (IoT) to develop HANs. A few of the most common techniques will be discussed briefly in the following subsections [ 38 ].
PLC is a technique that uses power lines to transmit both power and data via the same cable to customers simultaneously. Such wired schemes provide fast communication with low interference of data. Moreover, PLC provides many communication terminals, as all power plugs can be used for data transferring. As all electrical home devices are connected by power cables, PLC can communicate with all these devices via the same cable.
PLC set-up has a low cost, as it uses pre-installed power cables with minimum hardware requirements. With a PLC communication scheme, home controllers can also be integrated easily with a high speed of data transfer. On the other hand, PLC has a high probability of data-signal attenuation. Furthermore, data signals suffer from electromagnetic interference of transmitted power signals.
2.5.2 Zigbee
Zigbee is a wireless communication technique [ 37–46 ]. Zigbee follows the IEEE 802.15.4 standard as a radio-frequency wireless communication scheme. It does not require any licenses for limited zones such as homes [ 37 ]. Also, Zigbee is a low-power-consuming technique. Therefore, it is suitable for basic home appliances, such as lighting, alarm systems and air conditioners [ 39 , 40 ]. Zigbee usually considers all home devices as slaves with a master coordinator/controller, which is known as a master–slave architecture.
Zigbee provides highly secured transferred data [ 38 , 41 ] with high reliability and capacity [ 42 ]. It also has self-organizing capabilities [ 42 ]. Conversely, Zigbee is relatively expensive due to special hardware requirements with low data-transfer rates. Moreover, Zigbee is not compatible with many other protocols, such as internet-supported protocols and Wi-Fi.
2.5.3 Wi-Fi technology
Wi-Fi is a wireless communication technique that follows the IEEE 802.11 standard. Wi-Fi provides high-rate data transfer that is compatible with many information-based devices such as computers, laptops, etc. [ 43 , 44 ].
Wi-Fi is a highly secured scheme with many of the familiar internet capabilities and low data-transfer delays (<3 ms) [ 45 ]. On the contrary, it is a relatively high-power-consuming scheme compared to Zigbee schemes [ 45 ]. Also, home devices can affect transmitted data signals by their emitted electromagnetic fields [ 46 ]. Wi-Fi can also suffer from interference from other communication protocols such as Zigbee and Bluetooth [ 43 ].
RFID is a wireless communication technique that conforms to the electronic product code protocol [ 47–52 ]. It can coincide with other communication schemes such as Wi-Fi and Zigbee. It can be utilized for a relatively widespread range of frequencies, from 120 kHz to 10 GHz. It also covers a wide range of distances, from 10 cm to 200 m [ 48 ]. Many researchers are investigating RFID home applications, such as energy-management systems [ 49 ], door locks [ 50 ] and lighting controls [ 51 ].
RFID operates on tags and reader-identification systems with a high data-transfer rate. Nevertheless, RFID has expensive chips with low bandwidth. The possibility of tag collision within the same zone decreases the accuracy of the RFID scheme.
This scheme connects home devices, users and grid operators via the internet to monitor and manage smart homes [ 6 , 38 , 53–65 ]. Consequently, the IoT and cloud computing have proven to be cheap, popular and easy services for smart homes. Moreover, IoT schemes are compatible with many other communication protocols, such as Zigbee, Bluetooth, etc., as listed in Table 1 . Internet hacking is the main problem with IoT schemes. System security and privacy are critical challenges for such internet-based schemes.
IoT protocols features
Today, building energy-management systems (BEMS) are utilized within residential, commercial, administration and industrial buildings. Moreover, the integration of variable renewable-energy sources with proper ESSs deployed in buildings represents an essential need for reliable, efficient BEMS.
For small-scale residential buildings or ‘homes’, BEMS should deal with variable uncertain load behaviours according to the home occupants’ desires and requirements, which is known as SHEMS. Throughout recent decades, many SHEMS have been presented and defined in many research studies.
In [ 66 ], SHEMS are defined as services that efficiently monitor and manage electricity generation, storage and consumption in smart houses. Nazabal et al. [ 67 ] include a collaborative exchange between smart homes and the utility as a main function of SHEMS. In [ 68 ], SHEMS are defined from the electrical-grid point of view as important tools that provide several benefits such as flattening the load curve, a reduction in peak demand and meeting the demand-side requirements.
3.1 Functions of SHEMS
Adaptive SHEMS are required to conserve power, especially with the increasing evolution in home loads. SHEMS should control both home appliances and available energy resources according to the real-time tariff and home user’s requirements [ 4 ]. Home-management schemes should provide an interface platform between home occupants and the home controller to readjust occasionally the load priority [ 5 ].
As shown in Fig. 2 , the majority of smart-home centres can be summarized as having five main functions [ 5 ], as follows:

Functions of SHEMS
(i) Monitoring: provides home residents with visual instantaneous information about the consumed power of different appliances and the status of several home parameters such as temperature, lights, etc. Furthermore, it can guide users to available alternatives for saving energy according to the existing operating modes of different home appliances.
(ii) Logging: collects and saves data pertaining to the amount of electricity consumed by each appliance, generated out of energy-conservation states. This functionality includes analysing the demand response for real-time prices.
(iii) Control: both direct and remote-control schemes can be implemented in smart homes. Different home appliances are controlled directly by SHEMS to match the home users’ desires, whereas other management functions are controlled remotely via cell phones or laptops, such as logging and controlling the power consumption of interruptible devices.
(iv) Management: the main function of SHEMS. It concerns the coordination between installed energy sources such as PV modules, micro wind turbines, energy storage and home appliances to optimize the total system efficiency and/or increase economic benefits.
(v) Alarms: SHEMS should respond to specific threats or faults by generating proper alarms according to fault locations, types, etc.
3.2 Economic analysis
Economic factors affecting home-management systems are classified into two classes. First, sizing costs include expanses of smart-home planning. Second, operating costs consist of bills of consumed energy. These costs depend mainly on the electrical tariff.
3.2.1 Sizing costs
These include capital, maintenance and replacement costs of smart-home infrastructures, such as PV systems, wind turbines, batteries/fuel cells and communication systems. In most previous SHEMS, such planning costs usually are not taken into consideration, as management schemes usually concern the daily operating costs only [ 69 ].
3.2.2 Operating costs
The electricity tariff is the main factor that gives an indication of the value of saving energy, according to the governmental authority; there are many types of tariffs, as follows [ 70–74 ]:
(i) Flat tariffs: the cost of consumed energy is constant regardless of the continuous change in the load. Load-rescheduling schemes do not affect the electricity bills in this scheme. Therefore, homeowners are not encouraged to rearrange their consumed energy, as they have no any economic benefits from managing the consumption of their appliances.
(ii) Block-rate tariffs: in this scheme, the monthly consumed energy price is classified into different categories. Each category has its own flat-rate price. Therefore, the main target of SHEMS is minimizing the total monthly consumed energy to avoid the risk of high-priced categories.
(iii) Seasonal tariffs: in this scheme, the total grid-demand load is changed significantly from one season to another. Therefore, the utility grid applies a high flat-rate tariff in high-demand seasons and vice versa. SHEMS should minimize the total consumption in such high-priced seasons and get the benefit of consumption in low-priced seasons.
(iv) Time-of-use (TOU) tariff: there are two or three predefined categories of tariffs daily in this scheme. First, a high-priced-hours tariff is applied during high-demand hours, which is known as a peak-hours tariff. Second, an off-peak-hours tariff is applied during low-demand hours with low prices for energy consumption. Sometimes, three levels of pricing are defined by the utility grid during the day, i.e. off-, middle- and high-peak costs, as discussed in [ 75 ]. SHEMS shift interruptible loads with low priority to off-peak hours to minimize the bill.
(v) Super peak TOU: this can be considered as a special case of the previously described TOU tariff but with a short peak-hours period of ~4 hours daily.
(vi) Critical peak pricing (CPP): the utility grid uses this tariff scheme during expected critical events of increasing the gap between generation and power demand. The price is increased exceptionally during these critical events by a constant predefined rate.
(vii) Variable peak pricing: this is a subcategory of the CPP tariff in which the exceptional increase in the tariff is variable. The utility grid informs consumers of the exceptional dynamic price increase according to its initial expectations.
(viii) Real-time pricing (RTP): the price is changing continuously during pre-identified intervals that range from several minutes to an hour. This tariff is the riskiest pricing scheme for homeowners. The electricity bill can increase significantly without a proper management system. SHEMS should communicate with grid utility and reschedule both home appliances, sources and energy storage continuously to minimize the total bill.
(viii) Peak-time rebates (PTRs): a proper price discount is considered for low-consumption loads during peak hours, which can be refunded later by the grid.
Depending on the electricity tariff, SHEMS complexity varies dramatically. In the case of using a flat-rate tariff, the algorithm becomes simpler, as one value is recorded for selling or buying the electricity. Tariffs may be published from the proper authority or predicted according to historical data. Prediction of the dynamic tariff is a main step in any SHEMS. Many time frames of tariff prediction are proposed that vary from hourly, daily or even a yearly prediction. Many optimization techniques with various objective functions are proposed to handle different features of both smart-home infrastructures and electricity tariffs, as will be discussed in the following section.
3.3 Pre-proposed SHEMS
Different SHEMS may be classified according to four features: operational planning of load-scheduling techniques, system objective functions, optimization techniques and smart-home model characteristics, as will be discussed in the following subsections.
3.3.1 Load-scheduling techniques
SHEMS concern the generation/load power balance to provide a comfortable lifestyle with the minimum possible costs. Scheduling loads according to their priority and the periods of renewable energy (solar, wind and EV state) can help in reducing the overall energy consumption daily. According to data collected by the management system, an initial load schedule is suggested daily to minimize the daily cost of consumed energy [ 76 ].
By using a proper optimal scheduling algorithm, electricity bills can be reduced by shifting loads from high-priced to low-priced intervals [ 77 , 78 ]. Many techniques have been proposed for home load scheduling, as will be discussed in the following subsections:
(i) Rule-based scheduling: in this algorithm, all home appliances and resources are connected to smart data-collector taps. By processing the collected data, different appliances are scheduled according to their priorities and based on the if/then rule. Also, some high-priority loads are supplied by home renewable sources/storage to maintain their function during predicted peak hours [ 79 , 80 ].
(ii) Artificial intelligence (AI): many AI controllers have been proposed for home load scheduling, such as artificial neural networks (ANNs), fuzzy logic (FL) and adaptive neural fuzzy inference systems (ANFISs). Table 2 compares between the three types of scheduling scheme based on AI.
Optimization techniques for load scheduling
3.3.2 Objective functions
(i) Single-objective techniques: in these schemes, only one criterion is minimized or maximized according to the home-user requirements. Several minimization objective functions were proposed, as follows:
lifetime degradation [ 47–49 ];
life-cycle costs [ 93 ];
gas emissions [ 94–96 ];
both active and reactive losses [ 97 , 98 ].
On the other hand, some research defined other single maximizing objective functions, such as:
net present value [ 96 ].
economic profits [ 97 , 98 ].
increased system reliability: according to many well-known reliability indices, such as loss of power supply probability, loss of load probability and others [ 99 , 100 ].
generated power [ 101 , 102 ].
loadability [ 103 ];
Multi-objective techniques: homeowners may have several criteria to be optimized together. Multi-objective optimization (MOO) problems consider many functions simultaneously. MOO finds a proper coordination that moderately satisfies the considered objectives. In [ 102 ], SHEMS with MOO techniques are summarized. Table 3 lists some examples of such multi-objective functions.
Multi-objective functions of SHEMS
3.3.3 Optimization techniques
Optimization techniques aim usually to identify the best coordination taking into consideration predefined constraints. Many approaches are available for addressing optimization problems. These approaches can be classified into two categories: classical and AI-based techniques. Table 4 lists various SHEMS optimization techniques and their main features.
Optimization techniques in SHEMS
Classical methods, especially linear programming types, have been usually applied in the last decade for smart homes with limited objective functions and simple model characteristics of tariff and home appliances. Recently, AI-based techniques have been proposed to cover more complicated models of smart homes with multi-objective functions with high levels of comfortable lifestyles.
3.3.4 Home-model characteristics
The smart-home model differs significantly according to three factors: installed variable energy sources, applied tariff and EV deployment. PV systems have been applied for nearly all studied smart homes due to their low price, simplicity of installation, low maintenance requirements and easily predicted daily power profile. On the other hand, a few pieces of research have considered micro wind turbines in their home models, such as [ 120 ]. Wind turbines are limited by high-wind-speed zones that are usually located in rural areas. In addition, homeowners usually do not prefer wind turbines due to their high prices, mechanical maintenance requirements and the unpredictable variation in wind power.
Dynamic tariffs are applied in most smart-home research. Specifically, the TOU tariff is analysed in a lot of studies, such as [ 121 , 122 ], whereas little research uses RTP, such as [ 123 , 124 ]. EV is studied as an energy source in the parking period or vehicle-to-grid (V2G) mode. In [ 75 , 125 ], EV in V2G mode reduces the electricity bill in peak hours, whereas, in [ 126–130 ], ESSs are managed only to reduce the electricity usage from the grid.
Many technical challenges arise for modern grids due to the increasing mutual exchange between smart homes and utility grids, especially power-quality control. Electric-power-quality studies usually confirm the acceptable behaviour of electrical sources such as voltage limits and harmonics analysis. Recently, smart power grids have diverse generation sources from different technologies that depend mainly on power electronics devices that increase the difficulty in power-quality control. Power-quality constraints should be taken into consideration for any energy-management systems to provide harmony between modern sources and loads.
On the other hand, power-quality issues should not form an additional obstacle against the integration of new technologies in modern grids. Therefore, both advanced communication schemes and AI-based techniques make modern grids ‘smart’ enough to cope with selective power-quality management. Smart homes exchange power with utility grids. With the prospective increase in such smart homes, the effect of their behaviour should be studied and controlled. Smart homes affect the grid-power quality in three different areas, as will be discussed in the following paragraphs [ 154–156 ].
4.1 Generating equipment
Integrated micro generation schemes in smart homes are mainly single-phase sources based on inverters with high switching frequencies that reach to many kHz. Low-order harmonics of such a generation type can usually be disregarded. However, with the expected continuous increase in such micro generators, the harmonics of low-voltage networks may shift into a range of higher frequencies, perhaps from 2 to 9 kHz [ 157 ]. Therefore, more research is needed to re-evaluate the appropriate limits for generation equipment in smart homes. Moreover, single-phase generation increases the risk of an unbalanced voltage in low-voltage grids. Therefore, negative-sequence voltage limits should be re-evaluated particularly for weak distribution networks. Also, a need for zero-sequence voltage limits may arise [ 154 ].
4.2 Home appliances
Modern home appliances depend mainly on electronic devices, such as newer LED lighting systems, EV battery chargers, etc., with relatively low fundamental current and high harmonic contents compared to traditional ones. According to many power-system analysers, many harmonics will increase significantly to risky levels, particularly fifth-harmonic voltage, with increase in such new electronic appliances [ 155 ].
4.3 Distribution network
In future grids, significant unusual operating scenarios may be possible with high penetration of domestic generation, especially with the possibility of an islanded (self-balanced) operation of smart homes. Short-circuit power will differ significantly during different operating conditions compared to classical grids. Moreover, low-voltage networks may suffer from damping-stability problems due to the continuous decrease in resistive loads, in conjunction with the increase in capacitive loads of electronic equipment. In addition, resonance problems may occur with low frequencies according to the continuous change in the nature of the load [ 156 ].
Although smart homes have bad impacts on utility grids, there are no charges applied from the grid authority to homeowners based on their buildings’ effects on grid-power quality. Therefore, home planners and SHEMS designers are usually concerned only with the economic benefits of their proposed schemes.
Smart homes, using new revolutions in communication systems and AI, provide residential houses with electrical power of a dual nature, i.e. as producer and consumer or ‘prosumer’. The energy-management system includes many components that mainly depend on a suitable communication scheme to coordinate between available sources, loads and users’ desire. Among many proposed communication systems, the IoT has many advantages and was chosen in many studies. Besides the popularity of the IoT, it does not need any special equipment installation and is compatible with many other communications protocols.
Many functions are applied by management systems such as monitoring and logging to facilitate a proper interaction between home occupants and the management scheme. Home security also should be confirmed via the management scheme by using different alarms corresponding to preset threats. Home users control different home appliances according their desires by SHEMS and via cell phones or manually.
The electricity tariff plays an important role in defining management-system characteristics. Tariffs vary from simple fixed flat rates to complicated variable dynamic ones according to the electrical-grid authority’s rules for residential loads. According to the tariff and selected objective functions, pre-proposed optimization techniques vary significantly from simple classical linear programming to sophisticated AI ones.
Modern electronic-based home appliances increase power-grid-quality problems, such as high harmonic contents, unbalanced loading and unpredictable short-circuit currents. On the other hand, power-grid authorities do not charge homeowners according to their buildings’ effects on the power quality. Therefore, all proposed energy-management systems are concerned mainly with the economic profits from reducing electricity consumption or even selling electrical power to the utility grids. In the future, price-based power-quality constraints should be defined by the grid authorities to confirm proper power exchange between both smart homes and grids. A possible future direction is behaviour modelling of aggregated smart homes/smart cities in different operating scenarios to conclude probable power-grid scenarios for stability and quality.
This work was supported by the project entitled ‘Smart Homes Energy Management Strategies’, Project ID: 4915, JESOR-2015-Cycle 4, which is sponsored by the Egyptian Academy of Scientific Research and Technology (ASRT), Cairo, Egypt.
None declared.
Comité Européen de Normalisation . Energy Performance of Buildings—Impact of Building Automation, Control, and Building Management; European Technical Standard EN 15232 ; CEN: Brussels, Belgium . 2012 . http://www.cres.gr/greenbuilding/PDF/prend/set4/WI_22_TC-approval_version_prEN_15232_Integrated_Building_Automation_Systems.pdf ( 20 December 2020 , date last accessed).
European Parliament . Directive 2010/31/EU of the European Parliament and of the Council of 19 May 2010 on the Energy Performance of Buildings; Directive 2010/31/EU ; The European Parliament and the Council of the European Union: Brussels, Belgium . 2010 . https://eurlex.europa.eu/LexUriServ/LexUriServ.do?uri=OJ:L:2010:153:0013:0035:en:PDF ( 20 December 2020 , date last accessed).
European Climate Foundation . Roadmap 2050 Project . http://www.roadmap2050.eu/ ( 20 December 2020 , date last accessed).
Al-Ali AR , El-Hag A , Bahadiri M , et al. Smart home renewable energy management system . Energy Procedia , 2011 , 12 : 120 – 126 .
Google Scholar
van Dam S , Bakker CA , Buiter J . Do home energy management systems make sense? Assessing their overall lifecycle impact . Energy Policy, 2013 , 63 : 398 – 407 .
Kuzlu M , Pipattanasomporn M , Rahman S . Review of communication technologies for smart homes/building applications. In: 2015 IEEE Innovative Smart Grid Technologies—Asia (ISGT ASIA) , Bangkok, Thailand , 3–6 November 2015 , 1 – 6 .
Wilson C , Hargreaves T , Hauxwell-Baldwin R . Smart homes and their users: a systematic analysis and key challenges . Personal and Ubiquitous Computing , 2015 , 19 : 463 – 476 .
Schieweck A , Uhde E , Salthammer T , et al. . Smart homes and the control of indoor air quality . Renewable and Sustainable Energy Reviews, 2018 , 94 : 705 – 718 .
Nacer A , Marhic B , Delahoche L . Smart home, smart HEMS, smart heating: an overview of the latest products and trends. In: 2017 6th International Conference on Systems and Control (ICSC) , Batna, Algeria , 7–9 May 2017 , 90 – 95 .
Yuan X , Han P , Duan Y , et al. . Residential electrical load monitoring and modeling—state of the art and future trends for smart homes and grids . Electric Power Components and Systems , 2020 , 48 : 1125 – 1143 .
Wang Y , Chen Q , Hong H , et al. . Review of smart meter data analytics: applications, methodologies, and challenges . IEEE Transactions on Smart Grid , 2019 , 10 : 3125 – 3148 .
Sowah RA , Ofoli AR , Tetteh MK , et al. . Demand side management of smart homes using OpenHAB framework for interoperability of devices. In: 2018 IEEE 7th International Conference on Adaptive Science & Technology (ICAST) , Accra, Ghana , 22–24 August 2018 , 1 – 8 .
Robles RJ , Kim T . Applications, systems and methods in smart home technology: a review . Int. J. Adv. Sci. Technol. , 2010 , 15 : 37 – 48 .
Makhadmeh SN , Khader AT , Al-Betar MA , et al. . Optimization methods for power scheduling problems in smart home: survey . Renewable and Sustainable Energy Reviews , 2019 , 115 : 109362 .
Zhao Z , Lee WC , Shin Y , et al. . An optimal power scheduling method for demand response in home energy management system . IEEE Transactions on Smart Grid , 2013 , 4 : 1391 – 1400 .
Benzi F , Anglani N , Bassi E , et al. . Electricity smart meters interfacing the households . IEEE Transactions on Industrial Electronics , 2011 , 58 : 4487 – 4494 .
Depuru SR , Wang L , Devabhaktuni V , et al. . Smart meters for power grid — challenges, issues, advantages and status. In: 2011 IEEE/PES Power Systems Conference and Exposition , Phoenix, AZ (USA) , 20–23 March 2011 , 1 – 7 .
Zheng J , Gao W , Lin L . Smart meters in smart grid: an overview. In: IEEE Green Technologies Conference , Denver, Colorado, USA , 4–5 April 2013 , 57 – 64 .
Chavali P , Yang P , Nehorai A . A distributed algorithm of appliance scheduling for home energy management system . IEEE Transactions on Smart Grid , 2014 , 5 : 282 – 290 .
Ma Y , Houghton T , Cruden A , et al. . Modeling the benefits of vehicle-to-grid technology to a power system . IEEE Transactions on power systems , 2012 , 27 : 1012 – 1020 .
Yiyun T , Can L , Lin C , et al. . Research on vehicle-to-grid technology. In: International Conference on Computer Distributed Control and Intelligent Environmental Monitoring , Changsha, Hunan, China , 19–20 February 2011 , 1013 – 1016 .
Kempton W , Tomić J . Vehicle-to-grid power fundamentals: calculating capacity and net revenue . Journal of Power Sources , 2005 , 144 : 268 – 279 .
Makhadmeh SN , Khader AT , Al-Betar MA , et al. . A novel hybrid grey wolf optimizer with min-conflict algorithm for power scheduling problem in a smart home . Swarm and Evolutionary Computation , 2021 , 60 : 100793 .
Makhadmeh SN , Khader AT , Al-Betar MA , et al. . Multi-objective power scheduling problem in smart homes using grey wolf optimizer . Journal of Ambient Intelligence and Humanized Computing , 2019 , 10 : 3643 – 3667 .
Parida B , Iniyan S , Goic R . A review of solar photovoltaic technologies . Renewable and Sustainable Energy Reviews , 2011 , 15 : 1625 – 1636 .
Schwerin A . Analysis of the potential solar energy market in the Caribbean . REPOSITORIO NACIONAL CONACYT . 2010 . https://www.solarthermalworld.org/sites/default/files/story/2015-04-06/solar_market_analysis_caribbean.pdf ( 20 December 2020 , date last accessed).
Brown CE. World Energy Resources . Berlin : Springer Verlag , 2002 .
Google Preview
Wan C , Zhao J , Song Y , et al. . Photovoltaic and solar power forecasting for smart grid energy management . CSEE Journal of Power and Energy Systems , 2015 , 1 : 38 – 46 .
Ewais AM , El-Azab R , Adma MAA . Stand-alone microgrid energy storage schemes: comparative study. In: 2018 Twentieth International Middle East Power Systems Conference (MEPCON) , Cairo, Egypt , 18–20 December 2018 , 564 – 569 .
Fthenakis V , Kim HC . Land use and electricity generation: a life-cycle analysis . Renewable and Sustainable Energy Reviews , 2009 , 13 : 1465 – 1474 .
Lei M , Shiyan L , Chuanwen J , et al. . A review on the forecasting of wind speed and generated power . Renewable and Sustainable Energy Reviews , 2009 , 13 : 915 – 920 .
Mazen R , Radwan M , Abdel-Samiea M . Utilization of biomass energy in high-rise buildings. In: 2013 4th International Youth Conference on Energy (IYCE) , Siófok, Hungary , 6–8 June 2013 , 1 – 3 .
Carpio M , Zamorano M , Costa M . Impact of using biomass boilers on the energy rating and CO 2 emissions of Iberian Peninsula residential buildings . Energy and Buildings , 2013 , 66 : 732 – 744 .
Berković-Šubić M , Rauch M , Dović D , et al. . Primary energy consumption of the dwelling with solar hot water system and biomass boiler . Energy Conversion and Management , 2014 , 87 : 1151 – 1161 .
Huang Y , Wang Y , Rezvani S , et al. . Biomass fuelled trigeneration system in selected buildings . Energy Conversion and Management , 2011 , 52 : 2448 – 2454 .
Wu G , Yu X . Remote control system for energy efficient home. In: 2013 IEEE Energytech , Cleveland, OH, USA , 21–23 May 2013 , 1 – 5 .
Safaric S , Malaric K . ZigBee wireless standard. In: Proceedings ELMAR 2006 , Zadar, Croatia , 7–10 June 2006 , 259 – 262 .
Huq MZ , Islam S . Home Area Network technology assessment for demand response in smart grid environment. In: 2010 20th Australasian Universities Power Engineering Conference , Christchurch, New Zealand , 5–8 December 2010 , 1 – 6 .
Dou N , Mei Y , Yanjuan Z , Yan Z . The networking technology within smart home system—ZigBee technology. In: 2009 International Forum on Computer Science-Technology and Applications , Chongqing, China , 25–27 December 2009 , 29 – 33 .
Ajgaonkar P , Wang L , Alam M . Simulation studies on ZigBee communications for home automation and networking. In: 2010 IEEE AUTOTESTCON , Orlando, FL, USA , 13–16 September 2010 , 1 – 6 .
Li Y , Zhang K , Zhang X . Research on application of ZigBee technology in flammable and explosive environment . In: 2009 First International Conference on Information Science and Engineering , Nanjing, China , 26–28 December 2009 , 4074 – 4078 .
Khan SA , Khan FA , Shahid A , et al. . Zigbee based reconfigurable clustered home area network. In: 2009 Third International Conference on Sensor Technologies and Applications , Athens, Greece , 18–23 June 2009 , 32 – 37 .
Han T , Han B , Zhang L , et al. . Coexistence study for WiFi and ZigBee under smart home scenarios. In: 2012 3rd IEEE International Conference on Network Infrastructure and Digital Content , Beijing, China , 21–23 September 2012 , 669 – 674 .
Sarijari MA , Lo A , Abdullah MS , et al. . Coexistence of heterogeneous and homogeneous wireless technologies in smart grid-home area network. In: 2013 International Conference on Parallel and Distributed Systems , Seoul, South Korea , 15–18 December 2013 , 576 – 581 .
Kounev V , Tipper D . Advanced metering and demand response communication performance in Zigbee based HANs. In: 2013 IEEE Conference on Computer Communications Workshops (INFOCOM WKSHPS) , Turin, Italy , 14–19 April 2013 , 31 – 36 .
Batista NC , Melício R , Matias JCO , Catalão JPS . ZigBee wireless area network for home automation and energy management: field trials and installation approaches. In: 2012 3rd IEEE PES Innovative Smart Grid Technologies Europe (ISGT Europe) , Berlin, Germany , 14–17 October 2012 , 1 – 5 .
Salazar J. Wireless Networks . 1st edn. Prague : Czech Technical University of Prague Faculty of electrical engineering , 2017 .
Phillips T , Karygiannis T , Kuhn R . Security standards for the RFID market . IEEE Security & Privacy , 2005 , 3 : 85 – 89 .
Li Y . Design of a key establishment protocol for smart home energy management system. In: 2013 Fifth International Conference on Computational Intelligence, Communication Systems and Networks , Madrid, Spain , 5–7 June 2013 , 88 – 93 .
Park YT , Sthapit P , Pyun J . Smart digital door lock for the home automation. In: TENCON 2009—2009 IEEE Region 10 Conference , Singapore , 23–26 November 2009 , 1 – 6 .
Hussain S , Schaffner S , Moseychuck D . Applications of wireless sensor networks and RFID in a smart home environment. In: 2009 Seventh Annual Communication Networks and Services Research Conference , Moncton, NB, Canada , 11–13 May 2009 , 153 – 157 .
Yao W , Chu C , Li Z . The use of RFID in healthcare: benefits and barriers. In: 2010 IEEE International Conference on RFID-Technology and Applications , Guangzhou, China , 17–19 June 2010 , 128 – 134 .
Wang C , Li X , Liu Y , Wang H . The research on development direction and points in IoT in China power grid. In: 2014 International Conference on Information Science, Electronics and Electrical Engineering , Sapporo, Japan , 2–4 July 2014 , 245 – 248 .
Chettri L , Bera R . A comprehensive survey on Internet of Things (IoT) toward 5G wireless systems . IEEE Internet of Things Journal , 2020 , 7 : 16 – 32 .
Samuel SSI . A review of connectivity challenges in IoT-smart home. In: 2016 3rd MEC International Conference on Big Data and Smart City (ICBDSC) , Muscat, Oman , 15–16 March 2016 , 1 – 4 .
Withanage C , Ashok R , Yuen C , Otto K . A comparison of the popular home automation technologies. In: 2014 IEEE Innovative Smart Grid Technologies—Asia (ISGT ASIA) , Kuala Lumpur, Malaysia , 20–23 May 2014 , 600 – 605 .
Zhu YH , Qiu S , Chi K , Fang Y . Latency aware IPv6 packet delivery scheme over IEEE 802.15.4 based battery-free wireless sensor networks . IEEE Transactions on Mobile Computing , 2017 , 16 : 1691 – 1704 .
Yi P , Iwayemi A , Zhou C . Developing Zigbee deployment guideline under Wi-Fi interference for smart grid applications . IEEE Transactions on Smart Grid , 2011 , 2 : 110 – 120 .
Mincov N , Zanatta L , Lopes JA , Lôbo M . Communication technologies for differentiated smart grid environments. In: 2013 IEEE PES Conference on Innovative Smart Grid Technologies (ISGT Latin America) , Sao Paulo, Brazil , 24–27 February 2013 , 1 – 4 .
Chhaya L , Sharma P , Bhagwatikar G , Kumar A . Wireless sensor network based smart grid communications: cyber attacks, intrusion detection system and topology control . Electronics , 2017 , 6 : 5 .
Gungor VC , Hancke GP . Industrial wireless sensor networks: challenges, design principles, and technical approaches . IEEE Transactions on Industrial Electronics , 2009 , 56 : 4258 – 4265 .
Liu Y . Wireless sensor network applications in smart grid: recent trends and challenges . International Journal of Distributed Sensor Networks , 2012 , 8 : 492819 .
Al-Sarawi S , Anbar M , Alieyan K , Alzubaidi M . Internet of Things (IoT) communication protocols: review. In: 2017 8th International Conference on Information Technology (ICIT) , Amman, Jordan , 17–18 May 2017 , 685 – 690 .
Li Y , Cheng X , Cao Y , et al. Smart choice for the smart grid: narrowband Internet of Things (NB-IoT) . IEEE Internet of Things Journal , 2018 , 5 : 1505 – 1515 .
Ratasuk RR , Vejlgaard B , Mangalvedhe N , Ghosh A . NB-IoT system for M2M communication. In: 2016 IEEE Wireless Communications and Networking Conference Workshops (WCNCW) , Doha, Qatar , 3–6 April 2016 , 428 – 432 .
Son Y , Moon K . Home energy management system based on power line communication. In: 2010 Digest of Technical Papers International Conference on Consumer Electronics (ICCE) , Las Vegas, NV, USA , 9–13 January 2010 , 115 – 116 .
Nazabal JA , Falcone FJ , Fernandez-Valdivielso C , et al. Energy Management System proposal for efficient smart homes. In: 2013 International Conference on New Concepts in Smart Cities: Fostering Public and Private Alliances (SmartMILE) , Gijon, Spain , 11–13 December 2013 , 1 – 5 .
Boynuegri AR , Yagcitekin B , Baysal M , et al. Energy management algorithm for smart home with renewable energy sources. In: 4th International Conference on Power Engineering, Energy and Electrical Drives , Istanbul, Turkey , 13–17 May 2013 , 17531758 .
Torriti J . Price-based demand side management: assessing the impacts of time-of-use tariffs on residential electricity demand and peak shifting in Northern Italy . Energy , 2012 , 44 : 576 – 583 .
Asadinejad A , Tomsovic K . Optimal use of incentive and price based demand response to reduce costs and price volatility . Electric Power Systems Research , 2017 , 144 : 215 – 223 .
Hart DG . Using AMI to realize the Smart Grid. In: 2008 IEEE Power and Energy Society General Meeting-Conversion and Delivery of Electrical Energy in the 21st Century , Pittsburgh, PA, USA , 20–24 July 2008 , 1 – 2 .
Aigner DJ , Leamer EE . Estimation of time-of-use pricing response in the absence of experimental data: an application of the methodology of data transferability . Journal of Econometrics , 1984 , 26 : 205 – 222 .
de Sá Ferreira R , Barroso LA , Lino PR , et al. Time-of-use tariff design under uncertainty in price-elasticities of electricity demand: a stochastic optimization approach . IEEE Transactions on Smart Grid , 2013 , 4 : 2285 – 2295 .
Dutta G , Mitra K . A literature review on dynamic pricing of electricity . J Oper Res Soc , 2017 , 68 : 1131 – 1145 .
Erdinc O , Paterakis NG , Mendes TDP , et al. Smart household operation considering Bi-directional EV and ESS utilization by real-time pricing-based DR . IEEE Transactions on Smart Grid , 2015 , 6 : 1281 – 1291 .
Marzband M , Alavi H , Ghazimirsaeid S , et al. Optimal energy management system based on stochastic approach for a home microgrid with integrated responsive load demand and energy storage . Sustainable Cities and Society , 2017 , 28 : 256 – 264 .
Patel K , Khosla A . Home energy management systems in future smart grid networks: a systematic review. In: 2015 1st International Conference on Next Generation Computing Technologies (NGCT) , Dehradun, India , 4–5 September 2015 , 479 – 483 .
Zhou B , Li W , Chan KW , et al. Smart home energy management systems: concept, configurations, and scheduling strategies . Renewable and Sustainable Energy Reviews , 2016 , 61 : 30 – 40 .
Kawakami T , Yoshihisa T , Fujita N , Tsukamoto M . A rule-based home energy management system using the Rete algorithm. In: 2013 IEEE 2nd Global Conference on Consumer Electronics (GCCE) , Tokyo, Japan , 1–4 October 2013 , 162 – 163 .
Yoshihisa T , Fujita N , Tsukamoto M . A rule generation method for electrical appliances management systems with home EoD. In: The 1st IEEE Global Conference on Consumer Electronics , Tokyo, Japan , 2–5 October 2012 , 248 – 250 .
Yuce B , Rezgui Y , Mourshed M . ANN–GA smart appliance scheduling for optimised energy management in the domestic sector . Energy and Buildings , 2016 , 111 : 311 – 325 .
Gharghan SK , Nordin R , Ismail M , Abd Ali J . Accurate wireless sensor localization technique based on hybrid PSO-ANN algorithm for indoor and outdoor track cycling . IEEE Sensors Journal , 2015 , 16 : 529 – 541 .
Ahmed MS , Mohamed A , Homod RZ , Shareef H . Hybrid LSA-ANN based home energy management scheduling controller for residential demand response strategy . Energies , 2016 , 9 : 716 .
Onder Z , Sezer S , Çanak I . A Tauberian theorem for the weighted mean method of summability of sequences of fuzzy numbers . Journal of Intelligent and Fuzzy Systems , 2015 , 28 : 1403 – 1409 .
Wu Y , Zhang B , Lu J , et al. Fuzzy logic and neuro-fuzzy systems: a systematic introduction . International Journal of Artificial Intelligence and Expert Systems , 2011 , 2 : 2011 – 2047 .
Hong YY , Lin JK , Wu CP , Chuang CC . Multi-objective air-conditioning control considering fuzzy parameters using immune clonal selection programming . IEEE Transactions on Smart Grid , 2012 , 3 : 1603 – 1610 .
Mohsenzadeh A , Shariatkhah M , Haghifam MR . Applying fuzzy techniques to model customer comfort in a smart home control system. In: 22nd International Conference and Exhibition on Electricity Distribution (CIRED 2013) , Stockholm, Sweden , 10–13 June 2013 , 1 – 4 .
Ciabattoni L , Grisostomi M , Ippoliti G , et al. Home energy management benefits evaluation through fuzzy logic consumptions simulator. In: Proceedings of the International Joint Conference on Neural Networks . Beijing, China , 6–11 July 2014 .
Wu Z , Zhou S , Li J , et al. Real-time scheduling of residential appliances via conditional risk-at-value . IEEE Transactions on Smart Grid , 2014 , 5 : 1282 – 1291 .
Ainsworth N , Johnson B , Lundstrom B . A fuzzy-logic subsumption controller for Home Energy Management Systems. In: 2015 North American Power Symposium (NAPS) , Charlotte, NC, USA , 4–6 October 2015 , 1 – 7 .
Wu Z , Zhang XP , Brandt J , et al . Three control approaches for optimized energy flow with home energy management system . IEEE Power and Energy Technology Systems Journal , 2015 , 2 : 21 – 31 .
Choi IH , Yoo SH , Jung JH , et al. Design of Neuro-Fuzzy based intelligent inference algorithm for energy management system with legacy device . The Transactions of the Korean Institute of Electrical Engineers , 2015 , 64 : 779 – 785 .
Cao T , Hwang Y , Radermacher R . Development of an optimization based design framework for microgrid energy systems . Energy , 2017 , 140 : 340 – 351 .
Kanchev H , Francois B , Lazarov V . Unit commitment by dynamic programming for microgrid operational planning optimization and emission reduction. In: International Aegean Conference on Electrical Machines and Power Electronics and Electromotion, Joint Conference , Istanbul, Turkey , 8–10 September 2011 , 502 – 507 .
Tsikalakis AG , Hatziargyriou ND . Environmental benefits of distributed generation with and without emissions trading . Energy Policy , 2007 , 35 : 3395 – 3409 .
Moradi M , Eskandari M , Showkati H . A hybrid method for simultaneous optimization of DG capacity and operational strategy in microgrids utilizing renewable energy resources . International Journal of Electrical Power & Energy Systems , 2014 , 56 : 241 – 258 .
Kirthiga MV , Daniel SA , Gurunathan S . A methodology for transforming an existing distribution network into a sustainable autonomous micro-grid . IEEE Transactions on Sustainable Energy , 2012 , 4 : 31 – 41 .
Abou El-Ela AA , Allam SM , Shatla MM . Maximal optimal benefits of distributed generation using genetic algorithms . Electric power systems research , 2010 , 80 : 869 – 877 .
Oudalov A , Cherkaoui R , Beguin A . Sizing and optimal operation of battery energy storage system for peak shaving application. In: 2007 IEEE Lausanne Power Tech 2007 , Lausanne, Switzerland , 1–5 July 2007 , 621 – 625 .
Arefifar SA , Mohamed Y . DG mix, reactive sources and energy storage units for optimizing microgrid reliability and supply security . IEEE Transactions on smart grid , 2014 , 5 : 1835 – 1844 .
Luna R , Perea M , Vargas-Vázquez D , et al. Optimal sizing of renewable hybrids energy systems: a review of methodologies . Solar Energy , 2012 , 86 : 1077 – 1088 .
Iqbal M , Azam M , Naeem M , et al. Optimization classification, algorithms and tools for renewable energy: a review . Renewable and Sustainable Energy Reviews , 2014 , 39 : 640 – 654 .
Aman MM , Jasmon GB , Bakar AH , Mokhlis H . A new approach for optimum simultaneous multi-DG distributed generation Units placement and sizing based on maximization of system loadability using HPSO (hybrid particle swarm optimization) algorithm . Energy , 2014 , 66 : 202 – 215 .
Schwaegerl C , Tao L , Mancarella P , et al . A multi-objective optimization approach for assessment of technical, commercial and environmental performance of microgrids . European Transactions on Electrical Power , 2011 , 21 : 1271 – 1290 .
Boloukat MH , Foroud AA . Stochastic-based resource expansion planning for a grid-connected microgrid using interval linear programming . Energy , 2016 , 113 : 776 – 787 .
Zhao M , Chen Z , Blaabjerg F . Optimisation of electrical system for offshore wind farms via genetic algorithm . IET Renewable Power Generation , 2009 , 3 : 205 – 216 .
Lin N , Zhou B , Wang X . Optimal placement of distributed generators in micro-grid. In: 2011 International Conference on Consumer Electronics, Communications and Networks (CECNet) , Xianning, China , 16–18 April 2011 , 4239 – 4242 .
Zhang D , Evangelisti S , Lettieri P , et al. Economic and environmental scheduling of smart homes with microgrid: DER operation and electrical tasks . Energy Conversion and Management , 2016 , 110 : 113 – 124 .
Dey B , Roy SK , Bhattacharyya B . Solving multi-objective economic emission dispatch of a renewable integrated microgrid using latest bio-inspired algorithms . Engineering Science and Technology, an International Journal , 2019 , 22 : 55 – 66 .
Zhao B , Zhang X , Chen J , et al. Operation optimization of standalone microgrids considering lifetime characteristics of battery energy storage system . IEEE Transactions on Sustainable Energy , 2013 , 4 : 934 – 943 .
Pourmousavi SA , Sharma RK , Asghari B . A framework for real-time power management of a grid-tied microgrid to extend battery lifetime and reduce cost of energy. In: 2012 IEEE PES Innovative Smart Grid Technologies (ISGT) , Washington, DC, USA , 16–20 Jan. 2012 , 1 – 8 .
Shadmand MB , Balog RS . Multi-objective optimization and design of photovoltaic-wind hybrid system for community smart DC microgrid . IEEE Transactions on Smart Grid , 2014 , 5 : 2635 – 2643 .
Zhang M , Chen J . The energy management and optimized operation of electric vehicles based on microgrid . IEEE Transactions on Power Delivery , 2014 , 29 : 1427 – 1435 .
Shi L , Luo Y , Tu GY . Bidding strategy of microgrid with consideration of uncertainty for participating in power market . International Journal of Electrical Power & Energy Systems , 2014 , 59 : 1 – 13 .
Alsayed M , Cacciato M , Scelba G , et al . Optimal sizing of hybrid power generation systems based on multi criteria decision analysis. In: International Symposium on Power Electronics Power Electronics, Electrical Drives, Automation and Motion , Sorrento, Italy , 20–22 June 2012 , 1442 – 1447 .
Kaabeche A , Belhamel M , Ibtiouen R . Sizing optimization of grid-independent hybrid photovoltaic/wind power generation system . Energy , 2011 , 36 : 1214 – 1222 .
Arnette A , Zobel C . An optimization model for regional renewable energy development . Renewable and Sustainable Energy Reviews , 2012 , 16 : 4606 – 4615 .
Alsayed M , Cacciato M , Scarcella G , et al. Multicriteria optimal sizing of photovoltaic-wind turbine grid connected systems . IEEE Transactions on Energy Conversion , 2013 , 28 : 370 – 379 .
Bahramirad S , Reder W , Khodaei A . Reliability-constrained optimal sizing of energy storage system in a microgrid . IEEE Transactions on Smart Grid , 2012 , 3 : 2056 – 2062 .
Taşcıkaraoğlu A , Boynuegri AR , Uzunoglu M . A demand side management strategy based on forecasting of residential renewable sources: a smart home system in Turkey . Energy and Buildings , 2014 , 80 : 309 – 320 .
Erdinc O , Paterakis NG , Pappi IN , et al . A new perspective for sizing of distributed generation and energy storage for smart households under demand response . Applied Energy , 2015 , 143 : 26 – 37 .
Geng L , Wei Y , Lu Z , et al. A novel model for home energy management system based on Internet of Things. In: 2016 IEEE International Conference on Power and Renewable Energy (ICPRE) , Shanghai, China , 21–23 October 2016 , 474 – 480 .
Lee JY , Choi SG . Linear programming based hourly peak load shaving method at home area. In: 16th International Conference on Advanced Communication Technology , Pyeongchang, South Korea , 16–19 February 2014 , 310 – 313 .
Erdinc O , Paterakis NG , Catalão JPS , et al. Smart households and home energy management systems with innovative sizing of distributed generation and storage for customers. In: 2015 48th Hawaii International Conference on System Sciences , Kauai, HI, USA , 5–8 January 2015 , 1462 – 1471 .
Brahman F , Honarmand M , Jadid S . Optimal electrical and thermal energy management of a residential energy hub, integrating demand response and energy storage system . Energy and Buildings , 2015 , 90 : 65 – 75 .
Asare-Bediako B , Kling WL , Ribeiro PF . Integrated agent-based home energy management system for smart grids applications. In: IEEE PES ISGT Europe 2013 , Lyngby, Denmark , 6–9 October 2013 , 1 – 5 .
Hubert T , Grijalva S . Modeling for residential electricity optimization in dynamic pricing environments . IEEE Transactions on Smart Grid , 2012 , 3 : 2224 – 2231 .
Paterakis NG , Erdinç O , Bakirtzis AG , et al. Optimal household appliances scheduling under day-ahead pricing and load-shaping demand response strategies . IEEE Transactions on Industrial Informatics , 2015 , 11 : 1509 – 1519 .
Wang J , Sun Z , Zhou Y , et al . Optimal dispatching model of smart home energy management system. In: IEEE PES Innovative Smart Grid Technologies , Tianjin, China , 21–24 May 2012 , 1 – 5 .
Jo HC , Kim S , Joo SK . Smart heating and air conditioning scheduling method incorporating customer convenience for home energy management system . IEEE Transactions on Consumer Electronics , 2013 , 59 : 316 – 322 .
Rajasekharan J , Koivunen V . Optimal energy consumption model for smart grid households with energy storage . IEEE Journal of Selected Topics in Signal Processing , 2014 , 8 : 1154 – 1166 .
Xing H , Lin Z , Fu M . A new decentralized algorithm for optimal load shifting via electric vehicles . In: 2017 36th Chinese Control Conference (CCC) , Dalian, China , 26–28 July 2017 , 10708 – 10713 .
Chen Z , Xia B , You C , et al. A novel energy management method for series plug-in hybrid electric vehicles . Applied Energy , 2015 , 145 : 172 – 179 .
Wu X , Hu X , Teng Y , et al. Optimal integration of a hybrid solar-battery power source into smart home nanogrid with plug-in electric vehicle . Journal of Power Sources , 2017 , 363 : 277 – 283 .
Hu X , Martinez CM , Yang Y . Charging, power management, and battery degradation mitigation in plug-in hybrid electric vehicles: a unified cost-optimal approach . Mechanical Systems and Signal Processing , 2017 , 87 : 4 – 16 .
Henao-Muñoz AC , Saavedra-Montes AJ , Ramos-Paja CA . Energy management system for an isolated microgrid with photovoltaic generation . In: 2017 14th International Conference on Synthesis, Modeling, Analysis and Simulation Methods and Applications to Circuit Design (SMACD) , Giardini Naxos, Greece , 12–15 June 2017 , 1 – 4 .
Misra S , Bera S , Ojha T . D2P: distributed dynamic pricing policy in smart grid for PHEVs management . IEEE Transactions on Parallel and Distributed Systems , 2015 , 26 : 702 – 712 .
Setlhaolo D , Xia X . Combined residential demand side management strategies with coordination and economic analysis . International Journal of Electrical Power & Energy Systems , 2016 , 79 : 150 – 160 .
Akhavan-Rezai E , Shaaban MF , El-Saadany EF , et al . New EMS to incorporate smart parking lots into demand response . IEEE Transactions on Smart Grid , 2016 , 9 : 1376 – 1386 .
Zhang H , Hu Z , Xu Z , et al. Evaluation of achievable vehicle-to-grid capacity using aggregate PEV model . IEEE Transactions on Power Systems , 2017 , 32 : 784 – 794 .
Su W , Wang J , Roh J . Stochastic energy scheduling in microgrids with intermittent renewable energy resources . IEEE Transactions on Smart Grid , 2014 , 5 : 1876 – 1883 .
Khodakarami A , Farahani H , Aghaei J . Stochastic characterization of electricity energy markets including plug-in electric vehicles . Renewable and Sustainable Energy Reviews , 2017 , 69 : 112 – 122 .
Alipour M , Zare K , Abapour M . MINLP probabilistic scheduling model for demand response programs integrated energy hubs . IEEE Transactions on Industrial Informatics , 2018 , 14 : 79 – 88 .
Klein L , Kwak JY , Kavulya G , et al . Coordinating occupant behavior for building energy and comfort management using multi-agent systems . Automation in construction , 2012 , 22 : 525 – 536 .
Megahed TF , Abdelkader SM , Zakaria A . Energy management in zero-energy building using neural network predictive control . IEEE Internet of Things Journal , 2019 , 6 : 5336 – 5344 .
Ruiz-Cortes M , González-Romera E , Amaral-Lopes R , et al. Optimal charge/discharge scheduling of batteries in microgrids of prosumers . IEEE Transactions on Energy Conversion , 2018 , 34 : 468 – 477 .
Elsied M , Oukaour A , Youssef T , et al . An advanced real time energy management system for microgrids . Energy , 2016 , 114 : 742 – 752 .
Radosavljević J , Jevtić M , Klimenta D . Energy and operation management of a microgrid using particle swarm optimization . Engineering Optimization , 2016 , 48 : 811 – 830 .
Marzband M , Ghazimirsaeid SS , Uppal H , et al . A real-time evaluation of energy management systems for smart hybrid home microgrids . Electric Power Systems Research , 2017 , 143 : 624 – 633 .
Sousa T , Morais H , Vale Z , et al. Intelligent energy resource management considering vehicle-to-grid: a simulated annealing approach . IEEE Transactions on Smart Grid , 2012 , 3 : 535 – 542 .
Chaouachi A , Kamel RM , Andoulsi R , et al. Multiobjective intelligent energy management for a microgrid . IEEE Transactions on Industrial Electronics , 2013 , 60 : 1688 – 1699 .
Solanki BV , Bhattacharya K , Cañizares CA . A sustainable energy management system for isolated microgrids . IEEE Transactions on Sustainable Energy , 2017 , 8 : 1507 – 1517 .
Guo Y , Zhao C . Islanding-aware robust energy management for microgrids . IEEE Transactions on Smart Grid , 2018 , 9 : 1301 – 1309 .
Viciana E , Alcayde A , Montoya FG , et al . An open hardware design for Internet of Things power quality and energy saving solutions . Sensors , 2019 , 19 : 627 .
Bollen M , Zhong J , Zavoda F , et al . Power Quality aspects of smart grids. In: International Conference on Renewable Energies and Power Quality (ICREPQ’10) , Granada, Spain , 23–25 March 2010 , 1 – 6 .
Hartono BS , Mursid SP , Prajogo S . Home energy management system in a Smart Grid scheme to improve reliability of power systems . IOP Conference Series: Earth and Environmental Science , 2018 , 105 : 012081 .
Nguyen DT , Le LB . Risk-constrained profit maximization for microgrid aggregators with demand response . IEEE Transactions on smart grid , 2014 , 6 : 135 – 146 .
Email alerts
Citing articles via.
- Advertising and Corporate Services
Affiliations
- Online ISSN 2515-396X
- Print ISSN 2515-4230
- Copyright © 2024 National Institute of Clean-and-Low-Carbon Energy
- About Oxford Academic
- Publish journals with us
- University press partners
- What we publish
- New features
- Open access
- Institutional account management
- Rights and permissions
- Get help with access
- Accessibility
- Advertising
- Media enquiries
- Oxford University Press
- Oxford Languages
- University of Oxford
Oxford University Press is a department of the University of Oxford. It furthers the University's objective of excellence in research, scholarship, and education by publishing worldwide
- Copyright © 2024 Oxford University Press
- Cookie settings
- Cookie policy
- Privacy policy
- Legal notice
This Feature Is Available To Subscribers Only
Sign In or Create an Account
This PDF is available to Subscribers Only
For full access to this pdf, sign in to an existing account, or purchase an annual subscription.

- SUGGESTED TOPICS
- The Magazine
- Newsletters
- Managing Yourself
- Managing Teams
- Work-life Balance
- The Big Idea
- Data & Visuals
- Reading Lists
- Case Selections
- HBR Learning
- Topic Feeds
- Account Settings
- Email Preferences
Manage Your Energy, Not Your Time
- Tony Schwartz
- Catherine McCarthy

As the demands of the workplace keep rising, many people respond by putting in ever longer hours, which inevitably leads to burnout that costs both the organization and the employee. Meanwhile, people take for granted what fuels their capacity to work—their energy. Increasing that capacity is the best way to get more done faster and better.
Time is a finite resource, but energy is different. It has four wellsprings—the body, emotions, mind, and spirit—and in each, it can be systematically expanded and renewed. In this article, Schwartz, founder of the Energy Project, describes how to establish rituals that will build energy in the four key dimensions. For instance, harnessing the body’s ultradian rhythms by taking intermittent breaks restores physical energy. Rejecting the role of a victim and instead viewing events through three hopeful lenses defuses energy-draining negative emotions. Avoiding the constant distractions that technology has introduced increases mental energy. And participating in activities that give you a sense of meaning and purpose boosts the energy of the spirit.
The new workday rituals succeed only if leaders support their adoption, but when that happens, the results can be powerful. A group of Wachovia Bank employees who went through an energy management program outperformed a control group on important financial metrics like loans generated, and they reported substantially improved customer relationships, productivity, and personal satisfaction. These findings corroborated anecdotal evidence gathered about the effectiveness of this approach at other companies, including Ernst & Young, Sony, and Deutsche Bank. When organizations invest in all dimensions of their employees’ lives, individuals respond by bringing all their energy wholeheartedly to work—and both companies and their people grow in value.
The science of stamina has advanced to the point where individuals, teams, and whole organizations can, with some straightforward interventions, significantly increase their capacity to get things done.
Steve Wanner is a highly respected 37-year-old partner at Ernst & Young, married with four young children. When we met him a year ago, he was working 12- to 14-hour days, felt perpetually exhausted, and found it difficult to fully engage with his family in the evenings, which left him feeling guilty and dissatisfied. He slept poorly, made no time to exercise, and seldom ate healthy meals, instead grabbing a bite to eat on the run or while working at his desk.
- Tony Schwartz is the CEO of The Energy Project and the author of The Way We’re Working Isn’t Working . Become a fan of The Energy Project on Facebook .
- CM Catherine McCarthy ( [email protected] ) is a senior vice president at the Energy Project.

Partner Center
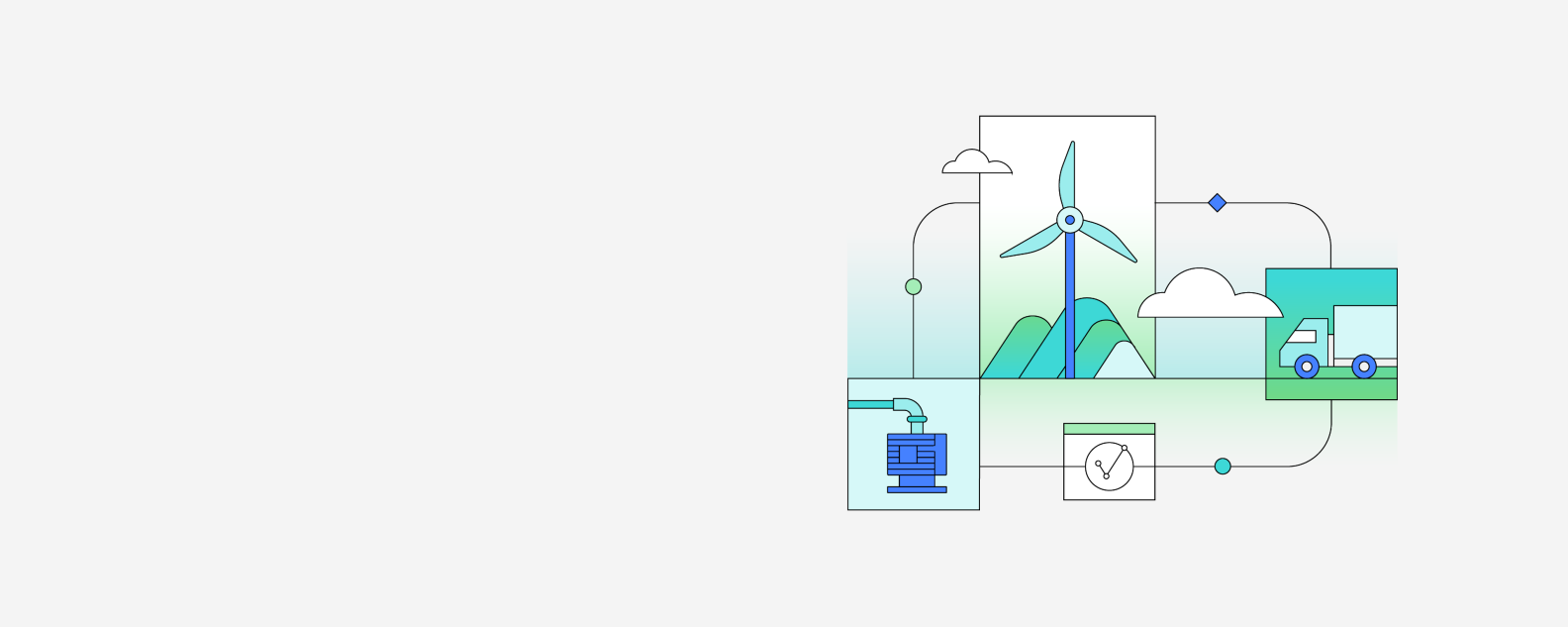
Energy management is the proactive and systematic monitoring, control, and optimization of an organization’s energy consumption to conserve use and decrease energy costs.
Energy management includes minor actions such as monitoring monthly energy bills and upgrading to energy-saving light bulbs. It can mean more extensive improvements like adding insulation, installing a reflective roof covering or improving HVAC (heating and cooling) equipment to optimize energy performance.
Energy management also includes more elaborate activities, such as creating financial projections for commissioning renewable energy services and making other improvements for clean energy consumption and reduced energy costs in coming years.
More sophisticated energy management programs take advantage of technology. For instance, utility tracking software predicts future energy usage and plans energy budgets. Which help a company’s strategic decision makers ensure its energy management strategy correlates with its objectives and financial planning. Enterprise management software uses IoT, advanced connectivity and big data, allowing a corporation to take advantage of energy data analytics for better facility management, and helps with energy consumption and energy management challenges.
With ESG disclosures starting as early as 2025 for some companies, make sure that you're prepared with our guide.
Register for the ebook on GHG emissions accounting
Around the globe, there is a great need to save energy , which impacts prices, emissions targets, and legislation that affects us all. Not only can energy management help reduce the carbon emissions that contribute to global warming, it also helps reduce our dependence on increasingly limited fossil fuels.
According to energystar.gov (link resides outside of IBM), energy use is a US commercial office building’s single largest operating expense. It represents about a third of an enterprise’s typical operating budget and accounts for almost 20% of the nation’s annual greenhouse gas emissions. Energy StarÒ says office buildings waste up to one-third of the energy they consume.
Energy management is even more important in Europe, where the energy supply (link resides outside of IBM) is especially vulnerable to cyberattacks. This is because, on average, EU corporations invest 41% less on information security than American companies. Therefore, European companies need more initiatives that implement energy security solutions and help them safeguard data, access, and networks.
In addition to helping mitigate global problems that result from carbon emissions, energy management programs also bring benefits to corporations.
Having energy management software in place helps control a corporation’s budget and reduce the risk that is associated with energy price increases that can impact a business’s ability to operate. Tracking utility costs and energy efficiency allows corporations to budget more efficiently and gain better insight into overall operational costs. According to Energy Star, decreasing energy use by 10% can lead to a 1.5% increase in net operating income.
Energy monitoring and management not only bring cost savings to a company’s bottom line through decreased usage and consumption but can also mean reduced reliance on sometimes volatile supply chains. Energy management programs can also help companies lower costs through competitive procurement.
Having a strong environmental, social and governance (ESG) foundation helps companies save energy, increase transparency and work toward better sustainability goals.
Energy management solutions that use a single system of record to reduce energy use, cost, time, and the burden of reporting allow clients to manage the impact of environmental risks . While also, identifying efficiency opportunities and assess sustainability risks, thus focusing on ESG strategic outcomes.
Besides saving energy costs and lowering carbon emissions, reducing your company’s carbon footprint also shows the company’s commitment to the environment, which promotes an image of greater sustainability and advocating for green energy. Reducing greenhouse gas emissions leads to having, and being recognized for, greater corporate social responsibility.
A strategic approach to consulting with sustainability experts on your sustainability strategy and roadmap leads to the most effective energy and ESG management . In addition to other benefits, consulting on efforts that can include decarbonization and transition to renewables can also help your business attract new and often younger employees who value the optimization of sustainable energy and renewable energy use and take corporate social responsibility seriously.
Intelligent asset management can create energy efficiency for several industry use cases. Some of these include:
- Buildings: Managing energy in your offices, factories and other facilities helps save energy and reduce carbon output in various ways. Intelligent asset management uses technology such as AI, IoT, and analytics to help you inspect and monitor a building’s efficiency, calculate potential impacts to the grid, anticipate failure, and better plan maintenance procedures. Companies that use this technology can increase their productivity and make their facilities more energy-efficient, reducing emissions, mitigating climate risk and extending asset lifecycles. They gain operational insights into clean energy sources, efficient waste management and decarbonization.
- Sustainable supply chains: Using AI and blockchain, intelligent supply chain automation can help reduce the impact that current supply chain weaknesses are having on your business. A more resilient, sustainable supply chain allows clients to act quickly and confidently and mitigate disruptions. Measuring Scope 3 emissions—indirect emissions that are not caused by a company directly but occurring within its supply chain, from warehousing, transportation and waste operations, among other areas—gives companies a competitive advantage in terms of sustainability. While Scope 3 emissions are out of a company’s direct control, measuring them identifies emission problems in their supply chain and allows them to perhaps affect change. Compared to Scope 1 (direct emissions) and Scope 2 (indirect), Scope 3 emissions generally represent the highest levels of greenhouse gases.
- Manufacturing: Manufacturing facilities burn numerous fossil fuels and are some of the largest energy consumers. Creating an energy management program to sustainably reduce energy use for manufacturing includes collecting and analyzing energy-efficiency data (from various meters, databases and multiple plant sites) and creating a project management plan. A more IT-based factory floor that uses the Industrial Internet of Things (IIoT) and analytics means better predictive maintenance and quality, which leads to smarter manufacturing. Case studies show that changing energy consumption patterns in manufacturing requires management personnel that are committed to reducing energy use because it requires change, infrastructure investment and possibly retraining.
Energy management also comes with its own set of challenges. Some of these include:
- Not enough data integrity, analysis, or clear benchmarks: Traditional building management systems and meters that collect data through manual energy audits don’t provide data that lets you see wasteful energy usage patterns. Using an energy management system makes it easier and more convenient to access and use more data about energy consumption. A strong energy management system automatically generates regular, reliable, and customized energy reports.
- Faulty systems, incorrect settings, and poorly maintained equipment: Scheduled checks that are conducted too infrequently mean wasted time and money. Equipment that breaks down unexpectedly thrusts you into reactive maintenance, which can create challenges and unexpected expenses. In contrast, intelligent energy systems alert you to equipment breakdown and energy wastage immediately. They provide real-time information on energy consumption, and you can set energy KPIs for consistent results. Having a proactive maintenance strategy, with routine and preventive maintenance schedules, means that equipment is serviced regularly and has longer lifespans.
- Failure to plan for energy upgrades: In-depth energy data lets you make smart decisions about energy retrofits or upgrade initiatives that bring cost savings and a good ROI.
Save energy and decarbonize with intelligent asset management.
Reduce energy and carbon emissions with efficient data centers and more sustainable, secure IT operations.
Accelerate sustainability by managing all your environmental, social, and governance (ESG) indicators in a single platform.
Optimize your real estate and facilities management operations for higher efficiency and sustainability.
Boost your sustainability journey and energy management efficiency by charting a sustainable and profitable path forward with open, AI-powered solutions and platforms plus deep industry expertise from IBM.
Automating application resource management should be your first step in the sustainability journey.
Learn how to minimize energy use and embed responsible computing across your IT environment.
Enterprises that want to reduce their carbon footprint should expand their sustainability goals to include green IT and responsible computing.
Unlock the full potential of your enterprise assets with IBM Maximo Application Suite by unifying maintenance, inspection and reliability systems into one platform. It’s an integrated cloud-based solution that harnesses the power of AI, IoT and advanced analytics to maximize asset performance, extend asset lifecycles, minimize operational costs and reduce downtime.
Academia.edu no longer supports Internet Explorer.
To browse Academia.edu and the wider internet faster and more securely, please take a few seconds to upgrade your browser .
Enter the email address you signed up with and we'll email you a reset link.
- We're Hiring!
- Help Center
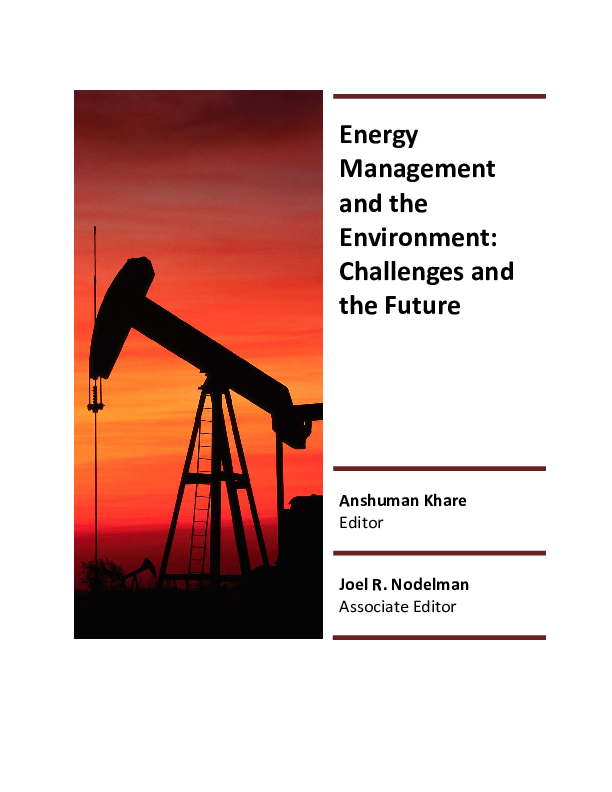
Energy Management and the Environment: Challenges and the Future

Related Papers
Mark C J Stoddart
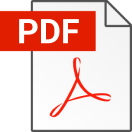
Nathan Lemphers
John Robinson
Michal C Moore
OECD Economics Department Working Papers
Annabelle Mourougane
Through this report, the BC Working Group on Sustainability Education is endeavouring to ‘take stock’ of institutions of higher learning in the province. We want to find out where our universities and colleges are in terms of policies, operations and academic research and teaching that support provincial and societal goals of sustainability.
Jean Kayira
Residents of Saskatchewan have a golden opportunity to consider whether and how to plan for the sustainability of the province’s northwestern region in the face of rapid environmental, economic, and social changes brought on by present and proposed resource development, including the possibility of large-scale oil sands extraction. Saskatchewan’s neighbour to the west, Alberta, has gained impressive wealth due to its oil sands developments, but the province has also experienced some undesirable environmental and social changes. Because oil sands development in Saskatchewan has not yet proceeded to full production, residents still have time to plan ahead. On September 23-25, 2009, the School of Environment and Sustainability at the University of Saskatchewan hosted a public forum and workshop to address the following questions: What lessons can we learn from the Alberta experience that will apply to the Saskatchewan context? What do we know about Saskatchewan’s environment, economy, ...
Byron Williston
Richard Mitchell
Mark Purdon
The development and release for consultation of the draft ‘Planning for a Sustainable Future: A Federal Sustainable Development Strategy for Canada’ is a positive step by the federal government, and is very much appreciated. As we prepare to take on the challenge of ensuring that our economy and society can be developed in a way that is environmentally sound, the government of Canada is to be congratulated for willingness to engage Canadians on these matters. The following SP Comments are provided from a perspective of academic leaders and policy experts who have come together through Sustainable Prosperity, to explore the policies that could make our markets work for the environment. Sustainable Prosperity is a national research and policy network which focuses on market-based approaches to build a stronger, greener economy. We bring together business, policy and academic leaders to foster the innovative thinking necessary to inform policy development. Our focus is on building a Canadian economy that values our natural world and is positioned to compete in the global, green economy of the future. We inject ideas into public dialogue in compelling and nonpartisan ways, by sharing advanced research, by facilitating innovative conversations, and by pioneering smart solutions - forward-looking initiatives to shift public policy toward sustainable economics. We enclose a brief set of reactions and proposals which we hope will be helpful to inform further federal deliberations on this Strategy, and to identify future directions for the elaboration of the Strategy. In these SP Comments, we seek to encourage the Draft Federal Sustainable Development Strategy to activate workable solutions that move us towards a future where the most ecologically restorative choices that Canadians make in their daily lives are also the choices that cost less.
RELATED PAPERS
Journal of the American Dietetic Association
Joyce Muhenge Olenja
Respiratory Medicine CME
Hayati Bilgic
Annals of PDE
Claudio Muñoz
Internal and emergency medicine
carmen sanclemente
Allergy, Asthma & Clinical Immunology
Bazir Serushago
Jurnal Kesejahteraan dan Pelayanan Sosial
Arie Surya Gutama
J Immigrant Minority Health
Phyllis Sharps
Journal of Sound and Vibration
Laurent de Ryck
The Cartel Network Party
Guillermo Colella
Magnus Sverke
HAL (Le Centre pour la Communication Scientifique Directe)
Laurent Houssin
Jozsef Sisa
IKRA-ITH ABDIMAS
Nourmayansa Vidya Anggraini
官方认证au毕业证 阿伯丁大学毕业证研究生学位证书毕业证认证原版一模一样
George Bray
Journal of Genetic Syndromes & Gene Therapy
Giovanna Cenacchi
Paola Rebora
Microprocessors and Microsystems
Daniel Chillet
- We're Hiring!
- Help Center
- Find new research papers in:
- Health Sciences
- Earth Sciences
- Cognitive Science
- Mathematics
- Computer Science
- Academia ©2024
Advertisement
Intelligent energy management systems: a review
- Open access
- Published: 13 March 2023
- Volume 56 , pages 11635–11674, ( 2023 )
Cite this article
You have full access to this open access article
- Stavros Mischos ORCID: orcid.org/0000-0002-6290-1133 1 ,
- Eleanna Dalagdi 1 &
- Dimitrios Vrakas 1
11k Accesses
12 Citations
1 Altmetric
Explore all metrics
Climate change has become a major problem for humanity in the last two decades. One of the reasons that caused it, is our daily energy waste. People consume electricity in order to use home/work appliances and devices and also reach certain levels of comfort while working or being at home. However, even though the environmental impact of this behavior is not immediately observed, it leads to increased CO2 emissions coming from energy generation from power plants. It has been shown that about 40% of these emissions come from the electricity consumption and also that about 20% of this percentage could have been saved if we started using energy more efficiently. Confronting such a problem efficiently will affect both the environment and our society. Monitoring energy consumption in real-time, changing energy wastage behavior of occupants and using automations with incorporated energy savings scenarios, are ways to decrease global energy footprint. In this review, we study intelligent systems for energy management in residential, commercial and educational buildings, classifying them in two major categories depending on whether they provide direct or indirect control. The article also discusses what the strengths and weaknesses are, which optimization techniques do they use and finally, provide insights about how these systems can be improved in the future.
Similar content being viewed by others
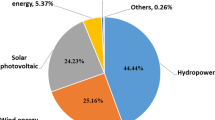
Cost, environmental impact, and resilience of renewable energy under a changing climate: a review
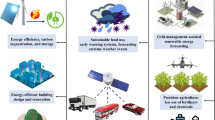
Artificial intelligence-based solutions for climate change: a review
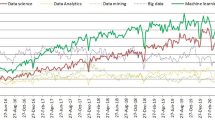
Data Science and Analytics: An Overview from Data-Driven Smart Computing, Decision-Making and Applications Perspective
Avoid common mistakes on your manuscript.
1 Introduction
Nowadays, electrical energy plays a vital role in various aspects of our life. However, the lack of ecological awareness along with the absence of energy-friendly infrastructures has led into increased energy consumption and waste. According to estimates of the United States Energy Information Administration USA ( 2019 ), 40% of the annual CO2 emissions are directly related to the electricity consumption. Out of these emissions, 40% of them concern residential and commercial consumers, and as Armel et al. ( 2013 ) and Darby ( 2006 ) mentioned, it is possible to achieve 20% savings if we use power more efficiently. Therefore, electricity consumption and wastage reduction can offer environmental and financial benefits to our society.
Different approaches and systems have been proposed in the literature that aim to reverse climate change and global warming. Intelligent energy management systems with incorporated automations is a promising approach towards the solution of these environmental problems. These systems convert a conventional home or building into a “smart” version of it. Smart Homes and Buildings, according to Energy ( 2021 ), include automations systems which provide the ability to monitor and control various services such as, lighting and heating-ventilation-airconditioning (HVAC), or devices such as fridges, ovens and washing machines. The set of installed sensors, actuators and smart devices constitute an Internet-of-Things (IoT) subsystem. When users are surrounded by microcontrollers and smart devices, they follow the paradigm of Ubiquitous or Pervasive Computing . When Artificial Intelligence (AI) methodologies enable the interaction of people with these devices, the environment embodies Ambient Intelligence (AmI) (Weiser 1993 ).
These environments play an important role in the Smart Grid . Smart grids consist of two parts, the supply-side and the demand-side , which optimize the energy production, transmission, distribution and consumption (Mir et al. 2021 ). Smart homes are a necessity for the demand-side of these grids because even if the supply-side is successfully optimized, a faulty demand-side, e.g. a conventional home/building, will decrease the total effectiveness of the system.
An immediate conversion of all residential and commercial buildings from conventional to smart, is a costly and time-consuming procedure. Even if governments around the world wanted to carry out this plan, the high deployment costs remain an impediment (García et al. 2017 ; Shigeyoshi et al. 2013 ). Therefore, research was expanded towards lower or no-cost energy saving solutions based on information and communication technologies (ICT) (Luo et al. 2017 ).
Research and development of energy management systems focused on new technologies that embody energy savings, and materials that decrease wastage of energy. However, the same attention wasn’t given at users’ behavioral change. Darby ( 2006 ), suggested that in order to improve the awareness on energy waste habits, consumers must firstly monitor their power consumption and then manage it after receiving appropriate advice. Governments and Non-Governmental Organizations support and facilitate energy-efficiency changes. However the impact of simple saving tips and peer devices’ comparison is low because of the wrong time and place these occur (Cattaneo 2019 ). End-users must alter their routine completely and adopt an environmental friendly behavior (Becchio et al. 2018 ).
Recommendation systems are information systems that assist users to discover personalized content, based on their preferences (Resnick and Varian 1997 ). They are used in many different real-world scenarios (Martin 2009 ) and recently some implementations were applied into energy profile reshaping (Alsalemi et al. 2020 ; Sardianos et al. 2019b ) using deep learning algorithms (Wei et al. 2020 ), data mining techniques (Ashouri et al. 2018 ), behavioral analytics and human decision-making processes to develop context-aware systems (Şimşek et al. 2016 , Sardianos et al. ( 2019a )). Despite the fact that these systems emerged in the mid 90’s (Resnick et al. 1994 ; Shardanand and Maes 1995 ), Himeur et al. ( 2021 ) reached the conclusion that the field of energy saving recommendation systems is still unexplored.
Intelligent Energy Management Systems (IEMS) are a necessary tool to reduce energy overconsumption in households, commercial, educational and industrial buildings and subsequently the total CO2 emissions that are produced. To be more precise, studies indicate that commercial buildings are consuming almost 40% of the total energy in most developed countries (Cao et al. 2016 ). Therefore, real-time energy usage monitoring, along with systems that can offer ways to manage energy consumption and, alternative sustainable energy sources (e.g. solar panels), are of the highest importance (Mir et al. 2021 ). This work provides a comprehensive review of IEMS of the literature over the last decade. Our goal in this article was first, to provide the readers an overview of the influential factors of energy overconsumption and also an overview of various approaches towards energy efficiency. Second, we present a high level architecture breakdown for these systems. Third, we provide a review of the state-of-the-art components of each module and we introduce a novel classification for the IEMS in Direct control systems, i.e. systems that provide automations to the environment in order to control functionalities and conditions, and in Indirect control systems, i.e. systems that aim in the behavioral modification of the occupants. Fourth, for these two novel classes, we discuss their respective advantages and disadvantages which class to conclude which class is more suitable for each environment. Finally, we provide a short discussion about their limitations and problematic aspects and also some future research orientations.
The remaining of our study is organized as follows. Section 2 presents our motivation towards studying and comparing these two types of energy management systems. Section 3 discusses related work on this topic and refers to surveys performed on smart environments and recommendation systems for energy efficiency. Section 4 provides background information of the energy efficiency topic from a researchers’ perspective. In Sect. 5 , we present necessary specifications for an intelligent energy management system and an overview of their architectures. Subsequently, Sect. 6 presents a discussion and an analysis of the advantages of each class, their problematic issues and some suggestions for future research. Finally, Sect. 8 concludes our findings.
2 Motivation
Energy management systems are a promising solution towards energy wastage reduction. The variety of studies on smart environments, and the plurality of algorithms and techniques developed over the last decade for automations and recommendations’ optimizations, are proofs of how important these systems are in our effort to reverse climate change and global warming. During our research, we noticed that in current literature, every discussion about smart environments involved mostly systems with integrated automations. Nevertheless, new systems emerged recently which incorporate recommendations mechanisms, aiming at occupants behavioral change rather than in automations. Therefore, we believe that a review was necessary in order to study both types of Intelligent Energy Management Systems.
From our perspective, studying, reviewing and eventually researching IEMS is an extremely important topic especially during the climate and energy crisis we have been experiencing in recent years. These systems can offer environmental solutions regarding efficient energy consumption in various building types, just by adjusting the type of actuation they will incorporate. Each type of building has different needs and capabilities to control its energy footprint and it is crucial for the community research to develop systems with the right approach for each case. For these reasons, a review of the state-of-the-art IEMS, an analysis and the extraction of useful insights is necessary in the literature. To the best of the authors knowledge, a review that include all these topics in the way this article does, does not exist and that is why we start this specific investigation and research. Almost every review until know was focusing either on systems that incorporated automations in the actuation module or systems that were focusing on behavioral management. Moreover, none of these articles was comparing these two types of systems in terms of their suitability on a specific building type. In order to successfully develop and choose an IEMS, a comparison of their advantages was necessary and will give the readers better perspective.
Before referring to IEMS, it would be useful to discuss about efficient energy consumption. There are multiple influential factors that cause energy overconsumption both in residential and in commercial environments. Moreover, a small discussion about various approaches towards energy efficiency. Our goal was to figure out which are the ways to achieve energy saving results and which implementations seem more promising for each installation environment.
Next, we wanted to proceed with the presentation of the IEMS architecture, and provide the reader with a categorization of their components and a classification of their sub-parts. During our research, a classification occurred for the IEMS in Direct Control IEMS and Indirect Control IEMS. Every IEMS can be classified in one of these classes based on the design of its actuation part. Besides various state-of-the-artcomponents we wanted also to show state-of-the-artcomplete prototypes that have been developed by research teams.
In the final parts of this article, we wanted to discuss about the advantages and disadvantages of each class of IEMS, compare their different aspects, investigate their major open problems and discuss about research gaps and future research orientations that will be helpful for researchers.
3 Related work
In this section, we present surveys and reviews that are related to IEMS (Table 1 ). Our intention is to provide the reader an extensive look in the field of Energy Management Systems in Residential, Educational and Commercial Buildings. One could read the work of De Paola et al. ( 2014 ) in order to understand which are the general approaches to energy efficiency and also the main architectural, technological and algorithmic aspects of an energy management system. Leitao et al. ( 2020 ) proposed a similar architecture that also incorporates smart appliances, while Lin et al. ( 2017 ) presented a more abstract architecture for IoT-based systems, consisting of three layers: a Perception layer , a Network layer and an Application layer. Boodi et al. ( 2018 ) dealt with a review of the state-of-the-art Building Energy Management Systems (BEMS) focusing on three model approaches: White box, Black box and Grey box models. They also performed a comparative analysis of the factors that have the highest impact in energy consumption. Himeur et al. ( 2020 ) surveyed a large number of databases with data that refer to building power consumption, with several features compared and examined, such as geographical location, number of monitored households, sampling rate, etc. Moreover, this research team presented a novel dataset for power consumption anomaly detection. Such a dataset will be very useful for training and testing models that aim to detect anomalies in order to reduce energy wastage. Finally, they performed review on current trends and new perspectives in the field of anomaly detection of energy consumption systems. According to them, detecting an anomaly in the energy data can help us prevent a problem before it gets big and spreads (Himeur et al. 2021 ).
When IEMS are installed, they provide AmI at the environment. According to Cook et al. ( 2009 ), there are various definitions about AmI depending on the features that are included. These environments offer environmental, comfort, safety and financial benefits. AmI is also an umbrella term which applies into technologies embedded into a physical space to create an invisible user interface augmented with AI (Dunne et al. 2021 ). They presented an comprehensive survey on Ambient Intelligence (AmI) and Ambient Assistive Living (AAL) while referring to the state-of-the-art AI techniques and methodologies to implement these systems.
An interesting study was performed by Shareef et al. ( 2018 ) reviewing load scheduling controllers which integrate AI techniques such as, artificial neural networks (ANNs), fuzzy logic, adaptive neural fuzzy inference and heuristic optimization. Al-Ani and Das ( 2022 ) surveyed the use of Reinforcement Learning (RL) and Deep Reinforcement Learning (DRL) techniques in home energy management systems (HEMS). They analyzed multiple RL algorithms, their objectives, and also their testing environments. RL and DRL seem like a very promising approach in simulation platforms but until now they are too slow during training and that is why only 12% of these approaches have been tested in the real world. Mason and Grijalva ( 2019 ) have also performed a review article about RL applications for autonomous building energy management. They showed that RL algorithms improve significantly the energy efficiency in domestic environments and also that DRL algorithms are usually preferred more than RL algorithms. Nevertheless, they found out that most of the RL approaches are tested mostly at simulation platforms and that an accurate simulation design is needed before these systems are installed in real world scenarios. An example of such a DRL algorithm was developed by Lissa et al. ( 2021 ). They proposed an algorithm for indoor and domestic hot water temperature control, aiming to reduce the total energy consumption by optimizing the way that solar produced energy is being used.
Leitao et al. ( 2020 ) conducted a survey about home energy management systems, where they discussed about the demand-side management, i.e. the collection of techniques applied to reduce energy costs on the consumption-side and improve energy efficiency. Furthermore, they discussed about dominant scheduling methodologies which are grouped into five categories. Additional energy saving techniques are presented by Mir et al. ( 2021 ), including statistical models, cloud computing-based solutions, fog computing, smart-metering-based architectures and also some IoT inspired solutions. Beaudin and Zareipour ( 2015 ) reviewed methods employed to model various aspects of residential energy management systems. Moreover, they discussed about complexity in such systems and presented also an overview of techniques for scheduling approaches, as well as a classification in mathematical programming, meta-heuristic search and heuristic scheduling techniques. Finally, a recent study by Ali et al. ( 2021 ) summarized research opportunities created by open issues in the field such as, blockchain-enabled IoT platforms for distributed energy management, deep learning models to handle, use and evaluate big energy data, peer-to-peer energy trading and demand-side energy management, context-aware pervasive future computing, resilience-oriented energy management, forecasting models, user comfort and real-time feedback systems as well as, Internet of Energy (IoE)-based energy management.
Multi-agent systems (MAS) is an approach used to model components of IEMS. An interesting article was published by González-Briones et al. ( 2018 ) reviewing state-of-the-art developments in MAS and how they are used to solve energy optimization problems. They discussed about the types of MAS architectures and also the reasons why they must be used as modeling tools. Asare-Bediako et al. ( 2013 ) proposed a multi-agent architecture of distributed intelligence to solve the complex and dynamic decision process of optimal energy management. Their architecture was based on four groups of agents: control and monitoring, information, application and management and optimization agents. According to the definition of MAS (Poole and Mackworth 2010 ) an agent is comprised by a coupling of perception, reasoning and acting components. Mekuria et al. ( 2018 ) conducted a comprehensive literature review aiming to identify and characterize the reasoning systems in MAS-based smart environments and also presented the strengths and limitations of them.
During the last decades, researchers studied means in order to transit into more sustainable energy usage. Because of their daily consumption, households are responsible for 72% of global greenhouse gas emissions (Hertwich and Peters 2009 ). Saving energy and being more efficient in our daily power consumption is important to reduce our greenhouse gas emissions. As previously mentioned, occupants’ behavior influences energy wastage, therefore behavioral changes of occupants remains a key objective for energy scientists. Steg et al. ( 2018 ) discussed of ways to promote the active engagement of people in a sustainable energy transition. According to them, some relevant behaviors must be managed and manipulated. Household consumption and behavioral decisions manipulation can offer a substantial reduction of greenhouse gas emissions if regulatory framework is set that supports behavioral changes (Dubois et al. 2019 ).
Recommendation systems are an important research field since the mid 90’s. Their goal is to help users find online content based on personalized preferences using collaborative or content-based filtering along with AI techniques such as, association rules, clustering, decision trees, k-nearest neighbor, neural networks, regression, etc.(Park et al. 2012 ). Over the last decade, the research community began to integrate recommendation modules into the components of smart environment systems to persuade users to adopt a more Eco-friendly behavior. Steg et al. ( 2018 ) discussed on ways to make people more engaged into actions and habits that are more energy sustainable. Furthermore, they analyzed how these actions will affect their daily lifestyle and comfort. Based on recent studies, Zhang et al. ( 2018a ) estimated that occupants behavior affect 10–25% of the consumption in residential buildings and around 5–30% in commercial buildings. Moreover, there are four topics where researchers must focus in order to identify the effects of occupants behavior in daily power consumption: understand occupants’ actions that affect space heating or cooling, develop methods and techniques to collect data on behavior and building performance, model quantitatively occupants’ behavior and building energy performance and finally, create an evaluation technique for the occupants to identify their energy saving potential.
For there recommendations systems and their applications, an extensive literature survey was conducted by Himeur et al. ( 2021 ) on energy saving recommendation systems in buildings, discussing how they evolved and also providing a taxonomy based on the nature of the recommender engine, their objectives, computing platforms and evaluation metrics. Furthermore, a critical analysis was also conducted to understand what the limitations of these systems are when they aim in energy efficiency. Additionally, Law et al. ( 2018 ) studied a set of recommendations published by companies and agencies, and designed micro-models to estimate how popular recommendations affect energy savings and conducted also a followup study to understand which types of recommendations were chosen and adopted more often.
From a theoretical point of view, Cattaneo ( 2019 ) analyzed the barriers towards the adoption of technologies for efficient energy usage and introduced ways to overcome them. Moreover, McIlvennie et al. ( 2020 ) indicated in their meta-review, that automation and control technologies are only “one piece of the puzzle”. The other one is systems that embed humans in the procedure of excessive consumption reduction using behavioral change techniques through recommendations and feedback. They suggested that the integration between techno-centric and user-centric approaches in more holistic implementations will be more effective.
In this section we mentioned various surveys that have been performed and are related to IEMS. In Table 2 we present a comparison between these surveys and our work. In the next sections, we will show that each IEMS, regardless of its type, follows a similar architecture. Our work breaks down their components and studies state-of-the-art designs, devices and algorithmic approaches. These IEMS implementations are based on requirements extracted from our need to tackle the factors that cause energy overconsumption. To the best of our knowledge, until now, no survey has been performed that both discusses approaches towards energy efficiency, factors that affect it and at the same time present a overview of the architecture, the components and their categories. Moreover, our work presents a novel classification on IEMS based on the type of actuation they incorporate and discusses major open problems of these classes and suggests research directions that will help community to develop better and more efficient IEMS, specialized for each specific installation environment.
4 Efficient energy consumption
4.1 influential factors.
The first step to achieve energy waste reduction is to understand where it originates from. According to Ashouri et al. ( 2018 ), there are four major influential factors of this phenomenon:
Building characteristics Construction materials and insulation levels are obvious factors that increase energy waste in all types of buildings. van den Brom et al. ( 2018 ) conducted a research on performance gaps in energy consumption, revealing that recent buildings, constructed with modern materials, consume less energy than recently renovated older buildings. Furthermore, a difference between actual and theoretical (simulated) consumption was also noticed.
Occupants behavior Occupants affect the overall energy consumption, especially in residential buildings (Bourgeois 2005 ). Even in buildings with the same energy labeling, discrepancies can occur in consumption, depending on heater/cooler set temperature, hot water wastage, requirements of indoor environmental quality, lighting usage, etc.
System efficiency and operation Many buildings or households are equipped either with low efficiency or outdated appliances and devices. Systems’ efficiency affects dramatically the total power consumption, as well as, neglected appliances such as oven, microwave, washing and drying machines.
Climatic conditions Outdoor temperature, solar radiation, humidity and wind velocity can affect energy consumption, especially combined with the aforementioned factors. Even though, these conditions cannot be managed, it is important to realize how they increase energy wastage in order to search for effective solutions.
4.2 Approaches towards energy efficiency
4.2.1 general approaches.
In order to confront climate change, society has to adopt a more energy efficient mindset. Corucci et al. ( 2011 ) identified four approaches towards energy efficiency: user awareness about energy consumption , reduction of standby consumptions , plan and scheduling of flexible activities and adaptive control .
Firstly, user awareness is a straightforward way to achieve energy wastage reduction (De Paola et al. 2014 ). Providing appropriate feedback, advice and recommendations along with detailed information about daily power consumption and total cost can encourage users to follow a more eco-friendly behavior (Darby 2006 ). However, aggregated measurements of energy consumptions make it difficult to understand which device or behavior causes the biggest waste (De Paola et al. 2014 ). Moreover, various studies (Himeur et al. 2021 ; Law et al. 2018 ; Jiang et al. 2009 ) show that behavioral change is still an ineffective strategy in the long term, therefore more research is required.
Secondly, standby devices and appliances are hidden sources of energy wastage. It was first identified as a new challenge in the early ’90 s (Sandberg 1993 ) when analysts began to study the number of appliances that were “leaking” electricity. According to Gram-Hanssen ( 2010 ), any plugged-in device in standby mode can consume some amount of energy, but the increased number of such devices in households and buildings lead to substantial increments in the total consumption. TVs, PCs, Coffee Machines and Printers, are some of the devices in every home, consuming energy without being used for long periods of time (De Paola et al. 2014 ). An interesting study was conducted by Hess et al. ( 2022 ), showing that education was positively correlated with the reduction of standby energy consumption. Moreover, households with children were using less multi-sockets and were less likely to waste standby energy, whereas high-income households were correlated with higher energy consumption.
Thirdly, activity planning and scheduling using modern smart automations systems can offer reduction of energy consumption during energy demand peak (De Paola et al. 2014 ). Scheduling activities, offers financial benefits when energy fares vary between day and night. Furthermore, as Bouakkaz et al. ( 2021 ) proposed, users can also save energy when a house is connected, through a hybrid energy system, into battery storage units.
Finally, another approach to reduce wasted energy is the installation of adaptive control mechanisms. HVAC and lightning systems waste energy in order to preserve user’s comfort. However, the incorporation of AI techniques such as user-presence detection, behavior prediction or reinforcement learning control can tune activation times of these services to avoid unnecessary consumption (De Paola et al. 2014 ; Eller et al. 2018 ).
4.2.2 Energy hubs and microgrids
Besides the four aforementioned approaches, another way to move towards energy efficiency, on a higher level, is the deployment of micro-grids infrastructures in community areas, commercial areas, etc. A micro-grid is a local electrical grid with defined electrical boundaries, acting as a single and controllable entity (Hu and Lanzon 2018 ). Microgrids are important to successfully transform existing grids into smart grids. These grids lead to decrease of the operational costs, reduced emissions and increase of energy efficiency system reliability (Bandeiras et al. 2020 ). These interoperable energy systems consist of local energy production units to increase self-sufficiency using solar cells, heat pumps and recycling of wasted water to achieve sustainability. Another approach towards sustainable energy consumption are the energy hubs which are multi-generation systems that supply different types of energy demands simultaneously by both converting energy carries and using energy storage systems (Nasir et al. 2022 ).
Various research works have been done in recent years on these systems In order to improve energy efficiency of energy hubs, decrease pollution and improve their reliability, Zhang et al. ( 2015 ) devised in a novel framework for the optimal planning of energy hub systems, whereas Mansouri et al. ( 2022 ) developed a two-stage stochastic model for the design and operation of an energy hub in the presence of electrical and thermal energy storage systems. As mentioned earlier, energy storage systems are crucial parts towards energy efficiency. Energy hubs incorporate these subsystems along with different energy carriers and demand response programs. Javadi et al. ( 2022 ) described in their study the joint operation and planning problem as a two-stage optimization problem to successfully design a model that will achieve optimal sizing and siting of an electrical energy storage device, along with electricity tariffs due to demand response program. In order to optimize the efficiency of these multi-systems and their emissions, Mansouri et al. ( 2022 ) developed a multi-objective model to design a hub considering the variable efficiency of its converters, the degradation of its equipment and the annual growth both in load and in energy prices. Nasir et al. ( 2022b ) studied the day-ahead scheduling of these infrastructures considering also the uncertainties on each energy carrier. Finally, Mansouri et al. ( 2021 ) designed also a scenario-based framework for an energy hub that includes a power-to-gas system, proving that the implementation of an integrated demand response program, along with renewable energy sources, reduced energy costs and CO2 emissions.
Similar frameworks have been developed also for microgrids aiming to reduce operating costs for users. An optimization framework for planning active distribution networks have been developed by Matin et al. ( 2022 ). Mansouri et al. ( 2022 ) presented a framework for the scheduling of microgrids considering also the load demand, the market prices and the renewable power generation level. Last but not least, another two-stage stochastic optimization problem was solved by the researchers to design successfully microgrids with dispatchable generators and wind turbines for energy production (Jordehi et al. 2022 ).
4.2.3 Energy saving based on behavioral change
Many research works are focusing into the modification of human habits and are looking for ways to manipulate them towards energy efficiency. A recent example is a system aiming to detect repeated usage patterns from consumption logs, developed by Sardianos et al. ( 2021 ). Another energy-saving recommendation system is presented in Varlamis et al. ( 2022a ), which fuses data from sensors, with users’ habits and their feedback, to provide personalized advises to occupants at the right moment. This system is implemented in the (EM) \(^3\) platform, which will be introduced in the next chapter. Two different edge-approaches were implemented by Sayed et al. ( 2021 ) and Alsalemi et al. ( 2021 ) that incorporate recommendation systems for energy efficiency into a home assistant and an edge-based custom device.
One major problem that energy related recommendations systems face is the user engagement. When actions are not automated and users must act, it is really difficult for them to retain engagement in the recommendations platform. Sardianos et al. ( 2020a ) implemented real-time personalized recommendations system that also provided energy saving facts that aim to increase the persuasiveness of the recommendations. Besides the aforementioned apporaches, another way to produce optimized recommendations is Reinforcement Learning. Shuvo and Yilmaz ( 2022 ) proposed a DRL method which integrated human feedback and activity in the decision process to optimize electricity cost and users’ comfort. This system was developed to be used in domestic environments. RecEnergy is recommender system aiming at energy consumption reduction in commercial buildings by human behavior modification (Wei et al. 2020 ). The overall testing over a four-week period showed energy reduction between 19% and 26%.
5 Intelligent energy management systems (IEMS)
A number of computer-aided tools and technologies were proposed in the last decade in order to effectively optimize energy consumption in our daily life. According to De Paola et al. ( 2014 ), each system or model that was developed must fulfill some basic functional and nonfunctional requirements. Each system has to perceive the environmental conditions of the place it will be installed, use the input data to learn users’ habits, behaviors, preferences, consumptions per device or appliance and also detect or predict existing context. Moreover, it must provide a way for the users to monitor the consumption and at the same time interact with them using notifications to gather feedback and commands. Finally, it should have the ability to modify its environment through actuation after planning optimized sequences of actions that will both reduce energy wastage and satisfy comfort preferences. In respect to the non-functional requirements, these systems have to maintain intrusiveness at low level in the matter of interaction with the user and the physical infrastructure. Furthermore, scalability and extensibility is desirable in such cases, meaning that the level of abstraction during the design should be high. Also, an intelligent system have to be easily deployed by the users and not not require installation from an expert. In the software engineering part of the implementation, the principle of modularity is really important to avoid problematic behaviors of the system. Finally, it is required to be interoperable, with respect to physical devices and other software components.
Energy management systems are developed in a unique way fulfilling the aforementioned requirements following the approaches of the previous section and also following a specific framework architecture (Leitao et al. 2020 ; De Paola et al. 2014 ). The main components of an IEMS are depicted in Fig. 1 :
Sensing and Measuring Infrastructure
Actuation mechanisms
Processing Engine
User Interfaces
Another classification is shown in Fig. 2 . Each IEMS requires at least one type of each component to work effectively.
Information that emerges from the sensing components is saved and processed by the Processing Engine. The engine is the specialized subsystem with components responsible for the process of all acquired data and also performs the optimization tasks based on the end-users preferences. It should also learn and recognize occupants’ activity patterns, communicate with the actuators and manage anomalies or outlier events. When decisions are made, they are transferred into the actuators to modify each appliance or device contextually. Along with the action commands, sometimes, the process engine provides recommendations to the end-users through the user interface to change behaviors that affect the total energy consumption. All these modifications are focused on the persuasion of a smaller energy footprint of our society, however economic impacts remain also a motive (Sardianos et al. 2020a ). Furthermore, through the user interface, users have access to graphs showing daily consumptions. The most common form of a user interface is a computer or smartphone application.
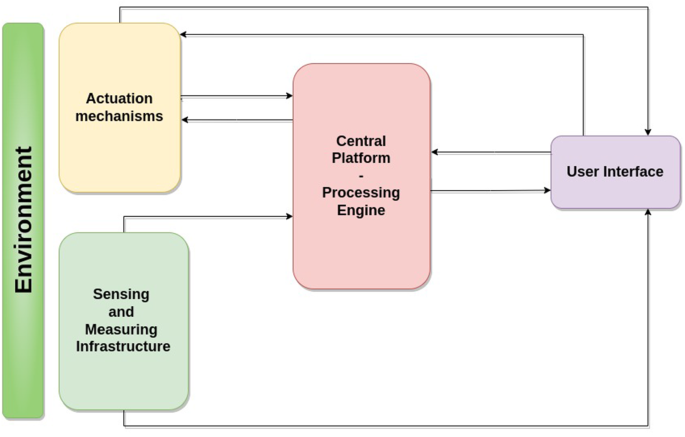
Intelligent energy management system
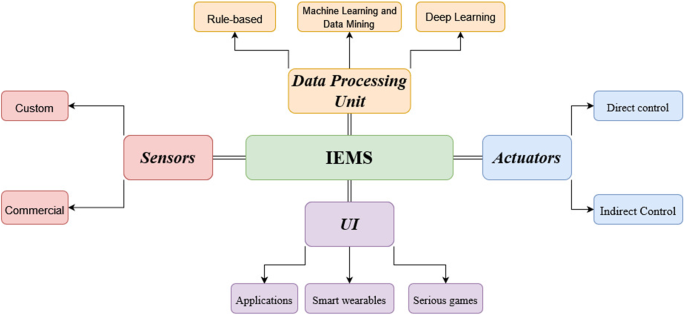
Classification figure of the components of an IEMS
A widespread approach to model state-of-the-art energy management systems is Multi-Agent Systems (MAS). MAS architecture is often used as a tool to model subsystems of an IEMS and is composed of multiple interacting intelligent agents (Hu et al. 2021 ). Each agent can be considered as “Intelligent” because it incorporated AI techniques such as decision-making or machine learning algorithms.
According to Wooldridge ( 2009 ), each agent in a multi-agent system has some important characteristics:
Autonomy The ability to be at least partially independent, self-aware and autonomous.
Local view The perception of the agent has boundaries and no agent has a global view. Otherwise, the agent will not be able to process that large amount of information.
Decentralization No control authority exists inside the MAS.
Wooldridge ( 2009 ) called the ability of an agent to act at a local level, “Sphere of Influence”. Each agent in a MAS has the ability to interact within a specific range. However, there are “spheres” which coincide, rising dependent relationships and creating a unified model. Because of that, MAS architectures are considered appropriate to model IEMS. González-Briones et al. ( 2018 ) argued that MAS are commonly used as models because of the communication, coordination and cooperation capabilities of the agents, and also because this design provides robustness to the system, when different tasks are assigned to each agent.
5.1 Sensors and measuring infrastructure
Sensors and measurement devices are installed on every smart environment, providing data about temperature, humidity and luminance levels, whereas different sensors are monitoring the presence of occupants. There are two types of IoT devices used for these tasks: Custom-made and Commercial . Arduino or Raspberry Pi microcontrollers are used by researchers to create custom modules that fit specific requirements, but in large-scale applications, commercial ones are a preferable option because of the default unified communication protocols.
5.1.1 Custom-made sensors
The most common sensors on these applications are the power consumption meters. Alsalemi et al. ( 2019a ) used SEN-11005 components on a microcontroller NodeMCU to build a custom energy monitoring device (Fig. 3 ). Eridani et al. ( 2021 ) built from scratch their own circuit for an electronic sensor consisting of several sub-circuits for, voltage and current metering, voltage regulation and operational amplification. This sensor was incorporated within an Arduino UNO that was processing the input data which were transferred through an ESP8266 chip to an application. Furthermore, Oberloier and Pearce ( 2018 ) created an open-source power monitoring system, designed around the Digital Universal Energy Logger (DUEL) Node. Ahmed et al. ( 2015 ) also designed their own smart plug using Zigbee protocol. Finally, Jamal et al. ( 2020 ) used ACS712 and ZMPT101B for current and voltage measurements, respectively.
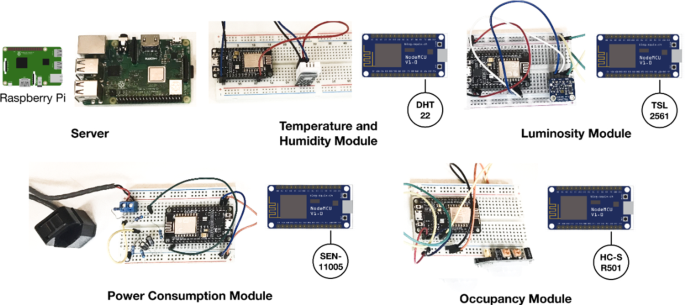
Custom sensors by Alsalemi et al. ( 2019a )
Temperature and humidity sensors were also commonly used in energy management systems. Mataloto et al. ( 2019 ), Sardianos et al. ( 2020a ) and Alsalemi et al. ( 2019a ) used DHT-22 sensors to receive real-time contextual information from the environment. DHT-22 sensor can measure both temperature and humidity levels. On the contrary, Reddy et al. ( 2016 ) used an LM35 temperature-only sensor. LM35 has an analog communication protocol, while DHT-22 has one-wire. Therefore, LM35 is faster in data transmission but it is more sensitive to noise. Also, Kodali et al. ( 2015 ) used LM35 in an ambient intelligent system with an Intel Galileo board.
Light and motion sensors are extremely important components for systems that aim to reduce energy wastage. Rooms and spaces that remain unoccupied tend to have increased consumptions due to switched on lights. Wei et al. ( 2020 ) and Alsalemi et al. ( 2019a ) used a TSL2561 Adafruit sensor for light monitoring and HC-SR501 for motion sensing. Mataloto et al. ( 2019 ) also included photo-resistor sensors and motion sensors with passive infrared (PIR) in their custom sensor-board and Reddy et al. ( 2016 ), used a light dependent resistor (LDR) that reduces its resistance when light hits the surface of it.
Gomes et al. ( 2017 ) created EnAPlug (Fig. 4 ), a multi-sensor smart plug with the ability to switch on/off devices, and monitor power, reactive power, voltage and current. It also included four sensors for temperature, humidity, outside temperature and a door opener detector.
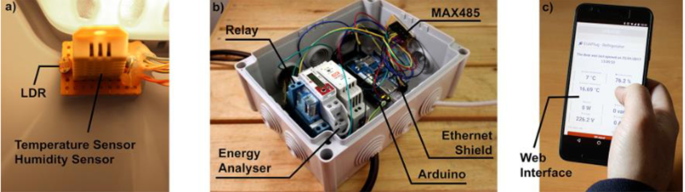
EnAPlug implementation, a custom multi-sensor smart plug by Gomes et al. ( 2017 )
5.1.2 Commercial sensors
Many companies develop smart plugs that are used in energy management systems for environmental sensing, containing usually multiple sensors on a single device. These devices are utilized in smart homes and buildings and are easier to be used by the average user. Furthermore, researchers are selecting these devices when performing large scale experiments to save time from building custom sensor boards.
In their work, Gomes et al. ( 2018 ) used the following smart plugs with metering abilities and on/off control: the DSP-W125 by D-Link, the SP-2101W by Edimax and the TP-link HS110. The DSP plug has also the ability to monitor temperature. Papaioannou et al. ( 2018 ) employed Fibaro 4-in-1 sensor (Fig. 5 ) at the site of the experiment to receive data about temperature, humidity, luminosity, motion and presence simultaneously. Schweizer et al. ( 2015 ) used digitalSTROM systems which were acting as power meters communicating with other nodes inside a smart environment.
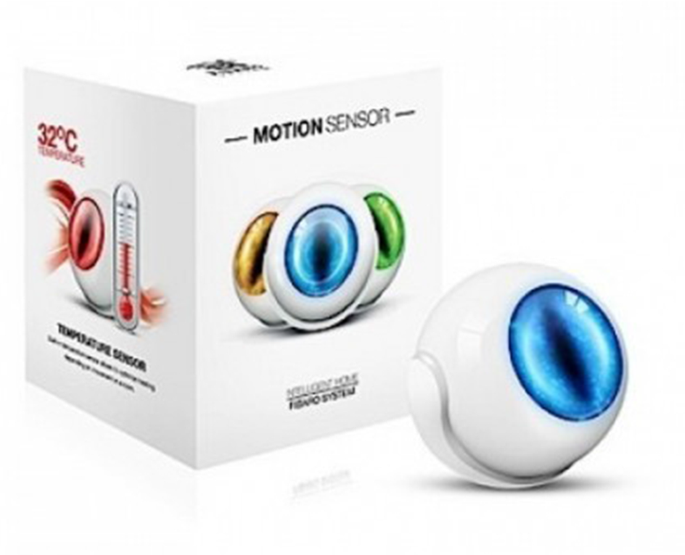
Fibaro 4-in-1 sensor
Popa et al. ( 2019 ) utilized three multi-sensors Aeon Gen5 to detect movement, read temperature, luminance and relative humidity values. Furthermore, they used a smart plug Aeon Smart Switch 6 to control devices and measure instant consumed power and energy and also provide with power consumption graphs for appliances. Last, another Aeon Gen6 multi-sensor was installed that could also provide ultraviolet light sensing data and also home energy meter was installed in the fuse box to measure instant consumption and energy for the entire home without noise.
5.2 Actuators
5.2.1 direct control.
Actuators are the components of an IEMS that execute decisions and commands in order to perform actions so as to optimize power consumption. There are two possible ways to interact with the devices and appliances. The first one is a set of electronic actuators. Actuators are electrical components that interact with the appliances following the decisions of the process engine after the optimization is performed. All systems with the ability to modify their environment using actuators are called Direct Control IEMS . This term encloses every system able to process data, take decisions and execute them on its own, without the intervention of an human being.
Elettra was an innovative system proposed by Cristani et al. ( 2014 , 2015 ), allowing users to monitor their power consumption. It incorporated AmI techniques and algorithms to successfully measure and forecast consumptions of devices providing also direct control to sockets using smart plugs and sensors. Stavropoulos et al. ( 2014 ) proposed the framework Smart IHU, which was deployed at the International Hellenic University (IHU), an application with two components, a Manager and a Rule app. Their actuation infrastructure implementation had custom sensor boards and Z-Wave devices providing automations based on preferable statistics selected by the users.
Chojecki et al. ( 2020 ) implemented an energy management system in a smart meter device. They incorporated a fuzzy logic controller to perform automated actions on the appliances which were divided into two groups, a group of low power devices such as, consumer electronics and multimedia equipment, and a group of medium and high power devices such as, HVAC, water heaters and washing machines.
Another platform with direct controls was implemented by Luo et al. ( 2019 ). Their system was designed to minimize the costs per day of a home by optimally scheduling operations. To achieve this, it included controllable household appliances (smart devices) such as pool pump, dish washer, washing machine, clothes dryer, coffee machine, dehumidifier and bread maker. The users were selecting preferred time range for operations and were providing information about their lifestyle.
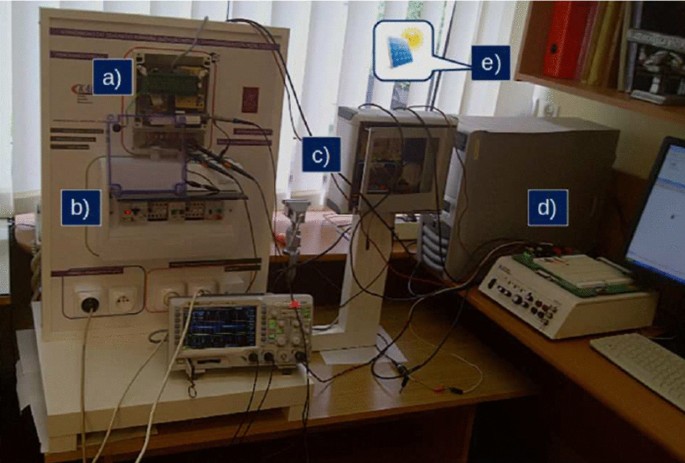
ReViCEE prototype by Kar et al. ( 2019 )
5.2.2 Indirect control
Some recent approaches put humans in the position of the actuator forming human-in-the-loop architectures. Their purpose is to change the behavior of the end-users to stop energy wasting habits. In order to accomplish that, they use recommendations engines to send suggestions and advice through interfaces in order to motivate people to act optimally. These systems can also be extended to propose replacements of inefficient devices and appliances that waste energy (Leitao et al. 2020 ). They are called Indirect Control IEMS.
There are different types of recommendations. The most typical of them are the personal resources recommendations which advise occupants to turn the lights or the HVAC appliances off in empty rooms or shut down idle devices such as, computers and printers. However, three more types of recommendations have been proposed by Wei et al. ( 2020 ). Move recommendations encourage occupants to change their working/living space to reduce services’ requirements. Schedule change recommendations are extensions of move recommendations aiming to shift the period of time an occupant spends within a space and not the duration. Finally, coerce recommendations suggest to the building managers when to force people to evacuate rooms if the occupancy is small in relation to the size of the space.
Alsalemi et al. ( 2019 ); Sardianos et al. ( 2020a , b ) designed (EM) \(^3\) , a framework aiming at occupants’ behavioral change. Using recommendations from its engine, REHAB-C, the human actuator is getting trained by repetition to behave efficiently. ReViCEE by Kar et al. ( 2019 ) follows the same logic by predicting energy consumption ratings and offering personalized recommendations, stimulating user-engagement towards energy conservation and sustainability. ReViCEE’s prototype implementation is show in Fig. 6 .
Popa et al. ( 2019 ) designed a modular platform named SHE (Smart Home Environment). The on-premises control was executed using some Z-Wave controllers, TKB Wall dimmer to control dimmable lights and the Aeon Z-Sticks, Gen-5 and S2, each designed to function on a specific location (US or Europe) depending on the allowed radio frequency for such devices. SHE was advising inhabitants about how they can improve their lifestyle and reduce the costs of energy consumption. Therefore, it provided notifications on a mobile device to motivate them to remotely turn on and off services.
García et al. ( 2013 ) used the framework CAFCLA to develop a recommendation system for usage in homes to promote efficient energy usage. The system was identifying behavioral patterns and along with CAFCLA’s real-time localization system and wireless sensor network it was used to provide personalized recommendations(García et al. 2017 ). KNOTES was a system developed by Shigeyoshi et al. ( 2011 ) that was proposing to the users how to change their life style using notifications, in order to save energy. The system was taking their personal data such as consumption, owned appliances, percentage of advice acceptance and evaluation history to find appropriate suggestions. Gamified approaches seemed also promising in terms of indirect control, especially in education facilities, due to the increased user engagement they provide, through an achievement system with rewards and leaderboards Papaioannou et al. ( 2018 , 2017 ).
5.3 Processing engine
Processing engine of an IEMS is designed to optimize the energy usage on each compartment of a smart environment and manage the actions that have to be performed. After years of research on this field, different techniques have been developed. The majority of the state-of-the-art systems employ Rule Engines, Data and Pattern mining algorithms, Machine Learning and Deep Learning models
5.3.1 Rule engine
The most frequently encountered technique on IEMSs is Rule Engines . Cuffaro et al. ( 2017 ) introduced a general-purpose Rule Engine that pushes notifications or reports to the end-users based on a resource graph model. In (EM) \(^3\) (Sardianos et al. 2020a ) a goal-based context-aware rule-based system (REHAB-C) was implemented with a rule mining algorithm, a process responsible to gather data about frequency of users actions. Papaioannou et al. ( 2018 ), developed an event-driven rule process on a gamified system aiming to reduce energy-wasting behaviors where each challenge assigned to the end-users is represented by a specific rule. Stavropoulos et al. ( 2014 , 2015 ), implemented a Rule App in their application in the form of a Hybrid Intelligent Agent. The agent had two interchangeable layers, the deliberative and the reactive. The reactive layer applies and maintains all energy-saving policies, while the deliberative layer incorporates a reasoner, based on defeasible logic to manage conflicting rules, responsible to optimize energy consumption while maintaining users’ comfort. A similar rule-based architecture using defeasible logic was also implemented by Cristani et al. ( 2016 ). Chojecki et al. ( 2020 ) designed a system that combined a rule-based implementation along with a fuzzy logic algorithm, incorporated on a smart meter to perform direct control. Last, Papaioannou et al. ( 2018 ) proposed an event-based rule engine to change energy waste behaviors in public buildings.
5.3.2 Machine learning and data mining
Smart environments produce a lot of data by the IoT components. Even though this data is processed in order to control the environment remotely, until recently they were rarely used from the system to train the models and achieve autonomy. Meurer et al. ( 2018 ), designed a system that takes advantage of contextual meta-data that originate from smart devices, using extra-trees classifiers, a technique that combines machine learning and data mining. That way, the dimensionality of the produced data was reduced, without loss of important features. Subsequently, an artificial neural network was trained to complete a context-aware engine, with a continuous learning capability based on feedback from the end-users.
Data mining techniques are also used to monitor inhabitants’ usage patterns. Schweizer et al. ( 2015 ), proposed a sequential pattern mining algorithm aiming on smart environments that predict future needs of their inhabitants. Thus, the system could avoid actions that lead to a comfort decrease.
Another system for energy management based on mining algorithms was developed by Dahihande et al. ( 2020 ). Their system provided personalized recommendations about turning on and off appliances at specific timestamps based on household profiles produced by association rule mining apporaches, such as Apriori and FP-Growth and also sequential pattern mining apporaches like RuleGrowth, TRuleGrowth, CMRules, ERMiner and CMDeo from a library created by Fournier-Viger et al. ( 2014 ).
5.3.3 Deep learning
On a smart environment where automated actions must be performed, human activities must be monitored. Recognizing patterns of a room occupant will provide necessary information to the back-end system, leading in more effective predictions. Deep learning techniques such as, convolutional and recurrent neural networks, showed great performance compared to others on human activity recognition Lentzas et al. ( 2019 ); Lentzas and Vrakas ( 2020 ). All this information combined with specific sensor measurements can also grant a context model. Using these models, anomalies and outliers can be detected which would affect energy consumption. Moreover, on more sophisticated systems, using all above can lead to residents’ identification. That way the system can initialize optimizations and actions based on resident’s profile. The aforementioned actions require complicated calculations, therefore Deep Neural Networks were employed (Popa et al. 2019 ).
The problem of the efficient energy consumption consists of two sub-problems, the non-intrusive load monitoring (NILM) and the energy load forecasting (ELF), which were resolved using deep learning models (Popa et al. 2019 ). NILM is a method used to monitor the energy profile of an environment and extract information about appliances consumption by disaggregating the total power consumption (Nalmpantis and Vrakas 2019 ). On the other hand, ELF is used to forecast patterns on energy consumption and detect anomalies that might increase energy consumption (Popa et al. 2019 ).
Another deep learning method used for energy saving is the deep reinforcement learning (DRL). Wei et al. ( 2020 ) used a DFL agent, trained along with the end-users’ decisions. For each successful reduction of consumption, the agent received a reward aimed at maximizing the amount of energy saved. Lissa et al. ( 2021 ) developed such a model, based on Markov Decision Processes (MDP) to control the temperature of domestic hot water. Their goal was to reduce the consumption by optimizing the usage of energy produced by photo-voltaic panels. Yu et al. ( 2019 ) suggested also a model using MDP to schedule optimally HVAC appliances and the energy storage system of a smart home. Finally, Shuvo and Yilmaz ( 2022 ), proposed a DFL model that incorporated human feedback in the objective function and human activity data in the reinforcement learning part of it to enhance optimization of energy.
5.4 User interface
IEM systems include necessarily a User Interface (UI) to allow interaction between them and the users. First of all, UI displays information about total power consumption or consumption per appliance. Secondly, it provides a mean for indirect or direct control of the devices in a smart space. Moreover, it is the only way for the users to change comfort parameters in direct control systems, schedule functions and set rules. Finally, the interface platform sends notifications stimulating recommended behaviors and receive feedback.
Nowadays, interfaces used by smart systems range from simple command-line environments, SMS texts to smartphone and smartwatch applications. There are also differences on the approaches of a user interface, meaning that, it could be a simple one just for system manipulation, or a complicated gamified environment, especially in systems aimed at behavioral changes.
5.4.1 Monitoring and management applications
A standard characteristic on every user interface application is the monitoring component. It usually consists of statistical graphs about consumptions or expenses. Zacharioudakis et al. ( 2017 ) designed a visualized performance graph of a building allowed the users to compare measurements from two different time periods. Moreover, the interface was used to provide alerts if outliers were detected. A simple monitoring agent was also introduced by González-Briones et al. ( 2018 ) providing statistical data about hourly consumptions and the ability to compare different days’ consumptions while it displayed estimated costs for the total kWh consumed and calculated CO2 emissions amount for a month. Another example of a monitoring application is shown in Fig. 7 , implemented by Sayed et al. ( 2021 ) for an intelligent edge-based recommendation system.
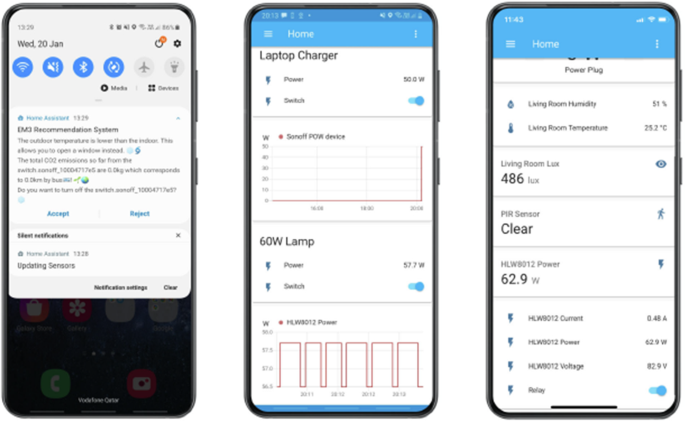
Monitoring application
A different approach in monitoring was given by Stavropoulos et al. ( 2014 ), where every room had its own section at the implemented Rule App, displaying statistics such as current power consumption, temperature, humidity, luminance and CO2 levels, and an indication about motion detection. However, their implementation allowed direct manipulation of these variables so that the agent could adjust comfort levels. Stavropoulos et al. ( 2015 ), a new agent with a GUI was introduced allowing the management of the rule engine through a user-friendly interface. In Figs. 8 , 9 and 10 , we present the user interface that allowed users of Smart IHU (Stavropoulos et al. 2014 ) to select preferred statistics, desired rule sets and monitor the environmental conditions. A similar approach was implemented also by Cristani et al. ( 2014 ), Tomazzoli et al. ( 2020 ) with three main menu choices, allowing manual activation of physical devices, setting of rules, actions and scenarios and also measurement of energy consumption and displaying along with real-time and stored data.
Alsalemi et al. ( 2019 ) implemented (EM) \(^3\) , a system with an end-user web application contained on-line daily consumption monitoring, displaying also indoor and outdoor levels of temperature and humidity, providing at the same time recommendations about energy efficiency. Additionally it had also a control menu with switches to modify devices’ activities. A similar user interface was prototyped also by Dahihande et al. ( 2020 ).
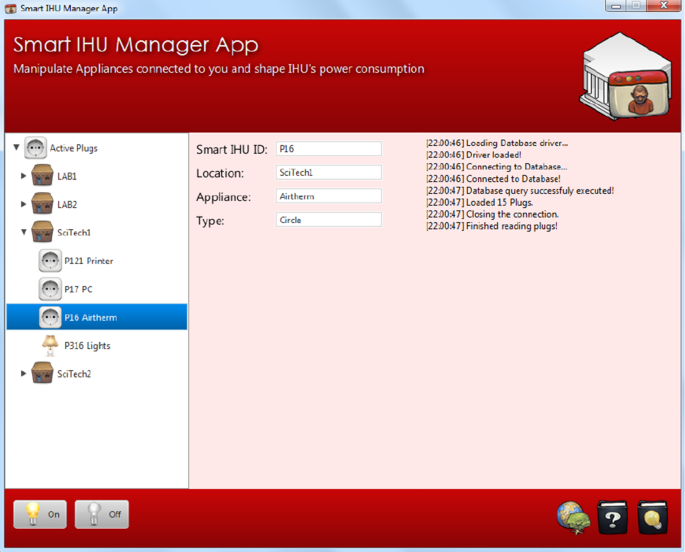
Smart IHU Manager App
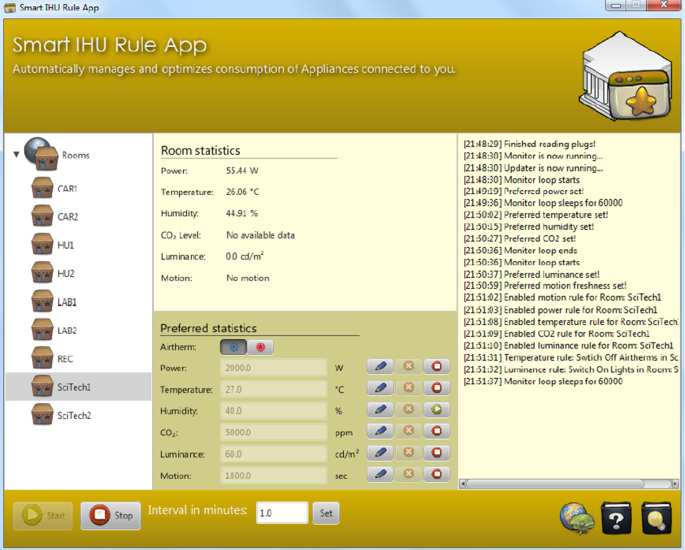
Smart IHU Rule App
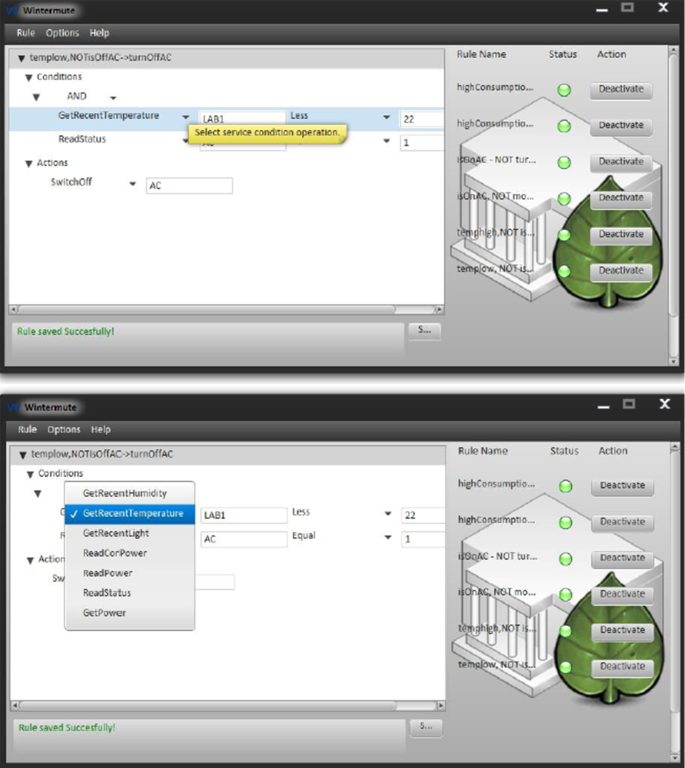
Smart IHU UI to set desired rule sets
5.4.2 Smart devices notifications
Many IEMS are using smartphones and smartwatches as their user interface. Mobile devices offer the convenience of monitoring and managing consumption on the go. Sardianos et al. ( 2020b ) and Schweizer et al. ( 2015 ), used the default text message services in order to push notifications with recommendations expecting feedback from the user to proceed in further actions.
Wei et al. ( 2017 ) proposed a real time energy usage tracking software, along with a web app, two mobile applications for iOS and Android devices and also one for Android wearables. The main dashboard of the system was in a more compressed form in the smartphone app, whereas the smartwatch app displayed only energy footprint breakdown and notifications when alarms were triggered. In Fig. 11 we show the user interface of the ePrints prototype.
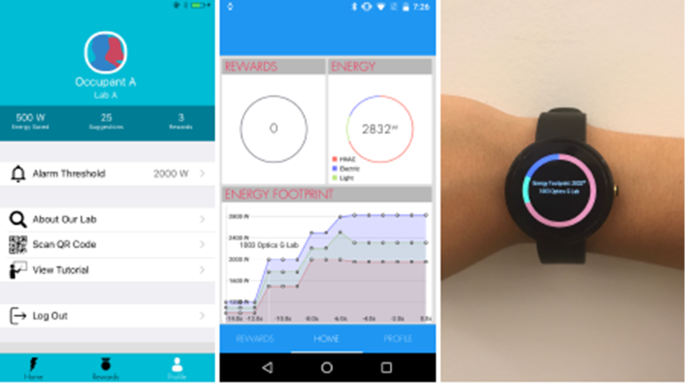
ePrints apps by Wei et al. ( 2017 )
5.4.3 Serious games: gamified approaches
For systems that aim at behavioral changes, gamified interfaces and applications seem to have impact. Papaioannou et al. ( 2018 ), Papaioannou et al. ( 2017 ) proposed a gamified approach was introduced based on challenges for public buildings. End-users had a mobile application plus an NFC chip installed on their smartphones that showed available challenges, e.g. use staircases instead of the elevator or turn off your PC before leaving your office, and rewarded points in case of completion. The user interface contained also a leaderboard, where users were competing each other (Fig. 12 ).
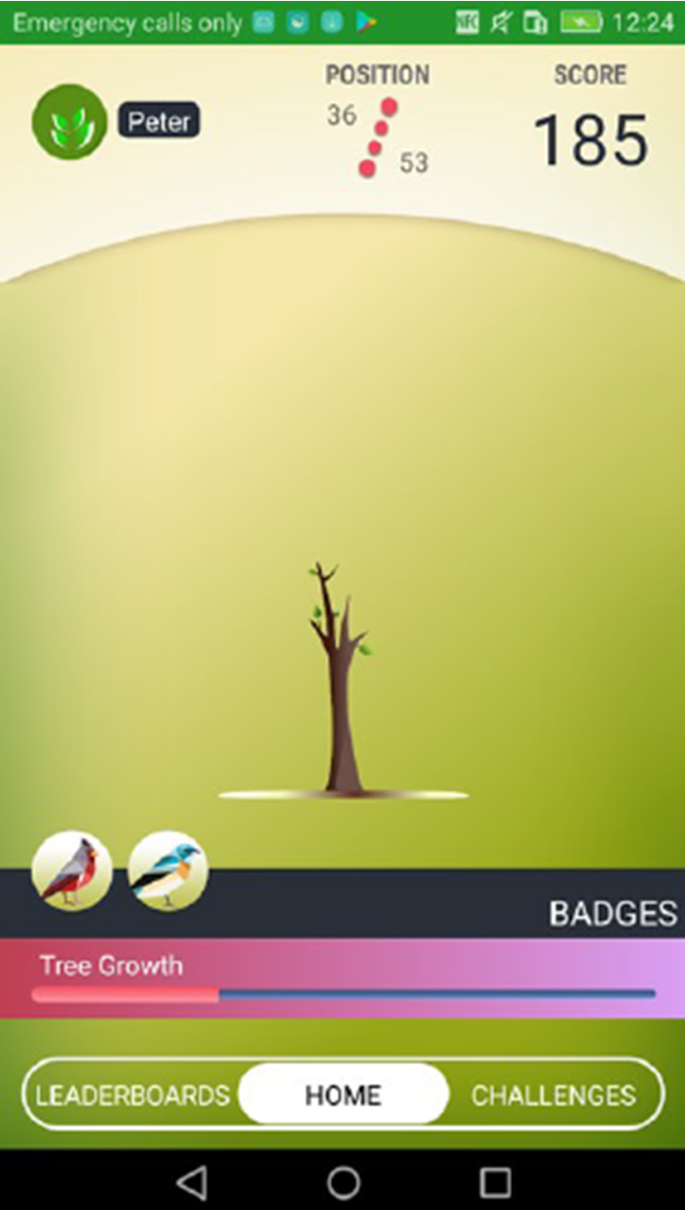
Charged gamified application by Papaioannou et al. ( 2017 )
6 Discussion
In our survey, we elaborated over the topic of Intelligent Energy Management Systems. Our goal in this work was to report state-of-the-art approaches of the area (Table 3 ). However, our work differs from previous surveys because it merges smart automation and recommendation systems under the umbrella term of IEM systems, considering them as two individual sub-classes, whereas in current literature, most articles about Home/Building Energy Management Systems (HEMS/BEMS) refer to systems with incorporated automations.
In this article, first, we referred to the major influential factors that increase energy consumption and wastage, and also presented some general approaches that should be followed in order to pursue energy efficiency. Second, we provided an IEMS architecture overview, based on some functional and non-functional requirements which arise from the aforementioned influential factors and discussed about the state of the art of these systems. Third, we showed that each IEMS can be classified as a Direct or Indirect Control IEMS based on the type of actuation it incorporates. When a system contains automation mechanics and the end-users choose only environmental preferences, the system is controlling the environment directly. On the contrary, when the end-users receive recommendations to perform actions, the performed control is indirect. More recent studies proved that recommendations systems provide improvements in terms of energy savings. For this reason, we classified automations and recommendation systems as Direct and Indirect Control IEMS, respectively, a novel classification that addresses the lack of a unified structure and helps new researchers to obtain a more accurate overview of the field. Following, we will discuss about the advantages of each IEMS control type (Table 4 ), as well as their major open issues, providing also some future research orientations.
6.1 Advantages of direct control IEMS
Direct control IEM systems have the ability to automate procedures and actions. Their major advantage is that the occupants of a smart environment are not obliged to alter their routine. Once preferences are set, the system receives input data, optimizes and sends action commands to all required appliances, without the need for further interaction. For example, when an occupant leaves a room and no human presence is detected, the lights can be turned off. Similarly, the TV can automatically be turned off if the room is empty.
Another argument in favour of direct control is the plan and scheduling ability, allowing users to set long-term ecological or economical goals on the system. Moreover, scheduling techniques can offer consumption shifting on various operations to find optimal time frames in order to reduce energy demand and cost.
Furthermore, smart environments with automations can protect occupants from emergency situations that can occur, e.g. an excessive power consumption caused by electricity “leakage” from a faulty appliance. A leaky device is wasting energy and is also hazardous for the occupants because it can cause accidents, such as fire or electrocution. Automated actuators have the ability to turn off appliances when necessary, reducing the energy wastage and offering also a safer environment.
Finally, fully automated IEMS can be applied in households where handicapped or elderly people live. A properly designed user interface along with ambient assisted living devices, such as Amazon’s Alexa, which support voice commands, will allow these people to modify easily the comfort parameters of their environment and the energy consumption will remain optimized.
6.2 Advantages of indirect control IEMS
The application of recommendation modules in IEMS is a very promising field of research. As it was mentioned in chapter 4, occupants’ behavior is one major influential factor of inefficient energy consumption. Therefore, recommendation systems are useful tools to confront this issue. There is one significant advantage towards these systems. When the occupants follow recommendations for a long time, they acquire an eco-friendlier mindset. Thus, people trained from a home recommendation system will also apply the same aware behavior on every other aspect of their life, e.g. their workplace. Therefore, indirect control will eventually have wider impact.
A major difference from direct control systems is the lack of actuation infrastructure, which provides three important advantages. Firstly, these systems are cheaper and easier to buy. Deducting the cost of smart devices and appliances from the total cost of an IEMS, these systems become more affordable. Secondly, a system with less electrical parts connected to the internet, is less vulnerable to cyberattacks. Even if a false data injection attack is performed on them, the worst case scenario is a system that produces irrational recommendations. Moreover, these systems can also run using a local area network or a Bluetooth area network, which are more secure. Thirdly, the architecture of indirect control systems allows non-controllable appliances to take part into the optimization procedures without any modification on their hardware.
Last, an additional feature of these systems is that it is possible to extend their design so that they can help consumers acquire appliances, based on input data from existing appliances in a household, power consumption of available models on the market, their price, manufacturer and other specifications (Himeur et al. 2021 ).
6.3 Open issues
There has been great progress in the development of energy management systems, both towards direct and indirect control. However, various issues and limitations hinder the wide installation of such systems in commercial, residential and educational buildings. We will discuss the most important problematic aspects of each class.
6.3.1 Security
Direct control IEMS convert conventional buildings into smart ones. Therefore, they can be part of the demand-side of a smart grid, one of the most complex cyber-physical systems. According to He and Yan ( 2016 ), every infrastructure based on cyber-physical systems is vulnerable to various types of attacks.
False Data Injection (FDI) is the most frequent type of attack. Sethi et al. ( 2020 ) proved that these attacks affect electricity bills and load consumption drastically, proposing afterwards a resilient scheduling algorithm to overcome these effects. Furthermore, Dayaratne et al. ( 2019 ) showed that small fluctuations in energy demand from FDI attacks significantly increased the unit price and provided financial benefits to the attacker. Because of the nature of the demand response scheme, these situations lead to inefficient energy consumptions by the system, increasing the energy footprint.
Additionally, another type of attack is the Denial of Service (DoS) attack. Yi et al. ( 2016a ) revealed a vulnerability of metering infrastructure using “Puppet Attack”, a DoS attack that exhausts the communication bandwidth, proposing also detection and prevention mechanisms. Moreover, Yi et al. ( 2016b ) demonstrated that some DoS attacks can cause disruption in the whole smart grid. However, they proposed an algorithmic solution to isolate the attacked nodes to continue data transmission.
Other types of attacks are, Control Signal Adulteration (Esfahani et al. 2010 ), which affects the automatic generation controller that regulates the frequency and power exchange between controlled areas and Information Leakage (Sankar et al. 2012 ). Data leakage of smart metering data can lead malicious user to detect the absence of occupants, a sensitive information that can offer chances for attacks on households.
He and Yan ( 2016 ) provided a classification of the attacks based on the part of the grid they occur.
Generation systems attacks Attacks against power generation and power lines of the smart grid damaging the balance between generation and supply.
Transmission systems attacks Attacks aiming to damage and interrupt the delivery of the generated energy through power stations and lines. These attacks can be classified as (a) Interdiction attacks and (b) Complex network attacks. (He and Yan 2016 )
End-user attacks Attacks on IoT devices and appliances at the end-user side, i.e. smart devices, appliances and electrical actuators. These attacks are serious, according to Pearson ( 2011 ), because in smart metering and monitoring devices there are stored private information about users, such as user’s activities, consumption and idle time and when user’s location is empty or not.
Electricity market attacks Attacks that exploit vulnerabilities in the transmission management that affect the price of the electricity. That way, they make illegal profits and cause congestion to the power lines.
6.3.2 Cold start problem and data sparsity
Cold Start Problem (CSP) refers to the lack of initial data on a recommendation system. This issue occurs for various reasons, mostly in collaborative filtering models. Content-based and knowledge-based systems tend to be more robust (Aggarwal 2016 ).
According to Bobadilla et al. ( 2012 ), there are three cases that cause CSP. First, the new community problem refers to the lack of ratings from the recommendation database. This usually occurs when there are not enough users to rate or vote for the proposed advice, therefore the precision of the recommendation can’t be calculated. Second, the new item problem. The new item problem occurs when new actions or recommendations are imported in the database of the system. These recommendations contain no rating, therefore it is rare for them to be chosen. However, if a recommendation remains unnoticed for a long time, it acts like it doesn’t exist at all, even though it could be useful. Finally, the greatest CS problem is the new user problem. Someone who uses the system for the first time has zero votes on contained recommendation. The filtering methods of the system have no prior information about the new user and no history of ratings to calculate a “neighborhood” of appropriate recommendations (Son 2016 ). Therefore, the performance of the system is negatively affected because it cannot produce meaningful recommendations (Safoury and Salah 2013 ).
Some recommendation systems are based in collaborative filtering (CF), which can be viewed as a classification and regression generalization (Aggarwal 2016 ) and it is the most mature and commonly implemented technique (Jain et al. 2020 ). This technique is making predictions about users’ preferences based on data collected from users with similar profiles. Ratings between users and items are stored in a matrix which is sparse, sometimes up to 99% (Guo 2012 ). This problem is known as Data Sparsity in CF recommendation systems.
Sparsity exists when there is lack of knowledge about new users who start using the system and also, because they ignore the evaluation process after a recommendation. Moreover, new users rarely report their feedback on received suggestions. In CF systems, data sparsity is what causes the cold start problem (Guo 2012 ).
To tackle these problems, some approaches have been proposed in the literature. Himeur et al. ( 2021 ) proposed that some explicitly stated preferences by the newcomers can be used as metrics to include them in some preexisting cluster of older users. Lika et al. ( 2014 ) proposed a model consisting of classification algorithms and similarity techniques that retrieved optimized recommendations. Furthermore, Liu et al. ( 2014 ) mentioned that promoting new items into new users is not very effective, but promoting new items into less active users showed some performance improvement. Jain et al. ( 2020 ) suggested an algorithm based on Sequence and Set Similarity Measure that utilized Singular Value Decomposition removes sparsity from the user-item-ratings matrix and Natarajan et al. ( 2020 ) proposed two methods to overcome sparsity using linked open data from the “DBpedia” knowledge base to create a recommendation system using Matrix Factorization.
6.4 Comparison of direct and indirect control IEMS
Every developed IEMS design has advantages and disadvantages that occur from the actuation type it incorporates. Both direct and indirect control can offer benefits to the end users, however, the installation environment that is most suitable for each class, varies. In Tables 5 and 6 we compare these systems with respect to the following characteristics: cost, daily life intrusiveness, security, comfort, efficiency, ease of installation, accessibility, radius of influence, planning and scheduling features.
In terms of cost, IEMS with automations require a greater number of equipment to control all necessary devices, appliances and services of the installation environment. Each one of these needs an exclusive actuator, which most of the times increases the cost considerably. Because of that, systems which produce recommendations are more affordable options for daily user.
Regarding the intrusiveness of such a system in users daily life, indirect control tend to be more intrusive. People must take actions every time a new recommendation arrives in order to get the best out of their system’s effectiveness. However, this means that the system is highly intrusive in their life, so in this case a system with automations is a better choice.
Security issues are a main concern for all users especially in recent years. Both direct and indirect control systems incorporate a sensing infrastructure, i.e. smart meters and the rest of the sensors that measure environmental variables. Data collected from these devices are extremely sensitive and multiple security measures should be taken into account. As mentioned earlier, absence detection from residential meter data is an extremely dangerous situation, if this information reaches in dangerous people. Nevertheless, the fact that indirect control systems do not incorporate digital actuators make them more resilient to cyber-attacks and no-one can interfere with the functionality of a device or a service.
In every aspect of their life, people are looking for conditions that increase their comfort, whether at home, at work or out for shopping. An energy management system tends to interfere with peoples comfort in all environments in order to achieve energy savings. A system with automations allows the end users to keep their daily routine intact, requiring only an initial setup and minor adjustments during its use. On the other hand, recommendation modules use humans as actuators, therefore their comfort is more affected.
The fact that indirect control systems have a human-in-the-loop design, decreases also their final efficiency. According to the aforementioned studies, user engagement is a major influential factor for the decrease of their efficiency, which makes systems with automations a better choice from this viewpoint.
In terms of ease of installation, direct control systems consist of many more different devices, such as metering, actuators and sensors rather than indirect control systems. On the contrary, an indirect control system can function even with one metering device in the power supply line and one application on a smartphone. Thus, these systems are easier to be installed and they usually are not requiring technical assistance.
The aspect of accessibility is rarely being considered during the design of an IEMS. Systems with automations are more suitable for environments where elderly or handicapped people live or work because usually it is more difficult for them to control the functionality of the services that need to be managed.
There are cases in our daily life where electricity can cause dangerous incidents. A typical example is the malfunction of an appliance. During the monitoring of the energy consumption an appliance can appear to consume energy, even though occupants know that it is not functioning. That might be a case of energy leakage from an old or faulty device. A direct control system can automatically turn of the power supply of this apparatus and protect users from getting electrocuted.
Another aspect IEMS is the planning and scheduling of energy saving actions. Direct control IEMS are capable of lowering HVAC temperature when temperature and humidity reach certain levels autonomously. Therefore, systems with automations have an advantage over recommendation IEMS.
Finally, energy awareness is another aspect of these systems. Usually, designers and developers of IEMS are focused only to achieve energy consumption reduction on the installation environment. However, by focusing on users behavioral management through recommendations systems, people can become more energy aware. When they learn to follow a specific pattern of actions at home, they can act similarly in any other place. This implies that IEMS with incorporated recommendation modules can have a long term effect in a broader set of environments.
7 Future research
7.1 research gap.
This subsection discusses some insights about the research gap in the field of Intelligent Energy Management Systems.
In order to better understand some topics that are related to IEMS, more reviews focused are necessary. First, we found out that Machine and Deep learning techniques are, in most cases, the state-of-the-artapproaches towards the solution of a problem. IEMS are IoT based systems which produce high volume of data, therefore fast and accurate processing is required. Furthermore, these data are, in some cases, collected and processed in real time and decisions must be taken. Therefore, an extensive survey on Machine and Deep learning techniques with applications in IEMS needs to be done so the research community has a better perspective about the limitations of these techniques and how they can be overcame.
Second, another important issue of the IEMS is security . As previously mentioned, security issues occur in IEMS and can be dangerous both for the users and for the energy providers and producers. Incidents such as false data injection attacks or DoS attacks are serious and must be taken into consideration during the development. Until now, due to different standards and communication stacks involved in the IoT technologies, traditional measures against cyberattacks are not always applicable (Sicari et al. 2015 ). Threats like systems’ failure, smart meters’ data corruption, infection by malware, spoofing of usernames and addresses and unauthorized access, show the necessity for research towards threat and risk modelling, IoT forensics, intrusion detection and prevention techniques (Kitchin and Dodge 2019 ; Atlam and Wills 2020 ). Also, Energy Theft is another recent problem that is mostly important for the Distribution System Operators which are managing and distributing produced energy into customers. During our research, we concluded that there is a gap on the area of energy related cyber-security issues and we consider it of great importance for the evolution of IEMS.
Finally, another topic that requires to be studied are ways to increase user engagement in recommendation systems. Indirect control IEMS which involve recommendations through notifications systems seem to be very promising. However, no such system can guarantee that users will remain engaged into the suggested actions and that they will act respectively. Moreover, the fact that recommendations systems suffer from the cold-start problem decreases more the chances for engagement, thus the effectiveness of these systems drops compared to systems with automations. Because of that, we consider it necessary for a study to be carried out that will investigate all problematic aspects of behavioral modification systems, focusing on user engagement.
7.2 Research opportunities
The field of IEMS is still developing, therefore many aspects of these systems need to be improved. Regarding indirect control systems, explainability of recommendations remains an issue that needs further study. The lack of explainable recommendations lead users to ignore advice, reducing the effectiveness of a system and the trustworthiness of it (Zhang et al. 2020 ). Each recommendation should be accompanied by answers to the questions “why to perform an action” and “what the benefits are” (Himeur et al. 2021 ). In (EM) \(^3\) system, (Sardianos et al. 2020a ; Varlamis et al. 2022b ), each recommendation had a reasoning and a persuasion feature, which resulted in 20% increase in the acceptance ratio. Moreover, Wilkinson et al. ( 2021 ) presented that it is more effective to provide justifications on why an action should be performed rather than why not.
Another research opportunity is towards gamified frameworks for systems with recommendation modules. They are more engaging than conventional ones when they aim to change certain behaviors (Papaioannou et al. 2017 ). These approaches are preferred for school buildings, in order to improve energy awareness in students. An example by Mylonas et al. ( 2018 ), was implemented through the GAIA project, where IoT lab kits where used along with a serious game resulting in acceptance of energy aware behaviors.
Reinforcement learning is a popular AI technique to develop smart frameworks that perform control actions. These frameworks can be incorporated in smart home energy management devices to offer higher levels of saved energy. According to Mason and Grijalva ( 2019 ), RL frameworks are capable of learning more complex policies than other neural networks implementations and because of the always increasing data volume, these frameworks will become eventually a necessity. Another opportunity is to develop multi-tasking RL frameworks that will be trained to follow different policies. Until now, many multi-objective optimization methods exists, but none of them utilizes Reinforcement learning. Furthermore, RL control frameworks are not tested yet in extreme condition changes. Minor changes at the environmental conditions have been handled until know (Mason and Grijalva 2019 ) but cases like extreme weather conditions, increase or decrease of occupants, solar panel failure, etc, have not been tested yet. Finally, RL apporaches in IEMS are relatively new (Al-Ani and Das 2022 ). Another research direction would be to perform a comparison between these new approaches with the traditional ones such as rule-based frameworks and other neural network based implementations.
Regarding privacy issues, protecting sensitive users’ information is essential, therefore some research directions could be towards the improvement of resilience of IEMS to cyberattacks by developing frameworks with less vulnerabilities. Until now, due to different standards and communication stacks involved in the IoT technologies, traditional measure against cyberattacks are not always applicable (Sicari et al. 2015 ). Threats like systems’ failure, smart meters’ data corruption, infection by malware, spoofing of usernames and addresses and unauthorized access, show the necessity for research towards threat and risk modelling, IoT forensics, intrusion detection and prevention techniques (Kitchin and Dodge 2019 ; Atlam and Wills 2020 ).
8 Conclusions
This paper has reviewed state-of-the-art approaches of Intelligent Energy Management Systems. Within the area of energy efficiency, IEMS are considered as a way to confront climate change. These systems follow a similar architecture consisting of four components: Sensors, Actuators, Processing Engine and a User Interface.
There are two types of sensing infrastructures in the literature, custom-made and commercial. Researchers choose their preferred type based on the goals and the scale of the application. In large-scale projects, commercial sensors provided convenience and sometimes a unified communication protocol, whereas custom made sensors were preferred for small-scale projects because they could embed more components.
Moreover, this review proposed a novel classification, based on the type of actuation. IEMS can be divided into direct and indirect control systems, depending on who is performing the actions to optimize energy consumption. IEMS with incorporated automation modules are controlling the consumption directly, whereas IEMS aimed at behavioral changes suggest actions and allow the users to decide about actions. Direct control provides convenience through automations and also safety in case of emergency situations. However, improving energy awareness through indirect control can bring about changes in larger scale.
Nevertheless, all of these systems have weak points and vulnerabilities. Systems with automations are mostly vulnerable against cyberattacks. False Data Injection attacks in such systems, can cause an increase of consumed energy. Systems with recommendations suffer from the Cold Start Problem which occurs when new users begin to use the system and when new actions are imported. These problems must be addressed to ensure the effectiveness of these applications.
Our intentions are to keep researching in the field of IEMS. We consider the area of Reinforcement Learning very promising for applications in energy management using direct control. Trying to overpass problems such as the slow training rate is one of our goals. Moreover, another research opportunity regarding the recommendations’ modules is to implement an application or a serious game that will drastically improve users’ engagement in order to create a more affordable and interesting way for people to become more energy aware.
To conclude, IEMS are going through a constant evolution. Direct control approaches seem like a better option for commercial buildings, where a large number of people is present. In that scale, recommendation systems are not very promising. On the contrary, indirect control seems an appropriate choice for educational buildings because eventually, they will increase the awareness of students and will provide long term advantages. Finally, for residential environments, systems with automations are currently more advanced, however, the installation of a complete smart home is still very expensive and unaffordable for the majority of households. Therefore, we suggest that more indirect control applications must be developed for domestic environments in the future.
Data availibility
Data sharing not applicable to this article as no datasets were generated or analysed during the current study
Aggarwal CC et al (2016) Recommender systems, vol 1. Springer, New York
Google Scholar
Ahmad T, Madonski R, Zhang D, Huang C, Mujeeb A (2022) Data-driven probabilistic machine learning in sustainable smart energy/smart energy systems: key developments, challenges, and future research opportunities in the context of smart grid paradigm. Renew Sustain Energy Rev 160:112128
Ahmed MS, Mohamed A, Homod RZ, Shareef H, Sabry AH, Khalid KB (2015) Smart plug prototype for monitoring electrical appliances in home energy management system. In: 2015 IEEE student conference on research and development (SCOReD). IEEE, pp 32–36
Al-Ani O, Das S (2022) Reinforcement learning: theory and applications in hems. Energies 15(17):6392
Ali M, Prakash K, Hossain MA, Pota HR (2021) Intelligent energy management: evolving developments, current challenges, and research directions for sustainable future. J Clean Prod 314:127904
Alsalemi A, Himeur Y, Bensaali F, Amira A (2021) Smart sensing and end-users’ behavioral change in residential buildings: an edge-based internet of energy perspective. IEEE Sens J 21(24):27623–27631
Alsalemi A, Himeur Y, Bensaali F, Amira A, Sardianos C, Varlamis I, Dimitrakopoulos G (2020) Achieving domestic energy efficiency using micro-moments and intelligent recommendations. IEEE Access 8:15047–15055
Alsalemi A, Ramadan M, Bensaali F, Amira A, Sardianos C, Varlamis I, Dimitrakopoulos G (2019) Endorsing domestic energy saving behavior using micro-moment classification. Appl Energy 250:1302–1311
Alsalemi A, Ramadan M, Bensaali F, Amira A, Sardianos C, Varlamis I, Dimitrakopoulos G (2019a) Boosting domestic energy efficiency through accurate consumption data collection. In 2019 IEEE SmartWorld, Ubiquitous Intelligence & Computing, Advanced & Trusted Computing, Scalable Computing & Communications, Cloud & Big Data Computing, Internet of People and Smart City Innovation (SmartWorld/SCALCOM/UIC/ATC/CBDCom/IOP/SCI). IEEE, pp 1468–1472
Armel KC, Gupta A, Shrimali G, Albert A (2013) Is disaggregation the holy grail of energy efficiency? The case of electricity. Energy Policy 52:213–234
Asare-Bediako B, Kling WL, Ribeiro PF (2013) Multi-agent system architecture for smart home energy management and optimization. In: IEEE PES ISGT Europe 2013. IEEE, pp 1–5
Ashouri M, Haghighat F, Fung BC, Lazrak A, Yoshino H (2018) Development of building energy saving advisory: a data mining approach. Energy Build 172:139–151
Atlam HF, Wills GB (2020) Iot security, privacy, safety and ethics. Digital twin technologies and smart cities. Springer, Berlin, pp 123–149
Bandeiras F, Pinheiro E, Gomes M, Coelho P, Fernandes J (2020) Review of the cooperation and operation of microgrid clusters. Renew Sustain Energy Rev 133:110311
Beaudin M, Zareipour H (2015) Home energy management systems: a review of modelling and complexity. Renew Sustain Energy Rev 45:318–335
Becchio C, Bertoncini M, Boggio A, Bottero M, Corgnati SP, Dell’Anna F (2018) The impact of users’ lifestyle in zero-energy and emission buildings: an application of cost-benefit analysis. International symposium on new metropolitan perspectives. Springer, Berlin, pp 123–131
Bobadilla J, Ortega F, Hernando A, Bernal J (2012) A collaborative filtering approach to mitigate the new user cold start problem. Knowl-Based Syst 26:225–238
Boodi A, Beddiar K, Benamour M, Amirat Y, Benbouzid M (2018) Intelligent systems for building energy and occupant comfort optimization: a state of the art review and recommendations. Energies 11(10):2604
Bouakkaz A, Mena AJG, Haddad S, Ferrari ML (2021) Efficient energy scheduling considering cost reduction and energy saving in hybrid energy system with energy storage. J Energy Storage 33:101887
Bourgeois DJ (2005) Detailed occupancy prediction, occupancy-sensing control and advanced behavioural modelling within whole-building energy simulation. PhD thesis, Citeseer
van den Brom P, Meijer A, Visscher H (2018) Performance gaps in energy consumption: household groups and building characteristics. Build Res Inf 46(1):54–70
Cao X, Dai X, Liu J (2016) Building energy-consumption status worldwide and the state-of-the-art technologies for zero-energy buildings during the past decade. Energy Build 128:198–213
Cattaneo C (2019) Internal and external barriers to energy efficiency: which role for policy interventions? Energy Efficiency 12(5):1293–1311
Chojecki A, Rodak M, Ambroziak A, Borkowski P (2020) Energy management system for residential buildings based on fuzzy logic: design and implementation in smart-meter. IET Smart Grid 3(2):254–266
Cook DJ, Augusto JC, Jakkula VR (2009) Ambient intelligence: technologies, applications, and opportunities. Pervasive Mobile Comput 5(4):277–298
Corucci F, Anastasi G, Marcelloni F (2011) A wsn-based testbed for energy efficiency in buildings. In: 2011 IEEE symposium on computers and communications (ISCC). IEEE, pp 990–993
Cristani M, Karafili E, Tomazzoli C (2014) Energy saving by ambient intelligence techniques. In: 2014 17th international conference on network-based information systems. IEEE, pp 157–164
Cristani M, Karafili E, Tomazzoli C (2015) Improving energy saving techniques by ambient intelligence scheduling. In: 2015 IEEE 29th international conference on advanced information networking and applications. IEEE, pp 324–331
Cristani M, Tomazzoli C, Karafili E, Olivieri F (2016) Defeasible reasoning about electric consumptions. In: 2016 IEEE 30th international conference on advanced information networking and applications (AINA). IEEE, pp 885–892
Cuffaro G, Paganelli F, Mylonas G (2017) A resource-based rule engine for energy savings recommendations in educational buildings. In: 2017 Global Internet of Things Summit (GIoTS). IEEE, pp 1–6
Dahihande J, Jaiswal A, Pagar AA, Thakare A, Eirinaki M, Varlamis I (2020) Reducing energy waste in households through real-time recommendations. In: Fourteenth ACM conference on recommender systems, pp 545–550
Darby S et al (2006) The effectiveness of feedback on energy consumption. A Review for DEFRA of the Literature on Metering, Billing and direct Displays 486(2006):26
Dayaratne T, Rudolph C, Liebman A, Salehi M, He S (2019) High impact false data injection attack against real-time pricing in smart grids. In: 2019 IEEE PES Innovative Smart Grid Technologies Europe (ISGT-Europe). IEEE, pp 1–5
Dubois G, Sovacool B, Aall C, Nilsson M, Barbier C, Herrmann A, Bruyère S, Andersson C, Skold B, Nadaud F et al (2019) It starts at home? Climate policies targeting household consumption and behavioral decisions are key to low-carbon futures. Energy Res Soc Sci 52:144–158
Dunne R, Morris T, Harper S (2021) A survey of ambient intelligence. ACM Comput Surv 54(4):1–27
Eller L, Siafara LC, Sauter T (2018) Adaptive control for building energy management using reinforcement learning. In 2018 IEEE International Conference on Industrial Technology (ICIT). IEEE, pp 1562–1567
Elnour M, Fadli F, Himeur Y, Petri I, Rezgui Y, Meskin N, Ahmad AM (2022) Performance and energy optimization of building automation and management systems: towards smart sustainable carbon-neutral sports facilities. Renew Sustain Energy Rev 162:112401
Energy SH (2021) What is a smart home?
Eridani D, Widianto E, Yulianto A (2021) Analysis of custom iot based electricity monitoring system. IOP Conf Ser 1071:012024
Esfahani PM, Vrakopoulou M, Margellos K, Lygeros J, Andersson G (2010) Cyber attack in a two-area power system: Impact identification using reachability. In: Proceedings of the 2010 American control conference. IEEE, pp 962–967
Fournier-Viger P, Gomariz A, Gueniche T, Soltani A, Wu C-W, Tseng VS et al (2014) Spmf: a java open-source pattern mining library. J. Mach. Learn. Res. 15(1):3389–3393
MATH Google Scholar
GAIA. Green awareness in action (gaia)
García Ó, Alonso RS, Tapia DI, Corchado JM (2013) Cafcla: an ami-based framework to design and develop context-aware collaborative learning activities. Ambient intelligence-software and applications. Springer, Berlin, pp 41–48
García Ó, Prieto J, Alonso RS, Corchado JM (2017) A framework to improve energy efficient behaviour at home through activity and context monitoring. Sensors 17(8):1749
Gomes L, Sousa F, Vale Z (2017) Enaplug-an environmental awareness plug to test energy management solutions for households. EPIA conference on artificial intelligence. Springer, New York, pp 253–259
Gomes L, Sousa F, Vale Z (2018) An agent-based iot system for intelligent energy monitoring in buildings. In: 2018 IEEE 87th vehicular technology conference (VTC Spring). IEEE, pp 1–5
González-Briones A, De La Prieta F, Mohamad MS, Omatu S, Corchado JM (2018) Multi-agent systems applications in energy optimization problems: a state-of-the-art review. Energies 11(8):1928
González-Briones A, Prieto J, De La Prieta F, Herrera-Viedma E, Corchado JM (2018) Energy optimization using a case-based reasoning strategy. Sensors 18(3):865
Gram-Hanssen K (2010) Standby consumption in households analyzed with a practice theory approach. J Ind Ecol 14(1):150–165
Guo G (2012) Resolving data sparsity and cold start in recommender systems. International conference on user modeling, adaptation, and personalization. Springer, New York, pp 361–364
He H, Yan J (2016) Cyber-physical attacks and defences in the smart grid: a survey. IET Cyber-Phys Syst 1(1):13–27
Hertwich EG, Peters GP (2009) Carbon footprint of nations: a global, trade-linked analysis. Environ Sci Technol 43(16):6414–6420
Hess A-K, Schubert I, Samuel R, Burger P (2022) Changing routinized household energy consumption using the example of washing, cooking, and standby: a randomized controlled field experiment of home energy advice. Cleaner and responsible consumption, p 100052
Himeur Y, Alsalemi A, Al-Kababji A, Bensaali F, Amira A (2020) Data fusion strategies for energy efficiency in buildings: overview, challenges and novel orientations. Inf Fusion 64:99–120
Himeur Y, Alsalemi A, Al-Kababji A, Bensaali F, Amira A, Sardianos C, Dimitrakopoulos G, Varlamis I (2021) A survey of recommender systems for energy efficiency in buildings: principles, challenges and prospects. Inf Fusion 72:1–21
Himeur Y, Alsalemi A, Bensaali F, Amira A (2020) Building power consumption datasets: survey, taxonomy and future directions. Energy Build 227:110404
Himeur Y, Ghanem K, Alsalemi A, Bensaali F, Amira A (2021) Artificial intelligence based anomaly detection of energy consumption in buildings: a review, current trends and new perspectives. Appl Energy 287:116601
Himeur Y, Elnour M, Fadli F, Meskin N, Petri I, Rezgui Y, Bensaali F, Amira A (2022a) Ai-big data analytics for building automation and management systems: a survey, actual challenges and future perspectives. Artif Intell Rev 1–93
Himeur Y, Elnour M, Fadli F, Meskin N, Petri I, Rezgui Y, Bensaali F, Amira A (2022b) Next-generation energy systems for sustainable smart cities: roles of transfer learning. Sustain Cities Soc 104059
Hu J, Bhowmick P, Jang I, Arvin F, Lanzon A (2021) A decentralized cluster formation containment framework for multirobot systems. IEEE Trans Robot 37(6):1936–1955
Hu J, Lanzon A (2018) Distributed finite-time consensus control for heterogeneous battery energy storage systems in droop-controlled microgrids. IEEE Trans Smart Grid 10(5):4751–4761
Jain AF, Vishwakarma SK, Jain P (2020) An efficient collaborative recommender system for removing sparsity problem. ICT analysis and applications. Springer, New York, pp 131–141
Jamal H, Arshad MN, Butt Y, Shafiq H, Manan A, Arif A, Janjua MK (2020) Design of an economical and reliable net-metering device for residential consumption measurement using iot. In: 2020 59th annual conference of the society of instrument and control engineers of Japan (SICE). IEEE, pp 1747–1752
Javadi MS, Gough M, Mansouri SA, Ahmarinejad A, Nematbakhsh E, Santos SF, Catalão JP (2022) A two-stage joint operation and planning model for sizing and siting of electrical energy storage devices considering demand response programs. Int J Electr Power Energy Syst 138:107912
Jiang X, Van Ly M, Taneja J, Dutta P, Culler D (2009) Experiences with a high-fidelity wireless building energy auditing network. In: Proceedings of the 7th ACM conference on embedded networked sensor systems, pp 113–126
Jordehi AR, Tabar VS, Mansouri S, Sheidaei F, Ahmarinejad A, Pirouzi S (2022) Two-stage stochastic programming for scheduling microgrids with high wind penetration including fast demand response providers and fast-start generators. Sustain Energy Grids Netw 31:100694
Kar P, Shareef A, Kumar A, Harn KT, Kalluri B, Panda SK (2019) Revicee: a recommendation based approach for personalized control, visual comfort & energy efficiency in buildings. Build Environ 152:135–144
Kitchin R, Dodge M (2019) The (in) security of smart cities: vulnerabilities, risks, mitigation, and prevention. J Urban Technol 26(2):47–65
Kodali RK, Swamy G, Lakshmi B (2015) An implementation of iot for healthcare. In: 2015 IEEE recent advances in intelligent computational systems (RAICS). IEEE, pp 411–416
Law M, Thirani M, Rollins S, Joshi A, Banerjee N (2018) Understanding home energy saving recommendations. International conference on persuasive technology. Springer, Berlin, pp 297–309
Leitao J, Gil P, Ribeiro B, Cardoso A (2020) A survey on home energy management. IEEE Access 8:5699–5722
Lentzas A, Vrakas D (2020) Non-intrusive human activity recognition and abnormal behavior detection on elderly people: A review. Artif Intell Rev 53(3):1975–2021
Lentzas A, Agapitos A, Vrakas D (2019) Evaluating state-of-the-art classifiers for human activity recognition using smartphones
Lika B, Kolomvatsos K, Hadjiefthymiades S (2014) Facing the cold start problem in recommender systems. Expert Syst Appl 41(4):2065–2073
Lin J, Yu W, Zhang N, Yang X, Zhang H, Zhao W (2017) A survey on internet of things: architecture, enabling technologies, security and privacy, and applications. IEEE Internet Things J 4(5):1125–1142
Lissa P, Deane C, Schukat M, Seri F, Keane M, Barrett E (2021) Deep reinforcement learning for home energy management system control. Energy AI 3:100043
Liu J-H, Zhou T, Zhang Z-K, Yang Z, Liu C, Li W-M (2014) Promoting cold-start items in recommender systems. PLoS ONE 9(12):e113457
Liu S, Zou Y, Ji W, Zhang Q, Ahmed A, Han X, Shen Y, Zhang S et al (2022) Energy-saving potential prediction models for large-scale building: a state-of-the-art review. Renew Sustain Energy Rev 156:111992
Luo F, Kong W, Ranzi G, Dong ZY (2019) Optimal home energy management system with demand charge tariff and appliance operational dependencies. IEEE Trans Smart Grid 11(1):4–14
Luo F, Ranzi G, Kong W, Dong ZY, Wang S, Zhao J (2017) Non-intrusive energy saving appliance recommender system for smart grid residential users. IET Gener Transm Distrib 11(7):1786–1793
Mansouri SA, Ahmarinejad A, Nematbakhsh E, Javadi MS, Nezhad AE, Catalão JP (2022) A sustainable framework for multi-microgrids energy management in automated distribution network by considering smart homes and high penetration of renewable energy resources. Energy 245:123228
Mansouri SA, Ahmarinejad A, Sheidaei F, Javadi MS, Jordehi AR, Nezhad AE, Catalao JP (2022) A multi-stage joint planning and operation model for energy hubs considering integrated demand response programs. Int J Electr Power Energy Syst 140:108103
Mansouri SA, Nematbakhsh E, Ahmarinejad A, Jordehi AR, Javadi MS, Matin SAA (2022) A multi-objective dynamic framework for design of energy hub by considering energy storage system, power-to-gas technology and integrated demand response program. J Energy Storage 50:104206
Mansouri SA, Ahmarinejad A, Nematbakhsh E, Javadi MS, Jordehi AR, Catalão JP (2021) Energy hub design in the presence of p2g system considering the variable efficiencies of gas-fired converters. In: 2021 international conference on smart energy systems and technologies (SEST). IEEE, pp 1–6
Martin FJ (2009) Recsys’ 09 industrial keynote: top 10 lessons learned developing deploying and operating real-world recommender systems. In: Proceedings of the third ACM conference on recommender systems, pp 1–2
Mason K, Grijalva S (2019) A review of reinforcement learning for autonomous building energy management. Comput Electr Eng 78:300–312
Mataloto B, Ferreira JC, Cruz N (2019) Lobems-iot for building and energy management systems. Electronics 8(7):763
Matin SAA, Mansouri SA, Bayat M, Jordehi AR, Radmehr P (2022) A multi-objective bi-level optimization framework for dynamic maintenance planning of active distribution networks in the presence of energy storage systems. J Energy Storage 52:104762
McIlvennie C, Sanguinetti A, Pritoni M (2020) Of impacts, agents, and functions: an interdisciplinary meta-review of smart home energy management systems research. Energy Res Soc Sci 68:101555
Mekuria DN, Sernani P, Falcionelli N, Dragoni AF (2018) Reasoning in multi-agent based smart homes: a systematic literature review. Italian forum of ambient assisted living. Springer, Berlin, pp 161–179
Meurer RS, Fröhlich AA, Hübner JF (2018) Ambient intelligence for the internet of things through context-awareness. In: 2018 international symposium on rapid system prototyping (RSP). IEEE, pp 83–89
Mir U, Abbasi U, Mir T, Kanwal S, Alamri S (2021) Energy management in smart buildings and homes: current approaches, a hypothetical solution, and open issues and challenges. IEEE Access 9:94132–94148
Mylonas G, Amaxilatis D, Pocero L, Markelis I, Hofstaetter J, Koulouris P (2018) Using an educational iot lab kit and gamification for energy awareness in european schools. In: Proceedings of the conference on creativity and making in education, pp 30–36
Nalmpantis C, Vrakas D (2019) Machine learning approaches for non-intrusive load monitoring: from qualitative to quantitative comparation. Artif Intell Rev 52(1):217–243
Nasir M, Jordehi AR, Matin SAA, Tabar VS, Tostado-Véliz M, Mansouri SA (2022) Optimal operation of energy hubs including parking lots for hydrogen vehicles and responsive demands. J Energy Storage 50:104630
Nasir M, Jordehi AR, Tostado-Veliz M, Tabar VS, Mansouri SA, Jurado F (2022b) Operation of energy hubs with storage systems, solar, wind and biomass units connected to demand response aggregators. Sustainable Cities and Society, p 103974
Natarajan S, Vairavasundaram S, Natarajan S, Gandomi AH (2020) Resolving data sparsity and cold start problem in collaborative filtering recommender system using linked open data. Expert Syst Appl 149:113248
Oberloier S, Pearce JM (2018) Open source low-cost power monitoring system. HardwareX 4:e00044
De Paola A, Ortolani M, Lo Re G, Anastasi G, Das SK (2014) Intelligent management systems for energy efficiency in buildings: a survey. ACM Comput Surv 47(1):1–38
Papaioannou TG, Vasilakis K, Dimitriou N, Garbi A, Schoofs A (2018) A sensor-enabled rule engine for changing energy-wasting behaviours in public buildings. In: 2018 IEEE international energy conference (ENERGYCON). IEEE, pp 1–6
Papaioannou TG, Kotsopoulos D, Bardaki C, Lounis S, Dimitriou N, Boultadakis G, Garbi A, Schoofs A (2017) Iot-enabled gamification for energy conservation in public buildings. In: 2017 Global Internet of Things Summit (GIoTS). IEEE, pp 1–6
Park DH, Kim HK, Choi IY, Kim JK (2012) A literature review and classification of recommender systems research. Expert Syst Appl 39(11):10059–10072
Pearson IL (2011) Smart grid cyber security for Europe. Energy Policy 39(9):5211–5218
Poole DL, Mackworth AK (2010) Artificial intelligence: foundations of computational agents. Cambridge University Press, Cambridge
Popa D, Pop F, Serbanescu C, Castiglione A (2019) Deep learning model for home automation and energy reduction in a smart home environment platform. Neural Comput Appl 31(5):1317–1337
Reddy PSN, Reddy KTK, Reddy PAK, Ramaiah GK, Kishor SN (2016) An iot based home automation using android application. In: 2016 international conference on signal processing, communication, power and embedded system (SCOPES). IEEE, pp 285–290
Resnick P, Varian HR (1997) Recommender systems. Commun ACM 40(3):56–58
Resnick P, Iacovou N, Suchak M, Bergstrom P, Riedl J (1994) Grouplens: An open architecture for collaborative filtering of netnews. In: Proceedings of the 1994 ACM conference on Computer supported cooperative work, pp 175–186
Safoury L, Salah A (2013) Exploiting user demographic attributes for solving cold-start problem in recommender system. Lect Notes Softw Eng 1(3):303–307
Sandberg E (1993) Electronic home equipment-leaking electricity. The energy efficiency challenge for Europe, 2
Sankar L, Rajagopalan SR, Mohajer S, Poor HV (2012) Smart meter privacy: a theoretical framework. IEEE Trans Smart Grid 4(2):837–846
Sardianos C, Varlamis I, Chronis C, Dimitrakopoulos G, Alsalemi A, Himeur Y, Bensaali F, Amira A (2019a) Reshaping consumption habits by exploiting energy-related micro-moment recommendations: a case study. Smart cities, green technologies and intelligent transport systems. Springer, Berlin, pp 65–84
Sardianos C, Varlamis I, Dimitrakopoulos G, Anagnostopoulos D, Alsalemi A, Bensaali F, Amira A (2019b) “ i want to... change”: Micro-moment based recommendations can change users’ energy habits. In: SMARTGREENS, pp 30–39
Sardianos C, Chronis C, Varlamis I, Dimitrakopoulos G, Himeur Y, Alsalemi A, Bensaali F, Amira A (2020a) Real-time personalised energy saving recommendations. In: 2020 International Conferences on Internet of Things (iThings) and IEEE Green Computing and Communications (GreenCom) and IEEE Cyber, Physical and Social Computing (CPSCom) and IEEE Smart Data (SmartData) and IEEE Congress on Cybermatics (Cybermatics). IEEE, pp 366–371
Sardianos C, Varlamis I, Dimitrakopoulos G, Anagnostopoulos D, Alsalemi A, Bensaali F, Himeur Y, Amira A (2020b) Rehab-c: recommendations for energy habits change. Future Gener Comput Syst 112:394–407
Sardianos C, Varlamis I, Chronis C, Dimitrakopoulos G, Alsalemi A, Himeur Y, Bensaali F, Amira A (2021) Reshaping consumption habits by exploiting energy-related micro-moment recommendations: a case study. In: Smart Cities, Green Technologies and Intelligent Transport Systems: 8th International Conference, SMARTGREENS 2019, and 5th International Conference, VEHITS 2019, Heraklion, Crete, Greece, May 3–5, 2019, Revised Selected Papers 8. Springer, pp 65–84
Sayed A, Himeur Y, Alsalemi A, Bensaali F, Amira A (2021) Intelligent edge-based recommender system for internet of energy applications. IEEE Syst J
Schweizer D, Zehnder M, Wache H, Witschel H-F, Zanatta D, Rodriguez M (2015) Using consumer behavior data to reduce energy consumption in smart homes: Applying machine learning to save energy without lowering comfort of inhabitants. In: 2015 IEEE 14th international conference on machine learning and applications (ICMLA). Ieee, pp 1123–1129
Sethi BK, Mukherjee D, Singh D, Misra RK, Mohanty S (2020) Smart home energy management system under false data injection attack. Int Trans Electr Energy Syst 30(7):e12411
Shardanand U, Maes P (1995) Social information filtering: Algorithms for automating “word of mouth”. In: Proceedings of the SIGCHI conference on human factors in computing systems, CHI ’95. ACM Press/Addison-Wesley Publishing Co, pp 210-217
Shareef H, Ahmed MS, Mohamed A, Al Hassan E (2018) Review on home energy management system considering demand responses, smart technologies, and intelligent controllers. Ieee Access 6:24498–24509
Shigeyoshi H, Inoue S, Tamano K, Aoki S, Tsuji H, Ueno T (2011) Knowledge and transaction based domestic energy saving support system. International conference on knowledge-based and intelligent information and engineering systems. Springer, Berlin, pp 242–251
Shigeyoshi H, Tamano K, Saga R, Tsuji H, Inoue S, Ueno T (2013) Social experiment on advisory recommender system for energy-saving. International conference on human interface and the management of information. Springer, New York, pp 545–554
Shuvo SS, Yilmaz Y (2022) Home energy recommendation system (hers): A deep reinforcement learning method based on residents’ feedback and activity. IEEE Transactions on Smart Grid
Sicari S, Rizzardi A, Grieco LA, Coen-Porisini A (2015) Security, privacy and trust in internet of things: the road ahead. Comput Netw 76:146–164
Şimşek U, Fensel A, Zafeiropoulos A, Fotopoulou E, Liapis P, Bouras T, Saenz FT, Gómez AFS (2016) A semantic approach towards implementing energy efficient lifestyles through behavioural change. In: Proceedings of the 12th international conference on semantic systems, pp 173–176
Son LH (2016) Dealing with the new user cold-start problem in recommender systems: a comparative review. Inf Syst 58:87–104
Stavropoulos TG, Kontopoulos E, Bassiliades N, Argyriou J, Bikakis A, Vrakas D, Vlahavas I (2015) Rule-based approaches for energy savings in an ambient intelligence environment. Pervasive Mobile Comput 19:1–23
Stavropoulos TG, Kontopoulos E, Sfounis D, Vrakas D, Bassiliades N, Vlahavas I (2014) An applied energy management approach in intelligent environments based on a hybrid agent architecture
Steg L, Shwom R, Dietz T (2018) What drives energy consumers?: Engaging people in a sustainable energy transition. IEEE Power Energy Mag 16(1):20–28
Tomazzoli C, Scannapieco S, Cristani M (2020) Internet of things and artificial intelligence enable energy efficiency. J Ambient Intell Hum Comput 2020:1–22
USA EIA (2019) Annual energy outlook 2019: With projections to 2050
Varlamis I, Sardianos C, Chronis C, Dimitrakopoulos G, Himeur Y, Alsalemi A, Bensaali F, Amira A (2022) Smart fusion of sensor data and human feedback for personalized energy-saving recommendations. Appl Energy 305:117775
Wei P, Xia S, Chen R, Qian J, Li C, Jiang X (2020) A deep-reinforcement-learning-based recommender system for occupant-driven energy optimization in commercial buildings. IEEE Internet Things J 7(7):6402–6413
Wei P, Chen X, Vega J, Xia S, Chandrasekaran R, Jiang X (2017) eprints: a real-time and scalable system for fair apportionment and tracking of personal energy footprints in commercial buildings. In: Proceedings of the 4th ACM international conference on systems for energy-efficient built environments, pp 1–10
Weiser M (1993) Some computer science issues in ubiquitous computing. Commun ACM 36(7):75–84
Wilkinson D, Alkan Ö, Liao QV, Mattetti M, Vejsbjerg I, Knijnenburg BP, Daly E (2021) Why or why not? the effect of justification styles on chatbot recommendations. ACM Trans Inf Syst 39(4):1–21
Wooldridge M (2009) An introduction to multiagent systems. Wiley, New York
Yi P, Zhu T, Zhang Q, Wu Y, Pan L (2016) Puppet attack: a denial of service attack in advanced metering infrastructure network. J Netw Comput Appl 59:325–332
Yu L, Xie W, Xie D, Zou Y, Zhang D, Sun Z, Zhang L, Zhang Y, Jiang T (2019) Deep reinforcement learning for smart home energy management. IEEE Internet Things J 7(4):2751–2762
Zacharioudakis E, Leligou HC, Papadopoulou A (2017) Energy efficiency tools for residential users. MATEC Web Conf 125:02066
Zhang Y, Bai X, Mills FP, Pezzey JC (2018) Rethinking the role of occupant behavior in building energy performance: a review. Energy Build 172:279–294
Zhang Y, Chen X et al (2020) Explainable recommendation: a survey and new perspectives. Found Trends Inf Retr 14(1):1–101
Zhang X, Shahidehpour M, Alabdulwahab A, Abusorrah A (2015) Optimal expansion planning of energy hub with multiple energy infrastructures. IEEE Trans Smart grid 6(5):2302–2311
Download references
Acknowledgements
This research has been co-financed by the European Regional Development Fund of the European Union and Greek national funds through the Operational Program Competitiveness, Entrepreneurship and Innovation, under the call RESEARCH–CREATE–INNOVATE (project code:95699 - Energy Controlling Voice Enabled Intelligent Smart Home Ecosystem).
Open access funding provided by HEAL-Link Greece.
Author information
Authors and affiliations.
School of Informatics, Aristotle University of Thessaloniki, Thessaloniki, Greece
Stavros Mischos, Eleanna Dalagdi & Dimitrios Vrakas
You can also search for this author in PubMed Google Scholar
Corresponding author
Correspondence to Stavros Mischos .
Additional information
Publisher's note.
Springer Nature remains neutral with regard to jurisdictional claims in published maps and institutional affiliations.
Rights and permissions
Open Access This article is licensed under a Creative Commons Attribution 4.0 International License, which permits use, sharing, adaptation, distribution and reproduction in any medium or format, as long as you give appropriate credit to the original author(s) and the source, provide a link to the Creative Commons licence, and indicate if changes were made. The images or other third party material in this article are included in the article's Creative Commons licence, unless indicated otherwise in a credit line to the material. If material is not included in the article's Creative Commons licence and your intended use is not permitted by statutory regulation or exceeds the permitted use, you will need to obtain permission directly from the copyright holder. To view a copy of this licence, visit http://creativecommons.org/licenses/by/4.0/ .
Reprints and permissions
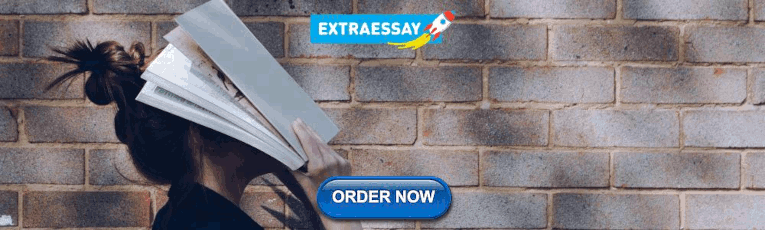
About this article
Mischos, S., Dalagdi, E. & Vrakas, D. Intelligent energy management systems: a review. Artif Intell Rev 56 , 11635–11674 (2023). https://doi.org/10.1007/s10462-023-10441-3
Download citation
Published : 13 March 2023
Issue Date : October 2023
DOI : https://doi.org/10.1007/s10462-023-10441-3
Share this article
Anyone you share the following link with will be able to read this content:
Sorry, a shareable link is not currently available for this article.
Provided by the Springer Nature SharedIt content-sharing initiative
- Energy management systems
- Recommendation systems
- Direct control
- Indirect control
- Automated control
- Find a journal
- Publish with us
- Track your research
Thank you for visiting nature.com. You are using a browser version with limited support for CSS. To obtain the best experience, we recommend you use a more up to date browser (or turn off compatibility mode in Internet Explorer). In the meantime, to ensure continued support, we are displaying the site without styles and JavaScript.
- View all journals
Energy management articles from across Nature Portfolio
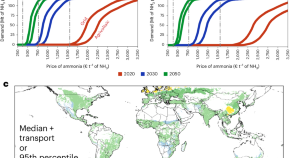
Small-scale decentralized ammonia production could become cost-competitive by 2030
With centralized production, the price of ammonia-based fertilizers is affected by the volatility of the fossil fuel market, complex supply chains and long-distance transportation costs. Now, an analysis of the cost-competitiveness of decentralized low-carbon ammonia production suggests that a substantial fraction of the global ammonia demand could be cost-competitively supplied by small-scale technologies by 2030.
Latest Research and Reviews
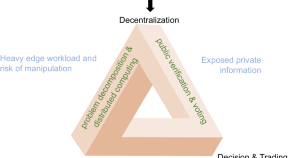
Break down the decentralization-security-privacy trilemma in management of distributed energy systems
Decentralized management of distributed energy sources for lower energy costs is of high interest. Here, authors show how privacy and security concerns are addressed under a decentralization framework through blockchain and parallelizable algorithms.
- Qinghan Sun
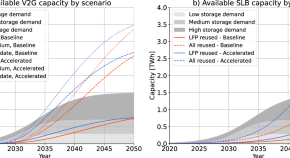
On the potential of vehicle-to-grid and second-life batteries to provide energy and material security
Europe is becoming increasingly dependent on battery material imports. Here, authors show that electric vehicle batteries could fully cover Europe’s need for stationary battery storage by 2040, through either vehicle-to-grid or second-life-batteries, and reduce battery material demand by up to 7.5%.
- Fernando Aguilar Lopez
- Dirk Lauinger
- Daniel B. Müller
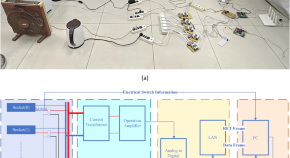
Transient dataset of household appliances with Intensive switching events
- Dongyang Zhang
- Xiaohu Zhang
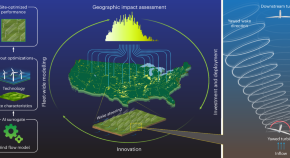
Artificial intelligence-aided wind plant optimization for nationwide evaluation of land use and economic benefits of wake steering
Wind farms would benefit from optimization of their design and operation. Harrison-Atlas et al. report an artificial intelligence-aided optimization approach that shows the potential of wake steering strategies to minimize land requirements and costs.
- Dylan Harrison-Atlas
- Andrew Glaws
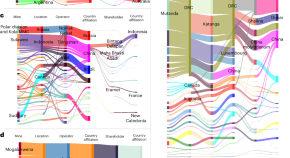
Reducing supply risk of critical materials for clean energy via foreign direct investment
Critical clean energy materials exhibit supply risks due to unbalanced cross-country production and consumption patterns. A study now maps the global distribution of mineral property rights, through foreign direct investment, to show its potential role in reducing critical materials’ supply risks.
- Fuquan Zhao
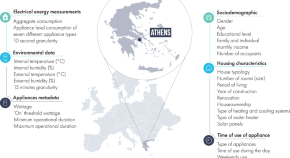
The Plegma dataset: Domestic appliance-level and aggregate electricity demand with metadata from Greece
- Sotirios Athanasoulias
- Fernanda Guasselli
- Vladimir Stankovic
News and Comment
Tourist donations.
- Silvana Lakeman
Energy demands of water distribution
Evidence of discrimination, extractive industry exploration.
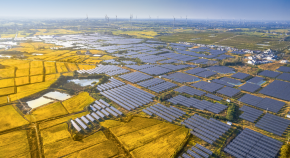
Booming solar energy is encroaching on cropland
The rapid spread of solar power plants onto cropland is having increasingly detrimental impacts. Targeted policy and technological solutions are urgently needed to resolve the tension between renewable energy and food production.
Quick links
- Explore articles by subject
- Guide to authors
- Editorial policies

Green Energy Sources: Issues and Challenges
Ieee account.
- Change Username/Password
- Update Address
Purchase Details
- Payment Options
- Order History
- View Purchased Documents
Profile Information
- Communications Preferences
- Profession and Education
- Technical Interests
- US & Canada: +1 800 678 4333
- Worldwide: +1 732 981 0060
- Contact & Support
- About IEEE Xplore
- Accessibility
- Terms of Use
- Nondiscrimination Policy
- Privacy & Opting Out of Cookies
A not-for-profit organization, IEEE is the world's largest technical professional organization dedicated to advancing technology for the benefit of humanity. © Copyright 2024 IEEE - All rights reserved. Use of this web site signifies your agreement to the terms and conditions.
- Open access
- Published: 13 March 2023
A comprehensive overview on demand side energy management towards smart grids: challenges, solutions, and future direction
- Mutiu Shola Bakare 1 ,
- Abubakar Abdulkarim 2 ,
- Mohammad Zeeshan 1 &
- Aliyu Nuhu Shuaibu 1
Energy Informatics volume 6 , Article number: 4 ( 2023 ) Cite this article
19k Accesses
25 Citations
10 Altmetric
Metrics details
Demand-side management, a new development in smart grid technology, has enabled communication between energy suppliers and consumers. Demand side energy management (DSM) reduces the cost of energy acquisition and the associated penalties by continuously monitoring energy use and managing appliance schedules. Demand response (DR), distributed energy resources (DER), and energy efficiency (EE) are three categories of DSM activities that are growing in popularity as a result of technological advancements in smart grids. During the last century, the energy demand has grown significantly in tandem with the increase in the global population. This is related to the expansion of business, industry, agriculture, and the increasing use of electric vehicles. Because of the sharp increase in global energy consumption, it is currently extremely difficult to manage problems such as the characterization of home appliances, integration of intermittent renewable energy sources, load categorization, various constraints, dynamic pricing, and consumer categorization. To address these issues, it is critical to examine demand-side management (DSM), which has the potential to be a practical solution in all energy demand sectors, including residential, commercial, industrial, and agricultural. This paper has provided a detailed analysis of the different challenges associated with DSM, including technical, economic, and regulatory challenges, and has proposed a range of potential solutions to overcome these challenges. The PRISMA reviewing methodology is adopted based on relevant literature to focus on the issues identified as barriers to improving DSM functioning. The optimization techniques used in the literature to address the problem of energy management were discussed, and the hybrid techniques have shown a better performance due to their faster convergence speed. Gaps in future research and prospective paths have been briefly discussed to provide a comprehensive understanding of the current DSM implementation and the potential benefits it can offer for an energy management system. This comprehensive review of DSM will assist all researchers in this field in improving energy management strategies and reducing the effects of system uncertainties, variances, and restrictions.
Introduction
The mechanism that allows electricity to be transmitted from power plants to energy customers is known as the “power grid”. This electricity goes from the power plant through the substations in one direction before it reaches the energy user when the voltage is changed via the transmission and distribution line (Piette et al. 2004 ).
The need for energy has expanded significantly along with the increase in the global population during the last century. The International Energy Agency predicted that by 2030, global electricity consumption will have increased by more than 50% (Freeman 2005 ). This is related to the growth of business, industry, agriculture, and the increasing use of electric vehicles (Martínez-Lao et al. 2017 ).
Due to the sharp increase in global energy consumption, it is currently extremely challenging to manage problems such as controlling power loss, dependability, efficiency, and security challenges. A “smart grid,” which combines self-monitoring, self-healing, pervasive control, adaptive, and islanding mode mechanisms, has been suggested to allow for energy transit from the point of production to the site of consumption to solve these problems (Fang et al. 2011 ; Xu et al. 2016b ).
The hardware and software components of smart grids provide the utilities the capacity to immediately identify and address any problems that could develop between the customers and the producing plants and endanger the consistency and quality of the power supply. The smart grid component is classified as shown in Table 1 .
Electrical energy management is used to reduce energy expenses and alter the load profile on both the supply and demand sides. The goal of supply side management (SSM) is to make energy generation, transmission, and distribution more operationally effective. SSM has many advantages, such as maximizing customer value by ensuring efficient energy production at the lowest practical cost, satisfying demand for electricity without the need for new infrastructure, and limiting environmental impact. However, supply-side management is affected by fuel price volatility because of its techniques for managing thermal generators (Haffaf et al. 2021 ).
Demand side energy management (DSM) reduces the cost of energy acquisition and the associated penalties by continuously monitoring energy use and managing appliance schedules (Dranka and Ferreira 2019 ). In order to lower peak loads, control time of use (TOU) levels of power demand, evaluate user profiles for electricity loads, lower carbon emissions, and provide consumers a choice of preferred energy source, the electrical industry originally developed the DSM in 1970 (Gellings 2017 ; Maharjan et al. 2014 ).
Several nations, including the UK (Warren 2014 ), China (Ming et al. 2013 ), North America (Wang et al. 2015 ), and Turkey (Alasseri et al. 2017 ), have adopted the Energy Management System (EMS), which is the most effective way to save energy costs while preserving system stability. However, there are still several constraints that prevent EMS from being fully implemented in underdeveloped nations. These components might be related to:
Adopting an EMS comes at a significant expense, and the long-term rate of return on investment is low.
Time-varying electricity tariffs are ideal. Making the switch from an older model to a newer one is tough for electrical companies and merchants.
Not all stakeholders benefit equally from the transformation;
Population knowledge has a significant impact on implementation speed.
Upgrading the network infrastructure could be very expensive for the system, and bidirectional power flow is still in the research stage, which could delay the idea of EMS.
Cappers et al. examined the prospective benefits of DSM to the electrical power system as illustrated in Fig. 1 . These enhancements have the potential to provide considerable secondary advantages, such as decreased losses and premature aging (Cappers et al. 2010 ).
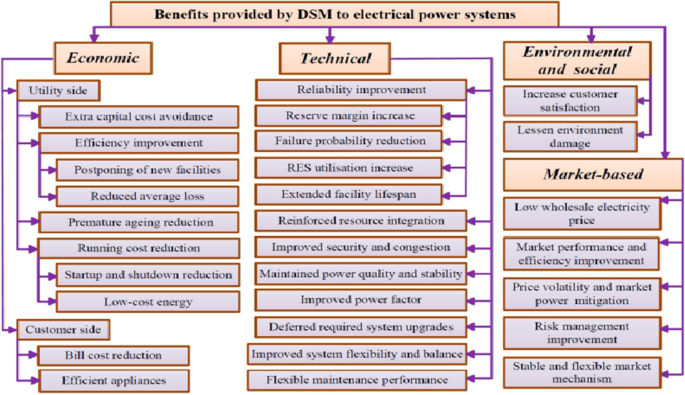
Benefit achieved by the DSM program (Cappers et al. 2010 )
To effectively reduce costs without the involvement of operators, a control system that selects the energy sources to power different loads according to the period of the energy demand is required. The most frequently used controllers in the literature to accomplish the aforementioned goal are programmable logic controllers (PLC), supervisory control and data acquisition (SCADA), building management systems (BMS), energy management systems (EMS), and automation systems (home automation systems, etc.) (Jabir et al. 2018 ).
Numerous studies have focused on the load control strategies used by DSM (Jabir et al. 2018 ), the roles played by DSM in the electricity market (Morgan and Talukdar 1979 ), the economic benefits of DS (Conchado and Linares 2012 ), the impacts of DSM on the commercial and residential sectors (Esther and Kumar 2016 ; Shoreh et al. 2016 ), the interactions between DSM and other smart grid technologies (Khan et al. 2015b ), the business strategies used by DSM (Behrangrad 2015 ), the impacts of DSM on the reliability of the power system (Kirby 2006 ), the optimization strategies used by DSM (Hussain et al. 2015 ; Vardakas et al. 2014 ), and the load control strategies (Khan et al. 2016 ).
The electrical market has just entered a phase of transformation where one of the primary objectives is to lower peak demand while making the greatest use of all resources available. Over the world, incentives have been created to motivate consumers by offering them a range of monetary benefits and different power rates at different load-dependent intervals. Dynamic pricing is an inherent aspect of the home energy scheduling problem in this situation since it encourages consumers to move their load from the on-peak to the off-peak period. Marginal cost, load pattern, social considerations, and the power utility’s capacity are the main variables utilized to define the energy tariff structure (Phuangpornpitak and Tia 2013 ).
All consumers must benefit from greater DSM effectiveness, which requires detailed consumer consumption data. With the advent of advanced metering infrastructure (AMI), utilities may collect all consumer consumption data, and various DSM programs may be developed depending on the data attributes. The scale, complexity, and unpredictability of smart meter data are addressed for use in load forecasting and DSM systems. When implementing DSM, it is important to consider some important factors, including the load profile of an appliance, the integration of renewable energy, load categorization, constraints, dynamic pricing, consumer categorization, optimization techniques, consumer behaviors, problems with electricity data, enough knowledge, a solid framework, and smart grid technology with its intelligent applications (Khan and Jayaweera 2019 ).
As the load profile of appliances heavily depends on the stochastic behavioral patterns of consumers and the surrounding environment, developing a universal DSM optimization method that works for all types of consumers is quite challenging. It is also difficult to develop a generic forecasting system that can accurately predict the power consumption of various appliances for different users. Thus, the load profile of the consumers’ appliances plays a crucial role in the development of a consumer-specific optimization algorithm that takes into consideration their preferences for comfort (Sharda et al. 2021 ). Different appliances have different characteristics, power requirements, and operating styles. For DSM optimization, the right grouping of home appliances based on consumer preferences or behavior is essential. Survey techniques, bottom-up models, top-down models, and hybrid methods have all been explored to do accurate appliance forecasting. Nonetheless, it is believed that utilizing smart appliances and meters is the best option (Proedrou 2021 ).
The effectiveness of demand scheduling optimization depends critically on customer classification. Customers should be made active DR participants by ensuring their comfort which is done by arranging various appliances within their own time and temperature ranges. likewise, customers may be grouped according to their behavior and demand (Liu et al. 2015 ). It is necessary to overcome consumers’ resistance to adopting and taking part in DSM programs, and this may be done by creating consumer awareness initiatives that will urge customers to use the DSM system. Increased expenses for installing and maintaining control devices must also be taken into account. It is necessary to address the impact of the accelerated development of storage systems brought on by the availability of cheap local storage. The majority of the increasing energy consumption is caused by thermostatically regulated equipment. Hence, there is a lot of room for energy savings via effective management of these devices. The following suggestions, which were emphasized in Ming et al. ( 2015 ) may truly aid in overcoming the difficulties associated with DSM.
The planning for the power sector and regional economic growth should all use DSM as a resource. To be properly implemented, rules, laws, and regulations need to be created by the governments and electricity grid businesses.
It is important to gradually establish the DSM’s assessment and monitoring methods. It might be put into practice by constructing a post evaluation system for DSM, an expert committee and oversight mechanisms for DSM, an energy efficiency evaluation system for performing energy inspections, and an analysis of the energy efficiency criteria for electrical equipment. It is also necessary to promote the creation and improvement of relevant supporting policies for DSM.
To fulfill the expanding energy demand and reduce the rising CO 2 emissions, energy generation from renewable energy sources has become more crucial. Several DSM methodologies are utilized to govern distributed energy resources, renewable energy resources, and storage devices to ensure the overall system operates as effectively as feasible. It is difficult to plan for optimal energy requirements since renewable energy sources and power costs are unpredictable. Each operating location must be thoroughly analyzed to pinpoint the areas where natural capital provides notable advantages for certain types of renewable energy consumption. Several optimization techniques, such as mixed-integer linear programming (MILP) (Erdinc et al. 2014 ), two-stage robust optimization (Liu and Hsu 2018 ), and heuristic optimization, have been proposed to enhance the scheduling of distributed energy sources (Luo et al. 2018 ). The ability of the electric vehicle to function as a battery energy storage system has also been researched for applications like vehicle-to-home (V2H) and vehicle-to-grid (V2G) (Erdinc et al. 2014 ).
An effective management system for scheduling various smart appliances and integrating renewable energy (RES) like solar, wind, distributed micro-generators, and energy storage devices, including plug-in electric automobiles and batteries, may be offered to DSM to provide an optimal management system (Qureshi et al. 2021 ; Wang et al. 2019 ; Wu et al. 2019 ). Electricity prices have a big impact on how much energy people use (Rahman and Miah 2017 ; Zhang and Peng 2017 ). But both the analysis and reshaping of the load profiles as well as the load market’s load patterns in SG may be handled by the DSM. This method lowers energy prices, carbon emissions, and grid running costs by lowering customer peak load demands. It also increases the system’s sustainability, security, and stability (Awais et al. 2015 ).
Numerous studies have been written about the DSM of SG, with the majority of them concentrating on distributed generation with renewable energy integration, optimal load scheduling of demand response (DR), and innovative enabling technologies and systems (Kakran and Chanana 2018 ; Lu et al. 2018 ). This paper reviews and examines carefully the DSM methods as well as the effects of distributed renewable energy generation and storage systems on SG. These strategies, seek to lessen peak load demands and uphold a highly developed synchronization between network operators and customers. This paper major contributions is shown below:
Challenges related to the full implementation of DSM in SG and their accompanying solution.
DSM policy, techniques, and their applications to lessen peak demands and price of electricity.
Recent trends of optimization techniques in the DSM.
The paper’s remaining section is shown as follows: The methodology used for this systematic DSM process and the existing work from the literature are also covered in depth in section “ Methodology ”. In section “ The demand side energy management policies ”, the DSM policy and related work done on these policies are examined. Section “ Demand side management techniques ” reviewed the DSM techniques extensively. The challenges related to the full implementation of DSM in SG are carefully examined in section “ Challenges of DSM ”. The future study is highlighted in chapter “ Future work ” with the concluding part shown in chapter “ Conclusion ”.
Methodology
PRISMA stands for Preferred Reporting Items for Systematic Reviews and Meta-Analyses. It is an evidence-based minimum set of guidelines meant to help scientific writers publish different kinds of systematic reviews and meta-analyses. PRISMA focuses on the methods through which authors may ensure accurate and comprehensive reporting of this type of research (Cortese et al. 2022 ). The PRISMA standard superseded the previous QUOROM standard by demonstrating the high review’s quality, allowing review process replication, and allowing readers to assess the review’s benefits and drawbacks. It offers the replication of a systematic literature review that will completely examine all papers published on the issue to identify the answers to a clearly defined research question. To do this, it will choose the reports to be included in the review using a range of inclusion and exclusion criteria, and it will then summarize the findings (Sarkis-Onofre et al. 2021 ).
Any research project’s main emphasis is centered on three crucial elements: the purpose, the research technique, and the output with potential future application. The planning, executing stage, and reporting are the three stages of the evaluation stage that are used. What are potential solutions to the problems encountered when implementing DSM in the smart grid? was one of the research questions that were developed in the initial step of planning the literature study. Which optimization method has recently become popular in DSM? How do DSM’s policies and methods affect peak demands and power costs in their use? The goal of the present research is to address these issues using the examined literature.
The second step of a systematic review, known as the “executing stage,” comprises the inclusive and exclusive criteria. Inclusive criteria give a full and in-depth assessment of current research papers, and an academic database is employed for this study, which comprises IEEE Explore, MDPI, ACM Digital Library, Springer, Science Direct, Google Scholar, and Taylor and Francis. These databases include reputable, excellent peer-reviewed materials including journal articles, conference papers, and review articles. To incorporate relevant terms in a single search, boolean operators are utilized. For instance, keywords and synonyms are combined using Boolean operators like “AND” and “OR.”. Hence, any article matching the keywords “Demand Side” Management,” “Demand Response,” “Load categorization,” “Optimization methods,” “Customer classification,” and “Distributed Energy Sources integration.” will show up in the search results. An organized approach based on PRISMA is used to cover the published material from the last 10 years. Which provides a guideline with features in the form of a checklist to improve openness and clarity in reviews (Page and Moher 2017 ) as shown in Fig. 2 . Based on keyword searches of published articles during the last 10 years, we found 95,736 review papers in the chosen database that were all authored in English.
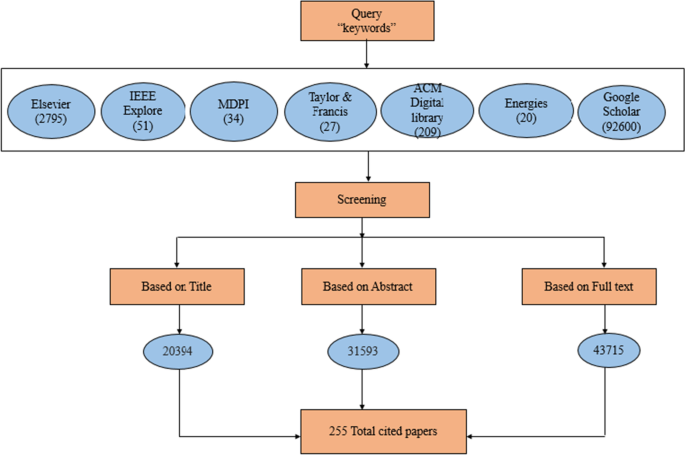
Overview of an articles search strategy
The Selection procedure was carried out based on the paper’s title, abstract, and English-written content. The publication should be published in an English journal or conference paper, feature a prominent DSM name, and make a significant contribution to the DSM’s practical application. Articles are not excluded based on their citation records, as is the case with traditional reviewing processes, and publications found in a general database like Google Scholar were tracked down to the relevant publishing journal and counted there rather than under Google Scholar to avoid duplicate entries. Parents or unpublished manuscripts are also excluded.
The final collection of papers is summarized, stored in Microsoft Word and Excel files, and then utilized in the R-Classify online tools, which help readers find the manuscript’s most important idea. In this last phase, the results are described together with any possible limits and prospective future study areas. The findings of earlier research on energy management systems are summarized in Table 2 . The total number of works considered and cited in the final analysis is 255. Of the 255 articles, 24 are peer-reviewed papers while the others are technical papers. The following details were obtained from each article included in this study: The DSM, demand response techniques, implementation challenges, customer-driven adoption, methodology, approaches, and upcoming optimization work. Table 3 indicated the relationship between the existing and current studies.
Table 3 shows that most review works focused on DSM policy, DSM techniques, and optimization techniques, with little or no consideration for the remaining work. As a result, this paper thoroughly analyzes optimization techniques while also providing future directions to bridge these existing gaps.
Demand side management (DSM) is the concept of allowing users to monitor their energy consumption while taking peak energy demand into account. This continuous monitoring and management of energy consumption aim to improve system reliability while lowering energy costs. Many studies have been conducted on demand side energy management due to its enormous complexity (Li et al. 2018 ). The following is a discussion of the principles, techniques, issues, optimization techniques, and future developments used in literature.
The demand side energy management policies
Energy Efficiency (EE), Demand Response (DR), and Distributed Energy Resources (DER) are three categories into which the strategies used to manage energy on the demand side are divided (Sharifi et al. 2017 ; Wu and Xia 2017 ).
Energy efficiency
Energy efficiency provides energy consumers with a comparable and superior service to lower the quantity of energy needed in an economically effective manner since these methods eliminate excessive power loss in the power network (Bukoski et al. 2016 ). Among the energy-efficient tactics are shown by (Jabir et al. 2018 ).
Using energy-efficient equipment and buildings, as well as promoting consumers’ energy-conscious behavior, to reduce energy usage. Typical instances are switching to energy-saving lights from incandescent bulbs and switching to variable-speed air conditioning from standard air conditioning.
Enhancing and performing routine maintenance on electrical equipment by recovering heat from waste, improving maintenance techniques, using contemporary equipment with optimum designs, and implementing cogeneration.
Increasing the efficiency of power transmission and distribution networks by utilizing distributed generation, advanced control systems for voltage regulation, three-phase balancing, power factor correction, data acquisition and analysis in supervisory control and data acquisition systems, and modern technologies such as low-loss transformers, gas installation substations, smart meters, fiber-optics for data acquisition, and high transmission voltages.
- Demand response
Customers’ energy expenses are reduced through demand response, an optional alteration to the load pattern in response to a change in the electricity tariff (Aghaei and Alizadeh 2013 ). However, it may create inconvenience during appliance waiting periods. Price-based and incentive-based DR policies are the two categories. The split and subdivision of the incentive-based DR are shown in Fig. 3 . The emergency demand response (EDR) program, which pays users for voluntarily decreasing power during crises, and the direct load control (DLC) program, which enables the utility to remotely regulate customers’ appliances to fulfill demand, are both components of the voluntary program. It should be emphasized that under the voluntary initiative, consumers who decide not to participate in energy adjustment will not suffer sanctions (Chen et al. 2014 ; Imani et al. 2018 ).
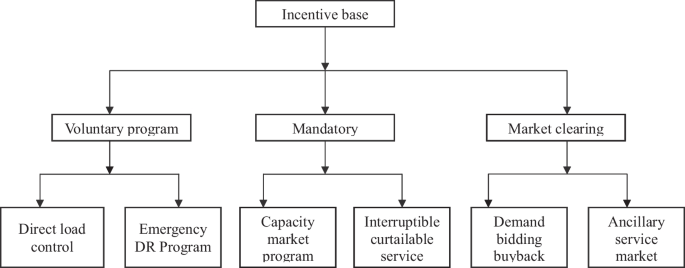
Incentive based Demand Response (Aalami et al. 2019 )
Energy consumers who violate utility company rules under the mandatory program, which consists of the Interruptible Curtailable Service (ICS) and the Capacity Market Program (CMP), are fined (ICS). Another scenario is where the utilities set a predetermined load reduction that the capacity market participants must strictly adhere to maintain a balance between supply, demand, and system dependability. Interruptible/curtailabe uses the emergency response paradigm to stabilize the system, but this paradigm is different from the latter in that users are still required to participate despite the inconvenience involved (Aalami et al. 2010 ; Conteh et al. 2019 ).
The last component of the incentive basis for DR is the market clearing scheme, in which users that participate are compensated with load reduction profits. When attempting to balance energy output and consumption in a market clearing program, procedures like demand bidding/buyback (DBB) and auxiliary service market service (ASM) programs are utilized (Aalami and Khatibzadeh 2016 ). Large energy users, such as industrial and commercial customers, favored this strategy because it gave them a way to bargain for the cost of energy for the load they would be prepared to reduce during a system outage. A negotiated quantity of load reduction with the related rates serves as the electric grid’s reserve energy in an ancillary service market program (Elma and Selamoğullari 2017 ; Yan et al. 2018 ).
Price-based DR is used to persuade energy users to participate in different electricity pricing signals with the aim of lowering energy usage. The primary goals of these regulations are to reduce energy prices and shift demand away from peak times. Several signs related to power price are shown in Fig. 4 .
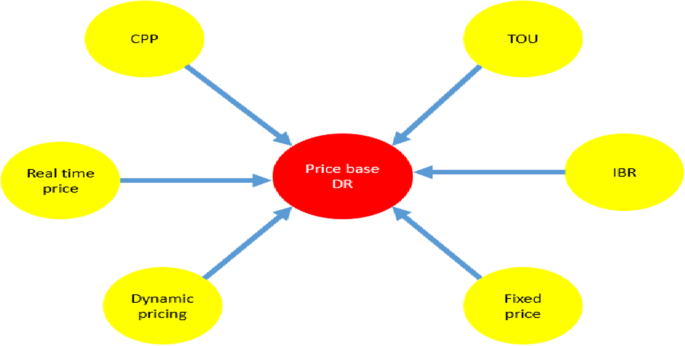
Price based Demand Response (Shewale et al. 2020 )
The cost of producing energy at a certain time of day depending on consumer demand is reflected in the time of use (TOU). The price signal of TOU, which is broken down into on-peak, mid-peak, and off-peak times, is determined by demand and cost. It has the excellent benefit of being simple for customers to follow, comprehend, and arrange for their schedule demands. Countries including China (Zeng et al. 2008 ), Ontario (Adepetu et al. 2013 ), Italy (Torriti 2012 ), USA (Faruqui and Sergici 2010 ) and Malaysia (Hussin et al. 2014 ) have implemented TOU after it was recommended in (Moon and Lee 2016 ; Vivekananthan et al. 2014 ) to minimize costs and energy consumption patterns in residential structures.
Critical peak pricing (CPP) is a price control signal that uses higher power charges to restrict energy usage at a peak time. It offers two time frames (the peak and off-peak). Customers were advised that CPP is granted on days that are predicted to have higher energy use in advance of this period. Since the system is not constantly subject to this constraint, CPP is not a daily DP, but it is also ineffectual at reducing energy costs and carbon emissions. Customers of energy have been urged to participate in DR via CPP, and significant energy and cost reductions have been noted (Kim et al. 2015 ; Yang et al. 2016 ). Most especially in countries like North America (Faruqui and Sergici 2010 ) and Sweden (Renner et al. 2011 ).
The real-time pricing (RTP) scheme is subject to frequent changes due to the utility price signal, which is made available to consumers an hour or day in advance. It is difficult for the consumers to actively participate in it due to its high level of intricacy and the fact that there are two lines of communication between the parties. This pricing strategy is recommended by (Yoon et al. 2014a , b ) as a way to increase system stability at a reduced cost and with favorable environmental impacts in a country like the USA (Yoon et al. 2014a , b ).
When Inclined Block Rate (IBR) is paired with RTP or TOU, both price signals may be utilized. Customers’ energy use and electricity prices are connected, thus if energy consumption falls below a certain point, so will the price. The RTP and TOU pricing scheme works well in terms of energy cost and stability when the IBR is utilized to boost its efficacy (Zhao et al. 2013 ).
A fixed price is a form of pricing indication that is consistent throughout the day or season and is not negotiable. Fixed power pricing in a nation like Nigeria makes it almost difficult to actively engage in any suggested fixed tariff to reduce the cost of energy (Faria et al. 2013 ; Pan et al. 2014 ).
Distributed renewable energy
An integrated decentralized power generating system that is connected to the electrical grid is known as a distributed energy resource (DER). With the increasing integration of DER into the grid, a variety of benefits and opportunities, including affordability, reliability, efficiency, power quality, and energy independence for the power system and its stakeholders emerge. The classification of DER into Distribution Generation (DG) and Electric Energy Storage is shown in Fig. 5 . The DER is powered by convection and renewable energy sources (RES). Conventional energy sources including diesel, gas, microturbines, and combustion turbines still make up the bulk of the energy market despite their limited availability. These sources, nevertheless, are constrained by high production costs, transmission loss, anthropogenic climate change, the greenhouse effect, and acid rain (Bongomin and Nziu 2022 ).
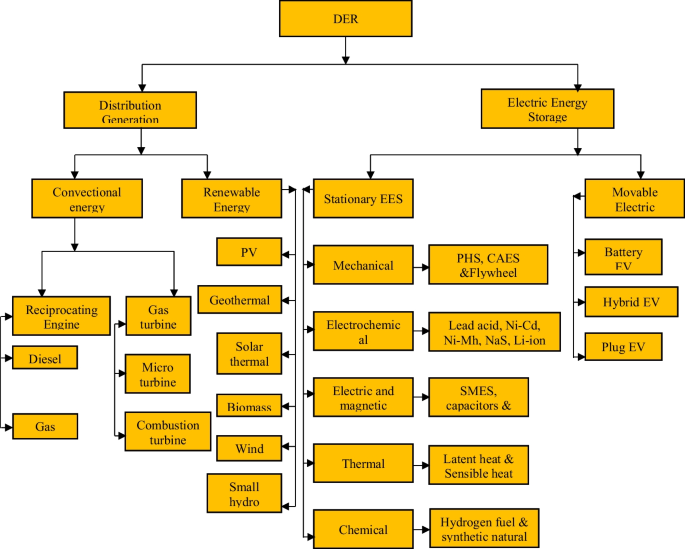
Classification of distributed energy resources (Oskouei et al. 2022 )
Despite being stochastic in nature, intermittent, unexpected, and uncontrolled, renewable energy sources (RES) including solar, biomass, wind, solar thermal, geothermal, and small hydro turbines have grown to be a popular source of energy (Platt et al. 2014 ). According to their storage concept, electrical energy may be transformed into mechanical, electrochemical, electromagnetic, thermodynamic, and chemical energy. The present energy storage methods, prices, guiding principles, benefits, and kinds of ESS applications can be found in Oskouei et al. ( 2022 ).
Demand side management techniques
As illustrated in Fig. 6 , Demand Side Management (DSM) techniques for load shaping include peak clipping, valley filling, load shifting, strategy conservation, strategic load growth, and variable load shape (Macedo et al. 2015 ).
Peak clipping is a concept used in poor countries to decrease the effect of peak demand during peak hours when the installation costs of additional power units are prohibitive. This strategy simultaneously reduced demand and the peak time by directly reducing user appliance loads (Al-enezi 2010 ).
Load shifting involves changing the demand for loads from peak hours to off-peak hours by applying filling and clipping strategies. The TOU and storage devices are used in this method with a constant level of total energy consumption (Chokpanyasuwan et al. 2015 ).
To preserve system balance, valley filling requires a structure during off-peak times, especially when the average cost is lower than the load cost. This often occurs when a plant’s energy production is not fully used and its running expenses are minimal. Even if the peak demand is unaltered, this leads to an increase in total energy usage. By using thermal storage to apply this technology, system efficiency is greatly raised at a reduced energy cost.
Strategic conservation reduces energy loss and consumption efficiency of seasonal energy consumption through technological change incentives. This technique is quite comprehensive and less considered as a technique in load management because it involves a reduction in sales that is not necessarily accompanied by peak reduction.
Strategic load growth increases peak demand in a particular season by managing the seasonal energy usage and a drastic rise in both effect of the energy usage and peak demand is recorded. However, the utilities make use of a more intelligent system to meet their target, especially in the electrification of industrial and commercial heating processes.
Flexible load shape uses load limiting devices to reduce energy consumption at the user’s end without affecting the actual system conditions, the utility interrupts the loads when necessary to reduce the peak demand and change the total energy consumption.
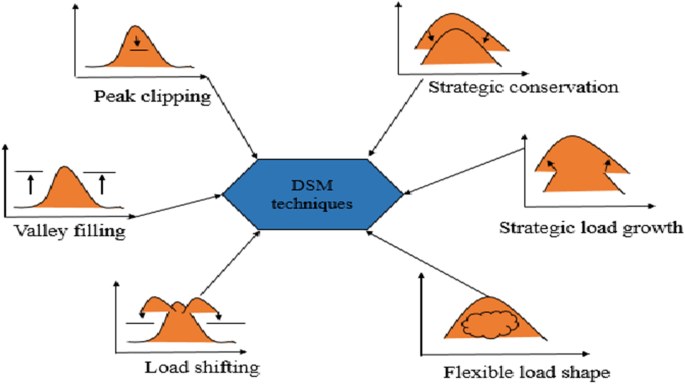
Demand side management techniques (Macedo et al. 2015 )
This paper reports some of the work on demand side energy management strategies and takes into account the three main categories of energy consumers, namely residential (R), commercial (C), and industrial (I) energy users. As indicated in Table 4 , certain authors in some of the examined works took into account all (A) energy users at once.
Challenges of DSM
Planning and managing decision parameters and operating constraints are necessary for the implementation of DSM and depend on several important factors, including the load profile of an appliance, the integration of renewable energy, load categorization, constraints, dynamic pricing, consumer categorization, optimization techniques, consumer behaviors, issues with electricity data, adequate knowledge, a reliable framework, technology-smart, and grid-intelligent appliances, appropriate control strategies, and these challenges encountered during the DSM’s deployment are briefly mentioned below:
Load profile of appliance
Smart appliances are an essential part of creating an accurate and efficient load management system since they come with built-in communication sensors that can link with the smart meter to analyze their energy usage. This is accomplished by collecting ambient data and operating in accordance with the power and tariff parameters provided to them. To create a more precise and trustworthy system, the energy profiles of smart appliances must be taken into consideration during the deployment phase. A normal survey load profile may take the role of smart meters, although it is less accurate. If you are aware of every piece of equipment your clients use, setting up a DR program is easy. To assess load profile management, a survey of various energy consumers is conducted, with an emphasis on quality of service (QoS) (Pilloni et al. 2016 ). Similar in approach, the authors (Vivekananthan et al. 2014 ) urge users to discuss their preferences for using controlled appliances and place greater emphasis on scheduling appliances according to time and preferences. According to a study published in (Yilmaz et al. 2019 ), the variables used to construct the experimental load profiles for 60 residential structures were consumer availability, occupant population, and age. The deployment of smart meters with specific devices, as well as the methodology for monitoring and analysis, are presented in Issi and Kaplan ( 2018 ), Teng and Yamazaki ( 2018 ). The writers in Yilmaz et al. ( 2020 ) investigate the major appliances that are responsible for this high energy consumption at the designated time of day to lower peak demand to 38% by implementing energy-efficient equipment. The stochastic ambient environment and user behavior, according to the currently available literature, make it challenging to develop a generalized load profile optimization algorithm that can accurately predict the energy consumption of various electrical appliances for various consumers.
In conclusion, compared to the usage of smart appliances and smart meters, load profiling assessment techniques like surveys, questionnaires, bottom-up, and top-down approaches are less technically complex, accurate, and time-consuming. However, performing this assessment comes at a far higher cost. By using the data produced by these smart devices, stakeholders may have a better knowledge of how they consume electricity. This is a crucial tactic to raise the power grids’ dependability and effectiveness.
Renewable energy integration
Since the use of renewable energy sources (RES) in the current power system seems to have a bright future, it is one of the factors considered while using DSM. Integration is very difficult, although encouraging, it may sometimes be irregular and intermittent (Elma et al. 2017 ). But in order to deal with the problems of power instability, power quality, and reliability brought on by RES’s intermittent nature, battery energy storage systems (BESS) are especially helpful (Elma et al. 2017 ). To address these difficulties, four battery consumption management techniques using centralized, decentralized, and distributed control structures have been investigated (Worthmann et al. 2015 ). The authors in (Yao et al. 2015 ) suggested an autonomous energy scheduling strategy to solve the problem of voltage escalation in HEMS. The DSM has recommended the optimal charging methods for plug-in electric cars (PHEV) and BESS to reduce the peak load demand (Mou et al. 2014 ). To assess how well the system uses its batteries, two metrics of battery efficiency factor and utilization factor have been created. It has been shown that system operating costs may decrease as battery efficiency increases (Nguyen et al. 2014 ). Since RES is rapidly evolving into one of the fundamental elements of DSM, it is imperative to develop cutting-edge optimization solutions for efficient load scheduling with the lowest cost while maintaining customer satisfaction.
By reducing system strain, which lowers the likelihood of power outages, diversifying the generation mix, and possibly improving power quality, it can be deduced from the literature that the integration of renewable energy can increase power network reliability. Moreover, it may help countries with climate change mitigation, energy cost reduction, and improving resistance to price volatility. Decentralized energy production, less environmental impact, and improved energy security are advantages of RES in DSM (Dincer and Bicer 2020 ). Yet, because the efficiency is lower than that of the conventional energy grid, synchronizing energy production and consumption is a significant issue for the energy sector. Nonetheless, the development of batteries has positively impacted the aforementioned constraint. The cost of production and the quantity of space needed for the use of this various energy are further barriers to the full integration of RES (Basit et al. 2020 ).
Load categorization
Electrical appliance classification is vital for efficient load management. These electrical loads may be categorized according to three standards:
Based on the appliances’ time of operation (Puente et al. 2020 ).
Based on power rating of appliances (Kim and Lee 2019 ).
Based on appliances’ total energy consumption (Ibrahim et al. 2023 ).
Deferrable and nondeferrable operated appliances make up the first standard’s loads, adjustable and nonadjustable operated appliances make up the second standard’s load, and basic and heavy operated appliances make up the third standard’s loads. It is important to note that there is presently no approved worldwide classification system for home appliances (Leitao et al. 2020 ). It should be noted that despite writers using the categorization suggested in Beaudin and Zareipour ( 2015 ), there is still no agreement on the appliances that belong to each group.
The literature classifies various smart home appliances based on user comfort and classification clarity. For scheduling home appliances, authors in the literature have used their own classification. Faisal et al. classified fifteen appliances as interruptible, non-interruptible, or base appliances. Among the interruptible appliances are the vacuum cleaner, sensors, PHEV, dishwasher, stove, microwave, and other intermittent loads. The clothes washer and spin dryer are non-interruptible appliances, while the oven, TV, PC, laptop, radio, and coffee maker are basic appliances (Faisal et al. 2019 ).
Shuja et al. classified fifteen appliances as shiftable, non-shiftable, or fixed. Water pumps, water heaters, vacuum cleaners, dishwashers, steam irons, air conditioners, and refrigerators are all shiftable appliances. Washing machines and tumble dryers are non-shiftable appliances, while TV, oven, desktops PC, blender, laptops, and ceiling fans are fixed appliances (Shuja et al. 2019 ). Thirteen smart home appliances were utilized (Rahim et al. 2016b ), including eight shiftable and five non-shiftable items. Shiftable appliances include an air conditioner, clothes dryer, washing machine, dishwasher, refrigerator, coffee maker, water heater, and space heater, whereas non-shiftable appliances include a fan, lamp, iron, toaster, and microwave oven. Abbasi et al. utilized eleven items divided into three categories: fixed appliances, shiftable appliances, and interruptible appliances. Fixed appliances include a lamp, oven, blender, and coffee maker. Shiftable appliances include the clothes dryer, washing machine, and dishwasher, whereas interruptible appliances include the water heater, iron, vacuum cleaner, and space heater (Abbasi et al. 2019 ). Eight shiftable appliances (dishwasher, refrigerator, air conditioner, clothes dryer, water heater, coffee maker, space heater, dishwasher) and six non-shiftable appliances (fan, light, blender, clothes iron, oven, and vacuum cleaner) were utilized (Rahim et al. 2018 ).
Deferrable and nondeferrable operated appliances
The time of operation of a deferrable appliance can be stopped, and restarted at other time slots. This is simply subdivided into interruptible and non-interruptible operated appliances (Abideen et al. 2017 ; Li et al. 2017 ).
Interruptible operated appliances may be stopped, interrupted, and resumed for a brief time without affecting the quality of the energy services provided, provided that it is completed before the deadline. Air conditioners, electric heaters, cold appliances, and hybrid electric automobiles are a few examples of interruptible operated equipment (PHEV). These appliances are also referred to as adjustable, shiftable, thermostatically controlled, and limitable operated equipment. These loads may be scheduled using a demand response system. Depending on the cost of the power or a financial incentive, they might be shifted from peak to off-peak hours, which will reduce the demand for peak load.
Non-interruptible operated appliances must finish their scheduled operation within a certain time frame. Non-interruptible appliances, also known as regular, fixed, non-adjustable, and non-controllable operated appliances, include lighting and kitchen systems. These loads are unsuitable for DR programs since they do not permit a time shift or interruption.
Adjustable and nonadjustable operated appliances
Most thermal loads are examples of adjustable operated appliances since they may be set to a lower level. These kinds of loads may actively take part in DR programs by reducing their total energy usage in line with energy pricing and financial incentives. However, it’s crucial to be informed that the DR software employed for these sorts of devices might make you uncomfortable while you wait. The overall consumption for non-adjustable loads is fixed (e.g., TVs and computers). An algorithm for demand response cannot plan for non-deferrable or non-adjustable loads (Li et al. 2017 ).
Basic and heavy operated appliances
An electrical appliance’s rating decides which categories it will fall under. Appliances with simple operating systems are those that use less energy. Lighting systems, televisions, laptops, and other basic operated appliances are just a few examples, and they hardly ever take part in DR programs. In contrast, appliances that require a lot of power consumption are more likely to be included in DR programs. The heavily operated appliances include things like air conditioners, electric cookers, and washing machines. The control of various appliances, particularly thermostatically controlled loads like air conditioning systems and electric water heaters, has already been the subject of several studies created by various authors (Du and Lu 2011 ; Goh and Apt 2004 ; Ibrahim et al. 2023 ; Ilic et al. 2002 ; Pedrasa et al. 2010 ).
The scheduling optimization problem involves many constraints. These restrictions apply to the system level as well as the appliance level. The restrictions listed below are addressed:
Electrical demand supply balance (Tasdighi et al. 2013 ):
The balance between the need for and supply of electricity at any given hour is shown in the equation below, which also accounts for power from batteries and the grid, load shifting, and both shiftable and non-shiftable load demands. Without considering load shifting
Considering Load Shifting
Temperature constraints (Tasdighi et al. 2013 ):
In this case, it is necessary to schedule thermostatically controllable loads (TCLs) with the understanding that the water and room temperatures must be maintained within a certain range.
The water temperature at the outlet is given as:
The HVAC room temperature is given as:
Battery constraints (Huang et al. 2016 ):
The manufacturer’s recommended range for battery level maintenance should be followed. As a result, the following constraints are put in place
Battery maximum charging and discharging power limit can be represented as:
Charge and discharge rate constraints for Electric vehicles (Zhao et al. 2012 )
Electric vehicles (EVs) are supposed to be charged and discharged at residential locations in this scenario. When parked at homes, EVs are typically wired into the residential metering systems.
During the charge cycle:
During the discharge cycle:
Grid constraints (Wong 1991 ):
Each time slot’s energy import from the grid must be upper bound by a predetermined limit to avoid overloading the utility.
User comfort-enabling constraints (Tamilarasu et al. 2021 ):
The wants and satisfaction of the users are given precedence in various circumstances. Certain limitations must be met to guarantee that the optimization process moves forward without significantly sacrificing comfort
Total daily load requirement:
Instantaneous power demand:
Idle constraint:
Phase wise energy requirement of appliances (Sou et al. 2011 ):
Since controllable appliances such as washing machines, and dishwashers have different power requirements at each operation cycle. This limitation guarantees each appliance’s operational cycle gets adequate energy for its functioning
Power safety (Sou et al. 2011 ):
This constraint places a maximum on the total energy allotted during any period, requiring that it always be less than the maximum energy from the grid.
Prioritization of appliance constraints (El-Metwally et al. 2006 ):
In this instance, the DSM optimization places a focus on the appliance priority. A priority index (PI), which is inversely proportional to the appliance’s load factor and proportionate to the peak demand of the appliance, is used to classify the loads
Up time required to finish a task (Paudyal and Ni 2019 ; Tasdighi et al. 2013 ):
When an appliance is switched on, it shouldn’t be shut off until the associated task is finished, for example, a dishwasher
where \(W_{n} (t)\) is the operation state of n th shiftable load at a time (t) 1: on, 0: off and \(TOP_{n}\) is the number of n th shiftable load’s time of operation.
Operation ordering of appliances (Paudyal and Ni 2019 ; Tasdighi et al. 2013 ):
The maintenance of the appliance’s operational ordering should be ensured. For instance, it is best to use the dryer after the washing machine has done its work. If shiftable load m is activated after shiftable load in such a scenario:
Dynamic pricing
Another element that exacerbates DSM challenges is dynamic pricing. One of the main goals of the reform of the energy market is to lower peak demand while increasing the use of all resources. Through various incentives provided by the utilities, customers are encouraged to participate in different dynamic pricing schemes. Since dynamic pricing encourages consumers to transfer their load from peak to off-peak periods, the scheduling issue for home energy usage must be addressed in this situation. The key elements influencing the structure of the electricity tariff are marginal cost, load pattern, societal considerations, and the profitability of the power company (Phuangpornpitak and Tia 2013 ). Numerous pricing strategies have been used, as can be shown in Fig. 6 to balance the supply and demand for energy. To preserve customer happiness and boost the system’s overall cost efficiency, advanced optimization algorithms must be developed to allow efficient energy consumption scheduling in addition to the reduction of dynamic tariffs (Panda et al. 2022 ).
Customer categorization
A thorough examination of numerous consumer categories may aid in a better understanding and design of DR. The customers are divided into four categories including the residential, commercial, industrial, and transportation sectors. In any of these categories, transportation is not a key problem for DR.
The residential sector is more challenging because of the diverse appliance consumption patterns, consumer dispersion, and individual user preferences. This suggests that rather than treating customers equally, each one is treated differently. Because the load profile and appliance use data are not readily available, DR adoption for industrial clients is quite challenging. Even with access to this data, the activities’ dependency on time makes it difficult to change energy use. Commercial users’ energy profiles may be modified with ease if they are identical. The most commonly used equipment, including air conditioners, heaters, ventilators, and lights, may be managed in line with the established specifications. It is crucial to remember that the DR is simple to deploy in the commercial and industrial sectors, allowing the system to react to DR fast.
Consumer behaviors
Some customers don’t respond well to price changes and it is unclear how people will respond to these programs. Customers have a variety of reactions to the price of electricity, and these reactions can be categorized as extremely flexible and unassuming behavior (Sharifi et al. 2017 ). Although there are many ways to implement DR and it offers many advantages, if the end user encounters any kind of difficulties, they may become disillusioned and leave the program or demand more money or incentives (Duncan and Hiskens 2011 ). The motivations behind these difficulties posed by each consumer’s decision to install microgeneration in their home are examined by the authors (Balcombe et al. 2014 ). They assert that inconveniencing people can prevent them from adopting technology.
The study by (Balcombe et al. 2014 ) does highlight an important aspect of end-use customers, namely that financial considerations are frequently more important than a desire to contribute to environmental change, even though micro-generation is a distinct but related problem. It is important to emphasize the importance of financial motivations, particularly in light of the high level of uncertainty previously mentioned regarding the potential financial benefits of enrolling in a DR program. The possibility is raised in (Boisvert and Neenan 2003 ), and raises a related financial concern, that the electricity bill savings from customers may not be sufficient to support equipment investment and make up for the inconvenience of continuously monitoring electricity prices when they may only need to react in exceptional circumstances. Naturally, this will depend on the type of software being used and the required level of customer interaction.
There will be little interest in DR if financial considerations are the primary factors influencing the adoption of DR programs and it is demonstrated that consumers will not be able to save money on their future power bills or recover their initial investment in DR technology. This dissuades people from using DR programs extensively. Despite receiving feedback on their energy use from in-home displays, most study participants continued with their regular routines and habits, according to research published in (Herrando et al. 2014 ). This is a great example of unanticipated or possibly irrational customer behavior, a challenge that needs to be taken into account when evaluating the DR implementation.
This study also emphasizes the importance of promoting greater DR knowledge and giving consumers the right information about DR programs for them to make informed decisions. As a result, utility companies won’t frequently send the DR resource (Cutter et al. 2012 ). This is a crucial factor to take into account when estimating the resource’s worth. It is crucial to take into account when estimating DR resources because it is connected to the traits and physical composition of electrical loads.
The main challenges are recognizing and properly accounting for the DR resource’s limitations as a result of end-user behavior and preferences in DR deployment. Understanding the variables that affect customers’ choices to accept or reject a DR program, as well as how these restrictions are reflected in the assessment study, is essential. Recognizing the potential effects that unanticipated consumer behavior may have on the DR features is essential as it successfully manages it throughout the evaluation process (Nolan and O’Malley 2015 ). Overall, different lifestyles and household activities have a significant influence on how much energy is used since it is predictable. Both long- and short-term trends are easily predicted. Participants reduce their electricity bills and Non-participating users may also save money since the programs shift power consumption from times when demand is highest to times when energy is least expensive.
- Optimization techniques
Numerous optimization strategies have been used to address the problems related to energy management. However, demand-side optimization methods are further divided into deterministic, stochastic, and hybrid approaches as illustrated in Fig. 7 .
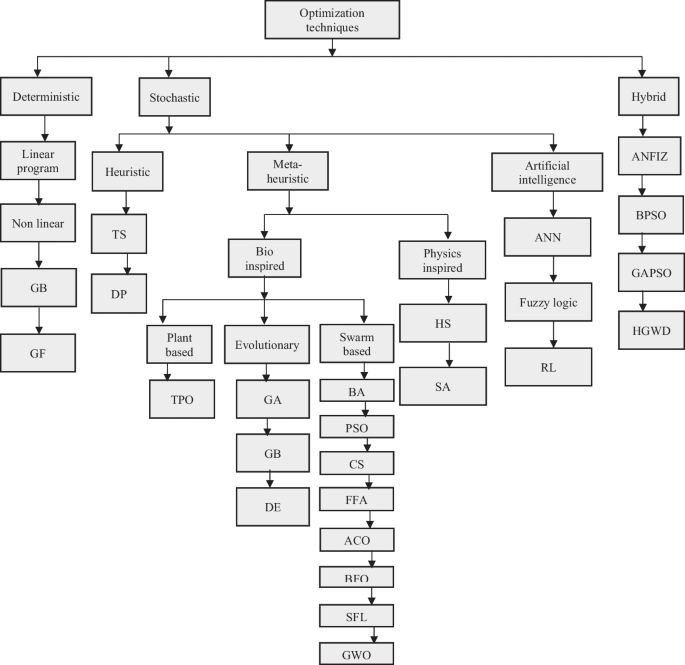
The goal of this method of optimization is to find a universally optimal solution by using the analytic properties of the problem. It is also important to note that as the problem constraint shrinks, the likelihood of discovering global solutions increases, as well as the assurance of the quality of the optimal solutions attained. Linear programming (LP) (Erol-Kantarci and Mouftah 2011 ; Zhu et al. 2012 ), nonlinear programming (NLP) (Althaher et al. 2015 ), gradient base (GB) (Huang et al. 2015 ), Lagrangian algorithms (Boyd; Gatsis and Giannakis 2011 ), Lagrange–Newton (Dong et al. 2012 ), interior point method (Samadi et al. 2012 ) and Lyapunov techniques (Guo et al. 2012 ), and mixed integer nonlinear programming (MINP) (Behrangrad et al. 2010 ) are few examples of deterministic methods used in energy management to reduce the amount of electricity used.
Zhu et al. ( 2012 ) proposed an integer LP system to schedule electrical appliances, together with power sources and operating time, in accordance with user preferences to decrease peak loads. Similarly to this, Wang et al. developed the ideal dispatching model for a smart HEMS with distributed energy resources and smart home appliances using the MINLP methodology (Wang et al. 2012 ). The cost of electricity and total energy used are both decreased. Due to consumers’ unexpected, impulsive, non-linear, and complex energy usage behaviors, the MINLP was unable to regulate some appliances. Existing work on Deterministic Optimization Techniques is shown in Table 5 .
Stochastic approach
The stochastic method is an iterative algorithm that makes use of the unpredictable nature to identify the optimal solution from the parent solution. It employs a variety of techniques to the problem in an attempt to identify the best answer conceivable because of the high dimensional nonlinear objectives issue; however, unlike the deterministic method, the optimal solution is not guaranteed. Even though the problem where determinism methods have several local solutions, its singularity makes it a powerful tool in engineering. This approach is broken down into heuristic, meta-heuristic, and artificial intelligence categories in Fig. 7 .
Every strategy has advantages and disadvantages that vary depending on the optimization problems. Because of this, there isn’t a perfect answer to every optimization problem. The fundamental weaknesses and advantages of each random method examined in this work are summarized in Table 6 . A fuzzy inference system (FIS) is recommended by Hasaranga et al. ( 2017 ) for the management of an energy storage system that utilizes renewable energy sources and a storage unit. Comparison with a rule-based control method demonstrated the recommended system’s efficiency in lowering fluctuation and prolonging the lifetime of energy storage devices (ESS).
Ambreen et al. published a heuristic technique for cost, PAR, and the load reduction in the smart grid in 2017. The recommended algorithms provide the appliances in a home with the best schedule possible, Cost savings, reduced PAR, and user comfort are all obtained when appliances are designed. Costs are cut by 52% using GA scheduling, while PAR is cut by 23% (Ambreen et al. 2017 ). Hsu et al. developed a DPbased optimization strategy to reduce the system’s energy-producing costs for the DLC dispatch. As a consequence, the dispatch DLC approaches and the unit commitment issue were integrated, and a DP strategy was developed to address both issues (Hsu and Su 1991 ).
A model predictive control strategy based on weather forecasts is offered to reduce the amount of energy required and improve the utilization of renewable energy sources for energy management in residential microgrids. The established MPC control approach is based on a constrained optimal control problem for a certain time horizon. The proposed approach was contrasted with conventional rule-based control logic. Primary fossil energy usage has dropped by 14.5% on average while home comfort levels have increased (Bruni et al. 2015 ).
Noor et al. proposed a GTA technique for a demand-side management model that includes storage components in distinct research. In addition to reducing the peak to average ratio for the benefit of the electric grid, the suggested model can smooth out dips in the demand profile caused by supply restrictions. This was decided by every player who took part, their strategies, and the awards they received. Customers are the participants in this strategy, and the reward is determined by the lowest cost (Noor et al. 2018 ).
For a variety of consumer loads, BFO was used to reduce peak load and energy expenditures by 7% and 10%, respectively. This method outperforms earlier evolutionary algorithms for controlling controlled devices (Priya Esther et al. 2016 ). Similarly to this, Bharathi et al. recommend combining GA with an appropriate load shifting technique to reduce and reconfigure the load needs of all sorts of energy consumers (Bharathi et al. 2017 ). Based on TOU and IBR, Rahim et al. employed ACO to decrease energy usage at the residential load. The recommended approach may dramatically lower peak load, PAR, and energy expenditures without affecting customer satisfaction (Rahim et al. 2016a ).
Mahmood et al. recommended a HEMC model to control the scheduling of appliances, lowering user comfort, PAR, and electricity costs. However, energy is wasted significantly when appliances are used unnecessarily, and environmental concerns are also disregarded (Mahmood et al. 2016 ).
Another study advises evaluating a HEMS’s ability to control its energy expenses using GWO and BFO. These proposed techniques resulted in 45% and 55% energy reductions respectively (Barolli et al. 2020 ). Furthermore, (Elmouatamid et al. 2020 ) evaluated the performance of a HEMS by using three meta-heuristic optimization techniques and the HS, BFO, and EDE algorithms. Existing work on Stochastic Optimization Techniques is shown in Table 7 .
Another sub-category of stochastic optimization techniques worth discussing due to its constantly evolving field is machine language. Machine learning (ML) is an evolving branch of computational algorithms that are designed to emulate human intelligence by learning from the surrounding environment. They are considered the working horse in the new era of the so-called big data, which has been used to address different issues in DSM as shown in Table 8 (Antonopoulos et al. 2020 ). The main types of machine learning are supervised learning, unsupervised learning, and reinforcement learning as stated (Murphy 2012 ). Figure 8 shows the subtypes of machine learning used in DR.
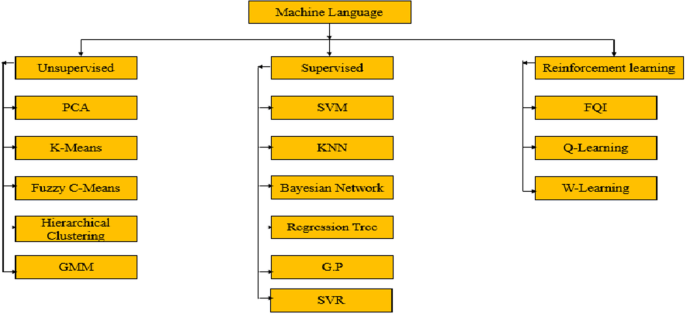
Machine language used in DSM (Antonopoulos et al. 2020 )
Supervised machine learning (SML) is the task of generating meaning from labeled training data that includes a set of training examples. In supervised learning, each example is a mainstay that contains an input object (typically a vector quantity) and an enforced output value (may also be referred to as a supervisory signal) (Praveena and Jaiganesh 2017 ). The authors in Giovanelli et al. ( 2017 ), Pal and Kumar ( 2016 ), Yang et al. ( 2018 ) proposed Support Vector Regression (SVR) to forecast the price of energy. This technique is also used for short time load forecasting for non-aggregated loads (Zhou et al. 2016 ).
Unsupervised machine learning (UML) approaches are very beneficial in description tasks because they try to discover links in a data structure without requiring a quantifiable output. Because there is no response variable to oversee the study, this kind of machine learning is referred to as unsupervised (Gareth et al. 2013 ). Cao et al. examine the clustering of 4000 households from the Irish CER dataset over 18 months using K-means, SOM, and hierarchical clustering algorithms with various distance calculations based on the 17 most significant PCA components (Cao et al. 2013 ).
Reinforcement learning (RL) is the task of determining how agents should perform actions in a given environment to maximize cumulative rewards. Q-learning is commonly used at the HEMS level to optimize appliance scheduling by using cost and user comfort as reward functions (O’Neill et al. 2010 ; Wen et al. 2015 ). O’Neill et al. consider pre-specified disutility functions for customers’ dissatisfaction with job scheduling (O’Neill et al. 2010 ), but Wen et al. address this limitation (Wen et al. 2015 ). A state in this context is made up of a price sequence from the retailer or aggregator, a vector that reflects the user’s consumption of specific appliances over time, and sometimes the priority of the considered device.
Hybrid approach
The hybrid approaches have been used in numerous engineering applications to get beyond the drawbacks of each optimization strategy and enhance their efficacy and accuracy to give a greater performance of the system (Tsipianitis & Tsompanakis). Several of the hybrid approaches used in DSM are briefly described below:
First, the teacher and learning-based optimization (TLBO) and the shuffling frog leap (SFL) methods of optimization are recommended. In this concept, the load is separated into three categories: shiftable, sheddable, and non-sheddable loads. The recommended strategy aimed to bring down the cost of electricity. This research employs ToU, RTP, and CPP as three alternative pricing models. The findings demonstrated that the recommended approach was successful in reducing consumption costs (Derakhshan et al. 2016 ).
Rahim et al. ( 2016a , b ) investigated the efficacy of binary particle swarm optimization (BPSO), ant colony optimization (ACO), and genetic algorithm (GA). Lowering power prices and the peak-to-average ratio (PAR) while taking into consideration RESs and storage systems is the main objective of the proposed effort (Rahim et al. 2016b ).
However, the validation results showed that GAPSO performed better than GA and BPSO in terms of cost and discomfort, lowering peak power use by 7.8532% and 27.7794%, respectively. While GA and BSPO reduced the cost of energy consumption by 24.0470% and 29.9702%, respectively, while GAPSO decreased peak power consumption (PAR) by 36.39%. While needing the least amount of waiting time, GAPSO was able to reduce consumption expenses by up to 25.2923% (Javaid et al. 2017a ).
In Küçüker et al. ( 2017 ), a hybrid energy management strategy is proposed by using a hierarchical genetic algorithm (HGA) to alter the fuzzy inference system’s rule base. The fuzzy-HGA method seems to be more effective than the conventional fuzzy-GA approach, even with just 47% of the total rules in the rule base. By purchasing a more basic fuzzy logic controller, the entire control system can be implemented in real time on low-cost embedded electronic devices. A fuzzy logic-based EMS is presented in Panwar et al. ( 2017 ) to lower the fluctuations and peak powers of a grid-tied microgrid. In a similar line, the study (Pascual et al. 2015 ) proposes the conventional fuzzy-genetic algorithm approach.
A hybrid power system for residential structures was the subject of an energy management strategy developed by Zenned et al. ( 2017 ). When compared to buying electricity from the grid, this plan’s results show a decrease in energy use, however, the modeling fails to take energy costs into account (Zenned et al. 2017 ).
A nonlinear MPC approach is recommended (Merabet et al. 2016 ). Using a synthetic NN, the loading trough was estimated. Voltage stability may be maintained by regulating the battery state of charge (SOC) and planning the load. Grid Connected based MPC EMS is used to reduce energy expenses (Arcos-Aviles et al. 2017 ).
Javaid et al. developed a hybrid genetic wind-driven (HGWD) technique to build a DSM controller for a residential area in an SG. The result shows that the HGWD algorithm performed the best. By lowering the cost of power use by 33% and 10%, respectively, when compared to the WDO algorithm and GA. To get the best results, the HGWD reduced user comfort by 40%, PAR by 17%, and electricity costs by 30% (Javaid et al. 2017b ). A hybrid method that combines PSO and Gray wolf optimization (GWO) is suggested using day-ahead scheduling (Hussain et al. 2016 ).
The hybrid GA/PSO method (HGPSO) was introduced by Ahmad et al. who also showed that it outperformed the GA, BPSO, BFO, and WDO algorithms. For the GA, BPSO, BFO, and WDO algorithms, the percentage of power bill decrease was 9.80%, 19.50%, 15.40%, and 15.80%, respectively. Each algorithm’s percentage of PAR reduction was 14.09%, 3.30%, 22.10%, and 33.54%. The PAR and the electric cost were reduced by 25.12% and 24.88% respectively by the HGPSO (Ahmad et al. 2017 ). In another investigation, the GA was put up against a more advanced PSO algorithm (IPSO). The peak load was reduced with the IPSO by about 30.26% while it was reduced with the GA by 25.78% (Yang et al. 2015 ).
The simulation results show how efficiently the proposed algorithm GHSA minimizes user discomfort while decreasing PAR and power costs. The GHSA reduces the peak load at 3.73 kWh in contrast to the present heuristic methods (13.84 kWh). According to the findings, smart home (SH) expenses have been decreased by WDO, HSA, GA, and GHSA to 2.61, 1.72, 1.12, and 1.34 cents/h, respectively (Javaid et al. 2017b ).
Manzoor et al. introduced the teacher learning genetic optimization (TLGO) method and compared it to the teacher learning-based optimization (TLBO) and GA for residential load scheduling with a day-ahead pricing scheme. Cost reductions of 31%, 31.5%, and 33% were produced by the GA, TLBO, and TLGO, respectively. User discomfort was lowest with TLGO when compared to GA and TLBO. User discomfort with the GA, TLBO, and TLGO had corresponding values of 2.37, 2.14, and 1.83 (Manzoor et al. 2017 ).
The hybrid algorithm known as the bat-crow search algorithm (BCSA) was developed by Javaid et al. by combining a meta-heuristic bat algorithm (BA) and a crow search algorithm (CSA). Using the critical peak pricing (CPP) system for HEMS, they compared the outcomes of BCSA with BA and CSA in terms of the amount of power cost reduction. According to the findings of optimization, the BCSA algorithm lowered power expenses by 31.19%, while the BA and CSA cut costs by 28.32% and 26.70%, respectively. The description above suggests that hybrid algorithms perform better than single algorithms because they are more adaptable and effective (Javaid et al. 2018 ). Existing work on Hybrid Optimization Techniques is shown in Table 9 .
Future work
The majority of the review focused on thermal comfort and appliance waiting time to address customer satisfaction. The user’s experience at a DR event, their social comfort, and other social variables should be taken into consideration as they can boost user satisfaction. It’s crucial to model EVs as both a load and a generator to make the most out of the system. Peer-to-peer trade between prosumers may result in flexible assets with lower costs. Most of the work that was examined represented EVs as interruptible or storage systems.
Fairness between users, standardization, and SG interoperability must be guaranteed while developing a DSM program. For the real-time synchronization and integration of security, safety, smart appliances, and monitoring, extensive research is needed to secure the security and privacy of customers’ data. In addition to this, the agencies, shareholders, and policymakers need to step up and enact new rules and policies to increase the trust of the public. A thorough evaluation of the technical, economic, and environmental performance of current and upcoming DSM systems is required. This is needed to compare DSM and conventional treatments fairly.
The convergence and computation times of DSM optimization problems are improved by the hybrid algorithms-based optimization models. However, while choosing an algorithm to solve DSM optimization issues, other factors such as problem types (such as single- or multi-objective), optimization types (such as local or global), robustness, and accuracy should be taken into account.
As DSM, as previously said, enables both system operation and system development, it offers versatile advantages and value. However, the business case for DSM has not been well established since there are no tools for weighing costs and advantages. There is still a lot of work to be done in this area.
The primary system operating variables will often determine the DSM value’s size (i.e., the value of demand controllability). The system stress, or how close the system is to being loaded to its full capacity and hence needing reinforcement, should be taken into account in this situation. Even though it is often low in systems with significant spare capacity, the value of DSM will be high in system components that need reinforcement.
This paper provides a comprehensive analysis of the different technologies, approaches used in DSM as well as the impact of distributed renewable energy generation and storage technologies in SG. The main goal of these methods is to decrease peak load demands and achieve advanced synchronization between network operators and customers via the development and application of power-saving technologies, financial incentives, the price of energy, and government rules. This research thoroughly investigated DSM implementation issues that must be overcome for DSM to be effectively integrated into the SG with some proposed solutions, DSM optimization methodologies, and their related solutions, which were not included in the earlier review article. As a consequence, a comprehensive comparison of many algorithms used in DSM optimization problems is provided in terms of a variety of factors such as energy cost reduction, PAR, waiting time, power scheduling, Voltage limitations, DR, risk management, client privacy, and carbon emission. We determined, after examining multiple DSM-based research, that a single strategy is not the best solution to handle the high complexity of the DSM optimization problem due to its poor performance and low convergence rate. As a consequence, hybrid algorithms may outperform single algorithms in terms of convergence rate, complexity, noisy environment, imprecision, uncertainty, and ambiguity. Furthermore, these tactics may be improved in the future to improve SG’s efficiency by balancing supply and demand. Even though these current breakthroughs in the use of optimization techniques in DSM are widely known, extra research is undoubtedly necessary to discover the optimal solutions in many real-world scenarios.
The power system’s functioning will become more difficult if corrective control is used. This is just another obstacle to the adoption of DSM. Yet, given that adaptability is increasingly seen as a key tool for coping with the unpredictability of future developments, together with the ongoing cost reductions of DSM technologies, it is anticipated that DSM will become noticeably more competitive in the near future. Increasing trust in the employment of DSM schemes for the provision of system security will benefit from the establishment of targeted trial schemes. This comprehensive review of DSM will assist all researchers in this field in improving energy management strategies and reducing the effects of system uncertainties, variances, and restrictions.
Availability of data and materials
Data sharing is not applicable to this articles as no datasets were generated or analysed during the current study.
Abbreviations
Software-Defined Network
Interdependent Networks
Field Area Networks
Wireless Sensor Networks
Neighborhood Area Networks
Advanced metering infrastructure
Supply side management
- Demand side management
Time of use
Critical peak pricing
Real time pricing
Renewable energy sources
Priority index
Thermal energy storage
Home energy management system
Direct load control
Capacity Market Program
Auxiliary service market
Battery energy storage system
Nonlinear programming
Mixed integer linear programming
Convex nonlinear programming
Peak to average ratio
Dynamic programming
Game theory algorithms
Particle swarm optimization
Grey wolf optimization
Harmony search algorithm
Binary particle swarm optimization
Satin bowerbird optimizer
Sine cosine algorithm
Crow search algorithm
Moth Fly Optimization
Cuckoo optimization algorithm
Firefly algorithm
Cat search algorithm
Differential evolution
Cultural algorithm
Artificial immune system
Earth Worm Algorithm
Shuffling frog leap
Adaptive neuro fuzzy logic
Improved particle swarm optimization
Genetic harmony search algorithms
Bat-crow search algorithm
Karush–Kuhn–Tucker
Gravitational Search Algorithm
Backtracking Search Optimization
Effective Differential Evolution
Hybrid genetic algorithm
Hybrid Effective Differential Evolution
Modified clonal selection algorithm
Hybrid genetic particle wind driven optimization
Hybrid genetic particle swarm optimization
Deep Reinforcement Learning
Elephant herding optimization
Unmanned aerial vehicle neural-fuzzy classification
Elephant herding optimization neuro fuzzy
Distributed automation
Distributed energy resources
Teleprotection
Anomaly detection
Substation automation
Privacy preserving
Inclined block rate
Energy management system
Programmable logic controller
Supervisory control and data acquisition
Building management system
Emergency demand response
Interruptible Curtailable Service
Demand bidding/buyback
Electric energy storage
Linear programming
Mixed integer nonlinear programming
Quadratic programming
Fuzzy logic interfere
Genetic algorithms
Model predictive control
Bat algorithm
Ant colony optimization
Artificial neural network
Reinforcement learning
Polar bear optimization
Whale optimization algorithm
Mosquito Host Seeking
Colliding body optimization
Social spider optimization
Biogeography based optimization
Imperialist competitive algorithm
Artificial bee colony
Bacterial foraging optimization
Candidate solution updation algorithm
Jaya Optimization Algorithm
Fuzzy logic
Teacher and learning-based optimization
Genetic algorithm particle swarm optimization
Hybrid genetic wind-driven
Teacher learning genetic optimization
Mixed grey wolf optimization
Runner Updation Optimization Algorithm
Enhanced leader particle swarm optimization
Expert advisors
Flower pollination algorithm
Math Kernel Library
Bacterial foraging optimization algorithm
Levy Whale Optimization Algorithm
Levy Whale Modified Crow Search Optimizer
Hybrid beamforming particle swarm optimization
Wind driven genetic algorithms
Wind driven grey wolf optimization
Wind driven binary particle swarm optimization
Power transferred from the grid at time (t) in kW
Power transferred from the battery at time (t) in kW
Total power consumption from non-shiftable loads at time (t)
Total power consumption from shiftable loads at time (t)
Shiftable loads
Minimum and maximum water outlet temperature in tank respectively
Mixed water temperature in the tank at interval i
Minimum and maximum room temperature respectively
Room temperature at interval i.
Minimum and maximum temperature
Minimum and maximum state of charge of battery at time (t)
Capacity of battery and the energy of battery at any time (t) in (kWh)
Battery’s charging and discharging power respectively at time (t)
Maximum battery’s charging and discharging power respectively
Battery’s charge efficiency
Charging and discharging power of EV at time (t) respectively
Maximum power level of EV at time (t).
Instantaneous and maximum instantaneous power demand (kW) respectively
Customer satisfaction
Energy requirement for energy phase j in appliance i.
Energy assigned to energy phase j of appliance i during the whole period of time slot
Total energy required by all running appliances at time (t)
Maximum energy from grid at that time (t).
Operation state of shift able load at time (t)
Number of shiftable load’s time of operation
Aalami HA, Khatibzadeh A (2016) Regulation of market clearing price based on nonlinear models of demand bidding and emergency demand response programs. Int Trans Electr Energy Syst 26(11):2463–2478
Article Google Scholar
Aalami H, Moghaddam MP, Yousefi G (2010) Demand response modeling considering interruptible/curtailable loads and capacity market programs. Appl Energy 87(1):243–250
Aalami HA, Pashaei-Didani H, Nojavan S (2019) Deriving nonlinear models for incentive-based demand response programs. Int J Electr Power Energy Syst 106:223–231
Abbasi RA, Javaid N, Khan S, Asif RM, Ahmad W (2019) Minimizing daily cost and maximizing user comfort using a new metaheuristic technique. Paper presented at the workshops of the international conference on advanced information networking and applications
Abideen ZU, Jamshaid F, Zahra A, Rehman AU, Razzaq S, Javaid N (2017) Meta-heuristic and nature inspired approaches for home energy management. Paper presented at the international conference on network-based information systems
Abualigah L, Diabat A (2021) Advances in sine cosine algorithm: a comprehensive survey. Artif Intell Rev 54(4):2567–2608
Adepetu A, Rezaei E, Lizotte D, Keshav S (2013) Critiquing time-of-use pricing in Ontario. Paper presented at the 2013 IEEE international conference on smart grid communications (SmartGridComm)
Aghaei J, Alizadeh M-I (2013) Demand response in smart electricity grids equipped with renewable energy sources: a review. Renew Sustain Energy Rev 18:64–72
Aghajani G, Shayanfar H, Shayeghi H (2015) Presenting a multi-objective generation scheduling model for pricing demand response rate in micro-grid energy management. Energy Convers Manag 106:308–321
Ahmad A, Khan A, Javaid N, Hussain HM, Abdul W, Almogren A, Alamri A, Azim Niaz I (2017) An optimized home energy management system with integrated renewable energy and storage resources. Energies 10(4):549
Ahmad MF, Isa NAM, Lim WH, Ang KM (2021) Differential evolution: a recent review based on state-of-the-art works. Alex Eng J 61:3831–3872
Ahmed MS, Mohamed A, Khatib T, Shareef H, Homod RZ, Abd Ali J (2017) Real time optimal schedule controller for home energy management system using new binary backtracking search algorithm. Energy Build 138:215–227
Ahmed AM, Rashid TA, Saeed SAM (2020) Cat swarm optimization algorithm: a survey and performance evaluation. Comput Intell Neurosci. https://doi.org/10.1155/2020/4854895
Al Essa MJM (2019) Home energy management of thermostatically controlled loads and photovoltaic-battery systems. Energy 176:742–752
Al Hasib A, Nikitin N, Natvig L (2014) Load scheduling in smart buildings with bidirectional energy trading. Paper presented at the 2014 IEEE 33rd international performance computing and communications conference (IPCCC)
Alasseri R, Tripathi A, Rao TJ, Sreekanth K (2017) A review on implementation strategies for demand side management (DSM) in Kuwait through incentive-based demand response programs. Renew Sustain Energy Rev 77:617–635
Al-enezi AN (2010) Demand side management (DSM) for efficient use of energy in the residential sector in Kuwait: analysis of options and priorities
Alipour M, Zare K, Abapour M (2017) MINLP probabilistic scheduling model for demand response programs integrated energy hubs. IEEE Trans Ind Inform 14(1):79–88
Althaher S, Mancarella P, Mutale J (2015) Automated demand response from home energy management system under dynamic pricing and power and comfort constraints. IEEE Trans Smart Grid 6(4):1874–1883
Amatullah A, Agung A, Arif A (2021) Minimizing power peaking factor of BEAVRS-based reactor using polar bear optimization algorithms. Paper presented at the IOP Conference Series: Earth and Environmental Science
Ambreen K, Khalid R, Maroof R, Khan HN, Asif S, Iftikhar H (2017) Implementing critical peak pricing in home energy management using biography based optimization and genetic algorithm in smart grid. Paper presented at the international conference on broadband and wireless computing, communication and applications
Amini M, Frye J, Ilić MD, Karabasoglu O (2015) Smart residential energy scheduling utilizing two stage mixed integer linear programming. Paper presented at the 2015 North American Power Symposium (NAPS)
Amrollahi MH, Bathaee SMT (2017) Techno-economic optimization of hybrid photovoltaic/wind generation together with energy storage system in a stand-alone micro-grid subjected to demand response. Appl Energy 202:66–77
Antonopoulos I, Robu V, Couraud B, Kirli D, Norbu S, Kiprakis A, Flynn D, Elizondo-Gonzalez S, Wattam S (2020) Artificial intelligence and machine learning approaches to energy demand-side response: a systematic review. Renew Sustain Energy Rev 130:109899
Anvari-Moghaddam A, Monsef H, Rahimi-Kian A (2014) Optimal smart home energy management considering energy saving and a comfortable lifestyle. IEEE Trans Smart Grid 6(1):324–332
Anvari-Moghaddam A, Rahimi-Kian A, Mirian MS, Guerrero JM (2017) A multi-agent based energy management solution for integrated buildings and microgrid system. Appl Energy 203:41–56
Arcos-Aviles D, Pascual J, Guinjoan F, Marroyo L, Sanchis P, Marietta MP (2017) Low complexity energy management strategy for grid profile smoothing of a residential grid-connected microgrid using generation and demand forecasting. Appl Energy 205:69–84
Arteconi A, Hewitt NJ, Polonara F (2012) State of the art of thermal storage for demand-side management. Appl Energy 93:371–389
Atia R, Yamada N (2016) Sizing and analysis of renewable energy and battery systems in residential microgrids. IEEE Trans Smart Grid 7(3):1204–1213
Awais M, Javaid N, Shaheen N, Iqbal Z, Rehman G, Muhammad K, Ahmad I (2015) An efficient genetic algorithm based demand side management scheme for smart grid. Paper presented at the 2015 18th international conference on network-based information systems
Awais M, Javaid N, Aurangzeb K, Haider SI, Khan ZA, Mahmood D (2018) Towards effective and efficient energy management of single home and a smart community exploiting heuristic optimization algorithms with critical peak and real-time pricing tariffs in smart grids. Energies 11(11):3125
Babaei Keshteli H, Rostamy-Malkhalifeh M, Hosseinzadeh Lotfi F (2021) Ranking of decision making units using the imperialist competitive algorithm in DEA. Meas Control 54(9–10):1326–1335
Bahamish HA, Al-Aidroos NM, Boraik AN (2021) Modified crow search algorithm for protein structure prediction
Balcombe P, Rigby D, Azapagic A (2014) Investigating the importance of motivations and barriers related to microgeneration uptake in the UK. Appl Energy 130:403–418
Barbato A, Capone A, Chen L, Martignon F, Paris S (2013) A power scheduling game for reducing the peak demand of residential users. Paper presented at the 2013 IEEE online conference on green communications (OnlineGreenComm)
Barolli L, Amato F, Moscato F, Enokido T, Takizawa M (2020) Web, artificial intelligence and network applications: proceedings of the workshops of the 34th international conference on advanced information networking and applications (WAINA-2020), vol 1150. Springer Nature
Basit MA, Dilshad S, Badar R, Sami ur Rehman SM (2020) Limitations, challenges, and solution approaches in grid-connected renewable energy systems. Int J Energy Res 44(6):4132–4162
Beaudin M, Zareipour H (2015) Home energy management systems: a review of modelling and complexity. Renew Sustain Energy Rev 45:318–335
Behrangrad M (2015) A review of demand side management business models in the electricity market. Renew Sustain Energy Rev 47:270–283
Behrangrad M, Sugihara H, Funaki T (2010) Analyzing the system effects of optimal demand response utilization for reserve procurement and peak clipping. Paper presented at the IEEE PES general meeting
Bharathi C, Rekha D, Vijayakumar V (2017) Genetic algorithm based demand side management for smart grid. Wirel Pers Commun 93(2):481–502
Bina MT, Ahmadi D (2015a) Stochastic modeling for the next day domestic demand response applications. IEEE Trans Power Syst 30(6):2880–2893
Bina VT, Ahmadi D (2015b) Stochastic modeling for scheduling the charging demand of EV in distribution systems using copulas. Int J Electr Power Energy Syst 71:15–25
Blake ST, O’Sullivan DT (2018) Optimization of distributed energy resources in an industrial microgrid. Procedia CIRP 67:104–109
Boisvert RN, Neenan BF (2003) Social welfare implications of demand response programs in competitive electricity markets. Lawrence Berkeley National Lab. (LBNL), Berkeley
Book Google Scholar
Bongomin O, Nziu P (2022) A critical review on the development and utilization of energy systems in Uganda
Boyd S. Dynamic network energy management via proximal message passing
Bruni G, Cordiner S, Mulone V, Rocco V, Spagnolo F (2015) A study on the energy management in domestic micro-grids based on model predictive control strategies. Energy Convers Manag 102:50–58
Bukoski JJ, Chaiwiwatworakul P, Gheewala SH (2016) Energy savings versus costs of implementation for demand side management strategies within an energy-efficient tropical residence. Energy Effic 9(2):473–485
Cao H-Â, Beckel C, Staake T (2013) Are domestic load profiles stable over time? An attempt to identify target households for demand side management campaigns. Paper presented at the IECON 2013—39th annual conference of the IEEE industrial electronics society
Cappers P, Goldman C, Kathan D (2010) Demand response in US electricity markets: empirical evidence. Energy 35(4):1526–1535
Chanal PM, Kakkasageri MS, Manvi SKS (2021) Security and privacy in the internet of things: computational intelligent techniques-based approaches. In: Recent trends in computational intelligence enabled research. Elsevier, Amsyerdam, pp 111–127
Chatziioannou K, Guštinčič J, Tjernberg LB (2013) On experience of smart grid projects in Europe and the Swedish demonstration projects. Chalmers University of Technology, Gothenburg
Google Scholar
Chen C, Wang J, Kishore S (2014) A distributed direct load control approach for large-scale residential demand response. IEEE Trans Power Syst 29(5):2219–2228
Chen H, Wang L, Di J, Ping S (2020) Bacterial foraging optimization based on self-adaptive chemotaxis strategy. Comput Intell Neurosci. https://doi.org/10.1155/2020/2630104
Chintam JR, Daniel M (2018) Real-power rescheduling of generators for congestion management using a novel satin bowerbird optimization algorithm. Energies 11(1):183
Chokpanyasuwan C, Bunnag T, Prommas R (2015) Ant colony optimization for load management based on load shifting in the textile industry. Am J Appl Sci 12(2):142
Conchado A, Linares P (2012) The economic impact of demand-response programs on power systems. A survey of the state of the art. Handb Netw Power Syst I:281–301
Conteh A, Lotfy ME, Kipngetich KM, Senjyu T, Mandal P, Chakraborty S (2019) An economic analysis of demand side management considering interruptible load and renewable energy integration: a case study of Freetown Sierra Leone. Sustainability 11(10):2828
Cortese T, Almeida J, Batista G, Storopoli J, Liu A, Yigitcanlar T (2022) Understanding sustainable energy in the context of smart cities: a PRISMA review. Energies 15:2382; s Note: MDPI stays neu-tral with regard to jurisdictional claims in ….
Cutter E, Woo CK, Kahrl F, Taylor A (2012) Maximizing the value of responsive load. Electr J 25(7):6–16
Derakhshan G, Shayanfar HA, Kazemi A (2016) The optimization of demand response programs in smart grids. Energy Policy 94:295–306
Dincer I, Bicer Y (2020) Integration of renewable energy systems for multigeneration. In: Integrated energy systems for multigeneration. pp 287–402
Dong Q, Yu L, Song W-Z, Tong L, Tang S (2012) Distributed demand and response algorithm for optimizing social-welfare in smart grid. Paper presented at the 2012 IEEE 26th international parallel and distributed processing symposium
Dranka GG, Ferreira P (2019) Review and assessment of the different categories of demand response potentials. Energy 179:280–294
Du P, Lu N (2011) Appliance commitment for household load scheduling. IEEE Trans Smart Grid 2(2):411–419
Duncan SC, Hiskens I (2011) Achieving controllability of electric loads’. Paper presented at the IEEE proceedings
Durairasan M, Ramprakash S, Balasubramanian D (2021) System modeling of micro-grid with hybrid energy sources for optimal energy management—a hybrid elephant herding optimization algorithm-adaptive neuro fuzzy inference system approach. Int J Numer Model Electron Netw Devices Fields 34(6):e2915
Elma O, Selamoğullari US (2017) Paper presented at the 2017 4th international conference on electrical and electronic engineering (ICEEE)
Elma O, Taşcıkaraoğlu A, Ince AT, Selamoğulları US (2017) Implementation of a dynamic energy management system using real time pricing and local renewable energy generation forecasts. Energy 134:206–220
El-Metwally M, El-Sobki M, Attia H, Wahdan S (2006) Paper presented at the 2006 eleventh international Middle East power systems conference
Elmouatamid A, Ouladsine R, Bakhouya M, El Kamoun N, Khaidar M, Zine-Dine K (2020) Review of control and energy management approaches in micro-grid systems. Energies 14(1):168
Eltaeib T, Mahmood A (2018) Differential evolution: a survey and analysis. Appl Sci 8(10):1945
Erdinc O, Paterakis NG, Mendes TD, Bakirtzis AG, Catalão JP (2014) Smart household operation considering bi-directional EV and ESS utilization by real-time pricing-based DR. IEEE Trans Smart Grid 6(3):1281–1291
Erol-Kantarci M, Mouftah HT (2011) Wireless sensor networks for cost-efficient residential energy management in the smart grid. IEEE Trans Smart Grid 2(2):314–325
Esther BP, Kumar KS (2016) A survey on residential demand side management architecture, approaches, optimization models and methods. Renew Sustain Energy Rev 59:342–351
Eusuff M, Lansey K, Pasha F (2006) Shuffled frog-leaping algorithm: a memetic meta-heuristic for discrete optimization. Eng Optim 38(2):129–154
Article MathSciNet Google Scholar
Faisal HM, Javaid N, Qasim U, Habib S, Iqbal Z, Mubarak H (2019) An efficient scheduling of user appliances using multi objective optimization in smart grid. Paper presented at the workshops of the international conference on advanced information networking and applications
Fang X, Misra S, Xue G, Yang D (2011) Smart grid—the new and improved power grid: a survey. IEEE Commun Surv Tutor 14(4):944–980
Fang G-H, Wu C-J, Liao T, Huang X-F, Qu B (2020) A two-layer improved invasive weed optimization algorithm for optimal operation of cascade reservoirs. Water Supply 20(6):2311–2323
Fanti MP, Mangini AM, Roccotelli M (2018) A simulation and control model for building energy management. Control Eng Pract 72:192–205
Faria P, Soares J, Vale Z, Morais H, Sousa T (2013) Modified particle swarm optimization applied to integrated demand response and DG resources scheduling. IEEE Trans Smart Grid 4(1):606–616
Faruqui A, Sergici S (2010) Household response to dynamic pricing of electricity: a survey of 15 experiments. J Regul Econ 38(2):193–225
Feng X, Liu X, Yu H (2016) Group mosquito host-seeking algorithm. Appl Intell 44(3):665–686
Freeman R (2005) Managing energy: reducing peak load and managing risk with demand response and demand side management. Refocus 6(5):53–55
Gaber M, El-Banna S, El-Dabah M, Hamad M (2021) Designing and implementation of an intelligent energy management system for electric ship power system based on adaptive neuro-fuzzy inference system (ANFIS). Adv Sci Technol Eng Syst J 6(2):195–203
Gareth J, Daniela W, Trevor H, Robert T (2013) An introduction to statistical learning: with applications in R. Spinger, Cham
MATH Google Scholar
Gatsis N, Giannakis GB (2011) Residential demand response with interruptible tasks: duality and algorithms. Paper presented at the 2011 50th IEEE conference on decision and control and European control conference
Gelazanskas L, Gamage KA (2014) Demand side management in smart grid: a review and proposals for future direction. Sustain Cities Soc 11:22–30
Gellings CW (2017) Evolving practice of demand-side management. J Mod Power Syst Clean Energy 5(1):1–9
Giovanelli C, Liu X, Sierla S, Vyatkin V, Ichise R (2017) Towards an aggregator that exploits big data to bid on frequency containment reserve market. Paper presented at the IECON 2017—43rd annual conference of the IEEE Industrial Electronics Society
GK JS (2020) MANFIS based SMART home energy management system to support SMART grid. Peer Peer Netw Appl 13(6):2177–2188
Goh CHK, Apt J (2004) Consumer strategies for controlling electric water heaters under dynamic pricing. Paper presented at the Carnegie Mellon Electricity Industry Center working paper
Goubko MV, Kuznetsov SO, Neznanov AA, Ignatov DI (2016) Bayesian learning of consumer preferences for residential demand response. IFAC-PapersOnLine 49(32):24–29
Guo Y, Pan M, Fang Y (2012) Optimal power management of residential customers in the smart grid. IEEE Trans Parallel Distrib Syst 23(9):1593–1606
Guo W, Liu T, Dai F, Xu P (2020) An improved whale optimization algorithm for feature selection. Comput Mater Contin 62:337–354
Gyamfi S, Krumdieck S, Urmee T (2013) Residential peak electricity demand response—highlights of some behavioural issues. Renew Sustain Energy Rev 25:71–77
Hafeez G, Javaid N, Iqbal S, Khan FA (2018) Optimal residential load scheduling under utility and rooftop photovoltaic units. Energies 11(3):611
Haffaf A, Lakdja F, Meziane R, Abdeslam DO (2021) Study of economic and sustainable energy supply for water irrigation system (WIS). Sustain Energy Grids Netw 25:100412
Haider HT, See OH, Elmenreich W (2016) Residential demand response scheme based on adaptive consumption level pricing. Energy 113:301–308
Hajibandeh N, Shafie-Khah M, Osório GJ, Aghaei J, Catalão JP (2018) A heuristic multi-objective multi-criteria demand response planning in a system with high penetration of wind power generators. Appl Energy 212:721–732
Harish V, Kumar A (2014) Demand side management in India: action plan, policies and regulations. Renew Sustain Energy Rev 33:613–624
Hasaranga W, Hemarathne R, Mahawithana M, Sandanuwan M, Hettiarachchi H, Hemapala K (2017) A fuzzy logic based battery SOC level control strategy for smart micro grid. Paper presented at the 2017 third international conference on advances in electrical, electronics, information, communication and bio-informatics (AEEICB)
Hernández-Ocana B, Mezura-Montes E, Pozos-Parra P (2013) A review of the bacterial foraging algorithm in constrained numerical optimization. Paper presented at the 2013 IEEE congress on evolutionary computation
Herrando M, Markides CN, Hellgardt K (2014) A UK-based assessment of hybrid PV and solar-thermal systems for domestic heating and power: system performance. Appl Energy 122:288–309
Hsu Y-Y, Su C-C (1991) Dispatch of direct load control using dynamic programming. IEEE Trans Power Syst 6(3):1056–1061
Huang Y, Tian H, Wang L (2015) Demand response for home energy management system. Int J Electr Power Energy Syst 73:448–455
Huang Y, Wang L, Guo W, Kang Q, Wu Q (2016) Chance constrained optimization in a home energy management system. IEEE Trans Smart Grid 9(1):252–260
Hussain I, Mohsin S, Basit A, Khan ZA, Qasim U, Javaid N (2015) A review on demand response: pricing, optimization, and appliance scheduling. Procedia Comput Sci 52:843–850
Hussain A, Bui V-H, Kim H-M (2016) A resilient and privacy-preserving energy management strategy for networked microgrids. IEEE Trans Smart Grid 9(3):2127–2139
Hussain HM, Javaid N, Iqbal S, Hasan QU, Aurangzeb K, Alhussein M (2018) An efficient demand side management system with a new optimized home energy management controller in smart grid. Energies 11(1):190
Hussin N, Abdullah M, Ali A, Hassan M, Hussin F (2014) Residential electricity time of use (ToU) pricing for Malaysia. Paper presented at the 2014 IEEE conference on energy conversion (CENCON)
Ibrahim O, Bakare MS, Amosa TI, Otuoze AO, Owonikoko WO, Ali EM, Adesina LM, Ogunbiyi O (2023) Development of fuzzy logic-based demand-side energy management system for hybrid energy sources. Energy Convers Manag 10:100354
Ilic M, Black JW, Watz JL (2002) Potential benefits of implementing load control. Paper presented at the 2002 IEEE power engineering society winter meeting. Conference proceedings (Cat. No. 02CH37309)
Imani MH, Niknejad P, Barzegaran M (2018) The impact of customers’ participation level and various incentive values on implementing emergency demand response program in microgrid operation. Int J Electr Power Energy Syst 96:114–125
Iqbal Z, Javaid N, Iqbal S, Aslam S, Khan ZA, Abdul W, Almogren A, Alamri A (2018) A domestic microgrid with optimized home energy management system. Energies 11(4):1002
Issi F, Kaplan O (2018) The determination of load profiles and power consumptions of home appliances. Energies 11(3):607
Jabir HJ, Teh J, Ishak D, Abunima H (2018) Impacts of demand-side management on electrical power systems: a review. Energies 11(5):1050
Jaiswal U, Aggarwal S (2011) Ant colony optimization. Int J Sci Eng Res 2(7):1–7
Javaid N, Ahmed F, Ullah I, Abid S, Abdul W, Alamri A, Almogren AS (2017a) Towards cost and comfort based hybrid optimization for residential load scheduling in a smart grid. Energies 10(10):1546
Javaid N, Javaid S, Abdul W, Ahmed I, Almogren A, Alamri A, Niaz I (2017b) A hybrid genetic wind driven heuristic optimization algorithm for demand side management in smart grid. Energies 10(3):319
Javaid N, Ullah I, Akbar M, Iqbal Z, Khan FA, Alrajeh N, Alabed MS (2017c) An intelligent load management system with renewable energy integration for smart homes. IEEE Access 5:13587–13600
Javaid N, Mohsin SM, Iqbal A, Yasmeen A, Ali I (2018) A hybrid bat-crow search algorithm based home energy management in smart grid. Paper presented at the conference on complex, intelligent, and software intensive systems
Jindal A, Bhambhu BS, Singh M, Kumar N, Naik K (2019) A heuristic-based appliance scheduling scheme for smart homes. IEEE Trans Ind Inform 16(5):3242–3255
Kakran S, Chanana S (2018) Smart operations of smart grids integrated with distributed generation: a review. Renew Sustain Energy Rev 81:524–535
Kannayeram G, Prakash N, Muniraj R (2020) Intelligent hybrid controller for power flow management of PV/battery/FC/SC system in smart grid applications. Int J Hydrogen Energy 45(41):21779–21795
Karaboga N, Cetinkaya B (2004) Performance comparison of genetic and differential evolution algorithms for digital FIR filter design. Paper presented at the International conference on advances in information systems
Keshtkar A, Arzanpour S, Keshtkar F, Ahmadi P (2015) Smart residential load reduction via fuzzy logic, wireless sensors, and smart grid incentives. Energy Build 104:165–180
Khalid A, Javaid N, Mateen A, Khalid B, Khan ZA, Qasim U (2016) Demand side management using hybrid bacterial foraging and genetic algorithm optimization techniques. Paper presented at the 2016 10th international conference on complex, intelligent, and software intensive systems (CISIS)
Khalid A, Javaid N, Guizani M, Alhussein M, Aurangzeb K, Ilahi M (2018) Towards dynamic coordination among home appliances using multi-objective energy optimization for demand side management in smart buildings. IEEE Access 6:19509–19529
Khan ZA, Jayaweera D (2019) Smart meter data based load forecasting and demand side management in distribution networks with embedded PV systems. IEEE Access 8:2631–2644
Khan AA, Razzaq S, Khan A, Khursheed F (2015a) HEMSs and enabled demand response in electricity market: an overview. Renew Sustain Energy Rev 42:773–785
Khan ZA, Ahmed S, Nawaz R, Mahmood A, Razzaq S (2015b) Optimization based individual and cooperative DSM in smart grids: a review. In: 2015b power generation system and renewable energy technologies (PGSRET). pp 1–6
Khan AR, Mahmood A, Safdar A, Khan ZA, Khan NA (2016) Load forecasting, dynamic pricing and DSM in smart grid: a review. Renew Sustain Energy Rev 54:1311–1322
Khan ZA, Zafar A, Javaid S, Aslam S, Rahim MH, Javaid N (2019) Hybrid meta-heuristic optimization based home energy management system in smart grid. J Ambient Intell Humaniz Comput 10(12):4837–4853
Kim J-G, Lee B (2019) Appliance classification by power signal analysis based on multi-feature combination multi-layer LSTM. Energies 12(14):2804
Kim B-G, Zhang Y, Van Der Schaar M, Lee J-W (2015) Dynamic pricing and energy consumption scheduling with reinforcement learning. IEEE Trans Smart Grid 7(5):2187–2198
Kirby BJ (2006) Demand response for power system reliability: FAQ. Citeseer
Küçüker A, Kamal T, Hassan SZ, Li H, Mufti GM, Waseem M (2017) Design and control of photovoltaic/wind/battery based microgrid system. Paper presented at the 2017 international conference on electrical engineering (ICEE)
Kumar A, Rizwan M, Nangia U (2022) A hybrid optimization technique for proficient energy management in smart grid environment. Int J Hydrogen Energy 47(8):5564–5576
Kuo H, Lin C (2013) Cultural evolution algorithm for global optimizations and its applications. J Appl Res Technol 11(4):510–522
Kwon S, Ntaimo L, Gautam N (2018) Demand response in data centers: integration of server provisioning and power procurement. IEEE Trans Smart Grid 10(5):4928–4938
Lee KY, Park J-B (2006) Application of particle swarm optimization to economic dispatch problem: advantages and disadvantages. Paper presented at the 2006 IEEE PES power systems conference and exposition
Leitao J, Gil P, Ribeiro B, Cardoso A (2020) A survey on home energy management. IEEE Access 8:5699–5722
Li Y, Ng BL, Trayer M, Liu L (2012) Automated residential demand response: algorithmic implications of pricing models. IEEE Trans Smart Grid 3(4):1712–1721
Li D, Chiu W-Y, Sun H (2017) Demand side management in microgrid control systems. In: Microgrid. Elsevier, pp 203–230
Chapter Google Scholar
Li W, Ng C, Logenthiran T, Phan V-T, Woo WL (2018) Smart Grid Distribution Management System (SGDMS) for optimised electricity bills. J Power Energy Eng 6(08):49
Li Y, Han T, Han B, Zhao H, Wei Z (2019) Whale optimization algorithm with chaos strategy and weight factor. Paper presented at the Journal of Physics: Conference Series
Li Y, Zhu X, Liu J (2020) An improved moth-flame optimization algorithm for engineering problems. Symmetry 12(8):1234
Li L, Qian S, Li Z, Li S (2022) Application of improved satin bowerbird optimizer in image segmentation. Front Plant Sci 1519
Lin Y-H (2018) Design and implementation of an IoT-oriented energy management system based on non-intrusive and self-organizing neuro-fuzzy classification as an electrical energy audit in smart homes. Appl Sci 8(12):2337
Liping L, Ning W, Peijun Z (2018) Modified cuckoo search algorithm with variational parameters and logistic map. MDPI J.
Liu R-S, Hsu Y-F (2018) A scalable and robust approach to demand side management for smart grids with uncertain renewable power generation and bi-directional energy trading. Int J Electr Power Energy Syst 97:396–407
Liu Y, Yuen C, Yu R, Zhang Y, Xie S (2015) Queuing-based energy consumption management for heterogeneous residential demands in smart grid. IEEE Trans Smart Grid 7(3):1650–1659
Liu D, Xu Y, Wei Q, Liu X (2017) Residential energy scheduling for variable weather solar energy based on adaptive dynamic programming. IEEE/CAA J Autom Sin 5(1):36–46
Liu H, Liu B, Li Y, Cui S (2021a) A rotation learning-based colliding bodies optimization algorithm. Paper presented at the Journal of Physics: Conference Series
Liu J, Ji H, Liu Q, Li Y (2021b) A bat optimization algorithm with moderate orientation and perturbation of trend. J Algorithms Comput Technol. https://doi.org/10.1177/17483026211008410
Liu J, Wei X, Huang H (2021c) An improved grey wolf optimization algorithm and its application in path planning. IEEE Access 9:121944–121956
Logenthiran T, Srinivasan D, Shun TZ (2012) Demand side management in smart grid using heuristic optimization. IEEE Trans Smart Grid 3(3):1244–1252
Logenthiran T, Srinivasan D, Phyu E (2015) Particle swarm optimization for demand side management in smart grid. Paper presented at the 2015 IEEE Innovative Smart Grid Technologies-Asia (ISGT ASIA)
Lokeshgupta B, Sivasubramani S (2019) Multi-objective home energy management with battery energy storage systems. Sustain Cities Soc 47:101458
Lowell J, Yoshimura H (2011) ISO New England: results of ancillary service pilot programs, alternative technology regulation pilot program and demand response reserves pilot program. ISO New England, Holyoke
Lu X, Zhou K, Chan FT, Yang S (2017) Optimal scheduling of household appliances for smart home energy management considering demand response. Nat Hazards 88(3):1639–1653
Lu X, Zhou K, Zhang X, Yang S (2018) A systematic review of supply and demand side optimal load scheduling in a smart grid environment. J Clean Prod 203:757–768
Luo F, Dong ZY, Xu Z, Kong W, Wang F (2018) Distributed residential energy resource scheduling with renewable uncertainties. IET Gener Transm Distrib 12(11):2770–2777
Luque-Chang A, Cuevas E, Fausto F, Zaldívar D, Pérez M (2018) Social spider optimization algorithm: modifications, applications, and perspectives. Math Probl Eng. https://doi.org/10.1155/2018/6843923
Macedo M, Galo J, De Almeida L, de C. Lima A (2015) Demand side management using artificial neural networks in a smart grid environment. Renew Sustain Energy Rev 41:128–133
Maharjan S, Zhang Y, Gjessing S, Tsang DH (2014) User-centric demand response management in the smart grid with multiple providers. IEEE Trans Emerg Top Comput 5(4):494–505
Mahmood D, Javaid N, Alrajeh N, Khan Z, Qasim U, Ahmed I, Ilahi M (2016) Realistic scheduling mechanism for smart homes. Energies 9(3):202
Makhadmeh SN, Khader AT, Al-Betar MA, Naim S (2018) An optimal power scheduling for smart home appliances with smart battery using grey wolf optimizer. Paper presented at the 2018 8th IEEE international conference on control system, computing and engineering (ICCSCE)
Manzoor A, Javaid N, Ullah I, Abdul W, Almogren A, Alamri A (2017) An intelligent hybrid heuristic scheme for smart metering based demand side management in smart homes. Energies 10(9):1258
Martínez-Lao J, Montoya FG, Montoya MG, Manzano-Agugliaro F (2017) Electric vehicles in Spain: an overview of charging systems. Renew Sustain Energy Rev 77:970–983
Martirano L, Parise G, Greco G, Manganelli M, Massarella F, Cianfrini M, Parise L, di Laura FP, Habib E (2018) Aggregation of users in a residential/commercial building managed by a building energy management system (BEMS). IEEE Trans Ind Appl 55(1):26–34
Mekhilef S, Saidur R, Kamalisarvestani M (2012) Effect of dust, humidity and air velocity on efficiency of photovoltaic cells. Renew Sustain Energy Rev 16(5):2920–2925
Menos-Aikateriniadis C, Lamprinos I, Georgilakis PS (2022) Particle swarm optimization in residential demand-side management: a review on scheduling and control algorithms for demand response provision. Energies 15(6):2211
Merabet A, Ahmed KT, Ibrahim H, Beguenane R, Ghias AM (2016) Energy management and control system for laboratory scale microgrid based wind-PV-battery. IEEE Trans Sustain Energy 8(1):145–154
Meyabadi AF, Deihimi MH (2017) A review of demand-side management: reconsidering theoretical framework. Renew Sustain Energy Rev 80:367–379
Ming Z, Song X, Mingjuan M, Lingyun L, Min C, Yuejin W (2013) Historical review of demand side management in China: management content, operation mode, results assessment and relative incentives. Renew Sustain Energy Rev 25:470–482
Ming Z, Li S, Yanying H (2015) Status, challenges and countermeasures of demand-side management development in China. Renew Sustain Energy Rev 47:284–294
Mirkhan A, Celebi N (2022) Binary representation of polar bear algorithm for feature selection. Comput Syst Sci Eng 43(2):767–783
Misaghi M, Yaghoobi M (2019) Improved invasive weed optimization algorithm (IWO) based on chaos theory for optimal design of PID controller. J Comput Des Eng 6(3):284–295
Mitras BA, Sultan JA (2013) A novel hybrid imperialist competitive algorithm for global optimization. Aust J Basic Appl Sci 7:330–341
Møller Andersen F, Grenaa Jensen S, Larsen HV, Meibom P, Ravn H, Skytte K, Togeby M (2006) Analyses of demand response in Denmark. Risoe National Lab.
Moon S, Lee J-W (2016) Multi-residential demand response scheduling with multi-class appliances in smart grid. IEEE Trans Smart Grid 9(4):2518–2528
Moreno Escobar JJ, Morales Matamoros O, Tejeida Padilla R, Lina Reyes I, Quintana Espinosa H (2021) A comprehensive review on smart grids: challenges and opportunities. Sensors 21(21):6978
Morgan MG, Talukdar SN (1979) Electric power load management: some technical, economic, regulatory and social issues. Proc IEEE 67(2):241–312
Mou Y, Xing H, Lin Z, Fu M (2014) Decentralized optimal demand-side management for PHEV charging in a smart grid. IEEE Trans Smart Grid 6(2):726–736
Muhamediyeva D (2020) Fuzzy cultural algorithm for solving optimization problems. Paper presented at the Journal of Physics: Conference Series
Muratori M, Schuelke-Leech B-A, Rizzoni G (2014) Role of residential demand response in modern electricity markets. Renew Sustain Energy Rev 33:546–553
Murphy KP (2012) Machine learning: a probabilistic perspective. MIT Press, Cambridge
Nadimi-Shahraki MH, Fatahi A, Zamani H, Mirjalili S, Abualigah L (2021) An improved moth-flame optimization algorithm with adaptation mechanism to solve numerical and mechanical engineering problems. Entropy 23(12):1637
Nawaz A, Hafeez G, Khan I, Jan KU, Li H, Khan SA, Wadud Z (2020) An intelligent integrated approach for efficient demand side management with forecaster and advanced metering infrastructure frameworks in smart grid. IEEE Access 8:132551–132581
Nguyen HT, Nguyen DT, Le LB (2014) Energy management for households with solar assisted thermal load considering renewable energy and price uncertainty. IEEE Trans Smart Grid 6(1):301–314
Nolan S, O’Malley M (2015) Challenges and barriers to demand response deployment and evaluation. Appl Energy 152:1–10
Noor S, Guo M, van Dam KH, Shah N, Wang X (2018) Energy demand side management with supply constraints: game theoretic approach. Energy Procedia 145:368–373
O’Neill D, Levorato M, Goldsmith A, Mitra U (2010) Residential demand response using reinforcement learning. Paper presented at the 2010 First IEEE international conference on smart grid communications
Oskouei MZ, Mohammadi-Ivatloo B, Abapour M, Ahmadian A, Piran MJ (2020) A novel economic structure to improve the energy label in smart residential buildings under energy efficiency programs. J Clean Prod 260:121059
Oskouei MZ, Şeker AA, Tunçel S, Demirbaş E, Gözel T, Hocaoğlu MH, Abapour M, Mohammadi-Ivatloo B (2022) A critical review on the impacts of energy storage systems and demand-side management strategies in the economic operation of renewable-based distribution network. Sustainability 14(4):2110
Page MJ, Moher D (2017) Evaluations of the uptake and impact of the Preferred Reporting Items for Systematic reviews and Meta-Analyses (PRISMA) Statement and extensions: a scoping review. Syst Rev 6(1):1–14
Pal S, Kumar R (2016) Price prediction techniques for residential demand response using support vector regression. Paper presented at the 2016 IEEE 7th power India international conference (PIICON)
Pan J, Jain R, Paul S (2014) A survey of energy efficiency in buildings and microgrids using networking technologies. IEEE Commun Surv Tutor 16(3):1709–1731
Panapakidis IP, Papadopoulos TA, Christoforidis GC, Papagiannis GK (2014) Pattern recognition algorithms for electricity load curve analysis of buildings. Energy Build 73:137–145
Panda S, Mohanty S, Rout PK, Sahu BK, Bajaj M, Zawbaa HM, Kamel S (2022) Residential Demand Side Management model, optimization and future perspective: a review. Energy Rep 8:3727–3766
Panwar LK, Konda SR, Verma A, Panigrahi BK, Kumar R (2017) Operation window constrained strategic energy management of microgrid with electric vehicle and distributed resources. IET Gener Transm Distrib 11(3):615–626
Pascual J, Barricarte J, Sanchis P, Marroyo L (2015) Energy management strategy for a renewable-based residential microgrid with generation and demand forecasting. Appl Energy 158:12–25
Paterakis NG, Erdinç O, Pappi IN, Bakirtzis AG, Catalão JP (2016) Coordinated operation of a neighborhood of smart households comprising electric vehicles, energy storage and distributed generation. IEEE Trans Smart Grid 7(6):2736–2747
Patyn C, Ruelens F, Deconinck G (2018) Comparing neural architectures for demand response through model-free reinforcement learning for heat pump control. Paper presented at the 2018 IEEE international energy conference (ENERGYCON)
Paudyal P, Ni Z (2019) Smart home energy optimization with incentives compensation from inconvenience for shifting electric appliances. Int J Electr Power Energy Syst 109:652–660
Pedrasa MAA, Spooner TD, MacGill IF (2010) Coordinated scheduling of residential distributed energy resources to optimize smart home energy services. IEEE Trans Smart Grid 1(2):134–143
Phuangpornpitak N, Tia S (2013) Opportunities and challenges of integrating renewable energy in smart grid system. Energy Procedia 34:282–290
Piette MA, Sezgen O, Watson DS, Motegi N, Shockman C, ten Hope L (2004) Development and evaluation of fully automated demand response in large facilities
Piette M, Sezgen O, Watson D, Motegi N, Shockman C, ten Hope L (2005) Development and evaluation of fully automated demand response in large facilities, California Energy Commission: CEC-500-2005-013, Jan
Pilloni V, Floris A, Meloni A, Atzori L (2016) Smart home energy management including renewable sources: a qoe-driven approach. IEEE Trans Smart Grid 9(3):2006–2018
Platt G, Paevere P, Higgins A, Grozev G (2014) Electric vehicles: new problem or distributed energy asset? In: Distributed generation and its implications for the utility industry. Elsevier, Amsterdam, pp 335–355
Praveena M, Jaiganesh V (2017) A literature review on supervised machine learning algorithms and boosting process. Int J Comput Appl 169(8):32–35
Priya Esther B, Shivarama Krishna K, Sathish Kumar K, Ravi K (2016) Demand side management using bacterial foraging optimization algorithm. In: Information systems design and intelligent applications. Springer, pp 657–666
Proedrou E (2021) A comprehensive review of residential electricity load profile models. IEEE Access 9:12114–12133
Puente C, Palacios R, González-Arechavala Y, Sánchez-Úbeda EF (2020) Non-intrusive load monitoring (NILM) for energy disaggregation using soft computing techniques. Energies 13(12):3117
Quiggin D, Cornell S, Tierney M, Buswell R (2012) A simulation and optimisation study: towards a decentralised microgrid, using real world fluctuation data. Energy 41(1):549–559
Qureshi WA, Nair N-KC, Farid MM (2021) Impact of energy storage in buildings on electricity demand side management. In: Thermal energy storage with phase change materials. CRC Press, Boca Raton, pp 176–197
Rahim S, Iqbal Z, Shaheen N, Khan ZA, Qasim U, Khan SA, Javaid N (2016a) Ant colony optimization based energy management controller for smart grid. Paper presented at the 2016a IEEE 30th international conference on advanced information networking and applications (AINA)
Rahim S, Javaid N, Ahmad A, Khan SA, Khan ZA, Alrajeh N, Qasim U (2016b) Exploiting heuristic algorithms to efficiently utilize energy management controllers with renewable energy sources. Energy Build 129:452–470
Rahim MH, Khalid A, Javaid N, Alhussein M, Aurangzeb K, Khan ZA (2018) Energy efficient smart buildings using coordination among appliances generating large data. IEEE Access 6:34670–34690
Rahman SM, Miah MD (2017) The impact of sources of energy production on globalization: evidence from panel data analysis. Renew Sustain Energy Rev 74:110–115
Rahman I, Vasant PM, Singh BSM, Abdullah-Al-Wadud M (2016) On the performance of accelerated particle swarm optimization for charging plug-in hybrid electric vehicles. Alex Eng J 55(1):419–426
Article MATH Google Scholar
Rahman MM, Arefi A, Shafiullah G, Hettiwatte S (2018) A new approach to voltage management in unbalanced low voltage networks using demand response and OLTC considering consumer preference. Int J Electr Power Energy Syst 99:11–27
Rajendhar P, Jeyaraj BE (2019) Application of DR and co-simulation approach for renewable integrated HEMS: a review. IET Gener Transm Distrib 13(16):3501–3512
Rehman AU, Wadud Z, Elavarasan RM, Hafeez G, Khan I, Shafiq Z, Alhelou HH (2021) An optimal power usage scheduling in smart grid integrated with renewable energy sources for energy management. IEEE Access 9:84619–84638
Renner S, Albu M, van Elburg H, Heinemann C, Lazicki A, Penttinen L, Puente F, Saele H (2011) European smart metering landscape report. Imprint 2:1–168
Roy C, Das DK (2021) A hybrid genetic algorithm (GA)–particle swarm optimization (PSO) algorithm for demand side management in smart grid considering wind power for cost optimization. Sādhanā 46(2):1–12
Ruelens F, Claessens BJ, Vandael S, Iacovella S, Vingerhoets P, Belmans R (2014) Demand response of a heterogeneous cluster of electric water heaters using batch reinforcement learning. Paper presented at the 2014 power systems computation conference
Saad W, Han Z, Poor HV, Basar T (2012) Game-theoretic methods for the smart grid: an overview of microgrid systems, demand-side management, and smart grid communications. IEEE Signal Process Mag 29(5):86–105
Sæle H, Grande OS (2011) Demand response from household customers: experiences from a pilot study in Norway. IEEE Trans Smart Grid 2(1):102–109
Safdarian A, Fotuhi-Firuzabad M, Lehtonen M (2015) Optimal residential load management in smart grids: a decentralized framework. IEEE Trans Smart Grid 7(4):1836–1845
Sala-Cardoso E, Delgado-Prieto M, Kampouropoulos K, Romeral L (2018) Activity-aware HVAC power demand forecasting. Energy Build 170:15–24
Samad T, Koch E, Stluka P (2016) Automated demand response for smart buildings and microgrids: the state of the practice and research challenges. Proc IEEE 104(4):726–744
Samadi P, Mohsenian-Rad H, Schober R, Wong VW (2012) Advanced demand side management for the future smart grid using mechanism design. IEEE Trans Smart Grid 3(3):1170–1180
Samadi P, Mohsenian-Rad H, Wong VW, Schober R (2014) Real-time pricing for demand response based on stochastic approximation. IEEE Trans Smart Grid 5(2):789–798
Samsuddin S, Othman MS, Yusuf LM (2018) A review of single and population-based metaheuristic algorithms solving multi depot vehicle routing problem. Int J Softw Eng Comput Syst 4(2):80–93
Sarker MR, Ortega-Vazquez MA, Kirschen DS (2014) Optimal coordination and scheduling of demand response via monetary incentives. IEEE Trans Smart Grid 6(3):1341–1352
Sarker E, Halder P, Seyedmahmoudian M, Jamei E, Horan B, Mekhilef S, Stojcevski A (2021) Progress on the demand side management in smart grid and optimization approaches. Int J Energy Res 45(1):36–64
Sarkis-Onofre R, Catalá-López F, Aromataris E, Lockwood C (2021) How to properly use the PRISMA statement. Syst Rev 10(1):1–3
Schwartz J (2012) Salt River Project: the persistence of consumer choice. Association for Demand Response and Smart Grid, June 15
Sehar F, Pipattanasomporn M, Rahman S (2017) Integrated automation for optimal demand management in commercial buildings considering occupant comfort. Sustain Cities Soc 28:16–29
Shafie-Khah M, Siano P (2017) A stochastic home energy management system considering satisfaction cost and response fatigue. IEEE Trans Ind Inform 14(2):629–638
Shafie-Khah M, Siano P, Aghaei J, Masoum MA, Li F, Catalão JP (2019) Comprehensive review of the recent advances in industrial and commercial DR. IEEE Trans Ind Inform 15(7):3757–3771
Sharda S, Singh M, Sharma K (2021) Demand side management through load shifting in IoT based HEMS: overview, challenges and opportunities. Sustain Cities Soc 65:102517
Shareef H, Ahmed MS, Mohamed A, Al Hassan E (2018) Review on home energy management system considering demand responses, smart technologies, and intelligent controllers. IEEE Access 6:24498–24509
Sharifi R, Fathi S, Vahidinasab V (2017) A review on demand-side tools in electricity market. Renew Sustain Energy Rev 72:565–572
Shen J, Jiang C, Liu Y, Qian J (2016) A microgrid energy management system with demand response for providing grid peak shaving. Electr Power Compon Syst 44(8):843–852
Shewale A, Mokhade A, Funde N, Bokde ND (2020) An overview of demand response in smart grid and optimization techniques for efficient residential appliance scheduling problem. Energies 13(16):4266
Shoreh MH, Siano P, Shafie-khah M, Loia V, Catalão JP (2016) A survey of industrial applications of Demand Response. Electr Power Syst Res 141:31–49
Shuja SM, Javaid N, Khan S, Akmal H, Hanif M, Fazalullah Q, Khan ZA (2019) Efficient scheduling of smart home appliances for energy management by cost and PAR optimization algorithm in smart grid. Paper presented at the workshops of the international conference on advanced information networking and applications
Simmhan Y, Aman S, Kumbhare A, Liu R, Stevens S, Zhou Q, Prasanna V (2013) Cloud-based software platform for big data analytics in smart grids. Comput Sci Eng 15(4):38–47
Simon D (2008) Biogeography-based optimization. IEEE Trans Evol Comput 12(6):702–713
Songyang L, Haipeng Y, Miao W (2022) Cat swarm optimization algorithm based on the information interaction of subgroup and the top-N learning strategy. J Intell Syst 31(1):489–500
Sou KC, Weimer J, Sandberg H, Johansson KH (2011) Scheduling smart home appliances using mixed integer linear programming. Paper presented at the 2011 50th IEEE conference on decision and control and European control conference
Suruli K, Ila V (2020) Social spider optimization algorithm-based optimized power management schemes. Electr Power Compon Syst 48(11):1111–1124
Tamilarasi K, Gogulkumar M, Velusamy K (2021) Enhancing the performance of social spider optimization with neighbourhood attraction algorithm. Paper presented at the Journal of Physics: Conference Series.
Tamilarasu K, Sathiasamuel CR, Joseph JDN, Madurai Elavarasan R, Mihet-Popa L (2021) Reinforced demand side management for educational institution with incorporation of user’s comfort. Energies 14(10):2855
Tang W-J, Wu Y-S, Yang H-T (2018) Adaptive segmentation and machine learning based potential DR capacity analysis. Paper presented at the 2018 18th international conference on harmonics and quality of power (ICHQP)
Tariq M, Khalid A, Ahmad I, Khan M, Zaheer B, Javaid N (2017) Load scheduling in home energy management system using meta-heuristic techniques and critical peak pricing tariff. Paper presented at the international conference on P2P, parallel, grid, cloud and internet computing
Tasdighi M, Ghasemi H, Rahimi-Kian A (2013) Residential microgrid scheduling based on smart meters data and temperature dependent thermal load modeling. IEEE Trans Smart Grid 5(1):349–357
Teng R, Yamazaki T (2018) Load profile-based coordination of appliances in a smart home. IEEE Trans Consum Electron 65(1):38–46
Tian Z, Zhang C (2018) An improved harmony search algorithm and its application in function optimization. J Inf Process Syst 14(5):1237–1253
Torriti J (2012) Price-based demand side management: assessing the impacts of time-of-use tariffs on residential electricity demand and peak shifting in Northern Italy. Energy 44(1):576–583
Tsipianitis AD, Tsompanakis Y. Solving engineering optimization problems with an efficient hybrid nature-inspired algorithm.
Tushar MHK, Assi C, Maier M, Uddin MF (2014) Smart microgrids: optimal joint scheduling for electric vehicles and home appliances. IEEE Trans Smart Grid 5(1):239–250
Vardakas JS, Zorba N, Verikoukis CV (2014) A survey on demand response programs in smart grids: pricing methods and optimization algorithms. IEEE Commun Surv Tutor 17(1):152–178
Vázquez-Canteli JR, Nagy Z (2019) Reinforcement learning for demand response: a review of algorithms and modeling techniques. Appl Energy 235:1072–1089
Vivekananthan C, Mishra Y, Ledwich G, Li F (2014) Demand response for residential appliances via customer reward scheme. IEEE Trans Smart Grid 5(2):809–820
Wang G, Lin Z (2017) Hybrid mosquito host-seeking algorithm for multi-depot vehicle routing problem. Paper presented at the 2017 9th international conference on measuring technology and mechatronics automation (ICMTMA)
Wang M, Lu G (2021) A modified sine cosine algorithm for solving optimization problems. IEEE Access 9:27434–27450
Wang Z, Paranjape R (2015) Optimal residential demand response for multiple heterogeneous homes with real-time price prediction in a multiagent framework. IEEE Trans Smart Grid 8(3):1173–1184
Wang J, Sun Z, Zhou Y, Dai J (2012) Optimal dispatching model of smart home energy management system. Paper presented at the IEEE PES innovative smart grid technologies
Wang Q, Zhang C, Ding Y, Xydis G, Wang J, Østergaard J (2015) Review of real-time electricity markets for integrating distributed energy resources and demand response. Appl Energy 138:695–706
Wang Y, Yang Z, Mourshed M, Guo Y, Niu Q, Zhu X (2019) Demand side management of plug-in electric vehicles and coordinated unit commitment: a novel parallel competitive swarm optimization method. Energy Convers Manag 196:935–949
Warren P (2014) A review of demand-side management policy in the UK. Renew Sustain Energy Rev 29:941–951
Wen Z, O’Neill D, Maei H (2015) Optimal demand response using device-based reinforcement learning. IEEE Trans Smart Grid 6(5):2312–2324
Weng Y, Rajagopal R (2015) Probabilistic baseline estimation via gaussian process. Paper presented at the 2015 IEEE power & energy society general meeting
Weng Y, Yu J, Rajagopal R (2018) Probabilistic baseline estimation based on load patterns for better residential customer rewards. Int J Electr Power Energy Syst 100:508–516
Wong MC-H (1991) Market-based systems of monetary control in developing countries: operating procedures and related issues
Worthmann K, Kellett CM, Braun P, Grüne L, Weller SR (2015) Distributed and decentralized control of residential energy systems incorporating battery storage. IEEE Trans Smart Grid 6(4):1914–1923
Wu Z, Xia X (2017) A portfolio approach of demand side management. IFAC-PapersOnLine 50(1):171–176
Wu Z, Tazvinga H, Xia X (2015) Demand side management of photovoltaic-battery hybrid system. Appl Energy 148:294–304
Wu Y, Ravey A, Chrenko D, Miraoui A (2019) Demand side energy management of EV charging stations by approximate dynamic programming. Energy Convers Manag 196:878–890
Xu B, Oudalov A, Ulbig A, Andersson G, Kirschen DS (2016a) Modeling of lithium-ion battery degradation for cell life assessment. IEEE Trans Smart Grid 9(2):1131–1140
Xu G, Yu W, Griffith D, Golmie N, Moulema P (2016b) Toward integrating distributed energy resources and storage devices in smart grid. IEEE Internet Things J 4(1):192–204
Yan X, Ozturk Y, Hu Z, Song Y (2018) A review on price-driven residential demand response. Renew Sustain Energy Rev 96:411–419
Yang X-S, He X (2013) Bat algorithm: literature review and applications. Int J Bio-Inspir Comput 5(3):141–149
Yang H-T, Yang C-T, Tsai C-C, Chen G-J, Chen S-Y (2015) Improved PSO based home energy management systems integrated with demand response in a smart grid. Paper presented at the 2015 IEEE congress on evolutionary computation (CEC)
Yang H, Zhang J, Qiu J, Zhang S, Lai M, Dong ZY (2016) A practical pricing approach to smart grid demand response based on load classification. IEEE Trans Smart Grid 9(1):179–190
Yang C, Cheng Q, Lai P, Liu J, Guo H (2018) Data-driven modeling for energy consumption estimation. In: Exergy for a better environment and improved sustainability, vol 2. Springer, pp 1057–1068
Yao E, Samadi P, Wong VW, Schober R (2015) Residential demand side management under high penetration of rooftop photovoltaic units. IEEE Trans Smart Grid 7(3):1597–1608
Yilmaz S, Weber S, Patel M (2019) Who is sensitive to DSM? Understanding the determinants of the shape of electricity load curves and demand shifting: socio-demographic characteristics, appliance use and attitudes. Energy Policy 133:110909
Yilmaz S, Rinaldi A, Patel MK (2020) DSM interactions: what is the impact of appliance energy efficiency measures on the demand response (peak load management)? Energy Policy 139:111323
Yoon JH, Baldick R, Novoselac A (2014a) Dynamic demand response controller based on real-time retail price for residential buildings. IEEE Trans Smart Grid 5(1):121–129
Yoon JH, Bladick R, Novoselac A (2014b) Demand response for residential buildings based on dynamic price of electricity. Energy Build 80:531–541
Yuce B, Packianather MS, Mastrocinque E, Pham DT, Lambiase A (2013) Honey bees inspired optimization method: the bees algorithm. Insects 4(4):646–662
Zeng S, Li J, Ren Y (2008) Research of time-of-use electricity pricing models in China: a survey. Paper presented at the 2008 IEEE international conference on industrial engineering and engineering management
Zenned S, Chaouali H, Mami A (2017) Fuzzy logic energy management strategy of a hybrid renewable energy system feeding a typical Tunisian house. Int J Adv Comput Sci Appl 8(12):206–212
Zhang Q, Grossmann IE (2016) Enterprise-wide optimization for industrial demand side management: fundamentals, advances, and perspectives. Chem Eng Res Des 116:114–131
Zhang Y-J, Peng H-R (2017) Exploring the direct rebound effect of residential electricity consumption: an empirical study in China. Appl Energy 196:132–141
Zhang L, Zhong Y, Li P (2004) Applications of artificial immune sysetms in remote sensing image classification.
Zhang D, Shah N, Papageorgiou LG (2013) Efficient energy consumption and operation management in a smart building with microgrid. Energy Convers Manag 74:209–222
Zhang L, Liu L, Yang X-S, Dai Y (2016) A novel hybrid firefly algorithm for global optimization. PLoS ONE 11(9):e0163230
Zhang X, Lu R, Jiang J, Hong SH, Song WS (2021) Testbed implementation of reinforcement learning-based demand response energy management system. Appl Energy 297:117131
Zhao J, Wen F, Dong ZY, Xue Y, Wong KP (2012) Optimal dispatch of electric vehicles and wind power using enhanced particle swarm optimization. IEEE Trans Ind Inform 8(4):889–899
Zhao Z, Lee WC, Shin Y, Song K-B (2013) An optimal power scheduling method for demand response in home energy management system. IEEE Trans Smart Grid 4(3):1391–1400
Zhao J, Lv L, Sun H (2015) Artificial bee colony using opposition-based learning. In: Genetic and evolutionary computing. Springer, pp 3–10
Zhou K, Yang S (2015) Demand side management in China: the context of China’s power industry reform. Renew Sustain Energy Rev 47:954–965
Zhou D, Balandat M, Tomlin C (2016) Residential demand response targeting using machine learning with observational data. Paper presented at the 2016 IEEE 55th conference on decision and control (CDC)
Zhu Z, Tang J, Lambotharan S, Chin WH, Fan Z (2012) An integer linear programming based optimization for home demand-side management in smart grid. Paper presented at the 2012 IEEE PES innovative smart grid technologies (ISGT)
Download references
Not applicable.
Author information
Authors and affiliations.
Department of Electrical Engineering, Kampala International University, 20000, Ishaka, Uganda
Mutiu Shola Bakare, Mohammad Zeeshan & Aliyu Nuhu Shuaibu
Department of Electrical Engineering, Faculty of Engineering, Ahmadu Bello University, Zaria, Nigeria
Abubakar Abdulkarim
You can also search for this author in PubMed Google Scholar
Contributions
All authors listed have significantly contributed to the development and writing of this manuscript. MSB conceptualized the idea, AA, MZ, and ANS supervise the research, MSB, AA and MZ revised and edited the final manuscript. All authors read and approved the final manuscript.
Corresponding author
Correspondence to Mutiu Shola Bakare .
Ethics declarations
Ethics approval and consent to participate, consent for publication, competing interests.
The authors declared that they have no competing interests.
Additional information
Publisher's note.
Springer Nature remains neutral with regard to jurisdictional claims in published maps and institutional affiliations.
Rights and permissions
Open Access This article is licensed under a Creative Commons Attribution 4.0 International License, which permits use, sharing, adaptation, distribution and reproduction in any medium or format, as long as you give appropriate credit to the original author(s) and the source, provide a link to the Creative Commons licence, and indicate if changes were made. The images or other third party material in this article are included in the article's Creative Commons licence, unless indicated otherwise in a credit line to the material. If material is not included in the article's Creative Commons licence and your intended use is not permitted by statutory regulation or exceeds the permitted use, you will need to obtain permission directly from the copyright holder. To view a copy of this licence, visit http://creativecommons.org/licenses/by/4.0/ .
Reprints and permissions
About this article
Cite this article.
Bakare, M.S., Abdulkarim, A., Zeeshan, M. et al. A comprehensive overview on demand side energy management towards smart grids: challenges, solutions, and future direction. Energy Inform 6 , 4 (2023). https://doi.org/10.1186/s42162-023-00262-7
Download citation
Received : 06 February 2023
Accepted : 05 March 2023
Published : 13 March 2023
DOI : https://doi.org/10.1186/s42162-023-00262-7
Share this article
Anyone you share the following link with will be able to read this content:
Sorry, a shareable link is not currently available for this article.
Provided by the Springer Nature SharedIt content-sharing initiative
- Machine language
This website uses cookies.
By clicking the "Accept" button or continuing to browse our site, you agree to first-party and session-only cookies being stored on your device to enhance site navigation and analyze site performance and traffic. For more information on our use of cookies, please see our Privacy Policy .
- AEA Papers and Proceedings
The Economics of the Global Energy Challenge
- Michael Greenstone
- Article Information
Additional Materials
- Replication Package
- Online Appendix (291.75 KB)
- Author Disclosure Statement(s) (11.24 KB)
JEL Classification
- Q58 Environmental Economics: Government Policy
- POWER Plant ID
- POWER Events
- Connected Plant
- Distributed Energy
- International
- COVID-19 Coverage
- Carbon Capture
- Climate change
- Cybersecurity
- Distributed Power
- Electric Vehicles
- Energy Storage
- Environmental
- Instrumentation & Controls
- Legal & Regulatory
- Legislative
- Ocean/Marine
- Physical security
- Plant Design
- Power Demand
- Research and Development
- Supply Chains
- Tidal Power
- Waste to Energy
- About POWER
- Privacy Policy
- Cookie Settings
- Diversity, Equity, Inclusion & Belonging
- Accessibility Statement
How Technology Helps Overcome 10 of the Top Operational Challenges in the Energy Sector
Recent government legislation and initiatives have prompted positive momentum in the energy industry. From regulatory matters to technology upgrades to renewable energy trends boosted by the Inflation Reduction Act (IRA) tax incentives, energy demand and projects are increasing across the sector.
However, every opportunity comes with its own set of issues, especially if an organization lacks the right data, analytics, or visibility when managing its operations. Let’s explore 10 of the top operational challenges the energy sector faces and how technology can help circumvent them.
Ensuring Contract Accuracy
The first three challenges are found in the fine print—or lack thereof: 1) vagueness in vendor contract terms and conditions, 2) incomplete or inaccurate field timesheets, and 3) unconfirmed labor charges.
Contract Vagueness. Problems could arise from ambiguities in how someone gets paid for weather delays, call-out pay vs. hours, vendor-supplied consumables, off-site training, and more. Insufficient details in contracts can snowball into uncertainty, doubt, and overpayments. Consider, for instance, if a contractor submits a timesheet for a weather delay and the person signing the timesheet doesn’t know if it’s an eligible expense but signs for the time anyway. The vendor most often includes this time when invoicing the client.
It’s hard to contest something like this if it isn’t spelled out in the contract. The right software platform can help owners improve vendor partnerships by ensuring the contract includes specifics. It can also prevent non-contractual charges from being presented to the client for approval. By putting all parties on the same page, it helps eliminate mistrust around overbilling.
Incomplete and Inaccurate Timesheets. Timesheets can create problems in many ways. Field supervisors don’t have access to gate information. Field supervisors seldom, if ever, see the contract terms and conditions for the vendors they are responsible for. Outage and maintenance leaders seldom, if ever, see the actual invoices prior to payment.
Using technology that audits who comes in and out of the gate in real-time connects everyone to the terms and conditions of vendor contracts, quickly giving management the information needed to pay the right amount for what the vendors have earned and accurately forecast future commitments.
Unconfirmed Labor Charges. Inaccurate billings often occur when an energy company thinks it thoroughly audits the gate but ultimately can’t confirm the information in its entirety. They may use Excel spreadsheets to compare gate logs and timesheets or use a similar manual system that’s rife with errors. Contractor spend management software takes real-time data from the access control system, which saves time, reduces paperwork, and ensures accuracy.
Tackling Finance-Related Issues
The next three challenges occur in the finance and accounting area: 4) unverified equipment and material charges, 5) mixing up time and material vs. lump sum, and 6) payments processed without invoice verification.
Unverified Equipment and Material Charges. Miscalculations can occur when a vendor submits an invoice for a piece of equipment or machinery that isn’t accompanied by a timesheet signed by management. When timesheets are used to confirm the vendors are adhering to agreed-upon payment terms and quantities, supervisors can’t verify their accuracy.
Technology that applies, for example, the lowest possible rate for each piece of equipment and markup on materials is accurate. It provides the client with a real-time view of the allocations and holds the vendor accountable for agreed-upon terms. Management can even adjust the amount of equipment needed to finish the job.
Mixing Up Time and Material Vs. Lump Sum. Mistakes can lead to paying a contractor twice. A common error when vendors are supporting both types of work at a site is for an employee to show up on multiple timesheets for the same time period. The right software platform can ensure all on-site contractors are paid for the exact hours they worked that day under net-billable time.
Payments Processed Without Invoice Verification. This can happen when maintenance managers, outage managers, and project managers responsible for budgets and approving bills don’t see invoices. Managers tend to focus controls on the front end of the process but fail to compare invoices that show up in a company’s system.
The opportunity is that the invoice might not match what the manager thought he or she approved. What if, instead, technology created an authorized timesheet that could double as a vendor invoice—so it’s an exact match of what should be invoiced? By requiring the vendor timesheet and invoice to match, discrepancies will become a thing of the past.
Conquering Human Error and Lack of Oversight
The final four challenges are comprised of: 7) inaccurate purchase orders (POs) for labor, equipment, and materials, 8) invoicing incorrect skill classifications and non-billables, 9) liabilities exceeding purchase order amounts, and 10) failure to update expired contracts and rates.
Inaccurate Purchase Order Allocation for Labor, Equipment, and Materials. Incorrect apportionment is a common occurrence when the proverbial right hand doesn’t know what the left hand is doing. Vendors often get their timesheets signed by the field supervisor responsible for the work. The field supervisor should ensure workers are allocated on the timesheet to a job number or cost object because it allows owners to know how much each line item costs, which is important for budgeting future projects.
Timesheets are forwarded to vendor timekeepers for invoice creation, unless noted on the timesheets, these timekeepers round craftsman hours to their assigned shifts. However, when allocations are made to the wrong job numbers or cost objects, the client’s invoices are incorrect.
Contractor spend management software not only knows which vendors are on-site but automatically applies all contractual terms and conditions to each individual. In doing so, managers know that labor, equipment, and material allocation go to the correct job number or cost object prior to approval.
Invoicing Incorrect Skill Classifications and Non-Billables. This takes place when craftsmen at different skill levels are paid at the highest level. For instance, a site could have four skill levels of crane operators, who are paid four different rates. When supervisors sign off on timesheets, skill classifications are not typically included. These crane operators might be paid the wrong, highest-possible skill rate, even if they have not been approved for the increase.
Contractor-specific technology empowers supervisors to see the vendor’s default skills classification so the people approving timesheets approve the right rate. The software blocks the approval of all skills that do not match pre-approved skill assignments. Non-billables are managed in the same way.
Liabilities Exceed Purchase Order Amounts. Charges may go over when vendors (typical process) don’t notify their clients in a timely manner that POs are about to reach their limit. The technology ensures energy companies track cost allocations and notify them as PO limits are nearing. It also eliminates late invoicing surprises because it accounts for all outstanding commitments.
Discrepancies Through Using Expired Contracts and Rates. Inconsistencies occur when terms and conditions change, but those processing times have not been notified of the changes. When procurement alters a contract, contractor spend management software automatically flags discrepancies between those changes and the time the vendor timekeeper allocates in real time. This eliminates audit findings and extra work to correct discrepancies.
Embracing Opportunities and Overcoming Challenges That Accompany Them
Too many energy organizations make operational decisions in the dark by using siloed, home-grown systems. If the above scenarios sound all too familiar, it’s time to consider alternative solutions for managing your vendors’ labor, equipment, and material spend. The right platform should measure, track, and help you make more informed decisions around routine maintenance, shutdowns, turnarounds, outages, and more—all in one place.
Contractor spend management software is a must for energy companies that want to comply with governmental regulations, such as the IRA tax incentives. It provides proof of compliance for things like journeyman-apprentice ratios and prevailing wage expectations.
Change is in the air for the energy sector, with the U.S. experiencing positive progress prompted by governmental regulation and efforts to reduce the country’s carbon footprint. Companies are discovering it is easier to embrace these new opportunities and challenges with the help of the right technology.
— Bruce Grissom is an outages and maintenance consultant with Management Controls .
SHARE this article
Getting it right: MLOps in energy and materials
Many energy and materials companies use artificial intelligence and machine learning (AI/ML) models to make difficult decisions about core processes or operations. In mining, for example, blast optimization models can help ensure safety while maximizing productivity and efficiency, and maintenance monitoring models can help predict and prevent equipment failure to ensure the safety of employees and minimize maintenance costs.
About the authors
A unified approach to managing machine learning models can help companies not only control risk but also make operations more reliable, efficient, and easy to scale. MLOps establishes key practices across the application life cycle that increase productivity, speed, and reliability while reducing risk. Our research shows that when MLOps receives the right support and encompasses the entire AI life cycle—including data management, model development and deployment, and live model operations—it can dramatically enhance the performance of energy and materials companies. 1 “ Scaling AI like a tech native: The CEO’s role ,” McKinsey, October 13, 2021.
Understanding risk: Quality and governance challenges
Governance practices can help reduce risk and ensure that the impact from analytical solutions is sustainable. But in our experience, many companies have limited or no AI or model management practices in place, and many lack company-wide standards for documenting, testing, implementing, and monitoring risk management (see sidebar “Differing levels of risk preparedness”).
Differing levels of risk preparedness
Companies implementing MLOps face different challenges based on the maturity of their machine learning models.
Limited digital adoption. Companies that don’t use the newest digital technology can get left behind as competitors achieve efficiency and productivity gains. However, deploying new models often requires a suite of digital tools that fundamentally change parts of the process, making them challenging to implement.
Adoption without supporting transformation. Some companies move machine learning tools into production but in doing so incur tech debt and unintentionally take on significant risk, particularly around environmental and safety issues. They may also have limited or no experience in AI and model management, which can increase the risk that use cases will go wrong.
Full digital transformation. Companies undergoing full digital transformations often work with machine learning–operations providers to help sustain the impact of newly implemented AI models. Hundreds of models can be centrally inventoried, executives can be given access to model performance in real time, and data science teams can stay abreast of model input or output deterioration and easily calibrate parameters or model methods.
Across industries, rigorous performance management is needed once AI systems are implemented , including frameworks for continuous monitoring. 2 On this point, McKinsey recently acquired Iguazio, a Tel Aviv–based leader in AI and machine learning, to help accelerate and scale AI deployments. For more, see “McKinsey & Company acquires Iguazio to accelerate and scale enterprise AI,” Iguazio, January 23, 2024. That said, implementing these frameworks can be challenging for companies that lack data science teams or the required digital tools. Although companies often have manual monitoring systems in place during the launch of new models, these can quickly become untenable as data sets grow in size and complexity, preventing systems from scaling and allowing warning signs to slip through the cracks.
Implementing model risk management practices
No matter how far along they are in digital implementation, companies that use AI systems grapple with a common set of risk issues.
- Mining companies use AI to optimize decision making in haul cycle and dispatch. Input to the models includes data from the fleet management system, sensors in haul trucks, and shovels and auxiliary equipment (such as temperature and pressure).
- Utility companies use predictive models to prevent equipment failure by monitoring the health of assets. Failure to make timely decisions based on model output can lead to higher maintenance costs and insufficient availability or use of equipment. For example, running an overheated processor could result in failures that disrupt the operation.
- Oil and gas companies use AI and digitalization to increase water treatment capacity and optimize the volumes of water discharge. Failure to make operational decisions in time would result in noncompliance with regulations and a negative environmental impact.
With these examples in mind, companies can implement four risk management practices to help ensure sustainable impact from digital tools: taking inventory, tiering, monitoring, and implementation.
Model inventory covers the main characteristics of the models
The model inventory serves as a summary of key information on all models, such as the relevant characteristics and other critical metadata. Thus, an inventory should be in place to begin the model life cycle management process.
As an example, an energy company with more than 200 advanced monitoring models and AI tools built a repository to save all relevant information about the model. For a predictive maintenance model, the inventory will likely capture the model type (scorecard, regression, ML, or AI), platform, status, and applicable locations. In addition, model dependency will include upstream and downstream models that feed into or from the model output. And key stakeholders can be listed to trace vendor selection, developer, reviewer, user, implementer, and business sponsor.
Model inventory should be comprehensive and include all models running in production processes that are used in business decision making. For example, a mining company could rely on an optimized truck allocation model at the beginning of each shift to decide how many trucks to deploy and where. The model considers the geology of the mine, the road conditions of the day, and truck drivers’ driving patterns to optimize throughput.
Across all models there should be one document that is continually updated to account for new models and updates to existing models, such as when they are decommissioned. This could occur with a model that has proved to be ineffective, such as a road-quality model that lacks variables to capture dynamic changes at the mine. Model managers can decommission such ineffective models and build new ones that consider more relevant variables.
With these points in mind, the preliminary structure of the model inventory includes the following dimensions: model characteristics, tier, governance, and reference documentation. Each of these dimensions is then further split into subdimensions, including item description, model use, model dependency, and key stakeholders.
Model tiering provides structure to assign risk ratings
The model tier determines review frequency, depth, and escalation pathways (Exhibit 1). The primary level consists of two parts: model materiality and model risk, both of which are informed by a number of dimensions that are typically assigned low, medium, or high ratings.
Model materiality serves as an indicator of the severity of consequences if the model does not perform as intended. It is based on the following three factors:
- External impact assesses the impact of the model on third parties or potential reputational loss. It assesses the impact caused by the failure of a model from a critical external-party perspective.
- Model criticality determines overall materiality, such as the size of the portfolio and the profit-and-loss (P&L) impact that the model addresses. Criticality assesses how much exposure the model is applied to or the potential loss that the model failure will lead to.
- Model reliance checks the degree of reliance on the model output for the overall business decision. This assesses the reliance placed on the model outcomes in determining the extent to which the model outcomes are relied upon within business decision processes.
Model risk indicates the probability that the model will not perform as designed. For example, models that typically require the manual input of temperature are prone to error and are more likely to make incorrect predictions. By contrast, models that rely on AI can get automatic readings from nearby weather stations. With this in mind, model risk is assigned based on the following four factors:
- Model input assesses the quality of the input data and whether its source was automated or manual.
- Model design assesses the complexity of the model methodology.
- Model implementation assesses the stability and controls of the implementation—for instance, model complexity and data validation checks along the process.
- Model use assesses the operational reliance on the model—such as whether it’s a closed loop or requires human interaction.
Both model materiality and risk tiering can be assessed using a questionnaire that covers all factors and aggregation rules (which define how a measure is integrated in relation to one or more dimensions). For example, a questionnaire could seek to assess external impact by asking, “Are there any regulatory requirements that are applicable to the operational or business decision based on the model output?”
Model monitoring enables risk tracking with metrics, frequency, and procedures to detect model performance
The life cycle of model development includes data management, development, deployment, and live model operations (Exhibit 2).
As part of the final steps of the life cycle, companies can regularly monitor or review model performance and business engagement to ensure they reflect business goals. With this in mind, the following topics can help companies monitor and review different models:
- Preproduction review. Models are reviewed before they are released to production. This covers dimensions such as data quality and backtesting model performance. Those that perform below standards go through more frequent periodical reviews. The review also includes validation of model implementation and review of model tier to ensure it is set appropriately.
- Continuous monitoring and periodic review. A verification process assesses whether the algorithm is working as intended and whether its use continues to be appropriate in the current environment.
Automated monitoring checks can capture potential issues in models and trigger root-cause analyses. In addition, trigger event thresholds can be adjusted for each model tier so that the level of monitoring matches the needs of the risk tier. Depending on the findings of the analysis, the model can then be recalibrated. Manual monitoring can also keep track of potential changes to model use, tier, and pipeline as well as reflect the changes of anything related to the model inventory.
Finally, a periodic review aims to identify potential issues before they arise in production, such as any changes to model usage that might affect model tier. Review frequency is determined by model tier and any performance issues identified during development (for instance, models that are identified as having poor performance can be reviewed more frequently).
Once the model is deployed and in use, it can be monitored and maintained. For example, after a predictive maintenance model is deployed, its input and output can be collected in real time, assumptions can be validated, and trigger warnings can let the user know when levels breach certain thresholds. Every recall of a piece of equipment to the factory for maintenance provides an opportunity to collect data and determine whether the model’s prediction was accurate. For instance, the model might say the tires of a vehicle have two months of use left and should be replaced to ensure safety. The accuracy of these predictions can then be entered into the machine learning tool to refresh for accuracy and set the schedule for the next maintenance check. Finally, recalibration or training might be needed to address any issues found in model reviews.
- Based on monitoring results, business leaders can set up continuous monitoring and take four potential actions. The default set of monitors and triggers for all models would likely cover the following elements: model output, data output, user feedback, model use, and regulatory. The resulting actions include sending notifications to take the model out from production, initiating immediate root-cause analysis, sending warning notifications with conservative thresholds, and sending warning notifications with more relaxed thresholds.
- Continuous-monitoring metrics are the same for all models, and the actions to be taken differ across materiality tiers. These metrics typically fall into two categories: automatic and manual. The former can track things such as output anomalies, missing data, and model acceptance rate, and the latter can help ensure that the model is used as intended and that regulatory changes are reflected in the model input, output, and assumptions.
- Root-cause analysis aims to understand issues identified during continuous monitoring or periodic review and find ways to mitigate them. Product owners are responsible for sharing consolidated and prioritized issues with model developers and for providing an expected time frame for each issue. Model developers are responsible for performing the root-cause analysis to understand the underlying reason for identified issues (see sidebar “Analysis outcomes”).
Analysis outcomes
Model developers can work with product owners to identify underlying issues. Based on the cause identified, they can respond in the following ways:
No action required. Issue was triggered by a one-time error in the model input or model pipeline and doesn’t require any additional actions.
Input or pipeline issues to be resolved by data engineers. Issue was triggered by the model input or any change or error in the pipeline.
Model recalibration. Issue was triggered by an error in model calculations, and model developers can mitigate it by performing a model recalibration.
Redeveloping the model. Issue was triggered by an error in model calculations. The model developers should mitigate it by redeveloping the model.
Model implementation enables ongoing monitoring
Once the model moves to the production environment, companies can adopt new tools and structures to support its use and ensure that results are accurate and up to date. Depending on the process, these tools can have complex interactions (Exhibit 3).
Monitoring processes—such as development and deployment, root-cause analysis, and even automated monitoring—require people to oversee them. These roles include a data science team and a data engineering team, as well as product owners, model inventory and tiering owners, and users. Current data science and engineering team members without a background in machine learning for operations can be upskilled to learn relevant practices.
Taking the first steps against risk, while getting the full potential from ML/AI models
Companies looking to implement model risk management can take a number of actions to improve practices and prevent risk.
They can start by cataloging, tiering, and monitoring their existing models to gain a better understanding of their technological strengths and weaknesses and their current risks.
Companies that already rely on advanced analytics to make product decisions can also upskill data science and data engineering teams to monitor models at scale using a unified set of tools.
More broadly, companies should also conduct continuous change management with users so that model managers can recognize early-warning signs of productivity degradation and other risks and then use model outputs as intended. Companies need to build awareness of risk across the organization so that more people feel empowered to notice and report instances in which the model has gone awry . 3 For more on the fundamental organization rewiring required by digital and AI transformations, see Eric Lamarre, Kate Smaje, and Rodney W. Zemmel, Rewired: The McKinsey guide to outcompeting in the age of digital and AI , Hoboken, NJ: John Wiley & Sons, 2023. Continuous change management is increasingly important in the era of generative AI. The increasing complexity of the tools and models at companies’ disposal heightens the importance of responsibly managing them.
For highly technical industries that rely on complex data sets and highly optimized processes, correct predictions can mean the difference between smooth performance and complete shutdown. Understanding and managing model risk is a critical element in overall operations—and it will only become more important as the technologies continue to evolve.
Alex Singla is a senior partner in McKinsey’s Chicago office, Ben Ellencweig is a senior partner in the Stamford office, Bryan Richardson is a partner in the Vancouver office, Lapo Mori is a partner in the Denver office, Rodney Zemmel is a senior partner in the New York office; and Xinru Cai is an associate partner in the New York office.
Explore a career with us
Related articles.

Using model risk management to address climate analytics: It’s a process, not a task

The future is now: Unlocking the promise of AI in industrials

Operationalizing machine learning in processes
- Undergraduate
- Short Courses
- Online Courses
- Masters/Postgraduate
- Postdoctoral
- Study in Africa
- Study in Asia
- Study in Australia
- Study in Europe
- Study in USA
- Fellowships
- Internships
- Volunteering
- OD Live Series
- Young Person of the Month
- Success Stories
- General Tips
- ODIC 2023 Application
- ODIC Judges
- ODIC 2022 Winners
- ODIC 2021 Winners
- ODIC 2019 Winners
- ODIC 2018 Winners
- ODIC 2017 Winners
- ODIC 2016 Winners
- Australia and Oceania

WFP Niger Innovation Challenge 2024: Energy for Food Security (up to $250,000)
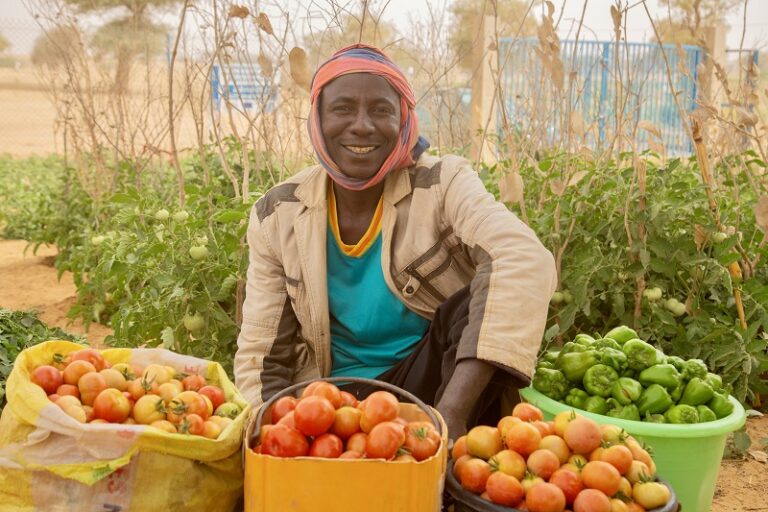
Deadline: June 14, 2024
Applications are open for the WFP Niger Innovation Challenge 2024: Energy for Food Security . Energy acts as a catalyst for socio-economic transformations, influencing all dimensions of sustainable development. Access to energy is a cornerstone in achieving food security and eliminating hunger. Not only is energy essential for food consumption—much of the food distributed by the World Food Programme (WFP) requires cooking—but it also plays a vital role throughout food systems in production, processing and preservation.
In Niger, communities face significant challenges of food insecurity and vulnerability due to climatic risks like droughts, floods and rising temperatures, coupled with environmental degradation and water scarcity. Heightened insecurity and the spillage of conflicts from neighboring countries into Niger exacerbate these issues, leading to forced displacements. A total of 3.3 million people are acutely food insecure.
To address these challenges, WFP Niger in collaboration with the WFP Innovation Accelerator is launching a call for applications for innovative solutions supporting the Energy for Food Security programme. This initiative aims to identify and scale cost-effective and sustainable energy solutions for cooking and agricultural practices across crisis-affected and stable regions of Niger.
They invite global and regional innovators, entrepreneurs and organizations to contribute impactful and fit-for-purpose solutions that can enhance energy access in vulnerable areas, fostering improved livelihoods and resilience. The WFP Innovation Accelerator and WFP Niger will provide comprehensive support to selected teams, facilitating the development and scaling of promising solutions. Their focus is on nurturing innovations that are not only novel but also sustainable and scalable, capable of bringing long-term change within challenging environments.
The three innovation thematic areas are listed below:
- Energizing Homes in Displacement Settings: They are looking for clean cooking solutions for households in rural areas, capable of powering electricity for cooking three meals a day, and household lighting systems that provide light inside the house during dark hours and surrounding areas.
- Energizing School Meals: They are looking for modern and efficient institutional cookstoves and energy systems that significantly reduce cooking time and improve air pollution within schools.
- Energizing Farmer Organizations: They are looking for solar-powered grain processing and preservation solutions that increase the production of primary processed products, including millet flour, millet couscous, cowpea flour and peanut oil.
Each selected team:
- will have the opportunity to take part in the WFP sprint programme, a six-month acceleration programme combined with piloting, business training and mentoring;
- will receive up to US$250,000 conditional funding towards project implementation. The specific amount will be determined based on the maturity stage of the solution and the three thematic areas listed below.
- will receive ongoing operational support from WFP Niger during piloting;
- may qualify for further support and fundraising opportunities in the future.
Eligibility
- Your organization must be an established legal entity (for-profit, not-for-profit, social business, NGO, INGO).
- Your organization should have an existing operational presence or willingness to establish a presence in Niger, through a subsidiary, in-country office, distributors or partnerships in the country.
- Your innovation must address one or more of the priority innovation areas listed.
- Your innovation must be at least at the Minimum Viable Product (MVP) stage. Proof-of-concept and initial traction are preferred.
- Your innovation should have a plan to implement in Niger within a six-month timeframe with the available funding and a clear pathway to scale.
Evaluation Criteria
- Impact: The solution has the potential to impact the people WFP Niger serves therefore contributing to WFP’s mission of achieving zero hunger.
- Level of innovation: The idea is a new technology or approach to applying existing technology, process, or business model to address the problem statements.
- Feasibility/Traction: The applying team has evidence of its ability to make and implement the proposed solution and has an indication of demand from the targeted users/customers of its solution.
- Financial sustainability: The solution has a robust plan to ensure sustainable revenue generation beyond WFP funding.
- Team quality: Team members have relevant experience and are committed to the project.
- Context relevance: The solution addresses at least one of the priority areas, and is suitable to implement in the Niger context.
Application
Click here to apply
For more information, see FAQ and visit WFP Niger .

Jude Ogar is an educator and youth development practitioner with years of experience working in the education and youth development space. He is passionate about the development of youth in Africa.
Related Posts
Malaysia international scholarship (mis) 2024/2025, bertha challenge fellowship 2025 for activists & investigative journalists (funded), ideation hub africa women in development summit 2024.
Type above and press Enter to search. Press Esc to cancel.
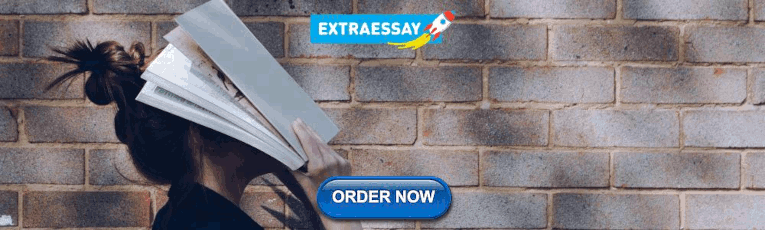
IMAGES
VIDEO
COMMENTS
Smart homes with a suitable sizing process and proper energy-management schemes can share in reducing the whole grid demand and even sell clean energy to the utility. Smart homes have been introduced recently as an alternative solution to classical power-system problems, such as the emissions of thermal plants and blackout hazards due to bulk ...
A group of Wachovia Bank employees who went through an energy management program outperformed a control group on important financial metrics like loans generated, and they reported substantially ...
8. Summary. This article has reviewed the issues associated with energy demand reduction, the dominant framings of that challenge, the current policy approaches to meeting that challenge and the insights offered by a broader socio-technical perspective on that challenge. There are three broad conclusions.
tackling energy management challenges. This essay explores various innovative solutions aimed at enhancing energy efficiency, optimizing resource utilization, and driving sustainable practices across different sectors. One of the most promising innovations in energy management is the implementation of smart grid technology.
demand side management, as well as a greater focus on the consumer as a buyer and seller 3of energy. ... synthesize the key challenges and consequences of the energy transition both for incumbent actors ... including many OIES papers on energy transition issues, which we hope will be of use to readers with a deeper interest in the various ...
Energy management is the proactive and systematic monitoring, control, and optimization of an organization's energy consumption to conserve use and decrease energy costs. Energy management includes minor actions such as monitoring monthly energy bills and upgrading to energy-saving light bulbs. It can mean more extensive improvements like ...
The structure of the paper Analyzing the papers published on energy management subject and introducing the challenges and limitations in this area, as well as discussing open questions for future ...
The results of this paper show that the available challenges in energy management to achieve use and generate energy in the most efficient way possible, and the development of a sustainable energy ...
Energy management includes planning and operation of energy production and energy consumption units as well as energy distribution and storage. Objectives are resource conservation, climate protection and cost savings, while the users have permanent access to the energy they need.It is connected closely to environmental management, production management, logistics and other established ...
Academia.edu is a platform for academics to share research papers. Energy Management and the Environment: Challenges and the Future . × ... As we prepare to take on the challenge of ensuring that our economy and society can be developed in a way that is environmentally sound, the government of Canada is to be congratulated for willingness to ...
Energy plays a pivotal role for economic development of a country. A reliable energy source is needed to improve the living standards of people. To achieve such a goal, governments and industries are trying to install a new energy infrastructure called the "Smart Grid". This helps to manage the electricity generation and distribution in an efficient manner. Buildings and other structures ...
The results of this paper show that the available challenges in energy management to achieve use and generate energy in the most efficient way possible, and the development of a sustainable energy structure will present both opportunities and solutions for this kind of challenges with the help of IoE. Efficient energy consumption is a key factor to World's ambitious goals for sustainable ...
Energy management in distribution systems has gained attention in recent years. Coordination of electricity generation and consumption is crucial to save energy, reduce energy prices and achieve global emission targets. Due to the importance of the subject, this paper provides a literature review on recent research on energy management systems and classifies the works based on several factors ...
Energy Management. Konark Sharma, Lalit Mohan Saini, in Comprehensive Energy Systems, 2018. 5.13.7 Future Directions. SGEM is a very fruitful and challenging research area. But presently it is still under development and is on the agenda of fabless semiconductor chip design and field-programmable-gate-arrays (FPGA) development companies in the world for a new democratic, sustainable and secure ...
Feature papers are submitted upon individual invitation or recommendation by the scientific editors and must receive positive feedback from the reviewers. ... The present study proposes a MILP model to address challenges concerning energy management and planning integrated operations for a smart seaport grid (such as a port MG) comprising PV ...
Essay # 1. Meaning of Energy Management: The use of energy has been a key in the development of the human society by helping it to control and adapt to the environment. Managing the use of energy is inevitable in any functional society. In the industrialized world the development of energy resources has become essential for agriculture ...
Climate change has become a major problem for humanity in the last two decades. One of the reasons that caused it, is our daily energy waste. People consume electricity in order to use home/work appliances and devices and also reach certain levels of comfort while working or being at home. However, even though the environmental impact of this behavior is not immediately observed, it leads to ...
Harrison-Atlas et al. report an artificial intelligence-aided optimization approach that shows the potential of wake steering strategies to minimize land requirements and costs. Dylan Harrison ...
Feature papers represent the most advanced research with significant potential for high impact in the field. ... high-quality images, and environmentally friendly nature. A major challenge in flexographic printing is the need to optimize energy usage, which requires diligent attention to resolve. ... This paper presents an energy management ...
Energy Management Systems: Challenges, Techniques and Opportunities. A special issue of Energies (ISSN 1996-1073). This special issue belongs to the section "A1: Smart Grids and Microgrids". Deadline for manuscript submissions: 25 September 2024 | Viewed by 7997.
Mobile offloading is a platform that facilitates the distribution of computationally intensive tasks from mobile devices to the cloud or other devices in order to conserve energy and improve performance. The concept is practically based on the idea of exchanging relatively low communication energy for high computation power utilization. Efforts have been channeled towards energy conservation ...
Demand-side management, a new development in smart grid technology, has enabled communication between energy suppliers and consumers. Demand side energy management (DSM) reduces the cost of energy acquisition and the associated penalties by continuously monitoring energy use and managing appliance schedules. Demand response (DR), distributed energy resources (DER), and energy efficiency (EE ...
The Economics of the Global Energy Challenge by Michael Greenstone. Published in volume 114, pages 1-30 of AEA Papers and Proceedings, May 2024, Abstract: Rather than facing an isolated climate change challenge, this paper argues that the world must confront the Global Energy Challenge (GEC) that r...
The next three challenges occur in the finance and accounting area: 4) unverified equipment and material charges, 5) mixing up time and material vs. lump sum, and 6) payments processed without ...
Given the steep rises in renewable energy's proportion in the global energy mix expected over several decades, a systematic way to plan the long-term deployment is needed. The main challenges are how to handle the significant uncertainties in technologies and market dynamics over a large time horizon. The problem is further complicated by the fast-timescale volatility of renewable energy ...
He noted that power-hungry and heat-producing GPUs will place unique challenges on the already overburdened power and cooling systems of many data centers. "In 2024, the global direction for data center operations is marked by a significant shift towards AI integration into the Ops function and the strategic deployment of GPUs at scale ...
Companies implementing MLOps face different challenges based on the maturity of their machine learning models. ... an inventory should be in place to begin the model life cycle management process. As an example, an energy company with more than 200 advanced monitoring models and AI tools built a repository to save all relevant information about ...
Electric vehicle (EV) fast charging systems are rapidly evolving to meet the demands of a growing electric mobility landscape. This paper provides a comprehensive overview of various fast charging techniques, advanced infrastructure, control strategies, and emerging challenges and future trends in EV fast charging. It discusses various fast charging techniques, including inductive charging ...
Heirs Insurance Essay Championship 2024 (Win N5,000,000 Scholarship) May 31, 2024. Search for: OD YPOM - Jiayuan Tian. Jiayuan Tian From China is OD Young Person of The Month - May 2024. Read more. Latest Posts. WFP Niger Innovation Challenge 2024: Energy for Food Security (up to $250,000) Ideation Hub Africa Women in Development Summit 2024;