Report | Wages, Incomes, and Wealth
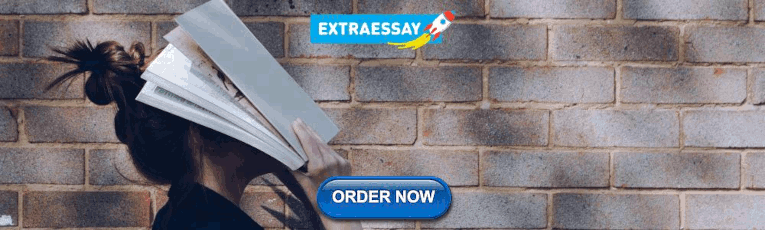
“Women’s work” and the gender pay gap : How discrimination, societal norms, and other forces affect women’s occupational choices—and their pay
Report • By Jessica Schieder and Elise Gould • July 20, 2016
Download PDF
Press release
Share this page:
What this report finds: Women are paid 79 cents for every dollar paid to men—despite the fact that over the last several decades millions more women have joined the workforce and made huge gains in their educational attainment. Too often it is assumed that this pay gap is not evidence of discrimination, but is instead a statistical artifact of failing to adjust for factors that could drive earnings differences between men and women. However, these factors—particularly occupational differences between women and men—are themselves often affected by gender bias. For example, by the time a woman earns her first dollar, her occupational choice is the culmination of years of education, guidance by mentors, expectations set by those who raised her, hiring practices of firms, and widespread norms and expectations about work–family balance held by employers, co-workers, and society. In other words, even though women disproportionately enter lower-paid, female-dominated occupations, this decision is shaped by discrimination, societal norms, and other forces beyond women’s control.
Why it matters, and how to fix it: The gender wage gap is real—and hurts women across the board by suppressing their earnings and making it harder to balance work and family. Serious attempts to understand the gender wage gap should not include shifting the blame to women for not earning more. Rather, these attempts should examine where our economy provides unequal opportunities for women at every point of their education, training, and career choices.
Introduction and key findings
Women are paid 79 cents for every dollar paid to men (Hegewisch and DuMonthier 2016). This is despite the fact that over the last several decades millions more women have joined the workforce and made huge gains in their educational attainment.
Critics of this widely cited statistic claim it is not solid evidence of economic discrimination against women because it is unadjusted for characteristics other than gender that can affect earnings, such as years of education, work experience, and location. Many of these skeptics contend that the gender wage gap is driven not by discrimination, but instead by voluntary choices made by men and women—particularly the choice of occupation in which they work. And occupational differences certainly do matter—occupation and industry account for about half of the overall gender wage gap (Blau and Kahn 2016).
To isolate the impact of overt gender discrimination—such as a woman being paid less than her male coworker for doing the exact same job—it is typical to adjust for such characteristics. But these adjusted statistics can radically understate the potential for gender discrimination to suppress women’s earnings. This is because gender discrimination does not occur only in employers’ pay-setting practices. It can happen at every stage leading to women’s labor market outcomes.
Take one key example: occupation of employment. While controlling for occupation does indeed reduce the measured gender wage gap, the sorting of genders into different occupations can itself be driven (at least in part) by discrimination. By the time a woman earns her first dollar, her occupational choice is the culmination of years of education, guidance by mentors, expectations set by those who raised her, hiring practices of firms, and widespread norms and expectations about work–family balance held by employers, co-workers, and society. In other words, even though women disproportionately enter lower-paid, female-dominated occupations, this decision is shaped by discrimination, societal norms, and other forces beyond women’s control.
This paper explains why gender occupational sorting is itself part of the discrimination women face, examines how this sorting is shaped by societal and economic forces, and explains that gender pay gaps are present even within occupations.
Key points include:
- Gender pay gaps within occupations persist, even after accounting for years of experience, hours worked, and education.
- Decisions women make about their occupation and career do not happen in a vacuum—they are also shaped by society.
- The long hours required by the highest-paid occupations can make it difficult for women to succeed, since women tend to shoulder the majority of family caretaking duties.
- Many professions dominated by women are low paid, and professions that have become female-dominated have become lower paid.
This report examines wages on an hourly basis. Technically, this is an adjusted gender wage gap measure. As opposed to weekly or annual earnings, hourly earnings ignore the fact that men work more hours on average throughout a week or year. Thus, the hourly gender wage gap is a bit smaller than the 79 percent figure cited earlier. This minor adjustment allows for a comparison of women’s and men’s wages without assuming that women, who still shoulder a disproportionate amount of responsibilities at home, would be able or willing to work as many hours as their male counterparts. Examining the hourly gender wage gap allows for a more thorough conversation about how many factors create the wage gap women experience when they cash their paychecks.
Within-occupation gender wage gaps are large—and persist after controlling for education and other factors
Those keen on downplaying the gender wage gap often claim women voluntarily choose lower pay by disproportionately going into stereotypically female professions or by seeking out lower-paid positions. But even when men and women work in the same occupation—whether as hairdressers, cosmetologists, nurses, teachers, computer engineers, mechanical engineers, or construction workers—men make more, on average, than women (CPS microdata 2011–2015).
As a thought experiment, imagine if women’s occupational distribution mirrored men’s. For example, if 2 percent of men are carpenters, suppose 2 percent of women become carpenters. What would this do to the wage gap? After controlling for differences in education and preferences for full-time work, Goldin (2014) finds that 32 percent of the gender pay gap would be closed.
However, leaving women in their current occupations and just closing the gaps between women and their male counterparts within occupations (e.g., if male and female civil engineers made the same per hour) would close 68 percent of the gap. This means examining why waiters and waitresses, for example, with the same education and work experience do not make the same amount per hour. To quote Goldin:
Another way to measure the effect of occupation is to ask what would happen to the aggregate gender gap if one equalized earnings by gender within each occupation or, instead, evened their proportions for each occupation. The answer is that equalizing earnings within each occupation matters far more than equalizing the proportions by each occupation. (Goldin 2014)
This phenomenon is not limited to low-skilled occupations, and women cannot educate themselves out of the gender wage gap (at least in terms of broad formal credentials). Indeed, women’s educational attainment outpaces men’s; 37.0 percent of women have a college or advanced degree, as compared with 32.5 percent of men (CPS ORG 2015). Furthermore, women earn less per hour at every education level, on average. As shown in Figure A , men with a college degree make more per hour than women with an advanced degree. Likewise, men with a high school degree make more per hour than women who attended college but did not graduate. Even straight out of college, women make $4 less per hour than men—a gap that has grown since 2000 (Kroeger, Cooke, and Gould 2016).
Women earn less than men at every education level : Average hourly wages, by gender and education, 2015
The data below can be saved or copied directly into Excel.
The data underlying the figure.
Source : EPI analysis of Current Population Survey Outgoing Rotation Group microdata
Copy the code below to embed this chart on your website.
Steering women to certain educational and professional career paths—as well as outright discrimination—can lead to different occupational outcomes
The gender pay gap is driven at least in part by the cumulative impact of many instances over the course of women’s lives when they are treated differently than their male peers. Girls can be steered toward gender-normative careers from a very early age. At a time when parental influence is key, parents are often more likely to expect their sons, rather than their daughters, to work in science, technology, engineering, or mathematics (STEM) fields, even when their daughters perform at the same level in mathematics (OECD 2015).
Expectations can become a self-fulfilling prophecy. A 2005 study found third-grade girls rated their math competency scores much lower than boys’, even when these girls’ performance did not lag behind that of their male counterparts (Herbert and Stipek 2005). Similarly, in states where people were more likely to say that “women [are] better suited for home” and “math is for boys,” girls were more likely to have lower math scores and higher reading scores (Pope and Sydnor 2010). While this only establishes a correlation, there is no reason to believe gender aptitude in reading and math would otherwise be related to geography. Parental expectations can impact performance by influencing their children’s self-confidence because self-confidence is associated with higher test scores (OECD 2015).
By the time young women graduate from high school and enter college, they already evaluate their career opportunities differently than young men do. Figure B shows college freshmen’s intended majors by gender. While women have increasingly gone into medical school and continue to dominate the nursing field, women are significantly less likely to arrive at college interested in engineering, computer science, or physics, as compared with their male counterparts.
Women arrive at college less interested in STEM fields as compared with their male counterparts : Intent of first-year college students to major in select STEM fields, by gender, 2014
Source: EPI adaptation of Corbett and Hill (2015) analysis of Eagan et al. (2014)
These decisions to allow doors to lucrative job opportunities to close do not take place in a vacuum. Many factors might make it difficult for a young woman to see herself working in computer science or a similarly remunerative field. A particularly depressing example is the well-publicized evidence of sexism in the tech industry (Hewlett et al. 2008). Unfortunately, tech isn’t the only STEM field with this problem.
Young women may be discouraged from certain career paths because of industry culture. Even for women who go against the grain and pursue STEM careers, if employers in the industry foster an environment hostile to women’s participation, the share of women in these occupations will be limited. One 2008 study found that “52 percent of highly qualified females working for SET [science, technology, and engineering] companies quit their jobs, driven out by hostile work environments and extreme job pressures” (Hewlett et al. 2008). Extreme job pressures are defined as working more than 100 hours per week, needing to be available 24/7, working with or managing colleagues in multiple time zones, and feeling pressure to put in extensive face time (Hewlett et al. 2008). As compared with men, more than twice as many women engage in housework on a daily basis, and women spend twice as much time caring for other household members (BLS 2015). Because of these cultural norms, women are less likely to be able to handle these extreme work pressures. In addition, 63 percent of women in SET workplaces experience sexual harassment (Hewlett et al. 2008). To make matters worse, 51 percent abandon their SET training when they quit their job. All of these factors play a role in steering women away from highly paid occupations, particularly in STEM fields.
The long hours required for some of the highest-paid occupations are incompatible with historically gendered family responsibilities
Those seeking to downplay the gender wage gap often suggest that women who work hard enough and reach the apex of their field will see the full fruits of their labor. In reality, however, the gender wage gap is wider for those with higher earnings. Women in the top 95th percentile of the wage distribution experience a much larger gender pay gap than lower-paid women.
Again, this large gender pay gap between the highest earners is partially driven by gender bias. Harvard economist Claudia Goldin (2014) posits that high-wage firms have adopted pay-setting practices that disproportionately reward individuals who work very long and very particular hours. This means that even if men and women are equally productive per hour, individuals—disproportionately men—who are more likely to work excessive hours and be available at particular off-hours are paid more highly (Hersch and Stratton 2002; Goldin 2014; Landers, Rebitzer, and Taylor 1996).
It is clear why this disadvantages women. Social norms and expectations exert pressure on women to bear a disproportionate share of domestic work—particularly caring for children and elderly parents. This can make it particularly difficult for them (relative to their male peers) to be available at the drop of a hat on a Sunday evening after working a 60-hour week. To the extent that availability to work long and particular hours makes the difference between getting a promotion or seeing one’s career stagnate, women are disadvantaged.
And this disadvantage is reinforced in a vicious circle. Imagine a household where both members of a male–female couple have similarly demanding jobs. One partner’s career is likely to be prioritized if a grandparent is hospitalized or a child’s babysitter is sick. If the past history of employer pay-setting practices that disadvantage women has led to an already-existing gender wage gap for this couple, it can be seen as “rational” for this couple to prioritize the male’s career. This perpetuates the expectation that it always makes sense for women to shoulder the majority of domestic work, and further exacerbates the gender wage gap.
Female-dominated professions pay less, but it’s a chicken-and-egg phenomenon
Many women do go into low-paying female-dominated industries. Home health aides, for example, are much more likely to be women. But research suggests that women are making a logical choice, given existing constraints . This is because they will likely not see a significant pay boost if they try to buck convention and enter male-dominated occupations. Exceptions certainly exist, particularly in the civil service or in unionized workplaces (Anderson, Hegewisch, and Hayes 2015). However, if women in female-dominated occupations were to go into male-dominated occupations, they would often have similar or lower expected wages as compared with their female counterparts in female-dominated occupations (Pitts 2002). Thus, many women going into female-dominated occupations are actually situating themselves to earn higher wages. These choices thereby maximize their wages (Pitts 2002). This holds true for all categories of women except for the most educated, who are more likely to earn more in a male profession than a female profession. There is also evidence that if it becomes more lucrative for women to move into male-dominated professions, women will do exactly this (Pitts 2002). In short, occupational choice is heavily influenced by existing constraints based on gender and pay-setting across occupations.
To make matters worse, when women increasingly enter a field, the average pay in that field tends to decline, relative to other fields. Levanon, England, and Allison (2009) found that when more women entered an industry, the relative pay of that industry 10 years later was lower. Specifically, they found evidence of devaluation—meaning the proportion of women in an occupation impacts the pay for that industry because work done by women is devalued.
Computer programming is an example of a field that has shifted from being a very mixed profession, often associated with secretarial work in the past, to being a lucrative, male-dominated profession (Miller 2016; Oldenziel 1999). While computer programming has evolved into a more technically demanding occupation in recent decades, there is no skills-based reason why the field needed to become such a male-dominated profession. When men flooded the field, pay went up. In contrast, when women became park rangers, pay in that field went down (Miller 2016).
Further compounding this problem is that many professions where pay is set too low by market forces, but which clearly provide enormous social benefits when done well, are female-dominated. Key examples range from home health workers who care for seniors, to teachers and child care workers who educate today’s children. If closing gender pay differences can help boost pay and professionalism in these key sectors, it would be a huge win for the economy and society.
The gender wage gap is real—and hurts women across the board. Too often it is assumed that this gap is not evidence of discrimination, but is instead a statistical artifact of failing to adjust for factors that could drive earnings differences between men and women. However, these factors—particularly occupational differences between women and men—are themselves affected by gender bias. Serious attempts to understand the gender wage gap should not include shifting the blame to women for not earning more. Rather, these attempts should examine where our economy provides unequal opportunities for women at every point of their education, training, and career choices.
— This paper was made possible by a grant from the Peter G. Peterson Foundation. The statements made and views expressed are solely the responsibility of the authors.
— The authors wish to thank Josh Bivens, Barbara Gault, and Heidi Hartman for their helpful comments.
About the authors
Jessica Schieder joined EPI in 2015. As a research assistant, she supports the research of EPI’s economists on topics such as the labor market, wage trends, executive compensation, and inequality. Prior to joining EPI, Jessica worked at the Center for Effective Government (formerly OMB Watch) as a revenue and spending policies analyst, where she examined how budget and tax policy decisions impact working families. She holds a bachelor’s degree in international political economy from Georgetown University.
Elise Gould , senior economist, joined EPI in 2003. Her research areas include wages, poverty, economic mobility, and health care. She is a co-author of The State of Working America, 12th Edition . In the past, she has authored a chapter on health in The State of Working America 2008/09; co-authored a book on health insurance coverage in retirement; published in venues such as The Chronicle of Higher Education , Challenge Magazine , and Tax Notes; and written for academic journals including Health Economics , Health Affairs, Journal of Aging and Social Policy, Risk Management & Insurance Review, Environmental Health Perspectives , and International Journal of Health Services . She holds a master’s in public affairs from the University of Texas at Austin and a Ph.D. in economics from the University of Wisconsin at Madison.
Anderson, Julie, Ariane Hegewisch, and Jeff Hayes 2015. The Union Advantage for Women . Institute for Women’s Policy Research.
Blau, Francine D., and Lawrence M. Kahn 2016. The Gender Wage Gap: Extent, Trends, and Explanations . National Bureau of Economic Research, Working Paper No. 21913.
Bureau of Labor Statistics (BLS). 2015. American Time Use Survey public data series. U.S. Census Bureau.
Corbett, Christianne, and Catherine Hill. 2015. Solving the Equation: The Variables for Women’s Success in Engineering and Computing . American Association of University Women (AAUW).
Current Population Survey Outgoing Rotation Group microdata (CPS ORG). 2011–2015. Survey conducted by the Bureau of the Census for the Bureau of Labor Statistics [ machine-readable microdata file ]. U.S. Census Bureau.
Goldin, Claudia. 2014. “ A Grand Gender Convergence: Its Last Chapter .” American Economic Review, vol. 104, no. 4, 1091–1119.
Hegewisch, Ariane, and Asha DuMonthier. 2016. The Gender Wage Gap: 2015; Earnings Differences by Race and Ethnicity . Institute for Women’s Policy Research.
Herbert, Jennifer, and Deborah Stipek. 2005. “The Emergence of Gender Difference in Children’s Perceptions of Their Academic Competence.” Journal of Applied Developmental Psychology , vol. 26, no. 3, 276–295.
Hersch, Joni, and Leslie S. Stratton. 2002. “ Housework and Wages .” The Journal of Human Resources , vol. 37, no. 1, 217–229.
Hewlett, Sylvia Ann, Carolyn Buck Luce, Lisa J. Servon, Laura Sherbin, Peggy Shiller, Eytan Sosnovich, and Karen Sumberg. 2008. The Athena Factor: Reversing the Brain Drain in Science, Engineering, and Technology . Harvard Business Review.
Kroeger, Teresa, Tanyell Cooke, and Elise Gould. 2016. The Class of 2016: The Labor Market Is Still Far from Ideal for Young Graduates . Economic Policy Institute.
Landers, Renee M., James B. Rebitzer, and Lowell J. Taylor. 1996. “ Rat Race Redux: Adverse Selection in the Determination of Work Hours in Law Firms .” American Economic Review , vol. 86, no. 3, 329–348.
Levanon, Asaf, Paula England, and Paul Allison. 2009. “Occupational Feminization and Pay: Assessing Causal Dynamics Using 1950-2000 U.S. Census Data.” Social Forces, vol. 88, no. 2, 865–892.
Miller, Claire Cain. 2016. “As Women Take Over a Male-Dominated Field, the Pay Drops.” New York Times , March 18.
Oldenziel, Ruth. 1999. Making Technology Masculine: Men, Women, and Modern Machines in America, 1870-1945 . Amsterdam: Amsterdam University Press.
Organisation for Economic Co-operation and Development (OECD). 2015. The ABC of Gender Equality in Education: Aptitude, Behavior, Confidence .
Pitts, Melissa M. 2002. Why Choose Women’s Work If It Pays Less? A Structural Model of Occupational Choice. Federal Reserve Bank of Atlanta, Working Paper 2002-30.
Pope, Devin G., and Justin R. Sydnor. 2010. “ Geographic Variation in the Gender Differences in Test Scores .” Journal of Economic Perspectives , vol. 24, no. 2, 95–108.
See related work on Wages, Incomes, and Wealth | Women
See more work by Jessica Schieder and Elise Gould
- Subject List
- Take a Tour
- For Authors
- Subscriber Services
- Publications
- African American Studies
- African Studies
- American Literature
- Anthropology
- Architecture Planning and Preservation
- Art History
- Atlantic History
- Biblical Studies
- British and Irish Literature
- Childhood Studies
- Chinese Studies
- Cinema and Media Studies
- Communication
- Criminology
- Environmental Science
- Evolutionary Biology
- International Law
- International Relations
- Islamic Studies
- Jewish Studies
- Latin American Studies
- Latino Studies
- Linguistics
- Literary and Critical Theory
- Medieval Studies
- Military History
- Political Science
- Public Health
- Renaissance and Reformation
- Social Work
- Urban Studies
- Victorian Literature
- Browse All Subjects
How to Subscribe
- Free Trials
In This Article Expand or collapse the "in this article" section Gender Pay Gap
Introduction, historic and contemporary trends.
- Intersectionality with Race
- Geographic Context
- Occupational Segregation
- Devaluation of Female-Typed Occupations
- Selection into Low-Paying Female-Dominated Occupations
- Career Discontinuity and Opting Out
- Marriage and Earnings
- Motherhood Penalty
- Fatherhood Premium
- Glass Ceiling/Glass Escalator
- Professionals
- Unstructured Work
- Closing the Gender Gap
Related Articles Expand or collapse the "related articles" section about
About related articles close popup.
Lorem Ipsum Sit Dolor Amet
Vestibulum ante ipsum primis in faucibus orci luctus et ultrices posuere cubilia Curae; Aliquam ligula odio, euismod ut aliquam et, vestibulum nec risus. Nulla viverra, arcu et iaculis consequat, justo diam ornare tellus, semper ultrices tellus nunc eu tellus.
- Gender and Work
- Labor Markets
- Occupations and Professions
Other Subject Areas
Forthcoming articles expand or collapse the "forthcoming articles" section.
- Consumer Credit and Debt
- Economic Globalization
- Transition to Parenthood in the Life Course
- Find more forthcoming articles...
- Export Citations
- Share This Facebook LinkedIn Twitter
Gender Pay Gap by JooHee Han , Michelle Budig LAST REVIEWED: 31 July 2019 LAST MODIFIED: 31 July 2019 DOI: 10.1093/obo/9780199756384-0226
The “gender pay gap” refers to the average difference in men’s and women’s earnings, and is typically adjusted for hours worked. The gender pay gap can refer to differences in mean or median annual earnings, weekly earnings, or hourly wage. Because women tend to work part-time at higher rates than do men, and because part-time work tends to pay lower hourly wages relative to full-time work, the size of the gender pay gap is affected by whether full- and part-time, full-year or seasonal, and very young and very old workers are included in the estimates. Among full-time, year-round American workers aged sixteen and above in 2017, the gender pay gap (median weekly earnings) was 18.2 percent, meaning that women earned 81.8 cents of every man’s dollar. In the United States, women of color earn less relative to white men than white women do, owing to racial gaps in pay among women; moreover, within-race gender pay gaps are often smaller among racial/ethnic minorities, reflecting the low earnings of minority men. The gender gap has narrowed considerably since the early 20th century, yet disparities in women’s and men’s earnings persist. Moreover, this narrowing has not proceeded in a linear fashion and the gap has occasionally increased. This entry first introduces important literatures on historic and contemporary trends in the gender pay gap and then discusses the various explanations for the persistence of, and changes in, the gap. These explanations highlight the role of occupational gender segregation; the devaluation of female-typed work; gender differences in experience; family structure, care responsibilities, and the gendered impact of parenthood; workplace structures of inequality; glass ceilings and glass escalators. This entry concludes with a discussion of narrowing the gap and what it will take to close the gap.
The gender pay gap has declined over time. The rapid attenuation of the gap occurred until the 1990s but the pace began to slow down in the 2000s. Scholars question how to understand the declining gender pay gap and the slower pace of the decline in recent decades. Blau, et al. 2008 explores the questions of whether the gender revolution is completely stalled and what factors are implicated in the declining pace of change. Because the gender pay gap refers to women’s pay level relative to men’s, the declining gender pay gap does not necessarily imply women’s progress in pay. Bernhardt, et al. 1995 points out that some part of the attenuation of gender gap is attributable to men’s wage decline rather than only women’s gain in wage. At the same time, Mandel 2013 and Mandel and Semyonov 2014 show that devaluation of female-dominated occupations and of feminized occupations may offset women’s progress in wage level, resulting in slow decline in the gender pay gap. Despite the attenuation of the gender pay gap, Blau and Kahn 2006 shows that residual difference by gender not explained by observed individual level human capital and the changes in the occupational structure became larger in the 1990s than 1980s, suggesting discrimination continues to produce gender gap at large.
Bernhardt, Annette, Martina Morris, and Mark S. Handcock. 1995. Women’s gains or men’s losses? A closer look at the shrinking gender gap in earnings. American Sociological Review 102.3: 1–37.
This study traces the source of the closing gender wage gaps in the 1967–1987 period. By decomposing distributional changes in this period, the authors find that the relative gain of women’s earning is attributed to the increasing wage inequality in men’s earnings, pushing women from the lower distribution of earnings. This suggests that women’s relative gains to men’s may be illusory.
Blau, Francine D., Mary C. Brinton, and David Grusky. 2008. The declining significance of gender? New York: Russell Sage Foundation.
The authors in this edited book try to answer whether closing the gender gap in the labor market is stalled or still on the process to complete parity.
Blau, Francine D., and Lawrence Kahn. 2006. The U.S. gender pay gap in the 1990s: Slowing convergence. Industrial & Labor Relations Review 60.1: 45–66.
The authors question why the convergence of the gender pay gap slowed down in the 1990s relative to the 1980s. They show the reduction of the unexplained residual between men and women’s pay gap was slower during the 1990s than the 1980s, which suggests that discrimination, selectivity, and differences in unmeasured characteristics may be the main causes of the slowdown.
Mandel, Hadas. 2013. Up the down staircase: Women’s upward mobility and the wage penalty for occupational feminization, 1970–2007. Social Forces 91.4: 1183–1207.
Mandel poses an insightful question from the two critical but separately analyzed trends: penalization of feminized occupations and increasing occupational mobility of women. Using the Integrated Public Use Microdata Series (IPUMS) data between 1970 and 2007, the author shows that, as an increasing number of women work in traditionally high-paying male-dominated occupations in management and the professions, they earn less than they otherwise would because the feminization of the occupations lowers their pay.
Mandel, Hadas, and Moshe Semyonov. 2014. Gender pay gap and employment sector: Sources of earnings disparities in the United States, 1970–2010. Demography 51:1597–1618.
Although an increasing number of women have entered into high-paying occupations, their occupational mobility is compromised/offset by devaluation of these traditionally male-dominated but newly feminized occupations. Such devaluation effect is stronger in high-wage male-typed occupations.
back to top
Users without a subscription are not able to see the full content on this page. Please subscribe or login .
Oxford Bibliographies Online is available by subscription and perpetual access to institutions. For more information or to contact an Oxford Sales Representative click here .
- About Sociology »
- Meet the Editorial Board »
- Actor-Network Theory
- Adolescence
- African Americans
- African Societies
- Agent-Based Modeling
- Analysis, Spatial
- Analysis, World-Systems
- Anomie and Strain Theory
- Arab Spring, Mobilization, and Contentious Politics in the...
- Asian Americans
- Assimilation
- Authority and Work
- Bell, Daniel
- Biosociology
- Bourdieu, Pierre
- Catholicism
- Causal Inference
- Chicago School of Sociology
- Chinese Cultural Revolution
- Chinese Society
- Citizenship
- Civil Rights
- Civil Society
- Cognitive Sociology
- Cohort Analysis
- Collective Efficacy
- Collective Memory
- Comparative Historical Sociology
- Comte, Auguste
- Conflict Theory
- Conservatism
- Consumer Culture
- Consumption
- Contemporary Family Issues
- Contingent Work
- Conversation Analysis
- Corrections
- Cosmopolitanism
- Crime, Cities and
- Cultural Capital
- Cultural Classification and Codes
- Cultural Economy
- Cultural Omnivorousness
- Cultural Production and Circulation
- Culture and Networks
- Culture, Sociology of
- Development
- Discrimination
- Doing Gender
- Du Bois, W.E.B.
- Durkheim, Émile
- Economic Institutions and Institutional Change
- Economic Sociology
- Education and Health
- Education Policy in the United States
- Educational Policy and Race
- Empires and Colonialism
- Entrepreneurship
- Environmental Sociology
- Epistemology
- Ethnic Enclaves
- Ethnomethodology and Conversation Analysis
- Exchange Theory
- Families, Postmodern
- Family Policies
- Feminist Theory
- Field, Bourdieu's Concept of
- Forced Migration
- Foucault, Michel
- Frankfurt School
- Gender and Bodies
- Gender and Crime
- Gender and Education
- Gender and Health
- Gender and Incarceration
- Gender and Professions
- Gender and Social Movements
- Gender Pay Gap
- Gender, Sexuality, and Migration
- Gender Stratification
- Gender, Welfare Policy and
- Gendered Sexuality
- Gentrification
- Gerontology
- Global Inequalities
- Globalization and Labor
- Goffman, Erving
- Historic Preservation
- Human Trafficking
- Immigration
- Indian Society, Contemporary
- Institutions
- Intellectuals
- Intersectionalities
- Interview Methodology
- Job Quality
- Knowledge, Critical Sociology of
- Latino/Latina Studies
- Law and Society
- Law, Sociology of
- LGBT Parenting and Family Formation
- LGBT Social Movements
- Life Course
- Lipset, S.M.
- Markets, Conventions and Categories in
- Marriage and Divorce
- Marxist Sociology
- Masculinity
- Mass Incarceration in the United States and its Collateral...
- Material Culture
- Mathematical Sociology
- Medical Sociology
- Mental Illness
- Methodological Individualism
- Middle Classes
- Military Sociology
- Money and Credit
- Multiculturalism
- Multilevel Models
- Multiracial, Mixed-Race, and Biracial Identities
- Nationalism
- Non-normative Sexuality Studies
- Organizations
- Panel Studies
- Parsons, Talcott
- Political Culture
- Political Economy
- Political Sociology
- Popular Culture
- Proletariat (Working Class)
- Protestantism
- Public Opinion
- Public Space
- Qualitative Comparative Analysis (QCA)
- Race and Sexuality
- Race and Violence
- Race and Youth
- Race in Global Perspective
- Race, Organizations, and Movements
- Rational Choice
- Relationships
- Religion and the Public Sphere
- Residential Segregation
- Revolutions
- Role Theory
- Rural Sociology
- Scientific Networks
- Secularization
- Sequence Analysis
- Sex versus Gender
- Sexual Identity
- Sexualities
- Sexuality Across the Life Course
- Simmel, Georg
- Single Parents in Context
- Small Cities
- Social Capital
- Social Change
- Social Closure
- Social Construction of Crime
- Social Control
- Social Darwinism
- Social Disorganization Theory
- Social Epidemiology
- Social History
- Social Indicators
- Social Mobility
- Social Movements
- Social Network Analysis
- Social Networks
- Social Policy
- Social Problems
- Social Psychology
- Social Stratification
- Social Theory
- Socialization, Sociological Perspectives on
- Sociolinguistics
- Sociological Approaches to Character
- Sociological Research on the Chinese Society
- Sociological Research, Qualitative Methods in
- Sociological Research, Quantitative Methods in
- Sociology, History of
- Sociology of Manners
- Sociology of Music
- Sociology of War, The
- Suburbanism
- Survey Methods
- Symbolic Boundaries
- Symbolic Interactionism
- The Division of Labor after Durkheim
- Tilly, Charles
- Time Use and Childcare
- Time Use and Time Diary Research
- Tourism, Sociology of
- Transnational Adoption
- Unions and Inequality
- Urban Ethnography
- Urban Growth Machine
- Urban Inequality in the United States
- Veblen, Thorstein
- Visual Arts, Music, and Aesthetic Experience
- Wallerstein, Immanuel
- Welfare, Race, and the American Imagination
- Welfare States
- Women’s Employment and Economic Inequality Between Househo...
- Work and Employment, Sociology of
- Work/Life Balance
- Workplace Flexibility
- Privacy Policy
- Cookie Policy
- Legal Notice
- Accessibility
Powered by:
- [66.249.64.20|91.193.111.216]
- 91.193.111.216
Numbers, Facts and Trends Shaping Your World
Read our research on:
Full Topic List
Regions & Countries
- Publications
- Our Methods
- Short Reads
- Tools & Resources
Read Our Research On:
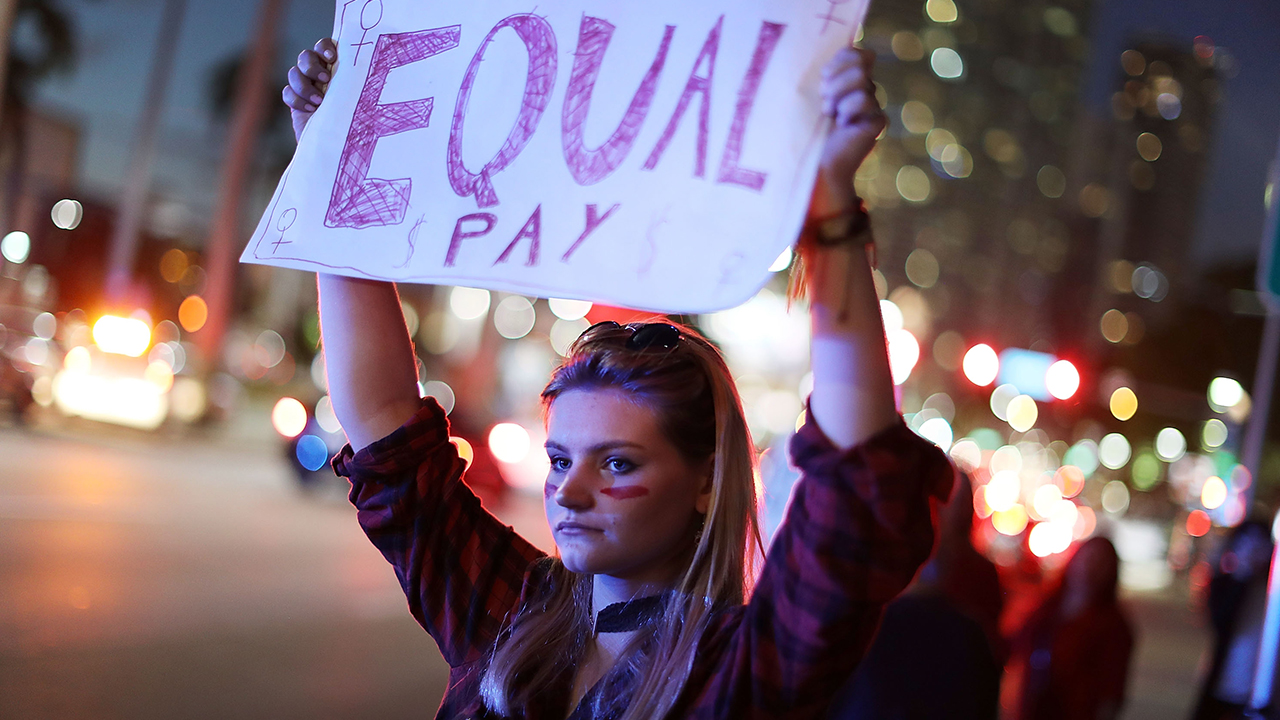
The Enduring Grip of the Gender Pay Gap
Table of contents, how the gender pay gap increases with age, mothers with children at home tend to be less engaged with the workplace, while fathers are more active, employed mothers earn about the same as similarly educated women without children at home; both groups earn less than fathers, progress in closing the gender pay gap has slowed despite gains in women’s education, gender pay gap differs widely by race and ethnicity, broader economic forces may impact men’s and women’s earnings in different ways, what’s next for the gender pay gap.
The gender pay gap – the difference between the earnings of men and women – has barely closed in the United States in the past two decades. In 2022, American women typically earned 82 cents for every dollar earned by men. That was about the same as in 2002, when they earned 80 cents to the dollar. The slow pace at which the gender pay gap has narrowed this century contrasts sharply with the progress in the preceding two decades: In 1982, women earned just 65 cents to each dollar earned by men.
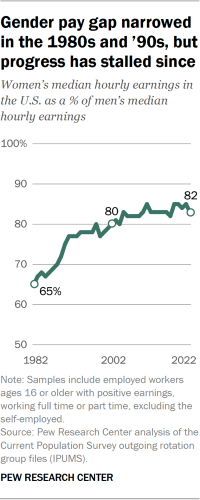
There is no single explanation for why progress toward narrowing the pay gap has all but stalled in the 21st century. Women generally begin their careers closer to wage parity with men, but they lose ground as they age and progress through their work lives, a pattern that has remained consistent over time. The pay gap persists even though women today are more likely than men to have graduated from college. In fact, the pay gap between college-educated women and men is not any narrower than the one between women and men who do not have a college degree. This points to the dominant role of other factors that still set women back or give men an advantage.
One of these factors is parenthood. Mothers ages 25 to 44 are less likely to be in the labor force than women of the same age who do not have children at home, and they tend to work fewer hours each week when employed. This can reduce the earnings of some mothers, although evidence suggests the effect is either modest overall or short-lived for many. On the other hand, fathers are more likely to be in the labor force – and to work more hours each week – than men without children at home. This is linked to an increase in the pay of fathers – a phenomenon referred to as the “ fatherhood wage premium ” – and tends to widen the gender pay gap.
Related: Gender pay gap in U.S. hasn’t changed much in two decades
Family needs can also influence the types of jobs women and men pursue , contributing to gender segregation across occupations. Differential treatment of women, including gender stereotypes and discrimination , may also play a role. And the gender wage gap varies widely by race and ethnicity.
Pew Research Center conducted this study to better understand how women’s pay compared with men’s pay in the U.S. in the economic aftermath of the COVID-19 outbreak .
The study is based on the analysis of monthly Current Population Survey (CPS) data from January 1982 to December 2022 monthly files ( IPUMS ). The CPS is the U.S. government’s official source for monthly estimates of unemployment . For a quarter of the sample each month, the CPS also records data on usual hourly earnings for hourly workers and usual weekly earnings and hours worked for other workers. In this report, monthly CPS files were combined to create annual files to boost sample sizes and to analyze the gender pay gap in greater detail.
The comparison between women’s and men’s pay is based on their median hourly earnings. For workers who are not hourly workers, hourly earnings were computed as the ratio of usual weekly earnings to usual weekly hours worked. The samples include employed workers ages 16 and older with positive earnings, working full time or part time, including those for whom earnings were imputed by the Census Bureau . Self-employed workers are excluded because their earnings are not recorded in the CPS.
The COVID-19 outbreak affected data collection efforts by the U.S. government in its surveys, especially in 2020 and 2021, limiting in-person data collection and affecting the response rate. It is possible that some measures of economic outcomes and how they vary across demographic groups are affected by these changes in data collection.
“Mothers” and “fathers” refer to women and men 16 and older who have an own child younger than 18 living in the household.
The U.S. labor force, used interchangeably with the workforce in this analysis, consists of people 16 and older who are either employed or actively looking for work.
White, Black and Asian workers include those who report being only one race and who are not Hispanic. Hispanics are of any race. Asian workers include Pacific Islanders. Other racial and ethnic groups are included in all totals but are not shown separately.
“High school graduate” refers to those who have a high school diploma or its equivalent, such as a General Education Development (GED) certificate, and those who had completed 12th grade, but their diploma status was unclear (those who had finished 12th grade but not received a diploma are excluded). “Some college” include workers with an associate degree and those who attended college but did not obtain a degree.
Younger women – those ages 25 to 34 and early in their work lives – have edged closer to wage parity with men in recent years. Starting in 2007, their earnings have consistently stood at about 90 cents to the dollar or more compared with men of the same age. But even as pay parity might appear in reach for women at the start of their careers, the wage gap tends to increase as they age.
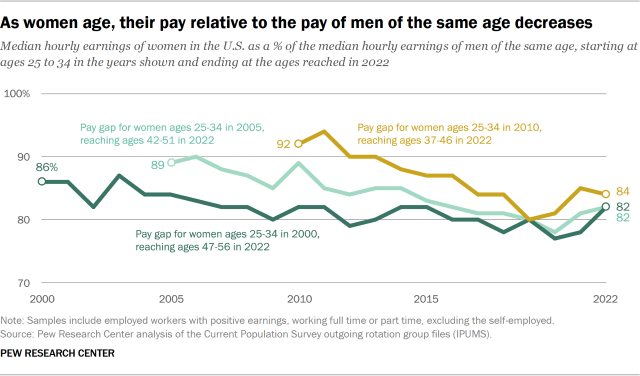
Consider, for example, women who were ages 25 to 34 in 2010. In that year, they earned 92% as much as men their age, compared with 83% for women overall. But by 2022, this group of women, now ages 37 to 46, earned only 84% as much as men of the same age. This pattern repeats itself for groups of women who were ages 25 to 34 in earlier years – say, 2005 or 2000 – and it may well be the future for women entering the workforce now.
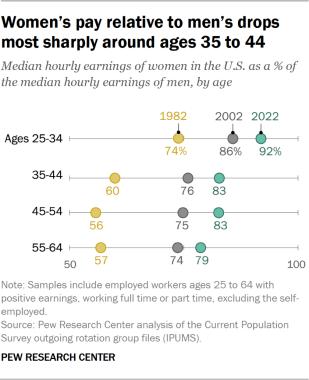
A good share of the increase in the gender pay gap takes place when women are between the ages of 35 and 44. In 2022, women ages 25 to 34 earned about 92% as much as men of the same ages, but women ages 35 to 44 and 45 to 54 earned 83% as much. The ratio dropped to 79% among those ages 55 to 64. This general pattern has not changed in at least four decades.
The increase in the pay gap coincides with the age at which women are more likely to have children under 18 at home. In 2022, 40% of employed women ages 25 to 34 had at least one child at home. The same was true for 66% of women ages 35 to 44 but for fewer – 39% – among women ages 45 to 54. Only 6% of employed women ages 55 to 64 had children at home in 2022.
Similarly, the share of employed men with children at home peaks between the ages of 35 to 44, standing at 58% in 2022. This is also when fathers tend to receive higher pay, even as the pay of employed mothers in same age group is unaffected.
Parenthood leads some women to put their careers on hold, whether by choice or necessity, but it has the opposite effect among men. In 2022, 70% of mothers ages 25 to 34 had a job or were looking for one, compared with 84% of women of the same age without children at home. This amounted to the withdrawal of 1.4 million younger mothers from the workforce. Moreover, when they are employed, younger mothers tend to put in a shorter workweek – by two hours per week, on average – than other women their age. Reduced engagement with the workplace among younger mothers is also a long-running phenomenon.
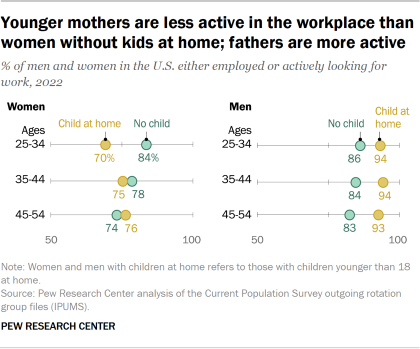
Fathers, however, are more likely to hold a job or be looking for one than men who don’t have children at home, and this is true throughout the prime of their working years , from ages 25 to 54. Among those who do have a job, fathers also work a bit more each week, on average, than men who do not have children at home.
As a result, the gender gap in workplace activity is greater among those who have children at home than among those who do not. For example, among those ages 35 to 44, 94% of fathers are active in the workforce, compared with 75% of mothers – a gap of 19 percentage points. But among those with no children at home in this age group, 84% of men and 78% of women are active in the workforce – a gap of 6 points.
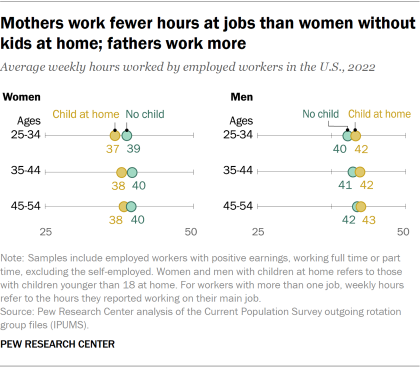
These patterns contribute to the gap in workplace activity between men and women overall. As of 2022, 68% of men ages 16 and older – with or without children at home – are either employed or seeking employment. That compares with 57% of women, a difference of 11 percentage points. This gap was as wide as 24 points in 1982, but it narrowed to 14 points by 2002. Men overall also worked about three hours more per week at a job than women in 2022, on average, down from a gap of about six hours per week in 1982.
Parenthood affects the hourly earnings of employed women and men in unexpected ways. While employed mothers overall appear to earn less than employed women without children at home, the gap is driven mainly by differences in educational attainment between the two groups. Among women with similar levels of education, there is little gap in the earnings of mothers and non-mothers. However, fathers earn more than other workers, including other men without children at home, regardless of education level. This phenomenon – known as the fatherhood wage premium – is one of the main ways that parenthood affects the gender pay gap among employed workers.
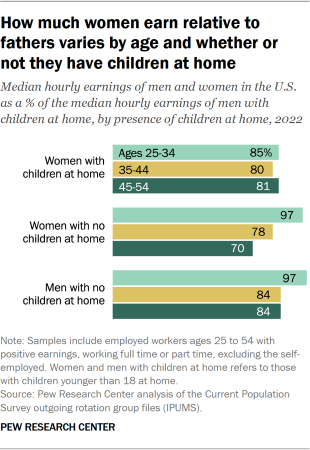
Motherhood does have important effects on the potential earnings of women. Women who experience breaks in their careers after becoming mothers sacrifice at least some of their earnings . Some mothers may never work for pay after having children, passing on earnings altogether. But it is difficult to know what the earnings of mothers might have been and, as a result, it is hard to know for certain what the full effect of motherhood is on women’s earnings. Estimates suggest that motherhood may account for much of the current shortfall in the earnings potential of women overall. 1
Among employed men and women, the impact of parenting is felt most among those ages 25 to 54, when they are most likely to have children under 18 at home. In 2022, mothers ages 25 to 34 earned 85% as much as fathers that age, but women without children at home earned 97% as much as fathers. In contrast, employed women ages 35 to 44 – with or without children – both earned about 80% as much as fathers. The table turns for women ages 45 to 54, with mothers earning more than women with no children at home. Among those ages 35 to 44 or 45 to 54, men without children earned only 84% as much as fathers.
But these patterns in the earnings of employed mothers and women with no children at home are influenced greatly by differences in education levels between the two. Among employed women ages 25 to 34, some 61% of women without children at home had a bachelor’s degree or higher level of education in 2022, compared with 37% of mothers. It follows that among women ages 25 to 34, those without children at home (a more highly educated group, on average) earned more than women with at least one child at home. Conversely, employed mothers ages 45 to 54 were more likely than other women to have at least a bachelor’s degree – 58% vs. 42%. For that reason, mothers ages 45 to 54 earned more than women without children. 2
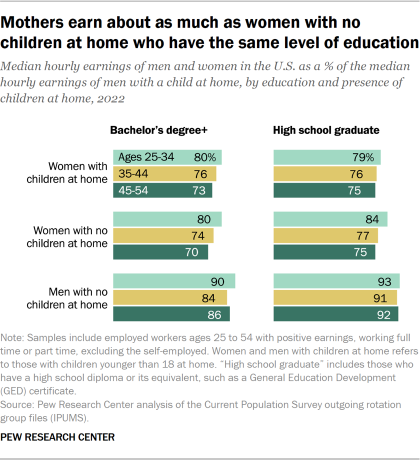
When the earnings of mothers are compared with those of women without children at home who have the same level of education, the differences either narrow or go away. Among employed women ages 25 to 34 with at least a bachelor’s degree, both mothers and women without children at home earned 80% as much as fathers in 2022. Among women ages 25 to 34 with a high school diploma and no further education, mothers earned 79% as much as fathers and women with no children at home earned 84% as much. The narrowing of the gap in earnings of mothers and women without children at home after controlling for education level also extends to other age groups.
Thus, among the employed, the effect of parenthood on the gender pay gap does not seem to be driven by a decrease in mothers’ earnings relative to women without children at home. Instead, the widening of the pay gap with parenthood appears to be driven more by an increase in the earnings of fathers. Fathers ages 25 to 54 not only earn more than mothers the same age, they also earn more than men with no children at home. Nonetheless, men without children at home still earn more than women with or without children at home.
Although there is little gap in the earnings of employed mothers and women with no children at home who have the same level of education, there is a lingering gap in workplace engagement between the two groups. Whether they had at least a bachelor’s degree or were high school graduates, mothers ages 25 to 34 are less likely to hold a job or be looking for one. Similarly, younger mothers on average work fewer hours than women without children at home each week, regardless of their education level. The opposite is true for fathers compared with men without children at home.
The share of women with at least a bachelor’s degree has increased steadily since 1982 – and faster than among men. In 1982, 20% of employed women ages 25 and older had a bachelor’s degree or higher level of education, compared with 26% of employed men. By 2022, 48% of employed women had at least a bachelor’s degree, compared with 41% of men. Still, women did not see the pay gap close to the same extent from 2002 to 2022 as they did from 1982 to 2002.
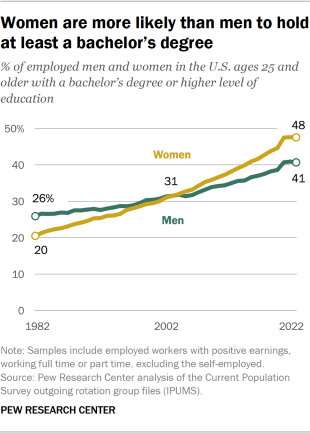
In part, this may be linked to how the gains from going to college have changed in recent decades, for women and men alike. The college wage premium – the boost in earnings workers get from a college degree – increased rapidly during the 1980s. But the rise in the premium slowed down over time and came to a halt around 2010. This likely reduced the relative growth in the earnings of women.
Although gains in education have raised the average earnings of women and have narrowed the gender pay gap overall, college-educated women are no closer to wage parity with their male counterparts than other women. In 2022, women with at least a bachelor’s degree earned 79% as much as men who were college graduates, and women who were high school graduates earned 81% as much as men with the same level of education. This underscores the challenges faced by women of all education levels in closing the pay gap.
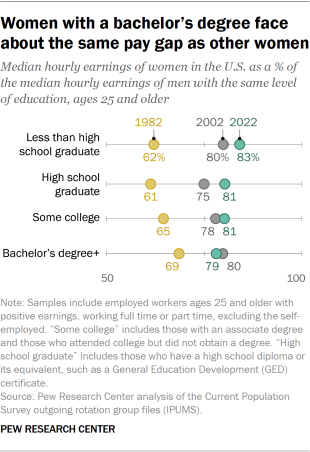
Notably, the gender wage gap has closed more among workers without a four-year college degree than among those who do have a bachelor’s degree or more education. For example, the wage gap for women without a high school diploma narrowed from 62% in 1982 to 83% in 2022 relative to men at the same education level. But it closed only from 69% to 79% among bachelor’s degree holders over the same period. This is because only men with at least a bachelor’s degree experienced positive wage growth from 1982 to 2022; all other men saw their real wages decrease. Meanwhile, the real earnings of women increased regardless of their level of education.
As women have improved their level of education in recent decades, they’ve also increased their share of employment in higher-paying occupations, such as managerial, business and finance, legal, and computer, science and engineering (STEM) occupations. In 1982, women accounted for only 26% of employment in managerial occupations. By 2022, their share had risen to 40%. Women also substantially increased their presence in social, arts and media occupations. Over the same period, the shares of women in several lower-paying fields, such as administrative support jobs and food preparation and serving occupations, fell significantly.
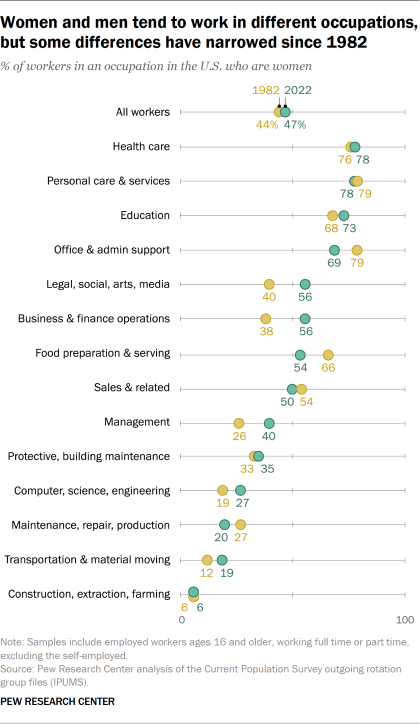
Even so, women are still underrepresented in managerial and STEM occupations – along with construction, repair and production, and transportation occupations – when compared with their share of employment overall. And there has been virtually no change in the degree to which women are over represented in education, health care, and personal care and services occupations – the last of which are lower paying than the average across all occupations. The distribution of women and men across occupations remains one of the drivers of the gender pay gap . But the degree to which this distribution is the result of personal choices or gender stereotypes is not entirely clear.
Looking across racial and ethnic groups, a wide gulf separates the earnings of Black and Hispanic women from the earnings of White men. 3 In 2022, Black women earned 70% as much as White men and Hispanic women earned only 65% as much. The ratio for White women stood at 83%, about the same as the earnings gap overall, while Asian women were closer to parity with White men, making 93% as much.
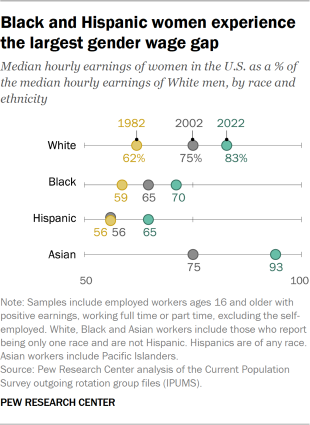
The pay gap narrowed for all groups of women from 1982 to 2022, but more so for White women than for Black and Hispanic women. The earnings gap for Asian women narrowed by about 17 percentage points from 2002 to 2022, but data for this group is not available for 1982.
To some extent, the gender wage gap varies by race and ethnicity because of differences in education, experience, occupation and other factors that drive the gender wage gap for women overall. But researchers have uncovered new evidence of hiring discrimination against various racial and ethnic groups, along with discrimination against other groups, such as LGBTQ and disabled workers. Discrimination in hiring may feed into differences in earnings by shutting out workers from opportunities.
Changes in the gender pay gap are also shaped by economic factors that sometimes drive men’s and women’s earnings in distinctive ways. Because men and women tend to work in different types of jobs and industries, their earnings may respond differently to external pressures.
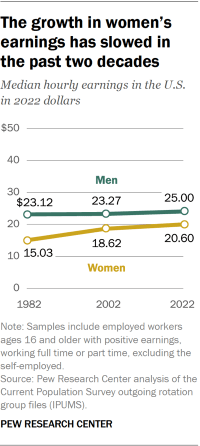
More specifically, men’s earnings essentially didn’t change from 1982 to 2002. Potential reasons for that include a more rapid decline in union membership among men, a shift away from jobs calling for more physical skills, and global competition that sharply reduced employment in manufacturing in the 1980s. At the same time, women’s earnings increased substantially as they raised their level of education and shifted toward higher-paying occupations.
But in some ways, the economic climate has proved less favorable for women this century. For reasons that are not entirely clear, women’s employment was slower to recover from the Great Recession of 2007-2009. More recently, the COVID-19 recession took on the moniker “ she-cession ” because of the pressure on jobs disproportionately held by women . Amid a broader slowdown in earnings growth from 2000 to 2015, the increase in women’s earnings from 2002 to 2022 was not much greater than the increase in men’s earnings, limiting the closure in the gender pay gap over the period.
Higher education, a shift to higher-paying occupations and more labor market experience have helped women narrow the gender pay gap since 1982. But even as women have continued to outpace men in educational attainment, the pay gap has been stuck in a holding pattern since 2002, ranging from 80 to 85 cents to the dollar.
More sustained progress in closing the pay gap may depend on deeper changes in societal and cultural norms and in workplace flexibility that affect how men and women balance their careers and family lives . Even in countries that have taken the lead in implementing family-friendly policies, such as Denmark, parenthood continues to drive a significant wedge in the earnings of men and women. New research suggests that family-friendly policies in the U.S. may be keeping the pay gap from closing. Gender stereotypes and discrimination, though difficult to quantify, also appear to be among the “last-mile” hurdles impeding further progress.
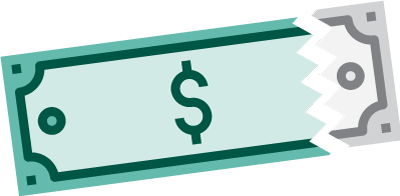
What is the gender wage gap in your metropolitan area? Find out with our pay gap calculator
1615 L St. NW, Suite 800 Washington, DC 20036 USA (+1) 202-419-4300 | Main (+1) 202-857-8562 | Fax (+1) 202-419-4372 | Media Inquiries
Research Topics
- Email Newsletters
ABOUT PEW RESEARCH CENTER Pew Research Center is a nonpartisan fact tank that informs the public about the issues, attitudes and trends shaping the world. It conducts public opinion polling, demographic research, media content analysis and other empirical social science research. Pew Research Center does not take policy positions. It is a subsidiary of The Pew Charitable Trusts .
© 2024 Pew Research Center
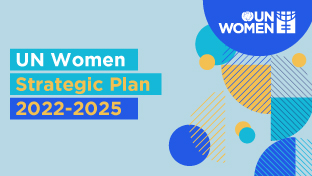
Everything you need to know about pushing for pay equity
- Share to Facebook
- Share to Twitter
- Share to LinkedIn
- Share to E-mail
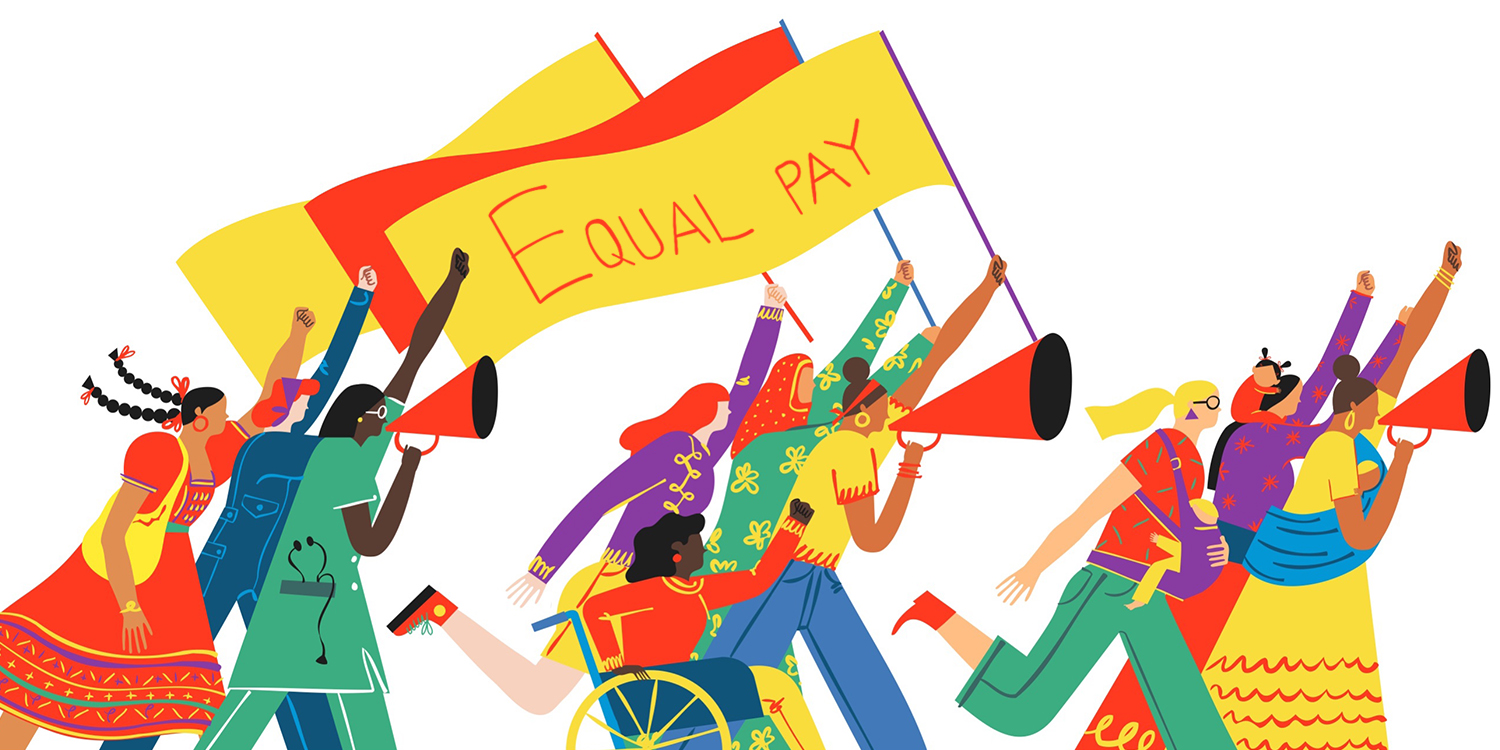
Workers worldwide look forward to payday. But while a paycheck may bring a sense of relief, satisfaction, or joy, it can also represent an injustice—a stark reminder of persistent inequalities between men and women in the workplace.
The gender pay gap stands at 20 per cent , meaning women workers earn 80 per cent of what men do. For women of colour, migrant women, those with disabilities, and women with children, the gap is even greater.
The cumulative effect of pay disparities has real, daily negative consequences for women, their families, and society, especially during crises. The widespread effects of COVID-19 have plunged up to 95 million people into extreme poverty, with one in every 10 women globally living in extreme poverty . If current trends continue, 342.4 million women and girls will be living on less than $2.15 a day by 2030.
What do we mean by equal pay for work of equal value?
Equal pay for work of equal value, as defined by the ILO Equal Remuneration Convention , means that all workers are entitled to receive equal remuneration not only for identical tasks but also for different work considered of equal value. This distinction is crucial because jobs held by women and men may involve varying qualifications, skills, responsibilities, or working conditions, yet hold equal value and warrant equal pay.
In 2020, New Zealand passed the Equal Pay Amendment Bill , ensuring that women and men are paid equally for work that’s different but has equal value, including in chronically underpaid female-dominated industries.
It is also important to recognize that remuneration is more than a basic wage; it encompasses all the elements of earnings. This includes overtime pay, bonuses, travel allowances, company shares, insurance, and other benefits.
Why does the gender pay gap persist?
The gender pay gap originates from ingrained inequalities. Women, particularly migrant women, are overrepresented in the informal sector. Look around you, from street vending to domestic service, from coffee shop attendants to subsistence farming. Women fill informal jobs that often fall outside the domains of labour laws, trapping them in low-paying, unsafe working environments, without social benefits. These poor conditions for women workers perpetuate the gender pay gap.
Women also do three more hours of daily care work than men , globally. This includes household tasks such as cooking, cleaning, fetching firewood and water, and taking care of children and the elderly. Although care work is the backbone of thriving families, communities, and economies, it remains undervalued and underrecognized. Try calculating your daily load with UN Women’s unpaid care calculator .
The motherhood penalty exacerbates pay inequity, with working mothers facing lower wages, a disparity that jumps as the number of children a woman has increases. Lower wages for mothers are linked to reduced working time, employment in more family-friendly jobs that tend to be lower paying, hiring and promotion decisions that penalize the careers of mothers, and a lack of programmes to support women’s return to work after time out of the labour market.
Restrictive, traditional gender roles are also spurring pay inequalities. Gender stereotypes steer women away from occupations traditionally dominated by men and push them toward care-focused work that is often regarded as “unskilled,” or “soft-skilled” and therefore, lower paid.
Furthermore, discriminatory hiring practices and promotion decisions that prevent women from gaining leadership roles and highly paid positions sustain the gender pay gap.
Why is pay equity an urgent issue?
Pay equity matters because it is a glaring injustice and subjects millions of women and families to lives of entrenched poverty and opportunity gaps. At the current rate, we risk leaving more than 340 million women and girls in abject poverty by 2030 , and an alarming 4 per cent could grapple with extreme food insecurity by that year.
Women also experience significantly lower social protection coverage than men, a discrepancy that largely reflects and reproduces their lower labour force participation rates, higher levels of temporary and precarious work, and informal employment. All these factors contribute to lower income , savings, and pensions of women and gendered poverty in old age.
What should be done?
As more women are plunged into poverty, the fight for equal pay and pay equity takes on a new sense of urgency because those who earn the least are most damaged by income discrepancy.
In the United States, Black women earn only 63.7 cents , Native women 59 cents , and Latinas 57 cents for every dollar that white men earn. Where money is tight, lower pay can prevent women and families from putting food on the table, securing safe housing, and accessing critical medical care and education—impacts that can perpetuate cycles of poverty across generations.
It is urgent that we put female workers on equal footing as male workers. In a world on the brink of a looming care deficit, women make up 67 per cent of workers providing essential health and social care services globally . Governments must address underpaid and undervalued jobs in the care sector, including in education, health care and social services, all jobs that women predominantly occupy.
What does the data say about pay equity around the world?
Unequal pay is a stubborn and universal problem. Despite significant progress in women’s education and labour market participation, progress in closing the gender pay gap has been too slow. At this pace, it will take almost 300 years to achieve economic gender parity .
Women workers’ average pay is generally lower than men’s in all countries and for all levels of education, and age groups, with women earning on average 80 per cent what men ear n. Women in male-dominated industries may earn more than those in female-dominated industries, but the gender pay gap persists across all sectors.
While gender pay gap estimates can vary substantially across regions and even within countries, higher income countries tend to have lower levels of wage inequality compared to low and middle-income countries. However, estimates of the gender pay gap understate the real extent of the issue, particularly in developing countries, because of a lack of information about informal economies, which are disproportionately made up of women workers, so the full picture is likely worse than what the available data shows us.
Explore UN Women’s report on the gender pay gap in Eastern and Southern Africa .
Closing the gender pay gap requires a set of measures that push for decent work for all people. This includes measures that promote the formalization of the informal economy, bringing informal workers under the umbrella of legal and effective protection and empowering them to better defend their interests.
Ensuring workers’ right to organize and bargain collectively is an important part of the solution. Women must be involved in employer and union leadership, enabling legislation that establishes comprehensive frameworks for gender equality in the workplace.
Economic empowerment Chief at UN Women Dr. Jemimah Njuki says that, “The gender pay gap requires all stakeholders, including employers, governments, trade unions take full responsibility and work side by side to address these challenges. Women deserve equal pay for work of equal value”.
[Last updated February 2024]
- Gender-responsive procurement
- Gender wage gap
Related content
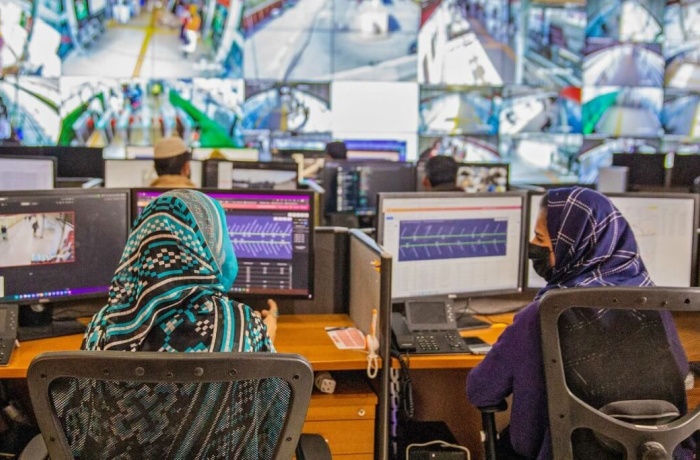
Op-ed: Improving women’s access to decent jobs
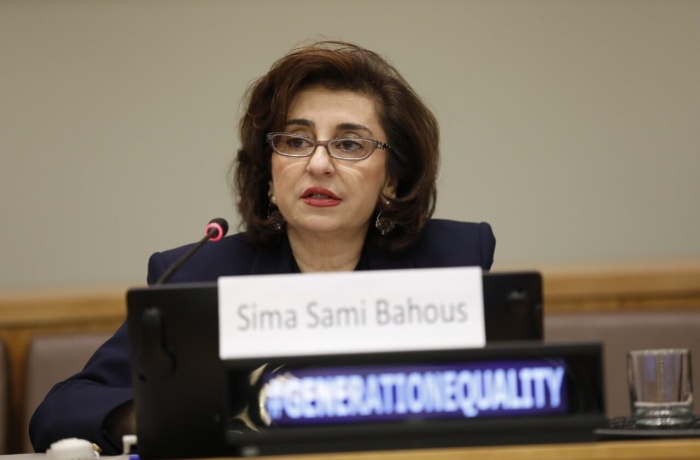
Speech: Ambitiously proactive – Generation Equality brings hope to stalled progress and financing
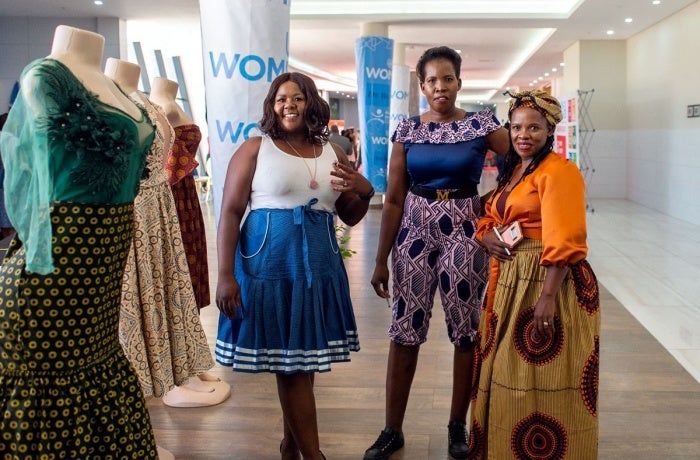
EntreprenHER programme empowers women entrepreneurs in Botswana, Namibia, and South Africa
Click through the PLOS taxonomy to find articles in your field.
For more information about PLOS Subject Areas, click here .
Loading metrics
Open Access
Peer-reviewed
Research Article
The persistence of pay inequality: The gender pay gap in an anonymous online labor market
Roles Conceptualization, Data curation, Formal analysis, Investigation, Methodology, Project administration, Resources, Supervision, Writing – original draft, Writing – review & editing
* E-mail: [email protected] (LL); [email protected] (LB)
Affiliation Department of Psychology, Lander College, Flushing, New York, United States of America
Roles Conceptualization, Data curation, Formal analysis, Investigation, Methodology, Project administration, Writing – original draft, Writing – review & editing
Affiliation Department of Computer Science, Lander College, Flushing, New York, United States of America
Roles Formal analysis, Writing – original draft, Writing – review & editing
Affiliation Department of Health Policy & Management, Mailman School of Public Health, Columbia University, New York, New York, United States of America
Roles Conceptualization, Writing – review & editing
Affiliation Department of Clinical Psychology, Columbia University, New York, New York, United States of America

Roles Formal analysis
Affiliation Department of Computer Science, Stern College for Women, New York, New York, United States of America
Roles Conceptualization, Methodology, Writing – original draft, Writing – review & editing
Affiliation Department of Epidemiology, Mailman School of Public Health, Columbia University New York, New York, United States of America
- Leib Litman,
- Jonathan Robinson,
- Zohn Rosen,
- Cheskie Rosenzweig,
- Joshua Waxman,
- Lisa M. Bates
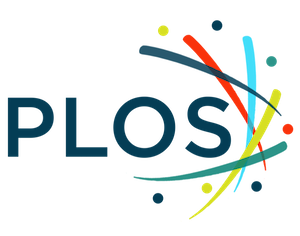
- Published: February 21, 2020
- https://doi.org/10.1371/journal.pone.0229383
- Reader Comments
Studies of the gender pay gap are seldom able to simultaneously account for the range of alternative putative mechanisms underlying it. Using CloudResearch, an online microtask platform connecting employers to workers who perform research-related tasks, we examine whether gender pay discrepancies are still evident in a labor market characterized by anonymity, relatively homogeneous work, and flexibility. For 22,271 Mechanical Turk workers who participated in nearly 5 million tasks, we analyze hourly earnings by gender, controlling for key covariates which have been shown previously to lead to differential pay for men and women. On average, women’s hourly earnings were 10.5% lower than men’s. Several factors contributed to the gender pay gap, including the tendency for women to select tasks that have a lower advertised hourly pay. This study provides evidence that gender pay gaps can arise despite the absence of overt discrimination, labor segregation, and inflexible work arrangements, even after experience, education, and other human capital factors are controlled for. Findings highlight the need to examine other possible causes of the gender pay gap. Potential strategies for reducing the pay gap on online labor markets are also discussed.
Citation: Litman L, Robinson J, Rosen Z, Rosenzweig C, Waxman J, Bates LM (2020) The persistence of pay inequality: The gender pay gap in an anonymous online labor market. PLoS ONE 15(2): e0229383. https://doi.org/10.1371/journal.pone.0229383
Editor: Luís A. Nunes Amaral, Northwestern University, UNITED STATES
Received: March 5, 2019; Accepted: February 5, 2020; Published: February 21, 2020
Copyright: © 2020 Litman et al. This is an open access article distributed under the terms of the Creative Commons Attribution License , which permits unrestricted use, distribution, and reproduction in any medium, provided the original author and source are credited.
Data Availability: Due to the sensitive nature of some of the data, and the terms of service of the websites used during data collection (including CloudResearch and MTurk), CloudResearch cannot release the full data set to make it publically available. The data are on CloudResearch's Sequel servers located at Queens College in the city of New York. CloudResearch makes data available to be accessed by researchers for replication purposes, on the CloudResearch premises, in the same way the data were accessed and analysed by the authors of this manuscript. The contact person at CloudResearch who can help researchers access the data set is Tzvi Abberbock, who can be reached at [email protected] .
Funding: The authors received no specific funding for this work.
Competing interests: We have read the journal's policy and the authors of this manuscript have the following potential competing interest: Several of the authors are employed at Cloud Research (previously TurkPrime), the database from which the data were queried. This does not alter our adherence to PLOS ONE policies on sharing data and materials.
Introduction
The gender pay gap, the disparity in earnings between male and female workers, has been the focus of empirical research in the US for decades, as well as legislative and executive action under the Obama administration [ 1 , 2 ]. Trends dating back to the 1960s show a long period in which women’s earnings were approximately 60% of their male counterparts, followed by increases in women’s earnings starting in the 1980s, which began to narrow, but not close, the gap which persists today [ 3 ]. More recent data from 2014 show that overall, the median weekly earnings of women working full time were 79–83% of what men earned [ 4 – 9 ].
The extensive literature seeking to explain the gender pay gap and its trajectory over time in traditional labor markets suggests it is a function of multiple structural and individual-level processes that reflect both the near-term and cumulative effects of gender relations and roles over the life course. Broadly speaking, the drivers of the gender pay gap can be categorized as: 1) human capital or productivity factors such as education, skills, and workforce experience; 2) industry or occupational segregation, which some estimates suggest accounts for approximately half of the pay gap; 3) gender-specific temporal flexibility constraints which can affect promotions and remuneration; and finally, 4) gender discrimination operating in hiring, promotion, task assignment, and/or compensation. The latter mechanism is often estimated by inference as a function of unexplained residual effects of gender on payment after accounting for other factors, an approach which is most persuasive in studies of narrowly restricted populations of workers such as lawyers [ 10 ] and academics of specific disciplines [ 11 ]. A recent estimate suggests this unexplained gender difference in earnings can account for approximately 40% of the pay gap [ 3 ]. However, more direct estimations of discriminatory processes are also available from experimental evidence, including field audit and lab-based studies [ 12 – 14 ]. Finally, gender pay gaps have also been attributed to differential discrimination encountered by men and women on the basis of parental status, often known as the ‘motherhood penalty’ [ 15 ].
Non-traditional ‘gig economy’ labor markets and the gender pay gap
In recent years there has been a dramatic rise in nontraditional ‘gig economy’ labor markets, which entail independent workers hired for single projects or tasks often on a short-term basis with minimal contractual engagement. “Microtask” platforms such as Amazon Mechanical Turk (MTurk) and Crowdflower have become a major sector of the gig economy, offering a source of easily accessible supplementary income through performance of small tasks online at a time and place convenient to the worker. Available tasks can range from categorizing receipts to transcription and proofreading services, and are posted online by the prospective employer. Workers registered with the platform then elect to perform the advertised tasks and receive compensation upon completion of satisfactory work [ 16 ]. An estimated 0.4% of US adults are currently receiving income from such platforms each month [ 17 ], and microtask work is a growing sector of the service economy in the United States [ 18 ]. Although still relatively small, these emerging labor market environments provide a unique opportunity to investigate the gender pay gap in ways not possible within traditional labor markets, due to features (described below) that allow researchers to simultaneously account for multiple putative mechanisms thought to underlie the pay gap.
The present study utilizes the Amazon Mechanical Turk (MTurk) platform as a case study to examine whether a gender pay gap remains evident when the main causes of the pay gap identified in the literature do not apply or can be accounted for in a single investigation. MTurk is an online microtask platform that connects employers (‘requesters’) to employees (‘workers’) who perform jobs called “Human Intelligence Tasks” (HITs). The platform allows requesters to post tasks on a dashboard with a short description of the HIT, the compensation being offered, and the time the HIT is expected to take. When complete, the requester either approves or rejects the work based on quality. If approved, payment is quickly accessible to workers. The gender of workers who complete these HITs is not known to the requesters, but was accessible to researchers for the present study (along with other sociodemographic information and pay rates) based on metadata collected through CloudResearch (formerly TurkPrime), a platform commonly used to conduct social and behavioral research on MTurk [ 19 ].
Evaluating pay rates of workers on MTurk requires estimating the pay per hour of each task that a worker accepts which can then be averaged together. All HITs posted on MTurk through CloudResearch display how much a HIT pays and an estimated time that it takes for that HIT to be completed. Workers use this information to determine what the corresponding hourly pay rate of a task is likely to be, and much of our analysis of the gender pay gap is based on this advertised pay rate of all completed surveys. We also calculate an estimate of the gender pay gap based on actual completion times to examine potential differences in task completion speed, which we refer to as estimated actual wages (see Methods section for details).
Previous studies have found that both task completion time and the selection of tasks influences the gender pay gap in at least some gig economy markets. For example, a gender pay gap was observed among Uber drivers, with men consistently earning higher pay than women [ 20 ]. Some of the contributing factors to this pay gap include that male Uber drivers selected different tasks than female drivers, including being more willing to work at night and to work in neighborhoods that were perceived to be more dangerous. Male drivers were also likely to drive faster than their female counterparts. These findings show that person-level factors like task selection, and speed can influence the gender pay gap within gig economy markets.
MTurk is uniquely suited to examine the gender pay gap because it is possible to account simultaneously for multiple structural and individual-level factors that have been shown to produce pay gaps. These include discrimination, work heterogeneity (leading to occupational segregation), and job flexibility, as well as human capital factors such as experience and education.
Discrimination.
When employers post their HITs on MTurk they have no way of knowing the demographic characteristics of the workers who accept those tasks, including their gender. While MTurk allows for selective recruitment of specific demographic groups, the MTurk tasks examined in this study are exclusively open to all workers, independent of their gender or other demographic characteristics. Therefore, features of the worker’s identity that might be the basis for discrimination cannot factor into an employer’s decision-making regarding hiring or pay.
Task heterogeneity.
Another factor making MTurk uniquely suited for the examination of the gender pay gap is the relative homogeneity of tasks performed by the workers, minimizing the potential influence of gender differences in the type of work pursued on earnings and the pay gap. Work on the MTurk platform consists mostly of short tasks such as 10–15 minute surveys and categorization tasks. In addition, the only information that workers have available to them to choose tasks, other than pay, is the tasks’ titles and descriptions. We additionally classified tasks based on similarity and accounted for possible task heterogeneity effects in our analyses.
Job flexibility.
MTurk is not characterized by the same inflexibilities as are often encountered in traditional labor markets. Workers can work at any time of the day or day of the week. This increased flexibility may be expected to provide more opportunities for participation in this labor market for those who are otherwise constrained by family or other obligations.
Human capital factors.
It is possible that the more experienced workers could learn over time how to identify higher paying tasks by virtue of, for example, identifying qualities of tasks that can be completed more quickly than the advertised required time estimate. Further, if experience is correlated with gender, it could contribute to a gender pay gap and thus needs to be controlled for. Using CloudResearch metadata, we are able to account for experience on the platform. Additionally, we account for multiple sociodemographic variables, including age, marital status, parental status, education, income (from all sources), and race using the sociodemographic data available through CloudResearch.
Expected gender pay gap findings on MTurk
Due to the aforementioned factors that are unique to the MTurk marketplace–e.g., anonymity, self-selection into tasks, relative homogeneity of the tasks performed, and flexible work scheduling–we did not expect a gender pay gap to be evident on the platform to the same extent as in traditional labor markets. However, potential gender differences in task selection and completion speed, which have implications for earnings, merit further consideration. For example, though we expect the relative homogeneity of the MTurk tasks to minimize gender differences in task selection that could mimic occupational segregation, we do account for potential subtle residual differences in tasks that could differentially attract male and female workers and indirectly lead to pay differentials if those tasks that are preferentially selected by men pay a higher rate. To do this we categorize all tasks based on their descriptions using K-clustering and add the clusters as covariates to our models. In addition, we separately examine the gender pay gap within each topic-cluster.
In addition, if workers who are experienced on the platform are better able to find higher paying HITs, and if experience is correlated with gender, it may lead to gender differences in earnings. Theoretically, other factors that may vary with gender could also influence task selection. Previous studies of the pay gap in traditional markets indicate that reservation wages, defined as the pay threshold at which a person is willing to accept work, may be lower among women with children compared to women without, and to that of men as well [ 21 ]. Thus, if women on MTurk are more likely to have young children than men, they may be more willing to accept available work even if it pays relatively poorly. Other factors such as income, education level, and age may similarly influence reservation wages if they are associated with opportunities to find work outside of microtask platforms. To the extent that these demographics correlate with gender they may give rise to a gender pay gap. Therefore we consider age, experience on MTurk, education, income, marital status, and parental status as covariates in our models.
Task completion speed may vary by gender for several reasons, including potential gender differences in past experience on the platform. We examine the estimated actual pay gap per hour based on HIT payment and estimated actual completion time to examine the effects of completion speed on the wage gap. We also examine the gender pay gap based on advertised pay rates, which are not dependent on completion speed and more directly measure how gender differences in task selection can lead to a pay gap. Below, we explain how these were calculated based on meta-data from CloudResearch.
To summarize, the overall goal of the present study was to explore whether gender pay differentials arise within a unique, non-traditional and anonymous online labor market, where known drivers of the gender pay gap either do not apply or can be accounted for statistically.
Materials and methods
Amazon mechanical turk and cloudresearch..
Started in 2005, the original purpose of the Amazon Mechanical Turk (MTurk) platform was to allow requesters to crowdsource tasks that could not easily be handled by existing technological solutions such as receipt copying, image categorization, and website testing. As of 2010, researchers increasingly began using MTurk for a wide variety of research tasks in the social, behavioral, and medical sciences, and it is currently used by thousands of academic researchers across hundreds of academic departments [ 22 ]. These research-related HITs are typically listed on the platform in generic terms such as, “Ten-minute social science study,” or “A study about public opinion attitudes.”
Because MTurk was not originally designed solely for research purposes, its interface is not optimized for some scientific applications. For this reason, third party add-on toolkits have been created that offer critical research tools for scientific use. One such platform, CloudResearch (formerly TurkPrime), allows requesters to manage multiple research functions, such as applying sampling criteria and facilitating longitudinal studies, through a link to their MTurk account. CloudResearch’s functionality has been described extensively elsewhere [ 19 ]. While the demographic characteristics of workers are not available to MTurk requesters, we were able to retroactively identify the gender and other demographic characteristics of workers through the CloudResearch platform. CloudResearch also facilitates access to data for each HIT, including pay, estimated length, and title.
The study was an analysis of previously collected metadata, which were analyzed anonymously. We complied with the terms of service for all data collected from CloudResearch, and MTurk. The approving institutional review board for this study was IntegReview.
Analytic sample.
We analyzed the nearly 5 million tasks completed during an 18-month period between January 2016 and June 2017 by 12,312 female and 9,959 male workers who had complete data on key demographic characteristics. To be included in the analysis a HIT had to be fully completed, not just accepted, by the worker, and had to be accepted (paid for) by the requester. Although the vast majority of HITs were open to both males and females, a small percentage of HITs are intended for a specific gender. Because our goal was to exclusively analyze HITs for which the requesters did not know the gender of workers, we excluded any HITs using gender-specific inclusion or exclusion criteria from the analyses. In addition, we removed from the analysis any HITs that were part of follow-up studies in which it would be possible for the requester to know the gender of the worker from the prior data collection. Finally, where possible, CloudResearch tracks demographic information on workers across multiple HITs over time. To minimize misclassification of gender, we excluded the 0.3% of assignments for which gender was unknown with at least 95% consistency across HITs.
The main exposure variable is worker gender and the outcome variables are estimated actual hourly pay accrued through completing HITs, and advertised hourly pay for completed HITs. Estimated actual hourly wages are based on the estimated length in minutes and compensation in dollars per HIT as posted on the dashboard by the requester. We refer to actual pay as estimated because sometimes people work multiple assignments at the same time (which is allowed on the platform), or may simultaneously perform other unrelated activities and therefore not work on the HIT the entire time the task is open. We also considered several covariates to approximate human capital factors that could potentially influence earnings on this platform, including marital status, education, household income, number of children, race/ethnicity, age, and experience (number of HITs previously completed). Additional covariates included task length, task cluster (see below), and the serial order with which workers accepted the HIT in order to account for potential differences in HIT acceptance speed that may relate to the pay gap.
Database and analytic approach.
Data were exported from CloudResearch’s database into Stata in long-form format to represent each task on a single row. For the purposes of this paper, we use “HIT” and “study” interchangeably to refer to a study put up on the MTurk dashboard which aims to collect data from multiple participants. A HIT or study consist of multiple “assignments” which is a single task completed by a single participant. Columns represented variables such as demographic information, payment, and estimated HIT length. Column variables also included unique IDs for workers, HITs (a single study posted by a requester), and requesters, allowing for a multi-level modeling analytic approach with assignments nested within workers. Individual assignments (a single task completed by a single worker) were the unit of analysis for all models.
Linear regression models were used to calculate the gender pay gap using two dependent variables 1) women’s estimated actual earnings relative to men’s and 2) women’s selection of tasks based on advertised earnings relative to men’s. We first examined the actual pay model, to see the gender pay gap when including an estimate of task completion speed, and then adjusted this model for advertised hourly pay to determine if and to what extent a propensity for men to select more remunerative tasks was evident and driving any observed gender pay gap. We additionally ran separate models using women’s advertised earnings relative to men’s as the dependent variable to examine task selection effects more directly. The fully adjusted models controlled for the human capital-related covariates, excluding household income and education which were balanced across genders. These models also tested for interactions between gender and each of the covariates by adding individual interaction terms to the adjusted model. To control for within-worker clustering, Huber-White standard error corrections were used in all models.
Cluster analysis.
To explore the potential influence of any residual task heterogeneity and gender preference for specific task type as the cause of the gender pay gap, we use K-means clustering analysis (seed = 0) to categorize the types of tasks into clusters based on the descriptions that workers use to choose the tasks they perform. We excluded from this clustering any tasks which contained certain gendered words (such as “male”, “female”, etc.) and any tasks which had fewer than 30 respondents. We stripped out all punctuation, symbols and digits from the titles, so as to remove any reference to estimated compensation or duration. The features we clustered on were the presence or absence of 5,140 distinct words that appeared across all titles. We then present the distribution of tasks across these clusters as well as average pay by gender and the gender pay gap within each cluster.
The demographics of the analytic sample are presented in Table 1 . Men and women completed comparable numbers of tasks during the study period; 2,396,978 (48.6%) for men and 2,539,229 (51.4%) for women.
- PPT PowerPoint slide
- PNG larger image
- TIFF original image
https://doi.org/10.1371/journal.pone.0229383.t001
In Table 2 we measure the differences in remuneration between genders, and then decompose any observed pay gap into task completion speed, task selection, and then demographic and structural factors. Model 1 shows the unadjusted regression model of gender differences in estimated actual pay, and indicates that, on average, tasks completed by women paid 60 (10.5%) cents less per hour compared to tasks completed by men (t = 17.4, p < .0001), with the mean estimated actual pay across genders being $5.70 per hour.
https://doi.org/10.1371/journal.pone.0229383.t002
In Model 2, adjusting for advertised hourly pay, the gender pay gap dropped to 46 cents indicating that 14 cents of the pay gap is attributable to gender differences in the selection of tasks (t = 8.6, p < .0001). Finally, after the inclusion of covariates and their interactions in Model 3, the gender pay differential was further attenuated to 32 cents (t = 6.7, p < .0001). The remaining 32 cent difference (56.6%) in earnings is inferred to be attributable to gender differences in HIT completion speed.
Task selection analyses
Although completion speed appears to account for a significant portion of the pay gap, of particular interest are gender differences in task selection. Beyond structural factors such as education, household composition and completion speed, task selection accounts for a meaningful portion of the gender pay gap. As a reminder, the pay rate and expected completion time are posted for every HIT, so why women would select less remunerative tasks on average than men do is an important question to explore. In the next section of the paper we perform a set of analyses to examine factors that could account for this observed gender difference in task selection.
Advertised hourly pay.
To examine gender differences in task selection, we used linear regression to directly examine whether the advertised hourly pay differed for tasks accepted by male and female workers. We first ran a simple model ( Table 3 ; Model 3A) on the full dataset of 4.93 million HITs, with gender as the predictor and advertised hourly pay as the outcome including no other covariates. The unadjusted regression results (Model 4) shown in Table 3 , indicates that, summed across all clusters and demographic groups, tasks completed by women were advertised as paying 28 cents (95% CI: $0.25-$0.31) less per hour (5.8%) compared to tasks completed by men (t = 21.8, p < .0001).
https://doi.org/10.1371/journal.pone.0229383.t003
Model 5 examines whether the remuneration differences for tasks selected by men and women remains significant in the presence of multiple covariates included in the previous model and their interactions. The advertised pay differential for tasks selected by women compared to men was attenuated to 21 cents (4.3%), and remained statistically significant (t = 9.9, p < .0001). This estimate closely corresponded to the inferred influence of task selection reported in Table 2 . Tests of gender by covariate interactions were significant only in the cases of age and marital status; the pay differential in tasks selected by men and women decreased with age and was more pronounced among single versus currently or previously married women.
To further examine what factors may account for the observed gender differences in task selection we plotted the observed pay gap within demographic and other covariate groups. Table 4 shows the distribution of tasks completed by men and women, as well as mean earnings and the pay gap across all demographic groups, based on the advertised (not actual) hourly pay for HITs selected (hereafter referred to as “advertised hourly pay” and the “advertised pay gap”). The average task was advertised to pay $4.88 per hour (95% CI $4.69, $5.10).
https://doi.org/10.1371/journal.pone.0229383.t004
The pattern across demographic characteristics shows that the advertised hourly pay gap between genders is pervasive. Notably, a significant advertised gender pay gap is evident in every level of each covariate considered in Table 4 , but more pronounced among some subgroups of workers. For example, the advertised pay gap was highest among the youngest workers ($0.31 per hour for workers age 18–29), and decreased linearly with age, declining to $0.13 per hour among workers age 60+. Advertised houry gender pay gaps were evident across all levels of education and income considered.
To further examine the potential influence of human capital factors on the advertised hourly pay gap, Table 5 presents the average advertised pay for selected tasks by level of experience on the CloudResearch platform. Workers were grouped into 4 experience levels, based on the number of prior HITs completed: Those who completed fewer than 100 HITs, between 100 and 500 HITs, between 500 and 1,000 HITs, and more than 1,000 HITs. A significant gender difference in advertised hourly pay was observed within each of these four experience groups. The advertised hourly pay for tasks selected by both male and female workers increased with experience, while the gender pay gap decreases. There was some evidence that male workers have more cumulative experience with the platform: 43% of male workers had the highest level of experience (previously completing 1,001–10,000 HITs) compared to only 33% of women.
https://doi.org/10.1371/journal.pone.0229383.t005
Table 5 also explores the influence of task heterogeneity upon HIT selection and the gender gap in advertised hourly pay. K-means clustering was used to group HITs into 20 clusters initially based on the presence or absence of 5,140 distinct words appearing in HIT titles. Clusters with fewer than 50,000 completed tasks were then excluded from analysis. This resulted in 13 clusters which accounted for 94.3% of submitted work assignments (HITs).
The themes of all clusters as well as the average hourly advertised pay for men and women within each cluster are presented in the second panel of Table 5 . The clusters included categories such as Games, Decision making, Product evaluation, Psychology studies, and Short Surveys. We did not observe a gender preference for any of the clusters. Specifically, for every cluster, the proportion of males was no smaller than 46.6% (consistent with the slightly lower proportion of males on the platform, see Table 1 ) and no larger than 50.2%. As shown in Table 5 , the gender pay gap was observed within each of the clusters. These results suggest that residual task heterogeneity, a proxy for occupational segregation, is not likely to contribute to a gender pay gap in this market.
Task length was defined as the advertised estimated duration of a HIT. Table 6 presents the advertised hourly gender pay gaps for five categories of HIT length, which ranged from a few minutes to over 1 hour. Again, a significant advertised hourly gender pay gap was observed in each category.
https://doi.org/10.1371/journal.pone.0229383.t006
Finally, we conducted additional supplementary analyses to determine if other plausible factors such as HIT timing could account for the gender pay gap. We explored temporal factors including hour of the day and day of the week. Each completed task was grouped based on the hour and day in which it was completed. A significant advertised gender pay gap was observed within each of the 24 hours of the day and for every day of the week demonstrating that HIT timing could not account for the observed gender gap (results available in Supplementary Materials).
In this study we examined the gender pay gap on an anonymous online platform across an 18-month period, during which close to five million tasks were completed by over 20,000 unique workers. Due to factors that are unique to the Mechanical Turk online marketplace–such as anonymity, self-selection into tasks, relative homogeneity of the tasks performed, and flexible work scheduling–we did not expect earnings to differ by gender on this platform. However, contrary to our expectations, a robust and persistent gender pay gap was observed.
The average estimated actual pay on MTurk over the course of the examined time period was $5.70 per hour, with the gender pay differential being 10.5%. Importantly, gig economy platforms differ from more traditional labor markets in that hourly pay largely depends on the speed with which tasks are completed. For this reason, an analysis of gender differences in actual earned pay will be affected by gender differences in task completion speed. Unfortunately, we were not able to directly measure the speed with which workers complete tasks and account for this factor in our analysis. This is because workers have the ability to accept multiple HITs at the same time and multiple HITs can sit dormant in a queue, waiting for workers to begin to work on them. Therefore, the actual time that many workers spend working on tasks is likely less than what is indicated in the metadata available. For this reason, the estimated average actual hourly rate of $5.70 is likely an underestimate and the gender gap in actual pay cannot be precisely measured. We infer however, by the residual gender pay gap after accounting for other factors, that as much as 57% (or $.32) of the pay differential may be attributable to task completion speed. There are multiple plausible explanations for gender differences in task completion speed. For example, women may be more meticulous at performing tasks and, thus, may take longer at completing them. There may also be a skill factor related to men’s greater experience on the platform (see Table 5 ), such that men may be faster on average at completing tasks than women.
However, our findings also revealed another component of a gender pay gap on this platform–gender differences in the selection of tasks based on their advertised pay. Because the speed with which workers complete tasks does not impact these estimates, we conducted extensive analyses to try to explain this gender gap and the reasons why women appear on average to be selecting tasks that pay less compared to men. These results pertaining to the advertised gender pay gap constitute the main focus of this study and the discussion that follows.
The overall advertised hourly pay was $4.88. The gender pay gap in the advertised hourly pay was $0.28, or 5.8% of the advertised pay. Once a gender earnings differential was observed based on advertised pay, we expected to fully explain it by controlling for key structural and individual-level covariates. The covariates that we examined included experience, age, income, education, family composition, race, number of children, task length, the speed of accepting a task, and thirteen types of subtasks. We additionally examined the time of day and day of the week as potential explanatory factors. Again, contrary to our expectations, we observed that the pay gap persisted even after these potential confounders were controlled for. Indeed, separate analyses that examined the advertised pay gap within each subcategory of the covariates showed that the pay gap is ubiquitous, and persisted within each of the ninety sub-groups examined. These findings allows us to rule out multiple mechanisms that are known drivers of the pay gap in traditional labor markets and other gig economy marketplaces. To our knowledge this is the only study that has observed a pay gap across such diverse categories of workers and conditions, in an anonymous marketplace, while simultaneously controlling for virtually all variables that are traditionally implicated as causes of the gender pay gap.
Individual-level factors
Individual-level factors such as parental status and family composition are a common source of the gender pay gap in traditional labor markets [ 15 ] . Single mothers have previously been shown to have lower reservation wages compared to other men and women [ 21 ]. In traditional labor markets lower reservation wages lead single mothers to be willing to accept lower-paying work, contributing to a larger gender pay gap in this group. This pattern may extend to gig economy markets, in which single mothers may look to online labor markets as a source of supplementary income to help take care of their children, potentially leading them to become less discriminating in their choice of tasks and more willing to work for lower pay. Since female MTurk workers are 20% more likely than men to have children (see Table 1 ), it was critical to examine whether the gender pay gap may be driven by factors associated with family composition.
An examination of the advertised gender pay gap among individuals who differed in their marital and parental status showed that while married workers and those with children are indeed willing to work for lower pay (suggesting that family circumstances do affect reservation wages and may thus affect the willingness of online workers to accept lower-paying online tasks), women’s hourly pay is consistently lower than men’s within both single and married subgroups of workers, and among workers who do and do not have children. Indeed, contrary to expectations, the advertised gender pay gap was highest among those workers who are single, and among those who do not have any children. This observation shows that it is not possible for parental and family status to account for the observed pay gap in the present study, since it is precisely among unmarried individuals and those without children that the largest pay gap is observed.
Age was another factor that we considered to potentially explain the gender pay gap. In the present sample, the hourly pay of older individuals is substantially lower than that of younger workers; and women on the platform are five years older on average compared to men (see Table 1 ). However, having examined the gender pay gap separately within five different age cohorts we found that the largest pay gap occurs in the two youngest cohort groups: those between 18 and 29, and between 30 and 39 years of age. These are also the largest cohorts, responsible for 64% of completed work in total.
Younger workers are also most likely to have never been married or to not have any children. Thus, taken together, the results of the subgroup analyses are consistent in showing that the largest pay gap does not emerge from factors relating to parental, family, or age-related person-level factors. Similar patterns were found for race, education, and income. Specifically, a significant gender pay gap was observed within each subgroup of every one of these variables, showing that person-level factors relating to demographics are not driving the pay gap on this platform.
Experience is a factor that has an influence on the pay gap in both traditional and gig economy labor markets [ 20 ] . As noted above, experienced workers may be faster and more efficient at completing tasks in this platform, but also potentially more savvy at selecting more remunerative tasks compared to less experienced workers if, for example, they are better at selecting tasks that will take less time to complete than estimated on the dashboard [ 20 ]. On MTurk, men are overall more experienced than women. However, experience does not account for the gender gap in advertised pay in the present study. Inexperienced workers comprise the vast majority of the Mechanical Turk workforce, accounting for 67% of all completed tasks (see Table 5 ). Yet within this inexperienced group, there is a consistent male earning advantage based on the advertised pay for tasks performed. Further, controlling for the effect of experience in our models has a minimal effect on attenuating the gender pay gap.
Task heterogeneity
Another important source of the gender pay gap in both traditional and gig economy labor markets is task heterogeneity. In traditional labor markets men are disproportionately represented in lucrative fields, such as those in the tech sector [ 23 ]. While the workspace within MTurk is relatively homogeneous compared to the traditional labor market, there is still some variety in the kinds of tasks that are available, and men and women may have been expected to have preferences that influence choices among these.
To examine whether there is a gender preference for specific tasks, we systematically analyzed the textual descriptions of all tasks included in this study. These textual descriptions were available for all workers to examine on their dashboards, along with information about pay. The clustering algorithm revealed thirteen categories of tasks such as games, decision making, several different kinds of survey tasks, and psychology studies.We did not observe any evidence of gender preference for any of the task types. Within each of the thirteen clusters the distribution of tasks was approximately equally split between men and women. Thus, there is no evidence that women as a group have an overall preference for specific tasks compared to men. Critically, the gender pay gap was also observed within each one of these thirteen clusters.
Another potential source of heterogeneity is task length. Based on traditional labor markets, one plausible hypothesis about what may drive women’s preferences for specific tasks is that women may select tasks that differ in their duration. For example, women may be more likely to use the platform for supplemental income, while men may be more likely to work on HITs as their primary income source. Women may thus select shorter tasks relative to their male counterparts. If the shorter tasks pay less money, this would result in what appears to be a gender pay gap.
However, we did not observe gender differences in task selection based on task duration. For example, having divided tasks into their advertised length, the tasks are preferred equally by men and women. Furthermore, the shorter tasks’ hourly pay is substantially higher on average compared to longer tasks.
Additional evidence that scheduling factors do not drive the gender pay gap is that it was observed within all hourly and daily intervals (See S1 and S2 Tables in Appendix). These data are consistent with the results presented above regarding personal level factors, showing that the majority of male and female Mechanical Turk workers are single, young, and have no children. Thus, while in traditional labor markets task heterogeneity and labor segmentation is often driven by family and other life circumstances, the cohort examined in this study does not appear to be affected by these factors.
Practical implications of a gender pay gap on online platforms for social and behavioral science research
The present findings have important implications for online participant recruitment in the social and behavioral sciences, and also have theoretical implications for understanding the mechanisms that give rise to the gender pay gap. The last ten years have seen a revolution in data collection practices in the social and behavioral sciences, as laboratory-based data collection has slowly and steadily been moving online [ 16 , 24 ]. Mechanical Turk is by far the most widely used source of human participants online, with thousands of published peer-reviewed papers utilizing Mechanical Turk to recruit at least some of their human participants [ 25 ]. The present findings suggest both a challenge and an opportunity for researchers utilizing online platforms for participant recruitment. Our findings clearly reveal for the first time that sampling research participants on anonymous online platforms tends to produce gender pay inequities, and that this happens independent of demographics or type of task. While it is not clear from our findings what the exact cause of this inequity is, what is clear is that the online sampling environment produces similar gender pay inequities as those observed in other more traditional labor markets, after controlling for relevant covariates.
This finding is inherently surprising since many mechanisms that are known to produce the gender pay gap in traditional labor markets are not at play in online microtasks environments. Regardless of what the generative mechanisms of the gender pay gap on online microtask platforms might be, researchers may wish to consider whether changes in their sampling practices may produce more equitable pay outcomes. Unlike traditional labor markets, online data collection platforms have built-in tools that can allow researchers to easily fix gender pay inequities. Researchers can simply utilize gender quotas, for example, to fix the ratio of male and female participants that they recruit. These simple fixes in sampling practices will not only produce more equitable pay outcomes but are also most likely advantageous for reducing sampling bias due to gender being correlated with pay. Thus, while our results point to a ubiquitous discrepancy in pay between men and women on online microtask platforms, such inequities have relatively easy fixes on online gig economy marketplaces such as MTurk, compared to traditional labor markets where gender-based pay inequities have often remained intractable.
Other gig economy markets
As discussed in the introduction, a gender wage gap has been demonstrated on Uber, a gig economy transportation marketplace [ 20 ], where men earn approximately 7% more than women. However, unlike in the present study, the gender wage gap on Uber was fully explained by three factors; a) driving speed predicted higher wages, with men driving faster than women, b) men were more likely than women to drive in congested locations which resulted in better pay, c) experience working for Uber predicted higher wages, with men being more experienced. Thus, contrary to our findings, the gender wage gap in gig economy markets studied thus far are fully explained by task heterogeneity, experience, and task completion speed. To our knowledge, the results presented in the present study are the first to show that the gender wage gap can emerge independent of these factors.
Generalizability
Every labor market is characterized by a unique population of workers that are almost by definition not a representation of the general population outside of that labor market. Likewise, Mechanical Turk is characterized by a unique population of workers that is known to differ from the general population in several ways. Mechanical Turk workers are younger, better educated, less likely to be married or have children, less likely to be religious, and more likely to have a lower income compared to the general United States population [ 24 ]. The goal of the present study was not to uncover universal mechanisms that generate the gender pay gap across all labor markets and demographic groups. Rather, the goal was to examine a highly unique labor environment, characterized by factors that should make this labor market immune to the emergence of a gender pay gap.
Previous theories accounting for the pay gap have identified specific generating mechanisms relating to structural and personal factors, in addition to discrimination, as playing a role in the emergence of the gender pay gap. This study examined the work of over 20,000 individuals completing over 5 million tasks, under conditions where standard mechanisms that generate the gender pay gap have been controlled for. Nevertheless, a gender pay gap emerged in this environment, which cannot be accounted for by structural factors, demographic background, task preferences, or discrimination. Thus, these results reveal that the gender pay gap can emerge—in at least some labor markets—in which discrimination is absent and other key factors are accounted for. These results show that factors which have been identified to date as giving rise to the gender pay gap are not sufficient to explain the pay gap in at least some labor markets.
Potential mechanisms
While we cannot know from the results of this study what the actual mechanism is that generates the gender pay gap on online platforms, we suggest that it may be coming from outside of the platform. The particular characteristics of this labor market—such as anonymity, relative task homogeneity, and flexibility—suggest that, everything else being equal, women working in this platform have a greater propensity to choose less remunerative opportunities relative to men. It may be that these choices are driven by women having a lower reservation wage compared to men [ 21 , 26 ]. Previous research among student populations and in traditional labor markets has shown that women report lower pay or reward expectations than men [ 27 – 29 ]. Lower pay expectations among women are attributed to justifiable anticipation of differential returns to labor due to factors such as gender discrimination and/or a systematic psychological bias toward pessimism relative to an overly optimistic propensity among men [ 30 ].
Our results show that even if the bias of employers is removed by hiding the gender of workers as happens on MTurk, it seems that women may select lower paying opportunities themselves because their lower reservation wage influences the types of tasks they are willing to work on. It may be that women do this because cumulative experiences of pervasive discrimination lead women to undervalue their labor. In turn, women’s experiences with earning lower pay compared to men on traditional labor markets may lower women’s pay expectations on gig economy markets. Thus, consistent with these lowered expectations, women lower their reservation wages and may thus be more likely than men to settle for lower paying tasks.
More broadly, gender norms, psychological attributes, and non-cognitive skills, have recently become the subject of investigation as a potential source for the gender pay gap [ 3 ], and the present findings indicate the importance of such mechanisms being further explored, particularly in the context of task selection. More research will be required to explore the potential psychological and antecedent structural mechanisms underlying differential task selection and expectations of compensation for time spent on microtask platforms, with potential relevance to the gender pay gap in traditional labor markets as well. What these results do show is that pay discrepancies can emerge despite the absence of discrimination in at least some circumstances. These results should be of particular interest for researchers who may wish to see a more equitable online labor market for academic research, and also suggest that novel and heretofore unexplored mechanisms may be at play in generating these pay discrepancies.
A final note about framing: we are aware that explanations of the gender pay gap that invoke elements of women’s agency and, more specifically, “choices” risk both; a) diminishing or distracting from important structural factors, and b) “naturalizing” the status quo of gender inequality [ 30 ] . As Connor and Fiske (2019) argue, causal attributions for the gender pay gap to “unconstrained choices” by women, common as part of human capital explanations, may have the effect, intended or otherwise, of reinforcing system-justifying ideologies that serve to perpetuate inequality. By explicitly locating women’s economic decision making on the MTurk platform in the broader context of inegalitarian gender norms and labor market experiences outside of it (as above), we seek to distance our interpretation of our findings from implicit endorsement of traditional gender roles and economic arrangements and to promote further investigation of how the observed gender pay gap in this niche of the gig economy may reflect both broader gender inequalities and opportunities for structural remedies.
Supporting information
S1 table. distribution of hits, average pays, and gender pay gaps by hour of day..
https://doi.org/10.1371/journal.pone.0229383.s001
S2 Table. Distribution of HITs, average pays, and gender pay gaps by day of the week.
https://doi.org/10.1371/journal.pone.0229383.s002
- 1. United States Equal Employment Opportunity Commission, Lily Ledbetter Fair Pay Act of 2009 (2009), available at https://www.eeoc.gov/eeoc/publications/brochure- equal_pay_and_ledbetter_act.cfm, accessed on 11/12/2018.
- 2. United States Department of Labor (DOL), Office of Federal Contract Compliance Programs (OFCCP), Pay Transparency Nondiscrimination Provision, available at https://www.dol.gov/ofccp/PayTransparencyNondiscrimination.html , accessed on 11/12/2018.
- View Article
- Google Scholar
- 4. United States Department of Labor (DOL), Bureau of Labor Statistics (BLS) (2016) Women’s earning 83 percent of men’s, but vary by occupation. TED Econ Dly , available at https://www.bls.gov/opub/ted/2016/womens-earnings-83-percent-of-mens-but-vary-by-occupation.htm , accessed on 11/12/2018.
- 5. Davis A (2015) Women still earn less than men across the board (Economic Policy Institute, 2015), available at http://www.epi.org/publication/women-still-earn-less-than-men-across-the-board/ , accessed on 11/12/2018.
- 6. “Gender Pay Inequality: Consequences for Women, Families and the Economy” (Joint Economic Committee, 2016). [no author]
- 7. Hartmann H, Hayes J, Clark J (2014) “How Equal Pay for Working Women would Reduce Poverty and Grow the American Economy” (Institute for Women’s Policy Research, 2014).
- 8. OECD (2015) In it together: Why Less Inequality Benefits All (OECD Publishing, Paris) available at http://www.oecd.org/els/soc/OECD2015-In-It-Together-Chapter1-Overview-Inequality.pdf , accessed on 11/12/2018.
- PubMed/NCBI
- 16. Litman L, Robinson J (In Press) Conducting Online Research on Amazon Mechanical Turk and Beyond. Sage Publications.
- 17. Farrell D, Greig F (2016) Paychecks, paydays, and the online platform economy: Big data on income volatility. JP Morgan Chase Institute.
- 18. Kuek SC, Paradi-Guilford C, Fayomi T, Imaizumi S, Ipeirotis P, Pina P, Singh M (2015) The global opportunity in online outsourcing (World Bank Group, 2015) Available at http://documents.worldbank.org/curated/en/138371468000900555/pdf/ACS14228-ESW-white-cover-P149016-Box391478B-PUBLIC-World-Bank-Global-OO-Study-WB-Rpt-FinalS.pdf , accessed on 11/12/2018.
- 23. Bureau of Labor Statistics, U.S. Department of Labor, Labor Force Statistics from the Current Population Survey, Household Data Annual Averages. Employed persons by detailed occupation, sex, race, and Hispanic or Latino ethnicity, on the Internet at https://www.bls.gov/cps/cpsaat11.htm (visited 9/3/18).
Home — Essay Samples — Social Issues — Gender Discrimination — Gender Pay Gap

Gender Pay Gap
- Categories: Gender Discrimination Income Inequality
About this sample

Words: 663 |
Published: Jan 29, 2024
Words: 663 | Page: 1 | 4 min read
Table of contents
Understanding the gender pay gap, causes of the gender pay gap, consequences of the gender pay gap, efforts to address the gender pay gap, current state and future prospects.
- Blau, F. D., & Kahn, L. M. (2017). The gender wage gap: Extent, trends, and explanations. Journal of Economic Literature, 55(3), 789-865.
- United States Census Bureau. (2021). Income and poverty in the United States : 2020. https://www.census.gov/library/publications/2021/demo/p60-272.html
- U.S. Equal Employment Opportunity Commission. (2021). Equal pay and the gender pay gap. https://www.eeoc.gov/equal-pay-and-gender-pay-gap
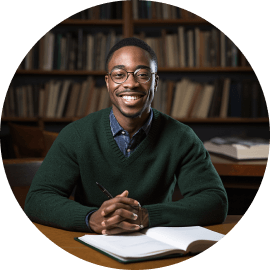
Cite this Essay
Let us write you an essay from scratch
- 450+ experts on 30 subjects ready to help
- Custom essay delivered in as few as 3 hours
Get high-quality help
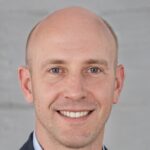
Prof. Kifaru
Verified writer
- Expert in: Social Issues Economics
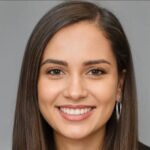
+ 120 experts online
By clicking “Check Writers’ Offers”, you agree to our terms of service and privacy policy . We’ll occasionally send you promo and account related email
No need to pay just yet!
Related Essays
2 pages / 911 words
3 pages / 1420 words
4 pages / 1597 words
2 pages / 769 words
Remember! This is just a sample.
You can get your custom paper by one of our expert writers.
121 writers online
Still can’t find what you need?
Browse our vast selection of original essay samples, each expertly formatted and styled
Related Essays on Gender Discrimination
Chinua Achebe's novel "Things Fall Apart" is a powerful portrayal of the complex character of Okonkwo, a man who struggles to maintain his traditional values in a rapidly changing world. Okonkwo is a dynamic character, whose [...]
Traditionally, the notion or concept of uniformed organizations such as the armed forces, navy, airforce and Police forces is marked with a strong masculine culture. In short, the presence of male dominance is often defined by [...]
In her thought-provoking book "Pink Think," Lynn Peril provides an insightful analysis of how gender stereotypes have been perpetuated and reinforced through the commercial and cultural messages of the postwar American era. [...]
Nathaniel Hawthorne's The Scarlet Letter is a renowned novel that explores the complex themes of sin, guilt, and redemption in Puritan society. One striking character in the narrative is Pearl, the illegitimate daughter of [...]
The “glass ceiling” has kept ladies away from specific positions and openings in the work environment. Ladies are stereotyped as low maintenance, lower grade workers with restricted open doors for preparing and headway due to [...]
Till this day, gender conflict in society is an on-going concern. Through generations men and women have been limited to their ability based on society’s labels that are attached to each gender in which the narrator experiences [...]
Related Topics
By clicking “Send”, you agree to our Terms of service and Privacy statement . We will occasionally send you account related emails.
Where do you want us to send this sample?
By clicking “Continue”, you agree to our terms of service and privacy policy.
Be careful. This essay is not unique
This essay was donated by a student and is likely to have been used and submitted before
Download this Sample
Free samples may contain mistakes and not unique parts
Sorry, we could not paraphrase this essay. Our professional writers can rewrite it and get you a unique paper.
Please check your inbox.
We can write you a custom essay that will follow your exact instructions and meet the deadlines. Let's fix your grades together!
Get Your Personalized Essay in 3 Hours or Less!
We use cookies to personalyze your web-site experience. By continuing we’ll assume you board with our cookie policy .
- Instructions Followed To The Letter
- Deadlines Met At Every Stage
- Unique And Plagiarism Free
- Share full article
Advertisement
Subscriber-only Newsletter
Jessica Grose
The gender pay gap is a culture problem.

By Jessica Grose
Opinion Writer
American women made significant progress toward closing the gender pay gap in the second half of the 20th century, but that gap has barely budged over the past two decades. In 2022, according to Pew Research , “American women typically earned 82 cents for every dollar earned by men. That was about the same as in 2002, when they earned 80 cents to the dollar.”
In a country where women are now a (slight) majority of the college-educated labor force and the annual earnings median for college degree holders is 55 percent more than that of those with high school diplomas, the stickiness of this gap is frustrating. While there are several factors at play, one of the key contributors to the gap is what’s known as the motherhood penalty and the corresponding fatherhood premium: Women’s pay decreases when they have children, while men’s pay increases.
This dynamic isn’t just an American phenomenon. “In general, women don’t recover. They don’t catch back up to men, even many years after first childbirth,” said Henrik Kleven, the lead author of a 2023 National Bureau of Economic Research working paper, “ The Child Penalty Atlas ,” in which he and his co-authors, Camille Landais and Gabriel Leite-Mariante, reviewed wage gap data from 134 countries. “Now, that basic pattern is true essentially everywhere, but the quantitative magnitudes of the effects vary greatly across countries,” he told me recently.
Somewhat surprisingly to me, his research, which builds on years of earlier scholarship, suggests that a country’s family policy has relatively little to do with how big the parenthood pay gap is. A society’s culture and norms seem to be much bigger factors in how big the motherhood penalty is: The more egalitarian the culture, the lower the gap.
Kleven told me that sometimes countries that seem superficially similar in terms of income levels, development, family policy and geography have very different pay gaps. (We see the same interplay in American states , with the child penalty 21 percent in Vermont and 61 percent in Utah.) Even countries right next to each other can have wildly different gaps. Spain’s child pay gap is much bigger than Portugal’s, and Germany’s is bigger than Denmark’s. Central European countries have “some of the highest child penalties we see anywhere in the world,” Kleven said. Scandinavian countries have some of the lowest.
Let’s look at Austria. It has generous family leave policies and child care subsidies , especially by American standards. But in a 2022 working paper, “Do Family Policies Reduce Gender Inequality? Evidence From 60 Years of Policy Experimentation,” Kleven and his co-authors’ analysis showed “that the enormous expansions of parental leave and child care subsidies have had virtually no impact on gender convergence.” Despite an influx of Austrian women into the work force in the past 50 or so years, the relatively large child penalty can be at least partly explained by gender attitudes and norms.
According to data from the 2012 wave of the International Social Survey Program that was analyzed in the paper, more than 60 percent of Austrians agreed that when a mother works for pay, her young children probably suffer. By comparison, those in the more egalitarian Scandinavian countries felt differently. Fewer than 20 percent of Danes agreed that children suffer when their mothers work outside the home. Though that data is more than a decade old, those kinds of attitudes die hard and are backed up by newer research .
Speaking of Danes: A new paper from economists at Lund University, the University of Amsterdam and Aarhus University found that for a subset of Danish women, the motherhood penalty disappeared in the long run and, in limited circumstances, turned into a premium. The paper followed the earnings trajectory of more than 18,000 child-free women who received in vitro fertilization treatment in Denmark, where, Time magazine reported in 2019, “the cost of three cycles of I.V.F. for a first child is covered by the tax-financed public health service” for women up to the age of 40. The study’s authors then compared the women who had successful first-round I.V.F. treatments and ended up with children and the women who didn’t.
The women who had successful I.V.F. treatments had a near-term child penalty, and their earnings dropped below those of the women with unsuccessful treatments. “By Year 10,” though, according to the study, “successfully treated women earn as much as unsuccessfully treated women. And by Year 15, successfully treated women earn slightly more. This earnings advantage persists throughout the remainder of the study period.” Men’s earnings weren’t affected, regardless of whether they became parents.
In my mind, one limitation in interpreting these findings is that I.V.F. pregnancies are planned, while over 40 percent of pregnancies in the United States, for example, are unplanned . One could imagine that the women pursuing I.V.F. at various income levels might be better set up to weather a career interruption than women who have surprise pregnancies. But it is still a thought-provoking finding that complicates previous child gap research.
In the United States, where gender norms are less progressive than in Scandinavia and the “costs for a single cycle of I.V.F. have recently been estimated to range from $15,000 to $20,000 and can exceed $30,000,” according to the Department of Health and Human Services, we find a very different experience with the motherhood pay gap than in Denmark. And it’s a much less happy picture.
A paper published last year in the scientific journal PNAS looked at 22 years of administrative data from the United States and found “surprisingly robust” motherhood penalties, even, unfortunately, in circumstances in which you might expect that the penalty would be slim, like in female-breadwinner families:
On average, women earn 57 percent more than men in these female-breadwinner families. Were couples simply seeking to maximize household income conditional on a certain amount of time investment in children, we would expect to see fatherhood penalties. Instead, we see one of the largest motherhood penalties in female-breadwinner families. Indeed, higher-earning women experience a 60 percent drop from prechildbirth earnings relative to their lower-earning male partner and the highest of our various sample stratifications. The pattern we find for the United States is the polar opposite of that for Sweden.
There was also no difference for mothers in companies that were female led or had a majority of female employees. “If anything,” according to the authors, “this motherhood penalty grows faster over time at firms headed by women. On the whole, our findings are discouraging even relative to the existing work on motherhood penalties.”
I asked one of the paper’s co-authors, Cecilia Machado , an economist at the Getulio Vargas Foundation, to summarize the state of the motherhood penalty in the United States. If we wanted to take steps to improve the pay gap as a society, what would we do? Via email, she said that there might be a limited scope of what public policy and workplace policy can do. But she added that federal and workplace policy that encouraged both men and women to take paid parental leave could help; creating the political conditions for involved fatherhood in a child’s first year can set egalitarian patterns that last a lifetime. Still, Machado said, “Both of these combined are important policies, but maybe them alone, by themselves, will not work if we don’t see culture and gender norms changing.”
My take is that we’re in a time when cultural norms around motherhood in the United States seem particularly contradictory and in flux. While a record high percentage of women with children under 5 work, a large subset of Americans still thinks society would be better off if they didn’t.
In an email, Jessica Calarco, a sociologist at the University of Wisconsin, Madison, and the author of “ Holding It Together: How Women Became America’s Safety Net, ” said:
I asked 2,000 parents from across the U.S., “Do you think children are better off if their mother is home and doesn’t hold a job, or are children just as well off if their mother works for pay?” Fifty-two percent of dads and 42 percent of moms said it’s better for kids if their moms aren’t working for pay. Those attitudes are somewhat more common among Republicans (60 percent of dads and 48 percent of moms), but they’re pretty common among Democrats, too (53 percent of dads and 41 percent of moms).
Until we reconcile our cultural ambivalence toward working mothers, I don’t think the gap is going to get any better. Maybe in another 20 years, we’ll get another two cents.
An earlier version of this article included a quotation from Jessica Calarco that misstated a finding from her work. The percentage of mothers surveyed who said it’s better for children if their mothers don’t work for pay is 42 percent, not 47 percent.
How we handle corrections
Jessica Grose is an Opinion writer for The Times, covering family, religion, education, culture and the way we live now.
- Skip to main content
- Skip to "About this site"
Language selection
- Français
- Search and menus
Studies on Gender and Intersecting Identities Measuring and Analyzing the Gender Pay Gap: A Conceptual and Methodological Overview
by Melissa Moyser , PhD

Skip to text
Acknowledgements
1. introduction, 2. measuring the gender pay gap, 3. analyzing the gender pay gap, 4. conclusion, appendix a: decomposing the gender pay gap, appendix b: correcting for selection bias, appendix c: data sources.
Text begins
This study is funded by the Department for Women and Gender Equality Canada.
Pay inequality between women and men is a persistent phenomenon in Canada and around the world. Note Note Focusing on Canada, female labour force participation has risen dramatically since the 1960s, driven simultaneously by an increase in the proportion of employed women at any given time, and a decrease in the propensity of employed women to withdraw from the workforce upon marriage or motherhood. Note Note Note Women are now better educated, on average, than their male counterparts, having made substantial gains in educational attainment over the past three decades by increasingly acquiring university degrees at the bachelor level or above, and doing so at a faster pace than men. Note Note Note At post-secondary institutions, women have diversified their fields of study, making inroads in lucrative science, technology, engineering, and mathematics (STEM) specialties. Note Note Recent cohorts of women also seem to have stronger attachments to the labour market than their predecessors, as they are more likely to return to employment after a work interruption (e.g., maternity/parental leave), and to be employed when they have young children. Note Note Although women remain the minority, their representation in leadership positions (i.e., senior management) and in the top 1% of income earners has grown since the 1980s. Note Note Finally, social-policy support for women’s employment, in the form of job-protected maternity/parental leave and partial income replacement for that leave, has expanded, along with legislation addressing unfair treatment in the labour market. Note Note Note Note
While these trends are associated with a reduction in gender differences in pay in Canada, women aged 15/16 years and older earned from $0.69 to $0.89 for every dollar earned by men in 2017, depending on how the gender pay gap is measured. Pay inequality between women and men is an important social, economic, and political issue for at least four reasons. First, earnings are the main determinant of economic well-being for employed individuals, and of potential gains to employment for those who are not currently in the workforce. Note In this way, earnings serve as a significant input into a variety of decisions, from labour supply to family formation, as well as a factor affecting bargaining power and relative status within the household. Note
Second, there have been dramatic changes in the structure of families in Canada: dual-earner families now outnumber single-male-earner families; single-female-earner families and lone-parent families (usually headed by mothers) have become increasingly common; and relationship dissolution is prevalent. Note Note Note Note While women’s contribution to household income has grown, gender differences in earnings make it harder for them to financially support themselves and their families. Note Further, a lifetime of pay inequality between women and men means that women are disproportionately retiring into financial insufficiency and even poverty. Note
Third, the gender pay gap is symbolic of gender-based discrimination and injustice. Women spend more time than men on unpaid housework, childrearing, and caregiving (i.e., domestic labour), and they often reduce their labour force participation accordingly, putting them at a disadvantage in the labour market and making them vulnerable to low income, particularly in the event of union dissolution and during old age. Note Note Women’s disproportionate responsibility for domestic labour enables men to prioritize paid work and spend more time doing it than women. Although both paid work and domestic labour are integral to “social reproduction”—that is, the interdependent processes by which societies organize themselves to produce and reproduce material life on a daily and intergenerational basis—only production for the market is economically rewarded. Note It follows that the unpaid work performed disproportionately by women creates broad social benefits, yet they pay the price for doing that work in the form of lower earnings, relative to men.
There is also a connection between the work that women do at home and their paid work, in that women’s employment is concentrated in industries and occupations that parallel their traditional gender roles. Note Note Female-dominated jobs tend to be underpaid, even when they involve the same level of skill as male-dominated jobs. Note Note
Fourth, pay inequality between women and men perpetuates the gender division of labour. When women earn less than their male counterparts, even when they work the same amount of time, it is economically disadvantageous for individual families to move toward greater sharing of paid and unpaid work.
For these reasons, reducing pay inequality between women and men is a key priority, both nationally and internationally, for achieving gender equality. In Budget 2018, the Government of Canada introduced the Gender Results Framework, which includes the gender pay gap as a measure of progress toward the strategic goal of women’s equal and full participation the economy. Internationally, the principle of equal pay for work of equal value is included in the 2030 Sustainable Development Agenda under Goal 8, “decent work and economic growth.”
Documenting gender inequality in pay and tracking progress in this regard for policy purposes requires at least one indicator. The gender pay gap—the difference between the employment earnings of women and men, expressed either as a proportion of men’s earnings (i.e., the “gender pay ratio”) or one minus the gender pay ratio—typically serves this purpose. At present, there are no internationally-recognized standards for measuring the gender pay gap, leaving considerable scope for political choice. Note The purpose of this paper, then, is to inform the development of international standards for measuring the gender pay gap by explaining the assumptions underlying, and the implications following from, various methods.
This paper has two additional purposes: One is to increase literacy about the meaning and interpretation of different estimates of the gender pay gap. Understanding the distinction between the unadjusted and adjusted gender pay gap is particularly important, as confusion in this regard contributes to debate as to the very existence of the gender pay gap. The other purpose is to bring together various explanations for the gender pay gap, and highlight the importance of taking context into account.
This paper is organized as follows: in Section 2, measurement of the gender pay gap in terms of who estimates are based upon, what type of earnings are counted, and how “typical” earnings are calculated are discussed. Section 3 addresses analysis of the gender pay gap, focusing on prevailing explanations for earnings inequality between women and men; the statistical technique used to determine the relative importance of different explanations (i.e., Oaxaca-Blinder decomposition); and considerations when studying trends in the gender pay gap. Section 4 concludes, providing an overview of key points.
Début de l’encadré
Text box 1 Sex, gender, gender identity, and gender expression
The complexity of sex and gender has become increasingly evident in the past decade, as growing numbers of people challenge binary and mutually-exclusive conceptualizations of female and male, feminine and masculine. For this reason, it is important to define these terms from the outset.
Sex is assigned to an individual at birth, typically based on his/her biology: anatomy, hormones, and physiology. Note In contrast, gender is a social category, entailing roles, behaviours, activities, and attributes that a society deems to be appropriate for a given sex. Note Gender refers to the social construction of female and male as oppositional categories with distinct characteristics and unequal social value and power. Note Individuals develop gendered selves by internalizing, through socialization beginning in childhood, social norms and cultural expectations regarding appropriate roles, behaviours, activities, and appearances for their sex. Note In their social interactions, individuals perform sex-appropriate gender roles (i.e., they “do gender”), thereby creating and sustaining the prevailing gender order.
There are also gender identity and gender expression. Gender identity refers to personal identification of one’s gender according to an internal awareness that can fall anywhere on the gender spectrum between female and male. Note Gender expression pertains to how an individual outwardly expresses or presents his/her gender. Note
Although sex, gender, gender identity, and gender expression are conceptually distinct, the terms tend to be conflated based on the understanding that most biological women are female/feminine, while most biological men are male/masculine (i.e., cisgender). Note In other words, gender, gender identity, and gender expression are equated with sex because they are associated, and generally align, with that biological base. Note This is necessary when it comes to measuring the gender pay gap, as information relevant to making distinctions between sex, gender, gender identity, and gender expression has not previously been collected. For the purposes of this paper, then, women and men are distinguished on the basis of sex, with the assumption that their gender, gender identity, and gender expression are consistent with expectations for people of their sex (i.e., they are cisgender). Note Note Importantly, Statistics Canada recently established new sex and gender standards (2018), which distinguish between sex assigned at birth and gender identity or expression. In alignment with these new standards, a new gender-inclusive question has been recently implemented on national surveys, including the 2019 Census of Population content test, allowing transgender persons and those with non-binary gender identities or expressions to report their gender.
Fin de l’encadré
Relative earnings often signify how different groups are valued socially and economically. Note For this reason, the unadjusted gender pay gap—the raw difference between the earnings acquired by women and men through their paid work, which favours the latter—has often been used as a call to action for gender equality and women’s economic empowerment. Not all women earn less than men; in fact, women’s representation among high-income earners has increased: 20.3% of high-income earners were women in 2016, compared with 8.5% in 1981. Note Note However, among those with earnings, most women earn less than men (Table 1). For this reason, women’s average annual earnings from wages, salaries, and commissions were lower than men’s in 2017: $40,600 vs. $59,200 (a difference of $18,600).
There are two ways of presenting the gender pay gap, whether unadjusted or adjusted, both of which are based on the gender pay ratio—that is, women’s earnings expressed as a proportion of men’s earnings. The gender pay ratio is calculated by dividing women’s earnings by men’s earnings (see Calculating the Gender Pay Gap ). It can be interpreted as the number of cents that women earn for every dollar earned by men. Alternatively, the gender pay ratio can be subtracted from one and then multiplied by 100, such that it represents how much less women earn than men in percentage terms.
Text box 2 Calculating the Gender Pay Gap
In 2017, the average hourly wages of all female and male workers aged 15 years and over in Canada were $24.28 and $28.00, respectively.
This section discusses how implicit or explicit decisions that are made when measuring the unadjusted gender pay gap affect its size, and therefore its perceived seriousness:
- Labour supply (i.e., hours and weeks worked)
- What type of earnings are counted (i.e., annual earnings or hourly wages)?
- How “typical” earnings are calculated (i.e., average or median)? Note Note
In addition to affecting the size of the gender pay gap, these decisions are important because they can either justify or challenge the status quo of gender differences in employment and earnings.
2.1. Who are estimates based upon?
For the purposes of measuring the gender pay gap, workers are generally defined as paid employees in their main job. Self-employed individuals are excluded because they typically work for themselves as a business owner, freelancer, or independent contractor for another company. Earnings of self-employed individuals usually come directly from the business, instead of from wages, salary or commission-based reimbursement, and they are therefore not comparable to earnings from paid employment.
Ideally, individuals who were enrolled as full-time students during the reference period or the past year would also be excluded, as work was not their primary activity.
Other considerations when defining the sample upon which estimates of the gender pay gap are based—namely, age group and labour supply (i.e., hours and weeks worked)—are discussed below.
2.1.1. Age group
Empirical studies of earnings often focus on a segment of the employed adult population in what are considered to be the core working ages, generally defined as 25 to 54. The logic of focusing on the core-working-age population is that younger and older adults are often full-time students or retired, respectively. To the extent that younger and older workers are systematically different from workers in the core-working ages (e.g., they have higher or lower socioeconomic status), excluding them from the analytical sample may bias estimates of the gender pay gap. “Selection bias” is discussed in more detail in Section 3.4.2.
In this paper, data pertain to the employed population aged 15 years and older, unless otherwise indicated, for comparability with international indicators of the gender pay gap. However, it is recognized that the gender pay gap differs by age group. As discussed in Section 3.4.3, earnings inequality between women and men tends to increase with age, both because women experience more employment interruptions related to family responsibilities than men, and because older women generally have lower levels of educational attainment and work experience than younger women (i.e., “cohort effects”). For these reasons, the gender pay gap is narrower among those aged 15/16 to 24 than it is among those aged 25 to 54 and 55 years and older.
Comparison of the average earnings of women and men aged 15/16 years and older and 25 to 54 over the past 30 years demonstrates that the gender pay gap is virtually the same for both age groups, whether measured in terms of annual earnings from wages, salaries and commissions or hourly wages (Chart 1). Given that gender-based earnings inequality tends to increase with age, as the workforce ages (due to both the aging of Canada’s population and the increasing labour force participation of older age groups, estimates of the gender pay gap based on those aged 15/16 years and older and those aged 25 to 54 may eventually diverge. Note

2.1.2. Labour supply
It is well established that women and men differ with respect to characteristics that determine earnings from employment, particularly work hours. According to data from the Labour Force Survey, employed women aged 15 years and older usually worked an average of 32.9 hours per week at their main job in 2017—5.5 fewer hours than employed men. Note Also, employed men were three times as likely as employed women to work long work hours—defined as 50 hours or more per week—at their main job (11.0% vs. 3.6%) .
Based on data from the 2016 General Social Survey on Canadians at Work and Home, employed women and men aged 15 years and older both worked an average of 44 weeks at all jobs in the past year. Note However, women were less likely than men to be employed on a full-time, full-year basis, defined as mostly working 30 or more hours per week for 49 to 52 weeks in a given year (43.6% vs. 56.4%).
Although women spend less time, on average, than men on paid work, they spend more time on housework, childrearing, and caregiving. Note Based on data from the 2015 General Social Survey on Time Use, women aged 15 years and older spent an average of 25.2 hours per week on domestic labour—8.4 hours more than men. Note Even when women were employed on a full-time basis, they spent more time on domestic labour than their male counterparts: 21.0 vs. 16.1 hours per week (a difference of nearly 5 hours).
Estimates of the gender pay gap can be based on either a sample consisting of all workers, regardless of hours and weeks worked, or a sample consisting of workers employed on a full-time, full-year basis. These samples imply different conceptualizations of women’s decision-making about their labour supply.
One perspective, coming from economics, is that individuals are rational, self-interested, and preference or “utility” maximizing, meaning that they will always select the course of action from which they derive the most satisfaction, given their relatively stable preferences. Note Note According to this perspective, women are biologically predisposed to place greater value on family than men and they are more efficient at performing housework, childrearing, and caregiving. For these reasons, women “choose” to perform less, if any, paid work, focusing instead on unpaid work. Note Viewed from this perspective, earnings inequality between women and men that is related to gender differences in labour supply is justified as the outcome of personal autonomy. Note It therefore makes sense to limit the sample from which the gender pay gap is estimated to full-time, full-year workers.
An alternative perspective, coming from sociology, is that individuals’ choices are constrained by social norms and cultural expectations related to gender, and the gendered structural conditions of everyday life. Different attitudes, appearances, and behaviours are communally deemed to be appropriate for biologically-based sex categories. Note Individuals are held morally responsible for “doing gender”—that is, conducting themselves in ways that are consistent with and demonstrate their sex category—lest their competence as members of society be questioned. Note When women specialize in housework, childrearing, and caregiving and men specialize in breadwinning, according to this perspective, they are doing gender. Note Individuals are also doing gender when they select the “right” field of study and occupation for their sex. Note Note Note Note Note Note Note
According to previous research, the gender division of labour is both reflected and reinforced in the labour market through employers’ discriminatory recruitment and promotion practices, which block women from stereotypically “male” occupations and limit their career advancement; gender-based pay inequality that makes it economically disadvantageous for couples to divide domestic labour and paid work more equitably; and work organizations’ preferences for employees who can prioritize paid work due to their limited family responsibilities (usually men). Note Note Note Note To the extent that women expect to experience gender-based discrimination and inequality in the labour market, it may affect their decisions regarding how much and which type of education and training to invest in and which occupations to pursue. Studies show that, through these feedback effects, the gender division of labour becomes self-reinforcing: women have lower-value human capital (e.g., education) and lower-status occupations, and they therefore earn less, which reduces their bargaining position relative to men in the household, making them more likely to be the one who performs a greater share of housework, childrearing, and caregiving. Note
Previous research supports this interpretation of women’s decisions about their labour supply, as it demonstrates that women’s employment status and work hours are negatively affected by their ideological support for gender-differentiated roles. Note Note Note Note Note Further, Pedualla and Thébaud find that the preferences of young, unmarried, childless women and men in the United States regarding their future gender division of labour are responsive to institutional constraints Note . Specifically, women and men generally prefer egalitarian relationships, but, in the absence of supportive work-family policies, women and men with higher levels of education and men with lower levels of education have a “fallback plan” of neo-traditional relationships (i.e., the female spouse/partner is primarily responsible for managing the household, while the male spouse/partner is primarily responsible for breadwinning). Note Less educated women have a fall-back plan of self-reliance (i.e., themselves as the primary breadwinner). Note
Under these circumstances, women (and men) make constrained choices about how much paid work they do. Note Estimates of the gender pay gap that are based on samples that are limited to full-time, full-year workers omit the gendered processes involved in women's decisions about their labour supply.
2.2. What type of earnings are counted?
There are three main ways of counting earnings: (1) the annual earnings of all workers; (2) the annual earnings of workers employed on a full-time, full-year basis; and (3) the hourly wages of all workers. Note Annual earnings are defined here as the sum of wages, salaries and commissions from all jobs in a given year before taxes among individuals whose main job was as a paid employee. Employees with zero annual earnings are excluded. Hourly wages are defined as the rate that an employer pays an employee per hour worked in his/her main job.
Three limitations characterize all three ways of counting earnings. First, earnings are only measured for individuals in paid employment. If employed women and men differ systematically from their counterparts who are not employed with respect to characteristics that affect earnings, the gender pay gap will be biased, irrespective of what type of earnings are counted (see Section 3.4.1).
Second, earnings derived from (self-)employment in the underground economy are excluded from all three measures. By definition, economic activities within the underground economy escape measurement because they are unreported, hidden or illegal. In 2016, the underground economy accounted for 2.5% (or $51.6 billion) of Canada’s Gross Domestic Product. Note It is unclear whether women and men in Canada participate equally in the underground economy, where workers are often poorly paid and lack access to promotional opportunities, legal protections, and employment benefits, because important illegal industries—namely, drugs and sex work—are excluded from national estimates. Note Note Also, national estimates of the underground economy rely on assumptions, weak indicative information, and various indirect methods because it is difficult to obtain information on hidden, illegal, and informal activities. Note With these caveats in mind, the three industries that accounted for more than half of measured underground economic activity in Canada in 2016 were residential construction (26.6%), retail trade (13.5%), and accommodation and food services (12.1%). Note These industries have been the main contributors since 1992, the first year of study. Note
Third, other sources of compensation related to employment, such as (supplementary) medical and dental benefits, pension plans, stock options, and use of company cars, are not counted as earnings. Previous research suggests that women may have less access to some of these employer-provided perks, relative to men, because they are more likely to work part-time, and they are less likely to hold higher-status occupations and work for large corporations. Note Note Note However, employed women in Canada were somewhat more likely than men to be covered by employer-sponsored pension plans in 2015 (40% vs. 36%), due to the fact that they are overrepresented in the three industries with the highest rates of pension coverage: education, health, and public administration. Note Consequently, women may have more discretionary income than men after saving for retirement—even with the same earnings. Note Gender inequality in non-monetary employee compensation is not reflected in any estimate of the gender pay gap that is currently available.
2.2.1 Comparing the annual earnings of all women and men, regardless of how much they work
The most inclusive, and therefore the largest, measure of the gender pay gap involves comparison of the annual earnings of all employed women and men, including those who worked on a part-time and/or part-year basis (Chart 2). By implication, this measure captures gender differences in both pay (i.e., the price of labour) and hours and weeks worked (i.e., labour supply). Note It can therefore be thought of as an unrestricted measure of the gender pay gap.

The typical criticism of the unrestricted measure of the gender pay gap is that it is “confounded” by gender differences in hours and weeks worked. The counterargument is that the unrestricted measure of the gender pay gap captures the full scope of the financial implications of gender, which partly result from women’s reduced labour supply, relative to men, given their greater family responsibilities.
Annual earnings have an advantage over hourly wages when it comes to conveying the economic significance of the gender pay gap, as it speaks to the women’s and men’s different command over goods and services. As Lips explains: “When a woman applies for a mortgage or a car loan, she is not asked about her hourly income. The income statistic that affects whether or not she gets the loan, and indeed what kind of life she is able to afford, is her annual income.” Note Measuring the gender pay gap in terms of annual earnings provides an index of gender differences in purchasing power and material well-being that the gender wage gap does not. In other words, the gender pay gap, as measured from annual earnings, speaks to women’s overall economic well-being.
2.2.2. Comparing the annual earnings of women and men employed on a full-time, full-year basis
Traditionally, the gender pay gap has been measured from the annual earnings of women and men who worked full-time, defined as a minimum of 35 hours per week, for 50 to 54 weeks in the reference year. Measured as such, the gender pay gap will be smaller than it would be if workers employed a part-time and/or part-year basis—who are predominantly women—are included in the estimate (Chart 2). However, gender differences in work hours are only partially removed when the gender pay gap is measured from the annual earnings of full-time, full-year workers. The reason is that women work fewer hours each week than men, on average, even when they are employed on full-time, full-year. Based on data from the Labour Force Survey, women aged 15 years and older who were employed on a full-time basis usually worked an average of 38.5 hours per week at their main job in 2017, while their male counterparts usually worked an average of 41.5 hours (a difference of three hours per week).
The exclusion of workers employed on a part-time and/or part-year basis from measurement of the gender pay gap implies the idealization of full-time, full-year employment—a pattern typified by men, both historically and currently. Note Studies show that the male pattern of employment itself depends on housework, childrearing, and caregiving disproportionately performed by women. Note Note Note Requiring full-time, full-year employment can therefore be construed as marginalizing mothers, who often experience work interruptions related to childrearing, and other women for whom it is impractical to fit that pattern, due to their greater family responsibilities, relative to men. Note Note Further, it renders invisible domestic labour, its financial cost to women, and its benefits to the economy. Note
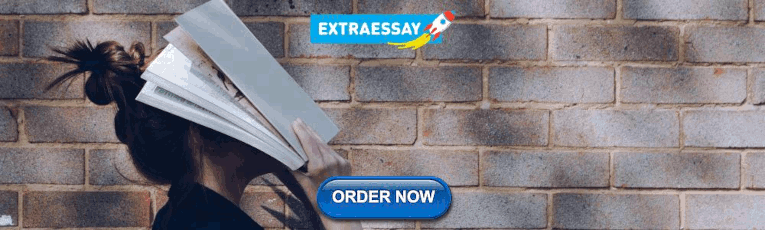
2.2.3 Comparing the hourly wages of women and men
Although there is no agreement internationally on a standard way of counting earnings for the purposes of measuring the gender pay gap, there is a strong preference for hourly wages. The ratio of women’s hourly wages to men’s hourly wages—known as the gender wage gap—is the most restricted measure of the gender pay gap, as it captures only the per-unit price of labour, and it is therefore unaffected by gender differences in labour supply. Note For this reason, hourly wages yield the smallest estimate of the gender pay gap (Chart 2). For example, between 1997 and 2017, the gender wage ratio in Canada ranged from 0.81 to 0.89, while the earnings-based gender pay ratio (all workers) ranged from 0.60 to 0.74. In many years the gender wage ratio was higher than the earnings-based gender pay ratio by nearly 20 percentage points.
In theory, it is not necessary to exclude part-time workers when estimating the gender wage gap in order to control for gender differences in work hours, as labour supply is irrelevant when earnings are measured on a per-unit basis. Indeed, the move from all workers to full-time workers makes little difference when the gender pay gap is measured in terms of hourly wages, as opposed to annual earnings (Chart 2).
The fact that the gender wage gap is not confounded by gender differences in labour supply can be viewed as a drawback. Treating gender differences in work hours as a separate issue from the gender pay gap rests on the assumption that women freely choose to work fewer hours than men, with the implication being that their lower earnings are deserved. In this way, the gendered processes that give rise to women’s reduced labour supply, relative to men, and the contribution of those processes to the gender wage gap are hidden from view.
Many workers are not paid on an hourly basis; instead, they are paid on the basis of an annual salary. Note According to the Labour Force Survey, 41.4% of women aged 15 years and older were salaried employees in 2017, as were 47.1% of men. For salaried employees, estimates of hourly wages are generally made by either assuming a standard 40 hour work week, or dividing their annual salary by their usual weekly work hours multiplied by the number of weeks in a year. However, 16.0% of salaried employees reported that their work hours varied from one week to the next in 2017, and 13.6% of salaried employees reported usual work hours in excess of 40 per week. It follows that, for many workers, estimates of hourly wages on the basis of an annual salary and usual work hours will be less accurate.
Notably, hourly wages do not reflect contingent or “pay-for-performance” that is included in annual earnings. Note Women are less likely than men to receive contingent pay for a number of reasons. Note First, they are less likely to be employed in leadership positions and higher-status occupations, particularly in the private sector, that offer contingent pay. Note Second, negotiation plays a greater role in determining bonuses and stock options than it does in fixed salaries. Note Note Women are less likely than men to negotiate more favorable employment terms with respect to base salary, stock options, and bonuses. Note Note Third, women tend to have lower risk tolerance in financial decision-making than men, with the implication that they are less likely to take jobs in which a significant component of compensation is dependent on performance. Note
In addition to women being less likely than men to receive contingent pay, previous research demonstrates that the gender pay gap is more pronounced for contingent pay than fixed salaries, possibly because the latitude for management discretion is greater in the case of contingent pay. Note Note Note Note For these reasons, the gender wage gap may underestimate to some extent earnings inequality between women and men.
2.3. How typical earnings are counted: Mean or median?
In addition to which workers are included in the samples from which the gender pay gap is estimated and what type of earnings are counted, the size of the gender pay gap depends on the statistic chosen to represent “typical” earnings: the mean (more commonly known as the average) or the median. To calculate the mean, the earnings of all workers of a given sex are summed and the resulting value is divided by the number of workers of that sex. The mean is interpreted as the pay that each worker of a given sex would receive, if the earnings of all workers of that sex were divided evenly among them.
To calculate the median, the earnings of all workers of a given sex are put in ascending/descending order, and the middle of that earnings distribution is identified. The median separates the half of workers who earn less than that amount from the other half of workers who earn more.
Averages have the advantage of taking into account the earnings of every worker, which medians do not. The downside of this characteristic is that averages can be skewed by workers with very low or very high earnings to a greater extent than medians. Note At the same time, it can be argued that the overrepresentation of women among workers with very low earnings, and the underrepresentation of women among workers with very high earnings, are central to the issue of gender-based pay inequality, and should therefore be reflected in the statistic used to measure central tendency. Note Further, previous research demonstrates that the gender pay gap increases over the earnings distribution, being greater at the top than the bottom. Note Note Note Note Note Note Note Note Measuring the gender pay gap at the median minimizes the impact of the segment of the workforce with the highest earnings. Note
Another advantage of the mean, over the median, is that the multivariate methods of data analysis that are typically used to examine the gender pay gap—ordinary least squares regression and standard decomposition techniques—are based on the mean. Note
Chart 3 shows the gender pay ratio calculated from average and median annual earnings (all workers) and hourly wages. Notably, since the late 2000s, the average and median gender pay ratios have generally been more similar when annual earnings are used, as opposed to their hourly wages.

2.4. Comparison of different measures over time
Different measures of the gender pay gap yield different stylized facts regarding the evolution of earnings inequality between women and men in Canada over time, and different implications for future progress. Based on the average annual earnings of full-time, full-year workers, the prevailing narrative highlights the fact that the gender pay ratio hovered around 0.70 between 1992 and 2008 (Chart 4). Note This contrasts with the preceding 15 years, during which time there was a steady narrowing of the gender pay gap (from 0.39 in 1976 to 0.32 in 1991). Note Since 2009, the gender pay ratio has been marginally higher than that observed between 1992 and 2008, averaging 0.73. Given similar findings in the United States, some scholars wonder whether progress toward equal pay between women and men has stalled. Note

Using the hourly wages of all female and male workers, instead of annual earnings, reveals that the pace of growth in the gender pay ratio slowed considerably between 1992 and 2000, but did not stall. Note The gender pay ratio picked up again in 2001, signalling the resumption of a strong, long-term trend towards convergence in women’s and men’s pay.
Text box 3 Selecting a measure of the gender pay gap
Although all of the previously-discussed measures of the gender pay gap are meaningful, each one approaches the issue from a different angle, and is therefore more or less informative, depending on the question/s being addressed. For example, pay equity legislation mandates equal pay for work of equal or comparable value to the employer, regardless of employees’ work hours and terms of employment (i.e., permanent, temporary, seasonal, or casual). It follows that the gender pay gap in hourly wages is more relevant to pay equity legislation than the gender pay gap in annual earnings, as the latter is confounded by gender differences in work hours. On the other hand, when used as a reflection of the realities of women’s and men’s lives, the gender pay gap is best measured in terms of the annual earnings of all workers, as doing so encompasses all of the different ways in which gender results in women having lower pay, and ultimately less purchasing power, than men.
For the purposes of monitoring progress toward gender equality, as per the Government of Canada’s Gender Results Framework , it is recommended that two measures of the gender pay gap be presented: one based on the annual earnings of all workers, and the other based on the hourly wages of all workers. Doing so highlights the contribution of gender differences in work hours to the gender pay gap, without implying that women’s route to pay equity with men lies in them adopting stereotypically-male patterns of work and labour-force attachment.
Similar contextual “fit” should guide the use of mean or median. In many cases, it makes sense that the gender pay gap be measured in terms of the mean, as opposed to the median. While the mean can be skewed by workers with either very high or very low earnings, the overrepresentation of women at the bottom of the earnings distribution and their underrepresentation at the top are central to the gender pay gap, as is the widening of the gender pay gap as one moves up the earnings distribution. Also, standard decomposition techniques used to identify the factors affecting the gender pay gap and the magnitude of their effects apply to the mean (see Appendix A). However, when undertaking a more thorough analysis of the gender pay gap, it is recommended that the earnings of women and men be examined at different points along the earnings distribution, including the median (see Appendix A).
No matter which measure is used, the unadjusted gender pay gap reflects, among other influences or “predictors,” gender differences in education, work experience, job tenure, occupation, and industry, as well as gender-based discrimination. Untangling the mechanisms that give rise to the gender pay gap requires the use of statistical control in the context of ordinary least squares regression or some other multivariate method of data analysis. Statistical control enables us to estimate earnings, holding constant the effects of various predictors. If the gender pay gap is calculated from these earnings, it is “adjusted.” Importantly, the adjusted gender pay gap reflects a hypothetical situation in which female and male workers are equally skilled and evenly distributed across occupations and industries. It should therefore not be interpreted as evidence that the gender pay gap is inconsequential or does not exist.
In this section, explanations for the gender pay gap, and changes in the relative importance of related predictors, are discussed. Considerations when studying trends in the gender pay gap are also presented. Although a brief overview of the statistical technique typically used to analyze the gender pay gap is provided here, readers are referred to Appendix A for more information.
3.1. Traditional economic explanations for the gender pay gap
Traditional economic explanations for the gender pay gap have largely focused on (1) gender differences in human capital, such as education, work experience and job tenture; (2) occupational/industrial gender segregation; and (3) gender differences in the treatment of similarly-qualified and similarly-positioned workers (i.e., discrimination). Note Note
3.1.1. Gender differences in human capital
Human capital refers to individuals’ stock of skills, knowledge, competencies, and other intangible assets, whether innate or acquired, that increase their productivity and therefore their economic value (i.e., earnings). Although many factors contribute to one’s human capital, education, work experience, and job tenure are among the most influential and amenable to measurement.
In the context of neoclassical economic theories of human capital and rational choice, women’s lower wages, relative to men’s, are understood as a consequence of their lower productivity. Note Women may be less productive in the workplace because of the traditional division of labour within the family, whereby women specialize in housework, childrearing, and caregiving, and men specialize in earning. Note Given these gender roles, women can rationally expect shorter and more discontinuous careers. Note Women therefore have less incentive to invest in market-oriented formal education and on-the-job training; they choose occupations and industries where such investments are less important and where pecuniary penalties for work interruptions are smaller; they avoid jobs that require significant investments in firm-specific skills, as returns to those investments accrue only as long as one remains with the firm; and they put less effort into their work. Note Note Note Also, these theories suggest that women’s human capital depreciates during their relatively frequent employment interruptions. Note Note
Contrary to the hypotheses of the human capital model, women in Canada have sustained a long-term trend toward higher education. Note Based on data from the Census of Population, the proportion of women aged 25 to 34 with at least a bachelor’s degree increased by 27.0 percentage points between 1986 and 2016, from 13.7% to 40.7%. Note The proportion of men in the same age group with at least a bachelor’s degree also increased, but to a lesser extent: 13.6 percentage points, from 15.5% in 1986 to 29.1% in 2016.
Although recent cohorts of women have surpassed the educational attainment of their male counterparts, gender differences persist in field of study. According to data from the 2016 Census of Population, women constituted the majority of Canadians aged 25 to 34 with at least a bachelor’s degree in all broad fields of study, with the exception of the lucrative engineering, computer science, and mathematics fields (Chart 5). Note

Data show, however, that women are more likely than men to experience work absences and interruptions—both long-term, scheduled absences related to childbearing and childrearing, and short-term, sporadic absences related to a child’s illness or a major household appliance in need of repair. Note Note Previous research documents the stigmatization of work absences and interruptions, as well as fewer subsequent promotion opportunities and earnings increases. Note Note
The 2011 General Social Survey on Families tracks respondents’ work history retrospectively, given its interrelationship with family transitions. In the context of this survey, a work interruption is defined as being away from work for more than three months since the respondent first started working for a period of six months or longer. A greater proportion of men aged 15 years and older than women had either no work interruptions (67.3% vs. 31.6%) or one work interruption (32.1% vs. 24.3%). Conversely, more women than men had two or more work interruptions (36.3% vs. 8.3%). Given women’s greater number of work interruptions, relative to men, they had an average of three fewer years of work experience (19.6 vs. 22.7 years).
In addition to having less work experience, women tend to have slightly less job tenure—that is, fewer consecutive months or years working for their current (or most recent) employer. Based on data from the Labour Force Survey, employed women aged 15 years and older had an average of 100.2 months with their current employer in 2017—6.2 months fewer than men.
3.1.2. Occupational/industrial gender segregation
Occupational gender segregation, also known simply as “gender segregation,” refers to the uneven distribution of women and men within and across occupations. It takes two forms—vertical segregation and horizontal segregation—both of which contribute to the gender pay gap. Vertical segregation describes the concentration of women at the bottom of the occupational hierarchy and men at the top, and it is suggestive of the extent to which women face obstacles to career advancement that ultimately prevent them from reaching highly-paid positions (i.e., the proverbial "glass ceiling"). Note Note Using data from the Labour Force Survey, occupations (four-digit National Occupational Classification) were sorted in ascending order by average hourly wages. In this context, 14.4% of women aged 15 and older had an occupation with average hourly wages in the bottom 20% of the occupational distribution in 2017, compared with 9.6% of men. On the other hand, 1.6% of women had an occupation with average hourly wages in the top 20% of the occupational distribution in 2017, compared with 2.7% of men.
In addition to women’s underrepresentation in higher-status occupations, the gender pay gap may be greater at the top of the occupational hierarchy than the bottom. Previous research demonstrates that wage inequality between women and men is greater and declined more slowly over time at the top of the wage distribution than the bottom. Note Note Note Note Note Note Note Based on data from the Labour Force Survey, the unadjusted gender pay ratio at the highest end of the wage distribution (i.e., the 95th percentile) was 0.86 in 2017, compared with 0.97 at the lowest end (i.e., the 5th percentile). These findings suggest that, even within higher-status occupations, women may encounter glass ceilings that prevent them from reaching the earnings of the highest-paid men.
Horizontal segregation refers to the concentration of women and men in different occupations and industries. It affects the gender pay gap to the extent that female-dominated occupations and industries are paid less than male-dominated occupations and industries, even when they involve the same skill levels.
Although breadwinning has become a central and enduring role for most women in Canada, their employment often parallels traditional female gender roles. Note In other words, what is typically designated as "women’s work" in the private sphere tends to be designated as such in the public sphere as well. Note
Many women in Canada are employed in traditionally-female occupations—teaching, nursing and related health occupations, social work, clerical or other administrative positions, or sales and services—in which women have been concentrated historically. This is reflected in Table 2, showing the proportion of women and men aged 15 years older in the 20 occupations (of 140, based on the three-digit National Occupational Classification) with the greatest concentration of women in 2017. About 27% of women were employed in these occupations, many of which involve the “5 Cs” of caring, clerical, catering, cashiering, and cleaning. In contrast, 5.2% of men were employed in the 20 occupations with the greatest concentration of women. Conversely, 11.5% of men were in the 20 occupations men in 2017, as were less than one percent of women (Table 3). In addition to illustrating how women’s paid work tends to parallel their unpaid work, these findings demonstrate that women are concentrated in sex-typed occupations to a greater extent than men (27.1% vs. 11.5%).
In a similar vein, in 2017, the three industries (of 20, based on the two-digit North American Industry Classification System) with the greatest share of women were (1) health care and social assistance, (2) educational services, and (3) accommodation and food services. The proportion of women aged 15 years and older who worked in these three industries combined was 40.1%, compared with 14.1% of men. By comparison, the three industries with the greatest share of men were (1) construction, (2) forestry, fishing, mining, oil and gas, and (3) manufacturing. The proportion of men who worked in these three industries combined was 23.2%, compared with 5.1% of women. As with occupation, women were concentrated in sex-typed industries to a greater extent than men (40.1% versus 23.2%).
Female-dominated occupations and industries tend to be compensated less than male-dominated ones—even when they involve the same skill level. Note For example, using data from the 2017 Labour Force Survey, the effect of being employed in a female-dominated occupation (at the 4-digit level of the National Occupational Classification) on the (log) hourly wages of workers aged 15 and older was estimated. Workers of both sexes employed in occupations in which 90% of incumbents were female earned an average of $6.60 less per hour than workers employed in occupations in which 10% of incumbents were female ($29.78 vs. $36.38). The negative effect of being employed in a female-dominated occupation on hourly wages remained the same when educational attainment was controlled.
Why do women end up in different occupations than men? There are both “supply (employee)-side” and “demand (employer)-side” processes at play. The former pertains to employees’ tastes and preferences for different kinds of work, while the latter pertains to employers’ greater demand for workers of a certain sex and differential valuation of occupations depending on their gender composition. Note
According to some theories, individuals act in rational ways, and therefore select courses of action that they believe will lead to the most desirable outcome. Note Individuals’ preferences influence the means that they choose to pursue their goals. Note Given social norms and cultural expectations that assign primary responsibility for housework, childrearing, and caregiving to women, and primary responsibility for breadwinning to men, women may place more importance on the non-pecuniary attributes of occupations than men. Note Note Note They may therefore trade off higher earnings for occupations that require less time, energy, and emotional input, in order to conserve these resources for their family responsibilities; occupations that engender greater intrinsic, altruistic, and social rewards; and/or occupations that offer amenities like temporal flexibility that facilitate work-family balance. Note Note Note Note
Gender differences in psychological attributes may also make some occupations more or less attractive to women, relative to men. Note For example, previous research demonstrates that women tend to be more risk averse and less competitive than men. Note Note These psychological attributes may lead women and men to choose occupations that differ with respect to job security, earnings stability, injury and fatality risk, performance evaluation, and competitiveness. Note To the extent that these occupational characteristics are associated with higher earnings, gender differences in risk aversion and competitiveness may contribute to the gender pay gap through choice of occupation. Note
Employers’ conscious or unconscious preferences for employees of a particular sex for certain occupations can also generate and maintain occupational segregation through their effects on hiring and promotion. Note Note However, formal human resource practices and policies may limit the extent to which employers’ discretion, and therefore gender bias come into play in occupational placement and advancement. Note
Why do occupations dominated by women pay less than those dominated by men? The two main alternative accounts of the relationship between gender segregation and gender-based wage inequality are (1) “crowding” and (2) the cultural devaluation of women’s work. Both imply discrimination by employers. The crowding hypothesis posits that the exclusion of women from stereotypically “male” occupations through employers’ discriminatory hiring and promotion practices results in an oversupply of labour for “female” occupations, thereby depressing the wages of equally-productive workers. Note Note
The theory of the cultural devaluation of women’s work posits that female-dominated occupations are under-rewarded because of their association with women. Note Note This theory suggests that women, as a group, tend to be valued less than men in the cultures of western industrialized countries, so anything associated with them, including occupations, are valued less by extension. Note Note Note Note Female-dominated occupations also tend to involve temporal flexibility (e.g., part-time work and telework) that does not conform to the organizational norms (i.e., full-time work, overtime, and a constant presence, except when travelling for work), which are predicated on male patterns. Note Note As England explains, this is a form of discrimination whereby “employers see the worth of predominantly female jobs through biased lenses and, as a result, set pay levels for both men and women in predominantly female jobs lower than they would be if the jobs had a more heavily male sex composition.” Note This account of the relationship between gender segregation and gender-based wage inequality is supported by previous research, which shows that the average earnings within occupations decrease as the proportion of women within occupations increases. Note Note
3.1.3. Gender differences in the treatment of similarly-qualified and similarly-positioned workers
Even with the same amount of human capital and employment in the same occupations and industries, women and men may be paid differently due to gender-based discrimination. Note Standard economic models suggest that discrimination can arise in a variety of ways. As per Becker, employers, coworkers, and customers or clients may have personal prejudice against members of particular groups, manifested in a desire to maintain social distance from them. Note Such prejudice will cause a wage differential between women and men when
Discriminatory employers will only hire women at a sufficient wage discount that compensates them for the disutility of employing women. Discriminatory male workers will demand a wage premium to work with women, thus raising men’s relative wages, and the reluctance of discriminatory customers or clients to buy goods or services provided by women will make women less productive in terms of revenue brought in, thus depressing their relative wages. Note
In theory, competitive forces should reduce or eliminate employer discrimination in the long run because the least discriminatory firms, which would hire more female workers because the price of their labour is less than that of male workers, would have lower costs of production and should therefore drive the more discriminatory firms out of business. Note However, discrimination has persisted, and “statistical discrimination” has been used to explain its persistence. Given employer uncertainty about either the productivity of prospective employees or the reliability with which their productivity can be predicted, employers observe prospective employees’ group identity (e.g., sex/gender, Aboriginal status, immigrant status, visible minority status, and/or disability status), and then make hiring, placement, or remuneration decisions on the basis of the expected or “average” level of productivity of that group. Note Employers may discriminate on the basis of that average. Note
3.2. Determining the relative importance of various explanations for the gender pay gap: Decomposition
Faced with the unadjusted gender pay gap, we usually want to know how much of that gap is attributable to gender differences in human capital, occupation, and industry. To make this determination, researchers often begin by using ordinary least square regression to model the logarithmic (log) earnings of workers as a function of sex—coded as one for women and zero for men—and other predictors. Note The baseline model usually includes only the indicator for sex, such that its coefficient represents the unadjusted difference in the log earnings of female and male workers, with a negative number indicating that the former earn less than the latter on average. Other predictors of log earnings are added in successive models. In these models, the regression coefficient for sex measures the extent to which the log earnings of female and male workers are different—all other predictors in the model being equal (i.e., statistically controlled). In this way, what the gender pay gap would be were it not for gender differences in various characteristics can be determined. For example, based on Labour Force Survey data, the unadjusted gender log wage gap among workers aged 15 years and older was -0.13 in 2017, while the adjusted gender log wage gap was -0.11 (net of province, human capital, union status, public-sector employment, occupation, and industry).
The contribution of human capital, occupation, and industry to explanation of the unadjusted gender pay gap can be then be more formally estimated using the Oaxaca-Blinder decomposition method (see Appendix A for a more technical discussion of this method). After estimating the log earnings of women and men separately as a function of human capital and other productivity-enhancing characteristics, a counterfactual exercise is undertaken, whereby one implicitly asks: what would women’s average earnings be if they received the same remuneration to their human capital, occupation, and industry as men? The portion of the gender pay gap that is “explained” by compositional differences between women and men in terms of their human capital, occupation, and industry is then calculated by subtracting women’s average earnings under the counterfactual scenario from men’s average earnings. The “unexplained” portion of the gender pay gap is the difference between the counterfactual earnings of women and their actual earnings, capturing different returns to women’s and men’s human capital, occupation, and industry. Although this portion of the gender pay gap is typically used as an estimate of gender-based discrimination in the labour market, it also includes the effects of omitted predictors of the gender pay gap. Note
An important caveat regarding these empirical methods for analyzing the gender pay gap is that the predictors do not “explain” earnings inequality between women and men in a social or cultural sense. Note Each predictor is embedded in several layers of context that shape its meaning and effect on earnings. Note In other words, each predictor is itself complicated and requires explanation. Note Also, seemingly neutral predictors may incorporate elements of the discriminatory gender system, and therefore the distinction between the explained and unexplained portion of the gender pay gap is imprecise. Note Gender differences in education and occupation, for example, themselves need to be understood as the outcomes of gendered processes that channel women and men toward different types of degrees, fields of study, and jobs.
What proportion of the gender pay gap in Canada is explained vs. unexplained? As seen in Section 2.4, the gender wage gap in Canada has narrowed over time, although the pace slowed considerably during the 1990s. There is no single, ongoing source of Canadian data on hourly wages for an extended period of time prior to 1997, when the Labour Force Survey began collecting these data. Note For this reason, the gender wage gap among all workers aged 15 years and older from the late 1990s onward is analyzed here (Table 4). Nearly half of the decrease in the gender wage gap that occurred between 1997 and 2017 was explained by changes in the relative characteristics of women and men. Particularly important in this regard were occupation and education. Specifically, the increased representation of women in high-paying managerial and professional occupations was the driving force behind the narrowing of the gender wage gap, followed by the increased proportion of women with a university degree at the bachelor level or above. These two factors are related, as a university degree is typically required for managerial and professional occupations. Note
While less important to explaining the decrease in the gender wage gap that occurred between 1997 and 2017, women’s increased job tenure, the reduced proportion of men with union coverage, and the increased proportion of women employed in the well-paid public sector and the decreased proportion of men employed there also played a role. Note
Notably, as the gender wage gap has narrowed over time, what remains is increasingly unexplained. Nearly 70% of the gender wage gap in 2017 could not be explained by gender differences in province, human capital, union status, public-sector employment, occupation, and industry. By comparison, focusing of full-time workers aged 25 to 54, Baker and Drolet find that 61% of the gender wage gap in 1981 was unexplained by a similar model specification. Note In effect, as women have surpassed men in terms of educational attainment and increased their representation in managerial and professional occupations, the remaining gender wage gap has largely become an issue of the unequal returns to such characteristics that women and men receive in the labour market.
3.3. Explanations for the remaining gender pay gap
As compositional differences between women and men in terms of human capital and occupation have been vastly reduced, if not eliminated (i.e., the gender education gap), it has become necessary to consider other explanations for the remaining gender pay gap. In addition to discrimination (previously discussed), motherhood earnings penalties, the high “price” of temporal flexibility in certain occupations, and gender differences in personality traits, psychological attributes, and non-cognitive skills are plausible candidates.
3.3.1. Motherhood earnings penalties
Previous research demonstrates that the gender pay gap is virtually non-existent during early adulthood, but increases with age thereafter (see Section 3.4.3). Note Note It also demonstrates that childless women have higher earnings than women with children; the earnings of childless women are almost equal to those of their male counterparts; and men with children earn as much, if not more, than childless men. Note Note Note Note Note Note Taken together, these findings are suggestive of gender differences in the effects of parenthood on earnings. Specifically, women with children experience “motherhood earnings penalties,” while men with children do not, and may even benefit from “fatherhood earnings premiums.” Note
There are a number of ways that motherhood can negatively affect women’s earnings, both before and after having children. Women may pre-emptively invest less in human capital or select family-friendly, but lower-paying, career paths in anticipation of becoming a mother. Note Note In response to becoming a mother, women may reduce their work hours or switch their occupation or firm to a more child-friendly one. Note Note Note Now that women invest in human capital to a similar extent as men and they have increased their representation in higher-status occupations, post-motherhood effects may be more important for understanding motherhood earnings penalties than pre-motherhood effects. In other words, women may largely be incurring earnings penalties after they have children, rather than before.
How does women’s employment change after they have children? The transition to parenthood tends to move previously-egalitarian couples toward a more traditional gendered division of labour. Note Note Note For this reason, mothers often adjust their labour supply in a downward fashion—leaving the workforce, temporarily or permanently, or reducing their work hours if they remain employed—to accommodate their greater responsibility for childrearing, relative to fathers. Note Note These strategies negatively affect earnings in both the short-term, by reducing time spent at work, and the long-term, by reducing work experience and job tenure. Note However, previous research demonstrates that motherhood earnings penalties persist after controlling for differences in overall work experience and full-time and part-time work experience. Note Note
Mothers may also be less productive at, or committed to, work—or perceived to be so by employers (i.e., statistical discrimination)—insofar as their family responsibilities leave them exhausted and/or distracted, or they put less effort forth at work in order to conserve their energy for later use at home. Note Note Exploring this issue, Kmec finds that mothers and fathers in the United States in the mid-2000s were similar on five out of seven “pro-work” behaviours and conditions: work effort; frequency with which the respondent’s responsibilities at home reduced his/her work effort; the extent to which the respondent’s home life helps with him/her relax and feel ready for the next day’s work; the frequency with which the respondent’s activities and chores at home prevented him/her from getting the needed amount of sleep to do his/her job well; and the frequency with which providing what was needed at home made the respondent work harder at his/her job. Note When they differed, mothers reported greater job engagement and work intensity than fathers. Note Further, mothers were no different from childless women and men on all outcomes. Note
Given mother’s disproportionate responsibility for childrearing, it may be the case that they trade off higher earnings for greater “non-pecuniary amenities” that enable to them balance work and family life, such as less demanding tasks, flexible work hours, few demands for travel or weekend or evening work, and/or on-site childcare. Note Note This mechanism, operating through occupation, industry and/or firm, is elaborated below.
3.3.2. Trading off earnings for temporal flexibility
The majority of the current gender pay gap in Canada derives from earnings inequality between women and men within occupations, as opposed to the uneven distribution of women and men across occupations. Note Based on similar findings for the United States, Goldin argues the gender pay gap is wider in some occupations than others, largely due to differences in the importance attributed to long work hours, set schedules and “face time,” and job tenure. Note Some occupations, particularly in the corporate, financial, and legal worlds, have high penalties for even short work interruptions, and earnings that increase disproportionately or “non-linearly” with hours worked. Note In these occupations, temporal flexibility—fewer and more discretionary work hours—comes at a high price in terms of earnings. Note Due to women’s greater family responsibilities, they may desire more temporal flexibility than men, and they are therefore willing to trade off earnings for that amenity, contributing to gender-based earnings inequality within occupations. Note
In a similar vein, Cha and Weeden suggest that the increasing prevalence of long work hours, defined as 50 or more hours per week, and rising earnings returns to overwork contribute to the gender pay gap, as men are more likely than women to perform long work hours. Note Notably, they demonstrate that the effects of overwork on trends in the gender pay gap in the United States between 1970 and 2009 were most pronounced in managerial and professional occupations, where long work hours are particularly common and the norm of overwork is deeply embedded in organizational practices and occupational cultures.
3.3.3. Gender differences in personality traits, psychological attributes, and non-cognitive skills
While it is unclear whether gender differences in personality traits, psychological attributes, and non-cognitive skills are the product of genetics, culture, or a combination of the two, studies have found that, compared with men, women tend to place a lower value on money; to be less willing to negotiate and compete; to be more risk averse; to have lower self-esteem; to be less self-confident; and to be more agreeable. Note Personality traits, psychological attributes, and non-cognitive skills may affect earnings directly, by increasing productivity like other forms of human capital, and/or indirectly, by influencing human capital, occupation, and industry. Note
Notably, the same personality trait, psychological attribute, or non-cognitive skill in women and men may be rewarded differently in the labour market. For example, Mueller and Plug find that women’s greater agreeableness (i.e., being more trusting, straightforward, altruistic, compliant, modest, and sympathetic), relative to men, is not related to their wages. Note However, men earned a premium for being disagreeable. Note There is also anecdotal and empirical evidence that women are often in a no-win situation at work, in that they face potential penalties for behaving in gender-stereotypical ways (e.g., not negotiating over salaries, raises, or promotions), but, if they try to act more like men, they may elicit negative or less positive responses than men, as they are behaving in “unfeminine” ways. Note Note
3.4. Considerations when studying trends in the gender pay gap
When analyzing and interpreting trends in the gender pay gap, whether using annual earnings or hourly wages, it is necessary to consider changes over time in both the characteristics of workers and the prevailing macroeconomic conditions. Specifically:
- Female labour force participation has increased dramatically in the past 60 years, and therefore the composition of the female workforce—that is, the characteristics of employed women—may have changed in non-random ways (i.e., selection into employment based on shared characteristics). Note
- Meaningful comparisons of earnings at different time points require that observed earnings be adjusted for inflation, such that they are expressed in constant or “real” dollars. Note
- Earnings are affected by the business cycle—the pattern of expansion, contraction, and recovery in the economy—in gendered ways. There is evidence from the United States that women’s earnings decrease, relative to men’s, during business-cycle expansions (i.e., the gender pay gap increases) and increase during recessions (i.e., the gender pay gap decreases). Note
- Technological changes, particularly advances in information and computer technologies, and globalization have affected the wage structure—that is, returns to human capital, occupation, and industry—and contributed to increasing earnings inequality in Canada since the 1980s. Note Note Note Given gender differences in human capital and occupational/industrial gender segregation, technological changes may have affect women’s and men’s earnings differently.
- The gender pay gap at a given time simultaneously reflects age, period, and cohort effects. Understanding trends in the gender pay gap therefore requires that these effects be distinguished.
This section explores the implications for trends in the gender pay gap of (1) selection into employment, (2) overall earnings inequality and the wage structure, and (3) age, period and cohort effects, as these issues have received considerable attention in the academic literature.
3.4.1. Selection into employment
Labour force participation is central to understanding the gender pay gap, both because receipt of earnings is conditional on employment, and because work experience is a predictor of earnings. Note Given that earnings data are only available for workers, and additional restrictions may be placed on the analytic sample of workers (i.e., workers employed on a full-time, full-year basis), it is possible that observed earnings reflect a non-random or self-selected group of individuals that chose paid work over staying home. Note Selection bias has generally been considered to be more influential for women’s earnings than men’s, as women are less likely than men to participate in the labour market. Note Note As Blau and Kahn explain, “…the closer the wage sample is to 100 percent of the underlying population, the smaller the selection bias.” Note
Women with the potential to earn more in the labour market due to their human capital, who therefore face higher opportunity costs to staying home in the form of foregone wages, may be more likely to be employed (i.e., positive selection). Note Note Furthermore, if women receive lower returns to their human capital, occupation, and industry than men, they may rationally and disproportionately choose not to enter the labour market. In this case, the observed gender pay gap will be understated or biased downward (and the gender pay ratio will be biased upward), relative to the corrected estimate.
Alternatively, women with the potential to earn more in the labour market may be less likely to be employed (i.e., negative selection). It is well-established that individuals tend to marry/cohabit with those who are similar to themselves in terms of education (i.e., “educational homogamy”), due to both preferences and greater opportunities to meet such spouses/partners by virtue of attending the same type of educational institutions. Note Note Note Note Note Given that earnings are positively associated with education, educational homogamy implies that women with more education are better positioned financially to remain home, insofar as their spouse/partner has relatively high earnings. In this case, the observed gender pay gap will be overstated or biased upward (and the gender pay ratio biased downward), relative to the corrected estimate.
As the labour force participation of women has increased and women have become more similar to men with respect to labour supply and human capital, scholars have questioned the continuing relevance of selection bias to the gender pay gap. Note Note Using a statistical technique to control for selectivity (i.e., Heckman’s method), as outlined in Appendix B, the gender wage gap among workers aged 15 and older in 2017 was estimated from the Labour Force Survey. Note There was no evidence that the gender wage gap was affected by selection bias.
When studying longer time series (or different geographies), correcting for selection bias may be necessary to make valid inferences. Note Note Women’s employment has increased dramatically since the 1960s, and therefore the characteristics of employed women may be different in 2017, as compared to say 1977, in ways that affect estimates of women’s earnings and the gender pay gap (Chart 6). Note The characteristics of employed women may also change over time, even if female labour force participation remains constant, in response to changes in earnings returns to human capital, occupation and industry (see Section 3.4.2). For example, Mulligan and Rubinstein provide evidence that the selection of women into full-time, full-year employment in the United States shifted from negative in the 1970s to positive in the 1990s. Note Further, they demonstrate that the majority of the apparent narrowing of the gender pay gap in the United States during that period reflected these changes in the characteristics of employed women. Note Baker, Benjamin, Cegep, and Grant reach the same conclusion regarding the gender pay gap in Canada between 1970 and 1990. Note

3.4.2. Overall earnings inequality and wage structure
Since 1980, earnings inequality (i.e., dispersion) in Canada has increased significantly, as the real earnings of individuals at the top of the earnings distribution have risen dramatically. Note Note At the same time, the earnings of middle-income earners have stagnated, and the earnings of low-income earners have fallen. Note Increasing earnings inequality tends to offset to some extent trends toward reducing the gender pay gap, as women are underrepresented at the top of the earnings distribution and overrepresented at the bottom. Note Note Note In effect, women have been “swimming upstream” by narrowing the gender pay gap despite economy-wide forces working against them. Note
Changes in the wage structure—that is, changes in returns to human capital, occupation, and industry—have contributed to increasing earnings inequality. Note New technologies, particularly computers in the workplace, tend to enhance productivity and therefore earnings in high-skill occupations involving cognitive tasks (e.g., managerial and professional occupations), while replacing workers who perform routine tasks (often found in middle-skill secretarial and clerical occupations), resulting in lower demand and earnings for those workers. Note Note Although low-skill sales and services occupations that entail non-routine tasks and personal interaction are less likely to be affected by greater use of computers, earnings in these occupations may decrease as job loss in middle-skilled occupations and manufacturing increases the supply of available workers. Note Note To the extent that these changes favor men over women or vice versa, given gender differences in human capital and occupational/industrial gender segregation, they may affect trends in the gender pay gap. Note
While weekly-wage returns to work experience have not changed in Canada since the 1980s, Foley and Green demonstrate that wage returns to education increased throughout the 1980s and 1990s, even as the supply of more educated workers grew. Note This pattern can be expected to have benefited women to a greater extent than men, as the share of university graduates rose faster among women than men after 1990. Note
In the 2000s, wage returns to university degrees, relative to high school diplomas, declined among men, and the trend toward increasing educational attainment stalled. Note Foley and Green attribute these findings to the resource (oil and gas) boom in western and Atlantic Canada, in that it increased demand for middle- and low-skilled workers, particularly young men, thereby increasing their wages. Note Notably, this shift in demand can be expected to have benefitted men, relative to women. However, in the wake of lower oil prices, Foley and Green predict that demand for workers in the resource sector will decline. Note
According to Lemieux and Riddell, certain occupations and industries drove the growth in top incomes in Canada (around 80% of which came from earnings) between 1981 and 2011. Note Specifically, senior managers in the finance and insurance industry, individuals in business and finance occupations, and individuals in natural and applied sciences occupations came to represent a much larger proportion of the top 1 percent, and their incomes grew much more rapidly than those of other top-income earners. Note The share of top earners also increased significantly in oil and gas extraction, while it decreased in manufacturing. Note Given women’s underrepresentation in leadership positions, STEM occupations, and resource extraction, increasing returns to these occupations and industries is increasingly unfavorable to women, and puts them at a growing disadvantage when it comes to narrowing the gender pay gap. Note
3.4.3. Age, period and cohort effects
It is well-established that the wages of young women and men are more equal than the wages of older women and men, and that the gradual convergence of the gender pay gap over time is driven by the replacement of older cohorts by younger ones. Note Note Note Note In order to understand these patterns, the effects of age, period, and cohort must be distinguished. In the simplest terms, age effects refer to variations produced by the physiological or social processes of aging. Note Studies consistently show that the gender pay gap is minimal at the time of entry into the labour market, but grows as women and men age and, in the process, form families (Table 5, reading across the rows). Note Since women’s employment is shaped to a greater extent by their caregiving roles and/or employers’ presumptions of these roles than men’s, women’s wages are more affected by growing older than men’s. Note As they age, women work fewer hours outside of the home than men, and they experience more work interruptions and absences related to childcare. Note Women are also less likely than men to have high-wage jobs with promotional opportunities, and they may face barriers to advancement. Note
There is evidence that the gender wage gap is not increasing with age among recent cohorts, as it did among their predecessors. Note In other words, the negative association between aging and the gender pay ratio is weakening over time. Note Based on data from the Labour Force Survey, in 1997, the gender wage ratio was 16.4 percentage points lower among seniors aged 65 years and older (0.78) than it was among 15-to-19-year-olds (0.94). By 2017, the difference between the gender wage ratio of younger and older workers was reduced to 5.6 percentage points. These findings suggest that cohort replacement drives the convergence of the gender pay gap over time: as younger cohorts replace older ones, the overall gender pay gap decreases simply because the gender pay gap is smaller among younger cohorts than older ones. Note
Period effects refer to variations produced by events that simultaneously affect all ages and cohorts. Note A number of dramatic social and economic changes have occurred in Canada since the 1970s, which may have contributed to the decrease in the gender pay gap over time. For example, the federal government has offered more legal protections against gender-based discrimination (e.g., Employment and Pay Equity legislation), and gender attitudes have become more egalitarian. Note At the same time, broad structural changes in the Canadian economy that have disproportionally affected men—such as the shift away from manufacturing toward the provision of services, and declining union coverage rates—have worsened their labour market position, thereby reducing the gender pay gap. Note
Period effects are difficult to separate from cohort effects: variations produced by being born at a particular time, within a particular social context. Note Recent cohorts of women have more education, work experience, and representation in higher-status occupations than their predecessors. They also have greater control over their fertility and more egalitarian personal relationships. Note For these reasons, the gender wage gap has decreased across successive cohorts (Table 5, reading down the columns), indicating that each new cohort of women has fared better than the previous one. Note
Although the narrowing of the gender pay gap is primarily related to the entry of new cohorts, within-cohort earnings growth has also played a role for older cohorts. Note The relatively high gender wage ratios for younger women (under the age of 35) tend to decline as they age (Table 5, reading diagonally), largely due to women’s tendency to interrupt or discontinue their labour force participation for family reasons. However, the gender wage ratios of older women (aged 35 years and older) tend to increase as they age, likely because the demands of motherhood ease as children mature.
The unadjusted gender pay gap is an important indicator of gender equality and women’s economic empowerment. Decisions made by analysts regarding who estimates are based upon (i.e., age and labour supply), what earnings are counted (i.e., annual earnings vs. hourly wages), and how typical earnings are calculated (i.e., average or median) affect the size of the gender pay gap and its perceived seriousness.
Whichever measure of the unadjusted gender pay gap is used, it reflects, among other predictors, gender differences in human capital, occupation, and industry, as well as gender-based discrimination. Statistical control in the context of ordinary least squares regression enables estimation of the gender pay gap adjusted for the effects of various predictors. The adjusted gender pay gap reflects a hypothetical situation in which female and male workers are equally skilled and evenly distributed across occupations and industries. It should therefore not be interpreted as evidence that the gender pay gap is inconsequential or does not exist.
Traditional economic explanations for the gender pay gap focus on gender differences in human capital, occupation and industry, and returns to these characteristics (i.e., discrimination). Women’s increased educational attainment, both overall and relative to men, stronger attachments to the labour market over the life course, and greater representation in managerial and professional occupations have contributed to the narrowing of the gender pay gap over time. However, the remaining gender pay gap is largely unexplained by gender differences in human capital, occupation, and industry, making it necessary to consider other explanations. In addition to gender-based discrimination, motherhood penalties, the high “price” of temporal flexibility in certain occupations, and gender differences in personality traits, psychological attributes, and non-cognitive skills are plausible candidates.
It is important to recognize that, in the context of ordinary least squares regression and Oaxaca-Blinder decomposition, predictors of the gender pay gap do not “explain” it in a social or cultural sense. Gender differences in education and occupation, for example, themselves need to be understood as the outcomes of gendered processes that channel women and men toward different types of degrees, fields of study, and jobs.
Also, when analyzing trends in the gender pay gap, it is necessary to consider changes over time in both the characteristics of workers and the prevailing macroeconomic conditions, including the degree and direction of selection bias, overall earnings inequality, and age, period and cohort effects.
Oaxaca-Blinder decomposition
Faced with an unadjusted pay gap between women and men (on average), researchers typically endeavor to determine how much of that gap can be explained by gender differences in human capital and other productivity-related characteristics. To do so, they use the Oaxaca-Blinder decomposition method in the context of an ordinary least squares regression, estimating the logarithmic (log) mean wages of women and men separately, as a function of productivity characteristics (equations a and b). After estimating an earnings equation for women and one for men, a counterfactual exercise is undertaken, whereby one implicitly asks: what would women’s average earnings be if they received the same remuneration to their human-capital characteristics as men (equation c)? The portion of the gender pay gap that is explained by compositional differences between women and men with respect to their human-capital characteristics is then calculated by subtracting women’s average earnings under the counterfactual scenario from men’s average earnings (equation d). The unexplained portion of the gender pay gap is the difference between the counterfactual earnings of women and their actual earnings, capturing different returns to women’s human capital (equation e).
- Earnings equation for women: E ¯ w = α w + β w X ¯ w + μ w MathType@MTEF@5@5@+= feaagKart1ev2aqatCvAUfKttLearuatH9givLearmWu51MyVXgatC vAUfeBSjuyZL2yd9gzLbvyNv2CaeHbd9wDYLwzYbItLDharyavP1wz ZbItLDhis9wBH5garqqtubsr4rNCHbGeaGqiVu0Je9sqqrpepC0xbb L8F4rqqrFfpeea0xe9Lq=Jc9vqaqpepm0xbba9pwe9Q8fs0=yqaqpe pae9pg0FirpepeKkFr0xfr=xfr=xb9adbaqaaeGaciGaaiaabeqaam aaeaqbaaGcbaaeaaaaaaaaa8qacuWGfbqrpaGbaebadaWgaaWcbaWd biabdEha3bWdaeqaaOWdbiabg2da9iabeg7aH9aadaWgaaWcbaWdbi abdEha3bWdaeqaaOWdbiabgUcaRiabek7aI9aadaWgaaWcbaWdbiab dEha3bWdaeqaaOWdbiqbdIfay9aagaqeamaaBaaaleaapeGaem4DaC hapaqabaGcpeGaey4kaSIaeqiVd02damaaBaaaleaapeGaem4DaCha paqabaaaaa@4E32@
- Earnings equation for men: E ¯ m = α m + β m X ¯ m + μ m MathType@MTEF@5@5@+= feaagKart1ev2aqatCvAUfKttLearuatH9givLearmWu51MyVXgatC vAUfeBSjuyZL2yd9gzLbvyNv2CaeHbd9wDYLwzYbItLDharyavP1wz ZbItLDhis9wBH5garqqtubsr4rNCHbGeaGqiVu0Je9sqqrpepC0xbb L8F4rqqrFfpeea0xe9Lq=Jc9vqaqpepm0xbba9pwe9Q8fs0=yqaqpe pae9pg0FirpepeKkFr0xfr=xfr=xb9adbaqaaeGaciGaaiaabeqaam aaeaqbaaGcbaaeaaaaaaaaa8qacuWGfbqrpaGbaebadaWgaaWcbaWd biabd2gaTbWdaeqaaOWdbiabg2da9iabeg7aH9aadaWgaaWcbaWdbi abd2gaTbWdaeqaaOWdbiabgUcaRiabek7aI9aadaWgaaWcbaWdbiab d2gaTbWdaeqaaOWdbiqbdIfay9aagaqeamaaBaaaleaapeGaemyBa0 gapaqabaGcpeGaey4kaSIaeqiVd02damaaBaaaleaapeGaemyBa0ga paqabaaaaa@4DCE@
- Counterfactual equation for women: E ¯ w * = α m + β m X ¯ w + μ w MathType@MTEF@5@5@+= feaagKart1ev2aqatCvAUfKttLearuatH9givLearmWu51MyVXgatC vAUfeBSjuyZL2yd9gzLbvyNv2CaeHbd9wDYLwzYbItLDharyavP1wz ZbItLDhis9wBH5garqqtubsr4rNCHbGeaGqiVu0Je9sqqrpepC0xbb L8F4rqqrFfpeea0xe9Lq=Jc9vqaqpepm0xbba9pwe9Q8fs0=yqaqpe pae9pg0FirpepeKkFr0xfr=xfr=xb9adbaqaaeGaciGaaiaabeqaam aaeaqbaaGcbaaeaaaaaaaaa8qacuWGfbqrpaGbaebadaqhaaWcbaWd biabdEha3bWdaeaapeGaeiOkaOcaaOGaeyypa0JaeqySde2damaaBa aaleaapeGaemyBa0gapaqabaGcpeGaey4kaSIaeqOSdi2damaaBaaa leaapeGaemyBa0gapaqabaGcpeGafmiwaG1dayaaraWaaSbaaSqaa8 qacqWG3bWDa8aabeaak8qacqGHRaWkcqaH8oqBpaWaaSbaaSqaa8qa cqWG3bWDa8aabeaaaaa@4EE7@
- “Explained” variation: E ¯ m − E ¯ w * MathType@MTEF@5@5@+= feaagKart1ev2aqatCvAUfKttLearuatH9givLearmWu51MyVXgatC vAUfeBSjuyZL2yd9gzLbvyNv2CaeHbd9wDYLwzYbItLDharyavP1wz ZbItLDhis9wBH5garqqtubsr4rNCHbGeaGqiVu0Je9sqqrpepC0xbb L8F4rqqrFfpeea0xe9Lq=Jc9vqaqpepm0xbba9pwe9Q8fs0=yqaqpe pae9pg0FirpepeKkFr0xfr=xfr=xb9adbaqaaeGaciGaaiaabeqaam aaeaqbaaGcbaaeaaaaaaaaa8qacuWGfbqrpaGbaebadaWgaaWcbaWd biabd2gaTbWdaeqaaOWdbiabgkHiTiqbdweaf9aagaqeamaaDaaale aapeGaem4DaChapaqaa8qacqGGQaGkaaaaaa@4251@
- “Unexplained” variation: ¯ E w * − ¯ E w MathType@MTEF@5@5@+= feaagKart1ev2aqatCvAUfKttLearuatH9givLearmWu51MyVXgatC vAUfeBSjuyZL2yd9gzLbvyNv2CaeHbd9wDYLwzYbItLDharyavP1wz ZbItLDhis9wBH5garqqtubsr4rNCHbGeaGqiVu0Je9sqqrpepC0xbb L8F4rqqrFfpeea0xe9Lq=Jc9vqaqpepm0xbba9pwe9Q8fs0=yqaqpe pae9pg0FirpepeKkFr0xfr=xfr=xb9adbaqaaeGaciGaaiaabeqaam aaeaqbaaGcbaGaeg4Ra8keaaaaaaaaa8qacqWGfbqrpaWaa0baaSqa a8qacqWG3bWDa8aabaWdbiabcQcaQaaakiabgkHiT8aacqyGVcWRpe Gaemyrau0damaaBaaaleaapeGaem4DaChapaqabaaaaa@470E@
Change in the unadjusted gender pay gap over time (equation f) can also be decomposed into an explained portion attributable to changes in the average human capital of women and men (equation g), and an unexplained portion attributable to changes in women’s and men’s returns to human capital (equation h). Note
- Change in the gender pay gap between two time points (t and t-1): ( E ¯ m t − E ¯ m t − 1 ) − ( E ¯ f t − E ¯ f t − 1 ) MathType@MTEF@5@5@+= feaagKart1ev2aqatCvAUfKttLearuatH9givLearmWu51MyVXgatC vAUfeBSjuyZL2yd9gzLbvyNv2CaeHbd9wDYLwzYbItLDharyavP1wz ZbItLDhis9wBH5garqqtubsr4rNCHbGeaGqiVu0Je9sqqrpepC0xbb L8F4rqqrFfpeea0xe9Lq=Jc9vqaqpepm0xbba9pwe9Q8fs0=yqaqpe pae9pg0FirpepeKkFr0xfr=xfr=xb9adbaqaaeGaciGaaiaabeqaam aaeaqbaaGcbaaeaaaaaaaaa8qadaqadaWdaeaapeGafmyrau0dayaa raWaaSbaaSqaa8qacqWGTbqBpaWaaSbaaWqaa8qacqWG0baDa8aabe aaaSqabaGcpeGaeyOeI0Iafmyrau0dayaaraWaaSbaaSqaa8qacqWG TbqBpaWaaSbaaWqaa8qacqWG0baDcqGHsislcqaIXaqma8aabeaaaS qabaaak8qacaGLOaGaayzkaaGaeyOeI0YaaeWaa8aabaWdbiqbdwea f9aagaqeamaaBaaaleaapeGaemOzay2damaaBaaameaapeGaemiDaq hapaqabaaaleqaaOWdbiabgkHiTiqbdweaf9aagaqeamaaBaaaleaa peGaemOzay2damaaBaaameaapeGaemiDaqNaeyOeI0IaeGymaedapa qabaaaleqaaaGcpeGaayjkaiaawMcaaaaa@5756@
- Explained variation between two time points: [ β m t ( X ¯ m t − X ¯ m t − 1 ) − β w t ( X ¯ w t − X ¯ w t − 1 ) ] MathType@MTEF@5@5@+= feaagKart1ev2aqatCvAUfKttLearuatH9givLearmWu51MyVXgatC vAUfeBSjuyZL2yd9gzLbvyNv2CaeHbd9wDYLwzYbItLDharyavP1wz ZbItLDhis9wBH5garqqtubsr4rNCHbGeaGqiVu0Je9sqqrpepC0xbb L8F4rqqrFfpeea0xe9Lq=Jc9vqaqpepm0xbba9pwe9Q8fs0=yqaqpe pae9pg0FirpepeKkFr0xfr=xfr=xb9adbaqaaeGaciGaaiaabeqaam aaeaqbaaGcbaaeaaaaaaaaa8qadaWadaWdaeaapeGaeqOSdi2damaa BaaaleaapeGaemyBa02damaaBaaameaapeGaemiDaqhapaqabaaale qaaOWdbmaabmaapaqaa8qacuWGybawpaGbaebadaWgaaWcbaWdbiab d2gaT9aadaWgaaadbaWdbiabdsha0bWdaeqaaaWcbeaak8qacqGHsi slcuWGybawpaGbaebadaWgaaWcbaWdbiabd2gaT9aadaWgaaadbaWd biabdsha0jabgkHiTiabigdaXaWdaeqaaaWcbeaaaOWdbiaawIcaca GLPaaacqGHsislcqaHYoGypaWaaSbaaSqaa8qacqWG3bWDpaWaaSba aWqaa8qacqWG0baDa8aabeaaaSqabaGcpeWaaeWaa8aabaWdbiqbdI fay9aagaqeamaaBaaaleaapeGaem4DaC3damaaBaaameaapeGaemiD aqhapaqabaaaleqaaOWdbiabgkHiTiqbdIfay9aagaqeamaaBaaale aapeGaem4DaC3damaaBaaameaapeGaemiDaqNaeyOeI0IaeGymaeda paqabaaaleqaaaGcpeGaayjkaiaawMcaaaGaay5waiaaw2faaaaa@64D7@
- Unexplained variation between two time points: [ X ¯ m t − 1 ( β m t − β m t − 1 ) − X ¯ f t − 1 ( β f t − β f t − 1 ) ] MathType@MTEF@5@5@+= feaagKart1ev2aqatCvAUfKttLearuatH9givLearmWu51MyVXgatC vAUfeBSjuyZL2yd9gzLbvyNv2CaeHbd9wDYLwzYbItLDharyavP1wz ZbItLDhis9wBH5garqqtubsr4rNCHbGeaGqiVu0Je9sqqrpepC0xbb L8F4rqqrFfpeea0xe9Lq=Jc9vqaqpepm0xbba9pwe9Q8fs0=yqaqpe pae9pg0FirpepeKkFr0xfr=xfr=xb9adbaqaaeGaciGaaiaabeqaam aaeaqbaaGcbaaeaaaaaaaaa8qadaWadaWdaeaapeGafmiwaG1dayaa raWaaSbaaSqaa8qacqWGTbqBpaWaaSbaaWqaa8qacqWG0baDcqGHsi slcqaIXaqma8aabeaaaSqabaGcpeWaaeWaa8aabaWdbiabek7aI9aa daWgaaWcbaWdbiabd2gaT9aadaWgaaadbaWdbiabdsha0bWdaeqaaa Wcbeaak8qacqGHsislcqaHYoGypaWaaSbaaSqaa8qacqWGTbqBpaWa aSbaaWqaa8qacqWG0baDcqGHsislcqaIXaqma8aabeaaaSqabaaak8 qacaGLOaGaayzkaaGaeyOeI0IafmiwaG1dayaaraWaaSbaaSqaa8qa cqWGMbGzpaWaaSbaaWqaa8qacqWG0baDcqGHsislcqaIXaqma8aabe aaaSqabaGcpeWaaeWaa8aabaWdbiabek7aI9aadaWgaaWcbaWdbiab dAgaM9aadaWgaaadbaWdbiabdsha0bWdaeqaaaWcbeaak8qacqGHsi slcqaHYoGypaWaaSbaaSqaa8qacqWGMbGzpaWaaSbaaWqaa8qacqWG 0baDcqGHsislcqaIXaqma8aabeaaaSqabaaak8qacaGLOaGaayzkaa aacaGLBbGaayzxaaaaaa@68CB@
Although there is no universally accepted set of predictors that should be included in a wage analysis, there are a number of widely-used predictors: personal characteristics, including presence of children in the household, age of the youngest child in the household, and province of residence; productivity-enhancing characteristics, including standardized test scores, highest level of educational attainment, work experience in quadratic terms, and job tenure; and work characteristics, including job permanence, class of worker (private or public sector), and union status. Note Note Presence of children and age of the youngest child in the household are intended to capture family responsibilities that can affect labour supply, but they may also be a harbinger of discrimination (i.e., motherhood earnings penalties).. These variables may also be considered to be endogenous to women’s decisions about their labour supply, and therefore exclude from analysis of the gender pay gap. Note
Province of residence controls for differences between local labour markets in wage structure and tightness (i.e., the number of job vacancies relative to the number of unemployed people). Note Standardized test scores , education, and work experience (typically approximated by age) are included in the gender wage gap decomposition as productivity-enhancing characteristics. Work experience squared captures diminishing returns to work experience as its duration lengthens. Job tenure with one’s current employer, as distinct from work experience, measures both job-specific knowledge and loyalty . Job permanence , class of worker, and union status account for differences in wage structures between different types of jobs (i.e., seasonal, temporary, and permanent jobs; private- and public-sector jobs; and unionized and non-unionized jobs).
Assuming that productivity-related differences between women and men are precisely measured, and that they are not themselves the product of discriminatory behavior by employers, the unexplained portion of the gender pay gap can be interpreted as evidence of discrimination. Note In effect, women and men with the same human capital are paid unequally on the grounds of a characteristic that has no direct effect on their productivity (i.e., gender). However, any analytic approach that relies on the statistical residual is open to question as to whether all of the relevant predictors were included in the regression. Note When human capital characteristics explain only a portion of the gender pay gap, the possibility exists that unmeasured productivity-related characteristics, such as effort, may be reflected in the residual. Note Note If men are more highly endowed with those characteristics than women, the extent of discrimination will be overestimated. Note Until recently, work experience has been particularly problematic in this regard. In the absence of retrospective data on work histories, as collected through the General Social Survey on Families until 2011, for example, “potential” experience is approximated by age or estimated by subtracting from respondents’ current age the number of years spent in school plus four (corresponding to the pre-school years). This solution tends to overestimate women’s actual experience because they have more frequent and longer lasting career interruptions than do men. Note However, as continuous labour force participation over the life course has become more typical among recent cohorts of women, relative to their predecessors, the accuracy of estimates of women’s potential experience has improved. Note Note
Additional and somewhat controversial predictors of the gender pay gap include field of study, occupation, and industry. Inclusion of any one of these characteristics (or all of them) in the analysis takes into account occupational/industrial gender segregation. Note However, if occupational/industrial gender segregation reflects gendered processes and gender-based discrimination, the unexplained portion of the gender pay gap will be underestimated when field of study, occupation, and/or industry are included. Given that these variables are controversial predictors of the gender pay gap, a worthwhile strategy is to present the results of a series of model specifications that alternately include and exclude them.
Gender pay gap at different points across the earnings distribution
Although most research focuses on the gender pay gap at the mean, it is worthwhile to examine the gender pay gap across the entire earnings distribution. Studies that have done so reveal that earnings inequality between women and men has decreased more at the bottom of the wage distribution than it has at the top of the wage distribution. Note Note Note Note Note Note Note
Decompositions can be performed at various points (i.e., percentiles) along the wage distribution. Doing so requires use of the unconditional quantile regression method, recently developed by Firpo, Fortin, and Lemieux Note Note and Fortin, Lemieux, and Firpo. Note This method involves finding women’s and men’s (log) wages at a given percentile (separately)—typically the 10th and/or 90th percentile, representing the bottom and top of the wage distribution, respectively. Each gender gap can then be decomposed as per the standard Oaxaca-Blinder approach, using a transformed dependent variable, known as the “re-centered influence function,” in the OLS regressions. For a given percentile ( j ), the recentered influence function (RIF) is computed for each individual ( i ) in the pooled male-female sample as such:
Where, w j MathType@MTEF@5@5@+= feaagKart1ev2aaatCvAUfKttLearuatH9givLearmWu51MyVXgatC vAUfeBSjuyZL2yd9gzLbvyNv2CaeHbd9wDYLwzYbItLDharyavP1wz ZbItLDhis9wBH5garqqtubsr4rNCHbGeaGqiVu0Je9sqqrpepC0xbb L8F4rqqrFfpeea0xe9Lq=Jc9vqaqpepm0xbba9pwe9Q8fs0=yqaqpe pae9pg0FirpepeKkFr0xfr=xfr=xb9adbaqaaeGaciGaaiaabeqaam aaeaqbaaGcbaaeaaaaaaaaa8qacqWG3bWDpaWaaSbaaSqaa8qacqWG QbGAa8aabeaaaaa@3DA6@ is the wage at a given percentile (j); { w i ≤ w j } MathType@MTEF@5@5@+= feaagKart1ev2aaatCvAUfKttLearuatH9givLearmWu51MyVXgatC vAUfeBSjuyZL2yd9gzLbvyNv2CaeHbd9wDYLwzYbItLDharyavP1wz ZbItLDhis9wBH5garqqtubsr4rNCHbGeaGqiVu0Je9sqqrpepC0xbb L8F4rqqrFfpeea0xe9Lq=Jc9vqaqpepm0xbba9pwe9Q8fs0=yqaqpe pae9pg0FirpepeKkFr0xfr=xfr=xb9adbaqaaeGaciGaaiaabeqaam aaeaqbaaGcbaWaaiWaaeaaqaaaaaaaaaWdbiabdEha39aadaWgaaWc baWdbiabdMgaPbWdaeqaaOWdbiabgsMiJkabdEha39aadaWgaaWcba WdbiabdQgaQbWdaeqaaaGccaGL7bGaayzFaaaaaa@44DC@ is an indicator function taking the value of one, if an individual’s wage is less than the wage at the j t h MathType@MTEF@5@5@+= feaagKart1ev2aaatCvAUfKttLearuatH9givLearmWu51MyVXgatC vAUfeBSjuyZL2yd9gzLbvyNv2CaeHbd9wDYLwzYbItLDharyavP1wz ZbItLDhis9wBH5garqqtubsr4rNCHbGeaGqiVu0Je9sqqrpepC0xbb L8F4rqqrFfpeea0xe9Lq=Jc9vqaqpepm0xbba9pwe9Q8fs0=yqaqpe pae9pg0FirpepeKkFr0xfr=xfr=xb9adbaqaaeGaciGaaiaabeqaam aaeaqbaaGcbaaeaaaaaaaaa8qacqWGQbGApaWaaWbaaSqabeaapeGa emiDaqNaemiAaGgaaaaa@3EEB@ percentile, and a value of zero otherwise; and f Y ( w j ) MathType@MTEF@5@5@+= feaagKart1ev2aaatCvAUfKttLearuatH9givLearmWu51MyVXgatC vAUfeBSjuyZL2yd9gzLbvyNv2CaeHbd9wDYLwzYbItLDharyavP1wz ZbItLDhis9wBH5garqqtubsr4rNCHbGeaGqiVu0Je9sqqrpepC0xbb L8F4rqqrFfpeea0xe9Lq=Jc9vqaqpepm0xbba9pwe9Q8fs0=yqaqpe pae9pg0FirpepeKkFr0xfr=xfr=xb9adbaqaaeGaciGaaiaabeqaam aaeaqbaaGcbaaeaaaaaaaaa8qacqWGMbGzpaWaaSbaaSqaa8qacqWG zbqwa8aabeaakmaabmaabaWdbiabdEha39aadaWgaaWcbaWdbiabdQ gaQbWdaeqaaaGccaGLOaGaayzkaaaaaa@423D@ is the density of the wage at the j t h MathType@MTEF@5@5@+= feaagKart1ev2aaatCvAUfKttLearuatH9givLearmWu51MyVXgatC vAUfeBSjuyZL2yd9gzLbvyNv2CaeHbd9wDYLwzYbItLDharyavP1wz ZbItLDhis9wBH5garqqtubsr4rNCHbGeaGqiVu0Je9sqqrpepC0xbb L8F4rqqrFfpeea0xe9Lq=Jc9vqaqpepm0xbba9pwe9Q8fs0=yqaqpe pae9pg0FirpepeKkFr0xfr=xfr=xb9adbaqaaeGaciGaaiaabeqaam aaeaqbaaGcbaaeaaaaaaaaa8qacqWGQbGApaWaaWbaaSqabeaapeGa emiDaqNaemiAaGgaaaaa@3EEB@ percentile, estimated by kernel density.
Simple method
The possibility that the gender pay gap reflects selection bias can be assessed using a technique delineated by Baker, Benjamin, Cegep, and Grant and Drolet. Note Note After estimating the earnings equations for employed women and men at a given time point (t), as per equations a and b in appendix A, the mean log wages of those who were not employed (and who were therefore not part of the analytic sample) at that time are estimated by substituting their mean values ( X ¯ w o r X ¯ m ) MathType@MTEF@5@5@+= feaagKart1ev2aqatCvAUfKttLearuatH9givLearmWu51MyVXgatC vAUfeBSjuyZL2yd9gzLbvyNv2CaeHbd9wDYLwzYbItLDharyavP1wz ZbItLDhis9wBH5garqqtubsr4rNCHbGeaGqiVu0Je9sqqrpepC0xbb L8F4rqqrFfpeea0xe9Lq=Jc9vqaqpepm0xbba9pwe9Q8fs0=yqaqpe pae9pg0FirpepeKkFr0xfr=xfr=xb9adbaqaaeGaciGaaiaabeqaam aaeaqbaaGcbaaeaaaaaaaaa8qadaqadaWdaeaapeGafmiwaG1dayaa raWaaSbaaSqaa8qacqWG3bWDcqGGGcaOa8aabeaak8qacqWGVbWBcq WGYbGCcqGGGcaOcuWGybawpaGbaebadaWgaaWcbaWdbiabd2gaTbWd aeqaaOWdbiabcckaGcGaayjkaiaawMcaaaaa@4AB1@ for the corresponding regression coefficients ( β w o r β m ) MathType@MTEF@5@5@+= feaagKart1ev2aqatCvAUfKttLearuatH9givLearmWu51MyVXgatC vAUfeBSjuyZL2yd9gzLbvyNv2CaeHbd9wDYLwzYbItLDharyavP1wz ZbItLDhis9wBH5garqqtubsr4rNCHbGeaGqiVu0Je9sqqrpepC0xbb L8F4rqqrFfpeea0xe9Lq=Jc9vqaqpepm0xbba9pwe9Q8fs0=yqaqpe pae9pg0FirpepeKkFr0xfr=xfr=xb9adbaqaaeGaciGaaiaabeqaam aaeaqbaaGcbaaeaaaaaaaaa8qadaqadaWdaeaapeGaeqOSdi2damaa BaaaleaapeGaem4DaChapaqabaGcpeGaeiiOaaQaem4Ba8MaemOCai NaeiiOaaQaeqOSdi2damaaBaaaleaapeGaemyBa0MaeiiOaakapaqa baaak8qacaGLOaGaayzkaaaaaa@4B51@ .
To look at changes over time, one year is designated as the base year (t = 0). For all of the subsequent years, a new weighted estimate of women’s log wages are calculated as:
New weighted estimates of men’s log wages would be similarly calculated.
Although the term, E ¯ n t w MathType@MTEF@5@5@+= feaagKart1ev2aqatCvAUfKttLearuatH9givLearmWu51MyVXgatC vAUfeBSjuyZL2yd9gzLbvyNv2CaeHbd9wDYLwzYbItLDharyavP1wz ZbItLDhis9wBH5garqqtubsr4rNCHbGeaGqiVu0Je9sqqrpepC0xbb L8F4rqqrFfpeea0xe9Lq=Jc9vqaqpepm0xbba9pwe9Q8fs0=yqaqpe pae9pg0FirpepeKkFr0xfr=xfr=xb9adbaqaaeGaciGaaiaabeqaam aaeaqbaaGcbaaeaaaaaaaaa8qacuWGfbqrpaGbaebadaqhaaWcbaWd biabd6gaUjabdsha0bWdaeaapeGaem4DaChaaaaa@405C@ , controls for observable differences between employed women and those who are not employed, it is also possible to control unobservable differences by multiplying that term by a scale factor (k). Note Assuming that those who are not employed would receive lower wage offers than those who are employed, a k value less than 1.0 would be appropriate. Baker and his colleagues present adjusted results for both k = 1.0 and k = 0.9.
Heckman two-step correction
Heckman’s two-step correction makes it possible to assess whether selection bias is present, identify its determinants, and control for it in estimating an outcome of interest. Note To begin, the substantive models of the outcomes of interest—in this case, women’s wages—are estimated by means of OLS regression. In the first step of the “Heckman,” a selection model is developed for women, which estimates the likelihood of participation vs. non-participation—in this case, (1) employed versus (0) not employed—by means of binary probit regression. Note Note Multiple predictors of women’s employment status will be included in the selection model, most of which will also be included in the substantive models of women’s wages. It is important that the selection model contain at least one variable that can be legitimately excluded from the substantive models, so as to safeguard against collinearity between these models and properly identify the effect of selection. In effect, such a variable (or “instrument”) must strongly predict women’s employment status, but not their wages: marital status, family type, and the availability or level of non-earned income within the household can play this role. Note
In the second step, the residuals (error terms) from the selection model are used to derive a new variable called the Inverse Mills Ratio (IMR). Note The IMR is then added to the substantive model to capture both observed and unobserved variables that affect selection. Here, two factors are of interest in terms of determining whether there is significant sample-selection bias that results from the exclusion of non-working respondents from the substantive models. First, if the IMR is significant, it is suggestive of sample-selection bias in the substantive models. A second factor to consider is whether there have been significant changes in the parameter estimates of the other predictors in the substantive models before and after correction.
Statistics Canada has a vast repository of data sources that can be used to examine the gender pay gap. Since the variables included in these data sources differ, the appropriateness and usefulness of a given dataset depends on the empirical questions being addressed. Below, an overview of relevant datasets is provided.
Census of Population
On one specific day every five years, Statistics Canada conducts the Census of Population in order to develop a statistical portrait the people in Canada by their demographic, social and economic characteristics. While all households receive a short-form questionnaire, a sample of approximately 25% of Canadian households receive a long-form questionnaire (with the exception of 2011). The short-form census enumerates the entire Canadian population, which consists of Canadian citizens (by birth and by naturalization), landed immigrants and non-permanent residents and their families living with them in Canada. Note The long-form census includes the same target population as the short-form census, with the exception of Canadian citizens living temporarily in other countries; full-time members of the Canadian Forces stationed outside Canada; persons living in institutional collective dwellings such as hospitals, nursing homes and penitentiaries; and persons living in non-institutional collective dwellings such as work camps, hotels and motels, and student residences.
For the first time, the 2016 Census included administrative data from the Canada Revenue Agency (CRA) on annual income from various sources in 2015 for those who completed the short-form census. Note From these data, the gender pay gap can be calculated in terms of annual earnings. Using information on the number of weeks worked in the year preceding the census day and the number hours worked in the week preceding the census day, annual income can be converted to hourly (or weekly) wages—if one assumes that both the number of weeks worked in the year preceding the census day, and the number of hours worked in the week preceding the census day, are typical. Even with that assumption, information on weeks worked and work hours is only available for a sub-sample of the Canadian population (i.e., those who completed the long-form census).
The long-form census includes a number of variables that correspond to key predictors of the gender pay gap, including: age, family composition, education, labour market activity, full-time and part-time weeks worked, class of worker, occupation, and industry. It also includes indicators of diversity characteristics beyond sex: Aboriginal status; immigrant status, birthplace, year of immigration, and immigrant generation and birthplace of parents; visible minority status; and religion (asked every five years, with the next time being in 2021). Importantly, the large sample size for long-form census enables analysts to produce reliable estimates of outcomes among small population groups or for low levels of geography.
Labour Force Survey (LFS)
The Labour Force Survey (LFS) is a household survey conducted monthly by Statistics Canada. It provides timely information on major labour market trends by dividing the working-age population (aged 15 and older) into three mutually exclusive categories—employed, unemployed and not in the labour force—and collecting data on a variety of demographic, socioeconomic, and employment characteristics.
The LFS is based on a sample of approximately 56,000 households (corresponding to around 100,000 individuals) each month. It uses a rotating panel sample design, meaning that households remain in the sample for six consecutive months, with one-sixth of the sample being replaced each month. Persons living on reserves and other Aboriginal settlements in the provinces, full-time members of the Canadian Armed Forces, the institutionalized population, and households in extremely remote areas with low population density are not covered by the LFS.
Since 1997, the LFS has collected data on weekly and hourly wages. It is the only data source to do so. The LFS contains a number of variables that correspond to key predictors of the gender pay gap, including: age, age of the youngest child in the household, education, labour force status, work hours, class of worker (i.e., self-employed, unpaid family worker, public-sector employee, and private-sector employee), job tenure, union status, occupation, and industry. However, the LFS is more limited when it comes to identity factors beyond sex. Whilethe LFS includes Aboriginal status, immigrant status, birthplace, and year of immigration, there is no variable pertaining to racialization.
It is often useful to work with annual estimates, instead of monthly ones, particularly for descriptive purposes. Calculated on the basis of 12 months of data, annual estimates avoid the problem of seasonality that affects unadjusted monthly estimates. Annualizing the monthly data can be done in three steps:
- There will now be one large dataset, containing a year’s worth of responses.
- The survey date (SYEAR and SMTH) in combination with household ID (HHLDID) and person ID (LINE) can be used to uniquely identify respondents, if need be.
- Divide the weight variable (FINALWT) by 12.
- Apply the weight variable, and calculate estimates as you would using a single month of data. Note
Canadian Income Survey (CIS)
The Canadian Income Survey (CIS) provides information on the annual income and income sources of Canadians, along with their individual and household characteristics. The CIS is administered to a sub-sample of LFS respondents, consisting of the outgoing rotation groups in January, February, March and April. Each of these rotation groups consists of approximately 8,400 respondents.
The CIS gathers information about labour market activity, school attendance, disability, support payments, child care expenses, inter-household transfers, personal income, and characteristics and costs of housing. This content is supplemented with information on individual and household characteristics from the LFS. CRA data on annual income and income sources are also combined with the CIS data.
Although the first CIS was conducted in 2012, the data series can be extended using its predecessors, including the Survey of Labour and Income Dynamics (SLID) and the Survey of Consumer Finances (SCF).
Longitudinal and International Study of Adults (LISA)
The Longitudinal and International Study of Adults (LISA) is a household survey that has collected data every two years since 2011 from the non-institutionalized population aged 15 or older living in Canada’s ten provinces. LISA is made up of two parts: (1) the interview portion, which collects information on respondents’ education, work, health, life events, pensions and finances, and (2) its linkage to administrative data from a variety of sources, as appropriate.
While the LISA is a relatively new data source, its longitudinal nature lends itself to being one of the richest data sources available in the study of the gender pay gap. In addition to personal and productivity-enhancing characteristics, the LISA includes other variables that may help us to better understand the gender pay gap: actual work experience, field of study, and variables pertaining skills used at work and job flexibility. It also includes indicators for identity factors beyond sex: Aboriginal status; immigrant status, year of immigration, birthplace, and birthplace of parents; and visible-minority status.
General Social Survey (GSS)
Since 1986, the General Social Survey (GSS) has gathered information on social trends in order to monitor changes in the living conditions and well-being of Canadians. The target population consists of all non-institutionalized persons 15 years of age or older, living in the 10 provinces of Canada. Survey content rotates, with themes generally recurring every five years. Notably, GSS cycles on “families” collect retrospective data on union formation and dissolution, childbearing, and work history. Although income data in the GSS pertain only to the reference year, Cycle 25 of the GSS (2011) has recently been linked to administrative tax data, enabling analysts to study the gender pay gap longitudinally in relation to time-varying variables. Standard predictors of the gender pay gap (i.e., demographic and productivity-enhancing characteristics, occupation, and industry), as well as a variety of identity factors, including sexual orientation, are available in the GSS.
Canadians at Work and Home , cycle 30 of the GSS (2016), provides information that is not typically found in labour/income surveys, yet which can yield greater knowledge of the challenges women face with respect to paid work: work load, work ethic, work environment, perceived unfair treatment at work, and division of chores within the household.
Longitudinal Worker File (LWF)
The Longitudinal Worker File (LWF) is an administrative data set consisting of a 10% random sample of all Canadian workers. It is constructed from four separate data sources: the T4 and T1 files from Canada Revenue Agency (CRA), the Record of Employment (ROE) files from Human Resources and Skills Development Canada (HRSDC), and the Longitudinal Employment Analysis Program (LEAP) file constructed by Statistics Canada. The current version of the LWF provides longitudinal information on individuals over the period from 1983 to 2015. In addition, an older, but comparable, version covers the period from 1978 to 1989.
The LWF provides the sample size necessary to examine the gender pay gap at the upper end of the earnings distribution. However, the lack of predictors other than sex and age limits its usefulness.
More information
Note of appreciation.
Canada owes the success of its statistical system to a long-standing partnership between Statistics Canada, the citizens of Canada, its businesses, governments and other institutions. Accurate and timely statistical information could not be produced without their continued co-operation and goodwill.
Standards of service to the public
Statistics Canada is committed to serving its clients in a prompt, reliable and courteous manner. To this end, the Agency has developed standards of service which its employees observe in serving its clients.
Published by authority of the Minister responsible for Statistics Canada.
© Her Majesty the Queen in Right of Canada as represented by the Minister of Industry, 2021
Use of this publication is governed by the Statistics Canada Open Licence Agreement .
Catalogue no. 45-20-0002
Frequency: Occasional

An official website of the United States government
The .gov means it’s official. Federal government websites often end in .gov or .mil. Before sharing sensitive information, make sure you’re on a federal government site.
The site is secure. The https:// ensures that you are connecting to the official website and that any information you provide is encrypted and transmitted securely.
- Publications
- Account settings
Preview improvements coming to the PMC website in October 2024. Learn More or Try it out now .
- Advanced Search
- Journal List

The persistence of pay inequality: The gender pay gap in an anonymous online labor market
Leib litman.
1 Department of Psychology, Lander College, Flushing, New York, United States of America
Jonathan Robinson
2 Department of Computer Science, Lander College, Flushing, New York, United States of America
3 Department of Health Policy & Management, Mailman School of Public Health, Columbia University, New York, New York, United States of America
Cheskie Rosenzweig
4 Department of Clinical Psychology, Columbia University, New York, New York, United States of America
Joshua Waxman
5 Department of Computer Science, Stern College for Women, New York, New York, United States of America
Lisa M. Bates
6 Department of Epidemiology, Mailman School of Public Health, Columbia University New York, New York, United States of America
Associated Data
Due to the sensitive nature of some of the data, and the terms of service of the websites used during data collection (including CloudResearch and MTurk), CloudResearch cannot release the full data set to make it publically available. The data are on CloudResearch's Sequel servers located at Queens College in the city of New York. CloudResearch makes data available to be accessed by researchers for replication purposes, on the CloudResearch premises, in the same way the data were accessed and analysed by the authors of this manuscript. The contact person at CloudResearch who can help researchers access the data set is Tzvi Abberbock, who can be reached at [email protected] .
Studies of the gender pay gap are seldom able to simultaneously account for the range of alternative putative mechanisms underlying it. Using CloudResearch, an online microtask platform connecting employers to workers who perform research-related tasks, we examine whether gender pay discrepancies are still evident in a labor market characterized by anonymity, relatively homogeneous work, and flexibility. For 22,271 Mechanical Turk workers who participated in nearly 5 million tasks, we analyze hourly earnings by gender, controlling for key covariates which have been shown previously to lead to differential pay for men and women. On average, women’s hourly earnings were 10.5% lower than men’s. Several factors contributed to the gender pay gap, including the tendency for women to select tasks that have a lower advertised hourly pay. This study provides evidence that gender pay gaps can arise despite the absence of overt discrimination, labor segregation, and inflexible work arrangements, even after experience, education, and other human capital factors are controlled for. Findings highlight the need to examine other possible causes of the gender pay gap. Potential strategies for reducing the pay gap on online labor markets are also discussed.
Introduction
The gender pay gap, the disparity in earnings between male and female workers, has been the focus of empirical research in the US for decades, as well as legislative and executive action under the Obama administration [ 1 , 2 ]. Trends dating back to the 1960s show a long period in which women’s earnings were approximately 60% of their male counterparts, followed by increases in women’s earnings starting in the 1980s, which began to narrow, but not close, the gap which persists today [ 3 ]. More recent data from 2014 show that overall, the median weekly earnings of women working full time were 79–83% of what men earned [ 4 – 9 ].
The extensive literature seeking to explain the gender pay gap and its trajectory over time in traditional labor markets suggests it is a function of multiple structural and individual-level processes that reflect both the near-term and cumulative effects of gender relations and roles over the life course. Broadly speaking, the drivers of the gender pay gap can be categorized as: 1) human capital or productivity factors such as education, skills, and workforce experience; 2) industry or occupational segregation, which some estimates suggest accounts for approximately half of the pay gap; 3) gender-specific temporal flexibility constraints which can affect promotions and remuneration; and finally, 4) gender discrimination operating in hiring, promotion, task assignment, and/or compensation. The latter mechanism is often estimated by inference as a function of unexplained residual effects of gender on payment after accounting for other factors, an approach which is most persuasive in studies of narrowly restricted populations of workers such as lawyers [ 10 ] and academics of specific disciplines [ 11 ]. A recent estimate suggests this unexplained gender difference in earnings can account for approximately 40% of the pay gap [ 3 ]. However, more direct estimations of discriminatory processes are also available from experimental evidence, including field audit and lab-based studies [ 12 – 14 ]. Finally, gender pay gaps have also been attributed to differential discrimination encountered by men and women on the basis of parental status, often known as the ‘motherhood penalty’ [ 15 ].
Non-traditional ‘gig economy’ labor markets and the gender pay gap
In recent years there has been a dramatic rise in nontraditional ‘gig economy’ labor markets, which entail independent workers hired for single projects or tasks often on a short-term basis with minimal contractual engagement. “Microtask” platforms such as Amazon Mechanical Turk (MTurk) and Crowdflower have become a major sector of the gig economy, offering a source of easily accessible supplementary income through performance of small tasks online at a time and place convenient to the worker. Available tasks can range from categorizing receipts to transcription and proofreading services, and are posted online by the prospective employer. Workers registered with the platform then elect to perform the advertised tasks and receive compensation upon completion of satisfactory work [ 16 ]. An estimated 0.4% of US adults are currently receiving income from such platforms each month [ 17 ], and microtask work is a growing sector of the service economy in the United States [ 18 ]. Although still relatively small, these emerging labor market environments provide a unique opportunity to investigate the gender pay gap in ways not possible within traditional labor markets, due to features (described below) that allow researchers to simultaneously account for multiple putative mechanisms thought to underlie the pay gap.
The present study utilizes the Amazon Mechanical Turk (MTurk) platform as a case study to examine whether a gender pay gap remains evident when the main causes of the pay gap identified in the literature do not apply or can be accounted for in a single investigation. MTurk is an online microtask platform that connects employers (‘requesters’) to employees (‘workers’) who perform jobs called “Human Intelligence Tasks” (HITs). The platform allows requesters to post tasks on a dashboard with a short description of the HIT, the compensation being offered, and the time the HIT is expected to take. When complete, the requester either approves or rejects the work based on quality. If approved, payment is quickly accessible to workers. The gender of workers who complete these HITs is not known to the requesters, but was accessible to researchers for the present study (along with other sociodemographic information and pay rates) based on metadata collected through CloudResearch (formerly TurkPrime), a platform commonly used to conduct social and behavioral research on MTurk [ 19 ].
Evaluating pay rates of workers on MTurk requires estimating the pay per hour of each task that a worker accepts which can then be averaged together. All HITs posted on MTurk through CloudResearch display how much a HIT pays and an estimated time that it takes for that HIT to be completed. Workers use this information to determine what the corresponding hourly pay rate of a task is likely to be, and much of our analysis of the gender pay gap is based on this advertised pay rate of all completed surveys. We also calculate an estimate of the gender pay gap based on actual completion times to examine potential differences in task completion speed, which we refer to as estimated actual wages (see Methods section for details).
Previous studies have found that both task completion time and the selection of tasks influences the gender pay gap in at least some gig economy markets. For example, a gender pay gap was observed among Uber drivers, with men consistently earning higher pay than women [ 20 ]. Some of the contributing factors to this pay gap include that male Uber drivers selected different tasks than female drivers, including being more willing to work at night and to work in neighborhoods that were perceived to be more dangerous. Male drivers were also likely to drive faster than their female counterparts. These findings show that person-level factors like task selection, and speed can influence the gender pay gap within gig economy markets.
MTurk is uniquely suited to examine the gender pay gap because it is possible to account simultaneously for multiple structural and individual-level factors that have been shown to produce pay gaps. These include discrimination, work heterogeneity (leading to occupational segregation), and job flexibility, as well as human capital factors such as experience and education.
Discrimination
When employers post their HITs on MTurk they have no way of knowing the demographic characteristics of the workers who accept those tasks, including their gender. While MTurk allows for selective recruitment of specific demographic groups, the MTurk tasks examined in this study are exclusively open to all workers, independent of their gender or other demographic characteristics. Therefore, features of the worker’s identity that might be the basis for discrimination cannot factor into an employer’s decision-making regarding hiring or pay.
Task heterogeneity
Another factor making MTurk uniquely suited for the examination of the gender pay gap is the relative homogeneity of tasks performed by the workers, minimizing the potential influence of gender differences in the type of work pursued on earnings and the pay gap. Work on the MTurk platform consists mostly of short tasks such as 10–15 minute surveys and categorization tasks. In addition, the only information that workers have available to them to choose tasks, other than pay, is the tasks’ titles and descriptions. We additionally classified tasks based on similarity and accounted for possible task heterogeneity effects in our analyses.
Job flexibility
MTurk is not characterized by the same inflexibilities as are often encountered in traditional labor markets. Workers can work at any time of the day or day of the week. This increased flexibility may be expected to provide more opportunities for participation in this labor market for those who are otherwise constrained by family or other obligations.
Human capital factors
It is possible that the more experienced workers could learn over time how to identify higher paying tasks by virtue of, for example, identifying qualities of tasks that can be completed more quickly than the advertised required time estimate. Further, if experience is correlated with gender, it could contribute to a gender pay gap and thus needs to be controlled for. Using CloudResearch metadata, we are able to account for experience on the platform. Additionally, we account for multiple sociodemographic variables, including age, marital status, parental status, education, income (from all sources), and race using the sociodemographic data available through CloudResearch.
Expected gender pay gap findings on MTurk
Due to the aforementioned factors that are unique to the MTurk marketplace–e.g., anonymity, self-selection into tasks, relative homogeneity of the tasks performed, and flexible work scheduling–we did not expect a gender pay gap to be evident on the platform to the same extent as in traditional labor markets. However, potential gender differences in task selection and completion speed, which have implications for earnings, merit further consideration. For example, though we expect the relative homogeneity of the MTurk tasks to minimize gender differences in task selection that could mimic occupational segregation, we do account for potential subtle residual differences in tasks that could differentially attract male and female workers and indirectly lead to pay differentials if those tasks that are preferentially selected by men pay a higher rate. To do this we categorize all tasks based on their descriptions using K-clustering and add the clusters as covariates to our models. In addition, we separately examine the gender pay gap within each topic-cluster.
In addition, if workers who are experienced on the platform are better able to find higher paying HITs, and if experience is correlated with gender, it may lead to gender differences in earnings. Theoretically, other factors that may vary with gender could also influence task selection. Previous studies of the pay gap in traditional markets indicate that reservation wages, defined as the pay threshold at which a person is willing to accept work, may be lower among women with children compared to women without, and to that of men as well [ 21 ]. Thus, if women on MTurk are more likely to have young children than men, they may be more willing to accept available work even if it pays relatively poorly. Other factors such as income, education level, and age may similarly influence reservation wages if they are associated with opportunities to find work outside of microtask platforms. To the extent that these demographics correlate with gender they may give rise to a gender pay gap. Therefore we consider age, experience on MTurk, education, income, marital status, and parental status as covariates in our models.
Task completion speed may vary by gender for several reasons, including potential gender differences in past experience on the platform. We examine the estimated actual pay gap per hour based on HIT payment and estimated actual completion time to examine the effects of completion speed on the wage gap. We also examine the gender pay gap based on advertised pay rates, which are not dependent on completion speed and more directly measure how gender differences in task selection can lead to a pay gap. Below, we explain how these were calculated based on meta-data from CloudResearch.
To summarize, the overall goal of the present study was to explore whether gender pay differentials arise within a unique, non-traditional and anonymous online labor market, where known drivers of the gender pay gap either do not apply or can be accounted for statistically.
Materials and methods
Amazon mechanical turk and cloudresearch.
Started in 2005, the original purpose of the Amazon Mechanical Turk (MTurk) platform was to allow requesters to crowdsource tasks that could not easily be handled by existing technological solutions such as receipt copying, image categorization, and website testing. As of 2010, researchers increasingly began using MTurk for a wide variety of research tasks in the social, behavioral, and medical sciences, and it is currently used by thousands of academic researchers across hundreds of academic departments [ 22 ]. These research-related HITs are typically listed on the platform in generic terms such as, “Ten-minute social science study,” or “A study about public opinion attitudes.”
Because MTurk was not originally designed solely for research purposes, its interface is not optimized for some scientific applications. For this reason, third party add-on toolkits have been created that offer critical research tools for scientific use. One such platform, CloudResearch (formerly TurkPrime), allows requesters to manage multiple research functions, such as applying sampling criteria and facilitating longitudinal studies, through a link to their MTurk account. CloudResearch’s functionality has been described extensively elsewhere [ 19 ]. While the demographic characteristics of workers are not available to MTurk requesters, we were able to retroactively identify the gender and other demographic characteristics of workers through the CloudResearch platform. CloudResearch also facilitates access to data for each HIT, including pay, estimated length, and title.
The study was an analysis of previously collected metadata, which were analyzed anonymously. We complied with the terms of service for all data collected from CloudResearch, and MTurk. The approving institutional review board for this study was IntegReview.
Analytic sample
We analyzed the nearly 5 million tasks completed during an 18-month period between January 2016 and June 2017 by 12,312 female and 9,959 male workers who had complete data on key demographic characteristics. To be included in the analysis a HIT had to be fully completed, not just accepted, by the worker, and had to be accepted (paid for) by the requester. Although the vast majority of HITs were open to both males and females, a small percentage of HITs are intended for a specific gender. Because our goal was to exclusively analyze HITs for which the requesters did not know the gender of workers, we excluded any HITs using gender-specific inclusion or exclusion criteria from the analyses. In addition, we removed from the analysis any HITs that were part of follow-up studies in which it would be possible for the requester to know the gender of the worker from the prior data collection. Finally, where possible, CloudResearch tracks demographic information on workers across multiple HITs over time. To minimize misclassification of gender, we excluded the 0.3% of assignments for which gender was unknown with at least 95% consistency across HITs.
The main exposure variable is worker gender and the outcome variables are estimated actual hourly pay accrued through completing HITs, and advertised hourly pay for completed HITs. Estimated actual hourly wages are based on the estimated length in minutes and compensation in dollars per HIT as posted on the dashboard by the requester. We refer to actual pay as estimated because sometimes people work multiple assignments at the same time (which is allowed on the platform), or may simultaneously perform other unrelated activities and therefore not work on the HIT the entire time the task is open. We also considered several covariates to approximate human capital factors that could potentially influence earnings on this platform, including marital status, education, household income, number of children, race/ethnicity, age, and experience (number of HITs previously completed). Additional covariates included task length, task cluster (see below), and the serial order with which workers accepted the HIT in order to account for potential differences in HIT acceptance speed that may relate to the pay gap.
Database and analytic approach
Data were exported from CloudResearch’s database into Stata in long-form format to represent each task on a single row. For the purposes of this paper, we use “HIT” and “study” interchangeably to refer to a study put up on the MTurk dashboard which aims to collect data from multiple participants. A HIT or study consist of multiple “assignments” which is a single task completed by a single participant. Columns represented variables such as demographic information, payment, and estimated HIT length. Column variables also included unique IDs for workers, HITs (a single study posted by a requester), and requesters, allowing for a multi-level modeling analytic approach with assignments nested within workers. Individual assignments (a single task completed by a single worker) were the unit of analysis for all models.
Linear regression models were used to calculate the gender pay gap using two dependent variables 1) women’s estimated actual earnings relative to men’s and 2) women’s selection of tasks based on advertised earnings relative to men’s. We first examined the actual pay model, to see the gender pay gap when including an estimate of task completion speed, and then adjusted this model for advertised hourly pay to determine if and to what extent a propensity for men to select more remunerative tasks was evident and driving any observed gender pay gap. We additionally ran separate models using women’s advertised earnings relative to men’s as the dependent variable to examine task selection effects more directly. The fully adjusted models controlled for the human capital-related covariates, excluding household income and education which were balanced across genders. These models also tested for interactions between gender and each of the covariates by adding individual interaction terms to the adjusted model. To control for within-worker clustering, Huber-White standard error corrections were used in all models.
Cluster analysis
To explore the potential influence of any residual task heterogeneity and gender preference for specific task type as the cause of the gender pay gap, we use K-means clustering analysis (seed = 0) to categorize the types of tasks into clusters based on the descriptions that workers use to choose the tasks they perform. We excluded from this clustering any tasks which contained certain gendered words (such as “male”, “female”, etc.) and any tasks which had fewer than 30 respondents. We stripped out all punctuation, symbols and digits from the titles, so as to remove any reference to estimated compensation or duration. The features we clustered on were the presence or absence of 5,140 distinct words that appeared across all titles. We then present the distribution of tasks across these clusters as well as average pay by gender and the gender pay gap within each cluster.
The demographics of the analytic sample are presented in Table 1 . Men and women completed comparable numbers of tasks during the study period; 2,396,978 (48.6%) for men and 2,539,229 (51.4%) for women.
In Table 2 we measure the differences in remuneration between genders, and then decompose any observed pay gap into task completion speed, task selection, and then demographic and structural factors. Model 1 shows the unadjusted regression model of gender differences in estimated actual pay, and indicates that, on average, tasks completed by women paid 60 (10.5%) cents less per hour compared to tasks completed by men (t = 17.4, p < .0001), with the mean estimated actual pay across genders being $5.70 per hour.
*Model adjusted for race, marital status, number of children and task clusters as categorical covariates, and age, HIT acceptance speed, and number of HITs as continuous covariates.
In Model 2, adjusting for advertised hourly pay, the gender pay gap dropped to 46 cents indicating that 14 cents of the pay gap is attributable to gender differences in the selection of tasks (t = 8.6, p < .0001). Finally, after the inclusion of covariates and their interactions in Model 3, the gender pay differential was further attenuated to 32 cents (t = 6.7, p < .0001). The remaining 32 cent difference (56.6%) in earnings is inferred to be attributable to gender differences in HIT completion speed.
Task selection analyses
Although completion speed appears to account for a significant portion of the pay gap, of particular interest are gender differences in task selection. Beyond structural factors such as education, household composition and completion speed, task selection accounts for a meaningful portion of the gender pay gap. As a reminder, the pay rate and expected completion time are posted for every HIT, so why women would select less remunerative tasks on average than men do is an important question to explore. In the next section of the paper we perform a set of analyses to examine factors that could account for this observed gender difference in task selection.
Advertised hourly pay
To examine gender differences in task selection, we used linear regression to directly examine whether the advertised hourly pay differed for tasks accepted by male and female workers. We first ran a simple model ( Table 3 ; Model 3A) on the full dataset of 4.93 million HITs, with gender as the predictor and advertised hourly pay as the outcome including no other covariates. The unadjusted regression results (Model 4) shown in Table 3 , indicates that, summed across all clusters and demographic groups, tasks completed by women were advertised as paying 28 cents (95% CI: $0.25-$0.31) less per hour (5.8%) compared to tasks completed by men (t = 21.8, p < .0001).
*Models adjusted for race, marital status, number of children, and task clusters as categorical covariates, and age, HIT acceptance speed, and number of HITs as continuous covariates.
Model 5 examines whether the remuneration differences for tasks selected by men and women remains significant in the presence of multiple covariates included in the previous model and their interactions. The advertised pay differential for tasks selected by women compared to men was attenuated to 21 cents (4.3%), and remained statistically significant (t = 9.9, p < .0001). This estimate closely corresponded to the inferred influence of task selection reported in Table 2 . Tests of gender by covariate interactions were significant only in the cases of age and marital status; the pay differential in tasks selected by men and women decreased with age and was more pronounced among single versus currently or previously married women.
To further examine what factors may account for the observed gender differences in task selection we plotted the observed pay gap within demographic and other covariate groups. Table 4 shows the distribution of tasks completed by men and women, as well as mean earnings and the pay gap across all demographic groups, based on the advertised (not actual) hourly pay for HITs selected (hereafter referred to as “advertised hourly pay” and the “advertised pay gap”). The average task was advertised to pay $4.88 per hour (95% CI $4.69, $5.10).
The pattern across demographic characteristics shows that the advertised hourly pay gap between genders is pervasive. Notably, a significant advertised gender pay gap is evident in every level of each covariate considered in Table 4 , but more pronounced among some subgroups of workers. For example, the advertised pay gap was highest among the youngest workers ($0.31 per hour for workers age 18–29), and decreased linearly with age, declining to $0.13 per hour among workers age 60+. Advertised houry gender pay gaps were evident across all levels of education and income considered.
To further examine the potential influence of human capital factors on the advertised hourly pay gap, Table 5 presents the average advertised pay for selected tasks by level of experience on the CloudResearch platform. Workers were grouped into 4 experience levels, based on the number of prior HITs completed: Those who completed fewer than 100 HITs, between 100 and 500 HITs, between 500 and 1,000 HITs, and more than 1,000 HITs. A significant gender difference in advertised hourly pay was observed within each of these four experience groups. The advertised hourly pay for tasks selected by both male and female workers increased with experience, while the gender pay gap decreases. There was some evidence that male workers have more cumulative experience with the platform: 43% of male workers had the highest level of experience (previously completing 1,001–10,000 HITs) compared to only 33% of women.
Table 5 also explores the influence of task heterogeneity upon HIT selection and the gender gap in advertised hourly pay. K-means clustering was used to group HITs into 20 clusters initially based on the presence or absence of 5,140 distinct words appearing in HIT titles. Clusters with fewer than 50,000 completed tasks were then excluded from analysis. This resulted in 13 clusters which accounted for 94.3% of submitted work assignments (HITs).
The themes of all clusters as well as the average hourly advertised pay for men and women within each cluster are presented in the second panel of Table 5 . The clusters included categories such as Games, Decision making, Product evaluation, Psychology studies, and Short Surveys. We did not observe a gender preference for any of the clusters. Specifically, for every cluster, the proportion of males was no smaller than 46.6% (consistent with the slightly lower proportion of males on the platform, see Table 1 ) and no larger than 50.2%. As shown in Table 5 , the gender pay gap was observed within each of the clusters. These results suggest that residual task heterogeneity, a proxy for occupational segregation, is not likely to contribute to a gender pay gap in this market.
Task length was defined as the advertised estimated duration of a HIT. Table 6 presents the advertised hourly gender pay gaps for five categories of HIT length, which ranged from a few minutes to over 1 hour. Again, a significant advertised hourly gender pay gap was observed in each category.
Finally, we conducted additional supplementary analyses to determine if other plausible factors such as HIT timing could account for the gender pay gap. We explored temporal factors including hour of the day and day of the week. Each completed task was grouped based on the hour and day in which it was completed. A significant advertised gender pay gap was observed within each of the 24 hours of the day and for every day of the week demonstrating that HIT timing could not account for the observed gender gap (results available in Supplementary Materials).
In this study we examined the gender pay gap on an anonymous online platform across an 18-month period, during which close to five million tasks were completed by over 20,000 unique workers. Due to factors that are unique to the Mechanical Turk online marketplace–such as anonymity, self-selection into tasks, relative homogeneity of the tasks performed, and flexible work scheduling–we did not expect earnings to differ by gender on this platform. However, contrary to our expectations, a robust and persistent gender pay gap was observed.
The average estimated actual pay on MTurk over the course of the examined time period was $5.70 per hour, with the gender pay differential being 10.5%. Importantly, gig economy platforms differ from more traditional labor markets in that hourly pay largely depends on the speed with which tasks are completed. For this reason, an analysis of gender differences in actual earned pay will be affected by gender differences in task completion speed. Unfortunately, we were not able to directly measure the speed with which workers complete tasks and account for this factor in our analysis. This is because workers have the ability to accept multiple HITs at the same time and multiple HITs can sit dormant in a queue, waiting for workers to begin to work on them. Therefore, the actual time that many workers spend working on tasks is likely less than what is indicated in the metadata available. For this reason, the estimated average actual hourly rate of $5.70 is likely an underestimate and the gender gap in actual pay cannot be precisely measured. We infer however, by the residual gender pay gap after accounting for other factors, that as much as 57% (or $.32) of the pay differential may be attributable to task completion speed. There are multiple plausible explanations for gender differences in task completion speed. For example, women may be more meticulous at performing tasks and, thus, may take longer at completing them. There may also be a skill factor related to men’s greater experience on the platform (see Table 5 ), such that men may be faster on average at completing tasks than women.
However, our findings also revealed another component of a gender pay gap on this platform–gender differences in the selection of tasks based on their advertised pay. Because the speed with which workers complete tasks does not impact these estimates, we conducted extensive analyses to try to explain this gender gap and the reasons why women appear on average to be selecting tasks that pay less compared to men. These results pertaining to the advertised gender pay gap constitute the main focus of this study and the discussion that follows.
The overall advertised hourly pay was $4.88. The gender pay gap in the advertised hourly pay was $0.28, or 5.8% of the advertised pay. Once a gender earnings differential was observed based on advertised pay, we expected to fully explain it by controlling for key structural and individual-level covariates. The covariates that we examined included experience, age, income, education, family composition, race, number of children, task length, the speed of accepting a task, and thirteen types of subtasks. We additionally examined the time of day and day of the week as potential explanatory factors. Again, contrary to our expectations, we observed that the pay gap persisted even after these potential confounders were controlled for. Indeed, separate analyses that examined the advertised pay gap within each subcategory of the covariates showed that the pay gap is ubiquitous, and persisted within each of the ninety sub-groups examined. These findings allows us to rule out multiple mechanisms that are known drivers of the pay gap in traditional labor markets and other gig economy marketplaces. To our knowledge this is the only study that has observed a pay gap across such diverse categories of workers and conditions, in an anonymous marketplace, while simultaneously controlling for virtually all variables that are traditionally implicated as causes of the gender pay gap.
Individual-level factors
Individual-level factors such as parental status and family composition are a common source of the gender pay gap in traditional labor markets [ 15 ] . Single mothers have previously been shown to have lower reservation wages compared to other men and women [ 21 ]. In traditional labor markets lower reservation wages lead single mothers to be willing to accept lower-paying work, contributing to a larger gender pay gap in this group. This pattern may extend to gig economy markets, in which single mothers may look to online labor markets as a source of supplementary income to help take care of their children, potentially leading them to become less discriminating in their choice of tasks and more willing to work for lower pay. Since female MTurk workers are 20% more likely than men to have children (see Table 1 ), it was critical to examine whether the gender pay gap may be driven by factors associated with family composition.
An examination of the advertised gender pay gap among individuals who differed in their marital and parental status showed that while married workers and those with children are indeed willing to work for lower pay (suggesting that family circumstances do affect reservation wages and may thus affect the willingness of online workers to accept lower-paying online tasks), women’s hourly pay is consistently lower than men’s within both single and married subgroups of workers, and among workers who do and do not have children. Indeed, contrary to expectations, the advertised gender pay gap was highest among those workers who are single, and among those who do not have any children. This observation shows that it is not possible for parental and family status to account for the observed pay gap in the present study, since it is precisely among unmarried individuals and those without children that the largest pay gap is observed.
Age was another factor that we considered to potentially explain the gender pay gap. In the present sample, the hourly pay of older individuals is substantially lower than that of younger workers; and women on the platform are five years older on average compared to men (see Table 1 ). However, having examined the gender pay gap separately within five different age cohorts we found that the largest pay gap occurs in the two youngest cohort groups: those between 18 and 29, and between 30 and 39 years of age. These are also the largest cohorts, responsible for 64% of completed work in total.
Younger workers are also most likely to have never been married or to not have any children. Thus, taken together, the results of the subgroup analyses are consistent in showing that the largest pay gap does not emerge from factors relating to parental, family, or age-related person-level factors. Similar patterns were found for race, education, and income. Specifically, a significant gender pay gap was observed within each subgroup of every one of these variables, showing that person-level factors relating to demographics are not driving the pay gap on this platform.
Experience is a factor that has an influence on the pay gap in both traditional and gig economy labor markets [ 20 ] . As noted above, experienced workers may be faster and more efficient at completing tasks in this platform, but also potentially more savvy at selecting more remunerative tasks compared to less experienced workers if, for example, they are better at selecting tasks that will take less time to complete than estimated on the dashboard [ 20 ]. On MTurk, men are overall more experienced than women. However, experience does not account for the gender gap in advertised pay in the present study. Inexperienced workers comprise the vast majority of the Mechanical Turk workforce, accounting for 67% of all completed tasks (see Table 5 ). Yet within this inexperienced group, there is a consistent male earning advantage based on the advertised pay for tasks performed. Further, controlling for the effect of experience in our models has a minimal effect on attenuating the gender pay gap.
Another important source of the gender pay gap in both traditional and gig economy labor markets is task heterogeneity. In traditional labor markets men are disproportionately represented in lucrative fields, such as those in the tech sector [ 23 ]. While the workspace within MTurk is relatively homogeneous compared to the traditional labor market, there is still some variety in the kinds of tasks that are available, and men and women may have been expected to have preferences that influence choices among these.
To examine whether there is a gender preference for specific tasks, we systematically analyzed the textual descriptions of all tasks included in this study. These textual descriptions were available for all workers to examine on their dashboards, along with information about pay. The clustering algorithm revealed thirteen categories of tasks such as games, decision making, several different kinds of survey tasks, and psychology studies.We did not observe any evidence of gender preference for any of the task types. Within each of the thirteen clusters the distribution of tasks was approximately equally split between men and women. Thus, there is no evidence that women as a group have an overall preference for specific tasks compared to men. Critically, the gender pay gap was also observed within each one of these thirteen clusters.
Another potential source of heterogeneity is task length. Based on traditional labor markets, one plausible hypothesis about what may drive women’s preferences for specific tasks is that women may select tasks that differ in their duration. For example, women may be more likely to use the platform for supplemental income, while men may be more likely to work on HITs as their primary income source. Women may thus select shorter tasks relative to their male counterparts. If the shorter tasks pay less money, this would result in what appears to be a gender pay gap.
However, we did not observe gender differences in task selection based on task duration. For example, having divided tasks into their advertised length, the tasks are preferred equally by men and women. Furthermore, the shorter tasks’ hourly pay is substantially higher on average compared to longer tasks.
Additional evidence that scheduling factors do not drive the gender pay gap is that it was observed within all hourly and daily intervals (See S1 and S2 Tables in Appendix). These data are consistent with the results presented above regarding personal level factors, showing that the majority of male and female Mechanical Turk workers are single, young, and have no children. Thus, while in traditional labor markets task heterogeneity and labor segmentation is often driven by family and other life circumstances, the cohort examined in this study does not appear to be affected by these factors.
Practical implications of a gender pay gap on online platforms for social and behavioral science research
The present findings have important implications for online participant recruitment in the social and behavioral sciences, and also have theoretical implications for understanding the mechanisms that give rise to the gender pay gap. The last ten years have seen a revolution in data collection practices in the social and behavioral sciences, as laboratory-based data collection has slowly and steadily been moving online [ 16 , 24 ]. Mechanical Turk is by far the most widely used source of human participants online, with thousands of published peer-reviewed papers utilizing Mechanical Turk to recruit at least some of their human participants [ 25 ]. The present findings suggest both a challenge and an opportunity for researchers utilizing online platforms for participant recruitment. Our findings clearly reveal for the first time that sampling research participants on anonymous online platforms tends to produce gender pay inequities, and that this happens independent of demographics or type of task. While it is not clear from our findings what the exact cause of this inequity is, what is clear is that the online sampling environment produces similar gender pay inequities as those observed in other more traditional labor markets, after controlling for relevant covariates.
This finding is inherently surprising since many mechanisms that are known to produce the gender pay gap in traditional labor markets are not at play in online microtasks environments. Regardless of what the generative mechanisms of the gender pay gap on online microtask platforms might be, researchers may wish to consider whether changes in their sampling practices may produce more equitable pay outcomes. Unlike traditional labor markets, online data collection platforms have built-in tools that can allow researchers to easily fix gender pay inequities. Researchers can simply utilize gender quotas, for example, to fix the ratio of male and female participants that they recruit. These simple fixes in sampling practices will not only produce more equitable pay outcomes but are also most likely advantageous for reducing sampling bias due to gender being correlated with pay. Thus, while our results point to a ubiquitous discrepancy in pay between men and women on online microtask platforms, such inequities have relatively easy fixes on online gig economy marketplaces such as MTurk, compared to traditional labor markets where gender-based pay inequities have often remained intractable.
Other gig economy markets
As discussed in the introduction, a gender wage gap has been demonstrated on Uber, a gig economy transportation marketplace [ 20 ], where men earn approximately 7% more than women. However, unlike in the present study, the gender wage gap on Uber was fully explained by three factors; a) driving speed predicted higher wages, with men driving faster than women, b) men were more likely than women to drive in congested locations which resulted in better pay, c) experience working for Uber predicted higher wages, with men being more experienced. Thus, contrary to our findings, the gender wage gap in gig economy markets studied thus far are fully explained by task heterogeneity, experience, and task completion speed. To our knowledge, the results presented in the present study are the first to show that the gender wage gap can emerge independent of these factors.
Generalizability
Every labor market is characterized by a unique population of workers that are almost by definition not a representation of the general population outside of that labor market. Likewise, Mechanical Turk is characterized by a unique population of workers that is known to differ from the general population in several ways. Mechanical Turk workers are younger, better educated, less likely to be married or have children, less likely to be religious, and more likely to have a lower income compared to the general United States population [ 24 ]. The goal of the present study was not to uncover universal mechanisms that generate the gender pay gap across all labor markets and demographic groups. Rather, the goal was to examine a highly unique labor environment, characterized by factors that should make this labor market immune to the emergence of a gender pay gap.
Previous theories accounting for the pay gap have identified specific generating mechanisms relating to structural and personal factors, in addition to discrimination, as playing a role in the emergence of the gender pay gap. This study examined the work of over 20,000 individuals completing over 5 million tasks, under conditions where standard mechanisms that generate the gender pay gap have been controlled for. Nevertheless, a gender pay gap emerged in this environment, which cannot be accounted for by structural factors, demographic background, task preferences, or discrimination. Thus, these results reveal that the gender pay gap can emerge—in at least some labor markets—in which discrimination is absent and other key factors are accounted for. These results show that factors which have been identified to date as giving rise to the gender pay gap are not sufficient to explain the pay gap in at least some labor markets.
Potential mechanisms
While we cannot know from the results of this study what the actual mechanism is that generates the gender pay gap on online platforms, we suggest that it may be coming from outside of the platform. The particular characteristics of this labor market—such as anonymity, relative task homogeneity, and flexibility—suggest that, everything else being equal, women working in this platform have a greater propensity to choose less remunerative opportunities relative to men. It may be that these choices are driven by women having a lower reservation wage compared to men [ 21 , 26 ]. Previous research among student populations and in traditional labor markets has shown that women report lower pay or reward expectations than men [ 27 – 29 ]. Lower pay expectations among women are attributed to justifiable anticipation of differential returns to labor due to factors such as gender discrimination and/or a systematic psychological bias toward pessimism relative to an overly optimistic propensity among men [ 30 ].
Our results show that even if the bias of employers is removed by hiding the gender of workers as happens on MTurk, it seems that women may select lower paying opportunities themselves because their lower reservation wage influences the types of tasks they are willing to work on. It may be that women do this because cumulative experiences of pervasive discrimination lead women to undervalue their labor. In turn, women’s experiences with earning lower pay compared to men on traditional labor markets may lower women’s pay expectations on gig economy markets. Thus, consistent with these lowered expectations, women lower their reservation wages and may thus be more likely than men to settle for lower paying tasks.
More broadly, gender norms, psychological attributes, and non-cognitive skills, have recently become the subject of investigation as a potential source for the gender pay gap [ 3 ], and the present findings indicate the importance of such mechanisms being further explored, particularly in the context of task selection. More research will be required to explore the potential psychological and antecedent structural mechanisms underlying differential task selection and expectations of compensation for time spent on microtask platforms, with potential relevance to the gender pay gap in traditional labor markets as well. What these results do show is that pay discrepancies can emerge despite the absence of discrimination in at least some circumstances. These results should be of particular interest for researchers who may wish to see a more equitable online labor market for academic research, and also suggest that novel and heretofore unexplored mechanisms may be at play in generating these pay discrepancies.
A final note about framing: we are aware that explanations of the gender pay gap that invoke elements of women’s agency and, more specifically, “choices” risk both; a) diminishing or distracting from important structural factors, and b) “naturalizing” the status quo of gender inequality [ 30 ] . As Connor and Fiske (2019) argue, causal attributions for the gender pay gap to “unconstrained choices” by women, common as part of human capital explanations, may have the effect, intended or otherwise, of reinforcing system-justifying ideologies that serve to perpetuate inequality. By explicitly locating women’s economic decision making on the MTurk platform in the broader context of inegalitarian gender norms and labor market experiences outside of it (as above), we seek to distance our interpretation of our findings from implicit endorsement of traditional gender roles and economic arrangements and to promote further investigation of how the observed gender pay gap in this niche of the gig economy may reflect both broader gender inequalities and opportunities for structural remedies.
Supporting information
Funding statement.
The authors received no specific funding for this work.
Data Availability
The Gender Pay Gap and the Equality
This essay will discuss the gender pay gap and its implications for gender equality. It will explore the causes of the pay gap, including discrimination, occupational segregation, and societal norms. The piece will also consider the economic and social consequences of the pay gap and the measures being taken to address this inequality, such as legislation, corporate policies, and advocacy efforts. You can also find more related free essay samples at PapersOwl about Discrimination.
How it works
- 1 Introduction
- 2 Pro Arguments
- 3 Con Arguments
- 4 Conclusion
Introduction
The gender pay gap and the equality of pay rates have always been topics of discussion in today’s society. Equal pay means that individuals accomplishing the same work should be compensated equivalently in regards to completion. Issues are raised between the earning differences between men and women due to the lack of equal pay between the two genders. By referring back to U.S. history on the subject, we found that the issue dates back over 100 years ago and grasps the concept around competencies and skills ultimately undervalued from women whose jobs are paid less than that of men, as a result, contradicting their effort in volume of production at work while also hindering their chances of potential growth in an organization.
The importance of unfair pay can be a detrimental factor when it comes to sustaining income necessary for present and future survival. Our position argues that the gender pay gap is not a myth, and must be taken seriously in order to ensure everyone has an equal chance of being recognized for the hard work they put into society.
Pro Arguments
One of the main elements to the gender pay gap being real is gender discrimination. Gender discrimination can result from women being excluded from meetings, promotions, and not being offered a job because the employer believes women are not as smart or determined enough as men in the workplace. As mentioned by Hessaramiri and Kleiner, male decision makers believe that men are superior, so they make decisions with that thought in mind (2001, p.44). Employers who do not hire women because they believe that a man will perform more efficiently is discriminating against women by stereotyping them. As discussed in The Complex Causes of the Gender Pay Gap, when employers are presented with identical resumes for male and female candidates, employers will more likely end up offering the job to a male (Wagner, 2015, p.17). Having identical qualifications to men, women are not considered at a man’s level, which is discrimination. Donna Kassman was overlooked for a promotion even though she was more than qualified (Widmer, p.23).
Unequal career advancement widens the pay gap for both females and males. According to a recent student founded by SHRM, women earn 0.79 cents for every dollar a man earns (Miller, S). These statistics have been unchanged for several years considering the similarities of jobs and qualifications. This highlights the underlying issue of gender pay gap that has still yet to be brought into major topic discussions to be acted upon. In addition, studies show 36% of men felt that women should not earn the same amount as they do if able to take family leave. However, this is a somewhat arguable point since there is indeed a discriminatory act towards pay differences due to personal matter. This could be a personal opinion looking into the negative sides of women taking time off to care for a child and not focusing on their work. The differences in how men and women are seen towards one another sets the stage for even further pressures in the workplace.
54% of women experience harassment in the workplace (Zetlin, 2018). Due to these pressures women receive from men, the concept of a wage gap between the two genders further defines itself. Women decide to leave these “male-dominated” industries to protect their values and themselves, but in turn hurt their chances of promotions and career advancement. The “leaving” of women in these jobs is more of them being pushed out of these roles due to their refusal of complying with their male co-workers’ ideas. According to The Cut, “Sexual harassment has been identified as one of the most damaging and ubiquitous barriers to career success and satisfaction for women,” (Covert, 2017, para. 2). Since the harassment of women is so common in the workplace, females experience lower incomes because they are shut out from certain promotions. This causes women to face even more obstacles when finding a job, thus earning less than their male co-workers.
Con Arguments
Women are willingly choosing low paying job as a result to the difference in pay wage. When speaking about the wage gap, people often tend to look at the same job with same skills to compare the differences each gender is getting pay for. Often times, this leads to findings that women are getting paid less than men per hour or annually for the same/similar job. However, research has shown that women are more likely to choose a career field that will result in lower pay for their earnings in total. Based on research by Harvard Business Review, “Many college majors that lead to high-paying roles in tech and engineering are male dominated, while majors that lead to lower-paying roles in social sciences and liberal arts tend to be female dominated, placing men in higher-paying career pathways, on average” (Carmichael, S). Yes, this is also a contributing factor adding up to wage gap. Overall, data does not account for the reason why women pick a career that lead to a lower pay but all comes down to their behaviors and choices leading up to the outcomes of their career. The argument here is that both women and men can be in the same major but it is the personal characteristics and determination that establish an outcome, or career, they want to achieve.
Women bringing the pay gap upon themselves by choosing to go into lower-paying jobs is a myth. Even though a large percentage of women are employed in lower paying jobs, their difference in industry choice compared to men still depicts a gap in wages per occupation, favoring the male gender. Regardless of the industry, women consistently earn less than their male colleagues. For example, it is fair to say that society views nursing and secretarial jobs as a more female-dominated industry; however, studies from Fortune Magazine depict that women still earn less than men in these fields. To further the argument, there is evidence that in most occupations across the board, wages fall when more women are present in the industry and in turn, the wages rise when there are more males present in the industry. In response to these unfair wages in the workplace, women ask for pay raises but only receive them 15% of the time while males receive their pay raises 20% of the time (Vagins, 2019) A reason for this is that females asking for a higher salary are seen as aggressive and are thus refused their request.
It is believed the gender pay gap is a myth considering the hours worked by both men and women, women make the choice to not work as many hours as men. Working overtime is not exclusive to only one gender, so when men decide to work more overtime, of course men will make more money than women. In a study by the Bureau of Labor Statistics, 27 percent of men compared to 15 percent of women work over 41 hours (Hymowitz, 2011, p.4). There is more than enough evidence to claim that women prefer to work fewer hours than men. As stated in Why the Gender Gap Won’t Go Away, despite the gap in earnings, women still decide to work fewer hours than men (Hymowitz, 2011). If women want the pay gap to be closed, all they must simply do is work the same hours as men. Their choice ends up causing the wage gap to show up with skewed results. There is a problem with this argument. Women do have a choice to work the hours they want, but the lack of work-family life balance programs lead women to choose lower paying jobs with more flexible hours.
Some believe the pay gap is due to women having children and leaving work to look after them. This myth is false because right off the bat, the gap in wages is present even before women create families, indicating that a distinction between male and female wages is already established. Fortune Magazine depicts that women before the age of 24 already earn less than their male co-workers; thus, proving that the differences in wages are present even before the main “child bearing” years experienced by women. When the hiring process is underworks, pregnant women are seen differently than men. Mothers-to-be as well as women already with children experience discrimination from employers and are “six times less likely” to be considered for a job when compared to childless women and men (Dunn, 2019, para. 11). While women are seen as less reliable to meet deadlines and scheduled appointments than men because of their “motherhood” tasks, men are seen as needing to be rewarded for having a new child and thus receive “daddy bonuses” to help their family out (Miller, 2018). SHRM explains that women who take a mid-career break from work earn less than men who also take similar mid-career breaks.
During our research to reveal that the gender pay gap is a myth, we found reasonable evidence to fortify our statements. Our analytical research proves that the lack of equal pay in society is indeed still in question by government entities as well as small sized companies to large sized corporations from across all job industries. Behavioral and work-life balance contributes to women earning less than men since it is found that they tend to work less according to the Bureau of Labor Statistics. We can conclude that personal factors such as lower paying jobs within respective career choices and degrees leads women to earn less. Even in so-called women dominated field, the research found relatively disproportionate annual pay between men and women. With the given evidence in our report, there should be a dramatic change in how equal pay is approached as well as the issue of the gender pay gap.”

Cite this page
The Gender Pay Gap and the Equality. (2021, May 20). Retrieved from https://papersowl.com/examples/the-gender-pay-gap-and-the-equality/
"The Gender Pay Gap and the Equality." PapersOwl.com , 20 May 2021, https://papersowl.com/examples/the-gender-pay-gap-and-the-equality/
PapersOwl.com. (2021). The Gender Pay Gap and the Equality . [Online]. Available at: https://papersowl.com/examples/the-gender-pay-gap-and-the-equality/ [Accessed: 5 Jun. 2024]
"The Gender Pay Gap and the Equality." PapersOwl.com, May 20, 2021. Accessed June 5, 2024. https://papersowl.com/examples/the-gender-pay-gap-and-the-equality/
"The Gender Pay Gap and the Equality," PapersOwl.com , 20-May-2021. [Online]. Available: https://papersowl.com/examples/the-gender-pay-gap-and-the-equality/. [Accessed: 5-Jun-2024]
PapersOwl.com. (2021). The Gender Pay Gap and the Equality . [Online]. Available at: https://papersowl.com/examples/the-gender-pay-gap-and-the-equality/ [Accessed: 5-Jun-2024]
Don't let plagiarism ruin your grade
Hire a writer to get a unique paper crafted to your needs.
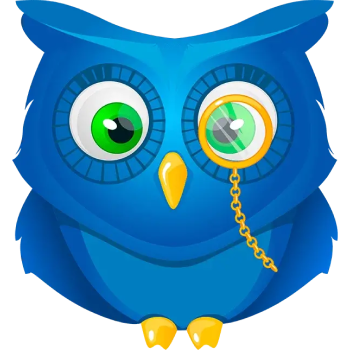
Our writers will help you fix any mistakes and get an A+!
Please check your inbox.
You can order an original essay written according to your instructions.
Trusted by over 1 million students worldwide
1. Tell Us Your Requirements
2. Pick your perfect writer
3. Get Your Paper and Pay
Hi! I'm Amy, your personal assistant!
Don't know where to start? Give me your paper requirements and I connect you to an academic expert.
short deadlines
100% Plagiarism-Free
Certified writers
Pay transparency and pay gap reporting may be rising but how effective are they?
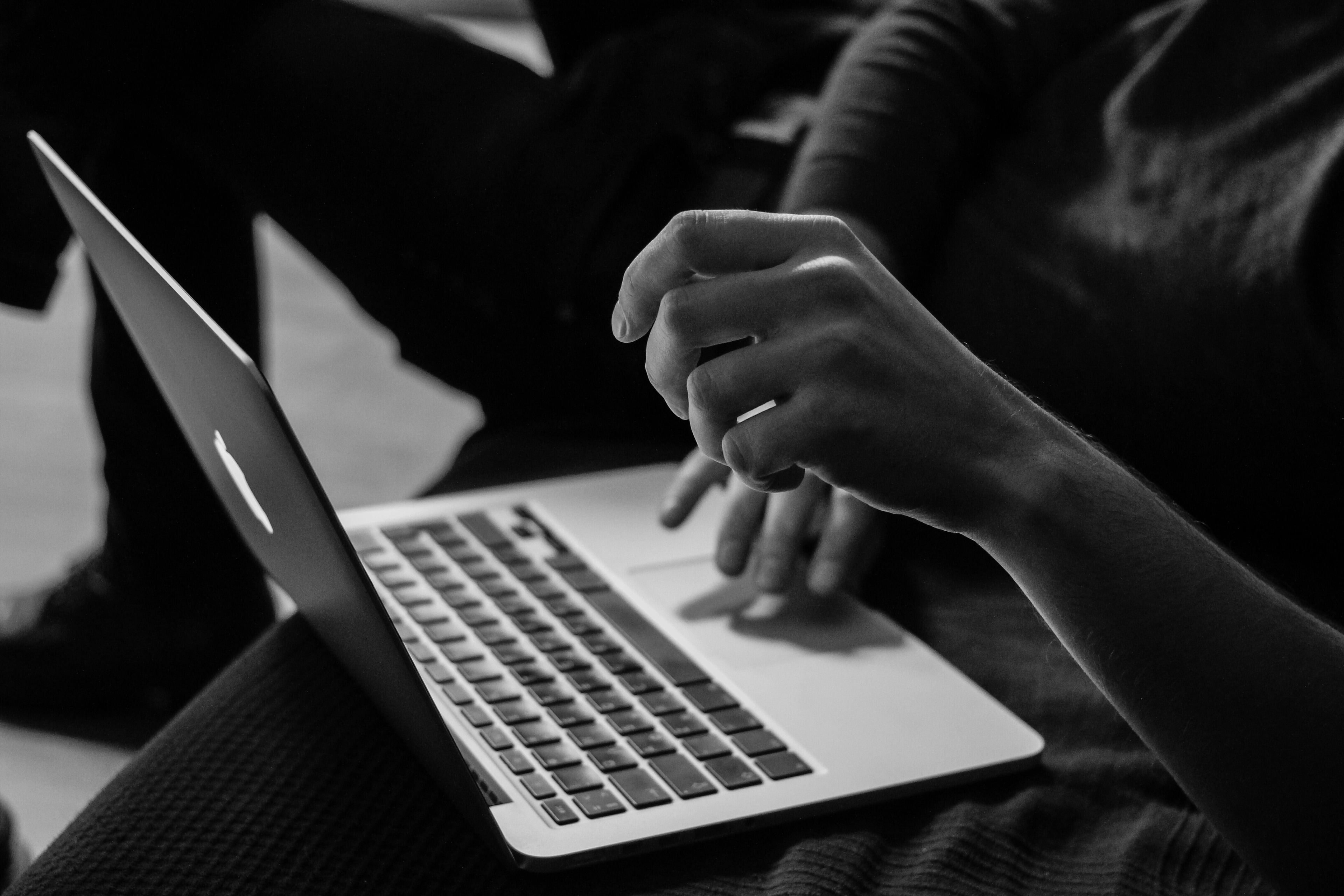
Gender pay gap and pay transparency has been scrutinized worldwide despite their rise. Image: Getty Images/iStockphoto
.chakra .wef-1c7l3mo{-webkit-transition:all 0.15s ease-out;transition:all 0.15s ease-out;cursor:pointer;-webkit-text-decoration:none;text-decoration:none;outline:none;color:inherit;}.chakra .wef-1c7l3mo:hover,.chakra .wef-1c7l3mo[data-hover]{-webkit-text-decoration:underline;text-decoration:underline;}.chakra .wef-1c7l3mo:focus,.chakra .wef-1c7l3mo[data-focus]{box-shadow:0 0 0 3px rgba(168,203,251,0.5);} Tom Heys
Emanuela nespoli.
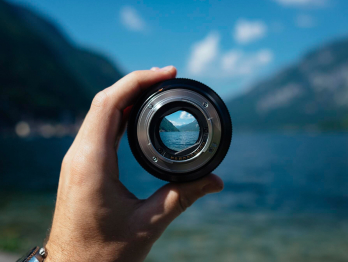
.chakra .wef-1nk5u5d{margin-top:16px;margin-bottom:16px;line-height:1.388;color:#2846F8;font-size:1.25rem;}@media screen and (min-width:56.5rem){.chakra .wef-1nk5u5d{font-size:1.125rem;}} Get involved .chakra .wef-9dduvl{margin-top:16px;margin-bottom:16px;line-height:1.388;font-size:1.25rem;}@media screen and (min-width:56.5rem){.chakra .wef-9dduvl{font-size:1.125rem;}} with our crowdsourced digital platform to deliver impact at scale
- Many countries have implemented or are moving towards implementing pay transparency and pay gap reporting laws, with mandatory requirements cemented in Europe.
- The comparability of pay gap reporting regimes varies according to the period analyzed, definitions of “pay,” the scope of entities that report and the specific statistics reported, making it difficult to compare and assess policy efficacy.
- The effectiveness of pay gap reporting has been scrutinized. For example, decreases in male pay rather than increased pay to women could explain gap reductions, while pay transparency may weaken bargaining power, meaning pay overall is reduced.
Nearly all countries have one thing in common: a persistent gender pay gap. The gender pay gap represents not just a moral quandary but an inefficient and untenable utilization of human capital. The International Monetary Fund reports that the estimated economic cost of economic disempowerment of women ranges from 10% of gross domestic product in advanced economies to more than 30% in South Asia, the Middle East and North Africa.
Yet, there appears to be a renewed determination to address this longstanding issue. Societal reckonings such as the #MeToo movement have laid bare entrenched inequalities and barriers that permeate workplaces. Governments worldwide are now keen to reduce pay gaps and are adopting pay transparency and reporting measures.
Have you read?
3 steps to tackling the global gender gap, global gender gap report 2023, legislative trends and global consensus.
“Pay transparency” encompasses various policies, from simply publishing pay ranges in job adverts to calculating and publicly reporting pay gaps. This last approach has gained increased traction in recent years.
In 1991, Italy introduced a reporting obligation for large companies concerning male and female employees’ work conditions and salaries. It was only in 2013, however, that the trend really began: Denmark introduced mandatory reporting on gender pay disparities for large companies, with the United Kingdom following suit in 2017.
Several other countries, including France, Spain, Italy , and Ireland , have since introduced similar reporting requirements. In May 2023, the European Union issued its Pay Transparency Directive , which requires all member states to implement gender pay gap reporting obligations and other mechanisms to reduce gender inequality.
Beyond Europe, more countries are joining this movement. In Australia, over 5,000 large employers have recently had their gender pay gaps published. In Brazil, new legislation will oblige companies with more than 100 employees to report salary differences between men and women in management and leadership positions. Japan implemented gender pay gap reporting in 2022. Twenty-one of 38 Organization for Economic Co-operation and Development countries now have a gender pay gap reporting regime.
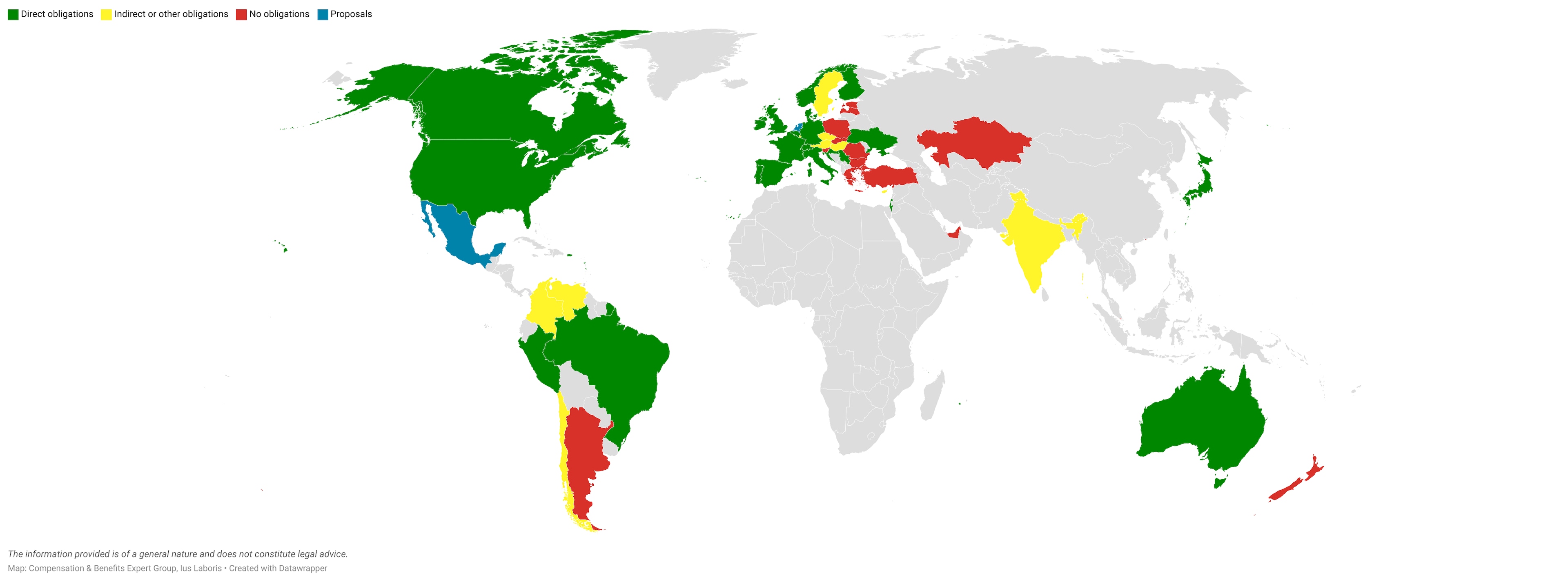
Devising a gender pay gap reporting regime
Pay reporting at the most basic level involves calculating and publishing statistics about men and women in the workforce. However, there is a high degree of variation between regimes, making comparison difficult.
Below are the questions that any policymaker must consider when devising a gender pay gap reporting regime. The aim is to motivate employers to increase gender diversity in their workplace without imposing too big a burden.
- What period is being analyzed? Using a whole year of data, including variable pay such as commissions, can cause gaps to vary annually due to performance and economic conditions. A snapshot might, therefore, miss significant seasonal differences, leading to incomplete reporting for employers with fluctuating headcounts.
- What is analyzed? Minor changes to the definition of “pay” affect comparability. For example, overtime paid at a premium can significantly impact total compensation, affecting statistics. Similarly, including salary sacrifice or flexible benefits can bias statistics based on personal choice rather than entitlement.
- Who has to report? Reporting obligations typically apply to individual legal entities, but when complex group structures divide a workforce, how many entities will meet the headcount threshold?
- What statistics are reported? The headline statistic always shows the gender pay gap. However, there can be a range of additional statistics relating to bonus gaps, the proportions of men and women who receive benefits, and the proportions within pay quartiles.
- What else is reported? In Ireland, employers must explain the causes of their gaps and any measures they are taking but do not need to take them – saying “We are not taking any measures” is still in statutory compliance. Mandatory explanations are common, but the obligatory proactive reduction is much rarer.
- How is pay reporting enforced? Enforcement tends to be very light touch with those employers that fail to report being “named and shamed” in the media rather than hit with a sanction.
Pay transparency is far from a proven policy.
Is pay gap reporting effective as a policy?
Gender pay gap reporting is borne from the idea that “sunlight is the best disinfectant.” Rather than legislative micromanagement, employers are left to decide what specific measures to take.
But gender pay gaps change slowly. Knowing whether this type of nudge is an effective policy will take time. Although plenty of examples of employers have been nudged into action, at the macro level, evidence of the effectiveness of pay gap reporting is still developing.
Using data from the United Kingdom, researchers at the London School of Economics found that gender pay gap reporting led to a 1.6% reduction in pay gaps . Similar conclusions have been made about the impact of legislation in Denmark ; however, this research found that gaps are reduced due to decreasing male pay rather than increased pay for women. Therefore, the primary motivation for pay reporting – the advancement of women in the labour market – was not achieved.
There is also evidence against pay transparency: one study found that pay transparency could reduce pay by 2% , as it reduces the bargaining position of workers. While this is a theoretical study of negotiation dynamics rather than an empirical study of outcomes, its conclusions shouldn’t be ignored.
These studies indicate that pay transparency is far from a proven policy. Legislators worldwide are, therefore, taking leaps of faith by creating new pay reporting regimes. Although the scale of potential economic, social and political benefits is significant, time will tell whether pay reporting can achieve these.
Don't miss any update on this topic
Create a free account and access your personalized content collection with our latest publications and analyses.
License and Republishing
World Economic Forum articles may be republished in accordance with the Creative Commons Attribution-NonCommercial-NoDerivatives 4.0 International Public License, and in accordance with our Terms of Use.
The views expressed in this article are those of the author alone and not the World Economic Forum.
Related topics:
The agenda .chakra .wef-n7bacu{margin-top:16px;margin-bottom:16px;line-height:1.388;font-weight:400;} weekly.
A weekly update of the most important issues driving the global agenda
.chakra .wef-1dtnjt5{display:-webkit-box;display:-webkit-flex;display:-ms-flexbox;display:flex;-webkit-align-items:center;-webkit-box-align:center;-ms-flex-align:center;align-items:center;-webkit-flex-wrap:wrap;-ms-flex-wrap:wrap;flex-wrap:wrap;} More on Equity, Diversity and Inclusion .chakra .wef-17xejub{-webkit-flex:1;-ms-flex:1;flex:1;justify-self:stretch;-webkit-align-self:stretch;-ms-flex-item-align:stretch;align-self:stretch;} .chakra .wef-nr1rr4{display:-webkit-inline-box;display:-webkit-inline-flex;display:-ms-inline-flexbox;display:inline-flex;white-space:normal;vertical-align:middle;text-transform:uppercase;font-size:0.75rem;border-radius:0.25rem;font-weight:700;-webkit-align-items:center;-webkit-box-align:center;-ms-flex-align:center;align-items:center;line-height:1.2;-webkit-letter-spacing:1.25px;-moz-letter-spacing:1.25px;-ms-letter-spacing:1.25px;letter-spacing:1.25px;background:none;padding:0px;color:#B3B3B3;-webkit-box-decoration-break:clone;box-decoration-break:clone;-webkit-box-decoration-break:clone;}@media screen and (min-width:37.5rem){.chakra .wef-nr1rr4{font-size:0.875rem;}}@media screen and (min-width:56.5rem){.chakra .wef-nr1rr4{font-size:1rem;}} See all
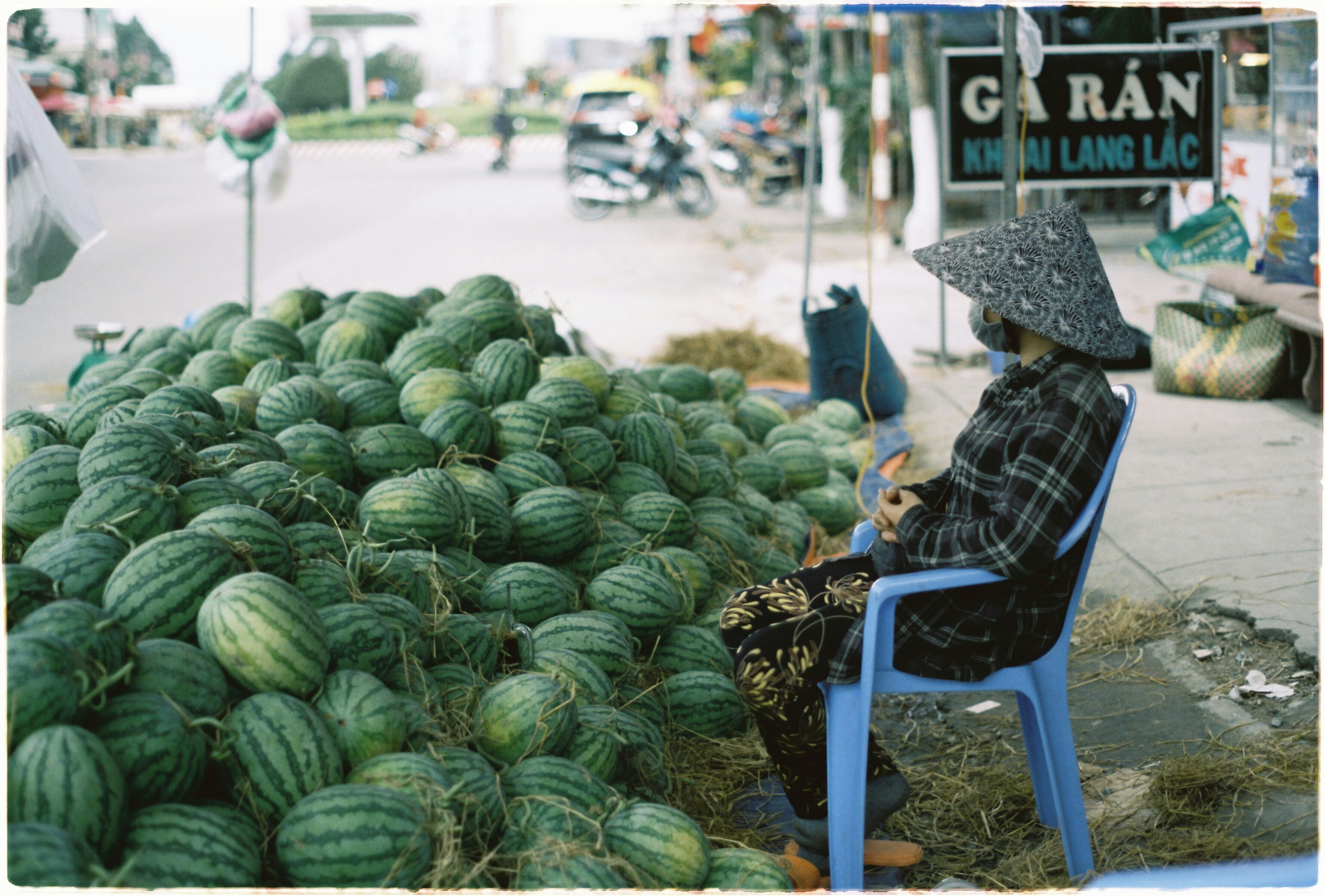
What is the informal economy and how many people work in it?
Simon Torkington
June 4, 2024
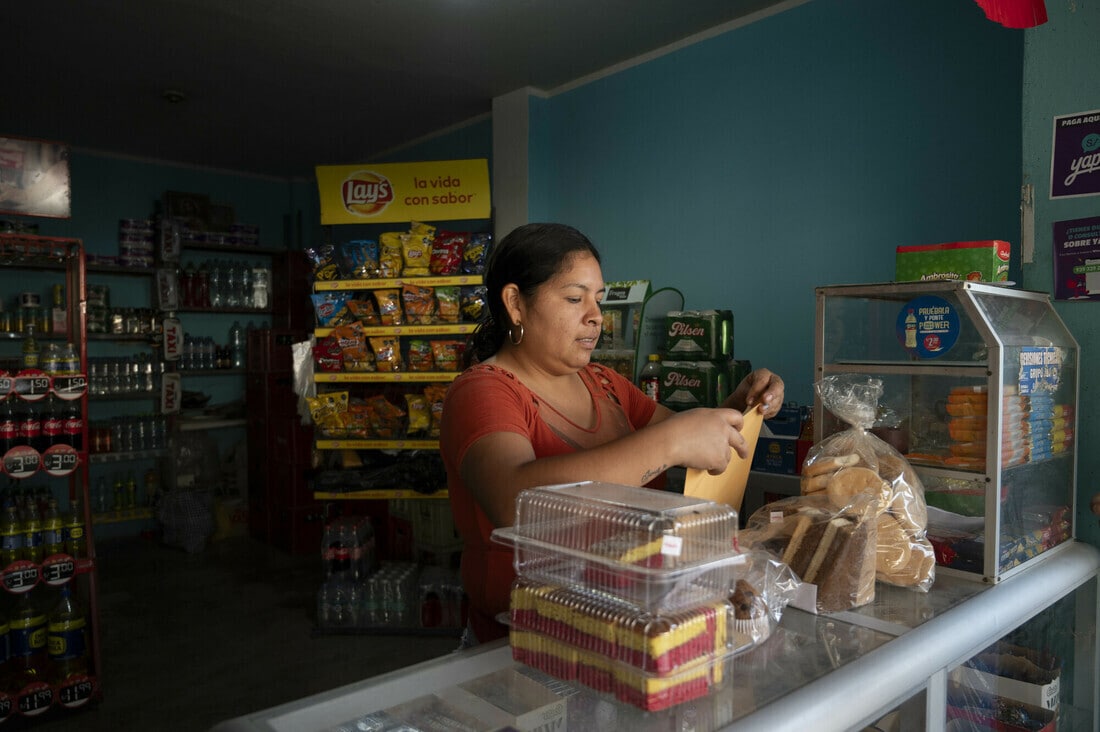
How focused giving can unlock billions and catapult women’s wealth
Mark Muckerheide
May 21, 2024
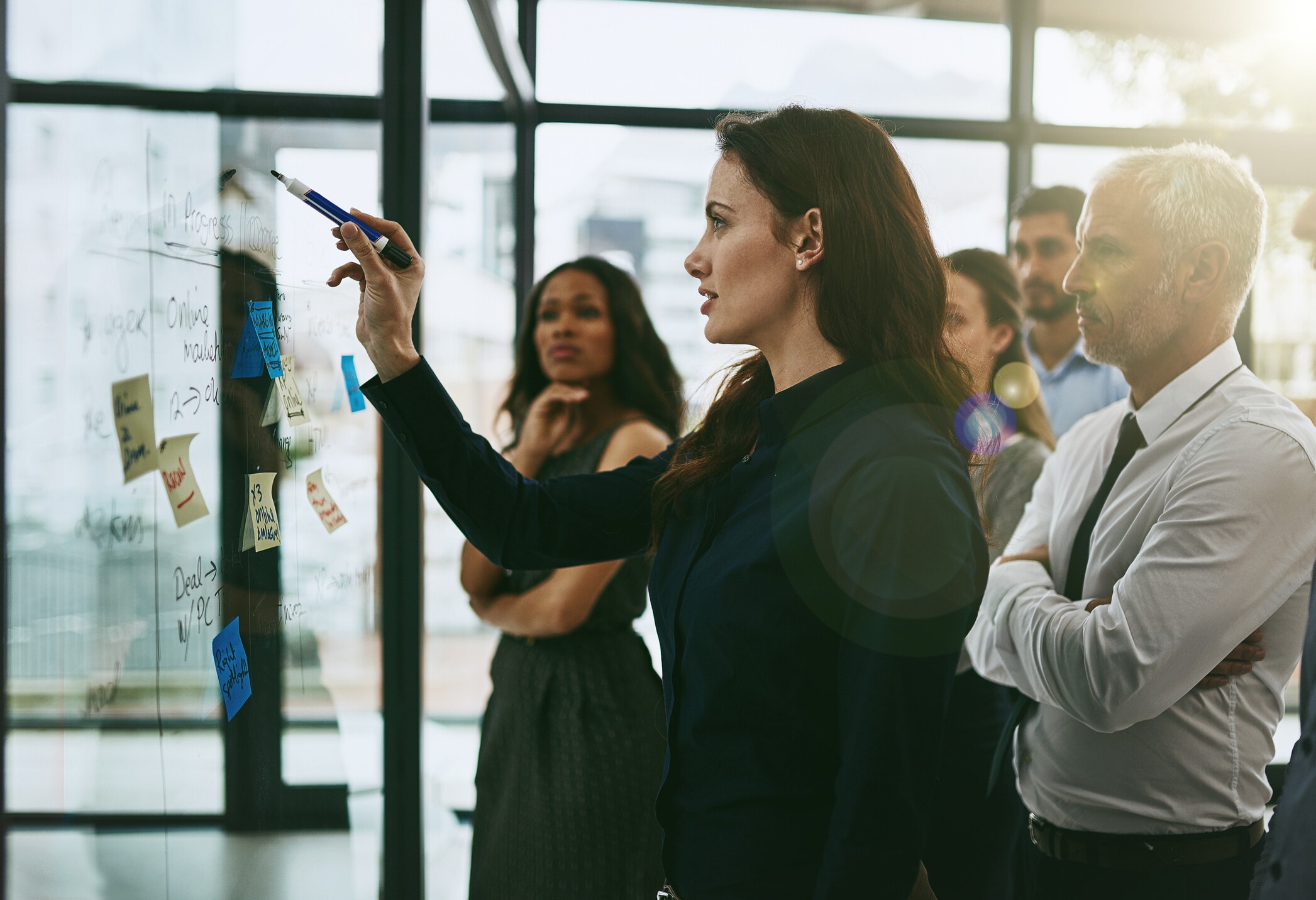
Progress for women in the workplace stagnating in four key areas, global study reveals
The care economy is one of humanity's most valuable assets. Here's how we secure its future
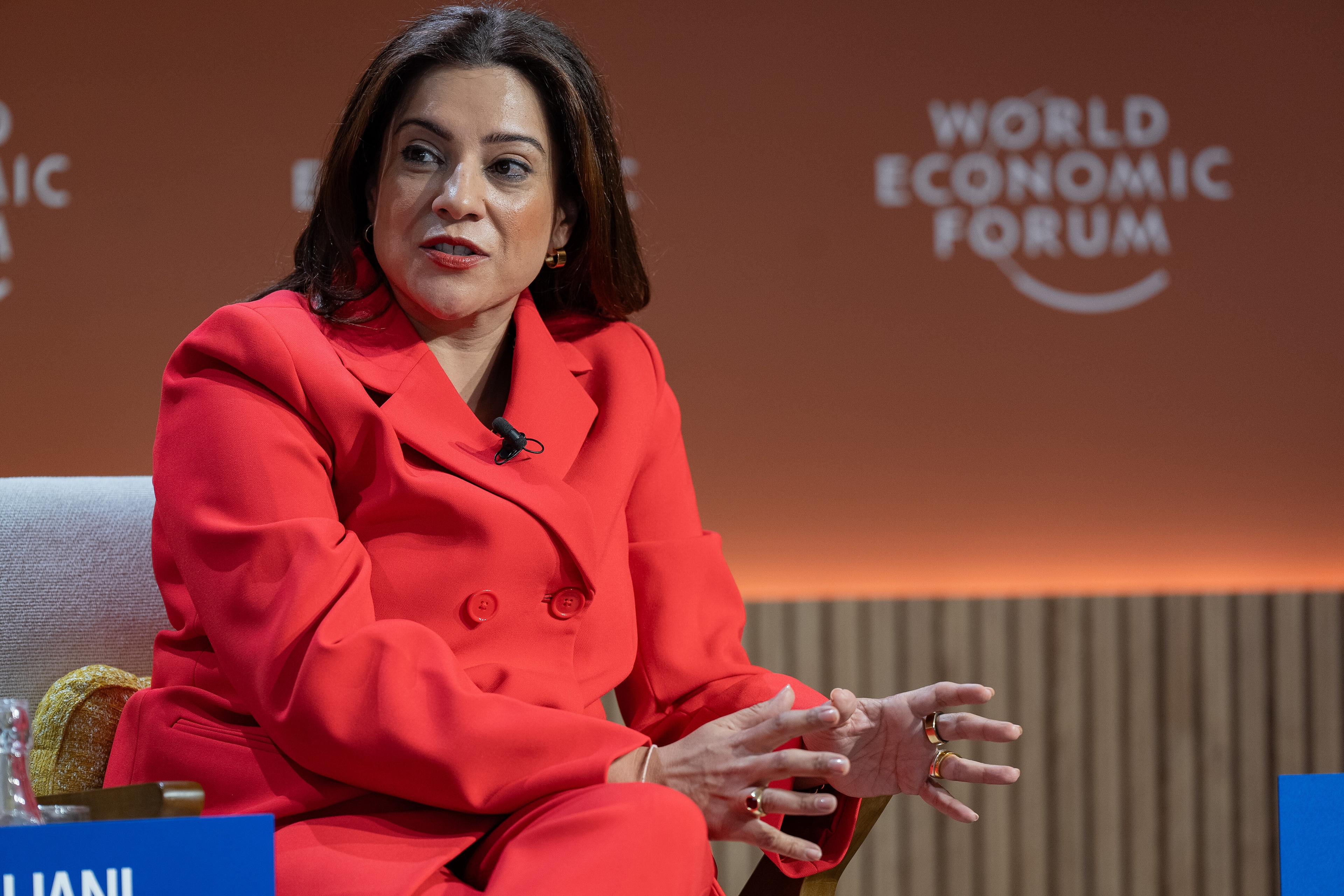
This is how AI can empower women and achieve gender equality, according to the founder of Girls Who Code and Moms First
Kate Whiting
May 14, 2024

Here's how rising air pollution threatens millions in the US
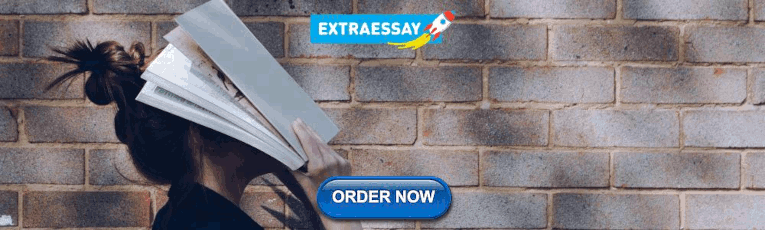
COMMENTS
Reviewing some essay samples of gender wage gap essay topics, like the ones below, will prove helpful. 19 essay samples found. Sort & filter. 1 The Issue of Gender Wage Gap in America . 2 pages / 789 words . ... Introduction The sexual category wage gap, the observed modification between salaries paid to women and incomes paid to men, has been ...
Table 4.4: The gender pay gap comparing married to unmarried people (£ per hour) Table 4.5: The gender pay gap by marital status and age (£ per hour) Table 4.6: The gender pay gap by presence of dependent children in household Table 4.7: Housework and paid work hours (UKHLS; 45,533 observations) Table 4.8: The pay gap by educational level
Introduction and key findings. Women are paid 79 cents for every dollar paid to men (Hegewisch and DuMonthier 2016). ... The gender pay gap is driven at least in part by the cumulative impact of many instances over the course of women's lives when they are treated differently than their male peers. Girls can be steered toward gender-normative ...
trends in the US gender wage gap and on their sources (in a descriptive sense). Accounting for the sources of the level and changes in the gender pay gap will provide guidance for understanding recent research studying gender and the labor market. Figure 1 shows the long-run trends in the gender pay gap over the 1955-2014 period based on two
Working to eliminate the gender wage gap requires looking beyond these statistics to explain why women's earnings . are lower even when they work full time, all year long. A recent report coauthored by the U.S. Census Bureau and . the Department of Labor's Women's Bureau provides what is currently the most comprehensive examination of the ...
Introduction. The "gender pay gap" refers to the average difference in men's and women's earnings, and is typically adjusted for hours worked. The gender pay gap can refer to differences in mean or median annual earnings, weekly earnings, or hourly wage. Because women tend to work part-time at higher rates than do men, and because part ...
A good share of the increase in the gender pay gap takes place when women are between the ages of 35 and 44. In 2022, women ages 25 to 34 earned about 92% as much as men of the same ages, but women ages 35 to 44 and 45 to 54 earned 83% as much. The ratio dropped to 79% among those ages 55 to 64.
The gender pay gap stands at 20 per cent, meaning women workers earn 80 per cent of what men do. For women of colour, migrant women, those with disabilities, and women with children, the gap is even greater. The cumulative effect of pay disparities has real, daily negative consequences for women, their families, and society, especially during ...
Introduction. The gender pay gap, the disparity in earnings between male and female workers, has been the focus of empirical research in the US for decades, as well as legislative and executive action under the Obama administration [1, 2].Trends dating back to the 1960s show a long period in which women's earnings were approximately 60% of their male counterparts, followed by increases in ...
Using the distribution of the male executives as the reference distribution, the decomposition shows that the basic covariates explain 82.4% of the log pay gap, while the log of the unexplained part is equal to 0.094. This leaves 17.6% of the log gap unexplained (equals 0.094 divided by 0.535).
The gender pay gap is a measurable indicator of inequality between women and men. Most govern- ments have legislated to guarantee equality of treatment between men and women in remuneration. The ILO Equal Remuneration Convention, 1951 (No. 100) is one of the most highly ratified conven- tions.
In this section, we provide a short introduction to the measurement of the gender wage gap based on several surveys and reports (among others Plantenga & Remery, 2006). The gender wage gap refers to the differences between the wages earned by women and men in comparable jobs that generate equal values (OECD 2021).
The findings suggest that the gender pay gap has a significant impact on women's economic empowerment, limiting their financial independence and autonomy. The study also highlights the need for ...
Introduction: The difference between the average gross earnings of female and male employees is known as the 'gender pay gap'. ("Gender Equality: Gender Pay Gap", 2019) Most commonly, it refers to the median annual pay of all women who work full time and year-round, compared to the pay of a similar cohort of men. Other estimates of the gender ...
The median gender pay gap in the United States, for example, is approximately 82 cents for every dollar earned by men. While there have been improvements in some sectors, the gender pay gap persists across industries and occupations. To address this issue, further action and improvement are needed, including the enforcement of existing laws ...
Adjusted basic pay is the sum of an employee's rate of basic pay plus any locality. comparability payment and/or special pay adjustment for law enforcement officers. Salaries are grouped by $10,000 intervals, except for an initial interval of less than. $20,000 and a final interval of $180,000 or more.
Gender pay gap refers to the difference in pay between men and women in the workforce. It is a persistent issue and has been widely acknowledged as a form of gender-based discrimination. Women tend to earn less than men, even when working in the same roles or with similar qualifications and experience. The gender pay gap can be attributed to ...
Opinion Writer. American women made significant progress toward closing the gender pay gap in the second half of the 20th century, but that gap has barely budged over the past two decades. In 2022 ...
Fin de l'encadré. 2. Measuring the gender pay gap. Relative earnings often signify how different groups are valued socially and economically. Note For this reason, the unadjusted gender pay gap—the raw difference between the earnings acquired by women and men through their paid work, which favours the latter—has often been used as a call to action for gender equality and women's ...
Introduction. The gender pay gap, the disparity in earnings between male and female workers, has been the focus of empirical research in the US for decades, as well as legislative and executive action under the Obama administration [1, 2].Trends dating back to the 1960s show a long period in which women's earnings were approximately 60% of their male counterparts, followed by increases in ...
The mean gender pay gap was about 54% for years from 2006 to 2011, but the level of difference had reduced from 70% to 40% in 2008 to 2011 (Shrivastava, 2016). It was found that the gender pay gap was maximum for the age group 50-60 years at 157% and least for the age group 20-30 years at 38%.
Median pay for all employees was 14.3% less for women than for men in April 2023. The full-time pay gap has been getting smaller since 1997 and the overall pay gap has also decreased over the period. The part-time pay gap has generally remained small and negative, with women earning more than men on average.
Introduction. The gender pay gap and the equality of pay rates have always been topics of discussion in today's society. Equal pay means that individuals accomplishing the same work should be compensated equivalently in regards to completion. Issues are raised between the earning differences between men and women due to the lack of equal pay ...
UK results found that 78% of organisations had a gender pay gap with 13% (1377 employers) of those having pay gaps over 30%, and some in excess of 40%. The results found that the majority of higher-paid jobs were held by men; there was no sector that paid women more; and men were paid higher bonuses than women.
The effectiveness of pay gap reporting has been scrutinized. For example, decreases in male pay rather than increased pay to women could explain gap reductions, while pay transparency may weaken bargaining power, meaning pay overall is reduced. Nearly all countries have one thing in common: a persistent gender pay gap.