- Comprehensive Learning Paths
- 150+ Hours of Videos
- Complete Access to Jupyter notebooks, Datasets, References.

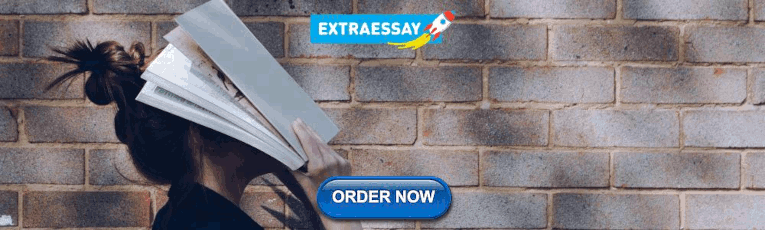
Hypothesis Testing ā A Deep Dive into Hypothesis Testing, The Backbone of Statistical Inference
- September 21, 2023
Explore the intricacies of hypothesis testing, a cornerstone of statistical analysis. Dive into methods, interpretations, and applications for making data-driven decisions.

In this Blog post we will learn:
- What is Hypothesis Testing?
- Steps in Hypothesis Testing 2.1. Set up Hypotheses: Null and Alternative 2.2. Choose a Significance Level (α) 2.3. Calculate a test statistic and P-Value 2.4. Make a Decision
- Example : Testing a new drug.
- Example in python
1. What is Hypothesis Testing?
In simple terms, hypothesis testing is a method used to make decisions or inferences about population parameters based on sample data. Imagine being handed a dice and asked if itās biased. By rolling it a few times and analyzing the outcomes, youād be engaging in the essence of hypothesis testing.
Think of hypothesis testing as the scientific method of the statistics world. Suppose you hear claims like āThis new drug works wonders!ā or āOur new website design boosts sales.ā How do you know if these statements hold water? Enter hypothesis testing.
2. Steps in Hypothesis Testing
- Set up Hypotheses : Begin with a null hypothesis (H0) and an alternative hypothesis (Ha).
- Choose a Significance Level (α) : Typically 0.05, this is the probability of rejecting the null hypothesis when itās actually true. Think of it as the chance of accusing an innocent person.
- Calculate Test statistic and P-Value : Gather evidence (data) and calculate a test statistic.
- p-value : This is the probability of observing the data, given that the null hypothesis is true. A small p-value (typically ⤠0.05) suggests the data is inconsistent with the null hypothesis.
- Decision Rule : If the p-value is less than or equal to α, you reject the null hypothesis in favor of the alternative.
2.1. Set up Hypotheses: Null and Alternative
Before diving into testing, we must formulate hypotheses. The null hypothesis (H0) represents the default assumption, while the alternative hypothesis (H1) challenges it.
For instance, in drug testing, H0 : āThe new drug is no better than the existing one,ā H1 : āThe new drug is superior .ā
2.2. Choose a Significance Level (α)
When You collect and analyze data to test H0 and H1 hypotheses. Based on your analysis, you decide whether to reject the null hypothesis in favor of the alternative, or fail to reject / Accept the null hypothesis.
The significance level, often denoted by $α$, represents the probability of rejecting the null hypothesis when it is actually true.
In other words, itās the risk youāre willing to take of making a Type I error (false positive).
Type I Error (False Positive) :
- Symbolized by the Greek letter alpha (α).
- Occurs when you incorrectly reject a true null hypothesis . In other words, you conclude that there is an effect or difference when, in reality, there isnāt.
- The probability of making a Type I error is denoted by the significance level of a test. Commonly, tests are conducted at the 0.05 significance level , which means thereās a 5% chance of making a Type I error .
- Commonly used significance levels are 0.01, 0.05, and 0.10, but the choice depends on the context of the study and the level of risk one is willing to accept.
Example : If a drug is not effective (truth), but a clinical trial incorrectly concludes that it is effective (based on the sample data), then a Type I error has occurred.
Type II Error (False Negative) :
- Symbolized by the Greek letter beta (β).
- Occurs when you accept a false null hypothesis . This means you conclude there is no effect or difference when, in reality, there is.
- The probability of making a Type II error is denoted by β. The power of a test (1 ā β) represents the probability of correctly rejecting a false null hypothesis.
Example : If a drug is effective (truth), but a clinical trial incorrectly concludes that it is not effective (based on the sample data), then a Type II error has occurred.
Balancing the Errors :
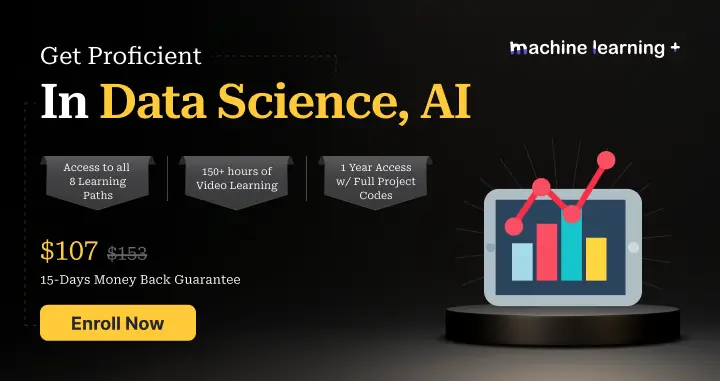
In practice, thereās a trade-off between Type I and Type II errors. Reducing the risk of one typically increases the risk of the other. For example, if you want to decrease the probability of a Type I error (by setting a lower significance level), you might increase the probability of a Type II error unless you compensate by collecting more data or making other adjustments.
Itās essential to understand the consequences of both types of errors in any given context. In some situations, a Type I error might be more severe, while in others, a Type II error might be of greater concern. This understanding guides researchers in designing their experiments and choosing appropriate significance levels.
2.3. Calculate a test statistic and P-Value
Test statistic : A test statistic is a single number that helps us understand how far our sample data is from what weād expect under a null hypothesis (a basic assumption weāre trying to test against). Generally, the larger the test statistic, the more evidence we have against our null hypothesis. It helps us decide whether the differences we observe in our data are due to random chance or if thereās an actual effect.
P-value : The P-value tells us how likely we would get our observed results (or something more extreme) if the null hypothesis were true. Itās a value between 0 and 1. ā A smaller P-value (typically below 0.05) means that the observation is rare under the null hypothesis, so we might reject the null hypothesis. ā A larger P-value suggests that what we observed could easily happen by random chance, so we might not reject the null hypothesis.
2.4. Make a Decision
Relationship between $α$ and P-Value
When conducting a hypothesis test:
We then calculate the p-value from our sample data and the test statistic.
Finally, we compare the p-value to our chosen $α$:
- If $pāvalueā¤Ī±$: We reject the null hypothesis in favor of the alternative hypothesis. The result is said to be statistically significant.
- If $pāvalue>α$: We fail to reject the null hypothesis. There isnāt enough statistical evidence to support the alternative hypothesis.
3. Example : Testing a new drug.
Imagine we are investigating whether a new drug is effective at treating headaches faster than drug B.
Setting Up the Experiment : You gather 100 people who suffer from headaches. Half of them (50 people) are given the new drug (letās call this the āDrug Groupā), and the other half are given a sugar pill, which doesnāt contain any medication.
- Set up Hypotheses : Before starting, you make a prediction:
- Null Hypothesis (H0): The new drug has no effect. Any difference in healing time between the two groups is just due to random chance.
- Alternative Hypothesis (H1): The new drug does have an effect. The difference in healing time between the two groups is significant and not just by chance.
Calculate Test statistic and P-Value : After the experiment, you analyze the data. The ātest statisticā is a number that helps you understand the difference between the two groups in terms of standard units.
For instance, letās say:
- The average healing time in the Drug Group is 2 hours.
- The average healing time in the Placebo Group is 3 hours.
The test statistic helps you understand how significant this 1-hour difference is. If the groups are large and the spread of healing times in each group is small, then this difference might be significant. But if thereās a huge variation in healing times, the 1-hour difference might not be so special.
Imagine the P-value as answering this question: āIf the new drug had NO real effect, whatās the probability that Iād see a difference as extreme (or more extreme) as the one I found, just by random chance?ā
For instance:
- P-value of 0.01 means thereās a 1% chance that the observed difference (or a more extreme difference) would occur if the drug had no effect. Thatās pretty rare, so we might consider the drug effective.
- P-value of 0.5 means thereās a 50% chance youād see this difference just by chance. Thatās pretty high, so we might not be convinced the drug is doing much.
- If the P-value is less than ($α$) 0.05: the results are āstatistically significant,ā and they might reject the null hypothesis , believing the new drug has an effect.
- If the P-value is greater than ($α$) 0.05: the results are not statistically significant, and they donāt reject the null hypothesis , remaining unsure if the drug has a genuine effect.
4. Example in python
For simplicity, letās say weāre using a t-test (common for comparing means). Letās dive into Python:
Making a Decision : āThe results are statistically significant! p-value < 0.05 , The drug seems to have an effect!ā If not, weād say, āLooks like the drug isnāt as miraculous as we thought.ā
5. Conclusion
Hypothesis testing is an indispensable tool in data science, allowing us to make data-driven decisions with confidence. By understanding its principles, conducting tests properly, and considering real-world applications, you can harness the power of hypothesis testing to unlock valuable insights from your data.
More Articles
Correlation ā connecting the dots, the role of correlation in data analysis, sampling and sampling distributions ā a comprehensive guide on sampling and sampling distributions, law of large numbers ā a deep dive into the world of statistics, central limit theorem ā a deep dive into central limit theorem and its significance in statistics, skewness and kurtosis ā peaks and tails, understanding data through skewness and kurtosisā, similar articles, complete introduction to linear regression in r, how to implement common statistical significance tests and find the p value, logistic regression ā a complete tutorial with examples in r.
Subscribe to Machine Learning Plus for high value data science content
Ā© Machinelearningplus. All rights reserved.

Machine Learning A-Zā¢: Hands-On Python & R In Data Science
Free sample videos:.

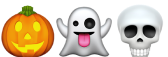
- Onsite training
3,000,000+ delegates
15,000+ clients
1,000+ locations
- KnowledgePass
- Log a ticket
01344203999 Available 24/7
Hypothesis Testing in Data Science: It's Usage and Types
Hypothesis Testing in Data Science is a crucial method for making informed decisions from data. This blog explores its essential usage in analysing trends and patterns, and the different types such as null, alternative, one-tailed, and two-tailed tests, providing a comprehensive understanding for both beginners and advanced practitioners.

Exclusive 40% OFF
Training Outcomes Within Your Budget!
We ensure quality, budget-alignment, and timely delivery by our expert instructors.
Share this Resource
- Advanced Data Science Certification
- Data Science and Blockchain Training
- Big Data Analysis
- Python Data Science Course
- Advanced Data Analytics Course {location}
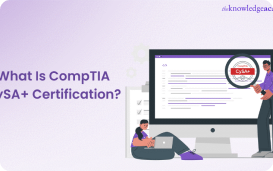
Table of Contents
1) What is Hypothesis Testing in Data Science?
2) Importance of Hypothesis Testing in Data Science
3) Types of Hypothesis Testing
4) Basic steps in Hypothesis Testing
5) Real-world use cases of Hypothesis Testing
6) Conclusion
What is Hypothesis Testing in Data Science?
Hypothesis Testing in Data Science is a statistical method used to assess the validity of assumptions or claims about a population based on sample data. It involves formulating two Hypotheses, the null Hypothesis (H0) and the alternative Hypothesis (Ha or H1), and then using statistical tests to find out if there is enough evidence to support the alternative Hypothesis.
Hypothetical Testing is a critical tool for making data-driven decisions, evaluating the significance of observed effects or differences, and drawing meaningful conclusions from data, allowing Data Scientists to uncover patterns, relationships, and insights that inform various domains, from medicine to business and beyond.
Unlock the power of data with our comprehensive Data Science & Analytics Training . Sign up now!
Importance of Hypothesis Testing in Data Science
The significance of Hypothesis Testing in Data Science cannot be overstated. It serves as the cornerstone of data-driven decision-making. By systematically testing Hypotheses, Data Scientists can:
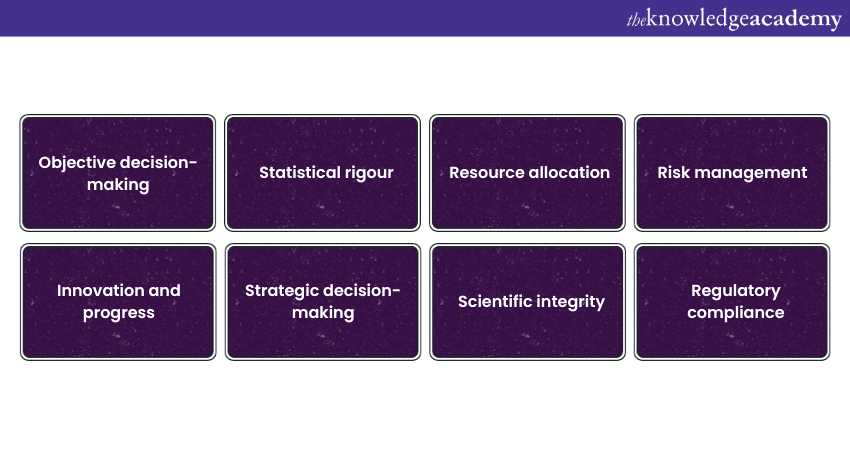
Objective decision-making
Hypothesis Testing provides a structured and impartial method for making decisions based on data. In a world where biases can skew perceptions, Data Scientists rely on this method to ensure that their conclusions are grounded in empirical evidence, making their decisions more objective and trustworthy.
Statistical rigour
Data Scientists deal with large amounts of data, and Hypothesis Testing helps them make sense of it. It quantifies the significance of observed patterns, differences, or relationships. This statistical rigour is essential in distinguishing between mere coincidences and meaningful findings, reducing the likelihood of making decisions based on random chance.
Resource allocation
Resources, whether they are financial, human, or time-related, are often limited. Hypothesis Testing enables efficient resource allocation by guiding Data Scientists towards strategies or interventions that are statistically significant. This ensures that efforts are directed where they are most likely to yield valuable results.
Risk management
In domains like healthcare and finance, where lives and livelihoods are at stake, Hypothesis Testing is a critical tool for risk assessment. For instance, in drug development, Hypothesis Testing is used to determine the safety and efficiency of new treatments, helping mitigate potential risks to patients.
Innovation and progress
Hypothesis Testing fosters innovation by providing a systematic framework to evaluate new ideas, products, or strategies. It encourages a cycle of experimentation, feedback, and improvement, driving continuous progress and innovation.
Strategic decision-making
Organisations base their strategies on data-driven insights. Hypothesis Testing enables them to make informed decisions about market trends, customer behaviour, and product development. These decisions are grounded in empirical evidence, increasing the likelihood of success.
Scientific integrity
In scientific research, Hypothesis Testing is integral to maintaining the integrity of research findings. It ensures that conclusions are drawn from rigorous statistical analysis rather than conjecture. This is essential for advancing knowledge and building upon existing research.
Regulatory compliance
Many industries, such as pharmaceuticals and aviation, operate under strict regulatory frameworks. Hypothesis Testing is essential for demonstrating compliance with safety and quality standards. It provides the statistical evidence required to meet regulatory requirements.
Supercharge your data skills with our Big Data and Analytics Training – register now!
Types of Hypothesis Testing
Hypothesis Testing can be seen in several different types. In total, we have five types of Hypothesis Testing. They are described below as follows:
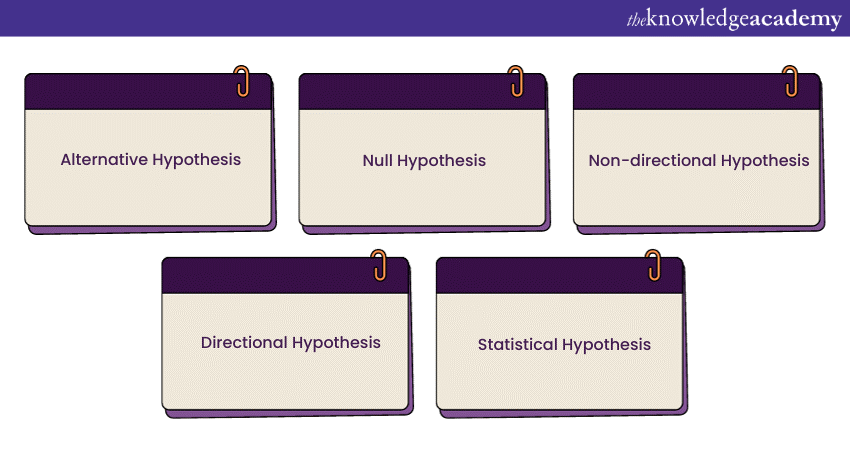
Alternative Hypothesis
The Alternative Hypothesis, denoted as Ha or H1, is the assertion or claim that researchers aim to support with their data analysis. It represents the opposite of the null Hypothesis (H0) and suggests that there is a significant effect, relationship, or difference in the population. In simpler terms, it's the statement that researchers hope to find evidence for during their analysis. For example, if you are testing a new drug's efficacy, the alternative Hypothesis might state that the drug has a measurable positive effect on patients' health.
Null Hypothesis
The Null Hypothesis, denoted as H0, is the default assumption in Hypothesis Testing. It posits that there is no significant effect, relationship, or difference in the population being studied. In other words, it represents the status quo or the absence of an effect. Researchers typically set out to challenge or disprove the Null Hypothesis by collecting and analysing data. Using the drug efficacy example again, the Null Hypothesis might state that the new drug has no effect on patients' health.
Non-directional Hypothesis
A Non-directional Hypothesis, also known as a two-tailed Hypothesis, is used when researchers are interested in whether there is any significant difference, effect, or relationship in either direction (positive or negative). This type of Hypothesis allows for the possibility of finding effects in both directions. For instance, in a study comparing the performance of two groups, a Non-directional Hypothesis would suggest that there is a significant difference between the groups, without specifying which group performs better.
Directional Hypothesis
A Directional Hypothesis, also called a one-tailed Hypothesis, is employed when researchers have a specific expectation about the direction of the effect, relationship, or difference they are investigating. In this case, the Hypothesis predicts an outcome in a particular direction—either positive or negative. For example, if you expect that a new teaching method will improve student test scores, a directional Hypothesis would state that the new method leads to higher test scores.
Statistical Hypothesis
A Statistical Hypothesis is a Hypothesis formulated in a way that it can be tested using statistical methods. It involves specific numerical values or parameters that can be measured or compared. Statistical Hypotheses are crucial for quantitative research and often involve means, proportions, variances, correlations, or other measurable quantities. These Hypotheses provide a precise framework for conducting statistical tests and drawing conclusions based on data analysis.
Want to unlock the power of Big Data Analysis? Join our Big Data Analysis Course today!
Basic steps in Hypothesis Testing
Hypothesis Testing is a systematic approach used in statistics to make informed decisions based on data. It is a critical tool in Data Science, research, and many other fields where data analysis is employed. The following are the basic steps involved in Hypothesis Testing:
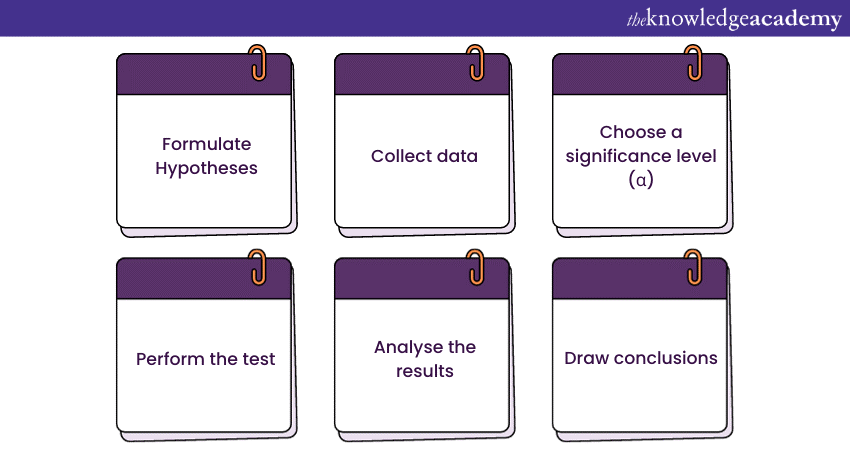
1) Formulate Hypotheses
The first step in Hypothesis Testing is to clearly define your research question and translate it into two mutually exclusive Hypotheses:
a) Null Hypothesis (H0): This is the default assumption, often representing the status quo or the absence of an effect. It states that there is no significant difference, relationship, or effect in the population.
b) Alternative Hypothesis (Ha or H1): This is the statement that contradicts the null Hypothesis. It suggests that there is a significant difference, relationship, or effect in the population.
The formulation of these Hypotheses is crucial, as they serve as the foundation for your entire Hypothesis Testing process.
2) Collect data
With your Hypotheses in place, the next step is to gather relevant data through surveys, experiments, observations, or any other suitable method. The data collected should be representative of the population you are studying. The quality and quantity of data are essential factors in the success of your Hypothesis Testing.
3) Choose a significance level (α)
Before conducting the statistical test, you need to decide on the level of significance, denoted as α. The significance level represents the threshold for statistical significance and determines how confident you want to be in your results. A common choice is α = 0.05, which implies a 5% chance of making a Type I error (rejecting the null Hypothesis when it's true). You can choose a different α value based on the specific requirements of your analysis.
4) Perform the test
Based on the nature of your data and the Hypotheses you've formulated, select the appropriate statistical test. There are various tests available, including t-tests, chi-squared tests, ANOVA, regression analysis, and more. The chosen test should align with the type of data (e.g., continuous or categorical) and the research question (e.g., comparing means or testing for independence).
Execute the selected statistical test on your data to obtain test statistics and p-values. The test statistics quantify the difference or effect you are investigating, while the p-value represents the probability of obtaining the observed results if the null Hypothesis were true.
5) Analyse the results
Once you have the test statistics and p-value, it's time to interpret the results. The primary focus is on the p-value:
a) If the p-value is less than or equal to your chosen significance level (α), typically 0.05, you have evidence to reject the null Hypothesis. This shows that there is a significant difference, relationship, or effect in the population.
b) If the p-value is more than α, you fail to reject the null Hypothesis, showing that there is insufficient evidence to support the alternative Hypothesis.
6) Draw conclusions
Based on the analysis of the p-value and the comparison to the significance level, you can draw conclusions about your research question:
a) In case you reject the null Hypothesis, you can accept the alternative Hypothesis and make inferences based on the evidence provided by your data.
b) In case you fail to reject the null Hypothesis, you do not accept the alternative Hypothesis, and you acknowledge that there is no significant evidence to support your claim.
It's important to communicate your findings clearly, including the implications and limitations of your analysis.
Real-world use cases of Hypothesis Testing
The following are some of the real-world use cases of Hypothesis Testing.
a) Medical research: Hypothesis Testing is crucial in determining the efficacy of new medications or treatments. For instance, in a clinical trial, researchers use Hypothesis Testing to assess whether a new drug is significantly more effective than a placebo in treating a particular condition.
b) Marketing and advertising: Businesses employ Hypothesis Testing to evaluate the impact of marketing campaigns. A company may test whether a new advertising strategy leads to a significant increase in sales compared to the previous approach.
c) Manufacturing and quality control: Manufacturing industries use Hypothesis Testing to ensure product quality. For example, in the automotive industry, Hypothesis Testing can be applied to test whether a new manufacturing process results in a significant reduction in defects.
d) Education: In the field of education, Hypothesis Testing can be used to assess the effectiveness of teaching methods. Researchers may test whether a new teaching approach leads to statistically significant improvements in student performance.
e) Finance and investment: Investment strategies are often evaluated using Hypothesis Testing. Investors may test whether a new investment strategy outperforms a benchmark index over a specified period.
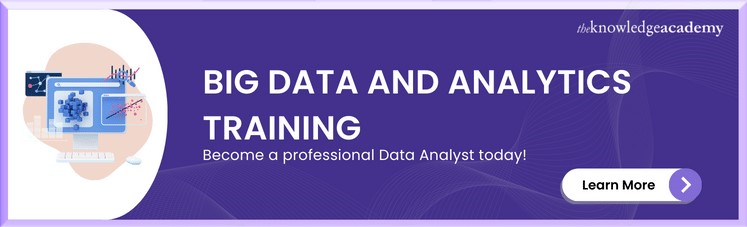
Conclusion
To sum it up, Hypothesis Testing in Data Science is a powerful tool that enables Data Scientists to make evidence-based decisions and draw meaningful conclusions from data. Understanding the types, methods, and steps involved in Hypothesis Testing is essential for any Data Scientist. By rigorously applying Hypothesis Testing techniques, you can gain valuable insights and drive informed decision-making in various domains.
Want to take your Data Science skills to the next level? Join our Big Data Analytics & Data Science Integration Course now!
Frequently Asked Questions
Upcoming data, analytics & ai resources batches & dates.
Fri 5th Jul 2024
Fri 1st Nov 2024
Get A Quote
WHO WILL BE FUNDING THE COURSE?
My employer
By submitting your details you agree to be contacted in order to respond to your enquiry
- Business Analysis
- Lean Six Sigma Certification
Share this course
Our biggest spring sale.

We cannot process your enquiry without contacting you, please tick to confirm your consent to us for contacting you about your enquiry.
By submitting your details you agree to be contacted in order to respond to your enquiry.
We may not have the course youāre looking for. If you enquire or give us a call on 01344203999 and speak to our training experts, we may still be able to help with your training requirements.
Or select from our popular topics
- ITILĀ® Certification
- Scrum Certification
- Change Management Certification
- Business Analysis Courses
- Microsoft Azure Certification
- Microsoft Excel Courses
- Microsoft Project
- Explore more courses
Press esc to close
Fill out your contact details below and our training experts will be in touch.
Fill out your contact details below
Thank you for your enquiry!
One of our training experts will be in touch shortly to go over your training requirements.
Back to Course Information
Fill out your contact details below so we can get in touch with you regarding your training requirements.
* WHO WILL BE FUNDING THE COURSE?
Preferred Contact Method
No preference
Back to course information
Fill out your training details below
Fill out your training details below so we have a better idea of what your training requirements are.
HOW MANY DELEGATES NEED TRAINING?
HOW DO YOU WANT THE COURSE DELIVERED?
Online Instructor-led
Online Self-paced
WHEN WOULD YOU LIKE TO TAKE THIS COURSE?
Next 2 - 4 months
WHAT IS YOUR REASON FOR ENQUIRING?
Looking for some information
Looking for a discount
I want to book but have questions
One of our training experts will be in touch shortly to go overy your training requirements.
Your privacy & cookies!
Like many websites we use cookies. We care about your data and experience, so to give you the best possible experience using our site, we store a very limited amount of your data. Continuing to use this site or clicking āAccept & closeā means that you agree to our use of cookies. Learn more about our privacy policy and cookie policy cookie policy .
We use cookies that are essential for our site to work. Please visit our cookie policy for more information. To accept all cookies click 'Accept & close'.
For enquiries call:
+1-469-442-0620

- Data Science
Hypothesis Testing in Data Science [Types, Process, Example]
Home Blog Data Science Hypothesis Testing in Data Science [Types, Process, Example]

In day-to-day life, we come across a lot of data lot of variety of content. Sometimes the information is too much that we get confused about whether the information provided is correct or not. At that moment, we get introduced to a word called āHypothesis testingā which helps in determining the proofs and pieces of evidence for some belief or information.
What is Hypothesis Testing?
Hypothesis testing is an integral part of statistical inference. It is used to decide whether the given sample data from the population parameter satisfies the given hypothetical condition. So, it will predict and decide using several factors whether the predictions satisfy the conditions or not. In simpler terms, trying to prove whether the facts or statements are true or not.
For example, if you predict that students who sit on the last bench are poorer and weaker than students sitting on 1st bench, then this is a hypothetical statement that needs to be clarified using different experiments. Another example we can see is implementing new business strategies to evaluate whether they will work for the business or not. All these things are very necessary when you work with data as a data scientist. If you are interested in learning about data science, visit this amazing⯠Data Science full course ⯠to learn data science. āÆ
How is Hypothesis Testing Used in Data Science?
It is important to know how and where we can use hypothesis testing techniques in the field of data science. Data scientists predict a lot of things in their day-to-day work, and to check the probability of whether that finding is certain or not, we use hypothesis testing. The main goal of hypothesis testing is to gauge how well the predictions perform based on the sample data provided by the population. If you are interested to know more about the applications of the data, then refer to this D ata Scien ce course in India which will give you more insights into application-based things. When data scientists work on model building using various machine learning algorithms, they need to have faith in their models and the forecasting of models. They then provide the sample data to the model for training purposes so that it can provide us with the significance of statistical data that will represent the entire population.
Where and When to Use Hypothesis Test?
Hypothesis testing is widely used when we need to compare our results based on predictions. So, it will compare before and after results. For example, someone claimed that students writing exams from blue pen always get above 90%; now this statement proves it correct, and experiments need to be done. So, the data will be collected based on the student's input, and then the test will be done on the final result later after various experiments and observations on students' marks vs pen used, final conclusions will be made which will determine the results. Now hypothesis testing will be done to compare the 1st and the 2nd result, to see the difference and closeness of both outputs. This is how hypothesis testing is done.
How Does Hypothesis Testing Work in Data Science?
In the whole data science life cycle, hypothesis testing is done in various stages, starting from the initial part, the 1st stage where the EDA, data pre-processing, and manipulation are done. In this stage, we will do our initial hypothesis testing to visualize the outcome in later stages. The next test will be done after we have built our model, once the model is ready and hypothesis testing is done, we will compare the results of the initial testing and the 2nd one to compare the results and significance of the results and to confirm the insights generated from the 1st cycle match with the 2nd one or not. This will help us know how the model responds to the sample training data. As we saw above, hypothesis testing is always needed when we are planning to contrast more than 2 groups. While checking on the results, it is important to check on the flexibility of the results for the sample and the population. Later, we can judge on the disagreement of the results are appropriate or vague. This is all we can do using hypothesis testing.
Different Types of Hypothesis Testing
Hypothesis testing can be seen in several types. In total, we have 5 types of hypothesis testing. They are described below:

1. Alternative Hypothesis
The alternative hypothesis explains and defines the relationship between two variables. It simply indicates a positive relationship between two variables which means they do have a statistical bond. It indicates that the sample observed is going to influence or affect the outcome. An alternative hypothesis is described using H a or H 1 . Ha indicates an alternative hypothesis and H 1 explains the possibility of influenced outcome which is 1. For example, children who study from the beginning of the class have fewer chances to fail. An alternate hypothesis will be accepted once the statistical predictions become significant. The alternative hypothesis can be further divided into 3 parts.
- Left-tailed: Left tailed hypothesis can be expected when the sample value is less than the true value.
- Right-tailed: Right-tailed hypothesis can be expected when the true value is greater than the outcome/predicted value.
- Two-tailed: Two-tailed hypothesis is defined when the true value is not equal to the sample value or the output.
2. Null Hypothesis
The null hypothesis simply states that there is no relation between statistical variables. If the facts presented at the start do not match with the outcomes, then we can say, the testing is null hypothesis testing. The null hypothesis is represented as H 0 . For example, children who study from the beginning of the class have no fewer chances to fail. There are types of Null Hypothesis described below:
Simple Hypothesis: It helps in denoting and indicating the distribution of the population.
Composite Hypothesis: It does not denote the population distribution
Exact Hypothesis: In the exact hypothesis, the value of the hypothesis is the same as the sample distribution. Example- μ= 10
Inexact Hypothesis: Here, the hypothesis values are not equal to the sample. It will denote a particular range of values.
3. Non-directional Hypothesis
The non-directional hypothesis is a tow-tailed hypothesis that indicates the true value does not equal the predicted value. In simpler terms, there is no direction between the 2 variables. For an example of a non-directional hypothesis, girls and boys have different methodologies to solve a problem. Here the example explains that the thinking methodologies of a girl and a boy is different, they donāt think alike.
4. Directional Hypothesis
In the Directional hypothesis, there is a direct relationship between two variables. Here any of the variables influence the other.
5. Statistical Hypothesis
Statistical hypothesis helps in understanding the nature and character of the population. It is a great method to decide whether the values and the data we have with us satisfy the given hypothesis or not. It helps us in making different probabilistic and certain statements to predict the outcome of the population... We have several types of tests which are the T-test, Z-test, and Anova tests.
Methods of Hypothesis Testing
1. frequentist hypothesis testing.
Frequentist hypotheses mostly work with the approach of making predictions and assumptions based on the current data which is real-time data. All the facts are based on current data. The most famous kind of frequentist approach is null hypothesis testing.
2. Bayesian Hypothesis Testing
Bayesian testing is a modern and latest way of hypothesis testing. It is known to be the test that works with past data to predict the future possibilities of the hypothesis. In Bayesian, it refers to the prior distribution or prior probability samples for the observed data. In the medical Industry, we observe that Doctors deal with patientsā diseases using past historical records. So, with this kind of record, it is helpful for them to understand and predict the current and upcoming health conditions of the patient.
Importance of Hypothesis Testing in Data Science
Most of the time, people assume that data science is all about applying machine learning algorithms and getting results, that is true but in addition to the fact that to work in the data science field, one needs to be well versed with statistics as most of the background work in Data science is done through statistics. When we deal with data for pre-processing, manipulating, and analyzing, statistics play. Specifically speaking Hypothesis testing helps in making confident decisions, predicting the correct outcomes, and finding insightful conclusions regarding the population. Hypothesis testing helps us resolve tough things easily. To get more familiar with Hypothesis testing and other prediction models attend the superb useful⯠KnowledgeHut Data Science full course āÆwhich will give you more domain knowledge and will assist you in working with industry-related projects. āÆāÆ
Basic Steps in Hypothesis Testing [Workflow]
1. null and alternative hypothesis.
After we have done our initial research about the predictions that we want to find out if true, it is important to mention whether the hypothesis done is a null hypothesis(H0) or an alternative hypothesis (Ha). Once we understand the type of hypothesis, it will be easy for us to do mathematical research on it. A null hypothesis will usually indicate the no-relationship between the variables whereas an alternative hypothesis describes the relationship between 2 variables.
- H0 ā Girls, on average, are not strong as boys
- Ha - Girls, on average are stronger than boys
2. Data Collection
To prove our statistical test validity, it is essential and critical to check the data and proceed with sampling them to get the correct hypothesis results. If the target data is not prepared and ready, it will become difficult to make the predictions or the statistical inference on the population that we are planning to make. It is important to prepare efficient data, so that hypothesis findings can be easy to predict.
3. Selection of an appropriate test statistic
To perform various analyses on the data, we need to choose a statistical test. There are various types of statistical tests available. Based on the wide spread of the data that is variance within the group or how different the data category is from one another that is variance without a group, we can proceed with our further research study.
4. Selection of the appropriate significant level
Once we get the result and outcome of the statistical test, we have to then proceed further to decide whether the reject or accept the null hypothesis. The significance level is indicated by alpha (α). It describes the probability of rejecting or accepting the null hypothesis. Example- Suppose the value of the significance level which is alpha is 0.05. Now, this value indicates the difference from the null hypothesis.
5. Calculation of the test statistics and the p-value
P value is simply the probability value and expected determined outcome which is at least as extreme and close as observed results of a hypothetical test. It helps in evaluating and verifying hypotheses against the sample data. This happens while assuming the null hypothesis is true. The lower the value of P, the higher and better will be the results of the significant value which is alpha (α). For example, if the P-value is 0.05 or even less than this, then it will be considered statistically significant. The main thing is these values are predicted based on the calculations done by deviating the values between the observed one and referenced one. The greater the difference between values, the lower the p-value will be.
6. Findings of the test
After knowing the P-value and statistical significance, we can determine our results and take the appropriate decision of whether to accept or reject the null hypothesis based on the facts and statistics presented to us.
How to Calculate Hypothesis Testing?
Hypothesis testing can be done using various statistical tests. One is Z-test. The formula for Z-test is given below:
Z = ( xĢ ā μ 0 ) / (Ļ /ān)
In the above equation, xĢ is the sample mean
- μ0 is the population mean
- Ļ is the standard deviation
- n is the sample size
Now depending on the Z-test result, the examination will be processed further. The result is either going to be a null hypothesis or it is going to be an alternative hypothesis. That can be measured through below formula-
- H0: μ=μ0
- Ha: μā μ0
- Here,
- H0 = null hypothesis
- Ha = alternate hypothesis
In this way, we calculate the hypothesis testing and can apply it to real-world scenarios.
Real-World Examples of Hypothesis Testing
Hypothesis testing has a wide variety of use cases that proves to be beneficial for various industries.
1. Healthcare
In the healthcare industry, all the research and experiments which are done to predict the success of any medicine or drug are done successfully with the help of Hypothesis testing.
2. Education sector
Hypothesis testing assists in experimenting with different teaching techniques to deal with the understanding capability of different students.
3. Mental Health
Hypothesis testing helps in indicating the factors that may cause some serious mental health issues.
4. Manufacturing
Testing whether the new change in the process of manufacturing helped in the improvement of the process as well as in the quantity or not. In the same way, there are many other use cases that we get to see in different sectors for hypothesis testing.
Error Terms in Hypothesis Testing
1. type-i error.
Type I error occurs during the process of hypothesis testing when the null hypothesis is rejected even though it is accurate. This kind of error is also known as False positive because even though the statement is positive or correct but results are given as false. For example, an innocent person still goes to jail because he is considered to be guilty.
2. Type-II error
Type II error occurs during the process of hypothesis testing when the null hypothesis is not rejected even though it is inaccurate. This Kind of error is also called a False-negative which means even though the statements are false and inaccurate, it still says it is correct and doesnāt reject it. For example, a person is guilty, but in court, he has been proven innocent where he is guilty, so this is a Type II error.
3. Level of Significance
The level of significance is majorly used to measure the confidence with which a null hypothesis can be rejected. It is the value with which one can reject the null hypothesis which is H0. The level of significance gauges whether the hypothesis testing is significant or not.
P-value stands for probability value, which tells us the probability or likelihood to find the set of observations when the null hypothesis is true using statistical tests. The main purpose is to check the significance of the statistical statement.
5. High P-Values
A higher P-value indicates that the testing is not statistically significant. For example, a P value greater than 0.05 is considered to be having higher P value. A higher P-value also means that our evidence and proofs are not strong enough to influence the population.
In hypothesis testing, each step is responsible for getting the outcomes and the results, whether it is the selection of statistical tests or working on data, each step contributes towards the better consequences of the hypothesis testing. It is always a recommendable step when planning for predicting the outcomes and trying to experiment with the sample; hypothesis testing is a useful concept to apply.
Frequently Asked Questions (FAQs)
We can test a hypothesis by selecting a correct hypothetical test and, based on those getting results.
Many statistical tests are used for hypothetical testing which includes Z-test, T-test, etc.
Hypothesis helps us in doing various experiments and working on a specific research topic to predict the results.
The null and alternative hypothesis, data collection, selecting a statistical test, selecting significance value, calculating p-value, check your findings.
In simple words, parametric tests are purely based on assumptions whereas non-parametric tests are based on data that is collected and acquired from a sample.

Gauri Guglani
Gauri Guglani works as a Data Analyst at Deloitte Consulting. She has done her major in Information Technology and holds great interest in the field of data science. She owns her technical skills as well as managerial skills and also is great at communicating. Since her undergraduate, Gauri has developed a profound interest in writing content and sharing her knowledge through the manual means of blog/article writing. She loves writing on topics affiliated with Statistics, Python Libraries, Machine Learning, Natural Language processes, and many more.
Avail your free 1:1 mentorship session.
Something went wrong
Upcoming Data Science Batches & Dates

Hypothesis Testing
Hypothesis Tests (or Significance Tests) are statistical tests to see if a difference we observe is due to chance.
There are many different types of hypothesis tests for different scenarios, but they all have the same basic ideas. Below are the general steps to performing a hypothesis test:
- Formulate your Null and Alternative Hypotheses.
- Ho- Null Hypothesis : The null hypothesis is the hypothesis of no effect. It's the dull, boring hypothesis that says that nothing interesting is going on. If we are trying to test if a difference we observe is due to chance, the null says it is!
- Ha- Alternative Hypothesis : The alternative hypothesis is the opposite of the null. It's what you are trying to test. If we are trying to test if a difference we observe is due to chance, the alternative says it is not!
Think about what you would expect to get if you randomly sampled from the population, assuming the null is true. Compare your observed data and expected data and calculate the test statistic .
Calculate the probability of getting the data you got or something even more extreme if the null were true. This is called the p-value .
- Make your conclusion and interpret it in the context of the problem. If p is very low, we say that the data support rejecting the null hypothesis.
How low is āvery lowā?
The convention is to reject the null when P < 5% (P < 0.05) and call the result āsignificantā. Thereās no particular justification for this value but itās commonly used.
The P-value cut-off is called the significance level and is often represented by the Greek letter alpha (α).
The One Sample Z Test: One-sided Hypothesis
The first type of hypothesis test we are going to look at is the one-sample z-test. You can do a z-test for means or for proportions. This is the most simple type of hypothesis test and it uses z-scores and the normal curve. Letās look at one below!
Hypothesis Test Example : Suppose a large university claims that the average ACT score of their incoming freshman class is 30, but we think the University may be inflating their average. To test the Universityās claim we take a simple random sample of 50 students and find their average to be only 28.3 with an SD of 4. Perform a hypothesis test to test the claim. Here are the 4 steps:
- This can be written in symbols as well: Ho: μ = 30
- μ is the symbol for the population mean
- This can be written in symbols as well: Ho: μ < 30
- Our test statistic for the one sample z test is z! We can calculate z using our z-score formula for random variables since we are dealing with a sample of 50 students.
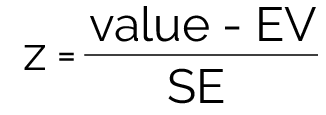
- In our case, the expected value (EV) is 30 since we are assuming our null hypothesis is true (until proven otherwise).
- Since we are dealing with means, our SE is found using the following formula:
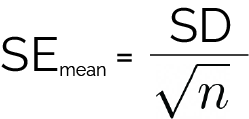
Our z-score is -3. See the calculation below:
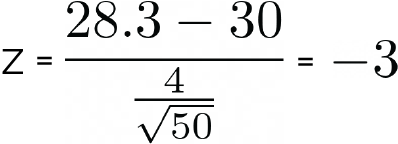
- Calculate the probability of getting the data you got or something even more extreme if the null were true. This is called the p-value . In this case, our p-value is going to be the area to the left of z = -3. We can use Python to calculate this by using norm.cdf(-3).
- We get that the p-value is 0.0013.
- This is the probability that we would get a sample average of 28.3 given that the null hypothesis was true (the true average was 30).
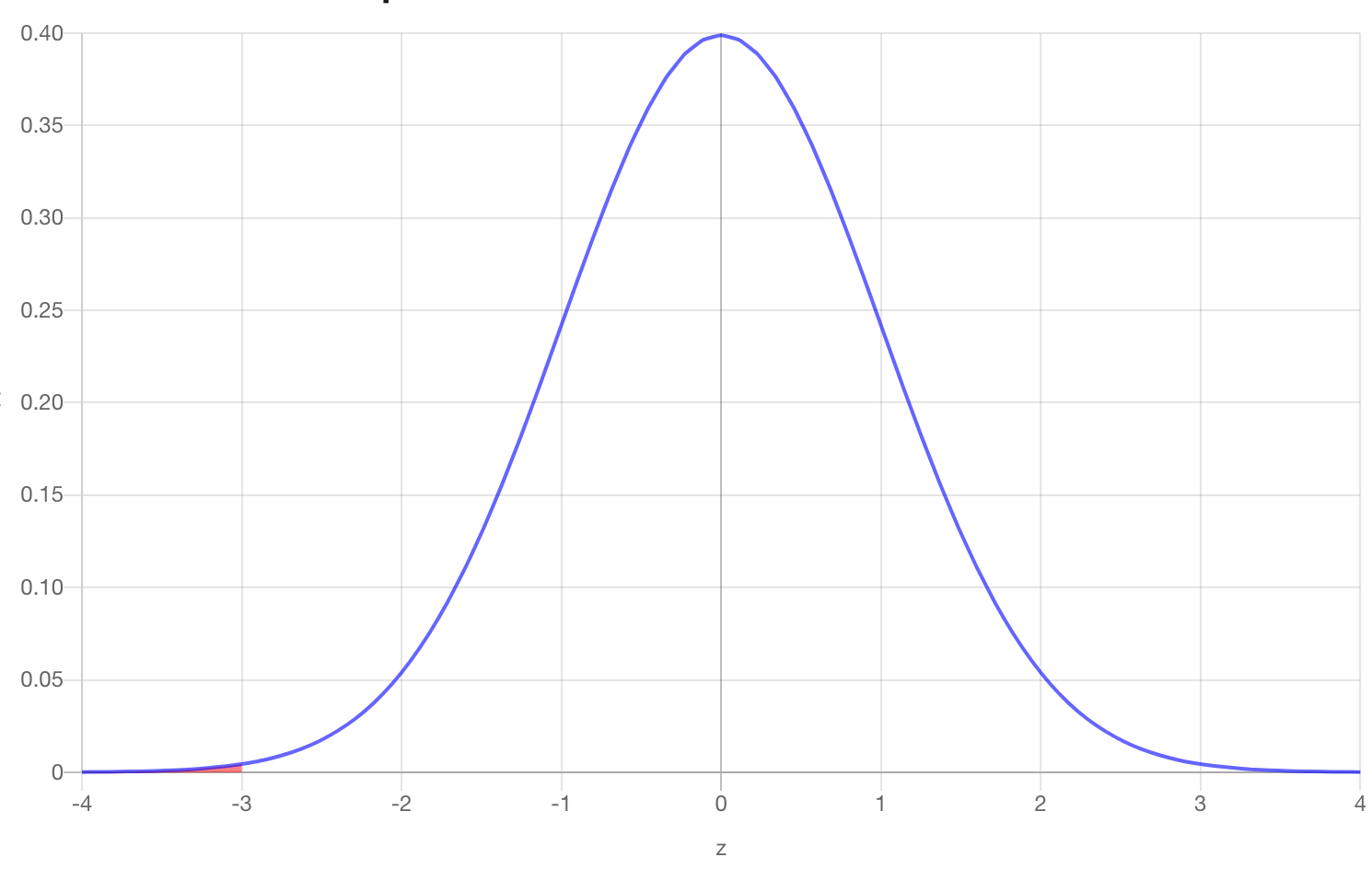
- Our p-value is less than 5% so we reject our Null Hypothesis. In other words, there is evidence of the Alternative Hypothesis (that the University is inflating their average).
The One Sample Z Test: Two-sided Hypothesis
Hypothesis Test Example : Now we're going to test the above claim but with a different alternative hypothesis. The large university still claims that the average ACT score of their incoming freshman class is 30, but now we think the University may be inflating or deflating their average. To test the Universityās claim we take a simple random sample of 50 students and find their average to be only 28.3 with an SD of 4. Perform a hypothesis test to test the claim with our new alternative hypothesis. Here are the 4 steps:
- This can be written in symbols as well: Ho: μ != 30
Step 2 is the same as the one-sided example, so our z score is still -3.
Calculate the probability of getting the data you got or something even more extreme if the null were true. This is called the p-value . In this case, our p-value is going to be the area to the left or right of z = -3. We can use Python to calculate this by using 2*norm.cdf(-3).
- We get that the p-value is 0.0027.
- Our p-value is less than 5% so we reject our Null Hypothesis. In other words, there is evidence of the Alternative Hypothesis (that the University is inflating or deflating their average).
Example Walk-Throughs with Worksheets
Video 1: one sample z-test examples.
- Download Blank Worksheet (PDF)
Video 2: Two Sample z-test Examples
Video 3: z-tests in Python
Video 4: One Sample t-test Examples
Video 5: t-tests in Python
- Prompt Library
- DS/AI Trends
- Stats Tools
- Interview Questions
- Generative AI
- Machine Learning
- Deep Learning
Hypothesis Testing Steps & Examples

Table of Contents
What is a Hypothesis testing?
As per the definition from Oxford languages, a hypothesis is a supposition or proposed explanation made on the basis of limited evidence as a starting point for further investigation. As per the Dictionary page on Hypothesis , Hypothesis means a proposition or set of propositions, set forth as an explanation for the occurrence of some specified group of phenomena, either asserted merely as a provisional conjecture to guide investigation (working hypothesis) or accepted as highly probable in the light of established facts.
The hypothesis can be defined as the claim that can either be related to the truth about something that exists in the world, or, truth about something that’s needs to be established a fresh . In simple words, another word for the hypothesis is the “claim” . Until the claim is proven to be true, it is called the hypothesis. Once the claim is proved, it becomes the new truth or new knowledge about the thing. For example , let’s say that a claim is made that students studying for more than 6 hours a day gets more than 90% of marks in their examination. Now, this is just a claim or a hypothesis and not the truth in the real world. However, in order for the claim to become the truth for widespread adoption, it needs to be proved using pieces of evidence, e.g., data.Ā In order to reject this claim or otherwise, one needs to do some empirical analysis by gathering data samples and evaluating the claim. The process of gathering data and evaluating the claims or hypotheses with the goal to reject or otherwise (failing to reject) can be called as hypothesis testing . Note the wordings – “failing to reject”. It means that we don’t have enough evidence to reject the claim. Thus, until the time that new evidence comes up, the claim can be considered the truth. There are different techniques to test the hypothesis in order to reach the conclusion of whether the hypothesis can be used to represent the truth of the world.
One must note that the hypothesis testing never constitutes a proof that the hypothesis is absolute truth based on the observations. It only provides added support to consider the hypothesis as truth until the time that new evidences can against the hypotheses can be gathered. We can never be 100% sure about truth related to those hypotheses based on the hypothesis testing.
Simply speaking, hypothesis testing is a framework that can be used to assert whether the claim or the hypothesis made about a real-world/real-life event can be seen as the truth or otherwise based on the given data (evidences).
Hypothesis Testing Examples
Before we get ahead and start understanding more details about hypothesis and hypothesis testing steps, lets take a look at someĀ real-world examplesĀ of how to think about hypothesis and hypothesis testing when dealing with real-world problems :
- Customers are churning because they ain’t getting response to their complaints or issues
- Customers are churning because there are other competitive services in the market which are providing these services at lower cost.
- Customers are churning because there are other competitive services which are providing more services at the same cost.
- It is claimed that a 500 gm sugar packet for a particular brand, say XYZA, contains sugar of less than 500 gm, say around 480gm.Ā Can this claim be taken as truth? How do we know that this claim is true? This is a hypothesis until proved.
- A group of doctors claims that quitting smoking increases lifespan. Can this claim be taken as new truth? The hypothesis is that quitting smoking results in an increase in lifespan.
- It is claimed that brisk walking for half an hour every day reverses diabetes. In order to accept this in your lifestyle, you may need evidence that supports this claim or hypothesis.
- It is claimed that doing Pranayama yoga for 30 minutes a day can help in easing stress by 50%. This can be termed as hypothesis and would require testing / validation for it to be established as a truth and recommended for widespread adoption.
- One common real-life example of hypothesis testing is election polling. In order to predict the outcome of an election, pollsters take a sample of the population and ask them who they plan to vote for. They then use hypothesis testing to assess whether their sample is representative of the population as a whole. If the results of the hypothesis test are significant, it means that the sample is representative and that the poll can be used to predict the outcome of the election. However, if the results are not significant, it means that the sample is not representative and that the poll should not be used to make predictions.
- Machine learning models make predictions based on the input data. Each of the machine learning model representing a function approximation can be taken as a hypothesis. All different models constitute what is called as hypothesis space .
- As part of a linear regression machine learning model , it is claimed that there is a relationship between the response variables and predictor variables? Can this hypothesis or claim be taken as truth? Let’s say, the hypothesis is that the housing price depends upon the average income of people already staying in the locality. How true is this hypothesis or claim? The relationship between response variable and each of the predictor variables can be evaluated using T-test and T-statistics .
- For linear regression model , one of the hypothesis is that there is no relationship between the response variable and any of the predictor variables. Thus, if b1, b2, b3 are three parameters, all of them is equal to 0. b1 = b2 = b3 = 0. This is where one performs F-test and use F-statistics to test this hypothesis.
You may note different hypotheses which are listed above. The next step would be validate some of these hypotheses. This is where data scientists will come into picture. One or more data scientists may be asked to work on different hypotheses. This would result in these data scientists looking for appropriate data related to the hypothesis they are working. This section will be detailed out in near future.
State the Hypothesis to begin Hypothesis Testing
The first step to hypothesis testing is defining or stating a hypothesis. Before the hypothesis can be tested, we need to formulate the hypothesis in terms of mathematical expressions. There are two important aspects to pay attention to, prior to the formulation of the hypothesis. The following represents different types of hypothesis that could be put to hypothesis testing:
- Claim made against the well-established fact : The case in which a fact is well-established, or accepted as truth or “knowledge” and a new claim is made about this well-established fact. For example , when you buy a packet of 500 gm of sugar, you assume that the packet does contain at the minimum 500 gm of sugar and not any less, based on the label of 500 gm on the packet. In this case, the fact is given or assumed to be the truth. A new claim can be made that the 500 gm sugar contains sugar weighing less than 500 gm. This claim needs to be tested before it is accepted as truth. Such cases could be considered for hypothesis testing if this is claimed that the assumption or the default state of being is not true. The claim to be established as new truth can be stated as “alternate hypothesis”. The opposite state can be stated as “null hypothesis”. Here the claim that the 500 gm packet consists of sugar less than 500 grams would be stated as alternate hypothesis. The opposite state which is the sugar packet consists 500 gm is null hypothesis.
- Claim to establish the new truth : The case in which there is some claim made about the reality that exists in the world (fact). For example , the fact that the housing price depends upon the average income of people already staying in the locality can be considered as a claim and not assumed to be true. Another example could be the claim that running 5 miles a day would result in a reduction of 10 kg of weight within a month. There could be varied such claims which when required to be proved as true have to go through hypothesis testing. The claim to be established as new truth can be stated as “alternate hypothesis”. The opposite state can be stated as “null hypothesis”. Running 5 miles a day would result in reduction of 10 kg within a month would be stated as alternate hypothesis.
Based on the above considerations, the following hypothesis can be stated for doing hypothesis testing.
- The packet of 500 gm of sugar contains sugar of weight less than 500 gm. (Claim made against the established fact). This is a new knowledge which requires hypothesis testing to get established and acted upon.
- The housing price depends upon the average income of the people staying in the locality. This is a new knowledge which requires hypothesis testing to get established and acted upon.
- Running 5 miles a day results in a reduction of 10 kg of weight within a month. This is a new knowledge which requires hypothesis testing to get established for widespread adoption.
Formulate Null & Alternate Hypothesis as Next Step
Once the hypothesis is defined or stated, the next step is to formulate the null and alternate hypothesis in order to begin hypothesis testing as described above.
What is a null hypothesis?
In the case where the given statement is a well-established fact or default state of being in the real world, one can call it a null hypothesis (in the simpler word, nothing new). Well-established facts don’t need any hypothesis testing and hence can be called the null hypothesis. In cases, when there are any new claims made which is not well established in the real world, the null hypothesis can be thought of as the default state or opposite state of that claim. For example , in the previous section, the claim or hypothesis is made that the students studying for more than 6 hours a day gets more than 90% of marks in their examination. The null hypothesis, in this case, will be that the claim is not true or real. The null hypothesis can be stated that there is no relationship or association between the students reading more than 6 hours a day and they getting 90% of the marks. Any occurrence is only a chance occurrence. Another example of hypothesis is when somebody is alleged that they have performed a crime.
Null hypothesis is denoted by letter H with 0, e.g., [latex]H_0[/latex]
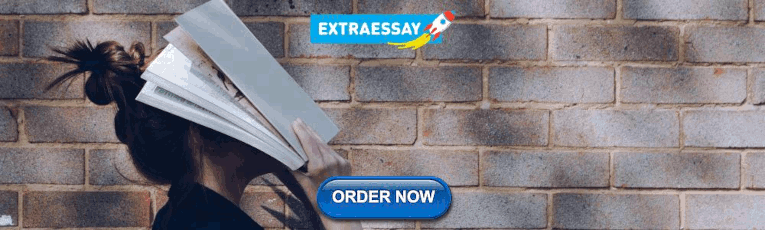
What is an alternate hypothesis?
When the given statement is a claim (unexpected event in the real world) and not yet proven, one can call/formulate it as an alternate hypothesis and accordingly define a null hypothesis which is the opposite state of the hypothesis. The alternate hypothesis is a new knowledge or truth that needs to be established. In simple words, the hypothesis or claim that needs to be tested against reality in the real world can be termed the alternate hypothesis. In order to reach a conclusion that the claim (alternate hypothesis) can be considered the new knowledge or truth (based on the available evidence), it would be important to reject the null hypothesis. It should be noted that null and alternate hypotheses are mutually exclusive and at the same time asymmetric. In the example given in the previous section, the claim that the students studying for more than 6 hours get more than 90% of marks can be termed as the alternate hypothesis.
Alternate hypothesis is denoted with H subscript a, e.g., [latex]H_a[/latex]
Once the hypothesis is formulated as null([latex]H_0[/latex]) and alternate hypothesis ([latex]H_a[/latex]), there are two possible outcomes that can happen from hypothesis testing. These outcomes are the following:
- Reject the null hypothesis : There is enough evidence based on which one can reject the null hypothesis. Let’s understand this with the help of an example provided earlier in this section. The null hypothesis is that there is no relationship between the students studying more than 6 hours a day and getting more than 90% marks. In a sample of 30 students studying more than 6 hours a day, it was found that they scored 91% marks. Given that the null hypothesis is true, this kind of hypothesis testing result will be highly unlikely. This kind of result can’t happen by chance. That would mean that the claim can be taken as the new truth or new knowledge in the real world. One can go and take further samples of 30 students to perform some more testing to validate the hypothesis. If similar results show up with other tests, it can be said with very high confidence that there is enough evidence to reject the null hypothesis that there is no relationship between the students studying more than 6 hours a day and getting more than 90% marks. In such cases, one can go to accept the claim as new truth that the students studying more than 6 hours a day get more than 90% marks. The hypothesis can be considered the new truth until the time that new tests provide evidence against this claim.
- Fail to reject the null hypothesis : There is not enough evidence-based on which one can reject the null hypothesis (well-established fact or reality). Thus, one would fail to reject the null hypothesis. In a sample of 30 students studying more than 6 hours a day, the students were found to score 75%. Given that the null hypothesis is true, this kind of result is fairly likely or expected. With the given sample, one can’t reject the null hypothesis that there is no relationship between the students studying more than 6 hours a day and getting more than 90% marks.
Examples of formulating the null and alternate hypothesis
The following are some examples of the null and alternate hypothesis.
Hypothesis Testing Steps
Here is the diagram which represents the workflow of Hypothesis Testing.

Figure 1. Hypothesis Testing Steps
Based on the above, the following are some of the Ā steps to be taken when doing hypothesis testing:
- State the hypothesis : First and foremost, the hypothesis needs to be stated. The hypothesis could either be the statement that is assumed to be true or the claim which is made to be true.
- Formulate the hypothesis : This step requires one to identify the Null and Alternate hypotheses or in simple words, formulate the hypothesis. Take an example of the canned sauce weighing 500 gm as the Null Hypothesis.
- Set the criteria for a decision : Identify test statistics that could be used to assess the Null Hypothesis. The test statistics with the above example would be the average weight of the sugar packet, and t-statistics would be used to determine the P-value. For different kinds of problems, different kinds of statistics including Z-statistics, T-statistics, F-statistics, etc can be used.
- Identify the level of significance (alpha) : Before starting the hypothesis testing, one would be required to set the significance level (also called asĀ alpha ) which represents the value for which a P-value less than or equal toĀ alpha Ā is considered statistically significant. Typical values ofĀ alpha Ā are 0.1, 0.05, and 0.01. In case the P-value is evaluated as statistically significant, the null hypothesis is rejected. In case, the P-value is more than theĀ alpha Ā value, the null hypothesis is failed to be rejected.
- Compute the test statistics : Next step is to calculate the test statistics (z-test, t-test, f-test, etc) to determine the P-value. If the sample size is more than 30, it is recommended to use z-statistics. Otherwise, t-statistics could be used. In the current example where 20 packets of canned sauce is selected for hypothesis testing, t-statistics will be calculated for the mean value of 505 gm (sample mean). The t-statistics would then be calculated as the difference of 505 gm (sample mean) and the population means (500 gm) divided by the sample standard deviation divided by the square root of sample size (20).
- Calculate the P-value of the test statistics : Once the test statistics have been calculated, find the P-value using either of t-table or a z-table. P-value is the probability of obtaining a test statistic (t-score or z-score) equal to or more extreme than the result obtained from the sample data, given that the null hypothesis H0 is true.
- Compare P-value with the level of significance : The significance level is set as the allowable range within which if the value appears, one will be failed to reject the Null Hypothesis. This region is also called as Non-rejection region . The value of alpha is compared with the p-value. If the p-value is less than the significance level, the test is statistically significant and hence, the null hypothesis will be rejected.
P-Value: Key to Statistical Hypothesis Testing
Once you formulate the hypotheses, there is the need to test those hypotheses. Meaning, say that the null hypothesis is stated as the statement that housing price does not depend upon the average income of people staying in the locality, it would be required to be tested by taking samples of housing prices and, based on the test results, this Null hypothesis could either be rejected or failed to be rejected . In hypothesis testing, the following two are the outcomes:
- Reject the Null hypothesis
- Fail to Reject the Null hypothesis
Take the above example of the sugar packet weighing 500 gm. The Null hypothesis is set as the statement that the sugar packet weighs 500 gm. After taking a sample of 20 sugar packets and testing/taking its weight, it was found that the average weight of the sugar packets came to 495 gm. The test statistics (t-statistics) were calculated for this sample and the P-value was determined. Let’s say the P-value was found to be 15%. Assuming that the level of significance is selected to be 5%, the test statistic is not statistically significant (P-value > 5%) and thus, the null hypothesis fails to get rejected. Thus, one could safely conclude that the sugar packet does weigh 500 gm. However, if the average weight of canned sauce would have found to be 465 gm, this is way beyond/away from the mean value of 500 gm and one could have ended up rejecting the Null Hypothesis based on the P-value .
Hypothesis Testing for Problem Analysis & Solution Implementation
Hypothesis testing can be applied in both problem analysis and solution implementation. The following represents method on how you can apply hypothesis testing technique for both problem and solution space:
- Problem Analysis : Hypothesis testing is a systematic way to validate assumptions or educated guesses during problem analysis. It allows for a structured investigation into the nature of a problem and its potential root causes. In this process, a null hypothesis and an alternative hypothesis are usually defined. The null hypothesis generally asserts that no significant change or effect exists, while the alternative hypothesis posits the opposite. Through controlled experiments, data collection, or statistical analysis, these hypotheses are then tested to determine their validity. For example, if a software company notices a sudden increase in user churn rate, they might hypothesize that the recent update to their application is the root cause. The null hypothesis could be that the update has no effect on churn rate, while the alternative hypothesis would assert that the update significantly impacts the churn rate. By analyzing user behavior and feedback before and after the update, and perhaps running A/B tests where one user group has the update and another doesn’t, the company can test these hypotheses. If the alternative hypothesis is confirmed, the company can then focus on identifying specific issues in the update that may be causing the increased churn, thereby moving closer to a solution.
- Solution Implementation : Hypothesis testing can also be a valuable tool during the solution implementation phase, serving as a method to evaluate the effectiveness of proposed remedies. By setting up a specific hypothesis about the expected outcome of a solution, organizations can create targeted metrics and KPIs to measure success. For example, if a retail business is facing low customer retention rates, they might implement a loyalty program as a solution. The hypothesis could be that introducing a loyalty program will increase customer retention by at least 15% within six months. The null hypothesis would state that the loyalty program has no significant effect on retention rates. To test this, the company can compare retention metrics from before and after the program’s implementation, possibly even setting up control groups for more robust analysis. By applying statistical tests to this data, the company can determine whether their hypothesis is confirmed or refuted, thereby gauging the effectiveness of their solution and making data-driven decisions for future actions.
- Tests of Significance
- Hypothesis testing for the Mean
- z-statistics vs t-statistics (Khan Academy)
Hypothesis testing quiz
The claim that needs to be established is set as ____________, the outcome of hypothesis testing is _________.
Please select 2 correct answers
P-value is defined as the probability of obtaining the result as extreme given the null hypothesis is true
There is a claim that doing pranayama yoga results in reversing diabetes. which of the following is true about null hypothesis.
In this post, you learned about hypothesis testing and related nuances such as the null and alternate hypothesis formulation techniques, ways to go about doing hypothesis testing etc. In data science, one of the reasons why one needs to understand the concepts of hypothesis testing is the need to verify the relationship between the dependent (response) and independent (predictor) variables. One would, thus, need to understand the related concepts such as hypothesis formulation into null and alternate hypothesis, level of significance, test statistics calculation, P-value, etc. Given that the relationship between dependent and independent variables is a sort of hypothesis or claim , the null hypothesis could be set as the scenario where there is no relationship between dependent and independent variables.
Recent Posts

- Gradient Descent in Machine Learning: Python Examples - April 19, 2024
- Loss Function vs Cost Function vs Objective Function: Examples - April 19, 2024
- Model Parallelism vs Data Parallelism: Examples - April 19, 2024
Ajitesh Kumar
Leave a reply cancel reply.
Your email address will not be published. Required fields are marked *
- Search for:
- Excellence Awaits: IITs, NITs & IIITs Journey
ChatGPT Prompts (250+)
- Generate Design Ideas for App
- Expand Feature Set of App
- Create a User Journey Map for App
- Generate Visual Design Ideas for App
- Generate a List of Competitors for App
- Gradient Descent in Machine Learning: Python Examples
- Loss Function vs Cost Function vs Objective Function: Examples
- Model Parallelism vs Data Parallelism: Examples
- Model Complexity & Overfitting in Machine Learning: How to Reduce
- 6 Game-Changing Features of ChatGPT’s Latest Upgrade
Data Science / AI Trends
- • Prepend any arxiv.org link with talk2 to load the paper into a responsive chat application
- • Custom LLM and AI Agents (RAG) On Structured + Unstructured Data - AI Brain For Your Organization
- • Guides, papers, lecture, notebooks and resources for prompt engineering
- • Common tricks to make LLMs efficient and stable
- • Machine learning in finance
Free Online Tools
- Create Scatter Plots Online for your Excel Data
- Histogram / Frequency Distribution Creation Tool
- Online Pie Chart Maker Tool
- Z-test vs T-test Decision Tool
- Independent samples t-test calculator
Recent Comments
I found it very helpful. However the differences are not too understandable for me
Very Nice Explaination. Thankyiu very much,
in your case E respresent Member or Oraganization which include on e or more peers?
Such a informative post. Keep it up
Thank you....for your support. you given a good solution for me.
9 Hypothesis testing
In scientific studies, youāll often see phrases like āthe results are statistically significantā. This points to a technique called hypothesis testing, where we use p-values, a type of probability, to test our initial assumption or hypothesis.
In hypothesis testing, rather than providing an estimate of the parameter weāre studying, we provide a probability that serves as evidence supporting or contradicting a specific hypothesis. The hypothesis usually involves whether a parameter is different from a predetermined value (often 0).
Hypothesis testing is used when you can phrase your research question in terms of whether a parameter differs from this predetermined value. Itās applied in various fields, asking questions such as: Does a medication extend the lives of cancer patients? Does an increase in gun sales correlate with more gun violence? Does class size affect test scores?
Take, for instance, the previously used example with colored beads. We might not be concerned about the exact proportion of blue beads, but instead ask: Are there more blue beads than red ones? This could be rephrased as asking if the proportion of blue beads is more than 0.5.
The initial hypothesis that the parameter equals the predetermined value is called the ānull hypothesisā. Itās popular because it allows us to focus on the dataās properties under this null scenario. Once data is collected, we estimate the parameter and calculate the p-value, which is the probability of the estimate being as extreme as observed if the null hypothesis is true. If the p-value is small, it indicates the null hypothesis is unlikely, providing evidence against it.
We will see more examples of hypothesis testing in Chapter 17 .
9.1 p-values
Suppose we take a random sample of \(N=100\) and we observe \(52\) blue beads, which gives us \(\bar{X} = 0.52\) . This seems to be pointing to the existence of more blue than red beads since 0.52 is larger than 0.5. However, we know there is chance involved in this process and we could get a 52 even when the actual \(p=0.5\) . We call the assumption that \(p = 0.5\) a null hypothesis . The null hypothesis is the skepticās hypothesis.
We have observed a random variable \(\bar{X} = 0.52\) , and the p-value is the answer to the question: How likely is it to see a value this large, when the null hypothesis is true? If the p-value is small enough, we reject the null hypothesis and say that the results are statistically significant .
The p-value of 0.05 as a threshold for statistical significance is conventionally used in many areas of research. A cutoff of 0.01 is also used to define highly significance . The choice of 0.05 is somewhat arbitrary and was popularized by the British statistician Ronald Fisher in the 1920s. We do not recommend using these cutoff without justification and recommend avoiding the phrase statistically significant .
To obtain a p-value for our example, we write:
\[\mbox{Pr}(\mid \bar{X} - 0.5 \mid > 0.02 ) \]
assuming the \(p=0.5\) . Under the null hypothesis we know that:
\[ \sqrt{N}\frac{\bar{X} - 0.5}{\sqrt{0.5(1-0.5)}} \]
is standard normal. We, therefore, can compute the probability above, which is the p-value.
\[\mbox{Pr}\left(\sqrt{N}\frac{\mid \bar{X} - 0.5\mid}{\sqrt{0.5(1-0.5)}} > \sqrt{N} \frac{0.02}{ \sqrt{0.5(1-0.5)}}\right)\]
In this case, there is actually a large chance of seeing 52 or larger under the null hypothesis.
Keep in mind that there is a close connection between p-values and confidence intervals. If a 95% confidence interval of the spread does not include 0, we know that the p-value must be smaller than 0.05.
To learn more about p-values, you can consult any statistics textbook. However, in general, we prefer reporting confidence intervals over p-values because it gives us an idea of the size of the estimate. If we just report the p-value, we provide no information about the significance of the finding in the context of the problem.
We can show mathematically that if a \((1-\alpha)\times 100\) % confidence interval does not contain the null hypothesis value, the null hypothesis is rejected with a p-value as smaller or smaller than \(\alpha\) . So statistical significance can be determined from confidence intervals. However, unlike the confidence interval, the p-value does not provide an estimate of the magnitude of the effect. For this reason, we recommend avoiding p-values whenever you can compute a confidence interval.
Pollsters are not successful at providing correct confidence intervals, but rather at predicting who will win. When we took a 25 bead sample size, the confidence interval for the spread:
included 0. If this were a poll and we were forced to make a declaration, we would have to say it was a ātoss-upā.
One problem with our poll results is that, given the sample size and the value of \(p\) , we would have to sacrifice the probability of an incorrect call to create an interval that does not include 0.
This does not mean that the election is close. It only means that we have a small sample size. In statistical textbooks, this is called lack of power . In the context of polls, power is the probability of detecting spreads different from 0.
By increasing our sample size, we lower our standard error, and thus, have a much better chance of detecting the direction of the spread.
9.3 Exercises
- Generate a sample of size \(N=1000\) from an urn model with 50% blue beads:
then, compute a p-value to test if \(p=0.5\) . Repeat this 10,000 times and report how often the p-value is lower than 0.05? How often is it lower than 0.01?
- Make a histogram of the p-values you generated in exercise 1. Which of the following seems to be true?
- The p-values are all 0.05.
- The p-values are normally distributed; CLT seems to hold.
- The p-values are uniformly distributed.
- The p-values are all less than 0.05.
Demonstrate, mathematically, why see the histogram we see in exercise 2.
Generate a sample of size \(N=1000\) from an urn model with 52% blue beads:
Compute a p-value to test if \(p=0.5\) . Repeat this 10,000 times and report how often the p-value is larger than 0.05? Note that you are computing 1 - power.
- Repeat exercise for but for the following values:
Plot power as a function of \(N\) with a different color curve for each value of p .

Interested in Personalized Training with Job Assistance? Know More
Data Science Course > Hypothesis Testing
Hypothesis Testing in Data Science
To view this video please enable JavaScript, and consider upgrading to a web browser that supports HTML5 video
Start a new search
To find content from modules and lessons
How hypothesis testing is used in data science?
How do you formulate a hypothesis in data science, what is meant by hypothesis testing, what are the types of hypothesis tests, what are the benefits of using hypothesis testing in data science, learner's ratings.
Overall Rating
Kashinath Myakale
All courses is very useful for which are looking free courses and gaining knowledge
ANKiT KUMAR BAMNiYA
Dogala udaykumar, nishant saxena.
great content
Aditya Purohit
Very good course & amazing cocepts & detailed explaination of each and every thing . Thanku soo much Learn Vern ...
Abinash prusty
Shivendra shahi.
Very good course for begineers.
good explanation
Recommended Courses
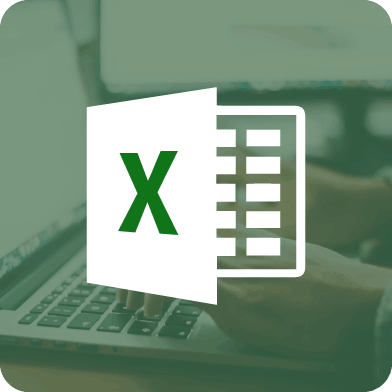
MS Excel Course
Course content.
Introduction to Data Science
Understanding of Data Science
How to use learnvern.
Data Measurement
Introduction to Data Measurement in Data Science
Types of variables in data science, levels of data measurement in data science.
Charts and Graphs
Charts and Graphs in Data Science
Frequency distribution in data science, bar graphs and pie charts in data science, summarizing quantitative data in data science, cummulative distribution in data science, histogram and scatter plot in data science, cross tabulation in data science.
Measures of Central Tendency and Variability
Measures of Central Tendency and Variability in Data Science
Mean and percentiles in data science, quartiles in data science, measures of variability in data science, variance and standard deviation in data science, application of standard deviation in data science, z-score and co-efficient of variation in data science, measures of central tendency for grouped data in data science, standard deviation and variance for grouped data in data science.
Measures of Shapes
Skewness in Data Science
Kurtosis in data science, box and whisker plots in data science.
Hypothesis Testing
Statistical Hypothesis in Data Science
Errors in statistical hypothesis in data science, statistical inference for single population in data science, testing example in data science, statistical inference with two population in data science.
Basics of R Programming
R Programming in Data Science
Data types in r in data science, understanding r console and r studio in data science.
Analysis of Variance
Analysis of Variance and Annova in Data Science
Assumption and variation in one-way anova in data science, two way anova in data science, summary of anova in data science, anova example in data science, one way anova example in data science, two way anova example in data science.
Introduction to Datamining
Understanding Datamining in Data Science
Regression Analysis and Correlation
Introduction to Simple Linear Regression and Correlation in Data Science
Least square and residual analysis in data science, test assumption of regression model in data science, hypothesis and co-efficient of determination in data science, regression example - creating model in data science, residual analaysis of model example in data science, residual analysis of model part 2 in data science, residual analysis of model part 3 in data science, understanding clustering in data science, hierarchical clustering in data science, example of hierarchial clustering in data science, k means clustering in data science, example of k means clustering in data science, theoretical concepts of dashboard, creating pivots and preparing charts, slicers in dashboard, preparing final dashboard, presenting dashboard, enjoying this course.
All the Course on LearnVern are Free. So Register/ Signup to have Access all the Course and Videos.
Data Science Course Code
- No Certificate
- Globally Recognized NSDC Certificate
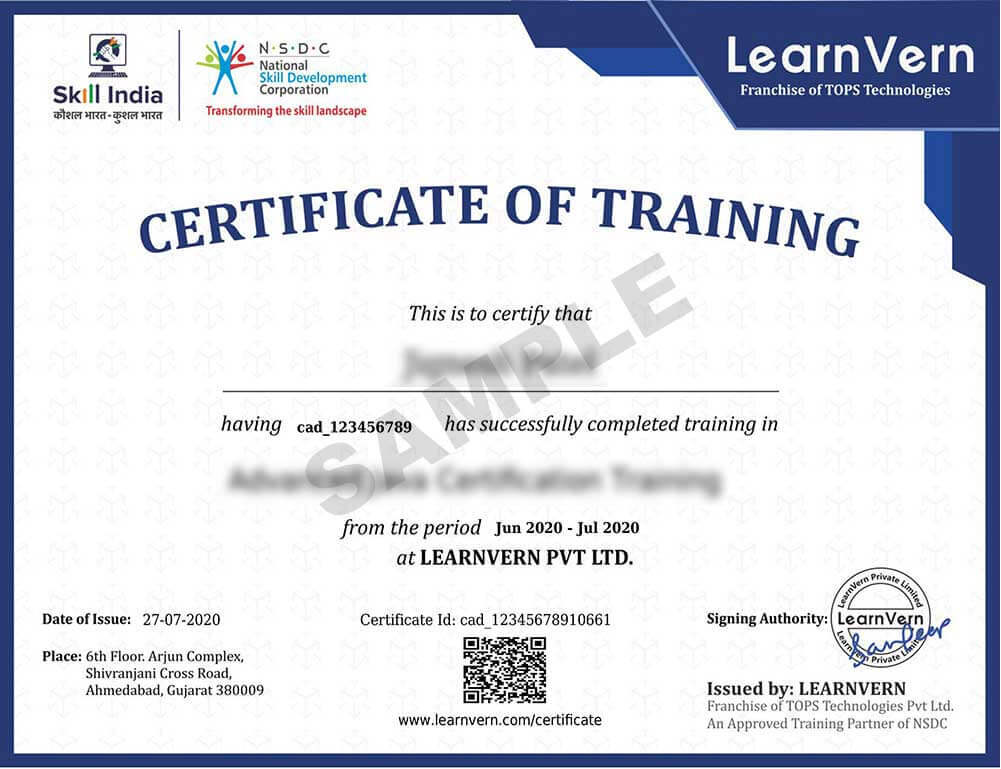
Share with your Friends
Copy this link and share it with your friends
Refer and win Exciting Gifts
Share learnvern with your friends and earn free rewards and certificates., share a personalized message with your friends..
Your Referred User Count
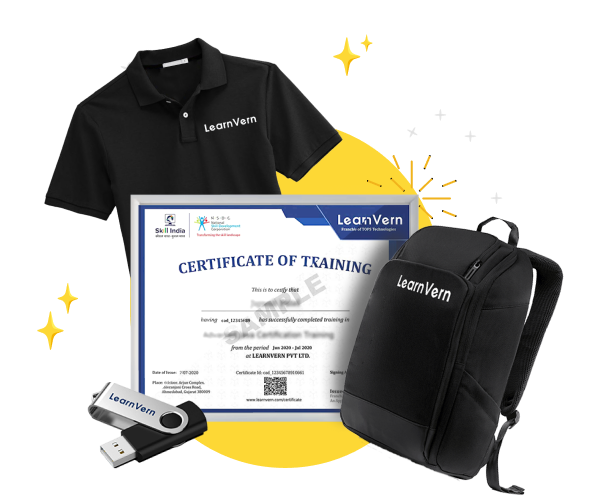
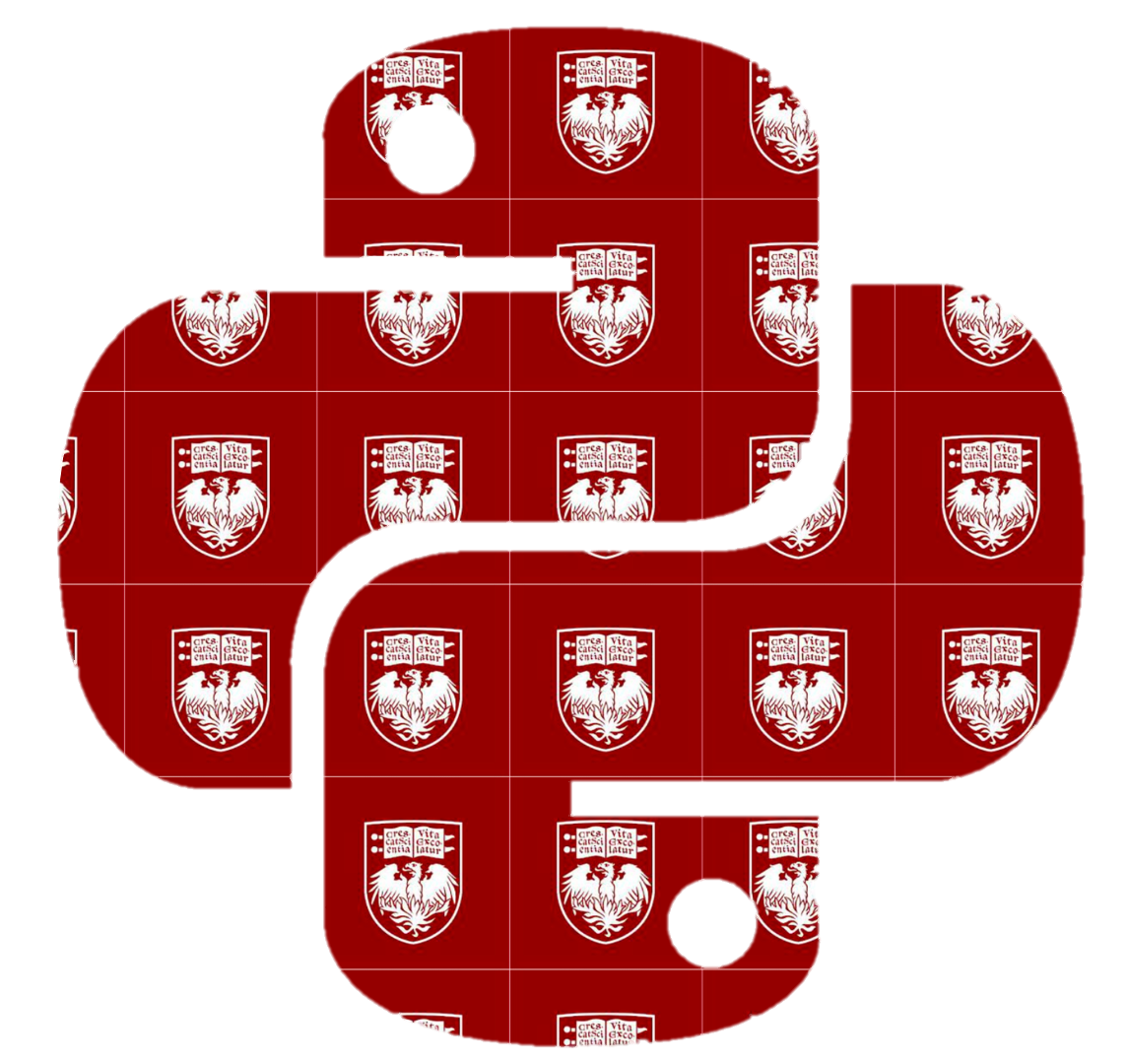
Introduction to Data Science I & II
Hypothesis testing, hypothesis testing #.
Dan L. Nicolae
Hypothesis testing can be thought of as a way to investigate the consistency of a dataset with a model, where a model is a set of rules that describe how data are generated. The consistency is evaluated using ideas from probability and probability distributions.
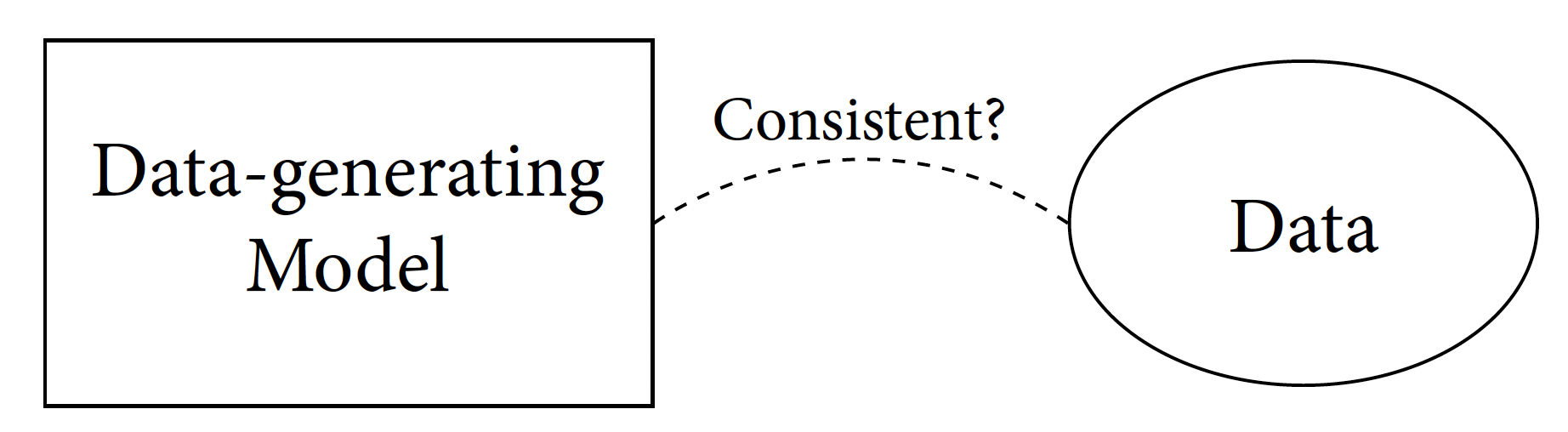
The consistency question in the above diagram is short for āIs it plausible that data was generated from this model?ā
We will use a simple example to illustrate this. Suppose that a friend is telling you that she has an urn with 6 blue and 4 red balls from which 5 balls are extracted without replacement. The description in the previous sentence is that of a model with four rules:
there is an urn with 10 balls: 6 blue and 4 red;
a total of 5 balls are extracted;
the balls are extracted without replacement (once a ball is out of the urn, it cannot be selected again);
at each extraction, every ball in the urn has the same chance of being selected (this assumption is implicit in urn problems).
Suppose your friend reports the results of a drawing (these are the data) and here are two hypothetical scenarios (datasets):
Scenario 1: outcome is 5 red balls . Is this outcome consistent with the model above? The answer is clearly no as it is not possible to obtain 5 red balls when the first 3 rules above are true.
Scenario 2: outcome is 2 blue and 3 red balls . The answer here is not as obvious as above, but we can use probability to get an evaluation of how likely this outcome is. We will formalize this process in this chapter.
We will use these ideas in the next sections to answer questions that are more complicated: Is pollution associated with risk of cancer? Are weights of babies different for older mothers?
We end this introduction with examples of other data-generating models (so you can gain more insight before learning how to evaluate them):
A simple random sample of 10 voters from a population of size 10,000 where 40% of the subjects vote for candidate A, 35% for candidate B and 25% for candidate C.
Data from a binomial setting; this was introduced in the previous chapter where the binomial distribution comes from a sequence of Bernoulli trials that follow 4 rules: (i) a fixed number of trials; (ii) two possible outcomes for each trial; (iii) trials are independent; and (iv) the probability of success is the same for each trial
A set of 100 observations generated independently from a Unif(1,5) distribution.
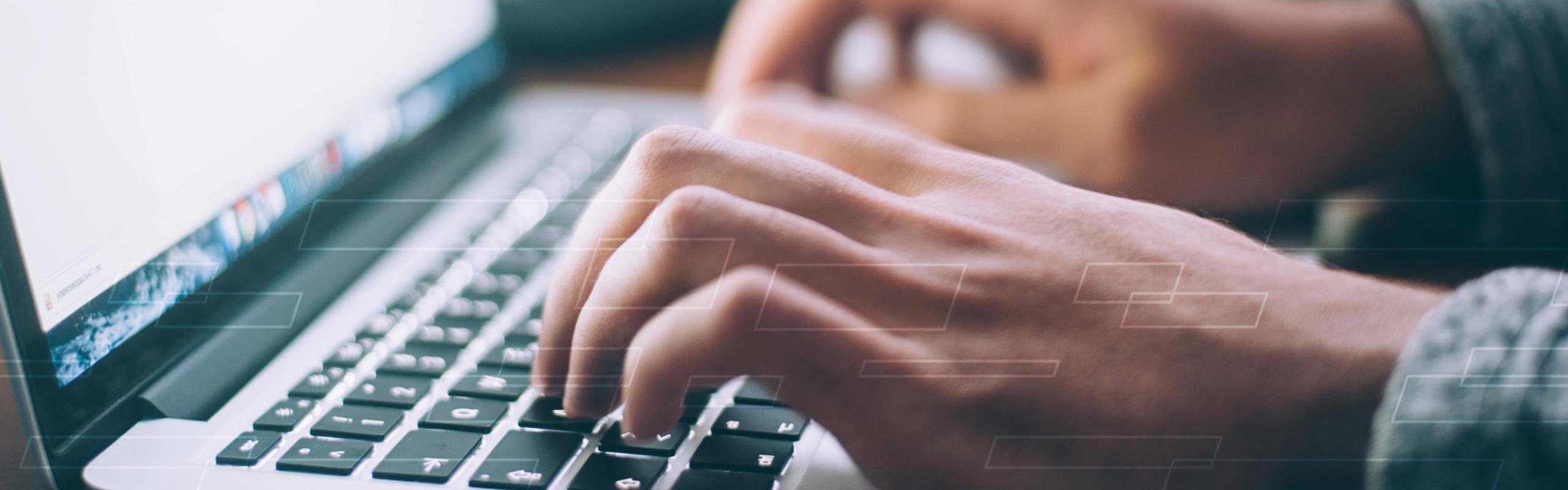
User Preferences
Content preview.
Arcu felis bibendum ut tristique et egestas quis:
- Ut enim ad minim veniam, quis nostrud exercitation ullamco laboris
- Duis aute irure dolor in reprehenderit in voluptate
- Excepteur sint occaecat cupidatat non proident
Keyboard Shortcuts
S.3.3 hypothesis testing examples.
- Example: Right-Tailed Test
- Example: Left-Tailed Test
- Example: Two-Tailed Test
Brinell Hardness Scores
An engineer measured the Brinell hardness of 25 pieces of ductile iron that were subcritically annealed. The resulting data were:
The engineer hypothesized that the mean Brinell hardness of all such ductile iron pieces is greater than 170. Therefore, he was interested in testing the hypotheses:
H 0 : μ = 170 H A : μ > 170
The engineer entered his data into Minitab and requested that the "one-sample t -test" be conducted for the above hypotheses. He obtained the following output:
Descriptive Statistics
$\mu$: mean of Brinelli
Null hypothesisĀ Ā Ā Hā: $\mu$ = 170 Alternative hypothesisĀ Ā Ā Hā: $\mu$ > 170
The output tells us that the average Brinell hardness of the n = 25 pieces of ductile iron was 172.52 with a standard deviation of 10.31. (The standard error of the mean "SE Mean", calculated by dividing the standard deviation 10.31 by the square root of n = 25, is 2.06). The test statistic t * is 1.22, and the P -value is 0.117.
If the engineer set his significance level α at 0.05 and used the critical value approach to conduct his hypothesis test, he would reject the null hypothesis if his test statistic t * were greater than 1.7109 (determined using statistical software or a t -table):
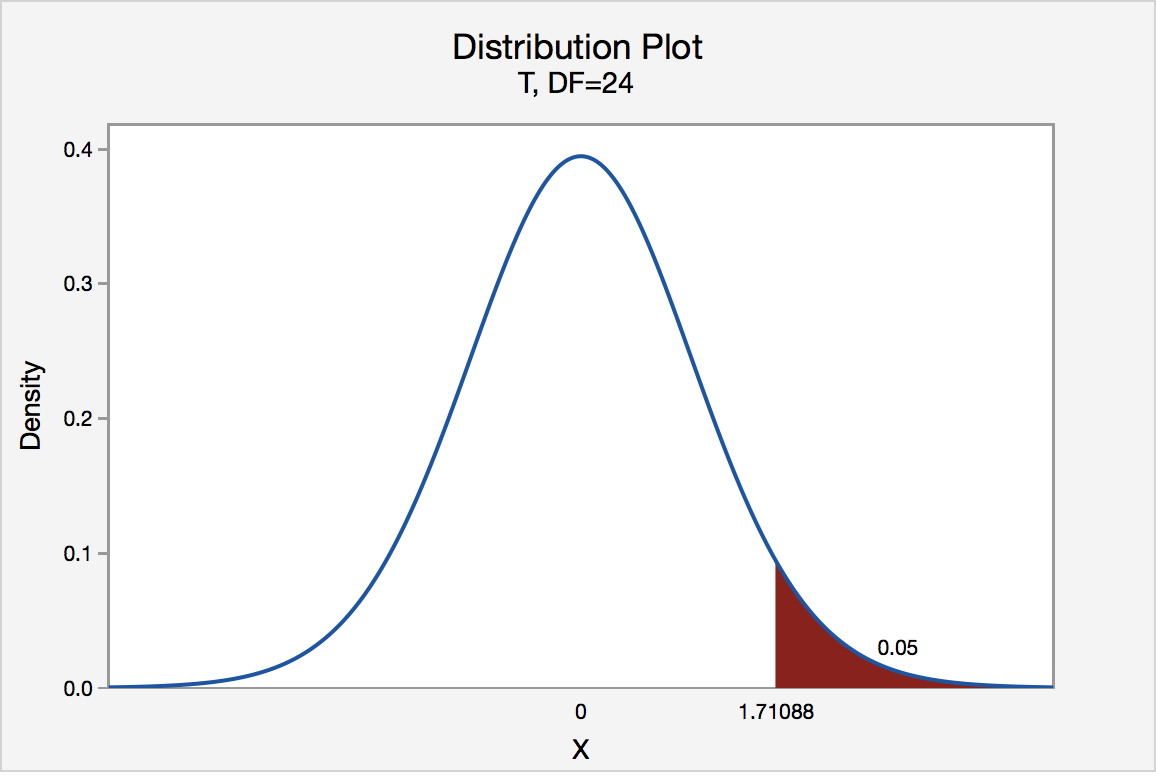
Since the engineer's test statistic, t * = 1.22, is not greater than 1.7109, the engineer fails to reject the null hypothesis. That is, the test statistic does not fall in the "critical region." There is insufficient evidence, at theĀ \(\alpha\) = 0.05 level, to conclude that the mean Brinell hardness of all such ductile iron pieces is greater than 170.
If the engineer used the P -value approach to conduct his hypothesis test, he would determine the area under a t n - 1 = t 24 curve and to the right of the test statistic t * = 1.22:
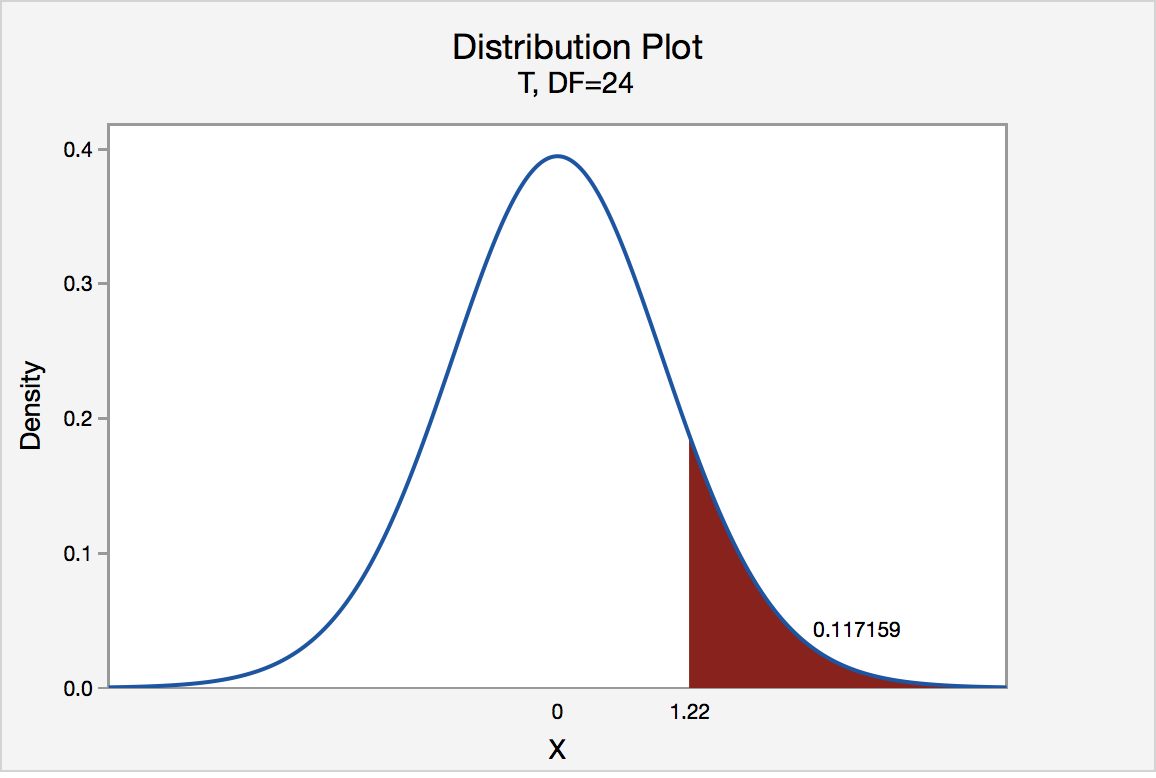
In the output above, Minitab reports that the P -value is 0.117. Since the P -value, 0.117, is greater thanĀ \(\alpha\) = 0.05, the engineer fails to reject the null hypothesis. There is insufficient evidence, at theĀ \(\alpha\) = 0.05 level, to conclude that the mean Brinell hardness of all such ductile iron pieces is greater than 170.
Note that the engineer obtains the same scientific conclusion regardless of the approach used. This will always be the case.
Height of Sunflowers
A biologist was interested in determining whether sunflower seedlings treated with an extract from Vinca minor roots resulted in a lower average height of sunflower seedlings than the standard height of 15.7 cm. The biologist treated a random sample of n = 33 seedlings with the extract and subsequently obtained the following heights:
The biologist's hypotheses are:
H 0 : μ = 15.7 H A : μ < 15.7
The biologist entered her data into Minitab and requested that the "one-sample t -test" be conducted for the above hypotheses. She obtained the following output:
$\mu$: mean of Height
Null hypothesisĀ Ā Ā Hā: $\mu$ = 15.7 Alternative hypothesisĀ Ā Ā Hā: $\mu$ < 15.7
The output tells us that the average height of the n = 33 sunflower seedlings was 13.664 with a standard deviation of 2.544. (The standard error of the mean "SE Mean", calculated by dividing the standard deviation 13.664 by the square root of n = 33, is 0.443). The test statistic t * is -4.60, and the P -value, 0.000, is to three decimal places.
Minitab Note. Minitab will always report P -values to only 3 decimal places. If Minitab reports the P -value as 0.000, it really means that the P -value is 0.000....something. Throughout this course (and your future research!), when you see that Minitab reports the P -value as 0.000, you should report the P -value as being "< 0.001."
If the biologist set her significance levelĀ \(\alpha\) at 0.05 and used the critical value approach to conduct her hypothesis test, she would reject the null hypothesis if her test statistic t * were less than -1.6939 (determined using statistical software or a t -table):s-3-3
Since the biologist's test statistic, t * = -4.60, is less than -1.6939, the biologist rejects the null hypothesis. That is, the test statistic falls in the "critical region." There is sufficient evidence, at the α = 0.05 level, to conclude that the mean height of all such sunflower seedlings is less than 15.7 cm.
If the biologist used the P -value approach to conduct her hypothesis test, she would determine the area under a t n - 1 = t 32 curve and to the left of the test statistic t * = -4.60:
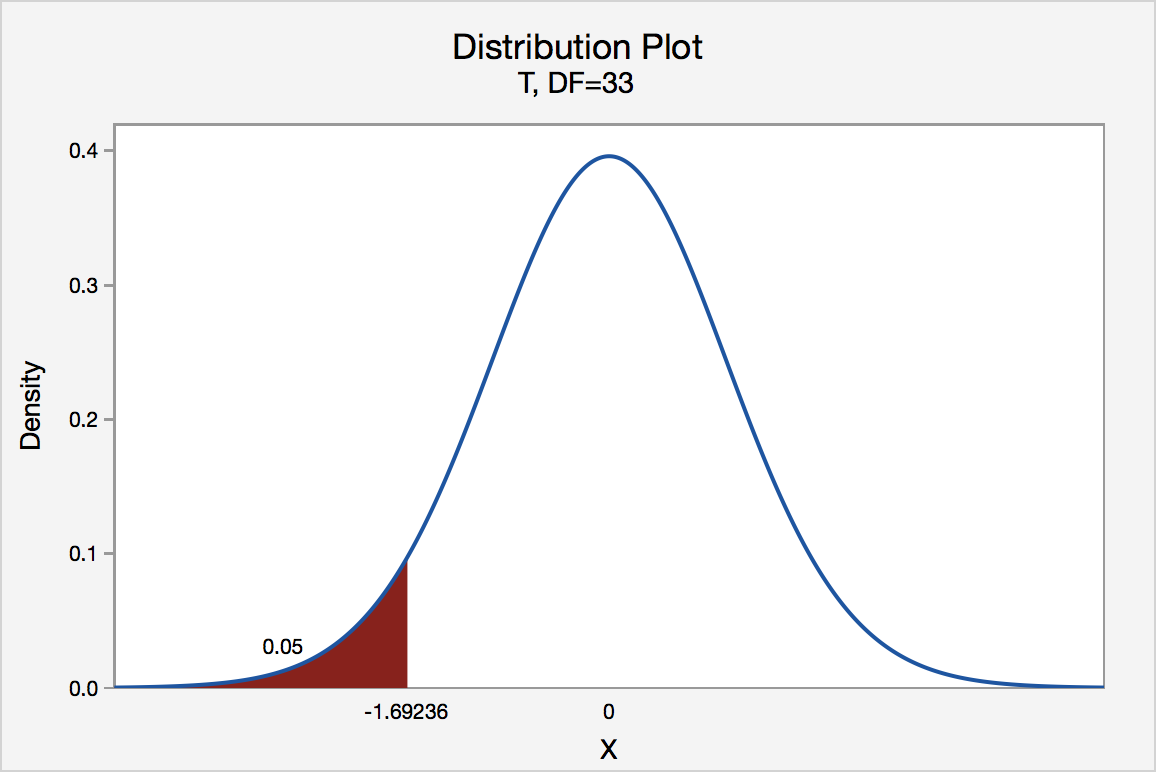
In the output above, Minitab reports that the P -value is 0.000, which we take to mean < 0.001. Since the P -value is less than 0.001, it is clearly less thanĀ \(\alpha\) = 0.05, and the biologist rejects the null hypothesis. There is sufficient evidence, at theĀ \(\alpha\) = 0.05 level, to conclude that the mean height of all such sunflower seedlings is less than 15.7 cm.
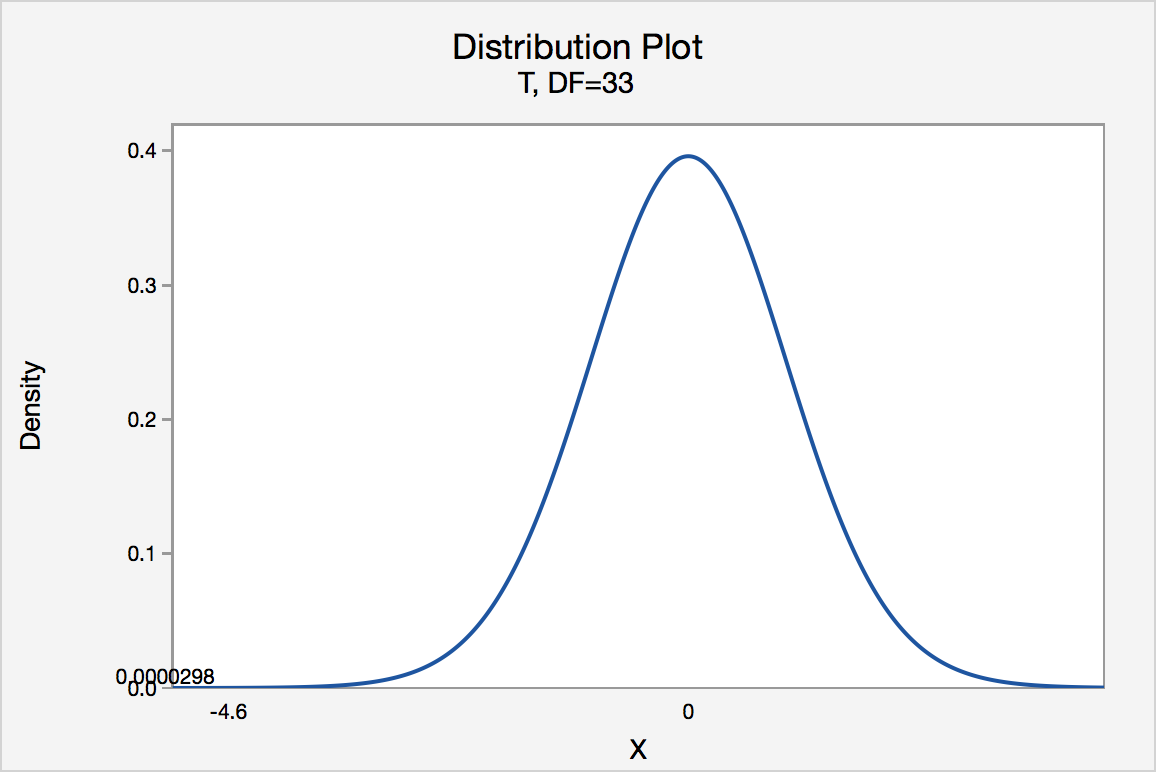
Note again that the biologist obtains the same scientific conclusion regardless of the approach used. This will always be the case.
Gum Thickness
A manufacturer claims that the thickness of the spearmint gum it produces is 7.5 one-hundredths of an inch. A quality control specialist regularly checks this claim. On one production run, he took a random sample of n = 10 pieces of gum and measured their thickness. He obtained:
The quality control specialist's hypotheses are:
H 0 : μ = 7.5 H A : μ ā 7.5
The quality control specialist entered his data into Minitab and requested that the "one-sample t -test" be conducted for the above hypotheses. He obtained the following output:
$\mu$: mean of Thickness
Null hypothesisĀ Ā Ā Hā: $\mu$ = 7.5 Alternative hypothesisĀ Ā Ā Hā: $\mu \ne$ 7.5
The output tells us that the average thickness of the n = 10 pieces of gums was 7.55 one-hundredths of an inch with a standard deviation of 0.1027. (The standard error of the mean "SE Mean", calculated by dividing the standard deviation 0.1027 by the square root of n = 10, is 0.0325). The test statistic t * is 1.54, and the P -value is 0.158.
If the quality control specialist sets his significance levelĀ \(\alpha\) at 0.05 and used the critical value approach to conduct his hypothesis test, he would reject the null hypothesis if his test statistic t * were less than -2.2616 or greater than 2.2616 (determined using statistical software or a t -table):
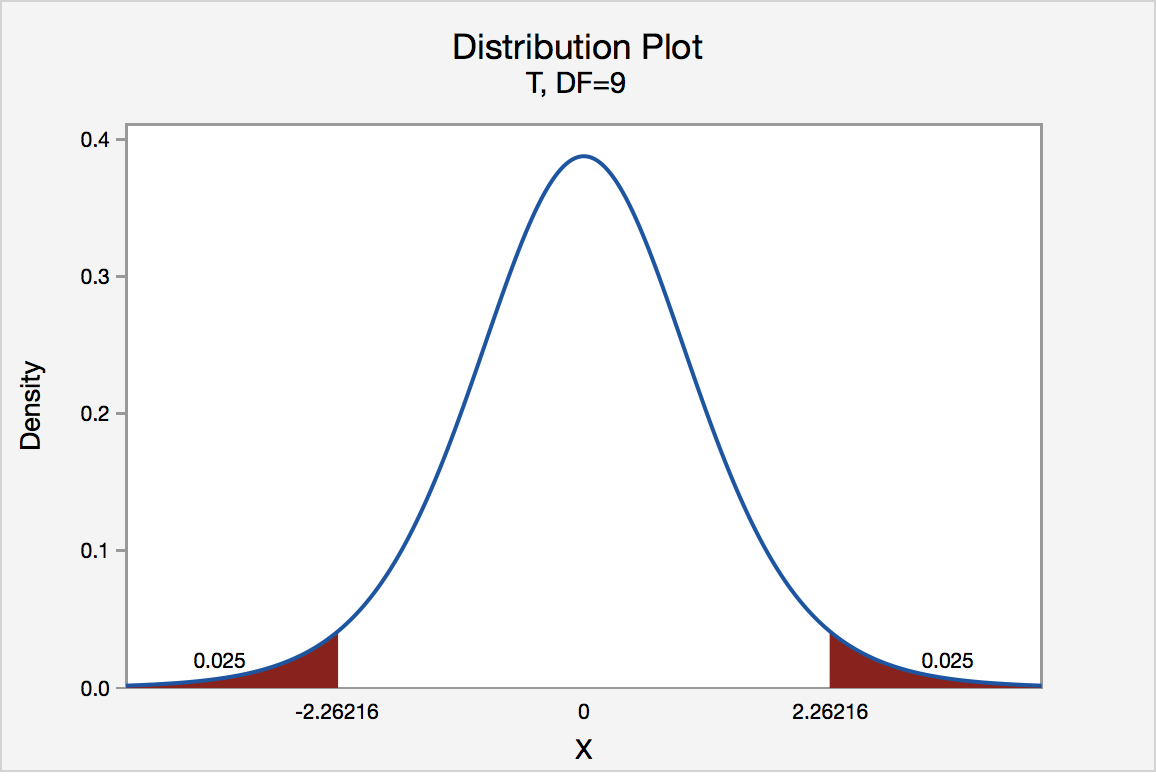
Since the quality control specialist's test statistic, t * = 1.54, is not less than -2.2616 nor greater than 2.2616, the quality control specialist fails to reject the null hypothesis. That is, the test statistic does not fall in the "critical region." There is insufficient evidence, at theĀ \(\alpha\) = 0.05 level, to conclude that the mean thickness of all of the manufacturer's spearmint gum differs from 7.5 one-hundredths of an inch.
If the quality control specialist used the P -value approach to conduct his hypothesis test, he would determine the area under a t n - 1 = t 9 curve, to the right of 1.54 and to the left of -1.54:
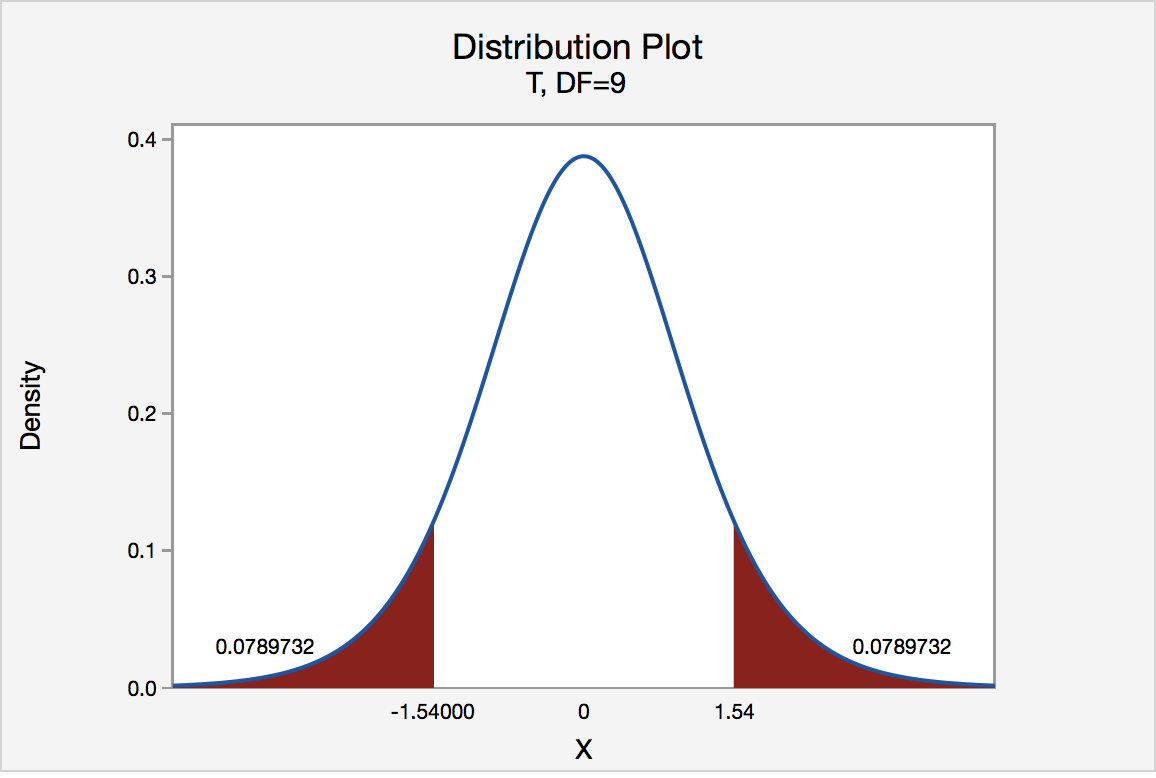
In the output above, Minitab reports that the P -value is 0.158. Since the P -value, 0.158, is greater thanĀ \(\alpha\) = 0.05, the quality control specialist fails to reject the null hypothesis. There is insufficient evidence, at theĀ \(\alpha\) = 0.05 level, to conclude that the mean thickness of all pieces of spearmint gum differs from 7.5 one-hundredths of an inch.
Note that the quality control specialist obtains the same scientific conclusion regardless of the approach used. This will always be the case.
In our review of hypothesis tests, we have focused on just one particular hypothesis test, namely that concerning the population mean \(\mu\). The important thing to recognize is that the topics discussed here ā the general idea of hypothesis tests, errors in hypothesis testing, the critical value approach, and the P -value approach ā generally extend to all of the hypothesis tests you will encounter.

Data Science Stunt
Join Our Stunt to Successful Product Strategies with a Data Mindset
What is hypothesis testing in data science?
Hypothesis testing is a statistical technique used to evaluate hypotheses about a population based on sample data. In data science, hypothesis testing is an essential tool used to make inferences about the population based on a representative sample. In this blog, we will discuss the key aspects of hypothesis testing, including null hypothesis, alternate hypothesis, significance level, type I and type II errors, p-value, region of acceptance, typical steps involved in p-value approach, and key terms around type I error and type II error.
Table of Contents
What is hypothesis testing in data science or AI or ML or Statistics?
Hypothesis testing is a statistical technique used to test whether a claim or hypothesis about a population is true or not. Imagine that you have a hypothesis that eating breakfast helps students perform better in school. To test this hypothesis, you would collect data from a sample of students and compare their performance in school to whether they ate breakfast or not. You would then compare the results to what would be expected by chance, assuming the null hypothesis is true, which means there is no difference in performance between those who ate breakfast and those who didn’t. If the results show a statistically significant difference, you can reject the null hypothesis and accept the alternative hypothesis, which in this case is that eating breakfast does, in fact, help students perform better in school. Hypothesis testing is important in many fields, including science and medicine, to help make decisions based on empirical evidence.
Hypothesis testing is a statistical tool that helps to test assumptions or claims about a population based on a sample of data. The process of hypothesis testing involves formulating a null hypothesis and an alternative hypothesis, selecting an appropriate test statistic, choosing a level of significance, calculating the p-value, and making a decision whether to reject or fail to reject the null hypothesis based on the p-value. Hypothesis testing is widely used in various fields, such as social sciences, medicine, engineering, and economics, to evaluate research questions and determine the statistical significance of results. It is a crucial component of data analysis that can help to make sound decisions based on empirical evidence.

What are the null hypothesis and alternative hypothesis with examples?
Hypothesis testing involves comparing two statements, the null hypothesis and the alternative hypothesis. The null hypothesis is the starting assumption we make about a population, which suggests there is no difference or relationship between two or more variables being tested. For instance, if we want to test whether there is a difference in the average height between boys and girls, the null hypothesis would state that there is no difference in height between the two groups.
On the other hand, the alternative hypothesis is a statement that assumes there is a difference or relationship between the variables being tested. In this example, the alternative hypothesis would propose that there is a difference in height between boys and girls.
To evaluate which hypothesis to accept or reject, we collect data from a sample and compare it to what would be expected by chance, assuming the null hypothesis is correct. If the results reveal a statistically significant difference, we reject the null hypothesis and accept the alternative hypothesis. However, if there is insufficient evidence to dismiss the null hypothesis, we fail to reject it. To sum up, the null hypothesis is the original assumption we test, and the alternative hypothesis is the statement we attempt to validate.
The null hypothesis is a statement of no effect or no difference between two groups. This statement is usually the starting point in hypothesis testing, and we assume it to be true. For example, if we want to test the efficacy of a new drug, the null hypothesis would state that the drug has no effect.
The alternate hypothesis is the opposite of the null hypothesis. It is the statement we want to test, and it suggests that there is an effect or difference between two groups. For example, if we want to test the efficacy of a new drug, the alternate hypothesis would suggest that the drug is effective.
Here are some more examples to help illustrate the concept of null and alternative hypotheses:
Example 1: Null hypothesis: There is no difference in exam scores between students who study alone versus students who study in groups. Alternative hypothesis: Students who study in groups score higher on exams than students who study alone.
Example 2: Null hypothesis: There is no difference in sales between two different advertising campaigns. Alternative hypothesis: One advertising campaign leads to more sales than the other.
Example 3: Null hypothesis: There is no relationship between the amount of sleep a person gets and their cognitive performance. Alternative hypothesis: The amount of sleep a person gets is positively correlated with their cognitive performance.
What is the significance level in hypothesis testing with examples?
In hypothesis testing, the significance level is a number that we pick before we do a test. It helps us know how sure we are about our results. It’s like saying, “I’m only going to say something is true if I’m really sure.”
For example, if we’re trying to find out if girls are taller than boys on average, we might set a significance level of 0.05. This means we’re only going to say girls are taller if we’re at least 95% sure that’s true. If our test gives us a result that is less than 95% sure, we won’t say for sure that girls are taller.
The significance level is like a safety net to make sure we’re not jumping to conclusions without enough evidence. It helps us be more careful and confident about what we say is true based on the data we have.
In hypothesis testing, the significance level is the probability threshold that we set for rejecting the null hypothesis. The significance level, also known as alpha (α), is usually chosen before the test is conducted and is typically set at 0.05, meaning that we are willing to accept a 5% chance of making a type I error, which is the probability of rejecting the null hypothesis when it is actually true.
For example, let’s say we’re testing the hypothesis that boys and girls have different average heights. We would start by setting a significance level of 0.05. If the results of our study indicate that there is a statistically significant difference in height between boys and girls, with a p-value less than 0.05, we would reject the null hypothesis and conclude that there is a significant difference in height between the two groups. However, if the p-value is greater than 0.05, we would fail to reject the null hypothesis and conclude that there is insufficient evidence to support the claim that there is a difference in height between boys and girls.
In summary, the significance level is the probability threshold we set for rejecting the null hypothesis, and it helps us determine how confident we are in our conclusions based on the data we have collected. It is a critical component of hypothesis testing and helps us make informed decisions based on empirical evidence.
The significance level is the probability of making a type I error, or the probability of rejecting the null hypothesis when it is actually true. It is denoted by alpha (α) and is typically set to 0.05 or 0.01.
What are Type I and Type II Errors in hypothesis testing with examples?
When we do a test to see if something is true or not, sometimes we can make mistakes. There are two kinds of mistakes we can make:
The first mistake is called a Type I error. This happens when we say something is true, but it’s really not true. It’s like thinking you found a diamond in the sand, but it’s just a piece of glass.
The second mistake is called a Type II error. This happens when we say something is not true, but it’s actually true. It’s like thinking there are no more cookies in the cookie jar, but there are still some left.
We use some special words to describe these mistakes. Type I errors are also called false positives, and Type II errors are also called false negatives.
To make things even more confusing, we use a letter called beta (β) to talk about the chance of making a Type II error. But don’t worry too much about that for now. Just remember that sometimes we can make mistakes when we do tests, and we have special names for those mistakes.
Type I error occurs when we reject the null hypothesis when it is actually true. It is also known as a false positive. Type II error occurs when we fail to reject the null hypothesis when it is actually false. It is also known as a false negative. The probability of making a type II error is denoted by beta (β).
We also have something called power. Power is like the opposite of a false negative. It’s the chance that we’ll figure out something is true, if it really is true.
Another thing we think about is the sample size. That means how many things we’re looking at to figure out if something is true or not.
And finally, we think about the effect size. That’s how big the difference is between two things we’re looking at. If the difference is really big, we might be more likely to find out if something is true or not.
So basically, when we do hypothesis testing, we try to figure out if something is true or not. But sometimes we make mistakes. We also think about how many things we’re looking at, how big the difference is between them, and how likely we are to figure out if something is true if it really is true.
What is the p-value in hypothesis testing with examples?
When we do a test to see if something is true or not, we get a number called the p-value. The p-value tells us how likely it is that we got the result we did just by chance.
Here’s an example: Let’s say we’re trying to find out if cats are smarter than dogs. We do a test and get a p-value of 0.03. This means there’s only a 3% chance that we got our result just by chance.
We also have something called a significance level, which is like a safety net to make sure we’re not jumping to conclusions without enough evidence. It’s like saying, “I’m only going to say something is true if I’m really sure.”
If the p-value is less than the significance level, we can say that we’re really sure our result is true, and we reject the idea that cats and dogs are equally smart. But if the p-value is greater than the significance level, we don’t have enough evidence to say for sure that cats are smarter than dogs.
So the p-value is a number that helps us decide if our test is giving us good evidence or if it’s just a fluke.
The p-value is the probability of obtaining a test statistic as extreme as the observed one, assuming that the null hypothesis is true. If the p-value is less than the significance level, we reject the null hypothesis. If the p-value is greater than the significance level, we fail to reject the null hypothesis.
What is the Region of Acceptance in hypothesis testing with examples?
When we do a test to see if something is true or not, we use a number called the test statistic. This number tells us how different our result is from what we would expect if the null hypothesis were true.
Sometimes, we get a test statistic that is in a certain range, and we say that it’s not different enough from what we would expect to be sure that our result is true. This range is called the region of acceptance.
For example, let’s say we’re trying to find out if a new medicine helps people sleep better. We do a test and get a test statistic of 1.2. We look at our chart and see that the region of acceptance is from -1.96 to 1.96. This means that our test statistic of 1.2 is in this range, so we can’t be sure that the medicine really works.
The region of acceptance is like a zone of uncertainty. It’s saying, “We’re not sure if this result is really different from what we would expect.”
The complement of the region of acceptance is called the critical region. This is the range of values that is different enough from what we would expect that we can be pretty sure our result is true.
So the region of acceptance is just the opposite of the critical region. If our test statistic is in the region of acceptance, we can’t say for sure if our result is true or not. But if it’s in the critical region, we can be pretty sure that it is.
The region of acceptance is the range of values of the test statistic that leads to failing to reject the null hypothesis. It is the complement of the critical region.
Typical Steps Involved in P-Value Approach
What are the 5 steps in hypothesis testing?
When we do a hypothesis test using the p-value approach, there are some typical steps we follow to find out if our hypothesis is true or not. Here are the steps we usually follow:
- We start by stating what we think is true (the null hypothesis) and what we think might be true instead (the alternate hypothesis).
- Then we pick a number called the test statistic. This number helps us figure out if our result is different enough from what we would expect to be sure it’s true.
- Next, we calculate something called the p-value. This is like a score that tells us how likely it is that our result is true, based on the test statistic.
- After that, we compare the p-value to another number called the significance level. If the p-value is smaller than the significance level, it means our result is very unlikely to be a coincidence, so we can say it’s true. If the p-value is bigger than the significance level, it means our result might just be a coincidence, so we can’t be sure it’s true.
- Finally, we make a decision about whether our hypothesis is true or not, based on the comparison we made in step 4. We also explain what our results mean in real-life terms.
So basically, when we do a hypothesis test using the p-value approach, we follow these steps to figure out if our hypothesis is true or not.
To summarize, hypothesis testing is a critical technique in data science that helps us draw conclusions about the population based on sample data. By setting up the null and alternate hypotheses, selecting an appropriate significance level, and computing the p-value, we can make informed judgments about whether to accept or reject the null hypothesis. It’s essential to recognize the kinds of errors that can happen during hypothesis testing and to choose a suitable sample size and effect size to reduce these errors.
Check out the table of contents for Product Management and Data Science to explore those topics.
Curious about how product managers can utilize Bhagwad Gitaās principles to tackle difficulties? Give this super short book a shot. This will certainly support my work.
Share this:
- Click to share on Twitter (Opens in new window)
- Click to share on Facebook (Opens in new window)
- Click to share on LinkedIn (Opens in new window)
- Click to share on Reddit (Opens in new window)
- Click to share on Tumblr (Opens in new window)
- Click to share on Pinterest (Opens in new window)
- Click to share on Telegram (Opens in new window)
- Click to share on WhatsApp (Opens in new window)
- Click to print (Opens in new window)
- Click to email a link to a friend (Opens in new window)
- Click to share on Pocket (Opens in new window)
- Click to share on Mastodon (Opens in new window)
Leave a Reply Cancel reply
Tutorial Playlist
Statistics tutorial, everything you need to know about the probability density function in statistics, the best guide to understand central limit theorem, an in-depth guide to measures of central tendency : mean, median and mode, the ultimate guide to understand conditional probability.
A Comprehensive Look at Percentile in Statistics
The Best Guide to Understand Bayes Theorem
Everything you need to know about the normal distribution, an in-depth explanation of cumulative distribution function, a complete guide to chi-square test, a complete guide on hypothesis testing in statistics, understanding the fundamentals of arithmetic and geometric progression, the definitive guide to understand spearmanās rank correlation, a comprehensive guide to understand mean squared error, all you need to know about the empirical rule in statistics, the complete guide to skewness and kurtosis, a holistic look at bernoulli distribution.
All You Need to Know About Bias in Statistics
A Complete Guide to Get a Grasp of Time Series Analysis
The Key Differences Between Z-Test Vs. T-Test
The Complete Guide to Understand Pearson's Correlation
A complete guide on the types of statistical studies, everything you need to know about poisson distribution, your best guide to understand correlation vs. regression, the most comprehensive guide for beginners on what is correlation, what is hypothesis testing in statistics types and examples.
Lesson 10 of 24 By Avijeet Biswal
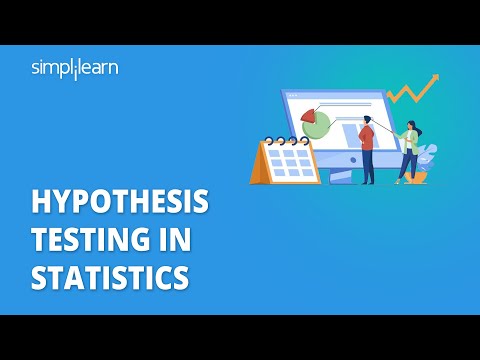
Table of Contents
In todayās data-driven world , decisions are based on data all the time. Hypothesis plays a crucial role in that process, whether it may be making business decisions, in the health sector, academia, or in quality improvement. Without hypothesis & hypothesis tests, you risk drawing the wrong conclusions and making bad decisions. In this tutorial, you will look at Hypothesis Testing in Statistics.
What Is Hypothesis Testing in Statistics?
Hypothesis Testing is a type of statistical analysis in which you put your assumptions about a population parameter to the test. It is used to estimate the relationship between 2 statistical variables.
Let's discuss few examples of statistical hypothesis from real-life -Ā
- A teacher assumes that 60% of his college's students come from lower-middle-class families.
- A doctor believes that 3D (Diet, Dose, and Discipline) is 90% effective for diabetic patients.
Now that you know about hypothesis testing, look at the two types of hypothesis testing in statistics.
Hypothesis Testing Formula
Z = ( xĢ ā μ0 ) / (Ļ /ān)
- Here, xĢ is the sample mean,
- μ0 is the population mean,
- Ļ is the standard deviation,
- n is the sample size.
How Hypothesis Testing Works?
An analyst performs hypothesis testing on a statistical sample to present evidence of the plausibility of the null hypothesis. Measurements and analyses are conducted on a random sample of the population to test a theory. Analysts use a random population sample to test two hypotheses: the null and alternative hypotheses.
The null hypothesis is typically an equality hypothesis between population parameters; for example, a null hypothesis may claim that the population means return equals zero. The alternate hypothesis is essentially the inverse of the null hypothesis (e.g., the population means the return is not equal to zero). As a result, they are mutually exclusive, and only one can be correct. One of the two possibilities, however, will always be correct.
Your Dream Career is Just Around The Corner!
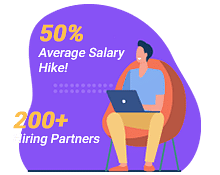
Null Hypothesis and Alternate Hypothesis
The Null Hypothesis is the assumption that the event will not occur. A null hypothesis has no bearing on the study's outcome unless it is rejected.
H0 is the symbol for it, and it is pronounced H-naught.
The Alternate Hypothesis is the logical opposite of the null hypothesis. The acceptance of the alternative hypothesis follows the rejection of the null hypothesis. H1 is the symbol for it.
Let's understand this with an example.
A sanitizer manufacturer claims that its product kills 95 percent of germs on average.Ā
To put this company's claim to the test, create a null and alternate hypothesis.
H0 (Null Hypothesis): Average = 95%.
Alternative Hypothesis (H1): The average is less than 95%.
Another straightforward example to understand this concept is determining whether or not a coin is fair and balanced. The null hypothesis states that the probability of a show of heads is equal to the likelihood of a show of tails. In contrast, the alternate theory states that the probability of a show of heads and tails would be very different.
Become a Data Scientist with Hands-on Training!
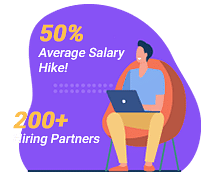
Hypothesis Testing Calculation With Examples
Let's consider a hypothesis test for the average height of women in the United States. Suppose our null hypothesis is that the average height is 5'4". We gather a sample of 100 women and determine that their average height is 5'5". The standard deviation of population is 2.
To calculate the z-score, we would use the following formula:
z = ( xĢ ā μ0 ) / (Ļ /ān)
z = (5'5" - 5'4") / (2" / ā100)
z = 0.5 / (0.045)
Ā We will reject the null hypothesis as the z-score of 11.11 is very large and conclude that there is evidence to suggest that the average height of women in the US is greater than 5'4".
Steps of Hypothesis Testing
Step 1: specify your null and alternate hypotheses.
It is critical to rephrase your original research hypothesis (the prediction that you wish to study) as a null (Ho) and alternative (Ha) hypothesis so that you can test it quantitatively. Your first hypothesis, which predicts a link between variables, is generally your alternate hypothesis. The null hypothesis predicts no link between the variables of interest.
Step 2: Gather Data
For a statistical test to be legitimate, sampling and data collection must be done in a way that is meant to test your hypothesis. You cannot draw statistical conclusions about the population you are interested in if your data is not representative.
Step 3: Conduct a Statistical Test
Other statistical tests are available, but they all compare within-group variance (how to spread out the data inside a category) against between-group variance (how different the categories are from one another). If the between-group variation is big enough that there is little or no overlap between groups, your statistical test will display a low p-value to represent this. This suggests that the disparities between these groups are unlikely to have occurred by accident. Alternatively, if there is a large within-group variance and a low between-group variance, your statistical test will show a high p-value. Any difference you find across groups is most likely attributable to chance. The variety of variables and the level of measurement of your obtained data will influence your statistical test selection.
Step 4: Determine Rejection Of Your Null Hypothesis
Your statistical test results must determine whether your null hypothesis should be rejected or not. In most circumstances, you will base your judgment on the p-value provided by the statistical test. In most circumstances, your preset level of significance for rejecting the null hypothesis will be 0.05 - that is, when there is less than a 5% likelihood that these data would be seen if the null hypothesis were true. In other circumstances, researchers use a lower level of significance, such as 0.01 (1%). This reduces the possibility of wrongly rejecting the null hypothesis.
Step 5: Present Your ResultsĀ
The findings of hypothesis testing will be discussed in the results and discussion portions of your research paper, dissertation, or thesis. You should include a concise overview of the data and a summary of the findings of your statistical test in the results section. You can talk about whether your results confirmed your initial hypothesis or not in the conversation. Rejecting or failing to reject the null hypothesis is a formal term used in hypothesis testing. This is likely a must for your statistics assignments.
Types of Hypothesis Testing
To determine whether a discovery or relationship is statistically significant, hypothesis testing uses a z-test. It usually checks to see if two means are the same (the null hypothesis). Only when the population standard deviation is known and the sample size is 30 data points or more, can a z-test be applied.
A statistical test called a t-test is employed to compare the means of two groups. To determine whether two groups differ or if a procedure or treatment affects the population of interest, it is frequently used in hypothesis testing.
Chi-SquareĀ
You utilize a Chi-square test for hypothesis testing concerning whether your data is as predicted. To determine if the expected and observed results are well-fitted, the Chi-square test analyzes the differences between categorical variables from a random sample. The test's fundamental premise is that the observed values in your data should be compared to the predicted values that would be present if the null hypothesis were true.
Hypothesis Testing and Confidence Intervals
Both confidence intervals and hypothesis tests are inferential techniques that depend on approximating the sample distribution. Data from a sample is used to estimate a population parameter using confidence intervals. Data from a sample is used in hypothesis testing to examine a given hypothesis. We must have a postulated parameter to conduct hypothesis testing.
Bootstrap distributions and randomization distributions are created using comparable simulation techniques. The observed sample statistic is the focal point of a bootstrap distribution, whereas the null hypothesis value is the focal point of a randomization distribution.
A variety of feasible population parameter estimates are included in confidence ranges. In this lesson, we created just two-tailed confidence intervals. There is a direct connection between these two-tail confidence intervals and these two-tail hypothesis tests. The results of a two-tailed hypothesis test and two-tailed confidence intervals typically provide the same results. In other words, a hypothesis test at the 0.05 level will virtually always fail to reject the null hypothesis if the 95% confidence interval contains the predicted value. A hypothesis test at the 0.05 level will nearly certainly reject the null hypothesis if the 95% confidence interval does not include the hypothesized parameter.
Simple and Composite Hypothesis Testing
Depending on the population distribution, you can classify the statistical hypothesis into two types.
Simple Hypothesis: A simple hypothesis specifies an exact value for the parameter.
Composite Hypothesis: A composite hypothesis specifies a range of values.
A company is claiming that their average sales for this quarter are 1000 units. This is an example of a simple hypothesis.
Suppose the company claims that the sales are in the range of 900 to 1000 units. Then this is a case of a composite hypothesis.
One-Tailed and Two-Tailed Hypothesis Testing
The One-Tailed test, also called a directional test, considers a critical region of data that would result in the null hypothesis being rejected if the test sample falls into it, inevitably meaning the acceptance of the alternate hypothesis.
In a one-tailed test, the critical distribution area is one-sided, meaning the test sample is either greater or lesser than a specific value.
In two tails, the test sample is checked to be greater or less than a range of values in a Two-Tailed test, implying that the critical distribution area is two-sided.
If the sample falls within this range, the alternate hypothesis will be accepted, and the null hypothesis will be rejected.
Become a Data Scientist With Real-World Experience
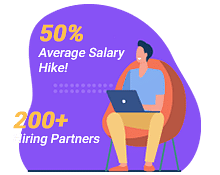
Right Tailed Hypothesis Testing
If the larger than (>) sign appears in your hypothesis statement, you are using a right-tailed test, also known as an upper test. Or, to put it another way, the disparity is to the right. For instance, you can contrast the battery life before and after a change in production. Your hypothesis statements can be the following if you want to know if the battery life is longer than the original (let's say 90 hours):
- The null hypothesis is (H0 <= 90) or less change.
- A possibility is that battery life has risen (H1) > 90.
The crucial point in this situation is that the alternate hypothesis (H1), not the null hypothesis, decides whether you get a right-tailed test.
Left Tailed Hypothesis Testing
Alternative hypotheses that assert the true value of a parameter is lower than the null hypothesis are tested with a left-tailed test; they are indicated by the asterisk "<".
Suppose H0: mean = 50 and H1: mean not equal to 50
According to the H1, the mean can be greater than or less than 50. This is an example of a Two-tailed test.
In a similar manner, if H0: mean >=50, then H1: mean <50
Here the mean is less than 50. It is called a One-tailed test.
Type 1 and Type 2 Error
A hypothesis test can result in two types of errors.
Type 1 Error: A Type-I error occurs when sample results reject the null hypothesis despite being true.
Type 2 Error: A Type-II error occurs when the null hypothesis is not rejected when it is false, unlike a Type-I error.
Suppose a teacher evaluates the examination paper to decide whether a student passes or fails.
H0: Student has passed
H1: Student has failed
Type I error will be the teacher failing the student [rejects H0] although the student scored the passing marks [H0 was true].Ā
Type II error will be the case where the teacher passes the student [do not reject H0] although the student did not score the passing marks [H1 is true].
Level of Significance
The alpha value is a criterion for determining whether a test statistic is statistically significant. In a statistical test, Alpha represents an acceptable probability of a Type I error. Because alpha is a probability, it can be anywhere between 0 and 1. In practice, the most commonly used alpha values are 0.01, 0.05, and 0.1, which represent a 1%, 5%, and 10% chance of a Type I error, respectively (i.e. rejecting the null hypothesis when it is in fact correct).
Future-Proof Your AI/ML Career: Top Dos and Don'ts
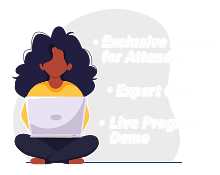
A p-value is a metric that expresses the likelihood that an observed difference could have occurred by chance. As the p-value decreases the statistical significance of the observed difference increases. If the p-value is too low, you reject the null hypothesis.
Here you have taken an example in which you are trying to test whether the new advertising campaign has increased the product's sales. The p-value is the likelihood that the null hypothesis, which states that there is no change in the sales due to the new advertising campaign, is true. If the p-value is .30, then there is a 30% chance that there is no increase or decrease in the product's sales.Ā If the p-value is 0.03, then there is a 3% probability that there is no increase or decrease in the sales value due to the new advertising campaign. As you can see, the lower the p-value, the chances of the alternate hypothesis being true increases, which means that the new advertising campaign causes an increase or decrease in sales.
Why is Hypothesis Testing Important in Research Methodology?
Hypothesis testing is crucial in research methodology for several reasons:
- Provides evidence-based conclusions: It allows researchers to make objective conclusions based on empirical data, providing evidence to support or refute their research hypotheses.
- Supports decision-making: It helps make informed decisions, such as accepting or rejecting a new treatment, implementing policy changes, or adopting new practices.
- Adds rigor and validity: It adds scientific rigor to research using statistical methods to analyze data, ensuring that conclusions are based on sound statistical evidence.
- Contributes to the advancement of knowledge: By testing hypotheses, researchers contribute to the growth of knowledge in their respective fields by confirming existing theories or discovering new patterns and relationships.
Limitations of Hypothesis Testing
Hypothesis testing has some limitations that researchers should be aware of:
- It cannot prove or establish the truth: Hypothesis testing provides evidence to support or reject a hypothesis, but it cannot confirm the absolute truth of the research question.
- Results are sample-specific: Hypothesis testing is based on analyzing a sample from a population, and the conclusions drawn are specific to that particular sample.
- Possible errors: During hypothesis testing, there is a chance of committing type I error (rejecting a true null hypothesis) or type II error (failing to reject a false null hypothesis).
- Assumptions and requirements: Different tests have specific assumptions and requirements that must be met to accurately interpret results.
After reading this tutorial, you would have a much better understanding of hypothesis testing, one of the most important concepts in the field of Data Science . The majority of hypotheses are based on speculation about observed behavior, natural phenomena, or established theories.
If you are interested in statistics of data science and skills needed for such a career, you ought to explore Simplilearnās Post Graduate Program in Data Science.
If you have any questions regarding this āHypothesis Testing In Statisticsā tutorial, do share them in the comment section. Our subject matter expert will respond to your queries. Happy learning!
1. What is hypothesis testing in statistics with example?
Hypothesis testing is a statistical method used to determine if there is enough evidence in a sample data to draw conclusions about a population. It involves formulating two competing hypotheses, the null hypothesis (H0) and the alternative hypothesis (Ha), and then collecting data to assess the evidence. An example: testing if a new drug improves patient recovery (Ha) compared to the standard treatment (H0) based on collected patient data.
2. What is hypothesis testing and its types?
Hypothesis testing is a statistical method used to make inferences about a population based on sample data. It involves formulating two hypotheses: the null hypothesis (H0), which represents the default assumption, and the alternative hypothesis (Ha), which contradicts H0. The goal is to assess the evidence and determine whether there is enough statistical significance to reject the null hypothesis in favor of the alternative hypothesis.
Types of hypothesis testing:
- One-sample test: Used to compare a sample to a known value or a hypothesized value.
- Two-sample test: Compares two independent samples to assess if there is a significant difference between their means or distributions.
- Paired-sample test: Compares two related samples, such as pre-test and post-test data, to evaluate changes within the same subjects over time or under different conditions.
- Chi-square test: Used to analyze categorical data and determine if there is a significant association between variables.
- ANOVA (Analysis of Variance): Compares means across multiple groups to check if there is a significant difference between them.
3. What are the steps of hypothesis testing?
The steps of hypothesis testing are as follows:
- Formulate the hypotheses: State the null hypothesis (H0) and the alternative hypothesis (Ha) based on the research question.
- Set the significance level: Determine the acceptable level of error (alpha) for making a decision.
- Collect and analyze data: Gather and process the sample data.
- Compute test statistic: Calculate the appropriate statistical test to assess the evidence.
- Make a decision: Compare the test statistic with critical values or p-values and determine whether to reject H0 in favor of Ha or not.
- Draw conclusions: Interpret the results and communicate the findings in the context of the research question.
4. What are the 2 types of hypothesis testing?
- One-tailed (or one-sided) test: Tests for the significance of an effect in only one direction, either positive or negative.
- Two-tailed (or two-sided) test: Tests for the significance of an effect in both directions, allowing for the possibility of a positive or negative effect.
The choice between one-tailed and two-tailed tests depends on the specific research question and the directionality of the expected effect.
5. What are the 3 major types of hypothesis?
The three major types of hypotheses are:
- Null Hypothesis (H0): Represents the default assumption, stating that there is no significant effect or relationship in the data.
- Alternative Hypothesis (Ha): Contradicts the null hypothesis and proposes a specific effect or relationship that researchers want to investigate.
- Nondirectional Hypothesis: An alternative hypothesis that doesn't specify the direction of the effect, leaving it open for both positive and negative possibilities.
Find our Data Analyst Online Bootcamp in top cities:
About the author.
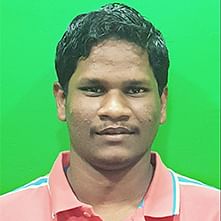
Avijeet is a Senior Research Analyst at Simplilearn. Passionate about Data Analytics, Machine Learning, and Deep Learning, Avijeet is also interested in politics, cricket, and football.
Recommended Resources

Free eBook: Top Programming Languages For A Data Scientist
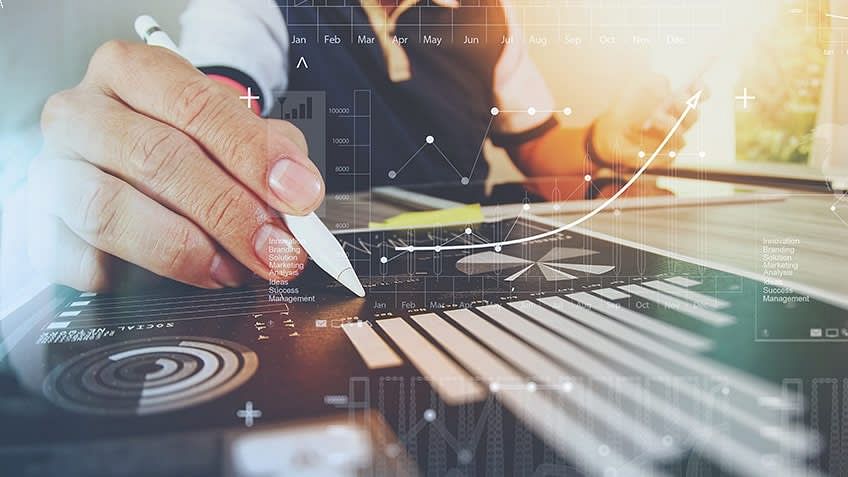
Normality Test in Minitab: Minitab with Statistics
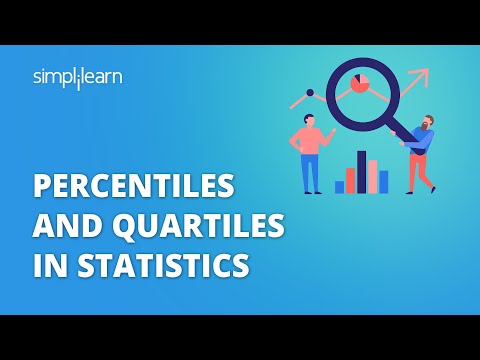
Machine Learning Career Guide: A Playbook to Becoming a Machine Learning Engineer
- PMP, PMI, PMBOK, CAPM, PgMP, PfMP, ACP, PBA, RMP, SP, and OPM3 are registered marks of the Project Management Institute, Inc.
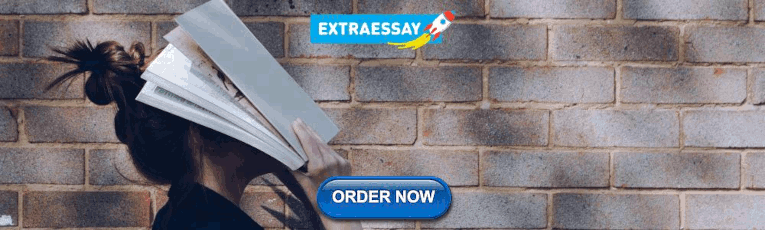
IMAGES
VIDEO
COMMENTS
4. Photo by Anna Nekrashevich from Pexels. Hypothesis testing is a common statistical tool used in research and data science to support the certainty of findings. The aim of testing is to answer how probable an apparent effect is detected by chance given a random data sample. This article provides a detailed explanation of the key concepts in ...
Likelihood ratio. In the likelihood ratio test, we reject the null hypothesis if the ratio is above a certain value i.e, reject the null hypothesis if L(X) > š, else accept it. š is called the critical ratio.. So this is how we can draw a decision boundary: we separate the observations for which the likelihood ratio is greater than the critical ratio from the observations for which it ...
Step 2: Collect data. For a statistical test to be valid, it is important to perform sampling and collect data in a way that is designed to test your hypothesis. If your data are not representative, then you cannot make statistical inferences about the population you are interested in. Hypothesis testing example.
Hypothesis testing is an indispensable tool in data science, allowing us to make data-driven decisions with confidence. By understanding its principles, conducting tests properly, and considering real-world applications, you can harness the power of hypothesis testing to unlock valuable insights from your data.
It tests the null hypothesis that the population variances are equal (called homogeneity of variance or homoscedasticity). Suppose the resulting p-value of Levene's test is less than the significance level (typically 0.05).In that case, the obtained differences in sample variances are unlikely to have occurred based on random sampling from a population with equal variances.
Hypothesis testing is a statistical method used to evaluate a claim or hypothesis about a population parameter based on sample data. It involves making decisions about the validity of a statement, often referred to as the null hypothesis, by assessing the likelihood of observing the sample data if the null hypothesis were true.
Hypothesis Testing in Data Science is a statistical method used to assess the validity of assumptions or claims about a population based on sample data. It involves formulating two Hypotheses, the null Hypothesis (H0) and the alternative Hypothesis (Ha or H1), and then using statistical tests to find out if there is enough evidence to support ...
In the whole data science life cycle, hypothesis testing is done in various stages, starting from the initial part, the 1st stage where the EDA, data pre-processing, and manipulation are done. ... It is always a recommendable step when planning for predicting the outcomes and trying to experiment with the sample; hypothesis testing is a useful ...
In simple words, t-tests are a statistical way of testing a hypothesis when: We do not know the population variance; Our sample size is small, n < 30; One-Sample T-Test. We perform a One-Sample t-test when we want to compare a sample mean with the population mean.
Hypothesis Test Example: Suppose a large university claims that the average ACT score of their incoming freshman class is 30, but we think the University may be inflating their average. To test the University's claim we take a simple random sample of 50 students and find their average to be only 28.3 with an SD of 4.
Hypothesis testing is a technique that helps scientists, researchers, or for that matter, anyone test the validity of their claims or hypotheses about real-world or real-life events in order to establish new knowledge. Hypothesis testing techniques are often used in statistics and data science to analyze whether the claims about the occurrence of the events are true, whether the results ...
Once data is collected, we estimate the parameter and calculate the p-value, which is the probability of the estimate being as extreme as observed if the null hypothesis is true. If the p-value is small, it indicates the null hypothesis is unlikely, providing evidence against it. We will see more examples of hypothesis testing in Chapter 17. 9. ...
Free ą¤¹ą¤æą¤Øą„ą¤¦ą„. 353894 4.4 Enroll For Free. Hypothesis Testing in Data Science: A Complete Guide with Examples In statistics and data analysis, hypothesis testing is crucial. Hypothesis testing is a statistical technique that involves putting your assumptions about a population parameter to the test.
Hypothesis testing#. Hypothesis testing is about choosing between two views, called hypotheses, on how data were generated (for example, SSR=1 or SSR =1.05 where SSR is the secondary sex ratio we defined in the previous section).Hypotheses, called null and alternative, should be specified before doing the analysis.Testing is a way to select the hypothesis that is better supported by the data.
In the world of Data Science, there are two parts to consider when putting together a hypothesis. Hypothesis Testing is when the team builds a strong hypothesis based on the available dataset. This will help direct the team and plan accordingly throughout the data science project. The hypothesis will then be tested with a complete dataset and ...
Hypothesis testing can be thought of as a way to investigate the consistency of a dataset with a model, where a model is a set of rules that describe how data are generated. The consistency is evaluated using ideas from probability and probability distributions. The consistency question in the above diagram is short for "Is it plausible that ...
If the biologist set her significance level \(\alpha\) at 0.05 and used the critical value approach to conduct her hypothesis test, she would reject the null hypothesis if her test statistic t* were less than -1.6939 (determined using statistical software or a t-table):s-3-3. Since the biologist's test statistic, t* = -4.60, is less than -1.6939, the biologist rejects the null hypothesis.
Hypothesis testing is a statistical technique used to evaluate hypotheses about a population based on sample data. In data science, hypothesis testing is an essential tool used to make inferences about the population based on a representative sample. In this blog, we will discuss the key aspects of hypothesis testing, including null hypothesis ...
Hypothesis testing is a statistical method used to determine if there is enough evidence in a sample data to draw conclusions about a population. It involves formulating two competing hypotheses, the null hypothesis (H0) and the alternative hypothesis (Ha), and then collecting data to assess the evidence.