- Research article
- Open access
- Published: 02 December 2020
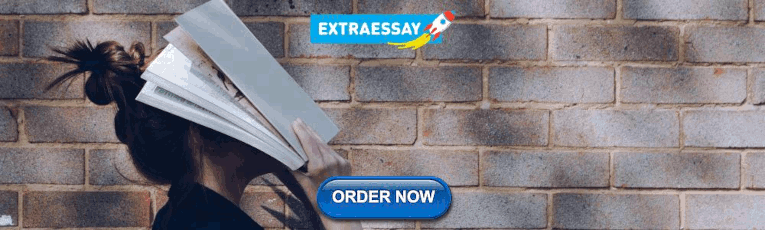
Integrating students’ perspectives about online learning: a hierarchy of factors
- Montgomery Van Wart 1 ,
- Anna Ni 1 ,
- Pamela Medina 1 ,
- Jesus Canelon 1 ,
- Melika Kordrostami 1 ,
- Jing Zhang 1 &
International Journal of Educational Technology in Higher Education volume 17 , Article number: 53 ( 2020 ) Cite this article
149k Accesses
51 Citations
24 Altmetric
Metrics details
This article reports on a large-scale ( n = 987), exploratory factor analysis study incorporating various concepts identified in the literature as critical success factors for online learning from the students’ perspective, and then determines their hierarchical significance. Seven factors--Basic Online Modality, Instructional Support, Teaching Presence, Cognitive Presence, Online Social Comfort, Online Interactive Modality, and Social Presence--were identified as significant and reliable. Regression analysis indicates the minimal factors for enrollment in future classes—when students consider convenience and scheduling—were Basic Online Modality, Cognitive Presence, and Online Social Comfort. Students who accepted or embraced online courses on their own merits wanted a minimum of Basic Online Modality, Teaching Presence, Cognitive Presence, Online Social Comfort, and Social Presence. Students, who preferred face-to-face classes and demanded a comparable experience, valued Online Interactive Modality and Instructional Support more highly. Recommendations for online course design, policy, and future research are provided.
Introduction
While there are different perspectives of the learning process such as learning achievement and faculty perspectives, students’ perspectives are especially critical since they are ultimately the raison d’être of the educational endeavor (Chickering & Gamson, 1987 ). More pragmatically, students’ perspectives provide invaluable, first-hand insights into their experiences and expectations (Dawson et al., 2019 ). The student perspective is especially important when new teaching approaches are used and when new technologies are being introduced (Arthur, 2009 ; Crews & Butterfield, 2014 ; Van Wart, Ni, Ready, Shayo, & Court, 2020 ). With the renewed interest in “active” education in general (Arruabarrena, Sánchez, Blanco, et al., 2019 ; Kay, MacDonald, & DiGiuseppe, 2019 ; Nouri, 2016 ; Vlachopoulos & Makri, 2017 ) and the flipped classroom approach in particular (Flores, del-Arco, & Silva, 2016 ; Gong, Yang, & Cai, 2020 ; Lundin, et al., 2018 ; Maycock, 2019 ; McGivney-Burelle, 2013 ; O’Flaherty & Phillips, 2015 ; Tucker , 2012 ) along with extraordinary shifts in the technology, the student perspective on online education is profoundly important. What shapes students’ perceptions of quality integrate are their own sense of learning achievement, satisfaction with the support they receive, technical proficiency of the process, intellectual and emotional stimulation, comfort with the process, and sense of learning community. The factors that students perceive as quality online teaching, however, has not been as clear as it might be for at least two reasons.
First, it is important to note that the overall online learning experience for students is also composed of non-teaching factors which we briefly mention. Three such factors are (1) convenience, (2) learner characteristics and readiness, and (3) antecedent conditions that may foster teaching quality but are not directly responsible for it. (1) Convenience is an enormous non-quality factor for students (Artino, 2010 ) which has driven up online demand around the world (Fidalgo, Thormann, Kulyk, et al., 2020 ; Inside Higher Education and Gallup, 2019 ; Legon & Garrett, 2019 ; Ortagus, 2017 ). This is important since satisfaction with online classes is frequently somewhat lower than face-to-face classes (Macon, 2011 ). However, the literature generally supports the relative equivalence of face-to-face and online modes regarding learning achievement criteria (Bernard et al., 2004 ; Nguyen, 2015 ; Ni, 2013 ; Sitzmann, Kraiger, Stewart, & Wisher, 2006 ; see Xu & Jaggars, 2014 for an alternate perspective). These contrasts are exemplified in a recent study of business students, in which online students using a flipped classroom approach outperformed their face-to-face peers, but ironically rated instructor performance lower (Harjoto, 2017 ). (2) Learner characteristics also affect the experience related to self-regulation in an active learning model, comfort with technology, and age, among others,which affect both receptiveness and readiness of online instruction. (Alqurashi, 2016 ; Cohen & Baruth, 2017 ; Kintu, Zhu, & Kagambe, 2017 ; Kuo, Walker, Schroder, & Belland, 2013 ; Ventura & Moscoloni, 2015 ) (3) Finally, numerous antecedent factors may lead to improved instruction, but are not themselves directly perceived by students such as instructor training (Brinkley-Etzkorn, 2018 ), and the sources of faculty motivation (e.g., incentives, recognition, social influence, and voluntariness) (Wingo, Ivankova, & Moss, 2017 ). Important as these factors are, mixing them with the perceptions of quality tends to obfuscate the quality factors directly perceived by students.
Second, while student perceptions of quality are used in innumerable studies, our overall understanding still needs to integrate them more holistically. Many studies use student perceptions of quality and overall effectiveness of individual tools and strategies in online contexts such as mobile devices (Drew & Mann, 2018 ), small groups (Choi, Land, & Turgeon, 2005 ), journals (Nair, Tay, & Koh, 2013 ), simulations (Vlachopoulos & Makri, 2017 ), video (Lange & Costley, 2020 ), etc. Such studies, however, cannot provide the overall context and comparative importance. Some studies have examined the overall learning experience of students with exploratory lists, but have mixed non-quality factors with quality of teaching factors making it difficult to discern the instructor’s versus contextual roles in quality (e.g., Asoodar, Vaezi, & Izanloo, 2016 ; Bollinger & Martindale, 2004 ; Farrell & Brunton, 2020 ; Hong, 2002 ; Song, Singleton, Hill, & Koh, 2004 ; Sun, Tsai, Finger, Chen, & Yeh, 2008 ). The application of technology adoption studies also fall into this category by essentially aggregating all teaching quality in the single category of performance ( Al-Gahtani, 2016 ; Artino, 2010 ). Some studies have used high-level teaching-oriented models, primarily the Community of Inquiry model (le Roux & Nagel, 2018 ), but empirical support has been mixed (Arbaugh et al., 2008 ); and its elegance (i.e., relying on only three factors) has not provided much insight to practitioners (Anderson, 2016 ; Cleveland-Innes & Campbell, 2012 ).
Research questions
Integration of studies and concepts explored continues to be fragmented and confusing despite the fact that the number of empirical studies related to student perceptions of quality factors has increased. It is important to have an empirical view of what students’ value in a single comprehensive study and, also, to know if there is a hierarchy of factors, ranging from students who are least to most critical of the online learning experience. This research study has two research questions.
The first research question is: What are the significant factors in creating a high-quality online learning experience from students’ perspectives? That is important to know because it should have a significant effect on the instructor’s design of online classes. The goal of this research question is identify a more articulated and empirically-supported set of factors capturing the full range of student expectations.
The second research question is: Is there a priority or hierarchy of factors related to students’ perceptions of online teaching quality that relate to their decisions to enroll in online classes? For example, is it possible to distinguish which factors are critical for enrollment decisions when students are primarily motivated by convenience and scheduling flexibility (minimum threshold)? Do these factors differ from students with a genuine acceptance of the general quality of online courses (a moderate threshold)? What are the factors that are important for the students who are the most critical of online course delivery (highest threshold)?
This article next reviews the literature on online education quality, focusing on the student perspective and reviews eight factors derived from it. The research methods section discusses the study structure and methods. Demographic data related to the sample are next, followed by the results, discussion, and conclusion.
Literature review
Online education is much discussed (Prinsloo, 2016 ; Van Wart et al., 2019 ; Zawacki-Richter & Naidu, 2016 ), but its perception is substantially influenced by where you stand and what you value (Otter et al., 2013 ; Tanner, Noser, & Totaro, 2009 ). Accrediting bodies care about meeting technical standards, proof of effectiveness, and consistency (Grandzol & Grandzol, 2006 ). Institutions care about reputation, rigor, student satisfaction, and institutional efficiency (Jung, 2011 ). Faculty care about subject coverage, student participation, faculty satisfaction, and faculty workload (Horvitz, Beach, Anderson, & Xia, 2015 ; Mansbach & Austin, 2018 ). For their part, students care about learning achievement (Marks, Sibley, & Arbaugh, 2005 ; O’Neill & Sai, 2014 ; Shen, Cho, Tsai, & Marra, 2013 ), but also view online education as a function of their enjoyment of classes, instructor capability and responsiveness, and comfort in the learning environment (e.g., Asoodar et al., 2016 ; Sebastianelli, Swift, & Tamimi, 2015 ). It is this last perspective, of students, upon which we focus.
It is important to note students do not sign up for online classes solely based on perceived quality. Perceptions of quality derive from notions of the capacity of online learning when ideal—relative to both learning achievement and satisfaction/enjoyment, and perceptions about the likelihood and experience of classes living up to expectations. Students also sign up because of convenience and flexibility, and personal notions of suitability about learning. Convenience and flexibility are enormous drivers of online registration (Lee, Stringer, & Du, 2017 ; Mann & Henneberry, 2012 ). Even when students say they prefer face-to-face classes to online, many enroll in online classes and re-enroll in the future if the experience meets minimum expectations. This study examines the threshold expectations of students when they are considering taking online classes.
When discussing students’ perceptions of quality, there is little clarity about the actual range of concepts because no integrated empirical studies exist comparing major factors found throughout the literature. Rather, there are practitioner-generated lists of micro-competencies such as the Quality Matters consortium for higher education (Quality Matters, 2018 ), or broad frameworks encompassing many aspects of quality beyond teaching (Open and Distant Learning Quality Council, 2012 ). While checklists are useful for practitioners and accreditation processes, they do not provide robust, theoretical bases for scholarly development. Overarching frameworks are heuristically useful, but not for pragmatic purposes or theory building arenas. The most prominent theoretical framework used in online literature is the Community of Inquiry (CoI) model (Arbaugh et al., 2008 ; Garrison, Anderson, & Archer, 2003 ), which divides instruction into teaching, cognitive, and social presence. Like deductive theories, however, the supportive evidence is mixed (Rourke & Kanuka, 2009 ), especially regarding the importance of social presence (Annand, 2011 ; Armellini and De Stefani, 2016 ). Conceptually, the problem is not so much with the narrow articulation of cognitive or social presence; cognitive presence is how the instructor provides opportunities for students to interact with material in robust, thought-provoking ways, and social presence refers to building a community of learning that incorporates student-to-student interactions. However, teaching presence includes everything else the instructor does—structuring the course, providing lectures, explaining assignments, creating rehearsal opportunities, supplying tests, grading, answering questions, and so on. These challenges become even more prominent in the online context. While the lecture as a single medium is paramount in face-to-face classes, it fades as the primary vehicle in online classes with increased use of detailed syllabi, electronic announcements, recorded and synchronous lectures, 24/7 communications related to student questions, etc. Amassing the pedagogical and technological elements related to teaching under a single concept provides little insight.
In addition to the CoI model, numerous concepts are suggested in single-factor empirical studies when focusing on quality from a student’s perspective, with overlapping conceptualizations and nonstandardized naming conventions. Seven distinct factors are derived here from the literature of student perceptions of online quality: Instructional Support, Teaching Presence, Basic Online Modality, Social Presence, Online Social Comfort, cognitive Presence, and Interactive Online Modality.
Instructional support
Instructional Support refers to students’ perceptions of techniques by the instructor used for input, rehearsal, feedback, and evaluation. Specifically, this entails providing detailed instructions, designed use of multimedia, and the balance between repetitive class features for ease of use, and techniques to prevent boredom. Instructional Support is often included as an element of Teaching Presence, but is also labeled “structure” (Lee & Rha, 2009 ; So & Brush, 2008 ) and instructor facilitation (Eom, Wen, & Ashill, 2006 ). A prime example of the difference between face-to-face and online education is the extensive use of the “flipped classroom” (Maycock, 2019 ; Wang, Huang, & Schunn, 2019 ) in which students move to rehearsal activities faster and more frequently than traditional classrooms, with less instructor lecture (Jung, 2011 ; Martin, Wang, & Sadaf, 2018 ). It has been consistently supported as an element of student perceptions of quality (Espasa & Meneses, 2010 ).
- Teaching presence
Teaching Presence refers to students’ perceptions about the quality of communication in lectures, directions, and individual feedback including encouragement (Jaggars & Xu, 2016 ; Marks et al., 2005 ). Specifically, instructor communication is clear, focused, and encouraging, and instructor feedback is customized and timely. If Instructional Support is what an instructor does before the course begins and in carrying out those plans, then Teaching Presence is what the instructor does while the class is conducted and in response to specific circumstances. For example, a course could be well designed but poorly delivered because the instructor is distracted; or a course could be poorly designed but an instructor might make up for the deficit by spending time and energy in elaborate communications and ad hoc teaching techniques. It is especially important in student satisfaction (Sebastianelli et al., 2015 ; Young, 2006 ) and also referred to as instructor presence (Asoodar et al., 2016 ), learner-instructor interaction (Marks et al., 2005 ), and staff support (Jung, 2011 ). As with Instructional Support, it has been consistently supported as an element of student perceptions of quality.
Basic online modality
Basic Online Modality refers to the competent use of basic online class tools—online grading, navigation methods, online grade book, and the announcements function. It is frequently clumped with instructional quality (Artino, 2010 ), service quality (Mohammadi, 2015 ), instructor expertise in e-teaching (Paechter, Maier, & Macher, 2010 ), and similar terms. As a narrowly defined concept, it is sometimes called technology (Asoodar et al., 2016 ; Bollinger & Martindale, 2004 ; Sun et al., 2008 ). The only empirical study that did not find Basic Online Modality significant, as technology, was Sun et al. ( 2008 ). Because Basic Online Modality is addressed with basic instructor training, some studies assert the importance of training (e.g., Asoodar et al., 2016 ).
Social presence
Social Presence refers to students’ perceptions of the quality of student-to-student interaction. Social Presence focuses on the quality of shared learning and collaboration among students, such as in threaded discussion responses (Garrison et al., 2003 ; Kehrwald, 2008 ). Much emphasized but challenged in the CoI literature (Rourke & Kanuka, 2009 ), it has mixed support in the online literature. While some studies found Social Presence or related concepts to be significant (e.g., Asoodar et al., 2016 ; Bollinger & Martindale, 2004 ; Eom et al., 2006 ; Richardson, Maeda, Lv, & Caskurlu, 2017 ), others found Social Presence insignificant (Joo, Lim, & Kim, 2011 ; So & Brush, 2008 ; Sun et al., 2008 ).
Online social comfort
Online Social Comfort refers to the instructor’s ability to provide an environment in which anxiety is low, and students feel comfortable interacting even when expressing opposing viewpoints. While numerous studies have examined anxiety (e.g., Liaw & Huang, 2013 ; Otter et al., 2013 ; Sun et al., 2008 ), only one found anxiety insignificant (Asoodar et al., 2016 ); many others have not examined the concept.
- Cognitive presence
Cognitive Presence refers to the engagement of students such that they perceive they are stimulated by the material and instructor to reflect deeply and critically, and seek to understand different perspectives (Garrison et al., 2003 ). The instructor provides instructional materials and facilitates an environment that piques interest, is reflective, and enhances inclusiveness of perspectives (Durabi, Arrastia, Nelson, Cornille, & Liang, 2011 ). Cognitive Presence includes enhancing the applicability of material for student’s potential or current careers. Cognitive Presence is supported as significant in many online studies (e.g., Artino, 2010 ; Asoodar et al., 2016 ; Joo et al., 2011 ; Marks et al., 2005 ; Sebastianelli et al., 2015 ; Sun et al., 2008 ). Further, while many instructors perceive that cognitive presence is diminished in online settings, neuroscientific studies indicate this need not be the case (Takamine, 2017 ). While numerous studies failed to examine Cognitive Presence, this review found no studies that lessened its significance for students.
Interactive online modality
Interactive Online Modality refers to the “high-end” usage of online functionality. That is, the instructor uses interactive online class tools—video lectures, videoconferencing, and small group discussions—well. It is often included in concepts such as instructional quality (Artino, 2010 ; Asoodar et al., 2016 ; Mohammadi, 2015 ; Otter et al., 2013 ; Paechter et al., 2010 ) or engagement (Clayton, Blumberg, & Anthony, 2018 ). While individual methods have been investigated (e.g. Durabi et al., 2011 ), high-end engagement methods have not.
Other independent variables affecting perceptions of quality include age, undergraduate versus graduate status, gender, ethnicity/race, discipline, educational motivation of students, and previous online experience. While age has been found to be small or insignificant, more notable effects have been reported at the level-of-study, with graduate students reporting higher “success” (Macon, 2011 ), and community college students having greater difficulty with online classes (Legon & Garrett, 2019 ; Xu & Jaggars, 2014 ). Ethnicity and race have also been small or insignificant. Some situational variations and student preferences can be captured by paying attention to disciplinary differences (Arbaugh, 2005 ; Macon, 2011 ). Motivation levels of students have been reported to be significant in completion and achievement, with better students doing as well across face-to-face and online modes, and weaker students having greater completion and achievement challenges (Clayton et al., 2018 ; Lu & Lemonde, 2013 ).
Research methods
To examine the various quality factors, we apply a critical success factor methodology, initially introduced to schools of business research in the 1970s. In 1981, Rockhart and Bullen codified an approach embodying principles of critical success factors (CSFs) as a way to identify the information needs of executives, detailing steps for the collection and analyzation of data to create a set of organizational CSFs (Rockhart & Bullen, 1981 ). CSFs describe the underlying or guiding principles which must be incorporated to ensure success.
Utilizing this methodology, CSFs in the context of this paper define key areas of instruction and design essential for an online class to be successful from a student’s perspective. Instructors implicitly know and consider these areas when setting up an online class and designing and directing activities and tasks important to achieving learning goals. CSFs make explicit those things good instructors may intuitively know and (should) do to enhance student learning. When made explicit, CSFs not only confirm the knowledge of successful instructors, but tap their intuition to guide and direct the accomplishment of quality instruction for entire programs. In addition, CSFs are linked with goals and objectives, helping generate a small number of truly important matters an instructor should focus attention on to achieve different thresholds of online success.
After a comprehensive literature review, an instrument was created to measure students’ perceptions about the importance of techniques and indicators leading to quality online classes. Items were designed to capture the major factors in the literature. The instrument was pilot studied during academic year 2017–18 with a 397 student sample, facilitating an exploratory factor analysis leading to important preliminary findings (reference withheld for review). Based on the pilot, survey items were added and refined to include seven groups of quality teaching factors and two groups of items related to students’ overall acceptance of online classes as well as a variable on their future online class enrollment. Demographic information was gathered to determine their effects on students’ levels of acceptance of online classes based on age, year in program, major, distance from university, number of online classes taken, high school experience with online classes, and communication preferences.
This paper draws evidence from a sample of students enrolled in educational programs at Jack H. Brown College of Business and Public Administration (JHBC), California State University San Bernardino (CSUSB). The JHBC offers a wide range of online courses for undergraduate and graduate programs. To ensure comparable learning outcomes, online classes and face-to-face classes of a certain subject are similar in size—undergraduate classes are generally capped at 60 and graduate classes at 30, and often taught by the same instructors. Students sometimes have the option to choose between both face-to-face and online modes of learning.
A Qualtrics survey link was sent out by 11 instructors to students who were unlikely to be cross-enrolled in classes during the 2018–19 academic year. 1 Approximately 2500 students were contacted, with some instructors providing class time to complete the anonymous survey. All students, whether they had taken an online class or not, were encouraged to respond. Nine hundred eighty-seven students responded, representing a 40% response rate. Although drawn from a single business school, it is a broad sample representing students from several disciplines—management, accounting and finance, marketing, information decision sciences, and public administration, as well as both graduate and undergraduate programs of study.
The sample age of students is young, with 78% being under 30. The sample has almost no lower division students (i.e., freshman and sophomore), 73% upper division students (i.e., junior and senior) and 24% graduate students (master’s level). Only 17% reported having taken a hybrid or online class in high school. There was a wide range of exposure to university level online courses, with 47% reporting having taken 1 to 4 classes, and 21% reporting no online class experience. As a Hispanic-serving institution, 54% self-identified as Latino, 18% White, and 13% Asian and Pacific Islander. The five largest majors were accounting & finance (25%), management (21%), master of public administration (16%), marketing (12%), and information decision sciences (10%). Seventy-four percent work full- or part-time. See Table 1 for demographic data.
Measures and procedure
To increase the reliability of evaluation scores, composite evaluation variables are formed after an exploratory factor analysis of individual evaluation items. A principle component method with Quartimin (oblique) rotation was applied to explore the factor construct of student perceptions of online teaching CSFs. The item correlations for student perceptions of importance coefficients greater than .30 were included, a commonly acceptable ratio in factor analysis. A simple least-squares regression analysis was applied to test the significance levels of factors on students’ impression of online classes.
Exploratory factor constructs
Using a threshold loading of 0.3 for items, 37 items loaded on seven factors. All factors were logically consistent. The first factor, with eight items, was labeled Teaching Presence. Items included providing clear instructions, staying on task, clear deadlines, and customized feedback on strengths and weaknesses. Teaching Presence items all related to instructor involvement during the course as a director, monitor, and learning facilitator. The second factor, with seven items, aligned with Cognitive Presence. Items included stimulating curiosity, opportunities for reflection, helping students construct explanations posed in online courses, and the applicability of material. The third factor, with six items, aligned with Social Presence defined as providing student-to-student learning opportunities. Items included getting to know course participants for sense of belonging, forming impressions of other students, and interacting with others. The fourth factor, with six new items as well as two (“interaction with other students” and “a sense of community in the class”) shared with the third factor, was Instructional Support which related to the instructor’s roles in providing students a cohesive learning experience. They included providing sufficient rehearsal, structured feedback, techniques for communication, navigation guide, detailed syllabus, and coordinating student interaction and creating a sense of online community. This factor also included enthusiasm which students generally interpreted as a robustly designed course, rather than animation in a traditional lecture. The fifth factor was labeled Basic Online Modality and focused on the basic technological requirements for a functional online course. Three items included allowing students to make online submissions, use of online gradebooks, and online grading. A fourth item is the use of online quizzes, viewed by students as mechanical practice opportunities rather than small tests and a fifth is navigation, a key component of Online Modality. The sixth factor, loaded on four items, was labeled Online Social Comfort. Items here included comfort discussing ideas online, comfort disagreeing, developing a sense of collaboration via discussion, and considering online communication as an excellent medium for social interaction. The final factor was called Interactive Online Modality because it included items for “richer” communications or interactions, no matter whether one- or two-way. Items included videoconferencing, instructor-generated videos, and small group discussions. Taken together, these seven explained 67% of the variance which is considered in the acceptable range in social science research for a robust model (Hair, Black, Babin, & Anderson, 2014 ). See Table 2 for the full list.
To test for factor reliability, the Cronbach alpha of variables were calculated. All produced values greater than 0.7, the standard threshold used for reliability, except for system trust which was therefore dropped. To gauge students’ sense of factor importance, all items were means averaged. Factor means (lower means indicating higher importance to students), ranged from 1.5 to 2.6 on a 5-point scale. Basic Online Modality was most important, followed by Instructional Support and Teaching Presence. Students deemed Cognitive Presence, Social Online Comfort, and Online Interactive Modality less important. The least important for this sample was Social Presence. Table 3 arrays the critical success factor means, standard deviations, and Cronbach alpha.
To determine whether particular subgroups of respondents viewed factors differently, a series of ANOVAs were conducted using factor means as dependent variables. Six demographic variables were used as independent variables: graduate vs. undergraduate, age, work status, ethnicity, discipline, and past online experience. To determine strength of association of the independent variables to each of the seven CSFs, eta squared was calculated for each ANOVA. Eta squared indicates the proportion of variance in the dependent variable explained by the independent variable. Eta squared values greater than .01, .06, and .14 are conventionally interpreted as small, medium, and large effect sizes, respectively (Green & Salkind, 2003 ). Table 4 summarizes the eta squared values for the ANOVA tests with Eta squared values less than .01 omitted.
While no significant differences in factor means among students in different disciplines in the College occur, all five other independent variables have some small effect on some or all CSFs. Graduate students tend to rate Online Interactive Modality, Instructional Support, Teaching Presence, and Cognitive Presence higher than undergraduates. Elder students value more Online Interactive Modality. Full-time working students rate all factors, except Social Online Comfort, slightly higher than part-timers and non-working students. Latino and White rate Basic Online Modality and Instructional Support higher; Asian and Pacific Islanders rate Social Presence higher. Students who have taken more online classes rate all factors higher.
In addition to factor scores, two variables are constructed to identify the resultant impressions labeled online experience. Both were logically consistent with a Cronbach’s α greater than 0.75. The first variable, with six items, labeled “online acceptance,” included items such as “I enjoy online learning,” “My overall impression of hybrid/online learning is very good,” and “the instructors of online/hybrid classes are generally responsive.” The second variable was labeled “face-to-face preference” and combines four items, including enjoying, learning, and communicating more in face-to-face classes, as well as perceiving greater fairness and equity. In addition to these two constructed variables, a one-item variable was also used subsequently in the regression analysis: “online enrollment.” That question asked: if hybrid/online classes are well taught and available, how much would online education make up your entire course selection going forward?
Regression results
As noted above, two constructed variables and one item were used as dependent variables for purposes of regression analysis. They were online acceptance, F2F preference, and the selection of online classes. In addition to seven quality-of-teaching factors identified by factor analysis, control variables included level of education (graduate versus undergraduate), age, ethnicity, work status, distance to university, and number of online/hybrid classes taken in the past. See Table 5 .
When the ETA squared values for ANOVA significance were measured for control factors, only one was close to a medium effect. Graduate versus undergraduate status had a .05 effect (considered medium) related to Online Interactive Modality, meaning graduate students were more sensitive to interactive modality than undergraduates. Multiple regression analysis of critical success factors and online impressions were conducted to compare under what conditions factors were significant. The only consistently significant control factor was number of online classes taken. The more classes students had taken online, the more inclined they were to take future classes. Level of program, age, ethnicity, and working status do not significantly affect students’ choice or overall acceptance of online classes.
The least restrictive condition was online enrollment (Table 6 ). That is, students might not feel online courses were ideal, but because of convenience and scheduling might enroll in them if minimum threshold expectations were met. When considering online enrollment three factors were significant and positive (at the 0.1 level): Basic Online Modality, Cognitive Presence, and Online Social Comfort. These least-demanding students expected classes to have basic technological functionality, provide good opportunities for knowledge acquisition, and provide comfortable interaction in small groups. Students who demand good Instructional Support (e.g., rehearsal opportunities, standardized feedback, clear syllabus) are less likely to enroll.
Online acceptance was more restrictive (see Table 7 ). This variable captured the idea that students not only enrolled in online classes out of necessity, but with an appreciation of the positive attributes of online instruction, which balanced the negative aspects. When this standard was applied, students expected not only Basic Online Modality, Cognitive Presence, and Online Social Comfort, but expected their instructors to be highly engaged virtually as the course progressed (Teaching Presence), and to create strong student-to-student dynamics (Social Presence). Students who rated Instructional Support higher are less accepting of online classes.
Another restrictive condition was catering to the needs of students who preferred face-to-face classes (see Table 8 ). That is, they preferred face-to-face classes even when online classes were well taught. Unlike students more accepting of, or more likely to enroll in, online classes, this group rates Instructional Support as critical to enrolling, rather than a negative factor when absent. Again different from the other two groups, these students demand appropriate interactive mechanisms (Online Interactive Modality) to enable richer communication (e.g., videoconferencing). Student-to-student collaboration (Social Presence) was also significant. This group also rated Cognitive Presence and Online Social Comfort as significant, but only in their absence. That is, these students were most attached to direct interaction with the instructor and other students rather than specific teaching methods. Interestingly, Basic Online Modality and Teaching Presence were not significant. Our interpretation here is this student group, most critical of online classes for its loss of physical interaction, are beyond being concerned with mechanical technical interaction and demand higher levels of interactivity and instructional sophistication.
Discussion and study limitations
Some past studies have used robust empirical methods to identify a single factor or a small number of factors related to quality from a student’s perspective, but have not sought to be relatively comprehensive. Others have used a longer series of itemized factors, but have less used less robust methods, and have not tied those factors back to the literature. This study has used the literature to develop a relatively comprehensive list of items focused on quality teaching in a single rigorous protocol. That is, while a Beta test had identified five coherent factors, substantial changes to the current survey that sharpened the focus on quality factors rather than antecedent factors, as well as better articulating the array of factors often lumped under the mantle of “teaching presence.” In addition, it has also examined them based on threshold expectations: from minimal, such as when flexibility is the driving consideration, to modest, such as when students want a “good” online class, to high, when students demand an interactive virtual experience equivalent to face-to-face.
Exploratory factor analysis identified seven factors that were reliable, coherent, and significant under different conditions. When considering students’ overall sense of importance, they are, in order: Basic Online Modality, Instructional Support, Teaching Presence, Cognitive Presence, Social Online Comfort, Interactive Online Modality, and Social Presence. Students are most concerned with the basics of a course first, that is the technological and instructor competence. Next they want engagement and virtual comfort. Social Presence, while valued, is the least critical from this overall perspective.
The factor analysis is quite consistent with the range of factors identified in the literature, pointing to the fact that students can differentiate among different aspects of what have been clumped as larger concepts, such as teaching presence. Essentially, the instructor’s role in quality can be divided into her/his command of basic online functionality, good design, and good presence during the class. The instructor’s command of basic functionality is paramount. Because so much of online classes must be built in advance of the class, quality of the class design is rated more highly than the instructor’s role in facilitating the class. Taken as a whole, the instructor’s role in traditional teaching elements is primary, as we would expect it to be. Cognitive presence, especially as pertinence of the instructional material and its applicability to student interests, has always been found significant when studied, and was highly rated as well in a single factor. Finally, the degree to which students feel comfortable with the online environment and enjoy the learner-learner aspect has been less supported in empirical studies, was found significant here, but rated the lowest among the factors of quality to students.
Regression analysis paints a more nuanced picture, depending on student focus. It also helps explain some of the heterogeneity of previous studies, depending on what the dependent variables were. If convenience and scheduling are critical and students are less demanding, minimum requirements are Basic Online Modality, Cognitive Presence, and Online Social Comfort. That is, students’ expect an instructor who knows how to use an online platform, delivers useful information, and who provides a comfortable learning environment. However, they do not expect to get poor design. They do not expect much in terms of the quality teaching presence, learner-to-learner interaction, or interactive teaching.
When students are signing up for critical classes, or they have both F2F and online options, they have a higher standard. That is, they not only expect the factors for decisions about enrolling in noncritical classes, but they also expect good Teaching and Social Presence. Students who simply need a class may be willing to teach themselves a bit more, but students who want a good class expect a highly present instructor in terms responsiveness and immediacy. “Good” classes must not only create a comfortable atmosphere, but in social science classes at least, must provide strong learner-to-learner interactions as well. At the time of the research, most students believe that you can have a good class without high interactivity via pre-recorded video and videoconference. That may, or may not, change over time as technology thresholds of various video media become easier to use, more reliable, and more commonplace.
The most demanding students are those who prefer F2F classes because of learning style preferences, poor past experiences, or both. Such students (seem to) assume that a worthwhile online class has basic functionality and that the instructor provides a strong presence. They are also critical of the absence of Cognitive Presence and Online Social Comfort. They want strong Instructional Support and Social Presence. But in addition, and uniquely, they expect Online Interactive Modality which provides the greatest verisimilitude to the traditional classroom as possible. More than the other two groups, these students crave human interaction in the learning process, both with the instructor and other students.
These findings shed light on the possible ramifications of the COVID-19 aftermath. Many universities around the world jumped from relatively low levels of online instruction in the beginning of spring 2020 to nearly 100% by mandate by the end of the spring term. The question becomes, what will happen after the mandate is removed? Will demand resume pre-crisis levels, will it increase modestly, or will it skyrocket? Time will be the best judge, but the findings here would suggest that the ability/interest of instructors and institutions to “rise to the occasion” with quality teaching will have as much effect on demand as students becoming more acclimated to online learning. If in the rush to get classes online many students experience shoddy basic functional competence, poor instructional design, sporadic teaching presence, and poorly implemented cognitive and social aspects, they may be quite willing to return to the traditional classroom. If faculty and institutions supporting them are able to increase the quality of classes despite time pressures, then most students may be interested in more hybrid and fully online classes. If instructors are able to introduce high quality interactive teaching, nearly the entire student population will be interested in more online classes. Of course students will have a variety of experiences, but this analysis suggests that those instructors, departments, and institutions that put greater effort into the temporary adjustment (and who resist less), will be substantially more likely to have increases in demand beyond what the modest national trajectory has been for the last decade or so.
There are several study limitations. First, the study does not include a sample of non-respondents. Non-responders may have a somewhat different profile. Second, the study draws from a single college and university. The profile derived here may vary significantly by type of student. Third, some survey statements may have led respondents to rate quality based upon experience rather than assess the general importance of online course elements. “I felt comfortable participating in the course discussions,” could be revised to “comfort in participating in course discussions.” The authors weighed differences among subgroups (e.g., among majors) as small and statistically insignificant. However, it is possible differences between biology and marketing students would be significant, leading factors to be differently ordered. Emphasis and ordering might vary at a community college versus research-oriented university (Gonzalez, 2009 ).
Availability of data and materials
We will make the data available.
Al-Gahtani, S. S. (2016). Empirical investigation of e-learning acceptance and assimilation: A structural equation model. Applied Comput Information , 12 , 27–50.
Google Scholar
Alqurashi, E. (2016). Self-efficacy in online learning environments: A literature review. Contemporary Issues Educ Res (CIER) , 9 (1), 45–52.
Anderson, T. (2016). A fourth presence for the Community of Inquiry model? Retrieved from https://virtualcanuck.ca/2016/01/04/a-fourth-presence-for-the-community-of-inquiry-model/ .
Annand, D. (2011). Social presence within the community of inquiry framework. The International Review of Research in Open and Distributed Learning , 12 (5), 40.
Arbaugh, J. B. (2005). How much does “subject matter” matter? A study of disciplinary effects in on-line MBA courses. Academy of Management Learning & Education , 4 (1), 57–73.
Arbaugh, J. B., Cleveland-Innes, M., Diaz, S. R., Garrison, D. R., Ice, P., Richardson, J. C., & Swan, K. P. (2008). Developing a community of inquiry instrument: Testing a measure of the Community of Inquiry framework using a multi-institutional sample. Internet and Higher Education , 11 , 133–136.
Armellini, A., & De Stefani, M. (2016). Social presence in the 21st century: An adjustment to the Community of Inquiry framework. British Journal of Educational Technology , 47 (6), 1202–1216.
Arruabarrena, R., Sánchez, A., Blanco, J. M., et al. (2019). Integration of good practices of active methodologies with the reuse of student-generated content. International Journal of Educational Technology in Higher Education , 16 , #10.
Arthur, L. (2009). From performativity to professionalism: Lecturers’ responses to student feedback. Teaching in Higher Education , 14 (4), 441–454.
Artino, A. R. (2010). Online or face-to-face learning? Exploring the personal factors that predict students’ choice of instructional format. Internet and Higher Education , 13 , 272–276.
Asoodar, M., Vaezi, S., & Izanloo, B. (2016). Framework to improve e-learner satisfaction and further strengthen e-learning implementation. Computers in Human Behavior , 63 , 704–716.
Bernard, R. M., et al. (2004). How does distance education compare with classroom instruction? A meta-analysis of the empirical literature. Review of Educational Research , 74 (3), 379–439.
Bollinger, D., & Martindale, T. (2004). Key factors for determining student satisfaction in online courses. Int J E-learning , 3 (1), 61–67.
Brinkley-Etzkorn, K. E. (2018). Learning to teach online: Measuring the influence of faculty development training on teaching effectiveness through a TPACK lens. The Internet and Higher Education , 38 , 28–35.
Chickering, A. W., & Gamson, Z. F. (1987). Seven principles for good practice in undergraduate education. AAHE Bulletin , 3 , 7.
Choi, I., Land, S. M., & Turgeon, A. J. (2005). Scaffolding peer-questioning strategies to facilitate metacognition during online small group discussion. Instructional Science , 33 , 483–511.
Clayton, K. E., Blumberg, F. C., & Anthony, J. A. (2018). Linkages between course status, perceived course value, and students’ preferences for traditional versus non-traditional learning environments. Computers & Education , 125 , 175–181.
Cleveland-Innes, M., & Campbell, P. (2012). Emotional presence, learning, and the online learning environment. The International Review of Research in Open and Distributed Learning , 13 (4), 269–292.
Cohen, A., & Baruth, O. (2017). Personality, learning, and satisfaction in fully online academic courses. Computers in Human Behavior , 72 , 1–12.
Crews, T., & Butterfield, J. (2014). Data for flipped classroom design: Using student feedback to identify the best components from online and face-to-face classes. Higher Education Studies , 4 (3), 38–47.
Dawson, P., Henderson, M., Mahoney, P., Phillips, M., Ryan, T., Boud, D., & Molloy, E. (2019). What makes for effective feedback: Staff and student perspectives. Assessment & Evaluation in Higher Education , 44 (1), 25–36.
Drew, C., & Mann, A. (2018). Unfitting, uncomfortable, unacademic: A sociological reading of an interactive mobile phone app in university lectures. International Journal of Educational Technology in Higher Education , 15 , #43.
Durabi, A., Arrastia, M., Nelson, D., Cornille, T., & Liang, X. (2011). Cognitive presence in asynchronous online learning: A comparison of four discussion strategies. Journal of Computer Assisted Learning , 27 (3), 216–227.
Eom, S. B., Wen, H. J., & Ashill, N. (2006). The determinants of students’ perceived learning outcomes and satisfaction in university online education: An empirical investigation. Decision Sciences Journal of Innovative Education , 4 (2), 215–235.
Espasa, A., & Meneses, J. (2010). Analysing feedback processes in an online teaching and learning environment: An exploratory study. Higher Education , 59 (3), 277–292.
Farrell, O., & Brunton, J. (2020). A balancing act: A window into online student engagement experiences. International Journal of Educational Technology in High Education , 17 , #25.
Fidalgo, P., Thormann, J., Kulyk, O., et al. (2020). Students’ perceptions on distance education: A multinational study. International Journal of Educational Technology in High Education , 17 , #18.
Flores, Ò., del-Arco, I., & Silva, P. (2016). The flipped classroom model at the university: Analysis based on professors’ and students’ assessment in the educational field. International Journal of Educational Technology in Higher Education , 13 , #21.
Garrison, D. R., Anderson, T., & Archer, W. (2003). A theory of critical inquiry in online distance education. Handbook of Distance Education , 1 , 113–127.
Gong, D., Yang, H. H., & Cai, J. (2020). Exploring the key influencing factors on college students’ computational thinking skills through flipped-classroom instruction. International Journal of Educational Technology in Higher Education , 17 , #19.
Gonzalez, C. (2009). Conceptions of, and approaches to, teaching online: A study of lecturers teaching postgraduate distance courses. Higher Education , 57 (3), 299–314.
Grandzol, J. R., & Grandzol, C. J. (2006). Best practices for online business Education. International Review of Research in Open and Distance Learning , 7 (1), 1–18.
Green, S. B., & Salkind, N. J. (2003). Using SPSS: Analyzing and understanding data , (3rd ed., ). Upper Saddle River: Prentice Hall.
Hair, J. F., Black, W. C., Babin, B. J., & Anderson, R. E. (2014). Multivariate data analysis: Pearson new international edition . Essex: Pearson Education Limited.
Harjoto, M. A. (2017). Blended versus face-to-face: Evidence from a graduate corporate finance class. Journal of Education for Business , 92 (3), 129–137.
Hong, K.-S. (2002). Relationships between students’ instructional variables with satisfaction and learning from a web-based course. The Internet and Higher Education , 5 , 267–281.
Horvitz, B. S., Beach, A. L., Anderson, M. L., & Xia, J. (2015). Examination of faculty self-efficacy related to online teaching. Innovation Higher Education , 40 , 305–316.
Inside Higher Education and Gallup. (2019). The 2019 survey of faculty attitudes on technology. Author .
Jaggars, S. S., & Xu, D. (2016). How do online course design features influence student performance? Computers and Education , 95 , 270–284.
Joo, Y. J., Lim, K. Y., & Kim, E. K. (2011). Online university students’ satisfaction and persistence: Examining perceived level of presence, usefulness and ease of use as predictor in a structural model. Computers & Education , 57 (2), 1654–1664.
Jung, I. (2011). The dimensions of e-learning quality: From the learner’s perspective. Educational Technology Research and Development , 59 (4), 445–464.
Kay, R., MacDonald, T., & DiGiuseppe, M. (2019). A comparison of lecture-based, active, and flipped classroom teaching approaches in higher education. Journal of Computing in Higher Education , 31 , 449–471.
Kehrwald, B. (2008). Understanding social presence in text-based online learning environments. Distance Education , 29 (1), 89–106.
Kintu, M. J., Zhu, C., & Kagambe, E. (2017). Blended learning effectiveness: The relationship between student characteristics, design features and outcomes. International Journal of Educational Technology in Higher Education , 14 , #7.
Kuo, Y.-C., Walker, A. E., Schroder, K. E., & Belland, B. R. (2013). Interaction, internet self-efficacy, and self-regulated learning as predictors of student satisfaction in online education courses. Internet and Education , 20 , 35–50.
Lange, C., & Costley, J. (2020). Improving online video lectures: Learning challenges created by media. International Journal of Educational Technology in Higher Education , 17 , #16.
le Roux, I., & Nagel, L. (2018). Seeking the best blend for deep learning in a flipped classroom – Viewing student perceptions through the Community of Inquiry lens. International Journal of Educational Technology in High Education , 15 , #16.
Lee, H.-J., & Rha, I. (2009). Influence of structure and interaction on student achievement and satisfaction in web-based distance learning. Educational Technology & Society , 12 (4), 372–382.
Lee, Y., Stringer, D., & Du, J. (2017). What determines students’ preference of online to F2F class? Business Education Innovation Journal , 9 (2), 97–102.
Legon, R., & Garrett, R. (2019). CHLOE 3: Behind the numbers . Published online by Quality Matters and Eduventures. https://www.qualitymatters.org/sites/default/files/research-docs-pdfs/CHLOE-3-Report-2019-Behind-the-Numbers.pdf
Liaw, S.-S., & Huang, H.-M. (2013). Perceived satisfaction, perceived usefulness and interactive learning environments as predictors of self-regulation in e-learning environments. Computers & Education , 60 (1), 14–24.
Lu, F., & Lemonde, M. (2013). A comparison of online versus face-to-face students teaching delivery in statistics instruction for undergraduate health science students. Advances in Health Science Education , 18 , 963–973.
Lundin, M., Bergviken Rensfeldt, A., Hillman, T., Lantz-Andersson, A., & Peterson, L. (2018). Higher education dominance and siloed knowledge: a systematic review of flipped classroom research. International Journal of Educational Technology in Higher Education , 15 (1).
Macon, D. K. (2011). Student satisfaction with online courses versus traditional courses: A meta-analysis . Disssertation: Northcentral University, CA.
Mann, J., & Henneberry, S. (2012). What characteristics of college students influence their decisions to select online courses? Online Journal of Distance Learning Administration , 15 (5), 1–14.
Mansbach, J., & Austin, A. E. (2018). Nuanced perspectives about online teaching: Mid-career senior faculty voices reflecting on academic work in the digital age. Innovative Higher Education , 43 (4), 257–272.
Marks, R. B., Sibley, S. D., & Arbaugh, J. B. (2005). A structural equation model of predictors for effective online learning. Journal of Management Education , 29 (4), 531–563.
Martin, F., Wang, C., & Sadaf, A. (2018). Student perception of facilitation strategies that enhance instructor presence, connectedness, engagement and learning in online courses. Internet and Higher Education , 37 , 52–65.
Maycock, K. W. (2019). Chalk and talk versus flipped learning: A case study. Journal of Computer Assisted Learning , 35 , 121–126.
McGivney-Burelle, J. (2013). Flipping Calculus. PRIMUS Problems, Resources, and Issues in Mathematics Undergraduate . Studies , 23 (5), 477–486.
Mohammadi, H. (2015). Investigating users’ perspectives on e-learning: An integration of TAM and IS success model. Computers in Human Behavior , 45 , 359–374.
Nair, S. S., Tay, L. Y., & Koh, J. H. L. (2013). Students’ motivation and teachers’ teaching practices towards the use of blogs for writing of online journals. Educational Media International , 50 (2), 108–119.
Nguyen, T. (2015). The effectiveness of online learning: Beyond no significant difference and future horizons. MERLOT Journal of Online Learning and Teaching , 11 (2), 309–319.
Ni, A. Y. (2013). Comparing the effectiveness of classroom and online learning: Teaching research methods. Journal of Public Affairs Education , 19 (2), 199–215.
Nouri, J. (2016). The flipped classroom: For active, effective and increased learning – Especially for low achievers. International Journal of Educational Technology in Higher Education , 13 , #33.
O’Neill, D. K., & Sai, T. H. (2014). Why not? Examining college students’ reasons for avoiding an online course. Higher Education , 68 (1), 1–14.
O'Flaherty, J., & Phillips, C. (2015). The use of flipped classrooms in higher education: A scoping review. The Internet and Higher Education , 25 , 85–95.
Open & Distant Learning Quality Council (2012). ODLQC standards . England: Author https://www.odlqc.org.uk/odlqc-standards .
Ortagus, J. C. (2017). From the periphery to prominence: An examination of the changing profile of online students in American higher education. Internet and Higher Education , 32 , 47–57.
Otter, R. R., Seipel, S., Graef, T., Alexander, B., Boraiko, C., Gray, J., … Sadler, K. (2013). Comparing student and faculty perceptions of online and traditional courses. Internet and Higher Education , 19 , 27–35.
Paechter, M., Maier, B., & Macher, D. (2010). Online or face-to-face? Students’ experiences and preferences in e-learning. Internet and Higher Education , 13 , 292–329.
Prinsloo, P. (2016). (re)considering distance education: Exploring its relevance, sustainability and value contribution. Distance Education , 37 (2), 139–145.
Quality Matters (2018). Specific review standards from the QM higher Education rubric , (6th ed., ). MD: MarylandOnline.
Richardson, J. C., Maeda, Y., Lv, J., & Caskurlu, S. (2017). Social presence in relation to students’ satisfaction and learning in the online environment: A meta-analysis. Computers in Human Behavior , 71 , 402–417.
Rockhart, J. F., & Bullen, C. V. (1981). A primer on critical success factors . Cambridge: Center for Information Systems Research, Massachusetts Institute of Technology.
Rourke, L., & Kanuka, H. (2009). Learning in Communities of Inquiry: A Review of the Literature. The Journal of Distance Education / Revue de l'ducation Distance , 23 (1), 19–48 Athabasca University Press. Retrieved August 2, 2020 from https://www.learntechlib.org/p/105542/ .
Sebastianelli, R., Swift, C., & Tamimi, N. (2015). Factors affecting perceived learning, satisfaction, and quality in the online MBA: A structural equation modeling approach. Journal of Education for Business , 90 (6), 296–305.
Shen, D., Cho, M.-H., Tsai, C.-L., & Marra, R. (2013). Unpacking online learning experiences: Online learning self-efficacy and learning satisfaction. Internet and Higher Education , 19 , 10–17.
Sitzmann, T., Kraiger, K., Stewart, D., & Wisher, R. (2006). The comparative effectiveness of web-based and classroom instruction: A meta-analysis. Personnel Psychology , 59 (3), 623–664.
So, H. J., & Brush, T. A. (2008). Student perceptions of collaborative learning, social presence and satisfaction in a blended learning environment: Relationships and critical factors. Computers & Education , 51 (1), 318–336.
Song, L., Singleton, E. S., Hill, J. R., & Koh, M. H. (2004). Improving online learning: Student perceptions of useful and challenging characteristics. The Internet and Higher Education , 7 (1), 59–70.
Sun, P. C., Tsai, R. J., Finger, G., Chen, Y. Y., & Yeh, D. (2008). What drives a successful e-learning? An empirical investigation of the critical factors influencing learner satisfaction. Computers & Education , 50 (4), 1183–1202.
Takamine, K. (2017). Michelle D. miller: Minds online: Teaching effectively with technology. Higher Education , 73 , 789–791.
Tanner, J. R., Noser, T. C., & Totaro, M. W. (2009). Business faculty and undergraduate students’ perceptions of online learning: A comparative study. Journal of Information Systems Education , 20 (1), 29.
Tucker, B. (2012). The flipped classroom. Education Next , 12 (1), 82–83.
Van Wart, M., Ni, A., Ready, D., Shayo, C., & Court, J. (2020). Factors leading to online learner satisfaction. Business Educational Innovation Journal , 12 (1), 15–24.
Van Wart, M., Ni, A., Rose, L., McWeeney, T., & Worrell, R. A. (2019). Literature review and model of online teaching effectiveness integrating concerns for learning achievement, student satisfaction, faculty satisfaction, and institutional results. Pan-Pacific . Journal of Business Research , 10 (1), 1–22.
Ventura, A. C., & Moscoloni, N. (2015). Learning styles and disciplinary differences: A cross-sectional study of undergraduate students. International Journal of Learning and Teaching , 1 (2), 88–93.
Vlachopoulos, D., & Makri, A. (2017). The effect of games and simulations on higher education: A systematic literature review. International Journal of Educational Technology in Higher Education , 14 , #22.
Wang, Y., Huang, X., & Schunn, C. D. (2019). Redesigning flipped classrooms: A learning model and its effects on student perceptions. Higher Education , 78 , 711–728.
Wingo, N. P., Ivankova, N. V., & Moss, J. A. (2017). Faculty perceptions about teaching online: Exploring the literature using the technology acceptance model as an organizing framework. Online Learning , 21 (1), 15–35.
Xu, D., & Jaggars, S. S. (2014). Performance gaps between online and face-to-face courses: Differences across types of students and academic subject areas. Journal of Higher Education , 85 (5), 633–659.
Young, S. (2006). Student views of effective online teaching in higher education. American Journal of Distance Education , 20 (2), 65–77.
Zawacki-Richter, O., & Naidu, S. (2016). Mapping research trends from 35 years of publications in distance Education. Distance Education , 37 (3), 245–269.
Download references
Acknowledgements
No external funding/ NA.
Author information
Authors and affiliations.
Development for the JHB College of Business and Public Administration, 5500 University Parkway, San Bernardino, California, 92407, USA
Montgomery Van Wart, Anna Ni, Pamela Medina, Jesus Canelon, Melika Kordrostami, Jing Zhang & Yu Liu
You can also search for this author in PubMed Google Scholar
Contributions
Equal. The author(s) read and approved the final manuscript.
Corresponding author
Correspondence to Montgomery Van Wart .
Ethics declarations
Competing interests.
We have no competing interests.
Additional information
Publisher’s note.
Springer Nature remains neutral with regard to jurisdictional claims in published maps and institutional affiliations.
Rights and permissions
Open Access This article is licensed under a Creative Commons Attribution 4.0 International License, which permits use, sharing, adaptation, distribution and reproduction in any medium or format, as long as you give appropriate credit to the original author(s) and the source, provide a link to the Creative Commons licence, and indicate if changes were made. The images or other third party material in this article are included in the article's Creative Commons licence, unless indicated otherwise in a credit line to the material. If material is not included in the article's Creative Commons licence and your intended use is not permitted by statutory regulation or exceeds the permitted use, you will need to obtain permission directly from the copyright holder. To view a copy of this licence, visit http://creativecommons.org/licenses/by/4.0/ .
Reprints and permissions
About this article
Cite this article.
Van Wart, M., Ni, A., Medina, P. et al. Integrating students’ perspectives about online learning: a hierarchy of factors. Int J Educ Technol High Educ 17 , 53 (2020). https://doi.org/10.1186/s41239-020-00229-8
Download citation
Received : 29 April 2020
Accepted : 30 July 2020
Published : 02 December 2020
DOI : https://doi.org/10.1186/s41239-020-00229-8
Share this article
Anyone you share the following link with will be able to read this content:
Sorry, a shareable link is not currently available for this article.
Provided by the Springer Nature SharedIt content-sharing initiative
- Online education
- Online teaching
- Student perceptions
- Online quality
- Student presence
- Open access
- Published: 14 July 2022
The impact of virtual learning on students’ educational behavior and pervasiveness of depression among university students due to the COVID-19 pandemic
- Fatima M. Azmi ORCID: orcid.org/0000-0001-9275-0965 1 ,
- Habib Nawaz Khan ORCID: orcid.org/0000-0003-3519-264X 2 &
- Aqil M. Azmi ORCID: orcid.org/0000-0002-0983-2861 3
Globalization and Health volume 18 , Article number: 70 ( 2022 ) Cite this article
52k Accesses
16 Citations
2 Altmetric
Metrics details
One of the worst pandemics of recent memory, COVID-19, severely impacted the public. In particular, students were physically and mentally affected by the lockdown and the shift from physical person-to-person classrooms to virtual learning (online classes). This increased the prevalence of psychological stress, anxiety, and depression among university students. In this study, we investigated the depression levels in Saudi Arabian university students who were learning virtually because of the COVID-19 pandemic and examined its impact on their educational proficiency.
The study focused on two points: first, examining the depression levels among undergraduate students in Saudi Arabia, by adapting the Zung (Self-Rating Depression Scale) questionnaire. Second, whether there is an association between the levels of depression and various distress factors associated with virtual (online) learning resulting from the COVID-19 pandemic and its impact on students’ educational behaviors. The questionnaire was prepared using a monkey survey and shared online, via email, and on WhatsApp groups, with participants in two universities, a public and private university in the largest city of Saudi Arabia. A total of 157 complete responses were received. Data were analyzed using SPSS-24, the chi-square test, descriptive statistics, and multilinear regression.
The results indicated that three-fourths of the university students suffered from different depressive symptoms, half of which had moderate to extreme levels of depression. Our study confirmed that a boring virtual (online) learning method, stress, fear of examinations, and decreased productivity were significantly associated with increased depression. In addition, 75% and 79% of the students suffered from stress and fear of examinations, respectively. About half of the students were associated with increased depression. The outcome also indicated that female students experienced extreme depression, stress, and fear of examinations more than males.
These findings can inform government agencies and representatives of the importance of making swift, effective decisions to address students’ depression levels. It is essential to provide training for students to change their educational experience mindset, which might help decrease "depression and stress-related growth." There is also a need to search for a better virtual teaching delivery method to lessen students' stress and fear of examinations.
On March 11, 2020, the World Health Organization (WHO) declared the highly contagious coronavirus (COVID-19) a global pandemic [ 1 ]. As the cases of COVID-19 increased, China, and many other countries practiced partial or complete lockdowns. It is estimated that this drastic measure helped save 3 million lives across 11 European nations [ 2 ]. Toward the end of January 2022, the total number of confirmed COVID-19 cases worldwide was 360,578,392, and 5,620,865 confirmed deaths. The number of people who received vaccination doses globally was 9,679,721,754 [ 3 ].
To contain the virus, the lockdown caused academic disruptions. This resulted in the indeterminate closure of schools, universities, various institutes, shopping malls, and centers of economic activities [ 4 , 5 ]. Repetitive activities, transfer of educational mode to distance (virtual) learning, and change in social life amplified the prevalence of psychological stress, anxiety, depression, and acute stress reactions among university students [ 6 ]. Sociodemographic factors associated with low mental health include financial constraints, old age, infection risk, and fear of losing a relative or friend. In addition, COVID-19 pandemic-related educational stress may be attributed to (in no particular order): (a) transformed teaching and assessment methods; (b) skepticism about university education; (c) technological worries about online courses [ 7 , 8 ]; (d) uncertainty about the future because of academic disruptions; (e) fear of failing examinations; (f) inability to concentrate during lectures, and many more factors. All these factors have been detected in universities across the world [ 9 , 10 ]. A global study that inspected students’ experiences in about 62 different countries, including a university in the United States, found that students expressed worries about their academic achievements and professional careers and feelings of dullness, anxiety, and frustration [ 9 ]. Students in China also reported increased sadness, anger, anxiety, and fear [ 11 ]. The occurrence of depression, psychological distress, and anxiety from pandemics differed from country to country. A study in Italy reported that 15.4% of Italians suffered from extremely high levels of depression, 12.6% were highly stressed, and 11.5% were highly anxious [ 12 ]. In Malaysia, it was reported that severe to extremely severe levels of depression and anxiety were found in 9.2% and 13.2% of the subjects, respectively. Moderate stress was found in 9.5% of subjects, and severe to highly severe stress was found in 6.6% of subjects [ 13 ]. Furthermore, students in Switzerland manifested a decrease in social interface and higher levels of stress, anxiety, and loneliness [ 14 ]. Adults have also reported declining physical activity, while food eating increased during pandemic quarantine periods compared to previous times [ 15 ].
The first COVID-19 case appeared in the Kingdom of Saudi Arabia (KSA) on March 2, 2020 [ 16 ], while the lockdown was imposed on March 8, 2020. To keep students on track due to the pandemic, the education delivery mode was switched to virtual learning. It has been over one year since teaching was transferred online, and many countries worldwide have tried to revert to the standard path of education by opening schools and universities. Although the COVID-19 vaccine is available worldwide, some countries are still practicing lockdown because of the appearance of several more contiguous variants of the coronavirus, such as Delta, a SARS-CoV-2 strain that was first spotted in India [ 17 ]. The spread of COVID-19 presents a serious risk; in mid-April 2022, the confirmed cases in KSA were 751,717, out of which 736,910 had recovered, and 9,055 deaths were recorded [ 18 ].
The psychological consequences of COVID-19 have been observed and described in KSA. Al-Hanawi et al. [ 19 ] reported different levels of distress in 40% of the general Saudi population because of COVID-19. Moreover, Alkhamees et al. [ 20 ] reported moderate to severe psychological effects in 23.6% of the general Saudi population. In another study of the influence of the COVID-19 pandemic on Saudi Arabian residents, Alyami et al. [ 21 ] stated that the percentages of mild, moderate, moderately severe, and severe levels of depression were 41%, 20%, 6.2%, and 3.2%, respectively. Furthermore, Khoshaim et al. [ 22 ] reported that about 35% of students experienced moderate to extreme anxiety levels. Azmi et al. [ 23 ] observed that 75% of students suffered from various levels of depression, while 41% suffered from low levels of self-esteem.
Likewise, another study found that 35% of students in the western and northern regions of KSA had high rates of distress [ 24 ]. Following the observed rise of psychological disorders, the authorities posted health messages and distributed procedures to the public. For example, during the pandemic, the Saudi Center for Disease Control and Prevention (CDC) [ 25 ] supplied a precautionary manual for mental and social health focused on prevention, pressure, and fear control. From the foregoing, the COVID-19 pandemic has had a severe impact on the physical and mental health of the public in general and students in particular, as university students are among those most severely affected by the COVID-19 pandemic.
In this study, we investigated the depression levels of university students in Riyadh, the capital and largest city in KSA, who were learning virtually because of the COVID-19 pandemic. We also assessed the impact of virtual learning on their educational behaviors. The following questions were explored during the investigation:
What are the levels of depression among university students?
What is the impact of virtual learning on students’ educational behaviors and what are the relationship between depressive symptoms they exhibited and virtual learning?
To answer the second question, we explored the relationship between the levels of depression and various distress factors associated with virtual learning because of the COVID-19 pandemic and its impact on students’ educational behaviors. These factors were divided into two main categories: Category 1 dealt with factors relating to how virtual learning has affected students’ feelings from an educational perspective. Category 2: dealt with factors relating to how virtual learning affected students’ understanding of subjects/learning materials.
Once we ascertain the current levels of depression and their impact on students’ educational behavior, we may embark on helping them cope with the extraordinary situation. Hopefully, this will help lower their elevated depression levels. Furthermore, we hope our study will guide policymakers in searching for innovative ways of online teaching to make learning less stressful and more productive.
Design and sampling procedure
This study examines depression levels and investigates virtual learning-related distress factors, which might predict the increased level of depressive symptoms among university students in Riyadh City during the COVID-19 pandemic.
Research design
We conducted a descriptive survey-based study to obtain responses from students at large universities in Riyadh, the capital of KSA. The total size of the target population of the city of Riyadh is about 7 million [ 26 ]. The sampled population of both universities’ undergraduate students was approximately 0.027 million (27,000). The male-to-female ratio of undergraduate students at King Saud University (KSU) is about 67%: 33%; the male-to-female ratio of undergraduate students at Prince Sultan University (PSU) is about 28%: 72%, as this is a female-dominated university. Since the sampled population was largely heterogeneous, we minimized the heterogeneity by dividing the given population into sub-populations to obtain sampling units that are homogeneous internally and heterogeneous externally. Hence, we used a stratified random sampling technique, which is more appropriate than other sampling techniques for obtaining better estimates of the parameters of interest. To ensure the efficiency of the estimates, we used the proportional allocation technique to determine the sample size.
A Monkey survey was used to prepare the questionnaire, following the approval of PSU’s Institutional Review Board. The questionnaire included demographic questions, such as gender, age, and college. Zung’s Self-Rating Depression Scale (ZSDS), with 20 items on a 4-point Likert scale, was used to measure depression. The questionnaire also had questions to address distress factors associated with virtual learning because of the COVID-19 pandemic. The students were asked to read all the questions carefully and answer them.
The survey was written in English and Arabic side by side. A subject expert translated the questionnaire from Arabic to English. Thereafter, five more experts checked the same questions for more corrections and authenticity. The actual online survey took place from March to April 2021. The survey was voluntary, and the informed consent of the students was sought. We received reasonable responses from the students; however, we also received some incomplete responses. The missing/incomplete responses were discarded from the study so that the estimated results were not compromised. The valid responses received from males and females were 49.7% and 50.3%, respectively.
Measuring instruments
Demographic data and personal characteristics, such as age, gender, and area of study, were recorded.
Depression measure
The ZSDS was used to measure the levels of depression. The tool is a 20-item self-reporting assessment device used for measuring depression levels [ 27 , 28 ]. This is divided into 10 positively worded and 10 negatively worded items. The latter items were reversely scored. Each item was scored on a Likert-type scale as follows: 1 = Never , 2 = Sometimes , 3 = Often/most of the time , and 4 = Always . The total raw scores ranged from 20–80, and when converted into the depression index (termed "ZSDS index"), the range becomes 25–100. To determine the level of depression, we classified the ZSDS index into four classes (levels). Therefore, ZSDS index scores were considered "normal" from 25–49, "Mildly Depressed,” from 50–59, “Moderately Depressed” from 60–69, and “Severely Depressed” from 70 and above [ 27 ]. In [ 29 ], the author translated the ZSDS measure into Arabic and further validated it. Question 6, “I still enjoy sex,” was deemed offensive religiously and culturally. Therefore, it was rephrased to “I enjoy looking at, talking to, and being with attractive women/men,” which is culturally more appropriate. The accuracy of the new version was verified in [ 29 ]. The Arabic and English languages were used side by side to prepare the questionnaire. The Cronbach’s alpha coefficient of this study was 0.87, showing high internal consistency.
Data on distress factors associated with virtual learning
Data on distress factors associated with virtual learning due to the COVID-19 pandemic were divided into two categories. The first category dealt with questions on how virtual learning due to the pandemic affected students’ feelings from an educational perspective and caused a) lack of motivation/boredom, b) stress, c) worry and fear of exams, and d) decreased productivity. The second category dealt with questions on virtual learning and its effect on students’ understanding of subjects/materials, such as a) It needs more self-effort to understand, b) It made learning and understanding harder for them, c) They need more time to understand the subject, i.e., the understanding pace became slower, d) Virtual learning is boring, and e) they had difficulty solving problems in academic subjects and writing down the solutions correctly. The answer to each question was either “Yes” or “No.”
Finally, the questionnaire had an open-ended question that offered students a chance to express in their own words how the lockdown and virtual teaching had affected their educational advancement.
Data analysis
Data were analyzed using IBM SPSS version 24 software. The categorical variable demographic data were analyzed descriptively to determine the essential characteristics of the sample and were presented as counts and percentages. The level of depression index among university students in Riyadh, and its association with gender, age, and their field of education, was analyzed using the chi-square test and descriptive statistics. Multilinear regression analysis was performed to investigate the connection between depression levels and various factors associated with virtual learning due to the COVID-19 pandemic. The statistically significant level was set at \(p \le 0.05.\)
Demographic characteristics
The total number of participants was 157 university students. Table 1 shows the demographic characteristics of the participants.
Students’ levels of depression and demographic variables
In the univariate analysis, chi-square tests were used to determine the associations between students’ demographic variables and the ZSDS level. Table 2 displays the association between depression levels with gender, age, and college. Among the demographic variables, only the association with gender was statistically significant at \({\chi }^{2}\) = 20.229, and p < 0.001, while the association with age and college was not significant. A total of 74.4% of the students had various levels of depression. Of these, 37%, 21.7%, and 16% had mild, moderate, and severe depression levels, respectively. In addition, females (28%) had more depressive symptoms than males (4%).
Educational distress factors associated with virtual learning and descriptive statistics
The factors related to virtual learning sequel to the COVID-19 pandemic, and its impact on students’ educational behaviors were divided into two categories. Questions on virtual learning's effect on students' feelings from an educational perspective (Category 1) had four items, each with a "Yes" or "No" answer. Likewise, questions on virtual learning and its effect on students’ understanding of the subjects/materials (Category 2) had five items, each with a “Yes” or “No” answer. Table 3 demonstrates the descriptive statistics. In the first category, the highest percentage was feeling worried and having a fear of exams (79%), followed by stress (75.2%), lack of motivation, and decreased productivity (70%, each). In the second category, the highest percentage was 78%, who felt they had to put extra self-effort into understanding and studying.
Furthermore, 74.5% felt that virtual learning was more challenging for them to understand than physical learning. In addition, 73% said virtual learning was slow and extra time was needed to understand and learn the concepts, while 64% found it boring. Finally, 58.6% had difficulty solving problems and submitting properly written answers (for math and computer science subjects).
Distress factors related to virtual learning and depressive symptoms
Multilinear regression analysis was used to study whether various distress factors related to virtual learning can influence depressive symptoms among students.
The first category, which dealt with students’ feelings from the educational point of view, hypothesized that lack of motivation, stress, worry/fear of examinations, and decreased productivity would significantly impact the development of depressive symptoms among students.
Multi-regression analysis was used to test the hypotheses, with the Zung depression index as a dependent variable. The results show that 24.6% of the variance in Zung’s depression index can be accounted for by four predictors, collectively \(, F(4, 152) = 12.414, p < 0.001\) . Looking at the unique individual contribution of the predictors, the result shows that worry and fear of exams ( \(\beta =0.290, t=3.589, p<0.001)\) , stress ( \(\beta =0.202, t=2.566, p=0.011<0.05)\) , and decreased learning amount and not being productive ( \(\beta =0.211, t=2.783, p=0.006<0.05)\) , statistically significantly contributed to worsening depressive symptoms. The predictor, feeling lack of motivation, did not significantly impact developing depressive symptoms.
The second category dealt with virtual learning and its effect on students’ understanding of the subjects/materials. It was hypothesized that the need for extra self-effort to understand the subject, learning became harder, learning became slower, learning was boring, and difficulty in solving problems and writing answers properly would have a statistically significant impact on developing depressive symptoms among students.
Multi-regression analysis was used to test the hypotheses, with Zung’s depression index as a dependent variable. The test showed that 13% of the variance in Zung's depression index can be accounted for by the five predictors, collectively \(, F(5, 151) = 4.505, p < 0.001\) . Looking at the unique individual contribution of the predictors, the result shows that learning is not much fun or exciting ( \(\beta =0.250, t=3.060, p=0.003<0.05)\) , and facing difficulty in solving questions and writing answers properly ( \(\beta =0.176, t=2.067, p=0.05<0.05)\) , were statistically significantly associated with worsening depressive symptoms. While the other three predictors, learning became harder, learning became slower, and the need to put extra self-effort did not contribute significantly to depressive symptoms, as shown in Table 4 .
Furthermore, we explored two distress factors, stress, and worry/fear of exams, which contributed statistically significantly to worsening depressive symptoms. Using the chi-square test, we examined the association of the distress factors with depression levels; that is, what extent does stress or worry/fear of exams contribute to moderate or severe depression. The results showed a statistically significant association between stress and moderate to severe levels of depression ( \({\chi }^{2}\) = 17.179, and p < 0.001). Likewise, there was a statistically significant association between worry/fear of exams and moderate to severe levels of depression ( \({\chi }^{2}\) = 30.236, and p < 0.001), Table 5 .
The association between stress or worry/fear of exams and gender was examined using the chi-square test. There was a statistically significant association between these two factors and gender, with more females having higher stress levels (54%) than males (41%). Also, worry/fear of exams manifested in 60% of females and 40% of males during virtual learning, sequel to the COVID-19 pandemic. The results are presented in Table 6 .
Open-ended questions
The questionnaire ended with an open-ended question, in which students were asked to write in their own words how the lockdown has affected their educational advancement. Excerpts of the negative comments from students are outlined below:
“Virtual teaching and exam resulted in increased cheating." “Virtual teaching caused difficulty in understanding the subject, which resulted in lowering my grades.” "I have to sit in the same room with my siblings while learning online, as my home is small. So, I cannot concentrate at all; it just makes me very frustrated.”
From their comments, it is clear that a virtual learning environment is entirely different from a physical classroom teaching environment where exams are conducted with invigilators proctoring.
Significantly few students provided positive comments.
"Virtual teaching made me understand better and increased productivity and my grades."
In this study, we investigated the severity of depressive symptoms among university students while learning virtually because of the COVID-19 pandemic and its impact on educational behaviors in KSA We collected samples from different universities in Riyadh. The total number of complete responses was 157. The Zung Self-Rating Depression measure was used to measure depression levels. Our results indicate that 75% of the students suffer from different levels of depression (37%, 22%, and 16% of the students reported mild, moderate, and extremely severe levels of depression, respectively). This result is consistent with an American study, which reported that 44% of students in the USA experienced an augmented level of depressive thoughts [ 30 ].
The association between the levels of depression and various distress factors associated with virtual learning due to the pandemic and its impact on students’ educational behaviors was explored using multilinear regression. These factors are divided into two main categories: Category 1: These factors relate to how virtual learning has affected students’ feelings from an educational perspective. This consists of four items: lack of motivation, stress, worry/fear of exam, and decreased productivity. Category 2 factors relate to how virtual learning has affected students’ understanding of the subjects/materials. This category has five items, including need of extra self-effort, need to study harder, learning is slower, virtually learning is boring, difficulty in solving problems, and writing properly.
Consistent with our hypotheses, we confirmed that stress, worry/fear of examinations, and decreased productivity were significantly associated with an increased level of depression. Another recognized factor that contributes significantly to a higher risk of developing depressive symptoms among university students is that virtual teaching and learning becomes boring. Furthermore, students faced difficulty in solving mathematics and science problems and writing the answers properly due to online teaching. A few other factors, such as lack of motivation, learning became more complex and slower, and the need to put extra self-effort contributed to developing depressive symptoms.
Our results indicate that 75% of the students suffer from stress, and about half (47%) have high levels of depression. This is consistent with the results in [ 13 ]. Our findings also indicate that 79% of the students suffer from fear of exams, and about half of them (47%) experience moderate to severe levels of depression. It is usual for some students to have worries and fear for exams; however, it is highly unusual for more than three-fourths of the students to experience fear and worry. This is a clear indication that the changed mode of lecture delivery and exam administration because of COVID-19 has a significant role in raising depression levels among university students. Our findings indicate that a higher percentage of females experience extreme levels of depression than males (28% of females compared to only 4% of males), stress (59% females, vs. 41% males), and worry/fear of exams (60% females, vs. 40% males). This finding is consistent with many studies concerning college students, in which females were at a higher risk of suffering psychologically during virtual learning because of the COVID-19 pandemic [ 9 , 31 , 32 , 33 ]. Another study showed that Vietnamese female students had a higher percentage of depression compared to male students [ 34 ]. Furthermore, Huange et al. [ 35 ] reasoned that Chinese females experienced more anxiety than males during the COVID-19 pandemic. Thus, we assert that feamles are more commonly inclined toward depression and anxiety disorders than males [ 36 ].
The results of the open-ended responses demonstrated the students’ frustration and stress relating to online learning. In contrast, very few students positively indicated that online learning and studying from home felt relaxing.
COVID-19 has been a catastrophic experience. Although it has largely subsided, new variants are causing apprehension among health officials. Our research found that 75% of university students in Saudi Arabia suffer from some degree of depression. Half of these students showed moderate to extreme levels of depression. This is greater than the expected depression level in the overall population. Our study confirms that stress, worry, and fear of examinations, decreased productivity, and the fact that virtual learning is boring are significantly associated with increased depression. Our findings also indicate that 75% (79%) of the students suffer from stress (fear of exams), and that about half of them have increased levels of depression. It should be noted that the students are 18–24 year olds. This is consistent with the study [ 22 ], which found that psychological distress, stress, and anxiety were higher in the younger age group during the COVID-19 pandemic.
Remarkably, more female students experienced extreme depression, stress, and fear of exams than male students. This result supports previous reports that females were at higher risk of psychological distress during the COVID-19 pandemic [ 9 , 31 , 32 , 33 ].
Our observation calls for instant attention and sustenance for students. There is a requirement to explore potential coping policies that have been shown to be effective during pandemics [ 37 ]. The results of our research might direct policymakers to develop distress management protocols as part of their policy for dealing with future pandemics [ 38 ]. It is essential to provide training for students to redirect their educational experience mindset to focus on the “bright side” and expand instances that may guide "depression and stress-related growth.” A flexible mindset can also help students adapt to new ways of learning and developing tremendous gratitude for life. In addition, there is a need to explore better online teaching delivery methods to lower students’ stress and fear of exams.
Study strengths and limitations
The strength of this study is that it was conducted after students had received virtual teaching for more than one year because of the Pandemic. Therefore, this study accurately reflects students’ depression levels and how these impact their educational behaviors in KSA.
Furthermore, the study was conducted in Riyadh, the capital of KSA, hence our study sample is more reflective of the Saudi student population. Moreover, the depression assessment tool for the study, the Zung Self-Rating Depression Scale, is a reliable, universally acceptable scale.
The limitation of our study is that the sample was not randomly selected from all university students, as a convenience sampling method was used.
Availability of data and materials
The raw data supporting the results of this study will be made available by the corresponding author without undue reservation.
WHO. Rolling updates on corona virus 2019 (covid-19). World Health Organization; 2020. https://www.who.int/emergencies/diseases/novel-coronavirus-2019/events-as-they-happen Retrieved on 14th Apr 2020.
Flaxman S, Mishra S, Gandy A, et al. Estimating the effects of non-pharmaceutical interventions on COVID-19 in Europe. Nature. 2020;584(7820):257–61. https://doi.org/10.1038/s41586-020-2405-7 .
Article CAS PubMed Google Scholar
World Health Organization. Coronavirus disease (COVID-19) (who.int) 2019. https://www.who.int/emergencies/diseases/novel-coronavirus-2019 Accessed 25 Jan 2022.
Viner RM, Russell SJ, Croker H, Packer J, Ward J, Stansfield C, et al. School closure and management practices during coronavirus outbreaks including COVID-19: a rapid systematic review. Lancet Child Adol Health. 2020;4:397–404. https://doi.org/10.1016/S2352-4642(20)30095-X .
Article CAS Google Scholar
Anderson RM, Heesterbeek H, Klinkenberg D, Hollingsworth TD. How will country-based mitigation measures influence the course of the COVID-19 epidemic? Lancet. 2020;395:931–4. https://doi.org/10.1016/S0140-6736(20)30567-5 .
Article CAS PubMed PubMed Central Google Scholar
Sankhi S, Marasine NR. Impact of COVID-19 pandemic on mental health of the general population, students, and health care workers: a review. 2020. https://doi.org/10.20944/preprints202007.0616.v1 (preprints.org.)
Book Google Scholar
Azmi F, Khoshaim H. The COVID-19 pandemic and the challenges of e-assessment of calculus courses in higher education: a case study in Saudi Arabia. Ijlter. 2021;20(3):265–81. https://doi.org/10.26803/ijlter.20.3.16 .
Article Google Scholar
Azmi F. Undergraduate students' preference regarding the type of online homework questions in math and science subjects and instructors' analysis. In proceedings of Conference IC4E 2021: 12th International Conference on E-Education, E-Business, E-Management, and E-Learning. Tokyo, Japan, January 10–13, 2021. https://doi.org/10.1145/3450148.3450209
Aristovnik A, Kerzˇič D, Ravsˇelj D, Tomazˇevič N, Umek L. Impacts of the COVID-19 pandemic on life of higher education students: a global perspective. preprints.org. 2020. https://doi.org/10.20944/preprints202008.0246.v2
Oerther DB, Peters CA. Educating heads, hands, and hearts in the COVID-19 classroom. Environ Eng Sci. 2020;37(5):303–303.
Cao W, Fang Z, Hou G, Han M, Xu X, Dong J, et al. The psychological impact of the COVID-19 epidemic on college students in China. Psychiatr Res. 2020;287:112934. https://doi.org/10.1016/j.psychres.2020.112934 (PMID: 32229390).
Mazza C, Ricci E, Biondi S, Colasanti M, Ferracuti S, Napoli C, et al. A nationwide survey of psychological distress among Italian people during the COVID-19 pandemic: immediate psychological responses and associated factors. Int J Environ Res Public Health. 2020;17(9):3165.
Article CAS PubMed Central Google Scholar
Woon LS-C, Leong Bin Abdullah MFI, Sidi H, Mansor NS, Nik Jaafar NR. Depression, anxiety, and the COVID-19 pandemic: Severity of symptoms and associated factors among university students after the end of the movement lockdown. PLoS One. 2021;16(5):e0252481. https://doi.org/10.1371/journal.pone.0252481 .
Elmer T, Mepham K, Stadtfeld C. Students under lockdown: comparisons of students’ social networks and mental health before and during the COVID-19 crisis in Switzerland. PLoS ONE. 2020;15:e0236337-e236422. https://doi.org/10.1371/journal.pone.0236337 (PMID: 32702065).
Ammar A, Brach M, Trabelsi K, Chtourou H, Boukhris O, Masmoudi L, et al. Effects of COVID-19 home confinement on physical activity and eating behaviour preliminary results of the ECLBCOVID19 international online-survey. medRxiv.org. 2020:1–24. https://doi.org/10.1101/2020.05.04.20072447
Ministry of Health. MOH reports 3,121 new COVID-19 cases, reiterates the importance of social distancing. 2020. https://www.moh.gov.sa/en/Ministry/MediaCenter/News/Pages/News-2020-06-06-001.aspx Accessed July.
Katella K. 5 things to know about the delta variant. Yale Medicine. 2022. https://www.yalemedicine.org/news/5-things-to-know-delta-variant-covid .
Worldometer. Total Coronavirus Cases in Saudi Arabia. 2022. https://www.worldometers.info/coronavirus/country/saudi-arabia/ .
Google Scholar
Al-Hanawi MK, Mwale ML, Alshareef N, Qattan AM, Angawi K, Almubark R, et al. Psychological distress amongst health workers and the general public during the COVID-19 pandemic in Saudi Arabia. Risk Manag Healthc Policy. 2020;13:733–42.
Article PubMed PubMed Central Google Scholar
Alkhamees AA, Alrashed SA, Alzunaydi AA, Almohimeed AS, Aljohani MS. The psychological impact of COVID-19 pandemic on the general population of Saudi Arabia. Compr Psychiatry. 2020;102:152192. https://doi.org/10.1016/j.comppsych.2020.152192 .
Alyami H, Naser A, Dahmash E, Alyami M, Alyami MS. Depression and anxiety during the COVID-19 pandemic in Saudi Arabia: a cross-sectional study. Int J Clin Pract. 2021;75:e14244. https://doi.org/10.1111/ijcp.14244 .
Khoshaim HB, Al-Sukayt A, Chinna K, Nurunnabi M, Sundarasen S, et al. Anxiety level of university students during COVID-19 in Saudi Arabia. Front Psychiatry. 2020;11:579750. https://doi.org/10.3389/fpsyt.2020.579750 .
Azmi FM, Khan HN, Azmi AM, Yaswi A, Jakovljevic M. Prevalence of COVID-19 pandemic, self-esteem and its effect on depression among university students in Saudi Arabia. Front Public Health. 2022;10:836688. https://doi.org/10.3389/fpubh.2022.836688 .
Elhessewi G, Almoayad F, et al. Psychological distress and its risk factors during COVID-19 pandemic in Saudi Arabia: a cross-sectional study. Middle East Curr Psychiatry. 2021;28:7. https://doi.org/10.1186/s43045-021-00089-6 .
Center for Disease prevention and control, Ministry of Health, Riyadh. Novel corona virus (2019-nCoV) infection guidelines V1.2. 2020.
Riyadh Population. Demographics, Maps, Graphs. 2020. https://worldpopulationreview.com/world-cities/riyadh-population .
Zung WW. A self-rating depression scale. Arch Gen Psychiatry. 1965;12:63–70.
Zung WWK. Factors influencing the Self-Rating Depression Scale. Arch Gen Psychiatry. 1967;16(5):543–7.
Kirkby R, AlSaif A, el-din Mohamed G. Validation of an Arabic translation of the Zung Self-Rating Depression Scale. Ann Saudi Med. 2005;25(3):205–8.
Son C, Hegde S, Smith A, Wang X, Sasangohar F. Effects of COVID-19 on college students’ mental health in the United States: interview survey study. J Med Internet Res. 2020;22(9):e21279.
da Silva ML, Rocha RSB, Buheji M, Jahrami H, Cunha KDC. A systematic review of the prevalence of anxiety symptoms during coronavirus epidemics. J Health Psychol. 2020;71:135910532095162–11. https://doi.org/10.1177/1359105320951620 (PMID: 32830577).
Xiong J, Lipsitz O, Nasri F, Lui LMW, Gill H, Phan L, et al. Impact of COVID-19 pandemic on mental health in the general population: a systematic review. J Affect Disord. 2020;277:55–64. https://doi.org/10.1016/j.jad.2020.08.001 (PMID: 32799105).
Sundarasen S, Chinna K, Kamaludin K, Nurunnabi M, Baloch GM, Khoshaim HB, et al. Psychological impact of COVID-19 and lockdown among university students in Malaysia: Implications and policy Recommendations. IJERPH. 2020;17:6206–13. https://doi.org/10.3390/ijerph17176206 (PMID:32867024).
Nguyen DT, Wright EP, Dedding C, Pham TT, Bunders J. Low self-esteem and its association with anxiety, depression, and suicidal ideation in Vietnamese secondary school students: a cross-sectional study. Front Psychiatry. 2019;10:698. https://doi.org/10.3389/fpsyt.2019.00698 .
Huang L, Xu F, Liu H. Emotional responses and coping strategies of nurses and nursing college students during COVID-19 outbreak. 2020. https://doi.org/10.1101/2020.03.05.20031898 .
Hunt J, Eisenberg D. Mental health problems and help-seeking behavior among college students. J Adolesc Health. 2010;46:3–10. https://doi.org/10.1016/j.jadohealth.2009.08.008 (PMID: 20123251).
Article PubMed Google Scholar
Wang H, Xia Q, Xiong Z, Li Z, Xiang W, Yuan Y, et al. The psychological distress and coping styles in the early stages of the 2019 Coronavirus disease (COVID-19) epidemic in the general mainland Chinese population: A web-based survey. PLoS ONE. 2020;15:e0233410. https://doi.org/10.1371/journal.pone.0233410 .
Ross AGP, Crowe SM, Tyndall MW. Planning for the next global Pandemic. Int J Infect Dis. 2015;38:89–94. https://doi.org/10.1016/j.ijid.2015.07.016 .
Download references
Acknowledgements
Fatima Azmi would like to thank Prince Sultan University for funding the project and covering the publication fees.
This work was supported by a research project grant [Grant number: COVID-19-DES-2020–43] from Prince Sultan University, KSA.
Author information
Authors and affiliations.
Department of Mathematics and Sciences, College of Humanities and Sciences, Prince Sultan University, Riyadh, Saudi Arabia
Fatima M. Azmi
Department of Statistics, University of Science and Technology, Bannu, KPK, Pakistan
Habib Nawaz Khan
Department of Computer Science, College of Computer and Information Sciences, King Saud University, Riyadh, Saudi Arabia
Aqil M. Azmi
You can also search for this author in PubMed Google Scholar
Contributions
Conceptualization: FMA; AMA. Data Curation: FMA; AMA. Formal Analysis: FMA. Methodology: FMA; HNK. Writing-Original Draft: FMA; AMA. Writing-Review and Editing: FMA; HNK; AMA. The author(s) read and approved the final manuscript.
Corresponding author
Correspondence to Fatima M. Azmi .
Ethics declarations
Ethics approval and consent to participate.
This study was approved by Prince Sultan University’s Institutional Review Board (IRB) (Reference Number: PSU IRB-2021–03-0077). Furthermore, informed consent was obtained from the participants.
Competing interests
The authors declare no conflict of interest.
Additional information
Publisher’s note.
Springer Nature remains neutral with regard to jurisdictional claims in published maps and institutional affiliations.
Rights and permissions
Open Access This article is licensed under a Creative Commons Attribution 4.0 International License, which permits use, sharing, adaptation, distribution and reproduction in any medium or format, as long as you give appropriate credit to the original author(s) and the source, provide a link to the Creative Commons licence, and indicate if changes were made. The images or other third party material in this article are included in the article's Creative Commons licence, unless indicated otherwise in a credit line to the material. If material is not included in the article's Creative Commons licence and your intended use is not permitted by statutory regulation or exceeds the permitted use, you will need to obtain permission directly from the copyright holder. To view a copy of this licence, visit http://creativecommons.org/licenses/by/4.0/ . The Creative Commons Public Domain Dedication waiver ( http://creativecommons.org/publicdomain/zero/1.0/ ) applies to the data made available in this article, unless otherwise stated in a credit line to the data.
Reprints and permissions
About this article
Cite this article.
Azmi, F.M., Khan, H.N. & Azmi, A.M. The impact of virtual learning on students’ educational behavior and pervasiveness of depression among university students due to the COVID-19 pandemic. Global Health 18 , 70 (2022). https://doi.org/10.1186/s12992-022-00863-z
Download citation
Received : 11 April 2022
Accepted : 29 June 2022
Published : 14 July 2022
DOI : https://doi.org/10.1186/s12992-022-00863-z
Share this article
Anyone you share the following link with will be able to read this content:
Sorry, a shareable link is not currently available for this article.
Provided by the Springer Nature SharedIt content-sharing initiative
- Saudi Arabia
- Higher education
- Quality education
Globalization and Health
ISSN: 1744-8603
- Submission enquiries: [email protected]
- General enquiries: [email protected]

An official website of the United States government
The .gov means it’s official. Federal government websites often end in .gov or .mil. Before sharing sensitive information, make sure you’re on a federal government site.
The site is secure. The https:// ensures that you are connecting to the official website and that any information you provide is encrypted and transmitted securely.
- Publications
- Account settings
Preview improvements coming to the PMC website in October 2024. Learn More or Try it out now .
- Advanced Search
- Journal List
- Wiley - PMC COVID-19 Collection

Online and face‐to‐face learning: Evidence from students’ performance during the Covid‐19 pandemic
Carolyn chisadza.
1 Department of Economics, University of Pretoria, Hatfield South Africa
Matthew Clance
Thulani mthembu.
2 Department of Education Innovation, University of Pretoria, Hatfield South Africa
Nicky Nicholls
Eleni yitbarek.
This study investigates the factors that predict students' performance after transitioning from face‐to‐face to online learning as a result of the Covid‐19 pandemic. It uses students' responses from survey questions and the difference in the average assessment grades between pre‐lockdown and post‐lockdown at a South African university. We find that students' performance was positively associated with good wifi access, relative to using mobile internet data. We also observe lower academic performance for students who found transitioning to online difficult and who expressed a preference for self‐study (i.e. reading through class slides and notes) over assisted study (i.e. joining live lectures or watching recorded lectures). The findings suggest that improving digital infrastructure and reducing the cost of internet access may be necessary for mitigating the impact of the Covid‐19 pandemic on education outcomes.
1. INTRODUCTION
The Covid‐19 pandemic has been a wake‐up call to many countries regarding their capacity to cater for mass online education. This situation has been further complicated in developing countries, such as South Africa, who lack the digital infrastructure for the majority of the population. The extended lockdown in South Africa saw most of the universities with mainly in‐person teaching scrambling to source hardware (e.g. laptops, internet access), software (e.g. Microsoft packages, data analysis packages) and internet data for disadvantaged students in order for the semester to recommence. Not only has the pandemic revealed the already stark inequality within the tertiary student population, but it has also revealed that high internet data costs in South Africa may perpetuate this inequality, making online education relatively inaccessible for disadvantaged students. 1
The lockdown in South Africa made it possible to investigate the changes in second‐year students' performance in the Economics department at the University of Pretoria. In particular, we are interested in assessing what factors predict changes in students' performance after transitioning from face‐to‐face (F2F) to online learning. Our main objectives in answering this study question are to establish what study materials the students were able to access (i.e. slides, recordings, or live sessions) and how students got access to these materials (i.e. the infrastructure they used).
The benefits of education on economic development are well established in the literature (Gyimah‐Brempong, 2011 ), ranging from health awareness (Glick et al., 2009 ), improved technological innovations, to increased capacity development and employment opportunities for the youth (Anyanwu, 2013 ; Emediegwu, 2021 ). One of the ways in which inequality is perpetuated in South Africa, and Africa as a whole, is through access to education (Anyanwu, 2016 ; Coetzee, 2014 ; Tchamyou et al., 2019 ); therefore, understanding the obstacles that students face in transitioning to online learning can be helpful in ensuring more equal access to education.
Using students' responses from survey questions and the difference in the average grades between pre‐lockdown and post‐lockdown, our findings indicate that students' performance in the online setting was positively associated with better internet access. Accessing assisted study material, such as narrated slides or recordings of the online lectures, also helped students. We also find lower academic performance for students who reported finding transitioning to online difficult and for those who expressed a preference for self‐study (i.e. reading through class slides and notes) over assisted study (i.e. joining live lectures or watching recorded lectures). The average grades between pre‐lockdown and post‐lockdown were about two points and three points lower for those who reported transitioning to online teaching difficult and for those who indicated a preference for self‐study, respectively. The findings suggest that improving the quality of internet infrastructure and providing assisted learning can be beneficial in reducing the adverse effects of the Covid‐19 pandemic on learning outcomes.
Our study contributes to the literature by examining the changes in the online (post‐lockdown) performance of students and their F2F (pre‐lockdown) performance. This approach differs from previous studies that, in most cases, use between‐subject designs where one group of students following online learning is compared to a different group of students attending F2F lectures (Almatra et al., 2015 ; Brown & Liedholm, 2002 ). This approach has a limitation in that that there may be unobserved characteristics unique to students choosing online learning that differ from those choosing F2F lectures. Our approach avoids this issue because we use a within‐subject design: we compare the performance of the same students who followed F2F learning Before lockdown and moved to online learning during lockdown due to the Covid‐19 pandemic. Moreover, the study contributes to the limited literature that compares F2F and online learning in developing countries.
Several studies that have also compared the effectiveness of online learning and F2F classes encounter methodological weaknesses, such as small samples, not controlling for demographic characteristics, and substantial differences in course materials and assessments between online and F2F contexts. To address these shortcomings, our study is based on a relatively large sample of students and includes demographic characteristics such as age, gender and perceived family income classification. The lecturer and course materials also remained similar in the online and F2F contexts. A significant proportion of our students indicated that they never had online learning experience before. Less than 20% of the students in the sample had previous experience with online learning. This highlights the fact that online education is still relatively new to most students in our sample.
Given the global experience of the fourth industrial revolution (4IR), 2 with rapidly accelerating technological progress, South Africa needs to be prepared for the possibility of online learning becoming the new norm in the education system. To this end, policymakers may consider engaging with various organizations (schools, universities, colleges, private sector, and research facilities) To adopt interventions that may facilitate the transition to online learning, while at the same time ensuring fair access to education for all students across different income levels. 3
1.1. Related literature
Online learning is a form of distance education which mainly involves internet‐based education where courses are offered synchronously (i.e. live sessions online) and/or asynchronously (i.e. students access course materials online in their own time, which is associated with the more traditional distance education). On the other hand, traditional F2F learning is real time or synchronous learning. In a physical classroom, instructors engage with the students in real time, while in the online format instructors can offer real time lectures through learning management systems (e.g. Blackboard Collaborate), or record the lectures for the students to watch later. Purely online courses are offered entirely over the internet, while blended learning combines traditional F2F classes with learning over the internet, and learning supported by other technologies (Nguyen, 2015 ).
Moreover, designing online courses requires several considerations. For example, the quality of the learning environment, the ease of using the learning platform, the learning outcomes to be achieved, instructor support to assist and motivate students to engage with the course material, peer interaction, class participation, type of assessments (Paechter & Maier, 2010 ), not to mention training of the instructor in adopting and introducing new teaching methods online (Lundberg et al., 2008 ). In online learning, instructors are more facilitators of learning. On the other hand, traditional F2F classes are structured in such a way that the instructor delivers knowledge, is better able to gauge understanding and interest of students, can engage in class activities, and can provide immediate feedback on clarifying questions during the class. Additionally, the designing of traditional F2F courses can be less time consuming for instructors compared to online courses (Navarro, 2000 ).
Online learning is also particularly suited for nontraditional students who require flexibility due to work or family commitments that are not usually associated with the undergraduate student population (Arias et al., 2018 ). Initially the nontraditional student belonged to the older adult age group, but with blended learning becoming more commonplace in high schools, colleges and universities, online learning has begun to traverse a wider range of age groups. However, traditional F2F classes are still more beneficial for learners that are not so self‐sufficient and lack discipline in working through the class material in the required time frame (Arias et al., 2018 ).
For the purpose of this literature review, both pure online and blended learning are considered to be online learning because much of the evidence in the literature compares these two types against the traditional F2F learning. The debate in the literature surrounding online learning versus F2F teaching continues to be a contentious one. A review of the literature reveals mixed findings when comparing the efficacy of online learning on student performance in relation to the traditional F2F medium of instruction (Lundberg et al., 2008 ; Nguyen, 2015 ). A number of studies conducted Before the 2000s find what is known today in the empirical literature as the “No Significant Difference” phenomenon (Russell & International Distance Education Certificate Center (IDECC), 1999 ). The seminal work from Russell and IDECC ( 1999 ) involved over 350 comparative studies on online/distance learning versus F2F learning, dating back to 1928. The author finds no significant difference overall between online and traditional F2F classroom education outcomes. Subsequent studies that followed find similar “no significant difference” outcomes (Arbaugh, 2000 ; Fallah & Ubell, 2000 ; Freeman & Capper, 1999 ; Johnson et al., 2000 ; Neuhauser, 2002 ). While Bernard et al. ( 2004 ) also find that overall there is no significant difference in achievement between online education and F2F education, the study does find significant heterogeneity in student performance for different activities. The findings show that students in F2F classes outperform the students participating in synchronous online classes (i.e. classes that require online students to participate in live sessions at specific times). However, asynchronous online classes (i.e. students access class materials at their own time online) outperform F2F classes.
More recent studies find significant results for online learning outcomes in relation to F2F outcomes. On the one hand, Shachar and Yoram ( 2003 ) and Shachar and Neumann ( 2010 ) conduct a meta‐analysis of studies from 1990 to 2009 and find that in 70% of the cases, students taking courses by online education outperformed students in traditionally instructed courses (i.e. F2F lectures). In addition, Navarro and Shoemaker ( 2000 ) observe that learning outcomes for online learners are as effective as or better than outcomes for F2F learners, regardless of background characteristics. In a study on computer science students, Dutton et al. ( 2002 ) find online students perform significantly better compared to the students who take the same course on campus. A meta‐analysis conducted by the US Department of Education finds that students who took all or part of their course online performed better, on average, than those taking the same course through traditional F2F instructions. The report also finds that the effect sizes are larger for studies in which the online learning was collaborative or instructor‐driven than in those studies where online learners worked independently (Means et al., 2010 ).
On the other hand, evidence by Brown and Liedholm ( 2002 ) based on test scores from macroeconomics students in the United States suggest that F2F students tend to outperform online students. These findings are supported by Coates et al. ( 2004 ) who base their study on macroeconomics students in the United States, and Xu and Jaggars ( 2014 ) who find negative effects for online students using a data set of about 500,000 courses taken by over 40,000 students in Washington. Furthermore, Almatra et al. ( 2015 ) compare overall course grades between online and F2F students for a Telecommunications course and find that F2F students significantly outperform online learning students. In an experimental study where students are randomly assigned to attend live lectures versus watching the same lectures online, Figlio et al. ( 2013 ) observe some evidence that the traditional format has a positive effect compared to online format. Interestingly, Callister and Love ( 2016 ) specifically compare the learning outcomes of online versus F2F skills‐based courses and find that F2F learners earned better outcomes than online learners even when using the same technology. This study highlights that some of the inconsistencies that we find in the results comparing online to F2F learning might be influenced by the nature of the course: theory‐based courses might be less impacted by in‐person interaction than skills‐based courses.
The fact that the reviewed studies on the effects of F2F versus online learning on student performance have been mainly focused in developed countries indicates the dearth of similar studies being conducted in developing countries. This gap in the literature may also highlight a salient point: online learning is still relatively underexplored in developing countries. The lockdown in South Africa therefore provides us with an opportunity to contribute to the existing literature from a developing country context.
2. CONTEXT OF STUDY
South Africa went into national lockdown in March 2020 due to the Covid‐19 pandemic. Like most universities in the country, the first semester for undergraduate courses at the University of Pretoria had already been running since the start of the academic year in February. Before the pandemic, a number of F2F lectures and assessments had already been conducted in most courses. The nationwide lockdown forced the university, which was mainly in‐person teaching, to move to full online learning for the remainder of the semester. This forced shift from F2F teaching to online learning allows us to investigate the changes in students' performance.
Before lockdown, classes were conducted on campus. During lockdown, these live classes were moved to an online platform, Blackboard Collaborate, which could be accessed by all registered students on the university intranet (“ClickUP”). However, these live online lectures involve substantial internet data costs for students. To ensure access to course content for those students who were unable to attend the live online lectures due to poor internet connections or internet data costs, several options for accessing course content were made available. These options included prerecorded narrated slides (which required less usage of internet data), recordings of the live online lectures, PowerPoint slides with explanatory notes and standard PDF lecture slides.
At the same time, the university managed to procure and loan out laptops to a number of disadvantaged students, and negotiated with major mobile internet data providers in the country for students to have free access to study material through the university's “connect” website (also referred to as the zero‐rated website). However, this free access excluded some video content and live online lectures (see Table 1 ). The university also provided between 10 and 20 gigabytes of mobile internet data per month, depending on the network provider, sent to students' mobile phones to assist with internet data costs.
Sites available on zero‐rated website
Note : The table summarizes the sites that were available on the zero‐rated website and those that incurred data costs.
High data costs continue to be a contentious issue in Africa where average incomes are low. Gilbert ( 2019 ) reports that South Africa ranked 16th of the 45 countries researched in terms of the most expensive internet data in Africa, at US$6.81 per gigabyte, in comparison to other Southern African countries such as Mozambique (US$1.97), Zambia (US$2.70), and Lesotho (US$4.09). Internet data prices have also been called into question in South Africa after the Competition Commission published a report from its Data Services Market Inquiry calling the country's internet data pricing “excessive” (Gilbert, 2019 ).
3. EMPIRICAL APPROACH
We use a sample of 395 s‐year students taking a macroeconomics module in the Economics department to compare the effects of F2F and online learning on students' performance using a range of assessments. The module was an introduction to the application of theoretical economic concepts. The content was both theory‐based (developing economic growth models using concepts and equations) and skill‐based (application involving the collection of data from online data sources and analyzing the data using statistical software). Both individual and group assignments formed part of the assessments. Before the end of the semester, during lockdown in June 2020, we asked the students to complete a survey with questions related to the transition from F2F to online learning and the difficulties that they may have faced. For example, we asked the students: (i) how easy or difficult they found the transition from F2F to online lectures; (ii) what internet options were available to them and which they used the most to access the online prescribed work; (iii) what format of content they accessed and which they preferred the most (i.e. self‐study material in the form of PDF and PowerPoint slides with notes vs. assisted study with narrated slides and lecture recordings); (iv) what difficulties they faced accessing the live online lectures, to name a few. Figure 1 summarizes the key survey questions that we asked the students regarding their transition from F2F to online learning.

Summary of survey data
Before the lockdown, the students had already attended several F2F classes and completed three assessments. We are therefore able to create a dependent variable that is comprised of the average grades of three assignments taken before lockdown and the average grades of three assignments taken after the start of the lockdown for each student. Specifically, we use the difference between the post‐ and pre‐lockdown average grades as the dependent variable. However, the number of student observations dropped to 275 due to some students missing one or more of the assessments. The lecturer, content and format of the assessments remain similar across the module. We estimate the following equation using ordinary least squares (OLS) with robust standard errors:
where Y i is the student's performance measured by the difference between the post and pre‐lockdown average grades. B represents the vector of determinants that measure the difficulty faced by students to transition from F2F to online learning. This vector includes access to the internet, study material preferred, quality of the online live lecture sessions and pre‐lockdown class attendance. X is the vector of student demographic controls such as race, gender and an indicator if the student's perceived family income is below average. The ε i is unobserved student characteristics.
4. ANALYSIS
4.1. descriptive statistics.
Table 2 gives an overview of the sample of students. We find that among the black students, a higher proportion of students reported finding the transition to online learning more difficult. On the other hand, more white students reported finding the transition moderately easy, as did the other races. According to Coetzee ( 2014 ), the quality of schools can vary significantly between higher income and lower‐income areas, with black South Africans far more likely to live in lower‐income areas with lower quality schools than white South Africans. As such, these differences in quality of education from secondary schooling can persist at tertiary level. Furthermore, persistent income inequality between races in South Africa likely means that many poorer black students might not be able to afford wifi connections or large internet data bundles which can make the transition difficult for black students compared to their white counterparts.
Descriptive statistics
Notes : The transition difficulty variable was ordered 1: Very Easy; 2: Moderately Easy; 3: Difficult; and 4: Impossible. Since we have few responses to the extremes, we combined Very Easy and Moderately as well as Difficult and Impossible to make the table easier to read. The table with a full breakdown is available upon request.
A higher proportion of students reported that wifi access made the transition to online learning moderately easy. However, relatively more students reported that mobile internet data and accessing the zero‐rated website made the transition difficult. Surprisingly, not many students made use of the zero‐rated website which was freely available. Figure 2 shows that students who reported difficulty transitioning to online learning did not perform as well in online learning versus F2F when compared to those that found it less difficult to transition.

Transition from F2F to online learning.
Notes : This graph shows the students' responses to the question “How easy did you find the transition from face‐to‐face lectures to online lectures?” in relation to the outcome variable for performance
In Figure 3 , the kernel density shows that students who had access to wifi performed better than those who used mobile internet data or the zero‐rated data.

Access to online learning.
Notes : This graph shows the students' responses to the question “What do you currently use the most to access most of your prescribed work?” in relation to the outcome variable for performance
The regression results are reported in Table 3 . We find that the change in students' performance from F2F to online is negatively associated with the difficulty they faced in transitioning from F2F to online learning. According to student survey responses, factors contributing to difficulty in transitioning included poor internet access, high internet data costs and lack of equipment such as laptops or tablets to access the study materials on the university website. Students who had access to wifi (i.e. fixed wireless broadband, Asymmetric Digital Subscriber Line (ADSL) or optic fiber) performed significantly better, with on average 4.5 points higher grade, in relation to students that had to use mobile internet data (i.e. personal mobile internet data, wifi at home using mobile internet data, or hotspot using mobile internet data) or the zero‐rated website to access the study materials. The insignificant results for the zero‐rated website are surprising given that the website was freely available and did not incur any internet data costs. However, most students in this sample complained that the internet connection on the zero‐rated website was slow, especially in uploading assignments. They also complained about being disconnected when they were in the middle of an assessment. This may have discouraged some students from making use of the zero‐rated website.
Results: Predictors for student performance using the difference on average assessment grades between pre‐ and post‐lockdown
Coefficients reported. Robust standard errors in parentheses.
∗∗∗ p < .01.
Students who expressed a preference for self‐study approaches (i.e. reading PDF slides or PowerPoint slides with explanatory notes) did not perform as well, on average, as students who preferred assisted study (i.e. listening to recorded narrated slides or lecture recordings). This result is in line with Means et al. ( 2010 ), where student performance was better for online learning that was collaborative or instructor‐driven than in cases where online learners worked independently. Interestingly, we also observe that the performance of students who often attended in‐person classes before the lockdown decreased. Perhaps these students found the F2F lectures particularly helpful in mastering the course material. From the survey responses, we find that a significant proportion of the students (about 70%) preferred F2F to online lectures. This preference for F2F lectures may also be linked to the factors contributing to the difficulty some students faced in transitioning to online learning.
We find that the performance of low‐income students decreased post‐lockdown, which highlights another potential challenge to transitioning to online learning. The picture and sound quality of the live online lectures also contributed to lower performance. Although this result is not statistically significant, it is worth noting as the implications are linked to the quality of infrastructure currently available for students to access online learning. We find no significant effects of race on changes in students' performance, though males appeared to struggle more with the shift to online teaching than females.
For the robustness check in Table 4 , we consider the average grades of the three assignments taken after the start of the lockdown as a dependent variable (i.e. the post‐lockdown average grades for each student). We then include the pre‐lockdown average grades as an explanatory variable. The findings and overall conclusions in Table 4 are consistent with the previous results.
Robustness check: Predictors for student performance using the average assessment grades for post‐lockdown
As a further robustness check in Table 5 , we create a panel for each student across the six assignment grades so we can control for individual heterogeneity. We create a post‐lockdown binary variable that takes the value of 1 for the lockdown period and 0 otherwise. We interact the post‐lockdown dummy variable with a measure for transition difficulty and internet access. The internet access variable is an indicator variable for mobile internet data, wifi, or zero‐rated access to class materials. The variable wifi is a binary variable taking the value of 1 if the student has access to wifi and 0 otherwise. The zero‐rated variable is a binary variable taking the value of 1 if the student used the university's free portal access and 0 otherwise. We also include assignment and student fixed effects. The results in Table 5 remain consistent with our previous findings that students who had wifi access performed significantly better than their peers.
Interaction model
Notes : Coefficients reported. Robust standard errors in parentheses. The dependent variable is the assessment grades for each student on each assignment. The number of observations include the pre‐post number of assessments multiplied by the number of students.
6. CONCLUSION
The Covid‐19 pandemic left many education institutions with no option but to transition to online learning. The University of Pretoria was no exception. We examine the effect of transitioning to online learning on the academic performance of second‐year economic students. We use assessment results from F2F lectures before lockdown, and online lectures post lockdown for the same group of students, together with responses from survey questions. We find that the main contributor to lower academic performance in the online setting was poor internet access, which made transitioning to online learning more difficult. In addition, opting to self‐study (read notes instead of joining online classes and/or watching recordings) did not help the students in their performance.
The implications of the results highlight the need for improved quality of internet infrastructure with affordable internet data pricing. Despite the university's best efforts not to leave any student behind with the zero‐rated website and free monthly internet data, the inequality dynamics in the country are such that invariably some students were negatively affected by this transition, not because the student was struggling academically, but because of inaccessibility of internet (wifi). While the zero‐rated website is a good collaborative initiative between universities and network providers, the infrastructure is not sufficient to accommodate mass students accessing it simultaneously.
This study's findings may highlight some shortcomings in the academic sector that need to be addressed by both the public and private sectors. There is potential for an increase in the digital divide gap resulting from the inequitable distribution of digital infrastructure. This may lead to reinforcement of current inequalities in accessing higher education in the long term. To prepare the country for online learning, some considerations might need to be made to make internet data tariffs more affordable and internet accessible to all. We hope that this study's findings will provide a platform (or will at least start the conversation for taking remedial action) for policy engagements in this regard.
We are aware of some limitations presented by our study. The sample we have at hand makes it difficult to extrapolate our findings to either all students at the University of Pretoria or other higher education students in South Africa. Despite this limitation, our findings highlight the negative effect of the digital divide on students' educational outcomes in the country. The transition to online learning and the high internet data costs in South Africa can also have adverse learning outcomes for low‐income students. With higher education institutions, such as the University of Pretoria, integrating online teaching to overcome the effect of the Covid‐19 pandemic, access to stable internet is vital for students' academic success.
It is also important to note that the data we have at hand does not allow us to isolate wifi's causal effect on students' performance post‐lockdown due to two main reasons. First, wifi access is not randomly assigned; for instance, there is a high chance that students with better‐off family backgrounds might have better access to wifi and other supplementary infrastructure than their poor counterparts. Second, due to the university's data access policy and consent, we could not merge the data at hand with the student's previous year's performance. Therefore, future research might involve examining the importance of these elements to document the causal impact of access to wifi on students' educational outcomes in the country.
ACKNOWLEDGMENT
The authors acknowledge the helpful comments received from the editor, the anonymous reviewers, and Elizabeth Asiedu.
Chisadza, C. , Clance, M. , Mthembu, T. , Nicholls, N. , & Yitbarek, E. (2021). Online and face‐to‐face learning: Evidence from students’ performance during the Covid‐19 pandemic . Afr Dev Rev , 33 , S114–S125. 10.1111/afdr.12520 [ CrossRef ] [ Google Scholar ]
1 https://mybroadband.co.za/news/cellular/309693-mobile-data-prices-south-africa-vs-the-world.html .
2 The 4IR is currently characterized by increased use of new technologies, such as advanced wireless technologies, artificial intelligence, cloud computing, robotics, among others. This era has also facilitated the use of different online learning platforms ( https://www.brookings.edu/research/the-fourth-industrialrevolution-and-digitization-will-transform-africa-into-a-global-powerhouse/ ).
3 Note that we control for income, but it is plausible to assume other unobservable factors such as parental preference and parenting style might also affect access to the internet of students.
- Almatra, O. , Johri, A. , Nagappan, K. , & Modanlu, A. (2015). An empirical study of face‐to‐face and distance learning sections of a core telecommunication course (Conference Proceedings Paper No. 12944). 122nd ASEE Annual Conference and Exposition, Seattle, Washington State.
- Anyanwu, J. C. (2013). Characteristics and macroeconomic determinants of youth employment in Africa . African Development Review , 25 ( 2 ), 107–129. [ Google Scholar ]
- Anyanwu, J. C. (2016). Accounting for gender equality in secondary school enrolment in Africa: Accounting for gender equality in secondary school enrolment . African Development Review , 28 ( 2 ), 170–191. [ Google Scholar ]
- Arbaugh, J. (2000). Virtual classroom versus physical classroom: An exploratory study of class discussion patterns and student learning in an asynchronous internet‐based MBA course . Journal of Management Education , 24 ( 2 ), 213–233. [ Google Scholar ]
- Arias, J. J. , Swinton, J. , & Anderson, K. (2018). On‐line vs. face‐to‐face: A comparison of student outcomes with random assignment . e‐Journal of Business Education and Scholarship of Teaching, , 12 ( 2 ), 1–23. [ Google Scholar ]
- Bernard, R. M. , Abrami, P. C. , Lou, Y. , Borokhovski, E. , Wade, A. , Wozney, L. , Wallet, P. A. , Fiset, M. , & Huang, B. (2004). How does distance education compare with classroom instruction? A meta‐analysis of the empirical literature . Review of Educational Research , 74 ( 3 ), 379–439. [ Google Scholar ]
- Brown, B. , & Liedholm, C. (2002). Can web courses replace the classroom in principles of microeconomics? American Economic Review , 92 ( 2 ), 444–448. [ Google Scholar ]
- Callister, R. R. , & Love, M. S. (2016). A comparison of learning outcomes in skills‐based courses: Online versus face‐to‐face formats . Decision Sciences Journal of Innovative Education , 14 ( 2 ), 243–256. [ Google Scholar ]
- Coates, D. , Humphreys, B. R. , Kane, J. , & Vachris, M. A. (2004). “No significant distance” between face‐to‐face and online instruction: Evidence from principles of economics . Economics of Education Review , 23 ( 5 ), 533–546. [ Google Scholar ]
- Coetzee, M. (2014). School quality and the performance of disadvantaged learners in South Africa (Working Paper No. 22). University of Stellenbosch Economics Department, Stellenbosch
- Dutton, J. , Dutton, M. , & Perry, J. (2002). How do online students differ from lecture students? Journal of Asynchronous Learning Networks , 6 ( 1 ), 1–20. [ Google Scholar ]
- Emediegwu, L. (2021). Does educational investment enhance capacity development for Nigerian youths? An autoregressive distributed lag approach . African Development Review , 32 ( S1 ), S45–S53. [ Google Scholar ]
- Fallah, M. H. , & Ubell, R. (2000). Blind scores in a graduate test. Conventional compared with web‐based outcomes . ALN Magazine , 4 ( 2 ). [ Google Scholar ]
- Figlio, D. , Rush, M. , & Yin, L. (2013). Is it live or is it internet? Experimental estimates of the effects of online instruction on student learning . Journal of Labor Economics , 31 ( 4 ), 763–784. [ Google Scholar ]
- Freeman, M. A. , & Capper, J. M. (1999). Exploiting the web for education: An anonymous asynchronous role simulation . Australasian Journal of Educational Technology , 15 ( 1 ), 95–116. [ Google Scholar ]
- Gilbert, P. (2019). The most expensive data prices in Africa . Connecting Africa. https://www.connectingafrica.com/author.asp?section_id=761%26doc_id=756372
- Glick, P. , Randriamamonjy, J. , & Sahn, D. (2009). Determinants of HIV knowledge and condom use among women in Madagascar: An analysis using matched household and community data . African Development Review , 21 ( 1 ), 147–179. [ Google Scholar ]
- Gyimah‐Brempong, K. (2011). Education and economic development in Africa . African Development Review , 23 ( 2 ), 219–236. [ Google Scholar ]
- Johnson, S. , Aragon, S. , Shaik, N. , & Palma‐Rivas, N. (2000). Comparative analysis of learner satisfaction and learning outcomes in online and face‐to‐face learning environments . Journal of Interactive Learning Research , 11 ( 1 ), 29–49. [ Google Scholar ]
- Lundberg, J. , Merino, D. , & Dahmani, M. (2008). Do online students perform better than face‐to‐face students? Reflections and a short review of some empirical findings . Revista de Universidad y Sociedad del Conocimiento , 5 ( 1 ), 35–44. [ Google Scholar ]
- Means, B. , Toyama, Y. , Murphy, R. , Bakia, M. , & Jones, K. (2010). Evaluation of evidence‐based practices in online learning: A meta‐analysis and review of online learning studies (Report No. ed‐04‐co‐0040 task 0006). U.S. Department of Education, Office of Planning, Evaluation, and Policy Development, Washington DC.
- Navarro, P. (2000). Economics in the cyber‐classroom . Journal of Economic Perspectives , 14 ( 2 ), 119–132. [ Google Scholar ]
- Navarro, P. , & Shoemaker, J. (2000). Performance and perceptions of distance learners in cyberspace . American Journal of Distance Education , 14 ( 2 ), 15–35. [ Google Scholar ]
- Neuhauser, C. (2002). Learning style and effectiveness of online and face‐to‐face instruction . American Journal of Distance Education , 16 ( 2 ), 99–113. [ Google Scholar ]
- Nguyen, T. (2015). The effectiveness of online learning: Beyond no significant difference and future horizons . MERLOT Journal of Online Teaching and Learning , 11 ( 2 ), 309–319. [ Google Scholar ]
- Paechter, M. , & Maier, B. (2010). Online or face‐to‐face? Students' experiences and preferences in e‐learning . Internet and Higher Education , 13 ( 4 ), 292–297. [ Google Scholar ]
- Russell, T. L. , & International Distance Education Certificate Center (IDECC) (1999). The no significant difference phenomenon: A comparative research annotated bibliography on technology for distance education: As reported in 355 research reports, summaries and papers . North Carolina State University. [ Google Scholar ]
- Shachar, M. , & Neumann, Y. (2010). Twenty years of research on the academic performance differences between traditional and distance learning: Summative meta‐analysis and trend examination . MERLOT Journal of Online Learning and Teaching , 6 ( 2 ), 318–334. [ Google Scholar ]
- Shachar, M. , & Yoram, N. (2003). Differences between traditional and distance education academic performances: A meta‐analytic approach . International Review of Research in Open and Distance Learning , 4 ( 2 ), 1–20. [ Google Scholar ]
- Tchamyou, V. S. , Asongu, S. , & Odhiambo, N. (2019). The role of ICT in modulating the effect of education and lifelong learning on income inequality and economic growth in Africa . African Development Review , 31 ( 3 ), 261–274. [ Google Scholar ]
- Xu, D. , & Jaggars, S. S. (2014). Performance gaps between online and face‐to‐face courses: Differences across types of students and academic subject areas . The Journal of Higher Education , 85 ( 5 ), 633–659. [ Google Scholar ]
- Open supplemental data
- Reference Manager
- Simple TEXT file
People also looked at
Original research article, factors and recommendations to support students’ enjoyment of online learning with fun: a mixed method study during covid-19.
- Rumpus Research Group, Faculty of Wellbeing, Education and Language Studies, The Open University, Milton Keynes, United Kingdom
Understanding components that influence students’ enjoyment of distance higher education is increasingly important to enhance academic performance and retention. Although there is a growing body of research about students’ engagement with online learning, a research gap exists concerning whether fun affect students’ enjoyment. A contributing factor to this situation is that the meaning of fun in learning is unclear, and its possible role is controversial. This research is original in examining students’ views about fun and online learning, and influential components and connections. This study investigated the beliefs and attitudes of a sample of 551 distance education students including pre-services and in-service teachers, consultants and education professionals using a mixed-method approach. Quantitative and Qualitative data were generated through a self-reflective instrument during the COVID-19 pandemic. The findings revealed that 88.77% of participants valued fun in online learning; linked to well-being, motivation and performance. However, 16.66% mentioned that fun within online learning could take the focus off their studies and result in distraction or loss of time. Principal component analysis revealed three groups of students who found (1) fun relevant in socio-constructivist learning (2) no fun in traditional transmissive learning and (3) disturbing fun in constructivist learning. This study also provides key recommendations extracted from participants’ views supported by consensual review for course teams, teaching staff and students to enhance online learning experiences with enjoyment and fun.
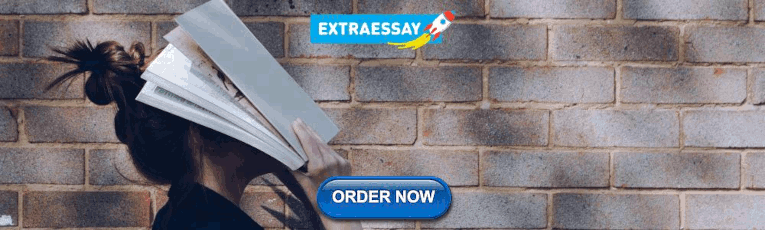
Introduction
Online learning has been considered vital in 21st century to provide flexible education for students as well to address the gap between demand for higher education and supply. Governments have advocated increasing rates of completion of secondary and higher education in the face of rapid population growth. However, they face financial pressure to support these larger numbers directly through additional infrastructure, in addition to scholarships and student loans ( Cooperman, 2014 :1).
In recent years, there has been an increasing interest in distance online learning not only to educate students who work but also who live too remotely or cannot access traditional campus universities for other reasons. However, literature shows that online distant education has dropout rates higher than traditional universities ( Xavier and Meneses, 2020 ). Studies also suggest that the students’ level of satisfaction about their online learning and own academic performance have significant correlation with their level of persistence toward completion ( Gortan and Jereb, 2007 ; Higher Education Academy (HEA), 2015 ).
Understanding components that influence students’ enjoyment in distance higher education is fundamental to promote student retention and success ( Higher Education Academy (HEA), 2015 ) during and post COVID-19 pandemic. There is a growing body of research about students’ engagement in virtual learning environments ( Arnone et al., 2011 ). However, there are key issues that whilst extensively researched in traditional teaching, remain relatively absent from research into distance education. For example, a long established body of research exists that demonstrates a link between students’ epistemological beliefs and their study, engagement, and outcomes ( Rodriguez and Cano, 2007 ; Richardson, 2013 ). The types of epistemological beliefs typically examined fall into two broad categories. The first is derived from Schommer’s research ( Schommer, 1990 ), in which she elicited dimensions that reflected students differing beliefs. This included “simple knowledge” (knowledge as isolated facts vs. knowledge as integrated conceptions) and “innate ability” (ability to learn is genetically determined vs. the ability to learn is enhanced through experience). The second category of research is more directly aligned with pedagogy. This has positioned epistemological beliefs in relation to traditional or constructivist beliefs. Traditional views of learning see learning occurring via the non-problematic transfer of untransformed knowledge from expert to student ( Chan and Elliott, 2004 ). This contrasts with constructivist beliefs in which knowledge arises through reasoning, which is facilitated by teaching ( Lee et al., 2013 ). This type of framing can be seen in large scale international comparative research, such as the Organization for Economic Co-operation and Development’s survey of teachers’ epistemological beliefs across 23 countries ( Organisation for Economic Co-operation and Development (OECD), 2010 , 2013 ). However, in relation to online and distance higher education, epistemological research is relatively absent ( Richardson, 2013 ; Knight et al., 2017 ). Given the impact of epistemological beliefs on students’ study experiences there is a need for greater epistemologically focused research in the context of online education.
Another underrepresented research area concerns fun in online learning; in particular, because the meaning of fun is unclear and controversial. There is no consensus about the value of fun in learning and what a fun learning experience means in higher education ( McManus and Furnham, 2010 ; Lesser et al., 2013 ; Tews et al., 2015 ; Whitton and Langan, 2018 ). Tews et al. (2015) argue that fun is a term used regularly in various contexts including education. Yet there is no clear agreement about its role and relationships with students’ learning experience. Congruently, McManus and Furnham (2010) highlight that fun has different meanings for different people and literature is limited about what generally comprises fun for learners. Similarly, Lesser et al. (2013) indicate that views about fun among educators are ambivalent as fun is perceived as too difficult or time-consuming to be implemented and it may distract students from serious learning. These three studies indicate that evidence about fun and learning are circumstantial and subjective for teaching staff to consider it as a compelling component for making their students’ experience more impactful. So that, further studies would be worthwhile to examine the practical meaning and educational value of fun on Distance Higher Education with a systematic and rigorous methodological approach.
To explore this challenge, this paper investigates students’ reflective views about fun and online learning and whether fun and enjoyment are interconnected components to enhance enthusiasm to learn and excel in online distant education. This investigation considers a critical question framed by the authors from Whitton and Langan (2018:11)’s work. How can we explore the impact of fun in higher education in view of the complexity of factors involved? To explore this question, this work is based on Responsible Research and Innovation (RRI) approach to understanding the what, how and why fun might be a valuable key in education with and for distinctive representatives: learners, educators, researchers, consultants, and policy makers. “For pedagogic innovation to succeed, learners must personally perceive the benefits of learning activities” designed to be fun and also “these gains must be translated into outcomes that are viewed positively within the institution quality monitoring by teaching staff.” Whitton and Langan (2018) also explain that there is a negative influence from the competitive job market that values “serious” performance – as the opposite of fun – so potentially this make course teams less likely to embed playful and fun approaches in the higher education curriculum.
The RRI approach implies that community-members and researchers interact together to better align both its process and outcomes with the values, needs and expectations of society ( European Commission, 2013 ; von Schomberg, 2013 ). The purpose of RRI is to promote greater involvement of societal members with research-authors in the process of research to increase knowledge, understanding and better decision-making about both societal needs and scientific research through eight principles: diversity and inclusion; transparency and openness, anticipation and reflexivity, adaptation and responsiveness ( RRI-Tools, 2016 ; European Commission, 2020 ). These principles were used to adapt, implement and refine a self-reflective instrument about learning and fun. So that, the following section-“Previous Studies about Fun and Learning” present Learning and Fun views from literature. Section-“Methodology” shows the self-reflective instrument, which was used integrated with the methodological approach. Section-“Findings” shows the findings and section-“Discussion and Final Remarks” discussion with final remarks.
Previous Studies about Fun and Learning
Studies that appear to research fun and learning, typically focus on types of activity and the extent to which these are seen as enjoyable and indicated as being fun, rather than drilling down to examine or define fun. While fun is consistently recognized as an important part of the lived experience of children, youth and adults, relatively few seek a deeper understanding of what the construct of fun means ( Kimiecik and Harris, 1996 ; Harmston, 2005 ; Garn and Cothran, 2006 ). This situation is in stark contrast to how fun is generally positioned with regard to the domain of learning and education.
There are different views in the literature about fun and learning, in terms of meanings and its effects. Negative perspectives describe fun as the opposite concept of meaningful “work” and consider it as an unnecessary distraction for learning.
Fun is a term that has changed over time. In the 1900s, it came to indicate an absence of seriousness, work, and labor. “Fun can be seen both as a resistance to the rigid demarcation between work and leisure and also as a means of reproducing that dichotomy” ( Blythe and Hassenzahl, 2018 , p92). As it took on these meanings, fun became a loaded term that challenges the status quo ( Beckman, 2014 ). It can be positioned as a challenge to the traditional split between fun and learning; welcomed by those who embrace social views of the learning process but seen as an unnecessary distraction for those who hold a traditional transmission view of how learning takes place.
The etymological meaning of fun ( fonne and fon from Germanic), which refers to “simple, foolish, silly, unwise” ( Etymonline, 2020 ) have still influence on the meanings attributed by people and researchers nowadays. The argument that fun can have a negative influence on learning was highlighted in newspaper reports of research by the Centre for Education Economics (CEE): “Making lessons fun does not help students to learn, a new report has found. The widely held belief that learners must be happy in order to do well is nothing more than a myth” ( Turner, 2018 ). Likewise, Whitton and Langan note in their analysis of fun in United Kingdom that many educators believe fun to be unsuitable in the “serious” business of higher education ( Whitton and Langan, 2018 , p3). They also highlight a need to research whether students believe that there is any place for fun in their university studies. So, for many, fun is seen as having little or no place within learning. Within the context of education, “fun” is often a derogatory term used to refer to a trivial experience ( Glaveanu, 2011 ).
Some researchers have identified a more positive relationship between fun and learning for children and adults. An analysis of outcomes from the United Kingdom’s “Excellence and Enjoyment” teaching initiative concluded that “Learning which is enjoyable (fun) and self-motivating is more effective than sterile (boring) solely teacher-directed learning” ( Elton-Chalcraft and Mills, 2015 , p482; Tews et al., 2015 ). In the context of informal adult learning, fun has been linked to positive learning outcomes, including job performance and learner engagement ( Francis and Kentel, 2008 ; Fine and Corte, 2017 ; Tews et al., 2017 ). This raises the question of why this conflict and controversy might exist.
The positive effect is not due to fun being an integral part of the learning process, but rather because it has physiological effects such as reducing stress and improving alertness which enhance “performance” ( Bisson and Luckner, 1996 ).
Similarly, Whitton and Langan (2018) describe fun as a “fluid state” ( Prouty, 2002 ) which makes learners feel good ( Koster, 2005 : 40) to engage with learning. This fluid state allows learners to take healthy risks beyond existing personal boundaries ( Ungar, 2007 ). This is because learners are attracted to participate in learning activities that they enjoy and can “fail forward” and feel safe. In addition, Feldberg (2011 :12) indicate that fun has a positive effect on the learning process for creating a state of “relaxed alertness” ( Bisson and Luckner, 1996 ) which enables the suspension of one’s social inhibitions and the reduction of stress. The author highlights fun may contribute to the maintenance of cognitive functioning and emotional growth ( Crosnoe et al., 2004 cited by Feldberg).
Dismore and Bailey’s (2011 , p.499) study indicates positive feelings associated with enjoyment, engagement and optimal experience. The authors described fun and enjoyment underpinned by the concept of “flow” ( Csikszentmihalyi, 2015 ) which refers to “ an optimum state of inner experience incorporating joy, creativity, total involvement and an exhilarating feeling of transcendence .” The optimum state is a key component to lead students to enjoyable accomplishment and optimal learning when their perceived skill and challenge are balanced and suitable. Flow is an important concept for educators to be aware that students’ anxiety caused when their challenge becomes higher compared to their skill, and boredom when challenge becomes too little compared to their skill will reduce their enjoyment and have a negative effect on their learning. Fun learning with flow experiences is relevant for learners to grow with positive opportunities where their skill meets their effort producing intrinsic rewards ( Dismore and Bailey, 2011 ; Chu et al., 2017 ; Whitton and Langan, 2018 ).
Literature about the meaning of fun in online learning is very limited. A set of studies about engaging e-learning games highlight that fun and challenge are essential for promoting students’ enjoyment and making them want to learn ( Fu et al., 2009 ). An engaging e-learning game facilitates the flow of experiences of students by increasing their attention, achieving learning goals and enjoyment with their learning experience ( Virvou et al., 2005 ; De Freitas and Oliver, 2006 ).
This study focuses on fun and learning in the context of Distance Higher Education supported by RRI. To explore what fun is, its meaning and the effects of the phenomenon need to be understood with learners. As a first step, there is a need to identify how the relationship between fun and online learning is conceived by learners based on their own learning experience. A second step is to examine whether this relationship connection has any connection with their epistemic views.
The aim of this study is to address the following questions:
• What are the relationships between fun and online learning practices identified by students?
• What are the connections between students’ epistemic views about online learning and fun?
• What are the recommendations for students, teaching staff and course teams?
Methodology
This work is part of a research program OLAF – Online Learning and Fun led by Rumpus Research Group. The methodology used in this study adopts the established epistemological questionnaire approach ( Feucht et al., 2017 ), and provides an opportunity to facilitate participants epistemic reflectivity ( Feucht et al., 2017 ). In this way the study is underpinned by the concept of reflective practitioners, by which participants “think in action” about principles and practices to share their reflective views ( Schon, 2015 ).
This study is based on a mixed-method approach. Quantitative and qualitative data were generated through a self-reflective instrument ( Feucht et al., 2017 ) constituted by two parts, both developed in Qualtrics. The first part was a Likert-scale survey with 25 statements about learning and fun. The second part was an open question (see “Instruments”).
The approach used for qualitative analysis was a systematic and novel multi methodical procedure that combined: word cloud visualization in Qualtrics ( Figure 2 ); automated thematic analysis map ( Figure 3 ) and sentiment analysis ( Figures 4 – 6 ) in NVivo 12. This integration of visualizations enabled us to identify seven themes to analyze the value of fun; and 26 themes of relationships between fun and learning. The quantitative analysis was supported by PCA – Principal Content Analysis (see “Relationships Between Fun and Learning Supported by Quantitative Analysis”). This approach enabled us to group our – multi-method qualitative analysis categorized by themes – into three groups (see “Relationships Between Fun and Learning Supported by Quantitative Analysis”) as well present our findings (section-“Findings”) with global recommendations underpinned by students’ needs, priorities and expectations, which were revealed in the qualitative data and grouped by quantitative analysis.
This study acknowledges 8 principles ( Box 1 ) of RRI ( von Schomberg, 2013 ; RRI-Tools, 2016 ) in the context of open educational research ( Okada and Sherborne, 2018 ) by which all participants reflect about practices and beliefs for better alignment between learners’ needs and research-based recommendations. The instrument with a special code to allow the withdrawal of participation without the collection of personal data was approved by the Ethics Committee and the Student Research Project Panel of the Open University-United Kingdom.
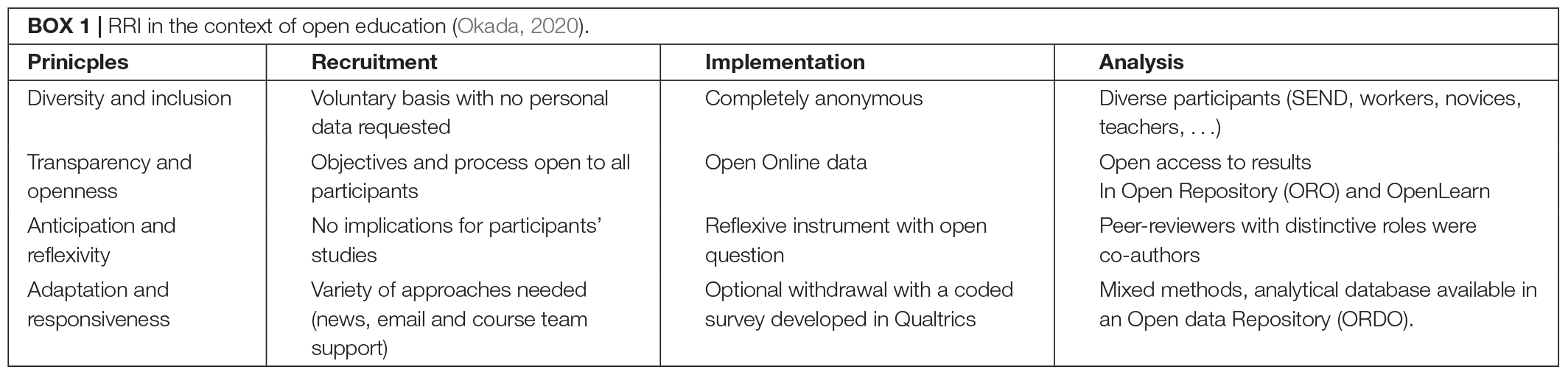
Participants
The OU offers flexible undergraduate and postgraduate courses and qualifications supported distance and open learning for 174,898 people from the United Kingdom, Europe and some worldwide. Approximately 76% of directly registered students work full or part-time during their studies; 23% of Open University United Kingdom undergraduates live in the 25% most deprived areas and 34% of new OU undergraduates are under 25, 14% with disabilities and 32% with lower qualification at entry.
This study focused on one of the largest introductory modules offered by the Wellbeing Education and Language Studies – WELS Faculty of The Open University. Currently this module has more than 4,300 students and is part of various qualifications. So that, participants were students from all levels and qualification’ interests with different occupations, include novices, undergraduates who had just completed secondary education, pre-service and in-service teachers; as well professionals interested in Education, Psychology and Social Care.
A balanced and representative sample were constituted by a total of 625 students who participated in this study as volunteers, 551 completed a self-reflective questionnaire to reflect about fun and learning and 206 provided their reflective views by answering an “optional” open question. The response rate (40%) for the open views about fun and learning was higher than expected.
In terms of students’ previous study experience 48.55% students completed pre-A levels or equivalent (secondary school), 26.81% had already finished other OU course modules (level 1, level 2, and level 3) and 24.64% reported other different experiences. In terms of qualification pathway targeted by students: 28.80% are interested in childhood studies; 34.24% in psychology; 27.17% Education primary, 4.53% Open and 1.81% do not know and 3.44 other qualification such as Social Care.
This study focuses on a 9-month-module course with twenty-four weekly units and four assessment activities. The course integrates reading materials, online audio-visual materials, a YouTube channel “The student hub live” and radio-style broadcast audio repository. Students have also access to a set of library resources, news and special “quick guides” to provide extra-support for developing activities successfully. Students’ interaction with peers and communication with tutors typically occur asynchronously in the online discussion forum and synchronously in online tutorials (in Adobe Connect) and face-to-face tutorials organized in a specific period and locations. In addition, the course provides a channel in social media (Twitter and Facebook) for students’ social engagement. This course module presentations are opened 3 weeks prior to the start in order to provide time for students to smoothly engage in their initial activities including a series of fun and friendly online workshops to promote interaction.
Recruitment
Students’ recruitment occurred at the middle of the online module. It was supported by the course chair and the module course tutors through an invitation shared in course news page and via central email sent to all students. Recruitment and data generation occurred during 5 weeks (February–March 2020) and was more effective after an email invitation sent to all students.
Instruments
The use of self-report questionnaires is well established as a methodology within research examining epistemological beliefs ( Feucht et al., 2017 ). The self-reflective instrument was underpinned by previous work led by the second author ( Sheehy et al., 2019b ) and adapted to the context of online learning and fun.
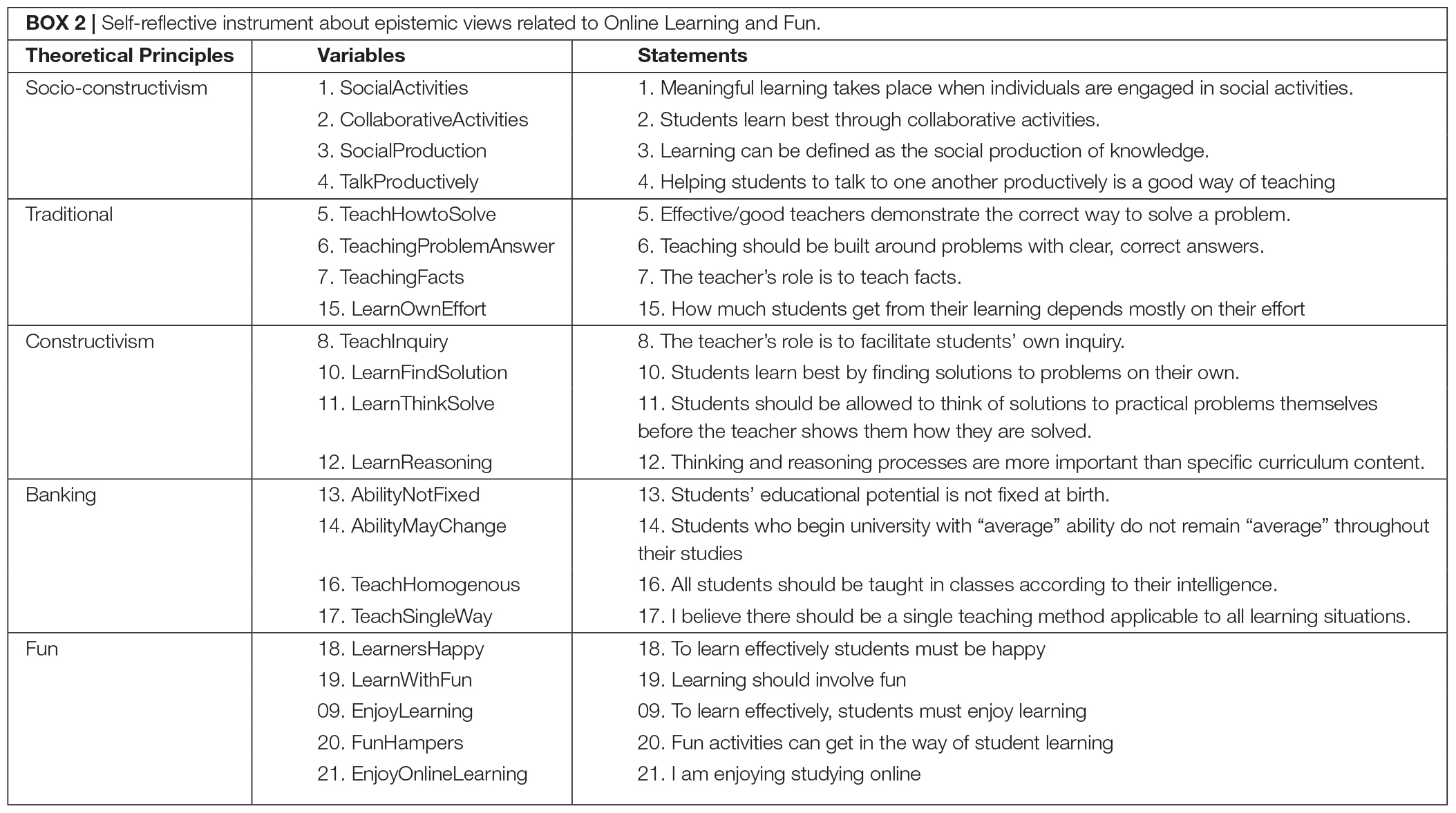
1. Statements 1–4, 13–17 relate to models of learning (Social Constructivist, and Banking) and are taken from Sheehy and Budiyanto’s (2015) development of the Theoretical Orientation Scale ( Hardman and Worthington, 2000 ).
2. Statements 5–7, 8, 10–12 relate to Constructivist and Traditional views of learning, from the OECD international survey ( Organisation for Economic Co-operation and Development (OECD), 2010 , 2013 ).
3. Statements 9, 18–21 elicit beliefs about fun and happiness and emerged as stable items from Budiyanto et al.’s (2017) epistemological research.
The adapted questionnaire was implemented in Qualtrics with consent forms, study objectives and a novel embedded code to enable students’ withdrawal. This is the first study that provides anonymous withdrawal in Qualtrics. It was then tested in two pre-pilots to check its reliability and the embedded code.
In the first phase of implementation, the self-reflective instrument was used by online students to reflect about the topic “Fun and Learning” through a series of 21 statements using Likert-scale to indicate the level of agreement.
In the second phase, students were invited to complete an optional open-ended question (What is your opinion about fun in online learning?) to provide their reflective views and freely express their feelings on this topic.
Preliminary outcomes of this study ( Figure 1 ) were presented to all participants through an article published in OpenLearn ( Okada, 2020 ) and also in a journal paper ( Okada and Sheehy, 2020 : 608). The framework ‘Butterfly of fun’ including four types of fun in online learning was developed underpinned by Piaget and Inhelder (1969) , Vygotsky et al. (1978) , Csikszentmihalyi (2020) , and Freire (1967 , 1984 , 1996 , 2009) and supported by students’ views. Optimal fun is the joy of being fully involved in learning, moving toward full capability and creativity. Individual fun is the happiness of fulfilling accomplishments, supported by clear goals and strategies. Collaborative fun is the happiness of making connections with others, creating social bonding and developing group identity. Emancipatory fun is the joy of being curious, able to search and discover whilst being critically aware ( Okada and Sheehy, 2020 ).
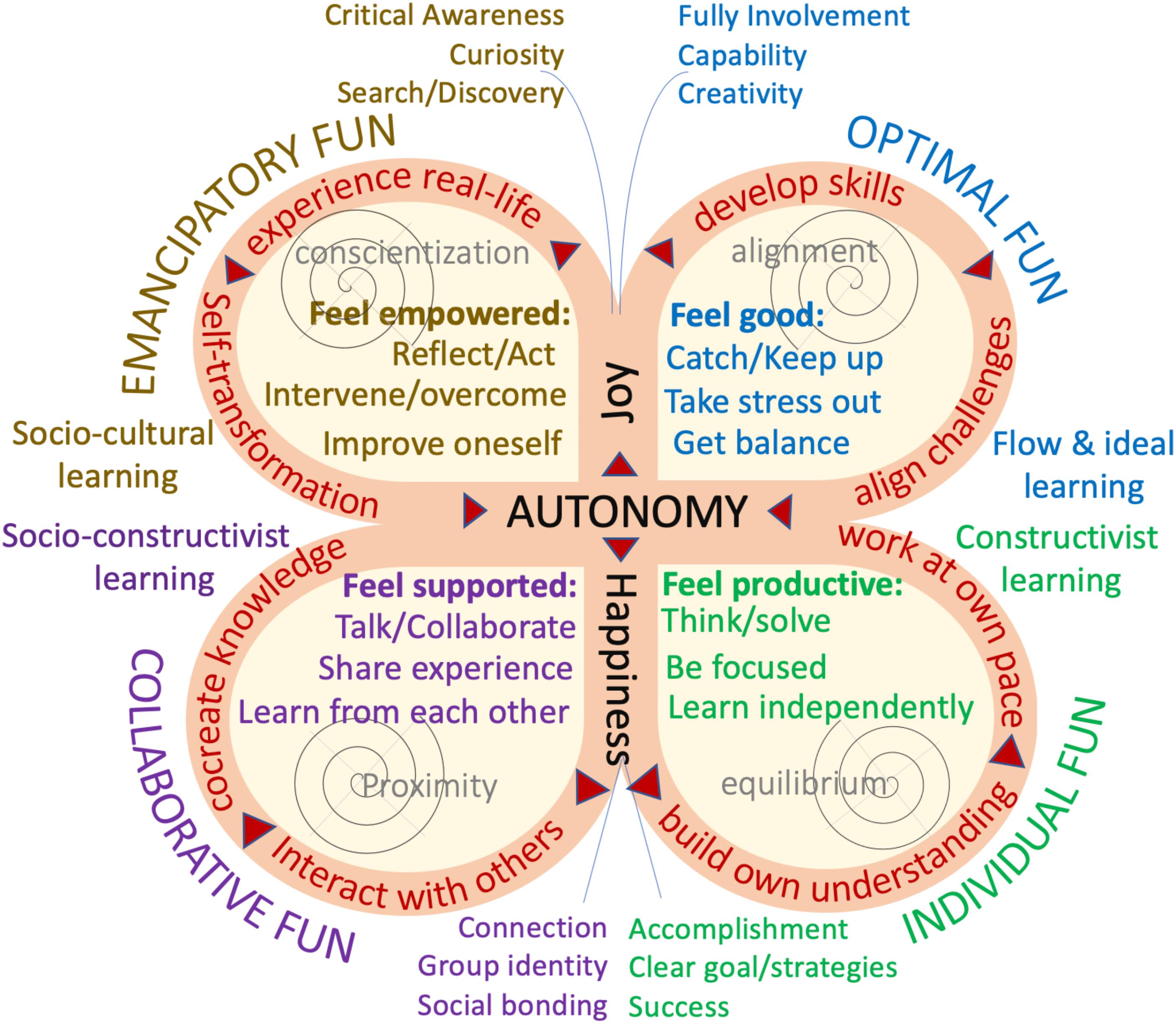
Figure 1. Four levels of Online Learning and Fun (Source: Okada, 2020 ).
Relationships Between Fun and Online Learning Supported by Qualitative Analysis
This study started with a content analysis in NVivo 12 after importing from Qualtrics a csv file with 206 responses about students’ views related to fun and learning (qualitative data). The word cloud visualization in Qualtrics ( Figure 2 ) about students’ views indicated the most frequent words: 148 fun, 123 learning, 50 enjoy/enjoyed/enjoyable/enjoyment, 45 students, 40 distance, 31 tutorials, 29 activity, and 26 time.
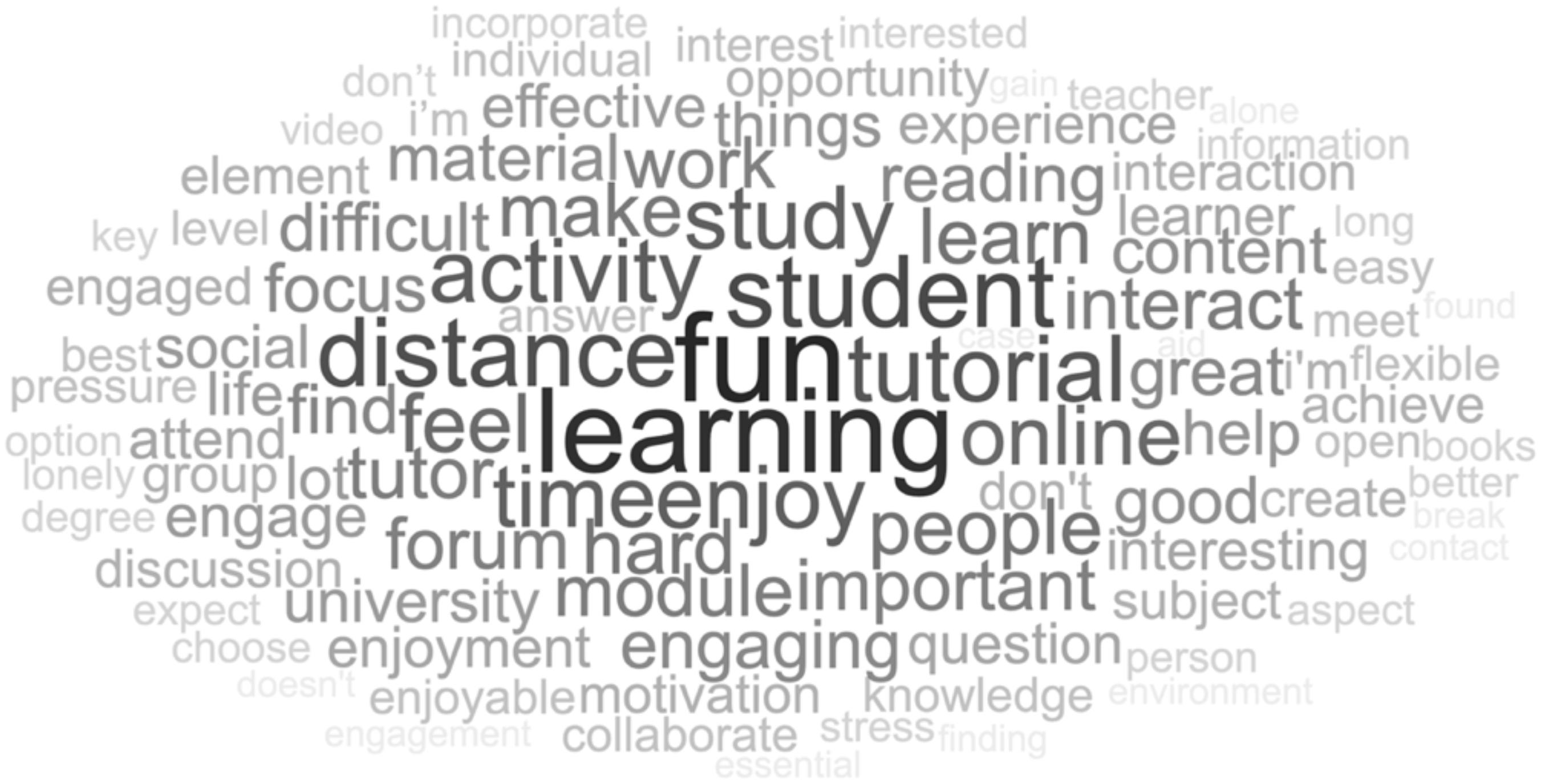
Figure 2. The word cloud visualization in Qualtrics about Online Learning and Fun.
The automated thematic analysis map ( Figure 3 ) in NVivo 12; represented in Cmap tools provided 89 codes grouped through seven themes: fun, learning, students, tutorials, material, online and activities, which enabled to identify connections between fun and learning presented as following.
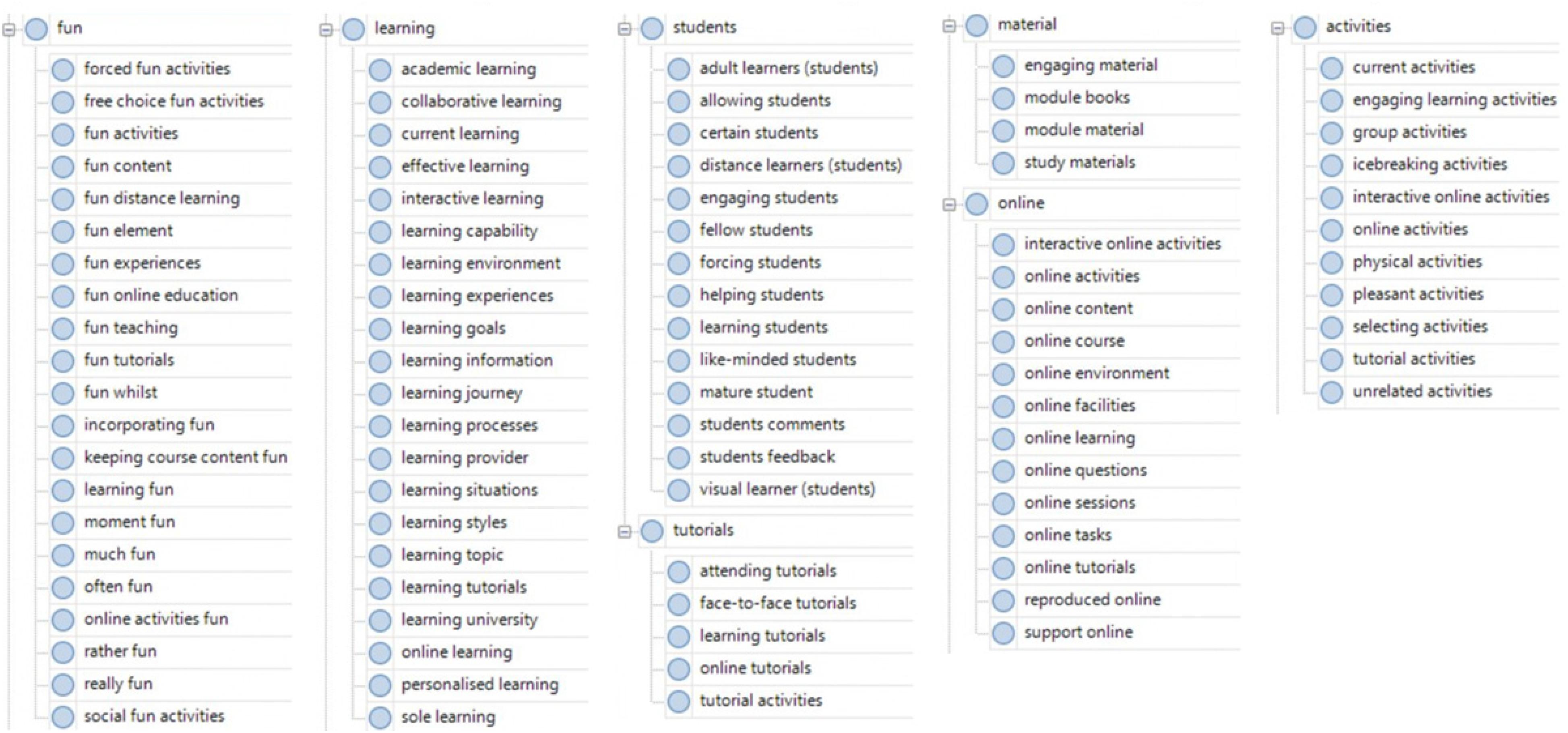
Figure 3. Thematic analysis map about Online Learning and Fun with codes generated by NVivo 12.
NVivo12 sentiment analysis tool ( Figure 4 ) indicated a significant amount of neutral and positive comments associated to narratives that included learning and fun. A small percentage of negative and mixed views emerged across all categories apart from course module “material.” Three largest clusters emerged focused on fun, learning and activities. Four medium clusters were online, tutorials, fun activities, and students. Two small clusters were material and group.
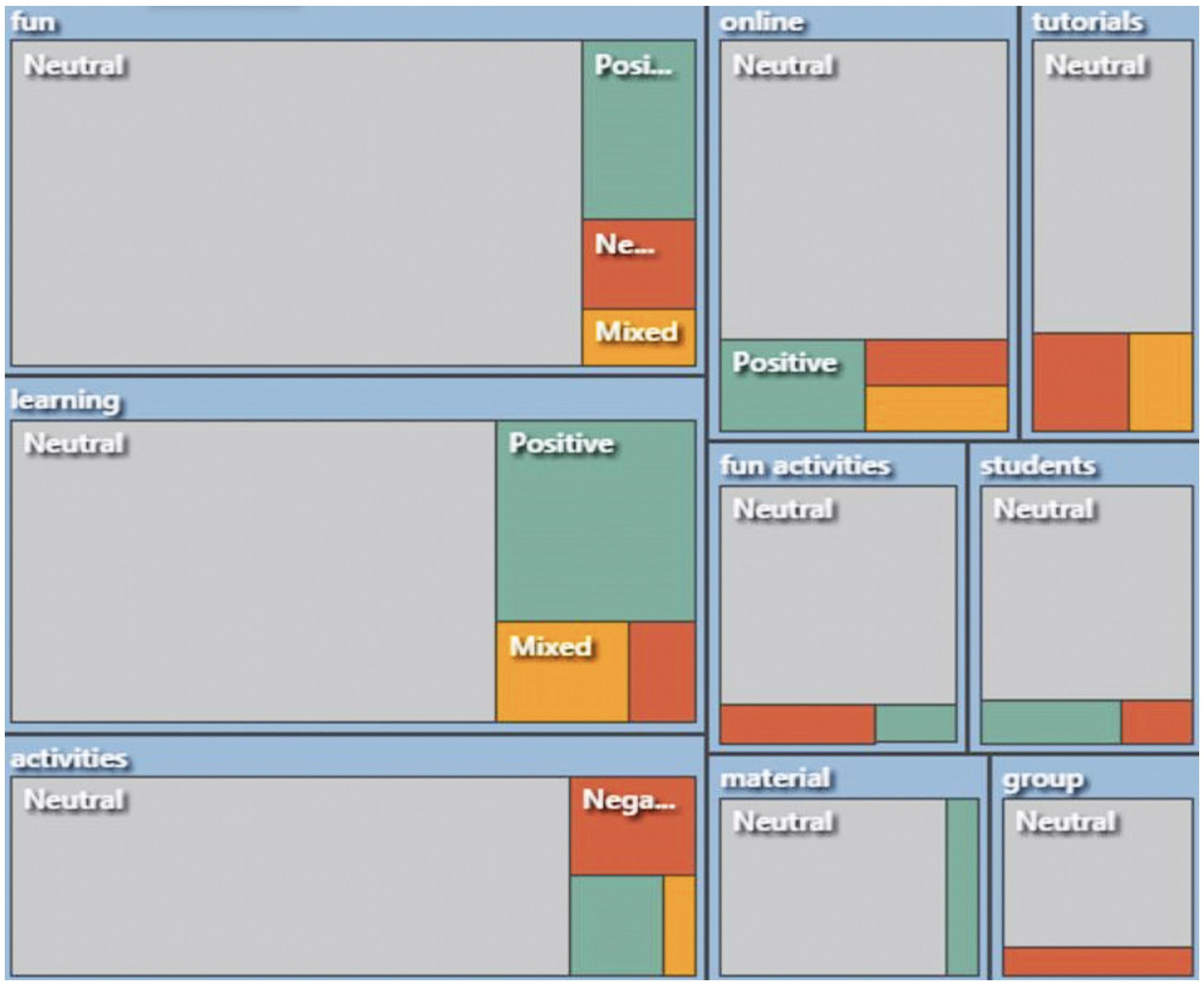
Figure 4. RRI sentiment analysis about Online Learning and Fun in NVivo 12.
NVivo 12 sentiment analysis were used to obtain an overview about students’ negative views ( Figure 5 ) and positive opinions ( Figure 6 ) which were highlighted in red and green by the authors to show the students’ responses with a significant narrative.
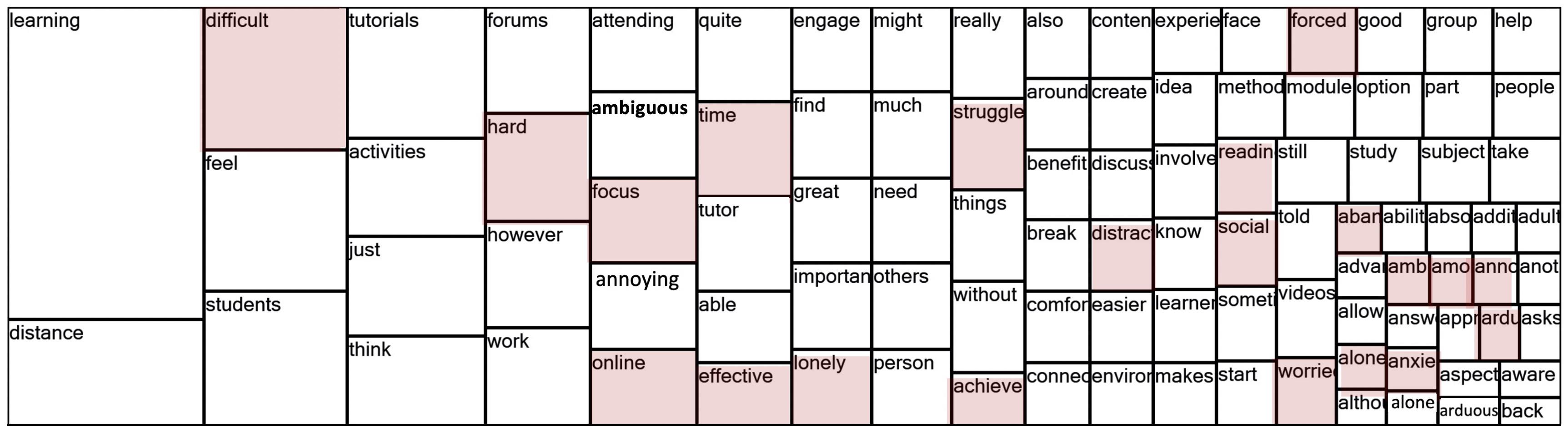
Figure 5. Sentiment analysis about students’ negative views related to Online Learning and Fun.
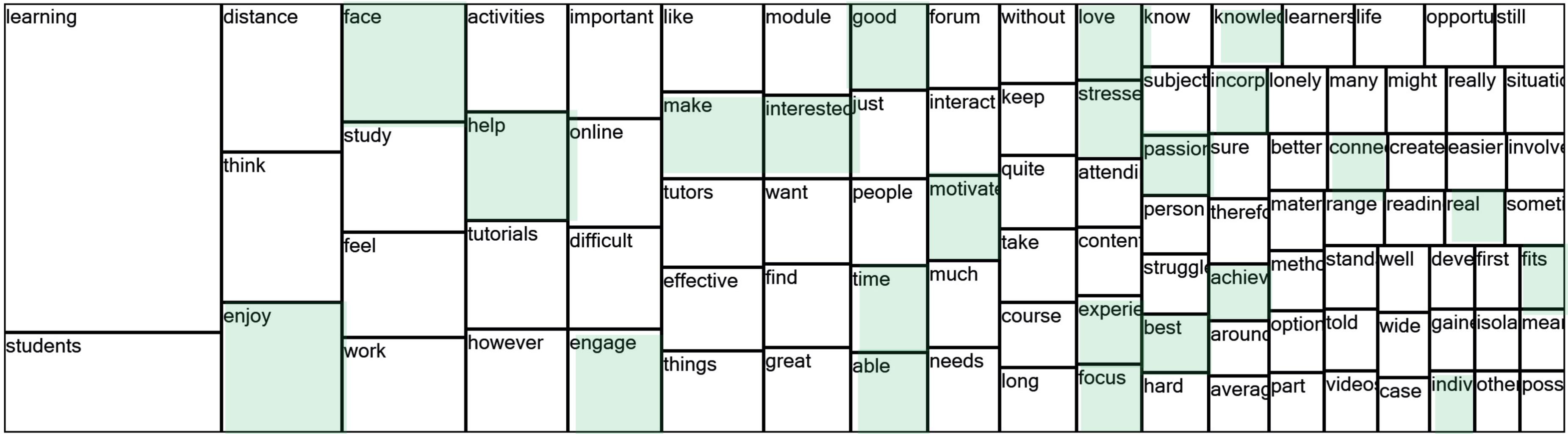
Figure 6. Sentiment analysis about students’ positive views related to Online Learning and Fun.
These visualizations were useful to identify two sets of themes and sub-themes ( Box 3 ) related to value and relationships between learning and fun as well review the automated sentiment analysis code manually to check nuances and recode it based on the meaning of narratives.
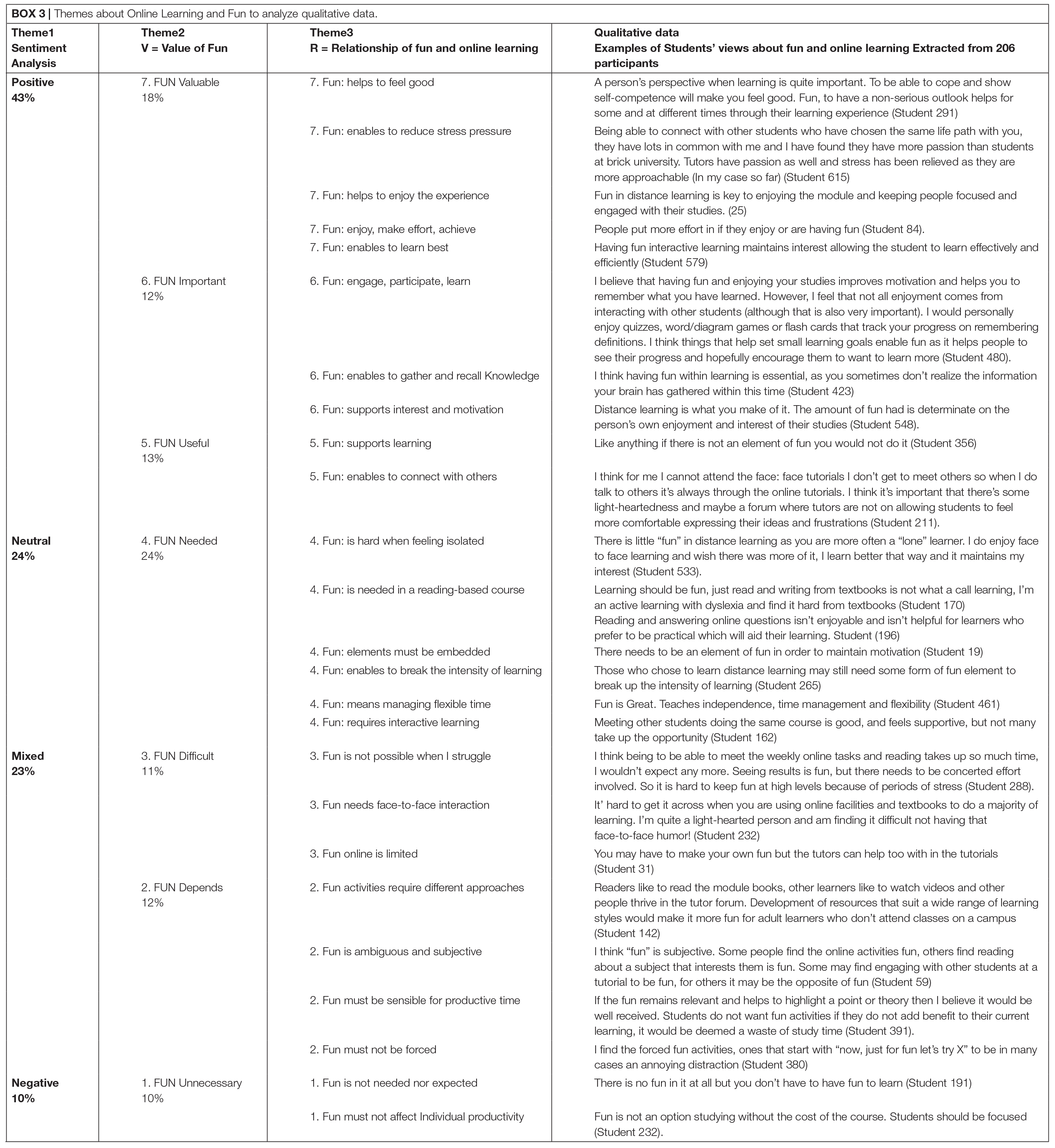
A total of 206 students’ testimonials were coded with these themes and the frequency of codes were represented by percentages ( Box 3 ). The first set of themes was used to code the value of fun for students; a total of 43% students indicated positive values about fun in learning, 24% indicated neutral, and 23% mixed. Only 10% indicated negative views about fun in learning. The second set of themes were used to explore the value and relationships about fun and learning. Approximately 18% of students indicated that fun is valuable, 12% fun is important, 13% fun is useful, 24% fun is needed, 11% fun is difficult, 12% fun depends, and 10% fun is unnecessary.
Relationships Between Fun and Learning Supported by Quantitative Analysis
Quantitative data analysis ( Graph 1 ) revealed largely positive views about fun and learning. Most students agreed that fun (as enjoyment) had value in supporting learning. The majority of students agreed with the following statements: 98% To learn effectively, students must enjoy learning; 91% To learn effectively, students must be happy to learn. 88.77% Learning should involve fun. However, a small group of students 16.66% beliefs that Fun activities can get in the way of student learning.
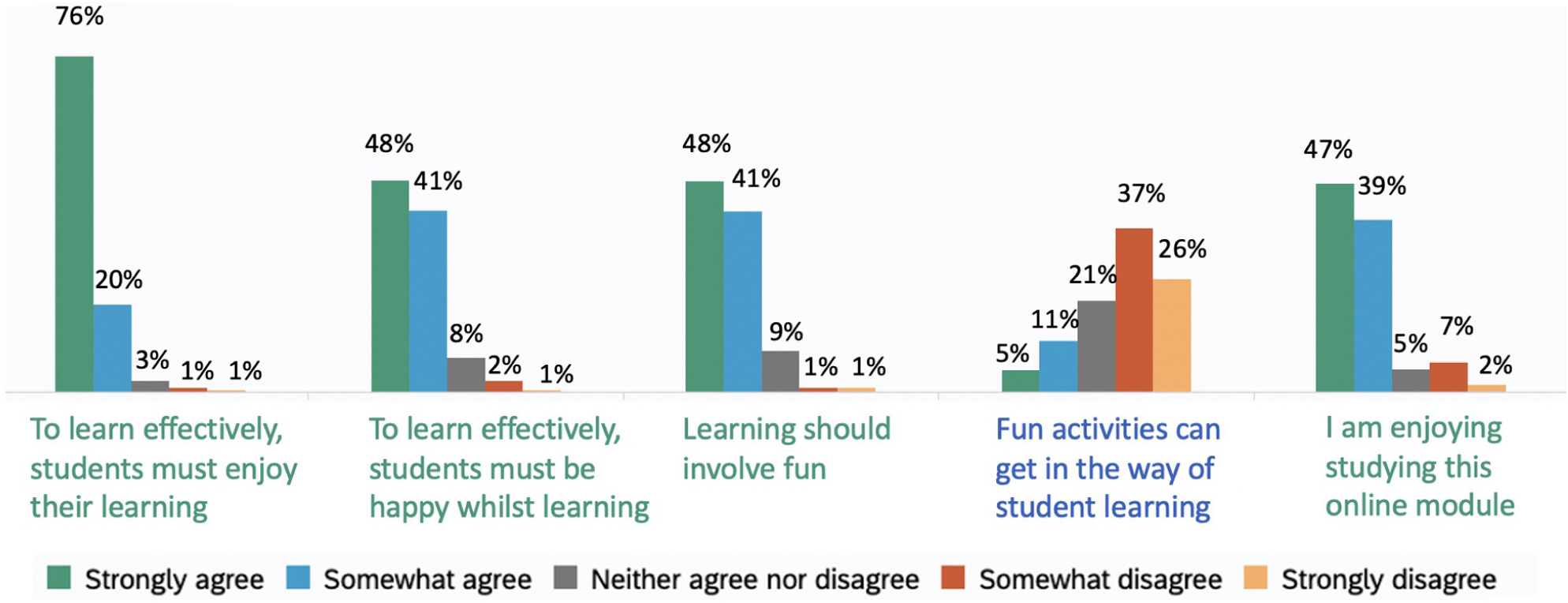
Graph 1. Descriptive analysis about Online Learning and Fun in Qualtrics.
The questionnaire data about 21 statements using Likert scale (1–5) were analyzed through SPSS 24. Cronbach’s alpha 0.717 confirmed that the principal components analysis (PCA) was supported ( Cohen et al., 2007 ). The instrument proved to be reliable for both PCAs ( Tavakol and Dennick, 2011 ). The Kaiser-Meyer-Olkin score of 0.756 indicated sample adequacy and the Bartlett’s sphericity test (Chi-square = 2329.046 with 210 degree of freedom, Sig. 0.000 < 0.5) confirmed consistency.
Table 2 illustrates factor analysis with principal components, with Varimax rotation and Kaiser Normalization indicated six groups emerged: (1) socio-constructivist perspective, (2)traditional perspective (3) fun and learning perspective, (4)constructivist perspective, (5) banking perspective, and (6) Emancipatory Learning. Table 1 using the same method but unrotated solution, indicated three relevant groups: (1) Socio-constructivist learning with traditional teaching and fun; (2) Banking model, transmissive learning and no fun and (4) Constructivist learning and disturbing fun; This approach was selected to examine students’ views and beliefs in order to develop recommendations. Therefore, based on the testimonies of the students grouped with PCA unrotated, twenty-one recommendations were listed and grouped according to three groups: apprentices, teaching professionals and the online course team. Three indexes were generated using the variables from the PCA to get an average among each group related to Fun, No Fun and Bad fun:
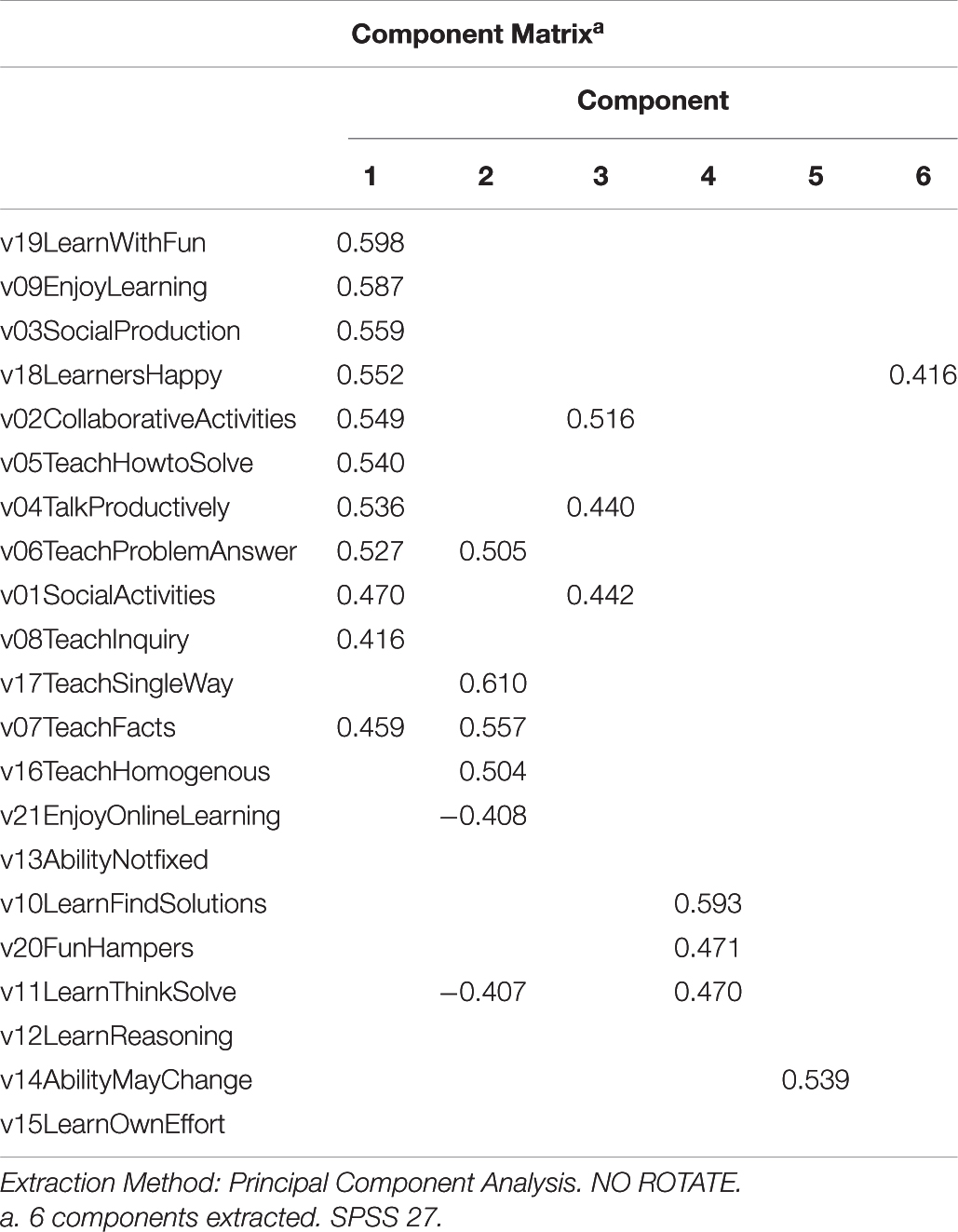
Table 1. FA Varimax without rotation in SPSS.
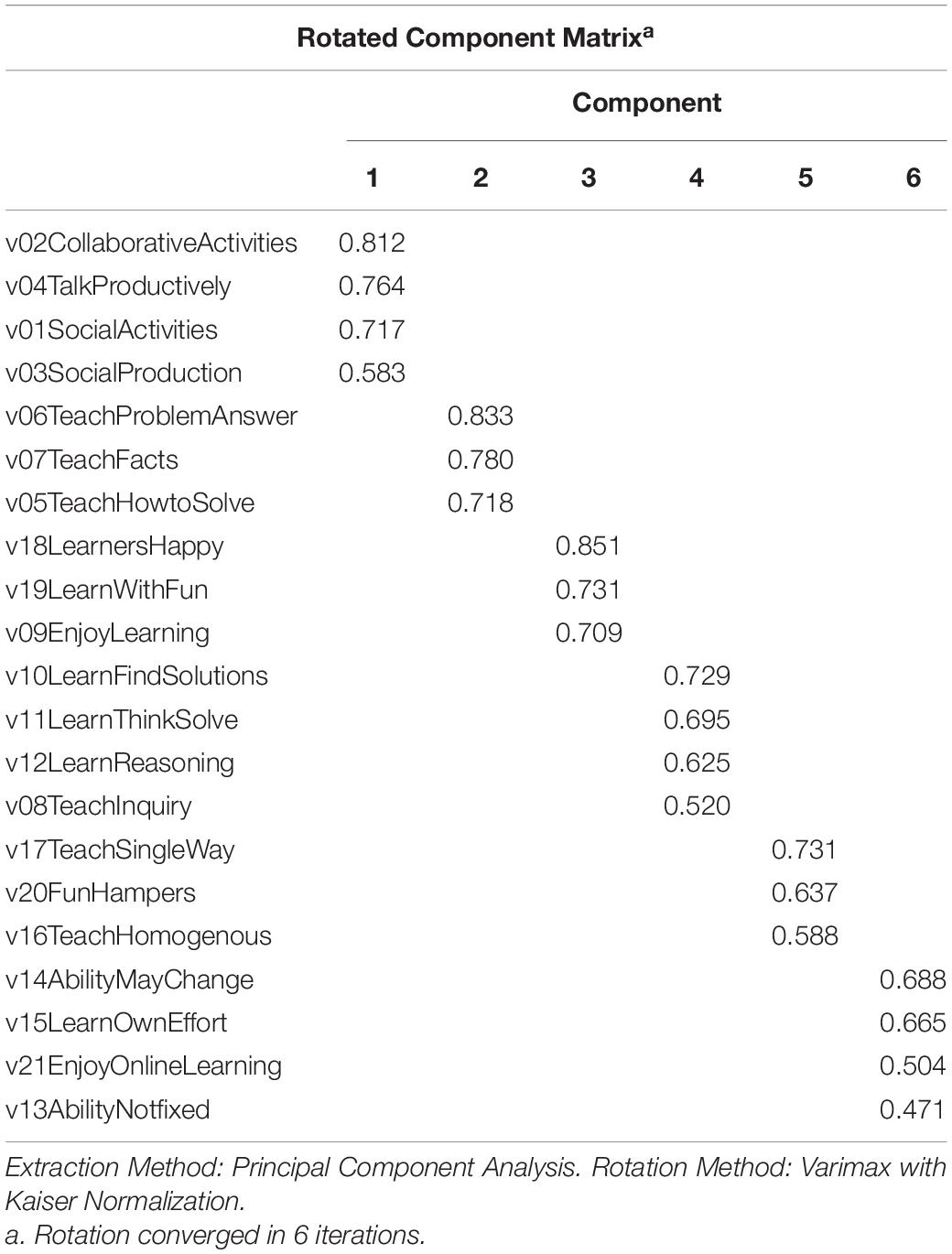
Table 2. FA with Varimax rotation in SPSS.
• C1 Fun = (V19 + V09 + V03 + V18 + V02 + V05 + V04 + V01 + V08)/9;
• C2 No fun = (V17 + V07 + V16 + V06 + -V21)/5;
• C3 Fun bad (hampers learning) = (V10 + V20 + V11)/3.
These indexes (above 3.5 – 5) allowed to group participants’ testimonies, select a variety of views and elaborate a representative list of recommendations to enhance students’ enjoyment with online learning. NVivo 12 was used to carry out a thematic qualitative analysis with an interpretative approach to extract 21 recommendations supported by inductive mapping ( Tables 3 – 5 ). A consensual review ( Hill et al., 1997 ) through three systematic checks between the recommendations against qualitative data were developed with two experts and a student: individually, in pairs and in group. Five types of feedback enabled reviewers to suggest improvements: 1. Reduce (too long, use short sentence), 2. Specify (very broad, use specific words), 3. Connect (unrelated, focus more on the data), 4. Simplify (complicated, use familiar vocabulary), 5. Clarify (confusing, revise the meaning). The results of the analysis from mixed methods are presented as follows.
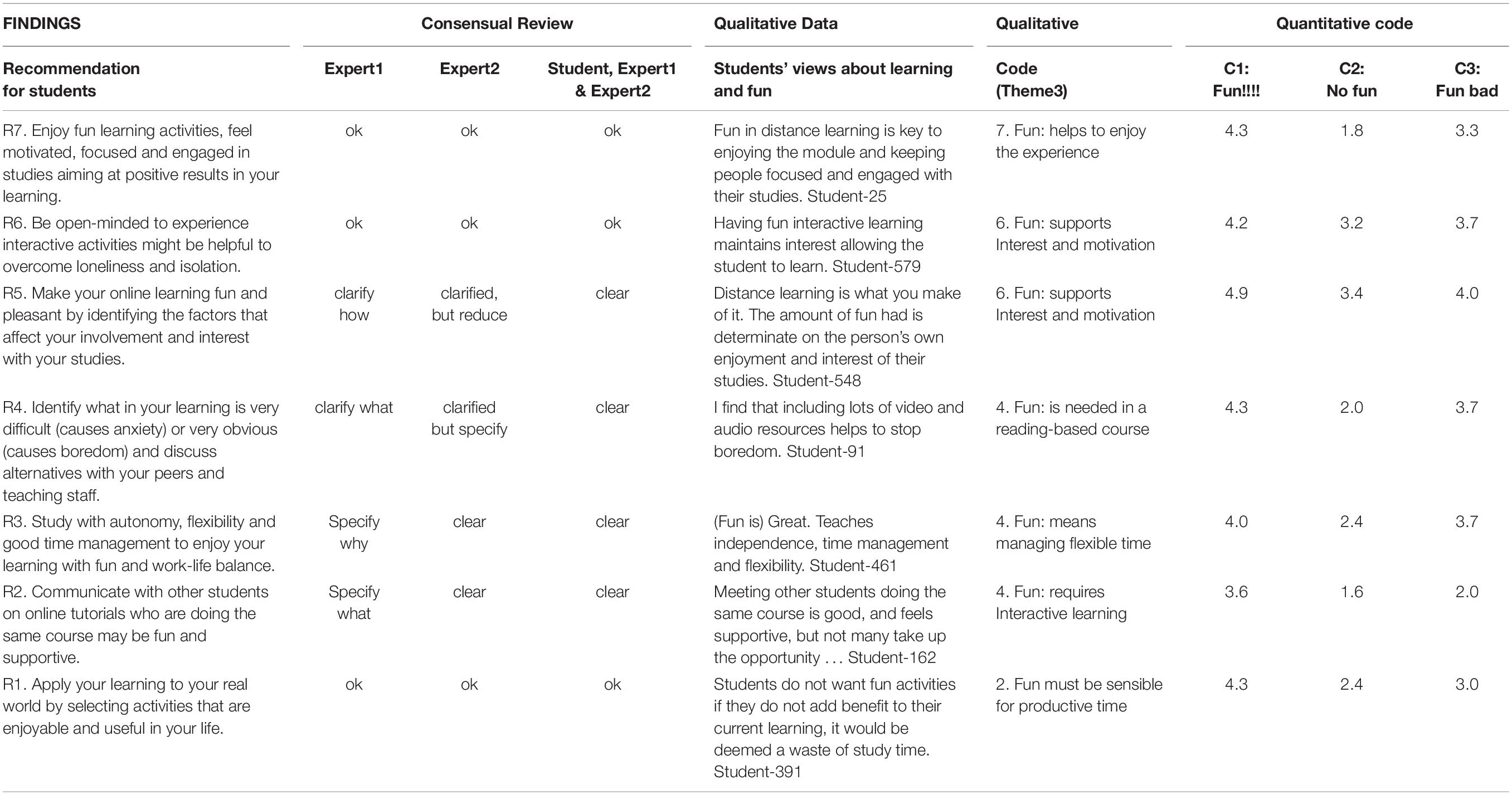
Table 3. Recommendations about Online Learning and Fun for students supported by mixed methods.
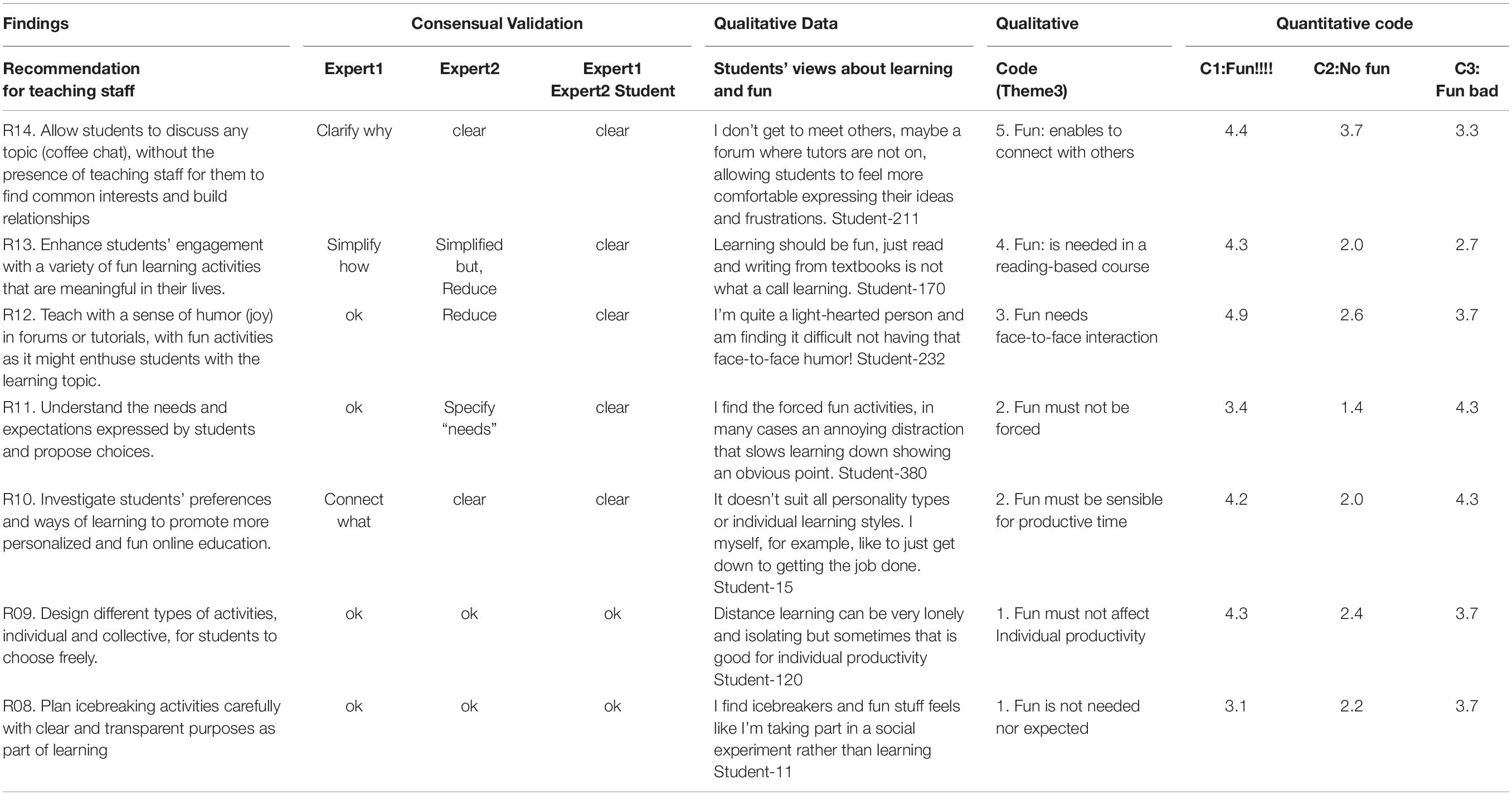
Table 4. Recommendations about Online Learning and Fun for teaching staff supported by mixed methods.
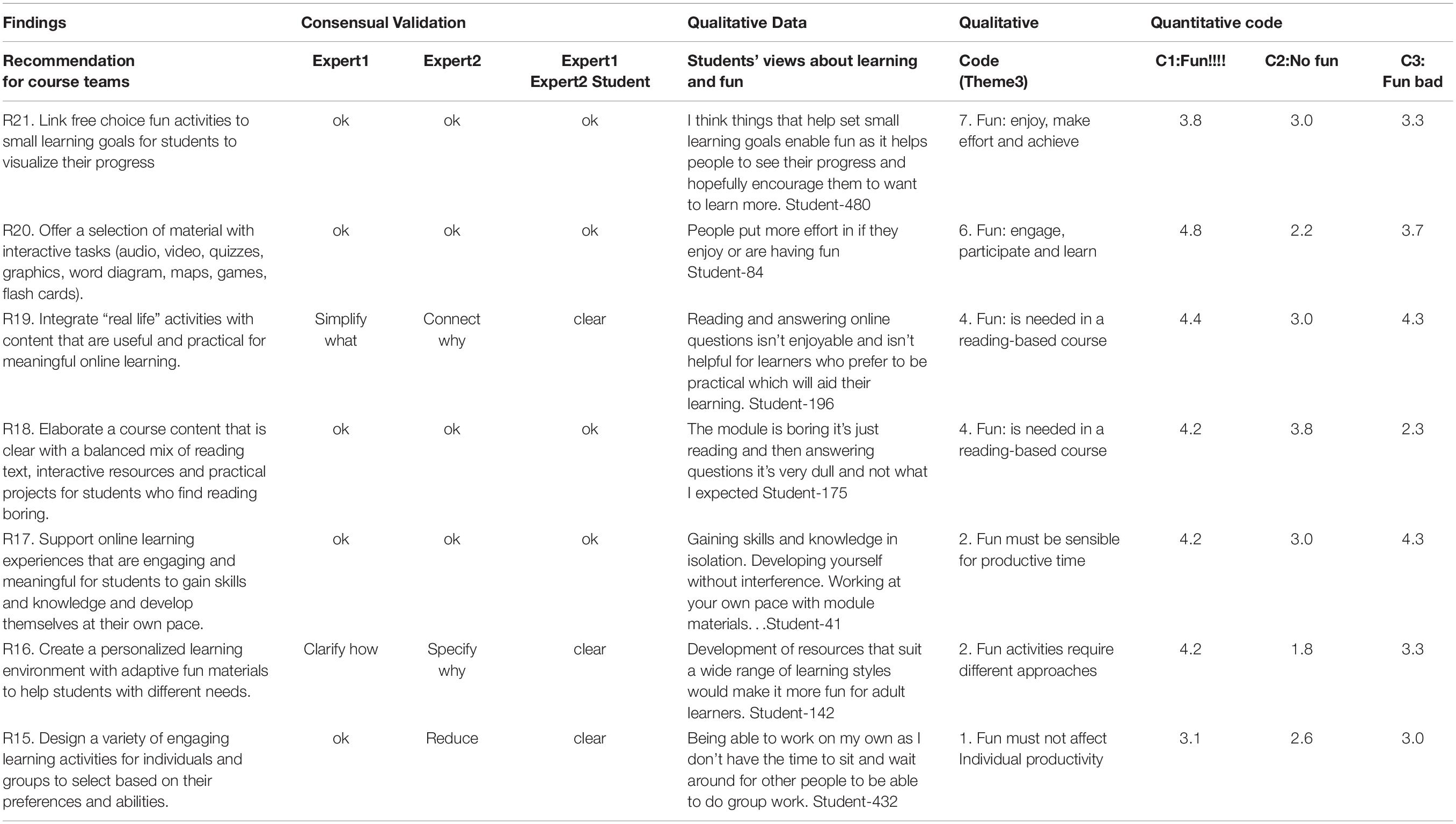
Table 5. Recommendations about Online Learning and Fun for course teams supported by mixed methods.
In addition, the graphical comparison between recommendations and full set of qualitative data both auto coded ( Figure 3 ) in NVivo 24 ( Graph 2 ) ensured diversity with a variety of views and consistency with a proportional representation among qualitative themes and quantitative components.
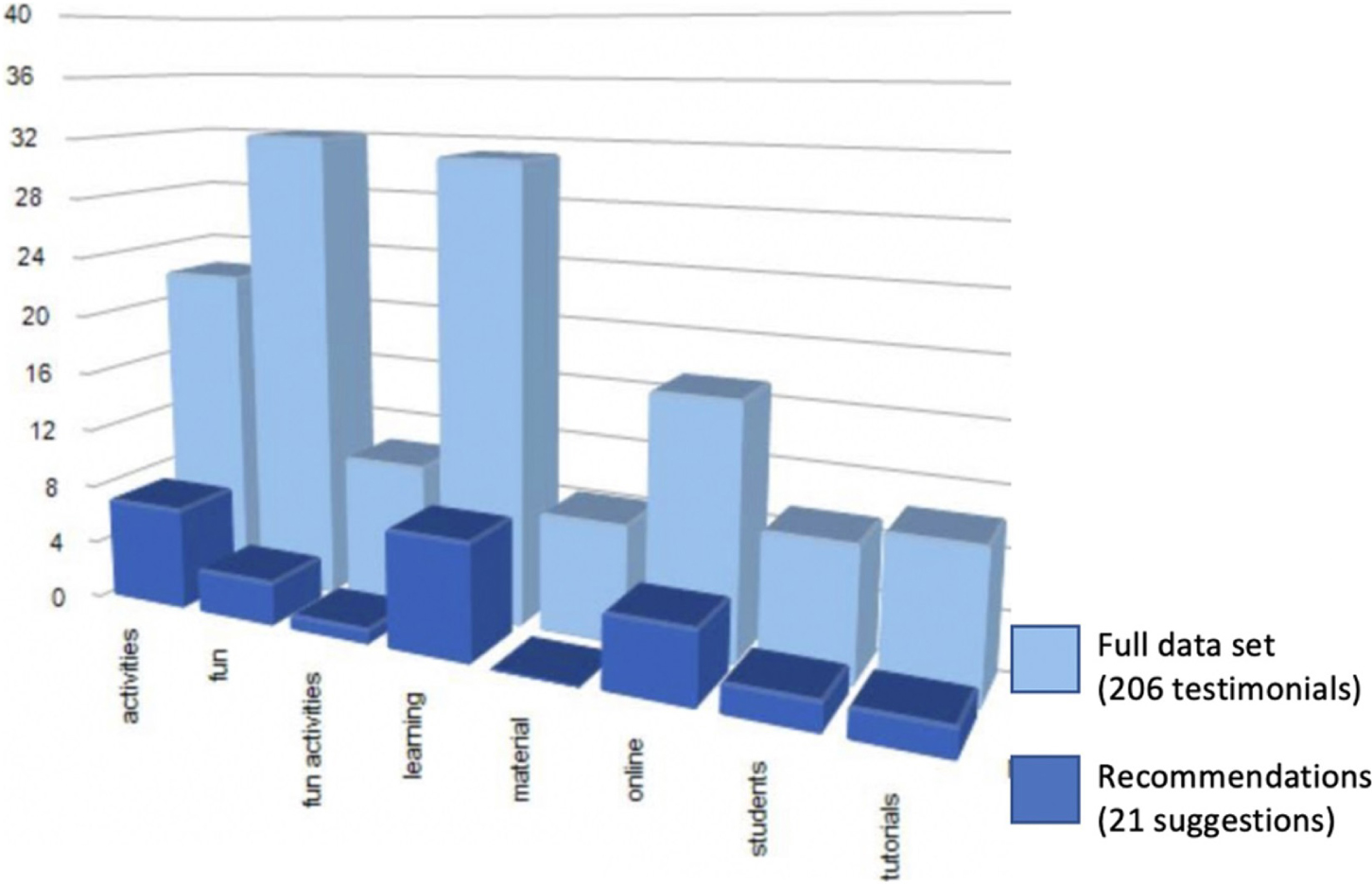
Graph 2. Evidence-based recommendations about Online Learning and Fun supported by consensual review.
Discussion and Final Remarks
The value of students’ enjoyment with online learning has become fundamental in today’s world. The World Bank (2020) and UNESCO (2020) emphasized that more than 160 countries are facing a crisis in education due to the COVID-19 pandemic with loss of learning and in human capital; and over the long term, the economic difficulties will increase inequalities. Various factors will affect educational systems; in particular, low learning outcomes and high dropout rates in secondary school and higher education.
Students’ confidence and satisfaction with online learning are highly relevant in a world in which distance education has rapidly become a necessary practice in response to the global the pandemic. This mixed-methods research revealed significant online students’ opinions about fun for enjoyable and meaningful learning. Fun is as an important part of the lived experience; however, its meaning is underexplored by literature.
This paper provided a methodology to examine fun in online learning supported by students’ epistemic beliefs, underpinned by RRI – Responsible Research and Innovation. A self-reflective instrument with valid and reliable measurement scales with epistemic constructs of online learning and fun helped participants to think about their views about how learning occurs and its relationship with fun. An open database with a three sets of code scheme was generated and shared with all participants during the covid-19 pandemic.
In this study, light is shed on the elements, meaning and relationships about fun and learning considering the students’ “nuanced views” that integrate fun and learning in different ways. Our results provided evidence that a large majority of higher education students (88.77%) value fun because they believe it has a positive social, cognitive and emotional effects on their distance online education. A small group (16.66%) highlighted that fun impairs learning.
This study confirmed that students should experience enjoyable learning so that learning should involve joy. Freire (1996) highlight that the joy of the “serious act” of learning does not refer to the easy joy of being inactive by doing nothing. “Emancipatory fun” ( Okada and Sheehy, 2020 ) underpinned by Freire’s pedagogy of autonomy is related to the hope and confidence that students can have fun by acting, reflecting and learning with enjoyment and consciousness. They can search, research and solve problems, identify and overcome obstacles as well transform and innovate their lives with knowledge, skills and resilience to shape a desirable future.
A key contribution of this study is that different epistemological beliefs are associated with different conceptualizations of the relationship between fun and learning ( Sheehy et al., 2019a ; Okada and Sheehy, 2020 ). Principal component analysis revealed three groups of students who found (1) fun relevant in socio-constructivist learning (2) no fun in traditional transmissive learning and (3) disturbing fun in constructivist learning. A set of 21 recommendations underpinned by systematic mixed methods and consensual review is provided for Higher Education community including course teams, teaching staff and students to enhance online learning experiences with optimal fun, emancipatory fun, collaborative fun and individual fun. Creating opportunities for students to voice and reflect on their own views and values is fundamental to develop more effective online course designs aligned with their needs.
Congruent with the positive effects of optimal experience in some online environments’ studies (e.g., Esteban-Millat et al., 2014 ; Sánchez-Franco et al., 2014 ), this study confirmed that fun creates an opportunity and expectation for students to experience positive feelings in learning such as good mood, enthusiasm, interest, satisfaction and enjoyment that are all relevant for “optimal” learning.
Researchers who see fun as having a close relationship with learning have proposed different types of fun. Lazzaro (2009) highlighted “easy fun” in activities such as games and role play that stimulate curiosity and exploration. Papert (2002) identified “hard fun” within goal-centered and challenging experiences, where the difficulty of the task is part of the fun. Tews et al. (2015 :17) examined fun in two contexts, fun in learning activities developed by students and fun in teaching delivery by the staff. The former was characterized as “hands-on” exercises and activities that promoted social engagement between students. The latter concerned instructor-focused teaching that included the use of humor, creative examples, and storytelling. Their findings indicated that fun delivery, and not fun activities, was positively associated with students’ motivation, interest and engagement.
Notably, their findings indicated fun delivery, but not fun activities, was positively related to student’ motivation, interest and engagement. Prior examining activities and delivery, our study highlights the importance of investigating students’ epistemic views. There is therefore the opportunity for novel research to examine factors and effects of fun and student learning experience including epistemic-guided learning design.
Our study highlights the importance of investigating students’ epistemic beliefs and its connections with the essence of their views. There is therefore the opportunity for novel research to examine factors and effects of fun and within student learning experience including the influence of epistemic-guided learning and teaching design.
A series of studies with Indonesian teachers ( Sheehy et al., 2019a ) suggested that their beliefs about how learning occurs are influenced by their views about happiness and, by implication, fun in relation to learning. These teachers often commented on the relationship between happiness and learning, and many saw happiness as an essential feature of good classroom teaching. However, they described a relationship between happiness and learning that was different in nature to that found in Western educational research. There is a tendency for Western educators to see happiness as “a tool for facilitating effective education” ( Fox et al., 2013 , p1), and as something that is promoted alongside educational excellence. In contrast, many Indonesian teachers see learning not as separate from happiness but as part of it ( Budiyanto et al., 2017 ; Budiyanto and Sheehy, 2019 ).
Other research has implied that this belief in separation arises when people see teaching as a simple transfer of “untransformed knowledge” from expert to student, in a traditional model of learning ( OECD, 2009 ) also known as the “banking model of education” Freire (2000) . This separation may be reflected in the balancing act between happiness with fun and academic achievement described in the CEE report mentioned above. In contrast, those who believe that learning is a social constructivist process are more likely to see happiness with fun as important to the process of learning. The situation remains that we have an incomplete understanding of fun in the domain of learning ( Tews et al., 2017 ) and it remains to be clarified by empirical research ( Iten and Petko, 2016 ); in particular under the lens of epistemological beliefs ( Sheehy et al., 2019a ) and practical experiences.
Our study also complemented a previous research about fun on traditional university’ campus whose students highlighted that fun in learning must integrate stimulating pedagogy; lecturer engagement; a safe learning space; shared experience; and a low-stress environment ( Whitton and Langan, 2018 ). Some key effects of fun, for example, pleasant communication and creation of a relaxed state to reduce stress ( Bisson and Luckner, 1996 ) are important factors to support learners during the isolation. Fun as an inner joy of wellbeing and engagement is an important component to propitiate learning with the creation of new patterns that are interesting, surprising and meaningful ( Schmidhuber, 2010 ) to involve students with formal education during uncertain time of post-pandemic.
As indicated by the research-authors and collaborators, further studies are important based on the RRI approach to construct new questions and also explore the issues indicated by preliminary studies ( Okada and Sheehy, 2020 ). New issues must be also examined on the effects of fun on online learning, also considering age, gender, socio-cultural aspects, accessibility, digital skills, and geographical differences. Developing further recommendations at broader institutional, national and international levels about effective and engaging online learning is also important to empower individuals and society to face, innovate and reconstruct a sustainable and enjoyable world.
Data Availability Statement
The open database can be accessed, downloaded and reused: Okada and Sheehy (2020) OLAF PROJECT data set. Open Research Data Online. The Open University. https://doi.org/10.21954/ou.rd.12670949 (November 2020). The Open Questionnaire can be accessed from the supplementary material Qualtrics Survey OLAF project.pdf.
Ethics Statement
The studies involving human participants were reviewed and approved by The Open University, HREC – Human Research and Ethics Committee. The patients/participants provided their written informed consent to participate in this study.
Author Contributions
AO wrote the first draft of the abstract and prepared the manuscript. KS provided the instrument and feedback about the final version. AO was responsible for the survey implementation in Qualtrics, data generation, instrument’s tests, data analysis through mixed methods, and validation supported by collaborators with consensual review. Additionally, AO created the figures, graphs, and tables. Both authors contributed to manuscript revision, read, and approved the submitted version.
Conflict of Interest
The authors declare that the research was conducted in the absence of any commercial or financial relationships that could be construed as a potential conflict of interest.
This study was funded by the Open University UK and is part of the international project OLAF – Online Learning and Fun. http://www.open.ac.uk/blogs/rumpus/index.php/projects/olaf/ .
Acknowledgments
We are grateful to our collaborators who supported the recruitment of participants, our expert colleagues Prof. Dr. Daniela Melaré Barros; Prof. Dr. Maria Elizabeth de Almeida; Dr. Victoria Cooper, and Miss Ana Beatriz Rocha who provided valuable feedback and our external reviewers for useful suggestions.
Arnone, M. P., Small, R. V., Chauncey, S. A., and McKenna, H. P. (2011). Curiosity, interest and engagement in technology-pervasive learning environments: a new research agenda. Educ. Technol. Res. Dev. 59, 181–198. doi: 10.1007/s11423-011-9190-9
CrossRef Full Text | Google Scholar
Beckman, J. (2014). American Fun: Four Centuries of Joyous Revolt. New York, NY: Knopf Doubleday Publishing Group.
Google Scholar
Bisson, C., and Luckner, J. (1996). Fun in learning: the pedagogical role of fun in adventure education. J. Exp. Educ. 19, 108–112. doi: 10.1177/105382599601900208
Blythe, M., and Hassenzahl, M. (2018). The semantics of fun: differentiating enjoyable experiences. Funology 2, 375–387. doi: 10.1007/978-3-319-68213-6_24
Budiyanto Sheehy, K. (2019). “Developing Signalong Indonesia: issues of politics, pedagogy and perceptions,” in Manual Sign Acquisition by Children with Developmental Disabilities , eds N. Grove and K. Launonen (Hauppauge, NY: Nova Science).
Budiyanto, Sheehy, K., Kaye, H., and Rofiah, K. (2017). Developing Signalong Indonesia: issues of happiness and pedagogy, training and stigmatisation. Int. J. Inclusive Educ. 22, 543–559. doi: 10.1080/13603116.2017.1390000
Chan, K. W., and Elliott, R. G. (2004). Relational analysis of personal epistemology and conceptions about teaching and learning. Teach. Teach. Edu. 20, 817–831. doi: 10.1016/j.tate.2004.09.002
Chu, S. L., Angello, G., Saenz, M., and Quek, F. (2017). Fun in Making: understanding the experience of fun and learning through curriculum-based Making in the elementary school classroom. Entertain. Comput. 18, 31–40. doi: 10.1016/j.entcom.2016.08.007
Cohen, L., Manion, L., and Morrison, K. (2007). Research Methods in Education , Sixth Edn. Abingdon: Routledge.
Cooperman, L. (2014). “Foreword,” in Open Educational Resources and Social Networks: Co-Learning and Professional Development , ed. A. Okada (London: Scholio Educational Research & Publishing).
Crosnoe, R., Johnson, M. K., and Elder, G. H. Jr. (2004). Intergenerational bonding in school: The behavioural and contextual correlates of student-teacher relationships. Sociol. Educ. 77, 60–81. doi: 10.1177/003804070407700103
Csikszentmihalyi, M. (2015). The Systems Model of Creativity: The Collected works of Mihaly Csikszentmihalyi. Springer.
Csikszentmihalyi, M. (2020) Finding Flow: The Psychology of Engagement with Everyday Life. Hachette.
De Freitas, S., and Oliver, M. (2006). How can exploratory learning with games and simulations within the curriculum be most effectively evaluated? Comput. Educ. 46, 249–264. doi: 10.1016/j.compedu.2005.11.007
Dismore, H., and Bailey, R. (2011). Fun and enjoyment in physical education: young people’s attitudes. Res. Papers Educ. 26, 499–516. doi: 10.1080/02671522.2010.484866
Elton-Chalcraft, S., and Mills, K. (2015). Measuring challenge, fun and sterility on a ‘phunometre’scale: evaluating creative teaching and learning with children and their student teachers in the primary school. Education 3-13 43, 482–497. doi: 10.1080/03004279.2013.822904
Esteban-Millat, I., Martínez-López, F. J., Huertas-García, R., Meseguer, A., and Rodríguez-Ardura, I. (2014). Modelling students’ flow experiences in an online learning environment. Comput. Educ. 71, 111–123. doi: 10.1016/j.compedu.2013.09.012
Etymonline. Dicionário etimológico . Available online at: https://www.etymonline.com/word/fun (accessed May 12, 2020).
European Commission (2013). Options for Strengthening Responsible Research and Innovation-Report of the Expert Group on the State of Art in Europe on Responsible Research and Innovation. Luxembourg: European Commission.
European Commission (2020). Responsible Research and Innovation. Available online at: https://ec.europa.eu/programmes/horizon2020/en/h2020-section/responsible-research-innovation (accessed July 10, 2020).
Feldberg, H. R. (2011). S’more then Just Fun and Games: Teachers’ Perceptions on the Educational Value of Camp Programs for School Groups. Master’s thesis, University of Waterloo, Waterloo.
Feucht, F. C., Lunn Brownlee, J., and Schraw, G. (2017). Moving beyond reflection: reflexivity and epistemic cognition in teaching and teacher education. Educ. Psychol. 52, 234–241. doi: 10.1080/00461520.2017.1350180
Fine, G., and Corte, U. (2017). Group pleasures: collaborative commitments, shared narrative, and the sociology of fun. Sociol. Theory 35, 64–86. doi: 10.1177/0735275117692836
Fox, E., Jennifer, M., Proctor, C., and Ashley, M. (2013). “Happiness in the classroom,” in Oxford Handbook of Happiness , eds A. C. Ayers, I. Boniwell, and S. David (Oxford: Oxford University Press).
Francis, N., and Kentel, J. (2008). The fun factor: adolescents’ self−regulated leisure activity and the implications for practitioners and researchers. Leisure/Loisir 32, 65–90. doi: 10.1080/14927713.2008.9651400
Freire, P. (1967). Papel da educação na humanização. Série Artigos.
Freire, P. (1984). Ação cultural para a liberdade , 7 Edn. Rio de Janeiro: Paz e Terra.
Freire, P. (1985). Pedagogia do oprimido , 14 Edn. Rio de Janeiro: Paz e Terra.
Freire, P. (1996). Pedagogia da autonomia: saberes necessários à prática educativa , 9 Edn. São Paulo: Paz e Terra.
Freire, P. (2000). Pedagogy of freedom: Ethics, democracy, and civic courage. Lanham, MD: Rowman & Littlefield Publishers.
Freire, P. (2009). Pedagogia da esperança: um reencontro com a pedagogia do oprimido. 16. ed. Rio de Janeiro: Paz e Terra.
Fu, F. L., Su, R. C., and Yu, S. C. (2009). EGameFlow: a scale to measure learners’ enjoyment of e-learning games. Comput. Educ. 52, 101–112. doi: 10.1016/j.compedu.2008.07.004
Garn, A. C., and Cothran, D. J. (2006). The fun factor in physical education. J. Teach. Phys. Educ. 25, 281–297. doi: 10.1123/jtpe.25.3.281
Glaveanu, V. P. (2011). Children and Creativity: a Most (Un)Likely Pair? Think. Skills Creat. 6, 122–131. doi: 10.1016/j.tsc.2011.03.002
Gortan, A., and Jereb, E. (2007). The dropout rate from e-learning courses and the satisfaction of students with e-learning. Organizacija 40.
Hardman, M., and Worthington, J. (2000). Educational Psychologists’ orientation to inclusion and assumptions about children’s learning. Educ. Psychol. Pract. 16, 349–360. doi: 10.1080/02667360020006417
Harmston, G. (2005). Sources of Enjoyment in Physical Activity Among Children and Adolescents. Los Angeles: California State University.
Higher Education Academy (HEA) (2015). Framework for Student Access, RETENTION, Attainment and Progression in Higher Education. Available online at: https://www.heacademy.ac.uk/system/files/downloads/studentaccess-retentionattainment-progression-in-he.pdf (accessed October 2020).
Hill, C. E., Thompson, B. J., and Williams, E. N. (1997). A guide to conducting consensual qualitative research. Couns. Psychol. 25, 517–572. doi: 10.1177/0011000097254001
Iten, N., and Petko, D. (2016). Learning with serious games: is fun playing the game a predictor of learning success? Br. J. Educ. Technol. 47, 151–163. doi: 10.1111/bjet.12226
Kimiecik, J. C., and Harris, A. T. (1996). What is enjoyment? A conceptual/definitional analysis with implications for sport and exercise psychology. J. Sport Exerc. Psychol. 18, 247–263. doi: 10.1123/jsep.20.3.247
Knight, S., Rienties, B., Littleton, K., Mitsui, M., Tempelaar, D., and Shah, C. (2017). The relationship of (perceived) epistemic cognition to interaction with resources on the internet. Comput. Human Behav. 73, 507–518. doi: 10.1016/j.chb.2017.04.014
Koster, R. (2005). Theory of Fun for Game Design. Scottsdale, AZ: Paraglyph Press.
Lazzaro, N. (2009). “Why we play: affect and the fun of games,” in Human-computer interaction: Designing for diverse users and domains , eds A. Sears and J. A. Jacko (Boca Raton, FLA: CRC Press).
Lee, J., Zhang, Z., Song, H., and Huang, X. (2013). Effects of epistemological and pedagogical beliefs on the instructional practices of teachers: a Chinese Perspective. Aust. J. Teach. Educ. 38, 119–146.
Lesser, L. M., Wall, A., Carver, R., Pearl, D. K., Martin, N., Kuiper, S., et al. (2013). Using fun in the statistics classroom: an exploratory study of college instructors’ hesitations and motivations. J. Stat. Educ. 21, 1–33.
McManus, I. C., and Furnham, A. (2010). “Fun, fun, fun”: types of fun, attitudes to fun, and their relation to personality and biographical factors. Psychology 1:159. doi: 10.4236/psych.2010.13021
Okada, A. (2020). Distance education: do students believe it should be fun? Available online at: https://www.open.edu/openlearn/education-development/learning/distance-education-do-students-believe-it-should-be-fun (accessed April 23, 2020).
Okada, A., and Sheehy, K. (2020). The value of fun in online learning: a study supported by responsible research and innovation and open data. Revista e-Curriculum 18, 319–343.
Okada, A., and Sherborne, T. (2018). Equipping the next generation for responsible research and innovation with open educational resources, open courses, open communities and open schooling: an impact case study in Brazil. J. Interact. Media Educ. 1, 1–15.
Organisation for Economic Co-operation and Development (OECD) (2010). Talis Technical Report. Available online at: http://www.oecd.org/education/school/44978960.pdf (accessed October 2020).
Organisation for Economic Co-operation and Development (OCED) (2013). Teaching and Learning International Survey TALIS 2013 Conceptual Framework. Available online at: http://www.oecd.org/edu/school/talis-2013-results.htm (accessed June 25, 2014).
Papert, S. (2002). Hard Fun. Bangor Daily News. Bangor. Available online at: http://www.papert.org/articles/HardFun.html (accessed July 10, 2020).
Piaget, J., and Inhelder, B. (1969). The Psychology of the Child. Basic Books.
Prouty, D. (2002). Courage, compassion, creativity: project adventure at thirty. Zip Lines Voice Adventure Educ. 44, 6–12.
Richardson, J. T. E. (2013). Epistemological development in higher education. Educ. Res. Rev. 9, 191–206.
Rodriguez, L., and Cano, F. (2007). The learning approaches and epistemological beliefs of university students: a cross-sectional and longitudinal study. Stud. High. Educ. 32, 647–667. doi: 10.1080/03075070701573807
RRI-Tools (2016). Self-Reflection tool. Available online at: https://www.rri-tools.eu/self-reflection-tool (accessed July 10, 2020).
Sánchez-Franco, M. J., Peral-Peral, B., and Villarejo-Ramos, ÁF. (2014). Users’ intrinsic and extrinsic drivers to use a web-based educational environment. Comput. Educ. 74, 81–97. doi: 10.1016/j.compedu.2014.02.001
Schmidhuber, J. (2010). Formal theory of creativity, fun, and intrinsic motivation (1990–2010). IEEE Trans. Auton. Ment. Dev. 2, 230–247. doi: 10.1109/tamd.2010.2056368
Schommer, M. (1990). Effects of beliefs about the nature of knowledge oncomprehension. J. Educ. Psychol. 82, 498–504. doi: 10.1037/0022-0663.82.3.498
Schon, D. (2015). Educating the reflective practitioner: toward a new design for teaching and learning in the professions (San Francisco: JosseyBass, 1987), and Ellen Schall,“learning to love the swamp: reshaping education for public service,” J. Policy Anal. Manage. 14:202. doi: 10.2307/3325150
Sheehy, K., and Budiyanto (2015). The pedagogic beliefs of Indonesian teachers in inclusive schools. Int. J. Disability Dev. Educ. 62, 469–485. doi: 10.1080/1034912X.2015.1061109
Sheehy, K., Budiyanto, Kaye, H., and Rofiah, K. (2019a). Indonesian teachers’ epistemological beliefs and inclusive education. J. Intellect. Disabil. 23, 39–56. doi: 10.1177/1744629517717613
PubMed Abstract | CrossRef Full Text | Google Scholar
Sheehy, K., Kasule, G. W., and Chamberlain, L. (2019b). Ugandan teachers epistemological beliefs and child-led research: implications for developing inclusive educational practice. Int. J. Disabil. Dev. Educ. (Early Access). doi: 10.1080/1034912X.2019.1699647
Tavakol, M., and Dennick, R. (2011). Making sense of Cronbach’s alpha. Int. J. Med. Educ. 2, 53–55. doi: 10.5116/ijme.4dfb.8dfd
Tews, M. J., Jackson, K., Ramsay, C., and Michel, J. W. (2015). Fun in the college classroom: examining its nature and relationship with student engagement. Coll. Teach. 63, 16–26. doi: 10.1080/87567555.2014.972318
Tews, M. J., Michel, J. W., and Noe, R. A. (2017). Does fun promote learning? The relationship between fun in the workplace and informal learning. J. Vocat. Behav. 98, 46–55. doi: 10.1016/j.jvb.2016.09.006
Turner, C. (2018). Making lessons fun does not help children to learn, new report finds. Available online at: https://www.telegraph.co.uk/education/2018/11/14/making-lessons-fun-does-not-help-children-learn-new-report-finds/ (accessed November 14, 2018).
UNESCO (2020). COVID-19 Educational Disruption and Response. Paris: UNESCO.
Ungar, M. (2007). Too Safe for their Own Good: How Risk and Responsibility Helpteens Thrive. Toronto, ON: McClelland & Stewart.
Virvou, M., Katsionis, G., and Manos, K. (2005). Combining software games with education: evaluation of its educational effectiveness. Educ. Technol. Soc. 8, 54–65.
von Schomberg, R. (2013). “A Vision of Responsible Research and Innovation,” in Responsible Innovation . Responsible Innovation: Managing the Responsible Emergence of Science and Innovation in Society , eds R. Owen, J. Bessant, and M. Heintz (Hoboken, NJ: John Wiley & Sons), 51–74. doi: 10.1002/9781118551424.ch3
Vygotsky, L. S. (1978). Mind in Society: The Development of Higher Psychological Processes. Cambridge, MA: Harvard University Press.
Whitton, N., and Langan, M. (2018). Fun and games in higher education: an analysis of UK student perspectives. Teach. High. Educ. 24, 1000–1013. doi: 10.1080/13562517.2018.1541885
World Bank (2020). The COVID-19 Pandemic: Shocks to Education and Policy Responses. Washington, DC: World Bank. Available online at: https://openknowledge.worldbank.org/handle/10986/33696 (accessed October 2020).
Xavier, M., and Meneses, J. (2020). Dropout in Online Higher Education: A Scoping Review from 2014 to 2018. Barcelona: eLearn Center, Universitat Oberta de Catalunya.
Keywords : COVID-19, online learning, fun, higher education, academic performance, epistemic views, responsible research and innovation, recommendations
Citation: Okada A and Sheehy K (2020) Factors and Recommendations to Support Students’ Enjoyment of Online Learning With Fun: A Mixed Method Study During COVID-19. Front. Educ. 5:584351. doi: 10.3389/feduc.2020.584351
Received: 17 July 2020; Accepted: 13 October 2020; Published: 11 December 2020.
Reviewed by:
Copyright © 2020 Okada and Sheehy. This is an open-access article distributed under the terms of the Creative Commons Attribution License (CC BY) . The use, distribution or reproduction in other forums is permitted, provided the original author(s) and the copyright owner(s) are credited and that the original publication in this journal is cited, in accordance with accepted academic practice. No use, distribution or reproduction is permitted which does not comply with these terms.
*Correspondence: Alexandra Okada, [email protected]
This article is part of the Research Topic
COVID-19 and the Educational Response: New Educational and Social Realities
Impact of online classes on the satisfaction and performance of students during the pandemic period of COVID 19
- Published: 21 April 2021
- Volume 26 , pages 6923–6947, ( 2021 )
Cite this article
- Ram Gopal 1 ,
- Varsha Singh 1 &
- Arun Aggarwal ORCID: orcid.org/0000-0003-3986-188X 2
625k Accesses
220 Citations
25 Altmetric
Explore all metrics
The aim of the study is to identify the factors affecting students’ satisfaction and performance regarding online classes during the pandemic period of COVID–19 and to establish the relationship between these variables. The study is quantitative in nature, and the data were collected from 544 respondents through online survey who were studying the business management (B.B.A or M.B.A) or hotel management courses in Indian universities. Structural equation modeling was used to analyze the proposed hypotheses. The results show that four independent factors used in the study viz. quality of instructor, course design, prompt feedback, and expectation of students positively impact students’ satisfaction and further student’s satisfaction positively impact students’ performance. For educational management, these four factors are essential to have a high level of satisfaction and performance for online courses. This study is being conducted during the epidemic period of COVID- 19 to check the effect of online teaching on students’ performance.
Avoid common mistakes on your manuscript.
1 Introduction
Coronavirus is a group of viruses that is the main root of diseases like cough, cold, sneezing, fever, and some respiratory symptoms (WHO, 2019 ). Coronavirus is a contagious disease, which is spreading very fast amongst the human beings. COVID-19 is a new sprain which was originated in Wuhan, China, in December 2019. Coronavirus circulates in animals, but some of these viruses can transmit between animals and humans (Perlman & Mclntosh, 2020 ). As of March 282,020, according to the MoHFW, a total of 909 confirmed COVID-19 cases (862 Indians and 47 foreign nationals) had been reported in India (Centers for Disease Control and Prevention, 2020 ). Officially, no vaccine or medicine is evaluated to cure the spread of COVID-19 (Yu et al., 2020 ). The influence of the COVID-19 pandemic on the education system leads to schools and colleges’ widespread closures worldwide. On March 24, India declared a country-wide lockdown of schools and colleges (NDTV, 2020 ) for preventing the transmission of the coronavirus amongst the students (Bayham & Fenichel, 2020 ). School closures in response to the COVID-19 pandemic have shed light on several issues affecting access to education. COVID-19 is soaring due to which the huge number of children, adults, and youths cannot attend schools and colleges (UNESCO, 2020 ). Lah and Botelho ( 2012 ) contended that the effect of school closing on students’ performance is hazy.
Similarly, school closing may also affect students because of disruption of teacher and students’ networks, leading to poor performance. Bridge ( 2020 ) reported that schools and colleges are moving towards educational technologies for student learning to avoid a strain during the pandemic season. Hence, the present study’s objective is to develop and test a conceptual model of student’s satisfaction pertaining to online teaching during COVID-19, where both students and teachers have no other option than to use the online platform uninterrupted learning and teaching.
UNESCO recommends distance learning programs and open educational applications during school closure caused by COVID-19 so that schools and teachers use to teach their pupils and bound the interruption of education. Therefore, many institutes go for the online classes (Shehzadi et al., 2020 ).
As a versatile platform for learning and teaching processes, the E-learning framework has been increasingly used (Salloum & Shaalan, 2018 ). E-learning is defined as a new paradigm of online learning based on information technology (Moore et al., 2011 ). In contrast to traditional learning academics, educators, and other practitioners are eager to know how e-learning can produce better outcomes and academic achievements. Only by analyzing student satisfaction and their performance can the answer be sought.
Many comparative studies have been carried out to prove the point to explore whether face-to-face or traditional teaching methods are more productive or whether online or hybrid learning is better (Lockman & Schirmer, 2020 ; Pei & Wu, 2019 ; González-Gómez et al., 2016 ; González-Gómez et al., 2016 ). Results of the studies show that the students perform much better in online learning than in traditional learning. Henriksen et al. ( 2020 ) highlighted the problems faced by educators while shifting from offline to online mode of teaching. In the past, several research studies had been carried out on online learning to explore student satisfaction, acceptance of e-learning, distance learning success factors, and learning efficiency (Sher, 2009 ; Lee, 2014 ; Yen et al., 2018 ). However, scant amount of literature is available on the factors that affect the students’ satisfaction and performance in online classes during the pandemic of Covid-19 (Rajabalee & Santally, 2020 ). In the present study, the authors proposed that course design, quality of the instructor, prompt feedback, and students’ expectations are the four prominent determinants of learning outcome and satisfaction of the students during online classes (Lee, 2014 ).
The Course Design refers to curriculum knowledge, program organization, instructional goals, and course structure (Wright, 2003 ). If well planned, course design increasing the satisfaction of pupils with the system (Almaiah & Alyoussef, 2019 ). Mtebe and Raisamo ( 2014 ) proposed that effective course design will help in improving the performance through learners knowledge and skills (Khan & Yildiz, 2020 ; Mohammed et al., 2020 ). However, if the course is not designed effectively then it might lead to low usage of e-learning platforms by the teachers and students (Almaiah & Almulhem, 2018 ). On the other hand, if the course is designed effectively then it will lead to higher acceptance of e-learning system by the students and their performance also increases (Mtebe & Raisamo, 2014 ). Hence, to prepare these courses for online learning, many instructors who are teaching blended courses for the first time are likely to require a complete overhaul of their courses (Bersin, 2004 ; Ho et al., 2006 ).
The second-factor, Instructor Quality, plays an essential role in affecting the students’ satisfaction in online classes. Instructor quality refers to a professional who understands the students’ educational needs, has unique teaching skills, and understands how to meet the students’ learning needs (Luekens et al., 2004 ). Marsh ( 1987 ) developed five instruments for measuring the instructor’s quality, in which the main method was Students’ Evaluation of Educational Quality (SEEQ), which delineated the instructor’s quality. SEEQ is considered one of the methods most commonly used and embraced unanimously (Grammatikopoulos et al., 2014 ). SEEQ was a very useful method of feedback by students to measure the instructor’s quality (Marsh, 1987 ).
The third factor that improves the student’s satisfaction level is prompt feedback (Kinicki et al., 2004 ). Feedback is defined as information given by lecturers and tutors about the performance of students. Within this context, feedback is a “consequence of performance” (Hattie & Timperley, 2007 , p. 81). In education, “prompt feedback can be described as knowing what you know and what you do not related to learning” (Simsek et al., 2017 , p.334). Christensen ( 2014 ) studied linking feedback to performance and introduced the positivity ratio concept, which is a mechanism that plays an important role in finding out the performance through feedback. It has been found that prompt feedback helps in developing a strong linkage between faculty and students which ultimately leads to better learning outcomes (Simsek et al., 2017 ; Chang, 2011 ).
The fourth factor is students’ expectation . Appleton-Knapp and Krentler ( 2006 ) measured the impact of student’s expectations on their performance. They pin pointed that the student expectation is important. When the expectations of the students are achieved then it lead to the higher satisfaction level of the student (Bates & Kaye, 2014 ). These findings were backed by previous research model “Student Satisfaction Index Model” (Zhang et al., 2008 ). However, when the expectations are students is not fulfilled then it might lead to lower leaning and satisfaction with the course. Student satisfaction is defined as students’ ability to compare the desired benefit with the observed effect of a particular product or service (Budur et al., 2019 ). Students’ whose grade expectation is high will show high satisfaction instead of those facing lower grade expectations.
The scrutiny of the literature show that although different researchers have examined the factors affecting student satisfaction but none of the study has examined the effect of course design, quality of the instructor, prompt feedback, and students’ expectations on students’ satisfaction with online classes during the pandemic period of Covid-19. Therefore, this study tries to explore the factors that affect students’ satisfaction and performance regarding online classes during the pandemic period of COVID–19. As the pandemic compelled educational institutions to move online with which they were not acquainted, including teachers and learners. The students were not mentally prepared for such a shift. Therefore, this research will be examined to understand what factors affect students and how students perceived these changes which are reflected through their satisfaction level.
This paper is structured as follows: The second section provides a description of theoretical framework and the linkage among different research variables and accordingly different research hypotheses were framed. The third section deals with the research methodology of the paper as per APA guideline. The outcomes and corresponding results of the empirical analysis are then discussed. Lastly, the paper concludes with a discussion and proposes implications for future studies.
2 Theoretical framework
Achievement goal theory (AGT) is commonly used to understand the student’s performance, and it is proposed by four scholars Carole Ames, Carol Dweck, Martin Maehr, and John Nicholls in the late 1970s (Elliot, 2005 ). Elliott & Dweck ( 1988 , p11) define that “an achievement goal involves a program of cognitive processes that have cognitive, affective and behavioral consequence”. This theory suggests that students’ motivation and achievement-related behaviors can be easily understood by the purpose and the reasons they adopted while they are engaged in the learning activities (Dweck & Leggett, 1988 ; Ames, 1992 ; Urdan, 1997 ). Some of the studies believe that there are four approaches to achieve a goal, i.e., mastery-approach, mastery avoidance, performance approach, and performance-avoidance (Pintrich, 1999 ; Elliot & McGregor, 2001 ; Schwinger & Stiensmeier-Pelster, 2011 , Hansen & Ringdal, 2018 ; Mouratidis et al., 2018 ). The environment also affects the performance of students (Ames & Archer, 1988 ). Traditionally, classroom teaching is an effective method to achieve the goal (Ames & Archer, 1988 ; Ames, 1992 ; Clayton et al., 2010 ) however in the modern era, the internet-based teaching is also one of the effective tools to deliver lectures, and web-based applications are becoming modern classrooms (Azlan et al., 2020 ). Hence, following section discuss about the relationship between different independent variables and dependent variables (Fig. 1 ).
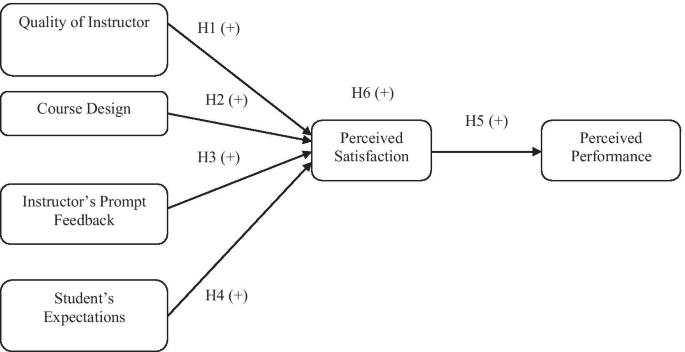
Proposed Model
3 Hypotheses development
3.1 quality of the instructor and satisfaction of the students.
Quality of instructor with high fanaticism on student’s learning has a positive impact on their satisfaction. Quality of instructor is one of the most critical measures for student satisfaction, leading to the education process’s outcome (Munteanu et al., 2010 ; Arambewela & Hall, 2009 ; Ramsden, 1991 ). Suppose the teacher delivers the course effectively and influence the students to do better in their studies. In that case, this process leads to student satisfaction and enhances the learning process (Ladyshewsky, 2013 ). Furthermore, understanding the need of learner by the instructor also ensures student satisfaction (Kauffman, 2015 ). Hence the hypothesis that the quality of instructor significantly affects the satisfaction of the students was included in this study.
H1: The quality of the instructor positively affects the satisfaction of the students.
3.2 Course design and satisfaction of students
The course’s technological design is highly persuading the students’ learning and satisfaction through their course expectations (Liaw, 2008 ; Lin et al., 2008 ). Active course design indicates the students’ effective outcomes compared to the traditional design (Black & Kassaye, 2014 ). Learning style is essential for effective course design (Wooldridge, 1995 ). While creating an online course design, it is essential to keep in mind that we generate an experience for students with different learning styles. Similarly, (Jenkins, 2015 ) highlighted that the course design attributes could be developed and employed to enhance student success. Hence the hypothesis that the course design significantly affects students’ satisfaction was included in this study.
H2: Course design positively affects the satisfaction of students.
3.3 Prompt feedback and satisfaction of students
The emphasis in this study is to understand the influence of prompt feedback on satisfaction. Feedback gives the information about the students’ effective performance (Chang, 2011 ; Grebennikov & Shah, 2013 ; Simsek et al., 2017 ). Prompt feedback enhances student learning experience (Brownlee et al., 2009 ) and boosts satisfaction (O'donovan, 2017 ). Prompt feedback is the self-evaluation tool for the students (Rogers, 1992 ) by which they can improve their performance. Eraut ( 2006 ) highlighted the impact of feedback on future practice and student learning development. Good feedback practice is beneficial for student learning and teachers to improve students’ learning experience (Yorke, 2003 ). Hence the hypothesis that prompt feedback significantly affects satisfaction was included in this study.
H3: Prompt feedback of the students positively affects the satisfaction.
3.4 Expectations and satisfaction of students
Expectation is a crucial factor that directly influences the satisfaction of the student. Expectation Disconfirmation Theory (EDT) (Oliver, 1980 ) was utilized to determine the level of satisfaction based on their expectations (Schwarz & Zhu, 2015 ). Student’s expectation is the best way to improve their satisfaction (Brown et al., 2014 ). It is possible to recognize student expectations to progress satisfaction level (ICSB, 2015 ). Finally, the positive approach used in many online learning classes has been shown to place a high expectation on learners (Gold, 2011 ) and has led to successful outcomes. Hence the hypothesis that expectations of the student significantly affect the satisfaction was included in this study.
H4: Expectations of the students positively affects the satisfaction.
3.5 Satisfaction and performance of the students
Zeithaml ( 1988 ) describes that satisfaction is the outcome result of the performance of any educational institute. According to Kotler and Clarke ( 1986 ), satisfaction is the desired outcome of any aim that amuses any individual’s admiration. Quality interactions between instructor and students lead to student satisfaction (Malik et al., 2010 ; Martínez-Argüelles et al., 2016 ). Teaching quality and course material enhances the student satisfaction by successful outcomes (Sanderson, 1995 ). Satisfaction relates to the student performance in terms of motivation, learning, assurance, and retention (Biner et al., 1996 ). Mensink and King ( 2020 ) described that performance is the conclusion of student-teacher efforts, and it shows the interest of students in the studies. The critical element in education is students’ academic performance (Rono, 2013 ). Therefore, it is considered as center pole, and the entire education system rotates around the student’s performance. Narad and Abdullah ( 2016 ) concluded that the students’ academic performance determines academic institutions’ success and failure.
Singh et al. ( 2016 ) asserted that the student academic performance directly influences the country’s socio-economic development. Farooq et al. ( 2011 ) highlights the students’ academic performance is the primary concern of all faculties. Additionally, the main foundation of knowledge gaining and improvement of skills is student’s academic performance. According to Narad and Abdullah ( 2016 ), regular evaluation or examinations is essential over a specific period of time in assessing students’ academic performance for better outcomes. Hence the hypothesis that satisfaction significantly affects the performance of the students was included in this study.
H5: Students’ satisfaction positively affects the performance of the students.
3.6 Satisfaction as mediator
Sibanda et al. ( 2015 ) applied the goal theory to examine the factors persuading students’ academic performance that enlightens students’ significance connected to their satisfaction and academic achievement. According to this theory, students perform well if they know about factors that impact on their performance. Regarding the above variables, institutional factors that influence student satisfaction through performance include course design and quality of the instructor (DeBourgh, 2003 ; Lado et al., 2003 ), prompt feedback, and expectation (Fredericksen et al., 2000 ). Hence the hypothesis that quality of the instructor, course design, prompts feedback, and student expectations significantly affect the students’ performance through satisfaction was included in this study.
H6: Quality of the instructor, course design, prompt feedback, and student’ expectations affect the students’ performance through satisfaction.
H6a: Students’ satisfaction mediates the relationship between quality of the instructor and student’s performance.
H6b: Students’ satisfaction mediates the relationship between course design and student’s performance.
H6c: Students’ satisfaction mediates the relationship between prompt feedback and student’s performance.
H6d: Students’ satisfaction mediates the relationship between student’ expectations and student’s performance.
4.1 Participants
In this cross-sectional study, the data were collected from 544 respondents who were studying the management (B.B.A or M.B.A) and hotel management courses. The purposive sampling technique was used to collect the data. Descriptive statistics shows that 48.35% of the respondents were either MBA or BBA and rests of the respondents were hotel management students. The percentages of male students were (71%) and female students were (29%). The percentage of male students is almost double in comparison to females. The ages of the students varied from 18 to 35. The dominant group was those aged from 18 to 22, and which was the under graduation student group and their ratio was (94%), and another set of students were from the post-graduation course, which was (6%) only.
4.2 Materials
The research instrument consists of two sections. The first section is related to demographical variables such as discipline, gender, age group, and education level (under-graduate or post-graduate). The second section measures the six factors viz. instructor’s quality, course design, prompt feedback, student expectations, satisfaction, and performance. These attributes were taken from previous studies (Yin & Wang, 2015 ; Bangert, 2004 ; Chickering & Gamson, 1987 ; Wilson et al., 1997 ). The “instructor quality” was measured through the scale developed by Bangert ( 2004 ). The scale consists of seven items. The “course design” and “prompt feedback” items were adapted from the research work of Bangert ( 2004 ). The “course design” scale consists of six items. The “prompt feedback” scale consists of five items. The “students’ expectation” scale consists of five items. Four items were adapted from Bangert, 2004 and one item was taken from Wilson et al. ( 1997 ). Students’ satisfaction was measure with six items taken from Bangert ( 2004 ); Wilson et al. ( 1997 ); Yin and Wang ( 2015 ). The “students’ performance” was measured through the scale developed by Wilson et al. ( 1997 ). The scale consists of six items. These variables were accessed on a five-point likert scale, ranging from 1(strongly disagree) to 5(strongly agree). Only the students from India have taken part in the survey. A total of thirty-four questions were asked in the study to check the effect of the first four variables on students’ satisfaction and performance. For full details of the questionnaire, kindly refer Appendix Tables 6 .
The study used a descriptive research design. The factors “instructor quality, course design, prompt feedback and students’ expectation” were independent variables. The students’ satisfaction was mediator and students’ performance was the dependent variable in the current study.
4.4 Procedure
In this cross-sectional research the respondents were selected through judgment sampling. They were informed about the objective of the study and information gathering process. They were assured about the confidentiality of the data and no incentive was given to then for participating in this study. The information utilizes for this study was gathered through an online survey. The questionnaire was built through Google forms, and then it was circulated through the mails. Students’ were also asked to write the name of their college, and fifteen colleges across India have taken part to fill the data. The data were collected in the pandemic period of COVID-19 during the total lockdown in India. This was the best time to collect the data related to the current research topic because all the colleges across India were involved in online classes. Therefore, students have enough time to understand the instrument and respondent to the questionnaire in an effective manner. A total of 615 questionnaires were circulated, out of which the students returned 574. Thirty responses were not included due to the unengaged responses. Finally, 544 questionnaires were utilized in the present investigation. Male and female students both have taken part to fill the survey, different age groups, and various courses, i.e., under graduation and post-graduation students of management and hotel management students were the part of the sample.
5.1 Exploratory factor analysis (EFA)
To analyze the data, SPSS and AMOS software were used. First, to extract the distinct factors, an exploratory factor analysis (EFA) was performed using VARIMAX rotation on a sample of 544. Results of the exploratory analysis rendered six distinct factors. Factor one was named as the quality of instructor, and some of the items were “The instructor communicated effectively”, “The instructor was enthusiastic about online teaching” and “The instructor was concerned about student learning” etc. Factor two was labeled as course design, and the items were “The course was well organized”, “The course was designed to allow assignments to be completed across different learning environments.” and “The instructor facilitated the course effectively” etc. Factor three was labeled as prompt feedback of students, and some of the items were “The instructor responded promptly to my questions about the use of Webinar”, “The instructor responded promptly to my questions about general course requirements” etc. The fourth factor was Student’s Expectations, and the items were “The instructor provided models that clearly communicated expectations for weekly group assignments”, “The instructor used good examples to explain statistical concepts” etc. The fifth factor was students’ satisfaction, and the items were “The online classes were valuable”, “Overall, I am satisfied with the quality of this course” etc. The sixth factor was performance of the student, and the items were “The online classes has sharpened my analytic skills”, “Online classes really tries to get the best out of all its students” etc. These six factors explained 67.784% of the total variance. To validate the factors extracted through EFA, the researcher performed confirmatory factor analysis (CFA) through AMOS. Finally, structural equation modeling (SEM) was used to test the hypothesized relationships.
5.2 Measurement model
The results of Table 1 summarize the findings of EFA and CFA. Results of the table showed that EFA renders six distinct factors, and CFA validated these factors. Table 2 shows that the proposed measurement model achieved good convergent validity (Aggarwal et al., 2018a , b ). Results of the confirmatory factor analysis showed that the values of standardized factor loadings were statistically significant at the 0.05 level. Further, the results of the measurement model also showed acceptable model fit indices such that CMIN = 710.709; df = 480; CMIN/df = 1.481 p < .000; Incremental Fit Index (IFI) = 0.979; Tucker-Lewis Index (TLI) = 0.976; Goodness of Fit index (GFI) = 0.928; Adjusted Goodness of Fit Index (AGFI) = 0.916; Comparative Fit Index (CFI) = 0.978; Root Mean Square Residual (RMR) = 0.042; Root Mean Squared Error of Approximation (RMSEA) = 0.030 is satisfactory.
The Average Variance Explained (AVE) according to the acceptable index should be higher than the value of squared correlations between the latent variables and all other variables. The discriminant validity is confirmed (Table 2 ) as the value of AVE’s square root is greater than the inter-construct correlations coefficient (Hair et al., 2006 ). Additionally, the discriminant validity existed when there was a low correlation between each variable measurement indicator with all other variables except with the one with which it must be theoretically associated (Aggarwal et al., 2018a , b ; Aggarwal et al., 2020 ). The results of Table 2 show that the measurement model achieved good discriminate validity.
5.3 Structural model
To test the proposed hypothesis, the researcher used the structural equation modeling technique. This is a multivariate statistical analysis technique, and it includes the amalgamation of factor analysis and multiple regression analysis. It is used to analyze the structural relationship between measured variables and latent constructs.
Table 3 represents the structural model’s model fitness indices where all variables put together when CMIN/DF is 2.479, and all the model fit values are within the particular range. That means the model has attained a good model fit. Furthermore, other fit indices as GFI = .982 and AGFI = 0.956 be all so supportive (Schumacker & Lomax, 1996 ; Marsh & Grayson, 1995 ; Kline, 2005 ).
Hence, the model fitted the data successfully. All co-variances among the variables and regression weights were statistically significant ( p < 0.001).
Table 4 represents the relationship between exogenous, mediator and endogenous variables viz—quality of instructor, prompt feedback, course design, students’ expectation, students’ satisfaction and students’ performance. The first four factors have a positive relationship with satisfaction, which further leads to students’ performance positively. Results show that the instructor’s quality has a positive relationship with the satisfaction of students for online classes (SE = 0.706, t-value = 24.196; p < 0.05). Hence, H1 was supported. The second factor is course design, which has a positive relationship with students’ satisfaction of students (SE = 0.064, t-value = 2.395; p < 0.05). Hence, H2 was supported. The third factor is Prompt feedback, and results show that feedback has a positive relationship with the satisfaction of the students (SE = 0.067, t-value = 2.520; p < 0.05). Hence, H3 was supported. The fourth factor is students’ expectations. The results show a positive relationship between students’ expectation and students’ satisfaction with online classes (SE = 0.149, t-value = 5.127; p < 0.05). Hence, H4 was supported. The results of SEM show that out of quality of instructor, prompt feedback, course design, and students’ expectation, the most influencing factor that affect the students’ satisfaction was instructor’s quality (SE = 0.706) followed by students’ expectation (SE =5.127), prompt feedback (SE = 2.520). The factor that least affects the students’ satisfaction was course design (2.395). The results of Table 4 finally depicts that students’ satisfaction has positive effect on students’ performance ((SE = 0.186, t-value = 2.800; p < 0.05). Hence H5 was supported.
Table 5 shows that students’ satisfaction partially mediates the positive relationship between the instructor’s quality and student performance. Hence, H6(a) was supported. Further, the mediation analysis results showed that satisfaction again partially mediates the positive relationship between course design and student’s performance. Hence, H6(b) was supported However, the mediation analysis results showed that satisfaction fully mediates the positive relationship between prompt feedback and student performance. Hence, H6(c) was supported. Finally, the results of the Table 5 showed that satisfaction partially mediates the positive relationship between expectations of the students and student’s performance. Hence, H6(d) was supported.
6 Discussion
In the present study, the authors evaluated the different factors directly linked with students’ satisfaction and performance with online classes during Covid-19. Due to the pandemic situation globally, all the colleges and universities were shifted to online mode by their respective governments. No one has the information that how long this pandemic will remain, and hence the teaching method was shifted to online mode. Even though some of the educators were not tech-savvy, they updated themselves to battle the unexpected circumstance (Pillai et al., 2021 ). The present study results will help the educators increase the student’s satisfaction and performance in online classes. The current research assists educators in understanding the different factors that are required for online teaching.
Comparing the current research with past studies, the past studies have examined the factors affecting the student’s satisfaction in the conventional schooling framework. However, the present study was conducted during India’s lockdown period to identify the prominent factors that derive the student’s satisfaction with online classes. The study also explored the direct linkage between student’s satisfaction and their performance. The present study’s findings indicated that instructor’s quality is the most prominent factor that affects the student’s satisfaction during online classes. This means that the instructor needs to be very efficient during the lectures. He needs to understand students’ psychology to deliver the course content prominently. If the teacher can deliver the course content properly, it affects the student’s satisfaction and performance. The teachers’ perspective is critical because their enthusiasm leads to a better online learning process quality.
The present study highlighted that the second most prominent factor affecting students’ satisfaction during online classes is the student’s expectations. Students might have some expectations during the classes. If the instructor understands that expectation and customizes his/her course design following the student’s expectations, then it is expected that the students will perform better in the examinations. The third factor that affects the student’s satisfaction is feedback. After delivering the course, appropriate feedback should be taken by the instructors to plan future courses. It also helps to make the future strategies (Tawafak et al., 2019 ). There must be a proper feedback system for improvement because feedback is the course content’s real image. The last factor that affects the student’s satisfaction is design. The course content needs to be designed in an effective manner so that students should easily understand it. If the instructor plans the course, so the students understand the content without any problems it effectively leads to satisfaction, and the student can perform better in the exams. In some situations, the course content is difficult to deliver in online teaching like the practical part i.e. recipes of dishes or practical demonstration in the lab. In such a situation, the instructor needs to be more creative in designing and delivering the course content so that it positively impacts the students’ overall satisfaction with online classes.
Overall, the students agreed that online teaching was valuable for them even though the online mode of classes was the first experience during the pandemic period of Covid-19 (Agarwal & Kaushik, 2020 ; Rajabalee & Santally, 2020 ). Some of the previous studies suggest that the technology-supported courses have a positive relationship with students’ performance (Cho & Schelzer, 2000 ; Harasim, 2000 ; Sigala, 2002 ). On the other hand, the demographic characteristic also plays a vital role in understanding the online course performance. According to APA Work Group of the Board of Educational Affairs ( 1997 ), the learner-centered principles suggest that students must be willing to invest the time required to complete individual course assignments. Online instructors must be enthusiastic about developing genuine instructional resources that actively connect learners and encourage them toward proficient performances. For better performance in studies, both teachers and students have equal responsibility. When the learner faces any problem to understand the concepts, he needs to make inquiries for the instructor’s solutions (Bangert, 2004 ). Thus, we can conclude that “instructor quality, student’s expectation, prompt feedback, and effective course design” significantly impact students’ online learning process.
7 Implications of the study
The results of this study have numerous significant practical implications for educators, students and researchers. It also contributes to the literature by demonstrating that multiple factors are responsible for student satisfaction and performance in the context of online classes during the period of the COVID-19 pandemic. This study was different from the previous studies (Baber, 2020 ; Ikhsan et al., 2019 ; Eom & Ashill, 2016 ). None of the studies had examined the effect of students’ satisfaction on their perceived academic performance. The previous empirical findings have highlighted the importance of examining the factors affecting student satisfaction (Maqableh & Jaradat, 2021 ; Yunusa & Umar, 2021 ). Still, none of the studies has examined the effect of course design, quality of instructor, prompt feedback, and students’ expectations on students’ satisfaction all together with online classes during the pandemic period. The present study tries to fill this research gap.
The first essential contribution of this study was the instructor’s facilitating role, and the competence he/she possesses affects the level of satisfaction of the students (Gray & DiLoreto, 2016 ). There was an extra obligation for instructors who taught online courses during the pandemic. They would have to adapt to a changing climate, polish their technical skills throughout the process, and foster new students’ technical knowledge in this environment. The present study’s findings indicate that instructor quality is a significant determinant of student satisfaction during online classes amid a pandemic. In higher education, the teacher’s standard referred to the instructor’s specific individual characteristics before entering the class (Darling-Hammond, 2010 ). These attributes include factors such as instructor content knowledge, pedagogical knowledge, inclination, and experience. More significantly, at that level, the amount of understanding could be given by those who have a significant amount of technical expertise in the areas they are teaching (Martin, 2021 ). Secondly, the present study results contribute to the profession of education by illustrating a realistic approach that can be used to recognize students’ expectations in their class effectively. The primary expectation of most students before joining a university is employment. Instructors have agreed that they should do more to fulfill students’ employment expectations (Gorgodze et al., 2020 ). The instructor can then use that to balance expectations to improve student satisfaction. Study results can be used to continually improve and build courses, as well as to make policy decisions to improve education programs. Thirdly, from result outcomes, online course design and instructors will delve deeper into how to structure online courses more efficiently, including design features that minimize adversely and maximize optimistic emotion, contributing to greater student satisfaction (Martin et al., 2018 ). The findings suggest that the course design has a substantial positive influence on the online class’s student performance. The findings indicate that the course design of online classes need to provide essential details like course content, educational goals, course structure, and course output in a consistent manner so that students would find the e-learning system beneficial for them; this situation will enable students to use the system and that leads to student performance (Almaiah & Alyoussef, 2019 ). Lastly, the results indicate that instructors respond to questions promptly and provide timely feedback on assignments to facilitate techniques that help students in online courses improve instructor participation, instructor interaction, understanding, and participation (Martin et al., 2018 ). Feedback can be beneficial for students to focus on the performance that enhances their learning.
Author information
Authors and affiliations.
Chitkara College of Hospitality Management, Chitkara University, Chandigarh, Punjab, India
Ram Gopal & Varsha Singh
Chitkara Business School, Chitkara University, Chandigarh, Punjab, India
Arun Aggarwal
You can also search for this author in PubMed Google Scholar
Corresponding author
Correspondence to Arun Aggarwal .
Ethics declarations
Ethics approval.
Not applicable.
Conflict of interest
The authors declare no conflict of interest, financial or otherwise.
Additional information
Publisher’s note.
Springer Nature remains neutral with regard to jurisdictional claims in published maps and institutional affiliations.
Rights and permissions
Reprints and permissions
About this article
Gopal, R., Singh, V. & Aggarwal, A. Impact of online classes on the satisfaction and performance of students during the pandemic period of COVID 19. Educ Inf Technol 26 , 6923–6947 (2021). https://doi.org/10.1007/s10639-021-10523-1
Download citation
Received : 07 December 2020
Accepted : 22 March 2021
Published : 21 April 2021
Issue Date : November 2021
DOI : https://doi.org/10.1007/s10639-021-10523-1
Share this article
Anyone you share the following link with will be able to read this content:
Sorry, a shareable link is not currently available for this article.
Provided by the Springer Nature SharedIt content-sharing initiative
- Quality of instructor
- Course design
- Instructor’s prompt feedback
- Expectations
- Student’s satisfaction
- Perceived performance
Advertisement
- Find a journal
- Publish with us
- Track your research
Shaping the Future of Online Learning
Published may 22, 2024.
If you’ve been enrolled in any educational course or postsecondary educational program since 2020, chances are you’ve witnessed the rise in online learning firsthand .
The COVID-19 global pandemic shuttered storefronts, theaters, and classrooms alike, causing major disruptions in how goods and services were delivered. As consumers adopted Instacart for their grocery needs and streamed new blockbuster movies from the comfort of their living rooms, students needed an innovative way to bring their classes home. A year into the pandemic over 60% of all undergraduate students were enrolled in at least one online course , with 28% exclusively enrolled in online courses, according to the National Center for Education Statistics.
There are other reasons for the widespread adoption, including accessibility. Rural and international students who may be far removed from traditional educational institutions can now attend Harvard classes anywhere there’s an internet connection. Or, consider working adults seeking to progress or switch careers. Life doesn’t stop for a class, and attending one in-person can be prohibitive. While still challenging, logging into a virtual classroom is far more manageable. Online education is for everyone.
Technological and pedagogical developments have helped online learning progress beyond the days of discussion boards and essay uploads. Now, students can enjoy a multimedia educational experience that is rooted in the latest research, all while participating in the community of their “virtual campus”.
If you’re one of the millions of learners who have experienced online education, you might be interested to learn where it’s going next. At Harvard Online, the question, “what is the future of online learning?” guides an ongoing conversation that drives us everyday.
In this blog, we sat down with Catherine Breen , Managing Director of Harvard Online. With more than two decades of senior executive leadership at Harvard University and oversight of Harvard Online, Breen has an invaluable perspective on the future of online learning, and the exciting role Harvard Online is playing in bringing the future into the present.
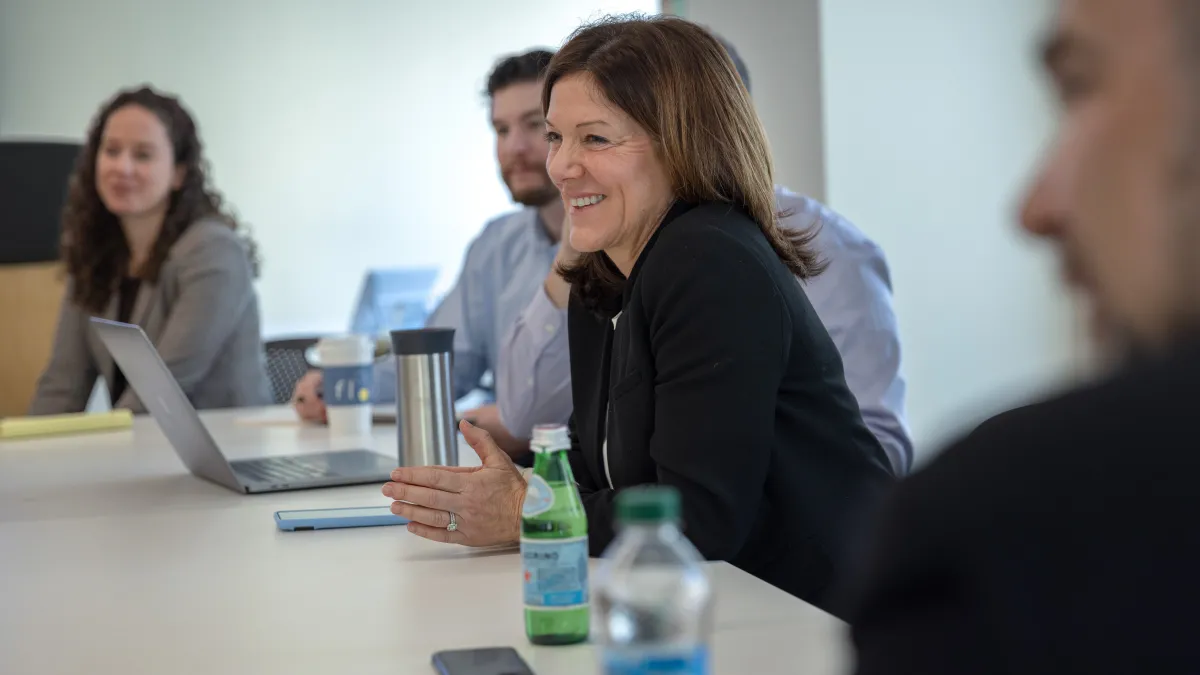
Catherine Breen, Managing Director of Harvard Online, in a team meeting.
Harvard Online (HO): How has the online learning landscape evolved in recent years?
Catherine Breen (CB): At the beginning of the COVID-19 lockdown, there was a massive escalation in demand for online learning. Demand began to recede slowly as the months wore on and by late 2022, it started to level out. But we observed two big changes: Internally, the demand for Harvard Online content was still almost three times higher than pre-pandemic. Externally, in reaction to the demand surge, there was significant and rapid growth of new online course offerings and companies that purveyed varying types of digital products.
HO: What is shaping the future of online learning today?
CB: Because of the rapid and massive shift to online that occurred around the globe in the spring of 2020, the landscape changed permanently. There are many things shaping the future but here are just a few that I can see from my perspective:
- Increased adoption of online learning across all ages and levels of education: Everyone expanded their online course catalogs; new companies and offerings sprung up everywhere.
- Greater tech investment across organizations and industries: Organizations are investing more time, money, and effort into technology infrastructure, tools, and platforms to support online learning and participants in these courses.
- New pedagogical methods to bridge the gap between traditional and novel learning methods: Instructors have adapted their teaching methods for online, hybrid, and blended environments.
- Enhanced accessibility to quality education and learning experiences: Efforts have been made to improve access for students of all types, abilities, geographies, and backgrounds so that everyone can participate effectively.
HO: What are the remaining challenges that online learning faces?
CB: While these changes have improved the online learning experience, challenges remain, including addressing the digital divide, maximizing student engagement, and refining the quality of online courses.
The pandemic accelerated the adoption of online learning and its impact will likely continue to shape higher education for many years to come.
HO: How does online learning contribute to Harvard's mission of promoting accessibility and inclusion in education, especially for learners who may not have traditional access to higher education?
CB: Online learning levels the playing field for learners in many ways.
Most students think that a Harvard-quality education is out of reach, for a variety of reasons. With online courses, however, learners from around the country and the world can take courses with Harvard instructors at their own pace at a more affordable price point.
Our online courses also typically incorporate a range of multimedia elements, allowing students with different learning styles to flourish. We also ensure that our online learning experiences are accessible to all learners, including those with disabilities. This commitment to inclusivity aligns with the broader goals of promoting equitable access to education.
Lastly, our online courses often include discussion forums and virtual communities where learners can connect and collaborate. This allows for interactions among students from diverse backgrounds and experiences, fostering a sense of belonging and inclusion.
It’s clear that online learning has a lot to offer everyone, and it’s only getting better. In our next blog in this series, we’ll hear more from Cathy on how institutions can implement online learning modalities effectively.
If you missed the first blog in this series detailing the future of online learning, you can check out the first blog here . To learn more about Harvard Online, explore our fully online course catalog here .
Related Blog Posts
Uniref brings harvard courses on web programming to syrian refugees.
Harvard Online is proud to provide access to education and experiences that help communities thrive.
Harvard Online in Your Workplace: Elevate Your Team's Professional Development
At Harvard Online we understand the value of an educated and skilled workforce.
A Decade of Innovation: Online Learning at Harvard
We are always asking, “What does the future look like for teaching and learning?”
Redding, HGSE Online Master’s Students to Headline Harvard Commencement Broadcast
- Posted May 22, 2024
- By Ryan Nagelhout
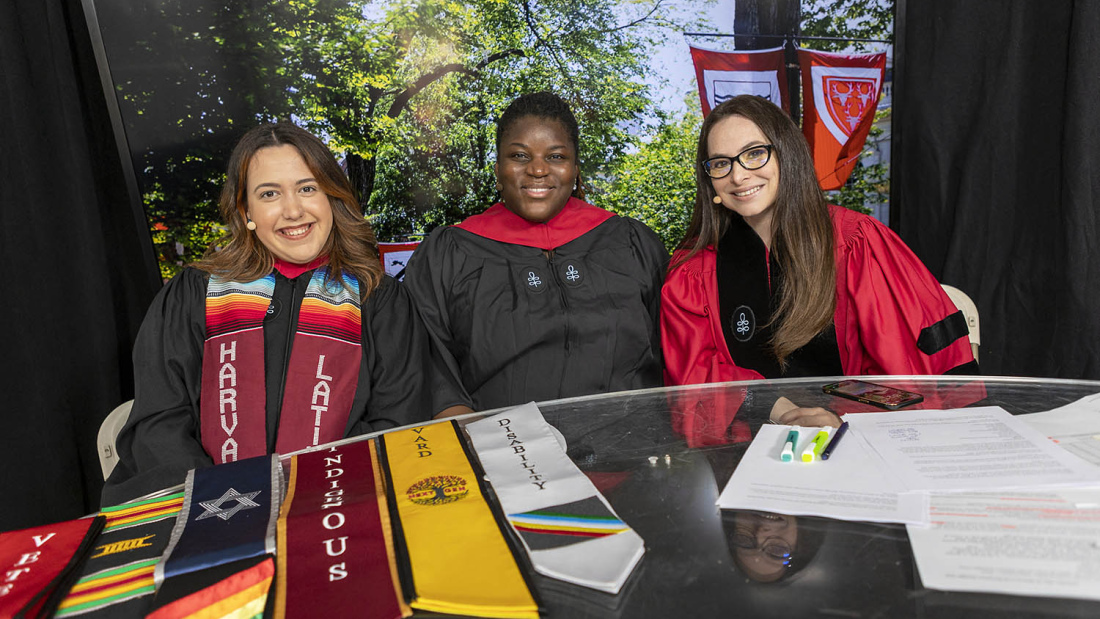
Harvard’s annual commencement activities are a highly orchestrated tradition that honors both the university’s centuries-long history as well as the accomplishments of those receiving diplomas.
Morning Exercises in Tercentenary Theater are an exclusive affair, with limited ticketing, so the university’s commencement broadcast is often the only glimpse into the proceedings for many, especially those not in Cambridge.
That’s a significant responsibility that HGSE Lecturer Alexis Redding will take on Thursday morning. The faculty co-chair of the Ed School’s Higher Education Concentration will serve as the lead commentator for Harvard’s Commencement broadcast this year, which will feature considerable contributions from the HGSE community.
“It is a privilege to be part of a program that provides access to loved ones from around the globe who cannot be with us on campus,” says Redding. “During the broadcast, I hope to give them a sense of the excitement and energy in the Yard and to share stories about our institution.”
The livestreamed broadcast of Harvard’s annual commencement celebration will feature speeches, orations, and the conferring of degrees upon the Class of 2024, along with significant insight into the history of Harvard and the unique stories of some of its newest alumni.
The biggest change in this year’s broadcast is the switch from two to one faculty broadcaster, as Redding will serve as the lone commentator. The break from precedent, Redding said, will allow for more time on air for guests from around the Harvard community.
“I am especially excited about this opportunity to uplift voices from across the university as a whole — graduating students, alumni, and administrators,” says Redding. “This will broaden representation and help our viewers at home get a more robust sense of what it means to be a Harvard student.”
Some of those voices will come from elsewhere in the HGSE community, including a pair of graduates — Adam Milano and Kamal James — making history as part of the first graduating cohort from the school’s Online Master's in Education Program . Redding noted that neither student would have been able to relocate for a campus-based degree program — Milano was a behavioral health officer in the U.S. Army on active duty, while James juggled work, school, and raising his children in North Carolina.
“Adam would Zoom into class from his army base while Kamal sometimes attended advising meetings from his car waiting for his daughter to finish dance class,” says Redding. “Their perspectives help to highlight different ways of being a Harvard student in the 21st century.”
The broadcast will also feature two Ed School alumni now working elsewhere at Harvard — Alysha Johnson Williams, Ed.M.’20 (resident dean of first-year students and assistant dean of Harvard College), and Anabella Morabito, Ed.M.’22 (associate director for Equity, Diversity & Inclusion Community Engagement at Harvard University).
Redding is no stranger to Harvard event broadcasts — she offered commentary on Claudine Gay’s inauguration broadcast in the fall of 2023. That was much more of a whirlwind experience, however, as she was a last-minute substitution due to another commentator's illness.
“I had less than 36 hours to prepare,” recalled Redding. “They handed me an enormous binder to study and brought me to the Archives to hold the keys to the university and see the charter, which was really special. But then, I feel like I blinked and I was live on air!”
Given much more time to prepare for commencement, Redding was able to use her own research and experience to help plan the broadcast’s vision. Redding’s work in recent years has included researching the student experience at Harvard and Radcliffe Colleges since 1940, a perspective she plans to include in her commentary on Thursday.
She was also able to hand-pick archival objects to share during the broadcast, which meant a trip to Pusey Library to look at some historic items that have shaped the history of the school. Redding recalled holding W.E.B. DuBois’ doctoral dissertation and exploring a box of daguerreotypes that represented the first yearbook of sorts for Harvard College. Some of those items were too fragile to leave the vault, but the experience offered her perspective on the vast history of the university and the magnitude of commencement festivities.
“I have spent a lot of time reflecting on what it means to celebrate our students and to give their loved ones at home a special experience,” says Redding, “While also acknowledging the complexity of hosting celebrations in our current moment and recognizing the challenging year we have all experienced here at the university and in higher education more broadly.”
Redding said the Ed School’s representation in the broadcast is a reflection of the important part it plays in the larger Harvard community.
“As a school of education, we think deeply about the themes that are celebrated in commencement — the student experience, institutional priorities, history of higher education, leadership, and community-building more broadly,” says Redding. “We also have a good number of higher ed students who find roles at the university after graduation, so the Ed School plays a key role in the day-to-day life of the institution and its students as well.”

The latest research, perspectives, and highlights from the Harvard Graduate School of Education
Related Articles

The Impact We Hope to Have
Kamal James and Inella Ray will be honored with the Intellectual Contribution Award for the Online Master's in Education Program

Paxton, Maheshwari-Kanoria to Receive 2024 Alumni Council Awards
Alums will be honored for their educational contributions at HGSE Convocation

HGSE Launches Online Master’s in Education Leadership
Why You Should Choose an Online Degree to Excel in Your Career?
Image Source
Technological advancements have made a huge impact on the education sector. As a result of it, online learning has become a major trend. It ensures a great level of convenience for students across the world to pursue a degree from anywhere.
Whether you want to earn a bachelor’s degree or want to continue further education to get an advanced degree, the option of an online degree is for everyone.
The trend of online learning was increased in different parts of the world, especially after the COVID-19 pandemic. It also increased the usage of different devices for distance learning in various parts of the world.
According to a statistical study, around 58% of Filipino students use different gadgets for online learning. It shows how education has evolved in different parts of the world, as now you can easily pursue your degree from the Philippines through remote learning.
Let’s find out why you should choose the online degree to excel in your career.
- Flexibility
One of the major benefits that online degree offers is flexibility. It allows you to plan your schedule according to your convenience and daily commitments.
Once you enroll in the online degree, you can also choose the timings of your classes. Most online degrees provide this option, but it is better to inquire beforehand about it.
Also, now you can enroll in the online accredited degrees of another country while staying in your own country. For example, if you are from the Philippines, then you can pursue a US-accredited degree from the Philippines. If you want have previously done majors in business, then you can enroll in an online MBA in the Philippines . In this way, you have the flexibility of earning a high-level degree from another country.
In on-campus programs, you have to follow a fixed schedule every week. But when it comes to distance learning, you can attend online lectures at any time, according to your convenience.
- Better Affordability
Another reason for investing in online degrees is better affordability. It allows you to save your cost big time, which can make your academic journey easier as a student.
You can save a good amount of money on tuition fees by enrolling in an online program. Comparing the fee structure of on-campus and online degrees will give you a better idea.
Apart from fees, there are also other ways in which an online degree can help you save money. By leveraging distance learning, you do not have to pay for transport, daily meals, and other expenses.
You also do not have to worry about the cost of commuting. The books and notes are also available in digital formats, which means you do not have to buy expensive books. So, opting for an online degree can be affordable in many ways.
- More Spare Time
When you do not have to commute to campus, it gives you more free time. You can utilize that time smartly to keep track of your different academic activities and manage your coursework efficiently.
When you have more spare time, you can also utilize it to develop useful skills which can go a long way in your professional journey.
It is also important to understand that when you are pursuing an online degree, procrastination can get in your way. Therefore, remote learning forces you to develop exceptional time management skills.
Successful online students have good time management skills that allow them to keep track of their academic activities. It helps them to meet deadlines for their assignments and projects, which positively impacts their academic performance. Try to manage your academic activities at fixed intervals during the week. It will be tough initially, but with time, you will develop the habit of studying regularly.
- Career Advancement Opportunities
Another benefit that online learning can provide you is career advancement opportunities. You can opt for the relevant master’s degree according to your field, and you do not have to leave your current job for it.
Additionally, earning an advanced degree will show your dedication to your current employer. It can improve your professional credentials, which will open the gates to better opportunities for you. It will make you eligible for the poisons with a higher pay scale and increase your chances of promotion. In short, it can help you to secure your dream job.
On the other hand, going for the traditional degree program does not provide you with such opportunities. Therefore, you have to make the right decision for a better future.
- Better Quality of Learning
When you are physically present in class, you can miss key points of the lecture when your mind drifts for a few seconds. In such situations, you would like to press a rewind button to catch those important points from the professor again.
In online learning, you have the luxury of catching anything that you missed previously. Many students have reported that it was easier for them to learn complex topics because they were able to watch lectures again and again.
The learning pace of every student is different, so online learning helps every student to excel. Furthermore, online courses also offer additional study guides and reading materials. It helps to engage students more effectively and to create a good learning environment.
The online programs also better address the changing needs of students. Universities offering distance learning are focused on providing market-driven programs and courses which can fulfill modern-day educational needs.
Most of the online programs are updated according to the latest technological trends, so it also significantly impacts the quality of learning. The leading-edge programs in any field laid the foundation for the successful careers of students. However, it is important to inquire about the previous track record of the particular institute before enrolling in a particular online program.
Choosing an online degree can provide you with different benefits. From flexible scheduling to better affordability, several benefits can positively impact your academic journey and help you to accomplish your career goals efficiently. The needs of every student are different, so you need to plan your academic life according to your requirements.
The post Why You Should Choose an Online Degree to Excel in Your Career? appeared first on Things That Make People Go Aww .
- Open Search box
- Master of Science in Business Analytics
- Admissions Overview
- Class Profile
- Prerequisites
- Holistic Career Services
- Constant Industry Infusion
- Student Outcomes & Placement
- Career Services FAQ
- Student Life
- For Companies Overview
- Meet Our Students
- Recruit MSBAs
- Capstone: Applied Analytics Project
- Class of 2018
- Class of 2019
- Class of 2020
- Class of 2021
- Class of 2022
- Class of 2023
- Class of 2024
- Meet Our Team Overview
- Admit Central Home
- Admit Checklist
- Prep Before You Start
- Program Calendar and Fees
- Campus Resources
- Student Health
- Transportation and Parking
- Housing and Utilities
- Career Services
- For International Students
- Meet Our Team
UCLA Anderson Master of Science in Business Analytics (MSBA)
Master of Science in Business Analytics (MSBA)
Ranked #1 in the World
by QS World University Rankings, 2023
Become a Master of Business Analytics (MSBA)
About our program, a day in the life of the msba program.
Trailblazing Faculty
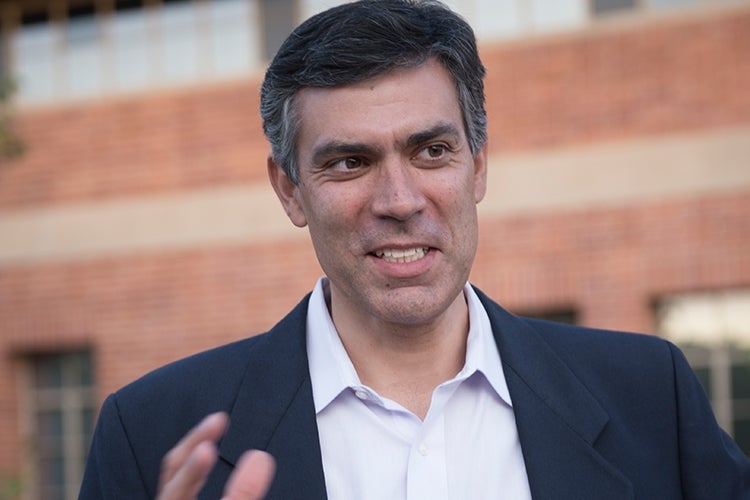
Faculty Spotlight
Felipe Caro Faculty Director of the MSBA Program, Professor of Decisions, Operations and Technology Management
Professor Caro, known for helping Zara re-engineer its supply chain to become a “fast fashion” global retailer (and more profitable), is a renowned researcher who is highly published and frequently awarded for his work. His design of the MSBA curriculum is greatly influenced by changing markets and is engineered to produce the critical, analytical thinkers that the organizations of tomorrow need most.
Listen to the Podcast
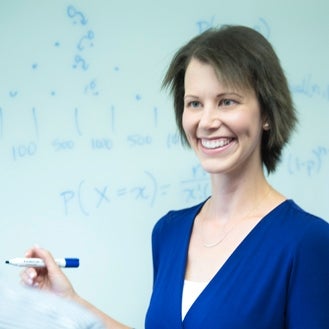
Professor Decisions, Operations and Technology Management
One of Long 's specialties includes applying quantitative analysis to the ambiguities of the health care industry.
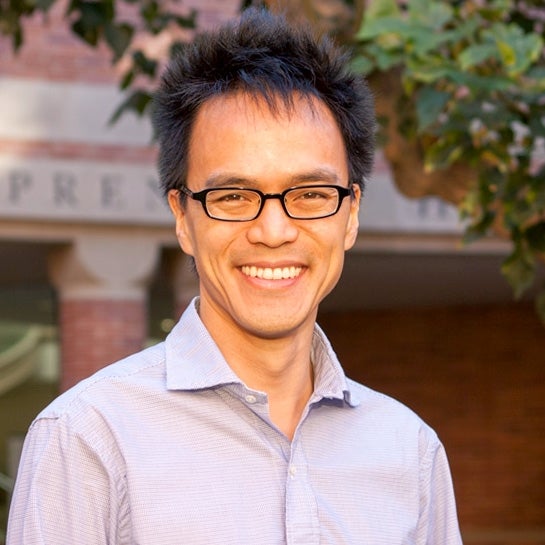
Professor of Behavioral Economics and Strategy
Beyond his award-winning teaching and research, Professor Chen advises companies on topics at the intersection of behavioral economics, business strategy and dynamic pricing. At Uber, he redesigned its dynamic “surge” pricing model.
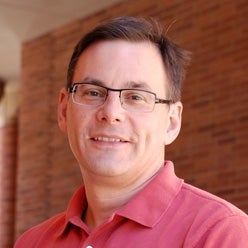
Distinguished Professor of Marketing, Economics and Statistics
While more recently focusing on consumer targeting and analytic pricing tools, Professor Rossi’s Bayesian hierarchical choice models created the most widely used methods for analysis of choice and conjoint data used today.
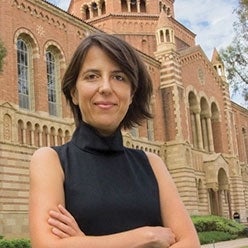
Professor of Economics
In her research, Professor Giuliano studies the nexus of culture, economics and political economy. She holds prestigious research positions at the National Bureau of Economic Research (Cambridge), the Centre for Economic Policy Research (London) and the Institute for the Study of Labor (Bonn).
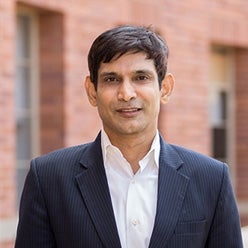
Associate Professor Marketing
Consistently awarded the MSBA faculty excellence award, Bodapati ’s Customer Analytics course addresses marketing problems in value creation, value communication, customer acquisition, customer development, customer retention and the assessment of customer response to marketing.

Assistant Professor Decisions, Operations and Technology Management
A multiple MSBA faculty excellence award winner, Mišić focuses on decisions in uncertainty, customer choice problems and machine learning-based optimization in his operations analytics course.
Follow the UCLA Anderson MSBA Program

- About UCLA Anderson
- Our Character
- Our Strategic Plan
- Our Leadership
- Our History
- Office of Development Home
- Impact Stories
- The Anderson Fund
- Student Fellowships
- Equity, Diversity and Inclusion
- Centers@Anderson
- Faculty Research
- Dean’s Society Leadership Giving
- Reunion Giving
- Anderson Affiliates
- Ways to Give
- Contact Development
- Our Centers Home
- Center for Global Management Home
- For Students Overview
- Specialize In Global Management
- On-Campus and/or Hybrid Global Management Courses
- Global Immersion Courses
- Global Nonprofit Capstone Projects
- MBA Research Assistants
- Career and Personal Development
- UCLA-NUS Executive MBA
- F/EMBA International Exchange
- EMBA International Business Residency
- Global Management Seminars
- International Exchange
- Events and Discussions Overview
- Global Conferences
- Greater China and LatAm Series
- Global Management Speaker Series
- Global Management Lecture Series
- Global Business & Policy Forums
- World Today Discussion Series
- Robertson Lecture Series on Global Business Leadership
- Lunch and Dinner Series
- External Collaborative Partnerships
- Upcoming Events
- Past Center Sponsored Events
- Other UCLA Events
- Faculty & Global Research
- Video Gallery
- Support The Center
- Center for Media, Entertainment & Sports Home
- Events Overview
- Pulse Conference Home
- Entertainment Case Competition
- Game Day Sports Case Competition
- Global Sports Business Forum
- INSIGHTS - Big Data Conference
- Real Madrid Global Sports Leadership
- Research & Insights
- Corporate Partnership
- Student Experience Overview
- Industry Network
- Undergraduate Summer Institute Overview
- Howard University Initiative
- High School Summer Discovery
- About The Center for Media, Entertainment & Sports
- Board of Directors
- Easton Technology Management Center
- Innovation Challenge Home
- Sustainability Track
- Healthcare Track
- Generative AI Track
- Mentors & Advisors
- Competition Details
- Past Events
- Easton Courses
- Specialization
- Innovate Conference
- Tech + Society Conference
- The Embracing AI Summit
- Easton Instructors
- Get Involved
- About The Easton Technology Management Center
- Board of Advisors
- Faculty Advisory Board
- Fink Center for Finance & Investments Home
- Career Impact
- Student Fellowships Overview
- Investment Banking Fellowship
- Kayne Investment Management Fellowship
- Brown Private Equity and Alternatives Fellowship
- Quantitative Finance Fellowship
- News and Events Overview
- Conference on Financial Markets
- Fink Investing Conference Home
- Private Equity Roundtable
- Fink Credit Pitch Competition
- Faculty & Research
- Meet Our Board
- Center for Impact Home
- Academics Overview
- Specializations and Certificates
- Impact Investing
- Social Impact Consulting
- Open For Good Transparency Index
- Environmental Metrics
- Social Metrics
- Governance Metrics
- Our Methodology
- State of Corporate Sustainability Disclosure
- 2023 Report
- 2022 Report
- Sustainability Workshops
- Corporate Partnership Program
- Faculty and Research
- Research and Seminars
- Research in Energy
- Research in Sustainability
- Research in Social Responsibility
- Alliance for Research on Corporate Sustainability ARCS
- Impact Week
- Morrison Center for Marketing & Data Analytics Home
- Gilbert Symposium
- Research Overview
- Funded Research
- Student Programs Overview
- Affiliated Student Organizations
- Case Competitions
- Ph.D. Students
- Morrison Center Advisory Board
- Price Center for Entrepreneurship & Innovation Home
- Fellowships
- Undergraduate Minor in Entrepreneurship
- Student Investment Fund
- For Professionals Overview
- Health Care Executive Program
- Entrepreneurship Bootcamp for Veterans
- UCLA Head Start Management Fellows Program
- Steinbeck Family Business Seminar
- Management Development for Entrepreneurs
- UCLA Health Care Institute
- Anderson Venture Accelerator Home
- Our Programs
- Our Companies
- Mentors and Advisors
- Showcase 2023
- Showcase 2022
- Showcase 2021
- Showcase 2020
- Knapp Venture Competition
- Entrepreneur Association (EA)
- Past Winners
- Hire an Anderson Intern
- UCLA Anderson Forecast Home
- Research and Reports Overview
- Forecast Direct Podcast
- Projects and Partnerships Overview
- Forecast Fellows Program
- Allen Matkins
- Cathay Bank
- City Human Capital Index
- Los Angeles City Employment
- Engage with Us Overview
- Become A Member
- Become A Sponsor
- Speaking Engagements
- Member Login
- Renew Membership
- Join Email List
- UCLA Ziman Center for Real Estate
- Howard and Irene Levine Fellows
- Peter Bren Fellows in Entrepreneurial Real Estate
- Corporate Concierge Recruiting
- Howard and Irene Levine Affordable Housing Development Program
- Alumni (UCLA REAG)
- UCLA Ziman Center Symposium
- Howard J. Levine Distinguished Lecture on Business Ethics & Social Responsibility
- UCLA Distinguished Speaker Series in Affordable Housing
- Faculty & Research Overview
- UCLA Gilbert Program in Real Estate, Finance and Urban Economics
- UCLA Economic Letter
- UCLA Affordable Housing Policy Brief
- Working Papers
- Eviction Moratoria and Other Rental Market COVID-19 Policy Interventions
- Mortgage Default Risk Index (MDRI)
- CRSP/Ziman REIT Data Series
- Conference on Low-Income Housing Supply and Housing Affordability
- Impact on Our Community Overview
- Housing as Health Care Initiative
- Howard and Irene Levine Program in Housing and Social Responsibility
- Board Leadership
- Clubs & Associations Home
- Anderson Student Association (ASA)
- Think in the Next Innovation Challenge
- Innovation & Design Case Competition
- Strategy and Operations Case Competition
- Health Care Business Case Competition
- Challenges in Energy Case Competition
- Professional Clubs
- Association of Veterans at Anderson (AVA)
- Association for Real Estate at Anderson (AREA)
- Energy and Cleantech Association (ECA) Home
- Energy Innovation Conference
- Entertainment Management Association (EMA) Home
- International Film Festival
- Food & Beverage Association (FABA)
- Healthcare Business Association (HBA) Home
- HBA VITALS Conference
- Innovation & Design at Anderson (IDeA) Home
- Innovation and Design Case Competition
- Investment Finance Association (IFA)
- Management Consulting Association (MCA)
- Marketing Association (MA)
- Net Impact (NI) Home
- High Impact Tea
- Retail Business Association (RBA) Home
- Evolve Conference
- Sports Business Association (SBA)
- Strategy & Operations Management Association (SOMA) Home
- Tech Business Association at Anderson (AnderTech) Home
- Unchained: Blockchain Business Forum
- Women’s Business Connection (WBC)
- Identity Clubs
- The Alliance for Latinx Management at Anderson (ALMA)
- Asian Management Student Association (AMSA)
- Black Business Students Association (BBSA) Home
- BHM Events - Better Together
- Christian Student Fellowship (CSF)
- European Business Association (EBA)
- Greater China Business Association (GCBA)
- Japan America Business Association (JABA)
- Jewish Business Students Association (JBSA)
- Joint Ventures (JV)
- Korean Business Student Association (KBSA)
- Latin American Business Association (LABA)
- Middle East & Africa Club
- Muslim Business Student Association (MBSA)
- Out@Anderson (O@A) Home
- LGBTQ Awareness Week
- South Asian Business Association (SABA)
- Southeast Asian Business Association (SEABA)
- Taiwanese Student Business Association (TSBA)
- Institutions Clubs
- Anderson Onboarding Committee (AOC)
- Admissions Ambassador Corps (AAC)
- Entrepreneurship Through Acquisition
- Challenge for Charity
- Interest Overview
- A Comedy Club (ACC)
- Adam Smith Society (SmithSoc)
- Craft Beer Club
- Creatives at Anderson (AnderCreative)
- Eats (AnderEats)
- Public Speaking Club at Anderson (PSC)
- Spirits @ Anderson
- Travel and Hospitality Association (THA)
- Wine Club at Anderson (WCA)
- Athletics Overview
- Basketball Club at Anderson (Anderball)
- John Anderson Golf Club
- Outdoor Adventure Club (OAC)
- Soccer Club (SC)
- Tennis Club at Anderson (TCA)
- Wellness Club
- Equity, Diversity & Inclusion
- Events and Spotlights
- Embracing Diversity Series
- Hear to Include
- Student EDI Council
- Key EDI Activities
- What You Can Do
- Pathway Guidance Program Overview
- Inclusive Ethics Initiative
- Asian@Anderson
- Black@Anderson
- Latinx@Anderson
- LGBTQ@Anderson
- Veterans@Anderson
- Women@Anderson
- Information & Technology Home
- New Faculty Information
- New PhD Information
- New Student Information
- Anderson Computing & Information Services (Intranet Portal)
- Rosenfeld Library Home
- Databases Overview
- Business Databases by Name
- Business Databases by Category Overview
- Analyst Reports
- Company Information
- Industry Information
- International Information
- Market Research
- Taxation & Accounting
- Books & Other Sources
- Anderson Proxy Server / Off-Campus Access
- Database Alerts (Under Revision)
- Discipline eSources Overview
- Decisions, Operations and Technology Management
- Global Economics and Management
- Information Systems
- Management and Organizations
- Working Papers, Cases
- Business Topics
- Government Information
- Search & Find
- Electronic Journals at UCLA
- New "Management" Titles at Rosenfeld and Other UCLA Libraries
- Citation Linker for Articles in (or Not in) UCLA-Licensed Online Content
- Career Management
- Company Ratios
- Industry Ratios
- Internet Search
- Special Collections
- UCLA Library Catalog
- Melvyl (UC Libraries)
- Citing Business Sources
- Assessing Global Issues
- Career Research in the Rosenfeld Library
- Competitive Intelligence
- Research Toolkit
- Services Overview
- Faculty Course Support
- Media & Technology Industry Information
- Ph.D. Research Support
- Consult a Business Research Librarian
- Borrowing Privileges
- Document Delivery
- Field Study Research Support: AMR/BCO/GAP/SMR/UCLA-NUS EMBA
- Course Reserves Overview
- Find Reserve Items
- Info for Faculty
- Hours of Operation
- Conduct in the UCLA Libraries
- External (Non-Anderson) Users of Rosenfeld Library
- New "Management" Titles RSS Feed
- UCLA Library
- User Rights and Responsibilities
- Degrees Home
- Full-Time MBA Home
- Admissions Home
- Request Information
- Requirements
- Admissions Events
- International Applicants
- Concurrent Degrees
- Admission Policies
- Consortium Candidates
- Academics Home
- Customizable Schedule
- Flexibility & Specializations
- Capstone Project
- Business Creation Program
- Anderson Student Asset Management (ASAM) Home
- Annual Report
- Fund Strategies and Resources
- Academic Centers
- Global Options
- Academic Calendar
- Consulting Career Path
- Marketing Career Path
- Entertainment Career Path
- Technology Career Path
- Finance Career Path
- Social Impact Career Path
- Health Care Career Path
- Entrepreneurship Career Path
- Real Estate Career Path
- Operations Career Path
- Energy Career Path
- Retail Career Path
- Sports Career Path
- Living in L.A.
- Family Life
- Clubs & Associations
- Embracing Diversity
- Financing Overview
- Financing Opportunities
- Financing Requirements
- Connect With Our Students
- Getting Here
- Admit Central
- Why UCLA Anderson
- Timeline & Email Archive
- Student Life Home
- Clubs & Extracurriculars
- Getting Settled Home
- International Students Home
- Student Visas
- Your Academic Experience
- Your Career Considerations
- International Students Onboarding Sessions
- Tips for International Students
- Anderson Onboarding Home
- Anderson Onboarding FAQ
- Curriculum & Academics Home
- Course Schedule
- Academic Preparation
- Career Services Home
- Career Preparation
- Industry Camps
- Paying for School
- Financing Your MBA Home
- Meet the Team Home
- Fully Employed MBA Home
- Assistant Dean's Advice
- Connect with a Student
- UC Transfers
- Exam Waiver
- Military and Veterans
- Admissions Policies
- Specializations
- Global Experience
- Flexible Options
- Drive Time Podcast
- Student Perspectives
- Costs & Financing
- Meet our Team
- Why UCLA Anderson?
- Accepting Admission
- Important Items & Official Onboarding
- Build Your Network
- Executive MBA Home
- Requirements and Deadlines
- Connect with an EMBAssador
- U.S. Military, Reservist, & Veterans
- Flexible Schedules
- Electives & Specializations
- Capstone Overview
- For Companies
- Culture Overview
- Equity, Diversity, & Inclusion
- Conferences and Special Events
- Clubs and Associations
- Meet the Team Overview
- EMBA Admit Central Home
- Finalizing Admission
- Pre-EMBA Academic Preparation
- Important Dates and Events
- Cost and Financing
- Directions and Accommodations
- Ph.D. Program Home
- Admissions FAQ
- Areas of Study Home
- Accounting Overview
- Meet the Students
- Courses and Seminars
- Behavioral Decision Making Overview
- Decisions, Operations and Technology Management Overview
- Finance Overview
- Global Economics and Management Overview
- Management and Organizations Overview
- Marketing Overview
- Strategy Overview
- Current Job Market Candidates
- Curriculum & Schedule
- Admissions Requirements
- UCLA NUS Alumni Connect
- Fees and Financing
- Meet the Team
- Visit UCLA-NUS Full Site
- Master of Financial Engineering
- Admissions Ambassadors
- Career Impact Overview
- Career Paths Overview
- Quant Trading and Sales Trading
- Data Science
- Quantitative Research and Analysis
- Strats and Modeling
- Portfolio Management
- Risk Management
- Consulting and Valuation
- Employment Report
- Alumni Coaches
- Advisory Board
- Recruit An MFE
- Meet our Team Overview
- MFE Admit Central Home
- Career Support
- Curriculum and Academics
- Executive Education Home
- Open Enrollment Overview
- Executive Program
- Corporate Governance
- Women's Leadership Institute
- Women In Governance Overview
- Board Ready Candidates
- Inclusive Leadership Program
- Strategic HR Program
- Leading High Performing Teams
- Customized Solutions
- Partner Programs Overview
- Accounting Minor Program Home
- Accounting Minor Admissions Requirements
- Enrolling In Classes
- Courses Overview
- Management 195
- Course Syllabus
- Useful Links
- Graduating Seniors
- Leaders in Sustainability Certificate Program
- Riordan Programs Home
- Riordan Scholars Program Overview
- Saturday Business Institute
- Riordan MBA Fellows Program Overview
- Riordan College to Career Program Overview
- Alumni Association
- Our Purpose
- Get Involved Overview
- Donor Honor Roll
- Volunteer Opportunities
- Spark Campaign
- Who We Are Overview
- Volunteers and Mentors
- Riordan Podcast
- Media Entertainment & Sports Summer Institute
- Venture Accelerator at UCLA Anderson Home
- HealthCare@Anderson
- Health Care and Behavioral Economics
- Women and Healthcare
- Research and Development
- Health Care Operations
- Healthcare Pricing and Financing
- Other Research
- Sector-Focused Programs for Professionals
- Faculty and Research Home
- Accounting Home
- Seminars and Events
- Ph.D. Program
- Behavioral Decision Making Home
- Decisions, Operations & Technology Management Home
- Meet The Ph.D. Students
- DOTM Supply Chain Blog
- Finance Home
- Global Economics and Management Home
- Meet the Ph.D. Students
- University of California GEM-BPP Research Workshop
- Management And Organizations Home
- Anderson Behavioral Lab
- HARRT at UCLA
- Marketing Home
- Strategy Home
- Information Systems Research Program Home
- Connections
- IS History Home
- Faculty Directory
- Faculty Awards
- Faculty Expertise Guide
- Open Positions
- Emeriti Faculty
- For Companies Home
- Hire an MBA
- Hire an MFE
- Hire an MSBA
- Engage a Student Consulting Team
- Applied Management Research Program Home
- Requirements & Schedule
- Benefits To Companies
- Application
- Student Experience
- Faculty Advisors
- Global Access Program Home
- Global Partner Network
- Meet the Advisors
- Past GAP Companies
- Executive Portal Home
- Key Dates and Schedules
- Event Registration
- Hotels and Directions
- Visa Information
- Explore Los Angeles
- Post-GAP Consulting Providers
- Strategic Management Research Program
- Applied Finance Project
- Applied Analytics Project
- Early-Stage Investment Fund
- Field Experiments in Strategy
- Management Practicum
- News and Events Home
- News Archive
- News Archive 2022-2023
- News Archive 2018-2021
- Virtual Events Archive
- Signature Events Overview
- Gerald Loeb Awards Home
- 2024 Loeb Awards Open Call For Entries
- Banquet and Ceremony
- Submit Entry
- Competition Categories
- Historical Winners
- Career Achievement Categories
- Eligibility and Rules Home
- Administration of Awards
- Final Judges
- Embracing Diversity Week
- Commencement Overview
- MBA, EMBA, FEMBA, Ph.D. Commencement Overview
- Commencement Speaker
- FAQ Students
- UCLA-NUS Commencement
- MFE Commencement Overview
- Parking & Directions
- MSBA Commencement Overview
- Hotel Information
- Video Archives
- John Wooden Global Leadership Awards Overview
- Fellowship Application
- John Wooden
- Anderson Speaker Series
- Dean's Distinguished Speaker Series
- Velocity Women's Summit
- 'Palooza
- Anderson Student Kickoff
- Alumni Home
- Alumni Directory
- All Chapters and Groups
- International
- Worldwide Welcome Weeks 2023
- Alumni Weekend 2024
- Friday Faculty Chats
- Alumni Weekend
- Alumni Weekend 2022
- Alumni Weekend 2021
- Alumni Weekend 2019
- Alumni Weekend 2018
- Worldwide Welcome Weeks 2022
- Worldwide Welcome Weeks 2021
- Worldwide Welcome Weeks 2018
- Worldwide Welcome Weeks 2017
- Career Re-LAUNCH
- UCLA Campus
- Career Services Overview
- Career Resources
- Stay Connected Overview
- Alumni Community
- Email Lists
- Class Notes
- News@Anderson
- Alumni Awards
- Board of Directors Overview
- Letter from the President
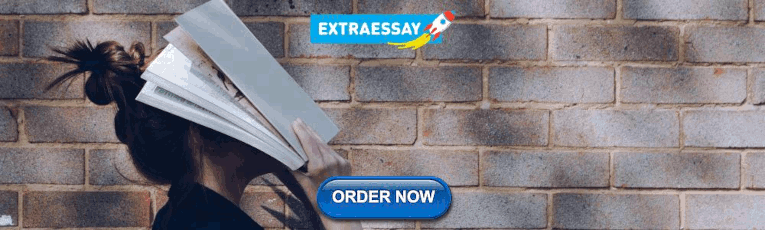
COMMENTS
The purpose of this study is to analyze the effect of online education, which has been extensively used on student achievement since the beginning of the pandemic. In line with this purpose, a meta-analysis of the related studies focusing on the effect of online education on students' academic achievement in several countries between the years 2010 and 2021 was carried out. Furthermore, this ...
online classes could affect the academic performance of students. This paper seeks to study the. impact of online learning on the academic performance of university students and to determine. whether education systems should increase the amount of online learning for traditional in-class. subjects.
The primary driving force behind the rapid integration of technology in education has been its emphasis on student performance (Lai and Bower, 2019).Over the past decade, numerous studies have ...
The Impact of Online Learning Strategies on Students' Academic Performance: A Systematic Literature Review ... this study recommends the continued use of online learning if both students and ...
According to the study of Bernard et al. ( 2004 ), this meta-analysis focuses on the activities done in online education lectures. As a result of the research, an overall effect size close to zero was found for online education utilizing more than one generation technology for students at different levels.
Turning to student response to online/blended learning, 50% of teachers in private schools reported they were receiving more than three-quarters of work back, compared with 27% in the most advantaged state schools, and just 8% in the least advantaged state schools. ... Sixth, it explores the impact of self-efficacy in learning outcomes. Seventh ...
Metrics. The coronavirus pandemic has forced students and educators across all levels of education to rapidly adapt to online learning. The impact of this — and the developments required to make ...
Distance learning has evolved over many generations into its newest form of what we commonly label as online learning. In this second-order meta-analysis, we analyze 19 first-order meta-analyses to examine the impact of distance learning and the special case of online learning on students' cognitive, affective and behavioral outcomes.
students in five core courses over the 2020 (in-person) and 2021 (online) academic years, the research provides empirical insights into the efficacy of online versus traditional edu- cation.
Online learning is one of the educational solutions for students during the COVID-19 pandemic. Worldwide, most universities have shifted much of their learning frameworks to an online learning model to limit physical interaction between people and slow the spread of COVID-19. The effectiveness of online learning depends on many factors, including student and instructor self-efficacy, attitudes ...
This article reports on a large-scale (n = 987), exploratory factor analysis study incorporating various concepts identified in the literature as critical success factors for online learning from the students' perspective, and then determines their hierarchical significance. Seven factors--Basic Online Modality, Instructional Support, Teaching Presence, Cognitive Presence, Online Social ...
The COVID-19 health crisis led to one of the largest disruptions in the history of American education. Beginning in March 2020, tens of millions of students attending school in person at all education levels abruptly shifted to online learning due to stay-at-home orders put in place to curb transmission of the virus.
This review enabled us to identify the online learning research themes examined from 2009 to 2018. In the section below, we review the most studied research themes, engagement and learner characteristics along with implications, limitations, and directions for future research. 5.1. Most studied research themes.
In summary, online learning provide students practical and flexible way in learning, it also make them more creative and active in learning. This study enlightment how the online learning bring out impact for students achievement. 20 students as participant in this study. The descriptive qualitative was used as method of the study. Based on the result of questionnare 80% of online learning is ...
Background One of the worst pandemics of recent memory, COVID-19, severely impacted the public. In particular, students were physically and mentally affected by the lockdown and the shift from physical person-to-person classrooms to virtual learning (online classes). This increased the prevalence of psychological stress, anxiety, and depression among university students. In this study, we ...
1.1. Related literature. Online learning is a form of distance education which mainly involves internet‐based education where courses are offered synchronously (i.e. live sessions online) and/or asynchronously (i.e. students access course materials online in their own time, which is associated with the more traditional distance education).
Understanding components that influence students' enjoyment of distance higher education is increasingly important to enhance academic performance and retention. Although there is a growing body of research about students' engagement with online learning, a research gap exists concerning whether fun affect students' enjoyment. A contributing factor to this situation is that the meaning ...
3.1 Quality of the instructor and satisfaction of the students. Quality of instructor with high fanaticism on student's learning has a positive impact on their satisfaction. Quality of instructor is one of the most critical measures for student satisfaction, leading to the education process's outcome (Munteanu et al., 2010; Arambewela & Hall, 2009; Ramsden, 1991).
discussion along the online learning. 75% of students feel more confidence joining. online learning that face to face in class. That's why 60% of students think online. learning can improve high ...
Online learning has both posi ve. and nega ve e ects on students, according to their percep ons. The. current research focuses on the e ects of online learning on students. It was discovered that ...
The literature has highlighted different models which provides the basic framework to understand the students perception regarding online education. Papers have also highlighted potential bottlenecks for success of the online learning. However, not many papers have attempted to understand the students perception and preference in Indian context.
peers. However, despite appearing to be more disrupted by the switch to online learning, the impact of COVID-19 on Honors students' academic outcomes is consistently smaller than the impact on non-Honors students. 3This approach has been used successfully in several other settings, such as to construct career and family returns to
CB: While these changes have improved the online learning experience, challenges remain, including addressing the digital divide, maximizing student engagement, and refining the quality of online courses. The pandemic accelerated the adoption of online learning and its impact will likely continue to shape higher education for many years to come.
Undeniably, COVID-19 disrupted higher education. The concepts of traditional learning were challenged, online learning was thrust into the mainstream in colleges and universities, and the student population was unavoidably affected. It became apparent that maintaining the status quo that existed prior to the pandemic was not the path to the future sustainability of higher education. As higher ...
Related work. Among these studies, we mention the one conducted by Göksün et al. (2017-2018) on a target group of 97 pre-service teachers. The study showed that the impact of Kahoot on academic achievement and student engagement was higher when compared to that of the control group and the reason students desired to use Kahoot and Quizizz applications in the classroom was due to students ...
There is no doubt that the COVID-19 pandemic and the resulting school closures had a large impact on student learning and test scores. School closures and the abrupt shift to online learning ...
Faculty-led programs to deepen your impact and build your effectiveness as an educator and leader. ... HGSE Online Master's Students to Headline Harvard Commencement Broadcast . Harvard-wide Commencement ceremony will feature Lecturer Alexis Redding, HGSE alumni, and graduates of the Online Master's in Education during the May 23 broadcast
Technological advancements have made a huge impact on the education sector. As a result of it, online learning has become a major trend. It ensures a great level of convenience for students across ...
Research was on online learning impact on the student of higher education. and what was the impact of COVID -19 on the student education. ... Journals, research papers and internet. Response rate .
About Our Program. Our MSBA students bridge the gap between the tech and business suites by analyzing data to arrive at solutions that can change an organization's strategy, and can even impact lives. To prepare students for these roles, we select faculty who are known authorities in research, modeling, communication and business practices.