Journal of Artificial Intelligence Research
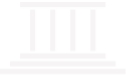
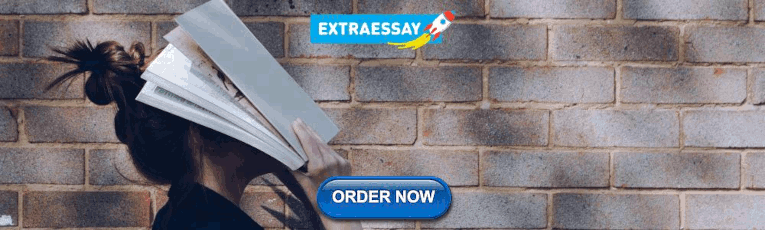
Subject Area and Category
- Artificial Intelligence
Morgan Kaufmann Publishers, Inc.
Publication type
1993, 1996-2023
Information
How to publish in this journal
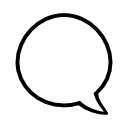
The set of journals have been ranked according to their SJR and divided into four equal groups, four quartiles. Q1 (green) comprises the quarter of the journals with the highest values, Q2 (yellow) the second highest values, Q3 (orange) the third highest values and Q4 (red) the lowest values.
The SJR is a size-independent prestige indicator that ranks journals by their 'average prestige per article'. It is based on the idea that 'all citations are not created equal'. SJR is a measure of scientific influence of journals that accounts for both the number of citations received by a journal and the importance or prestige of the journals where such citations come from It measures the scientific influence of the average article in a journal, it expresses how central to the global scientific discussion an average article of the journal is.
Evolution of the number of published documents. All types of documents are considered, including citable and non citable documents.
This indicator counts the number of citations received by documents from a journal and divides them by the total number of documents published in that journal. The chart shows the evolution of the average number of times documents published in a journal in the past two, three and four years have been cited in the current year. The two years line is equivalent to journal impact factor ™ (Thomson Reuters) metric.
Evolution of the total number of citations and journal's self-citations received by a journal's published documents during the three previous years. Journal Self-citation is defined as the number of citation from a journal citing article to articles published by the same journal.
Evolution of the number of total citation per document and external citation per document (i.e. journal self-citations removed) received by a journal's published documents during the three previous years. External citations are calculated by subtracting the number of self-citations from the total number of citations received by the journal’s documents.
International Collaboration accounts for the articles that have been produced by researchers from several countries. The chart shows the ratio of a journal's documents signed by researchers from more than one country; that is including more than one country address.
Not every article in a journal is considered primary research and therefore "citable", this chart shows the ratio of a journal's articles including substantial research (research articles, conference papers and reviews) in three year windows vs. those documents other than research articles, reviews and conference papers.
Ratio of a journal's items, grouped in three years windows, that have been cited at least once vs. those not cited during the following year.
Evolution of the percentage of female authors.
Evolution of the number of documents cited by public policy documents according to Overton database.
Evoution of the number of documents related to Sustainable Development Goals defined by United Nations. Available from 2018 onwards.
Leave a comment
Name * Required
Email (will not be published) * Required
* Required Cancel
The users of Scimago Journal & Country Rank have the possibility to dialogue through comments linked to a specific journal. The purpose is to have a forum in which general doubts about the processes of publication in the journal, experiences and other issues derived from the publication of papers are resolved. For topics on particular articles, maintain the dialogue through the usual channels with your editor.
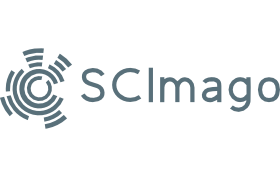
Follow us on @ScimagoJR Scimago Lab , Copyright 2007-2024. Data Source: Scopus®

Cookie settings
Cookie Policy
Legal Notice
Privacy Policy
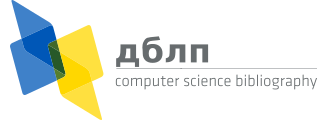
- solidarity - (ua) - (ru)
- news - (ua) - (ru)
- donate - donate - donate
for scientists:
- ERA4Ukraine
- Assistance in Germany
- Ukrainian Global University
- #ScienceForUkraine

default search action
- combined dblp search
- author search
- venue search
- publication search

Journal of Artificial Intelligence Research (JAIR)
- > Home > Journals
Venue statistics
records by year

frequent authors
Venue Information
- issn: 1076-9757 (print)

- 2024: Volumes 79 , 80 ,
- 2023: Volumes 76 , 77 , 78
- 2022: Volumes 73 , 74 , 75
- 2021: Volumes 70 , 71 , 72
- 2020: Volumes 67 , 68 , 69
- 2019: Volumes 64 , 65 , 66
- 2018: Volumes 61 , 62 , 63
- 2017: Volumes 58 , 59 , 60
- 2016: Volumes 55 , 56 , 57
- 2015: Volumes 52 , 53 , 54
- 2014: Volumes 49 , 50 , 51
- 2013: Volumes 46 , 47 , 48
- 2012: Volumes 43 , 44 , 45
- 2011: Volumes 40 , 41 , 42
- 2010: Volumes 37 , 38 , 39
- 2009: Volumes 34 , 35 , 36
- 2008: Volumes 31 , 32 , 33
- 2007: Volumes 28 , 29 , 30
- 2006: Volumes 25 , 26 , 27
- 2005: Volumes 23 , 24
- 2004: Volumes 21 , 22
- 2003: Volumes 18 , 19 , 20
- 2002: Volumes 16 , 17
- 2001: Volumes 14 , 15
- 2000: Volumes 12 , 13
- 1999: Volumes 10 , 11
- 1998: Volumes 8 , 9
- 1997: Volumes 6 , 7
- 1996: Volumes 4 , 5
- Volume 3: 1995
- Volume 2: 1994/1995
- Volume 1: 1993/1994

manage site settings
To protect your privacy, all features that rely on external API calls from your browser are turned off by default . You need to opt-in for them to become active. All settings here will be stored as cookies with your web browser. For more information see our F.A.Q.
Unpaywalled article links

load links from unpaywall.org
Privacy notice: By enabling the option above, your browser will contact the API of unpaywall.org to load hyperlinks to open access articles. Although we do not have any reason to believe that your call will be tracked, we do not have any control over how the remote server uses your data. So please proceed with care and consider checking the Unpaywall privacy policy .
Archived links via Wayback Machine

load content from archive.org
Privacy notice: By enabling the option above, your browser will contact the API of archive.org to check for archived content of web pages that are no longer available. Although we do not have any reason to believe that your call will be tracked, we do not have any control over how the remote server uses your data. So please proceed with care and consider checking the Internet Archive privacy policy .
Reference lists

load references from crossref.org and opencitations.net
Privacy notice: By enabling the option above, your browser will contact the APIs of crossref.org , opencitations.net , and semanticscholar.org to load article reference information. Although we do not have any reason to believe that your call will be tracked, we do not have any control over how the remote server uses your data. So please proceed with care and consider checking the Crossref privacy policy and the OpenCitations privacy policy , as well as the AI2 Privacy Policy covering Semantic Scholar.
Citation data
load citations from opencitations.net
Privacy notice: By enabling the option above, your browser will contact the API of opencitations.net and semanticscholar.org to load citation information. Although we do not have any reason to believe that your call will be tracked, we do not have any control over how the remote server uses your data. So please proceed with care and consider checking the OpenCitations privacy policy as well as the AI2 Privacy Policy covering Semantic Scholar.
OpenAlex data

load data from openalex.org
Privacy notice: By enabling the option above, your browser will contact the API of openalex.org to load additional information. Although we do not have any reason to believe that your call will be tracked, we do not have any control over how the remote server uses your data. So please proceed with care and consider checking the information given by OpenAlex .
last updated on 2024-06-03 20:32 CEST by the dblp team

see also: Terms of Use | Privacy Policy | Imprint
dblp was originally created in 1993 at:
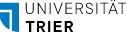
since 2018, dblp has been operated and maintained by:
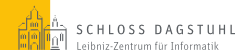
the dblp computer science bibliography is funded and supported by:
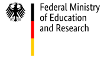

An official website of the United States government
Here’s how you know
Official websites use .gov A .gov website belongs to an official government organization in the United States.
Secure .gov websites use HTTPS A lock ( Lock A locked padlock ) or https:// means you’ve safely connected to the .gov website. Share sensitive information only on official, secure websites.
https://www.nist.gov/artificial-intelligence
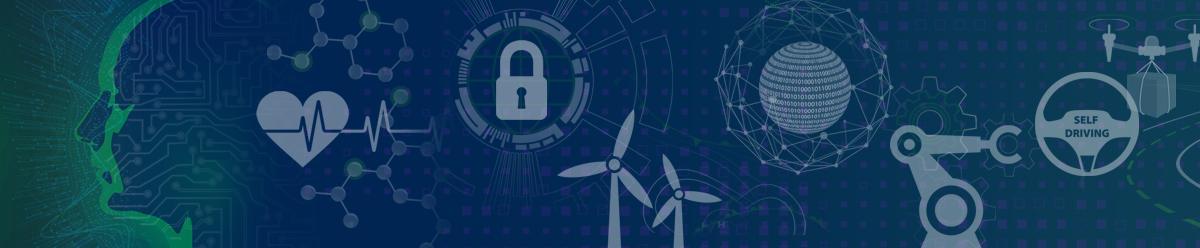
Artificial intelligence
NIST aims to cultivate trust in the design, development, use and governance of Artificial Intelligence (AI) technologies and systems in ways that enhance safety and security and improve quality of life. NIST focuses on improving measurement science, technology, standards and related tools — including evaluation and data.
With AI and Machine Learning (ML) changing how society addresses challenges and opportunities, the trustworthiness of AI technologies is critical. Trustworthy AI systems are those demonstrated to be valid and reliable; safe, secure and resilient; accountable and transparent; explainable and interpretable; privacy-enhanced; and fair with harmful bias managed. The agency’s AI goals and activities are driven by its statutory mandates, Presidential Executive Orders and policies, and the needs expressed by U.S. industry, the global research community, other federal agencies,and civil society.
NIST’s AI goals include:
- Conduct fundamental research to advance trustworthy AI technologies.
- Apply AI research and innovation across the NIST Laboratory Programs.
- Establish benchmarks, data and metrics to evaluate AI technologies.
- Lead and participate in development of technical AI standards.
- Contribute technical expertise to discussions and development of AI policies.
NIST’s AI efforts fall in several categories:
Fundamental AI Research
NIST’s AI portfolio includes fundamental research to advance the development of AI technologies — including software, hardware, architectures and the ways humans interact with AI technology and AI-generated information
Applied AI Research
AI approaches are increasingly an essential component in new research. NIST scientists and engineers use various machine learning and AI tools to gain a deeper understanding of and insight into their research. At the same time, NIST laboratory experiences with AI are leading to a better understanding of AI’s capabilities and limitations.
Test, Evaluation, Validation, and Verification (TEVV)
With a long history of working with the community to advance tools, standards and test beds, NIST increasingly is focusing on the sociotechnical evaluation of AI.
Voluntary Consensus-Based Standards
NIST leads and participates in the development of technical standards, including international standards, that promote innovation and public trust in systems that use AI. A broad spectrum of standards for AI data, performance and governance are a priority for the use and creation of trustworthy and responsible AI.
A fact sheet describes NIST's AI programs .
Featured Content
Artificial intelligence topics.
- AI Test, Evaluation, Validation and Verification (TEVV)
- Fundamental AI
- Hardware for AI
- Machine learning
- Trustworthy and Responsible AI
Stay in Touch
Sign up for our newsletter to stay up to date with the latest research, trends, and news for Artificial intelligence.
The Research
Projects & programs, deep learning for mri reconstruction and analysis.
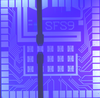
Emerging Hardware for Artificial Intelligence
Embodied ai and data generation for manufacturing robotics, deep generative modeling for communication systems testing and data sharing.
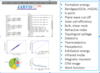
Additional Resources Links
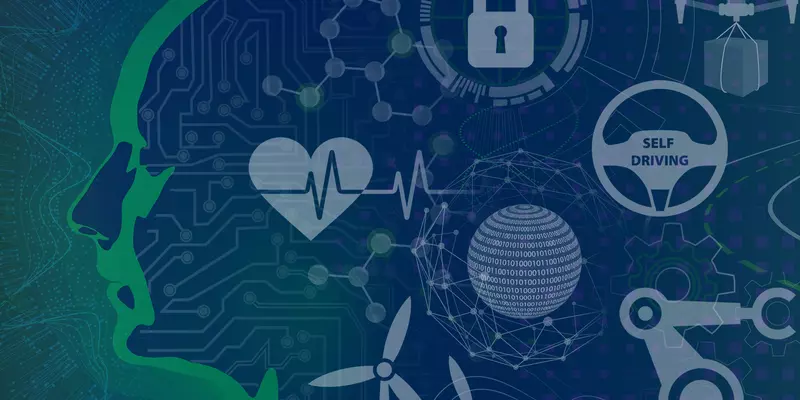
NIST Launches Trustworthy and Responsible AI Resource Center (AIRC)
One-stop shop offers industry, government and academic stakeholders knowledge of AI standards, measurement methods and metrics, data sets, and other resources.
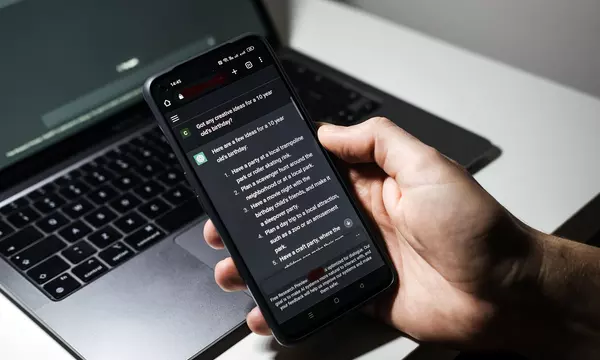
Minimizing Harms and Maximizing the Potential of Generative AI
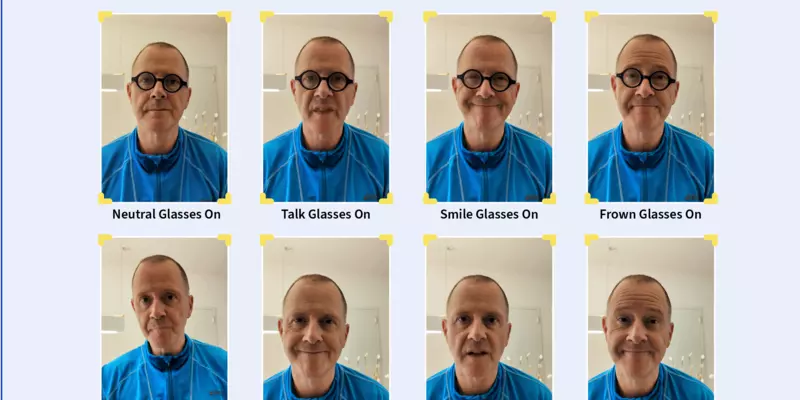
NIST Reports First Results From Age Estimation Software Evaluation
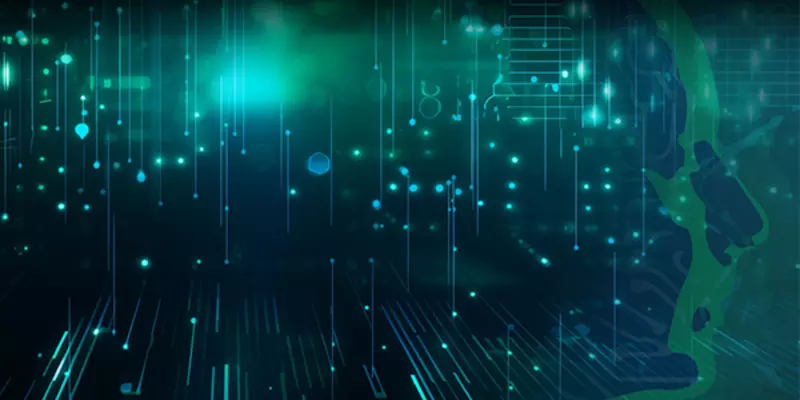
NIST Launches ARIA, a New Program to Advance Sociotechnical Testing and Evaluation for AI
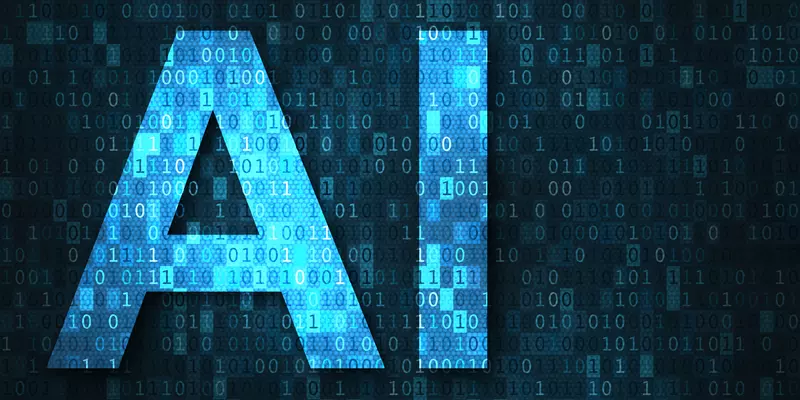
U.S. Secretary of Commerce Gina Raimondo Releases Strategic Vision on AI Safety, Announces Plan for Global Cooperation Among AI Safety Institutes
2024 Artificial Intelligence for Materials Science (AIMS) Workshop
Thank you for visiting nature.com. You are using a browser version with limited support for CSS. To obtain the best experience, we recommend you use a more up to date browser (or turn off compatibility mode in Internet Explorer). In the meantime, to ensure continued support, we are displaying the site without styles and JavaScript.
- View all journals
- Explore content
- About the journal
- Publish with us
- Sign up for alerts
- NEWS FEATURE
- 28 May 2024
- Correction 31 May 2024
The AI revolution is coming to robots: how will it change them?
- Elizabeth Gibney
You can also search for this author in PubMed Google Scholar
Humanoid robots developed by the US company Figure use OpenAI programming for language and vision. Credit: AP Photo/Jae C. Hong/Alamy
You have full access to this article via your institution.
For a generation of scientists raised watching Star Wars, there’s a disappointing lack of C-3PO-like droids wandering around our cities and homes. Where are the humanoid robots fuelled with common sense that can help around the house and workplace?
Rapid advances in artificial intelligence (AI) might be set to fill that hole. “I wouldn’t be surprised if we are the last generation for which those sci-fi scenes are not a reality,” says Alexander Khazatsky, a machine-learning and robotics researcher at Stanford University in California.
From OpenAI to Google DeepMind, almost every big technology firm with AI expertise is now working on bringing the versatile learning algorithms that power chatbots, known as foundation models, to robotics. The idea is to imbue robots with common-sense knowledge, letting them tackle a wide range of tasks. Many researchers think that robots could become really good, really fast. “We believe we are at the point of a step change in robotics,” says Gerard Andrews, a marketing manager focused on robotics at technology company Nvidia in Santa Clara, California, which in March launched a general-purpose AI model designed for humanoid robots.
At the same time, robots could help to improve AI. Many researchers hope that bringing an embodied experience to AI training could take them closer to the dream of ‘artificial general intelligence’ — AI that has human-like cognitive abilities across any task . “The last step to true intelligence has to be physical intelligence,” says Akshara Rai, an AI researcher at Meta in Menlo Park, California.
But although many researchers are excited about the latest injection of AI into robotics, they also caution that some of the more impressive demonstrations are just that — demonstrations, often by companies that are eager to generate buzz. It can be a long road from demonstration to deployment, says Rodney Brooks, a roboticist at the Massachusetts Institute of Technology in Cambridge, whose company iRobot invented the Roomba autonomous vacuum cleaner.
There are plenty of hurdles on this road, including scraping together enough of the right data for robots to learn from, dealing with temperamental hardware and tackling concerns about safety. Foundation models for robotics “should be explored”, says Harold Soh, a specialist in human–robot interactions at the National University of Singapore. But he is sceptical, he says, that this strategy will lead to the revolution in robotics that some researchers predict.
Firm foundations
The term robot covers a wide range of automated devices, from the robotic arms widely used in manufacturing, to self-driving cars and drones used in warfare and rescue missions. Most incorporate some sort of AI — to recognize objects, for example. But they are also programmed to carry out specific tasks, work in particular environments or rely on some level of human supervision, says Joyce Sidopoulos, co-founder of MassRobotics, an innovation hub for robotics companies in Boston, Massachusetts. Even Atlas — a robot made by Boston Dynamics, a robotics company in Waltham, Massachusetts, which famously showed off its parkour skills in 2018 — works by carefully mapping its environment and choosing the best actions to execute from a library of built-in templates.
For most AI researchers branching into robotics, the goal is to create something much more autonomous and adaptable across a wider range of circumstances. This might start with robot arms that can ‘pick and place’ any factory product, but evolve into humanoid robots that provide company and support for older people , for example. “There are so many applications,” says Sidopoulos.
The human form is complicated and not always optimized for specific physical tasks, but it has the huge benefit of being perfectly suited to the world that people have built. A human-shaped robot would be able to physically interact with the world in much the same way that a person does.
However, controlling any robot — let alone a human-shaped one — is incredibly hard. Apparently simple tasks, such as opening a door, are actually hugely complex, requiring a robot to understand how different door mechanisms work, how much force to apply to a handle and how to maintain balance while doing so. The real world is extremely varied and constantly changing.
The approach now gathering steam is to control a robot using the same type of AI foundation models that power image generators and chatbots such as ChatGPT. These models use brain-inspired neural networks to learn from huge swathes of generic data. They build associations between elements of their training data and, when asked for an output, tap these connections to generate appropriate words or images, often with uncannily good results.
Likewise, a robot foundation model is trained on text and images from the Internet, providing it with information about the nature of various objects and their contexts. It also learns from examples of robotic operations. It can be trained, for example, on videos of robot trial and error, or videos of robots that are being remotely operated by humans, alongside the instructions that pair with those actions. A trained robot foundation model can then observe a scenario and use its learnt associations to predict what action will lead to the best outcome.
Google DeepMind has built one of the most advanced robotic foundation models, known as Robotic Transformer 2 (RT-2), that can operate a mobile robot arm built by its sister company Everyday Robots in Mountain View, California. Like other robotic foundation models, it was trained on both the Internet and videos of robotic operation. Thanks to the online training, RT-2 can follow instructions even when those commands go beyond what the robot has seen another robot do before 1 . For example, it can move a drink can onto a picture of Taylor Swift when asked to do so — even though Swift’s image was not in any of the 130,000 demonstrations that RT-2 had been trained on.
In other words, knowledge gleaned from Internet trawling (such as what the singer Taylor Swift looks like) is being carried over into the robot’s actions. “A lot of Internet concepts just transfer,” says Keerthana Gopalakrishnan, an AI and robotics researcher at Google DeepMind in San Francisco, California. This radically reduces the amount of physical data that a robot needs to have absorbed to cope in different situations, she says.
But to fully understand the basics of movements and their consequences, robots still need to learn from lots of physical data. And therein lies a problem.
Data dearth
Although chatbots are being trained on billions of words from the Internet, there is no equivalently large data set for robotic activity. This lack of data has left robotics “in the dust”, says Khazatsky.
Pooling data is one way around this. Khazatsky and his colleagues have created DROID 2 , an open-source data set that brings together around 350 hours of video data from one type of robot arm (the Franka Panda 7DoF robot arm, built by Franka Robotics in Munich, Germany), as it was being remotely operated by people in 18 laboratories around the world. The robot-eye-view camera has recorded visual data in hundreds of environments, including bathrooms, laundry rooms, bedrooms and kitchens. This diversity helps robots to perform well on tasks with previously unencountered elements, says Khazatsky.
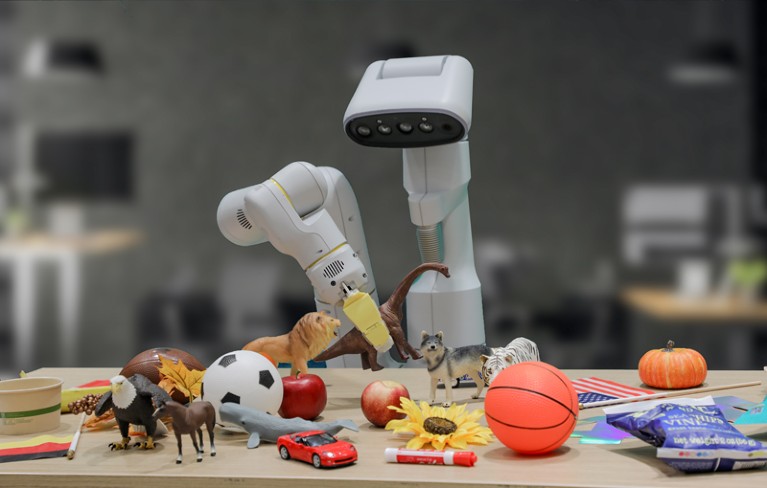
When prompted to ‘pick up extinct animal’, Google’s RT-2 model selects the dinosaur figurine from a crowded table. Credit: Google DeepMind
Gopalakrishnan is part of a collaboration of more than a dozen academic labs that is also bringing together robotic data, in its case from a diversity of robot forms, from single arms to quadrupeds. The collaborators’ theory is that learning about the physical world in one robot body should help an AI to operate another — in the same way that learning in English can help a language model to generate Chinese, because the underlying concepts about the world that the words describe are the same. This seems to work. The collaboration’s resulting foundation model, called RT-X, which was released in October 2023 3 , performed better on real-world tasks than did models the researchers trained on one robot architecture.
Many researchers say that having this kind of diversity is essential. “We believe that a true robotics foundation model should not be tied to only one embodiment,” says Peter Chen, an AI researcher and co-founder of Covariant, an AI firm in Emeryville, California.
Covariant is also working hard on scaling up robot data. The company, which was set up in part by former OpenAI researchers, began collecting data in 2018 from 30 variations of robot arms in warehouses across the world, which all run using Covariant software. Covariant’s Robotics Foundation Model 1 (RFM-1) goes beyond collecting video data to encompass sensor readings, such as how much weight was lifted or force applied. This kind of data should help a robot to perform tasks such as manipulating a squishy object, says Gopalakrishnan — in theory, helping a robot to know, for example, how not to bruise a banana.
Covariant has built up a proprietary database that includes hundreds of billions of ‘tokens’ — units of real-world robotic information — which Chen says is roughly on a par with the scale of data that trained GPT-3, the 2020 version of OpenAI's large language model. “We have way more real-world data than other people, because that’s what we have been focused on,” Chen says. RFM-1 is poised to roll out soon, says Chen, and should allow operators of robots running Covariant’s software to type or speak general instructions, such as “pick up apples from the bin”.
Another way to access large databases of movement is to focus on a humanoid robot form so that an AI can learn by watching videos of people — of which there are billions online. Nvidia’s Project GR00T foundation model, for example, is ingesting videos of people performing tasks, says Andrews. Although copying humans has huge potential for boosting robot skills, doing so well is hard, says Gopalakrishnan. For example, robot videos generally come with data about context and commands — the same isn’t true for human videos, she says.
Virtual reality
A final and promising way to find limitless supplies of physical data, researchers say, is through simulation. Many roboticists are working on building 3D virtual-reality environments, the physics of which mimic the real world, and then wiring those up to a robotic brain for training. Simulators can churn out huge quantities of data and allow humans and robots to interact virtually, without risk, in rare or dangerous situations, all without wearing out the mechanics. “If you had to get a farm of robotic hands and exercise them until they achieve [a high] level of dexterity, you will blow the motors,” says Nvidia’s Andrews.
But making a good simulator is a difficult task. “Simulators have good physics, but not perfect physics, and making diverse simulated environments is almost as hard as just collecting diverse data,” says Khazatsky.
Meta and Nvidia are both betting big on simulation to scale up robot data, and have built sophisticated simulated worlds: Habitat from Meta and Isaac Sim from Nvidia. In them, robots gain the equivalent of years of experience in a few hours, and, in trials, they then successfully apply what they have learnt to situations they have never encountered in the real world. “Simulation is an extremely powerful but underrated tool in robotics, and I am excited to see it gaining momentum,” says Rai.
Many researchers are optimistic that foundation models will help to create general-purpose robots that can replace human labour. In February, Figure, a robotics company in Sunnyvale, California, raised US$675 million in investment for its plan to use language and vision models developed by OpenAI in its general-purpose humanoid robot. A demonstration video shows a robot giving a person an apple in response to a general request for ‘something to eat’. The video on X (the platform formerly known as Twitter) has racked up 4.8 million views.
Exactly how this robot’s foundation model has been trained, along with any details about its performance across various settings, is unclear (neither OpenAI nor Figure responded to Nature ’s requests for an interview). Such demos should be taken with a pinch of salt, says Soh. The environment in the video is conspicuously sparse, he says. Adding a more complex environment could potentially confuse the robot — in the same way that such environments have fooled self-driving cars. “Roboticists are very sceptical of robot videos for good reason, because we make them and we know that out of 100 shots, there’s usually only one that works,” Soh says.
Hurdles ahead
As the AI research community forges ahead with robotic brains, many of those who actually build robots caution that the hardware also presents a challenge: robots are complicated and break a lot. Hardware has been advancing, Chen says, but “a lot of people looking at the promise of foundation models just don't know the other side of how difficult it is to deploy these types of robots”, he says.
Another issue is how far robot foundation models can get using the visual data that make up the vast majority of their physical training. Robots might need reams of other kinds of sensory data, for example from the sense of touch or proprioception — a sense of where their body is in space — say Soh. Those data sets don’t yet exist. “There’s all this stuff that’s missing, which I think is required for things like a humanoid to work efficiently in the world,” he says.
Releasing foundation models into the real world comes with another major challenge — safety. In the two years since they started proliferating, large language models have been shown to come up with false and biased information. They can also be tricked into doing things that they are programmed not to do, such as telling users how to make a bomb. Giving AI systems a body brings these types of mistake and threat to the physical world. “If a robot is wrong, it can actually physically harm you or break things or cause damage,” says Gopalakrishnan.
Valuable work going on in AI safety will transfer to the world of robotics, says Gopalakrishnan. In addition, her team has imbued some robot AI models with rules that layer on top of their learning, such as not to even attempt tasks that involve interacting with people, animals or other living organisms. “Until we have confidence in robots, we will need a lot of human supervision,” she says.
Despite the risks, there is a lot of momentum in using AI to improve robots — and using robots to improve AI. Gopalakrishnan thinks that hooking up AI brains to physical robots will improve the foundation models, for example giving them better spatial reasoning. Meta, says Rai, is among those pursuing the hypothesis that “true intelligence can only emerge when an agent can interact with its world”. That real-world interaction, some say, is what could take AI beyond learning patterns and making predictions, to truly understanding and reasoning about the world.
What the future holds depends on who you ask. Brooks says that robots will continue to improve and find new applications, but their eventual use “is nowhere near as sexy” as humanoids replacing human labour. But others think that developing a functional and safe humanoid robot that is capable of cooking dinner, running errands and folding the laundry is possible — but could just cost hundreds of millions of dollars. “I’m sure someone will do it,” says Khazatsky. “It’ll just be a lot of money, and time.”
Nature 630 , 22-24 (2024)
doi: https://doi.org/10.1038/d41586-024-01442-5
Updates & Corrections
Correction 31 May 2024 : An earlier version of this feature gave the wrong name for Nvidia’s simulated world.
Brohan, A. et al. Preprint at arXiv https://doi.org/10.48550/arXiv.2307.15818 (2023).
Khazatsky, A. et al. Preprint at arXiv https://doi.org/10.48550/arXiv.2403.12945 (2024).
Open X-Embodiment Collaboration et al. Preprint at arXiv https://doi.org/10.48550/arXiv.2310.08864 (2023).
Download references
Reprints and permissions
Related Articles
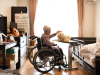
- Machine learning
Standardized metadata for biological samples could unlock the potential of collections
Correspondence 14 MAY 24
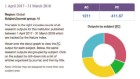
A guide to the Nature Index
Nature Index 13 MAR 24
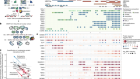
Decoding chromatin states by proteomic profiling of nucleosome readers
Article 06 MAR 24
Neurotechnologies that can read our mind could undermine international norms on freedom of thought
Correspondence 04 JUN 24
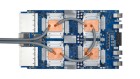
Accelerating AI: the cutting-edge chips powering the computing revolution
News Feature 04 JUN 24
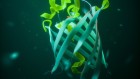
How to keep the lights on: the mission to make more photostable fluorophores
Technology Feature 03 JUN 24
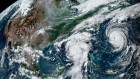
Super-fast Microsoft AI is first to predict air pollution for the whole world
News 04 JUN 24
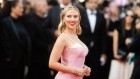
Who owns your voice? Scarlett Johansson OpenAI complaint raises questions
News Explainer 29 MAY 24
Post-Doctoral Fellow in Chemistry and Chemical Biology
We are seeking a highly motivated, interdisciplinary scientist to investigate the host-gut microbiota interactions that are associated with driving...
Cambridge, Massachusetts
Harvard University - Department of Chemistry and Chemical Biology
Postdoc Position (f/m/d) in “Building Healthcare Resilience Against Cyber-Attacks"
Karlsruhe Institute of Technology (KIT) – The Research University in the Helmholtz Association creates and imparts knowledge for the society and th...
76344, Eggenstein-Leopoldshafen (DE)
Karlsruher Institut für Technologie (KIT) Campus Nord
Research assistant (Praedoc) (m/f/d) - Department of Biology, Chemistry, Pharmacy
Department of Biology, Chemistry, Pharmacy - Institute of Chemistry and Biochemistry AG Absmeier Research assistant (Praedoc) (m/f/d) with 65%-pa...
14195, Berlin (DE)
Freie Universität Berlin
Professor, Associate Professor, Postdoctoral Fellow Recruitment
Candidate shall have an international academic vision, and have a high academic level and strong scientific research ability.
Shenzhen, Guangdong, China
Shenzhen University of Advanced Technology
Open Faculty Position in Mathematical and Information Security
We are now seeking outstanding candidates in all areas of mathematics and information security.
Dongguan, Guangdong, China
GREAT BAY INSTITUTE FOR ADVANCED STUDY: Institute of Mathematical and Information Security
Sign up for the Nature Briefing newsletter — what matters in science, free to your inbox daily.
Quick links
- Explore articles by subject
- Guide to authors
- Editorial policies
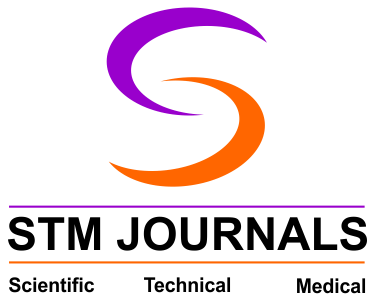
- STM Journals
- Special Issues
- Conferences
- Editorial Board Members
- Reviewers Board Members
- Advisory Panel
- Indexing Bodies
- For Authors
- For Reviewers
- For Editors
- For Advisory Board
- Special Issue Guidelines
- Peer-Review Policy
- Manuscript Submission Guidelines
- Publication Ethics and Virtue
- Article Processing Charge
- Editorial Policy
- Advertising Policy
- STM Website and Link Policy
- Distribution and dessemination of Research
- Informed consent Policy
- DOI Payment
"Connect with colleagues and showcase your academic achievements."
"Unleashing the potential of your words"
"Explore a vast collection of books and broaden your horizons."
"Empower yourself with the knowledge and skills needed to succeed."
"Collaborate with like-minded professionals and share your knowledge."
"Learn from experts and engage with a community of learners."
- ICDR Group of Companies
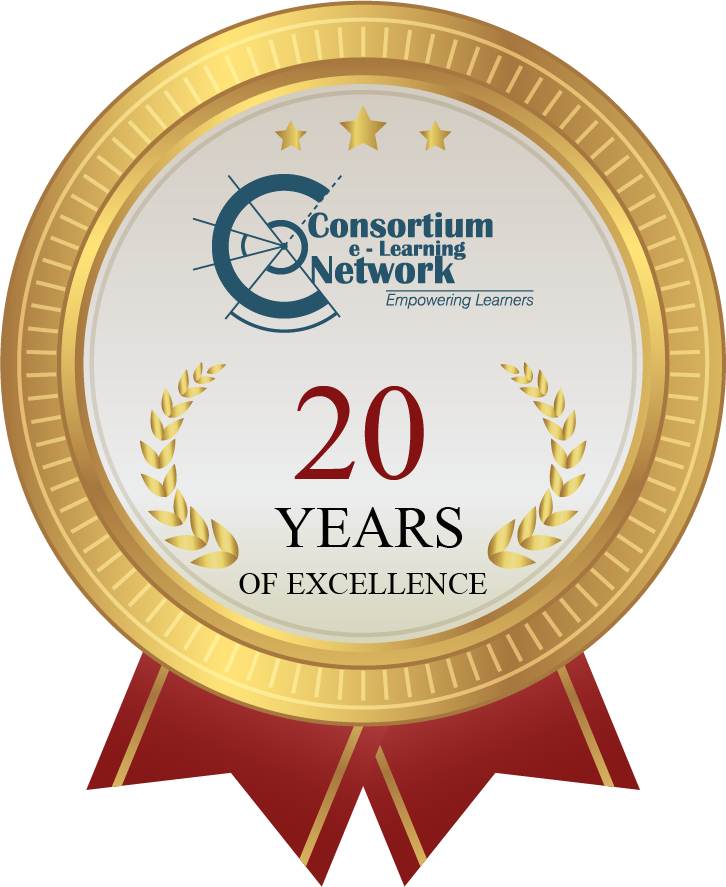
- Training Programs
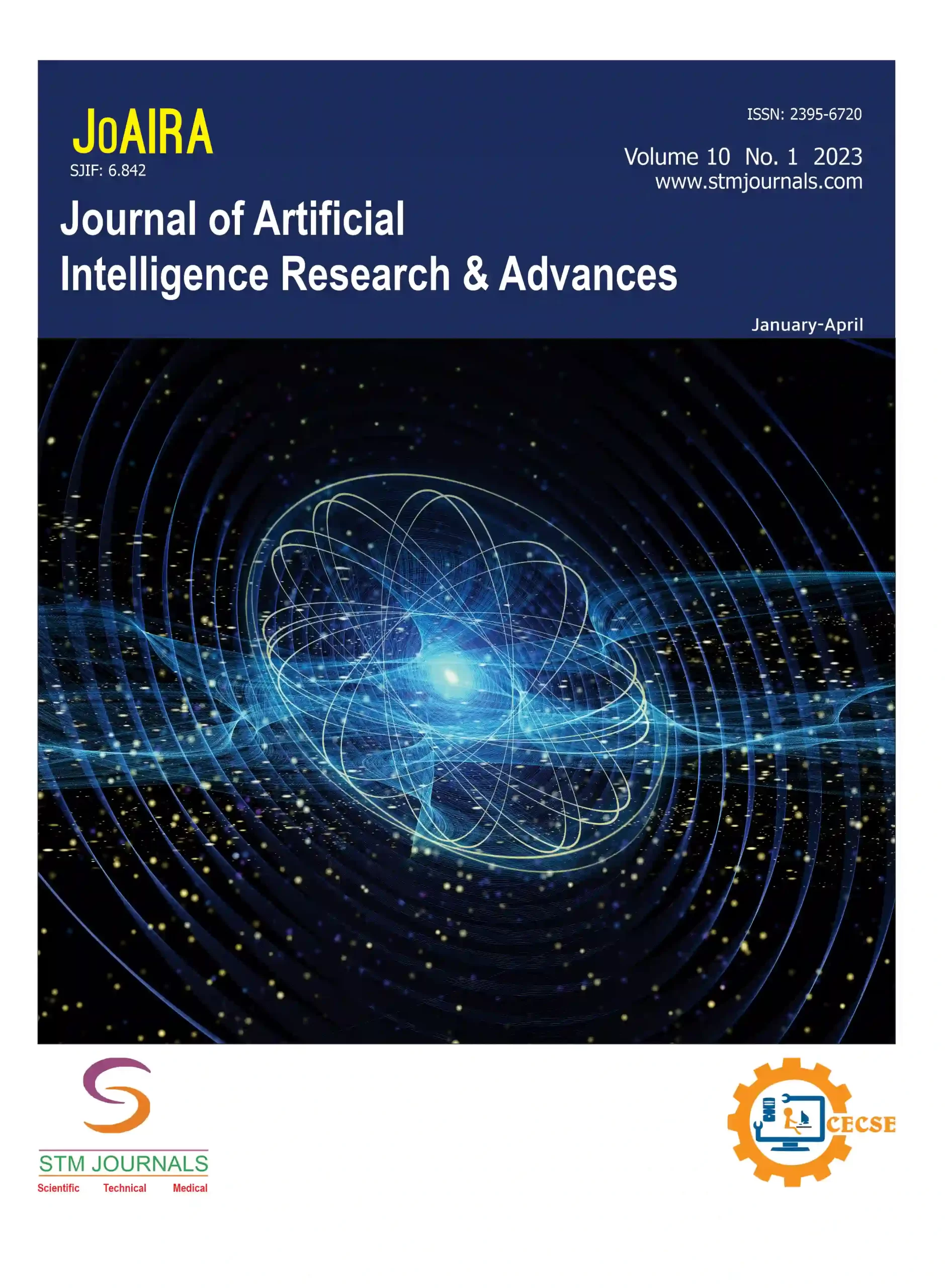
Journal of Artificial Intelligence Research & Advances
ISSN: 2395-6720
Journal Menu
Editors overview.
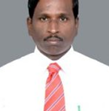
Prof. Gunasekaran Gurusamy
Institutional Profile Link : https://ww. . . APID Profile View Full Profile
STM Journals, An imprint of Consortium e-Learning Network Pvt. Ltd. A-118, 1st Floor, Sector-63, Noida, U.P. India, Pin – 201301 E-mail: [email protected] (Tel) (+91) 0120- 4781 200 (Mob) (+91) 9810078958, +919667725932
Experimental Study on Heart Disease Prediction Using Different Machine Learning Algorithms
Implementation of Data Mining Approach to Find the Adaptability of Students in Online Education during Covid-19 Pandemic
An Insight for Visually Impaired using AI Techniques
About the Journal
Journal of Artificial Intelligence Research & Advances (joaira) : 2395-6720(e) is a peer-reviewed online journal launched in 2014 that investigates the role of artificial in this rapidly progressing & challenging environment. View Full Focus and Scope…
Journal Particulars
[email protected]
Vol-11 Issue-01 2024
Country Cluster Visualization based on Agricultural Imports: Unsupervised Learning Approach R.S. Kamath, P.G. Naik, S.S. Jamsandekar Keywords: K-means, cluster visualization, unsupervised learning, artificial intelligence, cluster designs
Virtual Method to Predict Dental Disease Vardhineedi Likhitha, Vuriti Venkata Raviraju Keywords: Machine learning, deep learning, artificial intelligence, image recognition, oral diseases, python
Applications of Machine Learning Algorithms in Health Data Science (HDS) for Next Research Directions: A Survey Report Vinay Bhatt, Mayank Kumar Keywords: AI, ML, Data science, Health Data Science, Supervised Learning, Unsupervised Learning, Reinforcement Learning, Deep Learning, Deep-Reinforcement Learning, ANN
The Rise of AI in E-Commerce: Transforming Shopping Experiences Vamsi Krishna Thatikonda Keywords: Conversational AI, personalized recommendations, intelligent chatbots, virtual assistants, predictive analytics, inventory management, demand forecasting, dynamic pricing, promotions, visual search, image recognition, augmented reality.
Building a Smart, Secured and Sustainable Campus: A Self-Powered Wireless Network for Environmental Monitoring Qutaiba Ibrahim Keywords: Environmental monitoring, wireless sensor networks, campus buildings, self-powered network, energy harvesting, duty cycling, sustainable infrastructure, data analytics, real-time monitoring, Intrusion Detection System, Network security.
A Real-Time Visualization Framework to Enhance Prompt Accuracy and Result Outcomes Based on Number of Tokens Prashant D. Sawant Keywords: Real-time prompt analysis, Prompt optimization, AI interaction efficiency, Preventing prompt hallucination, Human-AI collaboration
Transformative Breakthroughs: Revolutionizing Potato Disease Detection through Machine Learning S. Shenbaha, V. Sreepriya Keywords: feature extraction, VGG16, CNN, transfer learning fine-tuning
Mindwell: A Psychological Guide for Well-being Ashwini Garole, Aditya Asabe, Mohini Jadhav, Shreepad Chavan, Shifa Gadiwale Keywords: Mental Health Therapy, Chatbot, Artificial intelligence, Machine learning, Power, Large Language Models
Comparison of K-Nearest Neighbor and Artificial Neural Network Classifiers for the Detection of Breast Cancer Jhumi Thapa, Anshu Ghimire Keywords: K-Nearest Neighbors (KNN), Artificial Neural Network (ANN), breast cancer, machine learning, prediction, Fine Needle Aspirate (FNA)
Analysing the Cognitive Proficiencies of Artificial Intelligence within the Legal Paradigm: Prospects within the Jurisdiction of India Indra Vijay Singh, Ranvijay Singh, Akash Singh, Sujit Tewari Keywords: Artificial Intelligence, Analysis, Ethical Scrutiny, Human Expertise, AI Capacities, Ramifications, Legal Practice.
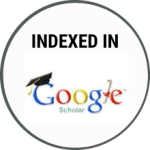
For Subscriber Access
Special Issue
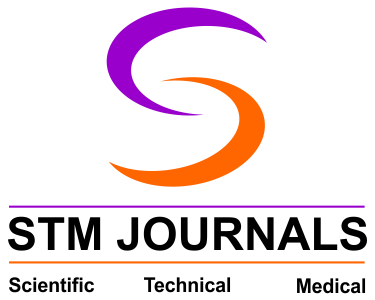
WEBSITE DISCLAIMER
Last updated: 2022-06-15
The information provided by STM Journals (“Company”, “we”, “our”, “us”) on https://journals.stmjournals.com / (the “Site”) is for general informational purposes only. All information on the Site is provided in good faith, however, we make no representation or warranty of any kind, express or implied, regarding the accuracy, adequacy, validity, reliability, availability, or completeness of any information on the Site.
UNDER NO CIRCUMSTANCE SHALL WE HAVE ANY LIABILITY TO YOU FOR ANY LOSS OR DAMAGE OF ANY KIND INCURRED AS A RESULT OF THE USE OF THE SITE OR RELIANCE ON ANY INFORMATION PROVIDED ON THE SITE. YOUR USE OF THE SITE AND YOUR RELIANCE ON ANY INFORMATION ON THE SITE IS SOLELY AT YOUR OWN RISK.
EXTERNAL LINKS DISCLAIMER
The Site may contain (or you may be sent through the Site) links to other websites or content belonging to or originating from third parties or links to websites and features. Such external links are not investigated, monitored, or checked for accuracy, adequacy, validity, reliability, availability, or completeness by us.
WE DO NOT WARRANT, ENDORSE, GUARANTEE, OR ASSUME RESPONSIBILITY FOR THE ACCURACY OR RELIABILITY OF ANY INFORMATION OFFERED BY THIRD-PARTY WEBSITES LINKED THROUGH THE SITE OR ANY WEBSITE OR FEATURE LINKED IN ANY BANNER OR OTHER ADVERTISING. WE WILL NOT BE A PARTY TO OR IN ANY WAY BE RESPONSIBLE FOR MONITORING ANY TRANSACTION BETWEEN YOU AND THIRD-PARTY PROVIDERS OF PRODUCTS OR SERVICES.
PROFESSIONAL DISCLAIMER
The Site can not and does not contain medical advice. The information is provided for general informational and educational purposes only and is not a substitute for professional medical advice. Accordingly, before taking any actions based on such information, we encourage you to consult with the appropriate professionals. We do not provide any kind of medical advice.
Content published on https://journals.stmjournals.com / is intended to be used and must be used for informational purposes only. It is very important to do your analysis before making any decision based on your circumstances. You should take independent medical advice from a professional or independently research and verify any information that you find on our Website and wish to rely upon.
THE USE OR RELIANCE OF ANY INFORMATION CONTAINED ON THIS SITE IS SOLELY AT YOUR OWN RISK.
AFFILIATES DISCLAIMER
The Site may contain links to affiliate websites, and we may receive an affiliate commission for any purchases or actions made by you on the affiliate websites using such links.
TESTIMONIALS DISCLAIMER
The Site may contain testimonials by users of our products and/or services. These testimonials reflect the real-life experiences and opinions of such users. However, the experiences are personal to those particular users, and may not necessarily be representative of all users of our products and/or services. We do not claim, and you should not assume that all users will have the same experiences.
YOUR RESULTS MAY VARY.
The testimonials on the Site are submitted in various forms such as text, audio, and/or video, and are reviewed by us before being posted. They appear on the Site verbatim as given by the users, except for the correction of grammar or typing errors. Some testimonials may have been shortened for the sake of brevity, where the full testimonial contained extraneous information not relevant to the general public.
The views and opinions contained in the testimonials belong solely to the individual user and do not reflect our views and opinions.
ERRORS AND OMISSIONS DISCLAIMER
While we have made every attempt to ensure that the information contained in this site has been obtained from reliable sources, STM Journals is not responsible for any errors or omissions or the results obtained from the use of this information. All information on this site is provided “as is”, with no guarantee of completeness, accuracy, timeliness, or of the results obtained from the use of this information, and without warranty of any kind, express or implied, including, but not limited to warranties of performance, merchantability, and fitness for a particular purpose.
In no event will STM Journals, its related partnerships or corporations, or the partners, agents, or employees thereof be liable to you or anyone else for any decision made or action taken in reliance on the information in this Site or for any consequential, special or similar damages, even if advised of the possibility of such damages.
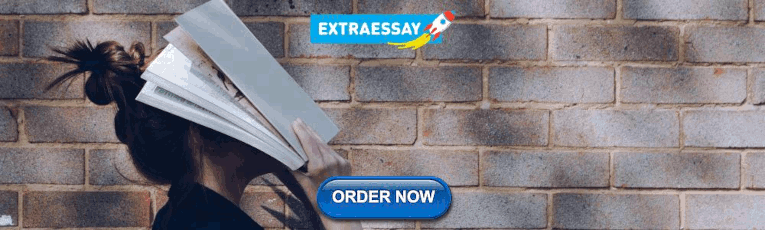
GUEST CONTRIBUTORS DISCLAIMER
This Site may include content from guest contributors and any views or opinions expressed in such posts are personal and do not represent those of STM Journals or any of its staff or affiliates unless explicitly stated.
LOGOS AND TRADEMARKS DISCLAIMER
All logos and trademarks of third parties referenced on https://journals.stmjournals.com / are the trademarks and logos of their respective owners. Any inclusion of such trademarks or logos does not imply or constitute any approval, endorsement, or sponsorship of STM Journals by such owners.
Should you have any feedback, comments, requests for technical support, or other inquiries, please contact us by email: [email protected] .
President Biden warns artificial intelligence could 'overtake human thinking'

WASHINGTON − President Joe Biden on Thursday amplified fears of scientists who say artificial intelligence could "overtake human thinking" in his most direct warning to date on growing concerns about the rise of AI .
Biden brought up AI during a commencement address to graduates of the Air Force Academy in Colorado Springs, Colo. while discussing the rapid transformation of technology that he said could "change the character" of future conflicts.
"It's not going to be easy decisions, guys," Biden said. "I met in the Oval Office with eight leading scientists in the area of AI. Some are very worried that AI can actually overtake human thinking and planning. So we've got a lot to deal with. An incredible opportunity, but a lot do deal with."
Scientists, tech execs warn of possible human extinction
Hundreds of scientists, tech industry executives and public figures – including leaders of Google, Microsoft and ChatGPT – sounded the alarm about artificial intelligence in a public statement Tuesday, arguing that fast-evolving AI technology could create as high a risk of killing off humankind as nuclear war and COVID-19-like pandemics.
Prep for the polls: See who is running for president and compare where they stand on key issues in our Voter Guide
"Mitigating the risk of extinction from AI should be a global priority alongside other societal-scale risks such as pandemics and nuclear war," said the one-sentence statement, which was released by the Center for AI Safety, or CAIS, a San Francisco-based nonprofit organization.
Biden met May 5 at the White House with CEOs of leading AI companies including Google, Microsoft and OpenA to discuss reforms that ensure AI products are safe before released to the public.
"It is one of the most powerful technologies that we see currently in our time," White House press secretary Karine Jean-Pierre said when asked about the extinction fears of scientists. "But in order to seize the opportunities it presents, we must first mitigate its risks, and that's what we're focused on in this administration."
White House launches $140 million in new AI research
The so-called “Godfather of AI” Geoffrey Hinton last month left his job as a Google vice president to speak freely about his concern that unexpectedly rapid advances could potentially endanger the human race. Others portrayed Hinton’s assessment as extreme and unwarranted.
Asked at a recent panel when asked what was the “worst case scenario that you think is conceivable,” Hinton replied without hesitation. “I think it's quite conceivable," he said, "that humanity is just a passing phase in the evolution of intelligence.”
The White House unveiled an initiative last month to promote responsible innovation in the field of artificial intelligence with the following actions:
- The National Science Foundation will fund $140 million to launch seven new National AI Research Institutes. This initiative aims to bring together federal agencies, private-sector developers and academia to pursue ethical, trustworthy and responsible development of AI that serves the public good.
- The new Institutes will advance AI R&D in critical areas, including climate change, agriculture, energy, public health, education, and cybersecurity.
- A commitment from leading AI developers to participate in a public evaluation of their technology systems to determine if they adhere to the principles outlined in the Biden administration’s October 2022 Blueprint for an AI Bill of Rights.
- The initiative includes new Office of Management and Budget (OMB)] policy guidance on the U.S. government’s use of AI systems in order to allow for public comment. This guidance will establish specific policies for federal agencies to ensure that their development, procurement, and use of AI systems centers on safeguarding the American people’s rights and safety.
Contributing: Josh Meyer and Maureen Groppe
Reach Joey Garrison on Twitter @joeygarrison.
- Reference Manager
- Simple TEXT file
People also looked at
Technology and code article, open and remotely accessible neuroplatform for research in wetware computing.
- FinalSpark, Rue du Clos 12, Vevey, Switzerland
Wetware computing and organoid intelligence is an emerging research field at the intersection of electrophysiology and artificial intelligence. The core concept involves using living neurons to perform computations, similar to how Artificial Neural Networks (ANNs) are used today. However, unlike ANNs, where updating digital tensors (weights) can instantly modify network responses, entirely new methods must be developed for neural networks using biological neurons. Discovering these methods is challenging and requires a system capable of conducting numerous experiments, ideally accessible to researchers worldwide. For this reason, we developed a hardware and software system that allows for electrophysiological experiments on an unmatched scale. The Neuroplatform enables researchers to run experiments on neural organoids with a lifetime of even more than 100 days. To do so, we streamlined the experimental process to quickly produce new organoids, monitor action potentials 24/7, and provide electrical stimulations. We also designed a microfluidic system that allows for fully automated medium flow and change, thus reducing the disruptions by physical interventions in the incubator and ensuring stable environmental conditions. Over the past three years, the Neuroplatform was utilized with over 1,000 brain organoids, enabling the collection of more than 18 terabytes of data. A dedicated Application Programming Interface (API) has been developed to conduct remote research directly via our Python library or using interactive compute such as Jupyter Notebooks. In addition to electrophysiological operations, our API also controls pumps, digital cameras and UV lights for molecule uncaging. This allows for the execution of complex 24/7 experiments, including closed-loop strategies and processing using the latest deep learning or reinforcement learning libraries. Furthermore, the infrastructure supports entirely remote use. Currently in 2024, the system is freely available for research purposes, and numerous research groups have begun using it for their experiments. This article outlines the system’s architecture and provides specific examples of experiments and results.
1 Introduction
The recent rise in wetware computing and consequently, artificial biological neural networks (BNNs), comes at a time when Artificial Neural Networks (ANNs) are more sophisticated than ever.
The latest generation of Large Language Models (LLMs), such as Meta’s Llama 2 or OpenAI’s GPT-4, fundamentally rely on ANNs.
The recent acceleration of ANN use in everyday life, such as in tools like ChatGPT or Perplexity combined with the explosion in complexity in the underlying ANN’s architectures, has had a significant impact on energy consumption. For instance, training a single LLM like GPT-3, a precursor to GPT-4, approximately required 10 GWh, which is about 6,000 times the energy a European citizen uses per year. According to a recent publication the energy consumption projected may increase faster than linearly ( De Vries, 2023 ). At the same time, the human brain operates with approximately 86 billion neurons while consuming only 20 W of power ( Clark and Sokoloff, 1999 ). Given these conditions, the prospect of replacing ANNs running on digital computers with real BNNs is enticing ( Smirnova et al., 2023 ). In addition to the substantial energy demands associated with training LLMs, the inference costs present a similarly pressing concern. Recent disclosures reveal that platforms like OpenAI generate over 100 billion words daily through services such as ChatGPT as reported by Sam Altman, the CEO of OpenAI. When we break down these figures, assuming an average of 1.5 tokens per word—a conservative estimate based on OpenAI’s own tokenizer data—the energy footprint becomes staggering. Preliminary calculations, using the LLaMA 65B model (precursor to Llama 2) as a reference point, suggest energy expenditures ranging from 450 to 600 billion Joules per day for word generation alone ( Samsi et al., 2023 ). While necessary for providing AI-driven insights and interactions to millions of users worldwide, this magnitude of energy use underscores the urgency for more energy-efficient computing paradigms.
Connecting probes to BNNs is not a new idea. In fact, the field of multi-unit electrophysiology has an established state of the art spanning easily over the past 40 years. As a result, there are already well-documented hardware and methods for performing functional electrical interfacing and micro-fluidics needed for nutrient delivery ( Gross et al., 1977 ; Pine, 1980 ; Wagenaar et al., 2005a ; Newman et al., 2013 ). Some systems are also specifically designed for brain organoids ( Yang et al., 2024 ). However, their research is mostly focused on exploring brain biology for biomedical applications (e.g., mechanisms and potential treatments of neurodegenerative diseases). The possibility of using these methods for making new computing hardware has not been extensively explored.
For this reason, there is comparatively less literature on methods that can be used to reliably program those BNNs in order to perform specific input–output functions (as this is essential for wetware computing, not for biomedical applications). To understand what we need for programming of BNNs, it is helpful to look at analogous problem for ANNs.
For ANNs, the programming task involves finding the network parameters, globally denoted as S below, that minimize the difference L computed between expected output E and actual output O , for given inputs I , given the transfer function T of the ANN. This can be written as:
L = f O E , with O = T I S
where f is typically a function that equals 0 when O = E .
The same equation applies to BNNs. However, the key differences compared to ANNs include the fact that the network parameters S cannot be individually adjusted in the case of BNNs, and the transfer function T is both unknown and non-stationary. Therefore, alternative heuristics must be developed, for instance based on spatiotemporal stimulation patterns ( Bakkum et al., 2008 ; Kagan et al., 2022 ; Cai et al., 2023a,b ). Such developments necessitate numerous electrophysiological experiments, including, for instance, complex closed-loop algorithms where stimulation is a function of the network’s prior responses. These experiments can sometimes span days or months.
To facilitate long-term experiments involving a global network of research groups, we designed an open innovation platform. This platform enables researchers to remotely perform experiments on a server interfaced with our hardware. For example, our Neuroplatform enhances the chances of discovering the abovementioned stimulation heuristics. It should be noted that, outside of the field of neuroplasticity, similar open platforms were already proposed in 2023 ( O’Leary et al., 2022 ; Armer et al., 2023 ; Elliott et al., 2023 ; Zhang et al., 2023 ). However, to our knowledge, there are no platforms specifically dedicated to research related to biocomputing.
2 Biological setup
The biological material used in our platform is made of brain spheroids [also called minibrains ( Govindan et al., 2021 ), brain organoids ( Qian et al., 2019 ), or neurospheres ( Brewer and Torricelli, 2007 )] developed from Human iPSC-derived Neural Stem Cells (NSCs), following the protocol of Prof. Roux Lab ( Govindan et al., 2021 ). Based on the recent guidelines to clarify the nomenclature for defining 3D cellular models of the nervous system ( Paşca et al., 2022 ), we can call those brain spheroids “forebrain organoids” (FOs). Generation of brain organoids from NSCs has been already described for both mouse ( Ciarpella et al., 2023 ), and human models ( Lee et al., 2020 ). Our protocol is based on the following steps: expansion phase of the NSCs, induction of the 3D structure, differentiation steps (using GDNF and BDNF), and maturation phase ( Figures 1A , B ). The Figure 1C is an image of the FO obtained using electronic microscope, it shows that it is a compact spheroid. The average shape of FOs obtained with this protocol are spheroids of a diameter around 500 μm ( Govindan et al., 2021 ). Our experiments show that the FOs obtained can be kept alive in an orbital shaker for years, as previously demonstrated ( Govindan et al., 2021 ).
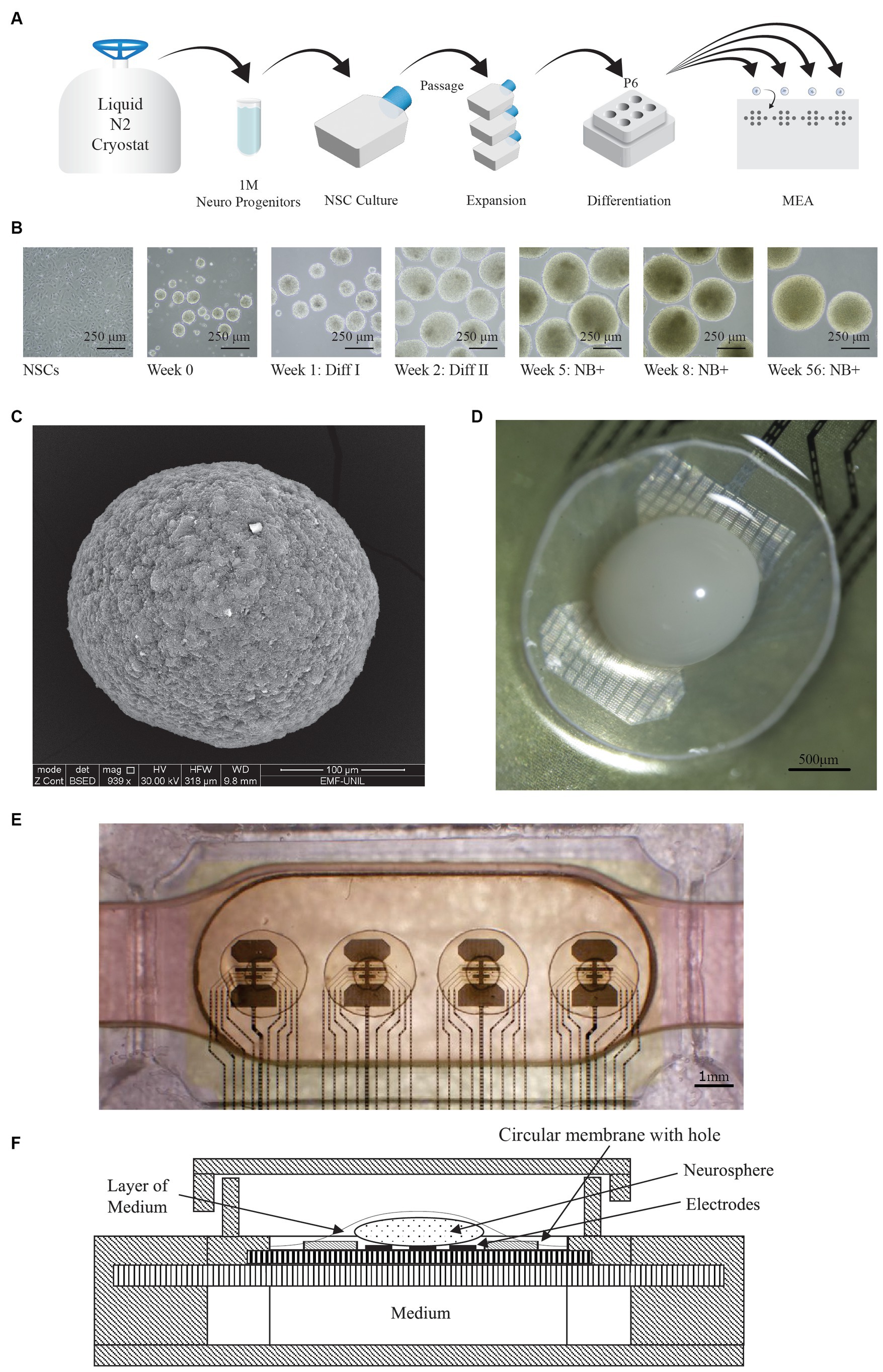
Figure 1 . FO generation and MEA setup. (A) Protocol used for the generation of forebrain organoids (FO). Neural progenitors are first thawed, plated and expanded in T25 flasks. They are then differentiated in P6 dishes on orbital shakers, and finally manually placed on the MEA. (B) Representative images showing various stages of FO formation and differentiation, taken at different time points. The scale bar represents 250 μm. (C) Image of a whole FO taken with scanning electron microscope. The scale bar represents 100 μm. (D) Microscope view of the FO (in white) sitting on the electrodes of the MEA, and the membrane. The hole in the membrane is not visible on the picture since it is hidden by the FO. The scale bar represents 500 μm (E) Overview of the MEA, where the 32 electrodes are visible as 4 sets of 8 electrodes each. An FO is placed atop of each set of 8 electrodes, visible as a darker area. For each FO, the 2 circles correspond to a 2.5 mm circular membrane with a central hole. The scale bar represents 1 mm. (F) Cross-sectional view of the MEA setup, illustrating the air-liquid interface. The medium covering the FO is supplied from the medium chamber through the porous membranes.
Gene expression analysis of mature FOs vs. NSCs showed a marked upregulation of genes characteristic to neurons, oligodendrocytes and astrocytes in FOs compare to NSCs. More precisely, FOs expressed genes typically enriched in the forebrain, such as striatum, sub pallium, and layer 6 of motor cortex ( Govindan et al., 2021 ). Pathway enrichment analysis of FOs vs. NSCs demonstrated activation of biological processes like synaptic activity, neuron differentiation and neurotransmitter release ( Govindan et al., 2021 ).
At the age of 12 weeks, FOs contain a high number of ramified neurons ( Govindan et al., 2021 ), and they are mature enough to be transferred to the electrophysiological measurement system ( Figure 1A ). In this setup, they have a life expectancy of several months, even with 24/7 experiments that include hours of electrical stimulations. This setup has a quick turnaround with occasional downtime – about 1 h – during organoid replacements. Therefore, the platform maintains a high availability for experiments.
3 Hardware architecture
3.1 introduction.
The remotely accessible hardware includes all the systems which are required to preserve homeostasis, monitor environmental parameters and perform electrophysiological experiments. These systems can be controlled interactively using our custom Graphical User Interface (GUI) or via Python scripts. All data is stored in a time-series database (InfluxDB), which can be accessed either via a GUI or via Python scripts. The users typically connect to the system using the Remote Desktop Protocol (RDP).
The platform is composed of several sub-systems, which can be accessed remotely via API calls over the internet, typically through Python.
3.2 Multi-Electrode Array (MEA)
Our current platform features 4 MEAs. The MEAs were designed by Prof. Roux’s Lab form Haute Ecole du Paysage, d’Ingénierie et d’Architecture (HEPIA) and are described in Wertenbroek et al. (2021) . Each MEA can accommodate 4 organoids, with 8 electrodes per organoid ( Figure 1E ).
The MEA setup utilizes an Air-Liquid-Interface (ALI) approach ( Stoppini et al., 1991 ), in which the organoids are directly placed on electrodes located atop of a permeable membrane ( Figure 1D ), with the medium flowing beneath this membrane in a 170 μL chamber. As a result, a thin layer of medium, created by surface tension, separates the upper side of the organoids from the humidified incubator air. This arrangement is further protected by a lid partially covering the MEA ( Figure 1F ). This ALI method enables a higher throughput and higher stability compared to submerged approaches, since no dedicated coating is required, and it is less prone to have the organoids detaching from the electrodes.
3.3 Electrophysiological stimulation and recording system
The electrodes in our system enable both stimulation and recording. The respective digital-to-analog and analog-to-digital conversions are performed by Intan RHS 32 headstages. Stimulations are executed using a current controller that ranges from 10 nA to 2.5 mA, and recordings are obtained by measuring the voltage on each electrode at a 30 kHz sampling frequency with a 16 bits resolution giving an accuracy of 0.15 μV. The headstages are connected to an Intan RHS controller, which in turn is connected to a computer via a USB port. The Figure 2A shows the electrical activity recorded for each of the 32 electrodes. It can be noticed that the recorded activity is different between each electrode. This difference comes from the facts that each set of 8 electrodes records a different FO and that for a given FO, electrodes record at a different location. This display is refreshed in real-time and also available 24/7 on our website at the URL https://finalspark.com/live/ . We compared the recording characteristics of this ALI setup to MCS MEA (60MEA200/30iR-Ti) monitoring a submerged FO, using the exact same Intan system for voltage conversion. The overlays of an action potential recorded, respectively, with the ALI and submerged versions are shown in Figures 2C , D and show similar signal characteristics.
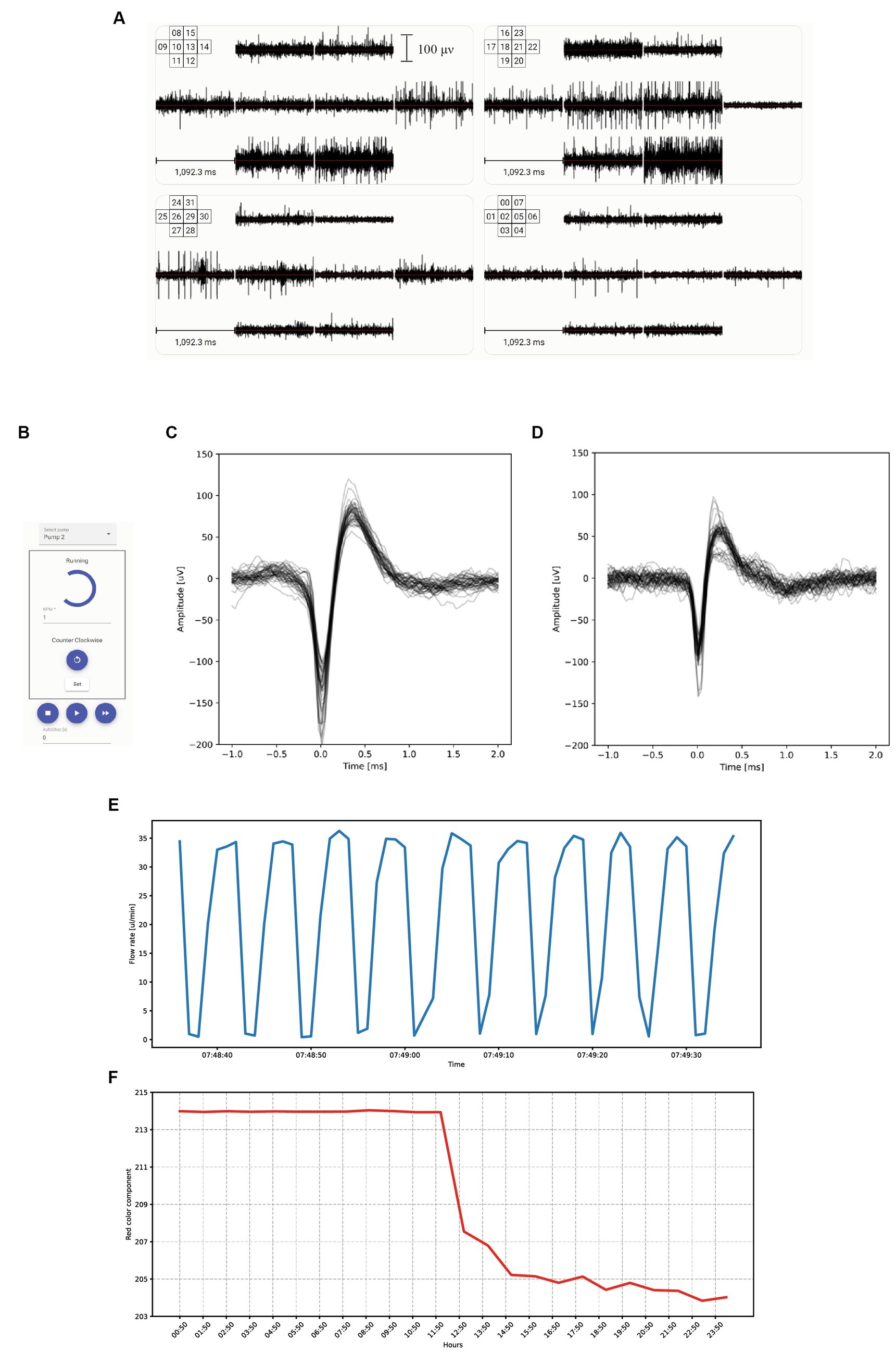
Figure 2 . Recording system and user interface. (A) Electrical activity measured in μV over one second for each of the 32 electrodes. Each set of 8 electrodes records a different FO. (B) Graphical User Interface for manually controlling each of the microfluidic pumps. (C) Overlays of FO action potential recorded by the ALI system of the Neuroplatform. (D) Overlays of FO action potentials recorded with an MCS system. (E) Fluctuations of the flowrate of the medium within the microfluidic system, illustrating the cyclic variations induced by the peristaltic pump operating at 1 round per minute with 10 cams. (F) Temporal variations of the red component of the medium color, triggered by a sudden change in medium acidity, resulting in phenol red color change.
3.4 Micro-fluidics
To sustain the life of the organoids on the MEA, Neuronal Medium (NM) needs to be constantly supplied. Our Neuroplatform is equipped with a closed-loop microfluidic system that allows for a 24/7 medium supply. The medium is circulating at a rate of 15uL/min. The medium flow rate is controlled by a BT-100 2 J peristaltic pump and is continuously adjusted according to needs, for instance during experimental runs. The peristaltic pump is connected to the PC-control software using an RS485 interface, for programmed (i.e., in Python) or manual operations ( Figure 2B ). Additionally, Figure 3A depicts this microfluidic closed-loop circuit.
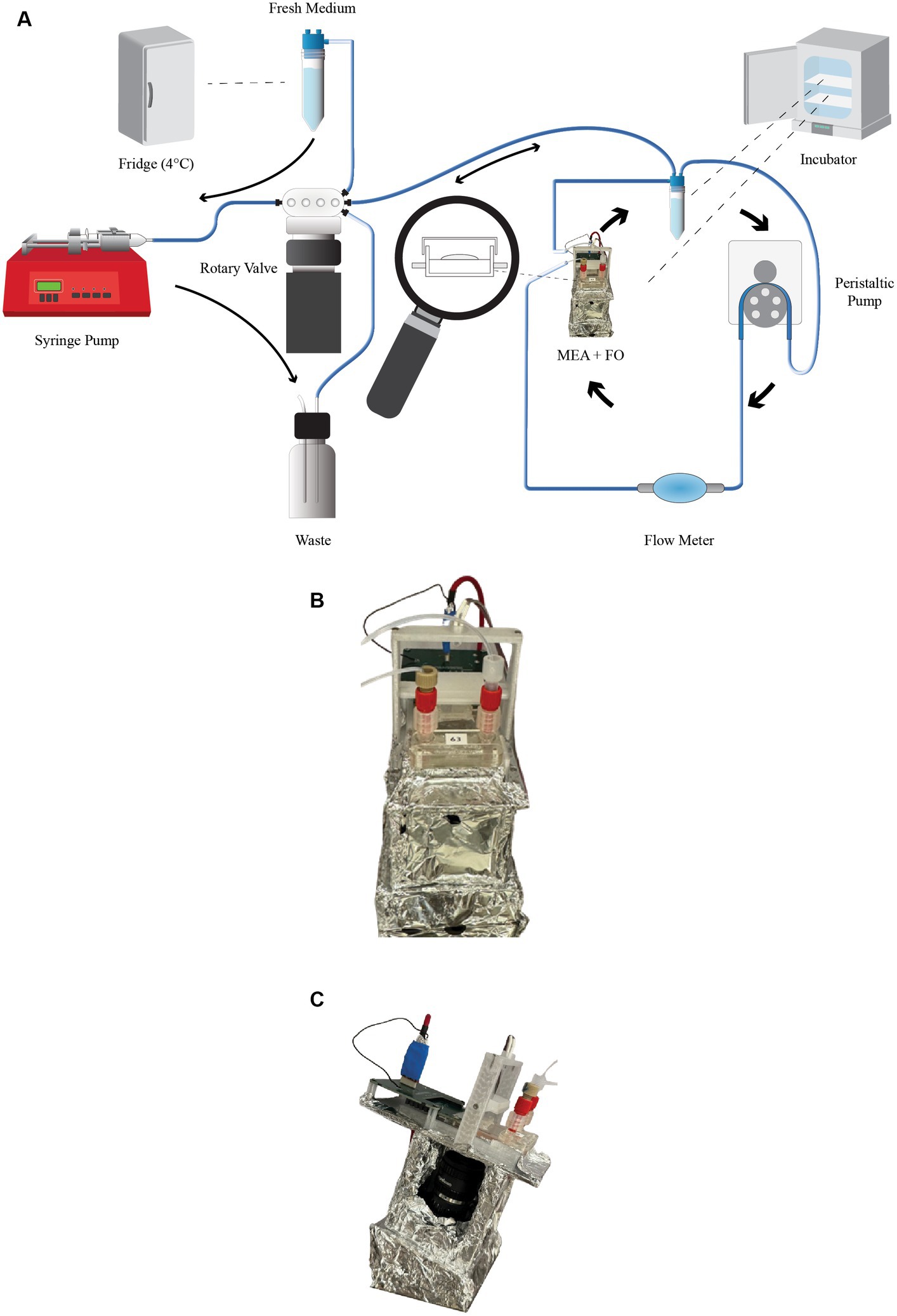
Figure 3 . Microfluidics. (A) Microfluidic system illustrating the continuously operating primary system, which ensures constant flow in the medium chamber, and the secondary system responsible for medium replacing every 48 h. (B) Side view of the assembly, featuring the camera and the MEA. The entire assembly is enclosed with aluminum foil to ensure the lowest possible noise level. (C) Front view of the assembly, showing the intake and outtake of the microfluidic system, as well as the LED used during image capture.
The microfluidic circuit is made of 0.8 mm (inside diameter, ID) tubing. Continuous monitoring of the microfluidic circuit and flow rate is achieved by using Fluigent flow-rate sensors, which connect to the Neuroplatform control center via USB. Data related to medium flow rate is stored in a database for later access. Figure 2E shows the cyclic variations in flow induced by the cams of the peristaltic pump.
A secondary microfluidic system is used to replace the medium in the closed-loop with fresh medium every 24 h, a process illustrated in Figure 3A . This replacement is fully automated through a Python script and performed in the following consecutive steps:
1. Set the rotary valve to select the path from the reservoir F50 to the syringe pump
2. Pump 2 mL of old medium using the syringe pump
3. Set the rotary valve to select the path from the syringe pump to the waste F50
4. Push 2 mL of old medium to the waste using the syringe pump
5. Set the rotary valve to select the path from the new medium in the F50 in the fridge to the syringe pump
6. Pump 2 mL of fresh medium using the syringe pump
7. Set the rotary valve to select the path from the syringe pump to the reservoir F50
8. Push 2 mL of fresh medium using the syringe pump
3.5 Cameras
Each MEA is equipped with a 12.3-megapixel camera that can be controlled interactively or programmatically (i.e., through a Raspberry Pi) for still image capture or video recording. The camera is positioned below the MEA, while illumination is provided by a remotely controlled LED situated above the MEA. Figures 3B , C illustrate this assembly (the aluminum wrapping is used in order to minimize the noise). This setup is particularly useful for detecting various changes, such as cell necrosis, possible organoid displacement caused by microfluidics, variations in medium acidity (using color analysis since our medium contains Phenol red), contamination, neuromelanin production (which can happen when uncaging dopamine), overflows (where the medium inadvertently fills the chamber above the membrane), or bubbles in the medium. For the latter two events, dedicated algorithms automatically detect these issues and trigger an alert to the on-site operator.
Changes of acidity, for example, can be detected by measuring the average color over a pre-defined window. Figure 2F shows the evolution of the medium’s red color component, with data points recorded hourly. The noticeable sudden drop is attributed to the pumping of medium with a slightly different acidity. This change in acidity results in a color alteration of the phenol red present in the medium.
3.6 UV light controlled uncaging
It is also possible to release molecules at specific timings using a process called uncaging. In this method, a specific wavelength of light is employed to break open a molecular “cage” that contains a neuroactive molecule, such as Glutamate, NMDA or Dopamine. A fiber optic of 1,500 μm core diameter and a numerical aperture of 0.5 is used to direct light in the medium within the MEA chamber. The current system, Prizmatix Silver-LED, operates at 365 nm with an optical power of 260 mW. The uncaging system is fully integrated into the Neuroplatform and can be programmatically controlled during experiment runs via our API (see section 5.3).
3.7 Environmental measurements
The environmental conditions are monitored within two incubators. In both incubators, the following parameters are recorded: CO2, O2 concentrations, humidity, atmospheric pressure and temperature. Door-opening events are also logged since they have a major impact on measurements. The primary purpose of this monitoring is to ensure that experiments are performed in stable and reproducible environmental conditions.
All these parameters are displayed in real-time in a graphic interface showing both instant values as well as variations versus time of noise and flowrates ( Figure 4A ).
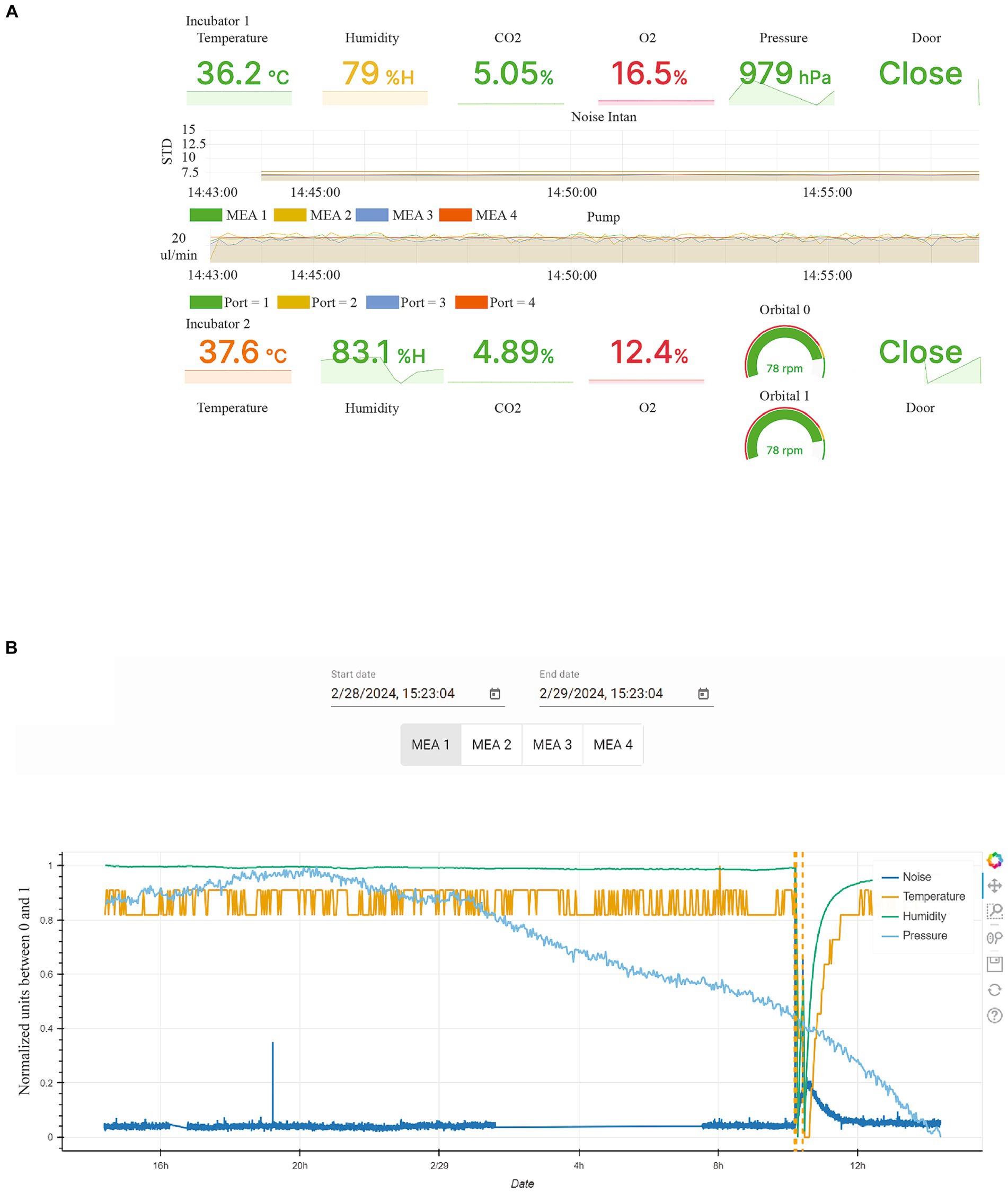
Figure 4 . Graphic user interface to monitor critical parameters in the incubators. (A) Graphical User Interface displaying critical environmental conditions for the incubator 1, where electrophysiological experiments are performed, as well as the incubator 2, where FO are maintained on an orbital shaker. (B) The display shows environmental data for incubator 1 for specific time periods, extracted from the database, with door opening events displayed as dashed line. Noise, Temperature, humidity and pressure are indicated by different colored lines. The units of each measurement are normalized between 0 and 1 for the selected time interval.
Incubator 1 houses the MEAs and the organoids used for electrophysiological experiments. In addition to the mentioned parameters, flowmeters are also utilized to report the actual flow rate of the microfluidic for each MEA, as depicted in the graph labelled “Pump” in Figure 4A . The system’s state is indirectly monitored through the noise level of each MEA, as shown in the graph labelled “Noise Intan” in Figure 4A . The noise level is calculated based on the standard deviation of the electrical signals recorded by the electrodes over a 30 ms period.
Incubator 2 houses the organoids which are kept in orbital shakers. Piezoelectric gyroscopes are used to measure the actual rotation speed of the orbital shakers.
Since all the data is logged in the database, it is also possible to access the historical measurements through a dedicated GUI ( Figure 4B ).
4.1 General architecture
The core of the system relies on a computational notebook which provides access to 3 resources ( Figure 5A ):
1. A database where all the information regarding the Neuroplatform system is stored
2. The Intan software running on a dedicated PC, which is used for:
• Recording the number of detected spikes in a 200 ms time window
• Setting stimulation parameters
3. A Raspberry Pi for triggering current stimulation according to stimulation parameters
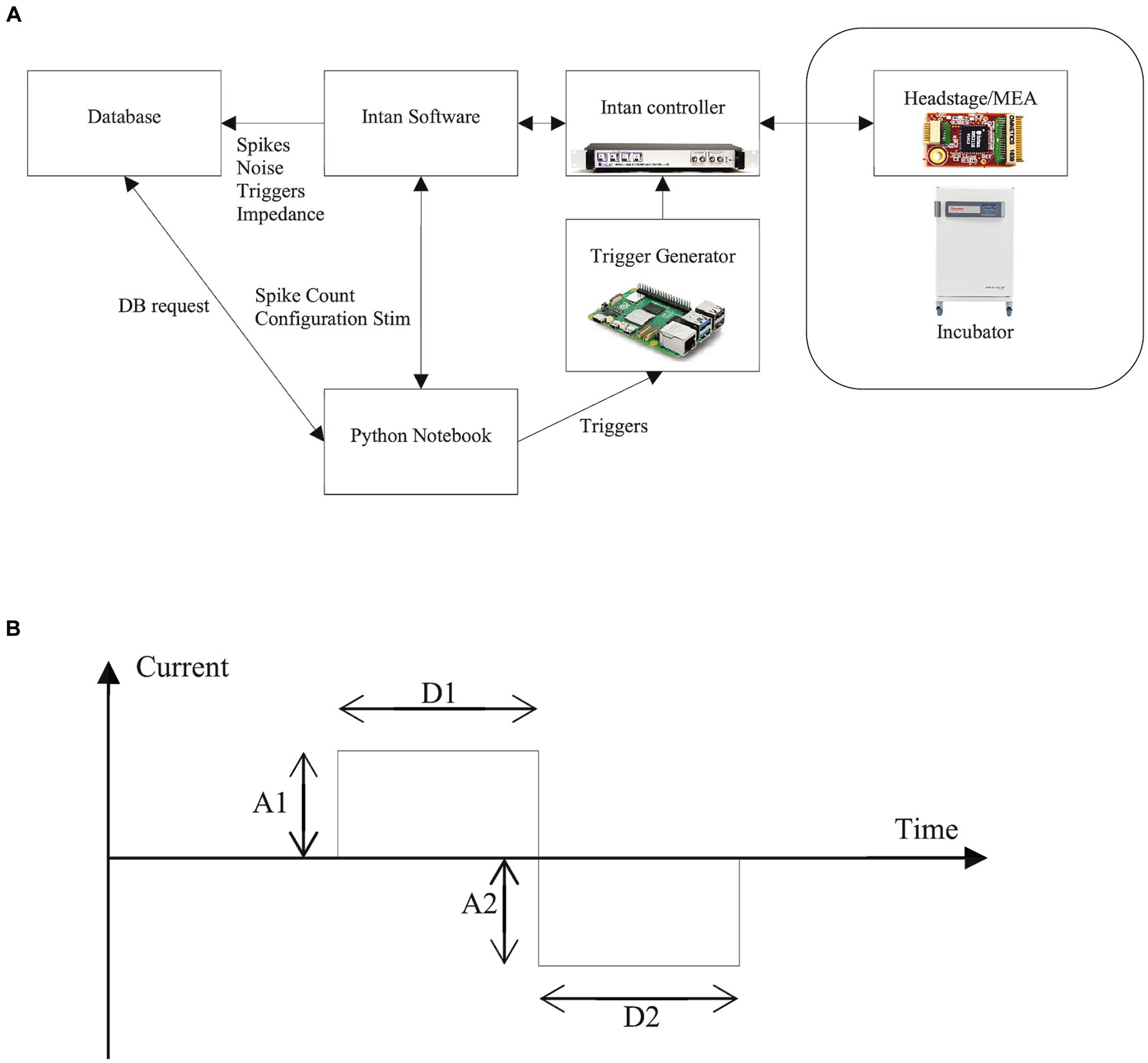
Figure 5 . Software setup and electrical stimulation. (A) General architecture of the Neuroplatform. The Jupyter Notebook serves as the main controller, enabling initiation and reading of spikes, configuration stimulation signals and access to database via, e.g., Python (B) Parameters of the stimulation current: settings optimally these parameters can elicit spikes. Through the Python API, parameters that can be adjusted for the bi-phasic stimulation signals include the duration (D1) and amplitude (A1) of the positive current phase, and, respectively, D2 and A2 for the negative current phase. Additionally, the polarity of the biphasic signal can be reversed to start with a negative current.
4.2 Database
The Neuroplatform records monitored data 24/7 using InfluxDB, a database designed for time series. Other options are also available.
This database contains all the data coming from the hardware listed in Section 3.
The electrical activity of the neurons is also recorded 24/7 at a sampling rate of 30 kHz. To minimize the volume of stored data, we designed a dedicated process that focuses on significant events, such as threshold crossings that are likely to be due to action potentials (spikes). The following pseudo code illustrates the implemented approach:
- Each 1-min write buffer to database
- Each 33 μs
- For each electrode
- If, at time t , the voltage exceeds a threshold T
- Store (in buffer) 3 ms of data [ t -1 ms, t + 2 ms]
- Each 3 s update T
Additionally, a timestamp corresponding to each detected event is also stored in the database, along with the maximum value of voltage during the 3 ms spike waveform recording.
The threshold T is computed directly from voltage values sampled each 33 μs, according to the following formula:
Where σ i is the standard deviation computed over a set i of 30 ms consecutive voltage values, and M d n represents the median function computed over 101 consecutive σ i values. The use of the median reduces the sensitivity to outliers, which is typically caused by action potentials. In our current setup, a multiplier of 6 on the median has proven to be a good compromise for achieving reliable spike detection.
Besides electric tension data, the number spikes recorded per minute is also computed and stored in the database every minute by a batch process.
4.3 Recording electrical activity
As previously discussed, the communication among neurons is captured by the MEA and converted into a voltage signal sampled at 30 kHz. The Neuroplatform offers two basic access modes to the recorded neural activity:
1. Raw: raw sampling values.
2. Optimized: waveforms of the raw signal near neuronal spikes, available directly from the database.
In addition to the aforementioned features, the Neuroplatform offers even more advanced methods. For instance, it includes counting spikes over a fixed time period of 200 ms following stimulation, with a 10 ms delay suppressing the stimulation artifact.
From a technical perspective, accessing the number of spikes can be accomplished in two different ways:
- Retrieving the number of spikes per minute from the database
- Through direct communication with the PC managing the Intan controller for spike count
The second approach is required when the stimulation protocol demands real-time responsiveness. This is typically the case for certain closed-loop strategies. For instance, closed-loop stimulation strategies have been deployed in primary cortical cultures for effective burst control ( Wagenaar et al., 2005a , b ) and for goal-directed learning ( Samsi et al., 2023 ).
4.4 Syntax for stimulations
Programmatically stimulating the FO on the Neuroplatform is accomplished by sending an electrical current to the MEA electrodes. The electrical current profile can be parameterized in a variety of ways, which is partly shown in Figure 5B . These parameters and controls include:
- Basic shape of stimulation signal:
o Bi-phasic
o Bi-phasic with interphase delay
o Tri-phasic
- Stimulation duration and intensity:
o Positive (A1) and negative (A2) electrical current intensity (typical 1uA, ranging from 0.1uA to 20uA)
o Duration of positive (D1) and negative (D2) stimulation currents
- Stimulation triggers
o Single start
o Table with collection of start triggers
o Pulse trains
- MEA electrodes
send_stim_param (electrodes, params)
5 Examples of electrophysiological experiments
To demonstrate the effectiveness of the Neuroplatform, the following sections will provide an overview of several experiments conducted on the Neuroplatform at FinalSpark’s Laboratories in Vevey, Switzerland.
5.1 Modification of spontaneous activity
The spontaneous electrical activity of the FO can be represented by the concept of “Center of Activity” (CA) ( Bakkum et al., 2008 ) which is defined as a virtual position C on the MEA described by:
Where X k Y k define the spatial position of the 8 electrodes and F k is the number of spontaneous spikes detected. The interest of the concept of CA is that its position provides statistical information about the average location of the activity over the surface of the FO. The ability to change the position of the CA is interesting because it also shows the ability to memorize information in the state of the FO.
The coordinates of the CA can be modified using a high frequency stimulation. In the following experiment we use the following protocol:
1) Compute the CA using the number of detected spikes over 500 ms
2) Goto 1,100x
3) Perform a 20 Hz stimulation during 500 ms using a bi-phasic current (negative first) of 2 μA of 200 μS, for both phases, on one electrode
4) Wait 1 s
5) Goto 5,100x
Figure 6A displays the 100 measured positions of the CA corresponding to the spontaneous activity before the 20 Hz stimulation in blue, and after the high-frequency stimulation in red (the average position is indicated by a cross). A close-up is shown in Figure 6B . The timestamps of the spontaneous activity, before and after stimulation, are presented in Figures 6C , D , respectively. Each graph shows one example of the 100 records of 500 ms used to compute the CA location (showing a decrease of spontaneous firing activity of electrodes 3, 4 and 6). A noticeable shift in the average position (shown by a cross) of the CA can be observed before and after the high-frequency stimulation (as seen in Figure 6A ), indicating a change of state of the biological network. A classifier based on a simple logistic regression is employed to predict if the network has received the 20 Hz stimulation. In this particular experiment, the classification accuracy, computed from the confusion matrix, is 95.5%.
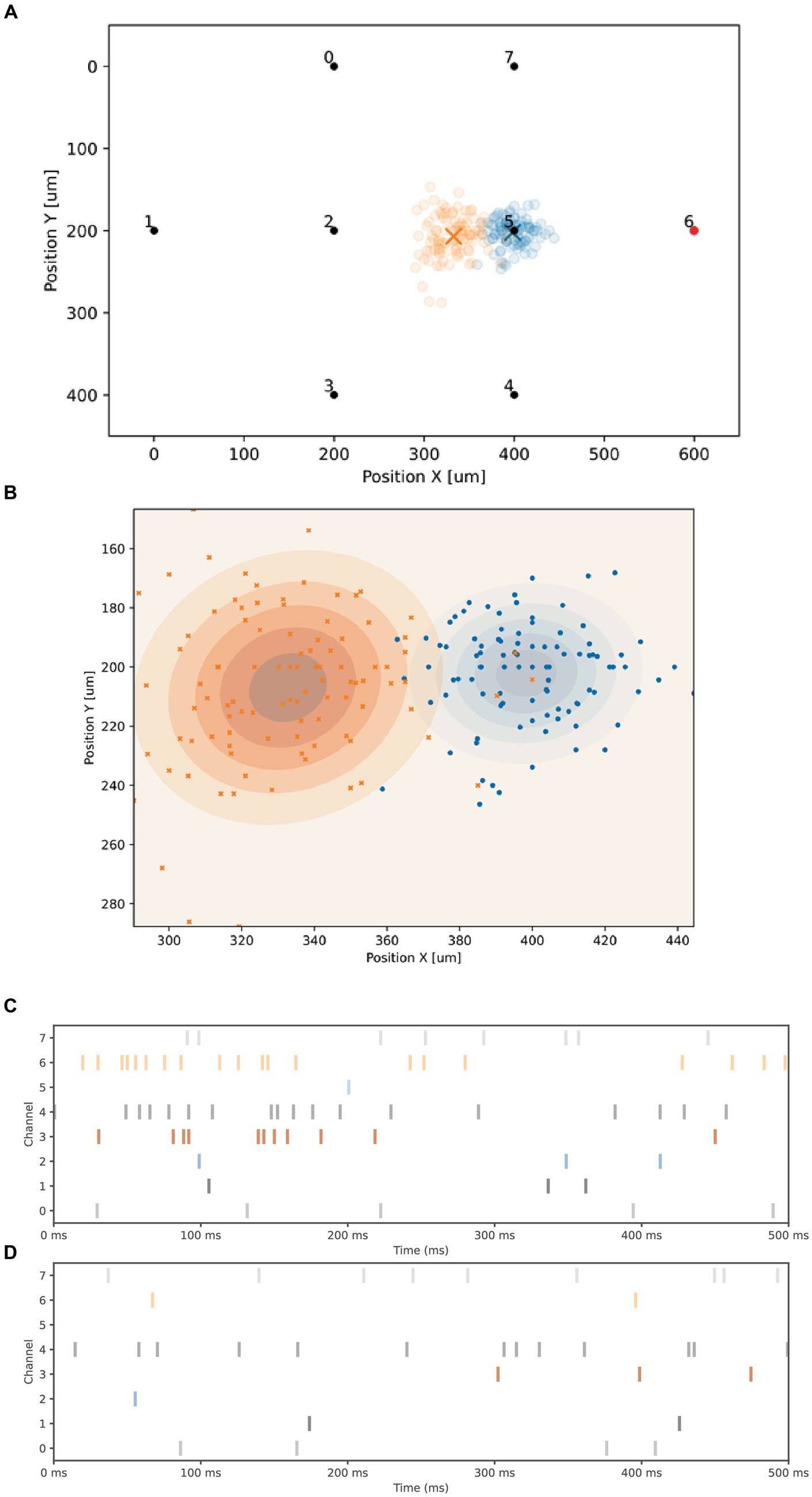
Figure 6 . Center of activity modification. (A) Graph showing the 2D layout of the 8 electrodes, the X and Y axis are normalized units showing the spatial coordinates of the electrodes. All electrodes can be used for both stimulation and reading. A 20 Hz stimulation signal is applied to electrode 6. The 100 blue circles represent the positions of the Center of Activity (CA) before 20 Hz stimulation, while the 100 red circles indicate the positions after the stimulation. The cross mark the average position. (B) A closer look at the two groups of CA. (C) Timestamps depicting the spontaneous activity over 500 ms for each of the 8 electrodes before the high-frequency stimulation. (D) Spontaneous activity observed after the high-frequency stimulation, showing a lower activity of electrodes 6, 4 and 3, compared to (C) .
The Neuroplatform allows users to perform both the experimental part (including stimulation and reading operations) and the visualization of the CA displacement within the same Python source code. The 500 ms 20 Hz signal is generated directly by the Python source code shown below. The first trigger.send instruction sends the trigger for the stimulation on a specific electrode and time.sleep pauses the execution for 50 ms.
Despite the common perception of Python as being less than ideal for real-time signal processing due to its inherent latency, our empirical data reveals a time accuracy of under 1 ms (on an Intel Xeon CPU E5-2690 v2 @ 3.00GHz), a level of precision that is satisfactory for the generation of tetanic signals.
5.2 Optimization of stimulation parameters
In this example, the objective is to identify the set of stimulation parameters that can elicit the maximum number of action potentials within 200 ms after a stimulation.
Depending on the FOs, their composition, and maturity, only specific combinations of electrodes and parameters can elicit spikes. In our experiment, we use an 8-electrode MEA and cycle through several stimulation signal parameters as shown in Figure 7A . Consequently, we need to test a total of 342 different parameter-electrode combinations. The following pseudo code illustrates the Python script used in this experiment.
1) For each set of stimulation parameters
2) For each stimulation electrode
3) For each recording electrode
4) During 15 s, every 250 ms
5) Decide between stimulating, or recording spontaneous activity, with a 50% probability
6) Record number of spikes during 200 ms
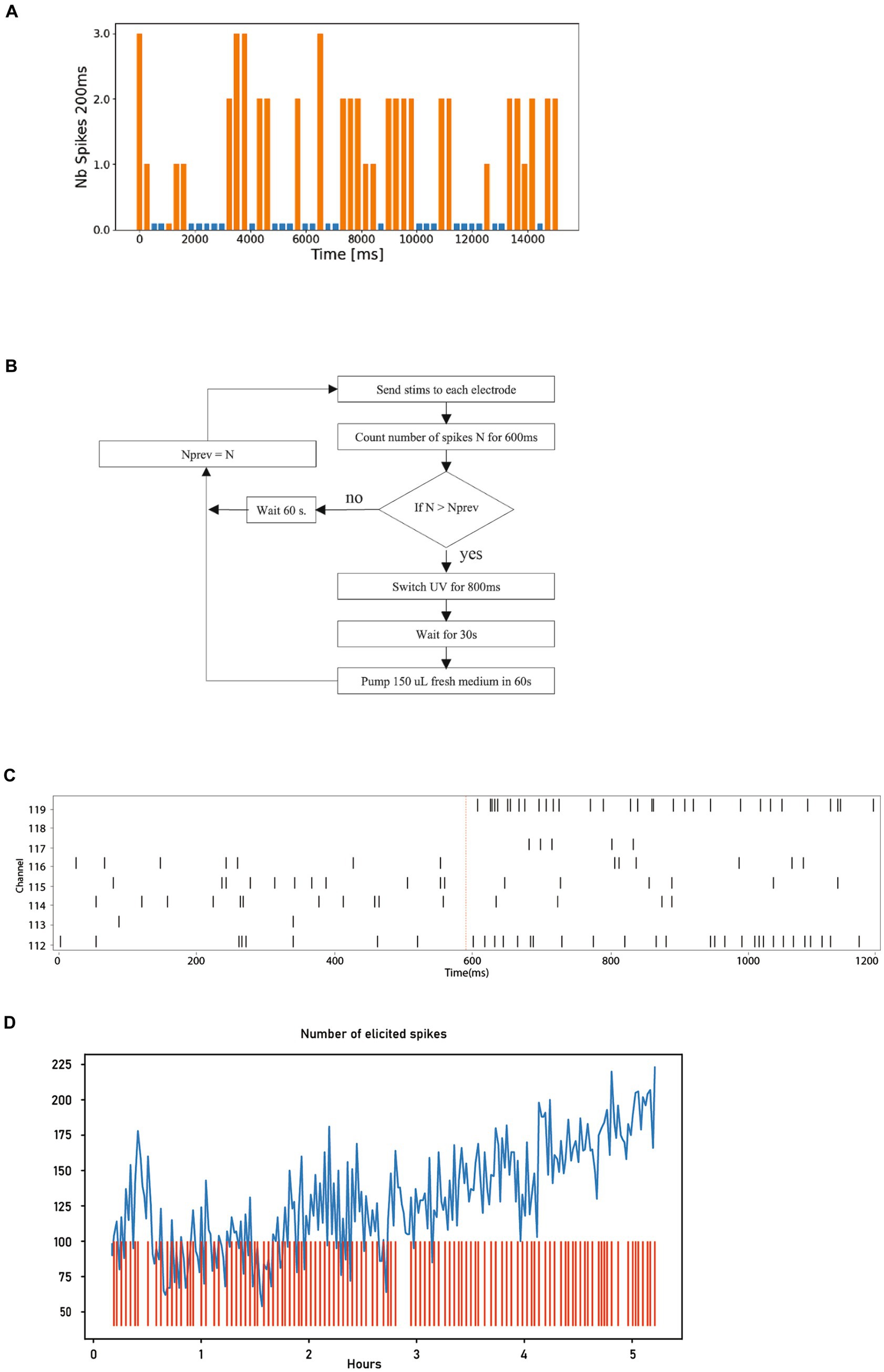
Figure 7 . Neural activity stimulation and dopamine uncaging. (A) Graph depicting the number of spikes recorded over 250 ms. The spike counts in orange were measured following a stimulation, while those in blue were measured during periods without stimulation. For clarity in visualization, a small bar is displayed even when no spikes are detected. (B) Diagram illustrating the different steps involved in the closed-loop uncaging process of dopamine, which is repeated 240 times. (C) Timestamps of action potentials from the 8 electrodes before and after stimulation (shown as red line), showcasing the elicited spikes. (D) Graph displaying the number of elicited spikes over the 240 steps of the closed-loop (in blue) alongside the activation events of the UV light source (red).
The aim of probabilistic stimulation and no stimulation in step 5 is to evaluate the difference between elicited and spontaneous spikes in a way that ensures there is no bias.
The bar chart in Figure 7A displays a segment of the experimental results. It shows a 15-s recording from a single electrode, corresponding to one execution of step 4 in the pseudo code above. Each bar represents the spike count during a 200 ms period, repeated every 250 ms. The orange bars in this plot are the result of the parameters selected in step 1 of the pseudo code. The blue bars represent no-stimulation periods, thus corresponding to the spontaneous activity of the neurons.
From Figure 7A , we can see that this particular combination of electrode and parameters reliably elicits responses.
In practice, the Python script can also be used to automatically display the 342 graphs similar to Figure 7A , allowing the operator to select the optimal set of parameters. Additionally, it can compute a scalar metric to characterize the “efficiency” of the parameters, and automatically identify the optimal parameters.
An example of a parameter maximization metric is given in the equation below. Let us denote μ r and μ s the average number of spikes recorded spontaneously or after a stimulation, respectively, and σ r and σ s as their standard deviations. The following metric is used:
The set of parameters that maximize this metric can then be utilized to perform other experiments requiring elicited spikes, such as investigating the effect of pharmacological agents on a biological network’s ability to react quickly to stimulation.
5.3 UV light-induced uncaging of molecules
‘Uncaging’ is a pivotal technique in cellular biology, enabling the precise control of molecular interactions within cells ( Gienger et al., 2020 ). It involves the use of photolabile caged compounds that are activated by specific light wavelengths, releasing bioactive molecules in a targeted and timely manner. This method is particularly valuable for studying dynamic processes in neural networks and intracellular signaling, offering real-time insights into complex biological mechanisms.
Our Neuroplatform is equipped with all necessary components to perform uncaging. In this example, we investigate closed-loop stimulation, where dopamine is used to reward the network when more spikes are elicited by the same stimulation. The release of the dopamine is achieved through the uncaging of CNV-dopamine using the UV system described in section 3.6.
Figure 7B shows the flow chart of the closed-loop uncaging process. The optimal stimulation parameters are first found using the technique shown in 5.2 (in this case, a current of 4uA, biphasic with 100uS per phase), which is sent successively to each of the 8 electrodes with a delay of 10 ms between each electrode.
Figure 7C shows the response timestamps of the 8 electrodes for a period of 1,200 ms, 600 ms before and after the stimulation. The stimulation event is indicated by the vertical red line. It is interesting to observe that in this particular case, most of the elicited spikes originate from 2 electrodes, specifically electrode 112 and electrode 119.
The Python source code implementing the closed-loop process illustrated in Figure 7B is provided below. We would like to highlight here how concise the code is. With only 13 lines of code, the entire closed-loop process has been implemented.
The graph in Figure 7D shows the variation in the number of spikes elicited during the execution of the script above across 5 h. A general increase in the number of elicited spikes can be observed. However, it is obviously not possible to establish causality between the closed-loop strategy and the observed increase with this single experiment alone. The primary purpose of this closed-loop experiment is to demonstrate the flexibility offered by the Neuroplatform.
6 External users of the Neuroplatform
Access to the Neuroplatform is freely available for research purposes. For researchers lacking lab infrastructure, the Neuroplatform provides the capability to conduct real-time experiments on biological networks. Additionally, it allows others to replicate results obtained in their own lab. The database is shared between all research groups, however the Python scripts and Jupyter Notebooks are in private sections.
In 2023, 36 academic groups proposed research projects, of which 8 were selected. At the time of writing, 4 of these have already yielded some results:
• University Côte d’Azure, CNRS, NeuroMod Institute and Laboratoire JA Dieudonné: investigates the functional connectivity of FO and how electrical stimulation can modify it.
• University of Michigan, investigates stimulation protocols that induce global changes in electrical activity of a FO.
• Free University of Berlin, investigates stimulation protocols that induce changes in the electrical activity of a FO. Additionally, this research employs machine learning tools to extract information from neural firing patterns and to develop well-conditioned responses. Moreover, it utilizes both shallow and deep reinforcement learning techniques to identify optimal training strategies, aiming to elicit reproducible behaviors in the FO.
• University of Exeter, Department of Mathematics and Statistics, Living Systems Institute, investigates storing and retrieving of spatiotemporal spiking patterns, using closed-loop experiments that combine mathematical models of synaptic communication with the Neuroplatform.
• Lancaster University Leipzig and University of York: characterizes computational properties of FOs under the reservoir computing model, with a view to building low-power environmental sensors.
• Oxford Brookes University, School of Engineering, Computing and Mathematics: investigating the properties of emerging dynamics and criticality within neural organizations using the FOs.
• University of Bath, ART-AI, IAH: using the free energy principle and active inference to study the learning capabilities of neurons, embodied in a virtual environment.
• University of Bristol: stimulating of FOs based on data gathered from an artificial tactile sensor. Use machine learning techniques to interpret the FO’s output, investigating their ability to process real-world data.
7 Discussion and conclusion
The Neuroplatform has now been operational 24/7 for the past 4 years. During this time, the organoids on the MEA have been replaced over 250 times. Considering that we place at least 4 organoids per MEA, and change all the organoids simultaneously, this amounts to testing over 1,000 organoids. Initially, their lifetime was only a few hours, but various improvements, especially related to the microfluidics setup, have extended this to up to 100 days in best cases. It is important to note that the spontaneous activity of the organoids can vary over their lifetime, a factor that must be taken into consideration when conducting experiments ( Wagenaar et al., 2006 ). Additionally, we observed that the minimum current required to elicit spikes, computed using the method described in section 5.2, is increasing over the lifetime of the organoid. This phenomenon may be linked to an impedance increase caused by glial encapsulation ( Salatino et al., 2017 ).
The 24/7 recording strategy as described in section 4.2, results in the constant growth of the database. As of this writing, its size has reached 18 terabytes. This volume encompasses the recording of over 20 billion individual action potentials, each sampled at a 30 kHz resolution for 3 ms. This extensive dataset is significant not only due to its size but also because it was all recorded in a similar in-vitro environment, as described in section 3.2. We are eager to share this data with any interested research group.
8 Future extensions
In the future, we plan to extend the capabilities of our platform to manage a broader range of experimental protocols relevant to wetware computing. For example, we aim to enable a remote control over the injection of specific molecules into the medium, facilitating remote experiments that involve pharmacological manipulation of neuronal activity. This expansion will provide additional degrees of freedom for the automatic optimization of parameters influencing neuroplasticity.
Currently, as detailed in Chapter 2, only one differentiation protocol is used for generating organoids. We plan to introduce additional types of organoid generation protocols soon, with the aim of exploring a broader range of possibilities.
Although 32 research groups requested to access to the Neuroplatform, our current infrastructure only allows us to accommodate 7 groups, considering our own research needs as well. We are in the process of scaling-up the AC/DC hardware system to support more users simultaneously. Additionally, we are currently limited to executing close-loop algorithms for neuroplasticity on one single FO, as these algorithms require sending in real-time adapted simulation signals to each FO. Our software is being updated to run closed-loops in parallel on up to 32 FO.
9.1 Brain organoid generation
Human forebrain organoids were originated as described in Govindan et al. (2021) . Briefly, Human Neural Stem Cells derived from the human induced pluripotent stem (hiPS) cell line (ThermoFisher), were plated in flasks coated with CellStart (Fisher Scientific) and amplified in Stempro NSC SFM kit (ThermoFischer) complete medium: KnockOut D-MEM/F12, 2 mM of GlutaMAX, 2% of StemPro Neural supplement, 20 ng/mL of Human FGF-basic (FGF-2/bFGF) Recombinant Protein, and 20 ng/mL of EGF Recombinant Human Protein (Fisher Scientific). Cells were then detached with StemPro ™ Accutase (Gibco) and plated in p6 at the concentration of 250,000 cells/well. The plates were sealed with breathable adhesive paper and leads, placed on an orbital shaker at 80 rpm, and culture for 7 days at 37°C 5% CO2. After one week the newly formed spheroids were put in differentiation medium I (Diff I), containing DMEM/F-12, GlutaMAX ™ supplement (Gibco), 2% BSA, 1X of Stempro® hESC Supplement, 20 ng/mL of BDNF Recombinant Human Protein (Invitrogen), 20 ng/mL of GDNF Recombinant Human Protein (Gibco), 100 mM of N6,2′-O-Dibutyryladenosine 3′,5′-cyclic monophosphate sodium salt, and 20 mM of 2-Phospho-L-ascorbic acid trisodium salt. After one week, brain spheroids were put in differentiation medium II (Diff II) made of 50% of Diff I and 50% of Neurobasal Plus (Invitrogen). After 3 weeks of culture in Diff II, brain organoids were plated in Neurobasal Plus and kept in the orbital shaker until the transfer on the MEA. Medium was change once per week.
9.2 Electron microscopy analysis of FOs
Mature FOs were fixed in 2.5% Glutaraldehyde in 0.1 M phosphate buffer pH 7.4, at RT. After 24 h the samples were processed as described in Cakir et al. (2019) at the Electron Microscopy Facility of University of Lausanne. The whole FO images were acquired with Quanta FEG 250 Scanning Electron Microscope.
9.3 Transfer of FOs on MEA
MEA connected with the microfluid system was moved from the incubator and placed on a 12.3-megapixel camera system (with an optical lens of 16 mm of focal, giving a magnification power of 21x) inside the cell culture hood. The lid was removed to access the top of the liquid/air interface. Sterile Hydrophilic PTFE MEMBRANE Hole ‘confetti’ (diameter 2.5 mm, diameter of the hole 0.7 mm) (HEPIA) were positioned on top of each electrode and left there 2 min to absorb the medium. FOs were collected from the plate using wide bore pipette tips (Axygen) and placed in the middle of confetti, in a 10 μL drop of medium. The position of the organoids was adjusted with the help of sterile forceps. After all the organoids were put on place, the chamber was covered with the plate sealer Greiner Bio-One ™ BREATHseal ™ Sealer (Fisher Scientific), and with the MEA lid. MEA containing the organoids were placed immediately back in the cell incubator and were ready to be used for recording and stimulation. A similar procedure was used for the positioning of organoids on MCS MEA (60MEA200/30iR-Ti). In this case the Hydrophilic PTFE MEMBRANE was not used and organoids were directly laid on the electrodes in a 30 μL drop of medium. Recording of organoid activity was performed immediately afterwards.
9.4 System design and assembly
Cell culture media was stored in a 50 mL Falcon tube with a multi-port delivery cap (ElveFlow) and stored at 4°C. Each reservoir delivery cap contained a single 0.8 mm ID × 1.6 mm OD PTFE tubing (Darwin Microfluidics), sealed by a two-piece PFA Fittings and ferrule threaded adapter (IDEX), extending from the bottom of the reservoir to an inlet port on the 4-port valve head of the RVM Rotary Valve (Advance Microfluidics SA). Sterile air is permitted to refill the reservoir through a 0.22-μm filter (Milian) fixed to the cap to compensate for syringe pump medium withdrawal. A similar PTFE tubing and PFA Fittings and adapters were used to connect the syringe pump to the 4-port valve head of the RVM Rotary Valve (Advance Microfluidics SA). Each PTFE tubing coming from the distribution valve connects with a 50 mL falcon tube inside the cell culture incubator (Binder) and to a borosilicate glass bottle (Milian) to collect discarded cell culture medium.
A secondary microfluid system made of 0.8 mm ID × 1.6 mm OD PTFE tubing, were used to connect each 50 mL falcon tube inside the cell culture incubator with its own MEA (HEPIA). The connection was through a precise peristaltic pump BT100-2 J (Darwin Microfluidics) containing 10 rollers. A compute module (Raspberry Pi 4) controlled the peristaltic pump and the Rotary Valve, through a custom application program interface (API), using RS485 interface and RS-232 interface, respectively. A Fluigent flow-rate sensor connected via USB to the Raspberry Pi 4 allowed the monitoring of the flow rate inside the microfluidic system between the peristaltic pump and the MEA. Python was used to develop the software required to carry out automation protocols.
9.5 Uncaging of dopamine
Carboxynitroveratryl (CNV)-caged dopamine (Tocris Bioscience) was dissolved in Neurobasal Plus at the concentration of 1 mM, and injected in the fluidic system. After 3 h from the injection, the uncaging experiment started as described in paragraph 5.3. UV Silver-LED fiber-coupled LED (Prizmatix) was used to uncage the dopamine at the wavelength of 365 nm for 800 ms each time.
Data availability statement
The raw data supporting the conclusions of this article will be made available by the authors, without undue reservation.
Ethics statement
Ethical approval was not required for the studies on humans in accordance with the local legislation and institutional requirements because only commercially available established cell lines were used.
Author contributions
FJ: Writing – original draft, Writing – review & editing. MK: Writing – original draft, Writing – review & editing. J-MC: Writing – original draft, Writing – review & editing. FB: Writing – original draft, Writing – review & editing. EK: Writing – original draft, Writing – review & editing.
The author(s) declare that no financial support was received for the research, authorship, and/or publication of this article.
Acknowledgments
We thank Steve M. Potter and Daniel Burger for their multiple advices and editing, as well as Mathias Reusser for the figures.
Conflict of interest
FJ, MK, J-MC, FB, and EK are employed at FinalSpark, Switzerland.
Publisher’s note
All claims expressed in this article are solely those of the authors and do not necessarily represent those of their affiliated organizations, or those of the publisher, the editors and the reviewers. Any product that may be evaluated in this article, or claim that may be made by its manufacturer, is not guaranteed or endorsed by the publisher.
Armer, C., Letronne, F., and DeBenedictis, E. (2023). Support academic access to automated cloud labs to improve reproducibility. PLoS Biol. 21:e3001919. doi: 10.1371/journal.pbio.3001919
PubMed Abstract | Crossref Full Text | Google Scholar
Bakkum, D. J., Chao, Z. C., and Potter, S. M. (2008). Spatio-temporal electrical stimuli shape behavior of an embodied cortical network in a goal-directed learning task. J. Neural Eng. 5, 310–323. doi: 10.1088/1741-2560/5/3/004
Brewer, G. J., and Torricelli, J. R. (2007). Isolation and culture of adult neurons and neurospheres. Nat. Protoc. 2, 1490–1498. doi: 10.1038/nprot.2007.207
Crossref Full Text | Google Scholar
Cai, H., Ao, Z., Tian, C., Wu, Z., Liu, H., Tchieu, Z., et al. (2023a). Brain organoid reservoir computing for artificial intelligence. Nat Electron 6, 1032–1039. doi: 10.1038/s41928-023-01069-w
Cai, H., Ao, Z., Tian, C., Wu, Z., Liu, H., et al. (2023b). Brain organoid computing for artificial intelligence. bioRxiv [Preprint]. doi: 10.1101/2023.02.28.530502
Cakir, B., Xiang, Y., Tanaka, Y., Kural, M. H., Parent, M., Kang, Y. J., et al. (2019). Engineering of human brain organoids with a functional vascular-like system. Nat. Methods 16, 1169–1175. doi: 10.1038/s41592-019-0586-5
Ciarpella, F., Zamfir, R. G., Campanelli, A., Pedrotti, G., Di Chio, M., Bottani, E., et al. (2023). Generation of mouse hippocampal brain organoids from primary embryonic neural stem cells. STAR Protoc. 4:102413. doi: 10.1016/j.xpro.2023.102413
Clark, D. D., and Sokoloff, L. (1999). “Circulation and energy metabolism of the brain” in Basic neurochemistry: Molecular, cellular and medical aspects . 6th ed (New York: Philadelphia Lippincott-Raven Publishers), 637–669.
Google Scholar
De Vries, A. (2023). The growing energy footprint of artificial intelligence. Joule 7, 2191–2194. doi: 10.1016/j.joule.2023.09.004
Elliott, M. A. T., Schweiger, H. E., Robbins, A., Vera-Choqqueccota, S., Ehrlich, D., Hernandez, S., et al. (2023). Internet-connected cortical organoids for project-based stem cell and neuroscience education. eNeuro 10. doi: 10.1523/ENEURO.0308-23.2023
Gienger, M., Hübner, H., Löber, S., König, B., and Gmeiner, P. (2020). Structure-based development of caged dopamine D2/D3 receptor antagonists. Sci. Rep. 10:829. doi: 10.1038/s41598-020-57770-9
Govindan, S., Batti, L., Osterop, S. F., Stoppini, L., and Roux, A. (2021). Mass generation, neuron labeling, and 3D imaging of Minibrains. Front. Bioeng. Biotechnol. 8:582650. doi: 10.3389/fbioe.2020.582650
Gross, G. W., Rieske, E., Kreutzberg, G. W., and Meyer, A. (1977). A new fixed-array multimicroelectrode system designed for long-term monitoring of extracellular single unit neuronal activity in vitro. Neurosci. Lett. 6, 101–105. doi: 10.1016/0304-3940(77)90003-9
Kagan, B. J., Kitchen, A. C., Tran, N. T., Habibollahi, F., Khajehnejad, M., Parker, B. J., et al. (2022). In vitro neurons learn and exhibit sentience when embodied in a simulated game-world. Neuron 110, 3952–3969.e8. doi: 10.1016/j.neuron.2022.09.001
Lee, S. E., Shin, N., Kook, G., Kong, D., Kim, N. G., Choi, S. W., et al. (2020). Human iNSC-derived brain organoid model of lysosomal storage disorder in Niemann–pick disease type C. Cell Death Dis. 11:1059. doi: 10.1038/s41419-020-03262-7
Newman, J. P., Zeller-Townson, R., Fong, M., Arcot Desai, S., Gross, R. E., and Potter, S. M. (2013). Closed-loop, multichannel experimentation using the open-source NeuroRighter electrophysiology platform. Front. Neur. Circ. 6:98. doi: 10.3389/fncir.2012.00098
O’Leary, G., Khramtsov, I., Ramesh, R., Perez-Ignacio, A., Shah, P., Chameh, H. M., et al. (2022). OpenMEA: open-source microelectrode Array platform for bioelectronic interfacing. BioRxiv . doi: 10.1101/2022.11.11.516234
Paşca, S. P., Arlotta, P., Bateup, H. S., Camp, G., Cappello, S., Gage, F. H., et al. (2022). A nomenclature consensus for nervous system organoids and assembloids. Nature 609, 907–910. doi: 10.1038/s41586-022-05219-6
Pine, J. (1980). Recording action potentials from cultured neurons with extracellular microcircuit electrodes. J Neurosci. Meth 2, 19–31. doi: 10.1016/0165-0270(80)90042-4
Qian, X., Song, H., and Ming, G. L. (2019). Brain organoids: advances, applications and challenges. Development 146:dev166074. doi: 10.1242/dev.166074
Salatino, J. W., Ludwig, K. A., Kozai, T. D. Y., and Purcell, E. K. (2017). Glial responses to implanted electrodes in the brain. Nat. Biomed. Eng. 1, 862–877. doi: 10.1038/s41551-017-0154-1
Samsi, S., Zhao, D., McDonald, J., Li, B., Michalea, A., Jones, M., et al. (2023). From words to Watts: benchmarking the energy costs of large language model inference. arXiv :2310.03003 [cs.CL] doi: 10.48550/arXiv.2310.03003
Smirnova, L., Caffo, B. S., Gracias, D. H., Huang, Q., Morales Pantoja, I. E., Tang, B., et al. (2023). Organoid intelligence (OI): the new frontier in biocomputing and intelligence-in-a-dish. Front. Sci. 1:1017235. doi: 10.3389/fsci.2023.1017235
Stoppini, L., Buchs, P.-A., and Muller, D. (1991). A simple method for organotypic cultures of nervous tissue. J. Neurosc. Methods 37, 173–182. doi: 10.1016/0165-0270(91)90128-M
Wagenaar, D. A., DeMarse, T. B., and Potter, S. M., "MeaBench: a toolset for multi-electrode data acquisition and on-line analysis.," In 2nd Intl. IEEE EMBS conference on neural Engigneering, Arlington, VA, USA, (2005a).
Wagenaar, D. A., Madhavan, R., Pine, J., and Potter, S. M. (2005b). Controlling bursting in cortical cultures with closed-loop multi-electrode stimulation. J. Neuroscience 25
Wagenaar, D. A., Pine, J., and Potter, S. M. (2006). An extremely rich repertoire of bursting patterns during the development of cortical cultures. BMC Neurosci. 7:11. doi: 10.1186/1471-2202-7-11
Wertenbroek, R., Thoma, Y., Mor, F. M., Grassi, S., Heuschkel, M. O., Roux, A., et al. (2021). SpikeOnChip: a custom embedded platform for neuronal activity recording and analysis. IEEE Trans. Biomed. Circuits Syst. 15, 743–755. doi: 10.1109/TBCAS.2021.3097833
Yang, X., Forró, C., Li, L. T., Miura, L., Zaluska, T. J., Tsai, C. T., et al. (2024). Kirigami electronics for long-term electrophysiological recording of human neural organoids and assembloids. Nat. Biotechnol. doi: 10.1038/s41587-023-02081-3
Zhang, X., Dou, Z., Kim, S. H., Upadhyay, G., Havert, D., Kang, S., et al. (2023). Mind in vitro platforms: versatile, scalable, robust, and open solutions to interfacing with living neurons. Adv. Sci. (Weinh) :e2306826. doi: 10.1002/advs.202306826. Online ahead of print.
Keywords: wetware computing, organoid intelligence, biocomputing, synthetic biology, AI, biological neural network, hybrot
Citation: Jordan FD, Kutter M, Comby J-M, Brozzi F and Kurtys E (2024) Open and remotely accessible Neuroplatform for research in wetware computing. Front. Artif. Intell . 7:1376042. doi: 10.3389/frai.2024.1376042
Received: 24 January 2024; Accepted: 11 March 2024; Published: 02 May 2024.
Reviewed by:
Copyright © 2024 Jordan, Kutter, Comby, Brozzi and Kurtys. This is an open-access article distributed under the terms of the Creative Commons Attribution License (CC BY) . The use, distribution or reproduction in other forums is permitted, provided the original author(s) and the copyright owner(s) are credited and that the original publication in this journal is cited, in accordance with accepted academic practice. No use, distribution or reproduction is permitted which does not comply with these terms.
*Correspondence: Fred D. Jordan, [email protected]
This article is part of the Research Topic
Intersection between the biological and digital: Synthetic Biological Intelligence and Organoid Intelligence
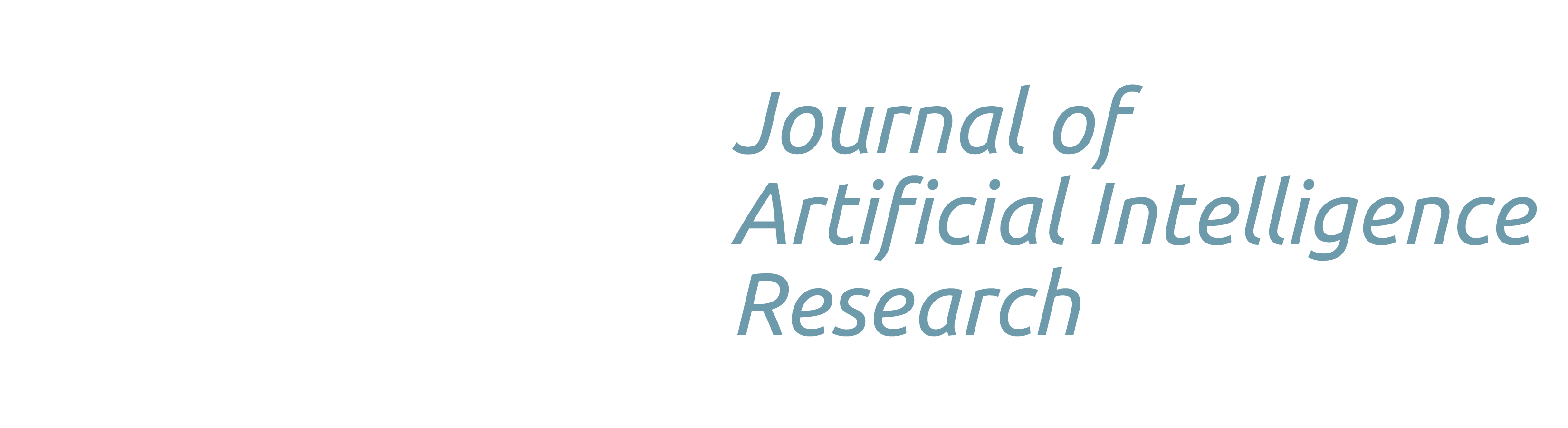
Editorial Team
Editor-in-chief, associate editor-in-chief.
J. Christopher Beck
Managing Editor
Electronic publishing editor, surveys editor, award track editor, editorial board chair.
- Mykel Kochenderfer
Editorial Board Associate Chair
Ijcai-jair best paper prize chair, associate editors.
- Eneko Agirre
- Andrea Aler Tubella
- Alessandro Artale
- James Bailey
- Adrien Bartoli
- Armin Biere
- Roberta Calegari
- Jesus Cerquides
- Kai-Wei Chang
- Alessandro Cimatti
- Stephen Clark
- Virginia Dignum
- Ran El-Yaniv
- Ulle Endriss
- Piotr Faliszewski
- Alessandro Farinelli
- Marcello Federico
- Ariel Felner
- Friedrich Fraundorfer
- Alex Fukunaga
- Nicola Gatti
- Martin Gebser
- Laura Giordano
- Davide Grossi
- Quanquan Gu
- Julia Handl
- Hannaneh Hajishirzi
- Patrik Haslum
- Julia Hockenmaier
- George Konidaris
- Anna Korhonen
- Sarit Kraus
- Akshat Kumar
- Thomas Lukasiewicz
- Sheila McIlraith
- Wendy E. Mackay
- Kuldeep S. Meel
- Thomas Meyer
- Miichela Milano
- Alessandro Moschitti
- Sriraam Natarajan
- Hwee Tou Ng
- Jakob Nordström
- Frans A. Oliehoek
- Rafael Peñaloza
- Maria Perez-Ortiz
- Gilles Pesant
- Marek Petrik
- Balaraman Ravindran
- Juan Rodriguez-Aguilar
- Scott Sanner
- Stefan Schlobach
- Renate Schmidt
- Laurent Simon
- Piotr Skowron
- Matthijs Spaan
- Siddharth Srivastava
- Nathan Sturtevant
- Stefan Szeider
- Myrthe L. Tielman
- Ivor W. Tsang
- Guy Van den Broeck
- Ivan Varzinczak
- Chang-Dong Wang
- Renata Wassermann
Editorial Board
- Christopher Amato
- Laura Barbulescu
- Roman Barták
- Maren Bennewitz
- Phil Blunsom
- Markus Brill
- Sylvain Bouveret
- Emma Brunskill
- Georgios Chalkiadakis
- Kyunghyun Cho
- Martin Cooper
- Marc Denecker
- Janardhan Doppa
- Kurt Driessens
- Yagil Engel
- Stefano Ermon
- Wolfgang Faber
- Artur d'Avila Garcez
- Enrico Gerding
- Dan Goldwasser
- Geoffrey J. Gordon
- Tamir Hazan
- Anthony Hunter
- Frank Hutter
- Albert Xin Jiang
- Erez Karpas
- George Katsirelos
- Gabriele Kern-Isberner
- Alexandre Klementiev
- Parisa Kordjamshidi
- Frederic Koriche
- Oren Kurland
- Philippe Laborie
- Hugo Larochelle
- Diane Litman
- Jongwoo Lim
- Carlos Linares Lopéz
- Alessio Lomuscio
- Sofus A. Macskassy
- Nicolas Maudet
- Aniello Murano
- Thomas Dyhre Nielsen
- Nardine Osman
- Patrice Perny
- Hoifung Poon
- Filip Radlinski
- Miquel Ramirez
- Gabriele Roger
- Alex Rogers
- Alla Rozovskaya
- Sasha Rubin
- Alessandro Saetti
- Chiaki Sakama
- Gerardo I. Simari
- Munindar P. Singh
- Parag Singla
- Kevin Small
- Thamar Solorio
- Yangqiu Song
- Aitor Soroa
- Vivek Srikumar
- Thomas Stützle
- Charles Sutton
- J.N. van Rijn
- Dan Vilenchi
- Martin Wehrle
- Stefan Woltran
- Pinar Yolum
- Neil Yorke-Smith
Advisory Board
- Craig Boutilier
- Ronen Brafman
- Vincent Conitzer
- Adnan Darwiche
- Marie desJardins
- Oren Etzioni
- Hector Geffner
- Holger Hoos
- Manfred Jaeger
- Thorsten Joachims
- Jerome Lang
- Kevin Leyton-Brown
- Shaul Markovitch
- Martha Pollack
- Prasad Tadepalli
- Moshe Tennenholtz
- Michael Wellman
- Michael Wooldridge
- Shlomo Zilberstein
Special Projects
Public relations, production supervisor, assistant managing editor, assistant electronic publishing editor.
Journal of Total Rewards Q2 2024
ANNOUNCEMENT - Thank you for your interest in WorldatWork. We are currently experiencing a temporary issue with e-commerce. Our team is working to resolve. For immediate order placement, please contact our Customer Support Team - email at [email protected] or Call U.S & Canada 1-877-951- 9191 , Outside U.S & Canada 1-480-951-9191. We apologize for the inconvenience.
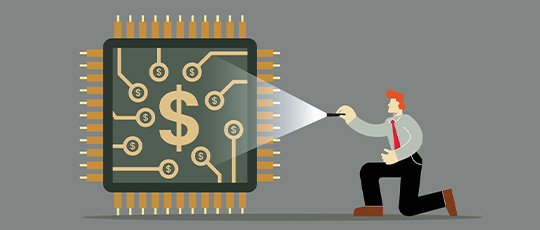
Please try a different combination of filters or categories.
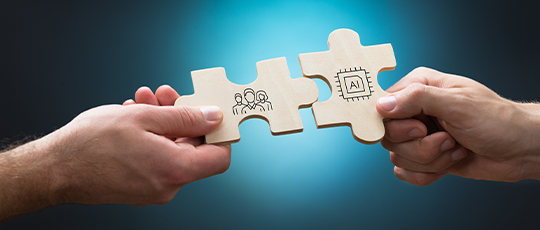
Artificial intelligence (AI) and its role in enhancing and improving total rewards is our focus in this issue of The Journal of Total Rewards.
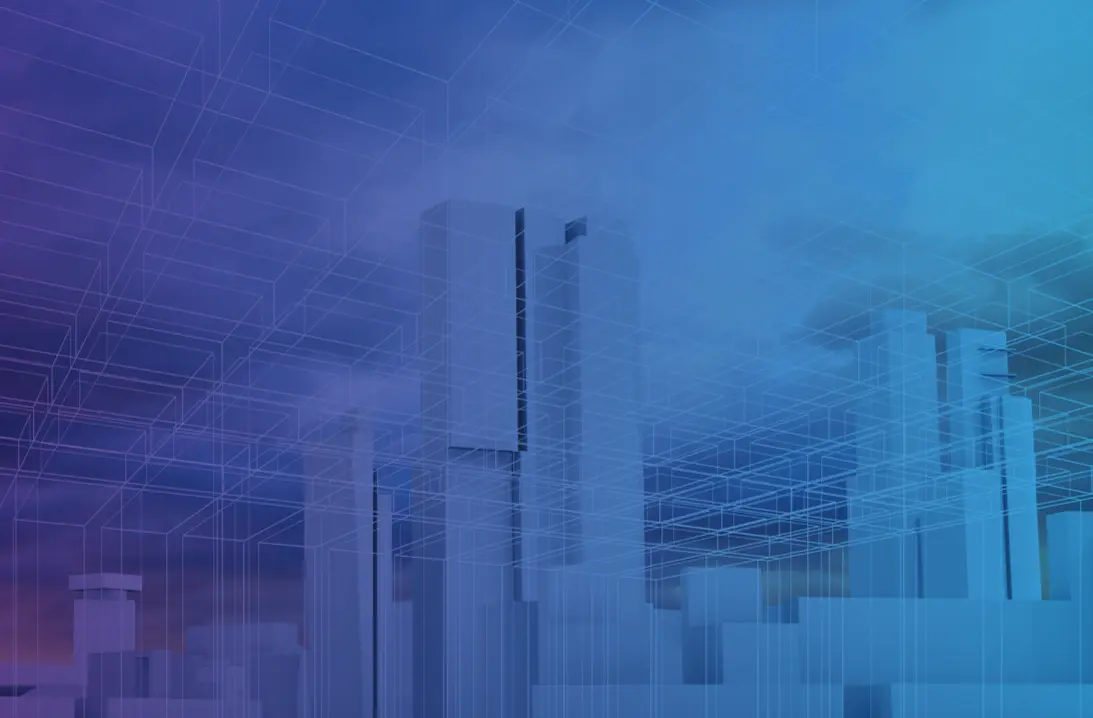
This issue of the Journal of Total Rewards focuses on teaching and communicating Total Rewards to employees.
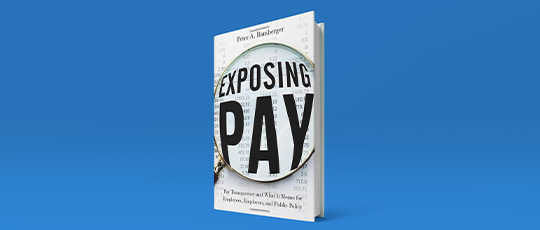
When deciding the theme for this issue of the Journal of Total Rewards, it was clear that pay transparency has become a growing area of focus for our readers.
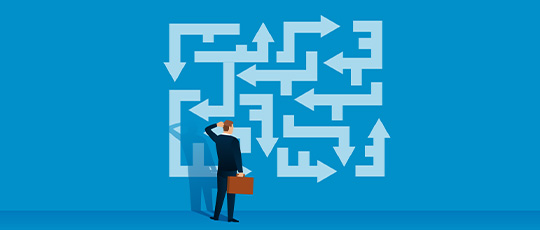
Future-looking research from the 8th Reward Conference in Brussels.
Stay current on hot topics, research, upcoming events and more. Unsubscribe at any time; view our privacy policy .
Please share your feedback!
- My View My View
- Following Following
- Saved Saved
Artificial Intelligence
How Artificial Intelligence is creating the next normal
Latest AI News
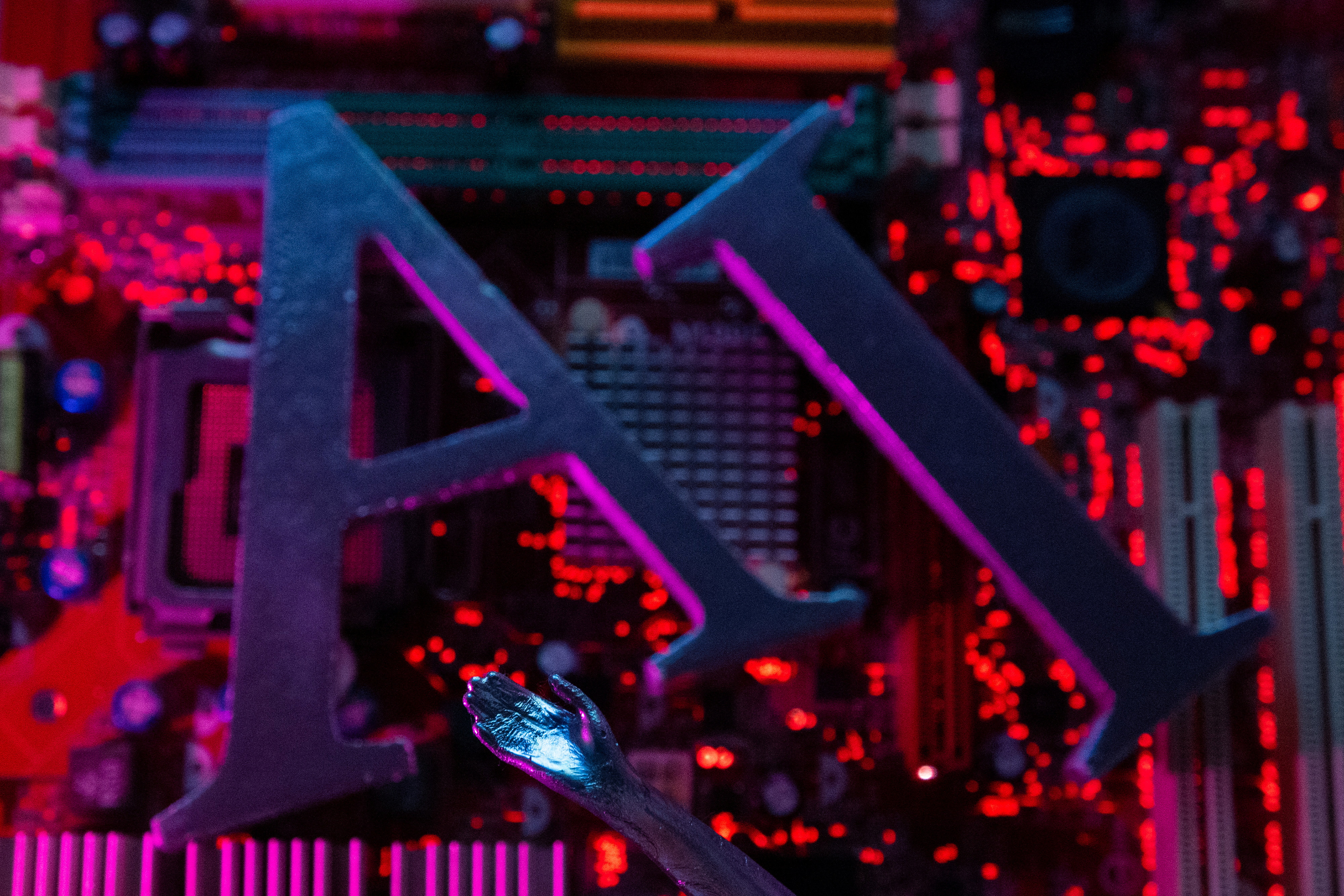
A group of current and former employees at artificial intelligence (AI) companies, including Microsoft-backed OpenAI and Alphabet's Google DeepMind on Tuesday raised concerns about risks posed by the emerging technology.
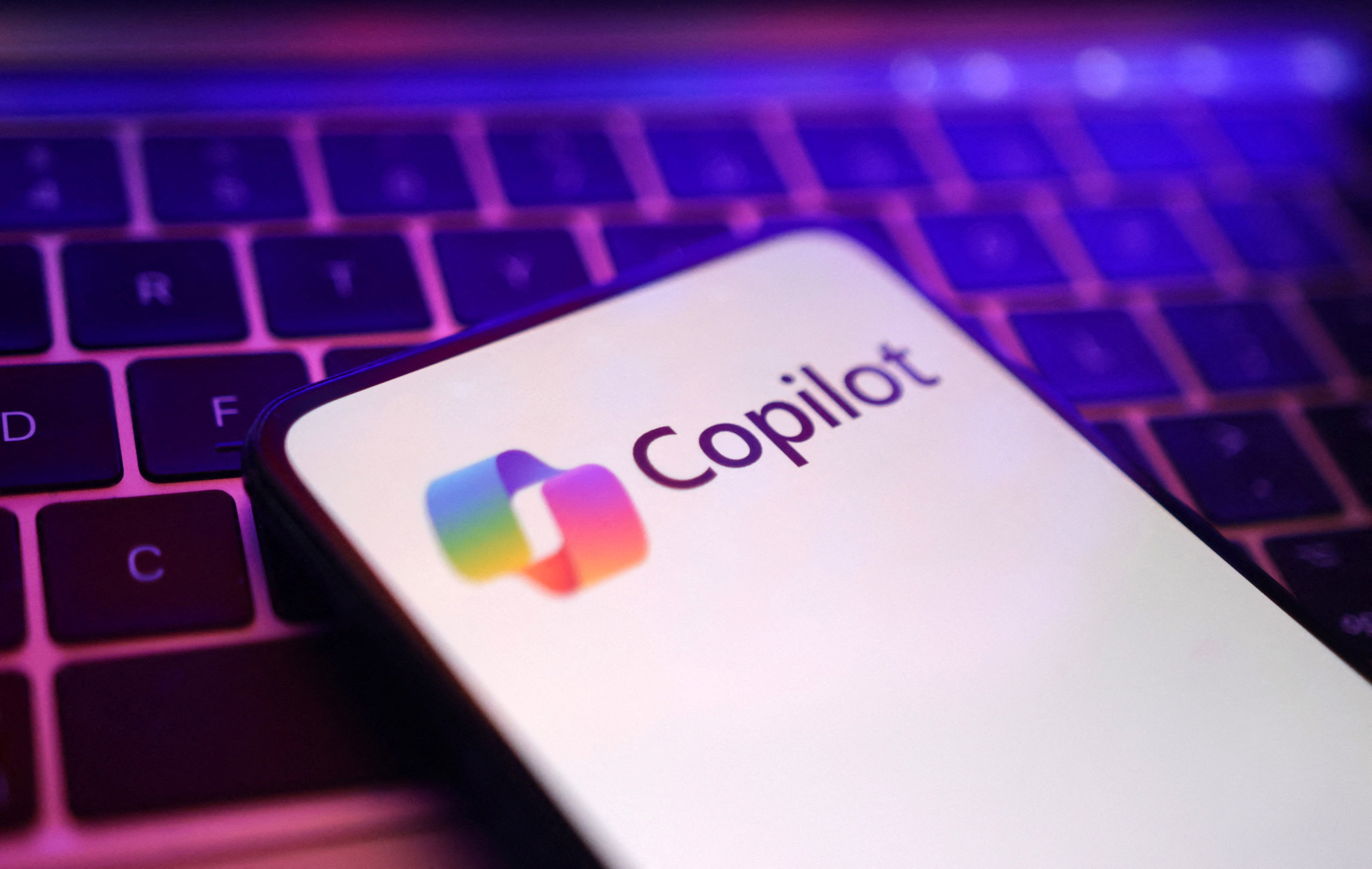
11-12 June 2024 · London
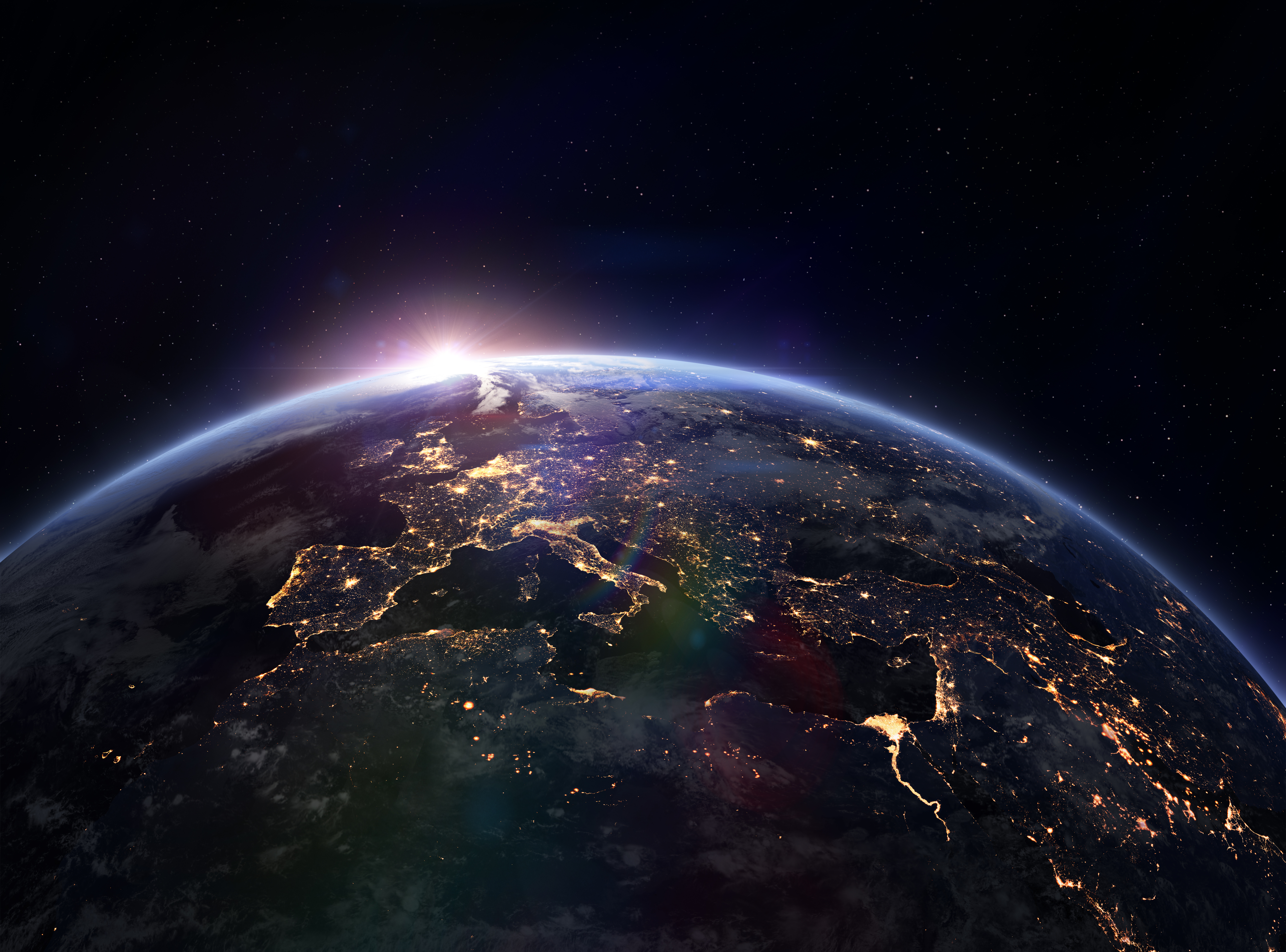
More AI News
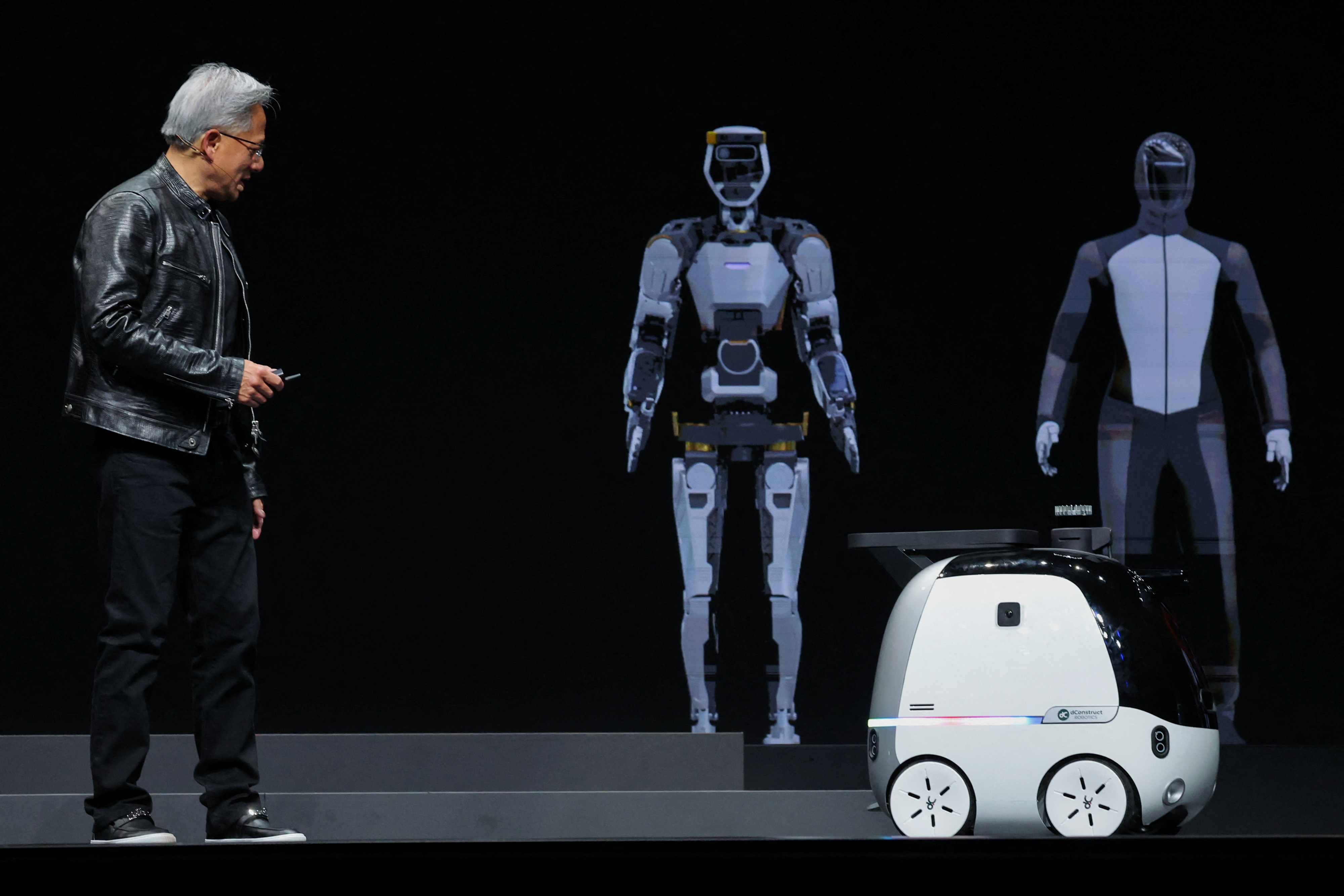
Taiwan's trade-reliant economy is expected to grow at a faster pace in 2024 than previously forecast, owing to high demand for artificial intelligence (AI) applications abroad and solid consumption at home, the statistics office said on Thursday.
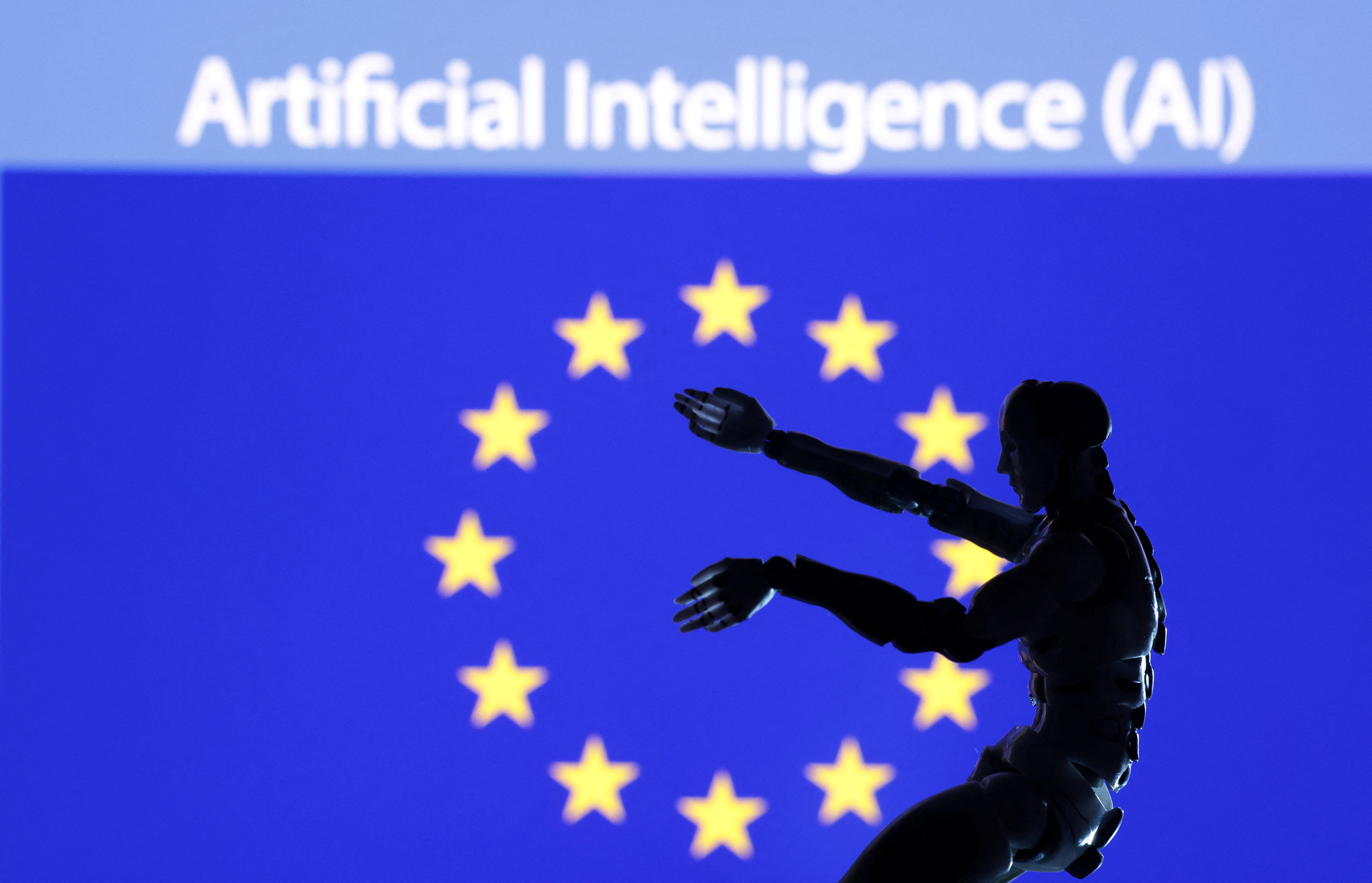
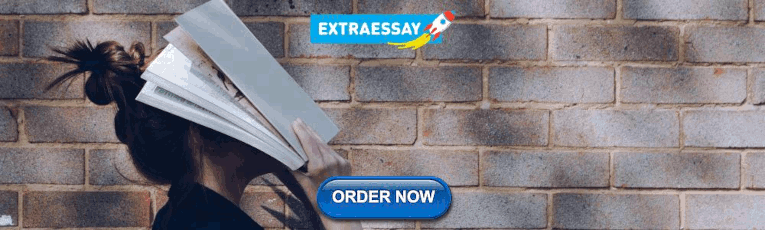
COMMENTS
The Journal of Artificial Intelligence Research (JAIR) publishes important research results in all areas of AI. The current issue (Vol. 79, 2024) features papers on topics such as fairness, explainability, anomaly detection, reinforcement learning, and more.
The Journal of Artificial Intelligence Research (www.jair.org) covers all areas of artificial intelligence, publishing refereed research articles, survey articles, and technical notes. JAIR was established in 1993 as one of the very first open access scientific journals on the Web. Since it began publication in 1993, JAIR has had a major impact on the field, and has been continuously ranked as ...
Learn how to submit your AI research to JAIR, a peer-reviewed journal that publishes original articles, research notes, survey articles, and special tracks. Find out the submission requirements, procedure, reviewing process, and formatting guidelines.
The Journal of Artificial Intelligence Research (JAIR) is dedicated to the rapid dissemination of important research results to the global artificial intelligence (AI) community. The journal's scope encompasses all areas of AI, including agents and multi-agent systems, automated reasoning, constraint processing and search, knowledge ...
Scimago Journal Rankings provides information and metrics about JAIR, a refereed journal covering all areas of artificial intelligence. See the journal's SJR, quartile, documents, citations, and other indicators over time.
The Journal of Artificial Intelligence Research (www.jair.org) covers all areas of artificial intelligence, publishing refereed research articles, survey articles, and technical notes. JAIR was established in 1993 as one of the very first open access scientific journals on the Web. Since it began publication in 1993, JAIR has had a major impact on the field, and has been continuously ranked as ...
A peer-reviewed, open access journal in artificial intelligence & computer science. Find out the journal's ISSN, website, publication fees, submission to publication time, and license terms.
Deep Reinforcement Learning (DRL) is an avenue of research in Artificial Intelligence (AI) that has received increasing attention within the research community in recent years, and is beginning to show potential for real-world application.
AIJ publishes papers on broad aspects of AI, including methods, applications, challenges, and competitions. It also features Research Notes, Reviews, and Position Papers on various topics of interest in the AI community.
Bibliographic content of Journal of Artificial Intelligence Research (JAIR) Stop the war! Остановите войну! solidarity - - news - - donate - donate - donate; for scientists: ERA4Ukraine; Assistance in Germany; Ukrainian Global University; #ScienceForUkraine; default search action.
Artificial intelligence (AI) is a rapidly evolving field that has transformed various domains of scientific research. This article provides an overview of the history, applications, challenges, and opportunities of AI in science. It also discusses how AI can enhance scientific creativity, collaboration, and communication. Learn more about the potential and impact of AI in science by reading ...
Artificial Intelligence (AI) is increasingly adopted by organizations to innovate, and this is ever more reflected in scholarly work. To illustrate, assess and map research at the intersection of AI and innovation, we performed a Systematic Literature Review (SLR) of published work indexed in the Clarivate Web of Science (WOS) and Elsevier Scopus databases (the final sample includes 1448 ...
1. Introduction. Artificial intelligence (AI) is now a major driver of societal acceleration (Gruetzemacher & Whittlestone, Citation 2022), making a significant impact on science (e.g. AAAS, Citation 2021) and science education (e.g. Alasadi & Baiz, Citation 2023; Cooper, Citation 2023) raising questions, for example about the use of AI tools in teaching (Talanquer, Citation 2023) and ...
Literature review. Artificial Intelligence (AI) has dramatically altered the landscape of academic research, acting as a catalyst for both methodological innovation and broader shifts in scholarly paradigms (Pal, Citation 2023).Its transformative power is evident across multiple disciplines, enabling researchers to engage with complex datasets and questions at a scale previously unimaginable ...
The purpose of this pre-recorded webinar, is to promote and more broadly share the release of NIST IR-8367, "Psychological Foundations of Explainability and Interpretability in Artificial Intelligence."
Journal of Artificial Intelligence Research Volume 78. Previous Article Next Article. Skip Abstract Section. Abstract. There has been a lot of activity in graph representation learning in recent years. Graph representation learning aims to produce graph representation vectors to represent the structure and characteristics of huge graphs ...
The melding of artificial intelligence and robotics could catapult both fields to new heights. ... As the AI research community forges ahead with robotic brains, many of those who actually build ...
About the Journal. Journal of Artificial Intelligence Research & Advances (joaira): 2395-6720(e) is a peer-reviewed online journal launched in 2014 that investigates the role of artificial in this View Full Focus and Scope… Journal Particulars
Browse the latest research papers on artificial intelligence from various domains and perspectives. Topics include superintelligence, reinforcement learning, planning, machine learning, multiagent systems, and more.
Research Article Artificial intelligence utilization in the healthcare setting: perceptions of the public in the UAE Anan S. Jarab a College of Pharmacy, Al Ain University, Abu Dhabi, United Arab Emirates;b Department of Clinical Pharmacy, Faculty of Pharmacy, Jordan University of Science and Technology, Irbid, Jordan Correspondence asjarab ...
11000 Background: Clinical outcomes such as response, progression, and metastasis represent crucial data for observational cancer research, but outside of clinical trials, such outcomes are usually recorded only in unstructured notes in electronic health records (EHRs). Manual EHR annotation is too resource-intensive to scale to large datasets. Individual cancer centers have trained artificial ...
WASHINGTON − President Joe Biden on Thursday amplified fears of scientists who say artificial intelligence could "overtake human thinking" in his most direct warning to date on growing concerns ...
In <i>International Conference on Artificial Intelligence and Statistics (AISTATS)</i>. Google Scholar; Shen, Y. and Sanghavi, S. (2019). Learning with bad training data via iterative trimmed loss minimization. In <i>International Conference on Machine Learning (ICML)</i>, volume 97 of <i>Proceedings of Machine Learning Research</i>. Google Scholar
Wetware computing and organoid intelligence is an emerging research field at the intersection of electrophysiology and artificial intelligence. The core concept involves using living neurons to perform computations, similar to how Artificial Neural Networks (ANNs) are used today. However, unlike ANNs, where updating digital tensors (weights) can instantly modify network responses, entirely new ...
JAIR is published by AI Access Foundation, a nonprofit public charity whose purpose is to facilitate the dissemination of scientific results in artificial intelligence. JAIR, established in 1993, was one of the first open-access scientific journals on the Web, and has been a leading publication venue since its inception.
Studies of a variety of topic rewards topics, from AI and pay transparency to career advancement to analyzing a pay gap.
Journal of Artificial Intelligence Research Volume 78. Previous Article Next Article. Skip Abstract Section. Abstract. A widely-studied deep reinforcement learning (RL) technique known as Prioritized Experience Replay (PER) allows agents to learn from transitions sampled with non-uniform probability proportional to their temporal-difference (TD ...
Taiwan's trade-reliant economy is expected to grow at a faster pace in 2024 than previously forecast, owing to high demand for artificial intelligence (AI) applications abroad and solid ...