On this page
When to see a doctor, risk factors, complications.
Malaria is a disease caused by a parasite. The parasite is spread to humans through the bites of infected mosquitoes. People who have malaria usually feel very sick with a high fever and shaking chills.
While the disease is uncommon in temperate climates, malaria is still common in tropical and subtropical countries. Each year nearly 290 million people are infected with malaria, and more than 400,000 people die of the disease.
To reduce malaria infections, world health programs distribute preventive drugs and insecticide-treated bed nets to protect people from mosquito bites. The World Health Organization has recommended a malaria vaccine for use in children who live in countries with high numbers of malaria cases.
Protective clothing, bed nets and insecticides can protect you while traveling. You also can take preventive medicine before, during and after a trip to a high-risk area. Many malaria parasites have developed resistance to common drugs used to treat the disease.
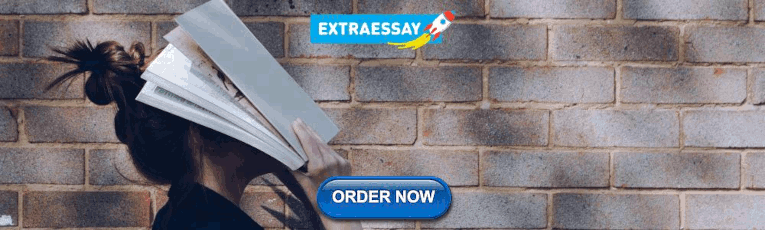
Products & Services
- A Book: Mayo Clinic Family Health Book, 5th Edition
- Newsletter: Mayo Clinic Health Letter — Digital Edition
Signs and symptoms of malaria may include:
- General feeling of discomfort
- Nausea and vomiting
- Abdominal pain
- Muscle or joint pain
- Rapid breathing
- Rapid heart rate
Some people who have malaria experience cycles of malaria "attacks." An attack usually starts with shivering and chills, followed by a high fever, followed by sweating and a return to normal temperature.
Malaria signs and symptoms typically begin within a few weeks after being bitten by an infected mosquito. However, some types of malaria parasites can lie dormant in your body for up to a year.
Talk to your doctor if you experience a fever while living in or after traveling to a high-risk malaria region. If you have severe symptoms, seek emergency medical attention.
From Mayo Clinic to your inbox
Malaria is caused by a single-celled parasite of the genus plasmodium. The parasite is transmitted to humans most commonly through mosquito bites.
Mosquito transmission cycle
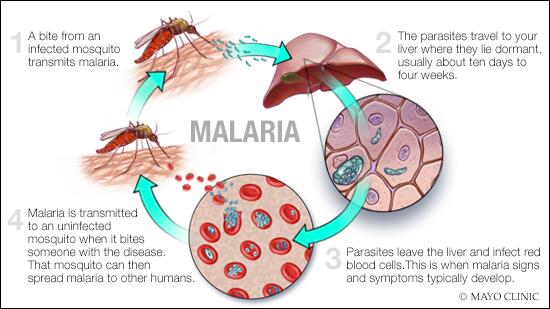
- Malaria transmission cycle
Malaria spreads when a mosquito becomes infected with the disease after biting an infected person, and the infected mosquito then bites a noninfected person. The malaria parasites enter that person's bloodstream and travel to the liver. When the parasites mature, they leave the liver and infect red blood cells.
- Uninfected mosquito. A mosquito becomes infected by feeding on a person who has malaria.
- Transmission of parasite. If this mosquito bites you in the future, it can transmit malaria parasites to you.
- In the liver. Once the parasites enter your body, they travel to your liver — where some types can lie dormant for as long as a year.
- Into the bloodstream. When the parasites mature, they leave the liver and infect your red blood cells. This is when people typically develop malaria symptoms.
- On to the next person. If an uninfected mosquito bites you at this point in the cycle, it will become infected with your malaria parasites and can spread them to the other people it bites.
Other modes of transmission
Because the parasites that cause malaria affect red blood cells, people can also catch malaria from exposure to infected blood, including:
- From mother to unborn child
- Through blood transfusions
- By sharing needles used to inject drugs
The greatest risk factor for developing malaria is to live in or to visit areas where the disease is common. These include the tropical and subtropical regions of:
- Sub-Saharan Africa
- South and Southeast Asia
- Pacific Islands
- Central America and northern South America
The degree of risk depends on local malaria control, seasonal changes in malaria rates and the precautions you take to prevent mosquito bites.
Risks of more-severe disease
People at increased risk of serious disease include:
- Young children and infants
- Older adults
- Travelers coming from areas with no malaria
- Pregnant women and their unborn children
In many countries with high malaria rates, the problem is worsened by lack of access to preventive measures, medical care and information.
Immunity can wane
Residents of a malaria region may be exposed to the disease enough to acquire a partial immunity, which can lessen the severity of malaria symptoms. However, this partial immunity can disappear if you move to a place where you're no longer frequently exposed to the parasite.
Malaria can be fatal, particularly when caused by the plasmodium species common in Africa. The World Health Organization estimates that about 94% of all malaria deaths occur in Africa — most commonly in children under the age of 5.
Malaria deaths are usually related to one or more serious complications, including:
- Cerebral malaria. If parasite-filled blood cells block small blood vessels to your brain (cerebral malaria), swelling of your brain or brain damage may occur. Cerebral malaria may cause seizures and coma.
- Breathing problems. Accumulated fluid in your lungs (pulmonary edema) can make it difficult to breathe.
- Organ failure. Malaria can damage the kidneys or liver or cause the spleen to rupture. Any of these conditions can be life-threatening.
- Anemia. Malaria may result in not having enough red blood cells for an adequate supply of oxygen to your body's tissues (anemia).
- Low blood sugar. Severe forms of malaria can cause low blood sugar (hypoglycemia), as can quinine — a common medication used to combat malaria. Very low blood sugar can result in coma or death.
Malaria may recur
Some varieties of the malaria parasite, which typically cause milder forms of the disease, can persist for years and cause relapses.
If you live in or are traveling to an area where malaria is common, take steps to avoid mosquito bites. Mosquitoes are most active between dusk and dawn. To protect yourself from mosquito bites, you should:
- Cover your skin. Wear pants and long-sleeved shirts. Tuck in your shirt, and tuck pant legs into socks.
- Apply insect repellent to skin. Use an insect repellent registered with the Environmental Protection Agency on any exposed skin. These include repellents that contain DEET, picaridin, IR3535, oil of lemon eucalyptus (OLE), para-menthane-3,8-diol (PMD) or 2-undecanone. Do not use a spray directly on your face. Do not use products with oil of lemon eucalyptus (OLE) or p-Menthane-3,8-diol (PMD) on children under age 3.
- Apply repellent to clothing. Sprays containing permethrin are safe to apply to clothing.
- Sleep under a net. Bed nets, particularly those treated with insecticides, such as permethrin, help prevent mosquito bites while you are sleeping.
Preventive medicine
If you'll be traveling to a location where malaria is common, talk to your doctor a few months ahead of time about whether you should take drugs before, during and after your trip to help protect you from malaria parasites.
In general, the drugs taken to prevent malaria are the same drugs used to treat the disease. What drug you take depends on where and how long you are traveling and your own health.
The World Health Organization has recommended a malaria vaccine for use in children who live in countries with high numbers of malaria cases.
Researchers are continuing to develop and study malaria vaccines to prevent infection.
Feb 09, 2023
- AskMayoExpert. Malaria. Rochester, Minn.: Mayo Foundation for Medical Education and Research; 2018.
- Jameson JL, et al., eds. Malaria. In: Harrison's Principles of Internal Medicine. 20th ed. New York, N.Y.: The McGraw-Hill Companies; 2018. https://accessmedicine.mhmedical.com. Accessed Oct. 9, 2018.
- Tintinalli JE, et al., eds. Malaria. In: Tintinalli's Emergency Medicine: A Comprehensive Study Guide. 8th ed. New York, N.Y.: McGraw-Hill Education; 2016. http://www.accessmedicine.mhmedical.com. Accessed Oct. 9, 2018.
- Malaria. Merck Manual Professional Version. http://www.merckmanuals.com/professional/infectious-diseases/extraintestinal-protozoa/malaria. Accessed Oct. 9, 2018.
- Malaria. Centers for Disease Control and Prevention. http://wwwnc.cdc.gov/travel/diseases/malaria. Accessed Nov. 6, 2015.
- Breman JG. Clinical manifestations of malaria in nonpregnant adults and children. https://www.uptodate.com/contents/search. Accessed Oct. 9, 2018.
- Daily J. Treatment of uncomplicated falciparum malaria in nonpregnant adults and children. https://www.uptodate.com/contents/search. Accessed Oct. 9, 2018.
- Key points: World malaria report 2017. World Health Organization. https://www.who.int/malaria/media/world-malaria-report-2017/en/. Accessed Oct. 9, 2018.
- Malaria. World Health Organization. https://www.who.int/malaria/en/. Accessed Oct. 9, 2018.
- Mutebi JP, et al. Protection against mosquitoes, ticks, & other arthropods. In: CDC Yellow Book 2018: Health Information for International Travelers. https://wwwnc.cdc.gov/travel/yellowbook/2018/the-pre-travel-consultation/protection-against-mosquitoes-ticks-other-arthropods. Accessed Oct. 27, 2018.
- Diseases & Conditions
- Malaria symptoms & causes
News from Mayo Clinic
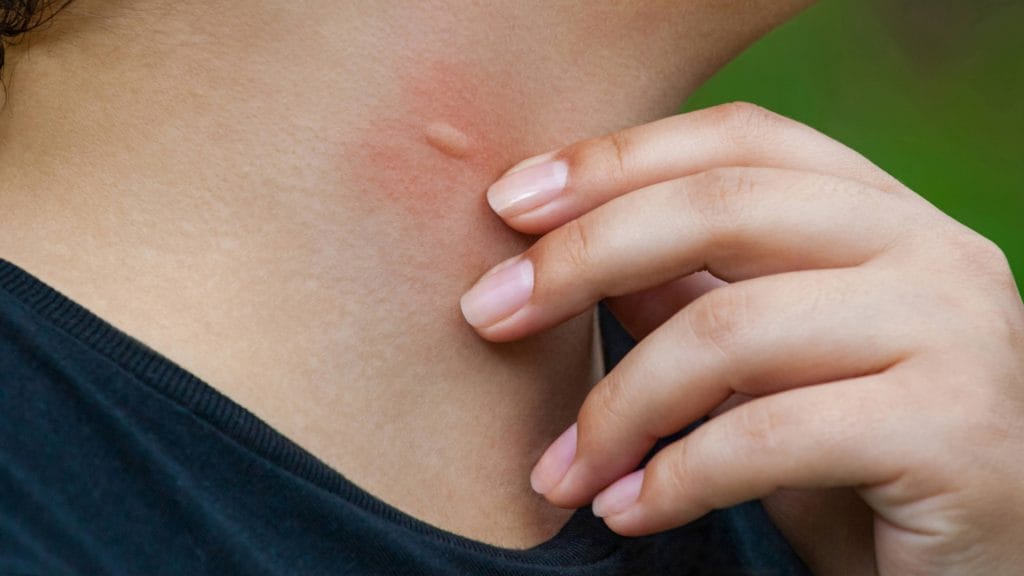
More Information
CON-XXXXXXXX
Your gift holds great power – donate today!
Make your tax-deductible gift and be a part of the cutting-edge research and care that's changing medicine.
Learn how UpToDate can help you.
Select the option that best describes you
- Medical Professional
- Resident, Fellow, or Student
- Hospital or Institution
- Group Practice
- Patient or Caregiver
- Find in topic
RELATED TOPICS
INTRODUCTION
The annual number of malaria cases decreased steadily between 2000 and 2015 but thereafter, malaria cases have increased. Between 2019 and 2021 the number of malaria cases increased by 13 million, significantly more than any inter-annual increase since 2000. This increase is attributed largely to interruption of services due to the coronavirus disease 2019 (COVID-19) pandemic [ 3 ]. The COVID-19 pandemic was also associated with an estimated increase of 63,000 deaths attributed to malaria between 2019 and 2021 [ 4 ].
In 2019, WHO revised its approach to calculating the number of deaths attributed to malaria. With the revised WHO calculation, malaria accounts globally for 7.8 percent of the fraction of deaths in children aged less than 5 years (rather than 4.8 percent as reported previously).
The emergence of Plasmodium falciparum artemisinin partial resistance in the African region is of great concern [ 4 ], as are increasing reports of Anopheles spp resistance to pyrethroid insecticides [ 2 ]. The expansion of the range of an Asian malaria vector, An. stephensi , to the African continent has prompted WHO to launch a new initiative aimed at stopping its spread. An additional threat to malaria control and elimination is the continued increase in parasites that have lost the genes that express proteins detected by rapid diagnostic tests [ 4 ].
In 2021, the WHO recommended the first malaria vaccine, RTS,S/AS01, for use in children who reside in regions with moderate to high P. falciparum transmission [ 3 ]. In 2023, 12 African countries (three countries that conducted a pilot introduction of the vaccine plus nine more nations) received approval to deploy the vaccine through their routine immunization programs [ 5 ]. WHO recommendation of a second malaria vaccine (R21/Matrix-M™) in 2023 is expected to expand available supply.
- More from M-W
- To save this word, you'll need to log in. Log In
Definition of malaria
Examples of malaria in a sentence.
These examples are programmatically compiled from various online sources to illustrate current usage of the word 'malaria.' Any opinions expressed in the examples do not represent those of Merriam-Webster or its editors. Send us feedback about these examples.
Word History
Italian, from mala aria bad air
1740, in the meaning defined at sense 2
Phrases Containing malaria
- anti - malaria
- falciparum malaria
- vivax malaria
Dictionary Entries Near malaria
Cite this entry.
“Malaria.” Merriam-Webster.com Dictionary , Merriam-Webster, https://www.merriam-webster.com/dictionary/malaria. Accessed 17 May. 2024.
Kids Definition
Kids definition of malaria, medical definition, medical definition of malaria, more from merriam-webster on malaria.
Nglish: Translation of malaria for Spanish Speakers
Britannica English: Translation of malaria for Arabic Speakers
Britannica.com: Encyclopedia article about malaria
Subscribe to America's largest dictionary and get thousands more definitions and advanced search—ad free!
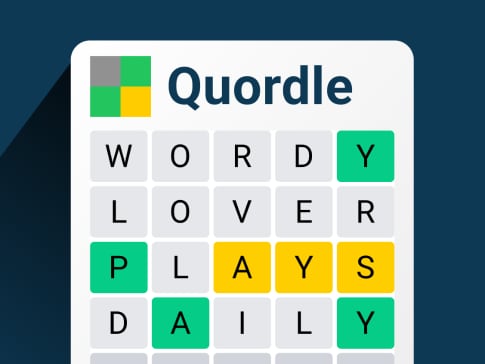
Can you solve 4 words at once?
Word of the day.
See Definitions and Examples »
Get Word of the Day daily email!
Popular in Grammar & Usage
More commonly misspelled words, your vs. you're: how to use them correctly, every letter is silent, sometimes: a-z list of examples, more commonly mispronounced words, how to use em dashes (—), en dashes (–) , and hyphens (-), popular in wordplay, the words of the week - may 17, birds say the darndest things, a great big list of bread words, 10 scrabble words without any vowels, 12 more bird names that sound like insults (and sometimes are), games & quizzes.
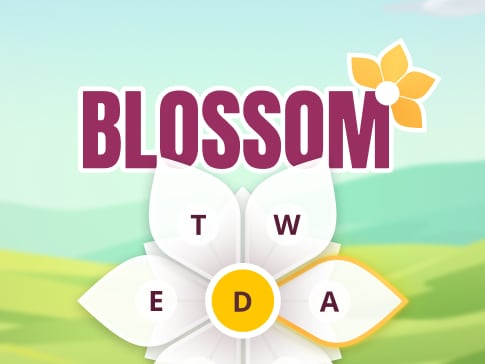
- Biology Article
- Common Diseases In Humans Malaria
Table of Contents
What is malaria, causes of malaria.
- Symptoms of Malaria
Malaria Life Cycle Diagram
- Prevention of Malaria
Malaria is a mosquito-borne infectious disease caused by various species of the parasitic protozoan microorganisms called Plasmodium . Malaria is a disease that man has battled with for a long time. The first evidence of this protozoan came from mosquitoes preserved in amber nearly 30 million years ago.
It is even thought to have brought the Roman Empire to its knees. Malaria was so prevalent during the Roman times that the disease is also called ‘Roman Fever’. Today, the credit for actually discovering the parasite is given to Charles Louis Alphonse Laveran, a French physician. He even won the Nobel Prize in 1907 for his findings.
Here, let’s learn more about malaria and the life cycle of malarial parasite with the help of a diagram.
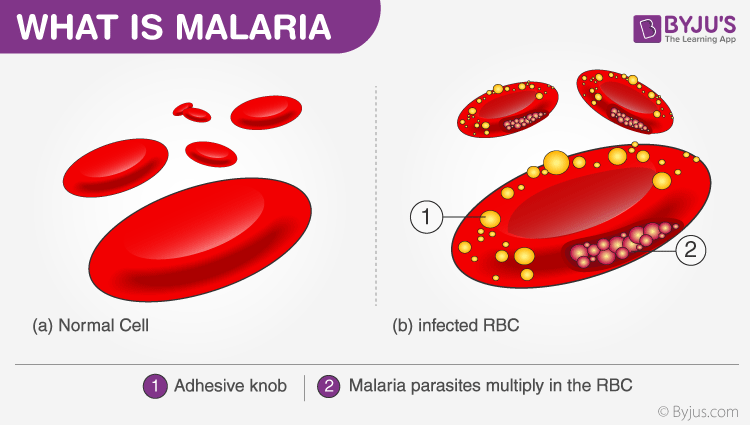
There are many factors that can cause malaria, such as –
- Bitten by a malarial vector (Anopheles stephensi)
- Use of shared and infected syringes.
- Organ transplantation.
- Transfusion.
- From an infected mother to her baby during birth.
Symptoms of malaria
Symptoms of malaria are exhibited within 7 to 18 days of being infected. Common symptoms include:
- Fever, fatigue, chills, vomiting, and headaches
- Diarrhoea, anaemia and muscle pain
- Profuse sweating and convulsions
- Bloody stools.
- In severe cases, malaria can be devastating; it can lead to seizures, coma and eventually, death.
Sir Ronald Ross and his study on the transmission of the disease helped carve the way for future scientists to effectively combat the disease. His deep research showed that specifically, the female Anopheles stephensi mosquito is the vector of the disease, and addressing this problem will prevent malaria and in turn, save countless lives.
Below is a labelled diagram of the malarial parasite to give you a better insight of their life cycle.
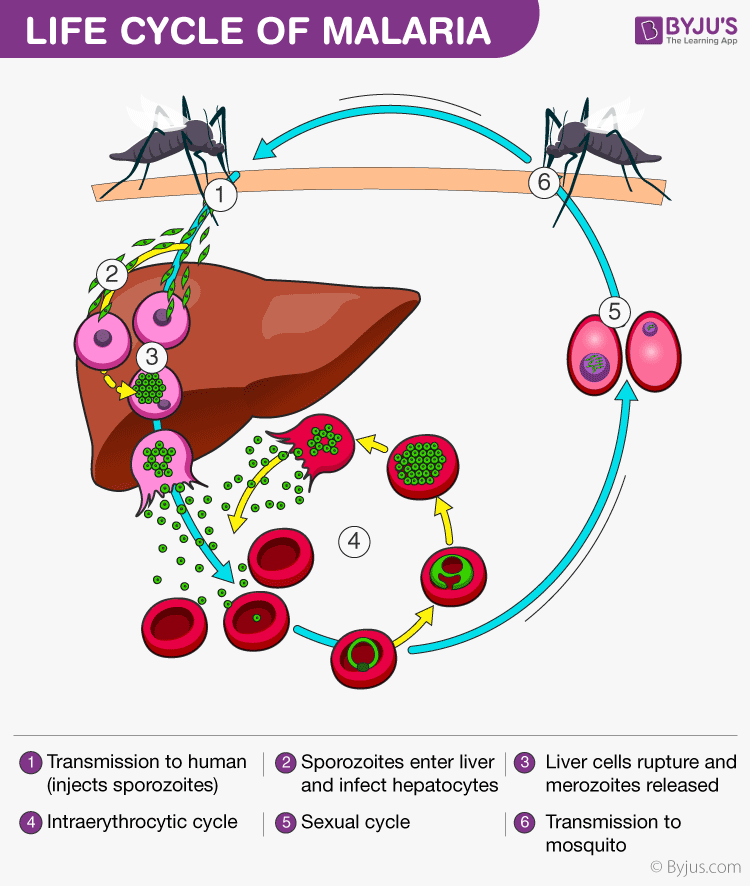
Malaria parasite exists in the form of a motile sporozoite. The vector of malaria i.e. the female Anopheles mosquito transmits the malarial sporozoites into the hosts. When an infected mosquito bites a human, the sporozoites are injected into the blood through the mosquito’s saliva.
The sporozoites travel into our body and accumulate in the liver. These parasites initially multiply within the liver, by damaging the liver and rupturing the blood cells in the body. Malaria kills by causing the destruction of the red blood cells in the host. The parasites reproduce asexually in the RBCs, bursting the cells and releasing more parasites to infect more cells. The rupture of red blood cells by the malaria parasite releases a toxin called hemozoin which causes the patient to experience a condition known as the chills.
When the female Anopheles mosquito bites an infected human, the parasites enter the mosquito’s body along the human blood it is drinking. It is inside the mosquito’s body that the actual development and maturing of the parasite happens. The parasites produced in the human body reach the intestine of the mosquito where the male and females cells fertilize each other to lead to the formation of a sporozoite. On maturing, the sporozoite breaks out the mosquito’s intestine and migrate to the salivary glands. Once they reach salivary glands, they wait till the mosquito bites another human and the process of infection and disease begins all over again. It is prudent however to observe that the complete development of the malaria parasite takes place in two different hosts; humans and mosquitoes.
Prevention of malaria
Malaria is one of the major causes of preventable death in the world today. It affects more than 500 million people worldwide and causes 1 to 2 million deaths every year. It is a tropical infectious disease and almost 90 per cent of the cases are from Sub-Saharan Africa.
There are two ways to deal with malaria – prevent the mosquito bite from happening (i.e. preventative steps) or attack the parasites once they have infected the body.
The first method advocates the use of mosquito nets and mosquito repellents such as permethrin to prevent mosquitoes from biting. The second form of treatment uses a chemical called Quinine present in the bark of a cinchona tree. A form of drug chloroquine has proven very effective against malaria even though it is not a vaccine.
To learn more about malaria or other related diseases, please visit BYJU’S.
Frequently Asked Questions
What is plasmodium.
Plasmodium is a genus of parasitic protozoans that are known to infect the red blood cells in mammals and causes an infectious disease called malaria. Some species of plasmodium such as P.ovale, P.vivax, P.malariae and P.knowlesi are known to cause malaria.
What are some symptoms of malaria?
The primary symptoms include fever, fatigue, vomiting, chills, headaches, anaemia and diarrhoea. Muscle pain, bloody stools and profuse sweating are also seen. In severe cases, it can lead to seizures, coma and eventually death.
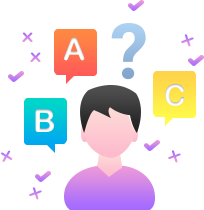
Put your understanding of this concept to test by answering a few MCQs. Click ‘Start Quiz’ to begin!
Select the correct answer and click on the “Finish” button Check your score and answers at the end of the quiz
Visit BYJU’S for all Biology related queries and study materials
Your result is as below
Request OTP on Voice Call
Leave a Comment Cancel reply
Your Mobile number and Email id will not be published. Required fields are marked *
Post My Comment

- Share Share
Register with BYJU'S & Download Free PDFs
Register with byju's & watch live videos.


An official website of the United States government
The .gov means it’s official. Federal government websites often end in .gov or .mil. Before sharing sensitive information, make sure you’re on a federal government site.
The site is secure. The https:// ensures that you are connecting to the official website and that any information you provide is encrypted and transmitted securely.
- Publications
- Account settings
Preview improvements coming to the PMC website in October 2024. Learn More or Try it out now .
- Advanced Search
- Journal List
- Med Sci (Basel)

An Overview of Malaria Transmission Mechanisms, Control, and Modeling
Merveille koissi savi.
1 Center for Development Research ZEF, University of Bonn, Genscherallee 3, 53113 Bonn, Germany; [email protected] or ude.dravrah.hpsh@ivaskm
2 T.H. Chan Harvard School of Public Health, Boston, MA 02115, USA
Associated Data
All data are generated from the content of publications mentioned in this manuscript and they are equally available from the corresponding authors on reasonable request.
In sub-Saharan Africa, malaria is a leading cause of mortality and morbidity. As a result of the interplay between many factors, the control of this disease can be challenging. However, few studies have demonstrated malaria’s complexity, control, and modeling although this perspective could lead to effective policy recommendations. This paper aims to be a didactic material providing the reader with an overview of malaria. More importantly, using a system approach lens, we intend to highlight the debated topics and the multifaceted thematic aspects of malaria transmission mechanisms, while showing the control approaches used as well as the model supporting the dynamics of malaria. As there is a large amount of information on each subject, we have attempted to provide a basic understanding of malaria that needs to be further developed. Nevertheless, this study illustrates the importance of using a multidisciplinary approach to designing next-generation malaria control policies.
1. Introduction
Approximately 2000 children die daily from malaria, with 90% of the victims located in sub-Saharan Africa (SSA) [ 1 , 2 ]. Transmission and control of malaria are mediated by complex interactions and feedback loops among humans, mosquitoes, parasites, their environments, healthcare systems, and policy implementation at a given period of time [ 3 ]. Therefore, malaria transmission can be described as complex, nonlinear, and dynamic. The endemism of malaria in West Africa, for example, has huge economic impacts [ 2 , 4 ]. In Ghana, the cost of treating a single episode of malaria can reach 34% of a household’s yearly income [ 5 ]. As a result of infected people being unable to produce wealth, and as wealth is low, the risk of exposure to malaria increases. There is a negative relationship between national economic growth and malaria control expenditures [ 2 , 6 ]. As a result, malaria can be considered both as a cause of poverty and as a consequence of it [ 7 ].
Furthermore, past interventions have been ineffective as they were unable to account for the nonlinear nature of malaria infection. Particularly, the unexpected consequences of interventions such as mosquito resistance to chemical compounds and parasite resistance to drugs led to their abandonment. This was the case with the eradication control program that was abandoned in 1969 and the Global Malaria Control Strategy in 1992 [ 8 ]. Scientists have widely acknowledged the complexity of malaria, but a depiction of this complexity is weakly documented, and further limits the scope of malaria control.
This short review embracing several topics or thematic areas provides the reader with a piece of basic knowledge of malaria transmission, control, and modeling while illustrating its complexity. Therefore, the manuscript intends to be a didactical material, providing a brief overview of the disease, reducing information asymmetry, while arousing the curiosity of readers who can refer to more specific literature.
2. Materials and Methods
We conducted an unsystematic narrative review of online literature to gain a deeper insight into malaria transmission mechanism, control strategy, and modeling approaches. Thus, we adopted the following approach ( Figure 1 ).

Synthesis of the method. In gray, the final objective of the review is shown, in green, the investigated databases are indicated, in yellow, the exclusion process is indicated, and in red, the clustering of the selected papers is shown.
2.1. Inclusion and Exclusion Criteria for Paper Eligibility
Our search for peer-reviewed papers on malaria in the online scientific database was restricted to the period from 1950 to 2020. The rationale for this time window lies in the fact that the first malaria model was developed in the 1950s and since then, most of the recommendations for malaria control are supported by quantitative analysis. Besides, we excluded all duplicated letters, narrative reviews, case reports, and commentary. Given the tremendous research done on malaria, we limited the scope of the study to only peer-reviewed published papers in either French or English. This was to avoid evidence that may be challenging to replicate and biased translation. Within the topic of interest, we exclude all case reports which happen in non-endemic areas.
2.2. Search Strategy
We searched three databases PUBMED, Google Scholar, and MEDLINE for relevant literature on malaria from 1950 to 2020.
2.3. Screening of Studies and Evidence Extraction
Our screening process focuses on the title of the study published in peer-reviewed journals. Our next step was to review the papers’ abstracts and remove the studies that did not fit under the umbrella of the keywords.
2.4. Analysis and Gaps in the Analysis
To get a comprehensive understanding of malaria, we categorized the list of 77 eligible peer-reviewed papers into topics of interest, such as mechanisms, prevention and treatment, mathematical modeling, and perspectives on modeling. The topical organization of the collected papers allowed us to get a broad perspective of the state of the art.
We found 2,150,000 studies after the search. A total of 150,000 studies were obtained after removing duplicates. We included only 300 studies after screening both titles and abstracts. Furthermore, we removed 50 case reports, 146 studies in malaria non-endemic areas, and 16 reports including 10 thesis and 6 NGO reports.
Using the remaining 77 eligible studies, we provided an overview of each topic and complemented our narrative with WHO, CDC reports, and 10 complimentary readings. There were 23 studies supporting malaria transmission, 19 on prevention and treatment, 23 on mathematical modeling, and 12 on future research on malaria modeling ( Figure 2 ).

Overview of the thematic distribution of the selected papers.
3.1. Malaria Transmission Mechanism
Malaria infection is caused by interactions between humans, mosquitoes, and the pathogen ( Figure 3 ). Female mosquitoes change during the egg-laying period when they experience a deficiency in protein and plant sucrose necessary for egg maturation. Consequently, they blood-feed on humans and can infect them after effective biting where infectious protozoans (of the genus Plasmodium ) are injected into the human bloodstream [ 9 , 10 ].

Complex interactions between humans, mosquitos, and parasites explaining the persistence of malaria. Plasmodium parasites, mosquitoes, and humans constitute three subsystems that interact with one another. The green cycle represents the human sub-system (SS). In addition to the micro-environment, which includes housing conditions, network connectivity, socioeconomic conditions, and the environment within the host (i.e., the individual’s genetic makeup), this SS is characterized by the social environment (social interaction, culture, and behavior regarding malaria risk-taking). The red indicates the SS of mosquitoes defined by the growth conditions favored by humans using or not either ITNs or IRS, and their adaptation to their living environment, including the harsh environmental conditions of cities, such as dirty water and weather conditions. In purple, Plasmodium parasite SS is characterized by interactions with the environment inside the host that may drive resistance to malaria drugs.
Female mosquitoes most found in West Africa belong to the Anopheles Giles genus [ 11 ]. Anopheles gambiae ( sensu stricto ) prefers to use human blood (anthropophilic) to complete its gonotrophic cycle, bites primarily indoors (endophagic), and rests indoors (endophilic). As far as its feeding habits are concerned, An . gambiae is the major malaria vector in West Africa [ 12 ]. In addition, there are other vectors, such as An. arabiensis , An. funestus and An. melas able to carry P. falciparum . Similar to An. gambiae , An. funestus is anthropophilic, endophagic, and endophilic [ 4 , 13 ]. On the other hand, An. arabiensis is zoophilic (prefers blood-feeding animals), exophilic (bites outdoors), and exophagic (rests outdoors) [ 11 ]. An. melas is also anthropophagic, exophagic, and exophilic [ 12 ]. Therefore, P. falciparum adapts well to Anopheles species, increasing malarial vulnerability in the host. The wide range of vector conditions, feeding habits, and geographical heterogeneity in malaria transmission make malaria modeling even more challenging [ 14 ].
A mosquito’s ability to adapt to environmental conditions such as humidity and temperature, is influenced by its sensitivity to those conditions. This adaptation can take the form of a change in the life cycle length, or the biting efficiency [ 15 , 16 , 17 , 18 , 19 ]. As a long-term study carried out in Kenya (1999–2010) has shown, vectors’ feeding habits and effectiveness can change over time [ 20 ].
The transmission intensity may differ significantly across host populations because vectors’ attraction to the host varies [ 21 ]. This variation is elicited by the difference in human bodies’ microflora and their reaction to mosquito bites [ 22 ]. For instance, an infected host is more attractive to other mosquitoes than a non-infected one [ 23 ]. Likewise, mosquitoes prefer the scent of children to that of the adults [ 21 , 24 ].
In SSA, infectious protozoans are, Plasmodium vivax, P. ovale, P. malariae, P. knowlesi, and P. falciparum where P. falciparum accounts for 95% of malaria cases [ 25 , 26 ]. P. falciparum ’s complete life cycle consists of an incubation period of 10 days in the vector and a period of 7 to 20 days in the human host [ 27 ]. When P. falciparum protozoans are injected into a host’s bloodstream via the vector saliva, the protozoan completes its life cycle. After entering the host bloodstream, the sporozoites (asexual protozoans) move into the liver, where they penetrate the hepatocytes (liver cells). In the host hepatocytes, the parasite divides asexually several times, a process known as schizogony [ 28 ]. Eventually, the hepatocytes break, releasing merozoites which infest red blood cells in the host bloodstream. The parasites can diverge into gametocytes after repeated divisions of the red blood cells [ 29 ]. Malaria can cause symptoms such as fever, chills, and sweat before and during the differentiation of P. falciparum into sexual stages. Even so, some hosts may not exhibit clinical symptoms and are considered asymptomatic [ 30 ]. Whenever mosquitoes bite infected people (symptomatic and asymptomatic), they primarily suck out the gametocytes [ 29 ]. Detecting asymptomatic individuals in endemic areas can be challenging, and mass testing may not always be cost-effective in detecting such individuals [ 31 ].
3.2. Malaria Prevention and Treatment
Due to recursive interactions between humans, mosquitoes, and parasites that cause malaria transmission, existing control methods might not be effective in the sense that they target either mosquitoes or parasites. Mosquitoes have been controlled mainly using the obliteration of larvae and adult stages while reducing the entomological inoculation rate. These controls include physical, biological, or chemical changes to the vector’s environment. As part of the physical modification, breeding sites are removed, and the sources of larvae are managed using drainage and weeding [ 32 ]. However, this method requires a constant working force needed for weeding and drain cleaning which led SSA governments to abandon it although it is among the most effective [ 33 ]. Chemical modification of larval environments includes the application of larvicides and insecticides. The downside of this method is that it is also costly, and mosquitoes often become resistant to insecticides [ 34 ]. Natural enemies of mosquito larvae, such as larvivorous fish and bacteria (e.g., Bacillus thuringiensis ), are used in the biological method. Nevertheless, this process is hindered by the temporary persistence of natural enemies [ 32 ].
The Global Malaria Action Plan (GMAP) and the World Health Organization (WHO) recommended the use of indoor residual spray (IRS) and insecticide-treated bed nets (ITNs) for controlling mosquito adult populations in the 2010s across Africa [ 35 ]. The main chemical component of ITNs and IRS is synthetic pyrethroid, a lethal compound, that repels mosquitoes, remains in the environment, and is harmless to mammals. From 2005 to 2010, malaria cases in Ghana declined by 41% due to the successful use of ITNs and IRS [ 36 ]. The residual effects of synthetic pyrethroids in the environment resulting from IRS, ITNs, and agricultural pest management force mosquitoes to develop coping mechanisms [ 37 ]. These include indoor and outdoor spray avoidance mechanisms, earlier biting, and insecticide resistance [ 38 ].
The control of malaria parasites is achieved through three lines of defense: antimalarial drugs, seasonal prevention, and vaccination. The first line of the curative measures adopted by GMAP is the Artemisinin-based Combination Therapies (ACT). ACTs are highly potent against P. falciparum with a higher clearance rate and symptoms resorption than other curative therapies [ 39 ]. Nevertheless, P. falciparum has progressively developed ACT resistance [ 40 ]. Malaria parasite resistance in SSA is exacerbated by using counterfeit malaria drugs [ 41 , 42 ]. Another preventive measure is intermittent prevention, which involves administering single-dose malaria therapy to pregnant women [ 43 ]. The treatment includes the combination of primaquine, sulfadoxine-pyrimethamine, amodiaquine, methylene blue, and dihydroartemisinin-piperaquine which have been proven to be able to prevent the transmission of P. falciparum [ 44 ]. A seasonal vaccine “RTS, S/AS01” is proven effective for children under 5 years old for a short period with an efficacy rate of 82% [ 45 , 46 ]. The authors demonstrated that RTS, S/AS01 induces an immune response to the proteins of infected sporozoites. Vaccine components protect against clinical malaria. The effectiveness of its protective action can wane over time and with advancing age. Particularly, children between the ages of 6 to 12 weeks benefit from the vaccine more than children between the ages of 5 to 17 months.
A less explored line of malaria treatment consists of phytotherapy. For example, a study on phytochemicals identified more than 20 local plants and herbs in central Africa that can heal and prevent malaria transmission with almost zero risks of P. falciparum developing resistance against these natural compounds [ 47 , 48 , 49 ]. According to the authors, the synergistic interplay between a wide range of chemical substances in the plants enables treating malaria holistically while preventing the development of resistance [ 48 , 50 ].
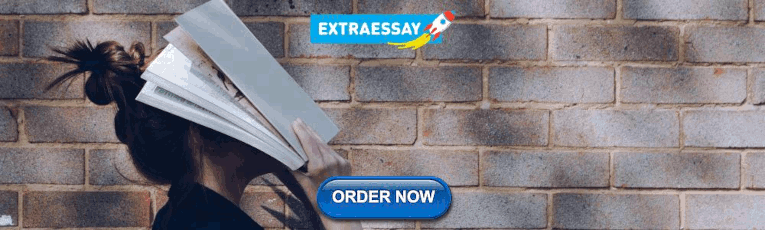
3.3. Mathematical Modeling of Malaria
Mathematical models developed to gain insight into disease transmission have influenced past and present interventions to prevent or treat diseases. Mathematical modeling of malaria transmission began in 1911 with the Susceptible-Infectious-Removed model (SIR), which compartmentalized the population of hosts and vectors into three groups [ 51 , 52 ]. The compartments were denoted susceptible (S), referring to the population likely to become infected, infectious (I), composed of the fraction of the infected population, and removed/ recovered (R), accounting for the fraction of the population that either died or healed from the disease. Several assumptions were assumed in the SIR model, including a closed and finite population, a homogeneous mosquito bite rate, and a well-mixed population. Although the Ross model (SIR) was unable to adjust to the new incidence data due to its limited predictability, SIR provides insights into the intricate relationship between infected hosts and mosquito densities. Because Ross’s model was theoretical and simulation-based, it failed to document proof for campaigns for mosquito eradication.
Subsequently, George Macdonald complemented the SIR model [ 51 ] by feeding the model with real data and embedding an additional compartment for the latency period between the mosquito bite and the onset of symptoms denoted Exposed (E). Additionally, Macdonald’s study argued for eradicating mosquitoes through a massive campaign based on the theory of superinfection [ 53 ]. Consequently, between 1955 and 1969, WHO implemented widespread and rigorous mosquito eradication campaigns as part of the Global Malaria Eradication Program (GMEP). In these campaigns, the pesticide Dichlorodiphenyltrichloroethane (DDT) was successfully used to control malaria in major European and American countries [ 54 ]. The GMEP, however, failed to eradicate malaria worldwide due to mosquito resistance [ 55 ]. Apart from mosquito insecticide resistance, the absence of basic healthcare services, high malaria transmission intensity, and other socio-ecological factors hindered the success of eradication campaigns in the SSA [ 56 ].
The mathematical epidemiology of malaria has evolved steadily in recent decades from “toy models” (which were not realistic but captured the key features of the disease) to “high-level models” (which are precise and sacrifice generality) [ 57 ]. As such, complex models display common features such as the interaction between many components [ 58 ]. For example, an earlier partial differential model split the infected population into age-dependent infections in the SIR framework [ 59 ].
An important metric that summarizes the transmission of a disease is the reproductive number (R 0 ). R 0 represents the expected number of infected human hosts after effective mosquito bites in a fully susceptible population [ 60 , 61 , 62 ]. Thus, R 0 provides a measurement for the intensity of transmission and contributes to the definition of disease-endemic areas when it is greater than one (R 0 > 1) [ 63 ]. Heterogeneity was therefore incorporated in populations for the computation of R 0 . These include, for example, the age structure of the host [ 64 ], migration of the vectors and hosts [ 65 ], host beliefs and practices [ 66 ], and host income classes [ 67 , 68 ]. R 0 also varies with the degree of complexity introduced into the compartmentalization of the population [ 66 ]. The more the modeling framework embodies the heterogeneity in the host population, the closer to real-life transmission dynamics the value obtained for R 0 becomes [ 63 , 68 , 69 , 70 ]. The pioneering models that provided insight into malaria dynamics and its controls were unrealistic for various reasons, and maximum control has hardly been achieved in [ 56 ]. Most specifically, a large R 0 indicates a higher density of mosquitoes that reduces the effectiveness of ITN in a large and likely heterogeneous host population [ 63 ].
Many mathematical models were developed combining the compartments S, E, I, and R (e.g., SIR, SIS, SI, SIRS, SEIR, SEIRS, SEI) and included the heterogeneity of the population (meta-population modeling approaches) [ 69 ]. Most of these models do not capture the dynamics of subpopulations because they tend to assume large and homogeneous populations that do not take into account the existence and specific characteristics of subpopulations [ 71 , 72 ]. Another issue is that they fail to account for host behavior, which influences the disease’s dynamics and control [ 73 , 74 ]. Besides, compartmental models are either knowledge or data-driven (e.g., Ross model, Macdonald models). The models cannot address the complex interactions between determinants that guide the disease dynamics in both cases. For example, socioeconomic determinants interfere with the parameters generally considered in the calculation of R 0 such as mortality, mobility, and birth rate [ 66 ]. Thus, models that capture, realistically, the transmission process of malaria are still missing.
3.4. Malaria Modeling: Present and Future
The ability to track epidemics became more accurate with the advancement in computing power and the availability of data. The deterministic framework uses in this context provides insight into disease dynamics, but usually fails to take uncertainties into account. Stochastic models, on the other hand, incorporate randomness that may occur during the epidemic and, most importantly, accounts for a large range of uncertainty that occurs during epidemics. For example, a stochastic model was used to predict the complex transmission pattern concerning environmental changes [ 75 ]. Like deterministic models, the stochastic models help to disclose interesting features of the transmission such as the impact of the network structure, and the properties of an outbreak most specifically get insights into the rate of the surge of vector-borne diseases in a new region in a context of climate change [ 76 , 77 ]. Furthermore, both stochastic and deterministic models are population-based and often fail to consider the feature of individuals which alternatively the individual-based models (IBMs) do [ 78 ]. IBMs can account for heterogeneity across individual agents and spatial gradients [ 79 , 80 ]. However, parametrizing them remains a daunting task, especially due to the lack and accuracy of data [ 81 ]. Beyond meta-population modeling (Ross-based models), within-host modeling opened new possibilities to address malaria infectiousness while examining the interplay between hosts’ immune systems and the dynamics of the parasite. However, modeling the superinfection of malaria while tracking each parasite strain and incorporating the diverse genetic makeup of the parasites remains extremely challenging, especially in terms of the model parameterization [ 82 , 83 ]. Most recently, the introduction of a new type of data such as human mobility collected from data providers (Facebook, Twitter, Google, etc.) refined the field of epidemiological surveillance. These data have been used to pinpoint malaria hotspots and predict their spread [ 84 , 85 , 86 ]. The use of fine-grained mobility data opens a new prospect of modeling and documenting evidence for policy recommendations; however, privacy concerns need to be addressed [ 87 ].
4. Discussion
Malaria remains a major health challenge in Sub-Saharan Africa, despite extensive research. Several factors contribute to malaria transmission, including socio-ecological factors and changes in the human host and vector behavior, which may be channeled by parasite genetic changes. Considering malaria’s complex nature, modeling it presents numerous challenges. As an example, it can be difficult to accurately parameterize a model with a compartment of asymptomatic individuals as there is a lack of reliable historical data. However, evidence suggests that malaria symptoms could progress rapidly from none to life-threatening while leading to a lifetime burden of cognitive impairments for some patients. It is thus necessary to develop a strategy that allows for the production at a low cost of reliable primary data on asymptomatic people with the capability of identifying infectious Plasmodium [ 88 ]. Additionally, the review highlighted that for each of the thematic areas discussed, there is a web of determinants that can be studied, using different perspectives including the use of a multidisciplinary lens to examine clinically relevant malaria issues.
A historical approach was used to illustrate the evolution of the malaria model from simplistic to more elaborate models. Moreover, this review outlines the challenges of modeling, where, for instance, introducing mobile data to a mathematical framework may pose a problem of re-identification. The number and scale of parameters involved in a complex modeling approach make model parameterization challenging.
Finally, this review demonstrates that malaria can efficiently be controlled by combining several strategies. Among them are constant awareness and education campaigns in communities at risk and among individuals whose resistance to non-pharmaceutical interventions (such as ITNs) can hinder malaria control efforts.
Given the wide range of thematic areas discussed, we acknowledge that we have only scratched the surface of the various cross-cutting topics. Consequently, we did not elaborate on the host immune response to malaria nor on technological advances in diagnosing and treating malaria since they are widely covered elsewhere [ 89 , 90 , 91 , 92 , 93 , 94 ]. Additionally, this paper faces two challenges, namely (1) a self-selection bias that might lead to a more prominent emphasis on some cross-cutting topics, and (2) not considering recently published papers [ 95 ]. Nonetheless, this review provides readers with an overview of malaria from a global perspective.
Acknowledgments
The author wants to thank Christian Borgemeister, Daniel Callo-Concha, Lauren Childs, Deguenon Jean Marcel, Henri Tonnang, and Joshua Ntajal for their edits, comments, and suggestions. In addition, we would like to extend our gratitude to the Center for Development Research (ZEF), Germany, for providing us with the logistics required for this review.
Abbreviations
Funding statement.
This research received no external funding.
Institutional Review Board Statement
This study was approved by the Center for Development Research (ZEF) ethics committees, University of Bonn.
Informed Consent Statement
Not applicable.
Data Availability Statement
Conflicts of interest.
The author declares no competing interests.
Disclaimer/Publisher’s Note: The statements, opinions and data contained in all publications are solely those of the individual author(s) and contributor(s) and not of MDPI and/or the editor(s). MDPI and/or the editor(s) disclaim responsibility for any injury to people or property resulting from any ideas, methods, instructions or products referred to in the content.
Thank you for visiting nature.com. You are using a browser version with limited support for CSS. To obtain the best experience, we recommend you use a more up to date browser (or turn off compatibility mode in Internet Explorer). In the meantime, to ensure continued support, we are displaying the site without styles and JavaScript.
- View all journals
- My Account Login
- Explore content
- About the journal
- Publish with us
- Sign up for alerts
- Open access
- Published: 14 May 2024
Severe outcomes of malaria in children under time-varying exposure
- Pablo M. De Salazar ORCID: orcid.org/0000-0002-8096-2001 1 , 2 ,
- Alice Kamau 3 ,
- Aurelien Cavelan 1 , 2 ,
- Samuel Akech 3 ,
- Arthur Mpimbaza 4 ,
- Robert W. Snow ORCID: orcid.org/0000-0003-3725-6088 3 , 5 na1 &
- Melissa A. Penny 1 , 2 , 6 , 7 na1
Nature Communications volume 15 , Article number: 4069 ( 2024 ) Cite this article
70 Accesses
Metrics details
- Epidemiology
- Policy and public health in microbiology
In malaria epidemiology, interpolation frameworks based on available observations are critical for policy decisions and interpreting disease burden. Updating our understanding of the empirical evidence across different populations, settings, and timeframes is crucial to improving inference for supporting public health. Here, via individual-based modeling, we evaluate a large, multicountry, contemporary Plasmodium falciparum severe malaria dataset to better understand the relationship between prevalence and incidence of malaria pediatric hospitalizations - a proxy of malaria severe outcomes- in East-Africa. We find that life-long exposure dynamics, and subsequent protection patterns in children, substantially determine the likelihood of malaria hospitalizations relative to ongoing prevalence at the population level. Unsteady transmission patterns over a lifetime in children -increasing or decreasing- lead to an exponential relationship of hospitalization rates versus prevalence rather than the asymptotic pattern observed under steady transmission. Addressing this increase in the complexity of malaria epidemiology is crucial to update burden assessments via inference models that guide current and future policy decisions.
Similar content being viewed by others
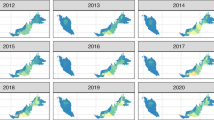
No evidence of sustained nonzoonotic Plasmodium knowlesi transmission in Malaysia from modelling malaria case data
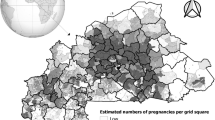
Bayesian Spatiotemporal Modeling of Routinely Collected Data to Assess the Effect of Health Programs in Malaria Incidence During Pregnancy in Burkina Faso
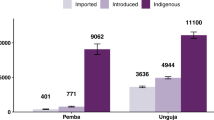
Modelling the impact of interventions on imported, introduced and indigenous malaria infections in Zanzibar, Tanzania
Introduction.
Assessing the burden of malaria life-threatening outcomes in populations at risk is a critically important step in evaluating and improving control efforts. Malaria mortality is challenging to measure accurately in the community 1 but remains a fundamental component of statistical-based interpolation from prevalence estimates, resulting in high uncertainty 2 . Inference of disease burden has been approached with different grades of sophistication, ranging from purely data-driven fits to multi-level mechanistic microsimulations 3 , 4 , 5 , 6 . Independent of the complexity of the approach, the ability of a model to generate accurate, robust, and valid malaria disease outcomes using exposure predictors, such as prevalence, requires (1) high-quality data as input from real-world observations, and (2) a comprehensive understanding and identification of the key factors determining the relationship between exposure and clinical outcomes.
Severe, life-threatening malaria syndromes presenting to hospitals are a valuable proxy for malaria-related death among communities. High exposure rates in children at a very young age are known to offset the risk of severe clinical outcomes at older ages 7 . This leads to a characteristic asymptotic pattern between exposure and disease risk at the population level, consistent with consensual malaria theory and historical observations 4 , 7 , 8 , 9 . Recent work has assessed the empirical relationship between community prevalence and the risk of severe malaria syndromes, namely severe anemia, cerebral malaria, and respiratory distress among children in East Africa, based on the largest standardized Plasmodium falciparum malaria pediatric dataset available to date 10 . Findings show that the occurrence of these severe malaria outcomes in the population may relate differently to increasing community prevalence. Particularly, an asymptotic relationship was observed when predicting severe anemia over community prevalence, while an exponential relationship was favored when predicting a combined outcome comprising the three syndromes. Evaluating new sources of standardized data, such as this contemporary dataset, contextualized with historical sources of data 9 , 11 improves our understanding of the accuracy, robustness, and validity of the inference frameworks.
Here, we use a previously validated multi-level individual-based malaria model 12 , OpenMalaria ( https://github.com/SwissTPH/openmalaria/wiki ), to systematically investigate clinical and epidemiological factors influencing the relationship between potentially life-threatening hospital malaria admissions among children upon a given observed community prevalence. We use community-based malaria hospitalization incidence rates in small catchment populations and adjusted for case under-ascertainment as an empirical proxy of the incidence of severe malaria outcomes. Our analysis framework interrogates standardized malaria data obtained in Sub-Saharan African time-sites within the 1990s throughout 2020 9 , 10 , 11 , 13 , aiming to improve and update our understanding of the dynamics from P. falciparum malaria infection to the occurrence of severe outcomes in children.
Malaria admissions were assembled from individual records of 21 hospitals representing 35 time-sites in Kenya, Uganda, and Tanzania, among children resident in specific catchment areas -within a defined distance radius from the hospital where surveillance took place- excluding urban settings where it was possible to estimate single-year censused population estimates. We assume that the hospitalization rates per time-site represent a lower limit of the hospitalization incidence and can be reasonably comparable when further adjusting for case ascertainment. Cases were included if malaria was the primary cause of hospitalization, and those with underlying conditions were excluded 10 , 13 . We further used data on malaria hospitalization incidence obtained with similar approaches between 1992 and 1997 at seven hospital time-site locations in Kenya, The Gambia, and Malawi 9 , 11 . This allows us to compare and interpret our findings with a dataset that has been classically used for informing malaria inference models 4 , 8 , 14 aiming to estimate severe outcomes of malaria across populations.
For each of the time-sites in the above datasets, the average number of hospitalizations due to malaria among children three months to 9 years old per 1000 children per year were paired with age-diagnostic method standardized community prevalence estimates, as empirical P. falciparum Parasite Rates among children 2–10 years old ( ePf PR 2–10 ). The data was obtained from community and school surveys undertaken during the period of hospital surveillance within the same catchment areas 9 , 10 , 11 . Further, we assessed the past exposure dynamics using catchment site-specific time-series of modeled age- and test-standardized parasite rates estimates, herein referred to as mPf PR 2–10 . For each time-site of the contemporary dataset, annual mPf PR 2–10 estimates were obtained using a Bayesian hierarchical geospatial model detailed elsewhere 13 , 15 . In those time sites where modeled estimates were available for at least 7 past years ( n = 27), and up to a maximum of 9 years, we computed the median mPf PR 2–10 of the time series. We assume that the median prevalence across the past 7–9 years roughly represents the cumulative past transmission to which the population of children up to 10 years of age have been long-life, which can then be compared to the empirical prevalence at the time of the survey to evaluate the gap between past and present-day transmission
Visual inspection of the empirical relationship between prevalence and hospitalization rates for the 35 time-sites included in the contemporary dataset does not suggest an asymptotic relationship of malaria hospitalization incidence across the ePf PR 2–10 range within the full dataset (Fig. 1a .). For illustration, we highlight four representative time-sites in Fig. 1a and alongside the mPf PR 2–10 time-series of these time-sites for the years prior to the collection of the empirical data (Fig. 1b ). Three major patterns of time-varying transmission are depicted, showing (1) a substantial increase in the mPf PR 2–10 (Apac A), (2) relative constant mPf PR 2–10 (e.g, Busia) (3) steady increase (Mubende B) and 4) substantial decrease in the Pf PR 2–10 (e.g., Jinja B). As depicted in Fig. 1c , there is substantial change between the ePf PR 2–10 and the median value of the mPf PR 2–10 over the previous years for each of the time-sites comparing at least seven years and up to ten years of modeled past exposure estimates. The difference between the ePf PR 2–10 and the median value of past mPf PR 2–10 can be interpreted as the gap in past exposure relative to ongoing exposure. For those time-sites at the higher end of the current ePf PR 2–10 range (i.e., higher than the median empirical prevalence, 20%), exposure had primarily substantially increased or remained relatively stable (12 out of 14 sites). For those time sites at the lower end of the range (lower than 20%), exposure had decreased or remained relatively stable (13 out of 13 sites). All available mPf PR 2–10 time series for the 35-time sites are shown in Fig. S1 13 , 15 .
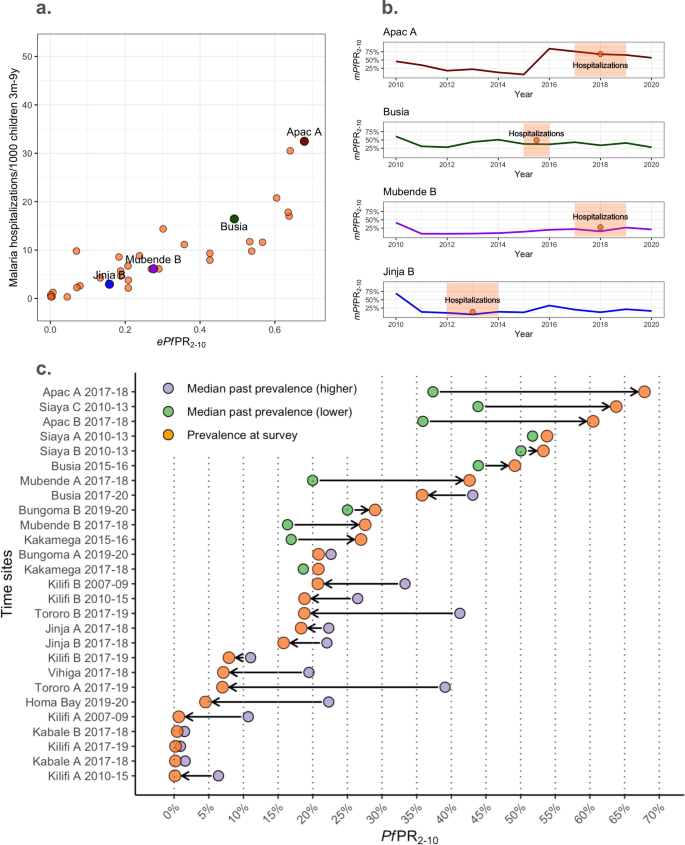
a The ePf PR 2–10 -severe malaria incidence empirical relationships highlighting four representative time-sites (red, green, purple, and blue colored dots) within all time-sites (orange dots). b Present day mPf PR 2–10 over time in four representative time-sites (red, green, purple, and blue colored lines) and time-site ePf PR 2–10 (orange) with highlighted time periods for which hospitalization incidence estimates were available for the empirical relations in ( a ). c Summarizing the prevalence trends over time estimated for each time-site as an increasing trend -when the estimated median mPf PR 2–10 in the past 7 to 9 years is lower than the ePf PR 2–10 at the time of assessment of severe outcomes incidence- or decreasing trend the estimated median mPf PR 2–10 in the past 7–9 years is lower than the ePf PR 2–10 at the time of assessment of severe outcomes incidence. The median past mPf PR 2–10 per time-site is plotted as yellow (for those with increasing trends) or red (for those time-sites with reducing trends), and ePf PR 2–10 per time-site is plotted in orange. Estimates have been computed among the 27-time sites with at least seven years of available past mPf PR 2–10 estimates.
Understanding the complex relationship between malaria exposure, immunity, and clinical outcomes across populations and time requires causal analytical frameworks that (a) can combine empirical observations with theory (b) can address multiple interacting causal effects, threshold dynamics, and interference (c) have generally accepted principles to build the models, populate and calibrate their parameters and test their predictions for avoiding misspecification. Individual-based models are amongst the few modeling tools that fulfill these requirements 1 , 2 .
Open-Malaria ( https://github.com/SwissTPH/openmalaria/wiki ) is an multi-level individual-based model that includes several key features that allow to generate counterfactuals of the effect of malaria exposure on clinical disease under different scenarios of population structure, changing transmission, health-access, diagnostic thresholds, drug-efficacy, and other major malaria control and prevention interventions 3 . Random effects can be incorporated into the modeled processes and allow the inclusion of uncertainty and heterogeneity in the simulations. Relevant to our analyses, the framework encompasses submodels specifically parameterized to empirical data including (1) population structure 4 , 5 ; (2) within-host dynamics of parasite burden and addressing the effect on single individuals of repeated infections in developing immunity and subsequent infections 6 , 7 , 8 ; (3) disease progression 6 , 7 and health-seeking behavior including rates of individuals accessing health services, as well as time to diagnostic and treatment 4 ; (4) efficacy of case-management including diagnostic sensitivity and specificity, first- and second-line treatment effectiveness and efficacy of hospitalization 4 ; and (5) the effect of age-structured comorbidities on severe malaria outcomes upon infection 5 . Further details are provided in the Supplementary Note 2 .
We iteratively interrogated the data under different sets of plausible parameterizations of our individual-based model, hereafter referred to as scenario analysis. The scenario analysis explores hypotheses of the impact of well-known determinants on disease risk and changes in contemporary disease risk compared to historical observations, including the deployment and availability of artemisinin-derivatives in primary- and hospital-care, improved treatment adherence, the reduction in the occurrence and progression of malaria-associated comorbidities. In addition to addressing the detailed major key changes between the historical and contemporary datasets, we further address the life-long exposure dynamics evidenced by the modeled community prevalence estimates (Fig. 1b and Fig. S1 ). The risk of malaria disease outcomes, including those severe, depends on immunity, which in turn depends on previous exposure dynamics. We hypothesize that the year-to-year variability of PfPR could strongly influence the risk of hospitalization. Thus, we further assessed the impact of this variability on malaria exposure on the ongoing hospital admission risk estimated from our individual-based model scenarios. Here, we define steady exposure as the exposure of a specific population that does not change substantially over the years, and we define unsteady exposure as an exposure that shows substantial increasing or decreasing dynamics over time. We performed simulations that included generic step-up and step-down exposure dynamics with differences between pre- and intra-survey prevalence within the range of those observed empirically. Further details are found in the Supplementary Note 3 . For each scenario analysis, we performed over 1000 individual-based simulations. We tested the model outputs— Pf PR 2–10 and incidence rate of malaria admissions—against three regression models, namely an intercept-only, a log-logistic, and a log-linear model, which was previously used to evaluate the prevalence-hospitalization relationship 10 .
The historical dataset shows higher levels of hospitalization rates at similar prevalence, consistent with the expected reduction of comorbidities and improved management effectiveness in the contemporary dataset (Fig. 2 and Supplementary Note 5 ). Figure 2a, b shows (1) the historical (Fig. 2a ) and contemporary (Fig. 2b ) empirical estimates, (2) their respective time-frame-specific simulations under the assumption of steady exposure, and (3) the regression-based predicted relationship, shown as the median and 50% and 95% prediction intervals. For both the historical and the contemporary predictions, the log-logistic regression model provides a better fit to the model outputs, with the asymptote reaching around 60% and 40% Pf PR 2–10 , respectively. However, while the prediction model based on steady transmission is consistent with the historical empirical data, it fails to recover the contemporary dataset relationship, with a substantial number of time-sites outlying from the predictions range, particularly for the highest ePf PR 2–10 values (i.e., over 60%).
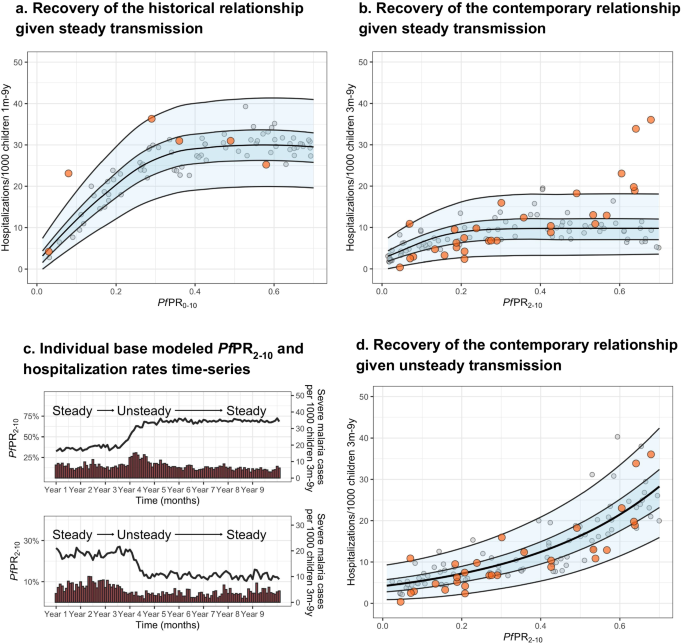
Showing the empirical Pf PR 2–10 -hospitalization rates relationship obtained from a the historical dataset (orange dots), and b the contemporary dataset (orange dots) overlapping respective Pf PR-hospitalization model-based estimates obtained through simulations consistent with steady transmission (gray dots, n = 100) and respective levels of health care access, treatment and comorbidities ( b ), and the best-fit model-based log-logistic regressions (median black line, blue ribbons 50% and 95% prediction intervals). c Representative simulation patterns of Pf PR 2–10 (black lines, increasing at the top and decreasing at the bottom) and subsequent malaria hospitalization incidence over time (red columns). d The empirical Pf PR 2–10 -hospitalization rates relationship obtained from the contemporary dataset (orange dots) overlapping Pf PR 2–10 -severity model-based estimates obtained through simulations scenarios consistent with time-varying exposure (gray dots, n = 100) and levels in health care access, treatment, and comorbidities, and the best-fit model-based log-linear regression (median black line, blue ribbons 50% and 95% prediction intervals).
Given that model predictions based on steady exposure do not capture the pattern of the empirical contemporary data, we further evaluated scenarios with unsteady past malaria transmission (either increasing or decreasing dynamics), and how these different trends affect the Pf PR 2–10 -hospitalization rate incidence relationship. Specifically, we performed simulations that included step-up and step-down exposure dynamics with differences between pre- and intra-survey prevalence within the range of those observed empirically (see Methods). Simulations captured representative patterns of time-varying exposure, with decreasing, steady or increasing transmission before computing severe disease incidence (Fig. 2c ) over the range of Pf PR 2–10 values. The best fit to a regression model is then obtained using the log-linear model (Fig. 2d ). Based on performance metrics 16 , simulations under the assumption of unsteady patterns of past exposure predict the relationship of the contemporary dataset more accurately than those based on steady exposure. Further, modeled predictions under unsteady exposure show hospitalization rates in different age groups increase towards higher ePf PR 2–10 in a similar way as observed in the empirical estimates (Fig. S2 ). However, this is opposite to the pattern in historical observations under steady state exposure; severe disease incidence among youngest children is typically higher in high transmission settings than in low transmission settings, whereas the opposite occurs among older children 4 , 7 , 8 , 9 . Consistent with these results, our model recovers more accurately the observed hospitalization age structure (Fig. S3 , left column for representative time-sites) under the unsteady transmission assumption (Fig. S3 , middle column) than under the steady-state assumption (Fig. S3 , right column). Further, when assessing the model estimates of hospitalization risk later in time (i.e., allowing the scenarios to maintain a steady-state level transmission over 5 years), the prevalence-hospitalization rates relationship transitions to the asymptotic pattern expected for steady transmission (Fig. S4 ).
Via scenario analysis, we have systematically evaluated the major clinical and epidemiological determinants influencing the occurrence of malaria hospitalization upon infection at the population level and thus proved a contemporary characterization of relationship trends and changes between malaria community prevalence and life-threatening disease risk in children. We found that the asymptotic relationship between prevalence and hospitalization disease risk, expected under a relatively steady transmission, is lost when children have been exposed to unstable, time-varying past malaria transmission. Overall, our analyses support the assumption that substantial fluctuations in malaria transmission over the years have led to a particular prevalence-hospitalization relationship observed among the East-African settings 10 , where increasing prevalence does not necessarily lead to saturating disease risk but increases toward the highest rates in an exponential manner. However, our analyses show that if transmission is further maintained at a steady-state level over sufficient time, disease risk would also eventually re-equilibrate back to the asymptotic relationship relative to the parasite rates (fig. S4 ). To date, inference frameworks aiming to estimate or predict severe outcomes of malaria used for policy decisions and public health action do not explicitly include past exposure as an independent variable 5 , 17 . Our analysis framework is capable of reconciling historical and contemporary observations encompassing three decades in sub-Saharan Africa and underscores the importance of taking the variability of past malaria exposure among children into account when predicting severe disease risk.
Our analyses have several limitations that need to be acknowledged. First, we use community-based hospitalization in non-urban settings as an empirical proxy of the incidence of severe outcomes of malaria. While these estimates could under- or over-estimate the true number of severe outcomes, the data was obtained aiming to standardized the under-ascertainment of cases, estimates would be affected by site specific treatment-seeking behaviors and therefore represent a lower limit for hospitalization rate at each time site. Also, the empirical contemporary dataset does not necessarily represent urban settings, where the referral pathways from infection to hospitalization might be more complex to understand or subject to other potential biases. Further, the curated data does not include cases where malaria was not the major syndrome for hospitalization. Our modeling approach allows access to health (i.e., access to diagnosis, treatment, and/or hospitalization) to randomly vary within the range of the rates estimated for the three countries, with sensitivity analysis showing that deviations from this assumption do not influence the overall relationship between prevalence and hospitalization. Nevertheless, if the data represents stronger deviations from these assumptions regarding case identification but remains relatively similar across time sites, the overall prevalence-severe disease trend will still hold. Second, the empirical Pf PR estimates obtained through community and school surveys might not necessarily reflect the underlying prevalence dynamics for the full catchment population, given how heterogeneous malaria exposure can be at a very granular spatial level. However, we obtain a similar prevalence-hospitalization relationship using estimates computed using the geospatial model (see Section “Discussion”, Fig. S10 ), thus supporting the assumption of the empirical values being a representative summarizing value for the catchment populations. Also, the time series of Pf PR values used to compute exposure steadiness can bear high uncertainty on the precise estimates. Nevertheless, given that we did not aim to replicate the prevalence changes over time but addressed this matter focusing on the relative change, our framework will remain well informed if the estimates approximate true trends. Third, our model OpenMalaria simulations are based on spatially homogeneous malaria transmission because the catchment populations in the empirical data are small. Assuming a heterogeneous transmission structure can affect the magnitude of the clinical outcomes, central estimates will not change 18 . Fourth, while parameterization of the hospitalization rates, efficacy of treatment, and comorbidities are consistent with the literature, for simplicity, we assumed similar ranges of values across time-sites with stochastic variation. In Supplementary Note 4 , we provide an uncertainty analysis of these mechanistic parameters, showing that our results hold under no major deviations from tenable assumptions. Last, we have applied parametric regression models to simulation data, which likely misspecifies the mechanical interpretation of the model. Still, we believe this is justified given this approach was originally used to determine the empirical relationship 10 and we aimed to replicate the trends under potential plausible mechanistic scenarios.
We have provided evidence that variation in malaria transmission and subsequent disease protection after life-long exposure can strongly influence severe disease risk estimates under otherwise equivalent ongoing force of transmission. Notably, frequent implementation and withdrawal of infection prevention strategies can strongly contribute to unsteady malaria exposure patterns and thus increase severe disease risk. Other potential sources of variability in the exposure include substantial movement of individuals from areas with different community prevalence (i.e., via increasing or reducing the overall population-level susceptibility to severe disease) or strong environmental changes influencing entomological inoculation rates such as urbanization or climatic drivers (i.e., prolonged drought or excessive rainfall).
Under constant, similar malaria exposure and health access rates, a population of children with higher immunity will substantially reduce their risk of severe malaria and, therefore, the number of severe cases. However, if malaria transmission has marked changes, either sudden reductions or increases, the resulting severe disease will be significantly lower or higher, respectively, than if the population had remained under constant transmission. This understanding is critical to evaluate the effects of interventions, and such mechanistic processes need to be included in future analytical approaches providing predictions of malaria disease burden. The processes that must be incorporated into disease burden estimates are best defined through differences in the age–structure patterns of the risk conditional to past exposure. For example, it is expected that following a strong reduction in malaria transmission, severe cases among age groups of children at a certain prevalence will be reduced earlier in time but will likely increase in later periods if malaria prevalence reaches steady levels. Similarly, the withdrawal of effective prevention and control strategies will lead to a higher number of severe cases than those expected when malaria prevalence has remained unchanged over time. In short, if past exposure and the dynamics described here are not accounted for in burden estimates, it will lead to long-term overestimation of severe malaria risk in places with recent effective interventions. And conversely, it will lead to long-term underestimation of severe malaria in places with deterioration of interventions. Finally, our findings underpin the need to build back rigorous clinical surveillance of severe malaria under the changing landscape of parasite exposure in Africa. It is striking that only two longitudinal clinical series exist since the launch of the Roll Back Malaria initiative in 2000 19 , 20 .
Overall, our findings provide evidence that inference in malaria epidemiology, such as the generation of counterfactual scenarios with predictions on clinical outcomes for policy decisions, should account from now on past exposure and subsequent protection to avoid substantial bias in such risk predictions and highlight the increase in the complexity of malaria epidemiology arising from unsteady transmission dynamics.
Contemporary malaria hospitalization incidence data and paired community prevalence estimates
The contemporary P. falciparum malaria hospitalization incidence data has been obtained from 35 time-sites in Kenya ( n = 18), Uganda ( n = 14), and Tanzania ( n = 3) between 2006 and 2020 and has been previously described elsewhere 10 . The data was collated from individual records of 21 hospitals, including hospitalized malaria cases in children resident in specific catchment areas within a 30 km range and excluding urban settings. Given potential differences in treatment-seeking behaviors at the different sites, the computed estimates represent the lower limit for hospitalization incidence, thus requiring further assumptions for adjusting to under ascertainment (see model parameterization in the Supplementary Text). for In the present analysis, cases were included if malaria was deemed the primary cause of hospitalization. Per each time-site, the number of hospitalizations among children 3 months to 9 years old per 1000 children per year was computed as a proxy of life-threatening disease incidence from the catchment population, obtained from census data and census data projections. Hospitalization data was paired with community surveys performed at the same periods of the hospital surveys for each time-site, adjusting for diagnostic accuracy (i.e., microscopy vs. rapid test) and standardized by computing the Pf PR 2–10 as described elsewhere 21 , 22 . Further details on hospitalization data, community prevalence, and estimates of hospital catchment population can be found in Supplementary Note 1 .
Modeled community prevalence time-series
We assessed the past exposure dynamics using catchment site-specific time series of modeled age- and test-standardized parasite rates estimates, referred to as mPf PR 2–10 13 , 15 . Modeled mPf PR 2–10 estimates were explicitly obtained for each time-site catchment population using a geospatial model detailed elsewhere 13 , 15 , a Bayesian hierarchical geostatistical framework based on more than 180000 geo-coded empirical prevalence survey data points from East Africa, interpolated in time to 1 × 1 km resolutions using climatic and ecological covariates. For a set of sites ( n = 11), annual mPf PR 2–10 estimates are available since 2000, while for the rest ( n = 15) available data begin in 2010. Time series of all the available mPf PR 2–10 per site are shown in Fig. S1 . Thus, for 27 out of the 35 present-day time sites, mPf PR 2–10 time series included at least seven-time points of past annual estimates. Further, to estimate the gap between past and present transmission, we first computed the median value of the annual mPf PR 2–10 time-series for each of the time-sites, under the assumption that it roughly represents the life-long transmission at which the surveyed population of children have been exposed in the previous years previous. This median mPf PR 2–10 can then be compared to the empirical Pf PR 2–10 that is estimated at the time of surveying the malaria hospitalization incidence.
Historical malaria hospitalization incidence data and paired community prevalence estimates
As an alternative source of data, we analyzed a historical dataset obtained between 1992 and 1997, encompassing the relation between severe disease incidence measured as malaria hospitalization rates and the Pf PR 2–10 up to 70% and published elsewhere 9 . Similar inclusion and exclusion criteria had been used to obtain both datasets explicitly to allow comparison. Thus, comparable approaches to those used in the contemporary dataset were used to estimate malaria hospitalization incidence at six hospital time-site locations in The Gambia ( n = 3), Kenya ( n = 2), and Malawi ( n = 1) and paired community prevalence estimates 7 , 9 , 11 . Further details can be found in Supplementary Note 1 .
Agent-based model of malaria transmission, immunity, and disease dynamics
To recover the relationship between hospitalization rates and Pf PR 2–10 , we used an individual-based model of P. falciparum malaria transmission and disease dynamics, OpenMalaria, previously described and calibrated elsewhere 12 . Briefly, OpenMalaria features within-host parasite dynamics, the progression of clinical disease, development of immunity, individual care-seeking behavior, vector exposure, and pharmaceutical and non-pharmaceutical antimalarial interventions at vector and human level ( https://github.com/SwissTPH/openmalaria/wiki ) 14 , 23 , 24 . The full model is calibrated to fit 23 parameters to 11 objectives representing different epidemiological outcomes, including age-specific prevalence and incidence patterns, age-specific mortality rates, and hospitalization rates, using a Bayesian optimization approach 12 . A detailed description of the model and references for the key technical details are provided in Supplementary Note 2 .
Model parameterization on disease management, comorbidities, and health access
In order to compare model predictions to empirical data, we first parameterized several key inputs of the individual-based model to be consistent with both historical and contemporary datasets and the corresponding existing clinical and epidemiological knowledge, including different sets of variables addressing (a) the individual probability of malaria exposure per time-step to cover a range of Pf PR 2–10 up to 70%, (b) the effectiveness in malaria case management, including the rate of access of uncomplicated and severe malaria cases to health-care (i.e., rate of accurate diagnostic of true occurrence and subsequent timely treatment) and the efficacy of the available malaria drugs at each period (e.g., the efficacy of artemisinin derivatives combination therapies in clearing malaria), and (c) the co-occurrence of other diseases with influence on malaria that affect the severe progression of malaria across age-groups.
To evaluate the contemporary dataset, model parameterization for the main analysis was as follows: (1) Pf PR 2–10 estimates ranged up to 70%; (2) drug efficacy ranged between 85 and 100% 25 , 26 ; (3) the rates of individuals suffering from malaria that required hospitalization who received diagnostic and treatment ranged from 60 to 90% while the rate of individuals with uncomplicated malaria accessing diagnostic and treatment ranged 20–60% 27 ; and (4) co-occurrence of diseases contributing to malaria hospitalization was substantially reduced by 60–80% since the 1990s 28 , 29 , 30 . For (2–4), we parameterized the model by randomly sampling values from a uniform distribution defined by the respective ranges. Sensitivity analysis for these assumptions can be found in the Supplementary Note 3 . To evaluate the historical dataset, we include the following: (1) Pf PR 2–10 estimates ranged up to 70% (2) first-line drug efficacy for uncomplicated malaria was negligible 31 ; (3) access rates of malaria requiring hospitalization ranged from 40–60% 8 , 32 , and (4) co-occurrence of diseases contributing to malaria hospitalization approximated a hyperbolic decay distribution over age-groups 8 . Details on key model parameters and submodels are provided in Supplementary Note 3 .
Time-varying malaria exposure on severity estimates
To evaluate how time-varying malaria exposure rates influence the ongoing Pf PR 2–10 -hospitalization rates relationship, we produced a set of plausible malaria-transmission scenarios consistent with time-varying exposure as for those obtained from a Bayesian hierarchical geostatistical framework 13 , 15 across all our time-sites. Particularly, we evaluated how a rapid reduction or increase of the Pf PR 2–10 level over 6 months (e.g., Pf PR 2–10 increasing from 10% to 70%) could affect the severe malaria incidence relative to a steady-state transmission assumption described before. This was performed by setting (1) the initial exposure rate (i.e., the individual probability of exposure to infectious bites pre-survey as a single rate over simulation time-steps), independent from (2) the final exposure rate (i.e., the probability of exposure during the survey period, also as a single rate over time steps). For simplicity, we set constant initial and final exposure rates. The relationship between the initial and final exposure rate was parameterized to reflect archetypical mPf PR 2–10 patterns,—depicted in Fig. 1b —showing (1) a substantial increase in the mPf PR 2–10 (e.g., Apac A), (2) relative constant mPf PR 2–10 (e.g., Busia), and (3) substantial decrease in the Pf PR 2–10 (e.g., Jinja B). Thus, our definition of time-varying (unsteady) exposure comprises both substantial increasing and decreasing dynamics. For each time site with retrospective data encompassing at least 7 years, we computed the average mPf PR 2–10 available up to 9 years prior to the date when the hospitalization data was available, used it as a proxy of the initial exposure rate, and computed the fold-change Pf PR 2–10 . We then performed a local polynomial regression model to obtain predictions of the relationship between the final exposure rate and the corresponding relative change per time site. See fig. S5 in Supplementary depicting the relationship between the contemporary community prevalence as ePf PR 2–10 , and estimated relative change at survey computed from the median value of mPf PR 2–10 of the past 7–9 years for each time-site. We then used these inputs of exposure to produce simulations using a combination of initial and final exposure rates to map a range of simulation-based Pf PR 2–10 -hospitalization rates.
Scenario analysis to recover the empirical relationship
To evaluate under which scenarios OpenMalaria can recover the empirical prevalence-hospitalization, we implemented an iterative 4-step procedure to explore hypotheses of the impact of the determinants on disease risk and changes in disease risk—see sections above for details. The procedure is represented schematically in the Supplementary (Fig. S6 ). For each scenario analysis, we performed four iterative steps as follows:
Using a high-performance computing framework ( http://scicore.unibas.ch/ ) we performed 1000 population-level individual-based model simulations of malaria transmission at a steady-state (i.e., same entomological inoculation rate for each simulation) over long periods of time (i.e., over 90 years which ensures that lifelong malaria exposure has occurred all the generations evaluated prospectively); computing hospitalization rates among children 3 months- 9 years per 1000 persons-year across values of Pf PR 2–10 within the input range. In each of the simulations, we parameterized the model, mapping the range of values set up for the major epidemiological determinants described in the previous section, namely disease management (which includes rates and efficacy of diagnostic and treatment of severe and uncomplicated malaria) and co-occurrence of comorbidities. We set the parameters according to the empirical dataset we were evaluating (i.e., historical or contemporary). At the same time, we evaluated the model outcomes simulating steady and unsteady transmission.
In order to assess how accurately the model simulations represent the empirical data, we evaluated the performance of two regression models to recover the prevalence-hospitalization relationship obtained via scenario analysis, namely a log-linear model and a log-logistic model. The approach was chosen to be consistent with the previous analysis framework of the contemporary dataset 10 , which analyses severe malaria syndrome-specific cases against prevalence. See Supplementary Note 3 for details on the regression models, model selection criteria, and computation of uncertainty estimates.
We used the best-fit regression model among the above to evaluate how the modeling framework predicted the empirical ePf PR 2–10 -hospitalization incidence relationship. Specifically, we evaluated the accuracy of the model predictions using prediction performance metrics 16 (see Supplementary Note 3 and Table S2 ).
We updated model assumptions based on the evaluation above and reparametrized the scenario(/s) accordingly.
Evaluation of key assumptions for scenario modeling
To evaluate the robustness of the simulation-based predictions of the Pf PR 2–10 -hospitalization relationship under time-varying exposure, we performed three major sensitivity analyses: (a) how increased or reduced rates of both uncomplicated case management and hospitalizations at the population level influence the severe disease incidence and prevalence relationship; (b) how the relationship changes over higher or lower drug efficacy estimates; (c) how changes on the incidence of comorbidities influence the severe disease risk. Details of the evaluation are provided in Supplementary Note 4 and Figs. S7 and S8 .
The age structure of the severity estimates and the effect of time-varying malaria
Last, to assess if the model framework recovers hospitalization risk over different age groups, we compared both empirical and simulation-based estimates disaggregated by age. We performed the 4-steps iterative analysis described above for the contemporary dataset under the steady and time-varying transmission assumptions and evaluated the Pf PR 2–10 -hospitalization relationship by age. More specifically, we computed hospitalization incidence over age groups at values of Pf PR2–10 equal to those estimated in the for four representative time sites Apac A, Busia, Mubende B, and Jinja B. Further details on the age-structured analysis are provided in Supplementary Note 5 .
Reporting summary
Further information on research design is available in the Nature Portfolio Reporting Summary linked to this article.
Data availability
Empirical contemporary data used in this analysis have been curated and uploaded to the Harvard Dataverse: https://dataverse.harvard.edu/dataset.xhtml?persistentId=doi:10.7910/DVN/XGDB3K . Correspondence and requests for materials should be addressed to the KEMRI Wellcome Data Governance Committee ([email protected]). These data are available through a formal requesting process to the KEMRI Institutional Data Access/Ethics Committee. Guideline details can be found on the KEMRI Wellcome website: https://kemri-wellcome.org/about-us/#ChildVerticalTab_15 .
Code availability
Model code, plotting code, and simulation data are available at https://github.com/PDeSalazarSwissTPH/SevereMalaria .
Snow, R. W. Sixty years trying to define the malaria burden in Africa: have we made any progress? BMC Med. 12 , 227 (2014).
Article PubMed PubMed Central Google Scholar
White, N. J., Day, N. P. J., Ashley, E. A., Smithuis, F. M. & Nosten, F. H. Have we really failed to roll back malaria? Lancet 399 , 799–800 (2022).
Camponovo, F., Bever, C. A., Galactionova, K., Smith, T. & Penny, M. A. Incidence and admission rates for severe malaria and their impact on mortality in Africa. Malar. J. 16 , 1 (2017).
Griffin, J. T. et al. Gradual acquisition of immunity to severe malaria with increasing exposure. Proc. Biol. Sci. 282 , 20142657 (2015).
PubMed PubMed Central Google Scholar
Weiss, D. J. et al. Mapping the global prevalence, incidence, and mortality of Plasmodium falciparum , 2000-17: a spatial and temporal modelling study. Lancet 394 , 322–331 (2019).
Bhatt, S. et al. The effect of malaria control on Plasmodium falciparum in Africa between 2000 and 2015. Nature 526 , 207–211 (2015).
Article ADS CAS PubMed PubMed Central Google Scholar
Snow, R. & Marsh, K. The consequences of reducing transmission of Plasmodium falciparum in Africa. Adv. Parasitol. https://doi.org/10.1016/s0065-308x(02)52013-3 (2002).
Ross, A., Maire, N., Molineaux, L. & Smith, T. An epidemiologic model of severe morbidity and mortality caused by Plasmodium falciparum . Am. J. Trop. Med. Hyg. 75 , 63–73 (2006).
Article PubMed Google Scholar
Marsh, K. & Snow, R. W. Malaria transmission and morbidity. Parassitologia 41 , 241–246 (1999).
Paton, R. S. et al. Malaria infection and severe disease risks in Africa. Science 373 , 926–931 (2021).
Snow, R. W. et al. Relation between severe malaria morbidity in children and level of Plasmodium falciparum transmission in Africa. The Lancet https://doi.org/10.1016/s0140-6736(97)02038-2 (1997).
Reiker, T. et al. Emulator-based Bayesian optimization for efficient multi-objective calibration of an individual-based model of malaria. Nat. Commun. 12 , 7212 (2021).
Kamau, A. et al. Malaria hospitalisation in East Africa: age, phenotype and transmission intensity. BMC Med. 20 , 28 (2022).
Smith, T. et al. Mathematical modeling of the impact of malaria vaccines on the clinical epidemiology and natural history of Plasmodium falciparum malaria: overview. Am. J. Trop. Med. Hyg. 75 , 1–10 (2006).
Alegana, V. A. et al. Plasmodium falciparum parasite prevalence in East Africa: Updating data for malaria stratification. PLOS Global Public Health https://doi.org/10.1371/journal.pgph.0000014 (2021).
Dziak, J. J., Coffman, D. L., Lanza, S. T., Li, R. & Jermiin, L. S. Sensitivity and specificity of information criteria. Brief. Bioinform. 21 , 553–565 (2020).
World malaria report. https://www.who.int/teams/global-malaria-programme/reports/world-malaria-report-2023 (2023).
Penny, M. A. et al. Public health impact and cost-effectiveness of the RTS,S/AS01 malaria vaccine: a systematic comparison of predictions from four mathematical models. Lancet 387 , 367–375 (2016).
Njuguna, P. et al. Observational study: 27 years of severe malaria surveillance in Kilifi, Kenya. BMC Med. 17 , 124 (2019).
Guinovart, C. et al. The epidemiology of severe malaria at Manhiça District Hospital, Mozambique: a retrospective analysis of 20 years of malaria admissions surveillance data. Lancet Glob. Health 10 , e873–e881 (2022).
Article CAS PubMed Google Scholar
Smith, D. L., Guerra, C. A., Snow, R. W. & Hay, S. I. Standardizing estimates of the Plasmodium falciparum parasite rate. Malar. J. 6 , 1–10 (2007).
Article Google Scholar
Mappin, B. et al. Standardizing Plasmodium falciparum infection prevalence measured via microscopy versus rapid diagnostic test. Malar. J. 14 , 460 (2015).
Smith, T. et al. Towards a comprehensive simulation model of malaria epidemiology and control. Parasitology 135 , 1507–1516 (2008).
Smith, T. et al. Ensemble modeling of the likely public health impact of a pre-erythrocytic malaria vaccine. PLoS Med. 9 , e1001157 (2012).
World Health Organization. Guidelines for the Treatment of Malaria . (World Health Organization, 2010).
World Health Organization. Guidelines for the Treatment of Malaria . Third Edition. (World Health Organization, 2015).
The DHS Program. https://dhsprogram.com/methodology/survey-types/mis.cfm .
GBD 2016 Causes of Death Collaborators. Global, regional, and national age-sex specific mortality for 264 causes of death, 1980-2016: a systematic analysis for the Global Burden of Disease Study 2016. Lancet 390 , 1151–1210 (2017).
Reiner, R. C. et al. Identifying residual hotspots and mapping lower respiratory infection morbidity and mortality in African children from 2000 to 2017. Nat. Microbiol. 4 , 2310–2318 (2019).
Reiner, R. C. Jr et al. Variation in childhood diarrheal morbidity and mortality in Africa, 2000-2015. N. Engl. J. Med. 379 , 1128–1138 (2018).
Amin, A. A. et al. The challenges of changing national malaria drug policy to artemisinin-based combinations in Kenya. Malar. J. 6 , 72 (2007).
Goodman, C. A., Coleman, P. G. & Mills, A. Economic analysis of malaria control in sub-Saharan Africa. (Global Forum for Health Research, Geneva, 2000).
Download references
Acknowledgements
Calculations were performed at sciCORE ( http://scicore.unibas.ch/ ) scientific computing center at the University of Basel. Funding : This study was partly funded by Bill & Melinda Gates Foundation (INV- 025569 to MAP) supporting M.A.P. and P.M.S. M.A.P. also received support via a Swiss National Science Foundation Professorship (PP00P3_203450 to M.A.P.); R.W.S. is supported under a Wellcome Trust Principal Research Fellowship (212176/Z/18/Z), which also provided support for A.K.; R.W.S., A.K. and S.A. are grateful for the support from the Wellcome Trust to the Core Award for the East Africa Major Overseas Program (203077/Z/16/Z). S.A. was supported by the CDC Foundation through funding from the World Health Organization for the RTS,S evaluation (project requisition number 2018/854999).
Author information
These authors contributed equally: Robert W. Snow, Melissa A. Penny.
Authors and Affiliations
Department of Epidemiology and Public Health, Swiss Tropical and Public Health Institute, Allschwil, Switzerland
Pablo M. De Salazar, Aurelien Cavelan & Melissa A. Penny
University of Basel, Basel, Switzerland
Kenya Medical Research Institute (KEMRI)-Wellcome Trust Research Programme, Nairobi, Kenya
Alice Kamau, Samuel Akech & Robert W. Snow
Child Health and Development Centre, College of Health Sciences, Makerere University, Kampala, Uganda
Arthur Mpimbaza
Centre for Tropical Medicine and Global Health, Nuffield Department of Clinical Medicine, University of Oxford, Oxford, UK
Robert W. Snow
Telethon Kids Institute, Nedlands, WA, Australia
Melissa A. Penny
Centre for Child Health Research, University of Western Australia, Crawley, WA, Australia
You can also search for this author in PubMed Google Scholar
Contributions
Conceptualization: P.M.S., M.A.P., A.K., R.W.S.; methodology: P.M.S., M.A.P., R.W.S.; investigation: P.M.S., S.A., A.M., A.K., A.C., R.W.S., M.A.P.; visualization: P.M.S., M.A.P.; funding acquisition: M.A.P., R.W.S.; writing—original draft: P.M.S., M.A.P., R.W.S.; writing—review & editing: P.M.S., M.A.P., R.W.S., A.K., A.C., S.A., A.M.
Corresponding authors
Correspondence to Pablo M. De Salazar or Melissa A. Penny .
Ethics declarations
Competing interests.
The authors declare no competing interests.
Ethics declaration
Kenya hospital surveillance: Kenya Medical Research Institute/ Scientific and Ethics Review Unit (KEMRI SERU) IRB numbers 1433, 3057, 3149; 1801, 2558, 2465, 3459, and 3771; Uganda hospital surveillance approved by the CDC as public health surveillance/non-research (NCEZID 031416), Tanzania hospital surveillance approved by the ethics committees of the National Institute for Medical Research, Tanzania, and the London School of Hygiene and Tropical Medicine. Kenya community parasite surveys KEMRI SERU IRB numbers 3149, 3592, 1801, 2558, 2675, 2801, and 3822; Uganda community prevalence surveys Vector Control Division Research Ethics Committee, MoH, Kampala: VCDREC/117.
Peer review
Peer review information.
Nature Communications thanks the anonymous reviewers for their contribution to the peer review of this work. A peer review file is available.
Additional information
Publisher’s note Springer Nature remains neutral with regard to jurisdictional claims in published maps and institutional affiliations.
Supplementary information
Supplementary information, peer review file, reporting summary, rights and permissions.
Open Access This article is licensed under a Creative Commons Attribution 4.0 International License, which permits use, sharing, adaptation, distribution and reproduction in any medium or format, as long as you give appropriate credit to the original author(s) and the source, provide a link to the Creative Commons licence, and indicate if changes were made. The images or other third party material in this article are included in the article’s Creative Commons licence, unless indicated otherwise in a credit line to the material. If material is not included in the article’s Creative Commons licence and your intended use is not permitted by statutory regulation or exceeds the permitted use, you will need to obtain permission directly from the copyright holder. To view a copy of this licence, visit http://creativecommons.org/licenses/by/4.0/ .
Reprints and permissions
About this article
Cite this article.
De Salazar, P.M., Kamau, A., Cavelan, A. et al. Severe outcomes of malaria in children under time-varying exposure. Nat Commun 15 , 4069 (2024). https://doi.org/10.1038/s41467-024-48191-7
Download citation
Received : 31 August 2023
Accepted : 22 April 2024
Published : 14 May 2024
DOI : https://doi.org/10.1038/s41467-024-48191-7
Share this article
Anyone you share the following link with will be able to read this content:
Sorry, a shareable link is not currently available for this article.
Provided by the Springer Nature SharedIt content-sharing initiative
By submitting a comment you agree to abide by our Terms and Community Guidelines . If you find something abusive or that does not comply with our terms or guidelines please flag it as inappropriate.
Quick links
- Explore articles by subject
- Guide to authors
- Editorial policies
Sign up for the Nature Briefing: Microbiology newsletter — what matters in microbiology research, free to your inbox weekly.

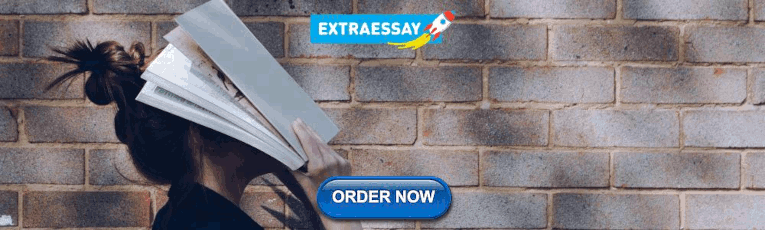
IMAGES
VIDEO
COMMENTS
Malaria is a life-threatening disease spread to humans by some types of mosquitoes. It is mostly found in tropical countries. It is preventable and curable. The infection is caused by a parasite and does not spread from person to person. Symptoms can be mild or life-threatening.
Headache and muscle aches. Fatigue. Chest pain, breathing problems and cough. Diarrhea, nausea and vomiting. As malaria gets worse, it can cause anemia and jaundice (yellowing of the skin and whites of the eyes). The most severe form of malaria, which may progress to a coma, is known as cerebral malaria.
malaria, serious relapsing infection in humans, characterized by periodic attacks of chills and fever, anemia, splenomegaly (enlargement of the spleen), and often fatal complications. It is caused by one-celled parasites of the genus Plasmodium that are transmitted to humans by the bite of Anopheles mosquitoes.Malaria can occur in temperate regions, but it is most common in the tropics and ...
Unlike other dangerous creatures, mosquitoes do their deadly work by spreading diseases - one of the worst of these is malaria. Malaria is an infectious disease caused by a parasite, called Plasmodium that invades red blood cells and liver cells. The parasites are transferred to humans by the bite of an infected Anopheles mosquito.
1. Introduction. Malaria affected an estimated 219 million people causing 435,000 deaths in 2017 globally. This burden of morbidity and mortality is a result of more than a century of global effort and research aimed at improving the prevention, diagnosis, and treatment of malaria [].Malaria is the most common disease in Africa and some countries in Asia with the highest number of indigenous ...
Malaria is a mosquito-borne infectious disease that affects vertebrates. Human malaria causes symptoms that typically include fever, fatigue, vomiting, and headaches. In severe cases, it can cause jaundice, seizures, coma, or death. Symptoms usually begin 10 to 15 days after being bitten by an infected Anopheles mosquito. If not properly treated, people may have recurrences of the disease ...
Malaria is a life-threatening disease primarily found in tropical countries. It is both preventable and curable. However, without prompt diagnosis and effective treatment, a case of uncomplicated malaria can progress to a severe form of the disease, which is often fatal without treatment.
Malaria is a disease caused by a parasite. The parasite is spread to humans through the bites of infected mosquitoes. People who have malaria usually feel very sick with a high fever and shaking chills. While the disease is uncommon in temperate climates, malaria is still common in tropical and subtropical countries.
Malaria is a mosquito-borne disease caused by a parasite. People with malaria often experience fever, chills, and flu-like illness. Left untreated, they may develop severe complications and die. A parasite is an organism that lives on or in a host and gets its food from or at the expense of its host. Parasites can cause disease in humans.
Malaria is a parasitic infection transmitted by the Anopheles mosquito that leads to acute life-threatening disease and poses a significant global health threat. Two billion people risk contracting malaria annually, including those in 90 endemic countries and 125 million travelers, and 1.5 to 2.7 million people die in a year.[1] The Plasmodium parasite has a multistage lifecycle, which leads ...
Malaria disease can be categorized as uncomplicated or severe (complicated). In general, malaria is a curable disease if diagnosed and treated promptly and correctly. All the clinical symptoms associated with malaria are caused by the asexual erythrocytic or blood stage parasites. When the parasite develops in the erythrocyte, numerous known ...
The malaria parasite life cycle involves two hosts. During a blood meal, a malaria-infected female Anopheles mosquito inoculates sporozoites into the human host .Sporozoites infect liver cells and mature into schizonts , which rupture and release merozoites . (Of note, in P. vivax and P. ovale a dormant stage [hypnozoites] can persist in the liver (if untreated) and cause relapses by invading ...
Malaria is a life-threatening disease caused by parasites that are transmitted to people through the bites of infected female mosquitoes. About 3.2 billion people - almost half of the world's population - are at risk of malaria. Young children, pregnant women and non-immune travelers from malaria-free areas are particularly vulnerable to ...
Malaria is a mosquito-borne disease caused by five protozoa: Plasmodium falciparum, P. vivax, P. malariae, P. ovale, and most recently implicated P.knowlesi. Infection with P. falciparum is being accounted for more than 90% of the world's malaria mortality and therefore remains an important threat to public health on a global scale.[1][2] The World Health Organization (WHO) World Malaria ...
In 2023, the World Health Organization (WHO) reported 247 million cases (up from 245 million in 2020) and 619 thousand deaths (down from 625,000 in 2020) due to malaria [ 2 ]. The annual number of malaria cases decreased steadily between 2000 and 2015 but thereafter, malaria cases have increased. Between 2019 and 2021 the number of malaria ...
Malaria is an infectious disease in tropical countries. It is spread by mosquitoes. It is manifested by fever along with chills and rigors. Unless it is diagnosed and treated promptly, it can be ...
The meaning of MALARIA is a human disease that is caused by sporozoan parasites (genus Plasmodium) in the red blood cells, is transmitted by the bite of anopheline mosquitoes, and is characterized by periodic attacks of chills and fever. How to use malaria in a sentence.
Malaria is a serious disease spread by mosquitoes . It affects people in tropical parts of the world. Most people who get malaria recover. Still, at least 1 million people die from malaria each year. Most of them are children in Africa.
The outlook for malaria control is grim. The disease, caused by mosquito-borne parasites, is present in 102 countries and is responsible for over 100 million clinical cases and 1 to 2 million deaths each year. Over the past two decades, efforts to control malaria have met with less and less success. In many regions where malaria transmission had been almost eliminated, the disease has made a ...
Common symptoms include: Fever, fatigue, chills, vomiting, and headaches. Diarrhoea, anaemia and muscle pain. Profuse sweating and convulsions. Bloody stools. In severe cases, malaria can be devastating; it can lead to seizures, coma and eventually, death. Sir Ronald Ross and his study on the transmission of the disease helped carve the way for ...
As a result of the interplay between many factors, the control of this disease can be challenging. However, few studies have demonstrated malaria's complexity, control, and modeling although this perspective could lead to effective policy recommendations. This paper aims to be a didactic material providing the reader with an overview of malaria.
Malaria is a disease caused by a parasite. How it spreads. Most people get malaria when bitten by a mosquito infected with the malaria parasite. Only female Anopheles mosquitoes can spread malaria. For the Anopheles mosquito to become infected, they must bite, or take a blood meal, from a person with the malaria parasites. About one week later ...
In malaria epidemiology, interpolation frameworks based on available observations are critical for policy decisions and interpreting disease burden. ... Thus, our definition of time-varying ...