- Open access
- Published: 14 November 2022
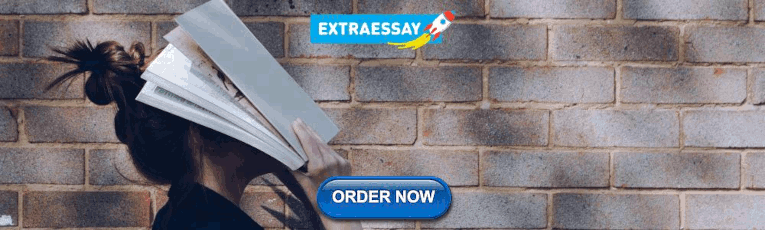
A systematic review of the prediction of consumer preference using EEG measures and machine-learning in neuromarketing research
- Adam Byrne ORCID: orcid.org/0000-0003-0761-3929 1 , 2 , 3 ,
- Emma Bonfiglio 2 ,
- Colin Rigby 1 &
- Nicky Edelstyn 4
Brain Informatics volume 9 , Article number: 27 ( 2022 ) Cite this article
7348 Accesses
9 Citations
9 Altmetric
Metrics details
Introduction
The present paper discusses the findings of a systematic review of EEG measures in neuromarketing, identifying which EEG measures are the most robust predictor of customer preference in neuromarketing. The review investigated which TF effect (e.g., theta-band power), and ERP component (e.g., N400) was most consistently reflective of self-reported preference. Machine-learning prediction also investigated, along with the use of EEG when combined with physiological measures such as eye-tracking.
Search terms ‘neuromarketing’ and ‘consumer neuroscience’ identified papers that used EEG measures. Publications were excluded if they were primarily written in a language other than English or were not published as journal articles (e.g., book chapters). 174 papers were included in the present review.
Frontal alpha asymmetry (FAA) was the most reliable TF signal of preference and was able to differentiate positive from negative consumer responses. Similarly, the late positive potential (LPP) was the most reliable ERP component, reflecting conscious emotional evaluation of products and advertising. However, there was limited consistency across papers, with each measure showing mixed results when related to preference and purchase behaviour.
Conclusions and implications
FAA and the LPP were the most consistent markers of emotional responses to marketing stimuli, consumer preference and purchase intention. Predictive accuracy of FAA and the LPP was greatly improved through the use of machine-learning prediction, especially when combined with eye-tracking or facial expression analyses.
1 Introduction
The present systematic review aimed to investigate the use of EEG measures in neuromarketing. We specifically focused on identifying which ERP and TF effects were most consistently associated with consumer preference and purchase intention, the best computational approaches to predict consumer behaviour, and which biometric measures are best combined with EEG measures to improve predictive accuracy.
Marketing is used to help a product inform, engage, and sustain its target audience by identifying and manipulating consumer preferences [ 167 , 188 , 288 ]. Conventional market research depends on self-report measures such as questionnaires, interviews, and focus-group discussions [ 167 , 188 , 288 ]. However, the traditional approach to marketing is usually only capable of conducting posteriori analysis of consumer preference towards marketing stimuli [ 288 ]. Self-report methods may also provide potentially unreliable or incomplete data due to participants misremembering their experiences or conforming to social desirability bias [ 288 ].
Neuromarketing overcomes the limitations of traditional marketing methods by capturing consumers’ unspoken cognitive and emotional responses to marketing stimuli using neuroimaging and biometric devices [ 11 , 21 , 30 , 213 , 304 ]. This allows for the concurrent recording of consumers’ emotional responses while engaging with marketing stimuli, and can detect emotional responses that consumers may be unwilling to report or may even be unware of [ 11 , 21 , 30 , 213 , 304 ]. The main techniques applied within this paradigm are neuroimaging measures, such as functional magnetic resonance imaging (fMRI) and electroencephalography (EEG); and biometric measures such as the galvanic skin response (GSR) and eye-tracking (ET) [ 163 ].
EEG measures and physiological measures (e.g., ET, GSR) are most commonly used in neuromarketing research due to them being relatively inexpensive and easy to implement [ 8 , 130 , 257 ]. EEG measurements are considered useful for their high temporal resolution and ability to adapt traditional experimental designs into neuroscience experiments [ 8 , 130 , 257 ], and are highly compatible with machine-learning algorithms due to the richness of data collected [ 59 , 144 , 164 ]. By comparison, physiological measures collect simpler data types, taken from bodily responses indexing changes in arousal, emotion and visual attention [ 18 , 214 , 221 , 257 , 290 ]. Heart-rate responses, GSR, and are typically used in neuromarketing stimuli to measure changes in physiological arousal in response to marketing stimuli, but cannot separate positive from negative responses [ 18 , 214 , 257 ]. Similarly, ET can be used to measure how marketing stimuli draw visual attention [ 10 , 168 , 221 , 290 ] and facial expression analysis can be used to detect specific emotional responses to stimuli such as disgust or anger [ 60 , 155 , 187 ].
In contrast, neuroimaging measures such as fMRI are favoured due to their ability to accurately localise neural activity through detection of haemodynamic blood flow, but has poor temporal resolution when compared to EEG [ 134 , 149 , 224 ]. fMRI may also be less favourable in neuromarketing research than physiological and EEG measures due to the high cost associated with fMRI measures, and their lack of portability [ 134 , 149 , 224 ].
However, despite the current popularity of neuromarketing, it remains unclear which measures are the most effective and in which context they are best used. For example, while fMRI is often preferred for its spatial resolution and EEG is preferred for its temporal resolution [ 200 ], the comparative effectiveness of these measures in neuromarketing research is yet to be systematically investigated. Further, the data recorded by neuromarketing devices can be analysed in several ways and situations. For example, EEG data can be considered in terms of event-related potentials (ERPs) occurring milliseconds after the presentation of a stimulus [ 172 , 184 , 238 ], or in terms of changes in relative power in specified frequency bands [ 197 , 256 ]. Recent developments in computer science have further allowed for machine-learning algorithms [ 2 ] to precisely predict consumer preference, purchasing behaviour, and remembered events [ 44 , 51 ].
We, therefore, conducted a large-scale systematic review on the field of neuromarketing, with the express purpose of investigating the use of differing neuroimaging and biometric measures to determine their best use in the context of marketing research. To ease analysis, the systematic review was divided into three subsets based on broad neuromarketing measures: EEG measures, functional imaging measures, and biometric measures. The subset discussed presently focused on the use of EEG measures in neuromarketing. Specifically, the effectiveness of phase-locked and time–frequency (TF) analysis methods were compared, as well as sub-analysis methods within these fields (e.g., alpha- compared to theta-band activity). EEG combined with other neuromarketing measures (e.g., EEG combined with eye-tracking) was further investigated. Finally, the effectiveness of algorithmic approaches (e.g., machine-learning) to analysing EEG data were compared to traditional statistical methods.
The present article, therefore, focused its investigations on the following research questions:
What different analysis methods are currently used for EEG research in neuromarketing?
In which conditions are differing ERP components and TF effects significantly modulated?
Which EEG effects are best used to predict consumer preference and emotional responses to marketing stimuli?
In which ways are EEG measures best combined with other measures, and which measures are best combined?
Does machine learning improve the predictive accuracy of EEG measures in neuromarketing?
The research questions were generated in descending order to first identify the TF and ERP measures currently used in neuromarketing research, and then to investigate which emotional processes each effect was most consistently associated with. Following the initial identification of relevant EEG measures, consumer preference was investigated, and the effects most consistently associated with preference and purchase intention were assessed. Finally, the use of other biometric measures (e.g., ET, GSR) when combined with EEG was investigated, as well as the best computational approaches to predict consumer preference and purchase intention (e.g., machine learning algorithms compared to regression analyses).
These questions will allow us to gain a comprehensive account of the use of EEG measures in neuromarketing, which components are the most useful, and in which situations they are best used. After the introduction, the systematic review methodology will be discussed, followed by the systematic review findings, and a broader discussion of these findings.
A systematic literature review was performed to collect and assess the measures used in neuromarketing. This method was selected due to systematic literature reviews' high degree of objectivity and replicability [ 104 ]. A systematic review uses pre-defined methods to collect, select, and analyse collected literature, with the purpose of unbiased evidence collection to reach an impartial conclusion [ 135 ].
The present systematic review aimed to examine measures used in neuromarketing (i.e., neuroimaging and physiological measures) and identify the best use of these measures. To ease analysis, literature was subdivided into three categories; EEG measures, physiological measures, and functional imaging measures. The present paper represents the first subset of the systematic review; EEG measures. The analysis of the literature collected in this subset focused on comparing different EEG analysis approaches (e.g., TF and ERP analysis) in terms of their effectiveness when used in different kinds of neuromarketing research.
2.1 Search terms
Studies were collected from relevant journals and databases using key search terms. The primary search terms were the simple terms' neuromarketing' and 'consumer neuroscience', intended to catch studies that self-identified as belonging to the field of neuromarketing. However, additional studies may have used neuroscience or physiological measures to investigate marketing-relevant behaviour without explicitly using these terms. For this reason, additional search terms were used to identify studies that used neuroscience or physiological measures (e.g., EEG, MRI, GSR, ET) combined with marketing or consumer investigation.
The key search terms were used as selection criteria for the titles, keywords, abstracts, and body of text in the selected databases and journals. Document types included in the search were ‘articles’, and time limits were not established. Therefore, the initial search resulted in a shortlist of relevant publications to be considered for inclusion in the review. Duplicate articles were excluded from subsequent analysis.
To ensure the maximum number of neuromarketing studies were collected, literature searches were conducted in multiple databases and individual journals. Databases were selected from those that are internationally recognised and widely used as a source of research for distinct post-graduate programmes. Table 1 presents the database sources and the search terms used, and the number of articles found within each database.
Twenty journals were searched to find any articles that were not found in the database search. These journals were selected due to their focus on consumer behaviour, marketing psychology, and neuroscience. Individual journals were searched using the keyword 'neuromarketing'. Table 2 presents the journals searched and the number of articles generated for each.
2.2 Inclusion/exclusion criteria
The inclusion criteria defined for the systematic review were as follows:
Primary study, published in a peer-reviewed journal.
Studies that explored marketing using brain or physiological mechanisms, underlying theories of marketing, consumer behaviour, psychology and neurology.
Research papers that used neuroimaging techniques such as EEG, fMRI, and positron emission tomography (PET) or physiological measures such as ET and GSR, to further understanding and application of marketing methods.
Research papers using exclusively human, non-clinical populations as participants.
Studies that explored new developments in neuromarketing or summarised current research were classed as review papers and not included in the review. However, review papers were screened for citations, and any relevant citations which were not included in the original search were added to the systematic review.
The exclusion criteria for the systematic review were defined as:
Any other literature review on Neuromarketing was excluded from the review.
Articles that were not published in peer-reviewed academic journal articles were excluded (e.g., book chapters, post-graduate theses, conference abstracts).
Articles written/published in any language other than English were excluded from the review.
2.3 Screening process
Overall, 2247 articles were found in the literature review. The titles and abstracts of these articles were individually analysed by multiple researchers, who screened the papers according to the pre-defined inclusion and exclusion criteria.
2.3.1 Process
Read all titles, exclude any duplicates and those that are clearly not relevant according to exclusion criteria.
Read abstracts of those remaining, exclude any that are not relevant according to exclusion criteria.
The remaining papers qualify for full-text screening.
Of those that qualify, search through their reference list and all published articles which cite them.
Compile this list and perform title and abstract screening (stages 1–3) again.
Repeat as many times as necessary until the compiled list from stage 5 yields no papers that qualify for full-text screening.
Following the first stage of article accumulation, articles were exhaustively screened by the authors through title and abstract reviews and were categorised according to whether they matched the inclusion or exclusion criteria. 2512 papers were excluded from the literature review, while 777 were included. Of the journals excluded from the review, 516 were duplicate articles, 268 were neuromarketing review papers, and 930 were removed according to the pre-defined exclusion criteria. 21 additional papers were extracted from the review papers, and these were added to the included from the initial literature search, meaning 798 papers were included in the literature review. A further 8 papers were included in the literature review due to a second search being conducted at a later date using the same search terms.
To aid the analysis of the collected data, papers were separated into four discrete categories based on the measures used. The first category included papers that used EEG measures to investigate consumer or marketing-related behaviour, in which 213 papers were identified. The second category included papers that used neuroimaging measures based on blood oxidation levels such as fMRI or FINRS, in which 158 papers were found. The third category described studies that used physiological measures such as ET or GSR, in which 410 studies were found.
All papers that used EEG or MEG measures were included in the current subsection of the systematic review. However, some of the papers included in the EEG subsection also used physiological methods such as ET or the GSR, and facial action coding and were therefore included in both the EEG and physiological subsections of the systematic review. EEG papers were categorised according to whether they primarily used ERP or TF analysis methods. Table 3 summarises the papers included in the EEG subset of the systematic review, along with the relevant measures used (ERP, TF, Mixed-Methods, Machine-learning).
2.3.2 Secondary literature search
In order to identify neuromarketing literature which used machine-learning algorithms to predict consumer behaviour based on EEG signals, a secondary literature search was conducted. Literature searches using the six journal databases used in the primary literature search were conducted using the search terms “consumer neuroscience”, “neuromarketing”, and “machine learning”. From this literature search, an additional eighteen studies were identified which used machine learning to predict consumer preference or purchase intention using EEG signals [ 4 , 6 , 23 , 24 , 87 , 96 , 185 , 185 , 186 , 186 , 206 , 210 , 237 , 263 , 287 , 291 , 294 , 307 , 308 , 313 ]. These additional studies have been included in the systematic review section.
2.4 Data synthesis
In the data synthesis step, an aggregative approach was used to summarise the conclusions of the literature. Such an approach depends on the subjective interpretation of the researchers concerning the reviewed articles and, considering this, a certain degree of subjective latitude should be given to enable researchers to evaluate and compare distinct studies, with the purpose of extracting shared meanings and abstracting the approaches that do not concern the purposes stated for the review [ 73 ].
The overall objective of the present subset is to provide a mapping of the consistency and direction of significant EEG effects found in neuromarketing research, identifying specific components showing strong effects or consistencies. The results were be analysed using pattern correspondence [ 64 ].
3 Systematic review
3.1 eeg introduction.
Cortical oscillatory activity can be analysed using three critical forms of information extracted from the waveform; amplitude, phase, and frequency [ 143 ]. Amplitude reflects the size of a peak in terms of its volts, while frequency measures how many oscillations occur per second (Hz), and phase represents the relative position of the wave in time. Using these forms of information, EEG data are typically analysed using one of two approaches; time–frequency analysis (TF) or event-related potential analysis (ERP).
3.2 Time–frequency analysis
EEG TF measures can expand on traditional marketing measures such as self-reports and behavioural willingness to pay by showing the underlying cognitive processes behind participant decision-making or the effect of specific changes to features of products or advertisements. EEG TF measures can further expand on physiological measures of arousal such as GSR by unveiling the underlying cognitive and emotional processes behind product and advertisement evaluation.
During time–frequency analysis, changes in the power of cortical oscillations are analysed according to pre-defined frequency bands, usually time-locked and averaged to a particular class of events [ 183 ]. Lower frequency bands generally exhibit larger amplitudes than higher frequency bands and usually reflect more extensive patterns of cortical activation [ 197 ]. Power changes can be analysed primarily according to a baseline condition (as is done in relative-band power or event-related desynchronisation analysis) across the scalp or relative to another region of the scalp (as is done in studies using measures of frontal asymmetry).
3.2.1 Frontal asymmetry
Consumer neuroscience is often focused on separating positive and negative responses to sales and marketing stimuli [ 63 ] to modify or predict consumer choices through behavioural, physiological, or neural measures [ 267 , 269 , 273 , 274 ]. The relative difference in power between the left and right prefrontal cortex, especially in the alpha frequency band, has emerged as a critical measure separating positive from negative responses [ 252 ]. This neural marker is generally interpreted as reflecting the motivational direction and preference towards a stimulus and often occurs just before the formation of behavioural intentions [ 108 , 112 , 275 ]. Frontal asymmetry is posited to reflect an approach response to stimuli when indicating an increase in cortical activity to the left side and an avoidance response when indicating an increase in cortical activity to the right side [ 108 , 112 ]. For this reason, frontal asymmetry is thought to be a more nuanced measure of preference than physiological measures such as GSR, which can only identify valence magnitude, not direction [ 108 , 112 ].
The relative degree of alpha frontal asymmetry is calculated using the following formula:
Frontal asymmetry was commonly found during positive elements of viewed advertisements [ 15 , 52 , 108 , 112 , 157 , 182 , 203 , 204 , 227 , 277 ], as well as proving useful in the prediction of advertisement preference and success [ 46 , 51 , 54 , 157 , 182 , 203 , 204 , 266 , 266 , 270 , 270 , 271 , 271 , 272 , 272 , 275 , 277 ]. Frontal asymmetry has also been shown to differentiate between emotional responses to advertisements by gender [ 267 , 269 , 273 , 274 ] and age [ 267 , 269 , 273 , 274 ] and shows approach/avoidance responses to marketing-related stimuli such as food [ 39 , 207 , 232 , 278 ], music [ 16 ] and sales/persuasion messaging [ 58 , 65 ].
Frontal asymmetry has also been found to be predictive self-reported preference [ 9 , 110 , 118 , 119 , 122 , 148 , 191 , 192 , 260 ], and emerged as the only EEG TF measure that is consistently associated with behavioural measures of willingness to pay (WTP) [ 50 , 138 , 148 , 217 , 218 , 219 , 239 ]. This suggests that frontal asymmetry may reflect actional/motivational responses to brands/products while evaluative ratings and recall may be better investigated using other TF measures such as relative-band power changes [ 26 , 87 , 99 , 115 , 117 , 138 , 145 , 226 , 237 ]. For example, Ramsøy et al. [ 217 ] and Ramsøy et al. [ 218 ] showed using a principal component analysis that prefrontal asymmetry best accounted for variance in WTP, while other TF measures best accounted for self-reported preference.
While frontal asymmetry is most commonly associated with alpha-band activity [ 108 , 112 , 275 ], effects were also reported in the theta and beta bands in the reviewed literature. Frontal asymmetry is most widely reported in the alpha band and appears to reflect WTP and advertisement effectiveness, therefore likely reflecting the motivational approach response most commonly associated with frontal asymmetry [ 44 , 45 , 46 , 50 , 65 , 148 , 157 , 182 , 191 , 192 , 203 , 204 , 218 , 219 , 227 , 237 , 239 , 267 , 267 , 269 , 269 , 273 , 273 , 274 , 274 , 275 , 277 , 278 ]. Frontal asymmetry reported in the theta-band mostly related to self-reported preference [ 16 , 138 , 266 , 270 , 271 , 272 , 275 ], and advertisement memorability [ 44 , 46 , 182 , 266 , 266 , 270 , 270 , 271 , 271 , 272 , 272 ]. Further, while two experiments reported significant changes in beta-band frontal asymmetry [ 9 , 182 , 217 ], the cognitive processes reflected by this response seem much less certain. Therefore, it is recommended that frontal asymmetry measures are primarily employed in the alpha band and are best used in neuromarketing when assessing approach/avoidance responses to advertisements and willingness to pay towards products.
3.2.2 Relative-band power changes
While frontal asymmetry is considered to be the best measure of motivational valence, relative-band power changes in specific frequency bands can be used to measure other cognitive and emotional responses to marketing stimuli. For example, a reduction in power in the alpha band (8–12 Hz) over occipital areas is generally considered to reflect visual processing. Occipital alpha-band power was found to be modulated by video but not print advertisements in early experiments [ 62 ], and has been used in more recent experiments to measure the visual processing of advertisement features [ 83 , 147 , 228 , 229 , 253 , 300 ].
Frontal alpha-band suppressions are linked to information processing, attentional orienting, decision-making and emotional regulation [ 55 , 143 , 190 , 309 ], and were shown in the reviewed literature to relate to advertisement recall and effectiveness [ 68 , 71 , 74 , 90 , 109 , 145 , 267 , 269 , 273 , 274 ] (Pozharliev 2022), TV viewership [ 237 ] and self-reported preference [ 26 , 82 , 83 , 118 , 119 ] as well as responding to preferred brands and pro-social products [ 146 , 161 , 244 ]. Power changes in the alpha band over frontal regions of the scalp can therefore be considered to be a helpful neuromarketing tool, especially when considering the saliency of advertisement and product features.
Theta rhythms describe slower and larger oscillatory frequencies within the 4–7 Hz range [ 235 ]. An increase in midline theta power is reliably associated with long-term memory encoding [ 95 , 142 , 234 ] and an increase in sustained effortful engagement [ 47 , 121 , 169 ]. The papers reviewed showed that theta-power was commonly associated with memorable elements of advertisements [ 68 , 70 , 74 , 132 , 145 , 266 , 266 , 267 , 267 , 269 , 269 , 270 , 270 , 271 , 271 , 272 , 272 , 273 , 273 , 274 , 274 ], recognised brands [ 68 , 70 , 74 , 132 , 145 , 146 , 171 , 191 , 192 , 195 , 245 , 266 , 266 , 267 , 267 , 269 , 269 , 270 , 270 , 271 , 271 , 272 , 272 , 273 , 273 , 274 , 274 ] and out-of- and within-sample success [ 44 , 46 , 68 , 70 , 74 , 83 , 90 , 109 , 117 , 132 , 145 , 146 , 158 , 171 , 191 , 192 , 195 , 237 , 245 , 258 , 266 , 266 , 267 , 267 , 269 , 269 , 270 , 270 , 271 , 271 , 272 , 272 , 273 , 273 , 274 , 274 ]. However, preference associations were less common [ 19 , 20 , 66 , 118 , 119 , 160 , 161 , 162 , 216 , 244 , 295 ]. Increases in theta-band power can be considered a valuable tool in advertising research, highlighting the memorability and out-of-sample effectiveness of tested advertisements, but may be less useful in investigating buying behaviour or preference.
Faster cortical oscillatory activity found in the beta (16–24 Hz) and gamma (30–45 Hz) ranges are less clearly interpreted in neuromarketing research. Beta-band power is traditionally associated with movement preparation and intention formation when suppressed over sensorimotor regions [ 72 , 208 , 209 ], as well as inhibition when increased over right frontal areas [ 40 , 276 ], while gamma-band over prefrontal areas is associated with visual attention [ 233 ], working memory [ 93 , 230 ], and language abilities [ 93 ]. However, in the literature, beta and gamma band changes were modulated by a range of stimuli, including advertisement memorability [ 13 , 14 , 17 , 74 , 194 ], preference [ 53 , 89 , 115 , 158 , 171 , 296 ], emotional valence [ 89 , 296 , 298 ], and changes in the shopping environment [ 27 , 28 , 116 ]. Therefore, relative-band power changes in the beta and gamma bands should be treated with caution when used in neuromarketing research. Further investigation is required to identify their exact role in buying and advertising behaviour.
Other EEG TF measures have been used in neuromarketing research, such as cross-brain correlations across two participants predicting advertisement preference and recall [ 24 ], or global field power and peak density function [ 13 , 14 , 94 , 105 , 106 , 266 , 266 , 266 , 267 , 269 , 270 , 270 , 270 , 271 , 271 , 271 , 272 , 272 , 272 , 273 , 274 ], and partial directed coherence [ 74 ]. Additionally, pre-defined emotion toolboxes have been used to gauge emotional responses to marketing stimuli based on EEG TF responses [ 21 , 63 , 115 , 199 , 231 ]. However, due to the limited research done, it is difficult to judge the consistency of these measures, so further research is required.
3.2.3 Mixed measures experiments
A subsection of the studies reviewed used a mix of EEG time–frequency measures and physiological measures; including measures of arousal, such as the GSR heart-rate variability (HRV) and pupil dilation (PD); ET measures of attentional orienting; and facial-expression analyses. The use of a mixed-measures design allows for the comparison of EEG TF measures with physiological measures, identifying the strengths and weaknesses of each and determining which measures should be used in which contexts [ 56 ].
Several studies have shown that measures of arousal and EEG TF responses are modulated by advertisement preference and memorability [ 1 , 44 , 46 , 58 , 83 , 203 , 266 , 266 , 267 , 267 , 269 , 269 , 270 , 270 , 271 , 271 , 272 , 272 , 273 , 273 , 274 , 274 ], as well as differences in product features [ 43 , 50 , 278 ]. However, physiological measures of arousal appear to be unable to differentiate between different emotional and cognitive responses, while EEG TF measures can [ 1 , 22 , 24 , 50 , 52 , 83 , 110 , 189 , 191 , 192 , 195 , 232 , 266 , 273 ]. For example, [ 50 ] Chen [ 49 ] showed that HRV measures of arousal could only differentiate between the intensity of mouthwash flavours, while FAA distinguished between flavours and was predictive of self-reported preference and purchase intention. It, therefore, appears that physiological measures of arousal are less useful when combined with EEG TF measures due to their lack of sensitivity.
ET, or the tracking of eye movements, is a measure that can easily be combined with EEG measures while participants view an advertisement or product [ 53 , 54 , 68 , 82 , 83 , 99 , 191 , 192 , 195 , 267 , 269 , 273 , 274 ]. The advantage of this measure over EEG TF measures is that it can be used to identify product or advertisement features that draw attention within a visual field [ 15 , 32 , 53 , 68 , 82 , 83 , 99 , 189 , 267 , 269 , 273 , 274 , 287 ] (Pozharliev et al. 2022). Further, Zhu et al. [ 314 ] found that, while EEG can be used to build more accurate machine learning models of customer preference than ET, the inclusion of ET data does improve the predictive accuracy of the model when compared to models using EEG data alone. ET, therefore, provides a useful and complementary measure to EEG TF measures. Some papers found significant attentional ET effects, but no significant EEG differences [ 32 , 82 ]. ET should therefore be considered for use with EEG measures.
Only eight studies identified have combined facial expression analysis or EMG with EEG TF analyses [ 15 , 35 , 36 , 54 , 115 , 203 , 278 ] (Berčík et al. 2021). However, this measure provided a useful complement to EEG TF measures, as facial expressions and micro-expressions can separate different emotional responses such as happiness and disgust. Although the combination of EEG TF and facial-expression analysis is currently not well employed in neuromarketing research, further exploration should be pursued.
3.2.4 Machine-learning prediction
In more recent years, neuromarketing researchers have begun to use machine-learning classification of EEG TF measures to improve the prediction of ‘like/dislike’ or pleasantness ratings. Early studies primarily used multivariate analysis methods, such as logistic regressions, to predict preference ratings [ 24 , 85 , 101 , 139 , 237 , 303 ]. The subsequent use of machine-learning algorithms has been shown to improve the predictive accuracy of a model above the use of traditional logistic regressions [ 31 , 85 , 87 , 96 , 102 , 103 , 151 , 185 , 185 , 186 , 186 , 251 , 255 , 287 , 291 , 294 , 313 ]. Most studies reviewed employed the use of multiple machine-learning algorithms, allowing for the direct comparison of these methods [ 2 , 3 , 4 , 6 , 23 , 87 , 98 , 102 , 103 , 206 , 210 , 251 , 254 , 263 , 287 , 294 , 307 , 308 ] (see Table 4 ).
Across the reviewed literature, the most commonly used classification methods were DNN [ 2 , 3 , 6 , 313 ], KNN [ 2 , 3 , 6 , 98 , 102 , 103 , 206 , 210 , 263 , 308 ], SVM [ 1 , 2 , 3 , 6 , 87 , 98 , 102 , 103 , 185 , 185 , 186 , 186 , 210 , 263 , 291 , 307 , 308 , 314 ], RF [ 1 , 2 , 3 , 6 , 23 , 85 , 98 , 151 , 206 , 307 , 308 , 314 ], and regressions [ 1 , 24 , 85 , 102 , 103 , 138 , 237 , 251 ].
The highest predictive accuracy reported was found by experiments using DNN algorithms, which achieved a binary classification accuracy of 85–94% (M = 0.89, SD = 0.06), followed by RF algorithms (M = 0.80, SD = 0.11), then SVM algorithms (M = 0.77, SD = 0.11), and KNN algorithms (M = 0.72, SD = 0.13). The worst classification accuracy was reported by studies using regression methods, which were only able to correctly classify consumer preference around 60% of the time (M = 0.59, SD = 0.24).
Machine-learning prediction appears to be a fruitful avenue of research within neuromarketing and can achieve very high predictive accuracy, potentially overcoming the reliability problems identified in earlier portions of the systematic review. However, there are several additional methodological considerations that researchers must take into account when using machine-learning prediction. Crucially, machine-learning algorithms may require a larger number of trials and greater computational power than traditional regression models, due to the complex calculations required [ 136 ]. Machine learning models are also vulnerable to ‘overfitting’, where models achieve high accuracy rates for training data, but perform poorly when predicting out-of-sample values [ 67 , 225 , 302 ]. Current neuromarketing research using machine-learning prediction also seems relatively limited, primarily focusing on product preference, so this method should be expanded in the future.
3.3 Event-related potential measures
ERPs reflect averaged transient effects to specific stimuli or a specific class of stimuli [ 172 , 183 , 238 ]. ERPs, therefore, measure phasic responses to advertising stimuli, occurring within hundreds of milliseconds following stimulus onset [ 172 , 238 ], and thus capitalise on the high temporal resolution of EEG recordings. It is generally considered that reactions that occur within the first 300 ms of decision-making are unconscious, while those occurring after 300 ms are related to conscious inclinations [ 165 , 193 ]. The consensus regarding whether the P300 and N400 components reflect conscious or unconscious reactions remains contentious, and these components may reflect a critical phase in the transition from unconscious to conscious mental processes [ 57 , 156 , 250 ].
3.3.1 N400/N200 ERP component
The N200 and N400 components are most commonly associated with conflict and unfamiliarity, especially brand extension and recognition [ 48 , 152 , 240 , 292 ]. The N200 is a negative potential peaking between 200 and 350 ms after stimulus onset, with an amplitude that is negatively related to familiarity and is generally considered to represent fast and unconscious conflict processing [ 48 , 152 , 240 , 292 ]. The N400, a negative potential peaking around 400 ms following stimulus onset, is commonly related to violations of grammatical rules and unexpected stimuli. N400 amplitude is thought to reflect the corresponding conscious processing of conflicting information [ 48 , 152 , 240 , 292 ]. These components are most commonly used in neuromarketing research to measure consumer familiarity with brands and products and the conflict between price and expected value. In the reviewed literature, fourteen studies using brand-extension paradigms reported modulations in N200/N400 amplitude [ 42 , 76 , 80 , 125 , 175 , 177 , 179 , 180 , 246 , 248 , 286 , 295 , 299 , 312 ], nine studies reporting N200/N400 modulations used conflict tasks such as oddball tasks [ 79 , 81 , 97 , 100 , 107 , 126 , 129 , 205 , 259 , 311 ], and nine used other tasks, such as auction tasks or advertising stimuli [ 91 , 92 , 105 , 106 , 124 , 128 , 137 , 279 , 279 , 282 , 282 , 284 , 284 , 285 , 285 , 310 ].
In the literature, significant N200 and N400 amplitude differences were most commonly found in experiments utilising brand extension paradigms. Brand extension tasks are used to investigate how generic product types (e.g., coffee) are associated with brand names or logos (e.g., Starbucks). Twelve out of the 15 studies which investigated brand extension in the reviewed literature found significant effects in N200 or N400 amplitude [ 76 , 80 , 125 , 177 , 179 , 180 , 246 , 248 , 286 , 297 , 299 , 312 ], while one used machine-learning and therefore did not investigate modulations in ERP amplitude directly [ 178 ]. Within the 11 studies that reported significant N200/N400 effects, nine reported significant effects of N400 amplitude [ 42 , 80 , 125 , 177 , 180 , 246 , 286 , 295 , 299 ], two reported significant N200 effects [ 76 , 179 ], while only two studies found significant effects in both N400 and N200 amplitude [ 80 , 299 ]. Further, two studies reported non-significant N2 amplitude modulations [ 297 , 299 ]. In contrast, six of the studies using brand extension experiments reported significant differences in P300 amplitude, and only two found significant LPP or LPC differences [ 76 , 246 ]. N200 and N400 amplitudes were generally interpreted as reflecting conflict processing and were negatively related to brand extension acceptance rates [ 42 , 76 , 80 , 177 , 179 , 180 , 246 , 286 , 295 ].
N400 and N200 amplitudes have also been associated with perceptions of brands and products, especially when conflicted with other relevant features such as reviews or previous experiences. It was found by nine of the reviewed studies that N200/N400 amplitudes were significantly modulated by participant awareness of a brand/product [ 105 , 106 , 107 , 175 , 279 , 282 , 284 , 285 , 310 , 311 ]. When elicited during oddness experiments, N200/N400 amplitudes were most commonly modulated by incongruence caused by negative framings or reviews during the viewing of a product or brand [ 49 , 81 , 97 , 100 , 126 , 166 , 205 ], as well as product preference [ 91 , 92 , 124 , 137 , 175 , 279 , 281 , 282 , 284 , 285 ]. Three studies investigated the effect of price on N400 and N200 amplitudes [ 79 , 129 , 137 ] and found that amplitudes were modulated by violations in price expectations and price deception.
Overall, the N200 and N400 ERP components are typically used in neuromarketing research to identify brand familiarity, extension, and conflict caused by negative attitudes and price violations. Reported effects of preference on N400/N200 amplitudes were less consistent and should be treated with caution. Significant effects were more commonly found in N400 than N200 amplitudes, suggesting that response conflict occurs more consciously in consumers.
3.3.2 P300/P200 ERP component
The P2 and P300 ERP components are positive potentials occurring between 200 and 400 ms after cue onset. It is generally considered that P300 amplitude is positively related to the attentional resources allocated towards a stimulus [ 133 , 140 , 196 ]. The P2 is a similar positive potential, which peaks approximately 200 ms after stimulus presentation [ 114 , 280 , 293 ], and is considered to reflect the rapid automatic activity of attention [ 113 , 114 , 280 , 293 ]. Based on the theoretical background of P300 and P200 modulations, it would be expected that their best use in neuromarketing would emerge in investigating how products and advertisements attract attention, and the best ways to draw attention.
In the present review, P300 and P2 amplitude were found to be especially effective in the investigation of advertisement [ 61 , 62 , 261 , 279 , 282 , 284 , 285 , 289 ] and marketing [ 212 , 215 , 259 , 264 , 315 ] effectiveness, with significant amplitude modulations found in all reviewed studies. P300 and P200 amplitude were also significantly modulated by preference [ 25 , 126 , 137 , 174 , 174 , 176 , 176 , 181 , 181 , 264 , 281 , 306 ], purchase intention [ 41 , 92 , 127 , 129 , 160 , 162 , 170 , 174 , 176 , 181 , 247 ], price [ 128 , 223 ], and brand or product features [ 75 , 81 , 105 , 106 , 107 , 311 ]. However, significant effects were not reported in all papers investigating these factors [ 37 , 49 , 79 , 91 , 92 , 111 , 131 , 166 , 205 , 249 , 258 ], so results may lack statistical power. Especially of note were the inconsistent effects found regarding preference on P200 amplitude, with some papers finding smaller amplitudes to preferred stimuli [ 107 , 124 , 126 , 129 , 170 , 315 ], and others finding the reverse [ 25 , 128 , 174 , 174 , 176 , 176 , 181 , 181 , 306 ].
Overall, P300 and P2 amplitudes were revealed to be especially effective when investigating advertising effectiveness. However, modulations in these ERP components should be treated with caution when examining consumer preference as it may lack statistical power and may only be reflective of attention drawn due to stimulus salience rather than valence.
3.3.3 LPP component
The LPP is a positive component, usually found later than 400 ms following stimulus onset, and is generally considered to reflect conscious emotional processing. The LPP is sensitive to emotional stimuli, both positively and negatively valanced [ 78 , 153 , 241 , 242 ], and has been proposed to represent emotional regulation processing or attention towards the emotional nature of stimuli. The LPP is commonly used in neuromarketing due to its relationship to conscious emotional evaluation, which is strongly related to purchase behaviour and brand perception [ 25 , 37 , 166 , 174 , 176 , 181 ].
In the reviewed literature, the LPP was most commonly associated with preference or emotional evaluation towards products and brands [ 25 , 37 , 75 , 79 , 91 , 92 , 126 , 128 , 166 , 174 , 174 , 174 , 176 , 176 , 176 , 181 , 181 , 181 , 198 , 215 , 247 , 249 , 306 , 310 , 315 ], while only six papers investigating participant preference did not report significant modulations in LPP amplitude [ 62 , 137 , 154 , 258 , 261 , 281 ]. However, the effect of emotional content on LPP amplitude appears to predominantly be reflective of valence strength rather than direction [ 128 , 249 , 315 ], meaning it may be unable to differentiate between positive and negative attitudes towards brands and products. The results reported by Goto et al. [ 92 ] further showed that when used to predict single-trial product preference, the LPP achieved the highest accuracy (70%) of all the ERP components investigated. However, it may be less sensitive under low trial numbers [ 107 ].
Taken together, the reviewed literature reveals the LPP as a key ERP component in neuromarketing research, as it directly reflects emotional evaluation of brands and products, rather than the correlated measures of attention and conflict. For this reason, the LPP may be a more appropriate measure in neuromarketing research for the investigation of preference. However, the LPP should be used with caution, as it may be unable to untangle emotional valence and may only be reflective of intensity.
3.3.4 Other ERP components
A limited number of studies identified in the literature review investigated further ERP components in relation to marketing stimuli, including the MMN [ 111 ], N1 [ 25 , 97 , 100 , 205 , 282 , 289 , 306 ], FRN [ 41 , 236 ], LPC [ 129 , 246 , 279 , 282 , 284 , 285 ], and PSW [ 92 ]. However, due to the limited number of studies investigating the effectiveness of these ERP components, it is difficult to make explicit judgments regarding their use and effectiveness.
3.3.5 ERP studies using machine learning/ICA
Three studies were identified that used independent component analysis or machine-learning on phase-locked EEG data rather than traditional ERP analysis. Tyson-Carr et al. [ 262 ] used an independent component analysis (ICA) to investigate ERP effects behind willingness-to-pay, finding that significant differences between EEG activity between the right and left parietal lobe at around 200 ms following stimulus onset were most predictive of willingness-to-pay. Similarly, Roberts et al. [ 223 ] differentiated between the phase-locked EEG activity found in response to high- and low-value items using ICA analysis. Ma and Zhuang [ 173 ] was the only study identified in the present systematic review which used machine-learning to investigate marketing-relevant stimuli based on phase-locked EEG activity. Using t-SNE machine learning, the researchers predicted brand-extension acceptance with an accuracy of 87.37%.
3.3.6 ERP conclusions
Taken together, the literature collected in the present review identified three ERP components that were most commonly used in neuromarketing research: N400, P300, and LPP. Modulations in N400 and P300 amplitude were best implemented when investigating specific neuromarketing effects such as conflict and attentional saliency. In contrast, LPP amplitude modulations appeared more suitable to measure preference and emotional evaluation, although it is only sensitive to magnitude, not valence. The use of alternate data analytic approaches such as machine learning and ICA is less common in ERP analysis than TF analysis. However, studies in this area seem promising. It is recommended that future ERP research in neuromarketing employs machine-learning and ICA analyses.
4 Discussion
The results of the present systematic review revealed several key recommendations that can be made regarding the use of EEG measures in neuromarketing. First, key ERP and TF components were identified as the most consistent markers of preference and emotional evaluation, namely the FAA and LPP. Second, the importance of machine-learning analysis in future neuromarketing research was highlighted. Finally, it was shown that EEG measures are best used in conjunction with ET and facial expression analysis rather than GSR or PD.
The core finding of the present systematic review was the identification of FAA and LPP as key TF and ERP components in the investigation of consumer preference. Overall, FAA was judged to be the optimal measure of preference due to its ability to disentangle positive from negative responses, while the LPP only indexed response magnitude. Further components were identified that were useful in indexing customer attention (P300 amplitude, alpha-band power, theta-band power), memorability (theta-band power) and emotional conflict (N400 amplitude). These components should be considered in future neuromarketing research but not used as principal measures of consumer preference.
Traditional marketing models assume that consumer decisions are mostly rational, and therefore ignore the role of implicit emotional responses in consumer preference and buying decisions [ 7 , 69 ]. Neuromarketing overcomes these limitations through the use of biometric and neuroimaging measures, which can detect implicit emotional responses traditionally ignored in marketing research [ 7 , 69 , 220 ]. The primary benefits of neuromarketing are therefore to improve the accuracy of models aiming to predict consumer preference and buying behaviour, and provide a greater understanding of the emotional impact of marketing stimuli on consumers [ 7 , 69 , 220 ]. Ultimately, neuromarketing research should be developed in ways that can be actively used to improve products or advertising campaigns. However, there was a large degree of inconsistency found in the reviewed literature regarding the significance and interpretation of different EEG effects in a marketing context, specifically when relating to consumer preference.
The present results, therefore contribute to the literature by demonstrating the most consistent EEG measures of consumer preference and willingness to pay, and these measures require greater focus in future research. Matching the theoretical literature, FAA appears to reflect approach/avoidance responses to stimuli [ 108 , 275 ] and was the only component identified in the current review that could untangle positive from negative emotional reactions. In contrast, the LPP ERP component appears to reflect conscious emotional processing of marketing stimuli [ 78 , 153 , 241 , 242 ] but cannot separate positive from negative responses. Future neuromarketing research should therefore focus on the use of the FAA and LPP when creating predictive models of consumer preference.
It would also be appropriate to use the other components identified as measures of factors that may indirectly relate to preference. For example, P300 amplitude and theta-band power appear to be effective measures when investigating advertisement effectiveness, reflecting attentional orienting [ 133 , 140 , 196 ] and memory encoding [ 95 , 143 ], respectively. In contrast, the N400 ERP component was most effective in investigating brand extension acceptance rates. Future research investigating these components should therefore build their hypotheses based on these findings and not use them as undifferentiated measures of preference.
When used in a mixed-measures design, EEG data were found to be best combined with eye-tracking and facial-expression analysis, as these provide data types that EEG cannot reveal. ET measures are useful in demonstrating which areas of a visual field (e.g., advertisement) draw customer attention [ 32 , 83 ]. Similarly, facial-expression analyses can reveal specific emotional responses to marketing stimuli such as joy or disgust [ 15 , 54 , 115 , 203 , 278 ]. In contrast, physiological measures of arousal such as GSR and PD provide less additional interpretive utility as they only reflect arousal intensity [ 1 , 24 , 50 , 52 , 83 , 189 , 191 , 192 , 195 , 232 ], which can be indexed by EEG measures such as the FAA or P300.
It is our hope that the interpretive framework provided in the present review will aid in the analysis and interpretation of future neuromarketing research, and provide a neuromarketing-specific interpretation of EEG data, preventing post hoc analysis of future results. Highlighting the importance of a clear interpretive framework, significant inconsistencies were found across several sections of the systematic review, and future researchers should be aware of the issues of replication in neuromarketing research. For example, several studies found a positive relationship between preference and P2 amplitude [ 107 , 124 , 126 , 129 , 170 , 315 ], while others found a negative relationship [ 25 , 128 , 174 , 174 , 176 , 176 , 181 , 181 , 306 ]. These inconsistencies reflect the larger replication crisis in psychology and may result from small effect sizes, cherry-picking of results, and the overuse of frequentist statistics.
Machine-learning algorithms are a potential solution to the replication problem, achieving high predictive accuracy in the reviewed literature, consistent across papers. The most effective machine-learning algorithms were DNNs, which reported accuracies as high as 94% in predicting consumer preference. The predictive accuracy of machine learning was further improved when conducted on EEG data combined with physiological measures. Therefore, the present results highlight the importance of machine-learning analyses in future neuromarketing research to improve the replicability and consistency of results.
While machine learning presents a promising avenue for neuromarketing research, care should be taken when designing machine learning models. First, due to the complex calculations made, machine learning requires more trials and computational power than traditional statistical models [ 136 , 222 ]. Machine learning models are also vulnerable to ‘overfitting’, where they may show high accuracy rates for the training data used, but perform poorly when predicting out-of-sample values. Overfitting can be solved in a number of ways, such as by splitting a dataset into ‘training’ and ‘testing’ data, or by using out-of-sample data to test machine-learning models [ 67 , 225 , 302 ].
In the literature reviewed presently, machine learning was used primarily to predict consumer self-reported preference or buying behaviour [ 1 , 2 , 3 , 4 , 6 , 23 , 24 , 31 , 85 , 87 , 96 , 98 , 101 , 102 , 103 , 139 , 151 , 178 , 185 , 185 , 186 , 186 , 206 , 210 , 223 , 254 , 262 , 263 , 287 , 294 , 307 , 308 , 314 ]. However, it has yet to be demonstrated how machine learning can be used to improve advertisement or product designs. For example, a machine learning approach could be used to suggest the shape or colour to use on product packaging. The integration of EEG machine learning methods with developing technologies such as VR headsets should also be investigated further (Fortunato 2014), as well as the use of ‘live’ machine-learning, which can actively update stimuli while a consumer is viewing them based on their patterns of brain activation (Robaina-Calderin 2021; Fortunato 2014).
5 Conclusion
The literature summarised in the present systematic review highlighted the effectiveness of FAA and the LPP as measures of consumer preference and pointed to the importance of machine learning to tackle problems of consistency and replicability existent in the current literature. It is recommended that in future research, investigators use LPP and FAA effects when investigating customer preference and only use other EEG components to investigate other specifically associated effects (e.g., memory encoding, attentional orienting). Further, the use of machine learning is encouraged in future research to improve the replicability of EEG measures of customer preference, and the scope of machine-learning should be expanded.
Availability of data and materials
The search terms and inclusion/exclusion criteria required for replicability of the present research are included in the manuscript text.
Abbreviations
Deep Neural Network
Electroencephalography
Event-related potential
Eye-tracking
Functional magnetic resonance imaging
Functional near-infrared spectroscopy
Galvanic skin response
Heart-rate variability
Independent component analysis
Frontal alpha asymmetry
Feedback related negativity
Late positive complex
Late positive potential
Mismatched negativity
Pupil dilation
Positron emission topography
Positive slow wave
- Time–frequency
Adrián CG, Fuentes-Hurtado F, Valery NO, Provinciale JG, Ausín JM, Mariano AR (2016) A comparison of physiological signal analysis techniques and classifiers for automatic emotional evaluation of audiovisual contents. Front Comput Neurosci 10:74. https://doi.org/10.3389/fncom.2016.00074
Article Google Scholar
Aldayel M, Ykhlef M, Al-Nafjan A (2020) Deep learning for EEG-based preference classification in neuromarketing. Applied Sci 10(4):1525. https://doi.org/10.3390/app10041525
Aldayel M, Ykhlef M, Al-Nafjan A (2021) Recognition of consumer preference by analysis and classification EEG signals. Front Hum Neurosci 14:604639. https://doi.org/10.3389/fnhum.2
Alimardani M and Kaba M (2021) Deep learning for neuromarketing; classification of user preference using EEG signals. In: Paper presented at the 12th Augmented Human International Conference, p 1–7. https://doi.org/10.1145/3460881.3460930020.604639
Alonso Dos Santos M, Calabuig Moreno F (2018) Assessing the effectiveness of sponsorship messaging: measuring the impact of congruence through electroencephalogram. Int J Sports Market Sponsors 19(1):25–40. https://doi.org/10.1108/IJSMS-09-2016-0067
Al-Nafjan A (2022) Feature selection of EEG signals in neuromarketing. PeerJ Comp Sci 8:e944. https://doi.org/10.7717/peerj-cs.944
Amran AS, Ibrahim SA, Malim NH, Hamzah N, Sumari P, Lutfi SL, Abdullah JM (2022) Data acquisition and data processing using electroencephalogram in neuromarketing: a review. J Sci Technol. https://doi.org/10.47836/pjst.30.1.02
Andrejevic M (2012) Brain whisperers: cutting through the clutter with neuromarketing. Somatechnics 2(2):198–215. https://doi.org/10.3366/soma.2012.0057
Aprilianty F, Purwanegara MS (2016) Effects of colour towards underwear choice based on electroencephalography (EEG). Australas Mark J 24(4):331–336. https://doi.org/10.1016/j.ausmj.2016.11.007
Arch DC (1979) Pupil dilation measures in consumer research: applications and limitations. N Am Adv 1:166–168
Google Scholar
Ariely D, Berns GS (2010) Neuromarketing: the hope and hype of neuroimaging in business. Nat Rev Neurosci 11(4):284–292. https://doi.org/10.1038/nrn2795
Astolfi L, De Vico Fallani F, Cincotti F, Mattia D, Bianchi L, Marciani MG, Salinari S, Colosimo A, Tocci A, Soranzo R, Babiloni F (2008) Neural basis for brain responses to TV commercials: a high-resolution EEG study. IEEE Trans Neural Syst Rehabilit Eng 16(6):522–531. https://doi.org/10.1109/TNSRE.2008.2009784
Astolfi L, Fallani FDV, Cincotti F, Mattia D, Bianchi L, Marciani MG, Salinari S, Gaudiano I, Scarano G, Soranzo R (2009) Brain activity during the memorization of visual scenes from TV commercials: an application of high resolution EEG and steady state somatosensory evoked potentials technologies. J Physiol 103(6):333–341. https://doi.org/10.1016/j.jphysparis.2009.07.002
Astolfi L, Vecchiato G, De Vico Fallani F, Salinari S, Cincotti F, Aloise F, Mattia D, Marciani MG, Bianchi L, Soranzo R, Babiloni F (2009) The track of brain activity during the observation of TV commercials with the high-resolution EEG technology. Comp Intell Neurosci. https://doi.org/10.1155/2009/652078
Ausin-Azofra JM, Bigne E, Ruiz C, Marín-Morales J, Guixeres J, Alcañiz M (2021) Do you see what i see? Effectiveness of 360-Degree vs. 2D video ads using a neuroscience approach. Front Psychol. https://doi.org/10.3389/fpsyg.2021.612717
Avinash T, Dikshant L, Seema S (2018) Methods of neuromarketing and implication of the frontal theta asymmetry induced due to musical stimulus as choice modeling. Proced Comp Sci 132:55–67. https://doi.org/10.1016/j.procs.2018.05.059
Babiloni F, Cincotti F, Mattia D, Mattiocco M, Bufalari S, Fallani FD, Tocci A, Bianchi L, Marciani MG, Meroni V, Astolfi L (2006) Neural basis for the brain responses to the marketing messages: an high resolution EEG study. In: Conference proceedings: annual International conference of the IEEE engineering in medicine and biology society. p 3676–9
Bagozzi RP, Verbeke WJ (2014) Biomarketing an emerging paradigm linking neuroscience, endocrinology, and genetics to buyer-seller behavior. In: Moutinho L, Bigne E, Manrai AK (eds) The Routledge companion to the future of marketing. Routledge, Milton Park
Balconi M, Sebastiani R, Angioletti L (2019) A neuroscientific approach to explore consumers’ intentions towards sustainability within the luxury fashion industry. Sustainability 11(18):5105. https://doi.org/10.3390/su11185105
Balconi M, Sebastiani R, Galeone AB, Angioletti L (2020) Sustainability in the fashion luxury branding. Using neuroscience to understand consumers’ intentions towards sustainable eco-luxury items. Neuropsychol Trends 27:65–74. https://doi.org/10.7358/neur-2020-027-ball
Baldo D, Parikh H, Piu Y, Müller K (2015) Brain waves predict success of new fashion products: a practical application for the footwear retailing industry. J Creating Value 1(1):61–71. https://doi.org/10.1177/2394964315569625
Baldo D, Viswanathan VS, Timpone RJ, Venkatraman V (2022) The heart, brain, and body of marketing: complementary roles of neurophysiological measures in tracking emotions, memory, and ad effectiveness. Psychol Market. https://doi.org/10.1002/mar.21697
Bandara SK, Wijesinghe UC, Jayalath BP, Bandara SK, Haddela PS, Wickramasinghe LM (2021) EEG Based Neuromarketing Recommender System for Video Commercials. In: Paper presented at the 2021 IEEE 16th International Conference on Industrial and Information Systems (ICIIS), p 11–16. https://doi.org/10.1109/ICIIS53135.2021.9660742
Barnett SB, Cerf M (2017) A ticket for your thoughts: method for predicting content recall and sales using neural similarity of moviegoers. J Cons Res 44(1):160–181. https://doi.org/10.1093/jcr/ucw083
Bastiaansen M, Straatman S, Driessen E, Mitas O, Stekelenburg J, Wang L (2018) My destination in your brain: a novel neuromarketing approach for evaluating the effectiveness of destination marketing. J Destin Market Manage 7:76–88. https://doi.org/10.1016/j.jdmm.2016.09.003
Bercik J, Horska H, Viragh R, Sulaj A (2017) Advanced mapping and evaluation of consumer perception and preferences on the car market based on eye-tracking. J Manag Stud 16(2):28–39. https://doi.org/10.17512/pjms.2017.16.2.03
Berčík J, Horská E, Gálová J, Margianti ES (2016) Consumer neuroscience in practice: the impact of store atmosphere on consumer behavior. Soc Manag Sci 24(2):96–101. https://doi.org/10.1108/EJM-02-2017-0122
Berčík J, Horská E, Wang RWY, Chen Y (2016) The impact of parameters of store illumination on food shopper response. Appetite 106:101–109. https://doi.org/10.1016/j.appet.2016.04.010
Berezka SM, Sheresheva MY (2019) Neurophysiological methods to study consumer perceptions of television advertising content. Vestn St Peterbg 18(2):175–203. https://doi.org/10.21638/11701/spbu08.2019.202
Berns GS, Moore SE (2012) A neural predictor of cultural popularity. J Cons Psychol 22(1):154–160. https://doi.org/10.1016/j.jcps.2011.05.001
Bhushan V, Saha G, Lindsen J, Shimojo S, Bhattacharya J (2012) How we choose one over another: predicting trial-by-trial preference decision. PLoS ONE 7(8):e43351. https://doi.org/10.1371/journal.pone.0043351
Bigne E, Chatzipanagiotou K, Ruiz C (2020) Pictorial content, sequence of conflicting online reviews and consumer decision-making: the stimulus-organism-response model revisited. J Bus Res 115:403–416. https://doi.org/10.1016/j.jbusres.2019.11.031
Bigne E, Simonetti A, Ruiz C, Kakaria S (2021) How online advertising competes with user-generated content in TripAdvisor. A neuroscientific approach. J Bus Res 123:279–288. https://doi.org/10.1016/j.jbusres.2020.10.010
Boksem MAS, Smidts A (2015) Brain responses to movie trailers predict individual preferences for movies and their population-wide commercial success. J Market Res 52(4):482–492. https://doi.org/10.1509/jmr.13.0572
Boshoff C (2012) A neurophysiological assessment of consumers’ emotional responses to service recovery behaviors: the impact of ethnic group and gender similarity. J Service Res 15(4):401–413. https://doi.org/10.1177/1094670512453879
Boshoff C (2016) The lady doth protest too much: a neurophysiological perspective on brand tarnishment. J Product Brand Manag 25(2):196–207. https://doi.org/10.1108/JPBM-08-2014-0697
Bosshard SS, Bourke JD, Kunaharan S, Koller M, Walla P (2016) Established liked versus disliked brands: brain activity, implicit associations and explicit responses. Cogent Psychol 3:1176691. https://doi.org/10.1080/23311908.2016.1176691
Braeutigam S, Rose SP, Swithenby SJ, Ambler T (2004) The distributed neuronal systems supporting choice-making in real-life situations: differences between men and women when choosing groceries detected using magnetoencephalography. Eur J Neurosci 20(1):293–302. https://doi.org/10.1111/j.1460-9568.2004.03467.x
Brown C, Randolph AB, Burkhalter JN (2012) The story of taste: using EEGs and self-reports to understand consumer choice. Kennesaw J Undergrad Res 2(1):5. https://doi.org/10.32727/25.2019.5
Buschman TJ, Denovellis EL, Diogo C, Bullock D, Miller EK (2012) Synchronous oscillatory neural ensembles for rules in the prefrontal cortex. Neuron 76(4):838–846. https://doi.org/10.1016/j.neuron.2012.09.029
Cai D, Zhu L, Zhang W, Ding H, Wang A, Lu Y, Jia J (2021) The impact of social crowding on consumers’ online mobile shopping: evidence from behavior and ERPs. Psychol Res Behav Manag 14:319–331. https://doi.org/10.2147/PRBM.S292360
Camarrone F, Van Hulle MM (2019) Measuring brand association strength with EEG: a single-trial N400 ERP study. PLoS ONE 14(6):e0217125. https://doi.org/10.1371/journal.pone.0217125
Caratu M, Cherubino P, Mattiacci A (2018) Application of neuro-marketing techniques to the wine tasting experience. In: Research advancements in national and global business theory and practice, p 299–307
Cartocci G, Caratu M, Modica E, Maglione AG, Rossi D, Cherubino P, Babiloni F (2017) Electroencephalographic, heart rate, and galvanic skin response assessment for an advertising perception study: application to antismoking public service announcements. J Vis Exper 126:e55872. https://doi.org/10.3791/55872
Cartocci G, Cherubino P, Rossi D, Modica E, Maglione AG, di Flumeri G, Babiloni F (2016) Gender and age related effects while watching TV advertisements: an EEG study. Comput Intell Neurosci. https://doi.org/10.1155/2016/3795325
Cartocci G, Modica E, Rossi D, Cherubino P, Maglione AG, Colosimo A, Trettel A, Mancini M, Babiloni F (2018) Neurophysiological measures of the perception of antismoking public service announcements among young population. Front Hum Neurosci. https://doi.org/10.3389/fnhum.2018.00231
Cavanagh JF, Frank MJ (2014) Frontal theta as a mechanism for cognitive control. Trends Cog Sci 18(8):414–421
Cheimariou S, Farmer TA, Gordon JK (2019) Lexical prediction in the aging brain: the effects of predictiveness and congruency on the N400 ERP component. Aging Neuropsychol Cogn 26(5):781–806. https://doi.org/10.1080/13825585.2018.1529733
Chen M, Ma Q, Li M, Lai H, Wang X, Shu L (2010) Cognitive and emotional conflicts of counter-conformity choice in purchasing books online: an event-related potentials study. Biol Psychol 85(3):437–445. https://doi.org/10.1016/j.biopsycho.2010.09.006
Chen Y, Gao Q, Lv Q, Qie N, Ma L (2018) Comparing measurements for emotion evoked by oral care products. Intern J Indust Ergon 66:119–129. https://doi.org/10.1016/j.ergon.2018.02.013
Cherubino P, Modica E, Cartocci G, Maglione AG, Trettel A, Mancini M, Rossi D, Di Flumeri G, Babiloni F (2017) Marketing meets neuroscience: useful insights for gender subgroups during the observation of TV ads. IGI Global. https://doi.org/10.4018/978-1-5225-1028-4.ch008
Cherubino P, Trettel A, Cartocci G, Rossi D, Modica E, Maglione AG, Mancini M, di Flumeri G, Babiloni F (2016) Neuroelectrical indexes for the study of the efficacy of TV advertising stimuli. Sel Issues Exp Econ. https://doi.org/10.1007/978-3-319-28419-4_22
Christoforou C, Papadopoulos TC, Constantinidou F, Theodorou M (2017) Your brain on the movies: a computational approach for predicting box-office performance from viewer’s brain responses to movie trailers. Front Neuroinform 11:72. https://doi.org/10.3389/fninf.2017.00072
Clark KR, Leslie KR, Garcia-Garcia M, Tullman ML (2018) How advertisers can keep mobile users engaged and reduce video-ad blocking best practices for video-ad placement and delivery based on consumer neuroscience measures. J Advert Res 58(3):311–325. https://doi.org/10.2501/JAR-2018-036
Clayton MS, Yeung N, Cohen Kadosh R (2018) The many characters of visual alpha oscillations. Eur J Neurosci 48(7):2498–2508. https://doi.org/10.1111/ejn.13747
Constantinescu M, Orindaru A, Pachitanu A, Rosca L, Caescu S, Orzan MC (2019) Attitude evaluation on using the neuromarketing approach in social media: matching company’s purposes and consumer’s benefits for sustainable business growth. Sustainability 11(24):7094. https://doi.org/10.3390/su11247094
Coronel JC, Federmeier KD (2016) The N400 reveals how personal semantics is processed: insights into the nature and organization of self-knowledge. Neuropsychologia 84:36–43. https://doi.org/10.1016/j.neuropsychologia.2016.01.029
Correa KA, Stone BT, Stikic M, Johnson RR, Berka C (2015) Characterizing donation behavior from psychophysiological indices of narrative experience. Front Neurosci. https://doi.org/10.3389/fnins.2015.00301
Dadebayev D, Goh WW, Tan EX (2021) EEG-based emotion recognition: review of commercial EEG devices and machine learning techniques. Comp Informat Sci. https://doi.org/10.1016/j.jksuci.2021.03.009
Danner L, Haindl S, Joechl M, Duerrschmid K (2014) Facial expressions and autonomous nervous system responses elicited by tasting different juices. Food Res Internat 64:81–90. https://doi.org/10.1016/j.foodres.2014.06.003
Daugherty T, Hoffman E, Kennedy K (2016) Research in reverse: ad testing using an inductive consumer neuroscience approach. J Bus Res. https://doi.org/10.1016/j.jbusres.2015.12.005
Daugherty T, Hoffman E, Kennedy K, Nolan M (2018) Measuring consumer neural activation to differentiate cognitive processing of advertising: revisiting Krugman. Eur J Market 52(1–2):182–198. https://doi.org/10.1108/EJM-10-2017-0657
Deitz GD, Royne MB, Peasley MC, Huang J, Coleman JT (2016) EEG-based measures versus panel ratings predicting social media-based behavioral response to super bowl ads. J Advertis Res 56(2):217–227. https://doi.org/10.2501/JAR-2016-030
Denyer D, Tranfield D (2009) Producing a systematic review. Sage Publications Ltd, Thousand Oaks
Di Gruttola F, Malizia AP, D’Arcangelo S, Lattanzi N, Ricciardi E, Orfei MD (2021) The relation between consumers’ frontal alpha asymmetry, attitude, and investment decision. Front Neurosci. https://doi.org/10.3389/fnins.2020.577978
Diao L, Li W, Zhang W, Ma Q, Jin J (2021) Electroencephalographic theta-band oscillatory dynamics represent attentional bias to subjective preferences in value-based decisions. Psychol Res Behav Manage 14:149–158. https://doi.org/10.2147/PRBM.S292172
Dietterich T (1995) Overfitting and undercomputing in machine learning. ACM Comput Surv 27(3):326–327. https://doi.org/10.1145/212094.212114
Dimpfel W (2015) Neuromarketing: neurocode-tracking in combination with eye-tracking for quantitative objective assessment of TV commercials. J Behav Brain Sci 5(04):137. https://doi.org/10.4236/jbbs.2015.54014
Duque-Hurtado P, Samboni-Rodriguez V, Castro-Garcia M, Montoya-Restrepo LA, Montoya-Restrepo IA (2020) Neuromarketing: its current status and research perspectives. Estud Gerenc 36(157):525–539. https://doi.org/10.18046/j.estger.2020.157.3890
Dulabh M, Vazquez D, Ryding D, Casson A (2018) Measuring consumer engagement in the brain to online interactive shopping environments. In: Claudia M (ed) Augmented reality virtual reality. Springer, Cham, pp 145–165. https://doi.org/10.1007/978-3-319-64027-3_11
Chapter Google Scholar
Eijlers E, Boksem MAS, Smidts A (2020) Measuring neural arousal for advertisements and its relationship with advertising success. Front Neurosci 14:736. https://doi.org/10.3389/fnins.2020.00736
Erbil N, Ungan P (2007) Changes in the alpha and beta amplitudes of the central EEG during the onset, continuation, and offset of long-duration repetitive hand movements. Brain Res 1169:44–56. https://doi.org/10.1016/j.brainres.2007.07.014
Evans D (2002) Systematic reviews of interpretive research: interpretive data synthesis of processed data. Aust J Adv Nurs 20(2):22
Fallani FDV, Astolfi L, Cincotti F, Mattia D, Marciani MG, Gao S, Salinari S, Soranzo R, Colosimo A, Babiloni F (2008) Structure of the cortical networks during successful memory encoding in TV commercials. Clin Neurophysiol 119(10):2231–2237. https://doi.org/10.1016/j.clinph.2008.06.018
Fan B, Li C, Jin J (2020) The brand scandal spillover effect at the Country level: evidence from event-related potentials. Front Neurosci. https://doi.org/10.3389/fnins.2019.01426
Fan B, Zhang Q (2019) Does the aura surrounding healthy-related imported products fade in China? ERP evidence for the country-of-origin stereotype. PLoS ONE 14(5):e0216866. https://doi.org/10.1371/journal.pone.0216866
Article MathSciNet Google Scholar
Fischer NL, Peres R, Fiorani M (2018) Frontal alpha asymmetry and theta oscillations associated with information sharing intention. Front Behav Neurosci. https://doi.org/10.3389/fnbeh.2018.00166
Flaisch T, Stockburger J, Schupp HT (2008) Affective prime and target picture processing: an ERP analysis of early and late interference effects. Brain Topogr 20(4):183–191. https://doi.org/10.1007/s10548-008-0045-6
Fu H, Ma H, Bian J, Wang C, Zhou J, Ma Q (2019) Don’t trick me: An event-related potentials investigation of how price deception decreases consumer purchase intention. Neurosci Lett 713:134522. https://doi.org/10.1016/j.neulet.2019.134522
Fudali-Czyz A, Ratomska M, Cudo A, Francuz P, Kopis N, Tuznik P (2016) Controlled categorisation processing in brand extension evaluation by Indo-European language speakers. An ERP study. Neurosci Lett 628:30–34. https://doi.org/10.1016/j.neulet.2016.06.005
Gajewski PD, Drizinsky J, Zuelch J, Falkenstein M (2016) ERP correlates of simulated purchase decisions. Front Neurosci 10:360. https://doi.org/10.3389/fnins.2016.00360
Garcia-Madariaga J, Blasco Lopez MF, Burgos IM, Virto NR (2019) Do isolated packaging variables influence consumers’ attention and preferences? Physiol Behav 200:96–103. https://doi.org/10.1016/j.physbeh.2018.04.030
Garcia-Madariaga J, Moya I, Recuero N, Blasco M (2020) Revealing unconscious consumer reactions to advertisements that include visual metaphors. A neurophysiological experiment. Front Psychol 11:760. https://doi.org/10.3389/fpsyg.2020.00760
Garczarek-Bak U, Disterheft A (2018) EEG frontal asymmetry predicts product purchase differently for national brands and private labels. J Neurosci Psychol Econ 11(3):182–195. https://doi.org/10.1037/npe0000094
Gauba H, Kumar P, Roy PP, Singh P, Dogra DP, Raman B (2017) Prediction of advertisement preference by fusing EEG response and sentiment analysis. Neural Netw 92:77–88. https://doi.org/10.1016/j.neunet.2017.01.013
Gkaintatzis A, van der Lubbe R, Karantinou K, Constantinides E (2019) Consumers’ cognitive, emotional and behavioral responses towards background music: an EEG study. Sci Tech Pub. https://doi.org/10.5220/0008346603140318
Golnar-Nik P, Farashi S, Safari M (2019) The application of EEG power for the prediction and interpretation of consumer decision-making: a neuromarketing study. Physiol Behav 207:90–98. https://doi.org/10.1016/j.physbeh.2019.04.025
González-Morales A (2020) Right evaluation of marketing stimuli with neuroscience. An electroencephalography experiment. Comput Hum Behav Rep 2:100030. https://doi.org/10.1016/j.chbr.2020.100030
Goode MR, Iwasa-Madge D (2019) The numbing effect of mortality salience in consumer settings. Psychol Market 36(6):630–641. https://doi.org/10.1002/mar.21201
Gordon R, Ciorciari J, van Laer T (2018) Using EEG to examine the role of attention, working memory, emotion, and imagination in narrative transportation. Eur J Market 52(1–2):92–117. https://doi.org/10.1108/EJM-12-2016-0881
Goto N, Mushtaq F, Shee D, Lim XL, Mortazavi M, Watabe M, Schaefer A (2017) Neural signals of selective attention are modulated by subjective preferences and buying decisions in a virtual shopping task. Biol Psychol 128:11–20
Goto N, Lim XL, Shee D, Hatano A, Khong KW, Buratto LG, Watabe M, Schaefer A (2019) Can brain waves really tell if a product will be purchased? Inferring consumer preferences from single-item brain potentials. Front Integr Neurosci 13:19. https://doi.org/10.3389/fnint.2019.00019
Gou Z, Choudhury N, Benasich AA (2011) Resting frontal gamma power at 16, 24 and 36 months predicts individual differences in language and cognition at 4 and 5 years. Behav Brain Res 220(2):263–270. https://doi.org/10.1016/j.bbr.2011.01.048
Gountas J, Gountas S, Ciorciari J, Sharma P (2019) Looking beyond traditional measures of advertising impact: using neuroscientific methods to evaluate social marketing messages. J Bus Res 105:121–135. https://doi.org/10.1016/j.jbusres.2019.07.011
Gruber T, Tsivilis D, Giabbiconi C, Müller MM (2008) Induced electroencephalogram oscillations during source memory: familiarity is reflected in the gamma band, recollection in the theta band. J Cog Neurosci 20(6):1043–1053. https://doi.org/10.1162/jocn.2008.20068
Guixeres J, Bigné E, Ausin Azofra JM, Alcaniz Raya M, Colomer Granero A, Fuentes Hurtado F, Naranjo Ornedo V (2017) Consumer neuroscience-based metrics predict recall, liking and viewing rates in online advertising. Front Psychol 8:1808. https://doi.org/10.3389/fpsyg.2017.01808
Guo F, Ding Y, Liu W, Liu C, Zhang X (2016) Can eye-tracking data be measured to assess product design?: Visual attention mechanism should be considered. Internat J Industrial Ergon 53:229–235. https://doi.org/10.1016/j.ergon.2015.12.001
Guo F, Li M, Hu M, Li F, Lin B (2019) Distinguishing and quantifying the visual aesthetics of a product: an integrated approach of eye-tracking and EEG. Internat J Industrial Ergon 71:47–56. https://doi.org/10.1016/j.ergon.2019.02.006
Guo F, Ye G, Duffy VG, Li M, Ding Y (2018) Applying eye tracking and electroencephalography to evaluate the effects of placement disclosures on brand responses. J Cons Behav 17(6):519–531. https://doi.org/10.1002/cb.1736
Guo F, Zhang X, Ding Y, Wang X (2016) Recommendation influence: differential neural responses of consumers during shopping online. J Neurosci Psychol Econ 9(1):29–37. https://doi.org/10.1037/npe0000051
Guo G, Elgendi M (2013) A new recommender system for 3D e-commerce: an EEG based approach. J Adv Manag Sci 1(1):61–65
Hakim A, Klorfeld S, Sela T, Friedman D, Shabat-Simon M, Levy DJ (2018) Pathways to consumers’ minds: using machine learning and multiple EEG metrics to increase preference prediction above and beyond traditional measurements. bioRxiv. https://doi.org/10.1101/317073
Hakim A, Klorfeld S, Sela T, Friedman D, Shabat-Simon M, Levy DJ (2020) Machines learn neuromarketing: improving preference prediction from self-reports using multiple EEG measures and machine learning. Internat J Res Market. https://doi.org/10.1016/j.ijresmar.2020.10.005
Hallinger P (2013) A conceptual framework for systematic reviews of research in educational leadership and management. J Educ Admin. https://doi.org/10.1108/09578231311304670
Han C, Lee J, Lim J, Kim Y, Im C (2017) Global electroencephalography synchronization as a new indicator for tracking emotional changes of a group of individuals during video watching. Front Hum Neurosci 11:577. https://doi.org/10.3389/fnhum.2017.00577
Han W, Zhang H, Wang J, Zhao M (2017) Neurological impact of the conflict between brand and product performance on consumer decision process. In: 2017 14th International conference on services systems and services management (Icsssm)
Handy TC, Smilek D, Geiger L, Liu C, Schooler JW (2010) ERP evidence for rapid hedonic evaluation of logos. J Cog Neurosci 22(1):124–138. https://doi.org/10.1162/jocn.2008.21180
Harmon-Jones E, Gable PA, Peterson CK (2010) The role of asymmetric frontal cortical activity in emotion-related phenomena: a review and update. Biol Psychol 84(3):451–462. https://doi.org/10.1016/j.biopsycho.2009.08.010
Harris JM, Ciorciari J, Gountas J (2019) Consumer neuroscience and digital/social media health/social cause advertisement effectiveness. Behav Sci. https://doi.org/10.3390/bs9040042
Herrando C, Jiménez-Martínez J, Martín-De Hoyos M, Constantinides E (2022) Emotional contagion triggered by online consumer reviews: evidence from a neuroscience study. J Retail Cons Serv 67:102973. https://doi.org/10.1016/j.jretconser.2022.102973
Herbes C, Friege C, Baldo D, Mueller K (2015) Willingness to pay lip service? Applying a neuroscience-based method to WTP for green electricity. Energy Policy 87:562–572. https://doi.org/10.1016/j.enpol.2015.10.001
Hewig J (2018) Intentionality in frontal asymmetry research. Psychophysiology 55(1):e12852. https://doi.org/10.1111/psyp.12852
Hoefer D, Handel M, Müller K, Hammer TR (2016) The buying brain, screens, and social media; vision of the future; electroencephalographic study showing that tactile stimulation by fabrics of different qualities elicit graded event-related potentials. Skin Res Technol 22(4):219. https://doi.org/10.1002/9781119200079.ch17
Hoefer D, Handel M, Muller KM, Hammer TR (2016) Electroencephalographic study showing that tactile stimulation by fabrics of different qualities elicit graded event-related potentials. Skin Res Technol 22(4):470–478. https://doi.org/10.1111/srt.12288
Horska E, Bercik J, Krasnodebski A, Matysik-Pejas R, Bakayova H (2016) Innovative approaches to examining consumer preferences when choosing wines. Agric Econ 62(3):124–133. https://doi.org/10.17221/290/2015-AGRICECON
Horská E, Berčík J (2014) The influence of light on consumer behavior at the food market. J Food Prod Market 20(4):429–440. https://doi.org/10.1080/10454446.2013.838531
Hsu L, Chen Y (2020) Neuromarketing, subliminal advertising, and hotel selection: an EEG study. Australas Market J (AMJ) 28(4):200–208. https://doi.org/10.1016/j.ausmj.2020.04.009
Hsu M (2017) Neuromarketing: inside the mind of the consumer. Calif Manag Rev 59(4):5–22. https://doi.org/10.1177/0008125617720208
Hsu W (2017) An integrated-mental brainwave system for analyses and judgments of consumer preference. Telemat Inform 34(5):518–526. https://doi.org/10.1016/j.tele.2016.11.002
Hungenberg E, Slavich M, Bailey A, Sawyer T (2020) Examining minor league baseball spectator nostalgia: a neuroscience perspective. Sport Manag Rev 23(5):824–837. https://doi.org/10.1016/j.smr.2020.04.001
Inanaga K (1998) Frontal midline theta rhythm and mental activity. Psychiatry Clin Neurosci 52(6):555–566. https://doi.org/10.1046/j.1440-1819.1998.00452.x
Janić M, Ćirović M, Dimitriadis N, Jovanović Dimitriadis N, Alevizou P (2022) Neuroscience and CSR: using EEG for assessing the effectiveness of branded videos related to environmental issues. Sustainability 14(3):1347. https://doi.org/10.3390/su14031347
Jeong YE, Kim JH (2017) A study of consumer’s emotional response on musical stimulus through EEG analysis: based on color perception of consumers. Korean J Bus Adm 30(12):2147–2172. https://doi.org/10.18032/kaaba.2017.30.9.2147
Jia J, Dou X, Liang M, Yu H (2018) Environmental-friendly eco-labeling matters: evidences from an ERPs study. Front Hum Neurosci. https://doi.org/10.3389/fnhum.2018.00417
Jin J, Wang C, Yu L, Ma Q (2015) Extending or creating a new brand: evidence from a study on event-related potentials. NeuroReport 26(10):572–577. https://doi.org/10.1097/WNR.0000000000000390
Jin J, Zhang W, Chen M (2017) How consumers are affected by product descriptions in online shopping: event-related potentials evidence of the attribute framing effect. Neurosci Res 125:21–28. https://doi.org/10.1016/j.neures.2017.07.006
Jin KIMHYUN, Cho S (2019) The effect of korean and chinese consumers’ expectancy disconfirmation in restaurants on complaining behavior: the moderating effect of neuroticism. J Product Res 37(5):181–192
Jing K, Mei Y, Song Z, Wang H, Shi R (2019) How do price and quantity promotions affect hedonic purchases? An ERPs study. Front Neurosci. https://doi.org/10.3389/fnins.2019.00526
Jones WJ, Childers TL, Jiang Y (2012) The shopping brain: math anxiety modulates brain responses to buying decisions. Biol Psychol 89(1):201–213. https://doi.org/10.1016/j.biopsycho.2011.10.011
Jordão IL, Souza MT, Oliveira JH, Giraldi JD (2017) Neuromarketing applied to consumer behaviour: an integrative literature review between 2010 and 2015. Internat J Bus Forecast Market Intell 3(3):270–288
Junghoefer M, Kissler J, Schupp HT, Putsche C, Elling L, Dobel C (2010) A fast neural signature of motivated attention to consumer goods separates the sexes. Front Hum Neurosci 4:179. https://doi.org/10.3389/fnhum.2010.00179
Kacaniova M, Vargova V (2017) Electroencephalography as a tool of advertising research in the context of Mac model. Eur J Sci Theol 13(6):145–155
Käthner I, Wriessnegger SC, Müller-Putz GR, Kübler A, Halder S (2014) Effects of mental workload and fatigue on the P300, alpha and theta band power during operation of an ERP (P300) brain–computer interface. Biol Psychol 102:118–129. https://doi.org/10.1016/j.biopsycho.2014.07.014
Kenning P, Plassmann H, Ahlert D (2007) Applications of functional magnetic resonance imaging for market research. Qualitat Market Res Internat J. https://doi.org/10.1108/13522750710740817
Khan KS, Kunz R, Kleijnen J, Antes G (2003) Five steps to conducting a systematic review. J Royal Soc Med 96(3):118–121. https://doi.org/10.1108/13522750710740817
Khurana V, Gahalawat M, Kumar P, Roy PP, Dogra DP, Scheme E, Soleymani M (2021) A survey on neuromarketing using EEG signals. IEEE Trans Cogn Dev Syst 13(4):732–749. https://doi.org/10.1109/TCDS.2021.3065200
Khushaba RN, Greenacre L, Al-Timemy A, Al-Jumaily A (2015) Event-related potentials of consumer preferences. Proced Comp Sci 76:68–73. https://doi.org/10.1016/j.procs.2015.12.277
Khushaba RN, Greenacre L, Kodagoda S, Louviere J, Burke S, Dissanayake G (2012) Choice modeling and the brain: a study on the Electroencephalogram (EEG) of preferences. Expert Syst Appl 39(16):12378–12388. https://doi.org/10.1016/j.eswa.2012.04.084
Khushaba RN, Wise C, Kodagoda S, Louviere J, Kahn BE, Townsend C (2013) Consumer neuroscience: assessing the brain response to marketing stimuli using electroencephalogram (EEG) and eye tracking. Expert Syst Appl 40(9):3803–3812. https://doi.org/10.1016/j.eswa.2012.12.095
Kim AE, Oines L, Miyake A (2018) Individual differences in verbal working memory underlie a tradeoff between semantic and structural processing difficulty during language comprehension: an ERP investigation. J Exp Psychol Learn Mem Cog 44(3):406. https://doi.org/10.1037/xlm0000457
Kim Y, Park K, Kim Y, Yang W, Han D, Kim W (2020) The impact of visual art and high affective arousal on heuristic decision-making in consumers. Front Psychol 11:565829. https://doi.org/10.3389/fpsyg.2020.565829
Klimesch W (1999) EEG alpha and theta oscillations reflect cognitive and memory performance: a review and analysis. Brain Res Rev 29(2–3):169–195. https://doi.org/10.1016/S0165-0173(98)00056-3
Klimesch W (2012) Alpha-band oscillations, attention, and controlled access to stored information. Trends Cog Sci 16(12):606–617. https://doi.org/10.1016/j.tics.2012.10.007
Knutson B, Genevsky A (2018) Neuroforecasting aggregate choice. Curr Direct Psychol Sci 27(2):110–115. https://doi.org/10.1177/0963721417737877
Kong W, Zhao X, Hu S, Vecchiato G, Babiloni F (2013) Electronic evaluation for video commercials by impression index. Cogn Neurodyn 7(6):531–535. https://doi.org/10.1007/s11571-013-9255-z
Kong W, Zhang X, Wang L, Fan Q, Dai Y, Miao Y (2019) Evaluation of product placement with attention on eye-tracking and EEG. J Beijing Instit Technol 39:792–793
Krugman DM, Fox RJ, Fletcher JE, Rojas TH (1994) Do adolescents attend to warnings in cigarette advertising? An eye-tracking approach. J Advert Res 34(6):39–53
Kuan KKY, Zhong Y, Chau PYK (2014) Informational and normative social influence in group-buying: evidence from self-reported and EEG data. J Manage Inf Syst 30(4):151–178. https://doi.org/10.2753/MIS0742-1222300406
Kühn S, Strelow E, Gallinat J (2016) Multiple “buy buttons” in the brain: forecasting chocolate sales at point-of-sale based on functional brain activation using fMRI. Neuroimage 136:122–128. https://doi.org/10.1016/j.neuroimage.2016.05.021
Kumagai Y, Arvaneh M, Tanaka T (2017) Familiarity affects entrainment of EEG in music listening. Front Hum Neurosci. https://doi.org/10.3389/fnhum.2017.00384
Kumar S, Yadava M, Roy PP (2019) Fusion of EEG response and sentiment analysis of products review to predict customer satisfaction. Informat Fusion 52:41–52. https://doi.org/10.1016/j.inffus.2018.11.001
Kutas M, Federmeier KD (2011) Thirty years and counting: finding meaning in the N400 component of the event-related brain potential (ERP). Annu Rev Psychol 62:621–647. https://doi.org/10.1146/annurev.psych.093008.131123
Kuzava S, Frost A, Perrone L, Kang E, Lindhiem O, Bernard K (2020) Adult processing of child emotional expressions: a meta-analysis of ERP studies. Dev Psychol 56(6):1170. https://doi.org/10.1037/dev0000928
Kytö E, Bult H, Aarts E, Wegman J, Ruijschop RM, Mustonen S (2019) Comparison of explicit vs. implicit measurements in predicting food purchases. Food Qual Prefer 78:103733. https://doi.org/10.1016/j.foodqual.2019.103733
Lajante MMP, Droulers O, Amarantini D (2017) How reliable are “State-of-the-Art” facial EMG processing methods? Guidelines for improving the assessment of emotional valence in advertising research. J Advert Res 57(1):28–37. https://doi.org/10.2501/JAR-2017-011
Lamy D, Salti M, Bar-Haim Y (2009) Neural correlates of subjective awareness and unconscious processing: an ERP study. J Cog Neurosci 21(7):1435–1446. https://doi.org/10.1162/jocn.2009.21064
Laurence J, Gerhold MM (2016) Saving the day: the relationship between emotion and purchase intent in television advertising. HeadSpace Neuromarket
Leanza F (2017) Consumer neuroscience: the traditional and VR TV commercial. Neuropsychol Trends 21:81–90. https://doi.org/10.7358/neur-2017-021-lean
Lee EJ, Shin HJ, Yang S, Kwon G, Suh M (2013) The conscious choice of homo evolutis: can fronto-parietal EEG activations predict the consumer choice of sustainable products? Korean Manag Rev 42(3):805–821
Lee E (2016) Empathy can increase customer equity related to pro-social brands. J Bus Res 69(9):3748–3754. https://doi.org/10.1016/j.jbusres.2015.05.018
Lee E, Kwon G, Shin HJ, Yang S, Lee S, Suh M (2014) The spell of green: can frontal EEG activations identify green consumers? J Bus Ethics 122(3):511–521. https://doi.org/10.1007/s10551-013-1775-2
Lee H (2016) Difference in P300 latency by levels of involvement to sport: Interdisciplinary approach to sport consumer behavior using embodied cognition theory and EEG/ERP technique. Korea Sports Ind Manag J 21(6):45–56
Lee N, Broderick AJ, Chamberlain L (2007) What is “neuromarketing”? A discussion and agenda for future research. Internat J Psychophysiol 63(2):199–204. https://doi.org/10.1016/j.ijpsycho.2006.03.007
Li B, Wang Y, Wang K (2016) Data fusion and analysis techniques of neuromarketing. WIT Transact Eng Sci 113:396–404. https://doi.org/10.2495/IWAMA150461
Li Z, Bu G (2013) Mechanism of Consumer's Brand Loyalty Based on Neuromarketing. In: 2013 3rd International Conference on Education and Education Management (Eem 2013), 25, p 235–238
Liao W, Zhang Y, Peng X (2019) Neurophysiological effect of exposure to gossip on product endorsement and willingness-to-pay. Neuropsychologia 132:107123. https://doi.org/10.1016/j.neuropsychologia.2019.107123
Lietz P (2010) Research into questionnaire design: a summary of the literature. Int J Market Res 52(2):249–272. https://doi.org/10.2501/5147078530920120X
Lim WM (2018) Demystifying neuromarketing. J Bus Res 91:205–220. https://doi.org/10.1016/j.jbusres.2018.05.036
Lin H, Saunders B, Hutcherson CA, Inzlicht M (2018) Midfrontal theta and pupil dilation parametrically track subjective conflict (but also surprise) during intertemporal choice. Neuroimage 172:838–852. https://doi.org/10.1016/j.neuroimage.2017.10.055
Luan J, Yao Z, Bai Y (2017) How social ties influence consumer: evidence from event-related potentials. PLoS ONE 12(1):e0169508. https://doi.org/10.1371/journal.pone.0169508
Lucchiari C, Pravettoni G (2012) The effect of brand on EEG modulation a study on mineral water. Swiss J Psychol 71(4):199–204. https://doi.org/10.1024/1421-0185/a000088
Luck SJ (2005) Ten simple rules for designing and interpreting ERP experiments. Event-related potentials: a methods handbook, 4
Ma G, Zhuang X (2021) Nutrition label processing in the past 10 years: contributions from eye tracking approach. Appetite 156:104859. https://doi.org/10.1016/j.appet.2020.104859
Ma H, Mo Z, Zhang H, Wang C, Fu H (2018) The temptation of zero price: event-related potentials evidence of how price framing influences the purchase of bundles. Front Neurosci. https://doi.org/10.3389/fnins.2018.00251
Ma Q, Abdeljelil HM, Hu L (2019) The influence of the consumer ethnocentrism and cultural familiarity on brand preference: evidence of event-related potential (ERP). Fronti Hum Neurosci 13:220. https://doi.org/10.3389/fnhum.2019.00220
Ma Q, Zhang L, Wang M (2018) “You win, you buy”-how continuous win effect influence consumers’ price perception: an ERP study. Front Neurosci 12:691. https://doi.org/10.3389/fnins.2018.00691
Ma Q, Wang C, Wang X (2014) Two-stage categorization in brand extension evaluation: electrophysiological time course evidence. PLoS ONE 9(12):e114150. https://doi.org/10.1371/journal.pone.0114150
Ma Q, Wang M, Hu L, Zhang L, Hua Z (2021) A novel recurrent neural network to classify EEG signals for customers’ decision-making behavior prediction in brand extension scenario. Front Hum Neurosci. https://doi.org/10.3389/fnhum.2021.610890
Ma Q, Wang X, Dai S, Shu L (2007) Event-related potential N270 correlates of brand extension. NeuroReport 18(10):1031–1034. https://doi.org/10.1097/WNR.0b013e3281667d59
Ma Q, Wang X, Shu L, Dai S (2008) P300 and categorization in brand extension. Neurosci Letters 431(1):57–61. https://doi.org/10.1016/j.neulet.2007.11.022
Ma Y, Jin J, Yu W, Zhang W, Xu Z, Ma Q (2018) How is the neural response to the design of experience goods related to personalized preference? An implicit view. Front Neurosci 12:760. https://doi.org/10.3389/fnins.2018.00760
Mahamad NA, Amin M, Mikami O (2019) Evaluating neuromarketing technique on consumer satisfaction using EEG imaging. J Adv Manufact Technol 13(2):2
Makeig S (1993) Auditory event-related dynamics of the EEG spectrum and effects of exposure to tones. Electroencephal Clin Neurophysiol 86(4):283–293. https://doi.org/10.1016/0013-4694(93)90110-H
Makeig S, Bell A, Jung T, Sejnowski TJ (1995) Independent component analysis of electroencephalographic data. Adv Neural Inform Process Syst, 8
Mashrur FR, Rahman KM, Miya MT, Vaidyanathan R, Anwar SF, Sarker F, Mamun KA (2022) BCI-based consumers’ choice prediction from EEG signals: an intelligent neuromarketing system. Front Hum Neurosci. https://doi.org/10.3389/fnhum.2022.861270
Mashrur FR, Rahman KM, Miya MT, Vaidyanathan R, Anwar SF, Sarker F, Mamun KA (2022) An Intelligent Neuromarketing System for Predicting Consumers’ Future Choice from Electroencephalography Signals. Physiol Behav. https://doi.org/10.1016/j.physbeh.2022.113847
McDuff D, El Kaliouby R, Cohn JF, Picard RW (2015) Predicting ad liking and purchase intent: large-scale analysis of facial responses to ads. IEEE Transact Affect Computi 6(3):223–235. https://doi.org/10.1109/TAFFC.2014.2384198
Mehrabian A (1995) Framework for a comprehensive description and measurement of emotional states. Genetic Soc Gen Psychol Monogr 121:339
Mengual-Recuerda A, Tur-Viñes V, Juárez-Varón D (2020) Neuromarketing in Haute cuisine gastronomic experiences. Front Psychol 11:1772. https://doi.org/10.3389/fpsyg.2020.01772
Misselhorn J, Friese U, Engel AK (2019) Frontal and parietal alpha oscillations reflect attentional modulation of cross-modal matching. Sci Rep 9(1):1–11. https://doi.org/10.1038/s41598-019-41636-w
Modica E, Cartocci G, Rossi D, Levy ACM, Cherubino P, Maglione AG, Di Flumeri G, Mancini M, Montanari M, Perrotta D, Di Feo P, Vozzi A, Ronca V, Arico P, Babiloni F (2018) Neurophysiological responses to different product experiences. Comput Intell Neurosci 2018:9616301. https://doi.org/10.1155/2018/9616301
Modica E, Rossi D, Cartocci G, Perrotta D, Di Feo P, Mancini M, Aricò P, Inguscio BMS, Babiloni F (2018) Neurophysiological profile of antismoking campaigns. Comput Intell Neurosci. https://doi.org/10.1155/2018/9721561
Moran RJ, Pinotsis DA, Friston KJ (2013) Neural masses and fields in dynamic causal modeling. Front Comp Neurosci 7:57
Morey AC (2017) Memory for positive and negative political TV ads: the role of partisanship and gamma power. Polit Commun 34(3):404–423. https://doi.org/10.1080/10584609.2016.1266534
Moya I, García-Madariaga J, Blasco M (2020) What can neuromarketing tell us about food packaging? Foods. https://doi.org/10.3390/foods9121856
Nie A, Yu Y (2021) External (versus internal) facial features contribute most to repetition priming in facial recognition: ERP evidence. Percept Motor Skills 128(1):15–47. https://doi.org/10.1177/0031512520957150
Niedermeyer E (2003) The clinical relevance of EEG interpretation. Clin Electroencephal 34(3):93–98. https://doi.org/10.1177/155005940303400303
Nittono H, Watari K (2017) Effects of food sampling on brain potential responses to food branding. Psychologia 60(1):3–15. https://doi.org/10.2117/psysoc.2017.3
Nomura T, Mitsukura Y (2015) EEG-based detection of TV commercials effects. Proced Comp Sci 60:131–140. https://doi.org/10.1016/j.procs.2015.08.112
Nunez PL, Srinivasan R, Westdorp AF, Wijesinghe RS, Tucker DM, Silberstein RB, Cadusch PJ (1997) EEG coherency: I: statistics, reference electrode, volume conduction, Laplacians, cortical imaging, and interpretation at multiple scales. Electroencephal Clin Neurophysiol 103(5):499–515. https://doi.org/10.1016/S0013-4694(97)00066-7
Ohme R, Matukin M (2012) A small frog that makes a big difference: brain wave testing of TV advertisements. IEEE Pulse 3(3):28–33. https://doi.org/10.1109/MPUL.2012.2189169
Ohme R, Matukin M, Pacula-Lesniak B (2011) Biometric measures for interactive advertising research. J Interact Advert 11(2):60–72. https://doi.org/10.1080/15252019.2011.10722185
Ohme R, Reykowska D, Wiener D, Choromanska A (2009) Analysis of neurophysiological reactions to advertising stimuli by means of EEG and galvanic skin response measures. J Neurosci Psychol Econ 2(1):21. https://doi.org/10.1037/a0015462
Ohme R, Reykowska D, Wiener D, Choromanska A (2010) Application of frontal EEG asymmetry to advertising research. J Econ Psychol 31(5):785–793. https://doi.org/10.1016/j.joep.2010.03.008
Ozkara BY, Bagozzi R (2021) The use of event related potentials brain methods in the study of Conscious and unconscious consumer decision making processes. J Retail Cons Serv 58:102202. https://doi.org/10.1016/j.jretconser.2020.102202
Pandey P, Swarnkar R, Kakaria S, Miyapuram KP (2020) Understanding consumer preferences for movie trailers from EEG using machine learning. arXiv Preprint. https://arxiv.org/abs/2007.10756
Pennanen K, Närväinen J, Vanhatalo S, Raisamo R, Sozer N (2020) Effect of virtual eating environment on consumers’ evaluations of healthy and unhealthy snacks. Food Qual Prefer 82:103871. https://doi.org/10.1016/j.foodqual.2020.103871
Pfurtscheller G (1981) Central beta rhythm during sensorimotor activities in man. Electroencephalogr Clin Neurophysiol 51(3):253–264. https://doi.org/10.1016/0013-4694(81)90139-5
Pfurtscheller G, Andrew C (1999) Event-related changes of band power and coherence: methodology and interpretation. J Clin Neurophysiol 16(6):512
Phutela N, Sreevathsan K, Krupa BN (2022) Intelligent analysis of EEG signals to assess consumer decisions: a Study on Neuromarketing. arXiv. https://arxiv.org/abs/2206.07484
Pileliene L, Grigaliunaite V (2017) The effect of female celebrity spokesperson in FMCG advertising: neuromarketing approach. J Consum Mark 34(3):202–213. https://doi.org/10.1108/JCM-02-2016-1723
Pileliene L, Grigaliunaite V (2017) Relationship between Spokesperson’s gender and advertising color temperature in a framework of advertising effectiveness. Sci Annals Econ Bus 64:1–13. https://doi.org/10.1515/saeb-2017-0036
Plassmann H, Ramsøy TZ, Milosavljevic M (2012) Branding the brain: a critical review and outlook. J Cons Psychol 22(1):18–36. https://doi.org/10.1016/j.jcps.2011.11.010
Poels K, Dewitte S (2006) How to capture the heart? Reviewing 20 years of emotion measurement in advertising. J Advert Res 46(1):18–37
Pozharliev R, Verbeke WJMI, Van Strien JW, Bagozzi RP (2015) Merely being with you increases my attention to luxury products: using EEG to understand consumers’ emotional experience with luxury branded products. J Market Res 52(4):546–558. https://doi.org/10.1509/jmr.13.0560
Raiesdana S, Mousakhani M (2022) An EEG-based neuromarketing approach for analyzing the preference of an electric car. Comput Intel Neurosci. https://doi.org/10.1155/2022/9002101
Ramsøy TZ, Skov M, Christensen MK, Stahlhut C (2018) Frontal brain asymmetry and willingness to pay. Front Neurosci 12:138. https://doi.org/10.3389/fnins.2018.00138
Ramsøy TZ, Noela M, Michael I (2019) A consumer neuroscience study of conscious and subconscious destination preference. Sci Rep 9:1–8. https://doi.org/10.1038/s41598-019-51567-1
Ravaja N, Somervuori O, Salminen M (2013) Predicting purchase decision: the role of hemispheric asymmetry over the frontal cortex. J Neurosci Psychol Econ 6(1):1–13. https://doi.org/10.1037/a0029949
Rawnaque FS, Rahman KM, Anwar SF, Vaidyanathan R, Chau T, Sarker F, Mamun KA (2020) Technological advancements and opportunities in neuromarketing: a systematic review. Brain Informat 7(1):1–19. https://doi.org/10.1186/s40708-020-00109-x
Rayner K, Castelhano MS (2008) Eye movements during reading, scene perception, visual search, and while looking at print advertisements. Taylor and Francis Group, Washington
Robaina-Calderín L, Martín-Santana J (2021) A review of research on neuromarketing using content analysis: key approaches and new avenues. Cog Neurodyn 15(6):923–938. https://doi.org/10.1007/s11571-021-09693-y
Roberts H, Soto V, Tyson-Carr J, Kokmotou K, Cook S, Fallon N, Giesbrecht T, Stancak A (2018) Tracking economic value of products in natural settings: a wireless EEG study. Front Neurosci. https://doi.org/10.3389/fnins.2018.00910
Robinson R (2004) fMRI beyond the clinic: will it ever be ready for prime time? PLoS Biol 2(6):e150. https://doi.org/10.1371/journal.pbio.0020150
Roelofs R, Shankar V, Recht B, Fridovich-Keil S, Hardt M, Miller J, Schmidt L (2019) A meta-analysis of overfitting in machine learning. Adv Neural Inform Proces Syst 10:32. https://doi.org/10.5555/3454287.3455110
Rosenbaum MS, Contreras Ramirez G, Matos N (2019) A neuroscientific perspective of consumer responses to retail greenery. Service Indust J 39(15–16):1034–1045. https://doi.org/10.1080/02642069.2018.1487406
Rothschild ML, Hyun YJ, Reeves B, Thorson E, Goldstein R (1988) Hemispherically lateralized EEG as a response to television commercials. J Cons Res 15(2):185–198. https://doi.org/10.1086/209156
Rothschild ML, Thorson E, Reeves B, Hirsch JE, Goldstein R (1986) EEG activity and the processing of television commercials. Commun Res 13(2):182–220. https://doi.org/10.1177/009365086013002003
Rothschild ML, Hyun YJ (1990) Predicting memory for components of Tv commercials from EEG. J Cons Res 16(4):472–478. https://doi.org/10.1086/209232
Roux F, Wibral M, Mohr HM, Singer W, Uhlhaas PJ (2012) Gamma-band activity in human prefrontal cortex codes for the number of relevant items maintained in working memory. J Neurosci 32(36):12411–12420. https://doi.org/10.1523/JNEUROSCI.0421-12.2012
Royo M, Chulvi V, Mulet E, Galan J (2018) Users’ reactions captured by means of an EEG headset on viewing the presentation of sustainable designs using verbal narrative. Eur J Market 52(1–2):159–181. https://doi.org/10.1108/EJM-12-2016-0837
Russo V, Songa G, Milani Marin LE, Balzaretti CM, Tedesco DEA (2020) Novel Food-Based Product Communication: A Neurophysiological Study. Nutrients. https://doi.org/10.3390/nu12072092
Sapountzis P, Gregoriou GG (2018) Neural signatures of attention: insights from decoding population activity patterns. Front Biosci 23:221–246
Sauseng P, Griesmayr B, Freunberger R, Klimesch W (2010) Control mechanisms in working memory: a possible function of EEG theta oscillations. Neurosci Biobehav Rev 34(7):1015–1022. https://doi.org/10.1016/j.neubiorev.2009.12.006
Schacter DL (1977) EEG theta waves and psychological phenomena: a review and analysis. Biol Psychol 5(1):47–82. https://doi.org/10.1016/0301-0511(77)90028-X
Schaefer A, Buratto LG, Goto N, Brotherhood EV (2016) The feedback-related negativity and the P300 brain potential are sensitive to price expectation violations in a virtual shopping task. PLoS ONE 11(9):e0163150. https://doi.org/10.1371/journal.pone.0163150
Shestyuk AY, Kasinathan K, Karapoondinott V, Knight RT, Gurumoorthy R (2019) Individual EEG measures of attention, memory, and motivation predict population level TV viewership and Twitter engagement. PLoS ONE 14(3):e0214507. https://doi.org/10.1371/journal.pone.0214507
Schneider S, Strüder HK (2012) EEG: Theoretical background and practical aspects. In: Henning B, Charles HH, Lukas S, Heiko KS (eds) Functional neuroimaging in exercise and sport sciences. Springer, New York, pp 197–212
Schoen F, Lochmann M, Prell J, Herfurth K, Rampp S (2018) Neuronal correlates of product feature attractiveness. Front Behav Neurosci. https://doi.org/10.3389/fnbeh.2018.00147
Schöne B, Köster M, Gruber T (2018) Coherence in general and personal semantic knowledge: functional differences of the posterior and centro-parietal N400 ERP component. Exp Brain Res 236(10):2649–2660. https://doi.org/10.1007/s00221-018-5324-1
Schupp HT, Öhman A, Junghöfer M, Weike AI, Stockburger J, Hamm AO (2004) The facilitated processing of threatening faces: an ERP analysis. Emotion 4(2):189. https://doi.org/10.1037/1528-3542.4.2.189
Schupp HT, Schmälzle R, Flaisch T, Weike AI, Hamm AO (2012) Affective picture processing as a function of preceding picture valence: an ERP analysis. Biol Psychol 91(1):81–87. https://doi.org/10.1016/j.biopsycho.2012.04.006
Senecal S, Fredette M, Leger P, Courtemanche F, Riedl R (2015) Consumers’ cognitive lock-in on websites: evidence from a neurophysiological study. J Internet Commer 14(3):277–293. https://doi.org/10.1080/15332861.2015.1028249
Senior C, Lee N (2013) The state of the art in organizational cognitive neuroscience: the therapeutic gap and possible implications for clinical practice. Front Hum Neurosci 7:808. https://doi.org/10.3389/fnhum.2013.00808
Shaari N, Syafiq M, Amin M, Mikami O (2019) Electroencephalography (EEG) application in neuromarketing-exploring the subconscious mind. J Adv Manuf Tech 13(2):2
Shang Q, Pei G, Jia J, Zhang W, Wang Y, Wang X (2018) ERP evidence for consumer evaluation of copycat brands. PLoS ONE 13(2):e0191475. https://doi.org/10.1371/journal.pone.0191475
Shang Q, Jin J, Pei G, Wang C, Wang X, Qiu J (2020) Low-order webpage layout in online shopping facilitates purchase decisions: evidence from event-related potentials. Psychol Res Behav Manage 13:29–39. https://doi.org/10.2147/PRBM.S238581
Shang Q, Pei G, Dai S, Wang X (2017) Logo effects on brand extension evaluations from the electrophysiological perspective. Front Neurosci 11:113. https://doi.org/10.3389/fnins.2017.00113
Shen Y, Shan W, Luan J (2018) Influence of aggregated ratings on purchase decisions: an event-related potential study. Eur J Market 52(1–2):147–158. https://doi.org/10.1108/EJM-12-2016-0871
Silverstein BH, Snodgrass M, Shevrin H, Kushwaha R (2015) P3b, consciousness, and complex unconscious processing. Cortex 73:216–227. https://doi.org/10.1016/j.cortex.2015.09.004
Slanzi G, Balazs JA, Velasquez JD (2017) Combining eye tracking, pupil dilation and EEG analysis for predicting web users click intention. Inform Fusion 35:51–57. https://doi.org/10.1016/j.inffus.2016.09.003
Smith EE, Reznik SJ, Stewart JL, Allen JJ (2017) Assessing and conceptualizing frontal EEG asymmetry: an updated primer on recording, processing, analyzing, and interpreting frontal alpha asymmetry. Internat J Psychophysiol 111:98–114. https://doi.org/10.1016/j.ijpsycho.2016.11.005
Smith ME, Gevins A (2004) Attention and brain activity while watching television: components of viewer engagement. Media Psychol 6(3):285–305. https://doi.org/10.1207/s1532785xmep0603_3
Soria Morillo LM, Alvarez-Garcia JA, Gonzalez-Abril L, Ortega Ramirez JA (2016) Discrete classification technique applied to TV advertisements liking recognition system based on low-cost EEG headsets. Biomed Eng Online 15(Suppl 1):75–82. https://doi.org/10.1186/s12938-016-0181-2
Soria Morillo LM, Alvarez Garcia JA, Gonzalez-Abril L, Ortega Ramirez JA (2015) Advertising liking recognition technique applied to neuromarketing by using low-cost EEG headset. Bioinform Biomed Eng 9044:701–709. https://doi.org/10.1007/978-3-319-16480-9_68
Steriade M (1999) Coherent oscillations and short-term plasticity in corticothalamic networks. Trends Neurosci 22(8):337–345. https://doi.org/10.1016/S0166-2236(99)01407-1
Stewart DW, Furse DH (1982) Applying psychophysiological measures to marketing and advertising research problems. Curr Issues Res Advert 5(1):1–38. https://doi.org/10.1080/01633392.1982.10505319
Telpaz A, Webb R, Levy DJ (2015) Using EEG to Predict Consumers’ Future Choices. J Market Res 52(4):511–529. https://doi.org/10.1509/jmr.13.0564
Thomas A, Hammer A, Beibst G, Muente TF (2013) An ERP-study of brand and no-name products. Bmc Neurosci 14:149. https://doi.org/10.1186/1471-2202-14-149
Touchette B, Lee S (2017) Measuring neural responses to apparel product attractiveness: an application of frontal asymmetry theory. Cloth Text Res J 35(1):3–15. https://doi.org/10.1177/0887302X16673157
Treleaven-Hassard S, Gold J, Bellman S, Schweda A, Ciorciari J, Critchley C, Varan D (2010) Using the P3a to gauge automatic attention to interactive television advertising. J Econ Psychol 31(5):777–784. https://doi.org/10.1016/j.joep.2010.03.007
Tyson-Carr J, Soto V, Kokmotou K, Roberts H, Fallon N, Byrne A, Giesbrecht T, Stancak A (2020) Neural underpinnings of value-guided choice during auction tasks: an eye-fixation related potentials study. Neuroimage. https://doi.org/10.1016/j.neuroimage.2019.116213
Ullah A, Baloch G, Ahmed A, Buriro AB, Junaid A, Ahmed B, Akhtar S (2022) Neuromarketing solutions based on EEG signal analysis using machine learning. Internat J Adv Comp Sci Appl. https://doi.org/10.14569/IJACSA.2022.0130137
Uva T, Freitas Paiva CLT (2015) Neuroscience technologies in marketing: a study of gender and TV advertisements using electroencephalography. Internat J Technol Market 10(4):362–380. https://doi.org/10.1504/IJTMKT.2015.072181
Varan D, Lang A, Barwise P, Weber R, Bellman S (2015) How reliable are neuromarketers’ measures of advertising effectiveness? Data from ongoing research holds no common truth among vendors. J Advert Res 55(2):176–191. https://doi.org/10.2501/JAR-55-2-176-191
Vecchiato G, Fallani FV, Astolfi L, Toppi J, Cincotti F, Mattia D, Salinari S, Babiloni F (2010) The issue of multiple univariate comparisons in the context of neuroelectric brain mapping: an application in a neuromarketing experiment. J Neurosci Methods 191(2):283–289. https://doi.org/10.1016/j.jneumeth.2010.07.009
Vecchiato G, Di Flumeri G, Maglione AG, Cherubino P, Kong W, Trettel A, Babiloni F (2014) An electroencephalographic peak density function to detect memorization during the observation of TV commercials. In: Vecchiato G (ed) Annual international conference of the IEEE engineering in medicine and biology society. IEEE, Chicago, pp 6969–6972. https://doi.org/10.1109/EMBC.2014.6945231
Vecchiato G, Kong W, Maglione AG, Wei D (2012) Understanding the impact of TV commercials: electrical neuroimaging. IEEE Pulse 3(3):42–47. https://doi.org/10.1109/MPUL.2012.2189171
Vecchiato G, Maglione AG, Cherubino P, Wasikowska B, Wawrzyniak A, Latuszynska A, Latuszynska M, Nermend K, Graziani I, Leucci MR, Trettel A, Babiloni F (2014) Neurophysiological tools to investigate consumer’s gender differences during the observation of TV commercials. Comput Math Methods Med 2014:912981. https://doi.org/10.1155/2014/912981
Vecchiato G, Astolfi L, Cincotti F, Fallani FDV, Sorrentino DM, Mattia D, Salinari S, Bianchi L, Toppi J, Aloise F (2010) Patterns of cortical activity during the observation of public service announcements and commercial advertisings. Nonlinear Biomed Phys 4(1):1–9
Vecchiato G, Astolfi L, De Vico Fallani F, Cincotti F, Mattia D, Salinari S, Soranzo R, Babiloni F (2010) Changes in brain activity during the observation of TV commercials by using EEG, GSR and HR measurements. Brain Topogr 23(2):165–179. https://doi.org/10.1007/s10548-009-0127-0
Vecchiato G, Astolfi L, Tabarrini A, Salinari S, Mattia D, Cincotti F, Bianchi L, Sorrentino D, Aloise F, Soranzo R (2010) EEG analysis of the brain activity during the observation of commercial, political, or public service announcements. Comput Intel Neurosci. https://doi.org/10.1155/2010/985867
Vecchiato G, Cherubino P, Maglione AG, Ezquierro MTH, Marinozzi F, Bini F, Trettel A, Babiloni F (2014) How to measure cerebral correlates of emotions in marketing relevant tasks. Cog Comput 6(4):856–871. https://doi.org/10.1007/s12559-014-9304-x
Vecchiato G, Kong W, Maglione AG, Cherubino P, Trettel A, Babiloni F (2014) Cross-cultural analysis of neuroelectrical cognitive and emotional variables during the appreciation of TV commercials. Neuropsychol Trends 16(16):23–29
Vecchiato G, Toppi J, Astolfi L, De Vico Fallani F, Cincotti F, Mattia D, Bez F, Babiloni F (2011) Spectral EEG frontal asymmetries correlate with the experienced pleasantness of TV commercial advertisements. Med Biol Eng Comp 49(5):579–583. https://doi.org/10.1007/s11517-011-0747-x
Wagner J, Makeig S, Gola M, Neuper C, Müller-Putz G (2016) Distinct β band oscillatory networks subserving motor and cognitive control during gait adaptation. J Neurosci 36(7):2212–2226. https://doi.org/10.1523/JNEUROSCI.3543-15.2016
Wajid A, Raziq MM, Ahmed QM, Ahmad M (2021) Observing viewers’ self-reported and neurophysiological responses to message appeal in social media advertisements. J Retail Cons Serv 59:102373. https://doi.org/10.1016/j.jretconser.2020.102373
Walsh AM, Duncan SE, Bell MA, O’Keefe SF, Gallagher DL (2017) Integrating implicit and explicit emotional assessment of food quality and safety concerns. Food Qual Prefer 56:212–224. https://doi.org/10.1016/j.foodqual.2016.11.002
Wang C, Li Y, Luo X, Ma Q, Fu W, Fu H (2018) The effects of money on fake rating behavior in E-commerce: electrophysiological time course evidence from consumers. Front Neurosci 12:156. https://doi.org/10.3389/fnins.2018.00156
Wang D, Zhou C, Chang Y (2015) Acute exercise ameliorates craving and inhibitory deficits in methamphetamine: an ERP study. Physiol Behav 147:38–46. https://doi.org/10.1016/j.physbeh.2015.04.008
Wang J, Han W (2014) The impact of perceived quality on online buying decisions: an event-related potentials perspective. NeuroReport 25(14):1091–1098. https://doi.org/10.1097/WNR.0000000000000233
Wang Q, Wedel M, Huang L, Liu X (2018) Effects of model eye gaze direction on consumer visual processing: evidence from China and America. Inform Manage 55(5):588–597. https://doi.org/10.1016/j.im.2017.12.003
Wang RWY, Chang Y, Chuang S (2016) EEG spectral dynamics of video commercials: impact of the narrative on the branding product preference. Sci Rep 6:36487. https://doi.org/10.1038/srep36487
Wang RW, Chen YC, Liu I, Chuang SW (2018) Temporal and spectral EEG dynamics can be indicators of stealth placement. Sci Rep 8:1–17. https://doi.org/10.1038/s41598-018-27294-4
Wang TC, Tsai CL, Tang TW (2018) Exploring advertising effectiveness of tourist hotels’ marketing images containing nature and performing arts: an eye-tracking analysis. Sustainability 10(9):3038. https://doi.org/10.3390/su10093038
Wang X, Ma Q, Wang C (2012) N400 as an index of uncontrolled categorization processing in brand extension. Neurosci Let 525(1):76–81. https://doi.org/10.1016/j.neulet.2012.07.043
Wang Y, Ma N, Wang J, Hu Z, Liu Z, He J (2020) Prediction of product design decision making: an investigation of eye movements and EEG features. Adv Eng Inform. https://doi.org/10.1016/j.aei.2020.101095
Wang YJ, Minor MS (2008) Validity, reliability, and applicability of psychophysiological techniques in marketing research. Psychol Market 25(2):197–232. https://doi.org/10.1002/mar.20206
Wang Y, Hsieh C (2018) Explore technology innovation and intelligence for IoT (Internet of Things) based eyewear technology. Technol Forecast Soc Change 127:281–290. https://doi.org/10.1016/j.techfore.2017.10.001
Wedel M, Pieters R (2017) A review of eye-tracking research in marketing. In: Naresh KM (ed) Review of marketing research. Routlege, London, pp 123–147
Wei Z, Wu C, Wang X, Supratak A, Wang P, Guo Y (2018) Using support vector machine on EEG for advertisement impact assessment. Front Neurosci 12:76. https://doi.org/10.3389/fnins.2018.00076
White KR, Crites SL Jr, Taylor JH, Corral G (2009) Wait, what? Assessing stereotype incongruities using the N400 ERP component. Soc Cognit Affect Neurosci 4(2):191–198. https://doi.org/10.1093/scan/nsp004
Wriessnegger SC, Hackhofer D, Müller-Putz GR (2015) Classification of unconscious like/dislike decisions: first results towards a novel application for BCI technology. In: 2015 37th Annual International Conference of the IEEE Engineering in Medicine and Biology Society (EMBC), 2331–2334
Yadava M, Kumar P, Saini R, Roy PP, Dogra DP (2017) Analysis of EEG signals and its application to neuromarketing. Multimed Tools Appl 76(18):19087–19111. https://doi.org/10.1007/s11042-017-4580-6
Yang D (2018) Exploratory neural reactions to framed advertisement messages of smoking cessation. Soc Market Quarter 24(3):216–232. https://doi.org/10.1177/1524500418788306
Yang S (2015) An eye-tracking study of the elaboration likelihood model in online shopping. Electron Comm Res Appl 14(4):233–240. https://doi.org/10.1016/j.elerap.2014.11.007
Yang T, Lee S, Seomoon E, Kim SP (2018) Characteristics of human brain activity during the evaluation of service-to-service brand extension. Front Hum Neurosci 12:44. https://doi.org/10.3389/fnhum.2018.00044
Yang T, Do-Young L, Kwak Y, Choi J, Kim C, Sung-Phil K (2015) Evaluation of TV commercials using neurophysiological responses. J Physiol Anthropol. https://doi.org/10.1186/s40101-015-0056-4
Yang T, Kim S (2019) Group-level neural responses to service-to-service brand extension. Front Neurosci 13:676. https://doi.org/10.3389/fnins.2019.00676
Yazid AF, Mohd SM, Khan AR, Ali KR, Kamarudin S, Jan NM (2020) Decision-making analysis using arduino-based electroencephalography (EEG): an exploratory study for marketing strategy. Internat J Adv Comput Sci Appl 11(9):236–243. https://doi.org/10.14569/IJACSA.2020.0110927
Yen C, Chiang M (2021) Examining the effect of online advertisement cues on human responses using eye-tracking, EEG, and MRI. Behav Brain Res 402:113128. https://doi.org/10.1016/j.bbr.2021.113128
Ying X (2019) An overview of overfitting and its solutions. J Phys 1168(2):022022. https://doi.org/10.1088/1742-6596/1168/2/022022
Yılmaz B, Korkmaz S, Arslan DB, Güngör E, Asyalı MH (2014) Like/dislike analysis using EEG: determination of most discriminative channels and frequencies. Comput Method Prog Biomed 113(2):705–713. https://doi.org/10.1016/j.cmpb.2013.11.010
Yoon C, Gonzalez R, Bechara A, Berns GS, Dagher AA, Dubé L, Huettel SA, Kable JW, Liberzon I, Plassmann H, Smidts A, Spence C (2012) Decision neuroscience and consumer decision making. Market Let 23(2):473–485. https://doi.org/10.1007/s11002-012-9188-z
Young C (2002) Brain waves, picture sorts (R), and branding moments. J Advert Res 42(4):42–53. https://doi.org/10.2501/JAR-42-4-42-53
Yu W, Sun Z, Xu T, Ma Q (2018) Things become appealing when i win: neural evidence of the influence of competition outcomes on brand preference. Front Neurosci 12:779. https://doi.org/10.3389/fnins.2018.00779
Zamani J, Naieni AB (2020) Best feature extraction and classification algorithms for EEG signals in neuromarketing. Front Biomed Technol 7(3):186–191. https://doi.org/10.18502/fbt.v7i3.4621
Zeng L, Lin M, Xiao K, Wang J, Zhou H (2021) Prediction of consumer preference for sports shoes with EEG: an application of neuromarketing. Front Hum Neurosci. p 775.
Zhang J, Tang C, Guo L, Xu H (2018) A longitudinal investigation of customer cooperation in services: The role of appraisal of cooperation behaviors. Psychol Market 35(12):957–967. https://doi.org/10.1002/mar.21148
Zhang W, Jin J, Wang A, Ma Q, Yu H (2019) Consumers’ implicit motivation of purchasing luxury brands: an EEG study. Psychol Res Behav Manage 12:913–929. https://doi.org/10.2147/PRBM.S215751
Zhang X (2020) The influences of brand awareness on consumers’ cognitive process: an event-related potentials study. Front Neurosci. https://doi.org/10.3389/fnins.2020.00549
Zhao M, Wang J, Zhang H, Zhao G (2019) ERP perspective analysis of PSS component and decision-making. Sustainability. https://doi.org/10.3390/su11041063
Zheng W, Liu W, Lu Y, Lu B, Cichocki A (2018) Emotionmeter: a multimodal framework for recognizing human emotions. IEEE Trans Cybern 49(3):1110–1122. https://doi.org/10.1109/TCYB.2018.2797176
Zhu S, Qi J, Hu J, Hao S (2022) A new approach for product evaluation based on integration of EEG and eye-tracking. Adv Eng Inform 52:101601. https://doi.org/10.1016/j.aei.2022.101601
Zubair M, Iqbal S, Usman SM, Awais M, Wang R, Wang X (2020) Message framing and self-conscious emotions help to understand pro-environment consumer purchase intention: an ERP study. Sci Rep. https://doi.org/10.1038/s41598-020-75343-8
Download references
Acknowledgements
Not applicable.
This work was funded by the Knowledge Transfer Partnership (KTP) scheme, [KTP Prog. No.: KTP 12421; KB ref. no.: R3200 C659], Innovate UK, UKRI, Keele University, Staffordshire, UK, and risual Limited, Staffordshire, UK.
Author information
Authors and affiliations.
Keele Business School, Denise Coates Foundation Building, Keele University, Staffordshire, ST5 5AA, UK
Adam Byrne & Colin Rigby
Faculty of Humanities and Social Sciences, Keele University, Staffordshire, UK
Adam Byrne & Emma Bonfiglio
Risual Limited, Staffordshire, UK
School of Psychology, Keele University, Staffordshire, UK
Nicky Edelstyn
You can also search for this author in PubMed Google Scholar
Contributions
AB was responsible for the primary data collection and the writing of the manuscript. EB administrated funding and reviewed the final manuscript. CR supervised the systematic review and reviewed the final manuscript. NE supervised the systematic review, aided in the writing of the manuscript and reviewed the final manuscript. All authors read and approved the final manuscript.
Corresponding author
Correspondence to Adam Byrne .
Ethics declarations
Competing interests.
The authors declare that they have no competing interests.
Additional information
Publisher's note.
Springer Nature remains neutral with regard to jurisdictional claims in published maps and institutional affiliations.
Rights and permissions
Open Access This article is licensed under a Creative Commons Attribution 4.0 International License, which permits use, sharing, adaptation, distribution and reproduction in any medium or format, as long as you give appropriate credit to the original author(s) and the source, provide a link to the Creative Commons licence, and indicate if changes were made. The images or other third party material in this article are included in the article's Creative Commons licence, unless indicated otherwise in a credit line to the material. If material is not included in the article's Creative Commons licence and your intended use is not permitted by statutory regulation or exceeds the permitted use, you will need to obtain permission directly from the copyright holder. To view a copy of this licence, visit http://creativecommons.org/licenses/by/4.0/ .
Reprints and permissions
About this article
Cite this article.
Byrne, A., Bonfiglio, E., Rigby, C. et al. A systematic review of the prediction of consumer preference using EEG measures and machine-learning in neuromarketing research. Brain Inf. 9 , 27 (2022). https://doi.org/10.1186/s40708-022-00175-3
Download citation
Received : 25 March 2022
Accepted : 15 September 2022
Published : 14 November 2022
DOI : https://doi.org/10.1186/s40708-022-00175-3
Share this article
Anyone you share the following link with will be able to read this content:
Sorry, a shareable link is not currently available for this article.
Provided by the Springer Nature SharedIt content-sharing initiative
- Neuromarketing
- Machine learning
- Systematic review

An official website of the United States government
The .gov means it’s official. Federal government websites often end in .gov or .mil. Before sharing sensitive information, make sure you’re on a federal government site.
The site is secure. The https:// ensures that you are connecting to the official website and that any information you provide is encrypted and transmitted securely.
- Publications
- Account settings
Preview improvements coming to the PMC website in October 2024. Learn More or Try it out now .
- Advanced Search
- Journal List
- Behav Sci (Basel)

Accessing Neuromarketing Scientific Performance: Research Gaps and Emerging Topics
Lucília cardoso.
1 CiTUR-Leiria, 2411-901 Leiria, Portugal; [email protected] (L.C.); [email protected] (F.D.)
Meng-Mei Chen
2 Ecole Hoteliere de Lausanne, HES-SO University of Applied Sciences and Arts Western, 2800 Delémont, Switzerland; [email protected]
Arthur Araújo
3 Transdisciplinary Research Center on Innovation & Entrepreneurship Ecosystems (TRIE), Lusofona University, 1749-024 Lisboa, Portugal; tp.plu.563osm@6075p
Giovana Goretti Feijó de Almeida
Francisco dias, luiz moutinho.
4 Visiting Professor of Marketing, Suffolk Business School, University of Suffolk, Suffolk IP4 1QJ, UK; moc.liamg@ohnituommaziul
(1) Background: Using neuroscience to understand and influence consumer behavior often leads to ethical controversy. Thus, it is necessary to demystify the use of neuroscience for marketing purposes; the present paper, by accessing the worldwide academic performance in this domain, fulfills this objective. (2) Methods: All extant literature on neuromarketing indexed to the Scopus database—318 articles—was subjected to a bibliometric analysis through a mixed-method approach. (3) Results: The results show that Spain leads the ranks of the most productive countries, while Italian researchers clearly dominate in terms of collaboration. Regarding the most prominent topics, the connection between “Neuroscience” and “Advertising” is highlighted. The findings provide a better understanding of the state-of-the-art in neuromarketing studies, research gaps, and emerging research topics, and additionally provide a new methodological contribution by including SciVal topic prominence in the bibliometric analysis. (4) Conclusions: As practical implications, this study provides useful insights for neuromarketing researchers seeking funding opportunities, which are normally associated with topics within the top prominence percentile or emerging topics. In terms of originality, this study is the first to apply SciVal topic prominence to a bibliometric analysis of neuromarketing, and provides a new bibliometric indicator for neuromarketing research.
1. Introduction
As a research field, neuromarketing emerged from the application of neuroscience methods and techniques for marketing purposes, i.e., consumer behavior as a response to a certain stimulus [ 1 ]. In this context, neuromarketing is an interdisciplinary domain with great of potential, as it allows researchers to understand and predict consumer choices and behavior. Therefore, it can lead to new marketing theories and good practices. However, the fulfillment of such potential is often limited by a mystified view of the use of neuroscience methods by marketing researchers. In order to mitigate this problem, Ref. [ 2 ] mapped the extant literature on neuromarketing, documenting the different methods employed and identifying the main researchers on the topic.
Mapping scientific literature through bibliometric studies is a useful method of providing an assessment of scientific production in a specific area over a given period [ 3 ]. Bibliometric studies allow for the assessment of research, including the themes sought, methods employed, and samples used. In this context, Koseoglu [ 4 ] argues that bibliometric studies highlight unknown patterns in research fields or disciplines, helping researchers to develop theories and test hypotheses, concluding that “these structural, dynamic, evaluative, and predictive models can be skillfully applied in the evaluation and prediction of a field” (p. 191).
Aware of this potential, several other studies have mapped scientific production on neuromarketing. Amongst these, Alsharif et al. [ 5 ] analysed studies indexed to the Web of Science database published between 2007 and 2019. In addition to being limited to this database and time period, the study did not include a key-theme analysis. Sousa and Lara [ 6 ], in turn, conducted a bibliometric study to identify the technologies applied in neuromarketing. This study, however, did not include articles indexed in the Scopus database. Finally, Wannyn [ 7 ] analysed studies from Web of Science covering the 2004–2015 period. In sum, to the best of the authors’ knowledge no previous work has mapped neuromarketing research production in the Scopus database. Moreover, no studies dealing with the mapping of co-word analysis and SCival topic prominence in neuromarketing were found. SCival topic prominence is provided by Scopus and combines three metrics that indicate the momentum of a topic: (1) Citation count in year n of papers published in n and n − 1; (2) Scopus view count in year n to papers published in n and n − 1; and (3) Average CiteScore for year n [ 8 , 9 , 10 ].
To contribute to the filling of these research gaps, the present study aims to assess the global performance of neuromarketing studies. To achieve this general goal, the following specific objectives were adopted:
- (1) To determine the overall performance of neuromarketing research;
- (2) To determine the performance of the most cited articles;
- (3) To identify the most prominent topics within the neuromarketing field;
- (4) To identify the prominent Scival topics on neuromarketing; and
- (5) To identify the best Scival topics on neuromarketing by percentile.
In order to fulfill these specific objectives, all of the extant literature on neuromarketing indexed to the Scopus database was analyzed. Said body of literature included a total of 318 articles published during the 2007–2020 period. The retrieved articles were subjected to a bibliometric analysis through a mixed-method approach, which encompassed a key-word analysis and a SCival topic prominence analysis. The findings revealed the overall structure of the neuromarketing topic as well as research gaps and emerging research areas, which is useful for both researchers and research institutions. Moreover, the authors with highest research performance were identified, and collaboration patterns were revealed.
Regarding the topic’s overall performance, the findings point to the most productive journals, countries, research institutions, and researchers, and provide a general picture of global collaboration. In terms of the most cited articles, the findings highlight the top ten papers in terms of views and citation, amongst which, as in other emergent topics, introductory works and systematic reviews are dominant. In terms of the most prominent topics, the findings point to the connection between “Neuroscience”, “Advertising”, “Television”, and several topics related to neuroscience techniques. The relationship between these topics is addressed in more detail in the conclusions. Finally, regarding the top 1% percentile, the findings show that these studies consist of audience response studies, methodology enhancement studies, and literature reviews. These findings provide a general picture of the most promising topics in neuromarketing, which is particularly useful for individual researchers and research centers aiming to increase their own impact factor and maximize their chances of obtaining funding for their projects.
2. Mapping Neuromarketing Science
2.1. bibliometric studies on neuromarketing.
Neuroscience methods use tools and techniques to measure, map and record brain or neural activity. In doing so, they generate neurological representations of this activity, which allows scientists to understand specific responses in the brain and nervous system upon exposure to a certain stimulus [ 1 ]. Due to their effectiveness in explaining human behavior, these methods are employed in several research fields. Recently, they have begun to be applied in order to “analyze and understand human behavior related to markets and marketing exchange” [ 11 ] (p. 3803), originating a new research field: neuromarketing [ 12 ]. In this context, neuromarketing is an interdisciplinary domain in which theories and methods from neuroscience and marketing, as well as from related disciplines such as economics, psychology and tourism, are combined in order to understand consumer choices and behavior. Neuromarketing is thus a promising field, as findings in the area can lead to new foundations and generate new marketing theories or complement existing ones [ 2 ]. The majority of neuromarketing studies focus on emotions, which play an important role in all human activities. Emotions relate to various affective states, both positive and negative [ 13 ], as well as to the psycho-behavioral aspects of consumers.
As argued by Lim [ 2 ], “neuromarketing, as a method of investigation, is important because it uses neuroscientific theories and methods to gain access to otherwise hidden information” (p. 1). However, the same author argues that neuromarketing needs to be demystified. Although there are many conceptual works and several bibliographical reviews on the topic, few studies have rigorously produced empirical findings. Therefore, Lim [ 2 ] points out a lack of effectiveness in the use of neuroscience measurement techniques to advance marketing theory and address ethical issues. Moreover, neuroscience can intimidate academics due to lack of knowledge regarding the data-gathering methods it uses. In this context, Lim [ 2 ] attempts to enhance understanding of the potential of neuromarketing by documenting the different neuroscientific methods used in neuromarketing, identifying the main researchers on the topic, and addressing their main contributions. In other words, the study mapped the extant literature on neuromarketing through a bibliometric study, the same method employed in the present study. Therefore, the next section is dedicated to addressing the main fundaments and contributions of the application of bibliometric methods to the mapping of research topics.
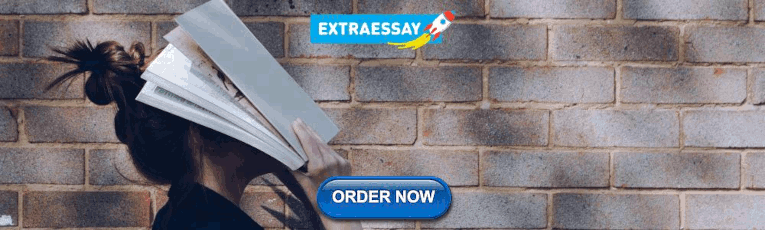
2.2. Mapping Research Topics through Bibliometric Studies
Bibliometric studies can analyze several aspects of a body of research, including the productivity of individual scholars and institutions, knowledge flow and social networks, topics’ long-term development trends and emerging topics, journal rankings and journal development, and the most frequently cited scholars and works [ 14 ]. Koseoglu et al. [ 4 ] and Huang et al. [ 15 ] identified three types of bibliometric methods: relational techniques, evaluative techniques, and review studies. Relational techniques explore relationships within research, such as the structure of research fields, the emergence of new research themes and methods, and co-citation and co-authorship patterns. These can be divided into four categories: co-citation analysis, co-word analysis, co-authorship analysis, and bibliographic coupling [ 4 ] (p. 182). Evaluative techniques assess the impact of scholarly work, usually aiming to compare the performance or scientific contributions of two or more individuals or groups. To this end, evaluative techniques employ productivity measures, impact metrics, and hybrid metrics. Finally, review studies simply perform thorough literature reviews on a given topic. Through these three sets of techniques, bibliometric studies allow researchers to analyze research topics and the publications of individuals and/or institutions, as well as to map the structure and dynamics of science [ 15 ]. In sum, they serve to assess research performance.
Research performance is characterized by metrics that measure certain variables considered to define academic excellence. The term is associated with many programs and departments of institutions that are recognized as having high-quality research output [ 16 ]. Quantitatively, research performance is assessed by a range of metrics such as citations per article [ 17 ] and other citation metrics [ 18 ], cooperation indicators [ 19 ], and institutional contribution [ 20 ]. Additionally, it can be assessed through qualitative performance aspects [ 21 ].
When applied to a country, research performance can be defined by the performance of its institutions, which in turn depends on the performance of its researchers and is frequently conveyed through rankings (i.e., authors, affiliations, journal scores, number of articles published) [ 22 , 23 ]. More recently, research productivity has been closely associated with collaboration between authors and institutions [ 23 ], which has been shown to be a tool for knowledge creation, acquisition, and dissemination [ 24 ]. In this context, techniques focused on cooperation, such as network analysis, have been incorporated into bibliometric studies.
Adopting a broader approach to bibliographic analysis, the resent studies of Cardoso et al. [ 8 , 9 , 10 ], and Lima Santos et al. [ 25 ] have added a qualitative aspect to the assessment of research performance. Specifically, these authors combined traditional quantitative variables with analysis of the pioneering authors in a scientific area or research topic, the consistency of research over time, the number of papers with first authorship, and Scival topic prominence (including top quartiles).
In traditional bibliometrics, when authors use citation analysis to assess the impact of an article (e.g., when analysing an author’s or a university’s performance), the main indicator applied is the average number of citations per publication. In topic prominence analysis, the indicator applied is percentile-based [ 26 ]; that is, the higher the percentile, the greater the impact of the article [ 27 ]. In this context, the percentile reflects “the proportion of frequently cited publications, for instance, the proportion of publications that belong to the top 10% most frequently cited of their field” [ 28 ] (p. 372). Scival topic prominence is used to make predictions regarding a field of research. This new research indicator emerges from technology-related areas, and has been employed in the analysis of social science and business studies (i.e., tourism and hospitality) by Cardoso et al. [ 8 , 9 , 10 ]. By identifying research topics and capturing emerging subjects/topics in a specific area, topic prominence acts as a new performance indicator. It is useful in identifying whether a research topic is growing or declining, predicting whether it will grow or decline in the future, and indicating emerging topics of research [ 26 ]. In this context, according to Elsevier Publisher [ 29 ], topic prominence is considered to be a pointer that explains the prevailing momentum of a topic.
Moreover, as it indicates the impact of a paper, article or journal, topic prominence is a useful tool for researchers seeking funding. As argued by Wang and Shapira [ 30 ], high-impact articles (i.e., articles positioned in 90th and 95th percentiles) are far more likely to be associated with acknowledged funding when compared with low-impact articles. Therefore, topic prominence is normally used to support research funding [ 26 ]. Moreover, institutions seek to position themselves and gain notoriety through the impact of their publications. Therefore, topic prominence is a promising metric and has been increasingly employed for mapping scientific performance [ 31 ]. Given the potential of topic prominence, it was deemed an adequate tool for the present investigation.
3. Materials and Methods
The present investigation aimed to assess the global performance of neuromarketing studies. This objective was achieved through a set of methodological procedures, including a systematic search on neuromarketing and a combination of bibliometric analysis techniques. The following sub-sections address each of these procedures in detail.
3.1. Indicators and Methods Used
The methodological procedures employed in the present study included a combination of qualitative and quantitative analysis methods employed in different phases of the investigation, as in in previous bibliographic analyses by Cardoso et al. [ 8 , 9 , 10 ] and Ohniwa et al. [ 31 ]. The decision to use this approach was based on the premise that quantitative methods are more appropriate for drawing statistical inferences and comparisons, while qualitative methods are more suitable for discovering and generating theories [ 25 ]. In this context, several indicators, based on the contributions of previous studies [ 8 , 10 ] were employed in order to assess the performance of neuromarketing studies.
One such indicator was Scival topic prominence. As it had not previously been employed in bibliographic studies on the domain of neuromarketing, its application in the present investigation relied on contributions from studies in other research areas such as Klavans and Boyack [ 26 ] and Small et al. [ 32 ]. Other indicators that must be clarified include word growth and worth network; the use of such indicators was based on the work of Small et al. [ 32 ]. The methodological procedures adopted and the specific research questions that these indicators were intended to tackle are summarized in Figure 1 .

Summary of research questions, indicators, and technics used.
3.2. Data Collection and Organization Procedures
The data collection procedures were carried out on 7 January 2021. The first step consisted of conducting a document search on Scopus for articles containing the exact key word (Limit to: exactkeywork) “neuromarketing” in the title, abstract, or keywords. The Scopus database is one of the largest high-quality abstract and citation datasets of peer-reviewed literature on the web, and is the only database that features Scival topic prominence [ 8 , 9 , 33 ]. Therefore, it was deemed an adequate source for the purposes of the present investigation.
A total of 318 articles were retrieved. The database was downloaded in Bibtex format from Scopus and subsequently screened with the help of R Studio software (version 1.2.5042) in order to eliminate duplicates and create a unified file. The database was later exported to R Bibliometrix 3.0., which was used for the network analysis in the same manner as previous studies [ 22 , 34 ]. The next step consisted of homogenizing the data and creating an Excel file to which SciVal topic prominence information was manually added, as Scopus does not include such data in the initial downloadable output. The Excel file was uploaded to DB Gnosis software, in which the content analysis was carried out. As argued by Cardoso et al. [ 8 , 10 ] when applied to bibliometric studies, content analysis allows for a clear view of the evolution of the literature on a given topic through the mapping of its scientific production. Therefore, it was deemed a useful technique in the context of the present investigation. Table 1 summarizes the main information obtained on the Scopus database.
Description of Scopus neuromarketing data.
3.3. Data Analysis Techniques and Procedures
Overall performance, analogous to the studies of Cardoso et al. [ 8 , 10 ], was accessed through rankings obtained via frequency counting using DB Gnosis software. The only exception to this was collaboration, which was assessed through network analysis carried out in R Bibliometrix, as proposed by Lima Santos et al. [ 25 ]. The performance of the most cited authors was assessed through DB Gnosis using citation frequency as an indicator. This body of literature was interpreted through categorical content analysis, which served to identify and describe the following variables: topic area, application area, neuroscience technology used, and research gaps addressed. The variables of analysis in the literature review analysis followed the results of Alsharif et al. [ 12 , 33 ]. Additionally, the affiliation of each author was checked during content analysis.
Following the methodology previously applied by Lima Santos et al. [ 25 ] and Cardoso et al. [ 10 ], performance of the prominent topics was accessed using two analyses in R Bibliometrix. The authors’ network and authors’ keyword network structures were performed in R Bibliometrix through Biblioshiny, which provides a web interface for R Bibliometrix. The authors’ network parameters used included normalization by inclusion. The cluster algorithm applied was betweenness. The analysis included 22 edges (excluding isolated nodes), and the output figure considered a minimum of two edges. The authors’ keyword network parameter used was normalization by association. Again, the applied cluster algorithm was betweenness, the analysis included 22 edges (excluding isolated nodes), and the output figure considered a minimum of two edges. Finally, Scival topic prominence and the prominence percentile were accessed using the methodology proposed by Cardoso et al. [ 8 , 9 , 10 ], that is, frequency rankings carried out using DB Gnosis.
4.1. Neuromarketing: Overall Research Performance
The retrieved articles are published in 212 different journals, with a high level of dispersion between them. As shown in Table 2 , two journals, “Frontiers in Neuroscience” and “Frontiers in Psychology”, stand out with nine articles each. Accordingly, four publishers stand out for having ten or more papers in neuromarketing field.
Top ten journals and publishers in neuromarketing research productivity.
In terms of publications by country, as detailed in Table 3 Spain leads the ranking of the most productive countries with 48% of neuromarketing publications, followed by the United States and China. Spanish researchers focus on advertising, particularly online advertising and TV Commercials, as well as on the role of image and sound effects on viewer behavior. The articles published by Spanish research centers use eye tracking, galvanic skin response, electroencephalography (EEG) and functional magnetic resonance imaging (fMRI) technology. The United States, a close second, is responsible for 39% of all Scopus publications on neuromarketing. American research centers focus on studying TV Commercials. With a considerable gap from the top two countries, China ranks third with 24% of all Scopus publications on neuromarketing. The most-researched topics in Chinese institutions are consumer association and branding strategy, both of which are linked to TV commercials, color association, and tourism.
Top twenty countries in neuromarketing research productivity.
Regarding the most productive Institutions in neuromarketing research, the results are summarized in Table 4 . Complutense Madrid University (Spain) leads the ranking with 21 papers, followed by Roma la Sapienza University (Italy) and Zhejiang University (China) with 19 articles each. As expected, and considering the results shown in Table 3 , in addition to the leading institution Spain has three other universities in the top ten: Granada University is ranked sixth with twelve papers, while Valencia Polytechnic University and Vigo University share the seventh position with two other institutions (eight papers each).
Top ten institutions in neuromarketing research productivity.
Regarding individual authors’ performance (using articles fractionalized/co-authorship and first authorship papers as indicators), as displayed in Table 5 , two authors share the first position in terms of total articles published. The first, Babiloni F., is Professor of Physiology and Director of the Industrial Neuroscience lab in Rome’s Sapienza University, Italy. He has an average fractional article participation items of 1.20 and is a co-author of nine neuromarketing papers (although not being the first author on any), which mostly address TV Commercials. The other author sharing the first position, Ma Q., is a Professor in the School of Management at Zhejiang University, China and a member of the Neuromanagement Lab. He is the first author on six papers, and a co-author of another three. His research encompasses the topics of neuromanagement, branding (consumer behavior), purchase intention, price perception, and emotion.
Top ten authors’ neuromarketing research productivity.
The third most prominent author is Vecchiato G., from Rome University. He is the first author of seven papers and researches TV Commercials, focusing on emotion and attention. The fourth most prominent author is Crespo-Pereira V., from the University of Ecuador. The first author of two papers (and co-author of another seven), his research is about the entertainment industry, mainly TV entertainment and the emotions it arouses in the audience. Regarding first authorships, Vecchiato G. stands out, as he is the first author on seven of the eight papers he published.
The analysis of the worldwide neuromarketing authors’ collaboration network, which is graphically represented in Figure 2 , reveals two strong connections between two groups of four authors. The first group is centered on Babiloni F. and Vecchiato G., both from Rome’s Sapienza University. This group shows a higher degree of connectivity and more consistent and direct connection. Vecchiato G., who is first author on most of the articles, acts as a broker by linking different clusters of Italian authors within the network. Amongst the authors brokered by Vecciato G., two stand out: Maglione A., from the Department of Economics and Marketing of the “IULM” University, and Cherubino P., from BrainSigns, Italy. Both are connected to Babiloni F. as well as with each other. This research group applies neuroelectrical, cognitive, and emotional variables to TV commercials.

Authors’ collaboration.
The second collaboration group stems from Astolfi L., of the Department of Computer Science at Rome’s Sapienza University. This author connects to Fallani F. from the Paris Brain Institute (ICM), France; Mattia D. from the Department of Neuroscience at the University Tor Vergata, Italy; and Cincotti F. from the Center di Ricerca de La Sapienza Per l’Analisi dei Modelli e dell’Informazione, Italy. This research group studies brain responses to TV commercials. Analogous to the first group, it consists exclusively of Italian researchers.
4.2. Performance of the Most Cited Neuromarketing Articles
Neuromarketing studies aim to better understand consumer preferences by gaining access to potentially unconscious neural and physiological responses [ 35 ]. They aim to predict or even manipulate consumer behavior and decision making [ 36 ]. Neuromarketing has been used for “neuro forecasting” [ 36 ], predicting marketplace responses [ 37 , 38 ], fine tuning segmentation [ 36 ], and nudging consumer behaviors [ 36 ]. Table 6 details the Top ten neuromarketing papers in terms of citations, and Table 7 presents the topic area, application area, neuroscience technology used, and research gap addressed by each of those papers.
Top ten authors in neuromarketing paper citations.
EEG—Electroencephalogram; EMG—Electromyography; fMRI—Functional magnetic resonance imaging.
Top ten: Scival Topic Prominence.
Ranking (R); Absolute Frequency (AF); Relative Frequency (RF); Scival Percentile (SP).
Among the top ten articles, three [ 39 , 40 , 41 ] consist of introductions to neuromarketing. The majority of articles focus on predicting consumer behavior and preferences in various industries (i.e., music, movies, snack foods) [ 11 , 37 , 38 , 42 , 43 , 44 ]. Another frequently-addressed topic is the generalizability of individual fMRI results to market sales data [ 31 , 37 ]. In terms of neuroscience techniques, these researchers rely on fMRI, EEG, skin conductance and eye tracking to collect data on participant responses to stimuli. The only exception in this regard is Lopes et al. [ 1 ], which was included due to the use of the keyword neural networks.
4.3. Performance of Most Prominent Neuromarketing Topics: Author Word Network
To explore the most prominent themes in neuromarketing research from 2007 to 2020, a word network structure was performed considering 22 nodes with a minimum of two edges, as represented in Figure 3 . “Neuroscience”, with a betweenness score of 6.14 of, maintains a network of interconnections with most of the themes in the network. Accordingly, “Advertising” is the topic with the highest proximity (2.32 betweenness). This analysis highlights the interconnections between “advertising”, “marketing”, “neuroscience”, “consumer neuroscience”, “EEG”, and “electroencephalography”, which define this thematic network.

Author keywords: co-word analysis.
Another strong thematic network (1.26 betweenness) is drawn through the theme of “attention”, with which several keywords are interconnected, including “emotion”, “decision-making”, “neuroeconomics”, “emotions”, “EEG”, and “electroencephalography”. Moreover, “emotions” is directly linked with “neuroeconomics” and “decision-making”, while “emotion” (with 0.2 betweenness) is directly linked with “attention” and “consumer”. These connections, however, are not strong. This low connectivity index is typical of emerging topics.
Other topics with low betweenness indexes (0), and therefore weak connectivity links, are “television”, “consumer behavior”, and “eye tracking”. Naturally, in the context of neuromarketing these are emerging topics. Regarding the nodes related to the technologies used in neuromarketing research, “EEG”, “FMRI”, and “eye tracking” relate to “consumer” and “advertising”, which reflects the use that is normally made of such techniques.
4.4. Performance of Prominent Scival Neuromarketing Topics
SciVal topic prominence performance is operationalized through the outputs of several variables, which are summarized in Table 7 . The most relevant result is that 55% of all scientific production on neuromarketing is clustered under the “Neuromarketing | Neurosciences | TV Commercial” Scival topic prominence, which has a 94.432 prominence percentile. This means that it is in the top 5% of all topics in the world, with high levels of CiteScore, citations, and views. The topic emerged in 2007, after which publications have consistently increased up to 2020. An analysis of this topic on the Scival platform (performed in 13 March 2021) reveals that 640 articles were published worldwide from 2010 to 2020, 27% of which were published in neuromarketing field. This is a high figure compared to overall world production. The leading country in this research topic is Spain, through Complutense University.
The other topics that follow the ranking of neuromarketing research mostly emerged after 2010. Most of these emerging topics have high prominence percentiles, which indicates that they generate high levels of research interest. For instance, the topic “Subjective Well-being | Happiness | Life Satisfaction” emerged in 2020, and has a 98.770 prominence percentile.
4.5. Neuromarketing
The 99th–100th percentile, that is, the 1% best percentile on the worldwide momentum and visibility of topics, includes 17 studies. Information on these topics, namely, the authors who publish on each topic, whether they got funding for their research, and first and second author affiliations, is summarized on Table 8 . Accordingly, information on the individual studies, namely, the topic area, application area, neuroscience technology used, and research objective/gap, are summarized on Table 9 .
Top ten Scival topics by best percentile: funding and author affiliations.
Ranking (R); Scival Percentile (SP); Number of papers (NP); Financing (F).
Articles within the top ten Scival topics by best percentile: topic area, application area, neuroscience technology, and research objectives.
EEG: Electroencephalogram; EMG: Electromyography; EOG: Electrooculography; fMRI: Functional magnetic resonance imaging; fNIRS: Functional near-infrared spectroscopy.
The articles within this percentile can be classified into three different categories: audience response studies, methodology improvement studies, and literature reviews. Audience response studies [ 45 , 46 , 47 , 48 , 49 , 50 , 51 , 52 ] explore the impact of marketing campaign pieces on the consumer decision-making process and examine consumer responses and preferences. These studies are applied to many consumer industries and specific markets, such as the coffee industry and hotel industry, and are applied to specific marketing functions, such as corporate communication and brand image.
Methodology improvement studies [ 1 , 53 , 54 , 55 , 56 , 57 , 58 ] propose methods for enhancing the accuracy of object recognition and classification by combining neuromarketing techniques with traditional marketing research methods. These studies are applied to specific goals of neuromarketing methods, such as emotional face retrieval and facial expression recognition. Others focus on certain consumer decision scenarios and situations, such as grocery shopping in supermarkets. Others focus on the media through which information is conveyed, such as audio, video, or still images. Finally, literature review articles [ 59 , 60 ] explore EEG applications in research, consumer privacy concerns, and the regulatory environment.
5. Conclusions
The present study aimed to examine the global performance of neuromarketing studies. To address this general goal, five specific objectives were adopted:
To achieve these objectives, scientific production on neuromarketing was mapped through a mixed-method bibliographic approach.
Regarding the first objective, 318 papers containing the word “neuromarketing” were published in the Scopus database between 2010 and 2020. In terms of leading journals, the ranking is led by Frontiers in Neuroscience and Frontiers in Psychology, closely followed by Profesional de la Informacion. In terms of publications by country, Spain leads the ranking, followed by the United States, China, and Italy. These results are reflected in those on the leading universities, as the Complutense Madrid University leads the ranking with 21 articles, followed by Rome’s la Sapienza University, Italy and Zhejiang University, China.
Although Italy is only fourth in the country ranking, Italian researchers stand out in terms of individual productivity as well as collaboration. The first, third and fourth most productive researchers are Italian and work in Italian research centers. Accordingly, the global collaboration network on neuromarketing is dominated by two very prominent groups of Italian researchers.
Regarding the second objective, the number of introductory articles within the top ten papers in terms of citation demands attention, as it indicates that neuromarketing remains a developing topic. This is somewhat expected, given how recently the subject has come to prominence. Another popular research goal is predicting consumer behavior and preferences in different scenarios, which is line with the general goals of neuromarketing studies, that is, to better understand consumer preferences by gaining access to potentially unconscious neural and physiological responses [ 36 ]. In neuromarketing studies, this goal is pursued via the application of neuroscience technologies. The present study’s findings point to fMRI, EEG, skin conductance, and eye tracking as the most employed neuroscience technologies in neuromarketing studies.
Regarding the third specific objective, most prominent topics within the subject are connected to “Neuroscience” and have some degree of approximation with “Advertising”. The network of prominent topics provides an overview of the main neuroscience techniques and technologies used in these studies, namely, EEG/electroencephalography. Once again, this reflects the very nature of neuromarketing studies, which apply neuroscience techniques to understand and predict consumer behavior in response to certain stimuli. Naturally, a popular source of such stimuli (as these results corroborate) is advertising, especially television advertising, as the topic is highlighted in the network. Therefore, these studies have the potential to unveil the effectiveness of advertising techniques and generate good practices on how best to generate the expected response from consumers in specific industries.
The findings stemming from the fourth objective largely corroborate those of the third, as they show that over half of the scientific production on neuromarketing is clustered under the “Neuromarketing | Neurosciences | TV Commercial” Scival topic prominence, which is within the top 5% of all topics in the world in terms of views and citations. Therefore, this cluster, and consequently neuromarketing research in general, arouses high levels of research interest and has significant potential for funding.
Finally, the findings from objective five provide a list of the very best rated topics in terms of visibility and momentum. Articles within the top 1% percentile include audience response studies, methodology improvement studies, and literature reviews. Audience response studies make up the bulk of neuromarketing research. They employ the technologies highlighted in the network analysis to analyse and predict consumer reactions, especially to advertising, as well as to real-world consumption situations such as hotel stays and grocery shopping.
Methodology improvement studies attempt to refine the methods applied in the previous category, providing best practices on how to analyse consumer reactions and behaviour more precisely and reliably. Finally, literature review studies, as in any other area, summarise and systematise the state of the art on the topic. The highlighted role they play in the neuromarketing field is (analogous to introductory studies) indicative of the fact that the topic is developing quickly, which calls for constant updating of systematic reviews.
Contributions, Implications, and Future Research
The present investigation consisted of a comprehensive bibliometric analysis of academic production on neuromarketing. To this end, in addition to traditional bibliometric indicators, SciVal Topic prominence was examined, which had not been carried out in previous neuromarketing reviews. Therefore, this study provides a more detailed understanding of the state of the art in this area as well as of trending and emerging topics and those with the highest potential for publication. For neuromarketing researchers, the present study reveals gaps in research as well as emerging subjects by identifying research topics that are growing, making it possible to identify future lines of research. This should be quite useful for researchers and research centers aiming to conduct investigatory projects in this area. Moreover, previous studies have shown that research impact and topic prominence are positively related to the proportion of financed research projects within a topic. Therefore, the findings of the present study are particularly useful for researchers seeking financing for such projects.
The present research provides a contribution for future bibliometric studies. It shows that the method proposed by Cardoso et. al. [ 10 ] to map a country’s research performance in a certain area of research—in that case, Switzerland, and Tourism and Hospitality—can be employed to map the worldwide scientific production in each area. Therefore, future studies are encouraged to employ this method, and particularly the novel measure used here (i.e., SciVal Topic prominence analysis), in order to assess research production in other areas and to update the state of the art on neuromarketing in the future.
Regarding future bibliographic studies on neuromarketing, the present research raises some questions that could be addressed by future works. In terms of worldwide collaboration, for instance, the present research highlights the clear domination of Italian research and research centres, although Italy is only fourth in the ranking of most productive countries. However, it was beyond the scope of this study to explore the reasons behind this phenomenon. In this context, future studies could further investigate these reasons, and determine whether research links aim to combine the expertise of researchers in each centre, the technologies owned by each institution, or whether they merely reflect the contact networks and academic histories of the involved researchers.
Author Contributions
Conceptualization, L.C., A.A., M.-M.C. and G.G.F.d.A.; methodology, L.C.; software, L.C.; formal analysis, L.C. and M.-M.C.; investigation, L.C., A.A. and M.-M.C.; resources, L.C. and G.G.F.d.A.; data curation, L.C.; writing, L.C., A.A., M.-M.C., G.G.F.d.A., F.D. and L.M.; writing—review and editing, L.C., A.A., M.-M.C., G.G.F.d.A., F.D. and L.M.; visualization, L.C., A.A., M.-M.C., G.G.F.d.A., F.D. and L.M.; supervision, L.C.; project administration, L.C.; funding acquisition, L.C. and G.G.F.d.A. All authors have read and agreed to the published version of the manuscript.
This research was financed by national funds through the FCT-Foundation for Science and Technology, I.P., within the scope of the UIDB/04470/2020 project, Portugal, and under the Scientific Employment Stimulus-Institutional Call-[CEECINST/00051/2018].
Conflicts of Interest
The authors declare no conflict of interest.
Publisher’s Note: MDPI stays neutral with regard to jurisdictional claims in published maps and institutional affiliations.
A review of research on neuromarketing using content analysis: key approaches and new avenues
- Research Article
- Published: 21 June 2021
- Volume 15 , pages 923–938, ( 2021 )
Cite this article
- Lorena Robaina-Calderín ORCID: orcid.org/0000-0001-7041-7336 1 &
- Josefa D. Martín-Santana ORCID: orcid.org/0000-0002-7078-0271 1
2231 Accesses
8 Citations
4 Altmetric
Explore all metrics
There is currently a growing interest in a deeper understanding of consumer behaviour. In this context, the union of different disciplines such as neuroscience and marketing has given birth to new fields of knowledge, e.g. neuromarketing. This study is mainly aimed at carrying out a systematic revision of the literature on neuromarketing from a holistic point of view, analysing its definition and processes, as well as more specific aspects such as its ethics and applications. Based on the results of our review, following a combined methodology with a base dictionary and text mining, our study presents both the current lines of research and the future lines of work.
This is a preview of subscription content, log in via an institution to check access.
Access this article
Price includes VAT (Russian Federation)
Instant access to the full article PDF.
Rent this article via DeepDyve
Institutional subscriptions
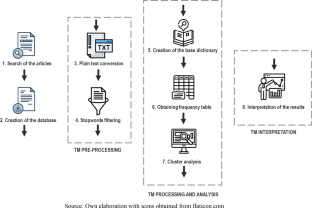
Similar content being viewed by others
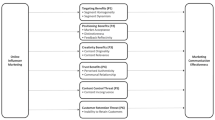
Online influencer marketing
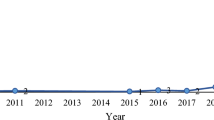
Social media influencer marketing: foundations, trends, and ways forward
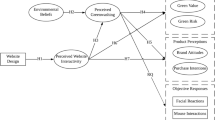
Perceived Greenwashing: The Effects of Green Marketing on Environmental and Product Perceptions
Data availability.
The authors are committed in making the data available if requested by the journal.
Agarwal S, Dutta T (2015) Neuromarketing and consumer neuroscience: current understanding and the way forward. Decision 42:457–462. https://doi.org/10.1007/s40622-015-0113-1
Article Google Scholar
Arens Z, Rust R (2012) The duality of decisions and the case for impulsiveness metrics. J Acad Mark Sci 40:468–479. https://doi.org/10.1007/s11747-011-0256-3
Bakardjieva E, Kimmel AJ (2017) Neuromarketing research practices: attitudes, ethics, and behavioral intentions. Ethics Behav 27:179–200. https://doi.org/10.1080/10508422.2016.1162719
Basaldella M, Furrer L, Colic N, et al (2016) Using a hybrid approach for entity recognition in the biomedical domain. In: Proceedings of the 7th international symposium on semantic mining in biomedicine. Postdam, p 9
Basaldella M, Furrer L, Tasso C, Rinaldi F (2017) Entity recognition in the biomedical domain using a hybrid approach. J Biomed Semant 8:51. https://doi.org/10.1186/s13326-017-0157-6
Berthoud H-R (2012) The neurobiology of food intake in an obesogenic environment. Proc Nutr Soc 71:478–487. https://doi.org/10.1017/S0029665112000602
Article PubMed PubMed Central Google Scholar
Bettiga D, Lamberti L, Noci G (2017) Do mind and body agree? Unconscious versus conscious arousal in product attitude formation. J Bus Res 75:108–117. https://doi.org/10.1016/j.jbusres.2017.02.008
Boksem M-AS, Smidts A (2015) Brain responses to movie trailers predict individual preferences for movies and their population-wide commercial success. J Mark Res 52:482–492
Butler MJR, O’Broin HLR, Lee N, Senior C (2016) How organizational cognitive neuroscience can deepen understanding of managerial decision-making: a review of the recent literature and future directions. Int J Manag Rev 18:542–559. https://doi.org/10.1111/ijmr.12071
Cartocci G, Cherubino P, Rossi D et al (2016) Gender and age related effects while watching TV advertisements: an EEG study. Comput Intell Neurosci 5:1–10. https://doi.org/10.1155/2016/3795325
Chen Y-P, Nelson LD, Hsu M (2015) From “Where” to “What”: Distributed representations of brand associations in the human brain. J Mark Res 52:453–466. https://doi.org/10.1509/jmr.14.0606
Chew LH, Teo J, Mountstephens J (2016) Aesthetic preference recognition of 3D shapes using EEG. Cogn Neurodyn 10:165–173. https://doi.org/10.1007/s11571-015-9363-z
Article PubMed Google Scholar
Christoforou C, Christou-Champi S, Constantinidou F, Theodorou M (2015) From the eyes and the heart: a novel eye-gaze metric that predicts video preferences of a large audience. Front Psychol. https://doi.org/10.3389/fpsyg.2015.00579
Christoforou C, Papadopoulos TC, Constantinidou F, Theodorou M (2017) Your brain on the movies: a computational approach for predicting box-office performance from viewer’s brain responses to movie trailers. Front Neuroinform. https://doi.org/10.3389/fninf.2017.00072
Couwenberg LE, Boksem MAS, Dietvorst RC et al (2017) Neural responses to functional and experiential ad appeals: explaining ad effectiveness. Int J Res Mark 34:355–366. https://doi.org/10.1016/j.ijresmar.2016.10.005
Cruz CML, de Medeiros JF, Hermes LCR et al (2016) Neuromarketing and the advances in the consumer behaviour studies: a systematic review of the literature. Int J Bus Glob 17:330–351. https://doi.org/10.1504/IJBG.2016.078842
Falk EB, Berkman ET, Lieberman MD (2012) From neural responses to population behavior: neural focus group predicts population-level media effects. Psychol Sci 23:439–445
Gillingwater D, Gillingwater TH (2011) A neuroanatomical approach to exploring organizational performance. Int J Bus Sci Appl Manag 4:35–52. https://doi.org/10.1201/b12879-7
Guerreiro J, Rita P, Trigueiros D (2016) A text mining-based review of cause-related marketing literature. J Bus Ethics 139:111–128. https://doi.org/10.1007/s10551-015-2622-4
Harris JM, Ciorciari J, Gountas J (2018a) Consumer neuroscience for marketing researchers. J Consum Behav 17:239–252. https://doi.org/10.1002/cb.1710
Harris JM, Ciorciari J, Gountas J (2018b) Public health social media communications and consumer neuroscience. Cogent Psychol 5:1434058. https://doi.org/10.1080/23311908.2018.1434058
He W, Zha S, Li L (2013) Social media competitive analysis and text mining: a case study in the pizza industry. Int J Inf Manag 33:464–472. https://doi.org/10.1016/j.ijinfomgt.2013.01.001
Hensel D, Iorga A, Wolter L, Znanewitz J (2017) Conducting neuromarketing studies ethically-practitioner perspectives. Cogent Psychol 4:1320858. https://doi.org/10.1080/23311908.2017.1320858
Hsu M (2017) Neuromarketing: Inside the mind of the consumer. Calif Manag Rev 59:5–22. https://doi.org/10.1177/0008125617720208
Hubert M (2010) Does neuroeconomics give new impetus to economic and consumer research? J Econ Psychol 31:812–817. https://doi.org/10.1016/j.joep.2010.03.009
Kenning P, Linzmajer M (2011) Consumer neuroscience: an overview of an emerging discipline with implications for consumer policy. J Verbr Lebensm 6:111–125. https://doi.org/10.1007/s00003-010-0652-5
Khushaba RN, Wise C, Kodagoda S et al (2013) Consumer neuroscience: assessing the brain response to marketing stimuli using electroencephalogram (EEG) and eye tracking. Expert Syst Appl 40:3803–3812. https://doi.org/10.1016/j.eswa.2012.12.095
Kumar BS, Ravi V (2016) A survey of the applications of text mining in financial domain. Knowl Based Syst 114:128–147. https://doi.org/10.1016/j.knosys.2016.10.003
Labrecque LI, Patrick VM, Milne GR (2013) The marketers’ prismatic palette: a review of color research and future directions. Psychol Mark 30:187–202. https://doi.org/10.1002/mar.20597
Laghari K ur R, Gupta R, Arndt S, et al (2014) Characterization of human emotions and preferences for text-to-speech systems using multimodal neuroimaging methods. In: 2014 IEEE 27th Canadian conference on electrical and computer engineering (CCECE),1–5. https://doi.org/10.1109/CCECE.2014.6901142
Lajante M, Droulers O, Dondaine T, Amarantini D (2012) Opening the “black box” of electrodermal activity in consumer neuroscience research. J Neuros Psychol Econ 5:238–249. https://doi.org/10.1037/a0030680
Lee E-J, Kwon G, Shin HJ et al (2014) The spell of green: can frontal EEG activations identify green consumers? J Bus Ethics 122:511–521
Lee N, Brandes L, Chamberlain L, Senior C (2017) This is your brain on neuromarketing: reflections on a decade of research. J Mark Manag 33:878–892. https://doi.org/10.1080/0267257X.2017.1327249
Lee N, Chamberlain L, Brandes L (2018) Welcome to the jungle! The neuromarketing literature through the eyes of a newcomer. Eur J Mark 52:4–38. https://doi.org/10.1108/EJM-02-2017-0122
Lim WM (2018) What will business-to-business marketers learn from neuro-marketing? Insights for business marketing practice. J Bus-Bus Mark 25:251–259. https://doi.org/10.1080/1051712X.2018.1488915
Lin C-H, Tuan H-P, Chiu Y-C (2010) Medial frontal activity in brand-loyal consumers: a behavior and near-infrared ray study. J Neuros Psychol Econ 3:59–73. https://doi.org/10.1037/a0015461
Lin Y-P, Yang Y-H, Jung T-P (2014) Fusion of electroencephalographic dynamics and musical contents for estimating emotional responses in music listening. Front Neurosci. https://doi.org/10.3389/fnins.2014.00094
Lin M-H, Cross SNN, Childers TL (2018a) Understanding olfaction and emotions and the moderating role of individual differences. Eur J Mark 52:811–836. https://doi.org/10.1108/EJM-05-2015-0284
Lin M-H, Cross SNN, Laczniak RN, Childers TL (2018b) The sniffing effect: olfactory sensitivity and olfactory imagery in advertising. J Advert 47:97–111. https://doi.org/10.1080/00913367.2017.1410739
Martín-Santana JD, Robaina-Calderín L, Reinares-Lara E, Romero-Domínguez L (2019) Knowing the blood nondonor to activate behaviour. Soc Sci 8:324. https://doi.org/10.3390/socsci8120324
Moro S, Pires G, Rita P, Cortez P (2019) A text mining and topic modelling perspective of ethnic marketing research. J Bus Res 103:275–285. https://doi.org/10.1016/j.jbusres.2019.01.053
Natarajan M (2005) Role of text mining in information extraction and information management. DESIDOC J Lib Inf Technol. https://doi.org/10.14429/djlit.25.4.3663
Neghina C, Henseler J, Aksoy L et al (2013) Data-driven services marketing in a connected world. J Serv Manag 24:330–352. https://doi.org/10.1108/09564231311327021
Nutt DJ, Attridge J (2014) CNS drug development in Europe—past progress and future challenges. Neurobiol Dis 61:6–20. https://doi.org/10.1016/j.nbd.2013.05.002
Ohme R, Reykowska D, Wiener D, Choromanska A (2010) Application of frontal EEG asymmetry to advertising research. J Econ Psychol 31:785–793. https://doi.org/10.1016/j.joep.2010.03.008
Petit O, Cheok AD, Spence C, et al (2015) Sensory marketing in light of new technologies. In: ACM international conference proceeding series 16–19-November-2015 https://doi.org/10.1145/2832932.2837006
Plassmann H, Ramsøy TZ, Milosavljevic M (2012) Branding the brain: a critical review and outlook. J Consum Psychol 22:18–36. https://doi.org/10.1016/j.jcps.2011.11.010
Plassmann H, Venkatraman V, Huettel S, Yoon C (2015) Consumer neuroscience: applications, challenges, and possible solutions. J Mark Res 52:427–435
Powell TC (2011) Neurostrategy. Strateg Manag J 32:1484–1499
Pykett J (2013) Neurocapitalism and the new neuros: using neuroeconomics, behavioural economics and picoeconomics for public policy. J Econ Geogr 13:845–869. https://doi.org/10.1093/jeg/lbs039
Rasch C, Louviere JJ, Teichert T (2015) Using facial EMG and eye tracking to study integral affect in discrete choice experiments. J Choice Model 14:32–47. https://doi.org/10.1016/j.jocm.2015.04.001
Reimann M, Zaichkowsky J, Neuhaus C et al (2010) Aesthetic package design: a behavioral, neural, and psychological investigation. J Consum Psychol 20:431–441. https://doi.org/10.1016/j.jcps.2010.06.009
Romero-Domínguez L, Martín-Santana JD, Sánchez-Medina AJ, Beerli-Palacio A (2019) Lines of scientific research in the study of blood donor behavior from a social marketing perspective. J Nonprofit Public Sector Mark. https://doi.org/10.1080/10495142.2019.1707741
Shi Z, Wang A-L, Aronowitz CA et al (2017) Individual differences in the processing of smoking-cessation video messages: an imaging genetics study. Biol Psychol 128:125–131. https://doi.org/10.1016/j.biopsycho.2017.07.019
Stasi A, Songa G, Mauri M et al (2018) Neuromarketing empirical approaches and food choice: a systematic review. Food Res Int 108:650–664. https://doi.org/10.1016/j.foodres.2017.11.049
Article CAS PubMed Google Scholar
Steele A, Jacobs D, Siefert C et al (2013) Leveraging synergy and emotion in a multi-platform world: a neuroscience-informed model of engagement. J Advert Res 53:417–430. https://doi.org/10.2501/JAR-53-4-417-430
Tarczynski T (2011) Document clustering—concepts, metrics and algorithms. Int J Electron Telecom 57:271–277. https://doi.org/10.2478/v10177-011-0036-5
Telpaz A, Webb R, Levy DJ (2015) Using EEG to predict consumers’ future choices. J Mark Res 52:511–529. https://doi.org/10.1509/jmr.13.0564
Teo J, Chew LH, Chia JT, Mountstephens J (2018) Classification of affective states via EEG and deep learning. Int J Adv Comp Sci Appl. https://doi.org/10.14569/IJACSA.2018.090517
Touchette B, Lee S-E (2017) Measuring neural responses to apparel product attractiveness: an application of frontal asymmetry theory. Cloth Text Res J 35:3–15. https://doi.org/10.1177/0887302X16673157
Vecchiato G, Babiloni F (2011) Neurophysiological measurements of memorization and pleasantness in neuromarketing experiments. In: Analysis of verbal and nonverbal communication and enactment. The processing issues. Springer, Berlin, pp 294–308 https://doi.org/10.1007/978-3-642-25775-9_28
Vecchiato G, Toppi J, Astolfi L et al (2012) The added value of the electrical neuroimaging for the evaluation of marketing stimuli. Bull Pol Acad Sci Tech Sci 60:419–426. https://doi.org/10.2478/v10175-012-0053-2
Vecchiato G, Toppi J, Astolfi L et al (2011) Spectral EEG frontal asymmetries correlate with the experienced pleasantness of TV commercial advertisements. Med Biol Eng Comput 49:579–583. https://doi.org/10.1007/s11517-011-0747-x
Wang Y, Chattaraman V, Kim H, Deshpande G (2015) Predicting purchase decisions based on spatio-temporal functional MRI features using machine learning. IEEE Trans Auton Ment Dev 7:248–255. https://doi.org/10.1109/TAMD.2015.2434733
Wang RWY, Chang Y, Chuang S (2016) EEG spectral dynamics of video commercials: impact of the narrative on the branding product preference. Sci Rep 6:36487. https://doi.org/10.1038/srep36487
Article CAS PubMed PubMed Central Google Scholar
Wang J, Zhao M, Zhao G (2017) The impact of customer cognitive competence on online service decision-making: an event-related potentials perspective. Serv Ind J 37:363–380. https://doi.org/10.1080/02642069.2017.1325467
Wang C, Wang C, Wang C et al (2018) The effects of money on fake rating behavior in E-commerce: electrophysiological time course evidence from consumers. Front Neurosci 12:156
Wei Z, Wu C, Wang X et al (2018) Using support vector machine on EEG for advertisement impact assessment. Front Neurosci 12:76. https://doi.org/10.3389/fnins.2018.00076
Yadava M, Kumar P, Saini R et al (2017) Analysis of EEG signals and its application to neuromarketing. Multimed Tools Appl 76:19087–19111. https://doi.org/10.1007/s11042-017-4580-6
Yang D-J (2018) Exploratory neural reactions to framed advertisement messages of smoking cessation. Soc Mark Q 24:216–232. https://doi.org/10.1177/1524500418788306
Yang T, Lee S, Seomoon E, Kim S-P (2018) Characteristics of human brain activity during the evaluation of service-to-service brand extension. Front Hum Neurosci 12:44. https://doi.org/10.3389/fnhum.2018.00044
Yilmaz B, Korkmaz S, Arslan DB et al (2014) Like/dislike analysis using EEG: determination of most discriminative channels and frequencies. Comput Methods Programs Biomed 113:705–713. https://doi.org/10.1016/j.cmpb.2013.11.010
Download references
This work has been co-financed by the Agencia Canaria de Investigación, Innovación y Sociedad de la Información (Canary Islands Agency on Research, Innovation and Information Society) of the Consejería de Economía, Industria, Comercio y Conocimiento (Department of Economy, Industry, Commerce and Knowledge) and the European Social Fund (ESF), Integrated Operational Programme ‘Canary Islands’ 2014–2020, Axis 3 Priority Topic 74 (85%).
Author information
Authors and affiliations.
Universidad de Las Palmas de Gran Canaria, Campus de Tafira s/n, 35017, Las Palmas de Gran Canaria, Las Palmas, Spain
Lorena Robaina-Calderín & Josefa D. Martín-Santana
You can also search for this author in PubMed Google Scholar
Contributions
LRC and JDMS contributed to the study conception and design, material preparation, data collection and analysis, and writing (draft preparation, review and editing). Both authors read and approved the final manuscript.
Corresponding author
Correspondence to Lorena Robaina-Calderín .
Ethics declarations
Conflict of interest.
The authors declare that they have no conflict of interest.
Ethical approval
Ethics approval was not required for this research.
Additional information
Publisher's note.
Springer Nature remains neutral with regard to jurisdictional claims in published maps and institutional affiliations.
Rights and permissions
Reprints and permissions
About this article
Robaina-Calderín, L., Martín-Santana, J.D. A review of research on neuromarketing using content analysis: key approaches and new avenues. Cogn Neurodyn 15 , 923–938 (2021). https://doi.org/10.1007/s11571-021-09693-y
Download citation
Received : 20 August 2020
Revised : 14 May 2021
Accepted : 11 June 2021
Published : 21 June 2021
Issue Date : December 2021
DOI : https://doi.org/10.1007/s11571-021-09693-y
Share this article
Anyone you share the following link with will be able to read this content:
Sorry, a shareable link is not currently available for this article.
Provided by the Springer Nature SharedIt content-sharing initiative
- Neuromarketing
- Consumer neuroscience
- Text mining
- Find a journal
- Publish with us
- Track your research
The state of AI in early 2024: Gen AI adoption spikes and starts to generate value
If 2023 was the year the world discovered generative AI (gen AI) , 2024 is the year organizations truly began using—and deriving business value from—this new technology. In the latest McKinsey Global Survey on AI, 65 percent of respondents report that their organizations are regularly using gen AI, nearly double the percentage from our previous survey just ten months ago. Respondents’ expectations for gen AI’s impact remain as high as they were last year , with three-quarters predicting that gen AI will lead to significant or disruptive change in their industries in the years ahead.
About the authors
This article is a collaborative effort by Alex Singla , Alexander Sukharevsky , Lareina Yee , and Michael Chui , with Bryce Hall , representing views from QuantumBlack, AI by McKinsey, and McKinsey Digital.
Organizations are already seeing material benefits from gen AI use, reporting both cost decreases and revenue jumps in the business units deploying the technology. The survey also provides insights into the kinds of risks presented by gen AI—most notably, inaccuracy—as well as the emerging practices of top performers to mitigate those challenges and capture value.
AI adoption surges
Interest in generative AI has also brightened the spotlight on a broader set of AI capabilities. For the past six years, AI adoption by respondents’ organizations has hovered at about 50 percent. This year, the survey finds that adoption has jumped to 72 percent (Exhibit 1). And the interest is truly global in scope. Our 2023 survey found that AI adoption did not reach 66 percent in any region; however, this year more than two-thirds of respondents in nearly every region say their organizations are using AI. 1 Organizations based in Central and South America are the exception, with 58 percent of respondents working for organizations based in Central and South America reporting AI adoption. Looking by industry, the biggest increase in adoption can be found in professional services. 2 Includes respondents working for organizations focused on human resources, legal services, management consulting, market research, R&D, tax preparation, and training.
Also, responses suggest that companies are now using AI in more parts of the business. Half of respondents say their organizations have adopted AI in two or more business functions, up from less than a third of respondents in 2023 (Exhibit 2).
Gen AI adoption is most common in the functions where it can create the most value
Most respondents now report that their organizations—and they as individuals—are using gen AI. Sixty-five percent of respondents say their organizations are regularly using gen AI in at least one business function, up from one-third last year. The average organization using gen AI is doing so in two functions, most often in marketing and sales and in product and service development—two functions in which previous research determined that gen AI adoption could generate the most value 3 “ The economic potential of generative AI: The next productivity frontier ,” McKinsey, June 14, 2023. —as well as in IT (Exhibit 3). The biggest increase from 2023 is found in marketing and sales, where reported adoption has more than doubled. Yet across functions, only two use cases, both within marketing and sales, are reported by 15 percent or more of respondents.
Gen AI also is weaving its way into respondents’ personal lives. Compared with 2023, respondents are much more likely to be using gen AI at work and even more likely to be using gen AI both at work and in their personal lives (Exhibit 4). The survey finds upticks in gen AI use across all regions, with the largest increases in Asia–Pacific and Greater China. Respondents at the highest seniority levels, meanwhile, show larger jumps in the use of gen Al tools for work and outside of work compared with their midlevel-management peers. Looking at specific industries, respondents working in energy and materials and in professional services report the largest increase in gen AI use.
Investments in gen AI and analytical AI are beginning to create value
The latest survey also shows how different industries are budgeting for gen AI. Responses suggest that, in many industries, organizations are about equally as likely to be investing more than 5 percent of their digital budgets in gen AI as they are in nongenerative, analytical-AI solutions (Exhibit 5). Yet in most industries, larger shares of respondents report that their organizations spend more than 20 percent on analytical AI than on gen AI. Looking ahead, most respondents—67 percent—expect their organizations to invest more in AI over the next three years.
Where are those investments paying off? For the first time, our latest survey explored the value created by gen AI use by business function. The function in which the largest share of respondents report seeing cost decreases is human resources. Respondents most commonly report meaningful revenue increases (of more than 5 percent) in supply chain and inventory management (Exhibit 6). For analytical AI, respondents most often report seeing cost benefits in service operations—in line with what we found last year —as well as meaningful revenue increases from AI use in marketing and sales.
Inaccuracy: The most recognized and experienced risk of gen AI use
As businesses begin to see the benefits of gen AI, they’re also recognizing the diverse risks associated with the technology. These can range from data management risks such as data privacy, bias, or intellectual property (IP) infringement to model management risks, which tend to focus on inaccurate output or lack of explainability. A third big risk category is security and incorrect use.
Respondents to the latest survey are more likely than they were last year to say their organizations consider inaccuracy and IP infringement to be relevant to their use of gen AI, and about half continue to view cybersecurity as a risk (Exhibit 7).
Conversely, respondents are less likely than they were last year to say their organizations consider workforce and labor displacement to be relevant risks and are not increasing efforts to mitigate them.
In fact, inaccuracy— which can affect use cases across the gen AI value chain , ranging from customer journeys and summarization to coding and creative content—is the only risk that respondents are significantly more likely than last year to say their organizations are actively working to mitigate.
Some organizations have already experienced negative consequences from the use of gen AI, with 44 percent of respondents saying their organizations have experienced at least one consequence (Exhibit 8). Respondents most often report inaccuracy as a risk that has affected their organizations, followed by cybersecurity and explainability.
Our previous research has found that there are several elements of governance that can help in scaling gen AI use responsibly, yet few respondents report having these risk-related practices in place. 4 “ Implementing generative AI with speed and safety ,” McKinsey Quarterly , March 13, 2024. For example, just 18 percent say their organizations have an enterprise-wide council or board with the authority to make decisions involving responsible AI governance, and only one-third say gen AI risk awareness and risk mitigation controls are required skill sets for technical talent.
Bringing gen AI capabilities to bear
The latest survey also sought to understand how, and how quickly, organizations are deploying these new gen AI tools. We have found three archetypes for implementing gen AI solutions : takers use off-the-shelf, publicly available solutions; shapers customize those tools with proprietary data and systems; and makers develop their own foundation models from scratch. 5 “ Technology’s generational moment with generative AI: A CIO and CTO guide ,” McKinsey, July 11, 2023. Across most industries, the survey results suggest that organizations are finding off-the-shelf offerings applicable to their business needs—though many are pursuing opportunities to customize models or even develop their own (Exhibit 9). About half of reported gen AI uses within respondents’ business functions are utilizing off-the-shelf, publicly available models or tools, with little or no customization. Respondents in energy and materials, technology, and media and telecommunications are more likely to report significant customization or tuning of publicly available models or developing their own proprietary models to address specific business needs.
Respondents most often report that their organizations required one to four months from the start of a project to put gen AI into production, though the time it takes varies by business function (Exhibit 10). It also depends upon the approach for acquiring those capabilities. Not surprisingly, reported uses of highly customized or proprietary models are 1.5 times more likely than off-the-shelf, publicly available models to take five months or more to implement.
Gen AI high performers are excelling despite facing challenges
Gen AI is a new technology, and organizations are still early in the journey of pursuing its opportunities and scaling it across functions. So it’s little surprise that only a small subset of respondents (46 out of 876) report that a meaningful share of their organizations’ EBIT can be attributed to their deployment of gen AI. Still, these gen AI leaders are worth examining closely. These, after all, are the early movers, who already attribute more than 10 percent of their organizations’ EBIT to their use of gen AI. Forty-two percent of these high performers say more than 20 percent of their EBIT is attributable to their use of nongenerative, analytical AI, and they span industries and regions—though most are at organizations with less than $1 billion in annual revenue. The AI-related practices at these organizations can offer guidance to those looking to create value from gen AI adoption at their own organizations.
To start, gen AI high performers are using gen AI in more business functions—an average of three functions, while others average two. They, like other organizations, are most likely to use gen AI in marketing and sales and product or service development, but they’re much more likely than others to use gen AI solutions in risk, legal, and compliance; in strategy and corporate finance; and in supply chain and inventory management. They’re more than three times as likely as others to be using gen AI in activities ranging from processing of accounting documents and risk assessment to R&D testing and pricing and promotions. While, overall, about half of reported gen AI applications within business functions are utilizing publicly available models or tools, gen AI high performers are less likely to use those off-the-shelf options than to either implement significantly customized versions of those tools or to develop their own proprietary foundation models.
What else are these high performers doing differently? For one thing, they are paying more attention to gen-AI-related risks. Perhaps because they are further along on their journeys, they are more likely than others to say their organizations have experienced every negative consequence from gen AI we asked about, from cybersecurity and personal privacy to explainability and IP infringement. Given that, they are more likely than others to report that their organizations consider those risks, as well as regulatory compliance, environmental impacts, and political stability, to be relevant to their gen AI use, and they say they take steps to mitigate more risks than others do.
Gen AI high performers are also much more likely to say their organizations follow a set of risk-related best practices (Exhibit 11). For example, they are nearly twice as likely as others to involve the legal function and embed risk reviews early on in the development of gen AI solutions—that is, to “ shift left .” They’re also much more likely than others to employ a wide range of other best practices, from strategy-related practices to those related to scaling.
In addition to experiencing the risks of gen AI adoption, high performers have encountered other challenges that can serve as warnings to others (Exhibit 12). Seventy percent say they have experienced difficulties with data, including defining processes for data governance, developing the ability to quickly integrate data into AI models, and an insufficient amount of training data, highlighting the essential role that data play in capturing value. High performers are also more likely than others to report experiencing challenges with their operating models, such as implementing agile ways of working and effective sprint performance management.
About the research
The online survey was in the field from February 22 to March 5, 2024, and garnered responses from 1,363 participants representing the full range of regions, industries, company sizes, functional specialties, and tenures. Of those respondents, 981 said their organizations had adopted AI in at least one business function, and 878 said their organizations were regularly using gen AI in at least one function. To adjust for differences in response rates, the data are weighted by the contribution of each respondent’s nation to global GDP.
Alex Singla and Alexander Sukharevsky are global coleaders of QuantumBlack, AI by McKinsey, and senior partners in McKinsey’s Chicago and London offices, respectively; Lareina Yee is a senior partner in the Bay Area office, where Michael Chui , a McKinsey Global Institute partner, is a partner; and Bryce Hall is an associate partner in the Washington, DC, office.
They wish to thank Kaitlin Noe, Larry Kanter, Mallika Jhamb, and Shinjini Srivastava for their contributions to this work.
This article was edited by Heather Hanselman, a senior editor in McKinsey’s Atlanta office.
Explore a career with us
Related articles.

Moving past gen AI’s honeymoon phase: Seven hard truths for CIOs to get from pilot to scale
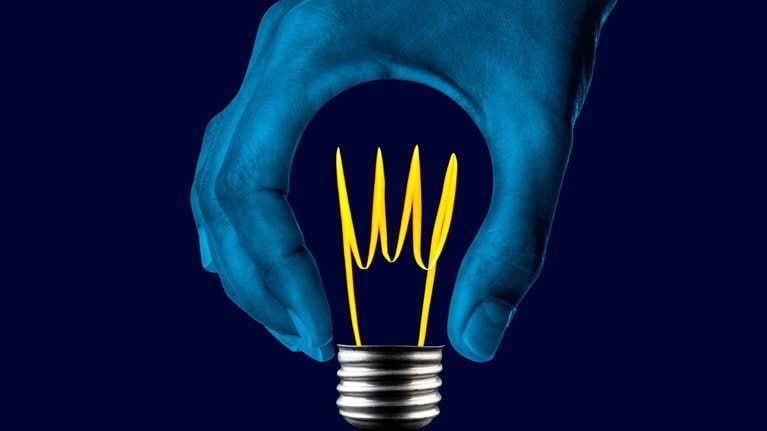
A generative AI reset: Rewiring to turn potential into value in 2024

Implementing generative AI with speed and safety
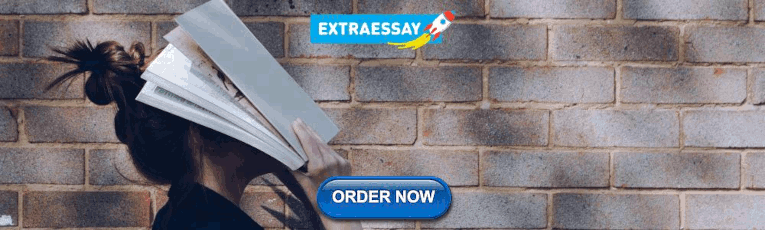
IMAGES
VIDEO
COMMENTS
View PDF View EPUB. Neuromarketing (NM) is an application of neuroimaging and physiological tools to record the neural correlates of consumers' behaviour (e.g., decision-making, emotion, attention, and memory) toward marketing stimuli such as brands and advertisements. This study aims to present the current tools employed in the empirical ...
Neuromarketing is the application of neuroscience to. marketing. Ne ur omarketing includes the direct use of brain. imaging, sca nning, or o ther brain ac tivity measur ement. technology to ...
Neuromarketing has become an academic and commercial area of interest, as the advancements in neural recording techniques and interpreting algorithms have made it an effective tool for recognizing the unspoken response of consumers to the marketing stimuli. This article presents the very first systematic review of the technological advancements in Neuromarketing field over the last 5 years ...
Neuromarketing relates brain sciences to the processes of buying and selling products. It is interested in knowing how the image of the brand, products, advertising, or marketing campaigns influence the human brain, since this organ is responsible for making decisions to buy any type of product offered [1, 2].In recent years the use of technology has been very beneficial in understanding the ...
Fortunato et al. [21] explored the techniques and applications of neuromarketing in businesses, whereas Bakardjieva and Kimmel [22] investigated the effectiveness of marketing-based services using ...
trends and co-citation network between references. This study differs from other review papers rele-vant to neuromarketing in terms of the discussion of the global trends of neuromarketing research. To this end, this review paper attempts to incorporate as many trends as possible, wherein this study tries
Abstract. Neuromarketing is an emerging field that bridges the study of consumer behavior with neuroscience. Controversial when it first emerged in 2002, the field is gaining rapid credibility and ...
voting behaviours, and neuromarketing is understood in a more practical and business way as a set of neurophysiological tools used by market research companies or practi-tioners [8]. In the same vein, Nemorin (2018, p. 56) understands "neuromarketing as a commercialised market research method for studying brain activity that combines the
impacted by Neuromarketing. The issue will consider theoretical and empirical research, qualitative and quantitative, perspective, viewpoints, case studies, and review papers. Topic Coverage: The topics must be based on a special issue but may include the following: • Neurogastronomy • Neuromarketing -challenges and opportunities
plements traditional ways of research based on the survey approach or classic experimental methods. the application of brain imaging techniques for resolving marketing-rela-ted questions is the core stream within "neuromarketing." However, this field of research also includes an intensified
One of the important areas that uses neuroscience methods is marketing field. Containing elements from the words "neuroscience" and "marketing" linguistically, neuromarketing can be described as a phenomenon that uses neuroscience methods to provide insights into marketing-related subjects. For better and efficient marketing programs ...
The present paper discusses the findings of a systematic review of EEG measures in neuromarketing, identifying which EEG measures are the most robust predictor of customer preference in neuromarketing. The review investigated which TF effect (e.g., theta-band power), and ERP component (e.g., N400) was most consistently reflective of self-reported preference.
Neuromarketing is the embryonic field of marketing science. Despite being controversial, it remains the most promising field to study genuine consumers' responses in front of the marketing stimuli ...
1. Introduction. As a research field, neuromarketing emerged from the application of neuroscience methods and techniques for marketing purposes, i.e., consumer behavior as a response to a certain stimulus [].In this context, neuromarketing is an interdisciplinary domain with great of potential, as it allows researchers to understand and predict consumer choices and behavior.
The purpose of a research conducted by (Mazhar, Daud, Mubin, & Bhutto, 2015) was to understand the success behind brands making it big. In a segment in Karachi, the paper analyses how product packaging affects the decisions in consumer buying behaviour. On the basis of primary research and the software, SPSS used, various constructive
research. Neuromarketing is the recent technique in market research that is attracting many eyeballs. As the word suggests, Neuromarketing is an unusual blend of Neuroscience and Marketing. The amalgamation of the two distinct branches of study has given rise to the scientific mapping of the brain for the purpose of analysis of
Neuromarketing is an emerging field that bridges the study of consumer behavior with neuroscience. Controversial when it first emerged in 2002, the field is gaining rapid credibility and adoption among advertising and marketing professionals. Each year, over 400 billion dollars is invested in advertising campaigns. Yet, conventional methods for testing and predicting the effectiveness of those ...
Neuromarketing is an emerging field in which academic and industry research scientists employ neuroscience techniques to study marketing practices and consumer behavior. The use of neuroscience techniques, it is argued, facilitates a more direct understanding of how brain states and other physiological mechanisms are related to consumer behavior and decision making.
Neuromarketing is a new field in marketing research. The area is based on the study of consumers' senses, cognitive, and affective responses to marketing stimuli. The human brain has a network of one hundred bill ion neurons. Nemorin (2017) stated in the research that the Consumer responds to the advertising stimuli.
NEUROSCIENCE RESEARCH NOTES. Neuromarketing: a historical review. Chiemelie Benneth Iloka* and Kenechi John Onyeke. Department of Marketing, Enugu State Universit y of Science and Technology (ESUT ...
2. Hedda Martina Sola, Original scientific paper "Neuromarketing - Science and Practice", December 2013. 3. Priyanka Singh, "Neuromarketing: An Emerging Tool of Market Research", December 2015. 4. Manu Melwin Joy, "The Potential and challenge of using Neuromarketing as a Marketing Tool", March 2018. 5.
In the last decade, researchers have become increasingly interested in neuromarketing (Lee et al. 2018).Neuromarketing is defined as the application of neuroscientific techniques (specialised in cognitive research) to the field of marketing (Cruz et al. 2016; Lee et al. 2017, 2018).This discipline aims to establish a sound neuropsychological theory that allows people to understand consumer ...
About the research. The online survey was in the field from February 22 to March 5, 2024, and garnered responses from 1,363 participants representing the full range of regions, industries, company sizes, functional specialties, and tenures. Of those respondents, 981 said their organizations had adopted AI in at least one business function, and ...
Neuromarketing research has emerged as a p romising field for developing effective. advertising strategies. This approach involves the use of different techniques and methods. such as recording of ...
Following the 2022 energy crisis, this paper investigates whether Europe's ongoing efforts to cut greenhouse gas emissions can also enhance its energy security. The global computational general equilibrium model analysis finds that individual policy tools, including carbon pricing, energy efficiency standards, and accelerated permitting procedures for renewables, tend to improve energy security.