Masks Strongly Recommended but Not Required in Maryland, Starting Immediately
Due to the downward trend in respiratory viruses in Maryland, masking is no longer required but remains strongly recommended in Johns Hopkins Medicine clinical locations in Maryland. Read more .
- Vaccines
- Masking Guidelines
- Visitor Guidelines
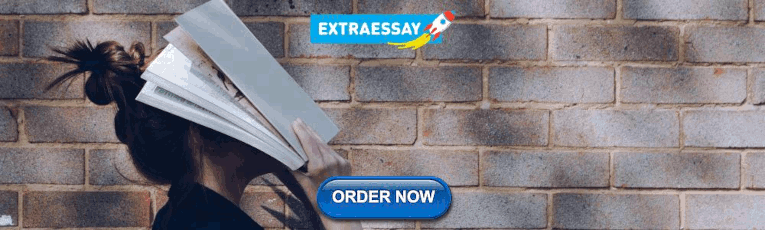
Division of General Internal Medicine
Research-in-progress (rip): tips.
Making the Most of RIP
Powerpoints by Fellowship Alumna, Neda Ratanawongsa, MD, MPH
What is RIP?
A forum where fellows can talk about their research idea, designs, and results and get feedback in an informal, comfortable setting.
At what stages of my research can I present?
All stages: starting a project, planning the design, analyzing the data, or getting ready to present your results at conferences or in manuscripts.
Why are we talking about this?
- Different objectives (& skills) for presenting research-in-progress vs. completed work
- Necessary to do this throughout your career
- It doesn’t have to be scary – can it even be enjoyable?!
- We want to encourage you to present often and feel more comfortable each time.
Setting Your Objectives
You will never get through as much as you thought you would – set a reasonable agenda.
Starting a research project
- Present what’s been done
- Identify the gap(s) in the literature
- Present/refine your conceptual framework
- Identify possible research questions/hypotheses
- Discuss pros/cons of research methodologies
- Identify potential datasets
- Solicit ideas on mentors/collaborators/funding sources
Research design or data analysis
- Briefly present what’s been done as it affects your design
- Identify your specific aim(s) and hypotheses
- Present your research design
- Focus on 1-2 issues / dilemmas – for example: Research design: • Are these appropriate inclusion / exclusion criteria? • How can I improve recruitment? • Please pilot my questionnaire. • How can I get my protocol through the IRB? • How can I collect data on other variables in my conceptual framework? Data analysis: • Do the variables in my model make sense? • Here’s an interesting finding – what do you think of my conclusions? • Are there other confounders I haven’t considered?
Presenting for conferences
- Explain the venue / audience / constraints for your future presentation
- My talk is too long.
- I talk too quickly.
- Do my slides make sense?
- I’m worried about fielding questions.
- Present as if you are at the conference (including Q&A time afterwards)
- Allow ample time to get feedback on the style, content, and your responses in Q&A
10 Ways to Make the Most of Your RIP Presentation
1. Present early and often.
- Better to reconsider your design before submitting the IRB, collecting data, or writing the manuscript
2. Present weeks or months before key deadlines.
- You'll be more willing to incorporate major changes and have time to present again
3. Invite faculty.
- Ask your mentor(s) to come.
- Invite faculty who are not working with you but who have experience with the methodology / content (use your mentors to help you identify and invite them)
- Allow enough lead time so you can coordinate with faculty schedules
4. Prepare for 20 minutes/20 slides.
- Allow enough time for questions while you present and discussion after
- Avoid the temptation to present lots of background
- Go over your slides and objectives with your project mentor in advance to optimize the structure of your talk
5. State upfront and explicitly your (one to three) objectives for the session (see above). 6. Consider how to manage your audience when they:
- If they question something upstream of your objective (e.g. research design), go with the flow for a period of time,
- But redirect your audience back to your objectives when necessary: "These are all great points, but I’d like to move on to …"
- You can ask the audience to hold questions during part/all of your talk,
- But try to practice managing interruptions (which may occur at a conference or job talk): "That’s an important question, and I think my next few slides will address that issue. If I don’t, please remind me to come back to that before we end."
7. Convert comments into constructive criticism.
- "That's a great point that I've struggled with - do you or does anyone else have suggestions on how I could do this differently?"
- Let the audience know in advance the type of feedback you want (content vs. style)
8. Assign a note-taker.
- Save your energy for thinking about and fielding questions
- Have someone bring a laptop to write down what others say and how you respond to their comments / questions
9. Set time aside that day to process the feedback.
- Look over the notes and/or talk about them with your project mentors
- Don’t necessarily act on every suggestion, but keep track of why you don’t (great for anticipating questions at future presentations and writing the limitations section)
10. Solicit feedback on how you present.
- Assign someone to take notes on how you can improve your format, speaking style, responses to questions, the way you redirect the audience
- Hand out a form asking for feedback on both content and presentation style.
When You’re Not Presenting in RIP
- You will learn by hearing others’ critiques and suggestions.
- You will learn by thinking critically about other presentations.
- Better to hear from a variety of viewpoints.
- If you don’t understand something from the presenter or from an audience member, chances are that someone else doesn’t understand either.
- You don’t have to have an answer to a concern that you raise; someone else may have one.
- Better to receive constructive criticism here now than everywhere else later.
- Consider writing down suggestions that don’t relate to the session’s objectives and are not immediately pressing.

Research Priorities for Airborne Particulate Matter: III. Early Research Progress (2001)
Chapter: 3. review of research progress and status, review of research progress and status, introduction.
I n this chapter, the committee reviews the progress made in implementing the particulate-matter (PM) research portfolio over the period from 1998 (the year in which the portfolio was first recommended by the committee) to the middle of 2000. Because that period represents the initial stage of the PM research program, the committee's assessment necessarily focused more on continuing and planned research projects than on published results.
For each of the 10 topics in the research portfolio, the committee first characterizes the status of relevant research and progress, including the approximate numbers of studies in progress on various subtopics (the committee did not attempt to list all relevant research projects but did attempt to capture the major studies across the spectrum of the research in progress), then considers the adequacy of the current research in addressing specific needs as identified in its first two reports, and finally applies the first three evaluation criteria discussed in Chapter 2 : scientific value, decisionmaking value, and feasibility and timing. The remaining three criteria—largely cross-cutting—are considered in more general terms in Chapter 4 . The committee's next report, due near the end 2002, will consider research in relation to these criteria in more detail.
RESEARCH TOPIC 1. OUTDOOR MEASURES VERSUS ACTUAL HUMAN EXPOSURES
What are the quantitative relationships between concentrations of partic ulate matter and gaseous copollutants measured at stationary outdoor air- monitoring sites and the contributions of these concentrations to actual personal exposures, especially for subpopulations and individuals?
In its first report (NRC 1998), the committee recommended that information be obtained on relationships between total personal exposures and outdoor concentrations of PM. Specifically, the committee recommended longitudinal panel studies, in which groups of 10-40 persons would be studied at successive times to examine the relationship between their exposures to PM and the corresponding outdoor concentrations. The studies were intended to focus not only on the general population, but also on subpopulations that could be susceptible 1 to the effects of PM exposures, such as the elderly, children, and persons with respiratory or cardiovascular disease. It was recommended that some of the exposure studies include measurements of PM with an aerodynamic diameter of 2.5 µ m or less (PM 2.5 ), PM with an aerodynamic diameter of 10 µ m or less (PM 10 ), and gaseous copollutants. It was expected that the investigations would quantify the contribution of outdoor sources to personal and indoor exposures. The design and execution of studies were to take about 3 years, and the suggestion was made to conduct the studies at various geographical locations in different seasons.
Research Progress and Status
Substantial research is in progress, and some studies, started before the committee's first report, have been completed. Results of
recent panel studies of personal exposure conducted in Wageningen, Netherlands (Janssen et al. 1999), Boston, MA (Rojas-Bracho et al. 2000), Baltimore, MD (Sarnat 2000; Williams et al. 2000), and other places suggest that 12-15 measurements per person are sufficient to examine relationships between personal exposures and outdoor PM concentrations. These longitudinal panel studies have increased the understanding of the relationships between personal exposures and outdoor concentrations more than did earlier cross-sectional exposure studies. Several additional longitudinal panel studies are going on in other U.S. cities, including New York, NY; Atlanta, GA; Los Angeles, CA; Research Triangle Park, NC; and Seattle, WA. A number of research and funding organizations—including academic institutions, the U.S. Environmental Protection Agency (EPA), the Health Effects Institute (HEI), the Electric Power Research Institute (EPRI), and the American Petroleum Institute (API)—already have been engaged in this effort. Collectively, the studies should provide an understanding of the relationships between personal exposures and outdoor pollutant concentrations in a large number of geographic areas in the United States.
Several insights have been gained from the results of completed studies (Janssen et al. 1999, 2000; Ebelt et al. 2000; Rojas-Bracho et al. 2000; Sarnat et al. 2000; Williams et al. 2000). These studies have observed significant differences among study participants in the relationship between personal exposures and outdoor concentrations. When such relationships were analyzed for each person, substantial variability was found. Because outdoor concentrations exhibited little spatial variability, the heterogeneity was attributed to differences in indoor concentrations. Indeed, indoor concentrations were found to be an excellent predictor of personal exposures for most study participants, independently of city (Baltimore or Boston), season (winter or summer), and panel (elderly; chronic obstructive pulmonary disease, or COPD; or children). The finding that indoor concentration is an excellent predictor of personal exposure is not surprising, in that people spend more than 80% of their time indoors (EPA 1996a). Apart from exposures to tobacco smoke and emissions from cooking, which produce long-term increases in PM exposures of around 30 µ g/m 3 (Spengler et al. 1981) and 15-20 µ g/m 3 (Ozkaynak 1996), respectively,
home activities that were expected to produce particles, such as vacuum-cleaning and dusting (EPA 1996; Ozkaynak 1996), were found to explain very little of the total variability in personal exposures (Rojas-Bracho et al. 2000). In general, indoor sources tend to operate intermittently and, when measured by continuous monitors, can produce indoor concentrations as high as several hundred micrograms per cubic meter (Abt et al. 2000). The impact of these indoor (or other microenvironmental) peak concentrations can be captured only by real-time or semicontinuous personal monitors (Howard-Reed et al. 2000). However, when such short-term increases in concentration are averaged, their contributions to the average 24-hr indoor concentrations or personal exposures are estimated to be small.
Analyses of data from the study of elderly people in Baltimore (Sarnat et al. 2000) and the study of COPD patients in Boston (Rojas-Bracho et al. 2000) have demonstrated that ventilation (rate of exchange of indoor with outdoor air) is the measure that most strongly influences the relationship of personal-exposure to outdoor concentration. Personal exposure data were classified into three groups based on reported home ventilation status, a surrogate for the rate of exchange of indoor with outdoor air. Homes were classified as “well,” “moderately,” or “poorly” ventilated, as defined by the distribution of the fraction of time that windows were open while a person was in an indoor environment. When the PM datasets were stratified into these ventilation groups and analyzed cross-sectionally, strong relationships between personal exposures and outdoor concentrations were observed for well-ventilated homes and, to a lesser extent, for moderately ventilated homes. However, a low correlation coefficient was found for the poorly ventilated homes. Those findings suggest that for homes with no smokers and little cooking activity most of the variability in indoor concentrations, as well as in personal exposures of occupants, is due to the varied impact of outdoor sources on the indoor environment. That effect is underscored by the influence of air-exchange rates on the relationship between indoor and outdoor concentrations when no activities are occurring in the homes. For instance, for well-ventilated homes, indoor-to-outdoor particle ratios are close to 1.0, whereas for homes with low rates of exchange and
no activities, indoor-to-outdoor ratios can be substantially lower (about 0.4-0.6) (Abt et al. 2000; Long et al. 2000).
Home ventilation rates are expected to vary with season, geographical location, and home characteristics; that implies that the relationship of human exposures to outdoor PM concentrations will also vary with these factors. Therefore, PM risk relationships estimated from epidemiological studies might differ by city, season, and overall home characteristics. However, the additional influence of personal activity patterns on the overall relationship between human exposure and outdoor PM concentrations is also relevant to interpretation of the results of observational studies. The pattern of reported findings is still based on a small number of studies, and replication of the results will be needed from current or recently completed studies in other cities before firm conclusions can be drawn.
Adequacy of Current Research in Addressing Research Needs
Considerable effort is going into examining the relationship between ambient particle concentrations and personal exposures. Several longitudinal panel studies are being conducted in various geographic locations, including New York, NY; Atlanta, GA; Los Angeles and Fresno, CA; and Seattle, WA (see Table 3.1 ). Collectively, these studies are assessing exposures of healthy subjects and susceptible subpopulations (such as those with COPD; myocardial infarction, or MI; or asthma) to PM and some gaseous copollutants (such as ozone, sulfur dioxide, and nitrogen dioxide). The studies are expected to greatly expand the database on personal exposures, indoor and outdoor concentrations, human activities, and home characteristics. They are also expected to improve understanding of factors that influence the relationship between ambient concentrations and personal exposures. Therefore, as new information from the panel studies accumulates, it appears that, in spite of the time needed to initiate them, many of the elements of research topic 1 are being addressed. Most of the studies have not been completed; their findings are expected to appear in the peer-reviewed literature in the next several years.
TABLE 3.1 Current Studies Relevant to Research Topic 1
Many of the recently completed and current studies examine the relationship between ambient concentrations of gaseous pollutants and personal exposures. Understanding that relationship will provide profiles of multipollutant exposures that can inform understanding of research topic 7 (combined effects of PM and gaseous pollutants). In
addition, understanding of differences between personal exposure and ambient concentrations for a suite of gaseous pollutants and PM will provide input into analyses of measurement error in a multi-pollutant context (see research topic 10 , analysis and measurement).
Application of Evaluation Criteria
Scientific value.
The current panel exposure studies are straightforward and have expanded on findings from previous investigations. They have used well-established research tools for conducting personal and micro-environmental measurements. They have also relied on field protocols developed as part of previous exposure studies, (such as the Particle Total Exposure Assessment Methodology (PTEAM) study (Pellizzari et al. 1993). The studies are generally designed to assess the range of exposures including those that occur in the home, in the workplace, and while traveling. To a large extent, the scientific value of these investigations will be judged by the appropriateness of their design. It appears that the study designs, (such as repeated measurements of a small number of people) can adequately address the scientific questions in research topic 1 .
Completed studies have indicated key factors that influence outdoor-personal relationships. Preliminary results suggest that for homes with no smokers and little cooking activity, home ventilation rate (or air-exchange rate) is the most important modifier of personal exposure. To a great extent, ventilation rate controls the impact of both outdoor and indoor sources on the indoor environment, where people spend most of their time. If correct, this observation implies that such entities as home characteristics, season, and location could be more important determinants of personal exposure than activities and type of susceptible subpopulation studied.
The panel studies will also produce a large set of data on human activities and home characteristics. These data will substantially enrich the existing information and will be available to other researchers
involved in human-exposure assessment investigations (such as EPA 's National Human Exposure Assessment Survey).
Decisionmaking Value
Exposure assessment is of paramount importance for understanding the effects of ambient particles and for developing cost-effective exposure-control strategies. The current studies should allow the scientific community and decisionmakers to understand the factors that affect the relationship between personal exposure and outdoor concentrations. That will be accomplished through the continued development of personal-exposure monitoring tools that allow a better understanding of the sources of exposure, physical and chemical properties of PM, and sampling durations that could be relevant to the subpopulations being studied. Although the panel studies are based on small numbers of participants (10-50 per panel), they are addressing factors that influence relationships between outdoor air and personal exposures. This is the first step in attempts to develop a comprehensive exposure model, which is a key research tool in the source-exposure-dose-response paradigm.
Feasibility and Timing
Sampling and analytical procedures, time-activity questionnaires, and other related methods necessary for conducting the panel studies have been adequately tested. They have been implemented successfully in various geographical locations by various research groups (such as Janssen et al. 1999, 2000; Ebelt et al. 2000; Rojas-Bracho et al. 2000; Sarnat et al. 2000; Williams et al. 2000). Therefore, it is expected that the current longitudinal panel studies will be completed without great difficulty. Although there was some delay in initiating some of the studies, abundant personal and microenvironmental measurements have been collected. Reporting of results from research related to this topic began during the summer of 2000, and the re-
maining studies should be reported.within about 2 years, a year later than originally planned.
RESEARCH TOPIC 2. EXPOSURES OF SUSCEPTIBLE SUBPOPULATIONS TO TOXIC PARTICULATE-MATTER COMPONENTS
What are the exposures to biologically important constituents and spe cific characteristics of particulate matter that cause responses in potentially susceptible subpopulations and the general population?
The committee recommended that after obtaining and interpreting results of studies from research topic 1 human exposure-assessment studies examine exposures to specific chemical constituents of PM considered relevant to health effects. To make research topic 2 investigations more practicable, it will be necessary to characterize susceptible subpopulations more fully, identify toxicologically important chemical constituents or particle-size fractions, develop and field-test exposure-measurement techniques for relevant properties of PM, and design comprehensive studies to determine population exposures.
Methods of measuring personal exposures to particles of various physical properties (such as particle number and size) or chemical properties (such as sulfate, nitrate, carbon, and other elements) are available and are being field-tested. Methods of measuring personal exposures to some gaseous copollutants—such as ozone, nitrogen dioxide, and sulfur dioxide—are also used. As interest in personal-exposure measurements increases, new sampling and analytical techniques will probably emerge.
The results of the longitudinal panel studies discussed under research topic 1 should facilitate the design of cost-effective protocols for future exposure studies that focus on PM components considered in determining toxicity. These studies will be based on toxicity and epidemiological studies that are successful in identifying particle properties of interest over the next few years; because they will prob-
ably not get under way for several years, the committee is planning to evaluate their progress in its next report.
RESEARCH TOPIC 3. CHARACTERIZATION OF EMISSION SOURCES
What are the size distribution, chemical composition, and mass-emission rates of particulate matter emitted from the collection of primary-particle sources in the United States, and what are the emissions of reactive gases that lead to secondary particle formation through atmospheric chemical reactions?
In its second report, the committee created a separate set of research recommendations that address measurement of the size distribution and chemical composition of PM emissions from sources. Characterization of the emission rates of reactive gases that can form particles on reaction in the atmosphere was also emphasized, including the need to maintain emission data on sulfur oxides, nitrogen oxides, ammonia, and volatile organic compounds (VOCs) (specifically those components that lead to particle formation).
The committee noted that traditional emission inventories have focused on representing PM mass emissions summed over all particles smaller than a given size, without detailed accounting of the particle-size distribution or chemical composition. Health-effects research recommended by the committee emphasized identification of the specific chemical components or size characteristics of the particles that are most directly related to the biological mechanisms that lead to the health effects of airborne particles. Detailed information on the size and composition of particle emissions from sources is important for this process of hazard identification and effective regulation. In the near term, toxicologists and epidemiologists need to know the size and composition of particles emitted from key emission sources to form hypotheses about the importance of particle characteristics and to give priority to their evaluation in laboratory- and field-based health-effects studies. In the longer term, detailed information on
particle size and composition will be needed for the design of effective air-quality control programs if those programs become more precisely targeted at the most biologically active components of the atmospheric particle mixture.
Detailed data on the particle size distribution and chemical composition of emissions from sources are also needed to support the application and evaluation of air-quality models that relate source emissions to ambient-air pollutant concentrations and chemical composition. These models are central to the process of evaluating emission-control strategies in advance of their adoption. Source-oriented models for particle transport and new particle formation can require detailed data on particle size and composition for use in condensation-evaporation calculations. Chemical mass-balance (CMB) receptor-oriented air-quality models determine source contributions to ambient particle concentrations by computing the best-fit linear combination of source chemical-composition profiles needed to reconstruct the chemical composition of atmospheric samples. These CMB models inherently require the use of accurate data on the chemical composition of particle emissions at their source. Finally, emissions data on particle chemical composition and size will be needed in the future to support detailed studies of air-quality model performance. Even when the regulated pollutant is fine-particle mass, assurances are needed that air-quality models are getting the right answers for the right reasons. Model-evaluation studies conducted in a way that tests a model's ability to account for ambient particle size and chemical composition can be used to confirm that the model has arrived at agreement between the predicted and observed mass-concentration values for the correct reasons.
In light of those needs for data on the size and chemical composition of particle emissions from sources, the committee's second report outlined the following set of research needs: establish standard source-test methods for measurement of particle size and chemical composition, characterize primary particle size and composition of emissions from the most important sources, develop new measurement methods and use of data to characterize sources of gas-phase ammonia and semivolatile organic vapors, and translate new source-
test procedures and source-test data into comprehensive national emission inventories.
Establish Standard Source-Test Methods for Measurement of Particle Size and Chemical Composition
Research into the establishment of new source-test methods for measurement of fine- particle chemical composition is under way at EPA. A dilution source sampler for measurement of emissions from stationary sources has been built and tested. It permits measurement of particle size distributions and elemental carbon, organic carbon, speciated organic compounds, inorganic ions, and trace elements. The inorganic ions typically would include sulfates, nitrates, ammonium, and chlorides. Catalytic trace metals are included among the more than 30 trace elements that will be measured. These measurements are aligned with many of the potentially hazardous characteristics of the particles that have been identified by the committee and include determination of size-fractionated PM mass, PM surface area, PM number concentration, transition metals, soot, polycyclic aromatic hydrocarbons (PAHs), sulfates, nitrates, and some copollutants. It is not clear whether plans are being made to measure strong acids, bioaerosols, peroxides, or free radicals, which constitute other categories of concern to the health-effects community in determining the toxicity of particles. The methods being developed can be used to collect data on volatile and semivolatile organic vapor emissions and could be adapted to measure ammonia emissions. Methods for dilution source sampling of diesel exhaust particles are also under development.
EPA has conducted field tests of these advanced emission-measurement methods for open biomass burning, residential wood stoves, heavy-duty diesel trucks, and small oil-fired boilers. Construction dust emissions have also been measured. Plans for the near future include measurement of PM emissions from diesel trucks, wood-
fired boilers, large residual oil-fired boilers, jet aircraft engines, and coal-fired boilers. In addition, dilution source sampling to determine particle size and composition by comparable methods is being supported by EPA through the Science to Achieve Results (STAR) grants program (biomass smoke), American Petroleum Institute (API) (petroleum-refinery processes), the Coordinating Research Council or CRC (diesel trucks), the California Air Resources Board, the National Park Service (NPS), the Department of Defense (motor vehicles, boilers, and so on), and the Department of Energy.
Those dilution source-sampling methods have been developed for research purposes and are being used to gather data to prepare accurate emission inventories. However, the new methods have not yet replaced earlier methods for testing to establish and enforce emission limits. EPA 's Office of Air and Radiation (OAR) is evaluating a dilution-based source-testing procedure for PM 2.5 compliance source-testing that might be proposed in the 2002 Federal Register.
Characterize Primary Particle Size and Composition of Emissions
In its second report, the committee advised EPA that development of new source-test methods would probably require substantial attention during FY 2000 and 2001. It was suggested that the new methods be used to characterize a larger number of sources over a 5-year period, beginning in FY 2002, because this information will be needed to revise the nation's emission inventories. EPA's method-development effort is well under way as recommended, but it is too early to expect large-scale application of the new methods.
In the course of development and testing of the new source-measurement methods, emissions from about six important source types have been characterized by EPA according to their particle size distributions and chemical composition, and another six will be characterized in the near future. Beyond those advances, EPA OAR reports that current resources will not support plans to conduct measurements of PM emissions from other stationary sources with either newly developed or more traditional source-test methods. Historically, few states
have devoted substantial resources to source testing for the purposes of emission-inventory development. Some source testing has been supported by government agencies other than EPA (such as CARB, the state of Colorado, NPS, and DOE) and by industry (for example, CRC, EPRI, and API). The committee located more than 150 projects related to source testing either under way or recently completed, with studies generally distributed as shown in Table 3.2 . However, few of these studies use methods, such as the dilution source-sampling system being developed by EPA, that fully characterize particle size and chemical composition.
The small number of sources scheduled for full characterization falls far short of a well-designed comprehensive testing program that would lead to more-accurate emission inventories. EPA has noted its reply to the committee's questions about the range of sources to be tested that “ORD can only test a limited number of source categories annually with currently available staff and funding. In addition, the ORD method development effort is unable to test sources within any one category under the full range of operating conditions typically encountered in the field. As previously stated, the number and diversity of sources means that, at any foreseeable resource level, many years would be needed to test a representative sample of all distinctive types of sources” (EPA response to questions from the committee
TABLE 3.2 PM Emissions-Related Research
dated June 2000). In its second report, the committee recommended that EPA plan to systematically achieve nearly complete characterization of emissions by particle size and composition for sources that contribute about 80% of the primary particle emissions nationally. The committee notes that now is the time to begin planning the selection of sources to be tested during the 5-year cycle beginning in FY 2002 to achieve that objective.
In its second report, the committee specifically recommended an expanded source-testing program at the level of an additional $5 million per year, beginning in FY 2002. That recommendation, if followed, will remove the program's current financial constraints. Therefore, it is appropriate to begin planning for a comprehensive source-testing program that will systematically measure the particle size distribution, particle chemical composition, and gaseous particle precursor emissions characteristics of a reasonably complete set of the relevant sources over a 5-year period. Consultations should be held with researchers in health effects, exposure, source-oriented air-quality modeling, and receptor-oriented air-quality modeling to solicit recommendations on sources to be tested and any additional chemical and physical dimensions that should be measured during the national source-testing program.
Develop New Measurement Methods and Use of Data to Characterize Sources of Gas-Phase Ammonia and Semivolatile Organic Vapors.
Methods for measurement of ammonia from nonpoint sources, such as hog-feeding facilities and highway operation of motor vehicles, have been tested by EPA ORD during the last year. Additional measurements of ammonia emissions from animal husbandry are planned for next year. Semivolatile organic compound emissions are among the dimensions listed as measurable by the research-grade dilution source-sampling procedures developed by ORD. As in the previous discussion of fine-particle emission characterization, there appears to be no program in place that will characterize more than a
handful of the relevant emission source types within the foreseeable future. Before FY 2002, a plan should be put into place for a comprehensive source-testing program that will lead to the creation of a national ammonia emission inventory based on credible and recent experimental data.
Translate New Source-Test Procedures and Source-Test Data into Comprehensive National Emission Inventories
EPA maintains a national regulatory emission inventory for PM 2.5 , PM 10 , and gases that act as particle precursors. The PM emission inventory is primarily a mass-emission inventory that does not extend to particle size distributions and particle chemical composition. EPA maintains a file of source chemical-composition profiles that can be used to estimate particle chemical-composition in many cases. These source chemical-composition profiles need to be brought up to date through a continuing program of literature review and additional source testing.
Funds appear to be available to incorporate data from new emission measurements into the national emission inventory. Although the new data are incorporated into the inventory continuously as they are collected, there is no specific date for completion of a truly new inventory. This process might appear to be one of continuous improvement, but that is not necessarily the case. Technologies used in various types of emission sources change over time. As a result, older emission data can become obsolete faster than the program of continuous improvement can keep up with the changes, especially if the emission inventory program does not have a systematic schedule for review and replacement of existing data. Highway diesel engines, for example, could be scheduled for new source-characterization experiments, but it is possible that many other diesel-engine types used in heavy-duty off-highway applications —such as construction equipment, railroad locomotives, and ships—are represented by obsolete source-test data as these technologies change over time.
The committee has recommended the compilation, beginning in
FY 2006, of a thoroughly revised national emission inventory for PM as a function of particle size and composition, and for gaseous particle precursors based on the new source-test data generated in accordance with the above recommendations. The infrastructure exists to support this work, and the committee has recommended new funds of $1 million per year to finance the effort over several years, beginning in FY 2006.
Application of Evaluation Criteria Scientific Value
There is great scientific value to the research under way to develop new source-test methods and demonstrate their capabilities to measure particle size, particle chemical composition, and rates of emission of ammonia and semivolatile organic compounds. This information is needed to guide exposure-assessment studies and help toxicologists and epidemiologists form potential hypotheses about components of PM that could be hazardous to human health. The emission data are also needed to support tests of advanced air-quality models that seek to relate pollutant emissions to ambient-air quality. Emission data that describe particle size and chemical composition are needed to permit the calculation of gas-to-particle conversion rates and support calculations of heterogeneous chemical reactions that occur clouds in clouds, haze, and fog. Furthermore, when emission data on particle size and composition are available, air-quality models that account for particle size and composition can be put to very demanding tests that ensure that they are producing the right answers for the right reasons.
Decisions about alternative emission-control policies have to be based on an accurate understanding of the relative strength and possible toxicity of emissions from various sources. Accurate emission
inventories are absolutely fundamental to the decisionmaking process. Although there is scientific merit in the work that is under way to develop new source-test methods, the potentially important benefits to the decisionmaking process of more-complete and accurate knowledge of particle emissions evaluated according to size and composition can be realized only if EPA proceeds to expand its present source-testing program substantially by FY 2002, in accordance with the committee 's recommendations. EPA should now develop a comprehensive plan for systematically translating the new source-test methods into a completed comprehensive national emissions inventory based on contemporary source tests of comparable quality. There is still ample opportunity to plan that future source-test program. The first step would involve the systematic creation of a master list of sources that most need to be tested over a specific period. The timeline for this testing must allow for the incorporation of revised and updated data into an overall emission inventory of predetermined quality and completeness by the time the next round of PM implementation plans must be drafted.
In the committee's second report, it was estimated that five to 15 source-testing campaigns would need to be directed at different source types each year for a 5-year period beginning in FY 2002 to bring new source-test methods to bear in creation of a reasonably complete emission inventory for particle size and composition based on contemporary data of high quality. EPA ORD is conducting about six such testing campaigns per year, at a cost of about $2.3 million per year while it is in the method-development phase that precedes the work of source testing for an emission inventory. That is reasonably consistent with the committee's recommendation that about $2.5 million per year should be spent during FY 2000 and 2001 on method-development research. On the basis of the observation that EPA ORD alone has been able to conduct about six source-test campaigns per year with an annual budget of $2.3 million, it seems reasonable that funds of $5-$7.5 million per year, as recommended by the committee
for FY 2002-2006, will be sufficient to support the proposed testing needed for a thorough upgrade of the emission inventory. With the FY 2002-2006 timeline, EPA has at least a year in which to draft a plan that identifies the sources to be tested in the future to ensure reasonably complete representation (a goal of about 80% coverage on a mass basis) of the national fine- particle emission inventory. Although some of the remarks by EPA in reply to committee questions appear to assume that a reasonably complete reworking of the emission inventory is beyond the planning horizon of the agency, the goal of a high-quality inventory for particle size and chemical composition is not out of reach. Drafting of a comprehensive plan that preselects sources to be tested and sets priorities for the work to be done over about a 5-year period will help to ensure the success of the research effort.
RESEARCH TOPIC 4. AIR-QUALITY MODEL DEVELOPMENT AND TESTING
What are the linkages between emission sources and ambient concentra tions of the biologically important components of particulate matter?
The focus of this research topic is development and testing of source-oriented and receptor-oriented models that represent the linkages between emission sources and ambient concentrations of the most biologically important components of PM. Comprehensive source-oriented models for PM are still under development; before they are ready for regulatory applications, they require more-certain emission inventories (see research topic 3 ) and an improved understanding of the chemical and physical processes that determine the size distribution and chemical composition of ambient particles. Receptor-oriented models have been used to apportion particle mass measurements to primary emission sources through a mathematical comparison of chemical profiles of ambient PM samples with the profiles of emission-source types. However, better mathematical tools and chemical tracers are needed to resolve additional sources and to handle secondary species. Before the models can be used with suffi-
cient confidence, both the receptor-oriented and source-oriented approaches need to be tested with observations from intensive field programs and then compared with each other.
Air-Quality Model Development
Source-oriented models.
EPA has developed its major new modeling platform, MODELS 3, over the last decade. MODELS 3 is just beginning to be deployed and has not yet been extensively tested. It has been developed in a specific configuration, Community Model for Air Quality (CMAQ), primarily for modeling ozone. Scientific reviews of MODELS 3 have focused primarily on its ability to provide adequate representations of chemical processes to estimate ozone. Only recently has there been active consideration of incorporating PM formation and transport into the model.
The atmospheric-science community had limited interaction with EPA during the development of MODELS 3. In EPA's response to the committee 's questions, the agency suggested that there was limited interaction because EPA faces relatively few major uncertainties about atmospheric processes and it is simply a matter of time before all the science that is needed to produce adequate estimates will be incorporated into the model. The committee did not get an indication as to whether MODELS 3 had been sufficiently tested with regard to PM formation and transport.
Table 3.3 presents a summary of the current studies identified by EPA and others as sources of information on atmospheric processes. These studies demonstrate the efforts under way to understand the processes governing atmospheric phenomena. However, the committee does not believe that current or planned efforts are sufficiently organized to effectively assess and use the information obtained through these studies.
EPA has developed a second model, the Regulatory Modeling System for Aerosols and Deposition (REMSAD), that is designed to simu-
TABLE 3.3 Summary of Current Studies Identified by EPA as Sources of Information on Atmospheric Processes
late the concentrations and chemical composition of primary and secondary PM 2.5 concentrations, PM 10 concentrations, and depositions of acids, nutrients, and toxic chemicals. To reduce computational time and costs, REMSAD uses simpler chemistry and physics modules than MODELS 3. REMSAD has been applied to model concentrations of total PM 2.5 and PM 2.5 species (sulfate, nitrate, organic carbon, elemental carbon, and other directly emitted PM 2.5 ) over the conterminous United States for every hour of every day in 1990. Annual, seasonal, and daily averages from the 1990 base case have been compared with data from the Interagency Monitoring of Protected Visual Environments (IMPROVE) network and the Clean Air Status and Trends Network (CASTNET). Sensitivity analyses have also been conducted for changes in SO x , NO x , ammonia, and directly emitted PM 2.5. Because of the lack or sparseness of available data on many areas of the United States (for example, IMPROVE provided only two 24-hour-average concentrations per week for a few dozen sites in 1990), there has not been an effective national evaluation of the model for PM. It is not clear whether REMSAD's simplified representations of chemistry
adequately capture the complex atmospheric processes that govern observed particle concentrations.
A number of other source-oriented PM models are being developed by individual investigators at universities or consulting companies. Seigneur et al. (1998, 1999) reviewed 10 Eulerian grid models: seven for episodic applications and three for long-term applications. The episodic models are the California Institute of Technology (CIT) model, the Denver Air Quality Model (DAQM), the Gas, Aerosol, Transport, and Radiation (GATOR) model, the Regional Particulate Model (RPM), the SARMAP Air Quality Model with Aerosols (SAQM-AERO), the Urban Airshed Model Version IV with Aerosols (UAM-AERO), and the Urban Airshed Model Version IV with an aerosol module based on the Aerosol Inorganic Model (UAM-AIM). The long-term models are the REMSAD, the Urban Airshed Model Version IV with Linearized Chemistry (UAM-LC), and the Visibility and Haze in the Western Atmosphere (VISHWA) model. In addition, several university groups are developing additional PM models that are primarily extensions of the CIT model to other areas of the country.
It appears that none of the models reviewed by Seigneur et al. (1998, 1999) is suitable for simulating PM ambient concentrations under a wide range of conditions. The following limitations were identified in both episodic and long-term models:
Most models need improvement, albeit to various extents, in their treatment of sulfate and nitrate formation in the presence of fog, haze, and/or clouds.
All models need improvement, albeit to various extents, in their treatment of secondary organic particle formation.
The urban-scale models will require modifications if they are to be applied to regional scales.
All models but one lack subgrid-scale treatment of point-source plumes.
In addition to the limitations identified above, the reliability of the simplified treatments of chemistry used for estimating the effect of emission changes on PM concentrations in the long-term models has
not been sufficiently tested. An alternative approach for predicting annual average PM concentrations has also not been adequately tested. This approach—to be used by EPA in applications of MODELS 3/CMAQ—is to approximate a full year by combining several typical meteorological scenarios with appropriate weighting factors and applying an episodic model separately to each scenario. The validity of the approach depends on, among other things, the meteorological representativeness of the selected scenarios. The approach has not yet been the subject of a comprehensive evaluation, so its validity is unknown.
In addition to the limitations indicated above regarding the formulations of PM source models, it must be noted that the application of PM source models requires input data for emission, meteorology, and ambient concentrations of PM and gases. For example, it might be possible to improve the models by incorporating more information on atmospheric processes, but any apparent improvements will need to be tested for their success in reproducing observations during specific meteorological situations. Substantial uncertainties are still associated with PM emission inventories, as described under research topic 3 . Ammonia and VOC emission inventories involve large gaps that will affect the predictions of secondary PM, and uncertainties in natural and anthropogenic emissions of mineral dust will affect the predictions of primary PM. Moreover, the most comprehensive modeling input databases are for California regions, and there are insufficient data on other states.
Emission and process data related to specific components of PM are notably lacking. A long-term research goal is to identify specific physical or chemical components in PM that are primarily responsible for the adverse health effects. EPA models focus on total mass concentrations and major PM constituents, such as sulfate and nitrate. However, future models could expand their focus to size distributions and other chemical constituents.
Efforts are under way at academic and other research institutions (such as EPRI) to improve air-quality models. Efforts are also under way to link and integrate air-quality models with exposure and dose
models. However, it appears that there is no coordinated effort to compare the various models with one another or to use improvements developed for one or another model to improve the others, particularly those earmarked for regulatory applications. It is not clear that the appropriate commitment is being made to have the best models available at the local air-quality management levels for use in PM planning efforts.
Receptor-Oriented Models
There has been very little support for the development and testing of new receptor-oriented models. Such models are used to identify and quantitatively appropriate an ambient PM sample from a given location (receptor) to its sources. The CMB model has been rewritten to run under the Windows operating system, and EPA has supported factor-analysis model development under a single STAR grant. Both products are now in the process of external review, with probable release at the end of 2000. A new version of the EPA source-profile library will run under modern PC operating systems. However, only 16 profiles have been added to the library since its revision in 1992—an indication of the lack of incorporation of recently published source profiles. Seigneur et al. (1998, 1999) reviewed existing receptor models, including back-trajectory-based analyses to locate candidate source areas, alternative factor-analysis models based on least-squares fitting, and alternative solution methods for the CMB. However, further development and testing are required before they can be widely distributed for air-quality management purposes.
There is a particular need for data-analysis tools to handle newly emerging monitoring technologies, such as aerosol mass spectroscopy. A number of approaches have been presented in the literature, but they are typically applied to only a single location or region. There has not been an extensive effort to test the effectiveness of these alternative methods or to review their potential use in future development of air-quality management strategies.
Air-Quality Model Testing
To test the predictive capability of models, it is necessary to have both the model input data and appropriately detailed sets of air-quality observations to compare with the model outputs. Earlier field campaigns —such as the Southern Oxidants Study (SOS; www2.ncsu.edu/ncsu/CIL/southern_oxidants/index.html ), the Northern Front Range Air Quality Study (NFRAQS; www.nfraqs.colostate.edu/index2.html ), the Southern California Air Quality Study (SCAQS; Lawson 1990), and the San Joaquin Valley Air Quality Study (SJVAQS; Lagarias and Sylte 1991; Chow et al. 1998)—provide the necessary data for model testing. (SOS, until recently, and SJVAQS have limited utility for PM models because they focused primarily on ozone.) Although those earlier efforts provided insights into basic atmospheric processes, only some of the supersite monitoring stations can possibly have sufficient data to validate regional-scale air-quality models. It is clear that more field campaigns are needed to provide data to test the predictive capability of state-of-the-science PM models.
A number of large studies are just starting, such as the supersite efforts, and continuing studies, such as SOS. However, there do not appear to be plans to use these databases fully for testing air-quality models. ORD personnel have suggested that EPA will use the data to test MODELS 3/CMAQ, but it is not clear to the committee that there will be effective internal and external review. The committee is aware of no defined plans to compare MODELS 3/CMAQ with any of the other similar-scale models. Such comparisons are needed, and a plan for evaluation and revision of the EPA model should be developed as part of EPA's PM research program.
This lack of effort to use available data effectively highlights the need for EPA to be active in defining the nature of the data needed to test Models 3/CMAQ fully and compare it with other independently developed models. There is now an agreement among the Baltimore, New York City, and Pittsburgh supersites and the NARSTO NE-OPS (Northeast Oxidant and Particle study) program in Philadelphia to operate in an intensive mode during July 2001. There will be only sparse upper-air measurements using LIDAR in Baltimore and Philadel-
phia. Although it might be too late to organize extensive additional upper-air measurements for those particular studies, this is one example of the kind of opportunity that EPA should be actively seeking, particularly in the eastern and southeastern United States, where such large-scale, detailed data are lacking.
It might be possible to build on the speciation network, once it is in place, to develop appropriate field campaigns. By operating these systems more intensively (more frequently than daily) and supplementing the speciation network monitors with particle-size measurement devices, it would be possible to provide a suitable database for regional-scale model testing.
The testing of receptor models is similarly incomplete. It appears that there has been no clear plan for development, testing, and deployment of additional receptor-modeling tools. CRC recently supported an effort to evaluate receptor models for VOCs, using grid models to produce test data. EPA has had a small effort to compare factor-analysis models, but a more extensive program will be needed to provide the full range of tools necessary for a comprehensive analysis, particularly for PM 2.5.
There is substantial support of current studies that are expected to make substantial contributions to the understanding of atmospheric processes. The development of source-oriented models represents the codification of a portion of new knowledge into an organized framework for application. The testing of individual models and the comparison of the results of multiple models can help to identify the effects of different approaches to incorporation of the knowledge into prediction based upon models. Such comparisons will help to refine the available knowledge. The development of better algorithms for source- and receptor-oriented models will also be substantial scientific advances.
Air-quality models are essential for making regulatory decisions. They provide the critical information required to develop the effective and efficient air-quality management strategies that are needed for state implementation plans (SIPs), which are developed when areas are found to be in nonattainment of the PM National Ambient Air Quality Standards (NAAQS). Regional-scale models are needed for reducing visibility impairment, acid precipitation, and other adverse environmental effects. Improved models would also provide critical exposure-related data that could be used in health studies to examine the relationships between ambient PM concentrations and health. There is insufficient effort to test the models developed by EPA and others or to use extensive comparison with other models to ascertain the differences and similarities in the results. Such efforts would provide further improvements in the models and greater confidence in the decisions based on the model results. In addition, it is important to link air-quality models with exposure models. EPA is collaborating with other organizations to develop a population exposure model for PM to provide such a linkage.
The development and testing of models is highly feasible. The increase in computational power permits the incorporation of greater numbers of observations and understanding of atmospheric processes into source-oriented models. The same computational power permits much more sophisticated methods for data analysis to be used in receptor-oriented models.
The new PM monitoring program provides a base from which data can be obtained for testing of the models. If research topic 3 is appropriately implemented, the necessary source data and source-oriented models will be readily available. The source profiles developed under research topic 3 will also provide data to introduce into recep-
tor-oriented models. Thus, the plan for testing models presented in the committee's second report can be used to provide the necessary tests and improvements for models. However, the planning process should be started immediately to allow time for important regional-scale field studies. It should be noted that the data needed for model testing and evaluation are not necessarily the same as data needed for developing exposure metrics, which is discussed later in this chapter.
There is also time to develop, test, and deploy advanced receptor models more fully before requirements arise from the SIP process. However, there must be a more concerted effort to recognize the need for improved models and improved data for existing models.
Integration and Planning
There appears to be insufficient effort in organizing and carrying out the field studies that would provide the data for thorough evaluation of existing models; only a small effort to leverage the investment is being made in the PM monitoring program to provide these data. There is a large body of historical data that would be of great value in model testing if they could be processed in a standard way into a central repository from which they can be easily accessed. It appears that EPA does not yet recognize the need for full model testing, so it has not mobilized the needed resources.
It appears that there has been no comprehensive planning for the development and deployment of receptor-oriented models. The current ad hoc approach to receptor-model development will not provide the additional tools essential to develop future state implementation plans. With the development of several new factor-analysis models, there has been some effort to compare them, but there is still no evidence of a plan for developing and implementing improved models in the context and timeframe of what will be needed for the PM 2.5 SIP process.
RESEARCH TOPIC 5. ASSESSMENT OF HAZARDOUS PARTICULATE-MATTER COMPONENTS
What is the role of physicochemical characteristics of particulate matter in eliciting adverse health effects?
The initial research portfolio (NRC 1998) outlined a research agenda designed to improve the understanding of the roles of specific characteristics of ambient PM (such as particle size distribution, particle shape, and chemical constituents) in determining the toxicity for underlying adverse health outcomes associated with PM exposure. The research plan indicated not only studies aimed at determining the relevance of those characteristics, but also work designed to evaluate the dose metrics that have been used to relate PM exposure to health effects in epidemiological and toxicological evaluations. Research was needed to develop PM surrogates, that is, material with specified characteristics for use in toxicity studies. In its second report, the committee (NRC 1999) reconfirmed the importance of this kind of investigation.
The nature of the chemical or physical characteristics of ambient PM that might account for its biological activity remains a critically important component of the PM research portfolio. In addition to providing mechanistic plausibility for epidemiological findings related to PM, an understanding of the relationship between mechanisms of biological action and specific PM characteristics will be a key element in selecting future control strategies. The following list of particle characteristics potentially relevant to health risk is large and possibly variable across health effects:
Size-fractionated PM mass concentration
PM surface area
PM number concentration
Transition metals
Soot and organic chemicals
Bioaerosols
Sulfate and nitrate
Peroxides and other free radicals
Those particle characteristics may be associated with cardiovascular disease, acute respiratory infection, chronic obstructive pulmonary disease, asthma, and morbidity. Inspection of this list, which could be expanded, makes clear the challenge that is faced by the investigative community and by research managers who need to focus resources toward the key relationships between particle characteristics and health effects.
In addressing this research topic, both toxicological and epidemiological approaches are needed. Hypotheses advanced from data in one domain need to be tested in the other, complementary domain. Greater certainty will be achieved as evidence from the laboratory and the population converges and as integrative research models merge the population and laboratory data into a common framework. For example, particles obtained from filters in the Utah Valley, the site of epidemiological studies of health risks posed by particles from a steel mill, have been assessed for toxicity in laboratory systems (Frampton et al. 1999; Soukup et al. 2000; Dye et al. in press). The availability of particle concentrators could also facilitate the implementation of integrative research models, in that animals and people can be exposed to a comparable mixture of real-world particles.
The general methodological issues arising in connection with this research topic are akin to the problem of assessing the toxicity of a mixture and determining the specific characteristics that are responsible for its toxicity. Particles, in fact, constitute a mixture: urban atmospheres are contaminated by diverse sources, and the characteristics of particles can change and vary among regions. The difficulties of studying mixtures have been addressed by numerous panels, including committees of the National Research Council (NRC 1988). Accepted and informative research models have not yet been developed, and even attempting to characterize several toxicity-determining characteristics of mixtures has proved challenging.
One objective of research related to this topic was to assess relevant dose metrics for PM to explain adverse health outcomes. EPA
routinely measures size-specific mass concentration, previously PM 10 and now PM 2.5 as well. The selection of these concentrations and the timeframe over which they are measured (24 hours) reflect technological feasibility to a greater extent than fit with time-exposure-response relationships of PM with health risk. Routine regulatory monitoring provides only 24-hour averaged mass concentrations, but special monitoring programs —including those instituted in support of epidemiological studies, the speciation sites, and the supersites program—offer the opportunity to explore alternative dose (exposure) metrics. Newer techniques to monitor PM 2.5 over shorter periods, and even continuously, are being developed and tested.
Another objective, to evaluate the role of particle size in toxicological responses to PM and that related to epidemiological outcomes, focuses on the size of particles that are relevant to the health effects observed in the epidemiological studies. To date, the associations of PM with both illness and death have been demonstrated in studies using indexes that incorporate particles with a large range of sizes (such as total suspended particles, or TSP, and PM 10 ). These studies have drawn on the available data. PM 10 , of course, includes particles in all smaller size categories and thus includes PM 2.5 and ultrafine particles (those smaller than 0.1 µ m in diameter). Ultrafine particles probably make up a very small fraction of PM 10 mass, but pathophysiological considerations and some initial toxicological findings have focused attention on the hypothesis that such smaller particles may be responsible for some toxicological responses that lead to the epidemiological findings.
New work directed at this research topic has been based largely on toxicological approaches. Forty-eight toxicology projects described in the HEI database were identified as potentially related to the topic.
Ambient PM is a complex mixture that contains various chemical components in various size fractions. Evaluation of whether biologi-
cal responses to PM are nonspecific—that is, are due merely to inhalation of any particle—or depend on specific PM properties is a critical focus of current research. Regarding the latter possibility, research performed in the recent past has indicated some specific potential characteristics that appear to be involved in PM-induced health effects. A compilation of these (Mauderly et al. 1998) is as follows: size-fractionated particle mass concentration; particle surface area; particle number concentration, which is generally related to the ultrafine component of PM; transition metals (especially the fraction soluble in vivo); acids; organic compounds (especially PAHs); bioaerosols; sulfates and nitrates, typically existing in ambient air as ammonium or sodium compounds; peroxides and free radicals that can accompany, and help to form, particles; soot (elemental carbon, black carbon, or light-absorbing carbon); and correlated cofactors (such as the presence of gaseous pollutants and variations in meteorology). The current toxicological research portfolio as reported in the HEI database was examined with regard to each of those specific chemical or physical characteristics.
Size-Fractionated Particle Mass Concentration. The mass concentration—the mass (weight) of collected PM per unit volume of sampled air, generally within some selected particle-size range or below some upper size cutoff—was the exposure metric most commonly evaluated in relation to health effects in the studies in the HEI database. More-recent epidemiological studies of ambient particles have generally focused on the 0.1- to 2.5- µ m size range, although there have been a few studies of coarse particles. In a number of cases, the particle-size cutoffs used in toxicity studies differed from those commonly used to define size fractions obtained with ambient monitoring networks, namely PM 2.5, coarse PM (PM 10 minus PM 2.5 ), and PM 10 , and TSP. For example, some toxicity studies have used mass in sizes termed PM 1.7 and PM 3.7-20 , whereas others have included particles of up to 4 µ m in the definition of “fine” particles. Definitions of specific size fractions, such as “fine” and “coarse,” used in toxicological research should be consistent with those used in ambient monitoring studies and in epidemiological studies.
PM Surface Area. A few studies in the HEI database address particle surface area in the context of particle size, specifically in terms of its relation to health effects. Surface area is also relevant to the absorption of gases onto particle surfaces.
PM Number Concentration. A few studies address the issue of particle number concentration, which is generally used to describe exposures to the ultrafine particles in ambient PM. These include in vivo and in vitro studies, the former including clinical-exposure studies and involving particles ranging from 0.01 to 0.1 µ m.
Transition Metals. The transition metals include titanium (Ti), vanadium (V), chromium (Cr), manganese (Mn), iron (Fe), cobalt (Co), nickel (Ni), copper (Cu), zinc (Zn), cadmium (Cd), and mercury (Hg). Some—Cr, Mn, Co, Ni, Cd, and Hg—are both transition metals and listed as EPA hazardous air pollutants. Although a number of studies address the issue of toxic metals, many use material containing a mix of various metals, and few specify single metals for evaluation. For example, residual-oil fly ash particles containing nickel and vanadium are commonly used in toxicity studies related to PM; such studies have involved both animal in vivo and in vitro study designs. Other studies have examined pulmonary inflammation related to Fe, V, Zn, and Ni; DNA damage related to Cr(VI); and oxidative stress after exposure to Fe. In general, however, exposure doses in these studies have been high and not relevant to ambient exposure. Metal concentrations found in ambient and source samples should serve as broad exposure guidelines for these experiments. Because in vitro studies often involve material extracted from ambient-air filters, methods of filter extraction (for example, water extraction vs. acid digestion) and analysis need to be standardized.
Acids. Health effects of exposure to acid aerosols have been extensively studied and are specifically addressed in the current controlled-exposure research portfolio in only a few studies reported in the HEI database. However, ambient acidity is generally not analyzed in filters obtained from studies that use ambient-particle concentrators. Such data would be valuable for comparison with pub-
lished epidemiological data on health effects. Furthermore, little information on specific types of acids is given in the project descriptions in the HEI database.
Soot, Organic Compounds, and Associated PAHs. The effects of PAHs are specifically addressed in only one in vivo study described in the HEI database. Characteristics of combustion-related organic chemicals will be explored further by the EPA-sponsored PM centers. Studies of diesel exhaust, diesel soot, and black carbon focus mainly on elemental carbon. More research with emphasis on organic speciation is needed to evaluate potential health effects. Because there are so many organic compounds in ambient PM, a subset specifically related to pollution sources needs to be defined for in vivo and in vitro studies.
Bioaerosols. One of the subjects clearly in need of evaluation is the role of biological agents in adverse health effects related to ambient PM exposure. Biological agents that might be involved in PM-induced response are diverse, and few are being evaluated. One class, endotoxins, has been identified as having the ability to induce or potentiate adverse health effects induced by PM, and some studies are addressing this issue. Another antigen being evaluated for its role in PM-related health effects is that derived from dust mites. Although exposure to dust mites largely occurs indoors, it may offer an informative example.
Sulfates and Nitrates. Sulfate has been examined in several studies, but nitrate and other nitrogen species have been largely ignored except as components of complex particle mixtures.
Peroxides and Other Free Radicals. One in vivo study addresses the role of peroxide in PM toxicity. This is a subject on which further research is needed.
Copollutants. There is an increasing effort in the research portfolio to evaluate the potential for interaction between PM and gaseous copollutants. The gases of potential concern include O 3 , NO 2 ,
SO 2 , CO, and irritant hydrocarbons. Data on precursor gases (especially HNO 3 , NH 3 , and SO 2 ) are important to relate ambient secondary particles to health effects. This subject is discussed further with regard to research topic 7 .
The committee is unable to identify any studies reported in the HEI database that address the issue of experimental PM surrogates that can mimic daily, seasonal, and regional particle characteristics. Specific in vivo and in vitro tests provide snapshots of adverse effect. Understanding of the role of PM characteristics in eliciting biological responses, measurements of PM components or analyses of aerosol filters should include extensive chemical speciation, consistent with the national PM 2.5 chemical-speciation network, in which mass, elements (40 elements from sodium to uranium), ions (nitrate, sulfate, ammonium, and water-soluble sodium and potassium), and carbon (organic and elemental) are determined. Furthermore, there needs to be a reconciliation of ambient concentrations with the exposures used in controlled studies. Concentrations of specific chemical compounds in PM vary widely. For example, V and Ni are often found at less than 0.01 µ g/m 3 in ambient samples, although most other metals are typically found at 0.01-0.5 µ g/m 3 . But crust-related materials (such as aluminum (Al), silicon (Si), calcium (Ca), Fe, and Mn) are often present at 0.5-10 µ g/m 3 , and many toxicity studies use concentrations as high as about 100-5,000 µ g/m 3 . The relevance of such high-exposure studies for materials present at much lower concentrations in ambient air must be considered in controlled-exposure studies. Furthermore, the ratio of specific chemical species in ambient air to those occurring in experimental atmospheres must be considered in experimental-study designs.
Epidemiology
Epidemiologists have approached the problem of mixtures or, in this instance, the toxicity-determining characteristics of particles, by evaluating risk in relation to heterogeneity in exposure, whether over time or across geographical regions. For example, time-series studies identified particles in urban air as a key determinant of morbidity and
mortality by evaluating risk for events on a day-by-day basis in relation to changing daily concentrations of particles and other pollutants. Statistical models, such as Poisson regression, are used to “separate” the effects of one pollutant from those of another. Comparisons have also been made across regions that have different pollution characteristics. Panel studies can also be used for this purpose.
In applying the epidemiological approach in investigating particle characteristics, there is a need to have measurements of particles in general and of the specific characteristics of interest over the period of the study. Because monitoring for particle characteristics of specific interest has been limited, opportunities for testing hypotheses related to those characteristics have also been somewhat limited, and few studies that incorporate substantial monitoring of both particle concentration and other specific characteristics have been carried out. One example is afforded by the work carried out in Erfurt, Germany, where particle mass, concentration, and numbers have been carefully tracked for a decade. The resulting data have been used to support several epidemiological studies of health effects in that community (Peters et al. 1997). EPA's supersite program will also offer a platform for carrying out observational studies on particle characteristics related to health risk.
Epidemiological data, if sufficiently abundant, can be used for testing alternative dose metrics. Statistical modeling approaches can be used to test which exposure metrics are most consistent with the data; with this general approach, the fit of the statistical model to the data is compared across exposure metrics, and the metric that best fits the data is given preference, assuming that its biological plausibility is at least equivalent to that of alternatives. For example, 2-day running averages might be compared with 24-hour averages, or peak values obtained with continuous monitoring might be contrasted with averages over longer periods. If a strong preference for one metric over alternatives is to be gained, the data requirements of this approach are substantial. Epidemiological studies relevant to this research topic need to be large and require data on the exposure metrics to be compared.
Table 3.4 shows that the number of potentially informative epidemiological studies is small. Studies in Erfurt, Germany, and in Atlanta
TABLE 3.4 Number of Epidemiological Studies Relating Health Outcomes to Target Pollutants a
capture mass concentrations, particle counts, and acidity; other studies are addressing ultrafine particles and risk of myocardial infarction in Augsburg, Germany, and in Atlanta. Several time-series studies include measurements of sulfate and acid aerosols, and a number of panel studies also incorporate measurements of a variety of particle characteristics. PM components are being considered in a small number of existing or planned studies. More data will probably be needed, particularly to obtain evidence related to the general issue of exposure metrics as applied to population risk. A number of current studies should provide information on the risks posed by ultrafine particles; these risks are the focus of one of the PM centers. Panel studies conducted by EPA will also contribute useful information.
There is considerable effort in evaluating physiochemical properties of PM in relation to biological effects. However, it has generally been concerned with only a few chemical characteristics; the largest body of work involves metals. Other potentially important PM characteristics as illustrated in Table 3.4 , have received less attention. Current work is beginning to address the issue of exposure or dose metrics other than mass concentration, although most studies continue to evaluate health effects in terms of total mass concentration during exposure. The relevance of high doses used in many controlled exposure studies to the low exposures to some components of ambient PM remains a subject that must be more adequately considered in study design than it is now.
In its second report, the committee noted that although most of the research activities recommended in its first report were being addressed or planned by EPA or other organizations, studies in one cross-cutting research topic of critical importance did not yet appear to be adequately under way or planned: studies of the effects of long-term exposure to PM and other major air pollutants. The committee recommended that efforts be undertaken to conduct epidemiological studies of the effects of long-term exposures to particle constituents, including ultrafine particles. There does not yet appear to be a sys-
tematic, sustained plan for implementing studies of human chronic exposure, including examining ultrafine particles.
This research topic is a key scientific question in the understanding of PM and health: Are effects of PM nonspecific—that is, determined only by the mass dose delivered to target sites—or do they depend on the specific physical and/or chemical characteristics of the particles? Data relevant to this question would be informative as to cardiopulmonary and/or systemic effects and therefore would guide mechanistic research. Thus, the scientific value of this research topic remains high. Identification of characteristics that produce adverse responses in controlled studies will allow comparison with PM properties obtained from epidemiological evaluations and will thus provide important confirmation of the role of specific properties in adverse health outcomes. There should be coordination between toxicological and epidemiological studies, including use of a consistent terminology for such PM characteristics as specific size fractions, so that study comparisons are possible not only between the two disciplines, but also among different controlled-exposure studies.
Integration across exposure assessment, toxicology, and epidemiology will be critical for obtaining a comprehensive body of evidence on this research topic that can guide decisionmakers from health effects back to responsible emission sources. Epidemiological studies need to include sufficient exposure assessment to guide toxicity studies of PM characteristics. Opportunities should be sought to apply hybrid research models that combine toxicological and epidemiological research.
Evidence on the particle characteristics that determine risk could have a profound influence on decisionmaking. At present, an ap-
proach of regulating particle mass in general is followed, in the recognition that particles vary substantially in size, makeup, and chemical properties. There are multiple sources of PM, and decisionmakers need guidance on whether some sources are producing more hazardous particles or whether all sources produce particles of equivalent toxicity.
Epidemiological research alone will not provide sufficiently certain evidence on this research topic; joint toxicological and epidemiological study is required. However, epidemiological data will be critical for decisionmakers, in that such data will confirm laboratory- based findings and hypotheses.
This is one of the most challenging research topics in the committee 's research portfolio. In the laboratory setting, characteristics of particles can be controlled through experimental design, so carefully measured studies of particles that have specific characteristics can be assessed. In the population setting, in contrast, participants in epidemiological studies inhale PM that has multiple sources and that changes in characteristics as participants move from location to location over the day and possibly even in one location at different times. Data on substantial numbers of persons will be needed to test hypotheses related to particle characteristics. Nonetheless, epidemiological studies can be carried out for this purpose; one of the most effective approaches is likely to be the panel study, with specific, tailored monitoring for particle characteristics of interest. Such studies are feasible, as shown, for example, by the studies in Erfurt (Peters et al. 1997).
RESEARCH TOPIC 6. DOSIMETRY: DEPOSITION AND FATE OF PARTICLES IN THE RESPIRATORY TRACT
What are the deposition patterns and fate of particles in the respiratory tract of individuals belonging to presumed susceptible subpopulations?
The committee's recommended research portfolio (NRC 1998) outlined research needed to improve understanding of the deposition of particles in the respiratory tract, their translocation, and their clearance. The recommendations encompassed the development of new data and predictive models and the validation of the models for respiratory-tract structure; respiratory variables; total, regional, and local deposition; and particle clearance. Also included were the micro-dosimetry of particles and particle-derived hazardous chemical species and metabolites in intrapulmonary and extrapulmonary tissues.
Information on dosimetry is important for decisionmaking because it is critical to understanding of the exposure-dose-response relationship that is key to setting the NAAQS. It is also important for understanding of how exposure-dose-response relationships differ between normal and especially susceptible subpopulations, if the standard is to be adjusted to protect sensitive people. Knowledge of interspecies differences is important for extrapolating results from animals to humans.
The committee's recommendations focused on dosimetry in people potentially more susceptible to particles because of respiratory abnormalities or age (children and the elderly). A large portion of the population is in one or more of the categories of concern. Most people spend at least one-fourth of their lives in stages during which lungs are developing or senescent. In 1997, an estimated 44.3 million adults were former smokers and 48 million were current smokers (ALA 2000a); many smokers develop some degree of airway abnormality. Asthma afflicts over 17 million Americans, including 5 million children whose lungs are still developing (ALA 2000b). COPD afflicts about 16.4 million people (ALA 2000c). All respiratory diseases together kill one of seven Americans (ALA 2000d). The focus of past dosimetry research —almost entirely on normal young adult humans and animals—leaves us with little ability to estimate exposure-dose-response relationships in the above subpopulations.
In its second report (NRC 1999), the committee confirmed its initial recommendations, added a recommendation for research to bolster interspecies dosimetry extrapolation models, and re-emphasized the need for dosimetric research in animals to focus on models of human susceptibility factors.
Several sources of information were examined to assess current research and recent research progress on the dosimetry of PM. The review of current research centered on the HEI-EPA database on PM research. The database was examined as of August 2000 for research projects and programs, including dosimetric research in the abstracts. Numerous additional past or current projects were evident from published reports, but because of uncertainty as to whether these projects were continuing, only those listed in the HEI database were included in Table 3.5 . In all, 22 project descriptions were identified as apparently responsive to the dosimetry research needs.
New information since the 1996 criteria document was assessed by examining published papers and abstracts from meetings. A search of the recent published literature was conducted by using numerous
TABLE 3.5 Summary of Dosimetry Projects and Reports
key words pertaining to the research recommendations. The first external review draft of the new criteria document for PM (EPA 1999) was examined for its portrayal of new information since the last criteria document, published in 1996. References added to the revised dosimetry chapter as of September 2000 were also reviewed. Published abstracts from 1999 and 2000 meetings of the American Thoracic Society (ATS 1999, 2000), HEI (HEI 1999, 2000), and the Society of Toxicology (SOT 1999, 2000) were examined for relevant research, as were the abstracts from the 1999 meeting of the International Society for Aerosols in Medicine (ISAM 1999). The abstracts and papers from the Third Colloquium on Particulate Air Pollution and Human Health in June 1999 (Phalen and Bell 1999) and from the “PM2000” conference in January 2000 (AWMA 2000) were also examined for relevant completed research. The evaluation of reports was limited to reviewing of abstracts. Published papers were not reviewed in detail, and authors were not queried.
In all, 62 papers and 59 presentation abstracts were identified as potentially relevant to the dosimetry research needs as set forth by the committee. On review of abstracts, some proved to fall outside the scope of the recommended research portfolio, and many more related to the recommendations only indirectly. A total of 96 reports were considered relevant to the dosimetry research needs. Although this review undoubtedly missed some potentially relevant reports, it was considered sufficient to provide a reasonable evaluation of the extent to which the recommendations are being addressed. The results of the review are summarized below, by categories according to the committee 's research recommendations (in italics). A numerical summary of the projects and reports is presented in Table 3.5 .
Conduct research on deposition of particles in the respiratory tracts of individuals having respiratory abnormalities presumed to increase suscepti bility to particles, and on the differences in deposition between these suscepti ble subpopulations and normals.
Obtain quantitative data on lung morphology and respiration for individuals of different ages and having respiratory abnormalities.
Research using advanced imaging and reconstruction techniques is producing new information on the effects of age, sex, and several types of abnormalities on airway dimensions. This information can serve as the foundation of mathematical models of deposition in abnormal airways. Some researchers are using stereolithography to construct physical models of airways from stereo images and computer-controlled etching of solid media. Other researchers are using magnetic resonance imaging to create airway images and develop digital data from which structures can be modeled or physical replicas can be machined. These techniques show promise for obtaining new morphological data useful for modeling deposition in a broad range of airway abnormalities. It is likely that, in some cases, these approaches will allow acquisition of data for more varied subjects and at a greater rate than is practical with traditional postmortem airway casting.
A modest amount of work is continuing with the more traditional methods of evaluating solid casts made from cadaver lungs and airways and measurements of airway dimensions with light microscopy of lung sections.
Information on the effect of age and respiratory abnormalities on breathing patterns and dosimetry in humans has been expanded substantially in the last 2 years. The EPA intramural program is the strongest contributor in this field. Laboratories working in this field are addressing the variables of age, sex, asthma, COPD, and cystic fibrosis. Inclusion of a broader range of susceptibility factors and particle types is needed. For example, there is little emphasis on people who have respiratory infections or edema related to cardiopulmonary failure. Most studies have measured only total particle uptake; information on regional and local dosimetry is also needed.
Determine the effects on deposition of particle size, hygroscopicity, and respiratory variables in individuals with respiratory abnormalities.
New information has been obtained on the influence of sex on regional (pulmonary vs. tracheobronchial and extrathoracic) fractional deposition and on differences between children and adults, and these data are being extended by current projects. Work on the effects of respiratory abnormalities on regional or local deposition has been limited largely to modeling or work with airway replicas. There has been little validation of the models with measurements of living subjects. An important advance has been the finding that total fractional deposition is greater in people who have asthma and COPD and in smokers than in people who have normal lungs. Total fractional deposition has been found to be similar in normal elderly people and young adults. More emphasis is needed on regional and local deposition in lungs and airways of susceptible subjects.
The influences of particle size and hygroscopicity on deposition have been addressed by some studies, but only a small portion of this work has included subjects or airway replicas that have abnormalities or different ages. There appears to be little emphasis on the influence of particle and respiratory variables on deposition in susceptible people or on the development of predictive models that incorporate these variables. In addition, only a few particle types and only a few of the many common combinations of ambient particle sizes and compositions have been studied.
As in the past, there continues to be only modest effort aimed at identifying the type and location of particles retained in lungs at autopsy. Although the locations are sometimes characterized as reflecting sites of particle deposition, the results typically reflect sites of retention of only the most biopersistent classes of deposited particles and might not reflect accurately the sites of deposition or the dose of the full spectrum of inhaled particles. When coupled with evaluations of accompanying tissue changes, this approach provides useful information on the relationship between long-term particle retention and disease.
Develop mathematical models for predicting particle deposition in susceptible individuals and validate the models by measurements in individuals having those conditions.
Several recently completed or current efforts have led, and will continue to lead, to the development and refinement of models for predicting deposition in abnormal lungs. Most efforts have focused on the effect of flow limitation in conducting airways and on the heterogeneity of local particle deposition.
Very few efforts have included validation of models by measurements in living subjects. Only two of the reports in this category involved validation experiments—one for deposition in asthmatics and one for the effect of particle size on deposition in rats. More emphasis is needed on model validation and on modeling a greater range of susceptibility factors.
Develop information on interspecies differences and similarities in the deposition of ultrafine particles in abnormal vs. normal respiratory tracts.
Although this recommendation focused on ultrafine particles, there is a dearth of information on deposition of particles of any size in animals that have respiratory abnormalities. As noted in the toxicology sections that follow, continued effort is needed to develop, refine, and validate animal models of human respiratory abnormalities. Progress has been made, but it has been accompanied by little effort to examine particle dosimetry in the models. Although a few laboratories are attempting to develop and refine mathematical models for interspecies adjustments in particle deposition, there is still little attempt to validate the models by comparing deposition in animals and humans directly, and only one group is generating comparative data on the deposition of ultrafine particles.
Several projects have developed models to predict comparative deposition in normal rats and humans, and most can be adapted for ultrafine particles. Other animal species have been largely ignored. The committee 's first report recommended increased development and use of animal models of human susceptibility factors, as described in other sections. Because differences in deposited dose can contribute substantially to differences in the models' response, there is a need for more work on particle deposition in animal models of respiratory abnormalities.
Translocation, Clearance, and Bioavailability
Conduct research on the translocation and clearance of particles and the bioavailability of particle-borne compounds in the respiratory tracts of individuals having respiratory abnormalities presumed to confer increased susceptibility. Determine differences in the disposition of particles between these susceptible subpopulations and normals.
New information is beginning to accumulate that shows that respiratory abnormalities can have variable effects on short-term clearance of inhaled particles deposited on conducting airways. As is the case for deposition, information on clearance is being developed in both the pharmaceutical and environmental fields. There are data on short-term airway clearance in adult humans who have asthma, chronic bronchitis, and COPD, including comparisons with normal subjects.
Although the available information is still sketchy, it reveals both the potential importance and the complexity of the issue. For example, Svartengren et al. (1996) did not find that clearance from small ciliated airways of unprovoked asthmatics differed from that of normal people, but later (Svartengren et al. 1999) found that more particles were retained in airways of asthmatics than of normal subjects when the allergic asthmatics were challenged with allergen before deposition of the particles. There is little information on the influence of respiratory abnormalities on longer-term clearance from the pulmonary region and little information on age-related differences. Some data suggest that there is little influence of age or sex on particle clearance in normal humans.
Several recent studies have demonstrated the importance of the bioavailability (solubility) of particleborne metals in eliciting adverse responses. A modest amount of work is being done on the bioavailability of particleborne organic compounds. Little if any effort is being expended to determine differences in bioavailability or the importance of bioavailability between normal and abnormal respiratory tracts.
It appears that differences in particle clearance are not yet being incorporated into models for predicting differences between normal
and susceptible people in the dosimetry of particles or particle-associated compounds.
Determine the disposition of ultrafine particles after deposition in th e respiratory tract, and whether respiratory abnormalities alter the disposition pathways or rates.
Despite the current interest in potential differences between the disposition of fine and ultrafine particles after deposition in the respiratory tract, little progress has been made, and little work appears to be under way. The technical difficulty of measuring small amounts of ultrafine particles in various intrapulmonary and extrapulmonary locations continues to be a deterrent to progress. The recent development of 13 C-labeled ultrafine carbon particles is likely to advance this field, and tracer technologies need to be developed and applied for use with other types of ultrafines.
Sufficient work has been done to confirm that solid ultrafine particles can penetrate into the circulatory system and reach other organs, but quantitative data are still lacking. There has been no apparent effort to study the dosimetry of nonsolid ultrafine condensates. Moreover, there has been no work on the disposition of ultrafines in either humans or animals that have respiratory abnormalities. As investigative techniques are developed, it is important that they be applied to both normal and abnormal subjects.
Develop information on interspecies differences and similarities in the translocation, bioavailability, and clearance of particles in abnormal vs. normal respiratory tracts.
Little research appears to have been completed recently or to be under way addressing interspecies differences in particle clearance, translocation, or bioavailability in either normal or abnormal respiratory tracts. Recent work demonstrated marked differences in the sites of retention of fine particles in lungs of normal rats and nonhuman primates, but at lung loadings much higher than would result from environmental exposures. There are some new data and reviews on
particle clearance in different species, but the committee is unable to identify any direct intercomparisons among species or comparisons in the presence of respiratory abnormalities.
Adequacy of Current Research in Addressing Information Needs
Although the volume of dosimetric work shown in Table 3.5 reflects a level of effort commensurate with the committee's recommendations, there is not yet an adequate focus on the specific information needs described by the committee. Only a portion of the work has addressed characteristics other than age and sex; there has been insufficient work on the impact of respiratory abnormalities. The committee called for development and validation of mathematical models for predicting deposition and clearance in abnormal lungs. There has been only modest advancement in the modeling of dosimetry in susceptible people and little effort to validate the models. Efforts to improve interspecies extrapolation models continue in a few laboratories but, again, little effort to validate the models. There has been little effort to assess dosimetry of any type in animal models of human respiratory abnormalities. Many potentially important aspects of respiratory abnormalities—such as microdosimetry in tissues and cells, bioavailability of particleborne compounds, translocation and clearance, and handling of diverse particle types—have been addressed little or not at all. Although the level of effort might appear adequate, the degree of focus is not yet adequate.
Among the many programs, studies, and recent reports contributing new information on the dosimetry of particles, only a portion are focused specifically on dosimetric issues. Much of the information was produced as a byproduct of research focused on health responses to inhaled particles, rather than on particle dosimetry. That is appropriate, but effort is needed to make investigators broadly aware of the need for dosimetric information to encourage them to develop and publish the data as a specific, albeit opportunistic, product of their research. In a related vein, our review demonstrated that relevant information is being produced as a byproduct of pharmaceutical re-
search. That suggests the importance of looking beyond the traditional environmental research community when searching for and summarizing information relevant to environmental dosimetric issues.
The information on particle deposition in potentially susceptible subgroups has grown since the 1996 PM criteria document; results have demonstrated important differences in total fractional deposition in some disease states. The findings support the importance of the committee's recommendations. Work is needed on a wider range of susceptibility conditions, and more emphasis is needed on regional and local deposition (deposition “hot spots”) in susceptible people.
Much less information has been, or is apparently being, produced on differences in the clearance and translocation of deposited particles and in bioavailability of and cellular response to particleborne compounds due to age or respiratory abnormalities. Although many adverse responses might be most strongly moderated by deposition, some might be more strongly influenced by the amount and location of retained dose. Translocation and bioavailability issues remain important for an understanding of response mechanisms.
The research recommendations noted ultrafine particles as a specific class on which more dosimetric information is needed. The effort focused on ultrafines is modest and addresses a narrow range of ultrafine-particle types. Like coarse and fine particles, ultrafines include diverse physiochemical classes that can be expected to behave differently when deposited.
There is not yet an adequate effort to determine the dosimetry of particles of any type in animals that are used to study characteristics of human susceptibility. If the animal models of susceptibility are to be useful, differences in particle deposition and disposition, as well as differences in response, must be considered. Not only might differences in dosimetry help to explain differences in response on a total or regional dose basis, but the models might also be useful for predicting the influences of abnormalities on local deposition in susceptible people on whom such data might never be obtained directly. Research sponsors need to explicitly encourage investigators to evaluate dosimetry as an integral component of the characterization of the responses of animal models.
The scientific value of this research is generally high. Nearly all the work noted above builds on previous knowledge in a logical way that will lead to a more integrated understanding of PM-related health effects. Most of the dosimetric data collected in response to PM research needs will also have high value for other purposes, such as understanding and predicting the dosimetry of inhaled pharmaceuticals in normal vs. abnormal respiratory tracts and in animal models vs. humans. Findings pointing toward differences in respiratory control, anatomy, and defenses are raising issues likely to lead to more studies that will provide a more complete understanding of respiratory-tract structure and function.
Although insufficient effort is being expended to evaluate dosimetry in animal models of respiratory abnormalities, the resulting data will have high scientific value for determining the extent to which differences in health responses between normal and susceptible people are due to differences in dose and differences in responsiveness. This information is important for the selection and interpretation of the animal models.
Considering the previous lack of data on dosimetry in people who have respiratory abnormalities or animal models of these conditions, almost any such data would have scientific value. As results accumulate, it will be important to focus on more-specific and more-detailed issues, for example, on local and regional deposition rather than total deposition.
The results of this research will have a direct bearing on the setting of air-quality standards in two principal ways: providing the dose component of dose-response information required to set the standard, and providing information on the dose component of susceptibility as input into the adjustment of the standard for protection of sensitive subpopulations.
Knowledge of differences between the deposited doses received by normal people and those who have respiratory abnormalities will play a direct role in the estimating of safe and hazardous PM exposures. In this role, dosimetry is an equal partner in the exposure-dose-response paradigm that is integral to risk assessment. In addition, knowledge of dosimetry in animal models of susceptibility will play an indirect role in decisionmaking by influencing the selection of appropriate models, the interpretation of results of the use of the models, and the understanding of the role of dose variables in the susceptibility of humans.
Lack of feasibility is not impeding the progress of dosimetric research. As noted in the committee's first report ( NRC 1998), there are few technical limitations on obtaining the needed data. An exception might be current technical limitations on detecting ultrafine particles in tissues and fluids.
The research gaps identified above result from inadequate coverage of topics, not from inadequate research tools or personnel. It remains true, as stated in the first report, that with the combination of modest funding and its direction toward key information gaps, most dosimetric issues could be resolved soon. It is clear that not all important topics are being covered, although most of the time originally projected for this work has been spent. Without greater attention to targeting particular gaps, key issues might not be adequately resolved.
RESEARCH TOPIC 7. COMBINED EFFECTS OF PARTICULATE MATTER AND GASEOUS POLLUTANTS
How can the effects of particulate matter be disentangled from the ef fects of other pollutants? How can the effects of long-term exposure to par ticulate matter and other pollutants be better understood?
PM exists in outdoor air in a pollutant mixture that also contains gases. Thus, biological effects attributed to PM alone in an observational study might also include those of other pollutants that arise independently or through interactions with PM. There might be chemical interactions between gases and PM, or gases can be adsorbed onto particles and thus carried into the lung. Interactions can also occur in the process of deposition on lung airway surfaces and later through lung injury. Research relevant to this topic includes toxicological and clinical studies that examine the effects of gaseous copollutants on the health impacts of PM.
The committee's first two reports (NRC 1998, 1999) indicated that it is important to consider the effects of combined exposures to particles and copollutants when characterizing health risks associated with PM exposure. This research topic remains of critical importance because epidemiological studies might not be able to characterize fully the specific contributions of PM and gases in causing health outcomes. Thus, mechanistic studies are needed to determine the relative roles that various components of ambient pollution play in observed health effects of exposure to atmospheric mixtures.
The HEI database was examined to determine the research status of this topic. A number of current studies involve pre-exposure to high levels of ambient gases (such as ozone and sulfur dioxide) to induce pulmonary pathology in animals so that effects of PM in a compromised host model can be assessed. However, those types of studies are not considered to fit this research theme. A number of studies are using concentrated ambient PM (CAP), and such exposure atmospheres might include ambient gases unless they are specifically scrubbed out before entering the exposure system. However, it was often not possible from a study description in the database to determine whether the effects of these gases on response to PM were being examined. One group of researchers is exposing animals specifically to highly complex emission atmospheres to determine the rela-
tive contributions of PM and gaseous copollutants to various health effects.
Studies of interactions of gaseous copollutants with PM are being conducted with both animal and controlled human-exposure studies. Fewer studies are examining such effects in vitro. Endpoints span the array of effects observed in populations but focus largely on cardiovascular effects, inflammatory response, and mediators. Some animal studies and some human studies also involve the use of compromised hosts to compare effects with those occurring in normal animals and humans. As with all animal toxicity studies, it is important to be able to relate responses to human responses. That is specifically addressed as a goal in only one study program being performed at one of the EPA-sponsored PM centers.
One of the gaseous copollutants of major concern with regard to interaction with PM is ozone, and this copollutant is the subject of the greatest research effort. That is evident in Table 3.6 , which shows the list of gaseous pollutants being studied and the number of research projects addressing them. However, some attention is also being given to other gases of potential concern, such as sulfur dioxide and nitrogen dioxide. Other suggested modulators of PM-induced effects are receiving little attention. The role of ambient gases should receive more attention in studies with CAP because these types of expo sures are the most realistic and do not require the generation of “sur-
TABLE 3.6 Gaseous-Copollutant Studies
rogate” atmospheres. Opportunities should be sought to augment CAP with concentrated gaseous pollutants or to scrub out specific residual gasses.
PM in outdoor air is one component of a complex mixture that varies over time and also geographically on both small and large spatial scales. PM is one of the six pollutants in outdoor air regulated as “criteria pollutants.” In part, driven by the needs of evidence-based regulation, epidemiologists and other researchers have attempted to separate the effects of PM from those of other pollutants, even though they are often components of the same mixtures and their concentrations are often correlated, reflecting their shared sources. The effects of the individual components of the mixture can be assessed in time-series approaches with multivariate statistical methods or in designs that incorporate contrasts in exposures to mixtures by drawing participants from locations that have different pollutant mixtures (for example, with higher and lower ozone concentrations).
In addressing the “combined effects” of PM and other pollutants, one of the scientific questions of interest is whether the risks to health associated with PM exposure vary with the concentrations of other pollutants. For example, are risks posed by PM to children who have asthma higher in communities that typically have higher background concentrations of ozone than in other communities? Epidemiologists refer to this phenomenon as “effect modification, ” and its presence is generally assessed with statistical methods that test for interaction in multivariable models. Effect modification that is positive, or synergistic, results in greater risks than would be predicted on the basis of estimates of risk posed by PM itself. Studies of effect modification need substantial sample sizes if statistical power is to be sufficient.
Studies on combined effects need to include information on PM and the copollutants of interest. Epidemiological studies of diverse design are potentially relevant to this topic. As for studies of mixtures
generally, a precise characterization of combined effects requires a substantial body of data.
Examination of the HEI research inventory shows that many studies in progress should provide relevant information on modification of PM risks by other pollutants. The range of PM indicators across the studies is broad, but most studies include monitoring results for the principal gaseous pollutants of concern. Samples range from too small and consequently uninformative to large enough to provide insights into combined effects.
Although attention to the issue of effects of gaseous copollutants on the toxicity of PM is increasing, the current controlled-exposure research portfolio aimed at assessing the role of gaseous pollutants in health effects of PM is not adequate. The use of CAP can provide valuable information on effects of exposure to complex mixtures. Furthermore, the research effort in evaluating the role of gases in influencing particle effects seems to be lagging behind the effort in studying of specific components of PM in the absence of gaseous copollutants. The epidemiological research portfolio on this topic is relatively substantial; as most epidemiological studies of PM include data on gaseous copollutants. There does not yet appear to be a systematic, sustained plan for implementing studies of chronic exposure.
The criteria pollutants have long been addressed as though their effects on health were independent, with recognition that they exist as components of complex mixtures in the air. Rather than seeking to characterize mixture toxicity overall, researchers have sought to determine, experimentally or in observational data, whether the pres-
ence of one pollutant changes the effect of another (a phenomenon referred to in epidemiology as “effect modification”). Findings on effect modification inform estimates of risk posed by mixtures and suggest hypotheses for followup laboratory investigation.
Present regulations are based on the tenet that effects of individual pollutants are independent and that public-health goals can be met by keeping individual pollutants at or below mandated concentrations. Epidemiological demonstration of effect modification for PM effects by other pollutants, such as ozone, would indicate that the regulatory structure does not fully reflect the actual risks to the population.
Epidemiological and controlled-exposure studies of effect modification or interaction can be carried out; in fact, most contemporary studies include the requisite data on other pollutants. Thus, studies could be readily carried out now to explore whether other prevalent pollutants affect risks posed by PM. Methods for experiments involving mixed atmospheres are available. Analytical information derived from evaluation of atmospheres in epidemiological studies can help to determine specific components of mixed atmospheres to be used in controlled-exposure protocols.
RESEARCH TOPIC 8. SUSCEPTIBLE SUBPOPULATIONS
What subpopulations are at increased risk of adverse health outcomes from particulate matter?
A number of subgroups within the population at large are postulated to be susceptible to the effects of inhaled PM. They include
people who have COPD, asthma, or coronary heart disease; the elderly; and infants. Also, fetuses are possibly susceptible. Those groups have long been assumed to be susceptible to the effects of air pollution, in general, and therefore assumed to be at risk from PM. Epidemiological data support that assumption, as does understanding of the compromised organ systems of people with chronic heart and lung diseases and of the physiologic and immunologic vulnerability of infants and the elderly. A number of epidemiological and controlled-exposure investigations are now directed at characterizing health effects of PM in those subpopulations. Other populations might also be at excess risk from PM, and the committee considers that this research topic includes both subpopulations already considered susceptible and others yet to be identified.
In susceptible subpopulations, there is likely to be a range of vulnerability reflecting the severity of underlying disease. For example, in persons with asthma, there is a broad distribution of level of lung function and of increased nonspecific airway responsiveness, a hallmark of the disease. The degree of susceptibility can also depend on the temporal exposure pattern. However, data to support such biologically based speculations are still notably lacking. For example, whether all children are equally at risk or only children who are exercising or who have specific predisposing factors, such as a history of atopy or asthma or other respiratory disease history, is unknown. In adults, the interplay among factors that determine susceptibility, such as the presence of both COPD and coronary heart disease, is not yet understood. Findings of both acute and chronic morbidity and mortality studies suggest that those with prior respiratory disease are more susceptible to acute changes in ambient PM concentrations.
Although from the early days of air-pollution research hypotheses have been proposed related to increased susceptibility of selected fractions of the population, much of that work has been directed at identifying acute morbid events during acute exposures. For example, research in London in the 1950s followed up on the observation that many of the excess deaths noted in the December 1952 fog were of persons who were already quite sick, many with heart or lung disease. Panels of people with chronic bronchitis were followed during the 1950s and 1960s with monitoring of pulmonary function and symp-
toms. Those studies followed a design now referred to as a panel study, which involves following a susceptible subpopulation with relatively detailed tracking of their status. This model is particularly useful for assessing acute effects of exposure and can provide evidence relevant from both the clinical and the public-health perspectives. More recently, work has been directed toward testing whether exposure to particles can contribute to initiation of disease, as well as exacerbating existing conditions. To date, the collective evidence indicates that there are susceptible subpopulations, particularly of people who have chronic heart or lung diseases.
Controlled-Exposure Studies
The committee identified 53 animal and human studies in the HEI database that specifically addressed the issue of subpopulations susceptible to PM-induced diseases ( Table 3.7 ). In several cases, a study identified more than one susceptible subpopulation; for these, each population group was entered into the table.
Almost all the studies concern with diseases of the respiratory and cardiac systems; only one concerns increased susceptibility to cancer induction. Twelve studies concern age as a risk factor. The disease states of concern include pulmonary allergies, asthma, bronchitis, emphysema, COPD, and cardiac disease. Twenty-four of the studies involve human subjects, and 29 use animal models intended to mimic human disease.
The particulate atmospheres most frequently being used for toxicity studies are those with CAPs, carbon black, and residual-oil fly ash delivered via inhalation or intratracheal instillation. The duration of the exposures is variable but typically only hours or a few days; this contrasts with epidemiological studies that involve chronically exposed populations.
A strength of the studies is their focus on the major human diseases that have been identified by epidemiological studies as placing
TABLE 3.7 Controlled-Exposure Studies on Effects of PM on Susceptible Subpopulations
people at risk from exposure to PM. An additional strength is that epidemiological studies in which exposures cannot be controlled are complemented with controlled-exposure studies of humans and laboratory animals.
There are difficulties in investigating susceptible populations. The effect of PM exposure is not large enough to be readily and precisely detected without carrying out fairly large studies. Identifying study participants can be difficult, particularly if emphasis is placed on the most susceptible persons. Frail elderly persons and persons with advanced heart and lung disease, for example, might be reluctant to participate if study protocols are demanding. In contrast, experimental studies involve very small populations and typically short observation periods. In laboratory-animal studies, investigators typically attempt to circumvent this issue of population size by increasing the level of exposure or dose. It is common for all the treated animals to manifest disease or some other response. However, a critical ques-
tion is whether the disease states observed and the underlying mechanisms of pathogenesis with short-term high exposures (doses) studied over periods of days, and occasionally weeks, are relevant to assessing the risks posed by exposure over long periods, even at high ambient doses.
Beyond the extrapolation issue, the adequacy of the design of each toxicity study must be addressed. For example, in most cases, the investigators are typically studying relatively young animals, usually in the first fourth of their normal life span, whereas in humans a substantial portion of the disease of concern occurs in the last fourth of the normal life span. Many of the human diseases of concern are chronic, with periods of acute exacerbation. It is crucial that additional effort be directed at evaluating the animal models to assess the degree to which they mimic human disease.
Rationales for selection of the exposure atmospheres, exposure concentrations, and exposure durations were not always readily apparent from the project descriptions. There is also a special need to articulate the relevance of using intratracheal instillation, which delivers a large dose of particles at once, in contrast with the chronic exposures of concern for human populations.
A general concern for the health effects of air pollution, including particles, on susceptible persons has permeated epidemiological research on air pollution. This concern has become increasingly focused as the body of evidence has expanded and led to hypothesis-driven studies of susceptible subpopulations. In addition, with the recognition that much of the morbidity and mortality associated with PM exposure appears to have been from cardiovascular diseases, the efforts to understand susceptibility have expanded greatly beyond considerations of chronic respiratory conditions, particularly asthma and COPD, to include persons with underlying heart disease.
About 70 funded studies using epidemiological databases were reviewed to identify those directed to understanding the impact of
particulate pollution on susceptible subjects, patients, or populations. In general, the studies can be divided into those related to people who have an underlying chronic condition (such as asthma, COPD, or pre-existing coronary arterial disease), those related to persons free of disease but considered to be at increased risk because of a relatively high pollution dose resulting from exertion or exercise, and those related to persons generally at risk for increased morbidity or mortality (such as the elderly). Across the studies, a wide variety of measures of exposure are used, and insights can be gained on some aspects of particle characteristics and toxicity. However, only a few of the strata of the matrix defined by subpopulation and particle characteristics are being addressed.
Taken together, the efforts under way indicate that a rigorous evaluation of risks posed by PM exposure of susceptible subpopulations with established diseases—such as asthma, COPD, coronary arterial disease, heart failure, and hypertension—can be expected. The evidence will be primarily in relation to PM mass as the exposure metric. The groups that have been or are being studied, as summarized in the examined HEI database, include subjects potentially at risk and patients. Few studies are identified specifically as targeted to ethnic minority populations.
Efforts are also under way to explore pathogenesis and intermediate markers of risk, including changes in blood concentrations of inflammatory markers and clotting factors; additional predictors of cardiac risk, including changes in heart-rate variability; and other risk factors for sudden death.
Several studies directed toward an understanding of mechanisms of putative cardiac effects in humans are being carried out in the EPA, at several of the EPA-sponsored PM centers, and through other funding agencies. These include panel studies and clinical studies of healthy persons and potentially high-risk persons exposed to ambient PM and to CAP. These studies are being conducted by multidisciplinary teams that include expertise in exposure assessment, epidemiology, and clinical toxicology. Investigating the role of PM in initiating disease is more challenging, and less progress can be expected in understanding how susceptibility plays a role in initiation of chronic
diseases, simply because the susceptible groups have been less well defined.
In all the studies mentioned above, most of the efforts are directed to explaining acute effects of relatively short-term modeled or directly measured exposures to ambient particles. In a few instances, copollutants or other gases are also being considered. In only a very few cases are effects of chronic exposure being considered; in those cases, long-term exposure is being modeled for relatively recently measured exposures and historical extrapolations of known industrial or ambient particles. Better modeling of past exposure is needed, to develop new efforts directed toward the understanding of chronic effects in potentially susceptible groups. Such data would also be useful in conjunction with studies of factors that determine the development of susceptibility.
There is increasing use of animal models and humans with chronic heart or lung disease in studies to evaluate effects of PM exposure. However, the animal studies need to mimic the human disease state of interest properly. Better modeling of past exposure is needed to develop new efforts to understand chronic effects in potentially susceptible subpopulations. Collection of such data in conjunction with studies of factors that determine the development of susceptibility would be useful.
The hypothesis that particular groups in the population have increased susceptibility has been long advanced and supported by substantial epidemiological evidence. In fact, a general acceptance of the hypothesis has led to focusing of effort in a large number of projects
on the assessment of acute air-pollution effects on morbidity and mortality in selected groups of potentially susceptible persons. The results have been relatively consistent in demonstrating modest effects of particles as measured by mass. The same susceptible subpopulations will need to be reinvestigated, and previously unrecognized subpopulations will need to be considered as hypotheses concerning toxicity-determining characteristics of particles are increasingly refined.
Data on susceptible populations are critical to decisionmakers because the Clean Air Act requires that protection against risks posed by air pollution be extended to almost all persons. Standards are, in fact, intended to provide protection with “an adequate margin of safety.” Sufficient studies are under way to identify and reduce uncertainty related to susceptible groups with respect to acute effects of particle mass. However, for each individual study and for the studies as a group, it is important to anticipate how the results will influence decisions in establishing a NAAQS for PM—that is, will the information obtained provide an improved scientific basis for a decision on appropriate standards for ambient PM? It appears that few of the investigators have adequately considered this matter in a critical manner, especially for the controlled-exposure studies.
The only practical way to increase the number of investigations with regard to either acute or chronic exposures is to undertake studies in conjunction with current supersite or speciation-site data collections or with the use of additional exposure-data sources in the future. There is continuing development of animal models that mimic various aspects of potentially susceptible human conditions. Thus, this field continues to evolve.
RESEARCH TOPIC 9. MECHANISMS OF INJURY
What are the underlying mechanisms (local pulmonary and systemic) that can explain the epidemiologicalal findings of mortality/morbidity associ ated with exposure to ambient particulate matter?
Epidemiological studies have associated various health outcomes with exposure to ambient PM. Controlled-exposure studies are attempting to provide plausible underlying biological mechanisms for these health effects. The results have indicated a number of potential biological responses by which PM could underly possible pulmonary or systemic responses to PM exposures, many of which have been related to specific particulate characteristics, such as chemical or particle size. The major potential biological responses which have been suggested as underlying the reported human health effects from ambient PM exposures include oxidative stress, pulmonary inflammation, airway hyperreactivity, and alterations in the cardiovascular system, such as changes in blood viscosity, rate and pattern of heartbeat, and heart-rate variability. The issue of mechanistic plausibility has been addressed with animal models, in vitro systems, and clinical models. Of the studies described in the HEI database, about 50% involve animal toxicology, and the other 50% are roughly evenly divided between in vitro and clinical and studies. The relative apportionment of research effort for specific mechanisms of PM-induced responses and the allocation of these efforts among the three research approaches are indicated in Table 3.8 .
Research Topic 9a. Animal Models
What are the appropriate animal models to use in studies of particulate matter toxicity?
As previously noted, epidemiological studies suggest that exposure to low concentrations of PM is associated with morbidity or mortality in susceptible people and not in normal healthy people.
TABLE 3.8 Mechanistic Studies
Experimental data show that healthy animals exposed to similar low concentrations of PM also show little to no effect. Animal models are needed to mimic susceptible human subpopulations, because, without supporting data from animal studies, it is difficult to identify individual toxic materials in ambient PM and the mechanisms by which they induce damage to human and pulmonary and cardiovascular systerms. The occurrence of some pathological conditions in an exposed population can establish the probability that some of or all the pollutants produce damages but, in any reasonable time frame, it cannot always differentiate the effects, if any, of specific pollutants or the mechanisms of their action. That will ultimately require controlled exposures of animals to individual pollutants and relevant mixtures and then
measurements of response. In the initial stages of investigating the toxicity of PM and copollutants, it was sufficient to determine a correlation between their presence in inspired air and disease. Now, however, animal models are clearly needed to establish causality, help to unravel cellular mechanisms, and help to elucidate specific PM components that produce responses.
In assessing progress toward the development of animal models, the committee found projects to be distinguished by their heterogeneity. Of the 47 relevant studies identified, most used young normal animals, which were not models for susceptible disease. Fewer studies used older animals as models to evaluate the effects of age, and others used animal models of disease, such as asthma and hypersensitivity, chronic lung diseases, and cardiac dysfunction. Normal or mutant animals were used in some studies.
There are a number of difficulties in developing animal models of human diseases. Deposition of particles in animal lungs differs in both rate and location from that in human lungs, and there is a need for detailed knowledge of the distribution of deposition in animal lungs so that it can be related to deposition in human lungs (see research topic 6 ). Advanced scaling and modeling of the lung airways in animals should be encouraged. The cellular mechanisms by which the pertinent lung and cardiovascular diseases are produced in humans and by which particles exacerbate or initiate these conditions are not understood so it is difficult to produce analogous pathological conditions in animals. The lung contains more types of cells than most other organs and thus provides the opportunity for numerous types of interactions between cells exposed to PM atmospheres and increases the complexity of particle-tissue interaction.
It has been possible to mimic some aspects of specific human diseases in animals. Therefore, it might be necessary to be satisfied with modeling and studying only part of a disease constellation at a time. For example, “asthma-like” allergic conditions have been mod-
eled by sensitizing animals to various foreign proteins. That might produce marked contraction of airway smooth muscle on appropriate challenge but not involve other aspects of human asthma, such as inflammation and mucus gland hypertrophy.
It is encouraging that numerous animal models are being used to measure the effects of exposure to PM. However, a substantial number of studies exposed healthy normal animals to particles, and this is not necessarily a useful model of exposure of susceptible humans. Even though animal models of cardiac and lung disease are being used to investigate the effect of particles, relevance to the human situation must be considered. Research to develop models that more closely mimic the natural history of human diseases caused by air pollution should be emphasized. Models need to be well characterized and validated before use.
The use of animal models that mimic susceptible human populations is important for the study of effects of ambient or surrogate PM. However, all models must be validated for their relevance to the human condition. Validated models will provide important insights into the mechanisms of action of ambient PM and associated pollutants.
Studies that use validated animal models will assist in the evaluation of particle characteristics that underlie human health effects of exposure to ambient PM. They will provide input into the standards-
setting process by contributing information needed to determine margins of safety for exposure.
Continued development and use of appropriate animal models are required. The necessary tools for such development are readily available.
Research Topic 9b. In Vitro Studies
What are the appropriate in vitro models to use in studies of particulate- matter toxicity?
In vitro studies are important in helping to determine underlying toxicological mechanisms. They remain a necessary complement to animal and clinical evaluations.
The HEI database and the proceedings of the PM 2000 meeting list 34 studies related to this research topic. However, three of the studies do not deal with in vitro methods, and four are not relevant to the PM issue, but rather address issues of occupational and fibrogenic particle exposure. Most in vitro studies with PM are still conducted without considering the important issue of relevant doses to be used or, at a minimum, the use of a study design incorporating dose-response assessments. Many studies also focus on only one particle type collected from different ambient sources without including any control particles; in general, this type of study design should be avoided.
Several in vitro studies reported in the database are based on findings of animal studies that use very high doses of a specific particle type. Although state-of-the-art methods of cellular and molecular
toxicology are applied, the lack of an adequate justification for doses, the lack of control particles, and the presence of insufficient discussion of these important issues make the interpretation of results difficult. The results, contrary to what investigators of those studies conclude, will not be directly applicable to an understanding of pathophysiological mechanisms of PM action, nor will they be useful for the validation of high-dose animal studies as models of human respiratory-tract responses to much lower doses. Conclusions that are based on high doses do not provide arguments for the biological plausibility of effects of ambient PM. At best, the studies could contribute mechanistic information on PM effects in occupationally exposed workers whose lungs are generally exposed to a particulate compound at several milligrams per cubic meter.
On the positive side, several studies that are under way do use appropriate dose-response designs. Recognizing the need to use lower doses, these studies compare the toxicity of different particle types and responses in animal vs. human cells; this facilitates extrapolation of in vivo responses in animals to humans. Although high doses are also delivered, the studies are valuable with respect to a toxicological evaluation of potentially reactive components, but will require followup studies with more realistic doses. Another well-designed study includes a comparison of responses in airway biopsy cells from normals and asthmatics for an in vitro determination of relative sensitivities to ambient PM. One study in this category of comparative in vitro studies evaluated the response of human bronchial epithelial cells to PM collected before and after a steel mill closure; the goal was to identify the importance of differing PM composition—in this case related to transition metals—for inducing adverse health effects.
Several other studies use methods of in vitro priming—for example, with lipopolysaccharides—of specific respiratory-tract cells, including alveolar macrophages and epithelial cells, to compare responses of oxidative stress induction by PM in sensitized cells and normal cells. These studies are aimed at assessing mechanistic concepts of PM toxicity and contribute to the establishment of a good basis for designing further in vivo studies.
Two planned in vitro studies are designed to investigate age differences by using cells from young and old animals and applying a variety
of doses down to very low ones. Plans of one group of investigators include delivery of particles in the airborne state to in vitro cell cultures so that the dosing will be similar to in vivo conditions. The importance of coculture of different cell types is realized in one study in which an in vitro lung-slice technology is used to compare responses to a variety of PM of different sources and to surrogate control particles. One in vitro study is aimed at evaluating mutagenic effects of airborne PM and associated organic compounds, addressing long-term effects. However, administered doses and the use of a dose-response design are not indicated, and it is necessary to consider these issues in studies addressing potential long-term effects.
The current and planned in vitro studies are designed to investigate several components of PM by using a number of end points, such as changes in the levels of inflammatory cytokines, and chemokines, release of oxidants, and oxidative stress responses. The issues of age-dependent responses and modulation of responses in cells from susceptible subjects are also being investigated. However, many current in vitro studies do not use or consider appropriate doses but, instead, are using unrealistic high doses; a dose-response design is still the exception in these types of studies. Despite those shortcomings, which need to be rectified, comparative in vitro toxicity studies to establish concepts and elucidate mechanistic events of PM toxicity are valuable additions to the database.
Specific mechanistic hypotheses related mainly to PM-induced effects are being tested at several laboratories. Although in vitro models are used for investigating mechanisms of PM-induced toxicity, the relevance of identified mechanistic pathways is highly question-
able when they are based on high doses, as is the case in most of the current studies. A major gap is a lack of testing of the validity of conclusions for specific mechanisms by using relevant low doses; this is due in large part to the lack of a demonstrated causal relationship between relatively low PM exposures and adverse effects in controlled in vivo studies. Thus, in vitro studies have their greatest scientific value when they are designed on the basis of results of controlled whole-animal or clinical studies, involve relatively realistic exposures, and test specific mechanistic hypotheses.
Mechanistic information at the cellular and molecular levels obtained from well-designed in vitro studies can contribute to the weight of evidence regarding a causal relationship between PM exposure and health effects. That will reduce uncertainties related to the plausibility of observed adverse PM effects. Knowledge gained about mechanisms of PM toxicity will contribute greatly to the scientific justification of the PM standards.
In vitro studies clearly are feasible in many laboratories. It is important for special attention to be directed toward the use of relevant doses. Moreover, the development of appropriate new methods for in vitro studies should be encouraged, including airborne-particle exposures of cell cultures, use of cells from compromised lungs, and use of genetically modified cells. Because the developmental phase of these models is potentially long, useful results might not become available very soon.
Research Topic 9c. Clinical Models
What are the appropriate clinical models to use in studies of particulate matter toxicity?
Clinical studies are controlled exposures of humans. In the case of PM, such studies are designed to use laboratory-generated surrogate particles or concentrated ambient-air particles. The use of human subjects avoids the need to extrapolate results from other species. Both normal and susceptible subpopulations can be studied, and physiologic, cellular, immunologic, electrocardiographic and vascular end points, as well as symptoms, can be assessed. Elucidation of responses in humans is key to understanding the importance of ambient pollution and determining the nature of adverse health effects of PM exposure.
Review of the HEI database and proceedings of the PM 2000 meeting identified about 10 active human-exposure studies. All are using particles of concern, which include CAP, ultrafine carbon, ultrafine acidic sulfates, diluted diesel exhaust, and smoke from burning of vegetable matter. Studies are under way in healthy volunteers, asthmatics, and atopic people. Studies in people who have COPD or cardiac disease are planned. The clinical studies focus on evaluation of pulmonary and systemic responses, such as pulmonary inflammation and injury to epithelial cells; cardiac rhythm, rate, and variability; initiation of the coagulation cascade; and symptoms.
Few laboratories are equipped to perform clinical studies of PM. However, the similarities in their protocols enhance the likelihood of obtaining useful data. For example, studies with CAP and ultrafine particles have incorporated prolonged electrocardiographic monitoring after exposure. All studies include physiologic assessments of lung function, and indicators of airway inflammation in nasal or bronchoalveolar lavage fluid, induced sputum, or exhaled air (such as nitric oxide). In addition, coagulation indexes in blood are examined in some of the studies. In selected cases, efforts have been made to centralize analytical studies in a core laboratory for standardization of techniques.
There are a number of difficulties in establishing clinical models to study PM. Although the particle concentrators allow exposure to
relevant atmospheres, the mixtures vary from day to day and, typically, minimal chemical analyses of the particles are performed. If responses to CAP are variable, it is not possible to determine whether the variability resulted from differences in human susceptibility or in particle chemistry. In contrast, studies with surrogate particles result in reproducible exposures but mimic only selected aspects of ambient particulate pollution. Furthermore, the epidemiological data suggest that the most severely ill are at risk of pollutant effects; these subgroups cannot be used in controlled clinical studies. Because clinical studies by design are limited to short-term exposures, they will rarely be able to contribute to an understanding of development of chronic disease secondary to exposure to particles.
The particle-exposure systems used in clinical studies include environmental chambers, facemasks, and mouthpieces. Each design offers specific advantages, but the mouthpiece studies with ultrafine particles have incorporated measurements of total particle deposition. One clinical study will investigate the interaction of particles with ozone, another plans to incorporate metals into the particles, and virtually all include some level of exercise to enhance minute ventilation, thus increasing the inhaled dose of pollutants.
The current and planned clinical studies are designed to investigate CAP and several specific components of PM (such as size, acids, metals, and diesel exhaust) with a number of pulmonary and systemic end points. Studies are under way in susceptible subpopulations and are planned in other subgroups with pre-existing disease. Despite the limited facilities available for clinical research, the array of studies under way should provide valuable information on PM toxicity.
Clinical studies present an opportunity to examine responses to
PM in both healthy and susceptible subpopulations. Carefully designed controlled exposures provide information on symptomatic, physiologic, and cellular responses in both healthy and at-risk groups. They also provide important insights into mechanisms of action of PM. Such studies can provide needed information on PM deposition and retention in healthy and susceptible subpopulations (see research topic 6 ).
Clinical studies often provide important information for regulatory decisions. Assessing acute responses in groups that have chronic diseases will establish important insights into plausible mechanistic pathways. In addition, they provide crucial data on relative differences in responsiveness between healthy and potentially at-risk populations.
Studies are under way in several laboratories. They should provide highly relevant information for the next review of PM for regulatory decisions.
RESEARCH TOPIC 10. ANALYSIS AND MEASUREMENT
To what extent does the choice of statistical methods in the analysis of data from epidemiological studies influence estimates of health risks from exposures to particulate matter? Can existing methods be improved? What is the effect of measurement error and misclassification on estimates of the association between air pollution and health?
The first report of this committee (NRC 1998) outlined several methodological issues that needed further study. These included the
choice of statistical methods for analyzing data obtained from other studies, especially epidemiologic studies. Because more than one method can be used to analyze data, it will be important to understand the extent to which alternative approaches can influence analytical results. In addition, new study designs will require new approaches to analyze the data. These include development of analytical methods to examine several constituents and fractions of PM in an effort to understand their associations with health end points and design of models and approaches to incorporate new biological insights. Specific attention was given to measurement error, an issue inherent in most epidemiological studies that use ambient-air data to characterize subjects' exposure. The committee's second report (NRC 1999) reiterated those needs and noted the existence of relevant research and papers nearing completion.
Review of scientific literature, meeting abstracts, and the HEI database identified extensive progress on several methodological subjects. The review was intended to evaluate the extent to which the research needs previously identified by the committee are being addressed and to stimulate further targeted research.
General Methodological Issues
Model development and evaluation.
Over the last several years, there has been considerable development of time-series data-analysis methods, which have provided much of the evidence on the association between PM exposures and health effects. The methods assess the variation in day-to-day mortality or morbidity counts with variation in PM concentrations on the same or previous day. Although systematic and comprehensive comparisons of alternative methods have not been reported, limited comparisons have suggested that results are relatively robust to the statistical approach used. However, the choices of input variables and data have been shown occasionally to influence results (Lipfert et al. 2000). That is particularly true with respect to the choice of pollution variables in
the statistical models. The presence of other variables in the models can influence the association between health measures and particulate air pollution.
The application of the time-series studies has been facilitated by recent advances in hardware and software and by the development of statistical approaches that can appropriately account for the data structure of the daily time series. Time-series analyses were initially conducted on a single location that had been selected primarily on the basis of data availability, rather than representing selection from a defined sampling frame. Meta-analysis was then used to summarize the data and to gain a more precise estimate of the effect of PM on mortality or morbidity. Recently, studies of more-formal, multicity designs have been conducted. These approaches have a priori plans for selecting locations and have standardized statistical methods across locations. The European Air Pollution and Health: A European Approach (APHEA) project (Katsouyanni et al. 1995) is a pioneering effort that initially analyzed routinely collected data from 15 European cities in 10 countries with a common statistical protocol, examining mortality and emergency hospitalizations in some cities. In the United States, the HEI has funded the National Morbidity, Mortality and Air Pollution Study (NMMAPS) (Samet et al. 2000, 2001). The NMMAPS includes analyses of mortality and morbidity separately; a joint analysis of morbidity and mortality is planned. For the mortality analysis, the NMMAPS investigators used a sampling frame defined by U.S. counties. The 90 largest urban areas (by population) were selected, and the daily mortality data for 1987-1994 were analyzed to assess associations with PM and other pollutants.
The methods used in the APHEA project and the NMMAPS show the potential power of multicity approaches. The potential selection bias of only a single or a few locations is avoided. Combining information across locations, increases power and heterogeneity. In addition, health effects can be compared between regions that have similar air-pollution levels.
Other research efforts involving model development are the exploration of distributed-lag models (Schwartz 2000a; Zanobetti et al. 2000), efforts to understand the dose-response relationship between PM exposure and health effects (Schwartz 2000b; Smith et al. 2000;
Schwartz and Zanobetti 2000), and examination of alternative ways of analyzing the relationship between air-quality data and health end points (Beer and Ricci 1999; Sunyer at al. 2000; Tu and Piegorsch 2000; Zhang et al. 2000). Other research efforts have also aimed at combining results from several studies, including those by Stroup et al. (2000) and Sutton et al. (2000).
Measurement Error
The difference between actual exposures and measured ambient-air concentrations is termed measurement error. Measurement error can occur when measures of ambient air pollution are used as an index of personal exposure. For PM, the three sources of measurement error are instrument error (the accuracy and precision of the monitoring instrument), error resulting from the nonrepresentativeness of a monitoring site (reflected by the spatial variability of the pollutant measured), and differences between the average personal exposure to a pollutant and the monitored concentration (influenced by microenvironmental exposures).
With regard to assessing the impact of outdoor exposures, the most important source of measurement error is related to the representativeness of the placement of monitors. In acute studies, other sources of error will not vary substantially from day to day. But in chronic studies, the most important errors are those associated with microenvironmental exposures. The presence of indoor sources of PM and the influence of home characteristics on penetration of outdoor particles into the indoor environment can be a source of substantial exposure error. The influence of home characteristics is important because it varies with geographical location, climate, socioeconomic factors, and season. Because those factors could introduce systematic errors, they must be considered in the analysis and interpretation of results of chronic epidemiological studies. They are often taken into account by using not direct measures of exposure, but surrogate measures that would influence the exposures, such as smoking in the household, the presence of gas stoves, and air conditioning.
Measurement error is of particular concern in studies intended to
isolate the effects of particles from those of gases or to distinguish the effects of individual particle species or size fractions from each other. When several population variables are included in the same analyses and the different variables have different magnitudes and types of measurement error, the issue of estimating the associations between health responses and specific variables is even more complicated. A well-measured but benign substance might serve as the best empirical predictor of community health effects, rather than a poorly measured but toxic substance that is similarly distributed in the atmosphere. The problem is that most pollutants tend to be similarly distributed, so collocated time series of pollutant measurements tend to covary because all pollutants are modulated by synoptic meteorological conditions. Long-term averages of pollutant concentrations tend to covary across cities because the rates of many categories of emissions tend to increase roughly with population. Various methods are available to adjust statistical analyses for the effects of differential measurement error (Fuller 1987; Carroll et al. 1995).
Several statistical issues must be considered in addressing measurement error. A full discussion of these issues is found in Fuller (1987) and Carroll et al. (1995). The most important is the type of model in which the measurement error is imbedded. Generally, in linear models, measurement error can be understood if it is assumed that errors are independent of each other and of other variables in the model and that they follow the same statistical distribution. However, it is common for measurement-error distribution and properties not to be readily apparent, for example in ambient-air quality data, because “true” measurements of personal exposure have not been available. Recent studies have generated data that will provide a better understanding of the properties of measurement error. Until its specific properties are understood, its consequences will be unclear. For instance, Stefanski (1997) cites examples from a linear model in which the regression coefficient could be biased in either direction or unbiased, depending on the characteristics of the measurement error. The issues are increasingly complex as one moves to multiple-regression models (Carroll and Galindo, 1998) and then to nonlinear models.
Development of a framework or method will be useful in consider-
ing the effects of measurement error on population-mortality relative risks (Zeger et al. 2000). The framework demonstrates that for a wide range of circumstances the impacts of measurement error will either lead to underestimates of association or have a negligible effect. Combined with some of the data now being generated, the framework promises considerable progress toward an understanding of measurement error.
Harvesting is an issue raised by time-series mortality studies. The term “harvesting” refers to the question of whether deaths from air pollution occur in people who are highly susceptible and near death (and die a few days earlier because of air pollution than they otherwise would have) or the air pollution leads to the death of people who are not otherwise near death.
Many studies have identified associations between daily mortality and air-quality variables measured at the same time or a few days before deaths, but none of them has been able to address fully the issue of harvesting, although several recent analyses (Zeger et al. 1999, Schwartz 2000c) suggest that the findings of daily time-series studies do not reflect mortality displacement alone. Several analytical approaches have been proposed to address harvesting, and they need to be tried on additional data sets and refined to quantify better the degree of life-shortening associated with PM and other pollutants. Four recent papers examine this issue from different perspectives (Smith et al. 1999; Zeger at al. 1999; Murray and Nelson 2000; Schwartz 2000c).
Spatial Analytical Methods
An important issue in the analysis of data from studies that examine the association between city-specific mortality and long-term average pollutant concentrations, is whether observations of individual subjects are independent or correlated. Spatial correlation in
mortality can result from common social and physical environments among residents of the same city. Air pollution can be spatially autocorrelated as a result of broad regional patterns stemming from source and dispersion patterns.
In a recent reanalysis of data from the study by Pope et al. (1995), which examined associations between mortality in 154 cities throughout the United States and fine-particle and sulfate concentrations, Krewski et al. (2000) developed and applied new methods to allow for the presence of spatial autocorrelation in the data. The methods included two-stage random-effects regression methods, which were used to account for spatial patterns in mortality data and between-and within-city specific-particle air pollution levels, and application of spatial filtering to remove regional patterns in the data. Taking spatial autocorrelation into account in this manner increased the estimate of the mortality ratios associated with exposure to PM and led to wider confidence limits than in the original analysis; it was assumed that all individuals in the study represented independent observations.
The initial work on the development of analytical methods for the analysis of community-level data that exhibit clear spatial patterns warrants further investigation. Failure to take such spatial patterns into account can lead to bias in the estimates of mortality associated with long-term exposure to fine particles and to inaccurate indications of statistical significance.
The recent research appears to address the research gaps and needs addressed by the committee. That is especially true for the measurement-error and harvesting issues. Because this research is new, it needs to be digested and applied to several data sets to increase our understanding. Data that are available or being collected allow further testing of the applications and methods. However, several subjects for further research are: elucidation of the statistical properties of the new spatial approaches discussed, consideration of
alternative ways of addressing spatial autocorrelation in the data, and application of such spatial analytical methods to additional data sets.
The research has been well conducted with strong statistical tools. In addition, it has taken advantage of the existing literature and statistical tools while applying them to new subjects. However, the statistical tools have been applied to few data sets. The value of the research will increase as it is applied to more data sets and as approaches and results from the various studies are compared, synthesized, and reconciled.
The research can contribute substantially to decisionmaking. Understanding of potential influence of model approaches on results is key to adequate use of the research findings. Because measurement error can affect the results, insights into the influence of measurement error will assist in the interpretation of the results and ultimately increase their influence in decisionmaking. Understanding of harvesting will help to place estimates of effects on mortality in a public-health perspective.
Feasibility is not a deterrent to the research in this field. It appears that extensive results will be available within the timeframe laid out by this committee.
Regulatory standards are already on the books at the the U.S. Environmental Protection Agency (EPA) to address health risks posed by inhaling tiny particles from smoke, vehicle exhaust, and other sources.
At the same time, Congress and EPA have initiated a multimillion dollar research effort to better understand the sources of these airborne particles, the levels of exposure to people, and the ways that these particles cause damage.
To provide independent guidance to the EPA, Congress asked the National Research Council to study the relevant issues. The result is a series of four reports on the particulate-matter research program. The first two books offered a conceptual framework for a national research program, identified the 10 most critical research needs, and described the recommended timing and estimated costs of such research.
This, the third volume, begins the task of assessing the progress made in implementing the research program. The National Research Council ultimately concludes that the ongoing program is appropriately addressing many of the key uncertainties. However, it also identifies a number of critical specific subjects that should be given greater attention. Research Priorities for Airborne Particulate Matter focuses on the most current and planned research projects with an eye toward the fourth and final report, which will contain an updated assessment.
READ FREE ONLINE
Welcome to OpenBook!
You're looking at OpenBook, NAP.edu's online reading room since 1999. Based on feedback from you, our users, we've made some improvements that make it easier than ever to read thousands of publications on our website.
Do you want to take a quick tour of the OpenBook's features?
Show this book's table of contents , where you can jump to any chapter by name.
...or use these buttons to go back to the previous chapter or skip to the next one.
Jump up to the previous page or down to the next one. Also, you can type in a page number and press Enter to go directly to that page in the book.
Switch between the Original Pages , where you can read the report as it appeared in print, and Text Pages for the web version, where you can highlight and search the text.
To search the entire text of this book, type in your search term here and press Enter .
Share a link to this book page on your preferred social network or via email.
View our suggested citation for this chapter.
Ready to take your reading offline? Click here to buy this book in print or download it as a free PDF, if available.
Get Email Updates
Do you enjoy reading reports from the Academies online for free ? Sign up for email notifications and we'll let you know about new publications in your areas of interest when they're released.

An official website of the United States government
The .gov means it's official. Federal government websites often end in .gov or .mil. Before sharing sensitive information, make sure you're on a federal government site.
The site is secure. The https:// ensures that you are connecting to the official website and that any information you provide is encrypted and transmitted securely.
- Publications
- Account settings
- Browse Titles
NCBI Bookshelf. A service of the National Library of Medicine, National Institutes of Health.
National Research Council (US) Committee on Assessing Behavioral and Social Science Research on Aging; Feller I, Stern PC, editors. A Strategy for Assessing Science: Behavioral and Social Research on Aging. Washington (DC): National Academies Press (US); 2007.

A Strategy for Assessing Science: Behavioral and Social Research on Aging.
- Hardcopy Version at National Academies Press
4 Progress in Science
This chapter examines theories and empirical findings on the overlapping topics of progress in science and the factors that contribute to scientific discoveries. It also considers the implications of these findings for behavioral and social science research on aging. The chapter first draws on contributions from the history and sociology of science to consider the nature of scientific progress and the paths that lead to realizing the potential scientific and societal outcomes of scientific activity. It considers indicators that might be used to assess progress toward these outcomes. The chapter then examines factors that contribute to scientific discovery, drawing eclectically on the history and sociology of science as well as on theories and findings from organizational behavior, policy analysis, and economics.
- THEORIES OF SCIENTIFIC PROGRESS
The history and sociology of science have produced extensive bodies of scholarship on some of these themes, generating in the process significant ongoing disagreements among scholars (see, e.g., Krige, 1980 ; Cole, 1992 ; Rule, 1997 ; Bowler and Morus, 2005 ). Most of this work focuses on processes and historical events in the physical and life sciences; relatively little of it addresses the social and behavioral sciences (or engineering, for that matter), except possibly subfields of psychology (e.g., Stigler, 1999 ). It is legitimate to ask whether this research even applies to the behavioral and social sciences ( Smelser, 2005 ). 1
We do not attempt an encyclopedic coverage nor a resolution of the debates, past and continuing, on such questions. Rather, we draw on this research to make more explicit the main issues underlying the tasks of prospective assessment of scientific fields for the purpose of setting priorities in federal research agencies, given the uncertain outcomes of research.
The history of science has produced several general theories about how science develops and evolves over long periods of time. A 19th century view is that of Auguste Comte, who argued that there is a hierarchy of the sciences, from the most general (astronomy), followed historically and in other ways by physics, chemistry, biology, and sociology. Sciences atop the hierarchy are characterized as having more highly developed theories; greater use of mathematical language to express ideas; higher levels of consensus on theory, methods, and the significance of problems and contributions to the field; more use of use theory to make verifiable predictions; faster obsolescence of research, to which citations drop off rapidly over time; and relatively fast progress. Sciences at the bottom of the hierarchy are said to exhibit the opposite characteristics ( Cole, 1983 ).
Many adherents to this hierarchical view place the natural sciences toward the top of the hierarchy and the social sciences toward the bottom. 2 In this view, advances in the “higher” sciences, conceived in terms of findings, concepts, methodologies, or technologies that are thought to be fundamental, are held to flow down to the “lower” sciences, while the reverse flow rarely occurs. Although evidence of such a unidirectional flow from donor to borrower disciplines does exist ( Losee, 1995 ), there are counterexamples. Historians and sociologists of science have offered evidence against several of these propositions, and particularly dispute the claimed association of natural science with the top of the hierarchy and social science with the bottom (e.g., Bourdieu, 1988 ; Cetina, 1999 ; Steinmetz, 2005 ). The picture is more complex, as noted below.
By far the best known modern theory of scientific progress is that of Thomas Kuhn (1962) , which focuses on the major innovations that have punctuated the history of science in the past 350 years, associated with such investigators as Copernicus, Galileo, Lavoisier, Darwin, and Einstein. Science, in Kuhn’s view, is usually a problem-solving activity within clear and accepted frameworks of theory and practice, or “paradigms.” Revolutions occur when disparities or anomalies arise between theoretical expectation and research findings that can be resolved only by changing fundamental rules of practice. These changes occur suddenly, Kuhn claims, in a process akin to Gestalt shifts: in a relative instant, the perceived relationships among the parts of a picture shift, and the whole takes on a new meaning. Canonical examples include the Copernican idea that the Earth revolves around the Sun, Darwin’s evolutionary theory, relativity in physics, and the helical model of DNA.
A quite different account is that of John Desmond Bernal (1939) . Inspired by Marxist social science and ideals of planned social progress, Bernal saw basic science progressing most vigorously when it was harnessed to practical efforts to serve humanity’s social and economic needs (material well-being, public health, social justice). Whereas in Kuhn’s view science progressed according to its inner logic, Bernal asserted that intellectual and practical advances could be engineered and managed.
Another tradition of thought, stemming from Derek Price’s (1963) vision of a quantitative “science of science,” has focused less on how innovations arise than on how they spread and how their full potential is exploited by small armies of scientists. Mainly pursued by sociologists of science, this line of analysis has focused on the social structure of research communities (e.g., Hagstrom, 1965 ), competition and cooperation in institutional systems ( Merton, 1965 ; Ben-David, 1971 ), and structured communication in schools of research or “invisible colleges” (e.g., Crane, 1972 ). These efforts, while focused mainly on how science works, may imply principles for stimulating scientific progress and innovation.
There are also evolutionary models of scientific development, such as that of the philosopher David Hull (1988) . Extending Darwin’s account of evolution by variation and selection, Hull argues that scientific concepts evolve in the same way, by social or communal selection of the diverse work of individual scientists. In evolutionary views, science continually produces new ideas, which, like genetic mutations, are essentially unpredictable. Their ability to survive and expand their niches depends on environmental factors.
Bruno Latour and Steve Woolgar (1979) also offer an account of a selective struggle for viability among scientific producers. The vast majority of scientific papers quickly disappear into the maw of the scientific literature. The few that are used by other scientists in their work are the ones that determine the general direction of science progress. In evolutionary and competitive models, a possible function of science managers is to shape the environment that selects for ideas so as to propagate research that is judged to promote the agency’s scientific and societal goals.
Stephen Cole (1992) emphasized a distinction between the frontier and the core of science that seems consistent with an evolutionary view. Work at the frontiers of sciences is characterized by considerable disagreement; as science progresses over time, disagreement decreases as processes such as empirical confirmation and paradigm shift select out certain ideas, while others become part of the received wisdom.
Although the view that different sciences have similar features at their respective frontiers is not unchallenged ( Hicks, 2004 ), we have found the idea of frontier and core science to be useful in examining the extent to which insights from the history and sociology of science, fields that have concentrated their attention predominantly on the natural sciences, also apply to the social and behavioral sciences.
Cole (1983 , 1992) reports considerable evidence to suggest that different fields of science have similar features at the frontier, even if they are very different at the core. In the review of research proposals and journal submissions, an activity at the frontier of knowledge, he concludes that consensus about the quality of research is not systematically higher in the natural sciences than in the social sciences, citing the standard deviations of reviewers’ ratings of proposals to the National Science Foundation, which were twice as large in meteorology as in economics.
In the core, represented by undergraduate textbooks, the situation appears to be quite different. Cole (1983) found that in textbooks published in the 1970s, the median publication date of the references cited in both physics and chemistry was before 1900, while the median publication date in sociology was post-1960. Sociology texts cited an average of about 800 references, while chemistry and physics texts generally cited only about 100. Moreover, a comparison of texts from the 1950s and the 1970s indicated that the material covered, as well as the sources cited, were much the same in both periods in physics and chemistry, whereas in sociology, the newer texts cited only a small proportion of the sources cited in the earlier texts.
Cole interpreted these findings as indicating that core knowledge in physics and chemistry was both more consensual and more stable over time than core knowledge in sociology. Such findings suggest that even though sciences may differ greatly at the core, for the purpose of assessing the progress of science at the frontiers of research fields, insights from the study of the natural sciences are likely to apply to the social sciences as well. They also point to the need to differentiate between “vitality,” as indicated by ferment at the frontier, and scientific progress as indicated by movement of knowledge from the frontier to the core. 3 These findings suggest that the policy challenges for research managers making prospective judgments at the frontiers of research fields are quite similar across the sciences.
- NATURE OF SCIENTIFIC PROGRESS
Scientific progress can be of various types—discoveries of phenomena, theoretical explanations or syntheses, tests of theories or hypotheses, acceptance or rejection of hypotheses or theories by the relevant scientific communities, development of new measurement or analytic techniques, application of general theory to specific theoretical or practical problems, development of technologies or useful interventions to improve human health and well-being from scientific efforts, and so forth. Consequently, many different developments might be taken as indicators, or measures, of progress in science.
Science policy decision makers need to consider the progress and potential of scientific fields in multiple dimensions, accepting that the absence of detectable advance on a particular dimension is not necessarily evidence of failure or poor performance. Drawing on Weinberg’s (1963) classification of internal and external criteria for formulating scientific choices, we make the practical distinction between internally defined types of scientific progress, that is, elements of progress defined by intellectual criteria, and externally defined types of progress, defined in terms of the contributions of science to society. Managers of public investments in science need to be concerned with both.
Scientific Progress Internally Defined
The literatures in the history of science and in science studies include various analyses and typologies of scientific and theoretical progress (e.g., Rule, 1997 ; Camic and Gross, 1998 ; Lamont, 2004 ). This section presents a distillation of insights from this research into a short checklist of major types of scientific progress. The list is intended as a reminder to participants in science policy decisions that assess the progress of scientific fields of the variety of kinds of progress science can make. Recognizing that these broad categories overlap and also that they are interdependent, with each kind of progress having the potential to influence the others, directly or indirectly, the list is intended to simplify a very complex phenomenon to a manageable level.
Types of Scientific Progress
Discovery. Science makes progress when it demonstrates the existence of previously unknown phenomena or relationships among phenomena, or when it discovers that widely shared understandings of phenomena are wrong or incomplete.
Analysis. Science makes progress when it develops concepts, typologies, frameworks of understanding, methods, techniques, or data that make it possible to uncover phenomena or test explanations of them. Thus, knowing where and how to look for discoveries and explanations is an important type of scientific progress. Improved theory, rigorous and replicable methods, measurement techniques, and databases all contribute to analysis.
Explanation. Science makes progress when it discovers regularities in the ways phenomena change over time or finds evidence that supports, rules out, or leads to qualifications of possible explanations of these regularities.
Integration. Science makes progress when it links theories or explanations across different domains or levels of organization. Thus, science progresses when it produces and provides support for theories and explanations that cover broader classes of phenomena or that link understandings emerging from different fields of research or levels of analysis.
Development. Science makes progress when it stimulates additional research in a field or discipline, including research critical of past conclusions, and when it stimulates research outside the original field, including interdisciplinary research and research on previously underresearched questions. It also develops when it attracts new people to work on an important research problem.
Recent scientific activities supported by the Behavioral and Social Research (BSR) Program of the National Institute on Aging (NIA) have yielded progress in the form of scientific advances of most of the above types. We cite only a few examples.
- Discovery: The improving health of elderly populations. An example is analyses of data from Sweden, which has the longest running national data set on longevity, that have shown that the maximum human life span has been increasing since the 1860s, that the rate of increase has accelerated since 1969, and that most of the change is due to improved probabilities of survival of individuals past age 70 ( Wilmoth et al., 2000 ). Parallel trends have been discovered among the elderly in the form of declining physical disability, which declined in the United States from 26 percent of the elderly population in 1982 to 20 percent in 1999 (e.g., Manton and Gu, 2001 ), and declining cognitive impairment (e.g., Freedman et al., 2001 , 2002 ). Such findings together suggest overall improvements in the health of elderly populations in high-income countries.
- Analysis: Longitudinal datasets for understanding processes of aging. The Health and Retirement Study ( Juster and Suzman, 1995 ), a major ongoing longitudinal study that assesses the health and socioeconomic condition of aging Americans in which BSR played a central entrepreneurial role, has provided data that made possible, among other things, some of the discoveries about declining disability already noted. International comparative data sets on health risk factors and health outcomes, such as the Global Burden of Disease dataset ( Ezzati et al., 2002 ), have also made significant scientific progress possible.
- Explanation: Questioning and refining understandings. Several BSR-funded research programs have yielded findings that called into question widely held views about aging processes. Examples include findings that question the beliefs that more health care spending leads to better health outcomes ( Fisher et al., 2003a , 2003b ), that increasing life expectancy implies increased health care expenditures ( Lubitz et al., 2003 ), that unequal access to health care is the main explanation for higher mortality rates among older people of lower socioeconomic status (e.g., Adda et al., 2003 ; Adams et al., 2003 ), and that aging is a purely biological process unaffected by personal or cultural beliefs ( Levy, 2003 ). Other BSR-sponsored research has provided evidence that a previously noted association of depression with heart disease may be explained in part by a process in which negative affect suppresses immune responses ( Rosenkranz et al., 2003 ).
- Integration and development: Creating a biodemography of aging. BSR supported and brought together “demographers, evolutionary theorists, genetic epidemiologists, anthropologists, and biologists from many different scientific taxa” ( National Research Council, 1997 :v) to seek coherent understandings of human longevity that are consistent with knowledge at levels from genes to populations and data from human and nonhuman species). This effort has helped to attract researchers from other fields into longevity studies, add vigor to this research field, and put the field on a broader and firmer interdisciplinary base of knowledge.
Paths to Scientific Progress
Scientific progress is widely recognized as nonlinear. Some new ideas have led to rapid revolutions, while other productive ideas have had lengthy gestation periods or met protracted resistance. Still other new ideas have achieved overly rapid, faddish acceptance followed by quick dismissal. An earlier generation of research in the history and sociology of science documented variety and surprise as characteristics of scientific progress, but it was not followed by broad transdisciplinary studies that developed and tested general theories of scientific progress.
No theory of scientific progress exists, or is on the horizon, that allows prediction of the future development of new scientific ideas or specifies how the different types of scientific progress influence each other—although they clearly are interdependent. Rather, recent studies by historians of science and practicing scientists typically emphasize the uncertainty surrounding which of a series of findings emerging at any point in time will be determinative of the most productive path for future scientific inquiries and indeed of the ways in which these findings will be used. Only in hindsight does the development of various experimental claims and theoretical generalizations appear to have the coherence that creates a sense of a linear, inexorable path.
Science policy seems to be in particular need of improved basic understanding of the apparently uncertain paths of scientific progress as a basis for making wiser, more efficient investments. Without this improved understanding, extensive investments into collecting and analyzing data on scientific outputs are unlikely to provide valid predictors of some of the most important kinds of scientific progress. Political and bureaucratic pressures to plan for steady progress and to assess it with reliable and valid performance indicators will not eliminate the gaps in basic knowledge that must be filled in order to develop such indicators.
Despite the incompleteness of knowledge, the findings of earlier research remain a suggestive and potentially useful resource for practical research managers. They suggest a variety of state-of-knowledge propositions that are consistent with our collective experience on multiple advisory and review panels across several federal science agencies. We consider the following propositions worthy of consideration in discussions of how science managers can best promote scientific progress:
- Scientific discoveries are initially the achievements of individuals or small groups and arise in varied and largely unpredictable ways: the larger and more important the discoveries, the less predictable they would have been.
- The great majority of scientific products have limited impact on their fields; there are only a few major or seminal outputs. Whether or not new scientific ideas or methods become productive research traditions depends on an uncertain process that may extend over considerable time. Sometimes the impacts of research are quite different from those anticipated by the initial research sponsors, the researchers, or the individuals or organizations that first make use of it. For example, the Internet, which was developed as a means of fostering scientific communication among geographically dispersed researchers, has now become a leading channel for entertainment and retail business, among other things.
- Existing procedures for allocating federal research funds are most effective at the mid-level of scientific innovation, where there is consensus among established fields about the importance of questions and the direction and content of emerging questions in those fields.
- The uncertainties of scientific discovery and the difficulties of accurately identifying turning points and sharp departures in scientific inquiry suggest that research managers will do best with a varied portfolio of projects, including both mainstream and discontinuous or exploratory research projects. These uncertainties also suggest that assessment of a program’s investments in research is most appropriately made at the portfolio rather than the project level.
- The portfolio concept also applies to a program’s investments in analysis: in advancing the state of theoretical understanding, tools, and databases. Scientific progress in both the natural and social sciences may either follow or precede the development of new tools (instruments, models, algorithms, databases) that apply to many problems. Contrary to simple models of scientific progress that have theory building as the grounding for empirical research or data collection as the foundation for theory building, the process is not linear or unidirectional. 4 Program investments in theory building, tool development, and data collection can all contribute to scientific progress, but it is very difficult to predict which kinds of investments will be most productive at any given time (see National Research Council, 1986 , 1988 ; Smelser, 1986 ).
- Scientific progress sometimes arises from efforts to solve technological or social problems in environments that combine concerns with basic research and with application. It can also arise in environments insulated from practical concerns. And progress can involve first one kind of setting and then the other (see Stokes, 1997 ).
Interdisciplinarity and Scientific Progress
The claim that the frontiers of science are generally located at the interstices between and intersections among disciplines deserves explicit attention because it is increasingly found in the conclusions and recommendations of national commissions and NRC committees (e.g., National Research Council, 2000b ; Committee on Science, Engineering, and Public Policy, 2004 ) and in statements by national science leaders. 5 Scholarship in the history and sociology of science is consistent with competing views on this claim. A considerable body of recent scholarship has noted that exciting developments often come at the edges of established research fields and at the boundaries between fields ( Dogan and Pahre, 1990 ; Galison, 1999 ; Boix-Mansilla and Gardner, 2003 ; National Research Council, 2005b ). Moreover, interdisciplinary thinking has become more integral to many areas of research because of the need to understand “the inherent complexity of nature and society” and “to solve societal problems” ( National Research Council, 2005b :2).
The idea is that scientific advances are most likely to arise, or are most easily promoted, when scientists from different disciplines are brought together and encouraged to free themselves from disciplinary constraints. A good example to support this idea is the rapid expansion and provocative results of research on the biodemography of aging that followed the 1996 NRC workshop on this topic ( National Research Council, 1997 ). The workshop occasioned serious efforts to develop and integrate related research fields.
To the extent that interdisciplinarity is important to scientific progress and for gaining the potential societal benefits of science, it is important for research managers to create favorable conditions for interdisciplinary contact and collaboration. In fact, for some time BSR has been seeking explicitly to promote both multidisciplinarity and interdisciplinarity ( Suzman, 2004 ). For example, when the Health and Retirement Study was started in 1990, it was explicitly designed to be useful to economists, demographers, epidemiologists, and psychologists, and explicit efforts were made to convince those research communities that the study was not for economists only. BSR has reorganized itself and redefined its areas of interest on issue-oriented, interdisciplinary lines; sought out leading researchers and funded them to do what was expected to be ground-breaking and highly visible research in interdisciplinary fields; supported workshops and studies to define new interdisciplinary fields (e.g., National Research Council, 1997 , 2000a , 2001c ); created broadly based multidisciplinary panels to review proposals in emerging interdisciplinary areas; and funded databases designed to be useful to researchers in multiple disciplines for addressing the same problems, thus creating pressure for communication across disciplines. Some of the results, such as those already mentioned, have been notably productive and potentially useful.
The available studies seem to support the following conclusions about the favorable conditions for interdisciplinary science ( Klein, 1996 ; Rhoten, 2003 ; National Research Council, 2005b ):
- Successful interdisciplinary research requires both disciplinary depth and breadth of interests, visions, and skills, integrated within research groups.
- The success of interdisciplinary research groups depends on institutional commitment and research leadership with clear vision and teambuilding skills.
- Interdisciplinary research requires communication among people from different backgrounds. This may take extra time and require special efforts by researchers to learn the languages of other fields and by team leaders to make sure that all participants both contribute and benefit.
- New modes of organization, new methods of recruitment, and modified reward structures may be necessary in universities and other research organizations to facilitate interdisciplinary interactions.
- Both problem-oriented organization of research organizations and the ability to reorganize as problems change facilitate interdisciplinary research.
- Funding organizations may need to design their proposal and review criteria to encourage interdisciplinary activities.
Several conditions favorable to interdisciplinary collaboration can be affected by the actions of funders of research. For example, science agencies can encourage or require interdisciplinary collaboration in the research they support, support activities that specifically bring researchers together from different disciplines to address a problem of common interest, provide additional funds or time to allow for the development of effective interdisciplinary communication in research groups or communities, and organize their programs internally and externally around interdisciplinary themes. They can ask review panels to consider how well groups and organizations that propose interdisciplinary research provide conditions, such as those above, that are commonly associated with successful interdisciplinary research. And they might also ensure that groups reviewing interdisciplinary proposals include individuals who have successfully led or participated in interdisciplinary projects.
Encouraging interdisciplinary research may have pitfalls, though. It is possible for funds to be offered but for researchers to fail to propose the kinds of interdisciplinary projects that were hoped for. Sometimes interdisciplinary efforts take hold, but they fail to produce important scientific advances or societal benefits. Interdisciplinarity can also become a mantra. If disciplines are at times presented as silos—independent units with no connections among them—interdisciplinary fields may also become silos that happen to straddle two fields. At any point in time, an observer can identify numerous new research trajectories, several involving novel combinations of existing disciplines. Thus, alongside recently institutionalized fields, such as biotechnology, materials science, information sciences, and cognitive (neuro)sciences, are claimants for scientific attention and programmatic support, such as vulnerability sciences, prevention science, and neuroeconomics.
Little is known about how to predict whether a new interdisciplinary field will take off in a productive way. Floral metaphors about budding fields are not always carried to the desired conclusion: many budding fields lack the intellectual or methodological germplasm to do more than pop up and quickly wither. It is at least as difficult to assess the prospects of interdisciplinary fields as of disciplinary ones, and probably more so ( Boix-Mansilla and Gardner, 2003 ; National Research Council, 2005b ). 6
Federal agency science managers can act as entrepreneurs of interdisciplinary fields, so that their expansion from an interest of a small number of researchers into a recognizable cluster of activity may reflect the level of external support from federal agencies and foundations. As a field develops, though, a good indicator of vitality may be the exchange of ideas with other fields and particularly the export of ideas from the new field to other scientific fields or to practical use. But progress in interdisciplinary fields may be hard to determine from recourse to such indicators alone. Fields can be vital without exporting ideas to other fields. Policy analysis, now a well-established academic field of instruction and research, engages researchers from several social science disciplines, but it is a net importer of ideas ( MacRae and Feller, 1998 ; Reuter and Smith-Ready, 2002 ).
It is worth noting that support for interdisciplinary research, although it has unique benefits, may be a relatively high-risk proposition because it requires high-level leadership skills and innovative organizational structures. These characteristics of interdisciplinary research may pose special challenges for research managers in times of tightening budgets, when pressures for risk aversion may conflict with the need to develop innovative approaches to scientific questions and societal needs.
Contributions of Science to Society
In government agencies with practical missions, investments in science are appropriately judged both on internal scientific grounds and on the basis of their contributions to societal objectives. In the case of NIA, these objectives largely concern the improved longevity, health, and well-being of older people ( National Institute on Aging, 2001 ). There are many ways research can contribute to these objectives. For simplicity, we group the societal objectives of science into four broad categories.
Identifying issues. Science can contribute to society by identifying problems relating to the health and well-being of older people that require societal action or sometimes showing that a problem is less serious than previously believed.
Finding solutions. Science can contribute to society by developing ways to address issues or solve problems, for example, by improving prevention or treatment of diseases, improving health care delivery systems, improving access to health care, or developing new products or services that contribute to the longevity, health, or quality of life for older people in America.
Informing choices. Science can contribute to society by providing accurate and compelling information to public officials, health care professionals, and the public and thus promoting better informed choices about life and health by older people and better informed policy decisions affecting them.
Educating the society. Science can contribute to society by producing fundamental knowledge and developing frameworks of understanding that are useful for people facing their own aging and the aging of family members, making decisions in the private sector, and participating as citizens in public policy decisions. Science can also contribute by educating the next generation of scientists.
Research on science utilization, a field that was most vital in the 1970s and that has seen some revival recently, has examined the ways in which scientific results, particularly social science results, may be used, particularly in government decisions (for recent reviews, see Landry et al., 2003 , and Romsdahl, 2005 , for some classic treatments, see Caplan, 1976 ; Weiss, 1977 , 1979 ; Lindblom and Cohen, 1979 ). In terms of the above typology, this research mainly examines the use or nonuse of research results for informing choices by public policy actors. It does not much address the use of results by ordinary citizens, medical practitioners, the mass media, or other users involved in identifying issues and finding solutions, other than policy solutions. The most general classification in this research tradition of the ways social science is used is for enlightenment (i.e., providing a broad conceptual base for decisions) and as instrumental input (e.g., providing specific policy-relevant data). In addition, researchers note that social science results may be used to provide justification or legitimization for decisions already reached or as a justification for postponing decisions ( Weiss, 1979 ; Oh, 1996 ; Romsdahl, 2005 ).
Federal science program managers face the challenges of establishing causal linkages between past research program activities and societal impacts and of projecting societal impacts from current and planned research activities. The challenges are substantial. Even when findings from social and behavioral science research influence policies and practices in the public and private sectors and may therefore be presumed to contribute to human well-being, they are seldom determinative. Indicators exist or could be created for many societal impacts of research ( Cozzens et al., 2002 ; Bozeman and Sarewitz, 2005 ). In addition, evidence that the results of research are used, for example, in government decisions, may be considered an interim indicator of ultimate societal benefit, presuming that the decisions promote people’s well-being.
Limits exist, however, to the ability of a mission agency to translate findings from the research it funds into practice. For the research findings of the National Institutes of Health (NIH) in general and NIA-BSR in particular, contributions to societal or individual well-being require the complementary actions of myriad other actors and organizations in government and the private sector, including state and local governments, insurance companies, nursing homes, physicians’ practices, and individuals. According to Balas and Boren (2000 :66), “studies suggest that it takes an average of 17 years for research evidence to reach clinical practice.” Similarly lengthy processes and circuitous connections link research findings to more enlightened or informed policy making ( Lynn, 1978 ).
A scientific development also may contribute to society in the above ways even if working scientists do not judge it to be a significant contribution on scientific grounds. For example, surveys sponsored by BSR produce data, for example on declining rates of disability among older people, that may be very useful for health care planning without, by themselves, contributing anything more to science than a phenomenon to be explained. Thus, it is appropriate for assessments of research progress to consider separately the effects of research activity on scientific and societal criteria. Scientific activities and outputs may contribute to either of these two kinds of desirable outcomes or to both.
Interpreting Scientific Progress
The extent to which particular scientific results constitute progress in knowledge or contribute to societal well-being is often contested. This is especially the case when scientific findings are uncertain or controversial and when they can be interpreted to support controversial policy choices. Many results in applied behavioral and social science have these characteristics. Disagreements arise over which research questions are important enough to deserve support (that is, over which issues constitute significant social problems), about whether or not a finding resolves a scientific dispute or has unambiguous policy implications, and about many other aspects of the significance of scientific outputs. The more controversial the underlying social issues, the further such disagreements are likely to penetrate into the details of scientific method. Interested parties may use their best rhetorical tools to “frame” science policy issues and may even attempt to exercise power by influencing legislative or administrative decision makers to support or curtail particular lines of research.
These aspects of the social context of science are relevant for the measurement and assessment of scientific progress and its societal impact. They underline the recognition that the meaning of assessments of scientific progress may not follow in any straightforward way from the evidence the assessments produce. Assessing science, no matter how rigorous the methods that may be used, is ultimately a matter of interpretation. The possibility of competing interpretations of evidence is ever-present when using science indicators or applying any other analytic method for measuring the progress and impact of science. In Chapter 5 , we discuss a strategy for assessing science that recognizes this social context while also seeking an appropriate role for indicators and other analytic approaches.
- INDICATORS OF SCIENTIFIC PROGRESS
Research managers understandably want early indicators of scientific progress to inform decisions that must be made before the above types of substantive progress can be definitively shown. Although scientific progress is sometimes demonstrable very quickly, recent histories of science, as noted above, tend to emphasize not only the length of time required for research findings to generate a new consensus but also the uncertainties at the time of discovery regarding what precisely constitutes the nature of the discovery. Time lag and impact may depend on various factors, including the type of research and publication and citation practices in the field. A longitudinal research project can be expected to take longer to yield demonstrable progress than a more conceptual project.
Research Vitality and Scientific Progress
Expressions of scientific interest and intellectual excitement, sometimes referred to as the vitality of a research field, have been suggested as a useful source of early indicators of scientific progress as defined from an internal perspective. Such indications of the development of science are of particular interest to science managers because many of them might potentially be converted into numerical indicators. They include the following:
- Established scientists begin to work in a new field.
- Students are increasingly attracted to a field, as indicated by enrollments in new courses and programs in the field.
- Highly promising junior scientists choose to pursue new concepts, methods, or lines of inquiry.
- The rate of publications in a field increases.
- Citations to publications in the field increase both in number and range across other scientific fields.
- Publications in the new field appear in prominent journals.
- New journals or societies appear.
- Ideas from a field are adopted in other fields.
- Researchers from different preexisting fields collaborate to work on a common set of problems.
Research on the nanoscale is an area that illustrates vitality by such indicators and that is beginning to have an impact on society and the economy. Zucker and Darby (2005 :9) point to the rate of increase in publishing and patenting in nanotechnology since 1986 as being of approximately the same order of magnitude as the “remarkable increase in publishing and patenting that occurred during the first twenty years of the biotechnology revolution…. Since 1990 the growth in nano S&T articles has been remarkable, and now exceeds 2.5 percent of all science and engineering articles.” Major scientific advances are often marked by flurries of research activity, and many observers expect that such indications of research vitality presage major progress in science and applications.
However, research vitality does not necessarily imply future scientific progress. For example, research on cold fusion was vital for a time precisely because most scientists believed it would not lead to progress. In the social sciences, many fields have shown great vitality for a period of time, as indicated by numbers of research papers and citations to the central works, only to decline rapidly in subsequent periods. Rule (1997) , in his study of progress in social science, discusses several examples from sociology, including the grand social theory of Talcott Parsons (1937 , 1964) , ethno-methodology (e.g., Garfinkel, 1967 ), and interaction process analysis (e.g., Bales, 1950 ). Although these fields were vital for a time, in longer retrospect many observers considered them to have been far less important to scientific progress than they had earlier appeared to be. Rule suggests several possible interpretations of this kind of historical trajectory: the fields that looked vital were in fact intellectual dead-ends; research in the fields did make important contributions that were so thoroughly integrated into thinking in the field that they became common knowledge and were no longer commonly cited; and the fields represented short-term intellectual tastes that lost currency with a shift in theoretical concerns. With enough hindsight, it may be possible to decide which interpretation is most correct, although disagreements remain in many specific cases. But the resource allocation challenge for a research manager, given multiple alternative fields whose aggregate claims for support exceed his or her program budget, is to make the correct interpretation of research vitality prospectively: that is, to project whether the field will be judged in hindsight to have produced valuable contributions or to have been no more than a fad or an intellectual dead-end.
Another trajectory of research is problematic for research managers who would use vitality as an indicator of future potential. Some research findings or fields lie dormant for considerable periods without showing signs of vitality, before the seminal contributions gain recognition as major scientific advances. Such findings have been labeled as “premature discoveries” ( Hook, 2002 ) and “sleeping beauties” ( van Raan, 2004b ). These are not findings that are resisted or rejected; rather, they are unappreciated, or their uses or implications are not initially recognized ( Stent, 2002 ). In effect, the contribution of such discoveries to scientific progress or societal needs or both lies dormant until there is some combination of independent discoveries that reveal the potency of the initial discovery. In such cases, vitality indicators focused predominately on the discovery and its related line of research would have been misleading as predictors of long-term scientific importance.
An instructive example of the limitations of vitality measures as early indicators in the social sciences is the intellectual history of John Nash’s approach to game theory—an approach that was recognized, applied, and then dismissed as having limited utility, only to reemerge again as a major construct (the Nash equilibrium), not only in the social and behavioral sciences but also in the natural sciences. As recounted by Nasar (1998) , the years following Nash’s seminal work at RAND in the early 1950s were a period of flagging interest in game theory. Luce and Raiffa’s authoritative overview of the field in 1957 observed: “We have the historical fact that many social scientists have become disillusioned with game theory. Initially there was a naïve band-wagon feeling that game theory solved innumerable problems of sociology and economics, or that, at least it made their solution a practical matter of a few years’ work. This has not turned out to be the case” (quoted in Nasar, 1998 :122). In later retrospect, game theory became widely influential in the social and natural sciences, and Nash was awarded the Nobel Memorial Prize in Economics in 1994.
The complexity of the relationship between the quantity of scientific activity being undertaken during a specific period and the pace of scientific progress (or the rate at which significant discoveries are made) can perhaps be illustrated by analogy to a bicycle race: a group of researchers, analogous to the peloton or pack in a bicycle race, proceeds along together over an extended period until a single individual or a small group attempts a breakaway to win the race. Some breakaways succeed and some fail, but because of the difficulties of making progress by working alone (wind resistance, in the bicycle race analogy), individuals need the cooperation of a group to make progress over the long run and to create the conditions for racing breakaways or scientific breakthroughs. When scientific progress follows this model, fairly intense activity is a necessary but not sufficient condition for progress. Alternatively, the pack may remain closely clustered together for extended periods of time, advancing apace yet with a sense that little progress toward victory, however specified, is being made ( Horan, 1996 ).
In our judgment, these various trajectories of scientific progress imply that quantitative indicators, such as citation counts, require interpretation if they are to be used as part of the prospective assessment of fields . Moreover, the implications of intensified activity in a research area may be quite different depending on the mission goals and the perspective of the agency funding the work. Significant research investments can create activity in a field by encouraging research and supporting communication among communities of researchers. But activity need not imply progress, at least not in terms of some of the indicators listed above, such as the export of ideas to other fields. If research managers conflate the concepts of scientific activity and progress, they can create self-fulfilling prophecies by simply creating scientific activity. These warnings become increasingly important as technical advances in data retrieval and mining make it easier to create and access quantitative indicators of research vitality and as precepts of performance assessment increase pressures on research managers to use quantitative indicators to assess the progress and value of the research they support.
Indicators of Societal Impact
A variety of events may indicate that scientific activities have generated results that are likely to have practical value, even though such value may not (yet) have been realized. Such events might function as leading indicators of the societal value of research. These events typically occur outside research communities. For example:
- Research is cited as the basis for patents that lead to licenses.
- Research is used to justify policies or laws or cited in court opinions.
- Research is prominently discussed in trade publications of groups that might apply it.
- Research is used as a basis for practice or training in medicine or other relevant fields of application.
- Research is cited and discussed in the popular press as having implications for personal decisions or for policy.
- Research attracts investments from other sources, such as philanthropic foundations.
Some of these potential indicators are readily quantifiable, so, like bibliometric indicators, they are attractive means by which science managers can document the value of their programs. But as with quantitative indicators of research vitality, the meaning of quantitative indicators of societal impact is subject to differing interpretations. For example, as studies of science utilization have emphasized, the use of research to justify policy changes may mean that the research has changed policy makers’ thinking or only that it provides legitimation for previously determined positions. Moreover, policy makers have been known to use research to justify a policy when the relevant scientific community is in fact sharply divided about the importance or even the validity of the cited research. Such research nevertheless has societal impact, even if not of the type the scientists may have expected.
- FACTORS THAT CONTRIBUTE TO SCIENTIFIC DISCOVERIES
Historically, analysis of the factors that contribute to scientific discoveries has occurred at least at three different levels of analysis. Macro-level studies have considered the effects of the structures of societies—their philosophical, social, political, religious, cultural, and economic systems ( Hart, 1999 ; Jones, 1988 ; Shapin, 1996 ). Meso-level analyses have examined the effects of functional and structural features of “national research and innovation systems”—for example, the relative apportionment of responsibility and public funding for scientific inquiry among government entities, quasi-independent research institutes, and universities ( Nelson, 1993 ). Microlevel studies have examined the associations between indicators of progress and such factors as the organization of research units and the age of the researcher ( Deutsch et al., 1971 ).
The programmatic latitude of any single federal science unit to adjust its actions to promote scientific discovery relates almost exclusively to micro-level factors. Even then, agency policies, legislation, and higher level executive branch policies may limit an agency’s options. For this reason, we look most closely at micro-level factors. It is nevertheless worth examining the larger structural factors affecting conditions for scientific discovery, if only to understand the implicit assumptrions likely to be accepted by BSR’s advisers and staff.
A convenient means of documenting contemporary thinking on the factors that contribute to scientific advances is to examine the series of “benchmarking” studies of the international standing of U.S. science in the fields of materials science, mathematics, and immunology made by panels of scientists under the auspices of the National Academies’ Committee on Science, Engineering, and Public Policy (COSEPUP). The benchmarking was conducted as a methodological experiment in response to a series of studies that had sought to establish national goals for U.S. science policy and to mesh these goals with the performance reporting requirements of the Government Performance and Results Act ( Committee on Science, Engineering, and Public Policy, 1993 , 1999a ; National Research Council, 1995a ).
The benchmarking reports covered the fields of mathematics ( Committee on Science, Engineering, and Public Policy, 1997 ), materials science ( Committee on Science, Engineering, and Public Policy, 1998 ), and immunology ( Committee on Science, Engineering, and Public Policy, 1999b ); they represented attempts to assess whether U.S. science was achieving the stated goals of the National Goals report ( Committee on Science, Engineering, and Public Policy, 1993 ) that the United States should be among the world leaders in all major areas of science and should maintain clear leadership in some major areas of science. These reports can be used to infer the collective beliefs across a broad range of the U.S. scientific community about the factors that contribute to U.S. scientific leadership, and implicitly to the factors that foster major scientific discoveries. The reports are also of interest because several of the factors they cite—for example, initiation of proposals by individual investigators, reliance on peer-based merit review—are the cynosures of proposals to modify the U.S. science system.
Across the three benchmarking reports, the core repeatedly cited as necessary for scientific progress was adequate facilities, quality and quantity of graduate students attracted to a field (and their subsequent early career successes in the field), diversity in funding sources, and adequate funding. In addition, with regard to the comparative international strength and the leadership position of U.S. science in these fields, the reports placed special emphasis on the “structure and financial-support mechanisms of the major research institutions in the United States” and on its organization of higher education research ( Committee on Science, Engineering and Public Policy, 1999b :35). Also highlighted as a contributing factor in “fostering innovation, creativity and rapid development of new technologies” was the “National Institutes of Health (NIH) model of research-grant allocation and funding: almost all research (except small projects funded by contracts) is initiated by individual investigators, and the decision as to merit is made by a dual review system of detailed peer review by experts in each subfield of biomedical science” (p. 36). 7
We accept the proposition that adequate funds to support research represents a necessary condition for sustained progress in a scientific field. Research progress also depends on the supply of researchers (including the number, age, and creativity of current and prospective researchers) and the organization of research, including the number and disciplinary mix of researchers engaged in a project or program and structure of the research team.
Supply of Researchers
The number, creativity, and age distribution of researchers in a field together affect the pace of scientific progress in the field. Numbers are important to the extent that the ability to generate scientific advances is randomly distributed through a population of comparably trained researchers. Fields with a larger number of active researchers can be expected to generate more scientific advance than fields with smaller such numbers. The pace of scientific advance across fields presumably also varies with their ability to attract the most able/creative/productive scientists. The attractiveness of a field at any point in time is likely to depend on its intellectual excitement (the challenges of the puzzles that it poses), its societal significance, the resources flowing to it to support research, and the prospects for longer term productive and gainful careers. Fields that exhibit these characteristics are likely to attract relatively larger cohorts of younger scientists; if scientific creativity is inversely correlated with age, such fields may be expected to exhibit greater vitality than those with aging cohorts of scientists.
This view is supported by much expert judgment and a number of empirical studies. For example, a study by the National Research Council (1998 :1) noted that “The continued success of the life-science research enterprise depends on the uninterrupted entry into the field of well-trained, skilled, and motivated young people. For this critical flow to be guaranteed, young aspirants must see that there are exciting challenges in life science research and they need to believe that they have a reasonable likelihood of becoming practicing independent scientists after their long years of training to prepare for their careers.”
Career opportunities for scientists affect the flow of young researchers into fields. Recent studies of career opportunities in the life sciences have noted that a “crisis of expectations” arises when career prospects fall short of scientific promise ( Freeman et al., 2001 ). Similar observations have been made at other times for the situations in physics, mathematics, computer science, and some fields of engineering. Studies also point, in general, to a decline in research productivity around midcareer. As detailed by Stephan and Levin (1992) , the decline reflects influences on both the willingness and ability of researchers to do scientific research. Older scientists are also seen to be slower to accept new ideas and techniques than are younger scientists. 8
Organization of Research
Since World War II, the social contract by which the federal government supports basic research has involved channeling large amounts of this support through awards to universities, much of that through grants to individual investigators. It is appropriate to consider whether such choices continue to be optimal and to consider related questions concerning the determinants of the research performance of individual faculty and of specific institutions or sets of institutions ( Guston and Keniston, 1994 ; Feller, 1996 ).
As detailed above, U.S. support of academic research across many fields, including aging research, is predicated on the proposition that “little science is the backbone of the scientific enterprise…. For those who believe that scientific discoveries are unpredictable, supporting many creative researchers who contribute to S&T, or the science base is prudent science policy” ( U.S. Congress Office of Technology Assessment, 1991 :146). Against this principle, trends toward “big science” and the requirements of interdisciplinary research have opened up the question of the optimal portfolio of funding mechanisms and award criteria to be employed by federal science agencies. Of special interest here as an alternative to the traditional model of single investigator–initiated research are what have been termed “industrial” models of research ( Ziman, 1984 ) or Mode II research; that is, research undertakings characterized by collaboration or teamwork among members of research groups participating in formally structured centers or institutes. Requests for proposals directed toward specific scientific, technological, and societal objectives; initiatives supporting collaborative, interdisciplinary modes of inquiry organized as centers rather than as single principal investigator projects; and use of selection criteria in addition to scientific merit are by now well-established parts of the research programs of federal science agencies, including NIH and the National Science Foundation. 9
A recurrent issue for federal science managers and for scientific communities is the relative rate of return to alternative arrangements, such as funding mechanisms. Making such comparisons is challenging. First, different research modes (e.g., single investigator–initiated proposals and multidisciplinary, center-based proposals submitted in response to a Request for Application) may produce different kinds of outputs. Single-investigator awards, typically described as the backbone of science, are intended cumulatively to build a knowledge base that affects clinical practice or public policy, to support the training of graduate students, to promote the development of networks of researchers and practitioners, and more—but no single awardee is expected to do all these things. Center awards also are expected to contribute to scientific progress—indeed to yield “value added” above the progress that can come from multiple single-investigator awards—but unlike single-investigator awards, they are typically expected to devote explicit attention to the other outcomes, such as translating the results of basic research into clinical practice. Because different modes of research support are expected to support different mixes of program objectives, direct comparisons of “performance” or “productivity” between or among them involves a complex set of weightings and assessments, both in terms of defining and measuring scientific progress and in assigning weights to the different kinds of scientific, programmatic, and societal objectives against which research is evaluated.
Little empirical evidence exists to inform comparisons among modes of research support. Empirical studies, most frequently in the form of bibliometric analyses, exist to compare the productivity of interdisciplinary research units, but these studies are not designed to answer the question of how much scientific progress would have been achieved had the funds allocated to such units been apportioned instead among a larger and more diverse number of single investigator awards ( Feller, 1992 ). Detailed criteria, for example, have been advanced to evaluate the performance of NIH’s center programs ( Institute of Medicine, 2004 ), and a number of center programs have been evaluated. However, these evaluations have not added up to a systematic assessment. 10
Expert judgment, historical assessment, and analysis of trends in science provide some support for core propositions about the sources of the vitality of U.S. science: adequate and sustainable funding; multiple, decentralized, funding streams; strong reliance on investigator-initiated proposals selected through competitive, merit-based review; coupling basic research with graduate education; and supplementary funding for capital-intensive modes of inquiry, interdisciplinary collaboration, targeted research objectives, and translation of basic research findings into clinical practice or technological innovations. Still, these principles may not provide wise guidance for the support of behavioral and social science research on aging, for three reasons. First, these observations come from experience with the life sciences, engineering sciences, and physical sciences, and it is not known whether the dynamics of scientific inquiry and progress are the same in the social and behavioral sciences. Second, it is not known whether recent trends in scientific inquiry, such as in the direction of interdisciplinarity, will continue, stop, or soon lead to a fundamental transformation in the way in which cutting-edge science (including in research on aging) is done. Third and perhaps most important, applying these principles presumes an environment of increasing total funds for research. In the more austere budget environment now projected for NIH and its subunits, it will not be possible to increase funding for all modes of support. Turning to existing research for guidance may prove of limited value for making trade-offs among competing funding paradigms.
- IMPLICATIONS FOR DECISION MAKING
- No theory exists that can reliably predict which research activities are most likely to lead to scientific advances or to societal benefit. The gulf between the decision-making environment of the research manager and the historian or other researcher retrospectively examining the emergence and subsequent development of a line of research is reflected in Weinberg’s (2001 :196) observation, “In judging the nature of scientific progress, we have to look at mature scientific theories, not theories at the moments when they are coming into being.” The history of science shows that evidence of past performance and current vitality, that is, of interest among scientists in a topic or line of research, are imperfect predictors of future progress. Thus, although it seems reasonable to expect that a newly developing field that generates excitement among scientists from other fields is a good bet to make progress in the near future, this expectation rests more on anecdote than on systematic empirical research. Notwithstanding the continuing search for improved quantitative measures and indicators for prospective assessment of scientific fields, practical choices about research investments will continue to depend on judgment. We address the prospects and potential roles of quantitative and other methods of science assessment in Chapter 5 .
- Science produces diverse kinds of benefits; consequently, assessing the potential of lines of research is a challenging task. Assessments should carefully apply multiple criteria of benefit. Science proceeds toward improving understanding and benefiting society on several fronts, but often at an uneven pace, so that a line of research may show rapid progress on one dimension or by one indicator while showing little or no progress on another. In setting research priorities among lines of research, it is important to consider evidence of past accomplishments on the several dimensions of scientific advances (discovery, analysis, explanation, integration, and development) and of contributions to society (e.g., identifying issues, finding solutions, informing choices). The policy implications of a finding that a line of research is not currently making much progress on one or more dimensions are not self-evident. Such an assessment might be used as a rationale for decreasing support (because the funds may be expected to be poorly spent), for increasing support (for example, if the poor performance is attributed to past underfunding), or for making investments to redirect the field so as to reinvigorate it. A field that appears unproductive may be stagnant, fallow, or pregnant. Telling which is not easy. Judgment can be aided by the assessments of people close to the field, although not just those so close as to have a vested interest in its survival or growth. The same kind of advice is useful for judging the proper timing for efforts to invest in fields in order to keep them alive or to reinvigorate them.
- Portfolio diversification strategies that involve investment in multiple fields and multiple kinds of research are appropriate for decision making, considering the inherent uncertainties of scientific progress. Through such strategies, research managers can minimize the consequences of overreliance on any single indicator of research quality or progress or any single presumption about what kinds of research are likely to be most productive. It is appropriate to diversify along several dimensions, including disciplines, modes of support, emphasis on theoretical or applied objectives, and so forth. Diversification is also advisable in terms of the kinds of evidence relied on to make decisions about what to support. For example, when quantitative indicators and informed peer judgment suggest supporting different lines of research, it is worth considering supporting some of each.
- Research managers should seek to emphasize investing where their investments are most likely to add value. This consideration may affect emphasis on types of scientific progress, research organizations and modes of support, and areas of support.
- Types of scientific progress. Even as they continue to pursue support of major scientific and programmatic advances, research managers may also find it productive to support improvements in databases and analytic techniques, efforts to integrate knowledge across fields and levels of analysis, efforts to examine underresearched questions, and the entry of new people to work on research problems.
- Research organizations and modes of support. Research managers should consider favoring support to research organizations or in modes that have been shown to have characteristics that are likely to promote progress, either generally or for specific fields or lines of scientific inquiry. NIH has multiple funding mechanisms available that would allow support for particular types of organizations ( Institute of Medicine, 2004 ). An ongoing study by Hollingsworth (2003 :8) identifies six organizational characteristics as “most important in facilitating the making of major discoveries” (see Box 4-1 ). Research managers might consider the findings of such studies in making choices about what kinds of organizations to support, especially in efforts to promote scientific innovation.
- Areas of support. Some fields may have sufficient other sources of funds that they do not need NIA support, or only need small investments from NIA to leverage funds from other sources. In other fields, however, BSR may be the only viable sponsor for the research. BSR managers may reasonably choose to emphasize supporting research in such fields because of the unlikelihood of leveraging funds. The value-added issue also affects decisions on modes of support and types of research to support.
- Interdisciplinary research. BSR should continue to support issue-focused interdisciplinary research to promote scientific activities and collaborations related to its mission that might not emerge from existing scientific communities and organizations structured around disciplines. Interdisciplinary research has significant potential to advance scientific objectives that research management can promote, such as scientific integration and development and scientists’ attention to societal objectives of science consistent with BSR’s mission. Moreover, BSR has a good track record of promoting these objectives through its support of selected areas of interdisciplinary, issue-focused research. BSR should continue to solicit research in areas that require interdisciplinary collaboration, to support data sets that can be used readily across disciplines, to fund interdisciplinary workshops and conferences, and to support cross-institution, issue-focused interdisciplinary research networks. Supporting such research requires special efforts and skills of research managers but holds the promise of yielding major advances that would not come from business-as-usual science.
Characteristics of Organizations That Produced Major Biomedical Discoveries: The Hollingsworth Study. Rogers Hollingsworth and colleagues (Hollingsworth and Hollingsworth, 2000; Hollingsworth, 2003) have been examining the characteristics of biomedical (more...)
It is often argued that progress in the behavioral and social sciences is qualitatively different from progress in the natural sciences. As noted in a National Research Council review of progress in the behavioral and social sciences ( Gerstein, 1986 :17), “Because they are embedded in social and technological change, subject to the unpredictable incidence of scientific ingenuity and driven by the competition of differing theoretical ideas, the achievements of behavioral and social science research are not rigidly predictable as to when they will occur, how they will appear, or what they might lead to.” The unstated (and untested) implication is that this unpredictability is more characteristic of the social sciences than the natural sciences. Another view states: “In the natural sciences, a sharp division of labor between the information-gathering and the theory-making functions is facilitated by an approximate consensus on the definition of research purposes and on the conceptual economizers guiding the systematic selection and organization of information. In the social sciences, where the subject matter of research and the comparatively lower level of theoretical agreement generally do not permit comparable consensus on the value and utility of information extracted from phenomena, sharp division of labor between empirical and theoretical tasks is less warranted” ( Ezrahi, 1978 :288). Even the same techniques are thought to have quite different roles in the social and natural sciences: “The role of statistics in social science is thus fundamentally different from its role in much of the physical science, in that it creates and defines the objects of study much more directly. Those objects are no less real than those of the physical science. They are even more often much better understood. But despite the unity of statistics—the same methods are useful in all areas—there are fundamental differences, and these have played a role in the historical development of all these fields” ( Stigler, 1999 :199).
Some observers even question the claims of the behavioral and social sciences to standing as sciences. As observed in a recent text on the history of science, “In the end, perhaps the most interesting question is: Did the drive to create a scientific approach to the study of human nature achieve its goal? For all the money and effort poured into creating a body of practical information on the topic, many scientists in better established areas remain suspicious, pointing to a lack of theoretical coherence that undermines the analogy with the ‘hard’ sciences” ( Bowler and Morus, 2005 :314–315).
According to Cole (2001 :37), “The problem with fields like sociology is that they have virtually no core knowledge. Sociology has a booming frontier but none of the activity at that frontier seems to enter the core.”
As noted by Galison (1999 :143), “Experimentalists … do not march in lockstep with theory…. Each subculture has its own rhythms of change, each has its own standards of demonstration, and each is embedded differently in the wider culture of institutions, practices, inventions and ideas.”
Rita Colwell, former director of the National Science Foundation, has stated that “Interdisciplinary connections are absolutely fundamental. They are synapses in this new capability to look over and beyond the horizon. Interfaces of the sciences are where the excitement will be the most intense” ( Colwell, 1998 ).
As stated in a recent National Research Council (2005b :150) report, “A remaining challenge is to determine what additional measures, if any, are needed to assess interdisciplinary research and teaching beyond those shown to be effective in disciplinary activities. Successful outcomes of an interdisciplinary research (IDR) program differ in several ways from those of a disciplinary program. First, a successful IDR program will have an impact on multiple fields or disciplines and produce results that feed back into and enhance disciplinary research. It will also create researchers and students with an expanded research vocabulary and abilities in more than one discipline and with an enhanced understanding of the interconnectedness inherent in complex problems.”
Consistent with the belief that competitive, merit-based review is key to creating the best possible conditions for scientific advance is the articulation of how “quality” is to be achieved and gauged under the Research and Development Investment Criteria established by the Office of Science and Technology Policy and the Office of Management and Budget on June 5, 2005: “A customary method for promoting quality is the use of a competitive, merit-based process” ( http://www .whitehouse .gov/omb/memoranda/m03-15.pdf , p. 7).
As Max Planck famously remarked, “a new scientific truth does not triumph by convincing its opponents and making them see the light, but because the its opponents eventually die, and a new generation grows up that is familiar with it.” Stephan and Levin (1992 :83) write: “empirical studies of Planck’s principle for the most part confirm the hypothesis that older scientists are slower than their younger colleagues are to accept new ideas and that eminent older scientists are the most likely to resist. The operative factor in resistance, however, is not age per se but, rather, the various indices of professional experience and prestige correlated with age …. [Y]oung scientists … may also be less likely to embrace new ideas, particularly if they assess such a course as being particularly risky.” Thus, a graying scientific community affects the rate of scientific innovation directly by being less productive and indirectly by being slow to accept new ideas as they emerge.
Interdisciplinary research and the industrial model of research are often found together, but they are not identical. One may organize centers based primarily on researchers from a single discipline, and researchers from several disciplines may collaborate, as co-principal investigators or as loosely coupled teams, on one-time awards. At NIH, research center grants “are awarded to extramural research institutions to provide support for long-term multidisciplinary programs of medical research. They also support the development of research resources, aim to integrate basic research with applied research and transfer activities, and promote research in areas of clinical applications with an emphasis on intervention, including prototype development and refinement of products, techniques, processes, methods, and practices” ( Institute of Medicine, 2004 ).
“NIH does not have formal regular procedures or criteria for evaluating center programs. From time to time, institutes conduct internal program reviews or appoint external review panels, but these ad hoc assessments are usually done in response to a perception that the program is no longer effective or appropriate rather than as part of a regular evaluation process. Most of these reviews rely on the judgment of experts rather than systematically collected objective data, although some formal program evaluations have been performed by outside firms using such data” ( Institute of Medicine, 2004 :121).
- Cite this Page National Research Council (US) Committee on Assessing Behavioral and Social Science Research on Aging; Feller I, Stern PC, editors. A Strategy for Assessing Science: Behavioral and Social Research on Aging. Washington (DC): National Academies Press (US); 2007. 4, Progress in Science.
- PDF version of this title (931K)
In this Page
Other titles in this collection.
- The National Academies Collection: Reports funded by National Institutes of Health
Recent Activity
- Progress in Science - A Strategy for Assessing Science Progress in Science - A Strategy for Assessing Science
Your browsing activity is empty.
Activity recording is turned off.
Turn recording back on
Connect with NLM
National Library of Medicine 8600 Rockville Pike Bethesda, MD 20894
Web Policies FOIA HHS Vulnerability Disclosure
Help Accessibility Careers
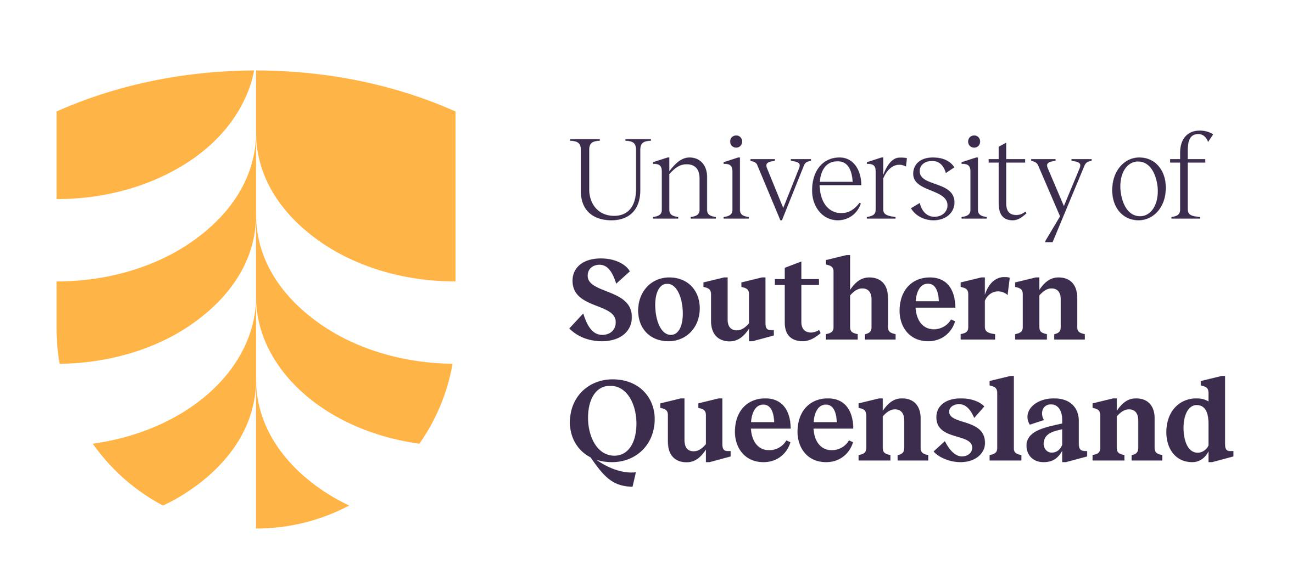
Want to create or adapt books like this? Learn more about how Pressbooks supports open publishing practices.
3 The research process
In Chapter 1, we saw that scientific research is the process of acquiring scientific knowledge using the scientific method. But how is such research conducted? This chapter delves into the process of scientific research, and the assumptions and outcomes of the research process.
Paradigms of social research
Our design and conduct of research is shaped by our mental models, or frames of reference that we use to organise our reasoning and observations. These mental models or frames (belief systems) are called paradigms . The word ‘paradigm’ was popularised by Thomas Kuhn (1962) [1] in his book The structure of scientific r evolutions , where he examined the history of the natural sciences to identify patterns of activities that shape the progress of science. Similar ideas are applicable to social sciences as well, where a social reality can be viewed by different people in different ways, which may constrain their thinking and reasoning about the observed phenomenon. For instance, conservatives and liberals tend to have very different perceptions of the role of government in people’s lives, and hence, have different opinions on how to solve social problems. Conservatives may believe that lowering taxes is the best way to stimulate a stagnant economy because it increases people’s disposable income and spending, which in turn expands business output and employment. In contrast, liberals may believe that governments should invest more directly in job creation programs such as public works and infrastructure projects, which will increase employment and people’s ability to consume and drive the economy. Likewise, Western societies place greater emphasis on individual rights, such as one’s right to privacy, right of free speech, and right to bear arms. In contrast, Asian societies tend to balance the rights of individuals against the rights of families, organisations, and the government, and therefore tend to be more communal and less individualistic in their policies. Such differences in perspective often lead Westerners to criticise Asian governments for being autocratic, while Asians criticise Western societies for being greedy, having high crime rates, and creating a ‘cult of the individual’. Our personal paradigms are like ‘coloured glasses’ that govern how we view the world and how we structure our thoughts about what we see in the world.
Paradigms are often hard to recognise, because they are implicit, assumed, and taken for granted. However, recognising these paradigms is key to making sense of and reconciling differences in people’s perceptions of the same social phenomenon. For instance, why do liberals believe that the best way to improve secondary education is to hire more teachers, while conservatives believe that privatising education (using such means as school vouchers) is more effective in achieving the same goal? Conservatives place more faith in competitive markets (i.e., in free competition between schools competing for education dollars), while liberals believe more in labour (i.e., in having more teachers and schools). Likewise, in social science research, to understand why a certain technology was successfully implemented in one organisation, but failed miserably in another, a researcher looking at the world through a ‘rational lens’ will look for rational explanations of the problem, such as inadequate technology or poor fit between technology and the task context where it is being utilised. Another researcher looking at the same problem through a ‘social lens’ may seek out social deficiencies such as inadequate user training or lack of management support. Those seeing it through a ‘political lens’ will look for instances of organisational politics that may subvert the technology implementation process. Hence, subconscious paradigms often constrain the concepts that researchers attempt to measure, their observations, and their subsequent interpretations of a phenomenon. However, given the complex nature of social phenomena, it is possible that all of the above paradigms are partially correct, and that a fuller understanding of the problem may require an understanding and application of multiple paradigms.
Two popular paradigms today among social science researchers are positivism and post-positivism. Positivism , based on the works of French philosopher Auguste Comte (1798–1857), was the dominant scientific paradigm until the mid-twentieth century. It holds that science or knowledge creation should be restricted to what can be observed and measured. Positivism tends to rely exclusively on theories that can be directly tested. Though positivism was originally an attempt to separate scientific inquiry from religion (where the precepts could not be objectively observed), positivism led to empiricism or a blind faith in observed data and a rejection of any attempt to extend or reason beyond observable facts. Since human thoughts and emotions could not be directly measured, they were not considered to be legitimate topics for scientific research. Frustrations with the strictly empirical nature of positivist philosophy led to the development of post-positivism (or postmodernism) during the mid-late twentieth century. Post-positivism argues that one can make reasonable inferences about a phenomenon by combining empirical observations with logical reasoning. Post-positivists view science as not certain but probabilistic (i.e., based on many contingencies), and often seek to explore these contingencies to understand social reality better. The post-positivist camp has further fragmented into subjectivists , who view the world as a subjective construction of our subjective minds rather than as an objective reality, and critical realists , who believe that there is an external reality that is independent of a person’s thinking but we can never know such reality with any degree of certainty.
Burrell and Morgan (1979), [2] in their seminal book Sociological p aradigms and organizational a nalysis , suggested that the way social science researchers view and study social phenomena is shaped by two fundamental sets of philosophical assumptions: ontology and epistemology. Ontology refers to our assumptions about how we see the world (e.g., does the world consist mostly of social order or constant change?). Epistemology refers to our assumptions about the best way to study the world (e.g., should we use an objective or subjective approach to study social reality?). Using these two sets of assumptions, we can categorise social science research as belonging to one of four categories (see Figure 3.1).
If researchers view the world as consisting mostly of social order (ontology) and hence seek to study patterns of ordered events or behaviours, and believe that the best way to study such a world is using an objective approach (epistemology) that is independent of the person conducting the observation or interpretation, such as by using standardised data collection tools like surveys, then they are adopting a paradigm of functionalism . However, if they believe that the best way to study social order is though the subjective interpretation of participants, such as by interviewing different participants and reconciling differences among their responses using their own subjective perspectives, then they are employing an interpretivism paradigm. If researchers believe that the world consists of radical change and seek to understand or enact change using an objectivist approach, then they are employing a radical structuralism paradigm. If they wish to understand social change using the subjective perspectives of the participants involved, then they are following a radical humanism paradigm.
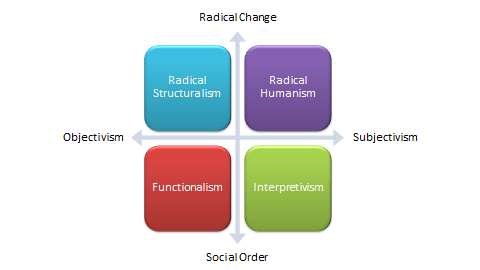
To date, the majority of social science research has emulated the natural sciences, and followed the functionalist paradigm. Functionalists believe that social order or patterns can be understood in terms of their functional components, and therefore attempt to break down a problem into small components and studying one or more components in detail using objectivist techniques such as surveys and experimental research. However, with the emergence of post-positivist thinking, a small but growing number of social science researchers are attempting to understand social order using subjectivist techniques such as interviews and ethnographic studies. Radical humanism and radical structuralism continues to represent a negligible proportion of social science research, because scientists are primarily concerned with understanding generalisable patterns of behaviour, events, or phenomena, rather than idiosyncratic or changing events. Nevertheless, if you wish to study social change, such as why democratic movements are increasingly emerging in Middle Eastern countries, or why this movement was successful in Tunisia, took a longer path to success in Libya, and is still not successful in Syria, then perhaps radical humanism is the right approach for such a study. Social and organisational phenomena generally consist of elements of both order and change. For instance, organisational success depends on formalised business processes, work procedures, and job responsibilities, while being simultaneously constrained by a constantly changing mix of competitors, competing products, suppliers, and customer base in the business environment. Hence, a holistic and more complete understanding of social phenomena such as why some organisations are more successful than others, requires an appreciation and application of a multi-paradigmatic approach to research.
Overview of the research process
So how do our mental paradigms shape social science research? At its core, all scientific research is an iterative process of observation, rationalisation, and validation. In the observation phase, we observe a natural or social phenomenon, event, or behaviour that interests us. In the rationalisation phase, we try to make sense of the observed phenomenon, event, or behaviour by logically connecting the different pieces of the puzzle that we observe, which in some cases, may lead to the construction of a theory. Finally, in the validation phase, we test our theories using a scientific method through a process of data collection and analysis, and in doing so, possibly modify or extend our initial theory. However, research designs vary based on whether the researcher starts at observation and attempts to rationalise the observations (inductive research), or whether the researcher starts at an ex ante rationalisation or a theory and attempts to validate the theory (deductive research). Hence, the observation-rationalisation-validation cycle is very similar to the induction-deduction cycle of research discussed in Chapter 1.
Most traditional research tends to be deductive and functionalistic in nature. Figure 3.2 provides a schematic view of such a research project. This figure depicts a series of activities to be performed in functionalist research, categorised into three phases: exploration, research design, and research execution. Note that this generalised design is not a roadmap or flowchart for all research. It applies only to functionalistic research, and it can and should be modified to fit the needs of a specific project.
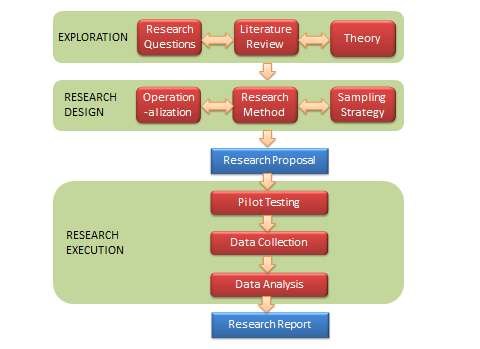
The first phase of research is exploration . This phase includes exploring and selecting research questions for further investigation, examining the published literature in the area of inquiry to understand the current state of knowledge in that area, and identifying theories that may help answer the research questions of interest.
The first step in the exploration phase is identifying one or more research questions dealing with a specific behaviour, event, or phenomena of interest. Research questions are specific questions about a behaviour, event, or phenomena of interest that you wish to seek answers for in your research. Examples include determining which factors motivate consumers to purchase goods and services online without knowing the vendors of these goods or services, how can we make high school students more creative, and why some people commit terrorist acts. Research questions can delve into issues of what, why, how, when, and so forth. More interesting research questions are those that appeal to a broader population (e.g., ‘how can firms innovate?’ is a more interesting research question than ‘how can Chinese firms innovate in the service-sector?’), address real and complex problems (in contrast to hypothetical or ‘toy’ problems), and where the answers are not obvious. Narrowly focused research questions (often with a binary yes/no answer) tend to be less useful and less interesting and less suited to capturing the subtle nuances of social phenomena. Uninteresting research questions generally lead to uninteresting and unpublishable research findings.
The next step is to conduct a literature review of the domain of interest. The purpose of a literature review is three-fold: one, to survey the current state of knowledge in the area of inquiry, two, to identify key authors, articles, theories, and findings in that area, and three, to identify gaps in knowledge in that research area. Literature review is commonly done today using computerised keyword searches in online databases. Keywords can be combined using Boolean operators such as ‘and’ and ‘or’ to narrow down or expand the search results. Once a shortlist of relevant articles is generated from the keyword search, the researcher must then manually browse through each article, or at least its abstract, to determine the suitability of that article for a detailed review. Literature reviews should be reasonably complete, and not restricted to a few journals, a few years, or a specific methodology. Reviewed articles may be summarised in the form of tables, and can be further structured using organising frameworks such as a concept matrix. A well-conducted literature review should indicate whether the initial research questions have already been addressed in the literature (which would obviate the need to study them again), whether there are newer or more interesting research questions available, and whether the original research questions should be modified or changed in light of the findings of the literature review. The review can also provide some intuitions or potential answers to the questions of interest and/or help identify theories that have previously been used to address similar questions.
Since functionalist (deductive) research involves theory-testing, the third step is to identify one or more theories can help address the desired research questions. While the literature review may uncover a wide range of concepts or constructs potentially related to the phenomenon of interest, a theory will help identify which of these constructs is logically relevant to the target phenomenon and how. Forgoing theories may result in measuring a wide range of less relevant, marginally relevant, or irrelevant constructs, while also minimising the chances of obtaining results that are meaningful and not by pure chance. In functionalist research, theories can be used as the logical basis for postulating hypotheses for empirical testing. Obviously, not all theories are well-suited for studying all social phenomena. Theories must be carefully selected based on their fit with the target problem and the extent to which their assumptions are consistent with that of the target problem. We will examine theories and the process of theorising in detail in the next chapter.
The next phase in the research process is research design . This process is concerned with creating a blueprint of the actions to take in order to satisfactorily answer the research questions identified in the exploration phase. This includes selecting a research method, operationalising constructs of interest, and devising an appropriate sampling strategy.
Operationalisation is the process of designing precise measures for abstract theoretical constructs. This is a major problem in social science research, given that many of the constructs, such as prejudice, alienation, and liberalism are hard to define, let alone measure accurately. Operationalisation starts with specifying an ‘operational definition’ (or ‘conceptualization’) of the constructs of interest. Next, the researcher can search the literature to see if there are existing pre-validated measures matching their operational definition that can be used directly or modified to measure their constructs of interest. If such measures are not available or if existing measures are poor or reflect a different conceptualisation than that intended by the researcher, new instruments may have to be designed for measuring those constructs. This means specifying exactly how exactly the desired construct will be measured (e.g., how many items, what items, and so forth). This can easily be a long and laborious process, with multiple rounds of pre-tests and modifications before the newly designed instrument can be accepted as ‘scientifically valid’. We will discuss operationalisation of constructs in a future chapter on measurement.
Simultaneously with operationalisation, the researcher must also decide what research method they wish to employ for collecting data to address their research questions of interest. Such methods may include quantitative methods such as experiments or survey research or qualitative methods such as case research or action research, or possibly a combination of both. If an experiment is desired, then what is the experimental design? If this is a survey, do you plan a mail survey, telephone survey, web survey, or a combination? For complex, uncertain, and multifaceted social phenomena, multi-method approaches may be more suitable, which may help leverage the unique strengths of each research method and generate insights that may not be obtained using a single method.
Researchers must also carefully choose the target population from which they wish to collect data, and a sampling strategy to select a sample from that population. For instance, should they survey individuals or firms or workgroups within firms? What types of individuals or firms do they wish to target? Sampling strategy is closely related to the unit of analysis in a research problem. While selecting a sample, reasonable care should be taken to avoid a biased sample (e.g., sample based on convenience) that may generate biased observations. Sampling is covered in depth in a later chapter.
At this stage, it is often a good idea to write a research proposal detailing all of the decisions made in the preceding stages of the research process and the rationale behind each decision. This multi-part proposal should address what research questions you wish to study and why, the prior state of knowledge in this area, theories you wish to employ along with hypotheses to be tested, how you intend to measure constructs, what research method is to be employed and why, and desired sampling strategy. Funding agencies typically require such a proposal in order to select the best proposals for funding. Even if funding is not sought for a research project, a proposal may serve as a useful vehicle for seeking feedback from other researchers and identifying potential problems with the research project (e.g., whether some important constructs were missing from the study) before starting data collection. This initial feedback is invaluable because it is often too late to correct critical problems after data is collected in a research study.
Having decided who to study (subjects), what to measure (concepts), and how to collect data (research method), the researcher is now ready to proceed to the research execution phase. This includes pilot testing the measurement instruments, data collection, and data analysis.
Pilot testing is an often overlooked but extremely important part of the research process. It helps detect potential problems in your research design and/or instrumentation (e.g., whether the questions asked are intelligible to the targeted sample), and to ensure that the measurement instruments used in the study are reliable and valid measures of the constructs of interest. The pilot sample is usually a small subset of the target population. After successful pilot testing, the researcher may then proceed with data collection using the sampled population. The data collected may be quantitative or qualitative, depending on the research method employed.
Following data collection, the data is analysed and interpreted for the purpose of drawing conclusions regarding the research questions of interest. Depending on the type of data collected (quantitative or qualitative), data analysis may be quantitative (e.g., employ statistical techniques such as regression or structural equation modelling) or qualitative (e.g., coding or content analysis).
The final phase of research involves preparing the final research report documenting the entire research process and its findings in the form of a research paper, dissertation, or monograph. This report should outline in detail all the choices made during the research process (e.g., theory used, constructs selected, measures used, research methods, sampling, etc.) and why, as well as the outcomes of each phase of the research process. The research process must be described in sufficient detail so as to allow other researchers to replicate your study, test the findings, or assess whether the inferences derived are scientifically acceptable. Of course, having a ready research proposal will greatly simplify and quicken the process of writing the finished report. Note that research is of no value unless the research process and outcomes are documented for future generations—such documentation is essential for the incremental progress of science.
Common mistakes in research
The research process is fraught with problems and pitfalls, and novice researchers often find, after investing substantial amounts of time and effort into a research project, that their research questions were not sufficiently answered, or that the findings were not interesting enough, or that the research was not of ‘acceptable’ scientific quality. Such problems typically result in research papers being rejected by journals. Some of the more frequent mistakes are described below.
Insufficiently motivated research questions. Often times, we choose our ‘pet’ problems that are interesting to us but not to the scientific community at large, i.e., it does not generate new knowledge or insight about the phenomenon being investigated. Because the research process involves a significant investment of time and effort on the researcher’s part, the researcher must be certain—and be able to convince others—that the research questions they seek to answer deal with real—and not hypothetical—problems that affect a substantial portion of a population and have not been adequately addressed in prior research.
Pursuing research fads. Another common mistake is pursuing ‘popular’ topics with limited shelf life. A typical example is studying technologies or practices that are popular today. Because research takes several years to complete and publish, it is possible that popular interest in these fads may die down by the time the research is completed and submitted for publication. A better strategy may be to study ‘timeless’ topics that have always persisted through the years.
Unresearchable problems. Some research problems may not be answered adequately based on observed evidence alone, or using currently accepted methods and procedures. Such problems are best avoided. However, some unresearchable, ambiguously defined problems may be modified or fine tuned into well-defined and useful researchable problems.
Favoured research methods. Many researchers have a tendency to recast a research problem so that it is amenable to their favourite research method (e.g., survey research). This is an unfortunate trend. Research methods should be chosen to best fit a research problem, and not the other way around.
Blind data mining. Some researchers have the tendency to collect data first (using instruments that are already available), and then figure out what to do with it. Note that data collection is only one step in a long and elaborate process of planning, designing, and executing research. In fact, a series of other activities are needed in a research process prior to data collection. If researchers jump into data collection without such elaborate planning, the data collected will likely be irrelevant, imperfect, or useless, and their data collection efforts may be entirely wasted. An abundance of data cannot make up for deficits in research planning and design, and particularly, for the lack of interesting research questions.
- Kuhn, T. (1962). The structure of scientific revolutions . Chicago: University of Chicago Press. ↵
- Burrell, G. & Morgan, G. (1979). Sociological paradigms and organisational analysis: elements of the sociology of corporate life . London: Heinemann Educational. ↵
Social Science Research: Principles, Methods and Practices (Revised edition) Copyright © 2019 by Anol Bhattacherjee is licensed under a Creative Commons Attribution-NonCommercial-ShareAlike 4.0 International License , except where otherwise noted.
Share This Book

- About Grants
Research Performance Progress Report (RPPR)
The RPPR is used by recipients to submit progress reports to NIH on their grant awards. This page provides an overview of the annual RPPR, the final RPPR and the interim RPPR and provides resources to help you understand how to submit a progress report.
Types of RPPRs
Progress reports document recipient accomplishments and compliance with terms of award. There are three types of RPPRs, all of which use the NIH RPPR Instruction Guide .
Annual RPPR – Use to describe a grant’s scientific progress, identify significant changes, report on personnel, and describe plans for the subsequent budget period or year.
Final RPPR – Use as part of the grant closeout process to submit project outcomes in addition to the information submitted on the annual RPPR, except budget and plans for the upcoming year.
Interim RPPR – Use when submitting a renewal (Type 2) application. If the Type 2 is not funded, the Interim RPPR will serve as the Final RPPR for the project. If the Type 2 is funded, the Interim RPPR will serve as the annual RPPR for the final year of the previous competitive segment. The data elements collected on the Interim RPPR are the same as for the Final RPPR, including project outcomes.
Submitting the RPPR
Only the project director/principal investigator (PD/PI) or their PD/PI delegate can initiate RPPRs. For multi-PD/PI grants only the Contact PI or the Contact PD/PI’s delegate can initiate the RPPR.
Signing Officials typically submit the annual RPPR, but may delegate preparation (Delegate Progress Report) to any PD/PI within the organization on behalf of the Contact PD/PI. Additionally, a Principal Investigator (PI) can delegate “Progress Report” to any eRA Commons user in their organization with the Assistant (ASST) role. This delegation provides the ASST with the ability to prepare Annual, Interim and Final RPPRs on behalf of the PI. However, only a Signing Official (SO) or PI (if delegated Submit by the SO) are allowed to submit the Annual, Interim, and Final RPPRs.
Follow the instructions in the RPPR User Guide to submit the RPPR, Interim RPPR or Final RPPR. The User Guide includes instructions for how to submit your RPPRs in the eRA Commons, how to complete the web-based forms, and what information is required. Instructions for completing the scientific portion of the report (see the elements below) may be found in Chapters 6 and 7.
The following resources may help with RPPR initiation and submission:
Annual RPPR Due Dates:
- Streamlined Non-Competing Award Process (SNAP) RPPRs are due approximately 45 days before the next budget period start date.
- Non-SNAP RPPRs are due approximately 60 days before the next budget period start date.
- Multi-year funded (MYF) RPPRs are due annually on or before the anniversary of the budget/project period start date of the award.
- The exact start date for a specific award may be found in grants status in eRA Commons.
Interim and Final RPPR Dues Dates:
- 120 days from period of performance end date for the competitive segment
The RPPR requests various types of information, including:
Accomplishments
What were the major goals and objectives of the project?
What was accomplished under these goals?
What opportunities for training and professional development did the project provide?
How were the results disseminated to communities of interest?
What do you plan to do during the next reporting period to accomplish the goals and objectives?
publications, conference papers, and presentations
website(s) or other Internet site(s)
technologies or techniques
inventions, patent applications, and/or licenses
other products, such as data or databases, physical collections, audio or video products, software, models, educational aids or curricula, instruments or equipment, research material, interventions (e.g., clinical or educational), or new business creation.
Participants and Other Collaborating Organizations
Changes/Problems (not required for Final or Interim RPPR)
Changes in approach and reasons for change
Actual or anticipated problems or delays and actions or plans to resolve them
Changes that have a significant impact on expenditures
Significant changes in use or care of human subjects, vertebrate animals, biohazards, and/or select agents
Budgetary Information (not required for Final or Interim RPPR)
Project Outcomes (only required on Final and Interim RPPR)
- Concise summary of the outcomes or findings of the award, written for the general public in clear and comprehensible language, without including any proprietary, confidential information or trade secrets
This page last updated on: November 2, 2022
- Bookmark & Share
- E-mail Updates
- Help Downloading Files
- Privacy Notice
- Accessibility
- National Institutes of Health (NIH), 9000 Rockville Pike, Bethesda, Maryland 20892
- NIH... Turning Discovery Into Health
This website may not work correctly because your browser is out of date. Please update your browser .
Interim reports
Interim (or progress) reports present the interim, preliminary, or initial evaluation findings.
Interim reports are scheduled according to the specific needs of your evaluation users, often halfway through the execution of a project. The interim report is necessary to let a project’s stakeholders know how an intervention is going. It provides information that will help the funders and other decision-makers determine whether to continue with the current direction, where to make adjustments if necessary, revise goals, add more resources or in the worst-case scenario, to shut it down.
An interim report is similar to a final report, in that it includes a summary, a brief description of the progress, the evaluation thus far, and an overview of the financial situation. Any delays or deviations to the plan are included and explained, as well as any comparison between actual compared to expected results.
Advice for using this method
To avoid critical issues being interpreted incorrectly, begin interim reports by stating the following:
- Which data collection activities are being reported on and which are not;
- When the final evaluation results will be available;
- Any cautions for readers in interpreting the findings.
Advice taken from Torres et al., 2005
This detailed example of a progress report describes Oxfam's work in Haiti following a large earthquake. It is intended to account to donors, partner organizations, allies, staff, and volunteers.
"Within every picture is a hidden language that conveys a message, whether it is intended or not. This language is based on the ways people perceive and process visual information.
This book from Torres, Preskill and Piontek has been designed to support evaluators to incorporate creative techniques in the design, conduct, communication and reporting of evaluation findings.
This guide is an IDRC publication with a module dedicated to writing a research report including information on layout and design.
This guide from the University of Wisconsin Cooperative Extension, provides a range of tips and advice for planning and writing evaluation reports that are concise and free of jargon.
Davies, L. (2012). Haiti Progress Report January-December 2011 . Oxford, UK: Oxfam GB. Retrieved from https://policy-practice.oxfam.org/resources/haiti-progress-report-january-december-2011-200732/
Oxfam GB Evaluation Guidelines (accessed 2012-05-08): https://www.alnap.org/help-library/oxfam-gb-evaluation-guidelines
Stetson, Valerie. (2008). Communicating and reporting on an evaluation: Guidelines and Tools. Catholic Relief Services and American Red Cross, Baltimore and Washington, USA. Retrieved from: https://www.alnap.org/help-library/communicating-and-reporting-on-an-evaluation-guidelines-and-tools
Torres, Rosalie T., Hallie Preskill and Mary E. Piontek. (2005). Evaluation Strategies for Communicating and Reporting: Enhancing Learning in Organizations (Second Edition). University of Mexico.
USAID. (2010). Performance monitoring & evaluation tips: Constructing an evaluation report. Retrieved from: https://pdf.usaid.gov/pdf_docs/pnadw117.pdf
Expand to view all resources related to 'Interim reports'
- Are you writing an evaluation report?
- Evaluation reporting: A guide to help ensure use of evaluation findings
- Quick tips for planning evaluation reports
'Interim reports' is referenced in:
Framework/guide.
- Rainbow Framework : Develop reporting media
Back to top
© 2022 BetterEvaluation. All right reserved.
Stages in the research process
Affiliation.
- 1 Faculty of Health, Social Care and Education, Anglia Ruskin University, Cambridge, England.
- PMID: 25736674
- DOI: 10.7748/ns.29.27.44.e8745
Research should be conducted in a systematic manner, allowing the researcher to progress from a general idea or clinical problem to scientifically rigorous research findings that enable new developments to improve clinical practice. Using a research process helps guide this process. This article is the first in a 26-part series on nursing research. It examines the process that is common to all research, and provides insights into ten different stages of this process: developing the research question, searching and evaluating the literature, selecting the research approach, selecting research methods, gaining access to the research site and data, pilot study, sampling and recruitment, data collection, data analysis, and dissemination of results and implementation of findings.
Keywords: Clinical nursing research; nursing research; qualitative research; quantitative research; research; research ethics; research methodology; research process; sampling.
- Data Collection / methods
- Pilot Projects
- Research Design / standards*
- Research Personnel / education*
- United Kingdom
- Privacy Policy

Home » Research Process – Steps, Examples and Tips
Research Process – Steps, Examples and Tips
Table of Contents

Research Process
Definition:
Research Process is a systematic and structured approach that involves the collection, analysis, and interpretation of data or information to answer a specific research question or solve a particular problem.
Research Process Steps
Research Process Steps are as follows:
Identify the Research Question or Problem
This is the first step in the research process. It involves identifying a problem or question that needs to be addressed. The research question should be specific, relevant, and focused on a particular area of interest.
Conduct a Literature Review
Once the research question has been identified, the next step is to conduct a literature review. This involves reviewing existing research and literature on the topic to identify any gaps in knowledge or areas where further research is needed. A literature review helps to provide a theoretical framework for the research and also ensures that the research is not duplicating previous work.
Formulate a Hypothesis or Research Objectives
Based on the research question and literature review, the researcher can formulate a hypothesis or research objectives. A hypothesis is a statement that can be tested to determine its validity, while research objectives are specific goals that the researcher aims to achieve through the research.
Design a Research Plan and Methodology
This step involves designing a research plan and methodology that will enable the researcher to collect and analyze data to test the hypothesis or achieve the research objectives. The research plan should include details on the sample size, data collection methods, and data analysis techniques that will be used.
Collect and Analyze Data
This step involves collecting and analyzing data according to the research plan and methodology. Data can be collected through various methods, including surveys, interviews, observations, or experiments. The data analysis process involves cleaning and organizing the data, applying statistical and analytical techniques to the data, and interpreting the results.
Interpret the Findings and Draw Conclusions
After analyzing the data, the researcher must interpret the findings and draw conclusions. This involves assessing the validity and reliability of the results and determining whether the hypothesis was supported or not. The researcher must also consider any limitations of the research and discuss the implications of the findings.
Communicate the Results
Finally, the researcher must communicate the results of the research through a research report, presentation, or publication. The research report should provide a detailed account of the research process, including the research question, literature review, research methodology, data analysis, findings, and conclusions. The report should also include recommendations for further research in the area.
Review and Revise
The research process is an iterative one, and it is important to review and revise the research plan and methodology as necessary. Researchers should assess the quality of their data and methods, reflect on their findings, and consider areas for improvement.
Ethical Considerations
Throughout the research process, ethical considerations must be taken into account. This includes ensuring that the research design protects the welfare of research participants, obtaining informed consent, maintaining confidentiality and privacy, and avoiding any potential harm to participants or their communities.
Dissemination and Application
The final step in the research process is to disseminate the findings and apply the research to real-world settings. Researchers can share their findings through academic publications, presentations at conferences, or media coverage. The research can be used to inform policy decisions, develop interventions, or improve practice in the relevant field.
Research Process Example
Following is a Research Process Example:
Research Question : What are the effects of a plant-based diet on athletic performance in high school athletes?
Step 1: Background Research Conduct a literature review to gain a better understanding of the existing research on the topic. Read academic articles and research studies related to plant-based diets, athletic performance, and high school athletes.
Step 2: Develop a Hypothesis Based on the literature review, develop a hypothesis that a plant-based diet positively affects athletic performance in high school athletes.
Step 3: Design the Study Design a study to test the hypothesis. Decide on the study population, sample size, and research methods. For this study, you could use a survey to collect data on dietary habits and athletic performance from a sample of high school athletes who follow a plant-based diet and a sample of high school athletes who do not follow a plant-based diet.
Step 4: Collect Data Distribute the survey to the selected sample and collect data on dietary habits and athletic performance.
Step 5: Analyze Data Use statistical analysis to compare the data from the two samples and determine if there is a significant difference in athletic performance between those who follow a plant-based diet and those who do not.
Step 6 : Interpret Results Interpret the results of the analysis in the context of the research question and hypothesis. Discuss any limitations or potential biases in the study design.
Step 7: Draw Conclusions Based on the results, draw conclusions about whether a plant-based diet has a significant effect on athletic performance in high school athletes. If the hypothesis is supported by the data, discuss potential implications and future research directions.
Step 8: Communicate Findings Communicate the findings of the study in a clear and concise manner. Use appropriate language, visuals, and formats to ensure that the findings are understood and valued.
Applications of Research Process
The research process has numerous applications across a wide range of fields and industries. Some examples of applications of the research process include:
- Scientific research: The research process is widely used in scientific research to investigate phenomena in the natural world and develop new theories or technologies. This includes fields such as biology, chemistry, physics, and environmental science.
- Social sciences : The research process is commonly used in social sciences to study human behavior, social structures, and institutions. This includes fields such as sociology, psychology, anthropology, and economics.
- Education: The research process is used in education to study learning processes, curriculum design, and teaching methodologies. This includes research on student achievement, teacher effectiveness, and educational policy.
- Healthcare: The research process is used in healthcare to investigate medical conditions, develop new treatments, and evaluate healthcare interventions. This includes fields such as medicine, nursing, and public health.
- Business and industry : The research process is used in business and industry to study consumer behavior, market trends, and develop new products or services. This includes market research, product development, and customer satisfaction research.
- Government and policy : The research process is used in government and policy to evaluate the effectiveness of policies and programs, and to inform policy decisions. This includes research on social welfare, crime prevention, and environmental policy.
Purpose of Research Process
The purpose of the research process is to systematically and scientifically investigate a problem or question in order to generate new knowledge or solve a problem. The research process enables researchers to:
- Identify gaps in existing knowledge: By conducting a thorough literature review, researchers can identify gaps in existing knowledge and develop research questions that address these gaps.
- Collect and analyze data : The research process provides a structured approach to collecting and analyzing data. Researchers can use a variety of research methods, including surveys, experiments, and interviews, to collect data that is valid and reliable.
- Test hypotheses : The research process allows researchers to test hypotheses and make evidence-based conclusions. Through the systematic analysis of data, researchers can draw conclusions about the relationships between variables and develop new theories or models.
- Solve problems: The research process can be used to solve practical problems and improve real-world outcomes. For example, researchers can develop interventions to address health or social problems, evaluate the effectiveness of policies or programs, and improve organizational processes.
- Generate new knowledge : The research process is a key way to generate new knowledge and advance understanding in a given field. By conducting rigorous and well-designed research, researchers can make significant contributions to their field and help to shape future research.
Tips for Research Process
Here are some tips for the research process:
- Start with a clear research question : A well-defined research question is the foundation of a successful research project. It should be specific, relevant, and achievable within the given time frame and resources.
- Conduct a thorough literature review: A comprehensive literature review will help you to identify gaps in existing knowledge, build on previous research, and avoid duplication. It will also provide a theoretical framework for your research.
- Choose appropriate research methods: Select research methods that are appropriate for your research question, objectives, and sample size. Ensure that your methods are valid, reliable, and ethical.
- Be organized and systematic: Keep detailed notes throughout the research process, including your research plan, methodology, data collection, and analysis. This will help you to stay organized and ensure that you don’t miss any important details.
- Analyze data rigorously: Use appropriate statistical and analytical techniques to analyze your data. Ensure that your analysis is valid, reliable, and transparent.
- I nterpret results carefully : Interpret your results in the context of your research question and objectives. Consider any limitations or potential biases in your research design, and be cautious in drawing conclusions.
- Communicate effectively: Communicate your research findings clearly and effectively to your target audience. Use appropriate language, visuals, and formats to ensure that your findings are understood and valued.
- Collaborate and seek feedback : Collaborate with other researchers, experts, or stakeholders in your field. Seek feedback on your research design, methods, and findings to ensure that they are relevant, meaningful, and impactful.
About the author
Muhammad Hassan
Researcher, Academic Writer, Web developer
You may also like

Data Collection – Methods Types and Examples

Delimitations in Research – Types, Examples and...

Research Design – Types, Methods and Examples

Institutional Review Board – Application Sample...

Evaluating Research – Process, Examples and...

Research Questions – Types, Examples and Writing...

The ‘Research in Progress’ article category
- Jo-Anne Kelder University of Tasmania https://orcid.org/0000-0002-8618-0537
ASRHE has introduced a novel article category named ‘Research in Progress’. While the journal’s website provides a succinct definition of this category, initial submissions indicate that further guidance is required to highlight requirements and opportunities. The editors have decided to approach this challenge by constructing an audio editorial, recorded in a conversational format, allowing for multiple voices and nuances to come across.
Important aspects of Research in Progress lie in facilitating publication of tentative results, sharing of research approaches and discussion of research designs. The editors emphasize the need for a strong research foundation, as in literature grounding or careful research question design, and open and honest discussion of successes and failures. Research in Progress is strongly placed to invite collaborations and authors are reminded to be explicit in specifying how they want to work with others to take research forward. The editorial also addresses the situation of research students and how a Research in Progress article might sit alongside thesis writing.
Research in Progress is a developing article category. The editors invite assistance from the higher education research community in shaping this category.
How to Cite
- Endnote/Zotero/Mendeley (RIS)
Copyright (c) 2021 Eva Heinrich; Geof Hill, Jo-Anne Kelder, Michelle Picard

This work is licensed under a Creative Commons Attribution-NonCommercial-NoDerivatives 4.0 International License .
Information
- For Readers
- For Authors
- For Librarians
Make a Submission


Why Are Progress Reports Important in Research?
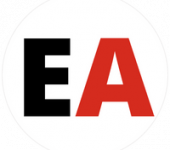
Did your supervisor ask you to write a progress report? A significant part of your supervisor’s job is to be a project manager and be accountable to funders. Remember, your research project is just one cog in the wheel of science in your lab. Therefore, your supervisor will need reports on the progress of each project. Their job is to evaluate your progress and adjust your action plan if things go wrong. These reports are not simply a report of your results.
Set SMART Goals
First, set “SMART” goals for your project (SMART = Specific, Measurable, Attainable, Realistic and Time-targeted goals). I like SMART goals because they are more than a list of things to achieve. They are part of an action plan. Your progress reports will help you check whether your goals are on track.
Progress Report Intervals
Your supervisor will tell you how often they need progress reports. The time interval depends on the nature of your work. I like to monitor my projects regularly , on a daily, weekly and monthly basis.
- Daily: this is an informal walk around the lab where I have a quick chat with my team members on what they are doing. I also set myself a daily “to do” list.
- Weekly: my team meets once a week for coffee and an informal journal club. After discussing a paper, we give a quick verbal report on our progress during the past week and any challenges that have arisen. If we find that someone is struggling or their work needs further discussion, we schedule a meeting.
- Monthly: once a month we write a formal progress report of our work and meet on a one-to-one basis to discuss it.
Purpose of Progress Reports
Your report should include your results obtained so far, experiments you are working on, plans for future work and any problems you might have with your work. It is a report on your overall plan. This plan needs constant assessment to ensure you reach your goals and to help you make informed decisions and justify any changes.
Progress reports also keep stakeholders informed. Anyone involved with your project wants to know:
- That you are working on the project and making progress.
- What findings you have made so far.
- That you are evaluating your work as you
- If you foresee any challenges.
- Any problems you encounter are being addressed.
- That you can manage your plan and schedule.
- That your project will be completed on time.
How to Write a Progress Report
Ask your supervisor if they have a template that they want you to use. Supervisors that manage many projects find it easier to keep track of all the information if it is presented in a consistent format. Write your report in concise, simple language. Progress report styles vary. However, most reports require the following sections :
- Project information . State the project name, any project ID codes, the names of all the researchers involved, report date and anticipated completion date.
- Introduction : This is a summary of your Write a short overview of the purpose of the project and its main objectives. You could add a summary of the results obtained so far, future goals, how much of the project has been completed, whether it will be completed on time, and whether you are within the budget.
- Progress: This section gives details of your objectives and how much you have completed so far. List your milestones, give details of your results, and include any tables and figures here. Some stakeholders like a completion rate which can be given as a percentage.
- Risks and Issues: Discuss any challenges that have arisen or that you Describe how you plan to solve them. If you need to make changes to your project, give reasons in this section.
- Round off with a reassuring paragraph that your research is on schedule. Give a summary of goals you will be working on next and when you expect to complete them.
Progress reports are an essential part of the research. They help to manage projects and secure funding. Many stakeholders need to know that you have completed certain stages of your project before releasing further funds.
Have you written any progress reports? Let us know how you manage your projects in the comments section below.
Rate this article Cancel Reply
Your email address will not be published.
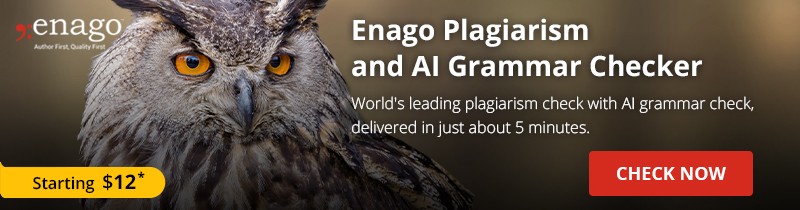
Enago Academy's Most Popular Articles
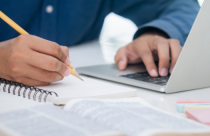
- Reporting Research
Academic Essay Writing Made Simple: 4 types and tips
The pen is mightier than the sword, they say, and nowhere is this more evident…
![research in progress meaning What is Academic Integrity and How to Uphold it [FREE CHECKLIST]](https://www.enago.com/academy/wp-content/uploads/2024/05/FeatureImages-59-210x136.png)
Ensuring Academic Integrity and Transparency in Academic Research: A comprehensive checklist for researchers
Academic integrity is the foundation upon which the credibility and value of scientific findings are…
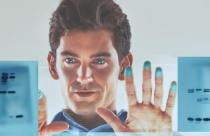
- AI in Academia
AI vs. AI: How to detect image manipulation and avoid academic misconduct
The scientific community is facing a new frontier of controversy as artificial intelligence (AI) is…
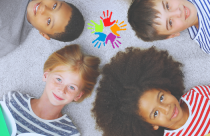
- Diversity and Inclusion
Need for Diversifying Academic Curricula: Embracing missing voices and marginalized perspectives
In classrooms worldwide, a single narrative often dominates, leaving many students feeling lost. These stories,…
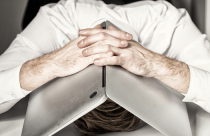
- Career Corner
- Trending Now
Recognizing the signs: A guide to overcoming academic burnout
As the sun set over the campus, casting long shadows through the library windows, Alex…
13 Behavioral Questions & Tips to Answer Them Like a Pro!
7 Steps of Writing an Excellent Academic Book Chapter
When Your Thesis Advisor Asks You to Quit

Sign-up to read more
Subscribe for free to get unrestricted access to all our resources on research writing and academic publishing including:
- 2000+ blog articles
- 50+ Webinars
- 10+ Expert podcasts
- 50+ Infographics
- 10+ Checklists
- Research Guides
We hate spam too. We promise to protect your privacy and never spam you.
I am looking for Editing/ Proofreading services for my manuscript Tentative date of next journal submission:
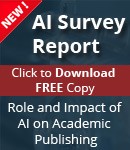
As a researcher, what do you consider most when choosing an image manipulation detector?

What is Research? Definition, Types, Methods and Process
By Nick Jain
Published on: July 25, 2023
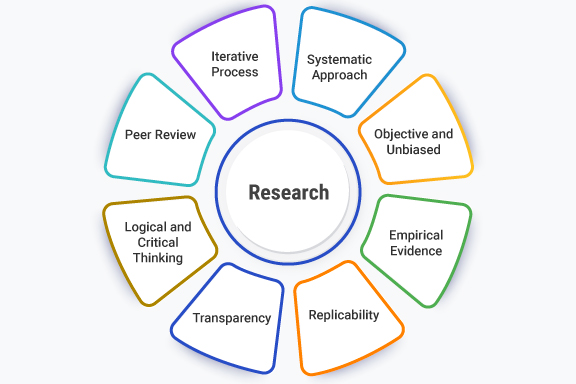
Table of Contents
Types of Research Methods
Research process: how to conduct research, top 10 best practices for conducting research in 2023, what is research.
Research is defined as a meticulous and systematic inquiry process designed to explore and unravel specific subjects or issues with precision. This methodical approach encompasses the thorough collection, rigorous analysis, and insightful interpretation of information, aiming to delve deep into the nuances of a chosen field of study. By adhering to established research methodologies, investigators can draw meaningful conclusions, fostering a profound understanding that contributes significantly to the existing knowledge base.
This dedication to systematic inquiry serves as the bedrock of progress, steering advancements across sciences, technology, social sciences, and diverse disciplines. Through the dissemination of meticulously gathered insights, scholars not only inspire collaboration and innovation but also catalyze positive societal change.
In the pursuit of knowledge, researchers embark on a journey of discovery, seeking to unravel the complexities of the world around us. By formulating clear research questions, researchers set the course for their investigations, carefully crafting methodologies to gather relevant data. Whether employing quantitative surveys or qualitative interviews, data collection lies at the heart of every research endeavor. Once the data is collected, researchers meticulously analyze it, employing statistical tools or thematic analysis to identify patterns and draw meaningful insights. These insights, often supported by empirical evidence, contribute to the collective pool of knowledge, enriching our understanding of various phenomena and guiding decision-making processes across diverse fields. Through research, we continually refine our understanding of the universe, laying the foundation for innovation and progress that shape the future.
Research embodies the spirit of curiosity and the pursuit of truth. Here are the key characteristics of research:
- Systematic Approach: Research follows a well-structured and organized approach, with clearly defined steps and methodologies. It is conducted in a systematic manner to ensure that data is collected, analyzed, and interpreted in a logical and coherent way.
- Objective and Unbiased: Research is objective and strives to be free from bias or personal opinions. Researchers aim to gather data and draw conclusions based on evidence rather than preconceived notions or beliefs.
- Empirical Evidence: Research relies on empirical evidence obtained through observations, experiments, surveys, or other data collection methods. This evidence serves as the foundation for drawing conclusions and making informed decisions.
- Clear Research Question or Problem: Every research study begins with a specific research question or problem that the researcher aims to address. This question provides focus and direction to the entire research process.
- Replicability: Good research should be replicable, meaning that other researchers should be able to conduct a similar study and obtain similar results when following the same methods.
- Transparency and Ethics: Research should be conducted with transparency, and researchers should adhere to ethical guidelines and principles. This includes obtaining informed consent from participants, ensuring confidentiality, and avoiding any harm to participants or the environment.
- Generalizability: Researchers often aim for their findings to be generalizable to a broader population or context. This means that the results of the study can be applied beyond the specific sample or situation studied.
- Logical and Critical Thinking: Research involves critical thinking to analyze and interpret data, identify patterns, and draw meaningful conclusions. Logical reasoning is essential in formulating hypotheses and designing the study.
- Contribution to Knowledge: The primary purpose of research is to contribute to the existing body of knowledge in a particular field. Researchers aim to expand understanding, challenge existing theories, or propose new ideas.
- Peer Review and Publication: Research findings are typically subject to peer review by experts in the field before being published in academic journals or presented at conferences. This process ensures the quality and validity of the research.
- Iterative Process: Research is often an iterative process, with findings from one study leading to new questions and further research. It is a continuous cycle of discovery and refinement.
- Practical Application: While some research is theoretical in nature, much of it aims to have practical applications and real-world implications. It can inform policy decisions, improve practices, or address societal challenges.
These key characteristics collectively define research as a rigorous and valuable endeavor that drives progress, knowledge, and innovation in various disciplines.
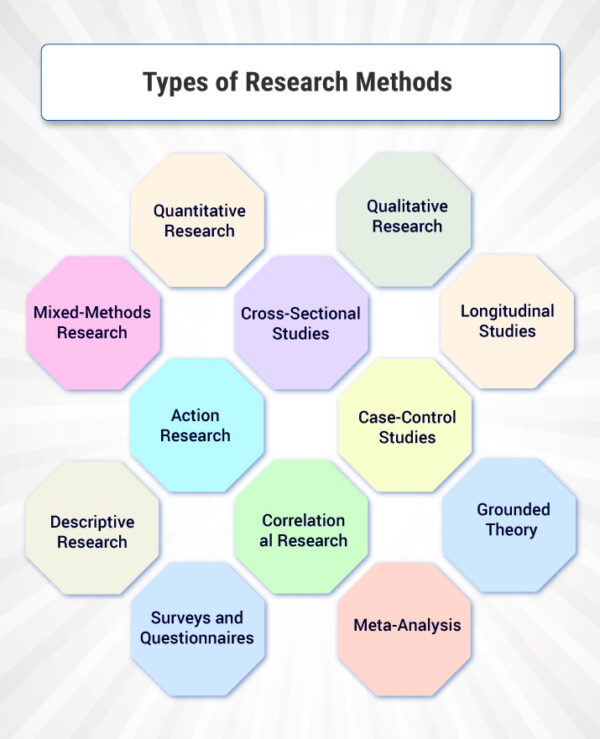
Research methods refer to the specific approaches and techniques used to collect and analyze data in a research study. There are various types of research methods, and researchers often choose the most appropriate method based on their research question, the nature of the data they want to collect, and the resources available to them. Some common types of research methods include:
1. Quantitative Research: Quantitative research methods focus on collecting and analyzing quantifiable data to draw conclusions. The key methods for conducting quantitative research are:
Surveys- Conducting structured questionnaires or interviews with a large number of participants to gather numerical data.
Experiments-Manipulating variables in a controlled environment to establish cause-and-effect relationships.
Observational Studies- Systematically observing and recording behaviors or phenomena without intervention.
Secondary Data Analysis- Analyzing existing datasets and records to draw new insights or conclusions.
2. Qualitative Research: Qualitative research employs a range of information-gathering methods that are non-numerical, and are instead intellectual in order to provide in-depth insights into the research topic. The key methods are:
Interviews- Conducting in-depth, semi-structured, or unstructured interviews to gain a deeper understanding of participants’ perspectives.
Focus Groups- Group discussions with selected participants to explore their attitudes, beliefs, and experiences on a specific topic.
Ethnography- Immersing in a particular culture or community to observe and understand their behaviors, customs, and beliefs.
Case Studies- In-depth examination of a single individual, group, organization, or event to gain comprehensive insights.
3. Mixed-Methods Research: Combining both quantitative and qualitative research methods in a single study to provide a more comprehensive understanding of the research question.
4. Cross-Sectional Studies: Gathering data from a sample of a population at a specific point in time to understand relationships or differences between variables.
5. Longitudinal Studies: Following a group of participants over an extended period to examine changes and developments over time.
6. Action Research: Collaboratively working with stakeholders to identify and implement solutions to practical problems in real-world settings.
7. Case-Control Studies: Comparing individuals with a particular outcome (cases) to those without the outcome (controls) to identify potential causes or risk factors.
8. Descriptive Research: Describing and summarizing characteristics, behaviors, or patterns without manipulating variables.
9. Correlational Research: Examining the relationship between two or more variables without inferring causation.
10. Grounded Theory: An approach to developing theory based on systematically gathering and analyzing data, allowing the theory to emerge from the data.
11. Surveys and Questionnaires: Administering structured sets of questions to a sample population to gather specific information.
12. Meta-Analysis: A statistical technique that combines the results of multiple studies on the same topic to draw more robust conclusions.
Researchers often choose a research method or a combination of methods that best aligns with their research objectives, resources, and the nature of the data they aim to collect. Each research method has its strengths and limitations, and the choice of method can significantly impact the findings and conclusions of a study.
Learn more: What is Research Design?
Conducting research involves a systematic and organized process that follows specific steps to ensure the collection of reliable and meaningful data. The research process typically consists of the following steps:
Step 1. Identify the Research Topic
Choose a research topic that interests you and aligns with your expertise and resources. Develop clear and focused research questions that you want to answer through your study.
Step 2. Review Existing Research
Conduct a thorough literature review to identify what research has already been done on your chosen topic. This will help you understand the current state of knowledge, identify gaps in the literature, and refine your research questions.
Step 3. Design the Research Methodology
Determine the appropriate research methodology that suits your research questions. Decide whether your study will be qualitative , quantitative , or a mix of both (mixed methods). Also, choose the data collection methods, such as surveys, interviews, experiments, observations, etc.
Step 4. Select the Sample and Participants
If your study involves human participants, decide on the sample size and selection criteria. Obtain ethical approval, if required, and ensure that participants’ rights and privacy are protected throughout the research process.
Step 5. Information Collection
Collect information and data based on your chosen research methodology. Qualitative research has more intellectual information, while quantitative research results are more data-oriented. Ensure that your data collection process is standardized and consistent to maintain the validity of the results.
Step 6. Data Analysis
Analyze the data you have collected using appropriate statistical or qualitative research methods . The type of analysis will depend on the nature of your data and research questions.
Step 7. Interpretation of Results
Interpret the findings of your data analysis. Relate the results to your research questions and consider how they contribute to the existing knowledge in the field.
Step 8. Draw Conclusions
Based on your interpretation of the results, draw meaningful conclusions that answer your research questions. Discuss the implications of your findings and how they align with the existing literature.
Step 9. Discuss Limitations
Acknowledge and discuss any limitations of your study. Addressing limitations demonstrates the validity and reliability of your research.
Step 10. Make Recommendations
If applicable, provide recommendations based on your research findings. These recommendations can be for future research, policy changes, or practical applications.
Step 11. Write the Research Report
Prepare a comprehensive research report detailing all aspects of your study, including the introduction, methodology, results, discussion, conclusion, and references.
Step 12. Peer Review and Revision
If you intend to publish your research, submit your report to peer-reviewed journals. Revise your research report based on the feedback received from reviewers.
Make sure to share your research findings with the broader community through conferences, seminars, or other appropriate channels, this will help contribute to the collective knowledge in your field of study.
Remember that conducting research is a dynamic process, and you may need to revisit and refine various steps as you progress. Good research requires attention to detail, critical thinking, and adherence to ethical principles to ensure the quality and validity of the study.
Learn more: What is Primary Market Research?
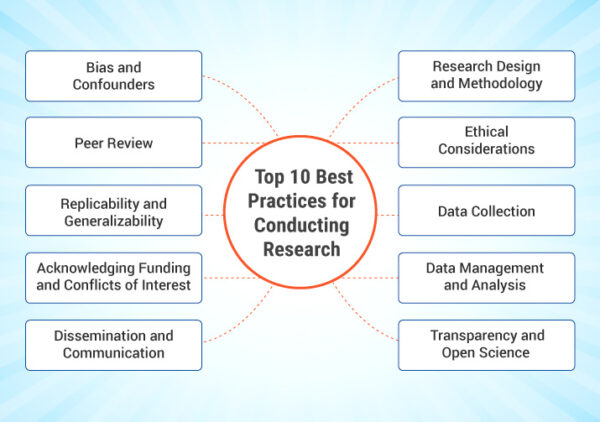
Best practices for conducting research remain rooted in the principles of rigor, transparency, and ethical considerations. Here are the essential best practices to follow when conducting research in 2023:
1. Research Design and Methodology
- Carefully select and justify the research design and methodology that aligns with your research questions and objectives.
- Ensure that the chosen methods are appropriate for the data you intend to collect and the type of analysis you plan to perform.
- Clearly document the research design and methodology to enhance the reproducibility and transparency of your study.
2. Ethical Considerations
- Obtain approval from relevant research ethics committees or institutional review boards, especially when involving human participants or sensitive data.
- Prioritize the protection of participants’ rights, privacy, and confidentiality throughout the research process.
- Provide informed consent to participants, ensuring they understand the study’s purpose, risks, and benefits.
3. Data Collection
- Ensure the reliability and validity of data collection instruments, such as surveys or interview protocols.
- Conduct pilot studies or pretests to identify and address any potential issues with data collection procedures.
4. Data Management and Analysis
- Implement robust data management practices to maintain the integrity and security of research data.
- Transparently document data analysis procedures, including software and statistical methods used.
- Use appropriate statistical techniques to analyze the data and avoid data manipulation or cherry-picking results.
5. Transparency and Open Science
- Embrace open science practices, such as pre-registration of research protocols and sharing data and code openly whenever possible.
- Clearly report all aspects of your research, including methods, results, and limitations, to enhance the reproducibility of your study.
6. Bias and Confounders
- Be aware of potential biases in the research process and take steps to minimize them.
- Consider and address potential confounding variables that could affect the validity of your results.
7. Peer Review
- Seek peer review from experts in your field before publishing or presenting your research findings.
- Be receptive to feedback and address any concerns raised by reviewers to improve the quality of your study.
8. Replicability and Generalizability
- Strive to make your research findings replicable, allowing other researchers to validate your results independently.
- Clearly state the limitations of your study and the extent to which the findings can be generalized to other populations or contexts.
9. Acknowledging Funding and Conflicts of Interest
- Disclose any funding sources and potential conflicts of interest that may influence your research or its outcomes.
10. Dissemination and Communication
- Effectively communicate your research findings to both academic and non-academic audiences using clear and accessible language.
- Share your research through reputable and open-access platforms to maximize its impact and reach.
By adhering to these best practices, researchers can ensure the integrity and value of their work, contributing to the advancement of knowledge and promoting trust in the research community.
Learn more: What is Consumer Research?
Enhance Your Research
Collect feedback and conduct research with IdeaScale’s award-winning software
Elevate Research And Feedback With Your IdeaScale Community!
IdeaScale is an innovation management solution that inspires people to take action on their ideas. Your community’s ideas can change lives, your business and the world. Connect to the ideas that matter and start co-creating the future.
Copyright © 2024 IdeaScale
Privacy Overview
What Is Research, and Why Do People Do It?
- Open Access
- First Online: 03 December 2022
Cite this chapter
You have full access to this open access chapter
- James Hiebert 6 ,
- Jinfa Cai 7 ,
- Stephen Hwang 7 ,
- Anne K Morris 6 &
- Charles Hohensee 6
Part of the book series: Research in Mathematics Education ((RME))
17k Accesses
Abstractspiepr Abs1
Every day people do research as they gather information to learn about something of interest. In the scientific world, however, research means something different than simply gathering information. Scientific research is characterized by its careful planning and observing, by its relentless efforts to understand and explain, and by its commitment to learn from everyone else seriously engaged in research. We call this kind of research scientific inquiry and define it as “formulating, testing, and revising hypotheses.” By “hypotheses” we do not mean the hypotheses you encounter in statistics courses. We mean predictions about what you expect to find and rationales for why you made these predictions. Throughout this and the remaining chapters we make clear that the process of scientific inquiry applies to all kinds of research studies and data, both qualitative and quantitative.
You have full access to this open access chapter, Download chapter PDF
Part I. What Is Research?
Have you ever studied something carefully because you wanted to know more about it? Maybe you wanted to know more about your grandmother’s life when she was younger so you asked her to tell you stories from her childhood, or maybe you wanted to know more about a fertilizer you were about to use in your garden so you read the ingredients on the package and looked them up online. According to the dictionary definition, you were doing research.
Recall your high school assignments asking you to “research” a topic. The assignment likely included consulting a variety of sources that discussed the topic, perhaps including some “original” sources. Often, the teacher referred to your product as a “research paper.”
Were you conducting research when you interviewed your grandmother or wrote high school papers reviewing a particular topic? Our view is that you were engaged in part of the research process, but only a small part. In this book, we reserve the word “research” for what it means in the scientific world, that is, for scientific research or, more pointedly, for scientific inquiry .
Exercise 1.1
Before you read any further, write a definition of what you think scientific inquiry is. Keep it short—Two to three sentences. You will periodically update this definition as you read this chapter and the remainder of the book.
This book is about scientific inquiry—what it is and how to do it. For starters, scientific inquiry is a process, a particular way of finding out about something that involves a number of phases. Each phase of the process constitutes one aspect of scientific inquiry. You are doing scientific inquiry as you engage in each phase, but you have not done scientific inquiry until you complete the full process. Each phase is necessary but not sufficient.
In this chapter, we set the stage by defining scientific inquiry—describing what it is and what it is not—and by discussing what it is good for and why people do it. The remaining chapters build directly on the ideas presented in this chapter.
A first thing to know is that scientific inquiry is not all or nothing. “Scientificness” is a continuum. Inquiries can be more scientific or less scientific. What makes an inquiry more scientific? You might be surprised there is no universally agreed upon answer to this question. None of the descriptors we know of are sufficient by themselves to define scientific inquiry. But all of them give you a way of thinking about some aspects of the process of scientific inquiry. Each one gives you different insights.
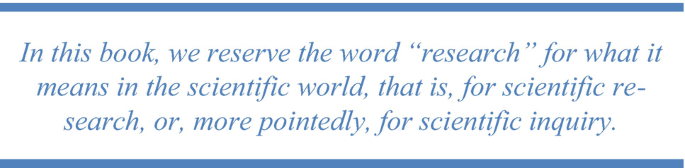
Exercise 1.2
As you read about each descriptor below, think about what would make an inquiry more or less scientific. If you think a descriptor is important, use it to revise your definition of scientific inquiry.
Creating an Image of Scientific Inquiry
We will present three descriptors of scientific inquiry. Each provides a different perspective and emphasizes a different aspect of scientific inquiry. We will draw on all three descriptors to compose our definition of scientific inquiry.
Descriptor 1. Experience Carefully Planned in Advance
Sir Ronald Fisher, often called the father of modern statistical design, once referred to research as “experience carefully planned in advance” (1935, p. 8). He said that humans are always learning from experience, from interacting with the world around them. Usually, this learning is haphazard rather than the result of a deliberate process carried out over an extended period of time. Research, Fisher said, was learning from experience, but experience carefully planned in advance.
This phrase can be fully appreciated by looking at each word. The fact that scientific inquiry is based on experience means that it is based on interacting with the world. These interactions could be thought of as the stuff of scientific inquiry. In addition, it is not just any experience that counts. The experience must be carefully planned . The interactions with the world must be conducted with an explicit, describable purpose, and steps must be taken to make the intended learning as likely as possible. This planning is an integral part of scientific inquiry; it is not just a preparation phase. It is one of the things that distinguishes scientific inquiry from many everyday learning experiences. Finally, these steps must be taken beforehand and the purpose of the inquiry must be articulated in advance of the experience. Clearly, scientific inquiry does not happen by accident, by just stumbling into something. Stumbling into something unexpected and interesting can happen while engaged in scientific inquiry, but learning does not depend on it and serendipity does not make the inquiry scientific.
Descriptor 2. Observing Something and Trying to Explain Why It Is the Way It Is
When we were writing this chapter and googled “scientific inquiry,” the first entry was: “Scientific inquiry refers to the diverse ways in which scientists study the natural world and propose explanations based on the evidence derived from their work.” The emphasis is on studying, or observing, and then explaining . This descriptor takes the image of scientific inquiry beyond carefully planned experience and includes explaining what was experienced.
According to the Merriam-Webster dictionary, “explain” means “(a) to make known, (b) to make plain or understandable, (c) to give the reason or cause of, and (d) to show the logical development or relations of” (Merriam-Webster, n.d. ). We will use all these definitions. Taken together, they suggest that to explain an observation means to understand it by finding reasons (or causes) for why it is as it is. In this sense of scientific inquiry, the following are synonyms: explaining why, understanding why, and reasoning about causes and effects. Our image of scientific inquiry now includes planning, observing, and explaining why.

We need to add a final note about this descriptor. We have phrased it in a way that suggests “observing something” means you are observing something in real time—observing the way things are or the way things are changing. This is often true. But, observing could mean observing data that already have been collected, maybe by someone else making the original observations (e.g., secondary analysis of NAEP data or analysis of existing video recordings of classroom instruction). We will address secondary analyses more fully in Chap. 4 . For now, what is important is that the process requires explaining why the data look like they do.
We must note that for us, the term “data” is not limited to numerical or quantitative data such as test scores. Data can also take many nonquantitative forms, including written survey responses, interview transcripts, journal entries, video recordings of students, teachers, and classrooms, text messages, and so forth.
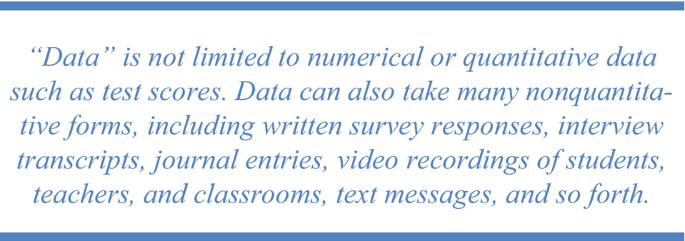
Exercise 1.3
What are the implications of the statement that just “observing” is not enough to count as scientific inquiry? Does this mean that a detailed description of a phenomenon is not scientific inquiry?
Find sources that define research in education that differ with our position, that say description alone, without explanation, counts as scientific research. Identify the precise points where the opinions differ. What are the best arguments for each of the positions? Which do you prefer? Why?
Descriptor 3. Updating Everyone’s Thinking in Response to More and Better Information
This descriptor focuses on a third aspect of scientific inquiry: updating and advancing the field’s understanding of phenomena that are investigated. This descriptor foregrounds a powerful characteristic of scientific inquiry: the reliability (or trustworthiness) of what is learned and the ultimate inevitability of this learning to advance human understanding of phenomena. Humans might choose not to learn from scientific inquiry, but history suggests that scientific inquiry always has the potential to advance understanding and that, eventually, humans take advantage of these new understandings.
Before exploring these bold claims a bit further, note that this descriptor uses “information” in the same way the previous two descriptors used “experience” and “observations.” These are the stuff of scientific inquiry and we will use them often, sometimes interchangeably. Frequently, we will use the term “data” to stand for all these terms.
An overriding goal of scientific inquiry is for everyone to learn from what one scientist does. Much of this book is about the methods you need to use so others have faith in what you report and can learn the same things you learned. This aspect of scientific inquiry has many implications.
One implication is that scientific inquiry is not a private practice. It is a public practice available for others to see and learn from. Notice how different this is from everyday learning. When you happen to learn something from your everyday experience, often only you gain from the experience. The fact that research is a public practice means it is also a social one. It is best conducted by interacting with others along the way: soliciting feedback at each phase, taking opportunities to present work-in-progress, and benefitting from the advice of others.
A second implication is that you, as the researcher, must be committed to sharing what you are doing and what you are learning in an open and transparent way. This allows all phases of your work to be scrutinized and critiqued. This is what gives your work credibility. The reliability or trustworthiness of your findings depends on your colleagues recognizing that you have used all appropriate methods to maximize the chances that your claims are justified by the data.
A third implication of viewing scientific inquiry as a collective enterprise is the reverse of the second—you must be committed to receiving comments from others. You must treat your colleagues as fair and honest critics even though it might sometimes feel otherwise. You must appreciate their job, which is to remain skeptical while scrutinizing what you have done in considerable detail. To provide the best help to you, they must remain skeptical about your conclusions (when, for example, the data are difficult for them to interpret) until you offer a convincing logical argument based on the information you share. A rather harsh but good-to-remember statement of the role of your friendly critics was voiced by Karl Popper, a well-known twentieth century philosopher of science: “. . . if you are interested in the problem which I tried to solve by my tentative assertion, you may help me by criticizing it as severely as you can” (Popper, 1968, p. 27).
A final implication of this third descriptor is that, as someone engaged in scientific inquiry, you have no choice but to update your thinking when the data support a different conclusion. This applies to your own data as well as to those of others. When data clearly point to a specific claim, even one that is quite different than you expected, you must reconsider your position. If the outcome is replicated multiple times, you need to adjust your thinking accordingly. Scientific inquiry does not let you pick and choose which data to believe; it mandates that everyone update their thinking when the data warrant an update.
Doing Scientific Inquiry
We define scientific inquiry in an operational sense—what does it mean to do scientific inquiry? What kind of process would satisfy all three descriptors: carefully planning an experience in advance; observing and trying to explain what you see; and, contributing to updating everyone’s thinking about an important phenomenon?
We define scientific inquiry as formulating , testing , and revising hypotheses about phenomena of interest.
Of course, we are not the only ones who define it in this way. The definition for the scientific method posted by the editors of Britannica is: “a researcher develops a hypothesis, tests it through various means, and then modifies the hypothesis on the basis of the outcome of the tests and experiments” (Britannica, n.d. ).

Notice how defining scientific inquiry this way satisfies each of the descriptors. “Carefully planning an experience in advance” is exactly what happens when formulating a hypothesis about a phenomenon of interest and thinking about how to test it. “ Observing a phenomenon” occurs when testing a hypothesis, and “ explaining ” what is found is required when revising a hypothesis based on the data. Finally, “updating everyone’s thinking” comes from comparing publicly the original with the revised hypothesis.
Doing scientific inquiry, as we have defined it, underscores the value of accumulating knowledge rather than generating random bits of knowledge. Formulating, testing, and revising hypotheses is an ongoing process, with each revised hypothesis begging for another test, whether by the same researcher or by new researchers. The editors of Britannica signaled this cyclic process by adding the following phrase to their definition of the scientific method: “The modified hypothesis is then retested, further modified, and tested again.” Scientific inquiry creates a process that encourages each study to build on the studies that have gone before. Through collective engagement in this process of building study on top of study, the scientific community works together to update its thinking.
Before exploring more fully the meaning of “formulating, testing, and revising hypotheses,” we need to acknowledge that this is not the only way researchers define research. Some researchers prefer a less formal definition, one that includes more serendipity, less planning, less explanation. You might have come across more open definitions such as “research is finding out about something.” We prefer the tighter hypothesis formulation, testing, and revision definition because we believe it provides a single, coherent map for conducting research that addresses many of the thorny problems educational researchers encounter. We believe it is the most useful orientation toward research and the most helpful to learn as a beginning researcher.
A final clarification of our definition is that it applies equally to qualitative and quantitative research. This is a familiar distinction in education that has generated much discussion. You might think our definition favors quantitative methods over qualitative methods because the language of hypothesis formulation and testing is often associated with quantitative methods. In fact, we do not favor one method over another. In Chap. 4 , we will illustrate how our definition fits research using a range of quantitative and qualitative methods.
Exercise 1.4
Look for ways to extend what the field knows in an area that has already received attention by other researchers. Specifically, you can search for a program of research carried out by more experienced researchers that has some revised hypotheses that remain untested. Identify a revised hypothesis that you might like to test.
Unpacking the Terms Formulating, Testing, and Revising Hypotheses
To get a full sense of the definition of scientific inquiry we will use throughout this book, it is helpful to spend a little time with each of the key terms.
We first want to make clear that we use the term “hypothesis” as it is defined in most dictionaries and as it used in many scientific fields rather than as it is usually defined in educational statistics courses. By “hypothesis,” we do not mean a null hypothesis that is accepted or rejected by statistical analysis. Rather, we use “hypothesis” in the sense conveyed by the following definitions: “An idea or explanation for something that is based on known facts but has not yet been proved” (Cambridge University Press, n.d. ), and “An unproved theory, proposition, or supposition, tentatively accepted to explain certain facts and to provide a basis for further investigation or argument” (Agnes & Guralnik, 2008 ).
We distinguish two parts to “hypotheses.” Hypotheses consist of predictions and rationales . Predictions are statements about what you expect to find when you inquire about something. Rationales are explanations for why you made the predictions you did, why you believe your predictions are correct. So, for us “formulating hypotheses” means making explicit predictions and developing rationales for the predictions.
“Testing hypotheses” means making observations that allow you to assess in what ways your predictions were correct and in what ways they were incorrect. In education research, it is rarely useful to think of your predictions as either right or wrong. Because of the complexity of most issues you will investigate, most predictions will be right in some ways and wrong in others.
By studying the observations you make (data you collect) to test your hypotheses, you can revise your hypotheses to better align with the observations. This means revising your predictions plus revising your rationales to justify your adjusted predictions. Even though you might not run another test, formulating revised hypotheses is an essential part of conducting a research study. Comparing your original and revised hypotheses informs everyone of what you learned by conducting your study. In addition, a revised hypothesis sets the stage for you or someone else to extend your study and accumulate more knowledge of the phenomenon.
We should note that not everyone makes a clear distinction between predictions and rationales as two aspects of hypotheses. In fact, common, non-scientific uses of the word “hypothesis” may limit it to only a prediction or only an explanation (or rationale). We choose to explicitly include both prediction and rationale in our definition of hypothesis, not because we assert this should be the universal definition, but because we want to foreground the importance of both parts acting in concert. Using “hypothesis” to represent both prediction and rationale could hide the two aspects, but we make them explicit because they provide different kinds of information. It is usually easier to make predictions than develop rationales because predictions can be guesses, hunches, or gut feelings about which you have little confidence. Developing a compelling rationale requires careful thought plus reading what other researchers have found plus talking with your colleagues. Often, while you are developing your rationale you will find good reasons to change your predictions. Developing good rationales is the engine that drives scientific inquiry. Rationales are essentially descriptions of how much you know about the phenomenon you are studying. Throughout this guide, we will elaborate on how developing good rationales drives scientific inquiry. For now, we simply note that it can sharpen your predictions and help you to interpret your data as you test your hypotheses.
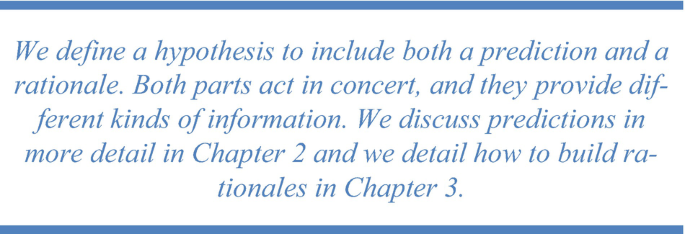
Hypotheses in education research take a variety of forms or types. This is because there are a variety of phenomena that can be investigated. Investigating educational phenomena is sometimes best done using qualitative methods, sometimes using quantitative methods, and most often using mixed methods (e.g., Hay, 2016 ; Weis et al. 2019a ; Weisner, 2005 ). This means that, given our definition, hypotheses are equally applicable to qualitative and quantitative investigations.
Hypotheses take different forms when they are used to investigate different kinds of phenomena. Two very different activities in education could be labeled conducting experiments and descriptions. In an experiment, a hypothesis makes a prediction about anticipated changes, say the changes that occur when a treatment or intervention is applied. You might investigate how students’ thinking changes during a particular kind of instruction.
A second type of hypothesis, relevant for descriptive research, makes a prediction about what you will find when you investigate and describe the nature of a situation. The goal is to understand a situation as it exists rather than to understand a change from one situation to another. In this case, your prediction is what you expect to observe. Your rationale is the set of reasons for making this prediction; it is your current explanation for why the situation will look like it does.
You will probably read, if you have not already, that some researchers say you do not need a prediction to conduct a descriptive study. We will discuss this point of view in Chap. 2 . For now, we simply claim that scientific inquiry, as we have defined it, applies to all kinds of research studies. Descriptive studies, like others, not only benefit from formulating, testing, and revising hypotheses, but also need hypothesis formulating, testing, and revising.
One reason we define research as formulating, testing, and revising hypotheses is that if you think of research in this way you are less likely to go wrong. It is a useful guide for the entire process, as we will describe in detail in the chapters ahead. For example, as you build the rationale for your predictions, you are constructing the theoretical framework for your study (Chap. 3 ). As you work out the methods you will use to test your hypothesis, every decision you make will be based on asking, “Will this help me formulate or test or revise my hypothesis?” (Chap. 4 ). As you interpret the results of testing your predictions, you will compare them to what you predicted and examine the differences, focusing on how you must revise your hypotheses (Chap. 5 ). By anchoring the process to formulating, testing, and revising hypotheses, you will make smart decisions that yield a coherent and well-designed study.
Exercise 1.5
Compare the concept of formulating, testing, and revising hypotheses with the descriptions of scientific inquiry contained in Scientific Research in Education (NRC, 2002 ). How are they similar or different?
Exercise 1.6
Provide an example to illustrate and emphasize the differences between everyday learning/thinking and scientific inquiry.
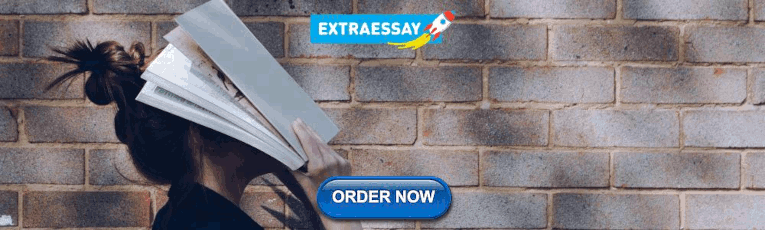
Learning from Doing Scientific Inquiry
We noted earlier that a measure of what you have learned by conducting a research study is found in the differences between your original hypothesis and your revised hypothesis based on the data you collected to test your hypothesis. We will elaborate this statement in later chapters, but we preview our argument here.
Even before collecting data, scientific inquiry requires cycles of making a prediction, developing a rationale, refining your predictions, reading and studying more to strengthen your rationale, refining your predictions again, and so forth. And, even if you have run through several such cycles, you still will likely find that when you test your prediction you will be partly right and partly wrong. The results will support some parts of your predictions but not others, or the results will “kind of” support your predictions. A critical part of scientific inquiry is making sense of your results by interpreting them against your predictions. Carefully describing what aspects of your data supported your predictions, what aspects did not, and what data fell outside of any predictions is not an easy task, but you cannot learn from your study without doing this analysis.
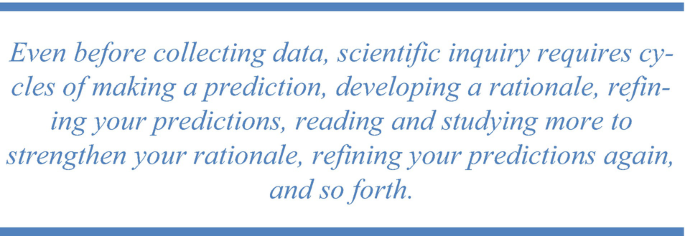
Analyzing the matches and mismatches between your predictions and your data allows you to formulate different rationales that would have accounted for more of the data. The best revised rationale is the one that accounts for the most data. Once you have revised your rationales, you can think about the predictions they best justify or explain. It is by comparing your original rationales to your new rationales that you can sort out what you learned from your study.
Suppose your study was an experiment. Maybe you were investigating the effects of a new instructional intervention on students’ learning. Your original rationale was your explanation for why the intervention would change the learning outcomes in a particular way. Your revised rationale explained why the changes that you observed occurred like they did and why your revised predictions are better. Maybe your original rationale focused on the potential of the activities if they were implemented in ideal ways and your revised rationale included the factors that are likely to affect how teachers implement them. By comparing the before and after rationales, you are describing what you learned—what you can explain now that you could not before. Another way of saying this is that you are describing how much more you understand now than before you conducted your study.
Revised predictions based on carefully planned and collected data usually exhibit some of the following features compared with the originals: more precision, more completeness, and broader scope. Revised rationales have more explanatory power and become more complete, more aligned with the new predictions, sharper, and overall more convincing.
Part II. Why Do Educators Do Research?
Doing scientific inquiry is a lot of work. Each phase of the process takes time, and you will often cycle back to improve earlier phases as you engage in later phases. Because of the significant effort required, you should make sure your study is worth it. So, from the beginning, you should think about the purpose of your study. Why do you want to do it? And, because research is a social practice, you should also think about whether the results of your study are likely to be important and significant to the education community.
If you are doing research in the way we have described—as scientific inquiry—then one purpose of your study is to understand , not just to describe or evaluate or report. As we noted earlier, when you formulate hypotheses, you are developing rationales that explain why things might be like they are. In our view, trying to understand and explain is what separates research from other kinds of activities, like evaluating or describing.
One reason understanding is so important is that it allows researchers to see how or why something works like it does. When you see how something works, you are better able to predict how it might work in other contexts, under other conditions. And, because conditions, or contextual factors, matter a lot in education, gaining insights into applying your findings to other contexts increases the contributions of your work and its importance to the broader education community.
Consequently, the purposes of research studies in education often include the more specific aim of identifying and understanding the conditions under which the phenomena being studied work like the observations suggest. A classic example of this kind of study in mathematics education was reported by William Brownell and Harold Moser in 1949 . They were trying to establish which method of subtracting whole numbers could be taught most effectively—the regrouping method or the equal additions method. However, they realized that effectiveness might depend on the conditions under which the methods were taught—“meaningfully” versus “mechanically.” So, they designed a study that crossed the two instructional approaches with the two different methods (regrouping and equal additions). Among other results, they found that these conditions did matter. The regrouping method was more effective under the meaningful condition than the mechanical condition, but the same was not true for the equal additions algorithm.
What do education researchers want to understand? In our view, the ultimate goal of education is to offer all students the best possible learning opportunities. So, we believe the ultimate purpose of scientific inquiry in education is to develop understanding that supports the improvement of learning opportunities for all students. We say “ultimate” because there are lots of issues that must be understood to improve learning opportunities for all students. Hypotheses about many aspects of education are connected, ultimately, to students’ learning. For example, formulating and testing a hypothesis that preservice teachers need to engage in particular kinds of activities in their coursework in order to teach particular topics well is, ultimately, connected to improving students’ learning opportunities. So is hypothesizing that school districts often devote relatively few resources to instructional leadership training or hypothesizing that positioning mathematics as a tool students can use to combat social injustice can help students see the relevance of mathematics to their lives.
We do not exclude the importance of research on educational issues more removed from improving students’ learning opportunities, but we do think the argument for their importance will be more difficult to make. If there is no way to imagine a connection between your hypothesis and improving learning opportunities for students, even a distant connection, we recommend you reconsider whether it is an important hypothesis within the education community.
Notice that we said the ultimate goal of education is to offer all students the best possible learning opportunities. For too long, educators have been satisfied with a goal of offering rich learning opportunities for lots of students, sometimes even for just the majority of students, but not necessarily for all students. Evaluations of success often are based on outcomes that show high averages. In other words, if many students have learned something, or even a smaller number have learned a lot, educators may have been satisfied. The problem is that there is usually a pattern in the groups of students who receive lower quality opportunities—students of color and students who live in poor areas, urban and rural. This is not acceptable. Consequently, we emphasize the premise that the purpose of education research is to offer rich learning opportunities to all students.
One way to make sure you will be able to convince others of the importance of your study is to consider investigating some aspect of teachers’ shared instructional problems. Historically, researchers in education have set their own research agendas, regardless of the problems teachers are facing in schools. It is increasingly recognized that teachers have had trouble applying to their own classrooms what researchers find. To address this problem, a researcher could partner with a teacher—better yet, a small group of teachers—and talk with them about instructional problems they all share. These discussions can create a rich pool of problems researchers can consider. If researchers pursued one of these problems (preferably alongside teachers), the connection to improving learning opportunities for all students could be direct and immediate. “Grounding a research question in instructional problems that are experienced across multiple teachers’ classrooms helps to ensure that the answer to the question will be of sufficient scope to be relevant and significant beyond the local context” (Cai et al., 2019b , p. 115).
As a beginning researcher, determining the relevance and importance of a research problem is especially challenging. We recommend talking with advisors, other experienced researchers, and peers to test the educational importance of possible research problems and topics of study. You will also learn much more about the issue of research importance when you read Chap. 5 .
Exercise 1.7
Identify a problem in education that is closely connected to improving learning opportunities and a problem that has a less close connection. For each problem, write a brief argument (like a logical sequence of if-then statements) that connects the problem to all students’ learning opportunities.
Part III. Conducting Research as a Practice of Failing Productively
Scientific inquiry involves formulating hypotheses about phenomena that are not fully understood—by you or anyone else. Even if you are able to inform your hypotheses with lots of knowledge that has already been accumulated, you are likely to find that your prediction is not entirely accurate. This is normal. Remember, scientific inquiry is a process of constantly updating your thinking. More and better information means revising your thinking, again, and again, and again. Because you never fully understand a complicated phenomenon and your hypotheses never produce completely accurate predictions, it is easy to believe you are somehow failing.
The trick is to fail upward, to fail to predict accurately in ways that inform your next hypothesis so you can make a better prediction. Some of the best-known researchers in education have been open and honest about the many times their predictions were wrong and, based on the results of their studies and those of others, they continuously updated their thinking and changed their hypotheses.
A striking example of publicly revising (actually reversing) hypotheses due to incorrect predictions is found in the work of Lee J. Cronbach, one of the most distinguished educational psychologists of the twentieth century. In 1955, Cronbach delivered his presidential address to the American Psychological Association. Titling it “Two Disciplines of Scientific Psychology,” Cronbach proposed a rapprochement between two research approaches—correlational studies that focused on individual differences and experimental studies that focused on instructional treatments controlling for individual differences. (We will examine different research approaches in Chap. 4 ). If these approaches could be brought together, reasoned Cronbach ( 1957 ), researchers could find interactions between individual characteristics and treatments (aptitude-treatment interactions or ATIs), fitting the best treatments to different individuals.
In 1975, after years of research by many researchers looking for ATIs, Cronbach acknowledged the evidence for simple, useful ATIs had not been found. Even when trying to find interactions between a few variables that could provide instructional guidance, the analysis, said Cronbach, creates “a hall of mirrors that extends to infinity, tormenting even the boldest investigators and defeating even ambitious designs” (Cronbach, 1975 , p. 119).
As he was reflecting back on his work, Cronbach ( 1986 ) recommended moving away from documenting instructional effects through statistical inference (an approach he had championed for much of his career) and toward approaches that probe the reasons for these effects, approaches that provide a “full account of events in a time, place, and context” (Cronbach, 1986 , p. 104). This is a remarkable change in hypotheses, a change based on data and made fully transparent. Cronbach understood the value of failing productively.
Closer to home, in a less dramatic example, one of us began a line of scientific inquiry into how to prepare elementary preservice teachers to teach early algebra. Teaching early algebra meant engaging elementary students in early forms of algebraic reasoning. Such reasoning should help them transition from arithmetic to algebra. To begin this line of inquiry, a set of activities for preservice teachers were developed. Even though the activities were based on well-supported hypotheses, they largely failed to engage preservice teachers as predicted because of unanticipated challenges the preservice teachers faced. To capitalize on this failure, follow-up studies were conducted, first to better understand elementary preservice teachers’ challenges with preparing to teach early algebra, and then to better support preservice teachers in navigating these challenges. In this example, the initial failure was a necessary step in the researchers’ scientific inquiry and furthered the researchers’ understanding of this issue.
We present another example of failing productively in Chap. 2 . That example emerges from recounting the history of a well-known research program in mathematics education.
Making mistakes is an inherent part of doing scientific research. Conducting a study is rarely a smooth path from beginning to end. We recommend that you keep the following things in mind as you begin a career of conducting research in education.
First, do not get discouraged when you make mistakes; do not fall into the trap of feeling like you are not capable of doing research because you make too many errors.
Second, learn from your mistakes. Do not ignore your mistakes or treat them as errors that you simply need to forget and move past. Mistakes are rich sites for learning—in research just as in other fields of study.
Third, by reflecting on your mistakes, you can learn to make better mistakes, mistakes that inform you about a productive next step. You will not be able to eliminate your mistakes, but you can set a goal of making better and better mistakes.
Exercise 1.8
How does scientific inquiry differ from everyday learning in giving you the tools to fail upward? You may find helpful perspectives on this question in other resources on science and scientific inquiry (e.g., Failure: Why Science is So Successful by Firestein, 2015).
Exercise 1.9
Use what you have learned in this chapter to write a new definition of scientific inquiry. Compare this definition with the one you wrote before reading this chapter. If you are reading this book as part of a course, compare your definition with your colleagues’ definitions. Develop a consensus definition with everyone in the course.
Part IV. Preview of Chap. 2
Now that you have a good idea of what research is, at least of what we believe research is, the next step is to think about how to actually begin doing research. This means how to begin formulating, testing, and revising hypotheses. As for all phases of scientific inquiry, there are lots of things to think about. Because it is critical to start well, we devote Chap. 2 to getting started with formulating hypotheses.
Agnes, M., & Guralnik, D. B. (Eds.). (2008). Hypothesis. In Webster’s new world college dictionary (4th ed.). Wiley.
Google Scholar
Britannica. (n.d.). Scientific method. In Encyclopaedia Britannica . Retrieved July 15, 2022 from https://www.britannica.com/science/scientific-method
Brownell, W. A., & Moser, H. E. (1949). Meaningful vs. mechanical learning: A study in grade III subtraction . Duke University Press..
Cai, J., Morris, A., Hohensee, C., Hwang, S., Robison, V., Cirillo, M., Kramer, S. L., & Hiebert, J. (2019b). Posing significant research questions. Journal for Research in Mathematics Education, 50 (2), 114–120. https://doi.org/10.5951/jresematheduc.50.2.0114
Article Google Scholar
Cambridge University Press. (n.d.). Hypothesis. In Cambridge dictionary . Retrieved July 15, 2022 from https://dictionary.cambridge.org/us/dictionary/english/hypothesis
Cronbach, J. L. (1957). The two disciplines of scientific psychology. American Psychologist, 12 , 671–684.
Cronbach, L. J. (1975). Beyond the two disciplines of scientific psychology. American Psychologist, 30 , 116–127.
Cronbach, L. J. (1986). Social inquiry by and for earthlings. In D. W. Fiske & R. A. Shweder (Eds.), Metatheory in social science: Pluralisms and subjectivities (pp. 83–107). University of Chicago Press.
Hay, C. M. (Ed.). (2016). Methods that matter: Integrating mixed methods for more effective social science research . University of Chicago Press.
Merriam-Webster. (n.d.). Explain. In Merriam-Webster.com dictionary . Retrieved July 15, 2022, from https://www.merriam-webster.com/dictionary/explain
National Research Council. (2002). Scientific research in education . National Academy Press.
Weis, L., Eisenhart, M., Duncan, G. J., Albro, E., Bueschel, A. C., Cobb, P., Eccles, J., Mendenhall, R., Moss, P., Penuel, W., Ream, R. K., Rumbaut, R. G., Sloane, F., Weisner, T. S., & Wilson, J. (2019a). Mixed methods for studies that address broad and enduring issues in education research. Teachers College Record, 121 , 100307.
Weisner, T. S. (Ed.). (2005). Discovering successful pathways in children’s development: Mixed methods in the study of childhood and family life . University of Chicago Press.
Download references
Author information
Authors and affiliations.
School of Education, University of Delaware, Newark, DE, USA
James Hiebert, Anne K Morris & Charles Hohensee
Department of Mathematical Sciences, University of Delaware, Newark, DE, USA
Jinfa Cai & Stephen Hwang
You can also search for this author in PubMed Google Scholar
Rights and permissions
Open Access This chapter is licensed under the terms of the Creative Commons Attribution 4.0 International License ( http://creativecommons.org/licenses/by/4.0/ ), which permits use, sharing, adaptation, distribution and reproduction in any medium or format, as long as you give appropriate credit to the original author(s) and the source, provide a link to the Creative Commons license and indicate if changes were made.
The images or other third party material in this chapter are included in the chapter's Creative Commons license, unless indicated otherwise in a credit line to the material. If material is not included in the chapter's Creative Commons license and your intended use is not permitted by statutory regulation or exceeds the permitted use, you will need to obtain permission directly from the copyright holder.
Reprints and permissions
Copyright information
© 2023 The Author(s)
About this chapter
Hiebert, J., Cai, J., Hwang, S., Morris, A.K., Hohensee, C. (2023). What Is Research, and Why Do People Do It?. In: Doing Research: A New Researcher’s Guide. Research in Mathematics Education. Springer, Cham. https://doi.org/10.1007/978-3-031-19078-0_1
Download citation
DOI : https://doi.org/10.1007/978-3-031-19078-0_1
Published : 03 December 2022
Publisher Name : Springer, Cham
Print ISBN : 978-3-031-19077-3
Online ISBN : 978-3-031-19078-0
eBook Packages : Education Education (R0)
Share this chapter
Anyone you share the following link with will be able to read this content:
Sorry, a shareable link is not currently available for this article.
Provided by the Springer Nature SharedIt content-sharing initiative
- Publish with us
Policies and ethics
- Find a journal
- Track your research
Research Process: 8 Steps in Research Process

The research process starts with identifying a research problem and conducting a literature review to understand the context. The researcher sets research questions, objectives, and hypotheses based on the research problem.
A research study design is formed to select a sample size and collect data after processing and analyzing the collected data and the research findings presented in a research report.
What is the Research Process?
There are a variety of approaches to research in any field of investigation, irrespective of whether it is applied research or basic research. Each research study will be unique in some ways because of the particular time, setting, environment, and place it is being undertaken.
Nevertheless, all research endeavors share a common goal of furthering our understanding of the problem, and thus, all traverse through certain primary stages, forming a process called the research process.
Understanding the research process is necessary to effectively carry out research and sequence the stages inherent in the process.
How Research Process Work?

Eight steps research process is, in essence, part and parcel of a research proposal. It is an outline of the commitment that you intend to follow in executing a research study.
A close examination of the above stages reveals that each of these stages, by and large, is dependent upon the others.
One cannot analyze data (step 7) unless he has collected data (step 6). One cannot write a report (step 8) unless he has collected and analyzed data (step 7).
Research then is a system of interdependent related stages. Violation of this sequence can cause irreparable harm to the study.
It is also true that several alternatives are available to the researcher during each stage stated above. A research process can be compared with a route map.
The map analogy is useful for the researcher because several alternatives exist at each stage of the research process.
Choosing the best alternative in terms of time constraints, money, and human resources in our research decision is our primary goal.
Before explaining the stages of the research process, we explain the term ‘iterative’ appearing within the oval-shaped diagram at the center of the schematic diagram.
The key to a successful research project ultimately lies in iteration: the process of returning again and again to the identification of the research problems, methodology, data collection, etc., which leads to new ideas, revisions, and improvements.
By discussing the research project with advisers and peers, one will often find that new research questions need to be added, variables to be omitted, added or redefined, and other changes to be made. As a proposed study is examined and reexamined from different perspectives, it may begin to transform and take a different shape.
This is expected and is an essential component of a good research study.
Besides, examining study methods and data collected from different viewpoints is important to ensure a comprehensive approach to the research question.
In conclusion, there is seldom any single strategy or formula for developing a successful research study, but it is essential to realize that the research process is cyclical and iterative.
What is the primary purpose of the research process?
The research process aims to identify a research problem, understand its context through a literature review, set research questions and objectives, design a research study, select a sample, collect data, analyze the data, and present the findings in a research report.
Why is the research design important in the research process?
The research design is the blueprint for fulfilling objectives and answering research questions. It specifies the methods and procedures for collecting, processing, and analyzing data, ensuring the study is structured and systematic.
8 Steps of Research Process
Identifying the research problem.

The first and foremost task in the entire process of scientific research is to identify a research problem .
A well-identified problem will lead the researcher to accomplish all-important phases of the research process, from setting objectives to selecting the research methodology .
But the core question is: whether all problems require research.
We have countless problems around us, but all we encounter do not qualify as research problems; thus, these do not need to be researched.
Keeping this point in mind, we must draw a line between research and non-research problems.
Intuitively, researchable problems are those that have a possibility of thorough verification investigation, which can be effected through the analysis and collection of data. In contrast, the non-research problems do not need to go through these processes.
Researchers need to identify both;
Non-Research Problems
Statement of the problem, justifying the problem, analyzing the problem.
A non-research problem does not require any research to arrive at a solution. Intuitively, a non-researchable problem consists of vague details and cannot be resolved through research.
It is a managerial or built-in problem that may be solved at the administrative or management level. The answer to any question raised in a non-research setting is almost always obvious.
The cholera outbreak, for example, following a severe flood, is a common phenomenon in many communities. The reason for this is known. It is thus not a research problem.
Similarly, the reasons for the sudden rise in prices of many essential commodities following the announcement of the budget by the Finance Minister need no investigation. Hence it is not a problem that needs research.
How is a research problem different from a non-research problem?
A research problem is a perceived difficulty that requires thorough verification and investigation through data analysis and collection. In contrast, a non-research problem does not require research for a solution, as the answer is often obvious or already known.
Non-Research Problems Examples
A recent survey in town- A found that 1000 women were continuous users of contraceptive pills.
But last month’s service statistics indicate that none of these women were using contraceptive pills (Fisher et al. 1991:4).
The discrepancy is that ‘all 1000 women should have been using a pill, but none is doing so. The question is: why the discrepancy exists?
Well, the fact is, a monsoon flood has prevented all new supplies of pills from reaching town- A, and all old supplies have been exhausted. Thus, although the problem situation exists, the reason for the problem is already known.
Therefore, assuming all the facts are correct, there is no reason to research the factors associated with pill discontinuation among women. This is, thus, a non-research problem.
A pilot survey by University students revealed that in Rural Town-A, the goiter prevalence among school children is as high as 80%, while in the neighboring Rural Town-A, it is only 30%. Why is a discrepancy?
Upon inquiry, it was seen that some three years back, UNICEF launched a lipiodol injection program in the neighboring Rural Town-A.
This attempt acted as a preventive measure against the goiter. The reason for the discrepancy is known; hence, we do not consider the problem a research problem.
A hospital treated a large number of cholera cases with penicillin, but the treatment with penicillin was not found to be effective. Do we need research to know the reason?
Here again, there is one single reason that Vibrio cholera is not sensitive to penicillin; therefore, this is not the drug of choice for this disease.
In this case, too, as the reasons are known, it is unwise to undertake any study to find out why penicillin does not improve the condition of cholera patients. This is also a non-research problem.
In the tea marketing system, buying and selling tea starts with bidders. Blenders purchase open tea from the bidders. Over the years, marketing cost has been the highest for bidders and the lowest for blenders. What makes this difference?
The bidders pay exorbitantly higher transport costs, which constitute about 30% of their total cost.
Blenders have significantly fewer marketing functions involving transportation, so their marketing cost remains minimal.
Hence no research is needed to identify the factors that make this difference.
Here are some of the problems we frequently encounter, which may well be considered non-research problems:
- Rises in the price of warm clothes during winter;
- Preferring admission to public universities over private universities;
- Crisis of accommodations in sea resorts during summer
- Traffic jams in the city street after office hours;
- High sales in department stores after an offer of a discount.
Research Problem
In contrast to a non-research problem, a research problem is of primary concern to a researcher.
A research problem is a perceived difficulty, a feeling of discomfort, or a discrepancy between a common belief and reality.
As noted by Fisher et al. (1993), a problem will qualify as a potential research problem when the following three conditions exist:
- There should be a perceived discrepancy between “what it is” and “what it should have been.” This implies that there should be a difference between “what exists” and the “ideal or planned situation”;
- A question about “why” the discrepancy exists. This implies that the reason(s) for this discrepancy is unclear to the researcher (so that it makes sense to develop a research question); and
- There should be at least two possible answers or solutions to the questions or problems.
The third point is important. If there is only one possible and plausible answer to the question about the discrepancy, then a research situation does not exist.
It is a non-research problem that can be tackled at the managerial or administrative level.
Research Problem Examples
Research problem – example #1.
While visiting a rural area, the UNICEF team observed that some villages have female school attendance rates as high as 75%, while some have as low as 10%, although all villages should have a nearly equal attendance rate. What factors are associated with this discrepancy?
We may enumerate several reasons for this:
- Villages differ in their socio-economic background.
- In some villages, the Muslim population constitutes a large proportion of the total population. Religion might play a vital role.
- Schools are far away from some villages. The distance thus may make this difference.
Because there is more than one answer to the problem, it is considered a research problem, and a study can be undertaken to find a solution.
Research Problem – Example #2
The Government has been making all-out efforts to ensure a regular flow of credit in rural areas at a concession rate through liberal lending policy and establishing many bank branches in rural areas.
Knowledgeable sources indicate that expected development in rural areas has not yet been achieved, mainly because of improper credit utilization.
More than one reason is suspected for such misuse or misdirection.
These include, among others:
- Diversion of credit money to some unproductive sectors
- Transfer of credit money to other people like money lenders, who exploit the rural people with this money
- Lack of knowledge of proper utilization of the credit.
Here too, reasons for misuse of loans are more than one. We thus consider this problem as a researchable problem.
Research Problem – Example #3
Let’s look at a new headline: Stock Exchange observes the steepest ever fall in stock prices: several injured as retail investors clash with police, vehicles ransacked .
Investors’ demonstration, protest and clash with police pause a problem. Still, it is certainly not a research problem since there is only one known reason for the problem: Stock Exchange experiences the steepest fall in stock prices. But what causes this unprecedented fall in the share market?
Experts felt that no single reason could be attributed to the problem. It is a mix of several factors and is a research problem. The following were assumed to be some of the possible reasons:
- The merchant banking system;
- Liquidity shortage because of the hike in the rate of cash reserve requirement (CRR);
- IMF’s warnings and prescriptions on the commercial banks’ exposure to the stock market;
- Increase in supply of new shares;
- Manipulation of share prices;
- Lack of knowledge of the investors on the company’s fundamentals.
The choice of a research problem is not as easy as it appears. The researchers generally guide it;
- own intellectual orientation,
- level of training,
- experience,
- knowledge on the subject matter, and
- intellectual curiosity.
Theoretical and practical considerations also play a vital role in choosing a research problem. Societal needs also guide in choosing a research problem.
Once we have chosen a research problem, a few more related steps must be followed before a decision is taken to undertake a research study.
These include, among others, the following:
- Statement of the problem.
- Justifying the problem.
- Analyzing the problem.
A detailed exposition of these issues is undertaken in chapter ten while discussing the proposal development.
A clear and well-defined problem statement is considered the foundation for developing the research proposal.
It enables the researcher to systematically point out why the proposed research on the problem should be undertaken and what he hopes to achieve with the study’s findings.
A well-defined statement of the problem will lead the researcher to formulate the research objectives, understand the background of the study, and choose a proper research methodology.
Once the problem situation has been identified and clearly stated, it is important to justify the importance of the problem.
In justifying the problems, we ask such questions as why the problem of the study is important, how large and widespread the problem is, and whether others can be convinced about the importance of the problem and the like.
Answers to the above questions should be reviewed and presented in one or two paragraphs that justify the importance of the problem.
As a first step in analyzing the problem, critical attention should be given to accommodate the viewpoints of the managers, users, and researchers to the problem through threadbare discussions.
The next step is identifying the factors that may have contributed to the perceived problems.
Issues of Research Problem Identification
There are several ways to identify, define, and analyze a problem, obtain insights, and get a clearer idea about these issues. Exploratory research is one of the ways of accomplishing this.
The purpose of the exploratory research process is to progressively narrow the scope of the topic and transform the undefined problems into defined ones, incorporating specific research objectives.
The exploratory study entails a few basic strategies for gaining insights into the problem. It is accomplished through such efforts as:
Pilot Survey
A pilot survey collects proxy data from the ultimate subjects of the study to serve as a guide for the large study. A pilot study generates primary data, usually for qualitative analysis.
This characteristic distinguishes a pilot survey from secondary data analysis, which gathers background information.
Case Studies
Case studies are quite helpful in diagnosing a problem and paving the way to defining the problem. It investigates one or a few situations identical to the researcher’s problem.
Focus Group Interviews
Focus group interviews, an unstructured free-flowing interview with a small group of people, may also be conducted to understand and define a research problem .
Experience Survey
Experience survey is another strategy to deal with the problem of identifying and defining the research problem.
It is an exploratory research endeavor in which individuals knowledgeable and experienced in a particular research problem are intimately consulted to understand the problem.
These persons are sometimes known as key informants, and an interview with them is popularly known as the Key Informant Interview (KII).
Reviewing of Literature

A review of relevant literature is an integral part of the research process. It enables the researcher to formulate his problem in terms of the specific aspects of the general area of his interest that has not been researched so far.
Such a review provides exposure to a larger body of knowledge and equips him with enhanced knowledge to efficiently follow the research process.
Through a proper review of the literature, the researcher may develop the coherence between the results of his study and those of the others.
A review of previous documents on similar or related phenomena is essential even for beginning researchers.
Ignoring the existing literature may lead to wasted effort on the part of the researchers.
Why spend time merely repeating what other investigators have already done?
Suppose the researcher is aware of earlier studies of his topic or related topics . In that case, he will be in a much better position to assess his work’s significance and convince others that it is important.
A confident and expert researcher is more crucial in questioning the others’ methodology, the choice of the data, and the quality of the inferences drawn from the study results.
In sum, we enumerate the following arguments in favor of reviewing the literature:
- It avoids duplication of the work that has been done in the recent past.
- It helps the researcher discover what others have learned and reported on the problem.
- It enables the researcher to become familiar with the methodology followed by others.
- It allows the researcher to understand what concepts and theories are relevant to his area of investigation.
- It helps the researcher to understand if there are any significant controversies, contradictions, and inconsistencies in the findings.
- It allows the researcher to understand if there are any unanswered research questions.
- It might help the researcher to develop an analytical framework.
- It will help the researcher consider including variables in his research that he might not have thought about.
Why is reviewing literature crucial in the research process?
Reviewing literature helps avoid duplicating previous work, discovers what others have learned about the problem, familiarizes the researcher with relevant concepts and theories, and ensures a comprehensive approach to the research question.
What is the significance of reviewing literature in the research process?
Reviewing relevant literature helps formulate the problem, understand the background of the study, choose a proper research methodology, and develop coherence between the study’s results and previous findings.
Setting Research Questions, Objectives, and Hypotheses

After discovering and defining the research problem, researchers should make a formal statement of the problem leading to research objectives .
An objective will precisely say what should be researched, delineate the type of information that should be collected, and provide a framework for the scope of the study. A well-formulated, testable research hypothesis is the best expression of a research objective.
A hypothesis is an unproven statement or proposition that can be refuted or supported by empirical data. Hypothetical statements assert a possible answer to a research question.
Step #4: Choosing the Study Design

The research design is the blueprint or framework for fulfilling objectives and answering research questions .
It is a master plan specifying the methods and procedures for collecting, processing, and analyzing the collected data. There are four basic research designs that a researcher can use to conduct their study;
- experiment,
- secondary data study, and
- observational study.
The type of research design to be chosen from among the above four methods depends primarily on four factors:
- The type of problem
- The objectives of the study,
- The existing state of knowledge about the problem that is being studied, and
- The resources are available for the study.
Deciding on the Sample Design

Sampling is an important and separate step in the research process. The basic idea of sampling is that it involves any procedure that uses a relatively small number of items or portions (called a sample) of a universe (called population) to conclude the whole population.
It contrasts with the process of complete enumeration, in which every member of the population is included.
Such a complete enumeration is referred to as a census.
A population is the total collection of elements we wish to make some inference or generalization.
A sample is a part of the population, carefully selected to represent that population. If certain statistical procedures are followed in selecting the sample, it should have the same characteristics as the population. These procedures are embedded in the sample design.
Sample design refers to the methods followed in selecting a sample from the population and the estimating technique vis-a-vis the formula for computing the sample statistics.
The fundamental question is, then, how to select a sample.
To answer this question, we must have acquaintance with the sampling methods.
These methods are basically of two types;
- probability sampling , and
- non-probability sampling .
Probability sampling ensures every unit has a known nonzero probability of selection within the target population.
If there is no feasible alternative, a non-probability sampling method may be employed.
The basis of such selection is entirely dependent on the researcher’s discretion. This approach is called judgment sampling, convenience sampling, accidental sampling, and purposive sampling.
The most widely used probability sampling methods are simple random sampling , stratified random sampling , cluster sampling , and systematic sampling . They have been classified by their representation basis and unit selection techniques.
Two other variations of the sampling methods that are in great use are multistage sampling and probability proportional to size (PPS) sampling .
Multistage sampling is most commonly used in drawing samples from very large and diverse populations.
The PPS sampling is a variation of multistage sampling in which the probability of selecting a cluster is proportional to its size, and an equal number of elements are sampled within each cluster.
Collecting Data From The Research Sample

Data gathering may range from simple observation to a large-scale survey in any defined population. There are many ways to collect data. The approach selected depends on the objectives of the study, the research design, and the availability of time, money, and personnel.
With the variation in the type of data (qualitative or quantitative) to be collected, the method of data collection also varies .
The most common means for collecting quantitative data is the structured interview .
Studies that obtain data by interviewing respondents are called surveys. Data can also be collected by using self-administered questionnaires . Telephone interviewing is another way in which data may be collected .
Other means of data collection include secondary sources, such as the census, vital registration records, official documents, previous surveys, etc.
Qualitative data are collected mainly through in-depth interviews, focus group discussions , Key Informant Interview ( KII), and observational studies.
Process and Analyze the Collected Research Data

Data processing generally begins with the editing and coding of data . Data are edited to ensure consistency across respondents and to locate omissions if any.
In survey data, editing reduces errors in the recording, improves legibility, and clarifies unclear and inappropriate responses. In addition to editing, the data also need coding.
Because it is impractical to place raw data into a report, alphanumeric codes are used to reduce the responses to a more manageable form for storage and future processing.
This coding process facilitates the processing of the data. The personal computer offers an excellent opportunity for data editing and coding processes.
Data analysis usually involves reducing accumulated data to a manageable size, developing summaries, searching for patterns, and applying statistical techniques for understanding and interpreting the findings in light of the research questions.
Further, based on his analysis, the researcher determines if his findings are consistent with the formulated hypotheses and theories.
The techniques used in analyzing data may range from simple graphical techniques to very complex multivariate analyses depending on the study’s objectives, the research design employed, and the nature of the data collected.
As in the case of data collection methods, an analytical technique appropriate in one situation may not be suitable for another.
Writing Research Report – Developing Research Proposal, Writing Report, Disseminating and Utilizing Results

The entire task of a research study is accumulated in a document called a proposal or research proposal.
A research proposal is a work plan, prospectus, outline, offer, and a statement of intent or commitment from an individual researcher or an organization to produce a product or render a service to a potential client or sponsor .
The proposal will be prepared to keep the sequence presented in the research process. The proposal tells us what, how, where, and to whom it will be done.
It must also show the benefit of doing it. It always includes an explanation of the purpose of the study (the research objectives) or a definition of the problem.
It systematically outlines the particular research methodology and details the procedures utilized at each stage of the research process.
The end goal of a scientific study is to interpret the results and draw conclusions.
To this end, it is necessary to prepare a report and transmit the findings and recommendations to administrators, policymakers, and program managers to make a decision.
There are various research reports: term papers, dissertations, journal articles , papers for presentation at professional conferences and seminars, books, thesis, and so on. The results of a research investigation prepared in any form are of little utility if they are not communicated to others.
The primary purpose of a dissemination strategy is to identify the most effective media channels to reach different audience groups with study findings most relevant to their needs.
The dissemination may be made through a conference, a seminar, a report, or an oral or poster presentation.
The style and organization of the report will differ according to the target audience, the occasion, and the purpose of the research. Reports should be developed from the client’s perspective.
A report is an excellent means that helps to establish the researcher’s credibility. At a bare minimum, a research report should contain sections on:
- An executive summary;
- Background of the problem;
- Literature review;
- Methodology;
- Discussion;
- Conclusions and
- Recommendations.
The study results can also be disseminated through peer-reviewed journals published by academic institutions and reputed publishers both at home and abroad. The report should be properly evaluated .
These journals have their format and editorial policies. The contributors can submit their manuscripts adhering to the policies and format for possible publication of their papers.
There are now ample opportunities for researchers to publish their work online.
The researchers have conducted many interesting studies without affecting actual settings. Ideally, the concluding step of a scientific study is to plan for its utilization in the real world.
Although researchers are often not in a position to implement a plan for utilizing research findings, they can contribute by including in their research reports a few recommendations regarding how the study results could be utilized for policy formulation and program intervention.
Why is the dissemination of research findings important?
Dissemination of research findings is crucial because the results of a research investigation have little utility if not communicated to others. Dissemination ensures that the findings reach relevant stakeholders, policymakers, and program managers to inform decisions.
How should a research report be structured?
A research report should contain sections on an executive summary, background of the problem, literature review, methodology, findings, discussion, conclusions, and recommendations.
Why is it essential to consider the target audience when preparing a research report?
The style and organization of a research report should differ based on the target audience, occasion, and research purpose. Tailoring the report to the audience ensures that the findings are communicated effectively and are relevant to their needs.

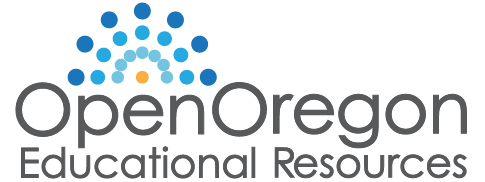
6.1 Functions and Contents of Progress Reports
In the progress report, you explain any or all of the following:
- How much of the work is complete
- What part of the work is currently in progress
- What work remains to be done
- What problems or unexpected things, if any, have arisen
- How the project is going in general
Progress reports have several important functions:
- Reassure recipients that you are making progress, that the project is going smoothly, and that it will be complete by the expected date.
- Provide recipients with a brief look at some of the findings or some of the work of the project.
- Give recipients a chance to evaluate your work on the project and to request changes.
- Give you a chance to discuss problems in the project and thus to forewarn recipients.
- Force you to establish a work schedule so that you’ll complete the project on time.
- Project a sense of professionalism to your work and your organization.
Chapter Attribution Information
This chapter was derived by Annemarie Hamlin, Chris Rubio, and Michele DeSilva, Central Oregon Community College, from Online Technical Writing by David McMurrey – CC: BY 4.0
Technical Writing Copyright © 2017 by Allison Gross, Annemarie Hamlin, Billy Merck, Chris Rubio, Jodi Naas, Megan Savage, and Michele DeSilva is licensed under a Creative Commons Attribution-NonCommercial-ShareAlike 4.0 International License , except where otherwise noted.
Share This Book

- Manuscript Status
Q: What does the status "Decision in progress" mean?
I have submitted my research article to a journal in June 2019. The status was "Under review" for 4 months, after which, on October 8, it changed to "Decision in progress." What does this statement mean? Will my paper rejected?
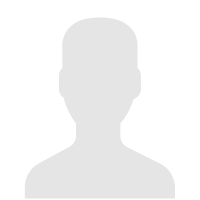
Asked by Pramod Soni on 12 Oct, 2019
You have not mentioned whether there were any other statuses before "Under review," so it's difficult to say if this is the internal editorial check or the external peer review. If the status changed to "Under review" directly after submission without any interim changes, it is likely to be the initial editorial screening. If that is the case, the status "Decision in progress" might be indicative of a rejection. However, if the "Under review" referred to the external peer review, you can be hopeful. Whatever it is, you should be informed of the decision soon.
All the best!
Related reading:
- Does a direct 'Decision in Process' status indicate rejection?
- Can the status change to "Decision in process" without a request for revisions?
- Why did my manuscript's status change from "With Editor" to "Decision in Process"?
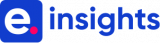
Answered by Editage Insights on 18 Oct, 2019
- Upvote this Answer
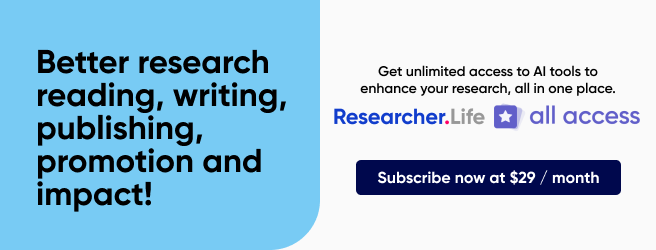
u will get message from editorial office soon...hope ur paper will accept
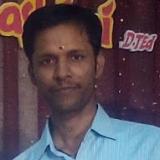
Answered by Raja Venkatesh on 21 Oct, 2019
This content belongs to the Journal submission & peer review Stage
Confirm that you would also like to sign up for free personalized email coaching for this stage.
Trending Searches
- Statement of the problem
- Background of study
- Scope of the study
- Types of qualitative research
- Rationale of the study
- Concept paper
- Literature review
- Introduction in research
- Under "Editor Evaluation"
- Ethics in research
Recent Searches
- Review paper
- Responding to reviewer comments
- Predatory publishers
- Scope and delimitations
- Open access
- Plagiarism in research
- Journal selection tips
- Editor assigned
- Types of articles
- "Reject and Resubmit" status
- Decision in process
- Conflict of interest

Progress Report: What is it & How to Write it? (Steps & Format)

Want to create a progress report to highlight the project’s achievements? No worries, we have got you covered! Read on…
A quick question – on a scale of 1 to 10, how important is it to regularly keep track and provide project updates to your supervisors, colleagues, or clients? The answer is 12! Simply, because nobody likes being left in the dark!
For any project in a company, people around it need to be well-informed about the project status, the research being done by the project team, their decisions, and the scope for improvement. These updates are an integral part of project management and ensure that every team member is operating efficiently with their goals being met on time.
One way to showcase the status of your project and keep track of it is to write a powerful progress report!
In fact, the American Society for Training and Development shows that having a specific place to check your progress increases the probability of meeting a goal by 95%.
Progress reports are a great place for project managers to inform and engage their supervisors, clients, or associates, about the progress they have made on a project over a certain period.
If executed well, progress reports provide a quick overview of how things are humming along, offering valuable insights to increase productivity, provide the necessary guidance, and quickly solve emerging difficulties.
However, writing a progress report can be a little daunting, especially, when you have a diverse team and various sub-projects to manage. Well, don’t fret! We’re going to fix that. In this blog post, we’ll teach you everything about progress reports, why they are important, and how you can write one that will make everyone say ‘wow’!
What is the Progress Report? (Definition)
A progress report is a document that explains in detail how much progress you have made towards the completion of your ongoing project.
A progress report is a management tool used in all types of organizations, that outlines the tasks completed, activities carried out, and target achieved vis-à-vis your project plan.
In a progress report, you explain any or all of the following:
- The amount of work complete?
- What part of the work is currently in progress?
- The problems or unexpected things that have occurred?
- What work is pending?
- How the project is going in general?
Read more: How To Write An Impressive Project Proposal?
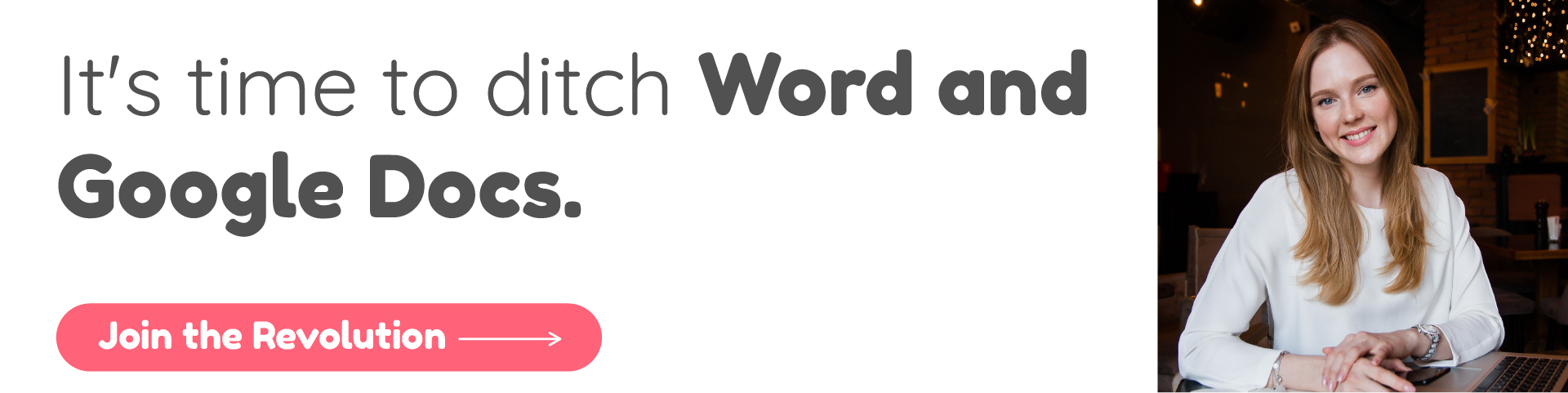
Why are Progress Reports Important?
No project manager wakes up thinking “ I wish I could make reports for my supervisor and team all day” ! We get it. Writing progress reports are not very fun.
However, you know that writing progress reports are part of the deal. Progressive reporting demands talking with your team or client to understand the goals and showcase the information that closely relates to the said goals.
Whether the report is about updating the investors, marketing performance, or resource management. These reports let everyone see what’s going well and what isn’t.
It also assists managers to see the overall success or failure of projects. Furthermore, progress reports help to:
1. Make Information Transparent
The glue that holds together any relationship is visibility and transparency. A well-defined progress report directly presents how your work affects the project’s bottom line and showcases the rights and wrongs!
By adding transparency to your project plan, you can build an unmatched level of credibility and trust with your team and clients.
2. Encourage Constant Interaction
Creating and discussing progress reports results in constant communication and keeps everyone in the loop. Being in constant contact with others on a weekly or monthly basis ensures a clear understanding of roles and responsibilities.
3. Improve Project Evaluation and Review
Previous progress reports will help you in clarifying loopholes, and systemic issues, and examine documents to find out what went wrong, what can be done right, and which area needs improvement.
4. Provides Insight for Future Planning
When a progress report shows all the delays that have occurred, the supervisor or a project manager can monitor and investigate the issue that hindered progress and take additional steps to prevent them from happening in the future.
Read more: How to Write Project Reports that ‘Wow’ Your Clients?
How to Write a Progress Report with 4 Simple Steps?
Progress reports are essential documents for tracking project plans and initiatives, but if the readers and writers are not in sync, these reports can be a hit-or-miss exercise for everyone involved.
Therefore, here are some steps to help you deliver the right information to the right people at the right time.
Step 1. Explain the purpose of your report
There are many reasons for someone to write a progress report. Obviously, for many of them, it’s to brief the progress and status of the project.
Readers might also want to know detailed information about the project’s purpose, its duration, and other important insights.
Step 2. Define your audience
Once you have sorted out the purpose of writing the progress report, consider the type of audience you will be targeting and the details that your readers are going to acknowledge in the report.
These can be, what decisions your readers are going to need to make after reading the progress report, the information they are going to need to know to oversee and participate in the project effectively, etc.
Step 3. Create a “work completed” section
In this section, you should describe everything that has already been done and the best way to do this is to mention the completed tasks chronologically.
You can specify dates, tasks you and your team were working on, information on key findings, etc.
Step 4. Summarize your progress report
In the summary section, provide the essential details about the to-do and completed work. Also, add a short description of the problems your team encountered, recommendations from your supervisor for their resolution, and whether any assistance on the project is required.
Read more: Business Report: What is it & How to Write it? (Steps & Format)
Creating a Progress Report that Stands Out with Bit.ai !
If you are planning to show a progress report that looks exactly like any other bland report, chances are your readers are just going to skim it along the way or won’t read it at all.
Well, to lure your reader’s attention and proudly display the work you have done on the project, you have to make the progress report irresistibly compelling!
How about awesome visuals, accompanied by quality content that could grab the reader’s interest and encourage them to read the whole thing? No doubt, everybody likes reading something easy to grasp and visually stunning!
Luckily, we have got the perfect tool for you that will provide a reading experience like never before and bring your grey-scale progress reports to come alive! A solution like Bit.ai
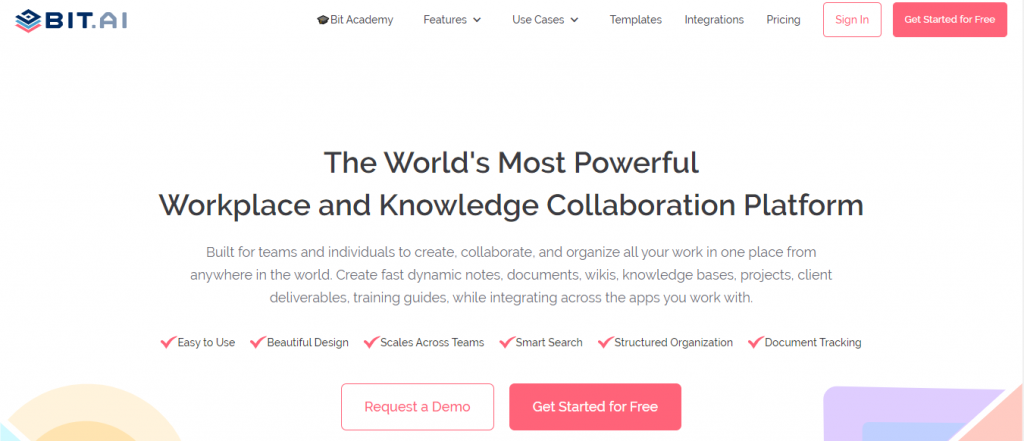
Bit is a new-age cloud-based document collaboration tool that helps teams create, share, manage, and track interactive workplace documents.
Bit helps you make sure your reports are more than just plain bland text and images. Thus, apart from allowing multiple users to collaborate on reports, Bit also allows users to share any sort of rich media like campaign video, tables, charts, One Drive files, Excel Spreadsheets, GIFs, Tweets, Pinterest boards, etc. Anything on the internet with a link can be shared and Bit will automatically turn it into visual content.
Bit has a very minimal design aesthetic which makes every design element pop, awesome readability, and rich features that will prevent collaborators from messing up any documents and help them rethink the way they work!
Besides writing progress reports, you can easily create other beautiful documents like the statement of work , project documentation, operational plan , roadmap, project charter , etc. in a common workplace for other team members to collaborate, document, share their knowledge, brainstorm ideas, store digital assets, and innovate together.
The best part is that this knowledge is safely secured in your workspaces and can be shared (or kept private) with anyone in your organization or the public!
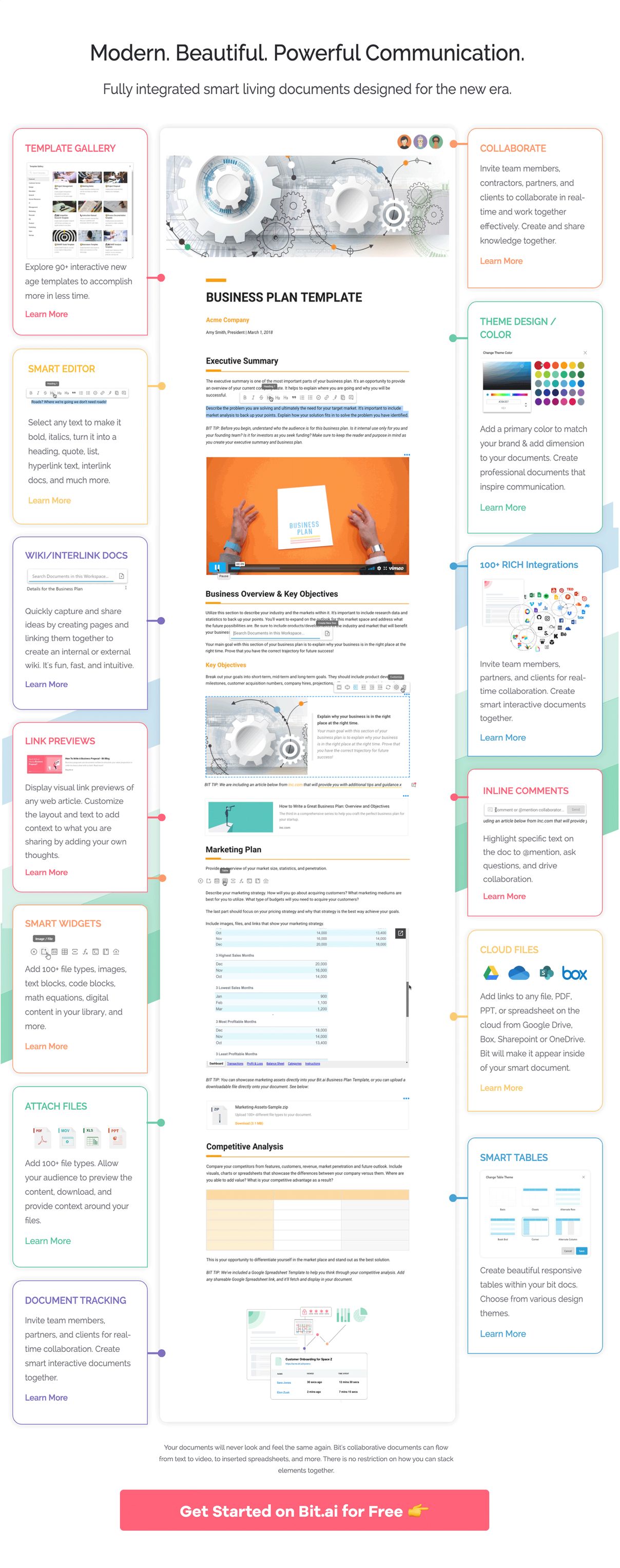
All-in-all Bit is like Google Docs on steroids! So, no more settling for those boring text editors when you have an excessively robust solution to walk you through!
Still, not sure how Bit can help you create that perfect progress report to woo your readers? Let’s see some more of Bit’s awesome capabilities!
Key Benefits of Creating Your Progress Reports on Bit.ai
Simple, clean UI: Bit has a very minimal design aesthetic to it, allowing a newbie to quickly get on board with the platform. Even though the platform is feature-rich, it does a great job as to not overwhelm a new user and provides a systematic approach to work.
Organization of information: Information is often scattered in cloud storage apps, emails, Slack channels, and more. Bit brings all your information in one place by allowing you to organize information in workspaces and folders. Bring all your documents, media files, and other important company data in one place.
Brand consistency: Focus on the content and let Bit help you with the design and formatting. Bit documents are completely responsive and look great on all devices. With amazing templates and themes, Bit docs provide you with the type of brand and design consistency that is unheard of in the documentation industry
Smart search: Bit has very robust search functionality that allows anyone to search and find their documents swiftly. You can search workspaces, folders, document titles, and the content inside of documents with Bit’s rich-text search.
Media integrations: Companies use an average of 34 SaaS apps! No wonder why most of our time is spent hopping from one app to the next, looking for information. This is why Bit.ai integrates with over 100+ popular applications (YouTube, Typeform, LucidChart, Loom, Google Drive, etc) to help teams weave information in their documents beyond just text and images.
Multiple ways of sharing : Bit documents can be shared in three different states :
- Live state : A live state meaning that all changes that you make to the document will update in real-time. If you are sharing your documents with clients, partners, or customers they will always get your most up-to-date changes.
- Embeds : You can embed Bit documents on any website or blog. Bit docs are fully responsive and render perfectly on your website.
- Tracking : You can track your documents and gather real-time insights to understand how users interact with your content. See how much time users spend viewing documents, scroll ratio, user information, and more.
Our team at bit.ai has created a few more templates to make your business processes more efficient. Make sure to check them out before you go, y our team might need them!
- Training Manual Template
- Brainstorming Template
- Meeting Minutes Template
- Employee Handbook Template
- Transition Plan Template
- Customer Service Training Manual Template
- Employee Contract Template
- Performance Improvement Plan Template
A well-defined progress report is like the pulse of a project! It determines your relationship with your readers, highlights all the updates- big or small, and keeps everyone on the same page. Remember, depending on the complexity and scope of the project, you might need to share your progress report on a weekly or monthly basis for better efficiency!
Once you follow all the steps that are mentioned above, your reports are surely going to feel like a breeze of fresh air to your readers, making you look credible and professional. So what are you waiting for?
Do you write such reports in your organization, if yes, which tool do you use? Let us know in the comments below or tweet us @bit_ai
Further reads:
- Technical Report: What is it & How to Write it? (Steps & Structure Included)
- 11 Amazing Goal Tracking Apps and Tools! (Free & Paid)
- 7 Types of Reports Your Business Certainly Needs!
- Performance Report: What is it & How to Create it? (Steps Included)
- Formal Reports: What are they & How to Create them!
- KPI Report: What it is & How to Create a Perfect One?
- How to Write a Project Charter Document?
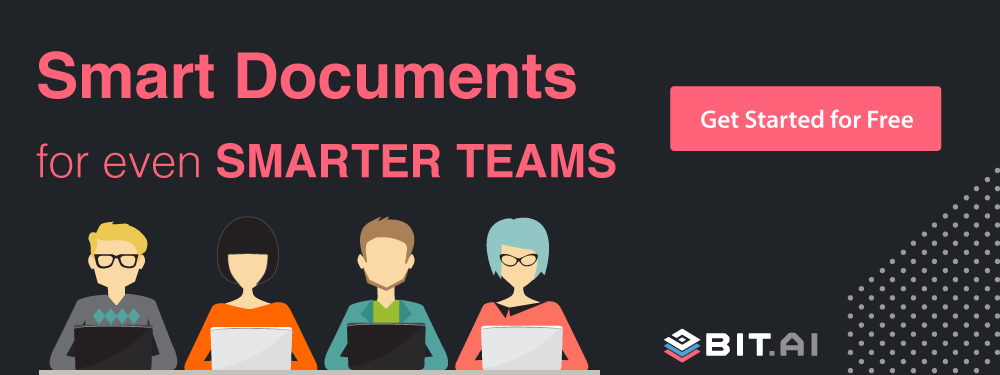
Document Creation: 12 Dos and Don'ts to Keep in Mind!
10 Best Apps for Writing a Book!
Related posts
6 ways digital transformation can fastrack your business growth in 2023, sales contract (agreement of sales): what is it & how to create one, 10 killer zoom alternatives and competitors worth checking out, how to embed figma designs inside your documents, how to embed gifs in your documents, how to create sales content assets for more leads.
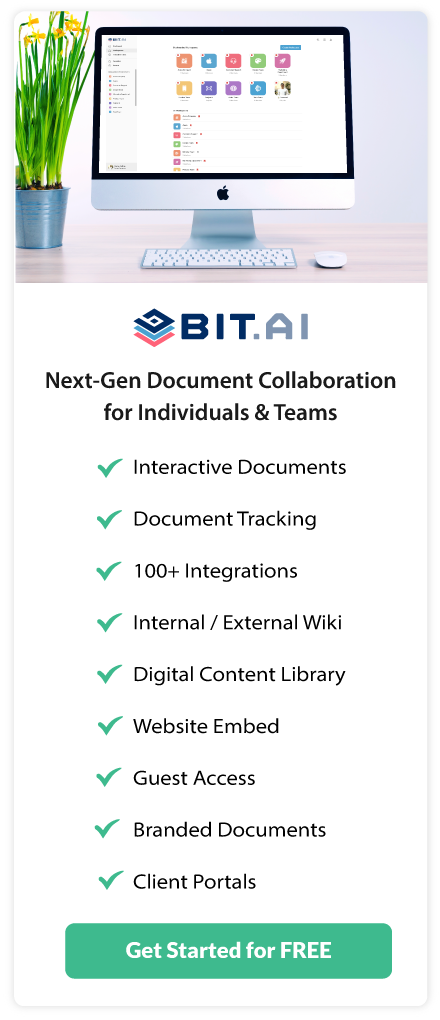
About Bit.ai
Bit.ai is the essential next-gen workplace and document collaboration platform. that helps teams share knowledge by connecting any type of digital content. With this intuitive, cloud-based solution, anyone can work visually and collaborate in real-time while creating internal notes, team projects, knowledge bases, client-facing content, and more.
The smartest online Google Docs and Word alternative, Bit.ai is used in over 100 countries by professionals everywhere, from IT teams creating internal documentation and knowledge bases, to sales and marketing teams sharing client materials and client portals.
👉👉Click Here to Check out Bit.ai.
Recent Posts
How to build an effective knowledge base for technical support, 9 knowledge base mistakes: what you need to know to avoid them, personal user manual: enhance professional profile & team productivity, 9 document management trends every business should know, ai for social media marketing: tools & tactics to boost engagement, a guide to building a client portal for your online course.
Thank you for visiting nature.com. You are using a browser version with limited support for CSS. To obtain the best experience, we recommend you use a more up to date browser (or turn off compatibility mode in Internet Explorer). In the meantime, to ensure continued support, we are displaying the site without styles and JavaScript.
- View all journals
- Explore content
- About the journal
- Publish with us
- Sign up for alerts
- 15 May 2024
‘Quantum internet’ demonstration in cities is most advanced yet
- Davide Castelvecchi
You can also search for this author in PubMed Google Scholar
You have full access to this article via your institution.
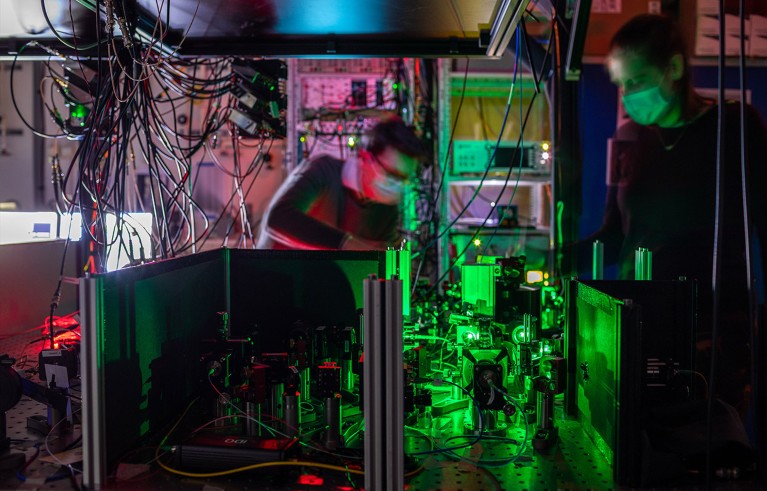
A quantum network node at Delft University of Technology in the Netherlands. Credit: Marieke de Lorijn for QuTech
Three separate research groups have demonstrated quantum entanglement — in which two or more objects are linked so that they contain the same information even if they are far apart — over several kilometres of existing optical fibres in real urban areas. The feat is a key step towards a future quantum internet , a network that could allow information to be exchanged while encoded in quantum states.
Together, the experiments are “the most advanced demonstrations so far” of the technology needed for a quantum internet, says physicist Tracy Northup at the University of Innsbruck in Austria. Each of the three research teams — based in the United States, China and the Netherlands — was able to connect parts of a network using photons in the optical-fibre-friendly infrared part of the spectrum, which is a “major milestone”, says fellow Innsbruck physicist Simon Baier.
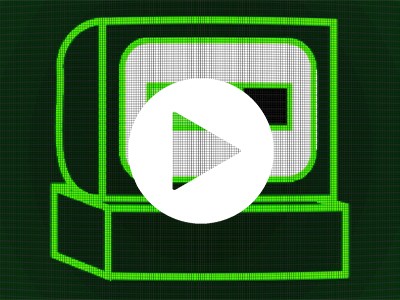
How to build a quantum internet
A quantum internet could enable any two users to establish almost unbreakable cryptographic keys to protect sensitive information . But full use of entanglement could do much more, such as connecting separate quantum computers into one larger, more powerful machine. The technology could also enable certain types of scientific experiment, for example by creating networks of optical telescopes that have the resolution of a single dish hundreds of kilometres wide.
Two of the studies 1 , 2 were published in Nature on 15 May. The third was described last month in a preprint posted on arXiv 3 , which has not yet been peer reviewed.
Impractical environment
Many of the technical steps for building a quantum internet have been demonstrated in the laboratory over the past decade or so. And researchers have shown that they can produce entangled photons using lasers in direct line of sight of each other, either in separate ground locations or on the ground and in space.
But going from the lab to a city environment is “a different beast”, says Ronald Hanson, a physicist who led the Dutch experiment 3 at the Delft University of Technology. To build a large-scale network, researchers agree that it will probably be necessary to use existing optical-fibre technology. The trouble is, quantum information is fragile and cannot be copied; it is often carried by individual photons, rather than by laser pulses that can be detected and then amplified and emitted again. This limits the entangled photons to travelling a few tens of kilometres before losses make the whole thing impractical. “They also are affected by temperature changes throughout the day — and even by wind, if they’re above ground,” says Northup. “That’s why generating entanglement across an actual city is a big deal.”
The three demonstrations each used different kinds of ‘quantum memory’ device to store a qubit, a physical system such as a photon or atom that can be in one of two states — akin to the ‘1’ or ‘0’ of ordinary computer bits — or in a combination, or ‘quantum superposition’, of the two possibilities.
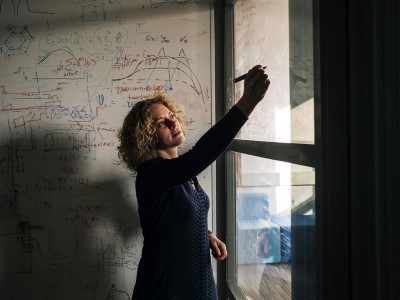
The quantum internet has arrived (and it hasn’t)
In one of the Nature studies, led by Pan Jian-Wei at the University of Science and Technology of China (USTC) in Hefei, qubits were encoded in the collective states of clouds of rubidium atoms 1 . The qubits’ quantum states can be set using a single photon, or can be read out by ‘tickling’ the atomic cloud to emit a photon. Pan’s team had such quantum memories set up in three separate labs in the Hefei area. Each lab was connected by optical fibres to a central ‘photonic server’ around 10 kilometres away. Any two of these nodes could be put in an entangled state if the photons from the two atom clouds arrived at the server at exactly the same time.
By contrast, Hanson and his team established a link between individual nitrogen atoms embedded in small diamond crystals with qubits encoded in the electron states of the nitrogen and in the nuclear states of nearby carbon atoms 3 . Their optical fibre went from the university in Delft through a tortuous 25-kilometre path across the suburbs of The Hague to reach a second laboratory in the city.
In the US experiment, Mikhail Lukin, a physicist at Harvard University in Cambridge, Massachusetts, and his collaborators also used diamond-based devices, but with silicon atoms instead of nitrogen, making use of the quantum states of both an electron and a silicon nucleus 2 . Single atoms are less efficient than atomic ensembles at emitting photons on demand, but they are more versatile, because they can perform rudimentary quantum computations. “Basically, we entangled two small quantum computers,” says Lukin. The two diamond-based devices were in the same building at Harvard, but to mimic the conditions of a metropolitan network, the researchers used an optical fibre that snaked around the local Boston area. “It crosses the Charles River six times,” Lukin says.
Challenges ahead
The entanglement procedure used by the Chinese and the Dutch teams required photons to arrive at a central server with exquisite timing precision, which was one of the main challenges in the experiments. Lukin’s team used a protocol that does not require such fine-tuning: instead of entangling the qubits by getting them to emit photons, the researchers sent one photon to entangle itself with the silicon atom at the first node. The same photon then went around the fibre-optic loop and came back to graze the second silicon atom, thereby entangling it with the first.
Pan has calculated that at the current pace of advance, by the end of the decade his team should be able to establish entanglement over 1,000 kilometres of optical fibres using ten or so intermediate nodes, with a procedure called entanglement swapping . (At first, such a link would be very slow, creating perhaps one entanglement per second, he adds.) Pan is the leading researcher for a project using the satellite Micius , which demonstrated the first quantum-enabled communications in space, and he says there are plans for a follow-up mission.
“The step has now really been made out of the lab and into the field,” says Hanson. “It doesn’t mean it’s commercially useful yet, but it’s a big step.”
Nature 629 , 734-735 (2024)
doi: https://doi.org/10.1038/d41586-024-01445-2
Knaut, C. M. et al. Nature 629 , 573–578 (2024).
Article PubMed Google Scholar
Liu, J. L. et al. Nature 629 , 579–585 (2024).
Stolk, A. J. et al. Preprint at arXiv https://doi.org/10.48550/arXiv.2404.03723 (2024).
Download references
Reprints and permissions
Related Articles
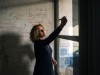
The AI–quantum computing mash-up: will it revolutionize science?
- Quantum physics
- Quantum information
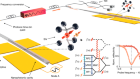
Entanglement of nanophotonic quantum memory nodes in a telecom network
Article 15 MAY 24
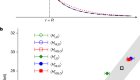
Wavefunction matching for solving quantum many-body problems
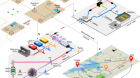
Creation of memory–memory entanglement in a metropolitan quantum network
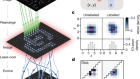
An atomic boson sampler
Article 08 MAY 24
Sir Run Run Shaw Hospital, School of Medicine, Zhejiang University, Warmly Welcomes Talents Abroad
“Qiushi” Distinguished Scholar, Zhejiang University, including Professor and Physician
No. 3, Qingchun East Road, Hangzhou, Zhejiang (CN)
Sir Run Run Shaw Hospital Affiliated with Zhejiang University School of Medicine
Associate Editor, Nature Briefing
Associate Editor, Nature Briefing Permanent, full time Location: London, UK Closing date: 10th June 2024 Nature, the world’s most authoritative s...
London (Central), London (Greater) (GB)
Springer Nature Ltd
Professor, Division Director, Translational and Clinical Pharmacology
Cincinnati Children’s seeks a director of the Division of Translational and Clinical Pharmacology.
Cincinnati, Ohio
Cincinnati Children's Hospital & Medical Center
Data Analyst for Gene Regulation as an Academic Functional Specialist
The Rheinische Friedrich-Wilhelms-Universität Bonn is an international research university with a broad spectrum of subjects. With 200 years of his...
53113, Bonn (DE)
Rheinische Friedrich-Wilhelms-Universität
Recruitment of Global Talent at the Institute of Zoology, Chinese Academy of Sciences (IOZ, CAS)
The Institute of Zoology (IOZ), Chinese Academy of Sciences (CAS), is seeking global talents around the world.
Beijing, China
Institute of Zoology, Chinese Academy of Sciences (IOZ, CAS)
Sign up for the Nature Briefing newsletter — what matters in science, free to your inbox daily.
Quick links
- Explore articles by subject
- Guide to authors
- Editorial policies
Home › Study Tips › Research Skills: What They Are and How They Benefit You
Research Skills: What They Are and How They Benefit You
- Published May 23, 2024
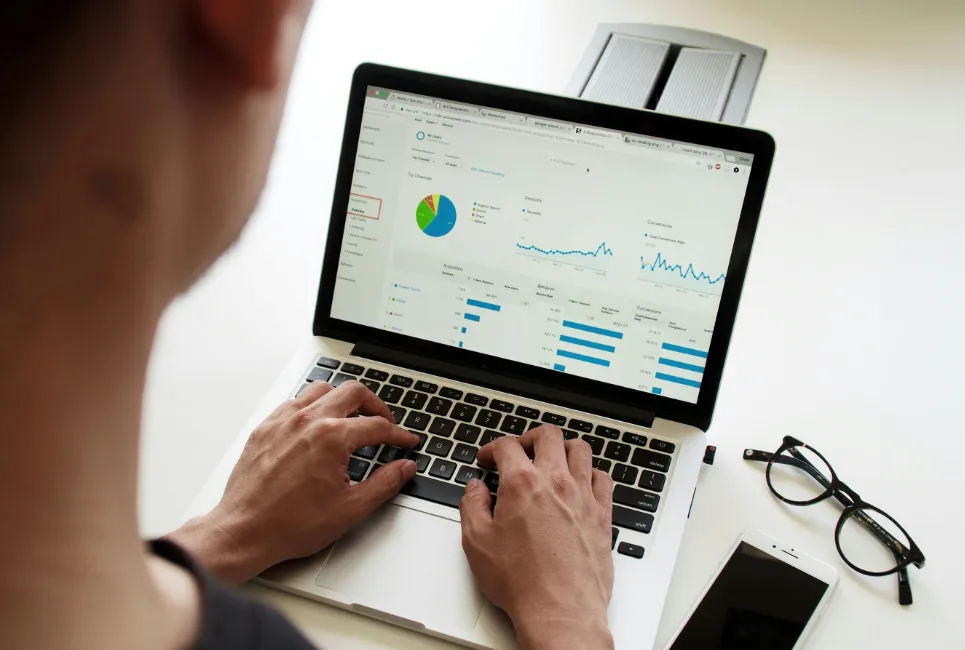
Table of Contents
Research skills give you the ability to gather relevant information from different sources and analyse it critically in order to develop a comprehensive understanding of a subject. Thus, research skills are fundamental to academic success.
Developing these skills will improve your studies, helping you understand subjects better and positioning you for academic success.
That said, how can you develop important research skills? This will explore what research skills are, identify the core ones, and explain how you can develop them.
What Are Research Skills?
Research skills are a set of abilities that allow individuals to find and gather reliable information and then evaluate the information to find answers to questions.
Good research skills are important in academic settings, as finding and critically evaluating relevant information can help you gain a deeper understanding of a subject.
These skills are also important in professional and personal settings. When you graduate and are working in a professional capacity, you’ll often need to analyse sets of data to identify issues and determine how to solve them.
In personal contexts, you’ll always need to assess relevant information to make an informed decision. Whether you’re deciding on a major purchase, choosing a healthcare provider, or planning to make an investment, you’ll need to evaluate options to ensure better decision outcomes.
Different Types of Research Skills
Research skills are categorised into different sub-skills. The most common types are:
Quantitative Skills
Quantitative skills refer to the ability to work with numerical data and perform mathematical and statistical analyses to extract meaningful insights and draw conclusions.
When you have quantitative skills, you’ll be able to apply mathematical concepts and operations in research design and data analysis.
You’ll also be proficient in using statistical methods to analyse data and interpreting numerical data to draw meaningful conclusions.
Analytical Skills
Analytical skills refer to the ability to gather data, evaluate it, and draw sound conclusions. When you have analytical skills, you’ll be able to systematically analyse information to reach a reasonable conclusion.
Analytical skills are important in problem-solving. They help you to break down complex problems into more manageable components, think critically about the information at hand, analyse root causes, and develop effective solutions.
Qualitative Skills
Qualitative skills refer to the ability to collect, analyse, and interpret non-numerical data. When you have qualitative skills, you’ll be proficient in observation, interviewing, and other methods for collecting qualitative research data.
You’ll also be able to analyse non-numerical data, such as documents and images, to identify themes, patterns, and meanings.
Research Skills Examples
The core research skills you need for success in academic, professional, and personal contexts include:
Data Collection
Data is at the centre of every research, as data is what you assess to find the answers you seek. Thus, research starts with collecting relevant data.
Depending on the research, there are two broad categories of data you can collect: primary and secondary.
Primary data is generated by the researcher, like data from interviews, observations, or experiments. Secondary data is pre-existing data obtained from different existing databases, like published literature, government reports, etc.
Thus, data collection is more than gathering information from the Internet. Depending on the research, it can require more advanced skills for conducting experiments to generate your own data.
Source Evaluation
When doing research on any subject (especially when using the Internet), you’ll be amazed at the volume of information you’ll find. And a lot is pure garbage that can compromise your research work.
Thus, an important research skill is being able to dig through the garbage to get to the real facts. This is where source evaluation comes in!
Good research skills call for being able to identify biases, assess the authority of the author, and determine the accuracy of information before using it.
Time Management Skills
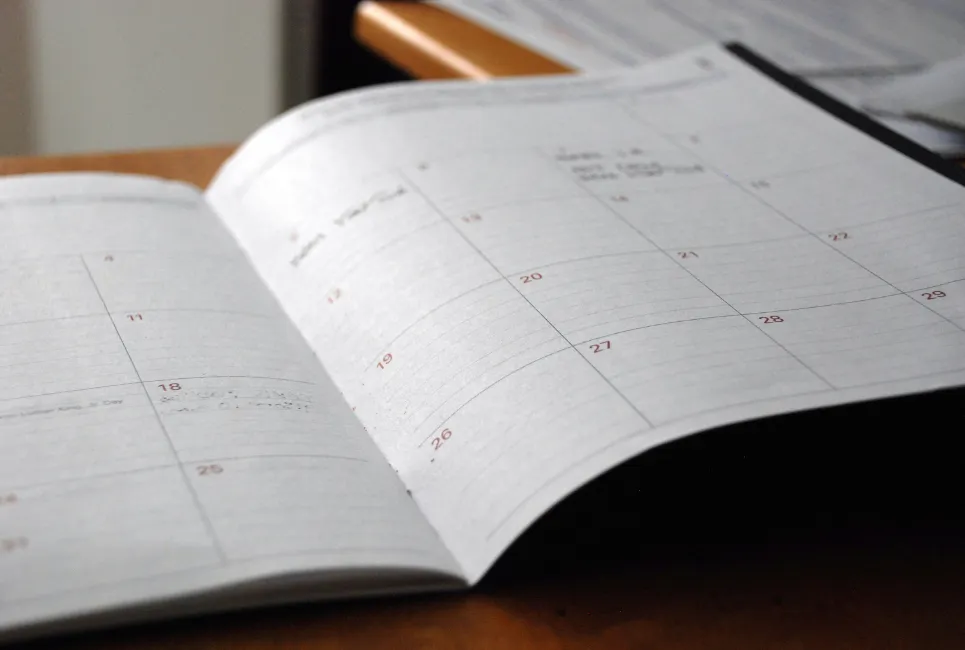
Have you ever felt that there is not enough time in a day for all that you need to do? When you already have so much to do, adding research can be overwhelming.
Good time management skills can help you find the time to do all you need to do, including relevant research work, making it an essential research skill.
Time management allows you to plan and manage your research project effectively. It includes breaking down research tasks into more manageable parts, setting priorities, and allocating time to the different stages of the research.
Communication Skills
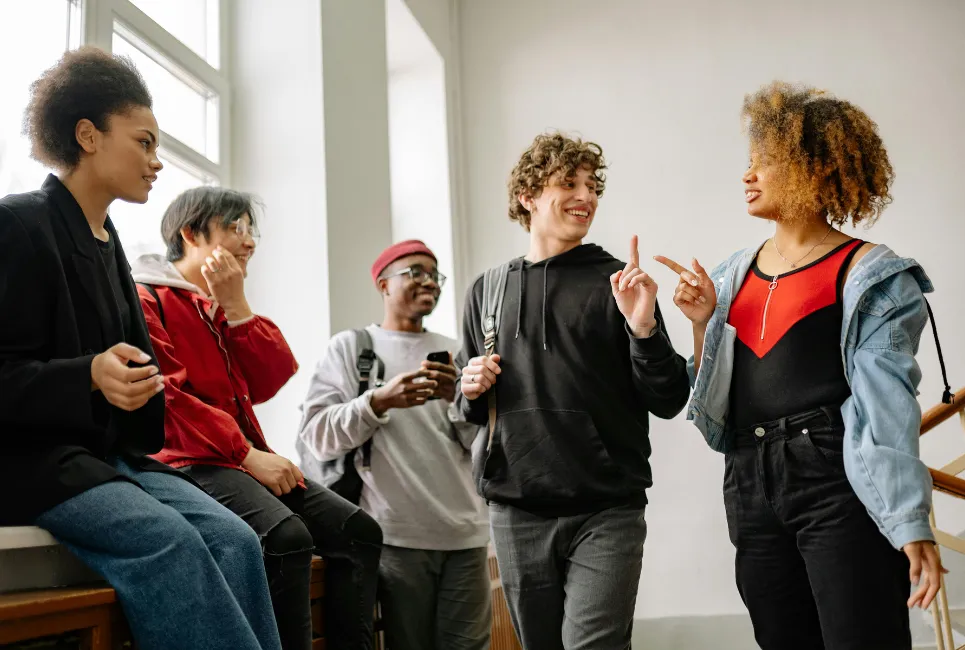
Communication is an important aspect of every research, as it aids in data collection and sharing research findings.
Important communication skills needed in research include active listening, active speaking, interviewing, report writing, data visualisation, and presentation, etc.
For example, when research involves collecting primary data via interviews, you must have sound speaking and listening skills.
When you conclude the research and need to share findings, you’ll need to write a research report and present key findings in easy-to-understand formats like charts.
Attention to Detail
Attention to detail is the ability to achieve thoroughness and accuracy when doing something. It requires focusing on every aspect of the tasks, even small ones.
Anything you miss during your research will affect the quality of your research findings. Thus, the ability to pay close attention to details is an important research skill.
You need attention to detail at every stage of the research process. During data collection, it helps you ensure reliable data.
During analysis, it reduces the risk of error to ensure your results are trustworthy. It also helps you express findings precisely to minimise ambiguity and facilitate understanding.
Note-Taking
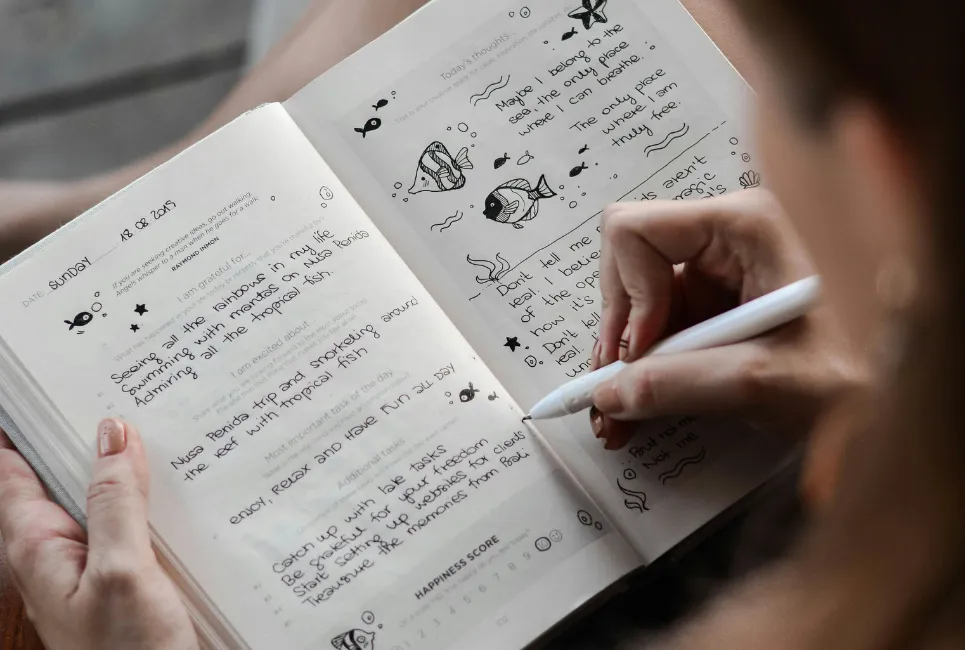
Note-taking is exactly what it sounds like—writing down key information during the research process.
Remember that research involves sifting through and taking in a lot of information. It’s impossible to take in all the information and recall it from memory. This is where note-taking comes in!
Note-taking helps you capture key information, making it easier to remember and utilise for the research later. It also involves writing down where to look for important information.
Critical Thinking
Critical thinking is the ability to think rationally and synthesise information in a thoughtful way. It is an important skill needed in virtually all stages of the research process.
For example, when collecting data, you need critical thinking to assess the quality and relevance of data. It can help you identify gaps in data to formulate your research question and hypothesis.
It can also help you to identify patterns and make reasonable connections when interpreting research findings.
Data Analysis
Data may not mean anything until you analyse it qualitatively or quantitatively (using techniques like Excel or SPSS). For this reason, data analysis analysis is an important research skill.
Researchers need to be able to build hypotheses and test these using appropriate research techniques. This helps to draw meaningful conclusions and gain a comprehensive understanding of research data.
Problem-Solving Skills
Research often involves addressing specific questions and solving problems. For this reason, problem-solving skills are important skills when conducting research.
Problem-solving skills refer to the ability to identify, analyse, and solve problems effectively.
With problem-solving skills, you’ll be able to assess a situation, consider various solutions, and choose the most appropriate course of action toward finding a solution.
Benefits of Research Skills
Research skills have many benefits, including:
Enhances Critical Thinking
Research skills and critical thinking are intertwined such that developing one enhances the other.
Research requires people to question assumptions, evaluate evidence, analyse information, and draw conclusions. These activities require you to think critically about the information at hand. Hence, engaging in research enhances critical thinking.
Develops Problem-Solving Skills
Research helps you acquire a set of critical skills that are directly transferable to problem-solving.
For example, research fosters creative thinking, as it often requires synthesising data from different sources and connecting different concepts. After developing creative thinking via research, you can apply the skill to generate innovative solutions in problem-solving situations.
Helps in Knowledge Acquisition
Engaging in research is a powerful way to acquire knowledge. Research involves exploring new ideas, and this helps you expand your breadth of knowledge.
It also involves applying research methods and methodologies. So, you’ll acquire knowledge about research methods, enhancing your ability to design and conduct studies in your higher education or professional life.
Why Are Research Skills Important?
Strong research skills offer numerous benefits, especially for students’ academic learning and development.
When you develop good research skills, you’ll reap great academic rewards that include:
In-Depth Understanding
Conducting research allows you to delve deep into specific topics, helping you gain a thorough understanding of the subject matter beyond what is covered in standard coursework.
Critical Thinking Development
Research involves critical evaluation of information and making informed decisions. This builds your ability to think critically.
This skill will not only help you solve academic problems better, but it’s also crucial to your personal and professional growth.
Encouragement of Independent Learning
Research encourages independent learning. When you engage in research, you seek answers independently. You take the initiative to find, retrieve, and evaluate information relevant to your research.
That helps you develop self-directed study habits. You’ll be able to take ownership of your education and actively seek out information for a better understanding of the subject matter.
Intellectual Curiosity Development
Research skills encourage intellectual curiosity and a love of learning, as they’ll make you explore topics you find intriguing or important. Thus, you’ll be more motivated to explore topics beyond the scope of your coursework.
Enhanced Communication Skills
Research helps you build better interpersonal skills as well as report-writing skills.
Research helps you sharpen your communication skills when you interact with research subjects during data collection. Communicating research findings to an audience also helps sharpen your presentation skills or report writing skills.
Assistance in Career Preparation
Many professions find people with good research skills. Whether you’ll pursue a career in academia, business, healthcare, or IT, being able to conduct research will make you a valuable asset.
So, researching skills for students prepares you for a successful career when you graduate.
Contribution to Personal Growth
Research also contributes to your personal growth. Know that research projects often come with setbacks, unexpected challenges, and moments of uncertainty. Navigating these difficulties helps you build resilience and confidence.
Acquisition of Time Management Skills
Research projects often come with deadlines. Such research projects force you to set goals, prioritise tasks, and manage your time effectively.
That helps you acquire important time management skills that you can use in other areas of academic life and your professional life when you graduate.
Ways to Improve Research Skills
The ways to improve your research skills involve a combination of learning and practice.
You should consider enrolling in research-related programmes, learning to use data analysis tools, practising summarising and synthesising information from multiple sources, collaborating with more experienced researchers, and more.
Looking to improve your research skills? Read our 11 ways to improve research skills article.
How Can I Learn Research Skills?
You can learn research skills using these simple three-point framework:
Clarifying the Objective
Start by articulating the purpose of your research. Identify the specific question you are trying to answer or the problem you are aiming to solve.
Then, determine the scope of your research to help you stay focused and avoid going after irrelevant information.
Cross-Referencing Sources
The next step is to search for existing research on the topic. Use academic databases, journals, books, and reputable online sources.
It’s important to compare information from multiple sources, taking note of consensus among studies and any conflicting findings.
Also, check the credibility of each source by looking at the author’s expertise, information recency, and reputation of the publication’s outlet.
Organise the Research
Develop a note-taking system to document key findings as you search for existing research. Create a research outline, then arrange your ideas logically, ensuring that each section aligns with your research objective.
As you progress, be adaptable. Be open to refining your research plan as new understanding evolves.
Enrolling in online research programmes can also help you build strong research skills. These programmes combine subject study with academic research project development to help you hone the skills you need to succeed in higher education.
Immerse Education is a foremost provider of online research programmes.
Acquire Research Skills with Immerse Education
Research skills are essential to academic success. They help you gain an in-depth understanding of subjects, enhance your critical thinking and problem-solving skills, improve your time management skills, and more.
In addition to boosting you academically, they contribute to your personal growth and prepare you for a successful professional career.
Thankfully, you can learn research skills and reap these benefits. There are different ways to improve research skills, including enrolling in research-based programmes. This is why you need Immerse Education!
Immerse Education provides participants aged 13-18 with unparalleled educational experience. All our programmes are designed by tutors from top global universities and help prepare participants for future success.
Our online research programme expertly combines subject study with academic research projects to help you gain subject matter knowledge and the important research skills you need to succeed in higher education. With one-on-one tutoring or group sessions from an expert academic from Oxford or Cambridge University and a flexible delivery mode, the programme is designed for you to succeed. Subsequently, enrolling in our accredited Online Research Programme will award students with 8 UCAS points upon completion.
Related Content
11 tips to improve your research skills for academic success.
Research suggests that plastic pollution must be reduced by at least 5% every year to make progress towards UN targets by the end of the century.
The study, a collaboration between researchers at Imperial College London and GNS Science, suggests that reducing plastic pollution by 5% per year would stabilize the level of microplastics – plastics less than 5 mm in length – in the surface oceans.
However, the modelling shows that even reducing pollution by 20% per year would not significantly reduce existing microplastics levels, meaning they will persist in our oceans beyond 2100.
Microplastics have been found to be circulating in all of the Earth’s oceans and some of the greatest concentrations of them are thousands of miles from land. These tiny particles of plastics can be hazardous to marine life and they find their way back from our oceans into human food systems.
The United Nations Environmental Assembly (UNEA) are aiming to adopt a legally binding resolution to completely eradicate the production of plastic pollution from 2040, including ocean microplastics.
Whilst our results show that microplastics will be in the oceans past the end of the century, stabilising their levels is the first step towards elimination. Zhenna Azimrayat Andrews Lead author and masters student at the Centre for Environmental Policy, Imperial College London
The researchers developed a model to predict the impact on ocean microplastics of eight different scenarios of plastic pollution reduction over the next century, starting from 2026 up to 2100.
The results, published in Environmental Research Letters , show that if countries reduce plastic pollution by more than 5% each year, the amount of microplastics in the ocean could stabilise, rather than continue to increase.
First author Zhenna Azimrayat Andrews, who completed the work for her MSc in Environmental Technology at the Centre for Environmental Policy , Imperial College London, said: “Plastic is now everywhere in the environment, and the ocean is no exception.
"Whilst our results show that microplastics will be around in the oceans past the end of the century, stabilising their levels is the first step towards elimination."
Removing microplastics from the ocean’s surface
Microplastics pose the greatest threat when they accumulate in the surface ocean, where they are consumed by ocean life, including fish that we may eat. One way microplastics can be removed from the surface ocean is by clumping together with tiny living organisms or waste material, like organic debris or animal droppings. These clumps can sink down into the deep ocean, taking the microplastics with them.
The team’s calculations, combined with real-world observations and testing of the model, suggest that the buoyancy of the microplastics prevents these clumps from sinking, trapping them near the surface. Understanding how these clumps affect the levels of microplastics in the ocean is important for setting goals to reduce plastic pollution.
As marine life holds onto microplastics near the surface, even if the level of pollution produced every year is reduced, there would still be microplastics in the surface ocean for centuries. When they do sink, they will subsequently last in the deeper levels of the ocean for much longer, where their impacts are not well known.
Azimrayat Andrews said: “There can never be a completely successful removal of microplastics from all depths of the ocean, we kind of just need to live with it now. But the current global output of plastic pollution is so great, that even a 1% annual reduction in pollution would make a big difference overall.”

Setting ambitious and realistic goals
The researchers’ model is the first study that examines the efficacy of plausible treaty reduction targets. The large reductions required to reduce contamination indicate that a more coordinated international policy is necessary, rather than the UN’s proposed goal of 0% plastic pollution by 2040.
“If we want to move towards a lower plastic society, change needs to happen at a higher level: an industrial level. No single individual should have the weight of the world on their shoulders. Zhenna Azimrayat Andrews Lead author and Masters student in Environmental Technology at the Centre for Environmental Policy
Azimrayat Andrews said: “If we want to move towards a lower plastic society, change needs to happen at a higher level: an industrial level. No single individual should have the weight of the world on their shoulders.
“Therefore, we need a more sustainable lifestyle integration, rather than people having to make individual choices, and so organisations like the NHS don’t have this pressure to become zero plastic in 10 years because the UN said so. National organisations will need to reduce their plastic use, but systemic change in industrial and commercial sectors could allow more grace for organisations like the NHS in the meantime.”
The researchers hope their analysis will help inform UN negotiations, which are planned throughout the year.
‘ Slow biological microplastics removal under ocean pollution phase-out trajectories ’ by Zhenna Azimrayat Andrews, Karin Kvale and Claire Hunt is published in Environmental Research Letters .
Article text (excluding photos or graphics) © Imperial College London.
Photos and graphics subject to third party copyright used with permission or © Imperial College London.

Alex Epshtein Centre for Languages, Culture and Communication
Climate-change , Environment , REF , Research , Pollution See more tags
Leave a comment
Your comment may be published, displaying your name as you provide it, unless you request otherwise. Your contact details will never be published.
Latest news
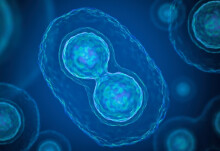
News in brief
Cell division decisions and cancer recommendations: news from imperial, impact of giving, impact of giving event to commemorate the impact of philanthropy, heartening news, imperial team awarded £5m from bhf to support world-leading heart research, most popular.
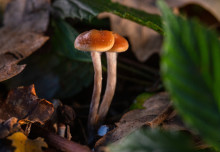
Assessing the interoperability of digital public services in the EU: the sooner, the better
JRC scientific evidence supporting the Interoperable Europe Act sheds light on benefits and challenges for connected European public administrations.

As national public services become more digitalised, interoperability assessments (IOPAs) have become an essential tool for improving cross-border interaction of public administrations. According to JRC research , early interoperability assessment can substantially reduce the investment, save resources and time, ease implementation and ensure higher quality of public services.
The interoperability assessments are introduced by the Interoperable Europe Act , a fresh legislation designed to help make interconnected digital public services a reality. Examples of services that can benefit from this include recognition of diplomas or professional qualifications, exchange of vehicle data for road safety, access to social security and health data, information exchange related to taxation, customs, public tender accreditation, digital driving licenses, or commercial registers.
The legislation aims to strengthen cross-border data exchange to benefit citizens, businesses, and public administrations. A JRC analysis found that improved interoperability would bring a 0.4% GDP increase to EU economy, save annually €543 million for citizens and €568 billion to businesses. These findings were recently cited in the report on the future of the single market (PDF) , prepared by former Italian Prime Minister Enrico Letta.
The Interoperable Europe Act, in force since 11 April, will become applicable as of 12 July 2024. It however envisages that European Institutions, bodies and agencies, and public entities start running interoperability assessments (IOPAs) as of January 2025. The assessments will help to detect and tackle barriers to cross-border interoperability early, in the design phase of policies and public services. According to the JRC analysis, a successful implementation of the IOPA requires cultural and organisational change.
The JRC has been at the forefront in supporting policy makers with robust evidence for the preparation, interinstitutional negotiations and, currently, implementation of the Act. JRC studies on interoperability highlight efforts, benefits and challenges for European public administrations, supporting their adoption of an “interoperable-by-design" approach.
Counting on innovation
The Interoperable Europe Act sets out measures to support the adoption of innovative solutions by the public sector. An in-depth JRC analysis of more than 180 use cases, Artificial Intelligence for Interoperability , finds that AI can offer numerous opportunities to improve service delivery and the overall efficiency of government operations. It also indicates further steps that policy makers may need to take to leverage AI use, such as raising awareness of the AI potential within the public institutions, and cross-organisational collaboration.
Tracking progress
The legislation establishes a monitoring scheme for ensuring the progress towards seamless functioning of cross-border digital services. A JRC study identified opportunities for streamlining the monitoring of European digital policies and presented key recommendations.
The authors highlighted elements that need attention, ranging from improving the timing of information requests to assessing if indicators are relevant.
They also pointed to the challenge of managing cases where EU countries move at different speeds in their digitalisation, and encouraging those still learning. It is important to reconsider monitoring beyond mere output measurement, and shift attention towards digital policy outcomes and impacts. Piloting alternative approaches should help understand whether minor adjustments or more fundamental changes are needed.
Among the recommendations, the study indicates the need to harmonise the monitoring of different digital policies to keep them coherent and aligned, including the Digital Decade targets for skills, governments, businesses and infrastructure.
GovTech, the government approach to modernise the public sector
The Interoperability Europe Act is also the first regulation to explicitly promote the design and deployment of GovTech solutions, where the public sector engages with private actors, especially with SMEs and start-ups, to procure innovative and interoperable technology solutions. The JRC reports contributed to deepen the understanding of the GovTech landscape and markets, bringing the attention to innovating procurement practices to exploit the full potential of GovTech.
The coming months will be dedicated to support the early implementation of the Interoperability Europe Act. The lines of investigations will focus on the support to interoperability assessments, regulatory sandboxing and learning, the competence building for digital skills of public servants, and the provision of human-centric digital public services. They will include investigating the adoption of AI solutions by public administrations, and the related citizen's acceptance, as well as the role of the private sector for the digital service development and delivery in the EU.
Related content
Interoperability Assessments: Exploring expected benefits, efforts and challenges
Artificial Intelligence for Interoperability in the European Public Sector
Quantifying the Benefits of Location Interoperability in the European Union
Identifying opportunities for streamlining European monitoring of digital policies
Scoping GovTech dynamics in the EU
- Digital transition
More news on a similar topic
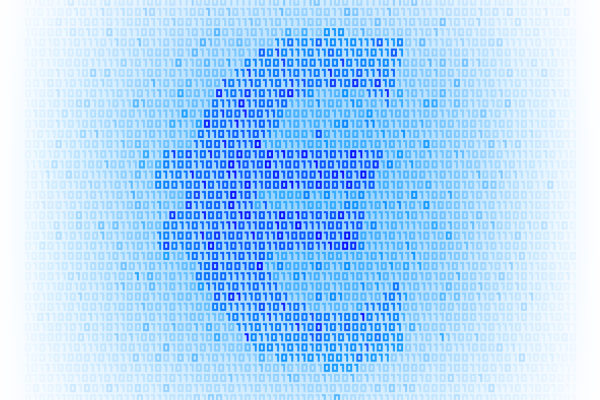
- General publications
- 20 March 2024
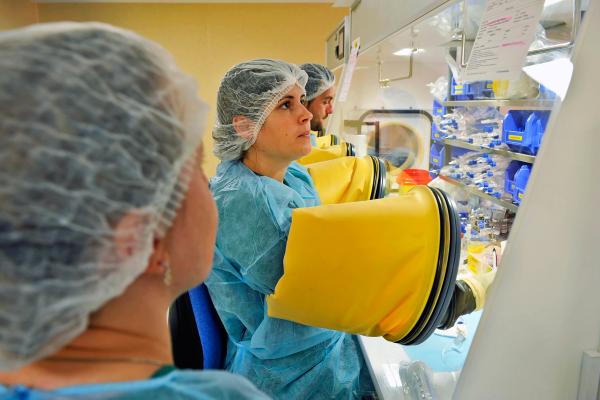
- News announcement
- 8 March 2024
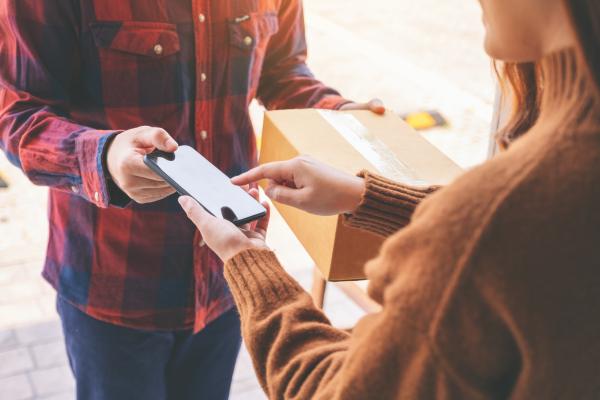
- 23 February 2024
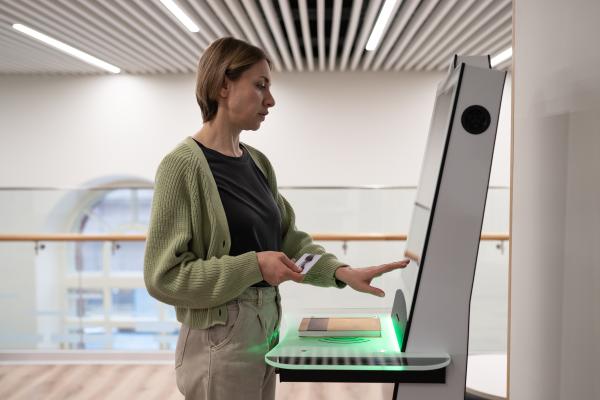
- 20 October 2023
Share this page
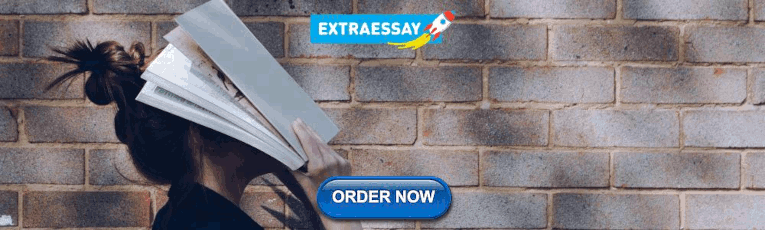
IMAGES
VIDEO
COMMENTS
A forum where fellows can talk about their research idea, designs, and results and get feedback in an informal, comfortable setting. All stages: starting a project, planning the design, analyzing the data, or getting ready to present your results at conferences or in manuscripts. Different ...
For each of the 10 topics in the research portfolio, the committee first characterizes the status of relevant research and progress, including the approximate numbers of studies in progress on various subtopics (the committee did not attempt to list all relevant research projects but did attempt to capture the major studies across the spectrum of the research in progress), then considers the ...
Progress in Science. This chapter examines theories and empirical findings on the overlapping topics of progress in science and the factors that contribute to scientific discoveries. It also considers the implications of these findings for behavioral and social science research on aging. The chapter first draws on contributions from the history ...
The research process often begins with a very broad idea for a topic you'd like to know more about. You do some preliminary research to identify a problem. After refining your research questions, you can lay out the foundations of your research design, leading to a proposal that outlines your ideas and plans.
Using this format as an example: Introduction This would probably be similar to the introduction on your final paper, but it should also give a bit of context on the progress of the project, so the reader knows how far along it is. Methods Depending on the progress of the project, talk about what have already done, what you plan to do, and/or ...
In Chapter 1, we saw that scientific research is the process of acquiring scientific knowledge using the scientific method. But how is such research conducted? This chapter delves into the process of scientific research, and the assumptions and outcomes of the research process.
Research is a rigorous problem-solving process whose ultimate goal is the discovery of new knowledge. Research may include the description of a new phenomenon, definition of a new relationship, development of a new model, or application of an existing principle or...
Research Performance Progress Report (RPPR) The RPPR is used by recipients to submit progress reports to NIH on their grant awards. This page provides an overview of the annual RPPR, the final RPPR and the interim RPPR and provides resources to help you understand how to submit a progress report.
Interim (or progress) reports present the interim, preliminary, or initial evaluation findings. Interim reports are scheduled according to the specific needs of your evaluation users, often halfway through the execution of a project. The interim report is necessary to let a project's stakeholders know how an intervention is going.
Here, we quantify relative progress in research topics of a nation from the time-series comparison of reference lists from papers, using 71 million published papers from Scopus. We discover a ...
Research should be conducted in a systematic manner, allowing the researcher to progress from a general idea or clinical problem to scientifically rigorous research findings that enable new developments to improve clinical practice. Using a research process helps guide this process. This article is the first in a 26-part series on nursing research. It examines the process that is common to all ...
Research Process is a systematic and structured approach that involves the collection, analysis, and interpretation of data or information to answer a specific research question or solve a particular problem.
Important aspects of Research in Progress lie in facilitating publication of tentative results, sharing of research approaches and discussion of research designs. The editors emphasize the need for a strong research foundation, as in literature grounding or careful research question design, and open and honest discussion of successes and failures.
The main objectives of progress reports are accountability and project monitoring. Project report summarizes project goal, the status of the target during the reporting period discusses significant costs and scheduling issues, and lists plans.
Research is defined as a meticulous and systematic inquiry process designed to explore and unravel specific subjects or issues with precision. Learn more about types of research, processes, and methods with best practices.
Abstractspiepr Abs1. Every day people do research as they gather information to learn about something of interest. In the scientific world, however, research means something different than simply gathering information. Scientific research is characterized by its careful planning and observing, by its relentless efforts to understand and explain ...
I currently work at a research org, and will be applying to PhD programs soon. For my CV, I am a co-author on a few publications that I'll be able to add, but have been recommended to add some in-progress or under review reports.
The research process identifies the research problem, sets the research design and sample size, collects and analyzes the data, and reports on the findings.
Another way to say Research In Progress? Synonyms for Research In Progress (other words and phrases for Research In Progress).
Nursing research is more than just an academic pursuit—it is a dynamic process that empowers nurses to question, explore, and innovate in the pursuit of better patient care. Through rigorous inquiry and scientific investigation, nurse researchers uncover insights that inform clinical practice, shape health policy, and drive transformational change within healthcare systems. From the bedside ...
6.1 Functions and Contents of Progress Reports. In the progress report, you explain any or all of the following: Progress reports have several important functions: Reassure recipients that you are making progress, that the project is going smoothly, and that it will be complete by the expected date. Provide recipients with a brief look at some ...
I have submitted my research article to a journal in June 2019. The status was "Under review" for 4 months, after which, on October 8, it changed to "Decision in progress." What does this statement mean? Will my paper rejected?
A progress report is a document that explains in detail how much progress you have made towards the completion of your ongoing project.
Learn how to effectively share research progress with your team using regular meetings, progress reports, digital tools, and more for successful collaboration.
Progress monitoring is an assessment technique that tells teachers how and when to adjust curriculum so that students meet benchmark goals by the end of the year. This research shows that progress monitoring is an effective way to set and meet academic goals.
Experiments generate quantum entanglement over optical fibres across three real cities, marking progress towards networks that could have revolutionary applications.
Research skills give you the ability to gather relevant information from different sources and analyse it critically in order to develop a comprehensive understanding of a subject. Thus, research skills are fundamental to academic success. Developing these skills will improve your studies, helping you understand subjects better and positioning ...
Sociology. Qualitative research is a type of research that aims to gather and analyse non-numerical (descriptive) data in order to gain an understanding of individuals' social reality, including understanding their attitudes, beliefs, and motivation. This type of research typically involves in-depth interviews, focus groups, or observations in ...
Research suggests that plastic pollution must be reduced by at least 5% every year to make progress towards UN targets by the end of the century.
The legislation establishes a monitoring scheme for ensuring the progress towards seamless functioning of cross-border digital services. A JRC study identified opportunities for streamlining the monitoring of European digital policies and presented key recommendations.