
An official website of the United States government
The .gov means it’s official. Federal government websites often end in .gov or .mil. Before sharing sensitive information, make sure you’re on a federal government site.
The site is secure. The https:// ensures that you are connecting to the official website and that any information you provide is encrypted and transmitted securely.
- Publications
- Account settings
Preview improvements coming to the PMC website in October 2024. Learn More or Try it out now .
- Advanced Search
- Journal List
- Can J Hosp Pharm
- v.68(4); Jul-Aug 2015

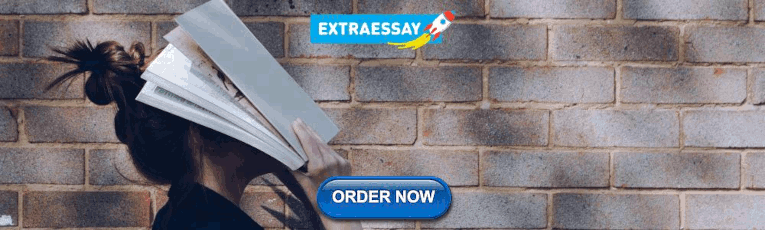
Creating a Data Analysis Plan: What to Consider When Choosing Statistics for a Study
There are three kinds of lies: lies, damned lies, and statistics. – Mark Twain 1
INTRODUCTION
Statistics represent an essential part of a study because, regardless of the study design, investigators need to summarize the collected information for interpretation and presentation to others. It is therefore important for us to heed Mr Twain’s concern when creating the data analysis plan. In fact, even before data collection begins, we need to have a clear analysis plan that will guide us from the initial stages of summarizing and describing the data through to testing our hypotheses.
The purpose of this article is to help you create a data analysis plan for a quantitative study. For those interested in conducting qualitative research, previous articles in this Research Primer series have provided information on the design and analysis of such studies. 2 , 3 Information in the current article is divided into 3 main sections: an overview of terms and concepts used in data analysis, a review of common methods used to summarize study data, and a process to help identify relevant statistical tests. My intention here is to introduce the main elements of data analysis and provide a place for you to start when planning this part of your study. Biostatistical experts, textbooks, statistical software packages, and other resources can certainly add more breadth and depth to this topic when you need additional information and advice.
TERMS AND CONCEPTS USED IN DATA ANALYSIS
When analyzing information from a quantitative study, we are often dealing with numbers; therefore, it is important to begin with an understanding of the source of the numbers. Let us start with the term variable , which defines a specific item of information collected in a study. Examples of variables include age, sex or gender, ethnicity, exercise frequency, weight, treatment group, and blood glucose. Each variable will have a group of categories, which are referred to as values , to help describe the characteristic of an individual study participant. For example, the variable “sex” would have values of “male” and “female”.
Although variables can be defined or grouped in various ways, I will focus on 2 methods at this introductory stage. First, variables can be defined according to the level of measurement. The categories in a nominal variable are names, for example, male and female for the variable “sex”; white, Aboriginal, black, Latin American, South Asian, and East Asian for the variable “ethnicity”; and intervention and control for the variable “treatment group”. Nominal variables with only 2 categories are also referred to as dichotomous variables because the study group can be divided into 2 subgroups based on information in the variable. For example, a study sample can be split into 2 groups (patients receiving the intervention and controls) using the dichotomous variable “treatment group”. An ordinal variable implies that the categories can be placed in a meaningful order, as would be the case for exercise frequency (never, sometimes, often, or always). Nominal-level and ordinal-level variables are also referred to as categorical variables, because each category in the variable can be completely separated from the others. The categories for an interval variable can be placed in a meaningful order, with the interval between consecutive categories also having meaning. Age, weight, and blood glucose can be considered as interval variables, but also as ratio variables, because the ratio between values has meaning (e.g., a 15-year-old is half the age of a 30-year-old). Interval-level and ratio-level variables are also referred to as continuous variables because of the underlying continuity among categories.
As we progress through the levels of measurement from nominal to ratio variables, we gather more information about the study participant. The amount of information that a variable provides will become important in the analysis stage, because we lose information when variables are reduced or aggregated—a common practice that is not recommended. 4 For example, if age is reduced from a ratio-level variable (measured in years) to an ordinal variable (categories of < 65 and ≥ 65 years) we lose the ability to make comparisons across the entire age range and introduce error into the data analysis. 4
A second method of defining variables is to consider them as either dependent or independent. As the terms imply, the value of a dependent variable depends on the value of other variables, whereas the value of an independent variable does not rely on other variables. In addition, an investigator can influence the value of an independent variable, such as treatment-group assignment. Independent variables are also referred to as predictors because we can use information from these variables to predict the value of a dependent variable. Building on the group of variables listed in the first paragraph of this section, blood glucose could be considered a dependent variable, because its value may depend on values of the independent variables age, sex, ethnicity, exercise frequency, weight, and treatment group.
Statistics are mathematical formulae that are used to organize and interpret the information that is collected through variables. There are 2 general categories of statistics, descriptive and inferential. Descriptive statistics are used to describe the collected information, such as the range of values, their average, and the most common category. Knowledge gained from descriptive statistics helps investigators learn more about the study sample. Inferential statistics are used to make comparisons and draw conclusions from the study data. Knowledge gained from inferential statistics allows investigators to make inferences and generalize beyond their study sample to other groups.
Before we move on to specific descriptive and inferential statistics, there are 2 more definitions to review. Parametric statistics are generally used when values in an interval-level or ratio-level variable are normally distributed (i.e., the entire group of values has a bell-shaped curve when plotted by frequency). These statistics are used because we can define parameters of the data, such as the centre and width of the normally distributed curve. In contrast, interval-level and ratio-level variables with values that are not normally distributed, as well as nominal-level and ordinal-level variables, are generally analyzed using nonparametric statistics.
METHODS FOR SUMMARIZING STUDY DATA: DESCRIPTIVE STATISTICS
The first step in a data analysis plan is to describe the data collected in the study. This can be done using figures to give a visual presentation of the data and statistics to generate numeric descriptions of the data.
Selection of an appropriate figure to represent a particular set of data depends on the measurement level of the variable. Data for nominal-level and ordinal-level variables may be interpreted using a pie graph or bar graph . Both options allow us to examine the relative number of participants within each category (by reporting the percentages within each category), whereas a bar graph can also be used to examine absolute numbers. For example, we could create a pie graph to illustrate the proportions of men and women in a study sample and a bar graph to illustrate the number of people who report exercising at each level of frequency (never, sometimes, often, or always).
Interval-level and ratio-level variables may also be interpreted using a pie graph or bar graph; however, these types of variables often have too many categories for such graphs to provide meaningful information. Instead, these variables may be better interpreted using a histogram . Unlike a bar graph, which displays the frequency for each distinct category, a histogram displays the frequency within a range of continuous categories. Information from this type of figure allows us to determine whether the data are normally distributed. In addition to pie graphs, bar graphs, and histograms, many other types of figures are available for the visual representation of data. Interested readers can find additional types of figures in the books recommended in the “Further Readings” section.
Figures are also useful for visualizing comparisons between variables or between subgroups within a variable (for example, the distribution of blood glucose according to sex). Box plots are useful for summarizing information for a variable that does not follow a normal distribution. The lower and upper limits of the box identify the interquartile range (or 25th and 75th percentiles), while the midline indicates the median value (or 50th percentile). Scatter plots provide information on how the categories for one continuous variable relate to categories in a second variable; they are often helpful in the analysis of correlations.
In addition to using figures to present a visual description of the data, investigators can use statistics to provide a numeric description. Regardless of the measurement level, we can find the mode by identifying the most frequent category within a variable. When summarizing nominal-level and ordinal-level variables, the simplest method is to report the proportion of participants within each category.
The choice of the most appropriate descriptive statistic for interval-level and ratio-level variables will depend on how the values are distributed. If the values are normally distributed, we can summarize the information using the parametric statistics of mean and standard deviation. The mean is the arithmetic average of all values within the variable, and the standard deviation tells us how widely the values are dispersed around the mean. When values of interval-level and ratio-level variables are not normally distributed, or we are summarizing information from an ordinal-level variable, it may be more appropriate to use the nonparametric statistics of median and range. The first step in identifying these descriptive statistics is to arrange study participants according to the variable categories from lowest value to highest value. The range is used to report the lowest and highest values. The median or 50th percentile is located by dividing the number of participants into 2 groups, such that half (50%) of the participants have values above the median and the other half (50%) have values below the median. Similarly, the 25th percentile is the value with 25% of the participants having values below and 75% of the participants having values above, and the 75th percentile is the value with 75% of participants having values below and 25% of participants having values above. Together, the 25th and 75th percentiles define the interquartile range .
PROCESS TO IDENTIFY RELEVANT STATISTICAL TESTS: INFERENTIAL STATISTICS
One caveat about the information provided in this section: selecting the most appropriate inferential statistic for a specific study should be a combination of following these suggestions, seeking advice from experts, and discussing with your co-investigators. My intention here is to give you a place to start a conversation with your colleagues about the options available as you develop your data analysis plan.
There are 3 key questions to consider when selecting an appropriate inferential statistic for a study: What is the research question? What is the study design? and What is the level of measurement? It is important for investigators to carefully consider these questions when developing the study protocol and creating the analysis plan. The figures that accompany these questions show decision trees that will help you to narrow down the list of inferential statistics that would be relevant to a particular study. Appendix 1 provides brief definitions of the inferential statistics named in these figures. Additional information, such as the formulae for various inferential statistics, can be obtained from textbooks, statistical software packages, and biostatisticians.
What Is the Research Question?
The first step in identifying relevant inferential statistics for a study is to consider the type of research question being asked. You can find more details about the different types of research questions in a previous article in this Research Primer series that covered questions and hypotheses. 5 A relational question seeks information about the relationship among variables; in this situation, investigators will be interested in determining whether there is an association ( Figure 1 ). A causal question seeks information about the effect of an intervention on an outcome; in this situation, the investigator will be interested in determining whether there is a difference ( Figure 2 ).

Decision tree to identify inferential statistics for an association.

Decision tree to identify inferential statistics for measuring a difference.
What Is the Study Design?
When considering a question of association, investigators will be interested in measuring the relationship between variables ( Figure 1 ). A study designed to determine whether there is consensus among different raters will be measuring agreement. For example, an investigator may be interested in determining whether 2 raters, using the same assessment tool, arrive at the same score. Correlation analyses examine the strength of a relationship or connection between 2 variables, like age and blood glucose. Regression analyses also examine the strength of a relationship or connection; however, in this type of analysis, one variable is considered an outcome (or dependent variable) and the other variable is considered a predictor (or independent variable). Regression analyses often consider the influence of multiple predictors on an outcome at the same time. For example, an investigator may be interested in examining the association between a treatment and blood glucose, while also considering other factors, like age, sex, ethnicity, exercise frequency, and weight.
When considering a question of difference, investigators must first determine how many groups they will be comparing. In some cases, investigators may be interested in comparing the characteristic of one group with that of an external reference group. For example, is the mean age of study participants similar to the mean age of all people in the target group? If more than one group is involved, then investigators must also determine whether there is an underlying connection between the sets of values (or samples ) to be compared. Samples are considered independent or unpaired when the information is taken from different groups. For example, we could use an unpaired t test to compare the mean age between 2 independent samples, such as the intervention and control groups in a study. Samples are considered related or paired if the information is taken from the same group of people, for example, measurement of blood glucose at the beginning and end of a study. Because blood glucose is measured in the same people at both time points, we could use a paired t test to determine whether there has been a significant change in blood glucose.
What Is the Level of Measurement?
As described in the first section of this article, variables can be grouped according to the level of measurement (nominal, ordinal, or interval). In most cases, the independent variable in an inferential statistic will be nominal; therefore, investigators need to know the level of measurement for the dependent variable before they can select the relevant inferential statistic. Two exceptions to this consideration are correlation analyses and regression analyses ( Figure 1 ). Because a correlation analysis measures the strength of association between 2 variables, we need to consider the level of measurement for both variables. Regression analyses can consider multiple independent variables, often with a variety of measurement levels. However, for these analyses, investigators still need to consider the level of measurement for the dependent variable.
Selection of inferential statistics to test interval-level variables must include consideration of how the data are distributed. An underlying assumption for parametric tests is that the data approximate a normal distribution. When the data are not normally distributed, information derived from a parametric test may be wrong. 6 When the assumption of normality is violated (for example, when the data are skewed), then investigators should use a nonparametric test. If the data are normally distributed, then investigators can use a parametric test.
ADDITIONAL CONSIDERATIONS
What is the level of significance.
An inferential statistic is used to calculate a p value, the probability of obtaining the observed data by chance. Investigators can then compare this p value against a prespecified level of significance, which is often chosen to be 0.05. This level of significance represents a 1 in 20 chance that the observation is wrong, which is considered an acceptable level of error.
What Are the Most Commonly Used Statistics?
In 1983, Emerson and Colditz 7 reported the first review of statistics used in original research articles published in the New England Journal of Medicine . This review of statistics used in the journal was updated in 1989 and 2005, 8 and this type of analysis has been replicated in many other journals. 9 – 13 Collectively, these reviews have identified 2 important observations. First, the overall sophistication of statistical methodology used and reported in studies has grown over time, with survival analyses and multivariable regression analyses becoming much more common. The second observation is that, despite this trend, 1 in 4 articles describe no statistical methods or report only simple descriptive statistics. When inferential statistics are used, the most common are t tests, contingency table tests (for example, χ 2 test and Fisher exact test), and simple correlation and regression analyses. This information is important for educators, investigators, reviewers, and readers because it suggests that a good foundational knowledge of descriptive statistics and common inferential statistics will enable us to correctly evaluate the majority of research articles. 11 – 13 However, to fully take advantage of all research published in high-impact journals, we need to become acquainted with some of the more complex methods, such as multivariable regression analyses. 8 , 13
What Are Some Additional Resources?
As an investigator and Associate Editor with CJHP , I have often relied on the advice of colleagues to help create my own analysis plans and review the plans of others. Biostatisticians have a wealth of knowledge in the field of statistical analysis and can provide advice on the correct selection, application, and interpretation of these methods. Colleagues who have “been there and done that” with their own data analysis plans are also valuable sources of information. Identify these individuals and consult with them early and often as you develop your analysis plan.
Another important resource to consider when creating your analysis plan is textbooks. Numerous statistical textbooks are available, differing in levels of complexity and scope. The titles listed in the “Further Reading” section are just a few suggestions. I encourage interested readers to look through these and other books to find resources that best fit their needs. However, one crucial book that I highly recommend to anyone wanting to be an investigator or peer reviewer is Lang and Secic’s How to Report Statistics in Medicine (see “Further Reading”). As the title implies, this book covers a wide range of statistics used in medical research and provides numerous examples of how to correctly report the results.
CONCLUSIONS
When it comes to creating an analysis plan for your project, I recommend following the sage advice of Douglas Adams in The Hitchhiker’s Guide to the Galaxy : Don’t panic! 14 Begin with simple methods to summarize and visualize your data, then use the key questions and decision trees provided in this article to identify relevant statistical tests. Information in this article will give you and your co-investigators a place to start discussing the elements necessary for developing an analysis plan. But do not stop there! Use advice from biostatisticians and more experienced colleagues, as well as information in textbooks, to help create your analysis plan and choose the most appropriate statistics for your study. Making careful, informed decisions about the statistics to use in your study should reduce the risk of confirming Mr Twain’s concern.
Appendix 1. Glossary of statistical terms * (part 1 of 2)
- 1-way ANOVA: Uses 1 variable to define the groups for comparing means. This is similar to the Student t test when comparing the means of 2 groups.
- Kruskall–Wallis 1-way ANOVA: Nonparametric alternative for the 1-way ANOVA. Used to determine the difference in medians between 3 or more groups.
- n -way ANOVA: Uses 2 or more variables to define groups when comparing means. Also called a “between-subjects factorial ANOVA”.
- Repeated-measures ANOVA: A method for analyzing whether the means of 3 or more measures from the same group of participants are different.
- Freidman ANOVA: Nonparametric alternative for the repeated-measures ANOVA. It is often used to compare rankings and preferences that are measured 3 or more times.
- Fisher exact: Variation of chi-square that accounts for cell counts < 5.
- McNemar: Variation of chi-square that tests statistical significance of changes in 2 paired measurements of dichotomous variables.
- Cochran Q: An extension of the McNemar test that provides a method for testing for differences between 3 or more matched sets of frequencies or proportions. Often used as a measure of heterogeneity in meta-analyses.
- 1-sample: Used to determine whether the mean of a sample is significantly different from a known or hypothesized value.
- Independent-samples t test (also referred to as the Student t test): Used when the independent variable is a nominal-level variable that identifies 2 groups and the dependent variable is an interval-level variable.
- Paired: Used to compare 2 pairs of scores between 2 groups (e.g., baseline and follow-up blood pressure in the intervention and control groups).
Lang TA, Secic M. How to report statistics in medicine: annotated guidelines for authors, editors, and reviewers. 2nd ed. Philadelphia (PA): American College of Physicians; 2006.
Norman GR, Streiner DL. PDQ statistics. 3rd ed. Hamilton (ON): B.C. Decker; 2003.
Plichta SB, Kelvin E. Munro’s statistical methods for health care research . 6th ed. Philadelphia (PA): Wolters Kluwer Health/ Lippincott, Williams & Wilkins; 2013.
This article is the 12th in the CJHP Research Primer Series, an initiative of the CJHP Editorial Board and the CSHP Research Committee. The planned 2-year series is intended to appeal to relatively inexperienced researchers, with the goal of building research capacity among practising pharmacists. The articles, presenting simple but rigorous guidance to encourage and support novice researchers, are being solicited from authors with appropriate expertise.
Previous articles in this series:
- Bond CM. The research jigsaw: how to get started. Can J Hosp Pharm . 2014;67(1):28–30.
- Tully MP. Research: articulating questions, generating hypotheses, and choosing study designs. Can J Hosp Pharm . 2014;67(1):31–4.
- Loewen P. Ethical issues in pharmacy practice research: an introductory guide. Can J Hosp Pharm. 2014;67(2):133–7.
- Tsuyuki RT. Designing pharmacy practice research trials. Can J Hosp Pharm . 2014;67(3):226–9.
- Bresee LC. An introduction to developing surveys for pharmacy practice research. Can J Hosp Pharm . 2014;67(4):286–91.
- Gamble JM. An introduction to the fundamentals of cohort and case–control studies. Can J Hosp Pharm . 2014;67(5):366–72.
- Austin Z, Sutton J. Qualitative research: getting started. C an J Hosp Pharm . 2014;67(6):436–40.
- Houle S. An introduction to the fundamentals of randomized controlled trials in pharmacy research. Can J Hosp Pharm . 2014; 68(1):28–32.
- Charrois TL. Systematic reviews: What do you need to know to get started? Can J Hosp Pharm . 2014;68(2):144–8.
- Sutton J, Austin Z. Qualitative research: data collection, analysis, and management. Can J Hosp Pharm . 2014;68(3):226–31.
- Cadarette SM, Wong L. An introduction to health care administrative data. Can J Hosp Pharm. 2014;68(3):232–7.
Competing interests: None declared.
Further Reading
- Devor J, Peck R. Statistics: the exploration and analysis of data. 7th ed. Boston (MA): Brooks/Cole Cengage Learning; 2012. [ Google Scholar ]
- Lang TA, Secic M. How to report statistics in medicine: annotated guidelines for authors, editors, and reviewers. 2nd ed. Philadelphia (PA): American College of Physicians; 2006. [ Google Scholar ]
- Mendenhall W, Beaver RJ, Beaver BM. Introduction to probability and statistics. 13th ed. Belmont (CA): Brooks/Cole Cengage Learning; 2009. [ Google Scholar ]
- Norman GR, Streiner DL. PDQ statistics. 3rd ed. Hamilton (ON): B.C. Decker; 2003. [ Google Scholar ]
- Plichta SB, Kelvin E. Munro’s statistical methods for health care research. 6th ed. Philadelphia (PA): Wolters Kluwer Health/Lippincott, Williams & Wilkins; 2013. [ Google Scholar ]
Customize Your Path
Filters Applied
Customize Your Experience.
Utilize the "Customize Your Path" feature to refine the information displayed in myRESEARCHpath based on your role, project inclusions, sponsor or funding, and management center.
Design the analysis plan
Need assistance with analysis planning?
Get help with analysis planning.
Contact the Biostatistics, Epidemiology, and Research Design (BERD) Methods Core:
- Submit a help request
- Top Courses
- Online Degrees
- Find your New Career
- Join for Free
What Is Data Analysis? (With Examples)
Data analysis is the practice of working with data to glean useful information, which can then be used to make informed decisions.
![research plan data analysis [Featured image] A female data analyst takes notes on her laptop at a standing desk in a modern office space](https://d3njjcbhbojbot.cloudfront.net/api/utilities/v1/imageproxy/https://images.ctfassets.net/wp1lcwdav1p1/2CUbULaq9mEfSSIq6lsCUu/b8ec58abf5106bf9bf75b17da09c39c0/What_is_data_analysis.png?w=1500&h=680&q=60&fit=fill&f=faces&fm=jpg&fl=progressive&auto=format%2Ccompress&dpr=1&w=1000)
"It is a capital mistake to theorize before one has data. Insensibly one begins to twist facts to suit theories, instead of theories to suit facts," Sherlock Holme's proclaims in Sir Arthur Conan Doyle's A Scandal in Bohemia.
This idea lies at the root of data analysis. When we can extract meaning from data, it empowers us to make better decisions. And we’re living in a time when we have more data than ever at our fingertips.
Companies are wisening up to the benefits of leveraging data. Data analysis can help a bank to personalize customer interactions, a health care system to predict future health needs, or an entertainment company to create the next big streaming hit.
The World Economic Forum Future of Jobs Report 2023 listed data analysts and scientists as one of the most in-demand jobs, alongside AI and machine learning specialists and big data specialists [ 1 ]. In this article, you'll learn more about the data analysis process, different types of data analysis, and recommended courses to help you get started in this exciting field.
Read more: How to Become a Data Analyst (with or Without a Degree)
Beginner-friendly data analysis courses
Interested in building your knowledge of data analysis today? Consider enrolling in one of these popular courses on Coursera:
In Google's Foundations: Data, Data, Everywhere course, you'll explore key data analysis concepts, tools, and jobs.
In Duke University's Data Analysis and Visualization course, you'll learn how to identify key components for data analytics projects, explore data visualization, and find out how to create a compelling data story.
Data analysis process
As the data available to companies continues to grow both in amount and complexity, so too does the need for an effective and efficient process by which to harness the value of that data. The data analysis process typically moves through several iterative phases. Let’s take a closer look at each.
Identify the business question you’d like to answer. What problem is the company trying to solve? What do you need to measure, and how will you measure it?
Collect the raw data sets you’ll need to help you answer the identified question. Data collection might come from internal sources, like a company’s client relationship management (CRM) software, or from secondary sources, like government records or social media application programming interfaces (APIs).
Clean the data to prepare it for analysis. This often involves purging duplicate and anomalous data, reconciling inconsistencies, standardizing data structure and format, and dealing with white spaces and other syntax errors.
Analyze the data. By manipulating the data using various data analysis techniques and tools, you can begin to find trends, correlations, outliers, and variations that tell a story. During this stage, you might use data mining to discover patterns within databases or data visualization software to help transform data into an easy-to-understand graphical format.
Interpret the results of your analysis to see how well the data answered your original question. What recommendations can you make based on the data? What are the limitations to your conclusions?
You can complete hands-on projects for your portfolio while practicing statistical analysis, data management, and programming with Meta's beginner-friendly Data Analyst Professional Certificate . Designed to prepare you for an entry-level role, this self-paced program can be completed in just 5 months.
Or, L earn more about data analysis in this lecture by Kevin, Director of Data Analytics at Google, from Google's Data Analytics Professional Certificate :
Read more: What Does a Data Analyst Do? A Career Guide
Types of data analysis (with examples)
Data can be used to answer questions and support decisions in many different ways. To identify the best way to analyze your date, it can help to familiarize yourself with the four types of data analysis commonly used in the field.
In this section, we’ll take a look at each of these data analysis methods, along with an example of how each might be applied in the real world.
Descriptive analysis
Descriptive analysis tells us what happened. This type of analysis helps describe or summarize quantitative data by presenting statistics. For example, descriptive statistical analysis could show the distribution of sales across a group of employees and the average sales figure per employee.
Descriptive analysis answers the question, “what happened?”
Diagnostic analysis
If the descriptive analysis determines the “what,” diagnostic analysis determines the “why.” Let’s say a descriptive analysis shows an unusual influx of patients in a hospital. Drilling into the data further might reveal that many of these patients shared symptoms of a particular virus. This diagnostic analysis can help you determine that an infectious agent—the “why”—led to the influx of patients.
Diagnostic analysis answers the question, “why did it happen?”
Predictive analysis
So far, we’ve looked at types of analysis that examine and draw conclusions about the past. Predictive analytics uses data to form projections about the future. Using predictive analysis, you might notice that a given product has had its best sales during the months of September and October each year, leading you to predict a similar high point during the upcoming year.
Predictive analysis answers the question, “what might happen in the future?”
Prescriptive analysis
Prescriptive analysis takes all the insights gathered from the first three types of analysis and uses them to form recommendations for how a company should act. Using our previous example, this type of analysis might suggest a market plan to build on the success of the high sales months and harness new growth opportunities in the slower months.
Prescriptive analysis answers the question, “what should we do about it?”
This last type is where the concept of data-driven decision-making comes into play.
Read more : Advanced Analytics: Definition, Benefits, and Use Cases
What is data-driven decision-making (DDDM)?
Data-driven decision-making, sometimes abbreviated to DDDM), can be defined as the process of making strategic business decisions based on facts, data, and metrics instead of intuition, emotion, or observation.
This might sound obvious, but in practice, not all organizations are as data-driven as they could be. According to global management consulting firm McKinsey Global Institute, data-driven companies are better at acquiring new customers, maintaining customer loyalty, and achieving above-average profitability [ 2 ].
Get started with Coursera
If you’re interested in a career in the high-growth field of data analytics, consider these top-rated courses on Coursera:
Begin building job-ready skills with the Google Data Analytics Professional Certificate . Prepare for an entry-level job as you learn from Google employees—no experience or degree required.
Practice working with data with Macquarie University's Excel Skills for Business Specialization . Learn how to use Microsoft Excel to analyze data and make data-informed business decisions.
Deepen your skill set with Google's Advanced Data Analytics Professional Certificate . In this advanced program, you'll continue exploring the concepts introduced in the beginner-level courses, plus learn Python, statistics, and Machine Learning concepts.
Frequently asked questions (FAQ)
Where is data analytics used .
Just about any business or organization can use data analytics to help inform their decisions and boost their performance. Some of the most successful companies across a range of industries — from Amazon and Netflix to Starbucks and General Electric — integrate data into their business plans to improve their overall business performance.
What are the top skills for a data analyst?
Data analysis makes use of a range of analysis tools and technologies. Some of the top skills for data analysts include SQL, data visualization, statistical programming languages (like R and Python), machine learning, and spreadsheets.
Read : 7 In-Demand Data Analyst Skills to Get Hired in 2022
What is a data analyst job salary?
Data from Glassdoor indicates that the average base salary for a data analyst in the United States is $75,349 as of March 2024 [ 3 ]. How much you make will depend on factors like your qualifications, experience, and location.
Do data analysts need to be good at math?
Data analytics tends to be less math-intensive than data science. While you probably won’t need to master any advanced mathematics, a foundation in basic math and statistical analysis can help set you up for success.
Learn more: Data Analyst vs. Data Scientist: What’s the Difference?
Article sources
World Economic Forum. " The Future of Jobs Report 2023 , https://www3.weforum.org/docs/WEF_Future_of_Jobs_2023.pdf." Accessed March 19, 2024.
McKinsey & Company. " Five facts: How customer analytics boosts corporate performance , https://www.mckinsey.com/business-functions/marketing-and-sales/our-insights/five-facts-how-customer-analytics-boosts-corporate-performance." Accessed March 19, 2024.
Glassdoor. " Data Analyst Salaries , https://www.glassdoor.com/Salaries/data-analyst-salary-SRCH_KO0,12.htm" Accessed March 19, 2024.
Keep reading
Coursera staff.
Editorial Team
Coursera’s editorial team is comprised of highly experienced professional editors, writers, and fact...
This content has been made available for informational purposes only. Learners are advised to conduct additional research to ensure that courses and other credentials pursued meet their personal, professional, and financial goals.
- Skip to main content
- Skip to primary sidebar
- Skip to footer
- QuestionPro

- Solutions Industries Gaming Automotive Sports and events Education Government Travel & Hospitality Financial Services Healthcare Cannabis Technology Use Case NPS+ Communities Audience Contactless surveys Mobile LivePolls Member Experience GDPR Positive People Science 360 Feedback Surveys
- Resources Blog eBooks Survey Templates Case Studies Training Help center

Home Market Research
Data Analysis in Research: Types & Methods
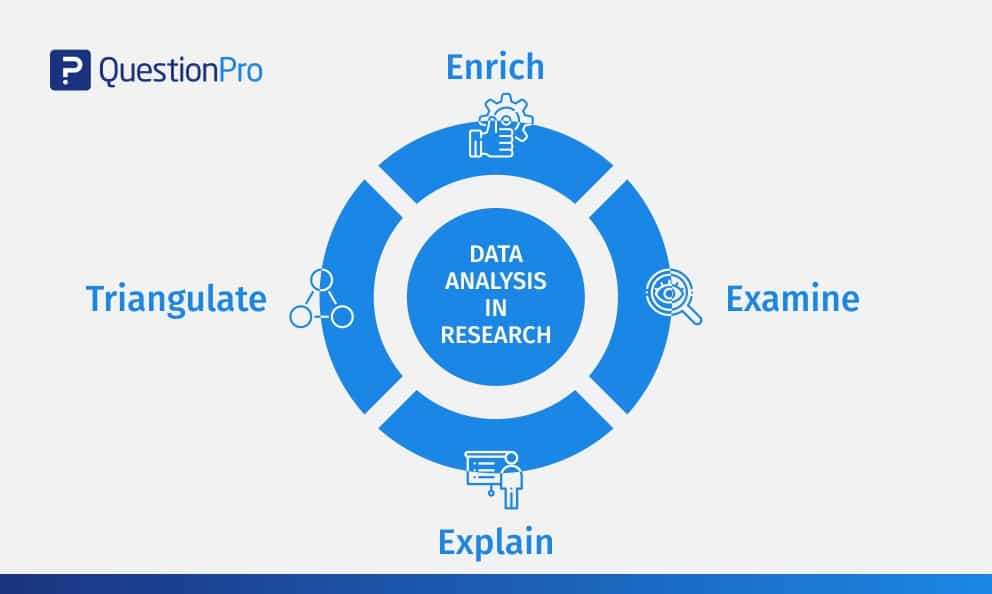
Content Index
Why analyze data in research?
Types of data in research, finding patterns in the qualitative data, methods used for data analysis in qualitative research, preparing data for analysis, methods used for data analysis in quantitative research, considerations in research data analysis, what is data analysis in research.
Definition of research in data analysis: According to LeCompte and Schensul, research data analysis is a process used by researchers to reduce data to a story and interpret it to derive insights. The data analysis process helps reduce a large chunk of data into smaller fragments, which makes sense.
Three essential things occur during the data analysis process — the first is data organization . Summarization and categorization together contribute to becoming the second known method used for data reduction. It helps find patterns and themes in the data for easy identification and linking. The third and last way is data analysis – researchers do it in both top-down and bottom-up fashion.
LEARN ABOUT: Research Process Steps
On the other hand, Marshall and Rossman describe data analysis as a messy, ambiguous, and time-consuming but creative and fascinating process through which a mass of collected data is brought to order, structure and meaning.
We can say that “the data analysis and data interpretation is a process representing the application of deductive and inductive logic to the research and data analysis.”
Researchers rely heavily on data as they have a story to tell or research problems to solve. It starts with a question, and data is nothing but an answer to that question. But, what if there is no question to ask? Well! It is possible to explore data even without a problem – we call it ‘Data Mining’, which often reveals some interesting patterns within the data that are worth exploring.
Irrelevant to the type of data researchers explore, their mission and audiences’ vision guide them to find the patterns to shape the story they want to tell. One of the essential things expected from researchers while analyzing data is to stay open and remain unbiased toward unexpected patterns, expressions, and results. Remember, sometimes, data analysis tells the most unforeseen yet exciting stories that were not expected when initiating data analysis. Therefore, rely on the data you have at hand and enjoy the journey of exploratory research.
Create a Free Account
Every kind of data has a rare quality of describing things after assigning a specific value to it. For analysis, you need to organize these values, processed and presented in a given context, to make it useful. Data can be in different forms; here are the primary data types.
- Qualitative data: When the data presented has words and descriptions, then we call it qualitative data . Although you can observe this data, it is subjective and harder to analyze data in research, especially for comparison. Example: Quality data represents everything describing taste, experience, texture, or an opinion that is considered quality data. This type of data is usually collected through focus groups, personal qualitative interviews , qualitative observation or using open-ended questions in surveys.
- Quantitative data: Any data expressed in numbers of numerical figures are called quantitative data . This type of data can be distinguished into categories, grouped, measured, calculated, or ranked. Example: questions such as age, rank, cost, length, weight, scores, etc. everything comes under this type of data. You can present such data in graphical format, charts, or apply statistical analysis methods to this data. The (Outcomes Measurement Systems) OMS questionnaires in surveys are a significant source of collecting numeric data.
- Categorical data: It is data presented in groups. However, an item included in the categorical data cannot belong to more than one group. Example: A person responding to a survey by telling his living style, marital status, smoking habit, or drinking habit comes under the categorical data. A chi-square test is a standard method used to analyze this data.
Learn More : Examples of Qualitative Data in Education
Data analysis in qualitative research
Data analysis and qualitative data research work a little differently from the numerical data as the quality data is made up of words, descriptions, images, objects, and sometimes symbols. Getting insight from such complicated information is a complicated process. Hence it is typically used for exploratory research and data analysis .
Although there are several ways to find patterns in the textual information, a word-based method is the most relied and widely used global technique for research and data analysis. Notably, the data analysis process in qualitative research is manual. Here the researchers usually read the available data and find repetitive or commonly used words.
For example, while studying data collected from African countries to understand the most pressing issues people face, researchers might find “food” and “hunger” are the most commonly used words and will highlight them for further analysis.
LEARN ABOUT: Level of Analysis
The keyword context is another widely used word-based technique. In this method, the researcher tries to understand the concept by analyzing the context in which the participants use a particular keyword.
For example , researchers conducting research and data analysis for studying the concept of ‘diabetes’ amongst respondents might analyze the context of when and how the respondent has used or referred to the word ‘diabetes.’
The scrutiny-based technique is also one of the highly recommended text analysis methods used to identify a quality data pattern. Compare and contrast is the widely used method under this technique to differentiate how a specific text is similar or different from each other.
For example: To find out the “importance of resident doctor in a company,” the collected data is divided into people who think it is necessary to hire a resident doctor and those who think it is unnecessary. Compare and contrast is the best method that can be used to analyze the polls having single-answer questions types .
Metaphors can be used to reduce the data pile and find patterns in it so that it becomes easier to connect data with theory.
Variable Partitioning is another technique used to split variables so that researchers can find more coherent descriptions and explanations from the enormous data.
LEARN ABOUT: Qualitative Research Questions and Questionnaires
There are several techniques to analyze the data in qualitative research, but here are some commonly used methods,
- Content Analysis: It is widely accepted and the most frequently employed technique for data analysis in research methodology. It can be used to analyze the documented information from text, images, and sometimes from the physical items. It depends on the research questions to predict when and where to use this method.
- Narrative Analysis: This method is used to analyze content gathered from various sources such as personal interviews, field observation, and surveys . The majority of times, stories, or opinions shared by people are focused on finding answers to the research questions.
- Discourse Analysis: Similar to narrative analysis, discourse analysis is used to analyze the interactions with people. Nevertheless, this particular method considers the social context under which or within which the communication between the researcher and respondent takes place. In addition to that, discourse analysis also focuses on the lifestyle and day-to-day environment while deriving any conclusion.
- Grounded Theory: When you want to explain why a particular phenomenon happened, then using grounded theory for analyzing quality data is the best resort. Grounded theory is applied to study data about the host of similar cases occurring in different settings. When researchers are using this method, they might alter explanations or produce new ones until they arrive at some conclusion.
LEARN ABOUT: 12 Best Tools for Researchers
Data analysis in quantitative research
The first stage in research and data analysis is to make it for the analysis so that the nominal data can be converted into something meaningful. Data preparation consists of the below phases.
Phase I: Data Validation
Data validation is done to understand if the collected data sample is per the pre-set standards, or it is a biased data sample again divided into four different stages
- Fraud: To ensure an actual human being records each response to the survey or the questionnaire
- Screening: To make sure each participant or respondent is selected or chosen in compliance with the research criteria
- Procedure: To ensure ethical standards were maintained while collecting the data sample
- Completeness: To ensure that the respondent has answered all the questions in an online survey. Else, the interviewer had asked all the questions devised in the questionnaire.
Phase II: Data Editing
More often, an extensive research data sample comes loaded with errors. Respondents sometimes fill in some fields incorrectly or sometimes skip them accidentally. Data editing is a process wherein the researchers have to confirm that the provided data is free of such errors. They need to conduct necessary checks and outlier checks to edit the raw edit and make it ready for analysis.
Phase III: Data Coding
Out of all three, this is the most critical phase of data preparation associated with grouping and assigning values to the survey responses . If a survey is completed with a 1000 sample size, the researcher will create an age bracket to distinguish the respondents based on their age. Thus, it becomes easier to analyze small data buckets rather than deal with the massive data pile.
LEARN ABOUT: Steps in Qualitative Research
After the data is prepared for analysis, researchers are open to using different research and data analysis methods to derive meaningful insights. For sure, statistical analysis plans are the most favored to analyze numerical data. In statistical analysis, distinguishing between categorical data and numerical data is essential, as categorical data involves distinct categories or labels, while numerical data consists of measurable quantities. The method is again classified into two groups. First, ‘Descriptive Statistics’ used to describe data. Second, ‘Inferential statistics’ that helps in comparing the data .
Descriptive statistics
This method is used to describe the basic features of versatile types of data in research. It presents the data in such a meaningful way that pattern in the data starts making sense. Nevertheless, the descriptive analysis does not go beyond making conclusions. The conclusions are again based on the hypothesis researchers have formulated so far. Here are a few major types of descriptive analysis methods.
Measures of Frequency
- Count, Percent, Frequency
- It is used to denote home often a particular event occurs.
- Researchers use it when they want to showcase how often a response is given.
Measures of Central Tendency
- Mean, Median, Mode
- The method is widely used to demonstrate distribution by various points.
- Researchers use this method when they want to showcase the most commonly or averagely indicated response.
Measures of Dispersion or Variation
- Range, Variance, Standard deviation
- Here the field equals high/low points.
- Variance standard deviation = difference between the observed score and mean
- It is used to identify the spread of scores by stating intervals.
- Researchers use this method to showcase data spread out. It helps them identify the depth until which the data is spread out that it directly affects the mean.
Measures of Position
- Percentile ranks, Quartile ranks
- It relies on standardized scores helping researchers to identify the relationship between different scores.
- It is often used when researchers want to compare scores with the average count.
For quantitative research use of descriptive analysis often give absolute numbers, but the in-depth analysis is never sufficient to demonstrate the rationale behind those numbers. Nevertheless, it is necessary to think of the best method for research and data analysis suiting your survey questionnaire and what story researchers want to tell. For example, the mean is the best way to demonstrate the students’ average scores in schools. It is better to rely on the descriptive statistics when the researchers intend to keep the research or outcome limited to the provided sample without generalizing it. For example, when you want to compare average voting done in two different cities, differential statistics are enough.
Descriptive analysis is also called a ‘univariate analysis’ since it is commonly used to analyze a single variable.
Inferential statistics
Inferential statistics are used to make predictions about a larger population after research and data analysis of the representing population’s collected sample. For example, you can ask some odd 100 audiences at a movie theater if they like the movie they are watching. Researchers then use inferential statistics on the collected sample to reason that about 80-90% of people like the movie.
Here are two significant areas of inferential statistics.
- Estimating parameters: It takes statistics from the sample research data and demonstrates something about the population parameter.
- Hypothesis test: I t’s about sampling research data to answer the survey research questions. For example, researchers might be interested to understand if the new shade of lipstick recently launched is good or not, or if the multivitamin capsules help children to perform better at games.
These are sophisticated analysis methods used to showcase the relationship between different variables instead of describing a single variable. It is often used when researchers want something beyond absolute numbers to understand the relationship between variables.
Here are some of the commonly used methods for data analysis in research.
- Correlation: When researchers are not conducting experimental research or quasi-experimental research wherein the researchers are interested to understand the relationship between two or more variables, they opt for correlational research methods.
- Cross-tabulation: Also called contingency tables, cross-tabulation is used to analyze the relationship between multiple variables. Suppose provided data has age and gender categories presented in rows and columns. A two-dimensional cross-tabulation helps for seamless data analysis and research by showing the number of males and females in each age category.
- Regression analysis: For understanding the strong relationship between two variables, researchers do not look beyond the primary and commonly used regression analysis method, which is also a type of predictive analysis used. In this method, you have an essential factor called the dependent variable. You also have multiple independent variables in regression analysis. You undertake efforts to find out the impact of independent variables on the dependent variable. The values of both independent and dependent variables are assumed as being ascertained in an error-free random manner.
- Frequency tables: The statistical procedure is used for testing the degree to which two or more vary or differ in an experiment. A considerable degree of variation means research findings were significant. In many contexts, ANOVA testing and variance analysis are similar.
- Analysis of variance: The statistical procedure is used for testing the degree to which two or more vary or differ in an experiment. A considerable degree of variation means research findings were significant. In many contexts, ANOVA testing and variance analysis are similar.
- Researchers must have the necessary research skills to analyze and manipulation the data , Getting trained to demonstrate a high standard of research practice. Ideally, researchers must possess more than a basic understanding of the rationale of selecting one statistical method over the other to obtain better data insights.
- Usually, research and data analytics projects differ by scientific discipline; therefore, getting statistical advice at the beginning of analysis helps design a survey questionnaire, select data collection methods , and choose samples.
LEARN ABOUT: Best Data Collection Tools
- The primary aim of data research and analysis is to derive ultimate insights that are unbiased. Any mistake in or keeping a biased mind to collect data, selecting an analysis method, or choosing audience sample il to draw a biased inference.
- Irrelevant to the sophistication used in research data and analysis is enough to rectify the poorly defined objective outcome measurements. It does not matter if the design is at fault or intentions are not clear, but lack of clarity might mislead readers, so avoid the practice.
- The motive behind data analysis in research is to present accurate and reliable data. As far as possible, avoid statistical errors, and find a way to deal with everyday challenges like outliers, missing data, data altering, data mining , or developing graphical representation.
LEARN MORE: Descriptive Research vs Correlational Research The sheer amount of data generated daily is frightening. Especially when data analysis has taken center stage. in 2018. In last year, the total data supply amounted to 2.8 trillion gigabytes. Hence, it is clear that the enterprises willing to survive in the hypercompetitive world must possess an excellent capability to analyze complex research data, derive actionable insights, and adapt to the new market needs.
LEARN ABOUT: Average Order Value
QuestionPro is an online survey platform that empowers organizations in data analysis and research and provides them a medium to collect data by creating appealing surveys.
MORE LIKE THIS
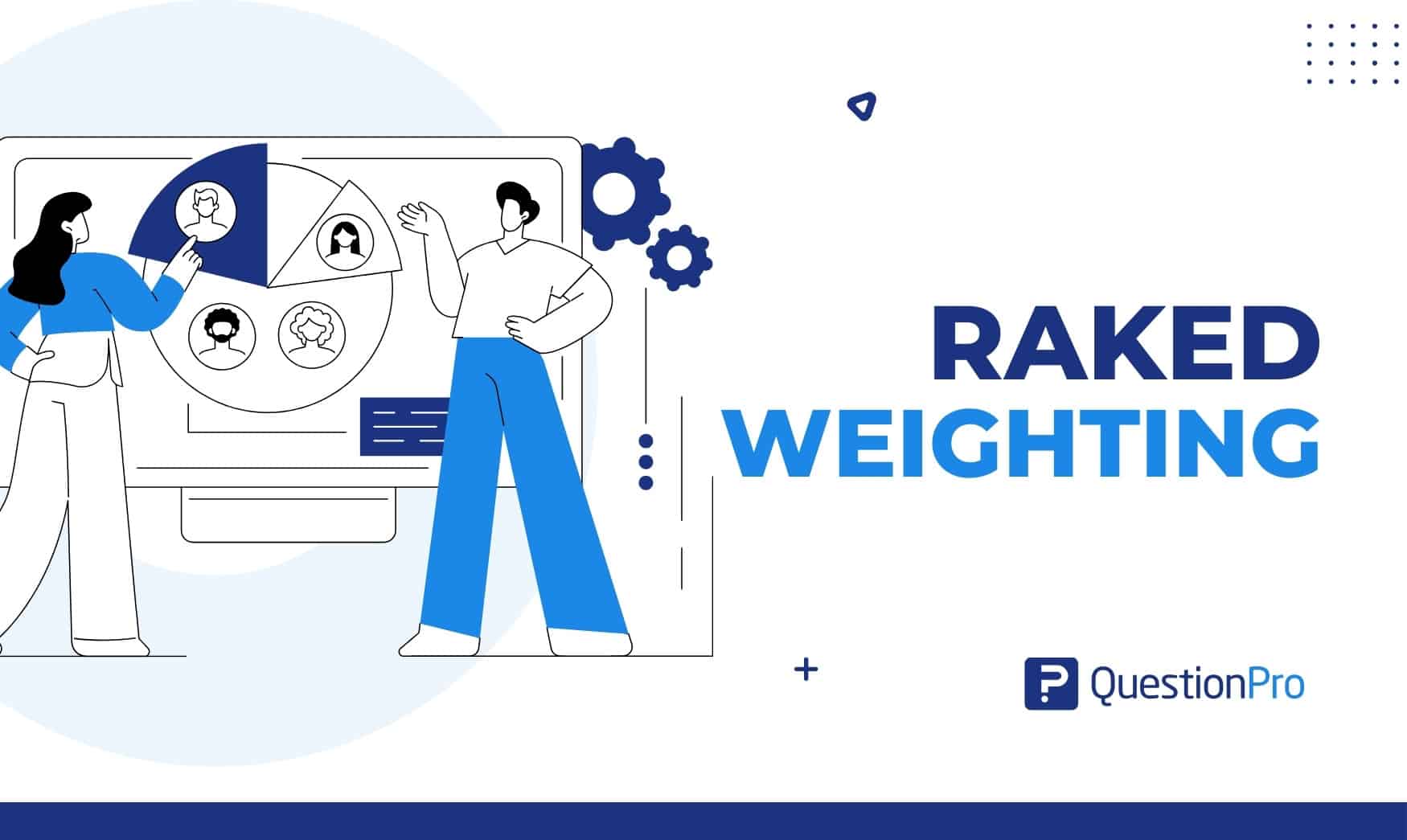
Raked Weighting: A Key Tool for Accurate Survey Results
May 31, 2024
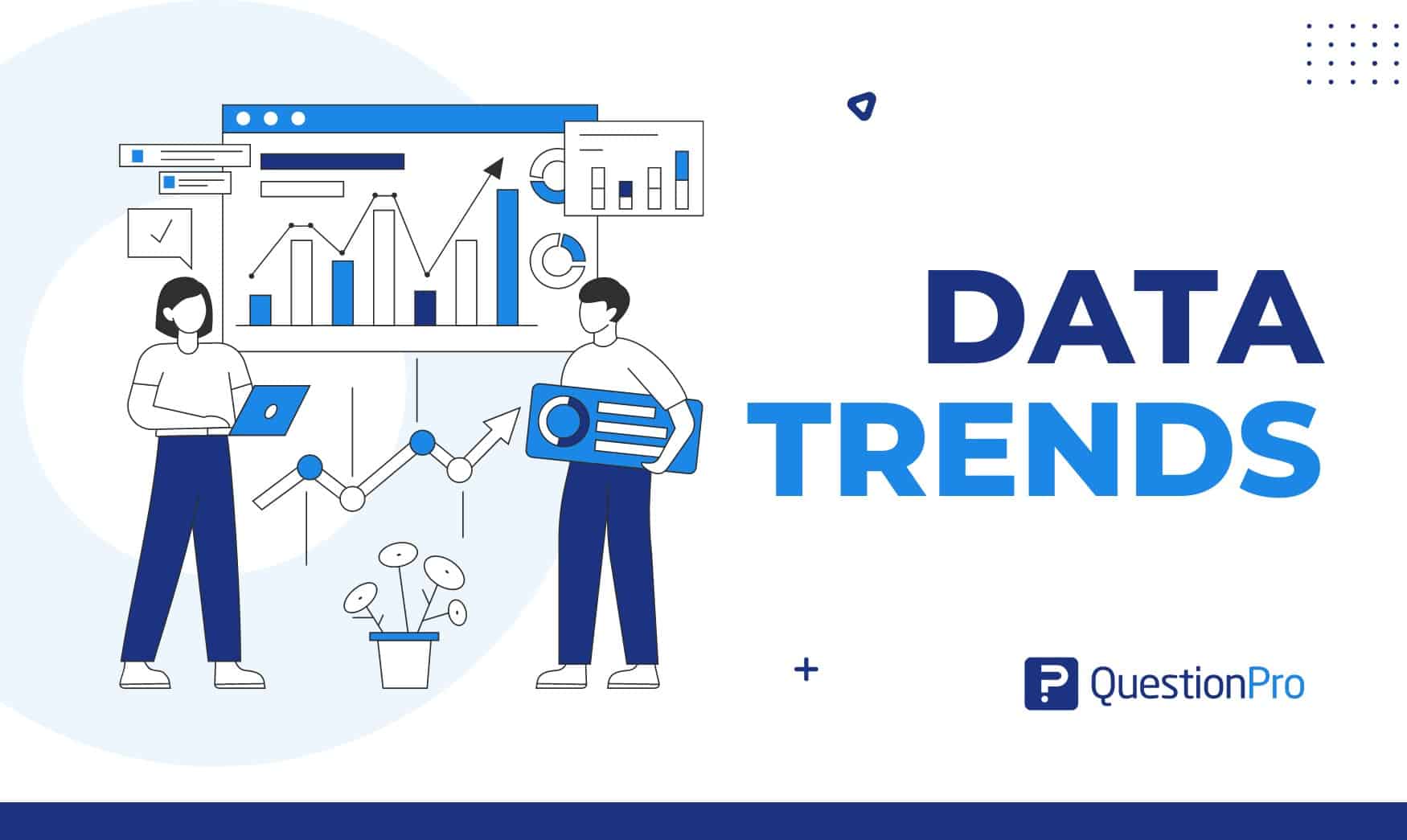
Top 8 Data Trends to Understand the Future of Data
May 30, 2024
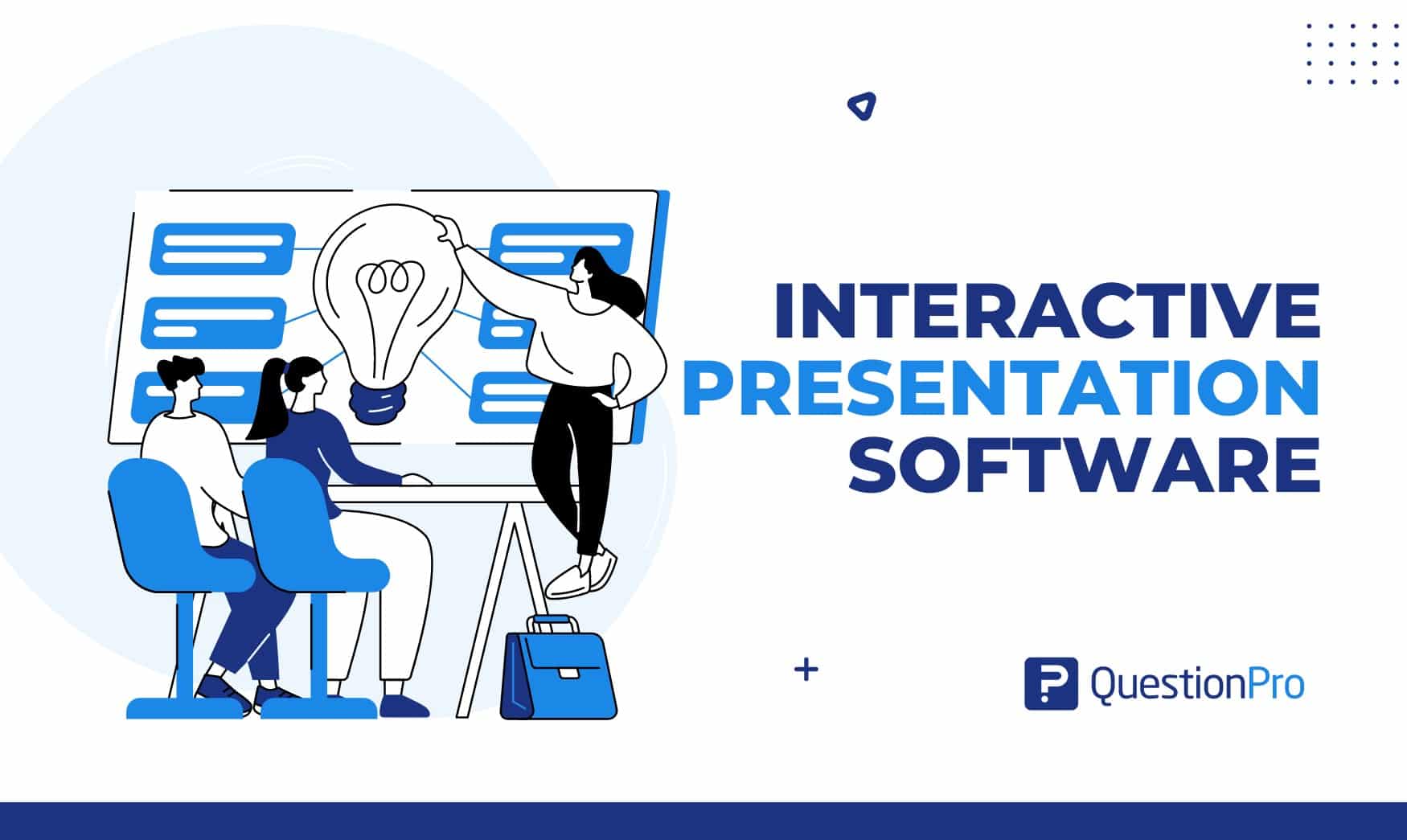
Top 12 Interactive Presentation Software to Engage Your User
May 29, 2024
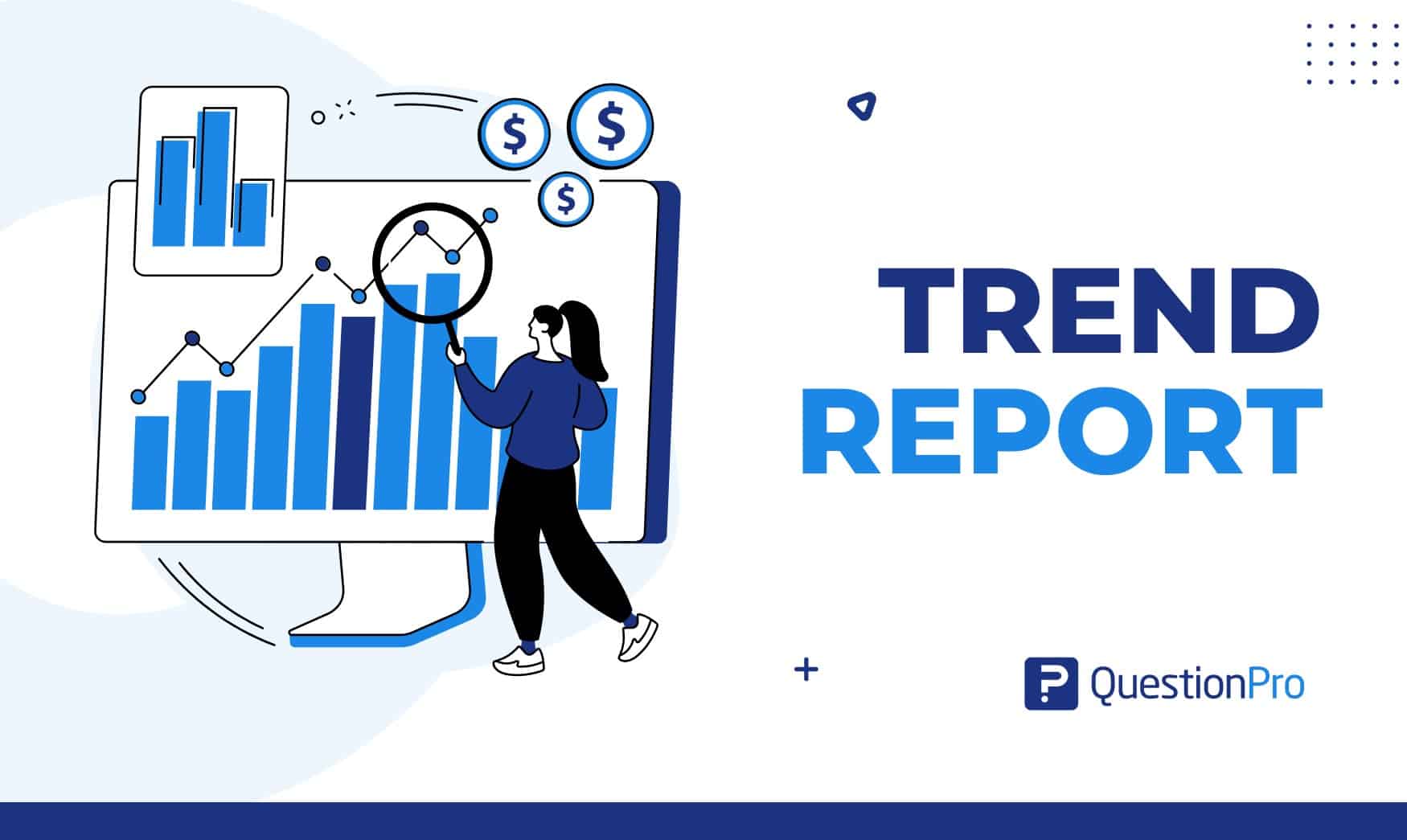
Trend Report: Guide for Market Dynamics & Strategic Analysis
Other categories.
- Academic Research
- Artificial Intelligence
- Assessments
- Brand Awareness
- Case Studies
- Communities
- Consumer Insights
- Customer effort score
- Customer Engagement
- Customer Experience
- Customer Loyalty
- Customer Research
- Customer Satisfaction
- Employee Benefits
- Employee Engagement
- Employee Retention
- Friday Five
- General Data Protection Regulation
- Insights Hub
- Life@QuestionPro
- Market Research
- Mobile diaries
- Mobile Surveys
- New Features
- Online Communities
- Question Types
- Questionnaire
- QuestionPro Products
- Release Notes
- Research Tools and Apps
- Revenue at Risk
- Survey Templates
- Training Tips
- Uncategorized
- Video Learning Series
- What’s Coming Up
- Workforce Intelligence
Data Analysis Plan: Ultimate Guide and Examples
Learn the post survey questions you need to ask attendees for valuable feedback.
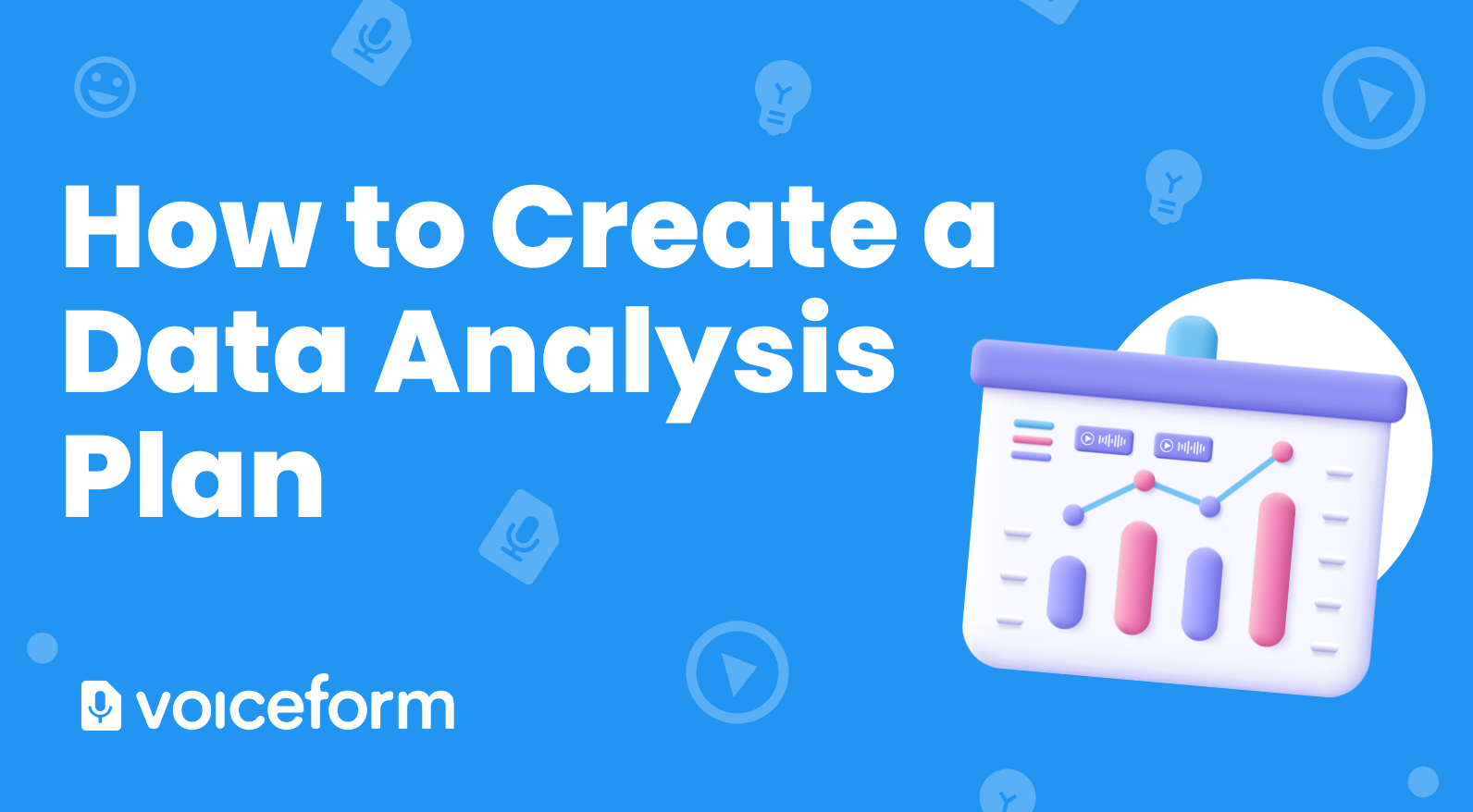
Once you get survey feedback , you might think that the job is done. The next step, however, is to analyze those results. Creating a data analysis plan will help guide you through how to analyze the data and come to logical conclusions.
So, how do you create a data analysis plan? It starts with the goals you set for your survey in the first place. This guide will help you create a data analysis plan that will effectively utilize the data your respondents provided.
What can a data analysis plan do?
Think of data analysis plans as a guide to your organization and analysis, which will help you accomplish your ultimate survey goals. A good plan will make sure that you get answers to your top questions, such as “how do customers feel about this new product?” through specific survey questions. It will also separate respondents to see how opinions among various demographics may differ.
Creating a data analysis plan
Follow these steps to create your own data analysis plan.
Review your goals
When you plan a survey, you typically have specific goals in mind. That might be measuring customer sentiment, answering an academic question, or achieving another purpose.
If you’re beta testing a new product, your survey goal might be “find out how potential customers feel about the new product.” You probably came up with several topics you wanted to address, such as:
- What is the typical experience with the product?
- Which demographics are responding most positively? How well does this match with our idea of the target market?
- Are there any specific pain points that need to be corrected before the product launches?
- Are there any features that should be added before the product launches?
Use these objectives to organize your survey data.
Evaluate the results for your top questions
Your survey questions probably included at least one or two questions that directly relate to your primary goals. For example, in the beta testing example above, your top two questions might be:
- How would you rate your overall satisfaction with the product?
- Would you consider purchasing this product?
Those questions offer a general overview of how your customers feel. Whether their sentiments are generally positive, negative, or neutral, this is the main data your company needs. The next goal is to determine why the beta testers feel the way they do.
Assign questions to specific goals
Next, you’ll organize your survey questions and responses by which research question they answer. For example, you might assign questions to the “overall satisfaction” section, like:
- How would you describe your experience with the product?
- Did you encounter any problems while using the product?
- What were your favorite/least favorite features?
- How useful was the product in achieving your goals?
Under demographics, you’d include responses to questions like:
- Education level
This helps you determine which questions and answers will answer larger questions, such as “which demographics are most likely to have had a positive experience?”
Pay special attention to demographics
Demographics are particularly important to a data analysis plan. Of course you’ll want to know what kind of experience your product testers are having with the product—but you also want to know who your target market should be. Separating responses based on demographics can be especially illuminating.
For example, you might find that users aged 25 to 45 find the product easier to use, but people over 65 find it too difficult. If you want to target the over-65 demographic, you can use that group’s survey data to refine the product before it launches.
Other demographic segregation can be helpful, too. You might find that your product is popular with people from the tech industry, who have an easier time with a user interface, while those from other industries, like education, struggle to use the tool effectively. If you’re targeting the tech industry, you may not need to make adjustments—but if it’s a technological tool designed primarily for educators, you’ll want to make appropriate changes.
Similarly, factors like location, education level, income bracket, and other demographics can help you compare experiences between the groups. Depending on your ultimate survey goals, you may want to compare multiple demographic types to get accurate insight into your results.
Consider correlation vs. causation
When creating your data analysis plan, remember to consider the difference between correlation and causation. For instance, being over 65 might correlate with a difficult user experience, but the cause of the experience might be something else entirely. You may find that your respondents over 65 are primarily from a specific educational background, or have issues reading the text in your user interface. It’s important to consider all the different data points, and how they might have an effect on the overall results.
Moving on to analysis
Once you’ve assigned survey questions to the overall research questions they’re designed to answer, you can move on to the actual data analysis. Depending on your survey tool, you may already have software that can perform quantitative and/or qualitative analysis. Choose the analysis types that suit your questions and goals, then use your analytic software to evaluate the data and create graphs or reports with your survey results.
At the end of the process, you should be able to answer your major research questions.
Power your data analysis with Voiceform
Once you have established your survey goals, Voiceform can power your data collection and analysis. Our feature-rich survey platform offers an easy-to-use interface, multi-channel survey tools, multimedia question types, and powerful analytics. We can help you create and work through a data analysis plan. Find out more about the product, and book a free demo today !
We make collecting, sharing and analyzing data a breeze
Get started for free. Get instant access to Voiceform features that get you amazing data in minutes.

Writing the Data Analysis Plan
- First Online: 01 January 2010
Cite this chapter
- A. T. Panter 4
5826 Accesses
3 Altmetric
You and your project statistician have one major goal for your data analysis plan: You need to convince all the reviewers reading your proposal that you would know what to do with your data once your project is funded and your data are in hand. The data analytic plan is a signal to the reviewers about your ability to score, describe, and thoughtfully synthesize a large number of variables into appropriately-selected quantitative models once the data are collected. Reviewers respond very well to plans with a clear elucidation of the data analysis steps – in an appropriate order, with an appropriate level of detail and reference to relevant literatures, and with statistical models and methods for that map well into your proposed aims. A successful data analysis plan produces reviews that either include no comments about the data analysis plan or better yet, compliments it for being comprehensive and logical given your aims. This chapter offers practical advice about developing and writing a compelling, “bullet-proof” data analytic plan for your grant application.
This is a preview of subscription content, log in via an institution to check access.
Access this chapter
- Available as PDF
- Read on any device
- Instant download
- Own it forever
- Available as EPUB and PDF
- Compact, lightweight edition
- Dispatched in 3 to 5 business days
- Free shipping worldwide - see info
Tax calculation will be finalised at checkout
Purchases are for personal use only
Institutional subscriptions
Similar content being viewed by others
A Holistic Approach to Empirical Analysis: The Insignificance of P, Hypothesis Testing and Statistical Significance*
Meta-Analysis
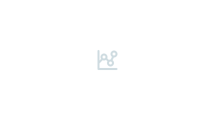
Researchers’ data analysis choices: an excess of false positives?
Aiken, L. S. & West, S. G. (1991). Multiple regression: testing and interpreting interactions . Newbury Park, CA: Sage.
Google Scholar
Aiken, L. S., West, S. G., & Millsap, R. E. (2008). Doctoral training in statistics, measurement, and methodology in psychology: Replication and extension of Aiken, West, Sechrest and Reno’s (1990) survey of PhD programs in North America. American Psychologist , 63 , 32–50.
Article PubMed Google Scholar
Allison, P. D. (2003). Missing data techniques for structural equation modeling. Journal of Abnormal Psychology , 112 , 545–557.
American Psychological Association (APA) Task Force to Increase the Quantitative Pipeline (2009). Report of the task force to increase the quantitative pipeline . Washington, DC: American Psychological Association.
Bauer, D. & Curran, P. J. (2004). The integration of continuous and discrete latent variables: Potential problems and promising opportunities. Psychological Methods , 9 , 3–29.
Bollen, K. A. (1989). Structural equations with latent variables . New York: Wiley.
Bollen, K. A. & Curran, P. J. (2007). Latent curve models: A structural equation modeling approach . New York: Wiley.
Cohen, J., Cohen, P., West, S. G., & Aiken, L. S. (2003). Multiple correlation/regression for the behavioral sciences (3rd ed.). Mahwah, NJ: Erlbaum.
Curran, P. J., Bauer, D. J., & Willoughby, M. T. (2004). Testing main effects and interactions in hierarchical linear growth models. Psychological Methods , 9 , 220–237.
Embretson, S. E. & Reise, S. P. (2000). Item response theory for psychologists . Mahwah, NJ: Erlbaum.
Enders, C. K. (2006). Analyzing structural equation models with missing data. In G. R. Hancock & R. O. Mueller (Eds.), Structural equation modeling: A second course (pp. 313–342). Greenwich, CT: Information Age.
Hosmer, D. & Lemeshow, S. (1989). Applied logistic regression . New York: Wiley.
Hoyle, R. H. & Panter, A. T. (1995). Writing about structural equation models. In R. H. Hoyle (Ed.), Structural equation modeling: Concepts, issues, and applications (pp. 158–176). Thousand Oaks: Sage.
Kaplan, D. & Elliott, P. R. (1997). A didactic example of multilevel structural equation modeling applicable to the study of organizations. Structural Equation Modeling , 4 , 1–23.
Article Google Scholar
Lanza, S. T., Collins, L. M., Schafer, J. L., & Flaherty, B. P. (2005). Using data augmentation to obtain standard errors and conduct hypothesis tests in latent class and latent transition analysis. Psychological Methods , 10 , 84–100.
MacKinnon, D. P. (2008). Introduction to statistical mediation analysis . Mahwah, NJ: Erlbaum.
Maxwell, S. E. (2004). The persistence of underpowered studies in psychological research: Causes, consequences, and remedies. Psychological Methods , 9 , 147–163.
McCullagh, P. & Nelder, J. (1989). Generalized linear models . London: Chapman and Hall.
McDonald, R. P. & Ho, M. R. (2002). Principles and practices in reporting structural equation modeling analyses. Psychological Methods , 7 , 64–82.
Messick, S. (1989). Validity. In R. L. Linn (Ed.), Educational measurement (3rd ed., pp. 13–103). New York: Macmillan.
Muthén, B. O. (1994). Multilevel covariance structure analysis. Sociological Methods & Research , 22 , 376–398.
Muthén, B. (2008). Latent variable hybrids: overview of old and new models. In G. R. Hancock & K. M. Samuelsen (Eds.), Advances in latent variable mixture models (pp. 1–24). Charlotte, NC: Information Age.
Muthén, B. & Masyn, K. (2004). Discrete-time survival mixture analysis. Journal of Educational and Behavioral Statistics , 30 , 27–58.
Muthén, L. K. & Muthén, B. O. (2004). Mplus, statistical analysis with latent variables: User’s guide . Los Angeles, CA: Muthén &Muthén.
Peugh, J. L. & Enders, C. K. (2004). Missing data in educational research: a review of reporting practices and suggestions for improvement. Review of Educational Research , 74 , 525–556.
Preacher, K. J., Curran, P. J., & Bauer, D. J. (2006). Computational tools for probing interaction effects in multiple linear regression, multilevel modeling, and latent curve analysis. Journal of Educational and Behavioral Statistics , 31 , 437–448.
Preacher, K. J., Curran, P. J., & Bauer, D. J. (2003, September). Probing interactions in multiple linear regression, latent curve analysis, and hierarchical linear modeling: Interactive calculation tools for establishing simple intercepts, simple slopes, and regions of significance [Computer software]. Available from http://www.quantpsy.org .
Preacher, K. J., Rucker, D. D., & Hayes, A. F. (2007). Addressing moderated mediation hypotheses: Theory, methods, and prescriptions. Multivariate Behavioral Research , 42 , 185–227.
Raudenbush, S. W. & Bryk, A. S. (2002). Hierarchical linear models: Applications and data analysis methods (2nd ed.). Thousand Oaks, CA: Sage.
Radloff, L. (1977). The CES-D scale: A self-report depression scale for research in the general population. Applied Psychological Measurement , 1 , 385–401.
Rosenberg, M. (1965). Society and the adolescent self-image . Princeton, NJ: Princeton University Press.
Schafer. J. L. & Graham, J. W. (2002). Missing data: Our view of the state of the art. Psychological Methods , 7 , 147–177.
Schumacker, R. E. (2002). Latent variable interaction modeling. Structural Equation Modeling , 9 , 40–54.
Schumacker, R. E. & Lomax, R. G. (2004). A beginner’s guide to structural equation modeling . Mahwah, NJ: Erlbaum.
Selig, J. P. & Preacher, K. J. (2008, June). Monte Carlo method for assessing mediation: An interactive tool for creating confidence intervals for indirect effects [Computer software]. Available from http://www.quantpsy.org .
Singer, J. D. & Willett, J. B. (1991). Modeling the days of our lives: Using survival analysis when designing and analyzing longitudinal studies of duration and the timing of events. Psychological Bulletin , 110 , 268–290.
Singer, J. D. & Willett, J. B. (1993). It’s about time: Using discrete-time survival analysis to study duration and the timing of events. Journal of Educational Statistics , 18 , 155–195.
Singer, J. D. & Willett, J. B. (2003). Applied longitudinal data analysis: Modeling change and event occurrence . New York: Oxford University.
Book Google Scholar
Vandenberg, R. J. & Lance, C. E. (2000). A review and synthesis of the measurement invariance literature: Suggestions, practices, and recommendations for organizational research. Organizational Research Methods , 3 , 4–69.
Wirth, R. J. & Edwards, M. C. (2007). Item factor analysis: Current approaches and future directions. Psychological Methods , 12 , 58–79.
Article PubMed CAS Google Scholar
Download references
Author information
Authors and affiliations.
L. L. Thurstone Psychometric Laboratory, Department of Psychology, University of North Carolina, Chapel Hill, NC, USA
A. T. Panter
You can also search for this author in PubMed Google Scholar
Corresponding author
Correspondence to A. T. Panter .
Editor information
Editors and affiliations.
National Institute of Mental Health, Executive Blvd. 6001, Bethesda, 20892-9641, Maryland, USA
Willo Pequegnat
Ellen Stover
Delafield Place, N.W. 1413, Washington, 20011, District of Columbia, USA
Cheryl Anne Boyce
Rights and permissions
Reprints and permissions
Copyright information
© 2010 Springer Science+Business Media, LLC
About this chapter
Panter, A.T. (2010). Writing the Data Analysis Plan. In: Pequegnat, W., Stover, E., Boyce, C. (eds) How to Write a Successful Research Grant Application. Springer, Boston, MA. https://doi.org/10.1007/978-1-4419-1454-5_22
Download citation
DOI : https://doi.org/10.1007/978-1-4419-1454-5_22
Published : 20 August 2010
Publisher Name : Springer, Boston, MA
Print ISBN : 978-1-4419-1453-8
Online ISBN : 978-1-4419-1454-5
eBook Packages : Medicine Medicine (R0)
Share this chapter
Anyone you share the following link with will be able to read this content:
Sorry, a shareable link is not currently available for this article.
Provided by the Springer Nature SharedIt content-sharing initiative
- Publish with us
Policies and ethics
- Find a journal
- Track your research
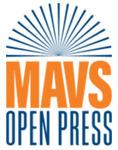
Want to create or adapt books like this? Learn more about how Pressbooks supports open publishing practices.
2.3 Data management and analysis
Learning objectives.
Learners will be able to…
- Define and construct a data analysis plan
- Define key quantitative data management terms—variable name, data dictionary, and observations/cases
- Differentiate between univariate and bivariate quantitative analysis
- Explain when we might use quantitative bivariate analysis in social work research
- Identify how your qualitative research question, research aim, and type of data may influence your choice of analytic methods
- Outline the steps you will take in preparation for conducting qualitative data analysis
After you have your raw data, whether this is secondary data or data you collected yourself, you will need to analyze it. While the specific steps to follow in quantitative or qualitative data analysis are beyond the scope of this chapter, we are going to address some basic concepts in this section to help you create a data analysis plan. A data analysis plan is an ordered outline that includes your research question, a description of the data you are going to use to answer it, and the exact step-by-step analyses that you plan to run to answer your research question. If you look back at Table 2.1, you will see that creating a data analysis plan is a part of the study design process. The data analysis plan flows from the research question, is integral to the study design, and should be well conceptualized prior to beginning data collection. In this section, we will walk through the basics of quantitative and qualitative data analysis to help you understand the fundamentals of creating a data analysis plan.
Quantitative Data: Management
When considering what data you might want to collect as part of your project, there are two important considerations that can create dilemmas for researchers. You might only get one chance to interact with your participants, so you must think comprehensively in your planning phase about what information you need and collect as much relevant data as possible. At the same time, though, especially when collecting sensitive information, you need to consider how onerous the data collection is for participants and whether you really need them to share that information. Just because something is interesting to us doesn’t mean it’s related enough to our research question to chase it down. Work with your research team and/or faculty early in your project to talk through these issues before you get to this point. And if you’re using secondary data, make sure you have access to all the information you need in that data before you use it.
Once you’ve collected your quantitative data, you need to make sure it is well-organized in a database in a way that’s actually usable. “Database” can be kind of a scary word, but really, it can be as simple as an Excel spreadsheet or a data file in whatever program you’re using to analyze your data. You may want to avoid Excel and use a formal database such as Microsoft Access or MySQL if you’ve got a large or complicated data set. But if your data set is smaller and you plan to keep your analyses simple, you can definitely get away with Excel. A typical data set is organized with variables as columns and observations/cases as rows. For example, let’s say we did a survey on ice cream preferences and collected the following information in Table 2.3:
There are a few key data management terms to understand:
- Variable name : Just what it sounds like—the name of your variable. Make sure this is something useful, short and, if you’re using something other than Excel, all one word. Most statistical programs will automatically rename variables for you if they aren’t one word, but the names can be a little ridiculous and long.
- Observations/cases : The rows in your data set. In social work, these are often your study participants (people), but can be anything from census tracts to black bears to trains. When we talk about sample size, we’re talking about the number of observations/cases. In our mini data set, each person is an observation/case.
- Data dictionary (also called a code book or metadata) : This is the document where you list your variable names, what the variables actually measure or represent, what each of the values of the variable mean if the meaning isn’t obvious (i.e., if there are numbers assigned to gender), the level of measurement and anything special to know about the variables (for instance, the source if you mashed two data sets together). If you’re using secondary data, the researchers sharing the data should make the data dictionary available.
Let’s take that mini data set we’ve got up above and we’ll show you what your data dictionary might look like in Table 2.4.
Quantitative Data: Univariate Analysis
As part of planning for your research, you should come up with a data analysis plan. Remember, a data analysis plan is an ordered outline that includes your research question, a description of the data you are going to use to answer it, and the exact step-by-step analyses that you plan to run to answer your research question. A basic data analysis plan might look something like what you see in Table 2.5. Don’t panic if you don’t yet understand some of the statistical terms in the plan; we’re going to delve into some of them in this section, and others will be covered in more depth in your statistics courses. Note here also that this is what operationalizing your variables and moving through your research with them looks like on a basic level. We will cover operationalization in more depth in Chapter 10.
An important point to remember is that you should never get stuck on using a particular statistical method because you or one of your co-researchers thinks it’s cool or it’s the hot thing in your field right now. You should certainly go into your data analysis plan with ideas, but in the end, you need to let your research question guide what statistical tests you plan to use. Be prepared to be flexible if your plan doesn’t pan out because the data is behaving in unexpected ways.
You’ll notice that the first step in the quantitative data analysis plan is univariate and descriptive statistics. Univariate data analysis is a quantitative method in which a variable is examined individually to determine its distribution , or the way the scores are distributed across the levels, or values, of that variable. When we talk about levels , what we are talking about are the possible values of the variable—like a participant’s age, income or gender. (Note that this is different from levels of measurement , which will be discussed in Chapter 11, but the level of measurement of your variables absolutely affects what kinds of analyses you can do with it.) Univariate analysis is non-relational , which just means that we’re not looking into how our variables relate to each other. Instead, we’re looking at variables in isolation to try to understand them better. For this reason, univariate analysis is used for descriptive research questions.
So when do you use univariate data analysis? Always! It should be the first thing you do with your quantitative data, whether you are planning to move on to more sophisticated statistical analyses or are conducting a study to describe a new phenomenon. You need to understand what the values of each variable look like—what if one of your variables has a lot of missing data because participants didn’t answer that question on your survey? What if there isn’t much variation in the gender of your sample? These are things you’ll learn through univariate analysis.
Quantitative Data: Bivariate Analysis
Did you know that ice cream causes shark attacks? It’s true! When ice cream sales go up in the summer, so does the rate of shark attacks. So you’d better put down that ice cream cone, unless you want to make yourself look more delicious to a shark.
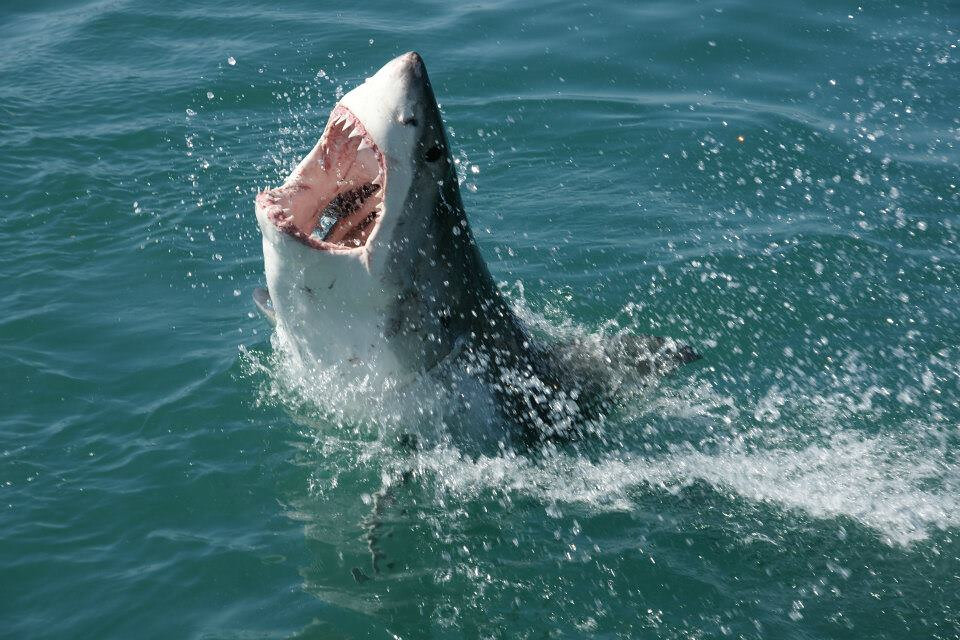
Ok, so it’s quite obviously not true that ice cream causes shark attacks. But if you looked at these two variables and how they’re related, you’d notice that during times of the year with high ice cream sales, there are also the most shark attacks. This is a classic example of the difference between correlation and causation. Despite the fact that the conclusion we drew about causation was wrong, it’s nonetheless true that these two variables appear related, and researchers figured that out through the use of bivariate analysis.
Bivariate analysis consists of a group of statistical techniques that examine the association between two variables. We could look at how anti-depressant medications and appetite are related, whether there is a relation between having a pet and emotional well-being, or if a policy-maker’s level of education is related to how they vote on bills related to environmental issues.
Bivariate analysis forms the foundation of multivariate analysis, which we don’t get to in this book. All you really need to know here is that there are steps beyond bivariate analysis, which you’ve undoubtedly seen in scholarly literature already! But before we can move forward with multivariate analysis, we need to understand the associations between the variables in our study.
Throughout your PhD program, you will learn much more about quantitative data analysis techniques, including more sophisticated multivariate analysis methods. Hopefully this section has provided you with some initial insights into how data is analyzed, and the importance of creating a data analysis plan prior to collecting data. Next, we will discuss some basic strategies for creating a qualitative data analysis plan.
Resources for Quantitative Data Analysis
While you are affiliated with a university, it is likely that you will have access to some kind of commercial statistics software. Examples in the previous section uses SPSS, the most common one our authoring team has seen in social work education. Like its competitors SAS and STATA, SPSS is expensive and your license to the software must be renewed every year (like a subscription). Even if you are able to install commercial statistics software on your computer, once your license expires, your program will no longer work. We believe that forcing students to learn software they will never use is wasteful and contributes to the (accurate, in many cases) perception from students that research class is unrelated to real-world practice. SPSS is more accessible due to its graphical user interface and does not require researchers to learn basic computer programming, but it is prohibitively costly if a student wanted to use it to measure practice data in their agency post-graduation.
Instead, we suggest getting familiar with JASP Statistics , a free and open-source alternative to SPSS developed and supported by the University of Amsterdam. It has a similar user interface as SPSS, and should be similarly easy to learn. Moreover, usability upgrades from SPSS like generating APA formatted tables make it a compelling option. While a great many of my students will rely on statistical analyses of their programs and practices in reports to funders, it is unlikely that any will use SPSS. Browse JASP’s how-to guide or consult this textbook Learning Statistics with JASP: A Tutorial for Psychology Students and Other Beginners , written by Danielle J. Navarro , David R. Foxcroft , and Thomas J. Faulkenberry .
Another open source statistics software package is R (a.k.a. The R Project for Statistical Computing ). R uses a command line interface, so you will need some coding knowledge in order to use it. Luckily, R is the most commonly used statistics software in the world, and the community of support and guides for using R are omnipresent online. For beginning researchers, consult the textbook Learning Statistics with R: A tutorial for psychology students and other beginners by Danielle J. Navarro .
While statistics software is sometimes needed to perform advanced statistical tests, most univariate and bivariate tests can be performed in spreadsheet software like Microsoft Excel, Google Sheets, or the free and open source LibreOffice Calc . Microsoft includes a ToolPak to perform complex data analysis as an add-on to Excel. For more information on using spreadsheet software to perform statistics, the open textbook Collaborative Statistics Using Spreadsheets by Susan Dean, Irene Mary Duranczyk, Barbara Illowsky, Suzanne Loch, and Janet Stottlemyer.
Statistical analysis is performed in just about every discipline, and as a result, there are a lot of openly licensed, free resources to assist you with your data analysis. We have endeavored to provide you the basics in the past few chapters, but ultimately, you will likely need additional support in completing quantitative data analysis from an instructor, textbook, or other resource. Browse the Open Textbook Library for statistics resources or look for video tutorials from reputable instructors like this video textbook on statistics by Bryan Koenig .
Qualitative Data: Management
Qualitative research often involves human participants and qualitative data can include of recordings or transcripts of their words, photographs or images, or diaries and documents. The personal nature of qualitative data poses the challenge of recognizability of sensitive information on individuals, communities, and places. If you choose this methodology for your research, you should familiarize yourself with policies, procedures, and rules to ensure safety and security of data in the documentation and dissemination process.
In any research involving primary data, a researcher is not only entrusted with the responsibility of upholding privacy of their participants but also accountable to them, making confidentiality and human subjects’ protection front and center of qualitative data management. Data such as audiotapes, videotapes, transcripts, notes, and other records should be stored and secured in locations where only authorized persons have access to them.
Sometimes in qualitative research, you will learn intimate details about people’s lives. Often, qualitative data contain personal identifiers. A helpful practice to ensure that participants confidentiality is to replace personal information in transcripts with pseudonyms or descriptive language (e.g., “[the participant’s sister]” instead of the sister’s name). Once audio and video recordings have been accurately transcribed with the de-identification of personal identifiers, the original recordings should be destroyed.
Qualitative Data: Analysis
There are many different types of qualitative data, including transcripts of interviews and focus groups, observational data, documents and other artifacts, and more. Your qualitative data analysis plan should be anchored in the type of data collected and the purpose of your study. Qualitative research can serve a range of purposes. Below is a brief list of general purposes we might consider when using a qualitative approach.
- Are you trying to understand how a particular group is affected by an issue?
- Are you trying to uncover how people arrive at a decision in a given situation?
- Are you trying to examine different points of view on the impact of a recent event?
- Are you trying to summarize how people understand or make sense of a condition?
- Are you trying to describe the needs of your target population?
If you don’t see the general aim of your research question reflected in one of these areas, don’t fret! This is only a small sampling of what you might be trying to accomplish with your qualitative study. Whatever your aim, you need to have a plan for what you will do once you have collected your data.
Iterative or Linear
Some qualitative research is linear , meaning it follows more of a traditionally quantitative process: create a plan, gather data, and analyze data; each step is completed before we proceed to the next. You can think of this like how information is presented in this book. We discuss each topic, one after another.
However, many times qualitative research is iterative , or evolving in cycles. An iterative approach means that once we begin collecting data, we also begin analyzing data as it is coming in. This early and ongoing analysis of our (incomplete) data then impacts our continued planning, data gathering and future analysis. Again, coming back to this book, while it may be written linear, we hope that you engage with it iteratively as you design and conduct your own research. By this we mean that you will revisit previous sections so you can understand how they fit together and you are in continuous process of building and revising how you think about the concepts you are learning about.
As you may have guessed, there are benefits and challenges to both linear and iterative approaches. A linear approach is much more straightforward, each step being fairly defined. However, linear research being more defined and rigid also presents certain challenges. A linear approach assumes that we know what we need to ask or look for at the very beginning of data collection, which often is not the case. Figure 2.1 contrasts the two approaches.
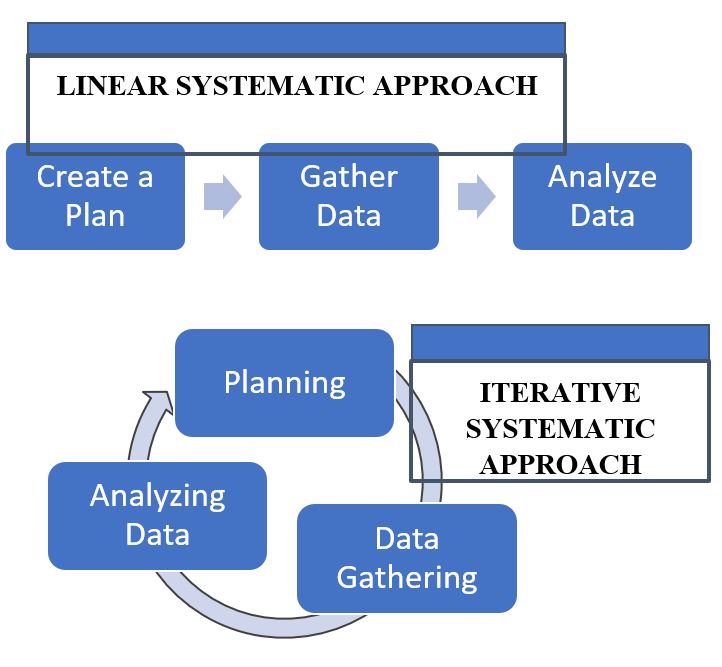
With iterative research, we have more flexibility to adapt our approach as we learn new things. We still need to keep our approach systematic and organized, however, so that our work doesn’t become a free-for-all. As we adapt, we do not want to stray too far from the original premise of our study. It’s also important to remember with an iterative approach that we may risk ethical concerns if our work extends beyond the original boundaries of our informed consent and institutional review board agreement (IRB; see Chapter 3 for more on IRBs). If you feel that you do need to modify your original research plan in a significant way as you learn more about the topic, you can submit an addendum to modify your original application that was submitted. Make sure to keep detailed notes of the decisions that you are making and what is informing these choices. This helps to support transparency and your credibility throughout the research process.
Acquainting yourself with your data
As you begin your analysis, you need to get to know your data. This often means reading through your data prior to any attempt at breaking it apart and labeling it. You might read through a couple of times, in fact. This helps give you a more comprehensive feel for each piece of data and the data as a whole, again, before you start to break it down into smaller units or deconstruct it. This is especially important if others assisted us in the data collection process. We often gather data as part of team and everyone involved in the analysis needs to be very familiar with all of the data.
Capturing your emerging understanding of the data
During your reviewing you will start to develop and evolve your understanding of what the data means. Coding is a part of the qualitative data analysis process where we begin to interpret and assign meaning to the data. It represents one of the first steps as we begin to filter the data through our own subjective lens as the researcher. This understanding of the data should be dynamic and flexible, but you want to have a way to capture this understanding as it evolves. You may include this as part of your qualitative codebook where you are tracking the main ideas that are emerging and what they mean. Table 2.6 is an example of how your thinking might change about a code and how you can go about capturing it.
There are a variety of different approaches to qualitative analysis, including thematic analysis, content analysis, grounded theory, phenomenology, photovoice, and more. The specific steps you will take to code your qualitative data, and to generate themes from these codes, will vary based on the analytic strategy you are employing. In designing your qualitative study, you would identify an analytical approach as you plan out your project. The one you select would depend on the type of data you have and what you want to accomplish with it. In Chapter 19, we will go into more detail about various types of qualitative data analysis. Each qualitative approach has specific techniques and methods that take substantial study and practice to master.
Key Takeaways
- Getting organized at the beginning of your project with a data analysis plan will help keep you on track. Data analysis plans should include your research question, a description of your data, and a step-by-step outline of what you’re going to do with it. [chapter 14.1]
- Be flexible with your data analysis plan—sometimes data surprises us and we have to adjust the statistical tests we are using. [chapter 14.1]
- Always make a data dictionary or, if using secondary data, get a copy of the data dictionary so you (or someone else) can understand the basics of your data. [chapter 14.1]
- Bivariate analysis is a group of statistical techniques that examine the relationship between two variables. [chapter 15.1]
- You need to conduct bivariate analyses before you can begin to draw conclusions from your data, including in future multivariate analyses. [chapter 15.1]
- There are a lot of high quality and free online resources to learn and perform statistical analysis.
- Qualitative research analysis requires preparation and careful planning. You will need to take time to familiarize yourself with the data in a general sense before you begin analyzing. [chapter 19.3]
- The specific steps you will take to code your qualitative data and generate final themes will depend on the qualitative analytic approach you select.
TRACK 1 (IF YOU ARE CREATING A RESEARCH PROPOSAL FOR THIS CLASS)
- Make a data analysis plan for your project. Remember this should include your research question, a description of the data you will use, and a step-by-step outline of what you’re going to do with your data once you have it, including statistical tests (non-relational and relational) that you plan to use. You can do this exercise whether you’re using quantitative or qualitative data! The same principles apply.
- Make a data dictionary for the data you are proposing to collect as part of your study. You can use the example above as a template.
TRACK 2 (IF YOU AREN’T CREATING A RESEARCH PROPOSAL FOR THIS CLASS)
You are researching the impact of your city’s recent harm reduction interventions for intravenous drug users (e.g., sterile injection kits, monitored use, overdose prevention, naloxone provision, etc.).
- Make a draft quantitative data analysis plan for your project. Remember this should include your research question, a description of the data you will use, and a step-by-step outline of what you’re going to do with your data once you have it, including statistical tests (non-relational and relational) that you plan to use. It’s okay if you don’t yet have a complete idea of the types of statistical analyses you might use.
An ordered outline that includes your research question, a description of the data you are going to use to answer it, and the exact analyses, step-by-step, that you plan to run to answer your research question.
The name of your variable.
The rows in your data set. In social work, these are often your study participants (people), but can be anything from census tracts to black bears to trains.
This is the document where you list your variable names, what the variables actually measure or represent, what each of the values of the variable mean if the meaning isn't obvious.
process by which researchers spell out precisely how a concept will be measured in their study
A group of statistical techniques that examines the relationship between at least three variables
Univariate data analysis is a quantitative method in which a variable is examined individually to determine its distribution.
the way the scores are distributed across the levels of that variable.
Chapter Outline
- Practical and ethical considerations ( 14 minute read)
- Raw data (10 minute read)
- Creating a data analysis plan (?? minute read)
- Critical considerations (3 minute read)
Content warning: Examples in this chapter discuss substance use disorders, mental health disorders and therapies, obesity, poverty, gun violence, gang violence, school discipline, racism and hate groups, domestic violence, trauma and triggers, incarceration, child neglect and abuse, bullying, self-harm and suicide, racial discrimination in housing, burnout in helping professions, and sex trafficking of indigenous women.
2.1 Practical and ethical considerations
Learners will be able to...
- Identify potential stakeholders and gatekeepers
- Differentiate between raw data and the results of scientific studies
- Evaluate whether you can feasibly complete your project
Pre-awareness check (Knowledge)
Similar to practice settings, research has ethical considerations that must be taken to ensure the safety of participants. What ethical considerations were relevant to your practice experience that may have impacted the delivery of services?
As a PhD student, you will have many opportunities to conduct research. You may be asked to be a part of a research team led by the faculty at your institution. You will also conduct your own research for your dissertation. As you will learn, research can take many forms. For example, you may want to focus qualitatively on individuals’ lived experiences, or perhaps you will quantitatively assess the impact of interventions on research subjects. You may work with large, already-existing datasets, or you may create your own data. Though social work research can vary widely from project to project, researchers typically follow the same general process, even if their specific research questions and methodologies differ. Table 2.1 outlines the major components of the research process covered in this textbook, and indicates the chapters where you will find more information on each subject. You will notice that your research paradigm is an organizing framework that guides each component of the research process.
Table 2.1 Components of the Research Process
Feasibility
Feasibility refers to whether you can practically conduct the study you plan to do, given the resources and ethical obligations you have. In this chapter, we will review some important practical and ethical considerations researchers should start thinking about from the beginning of a research project. These considerations apply to all research, but it is important to also consider the context of research and researchers when thinking about feasibility.
For example, as a doctoral student, you likely have a unique set of circumstances that inspire and constrain your research. Some students have the ability to engage in independent studies where they can gain skills and expertise in specialized research methods to prepare them for a research-intensive career. Others may have reasons, such as a limited amount of funding or family concerns, that encourage them to complete their dissertation research as quickly as possible. These circumstances relate to the feasibility of a research project. Regardless of the potential societal importance of a 10-year longitudinal study, it’s not feasible for a student to conduct it in time to graduate! Your dissertation chair, doctoral program director, and other faculty mentors can help you navigate the many decisions you will face as a doctoral student about conducting independent research or joining research projects.
The context and role of the researcher continue to affect feasibility even after a doctoral student graduates. Many will continue in their careers to become tenure track faculty with research expectations to obtain tenure. Some funders expect faculty members to have a track record of successful projects before trusting them to lead expensive or long-term studies. Realistically, these expectations will influence what research is feasible for a junior faculty member to conduct. Just like for doctoral students, mentorship is incredibly valuable for junior faculty to make informed decisions about what research to conduct. Senior faculty, associate deans of research, chairs, and deans can help junior faculty decide what projects to pursue to ensure they meet the expectations placed on them without losing sight of the reasons they became a researcher in the first place.
As you read about other feasibility considerations such as gaining access, consent, and collecting data, consider the ways in which context and roles also influence feasibility.
Access, consent, and ethical obligations
One of the most important feasibility issues is gaining access to your target population. For example, let’s say you wanted to better understand middle-school students who engaged in self-harm behaviors. That is a topic of social importance, but what challenges might you face in accessing this population? Let's say you proposed to identify students from a local middle school and interview them about self-harm. Methodologically, that sounds great since you are getting data from those with the most knowledge about the topic, the students themselves. But practically, that sounds challenging. Think about the ethical obligations a social work practitioner has to adolescents who are engaging in self-harm (e.g., competence, respect). In research, we are similarly concerned mostly with the benefits and harms of what you propose to do as well as the openness and honesty with which you share your project publicly.
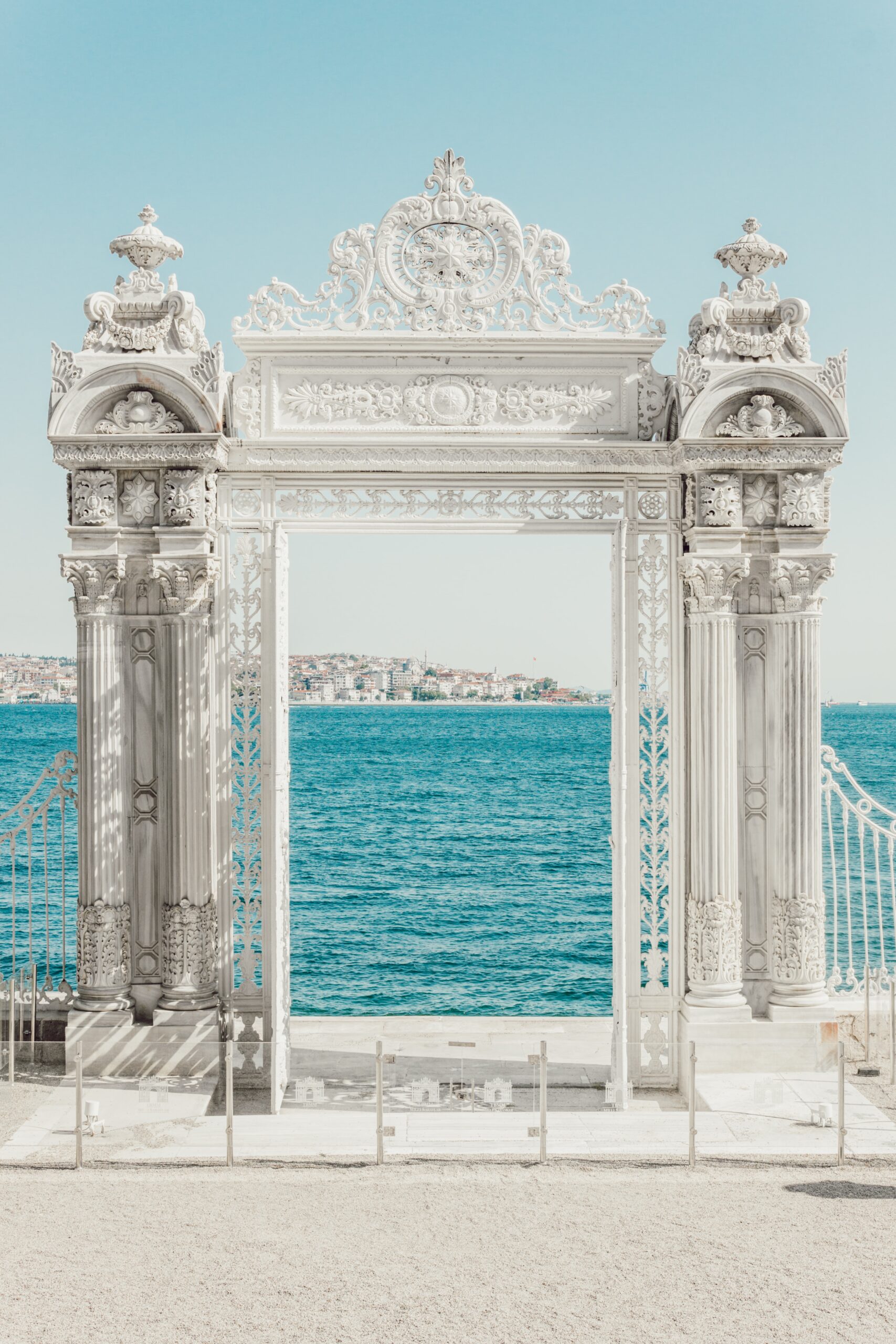
Gatekeepers
If you were the principal at your local middle school, would you allow researchers to interview kids in your schools about self-harm? What if the results of the study showed that self-harm was a big problem that your school was not addressing? What if the researcher's interviews themselves caused an increase in self-harming behaviors among the children? The principal in this situation is a gatekeeper . Gatekeepers are the individuals or organizations who control access to the population you want to study. The school board would also likely need to give consent for the research to take place at their institution. Gatekeepers must weigh their ethical questions because they have a responsibility to protect the safety of the people at their organization, just as you have an ethical obligation to protect the people in your research study.
For vulnerable populations, it can be a challenge to get consent from gatekeepers to conduct your research project. As a result, researchers often conduct research projects in places where they have established trust with gatekeepers. In the case where the population (children who self-harm) are too vulnerable, researchers may collect data from people who have secondary knowledge about the topic. For example, the principal may be more willing to let you talk to teachers or staff, rather than children.
Stakeholders
In some cases, researchers and gatekeepers partner on a research project. When this happens, the gatekeepers become stakeholders . Stakeholders are individuals or groups who have an interest in the outcome of the study you conduct. As you think about your project, consider whether there are formal advisory groups or boards (like a school board) or advocacy organizations who already serve or work with your target population. Approach them as experts and ask for their review of your study to see if there are any perspectives or details you missed that would make your project stronger.
There are many advantages to partnering with stakeholders to complete a research project together. Continuing with our example on self-harm in schools, in order to obtain access to interview children at a middle school, you will have to consider other stakeholders' goals. School administrators also want to help students struggling with self-harm, so they may want to use the results to form new programs. But they may also need to avoid scandal and panic if the results show high levels of self-harm. Most likely, they want to provide support to students without making the problem worse. By bringing in school administrators as stakeholders, you can better understand what the school is currently doing to address the issue and get an informed perspective on your project's questions. Negotiating the boundaries of a stakeholder relationship requires strong meso-level practice skills.
Of course, partnering with administrators probably sounds quite a bit easier than bringing on board the next group of stakeholders—parents. It's not ethical to ask children to participate in a study without their parents' consent. We will review the parameters of parental and child consent in Chapter 5 . Parents may be understandably skeptical of a researcher who wants to talk to their child about self-harm, and they may fear potential harm to the child and family from your study. Would you let a researcher you didn't know interview your children about a very sensitive issue?
Social work research must often satisfy multiple stakeholders. This is especially true if a researcher receives a grant to support the project, as the funder has goals it wants to accomplish by funding the research project. Your university is also a stakeholder in your project. When you conduct research, it reflects on your school. If you discover something of great importance, your school looks good. If you harm someone, they may be liable. Your university likely has opportunities for you to share your research with the campus community, and may have incentives or grant programs for researchers. Your school also provides you with support and access to resources like the library and data analysis software.
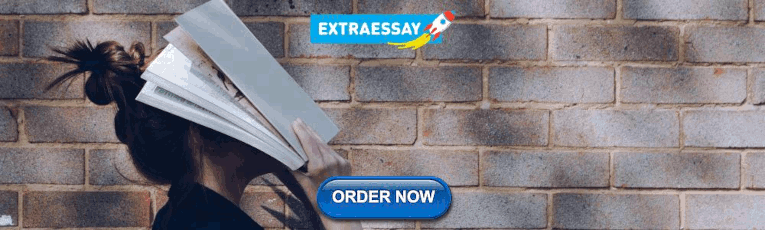
Target population
So far, we've talked about access in terms of gatekeepers and stakeholders. Let's assume all of those people agree that your study should proceed. But what about the people in the target population? They are the most important stakeholder of all! Think about the children in our proposed study on self-harm. How open do you think they would be to talking to you about such a sensitive issue? Would they consent to talk to you at all?
Maybe you are thinking about simply asking clients on your caseload. As we talked about before, leveraging existing relationships created through field work can help with accessing your target population. However, they introduce other ethical issues for researchers. Asking clients on your caseload or at your agency to participate in your project creates a dual relationship between you and your client. What if you learn something in the research project that you want to share with your clinical team? More importantly, would your client feel uncomfortable if they do not consent to your study? Social workers have power over clients, and any dual relationship would require strict supervision in the rare case it was allowed.
Resources and scope
Let's assume everyone consented to your project and you have adequately addressed any ethical issues with gatekeepers, stakeholders, and your target population. That means everything is ready to go, right? Not quite yet. As a researcher, you will need to carry out the study you propose to do. Depending on how big or how small your proposed project is, you’ll need a little or a lot of resources.
One thing that all projects need is raw data . Raw data can come in may forms. Very often in social science research, raw data includes the responses to a survey or transcripts of interviews and focus groups, but raw data can also include experimental results, diary entries, art, or other data points that social scientists use in analyzing the world. Primary data is data you have collected yourself. Sometimes, social work researchers do not collect raw data of their own, but instead use secondary data analysis to analyze raw data that has been shared by other researchers. Secondary data is data someone else has collected that you have permission to use in your research. For example, you could use data from a local probation program to determine if a shoplifting prevention group was reducing the rate at which people were re-offending. You would need data on who participated in the program and their criminal history six months after the end of their probation period. This is secondary data you could use to determine whether the shoplifting prevention group had any effect on an individual's likelihood of re-offending. Whether a researcher should use secondary data or collect their own raw data is an important choice which we will discuss in greater detail in section 2.2. Collecting raw data or obtaining secondary data can be time consuming or expensive, but without raw data there can be no research project.
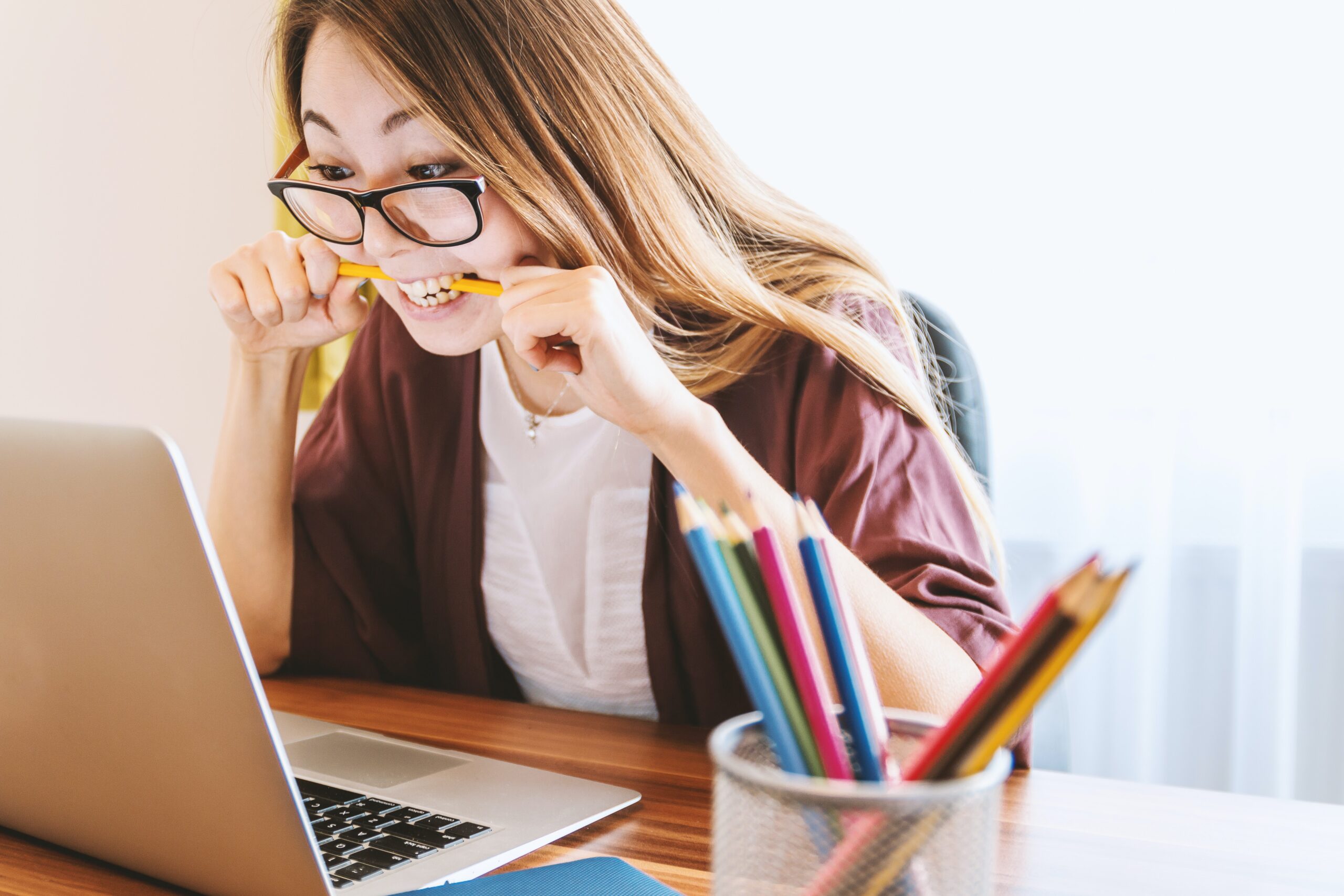
Time is an important resource to consider when designing research projects. Make sure that your proposal won't require you to spend more time than you have to collect and analyze data. Think realistically about the timeline for your research project. If you propose to interview fifty mental health professionals in their offices in your community about your topic, make sure you can dedicate fifty hours to conduct those interviews, account for travel time, and think about how long it will take to transcribe and analyze those interviews.
- What is reasonable for you to do in your timeframe?
- How many hours each week can the research team dedicate to this project?
One thing that can delay a research project is receiving approval from the institutional review board (IRB), the research ethics committee at your university. If your study involves human subjects , you may have to formally propose your study to the IRB and get their approval before gathering your data. A well-prepared study is likely to gain IRB approval with minimal revisions needed, but the process can take weeks to complete and must be done before data collection can begin. We will address the ethical obligations of researchers in greater detail in Chapter 5 .
Most research projects cost some amount of money. Potential expenses include wages for members of the research team, incentives for research participants, travel expenses, and licensing costs for standardized instruments. Most researchers seek grant funding to support the research. Grant applications can be time consuming to write and grant funding can be competitive to receive.
Knowledge, competence, and skills
For social work researchers, the social work value of competence is key in their research ethics.
Clearly, researchers need to be skilled in working with their target population in order to conduct ethical research. Some research addresses this challenge by collecting data from competent practitioners or administrators who have second-hand knowledge of target populations based on professional relationships. Members of the research team delivering an intervention also need to have training and skills in the intervention. For example, if a research study examines the effectiveness of dialectical behavioral therapy (DBT) in a particular context, the person delivering the DBT must be certified in DBT. Another idea to keep in mind is the level of data collection and analysis skills needed to complete the project. Some assessments require training to administer. Analyses may be complex or require statistical consultation or advanced training.
In summary, here are a few questions you should ask yourself about your project to make sure it's feasible. While we present them early on in the research process (we're only in Chapter 2), these are certainly questions you should ask yourself throughout the proposal writing process. We will revisit feasibility again in Chapter 9 when we work on finalizing your research question .
- Do you have access to the data you need or can you collect the data you need?
- Will you be able to get consent from stakeholders, gatekeepers, and your target population?
- Does your project pose risk to individuals through direct harm, dual relationships, or breaches in confidentiality?
- Are you competent enough to complete the study?
- Do you have the resources and time needed to carry out the project?
- People will have to say “yes” to your research project. Evaluate whether your project might have gatekeepers or potential stakeholders. They may control access to data or potential participants.
- Researchers need raw data such as survey responses, interview transcripts, or client charts. Your research project must involve more than looking at the analyses conducted by other researchers, as the literature review is only the first step of a research project.
- Make sure you have enough resources (time, money, and knowledge) to complete your research project.
Post-awareness check (Emotion)
What factors have created your passion toward assisting your target population? How can this connection enhance your ability to receive a “yes” from potential participants? What are the anticipated challenges to receiving a “yes” from potential participants?
Think about how you might answer your question by collecting your own data.
- Identify any gatekeepers and stakeholders you might need to contact.
- How can you increase the likelihood you will get access to the people or records you need for your study?
Describe the resources you will need for your project.
- Do you have concerns about feasibility?
TRACK 2 (IF YOU AREN'T CREATING A RESEARCH PROPOSAL FOR THIS CLASS)
You are researching the impact of your city's recent harm reduction interventions for intravenous drug users (e.g., sterile injection kits, monitored use, overdose prevention, naloxone provision, etc.).
- Thinking about the services related to this issue in your own city, identify any gatekeepers and stakeholders you might need to contact.
- How might you approach these gatekeepers and stakeholders? How would you explain your study?
2.2 Raw data
- Identify potential sources of available data
- Weigh the challenges and benefits of collecting your own data
In our previous section, we addressed some of the challenges researchers face in collecting and analyzing raw data. Just as a reminder, raw data are unprocessed, unanalyzed data that researchers analyze using social science research methods. It is not just the statistics or qualitative themes in journal articles. It is the actual data from which those statistical outputs or themes are derived (e.g., interview transcripts or survey responses).
There are two approaches to getting raw data. First, students can analyze data that are publicly available or from agency records. Using secondary data like this can make projects more feasible, but you may not find existing data that are useful for answering your working question. For that reason, many students gather their own raw data. As we discussed in the previous section, potential harms that come from addressing sensitive topics mean that surveys and interviews of practitioners or other less-vulnerable populations may be the most feasible and ethical way to approach data collection.
Using secondary data
Within the agency setting, there are two main sources of raw data. One option is to examine client charts. For example, if you wanted to know if substance use was related to parental reunification for youth in foster care, you could look at client files and compare how long it took for families with differing levels of substance use to be reunified. You will have to negotiate with the agency the degree to which your analysis can be public. Agencies may be okay with you using client files for a class project but less comfortable with you presenting your findings at a city council meeting. When analyzing data from your agency, you will have to manage a stakeholder relationship.
Another great example of agency-based raw data comes from program evaluations. If you are working with a grant funded agency, administrators and clinicians are likely producing data for grant reporting. The agency may consent to have you look at the raw data and run your own analysis. Larger agencies may also conduct internal research—for example, surveying employees or clients about new initiatives. These, too, can be good sources of available data. Generally, if the agency has already collected the data, you can ask to use them. Again, it is important to be clear on the boundaries and expectations of the agency. And don't be angry if they say no!
Some agencies, usually government agencies, publish their data in formal reports. You could take a look at some of the websites for county or state agencies to see if there are any publicly available data relevant to your research topic. As an example, perhaps there are annual reports from the state department of education that show how seclusion and restraint is disproportionately applied to Black children with disabilities , as students found in Virginia. In another example, one student matched public data from their city's map of criminal incidents with historically redlined neighborhoods. For this project, she is using publicly available data from Mapping Inequality , which digitized historical records of redlined housing communities and the Roanoke, VA crime mapping webpage . By matching historical data on housing redlining with current crime records, she is testing whether redlining still impacts crime to this day.
Not all public data are easily accessible, though. The student in the previous example was lucky that scholars had digitized the records of how Virginia cities were redlined by race. Sources of historical data are often located in physical archives, rather than digital archives. If your project uses historical data in an archive, it would require you to physically go to the archive in order to review the data. Unless you have a travel budget, you may be limited to the archival data in your local libraries and government offices. Similarly, government data may have to be requested from an agency, which can take time. If the data are particularly sensitive or if the department would have to dedicate a lot of time to your request, you may have to file a Freedom of Information Act request. This process can be time-consuming, and in some cases, it will add financial cost to your study.
Another source of secondary data is shared by researchers as part of the publication and review process. There is a growing trend in research to publicly share data so others can verify your results and attempt to replicate your study. In more recent articles, you may notice links to data provided by the researcher. Often, these have been de-identified by eliminating some information that could lead to violations of confidentiality. You can browse through the data repositories in Table 2.1 to find raw data to analyze. Make sure that you pick a data set with thorough and easy to understand documentation. You may also want to use Google's dataset search which indexes some of the websites below as well as others in a very intuitive and easy to use way.
Ultimately, you will have to weigh the strengths and limitations of using secondary data on your own. Engel and Schutt (2016, p. 327) [1] propose six questions to ask before using secondary data:
- What were the agency’s or researcher’s goals in collecting the data?
- What data were collected, and what were they intended to measure?
- When was the information collected?
- What methods were used for data collection? Who was responsible for data collection, and what were their qualifications? Are they available to answer questions about the data?
- How is the information organized (by date, individual, family, event, etc.)? Are identifiers used to indicate different types of data available?
- What is known about the success of the data collection effort? How are missing data indicated and treated? What kind of documentation is available? How consistent are the data with data available from other sources?
In this section, we've talked about data as though it is always collected by scientists and professionals. But that's definitely not the case! Think more broadly about sources of data that are already out there in the world. Perhaps you want to examine the different topics mentioned in the past 10 State of the Union addresses by the President. Or maybe you want to examine whether the websites and public information about local health and mental health agencies use gender-inclusive language. People share their experiences through blogs, social media posts, videos, performances, among countless other sources of data. When you think broadly about data, you'll be surprised how much you can answer with available data.
Collecting your own raw data
The primary benefit of collecting your own data is that it allows you to collect and analyze the specific data you are looking for, rather than relying on what other people have shared. You can make sure the right questions are asked to the right people. Your early research projects may be smaller in scope. This isn't necessarily a limitation. Early projects are often the first step in a long research trajectory in which the same topic is studied in increasing detail and sophistication over time.
Student researchers often propose to survey or interview practitioners. The focus of these projects should be about the practice of social work and the study will uncover how practitioners understand what they do. Surveys of practitioners often test whether responses to questions are related to each other. For example, you could propose to examine whether someone's length of time in practice was related to the type of therapy they use or their level of burnout. Interviews or focus groups can also illuminate areas of practice. One student proposed to conduct focus groups of individuals in different helping professions in order to understand how they viewed the process of leaving an abusive partner. She suspected that people from different disciplines would make unique assumptions about the survivor's choices.
It's worth remembering here that you need to have access to practitioners, as we discussed in the previous section. Resourceful researchers will look at publicly available databases of practitioners, draw from agency and personal contacts, or post in public forums like Facebook groups. Consent from gatekeepers is important, and as we described earlier, you and your agency may be interested in collaborating on a project. Bringing your agency on board as a stakeholder in your project may allow you access to company email lists or time at staff meetings as well as access to practitioners. One student partnered with her internship placement at a local hospital to measure the burnout that nurses experienced in their department. Her project helped the agency identify which departments may need additional support.
Another possible way you could collect data is by partnering with your agency on evaluating an existing program. Perhaps they want you to evaluate the early stage of a program to see if it's going as planned and if any changes need to be made. Maybe there is an aspect of the program they haven't measured but would like to, and you can fill that gap for them. Collaborating with agency partners in this way can be a challenge, as you must negotiate roles, get stakeholder buy-in, and manage the conflicting time schedules of field work and research work. At the same time, it allows you to make your work immediately relevant to your specific practice and client population.
In summary, many early projects fall into one of the following categories. These aren't your only options! But they may be helpful in thinking about what research projects can look like.
- Analyzing charts or program evaluations at an agency
- Analyzing existing data from an agency, government body, or other public source
- Analyzing popular media or cultural artifacts
- Surveying or interviewing practitioners, administrators, or other less-vulnerable groups
- Conducting a program evaluation in collaboration with an agency
- All research projects require analyzing raw data.
- Research projects often analyze available data from agencies, government, or public sources. Doing so allows researchers to avoid the process of recruiting people to participate in their study. This makes projects more feasible but limits what you can study to the data that are already available to you.
- Think through the potential harm of discussing sensitive topics when surveying or interviewing clients and other vulnerable populations. Since many social work topics are sensitive, researchers often collect data from less-vulnerable populations such as practitioners and administrators.
Post-awareness check (Environment)
In what environment are you most comfortable in data collection (phone calls, face to face recruitment, etc)? Consider your preferred method of data collection that may align with both your personality and your target population.
- Describe the difference between raw data and the results of research articles.
- Consider browsing around the data repositories in Table 2.1.
- Identify a common type of project (e.g., surveys of practitioners) and how conducting a similar project might help you answer your working question.
- What kind of raw data might you collect yourself for your study?
2.3 Creating a data analysis plan
- Define and construct a data analysis plan.
- Define key quantitative data management terms—variable name, data dictionary, primary and secondary data, observations/cases.
- Differentiate between univariate and bivariate quantitative analysis.
- Explain when we might use quantitative bivariate analysis in social work research.
- Identify how your qualitative research question, research aim, and type of data may influence your choice of analytic methods.
- Outline the steps you will take in preparation for conducting qualitative data analysis.
After you have your raw data , whether this is secondary data or data you collected yourself, you will need to analyze it. While the specific steps to follow in quantitative or qualitative data analysis are beyond the scope of this chapter, we are going to address some basic concepts in this section to help you create a data analysis plan. A data analysis plan is an ordered outline that includes your research question, a description of the data you are going to use to answer it, and the exact step-by-step analyses that you plan to run to answer your research question. If you look back at Table 2.1, you will see that creating a data analysis plan is a part of the study design process. The data analysis plan flows from the research question, is integral to the study desig n, and should be well conceptualized prior to beginning data collection. In this section, we will walk through the basics of quantitative and qualitative data analysis to help you understand the fundamentals of creating a data analysis plan.
When considering what data you might want to collect as part of your project, there are two important considerations that can create dilemmas for researchers. You might only get one chance to interact with your participants, so you must think comprehensively in your planning phase about what information you need and collect as much relevant data as possible. At the same time, though, especially when collecting sensitive information, you need to consider how onerous the data collection is for participants and whether you really need them to share that information. Just because something is interesting to us doesn't mean it's related enough to our research question to chase it down. Work with your research team and/or faculty early in your project to talk through these issues before you get to this point. And if you're using secondary data, make sure you have access to all the information you need in that data before you use it.
Once you've collected your quantitative data, you need to make sure it is well- organized in a database in a way that's actually usable. "Database" can be kind of a scary word, but really, it can be as simple as an Excel spreadsheet or a data file in whatever program you're using to analyze your data. You may want to avoid Excel and use a formal database such as Microsoft Access or MySQL if you've got a large or complicated data set. But if your data set is smaller and you plan to keep your analyses simple, you can definitely get away with Excel. A typical data set is organized with variables as columns and observations/cases as rows. For example, let's say we did a survey on ice cream preferences and collected the following information in Table 2.3:
- Variable name : Just what it sounds like—the name of your variable. Make sure this is something useful, short and, if you're using something other than Excel, all one word. Most statistical programs will automatically rename variables for you if they aren't one word, but the names can be a little ridiculous and long.
- Observations/cases : The rows in your data set. In social work, these are often your study participants (people), but can be anything from census tracts to black bears to trains. When we talk about sample size, we're talking about the number of observations/cases. In our mini data set, each person is an observation/case.
- Data dictionary (sometimes called a code book or metadata) : This is the document where you list your variable names, what the variables actually measure or represent, what each of the values of the variable mean if the meaning isn't obvious (i.e., if there are numbers assigned to gender), the level of measurement and anything special to know about the variables (for instance, the source if you mashed two data sets together). If you're using secondary data, the researchers sharing the data should make the data dictionary available .
Let's take that mini data set we've got up above and we'll show you what your data dictionary might look like in Table 2.4.
As part of planning for your research, you should come up with a data analysis plan. Remember, a data analysis plan is an ordered outline that includes your research question, a description of the data you are going to use to answer it, and the exact step-by-step analyses that you plan to run to answer your research question. A basic data analysis plan might look something like what you see in Table 2.5. Don't panic if you don't yet understand some of the statistical terms in the plan; we're going to delve into some of them in this section, and others will be covered in more depth in your statistics courses. Note here also that this is what operationalizing your variables and moving through your research with them looks like on a basic level. We will cover operationalization in more depth in Chapter 11.
An important point to remember is that you should never get stuck on using a particular statistical method because you or one of your co-researchers thinks it's cool or it's the hot thing in your field right now. You should certainly go into your data analysis plan with ideas, but in the end, you need to let your research question guide what statistical tests you plan to use. Be prepared to be flexible if your plan doesn't pan out because the data is behaving in unexpected ways.
You'll notice that the first step in the quantitative data analysis plan is univariate and descriptive statistics. Univariate data analysis is a quantitative method in which a variable is examined individually to determine its distribution , or the way the scores are distributed across the levels, or values, of that variable. When we talk about levels , what we are talking about are the possible values of the variable—like a participant's age, income or gender. (Note that this is different from levels of measurement , which will be discussed in Chapter 11, but the level of measurement of your variables absolutely affects what kinds of analyses you can do with it.) Univariate analysis is n on-relational , which just means that we're not looking into how our variables relate to each other. Instead, we're looking at variables in isolation to try to understand them better. For this reason, univariate analysis is used for descriptive research questions.
So when do you use univariate data analysis? Always! It should be the first thing you do with your quantitative data, whether you are planning to move on to more sophisticated statistical analyses or are conducting a study to describe a new phenomenon. You need to understand what the values of each variable look like—what if one of your variables has a lot of missing data because participants didn't answer that question on your survey? What if there isn't much variation in the gender of your sample? These are things you'll learn through univariate analysis.
Did you know that ice cream causes shark attacks? It's true! When ice cream sales go up in the summer, so does the rate of shark attacks. So you'd better put down that ice cream cone, unless you want to make yourself look more delicious to a shark.
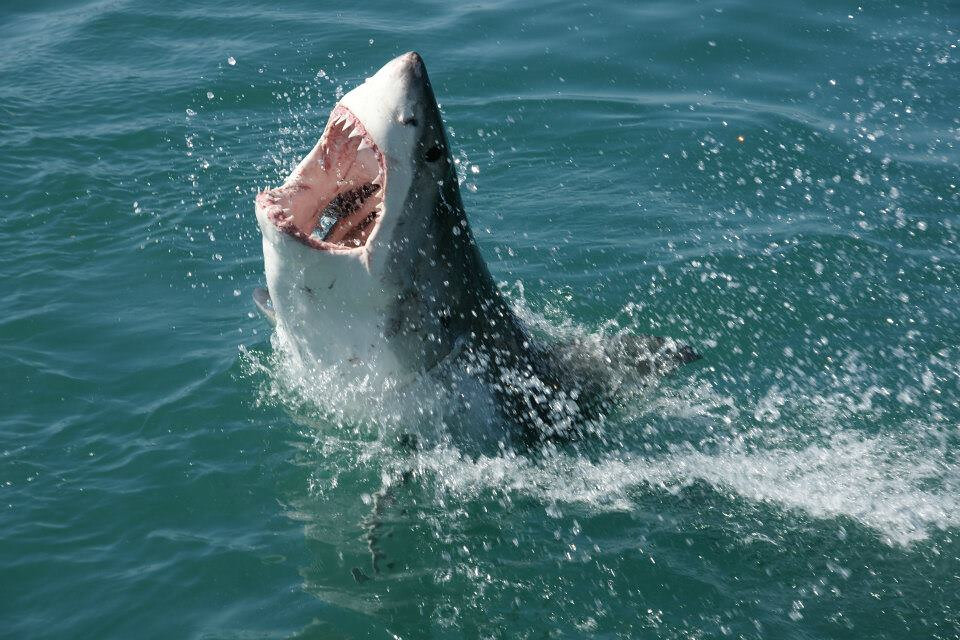
Ok, so it's quite obviously not true that ice cream causes shark attacks. But if you looked at these two variables and how they're related, you'd notice that during times of the year with high ice cream sales, there are also the most shark attacks. Despite the fact that the conclusion we drew about the relationship was wrong, it's nonetheless true that these two variables appear related, and researchers figured that out through the use of bivariate analysis. (You will learn about correlation versus causation in Chapter 8 .)
Bivariate analysis consists of a group of statistical techniques that examine the association between two variables. We could look at how anti-depressant medications and appetite are related, whether there is a relation between having a pet and emotional well-being, or if a policy-maker's level of education is related to how they vote on bills related to environmental issues.
Bivariate analysis forms the foundation of multivariate analysis, which we don't get to in this book. All you really need to know here is that there are steps beyond bivariate analysis, which you've undoubtedly seen in scholarly literature already! But before we can move forward with multivariate analysis, we need to understand the associations between the variables in our study .
[MADE THIS UP] Throughout your PhD program, you will learn more about quantitative data analysis techniques. Hopefully this section has provided you with some initial insights into how data is analyzed, and the importance of creating a data analysis plan prior to collecting data. Next, we will discuss some basic strategies for creating a qualitative data analysis plan.
If you don't see the general aim of your research question reflected in one of these areas, don't fret! This is only a small sampling of what you might be trying to accomplish with your qualitative study. Whatever your aim, you need to have a plan for what you will do once you have collected your data.
Iterative or linear
Some qualitative research is linear , meaning it follows more of a tra ditionally quantitative process: create a plan, gather data, and analyze data; each step is completed before we proceed to the next. You can think of this like how information is presented in this book. We discuss each topic, one after another.
However, many times qualitative research is iterative , or evolving in cycles. An iterative approach means that once we begin collecting data, we also begin analyzing data as it is coming in. This early and ongoing analysis of our (incomplete) data then impacts our continued planning, data gathering and future analysis. Again, coming back to this book, while it may be written linear, we hope that you engage with it iteratively as you design and conduct your own research. By this we mean that you will revisit previous sections so you can understand how they fit together and you are in continuous process of building and revising how you think about the concepts you are learning about.
As you may have guessed, there are benefits and challenges to both linear and iterative approaches. A linear approach is much more straightforward, each step being fairly defined. However, linear research being more defined and rigid also presents certain challenges. A linear approach assumes that we know what we need to ask or look for at the very beginning of data collection, which often is not the case.
With iterative research, we have more flexibility to adapt our approach as we learn new things. We still need to keep our approach systematic and organized, however, so that our work doesn't become a free-for-all. As we adapt, we do not want to stray too far from the original premise of our study. It's also important to remember with an iterative approach that we may risk ethical concerns if our work extends beyond the original boundaries of our informed consent and institutional review board agreement (IRB; see Chapter 6 for more on IRBs). If you feel that you do need to modify your original research plan in a significant way as you learn more about the topic, you can submit an addendum to modify your original application that was submitted. Make sure to keep detailed notes of the decisions that you are making and what is informing these choices. This helps to support transparency and your credibility throughout the research process.
As y ou begin your analysis, y ou need to get to know your data. This often means reading through your data prior to any attempt at breaking it apart and labeling it. You mig ht read through a couple of times, in fact. This helps give you a more comprehensive feel for each piece of data and the data as a whole, again, before you start to break it down into smaller units or deconstruct it. This is especially important if others assisted us in the data collection process. We often gather data as part of team and everyone involved in the analysis needs to be very familiar with all of the data.
During your reviewing you will start to develop and evolve your understanding of what the data means. Coding is a part of the qualitative data analysis process where we begin to interpret and assign meaning to the data. It represents one of the first steps as we begin to filter the data through our own subjective lens as the researcher. This understanding of the data should be dynamic and flexible, but you want to have a way to capture this understanding as it evolves. You may include this as part of your qualitative codebook where you are tracking the main ideas that are emerging and what they mean. Figure 2.2 is an example of how your thinking might change about a code and how you can go about capturing it.
There are a variety of different approaches to qualitative analysis, including thematic analysis, content analysis, grounded theory, phenomenology, photovoice, and more. The specific steps you will take to code your qualitative data, and to generate themes from these codes, will vary based on the analytic strategy you are employing. In designing your qualitative study, you would identify an analytical approach as you plan out your project. The one you select would depend on the type of data you have and what you want to accomplish with it.
- Getting organized at the beginning of your project with a data analysis plan will help keep you on track. Data analysis plans should include your research question, a description of your data, and a step-by-step outline of what you're going to do with it. [chapter 14.1]
Exercises [from chapter 14.1]
- Make a data analysis plan for your project. Remember this should include your research question, a description of the data you will use, and a step-by-step outline of what you're going to do with your data once you have it, including statistical tests (non-relational and relational) that you plan to use. You can do this exercise whether you're using quantitative or qualitative data! The same principles apply.
- Make a draft quantitative data analysis plan for your project. Remember this should include your research question, a description of the data you will use, and a step-by-step outline of what you're going to do with your data once you have it, including statistical tests (non-relational and relational) that you plan to use. It's okay if you don't yet have a complete idea of the types of statistical analyses you might use.
2.4 Critical considerations
- Critique the traditional role of researchers and identify how action research addresses these issues
So far in this chapter, we have presented the steps of research projects as follows:
- Find a topic that is important to you and read about it.
- Pose a question that is important to the literature and to your community.
- Propose to use specific research methods and data analysis techniques to answer your question.
- Carry out your project and report the results.
These were depicted in more detail in Table 2.1 earlier in this chapter. There are important limitations to this approach. This section examines those problems and how to address them.
Whose knowledge is privileged?
First, let's critically examine your role as the researcher. Following along with the steps in a research project, you start studying the literature on your topic, find a place where you can add to scientific knowledge, and conduct your study. But why are you the person who gets to decide what is important? Just as clients are the experts on their lives, members of your target population are the experts on their lives. What does it mean for a group of people to be researched on, rather than researched with? How can we better respect the knowledge and self-determination of community members?
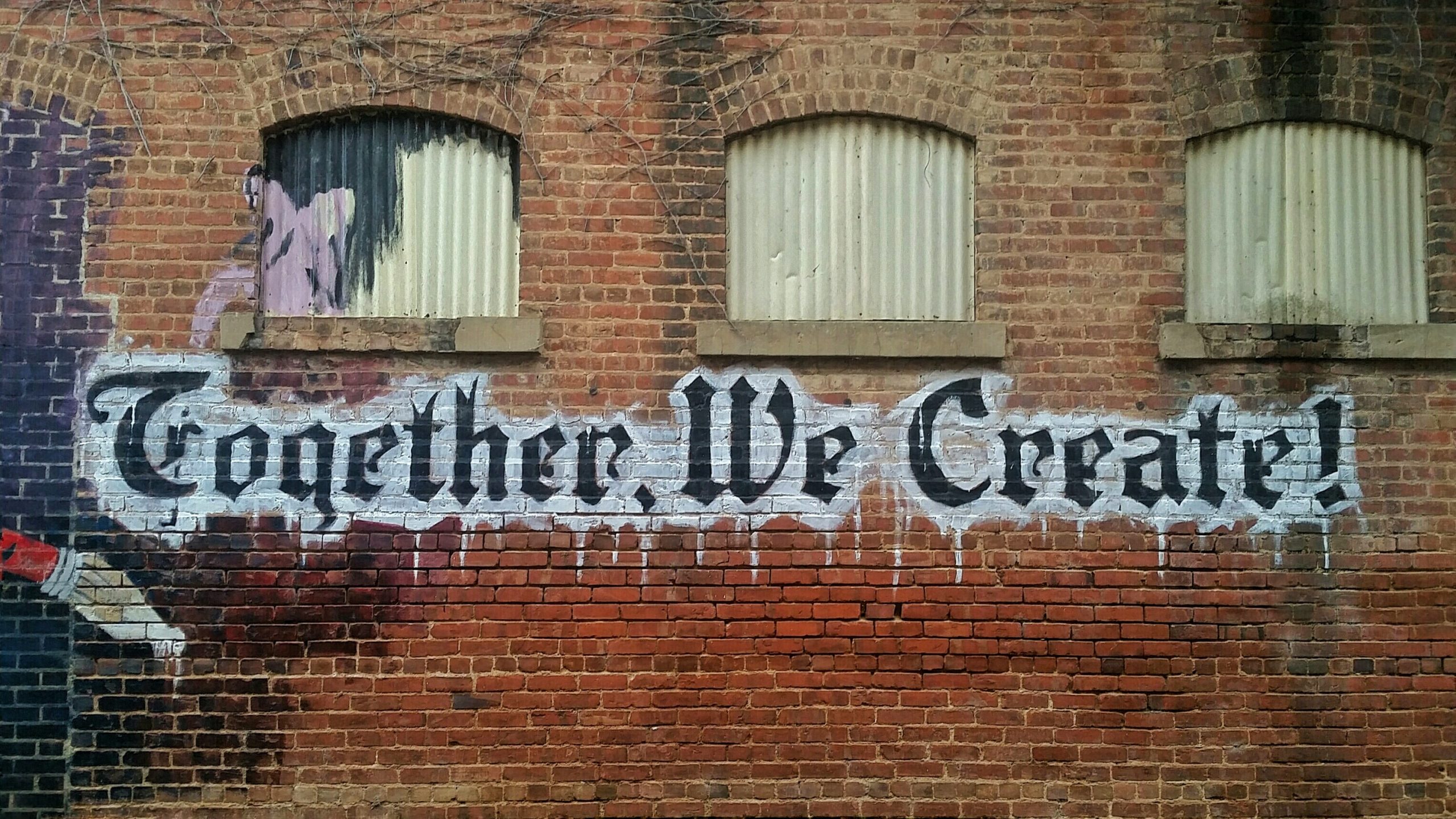
A different way of approaching your research project is to start by talking with members of the target population and those who are knowledgeable about that community. Perhaps there is a community-led organization you can partner with on a research project. The researcher's role in this case would be more similar to a consultant, someone with specialized knowledge about research who can help communities study problems they consider to be important. The social worker is a co-investigator, and community members are equal partners in the research project. Each has a type of knowledge—scientific expertise vs. lived experience—that should inform the research process.
The community focus highlights something important: they are localized. These projects can dedicate themselves to issues at a single agency or within a service area. With a local scope, researchers can bring about change in their community. This is the purpose behind action research.
Action research
Action research is research that is conducted for the purpose of creating social change. When engaging in action research, scholars collaborate with community stakeholders to conduct research that will be relevant to the community. Social workers who engage in action research don't just go it alone; instead, they collaborate with the people who are affected by the research at each stage in the process. Stakeholders, particularly those with the least power, should be consulted on the purpose of the research project, research questions, design, and reporting of results.
Action research also distinguishes itself from other research in that its purpose is to create change on an individual and community level. Kristin Esterberg puts it quite eloquently when she says, “At heart, all action researchers are concerned that research not simply contribute to knowledge but also lead to positive changes in people’s lives” (2002, p. 137). [2] Action research has multiple origins across the globe, including Kurt Lewin’s psychological experiments in the US and Paulo Friere’s literacy and education programs (Adelman, 1993; Reason, 1994). [3] Over the years, action research has become increasingly popular among scholars who wish for their work to have tangible outcomes that benefit the groups they study.
A traditional scientist might look at the literature or use their practice wisdom to formulate a question for quantitative or qualitative research, as we suggested earlier in this chapter. An action researcher, on the other hand, would consult with people in the target population and community to see what they believe the most pressing issues are and what their proposed solutions may be. In this way, action research flips traditional research on its head. Scientists are not the experts on the research topic. Instead, they are more like consultants who provide the tools and resources necessary for a target population to achieve their goals and to address social problems using social science research.
According to Healy (2001), [4] the assumptions of participatory-action research are that (a) oppression is caused by macro-level structures such as patriarchy and capitalism; (b) research should expose and confront the powerful; (c) researcher and participant relationships should be equal, with equitable distribution of research tasks and roles; and (d) research should result in consciousness-raising and collective action. Consistent with social work values, action research supports the self-determination of oppressed groups and privileges their voice and understanding through the conceptualization, design, data collection, data analysis, and dissemination processes of research. We will return to similar ideas in Part 4 of the textbook when we discuss qualitative research methods, though action research can certainly be used with quantitative research methods, as well.
- Traditionally, researchers did not consult target populations and communities prior to formulating a research question. Action research proposes a more community-engaged model in which researchers are consultants that help communities research topics of import to them.
Post- awareness check (Knowledge)
Based on what you know of your target population, what are a few ways to receive their “buy-in” to participate in your proposed research study?
- Apply the key concepts of action research to your project. How might you incorporate the perspectives and expertise of community members in your project?
The level that describes how data for variables are recorded. The level of measurement defines the type of operations can be conducted with your data. There are four levels: nominal, ordinal, interval, and ratio.
Referring to data analysis that doesn't examine how variables relate to each other.
a group of statistical techniques that examines the relationship between two variables
A research process where you create a plan, you gather your data, you analyze your data and each step is completed before you proceed to the next.
An iterative approach means that after planning and once we begin collecting data, we begin analyzing as data as it is coming in. This early analysis of our (incomplete) data, then impacts our planning, ongoing data gathering and future analysis as it progresses.
Part of the qualitative data analysis process where we begin to interpret and assign meaning to the data.
A document that we use to keep track of and define the codes that we have identified (or are using) in our qualitative data analysis.
Doctoral Research Methods in Social Work Copyright © by Mavs Open Press. All Rights Reserved.
Share This Book
educational research techniques
Research techniques and education.

Developing a Data Analysis Plan
It is extremely common for beginners and perhaps even experience researchers to lose track of what they are trying to achieve or do when trying to complete a research project. The open nature of research allows for a multitude of equally acceptable ways to complete a project. This leads to an inability to make a decision and or stay on course when doing research.
Data Analysis Plan
A data analysis plan includes many features of a research project in it with a particular emphasis on mapping out how research questions will be answered and what is necessary to answer the question. Below is a sample template of the analysis plan.

The majority of this diagram should be familiar to someone who has ever done research. At the top, you state the problem , this is the overall focus of the paper. Next, comes the purpose , the purpose is the over-arching goal of a research project.
After purpose comes the research questions . The research questions are questions about the problem that are answerable. People struggle with developing clear and answerable research questions. It is critical that research questions are written in a way that they can be answered and that the questions are clearly derived from the problem. Poor questions means poor or even no answers.
After the research questions, it is important to know what variables are available for the entire study and specifically what variables can be used to answer each research question. Lastly, you must indicate what analysis or visual you will develop in order to answer your research questions about your problem. This requires you to know how you will answer your research questions
Below is an example of a completed analysis plan for simple undergraduate level research paper

In the example above, the student wants to understand the perceptions of university students about the cafeteria food quality and their satisfaction with the university. There were four research questions, a demographic descriptive question, a descriptive question about the two main variables, a comparison question, and lastly a relationship question.
The variables available for answering the questions are listed off to the left side. Under that, the student indicates the variables needed to answer each question. For example, the demographic variables of sex, class level, and major are needed to answer the question about the demographic profile.
The last section is the analysis. For the demographic profile, the student found the percentage of the population in each sub group of the demographic variables.
A data analysis plan provides an excellent way to determine what needs to be done to complete a study. It also helps a researcher to clearly understand what they are trying to do and provides a visual for those who the research wants to communicate with about the progress of a study.
Share this:
Leave a reply cancel reply, discover more from educational research techniques.
Subscribe now to keep reading and get access to the full archive.
Type your email…
Continue reading

Data analysis plan
Data analysis plan refers to a roadmap for how the data will be organized and analyzed and how results will be presented. A data analysis plan should be established when planning a research study (i.e., before data collection begins). Among other things, the data analysis plan should describe: (a) the data to be collected; (b) the analyses to be conducted to address the research objectives, including assumptions required by said analyses; (c) data cleaning and management procedures; (d) data transformations, if applicable; and (e) how the study results will be presented (e.g., graphs, tables).
Sourced From U.S. Food and Drug Administration (FDA) Patient-Focused Drug Development Glossary
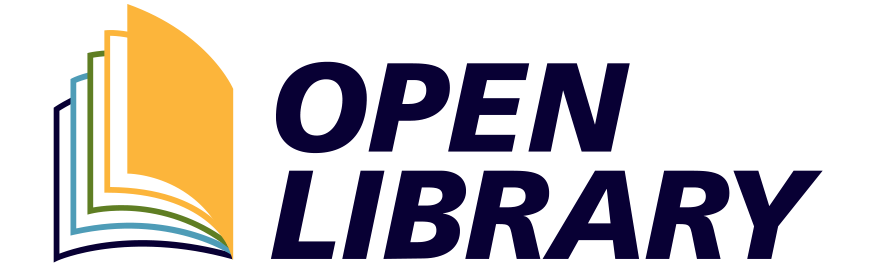
Want to create or adapt books like this? Learn more about how Pressbooks supports open publishing practices.
Data Analysis
Module 5: Data Analysis & Reciprocity
At this stage, you’re probably carrying out your planned intervention or action and gathering data to address your research question. Many newcomers to action research believe that analysis should only start after all the data has been collected.
An interim analysis is part of the continuous, ongoing data analysis. It is part of the ongoing reflective planning process of action research (Hendricks, 2013).
Your action research projects will typically involve both quantitative and qualitative data. The methods for simplifying quantitative data, such as reporting, comparing , and displaying data, differ significantly from those used for qualitative data, which include analyzing the data to identify patterns and themes.
New researchers often feel disappointed when their interventions don’t lead to the anticipated results. However, even in these situations, exploring the data to understand why things didn’t work as expected can provide valuable insights. This process can guide you in refining your intervention to achieve better results in the future.
Remember! Action research is an iterative process so what you learn from this cycle of your research project will inform your next iteration of action research.
Analysis of Quantitative Data: Reporting & Comparing
Quantitative data is usually gathered via:
- Test scores
- Rubric-scored work
- Tally sheets
- Behavioural scales
- Attitude scales
- Closed-ended survey items
For example: Counting or averaging the number of responses for each item.
- Closed-ended responses (strong, average, weak) can reflect counts for the number of respondents who chose each response.
- For the behavioural scale item, which includes numerical responses, the actual number chosen for each item could be tallied and the numbers could be averaged to describe results (Hendricks, 2013).
Quick Tips to Analyze Quantitative Data
“According to Shank (ibid) “themes do not emerge from data. What emerges after much hard work and creative thought, is an awareness in the mind of the researcher that there are patterns of order that seem to cut across various aspects of the data. When these patterns become organized, and when they characterize different segments of data, then we can call them ‘themes’.”
(Hendricks 2013)
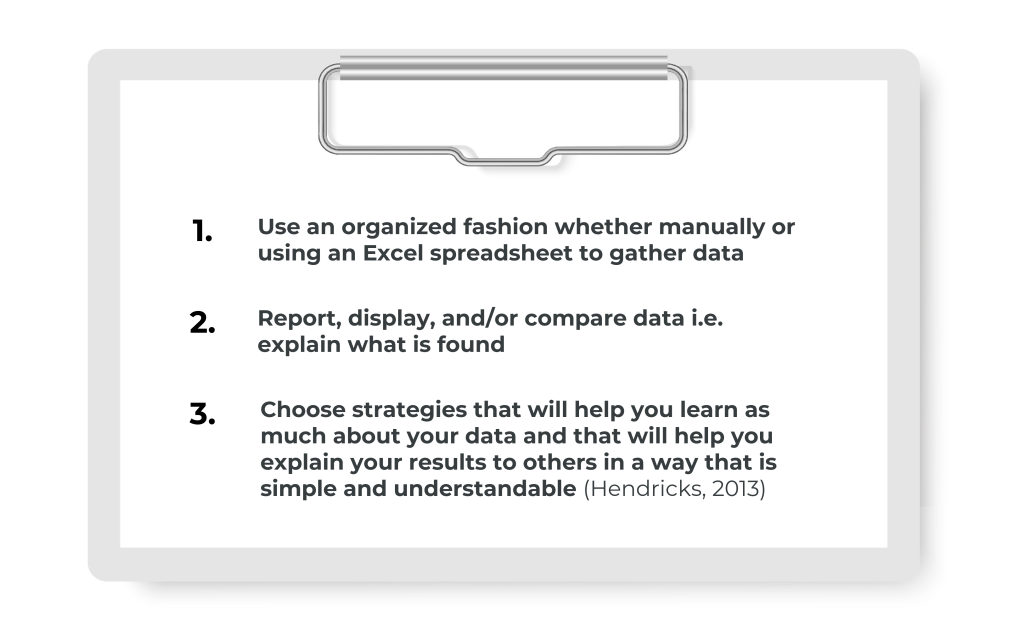
Analysis of Qualitative Data: Looking for Themes & Patterns
Analysis of qualitative data is a process of making meaning from data sources that can be interpreted in several ways and helps answer the why questions .
These data sources can be explained and used to answer your research question only after they have been interpreted. This process requires a deeper analysis of data than those processes used to explain quantitative data sources (Hendricks 2013).
Verification
Verification is knowing when you “got it right.” Reaching valid conclusions in your study is a critical step in the action research cycle. Conclusions must be reasonable in light of the results obtained.
Quick Tips to Analyze Qualitative Data
Action Research Handbook Copyright © by Dr. Zabedia Nazim and Dr. Sowmya Venkat-Kishore is licensed under a Creative Commons Attribution-NonCommercial-ShareAlike 4.0 International License , except where otherwise noted.
Share This Book
This paper is in the following e-collection/theme issue:
Published on 30.5.2024 in Vol 26 (2024)
An Infrastructure Framework for Remote Patient Monitoring Interventions and Research
Authors of this article:

- Jennifer Claggett 1, 2 , PhD ;
- Stacie Petter 1 , PhD ;
- Amol Joshi 1, 2 , PhD ;
- Todd Ponzio 3 , PhD ;
- Eric Kirkendall 2 , MD
1 School of Business, Wake Forest University, Winston-Salem, NC, United States
2 Center for Healthcare Innovation, School of Medicine, Wake Forest University, Winston-Salem, NC, United States
3 Health Science Center, University of Tennessee, Memphis, TN, United States
Corresponding Author:
Jennifer Claggett, PhD
School of Business
Wake Forest University
1834 Wake Forest Rd
Winston-Salem, NC, 27109-6000
United States
Phone: 1 3363027991
Email: [email protected]
Remote patient monitoring (RPM) enables clinicians to maintain and adjust their patients’ plan of care by using remotely gathered data, such as vital signs, to proactively make medical decisions about a patient’s care. RPM interventions have been touted as a means to improve patient care and well-being while reducing costs and resource needs within the health care ecosystem. However, multiple interworking components must be successfully implemented for an RPM intervention to yield the desired outcomes, and the design and key driver of each component can vary depending on the medical context. This viewpoint and perspective paper presents a 4-component RPM infrastructure framework based on a synthesis of existing literature and practice related to RPM. Specifically, these components are identified and considered: (1) data collection, (2) data transmission and storage, (3) data analysis, and (4) information presentation. Interaction points to consider between components include transmission, interoperability, accessibility, workflow integration, and transparency. Within each of the 4 components, questions affecting research and practice emerge that can affect the outcomes of RPM interventions. This framework provides a holistic perspective of the technologies involved in RPM interventions and how these core elements interact to provide an appropriate infrastructure for deploying RPM in health systems. Further, it provides a common vocabulary to compare and contrast RPM solutions across health contexts and may stimulate new research and intervention opportunities.
Introduction
Remote patient monitoring (RPM; sometimes referred to as eHealth, telehealth, telemonitoring, or telemedicine) involves the capture of patient data through sensors or devices outside of a clinical setting, such as at the patient’s home or work while the patient is engaging in everyday activities. Ideally, the data captured through RPM devices are analyzed and used to inform clinicians’ decisions on patient care. For example, typical decisions include adjusting the recommended dosage or timing of a patient’s medication based on observed changes in the patient’s vital signs or patterns of activity.
RPM interventions have increased exponentially in the United States of America since 2020 [ 1 ]. The COVID-19 pandemic exacerbated the need for remote patient care solutions when there were severe resource shortages of clinicians, equipment, and capacity within health care systems [ 2 - 4 ] and patients were required to socially distance themselves to mitigate the spread of COVID-19. As the United States of America eased regulations and made changes to encourage reimbursements for RPM interventions, health care providers sought to reap RPM’s potential benefits along three main dimensions: (1) enhancing quality by offering more personalized care; (2) achieving scale by growing their customer (patient) base; and (3) securing new reimbursement opportunities by evolving in response to shifts in payment policies [ 1 , 3 ].
The excitement and promise of the benefits of RPM to improve patient care while also expanding a health system’s market are well-documented in meta-analyses that find evidence of RPM reducing hospital admissions and length of stay for certain conditions, such as cardiovascular disease or chronic obstructive pulmonary disease [ 5 , 6 ]. Decreased travel time, cost savings, and increased access to services are commonly ascribed as benefits for patients, and most eHealth interventions are described as successes [ 7 ]. However, other scholars counter that RPM interventions may not live up to the hype. One study finds that RPM interventions do not impact patient health factors, such as weight, body fat percentage, and blood pressure [ 8 ], and other related studies raise concerns about the limited evidence that RPM interventions can indeed adequately scale to meaningfully improve patient outcomes and demonstrably reduce health care costs [ 3 ].
These mixed results regarding the impact of RPM interventions showcase the current challenge of understanding how to design and effectively implement the infrastructure to support successful RPM programs. Successful RPM programs should meet at least one, but ideally both, of the following standards: (1) improved management of symptoms (evaluated using population-normalized values or patient feedback) and (2) reduced financial costs (evaluated in terms of the health system, payers, and patient out-of-pocket expenses). Previous work reporting on RPM interventions tends to report details on isolated projects and is focused, understandably, on a specific medical condition without offering generalizable advice to a broader audience or a catalog of best practices. Although RPM has been implemented in many different types of contexts, we contend that the key infrastructure points are consistent across interventions. Therefore, we present a framework consisting of 4 core infrastructure components necessary for any RPM intervention and identify common questions across contexts that should influence the RPM intervention design and results. This RPM infrastructure framework is useful to scholars and clinicians implementing RPM projects in that it (1) presents a shared vocabulary and reference point, (2) serves as a resource to guide some of the major decisions associated with an RPM implementation, and (3) provides a logical scaffolding to categorize and disseminate lessons learned within RPM projects to leverage them in other contexts. While the set of considerations nested within the four infrastructure components is not exhaustive, these considerations serve as a useful starting point as RPM research and interventions are planned and developed in the future.
RPM Infrastructure Framework
As an information technology (IT), RPM relies on a combined and layered infrastructure of hardware, software, and networks to support the collection, storage, processing, and management of data. By considering emergent patterns and themes from the literature, cases, and reports, discussing this topic in various panels and workshops, and reflecting on our experiences designing and assessing RPM projects, we propose a four-component infrastructure framework that is necessary in any RPM infrastructure project: (1) data collection, (2) data transmission and storage, (3) algorithmic data analysis, and (4) information presentation. The first RPM infrastructure component, data collection, collects a patient’s vital signs and other biometric data remotely through a measurement device such as a wearable sensor. Data transmission and storage, the second infrastructure component, leverages software interface services, networking, and hardware to transfer the data from the patient’s device to a centralized data architecture [ 9 , 10 ]. Third, software-based algorithms analyze the stored remote patient data to identify patterns and outliers for a single patient or for a patient population. The final RPM infrastructure component is to present information obtained from the analysis to support clinicians’ decision-making processes [ 11 , 12 ]. Figure 1 depicts the RPM infrastructure framework, and each of the following sections describes key considerations for each component.

Component 1: Remote Patient Data Collection
Patients interact with an RPM device to enable the collection of data outside of clinical settings. Some devices are worn continuously throughout a person’s day, while other devices are used at specific times to capture health indicators periodically based on the patient’s medical condition and the provider’s care protocol. Patients may use a specialized RPM device that registers a single form of biometric data (eg, a continuous glucose monitor capturing blood glucose levels) or a device that captures multiple data types (eg, a blood pressure cuff that measures blood pressure, pulse rate, and oxygen saturation). Given the growing number of technologies capable of collecting patient health data along with the need for patients to interact with a device for data collection, several questions must be carefully addressed when considering how to best collect data for an RPM intervention.
How Should Patients be Selected for RPM?
While RPM has the potential to improve patients’ quality of care and reduce clinic costs, successful implementation relies on the effective use of the device and the fidelity of the collected data. The existing literature highlights several key considerations and components for identifying patients who are a good match for remote monitoring. Of paramount importance is suitability—is the patient’s medical condition one that is likely to actually benefit from the collection and analysis of more data? Patients with chronic diseases such as diabetes, heart failure, hypertension, or chronic obstructive pulmonary disease are often more likely to benefit from RPM, as it can help them better manage their health status and condition over the long-term [ 13 ]. Comorbidities also play a significant role in patient selection, as those with multiple chronic conditions or complex health situations might require more comprehensive monitoring [ 14 ]. RPM can provide a more holistic view of their health, making it a potentially valuable tool for these patients; however, the complexity of their medical conditions may limit their ability to adhere to the monitoring program and necessitate more immediate and direct medical interventions.
Patients who are noncompliant or have a history of difficulty adhering to their treatment plans might benefit from RPM, as it can help improve compliance and provide additional support [ 15 ]. RPM solutions may make a patient feel more engaged, empowered, and informed through messaging systems that interact with patients on a routinely structured basis [ 16 , 17 ]. Patient motivation and engagement are key factors, as patients who are motivated and engaged in managing their health are more likely to actively participate in and adhere to the RPM program [ 18 ].
Other patient-specific factors—commonly referred to as the social determinants of health—such as socioeconomic status, age, and social support should be considered when designing RPM interventions [ 19 ]. For instance, patients with lower socioeconomic status might benefit from RPM the most, as it can help reduce health care disparities and provide better access to care [ 20 - 22 ]. A disproportionally large number of people affected by chronic conditions are from socioeconomically disadvantaged groups [ 23 ]. Communities of color, immigrants, and women are particularly likely to be in distress from undiagnosed chronic diseases, and even when diagnosed, these populations are more likely than their counterparts to face structural and logistical obstacles to obtaining the appropriate level of intermittent care. So long as they have reliable connectivity to the internet, patients who live in remote or rural areas or have limited access to transportation might benefit from RPM, as it can help overcome geographical barriers to care [ 18 , 24 ]. Age can also play a role in identifying suitable patients for RPM, in that elderly patients or those with age-related conditions may benefit from RPM. The patient’s living situation is another important factor. A strong support system, such as family or caregivers, can facilitate device use, data collection, and overall engagement, making these patients more suitable for RPM [ 25 ].
Finally, technological competence plays a crucial role in a patient’s ability to engage with an RPM device. Patients with some level of technology literacy (eg, “digital natives”) are more likely to engage with and effectively use RPM devices and systems [ 26 ]. However, patients with lower socioeconomic status or those who are elderly may have lower levels of technological competence or may have other barriers that could limit the effectiveness of an RPM program [ 27 - 29 ]. There is a natural continuum of sophistication and familiarity with devices and the inevitable troubleshooting they often require, and a more “set and forget” approach may be advisable for certain populations.
Which Device and Which Types of Data?
A fundamental characteristic of RPM is the acquisition of data outside of conventional clinical environments. Consequently, patient data must be collected remotely using sensors and equipment such as wearable devices, mobile phones, or portable devices installed at a patient’s residence or other environments [ 30 ]. One strategy involves using data from off-the-shelf, general-purpose smart health consumer electronics purchased by the patient, while another option is to rely on data from specialized devices or software prescribed or supplied by the health care provider. Technological advancements enable the collection, through devices within the RPM infrastructure, of various types of data, such as electrocardiograms, electroencephalograms, heartbeats and respiration rates, oxygen saturation in the blood or pulse oximetry, nervous system signals, blood pressure, body or skin temperature, blood glucose levels, patient weight, and sleep patterns, among others [ 31 ].
A crucial consideration is the optimal combination of metrics to be collected for a specific patient. The US Centers for Disease Control report that 51.8% of US adults have at least one chronic condition, and 27.2% have multiple chronic conditions such as obesity, diabetes, and cardiovascular disease [ 32 ]. Emerging evidence indicates that RPM initiatives are more likely to succeed when multiple metrics are evaluated concurrently [ 33 ]. For instance, compiling data from various physiological sensors measuring heart rate, blood oxygen saturation, and blood glucose levels simultaneously can offer a more comprehensive overview of a patient’s health, which is particularly significant for patients with comorbidities and additional complications. This suggests that the diagnostic value of data can be enhanced by carefully considering what health indicators are needed to manage a patient’s care.
How Frequently are Data Collected?
Determining the optimal frequency of data collection in RPM scenarios is a critical consideration, as it can significantly impact the effectiveness of patient care and the efficient use of health care resources. The appropriate frequency for data collection depends on various factors, including the severity and type of the patient’s condition, the objectives of monitoring, and the required patient involvement in data collection [ 34 ]. For instance, some conditions may necessitate multiple data readings per day, while others may only require weekly monitoring [ 35 ]. Passive data collection methods, such as continuous monitoring of vital signs using wearable sensors, can be advantageous for patients requiring frequent monitoring, whereas active data collection methods, which involve patient involvement and interaction, may be suitable for other conditions [ 16 , 36 ]. Passive methods are usually less likely to cause patient burnout and abandonment [ 37 ].
Health care providers should consider adopting several best practices to ensure that patients remain engaged and compliant with RPM protocols. These typically include providing personalized and clear instructions, offering training and support to ensure device functionality, improving patients’ understanding and comfort with the technology, and fostering regular remote communication between patients and health care providers [ 38 ]. Furthermore, involving patients in the decision-making process regarding their monitoring plans and adjusting the frequency and type of data collection based on their individual needs and preferences can lead to increased patient engagement and satisfaction [ 13 , 39 ].
Component 2: Remote Patient Data Transmission and Storage
Once remote patient data are collected by one or more devices, the data must be transmitted and shared with clinicians, and stored in a data architecture. The manner in which the data are transmitted from an RPM device is dependent on the device and the network access of the patient. RPM data transmission may occur through a network using a wired link, or high-speed wireless link with or without human intervention. In some cases, patients or caregivers may be asked to record readings or values from devices into an app available on their smartphone or computer that will transmit data to the medical provider. Another option could be that a patient must physically visit a clinician’s office with the device to upload the data to the patient’s electronic medical record. The storage of remote patient data may be in a system that is managed by the device manufacturer and accessed through a web portal, and the data may or may not be integrated within the patient’s electronic medical record.
Is There Sufficient Connectivity?
Connectivity plays a vital role in the successful implementation of RPM, as it enables the transmission of patient data from monitoring devices to health care providers and fosters timely interventions and informed decision-making. Addressing the digital divide is crucial to ensuring equitable access to RPM services, as patients with limited internet access or low digital literacy may face barriers to fully benefiting from RPM [ 40 ]. This disparity is particularly concerning for patients from socioeconomically disadvantaged backgrounds, who may experience greater difficulties in accessing health care services and could benefit the most from RPM [ 40 , 41 ]. Some patients may have access to home internet solutions through local internet service providers that include Wi-Fi networks at home, while others may be limited to cellular network access through mobile devices. Often, the latter is subject to slower connections and data caps that place constraints on the patient’s connectivity.
Strategies for addressing connectivity for RPM interventions should consider alternatives, such as the constant connectivity approach or using batch or episodic data uploads when data connections are available [ 42 ]. Constant connectivity can facilitate real-time monitoring and immediate interventions, which may be especially beneficial for patients with critical or rapidly changing health conditions [ 43 ]. However, this approach may not be feasible for patients living in areas with limited or unreliable internet access or for those who cannot afford consistent connectivity. In these cases, episodic data uploads when a connection is possible may provide a more accessible and cost-effective solution, allowing health care providers to track patient progress and identify potential issues while accommodating the patients’ connectivity limitations [ 44 ]. Additionally, some RPM hardware solutions may include direct cellular network connectivity, where the device sends the data through a connection provided by the wearable device to the provider, bypassing the need for a patient home network. These solutions will incur additional costs related to data transmission and may not naturally provide a patient dashboard or a way for patients to easily view data that may traditionally be housed in a patient application.
Is the Transmission Secure?
The sensitive nature of medical data necessitates robust protection measures to maintain patient privacy and prevent unauthorized access. Data breaches and cyberattacks can have severe consequences for patients and their health care providers, including identity theft, financial loss, and reputational damage [ 45 ]. The increasing connectivity of medical devices and the use of cloud-based data storage have created new opportunities for cybercriminals, leading to the emergence of threats such as medjacking [ 46 ]. Medjacking, a term coined from “medical device hijacking,” refers to the unauthorized access and manipulation of medical devices, such as pacemakers or insulin pumps, to cause harm to patients or extract sensitive data [ 47 ]. As RPM technologies rely on a variety of connected devices for data collection across multiple networks, they can be vulnerable to medjacking and other cybersecurity risks. Furthermore, the rapid expansion of the internet of things in health care has amplified these risks, as a larger number of interconnected devices create more potential entry points for attackers [ 48 , 49 ].
Health care providers and technology developers should prioritize the implementation of robust security measures to mitigate the risks associated with medjacking and other security threats in RPM. These may include strong encryption protocols for data transmission (“in flight”) and storage (“at rest”), regular security updates, and the development of secure communication channels between devices and health care providers [ 45 , 48 ]. Additionally, health care organizations should adopt a proactive approach to security by conducting regular risk assessments, promoting cybersecurity awareness and training among staff, and fostering a culture of security-mindedness [ 50 ].
Can Data Move Across Health Systems Software?
Interoperability is a crucial aspect of RPM projects, as it enables seamless communication and data sharing among different health information systems, devices, and providers. This encompasses not only the technical aspects of data exchange but also the semantic understanding and interpretation of shared data, ensuring that the information can be effectively used by health care providers, patients, and other stakeholders. Effective interoperability contributes to improved patient care by ensuring that clinicians have access to comprehensive and up-to-date medical information, allowing for better decision-making and coordination of care [ 51 ]. However, achieving interoperability in RPM poses several challenges, including the need to balance data accessibility with patient privacy and maintain control over personal health information.
One of the primary challenges in achieving interoperability in RPM is the heterogeneity of health information systems and devices used by health care providers. These systems often rely on different (often proprietary) data formats, communication protocols, and standards, which can create barriers to effective information exchange. To address this issue, several major standards have been developed to facilitate interoperability in health IT (eg, [ 52 , 53 ]). For example, the US Department of Health and Human Services Office of the National Coordinator for Health IT released the third version (V3) of the US Core Data for Interoperability in 2022 [ 54 ].
Another challenge in achieving interoperability is protecting patient privacy while sharing data freely among authorized health care providers [ 44 ]. Using privacy-preserving techniques, such as pseudonymization, which replaces personally identifiable information with unique identifiers to maintain patient anonymity, may reinforce privacy during the transmission of data between systems. However, these approaches must be rigorously tested to systematically mitigate privacy risks [ 55 ]. One-way hashing of sensitive identifiers is another technique that can reduce the risk of leakage of personal health identifiers. Additionally, the implementation of access control mechanisms can help ensure that only authorized users can access and share patient data, further safeguarding privacy [ 56 ].
A related issue to moving data across health systems is determining the appropriate granularity to share between stakeholders and systems. For example, in a remote blood pressure monitoring project, should each reading be recorded, transmitted, and made available, including any relevant metadata about time, place, and cuff placement, or should only summary data about daily or weekly averages be shared between systems? Like any sensor-based technology, the amount of raw data generated by RPM initiatives may be overwhelming [ 57 ]; however, providing only summarized data limits the transparency and future uses of the data.
Component 3: Algorithmic Analysis of Remote Patient Data
Remote patient data that are stored within an information system and are not analyzed provide no value to the patient or the clinician. After transmitting and storing RPM data, they should be processed and analyzed to identify and summarize patterns and trends in individual patients and patient populations [ 58 ]. The process of analyzing raw data to deliver actionable insights could also form the basis for financial reimbursement, which is fundamental to any sustainable RPM program.
What Analysis Techniques are Appropriate?
Data analysis involves the use of algorithms, or a series of steps, to process the data in a meaningful way. Algorithms may use static rule logic, which can be used to draw attention to results over a certain threshold, or they may leverage machine learning techniques to dynamically adapt and learn from large sets of patient data, such as adjusting the threshold based on similar patients with similar conditions recorded in the data [ 59 ]. The distinction between static and dynamic rules has implications that need to be explored.
Static rules can be based on established medical guidelines, such as thresholds for vital signs or other clinical parameters, which can help health care providers identify potential health issues and take appropriate actions [ 60 ]. While this method can be effective in some cases, it may not account for the unique characteristics and complexities of individual patients, which may limit its ability to provide personalized care [ 61 ].
Alternatively, machine learning techniques offer more advanced and adaptable solutions for analyzing RPM data [ 62 ]. These techniques use algorithms that can learn from data patterns and make predictions or decisions without being explicitly programmed [ 63 , 64 ]. Machine learning can be used to identify trends, anomalies, and correlations in patient data, enabling health care providers to make more informed decisions and deliver personalized care [ 65 , 66 ]. Adaptive interpretation techniques take RPM data analysis a step further by dynamically adjusting their approach based on real-time patient data. These methods, which often rely on artificial intelligence and machine learning algorithms, can continuously refine their analysis and predictions to better understand the evolving health status of individual patients [ 63 ]. This adaptive approach can help health care providers identify subtle changes in patients’ conditions that may not be evident through traditional analysis techniques, leading to more proactive and personalized care [ 67 ].
Which Comorbidities Should be Included in the Analysis?
This question centers around the appropriate complexity level of analyses of RPM solutions. Incorporating comorbidities into the analysis of RPM data can help health care providers better understand the complex interactions between various conditions and their impact on patients’ health. This, in turn, can lead to more accurate and personalized treatment recommendations. Static rules that solely focus on a single condition, such as high blood pressure, may not adequately account for the impact of comorbidities on patients’ overall health status. For instance, a patient with both diabetes and hypertension may require a different treatment approach than a patient with hypertension alone, which is why any given individual should be managed holistically with a consolidated approach, rather than divided by symptoms and specialty [ 68 ].
This comprehensive monitoring can provide a more accurate representation of the patient’s health status, allowing health care providers to make more informed decisions regarding treatment and care management [ 69 , 70 ]. However, these solutions may be so patient-specific that cognitive efficiencies and the ability to scale the solution are compromised in the absence of built-in coordination systems with well-defined decision-making heuristics and robust care protocols.
What Biases Exist Within the Analysis and How Should They be Mitigated?
Biases in the analysis of remote patient data can have a significant impact on the accuracy and effectiveness of health care services. Particularly in machine learning-based analysis techniques, biases can arise from various sources, such as data sampling, measurement errors, or algorithmic design, leading to potentially biased predictions or recommendations [ 71 , 72 ]. It is essential to detect and account for biases to ensure that the solutions provided are equitable and reliable for all patients.
One primary source of bias in data analysis is the data itself. If the training data used to develop machine learning models do not accurately represent the diverse patient population, the resulting models may be skewed toward specific subgroups, leading to suboptimal or even harmful recommendations for other groups [ 73 , 74 ]. For instance, if a model is trained predominantly on data from patients of a particular age, gender, or ethnicity, it may not perform well on patients from other demographics. To mitigate such biases, it is crucial to ensure that the training data are representative of the target patient population, considering factors such as age, gender, ethnicity, and socioeconomic status [ 75 ].
Another source of bias can arise from the choice of features or variables used in the analysis. If certain relevant variables are not included, or if irrelevant variables are considered, the resulting predictions or recommendations may be biased or even spurious [ 76 ]. Careful feature selection, based on domain knowledge and a thorough understanding of the underlying data, can help address this issue.
Algorithmic biases can also emerge from the choice of machine learning methods or algorithms, as well as their specific implementations. To address this, it is essential to evaluate and compare multiple algorithms and implementations to identify potential biases and select the most appropriate method for the specific application [ 77 ]. Patients themselves can serve as their own baselines too, particularly for measurements that do not lend themselves as easily to a population approach (eg, mood and gastric motility).
Lastly, ongoing monitoring and evaluation of the performance of data analysis solutions, including machine learning models, is critical to detecting and addressing biases. Regular assessments of model performance, particularly with respect to various subgroups within the patient population, can help identify potential biases and ensure that the solutions remain equitable and effective for all patients [ 78 ].
Component 4: Presentation of RPM Data to a Clinician
Once the data have been analyzed, the results need to be presented as information to support clinicians’ decision-making. Unless the RPM data are used to inform patient care, the RPM intervention will not yield the intended results. Therefore, it is critical that the information is presented in a manner that is likely to inform clinicians as they make decisions that affect specific patients and patient populations.
Is RPM Information Accessible in the Right Electronic Health Record Software?
Physicians and other clinical decision makers often face significant time constraints and high cognitive workloads in their daily practice, making it challenging for them to manage and monitor patient data effectively. A study by Sinsky et al [ 79 ] found that primary care physicians spent nearly half of their workday interacting with EHR systems, leaving them with limited time for direct patient care. The high volume of clinical tasks and responsibilities can lead to cognitive overload, increasing the risk of burnout and negatively impacting the quality of care provided [ 80 ]. Given these constraints, it is critical to ensure that RPM data are easily accessible within the existing EHR systems without requiring clinicians to log into additional platforms or apps. Integrating RPM data into EHRs can help streamline clinical workflows and reduce the cognitive burden on health care providers, enabling them to focus on essential tasks such as patient evaluation, diagnosis, and treatment planning [ 81 ]. This underscores the importance of seamless integration and interoperability between RPM solutions and EHR systems, ultimately supporting more efficient and effective patient care by easing the pathway of the information being used in decision-making.
One of the key benefits of integrating RPM data into EHR systems is the ability to provide a comprehensive and up-to-date view of a patient’s health status. By combining RPM data with other health information such as medical history, laboratory results, and imaging studies, clinicians can gain a more holistic understanding of a patient’s condition, enabling them to make more informed decisions about treatment plans and care management strategies [ 82 ].
Integration of RPM data into EHR systems can also support the development and implementation of clinical decision support (CDS) tools, which can help health care providers make more informed, evidence-based decisions about patient care [ 83 ]. By leveraging RPM data, CDS tools can provide real-time alerts or recommendations to clinicians, assisting them in diagnosing, treating, or managing a patient’s condition more effectively.
How Should the Decision Maker Receive Information?
In the context of RPM solutions, there is a delicate balance between providing exception reporting and summary data reporting. Exception reporting involves the generation of alerts or notifications only when specific events or abnormal values are detected, which require immediate attention from health care providers. This yields the advantage of focusing health care providers’ attention on situations that need prompt intervention, potentially improving the efficiency and timeliness of care and reducing the number of alerts [ 84 ]. However, exception reporting may not always provide sufficient context or information about a patient’s overall health status, making it difficult for clinicians to assess the impact of treatment strategies or identify more subtle changes in condition over time. On the other hand, summary data reporting provides a broader overview of a patient’s progress over time, allowing clinicians to evaluate trends and assess the overall effectiveness of treatment plans. Both approaches have their merits and challenges, making the choice between them a critical consideration in RPM projects.
Alert fatigue is a critical concern in the context of RPM solutions, as it can have significant implications for the effectiveness of the system and the quality of patient care. Alert fatigue occurs when health care providers are exposed to a high volume of alerts, leading to desensitization and potentially reduced responsiveness to these notifications [ 85 - 87 ]. This phenomenon has been observed in various clinical settings, including electronic health record systems and CDS tools, where excessive alerts can contribute to cognitive overload, increased stress, and the risk of overlooking critical information [ 88 ].
In RPM systems, balancing the type and frequency of messaging is essential to minimize alert fatigue. The choice between push and pull messaging strategies can play a significant role in this regard. Push messaging involves automatically sending alerts or notifications to health care providers, whereas pull messaging requires providers to actively request or retrieve the information. Although push messaging can ensure timely delivery of critical information, it may also contribute to alert fatigue if used indiscriminately or too frequently. Solutions to alleviate this tension may involve tailoring alert thresholds based on individual patient needs, incorporating CDS algorithms to filter and prioritize alerts, and using a combination of push and pull messaging to strike the right balance between proactively notifying providers and allowing them to access information on demand.
What is the Right Amount of Information to Provide to Decision Makers?
Balancing transparency and detail in the presentation of RPM data with cognitive ease is crucial for ensuring that health care providers effectively use the information in their decision-making processes. While transparency is essential for building trust and understanding of the underlying data analysis, providing excessive detail can overwhelm clinicians and hinder their ability to quickly assimilate the information [ 89 ]. Consequently, it is vital to strike an optimal balance between presenting comprehensive information and ensuring cognitive ease for end users.
One approach to achieving this balance is to use a tiered or “drill-down” presentation of data, which allows health care providers to access additional layers of detail only if they require it [ 90 ]. This design can present a high-level summary of the patient’s condition and only flag critical alerts, while enabling providers to delve deeper into the data if they desire further context or clarification. This, in turn, helps mitigate information overload and supports more efficient decision-making by prioritizing the most relevant and actionable insights [ 91 ]. Moreover, incorporating the principles of cognitive ergonomics and human-centered design can further enhance the usability of RPM solutions. This may involve the use of visual aids, such as graphs, charts, and color-coding, to facilitate rapid comprehension of complex data and even presenting proposed treatment plans based on the algorithmic analysis of the patient’s full record [ 92 ] and providing reference statistics from the health system’s relevant patient population.
The mixed results with RPM interventions have raised concerns about the scalability and value of this technology. This viewpoint paper highlights some of the key questions and core considerations that affect the various infrastructure components of an RPM intervention. Differences between health conditions, metrics, devices, storage, analysis, and information presentation across RPM implementations result in countless permutations. If scholars fail to document and clearly explain the RPM infrastructure and choices made for an RPM implementation, it will be difficult to create an evidence-based research tradition. Having a shared vocabulary and more consistent documentation of the RPM infrastructure can support future literature reviews and meta-analyses seeking to evaluate the outcomes of RPM interventions. The RPM infrastructure framework presented in this article offers scholars a means to describe the different choices and constraints associated with their RPM interventions.
We also identify how each of the infrastructure components can stimulate new research and intervention opportunities in Table 1 . While not exhaustive, the list offers a sampling of the many research questions that could be studied to further increase the understanding associated with RPM interventions. The RPM framework offers scholars and clinicians a more comprehensive guide to exploring various aspects of RPM implementation. As a result, they can further optimize the design and functionality of RPM solutions for improved patient care and health care provider experiences.
Acknowledgments
This research is supported in part by funding from Wake Forest University through the School of Business and the School of Medicine Center for Healthcare Innovation. These sponsors had no involvement in the research.
Conflicts of Interest
None declared.
- Tang M, Mehrotra A, Stern AD. Rapid growth of remote patient monitoring is driven by a small number of primary care providers. Health Aff (Millwood). 2022;41(9):1248-1254. [ CrossRef ] [ Medline ]
- Birkmeyer JD, Barnato A, Birkmeyer N, Bessler R, Skinner J. The impact of the COVID-19 pandemic on hospital admissions in the United States. Health Aff (Millwood). 2020;39(11):2010-2017. [ FREE Full text ] [ CrossRef ] [ Medline ]
- Mecklai K, Smith N, Stern AD, Kramer DB. Remote patient monitoring: overdue or overused? N Engl J Med. 2021;384(15):1384-1386. [ CrossRef ] [ Medline ]
- Mann DM, Chen J, Chunara R, Testa PA, Nov O. COVID-19 transforms health care through telemedicine: evidence from the field. J Am Med Inform Assoc. 2020;27(7):1132-1135. [ FREE Full text ] [ CrossRef ] [ Medline ]
- de Farias FAC, Dagostini CM, de Assunção Bicca Y, Falavigna VF, Falavigna A. Remote patient monitoring: a systematic review. Telemed J E Health. 2020;26(5):576-583. [ CrossRef ] [ Medline ]
- Taylor ML, Thomas EE, Snoswell CL, Smith AC, Caffery LJ. Does remote patient monitoring reduce acute care use? A systematic review. BMJ Open. 2021;11(3):e040232. [ FREE Full text ] [ CrossRef ] [ Medline ]
- LeBlanc M, Petrie S, Paskaran S, Carson DB, Peters PA. Patient and provider perspectives on eHealth interventions in Canada and Australia: a scoping review. Rural Remote Health. 2020;20(3):5754. [ FREE Full text ] [ CrossRef ] [ Medline ]
- Noah B, Keller MS, Mosadeghi S, Stein L, Johl S, Delshad S, et al. Impact of remote patient monitoring on clinical outcomes: an updated meta-analysis of randomized controlled trials. NPJ Digit Med. 2018;1(1):20172. [ FREE Full text ] [ CrossRef ] [ Medline ]
- Silva BMC, Rodrigues JJPC, de la Torre Díez I, López-Coronado M, Saleem K. Mobile-health: a review of current state in 2015. J Biomed Inform. 2015;56:265-272. [ FREE Full text ] [ CrossRef ] [ Medline ]
- Verma P, Sood SK. Fog assisted-IoT enabled patient health monitoring in smart homes. IEEE Internet Things J. 2018;5(3):1789-1796. [ CrossRef ]
- Gold R, Bunce A, Cowburn S, Dambrun K, Dearing M, Middendorf M, et al. Adoption of social determinants of health EHR tools by community health centers. Ann Fam Med. 2018;16(5):399-407. [ FREE Full text ] [ CrossRef ] [ Medline ]
- Kwon BC, Choi MJ, Kim JT, Choi E, Kim YB, Kwon S, et al. RetainVis: visual analytics with interpretable and interactive recurrent neural networks on electronic medical records. IEEE Trans Vis Comput Graph. 2019;25(1):299-309. [ CrossRef ] [ Medline ]
- Kvedar J, Coye MJ, Everett W. Connected health: a review of technologies and strategies to improve patient care with telemedicine and telehealth. Health Aff (Millwood). 2014;33(2):194-199. [ CrossRef ] [ Medline ]
- Bogyi P, Vamos M, Bari Z, Polgar B, Muk B, Nyolczas N, et al. Association of remote monitoring with survival in heart failure patients undergoing cardiac resynchronization therapy: retrospective observational study. J Med Internet Res. 2019;21(7):e14142. [ FREE Full text ] [ CrossRef ] [ Medline ]
- Lippke S, Gao L, Keller FM, Becker P, Dahmen A. Adherence with online therapy vs face-to-face therapy and with online therapy vs care as usual: secondary analysis of two randomized controlled trials. J Med Internet Res. 2021;23(11):e31274. [ FREE Full text ] [ CrossRef ] [ Medline ]
- Midaglia L, Mulero P, Montalban X, Graves J, Hauser SL, Julian L, et al. Adherence and satisfaction of smartphone- and smartwatch-based remote active testing and passive monitoring in people with multiple sclerosis: nonrandomized interventional feasibility study. J Med Internet Res. 2019;21(8):e14863. [ FREE Full text ] [ CrossRef ] [ Medline ]
- Rojas G, Guajardo V, Martínez P, Castro A, Fritsch R, Moessner M, et al. A remote collaborative care program for patients with depression living in rural areas: open-label trial. J Med Internet Res. 2018;20(4):e158. [ FREE Full text ] [ CrossRef ] [ Medline ]
- Seto E. Cost comparison between telemonitoring and usual care of heart failure: a systematic review. Telemed J E Health. 2008;14(7):679-686. [ CrossRef ] [ Medline ]
- NEJM Catalyst. Social Determinants of Health (SDOH). NEJM Catalyst. 2017;3(6). [ FREE Full text ]
- Najarian M, Goudie A, Bona JP, Rezaeiahari M, Young SG, Bogulski CA, et al. Socioeconomic determinants of remote patient monitoring implementation among rural and urban hospitals. Telemed J E Health. 2023;29(11):1624-1633. [ CrossRef ] [ Medline ]
- Bailey JE, Gurgol C, Pan E, Njie S, Emmett S, Gatwood J, et al. Early patient-centered outcomes research experience with the use of telehealth to address disparities: scoping review. J Med Internet Res. 2021;23(12):e28503. [ FREE Full text ] [ CrossRef ] [ Medline ]
- Yong J, Yang O. Does socioeconomic status affect hospital utilization and health outcomes of chronic disease patients? Eur J Health Econ. 2021;22(2):329-339. [ CrossRef ] [ Medline ]
- Veisani Y, Jenabi E, Nematollahi S, Delpisheh A, Khazaei S. The role of socio-economic inequality in the prevalence of hypertension in adults. J Cardiovasc Thorac Res. 2019;11(2):116-120. [ FREE Full text ] [ CrossRef ] [ Medline ]
- Kruse CS, Stein A, Thomas H, Kaur H. The use of electronic health records to support population health: a systematic review of the literature. J Med Syst. 2018;42(11):214. [ FREE Full text ] [ CrossRef ] [ Medline ]
- Demiris G, Speedie SM, Finkelstein S. Change of patients' perceptions of TeleHomeCare. Telemed J E Health. 2001;7(3):241-248. [ CrossRef ] [ Medline ]
- Birati Y, Yefet E, Perlitz Y, Shehadeh N, Spitzer S. Cultural and digital health literacy appropriateness of app- and web-based systems designed for pregnant women with gestational diabetes mellitus: scoping review. J Med Internet Res. 2022;24(10):e37844. [ FREE Full text ] [ CrossRef ] [ Medline ]
- Tseng KC, Hsu CL, Chuang YH. Designing an intelligent health monitoring system and exploring user acceptance for the elderly. J Med Syst. 2013;37(6):9967. [ CrossRef ] [ Medline ]
- Martínez A, Everss E, Rojo-Alvarez JL, Figal DP, García-Alberola A. A systematic review of the literature on home monitoring for patients with heart failure. J Telemed Telecare. 2006;12(5):234-241. [ CrossRef ] [ Medline ]
- Rodríguez I, Herskovic V, Gerea C, Fuentes C, Rossel PO, Marques M, et al. Understanding monitoring technologies for adults with pain: systematic literature review. J Med Internet Res. 2017;19(10):e364. [ FREE Full text ] [ CrossRef ] [ Medline ]
- Baig MM, GholamHosseini H, Moqeem AA, Mirza F, Lindén M. A systematic review of wearable patient monitoring systems: current challenges and opportunities for clinical adoption. J Med Syst. 2017;41(7):115. [ CrossRef ] [ Medline ]
- Jagadeeswari V, Subramaniyaswamy V, Logesh R, Vijayakumar V. A study on medical internet of things and big data in personalized healthcare system. Health Inf Sci Syst. 2018;6(1):14. [ FREE Full text ] [ CrossRef ] [ Medline ]
- Nguyen T, Barefield A, Nguyen GT. Social determinants of health associated with the use of screenings for hypertension, hypercholesterolemia, and hyperglycemia among American adults. Med Sci (Basel). 2021;9(1):19. [ FREE Full text ] [ CrossRef ] [ Medline ]
- Kalid N, Zaidan AA, Zaidan BB, Salman OH, Hashim M, Muzammil H. Based real time remote health monitoring systems: a review on patients prioritization and related "big data" using body sensors information and communication technology. J Med Syst. 2018;42(2):30. [ CrossRef ] [ Medline ]
- Matthews M, Abdullah S, Gay G, Choudhury T. Tracking mental well-being: balancing rich sensing and patient needs. Computer. 2014;47(4):36-43. [ CrossRef ]
- Maguire R, Connaghan J, Arber A, Klepacz N, Blyth KG, McPhelim J, et al. Advanced symptom management system for patients with malignant pleural mesothelioma (ASyMSmeso): mixed methods study. J Med Internet Res. 2020;22(11):e19180. [ FREE Full text ] [ CrossRef ] [ Medline ]
- Simblett S, Greer B, Matcham F, Curtis H, Polhemus A, Ferrão J, et al. Barriers to and facilitators of engagement with remote measurement technology for managing health: systematic review and content analysis of findings. J Med Internet Res. 2018;20(7):e10480. [ FREE Full text ] [ CrossRef ] [ Medline ]
- Hunt D. Passive analytics and the future of RPM (remote patient monitoring). Healthcare IT Today. 2021. URL: https://www.healthcareittoday.com/2021/02/12/passive-analytics-and-the-future-of-rpm-remote-patient-monitoring/ [accessed 2023-05-29]
- Greenhalgh T, Wherton J, Papoutsi C, Lynch J, Hughes G, A'Court C, et al. Analysing the role of complexity in explaining the fortunes of technology programmes: empirical application of the NASSS framework. BMC Med. 2018;16(1):66. [ FREE Full text ] [ CrossRef ] [ Medline ]
- Su D, Michaud TL, Estabrooks P, Schwab RJ, Eiland LA, Hansen G, et al. Diabetes management through remote patient monitoring: the importance of patient activation and engagement with the technology. Telemed J E Health. 2019;25(10):952-959. [ CrossRef ] [ Medline ]
- Choi NG, Dinitto DM. The digital divide among low-income homebound older adults: internet use patterns, eHealth literacy, and attitudes toward computer/internet use. J Med Internet Res. 2013;15(5):e93. [ FREE Full text ] [ CrossRef ] [ Medline ]
- Leidig M, Teeuw RM. Quantifying and mapping global data poverty. PLoS One. 2015;10(11):e0142076. [ FREE Full text ] [ CrossRef ] [ Medline ]
- Pappas G. Planning for internet connectivity in remote patient monitoring. Telemed J E Health. 2010;16(5):639-641. [ CrossRef ] [ Medline ]
- McConnell MV, Turakhia MP, Harrington RA, King AC, Ashley EA. Mobile health advances in physical activity, fitness, and atrial fibrillation: moving hearts. J Am Coll Cardiol. 2018;71(23):2691-2701. [ FREE Full text ] [ CrossRef ] [ Medline ]
- Ancker JS, Witteman HO, Hafeez B, Provencher T, Van de Graaf M, Wei E. The invisible work of personal health information management among people with multiple chronic conditions: qualitative interview study among patients and providers. J Med Internet Res. 2015;17(6):e137. [ FREE Full text ] [ CrossRef ] [ Medline ]
- Kramer DB, Baker M, Ransford B, Molina-Markham A, Stewart Q, Fu K, et al. Security and privacy qualities of medical devices: an analysis of FDA postmarket surveillance. PLoS One. 2012;7(7):e40200. [ FREE Full text ] [ CrossRef ] [ Medline ]
- Cuningkin V, Riley E, Rainey L. Preventing medjacking. Am J Nurs. 2021;121(10):46-50. [ CrossRef ] [ Medline ]
- Williams PAH, Woodward AJ. Cybersecurity vulnerabilities in medical devices: a complex environment and multifaceted problem. Med Devices (Auckl). 2015;8:305-316. [ FREE Full text ] [ CrossRef ] [ Medline ]
- Kelly JT, Campbell KL, Gong E, Scuffham P. The Internet of Things: impact and implications for health care delivery. J Med Internet Res. 2020;22(11):e20135. [ FREE Full text ] [ CrossRef ] [ Medline ]
- Djenna A, Harous S, Saidouni DE. Internet of Things meet internet of threats: new concern cyber security issues of critical cyber infrastructure. Appl Sci. 2021;11(10):4580. [ FREE Full text ] [ CrossRef ]
- Roman R, Zhou J, Lopez J. On the features and challenges of security and privacy in distributed internet of things. Comput Netw. 2013;57(10):2266-2279. [ CrossRef ]
- Adler-Milstein J, Jha AK. HITECH act drove large gains in hospital electronic health record adoption. Health Aff (Millwood). 2017;36(8):1416-1422. [ FREE Full text ] [ CrossRef ] [ Medline ]
- Pathak N, Misra S, Mukherjee A, Kumar N. HeDI: healthcare device interoperability for IoT-based e-Health platforms. IEEE Internet Things J. 2021;8(23):16845-16852. [ CrossRef ]
- Finet P, Gibaud B, Dameron O, Le Bouquin Jeannès R. Interoperable infrastructure and implementation of a health data model for remote monitoring of chronic diseases with comorbidities. IRBM. 2018;39(3):151-159. [ CrossRef ]
- United States Core Data for Interoperability (USCDI). Office of the National Coordinator for Health Information Technology. 2022. URL: https://www.healthit.gov/isa/united-states-core-data-interoperability-uscdi [accessed 2024-04-19]
- El Emam K, Jonker E, Arbuckle L, Malin B. A systematic review of re-identification attacks on health data. PLoS One. 2011;6(12):e28071. [ FREE Full text ] [ CrossRef ] [ Medline ]
- Fernández-Alemán JL, Señor IC, Lozoya PÁO, Toval A. Security and privacy in electronic health records: a systematic literature review. J Biomed Inform. 2013;46(3):541-562. [ FREE Full text ] [ CrossRef ] [ Medline ]
- Azodo I, Williams R, Sheikh A, Cresswell K. Opportunities and challenges surrounding the use of data from wearable sensor devices in health care: qualitative interview study. J Med Internet Res. 2020;22(10):e19542. [ FREE Full text ] [ CrossRef ] [ Medline ]
- Lowery C. What is digital health and what do i need to know about it? Obstet Gynecol Clin North Am. 2020;47(2):215-225. [ CrossRef ] [ Medline ]
- El-Rashidy N, El-Sappagh S, Islam SMR, El-Bakry HM, Abdelrazek S. Mobile health in remote patient monitoring for chronic diseases: principles, trends, and challenges. Diagnostics (Basel). 2021;11(4):607. [ FREE Full text ] [ CrossRef ] [ Medline ]
- Zhou N, Wang L, Almirall D. Estimating tree-based dynamic treatment regimes using observational data with restricted treatment sequences. Biometrics. 2023;79(3):2260-2271. [ CrossRef ] [ Medline ]
- Norton EH, Fleming SM, Daw ND, Landy MS. Suboptimal criterion learning in static and dynamic environments. PLoS Comput Biol. 2017;13(1):e1005304. [ FREE Full text ] [ CrossRef ] [ Medline ]
- Kumari J, Kumar E, Kumar D. A structured analysis to study the role of machine learning and deep learning in the healthcare sector with big data analytics. Arch Comput Methods Eng. 2023;30:3673-3701. [ FREE Full text ] [ CrossRef ] [ Medline ]
- Jiang F, Jiang Y, Zhi H, Dong Y, Li H, Ma S, et al. Artificial intelligence in healthcare: past, present and future. Stroke Vasc Neurol. 2017;2(4):230-243. [ FREE Full text ] [ CrossRef ] [ Medline ]
- Gomez-Garcia CA, Askar-Rodriguez M, Velasco-Medina J. Platform for healthcare promotion and cardiovascular disease prevention. IEEE J Biomed Health Inform. 2021;25(7):2758-2767. [ CrossRef ] [ Medline ]
- Marimuthu P, Perumal V, Vijayakumar V. Intelligent personalized abnormality detection for remote health monitoring. Int J Intell Inf Technol. 2020;16(2):87-109. [ CrossRef ]
- Ukil A, Jara AJ, Marin L. Data-driven automated cardiac health management with robust edge analytics and de-risking. Sensors (Basel). 2019;19(12):2733. [ FREE Full text ] [ CrossRef ] [ Medline ]
- Trohman RG, Huang HD, Larsen T, Krishnan K, Sharma PS. Sensors for rate-adaptive pacing: how they work, strengths, and limitations. J Cardiovasc Electrophysiol. 2020;31(11):3009-3027. [ CrossRef ] [ Medline ]
- Sloan FA, Bethel MA, Ruiz D, Shea AM, Feinglos MN. The growing burden of diabetes mellitus in the US elderly population. Arch Intern Med. 2008;168(2):192-199; discussion 199. [ FREE Full text ] [ CrossRef ] [ Medline ]
- Mancia G, Grassi G. The autonomic nervous system and hypertension. Circ Res. 2014;114(11):1804-1814. [ FREE Full text ] [ CrossRef ] [ Medline ]
- Shimada SL, Brandt CA, Feng H, McInnes DK, Rao SR, Rothendler JA, et al. Personal health record reach in the veterans health administration: a cross-sectional analysis. J Med Internet Res. 2014;16(12):e272. [ FREE Full text ] [ CrossRef ] [ Medline ]
- Gianfrancesco MA, Tamang S, Yazdany J, Schmajuk G. Potential biases in machine learning algorithms using electronic health record data. JAMA Intern Med. 2018;178(11):1544-1547. [ FREE Full text ] [ CrossRef ] [ Medline ]
- Shaw J, Rudzicz F, Jamieson T, Goldfarb A. Artificial intelligence and the implementation challenge. J Med Internet Res. 2019;21(7):e13659. [ FREE Full text ] [ CrossRef ] [ Medline ]
- Rajkomar A, Hardt M, Howell MD, Corrado G, Chin MH. Ensuring fairness in machine learning to advance health equity. Ann Intern Med. 2018;169(12):866-872. [ FREE Full text ] [ CrossRef ] [ Medline ]
- Panch T, Mattie H, Atun R. Artificial intelligence and algorithmic bias: implications for health systems. J Glob Health. 2019;9(2):010318. [ FREE Full text ] [ CrossRef ] [ Medline ]
- Obermeyer Z, Powers B, Vogeli C, Mullainathan S. Dissecting racial bias in an algorithm used to manage the health of populations. Science. 2019;366(6464):447-453. [ FREE Full text ] [ CrossRef ] [ Medline ]
- Seyyed-Kalantari L, Zhang H, McDermott MBA, Chen IY, Ghassemi M. Underdiagnosis bias of artificial intelligence algorithms applied to chest radiographs in under-served patient populations. Nat Med. 2021;27(12):2176-2182. [ FREE Full text ] [ CrossRef ] [ Medline ]
- Wiens J, Saria S, Sendak M, Ghassemi M, Liu VX, Doshi-Velez F, et al. Do no harm: a roadmap for responsible machine learning for health care. Nat Med. 2019;25(9):1337-1340. [ CrossRef ] [ Medline ]
- Vyas DA, Eisenstein LG, Jones DS. Hidden in plain sight: reconsidering the use of race correction in clinical algorithms. N Engl J Med. 2020;383(9):874-882. [ FREE Full text ] [ CrossRef ] [ Medline ]
- Sinsky C, Colligan L, Li L, Prgomet M, Reynolds S, Goeders L, et al. Allocation of physician time in ambulatory practice: a time and motion study in 4 specialties. Ann Intern Med. 2016;165(11):753-760. [ CrossRef ] [ Medline ]
- West CP, Dyrbye LN, Shanafelt TD. Physician burnout: contributors, consequences and solutions. J Intern Med. 2018;283(6):516-529. [ FREE Full text ] [ CrossRef ] [ Medline ]
- Scott Kruse C, Karem P, Shifflett K, Vegi L, Ravi K, Brooks M. Evaluating barriers to adopting telemedicine worldwide: a systematic review. J Telemed Telecare. 2018;24(1):4-12. [ FREE Full text ] [ CrossRef ] [ Medline ]
- Menachemi N, Rahurkar S, Harle CA, Vest JR. The benefits of health information exchange: an updated systematic review. J Am Med Inform Assoc. 2018;25(9):1259-1265. [ FREE Full text ] [ CrossRef ] [ Medline ]
- Osheroff JA, Teich J, Levick D, Saldana L, Velasco F, Sittig D, et al. Improving Outcomes with Clinical Decision Support: An Implementer's Guide, Second Edition. New York. HIMSS Publishing; 2012.
- Weenk M, van Goor H, Frietman B, Engelen LJLPG, van Laarhoven CJHM, Smit J, et al. Continuous monitoring of vital signs using wearable devices on the general ward: pilot study. JMIR Mhealth Uhealth. 2017;5(7):e91. [ FREE Full text ] [ CrossRef ] [ Medline ]
- Sendelbach S, Funk M. Alarm fatigue: a patient safety concern. AACN Adv Crit Care. 2013;24(4):378-386; quiz 387-388. [ CrossRef ] [ Medline ]
- van der Sijs H, Baboe I, Phansalkar S. Human factors considerations for contraindication alerts. Stud Health Technol Inform. 2013;192:132-136. [ Medline ]
- van der Sijs H, van Gelder T, Vulto A, Berg M, Aarts J. Understanding handling of drug safety alerts: a simulation study. Int J Med Inform. 2010;79(5):361-369. [ CrossRef ] [ Medline ]
- Ancker JS, Edwards A, Nosal S, Hauser D, Mauer E, Kaushal R, et al. HITEC Investigators. Effects of workload, work complexity, and repeated alerts on alert fatigue in a clinical decision support system. BMC Med Inform Decis Mak. 2017;17(1):36. [ FREE Full text ] [ CrossRef ] [ Medline ]
- Kawamoto K, Houlihan CA, Balas EA, Lobach DF. Improving clinical practice using clinical decision support systems: a systematic review of trials to identify features critical to success. BMJ. 2005;330(7494):765. [ FREE Full text ] [ CrossRef ] [ Medline ]
- Miller K, Mosby D, Capan M, Kowalski R, Ratwani R, Noaiseh Y, et al. Interface, information, interaction: a narrative review of design and functional requirements for clinical decision support. J Am Med Inform Assoc. 2018;25(5):585-592. [ FREE Full text ] [ CrossRef ] [ Medline ]
- Rand V, Coleman C, Park R, Karar A, Khairat S. Towards understanding the impact of EHR-related information overload on provider cognition. Stud Health Technol Inform. 2018;251:277-280. [ Medline ]
- Baker J, Jones D, Burkman J. Using visual representations of data to enhance sensemaking in data exploration tasks. J Assoc Inf Syst. 2009;10(7):533-559. [ FREE Full text ] [ CrossRef ]
Abbreviations
Edited by T de Azevedo Cardoso; submitted 26.07.23; peer-reviewed by M Baucum, H Ewald, E Vashishtha, R Williams, A Georgiou, R Bidkar; comments to author 24.08.23; revised version received 12.10.23; accepted 09.04.24; published 30.05.24.
©Jennifer Claggett, Stacie Petter, Amol Joshi, Todd Ponzio, Eric Kirkendall. Originally published in the Journal of Medical Internet Research (https://www.jmir.org), 30.05.2024.
This is an open-access article distributed under the terms of the Creative Commons Attribution License (https://creativecommons.org/licenses/by/4.0/), which permits unrestricted use, distribution, and reproduction in any medium, provided the original work, first published in the Journal of Medical Internet Research, is properly cited. The complete bibliographic information, a link to the original publication on https://www.jmir.org/, as well as this copyright and license information must be included.
Uncertainty about young voters stems from the age group’s complexity
Young Americans are definitionally the country’s future. That’s particularly true in the realm of politics.

Barack Obama’s election in 2008 heralded a new era in American politics. Younger Americans turned out heavily for the popular Democrat, facilitating his easy win over Republican John McCain and ripping open a partisan divide between their voting patterns and those of older Americans.
But that was 16 years ago, and voters who were under 30 in 2008 are now between 34 and 45 years old. America’s young voters today hold different views than young voters used to — and look different from the Obama-coalition young voters who have driven so much analysis in the ensuing years. Those differences, in fact, are central to understanding why there’s so much uncertainty about how young voters will vote in November — if they do at all.
Sign up for the How to Read This Chart newsletter
For one thing, we often talk about President Biden ’s weak polling with young voters and his weak polling with non-White voters as separate things. But they are related, given that young voters are more likely to be non-White.
Using data from the two most recent General Social Surveys (national polls completed in 2021 and 2022), we get a sense of how the U.S. adult population breaks down. Among Americans 18-30, there were about twice as many White people as non-White people. Among Americans ages 65 and older, there were about six White respondents for every non-White respondent. Many of the younger non-White respondents were Hispanic; part of the reason that older populations are more heavily White is that immigration was restricted during the mid-20th century baby boom.
At the same time, younger Americans are more likely to identify as independent than older Americans. That’s particularly true of young non-White people. In the GSS, about half of Whites under 30 were independent or independents who lean to one party or the other. Six in 10 non-Whites were. Among the oldest respondents, about a third of Whites were independents but only 3 in 10 non-Whites.
This is a central issue. As I wrote in November , the fact that younger Americans are often not actually Democrats means there isn’t a sense of institutional loyalty to the party or its candidates. That they are independents who often vote for Democrats has been good enough for a lot of Democrats in a lot of elections, but when the question at hand centers on a particular person — in November, President Biden — that is disadvantageous. In statewide races, younger voters (like lots of voters) will recognize the (D) or (R) before the name. They know Biden’s name and that of his opponent … which we’ll come back to in a moment.
We can see how this question of institutional association manifests in participation. Earlier this year, Pew Research Center published data showing that there was still a wide gap between younger and older Americans in their political views.
This overlaps with race, too. Non-White respondents in Pew’s data were much more likely to identify as Democrats or Democratic-leaning independents and, as noted above, younger Americans are more likely to be non-White. The GSS data also shows that 4 in 5 of those under 30 who identify as Republican or Republican-leaning independents are White.
The Pew analysis, though, looked only at registered voters — people who were participating in the political process to at least some extent. Gallup data released in February considered the political views of adults regardless of registration status. It found, unlike Pew, that there was a significant shift toward Republican identification among younger and non-White Americans, even if they didn’t register as Republicans.
There is some indication that younger voters are more frequently registering as Republicans than in the past. That my be a reflection of Biden’s increased unpopularity and its corollary: Donald Trump’s decreased unpopularity.
Earlier this year, I used YouGov data to show that views of Trump now are less negative among several groups than during his presidency. Compare the percentage of respondents each month who say they view him unfavorably with the average during his presidency.
Democratic, Black and Hispanic respondents still generally view Trump negatively, but less than they used to.
That analysis didn’t break down age, so I asked YouGov for data by age and gender. In part, this was a function of recent Brookings analysis documenting the divergence in values between young men and young women. What the YouGov polling shows is that, in every age group, men view Trump less negatively than women. But notice the annual averages broken out at the bottom of the chart below: Views among younger Americans have grown more positive since he first took office while views among older Americans sank before rebounding slightly.
In 2024, young men view Trump about as negatively as older women do. The 2017 annual average showed a nearly 50-point gap.
The silver lining for Biden is that he fares much better among those more-engaged voters. The best encapsulation of this phenomenon comes from the Harvard Youth Poll released earlier this year. Among all respondents under 30, Biden gets about 45 percent. Among those most likely to vote, he’s closer to 60 percent. Support for Trump, meanwhile, doesn’t change.
Historically, of course, younger people are less likely to vote anyway. That was one reason the Obama result was so striking: Young people actually turned out! Siena College polling conducted for the New York Times and Philadelphia Inquirer in six battleground states found that younger people were less likely to say they were likely to vote than respondents overall.
That same poll also showed that younger people were a bit more skeptical about the political and economic system than older Americans. More than three-quarters said the system needs to be torn down completely or changed significantly. Such sentiments turn up elsewhere , too — and in states where young people were more likely to view Trump’s interest in upending the system as a positive, Trump fared better.
Here we could segue into other areas in which younger Americans diverge from older Americans, like media consumption. New research from Pew shows that younger Americans are less likely to get news about the election from journalists and news organizations than older Americans, while they are more likely than older people to get it from friends, celebrities, social media personalities and other ordinary people they don’t know.
But this is probably to a significant extent a function of whether those groups seek out election news. If you aren’t trying to stay up to speed on the election, it makes sense that you would get more information passively from non-news sources. Consider that the Harvard Youth Poll found that younger Americans had the same general concerns about the election as older Americans, suggesting that the differences in consumption (or reception) of political news didn’t lead to a divergence in priorities.
So what do we have? A less engaged, more diverse population that isn’t as hostile to Trump as it used to be even as it has grown more skeptical of Biden — and lacks the institutional ties to the Democratic Party that might incline them to vote for the incumbent president anyway. There are a lot of fringes and asterisks that can modify those descriptors, but that appears to be the important distillation.
What will matter in November, then, is who turns out to vote. Which, of course, is what matters every November.
Election 2024
Get the latest news on the 2024 election from our reporters on the campaign trail and in Washington.
Who is running?: President Biden and Donald Trump secured their parties’ nominations for the presidency . Here’s how we ended up with a Trump-Biden rematch .
Presidential debates: Biden and Trump agreed to a June 27 debate on CNN and a Sept. 10 debate broadcast by ABC News.
Key dates and events: From January to June, voters in all states and U.S. territories will pick their party’s nominee for president ahead of the summer conventions. Here are key dates and events on the 2024 election calendar .
Abortion and the election: Voters in about a dozen states could decide the fate of abortion rights with constitutional amendments on the ballot in a pivotal election year. Biden supports legal access to abortion , and he has encouraged Congress to pass a law that would codify abortion rights nationwide. After months of mixed signals about his position, Trump said the issue should be left to states . Here’s how Biden’s and Trump’s abortion stances have shifted over the years.

Sorry, there’s no results for ' '
Senior Data Scientist
Company description.
Digitas is the Networked Experience Agency, built on the vision that we create magnetic experiences that earn the right for brands to exist in human networks. Today, and tomorrow. We deliver Networked Experiences by leveraging comprehensive data, technology, creative, media and strategy capabilities. Digitas delivers ambitious outcomes via unique solutions that include Creative Experiences, Integrated Media, Addressable Relationships, Social Marketing and Total Commerce. Celebrated by Ad Age as Data and Insights Agency of the Year, U.S Campaign’s Brand Experience Agency of the Year, Media Network of the Year and celebrated by Forrester and Gartner, Digitas serves the world’s leading brands through a global network comprised of more than 5,500 employees across over 65 offices in 43 countries.
What you’ll do:
Our Data Scientists deliver analytic solutions across a wide variety of client applications. We build inferential and predictive models, including machine learning algorithms and AI; we process, integrate and manipulate big data with distributed systems and customer data pipelines; we synthesize results and translate findings into compelling stories that resonate with clients.
As a Senior Data Scientist , you’ll solve complex marketing and business challenges—from cross-channel media and customer experience optimization to segmentation, targeting and business strategy—by accessing, integrating, manipulating, mining and modeling a wide array of data sources.
Job Responsibilities:
- Translating and reframing marketing and business questions into analytical plans.
- Using distributed computing systems to ingest, access and integrate disparate big data sources.
- Conducting extensive exploratory analysis to identify relevant insights, useful transformations and analytical applications.
- Applying quantitative techniques, including statistical and machine learning, to uncover latent patterns in the data.
- Building and testing scalable data pipelines or models for real-time applications.
- Summarizing, visualizing, communicating and documenting analytic concepts, processes and results for technical and non-technical audiences.
- Collaborating with internal and external stakeholders to establish clear analytical objectives, approaches and timelines.
- Sharing knowledge, debating techniques, and conducting research to advance the collective knowledge and skills of our Data Science practice.
Qualifications
We’re looking for rigorous analytic training and 3+ years professional experience in a data science or analytics role, which typically includes:
- A Bachelor’s or Master’s degree in a quantitative field such as statistics, mathematics, econometrics, operations research, data science, computer science, engineering, marketing or social science methods.
- Hands-on experience mining data for decision-focused insights.
- Hands-on experience running common statistical or machine learning procedures, such as descriptive statistics, hypothesis testing, dimension reduction, feature transformation, supervised or unsupervised learning.
- Hands-on experience using Python or R, SQL, and distributed computing systems such as Hadoop or AWS. Familiarity with Linux and/or Spark preferred.
- Demonstrated interest in marketing analytical applications.
- Demonstrated self-starter who thrives in a fast-paced environment with flat structure.
Got what it takes? We’d love to hear from you.
Additional Information
Digitas is an equal opportunity employer.
“Compensation Range: $70,000 - $105,000 annually. This is the pay range the Company believes it will pay for this position at the time of this posting. Consistent with applicable law, compensation will be determined based on the skills, qualifications, and experience of the applicant along with the requirements of the position, and the Company reserves the right to modify this pay range at any time. For this role, the Company will offer medical coverage, dental, vision, disability, 401k, and paid time off.” If your requirements fall outside of this range, you are still welcome to apply. The Company anticipates the application window for this job posting will end 07/04/2024.
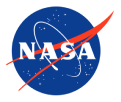
Suggested Searches
- Climate Change
- Expedition 64
- Mars perseverance
- SpaceX Crew-2
- International Space Station
- View All Topics A-Z
Humans in Space
Earth & climate, the solar system, the universe, aeronautics, learning resources, news & events.
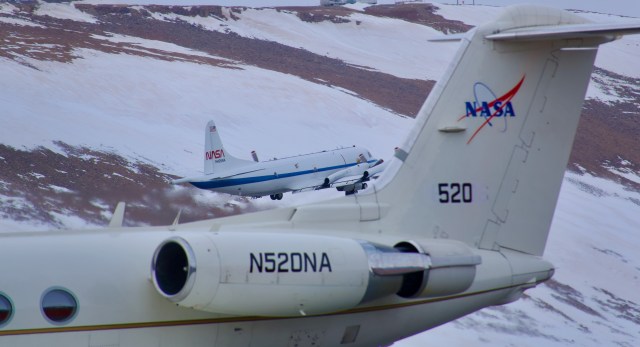
NASA Mission Flies Over Arctic to Study Sea Ice Melt Causes
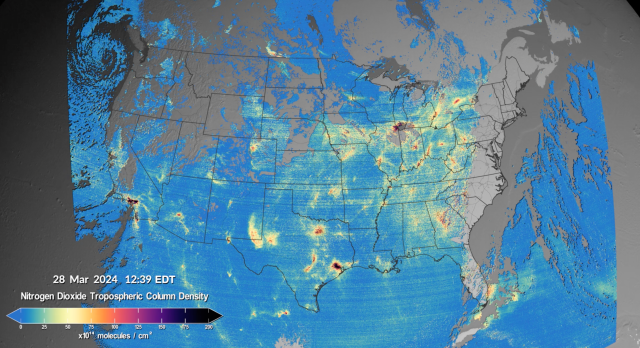
NASA Releases New High-Quality, Near Real-Time Air Quality Data
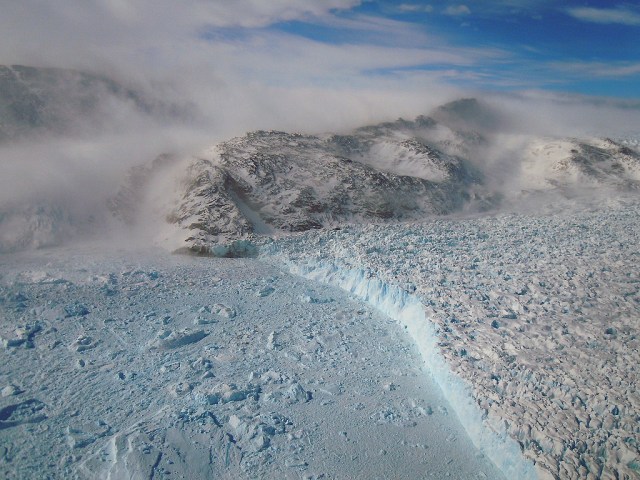
Twin NASA Satellites Ready to Help Gauge Earth’s Energy Balance
- Search All NASA Missions
- A to Z List of Missions
- Upcoming Launches and Landings
- Spaceships and Rockets
- Communicating with Missions
- James Webb Space Telescope
- Hubble Space Telescope
- Why Go to Space
- Astronauts Home
- Commercial Space
- Destinations
- Living in Space
- Explore Earth Science
- Earth, Our Planet
- Earth Science in Action
- Earth Multimedia
- Earth Science Researchers
- Pluto & Dwarf Planets
- Asteroids, Comets & Meteors
- The Kuiper Belt
- The Oort Cloud
- Skywatching
- The Search for Life in the Universe
- Black Holes
- The Big Bang
- Dark Energy & Dark Matter
- Earth Science
- Planetary Science
- Astrophysics & Space Science
- The Sun & Heliophysics
- Biological & Physical Sciences
- Lunar Science
- Citizen Science
- Astromaterials
- Aeronautics Research
- Human Space Travel Research
- Science in the Air
- NASA Aircraft
- Flight Innovation
- Supersonic Flight
- Air Traffic Solutions
- Green Aviation Tech
- Drones & You
- Technology Transfer & Spinoffs
- Space Travel Technology
- Technology Living in Space
- Manufacturing and Materials
- Science Instruments
- For Kids and Students
- For Educators
- For Colleges and Universities
- For Professionals
- Science for Everyone
- Requests for Exhibits, Artifacts, or Speakers
- STEM Engagement at NASA
- NASA's Impacts
- Centers and Facilities
- Directorates
- Organizations
- People of NASA
- Internships
- Our History
- Doing Business with NASA
- Get Involved
- Aeronáutica
- Ciencias Terrestres
- Sistema Solar
- All NASA News
- Video Series on NASA+
- Newsletters
- Social Media
- Media Resources
- Upcoming Launches & Landings
- Virtual Events
- Sounds and Ringtones
- Interactives
- STEM Multimedia
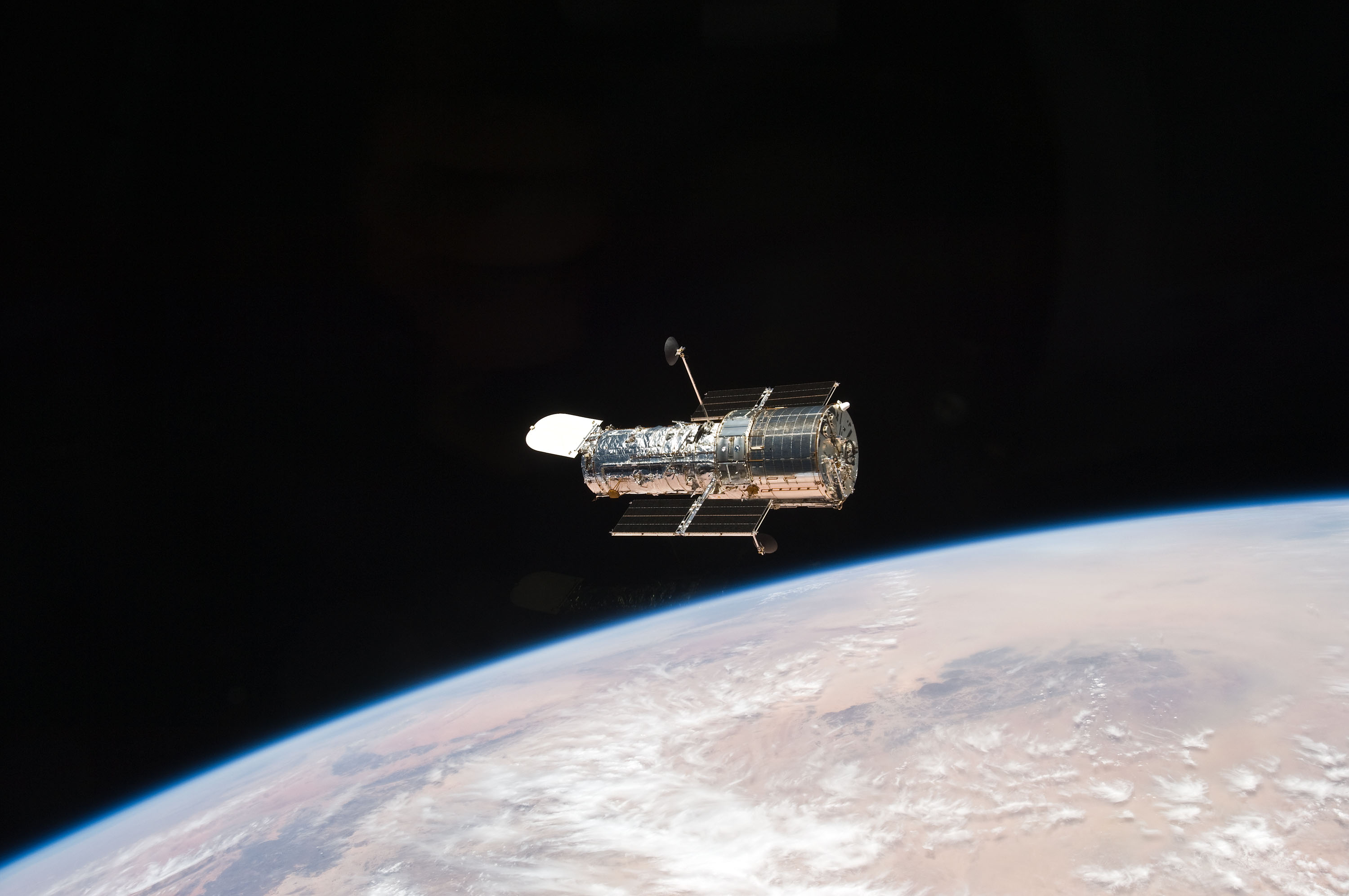
NASA’s Hubble Temporarily Pauses Science
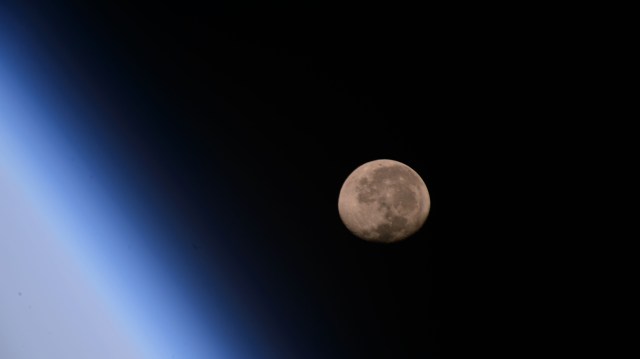
Space Station Research Advances NASA’s Plans to Explore the Moon, Mars
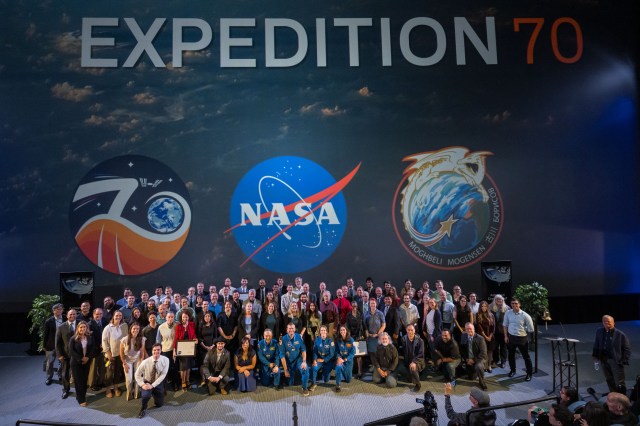
Welcome Back to Planet Earth, Expedition 70 Crew!
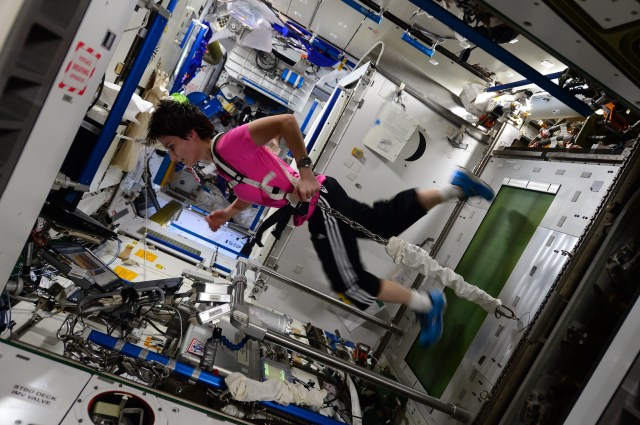
Astronaut Exercise
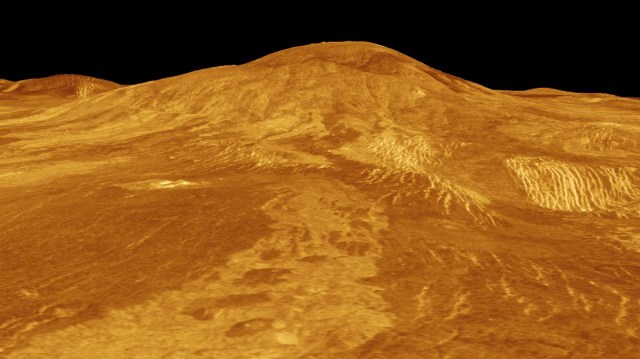
Ongoing Venus Volcanic Activity Discovered With NASA’s Magellan Data
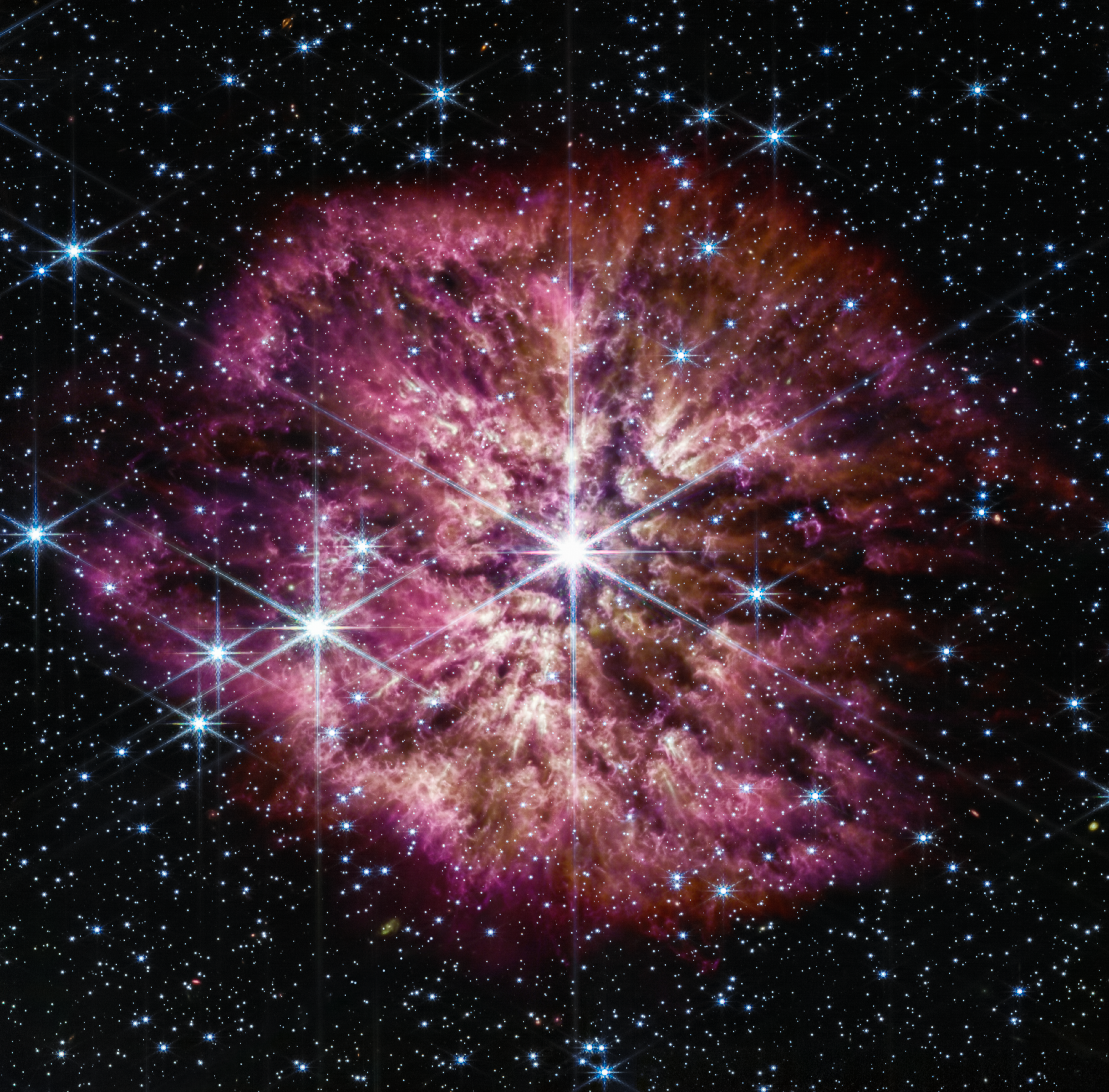
C.12 Planetary Instrument Concepts for the Advancement of Solar System Observations POC Change
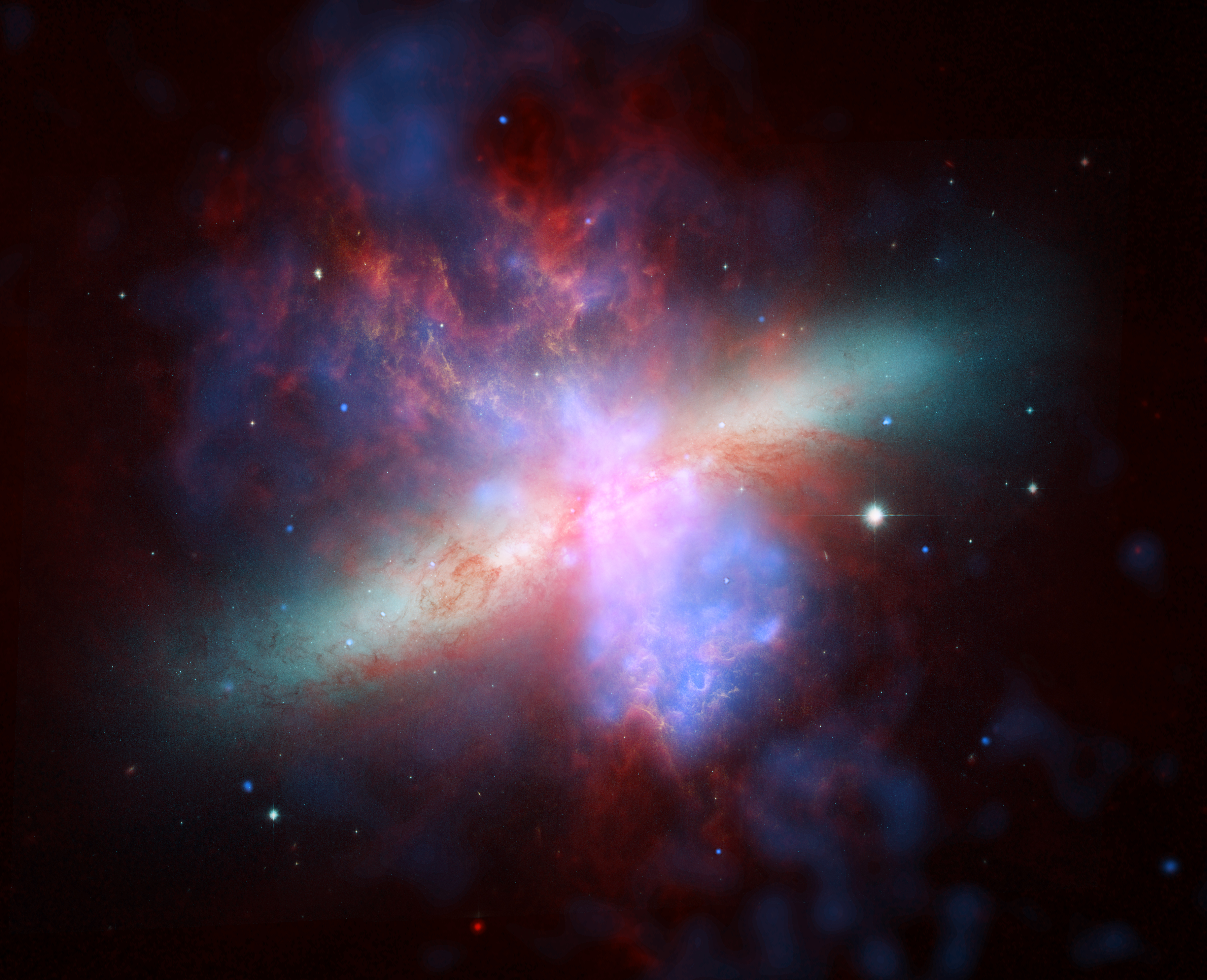
June’s Night Sky Notes: Constant Companions: Circumpolar Constellations, Part III
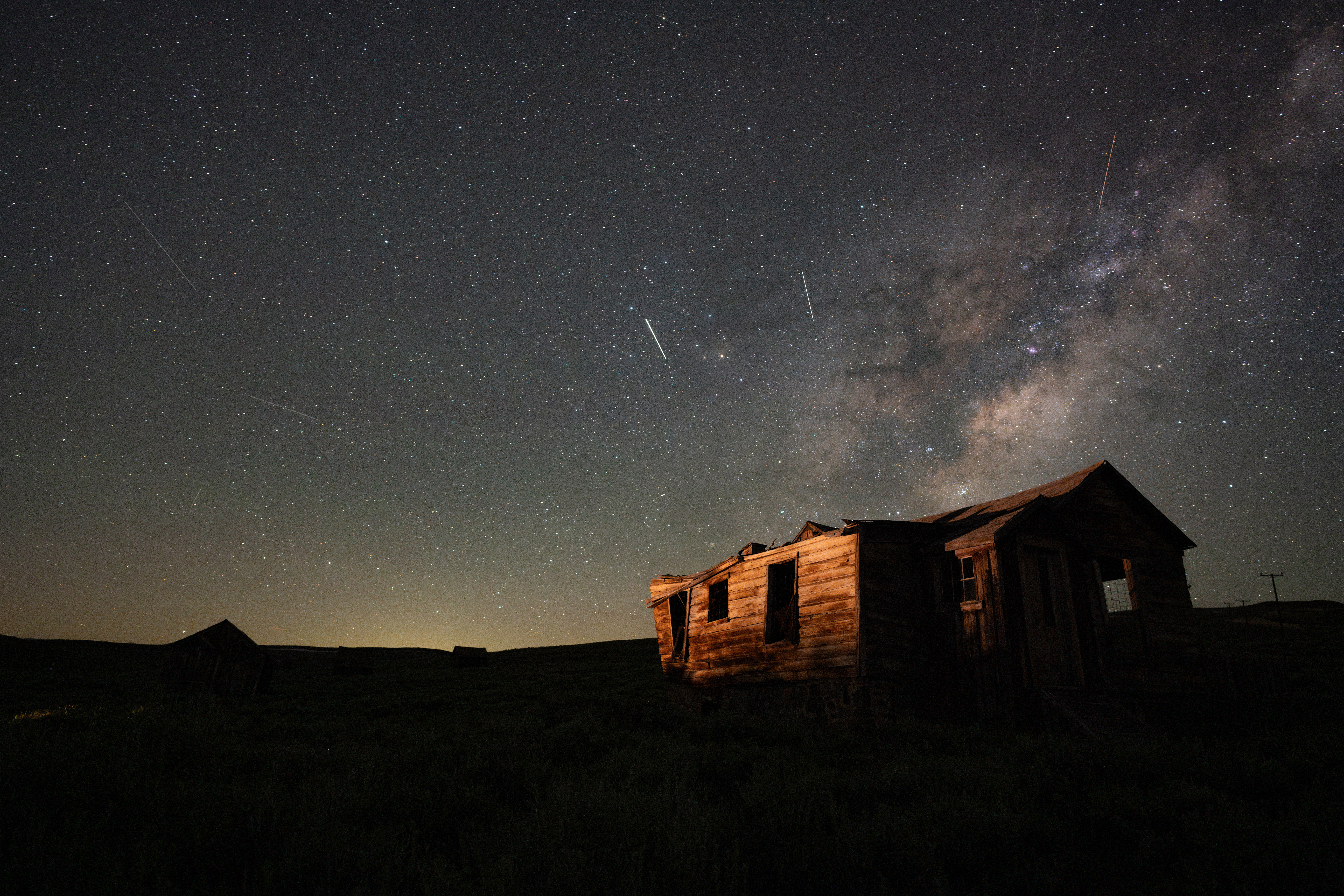
What’s Up: June 2024 Skywatching Tips from NASA
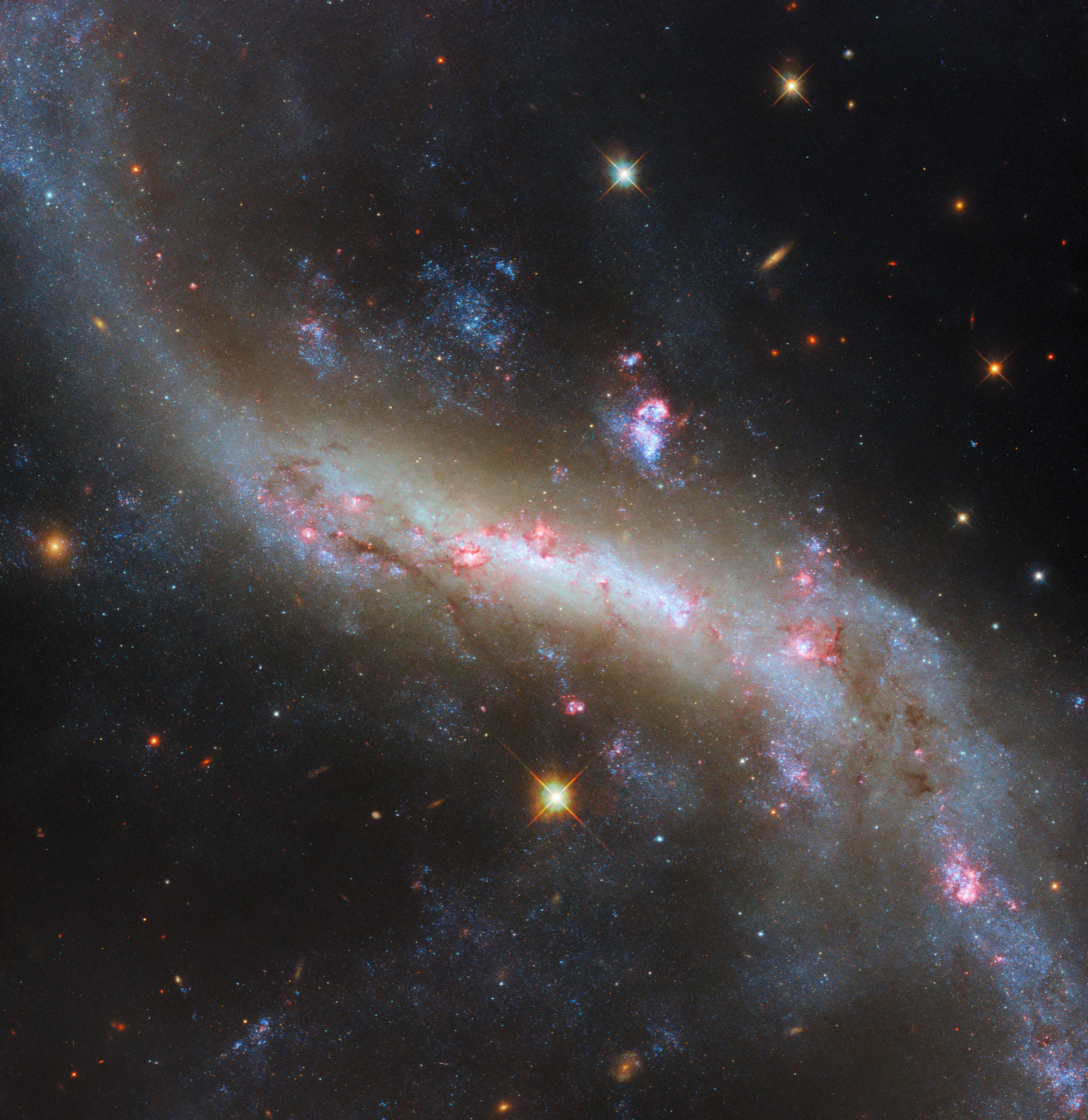
Hubble Views the Lights of a Galactic Bar
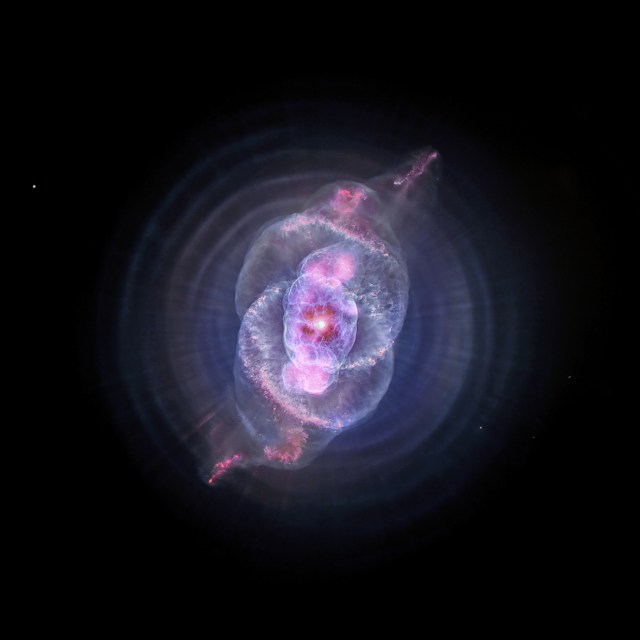
Travel Through Data From Space in New 3D Instagram Experiences
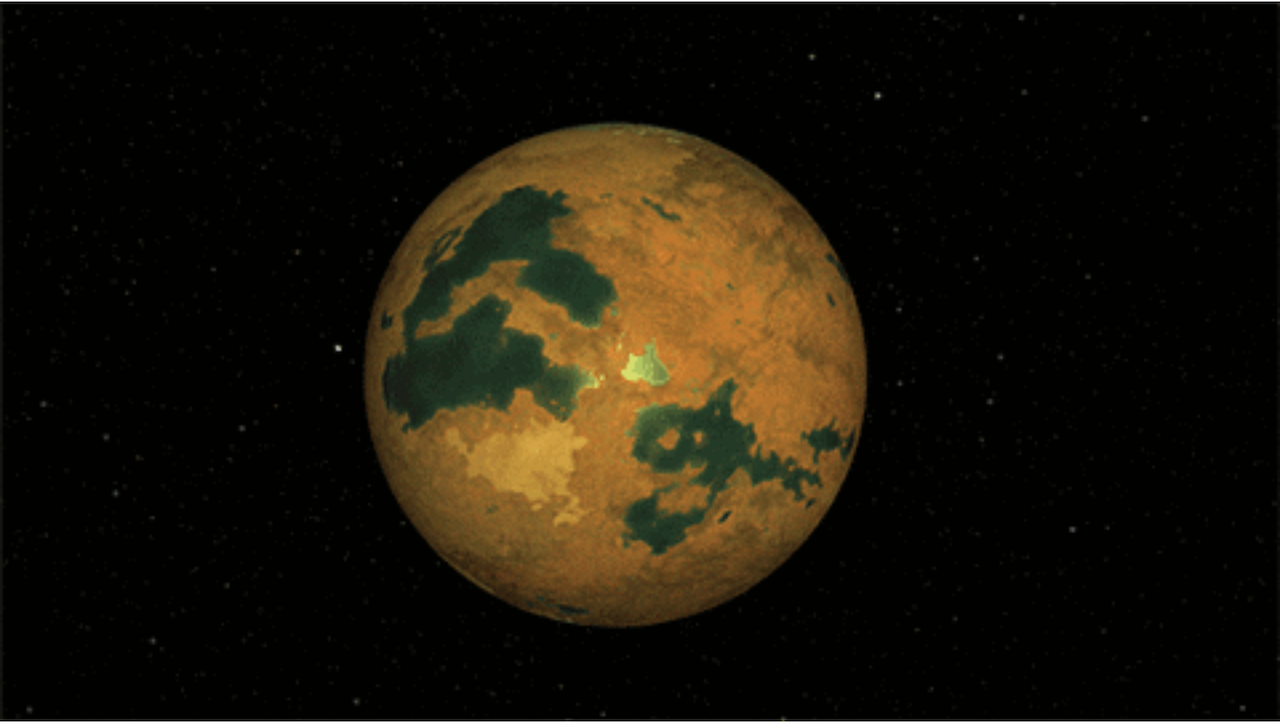
Discovery Alert: Spock’s Home Planet Goes ‘Poof’
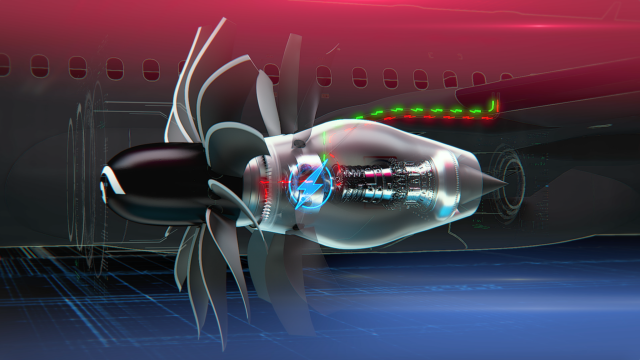
NASA, Industry to Start Designing More Sustainable Jet Engine Core
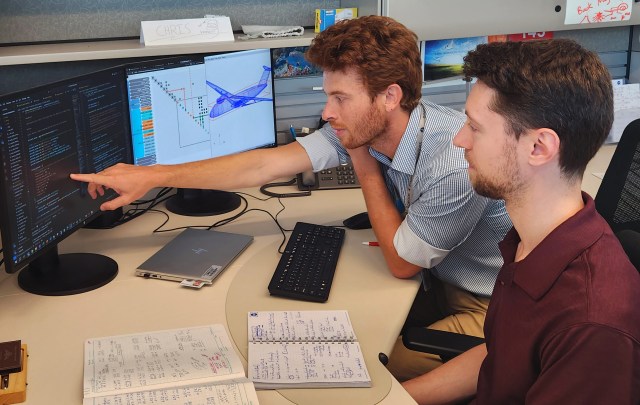
Aviary: A New NASA Software Platform for Aircraft Modelling
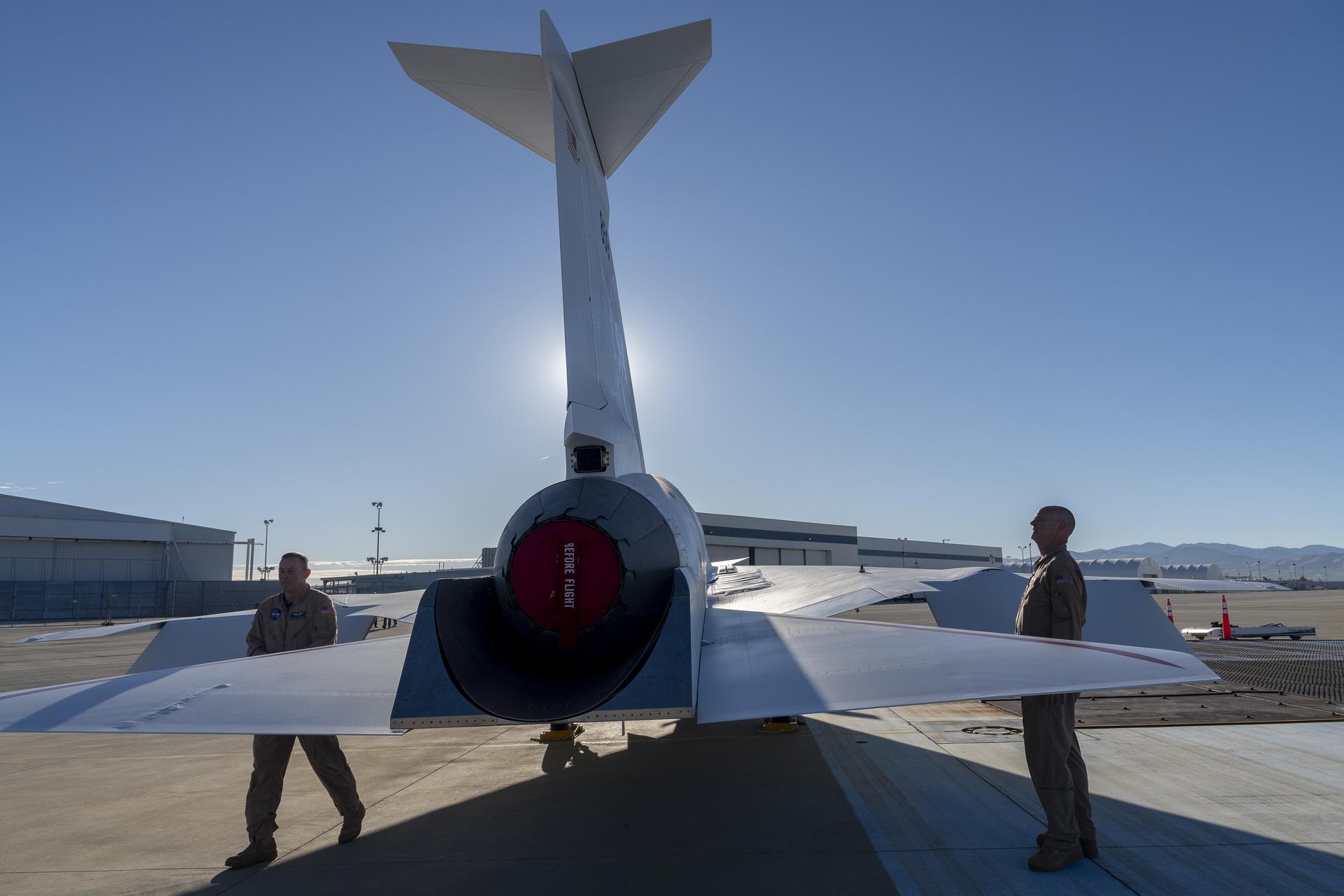
NASA’s X-59 Passes Milestone Toward Safe First Flight
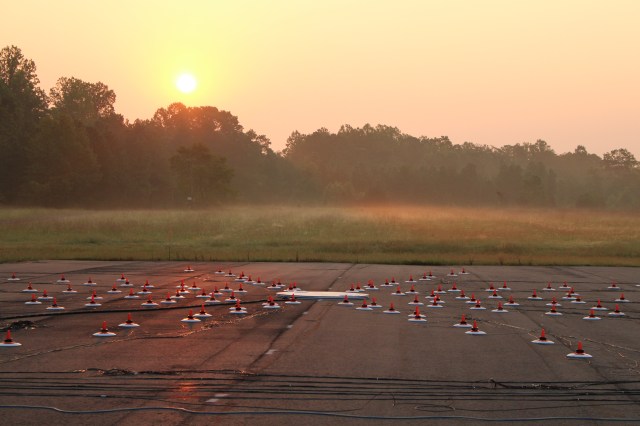
Tech Today: Measuring the Buzz, Hum, and Rattle
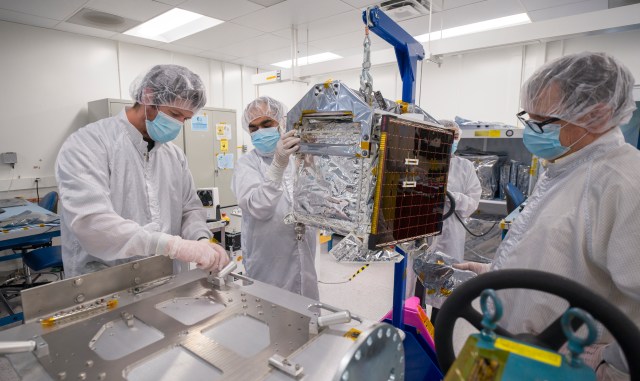
NASA to Measure Moonquakes With Help From InSight Mars Mission
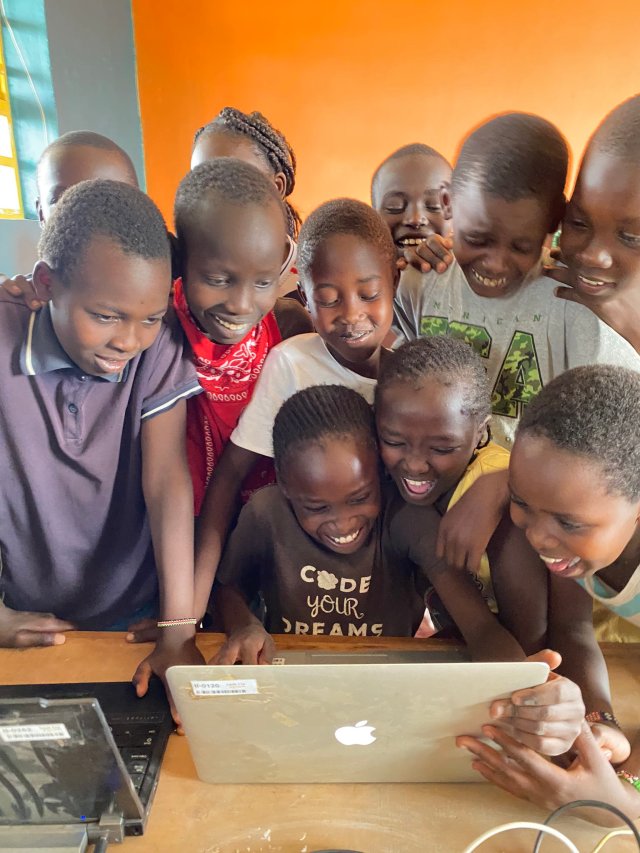
NASA Around the World: Interns Teach Virtual Lessons in Kenya
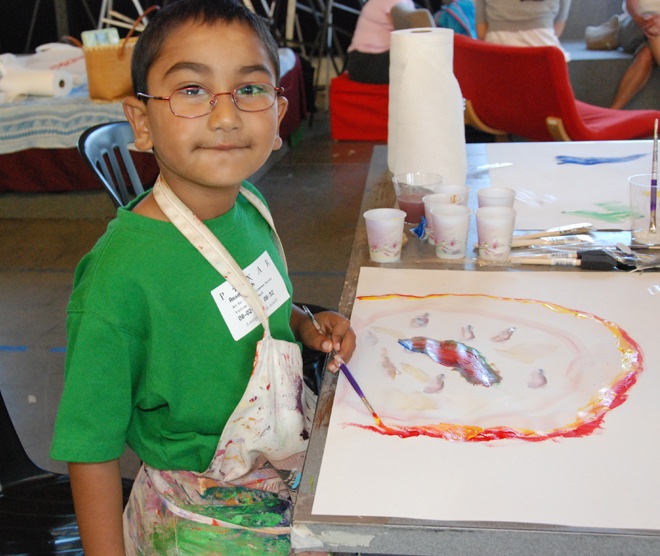
The Moon and Amaey Shah
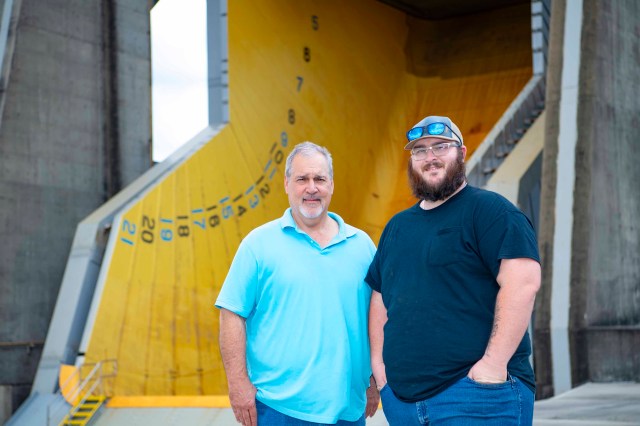
NASA Stennis Helps Family Build a Generational Legacy
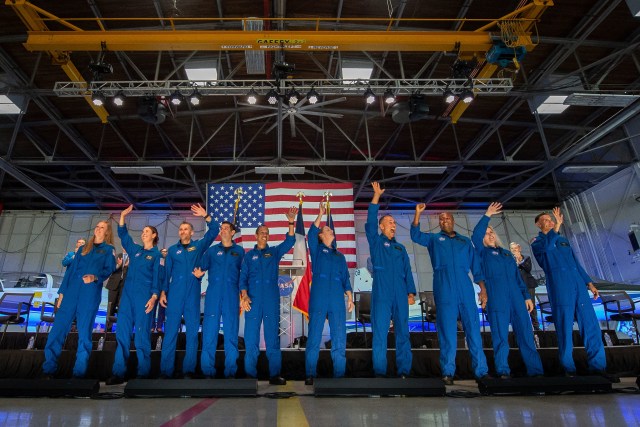
Diez maneras en que los estudiantes pueden prepararse para ser astronautas
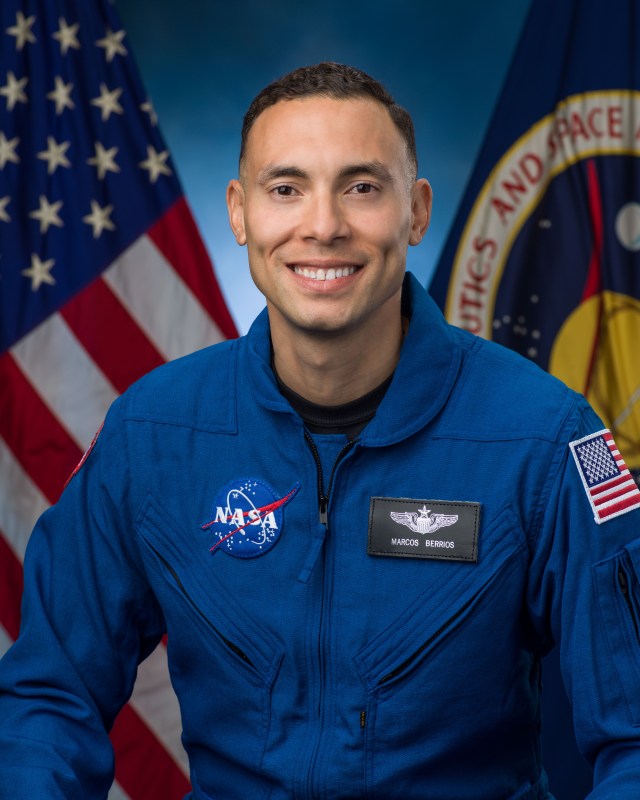
Astronauta de la NASA Marcos Berríos

Resultados científicos revolucionarios en la estación espacial de 2023
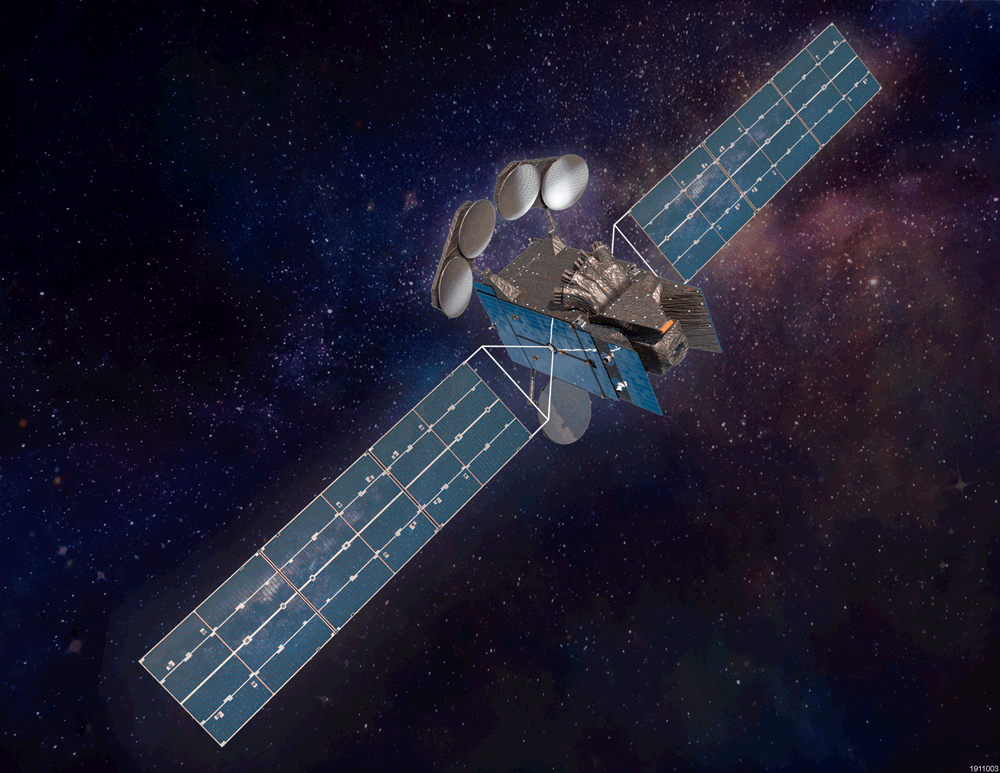
Charles G. Hatfield
Earth science public affairs officer, nasa langley research center.
NASA has made new data available that can provide air pollution observations at unprecedented resolutions – down to the scale of individual neighborhoods. The near real-time data comes from the agency’s TEMPO (Tropospheric Emissions: Monitoring of Pollution) instrument, which launched last year to improve life on Earth by revolutionizing the way scientists observe air quality from space. This new data is available from the Atmospheric Science Data Center at NASA’s Langley Research Center in Hampton, Virginia.
“TEMPO is one of NASA’s Earth observing instruments making giant leaps to improve life on our home planet,” said NASA Administrator Bill Nelson. “NASA and the Biden-Harris Administration are committed to addressing the climate crisis and making climate data more open and available to all. The air we breathe affects everyone, and this new data is revolutionizing the way we track air quality for the benefit of humanity.”
To view this video please enable JavaScript, and consider upgrading to a web browser that supports HTML5 video
The TEMPO mission gathers hourly daytime scans of the atmosphere over North America from the Atlantic Ocean to the Pacific Coast, and from Mexico City to central Canada. The instrument detects pollution by observing how sunlight is absorbed and scattered by gases and particles in the troposphere, the lowest layer of Earth’s atmosphere.
“All the pollutants that TEMPO is measuring cause health issues,” said Hazem Mahmoud, science lead at NASA Langley’s Atmospheric Science Data Center. “We have more than 500 early adopters using these datasets right away. We expect to see epidemiologists and health experts using this data in the near future. Researchers studying the respiratory system and the impact of these pollutants on people’s health will find TEMPO’s measurements invaluable.”
An early adopter program has allowed policymakers and other air quality stakeholders to understand the capabilities and benefits of TEMPO’s measurements . Since October 2023, the TEMPO calibration and validation team has been working to evaluate and improve TEMPO data products.
We have more than 500 early adopters that will be using these datasets right away.
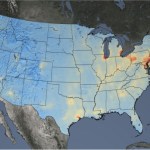
hazem mahmoud
NASA Data Scientist
“Data gathered by TEMPO will play an important role in the scientific analysis of pollution,” said Xiong Liu, senior physicist at the Smithsonian Astrophysical Observatory and principal investigator for the mission. “For example, we will be able to conduct studies of rush hour pollution, linkages of diseases and health issues to acute exposure of air pollution, how air pollution disproportionately impacts underserved communities, the potential for improved air quality alerts, the effects of lightning on ozone, and the movement of pollution from forest fires and volcanoes.”
Measurements by TEMPO include air pollutants such as nitrogen dioxide, formaldehyde, and ground-level ozone.
“Poor air quality exacerbates pre-existing health issues, which leads to more hospitalizations,” said Jesse Bell, executive director at the University of Nebraska Medical Center’s Water, Climate, and Health Program. Bell is an early adopter of TEMPO’s data.
Bell noted that there is a lack of air quality data in rural areas since monitoring stations are often hundreds of miles apart. There is also an observable disparity in air quality from neighborhood to neighborhood.
“Low-income communities, on average, have poorer air quality than more affluent communities,” said Bell. “For example, we’ve conducted studies and found that in Douglas County, which surrounds Omaha, the eastern side of the county has higher rates of pediatric asthma hospitalizations. When we identify what populations are going to the hospital at a higher rate than others, it’s communities of color and people with indicators of poverty. Data gathered by TEMPO is going to be incredibly important because you can get better spatial and temporal resolution of air quality across places like Douglas County.”
Determining sources of air pollution can be difficult as smoke from wildfires or pollutants from industry and traffic congestion drift on winds. The TEMPO instrument will make it easier to trace the origin of some pollutants.
“The National Park Service is using TEMPO data to gain new insight into emerging air quality issues at parks in southeast New Mexico,” explained National Park Service chemist, Barkley Sive. “Oil and gas emissions from the Permian Basin have affected air quality at Carlsbad Caverns and other parks and their surrounding communities. While pollution control strategies have successfully decreased ozone levels across most of the United States, the data helps us understand degrading air quality in the region.”
The TEMPO instrument was built by BAE Systems, Inc., Space & Mission Systems (formerly Ball Aerospace) and flies aboard the Intelsat 40e satellite built by Maxar Technologies. The TEMPO Ground System, including the Instrument Operations Center and the Science Data Processing Center, are operated by the Smithsonian Astrophysical Organization, part of the Center for Astrophysics | Harvard & Smithsonian.
To learn more about TEMPO visit: https://nasa.gov/tempo
Related Terms
- Tropospheric Emissions: Monitoring of Pollution (TEMPO)
- Langley Research Center
Explore More
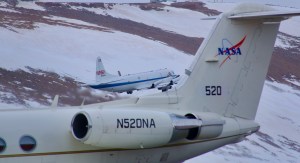
NASA-supported wireless microphone array quickly, cheaply, and accurately maps noise from aircraft, animals, and more.
Create an account
Create a free IEA account to download our reports or subcribe to a paid service.
SUVs are setting new sales records each year – and so are their emissions

Cite commentary
IEA (2024), SUVs are setting new sales records each year – and so are their emissions , IEA, Paris https://www.iea.org/commentaries/suvs-are-setting-new-sales-records-each-year-and-so-are-their-emissions, Licence: CC BY 4.0
Share this commentary
- Share on Twitter Twitter
- Share on Facebook Facebook
- Share on LinkedIn LinkedIn
- Share on Email Email
- Share on Print Print
The large, heavy passenger vehicles were responsible for over 20% of the growth in global energy-related CO2 emissions last year
SUVs accounted for 48% of global car sales in 2023, reaching a new record and further strengthening the defining automobile trend of the early 21 st century – the shift towards ever larger and heavier cars. There are various driving forces behind this trend, from the appeal of SUVs as a status symbol and their potential enhancements in comfort, to the marketing strategies of leading automakers.
New car registrations by size and powertrain, 2010-2023
In advanced economies, SUV sales reached around 20 million last year, surpassing a market share of 50% for the first time. This preference for larger vehicles extends to emerging and developing economies, too, where the share of SUVs in total car sales mirrors this trend. Today, more than one in four cars on the road worldwide are SUVs, the majority of which are conventional internal combustion engine (ICE) vehicles. While only 5% of SUVs currently on the road are electric, they account for a growing share of electric car sales. In 2023, more than 55% of new electric car registrations were SUVs.
If SUVs were a country, they would be the world’s fifth largest emitter of CO2
SUVs weigh 200-300 kg more than an average medium-sized car, and typically take up nearly 0.3 m 2 more space – emitting roughly 20% more carbon dioxide (CO 2 ) emissions. The trend towards heavier and less fuel-efficient cars increases energy demand, including oil and electricity use, as well as demand for basic metals and critical minerals needed for battery production. Over the course of 2022 and 2023, global oil consumption directly related to SUVs rose by a total of over 600 000 barrels per day, accounting for more than a quarter of the overall annual growth in oil demand.
Combustion-related CO2 emissions from SUVs and the 10 highest-emitting countries, 2023
In 2023, there were more than 360 million SUVs on the roads worldwide, resulting in combustion-related CO 2 emissions of 1 billion tonnes, an increase of around 100 million tonnes from the previous year. This accounted for more than 20% of the growth in global energy-related CO 2 emissions last year. The annual increase in CO 2 emissions attributed to the rise of SUVs is equivalent to about half of the emissions growth stemming from the global electricity sector. Compared with smaller cars, SUVs are also associated with higher indirect emissions resulting from producing the materials used to manufacture them. If ranked among countries, the global fleet of SUVs would be the world’s fifth largest emitter of CO 2 , exceeding the emissions of Japan and various other major economies.
Global electric vehicles fleet and sales of SUVs, 2023
Around 30 million SUVs with internal combustion engines were added to the global fleet in 2023, comparable to the total number of electric cars on the roads today. In 2023, there were 500 electric car models available worldwide, of which 60% fell under the SUV category, marking a significant increase from previous years. This trend is further reinforced as automakers plan to introduce a greater number of electrified SUV models in the near future.
Globally, SUVs now account for approximately 45% of the electric car fleet, a share that would be even higher were it not for the strong growth of small electric cars in urban areas in China. In advanced economies, the share of SUVs among electric cars is even higher at 55%. This is due to limited availability of smaller and more affordable compact models.
Despite advances in fuel efficiency and electrification, the trend toward heavier and less efficient vehicles such as SUVs, which emit roughly 20% more emissions than an average medium-sized car, has largely nullified the improvements in energy consumption and emissions achieved elsewhere in the world’s passenger car fleet in recent decades. Larger vehicles also pose challenges related to their increased use of critical minerals, as they are equipped with larger batteries. Additionally, they raise questions around pedestrian safety in constrained urban environments due to their higher front ends. On top of this, their greater parking space requirements, approximately 10% more than for medium-sized cars, can limit the use of valuable space in dense urban areas for other purposes.
To respond to some of these challenges, countries such as France, Norway, and Ireland have either established or are exploring legislative frameworks to reign in demand for SUVs. Major cities like Paris and Lyon are implementing higher parking charges specifically targeting SUVs in urban areas.
Shifting from fossil-fuelled cars to electric vehicles is a key strategy for reaching international energy and climate goals. However, measures such as rightsizing EV battery packs, tailoring fuel efficiency standards based on car size, and investing in innovative battery technologies with enhanced performance and durability, as well as lower material demand requirements, are also essential for a sustainable future.
This analysis was supported by the work of IEA Energy Analysts Mathilde Huismans and Jules Sery.
Subscription successful
Thank you for subscribing. You can unsubscribe at any time by clicking the link at the bottom of any IEA newsletter.
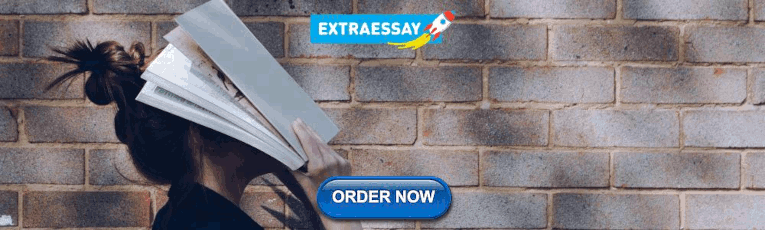
IMAGES
VIDEO
COMMENTS
The first step in a data analysis plan is to describe the data collected in the study. This can be done using figures to give a visual presentation of the data and statistics to generate numeric descriptions of the data. ... Sutton J, Austin Z. Qualitative research: data collection, analysis, and management. Can J Hosp Pharm. 2014;68(3):226 ...
In this blog article, we will explore how to create a data analysis plan: the content and structure. This data analysis plan serves as a roadmap to how data collected will be organised and analysed. It includes the following aspects: Clearly states the research objectives and hypothesis. Identifies the dataset to be used.
A Data Analysis Plan (DAP) is about putting thoughts into a plan of action. Research questions are often framed broadly and need to be clarified and funnelled down into testable hypotheses and action steps. The DAP provides an opportunity for input from collaborators and provides a platform for training. Having a clear plan of action is also ...
analysis plan: example. • The primary endpoint is free testosterone level, measured at baseline and after the diet intervention (6 mo). • We expect the distribution of free T levels to be skewed and will log-transform the data for analysis. Values below the detectable limit for the assay will be imputed with one-half the limit.
Analysis Plan and Manage Data. The main tasks are as follows: 1. Create an analysis plan • Identify research questions and/or hypotheses. • Select and access a dataset. • List inclusion/exclusion criteria. • Review the data to determine the variables to be used in the main analysis. • Select the appropriate statistical methods and ...
A data analysis plan is a roadmap for how you're going to organize and analyze your survey data—and it should help you achieve three objectives that relate to the goal you set before you started your survey: Answer your top research questions. Use more specific survey questions to understand those answers. Segment survey respondents to ...
22.1 Writing the Data Analysis Plan. Congratulations! You have now arrived at one of the most creative and straightforward, sections of your grant proposal. You and your project statistician have one major goal for your data analysis plan: You need to convince all the reviewers reading your proposal that you would know what to do with your data ...
Get Help. Designing an analysis plan ensures data collection methods meet the needs of the research question, and that the study is accurately powered to produce meaningful results. Based on investigator affiliations and the type of analysis, consultative services are available to discuss statistical methods, analysis software, or potential ...
One example of this is Sentiment Analysis, which is a way of identifying the emotion behind people's comments. When this functionality is enabled in SurveyMonkey, your survey answers will be categorised as Positive, Neutral, Negative or Undetected. Meanwhile, grounded theory involves looking at the qualitative data to explain a pattern.
Written by Coursera Staff • Updated on Apr 19, 2024. Data analysis is the practice of working with data to glean useful information, which can then be used to make informed decisions. "It is a capital mistake to theorize before one has data. Insensibly one begins to twist facts to suit theories, instead of theories to suit facts," Sherlock ...
Definition of research in data analysis: According to LeCompte and Schensul, research data analysis is a process used by researchers to reduce data to a story and interpret it to derive insights. The data analysis process helps reduce a large chunk of data into smaller fragments, which makes sense. Three essential things occur during the data ...
Data Analysis Plan: Ultimate Guide and Examples. Learn the post survey questions you need to ask attendees for valuable feedback. Once you get survey feedback, you might think that the job is done. The next step, however, is to analyze those results. Creating a data analysis plan will help guide you through how to analyze the data and come to ...
analytic plan for your grant application. Your data analytic plan has a story line with a beginning, middle, and end. The reviewers who will be evaluating your work will want to hear your complete story of what you plan to do given the many different assessments you will collect. 22.2 Before You Begin Writing Your challenge is to demonstrate to ...
Step 1: Consider your aims and approach. Step 2: Choose a type of research design. Step 3: Identify your population and sampling method. Step 4: Choose your data collection methods. Step 5: Plan your data collection procedures. Step 6: Decide on your data analysis strategies. Other interesting articles.
The data analysis plan flows from the research question, is integral to the study design, and should be well conceptualized prior to beginning data collection. In this section, we will walk through the basics of quantitative and qualitative data analysis to help you understand the fundamentals of creating a data analysis plan.
A research proposal serves as a blueprint and guide for your research plan, helping you get organized and feel confident in the path forward you choose to take. Table of contents. Research proposal purpose; ... Finalize sampling methods and data analysis methods; 13th February: 3. Data collection and preparation:
The first step in developing an effective analysis plan is to establish clear analytic objectives. The approach taken in developing the plan will be somewhat deter-mined by whether the data will be analyzed in real time, as they are generated, or if you are developing an analysis plan after the data have been collected, pro-cessed, and cleaned.
This article is a practical guide to conducting data analysis in general literature reviews. The general literature review is a synthesis and analysis of published research on a relevant clinical issue, and is a common format for academic theses at the bachelor's and master's levels in nursing, physiotherapy, occupational therapy, public health and other related fields.
For many researchers unfamiliar with qualitative research, determining how to conduct qualitative analyses is often quite challenging. Part of this challenge is due to the seemingly limitless approaches that a qualitative researcher might leverage, as well as simply learning to think like a qualitative researcher when analyzing data. From framework analysis (Ritchie & Spencer, 1994) to content ...
A data analysis plan includes many features of a research project in it with a particular emphasis on mapping out how research questions will be answered and what is necessary to answer the question. Below is a sample template of the analysis plan. The majority of this diagram should be familiar to someone who has ever done research.
A data analysis plan should be established when planning a research study (i.e., before data collection begins). Among other things, the data analysis plan should describe: (a) the data to be collected; (b) the analyses to be conducted to address the research objectives, including assumptions required by said analyses; (c) data cleaning and ...
An interim analysis is part of the continuous, ongoing data analysis. It is part of the ongoing reflective planning process of action research (Hendricks, 2013). Your action research projects will typically involve both quantitative and qualitative data. The methods for simplifying quantitative data, such as reporting, comparing, and displaying ...
Data collection was carried out by technicians hired by the project for this purpose. A total of 37 people participated in the process. The technicians underwent an intensive training programme developed by the research team. Qualitative data were collected by two members of the research team with expertise in qualitative methodology.
Methods: We conducted a secondary data analysis of the E-COMPARED (European Comparative Effectiveness Research on Blended Depression Treatment versus Treatment-as-usual) trial, which compared bCBT with TAU across 9 European countries. Data were collected in primary care and specialized services between April 2015 and December 2017.
Remote patient monitoring (RPM) enables clinicians to maintain and adjust their patients' plan of care by using remotely gathered data, such as vital signs, to proactively make medical decisions about a patient's care. RPM interventions have been touted as a means to improve patient care and well-being while reducing costs and resource needs within the health care ecosystem.
Using data from the two most recent General Social Surveys (national polls completed in 2021 and 2022), we get a sense of how the U.S. adult population breaks down.
Conducting extensive exploratory analysis to identify relevant insights, useful transformations and analytical applications. Applying quantitative techniques, including statistical and machine learning, to uncover latent patterns in the data. Building and testing scalable data pipelines or models for real-time applications.
In addition to raising its quarterly dividend, Apple also increased its stock buyback plan. While generating $90.8 billion for Q2 of fiscal 2024, the King of Cupertino delivered a slight 1% ...
The near real-time data comes from the agency's TEMPO (Tropospheric Emissions: Monitoring of Pollution) instrument, which launched last year to improve life on Earth by revolutionizing the way scientists observe air quality from space. This new data is available from the Atmospheric Science Data Center at NASA's Langley Research Center in ...
SUVs accounted for 48% of global car sales in 2023, reaching a new record and further strengthening the defining automobile trend of the early 21 st century - the shift towards ever larger and heavier cars. There are various driving forces behind this trend, from the appeal of SUVs as a status symbol and their potential enhancements in comfort, to the marketing strategies of leading automakers.