
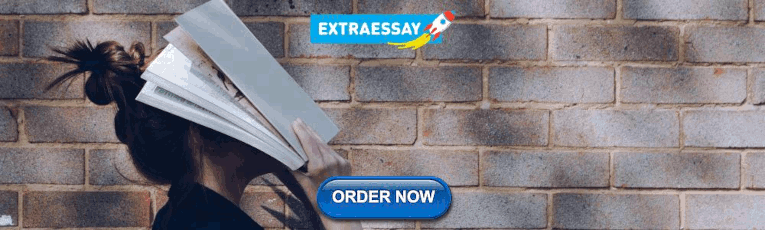
Research Topics & Ideas: Data Science
50 Topic Ideas To Kickstart Your Research Project
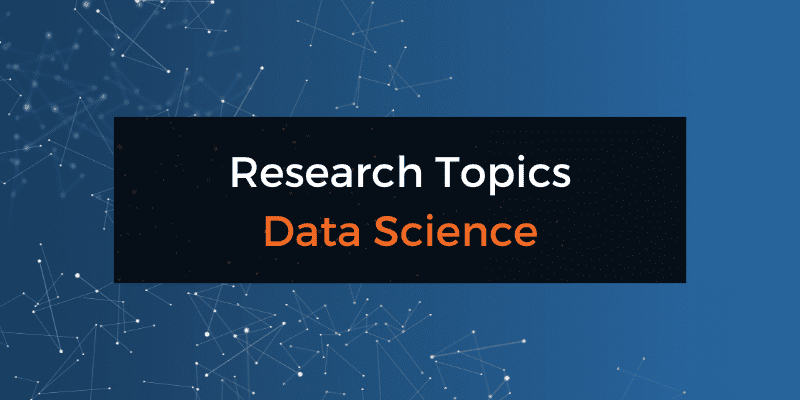
If you’re just starting out exploring data science-related topics for your dissertation, thesis or research project, you’ve come to the right place. In this post, we’ll help kickstart your research by providing a hearty list of data science and analytics-related research ideas , including examples from recent studies.
PS – This is just the start…
We know it’s exciting to run through a list of research topics, but please keep in mind that this list is just a starting point . These topic ideas provided here are intentionally broad and generic , so keep in mind that you will need to develop them further. Nevertheless, they should inspire some ideas for your project.
To develop a suitable research topic, you’ll need to identify a clear and convincing research gap , and a viable plan to fill that gap. If this sounds foreign to you, check out our free research topic webinar that explores how to find and refine a high-quality research topic, from scratch. Alternatively, consider our 1-on-1 coaching service .
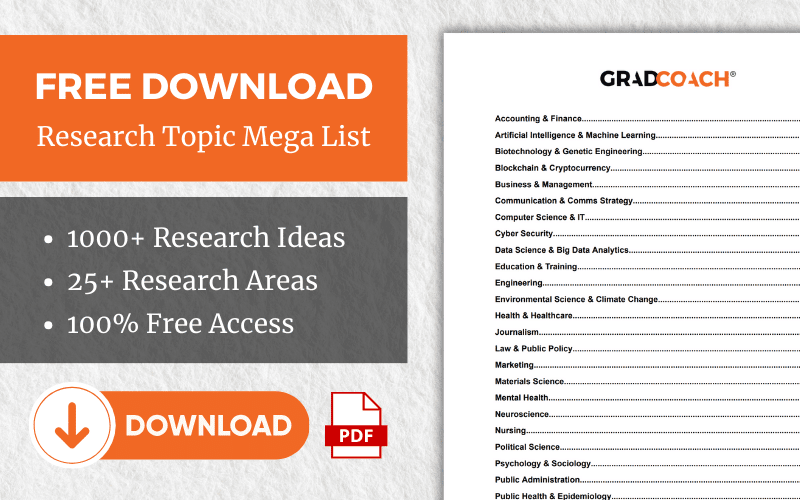
Data Science-Related Research Topics
- Developing machine learning models for real-time fraud detection in online transactions.
- The use of big data analytics in predicting and managing urban traffic flow.
- Investigating the effectiveness of data mining techniques in identifying early signs of mental health issues from social media usage.
- The application of predictive analytics in personalizing cancer treatment plans.
- Analyzing consumer behavior through big data to enhance retail marketing strategies.
- The role of data science in optimizing renewable energy generation from wind farms.
- Developing natural language processing algorithms for real-time news aggregation and summarization.
- The application of big data in monitoring and predicting epidemic outbreaks.
- Investigating the use of machine learning in automating credit scoring for microfinance.
- The role of data analytics in improving patient care in telemedicine.
- Developing AI-driven models for predictive maintenance in the manufacturing industry.
- The use of big data analytics in enhancing cybersecurity threat intelligence.
- Investigating the impact of sentiment analysis on brand reputation management.
- The application of data science in optimizing logistics and supply chain operations.
- Developing deep learning techniques for image recognition in medical diagnostics.
- The role of big data in analyzing climate change impacts on agricultural productivity.
- Investigating the use of data analytics in optimizing energy consumption in smart buildings.
- The application of machine learning in detecting plagiarism in academic works.
- Analyzing social media data for trends in political opinion and electoral predictions.
- The role of big data in enhancing sports performance analytics.
- Developing data-driven strategies for effective water resource management.
- The use of big data in improving customer experience in the banking sector.
- Investigating the application of data science in fraud detection in insurance claims.
- The role of predictive analytics in financial market risk assessment.
- Developing AI models for early detection of network vulnerabilities.
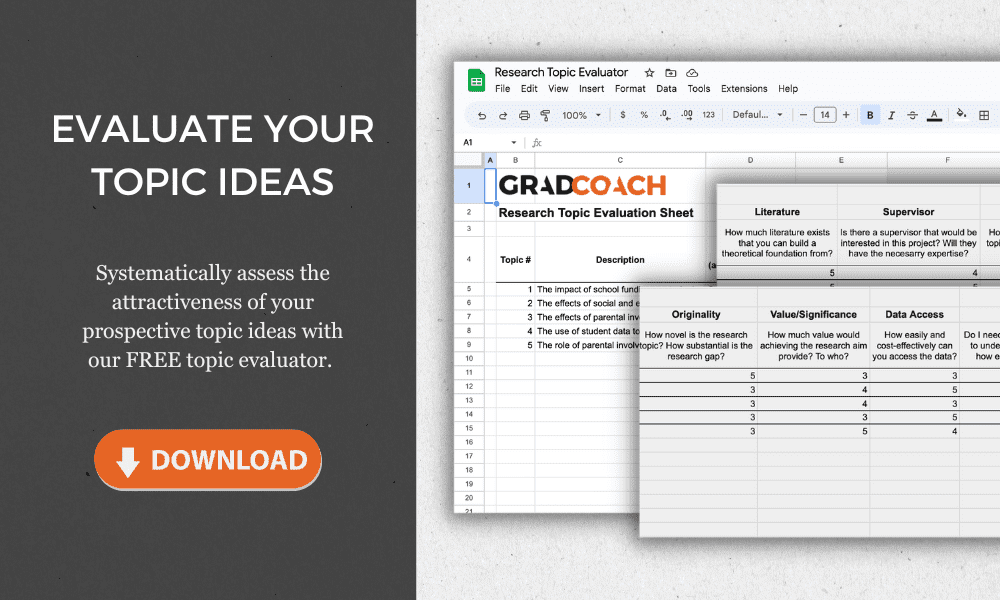
Data Science Research Ideas (Continued)
- The application of big data in public transportation systems for route optimization.
- Investigating the impact of big data analytics on e-commerce recommendation systems.
- The use of data mining techniques in understanding consumer preferences in the entertainment industry.
- Developing predictive models for real estate pricing and market trends.
- The role of big data in tracking and managing environmental pollution.
- Investigating the use of data analytics in improving airline operational efficiency.
- The application of machine learning in optimizing pharmaceutical drug discovery.
- Analyzing online customer reviews to inform product development in the tech industry.
- The role of data science in crime prediction and prevention strategies.
- Developing models for analyzing financial time series data for investment strategies.
- The use of big data in assessing the impact of educational policies on student performance.
- Investigating the effectiveness of data visualization techniques in business reporting.
- The application of data analytics in human resource management and talent acquisition.
- Developing algorithms for anomaly detection in network traffic data.
- The role of machine learning in enhancing personalized online learning experiences.
- Investigating the use of big data in urban planning and smart city development.
- The application of predictive analytics in weather forecasting and disaster management.
- Analyzing consumer data to drive innovations in the automotive industry.
- The role of data science in optimizing content delivery networks for streaming services.
- Developing machine learning models for automated text classification in legal documents.
- The use of big data in tracking global supply chain disruptions.
- Investigating the application of data analytics in personalized nutrition and fitness.
- The role of big data in enhancing the accuracy of geological surveying for natural resource exploration.
- Developing predictive models for customer churn in the telecommunications industry.
- The application of data science in optimizing advertisement placement and reach.
Recent Data Science-Related Studies
While the ideas we’ve presented above are a decent starting point for finding a research topic, they are fairly generic and non-specific. So, it helps to look at actual studies in the data science and analytics space to see how this all comes together in practice.
Below, we’ve included a selection of recent studies to help refine your thinking. These are actual studies, so they can provide some useful insight as to what a research topic looks like in practice.
- Data Science in Healthcare: COVID-19 and Beyond (Hulsen, 2022)
- Auto-ML Web-application for Automated Machine Learning Algorithm Training and evaluation (Mukherjee & Rao, 2022)
- Survey on Statistics and ML in Data Science and Effect in Businesses (Reddy et al., 2022)
- Visualization in Data Science VDS @ KDD 2022 (Plant et al., 2022)
- An Essay on How Data Science Can Strengthen Business (Santos, 2023)
- A Deep study of Data science related problems, application and machine learning algorithms utilized in Data science (Ranjani et al., 2022)
- You Teach WHAT in Your Data Science Course?!? (Posner & Kerby-Helm, 2022)
- Statistical Analysis for the Traffic Police Activity: Nashville, Tennessee, USA (Tufail & Gul, 2022)
- Data Management and Visual Information Processing in Financial Organization using Machine Learning (Balamurugan et al., 2022)
- A Proposal of an Interactive Web Application Tool QuickViz: To Automate Exploratory Data Analysis (Pitroda, 2022)
- Applications of Data Science in Respective Engineering Domains (Rasool & Chaudhary, 2022)
- Jupyter Notebooks for Introducing Data Science to Novice Users (Fruchart et al., 2022)
- Towards a Systematic Review of Data Science Programs: Themes, Courses, and Ethics (Nellore & Zimmer, 2022)
- Application of data science and bioinformatics in healthcare technologies (Veeranki & Varshney, 2022)
- TAPS Responsibility Matrix: A tool for responsible data science by design (Urovi et al., 2023)
- Data Detectives: A Data Science Program for Middle Grade Learners (Thompson & Irgens, 2022)
- MACHINE LEARNING FOR NON-MAJORS: A WHITE BOX APPROACH (Mike & Hazzan, 2022)
- COMPONENTS OF DATA SCIENCE AND ITS APPLICATIONS (Paul et al., 2022)
- Analysis on the Application of Data Science in Business Analytics (Wang, 2022)
As you can see, these research topics are a lot more focused than the generic topic ideas we presented earlier. So, for you to develop a high-quality research topic, you’ll need to get specific and laser-focused on a specific context with specific variables of interest. In the video below, we explore some other important things you’ll need to consider when crafting your research topic.
Get 1-On-1 Help
If you’re still unsure about how to find a quality research topic, check out our Research Topic Kickstarter service, which is the perfect starting point for developing a unique, well-justified research topic.
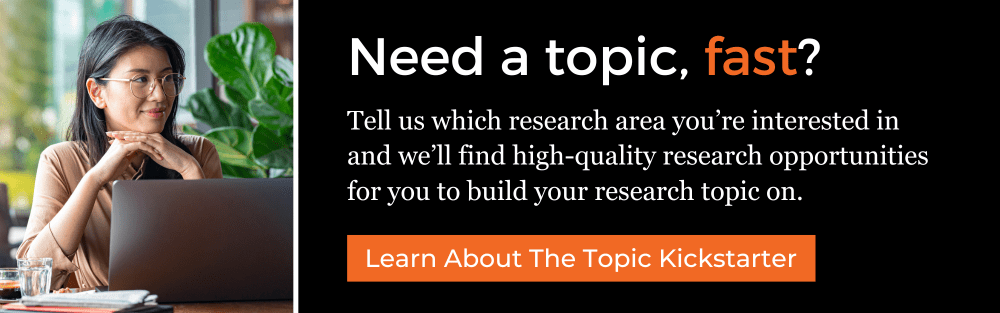
You Might Also Like:
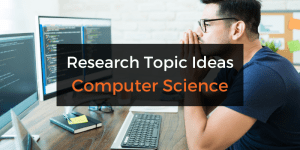
Submit a Comment Cancel reply
Your email address will not be published. Required fields are marked *
Save my name, email, and website in this browser for the next time I comment.
- Print Friendly
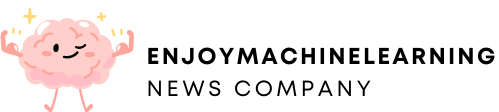
37 Research Topics In Data Science To Stay On Top Of
- February 22, 2024
As a data scientist, staying on top of the latest research in your field is essential.
The data science landscape changes rapidly, and new techniques and tools are constantly being developed.
To keep up with the competition, you need to be aware of the latest trends and topics in data science research.
In this article, we will provide an overview of 37 hot research topics in data science.
We will discuss each topic in detail, including its significance and potential applications.
These topics could be an idea for a thesis or simply topics you can research independently.
Stay tuned – this is one blog post you don’t want to miss!
37 Research Topics in Data Science
1.) predictive modeling.
Predictive modeling is a significant portion of data science and a topic you must be aware of.
Simply put, it is the process of using historical data to build models that can predict future outcomes.
Predictive modeling has many applications, from marketing and sales to financial forecasting and risk management.
As businesses increasingly rely on data to make decisions, predictive modeling is becoming more and more important.
While it can be complex, predictive modeling is a powerful tool that gives businesses a competitive advantage.
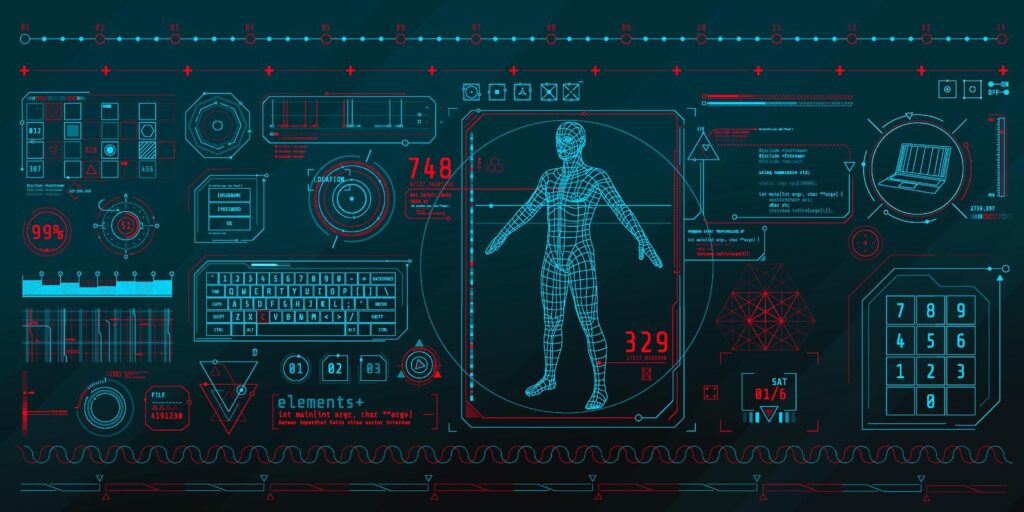
2.) Big Data Analytics
These days, it seems like everyone is talking about big data.
And with good reason – organizations of all sizes are sitting on mountains of data, and they’re increasingly turning to data scientists to help them make sense of it all.
But what exactly is big data? And what does it mean for data science?
Simply put, big data is a term used to describe datasets that are too large and complex for traditional data processing techniques.
Big data typically refers to datasets of a few terabytes or more.
But size isn’t the only defining characteristic – big data is also characterized by its high Velocity (the speed at which data is generated), Variety (the different types of data), and Volume (the amount of the information).
Given the enormity of big data, it’s not surprising that organizations are struggling to make sense of it all.
That’s where data science comes in.
Data scientists use various methods to wrangle big data, including distributed computing and other decentralized technologies.
With the help of data science, organizations are beginning to unlock the hidden value in their big data.
By harnessing the power of big data analytics, they can improve their decision-making, better understand their customers, and develop new products and services.
3.) Auto Machine Learning
Auto machine learning is a research topic in data science concerned with developing algorithms that can automatically learn from data without intervention.
This area of research is vital because it allows data scientists to automate the process of writing code for every dataset.
This allows us to focus on other tasks, such as model selection and validation.
Auto machine learning algorithms can learn from data in a hands-off way for the data scientist – while still providing incredible insights.
This makes them a valuable tool for data scientists who either don’t have the skills to do their own analysis or are struggling.
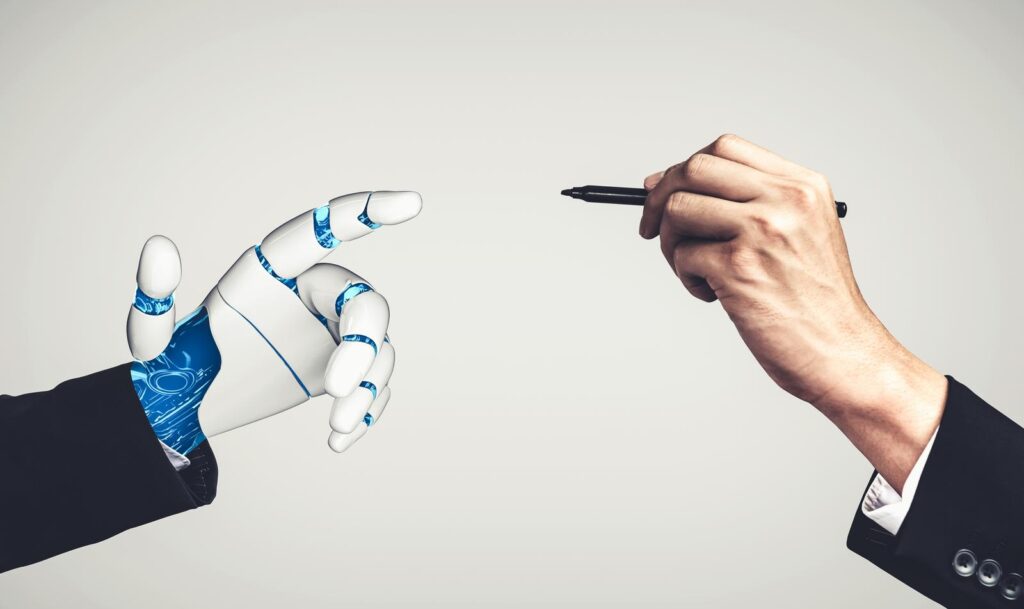
4.) Text Mining
Text mining is a research topic in data science that deals with text data extraction.
This area of research is important because it allows us to get as much information as possible from the vast amount of text data available today.
Text mining techniques can extract information from text data, such as keywords, sentiments, and relationships.
This information can be used for various purposes, such as model building and predictive analytics.
5.) Natural Language Processing
Natural language processing is a data science research topic that analyzes human language data.
This area of research is important because it allows us to understand and make sense of the vast amount of text data available today.
Natural language processing techniques can build predictive and interactive models from any language data.
Natural Language processing is pretty broad, and recent advances like GPT-3 have pushed this topic to the forefront.
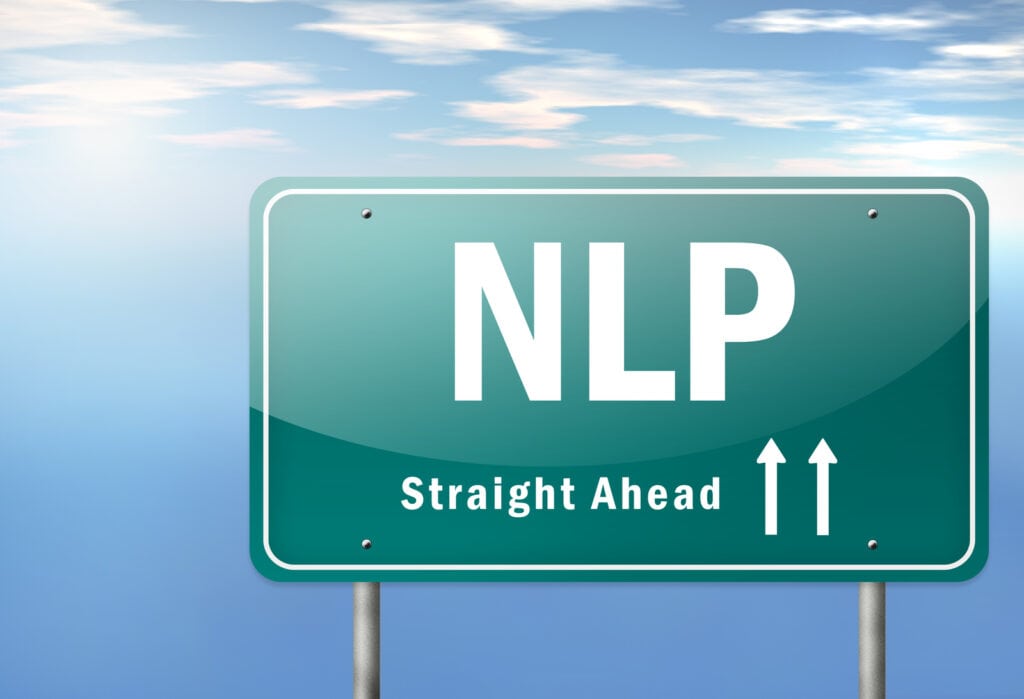
6.) Recommender Systems
Recommender systems are an exciting topic in data science because they allow us to make better products, services, and content recommendations.
Businesses can better understand their customers and their needs by using recommender systems.
This, in turn, allows them to develop better products and services that meet the needs of their customers.
Recommender systems are also used to recommend content to users.
This can be done on an individual level or at a group level.
Think about Netflix, for example, always knowing what you want to watch!
Recommender systems are a valuable tool for businesses and users alike.
7.) Deep Learning
Deep learning is a research topic in data science that deals with artificial neural networks.
These networks are composed of multiple layers, and each layer is formed from various nodes.
Deep learning networks can learn from data similarly to how humans learn, irrespective of the data distribution.
This makes them a valuable tool for data scientists looking to build models that can learn from data independently.
The deep learning network has become very popular in recent years because of its ability to achieve state-of-the-art results on various tasks.
There seems to be a new SOTA deep learning algorithm research paper on https://arxiv.org/ every single day!
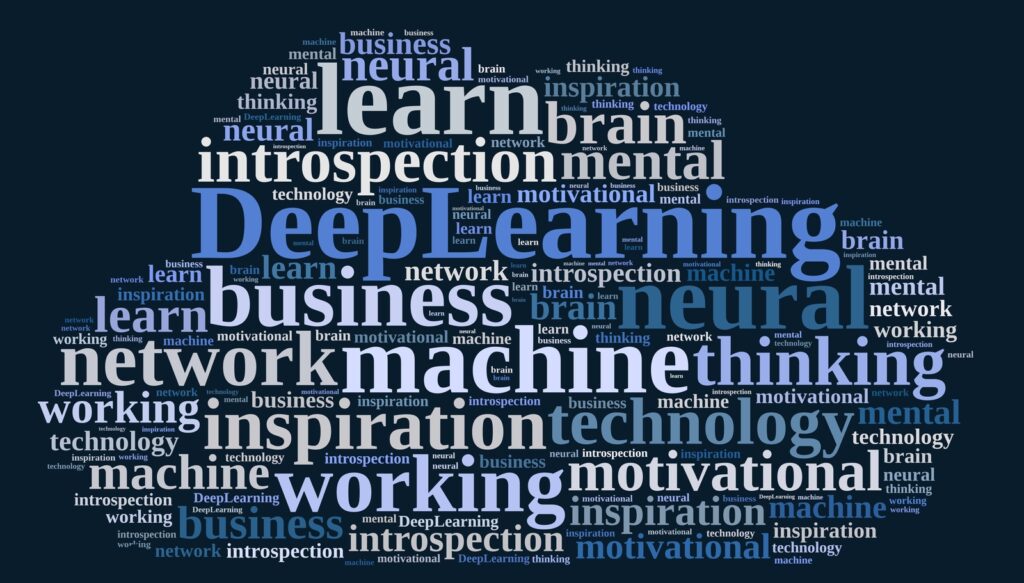
8.) Reinforcement Learning
Reinforcement learning is a research topic in data science that deals with algorithms that can learn on multiple levels from interactions with their environment.
This area of research is essential because it allows us to develop algorithms that can learn non-greedy approaches to decision-making, allowing businesses and companies to win in the long term compared to the short.
9.) Data Visualization
Data visualization is an excellent research topic in data science because it allows us to see our data in a way that is easy to understand.
Data visualization techniques can be used to create charts, graphs, and other visual representations of data.
This allows us to see the patterns and trends hidden in our data.
Data visualization is also used to communicate results to others.
This allows us to share our findings with others in a way that is easy to understand.
There are many ways to contribute to and learn about data visualization.
Some ways include attending conferences, reading papers, and contributing to open-source projects.
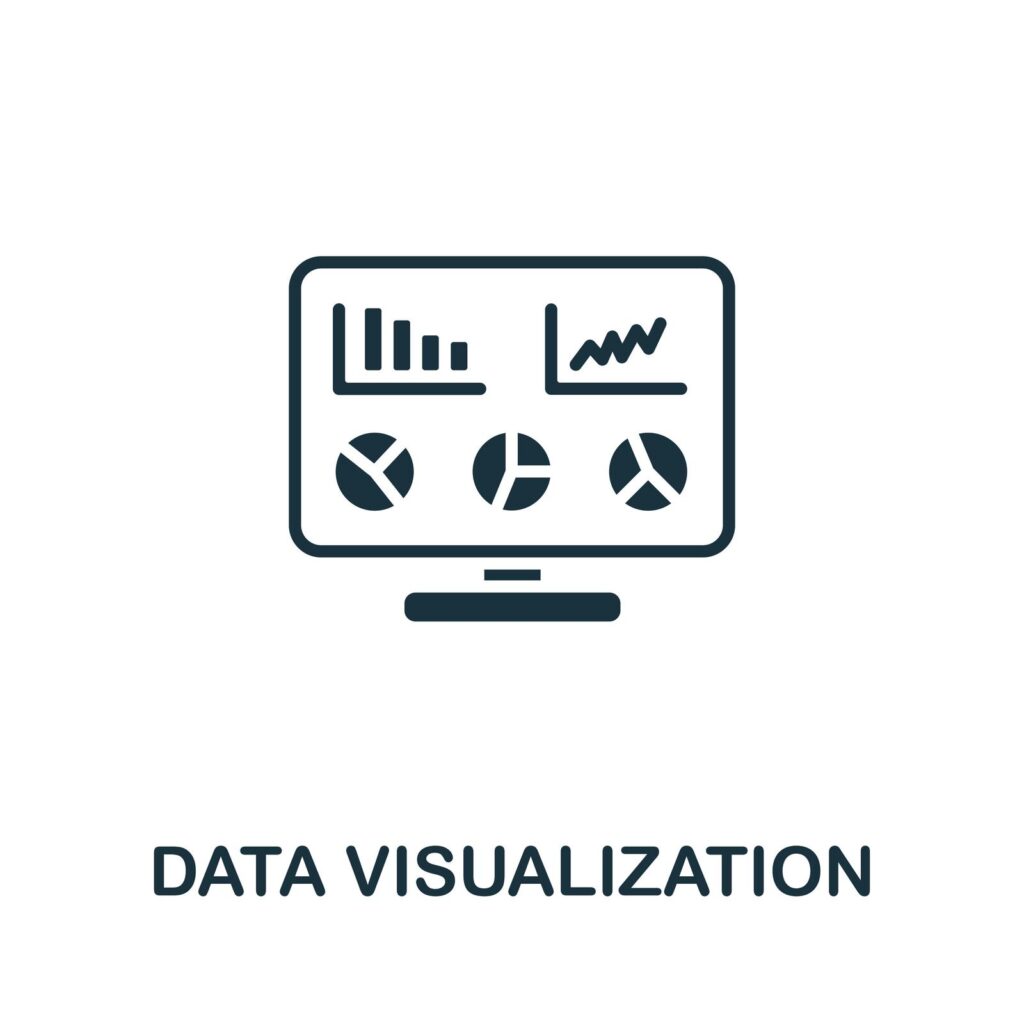
10.) Predictive Maintenance
Predictive maintenance is a hot topic in data science because it allows us to prevent failures before they happen.
This is done using data analytics to predict when a failure will occur.
This allows us to take corrective action before the failure actually happens.
While this sounds simple, avoiding false positives while keeping recall is challenging and an area wide open for advancement.
11.) Financial Analysis
Financial analysis is an older topic that has been around for a while but is still a great field where contributions can be felt.
Current researchers are focused on analyzing macroeconomic data to make better financial decisions.
This is done by analyzing the data to identify trends and patterns.
Financial analysts can use this information to make informed decisions about where to invest their money.
Financial analysis is also used to predict future economic trends.
This allows businesses and individuals to prepare for potential financial hardships and enable companies to be cash-heavy during good economic conditions.
Overall, financial analysis is a valuable tool for anyone looking to make better financial decisions.
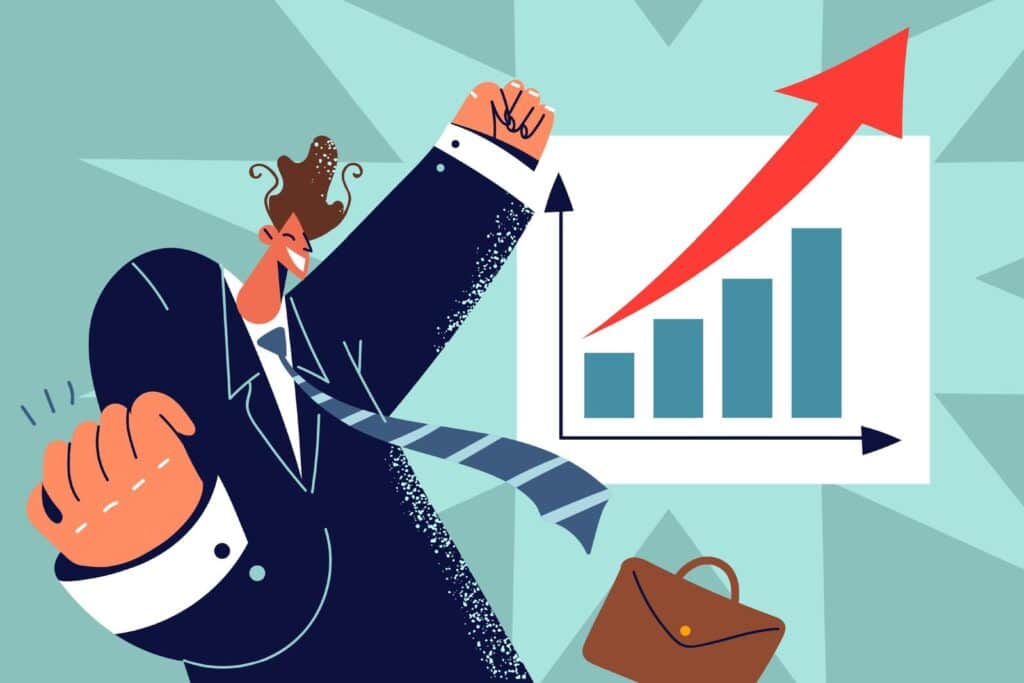
12.) Image Recognition
Image recognition is one of the hottest topics in data science because it allows us to identify objects in images.
This is done using artificial intelligence algorithms that can learn from data and understand what objects you’re looking for.
This allows us to build models that can accurately recognize objects in images and video.
This is a valuable tool for businesses and individuals who want to be able to identify objects in images.
Think about security, identification, routing, traffic, etc.
Image Recognition has gained a ton of momentum recently – for a good reason.
13.) Fraud Detection
Fraud detection is a great topic in data science because it allows us to identify fraudulent activity before it happens.
This is done by analyzing data to look for patterns and trends that may be associated with the fraud.
Once our machine learning model recognizes some of these patterns in real time, it immediately detects fraud.
This allows us to take corrective action before the fraud actually happens.
Fraud detection is a valuable tool for anyone who wants to protect themselves from potential fraudulent activity.
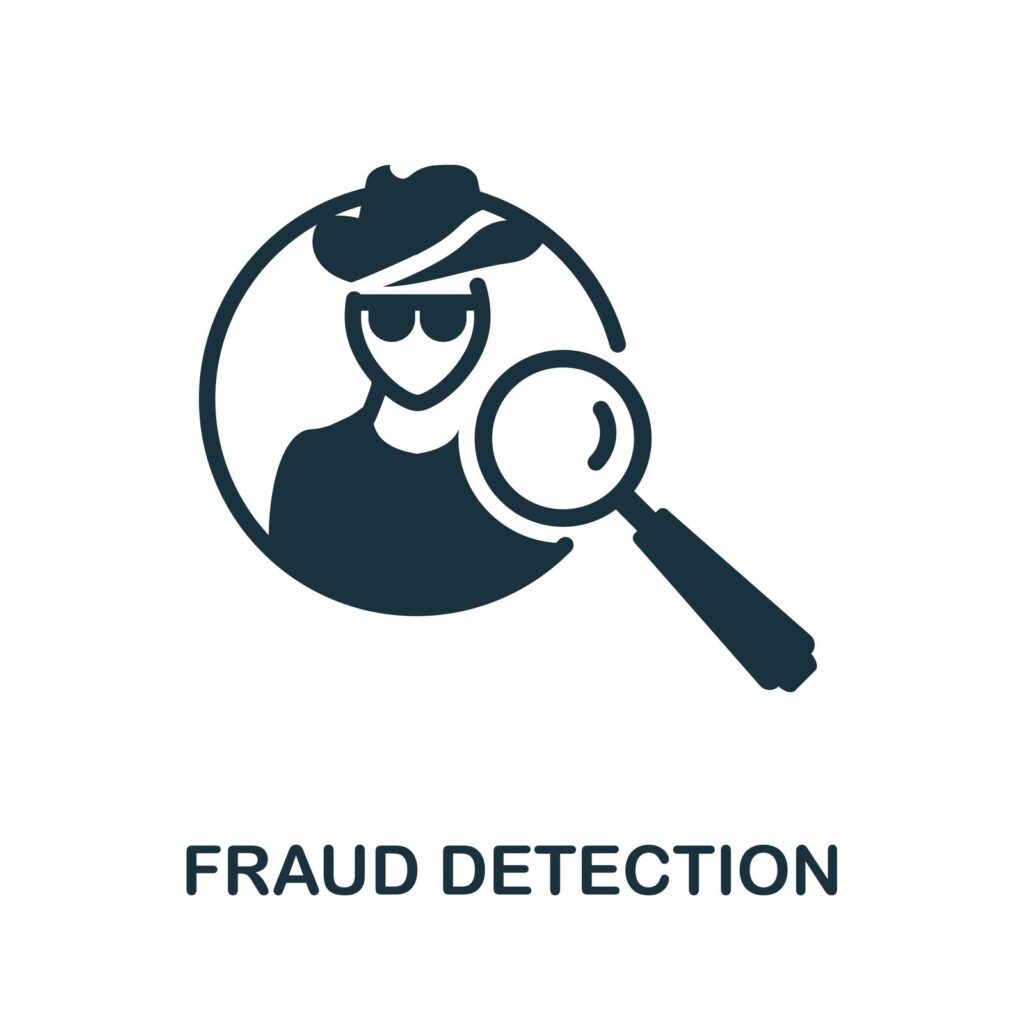
14.) Web Scraping
Web scraping is a controversial topic in data science because it allows us to collect data from the web, which is usually data you do not own.
This is done by extracting data from websites using scraping tools that are usually custom-programmed.
This allows us to collect data that would otherwise be inaccessible.
For obvious reasons, web scraping is a unique tool – giving you data your competitors would have no chance of getting.
I think there is an excellent opportunity to create new and innovative ways to make scraping accessible for everyone, not just those who understand Selenium and Beautiful Soup.
15.) Social Media Analysis
Social media analysis is not new; many people have already created exciting and innovative algorithms to study this.
However, it is still a great data science research topic because it allows us to understand how people interact on social media.
This is done by analyzing data from social media platforms to look for insights, bots, and recent societal trends.
Once we understand these practices, we can use this information to improve our marketing efforts.
For example, if we know that a particular demographic prefers a specific type of content, we can create more content that appeals to them.
Social media analysis is also used to understand how people interact with brands on social media.
This allows businesses to understand better what their customers want and need.
Overall, social media analysis is valuable for anyone who wants to improve their marketing efforts or understand how customers interact with brands.
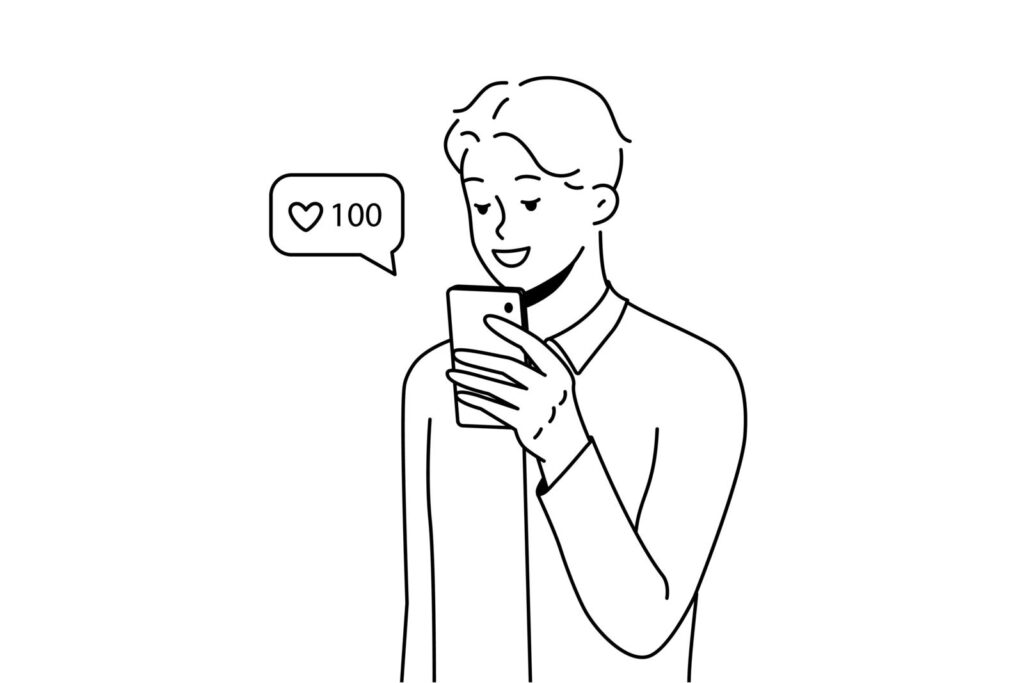
16.) GPU Computing
GPU computing is a fun new research topic in data science because it allows us to process data much faster than traditional CPUs .
Due to how GPUs are made, they’re incredibly proficient at intense matrix operations, outperforming traditional CPUs by very high margins.
While the computation is fast, the coding is still tricky.
There is an excellent research opportunity to bring these innovations to non-traditional modules, allowing data science to take advantage of GPU computing outside of deep learning.
17.) Quantum Computing
Quantum computing is a new research topic in data science and physics because it allows us to process data much faster than traditional computers.
It also opens the door to new types of data.
There are just some problems that can’t be solved utilizing outside of the classical computer.
For example, if you wanted to understand how a single atom moved around, a classical computer couldn’t handle this problem.
You’ll need to utilize a quantum computer to handle quantum mechanics problems.
This may be the “hottest” research topic on the planet right now, with some of the top researchers in computer science and physics worldwide working on it.
You could be too.
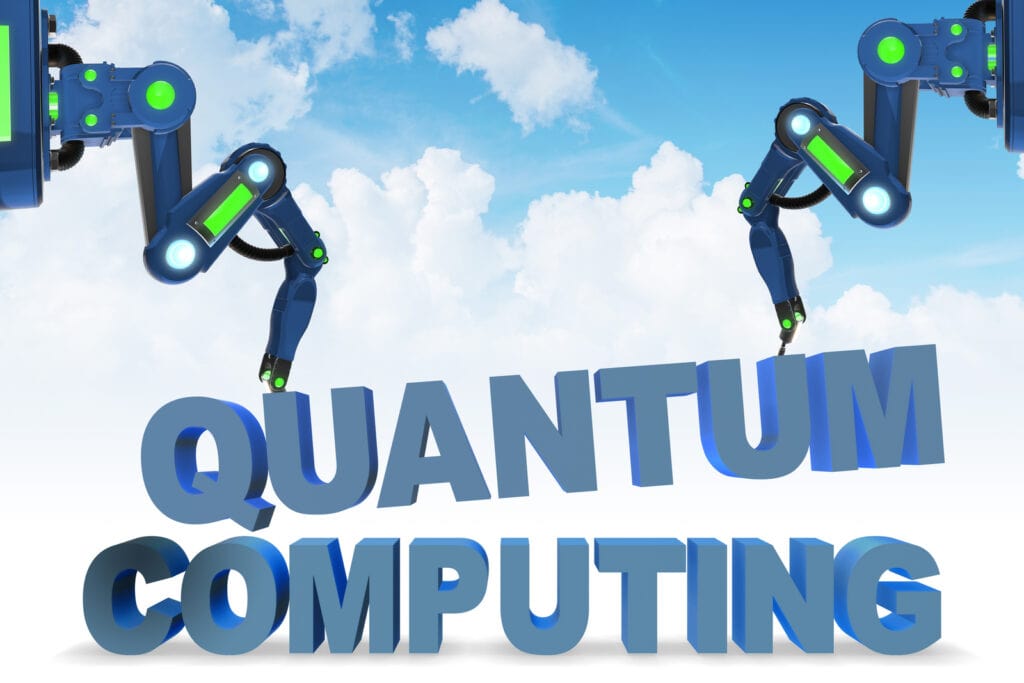
18.) Genomics
Genomics may be the only research topic that can compete with quantum computing regarding the “number of top researchers working on it.”
Genomics is a fantastic intersection of data science because it allows us to understand how genes work.
This is done by sequencing the DNA of different organisms to look for insights into our and other species.
Once we understand these patterns, we can use this information to improve our understanding of diseases and create new and innovative treatments for them.
Genomics is also used to study the evolution of different species.
Genomics is the future and a field begging for new and exciting research professionals to take it to the next step.
19.) Location-based services
Location-based services are an old and time-tested research topic in data science.
Since GPS and 4g cell phone reception became a thing, we’ve been trying to stay informed about how humans interact with their environment.
This is done by analyzing data from GPS tracking devices, cell phone towers, and Wi-Fi routers to look for insights into how humans interact.
Once we understand these practices, we can use this information to improve our geotargeting efforts, improve maps, find faster routes, and improve cohesion throughout a community.
Location-based services are used to understand the user, something every business could always use a little bit more of.
While a seemingly “stale” field, location-based services have seen a revival period with self-driving cars.
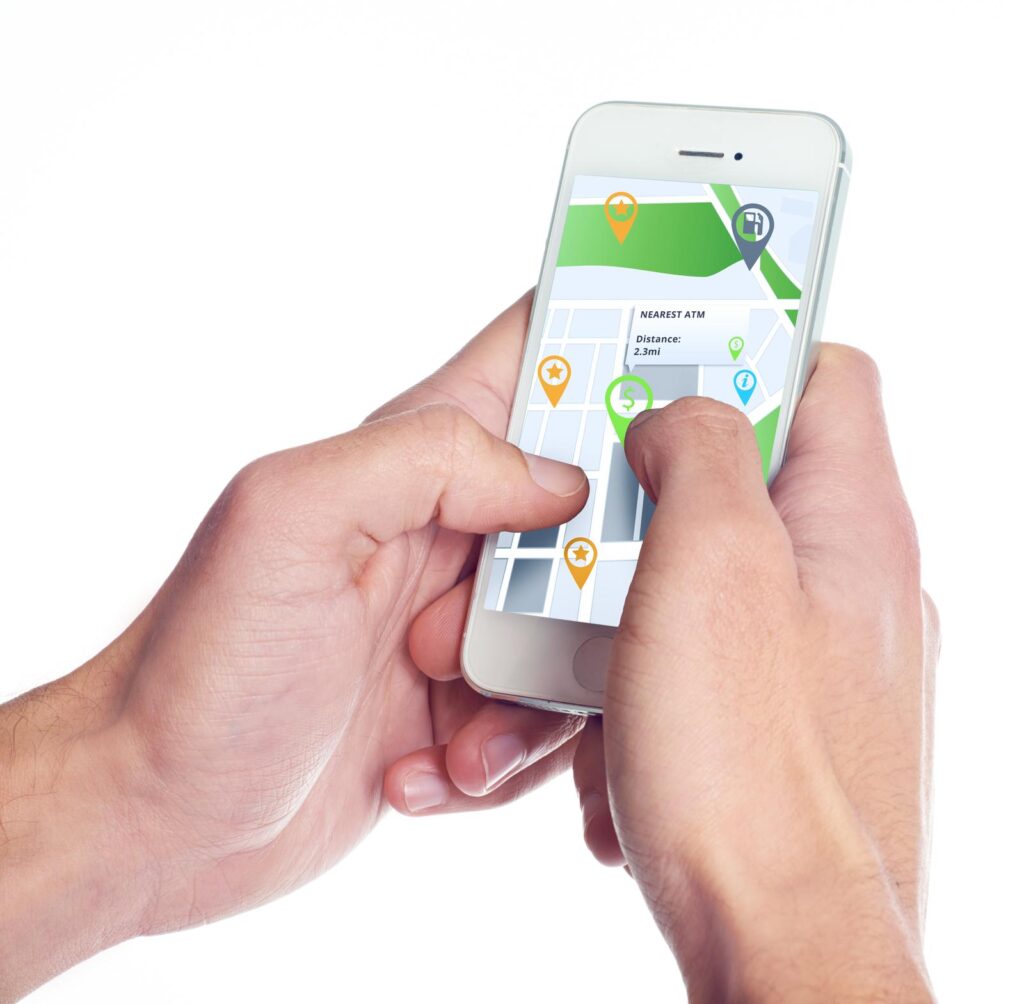
20.) Smart City Applications
Smart city applications are all the rage in data science research right now.
By harnessing the power of data, cities can become more efficient and sustainable.
But what exactly are smart city applications?
In short, they are systems that use data to improve city infrastructure and services.
This can include anything from traffic management and energy use to waste management and public safety.
Data is collected from various sources, including sensors, cameras, and social media.
It is then analyzed to identify tendencies and habits.
This information can make predictions about future needs and optimize city resources.
As more and more cities strive to become “smart,” the demand for data scientists with expertise in smart city applications is only growing.
21.) Internet Of Things (IoT)
The Internet of Things, or IoT, is exciting and new data science and sustainability research topic.
IoT is a network of physical objects embedded with sensors and connected to the internet.
These objects can include everything from alarm clocks to refrigerators; they’re all connected to the internet.
That means that they can share data with computers.
And that’s where data science comes in.
Data scientists are using IoT data to learn everything from how people use energy to how traffic flows through a city.
They’re also using IoT data to predict when an appliance will break down or when a road will be congested.
Really, the possibilities are endless.
With such a wide-open field, it’s easy to see why IoT is being researched by some of the top professionals in the world.
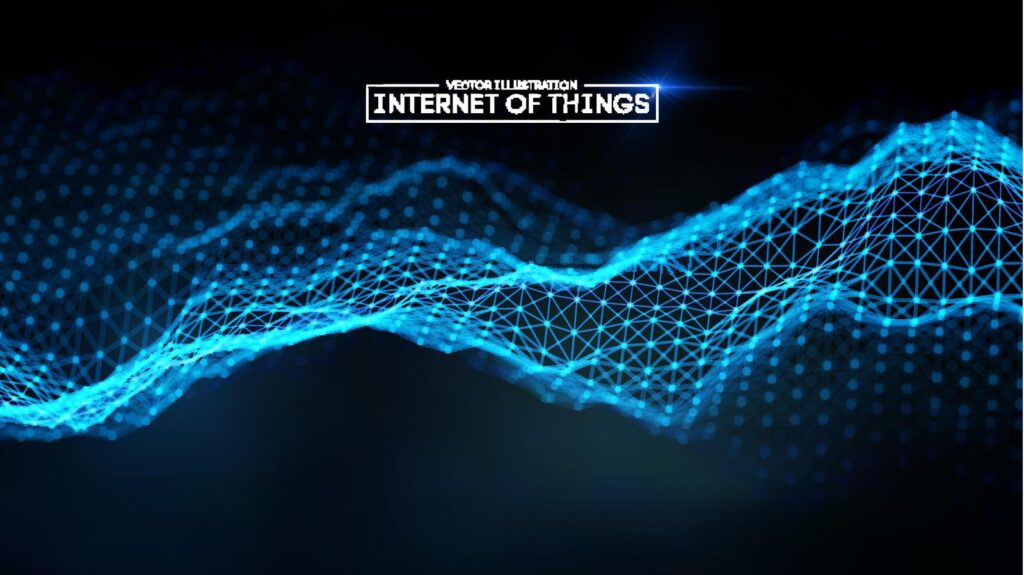
22.) Cybersecurity
Cybersecurity is a relatively new research topic in data science and in general, but it’s already garnering a lot of attention from businesses and organizations.
After all, with the increasing number of cyber attacks in recent years, it’s clear that we need to find better ways to protect our data.
While most of cybersecurity focuses on infrastructure, data scientists can leverage historical events to find potential exploits to protect their companies.
Sometimes, looking at a problem from a different angle helps, and that’s what data science brings to cybersecurity.
Also, data science can help to develop new security technologies and protocols.
As a result, cybersecurity is a crucial data science research area and one that will only become more important in the years to come.
23.) Blockchain
Blockchain is an incredible new research topic in data science for several reasons.
First, it is a distributed database technology that enables secure, transparent, and tamper-proof transactions.
Did someone say transmitting data?
This makes it an ideal platform for tracking data and transactions in various industries.
Second, blockchain is powered by cryptography, which not only makes it highly secure – but is a familiar foe for data scientists.
Finally, blockchain is still in its early stages of development, so there is much room for research and innovation.
As a result, blockchain is a great new research topic in data science that vows to revolutionize how we store, transmit and manage data.
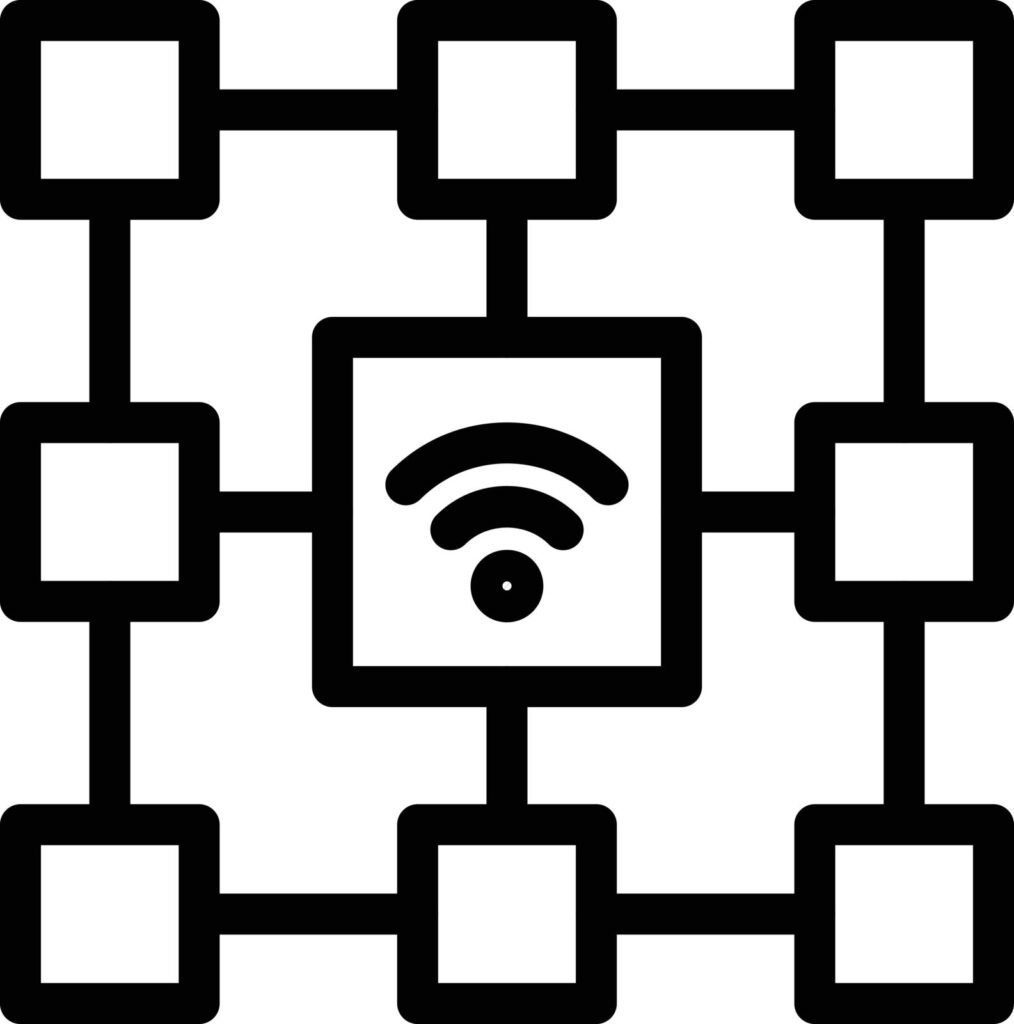
24.) Sustainability
Sustainability is a relatively new research topic in data science, but it is gaining traction quickly.
To keep up with this demand, The Wharton School of the University of Pennsylvania has started to offer an MBA in Sustainability .
This demand isn’t shocking, and some of the reasons include the following:
Sustainability is an important issue that is relevant to everyone.
Datasets on sustainability are constantly growing and changing, making it an exciting challenge for data scientists.
There hasn’t been a “set way” to approach sustainability from a data perspective, making it an excellent opportunity for interdisciplinary research.
As data science grows, sustainability will likely become an increasingly important research topic.
25.) Educational Data
Education has always been a great topic for research, and with the advent of big data, educational data has become an even richer source of information.
By studying educational data, researchers can gain insights into how students learn, what motivates them, and what barriers these students may face.
Besides, data science can be used to develop educational interventions tailored to individual students’ needs.
Imagine being the researcher that helps that high schooler pass mathematics; what an incredible feeling.
With the increasing availability of educational data, data science has enormous potential to improve the quality of education.
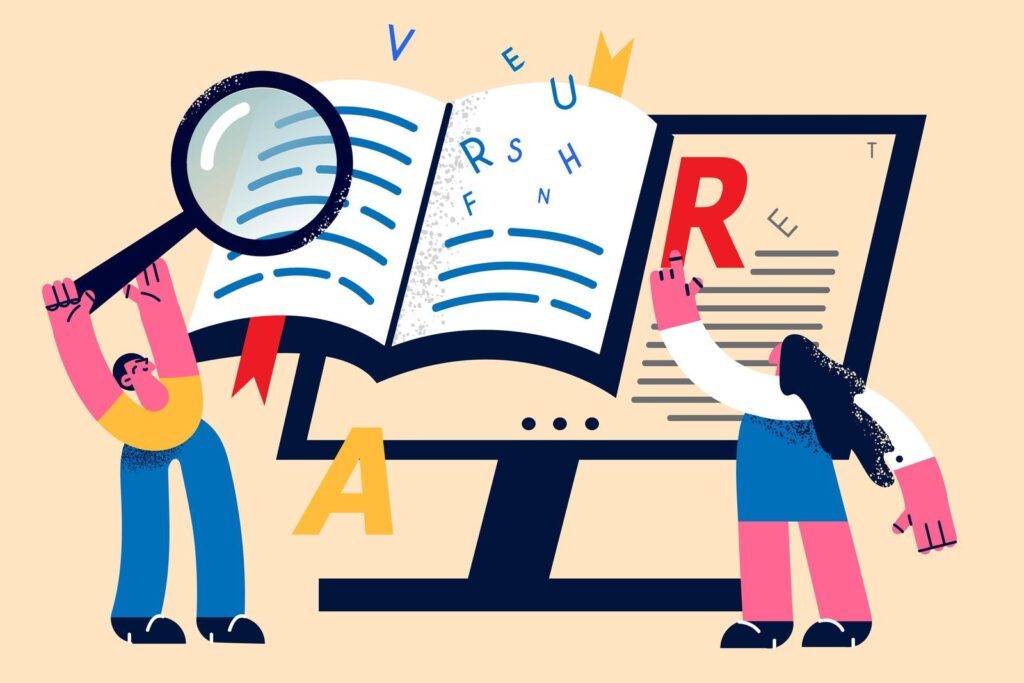
26.) Politics
As data science continues to evolve, so does the scope of its applications.
Originally used primarily for business intelligence and marketing, data science is now applied to various fields, including politics.
By analyzing large data sets, political scientists (data scientists with a cooler name) can gain valuable insights into voting patterns, campaign strategies, and more.
Further, data science can be used to forecast election results and understand the effects of political events on public opinion.
With the wealth of data available, there is no shortage of research opportunities in this field.
As data science evolves, so does our understanding of politics and its role in our world.
27.) Cloud Technologies
Cloud technologies are a great research topic.
It allows for the outsourcing and sharing of computer resources and applications all over the internet.
This lets organizations save money on hardware and maintenance costs while providing employees access to the latest and greatest software and applications.
I believe there is an argument that AWS could be the greatest and most technologically advanced business ever built (Yes, I know it’s only part of the company).
Besides, cloud technologies can help improve team members’ collaboration by allowing them to share files and work on projects together in real-time.
As more businesses adopt cloud technologies, data scientists must stay up-to-date on the latest trends in this area.
By researching cloud technologies, data scientists can help organizations to make the most of this new and exciting technology.
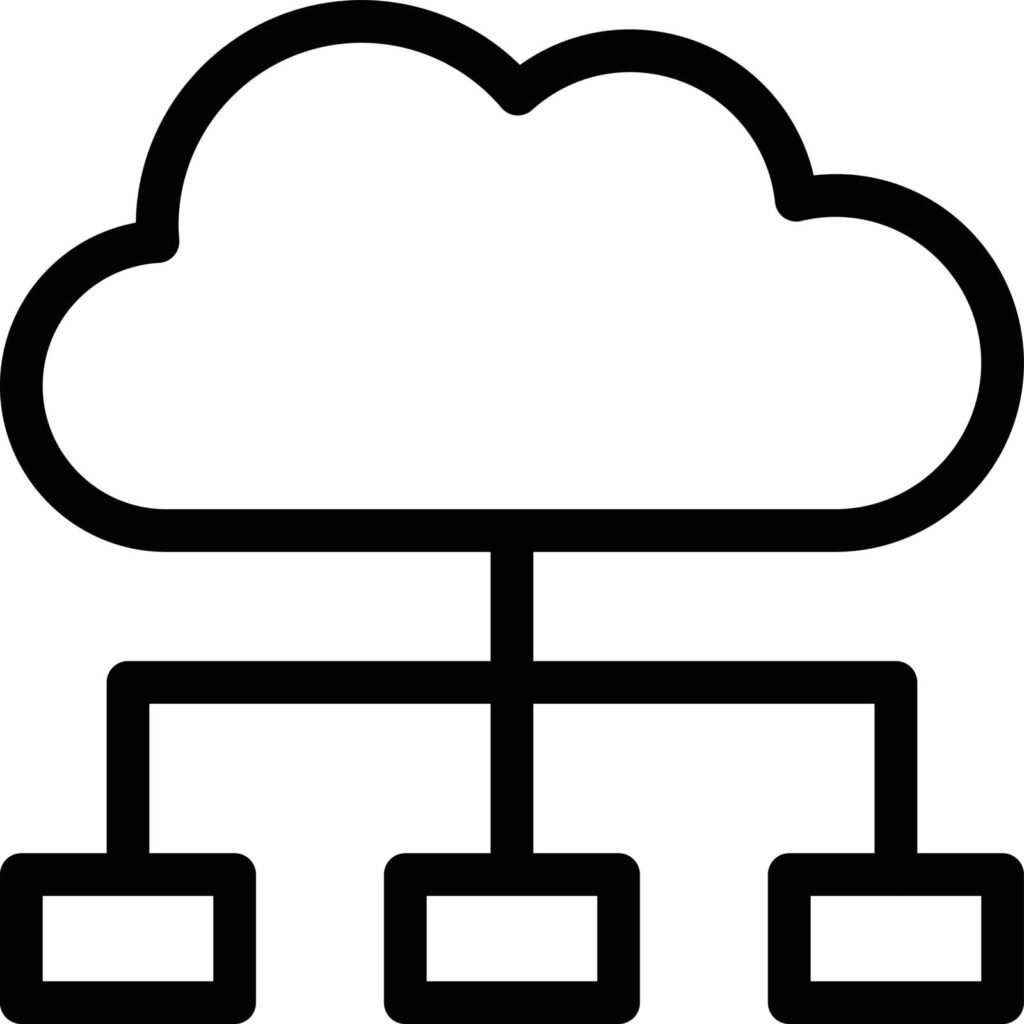
28.) Robotics
Robotics has recently become a household name, and it’s for a good reason.
First, robotics deals with controlling and planning physical systems, an inherently complex problem.
Second, robotics requires various sensors and actuators to interact with the world, making it an ideal application for machine learning techniques.
Finally, robotics is an interdisciplinary field that draws on various disciplines, such as computer science, mechanical engineering, and electrical engineering.
As a result, robotics is a rich source of research problems for data scientists.
29.) HealthCare
Healthcare is an industry that is ripe for data-driven innovation.
Hospitals, clinics, and health insurance companies generate a tremendous amount of data daily.
This data can be used to improve the quality of care and outcomes for patients.
This is perfect timing, as the healthcare industry is undergoing a significant shift towards value-based care, which means there is a greater need than ever for data-driven decision-making.
As a result, healthcare is an exciting new research topic for data scientists.
There are many different ways in which data can be used to improve healthcare, and there is a ton of room for newcomers to make discoveries.
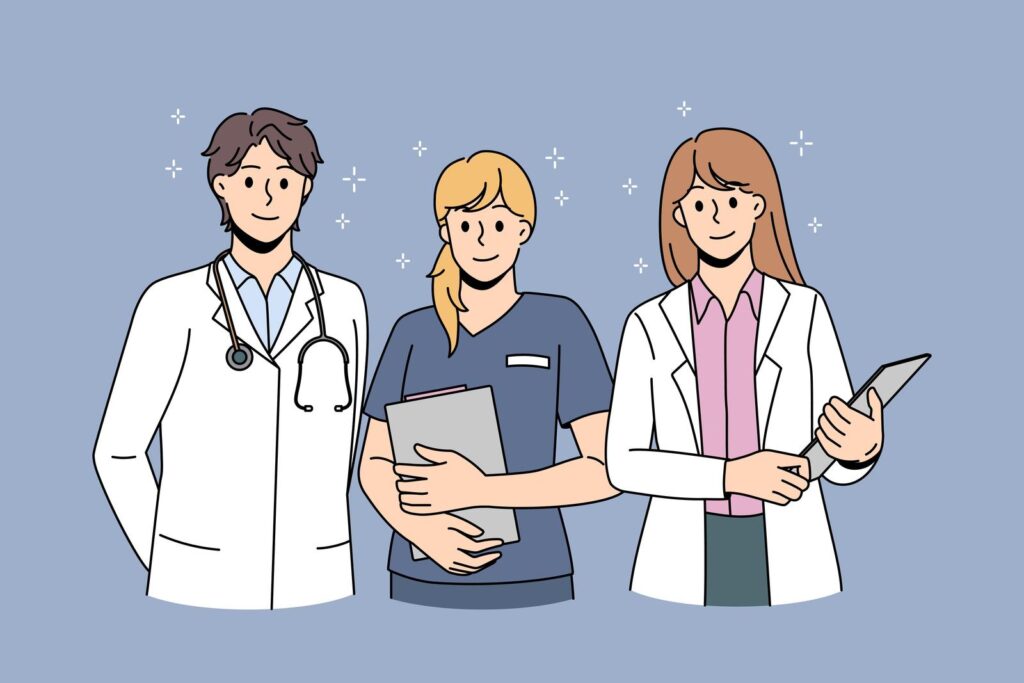
30.) Remote Work
There’s no doubt that remote work is on the rise.
In today’s global economy, more and more businesses are allowing their employees to work from home or anywhere else they can get a stable internet connection.
But what does this mean for data science? Well, for one thing, it opens up a whole new field of research.
For example, how does remote work impact employee productivity?
What are the best ways to manage and collaborate on data science projects when team members are spread across the globe?
And what are the cybersecurity risks associated with working remotely?
These are just a few of the questions that data scientists will be able to answer with further research.
So if you’re looking for a new topic to sink your teeth into, remote work in data science is a great option.
31.) Data-Driven Journalism
Data-driven journalism is an exciting new field of research that combines the best of both worlds: the rigor of data science with the creativity of journalism.
By applying data analytics to large datasets, journalists can uncover stories that would otherwise be hidden.
And telling these stories compellingly can help people better understand the world around them.
Data-driven journalism is still in its infancy, but it has already had a major impact on how news is reported.
In the future, it will only become more important as data becomes increasingly fluid among journalists.
It is an exciting new topic and research field for data scientists to explore.
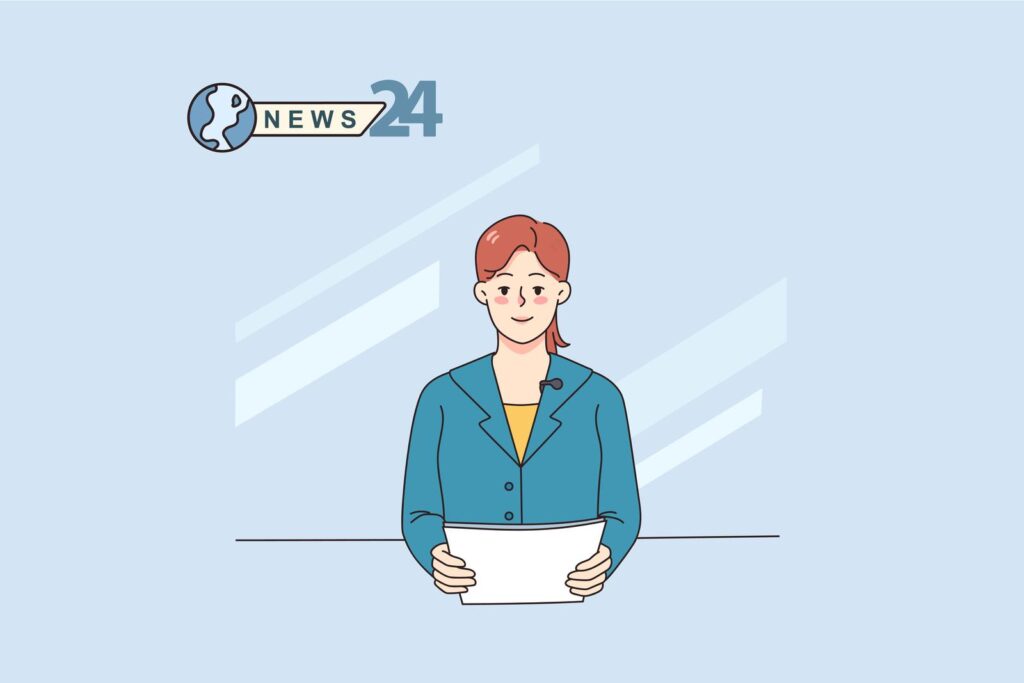
32.) Data Engineering
Data engineering is a staple in data science, focusing on efficiently managing data.
Data engineers are responsible for developing and maintaining the systems that collect, process, and store data.
In recent years, there has been an increasing demand for data engineers as the volume of data generated by businesses and organizations has grown exponentially.
Data engineers must be able to design and implement efficient data-processing pipelines and have the skills to optimize and troubleshoot existing systems.
If you are looking for a challenging research topic that would immediately impact you worldwide, then improving or innovating a new approach in data engineering would be a good start.
33.) Data Curation
Data curation has been a hot topic in the data science community for some time now.
Curating data involves organizing, managing, and preserving data so researchers can use it.
Data curation can help to ensure that data is accurate, reliable, and accessible.
It can also help to prevent research duplication and to facilitate the sharing of data between researchers.
Data curation is a vital part of data science. In recent years, there has been an increasing focus on data curation, as it has become clear that it is essential for ensuring data quality.
As a result, data curation is now a major research topic in data science.
There are numerous books and articles on the subject, and many universities offer courses on data curation.
Data curation is an integral part of data science and will only become more important in the future.
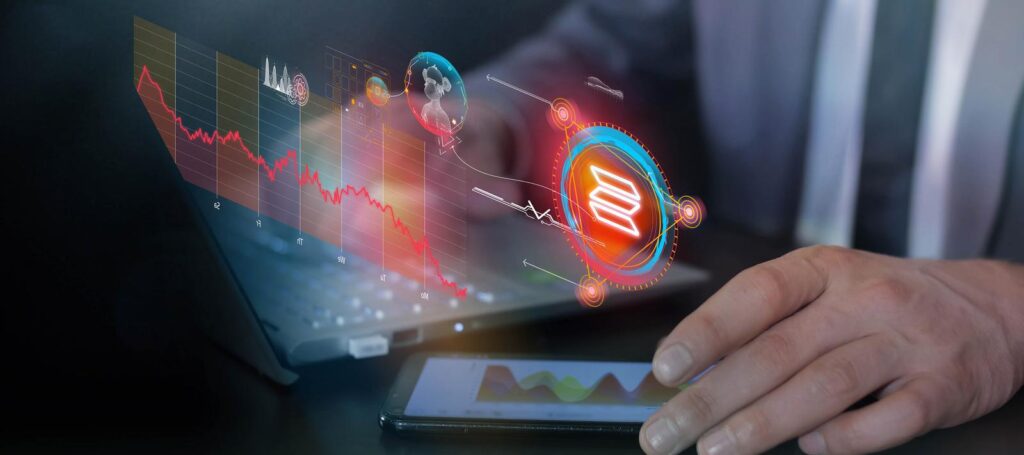
34.) Meta-Learning
Meta-learning is gaining a ton of steam in data science. It’s learning how to learn.
So, if you can learn how to learn, you can learn anything much faster.
Meta-learning is mainly used in deep learning, as applications outside of this are generally pretty hard.
In deep learning, many parameters need to be tuned for a good model, and there’s usually a lot of data.
You can save time and effort if you can automatically and quickly do this tuning.
In machine learning, meta-learning can improve models’ performance by sharing knowledge between different models.
For example, if you have a bunch of different models that all solve the same problem, then you can use meta-learning to share the knowledge between them to improve the cluster (groups) overall performance.
I don’t know how anyone looking for a research topic could stay away from this field; it’s what the Terminator warned us about!
35.) Data Warehousing
A data warehouse is a system used for data analysis and reporting.
It is a central data repository created by combining data from multiple sources.
Data warehouses are often used to store historical data, such as sales data, financial data, and customer data.
This data type can be used to create reports and perform statistical analysis.
Data warehouses also store data that the organization is not currently using.
This type of data can be used for future research projects.
Data warehousing is an incredible research topic in data science because it offers a variety of benefits.
Data warehouses help organizations to save time and money by reducing the need for manual data entry.
They also help to improve the accuracy of reports and provide a complete picture of the organization’s performance.
Data warehousing feels like one of the weakest parts of the Data Science Technology Stack; if you want a research topic that could have a monumental impact – data warehousing is an excellent place to look.
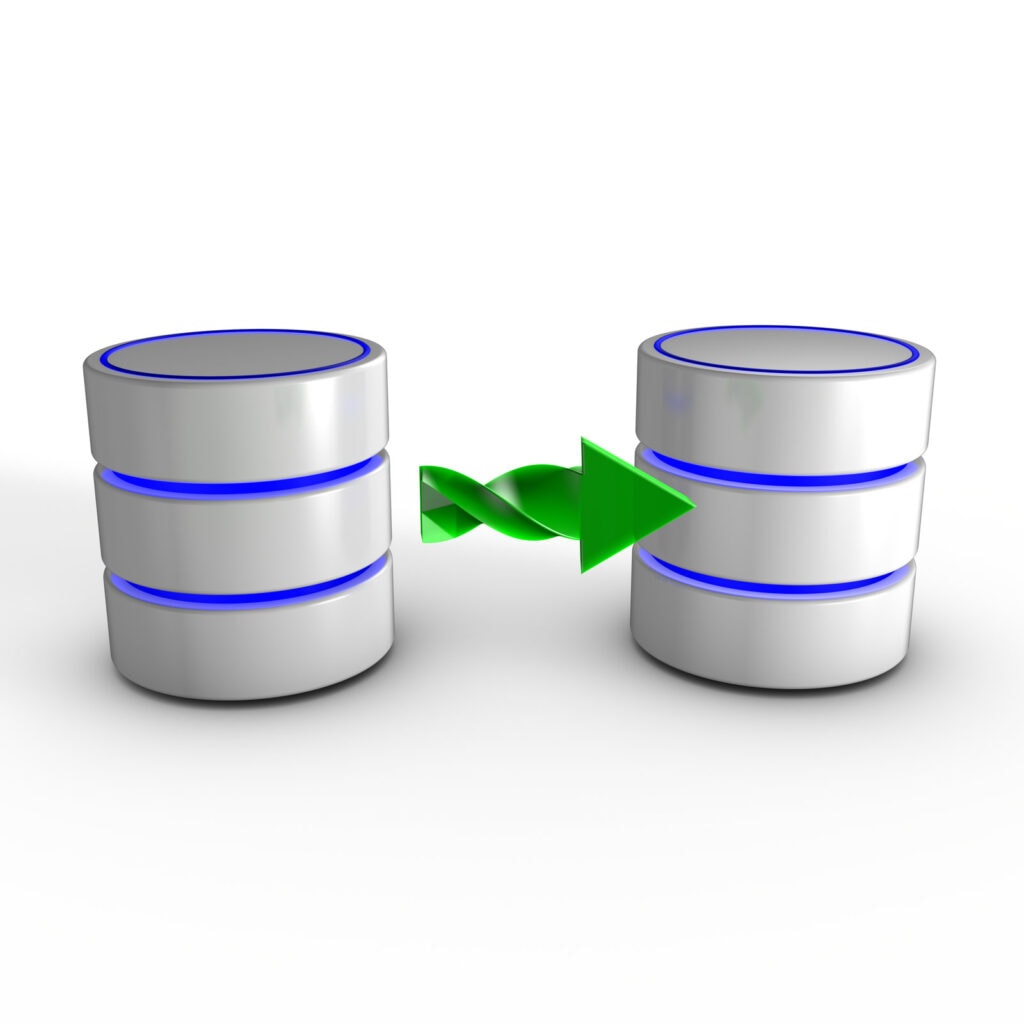
36.) Business Intelligence
Business intelligence aims to collect, process, and analyze data to help businesses make better decisions.
Business intelligence can improve marketing, sales, customer service, and operations.
It can also be used to identify new business opportunities and track competition.
BI is business and another tool in your company’s toolbox to continue dominating your area.
Data science is the perfect tool for business intelligence because it combines statistics, computer science, and machine learning.
Data scientists can use business intelligence to answer questions like, “What are our customers buying?” or “What are our competitors doing?” or “How can we increase sales?”
Business intelligence is a great way to improve your business’s bottom line and an excellent opportunity to dive deep into a well-respected research topic.
37.) Crowdsourcing
One of the newest areas of research in data science is crowdsourcing.
Crowdsourcing is a process of sourcing tasks or projects to a large group of people, typically via the internet.
This can be done for various purposes, such as gathering data, developing new algorithms, or even just for fun (think: online quizzes and surveys).
But what makes crowdsourcing so powerful is that it allows businesses and organizations to tap into a vast pool of talent and resources they wouldn’t otherwise have access to.
And with the rise of social media, it’s easier than ever to connect with potential crowdsource workers worldwide.
Imagine if you could effect that, finding innovative ways to improve how people work together.
That would have a huge effect.
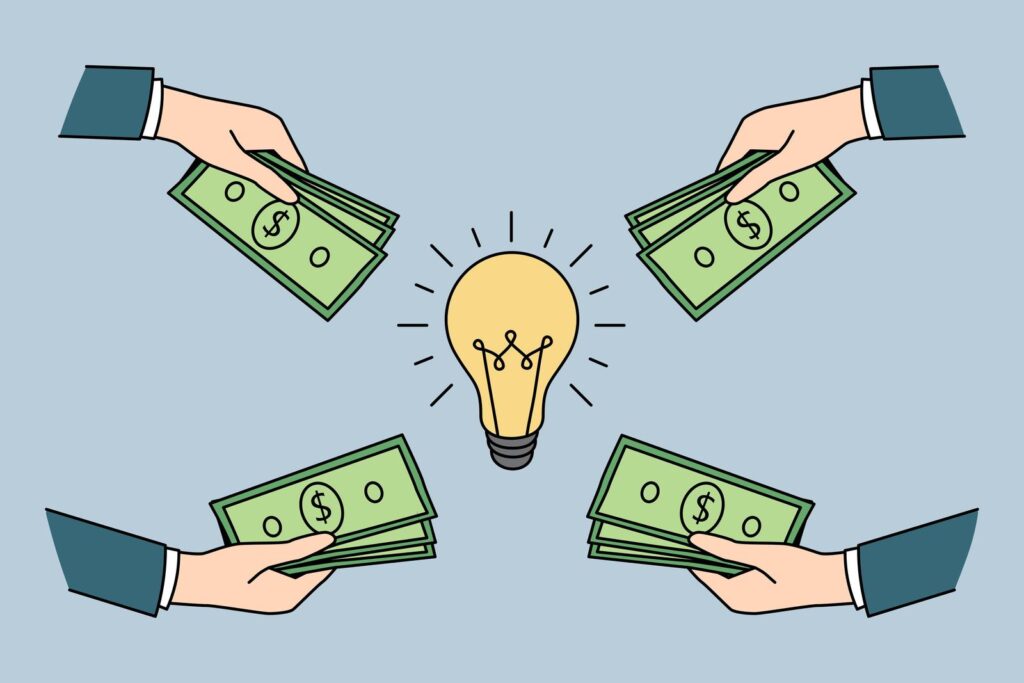
Final Thoughts, Are These Research Topics In Data Science For You?
Thirty-seven different research topics in data science are a lot to take in, but we hope you found a research topic that interests you.
If not, don’t worry – there are plenty of other great topics to explore.
The important thing is to get started with your research and find ways to apply what you learn to real-world problems.
We wish you the best of luck as you begin your data science journey!
Other Data Science Articles
We love talking about data science; here are a couple of our favorite articles:
- Why Are You Interested In Data Science?
- Recent Posts
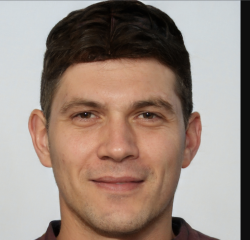
- Unlocking the Power of Line Graphs in Machine Learning [Boost Your Predictions] - May 31, 2024
- Software Engineer Salary at Wells Fargo Charlotte [Revealed Inside] - May 31, 2024
- Do I have to use AMD Radeon software? [Find Out Why It’s Essential] - May 31, 2024

Research Areas
Main navigation.
The world is being transformed by data and data-driven analysis is rapidly becoming an integral part of science and society. Stanford Data Science is a collaborative effort across many departments in all seven schools. We strive to unite existing data science research initiatives and create interdisciplinary collaborations, connecting the data science and related methodologists with disciplines that are being transformed by data science and computation.
Our work supports research in a variety of fields where incredible advances are being made through the facilitation of meaningful collaborations between domain researchers, with deep expertise in societal and fundamental research challenges, and methods researchers that are developing next-generation computational tools and techniques, including:
Data Science for Wildland Fire Research
In recent years, wildfire has gone from an infrequent and distant news item to a centerstage isssue spanning many consecutive weeks for urban and suburban communities. Frequent wildfires are changing everyday lives for California in numerous ways -- from public safety power shutoffs to hazardous air quality -- that seemed inconceivable as recently as 2015. Moreover, elevated wildfire risk in the western United States (and similar climates globally) is here to stay into the foreseeable future. There is a plethora of problems that need solutions in the wildland fire arena; many of them are well suited to a data-driven approach.
Seminar Series
Data Science for Physics
Astrophysicists and particle physicists at Stanford and at the SLAC National Accelerator Laboratory are deeply engaged in studying the Universe at both the largest and smallest scales, with state-of-the-art instrumentation at telescopes and accelerator facilities
Data Science for Economics
Many of the most pressing questions in empirical economics concern causal questions, such as the impact, both short and long run, of educational choices on labor market outcomes, and of economic policies on distributions of outcomes. This makes them conceptually quite different from the predictive type of questions that many of the recently developed methods in machine learning are primarily designed for.
Data Science for Education
Educational data spans K-12 school and district records, digital archives of instructional materials and gradebooks, as well as student responses on course surveys. Data science of actual classroom interaction is also of increasing interest and reality.
Data Science for Human Health
It is clear that data science will be a driving force in transitioning the world’s healthcare systems from reactive “sick-based” care to proactive, preventive care.
Data Science for Humanity
Our modern era is characterized by massive amounts of data documenting the behaviors of individuals, groups, organizations, cultures, and indeed entire societies. This wealth of data on modern humanity is accompanied by massive digitization of historical data, both textual and numeric, in the form of historic newspapers, literary and linguistic corpora, economic data, censuses, and other government data, gathered and preserved over centuries, and newly digitized, acquired, and provisioned by libraries, scholars, and commercial entities.
Data Science for Linguistics
The impact of data science on linguistics has been profound. All areas of the field depend on having a rich picture of the true range of variation, within dialects, across dialects, and among different languages. The subfield of corpus linguistics is arguably as old as the field itself and, with the advent of computers, gave rise to many core techniques in data science.
Data Science for Nature and Sustainability
Many key sustainability issues translate into decision and optimization problems and could greatly benefit from data-driven decision making tools. In fact, the impact of modern information technology has been highly uneven, mainly benefiting large firms in profitable sectors, with little or no benefit in terms of the environment. Our vision is that data-driven methods can — and should — play a key role in increasing the efficiency and effectiveness of the way we manage and allocate our natural resources.
Ethics and Data Science
With the emergence of new techniques of machine learning, and the possibility of using algorithms to perform tasks previously done by human beings, as well as to generate new knowledge, we again face a set of new ethical questions.
The Science of Data Science
The practice of data analysis has changed enormously. Data science needs to find new inferential paradigms that allow data exploration prior to the formulation of hypotheses.
Emerging trends and global scope of big data analytics: a scientometric analysis
- Published: 27 October 2020
- Volume 55 , pages 1371–1396, ( 2021 )
Cite this article
- Keshav Singh Rawat ORCID: orcid.org/0000-0002-1497-985X 1 &
- Sandeep Kumar Sood 1
1151 Accesses
22 Citations
Explore all metrics
The primary sources of big data nowadays are from cloud computing, social networks, and the internet of things, and henceforth the data analytics has gained popularity these days, with the increasing demand for these technologies. This study presents scientometric analysis to identify overall growth, emerging trends, and global scope of data analytics research during 2010–2019. This study uses a bibliometric database retrieved from the Scopus in CSV files that contain bibliographic information. This study provides a detailed look of bibliometric features of Scopus indexed documents and analyses bibliometric networks to identify the hidden information from the downloaded dataset. This study focuses on the research publication growth, subject categories, geographical distribution, citation, and productivity parameters of bibliometric data. Furthermore, it identifies significant major contributors, highly cited publications, prominent journals, influential institutes, and research collaborations. This study also reveals research frontiers,and hotspots of data analytics research by analyzing keyword co-occurrence using VOSviewer. The outcomes of this study present the applications, emerging trends, and global research landscape over the last decade that help to understand fundamental research and the directions of future research in this field.
This is a preview of subscription content, log in via an institution to check access.
Access this article
Price includes VAT (Russian Federation)
Instant access to the full article PDF.
Rent this article via DeepDyve
Institutional subscriptions
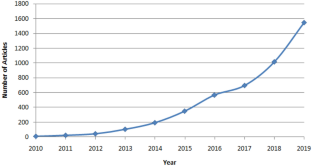
Similar content being viewed by others
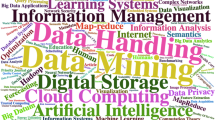
A decade of big data literature: analysis of trends in light of bibliometrics
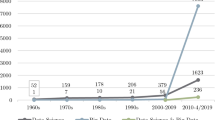
The evolution of data science and big data research: A bibliometric analysis
A bibliometric approach to tracking big data research trends.
Ahmed, E., Yaqoob, I., Hashem, I., Khan, I., Ahmed, A., Imran, M., Vasilakos, A.: The role of big data analytics in internet of things. Comput. Netw. 129 (2), 459–471 (2017). https://doi.org/10.1016/j.comnet.2017.06.013
Article Google Scholar
Al-Fuqaha, A., guizani, m, Mohammadi, M., Aledhari, M., Ayyash, M.: Internet of things: a survey on enabling technologies, protocols, and applications. IEEE Commun. Surv. Tutor. 17 (4), 2347–2376 (2015). https://doi.org/10.1109/COMST.2015.2444095
Aldowah, H., Al-Samarraie, H., Fauzy, W.M.: Educational data mining and learning analytics for 21st century higher education: A review and synthesis. Telematics Inform. 37 , 13–49 (2019). https://doi.org/10.1016/j.tele.2019.01.007
Alexandrov, A., Bergmann, R., Ewen, S., Freytag, J.C., Hueske, F., Heise, A., Kao, O., Leich, M., Leser, U., Markl, V., Naumann, F., Peters, M., Rheinlander, A., Sax, M.J., Schelter, S., Hoger, M., Tzoumas, K., Warneke, D.: The stratosphere platform for big data analytics. VLDB J. 23 (6), 939–964 (2014). https://doi.org/10.1007/s00778-014-0357-y
Attaran, M., Stark, J., Stotler, D.: Opportunities and challenges for big data analytics in us higher education: A conceptual model for implementation. Ind. Higher Educ. 32 (3), 169–182 (2018). https://doi.org/10.1177/0950422218770937
Baker, R.S., Inventado, P.S.: Educational Data Mining and Learning Analytics, pp. 61–75. Springer New York (2014). https://doi.org/10.1007/978-1-4614-3305-7_4
Banerjee, A., Chakraborty, C., Kumar, A., Biswas, D.: Emerging trends in iot and big data analytics for biomedical and health care technologies. In: Handbook of Data Science Approaches for Biomedical Engineering, chap. 5, pp. 121 – 152. Academic Press (2020). https://doi.org/10.1016/B978-0-12-818318-2.00005-2
Batrinca, B., Treleaven, P.C.: Social media analytics: a survey of techniques, tools and platforms. AI Soc. 30 , 89–116 (2015). https://doi.org/10.1007/s00146-014-0549-4
Biljecki, F.: A scientometric analysis of selected giscience journals. Int. J. Geogr. Inf. Sci. 30 (7), 1302–1335 (2016). https://doi.org/10.1080/13658816.2015.1130831
Botta, A., de Donato, W., Persico, V., Pescape, A.: Integration of cloud computing and internet of things: a survey. Future Gener. Comput. Syst. 56 , 684–700 (2016). https://doi.org/10.1016/j.future.2015.09.021
Boyd, D., Crawford, K.: Critical questions for big data. Inf. Commun. Soc. 15 (5), 662–679 (2012). https://doi.org/10.1080/1369118X.2012.678878
Chadegani, A.A., Salehi, H., Yunus, M.M., Farhadi, H., Fooladi, M., Farhadi, M., Ebrahim, N.A.: A comparison between two main academic literature collections: Web of science and scopus databases. Asian Soc. Sci. 9 , 18–26 (2013). https://doi.org/10.5539/ass.v9n5p18
Chen, H., Chiang, R.H.L., Storey, V.C.: Business intelligence and analytics: From big data to big impact. MIS Q. 36 (4), 1165–1188 (2012). https://doi.org/10.2307/41703503
Cobo, M., Lopez-Herrera, A.G., Herrera-Viedma, E., Herrera, F.: Scimat: A new science mapping analysis software tool. J. Am. Soc. Inform. Sci. Technol. 63 , 1609–1630 (2012). https://doi.org/10.1002/asi.22688
Dash, S., Shakyawar, S., Sharma, M., Kaushik, S.: Big data in healthcare: management, analysis and future prospects. J. Big Data. (2019). https://doi.org/10.1186/s40537-019-0217-0
Erevelles, S., Fukawa, N., Swayne, L.: Big data consumer analytics and the transformation of marketing. J. Bus. Res. 69 (2), 897–904 (2016). https://doi.org/10.1016/j.jbusres.2015.07.001
Fayyad, U.M., Piatetsky-Shapiro, G., Smyth, P.: From data mining to knowledge discovery in databases. AI Magaz. 17 (3), 37–54 (1996). https://doi.org/10.1609/aimag.v17i3.1230
Galetsi, P., Katsaliaki, K.: A review of the literature on big data analytics in healthcare. J. Oper. Res. Soc. (2019). https://doi.org/10.1080/01605682.2019.1630328
Gandomi, A., Haider, M.: Beyond the hype: Big data concepts, methods, and analytics. Int. J. Inf. Manage. 35 (2), 137–144 (2015). https://doi.org/10.1016/j.ijinfomgt.2014.10.007
Ge, M., Bangui, H., Buhnova, B.: Big data for internet of things: a survey. Future Gener. Comput. Syst. 87 , 601–614 (2018). https://doi.org/10.1016/j.future.2018.04.053
Ge, Z., Song, Z., Ding, S.X., Huang, B.: Data mining and analytics in the process industry: The role of machine learning. IEEE Access 5 , 20590–20616 (2017). https://doi.org/10.1109/ACCESS.2017.2756872
Ghani, N., Hamid, S., Hashem, I., Ahmed, E.: Social media big data analytics: a survey. Comput. Human Behav. (2019). https://doi.org/10.1016/j.chb.2018.08.039
Hariri, R.H., Fredericks, E.M., Bowers, K.M.: Uncertainty in big data analytics: survey, opportunities, and challenges. J. Big Data (2019). https://doi.org/10.1186/s40537-019-0206-3
Hashem, I.A.T., Chang, V., Anuar, N.B., Adewole, K., Yaqoob, I., Gani, A., Ahmed, E., Chiroma, H.: The role of big data in smart city. Int. J. Inf. Manage. 36 (5), 748–758 (2016). https://doi.org/10.1016/j.ijinfomgt.2016.05.002
Hassan, M.D., Castanha, R.C.G., Wolfram, D.: Scientometric analysis of global trypanosomiasis research: 1988–2017. J. Infect. Public Health 13 (4), 514–520 (2020). https://doi.org/10.1016/j.jiph.2019.10.006
Hassan, S.U., Haddawy, P., Zhu, J.: A bibliometric study of the world’s research activity in sustainable development and its sub-areas using scientific literature. Scientometrics 99 , 549–579 (2014). https://doi.org/10.1007/s11192-013-1193-3
Heilig, L., Voss, S.: A scientometric analysis of cloud computing literature. IEEE Trans. Cloud Comput. 2 (3), 266–278 (2014). https://doi.org/10.1109/TCC.2014.2321168
Herodotou, H., Babu, S.: Pro ling, what-if analysis, and cost-based optimization of mapreduce programs. Proc. VLDB Endow. 4 (11), 1111–1122 (2011). https://doi.org/10.14778/3402707.3402746
Hirsch, J.E.: An index to quantify an individual’s scientific research output. Proc. Nat. Acad. Sci. USA 102 (46), 16569–16572 (2005). https://doi.org/10.1073/pnas.0507655102
Hu, H., Wen, Y., Chua, T.S., Li, X.: Toward scalable systems for big data analytics: A technology tutorial. IEEE Access 2 , 652–687 (2014). https://doi.org/10.1109/ACCESS.2014.2332453
Huang, L., Chen, K., Zhou, M.: Climate change and carbon sink: a bibliometric analysis. Environ. Sci. Pollut. Res. 27 , 8740–8758 (2020). https://doi.org/10.1007/s11356-019-07489-6
Imran, A., Zoha, A., Abu-Dayya, A.: Challenges in 5g: how to empower son with big data for enabling 5g. IEEE Netw. 28 (6), 27–33 (2014). https://doi.org/10.1109/MNET.2014.6963801
Jacomy, M., Venturini, T., Heymann, S., Bastian, M.: Forceatlas2, a continuous graph layout algorithm for handy network visualization designed for the gephi software. PLoS ONE 9 (6), 1–12 (2014). https://doi.org/10.1371/journal.pone.0098679
Jalali, S.M.J., Mahdizadeh, E., Mahmoudi, M., Moro, S.: Analytical assessment process of e-learning domain research between 1980 and 2014. Int. J. Manage. Educ. 12 , 43 (2018). https://doi.org/10.1504/IJMIE.2018.10008710
Jalali, S.M.J., Park, H.W.: State of the art in business analytics: themes and collaborations. Qual. Quant. 52 , 627–633 (2018). https://doi.org/10.1007/s11135-017-0522-7
Jalali, S.M.J., Park, H.W., Raeesi Vanani, I., Kim Hung, P.: Research trends on big data domain using text mining algorithms. Digital Scholarship in the Humanities (2020). https://doi.org/10.1093/llc/fqaa012
Ji, Q., Pang, X., Zhao, X.: A bibliometric analysis of research on antarctica during 1993–2012. Scientometrics 101 , 1925–1939 (2014). https://doi.org/10.1007/s11192-014-1332-5
Kambatla, K., Kollias, G., Kumar, V., Grama, A.: Trends in big data analytics. J. Parallel Distrib. Comput. 74 (7), 2561–2573 (2014). https://doi.org/10.1016/j.jpdc.2014.01.003
Kaur, A., Sood, S.K.: Ten years of disaster management and use of ict: a scientometric analysis. Earth Sci. Inf. 13 , 1–27 (2020). https://doi.org/10.1007/s12145-019-00408-w
Khan, M., Saqib, S., Alyas, T., Rehman, A., Saeed, Y., Zeb, A., Zareei, M., Mohamed, E.: Effective demand forecasting model using business intelligence empowered with machine learning. IEEE Access 8 , 116013–116023 (2020). https://doi.org/10.1109/ACCESS.2020.3003790
Kitchin, R.: The real-time city? Big data and smart urbanism. GeoJournal 79 , 1–14 (2014a). https://doi.org/10.1007/s10708-013-9516-8
Kitchin, R.: Big data, new epistemologies and paradigm shifts. Big Data Soc. (2014b). https://doi.org/10.1177/2053951714528481
Klievink, B., Romijn, B.J., Cunningham, S., Bruijn, H.: Big data in the public sector: uncertainties and readiness. Inf/ Syst. Front. 19 , 267–283 (2017). https://doi.org/10.1007/s10796-016-9686-2
Kwon, O., Lee, N., Shin, B.: Data quality management, data usage experience and acquisition intention of big data analytics. Int. J. Inf. Manage. 34 (3), 387–394 (2014). https://doi.org/10.1016/j.ijinfomgt.2014.02.002
Liang, T.P., Liu, Y.H.: Research landscape of business intelligence and big data analytics: a bibliometrics study. Exp. Syst. Appl. 111 , 2–10 (2018). https://doi.org/10.1016/j.eswa.2018.05.018 . Big Data Analytics for Business Intelligence
Liu, J., Tian, J., Kong, X., Lee, I., Xia, F.: Two decades of information systems: a bibliometric review. Scientometrics 118 , 617–643 (2019). https://doi.org/10.1007/s11192-018-2974-5
Mehta, N., Pandit, A.: Concurrence of big data analytics and healthcare: a systematic review. Int. J. Med. Inf. 114 , 57–65 (2018). https://doi.org/10.1016/j.ijmedinf.2018.03.013
Najafabadi, M.M., Villanustre, F., Seliya, T.M.K.N., Wald, R., Muharemagic, E.: Deep learning applications and challenges in big data analytics. J Big Data (2015). https://doi.org/10.1186/s40537-014-0007-7
Nguyen, A., Gardner, L., Sheridan, D.: Data analytics in higher education: an integrated view. J. Inf. Syst. Educ. 31 , 61–71 (2020). https://aisel.aisnet.org/jise/vol31/iss1/5
Nuaimi, E.A., Neyadi, H.A., Mohamed, N., Al-Jaroodi, J.: Applications of big data to smart cities. J. Internet Serv. Appl. (2015). https://doi.org/10.1186/s13174-015-0041-5
Ozturk, G.B.: Interoperability in building information modeling for aeco/fm industry. Autom. Constr. 113 , 103122 (2020). https://doi.org/10.1016/j.autcon.2020.103122
Prinsloo, P., Slade, S.: Big Data, Higher Education and Learning Analytics: Beyond Justice, Towards an Ethics of Care, pp. 109–124. Springer International Publishing, Cham (2017). https://doi.org/10.1007/978-3-319-06520-5_8
Rathore, M.M., Ahmad, A., Paul, A., Rho, S.: Urban planning and building smart cities based on the internet of things using big data analytics. Comput. Netw. 101 , 63–80 (2016). https://doi.org/10.1016/j.comnet.2015.12.023
Ravi, D., Wong, C., Deligianni, F., Berthelot, M., Andreu-Perez, J., Lo, B., Yang, G.Z.: Deep learning for health informatics. IEEE J. Biomed. Health Inf. 21 (1), 4–21 (2017). https://doi.org/10.1109/JBHI.2016.2636665
Sahil, Sood, S.K.: Bibliometric monitoring of research performance in ict-based disaster management literature. Qual. Quant. (2020). https://doi.org/10.1007/s11135-020-00991-x
Santhakumar, R., Kaliyaperumal, K.: A scientometric analysis of mobile technology publications. Scientometrics 105 , 921–939 (2015). https://doi.org/10.1007/s11192-015-1710-7
Shayaa, S., Jaafar, N.I., Bahri, S., Sulaiman, A., Phoong, S.W., Chung, Y., Piprani, A., al garadi, M.: Sentiment analysis of big data: Methods, applications, and open challenges. IEEE Access 6 , 37807–37827 (2018). https://doi.org/10.1109/ACCESS.2018.2851311
Sidiropoulos, N.D., Lathauwer, L.D., Fu, X., Huang, K., Papalexakis, E.E., Faloutsos, C.: Tensor decomposition for signal processing and machine learning. IEEE Trans. Signal Process. 65 (13), 3551–3582 (2017). https://doi.org/10.1109/TSP.2017.2690524
Siemens, G.: Learning analytics: the emergence of a discipline. Am. Behav. Sci. 57 (10), 1380–1400 (2013). https://doi.org/10.1177/0002764213498851
Sivarajah, U., Kamal, M.M., Irani, Z., Weerakkody, V.: Critical analysis of big data challenges and analytical methods. J. Bus. Res. 70 , 263–286 (2017). https://doi.org/10.1016/j.jbusres.2016.08.001
Soleimani-Roozbahani, F., Ghatari, A.R., Radfar, R.: Knowledge discovery from a more than a decade studies on healthcare big data systems: a scientometrics study. J. Big Data (2019). https://doi.org/10.1186/s40537-018-0167-y
Sun, Y., Song, H., Jara, A.J., Bie, R.: Internet of things and big data analytics for smart and connected communities. IEEE Access 4 , 766–773 (2016). https://doi.org/10.1109/ACCESS.2016.2529723
Tsai, C.W., Lai, C.F., Chao, H.C., Vasilakos, A.V.: Big data analytics: a survey. J. Big Data 2 (1), 1–32 (2015). https://doi.org/10.1186/s40537-015-0030-3
van Eck, N.J., Waltman, L.: Software survey: Vosviewer, a computer program for bibliometric mapping. Scientometrics 84 , 523–538 (2010). https://doi.org/10.1007/s11192-009-0146-3
van Eck, N.J., Waltman, L.: Visualizing Bibliometric Networks, pp. 285–320. Springer International Publishing, Cham (2014). https://doi.org/10.1007/978-3-319-10377-8_13
Vanani, I.R., Jalali, S.M.J.: A comparative analysis of emerging scientific themes in business analytics. Int. J. Bus. Inf. Syst. 29 (2), 183–206 (2018). https://doi.org/10.1504/IJBIS.2018.10009115
Waheed, H., Hassan, S.U., Aljohani, N.R., Wasif, M.: A bibliometric perspective of learning analytics research landscape. Behav. Inf. Technol. 37 (10–11), 941–957 (2018). https://doi.org/10.1080/0144929X.2018.1467967
Wamba, S.F., Gunasekaran, A., Akter, S., fan Ren, S.J., Dubey, R., Childe, S.J.: Big data analytics and rm performance: E ects of dynamic capabilities. J. Bus. Res. 70 , 356–365 (2017). https://doi.org/10.1016/j.jbusres.2016.08.009
Wang, S., Wan, J., Li, D., Zhang, C.: Implementing smart factory of industrie 4.0: An outlook. Int. J. Distrib. Sens. Netw. 12 (1), 3159805 (2016). https://doi.org/10.1155/2016/3159805
Wang, W., Lu, C.: Visualization analysis of big data research based on citespace. Soft. Comput. 24 , 8173–8186 (2020). https://doi.org/10.1007/s00500-019-04384-7
Wu, Q., Ding, G., Xu, Y., Feng, S., Du, Z., Wang, J., Long, K.: Cognitive internet of things: A new paradigm beyond connection. IEEE Internet Things J. 1 (2), 129–143 (2014). https://doi.org/10.1109/JIOT.2014.2311513
Wu, Y., Duan, Z.: Social network analysis of international scientific collaboration on psychiatry research. Int. J. Ment. Health Syst. 9 , 2 (2015). https://doi.org/10.1186/1752-4458-9-2
Xiang, Z., Schwartz, Z., Gerdes, J.H., Uysal, M.: What can big data and text analytics tell us about hotel guest experience and satisfaction? Int. J. Hospitality Manage. 44 , 120–130 (2015). https://doi.org/10.1016/j.ijhm.2014.10.013
Xu, Z., Yu, D.: A bibliometrics analysis on big data research (2009–2018). J. Data Inf. Manage. 1 , 3–15 (2019). https://doi.org/10.1007/s42488-019-00001-2
Zeng, L., Li, Z., Wu, T., Yang, L.: Mapping knowledge domain research in big data: From 2006 to 2016. In: Data Mining and Big Data, pp. 234–246. Springer International Publishing, Cham (2017). https://doi.org/10.1007/978-3-319-61845-6_24
Zhang, Y., Qiu, M., Tsai, C., Hassan, M.M., Alamri, A.: Health-cps: Healthcare cyber-physical system assisted by cloud and big data. IEEE Syst. J. 11 (1), 88–95 (2017). https://doi.org/10.1109/JSYST.2015.2460747
Zheng, Y., Capra, L., Wolfson, O., Yang, H.: Urban computing: Concepts, methodologies, and applications. ACM Trans. Intell. Syst. Technol. (2014). https://doi.org/10.1145/2629592
Zhong, R.Y., Xu, X., Klotz, E., Newman, S.T. (2017) Intelligent manufacturing in the context of industry 4.0: a review. Engineering 3 (5):616–630 (2017). https://doi.org/10.1016/J.ENG.2017.05.015
Download references
Author information
Authors and affiliations.
Department of Computer Science and Informatics, Central University of Himachal Pradesh, Dharamshala, India
Keshav Singh Rawat & Sandeep Kumar Sood
You can also search for this author in PubMed Google Scholar
Corresponding author
Correspondence to Keshav Singh Rawat .
Additional information
Publisher's note.
Springer Nature remains neutral with regard to jurisdictional claims in published maps and institutional affiliations.
Rights and permissions
Reprints and permissions
About this article
Rawat, K.S., Sood, S.K. Emerging trends and global scope of big data analytics: a scientometric analysis. Qual Quant 55 , 1371–1396 (2021). https://doi.org/10.1007/s11135-020-01061-y
Download citation
Accepted : 14 October 2020
Published : 27 October 2020
Issue Date : August 2021
DOI : https://doi.org/10.1007/s11135-020-01061-y
Share this article
Anyone you share the following link with will be able to read this content:
Sorry, a shareable link is not currently available for this article.
Provided by the Springer Nature SharedIt content-sharing initiative
- Data analytics
- Scientometric
- Citation analysis
- Emerging trends
- Find a journal
- Publish with us
- Track your research
- How It Works
- PhD thesis writing
- Master thesis writing
- Bachelor thesis writing
- Dissertation writing service
- Dissertation abstract writing
- Thesis proposal writing
- Thesis editing service
- Thesis proofreading service
- Thesis formatting service
- Coursework writing service
- Research paper writing service
- Architecture thesis writing
- Computer science thesis writing
- Engineering thesis writing
- History thesis writing
- MBA thesis writing
- Nursing dissertation writing
- Psychology dissertation writing
- Sociology thesis writing
- Statistics dissertation writing
- Buy dissertation online
- Write my dissertation
- Cheap thesis
- Cheap dissertation
- Custom dissertation
- Dissertation help
- Pay for thesis
- Pay for dissertation
- Senior thesis
- Write my thesis
214 Best Big Data Research Topics for Your Thesis Paper
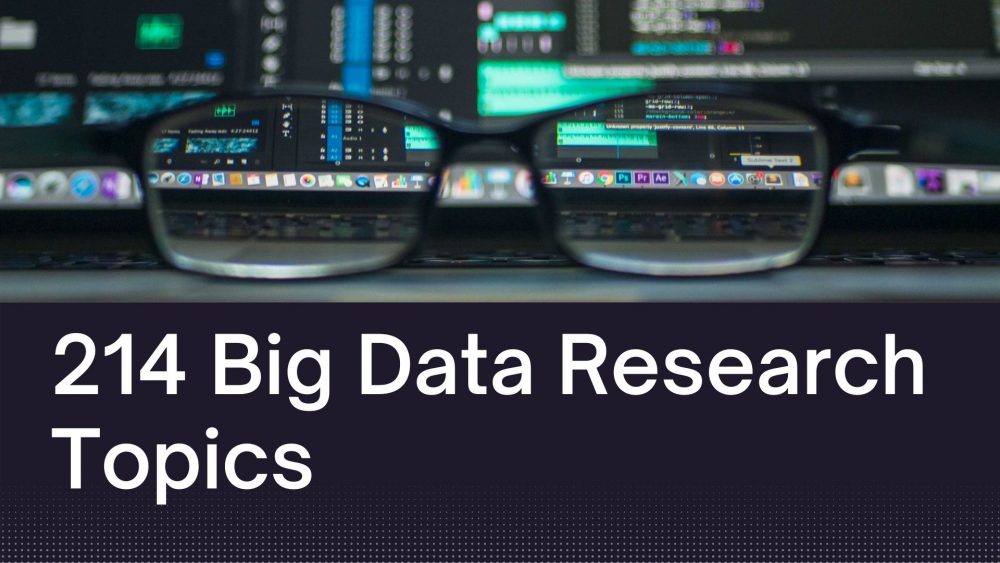
Finding an ideal big data research topic can take you a long time. Big data, IoT, and robotics have evolved. The future generations will be immersed in major technologies that will make work easier. Work that was done by 10 people will now be done by one person or a machine. This is amazing because, in as much as there will be job loss, more jobs will be created. It is a win-win for everyone.
Big data is a major topic that is being embraced globally. Data science and analytics are helping institutions, governments, and the private sector. We will share with you the best big data research topics.
On top of that, we can offer you the best writing tips to ensure you prosper well in your academics. As students in the university, you need to do proper research to get top grades. Hence, you can consult us if in need of research paper writing services.
Big Data Analytics Research Topics for your Research Project
Are you looking for an ideal big data analytics research topic? Once you choose a topic, consult your professor to evaluate whether it is a great topic. This will help you to get good grades.
- Which are the best tools and software for big data processing?
- Evaluate the security issues that face big data.
- An analysis of large-scale data for social networks globally.
- The influence of big data storage systems.
- The best platforms for big data computing.
- The relation between business intelligence and big data analytics.
- The importance of semantics and visualization of big data.
- Analysis of big data technologies for businesses.
- The common methods used for machine learning in big data.
- The difference between self-turning and symmetrical spectral clustering.
- The importance of information-based clustering.
- Evaluate the hierarchical clustering and density-based clustering application.
- How is data mining used to analyze transaction data?
- The major importance of dependency modeling.
- The influence of probabilistic classification in data mining.
Interesting Big Data Analytics Topics
Who said big data had to be boring? Here are some interesting big data analytics topics that you can try. They are based on how some phenomena are done to make the world a better place.
- Discuss the privacy issues in big data.
- Evaluate the storage systems of scalable in big data.
- The best big data processing software and tools.
- Data mining tools and techniques are popularly used.
- Evaluate the scalable architectures for parallel data processing.
- The major natural language processing methods.
- Which are the best big data tools and deployment platforms?
- The best algorithms for data visualization.
- Analyze the anomaly detection in cloud servers
- The scrutiny normally done for the recruitment of big data job profiles.
- The malicious user detection in big data collection.
- Learning long-term dependencies via the Fourier recurrent units.
- Nomadic computing for big data analytics.
- The elementary estimators for graphical models.
- The memory-efficient kernel approximation.
Big Data Latest Research Topics
Do you know the latest research topics at the moment? These 15 topics will help you to dive into interesting research. You may even build on research done by other scholars.
- Evaluate the data mining process.
- The influence of the various dimension reduction methods and techniques.
- The best data classification methods.
- The simple linear regression modeling methods.
- Evaluate the logistic regression modeling.
- What are the commonly used theorems?
- The influence of cluster analysis methods in big data.
- The importance of smoothing methods analysis in big data.
- How is fraud detection done through AI?
- Analyze the use of GIS and spatial data.
- How important is artificial intelligence in the modern world?
- What is agile data science?
- Analyze the behavioral analytics process.
- Semantic analytics distribution.
- How is domain knowledge important in data analysis?
Big Data Debate Topics
If you want to prosper in the field of big data, you need to try even hard topics. These big data debate topics are interesting and will help you to get a better understanding.
- The difference between big data analytics and traditional data analytics methods.
- Why do you think the organization should think beyond the Hadoop hype?
- Does the size of the data matter more than how recent the data is?
- Is it true that bigger data are not always better?
- The debate of privacy and personalization in maintaining ethics in big data.
- The relation between data science and privacy.
- Do you think data science is a rebranding of statistics?
- Who delivers better results between data scientists and domain experts?
- According to your view, is data science dead?
- Do you think analytics teams need to be centralized or decentralized?
- The best methods to resource an analytics team.
- The best business case for investing in analytics.
- The societal implications of the use of predictive analytics within Education.
- Is there a need for greater control to prevent experimentation on social media users without their consent?
- How is the government using big data; for the improvement of public statistics or to control the population?
University Dissertation Topics on Big Data
Are you doing your Masters or Ph.D. and wondering the best dissertation topic or thesis to do? Why not try any of these? They are interesting and based on various phenomena. While doing the research ensure you relate the phenomenon with the current modern society.
- The machine learning algorithms are used for fall recognition.
- The divergence and convergence of the internet of things.
- The reliable data movements using bandwidth provision strategies.
- How is big data analytics using artificial neural networks in cloud gaming?
- How is Twitter accounts classification done using network-based features?
- How is online anomaly detection done in the cloud collaborative environment?
- Evaluate the public transportation insights provided by big data.
- Evaluate the paradigm for cancer patients using the nursing EHR to predict the outcome.
- Discuss the current data lossless compression in the smart grid.
- How does online advertising traffic prediction helps in boosting businesses?
- How is the hyperspectral classification done using the multiple kernel learning paradigm?
- The analysis of large data sets downloaded from websites.
- How does social media data help advertising companies globally?
- Which are the systems recognizing and enforcing ownership of data records?
- The alternate possibilities emerging for edge computing.
The Best Big Data Analysis Research Topics and Essays
There are a lot of issues that are associated with big data. Here are some of the research topics that you can use in your essays. These topics are ideal whether in high school or college.
- The various errors and uncertainty in making data decisions.
- The application of big data on tourism.
- The automation innovation with big data or related technology
- The business models of big data ecosystems.
- Privacy awareness in the era of big data and machine learning.
- The data privacy for big automotive data.
- How is traffic managed in defined data center networks?
- Big data analytics for fault detection.
- The need for machine learning with big data.
- The innovative big data processing used in health care institutions.
- The money normalization and extraction from texts.
- How is text categorization done in AI?
- The opportunistic development of data-driven interactive applications.
- The use of data science and big data towards personalized medicine.
- The programming and optimization of big data applications.
The Latest Big Data Research Topics for your Research Proposal
Doing a research proposal can be hard at first unless you choose an ideal topic. If you are just diving into the big data field, you can use any of these topics to get a deeper understanding.
- The data-centric network of things.
- Big data management using artificial intelligence supply chain.
- The big data analytics for maintenance.
- The high confidence network predictions for big biological data.
- The performance optimization techniques and tools for data-intensive computation platforms.
- The predictive modeling in the legal context.
- Analysis of large data sets in life sciences.
- How to understand the mobility and transport modal disparities sing emerging data sources?
- How do you think data analytics can support asset management decisions?
- An analysis of travel patterns for cellular network data.
- The data-driven strategic planning for citywide building retrofitting.
- How is money normalization done in data analytics?
- Major techniques used in data mining.
- The big data adaptation and analytics of cloud computing.
- The predictive data maintenance for fault diagnosis.
Interesting Research Topics on A/B Testing In Big Data
A/B testing topics are different from the normal big data topics. However, you use an almost similar methodology to find the reasons behind the issues. These topics are interesting and will help you to get a deeper understanding.
- How is ultra-targeted marketing done?
- The transition of A/B testing from digital to offline.
- How can big data and A/B testing be done to win an election?
- Evaluate the use of A/B testing on big data
- Evaluate A/B testing as a randomized control experiment.
- How does A/B testing work?
- The mistakes to avoid while conducting the A/B testing.
- The most ideal time to use A/B testing.
- The best way to interpret results for an A/B test.
- The major principles of A/B tests.
- Evaluate the cluster randomization in big data
- The best way to analyze A/B test results and the statistical significance.
- How is A/B testing used in boosting businesses?
- The importance of data analysis in conversion research
- The importance of A/B testing in data science.
Amazing Research Topics on Big Data and Local Governments
Governments are now using big data to make the lives of the citizens better. This is in the government and the various institutions. They are based on real-life experiences and making the world better.
- Assess the benefits and barriers of big data in the public sector.
- The best approach to smart city data ecosystems.
- The big analytics used for policymaking.
- Evaluate the smart technology and emergence algorithm bureaucracy.
- Evaluate the use of citizen scoring in public services.
- An analysis of the government administrative data globally.
- The public values are found in the era of big data.
- Public engagement on local government data use.
- Data analytics use in policymaking.
- How are algorithms used in public sector decision-making?
- The democratic governance in the big data era.
- The best business model innovation to be used in sustainable organizations.
- How does the government use the collected data from various sources?
- The role of big data for smart cities.
- How does big data play a role in policymaking?
Easy Research Topics on Big Data
Who said big data topics had to be hard? Here are some of the easiest research topics. They are based on data management, research, and data retention. Pick one and try it!
- Who uses big data analytics?
- Evaluate structure machine learning.
- Explain the whole deep learning process.
- Which are the best ways to manage platforms for enterprise analytics?
- Which are the new technologies used in data management?
- What is the importance of data retention?
- The best way to work with images is when doing research.
- The best way to promote research outreach is through data management.
- The best way to source and manage external data.
- Does machine learning improve the quality of data?
- Describe the security technologies that can be used in data protection.
- Evaluate token-based authentication and its importance.
- How can poor data security lead to the loss of information?
- How to determine secure data.
- What is the importance of centralized key management?
Unique IoT and Big Data Research Topics
Internet of Things has evolved and many devices are now using it. There are smart devices, smart cities, smart locks, and much more. Things can now be controlled by the touch of a button.
- Evaluate the 5G networks and IoT.
- Analyze the use of Artificial intelligence in the modern world.
- How do ultra-power IoT technologies work?
- Evaluate the adaptive systems and models at runtime.
- How have smart cities and smart environments improved the living space?
- The importance of the IoT-based supply chains.
- How does smart agriculture influence water management?
- The internet applications naming and identifiers.
- How does the smart grid influence energy management?
- Which are the best design principles for IoT application development?
- The best human-device interactions for the Internet of Things.
- The relation between urban dynamics and crowdsourcing services.
- The best wireless sensor network for IoT security.
- The best intrusion detection in IoT.
- The importance of big data on the Internet of Things.
Big Data Database Research Topics You Should Try
Big data is broad and interesting. These big data database research topics will put you in a better place in your research. You also get to evaluate the roles of various phenomena.
- The best cloud computing platforms for big data analytics.
- The parallel programming techniques for big data processing.
- The importance of big data models and algorithms in research.
- Evaluate the role of big data analytics for smart healthcare.
- How is big data analytics used in business intelligence?
- The best machine learning methods for big data.
- Evaluate the Hadoop programming in big data analytics.
- What is privacy-preserving to big data analytics?
- The best tools for massive big data processing
- IoT deployment in Governments and Internet service providers.
- How will IoT be used for future internet architectures?
- How does big data close the gap between research and implementation?
- What are the cross-layer attacks in IoT?
- The influence of big data and smart city planning in society.
- Why do you think user access control is important?
Big Data Scala Research Topics
Scala is a programming language that is used in data management. It is closely related to other data programming languages. Here are some of the best scala questions that you can research.
- Which are the most used languages in big data?
- How is scala used in big data research?
- Is scala better than Java in big data?
- How is scala a concise programming language?
- How does the scala language stream process in real-time?
- Which are the various libraries for data science and data analysis?
- How does scala allow imperative programming in data collection?
- Evaluate how scala includes a useful REPL for interaction.
- Evaluate scala’s IDE support.
- The data catalog reference model.
- Evaluate the basics of data management and its influence on research.
- Discuss the behavioral analytics process.
- What can you term as the experience economy?
- The difference between agile data science and scala language.
- Explain the graph analytics process.
Independent Research Topics for Big Data
These independent research topics for big data are based on the various technologies and how they are related. Big data will greatly be important for modern society.
- The biggest investment is in big data analysis.
- How are multi-cloud and hybrid settings deep roots?
- Why do you think machine learning will be in focus for a long while?
- Discuss in-memory computing.
- What is the difference between edge computing and in-memory computing?
- The relation between the Internet of things and big data.
- How will digital transformation make the world a better place?
- How does data analysis help in social network optimization?
- How will complex big data be essential for future enterprises?
- Compare the various big data frameworks.
- The best way to gather and monitor traffic information using the CCTV images
- Evaluate the hierarchical structure of groups and clusters in the decision tree.
- Which are the 3D mapping techniques for live streaming data.
- How does machine learning help to improve data analysis?
- Evaluate DataStream management in task allocation.
- How is big data provisioned through edge computing?
- The model-based clustering of texts.
- The best ways to manage big data.
- The use of machine learning in big data.
Is Your Big Data Thesis Giving You Problems?
These are some of the best topics that you can use to prosper in your studies. Not only are they easy to research but also reflect on real-time issues. Whether in University or college, you need to put enough effort into your studies to prosper. However, if you have time constraints, we can provide professional writing help. Are you looking for online expert writers? Look no further, we will provide quality work at a cheap price.
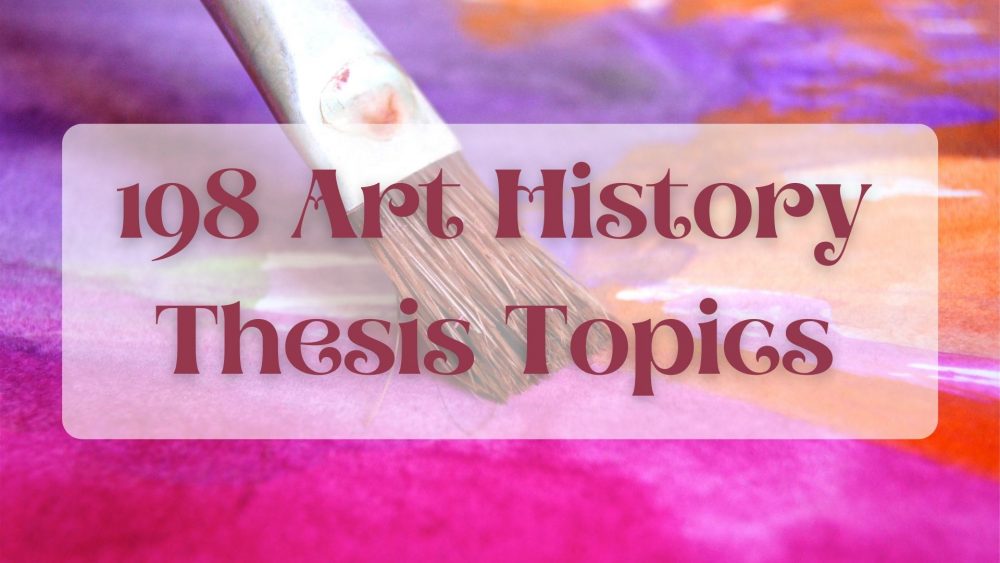
Leave a Reply Cancel reply
Your email address will not be published. Required fields are marked *
Comment * Error message
Name * Error message
Email * Error message
Save my name, email, and website in this browser for the next time I comment.
As Putin continues killing civilians, bombing kindergartens, and threatening WWIII, Ukraine fights for the world's peaceful future.
Ukraine Live Updates

99+ Interesting Data Science Research Topics For Students In 2024
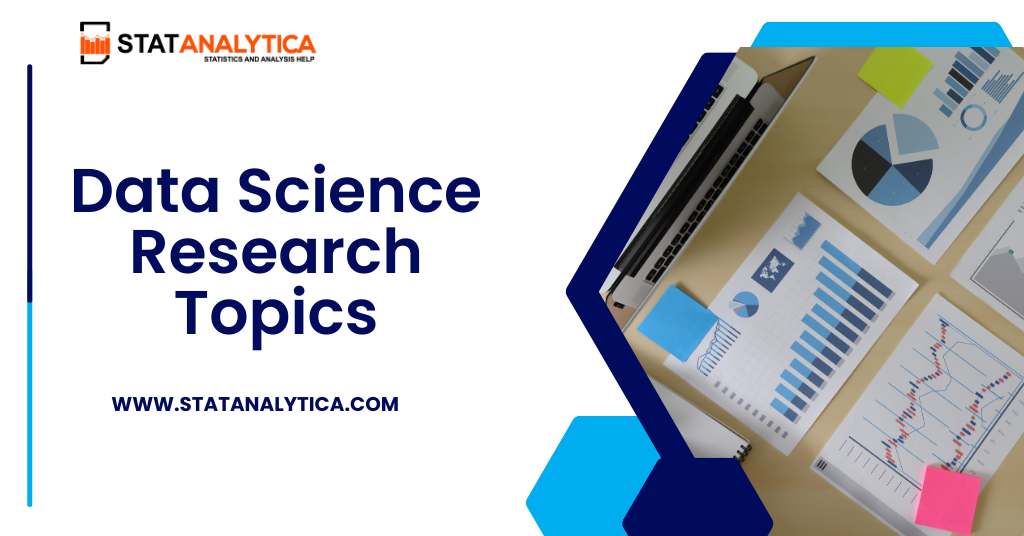
In today’s information-driven world, data science research stands as a pivotal domain shaping our understanding and application of vast data sets. It amalgamates statistics, computer science, and domain knowledge to extract valuable insights from data. Understanding ‘What Is Data Science?’ is fundamental—a field exploring patterns, trends, and solutions embedded within data.
However, the significance of data science research papers in a student’s life cannot be overstated. They foster critical thinking, analytical skills, and a deeper comprehension of the subject matter. To aid students in navigating this realm effectively, this blog dives into essential elements integral to a data science research paper, while also offering a goldmine of 99+ engaging and timely data science research topics for 2024.
Unraveling tips for crafting an impactful research paper and insights on choosing the right topic, this blog is a compass for students exploring data science research topics. Stay tuned to unearth more about ‘data science research topics’ and refine your academic journey.
What Is Data Science?
Table of Contents
Data Science is like a detective for information! It’s all about uncovering secrets and finding valuable stuff in heaps of data. Imagine you have a giant puzzle with tons of pieces scattered around. Data Science helps in sorting these pieces and figuring out the picture they create. It uses tools and skills from math, computer science, and knowledge about different fields to solve real-world problems.
In simpler terms, Data Science is like a chef in a kitchen, blending ingredients to create a perfect dish. Instead of food, it combines data—numbers, words, pictures—to cook up solutions. It helps in understanding patterns, making predictions, and answering tricky questions by exploring data from various sources. In essence, Data Science is the magic that turns data chaos into meaningful insights that can guide decisions and make life better.
Importance Of Data Science Research Paper In Student’s Life
Data Science research papers are like treasure maps for students! They’re super important because they teach students how to explore and understand the world of data. Writing these papers helps students develop problem-solving skills, think critically, and become better at analyzing information. It’s like a fun adventure where they learn how to dig into data and uncover valuable insights that can solve real-world problems.
- Enhances critical thinking: Research papers challenge students to analyze and interpret data critically, honing their thinking skills.
- Fosters analytical abilities: Students learn to sift through vast amounts of data, extracting meaningful patterns and information.
- Encourages exploration: Engaging in research encourages students to explore diverse data sources, broadening their knowledge horizon.
- Develops communication skills: Writing research papers hones students’ ability to articulate complex findings and ideas clearly.
- Prepares for real-world challenges: Through research, students learn to apply theoretical knowledge to practical problems, preparing them for future endeavors.
Elements That Must Be Present In Data Science Research Paper
Here are some elements that must be present in data science research paper:
1. Clear Objective
A data science research paper should start with a clear goal, stating what the study aims to investigate or achieve. This objective guides the entire paper, helping readers understand the purpose and direction of the research.
2. Detailed Methodology
Explaining how the research was conducted is crucial. The paper should outline the tools, techniques, and steps used to collect, analyze, and interpret data. This section allows others to replicate the study and validate its findings.
3. Accurate Data Presentation
Presenting data in an organized and understandable manner is key. Graphs, charts, and tables should be used to illustrate findings clearly, aiding readers’ comprehension of the results.
4. Thorough Analysis and Interpretation
Simply presenting data isn’t enough; the paper should delve into a deep analysis, explaining the meaning behind the numbers. Interpretation helps draw conclusions and insights from the data.
5. Conclusive Findings and Recommendations
A strong conclusion summarizes the key findings of the research. It should also offer suggestions or recommendations based on the study’s outcomes, indicating potential avenues for future exploration.
Here are some interesting data science research topics for students in 2024:
Natural Language Processing (NLP)
- Multi-modal Contextual Understanding: Integrating text, images, and audio to enhance NLP models’ comprehension abilities.
- Cross-lingual Transfer Learning: Investigating methods to transfer knowledge from one language to another for improved translation and understanding.
- Emotion Detection in Text: Developing models to accurately detect and interpret emotions conveyed in textual content.
- Sarcasm Detection in Social Media: Building algorithms that can identify and understand sarcastic remarks in online conversations.
- Language Generation for Code: Generating code snippets and scripts from natural language descriptions using NLP techniques.
- Bias Mitigation in Language Models: Developing strategies to mitigate biases present in large language models and ensure fairness in generated content.
- Dialogue Systems for Personalized Assistance: Creating intelligent conversational agents that provide personalized assistance based on user preferences and history.
- Summarization of Legal Documents: Developing NLP models capable of summarizing lengthy legal documents for quick understanding and analysis.
- Understanding Contextual Nuances in Sentiment Analysis: Enhancing sentiment analysis models to better comprehend contextual nuances and sarcasm in text.
- Hate Speech Detection and Moderation: Building systems to detect and moderate hate speech and offensive language in online content.
Computer Vision
- Weakly Supervised Object Detection: Exploring methods to train object detection models with limited annotated data.
- Video Action Recognition in Uncontrolled Environments: Developing models that can recognize human actions in videos captured in uncontrolled settings.
- Image Generation and Translation: Investigating techniques to generate realistic images from textual descriptions and translate images across different domains.
- Scene Understanding in Autonomous Systems: Enhancing computer vision algorithms for better scene understanding in autonomous vehicles and robotics.
- Fine-grained Visual Classification: Improving models to classify objects at a more granular level, distinguishing subtle differences within similar categories.
- Visual Question Answering (VQA): Creating systems capable of answering questions based on visual input, requiring reasoning and comprehension abilities.
- Medical Image Analysis for Disease Diagnosis: Developing computer vision models for accurate and early diagnosis of diseases from medical images.
- Action Localization in Videos: Building models to precisely localize and recognize specific actions within video sequences.
- Image Captioning with Contextual Understanding: Generating captions for images considering the context and relationships between objects.
- Human Pose Estimation in Real-time: Improving algorithms for real-time estimation of human poses in videos for applications like motion analysis and gaming.
Machine Learning Algorithms
- Self-supervised Learning Techniques: Exploring novel methods for training machine learning models without explicit supervision.
- Continual Learning in Dynamic Environments: Investigating algorithms that can continuously learn and adapt to changing data distributions and tasks.
- Explainable AI for Model Interpretability: Developing techniques to explain the decisions and predictions made by complex machine learning models.
- Transfer Learning for Small Datasets: Techniques to effectively transfer knowledge from large datasets to small or domain-specific datasets.
- Adaptive Learning Rate Optimization: Enhancing optimization algorithms to dynamically adjust learning rates based on data characteristics.
- Robustness to Adversarial Attacks: Building models resistant to adversarial attacks, ensuring stability and security in machine learning applications.
- Active Learning Strategies: Investigating methods to select and label the most informative data points for model training to minimize labeling efforts.
- Privacy-preserving Machine Learning: Developing algorithms that can train models on sensitive data while preserving individual privacy.
- Fairness-aware Machine Learning: Techniques to ensure fairness and mitigate biases in machine learning models across different demographics.
- Multi-task Learning for Jointly Learning Tasks: Exploring approaches to jointly train models on multiple related tasks to improve overall performance.
Deep Learning
- Graph Neural Networks for Representation Learning: Using deep learning techniques to learn representations from graph-structured data.
- Transformer Models for Image Processing: Adapting transformer architectures for image-related tasks, such as image classification and generation.
- Few-shot Learning Strategies: Investigating methods to enable deep learning models to learn from a few examples in new categories.
- Memory-Augmented Neural Networks: Enhancing neural networks with external memory for improved learning and reasoning capabilities.
- Neural Architecture Search (NAS): Automating the design of neural network architectures for specific tasks or constraints.
- Meta-learning for Fast Adaptation: Developing models capable of quickly adapting to new tasks or domains with minimal data.
- Deep Reinforcement Learning for Robotics: Utilizing deep RL techniques for training robots to perform complex tasks in real-world environments.
- Generative Adversarial Networks (GANs) for Data Augmentation: Using GANs to generate synthetic data for enhancing training datasets.
- Variational Autoencoders for Unsupervised Learning: Exploring VAEs for learning latent representations of data without explicit supervision.
- Lifelong Learning in Deep Networks: Strategies to enable deep networks to continually learn from new data while retaining past knowledge.
Big Data Analytics
- Streaming Data Analysis for Real-time Insights: Techniques to analyze and derive insights from continuous streams of data in real-time.
- Scalable Algorithms for Massive Graphs: Developing algorithms that can efficiently process and analyze large-scale graph-structured data.
- Anomaly Detection in High-dimensional Data: Detecting anomalies and outliers in high-dimensional datasets using advanced statistical methods and machine learning.
- Personalization and Recommendation Systems: Enhancing recommendation algorithms for providing personalized and relevant suggestions to users.
- Data Quality Assessment and Improvement: Methods to assess, clean, and enhance the quality of big data to improve analysis and decision-making.
- Time-to-Event Prediction in Time-series Data: Predicting future events or occurrences based on historical time-series data.
- Geospatial Data Analysis and Visualization: Analyzing and visualizing large-scale geospatial data for various applications such as urban planning, disaster management, etc.
- Privacy-preserving Big Data Analytics: Ensuring data privacy while performing analytics on large-scale datasets in distributed environments.
- Graph-based Deep Learning for Network Analysis: Leveraging deep learning techniques for network analysis and community detection in large-scale networks.
- Dynamic Data Compression Techniques: Developing methods to compress and store large volumes of data efficiently without losing critical information.
Healthcare Analytics
- Predictive Modeling for Patient Outcomes: Using machine learning to predict patient outcomes and personalize treatments based on individual health data.
- Clinical Natural Language Processing for Electronic Health Records (EHR): Extracting valuable information from unstructured EHR data to improve healthcare delivery.
- Wearable Devices and Health Monitoring: Analyzing data from wearable devices to monitor and predict health conditions in real-time.
- Drug Discovery and Development using AI: Utilizing machine learning and AI for efficient drug discovery and development processes.
- Predictive Maintenance in Healthcare Equipment: Developing models to predict and prevent equipment failures in healthcare settings.
- Disease Clustering and Stratification: Grouping diseases based on similarities in symptoms, genetic markers, and response to treatments.
- Telemedicine Analytics: Analyzing data from telemedicine platforms to improve remote healthcare delivery and patient outcomes.
- AI-driven Radiomics for Medical Imaging: Using AI to extract quantitative features from medical images for improved diagnosis and treatment planning.
- Healthcare Resource Optimization: Optimizing resource allocation in healthcare facilities using predictive analytics and operational research techniques.
- Patient Journey Analysis and Personalized Care Pathways: Analyzing patient trajectories to create personalized care pathways and improve healthcare outcomes.
Time Series Analysis
- Forecasting Volatility in Financial Markets: Predicting and modeling volatility in stock prices and financial markets using time series analysis.
- Dynamic Time Warping for Similarity Analysis: Utilizing DTW to measure similarities between time series data, especially in scenarios with temporal distortions.
- Seasonal Pattern Detection and Analysis: Identifying and modeling seasonal patterns in time series data for better forecasting.
- Time Series Anomaly Detection in Industrial IoT: Detecting anomalies in industrial sensor data streams to prevent equipment failures and improve maintenance.
- Multivariate Time Series Forecasting: Developing models to forecast multiple related time series simultaneously, considering interdependencies.
- Non-linear Time Series Analysis Techniques: Exploring non-linear models and methods for analyzing complex time series data.
- Time Series Data Compression for Efficient Storage: Techniques to compress and store time series data efficiently without losing crucial information.
- Event Detection and Classification in Time Series: Identifying and categorizing specific events or patterns within time series data.
- Time Series Forecasting with Uncertainty Estimation: Incorporating uncertainty estimation into time series forecasting models for better decision-making.
- Dynamic Time Series Graphs for Network Analysis: Representing and analyzing dynamic relationships between entities over time using time series graphs.
Reinforcement Learning
- Multi-agent Reinforcement Learning for Collaboration: Developing strategies for multiple agents to collaborate and solve complex tasks together.
- Hierarchical Reinforcement Learning: Utilizing hierarchical structures in RL for solving tasks with varying levels of abstraction and complexity.
- Model-based Reinforcement Learning for Sample Efficiency: Incorporating learned models into RL for efficient exploration and planning.
- Robotic Manipulation with Reinforcement Learning: Training robots to perform dexterous manipulation tasks using RL algorithms.
- Safe Reinforcement Learning: Ensuring that RL agents operate safely and ethically in real-world environments, minimizing risks.
- Transfer Learning in Reinforcement Learning: Transferring knowledge from previously learned tasks to expedite learning in new but related tasks.
- Curriculum Learning Strategies in RL: Designing learning curricula to gradually expose RL agents to increasingly complex tasks.
- Continuous Control in Reinforcement Learning: Exploring techniques for continuous control tasks that require precise actions in a continuous action space.
- Reinforcement Learning for Adaptive Personalization: Utilizing RL to personalize experiences or recommendations for individuals in dynamic environments.
- Reinforcement Learning in Healthcare Decision-making: Using RL to optimize treatment strategies and decision-making in healthcare settings.
Data Mining
- Graph Mining for Social Network Analysis: Extracting valuable insights from social network data using graph mining techniques.
- Sequential Pattern Mining for Market Basket Analysis: Discovering sequential patterns in customer purchase behaviors for market basket analysis.
- Clustering Algorithms for High-dimensional Data: Developing clustering techniques suitable for high-dimensional datasets.
- Frequent Pattern Mining in Healthcare Datasets: Identifying frequent patterns in healthcare data for actionable insights and decision support.
- Outlier Detection and Fraud Analysis: Detecting anomalies and fraudulent activities in various domains using data mining approaches.
- Opinion Mining and Sentiment Analysis in Reviews: Analyzing opinions and sentiments expressed in product or service reviews to derive insights.
- Data Mining for Personalized Learning: Mining educational data to personalize learning experiences and adapt teaching methods.
- Association Rule Mining in Internet of Things (IoT) Data: Discovering meaningful associations and patterns in IoT-generated data streams.
- Multi-modal Data Fusion for Comprehensive Analysis: Integrating information from multiple data modalities for a holistic understanding and analysis.
- Data Mining for Energy Consumption Patterns: Analyzing energy usage data to identify patterns and optimize energy consumption in various sectors.
Ethical AI and Bias Mitigation
- Fairness Metrics and Evaluation in AI Systems: Developing metrics and evaluation frameworks to assess the fairness of AI models.
- Bias Detection and Mitigation in Facial Recognition: Addressing biases present in facial recognition systems to ensure equitable performance across demographics.
- Algorithmic Transparency and Explainability: Designing methods to make AI algorithms more transparent and understandable to stakeholders.
- Fair Representation Learning in Unbalanced Datasets: Learning fair representations from imbalanced data to reduce biases in downstream tasks.
- Fairness-aware Recommender Systems: Ensuring fairness and reducing biases in recommendation algorithms across diverse user groups.
- Ethical Considerations in AI for Criminal Justice: Investigating the ethical implications of AI-based decision-making in criminal justice systems.
- Debiasing Techniques in Natural Language Processing: Developing methods to mitigate biases in language models and text generation.
- Diversity and Fairness in Hiring Algorithms: Ensuring diversity and fairness in AI-based hiring systems to minimize biases in candidate selection.
- Ethical AI Governance and Policy: Examining the role of governance and policy frameworks in regulating the development and deployment of AI systems.
- AI Accountability and Responsibility: Addressing ethical dilemmas and defining responsibilities concerning AI system behaviors and decision-making processes.
Tips For Writing An Effective Data Science Research Paper
Here are some tips for writing an effective data science research paper:
Tip 1: Thorough Planning and Organization
Begin by planning your research paper carefully. Outline the sections and information you’ll include, ensuring a logical flow from introduction to conclusion. This organized approach makes writing easier and helps maintain coherence in your paper.
Tip 2: Clarity in Writing Style
Use clear and simple language to communicate your ideas. Avoid jargon or complex terms that might confuse readers. Write in a way that is easy to understand, ensuring your message is effectively conveyed.
Tip 3: Precise and Relevant Information
Include only information directly related to your research topic. Ensure the data, explanations, and examples you use are precise and contribute directly to supporting your arguments or findings.
Tip 4: Effective Data Visualization
Utilize graphs, charts, and tables to present your data visually. Visual aids make complex information easier to comprehend and can enhance the overall presentation of your research findings.
Tip 5: Review and Revise
Before submitting your paper, review it thoroughly. Check for any errors in grammar, spelling, or formatting. Revise sections if necessary to ensure clarity and coherence in your writing. Asking someone else to review it can also provide valuable feedback.
- Hospitality Management Research Topics
Things To Remember While Choosing The Data Science Research Topic
When selecting a data science research topic, consider your interests and its relevance to the field. Ensure the topic is neither too broad nor too narrow, striking a balance that allows for in-depth exploration while staying manageable.
- Relevance and Significance: Choose a topic that aligns with current trends or addresses a significant issue in the field of data science.
- Feasibility : Ensure the topic is researchable within the resources and time available. It should be practical and manageable for the scope of your study.
- Your Interest and Passion: Select a topic that genuinely interests you. Your enthusiasm will drive your motivation and engagement throughout the research process.
- Availability of Data: Check if there’s sufficient data available for analysis related to your chosen topic. Accessible and reliable data sources are vital for thorough research.
- Potential Contribution: Consider how your chosen topic can contribute to existing knowledge or fill a gap in the field. Aim for a topic that adds value and insights to the data science domain.
In wrapping up our exploration of data science research topics, we’ve uncovered a world of importance and guidance for students. From defining data science to understanding its impact on student life, identifying essential elements in research papers, offering a multitude of intriguing topics for 2024, to providing tips for crafting effective papers—the journey has been insightful.
Remembering the significance of topic selection and the key components of a well-structured paper, this voyage emphasizes how data science opens doors to endless opportunities. It’s not just a subject; it’s the compass guiding tomorrow’s discoveries and innovations in our digital landscape.
Related Posts
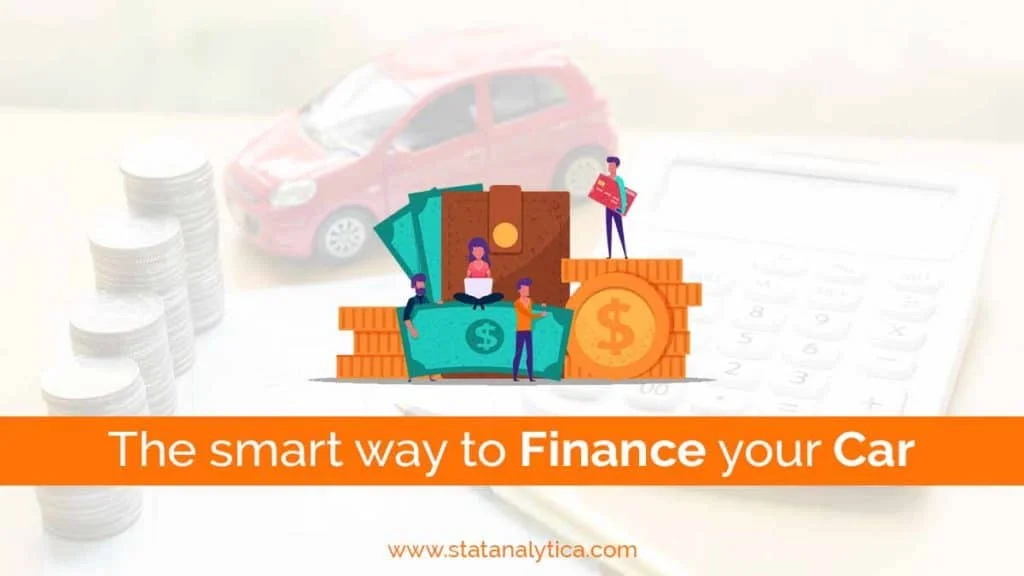
Step by Step Guide on The Best Way to Finance Car
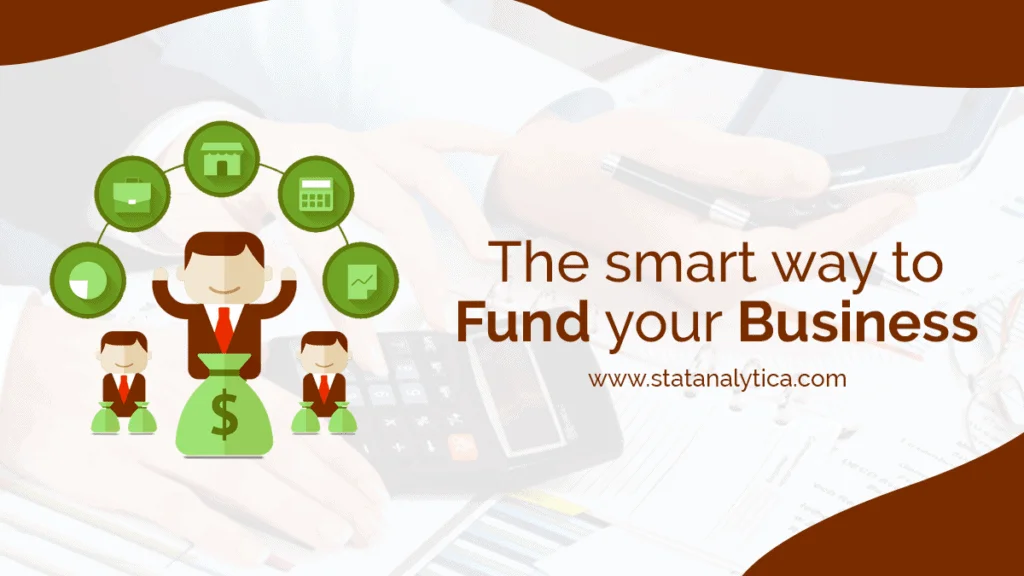
The Best Way on How to Get Fund For Business to Grow it Efficiently

An official website of the United States government
The .gov means it’s official. Federal government websites often end in .gov or .mil. Before sharing sensitive information, make sure you’re on a federal government site.
The site is secure. The https:// ensures that you are connecting to the official website and that any information you provide is encrypted and transmitted securely.
- Publications
- Account settings
Preview improvements coming to the PMC website in October 2024. Learn More or Try it out now .
- Advanced Search
- Journal List
- Springer Nature - PMC COVID-19 Collection

Data Analytics in Healthcare: A Tertiary Study
Toni taipalus.
Faculty of Information Technology, University of Jyväskylä, P.O. Box 35, FI-40014 Jyvaskyla, Finland
Ville Isomöttönen
Hanna erkkilä, sami Äyrämö, associated data.
The datasets generated during and/or analysed during the current study are available from the corresponding author on reasonable request.
The field of healthcare has seen a rapid increase in the applications of data analytics during the last decades. By utilizing different data analytic solutions, healthcare areas such as medical image analysis, disease recognition, outbreak monitoring, and clinical decision support have been automated to various degrees. Consequently, the intersection of healthcare and data analytics has received scientific attention to the point of numerous secondary studies. We analyze studies on healthcare data analytics, and provide a wide overview of the subject. This is a tertiary study, i.e., a systematic review of systematic reviews. We identified 45 systematic secondary studies on data analytics applications in different healthcare sectors, including diagnosis and disease profiling, diabetes, Alzheimer’s disease, and sepsis. Machine learning and data mining were the most widely used data analytics techniques in healthcare applications, with a rising trend in popularity. Healthcare data analytics studies often utilize four popular databases in their primary study search, typically select 25–100 primary studies, and the use of research guidelines such as PRISMA is growing. The results may help both data analytics and healthcare researchers towards relevant and timely literature reviews and systematic mappings, and consequently, towards respective empirical studies. In addition, the meta-analysis presents a high-level perspective on prominent data analytics applications in healthcare, indicating the most popular topics in the intersection of data analytics and healthcare, and provides a big picture on a topic that has seen dozens of secondary studies in the last 2 decades.
Introduction
The purpose of data analytics in healthcare is to find new insights in data, at least partially automate tasks such as diagnosing, and to facilitate clinical decision-making [ 1 , 2 ]. Higher hardware cost-efficiency and the popularization and advancement of data analysis techniques have led to data analytics gaining increasing scholarly and practical footing in the healthcare sector in recent decades [ 3 ]. Some data analytics solutions have also been demonstrated to surpass human effort [ 4 ]. As healthcare data is often characterized as diverse and plentiful, especially big data analysis techniques, prospects, and challenges have been discussed in scientific literature [ 5 ]. Other related concepts such as data mining, machine learning, and artificial intelligence have also been used either as buzzwords to promote data analytics applications or as genuine novel innovations or combinations of previously tested solutions.
The terms big data , big data analytics , and data analytics are often used interchangeably, which makes the search for related scientific works difficult. Especially, big data is often used as a synonym for analytics [ 6 ], a view contested in multiple sources [ 7 – 9 ]. In addition, the term data analytics is wide and usually at least partly subsumes concepts such as statistical analyses, machine learning, data mining, and artificial intelligence, many of which overlap with each other as well in terms of, e.g., using similar algorithms for different purposes. Finally, it is not uncommon that scientific works that are not focused on technical details discuss concepts such as machine learning at different levels of specificity. For example, some studies consider merely high-level paradigms such as supervised on unsupervised learning, while some discuss different tasks such as classification or clustering, and others focus on specific modeling techniques such as decision trees, kernel methods, or different types of artificial neural networks. These concerns of nomenclature and terminology apply to healthcare as well, and we adapt the broad view of both healthcare and data analytics in this study. In other words, with data analytics we refer to general data analytics encompassing terms such as data mining, machine learning, and big data analytics, and with healthcare we refer to different fields of medicine such as oncology and cardiology, some closely related concepts such as diagnosis and disease profiling, and diseases in the broad sense of the word, including but not limited to symptoms, injuries, and infections.
Naturally, because of growing interest in the intersection of data analytics and healthcare, the scientific field has seen numerous secondary studies on the applications of different data analysis techniques to different healthcare subfields such as disease profiling, epidemiology, oncology, and mental health. As the purpose of systematic reviews and mapping studies is to summarize and synthesize literature for easier conceptualization and a higher level view [ 10 , 11 ], when the number of secondary studies renders the subjective point of understanding a phenomenon on a high level arduous, a tertiary study is arguably warranted. In fact, we deemed the number of secondary studies high enough to conduct a tertiary study. In this study, we review systematic secondary studies on healthcare data analytics during 2000–2021, with the research goals to map publication fora, publication years, numbers of primary studies utilized, scientific databases utilized, healthcare subfields, data analytics subfields, and the intersection of healthcare and data analytics. The results indicate that the number of secondary studies is rising steadily, that data analytics is widely applied in a myriad of healthcare subfields, and that machine learning techniques are the most widely utilized data analytics subfield in healthcare. The relatively high number of secondary studies appears to be the consequence of over 6800 primary studies utilized by the secondary studies included in our review. Our results present a high-level overview of healthcare data analytics: specific and general data analytics and healthcare subfields and the intersection thereof, publication trends, as well as synthesis on the challenges and opportunities of healthcare data analytics presented by the secondary studies.
The rest of the study is structured as follows. In the next section, we describe the systematic method behind secondary study search and selection. In Section “ Results ” we present the results of this tertiary study, and in Section “ Discussion ” discuss the practical implications of the results as well as threats to validity. Section “ Conclusion ” concludes the study.
Search Strategy
We searched for eligible secondary studies using five databases: ACM Digital Library (ACM DL), IEEExplore, ScienceDirect, Scopus, and PubMed. In addition, we utilized Google Scholar, but the search returned too many results to be considered in a feasible timeframe. The search strings and publications returned from the respective databases are detailed in Table 1 . Because the relevant terms healthcare , big data and data analytics have been used in an ambiguous manner in the literature, we performed two rounds of backward snowballing, i.e., followed the reference lists of included articles to capture works not found by the database searches. The search and selection processes are detailed in Fig. 1 .
Search strings—Scopus database search returned 16,135 results which were sorted by relevance, and the first 2,000 papers were selected for further inspection

Study selection process showing the process step by step as well as the numbers of secondary studies in each step—A1, A2 and A3 refer to the authors responsible for each step, E refers to an exclusion criterion described in Table 2 , and n indicates the number of included papers after a step was completed
Study Selection
After the secondary studies were searched for closer eligibility inspection, the first author applied the exclusion criteria listed in Table 2 . In case the first author was unsure about a study, the second author was consulted. In case a consensus was not reached, the third author was consulted with the final decision on whether to include or exclude the study. Regarding exclusion criterion E5, we only considered secondary studies, i.e., mapping studies and different types of literature reviews. Furthermore, due to different levels of systematic approaches, we deemed a study systematic if (i) the utilized databases were explicitly stated (i.e., stated with more detail than “we used databases such as...” or “we mainly used Scopus”), (ii) search terms were explicitly stated, and (iii) inclusion or exclusion criteria or both were explicitly stated. Regarding exclusion criteria E6, E7 and E8, several studies considered healthcare in related fields such as healthcare from administrative perspectives [ 12 ], healthcare data privacy [ 13 , 14 ], data quality [ 15 ], and comparing human performance with data analytics solutions [ 4 ]. Such studies were excluded. Similarly, studies returned by the database searches on data analytics related fields such as big data and its challenges [ 16 ], Internet-of-Things [ 17 ], and studies with a focus on software or hardware architectures behind analytics platforms [ 18 , 19 ] rather than on the process of analysis were also excluded.
Exclusion (E) criteria
It is worth noting that we followed the respective secondary study authors’ classification of techniques, e.g., whether a technique is considered machine learning or deep learning. In the case a study considered more than one data analytics or healthcare subfield, we categorized the study according to what was to our understanding the primary focus. This is the reason we have refrained from defining terms such as deep learning in this study—the definitions are numerous and by defining the terms, we might give the reader the impression that we have judged whether a secondary study is concerned with, e.g., machine learning or deep learning.
Publication Fora and Years
We included 45 secondary studies (abbreviated SE in the figures, cf. 7 for full bibliographic details). A total of 34 (76%) of the selected secondary studies were published in academic journals, nine (20%) in conference proceedings, and two (4%) were book chapters. Most of the studies were published in distinct fora (cf. Table 3 ), and fora with more than one selected secondary study consisted of Journal of Medical Systems , International Journal of Medical Informatics , Journal of Biomedical Informatics , and IEEE Access . As expected, the publication fora were aimed at either computer science, healthcare, or both. Finally, as can be observed in Fig. 2 , the trend of systematic secondary studies in the intersection of data analytics and healthcare is growing.
Publication fora

Number of included secondary studies by publication year (bars, left y-axis), and the number of included primary studies by publication year (dots, right y-axis)—the year 2021 was only considered from January to April; the figure shows that the number of secondary studies is rising
Secondary Study Qualities
The selected secondary studies utilized a total of 37 different databases. The most frequently used databases were PubMed, Scopus, IEEExplore, and Web of Science, respectively. Other relatively frequently used databases were ACM Digital Library, Google Scholar, and Springer Link. Most of the secondary studies (33, or 73%) utilized four or fewer databases ( M = 3.6, Mdn = 3). However, many bibliographic databases subsume others, and the number of utilized databases should not be taken as a metric for a systematic review quality. For example, a PubMed search implicitly searches MEDLINE records, and Google Scholar indexes works from most other scientific databases. The extended coverage of a wider range of academic works naturally results in numerous studies to further inspect, posing a challenge in the amount of work required. The most popular databases used in the secondary studies are visualized in Fig. 3 .

Four most popular databases used by the secondary studies were PubMed, IEEEXplore, Scopus and Web of Science—4 studies did not use any of these four databases, and other databases are not considered, e.g., the secondary study SE14, in addition to IEEExplore, might have also utilized other databases not visualized here
The secondary studies reported an average of 155 selected primary studies ( Mdn = 63, SD = 379.2), with a minimum of 6 (SE44) and a maximum of 2,421 primary studies (SE31). Five secondary studies selected more than 200 primary studies (cf. Fig 5 ). In total, the secondary studies utilized 6,838 primary studies. The number of secondary and primary studies categorized by the data analytics approach is summarized in Fig. 4 .

Number of secondary studies included in this tertiary study, and the number of primary studies utilized by the secondary studies, categorized by data analytics approach; DA general data analytics, TA text analytics, INF informatics, NA network analytics, DL deep learning, PM process mining, BDA big data analytics, DM data mining, ML machine learning; the figure shows that the general term data analytics was the most popular in the secondary studies

Number of primary studies (x-axis) selected for final inclusion in the secondary studies (y-axis), e.g., the chart shows that six secondary studies included 0–24 primary studies—one study (SE6) did not disclose the number of primary studies, and one study (SE15) reported two numbers: 24 primary studies for a quantitative analysis, and 28 primary studies for a qualitative analysis, and we reported that study using the latter number
Some secondary studies reported similar details on their respective primary studies, such as visualizations of publication years (22 studies), research approach summaries such as the number of qualitative and quantitative studies (8 studies), research field summaries (4 studies), and details on the geographic distribution of the primary study authors (5 studies). The use of PRISMA (preferred reporting items for systematic reviews and meta-analyses) [ 41 ] guidelines was reported in 15 studies.
Subject Areas Identified
Some selected studies considered the relationship between healthcare in general and a specific data analysis technique, while other studies considered the relationship between data analytics in general and a specific healthcare subfield. Most of the studies, however, considered the relationship between a specific data analysis technique and a specific healthcare subfield. These considerations are summarized in Fig. 6 . Readers interested mainly in general healthcare in the context of a specific analysis topic should refer to the secondary studies on the left-hand side, readers interested in general data analytics in the context of a specific healthcare topic should refer to secondary studies on the right-hand side, readers interested in a specific analysis topic applied to a specific healthcare topic should consider the studies in the middle, and readers interested in the applicability of analytics techniques in general to healthcare in general should consider the studies in the top row. Additional information on the secondary studies is presented in 6 .

Selected secondary studies and whether they consider only specific data analytics techniques (left side), only specific healthcare subfields (right side), both (center), or neither (top); the figure may be utilized in finding relevant secondary studies on desired subfields
Implications
Considering the number of primary studies utilized, only 12 studies (27%) used more than a hundred primary studies. Figure 5 seems to indicate that the threshold for conducting a literature review or a mapping study in healthcare data analytics is typically between 25 and 100 studies. Furthermore, and on the basis of the evidence currently available, it seems reasonable to argue that at least 25 primary studies (84% of the secondary studies) warrant a systematic review, and the results of systematic reviews can be seen as valuable synthesizing contributions to the field. This observation arguably also supports the relevance of this study, although this study covers a relatively large intersection of the two research areas.
The earliest included secondary study was published in 2009, which might be explained by the relative novelty of data analysis in healthcare, at least with computerized automation rather than merely applying statistical analyses. In addition, although systematic reviews are relatively common in medicine, they have only recently gained popularity and visibility in information technology [ 10 ]. As may be observed in Fig. 2 , the trend of secondary studies is growing, which consequently indicates that the number of primary studies in the intersection of data analytics and healthcare is gaining research interest. The rising popularity of machine learning algorithms may be explained by the rising popularity of unstructured data, the growing utilization of graphics processing units, and the development of different machine learning tools and software libraries. Indeed, many of the techniques behind modern machine learning implementations have been around since the 1980s, but only the combination of large amounts of data, and developments in methods and computer hardware in recent years have made such implementations more cost-effective. The development of trends illustrated in, e.g., Fig. 2 propounds the view that machine learning algorithms will gain more and more practical applications in healthcare and related fields, such as molecular biology [ 42 ]. Finally, some studies have argued [ 43 ] as well as demonstrated [ 44 , 45 ] that the evolution of machine learning is changing the way research hypotheses are formulated. Instead of theory-driven hypothesis formulation, machine learning can be used to facilitate the formulation of data-driven hypotheses, also in the field of medicine.
Secondary study publication fora were numerous and focused either on information technology, healthcare, or both, without obvious anomalies. The secondary studies utilized dozens of different databases in their primary study searches. It seems that the coverage of these databases is not always understood, or it is disregarded, regardless that utilizing non-overlapping databases results in less work in duplicate publication removal. For example, Scopus indexes some of ACM DL, some of Web of Science, and all of IEEExplore, effectively rendering IEEExplore search redundant if Scopus is utilized—a fact we as well understood only after conducting our searches. In addition, Google Scholar appears to index the bibliographic details of effectively all published research, yet the number of search results returned may be overwhelming for a systematic review. In practice, the selection of databases is balanced by the amount of work needed to examine the results on one end of the scales, and coverage on the other. Backward or forward snowballing may be utilized to limit the amount of work and to extend coverage.
Secondary study topics summarized in Fig. 6 give some implications for subject areas of healthcare data analytics that are mature enough to warrant a secondary study. As the figure shows, these areas are aplenty, and the most frequent data analytics techniques applied seem to be machine learning (13 secondary studies) and data mining (7 secondary studies). It is worth noting that the nomenclature we applied in this study reflects that of the secondary study authors. As explained earlier in this study, attempts at defining, e.g., machine learning and data mining in this study would inevitably contradict the definitions given in some of the included secondary studies. For further reading, Cabatuan and Maguerra [ 46 ] provide a high-level overview of machine learning and deep learning, and Shukla, Patel and Sen [ 47 ] on data mining. For more technical approaches, both Ahmad, Qamar and Rizvi [ 30 ] and Harper [ 48 ] review data mining techniques and algorithms in healthcare.
Opportunities and Challenges in Healthcare Data Analytics
Many of the selected secondary studies provided syntheses on the current challenges and opportunities in healthcare data analytics. As the secondary studies inspected over 6800 studies of healthcare data analytics, we have summarized recurring insights here.
It was a generally accepted view in the secondary studies that healthcare data analytics is an opportunity that has already been partly realized, yet needs to be more studied and applied in more diverse contexts and in-depth scenarios [ 49 – 51 ]. For example, it has been noted that while big data applications are relatively mature in bio-informatics, this is not necessarily the case in other biomedical fields [ 52 ]. In general, healthcare data analytics is rather uniformly perceived as an opportunity for more cost-efficient healthcare [ 52 , 53 ] through many applications such as automating a specialist’s routine tasks so that they may focus on tasks more crucial in a patient’s treatment. The cost-efficiency is likely to be more concretized by novel deep learning techniques such as large language models [ 54 ], which are also offered through implementations that perform tasks faster while consuming less resources [ 55 ]. In addition to faster diagnoses, data analytics solutions may also offer more objective diagnoses in, e.g., pathology, if the models are trained with data from multiple pathologists.
Challenges regarding healthcare data analytics are more diverse. Perhaps the most discussed challenge was the nature of the data and how it can be treated. Many secondary studies highlighted problems with missing data [ 56 , 57 ], low-quality data [ 54 ], and datasets stored in various formats which are not interoperable with each other [ 52 , 55 , 56 ]. Furthermore, some studies raised the concern of missing techniques to visualize the outputs given by different data analyses [ 56 , 58 ]. Rather intuitively, many new implementations and the increases in the amount of data require new computational infrastructure for feasible use [ 54 , 58 – 60 ]. Some studies raised ethical concerns regarding data collection, merging, and sharing, as data privacy is a multifaceted concept [ 52 , 54 , 58 , 59 ], especially when the datasets cover multiple countries with different legislations. Many studies also called for multidisciplinary collaboration between medical and computing experts, stating that it is crucial that the analytics implementations are based on the same vocabulary and rules as medical experts use [ 49 , 57 , 61 – 64 ], and that the technical experts understand, e.g., how feasible it is to collect training data for a model to find patterns in medical images. Closely related, many of the more complex analytics solutions operate on a black box principle, meaning that it is not obvious how the implementation reaches the conclusion it reaches [ 56 , 59 , 65 – 67 ]. Open solutions, on the other hand, are typically understandable only for technical experts and may be outperformed by the more complex black box solutions. Finally, it has been observed that the already existing analytics solutions implemented in different environments, e.g., different hospitals [ 56 , 59 , 64 ], are not portable into other environments. In addition, it may be that the existing solutions are not fully integrated into actual day-to-day work [ 57 ]. Fleuren et al. [ 68 ] summarize the issue aptly, urging “ to bridge the gap between bytes and bedside. ”
Threats to Validity
As is typical for studies involving human judgment, it is possible for another group of researchers to select at least a slightly different group of studies. Furthermore, the categorization of studies into specific healthcare and data analytics topics is a likely candidate for the subject of change. We tried to mitigate the effect of human judgment by following the systematic mapping study guidelines, such as utilizing and reporting explicit exclusion criteria and search terms [ 11 ], following the PRISMA flow of information guidelines [ 41 ], and discussing discrepancies and disagreements among the authors until consensus was reached. Regarding the challenges related to the wide and rather ambiguous subject areas of data analytics and healthcare, we utilized two rounds of backward snowballing to mitigate the threat of missing relevant studies.
In this study, we systematically mapped systematic secondary studies on healthcare data analytics. The results implicate that the number of secondary—and naturally primary—studies are rising, and the scientific publication fora around the topics are numerous. We also discovered that the number of primary studies included in the secondary studies varies greatly, as do the scientific databases used in primary study search. The results also show that while machine learning and data mining seem to be the most popular data analytics subfields in healthcare, specific healthcare topics are more diverse. This meta-analysis provides researchers with a high-level overview of the intersection of data analytics and healthcare, and an accessible starting point towards specific studies. What was not considered in this study is whether or not and how much the selected secondary studies overlap in their primary study selection, which could indicate the level of either deliberate or unaware overlap of similar work.
Appendix A. Secondary Study Qualities
See Table Table4 4 .
Detailed information on the secondary studies— PS = number of primary studies initially considered and finally included, PRISMA = whether the guidelines were used, Fields = whether the study reports the fields of primary studies, e.g., information systems, computer science, Years = whether the study reports and visualizes the distribution of publication years, Approach = whether the study reports primary study approaches, e.g., case study, qualitative study, philosophical, Geographic = whether the study reports the geographic distribution of primary study authors
Appendix B. Secondary Studies
- ] Albahri AS, Hamid RA, Alwan Jk, Al-qays ZT, Zaidan AA, Zaidan BB, Albahri AOS, AlAmoodi AH, Khlaf JM, Almahdi EM, Thabet E, Hadi SM, Mohammed KI, Alsalem MA, Al-Obaidi JR, Madhloom HT. Role of biological data mining and machine learning techniques in detecting and diagnosing the novel coronavirus (COVID-19): a systematic review. J Med Syst. 2020;44(7).
- ] Alkhatib M, Talaei-Khoei A, Ghapanchi A. Analysis of research in healthcare data analytics. In: Australasian Conference on Information Systems, 2016.
- ] Alonso SG, de la Torre-Díez I, Hamrioui S, López-Coronado M, Barreno DC, Nozaleda LM, Franco M. Data mining algorithms and techniques in mental health: a systematic review. J Med Syst. 2018;42(9):1–15.
- ] Alonso SG, de la Torre Diez I, Rodrigues JJPC, Hamrioui S, Lopez-Coronado M. A systematic review of techniques and sources of big data in the healthcare sector. J Med Syst. 2017;41(11):1–9.
- ] Behera RK, Bala PK, Dhir A. The emerging role of cognitive computing in healthcare: a systematic literature review. Int J Med Inf. 2019;129:154–166.
- ] Buettner R, Bilo M, Bay N, Zubac T. A systematic literature review of medical image analysis using deep learning. In: 2020 IEEE Symposium on Industrial Electronics & Applications (ISIEA). IEEE, 2020.
- ] Buettner R, Klenk F, Ebert M. A systematic literature review of machine learning-based disease profiling and personalized treatment. In: 2020 IEEE 44th Annual Computers, Software, and Applications Conference (COMPSAC). IEEE, 2020.
- ] Cabatuan M, Manguerra M. Machine learning for disease surveillance or outbreak monitoring: a review. In: 2020 IEEE 12th International Conference on Humanoid, Nanotechnology, Information Technology, Communication and Control, Environment, and Management (HNICEM). IEEE, 2020.
- ] Carroll LN, Au AP, Detwiler LT, Fu Tc, Painter IS, Abernethy NF. Visualization and analytics tools for infectious disease epidemiology: a systematic review. J Biomed Inf. 2014;51:287–298.
- ] Choudhury A, Asan O. Role of artificial intelligence in patient safety outcomes: systematic literature review. JMIR Med Inf. 2020;8(7):e18599.
- ] Choudhury A, Renjilian E, Asan O. Use of machine learning in geriatric clinical care for chronic diseases: a systematic literature review. JAMIA Open. 2020;3(3):459–471.
- ] Dallora AL, Eivazzadeh S, Mendes E, Berglund J, Anderberg P. Prognosis of dementia employing machine learning and microsimulation techniques: a systematic literature review. Proc Comput Sci. 2016;100:480–8.
- ] de la Torre Díez I, Cosgaya HM, Garcia-Zapirain B, López-Coronado M. Big data in health: a literature review from the year 2005. J Med Syst. 2016;40(9).
- ] Elbattah M, Arnaud E, Gignon M, Dequen G. The role of text analytics in healthcare: a review of recent developments and applications. In: Proceedings of the 14th International Joint Conference on Biomedical Engineering Systems and Technologies. SCITEPRESS—Science and Technology Publications, 2021.
- ] Fleuren LM, Klausch TLT, Zwager CL, Schoonmade LJ, Guo T, Roggeveen LF, Swart EL, Girbes ARJ, Thoral P, Ercole A, et al. Machine learning for the prediction of sepsis: a systematic review and meta-analysis of diagnostic test accuracy. Intensive Care Med. 2020;46(3):383–400.
- ] Gaitanou P, Garoufallou E, Balatsoukas P. The effectiveness of big data in health care: A systematic review. In: Communications in Computer and Information Science, pp. 141–153. Springer International Publishing; 2014.
- ] Galetsi P, Katsaliaki K. A review of the literature on big data analytics in healthcare. J Oper Res Soc. 2020;71(10):1511–1529.
- ] Gesicho MB, Babic A. Analysis of usage of indicators by leveraging health data warehouses: A literature review. In: Studies in Health Technology and Informatics, pages 184–187. IOS Press; 2019.
- ] Iavindrasana J, Cohen G, Depeursinge A, Müller H, Meyer R, Geissbuhler A. Clinical data mining: a review. Yearb Med Inf. 2009;18(01):121–133.
- ] Islam Md, Hasan Md, Wang X, Germack H, Noor-E-Alam Md. A systematic review on healthcare analytics: Application and theoretical perspective of data mining. Healthcare. 2018;6(2):54.
- ] Kamble SS, Gunasekaran A, Goswami M, Manda J. A systematic perspective on the applications of big data analytics in healthcare management. Int J Healthc Manag. 2018;12(3):226–240.
- ] Kavakiotis I, Tsave O, Salifoglou A, Maglaveras N, Vlahavas I, Chouvarda I. Machine learning and data mining methods in diabetes research. Comput Struct Biotechnol J. 2017;15:104–116.
- ] Khanra S, Dhir A, Najmul Islam AKM, Mäntymäki M. Big data analytics in healthcare: a systematic literature review. Enterp Inf Syst. 2020;14(7):878–912.
- ] Sudheer Kumar E, Shoba Bindu C. Medical image analysis using deep learning: a systematic literature review. In: International Conference on Emerging Technologies in Computer Engineering, pages 81–97. Springer; 2019.
- ] Kurniati AP, Johnson O, Hogg D, Hall G. Process mining in oncology: a literature review. In: 2016 6th International Conference on Information Communication and Management (ICICM). IEEE, 2016.
- ] Li J, Ding W, Cheng H, Chen P, Di D, Huang W. A comprehensive literature review on big data in healthcare. In: Twenty-second Americas Conference on Information Systems (AMCIS), 2016.
- ] Luo J, Wu M, Gopukumar D, Zhao Y. Big data application in biomedical research and health care: A literature review. Biomed Inf Insights. 2016;8:BII.S31559.
- ] Malik MM, Abdallah S, Ala’raj M. Data mining and predictive analytics applications for the delivery of healthcare services: a systematic literature review. Ann Oper Res. 2016;270(1-2):287–312.
- ] Marinov M, Mohammad Mosa AS, Yoo I, Boren SA. Data-mining technologies for diabetes: A systematic review. J Diabetes Sci Technol. 2011;5(6):1549–1556.
- ] Mehta N, Pandit A. Concurrence of big data analytics and healthcare: a systematic review. Int J Med Inf. 2018;114:57–65.
- ] Mehta N, Pandit A, Shukla S. Transforming healthcare with big data analytics and artificial intelligence: a systematic mapping study. J Biomed Inf. 2019;100:103311.
- ] Nazir S, Khan S, Khan HU, Ali S, Garcia-Magarino I, Atan RB, Nawaz M. A comprehensive analysis of healthcare big data management, analytics and scientific programming. IEEE Access. 2020;8:95714–95733.
- ] Nazir S, Nawaz M, Adnan A, Shahzad S, Asadi S. Big data features, applications, and analytics in cardiology—a systematic literature review. IEEE Access. 2019;7:143742–143771.
- ] Peiffer-Smadja N, Rawson TM, Ahmad R, Buchard A, Georgiou P, Lescure F-X, Birgand G, Holmes AH. Machine learning for clinical decision support in infectious diseases: a narrative review of current applications. Clin Microbiol Infect. 2020;26(5):584–595.
- ] Raja R, Mukherjee I, Sarkar BK. A systematic review of healthcare big data. Sci Programm. 2020;2020.
- ] Rojas E, Munoz-Gama J, Sepúlveda M, Capurro D. Process mining in healthcare: a literature review. J Biomed Inform. 2016;61:224–236.
- ] Salazar-Reyna R, Gonzalez-Aleu F, Granda-Gutierrez EMA, Diaz-Ramirez J, Garza-Reyes JA, Kumar A. A systematic literature review of data science, data analytics and machine learning applied to healthcare engineering systems. Manag Decis. 2020.
- ] Secinaro S, Calandra D, Secinaro A, Muthurangu V, Biancone P. The role of artificial intelligence in healthcare: a structured literature review. BMC Med Inf Decis Making. 2021;21(1).
- ] Stafford IS, Kellermann M, Mossotto E, Beattie RM, MacArthur BD, Ennis S. A systematic review of the applications of artificial intelligence and machine learning in autoimmune diseases. NPJ Digit Med. 2020;3(1):1–11.
- ] Teng AK, Wilcox AB. A review of predictive analytics solutions for sepsis patients. Appl Clin Inf. 2020;11(03):387–398.
- ] Toor R, Chana I. Network analysis as a computational technique and its benefaction for predictive analysis of healthcare data: a systematic review. Arch Comput Methods Eng. 2020;28(3):1689–1711.
- ] Tsang G, Xie X, Zhou S-M. Harnessing the power of machine learning in dementia informatics research: Issues, opportunities, and challenges. Rev Biomed Eng. 2020;13:113–129.
- ] Waring J, Lindvall C, Umeton R. Automated machine learning: review of the state-of-the-art and opportunities for healthcare. Artif Intell Med. 2020;104:101822.
- ] Waschkau A, Wilfling D, Steinhäuser J. Are big data analytics helpful in caring for multimorbid patients in general practice?—a scoping review. Family Pract. 2016;20(1).
- ] Zhang R, Simon G, Yu F. Advancing Alzheimer’s research: a review of big data promises. Int J Med Inf. 2017;106:48–56.
Open Access funding provided by University of Jyväskylä (JYU). This research did not receive any specific grant from funding agencies in the public, commercial, or not-for-profit sectors.
Data availability
Declarations.
On behalf of all authors, the corresponding author states that there is no conflict of interest.
Publisher's Note
Springer Nature remains neutral with regard to jurisdictional claims in published maps and institutional affiliations.
- Frontiers in Network Physiology
- Information Theory
- Research Topics
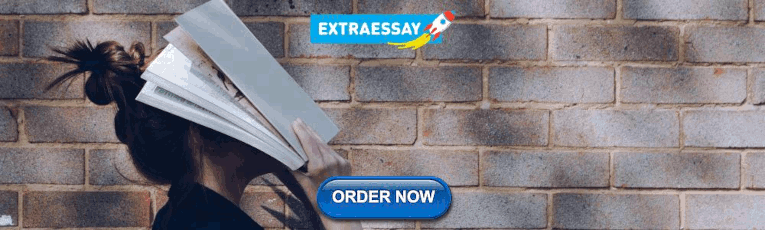
Machine Learning and Deep Learning in Data Analytics and Predictive Analytics of Physiological Data
Total Downloads
Total Views and Downloads
About this Research Topic
Complexity is an intrinsic feature of physiological data where results are often interpreted based on individual chemical and physical functions, and thus it is prone to two main limitations: failure of detection through standard testing, and lack of capacity to include a large number of potential predictors. Artificial intelligence, machine learning, and deep learning have been used effectively not only to understand diverse features of complex physiological data but also to predict and forecast likely future outcomes on unseen data. In the past few years, there was an exponential growth in implementing AI and machine learning in basic, translational and clinical research. In this Research Topic, we seek to facilitate the communication of up-to-date knowledge on AI in cellular and systems physiology. This Research topic will focus on AI and machine learning tools in exploratory analysis of multi-featured physiological data and in and prediction of physiological states and conditions in health and disease. This is closely connected with Frontiers in Network Physiology scope on how diverse physiological components interact during physiological and pathological conditions. This Research Topic provides a platform for AI-powered basic, translational, and clinical biomedical research by featuring recent innovations made by applying AI and machine learning in physiology, in particular cellular, molecular, and systems physiology. The focus of this topic is to create a collection of high-quality papers of innovative studies combining artificial intelligence, cellular and molecular physiology, and systems physiology. This Research Topic will be of high interest for Frontiers in Network Physiology readers as it focuses on the use of AI and machine learning tools in data analysis and defining new features in physiological data as well as model prediction which can assist translational and clinical research in the field of physiology. This Research Topic on ”Machine Learning and Deep Learning in Data Analytics and Predictive Analytics of Physiological Data” focuses on basic and translational research targeting AI and machine learning in physiological and pathophysiological states. In this Research Topic we gather high-quality papers including Original Research, Review, Mini Review, and commentary articles covering research in machine learning and deep learning in molecular, cellular ,and systems biology. The scope of this issue Topics of interests encompass but are not limited to: Supervised and unsupervised learning for physiological data Machine learning and deep learning for prognosis and diagnosis Deep learning for cellular activity Deep learning for systems biology Machine learning in molecular biology and physiology Machine learning for multi-omics data
Keywords : Network Physiology, Machine Learning, Deep learning, prediction model, physiology, systems biology, cellular and molecular biology, Data processing
Important Note : All contributions to this Research Topic must be within the scope of the section and journal to which they are submitted, as defined in their mission statements. Frontiers reserves the right to guide an out-of-scope manuscript to a more suitable section or journal at any stage of peer review.
Topic Editors
Topic coordinators, submission deadlines, participating journals.
Manuscripts can be submitted to this Research Topic via the following journals:
total views
- Demographics
No records found
total views article views downloads topic views
Top countries
Top referring sites, about frontiers research topics.
With their unique mixes of varied contributions from Original Research to Review Articles, Research Topics unify the most influential researchers, the latest key findings and historical advances in a hot research area! Find out more on how to host your own Frontiers Research Topic or contribute to one as an author.
Center for Big Data Analytics
Research topics.
- Alternating Minimization
- Bioinformatics
- Bregman Divergence
- Co-Clustering
- Compressed Sensing
- Computer Vision
- Coordinate Descent
- Covariance Estimation
- Crowd Computing
- Data Clustering
- Deep Learning
- Divide-and-Conquer Methods
- Eigenvalue Decomposition
- Empirical Risk Minimization
- Gene-Disease Prediction
- Graph Clustering
- Graphical Models
- Greedy Method
- High-Dimensional Statistics
- Information-Theoretic Analysis
- Kernel Methods
- Learning Theory
- Link Prediction
- Matrix Approximation
- Matrix Completion
- Metric Learning
- Multi-label Learning
- Newton Methods
- Nonnegative Matrix Factorization
- Numerical Linear Algebra
- Online Learning
- Recommender Systems
- Robust Learning
- Signed Networks
- Social Network Analysis
- Spectral Clustering
- Stochastic Gradient Methods
- Support Vector Machines
- Tight Frames
- Topic Models
University Libraries
Advanced data analytics.
- Advanced Data Analytics Course Libguide
- Data sources
- Learning resources
- Analytic tools
- Research and data services at UNT
- Research on the topic of data analytics
Finding research on data analytics
Data analytics is a very new area of work and not really a discipline of its own. This makes finding scholarly work about data analytics kind of tricky.
Much of the work is done by computer scientists, so a good bit of the literature can be found in the these databases:
- IEEE Xplore EL (IEEE Xplore) Full text access to IEEE Journals, Transactions, Magazines, Letters, Conference Proceedings, Standards (current only), and IEE Journals and Conferences. Dates of Coverage: 1988 to current, with some journals and conference titles available from 1950.
- ACM digital library ACM Digital Library bibliographic citations, abstracts, reviews, and full text for articles published in ACM proceedings and periodicals, covering computer science and engineering, as well as more general computing topics. Dates of Coverage: 1954 - Present
Otherwise, you'll want to search a database related to the domain in which you want to apply data analytics: see one of the UNT Libraries' subject guides to find relevant database.
Or you can explore the literature across fields, such as by using the Books & More (for ebooks) and Online Article Search (for journal articles) features, both on the homepage of the UNT Libraries . (On a mobile device, click the magnifying glass icon and then choose Library Catalog for ebooks or Full Text Online for articles.)
- << Previous: Research and data services at UNT
- Last Updated: Mar 18, 2024 12:32 PM
- URL: https://guides.library.unt.edu/data-analytics
Additional Links
UNT: Apply now UNT: Schedule a tour UNT: Get more info about the University of North Texas
UNT: Disclaimer | UNT: AA/EOE/ADA | UNT: Privacy | UNT: Electronic Accessibility | UNT: Required Links | UNT: UNT Home
- Enroll & Pay
- Prospective Students
- Current Students
Analytics, Information, Operations research

Research information
The Analytics, Information, Operations (AIO) academic area includes faculty who are experts in topics such as business analytics, information systems and supply chain management, as well as former industry professionals whose experience supplements our curriculum.
AIO faculty regularly publish in leading academic journals and lend their expertise to regional and national media. Their intellectual contributions generate new knowledge and understanding, particularly as industries continue to rely more heavily on data-driven decision making.
Area directors
- Debabrata Dey, Davis Area Director
- Detelina Stoyanova, Assistant Area Director
Degree programs
- Business analytics B.S.B.
- Information systems B.S.B.
- Supply chain management B.S.B.
- Master's in Business Analytics
- Master's in Supply Chain Management & Logistics
- Ph.D. in Analytics and Operations
Research topics
Data mining
Machine learning
Information security
Supply chain management
Healthcare information systems
In the Media
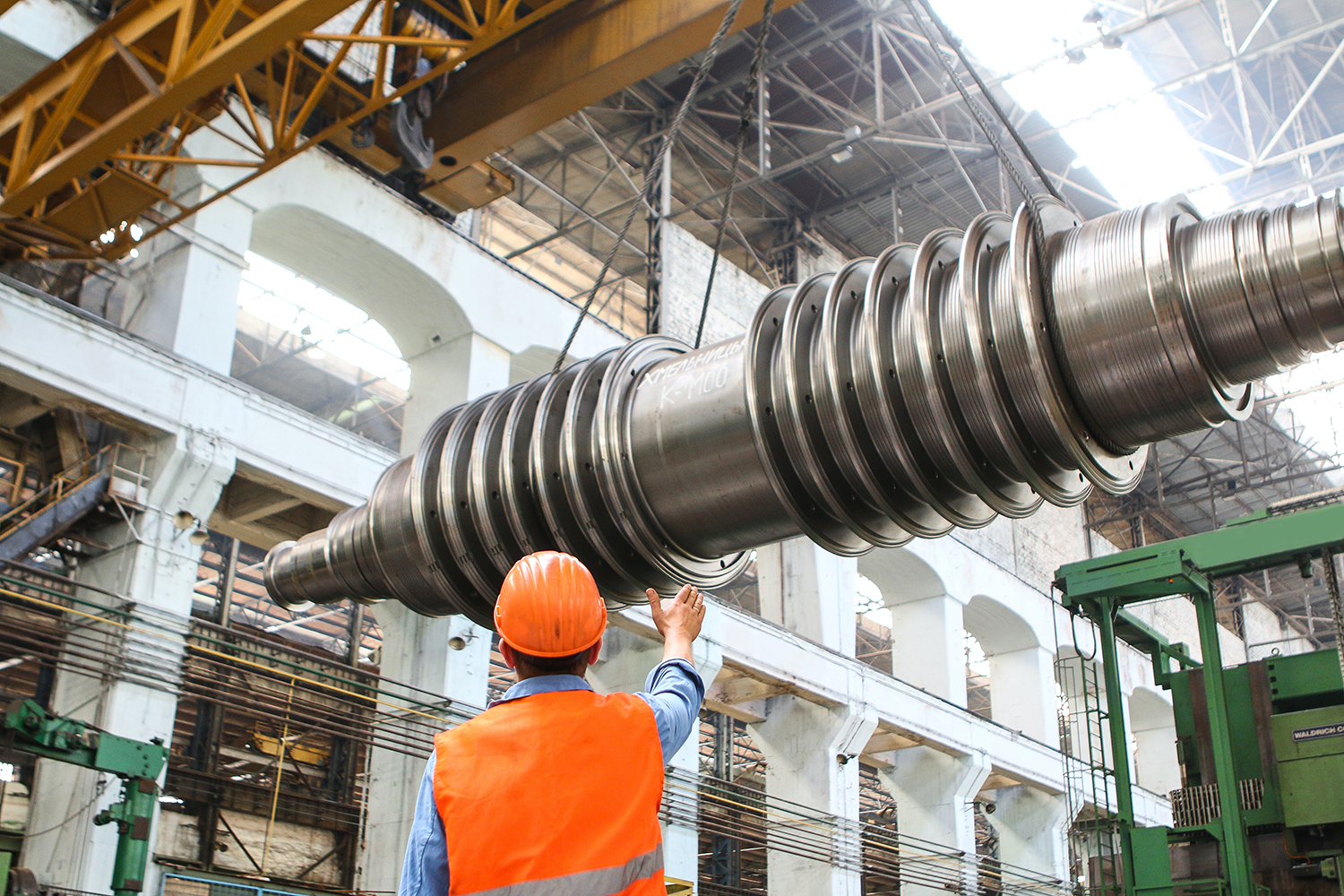
How to address the supply-chain staffing crisis
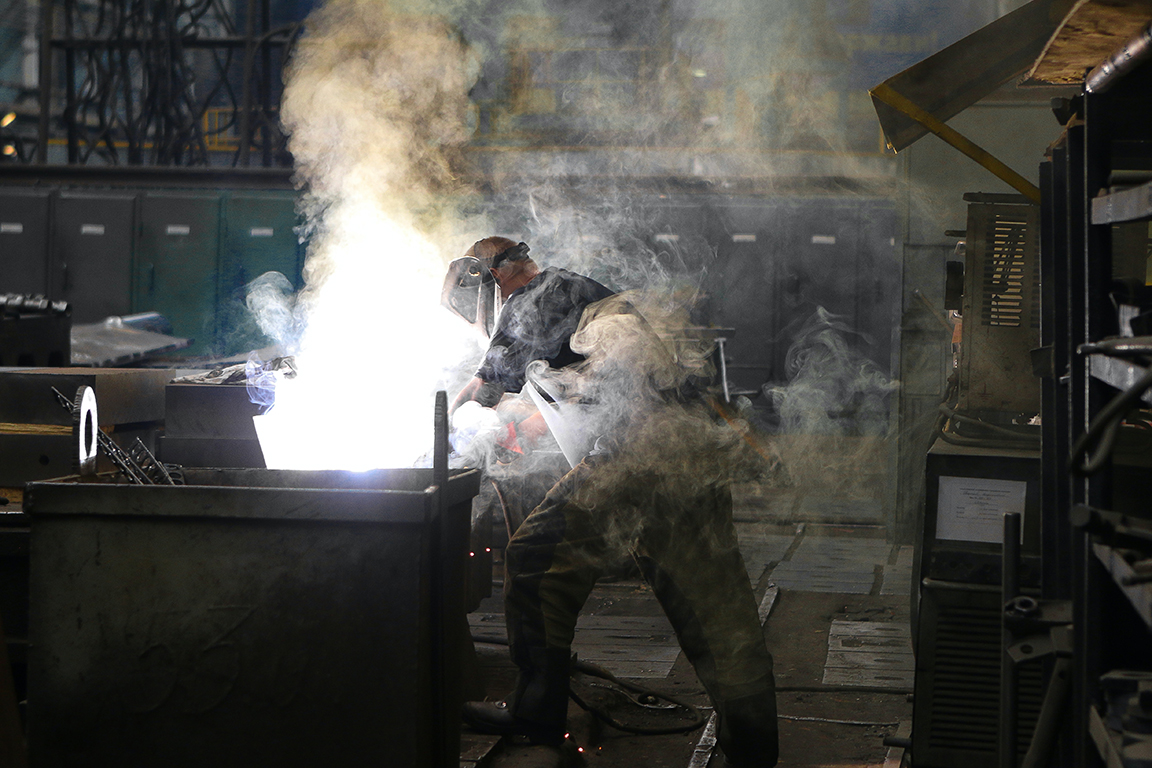
Disruptor Daily
What is the future of supply chain management 16 experts share insights.
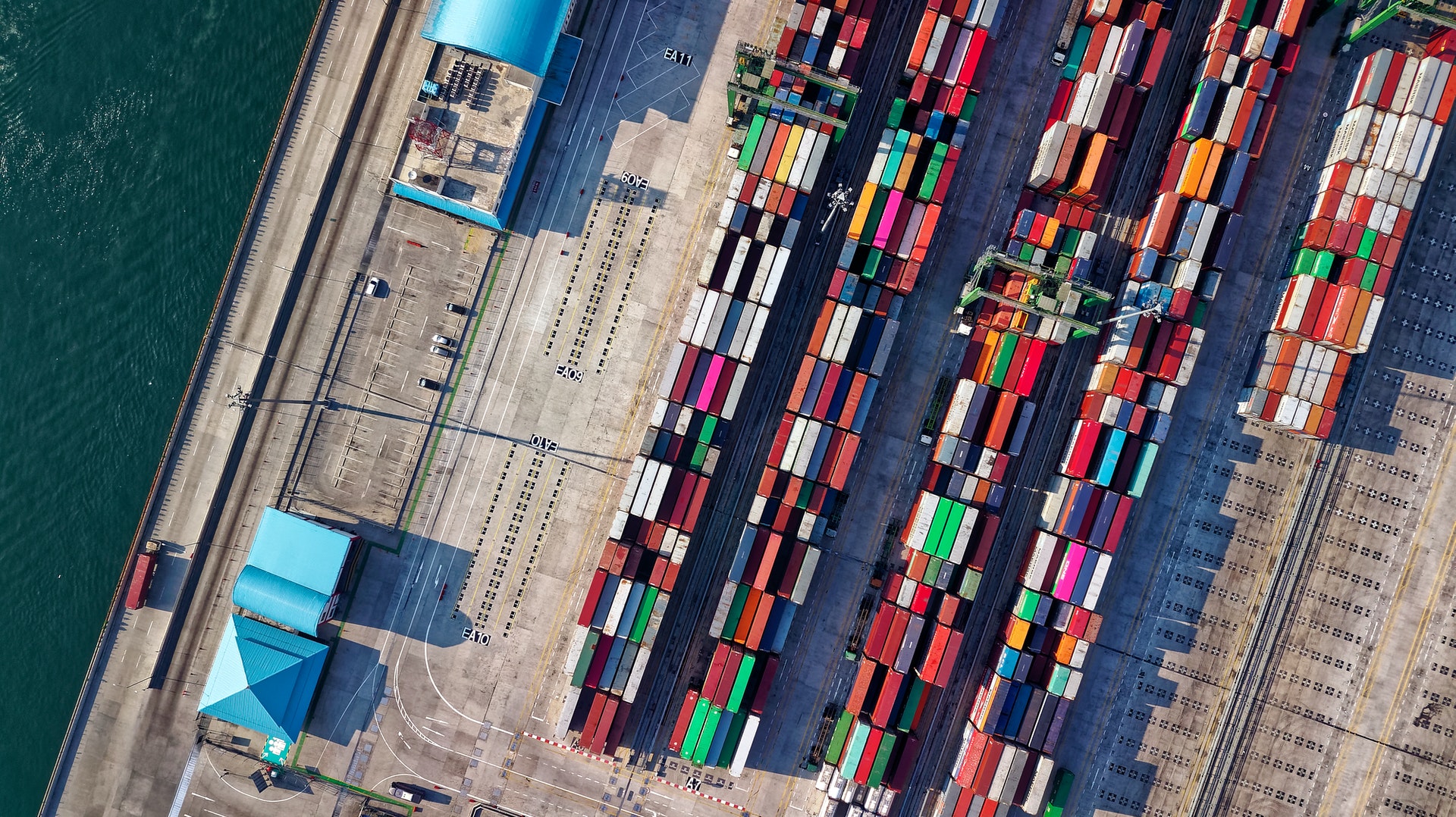
Inbound Logistics
What's the difference between supply chain visibility and transparency.
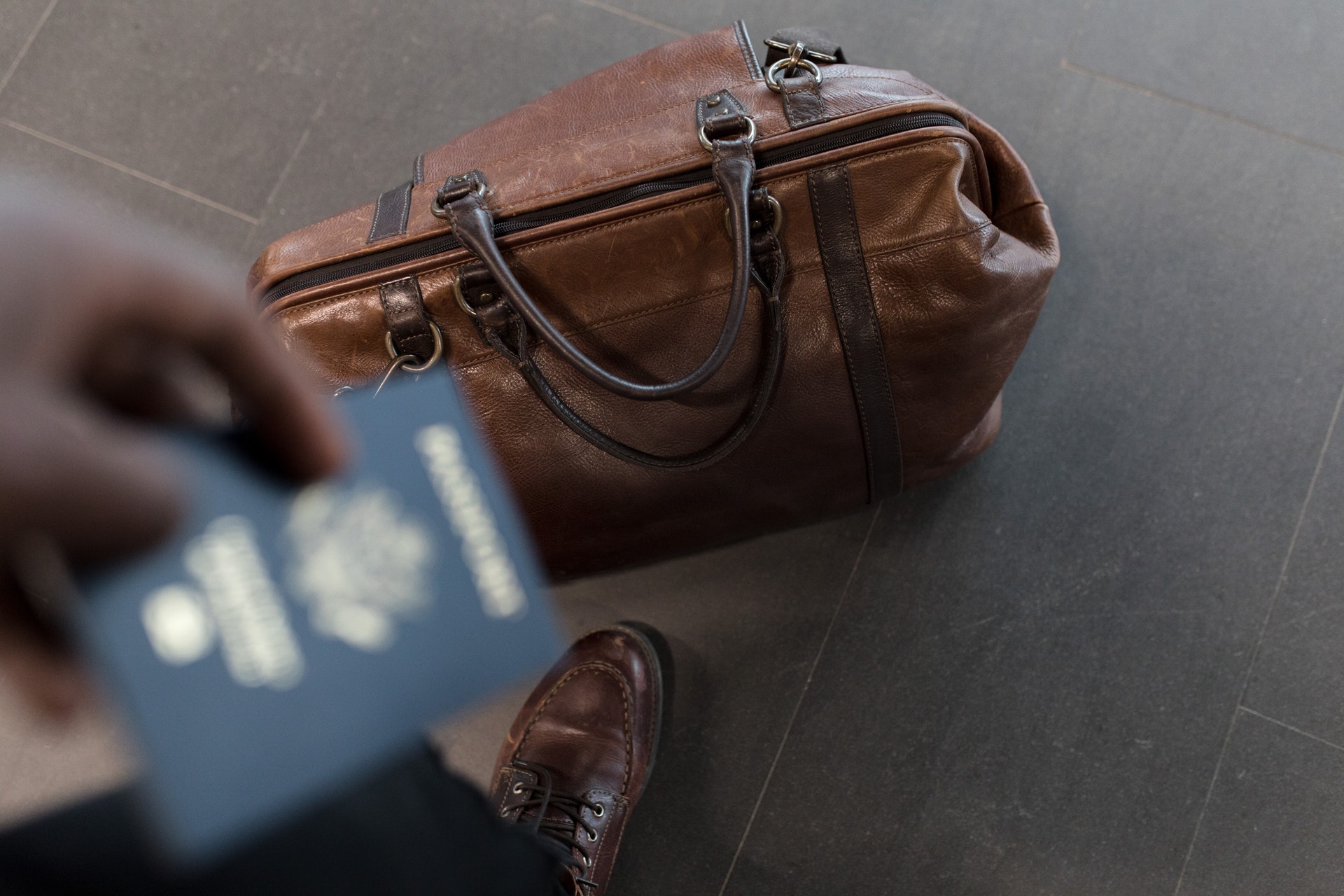
Academic Minute
Mazhar arikan, university of kansas, talks baggage fees, recent research & news.
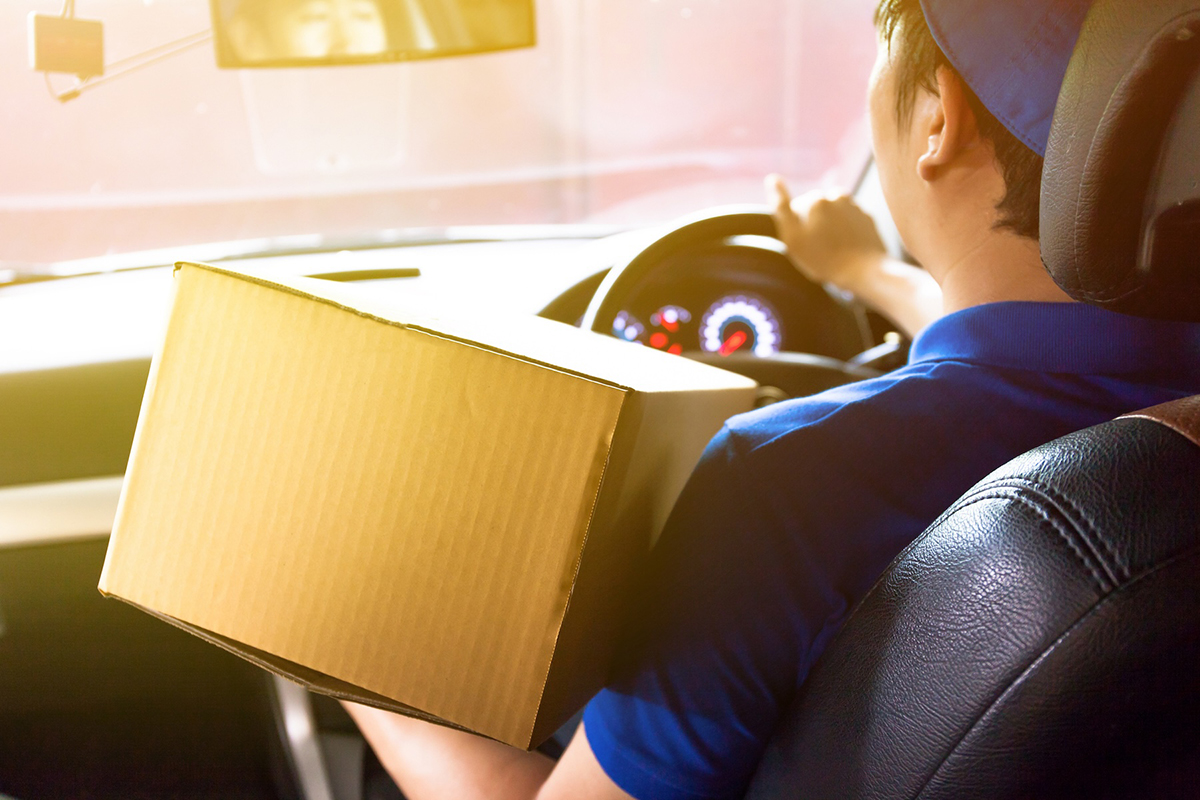
Study examines strategies to improve effect of parking time on last-mile deliveries
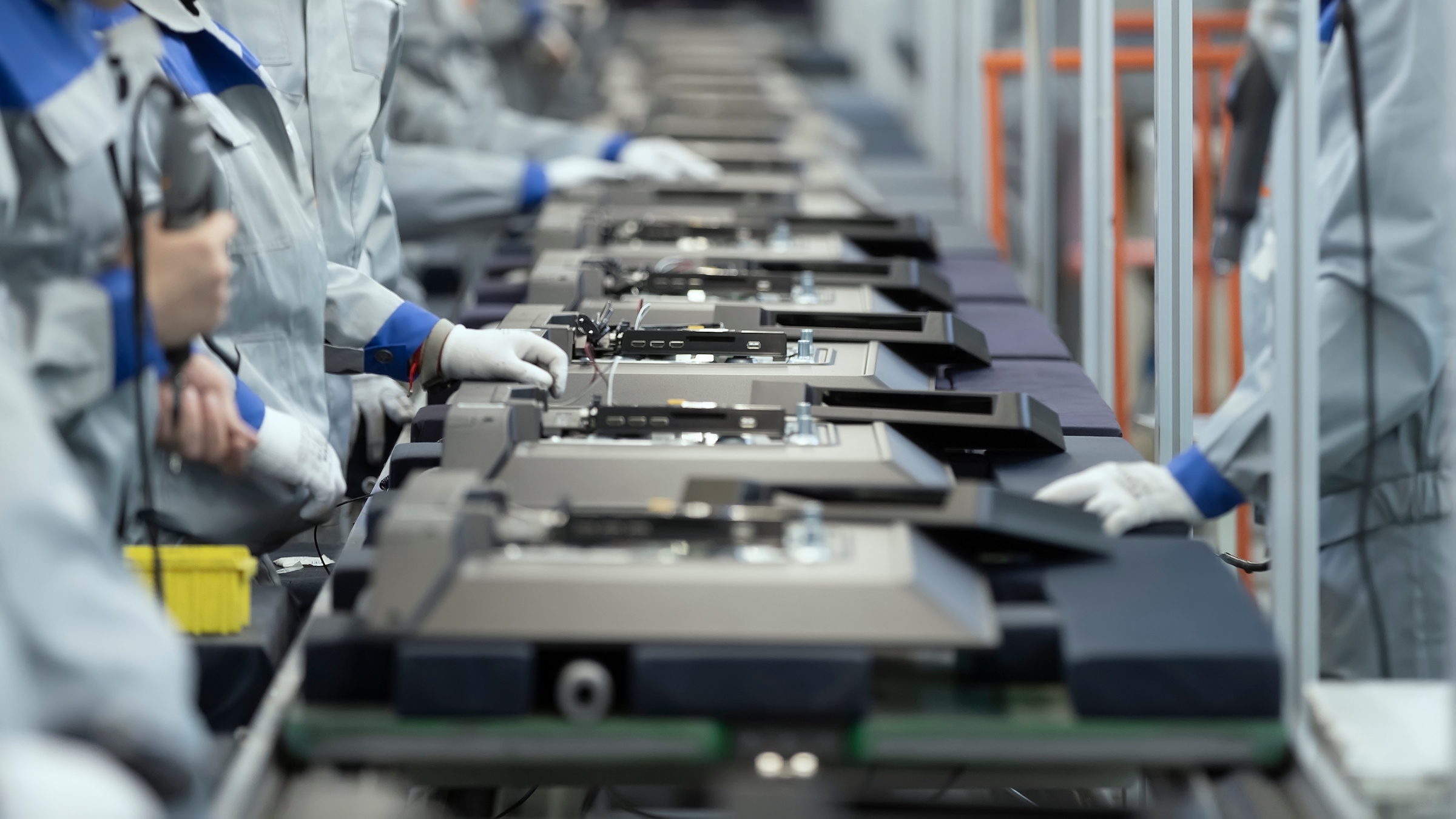
Workplace 'slavery' still embedded in supply chain, researcher says
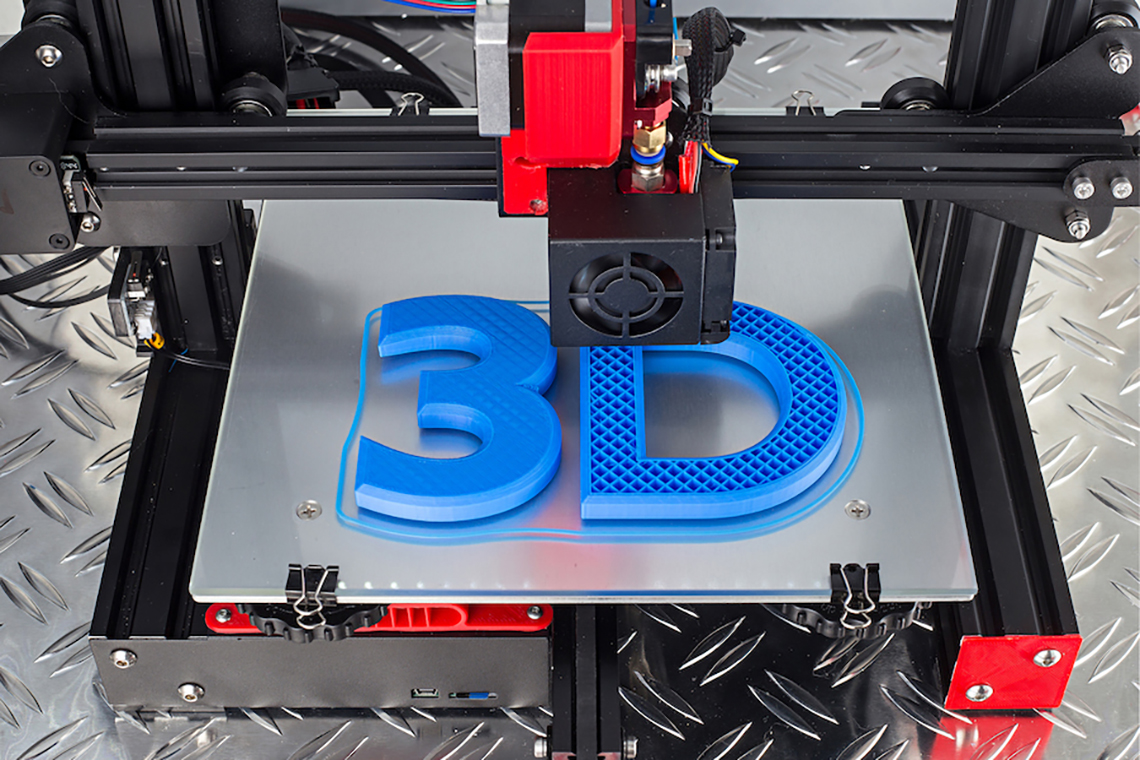
Business advantages of customers using 'personal fabrication' in 3D printing
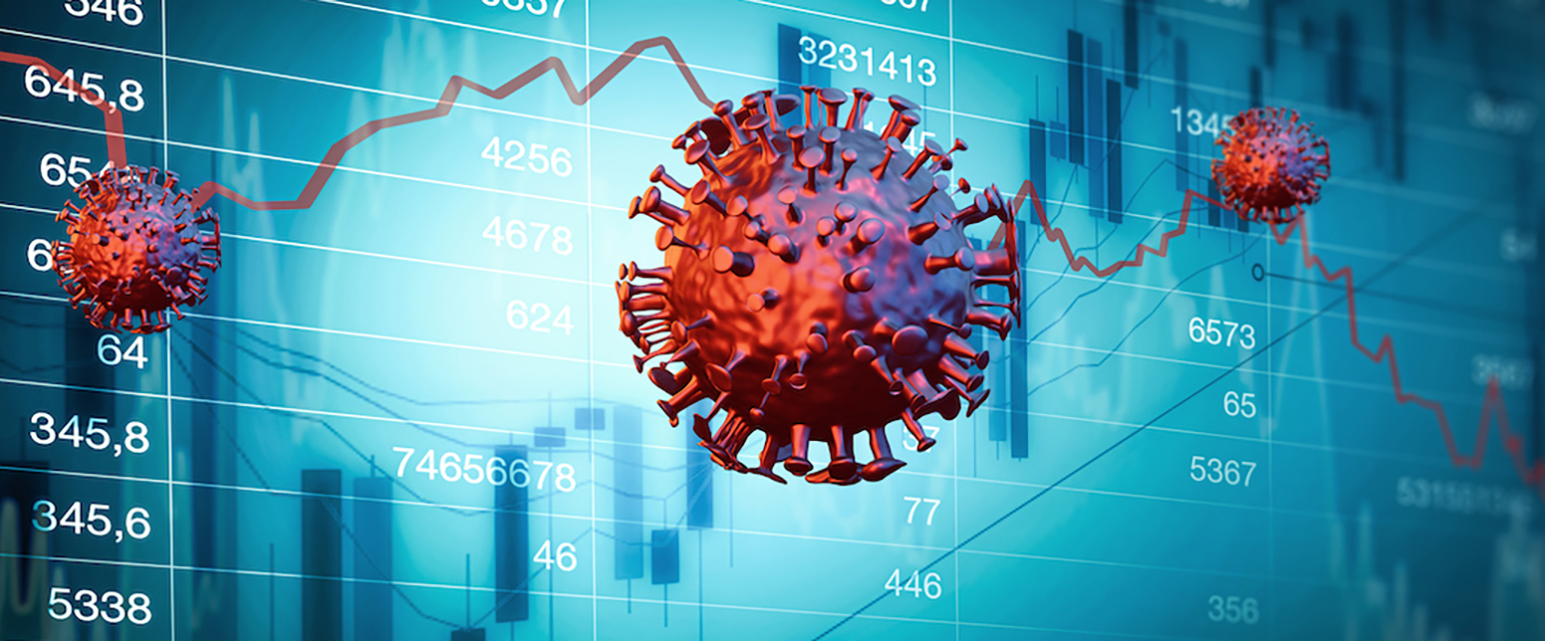
Pandemic altered predictability of stock market, according to social media data
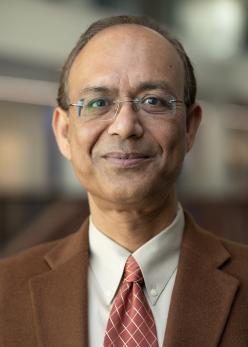
Analytics, Information, Operations faculty
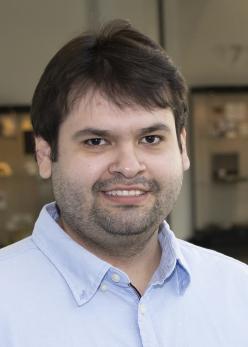
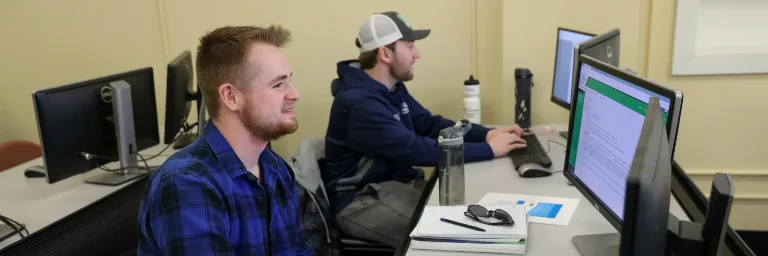
Data Science vs. Data Analytics: What’s the Difference?
Author: University of North Dakota May 22, 2024
The origins of data science are deeply rooted in the convergence of computer science and statistics.
Request Information
In the early days of computing, statisticians quickly recognized the profound potential of computers to enhance data analysis. Then, as data science continued to evolve, a complementary discipline began to take shape—data analytics.
While both fields deal with data, they exhibit distinctive characteristics and cater to different objectives, ultimately leading to divergent career paths. Continue reading to explore the key distinctions between data science vs. data analytics and determine which path aligns with your career aspirations.
What is Data Science?
Data science is an interdisciplinary field that focuses on extracting knowledge and insights from structured and unstructured data. Using various techniques, from statistics, machine learning and data mining, data scientists collect, clean and analyze data to make predictions and inform strategic decision-making. The process typically includes data collection, rigorous data cleaning, deep analysis and the interpretation of the results.
This field applies to virtually every industry, including finance, healthcare and technology, showcasing its wide-ranging utility and importance in solving complex problems.
What is Data Analytics?
Data analytics is the systematic examination of datasets to uncover valuable insights and informed conclusions, crucial for strategic decision-making in organizations.
The discipline includes several distinct approaches. Descriptive analytics summarizes past data to clarify what has occurred, setting the stage for diagnostic analytics, which probes into the causes behind these events. Building on this understanding, predictive analytics uses historical data to anticipate future outcomes. Finally, prescriptive analytics offers targeted strategies based on these forecasts, helping organizations achieve optimal results.
This progression from understanding what has happened to shaping future outcomes is vital for enhancing performance and sparking innovation across various industries.
Education Requirements
Pursuing a data science or analytics career requires a solid educational foundation in relevant fields. Each area has its specific educational paths that help aspirants acquire the necessary skills and knowledge.
Data Science Education
The journey into data science typically begins with an undergraduate degree in Data Science , Computer Science , statistics, Mathematics or a related field. These programs lay the groundwork by equipping students with essential mathematical and computational skills crucial for handling and analyzing complex data sets.
To deepen their knowledge and specialize further, many individuals pursue graduate degrees, such as a master's degree in Data Science or related fields. These programs often focus on advanced topics like machine learning, big data technologies, artificial intelligence and sophisticated statistical methods.
Those looking to push the boundaries of data science may even opt for Ph.D. programs, which are research-focused and allow for specialization in cutting-edge areas such as deep learning, natural language processing or cognitive computing.
Data Analytics Education
Aspiring data analysts often start with undergraduate degrees in Business Analytics, Information Systems , Statistics or similar fields. These degrees provide a solid foundation for understanding how to collect, process and analyze data as well as apply it in a business context to solve problems and support decision-making.
Advancing in data analytics might involve pursuing a master's degree in Data Analytics, Business Intelligence or Applied Statistics . These programs typically focus on more strategic aspects of data analytics, including advanced statistical methods, predictive analytics and the use of analytics in business strategy. Post-graduate certifications in tools and technologies such as SQL, Tableau, SAS or Power BI are also highly valued in the industry.
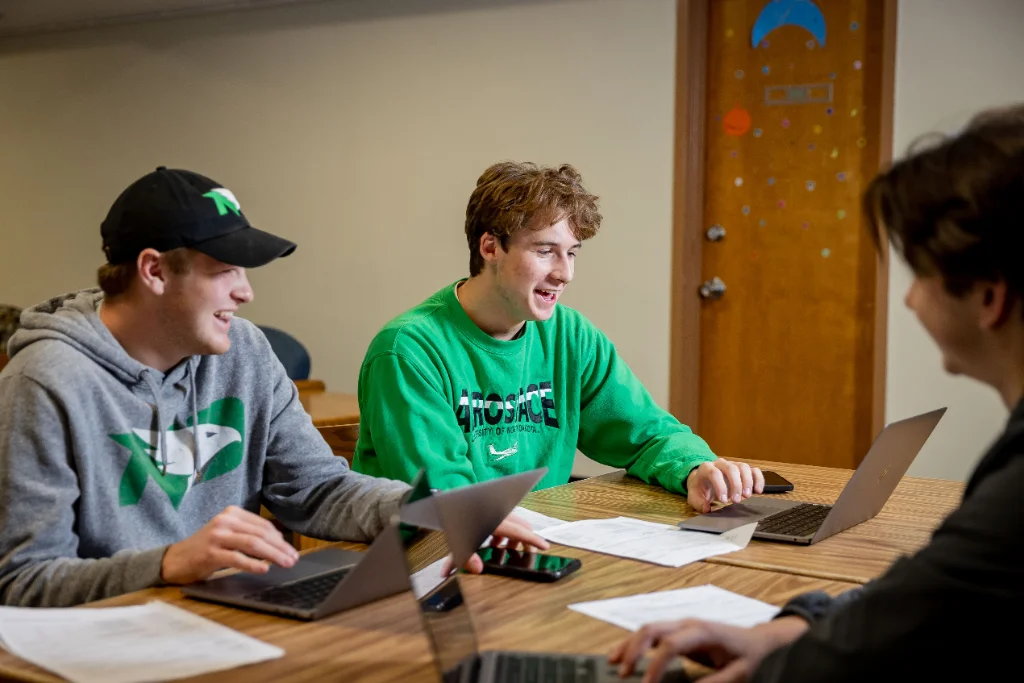
Skill Set Requirements
Both data science and data analytics professionals require a specific set of skills; however, some of these skills overlap, reflecting the integrated nature of these disciplines.
Data Science Skills
Data scientists are expected to possess a wide array of skills, each critical for various aspects of their role:
- Programming languages: Python and R for coding and data manipulation
- Machine learning: Application of algorithms and models for complex problem-solving
- Data visualization: Creation of visual representations to clarify and highlight data trends
- Domain expertise: Deep industry knowledge to effectively apply data insights
- Data analysis: Interpretation of complex datasets to extract actionable information
- Big data tools: Proficiency with platforms like Hadoop and Spark for handling large data volumes
- Deep learning frameworks: Familiarity with sophisticated models for advanced data analysis
- Statistical analysis: Application of statistical methods for meaningful data interpretation
Data Analytics Skills
Data analysts require several core competencies to effectively handle data:
- Data manipulation: Proficiency in cleaning, sorting, and organizing data
- Database management: Skilled in using SQL for efficient data retrieval and management
- Data visualization: Ability to create clear and impactful visual representations of data
- Data interpretation: Competence in analyzing large datasets to extract meaningful insights
- Analytical thinking: Strong critical thinking skills to evaluate data and assess implications
- Communication: Capability to translate technical data findings into understandable and actionable business insights
- Statistical analysis: Solid understanding of statistical methods to conduct accurate data evaluations
Career Opportunities
The fields of data science and data analytics offer varied career opportunities, some overlapping while others are unique to each field.
Data Science Careers
Data science offers a range of career paths, including:
- Data scientist
- Machine learning engineer
- Artificial intelligence specialist
- Deep learning engineer
- Big data engineer
- Algorithm developer
These roles typically focus on the development of new algorithms, predictive models and systems for data analysis and interpretation, pushing the boundaries of what organizations can achieve with data.
Data Analytics Careers
In data analytics, the roles are often more directly linked to business applications and include:
- Data analyst
- Business intelligence analyst
- Data engineer
- Marketing analyst
- Financial analyst
These professionals are crucial in analyzing data to inform business strategies, improve operational efficiency and enhance market understanding across various industries, such as finance, marketing and healthcare.
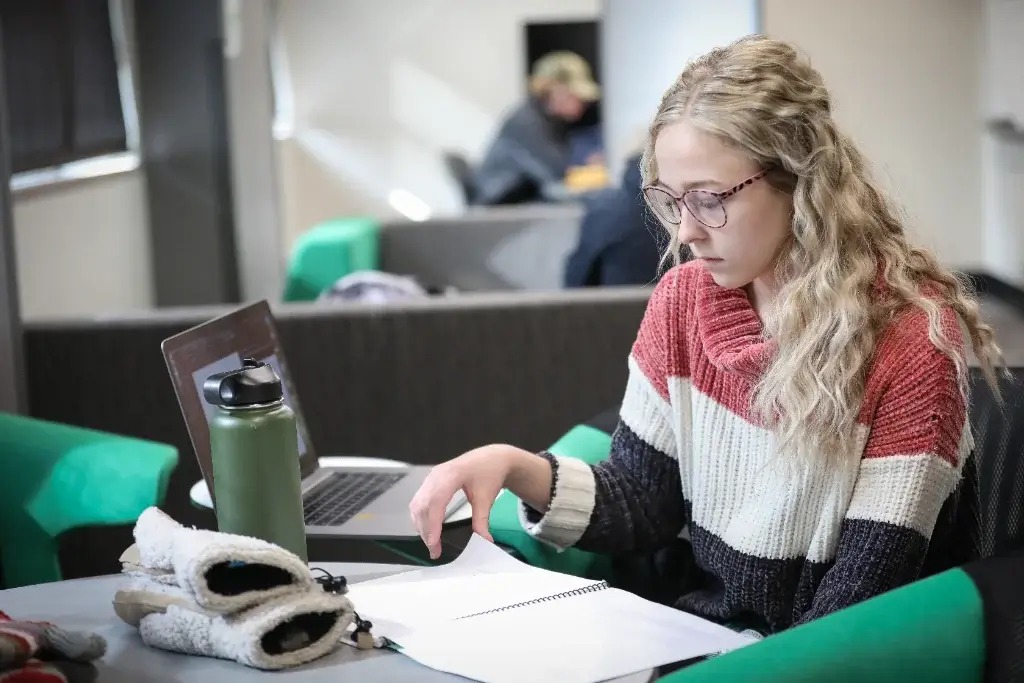
Salary and Job Outlook
The growing emphasis on data has propelled the fields of data science and data analytics into the spotlight, predicting a promising future for both domains. Not only are these roles expected to remain in high demand, but they also offer attractive salaries and substantial career growth opportunities.
Data Dcience Jobs
The role of a data scientist is not only intellectually rewarding but also financially lucrative, with an average salary of approximately $108,020 per year . The job market for data scientists is robust, boasting about 168,900 professionals engaged in this dynamic field. Looking ahead, the outlook for data science is exceptionally bright, with employment projected to grow at a rate of 35% from 2022 to 2032, a pace much faster than the average for all occupations.
Data Analytics Jobs
Similarly, data analytics professionals enjoy competitive salaries, with an average annual income of around $77,445 . The salary can vary depending on factors such as experience, location and the specific industry in which one works.
The job market for data analysts is also experiencing a significant upswing and is projected to grow by 25% by 2030. This expansion is a testament to the growing reliance on data analytics to inform and shape business decisions.
Challenges and Future Trends
Professionals in data science and analytics face numerous challenges, including maintaining data quality, managing privacy concerns and overcoming scalability issues. As data volumes continue to grow, keeping data accurate and clean becomes even more challenging.
Privacy concerns are also significant as both fields handle sensitive information, requiring compliance with increasingly stringent regulations. Scalability is another hurdle, as systems must be designed to handle large-scale data analysis.
Several trends and technologies are shaping the future of data science and data analytics. For instance, the advancement of AI and machine learning is expanding the capabilities and efficiency of data analysis. Big data analytics is also becoming more sophisticated, providing deeper insights and predictive capabilities. Additionally, there is a growing focus on the ethical aspects of data usage, pushing for transparency and fairness in data-driven decision-making.
Data Science vs. Data Analytics: Which One Should You Choose?
Choosing between a career in data science and analytics depends largely on your interests, strengths and aspirations. Data science might be the right path if you are drawn to complex mathematical models, deep statistical analysis and building algorithms.
On the other hand, if you are interested in using data to solve business problems, improve operational efficiencies and support decision-making, then a career in data analytics could be more suitable.
To make an informed decision, assess your skills in programming, mathematical analysis and interest in business applications. Reflect on whether you thrive in abstract mathematical concepts or prefer the tangible impact of data-driven solutions in business contexts. Also, consider your long-term career aspirations and how each path aligns with your goals.
Keep in mind that the fields of data science and data analytics are not mutually exclusive, and there is often overlap between the two. Ultimately, choose the path that resonates most with your passion, strengths and professional aspirations.
All in all, while both data science and data analytics revolve around the use of data, they differ significantly in scope and application. But, regardless of which path you choose, each offers a wealth of opportunities and the potential for a rewarding career in a field that is only expected to grow in relevance and demand.
Remember, in a world increasingly driven by data, your ability to analyze and interpret this vital resource can set you apart and propel you to success. Let UND help you unlock the full potential of data in your professional journey. Explore our cutting-edge programs to gain the expertise and skills necessary to thrive in these fields.
What tools and technologies are commonly used in data science compared to data analytics? ( Open this section)
Data science often utilizes tools such as Python, R, TensorFlow and Apache Hadoop, focusing on machine learning, deep learning and big data technologies. In contrast, data analytics frequently employs SQL, Tableau, Power BI and Excel, emphasizing data manipulation, visualization and reporting.
Are there any specific industries where data science is more prevalent compared to data analytics and vice versa? ( Open this section)
Data science is particularly prevalent in industries like technology, healthcare and finance, where predictive modeling and deep learning are crucial. Data analytics is more widespread in business sectors such as retail, e-commerce and marketing, where operational decisions and consumer behavior analysis are key.
How do data science and data analytics teams collaborate with other departments such as marketing, finance and operations? ( Open this section)
Data science and analytics teams work closely with marketing, finance and operations to provide insights that inform strategies and improve efficiency. They help these departments by analyzing trends, forecasting outcomes and optimizing processes based on data-driven evidence.
By clicking any link on this page you are giving your consent for us to set cookies, Privacy Information .
- Software Development
- Data Science and Business Analytics
- Press Announcements
- Scaler Academy Experience
25 Data Science Tools to Consider in 2024
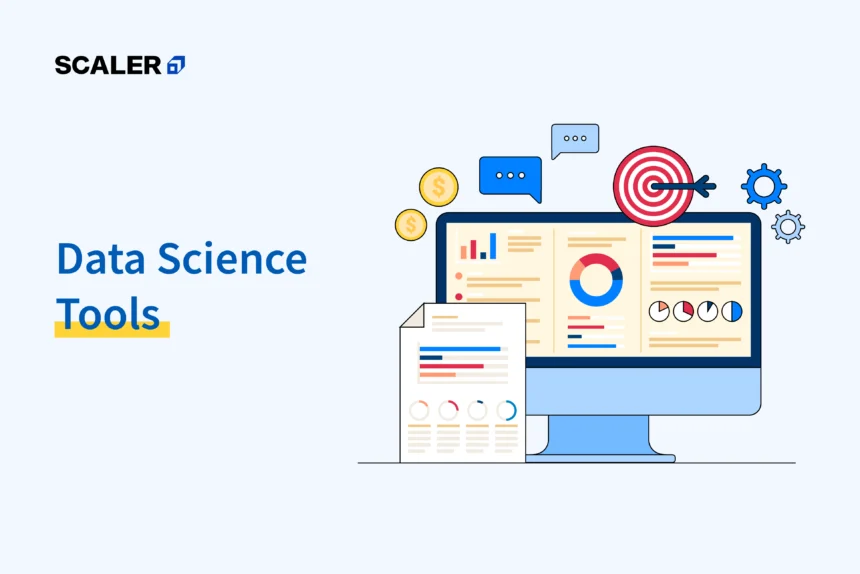
#ezw_tco-2 .ez-toc-title{ font-size: 120%; font-weight: 500; color: #000; } #ezw_tco-2 .ez-toc-widget-container ul.ez-toc-list li.active{ background-color: #ededed; } Contents
Data science has emerged as a transformative force, revolutionizing industries and reshaping how we understand and interact with the world. Yet, a potent toolkit of technologies and tools that enable data scientists to gather, examine, visualize, and model data powers this magic in the background. Imagine trying to build a house without a hammer, saw, or screwdriver. It would be nearly impossible! Similarly, data scientists rely on specialized tools to tackle the complexities of data analysis and extract meaningful insights. Just as a carpenter needs tools, a data scientist needs a powerful toolkit. Scaler’s Data Science Course provides you with the essential toolkit for success. These tools, which range from machine learning frameworks that create predictive models to programming languages that facilitate data manipulation, are the foundation of the data science process. In this comprehensive guide, we will look at the top 25 data science tools shaping the landscape in 2024. We’ll explore their unique features, capabilities, and applications, empowering you to choose the right tools for your specific needs and level up your data science game. To fully utilize data and spur innovation in your industry, regardless of experience level, you must comprehend and become proficient with these tools.
Why Use Data Science Tools?
Data science tools are a necessity in today’s data-driven world. They provide you the ability to solve challenging problems quickly, find undiscovered information, and come to wise judgments. Let’s explore why these tools are essential for anyone working with data:
- Enhanced Productivity: Data science tools automate repetitive and time-consuming tasks, freeing you up to focus on higher-level analysis and decision-making. Instead of manually cleaning data or performing calculations, you can leverage these tools to streamline your workflow and accelerate your projects.
- Simplified Complex Processes: Data science involves complex algorithms, statistical models, and massive datasets. By offering user-friendly interfaces and pre-built functions that facilitate data exploration, analysis, and visualization, specialized tools streamline these procedures.
- Improved Accuracy and Efficiency: Manual data analysis is prone to human error. Data science tools automate calculations and statistical analysis, ensuring greater accuracy and reducing the risk of mistakes. Additionally, by processing large datasets more quickly, these tools improve the effectiveness and timeliness of your analysis.
In addition to these core benefits, data science tools also offer features like collaboration, reproducibility, and scalability, making them indispensable for teams working on data-driven projects. You can increase productivity, improve the caliber of your analysis, and ultimately make better decisions based on insights from data by implementing these tools into your workflow.
Evolution of Data Science Tools
The tools that data scientists use today are a far cry from the early days of punch cards and mainframe computers. Over the decades, data science tools have evolved dramatically, becoming more powerful, accessible, and user-friendly. Let’s journey through the key milestones in this evolution:
1. Early Statistical and Analytical Tools (1960s-1990s)
The foundation of data science tools began with statistical packages like SPSS (Statistical Package for the Social Sciences) and SAS (Statistical Analysis System). These tools were primarily used for academic research and business analytics, offering capabilities for basic statistical analysis, data management, and reporting.
2. Emergence of Open Source Programming Languages (2000s)
The early 2000s saw the rise of open-source programming languages like Python and R, which democratized access to data analysis tools and fostered a vibrant community of developers and users. These languages offered flexibility, customizability, and a vast array of libraries for data manipulation, analysis, and visualization.
3. Big Data and Scalable Computing (2010s)
The explosion of big data in the 2010s necessitated tools that could process and analyze massive datasets efficiently. Hadoop, an open-source framework for distributed storage and processing, emerged as a game-changer. Later, Apache Spark, with its in-memory computing capabilities, further accelerated big data processing.
4. Development of Machine Learning Libraries (2010s-Present)
The development of machine learning libraries like scikit-learn (Python) and caret (R) made it easier for data scientists to build and deploy complex machine learning models. These libraries provided a wide range of algorithms, model evaluation metrics, and tools for data preprocessing, democratizing access to powerful machine learning capabilities.
5. Interactive Data Science and Visualization Tools (2010s-Present)
The rise of interactive data science environments like Jupyter Notebook and RStudio revolutionized the way data scientists work. These tools allowed for seamless integration of code, visualizations, and narrative text, facilitating exploration, experimentation, and collaboration. Additionally, data visualization tools like Tableau and Power BI emerged, empowering users to create interactive and visually appealing dashboards to communicate insights effectively.
6. AutoML and Cloud-Based Data Science Platforms (2020s)
The 2020s have seen the rise of AutoML (Automated Machine Learning) platforms that automate various aspects of the machine learning process, from data preprocessing and feature engineering to model selection and hyperparameter tuning. Cloud-based data science platforms like Amazon SageMaker, Google Cloud AI Platform, and Microsoft Azure Machine Learning have also gained prominence, offering scalable and accessible infrastructure for data storage, processing, and model deployment.
7. Integrated Data Science Tools (Emerging Trend)
We’re now witnessing the emergence of integrated data science platforms that combine multiple tools and capabilities into a single, unified environment. These platforms aim to streamline the data science workflow by providing seamless integration between data collection, cleaning, analysis, modeling, and deployment. The evolution of data science tools is far from over. As technology continues to advance at an unprecedented pace, we can expect even more powerful, intuitive, and accessible tools to emerge, empowering data scientists to tackle complex challenges and unlock the full potential of data.
Top 25 Essential Data Science Tools
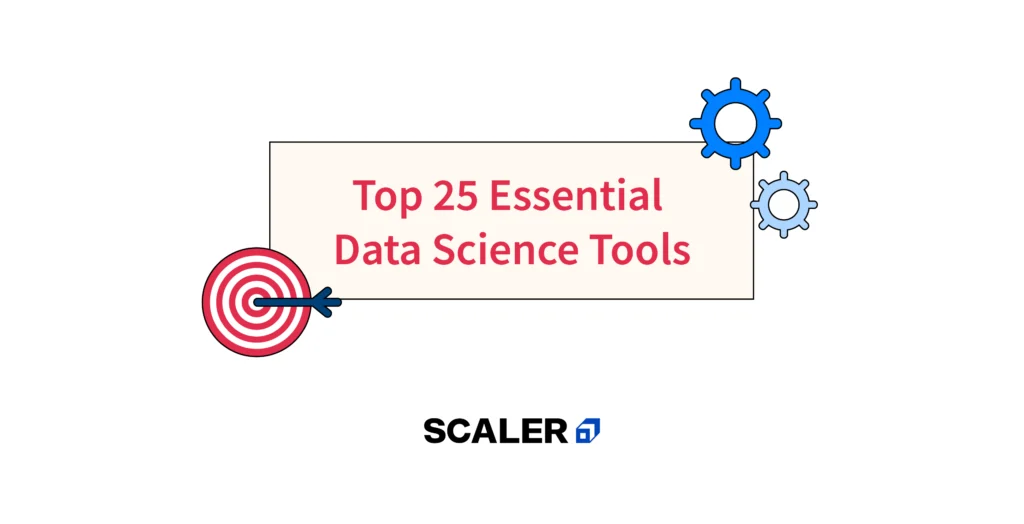
This curated list explores 25 essential tools empowering data scientists to tackle complex challenges, unlock hidden insights, and drive innovation across industries:
1. Apache Spark
This powerful open-source framework is a game-changer for big data processing and analytics. It offers lightning-fast speeds by distributing computations across clusters of computers, making it ideal for handling massive datasets that would overwhelm traditional tools. Spark’s versatility shines through its support for diverse tasks like machine learning, real-time stream processing, interactive queries, and even graph processing. With its easy-to-use APIs and integration with popular programming languages like Python, Scala, and Java, Spark has become a staple for data engineers and scientists alike.
- Blazing-fast speed due to in-memory processing and optimized execution
- Ease of use with high-level APIs
- Versatile support for various data processing tasks
- Vibrant community with extensive resources and support.
- Real-time analytics of streaming data (e.g., fraud detection)
- Machine learning on large datasets (e.g., recommendation systems)
- Interactive data exploration
- Graph analysis (e.g., social network analysis).
This JavaScript library is your go-to tool for crafting dynamic and interactive data visualizations on the web. It provides a rich set of features for creating custom charts, graphs, maps, and infographics that respond to user interactions. D3.js is highly flexible and customizable, allowing you to tailor visualizations to your specific needs and data.
- Unparalleled flexibility and customization options.
- Ability to create highly interactive and engaging visualizations.
- Vast community of users and resources.
- Building interactive dashboards for data exploration.
- Creating data-driven stories for websites and blogs.
- Generating custom visualizations for scientific publications or reports.
3. IBM SPSS
A stalwart in the field of statistical analysis, IBM SPSS is a comprehensive software suite used by researchers, analysts, and businesses for decades. It offers a wide range of statistical procedures, from basic descriptive statistics to advanced modeling and forecasting techniques. With its user-friendly interface and extensive documentation, SPSS is a great option for both beginners and experienced statisticians.
- User-friendly interface with menus and dialog boxes.
- Comprehensive set of statistical tools and procedures.
- Integration with other IBM products, and extensive documentation and support.
- Social science research (e.g., survey analysis, experimental design).
- Market research, customer analytics, healthcare analytics, and quality control in manufacturing.
This relatively new programming language is making waves in the data science community. Julia is designed for high-performance numerical and scientific computing, offering a combination of speed, ease of use, and a familiar syntax reminiscent of Python. It’s quickly gaining popularity for its ability to bridge the gap between research and production, allowing for rapid prototyping and efficient deployment of data science applications.
- High performance comparable to C and Fortran.
- Dynamic typing with optional type declarations.
- Familiar syntax similar to Python.
- Support for parallel and distributed computing.
- Scientific computing (e.g., simulations, numerical analysis).
- Machine learning (especially deep learning).Data visualization.
- High-performance computing applications.
5. Jupyter Notebook
Think of Jupyter Notebook as your interactive data science diary. It’s a web-based platform that allows you to create and share documents that combine live code, equations, visualizations, and narrative text. This makes it an ideal tool for data exploration, analysis, and collaboration. Jupyter Notebook supports multiple programming languages, including Python and R, making it a versatile tool for data scientists of all stripes.
- Interactive environment for real-time feedback.
- Supports multiple programming languages.
- Easy to share and collaborate on notebooks.
- Ideal for documentation and reproducibility.
- Data cleaning and preparation.
- Exploratory data analysis.
- Statistical modeling.
- Machine learning experiments.
- Creating educational tutorials or presentations.
Deep learning can seem daunting, but Keras makes it accessible to everyone. This high-level neural network API simplifies building and training deep learning models. Its user-friendly interface and modular design allow you to quickly experiment with different architectures and parameters. Plus, Keras runs on top of popular deep learning frameworks like TensorFlow and Theano, giving you the best of both worlds: ease of use and powerful performance.
- User-friendly interface.
- Modular and extensible design.
- Support for multiple backends (TensorFlow, Theano, CNTK).
- Rapid prototyping capabilities.
- Image and speech recognition.
- Natural language processing.
- Recommendation systems.
- Generative models.
- Other deep learning applications.
This proprietary programming language and numeric computing environment is widely used in engineering, science, and academia. MATLAB offers a comprehensive set of mathematical and engineering tools, along with powerful visualization capabilities. Its extensive library of toolboxes caters to specific applications, making it a valuable asset for researchers and engineers working with complex data and simulations.
- Extensive library of mathematical and engineering functions.
- Advanced visualization tools.
- Interactive environment for algorithm development and testing.
- Support for parallel computing.
- Numerical analysis, signal processing.
- Image and video processing.
- Control systems.
- Financial modeling
- Simulations in various engineering and scientific domains.
8. Matplotlib
If you’re working with Python and need to create eye-catching plots, charts, or graphs, Matplotlib is your go-to library. It offers extensive flexibility and customization options, allowing you to tailor your visualizations to perfection. Whether you need a simple line plot or a complex 3D visualization, Matplotlib has the tools to bring your data to life.
- Highly customizable plots and charts.
- Supports various output formats (PNG, PDF, SVG).
- Integrates well with other Python libraries like NumPy and pandas.
- Creating publication-quality plots for scientific papers.
- Generating interactive visualizations for web applications.
- Exploring data relationships through exploratory data analysis.
This fundamental package is the backbone of numerical computing in Python. It provides support for large, multi-dimensional arrays and matrices, along with a vast collection of high-level mathematical functions to operate on these arrays. NumPy is essential for scientific computing, data analysis, and machine learning tasks, serving as the foundation for many other data science libraries.
- Efficient array operations.
- Broadcasting capabilities for element-wise operations.
- Support for linear algebra.
- Random number generation.
- Fourier transforms.
- Other mathematical operations.
- Numerical simulations.
- Image and signal processing.
- Linear algebra computations.
- Random number generation.
- Building block for other data science libraries.
Elevate your numerical computing skills with Scaler. Explore our Data Science Course Now!
This powerful library is your Swiss Army knife for data manipulation and analysis in Python. It introduces two key data structures: Series (one-dimensional arrays) and DataFrames (two-dimensional tables), making it incredibly easy to work with structured data. Pandas provides a wide range of tools for reading and writing data, cleaning and transforming data, handling missing values, merging and joining datasets, and performing exploratory data analysis.
- Intuitive data structures.
- Expressive and flexible syntax.
- Powerful data manipulation capabilities.
- Seamless integration with other Python libraries.
- Data cleaning and preparation.
- Exploratory data analysis.
- Feature engineering.
- Time series analysis.
- Data wrangling for machine learning.
The undisputed king of data science languages, Python boasts a simple syntax and a vast ecosystem of libraries that make it the go-to choice for beginners and seasoned professionals alike. Its user-friendly nature, coupled with powerful tools like pandas for data manipulation and scikit-learn for machine learning, empower data scientists to efficiently clean, analyze, and model data, regardless of their experience level.
- Easy-to-learn syntax.
- Extensive libraries for data analysis.
- Machine learning, and visualization.
- Large and active community with abundant resources and support.
- Machine learning.
- Web scraping.
- Automation.
- General-purpose programming.
12. PyTorch
Developed by Facebook’s AI Research Lab, PyTorch is a dynamic and flexible deep learning framework that has gained immense popularity in the research community. Its intuitive interface and Pythonic nature make it easy to learn and use. PyTorch’s dynamic computation graph allows for on-the-fly modifications, making it ideal for experimenting with new architectures and algorithms.
- Dynamic computation graph for flexibility.
- Pythonic syntax for ease of use.
- Strong community support.
- Efficient GPU acceleration for training large models.
- Natural language processing (NLP) tasks like language modeling and translation.
- Computer vision applications like image recognition and object detection.
- Reinforcement learning.
- Research in deep learning.
A powerful statistical programming language, R is a treasure trove of tools and packages for data analysis, statistics, and visualization. It boasts a vast and active community of users and developers who contribute to its extensive library of packages, making it a comprehensive solution for data science tasks.
- Extensive statistical capabilities.
- Powerful data visualization libraries (ggplot2).
- Comprehensive packages for specific domains (e.g., bioinformatics, finance).
- Strong community for support and collaboration.
- Hypothesis testing.
- Creating publication-quality plots, and specialized analysis in fields like bioinformatics.
- Econometrics.
- Social sciences.
This comprehensive analytics platform is a staple in the corporate world, providing a wide array of tools for data management, advanced analytics, business intelligence, and predictive modeling. SAS is known for its robustness, reliability, and scalability, making it a trusted choice for enterprise-level data analysis.
- Comprehensive suite of tools for data management.
- Analysis.
- Reporting
- Strong reputation for reliability and security.
- Extensive documentation and support.
- Industry-specific solutions for various sectors.
- Business intelligence and reporting.
- Customer analytics.
- Risk management.
- Fraud detection.
- Clinical trial analysis.
- Other enterprise-level data analysis tasks.
15. Scikit-learn
This user-friendly machine learning library for Python is a favorite among beginners and experienced data scientists alike. It offers a wide range of algorithms for classification, regression, clustering, and dimensionality reduction, along with tools for model evaluation, selection, and tuning.
- Simple and consistent API.
- Extensive documentation and examples.
- Efficient implementations of various algorithms.
- Active community support.
- Building predictive models for various tasks.
- Including spam detection.
- Image classification.
- Customer segmentation.
- Sentiment analysis.
Building upon the foundation of NumPy, SciPy is a powerful library for scientific and technical computing in Python. It provides modules for optimization, linear algebra, integration, interpolation, special functions, FFT, signal and image processing, ODE solvers, and other tasks common in science and engineering.
- Extensive collection of scientific computing tools.
- Seamless integration with NumPy.
- Active development community.
- Scientific research.
- Engineering simulations.
- Signal processing.
- Image analysis.
- Optimization problems.
- Numerical calculations in various domains.
17. TensorFlow
Developed by Google, TensorFlow is a leading open-source deep learning framework used for building and training a wide range of machine learning models. It offers flexibility and scalability, making it suitable for both research and production environments.
- Flexible architecture for building complex models.
- Efficient computation graphs for faster training.
- Support for distributed training across multiple devices.
- Extensive community and resources.
- Image and speech recognition.
- Recommender systems.
- Time series forecasting.
- Other cutting-edge applications in deep learning.
This open-source machine learning software is widely used for research and education. It offers a collection of machine learning algorithms for data mining tasks, including classification, regression, clustering, and association rule mining. Weka’s graphical user interface makes it accessible to non-programmers, while its Java API allows for customization and integration with other systems.
- User-friendly graphical interface
- Extensive collection of machine learning algorithms
- Support for various data mining tasks.
- Educational and research environments for learning data mining concepts
- Experimenting with different algorithms
- Analyzing small to medium-sized datasets.
19. Tableau
A visual analytics platform that enables users to create interactive dashboards and reports with ease. Its drag-and-drop interface makes it accessible to non-technical users, while its powerful analytical capabilities cater to data scientists and analysts alike.
- Intuitive drag-and-drop interface.
- wide range of visualizations.
- Powerful data blending and preparation capabilities.
- Ability to connect to various data sources.
- Business intelligence reporting.
- Data exploration and discovery.
- Creating interactive dashboards for stakeholders.
- Data storytelling.
Transform Data into Insights with Tableau Expertise. Enroll in Scaler’s Data Science Course Now!
20. Microsoft Excel
A ubiquitous spreadsheet software that remains a valuable tool for data analysis and manipulation. While not as powerful as specialized data science tools, Excel’s familiarity and ease of use make it a great starting point for beginners and a handy tool for quick calculations and basic data cleaning.
- Widely available and familiar interface.
- Built-in functions for basic statistical analysis and data manipulation.
- Ability to create simple visualizations.
- Simple data analysis.
- Creating basic charts and graphs.
- Data entry and management.
21. RapidMiner
A comprehensive data science platform that covers the entire data science lifecycle, from data preparation and modeling to deployment and monitoring. It offers a visual workflow designer, a wide range of algorithms, and integration with various data sources.
- User-friendly visual interface
- Comprehensive set of data science tools,
- Support for various data types and sources
- Ability to automate repetitive tasks.
- Predictive modeling
- Text mining
- Sentiment analysis
- Recommendation systems
- Data-driven decision-making
An open-source data analytics platform that enables users to create visual workflows for data preprocessing, analysis, and visualization. It offers a wide range of nodes for various tasks and supports integration with other tools and platforms.
- Free and open-source.
- Visual workflow designer.
- Extensive collection of nodes for various tasks.
- Data integration.
- Data cleaning and transformation.
- Machine learning
- Data visualization.
An open-source platform for building and deploying machine learning models at scale. It offers a variety of algorithms for supervised and unsupervised learning, as well as automated machine-learning capabilities.
- Scalable and distributed architecture
- automated machine learning features
- support for various data types and formats
- integration with popular programming languages like Python and R
- Building and deploying machine learning models for various tasks
- Including fraud detection
- Churn prediction
An open-source data mining and machine learning software suite with a visual programming interface. It offers a wide range of widgets for data visualization, preprocessing, modeling, and evaluation.
- Visual programming interface.
- Intuitive and easy to use.
- Wide range of widgets for various tasks.
- Interactive data exploration capabilities.
- Educational and research environments for learning data mining and machine learning concepts.
- Prototyping models.
- Exploring data.
25. Google Cloud AI
A suite of cloud-based tools and services for building and deploying machine learning and AI applications. It offers various products, including AutoML, Vertex AI, and BigQuery ML, for tasks like image and video analysis, natural language processing, and structured data analysis.
- Scalable and managed infrastructure.
- Access to Google’s powerful AI models and algorithms.
- Integration with other Google Cloud services.
- Pay-as-you-go pricing.
- Building and deploying AI-powered applications in various domains, such as healthcare, finance, retail, and manufacturing.
Unleash the Potential of Google Cloud AI: Enroll in Scaler’s Data Science Course Today!
Data Science and Machine Learning Platforms
As data science projects grow in complexity, managing the entire workflow—from data preparation to model deployment—can become a daunting task. This is where integrated data science and machine learning platforms come to the rescue. These platforms provide an integrated environment that simplifies and expedites the whole data science lifecycle, along with a range of tools and functionalities.
Overview of Integrated Platforms
Platforms for data science and machine learning are made to be complete, end-to-end solutions that cover every phase of the data science workflow. They typically include:
- Data Integration and Management: Tools for connecting to various data sources, cleaning and transforming data, and managing data pipelines.
- Exploratory Data Analysis (EDA): Interactive environments for data exploration, visualization, and statistical analysis.
- Model Building and Deployment: Tools for developing, training, evaluating, and deploying machine learning models.
- Collaboration and Governance: Features that enable teams to collaborate on projects, share knowledge, and ensure compliance with data governance policies.
Popular Examples and Their Benefits
Let’s see some of the Popular Examples of integrated platforms and their benefits.
- Databricks: A unified platform for data engineering, data science, and machine learning. It provides a collaborative environment, scalable infrastructure, and support for various programming languages and libraries.
- Amazon SageMaker: A fully managed service that provides tools for building, training, and deploying machine learning models at scale. It offers a wide range of built-in algorithms and frameworks, making it easy to get started with machine learning.
- Google Cloud AI Platform: A suite of tools and services for building and deploying AI applications on Google Cloud. It offers a wide range of features, including data labeling, model training, and model serving.
- Microsoft Azure Machine Learning: A cloud-based service for building, training, and deploying machine learning models. It provides a drag-and-drop interface for building models and offers a variety of pre-built algorithms and templates.
Benefits of Integrated Platforms
- Streamlined Workflow: By offering a single environment for every step of the procedure, integrated platforms streamline the data science workflow.
- Improved Collaboration: These platforms facilitate collaboration among team members, enabling them to share data, code, and models seamlessly.
- Scalability: Cloud-based platforms ensure that your projects can handle complex computations and large datasets by providing the flexibility to scale resources up or down as needed.
- Faster Time to Market: By automating repetitive tasks and providing pre-built tools and templates, these platforms accelerate the development and deployment of data science solutions.
- Reduced Complexity: Integrated platforms abstract away many of the technical complexities associated with data science, making it accessible to a wider range of users.
Criteria for Choosing the Right Data Science Tools
Selecting the best data science tools for your needs can be overwhelming, given the variety of options available. But don’t worry, we’ve got your back! Here are some key factors to consider when making your selection:
- Ease of Use: If you’re just starting, look for tools with user-friendly interfaces and intuitive workflows. Sophisticated instruments with steep learning curves can be intimidating and impede your development. However, if you’re an experienced data scientist, you might prioritize more advanced features and customization options, even if they come with a steeper learning curve.
- Community Support: A strong and active community is invaluable. It means you’ll have access to forums, tutorials, documentation, and help from fellow users when you encounter challenges. Tools with large communities often have better documentation, more extensive libraries, and faster updates.
- Scalability: Consider the size and complexity of your datasets. Some tools are better suited for smaller datasets, while others can handle massive amounts of data with ease. Choose tools that can scale with your needs as your projects grow.
- Cost: Data science tools range from free and open-source options to expensive enterprise-level solutions. Sort the tools that provide the best return on investment based on your budget.
- Functionality: Different tools excel at different tasks. Some are great for data visualization, while others are specialized for machine learning or statistical modeling. Determine your specific needs and choose tools that align with your goals.
- Integration: If you’re using multiple tools, ensure they can seamlessly integrate with each other. This will streamline your workflow and avoid compatibility issues.
- Support and Maintenance: Reliable customer support and regular updates are essential, especially for complex tools. Check for vendor support options and community forums to ensure you’ll have assistance when needed.
By carefully considering these factors, you can select the right tools that empower you to tackle your data science projects with confidence and efficiency. Remember, the best tools are the ones that fit your specific needs, skill level, and budget. So take your time, explore different options, and choose the ones that will help you unlock the full potential of your data.
Usage Scenarios and Case Studies
To truly appreciate the power of data science tools, let’s explore how they’re being leveraged by industry giants to drive innovation and transform business practices:
1. Netflix: Recommending Your Next Binge-Watch (Python, TensorFlow)
Netflix’s personalized recommendation engine is a classic example of data science in action. It utilizes Python for data processing and TensorFlow for building sophisticated machine learning models that analyze viewer behavior, preferences, and ratings to suggest content tailored to individual tastes. This not only keeps users engaged but also contributes significantly to Netflix’s bottom line.
2. Airbnb: Optimizing Pricing and Demand Forecasting (R, Python)
Airbnb uses R and Python extensively for analyzing market trends, predicting demand fluctuations, and optimizing pricing strategies. By leveraging data science models, Airbnb can dynamically adjust prices based on factors like seasonality, local events, and competitor pricing, ensuring optimal occupancy rates and revenue generation.
3. Facebook: Understanding User Behavior and Ad Targeting (PyTorch, Spark)
Facebook relies on PyTorch to power its machine learning models, which are trained on massive datasets to understand user behavior, preferences, and interests. This information is then used to deliver targeted advertising, ensuring that users see ads that are relevant to their needs and interests, thus maximizing ad revenue. Spark is also utilized for processing the vast amounts of data generated by the platform.
4. Amazon: Enhancing Product Recommendations and Supply Chain Management (Python, AWS)
Amazon’s recommendation engine is powered by a sophisticated blend of Python-based algorithms and Amazon Web Services (AWS) infrastructure. By analyzing vast amounts of customer data, including purchase history, browsing behavior, and product reviews, Amazon can deliver personalized product recommendations that drive sales and improve customer satisfaction. Additionally, Amazon uses data science to optimize its complex supply chain, forecasting demand, managing inventory, and ensuring efficient delivery.
5. Tesla: Building Autonomous Vehicles (Python, TensorFlow, PyTorch)
Tesla’s self-driving cars are a testament to the power of data science and machine learning. Python is used extensively for data processing and analysis, while TensorFlow and PyTorch are leveraged for building the complex deep learning models that power the cars’ perception, decision-making, and control systems. These models are trained on massive amounts of real-world driving data, continuously learning and improving their ability to navigate roads and avoid obstacles.
The Takeaway:
These are just a few instances of how business executives are using data science tools to obtain a competitive advantage, spur innovation, and provide individualized experiences. By understanding the capabilities and applications of these tools, you can identify the right ones for your projects and unleash the power of data to transform your business or research.
Scaler’s Data Science Course: Your Comprehensive Toolkit
If you’re looking for a structured path to mastering the essential data science tools and techniques, consider exploring Scaler’s comprehensive Data Science Course . With its industry-vetted curriculum, hands-on projects, and expert mentorship, Scaler equips you with the skills and knowledge needed to excel in this dynamic field.
With Scaler, you’ll gain a deep understanding of Python, SQL, machine learning libraries, and data visualization tools, all while working on real-world projects that build your portfolio and prepare you for a rewarding career in data science.
Read More Articles on Data Science
- Data Science Roadmap
- How to Become a Data Scientist
- Career Transition to Data Science
- Career in Data Science
- Data Science Career Opportunities
In the ever-evolving landscape of data science, the tools you choose can be the difference between uncovering groundbreaking insights and getting lost in a sea of information. With the correct tools, you can not only expedite your workflow but also take on challenging tasks, obtain a deeper understanding, and effectively present your findings.
Whether you’re a seasoned data scientist or just starting your journey, it’s crucial to explore and experiment with different tools to find the ones that best suit your needs and working style. From versatile programming languages like Python and R to specialized machine learning frameworks like TensorFlow and PyTorch, each tool brings its own unique strengths to the table.
Remember, the world of data science tools is constantly evolving. New technologies and platforms emerge regularly, offering innovative ways to analyze, visualize, and model data. By staying curious and embracing new tools, you can stay at the forefront of this exciting field and unlock the full potential of data.
What is the best tool for data science?
There’s no single “best” tool, as the ideal choice depends on your specific needs and skill level. Because of its adaptability and simplicity of use, Python is frequently suggested for novices, whereas R is preferred by individuals with a solid background in statistics. Ultimately, the best tool is the one that best suits your individual needs and preferences.
How do I choose the right data science tool?
Consider factors like ease of use, community support, scalability, cost, functionality, integration with other tools, and the specific tasks you need to accomplish. Research different options, try out free trials, and seek recommendations from experienced data scientists to find the tools that best fit your workflow.
Can I learn data science tools online?
Absolutely! Many online platforms offer courses, tutorials, and resources for learning data science tools. You can find comprehensive programs, like Scaler’s Data Science Course, or specialized courses on specific tools like Python, R, or Tableau.
What tools should a beginner in data science learn first?
Python is a great starting point for beginners, as it’s relatively easy to learn and offers a wide range of libraries for data analysis and machine learning. SQL is also essential for working with databases, and a basic understanding of data visualization tools like Tableau or Matplotlib can be helpful.
Are there any free data science tools?
Yes, many free and open-source data science tools are available, such as Python, R, Jupyter Notebook, and Weka. These tools offer powerful capabilities and are widely used in the data science community.
Leave a Reply Cancel reply
Your email address will not be published. Required fields are marked *
Save my name, email, and website in this browser for the next time I comment.
Get Free Career Counselling
By continuing, I have read and agree to Scaler’s Terms and Privacy Policy
Get Free Career Counselling ➞
- Go back to Main Menu
- Client Log In
- MSCI Client Support Site
- Barra PortfolioManager
- MSCI ESG Manager
- MSCI ESG Direct
- Global Index Lens
- MSCI Real Assets Analytics Portal
- RiskManager 3
- CreditManager
- RiskManager 4
- Index Monitor
- MSCI Datscha
- MSCI Real Capital Analytics
- Total Plan/Caissa
- MSCI Fabric
- MSCI Carbon Markets
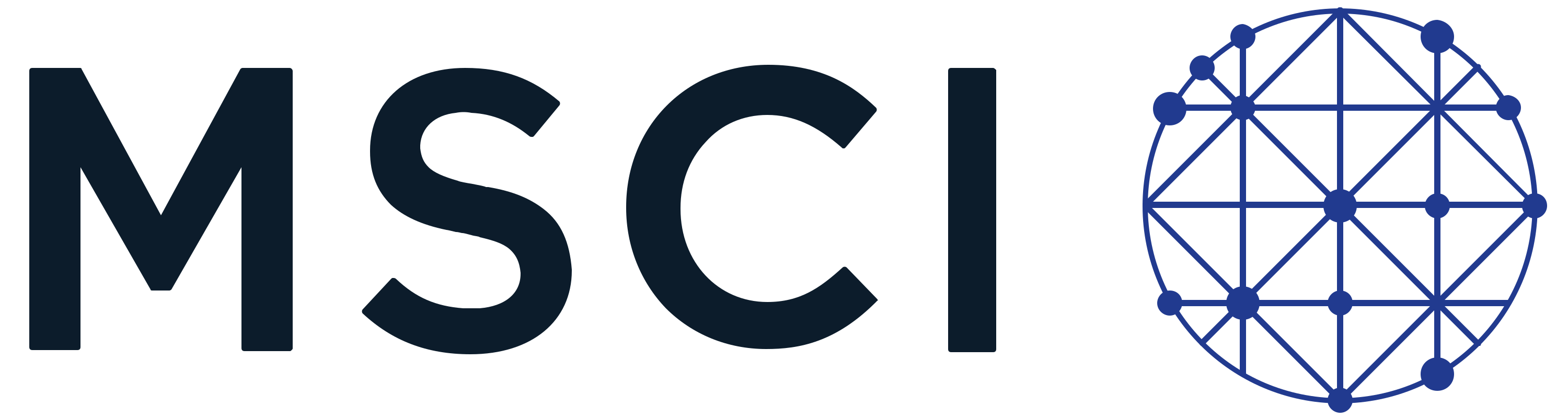
Navigation Menu
- Our Clients
Insights on MSCI One
Institutional client designed indexes (icdis), total portfolio footprinting, esg trends to watch, factor models, visualizing investment data.
- Our Solutions
- Go back to Our Solutions
- Analytics Overview
- Crowding Solutions
- Fixed Income Analytics
- Managed Solutions
- Multi-asset Class Factor Models
- Portfolio Management
- Quantitative Investment Solutions
- Regulatory Solutions
- Risk Insights
- Climate Investing
- Climate Investing Overview
Implied Temperature Rise
Trends 2024.
- Biodiversity
- Carbon Markets
- Climate Lab Enterprise
- Real Estate Climate Solutions
- Sustainable Investing
- Sustainable Investing Overview
ESG and Climate Funds in Focus
What is esg, role of capital in the net-zero revolution.
- Sustainability Reporting Services
- Factor Investing
- Factor Investing Overview
MSCI Japan Equity Factor Model
- Equity Factor Models
- Factor Indexes
- Indexes Overview
Index Education
Msci climate action corporate bond indexes.
- Client-Designed
- Direct Indexing
- Fixed Income
- Private Real Assets
Thematic Exposure Standard
- Go back to Indexes
- Resources Overview
MSCI Indexes Underlying Exchange Traded Products
- Communications
- Equity Factsheets
- Derivatives
- Methodology
- Performance
- Private Capital
- Private Capital Overview
Global Private Capital Performance Review
- Total Plan (formerly Caissa)
- Carbon Footprinting
- Private Capital Indexes
- Private Company Data Connect
- Real Assets
- Real Assets Overview
2024 Trends to Watch in Real Assets
- Index Intel
- Portfolio Services
- Property Intel
- Private Real Assets Indexes
- Real Capital Analytics
- Research & Insights
- Go back to Research & Insights
- Research & Insights Overview
- Multi-Asset Class
- Real Estate
- Sustainability
- Events Overview
Capital for Climate Action Conference
- Data Explorer
- Developer Community
- Technology and Data
2022 Annual Report
- Go back to Who We Are
- Corporate Responsibility
- Corporate Responsibility Overview
- Enabling Sustainable Investing
- Environmental Sustainability
- Governance Practices
- Social Practices
- Sustainability Reports and Policies
- Diversity, Equity and Inclusion
Henry A. Fernandez
- Recognition
Main Search
Esg rating hero banner, esg ratings.
Measuring a company’s resilience to long-term, financially relevant ESG risks
Social Sharing
Esg rating intro para, what is an msci esg rating.
MSCI ESG Ratings aim to measure a company’s management of financially relevant ESG risks and opportunities. We use a rules-based methodology to identify industry leaders and laggards according to their exposure to ESG risks and how well they manage those risks relative to peers. Our ESG Ratings range from leader (AAA, AA), average (A, BBB, BB) to laggard (B, CCC). We also rate equity and fixed income securities, loans, mutual funds, ETFs and countries.
ESG Ratings video
How do MSCI ESG Ratings work? What are significant ESG risks? What does a poor rating look like? How can you use them?
Download Transcript (PDF, 120 KB) (opens in a new tab)
ESG ratings
Download brochure (PDF, 1.08 MB) (opens in a new tab)
How do MSCI ESG Ratings work?
How does msci esg ratings work.
ESG risks and opportunities can vary by industry and company. Our MSCI ESG Ratings model identifies the ESG risks, (what we call Key Issues), that are most material to a GICS® sub-industry or sector. With over 13 years of live track history we have been able to examine and refine our model to identify the E, S, and G Key Issues which are most material to an industry.
View our Key Issues framework | ESG Methodologies (opens in a new tab) | What MSCI’s ESG Ratings are and are not
ESG Ratings module
A company lagging its industry based on its high exposure and failure to manage significant ESG risks
A company with a mixed or unexceptional track record of managing the most significant ESG risks and opportunities relative to industry peers
A company leading its industry in managing the most significant ESG risks and opportunities
Explore our ESG transparency tools
Contact sales
Explore our ESG Transparency Tools content - part 1
Explore the Implied Temperature Rise, Decarbonization Targets, MSCI ESG Rating and Key ESG Issues of over 2,900 companies.
Explore E, S & G Key Issues by GICS® sub-industry or sector and their contribution to companies' ESG Ratings.
Example: Explore the data metrics and sources used to determine the MSCI ESG Rating of a US-based producer of paper products.
Explore our ESG Transparency Tools content - part 2
ESG Fund Ratings aim to measure the resilience of mutual funds and ETFs to long term risks and opportunities.
Explore ESG and climate metrics for all MSCI equity, fixed income and blended indexes regulated by the EU.
ESG ratings Tabs
Integrating esg ratings into the investment process: key features.
A growing body of client, industry and MSCI research has shown the value of integrating MSCI ESG Ratings to manage and mitigate risks and identify opportunities. We are proud to work with over 1,700 clients worldwide that help inform and improve our ESG Research, including our ESG Ratings methodology and coverage. Investor clients use MSCI ESG Ratings as follows.
Fundamental / quant analyses
Portfolio construction / risk management, benchmarking / index-based product development, disclosure and reporting for regulators and stakeholders, engagement & thought leadership.
- Stock analysis
- ESG Ratings used for security selection or within systematic strategies
- ESG Factor in quant model- identify long term trends and arbitrage opportunities
- Adjust discounted cashflow models
- Identify leaders and laggards to support construction
- Use ratings and underlying scores to inform asset allocation
- Stress testing, and risk and performance attribution analysis
- ESG as a Factor in Global Equity Models
- MSCI ESG Ratings are used in many of MSCI’s 1,500 equity and fixed indexes
- Select policy or performance benchmark
- Develop Exchange-Traded-Funds and other index-based products
- Make regulatory disclosures
- Report to clients & stakeholders
- Demonstrate ESG transparency and leadership
- Engage companies and external stakeholders
- Provide transparency through client reporting
- Conduct thematic or industry research
ESG rating Key benefits
Key product features:.
We rate over 8,500 companies (14,000 issuers including subsidiaries) and more than 680,000 equity and fixed income securities globally (as of October 2020), collecting thousands of data points for each company.
MSCI ESG Research Experience and Leadership
Msci esg research experience and leadership.
- We have over 40 years 2 of experience measuring and modelling ESG performance of companies. We are recognized as a ‘Gold Standard data provider’3 and voted 'Best Firm for SRI research' and ‘Best Firm for Corporate Governance research' for the last four years 3
- We were the first ESG provider to assess companies based on industry materiality, dating back to 1999. Only dataset with live history (13+ years) demonstrating economic relevance
- Objective rules based ESG ratings, with an average 45% of data, 5 coming from alternative data sources, utilizing AI tech to extract and verify unstructured data
- First ESG ratings provider to measure and embed companies’ ESG risk exposure 4
ESG Ratings Related Content
Related content, .rel-cont-head{ font-size: 31px important; line-height: 38px important; } sustainable investing.
Companies with strong MSCI ESG Ratings profiles may be better positioned for future challenges and experience fewer instances of bribery, corruption and fraud. Learn how our sustainability solutions can provide insights into risks and opportunities.
Climate and Net-Zero Solutions
To empower investors to analyze and report on their portfolios’ exposures to transition and physical climate risk. 1 .
Sustainable Finance
ESG and climate regulation and disclosure resource center for institutional investors, managers and advisors.
ESG ratings footnotes
MSCI ESG Research LLC. is a Registered Investment Adviser under the Investment Adviser Act of 1940. The most recent SEC Form ADV filing, including Form ADV Part 2A, is available on the U.S. SEC’s website at www.adviserinfo.sec.gov (opens in a new tab) .
MIFID2/MIFIR notice: MSCI ESG Research LLC does not distribute or act as an intermediary for financial instruments or structured deposits, nor does it deal on its own account, provide execution services for others or manage client accounts. No MSCI ESG Research product or service supports, promotes or is intended to support or promote any such activity. MSCI ESG Research is an independent provider of ESG data, reports and ratings based on published methodologies and available to clients on a subscription basis.
ESG ADV 2A (PDF, 354 KB) (opens in a new tab) ESG ADV 2B (brochure supplement) (PDF, 232 KB) (opens in a new tab)
1 GICS®, the global industry classification standard jointly developed by MSCI Inc. and S&P Global.
2 Through our legacy companies KLD, Innovest, IRRC, and GMI Ratings.
3 Deep Data Delivery Standard http://www.deepdata.ai/
4 Through our legacy companies KLD, Innovest, IRRC, and GMI Ratings. Origins of MSCI ESG Ratings established in 1999. Produced time series data since 2007.
5 Source: MSCI ESG Research 2,434 constituents of the MSCI ACWI Index as of November 30, 2017.
UtmAnalytics
Articles on Australian budget 2024
Displaying all articles.
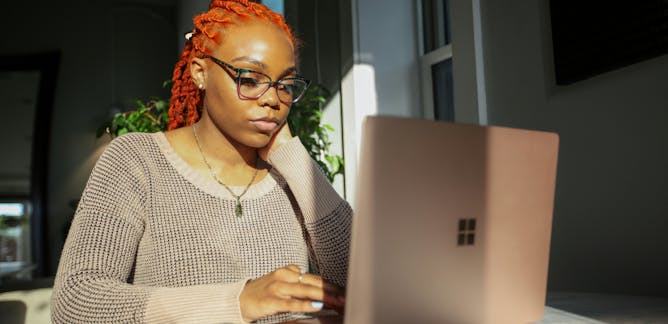
Australia is getting a new digital mental health service. Will it help? Here’s what the evidence says
Peter Baldwin , UNSW Sydney
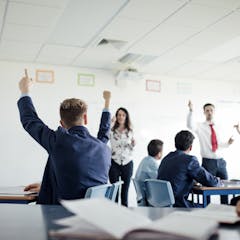
There’s $110 million for Indigenous education in the budget. But where’s the evidence it will work?
Marnee Shay , The University of Queensland and Grace Sarra , Queensland University of Technology
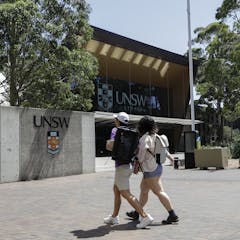
Why is the government proposing caps on international students and how did we get here?
Christopher Ziguras , The University of Melbourne
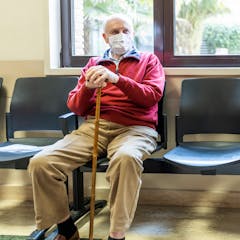
It’s so hard to see a doctor right now. What are my options?
Anthony Scott , Monash University
Related Topics
- Aboriginal and Torres Strait Islander peoples
- Clare O'Neil
- Federal budget 2024
- Health analysis
- Health budget
- Health economics
- Health policy

Data Manager

Research Support Officer

Director, Social Policy

Head, School of Psychology

Senior Research Fellow - Women's Health Services
Top contributors.
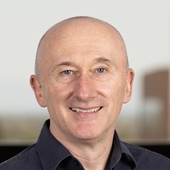
Professor of Health Economics and Director, Centre for Health Economics, Monash University, Monash University
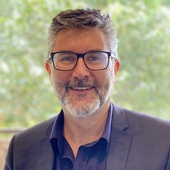
Director, Centre for the Study of Higher Education, The University of Melbourne
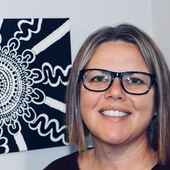
Associate Professor, Principal Research Fellow, The University of Queensland
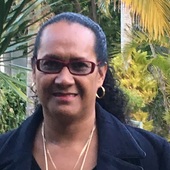
Professor, Faculty of Creative Industries, Education and Social Justice, Queensland University of Technology
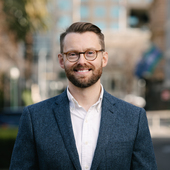
Senior Research Fellow and Clinical Psychologist at the Black Dog Institute , UNSW Sydney
- X (Twitter)
- Unfollow topic Follow topic
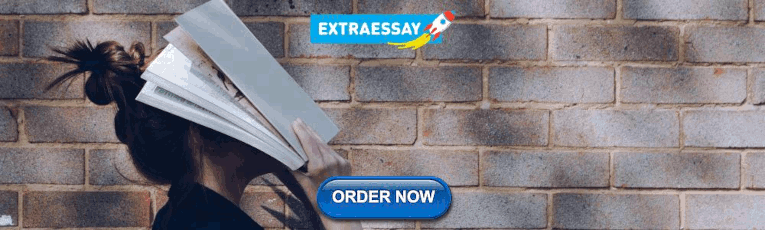
IMAGES
VIDEO
COMMENTS
Data Science-Related Research Topics. Developing machine learning models for real-time fraud detection in online transactions. The use of big data analytics in predicting and managing urban traffic flow. Investigating the effectiveness of data mining techniques in identifying early signs of mental health issues from social media usage.
By harnessing the power of big data analytics, they can improve their decision-making, better understand their customers, and develop new products and services. 3.) Auto Machine Learning. Auto machine learning is a research topic in data science concerned with developing algorithms that can automatically learn from data without intervention.
The top level of analytics in Figure 1, prescriptive analytics for decision making, tends to be under-focused in statistics and data science programs. Recall the marketing 4P's in Table 1, if we can answer these questions causally , we can use the results to optimize , as listed in the last column of the table, linking predictive and ...
General big data research topics [3] are in the lines of: Scalability — Scalable Architectures for parallel data processing; Real-time big data analytics — Stream data processing of text, image, and video; Cloud Computing Platforms for Big Data Adoption and Analytics — Reducing the cost of complex analytics in the cloud; Security and ...
99+ Data Science Research Topics: A Path to Innovation. In today's rapidly advancing digital age, data science research plays a pivotal role in driving innovation, solving complex problems, and shaping the future of technology. Choosing the right data science research topics is paramount to making a meaningful impact in this field.
Abstract. To drive progress in the field of data science, we propose 10 challenge areas for the research community to pursue. Since data science is broad, with methods drawing from computer science, statistics, and other disciplines, and with applications appearing in all sectors, these challenge areas speak to the breadth of issues spanning ...
Machine Learning and Cutting-Edge Tools for Prediction and Treatment Strategies of Dementia and Associated Diseases. Ahmed Alsayat. Saad Alanazi. Ankit Ganeshpurkar. Ayman Mostafa. 474 views. This innovative journal focuses on the power of big data - its role in machine learning, AI, and data mining, and its practical application from ...
The world is being transformed by data and data-driven analysis is rapidly becoming an integral part of science and society. Stanford Data Science is a collaborative effort across many departments in all seven schools. We strive to unite existing data science research initiatives and create interdisciplinary collaborations, connecting the data ...
Data analytics jobs. Typically, data analytics professionals make higher-than-average salaries and are in high demand within the labor market. The US Bureau of Labor Statistics (BLS) projects that careers in data analytics fields will grow by 23 percent between 2022 and 2032—much faster than average—and are estimated to pay a higher-than-average annual income of $85,720 [].
Recent topics of research related to data analytics were published from 2018 to 2019. In this period, the publications related to the research area have gradually focused on the learning methods, issues, and data analytics challenges. Some recent topics that rapidly developed in this period are machine learning, IoT, health care, deep learning ...
The importance of data analysis in conversion research; The importance of A/B testing in data science. Amazing Research Topics on Big Data and Local Governments . Governments are now using big data to make the lives of the citizens better. This is in the government and the various institutions.
A data science research paper should start with a clear goal, stating what the study aims to investigate or achieve. This objective guides the entire paper, helping readers understand the purpose and direction of the research. 2. Detailed Methodology. Explaining how the research was conducted is crucial.
About the journal. The journal aims to promote and communicate advances in big data research by providing a fast and high quality forum for researchers, practitioners and policy makers from the very many different communities working on, and with, this topic. The journal will accept papers on foundational aspects in dealing with big data, as ...
In this article, we have listed 10 such research and thesis topic ideas to take up as data science projects in 2022. Handling practical video analytics in a distributed cloud: With increased dependency on the internet, sharing videos has become a mode of data and information exchange. The role of the implementation of the Internet of Things ...
The role of data and analytics is to equip businesses, their employees and leaders to make better decisions and improve decision outcomes. This applies to all types of decisions, including macro, micro, real-time, cyclical, strategic, tactical and operational. At the same time, D&A can unearth new questions, as well as innovative solutions and ...
Big data analytics (BDA) has had a considerable influence across healthcare functions (Gu et al. Citation 2017; ... indicating that the research topic is relevant to the recent literature on BDA and healthcare. RQ2 is answered by analysing the contexts of the reviewed studies. The synthesis of the findings of prior studies addresses RQ3 ...
There are four key types of data analytics: descriptive, diagnostic, predictive, and prescriptive. These four types of data analytics can help an organisation make data-driven decisions. At a glance, each of them tells us the following: Descriptive analytics tell us what happened. Diagnostic analytics tell us why something happened.
Introduction. The purpose of data analytics in healthcare is to find new insights in data, at least partially automate tasks such as diagnosing, and to facilitate clinical decision-making [1, 2].Higher hardware cost-efficiency and the popularization and advancement of data analysis techniques have led to data analytics gaining increasing scholarly and practical footing in the healthcare sector ...
In this Research Topic, we seek to facilitate the communication of up-to-date knowledge on AI in cellular and systems physiology. This Research topic will focus on AI and machine learning tools in exploratory analysis of multi-featured physiological data and in and prediction of physiological states and conditions in health and disease.
Coordinate Descent. Covariance Estimation. Crowd Computing. Data Clustering. Deep Learning. Divide-and-Conquer Methods. Eigenvalue Decomposition. Empirical Risk Minimization. Gene-Disease Prediction.
Research on the topic of data analytics; Finding research on data analytics. Data analytics is a very new area of work and not really a discipline of its own. This makes finding scholarly work about data analytics kind of tricky. Much of the work is done by computer scientists, so a good bit of the literature can be found in the these databases
Today, information can be captured from many different sources, and technology to extract insights is becoming increasingly accessible. The Top 5 Data Science And Analytics Trends In 2023. Adobe ...
The Analytics, Information, Operations (AIO) academic area includes faculty who are experts in topics such as business analytics, information systems and supply chain management, as well as former industry professionals whose experience supplements our curriculum. AIO faculty regularly publish in leading academic journals and lend their ...
Whether through augmented analytics or generative AI, AI is making it easier for users to find and analyze the data they need without the manual processes that typically deter broader BI and analytics adoption. To assess the state of analytics and BI platforms in today's organizations, Enterprise Strategy Group surveyed 375 data and IT ...
Open. Data science often utilizes tools such as Python, R, TensorFlow and Apache Hadoop, focusing on machine learning, deep learning and big data technologies. In contrast, data analytics frequently employs SQL, Tableau, Power BI and Excel, emphasizing data manipulation, visualization and reporting.
The foundation of data science tools began with statistical packages like SPSS (Statistical Package for the Social Sciences) and SAS (Statistical Analysis System). These tools were primarily used for academic research and business analytics, offering capabilities for basic statistical analysis, data management, and reporting. 2.
On balance more consumers have adopted a sustainable lifestyle in 2023 compared with a year ago. In the past 12 months there has been an increase in the proportion of consumers saying they have adopted a more sustainable lifestyle across 11 of the 23 sustainable behaviours we track in our research. In contrast, there was a fall in six of them.
Objective rules based ESG ratings, with an average 45% of data, 5 coming from alternative data sources, utilizing AI tech to extract and verify unstructured data. First ESG ratings provider to measure and embed companies' ESG risk exposure 4. MSCI ESG Research LLC. is a Registered Investment Adviser under the Investment Adviser Act of 1940.
Here's what the evidence says. Peter Baldwin, UNSW Sydney. It sounds good in theory, but it doesn't always work in practice. Here's what the evidence from the UK and elsewhere shows so far ...