
An official website of the United States government
The .gov means it’s official. Federal government websites often end in .gov or .mil. Before sharing sensitive information, make sure you’re on a federal government site.
The site is secure. The https:// ensures that you are connecting to the official website and that any information you provide is encrypted and transmitted securely.
- Publications
- Account settings
Preview improvements coming to the PMC website in October 2024. Learn More or Try it out now .
- Advanced Search
- Journal List
- v.37(1); 2014 May
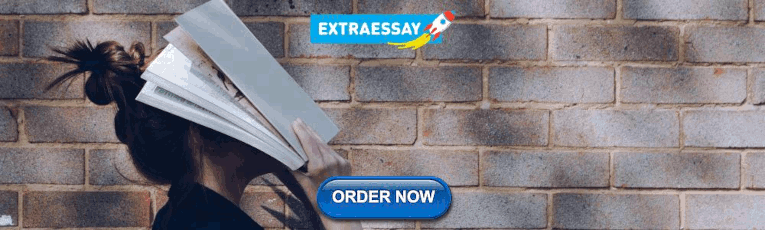
The Evidence-Based Practice of Applied Behavior Analysis
Timothy a. slocum.
Utah State University, Logan, UT USA
Ronnie Detrich
Wing Institute, Oakland, CA USA
Susan M. Wilczynski
Ball State University, Muncie, IN USA
Trina D. Spencer
Northern Arizona University, Flagstaff, AZ USA
Oregon State University, Corvallis, OR USA
Katie Wolfe
University of South Carolina, Columbia, SC USA
Evidence-based practice (EBP) is a model of professional decision-making in which practitioners integrate the best available evidence with client values/context and clinical expertise in order to provide services for their clients. This framework provides behavior analysts with a structure for pervasive use of the best available evidence in the complex settings in which they work. This structure recognizes the need for clear and explicit understanding of the strength of evidence supporting intervention options, the important contextual factors including client values that contribute to decision making, and the key role of clinical expertise in the conceptualization, intervention, and evaluation of cases. Opening the discussion of EBP in this journal, Smith ( The Behavior Analyst, 36 , 7–33, 2013 ) raised several key issues related to EBP and applied behavior analysis (ABA). The purpose of this paper is to respond to Smith’s arguments and extend the discussion of the relevant issues. Although we support many of Smith’s ( The Behavior Analyst, 36 , 7–33, 2013 ) points, we contend that Smith’s definition of EBP is significantly narrower than definitions that are used in professions with long histories of EBP and that this narrowness conflicts with the principles that drive applied behavior analytic practice. We offer a definition and framework for EBP that aligns with the foundations of ABA and is consistent with well-established definitions of EBP in medicine, psychology, and other professions. In addition to supporting the systematic use of research evidence in behavior analytic decision making, this definition can promote clear communication about treatment decisions across disciplines and with important outside institutions such as insurance companies and granting agencies.
Almost 45 years ago, Baer et al. ( 1968 ) described a new discipline—applied behavior analysis (ABA). This discipline was distinguished from the experimental analysis of behavior by its focus on social impact (i.e., solving socially important problems in socially important settings). ABA has produced remarkably powerful interventions in fields such as education, developmental disabilities and autism, clinical psychology, behavioral medicine, organizational behavior management, and a host of other fields and populations. Behavior analysts have long recognized that developing interventions capable of improving client behavior solves only one part of the problem. The problem of broad social impact must be solved by having interventions implemented effectively in socially important settings and at scales of social importance (Baer et al. 1987 ; Horner et al. 2005b ; McIntosh et al. 2010 ). This latter set of challenges has proved to be more difficult. In many cases, demonstrations of effectiveness are not sufficient to produce broad adoption and careful implementation of these procedures. Key decision makers may be more influenced by variables other than the increases and decreases in the behaviors of our clients. In addition, even when client behavior is a very powerful factor in decision making, it does not guarantee that empirical data will be the basis for treatment selection; anecdotes, appeals to philosophy, or marketing have been given priority over evidence of outcomes (Carnine 1992 ; Polsgrove 2003 ).
Across settings in which behavior analysts work, there has been a persistent gap between what is known from research and what is actually implemented in practice. Behavior analysts have been concerned with the failed adoption of research-based practices for years (Baer et al. 1987 ). Even in the fields in which behavior analysts have produced powerful interventions, the vast majority of current practice fails to take advantage of them.
Behavior analysts have not been alone in recognizing serious problems with the quality of interventions used employed in practice settings. In the 1960s, many within the medical field recognized a serious research-to-practice gap. Studies suggested that a relatively small percentage (estimates range from 10 to 25 %) of medical treatment decisions were based on high-quality evidence (Goodman 2003 ). This raised the troubling question of what basis was used for the remaining decisions if it was not high-quality evidence. These concerns led to the development of evidence-based practice (EBP) of medicine (Goodman 2003 ; Sackett et al. 1996 ).
The research-to-practice gap appears to be universal across professions. For example, Kazdin ( 2000 ) has reported that less than 10 % of the child and adolescent mental health treatments reported in the professional literature have been systematically evaluated and found to be effective and those that have not been evaluated are more likely to be adopted in practice settings. In recognition of their own research-to-practice gaps, numerous professions have adopted an EBP framework. Nursing and other areas of health care, social work, clinical and educational psychology, speech and language pathology, and many others have adopted this framework and adapted it to the specific needs of their discipline to help guide decision-making. Not only have EBP frameworks been helping to structure professional practice, but they have also been used to guide federal policy. With the passage of No Child Left Behind ( 2002 ) and the reauthorization of the Individuals with Disabilities Education Improvement Act ( 2005 ), the federal department of education has aligned itself with the EBP movement. A recent memorandum from the federal Office of Management and Budget instructed agencies to consider evidence of effectiveness when awarding funds, increase the use of evidence in competitions, and to encourage widespread program evaluation (Zients 2012 ). The memo, which used the term evidence-based practice extensively, stated: “Where evidence is strong, we should act on it. Where evidence is suggestive, we should consider it. Where evidence is weak, we should build the knowledge to support better decisions in the future” (Zients 2012 , p. 1).
EBP is more broadly an effort to improve decision-making in applied settings by explicitly articulating the central role of evidence in these decisions and thereby improving outcomes. It addresses one of the long-standing challenges for ABA; the need to effectively support and disseminate interventions in the larger social systems in which our work is embedded. In particular, EBP addresses the fact that many decision-makers are not sufficiently influenced by the best evidence that is relevant to important decisions. EBP is an explicit statement of one of ABA’s core tenets—a commitment to evidence-based decision-making. Given that the EBP framework is well established in many disciplines closely related to ABA and in the larger institutional contexts in which we operate (e.g., federal policy and funding agencies), aligning ABA with EBP offers an opportunity for behavior analysts to work more effectively within broader social systems.
Discussion of issues related to EBP in ABA has taken place across several years. Researchers have extensively discussed methods for identifying well-supported treatments (e.g., Horner et al. 2005a ; Kratochwill et al. 2010 ), and systematically reviewed the evidence to identify these treatments (e.g., Maggin et al. 2011 ; National Autism Center 2009 ). However, until recently, discussion of an explicit definition of EBP in ABA has been limited to conference papers (e.g., Detrich 2009 ). Smith ( 2013 ) opened a discussion of the definition and critical features of EBP of ABA in the pages of The Behavior Analyst . In his thought-provoking article, Smith raised many important points that deserve serious discussion as the field moves toward a clear vision of EBP of ABA. Most importantly, Smith ( 2013 ) argued that behavior analysts must carefully consider how EBP is to be defined and understood by researchers and practitioners of behavior analysis.
Definitions Matter
We find much to agree with in Smith’s paper, and we will describe these points of agreement below. However, we have a core disagreement with Smith concerning the vision of what EBP is and how it might enhance and expand the effective practice of ABA. As behavior analysts know, definitions matter. A well-conceived definition can promote conceptual understanding and set the context for effective action. Conversely, a poor definition or confusion about definitions hinders clear understanding, communication, and action.
In providing a basis for his definition of EBP, Smith refers to definitions in professions that have well-developed conceptions of EBP. He quotes the American Psychological Association (APA) ( 2005 ) definition (which we quote here more extensively than he did):
Evidence-based practice in psychology (EBPP) is the integration of the best available research with clinical expertise in the context of patient characteristics, culture, and preferences. This definition of EBPP closely parallels the definition of evidence-based practice adopted by the Institute of Medicine ( 2001 , p. 147) as adapted from Sackett et al. ( 2000 ): “Evidence-based practice is the integration of best research evidence with clinical expertise and patient values.” The purpose of EBPP is to promote effective psychological practice and enhance public health by applying empirically supported principles of psychological assessment, case formulation, therapeutic relationship, and intervention.
The key to understanding this definition is to note how APA and the Institute of Medicine use the word practice . Clearly, practice does not refer to an intervention; instead, it references one’s professional behavior. This is the sense in which one might speak of the professional practice of behavior analysis. American Psychological Association Presidential Task Force of Evidence-Based Practice ( 2006 ) further elaborates this point:
It is important to clarify the relation between EBPP and empirically supported treatments (ESTs)…. ESTs are specific psychological treatments that have been shown to be efficacious in controlled clinical trials, whereas EBPP encompasses a broader range of clinical activities (e.g., psychological assessment, case formulation, therapy relationships). As such, EBPP articulates a decision-making process for integrating multiple streams of research evidence—including but not limited to RCTs—into the intervention process. (p. 273)
In contrast, Smith defined EBP not as a decision-making process but as a set of interventions that have been shown to be efficacious through rigorous research. He stated:
An evidence-based practice is a service that helps solve a consumer’s problem. Thus it is likely to be an integrated package of procedures, operationalized in a manual, and validated in studies of socially meaningful outcomes, usually with group designs. (p. 27).
Smith’s EBP is what APA has clearly labeled an empirically supported treatment . This is a common misconception found in conversation and in published articles (e.g., Cook and Cook 2013 ) but at odds with formal definitions provided by many professional organizations; definitions which result from extensive consideration and debate by representative leaders of each professional field (e.g., APA 2005 ; American Occupational Therapy Association 2008 ; American Speech-Language Hearing Association 2005 ; Institute of Medicine 2001 ).
Before entering into the discussion of a useful definition of EBP of ABA, we should clarify the functions that we believe a useful definition of EBP should perform. First, a useful definition should align with the philosophical tenets of ABA, support the most effective current practice of ABA, and contribute to further improvement of ABA practice. A definition that is in conflict with the foundations of ABA or detracts from effective practice clearly would be counterproductive. Second, a useful definition of EBP of ABA should enhance social support for ABA practice by describing its empirical basis and decision-making processes in a way that is understandable to professions that already have well-established definitions of EBP. A definition that corresponds with the fundamental components of EBP in other fields would promote ABA practice by improving communication with external audiences. This improved communication is critical in the interdisciplinary contexts in which behavior analysts often practice and for legitimacy among those familiar with EBP who often control local contingencies (e.g., policy makers and funding agencies).
Based on these functions, we propose the following definition: Evidence-based practice of applied behavior analysis is a decision-making process that integrates (a) the best available evidence with (b) clinical expertise and (c) client values and context. This definition positions EBP as a pervasive feature of all professional decision-making by a behavior analyst with respect to client services; it is not limited to a narrowly restricted set of situations or decisions. The definition asserts that the best available evidence should be a primary influence on all decision-making related to services for clients (e.g., intervention selection, progress monitoring, etc.). It also recognizes that evidence cannot be the sole basis for a decision; effective decision-making in a discipline as complex as ABA requires clinical expertise in identifying, defining, and analyzing problems, determining what evidence is relevant, and deciding how it should be applied. In the absence of this decision-making framework, practitioners of ABA would be conceptualized as behavioral technicians rather than analysts. Further, the definition of EBP of ABA includes client values and context. Decision-making is necessarily based on a set of values that determine the goals that are to be pursued and the means that are appropriate to achieve them. Context is included in recognition of the fact that the effectiveness of an intervention is highly dependent upon the context in which it is implemented. The definition asserts that effective decision-making must be informed by important contextual factors. We elaborate on each component of the definition below, but first we contrast our definition with that offered by Smith ( 2013 ).
Although Smith ( 2013 ) made brief reference to the other critical components of EBP, he framed EBP as a list of multicomponent interventions that can claim a sufficient level of research support. We agree with his argument that such lists are valuable resources for practitioners and therefore developing them should be a goal of researchers. However, such lists are not, by themselves , a powerful means of improving the effectiveness of behavior analytic practice. The vast majority of decisions faced in the practice of behavior analysis cannot be made by implementing the kind of manualized, multicomponent treatment packages described by Smith.
There are a number of reasons a list of interventions is not an adequate basis for EBP of ABA. First, there are few interventions that qualify as “practices” under Smith’s definition. For example, when discussing the importance of manuals for operationalizing treatments, Smith stated that the requirement that a “practice” be based on a manual, “sharply reduces the number of ABA approaches that can be regarded as evidence based. Of the 11 interventions for ASD identified in the NAC ( 2009 ) report, only the three that have been standardized in manuals might be considered to be practices, and even these may be incomplete” (p. 18). Thus, although the example referenced the autism treatment literature, it seems apparent that even a loose interpretation of this particular criterion would leave all practitioners with a highly restricted number of intervention options.
Second, even if more “practices” were developed and validated, many consumers cannot be well served with existing multicomponent packages. In order to meet their clients’ needs, behavior analysts must be able to selectively implement focused interventions alone or in combination. This flexibility is necessary to meet the diverse needs of their clients and to minimize the response demands on direct care providers or staff, who are less likely to implement a complicated intervention with fidelity (Riley-Tillman and Chafouleas 2003 ).
Third, the strategy of assembling a list of treatments and describing these as “practices” severely limits the ways in which research findings are used by practitioners. With the list approach to defining EBP, research only impacts practice by placing an intervention on a list when a specific criteria has been met. Thus, any research on an intervention that is not sufficiently broad or manualized to qualify as a “practice” has no influence on EBP. Similarly, a research study that shows clear results but is not part of a sufficient body of support for an intervention would also have no influence. A study that provides suggestive results but is not methodologically strong enough to be definitive would have no influence, even if it were the only study that is relevant to a given problem.
The primary problem with a list approach is that it does not provide a strong framework that directs practitioners to include the best available evidence in all of their professional decision-making. Too often, practitioners who consult such lists find that no interventions relevant to their specific case have been validated as “evidence-based” and therefore EBP is irrelevant. In contrast, definitions of EBP as a decision-making process can provide a robust framework for including research evidence along with clinical expertise and client values and context in the practice of behavior analysis. In the next sections, we explore the components of this definition in more detail.
Best Available Evidence
The term “best available evidence” occupies a critical and central place in the definition and concept of EBP; this aligns with the fundamental reliance on scientific research that is one of the core tenets of ABA. The Behavior Analyst Certification Board ( 2010 ) Guidelines for Responsible Conduct for Behavior Analysts repeatedly affirm ways in which behavior analysts should base their professional conduct on the best available evidence. For example:
Behavior analysts rely on scientifically and professionally derived knowledge when making scientific or professional judgments in human service provision, or when engaging in scholarly or professional endeavors.
- The behavior analyst always has the responsibility to recommend scientifically supported most effective treatment procedures. Effective treatment procedures have been validated as having both long-term and short-term benefits to clients and society.
- Clients have a right to effective treatment (i.e., based on the research literature and adapted to the individual client).
A Continuum of Evidence Quality
The term best implies that evidence can be of varying quality, and that better quality evidence is preferred over lower quality evidence. Quality of evidence for informing a specific practical question involves two dimensions: (a) relevance of the evidence and (b) certainty of the evidence.
The dimension of relevance recognizes that some evidence is more germane to a particular decision than is other evidence. This idea is similar to the concept of external validity. External validity refers to the degree to which research results apply to a range of applied situations whereas relevance refers to the degree to which research results apply to a specific applied situation. In general, evidence is more relevant when it matches the particular situation in terms of (a) important characteristics of the clients, (b) specific treatments or interventions under consideration, (c) outcomes or target behaviors including their functions, and (d) contextual variables such as the physical and social environment, staff skills, and the capacity of the organization. Unless all conditions match perfectly, behavior analysts are necessarily required to use their expertise to determine the applicability of the scientific evidence to each unique clinical situation. Evidence based on functionally similar situations is preferred over evidence based on situations that share fewer important characteristics with the specific practice situation. However, functional similarity between a study or set of studies and a particular applied problem is not always obvious.
The dimension of certainty of evidence recognizes that some evidence provides stronger support for claims that a particular intervention produced a specific result. Any instance of evidence can be evaluated for its methodological rigor or internal validity (i.e., the degree to which it provides strong support for the claim of effectiveness and rules out alternative explanations). Anecdotes are clearly weaker than more systematic observations, and well-controlled experiments provide the strongest evidence. Methodological rigor extends to the quality of the dependent measure, treatment fidelity, and other variables of interest (e.g., maintenance of skill acquisition), all of which influence the certainty of evidence. But the internal validity of any particular study is not the only variable influencing the certainty of evidence; the quantity of evidence supporting a claim is also critical to its certainty. Both systematic and direct replication are vital for strengthening claims of effectiveness (Johnston and Pennypacker 1993 ; Sidman 1960 ). Certainty of evidence is based on both the rigor of each bit of evidence and the degree to which the findings have been consistently replicated. Although these issues are simple in principle, operationalizing and measuring rigor of research is extremely complex. Numerous quality appraisal systems for both group and single-subject research have been proposed and used in systematic reviews (see below for more detail).
Under ideal circumstances, consistently high-quality evidence that closely matches the specifics of the practice situation is available; unfortunately, this is not always the case, and evidence-based practitioners of ABA must proceed despite an imperfect evidence base. The mandate to use the best available evidence specifies that the practitioner make decisions based on the best evidence that is available. Although this statement may seem rather obvious, the point is worth underscoring because the implications are highly relevant to behavior analysts. In an area with considerable high-quality relevant research, the standards for evidence should be quite high. But in an area with more limited research, the practitioner should take advantage of the best evidence that is available. This may require tentative reliance on research that is somewhat weaker or is only indirectly relevant to the specific situation at hand. For example, ideally, evidence-based practitioners of ABA would rely on well-controlled experimental results that have been replicated with the precise population with whom they are working. However, if this kind of evidence is not available, they might have to make decisions based on a single study that involves a similar but not identical population.
This idea of using the best of the available evidence is very different from one of using only extremely high-quality evidence (i.e., empirically supported treatments). If we limit EBP to considering only the highest quality evidence, we leave the practitioner with no guidance in the numerous situations in which high-quality and directly relevant evidence (i.e., precise matching of setting, function, behavior, motivating operations and precise procedures) simply does not exist. This approach would lead to a form of EBP that is irrelevant to the majority of decisions that a behavior analyst must make on a daily basis. Instead, our proposed definition of EBP asserts that the practitioner should be informed by the best evidence that is available.
Expanding Research on Utility of Treatments
Smith ( 2013 ) argued that the research methods used by behavior analysts to evaluate these treatments should be expanded to more comprehensively describe the utility of interventions. He suggested that too much ABA research is conducted in settings that do not approximate typical service settings, optimizing experimental control at the expense of external validity. Along this same line of reasoning, he noted that it is important to test the generality of effects across clients and identify variables that predict differential effectiveness. He suggested systematically reporting results from all research participants (e.g., the intent-to-treat model), and purposive selection of participants would provide a more complete account of the situations in which treatments are successful and those in which they are unsuccessful. Smith argued that researchers should include more distal and socially important outcomes because with a narrow target “behavior may change, but remain a problem for the individual or may be only a small component of a much larger cluster of problems such as addiction or delinquency.” He pointed out that in order to best support effective practice, research must demonstrate that an intervention produces or contributes to producing the socially important outcomes that would cause a consumer to say that the problem is solved.
Further, Smith argues that many of the questions most relevant to EBP—questions about the likely outcomes of a treatment when applied in a particular type of situation—are well suited to group research designs. He argued that RCTs are likely to be necessary within a program of research because:
most problems pose important actuarial questions (e.g., determining whether an intervention package is more effective than community treatment as usual; deciding whether to invest in one intervention package or another, both, or neither; and determining whether the long-term benefits justify the resources devoted to the intervention)…. A particularly important actuarial issue centers on the identification of the conditions under which the intervention is most likely to be effective. (p. 23)
We agree that selection of research methods should be driven by the kinds of questions being asked and that group research designs are the methods of choice for some types of questions that are central to EBP. Therefore, we support Smith’s call for increased use of group research designs within ABA. If practice decisions are to be informed by the best available evidence, we must take advantage of both group and single-subject designs. However, we disagree with Smith’s statement that EBP should be limited to treatments that are validated “usually with group designs” (Smith, p. 27). Practitioners should be supported by reviews of research that draw from all of the available evidence and provide the best recommendations possible given the state of knowledge on the particular question. In most areas of behavior analytic practice, single-subject research makes up a large portion of the best available evidence. The Institute for Education Science (IES) has recognized the contribution single case designs can make toward identifying effective practices and has recently established standards for evaluating the quality of single case design studies (Institute of Educational Sciences, n.d. ; Kratochwill et al. 2013 ).
Classes of Evidence
Identifying the best available evidence to inform specific practice decisions is extremely complex, and no single currently available source of evidence can adequately inform all aspects of practice. Therefore, we outline a number of strategies for identifying and summarizing evidence in ways that can support the EBP of ABA. We do not intend to cover all sources of evidence comprehensively, but merely outline some of the options available to behavior analysts.
Empirically Supported Treatment Reviews
Empirically supported treatments (EST) are identified through a particular form of systematic literature review. Systematic reviews bring a rigorous methodology to the process of reviewing research. The development and use of these methods are, in part, a response to the recognition that the process of reviewing the literature is subject to threats to validity. The systematic review process is characterized by explicitly stated and replicable methods for (a) searching for studies, (b) screening studies for relevance to the review question, (c) appraising the methodological quality of studies, (d) describing outcomes from each study, and (e) determining the degree to which the treatment (or treatments) is supported by the research. When the evidence in support of a treatment is plentiful and of high quality, the treatment generally earns the status of an EST. Many systematic reviews, however, find that no intervention for a particular problem has sufficient evidence to qualify as an EST.
Well-known organizations in medicine (e.g., Cochrane Collaboration), education (e.g., What Works Clearinghouse), and mental health (e.g., National Registry of Evidence-based Programs and Practices) conduct EST reviews. Until recently, systematic reviews have focused nearly exclusively on group research; however, systematic reviews of single-subject research are quickly becoming more common and more sophisticated (e.g., Carr 2009 ; NAC 2009 ; Maggin et al. 2012 ).
Systematic reviews for EST status is one important way to summarize the best available evidence because it can give a relatively objective evaluation of the strength of the research literature supporting a particular intervention. But systematic reviews are not infallible; as with all other research and evaluation methods, they require skillful application and are subject to threats to validity. The results of reviews can change dramatically based on seemingly minor changes in operational definitions and procedures for locating articles, screening for relevance, describing treatments, appraising methodological quality, describing outcomes, summarizing outcomes for the body of research as a whole, and rating the degree to which an intervention is sufficiently supported (Slocum et al. 2012a ; Wilczynski 2012 ). Systematic reviews and claims based upon them must be examined critically with full recognition of their limitations just as one examines primary research reports.
Behavior analysts encounter many situations in which no ESTs have been established for the particular combination of client characteristics, target behaviors, functions, contexts, and other parameters for decision-making. This dearth may exist because no systematic review has addressed the particular problem or because a systematic review has been conducted but failed to find any well-supported treatments for the particular problem. For example, in a recent review of all of the recommendations in the empirically supported practice guides published by the IES, 45 % of the recommendations had minimal support (Slocum et al. 2012b ). As Smith noted ( 2013 ), only 3 of the 11 interventions that the NAC identified as meeting quality standards might be considered practices in the sense that they are manualized. In these common situations, a behavior analyst cannot respond by simply selecting an intervention from a list of ESTs. A comprehensive EBP of ABA requires additional strategies for reviewing research evidence and drawing practice recommendations from existing evidence—strategies that can glean the best available evidence from an imperfect research base and formulate practice recommendations that are most likely to lead to favorable outcomes under conditions of uncertainty.
Other Methods for Reviewing Research Literature
The three strategies outlined below may complement systematic reviews in guiding behavior analysts toward effective decision-making.
Narrative Reviews of the Literature
There has been a long tradition across disciplines of relying on narrative reviews to summarize what is known with respect to treatments for a class of problems (e.g., aggression) or what is known about a particular treatment (e.g., token economy). The author of the review, presumably an expert, selects the theme and synthesizes the research literature that he or she considers most relevant. Narrative reviews allow the author to consider a wide range of research including studies that are indirectly relevant (e.g., those studying a given problem with a different population or demonstrating general principles) and studies that may not qualify for systematic reviews because of methodological limitations but which illustrate important points nonetheless. Narrative reviews can consider a broader array of evidence and have greater interpretive flexibility than most systematic reviews.
As with all sources of evidence, there are difficulties with narrative reviews. The selection of the literature is left up to the author’s discretion; there are no methodological guidelines and little transparency about how the author decided which literature to include and which to exclude. There is always the risk of confirmation bias that the author emphasized literature that is consistent with her preconceived opinions. Even with a peer-review process, it is always possible that the author neglected or misinterpreted research relevant to the discussion. These concerns not withstanding, narrative reviews may provide the best available evidence when no systematic reviews exist or when substantial generalizations from the systematic review to the practice context are needed. Many textbooks (e.g., Cooper et al. 2007 ) and handbooks (e.g., Fisher et al. 2011 ; Madden et al. 2013 ) provide excellent examples of narrative reviews that can provide important guidance for evidence-based practitioners of ABA.
Best Practice Guides
Best practice guides are another source of evidence that can inform decisions in the absence of available and relevant systematic reviews. Best practice guides provide recommendations that reflect the collective wisdom of an expert panel. It is presumed that the recommendations reflect what is known from the research literature, but the validity of recommendations is largely derived from the panel’s expertise rather than from the rigor of their methodology. Recommendations from best practice panels are usually much broader than the recommendations from systematic reviews. The recommendations from these guides can provide important information about how to implement a treatment, how to adapt the treatment for specific circumstances, and what is necessary for broad scale or system-wide implementation.
The limitations to best practice guides are similar to those for narrative reviews; specifically, potential bias and lack of transparency are significant concerns. Panel members are typically not selected using a specific set of operationalized criteria. Bias is possible if the panel is drawn too narrowly. If the panel is drawn too broadly; however, the panel may have difficulty reaching a consensus (Wilczynski 2012 ).
Empirically Supported Practice Guides
Empirically supported practice guides, a more recently developed strategy, integrate the strengths of systematic reviews and best practice panels. In this type of review, an expert panel is charged with developing recommendations on a topic. As part of the process, a systematic review of the literature is conducted. Following the systematic review, the panel generates a set of recommendations and objectively determines the strength of evidence for the recommendation and assigns an evidence rating. When there is little empirical evidence directly related to a specific issue, the panel’s recommendations may have weak research support but nonetheless may be based on the best evidence that is available. The obvious advantage of empirically supported practice guides is that there is greater transparency about the review process and certainty of recommendations. Practice recommendations are usually broader than those derived from systematic reviews and address issues related to implementation and acceptable variations to enhance the treatment’s contextual fit (Shanahan et al. 2010 ; Slocum et al. 2012b ). Although empirically supported practice guides offer the objectivity of a systematic review and the flexibility of best practice guidelines, they also face potential sources of error from both methods. Systematic and explicit criteria are used to review the research and rate the level of evidence for each recommendation; however, it is the panel that formulates recommendations. Thus, results of these reviews are influenced by the selection of panel members. When research evidence is incomplete or equivocal, panelists must exercise judgment in interpreting the evidence and drawing conclusions (Shanahan et al. 2010 ).
Other Units of Analysis
Smith ( 2013 ) weighed in on the critical issue of the unit of analysis when describing and evaluating treatments (Slocum and Wilczynski 2008 ). The unit of analysis refers to whether EBP should focus on (a) principles, such as reinforcement; (b) tactics, such as backward chaining; (c) multicomponent packages, such as Functional Communication Training; or (d) even more comprehensive systems, such as Early Intensive Behavioral Intervention. After reviewing the ongoing debate between those favoring a smaller unit of analysis that focuses on specific procedures and those favoring a larger unit of analysis that evaluates the effects of multicomponent packages, Smith made a case that the multicomponent treatment package is the key unit in EBP. Smith noted that practitioners rarely solve a client’s problem with a single procedure; instead, solutions typically involve combinations of procedures. He argued that the unit should be “a service aimed at solving people’s problems” and procedures that are merely components of such services are not sufficiently complete to be the proper unit of analysis for EBP. He further stated that these treatment packages should include strategies for implementation in typical service settings and an intervention manual.
We concur that the multicomponent treatment package is a particularly significant and strategic unit of treatment because it specifies a suite of procedures and exactly how they are to be used together to solve a problem. Validated treatment packages are far more than the sum of their parts. A well-developed treatment package can be revised and optimized over many iterations in a way that would be difficult or impossible for a practitioner to accomplish independently. In addition, research outcomes from implementation of treatment packages reflect the interaction of the components, and these interactions may not be evident in the research literature on the individual components. Further, research on the outcomes from multicomponent packages can evaluate broader and more socially important outcomes than is generally possible when evaluating more narrowly defined treatments. For example, in the case of teaching a child with autism to communicate, research on a focused procedure such as time delay may indicate that its use leads to more independent communicative responses; however, research on a comprehensive Early Intensive Behavioral Intervention can evaluate the impact of the program on children’s global development or intellectual functioning.
Having recognized our agreement with Smith ( 2013 ) on the special importance of multicomponent treatment packages for EBP, we hasten to add that this type of intervention is not enough to support a broad and robust EBP of ABA. EBP must also provide guidance to the practitioner in the frequently encountered situations in which well-established treatment packages are not available. In these situations, problems may be best addressed by building an intervention from a set of elemental components. These components, referred to as practice elements (Chorpita et al. 2005 , 2007 ) or kernels (Embry 2004 ; Embry and Biglan 2008 ), may be validated either directly or indirectly. The practitioner assembles a particular combination of components to solve a specific problem. Because this newly constructed package has not been evaluated as a whole, there is additional uncertainty about the effectiveness of the package, and the quality of evidence may be considered lower than a well-supported treatment package (Slocum et al. 2012b ; Smith 2013 ; however, see Chorpita ( 2003 ) for a differing view). Nonetheless, treatment components that are supported by strong evidence provide the practitioner with tools to solve practical problems when EST packages are not relevant.
In some cases, behavior analysts are presented with problems that cannot be addressed even by assembling established components. In these cases, the ABA practitioner must apply principles of behavior to construct an intervention and must depend on these principles to guide sensible modifications of interventions in response to client needs and to support sensible implementation of interventions. Principles of behavior are broadly generalized statements describing behavioral relations. Their empirical base is extremely large and diverse including both human and nonhuman participants across numerous contexts, behaviors, and consequences. Although principles of behavior are based on an extremely broad research literature, they are also stated at a broad level. As a result, the behavior analyst must use a great deal of judgment in applying principles to particular problems and a particular attempt to apply a principle to solve a problem may not be successful. Thus, although behavioral principles are supported by evidence, newly constructed interventions based on these principles have not yet been evaluated. These interventions must be considered less certain or validated than treatment packages or elements that have been demonstrated to be effective for specific problems, populations, and context (Slocum et al. 2012b ).
Evidence-based practitioners of ABA recognize that the process of selecting and implementing treatments always includes some level of uncertainty (Detrich et al. 2013 ). One of the fundamental tenets of ABA shared with many other professions is that the best evidence regarding the effectiveness of an intervention does not come from systematic literature reviews, best practice guides, or principles of behavior, but from close continual contact with the relevant outcomes (Bushell and Baer 1994 ). The BACB guidelines ( 2010 ) state that, “behavior analysts recognize limits to the certainty with which judgments or predictions can be made about individuals” (item 3.0 [c]). As a result, “the behavior analyst collects data…needed to assess progress within the program” (item 4.07) and “modifies the program on the basis of data” (item 4.08). Thus, an important feature of the EBP of ABA is that professional decision-making does not end with the selection of an initial intervention. The process continues with ongoing progress monitoring and adjustments to the treatment plan as needed to achieve the targeted outcomes. Progress monitoring and data-based decision-making are the ultimate hedge against the inherent uncertainties of imperfect knowledge derived from research. As the quality of the best available evidence decreases, the importance of frequent direct measurement of client progress increases.
Practice decisions are always accompanied by some degree of uncertainty; however, better decisions are likely when multiple of sources of evidence are integrated. For example, a multicomponent treatment package may be an EST for clients who differ slightly from those the practitioner currently serves. Confidence in the use of this treatment may be increased if there is evidence showing the central components are effective with clients belonging to the population of interest. The principles of behavior might further inform sensible variations appropriate for the specific context of practice. When considered together, numerous sources of evidence increase the confidence the behavior analyst can have in the intervention. And when the plan is implemented, progress monitoring may reveal the need for additional adjustments. Each of these different classes of evidence provides answers to different questions for the practitioner, resulting in a more fine-grained analysis of the clinical problem and solutions to it (Detrich et al. 2013 ).
Client Values and Context
In order to be compatible with the underlying tenets of ABA, parallel with other professions, and to promote effective practice, a definition of EBP of ABA must include client values and context among the primary contributors to professional decision-making. Baer et al. ( 1968 ) suggested that the word applied refers to an immediate and important change in behavior that has practical value and that this value is determined “by the interest which society shows in the problems” (p. 92)—that is, by social values. Wolf ( 1978 ) went on to specify that behavior analytic practice can only be termed successful if it addresses goals that are meaningful to our clients, uses procedures that are judged appropriate by our clients, and produces effects that are valued by our clients. These foundational tenets of ABA correspond with the centrality of client values in classic definitions of EBP (e.g., Institute of Medicine 2001 ). Like medical professionals and those in the many other fields that have adopted similar conceptualizations of EBP, behavior analysts have long recognized that client values are critical contributors to responsible decision-making.
Behavior analysts have defined the client as the individual who is the focus of the behavior change, other individuals who are critical to the behavior change process (Baer et al. 1968 ; Heward et al. 2005 ), as well as outside individuals or groups who may have a stake in the target behavior or improved outcomes (Baer et al. 1987 ; Wolf 1978 ). Wolf ( 1978 ) argued that only our clients can judge the social validity of our work and suggested that behavior analysts address three levels of social validity: (a) the social significance of the goals, (b) the social desirability of the procedures, and (c) the social importance of the outcomes. With respect to selection of interventions, Wolf noted, “not only is it important to determine the acceptability of treatment procedures to participants for ethical reasons, it may also be that the acceptability of the program is related to effectiveness, as well as to the likelihood that the program will be adopted and supported by others” (p. 210). He further maintained that clients are the ultimate arbiters of whether or not the effects of a program are sufficiently helpful to be termed successful.
The concept of social validity directs our attention to some of the important aspects of the context of intervention. Intervention always occurs in some context and features of that context can directly influence the fidelity with which the intervention is implemented and its effectiveness. Albin et al. ( 1996 ) expanded further on the contextual variables that might be critical for designing and implementing effective interventions. They described the concept of contextual fit or the congruence of a behavioral support plan and the context and indicate that this fit will determine its implementation, effectiveness, and maintenance.
Contextual fit includes the issues of social validity, but also explicitly encompasses issues associated with the individuals who implement treatments and manage other aspects of the environments within which treatments are implemented. Behavioral intervention plans prescribe the behavior of implementers. These implementers may include professionals, such as therapists and teachers, as well as nonprofessionals, such as family and community members. It is important to consider characteristics of these implementers when developing plans because the success of a plan may hinge on how it corresponds with the values, skills, goals, and stressors of the implementers. Effective plans must be within the skill repertoire of the implementers, or training to fidelity must occur to introduce the plan components into that repertoire. Values, goals, and stressors refer to motivating operations that determine the reinforcing or punishing value of implementing the plan. Plans that provide little reinforcement and substantial punishment in the process of implementation or outcomes are unlikely to be implemented with fidelity or maintained over time. The effectiveness of behavioral interventions is also influenced by their compatibility with other aspects of their context. Plans that are compatible with ongoing routines are more likely to be implemented than those that conflict (Riley-Tillman and Chafouleas 2003 ). Interventions require various kinds of resources to be implemented and sustained. For example, financial resources may be necessary to purchase curricula, equipment, or other goods. Interventions may require human resources such as direct service staff, training, supervision, administration, and consultation. Fixsen et al. ( 2005 ) have completed an extensive review of contextual variables that can potentially influence the quality of intervention implementation. Behavior analytic practice is unlikely to be effective if it does not consider the context in which interventions will be implemented.
Extensive behavior analytic research has documented the importance of social validity and other contextual factors in producing behavioral changes with practical value. This research tradition is as old as our field (e.g., Jones and Azrin 1969 ) and continues through the present day. For example, Strain et al. ( 2012 ) provided multiple examples of the impact of social validity considerations on relevant outcomes. They reported that integrating client values, preferences, and characteristics in the selection and implementation of an intervention can successfully inform decisions regarding (a) how to design service delivery systems, (b) how to support implementers with complex strategies, (c) when to fade support, (e) how to identify important and unanticipated effects, and (f) how to focus on future research efforts.
Benazzi et al. ( 2006 ) examined the effect of stakeholder participation in intervention planning on the acceptability and usability of behavior intervention plans (BIP) based on descriptive functional behavior assessments (FBA). Plans developed by behavior experts were rated as high in technical adequacy, but low in acceptability. Conversely, plans developed by key stakeholders were highly acceptable, but lacked technical adequacy. However, when the process included both behavior experts and key stakeholders, BIPs were considered both acceptable and technically adequate. Thus, the BIPs developed by behavior analysts may be marginalized and implementation may be less likely to occur in the absence of key stakeholder input. Thus, a practical commitment to effective interventions that are implemented and maintained with integrity over time requires that behavior analysts consider motivational variables such as the alignment of interventions with the values, reinforcers, and punishers of relevant stakeholders.
Clinical Expertise
All of the key components for expert behavior analytic practice (i.e., identification of important behavioral problems, recognition of underlying behavioral processes, weighing of evidence supporting various treatment options, selecting and implementing treatments in complex social contexts, engaging in ongoing data-based decision making, and being responsive to client values and context) require clinical expertise. Clinical expertise refers to the competence attained by practitioners through education, training, and experience that results in effective practice (American Psychological Association Presidential Task Force of Evidence-Based Practice 2006 ). Clinical expertise is the means by which the best available evidence is applied to individual cases in all their complexity. Based on the work of Goodheart ( 2006 ), we suggest that clinical expertise in EBP of ABA includes (a) knowledge of the research literature and its applicability to particular clients, (b) incorporation of the conceptual system of ABA, (c) breadth and depth of clinical and interpersonal skills, (d) integration of client values and context, (e) recognition of the need for outside consultation, (f) data-based decision making, and (g) ongoing professional development. In the sections that follow, we describe each component of clinical expertise in ABA.
Knowledge and Application of the Research Literature
ABA practitioners must be skilled in applying the best available evidence to unique cases in specific contexts. The role of the best available evidence in EBP of ABA was discussed above. Practitioners need to be knowledgeable about the scientific literature and able to appropriately apply the literature to behaviors, clients, and contexts that are rarely a perfect match to the behaviors, clients, and contexts in any particular study. This confluence of knowledge and skillful application requires that the behavior analyst respond to the functionally important features of cases. A great deal of training is necessary to build the expertise required to discriminate critical functional features from those that are incidental. These discriminations must be made with respect to the presenting problem (i.e., the behavioral patterns that have been identified as problematic, their antecedent stimuli, motivating operations, and consequences); client variables such as histories, skills, and preferences; and contextual variables that may impact the effectiveness of various treatment options as applied to the particular case. These skills are reflected in BACB Guidelines 1.01 and 2.10 cited above.
Incorporation of the Conceptual System
The critical features of a case must be identified and mapped onto the conceptual system of ABA. It is not enough to recognize that a particular feature of the environment is important; it must also be understood in terms of its likely behavioral function. This initial conceptualization is necessary in order to generate reasonable hypotheses that may be tested in more thorough analyses. Developing the skill of describing cases in terms of likely behavioral functions typically requires a great deal of formal and informal training as well as ongoing learning from experience. These repertoires are usually acquired through extensive training, supervised practice, and the ongoing feedback of client outcomes. This is recognized in BACB Guidelines; for example, 4.0 states that “the behavior analyst designs programs that are based on behavior analytic principles” (BACB 2010 ).
Breadth and Depth of Clinical and Interpersonal Skills
Evidence-based practitioners of behavior analysis must be able to implement various assessment and intervention procedures with fidelity, and often to train and supervise others to implement such procedures with fidelity. Further, clinical expertise in ABA requires that the practitioner have effective interpersonal skills. For example, he must be able to explain the behavioral philosophy and approach, in nonbehavioral terms, to various audiences who may have different theoretical orientations. BCBA Guidelines 1.05 specifies that behavior analysts “use language that is fully understandable to the recipient of those services” (BACB 2010 ).
Integration of Client Values and Context
In all aspects of their work, practitioners of evidence-based ABA must integrate the values and preferences of the client and other stakeholders as well as the features of the specific context that may impact the effectiveness of an intervention. These factors can be considered additional variables that the behavior analyst must attend to when planning and providing behavior-analytic services. For example, when assessment data suggest behavior serves a particular function, a range of intervention alternatives may be considered (see Geiger, Carr, and LeBlanc for an example of a model for selecting treatments for escape-maintained problem behavior). A caregiver’s statements might suggest that one type of intervention may not be viable due to limited resources while another treatment may be acceptable based on financial considerations, available resources, or other practical factors; the behavior analyst must have the training and expertise to evaluate and incorporate these factors into initial treatment selection and to re-evaluate these concerns as a part of progress monitoring for both treatment integrity and client improvement. BACB Guideline 4.0 states that the behavior analyst “involves the client … in the planning of … programs, [and] obtains the consent of the client” and 4.1 states that “if environmental conditions hamper implementation of the behavior analytic program, the behavior analyst seeks to eliminate the environmental constraints, or identifies in writing the obstacles to doing so” (BACB 2010 ).
Recognition of Need for Outside Consultation
Behavior analysts engaging in responsible evidence-based practice discriminate between behaviors and contexts that are within the scope of their training and those that are not, and respond differently based on this discrimination. For example, a behavior analyst who has been trained to provide assessment and intervention for severe problem behavior may not have the specific training to provide organizational behavior management services to a corporation; in this case, a behavior analyst with clinical expertise would make this discrimination and seek additional consultation or make appropriate referrals. This aspect of expertise is described in BACB ( 2010 ) Guidelines 1.02 and 2.02.
Data-Based Decision Making
Data-based decision making plays a central role in the practice of ABA and is an indispensable feature of clinical expertise. The process of data-based decision making includes identifying useful measurement pinpoints, constructing measurement systems, and graphing results, as well as identifying meaningful patterns in data, interpreting these patterns, and making appropriate responses to them (e.g., maintaining, modifying, replacing, or ending a program). The functional features of the case, the best available research evidence, and the new evidence obtained through progress monitoring must inform these judgments and are central to this model of EBP of ABA. BACB ( 2010 ) Guidelines 4.07 and 4.08 specify that behavior analysts collect data to assess progress and modify programs on the basis of data.
Ongoing Professional Development
Clinical expertise is not static; rather, it requires ongoing professional development. Clinical expertise in ABA requires ongoing contact with the research literature to ensure that practice reflects current knowledge about the most effective and efficient assessment and intervention procedures. The critical literature includes primary empirical research as well as reviews and syntheses such as those described in the section on “ Best Available Evidence ”. In addition, professional consensus on important topics for professional practice evolves over time. For example, in ABA, there has been increased emphasis recently on ethics and supervision competence. All of these dynamics point to the need for ongoing professional development. This is reflected in the requirement that certified behavior analysts “undertake ongoing efforts to maintain competence in the skills they use by reading the appropriate literature, attending conferences and conventions, participating in workshops, and/or obtaining Behavior Analyst Certification Board certification” (Guideline 1.03, BACB 2010 ).
Conclusions
We propose that EBP of ABA be understood as a professional decision-making framework that draws on the best available evidence, client values and context, and clinical expertise. We argue that this conception of EBP of ABA is more compatible with the basic tenets of ABA and more closely aligned with definitions of EBP in other fields than that provided by Smith ( 2013 ). It is noteworthy that this notion of EBP is not necessarily in conflict with many of the observations and arguments put forth by Smith ( 2013 ). His concerns were primarily about how to define and validate EST, which is an important way to inform practitioners about the best available evidence to integrate into their overall EBP.
Given the close alignment between the proposed framework of EBP of ABA and broadly accepted descriptions of behavior analytic practice, one might wonder whether EBP offers anything new. We believe that the EBP of ABA framework, offered here, has several important implications for our field. First, this framework draws together numerous elements of ABA practice into a single coherent system, which can help behavior analysts provide an explicit rationale for their decision-making to clients and other stakeholders. The EBP of ABA provides a decision-making framework that supports a cogent and transparent description of (a) the evidence considered, including direct and frequent measurement of the client’s behavior; (b) why this evidence was identified as the “best available” for the particular case; (c) how client values and contextual factors influenced the process; and (d) the ways in which clinical expertise was used to conceptualize the case and integrate the various considerations. This transparency and explicitness allows the behavior analyst to offer empirically based treatment recommendations while addressing the concerns raised by stakeholders. It also highlights the critical analysis required to be an effective behavior analyst. For example, if an EST is available and appropriate, the behavior analyst can describe the relevance and certainty of the evidence for this intervention. If no relevant EST is available, the behavior analyst can describe how the best available evidence supports the intervention and emphasize the importance of progress monitoring.
Second, the EBP framework prompts the behavior analyst to refer to the important client values that underlie the goals of intervention, the specific methods of intervention, and describe how the intervention is supported by features of the context. This requires the behavior analyst to explicitly recognize that the effectiveness of an intervention is always context dependent. By serving as a prompt, the EBP framework should increase behavior analysts’ adherence to this central tenet of ABA.
Third, by explicitly recognizing the role of clinical expertise, the framework gives the behavior analyst a way to talk about the complex skills required to make appropriate decisions about client needs. In addition, the fact that the proposed definition of EBP of ABA is so closely aligned with definitions in other professions such as medicine and psychology that it provides a common framework and language for communicating about a particular case that can enhance collaboration between behavior analysts and other professionals.
Fourth, this framework for EBP of ABA suggests further development of behavior analysis as well. Examination of the meaning of best available evidence encourages behavior analysts to continue to refine methods for systematically reviewing research literature and identifying ESTs. Further, behavior analysts could better support EBP if we developed methods for validating other units of intervention such as practice elements, kernels, and even the principles of behavior; when these are invoked to support interventions, they must be supported by a clearly specified research base.
Finally, the explicit recognition of the role of clinical expertise in the EBP of ABA has important implications for training behavior analysts. This framework suggests that decision-making is at the heart of EBP of ABA and could be an organizing theme for ABA training programs. Training programs could systematically teach students to articulate the chain of logic that is the basis for their treatment recommendations. The chain of logic would include statements about which research was considered and why, how the client’s values influenced decision-making, and how contextual factors influenced the selection and adaptation (if necessary) of the treatment. This type of training could be embedded in all instructional activities. Formally requiring students to articulate a rationale for the decisions and receiving feedback about their decisions would sharpen their clinical expertise.
In addition to influencing our behavior analytic practice, the EBP of ABA framework impacts our relationship with other members of the broader human service field as well as individuals and agencies that control contingencies relevant to practitioners and scientists. Methodologically rigorous reviews that identify ESTs and other treatments supported by the best available evidence are extremely important for working with organizations that control funding for behavior analytic research and practice. Federal funding for research and service provision is moving strongly towards EBP and ESTs. This trend is clear in education through the No Child Left Behind Act of 2001 , the Individuals with Disabilities Education Act of 2004 , the funding policies of IES, and the What Works Clearinghouse. The recent memorandum by the Director of the Office of Management and Budget (Zients 2012 ) makes it clear that the importance of EBP is not limited to a single discipline or to one political party. In addition, insurance companies are increasingly making reimbursement decisions based, in part, on whether or not credible scientific evidence supports the use of the treatment (Small 2004 ). The insurance companies have consistently adopted criteria for scientific evidence that are closely related to EST (Bogduk and Fraifeld 2010 ). As a result, reimbursement for ABA services may depend on the scientific credibility of EST reviews, a critical component of EBP. Methodologically rigorous reviews that identify ESTs within a broader framework of EBP appear to be critical for ABA to maintain and expand its access to federal funding and insurance reimbursement for services. Establishment of this literature base will require behavior analysts to develop appropriate methods for reviewing and summarizing research based on single-subject designs. IES has established such standards for reviewing studies, but to date, there are no accepted methods for calculating a measure of effect size as an objective basis for combining result across studies (Kratochwill et al. 2013 ). If behavior analysts develop such a measure, it would reflect a significant methodological advance as a field and it would increase the credibility of behavior analytic research with agencies that fund research and services.
EBP of ABA emphasizes the research-supported selection of treatments and data-driven decisions about treatment progress that have always been at the core of ABA. ABA’s long-standing recognition of the importance of social validity is reflected in the definition of EBP. This framework for EBP of ABA offers many positive professional consequences for scientists and practitioners while promoting the best of the behavior analytic tradition and making contact with developments in other disciplines and the larger context in which behavior analysts work.
- Albin RW, Lucyshyn JM, Horner RH, Flannery KB. Contextual fit for behavior support plans. In: Koegel LK, Koegel RL, Dunlap G, editors. Positive behavioral support: Including people with difficult behaviors in the community. Baltimore: Brookes; 1996. pp. 81–92. [ Google Scholar ]
- American Occupational Therapy Association Occupational therapy practice framework: domain and process (2nd ed.) American Journal of Occupational Therapy. 2008; 62 :625–683. doi: 10.5014/ajot.62.6.625. [ PubMed ] [ CrossRef ] [ Google Scholar ]
- American Psychological Association (2005). Policy statement on evidence-based practice in psychology. http://www.apa.org/practice/resources/evidence/evidence-based-statement.pdf .
- American Psychological Association Presidential Task Force of Evidence-Based Practice Evidence-based practice in psychology. American Psychologist. 2006; 61 :271–285. doi: 10.1037/0003-066X.61.4.271. [ PubMed ] [ CrossRef ] [ Google Scholar ]
- American Speech-Language-Hearing Association (2005). Evidence-based practice in communication disorders [position statement]. www.asha.org/policy .
- Baer DM, Wolf MM, Risley TR. Some current dimensions of applied behavior analysis. Journal of Applied Behavior Analysis. 1968; 1 :91–97. doi: 10.1901/jaba.1968.1-91. [ PMC free article ] [ PubMed ] [ CrossRef ] [ Google Scholar ]
- Baer DM, Wolf MM, Risley TR. Some still-current dimensions of applied behavior analysis. Journal of Applied Behavior Analysis. 1987; 20 :313–327. doi: 10.1901/jaba.1987.20-313. [ PMC free article ] [ PubMed ] [ CrossRef ] [ Google Scholar ]
- Behavior Analyst Certification Board (2010). Guidelines for responsible conduct for behavior analysts. http://www.bacb.com/index.php?page=57 .
- Benazzi L, Horner RH, Good RH. Effects of behavior support team composition on the technical adequacy and contextual-fit of behavior support plans. The Journal of Special Education. 2006; 40 (3):160–170. doi: 10.1177/00224669060400030401. [ CrossRef ] [ Google Scholar ]
- Bogduk N, Fraifeld EM. Proof or consequences: who shall pay for the evidence in pain medicine? Pain Medicine. 2010; 11 (1):1–2. doi: 10.1111/j.1526-4637.2009.00770.x. [ PubMed ] [ CrossRef ] [ Google Scholar ]
- Bushell D, Jr, Baer DM. Measurably superior instruction means close, continual contact with the relevant outcome data. Revolutionary! In: Gardner R III, Sainato DM, Cooper JO, Heron TE, Heward WL, Eshleman J, Grossi TA, editors. Behavior analysis in education: Focus on measurably superior instruction. Pacific Grove: Brooks; 1994. pp. 3–10. [ Google Scholar ]
- Carnine D. Expanding the notion of teachers’ rights: access to tools that work. Journal of Applied Behavior Analysis. 1992; 25 (1):13–19. doi: 10.1901/jaba.1992.25-13. [ PMC free article ] [ PubMed ] [ CrossRef ] [ Google Scholar ]
- Carr JE, Severtson JM, Lepper TL. Noncontingent reinforcement is an empirically supported treatment for problem behavior exhibited by individuals with developmental disabilities. Research in Developmental Disabilities. 2009; 30 :44–57. doi: 10.1016/j.ridd.2008.03.002. [ PubMed ] [ CrossRef ] [ Google Scholar ]
- Chorpita BF. The frontier of evidence-based practice. In: Kazdin AE, Weisz JR, editors. Evidence-based psychotherapies for children and adolescents. New York: Oxford; 2003. pp. 42–59. [ Google Scholar ]
- Chorpita BF, Daleiden EL, Weisz JR. Identifying and selecting the common elements of evidence based interventions: a distillation and matching model. Mental Health Services Research. 2005; 7 :5–20. doi: 10.1007/s11020-005-1962-6. [ PubMed ] [ CrossRef ] [ Google Scholar ]
- Chorpita BF, Becker KD, Daleiden EL. Understanding the common elements of evidence-based practice: misconceptions and clinical examples. Journal of the American Academy of Child and Adolescent Psychiatry. 2007; 46 :647–652. doi: 10.1097/chi.0b013e318033ff71. [ PubMed ] [ CrossRef ] [ Google Scholar ]
- Cook BG, Cook SC. Unraveling evidence-based practices in special education. Journal of Special Education. 2013; 47 (2):71–82. doi: 10.1177/0022466911420877. [ CrossRef ] [ Google Scholar ]
- Cooper JO, Heron TE, Heward WL. Applied behavior analysis. 2. Upper Saddle River: Pearson; 2007. [ Google Scholar ]
- Detrich, R. (Chair) (2009). Evidence-based, empirically supported, best practice: What does it all mean? Symposium conducted at the annual meeting of the Association for Behavior Analysis International, Phoenix, AZ.
- Detrich R, Slocum TA, Spencer TD. Evidence-based education and best available evidence: Decision-making under conditions of uncertainty. In: Cook BG, Tankersley M, Landrum TJ, editors. Advances in learning and behavioral disabilities, 26. Bingly, UK: Emerald; 2013. pp. 21–44. [ Google Scholar ]
- Embry DD. Community-based prevention using simple, low-cost, evidence-based kernels and behavior vaccines. Journal of Community Psychology. 2004; 32 :575–591. doi: 10.1002/jcop.20020. [ CrossRef ] [ Google Scholar ]
- Embry DD, Biglan A. Evidence-based kernels: fundamental units of behavioral influence. Clinical Child and Family Psychology Review. 2008; 11 :75–113. doi: 10.1007/s10567-008-0036-x. [ PMC free article ] [ PubMed ] [ CrossRef ] [ Google Scholar ]
- Fisher WW, Piazza CC, Roane HS, editors. Handbook of applied behavior analysis. New York: Guilford Press; 2011. [ Google Scholar ]
- Fixsen DL, Naoom SF, Blase KA, Friedman RM, Wallace F. Implementation research: A synthesis of the literature (FMHI publication #231) Tampa: University of South Florida, Louis de la Parte Florida Mental Health Institute, The National Implementation Research Network; 2005. [ Google Scholar ]
- Goodheart CD. Evidence, endeavor, and expertise in psychology practice. In: Goodheart CD, Kazdin AE, Sternberg RJ, editors. Evidence-based psychotherapy: Where practice and research meet. Washington, D.C.: APA; 2006. pp. 37–61. [ Google Scholar ]
- Goodman KW. Ethics and evidence-based education: Fallibility and responsibility in clinical science. New York: Cambridge University Press; 2003. [ Google Scholar ]
- Heward WL, et al., editors. Focus on behavior analysis in education: Achievements, challenges, and opportunities. Upper Saddle River: Prentice Hall; 2005. [ Google Scholar ]
- Horner RH, Carr EG, Halle J, McGee G, Odom S, Wolery M. The use of single-subject research to identify evidence-based practice in special education. Exceptional Children. 2005; 71 (2):165–179. doi: 10.1177/001440290507100203. [ CrossRef ] [ Google Scholar ]
- Horner RH, Sugai G, Todd AW, Lewis-Palmer T. Schoolwide positive behavior support. In: Bambera LM, Kern L, editors. Individualized supports for students with problem behaviors: Designing positive behavior plans. New York: Guilford Press; 2005. pp. 359–390. [ Google Scholar ]
- Individuals with Disabilities Education Improvement Act of 2004, 70, Fed. Reg., (2005).
- Institute of Education Sciences, US. Department of Education. (n.d.). What Works Clearinghouse Procedures and Standards Handbook (No. Version 3.0). Washington DC. Retrieved from http://ies.ed.gov/ncee/wwc/pdf/reference_resources/wwc_procedures_v3_0_standards_handbook.pdf .
- Institute of Medicine . Crossing the quality chasm: A new health system for the 21st century. Washington, DC: National Academies Press; 2001. [ PubMed ] [ Google Scholar ]
- Johnston JM, Pennypacker HS. Strategies and tactics of behavioral research. 2. Hillsdale: Erlbaum; 1993. [ Google Scholar ]
- Jones RJ, Azrin NH. Behavioral engineering: stuttering as a function of stimulus duration during speech synchronization. Journal of Applied Behavior Analysis. 1969; 2 :223–229. doi: 10.1901/jaba.1969.2-223. [ PMC free article ] [ PubMed ] [ CrossRef ] [ Google Scholar ]
- Kazdin AE. Psychotherapy for children and adolescents: Directions for research and practice. New York: Oxford University Press; 2000. [ Google Scholar ]
- Kratochwill, T. R., Hitchcock, J., Horner, R. H., Levin, J. R., Odom, S. L., Rindskopf, D. M., & Shadish, W. R. (2010). Single-case designs technical documentation. Retrieved from http://ies.ed.gov/ncee/wwc/pdf/wwc_scd.pdf .
- Kratochwill TR, Hitchcock JH, Horner RH, Levin JR, Odom SL, Rindskopf DM, et al. Single-case intervention research design standards. Remedial & Special Education. 2013; 34 (1):26–38. doi: 10.1177/0741932512452794. [ CrossRef ] [ Google Scholar ]
- Madden GJ, Dube WV, Hackenberg TD, Hanley GP, Lattal KA, editors. American Psychological Association handbook of behavior analysis. Washington, DC: American Psychological Association; 2013. [ Google Scholar ]
- Maggin DM, O’Keeffe BV, Johnson AH. A quantitative synthesis of single-subject meta-analyses in special education, 1985–2009. Exceptionality. 2011; 19 :109–135. doi: 10.1080/09362835.2011.565725. [ CrossRef ] [ Google Scholar ]
- Maggin DM, Johnson AH, Chafouleas SM, Ruberto LM, Berggren M. A systematic evidence review of school-based group contingency interventions for students with challenging behavior. Journal of School Psychology. 2012; 50 :625–654. doi: 10.1016/j.jsp.2012.06.001. [ PubMed ] [ CrossRef ] [ Google Scholar ]
- McIntosh K, Filter KJ, Bennett JL, Ryan C, Sugai G. Principles of sustainable prevention: designing scale–up of School–wide Positive Behavior Support to promote durable systems. Psychology in the Schools. 2010; 47 (1):5–21. [ Google Scholar ]
- National Autism Center . National Standards Project: Findings and conclusions. Randolph: National Autism Center; 2009. [ Google Scholar ]
- No Child Left Behind Act of 2001, Pub. L. No. 107-110. (2002).
- Polsgrove L. Reflections on the past and future. Education and Treatment of Children. 2003; 26 :337–344. [ Google Scholar ]
- Riley-Tillman TC, Chafouleas SM. Using interventions that exist in the natural environment to increase treatment integrity and social influence in consultation. Journal of Educational & Psychological Consultation. 2003; 14 (2):139–156. doi: 10.1207/s1532768xjepc1402_3. [ CrossRef ] [ Google Scholar ]
- Sackett DL, Rosenberg WM, Gray JA, Haynes RB, Richardson WS. Evidence based medicine: what it is and what it isn’t. British Medical Journal. 1996; 312 (7023):71. doi: 10.1136/bmj.312.7023.71. [ PMC free article ] [ PubMed ] [ CrossRef ] [ Google Scholar ]
- Sackett DL, Straus SE, Richardson WS, Rosenberg W, Haynes RB, editors. Evidence-based medicine: How to teach and practice EBM. Edinburgh: Livingstone; 2000. [ Google Scholar ]
- Shanahan, T., Callison, K., Carriere, C., Duke, N.K., Pearson, P.D., Schatschneider, C., et al. (2010). Improving reading comprehension in kindergarten through 3rd grade: A practice guide (NCEE 2010-4038). Washington, DC: National Center for Education Evaluation and Regional Assistance, Institute of Education Sciences, U.S. Department of Education. http://ies.ed.gov/ncee/wwc/publications/practiceguides . Accessed 12 Sept 2013
- Sidman M. Tactics of scientific research: Evaluating experimental data in psychology. New York: Basic Books; 1960. [ Google Scholar ]
- Slocum, T. A., & Wilczynski, S. (2008). The unit of analysis in evidence-based practice . Invited paper presented at the meeting the Association for Behavior Analysis International, Chicago, Il.
- Slocum TA, Detrich R, Spencer TD. Evaluating the validity of systematic reviews to identify empirically supported treatments. Education and Treatment of Children. 2012; 35 :201–234. doi: 10.1353/etc.2012.0009. [ CrossRef ] [ Google Scholar ]
- Slocum TA, Spencer TD, Detrich R. Best available evidence: three complementary approaches. Education and Treatment of Children. 2012; 35 :27–55. [ Google Scholar ]
- Small RH. Maximize the likelihood of reimbursement when appealing managed care medical necessity denials. Getting Paid in Behavioral Healthcare. 2004; 9 (12):1–3. [ Google Scholar ]
- Smith T. What is evidence-based behavior analysis? The Behavior Analyst. 2013; 36 :7–33. [ PMC free article ] [ PubMed ] [ Google Scholar ]
- Strain PS, Barton EE, Dunap G. Lessons learned about the utility of social validity. Education and Treatment of Children. 2012; 35 (2):183–200. doi: 10.1353/etc.2012.0007. [ CrossRef ] [ Google Scholar ]
- Wilczynski SM. Risk and strategic decision-making in developing evidence-based practice guidelines. Education and Treatment of Children. 2012; 35 :291–311. doi: 10.1353/etc.2012.0012. [ CrossRef ] [ Google Scholar ]
- Wolf M. Social validity: the case for subjective measurement, or how applied behavior analysis is finding its heart. Journal of Applied Behavior Analysis. 1978; 11 :203–214. doi: 10.1901/jaba.1978.11-203. [ PMC free article ] [ PubMed ] [ CrossRef ] [ Google Scholar ]
- Zients, J. D. (2012). M-12-14. Memorandum to the heads of executive departs. From: Jeffrey D. Zients, Acting Director. Subject: use of evidence and evaluation in the 2014 Budget. www.whitehouse.gov/sites/default/files/omb/…/2012/m-12-14.pdf . Accessed 30 Sept 2012
ADVANCES IN DECISION ANALYSIS
Decision analysis consists of a prescriptive theory and associated models and tools that aid individuals or groups confronted with complex decision problems in a wide variety of contexts. Decision analysis can offer workable solutions in domains such as the environment, health and medicine, engineering, public policy, and business. This book extends traditional textbook knowledge of decision analysis to today’s state of the art. Part I covers the history and foundations of decision analysis. Part II discusses structuring decision problems, including the development of objectives and their attributes, and influence diagrams. Part III provides an overview of the elicitation and aggregation of probabilities and advances in model building with influence diagrams and belief networks. Part IV discusses utility and risk preferences, practical value models, and extensions of the subjective expected utility model. Part V consists of an overview of advances in risk analysis. Part VI puts decision analysis in a behavioral and organizational context. Part VII presents a selection of major applications.
Ward Edwards (1927–2005) received his Ph.D. in psychology from Harvard University. He was the recipient of many awards, including the Frank P. Ramsey Medal from the Decision Analysis Society of INFORMS in 1988 and the Distinguished Scientific Contributions Award in Applied Psychology from the American Psychological Association in 1996. He wrote more than 100 journal articles and books, including Decision Analysis and Behavioral Research and Utility Theories: Measurement and Applications.
Ralph F. Miles, Jr., received his Ph.D. in physics from the California Institute of Technology and is a consultant in risk and decision analysis. He was the editor and coauthor of Systems Concepts and has written many articles. Until 1991, he worked as an engineer, supervisor, and manager in the Jet Propulsion Laboratory ( JPL ) at the California Institute of Technology. At JPL he was the Spacecraft System Engineer for two flights to Mars. He was awarded the NASA Exceptional Service Medal for his contributions to early mission design and spacecraft development in the role of Mission Analysis and Engineering Manager for the Voyager Mission to Jupiter, Saturn, Uranus, and Neptune.
Detlof von Winterfeldt is Professor of Industrial and Systems Engineering and of Public Policy and Management at the University of Southern California ( USC ). He is also Director of the Homeland Security Center for Risk and Economic Analysis of Terrorist Events at USC . He received his Ph.D. in psychology from the University of Michigan. He cowrote Decision Analysis and Behavioral Research with Ward Edwards and has published more than 100 articles and reports on decision and risk analysis. He is a Fellow of the Institute for Operations Research and the Management Sciences ( INFORMS ) and of the Society for Risk Analysis. In 2000 he received the Frank P. Ramsey Medal for distinguished contributions to decision analysis from the Decision Analysis Society of INFORMS .
Advances in Decision Analysis
from foundations to applications.
Edited by
Ward Edwards University of Southern California
Ralph F. Miles, Jr.
Detlof von Winterfeldt University of Southern California
CAMBRIDGE UNIVERSITY PRESS Cambridge, New York, Melbourne, Madrid, Cape Town, Singapore, São Paulo, Delhi
Cambridge University Press 32 Avenue of the Americas, New York, NY 10013-2473, USA
www.cambridge.org Information on this title: www.cambridge.org/9780521863681
© Cambridge University Press 2007
This publication is in copyright. Subject to statutory exception and to the provisions of relevant collective licensing agreements, no reproduction of any part may take place without the written permission of Cambridge University Press.
First published 2007
Printed in the United States of America
A catalog record for this publication is available from the British Library.
Library of Congress Cataloging in Publication Data Advances in decision analysis : from foundations to applications / edited by Ward Edwards, Ralph F. Miles, Jr., Detlof von Winterfeldt. p. cm. Includes bibliographical references and index. ISBN -13: 978-0-521-86368-1 (hardback) ISBN -10: 0-521-86368-6 (hardback) ISBN -13: 978-0-521-68230-5 (pbk.) ISBN -10: 0-521-68230-4 (pbk.) 1. Decision making. I. Edwards, Ward. II. Miles, Ralph F., 1933– III. von Winterfeldt, Detlof. IV. Title. BF 448.A38 2007 153.8′3 – dc22 2006025610
ISBN 978-0-521-86368-1 hardback ISBN 978-0-521-68230-5 paperback
Cambridge University Press has no responsibility for the persistence or accuracy of URL s for external or third-party Internet Web sites referred to in this publication and does not guarantee that any content on such Web sites is, or will remain, accurate or appropriate.
List of Contributors
PHILLIP C. BECCUE Strategy, Baxter BioScience
DAVID E. BELL Harvard Business School, Harvard University
VICKI M. BIER Department of Industrial and Systems Engineering, University of Wisconsin-Madison
TERRY A. BRESNICK Innovative Decisions, Inc.
DENNIS M. BUEDE Innovative Decisions, Inc.
JOHN C. BUTLER A.B. Freeman School of Business, Tulane University
ALEXANDER N. CHEBESKOV State Scientific Center of Russian Federation, Institute for Physics and Power Engineering
ROBERT T. CLEMEN Fuqua School of Business, Duke University
JAMES L. CORNER Waikato Management School, University of Waikato
LOUIS ANTHONY COX, JR. Cox Associates and University of Colorado
JAMES S. DYER McCombs School of Business, University of Texas-Austin
THOMAS A. EDMUNDS Systems and Decision Sciences Section, Lawrence Livermore National Laboratory
WARD EDWARDS Department of Psychology, University of Southern California (deceased)
DAVID HECKERMAN Machine Learning and Applied Statistics Group, Microsoft Research
STEPHEN C. HORA College of Business and Economics, University of Hawaii-Hilo
RONALD A. HOWARD Department of Management Science and Engineering, Stanford University
JIANMIN JIA Faculty of Business Administration, The Chinese University of Hong Kong
DONALD L. KEEFER W. P. Carey School of Business, Arizona State University
RALPH L. KEENEY Fuqua School of Business, Duke University
CRAIG W. KIRKWOOD W. P. Carey School of Business, Arizona State University
DON N. KLEINMUNTZ School of Policy, Planning, and Development, University of Southern California
CONNSON LOCKE Haas School of Business, University of California, Berkeley
DAVID MATHESON SmartOrg, Inc.
JAMES E. MATHESON SmartOrg, Inc., and Department of Management Science and Engineering, Stanford University
BARBARA MELLERS Haas School of Business, University of California, Berkeley
RALPH F. MILES, JR. Jet Propulsion Laboratory, California Institute of Technology (retired)
ROBERT F. NAU Fuqua School of Business, Duke University
VLADIMIR I. OUSSANOV State Scientific Center of Russian Federation, Institute for Physics and Power Engineering
M. ELISABETH PATÉ-CORNELL Department of Management Science and Engineering, Stanford University
LAWRENCE D. PHILLIPS Department of Operational Research, London School of Economics and Political Science
HOWARD RAIFFA Harvard Business School and the Kennedy School of Government, Harvard University
JAMES K. SEBENIUS Harvard Business School, Harvard University
ROSS D. SHACHTER Department of Management Science and Engineering, Stanford University
CARL S. SPETZLER Strategic Decisions Group
JEFFREY S. STONEBRAKER Decision Sciences, GlaxoSmithKline
ROBERT L. WINKLER Fuqua School of Business, Duke University
DETLOF VON WINTERFELDT Viterbi School of Engineering, University of Southern California
Decision analysis consists of models and tools to improve decision making. It is especially useful when decisions have multiple conflicting objectives and when their consequences are uncertain. The theoretical foundations of decision analysis can be found in the three areas of decision theory: individual decision theory , social choice theory , and game theory . In addition, disciplines including the behavioral sciences, economics, operations research, philosophy of science, and statistics have contributed to the foundations of decision analysis.
Several introductory decision analysis texts have been written since Raiffa’s Decision Analysis (1968); including Bell and Schleifer (1995); Brown (2005); Brown, Kahr, and Peterson (1974); Clemen (1996); Keeney and Raiffa (1976); Kirkwood (1997); Skinner (1999); von Winterfeldt and Edwards (1986); Watson and Buede (1987); and Winkler (2003). Nevertheless, none of these books cover all of the recent developments in decision analysis. These developments include advances in risk analysis, the use of Bayesian networks and influence diagrams, analysis with multiple stakeholders, generalizations of the expected utility model, and much more. The purpose of this book is to fill this gap with the present state of the art of decision analysis.
This book is intended for two audiences: (1) students who are taking a second course in decision analysis, following an introductory course using any of the texts listed above; and (2) decision analysts or managers who are already familiar with the subject and wish to know the present state of the art. The word “Advances” in the title is meant to convey the significant advances since the first decision analysis books were published, but that we also expect there to be many more to follow in the twenty-first century.
This book came about as a result of weekly meetings between the editors while discussing a future paper on the distinction between normative, prescriptive, and descriptive decision analysis (see Chapter 1: “Introduction” for our resolution) and putting forth our praise of prescriptive decision analysis in support of decision making. We were also discussing possible revisions for a second edition of Detlof’s and Ward’s Decision Analysis and Behavioral Research (von Winterfeldt and Edwards, 1986). It became clear that expanding Detlof and Ward’s book to cover recent advances was just not possible. Decision analysis has expanded to encompass such a wide range of theories and applications that we lacked the intellectual resources to master it. Nevertheless, we recognized the need for such material in text form. Thus the idea for this book was born.
Our first task was to identify the advanced areas of decision analysis that should be included in this book. We identified roughly twenty advanced topics and developed an outline in the form of chapters. We also thought that some chapters on the foundations and applications of decision analysis were important and included several chapters on these topics. We then organized the chapters into the seven principal parts of the book. Our second task was to find the most knowledgeable authors for each of the chapters. Much to our delight, nearly every author we approached agreed to participate, and all recognized the need for this book. Almost everyone followed through and delivered their chapters by our deadlines. With few exceptions, if these authors were asked if they were, along with other talents, a decision analyst, the answer would firmly be “yes.” Finally, Cambridge University Press agreed to publish this book.
Following are the seven principal parts. Part I covers the history and foundations of decision analysis. Part II discusses structuring decision problems, including the development of objectives and their attributes. Part III presents an overview of probabilities and their elicitation and aggregation across experts, model building with belief nets and influence diagrams, and learning causal networks. Part IV discusses utility and risk preferences, practical value models, and extensions of the subjective expected utility model. Part V reviews engineering risk analysis and risk analysis for health risk management. Part VI puts decision analysis in a behavioral and organizational context, including behavioral research, decision conferencing, resource allocation decisions, transitioning from decision analysis to the decision organization, and negotiation analysis. Part VII presents case studies of applications based on the discussions of decision analysis throughout the book.
Some subjects are conspicuous by their limited appearance. Social choice theory has its own disciples and many excellent texts. It does appear in the context of Part VI: “Decision Analysis in a Behavioral and Organizational Context.” Likewise for game theory, which provides theoretical support for Sebenius’ Chapter 23,” Negotiation Analysis: Between Decisions and Games.” The reader will find little on nonexpected utility theory, except as it has a bearing on decision analysis – see Nau’s Chapter 14, “Extensions of the Subjective Expected Utility Model.” We believe that the theoretical foundations for decision-making support should come from expected utility theory, and that the role of nonexpected utility theory lies in understanding the heuristics and biases that one encounters in decision making (Kahneman and Tversky 1982; Kahneman, Slovic, and Tversky 2000; Gilovich, Griffin, and Kahneman 2002) and in understanding market behavior (Glaser, Nöth, and Weber 2004). We had hoped for chapters on the growing use of decision analysis in the medical field and on the subjects of options pricing and decision analysis innovations in finance, but for various reasons these chapters were not written.
Finally, decision analysis is an emerging discipline, having existed by name only since 1966 (Howard 1966). As a result there remains much in contention among decision analysts. Even the nomenclature is in debate. See the June 2004 (Vol. 1, No. 2) issue of Decision Analysis and the articles by Brown (2004), Clemen and Kleinmuntz (2004), Howard (2004, 2004a), Keeney (2004), Kirkwood (2004), and Smith (2004).
It is interesting to reflect on the views of the three founders of decision analysis, as each provides a different perspective and emphasizes different aspects of decision analysis. Ronald Howard emphasizes the uncertainty part of decision ana-lysis over the multiple objective part, criticizing some of the simpler applications of multiattribute utility theory. Ward Edwards considered multiple objectives as the key problems in decision analysis, while he worked on probability problems in the 1960s and in the 1980s. Howard Raiffa, who pioneered multiattribute utility theory with Ralph Keeney, also sees a major role of decision analysis as solving multiattribute problems. In addition, Howard Raiffa always had a strong interest in expanding decision analysis to bargaining and negotiation problems, and he expressed regret that these aspects of decision analysis have not become more prominent.
There are even different views about history. Ronald Howard traces the history of subjective probability back to Laplace (1814), who introduced probability by partitioning an event space into equally likely events. Many other decision analysts and philosophers have criticized Laplace’s definition of probability and instead see the foundations of subjective probability in the works of Bayes (1763), Ramsey (1926), de Finetti (1931, 1937), and Savage (1954).
As editors, we believe that taking a position on these issues is not our role. Thus all opinions of the authors are presented without restriction in this edited book. We leave it to future generations to resolve these issues. Quoting Kirkwood (2004), “In the long run, the answers to these questions will be determined by the research, teaching, and practice of those who view themselves to be in the field.”
Ralph Miles wishes to thank Ron Howard and James Matheson for introducing an engineer to decision analysis in a course taught for Caltech’s Jet Propulsion Laboratory in the mid-1960s. Detlof von Winterfeldt wishes to thank Ralph Keeney for many years of friendship and collaborations on decision analysis projects. He also acknowledges the support of the Department of Homeland Security under grants EMW -2004- GR -0112 ( FEMA ) and N0014-05-0630 ( ONR ). However, any opinions, findings, and conclusions or recommendations in this document are those of the authors and do not necessarily reflect the views of the United States Department of Homeland Security. Unfortunately, Ward Edwards died on February 1, 2005, before this book could be completed. We believe he would be proud of the product that he initiated.
R. F. Miles, Jr. D. v. W.
Bayes, T. (1763). An essay toward solving a problem in the doctrine of chances. Philosophical Transactions of the Royal Society of London , 53, 370–418. Reprinted in 1958 with biographical note by G. A. Barnard in Biometrika , 45 , 293–315.
Bell, D. E., and Schleifer, A. Jr. (1995). Decision making under uncertainty . Boston, MA : Harvard Business School.
Brown, R. (2004). Naming concepts worth naming. Decision Analysis, 1, 86–88.
Brown, R. (2005). Rational choice and judgment: Decision analysis for the decider . New York: John Wiley.
Brown, R., Kahr, A., and Peterson, C. (1974). Decision analysis for the manager . New York: Holt, Reinhart & Winston.
Clemen, R. T. (1996). Making hard decisions: An introduction to decision analysis (2nd ed.). Belmont, CA : Duxbury Press.
Clemen, R. T., and Kleinmuntz, D. N. (2004). From the editors…. Decision Analysis, 1, 69–70.
de Finetti, B. (1931). Sul Significato Soggettivo della Probabilità. Fundamenta Mathematicae, 17, 298–329. Translated in 1993 in P. Monari and D. Cocchi (Eds.), On the Subjective Meaning of Probability. Probabilità e Induxione. Bologna: Clueb, pp. 291–321.
de Finetti, B. (1937). La prévision: Ses lois logiques, ses sources subjectives. Annales de l’Institut Henri Poincaré, 7, 1–68. Translated in 1980 by H. E. Kyburg, Jr., Foresight. Its logical laws, its subjective sources. In H. E. Kyburg, Jr. and H. E. Smokler (Eds.), Studies in subjective probability (2nd ed.). Huntington, NY : Robert E. Krieger, pp. 53–118.
Gilovich, T., Griffin, D., and Kahneman, D. (Eds.). (2002). Heuristics and biases: The psychology of intuitive judgment . Cambridge, UK : Cambridge University Press.
Glaser, M., Nöth, M., and Weber, M. (2004). Behavioral finance. In D. J. Koehler and N. Harvey (Eds.), Blackwell handbook of judgment and decision making . Malden, MA : Blackwell Publishing, pp. 527–546.
Howard, R. A. (1966). Decision analysis: Applied decision theory. In Proceedings of the Fourth International Conference on Operational Research. New York: John Wiley, pp. 55–71.
Howard, R. A. (2004). Speaking of decisions: Precise decision language. Decision Analysis, 1, 71–78.
Howard, R. A. (2004a). Response to comments on Howard. Decision Analysis, 1, 89–92.
Kahneman, D., and Tversky, A. (Eds.). (1982). Judgment under uncertainty: Heuristics and biases . New York: Cambridge University Press.
Kahneman, D., Slovic, P., and Tversky, A. (Eds.). (2000). Choices, values, and frames . Cambridge, UK : Cambridge University Press.
Keeney, R. L. (2004). Communicating about decisions. Decision Analysis, 1, 84–85.
Keeney, R. L., and Raiffa, H. (1976). Decisions with multiple objectives: Preferences and value tradeoffs . New York: John Wiley.
Kirkwood, C. W. (1997). Strategic decision making: Multiobjective decision analysis with spreadsheets . Belmont, CA : Duxbury Press.
Kirkwood, C. W. (2004). Prospects for a decision language. Decision Analysis, 1, 84–85.
Laplace (P. Simon, Marquis de Laplace). (1814). Essai philosophique sur les probabilities . Translated by F. W. Truscott and F. L. Emory and reprinted in 1951 with an introductory note by E. T. Bell as A philosophical essay on probabilities . New York: Dover Publications.
Raiffa, H. (1968). Decision analysis: Introductory lectures on choices under uncertainty. Reading, MA : Addison-Wesley.
Ramsey, F. P. (1926). Truth and probability. In R. B. Braithwaite (Ed.). 1931. F. P. Ramsey, The foundations of mathematics and other logical essays . London: Routledge and Kegan Paul. Reprinted in 1980 in H. E. Kyburg, Jr., and H. E. Smokler, Studies in subjective probability (2nd ed.). New York: John Wiley. Reprinted in 1990 in D. H. Mellor, Philosophical papers: F. P. Ramsey . Cambridge, UK : Cambridge University Press.
Savage, L. J. (1954). The foundations of statistics . New York: John Wiley. Revised 2nd ed. in 1972. New York: Dover Publications.
Skinner, D. C. (1999). Introduction to decision analysis: A practitioner’s guide to improving decision quality (2nd ed.). Gainesville, FL : Probabilistic Publishing,
Smith, J. E. (2004). Precise decision language. Decision Analysis, 1, 79–81.
von Winterfeldt, D., and Edwards, W. (1986). Decision analysis and behavioral research . Cambridge, UK : Cambridge University Press.
Watson, S. R., and Buede, D. M. (1987). Decision synthesis: The principles and practice of decision analysis . Cambridge, UK : Cambridge University Press.
Winkler, R. L. (2003). An introduction to Bayesian inference and decision (2nd ed.). Gainesville, FL : Probabilistic Publishing.
A business journal from the Wharton School of the University of Pennsylvania
Four Pillars of Decision-driven Analytics
May 14, 2024 • 5 min read.
In an excerpt from their new book, Wharton’s Stefano Puntoni and co-author Bart De Langhe argue that the power of data can only be realized by leveraging human intelligence.
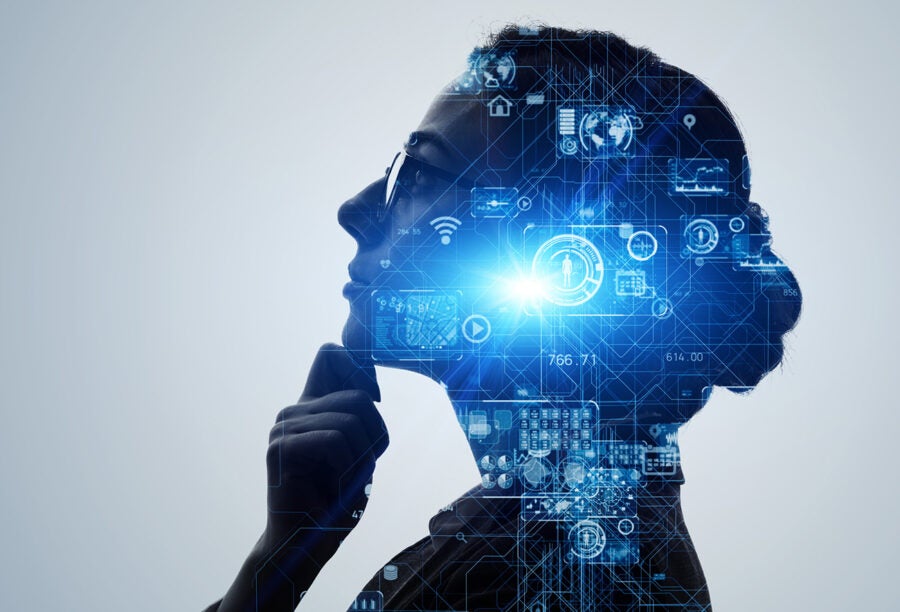
In a new book titled Decision-Driven Analytics: Leveraging Human Intelligence to Unlock the Power of Data , professors and behavioral scientists Bart De Langhe and Stefano Puntoni challenge the idea that our decisions should be driven by data. Rather, they argue that the power of data can only be realized by putting data in the background.
In this excerpt from their book, De Langhe and Puntoni draw from their own research and teaching to offer four pillars of decision-driven analytics.
In the mid-1850s, astronomers figured that the orbit of the planet Uranus was not like it should be according to the laws of physics. A French astronomer, Alexis Bouvard, thought that perhaps that was because we didn’t know about a planet further out in the solar system that exerted an influence on Uranus’s orbit. People started searching the sky for it. Soon enough, Urbain Le Verrier, another Frenchman, found the missing planet. It was named Neptune.
This was a great victory for the power of observation. Investing in data collection saved the day. It taught astronomers that the key to unraveling the mysteries of the cosmos was more and better data.
The rationale for making decisions with input from analytics rests on similar principles. Without data we navigate blind, while with data we can make decisions rooted in evidence. The implication is that good thinking means thinking with data.
The story doesn’t end here, though. An anomaly was soon observed also in the orbit of another planet: Mercury. The same Urbain Le Verrier who had found Neptune now hypothesized the existence of a missing planet lying between Mercury and the Sun. He called this missing planet Vulcan. Again, people started looking for it, only this time nobody could find it. Astronomers kept looking for Vulcan in the subsequent decades but the missing planet remained missing, and the mystery of Mercury unsolved.
The anomaly in the orbit of Mercury could be explained only half a century later. The explanation had to wait for Albert Einstein’s publication of a new theory of gravitation, called the theory of general relativity. This theory revolutionized our understanding of the universe by placing space and time in a four-dimensional continuum.
Although nobody knew that before Einstein entered the scene, all planetary orbits were in fact not conforming to Isaac Newton’s laws. Nobody knew that because the difference between the predictions of the two theories are smaller and smaller as you move away from the Sun. Only in the case of Mercury, which is the planet closest to the Sun, the curvature in space-time caused by the mass of the Sun was large enough for the divergence between the predictions based on Newton’s and Einstein’s theories to be detected by the telescopes of the time.
The mystery of Mercury was solved in a very different way from the mystery of Uranus. While the latter could be solved with better observations, the former could only be solved with better theory, by thinking without data.
Managers are like astronomers, looking to solve problems and find solutions in a complex world, where data is abundant but often hard to make sense of. The message is clear: Data and algorithms are crucial to making good decisions. But human judgment and intelligence are crucial, too.
The Four Pillars of Decision-driven Analytics
Many companies are witnessing an expanding gap between data and decisions, even with the goal of being a “data-driven organization.” The increasing complexity of data and algorithms can make it harder for decision-makers to collaborate with data analysts. For a business to thrive, it’s essential for both groups to understand and value each other’s expertise.
Many businesses find themselves overwhelmed by the sheer volume of data at their disposal. Putting decisions firmly at the center of the analytics process can be transformative. Starting with decisions and working back to the data will improve the quality of decision-making, improve the collaboration between managers and data analysts, and ultimately foster an organizational culture that is action oriented and that prizes the quality of decisions over ego or politics.
Here are the four core principles of decision-driven analytics:
- Decisions. Identify controllable, relevant decision alternatives. Consider diverse perspectives and a wide array of solutions. Prioritize feasible and impactful alternatives to achieve important business outcomes.
- Questions. Formulate precise questions that will help rank the identified decision alternatives. Ambiguous questions can lead to miscommunication and poor decisions.
- Data. Evaluate the data-generating mechanism. While Big Data can be tempting, the emphasis should be on collecting relevant data.
- Answers. When the earlier steps are done right, determining the best action becomes straightforward. Remember, acknowledging uncertainty and sidestepping overconfidence are key for informed decisions.
Decision-driven analytics is about making informed choices, not just processing data or flooding presentations with graphs. It emphasizes gleaning actionable insights from pertinent data. Embracing this approach means letting go of the notion that every data point is vital and not being distracted by the newest tools.
Data is just a means to an end. What matters is the decisions we make.
Excerpted and adapted from Decision-Driven Analytics: Leveraging Human Intelligence to Unlock the Power of Data, by Bart De Langhe and Stefano Puntoni, copyright 2024. Reprinted by permission of Wharton School Press.
More From Knowledge at Wharton
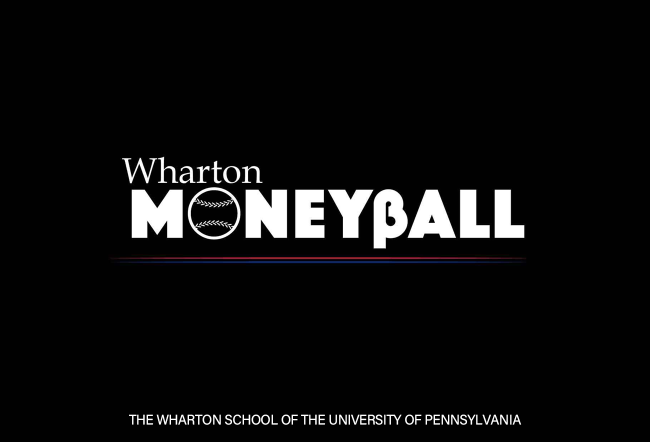
NBA Motion Tracking Data
Nba playing time statistics, 2024 kentucky derby with jeff seder, looking for more insights.
Sign up to stay informed about our latest article releases.
We will keep fighting for all libraries - stand with us!
Internet Archive Audio
- This Just In
- Grateful Dead
- Old Time Radio
- 78 RPMs and Cylinder Recordings
- Audio Books & Poetry
- Computers, Technology and Science
- Music, Arts & Culture
- News & Public Affairs
- Spirituality & Religion
- Radio News Archive
- Flickr Commons
- Occupy Wall Street Flickr
- NASA Images
- Solar System Collection
- Ames Research Center
- All Software
- Old School Emulation
- MS-DOS Games
- Historical Software
- Classic PC Games
- Software Library
- Kodi Archive and Support File
- Vintage Software
- CD-ROM Software
- CD-ROM Software Library
- Software Sites
- Tucows Software Library
- Shareware CD-ROMs
- Software Capsules Compilation
- CD-ROM Images
- ZX Spectrum
- DOOM Level CD

- Smithsonian Libraries
- FEDLINK (US)
- Lincoln Collection
- American Libraries
- Canadian Libraries
- Universal Library
- Project Gutenberg
- Children's Library
- Biodiversity Heritage Library
- Books by Language
- Additional Collections
- Prelinger Archives
- Democracy Now!
- Occupy Wall Street
- TV NSA Clip Library
- Animation & Cartoons
- Arts & Music
- Computers & Technology
- Cultural & Academic Films
- Ephemeral Films
- Sports Videos
- Videogame Videos
- Youth Media
Search the history of over 866 billion web pages on the Internet.
Mobile Apps
- Wayback Machine (iOS)
- Wayback Machine (Android)
Browser Extensions
Archive-it subscription.
- Explore the Collections
- Build Collections
Save Page Now
Capture a web page as it appears now for use as a trusted citation in the future.
Please enter a valid web address
- Donate Donate icon An illustration of a heart shape
Decision analysis and behavioral research
Bookreader item preview, share or embed this item, flag this item for.
- Graphic Violence
- Explicit Sexual Content
- Hate Speech
- Misinformation/Disinformation
- Marketing/Phishing/Advertising
- Misleading/Inaccurate/Missing Metadata
![[WorldCat (this item)] [WorldCat (this item)]](https://archive.org/images/worldcat-small.png)
plus-circle Add Review comment Reviews
49 Previews
2 Favorites
DOWNLOAD OPTIONS
No suitable files to display here.
PDF access not available for this item.
IN COLLECTIONS
Uploaded by station50.cebu on June 14, 2022
Improving and Measuring the Effectiveness of Decision Analysis: Linking Decision Analysis and Behavioral Decision Research
Cite this chapter.
- Robert T. Clemen 6
Part of the book series: Springer Optimization and Its Applications ((SOIA,volume 21))
1816 Accesses
5 Citations
Although behavioral research and decision analysis began with a close connection, that connection appears to have diminished over time. This chapter discusses how to re-establish the connection between the disciplines in two distinct ways. First, theoretical and empirical results in behavioral research in many cases provide a basis for crafting improved prescriptive decision analysis methods. Several productive applications of behavioral results to decision analysis are reviewed, and suggestions are made for additional areas in which behavioral results can be brought to bear on decision analysis methods in precise ways. Pursuing behaviorally based improvements in prescriptive techniques will go a long way toward re-establishing the link between the two fields.
The second way to reconnect behavioral research and decision analysis involves the development of new empirical methods for evaluating the effectiveness of prescriptive techniques. New techniques, including behaviorally based ones such as those proposed above, will undoubtedly be subjected to validation studies as part of the development process. However, validation studies typically focus on specific aspects of the decision-making process and do not answer a more fundamental question. Are the proposed methods effective in helping people achieve their objectives? More generally, if we use decision analysis techniques, will we do a better job of getting what we want over the long run than we would if we used some other decisionmaking method? In order to answer these questions, we must develop methods that will allow us to measure the effectiveness of decision-making methods. In our framework, we identify two types of effectiveness. We begin with the idea that individuals typically make choices based on their own preferences and often before all uncertainties are resolved. A decision-making method is said to be weakly effective if it leads to choices that can be shown to be preferred (in a way that we make precise) before consequences are experienced. In contrast, when the decision maker actually experiences his or her consequences, the question is whether decision analysis helps individuals do a better job of achieving their objectives in the long run. A decisionmaking method that does so is called strongly effective .We propose some methods for measuring effectiveness, discuss potential research paradigms, and suggest possible research projects. The chapter concludes with a discussion of the beneficial interplay between research on specific prescriptive methods and effectiveness studies.
This is a preview of subscription content, log in via an institution to check access.
Access this chapter
- Available as PDF
- Read on any device
- Instant download
- Own it forever
- Compact, lightweight edition
- Dispatched in 3 to 5 business days
- Free shipping worldwide - see info
- Durable hardcover edition
Tax calculation will be finalised at checkout
Purchases are for personal use only
Institutional subscriptions
Unable to display preview. Download preview PDF.
Similar content being viewed by others
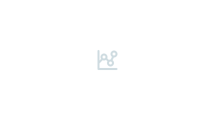
Empirical content as a criterion for evaluating models
Behavioral Economics, Public Policy, and Basic Decision-Making: A Critical Narrative
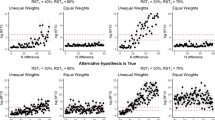
A robust Bayesian test for identifying context effects in multiattribute decision-making
M. Allais. Le comportement de l’homme rationnel devant le risque: Critique des postulats et axiomes de l’ecole americaine. Econometrica , 21:503–546, 1953.
Article MATH MathSciNet Google Scholar
M. Allais and J. Hagen. Expected Utility Hypotheses and the Allais Paradox . Reidel, Dordrecht, The Netherlands, 1979.
MATH Google Scholar
R. M. Anderson and B. F. Hobbs. Using a Bayesian approach to quantify scale compatibility bias. Management Science , 48:1555–1568, 2002.
Article Google Scholar
F. G. Ashby, A. M. Eisen, and A. U. Turken. A neuropsychological theory of positive affect and its influence on cognition. Psychological Review , 106:529–550, 1999.
R. F. Baumeister and T. F. Heatherton. Self-regulation failure: An overview. Psychological Inquiry , 7:1–15, 1996.
H. Bleichrodt, J. L. Pinto, and P. P. Wakker. Making descriptive use of prospect theory to improve the prescriptive use of expected utility. Management Science , 47:1498–1514, 2001.
L. G. Boiney. When efficient is insufficient: Fairness in decisions affecting a group. Management Science , 41:1523–1537, 1995.
Article MATH Google Scholar
D. Bunn. Applied Decision Analysis . McGraw-Hill, New York, 1984.
C. M. Clancy, R. D. Cebul, and S. V. Williams. Guiding individual decisions: A randomized, controlled trial of decision analysis. American Journal of Medicine , 84:283–288, 1988.
R. Clemen, S. K. Jones, and R. L. Winkler. Aggregating forecasts: An empirical evaluation of some Bayesian methods. In D. Berry, K. M. Chaloner, and J. K. Geweke, editors, Bayesian Analysis in Statistics and Econometrics , pages 3–14. Wiley, New York, 1996.
Google Scholar
R. T. Clemen. Making Hard Decisions: An Introduction to Decision Analysis . Duxbury, Belmont, CA, second edition, 1996.
R. T. Clemen and R. C. Kwit. The value of decision analysis at Eastman Kodak Company, 1990–1999. Interfaces , 31:74–92, 2001.
R. T. Clemen and C. Ulu. Interior additivity and subjective probability assessment of continuous variables. Unpublished manuscript, Duke University, 2006.
P. Delquié. “Bimatching”: A new preference assessment method to reduce compatibility effects. Management Science , 43:640–658, 1997.
A. Dijksterhuis, M. W. Bos, L. F. Nordgren, and R. B. van Baaren. On making the right choice: The deliberation-without-attention effect. Science , 311:1005–1007, 2006.
G. W. Fischer. Utility models for multiple objective decisions: Do they accurately represent human preferences? Decision Sciences , 10:451–479, 1979.
B. Fischhoff. Debiasing. In D. Kahneman, P. Slovic, and A. Tversky, editors, Judgment Under Uncertainty: Heuristics and Biases , pages 422–444. Cambridge University Press, Cambridge, UK, 1982.
P. C. Fishburn and R. K. Sarin. Fairness and social risk I: Unaggregated analyses. Management Science , 40:1174–1188, 1994.
P. C. Fishburn and R. K. Sarin. Fairness and social risk II: Aggregated analyses. Management Science , 43:115–126, 1997.
R. Folger. Distributive and procedural justice: Combined impact of “voice” and improvement on experienced inequity. Journal of Personality and Social Psychology , 35:108–119, 1977.
C. R. Fox and R. T. Clemen. Subjective probability assessment in decision analysis: Partition dependence and bias toward the ignorance prior. Management Science , 51:1417–1432, 2005.
C. R. Fox and Y. Rottenstreich. Partition priming in judgment under uncertainty. Psychological Science , 14:195–200, 2003.
C. R. Fox and A. Tversky. A belief-based account of decision under uncertainty. Management Science , 44:879–895, 1998.
D. Frisch and R. T. Clemen. Beyond expected utility: Rethinking behavioral decision research. Psychological Bulletin , 116:46–54, 1994.
D. G. Fryback and J. R. Thornbury. Informal use of decision theory to improve radiological patient management. Radiology , 129:385–388, 1978.
G. Gigerenzer. How to make cognitive illusions disappear: Beyond heuristics and biases. European Review of Social Psychology , 2:83–115, 1991.
G. Gigerenzer, U. Hoffrage, and H. Kleinbölting. Probabilistic mental models: A Brunswikian theory of confidence. Psychological Review , 98:506–528, 1991.
T. Gilovich, D. Griffin, and D. Kahneman, editors. Heuristics and Biases: The Psychology of Intuitive Judgment . Cambridge University Press, Cambridge, UK, 2002.
R. Gregory, S. Lichtenstein, and P. Slovic. Valuing environmental resources: A constructive approach. Journal of Risk and Uncertainty , 7:177–197, 1993.
J. Hershey, H. C. Kunreuther, and P. J. Schoemaker. Sources of bias in assessment of utility functions. Management Science , 28:936–954, 1982.
S. C. Hora, N. G. Dodd, and J. A. Hora. The use of decomposition in probability assessments on continuous variables. Journal of Behavioral Decision Making , 6:133–147, 1993.
C. K. Hsee and Y. Rottenstreich. Music, pandas, and muggers: On the affective psychology of value. Journal of Experimental Psychology: General , 133:23–30, 2004.
S. K. Jacobi and B. F. Hobbs. Quantifying and mitigating splitting biases in value trees. Unpublished manuscript, Johns Hopkins University, Baltimore, MD, 2006.
D. Kahneman. Maps of bounded rationality: Psychology for behavioral economics. American Economic Review , 93:1449–1475, 2003.
D. Kahneman and S. Frederick. Representativeness revisited: Attribute substitution in intuitive judgment. In T. Gilovich, D. Griffin, and D. Kahneman, editors, Heuristics and Biases: The Psychology of Intuitive Judgment , pages 49–81. Cambridge University Press, New York, 2002.
D. Kahneman, P. Slovic, and A. Tversky, editors. Judgment Under Uncertainty: Heuristics and Biases . Cambridge University Press, Cambridge, UK, 1982.
D. Kahneman and A. Tversky. Prospect theory: An analysis of decision under risk. Econometrica , 47:263–291, 1979.
D. Kahneman and A. Tversky. Choices, Values, and Frames . Cambridge University Press, Cambridge, UK, 2000.
R. Keeney and D. von Winterfeldt. Eliciting probabilities from experts in complex technical problems. IEEE Transactions on Engineering Management , 38:191–201, 1991.
R. L. Keeney. Value-Focused Thinking: A Path to Creative Decision Making . Harvard University Press, Cambridge, MA, 1992.
S. Lichtenstein, B. Fischhoff, and L. D. Phillips. Calibration of probabilities: The state of the art to 1980. In D. Kahneman, P. Slovic, and A. Tversky, editors, Judgment Under Uncertainty: Heuristics and Biases , pages 306–334. Cambridge University Press, Cambridge, UK, 1982.
S. Lichtenstein and P. Slovic. Reversals of preference between bids and choices in gambling decisions. Journal of Experimental Psychology , 89:46–55, 1971.
G. F. Loewenstein, C. K. Hsee, E. U. Weber, and N. Welch. Risk as feelings. Psychological Bulletin , 127:267–286, 2001.
M. F. Luce, J. R. Bettman, and J. W. Payne. Choice processing in emotionally difficult decisions. Journal of Experimental Psychology: Learning, Memory, and Cognition , 23:384–405, 1997.
S. Makridakis, A. Andersen, R. Carbone, R. Fildes, M. Hibon, R. Lewandowski, J. Newton, E. Parzen, and R. Winkler. The accuracy of extrapolation (time series) methods: Results of a forecasting competition. Journal of Forecasting , 1:111–153, 1982.
S. Makridakis, C. Chatfield, M. Hibon, M. Lawrence, T. Mills, K. Ord, and L. Simmons. The M-2 competition: A real-time judgmentally based forecasting study. International Journal of Forecasting , 9:5–22, 1993.
S. Makridakis and M. Hibon. The M3-competition. International Journal of Forecasting , 16:451–476, 2000.
M. McCord and R. de Neufville. Lottery equivalents: Reduction of the certainty effect problem in utility assessment. Management Science , 32:56–60, 1986.
M. W. Merkhofer. Quantifying judgmental uncertainty: Methodology, experiences, and insights. IEEE Transactions on Systems, Man, and Cybernetics , 17:741–752, 1987.
M. G. Morgan and M. Henrion. Uncertainty: A Guide to Dealing with Uncertainty in Quantitative Risk and Policy Analysis . Cambridge University Press, Cambridge, UK, 1990.
M. Muraven and R. F. Baumeister. Self-regulation and depletion of limited resources: Does self-control resemble a muscle? Psychological Bulletin , 126:247–259, 2000.
A. H. Murphy and R. L. Winkler. Scoring rules in probability assessment and evaluation. Acta Psychologica , 34:273–286, 1970.
L. D. Ordóñez, B. A. Mellers, S.-J. Chang, and J. Roberts. Are preference reversals reduced when made explicit? Journal of Behavioral Decision Making , 8:265–277, 1995.
J. W. Payne, J. R. Bettman, and E. J. Johnson. The Adaptive Decision Maker . Cambridge University Press, Cambridge, UK, 1993.
J. W. Payne, J. R. Bettman, and D. A. Schkade. Measuring constructed preferences: Towards a building code. Journal of Risk and Uncertainty , 19:243–270, 1999.
J. Protheroe, T. Fahey, A. A. Montgomery, and T. J. Peters. The impact of patients’ preferences on the treatment of atrial fibrillation: Observational study of patient based decision analysis. British Medical Journal , 320:1380–1384, 2000.
H. Raiffa. Decision Analysis . Addison-Wesley, Reading, MA, 1968.
Y. Rottenstreich and A. Tversky. Unpacking, repacking, and anchoring: Advances in support theory. Psychological Review , 2:406–415, 1997.
T. Saaty. The Analytic Hierarchy Process . McGraw-Hill, New York, 1980.
R. E. Schaefer and K. Borcherding. The assessment of subjective probability distributions: A training experiment. Acta Psychologica , 37:117–129, 1973.
B. J. Schmeichel, K. D. Vohs, and R. F. Baumeister. Intellectual performance and ego depletion: Role of the self in logical reasoning and other information processing. Journal of Personality and Social Psychology , 85:33–46, 2003.
K. E. See, C. R. Fox, and Y. Rottenstreich. Between ignorance and truth: Partition dependence and learning in judgment under uncertainty. Unpublished manuscript, University of Pennsylvania, 2006.
S. Sloman. The empirical case for two systems of reasoning. Psychological Bulletin , 119:3–22, 1996.
P. Slovic. The construction of preferences. American Psychologist , 50:364–371, 1995.
P. Slovic, M. Finucane, E. Peters, and D. G. MacGregor. The affect heuristic. In T. Gilovich, D. Griffin, and D. Kahneman, editors, Heuristics and Biases: The Psychology of Intuitive Judgment , pages 397–420. Cambridge University Press, Cambridge, UK, 2002.
P. Slovic, D. Griffin, and A. Tversky. Compatibility effects in judgment and choice. In R. Hogarth, editor, Insights in Decision Making: A Tribute to Hillel J. Einhorn , pages 5–27. University of Chicago Press, IL, 1990.
C. S. Spetzler and C.-A. S. Staël Von Holstein. Probability encoding in decision analysis. Management Science , 22:340–352, 1975.
C.-A. S. Staël Von Holstein. The effect of learning on the assessment of subjective probability distributions. Organizational Behavior and Human Decision Processes , 6:304–315, 1971.
C.-A. S. Staël Von Holstein. Two techniques for assessment of subjective probability distributions: An experimental study. Acta Psychologica , 35:478–494, 1971.
A. Tversky and D. Kahneman. The framing of decisions and the psychology of choice. Science , 211:453–458, 1981.
Article MathSciNet Google Scholar
A. Tversky and D. Kahneman. Advances in prospect theory: Cumulative representation of uncertainty. Journal of Risk and Uncertainty , 26:297–323, 1992.
A. Tversky and D. J. Koehler. Support theory: A nonextensional representation of subjective probability. Psychological Review , 101:547–567, 1994.
A. Tversky, S. Sattath, and P. Slovic. Contingent weighting in judgment and choice. Psychological Review , 95:371–84, 1988.
A. Tversky, P. Slovic, and D. Kahneman. The causes of preference reversal. The American Economic Review , 80:204–217, 1990.
D. von Winterfeldt and W. Edwards. Decision Analysis and Behavioral Research . Cambridge University Press, Cambridge, UK, 1986.
P. Wakker and D. Deneffe. Eliciting von Neumann-Morgenstern utilities when probabilities are distorted or unknown. Management Science , 42:1131–1150, 1996.
M. Weber, F. Eisenführ, and D. von Winterfeldt. The effects of splitting attributes on weights in multiattribute utility measurement. Management Science , 34:431–445, 1988.
G. Wu and R. Gonzalez. Nonlinear decision weights in choice under uncertainty. Management Science , 45:74–85, 1999.
Download references
Author information
Authors and affiliations.
Fuqua School of Business, Duke University, 27708-1208, Durham, NC, USA
Robert T. Clemen
You can also search for this author in PubMed Google Scholar
Editor information
Editors and affiliations.
Management and Organizations, University of Arizona, 85721, Tucson, AZ, USA
Tamar Kugler
Dept. Industrial & Systems Engineering, University of Florida, 303 Weil Hall, 32611-6595, Gainesville, FL, USA
J. Cole Smith
Eller College of Management, University of Arizona, 1130 E. Helen St, 85721, Tucson, AZ, USA
Terry Connolly
College of Engineering Dept. Systems & Industrial Engineering, University of Arizona, 1127 E. James E. Rogers Way, 85721-0020, Tucson, AZ, USA
Young-Jun Son
Rights and permissions
Reprints and permissions
Copyright information
© 2008 Springer Science+Business Media, LLC
About this chapter
Clemen, R.T. (2008). Improving and Measuring the Effectiveness of Decision Analysis: Linking Decision Analysis and Behavioral Decision Research. In: Kugler, T., Smith, J.C., Connolly, T., Son, YJ. (eds) Decision Modeling and Behavior in Complex and Uncertain Environments. Springer Optimization and Its Applications, vol 21. Springer, New York, NY. https://doi.org/10.1007/978-0-387-77131-1_1
Download citation
DOI : https://doi.org/10.1007/978-0-387-77131-1_1
Publisher Name : Springer, New York, NY
Print ISBN : 978-0-387-77130-4
Online ISBN : 978-0-387-77131-1
eBook Packages : Mathematics and Statistics Mathematics and Statistics (R0)
Decision analysis and behavioral research
By detlof von winterfeldt.
- 1 Want to read
- 0 Currently reading
- 0 Have read

Preview Book
My Reading Lists:
Use this Work
Create a new list
My book notes.
My private notes about this edition:
Check nearby libraries
- Library.link
Buy this book
This edition doesn't have a description yet. Can you add one ?
Previews available in: English
Showing 1 featured edition. View all 1 editions?
Add another edition?
Book Details
Published in.
Cambridge [Cambridgeshire], New York
Edition Notes
Bibliography: p. 575-594. Includes indexes.
Classifications
External links.
- Publisher description
The Physical Object
Community reviews (0).
- Created April 1, 2008
- 17 revisions
Wikipedia citation
Copy and paste this code into your Wikipedia page. Need help?

- Professional & Technical
- Professional Science

Download the free Kindle app and start reading Kindle books instantly on your smartphone, tablet or computer – no Kindle device required .
Read instantly on your browser with Kindle for Web.
Using your mobile phone camera, scan the code below and download the Kindle app.

Follow the author

Image Unavailable

- To view this video, download Flash Player
Decision Analysis and Behavioral Research Paperback – Sept. 26 1986
- ISBN-10 0521273048
- ISBN-13 978-0521273046
- Publisher Cambridge University Press
- Publication date Sept. 26 1986
- Language English
- Dimensions 15.88 x 3.18 x 24.13 cm
- Print length 624 pages
- See all details
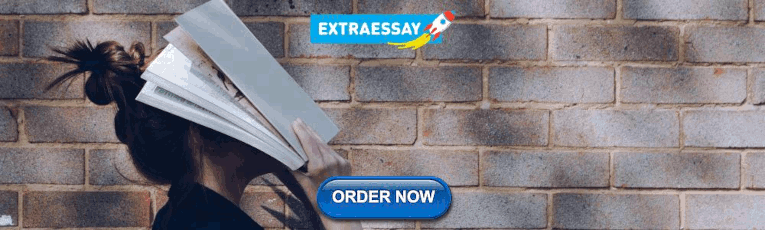
Product details
- Publisher : Cambridge University Press (Sept. 26 1986)
- Language : English
- Paperback : 624 pages
- ISBN-10 : 0521273048
- ISBN-13 : 978-0521273046
- Item weight : 827 g
- Dimensions : 15.88 x 3.18 x 24.13 cm
- #2,143 in Cognitive Psychology Books
- #2,561 in Cognitive Science
- #2,568 in Cognitive Psychology in Professional Science
About the author
Detlof von winterfeldt.
Discover more of the author’s books, see similar authors, read author blogs and more
Customer reviews
- Sort reviews by Top reviews Most recent Top reviews
Top reviews from Canada
Top reviews from other countries.

- Amazon and Our Planet
- Investor Relations
- Press Releases
- Amazon Science
- Sell on Amazon
- Supply to Amazon
- Become an Affiliate
- Protect & Build Your Brand
- Sell on Amazon Handmade
- Advertise Your Products
- Independently Publish with Us
- Host an Amazon Hub
- Amazon.ca Rewards Mastercard
- Shop with Points
- Reload Your Balance
- Amazon Currency Converter
- Amazon Cash
- Shipping Rates & Policies
- Amazon Prime
- Returns Are Easy
- Manage your Content and Devices
- Recalls and Product Safety Alerts
- Customer Service
- Conditions of Use
- Privacy Notice
- Interest-Based Ads
- Amazon.com.ca ULC | 40 King Street W 47th Floor, Toronto, Ontario, Canada, M5H 3Y2 |1-877-586-3230
Loading metrics
Open Access
Peer-reviewed
Artificial neural networks for model identification and parameter estimation in computational cognitive models
Roles Conceptualization, Data curation, Formal analysis, Investigation, Methodology, Software, Validation, Visualization, Writing – original draft, Writing – review & editing
* E-mail: [email protected]
Affiliation Department of Psychology, University of California, Berkeley, Berkeley, California, United States of America

Roles Data curation, Formal analysis, Methodology, Software, Validation, Visualization, Writing – original draft, Writing – review & editing
Roles Conceptualization, Investigation, Methodology, Software, Writing – original draft, Writing – review & editing
Affiliation Department of Mathematics, University of California, Berkeley, Berkeley, California, United States of America
Roles Conceptualization, Funding acquisition, Investigation, Project administration, Supervision, Writing – original draft, Writing – review & editing
Affiliations Department of Psychology, University of California, Berkeley, Berkeley, California, United States of America, Helen Wills Neuroscience Institute, University of California, Berkeley, Berkeley, California, United States of America
- Milena Rmus,
- Ti-Fen Pan,
- Liyu Xia,
- Anne G. E. Collins
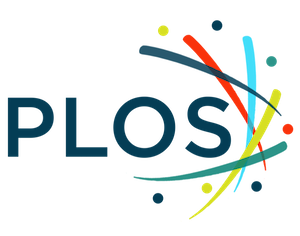
- Published: May 15, 2024
- https://doi.org/10.1371/journal.pcbi.1012119
- Peer Review
- Reader Comments
This is an uncorrected proof.
Computational cognitive models have been used extensively to formalize cognitive processes. Model parameters offer a simple way to quantify individual differences in how humans process information. Similarly, model comparison allows researchers to identify which theories, embedded in different models, provide the best accounts of the data. Cognitive modeling uses statistical tools to quantitatively relate models to data that often rely on computing/estimating the likelihood of the data under the model. However, this likelihood is computationally intractable for a substantial number of models. These relevant models may embody reasonable theories of cognition, but are often under-explored due to the limited range of tools available to relate them to data. We contribute to filling this gap in a simple way using artificial neural networks (ANNs) to map data directly onto model identity and parameters, bypassing the likelihood estimation. We test our instantiation of an ANN as a cognitive model fitting tool on classes of cognitive models with strong inter-trial dependencies (such as reinforcement learning models), which offer unique challenges to most methods. We show that we can adequately perform both parameter estimation and model identification using our ANN approach, including for models that cannot be fit using traditional likelihood-based methods. We further discuss our work in the context of the ongoing research leveraging simulation-based approaches to parameter estimation and model identification, and how these approaches broaden the class of cognitive models researchers can quantitatively investigate.
Author summary
Computational cognitive models occupy an important position in cognitive science research, as they offer a simple way of quantifying cognitive processes (such as how fast someone learns, or how noisy they are in choice selection), and testing which cognitive theories offer a better explanation of the behavior. To relate cognitive models to the behavioral data, researchers rely on statistical tools that require estimating the likelihood of observed data under the assumptions of the cognitive model. This is, however, not possible to do for all models as some models present significant challenges to likelihood computation. In this work, we use artificial neural networks (ANNs) to bypass likelihood computation and approximation altogether, and demonstrate the success of this approach applied to model parameter estimation and model comparison. The proposed method is a contribution to ongoing development of modeling tools which will enable cognitive researchers to test a broader range of theories of cognition.
Citation: Rmus M, Pan T-F, Xia L, Collins AGE (2024) Artificial neural networks for model identification and parameter estimation in computational cognitive models. PLoS Comput Biol 20(5): e1012119. https://doi.org/10.1371/journal.pcbi.1012119
Editor: Ming Bo Cai, University of Miami, UNITED STATES
Received: September 22, 2023; Accepted: April 27, 2024; Published: May 15, 2024
Copyright: © 2024 Rmus et al. This is an open access article distributed under the terms of the Creative Commons Attribution License , which permits unrestricted use, distribution, and reproduction in any medium, provided the original author and source are credited.
Data Availability: The data in this manuscript is simulated using the code stored in the referenced GitHub repository, and can be generated by running the simulation code. Accessible at: https://github.com/MilenaCCNlab/MI-PEstNets.git .
Funding: This work was supported by the National Institutes of Health (NIH R21MH132974 to AGEC). The funders had no role in study design, data collection and analysis, decision to publish, or preparation of the manuscript.
Competing interests: The authors have declared that no competing interests exist.
This is a PLOS Computational Biology Methods paper.
Introduction
Computational modeling is an important tool for studying behavior, cognition, and neural processes. Computational cognitive models translate scientific theories into algorithms using simple equations with a small number of interpretable parameters to make predictions about the cognitive or neural processes that underlie observable behavioral or neural measures. These models have been widely used to test different theories about cognitive processes that shape behavior and relate to neural mechanisms [ 1 – 4 ]. By specifying model equations, researchers can inject different theoretical assumptions into most models, and simulate synthetic data to make predictions and compare against observed behavior. Researchers can quantitatively arbitrate between different theories by comparing goodness of fit [ 5 , 6 ], across different models. Furthermore, by estimating model parameters that quantify underlying cognitive processes, researchers have been able to characterize important individual differences (e.g. developmental: [ 7 – 10 ]; clinical: [ 11 – 15 ]) as well as condition effects [ 16 , 17 ].
Researchers’ ability to benefit from computational modeling crucially depends on the availability of methods for model fitting and comparison. Such tools are available for a large group of cognitive models (such as, for example, reinforcement learning and drift diffusion models). Examples of commonly used model parameter fitting tools include maximum likelihood estimation (MLE, [ 18 ]), maximum a-posteriori (MAP, [ 19 ]), and sampling approaches ([ 20 , 21 ]). Examples of model comparison tools include information criteria such as AIC and BIC [ 5 , 22 ], and Bayesian group level approaches, including protected exceedance probability [ 23 , 24 ]. These methods all have one important thing in common—they necessitate computing the likelihood of the data conditioned on models and parameters, thus limiting their use to models with tractable likelihood. However, many models do not have a tractable likelihood. This severely limits the types of inferences researchers can make about cognitive processes, as many models with intractable likelihood might offer better theoretical accounts of the observed data. Examples of such models include cases where observed data (e.g. choices) might depend on latent variables—such as the unobserved rules that govern the choices [ 25 – 27 ], or a latent state of engagement (e.g. attentive/distracted, [ 28 , 29 ]) a participant/agent might be in during the task. In these cases, computing the likelihood of the data often demands integrating over the latent variables (rules/states) across all trials, which grows exponentially and thus is computationally intractable. This highlights an important challenge—computing likelihoods is essential for estimating model parameters, and performing fitness comparison/model identification, and alternative models are less likely to be considered or taken advantage of to a greater extent.
Some existing techniques attempt to bridge this gap. For example, Inverse Binomial Sampling [ 30 ], particle filtering [ 31 ], and assumed density estimation [ 32 ] provide approximate solutions to the Bayesian inference process in specific cases. Many of these methods, however, require advanced mathematical expertise for effective use and adaptation beyond specific cases they were developed for, making them less accessible many researchers. Approximate Bayesian Computation (ABC, [ 33 – 37 ]) offers a more accessible avenue for estimating parameters in models limited by intractable likelihoods. More widely employed in cognitive modeling, the approach of basic ABC rejection algorithms involves translating trial-level data into summary statistics. Parameter values of the candidate model are then selected based on their ability to produce simulated data that is closely aligned with summarized data, guided by some predefined rejection criterion.
While ABC rejection algorithms provide a useful workaround solution, it’s important to acknowledge their inherent limitations. Specifically, ABC results are sensitive to the choice of summary statistics (and rejection criteria) and sample efficiency of ABC demonstrates scales poorly in cases of high-dimensional data [ 33 , 38 , 39 ]. Recent strides in the field of simulation-based inference/likelihood-free inference have addressed these limitations by using artificial neural network(ANN) structures designed to optimize summary statistics, and consequently infer parameters. These methods enable automated (or semi-automated) construction of summary statistics, minimizing the effect the choice of summary statistics may have on the accuracy of parameter estimation [ 38 , 40 – 44 ]. This innovative approach serves to amortize the computational cost of simulation-based inference, opening new frontiers in terms of scalability and performance [ 40 , 41 , 45 – 50 ].
Here, we test a related, general approach that leverage advances in artificial neural networks (ANNs) to estimate parameters and perform model identification for models with and without tractable likelihood, entirely bypassing the likelihood estimation (or approximation) step. ANNs have been successfully used to fit intractable models in different fields, including weather models [ 51 ] and econometric models [ 6 ], and more recently cognitive models of decision making [ 40 , 41 ]. We develop similar approaches to specifically target the intractability estimation problem in the field of computational cognitive science, including both parameter estimation and model identification, and thoroughly test it in a challenging class of models where there are strong dependencies between trials (e.g. learning experiments).
Our approach relies on the property of ANNs as universal function approximators. The ANN structure we implemented was a recurrent neural network (RNN) with feed-forward layers inspired by [ 52 ] ( Fig 1 ) that is trained to estimate model parameters, or identify which model most likely generated the data based on input data sequences simulated by the cognitive model. Our approach is similar to previous work in the domain of simulation-based inference [ 40 , 41 ], with a difference that such architectures are specifically designed to optimize explicit summary statistics that describe the data patterns (e.g. invertible networks). Here, rather than emphasizing steps involving the reduction of data dimensionality through the creation (and selection) of summary statistic vectors and subsequent inference based on parameter value samples, our focus is on the direct translation of raw data sequences into precise parameter estimates or the identification of the source model (via implicit summary statistics in network layers).
- PPT PowerPoint slide
- PNG larger image
- TIFF original image
A) Traditional methods rely on computing log-likelihood (LLH) of the data under the given model, and optimizing the likelihood to derive model parameter estimates. B) The ANN is trained to map parameter values onto data sequences using a large simulated data set; the trained network can then be used to estimate cognitive model parameters based on new data without the need to compute or approximate likelihood. C) The ANN structure inspired by [ 52 ] is suitable for data with strong inter-trial dependencies: it consists of an RNN and fully connected feed-forward network, with an output containing ANN estimates of parameter values the data was simulated from for each agent. D) As in parameter estimation, traditional tools for model identification rely on likelihood to derive model comparison metrics (e.g. AIC, BIC) that are used to determine which model likely generated the data. E) ANN is instead trained to learn the mapping between data sequences and respective cognitive models the data was simulated from. F) Structure of the ANN follows the structure introduced for parameter estimation, with the key difference of final layer containing the probability distribution over classes representing model candidates, with highest probability class corresponding to the model the network identified as the one that likely generated the agent’s data.
https://doi.org/10.1371/journal.pcbi.1012119.g001
To validate and benchmark our approach, we first compared it against standard model parameter fitting methods most commonly used by cognitive researchers (MLE, MAP, rejection ABC) in cognitive models from different families (reinforcement learning, Bayesian Inference) with tractable likelihood. Next, we demonstrated that neural networks can be used for parameter estimation of models with intractable likelihood, and compared it to standard approximation method (ABC). Finally, we showed that our approach can also be used for model identification.
Our results showed that our method is highly successful and robust at parameter and model identification while remaining technically lightweight and accessible. We highlight the fact that our method can be applied to standard cognitive data sets (i.e. with arbitrarily small number of participants, and normal number of trials per participant), as the ANN training is fully done on a large simulated data set. Our work contributes to the ongoing research focusing on leveraging artificial neural networks to advance the field of computational modeling, and provides multiple new avenues for maximizing the utility of computational cognitive models.
We focused on two distinct artificial neural network (ANNs) applications in cognitive modeling: parameter estimation and model identification. Specifically, we built a network with a structure suitable for sequential data/data with time dependencies (e.g. recurrent neural network (RNN); [ 52 ]). Training deep ANNs requires large training data sets. We generated such a data set at minimal cost by simulating a cognitive computational model on a cognitive task a large number of times. Model behavior in the cognitive task (e.g. a few hundred trials of stimulus-action pairs or stimulus-action-outcome triplets (depending on the task) for each simulated agent) constituted ANN’s training input; true known parameter values (or identity of the model) from which the data was simulated constituted ANNs’ training targets. We evaluated the network’s training performance in predicting parameter values/identity of the model in a separate validation set, and tested the trained network on a held out test set. We tested RNN variants and compared their accuracy against traditional likelihood-based model fitting/identification methods using both likelihood-tractable and likelihood-intractable cognitive models. See Methods section for details on the ANN training and testing process.
Parameter recovery
Benchmark comparison..
First, we sought to validate our ANN method and compare its performance to existing methods by testing it on standard likelihood-tractable cognitive models of different levels of complexity in the same task: 2-parameter (2 P − RL ) and 4-parameter (4 P − RL ) reinforcement learning models commonly used to model behavior on reversal tasks [ 7 , 14 , 53 , 54 ], as well as Bayesian Inference model ( BI ) and Bayesian Inference with Stickiness ( S − BI ) as an alternative model family that has been found to outperform RL in some cases [ 55 – 57 ]. We estimated model parameters using multiple traditional methods for computing (maximum likelihood and maximum a-posteriori estimation; MLE and MAP) and approximating (Approximate Bayesian Computation; ABC) likelihood. We used the results of these tools as a benchmark for evaluating the neural network approach. Next, we estimated parameters of these models using two variants of RNNs: with gated recurrent units (GRUs) or Long-Short-Term-Memory units (LSTM).
We used the same held out data set to evaluate all methods (the test set the ANN has not observed yet, see simulation details). For each of the methods we extracted the best fit parameters, and then quantitatively estimated the method’s performance as the mean squared error (MSE) between estimated and true parameters across all agents. Methods with lower MSE indicated better relative performance. All of the parameters were scaled for the purpose of loss computation, to ensure comparable contribution to loss across different parameters. To quantify overall loss for a cognitive model we averaged across all individual parameter MSE scores; to calculate fitting method’s MSE score for a class of cognitive models (e.g. likelihood tractable models) we averaged across respective method’s MSE scores for those models (See Methods for details about method evaluation).
First, we examined the performance of standard model-fitting tools (MLE, MAP and ABC). The standard tools yielded a pattern of results that are expected based on noisy, realistic-size data sets (with several-hundred trials per agent). Specifically, we found that MAP outperformed MLE ( Fig 2A , average MSEs: MLE =.67, MAP =.35), since the parameter prior applied in MAP regularizes the fitting process. ABC was also worse compared to MAP ( Fig 2A , average MSE: ABC =.53). While fitting process is also regularized in ABC, worse performance in some models can be attributed to signal loss that arises from approximation to the likelihood. Next, we focused on the ANN performance; our results showed that for each of the models, ANN performed better than or just as well as the traditional methods ( Fig 2A , average MSEs for different RNN variants: GRU =.32, LSTM =.35). Better network performance was more evident for parameter estimation in more complex models (e.g. models with higher number of parameters such as 4P-RL and S-BI; average MSE across these 2 models: MLE =.95, MAP =.43, ABC =.71, GRU =.38, LSTM =.44).
A) Parameter recovery loss from the held out test set for the tractable-likelihood models (2P-RL, 4P-RL, BI, S-BI) using each of the tested methods. Loss is quantified as the mean squared error (MSE) based on the discrepancy between true and estimated parameters. Bars represent loss average for each parameter across all agents, with errorbars representing standard error across agents. B) Parameter recovery from the 4P-RL model using MAP and GRU. ρ values represent Spearman ρ correlation between true and estimated parameters. Red line represents a unity line ( x = y ) and black line represents a least squares regression line. All correlations were significant at p <.001.
https://doi.org/10.1371/journal.pcbi.1012119.g002
Next, we visualized parameter recovery. We found that for each of the cognitive models the parameter recovery was largely successful (Spearman ρ correlations between true parameter values and estimated values: β ρ MAP , ρ GRU = [.90, .91], α + ρ MAP , ρ GRU = [.53, .52], α − ρ MAP , ρ GRU = [.88, .89], κ : ρ MAP , ρ GRU = [.78, .79], Fig 2B ; all correlations were significant at p <.001). For conciseness, we only show recovery of the more complex model parameters from the RL model family (and only MAP method as it performed better compared to ABC and MLE, as well as only GRU since it performed better than LSTM), as we would expect a more complex model to emphasize superiority of a fitting method more clearly compared to simpler models. Recovery plots of the remaining models (and respective fitting methods) can be found in S2 – S5 Figs. Our results suggest that 1) ANN performed as well as traditional methods in parameter estimation based on the MSE loss; 2) more complex models may limit accuracy of parameter estimation in traditional methods that neural networks appear to be more robust against. We note that for the 4 P − RL model, parameter recovery was noisy for all methods, with some parameters being less recoverable than others (e.g. α + , Fig 2B ). This is an expected property of cognitive models applied to realistic-sized experimental data as found in most human experiments (i.e. a few hundred trials per participant). To check whether the limited recovery can be attributed to parameter identifiability rather than pitfalls of any specific method, we looked at the correlation between parameter estimates obtained using the standard model fitting method (MAP) and the ANN (GRU) ( S10 Fig )—with parameters that are not well recovered (e.g. α + in 4P-RL model) being of particular interest. High correlation between estimated parameters obtained via 2 methods imply systematic errors in parameter identification that apply to both methods—thus suggesting that the weaker correlation between true and fit parameters for some parameters is more likely due to limitations in the model applied to the data set than method specifications such as poor optimization performance. We further discuss the implications in discussion section—highlighting that computational models should be carefully crafted and specified regardless of the tools used for model fitting.
Testing in cognitive models with intractable likelihood.
Next, we tested our method in two examples of computational models with intractable likelihood. As a comparison method, we implemented Approximate Bayesian Computation (ABC), alongside our ANN approach to estimate parameters. The two example likelihood-intractable models we used had in common the presence of a latent state which conditioned sequential updates: RL with latent attentive state ( RL − LAS ), and a form of non-temporal hierarchical reinforcement learning ( HRL , [ 27 ]). Since we cannot fit these models using MAP or MLE we used only ABC as a benchmark. Because we found LSTM RNN to be more challenging to train and achieve similar results when compared to GRU, we focused on GRU for the remainder of comparisons. We found that average MSE was much lower for the neural network compared to ABC for both RL-LAS ( Fig 3A , average MSEs: ABC =.62, GRU =.21) and HRL ( Fig 3A , average MSEs: ABC =.28, GRU =.19). Spearman correlations were noisier for ABC compared to GRU in both models ( Fig 3B , RL-LAS : β ρ ABC , ρ GRU = [.72, .91], α ρ ABC , ρ GRU = [.83, .95], T ρ ABC , ρ GRU = [.5, .81]; HRL : β ρ ABC , ρ GRU = [.86, .89], α ρ ABC , ρ GRU = [.85, .9]; all correlations were significant at p <.001). Furthermore, some parameters were less recoverable than others (e.g. the T parameter in RL-LAS model, which indexed how long participants remained in an inattentive state); this might be in part due to less straightforward effect of T on behavior ( S6 Fig ). Note that in order to obtain our ABC results we had to perform an extensive exploration procedure to select summary statistics—ensuring reasonable ABC results. Indeed, the choice of summary statistics is not trivial and represents an important difficulty of applying basic rejection ABC [ 33 , 38 ], that we can entirely bypass using our new neural network approach. We acknowledge that recent methods that rely on ANNs replaced standard ABC methods by automating (or semi-automating) construction of summary statistics [ 38 , 40 – 44 , 51 ]. However, we aimed to explore an alternative approach, independent of explicit optimization of summary statistics, and focused on the ABC instantiation that has been most frequently implemented in the field of cognitive science as a benchmark [ 33 – 35 ].
A) Parameter recovery loss from the held out test set for the intractable-likelihood models (RL-LAS, HRL) using ABC and GRU network. Loss is quantified as the mean squared error (MSE) based on the discrepancy between true and estimated parameters. Bars represent MSE average for each parameter across all agents, with errorbars representing standard error across agents ( S17 Fig ) shows variability across seeds). B) Parameter recovery from the RL-LAS and HRL models using ABC (green) and GRU network (yellow). ρ values represent Spearman ρ correlation between true and estimated parameters. Red line represents a unity line ( x = y ) and black line represents a least squares regression line.All correlations were significant at p <.001.
https://doi.org/10.1371/journal.pcbi.1012119.g003
Uncertainty of parameter estimates.
Thus far, we have outlined a method that provides point estimates of parameters based on input data sequences, as is typically the use for much lightweight cognitive modeling (e.g. maximum likelihood estimation or MAP). However, it is sometimes also valuable to compute the uncertainty associated with these estimates [ 21 ]. It is possible to extend our approach to add this capability. While there are various alternative ways to do so (e.g. Bayesian neural networks), the approach we have opted for is incorporating evidential learning into our method [ 58 ]. Evidential learning differs from Bayesian networks in that it places priors over likelihood function, rather than network weights. The network leverages this property to learn both statistical (aleatoric) and systematic (epistemic) uncertainty during the process of estimating a continuous target based on the input data sequences. This marks a shift from optimizing a network to minimize errors based on average prediction, without considering uncertainty.
We applied our method with integrated evidential learning to tractable and intractable versions of the RL models (2P-RL and RL-LAS, Fig 4 ). We found that incorporating this modification did not compromise the point estimate parameter recovery (e.g. compared to our baseline method focused only on maximizing the accuracy of the predictions). Additionally, it enabled the estimation of the uncertainty around the point estimate, as demonstrated by [ 58 ]. This extension appears to be more computationally expensive (with longer training periods) than our original method, but not to a prohibitive extent.
Using evidential learning to evaluate uncertainty of parameter estimates for A) 2-parameter RL model (tractable likelihood) and B) RL model with latent attention states (intractable likelihood). Vertical lines around point estimates illustrate model uncertainty. We are showing only 100 data points for the purpose of cleaner visualization, Spearman ρ values are computed based on the total number of agents in the held-out test data (3k).
https://doi.org/10.1371/journal.pcbi.1012119.g004
Model identification
We also tested the use of our ANN approach for model identification. Specifically, we simulated data from different cognitive models, and trained the network to make a prediction regarding which model most likely generated the data out of all model candidates. The network architecture was identical to the network used for parameter estimation, except that the last layer became a classification layer (with one output unit per model category) instead of a regression layer (with one output unit per target parameter).
For models with tractable likelihood, we performed the same model identification process using AIC [ 5 ] that relies on likelihood computation, penalized by number of parameters, to quantify model fitness as a benchmark. We note that another common criterion, BIC [ 6 ], performed more poorly than AIC in our case. The best fitting model is identified based on the lowest AIC score—a successful model recovery would indicate that the true model has the lowest AIC score compared to other models fit to that data. To construct the confusion matrix, we computed best AIC score proportions for all models, across all agents, for data sets simulated from each cognitive model ( Fig 5 ; see Methods ).
A) Confusion matrix of likelihood-tractable models from PRL task based on 1) likelihood/AIC metric, and 2) ANN identification. AIC confusion matrix revealed a much higher degree of misclassification (e.g. true simulated model being incorrectly identified as a different model). B) Confusion matrix of likelihood-intractable models using ANN (2P-RL and RL-LAS models were simulated on the PRL task; HRL, BI and S-BI models were simulated on the HRL task).
https://doi.org/10.1371/journal.pcbi.1012119.g005
As shown in Fig 5A , model identification performed using our ANN approach was better compared to the AIC confusion matrix, with less “confusion”—lower off-diagonal proportions compared to diagonal proportions (correct identification). Model identification using AIC is likely in part less successful due to some models being nested in others (e.g. 2 P − RL in 4 P − RL , BI in S − BI ). Specifically, since AIC score represents a combination of likelihood and penalty incurred by the number of parameters it is possible that the data from more complex models is incorrectly identified as better fit by a simpler version of that model (e.g. the model with fewer parameters; an issue which would be more pronounced if we used a BIC confusion matrix). The same phenomenon is observed with the network, but to a much lesser extent, showing better identification out of sample—even for nested models. Furthermore, the higher degree of ANN misclassification observed for BI / S − BI was driven by S − BI simulations with stickiness parameter close to 0, which would render the BI and S − BI non-distinguishable ( S7 Fig ).
Because we cannot compute the likelihood for our likelihood-intractable models based on closed-form solutions via MAP, we only report the confusion matrices obtained from our ANN approach In the first confusion matrix we performed model identification for 2 P − RL and RL − LAS , as we reasoned these two models differ by only one mechanism (occasional inattentive state), and thus could potentially pose the biggest challenge to model identification. In the second confusion matrix, we included all models used to simulate data on the HRL task ( HRL model , Bayesian inference model , Bayesian inference with stickiness model ). In both cases, the network successfully identified the correct models as true models, with a very small degree of misidentification, mostly in the nested models. Based on our benchmark comparison to AIC, and the proof of concept identification for likelihood intractable models, our results indicate that the ANN can be leveraged as a valuable tool in model identification.
Robustness tests
Robustness tests: influence of different input trial sequence lengths..
ANNs are sometimes known to fail catastrophically when data is different from the training distribution in minor ways [ 59 – 62 ]. Thus, we investigated the robustness of our method to differences in data format we might expect in empirical data, such as different numbers of trials across participants. Specifically, we conducted robustness experiments by varying the number of trials in each individual simulation contributing to training or test sets, fixing the number of agents in the training set.
To evaluate the quality of parameter recovery, we used the coefficient of determination score ( R 2 ) which normalizes different parameter ranges. We found that the ANNs trained with a higher trial number reach high R 2 scores in long test trials. However, their performance suffers significantly with smaller number of test trials. The results also show a similar trend in model identification tasks except that training with higher trial number doesn’t guarantee a better performance. For instance, the classification accuracy between HRL task models of the ANN trained with 300 trials reaches 87% while the ANN trained with 500 trials is 84%.
Data-augmentation practices in machine learning increase robustness of models during training [ 63 ] by introducing different types of variability in the training data set (e.g. adding noise, different data sizes). Specifically, slicing time-series data into sub-series is a data-augmentation practice that increases accuracy [ 64 ]. Thus, we trained our ANN with the fixed number of simulations of different trial numbers. As predicted, we found that the ANNs trained with a mixture of trial sequence lengths across simulations (purple line) consistently yielded better performance across different numbers of test trials for both parameter recovery and model identification ( Fig 6A and 6B ).
A) Parameter estimation in both RL-LAS and HRL show that training with a mixture of trial sequence lengths (purple line) yields more robust out-of-sample parameter value prediction compared to fixed trial sequence lengths. B) Best model identification results, performed on different combinations of model candidates, were also yielded by mixed trial sequence length training. The number of agents/simulations used for training was kept constant across all the tests (N agents = 30k).
https://doi.org/10.1371/journal.pcbi.1012119.g006
Robustness tests: Prior parameter assumptions.
We also tested the effects of incorrect prior assumptions about the parameter range on method performance. Specifically we 1) trained the network using data simulated from a narrow range of parameters (theoretically informed) and 2) trained the network based on broader range of parameter values. Next, we tested both networks in making out-of-sample predictions for test data sets that were simulated from narrow and broad parameter ranges respectively. The network trained using a narrow parameter range made large errors at estimating parameters for data simulated outside of the range it was trained on; training the network on a broader range overall resulted in smaller error, with some loss of precision for the parameter values in range of most interest (e.g. the narrow range of parameters the alternative network is trained on). We observed similar results with MAP, where we specified narrow/broad prior (where narrow prior would place high density on a specific parameter range). Notably, training the network using a broader range of parameters while oversampling from a range of interest yielded more accurate parameter estimation compared to MAP with broad priors (Approach described in S9 Fig ).
Robustness tests: Model misspecification.
In addition to testing the effects of incorrect priors, we also tested the effect of model misspecification on standard method and ANN performance (focusing on MAP and GRU network, as they performed the best in parameter recovery tests on benchmark models). We fit the Bayesian inference model (without stickiness) to the data simulated from the Bayesian inference model with stickiness using MAP. For the ANN, we trained the neural network to estimate parameters of the Bayesian inference model, and tested it on the separate test set data simulated from the Bayesian inference model with stickiness. For each method, we looked at the correlation between the ground truth Bayesian inference with stickiness model parameters, and the method’s parameter estimates ( S13 Fig ). Our results suggest that the parameters shared between the 2 models are reasonably recoverable using both MAP and ANN (e.g. the recovery is noisier but comparable to that of parameters in Bayesian models without model misspecification ( S4 and S5 Figs); furthermore, the correlation between ground truth and estimated values is similar for the two methods.
To make the model misspecification more extreme, we additionally simulated data from a Bayesian inference model, and estimated RL model parameters from the simulated data. We did this using standard methods (MAP) and ANN, and repeated the same process in reverse (simulating data from an RL model, and fitting Bayesian inference model parameters). We found that both MAP and ANN exhibited similar patterns. That is, in the case of simulating Bayesian inference model and fitting RL model parameters, the estimated β captured the variance from the true β and p switch , while the estimated α parameter captured the variance driven by the Bayesian updating parameters p reward and p switch ( S14 Fig ). In the case of simulating RL model and fitting Bayesian inference model parameters, p switch parameter captured the noise in the simulated data coming from the β parameter, and the variance from the α parameter was attributed to the p reward parameter ( S15 Fig ). We also correlated parameter estimates generated by the two methods. High correlation implies that MAP and GRU generate similar parameter estimates, suggesting that they are impacted by model misspecification in a similar way ( S11 Fig ).
Our results demonstrate that artificial neural networks (ANNs) can be successfully and efficiently used to estimate best fitting free parameters of likelihood-intractable cognitive models, in a way that is independent of likelihood approximation. ANNs also show remarkable promise in successfully arbitrating between competing cognitive models. While our method leverages “big data” techniques, it does not require large experimental data sets: indeed, the large training set used to train the ANNs is obtained purely through efficient and fast model simulation. Thus, our method is applicable to any standard cognitive data set with a normal number of participants and trials per participants. Furthermore, while our method requires some ability to work with ANNs, it does not require any advanced mathematical skills, making it largely accessible to the broad computational cognitive modeling community.
Our method adds to a family of approaches from other attempts at using neural networks for fitting computational cognitive models. Specifically, previous work leveraging amortized inference has focused on taking advantage of large-scale simulations and invertible networks. This approach involves training the summary segment of the network to adeptly learn relevant summary statistic vectors, while concurrently training the inference segment of the network to approximate the posterior distribution of model parameters based on the outputs generated by the summary network [ 40 , 41 , 46 ]. This method has successfully been applied to both parameter estimation and model identification (and performs in a similar range as our method when applied to intractable models we implemented in this paper), bypassing many issues of ABC. In parallel, work by [ 47 ] showcased Likelihood Approximation Networks (LANs) as a method that approximates likelihood of sequential sampling models (but requires ABC-like approaches for training), and recovers posterior parameter distributions with high accuracy for a specific class of models (e.g. drift diffusion models); more recently, [ 48 ] used a similar approach with higher training data efficiency. Work by [ 65 ] used Approximate Bayesian Computation (ABC) in conjunction with mixture density networks to map data to parameter posterior distributions. Unlike most of these approaches our architecture is not dependent on [ 47 , 48 ] or explicitly designed to optimize [ 40 , 41 , 46 ] summary statistics. By necessity, hidden layers of our network do implicitly compute a form of summary statistic that are translated into estimated parameters/model class in the output layer; however, we do not optimize for such statistics explicitly, beyond their ability to support parameter/model recovery.
Other approaches have used ANNs for different purposes than fitting cognitive models [ 66 ]. For example, [ 52 ] leveraged flexibility of RNNs (which inspired our network design) to map data sequences onto separable latent dimensions that have different effects on decision-making behavior of agents, as an alternative to cognitive models that make more restrictive assumptions. Similarly, work by [ 67 ] also used RNNs to estimate RL parameters and make predictions about behavior of RL agents. Our work goes further than this approach in that it focuses on both parameter recovery and model identification of models with intractable likelihood, without relying on likelihood approximation. Furthermore, multiple recent papers [ 68 , 69 ] use ANNs as a replacement for cognitive models, rather than as a tool for supporting cognitive modeling as we do, demonstrating the number of different ways ANNs are taking a place in computational cognitive science.
It is important to note that while ANNs may prove to be a useful tool for cognitive modeling, one should not expect that their use immediately fixes or overrides all issues that may arise in parameter estimation and model identification. For instance, we have observed that while ANNs outperformed many of the traditional likelihood-based methods, recovery for some model parameters was still noisy (e.g. learning rate α in the 4P-RL model, Fig 2 ). This is a property of cognitive models when applied to experimental applied to data sets that range in hundreds of trials. Standard methods (e.g. MAP) fail in a similar way—as shown by the high correlation between MAP and ANN parameter estimates ( S10 Fig ), which suggests that parameter recovery issues have more to do with identifiability limitations of the data and model, rather than other issues such as optimization method. Similarly, often times model parameters are not meaningful in certain numerical ranges, and sometimes model parameters trade off in how they impact behavior through mathematical equations that define the models—making the parameter recovery more challenging. Furthermore, when it comes to model identification, particularly with nested models, the specific parameter ranges can influence the outcome of model identification, favoring simpler models over more complex ones (or vice versa). This was evident in our observations regarding the confusion between Bayesian inference models with and without stickiness, wherein the ground truth values of stickiness played a decisive role in the model identification. This is to say ANNs should be treated as a useful tool that is only useful if the researchers apply significant forethought to developing appropriate, identifiable cognitive models.
In a similar vein, it is important to recognize that the potential negative implications of model misspecification extend to neural networks, much like they impact traditional model-fitting approaches. For instance, our estimation of parameters may be conducted under the assumption of model X, whereas, in reality, model Y might be the most suitable for explaining the data—leading to poor parameter estimation and model predictions. Our test of the systematic effects of model misspecification involved utilizing a network trained to estimate parameters from one model (e.g. Bayesian Inference) to predict parameters for the held-out test set data simulated from a different model (e.g. Bayesian Inference with stickiness, or RL). We compared this to model misspecification with a standard MAP approach. Notably, neither method exhibited significant adverse effects. When models were nested, the parameters shared between the two models were reasonably well recovered. When the model misspecificpation was more extreme (with models from different families), we again observed similar effects on the two methods, where variance driven by one parameter tended to be recovered similarly. Thus, our approach appears equally (but not worse) subject to the risk of model misspecification as other fitting methods. In light of these findings, our key takeaway is to exercise caution against assuming that the use of a neural network remedies all issues typically associated with modeling. Instead, we advocate for the application of conventional diagnostics (e.g., model comparison, predictive checks) that are commonly employed in standard methods to ensure robust and accurate results.
Relatedly, we have shown that the parameter estimation accuracy varies greatly as a function of the parameter range the network was trained on, along with whether the underlying parameter distribution of the held out test-set is included in that range or not. This is an expected property of ANNs that are known to underperform when the test data systematically differs from training examples [ 59 – 61 ]. As such, the range of parameters/models used for inputs constitutes a form of prior that constrains the fit, and it is important to carefully specify it with informed priors (as is done with other methods, such as MAP). We found that training the network using a broader parameter range, while heavily sampling from a range of interest (e.g. plausible parameter values based on previous research) affords both accurate prediction for data generated outside of the main expected range, with limited loss of precision within the range of interest ( S9 Fig ). This kind of practice is also consistent with practices in computational cognitive modeling, where a researcher might specify (e.g. using a prior) that parameter might range between two values, with most falling within a certain, more narrow range.
One factor that is specific to ANN-based methods (as opposed to standard methods) is the effect different hyperparameters (e.g. size of the neural network, choice of the learning rate, dropout values, etc.) may have on network performance—commonly resulting in overfitting or underfitting. We observed that the network performance, particularly in parameter recovery, is most significantly influenced by the number of units in the GRU layer and the chosen dropout rate. A suitable range for the number of GRU units is typically between 90 and 256, covering the needs of most cognitive models. A dropout rate within the range of 0.1 to 0.2 is generally sufficient. We have outlined the details of parameter ranges we tested in the table ( S1 Table ). To address this challenge, we employed an automated hyperparameter tuning approach, as outlined by Bergstra, Yamins, and Cox (2013). This Bayesian optimization for tuning hyper-parameters helps reduce the time required to obtain an optimal parameter set by learning from previous iterations. Additionally, in the process of training a neural network, the initialized random weights play a significant role in determining the network’s convergence and the final performance. Different random seeds can result in different initializations of the network weights, which may affect the optimization process downstream, and potentially yield different final solutions. It is important to be mindful of this; we have inspected effects of setting different seeds on our network performance ( S17 Fig ), and found that overall network performance was stable across different seeds, with slight variations (1 seed) for both parameter estimation and model identification—showcasing the need for cautious practice of inspecting network’s performance under multiple seeds.
We compared our artificial neural network approach against existing methods that are commonly used to estimate parameters of likelihood-intractable models (e.g. ABC, [ 33 , 70 ]). While traditional rejection ABC provides a workaround solution, it also imposes certain constraints. Specifically, it is more suitable for data with no sequential-dependencies, and the accuracy of parameter recovery is largely contingent on selection of appropriate summary statistics, which is not always a straightforward problem. More recent advances in the domain of simulation-based inference [ 38 , 40 , 42 , 44 ] solve many ABC-issues by automating the process of construction of summary statistics. For the purpose of this project we have focused on the methods that are most commonly used in cognitive modeling (e.g. maximum likelihood/maximum a posteriori), but future work should extend to conducting the same benchmarking procedure involving these inference methods.
Alternative approximation methods (e.g. particle filtering [ 31 ]; density estimation [ 32 ]); inverse binomial sampling [ 30 ] may prove to be more robust, but frequently require more advanced mathematical knowledge and model case-based adaptations, or are more computationally expensive; indeed, some of them may not be usable or tractable in our type of data and models where there are sequential dependencies between trials [ 30 , 71 ]. ANN-based methods such as ours or others’ [ 40 , 41 , 49 ], on the other hand, offers a more straightforward and time-efficient path to both parameter estimation and model identification. Developing more accessible and robust methods is critical for advances in computational modeling and cognitive science, and the rising popularity of deep learning puts neural networks forward as useful tools for this purpose. Our method also offers an advantage of requiring very little computational power. The aim of the project at its current state was not to optimize our ANN training in terms of time and computing resources; nevertheless, we used Nvidia V100 GPUs with 25 GB memory and required at most 1 hour for model training and predictions. This makes the ANN tool useful, as it requires a low amount of computing resources and can be done fast and inexpensively. All of our code is shared on GitHub .
We primarily focused on extensive tests using synthetic data, in particular in the context of learning experiments that present important challenges for some methods (such as BADS [ 71 ] or ABC [ 33 – 35 ]) due to the dependency between trials, and have not been thoroughly investigated with other ANN-based approaches. A critical next step will be to further validate our approach using empirical data (e.g. participant data from the tasks). Similarly, we relied on RNNs due to their flexibility and capacity to handle sequential data. However, it will be important to explore different structures, such as transformers [ 72 ], for potentially improved accuracy in parameter recovery/model identification, as well as alternative uses in cognitive modeling.
In addition, our baseline approach lacks the capability to quantify the complete uncertainty in parameter estimation, offering only point estimates. This is similar to many lightweight cognitive modeling approaches (such as MAP and LLH), but stands in contrast to other methods that integrate simulation-based inference with neural network structures [ 40 , 41 , 45 , 47 , 48 ], where the ability to capture full uncertainty represents a notable strength. Nevertheless, we have showcased that our method can easily be extended to provide uncertainty estimates by incorporating evidential learning techniques [ 58 ], at a slight computational cost, but minimal impact on point estimates’ accuracy. Furthermore, we included both RL and Bayesian inference models to demonstrate our approach can work with different classes of computational models. Future work will include additional models (e.g. sequential decision making models) to further test robustness of our approach.
In conclusion, we propose an accessible ANN-based method to perform parameter and model identification across a broad class of computational cognitive models for which application of existing methods is challenging. Our work should contribute to a growing literature focused on developing new methods that will allow researchers to quantitatively test a broader family of theories than previously possible.
Materials and methods
Probabilistic reversal learning task..
We have simulated data from different models (see the Models section) on a simple probabilistic reversal learning task (PRL; [ 73 , 74 ]). In the task, an agent chooses between two actions on each trial, and receives binary outcome ( r = 1 [reward] or r = 0 [no reward]). One of the two actions is correct for a number of trials; a correct action is defined as the action that gets rewarded with higher probability (e.g. p ( r = 1| action = correct ) = 0.80), with 1 − p probability of getting no reward if selected. After a certain number of trials, the correct action reverses; thus the action that was previously rewarded with low probability becomes the more frequently rewarded one ( S1 Fig ). This simple task (and its variants) have been extensively used to provide mechanistic insights into learning from reinforcement, inferring probabilistic structure of the environment, and people’s ability (or failure) to update the representation of a correct choice.
Hierarchical reinforcement learning task.
We developed a novel task environment that can be solved using a simple but plausible model with intractable likelihood. In this task, an agent observes N arrows (in different colors), each pointing at either left or right direction. The agent needs to learn which arrow is the correct one, by selecting an action that corresponds to either left or right side (consistent with the direction the arrow is pointing at) in order to get rewarded. Selecting the side the correct arrow is pointing at rewards the agent with high probability ( p =.9); choosing an action by following direction of other arrows leads to no reward ( r = 0) with same high probability. The correct arrow changes unpredictably in the task, which means that the agent must keep track of which arrow most reliably leads to the reward, and update accordingly upon the change. We refer to this task structure as hierarchical because the choice policy (left/right) depends on the higher-level rule (color) agents choose to follow.
Cognitive models
Prl task models..
We implemented multiple models of the PRL task to test the artificial neural network (ANN) approach to parameter estimation. First, we cover the benchmark models; these are the models that we can fit using traditional methods (MLE, MAP), as well as the ANN, to ensure that we can justify using the ANN if it performs at least just as well as (or better than) the traditional methods.
Reinforcement learning models family.
Two-parameter reinforcement learning model . We simulated artificial data on the PRL task using a simple 2-parameter reinforcement learning model (2P-RL). The model assumes that the agent tracks the value of each action contingent on the reward history, and uses these values to inform the action selection on each trial.
The 2p-RL model contained following free parameters: learning rate ( α ) and softmax beta ( β ).
Like in the 2P-RL we also included counterfactual updating of values for non-selected actions. The 4P-RL model included following free parameters: positive learning rate ( α + ), negative learning rate ( α − ), softmax beta ( β ) and stickiness ( κ ).
Bayesian models family.
The BI model included following parameters: inferred probability of reward given the action determined by the current belief ( p reward ), likelihood of the correct action reversing ( p switch ) and softmax beta ( β ).
Intractable likelihood.
As a proof of concept, we implemented a simple model that assumes a latent state of agent’s attention (engaged/disengaged). This model can’t be fit using methods that rely on computing likelihood. While models can have intractable likelihood for a variety of reasons, we focused on leveraging latent variables (e.g. attention state), that are not readily observable in the data. Thus, in the data that is being modeled, only the choices are observed—but not the state the agent was in while executing the choices. The learned choice value which affects the choice likelihood depends on the trial history, including which state the agent was in. Thus, if there are 2 such states, there are 2 N possible sequences that may result in different choice value estimates after N trials. To estimate choice values and likelihood on any given trial one must integrate over the uncertainty of an exponentially increasing latent variable—thus making the likelihood intractable.
RL and latent engagement state.
We simulated a version of a 2p-RL model for a probabilistic reversal learning (PRL) task that also assumes that an agent might occupy two of the latent attention states—engaged or disengaged— during the task (RL-LAS). The model assumes that in the engaged state an agent behaves in accordance with the task structure (e.g. tracks and updates values of actions, and uses action values to inform action selection). In the disengaged state, an agent behaves in a noisy way, in that 1) it does not update the Q value of actions, and 2) chooses between the two actions randomly (e.g. uniform policy) instead of based on their value (e.g. through softmax). Note that assumption 1) is different from a previous version of the model our group considered [ 78 , 79 ], and is the core assumption that renders the likelihood intractable. The agent can shift between different engagement states at any point throughout the task, and the transition between the states is controlled by a parameter τ . Specifically, for each agent we initialized a random value T between 10 and 30 (which roughly maps onto approximately how many trials an agent spends in a latent attention state), and then used a non-linear transformation to compute τ : 1-(1/T). The value of τ , thus quantifies the probability of transitioning between the two states. The agent was initialized to be in an attentive state at the onset of trials.
Cognitive models of the HRL task.
Bayesian models of the HRL task . Bayesian models of the HRL task assume an inference process over the latent variable of which arrow is currently the valid arrow, and thus which side (R/L) (given the current trial’s set of arrows) is most likely to result in positive outcome. The inference relies on the generative model of the task determined by parameters p switch and p reward , history of trial observations O t , set of arrows and stochastic choice based on this inference. Initial prior belief over arrows is initialized uniformly prior = 1/ nA , where nA corresponds to the number of arrows.
This belief is subsequently used to inform arrow choices on the next trial. This model differs from the Bayesian Inference model for the probabilistic task in that 1) p reward and p switch parameters are not free/inferred and 2) the choice of the side is stochastic, allowing for a potential lapse in selecting the side that is not consistent with the selected arrow. This model, thus has following free parameters: decision parameter β and noise parameter ϵ . Like in the in Bayesian inference model for the PRL task, we also tested the model variant with stickiness κ parameter that biases beliefs associated with the arrow/side chosen on the previous trial. Both models have tractable likelihoods.
Hierarchical reinforcement learning.
Likelihood-dependent methods
Maximum likelihood and maximum a posteriori estimation..
As a prior for the MAP approach, we used an empirical prior derived from the true simulating distribution of parameters. We note that this gives an advantage to the MAP method above what would be available for empirical data, allowing MAP to provide a ceiling performance on the test set.
Because MAP and MLE rely on likelihood computation, their use is essentially limited to models with tractable likelihood. We used MAP and MLE to estimate parameters of tractable-likelihood models as one of the benchmarks against which we compared our ANN approach. Specifically, we fit the models to the test-set data used to compute the MSE of the ANN, and compared fit using the same metric across methods (see main text).
Likelihood approximation methods.
Because models with tractable likelihood comprise only a small subset of all possible (and likely more plausible) models, researchers have handled the issue of likelihood intractability by implementing various likelihood approximation methods. While there are different likelihood approximation tools, such as particle filtering [ 31 ] and assumed density estimation [ 32 ], we focus on Approximate Bayesian Computation (ABC; [ 33 , 36 , 37 , 70 ]), as it is more widely accessible and does not require more extensive mathematical expertise. ABC leverages large scale model simulation to approximate likelihood. Specifically, a large synthetic data set is simulated from a model, with parameters randomly sampled from a specific range for each agent. Summary statistics that describe the data (e.g average accuracy or variance in accuracy) are used to construct the empirical likelihood that can be used in combination with classic methods.
The distance metric, like the rejection criterion, is determined by the researcher. The samples that are accepted are the samples with distance to the real data smaller than the criterion, resulting in the conclusion that parameters used to generate the sample data set can plausibly be the ones that capture the target data. Thus, the result of the ABC for each data set is a distribution of plausible parameter values which can be used to obtain point estimates via the mean, median, etc.
ABC is a valuable tool,but standard ABC has serious limitations [ 33 ]. For instance, the choice of summary statistics is not a trivial problem, and different summary statistics can yield significantly different results. Similarly, in the case of rejection algorithm ABC, researchers must choose the rejection criterion which can also affect the parameter estimates. A possible way to address this is using cross validation to determine which rejection criterion is the best, but this also requires specification of the set of possible criteria values for the cross validation algorithm to choose from. Furthermore, one of ABC assumptions is independence of data points, which is violated in many sequential decision making models (e.g. reinforcement learning).
To compare our approach to ABC, we used network training set data as a large scale simulation data set, and then estimated parameters of the held out test set also used to evaluate the ANN.
To apply ABC in our case, we needed to select summary statistics that adequately describe performance on the task. We used the following summary statistics to quantify the agent for the models simulated on the PRL task:
- Learning curves: We computed agents’ probability of selecting the correct action, aligned to the number of trials with reference to the reversal point. Specifically, for each agent we computed an average proportion of trials where a correct action was selected N trials before and N trials after the correct action reversal point, for all reversal points throughout the task. This summary statistic should capture learning dynamics, as the agent learns to select the correct action, and then experiences dip in accuracy once the correct actions switch, subsequently learning to adjust based on feedback after several trials.
- 3-back feedback integration: The 3-back analysis quantifies learning as well; however, instead of aligning the performance to reversal points, it allowed us to examine agents’ tendency to repeat action selection from the previous trial contingent on reward history—specifically the outcome they observed on the most recent 3 trials. Higher probability of repeating the same action following more positive feedback indicates learning/sensitivity to reward as reported in [ 11 ]
- Ab-analysis: The Ab-analysis allowed us to quantify probability of selecting an action at trial t ,contingent both on previous reward and action selection history (trials t − 2 and t − 1, [ 11 , 80 ]).
For the models simulated on a hierarchical task we used the learning curves as summary statistics (same as for the PRL), where reversal points were defined as the switch of the correct rule/arrow to follow. In addition, we quantified agent’s propensity to stick with the previously correct rule/arrow, where the agent should be increasingly less likely to select the side consistent with the arrow that was correct before the switch as the number of trials since the switch increases. Similarly, we used a version of the 3-back analysis where the probability of staying contingent on the reward history referred to the probability of potentially selecting the same cue across the trial window, based on observed choices of the agent. All summary statistics are visualized in S8 Fig .
Model comparison.
We used AIC score as it outperformed BIC model comparison, and thus provided us with ceiling benchmark to evaluate the ANN.
To perform proper model comparison, it is essential to not only evaluate the model fitness (overall AIC/BIC score), but also to test how reliably the true models (that generated the data) can be identified/successfully distinguished from others. To do so, we constructed a confusion matrix based on the AIC score ( Fig 5A ). We used the test set data simulated from each model, and then fit all candidate models to each of the data sets while also computing the AIC score for each fit. If the models are identifiable, we should observe that AIC scores for true models (e.g. the models the data was simulated from) should be the lowest for that model when it’s fit to the data compared to other model candidates.
Artificial neural network-based method
Parameter recovery..
To implement ANNs for parameter estimation we have used the relatively simple neural network structure inspired by the previous work [ 52 ]. In all experiments, we used 1 recurrent GRU layer followed by 3 fully connected dense layers with 2000 dimensional input embeddings ( S1 Table ). To train the network, we simulated a training data set using known parameters. For each model, we used 30000 training samples, 3000 validation samples, and 3000 test samples that are generated from simulations separately. For probabilistic RL, the input sequence consisted of rewards and actions. For hierarchical RL, the sides (left/right) of three arrow stimuli are added to the rewards and actions sequences. The network output dimension was proportional to the number of model parameters. We used a tanh activation in the GRU layer, reLu activations in 2 dense layers, and a linear activation at the final output. Additional training details are given below:
- We used He normal initialization to initialize GRU parameters [ 81 ].
- We used the Adam optimizer with mean square error (MSE) loss and a fixed learning rate of 0.003. Early stopping (e.g. network training was terminated if validation loss failed to decrease after 10 epochs) was applied with a maximum of 200 epochs.
- We selected network hyperparameters with Bayesian optimization algorithms [ 82 ] applied on a validation set. Details of the selected values are shown in S1 Table .
All of the training/validation was run using TensorFlow [ 83 ]. The training was performed on Nvidia V100 GPUs with 25 GB memory.
Network evaluation.
The network predicted the values of parameter on the test set that is unseen in the training and validation. We also conducted robustness tests by varying trial numbers (input size).
To evaluate the output of both ANN and traditional tools we used the following metrics (ensuring our results are robust to the choice of performance quantification):
- Mean squared error (MSE): To evaluate parameter estimation accuracy we calculated a mean squared error between true and estimated model parameter across all agents. Prior to calculating MSE all parameters were normalized, to ensure comparable contribution to MSE across all parameters. Overall loss for a cognitive model (across all parameters) was an average of individual parameter MSE scores. Overall loss for a class of models (e.g. likelihood-tractable models) was an average across all model MSE scores.
- Spearman correlation ( ρ ): We used Spearman correlation as an additional metric for examining how estimated parameter values relate to true parameter values, with higher Spearman ρ values indicating higher accuracy. We paired Spearman correlations with scatter plots, to visualize patterns in parameter recoverability (e.g. whether there are specific parameter ranges where parameters are more/less recoverable).
- R-Squared ( R 2 or the coefficient of determination): R-Squared represents the proportion of variance in true parameters that can be explained by a linear regression between true and predicted values. It thus indicates the goodness of fit of an ANN model. We calculated an R-Squared score for each individual parameters across all agents and used it as an additional evaluation for how well the data fit the regression model.
Uncertainty estimation.
To compute uncertainty of parameter estimates we have incorporated evidential learning into our method [ 58 ]. In the application of evidential learning to continuous regression [ 58 ] observed targets follow a Gaussian distribution, characterized by its mean and variance. Conjugate Gaussian prior, normal inverse-gamma distribution, is created by placing a prior on both the mean (Gaussian distribution) and the variance (inverse-gamma distribution). By sampling from this distribution, specific instance of the likelihood function is obtained (based on both mean and the variance). This approach not only aims for accurate target predictions but also takes into account the uncertainty (quantified by the variance term). For more insights and details into evidential learning, refer to the work by [ 58 ]. Their research also introduces a regularization term, which is useful for penalizing incorrect evidence and data that falls outside the expected distribution.
For the purpose of visualization ( Fig 4 ) we have created upper and lower bounds of targets by adding/subtracting variance from the predicted target values. We then re-scaled these values by applying the inverse scaler (e.g. from the scaler applied to normalize parameters for network training). This provides a scale-appropriate and more interpretable visualization of parameter recovery and uncertainty for each parameter.
Alternative models.
We have also tested the network with long short-term memory (LSTM) units since LSTM units are more complex and expressive than GRU units; nevertheless they achieved the similar performance as GRU units but are more computationally expensive, and thus we mostly focused on the GRU version of the model. Since LSTM worked, but not better than GRU, the LSTM results are reported in S2 – S5 Figs.
Model identification.
The network structure and training process were similar to that of the network used for parameter recovery, with an exception of the output layer that utilized categorical cross-entropy loss and a softmax activation function. The network validation approach was the same as the one we used for parameter recovery (e.g. based on the held-out test set). We also observed a better performance when training with various trial numbers.
Robustness test: Influence of different input trial numbers.
For all robustness experiments, we followed the same training procedures as described previously while varying the training data. The details of training data generation are given below:
We simulated 30,000 training samples with 2000 trials per simulation in the probabilistic reversal learning task. For shorter fixed trial sequence lengths per training samples (e.g 500), we used the same training set truncated to the first 500 trials. To generate the training data with different trial numbers across training samples, we reused the same training set, with sequences of trials truncated to a given number. There were 6000 training samples of 50, 100, 500, 1000, 1500, and 2000 trials, each.
The process of data generation for model identification robustness checks was similar to parameter recovery. However, we only simulated 500 trials for each model because we found no significant increase in accuracy with higher trial numbers.
Supporting information
S1 fig. tasks..
A) Probabilistic reversal learning task. We simulated artificial agents using cognitive models of behavior on a Probabilistic reversal learning (PRL) task, which provides a dynamic context for studying reward-driven learning. In this task, an agent chooses between two actions, where one of the actions gets rewarded with higher probability ( p ( r ) =.80) and one with lower (1 − p ). After a certain number of correct trials, the reward probabilities of the two actions reverse. The task provides an opportunity to observe how agents update their model of the task (e.g. correct actions) based on observed feedback. B) Hierarchical reinforcement learning task. In this task, three differently colored arrows represent three potential rules an agent can follow when selecting one of the two actions (left/right) corresponding to the side the chosen arrow is pointing at. Selecting a side consistent with correct arrow is rewarded with probability p =.90. Correct arrow switches after a certain number of trials. The task provides a possibility to examine how following latent rules may shape agents’ learning behavior.
https://doi.org/10.1371/journal.pcbi.1012119.s001
S2 Fig. 2 Parameter RL (2P-RL) model parameter recovery using different fitting methods.
ρ corresponds to Spearman correlation coefficient, red line represents a unity line (x = y), and black line represents a least squares regression line.
https://doi.org/10.1371/journal.pcbi.1012119.s002
S3 Fig. 4 Parameter RL model (4P-RL) parameter recovery using different fitting methods.
https://doi.org/10.1371/journal.pcbi.1012119.s003
S4 Fig. Bayesian Inference (BI) model parameter recovery using different fitting methods.
https://doi.org/10.1371/journal.pcbi.1012119.s004
S5 Fig. Bayesian Inference with stickiness (S-BI) model parameter recovery using different fitting methods.
https://doi.org/10.1371/journal.pcbi.1012119.s005
S6 Fig. Bayesian Inference with stickiness (S-BI) model parameter recovery using different fitting methods.
Correlation between the average experienced time intervals in attentive state and the τ parameter in RL-LAS model that captures transition between disengaged/engaged attention states estimated by the ANN.
https://doi.org/10.1371/journal.pcbi.1012119.s006
S7 Fig. Misclassification of Bayes and sticky Bayes model.
Misclassification of Bayes and sticky Bayes model is contingent on the value of the stickiness parameter κ . The misclassification percentage is higher at κ values closer to 0.
https://doi.org/10.1371/journal.pcbi.1012119.s007
S8 Fig. Summary statistics for Approximate Bayesian Computation (ABC).
Top row shows summary statistics computed for all models simulated on a probabilistic reversal learning task; the figure only shows agents simulated using a 4-parameter RL model. Bottom row shows summary statistics computed for all models simulated on a hierarchical reversal learning task; the figure only shows performance of HRL model agents. Both rows depict 200 out of 3000 test set agents. Gray lines represent individual agents; black line represents an average across the agents.
https://doi.org/10.1371/journal.pcbi.1012119.s008
S9 Fig. Effect of prior misspecification on parameter estimation in MAP and our ANN approach.
A) Applying too narrow prior specification to the fitting procedure (prior in MAP, training samples in ANN) results in difficulty estimating out-of-range parameters for both MAP and ANN. Broader prior specification addresses this issue, with only a slight loss of precision in specific target ranges. Training the network with a broad range of parameters while oversampling parameters from regions of interest yields most robust results. B) Visualization of fitting with MAP and ANN with a wide prior, tested on a full range/wide range data set—training the network with broader range while oversampling from the most plausible range yields less noisy performance in the range compared to MAP. Red lines delineate the range of the narrow prior, which corresponds to the main text results. C) The broad prior was designed by sampling from the full broader range ( β ∈ [0, 10], α ∈ [0, 1]), with the constraint that 70% of samples are in the expected narrow range ( β ∈ [2, 6], α ∈ [0.5, 1], and 30% outside).
https://doi.org/10.1371/journal.pcbi.1012119.s009
S10 Fig. Consistency between methods for parameter estimation.
A) The correlation between parameter estimates in the 4P-RL model derived using MAP and ANN, is high, and indeed stronger than the correlation between true and derived parameters (see Fig 2 ), showing that both methods systematically misidentify some parameters similarly, likely due to specific data patterns. B) The correlation between parameter estimates in the Bayesian inference model derived using MAP and ANN shows similar results.
https://doi.org/10.1371/journal.pcbi.1012119.s010
S11 Fig. Consistency between methods for parameter estimation in two model misspecification cases.
A) The correlation between MAP and GRU RL model parameter estimates, fit to data simulated from Bayesian Inference model. B) The correlation between MAP and GRU Bayes model parameter estimates, fit to data simulated from the RL model. High correlation would imply similarities in estimates between MAP and GRU, suggesting that ANNs are similarly impacted by model misspecification as traditional methods such as MAP.
https://doi.org/10.1371/journal.pcbi.1012119.s011
S12 Fig. Comparison of model predictions of ground truth simulated behavior (black line) and choices simulated using A) MAP and B) GRU estimated parameters (gray line) of the 4P-RL model.
We randomly sampled 100 agents from the test set, and the respective parameter estimates for each of the methods. We simulated data from the model and compared it to ground truth. Both methods successfully recover choices from the ground truth agents.
https://doi.org/10.1371/journal.pcbi.1012119.s012
S13 Fig. Effect of model misspecification on standard method and ANN performance.
We randomly sampled 100 agents from the test set, and the respective parameter estimates for each of the methods. We simulated data from the model and compared it to ground truth. Both methods successfully recover choices from the ground truth agents. A) We fit the Bayesian Inference model (without stickiness) to the data simulated from the Bayesian Inference Model with stickiness using MAP. We correlated the estimated Bayesian inference model parameters (y axis) with the ground truth parameters from the model with stickiness (x-axis). B) For the ANN, we trained the neural network to estimate parameters of the Bayesian inference model, and tested it on the data simulated from the Bayesian inference model with stickiness. We looked at the correlation between the ground truth parameters (from a separate test set), and the predictions of the network trained on the model without stickiness. Both methods show that parameters shared between the misspecified models can be reasonably and similarly recovered. Both ANN and MAP generated some non-zero estimates of stickiness when data simulated from model without stickiness was fit using the model/network that assumes presence of stickiness in the model; however, these values were closely clustered around 0, to a similar degree between methods ( S16 Fig ).
https://doi.org/10.1371/journal.pcbi.1012119.s013
S14 Fig. Effect of model class misspecification on standard method and ANN performance.
We fit the RL model to the data simulated from the Bayesian Inference model in the probabilistic reversal learning task (see Methods section on Tasks and Cognitive Models) using A) MAP and B) GRU. We correlated the estimated RL model parameters (y axis) with the ground truth parameters from the Bayesian inference model (x-axis). MAP and GRU show similar patterns between estimated and true parameters, such that variance driven by true parameter β p switch are both captured in the fit β parameters, while the fit learning rate parameter α captures behavioral variance driven by the Bayesian update parameters p reward and p switch .
https://doi.org/10.1371/journal.pcbi.1012119.s014
S15 Fig. Effect of model class misspecification on standard method and ANN performance.
We fit the Bayesian Inference model to the data simulated from the RL model in the probabilistic reversal learning task (see Methods section on Tasks and Cognitive Models) using A) MAP and B) GRU. We correlated the estimated Bayesian inference model parameters (y axis) with the ground truth parameters from the RL model (x-axis). MAP and GRU again show similar patterns between estimated and true parameters. In particular, we see that in both cases, noise in behavior due to β in the RL model tends to be attributed to the p switch fit parameter rather than the fit Bayesian model β parameter. Effect of learning rate parameter α are attributed to p reward by both methods.
https://doi.org/10.1371/journal.pcbi.1012119.s015
S16 Fig. Stickiness parameter estimates.
Stickiness parameter estimates from the data simulated from the Bayesian inference model without stickiness from A) fitting the Bayesian inference model with stickiness using MAP, and B) utilizing the ANN trained to estimate parameters of the model with stickiness. Despite both methods producing non-zero estimates of stickiness, they tend to cluster around the value of 0.
https://doi.org/10.1371/journal.pcbi.1012119.s016
S17 Fig. Neural network performance variability by different seeds for model identification and parameter estimation.
For conciseness, we show tests from 10 different seeds on model identification with 4 models simulated on the PRL task (e.g. same as Fig 5 ) and parameter estimation of one of the likelihood-intractable models (e.g. RL-LAS model). We found that overall both model identification and parameter estimation had relatively stable results across different seeds, with an exception of one seed value in both cases.
https://doi.org/10.1371/journal.pcbi.1012119.s017
S1 Table. Summary of hyper-parameter values selected from Bayesian optimization algorithms.
https://doi.org/10.1371/journal.pcbi.1012119.s018
Acknowledgments
We thank Jasmine Collins, Kshitiz Gupta, Yi Liu and Jaeyoung Park for their contributions to the project. We thank Bill Thompson and all CCN lab members for useful feedback on the project.
- View Article
- PubMed/NCBI
- Google Scholar
- 3. Shultz TR. Computational developmental psychology. Mit Press; 2003.
- 32. Minka TP. Expectation propagation for approximate Bayesian inference. arXiv preprint arXiv:13012294. 2013;.
- 36. Palestro JJ, Sederberg PB, Osth AF, Van Zandt T, Turner BM. Likelihood-free methods for cognitive science. Springer; 2018.
- 38. Lavin A, Krakauer D, Zenil H, Gottschlich J, Mattson T, Brehmer J, et al. Simulation intelligence: Towards a new generation of scientific methods. arXiv preprint arXiv:211203235. 2021;.
- 41. Radev ST, Voss A, Wieschen EM, Bürkner PC. Amortized Bayesian Inference for Models of Cognition. arXiv preprint arXiv:200503899. 2020;.
- 43. Chen Y, Zhang D, Gutmann M, Courville A, Zhu Z. Neural approximate sufficient statistics for implicit models. arXiv preprint arXiv:201010079. 2020;.
- 46. Schmitt M, Bürkner PC, Köthe U, Radev ST. Detecting model misspecification in amortized Bayesian inference with neural networks. arXiv preprint arXiv:211208866. 2021;.
- 55. Särkkä S, Svensson L. Bayesian filtering and smoothing. vol. 17. Cambridge university press; 2023.
- 59. Nguyen A, Yosinski J, Clune J. Deep neural networks are easily fooled: High confidence predictions for unrecognizable images. In: Proceedings of the IEEE conference on computer vision and pattern recognition; 2015. p. 427–436.
- 60. Szegedy C, Zaremba W, Sutskever I, Bruna J, Erhan D, Goodfellow I, et al. Intriguing properties of neural networks. arXiv preprint arXiv:13126199. 2013;.
- 61. Liang S, Li Y, Srikant R. Enhancing the reliability of out-of-distribution image detection in neural networks. arXiv preprint arXiv:170602690. 2017;.
- 62. Moosavi-Dezfooli SM, Alhussein Fawzi OF. Pascal Frossard.”. In: Universal adversarial perturbations.” 2017 IEEE Conference on Computer Vision and Pattern Recognition (CVPR); 2017.
- 70. Sisson SA, Fan Y, Beaumont M. Handbook of approximate Bayesian computation. CRC Press; 2018.
- 72. Devlin J, Chang MW, Lee K, Toutanova K. Bert: Pre-training of deep bidirectional transformers for language understanding. arXiv preprint arXiv:181004805. 2018;.
- 75. Sutton RS, Barto AG. Reinforcement learning: An introduction. MIT press; 2018.
- 78. Li JJ, Shi C, Li L, Collins AG. A generalized method for dynamic noise inference in modeling sequential decision-making. In: Proceedings of the Annual Meeting of the Cognitive Science Society. vol. 45; 2023.
- 81. He K, Zhang X, Ren S, Sun J. Delving deep into rectifiers: Surpassing human-level performance on imagenet classification. In: Proceedings of the IEEE international conference on computer vision; 2015. p. 1026–1034.
- 82. Bergstra J, Yamins D, Cox D. Making a science of model search: Hyperparameter optimization in hundreds of dimensions for vision architectures. In: International conference on machine learning. PMLR; 2013. p. 115–123.
- 83. Abadi M, Barham P, Chen J, Chen Z, Davis A, Dean J, et al. {TensorFlow}: a system for {Large-Scale} machine learning. In: 12th USENIX symposium on operating systems design and implementation (OSDI 16); 2016. p. 265–283.

- Health, Fitness & Dieting
- Psychology & Counseling

Download the free Kindle app and start reading Kindle books instantly on your smartphone, tablet, or computer - no Kindle device required .
Read instantly on your browser with Kindle for Web.
Using your mobile phone camera - scan the code below and download the Kindle app.

Follow the author

Image Unavailable

- To view this video download Flash Player
Decision Analysis and Behavioral Research
- ISBN-10 0521273048
- ISBN-13 978-0521273046
- Publisher Cambridge University Press
- Publication date September 26, 1986
- Language English
- Dimensions 6.25 x 1.25 x 9.5 inches
- Print length 624 pages
- See all details

Product details
- Publisher : Cambridge University Press (September 26, 1986)
- Language : English
- Paperback : 624 pages
- ISBN-10 : 0521273048
- ISBN-13 : 978-0521273046
- Item Weight : 1.82 pounds
- Dimensions : 6.25 x 1.25 x 9.5 inches
- #3,547 in Medical Applied Psychology
- #4,606 in Popular Applied Psychology
- #15,137 in Psychology (Books)
About the author
Detlof von winterfeldt.
Discover more of the author’s books, see similar authors, read author blogs and more
Customer reviews
Customer Reviews, including Product Star Ratings help customers to learn more about the product and decide whether it is the right product for them.
To calculate the overall star rating and percentage breakdown by star, we don’t use a simple average. Instead, our system considers things like how recent a review is and if the reviewer bought the item on Amazon. It also analyzed reviews to verify trustworthiness.
- Sort reviews by Top reviews Most recent Top reviews
Top review from the United States
There was a problem filtering reviews right now. please try again later..

- Amazon Newsletter
- About Amazon
- Accessibility
- Sustainability
- Press Center
- Investor Relations
- Amazon Devices
- Amazon Science
- Sell on Amazon
- Sell apps on Amazon
- Supply to Amazon
- Protect & Build Your Brand
- Become an Affiliate
- Become a Delivery Driver
- Start a Package Delivery Business
- Advertise Your Products
- Self-Publish with Us
- Become an Amazon Hub Partner
- › See More Ways to Make Money
- Amazon Visa
- Amazon Store Card
- Amazon Secured Card
- Amazon Business Card
- Shop with Points
- Credit Card Marketplace
- Reload Your Balance
- Amazon Currency Converter
- Your Account
- Your Orders
- Shipping Rates & Policies
- Amazon Prime
- Returns & Replacements
- Manage Your Content and Devices
- Recalls and Product Safety Alerts
- Conditions of Use
- Privacy Notice
- Consumer Health Data Privacy Disclosure
- Your Ads Privacy Choices
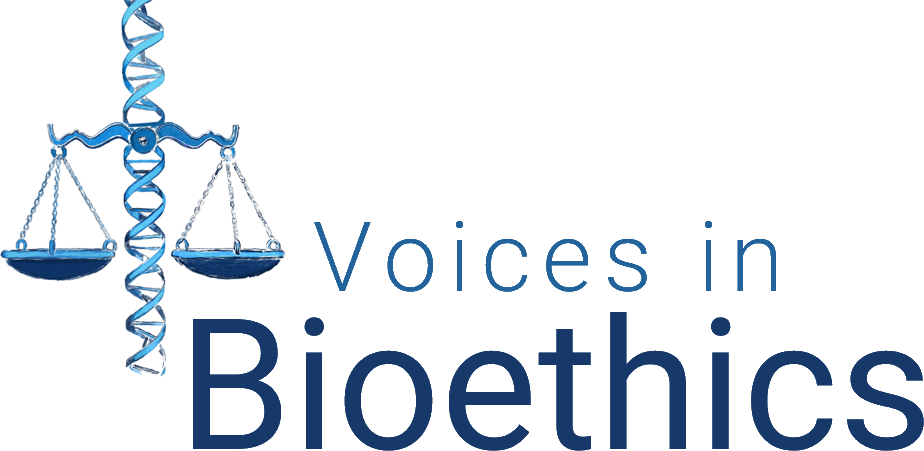
Cultural Relativity and Acceptance of Embryonic Stem Cell Research
Article sidebar.
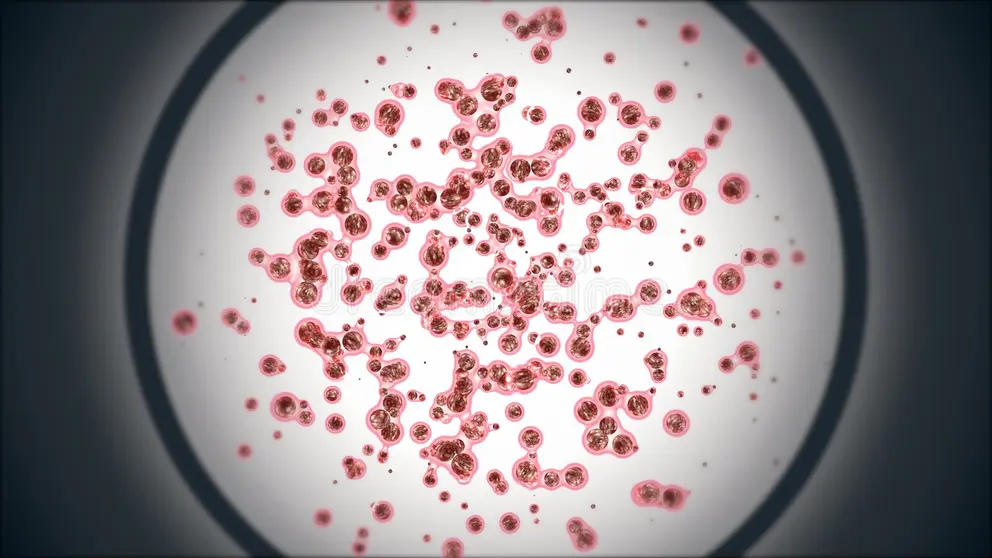
Main Article Content
There is a debate about the ethical implications of using human embryos in stem cell research, which can be influenced by cultural, moral, and social values. This paper argues for an adaptable framework to accommodate diverse cultural and religious perspectives. By using an adaptive ethics model, research protections can reflect various populations and foster growth in stem cell research possibilities.
INTRODUCTION
Stem cell research combines biology, medicine, and technology, promising to alter health care and the understanding of human development. Yet, ethical contention exists because of individuals’ perceptions of using human embryos based on their various cultural, moral, and social values. While these disagreements concerning policy, use, and general acceptance have prompted the development of an international ethics policy, such a uniform approach can overlook the nuanced ethical landscapes between cultures. With diverse viewpoints in public health, a single global policy, especially one reflecting Western ethics or the ethics prevalent in high-income countries, is impractical. This paper argues for a culturally sensitive, adaptable framework for the use of embryonic stem cells. Stem cell policy should accommodate varying ethical viewpoints and promote an effective global dialogue. With an extension of an ethics model that can adapt to various cultures, we recommend localized guidelines that reflect the moral views of the people those guidelines serve.
Stem cells, characterized by their unique ability to differentiate into various cell types, enable the repair or replacement of damaged tissues. Two primary types of stem cells are somatic stem cells (adult stem cells) and embryonic stem cells. Adult stem cells exist in developed tissues and maintain the body’s repair processes. [1] Embryonic stem cells (ESC) are remarkably pluripotent or versatile, making them valuable in research. [2] However, the use of ESCs has sparked ethics debates. Considering the potential of embryonic stem cells, research guidelines are essential. The International Society for Stem Cell Research (ISSCR) provides international stem cell research guidelines. They call for “public conversations touching on the scientific significance as well as the societal and ethical issues raised by ESC research.” [3] The ISSCR also publishes updates about culturing human embryos 14 days post fertilization, suggesting local policies and regulations should continue to evolve as ESC research develops. [4] Like the ISSCR, which calls for local law and policy to adapt to developing stem cell research given cultural acceptance, this paper highlights the importance of local social factors such as religion and culture.
I. Global Cultural Perspective of Embryonic Stem Cells
Views on ESCs vary throughout the world. Some countries readily embrace stem cell research and therapies, while others have stricter regulations due to ethical concerns surrounding embryonic stem cells and when an embryo becomes entitled to moral consideration. The philosophical issue of when the “someone” begins to be a human after fertilization, in the morally relevant sense, [5] impacts when an embryo becomes not just worthy of protection but morally entitled to it. The process of creating embryonic stem cell lines involves the destruction of the embryos for research. [6] Consequently, global engagement in ESC research depends on social-cultural acceptability.
a. US and Rights-Based Cultures
In the United States, attitudes toward stem cell therapies are diverse. The ethics and social approaches, which value individualism, [7] trigger debates regarding the destruction of human embryos, creating a complex regulatory environment. For example, the 1996 Dickey-Wicker Amendment prohibited federal funding for the creation of embryos for research and the destruction of embryos for “more than allowed for research on fetuses in utero.” [8] Following suit, in 2001, the Bush Administration heavily restricted stem cell lines for research. However, the Stem Cell Research Enhancement Act of 2005 was proposed to help develop ESC research but was ultimately vetoed. [9] Under the Obama administration, in 2009, an executive order lifted restrictions allowing for more development in this field. [10] The flux of research capacity and funding parallels the different cultural perceptions of human dignity of the embryo and how it is socially presented within the country’s research culture. [11]
b. Ubuntu and Collective Cultures
African bioethics differs from Western individualism because of the different traditions and values. African traditions, as described by individuals from South Africa and supported by some studies in other African countries, including Ghana and Kenya, follow the African moral philosophies of Ubuntu or Botho and Ukama , which “advocates for a form of wholeness that comes through one’s relationship and connectedness with other people in the society,” [12] making autonomy a socially collective concept. In this context, for the community to act autonomously, individuals would come together to decide what is best for the collective. Thus, stem cell research would require examining the value of the research to society as a whole and the use of the embryos as a collective societal resource. If society views the source as part of the collective whole, and opposes using stem cells, compromising the cultural values to pursue research may cause social detachment and stunt research growth. [13] Based on local culture and moral philosophy, the permissibility of stem cell research depends on how embryo, stem cell, and cell line therapies relate to the community as a whole . Ubuntu is the expression of humanness, with the person’s identity drawn from the “’I am because we are’” value. [14] The decision in a collectivistic culture becomes one born of cultural context, and individual decisions give deference to others in the society.
Consent differs in cultures where thought and moral philosophy are based on a collective paradigm. So, applying Western bioethical concepts is unrealistic. For one, Africa is a diverse continent with many countries with different belief systems, access to health care, and reliance on traditional or Western medicines. Where traditional medicine is the primary treatment, the “’restrictive focus on biomedically-related bioethics’” [is] problematic in African contexts because it neglects bioethical issues raised by traditional systems.” [15] No single approach applies in all areas or contexts. Rather than evaluating the permissibility of ESC research according to Western concepts such as the four principles approach, different ethics approaches should prevail.
Another consideration is the socio-economic standing of countries. In parts of South Africa, researchers have not focused heavily on contributing to the stem cell discourse, either because it is not considered health care or a health science priority or because resources are unavailable. [16] Each country’s priorities differ given different social, political, and economic factors. In South Africa, for instance, areas such as maternal mortality, non-communicable diseases, telemedicine, and the strength of health systems need improvement and require more focus. [17] Stem cell research could benefit the population, but it also could divert resources from basic medical care. Researchers in South Africa adhere to the National Health Act and Medicines Control Act in South Africa and international guidelines; however, the Act is not strictly enforced, and there is no clear legislation for research conduct or ethical guidelines. [18]
Some parts of Africa condemn stem cell research. For example, 98.2 percent of the Tunisian population is Muslim. [19] Tunisia does not permit stem cell research because of moral conflict with a Fatwa. Religion heavily saturates the regulation and direction of research. [20] Stem cell use became permissible for reproductive purposes only recently, with tight restrictions preventing cells from being used in any research other than procedures concerning ART/IVF. Their use is conditioned on consent, and available only to married couples. [21] The community's receptiveness to stem cell research depends on including communitarian African ethics.
c. Asia
Some Asian countries also have a collective model of ethics and decision making. [22] In China, the ethics model promotes a sincere respect for life or human dignity, [23] based on protective medicine. This model, influenced by Traditional Chinese Medicine (TCM), [24] recognizes Qi as the vital energy delivered via the meridians of the body; it connects illness to body systems, the body’s entire constitution, and the universe for a holistic bond of nature, health, and quality of life. [25] Following a protective ethics model, and traditional customs of wholeness, investment in stem cell research is heavily desired for its applications in regenerative therapies, disease modeling, and protective medicines. In a survey of medical students and healthcare practitioners, 30.8 percent considered stem cell research morally unacceptable while 63.5 percent accepted medical research using human embryonic stem cells. Of these individuals, 89.9 percent supported increased funding for stem cell research. [26] The scientific community might not reflect the overall population. From 1997 to 2019, China spent a total of $576 million (USD) on stem cell research at 8,050 stem cell programs, increased published presence from 0.6 percent to 14.01 percent of total global stem cell publications as of 2014, and made significant strides in cell-based therapies for various medical conditions. [27] However, while China has made substantial investments in stem cell research and achieved notable progress in clinical applications, concerns linger regarding ethical oversight and transparency. [28] For example, the China Biosecurity Law, promoted by the National Health Commission and China Hospital Association, attempted to mitigate risks by introducing an institutional review board (IRB) in the regulatory bodies. 5800 IRBs registered with the Chinese Clinical Trial Registry since 2021. [29] However, issues still need to be addressed in implementing effective IRB review and approval procedures.
The substantial government funding and focus on scientific advancement have sometimes overshadowed considerations of regional cultures, ethnic minorities, and individual perspectives, particularly evident during the one-child policy era. As government policy adapts to promote public stability, such as the change from the one-child to the two-child policy, [30] research ethics should also adapt to ensure respect for the values of its represented peoples.
Japan is also relatively supportive of stem cell research and therapies. Japan has a more transparent regulatory framework, allowing for faster approval of regenerative medicine products, which has led to several advanced clinical trials and therapies. [31] South Korea is also actively engaged in stem cell research and has a history of breakthroughs in cloning and embryonic stem cells. [32] However, the field is controversial, and there are issues of scientific integrity. For example, the Korean FDA fast-tracked products for approval, [33] and in another instance, the oocyte source was unclear and possibly violated ethical standards. [34] Trust is important in research, as it builds collaborative foundations between colleagues, trial participant comfort, open-mindedness for complicated and sensitive discussions, and supports regulatory procedures for stakeholders. There is a need to respect the culture’s interest, engagement, and for research and clinical trials to be transparent and have ethical oversight to promote global research discourse and trust.
d. Middle East
Countries in the Middle East have varying degrees of acceptance of or restrictions to policies related to using embryonic stem cells due to cultural and religious influences. Saudi Arabia has made significant contributions to stem cell research, and conducts research based on international guidelines for ethical conduct and under strict adherence to guidelines in accordance with Islamic principles. Specifically, the Saudi government and people require ESC research to adhere to Sharia law. In addition to umbilical and placental stem cells, [35] Saudi Arabia permits the use of embryonic stem cells as long as they come from miscarriages, therapeutic abortions permissible by Sharia law, or are left over from in vitro fertilization and donated to research. [36] Laws and ethical guidelines for stem cell research allow the development of research institutions such as the King Abdullah International Medical Research Center, which has a cord blood bank and a stem cell registry with nearly 10,000 donors. [37] Such volume and acceptance are due to the ethical ‘permissibility’ of the donor sources, which do not conflict with religious pillars. However, some researchers err on the side of caution, choosing not to use embryos or fetal tissue as they feel it is unethical to do so. [38]
Jordan has a positive research ethics culture. [39] However, there is a significant issue of lack of trust in researchers, with 45.23 percent (38.66 percent agreeing and 6.57 percent strongly agreeing) of Jordanians holding a low level of trust in researchers, compared to 81.34 percent of Jordanians agreeing that they feel safe to participate in a research trial. [40] Safety testifies to the feeling of confidence that adequate measures are in place to protect participants from harm, whereas trust in researchers could represent the confidence in researchers to act in the participants’ best interests, adhere to ethical guidelines, provide accurate information, and respect participants’ rights and dignity. One method to improve trust would be to address communication issues relevant to ESC. Legislation surrounding stem cell research has adopted specific language, especially concerning clarification “between ‘stem cells’ and ‘embryonic stem cells’” in translation. [41] Furthermore, legislation “mandates the creation of a national committee… laying out specific regulations for stem-cell banking in accordance with international standards.” [42] This broad regulation opens the door for future global engagement and maintains transparency. However, these regulations may also constrain the influence of research direction, pace, and accessibility of research outcomes.
e. Europe
In the European Union (EU), ethics is also principle-based, but the principles of autonomy, dignity, integrity, and vulnerability are interconnected. [43] As such, the opportunity for cohesion and concessions between individuals’ thoughts and ideals allows for a more adaptable ethics model due to the flexible principles that relate to the human experience The EU has put forth a framework in its Convention for the Protection of Human Rights and Dignity of the Human Being allowing member states to take different approaches. Each European state applies these principles to its specific conventions, leading to or reflecting different acceptance levels of stem cell research. [44]
For example, in Germany, Lebenzusammenhang , or the coherence of life, references integrity in the unity of human culture. Namely, the personal sphere “should not be subject to external intervention.” [45] Stem cell interventions could affect this concept of bodily completeness, leading to heavy restrictions. Under the Grundgesetz, human dignity and the right to life with physical integrity are paramount. [46] The Embryo Protection Act of 1991 made producing cell lines illegal. Cell lines can be imported if approved by the Central Ethics Commission for Stem Cell Research only if they were derived before May 2007. [47] Stem cell research respects the integrity of life for the embryo with heavy specifications and intense oversight. This is vastly different in Finland, where the regulatory bodies find research more permissible in IVF excess, but only up to 14 days after fertilization. [48] Spain’s approach differs still, with a comprehensive regulatory framework. [49] Thus, research regulation can be culture-specific due to variations in applied principles. Diverse cultures call for various approaches to ethical permissibility. [50] Only an adaptive-deliberative model can address the cultural constructions of self and achieve positive, culturally sensitive stem cell research practices. [51]
II. Religious Perspectives on ESC
Embryonic stem cell sources are the main consideration within religious contexts. While individuals may not regard their own religious texts as authoritative or factual, religion can shape their foundations or perspectives.
The Qur'an states:
“And indeed We created man from a quintessence of clay. Then We placed within him a small quantity of nutfa (sperm to fertilize) in a safe place. Then We have fashioned the nutfa into an ‘alaqa (clinging clot or cell cluster), then We developed the ‘alaqa into mudgha (a lump of flesh), and We made mudgha into bones, and clothed the bones with flesh, then We brought it into being as a new creation. So Blessed is Allah, the Best of Creators.” [52]
Many scholars of Islam estimate the time of soul installment, marked by the angel breathing in the soul to bring the individual into creation, as 120 days from conception. [53] Personhood begins at this point, and the value of life would prohibit research or experimentation that could harm the individual. If the fetus is more than 120 days old, the time ensoulment is interpreted to occur according to Islamic law, abortion is no longer permissible. [54] There are a few opposing opinions about early embryos in Islamic traditions. According to some Islamic theologians, there is no ensoulment of the early embryo, which is the source of stem cells for ESC research. [55]
In Buddhism, the stance on stem cell research is not settled. The main tenets, the prohibition against harming or destroying others (ahimsa) and the pursuit of knowledge (prajña) and compassion (karuna), leave Buddhist scholars and communities divided. [56] Some scholars argue stem cell research is in accordance with the Buddhist tenet of seeking knowledge and ending human suffering. Others feel it violates the principle of not harming others. Finding the balance between these two points relies on the karmic burden of Buddhist morality. In trying to prevent ahimsa towards the embryo, Buddhist scholars suggest that to comply with Buddhist tenets, research cannot be done as the embryo has personhood at the moment of conception and would reincarnate immediately, harming the individual's ability to build their karmic burden. [57] On the other hand, the Bodhisattvas, those considered to be on the path to enlightenment or Nirvana, have given organs and flesh to others to help alleviate grieving and to benefit all. [58] Acceptance varies on applied beliefs and interpretations.
Catholicism does not support embryonic stem cell research, as it entails creation or destruction of human embryos. This destruction conflicts with the belief in the sanctity of life. For example, in the Old Testament, Genesis describes humanity as being created in God’s image and multiplying on the Earth, referencing the sacred rights to human conception and the purpose of development and life. In the Ten Commandments, the tenet that one should not kill has numerous interpretations where killing could mean murder or shedding of the sanctity of life, demonstrating the high value of human personhood. In other books, the theological conception of when life begins is interpreted as in utero, [59] highlighting the inviolability of life and its formation in vivo to make a religious point for accepting such research as relatively limited, if at all. [60] The Vatican has released ethical directives to help apply a theological basis to modern-day conflicts. The Magisterium of the Church states that “unless there is a moral certainty of not causing harm,” experimentation on fetuses, fertilized cells, stem cells, or embryos constitutes a crime. [61] Such procedures would not respect the human person who exists at these stages, according to Catholicism. Damages to the embryo are considered gravely immoral and illicit. [62] Although the Catholic Church officially opposes abortion, surveys demonstrate that many Catholic people hold pro-choice views, whether due to the context of conception, stage of pregnancy, threat to the mother’s life, or for other reasons, demonstrating that practicing members can also accept some but not all tenets. [63]
Some major Jewish denominations, such as the Reform, Conservative, and Reconstructionist movements, are open to supporting ESC use or research as long as it is for saving a life. [64] Within Judaism, the Talmud, or study, gives personhood to the child at birth and emphasizes that life does not begin at conception: [65]
“If she is found pregnant, until the fortieth day it is mere fluid,” [66]
Whereas most religions prioritize the status of human embryos, the Halakah (Jewish religious law) states that to save one life, most other religious laws can be ignored because it is in pursuit of preservation. [67] Stem cell research is accepted due to application of these religious laws.
We recognize that all religions contain subsets and sects. The variety of environmental and cultural differences within religious groups requires further analysis to respect the flexibility of religious thoughts and practices. We make no presumptions that all cultures require notions of autonomy or morality as under the common morality theory , which asserts a set of universal moral norms that all individuals share provides moral reasoning and guides ethical decisions. [68] We only wish to show that the interaction with morality varies between cultures and countries.
III. A Flexible Ethical Approach
The plurality of different moral approaches described above demonstrates that there can be no universally acceptable uniform law for ESC on a global scale. Instead of developing one standard, flexible ethical applications must be continued. We recommend local guidelines that incorporate important cultural and ethical priorities.
While the Declaration of Helsinki is more relevant to people in clinical trials receiving ESC products, in keeping with the tradition of protections for research subjects, consent of the donor is an ethical requirement for ESC donation in many jurisdictions including the US, Canada, and Europe. [69] The Declaration of Helsinki provides a reference point for regulatory standards and could potentially be used as a universal baseline for obtaining consent prior to gamete or embryo donation.
For instance, in Columbia University’s egg donor program for stem cell research, donors followed standard screening protocols and “underwent counseling sessions that included information as to the purpose of oocyte donation for research, what the oocytes would be used for, the risks and benefits of donation, and process of oocyte stimulation” to ensure transparency for consent. [70] The program helped advance stem cell research and provided clear and safe research methods with paid participants. Though paid participation or covering costs of incidental expenses may not be socially acceptable in every culture or context, [71] and creating embryos for ESC research is illegal in many jurisdictions, Columbia’s program was effective because of the clear and honest communications with donors, IRBs, and related stakeholders. This example demonstrates that cultural acceptance of scientific research and of the idea that an egg or embryo does not have personhood is likely behind societal acceptance of donating eggs for ESC research. As noted, many countries do not permit the creation of embryos for research.
Proper communication and education regarding the process and purpose of stem cell research may bolster comprehension and garner more acceptance. “Given the sensitive subject material, a complete consent process can support voluntary participation through trust, understanding, and ethical norms from the cultures and morals participants value. This can be hard for researchers entering countries of different socioeconomic stability, with different languages and different societal values. [72]
An adequate moral foundation in medical ethics is derived from the cultural and religious basis that informs knowledge and actions. [73] Understanding local cultural and religious values and their impact on research could help researchers develop humility and promote inclusion.
IV. Concerns
Some may argue that if researchers all adhere to one ethics standard, protection will be satisfied across all borders, and the global public will trust researchers. However, defining what needs to be protected and how to define such research standards is very specific to the people to which standards are applied. We suggest that applying one uniform guide cannot accurately protect each individual because we all possess our own perceptions and interpretations of social values. [74] Therefore, the issue of not adjusting to the moral pluralism between peoples in applying one standard of ethics can be resolved by building out ethics models that can be adapted to different cultures and religions.
Other concerns include medical tourism, which may promote health inequities. [75] Some countries may develop and approve products derived from ESC research before others, compromising research ethics or drug approval processes. There are also concerns about the sale of unauthorized stem cell treatments, for example, those without FDA approval in the United States. Countries with robust research infrastructures may be tempted to attract medical tourists, and some customers will have false hopes based on aggressive publicity of unproven treatments. [76]
For example, in China, stem cell clinics can market to foreign clients who are not protected under the regulatory regimes. Companies employ a marketing strategy of “ethically friendly” therapies. Specifically, in the case of Beike, China’s leading stem cell tourism company and sprouting network, ethical oversight of administrators or health bureaus at one site has “the unintended consequence of shifting questionable activities to another node in Beike's diffuse network.” [77] In contrast, Jordan is aware of stem cell research’s potential abuse and its own status as a “health-care hub.” Jordan’s expanded regulations include preserving the interests of individuals in clinical trials and banning private companies from ESC research to preserve transparency and the integrity of research practices. [78]
The social priorities of the community are also a concern. The ISSCR explicitly states that guidelines “should be periodically revised to accommodate scientific advances, new challenges, and evolving social priorities.” [79] The adaptable ethics model extends this consideration further by addressing whether research is warranted given the varying degrees of socioeconomic conditions, political stability, and healthcare accessibilities and limitations. An ethical approach would require discussion about resource allocation and appropriate distribution of funds. [80]
While some religions emphasize the sanctity of life from conception, which may lead to public opposition to ESC research, others encourage ESC research due to its potential for healing and alleviating human pain. Many countries have special regulations that balance local views on embryonic personhood, the benefits of research as individual or societal goods, and the protection of human research subjects. To foster understanding and constructive dialogue, global policy frameworks should prioritize the protection of universal human rights, transparency, and informed consent. In addition to these foundational global policies, we recommend tailoring local guidelines to reflect the diverse cultural and religious perspectives of the populations they govern. Ethics models should be adapted to local populations to effectively establish research protections, growth, and possibilities of stem cell research.
For example, in countries with strong beliefs in the moral sanctity of embryos or heavy religious restrictions, an adaptive model can allow for discussion instead of immediate rejection. In countries with limited individual rights and voice in science policy, an adaptive model ensures cultural, moral, and religious views are taken into consideration, thereby building social inclusion. While this ethical consideration by the government may not give a complete voice to every individual, it will help balance policies and maintain the diverse perspectives of those it affects. Embracing an adaptive ethics model of ESC research promotes open-minded dialogue and respect for the importance of human belief and tradition. By actively engaging with cultural and religious values, researchers can better handle disagreements and promote ethical research practices that benefit each society.
This brief exploration of the religious and cultural differences that impact ESC research reveals the nuances of relative ethics and highlights a need for local policymakers to apply a more intense adaptive model.
[1] Poliwoda, S., Noor, N., Downs, E., Schaaf, A., Cantwell, A., Ganti, L., Kaye, A. D., Mosel, L. I., Carroll, C. B., Viswanath, O., & Urits, I. (2022). Stem cells: a comprehensive review of origins and emerging clinical roles in medical practice. Orthopedic reviews , 14 (3), 37498. https://doi.org/10.52965/001c.37498
[2] Poliwoda, S., Noor, N., Downs, E., Schaaf, A., Cantwell, A., Ganti, L., Kaye, A. D., Mosel, L. I., Carroll, C. B., Viswanath, O., & Urits, I. (2022). Stem cells: a comprehensive review of origins and emerging clinical roles in medical practice. Orthopedic reviews , 14 (3), 37498. https://doi.org/10.52965/001c.37498
[3] International Society for Stem Cell Research. (2023). Laboratory-based human embryonic stem cell research, embryo research, and related research activities . International Society for Stem Cell Research. https://www.isscr.org/guidelines/blog-post-title-one-ed2td-6fcdk ; Kimmelman, J., Hyun, I., Benvenisty, N. et al. Policy: Global standards for stem-cell research. Nature 533 , 311–313 (2016). https://doi.org/10.1038/533311a
[4] International Society for Stem Cell Research. (2023). Laboratory-based human embryonic stem cell research, embryo research, and related research activities . International Society for Stem Cell Research. https://www.isscr.org/guidelines/blog-post-title-one-ed2td-6fcdk
[5] Concerning the moral philosophies of stem cell research, our paper does not posit a personal moral stance nor delve into the “when” of human life begins. To read further about the philosophical debate, consider the following sources:
Sandel M. J. (2004). Embryo ethics--the moral logic of stem-cell research. The New England journal of medicine , 351 (3), 207–209. https://doi.org/10.1056/NEJMp048145 ; George, R. P., & Lee, P. (2020, September 26). Acorns and Embryos . The New Atlantis. https://www.thenewatlantis.com/publications/acorns-and-embryos ; Sagan, A., & Singer, P. (2007). The moral status of stem cells. Metaphilosophy , 38 (2/3), 264–284. http://www.jstor.org/stable/24439776 ; McHugh P. R. (2004). Zygote and "clonote"--the ethical use of embryonic stem cells. The New England journal of medicine , 351 (3), 209–211. https://doi.org/10.1056/NEJMp048147 ; Kurjak, A., & Tripalo, A. (2004). The facts and doubts about beginning of the human life and personality. Bosnian journal of basic medical sciences , 4 (1), 5–14. https://doi.org/10.17305/bjbms.2004.3453
[6] Vazin, T., & Freed, W. J. (2010). Human embryonic stem cells: derivation, culture, and differentiation: a review. Restorative neurology and neuroscience , 28 (4), 589–603. https://doi.org/10.3233/RNN-2010-0543
[7] Socially, at its core, the Western approach to ethics is widely principle-based, autonomy being one of the key factors to ensure a fundamental respect for persons within research. For information regarding autonomy in research, see: Department of Health, Education, and Welfare, & National Commission for the Protection of Human Subjects of Biomedical and Behavioral Research (1978). The Belmont Report. Ethical principles and guidelines for the protection of human subjects of research.; For a more in-depth review of autonomy within the US, see: Beauchamp, T. L., & Childress, J. F. (1994). Principles of Biomedical Ethics . Oxford University Press.
[8] Sherley v. Sebelius , 644 F.3d 388 (D.C. Cir. 2011), citing 45 C.F.R. 46.204(b) and [42 U.S.C. § 289g(b)]. https://www.cadc.uscourts.gov/internet/opinions.nsf/6c690438a9b43dd685257a64004ebf99/$file/11-5241-1391178.pdf
[9] Stem Cell Research Enhancement Act of 2005, H. R. 810, 109 th Cong. (2001). https://www.govtrack.us/congress/bills/109/hr810/text ; Bush, G. W. (2006, July 19). Message to the House of Representatives . National Archives and Records Administration. https://georgewbush-whitehouse.archives.gov/news/releases/2006/07/20060719-5.html
[10] National Archives and Records Administration. (2009, March 9). Executive order 13505 -- removing barriers to responsible scientific research involving human stem cells . National Archives and Records Administration. https://obamawhitehouse.archives.gov/the-press-office/removing-barriers-responsible-scientific-research-involving-human-stem-cells
[11] Hurlbut, W. B. (2006). Science, Religion, and the Politics of Stem Cells. Social Research , 73 (3), 819–834. http://www.jstor.org/stable/40971854
[12] Akpa-Inyang, Francis & Chima, Sylvester. (2021). South African traditional values and beliefs regarding informed consent and limitations of the principle of respect for autonomy in African communities: a cross-cultural qualitative study. BMC Medical Ethics . 22. 10.1186/s12910-021-00678-4.
[13] Source for further reading: Tangwa G. B. (2007). Moral status of embryonic stem cells: perspective of an African villager. Bioethics , 21(8), 449–457. https://doi.org/10.1111/j.1467-8519.2007.00582.x , see also Mnisi, F. M. (2020). An African analysis based on ethics of Ubuntu - are human embryonic stem cell patents morally justifiable? African Insight , 49 (4).
[14] Jecker, N. S., & Atuire, C. (2021). Bioethics in Africa: A contextually enlightened analysis of three cases. Developing World Bioethics , 22 (2), 112–122. https://doi.org/10.1111/dewb.12324
[15] Jecker, N. S., & Atuire, C. (2021). Bioethics in Africa: A contextually enlightened analysis of three cases. Developing World Bioethics, 22(2), 112–122. https://doi.org/10.1111/dewb.12324
[16] Jackson, C.S., Pepper, M.S. Opportunities and barriers to establishing a cell therapy programme in South Africa. Stem Cell Res Ther 4 , 54 (2013). https://doi.org/10.1186/scrt204 ; Pew Research Center. (2014, May 1). Public health a major priority in African nations . Pew Research Center’s Global Attitudes Project. https://www.pewresearch.org/global/2014/05/01/public-health-a-major-priority-in-african-nations/
[17] Department of Health Republic of South Africa. (2021). Health Research Priorities (revised) for South Africa 2021-2024 . National Health Research Strategy. https://www.health.gov.za/wp-content/uploads/2022/05/National-Health-Research-Priorities-2021-2024.pdf
[18] Oosthuizen, H. (2013). Legal and Ethical Issues in Stem Cell Research in South Africa. In: Beran, R. (eds) Legal and Forensic Medicine. Springer, Berlin, Heidelberg. https://doi.org/10.1007/978-3-642-32338-6_80 , see also: Gaobotse G (2018) Stem Cell Research in Africa: Legislation and Challenges. J Regen Med 7:1. doi: 10.4172/2325-9620.1000142
[19] United States Bureau of Citizenship and Immigration Services. (1998). Tunisia: Information on the status of Christian conversions in Tunisia . UNHCR Web Archive. https://webarchive.archive.unhcr.org/20230522142618/https://www.refworld.org/docid/3df0be9a2.html
[20] Gaobotse, G. (2018) Stem Cell Research in Africa: Legislation and Challenges. J Regen Med 7:1. doi: 10.4172/2325-9620.1000142
[21] Kooli, C. Review of assisted reproduction techniques, laws, and regulations in Muslim countries. Middle East Fertil Soc J 24 , 8 (2020). https://doi.org/10.1186/s43043-019-0011-0 ; Gaobotse, G. (2018) Stem Cell Research in Africa: Legislation and Challenges. J Regen Med 7:1. doi: 10.4172/2325-9620.1000142
[22] Pang M. C. (1999). Protective truthfulness: the Chinese way of safeguarding patients in informed treatment decisions. Journal of medical ethics , 25(3), 247–253. https://doi.org/10.1136/jme.25.3.247
[23] Wang, L., Wang, F., & Zhang, W. (2021). Bioethics in China’s biosecurity law: Forms, effects, and unsettled issues. Journal of law and the biosciences , 8(1). https://doi.org/10.1093/jlb/lsab019 https://academic.oup.com/jlb/article/8/1/lsab019/6299199
[24] Wang, Y., Xue, Y., & Guo, H. D. (2022). Intervention effects of traditional Chinese medicine on stem cell therapy of myocardial infarction. Frontiers in pharmacology , 13 , 1013740. https://doi.org/10.3389/fphar.2022.1013740
[25] Li, X.-T., & Zhao, J. (2012). Chapter 4: An Approach to the Nature of Qi in TCM- Qi and Bioenergy. In Recent Advances in Theories and Practice of Chinese Medicine (p. 79). InTech.
[26] Luo, D., Xu, Z., Wang, Z., & Ran, W. (2021). China's Stem Cell Research and Knowledge Levels of Medical Practitioners and Students. Stem cells international , 2021 , 6667743. https://doi.org/10.1155/2021/6667743
[27] Luo, D., Xu, Z., Wang, Z., & Ran, W. (2021). China's Stem Cell Research and Knowledge Levels of Medical Practitioners and Students. Stem cells international , 2021 , 6667743. https://doi.org/10.1155/2021/6667743
[28] Zhang, J. Y. (2017). Lost in translation? accountability and governance of Clinical Stem Cell Research in China. Regenerative Medicine , 12 (6), 647–656. https://doi.org/10.2217/rme-2017-0035
[29] Wang, L., Wang, F., & Zhang, W. (2021). Bioethics in China’s biosecurity law: Forms, effects, and unsettled issues. Journal of law and the biosciences , 8(1). https://doi.org/10.1093/jlb/lsab019 https://academic.oup.com/jlb/article/8/1/lsab019/6299199
[30] Chen, H., Wei, T., Wang, H. et al. Association of China’s two-child policy with changes in number of births and birth defects rate, 2008–2017. BMC Public Health 22 , 434 (2022). https://doi.org/10.1186/s12889-022-12839-0
[31] Azuma, K. Regulatory Landscape of Regenerative Medicine in Japan. Curr Stem Cell Rep 1 , 118–128 (2015). https://doi.org/10.1007/s40778-015-0012-6
[32] Harris, R. (2005, May 19). Researchers Report Advance in Stem Cell Production . NPR. https://www.npr.org/2005/05/19/4658967/researchers-report-advance-in-stem-cell-production
[33] Park, S. (2012). South Korea steps up stem-cell work. Nature . https://doi.org/10.1038/nature.2012.10565
[34] Resnik, D. B., Shamoo, A. E., & Krimsky, S. (2006). Fraudulent human embryonic stem cell research in South Korea: lessons learned. Accountability in research , 13 (1), 101–109. https://doi.org/10.1080/08989620600634193 .
[35] Alahmad, G., Aljohani, S., & Najjar, M. F. (2020). Ethical challenges regarding the use of stem cells: interviews with researchers from Saudi Arabia. BMC medical ethics, 21(1), 35. https://doi.org/10.1186/s12910-020-00482-6
[36] Association for the Advancement of Blood and Biotherapies. https://www.aabb.org/regulatory-and-advocacy/regulatory-affairs/regulatory-for-cellular-therapies/international-competent-authorities/saudi-arabia
[37] Alahmad, G., Aljohani, S., & Najjar, M. F. (2020). Ethical challenges regarding the use of stem cells: Interviews with researchers from Saudi Arabia. BMC medical ethics , 21 (1), 35. https://doi.org/10.1186/s12910-020-00482-6
[38] Alahmad, G., Aljohani, S., & Najjar, M. F. (2020). Ethical challenges regarding the use of stem cells: Interviews with researchers from Saudi Arabia. BMC medical ethics , 21(1), 35. https://doi.org/10.1186/s12910-020-00482-6
Culturally, autonomy practices follow a relational autonomy approach based on a paternalistic deontological health care model. The adherence to strict international research policies and religious pillars within the regulatory environment is a great foundation for research ethics. However, there is a need to develop locally targeted ethics approaches for research (as called for in Alahmad, G., Aljohani, S., & Najjar, M. F. (2020). Ethical challenges regarding the use of stem cells: interviews with researchers from Saudi Arabia. BMC medical ethics, 21(1), 35. https://doi.org/10.1186/s12910-020-00482-6), this decision-making approach may help advise a research decision model. For more on the clinical cultural autonomy approaches, see: Alabdullah, Y. Y., Alzaid, E., Alsaad, S., Alamri, T., Alolayan, S. W., Bah, S., & Aljoudi, A. S. (2022). Autonomy and paternalism in Shared decision‐making in a Saudi Arabian tertiary hospital: A cross‐sectional study. Developing World Bioethics , 23 (3), 260–268. https://doi.org/10.1111/dewb.12355 ; Bukhari, A. A. (2017). Universal Principles of Bioethics and Patient Rights in Saudi Arabia (Doctoral dissertation, Duquesne University). https://dsc.duq.edu/etd/124; Ladha, S., Nakshawani, S. A., Alzaidy, A., & Tarab, B. (2023, October 26). Islam and Bioethics: What We All Need to Know . Columbia University School of Professional Studies. https://sps.columbia.edu/events/islam-and-bioethics-what-we-all-need-know
[39] Ababneh, M. A., Al-Azzam, S. I., Alzoubi, K., Rababa’h, A., & Al Demour, S. (2021). Understanding and attitudes of the Jordanian public about clinical research ethics. Research Ethics , 17 (2), 228-241. https://doi.org/10.1177/1747016120966779
[40] Ababneh, M. A., Al-Azzam, S. I., Alzoubi, K., Rababa’h, A., & Al Demour, S. (2021). Understanding and attitudes of the Jordanian public about clinical research ethics. Research Ethics , 17 (2), 228-241. https://doi.org/10.1177/1747016120966779
[41] Dajani, R. (2014). Jordan’s stem-cell law can guide the Middle East. Nature 510, 189. https://doi.org/10.1038/510189a
[42] Dajani, R. (2014). Jordan’s stem-cell law can guide the Middle East. Nature 510, 189. https://doi.org/10.1038/510189a
[43] The EU’s definition of autonomy relates to the capacity for creating ideas, moral insight, decisions, and actions without constraint, personal responsibility, and informed consent. However, the EU views autonomy as not completely able to protect individuals and depends on other principles, such as dignity, which “expresses the intrinsic worth and fundamental equality of all human beings.” Rendtorff, J.D., Kemp, P. (2019). Four Ethical Principles in European Bioethics and Biolaw: Autonomy, Dignity, Integrity and Vulnerability. In: Valdés, E., Lecaros, J. (eds) Biolaw and Policy in the Twenty-First Century. International Library of Ethics, Law, and the New Medicine, vol 78. Springer, Cham. https://doi.org/10.1007/978-3-030-05903-3_3
[44] Council of Europe. Convention for the protection of Human Rights and Dignity of the Human Being with regard to the Application of Biology and Medicine: Convention on Human Rights and Biomedicine (ETS No. 164) https://www.coe.int/en/web/conventions/full-list?module=treaty-detail&treatynum=164 (forbidding the creation of embryos for research purposes only, and suggests embryos in vitro have protections.); Also see Drabiak-Syed B. K. (2013). New President, New Human Embryonic Stem Cell Research Policy: Comparative International Perspectives and Embryonic Stem Cell Research Laws in France. Biotechnology Law Report , 32 (6), 349–356. https://doi.org/10.1089/blr.2013.9865
[45] Rendtorff, J.D., Kemp, P. (2019). Four Ethical Principles in European Bioethics and Biolaw: Autonomy, Dignity, Integrity and Vulnerability. In: Valdés, E., Lecaros, J. (eds) Biolaw and Policy in the Twenty-First Century. International Library of Ethics, Law, and the New Medicine, vol 78. Springer, Cham. https://doi.org/10.1007/978-3-030-05903-3_3
[46] Tomuschat, C., Currie, D. P., Kommers, D. P., & Kerr, R. (Trans.). (1949, May 23). Basic law for the Federal Republic of Germany. https://www.btg-bestellservice.de/pdf/80201000.pdf
[47] Regulation of Stem Cell Research in Germany . Eurostemcell. (2017, April 26). https://www.eurostemcell.org/regulation-stem-cell-research-germany
[48] Regulation of Stem Cell Research in Finland . Eurostemcell. (2017, April 26). https://www.eurostemcell.org/regulation-stem-cell-research-finland
[49] Regulation of Stem Cell Research in Spain . Eurostemcell. (2017, April 26). https://www.eurostemcell.org/regulation-stem-cell-research-spain
[50] Some sources to consider regarding ethics models or regulatory oversights of other cultures not covered:
Kara MA. Applicability of the principle of respect for autonomy: the perspective of Turkey. J Med Ethics. 2007 Nov;33(11):627-30. doi: 10.1136/jme.2006.017400. PMID: 17971462; PMCID: PMC2598110.
Ugarte, O. N., & Acioly, M. A. (2014). The principle of autonomy in Brazil: one needs to discuss it ... Revista do Colegio Brasileiro de Cirurgioes , 41 (5), 374–377. https://doi.org/10.1590/0100-69912014005013
Bharadwaj, A., & Glasner, P. E. (2012). Local cells, global science: The rise of embryonic stem cell research in India . Routledge.
For further research on specific European countries regarding ethical and regulatory framework, we recommend this database: Regulation of Stem Cell Research in Europe . Eurostemcell. (2017, April 26). https://www.eurostemcell.org/regulation-stem-cell-research-europe
[51] Klitzman, R. (2006). Complications of culture in obtaining informed consent. The American Journal of Bioethics, 6(1), 20–21. https://doi.org/10.1080/15265160500394671 see also: Ekmekci, P. E., & Arda, B. (2017). Interculturalism and Informed Consent: Respecting Cultural Differences without Breaching Human Rights. Cultura (Iasi, Romania) , 14 (2), 159–172.; For why trust is important in research, see also: Gray, B., Hilder, J., Macdonald, L., Tester, R., Dowell, A., & Stubbe, M. (2017). Are research ethics guidelines culturally competent? Research Ethics , 13 (1), 23-41. https://doi.org/10.1177/1747016116650235
[52] The Qur'an (M. Khattab, Trans.). (1965). Al-Mu’minun, 23: 12-14. https://quran.com/23
[53] Lenfest, Y. (2017, December 8). Islam and the beginning of human life . Bill of Health. https://blog.petrieflom.law.harvard.edu/2017/12/08/islam-and-the-beginning-of-human-life/
[54] Aksoy, S. (2005). Making regulations and drawing up legislation in Islamic countries under conditions of uncertainty, with special reference to embryonic stem cell research. Journal of Medical Ethics , 31: 399-403.; see also: Mahmoud, Azza. "Islamic Bioethics: National Regulations and Guidelines of Human Stem Cell Research in the Muslim World." Master's thesis, Chapman University, 2022. https://doi.org/10.36837/ chapman.000386
[55] Rashid, R. (2022). When does Ensoulment occur in the Human Foetus. Journal of the British Islamic Medical Association , 12 (4). ISSN 2634 8071. https://www.jbima.com/wp-content/uploads/2023/01/2-Ethics-3_-Ensoulment_Rafaqat.pdf.
[56] Sivaraman, M. & Noor, S. (2017). Ethics of embryonic stem cell research according to Buddhist, Hindu, Catholic, and Islamic religions: perspective from Malaysia. Asian Biomedicine,8(1) 43-52. https://doi.org/10.5372/1905-7415.0801.260
[57] Jafari, M., Elahi, F., Ozyurt, S. & Wrigley, T. (2007). 4. Religious Perspectives on Embryonic Stem Cell Research. In K. Monroe, R. Miller & J. Tobis (Ed.), Fundamentals of the Stem Cell Debate: The Scientific, Religious, Ethical, and Political Issues (pp. 79-94). Berkeley: University of California Press. https://escholarship.org/content/qt9rj0k7s3/qt9rj0k7s3_noSplash_f9aca2e02c3777c7fb76ea768ba458f0.pdf https://doi.org/10.1525/9780520940994-005
[58] Lecso, P. A. (1991). The Bodhisattva Ideal and Organ Transplantation. Journal of Religion and Health , 30 (1), 35–41. http://www.jstor.org/stable/27510629 ; Bodhisattva, S. (n.d.). The Key of Becoming a Bodhisattva . A Guide to the Bodhisattva Way of Life. http://www.buddhism.org/Sutras/2/BodhisattvaWay.htm
[59] There is no explicit religious reference to when life begins or how to conduct research that interacts with the concept of life. However, these are relevant verses pertaining to how the fetus is viewed. (( King James Bible . (1999). Oxford University Press. (original work published 1769))
Jerimiah 1: 5 “Before I formed thee in the belly I knew thee; and before thou camest forth out of the womb I sanctified thee…”
In prophet Jerimiah’s insight, God set him apart as a person known before childbirth, a theme carried within the Psalm of David.
Psalm 139: 13-14 “…Thou hast covered me in my mother's womb. I will praise thee; for I am fearfully and wonderfully made…”
These verses demonstrate David’s respect for God as an entity that would know of all man’s thoughts and doings even before birth.
[60] It should be noted that abortion is not supported as well.
[61] The Vatican. (1987, February 22). Instruction on Respect for Human Life in Its Origin and on the Dignity of Procreation Replies to Certain Questions of the Day . Congregation For the Doctrine of the Faith. https://www.vatican.va/roman_curia/congregations/cfaith/documents/rc_con_cfaith_doc_19870222_respect-for-human-life_en.html
[62] The Vatican. (2000, August 25). Declaration On the Production and the Scientific and Therapeutic Use of Human Embryonic Stem Cells . Pontifical Academy for Life. https://www.vatican.va/roman_curia/pontifical_academies/acdlife/documents/rc_pa_acdlife_doc_20000824_cellule-staminali_en.html ; Ohara, N. (2003). Ethical Consideration of Experimentation Using Living Human Embryos: The Catholic Church’s Position on Human Embryonic Stem Cell Research and Human Cloning. Department of Obstetrics and Gynecology . Retrieved from https://article.imrpress.com/journal/CEOG/30/2-3/pii/2003018/77-81.pdf.
[63] Smith, G. A. (2022, May 23). Like Americans overall, Catholics vary in their abortion views, with regular mass attenders most opposed . Pew Research Center. https://www.pewresearch.org/short-reads/2022/05/23/like-americans-overall-catholics-vary-in-their-abortion-views-with-regular-mass-attenders-most-opposed/
[64] Rosner, F., & Reichman, E. (2002). Embryonic stem cell research in Jewish law. Journal of halacha and contemporary society , (43), 49–68.; Jafari, M., Elahi, F., Ozyurt, S. & Wrigley, T. (2007). 4. Religious Perspectives on Embryonic Stem Cell Research. In K. Monroe, R. Miller & J. Tobis (Ed.), Fundamentals of the Stem Cell Debate: The Scientific, Religious, Ethical, and Political Issues (pp. 79-94). Berkeley: University of California Press. https://escholarship.org/content/qt9rj0k7s3/qt9rj0k7s3_noSplash_f9aca2e02c3777c7fb76ea768ba458f0.pdf https://doi.org/10.1525/9780520940994-005
[65] Schenker J. G. (2008). The beginning of human life: status of embryo. Perspectives in Halakha (Jewish Religious Law). Journal of assisted reproduction and genetics , 25 (6), 271–276. https://doi.org/10.1007/s10815-008-9221-6
[66] Ruttenberg, D. (2020, May 5). The Torah of Abortion Justice (annotated source sheet) . Sefaria. https://www.sefaria.org/sheets/234926.7?lang=bi&with=all&lang2=en
[67] Jafari, M., Elahi, F., Ozyurt, S. & Wrigley, T. (2007). 4. Religious Perspectives on Embryonic Stem Cell Research. In K. Monroe, R. Miller & J. Tobis (Ed.), Fundamentals of the Stem Cell Debate: The Scientific, Religious, Ethical, and Political Issues (pp. 79-94). Berkeley: University of California Press. https://escholarship.org/content/qt9rj0k7s3/qt9rj0k7s3_noSplash_f9aca2e02c3777c7fb76ea768ba458f0.pdf https://doi.org/10.1525/9780520940994-005
[68] Gert, B. (2007). Common morality: Deciding what to do . Oxford Univ. Press.
[69] World Medical Association (2013). World Medical Association Declaration of Helsinki: ethical principles for medical research involving human subjects. JAMA , 310(20), 2191–2194. https://doi.org/10.1001/jama.2013.281053 Declaration of Helsinki – WMA – The World Medical Association .; see also: National Commission for the Protection of Human Subjects of Biomedical and Behavioral Research. (1979). The Belmont report: Ethical principles and guidelines for the protection of human subjects of research . U.S. Department of Health and Human Services. https://www.hhs.gov/ohrp/regulations-and-policy/belmont-report/read-the-belmont-report/index.html
[70] Zakarin Safier, L., Gumer, A., Kline, M., Egli, D., & Sauer, M. V. (2018). Compensating human subjects providing oocytes for stem cell research: 9-year experience and outcomes. Journal of assisted reproduction and genetics , 35 (7), 1219–1225. https://doi.org/10.1007/s10815-018-1171-z https://www.ncbi.nlm.nih.gov/pmc/articles/PMC6063839/ see also: Riordan, N. H., & Paz Rodríguez, J. (2021). Addressing concerns regarding associated costs, transparency, and integrity of research in recent stem cell trial. Stem Cells Translational Medicine , 10 (12), 1715–1716. https://doi.org/10.1002/sctm.21-0234
[71] Klitzman, R., & Sauer, M. V. (2009). Payment of egg donors in stem cell research in the USA. Reproductive biomedicine online , 18 (5), 603–608. https://doi.org/10.1016/s1472-6483(10)60002-8
[72] Krosin, M. T., Klitzman, R., Levin, B., Cheng, J., & Ranney, M. L. (2006). Problems in comprehension of informed consent in rural and peri-urban Mali, West Africa. Clinical trials (London, England) , 3 (3), 306–313. https://doi.org/10.1191/1740774506cn150oa
[73] Veatch, Robert M. Hippocratic, Religious, and Secular Medical Ethics: The Points of Conflict . Georgetown University Press, 2012.
[74] Msoroka, M. S., & Amundsen, D. (2018). One size fits not quite all: Universal research ethics with diversity. Research Ethics , 14 (3), 1-17. https://doi.org/10.1177/1747016117739939
[75] Pirzada, N. (2022). The Expansion of Turkey’s Medical Tourism Industry. Voices in Bioethics , 8 . https://doi.org/10.52214/vib.v8i.9894
[76] Stem Cell Tourism: False Hope for Real Money . Harvard Stem Cell Institute (HSCI). (2023). https://hsci.harvard.edu/stem-cell-tourism , See also: Bissassar, M. (2017). Transnational Stem Cell Tourism: An ethical analysis. Voices in Bioethics , 3 . https://doi.org/10.7916/vib.v3i.6027
[77] Song, P. (2011) The proliferation of stem cell therapies in post-Mao China: problematizing ethical regulation, New Genetics and Society , 30:2, 141-153, DOI: 10.1080/14636778.2011.574375
[78] Dajani, R. (2014). Jordan’s stem-cell law can guide the Middle East. Nature 510, 189. https://doi.org/10.1038/510189a
[79] International Society for Stem Cell Research. (2024). Standards in stem cell research . International Society for Stem Cell Research. https://www.isscr.org/guidelines/5-standards-in-stem-cell-research
[80] Benjamin, R. (2013). People’s science bodies and rights on the Stem Cell Frontier . Stanford University Press.
Mifrah Hayath
SM Candidate Harvard Medical School, MS Biotechnology Johns Hopkins University
Olivia Bowers
MS Bioethics Columbia University (Disclosure: affiliated with Voices in Bioethics)
Article Details

This work is licensed under a Creative Commons Attribution 4.0 International License .
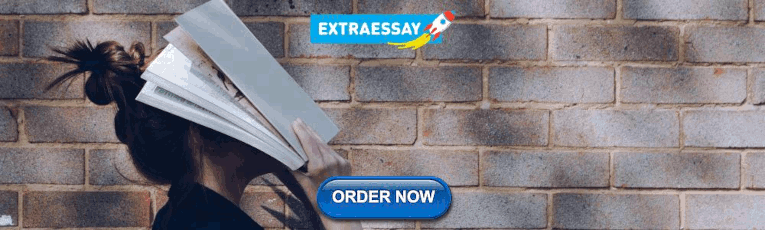
COMMENTS
Decision-making is a crucial skill that has a central role in everyday life and is necessary for adaptation to the environment and autonomy. It is the ability to choose between two or more options, and it has been studied through several theoretical approaches and by different disciplines. In this overview article, we contend a theoretical review regarding most theorizing and research on ...
Decision analysis is a technology designed to help individuals and organizations make wise inferences and decisions. It synthesises ideas from economics, statistics, psychology, operations research, and other disciplines. A great deal of behavioural research is relevant to decision analysis; behavioural scientists have both suggested easy and natural ways to describe and quantify problems and ...
The science of judgment and decision making involves three interrelated forms of research: analysis of the decisions people face, description of their natural responses, and interventions meant to help them do better. After briefly introducing the field's intellectual foundations, we review recent basic research into the three core elements of decision making: judgment, or how people predict ...
Evidence-based practice (EBP) is a model of professional decision-making in which practitioners integrate the best available evidence with client values/context and clinical expertise in order to provide services for their clients. This framework provides behavior analysts with a structure for pervasive use of the best available evidence in the ...
Part V reviews engineering risk analysis and risk analysis for health risk management. Part VI puts decision analysis in a behavioral and organizational context, including behavioral research, decision conferencing, resource allocation decisions, transitioning from decision analysis to the decision organization, and negotiation analysis.
A ONE-OF-A-KIND GUIDE TO THE BEST PRACTICES IN DECISION ANALYSIS Decision analysis provides powerful tools for addressing complex decisions that involve uncertainty and multiple objectives, yet most training materials on the subject overlook the soft skills that are essential for success in the field. This unique resource fills this gap in the decision analysis literature and features both ...
Decision analysis is a technology designed to help individuals and organizations make wise inferences and decisions. It synthesises ideas from economics, statistics, psychology, operations research, and other disciplines. A great deal of behavioural research is relevant to decision analysis; behavioural scientists have both suggested easy and ...
Decision analysis is a technology designed to help individuals and organizations make wise inferences and decisions. It synthesises ideas from economics, statistics, psychology, operations research, and other disciplines. A great deal of behavioural research is relevant to decision analysis; behavioural scientists have both suggested easy and natural ways to describe and quantify problems and ...
Here are the four core principles of decision-driven analytics: Decisions. Identify controllable, relevant decision alternatives. Consider diverse perspectives and a wide array of solutions ...
Decision analysis and behavioral research by Von Winterfeldt, Detlof. Publication date 1986 Topics Decision making -- Research, Statistical decision, Psychology -- Research, Psychometrics, Psychophysics Publisher Cambridge [Cambridgeshire] ; New York : Cambridge University Press Collection
Decision Analysis and Behavioral Research. Detlof Von Winterfeldt, Ward Edwards. Cambridge University Press, 1986 - Decision making - 604 pages. Bibliographic information. Title: Decision Analysis and Behavioral Research: Authors: Detlof Von Winterfeldt, Ward Edwards: Publisher: Cambridge University Press, 1986:
Behavioral Decision Research: A Constructive Processing Perspective, Page 1 of 1 < Previous page ... Mediation Analysis David P. MacKinnon, Amanda J. Fairchild, and Matthew S. Fritz. Vol. 58 (2007), pp. 593-614 Missing Data Analysis: Making It Work in the Real World John W. Graham.
Ward Edwards: Founder of Behavioral Decision Theory. In 1954 and 1961 Ward Edwards published two seminal articles that created behavioral decision research as a new field in psychology (Edwards 1954, 1961). The topics of this research include how people make decisions and how these decisions can be improved with tools and training.
It synthesises ideas from economics, statistics, psychology, operations research, and other disciplines. A great deal of behavioural research is relevant to decision analysis; behavioural scientists have both suggested easy and natural ways to describe and quantify problems and shown the kind of errors to which unaided intuitive judgements can ...
Decision analysis is a technology designed to help individuals and organizations make wise inferences and decisions. It synthesises ideas from economics, statistics, psychology, operations research, and other disciplines. A great deal of behavioural research is relevant to decision analysis; behavioural scientists have both suggested easy and natural ways to describe and quantify problems and ...
Decision Analysis: Introductory Lectures on Choices under Uncertainty, Addison-Wesley, 309 pp.Google Scholar Raiffa , H. and Schlaifer , R. ( 1961 ). Applied Statistical Decision Theory , Division of Research, Graduate School of Business Administration, Harvard University , 356 pp. Google Scholar
Although behavioral research and decision analysis began with a close connection, that connection appears to have diminished over time. This chapter discusses how to re-establish the connection between the disciplines in two distinct ways. First, theoretical and...
December 7, 2022. Edited by ImportBot. import existing book. April 1, 2008. Created by an anonymous user. Imported from Scriblio MARC record . Decision analysis and behavioral research by Detlof Von Winterfeldt, 1986, Cambridge University Press edition, in English.
A Review of: "DECISION ANALYSIS AND BEHAVIORAL RESEARCH" by Detlof von Winterfeldt and Ward Edwards. Cambridge University Press, New York, 1986, xv + 604 pp., ISBN -521-27304-8. List $19.95 (paperback).
1 Basic concepts of decision analysis and their relation to behavior 1 1.1. Introduction 1 1.2. The central topics of decision analysis 4 1.3. Rationality and expected quantities 18 1.4. Other purposes of decision-analytic procedures 22 1.5. Decision aids, artificial intelligence, and other aspects of intellectual technology 23 2 Structuring ...
Decision analysis is a technology designed to help individuals and organizations make wise inferences and decisions. It synthesises ideas from economics, statistics, psychology, operations research, and other disciplines. A great deal of behavioural research is relevant to decision analysis; behavioural scientists have both suggested easy and ...
Current theories of decision-making propose that decisions arise through competition between choice options. Computational models of the decision process estimate how quickly information about choice options is integrated and how much information is needed to trigger a choice. Experiments using this approach typically report data from well-trained participants. As such, we do not know how the ...
Author summary Computational cognitive models occupy an important position in cognitive science research, as they offer a simple way of quantifying cognitive processes (such as how fast someone learns, or how noisy they are in choice selection), and testing which cognitive theories offer a better explanation of the behavior. To relate cognitive models to the behavioral data, researchers rely ...
Decision analysis is a technology designed to help individuals and organizations make wise inferences and decisions. It synthesises ideas from economics, statistics, psychology, operations research, and other disciplines. A great deal of behavioural research is relevant to decision analysis; behavioural scientists have both suggested easy and ...
Supply Chain Management. Economics. Purchasing. Article. Unveiling the Impact: A Comprehensive Analysis of Influencer Marketing on Consumer Purchase Decisions. April 2024. International Journal of ...
Voices in Bioethics is currently seeking submissions on philosophical and practical topics, both current and timeless. Papers addressing access to healthcare, the bioethical implications of recent Supreme Court rulings, environmental ethics, data privacy, cybersecurity, law and bioethics, economics and bioethics, reproductive ethics, research ethics, and pediatric bioethics are sought.