- The Open University
- Guest user / Sign out
- Study with The Open University
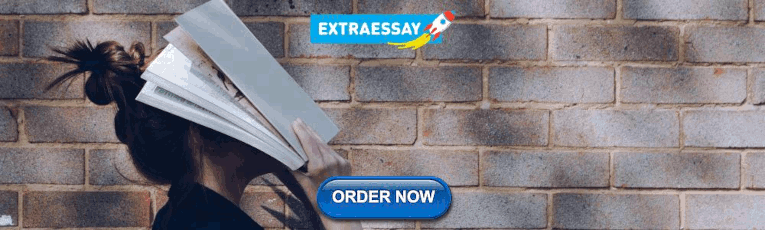
My OpenLearn Profile
Personalise your OpenLearn profile, save your favourite content and get recognition for your learning

Addressing ethical issues in your research proposal
This article explores the ethical issues that may arise in your proposed study during your doctoral research degree.
What ethical principles apply when planning and conducting research?
Research ethics are the moral principles that govern how researchers conduct their studies (Wellcome Trust, 2014). As there are elements of uncertainty and risk involved in any study, every researcher has to consider how they can uphold these ethical principles and conduct the research in a way that protects the interests and welfare of participants and other stakeholders (such as organisations).
You will need to consider the ethical issues that might arise in your proposed study. Consideration of the fundamental ethical principles that underpin all research will help you to identify the key issues and how these could be addressed. As you are probably a practitioner who wants to undertake research within your workplace, consider how your role as an ‘insider’ influences how you will conduct your study. Think about the ethical issues that might arise when you become an insider researcher (for example, relating to trust, confidentiality and anonymity).
What key ethical principles do you think will be important when planning or conducting your research, particularly as an insider? Principles that come to mind might include autonomy, respect, dignity, privacy, informed consent and confidentiality. You may also have identified principles such as competence, integrity, wellbeing, justice and non-discrimination.
Key ethical issues that you will address as an insider researcher include:
- Gaining trust
- Avoiding coercion when recruiting colleagues or other participants (such as students or service users)
- Practical challenges relating to ensuring the confidentiality and anonymity of organisations and staff or other participants.
(Heslop et al, 2018)
A fuller discussion of ethical principles is available from the British Psychological Society’s Code of Human Research Ethics (BPS, 2021).
You can also refer to guidance from the British Educational Research Association and the British Association for Applied Linguistics .
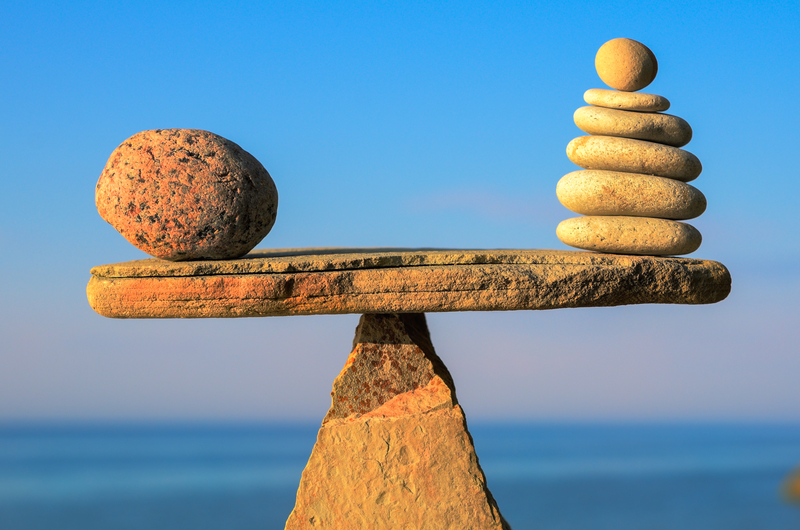
Ethical principles are essential for protecting the interests of research participants, including maximising the benefits and minimising any risks associated with taking part in a study. These principles describe ethical conduct which reflects the integrity of the researcher, promotes the wellbeing of participants and ensures high-quality research is conducted (Health Research Authority, 2022).
Research ethics is therefore not simply about gaining ethical approval for your study to be conducted. Research ethics relates to your moral conduct as a doctoral researcher and will apply throughout your study from design to dissemination (British Psychological Society, 2021). When you apply to undertake a doctorate, you will need to clearly indicate in your proposal that you understand these ethical principles and are committed to upholding them.
Where can I find ethical guidance and resources?
Professional bodies, learned societies, health and social care authorities, academic publications, Research Ethics Committees and research organisations provide a range of ethical guidance and resources. International codes such as the Universal Declaration of Human Rights underpin ethical frameworks (United Nations, 1948).
You may be aware of key legislation in your own country or the country where you plan to undertake the research, including laws relating to consent, data protection and decision-making capacity, for example, the Data Protection Act, 2018 (UK). If you want to find out more about becoming an ethical researcher, check out this Open University short course: Becoming an ethical researcher: Introduction and guidance: What is a badged course? - OpenLearn - Open University
You should be able to justify the research decisions you make. Utilising these resources will guide your ethical judgements when writing your proposal and ultimately when designing and conducting your research study. The Ethical Guidelines for Educational Research (British Educational Research Association, 2018) identifies the key responsibilities you will have when you conduct your research, including the range of stakeholders that you will have responsibilities to, as follows:
- to your participants (e.g. to appropriately inform them, facilitate their participation and support them)
- clients, stakeholders and sponsors
- the community of educational or health and social care researchers
- for publication and dissemination
- your wellbeing and development
The National Institute for Health and Care Research (no date) has emphasised the need to promote equality, diversity and inclusion when undertaking research, particularly to address long-standing social and health inequalities. Research should be informed by the diversity of people’s experiences and insights, so that it will lead to the development of practice that addresses genuine need. A commitment to equality, diversity and inclusion aims to eradicate prejudice and discrimination on the basis of an individual or group of individuals' protected characteristics such as sex (gender), disability, race, sexual orientation, in line with the Equality Act 2010.
The NIHR has produced guidance for enhancing the inclusion of ‘under-served groups’ when designing a research study (2020). Although the guidance refers to clinical research it is relevant to research more broadly.
You should consider how you will promote equality and diversity in your planned study, including through aspects such as your research topic or question, the methodology you will use, the participants you plan to recruit and how you will analyse and interpret your data.
What ethical issues do I need to consider when writing my research proposal?
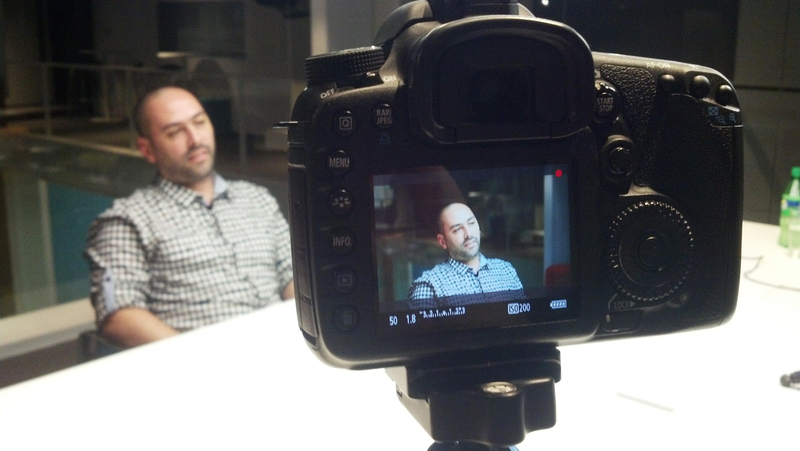
You might be planning to undertake research in a health, social care, educational or other setting, including observations and interviews. The following prompts should help you to identify key ethical issues that you need to bear in mind when undertaking research in such settings.
1. Imagine you are a potential participant. Think about the questions and concerns that you might have:
- How would you feel if a researcher sat in your space and took notes, completed a checklist, or made an audio or film recording?
- What harm might a researcher cause by observing or interviewing you and others?
- What would you want to know about the researcher and ask them about the study before giving consent?
- When imagining you are the participant, how could the researcher make you feel more comfortable to be observed or interviewed?
2. Having considered the perspective of your potential participant, how would you take account of concerns such as privacy, consent, wellbeing and power in your research proposal?
[Adapted from OpenLearn course: Becoming an ethical researcher, Week 2 Activity 3: Becoming an ethical researcher - OpenLearn - Open University ]
The ethical issues to be considered will vary depending on your organisational context/role, the types of participants you plan to recruit (for example, children, adults with mental health problems), the research methods you will use, and the types of data you will collect. You will need to decide how to recruit your participants so you do not inappropriately exclude anyone. Consider what methods may be necessary to facilitate their voice and how you can obtain their consent to taking part or ensure that consent is obtained from someone else as necessary, for example, a parent in the case of a child.
You should also think about how to avoid imposing an unnecessary burden or costs on your participants. For example, by minimising the length of time they will have to commit to the study and by providing travel or other expenses. Identify the measures that you will take to store your participants’ data safely and maintain their confidentiality and anonymity when you report your findings. You could do this by storing interview and video recordings in a secure server and anonymising their names and those of their organisations using pseudonyms.
Professional codes such as the Code of Human Research Ethics (BPS, 2021) provide guidance on undertaking research with children. Being an ‘insider’ researching within your own organisation has advantages. However, you should also consider how this might impact on your research, such as power dynamics, consent, potential bias and any conflict of interest between your professional and researcher roles (Sapiro and Matthews, 2020).
How have other researchers addressed any ethical challenges?
The literature provides researchers’ accounts explaining how they addressed ethical challenges when undertaking studies. For example, Turcotte-Tremblay and McSween-Cadieux (2018) discuss strategies for protecting participants’ confidentiality when disseminating findings locally, such as undertaking fieldwork in multiple sites and providing findings in a generalised form. In addition, professional guidance includes case studies illustrating how ethical issues can be addressed, including when researching online forums (British Sociological Association, no date).
Watch the videos below and consider what insights the postgraduate researcher and supervisor provide regarding issues such as being an ‘insider researcher’, power relations, avoiding intrusion, maintaining participant anonymity and complying with research ethics and professional standards. How might their experiences inform the design and conduct of your own study?
Postgraduate researcher and supervisor talk about ethical considerations
Your thoughtful consideration of the ethical issues that might arise and how you would address these should enable you to propose an ethically informed study and conduct it in a responsible, fair and sensitive manner.
British Educational Research Association (2018) Ethical Guidelines for Educational Research. Available at: https://www.bera.ac.uk/publication/ethical-guidelines-for-educational-research-2018 (Accessed: 9 June 2023).
British Psychological Society (2021) Code of Human Research Ethics . Available at: https://cms.bps.org.uk/sites/default/files/2022-06/BPS%20Code%20of%20Human%20Research%20Ethics%20%281%29.pdf (Accessed: 9 June 2023).
British Sociological Association (2016) Researching online forums . Available at: https://www.britsoc.co.uk/media/24834/j000208_researching_online_forums_-cs1-_v3.pdf (Accessed: 9 June 2023).
Health Research Authority (2022) UK Policy Framework for Health and Social Care Research . Available at: https://www.hra.nhs.uk/planning-and-improving-research/policies-standards-legislation/uk-policy-framework-health-social-care-research/uk-policy-framework-health-and-social-care-research/#chiefinvestigators (Accessed: 9 June 2023).
Heslop, C., Burns, S., Lobo, R. (2018) ‘Managing qualitative research as insider-research in small rural communities’, Rural and Remote Health , 18: pp. 4576.
Equality Act 2010, c. 15. Available at: https://www.legislation.gov.uk/ukpga/2010/15/introduction (Accessed: 9 June 2023).
National Institute for Health and Care Research (no date) Equality, Diversity and Inclusion (EDI) . Available at: https://arc-kss.nihr.ac.uk/public-and-community-involvement/pcie-guide/how-to-do-pcie/equality-diversity-and-inclusion-edi (Accessed: 9 June 2023).
National Institute for Health and Care Research (2020) Improving inclusion of under-served groups in clinical research: Guidance from INCLUDE project. Available at: https://www.nihr.ac.uk/documents/improving-inclusion-of-under-served-groups-in-clinical-research-guidance-from-include-project/25435 (Accessed: 9 June 2023).
Sapiro, B. and Matthews, E. (2020) ‘Both Insider and Outsider. On Conducting Social Work Research in Mental Health Settings’, Advances in Social Work , 20(3). Available at: https://doi.org/10.18060/23926
Turcotte-Tremblay, A. and McSween-Cadieux, E. (2018) ‘A reflection on the challenge of protecting confidentiality of participants when disseminating research results locally’, BMC Medical Ethics, 19(supplement 1), no. 45. Available at: https://bmcmedethics.biomedcentral.com/articles/10.1186/s12910-018-0279-0
United Nations General Assembly (1948) The Universal Declaration of Human Rights . Resolution A/RES/217/A. Available at: https://www.un.org/en/about-us/universal-declaration-of-human-rights#:~:text=Drafted%20by%20representatives%20with%20different,all%20peoples%20and%20all%20nations . (Accessed: 9 June 2023).
Wellcome Trust (2014) Ensuring your research is ethical: A guide for Extended Project Qualification students . Available at: https://wellcome.org/sites/default/files/wtp057673_0.pdf (Accessed: 9 June 2023).
More articles from the research proposal collection
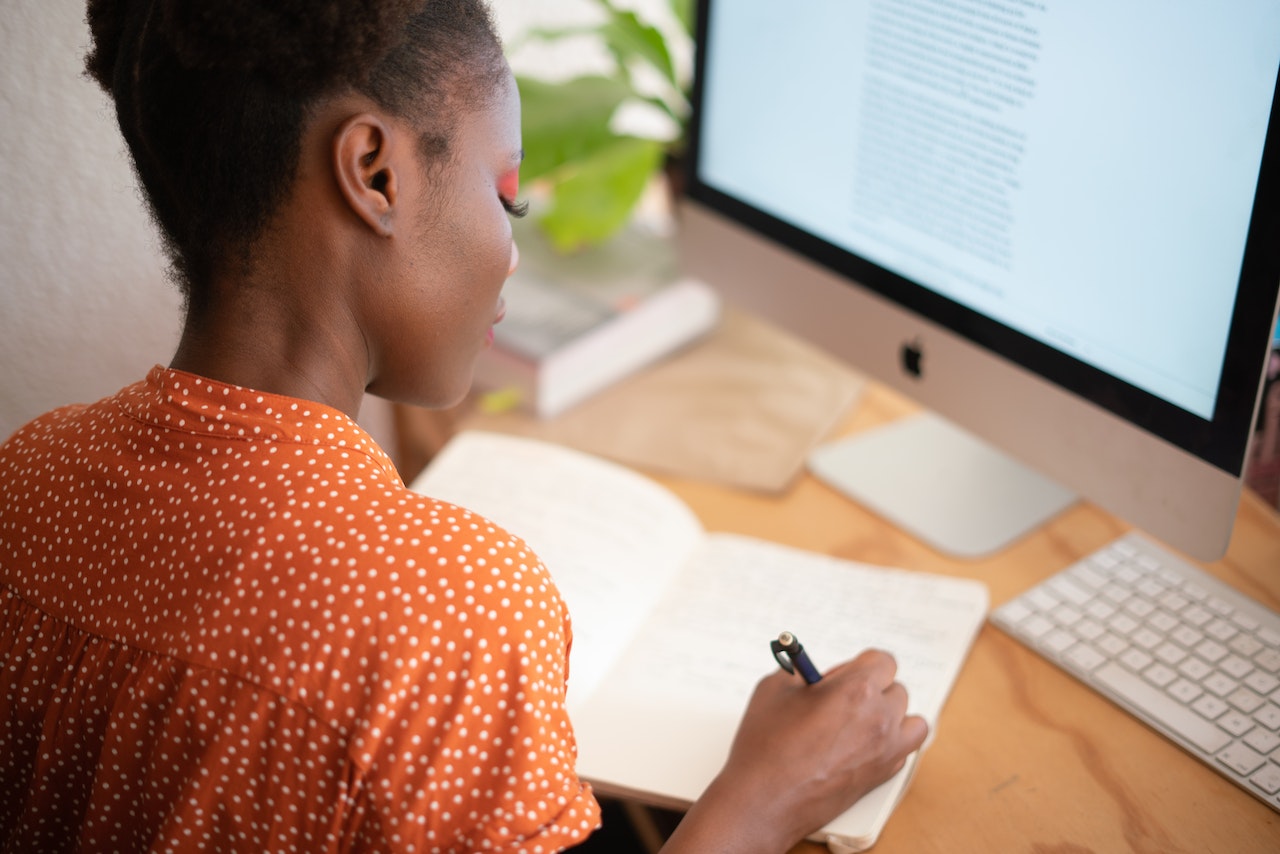
Writing your research proposal
A doctoral research degree is the highest academic qualification that a student can achieve. The guidance provided in these articles will help you apply for one of the two main types of research degree offered by The Open University.
Level: 1 Introductory
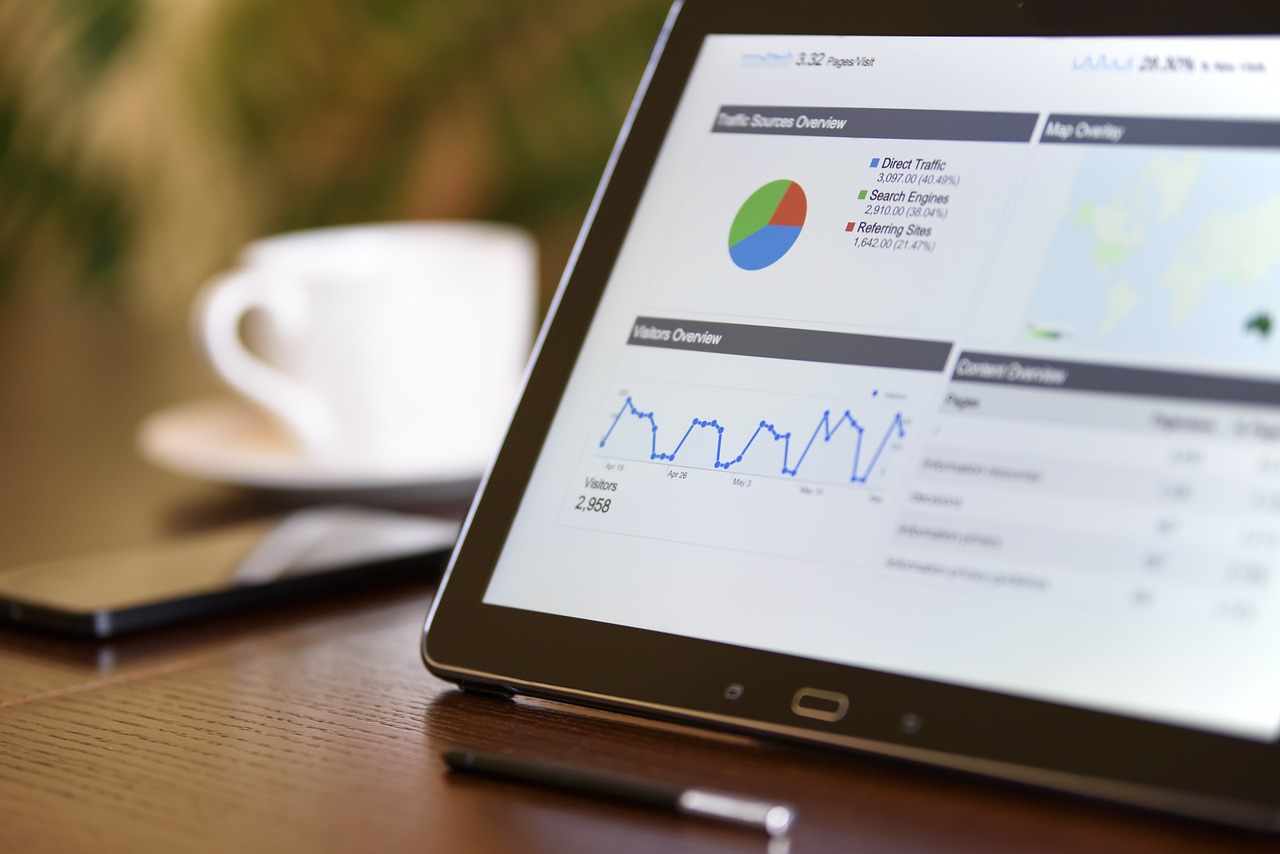
Defining your research methodology
Your research methodology is the approach you will take to guide your research process and explain why you use particular methods. This article explains more.
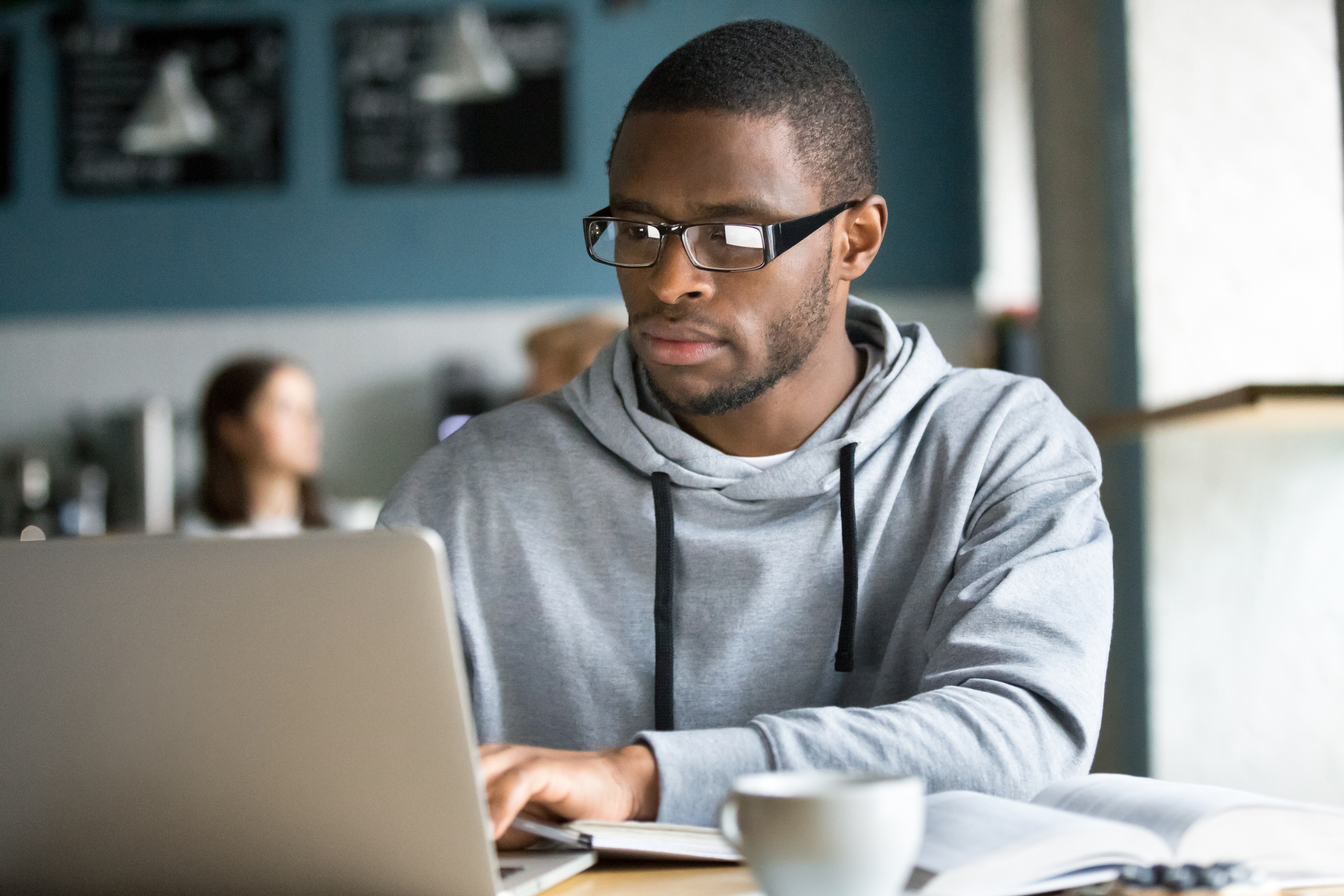
Writing your proposal and preparing for your interview
The final article looks at writing your research proposal - from the introduction through to citations and referencing - as well as preparing for your interview.
Free courses on postgraduate study
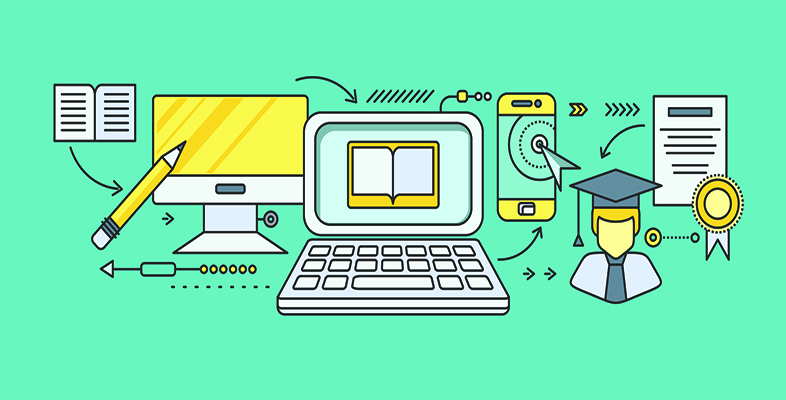
Are you ready for postgraduate study?
This free course, Are you ready for postgraduate study, will help you to become familiar with the requirements and demands of postgraduate study and ensure you are ready to develop the skills and confidence to pursue your learning further.

Succeeding in postgraduate study
This free course, Succeeding in postgraduate study, will help you to become familiar with the requirements and demands of postgraduate study and to develop the skills and confidence to pursue your learning further.
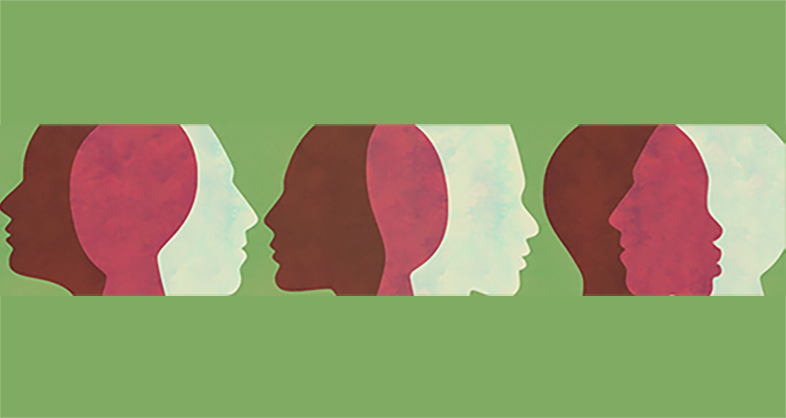
Applying to study for a PhD in psychology
This free OpenLearn course is for psychology students and graduates who are interested in PhD study at some future point. Even if you have met PhD students and heard about their projects, it is likely that you have only a vague idea of what PhD study entails. This course is intended to give you more information.
Become an OU student
Ratings & comments, share this free course, copyright information, publication details.
- Originally published: Tuesday, 27 June 2023
- Body text - Creative Commons BY-NC-SA 4.0 : The Open University
- Image 'Pebbles balance on a stone see-saw' - Copyright: Photo 51106733 / Balance © Anatoli Styf | Dreamstime.com
- Image 'Camera equipment set up filming a man talking' - Copyright: Photo 42631221 © Gabriel Robledo | Dreamstime.com
- Image 'Applying to study for a PhD in psychology' - Copyright free
- Image 'Succeeding in postgraduate study' - Copyright: © Everste/Getty Images
- Image 'Addressing ethical issues in your research proposal' - Copyright: Photo 50384175 / Children Playing © Lenutaidi | Dreamstime.com
- Image 'Writing your proposal and preparing for your interview' - Copyright: Photo 133038259 / Black Student © Fizkes | Dreamstime.com
- Image 'Defining your research methodology' - Copyright free
- Image 'Writing your research proposal' - Copyright free
- Image 'Are you ready for postgraduate study?' - Copyright free
Rate and Review
Rate this article, review this article.
Log into OpenLearn to leave reviews and join in the conversation.
Article reviews
For further information, take a look at our frequently asked questions which may give you the support you need.
- Privacy Policy

Home » Ethical Considerations – Types, Examples and Writing Guide
Ethical Considerations – Types, Examples and Writing Guide
Table of Contents

Ethical Considerations
Ethical considerations in research refer to the principles and guidelines that researchers must follow to ensure that their studies are conducted in an ethical and responsible manner. These considerations are designed to protect the rights, safety, and well-being of research participants, as well as the integrity and credibility of the research itself
Some of the key ethical considerations in research include:
- Informed consent: Researchers must obtain informed consent from study participants, which means they must inform participants about the study’s purpose, procedures, risks, benefits, and their right to withdraw at any time.
- Privacy and confidentiality : Researchers must ensure that participants’ privacy and confidentiality are protected. This means that personal information should be kept confidential and not shared without the participant’s consent.
- Harm reduction : Researchers must ensure that the study does not harm the participants physically or psychologically. They must take steps to minimize the risks associated with the study.
- Fairness and equity : Researchers must ensure that the study does not discriminate against any particular group or individual. They should treat all participants equally and fairly.
- Use of deception: Researchers must use deception only if it is necessary to achieve the study’s objectives. They must inform participants of the deception as soon as possible.
- Use of vulnerable populations : Researchers must be especially cautious when working with vulnerable populations, such as children, pregnant women, prisoners, and individuals with cognitive or intellectual disabilities.
- Conflict of interest : Researchers must disclose any potential conflicts of interest that may affect the study’s integrity. This includes financial or personal relationships that could influence the study’s results.
- Data manipulation: Researchers must not manipulate data to support a particular hypothesis or agenda. They should report the results of the study objectively, even if the findings are not consistent with their expectations.
- Intellectual property: Researchers must respect intellectual property rights and give credit to previous studies and research.
- Cultural sensitivity : Researchers must be sensitive to the cultural norms and beliefs of the participants. They should avoid imposing their values and beliefs on the participants and should be respectful of their cultural practices.
Types of Ethical Considerations
Types of Ethical Considerations are as follows:
Research Ethics:
This includes ethical principles and guidelines that govern research involving human or animal subjects, ensuring that the research is conducted in an ethical and responsible manner.
Business Ethics :
This refers to ethical principles and standards that guide business practices and decision-making, such as transparency, honesty, fairness, and social responsibility.
Medical Ethics :
This refers to ethical principles and standards that govern the practice of medicine, including the duty to protect patient autonomy, informed consent, confidentiality, and non-maleficence.
Environmental Ethics :
This involves ethical principles and values that guide our interactions with the natural world, including the obligation to protect the environment, minimize harm, and promote sustainability.
Legal Ethics
This involves ethical principles and standards that guide the conduct of legal professionals, including issues such as confidentiality, conflicts of interest, and professional competence.
Social Ethics
This involves ethical principles and values that guide our interactions with other individuals and society as a whole, including issues such as justice, fairness, and human rights.
Information Ethics
This involves ethical principles and values that govern the use and dissemination of information, including issues such as privacy, accuracy, and intellectual property.
Cultural Ethics
This involves ethical principles and values that govern the relationship between different cultures and communities, including issues such as respect for diversity, cultural sensitivity, and inclusivity.
Technological Ethics
This refers to ethical principles and guidelines that govern the development, use, and impact of technology, including issues such as privacy, security, and social responsibility.
Journalism Ethics
This involves ethical principles and standards that guide the practice of journalism, including issues such as accuracy, fairness, and the public interest.
Educational Ethics
This refers to ethical principles and standards that guide the practice of education, including issues such as academic integrity, fairness, and respect for diversity.
Political Ethics
This involves ethical principles and values that guide political decision-making and behavior, including issues such as accountability, transparency, and the protection of civil liberties.
Professional Ethics
This refers to ethical principles and standards that guide the conduct of professionals in various fields, including issues such as honesty, integrity, and competence.
Personal Ethics
This involves ethical principles and values that guide individual behavior and decision-making, including issues such as personal responsibility, honesty, and respect for others.
Global Ethics
This involves ethical principles and values that guide our interactions with other nations and the global community, including issues such as human rights, environmental protection, and social justice.
Applications of Ethical Considerations
Ethical considerations are important in many areas of society, including medicine, business, law, and technology. Here are some specific applications of ethical considerations:
- Medical research : Ethical considerations are crucial in medical research, particularly when human subjects are involved. Researchers must ensure that their studies are conducted in a way that does not harm participants and that participants give informed consent before participating.
- Business practices: Ethical considerations are also important in business, where companies must make decisions that are socially responsible and avoid activities that are harmful to society. For example, companies must ensure that their products are safe for consumers and that they do not engage in exploitative labor practices.
- Environmental protection: Ethical considerations play a crucial role in environmental protection, as companies and governments must weigh the benefits of economic development against the potential harm to the environment. Decisions about land use, resource allocation, and pollution must be made in an ethical manner that takes into account the long-term consequences for the planet and future generations.
- Technology development : As technology continues to advance rapidly, ethical considerations become increasingly important in areas such as artificial intelligence, robotics, and genetic engineering. Developers must ensure that their creations do not harm humans or the environment and that they are developed in a way that is fair and equitable.
- Legal system : The legal system relies on ethical considerations to ensure that justice is served and that individuals are treated fairly. Lawyers and judges must abide by ethical standards to maintain the integrity of the legal system and to protect the rights of all individuals involved.
Examples of Ethical Considerations
Here are a few examples of ethical considerations in different contexts:
- In healthcare : A doctor must ensure that they provide the best possible care to their patients and avoid causing them harm. They must respect the autonomy of their patients, and obtain informed consent before administering any treatment or procedure. They must also ensure that they maintain patient confidentiality and avoid any conflicts of interest.
- In the workplace: An employer must ensure that they treat their employees fairly and with respect, provide them with a safe working environment, and pay them a fair wage. They must also avoid any discrimination based on race, gender, religion, or any other characteristic protected by law.
- In the media : Journalists must ensure that they report the news accurately and without bias. They must respect the privacy of individuals and avoid causing harm or distress. They must also be transparent about their sources and avoid any conflicts of interest.
- In research: Researchers must ensure that they conduct their studies ethically and with integrity. They must obtain informed consent from participants, protect their privacy, and avoid any harm or discomfort. They must also ensure that their findings are reported accurately and without bias.
- In personal relationships : People must ensure that they treat others with respect and kindness, and avoid causing harm or distress. They must respect the autonomy of others and avoid any actions that would be considered unethical, such as lying or cheating. They must also respect the confidentiality of others and maintain their privacy.
How to Write Ethical Considerations
When writing about research involving human subjects or animals, it is essential to include ethical considerations to ensure that the study is conducted in a manner that is morally responsible and in accordance with professional standards. Here are some steps to help you write ethical considerations:
- Describe the ethical principles: Start by explaining the ethical principles that will guide the research. These could include principles such as respect for persons, beneficence, and justice.
- Discuss informed consent : Informed consent is a critical ethical consideration when conducting research. Explain how you will obtain informed consent from participants, including how you will explain the purpose of the study, potential risks and benefits, and how you will protect their privacy.
- Address confidentiality : Describe how you will protect the confidentiality of the participants’ personal information and data, including any measures you will take to ensure that the data is kept secure and confidential.
- Consider potential risks and benefits : Describe any potential risks or harms to participants that could result from the study and how you will minimize those risks. Also, discuss the potential benefits of the study, both to the participants and to society.
- Discuss the use of animals : If the research involves the use of animals, address the ethical considerations related to animal welfare. Explain how you will minimize any potential harm to the animals and ensure that they are treated ethically.
- Mention the ethical approval : Finally, it’s essential to acknowledge that the research has received ethical approval from the relevant institutional review board or ethics committee. State the name of the committee, the date of approval, and any specific conditions or requirements that were imposed.
When to Write Ethical Considerations
Ethical considerations should be written whenever research involves human subjects or has the potential to impact human beings, animals, or the environment in some way. Ethical considerations are also important when research involves sensitive topics, such as mental health, sexuality, or religion.
In general, ethical considerations should be an integral part of any research project, regardless of the field or subject matter. This means that they should be considered at every stage of the research process, from the initial planning and design phase to data collection, analysis, and dissemination.
Ethical considerations should also be written in accordance with the guidelines and standards set by the relevant regulatory bodies and professional associations. These guidelines may vary depending on the discipline, so it is important to be familiar with the specific requirements of your field.
Purpose of Ethical Considerations
Ethical considerations are an essential aspect of many areas of life, including business, healthcare, research, and social interactions. The primary purposes of ethical considerations are:
- Protection of human rights: Ethical considerations help ensure that people’s rights are respected and protected. This includes respecting their autonomy, ensuring their privacy is respected, and ensuring that they are not subjected to harm or exploitation.
- Promoting fairness and justice: Ethical considerations help ensure that people are treated fairly and justly, without discrimination or bias. This includes ensuring that everyone has equal access to resources and opportunities, and that decisions are made based on merit rather than personal biases or prejudices.
- Promoting honesty and transparency : Ethical considerations help ensure that people are truthful and transparent in their actions and decisions. This includes being open and honest about conflicts of interest, disclosing potential risks, and communicating clearly with others.
- Maintaining public trust: Ethical considerations help maintain public trust in institutions and individuals. This is important for building and maintaining relationships with customers, patients, colleagues, and other stakeholders.
- Ensuring responsible conduct: Ethical considerations help ensure that people act responsibly and are accountable for their actions. This includes adhering to professional standards and codes of conduct, following laws and regulations, and avoiding behaviors that could harm others or damage the environment.
Advantages of Ethical Considerations
Here are some of the advantages of ethical considerations:
- Builds Trust : When individuals or organizations follow ethical considerations, it creates a sense of trust among stakeholders, including customers, clients, and employees. This trust can lead to stronger relationships and long-term loyalty.
- Reputation and Brand Image : Ethical considerations are often linked to a company’s brand image and reputation. By following ethical practices, a company can establish a positive image and reputation that can enhance its brand value.
- Avoids Legal Issues: Ethical considerations can help individuals and organizations avoid legal issues and penalties. By adhering to ethical principles, companies can reduce the risk of facing lawsuits, regulatory investigations, and fines.
- Increases Employee Retention and Motivation: Employees tend to be more satisfied and motivated when they work for an organization that values ethics. Companies that prioritize ethical considerations tend to have higher employee retention rates, leading to lower recruitment costs.
- Enhances Decision-making: Ethical considerations help individuals and organizations make better decisions. By considering the ethical implications of their actions, decision-makers can evaluate the potential consequences and choose the best course of action.
- Positive Impact on Society: Ethical considerations have a positive impact on society as a whole. By following ethical practices, companies can contribute to social and environmental causes, leading to a more sustainable and equitable society.
About the author
Muhammad Hassan
Researcher, Academic Writer, Web developer
You may also like

How to Cite Research Paper – All Formats and...

Thesis Statement – Examples, Writing Guide

Chapter Summary & Overview – Writing Guide...

Dissertation vs Thesis – Key Differences

Tables in Research Paper – Types, Creating Guide...

Informed Consent in Research – Types, Templates...
Ethical considerations in research: Best practices and examples
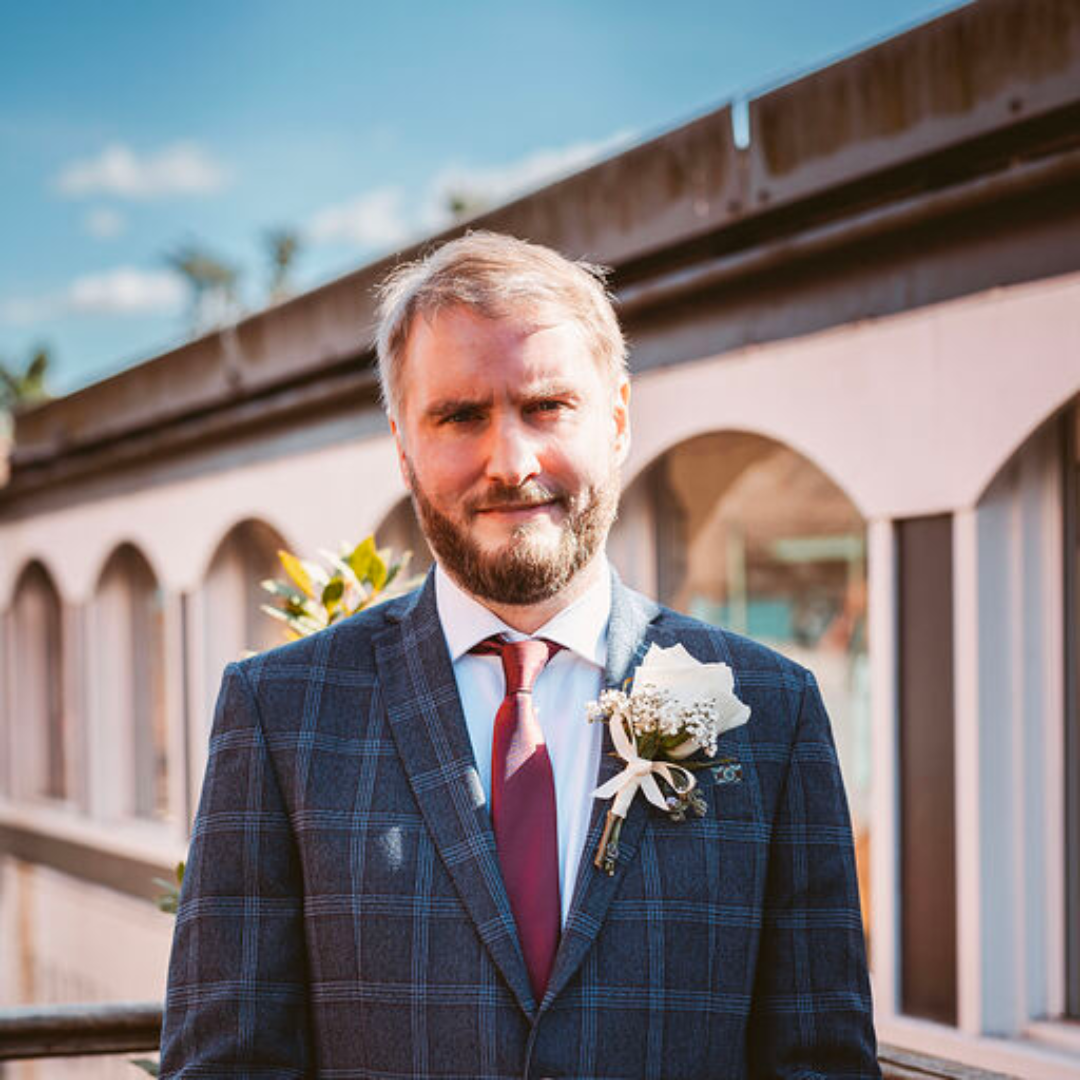
To conduct responsible research, you’ve got to think about ethics. They protect participants’ rights and their well-being - and they ensure your findings are valid and reliable. This isn’t just a box for you to tick. It’s a crucial consideration that can make all the difference to the outcome of your research.
In this article, we'll explore the meaning and importance of research ethics in today's research landscape. You'll learn best practices to conduct ethical and impactful research.
Examples of ethical considerations in research
As a researcher, you're responsible for ethical research alongside your organization. Fulfilling ethical guidelines is critical. Organizations must ensure employees follow best practices to protect participants' rights and well-being.
Keep these things in mind when it comes to ethical considerations in research:
Voluntary participation
Voluntary participation is key. Nobody should feel like they're being forced to participate or pressured into doing anything they don't want to. That means giving people a choice and the ability to opt out at any time, even if they've already agreed to take part in the study.
Informed consent
Informed consent isn't just an ethical consideration. It's a legal requirement as well. Participants must fully understand what they're agreeing to, including potential risks and benefits.
The best way to go about this is by using a consent form. Make sure you include:
- A brief description of the study and research methods.
- The potential benefits and risks of participating.
- The length of the study.
- Contact information for the researcher and/or sponsor.
- Reiteration of the participant’s right to withdraw from the research project at any time without penalty.
Anonymity means that participants aren't identifiable in any way. This includes:
- Email address
- Photographs
- Video footage
You need a way to anonymize research data so that it can't be traced back to individual participants. This may involve creating a new digital ID for participants that can’t be linked back to their original identity using numerical codes.
Confidentiality
Information gathered during a study must be kept confidential. Confidentiality helps to protect the privacy of research participants. It also ensures that their information isn't disclosed to unauthorized individuals.
Some ways to ensure confidentiality include:
- Using a secure server to store data.
- Removing identifying information from databases that contain sensitive data.
- Using a third-party company to process and manage research participant data.
- Not keeping participant records for longer than necessary.
- Avoiding discussion of research findings in public forums.
Potential for harm
The potential for harm is a crucial factor in deciding whether a research study should proceed. It can manifest in various forms, such as:
- Psychological harm
- Social harm
- Physical harm
Conduct an ethical review to identify possible harms. Be prepared to explain how you’ll minimize these harms and what support is available in case they do happen.
Fair payment
One of the most crucial aspects of setting up a research study is deciding on fair compensation for your participants. Underpayment is a common ethical issue that shouldn't be overlooked. Properly rewarding participants' time is critical for boosting engagement and obtaining high-quality data. While Prolific requires a minimum payment of £6.00 / $8.00 per hour, there are other factors you need to consider when deciding on a fair payment.
First, check your institution's reimbursement guidelines to see if they already have a minimum or maximum hourly rate. You can also use the national minimum wage as a reference point.
Next, think about the amount of work you're asking participants to do. The level of effort required for a task, such as producing a video recording versus a short survey, should correspond with the reward offered.
You also need to consider the population you're targeting. To attract research subjects with specific characteristics or high-paying jobs, you may need to offer more as an incentive.
We recommend a minimum payment of £9.00 / $12.00 per hour, but we understand that payment rates can vary depending on a range of factors. Whatever payment you choose should reflect the amount of effort participants are required to put in and be fair to everyone involved.
Ethical research made easy with Prolific
At Prolific, we believe in making ethical research easy and accessible. The findings from the Fairwork Cloudwork report speak for themselves. Prolific was given the top score out of all competitors for minimum standards of fair work.
With over 25,000 researchers in our community, we're leading the way in revolutionizing the research industry. If you're interested in learning more about how we can support your research journey, sign up to get started now.
You might also like
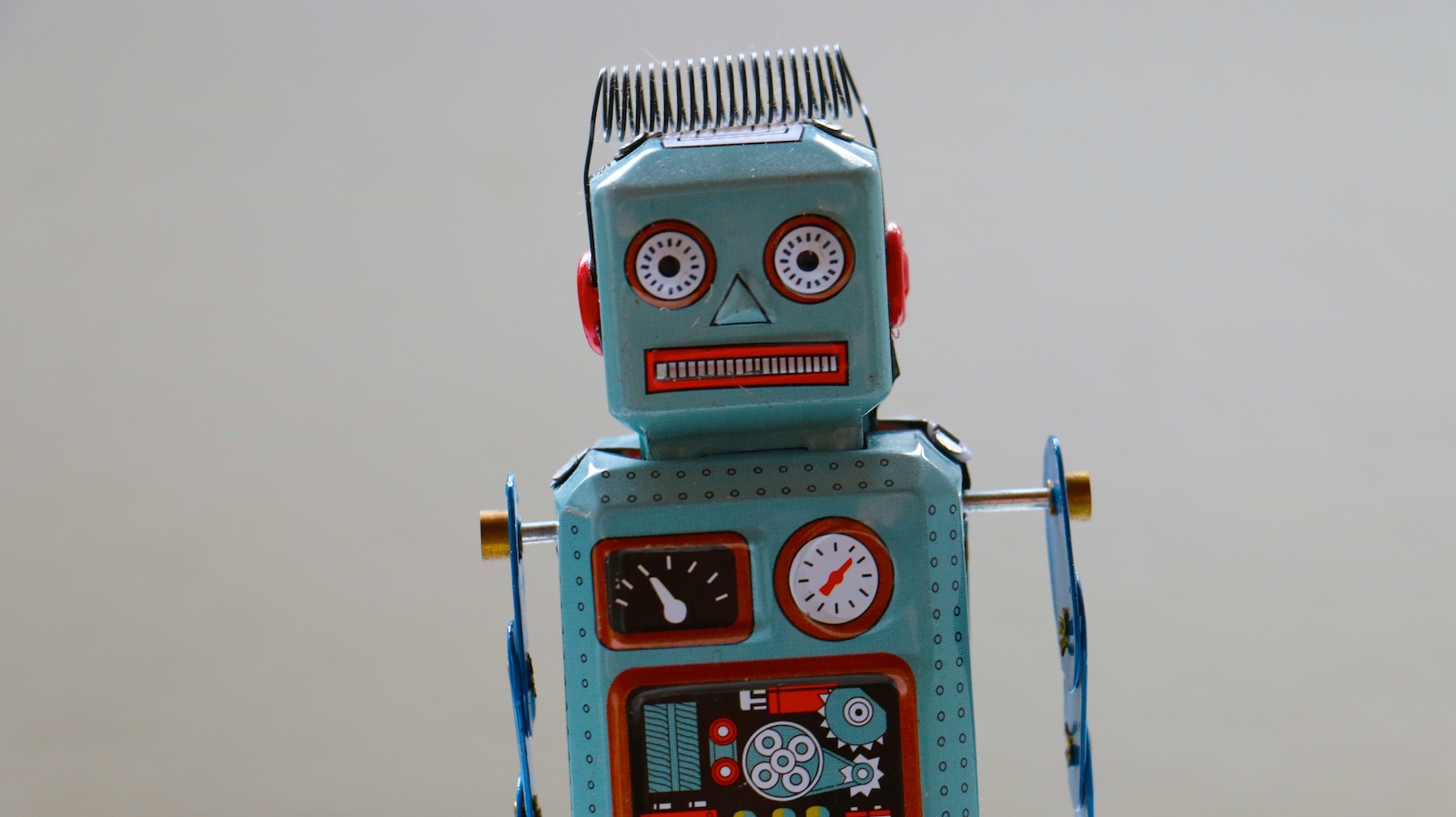
High-quality human data to deliver world-leading research and AIs.
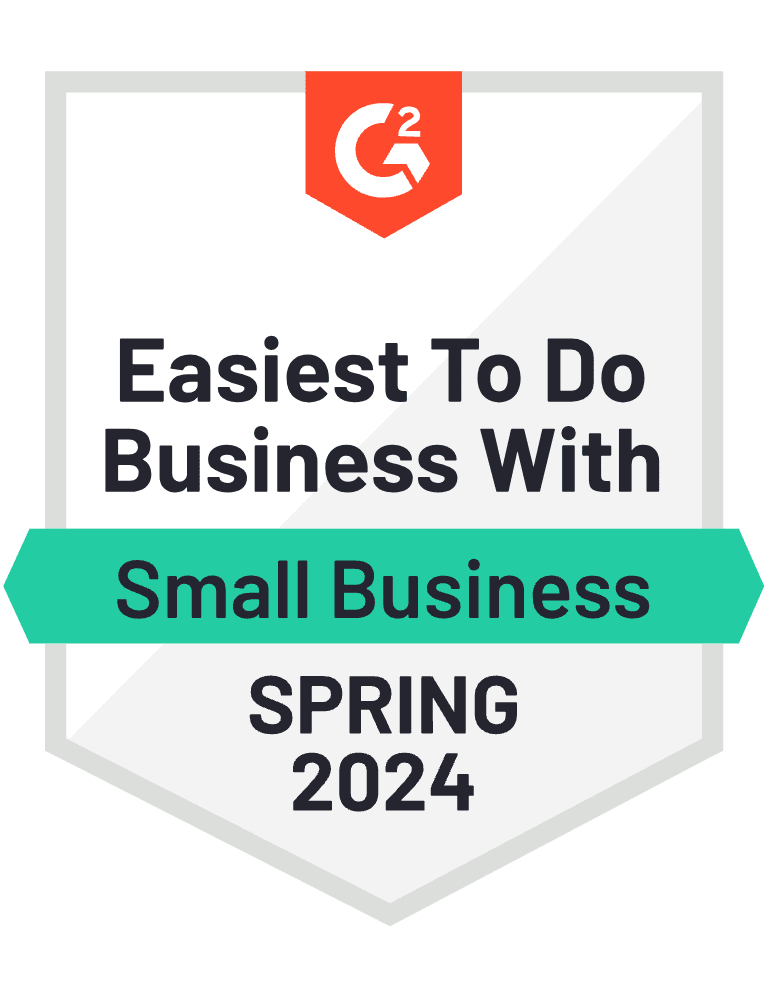
Follow us on
All Rights Reserved Prolific 2024

What Are the Ethical Considerations in Research Design?
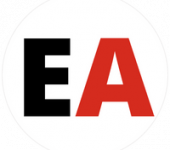
When I began my work on the thesis I was always focused on my research. However, once I began to make my way through research, I realized that research ethics is a core aspect of the research work and the foundation of research design.
Research ethics play a crucial role in ensuring the responsible conduct of research. Here are some key reasons why research ethics matter:
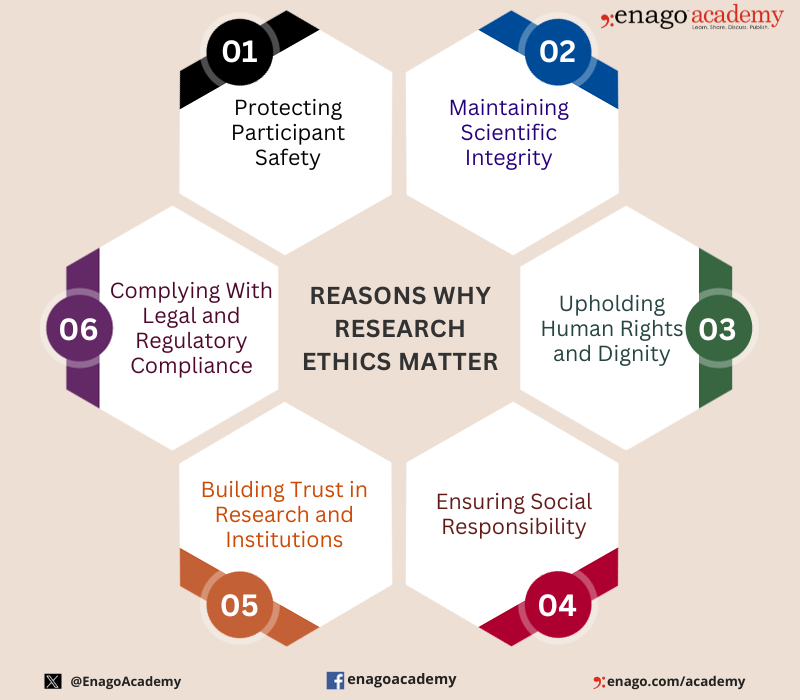
Let us look into some of the major ethical considerations in research design.
Ethical Issues in Research
There are many organizations, like the Committee on Publication Ethics , dedicated to promoting ethics in scientific research. These organizations agree that ethics is not an afterthought or side note to the research study. It is an integral aspect of research that needs to remain at the forefront of our work.
The research design must address specific research questions. Hence, the conclusions of the study must correlate to the questions posed and the results. Also, research ethics demands that the methods used must relate specifically to the research questions.
Voluntary Participation and Consent
An individual should at no point feel any coercion to participate in a study. This includes any type of persuasion or deception in attempting to gain an individual’s trust.
Informed consent states that an individual must give their explicit consent to participate in the study. You can think of consent form as an agreement of trust between the researcher and the participants.
Sampling is the first step in research design . You will need to explain why you want a particular group of participants. You will have to explain why you left out certain people or groups. In addition, if your sample includes children or special needs individuals, you will have additional requirements to address like parental permission.
Confidentiality
The third ethics principle of the Economic and Social Research Council (ESRC) states that: “The confidentiality of the information supplied by research subjects and the anonymity of respondents must be respected.” However, sometimes confidentiality is limited. For example, if a participant is at risk of harm, we must protect them. This might require releasing confidential information.
Risk of Harm
We should do everything in our power to protect study participants. For this, we should focus on the risk to benefit ratio. If possible risks outweigh the benefits, then we should abandon or redesign the study. Risk of harm also requires us to measure the risk to benefit ratio as the study progresses.
Research Methods
We know there are numerous research methods. However, when it comes to ethical considerations, some key questions can help us find the right approach for our studies.
i. Which methods most effectively fit the aims of your research?
ii. What are the strengths and restrictions of a particular method?
iii. Are there potential risks when using a particular research method?
For more guidance, you can refer to the ESRC Framework for Research Ethics .
Ethical issues in research can arise at various stages of the research process and involve different aspects of the study. Here are some common examples of ethical issues in research:
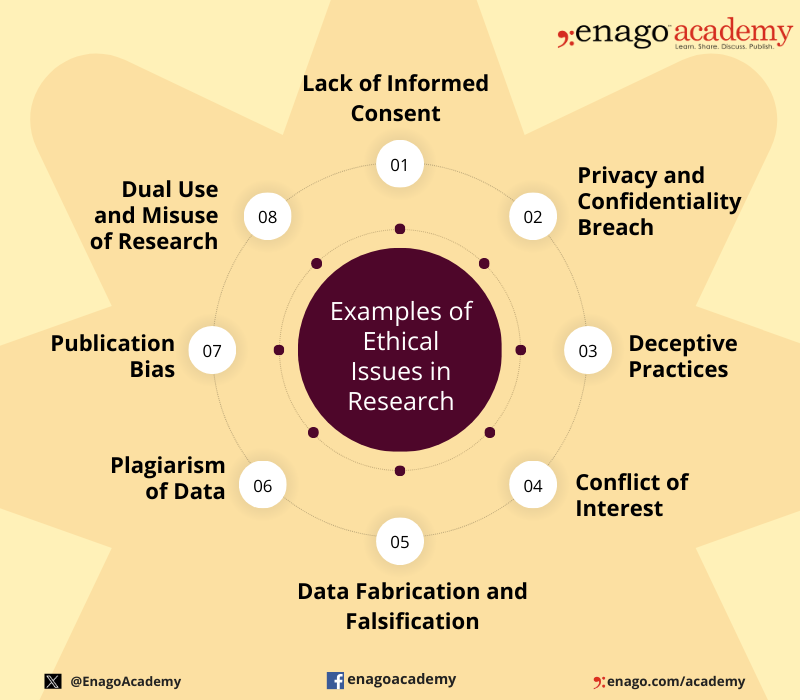
Institutional Review Boards
The importance of ethics in research cannot be understated. Following ethical guidelines will ensure your study’s validity and promote its contribution to scientific study. On a personal level, you will strengthen your research and increase your opportunities to gain funding.
To address the need for ethical considerations, most institutions have their own Institutional Review Board (IRB). An IRB secures the safety of human participants and prevents violation of human rights. It reviews the research aims and methodologies to ensure ethical practices are followed. If a research design does not follow the set ethical guidelines, then the researcher will have to amend their study.
Applying for Ethical Approval
Applications for ethical approval will differ across institutions. Regardless, they focus on the benefits of your research and the risk to benefit ratio concerning participants. Therefore, you need to effectively address both in order to get ethical clearence.
Participants
It is vital that you make it clear that individuals are provided with sufficient information in order to make an informed decision on their participation. In addition, you need to demonstrate that the ethical issues of consent, risk of harm, and confidentiality are clearly defined.
Benefits of the Study
You need to prove to the panel that your work is essential and will yield results that contribute to the scientific community. For this, you should demonstrate the following:
i. The conduct of research guarantees the quality and integrity of results.
ii. The research will be properly distributed.
iii. The aims of the research are clear and the methodology is appropriate.
Integrity and transparency are vital in the research. Ethics committees expect you to share any actual or potential conflicts of interest that could affect your work. In addition, you have to be honest and transparent throughout the approval process and the research process.
The Dangers of Unethical Practices
There is a reason to follow ethical guidelines. Without these guidelines, our research will suffer. Moreover, more importantly, people could suffer.
The following are just two examples of infamous cases of unethical research practices that demonstrate the importance of adhering to ethical standards:
- The Stanford Prison Experiment (1971) aimed to investigate the psychological effects of power using the relationship between prisoners and prison officers. Those assigned the role of “prison officers” embraced measures that exposed “prisoners” to psychological and physical harm. In this case, there was voluntary participation. However, there was disregard for welfare of the participants.
- Recently, Chinese scientist He Jiankui announced his work on genetically edited babies . Over 100 Chinese scientists denounced this research, calling it “crazy” and “shocking and unacceptable.” This research shows a troubling attitude of “do first, debate later” and a disregard for the ethical concerns of manipulating the human body Wang Yuedan, a professor of immunology at Peking University, calls this “an ethics disaster for the world” and demands strict punishments for this type of ethics violation.
What are your experiences with research ethics? How have you developed an ethical approach to research design? Please share your thoughts with us in the comments section below.

I love the articulation of reasoning and practical examples of unethical research
Rate this article Cancel Reply
Your email address will not be published.
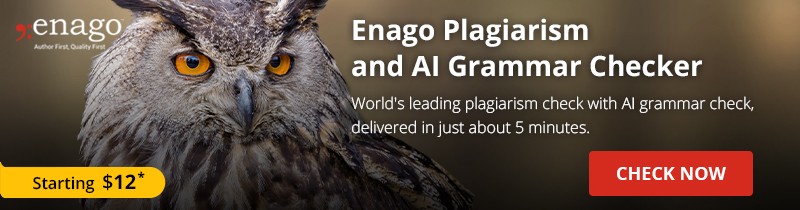
Enago Academy's Most Popular Articles
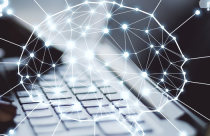
- AI in Academia
- Trending Now
6 Leading AI Detection Tools for Academic Writing — A comparative analysis
The advent of AI content generators, exemplified by advanced models like ChatGPT, Claude AI, and…
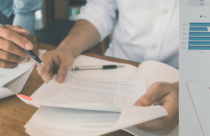
- Reporting Research
Choosing the Right Analytical Approach: Thematic analysis vs. content analysis for data interpretation
In research, choosing the right approach to understand data is crucial for deriving meaningful insights.…
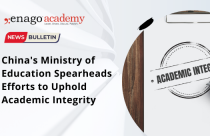
- Industry News
China’s Ministry of Education Spearheads Efforts to Uphold Academic Integrity
In response to the increase in retractions of research papers submitted by Chinese scholars to…
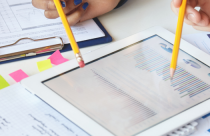
Comparing Cross Sectional and Longitudinal Studies: 5 steps for choosing the right approach
The process of choosing the right research design can put ourselves at the crossroads of…
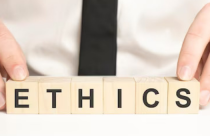
- Publishing Research
- Understanding Ethics
Understanding the Difference Between Research Ethics and Compliance
Ethics refers to the principles, values, and moral guidelines that guide individual or group behavior…
Unlocking the Power of Networking in Academic Conferences
Intersectionality in Academia: Dealing with diverse perspectives
Meritocracy and Diversity in Science: Increasing inclusivity in STEM education
Avoiding the AI Trap: Pitfalls of relying on ChatGPT for PhD applications

Sign-up to read more
Subscribe for free to get unrestricted access to all our resources on research writing and academic publishing including:
- 2000+ blog articles
- 50+ Webinars
- 10+ Expert podcasts
- 50+ Infographics
- 10+ Checklists
- Research Guides
We hate spam too. We promise to protect your privacy and never spam you.
I am looking for Editing/ Proofreading services for my manuscript Tentative date of next journal submission:
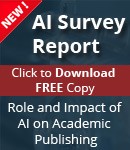
As a researcher, what do you consider most when choosing an image manipulation detector?
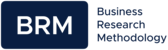
Ethical Considerations
Ethical Considerations can be specified as one of the most important parts of the research. Dissertations may even be doomed to failure if this part is missing.
According to Bryman and Bell (2007) [1] the following ten points represent the most important principles related to ethical considerations in dissertations:
- Research participants should not be subjected to harm in any ways whatsoever.
- Respect for the dignity of research participants should be prioritised.
- Full consent should be obtained from the participants prior to the study.
- The protection of the privacy of research participants has to be ensured.
- Adequate level of confidentiality of the research data should be ensured.
- Anonymity of individuals and organisations participating in the research has to be ensured.
- Any deception or exaggeration about the aims and objectives of the research must be avoided.
- Affiliations in any forms, sources of funding, as well as any possible conflicts of interests have to be declared.
- Any type of communication in relation to the research should be done with honesty and transparency.
- Any type of misleading information, as well as representation of primary data findings in a biased way must be avoided.
In order to address ethical considerations aspect of your dissertation in an effective manner, you will need to expand discussions of each of the following points to at least one paragraph:
1. Voluntary participation of respondents in the research is important. Moreover, participants have rights to withdraw from the study at any stage if they wish to do so.
2. Respondents should participate on the basis of informed consent. The principle of informed consent involves researchers providing sufficient information and assurances about taking part to allow individuals to understand the implications of participation and to reach a fully informed, considered and freely given decision about whether or not to do so, without the exercise of any pressure or coercion. [2]
3. The use of offensive, discriminatory, or other unacceptable language needs to be avoided in the formulation of Questionnaire/Interview/Focus group questions.
4. Privacy and anonymity or respondents is of a paramount importance.
5. Acknowledgement of works of other authors used in any part of the dissertation with the use of Harvard/APA/Vancouver referencing system according to the Dissertation Handbook
6. Maintenance of the highest level of objectivity in discussions and analyses throughout the research
7. Adherence to Data Protection Act (1998) if you are studying in the UK
In studies that do not involve primary data collection, on the other hand, ethical issues are going to be limited to the points d) and e) above.
Most universities have their own Code of Ethical Practice. It is critically important for you to thoroughly adhere to this code in every aspect of your research and declare your adherence in ethical considerations part of your dissertation.
My e-book, The Ultimate Guide to Writing a Dissertation in Business Studies: a step by step assistance offers practical assistance to complete a dissertation with minimum or no stress. The e-book covers all stages of writing a dissertation starting from the selection to the research area to submitting the completed version of the work within the deadline. John Dudovskiy
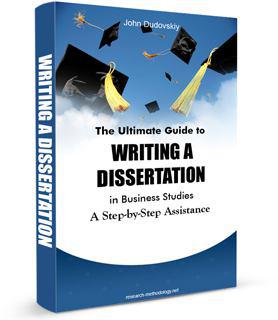
[1] Bryman, A. & Bell, E. (2007) “Business Research Methods”, 2nd edition. Oxford University Press.
[2] Saunders, M., Lewis, P. & Thornhill, A. (2012) “Research Methods for Business Students” 6th edition, Pearson Education Limited.
- U.S. Department of Health & Human Services

- Virtual Tour
- Staff Directory
- En Español
You are here
Nih clinical research trials and you, guiding principles for ethical research.
Pursuing Potential Research Participants Protections

“When people are invited to participate in research, there is a strong belief that it should be their choice based on their understanding of what the study is about, and what the risks and benefits of the study are,” said Dr. Christine Grady, chief of the NIH Clinical Center Department of Bioethics, to Clinical Center Radio in a podcast.
Clinical research advances the understanding of science and promotes human health. However, it is important to remember the individuals who volunteer to participate in research. There are precautions researchers can take – in the planning, implementation and follow-up of studies – to protect these participants in research. Ethical guidelines are established for clinical research to protect patient volunteers and to preserve the integrity of the science.
NIH Clinical Center researchers published seven main principles to guide the conduct of ethical research:
Social and clinical value
Scientific validity, fair subject selection, favorable risk-benefit ratio, independent review, informed consent.
- Respect for potential and enrolled subjects
Every research study is designed to answer a specific question. The answer should be important enough to justify asking people to accept some risk or inconvenience for others. In other words, answers to the research question should contribute to scientific understanding of health or improve our ways of preventing, treating, or caring for people with a given disease to justify exposing participants to the risk and burden of research.
A study should be designed in a way that will get an understandable answer to the important research question. This includes considering whether the question asked is answerable, whether the research methods are valid and feasible, and whether the study is designed with accepted principles, clear methods, and reliable practices. Invalid research is unethical because it is a waste of resources and exposes people to risk for no purpose
The primary basis for recruiting participants should be the scientific goals of the study — not vulnerability, privilege, or other unrelated factors. Participants who accept the risks of research should be in a position to enjoy its benefits. Specific groups of participants (for example, women or children) should not be excluded from the research opportunities without a good scientific reason or a particular susceptibility to risk.
Uncertainty about the degree of risks and benefits associated with a clinical research study is inherent. Research risks may be trivial or serious, transient or long-term. Risks can be physical, psychological, economic, or social. Everything should be done to minimize the risks and inconvenience to research participants to maximize the potential benefits, and to determine that the potential benefits are proportionate to, or outweigh, the risks.
To minimize potential conflicts of interest and make sure a study is ethically acceptable before it starts, an independent review panel should review the proposal and ask important questions, including: Are those conducting the trial sufficiently free of bias? Is the study doing all it can to protect research participants? Has the trial been ethically designed and is the risk–benefit ratio favorable? The panel also monitors a study while it is ongoing.
Potential participants should make their own decision about whether they want to participate or continue participating in research. This is done through a process of informed consent in which individuals (1) are accurately informed of the purpose, methods, risks, benefits, and alternatives to the research, (2) understand this information and how it relates to their own clinical situation or interests, and (3) make a voluntary decision about whether to participate.
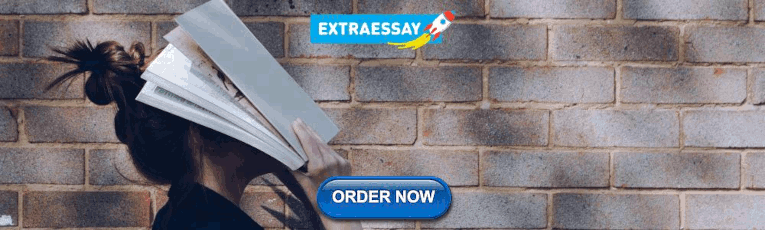
Respect for potential and enrolled participants
Individuals should be treated with respect from the time they are approached for possible participation — even if they refuse enrollment in a study — throughout their participation and after their participation ends. This includes:
- respecting their privacy and keeping their private information confidential
- respecting their right to change their mind, to decide that the research does not match their interests, and to withdraw without a penalty
- informing them of new information that might emerge in the course of research, which might change their assessment of the risks and benefits of participating
- monitoring their welfare and, if they experience adverse reactions, unexpected effects, or changes in clinical status, ensuring appropriate treatment and, when necessary, removal from the study
- informing them about what was learned from the research
More information on these seven guiding principles and on bioethics in general
This page last reviewed on March 16, 2016
Connect with Us
- More Social Media from NIH
Ethical consideration of the research proposal and the informed-consent process: An online survey of researchers and ethics committee members in Thailand
Affiliations.
- 1 a Department of Tropical Hygiene, Faculty of Tropical Medicine , Mahidol University , Bangkok , Thailand.
- 2 b Office of Research Services, Faculty of Tropical Medicine , Mahidol University , Bangkok , Thailand.
- PMID: 30987450
- DOI: 10.1080/08989621.2019.1608190
Researchers designing and conducting studies using human data should consider the values and principles of ethical conduct. Research ethics committees (RECs) typically evaluate the ethical acceptability of research proposals. Sometimes, differences arise between how researchers and RECs interpret ethical principles, and how they decide what constitutes ethical conduct. This study aimed to explore the opinions of these two groups about the importance of core ethical issues in the proposal and in the informed-consent process. An anonymous online questionnaire was distributed to a target population in health-related academic/research institutes across Thailand; 219 researchers and 72 REC members participated. Significantly, more REC members than researchers attributed the highest importance to three core ethical considerations - risk/benefit, vulnerability, and confidentiality/privacy. For the informed-consent process, significant differences were found for communication of risks, decision-making authority for consent, process for approaching study participants, and availability of a contact for study deviations/violations. The different ratings indicate differences in the groups' perspectives on ethical principles, which may affect focal congruence on ethical issues in the proposal. Communication of these findings should help close gaps between REC and researcher perceptions. Further study should investigate how RECs and researchers perceive equivocal ethics terms.
Keywords: Ethical consideration; ethics committee; informed-consent form; informed-consent process; perception.
- Committee Membership*
- Ethics Committees, Research*
- Informed Consent / ethics*
- Research Design
- Research Personnel / psychology*
- Surveys and Questionnaires
Have a language expert improve your writing
Run a free plagiarism check in 10 minutes, automatically generate references for free.
- Knowledge Base
- Methodology
- Ethical Considerations in Research | Types & Examples
Ethical Considerations in Research | Types & Examples
Published on 7 May 2022 by Pritha Bhandari .
Ethical considerations in research are a set of principles that guide your research designs and practices. Scientists and researchers must always adhere to a certain code of conduct when collecting data from people.
The goals of human research often include understanding real-life phenomena, studying effective treatments, investigating behaviours, and improving lives in other ways. What you decide to research and how you conduct that research involve key ethical considerations.
These considerations work to:
- Protect the rights of research participants
- Enhance research validity
- Maintain scientific integrity
Table of contents
Why do research ethics matter, getting ethical approval for your study, types of ethical issues, voluntary participation, informed consent, confidentiality, potential for harm, results communication, examples of ethical failures, frequently asked questions about research ethics.
Research ethics matter for scientific integrity, human rights and dignity, and collaboration between science and society. These principles make sure that participation in studies is voluntary, informed, and safe for research subjects.
You’ll balance pursuing important research aims with using ethical research methods and procedures. It’s always necessary to prevent permanent or excessive harm to participants, whether inadvertent or not.
Defying research ethics will also lower the credibility of your research because it’s hard for others to trust your data if your methods are morally questionable.
Even if a research idea is valuable to society, it doesn’t justify violating the human rights or dignity of your study participants.
Prevent plagiarism, run a free check.
Before you start any study involving data collection with people, you’ll submit your research proposal to an institutional review board (IRB) .
An IRB is a committee that checks whether your research aims and research design are ethically acceptable and follow your institution’s code of conduct. They check that your research materials and procedures are up to code.
If successful, you’ll receive IRB approval, and you can begin collecting data according to the approved procedures. If you want to make any changes to your procedures or materials, you’ll need to submit a modification application to the IRB for approval.
If unsuccessful, you may be asked to re-submit with modifications or your research proposal may receive a rejection. To get IRB approval, it’s important to explicitly note how you’ll tackle each of the ethical issues that may arise in your study.
There are several ethical issues you should always pay attention to in your research design, and these issues can overlap with each other.
You’ll usually outline ways you’ll deal with each issue in your research proposal if you plan to collect data from participants.
Voluntary participation means that all research subjects are free to choose to participate without any pressure or coercion.
All participants are able to withdraw from, or leave, the study at any point without feeling an obligation to continue. Your participants don’t need to provide a reason for leaving the study.
It’s important to make it clear to participants that there are no negative consequences or repercussions to their refusal to participate. After all, they’re taking the time to help you in the research process, so you should respect their decisions without trying to change their minds.
Voluntary participation is an ethical principle protected by international law and many scientific codes of conduct.
Take special care to ensure there’s no pressure on participants when you’re working with vulnerable groups of people who may find it hard to stop the study even when they want to.
Informed consent refers to a situation in which all potential participants receive and understand all the information they need to decide whether they want to participate. This includes information about the study’s benefits, risks, funding, and institutional approval.
- What the study is about
- The risks and benefits of taking part
- How long the study will take
- Your supervisor’s contact information and the institution’s approval number
Usually, you’ll provide participants with a text for them to read and ask them if they have any questions. If they agree to participate, they can sign or initial the consent form. Note that this may not be sufficient for informed consent when you work with particularly vulnerable groups of people.
If you’re collecting data from people with low literacy, make sure to verbally explain the consent form to them before they agree to participate.
For participants with very limited English proficiency, you should always translate the study materials or work with an interpreter so they have all the information in their first language.
In research with children, you’ll often need informed permission for their participation from their parents or guardians. Although children cannot give informed consent, it’s best to also ask for their assent (agreement) to participate, depending on their age and maturity level.
Anonymity means that you don’t know who the participants are and you can’t link any individual participant to their data.
You can only guarantee anonymity by not collecting any personally identifying information – for example, names, phone numbers, email addresses, IP addresses, physical characteristics, photos, and videos.
In many cases, it may be impossible to truly anonymise data collection. For example, data collected in person or by phone cannot be considered fully anonymous because some personal identifiers (demographic information or phone numbers) are impossible to hide.
You’ll also need to collect some identifying information if you give your participants the option to withdraw their data at a later stage.
Data pseudonymisation is an alternative method where you replace identifying information about participants with pseudonymous, or fake, identifiers. The data can still be linked to participants, but it’s harder to do so because you separate personal information from the study data.
Confidentiality means that you know who the participants are, but you remove all identifying information from your report.
All participants have a right to privacy, so you should protect their personal data for as long as you store or use it. Even when you can’t collect data anonymously, you should secure confidentiality whenever you can.
Some research designs aren’t conducive to confidentiality, but it’s important to make all attempts and inform participants of the risks involved.
As a researcher, you have to consider all possible sources of harm to participants. Harm can come in many different forms.
- Psychological harm: Sensitive questions or tasks may trigger negative emotions such as shame or anxiety.
- Social harm: Participation can involve social risks, public embarrassment, or stigma.
- Physical harm: Pain or injury can result from the study procedures.
- Legal harm: Reporting sensitive data could lead to legal risks or a breach of privacy.
It’s best to consider every possible source of harm in your study, as well as concrete ways to mitigate them. Involve your supervisor to discuss steps for harm reduction.
Make sure to disclose all possible risks of harm to participants before the study to get informed consent. If there is a risk of harm, prepare to provide participants with resources, counselling, or medical services if needed.
Some of these questions may bring up negative emotions, so you inform participants about the sensitive nature of the survey and assure them that their responses will be confidential.
The way you communicate your research results can sometimes involve ethical issues. Good science communication is honest, reliable, and credible. It’s best to make your results as transparent as possible.
Take steps to actively avoid plagiarism and research misconduct wherever possible.
Plagiarism means submitting others’ works as your own. Although it can be unintentional, copying someone else’s work without proper credit amounts to stealing. It’s an ethical problem in research communication because you may benefit by harming other researchers.
Self-plagiarism is when you republish or re-submit parts of your own papers or reports without properly citing your original work.
This is problematic because you may benefit from presenting your ideas as new and original even though they’ve already been published elsewhere in the past. You may also be infringing on your previous publisher’s copyright, violating an ethical code, or wasting time and resources by doing so.
In extreme cases of self-plagiarism, entire datasets or papers are sometimes duplicated. These are major ethical violations because they can skew research findings if taken as original data.
You notice that two published studies have similar characteristics even though they are from different years. Their sample sizes, locations, treatments, and results are highly similar, and the studies share one author in common.
Research misconduct
Research misconduct means making up or falsifying data, manipulating data analyses, or misrepresenting results in research reports. It’s a form of academic fraud.
These actions are committed intentionally and can have serious consequences; research misconduct is not a simple mistake or a point of disagreement about data analyses.
Research misconduct is a serious ethical issue because it can undermine scientific integrity and institutional credibility. It leads to a waste of funding and resources that could have been used for alternative research.
Later investigations revealed that they fabricated and manipulated their data to show a nonexistent link between vaccines and autism. Wakefield also neglected to disclose important conflicts of interest, and his medical license was taken away.
This fraudulent work sparked vaccine hesitancy among parents and caregivers. The rate of MMR vaccinations in children fell sharply, and measles outbreaks became more common due to a lack of herd immunity.
Research scandals with ethical failures are littered throughout history, but some took place not that long ago.
Some scientists in positions of power have historically mistreated or even abused research participants to investigate research problems at any cost. These participants were prisoners, under their care, or otherwise trusted them to treat them with dignity.
To demonstrate the importance of research ethics, we’ll briefly review two research studies that violated human rights in modern history.
These experiments were inhumane and resulted in trauma, permanent disabilities, or death in many cases.
After some Nazi doctors were put on trial for their crimes, the Nuremberg Code of research ethics for human experimentation was developed in 1947 to establish a new standard for human experimentation in medical research.
In reality, the actual goal was to study the effects of the disease when left untreated, and the researchers never informed participants about their diagnoses or the research aims.
Although participants experienced severe health problems, including blindness and other complications, the researchers only pretended to provide medical care.
When treatment became possible in 1943, 11 years after the study began, none of the participants were offered it, despite their health conditions and high risk of death.
Ethical failures like these resulted in severe harm to participants, wasted resources, and lower trust in science and scientists. This is why all research institutions have strict ethical guidelines for performing research.
Ethical considerations in research are a set of principles that guide your research designs and practices. These principles include voluntary participation, informed consent, anonymity, confidentiality, potential for harm, and results communication.
Scientists and researchers must always adhere to a certain code of conduct when collecting data from others .
These considerations protect the rights of research participants, enhance research validity , and maintain scientific integrity.
Research ethics matter for scientific integrity, human rights and dignity, and collaboration between science and society. These principles make sure that participation in studies is voluntary, informed, and safe.
Anonymity means you don’t know who the participants are, while confidentiality means you know who they are but remove identifying information from your research report. Both are important ethical considerations .
You can only guarantee anonymity by not collecting any personally identifying information – for example, names, phone numbers, email addresses, IP addresses, physical characteristics, photos, or videos.
You can keep data confidential by using aggregate information in your research report, so that you only refer to groups of participants rather than individuals.
These actions are committed intentionally and can have serious consequences; research misconduct is not a simple mistake or a point of disagreement but a serious ethical failure.
Cite this Scribbr article
If you want to cite this source, you can copy and paste the citation or click the ‘Cite this Scribbr article’ button to automatically add the citation to our free Reference Generator.
Bhandari, P. (2022, May 07). Ethical Considerations in Research | Types & Examples. Scribbr. Retrieved 7 June 2024, from https://www.scribbr.co.uk/research-methods/ethical-considerations/
Is this article helpful?
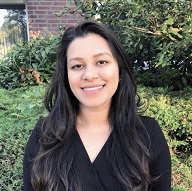
Pritha Bhandari
Other students also liked, a quick guide to experimental design | 5 steps & examples, data collection methods | step-by-step guide & examples, how to avoid plagiarism | tips on citing sources.

An official website of the United States government
The .gov means it’s official. Federal government websites often end in .gov or .mil. Before sharing sensitive information, make sure you’re on a federal government site.
The site is secure. The https:// ensures that you are connecting to the official website and that any information you provide is encrypted and transmitted securely.
- Publications
- Account settings
Preview improvements coming to the PMC website in October 2024. Learn More or Try it out now .
- Advanced Search
- Journal List
- Indian J Anaesth
- v.60(9); 2016 Sep
Legal and ethical issues in research
Camille yip.
1 Department of Women's Anaesthesia, KK Women's and Children's Hospital, Bukit Timah, Singapore
Nian-Lin Reena Han
2 Division of Clinical Support Services, KK Women's and Children's Hospital, Bukit Timah, Singapore
Ban Leong Sng
3 Anesthesiology and Perioperative Sciences Academic Clinical Program, Duke-NUS Medical School, Singapore
Legal and ethical issues form an important component of modern research, related to the subject and researcher. This article seeks to briefly review the various international guidelines and regulations that exist on issues related to informed consent, confidentiality, providing incentives and various forms of research misconduct. Relevant original publications (The Declaration of Helsinki, Belmont Report, Council for International Organisations of Medical Sciences/World Health Organisation International Guidelines for Biomedical Research Involving Human Subjects, World Association of Medical Editors Recommendations on Publication Ethics Policies, International Committee of Medical Journal Editors, CoSE White Paper, International Conference on Harmonisation of Technical Requirements for Registration of Pharmaceuticals for Human Use-Good Clinical Practice) form the literature that are relevant to the ethical and legal aspects of conducting research that researchers should abide by when conducting translational and clinical research. Researchers should note the major international guidelines and regional differences in legislation. Hence, specific ethical advice should be sought at local Ethics Review Committees.
INTRODUCTION
The ethical and legal issues relating to the conduct of clinical research involving human participants had raised the concerns of policy makers, lawyers, scientists and clinicians for many years. The Declaration of Helsinki established ethical principles applied to clinical research involving human participants. The purpose of a clinical research is to systematically collect and analyse data from which conclusions are drawn, that may be generalisable, so as to improve the clinical practice and benefit patients in future. Therefore, it is important to be familiar with Good Clinical Practice (GCP), an international quality standard that is provided by the International Conference on Harmonisation of Technical Requirements for Registration of Pharmaceuticals for Human Use (ICH),[ 1 ] or the local version, GCP of the Central Drugs Standard Control Organization (India's equivalent of US Food and Drug Administration)[ 2 ] and local regulatory policy to ensure that the research is conducted both ethically and legally. In this article, we will briefly review the legal and ethical issues pertaining to recruitment of human subjects, basic principles of informed consent and precautions to be taken during data and clinical research publications. Some of the core principles of GCP in research include defining responsibilities of sponsors, investigators, consent process monitoring and auditing procedures and protection of human subjects.[ 3 ]
ISSUES RELATED TO THE RESEARCH PARTICIPANTS
The main role of human participants in research is to serve as sources of data. Researchers have a duty to ‘protect the life, health, dignity, integrity, right to self-determination, privacy and confidentiality of personal information of research subjects’.[ 4 ] The Belmont Report also provides an analytical framework for evaluating research using three ethical principles:[ 5 ]
- Respect for persons – the requirement to acknowledge autonomy and protect those with diminished autonomy
- Beneficence – first do no harm, maximise possible benefits and minimise possible harms
- Justice – on individual and societal level.
Mistreatment of research subjects is considered research misconduct (no ethical review approval, failure to follow approved protocol, absent or inadequate informed consent, exposure of subjects to physical or psychological harm, exposure of subjects to harm due to unacceptable research practices or failure to maintain confidentiality).[ 6 ] There is also scientific misconduct involving fraud and deception.
Consent, possibility of causing harm
Based on ICH definition, ‘informed consent is a process by which a subject voluntarily confirms his or her willingness to participate in a particular trial, after having been informed of all aspects of the trial that are relevant to the subject's decision to participate’. As for a standard (therapeutic) intervention that carries certain risks, informed consent – that is voluntary, given freely and adequately informed – must be sought from participants. However, due to the research-centred, rather than patient-centred primary purpose, additional relevant information must be provided in clinical trials or research studies in informed consent form. The essential components of informed consent are listed in Table 1 [Adapted from ICH Harmonised Tripartite Guideline, Guideline for Good Clinical Practice E6(R1)].[ 1 ] This information should be delivered in the language and method that individual potential subjects can understand,[ 4 ] commonly in the form of a printed Participant Information Sheet. Informed consent is documented by means of written, signed and dated informed consent form.[ 1 ] The potential subjects must be informed of the right to refuse to participate or withdraw consent to participate at any time without reprisal and without affecting the patient–physician relationship. There are also general principles regarding risk assessment, scientific requirements, research protocols and registration, function of ethics committees, use of placebo, post-trial provisions and research publication.[ 4 ]
Essential components of an informed consent

Special populations
Informed consent may be sought from a legally authorised representative if a potential research subject is incapable of giving informed consent[ 4 ] (children, intellectual impairment). The involvement of such populations must fulfil the requirement that they stand to benefit from the research outcome.[ 4 ] The ‘legally authorised representative’ may be a spouse, close relative, parent, power of attorney or legally appointed guardian. The hierarchy of priority of the representative may be different between different countries and different regions within the same country; hence, local guidelines should be consulted.
Special case: Emergency research
Emergency research studies occur where potential subjects are incapacitated and unable to give informed consent (acute head trauma, cardiac arrest). The Council for International Organisations of Medical Sciences/World Health Organisation guidelines and Declaration of Helsinki make exceptions to the requirement for informed consent in these situations.[ 4 , 7 ] There are minor variations in laws governing the extent to which the exceptions apply.[ 8 ]
Reasonable efforts should have been made to find a legal authority to consent. If there is not enough time, an ‘exception to informed consent’ may allow the subject to be enrolled with prior approval of an ethical committee.[ 7 ] Researchers must obtain deferred informed consent as soon as possible from the subject (when regains capacity), or their legally authorised representative, for continued participation.[ 4 , 7 ]
Collecting patient information and sensitive personal information, confidentiality maintenance
The Health Insurance Portability and Accountability Act has requirements for informed consent disclosure and standards for electronic exchange, privacy and information security. In the UK, generic legislation is found in the Data Protection Act.[ 9 ]
The International Committee of Medical Journal Editors (ICMJE) recommendations suggest that authors must ensure that non-essential identifying information (names, initials, hospital record numbers) are omitted during data collection and storage wherever possible. Where identifying information is essential for scientific purposes (clinical photographs), written informed consent must be obtained and the patient must be shown the manuscript before publication. Subjects should also be informed if any potential identifiable material might be available through media access.
Providing incentives
Cash or other benefits ‘in-kind’ (financial, medical, educational, community benefits) should be made known to subjects when obtaining informed consent without emphasising too much on it.[ 7 ] Benefits may serve as appreciation or compensation for time and effort but should not result in the inducement to participation.[ 10 ] The amount and nature of remuneration should be compared to norms, cultural traditions and are subjected to the Ethical Committee Review.[ 7 ]
ISSUES RELATED TO THE RESEARCHER
Legal issues pertaining to regulatory bodies.
Various regulatory bodies have been constituted to uphold the safety of subjects involved in research. It is imperative to obtain approval from the appropriate regulatory authorities before proceeding to any research. The constitution and the types of these bodies vary nation-wise. The researchers are expected to be aware of these authorities and the list of various bodies pertinent to India are listed in the article “Research methodology II” of this issue.
Avoiding bias, inappropriate research methodology, incorrect reporting and inappropriate use of information
Good, well-designed studies advance medical science development. Poorly conducted studies violate the principle of justice, as there are time and resources wastage for research sponsors, researchers and subjects, and undermine the societal trust on scientific enquiry.[ 11 ] The Guidelines for GCP is an international ethical and scientific quality standard for designing, conducting, recording and reporting trials.[ 1 ]
Fraud in research and publication
De novo data invention (fabrication) and manipulation of data (falsification)[ 6 ] constitute serious scientific misconduct. The true prevalence of scientific fraud is difficult to measure (2%–14%).[ 12 ]
Plagiarism and its checking
Plagiarism is the use of others' published and unpublished ideas or intellectual property without attribution or permission and presenting them as new and original rather than derived from an existing source.[ 13 ] Tools such as similarity check[ 14 ] are available to aid researchers detect similarities between manuscripts, and such checks should be done before submission.[ 15 ]
Overlapping publications
Duplicate publications violate international copyright laws and waste valuable resources.[ 16 , 17 ] Such publications can distort evidence-based medicine by double-counting of data when inadvertently included in meta-analyses.[ 16 ] This practice could artificially enlarge one's scientific work, distorting apparent productivity and may give an undue advantage when competing for research funding or career advancement.[ 17 ] Examples of these practices include:
Duplicate publication, redundant publication
Publication of a paper that overlaps substantially with one already published, without reference to the previous publication.[ 11 ]
Salami publication
Slicing of data from a single research process into different pieces creating individual manuscripts from each piece to artificially increase the publication volume.[ 16 ]
Such misconduct may lead to retraction of articles. Transparent disclosure is important when submitting papers to journals to declare if the manuscript or related material has been published or submitted elsewhere, so that the editor can decide how to handle the submission or to seek further clarification. Further information on acceptable secondary publication can be found in the ICMJE ‘Recommendations for the Conduct, Reporting, Editing, and Publishing of Scholarly Work in Medical Journals’.
Usually, sponsors and authors are required to sign over certain publication rights to the journal through copyright transfer or a licensing agreement; thereafter, authors should obtain written permission from the journal/publisher if they wish to reuse the published material elsewhere.[ 6 ]
Authorship and its various associations
The ICMJE recommendation lists four criteria of authorship:
- Substantial contributions to the conception of design of the work, or the acquisition, analysis or interpretation of data for the work
- Drafting the work or revising it critically for important intellectual content
- Final approval of the version to be published
- Agreement to be accountable for all aspects of the work in ensuring that questions related to the accuracy or integrity of any part of the work are appropriately investigated and resolved.
Authors and researchers have an ethical obligation to ensure the accuracy, publication and dissemination of the result of research,[ 4 ] as well as disclosing to publishers relevant corrections, retractions and errata, to protect scientific integrity of published evidence. Every research study involving human subjects must be registered in a publicly accessible database (e.g., ANZCTR [Australia and NZ], ClinicalTrials.gov [US and non-US], CTRI [India]) and the results made publicly available.[ 4 ] Sponsors of clinical trials must allow all study investigators and manuscript authors access to the full study data set and the right to use all study data for publication.[ 5 ] Source documents (containing trial data) and clinical study report (results and interpretation of trial) form part of the essential documentation that must be retained for a length of time prescribed by the applicable local legislation.[ 1 ] The ICMJE is currently proposing a requirement of authors to share with others de-identified individual patient data underlying the results presented in articles published in member journals.[ 18 ]
Those who have contributed to the work but do not meet all four criteria should be acknowledged; some of these activities include provision of administrative support, writing assistance and proofreading. They should have their written permission sought for their names to be published and disclose any potential conflicts of interest.[ 6 ] The Council of Scientific Editors has identified several inappropriate types of authorship, such as guest authorship, honorary or gift authorship and ghost authorship.[ 6 ] Various interventions should be put in place to prevent such fraudulent practices in research.[ 19 ] The list of essential documents for the conduct of a clinical trial is included in other articles of the same issue.
The recent increase in research activities has led to concerns regarding ethical and legal issues. Various guidelines have been formulated by organisations and authorities, which serve as a guide to promote integrity, compliance and ethical standards in the conduct of research. Fraud in research undermines the quality of establishing evidence-based medicine, and interventions should be put in place to prevent such practices. A general overview of ethical and legal principles will enable research to be conducted in accordance with the best practices.
Financial support and sponsorship
Conflicts of interest.
There are no conflicts of interest.
The ethics of using artificial intelligence in scientific research: new guidance needed for a new tool
- Original Research
- Open access
- Published: 27 May 2024
Cite this article
You have full access to this open access article
- David B. Resnik ORCID: orcid.org/0000-0002-5139-9555 1 &
- Mohammad Hosseini 2 , 3
821 Accesses
8 Altmetric
Explore all metrics
Using artificial intelligence (AI) in research offers many important benefits for science and society but also creates novel and complex ethical issues. While these ethical issues do not necessitate changing established ethical norms of science, they require the scientific community to develop new guidance for the appropriate use of AI. In this article, we briefly introduce AI and explain how it can be used in research, examine some of the ethical issues raised when using it, and offer nine recommendations for responsible use, including: (1) Researchers are responsible for identifying, describing, reducing, and controlling AI-related biases and random errors; (2) Researchers should disclose, describe, and explain their use of AI in research, including its limitations, in language that can be understood by non-experts; (3) Researchers should engage with impacted communities, populations, and other stakeholders concerning the use of AI in research to obtain their advice and assistance and address their interests and concerns, such as issues related to bias; (4) Researchers who use synthetic data should (a) indicate which parts of the data are synthetic; (b) clearly label the synthetic data; (c) describe how the data were generated; and (d) explain how and why the data were used; (5) AI systems should not be named as authors, inventors, or copyright holders but their contributions to research should be disclosed and described; (6) Education and mentoring in responsible conduct of research should include discussion of ethical use of AI.
Similar content being viewed by others
Biases and Ethical Considerations for Machine Learning Pipelines in the Computational Social Sciences
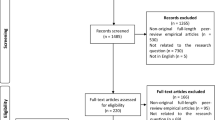
Publics’ views on ethical challenges of artificial intelligence: a scoping review
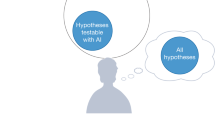
Artificial intelligence and illusions of understanding in scientific research
Avoid common mistakes on your manuscript.
1 Introduction: exponential growth in the use of artificial intelligence in scientific research
In just a few years, artificial intelligence (AI) has taken the world of scientific research by storm. AI tools have been used to perform or augment a variety of scientific tasks, including Footnote 1 :
Analyzing data and images [ 34 , 43 , 65 , 88 , 106 , 115 , 122 , 124 , 149 , 161 ].
Interpreting data and images [ 13 , 14 , 21 , 41 ].
Generating hypotheses [ 32 , 37 , 41 , 107 , 149 ].
Modelling complex phenomena [ 32 , 41 , 43 , 122 , 129 ].
Designing molecules and materials [ 15 , 37 , 43 , 205 ].
Generating data for use in validation of hypotheses and models [ 50 , 200 ].
Searching and reviewing the scientific literature [ 30 , 72 ].
Writing and editing scientific papers, grant proposals, consent forms, and institutional review board applications [ 3 , 53 , 54 , 82 , 163 ].
Reviewing scientific papers and other research outputs [ 53 , 54 , 98 , 178 , 212 ].
The applications of AI in scientific research appears to be limitless, and in the next decade AI is likely to completely transform the process of scientific discovery and innovation [ 6 , 7 , 8 , 9 , 105 , 201 ].
Although using AI in scientific research has steadily grown, ethical guidance has lagged far behind. With the exception of using AI to draft or edit scientific papers (see discussion in Sect. 7.6 ), most codes and policies do not explicitly address ethical issues related to using AI in scientific research. For example, the 2023 revision of the European Code of Conduct for Research Integrity [ 4 ] briefly discusses the importance of transparency. The code stipulates that researchers should report “their results and methods including the use of external services or AI and automated tools” (Ibid., p. 7) and considers “hiding the use of AI or automated tools in the creation of content or drafting of publications” as a violation of research integrity (Ibid. p. 10). One of the most thorough and up-to-date institutional documents, the National Institutes of Health Guidelines and Policies for the Conduct of Research provides guidance for using AI to write and edit manuscripts but not for other tasks [ 158 ]. Footnote 2 Codes of AI ethics, such as UNESCO’s [ 223 ] Ethics of Artificial Intelligence and the Office of Science and Technology Policy’s [ 168 , 169 ] Blueprint for an AI Bill of Rights, provide useful guidance for the development and use of AI in general without including specific guidance concerning the development and use of AI in scientific research [ 215 ].
There is therefore a gap in ethical and policy guidance concerning AI use in scientific research that needs to be filled to promote its appropriate use. Moreover, the need for guidance is urgent because using AI raises novel epistemological and ethical issues related to objectivity, reproducibility, transparency, accountability, responsibility, and trust in science [ 9 , 102 ]. In this paper, we will examine important questions related to AI’s impact on ethics of science. We will argue that while the use of AI does not require a radical change in the ethical norms of science, it will require the scientific community to develop new guidance for the appropriate use of AI. To defend this thesis, we will provide an overview of AI and an account of ethical norms of science, and then we will discuss the implications of AI for ethical norms of science and offer recommendations for its appropriate use.
2 What is AI?
AI can be defined as “a technical and scientific field devoted to the engineered system that generates outputs such as content, forecasts, recommendations or decisions for a given set of human-defined objectives [ 114 ].” AI is a subfield within the discipline of computer science [ 144 ]. However, the term ‘AI’ is also commonly used to refer to technologies (or tools) that can perform human tasks that require intelligence, such as perception, judgment, reasoning, or decision-making. We will use both senses of ‘AI’ in this paper, depending on the context.
While electronic calculators, cell phone apps, and programs that run on personal computers can perform functions associated with intelligence, they are not generally considered to be AI because they do not “learn” from the data [ 108 ]. As discussed below, AI systems can learn from the data insofar as they can adapt their programming in response to input data. While applying the term ‘learning’ to a machine may seem misleadingly anthropomorphic, it does make sense to say that a machine can learn if learning is regarded as a change in response to information about the environment [ 151 ]. Many different entities can learn in this sense of the term, including the immune system, which changes after being exposed to molecular information about pathogens, foreign objects, and other things that provoke an immune response.
This paper will focus on what is commonly referred to as narrow (or weak) AI, which is already being extensively used in science. Narrow AI has been designed and developed to do a specific task, such as playing chess, modelling complex phenomena, or identifying possible brain tumors in diagnostic images [ 151 ]. See Fig. 1 . Footnote 3 Other types of AI discussed in the literature include broad AI (also known as artificial general intelligence or AGI), which is a machine than can perform multiple tasks requiring human-like intelligence; and artificial consciousness (AC), which is a form of AGI with characteristics widely considered to be essential for consciousness [ 162 , 219 ]. Because there are significant technical and conceptual obstacles to developing AGI and AC, it may be years before machines have this degree of human-like intelligence [ 206 , 227 ]. Footnote 4
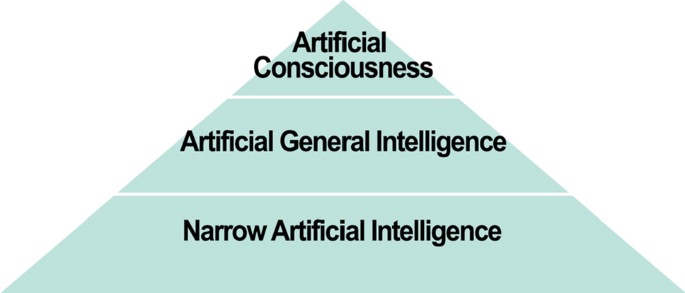
Levels of Artificial Intelligence, according to Turing [ 219 ]
3 What is machine learning?
Machine learning (ML) can be defined as a branch of AI “that focuses on the using data and algorithms to enable AI to imitate the way that humans learn, gradually improving its accuracy [ 112 ].” There are several types of ML, including support vector machines, decisions trees, and neural networks. In this paper we will focus on ML that uses artificial neural networks (ANNs).
An ANN is composed of artificial neurons, which are modelled after biological neurons. An artificial neuron receives a series of computational inputs, Footnote 5 applies a function, and produces an output. The inputs have different weightings. In most applications, a specific output is generated only when a certain threshold value for the inputs is reached. In the example below, an output of ‘1’ would be produced if the threshold is reached; otherwise, the output would be ‘0’. See Fig. 2 . A pair statements describing how a very simple artificial neuron processes inputs could be as follows:
where x1, x2, x3, and x4 are inputs; w1, w2, w3, and w4 are weightings, T is a threshold value; and U is an output value (1 or 0). An artificial neuron is represented schematically in Fig. 2 , below.
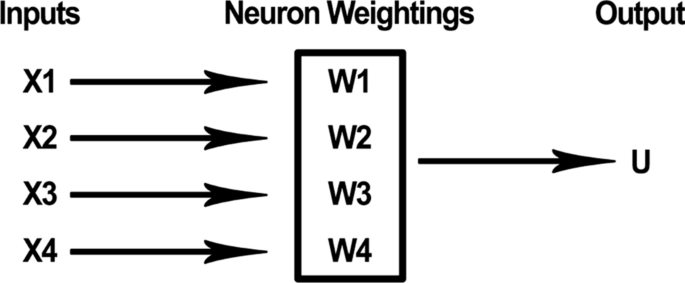
Artificial neuron
A single neuron may have dozens of inputs. An ANN may consist of thousands of interconnected neurons. In a deep learning ANN, there may be many hidden layers of neurons between the input and output layers. See Fig. 3 .
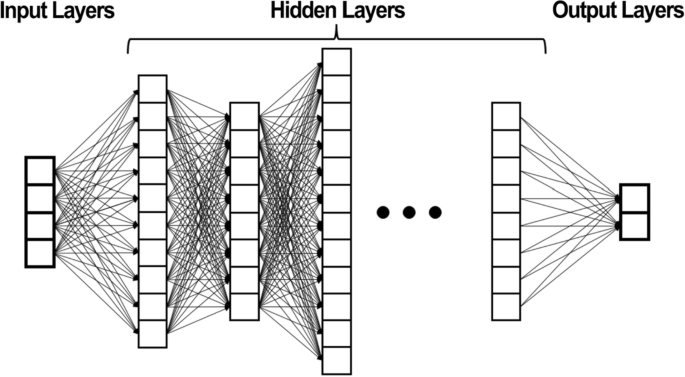
Deep learning artificial neural network [ 38 ]
Training (or reinforcement) occurs when the weightings on inputs are changed in response to system’s output. Changes in the weightings are based on their contribution to the neuron’s error, which can be understood as the difference between the output value and the correct value as determined by the human trainers (see discussion of error in Sect. 5 ). Training can occur via supervised or unsupervised learning. In supervised learning, the ANN works with labelled data and becomes adept at correctly representing structures in the data recognized by human trainers. In unsupervised learning, the ANN works with unlabeled data and discovers structures inherent in the data that might not have been recognized by humans [ 59 , 151 ]. For example, to use supervised learning to train an ANN to recognize dogs, human beings could present the system with various images and evaluate the accuracy of its output accordingly. If the ANN labels an image a “dog” that human beings recognize as a dog, then its output would be correct, otherwise, it would be incorrect (see discussion of error in Sects. 5.1 and 5.5). In unsupervised learning, the ANN would be presented with images and would be reinforced for accurately modelling structures inherent in the data, which may or may not correspond to patterns, properties, or relationships that humans would recognize or conceive of.
For an example of the disconnect between ML and human processing of information, consider research conducted by Roberts et al. [ 195 ]. In this study, researchers trained an ML system on radiologic images from hospital patients so that it would learn to identify patients with COVID-19 and predict the course of their illness. Since the patients who were sicker tended to laying down when their images were taken, the ML system identified laying down as a diagnostic criterion and disease predictor [ 195 ]. However, laying down is a confounding factor that has nothing to do with the likelihood of having COVID-19 or getting very sick from it [ 170 ]. The error occurred because the ML system did not account for this fundamental fact of clinical medicine.
Despite problems like the one discovered by Roberts et al. [ 195 ], the fact that ML systems process and analyze data differently from human beings can be a great benefit to science and society because these systems may be able to identify useful and innovative structures, properties, patterns, and relationships that human beings would not recognize. For example, ML systems have been able to design novel compounds and materials that human beings might not be able to conceive of [ 15 ]. That said, the disconnect between AI/ML and human information processing can also make it difficult to anticipate, understand, control, and reduce errors produced by ML systems. (See discussion of error in Sects. 5.1–5.5).
Training ANNs is a resource-intensive activity that involves gigabytes of data, thousands of computers, and hundreds of thousands of hours of human labor [ 182 , 229 ]. A system can continue to learn after the initial training period as it processes new data [ 151 ]. ML systems can be applied to any dataset that has been properly prepared for manipulation by computer algorithms, including digital images, audio and video recordings, natural language, medical records, chemical formulas, electromagnetic radiation, business transactions, stock prices, and games [ 151 ].
One of the most impressive feats accomplished by ML systems is their contribution to solving the protein folding problem [ 41 ]. See Fig. 4 . A protein is composed of one or more long chains of amino acids known as polypeptides. The three-dimensional (3-D) structure of the protein is produced by folding of the polypeptide(s), which is caused by the interplay of hydrogen bonds, Van der Waals attractive forces, and conformational entropy between different parts of the polypeptide [ 2 ]. Molecular biologists and biochemists have been trying to develop rules for predicting the 3-D structures of proteins from amino acid sequences since the 1960s, but this is, computationally speaking, a very hard problem, due to the immense number of possible ways that polypeptides can fold [ 52 , 76 ]. Tremendous progress on the protein-folding problem was made in 2022, when scientists demonstrated that an ML system, DeepMind’s AlphaFold, can predict 3-D structures from amino acid sequences with 92.4% accuracy [ 118 , 204 ]. AlphaFold, which built upon available knowledge of protein chemistry [ 176 ], was trained on thousands of amino acids sequences and their corresponding 3-D structures. Although human researchers still needed to test and refine AlphaFold’s output to ensure that the proposed structure is 100% accurate, the ML system greatly improves the efficiency of protein chemistry research [ 216 ]. Recently developed ML systems can generate new proteins by going in the opposite direction and predicting amino acids sequences from 3-D protein structures [ 156 ]. Since proteins play a key role in the structure and function of all living things, these advances in protein science are likely to have important applications in different areas of biology and medicine [ 204 ].
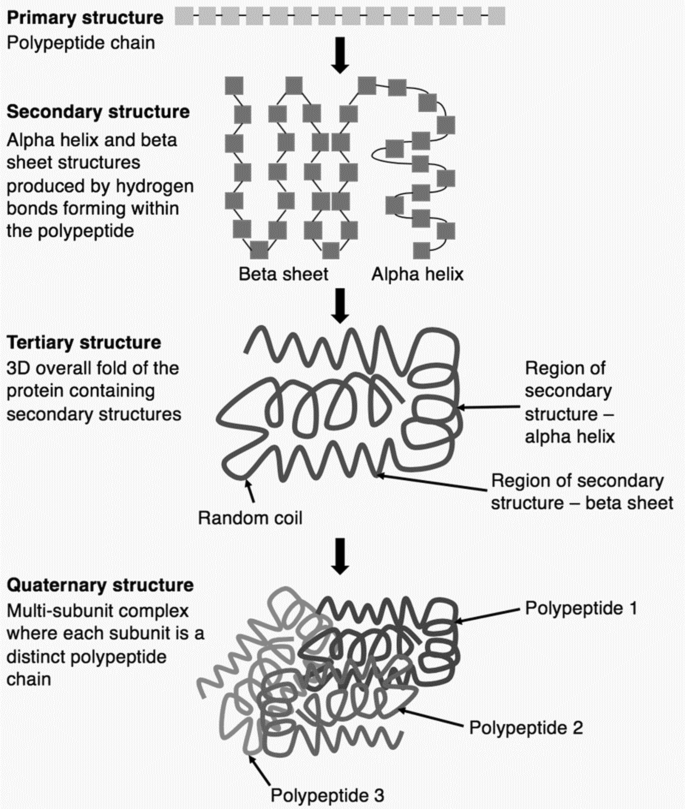
Protein folding. CC BY-SA 4.0 DEED [ 45 ]
4 What is generative AI?
Not only can ML image processing systems recognize patterns in the data that correspond to objects (e.g., cat, dog, car), when coupled with appropriate algorithms they can also generate images in response to visual or linguistic prompts [ 87 ]. The term ‘generative AI’ refers to “deep-learning models that can generate high-quality text, images, and other content based on the data they were trained on” [ 111 ].
Perhaps the most well-known types of generative AI are those that are based on large language models (LLMs), such as chatbots like OpenAI’s ChatGPT and Google’s Gemini, which analyze, paraphrase, edit, translate, and generate text, images and other types of content. LLMs are statistical algorithms trained on huge sets of natural language data, such as text from the internet, books, journal articles, and magazines. By processing this data, LLMs can learn to estimate probabilities associated with possible responses to text and can rank responses according to the probability that they will be judged to be correct by human beings [ 151 ]. In just a few years, some types of generative AI, such as ChatGPT, have become astonishingly proficient at responding to text data. ChatGPT has passed licensing exams for medicine and law and scored in the 93rd percentile on the Scholastic Aptitude Test reading exam and in the 89th percentile on the math exam [ 133 , 138 , 232 ]. Some researchers have used ChatGPT to write scientific papers and have even named them as authors [ 48 , 53 , 54 , 167 ]. Footnote 6 Some LLMs are so adept at mimicking the type of discourse associated with conscious thought that computer scientists, philosophers, and cognitive psychologists are updating the Turing test (see Fig. 5 ) to more reliably distinguish between humans and machines [ 5 , 22 ].
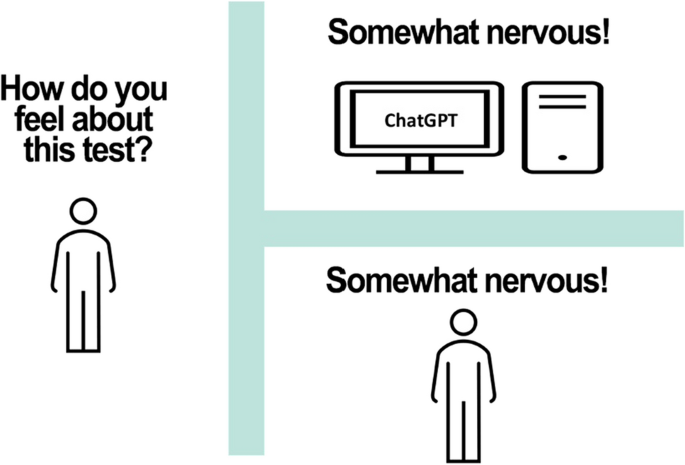
The Turing test. Computer scientist Alan Turing [ 220 ] proposed a famous test for determining whether a machine can think. The test involves a human interrogating another person, and a computer. The interrogator poses questions to the interviewees, who are in different rooms, so that interrogator cannot see where the answers are coming from. If the interrogator cannot distinguish between answers to questions given by another person and answers provided by a computer, then the computer passes the Turing test
5 Challenges of using AI
It has been long known that any AI systems are not error-free. To understand this topic, it is important to define ‘error’ and distinguish between systemic errors and random errors. The word ‘error’ has various meanings: we speak of grammatical errors, reasoning errors, typographical errors, measurement errors, etc. What these different senses of ‘error’ have in common is (1) errors involve divergence from a standard of correctness; and (2) errors, when committed by conscious beings, are unintentional; that is, they are accidents or mistakes and different from frauds, deceptions, or jokes.
If we set aside questions related to intent on the grounds that AI systems are not moral agents (see discussion in Sect. 7.6), we can think of AI error as the difference between the output of an AI system and the correct output . The difference between an AI output and the correct output can be measured quantitatively or qualitatively, depending on what is being measured and the purpose of the measurement [ 151 ]. For example, if a ML image recognition tool is presented with 50 images of wolves and 50 images of dogs, and it labels 98 of them correctly, we could measure its error quantitatively (i.e., 2%). In other cases, we might measure (or describe) error qualitatively. For example, if we ask ChatGPT to write a 12-line poem about a microwave oven in the style Edgar Allan Poe, we could rate its performance as ‘excellent,’ ‘very good,’ ‘good,’ ‘fair,’ or ‘poor.’ We could also assign numbers to these ratings to convert qualitative measurements into quantitative assessments (e.g., 5 = excellent, 4 = very good).
The correct output of an AI system is ultimately defined by its users and others who may be affected. For example, radiologists define correctness for reading diagnostic images; biochemists define the standard for modeling proteins; and attorneys, judges, clients, and law professors define the standard for writing legal briefs. In some contexts, such as testing hypotheses or reading radiologic images, ‘correct’ may mean ‘true’; in other contexts, such as generating text or creating models, it may simply mean ‘acceptable’ or ‘desirable.’ Footnote 7 While AI systems can play a key role in providing information that is used to define correct outputs (for example, when a system is used to discover new chemical compounds or solve complex math problems), human beings are ultimately responsible for determining whether outputs are correct (see discussion of moral agency in Sect. 7.6 ).
5.2 Random versus systemic errors ( Bias )
We can use an analogy with target shooting to think about the difference between random and systemic errors [ 94 ]. If error is understood as the distance of a bullet hole from a target, then random error would be a set of holes distributed randomly around the target without a discernable pattern (Fig. 6 A), while systemic error (or bias) would be a set of holes with a discernable pattern, for example holes skewed in a particular direction (Fig. 6 B). The accuracy of a set of bullet holes would be a function of their distance from the target, while their precision would be a function of their distance from each other [ 27 , 172 , 184 ].
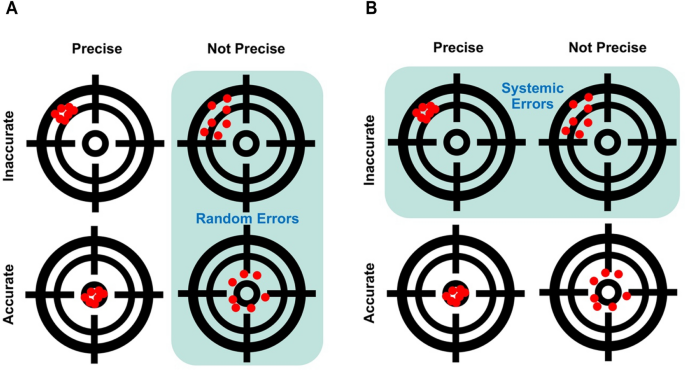
Random errors versus systemic errors
The difference between systemic and random errors can be ambiguous because errors that appear to be random may be shown to be systemic when one acquires more information about how they were generated or once a pattern is discerned. Footnote 8 Nevertheless, the distinction is useful. Systemic errors are often more detrimental to science and society than random ones, because they may negatively affect many different decisions involving people, projects, and paradigms. For example, racist biases distorted most research on human intelligence from the 1850s to the 1960s, including educational policies based on the applications of intelligence research. As will be discussed below, AI systems can make systemic and random errors [ 70 , 174 ].
5.3 AI biases
Since AI systems are designed to accurately represent the data on which they are trained, they can reproduce or even amplify racial, ethnic, gender, political, or other biases in the training data and subsequent data received [ 131 ]. The computer science maxim “garbage in, garbage out” applies here. Studies have shown that racial and ethnic biases impact the use of AI/ML in medical imaging, diagnosis, and prognosis due to biases in healthcare databases [ 78 , 154 ]. Bias is also a problem in using AI systems to find relationships between genomics and disease due to racial and ethnic prejudices in genomic databases [ 55 ]. LLMs are also impacted by various biases inherent in their training data, and when used in generative AI models like ChatGPT, can propagate biases related to race, ethnicity, nationality, gender, sexuality, age, and politics [ 25 , 171 ]. Footnote 9
Because scientific theories, hypotheses, and models are based on human perceptual categories, concepts, and assumptions, bias-free research is not possible [ 121 , 125 , 137 ]. Nevertheless, scientists can (and should) take steps to understand sources of bias and control them, especially those that can lead to discrimination, stigmatization, harm, or injustice [ 89 , 154 , 188 ]. Indeed, bias reduction and management is essential to promoting public trust in AI (discussed in Sects. 5.5 and 5.7 ).
Scientists have dealt with bias in research for years and have developed methods and strategies for minimizing and controlling bias in experimental design, data analysis, model building, and theory construction [ 79 , 89 , 104 ]. However, bias related to using AI in science can be subtle and difficult to detect due to the size and complexity of research data and interactions between data, algorithms, and applications [ 131 ]. See Fig. 7 . Scientists who use AI systems in research should take appropriate steps to anticipate, identify, control, and minimize biases by ensuring that datasets reflect the diversity of the investigated phenomena and disclosing the variables, algorithms, models, and parameters used in data analysis [ 56 ]. Managing bias related to the use of AI should involve continuous testing of the outputs in real world applications and adjusting systems accordingly [ 70 , 131 ]. For example, if a ML tool is used to read radiologic images, software developers, radiologists, and other stakeholders should continually evaluate the tool and its output to improve accuracy and precision.
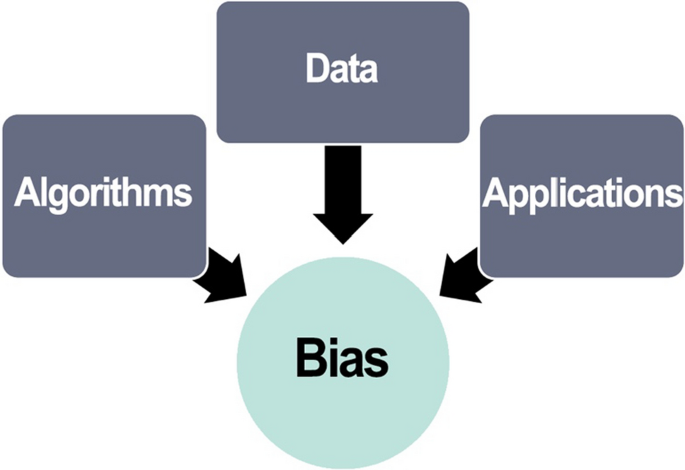
Sources of bias in AI/ML
5.4 Random errors in AI
AI systems can make random errors even after extensive training [ 51 , 151 ]. Nowhere has this problem been more apparent and concerning than in the use of LLMs in business, law, and scientific research. ChatGPT, for example, is prone to making random factual and citation errors. For example, Bhattacharyya et al. [ 24 ] used ChatGPT 3.5 to generate 30 short papers (200 words or less) on medical topics. 47% of the references produced by the chatbot were fabricated, 46% were authentic but inaccurately used, and only 7% were correct. Although ChatGPT 4.0 performs significantly better than ChatGPT 3.5, it still produces fabricated and inaccurate citations [ 230 ]. Another example of a random error was seen in a now-retracted paper published in Frontiers in Cell Development and Biology , which included an AI-generated image of a rat with unreal genitals [ 179 ]. Concerns raised by researchers led to OpenAI [ 173 ] warning users that “ChatGPT may produce inaccurate information about people, places, or facts.” The current interface includes the following disclaimer underneath the input box “ChatGPT can make mistakes. Consider checking important information.” Two US lawyers learned this lesson the hard way after a judge fined them $5000 for submitting court filing prepared by ChatGPT that included fake citations. The judge said that there was nothing improper about using ChatGPT but that the lawyers should exhibit due care in checking its work for accuracy [ 150 ].
An example of random errors made by generative AI discussed in the literature pertains to fake citations. Footnote 10 One reason why LLM-based systems, such as ChatGPT produce fake, but realistic-looking citations is that they process text data differently from human beings. Researchers produce citations by reading a specific text and citating it, but ChatGPT produces citations by processing a huge amount of text data and generating a highly probable response to a request for a citation. Software developers at OpenAI, Google, and other chatbot companies have been trying to fix this problem, but it is not easy to solve, due to differences between human and LLM processing of language [ 24 , 230 ]. AI companies advise users to use context-specific GPTs installed on top of ChatGPT. For instance, by using the Consensus.ai GPT ( https://consensus.app/ ), which claims to be connected to “200M + scientific papers”, users can ask for specific citations for a given input (e.g., “coffee is good for human health”). While the offered citations are likely to be correct bibliometrically, errors and biases may not be fully removed because it is unclear how these systems come to their conclusions and offer specific citations (see discussion of the black box problem in Sect. 5.7 ). Footnote 11
5.5 Prospects for reducing AI errors
If AI systems follow the path taken by most other technologies, it is likely that errors will decrease over time as improvements are made [ 151 ]. For example, early versions of ChatGPT were very bad at solving math problems but newer versions are much better math because they include special GPTs for performing this task [ 210 ]. AI systems also make errors in reading, classifying, and reconstructing radiological images, but the error rate is decreasing, and AI systems will soon outperform humans in terms of speed and accuracy of image reading [ 12 , 17 , 103 , 228 ]. However, it is also possible that AI systems will make different types of errors as they evolve or that there will be limits to their improvement. For example, newer versions of ChatGPT are prone to reasoning errors associated with intuitive thinking but older versions did not make these errors [ 91 ]. Also, studies have shown that LLMs are not good at self-correcting and need human supervision and fine-tuning to perform this task well [ 61 ].
Some types of errors may be difficult to eliminate due to differences between human perception/understanding and AI data processing. As discussed previously, AI systems, such as the system that generated the implausible hypothesis that laying down when having a radiologic image taken is a COVID-19 risk factor, make errors because they process information differently from humans. The AI system made this implausible inference because it did not factor basic biological and medical facts that would be obvious to doctors and scientists [ 170 ]. Another salient example of this phenomenon occurred when an image recognition AI was trained to distinguish between wolves and huskies, but it had difficulty recognizing huskies in the snow or wolves on the grass, because it had learned to distinguish between wolves and huskies by attending to the background of the images [ 222 ]. Humans are less prone to this kind of error because they use concepts to process perceptions and can therefore recognize objects in different settings. Consider, for example, captchas (Completely Automated Public Turing test to tell Computers and Humans Apart), which are used by many websites for security purposes and take advantage of some AI image processing deficiencies to authenticate whether a user is human [ 109 ]. Humans can pass Captchas tests because they learn to recognize images in various contexts and can apply what they know to novel situations [ 23 ].
Some of the factual and reasoning errors made by LLM-based systems occur because they lack human-like understanding of language [ 29 , 135 , 152 , 153 ]. ChatGPT, for example, can perform well when it comes to processing language that has already been curated by humans, such as describing the organelles in a cell or explaining known facts about photosynthesis, but they may perform sub-optimally (and sometimes very badly) when dealing with novel text that requires reasoning and problem-solving because it does do not have a human-like understanding of language. When a person processes language, they usually form a mental model that provides meaning and context for the words [ 29 ]. This mental model is based on implicit facts and assumptions about the natural world, human psychology, society, and culture, or what we might call commonsense [ 119 , 152 , 153 , 197 ]. LLMs do not do this,they only process symbols and predict the most likely string of symbols from linguistic prompts. Thus, to perform optimally, LLMs often need human supervision and input to provide the necessary meaning and context for language [ 61 ].
As discussed in Sect. 4 , because AI systems do not process information in the way that humans do, it can be difficult to anticipate, understand and detect the errors these tools make. For this reason, continual monitoring of AI performance in real-world applications, including feedback from end-users, developers, and other stakeholders, is essential to AI quality control and quality improvement and public trust in AI [ 131 , 174 ].
5.6 Lack of moral agency
As mentioned in Sect. 2 , narrow AI systems, such as LLMs, lack the capacities regarded as essential for moral agency, such as consciousness, self-concepts, personal memory, life experiences, goals, and emotions [ 18 , 139 , 151 ]. While this is not a problem for most technologies, it is for AI systems because they may be used to perform activities with significant moral and social consequences, such as reading radiological images or writing scientific papers (see discussion in Sect. 7.6 ), even though AI cannot be held morally or legally responsible or accountable. The lack of moral agency, when combined with other AI limitations, such as lack of a meaningful and human-like connection to the physical world, can produce dangerous results. For example, in 2021, Alexa, Amazon’s LLM-based voice-assistant, instructed a 10-year-old girl to stick a penny into an electric outlet when she asked it for a challenge to do [ 20 ]. In 2023, the widow of a Belgian man who committed suicide claimed that he had been depressed and was chatting with an LLM that encouraged him to kill himself [ 44 , 69 ]). OpenAI and other companies have tried to put guardrails in place to prevent their systems from giving dangerous advice, but this is not easy to fix. A recent study found that while ChatGPT can pass medical boards, it can give dangerous medical advice due to its tendency to make factual errors and its lack of understanding of the meaning and context of language [ 51 ].
5.7 The black box problem
Suppose ChatGPT produces erroneous output, and a computer scientist or engineer wants to know why. As a first step, they could examine the training data and algorithms to determine the source of the problem. Footnote 12 However, to fully understand what ChatGPT is doing they need to probe deeply into the system and examine not only the code but also the weightings attached to inputs in the ANN layers and the mathematical computations produced from the inputs. While an expert computer scientist or engineer could troubleshoot the code, they will not be able to interpret the thousands of numbers used in the weightings and the billions of calculations from those numbers [ 110 , 151 , 199 ]. This is what is meant when an AI system is described as a “black box.” See Fig. 8 . Trying to understand the meaning of the weightings and calculations in ML is very different from trying to understand other types of computer programs, such as those used in most cell phones or personal computers, in which an expert could examine the system (as a whole) to determine what it is doing and why [ 151 , 199 ]. Footnote 13
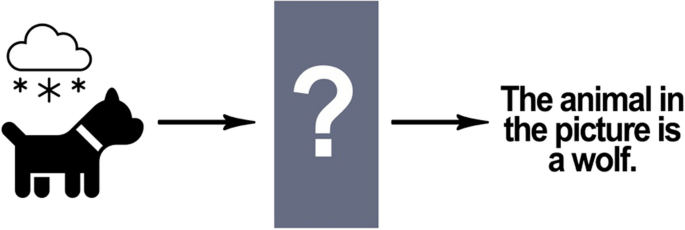
The black box: AI incorrectly labels a picture of a dog as a picture of a wolf but a complete investigation of this error is not possible due to a “black box” in the system
The opacity of AI systems is ethically problematic because one might argue that we should not use these devices if we cannot trust them, and we cannot trust them if even the best experts do not completely understand how they work [ 6 , 7 , 39 , 47 , 63 , 186 ]. Trust in a technology is partially based on understanding that technology. If we do not understand how a telescope works, then we should not trust in what we see with it. Footnote 14 Likewise, if computer experts do not completely understand how an AI/ML system works, then perhaps we should not use them for important tasks, such as making hiring decisions, diagnosing diseases, analyzing data, or generating scientific hypotheses or theories [ 63 , 74 ].
The black box problem raises important ethical issues for science (discussed further in Sect. 7.4 ), because it can undermine public trust in science, which is already in decline, due primarily to the politicization of topics with significant social implications, such as climate change, COVID-19 vaccines and public health measures [ 123 , 189 ].
One way of responding to the black box problem is to argue that we do not need to completely understand AI systems to trust them; what matters is an acceptably low rate of error [ 136 , 186 ]. Proponents of this view draw an analogy between using AI systems and using other artifacts, such as using aspirin for pain relief, without fully understanding how they work. All that really matters for trusting a machine or tool is that we have evidence that it works well for our purposes, not that we completely understand how it works. This line of argument implies that it is justifiable to use AI systems to read radiological images, model the 3-D structures of proteins, or write scientific papers provided that we have evidence that they perform these tasks as well as human beings [ 136 ].
This response to the black box problem does not solve the problem but simply tells us not to worry about it [ 63 ]. Footnote 15 There are several reasons to be concerned about the black box problem. First, if something goes wrong with a tool or technology, regulatory agencies, injured parties, insurers, politicians, and others want to know precisely how it works to prevent similar problems in the future and hold people and organizations legally accountable [ 141 ]. For example, when the National Transportation Safety Board [ 160 ] investigates an airplane crash, they want to know what precisely went wrong. Was the crash due to human error? Bad weather? A design flaw? A defective part? The NTSB will not be satisfied with an explanation that appeals to a mysterious technology within the airplane.
Second, when regulatory agencies, such as the Food and Drug Administration (FDA), make decisions concerning the approval of new products, they want to know how the products work, so they can make well-informed, publicly-defendable decisions and inform the consumers about risks. To obtain FDA approval for a new drug, a manufacturer must submit a vast amount of information to the agency, including information about the drug’s chemistry, pharmacology, and toxicology; the results of pre-clinical and clinical trials; processes for manufacturing the drug; and proposed labelling and advice to healthcare providers [ 75 ]. Indeed, dealing with the black box problem has been a key issue in FDA approval of medical devices that use AI/ML [ 74 , 183 ].
Third, end-users of technologies, such as consumers, professionals, researchers, government officials, and business leaders may not be satisfied with black boxes. Although most laypeople comfortably use technologies without fully understanding their innerworkings, they usually assume that experts who understand how these technologies work have assessed them and deemed them to be safe. End-users may become highly dissatisfied with a technology when it fails to perform its function, especially when not even the experts can explain why. Public dissatisfaction with responses to the black box problem may undermine the adoption of AI/ML technologies, especially when these technologies cause harm, invade privacy, or produce biased claims and results [ 60 , 85 , 134 , 175 ].
5.8 Explainable AI
An alternative to the non-solution approach is to make AI explainable [ 11 , 96 , 151 , 186 ]. The basic idea behind explainability is to develop “processes and methods that allows human users to comprehend and trust the results and output created by machine learning algorithms” [ 110 ]. Transparency of algorithms, models, parameters, and data is essential to making AI explainable, so that users can understand an AI system’s accuracy and precision and the types of errors it is prone to making. Explainable AI does not attempt to “peer inside” the black box, but it can make AI behavior more understandable to developers, users, and other stakeholders. Explainability, according to proponents of this approach, helps to promote trust in AI because it allows users and other stakeholders to make rational and informed decisions about it [ 77 , 83 , 110 , 186 ].
While the explainable AI approach is preferable to the non-solution approach, it still has some shortcomings. First, it is unclear whether making AI explainable will satisfy non-experts because considerable expertise in computer science and/or data analytics may be required to understand what is being explained [ 120 , 186 ]. For transparency to be effective, it must address the audience’s informational needs [ 68 ]. Explainable AI, at least in its current form, may not address the informational needs of laypeople, politicians, professionals, or scientists because the information is too technical [ 58 ]. To be explainable to non-experts, the information should be expressed in plain, jargon-free language that describes what the AI did and why [ 96 ].
Second, it is unclear whether explainable AI completely solves issues related to accountability and legal liability because we are yet to witness how legal systems will deal with AI lawsuits in which information pertaining to explainability (or lack thereof) is used as evidence in a court [ 141 ]. However, it is conceivable that the information conveyed to make AI explainable will satisfy the courts in some cases and set judicial precedent, so that legal doctrines and practices related to liability for AI-caused harms will emerge, much in the same way that doctrines and practices for medical technologies emerged.
Third, there is also the issue of whether explainable AI will satisfy the requirements of regulatory agencies, such as the FDA. However, regulatory agencies have been making some progress toward addressing the black box problem and explainability is likely to play a key role in these efforts [ 183 ].
Fourth, private companies uninterested in sharing information about their systems may not comply with explainable AI requirements or they may “game” the requirements to resemble compliance without actually complying. ChatGPT, for example, is a highly opaque system that is yet to disclose its training data and it is unclear whether/when OpenAI would open up its technology to external scrutiny [ 28 , 66 , 130 ].
Despite these shortcomings, the explainable AI approach is a reasonable way of dealing with transparency issues, and we encourage its continued development and application to AI/ML systems.
6 Ethical norms of science
With this overview of AI in mind, we can now consider how using AI in research impacts the ethical norms of science. But first, we need to describe these norms. Ethical norms of science are principles, values, or virtues that are essential for conducting good research [ 147 , 180 , 187 , 191 ]. See Table 1 . These norms apply to various practices, including research design; experimentation and testing; modelling; concept formation; data collection and storage; data analysis and interpretation; data sharing; publication; peer review; hypothesis/theory formulation and acceptance; communication with the public; as well as mentoring and education [ 207 ]. Many of these norms are expressed in codes of conduct, professional guidelines, institutional or journal policies, or books and papers on scientific methodology [ 4 , 10 , 113 , 235 ]. Others, like collegiality, might not be codified but are implicit in the practice of science. Some norms, such as testability, rigor, and reproducibility, are primarily epistemic, while others, such as fair attribution of credit, protection of research subjects, and social responsibility, are primarily moral (when enshrined in law, like instance of fraud, these norms become legal but here we only focus on ethical norms). There are also some like honesty, openness, and transparency, which have both epistemic and moral dimensions [ 191 , 192 ].
Scholars from different fields, including philosophy, sociology, history, logic, decision theory, and statistics have studied ethical norms of science [ 84 , 89 , 104 , 125 , 128 , 137 , 147 , 180 , 208 , 209 , 237 ]. Sociologists such as Merton [ 147 ] and Shapin [ 208 ], tend to view ethical norms as generalizations that accurately describe the practice of science, while philosophers, such as Kitcher [ 125 ] and Haack [ 89 ], conceive of these norms as prescriptive standards that scientists ought to follow. These approaches need not be mutually exclusive, and both can offer useful insights about ethical norms of science. Clearly, the study of norms must take the practice of science as its starting point, otherwise our understanding of norms would have no factual basis. However, one cannot simply infer the ethical norms of science from the practice of science because scientists may endorse and defend norms without always following them. For example, most scientists would agree that they should report data honestly, disclose significant conflicting interests, and keep good research records, but evidence indicates that they sometimes fail to do so [ 140 ].
One way of bridging the gap between descriptive and prescriptive accounts of ethical norms of science is to reflect on the social and epistemological foundations (or justifications) of these norms. Ethical norms of science can be justified in at least three ways [ 191 ].
First, these norms help the scientific community achieve its epistemic and practical goals, such as understanding, predicting, and controlling nature. It is nearly impossible to understand how a natural or social process works or make accurate predictions about it without standards pertaining to honesty, logical consistency, empirical support, and reproducibility of data and results. These and other epistemic standards distinguish science form superstition, pseudoscience, and sophistry [ 89 ].
Second, ethical norms promote trust among scientists, which is essential for collaboration, peer review, publication, sharing of data and resources, mentoring, education, and other scientific activities. Scientists need to be able to trust that the data and results reported in papers have not been fabricated, falsified, or manipulated; that reviewers for journals and funding agencies will maintain confidentiality; that colleagues or mentors will not steal their ideas and other forms of intellectual property; and that credit for collaborative work will be distributed fairly [ 26 , 233 ].
Third, ethical norms are important for fostering public support for science. The public is not likely to financially, legally, or socially support research that is perceived as corrupt, incompetent, untrustworthy, or unethical [ 191 ]. Taken together, these three modes of justification link ethical norms to science’s social foundations; that is, ethical norms are standards that govern the scientific community, which itself operates within and interacts with a larger community, namely society [ 137 , 187 , 209 ].
Although vital for conducting science, ethical norms are not rigid rules. Norms sometimes conflict, and when they do, scientists must make decisions concerning epistemic or moral priorities [ 191 ]. For example, model-building in science may involve tradeoffs among various epistemic norms, including generality, precision, realism, simplicity, and explanatory power [ 143 ]. Research with human subjects often involves tradeoffs between rigor and protection of participants. For example, placebo control groups are not used in clinical trials when receiving a placebo instead of an effective treatment would cause serious harm to the participant [ 207 ].
Although the norms can be understood as guidelines, some have higher priority than others. For example, honesty is the hallmark of good science, and there are very few situations in which scientists are justified in deviating from this norm. Footnote 16 Openness, on the other hand, can be deemphasized to protect research participants’ privacy, intellectual property, classified information, or unpublished research [ 207 ].
Finally, science’s ethical norms have changed over time, and they are likely to continue to evolve [ 80 , 128 , 147 , 237 ]. While norms such as empiricism, objectivity, and consistency originated in ancient Greek science, others, such as reproducibility and openness, developed during the 1500s; and many, such as protection of research subjects and social responsibility, did not emerge as formalized norms until the twentieth century. This evolution is in response to changes in science’s social, institutional, economic, and political environment and advancements in scientific instruments, tools, and methods [ 100 ]. For example, the funding of science by private companies and their requirements concerning data access and release policies have led to changes in norms related to open sharing of data and materials [ 188 ]. The increased presence of women and racial and ethnic minorities in science has led to the development of policies for preventing sexual and other forms of harassment [ 185 ]. The use of computer software to analyze large sets of complex data has challenged traditional views about norms related to hypothesis testing [ 193 , 194 ].
7 AI and the ethical norms of science
We will divide our discussion of AI and the ethics of science into six topics corresponding to the problems and issues previously identified in this paper and seventh topic related to scientific education. While these topics may seem somewhat disconnected, they all involve ethical issues that scientists who use AI in research are currently dealing with.
7.1 AI biases and the ethical norms of science
Bias can undermine the quality and trustworthiness of science and its social impacts [ 207 ]. While reducing and managing bias are widely recognized as essential to good scientific methodology and practice [ 79 , 89 ], they become crucial when AI is employed in research because AI can reproduce and amplify biases inherent in the data and generate results that lend support to policies that are discriminatory, unfair, harmful, or ineffective [ 16 , 202 ]. Moreover, by taking machines’ disinterestedness in findings as a necessary and sufficient condition of objectivity, users of AI in research may overestimate the objectivity of their findings. AI biases in medical research have generated considerable concern, since biases related to race, ethnicity, gender, sexuality, age, nationality, and socioeconomic status in health-related datasets can perpetuate health disparities by supporting biased hypotheses, models, theories, and policies [ 177 , 198 , 211 ]. Biases also negatively impact areas of science outside the health sphere, including ecology, forestry, urban planning, economics, wildlife management, geography, and agriculture [ 142 , 164 , 165 ].
OpenAI, Google, and other generative AI developers have been using filters that prevent their systems from generating text that is outright racist, sexist, homophobic, pornographic, offensive, or dangerous [ 93 ]. While bias reduction is a necessary step to make AI safe for human use, there are reasons to be skeptical of the idea that AI can be appropriately sanitized. First, the biases inherent in data are so pervasive that no amount of filtering can remove all of them [ 44 , 69 ]. Second, AI systems may also have political and social biases that are difficult to identify or control [ 19 ]. Even in the case of generative AI models where some filtering has happened, changing the inputted prompt may simply confuse and push a system to generate biased content anyway [ 98 ].
Third, by removing, reducing and controlling some biases, AI developers may create other biases, which are difficult to anticipate, identify or describe at this point. For example, LLMs have been trained using data gleaned from the Internet, scholarly articles and Wikipedia [ 90 ], all of which consist of the broad spectrum of human behavior and experience, from good to bad and virtuous to sinister. If we try to weed undesirable features of this data, we will eliminate parts of our language and culture, and ultimately, parts of us. Footnote 17 If we want to use LLMs to make sound moral and political judgments, sanitizing their data processing and output may hinder their ability to excel at this task, because the ability to make sound moral judgements or anticipate harm may depend, in part, on some familiarity with immoral choices and the darker side of humanity. It is only by understanding evil that we can freely and rationally choose the good [ 40 ]. We admit this last point is highly speculative, but it is worth considering. Clearly, the effects of LLM bias-management bear watching.
While the problem of AI bias does not require a radical revision of scientific norms, it does imply that scientists who use AI systems in research have special obligations to identify, describe, reduce, and control bias [ 132 ]. To fulfill these obligations, scientists must not only attend to matters of research design, data analysis and interpretation, but also address issues related to data diversity, sampling, and representativeness [ 70 ]. They must also realize that they are ultimately accountable for AI biases, both to other scientists and to members of the public. As such, they should only use AI in contexts where their expertise and judgement are sufficient to identify and remove biases [ 97 ]. This is important because given the accessibility of AI systems and the fact that they can exploit our cognitive shortcomings, they are creating an illusion of understanding [ 148 ].
Furthermore, to build public trust in AI and promote transparency and accountability, scientists who use AI should engage with impacted populations, communities and other stakeholders to address their needs and concerns and seek their assistance in identifying and reducing potential biases [ 132 , 181 , 202 ]. Footnote 18 During the engagement process, researchers should help populations and communities understand how their AI system works, why they are using it, and how it may produce bias. To address the problem of AI bias, the Biden Administration recently signed an executive order that directs federal agencies to identify and reduce bias and protect the public from algorithmic discrimination [ 217 ].
7.2 AI random errors and the ethical norms of science
Like bias, random errors can undermine the validity and reliability of scientific knowledge and have disastrous consequences for public health, safety, and social policy [ 207 ]. For example, random errors in the processing of radiologic images in a clinical trial of a new cancer drug could harm patients in the trial and future patients who take an approved drug, and errors related to the modeling of the transmission of an infectious disease could undermine efforts to control an epidemic. Although some random errors are unavoidable in science, an excessive amount when using AI could be considered carelessness or recklessness when using AI (see discussion of misconduct in Sect. 7.3 ).
Reduction of random errors, like reduction of bias, is widely recognized as essential to good scientific methodology and practice [ 207 ]. Although some random errors are unavoidable in research, scientists have obligations to identify, describe, reduce, and correct them because they are ultimately accountable for both human and AI errors. Scientists who use AI in their research should disclose and discuss potential limitations and (known) AI-related errors. Transparency about these is important for making research trustworthy and reproducible [ 16 ].
Strategies for reducing errors in science include time-honored quality assurance and quality improvement techniques, such as auditing data, instruments, and systems; validating and testing instruments that analyze or process data; and investigating and analyzing errors [ 1 ]. Replication of results by independent researchers, journal peer review, and post-publication peer review also play a major role in error reduction [ 207 ]. However, given that content generated by AI systems is not always reproducible [ 98 ], identifying and adopting measures to reduce errors is extremely complicated. Either way, accountability requires that scientists take responsibility for errors produced by AI/ML systems, that they can explain why errors have occurred, and that they transparently share their limitations of their knowledge related to these errors.
7.3 AI and research misconduct
Failure to appropriately control AI-related errors could make scientists liable for research misconduct, if they intentionally, knowingly, or recklessly disseminate false data or plagiarize [ 207 ]. Footnote 19 Although most misconduct regulations and policies distinguish between misconduct and honest error, scientists may still be liable for misconduct due to recklessness [ 42 , 193 , 194 ], which may have consequences for using AI. Footnote 20 For example, a person who uses ChatGPT to write a paper without carefully checking its output for errors or plagiarism could be liable for research misconduct for reckless use of AI. Potential liability for misconduct is yet another reason why using AI in research requires taking appropriate steps to minimize and control errors.
It is also possible that some scientists will use AI to fabricate data or images presented in scientific papers, grant proposals, or other documents. This unethical use of AI is becoming increasingly likely since generative models can be used to create synthetic datasets from scratch or make alternative versions of existing datasets [ 50 , 155 , 200 , 214 ]. Synthetic data are playing an increasingly important role in some areas of science. For example, researchers can use synthetic data to develop and validate models and enhance statistical analysis. Also, because synthetic data are similar to but not the same as real data, they can be used to eliminate or mask personal identifiers and protect the confidentiality of human participants [ 31 , 81 , 200 ].
Although we do not know of any cases where scientists have been charged with research misconduct for presenting synthetic data as real data, it is only a matter of time until this happens, given the pressures to produce results, publish, and obtain grants, and the temptations to cheat or cut corners. Footnote 21 This speculation is further corroborated by the fact that a small proportion of scientists deliberately fabricate or falsify data at some point in their careers [ 73 , 140 ]. Also, using synthetic data in research, even appropriately, may blur the line between real and fake data and undermine data integrity. Researchers who use synthetic data should (1) indicate which parts of data are synthetic, (2) describe how the data were generated; (3) explain how and why they were used [ 221 ].
7.4 The black box problem and the ethical norms of science
The black box problem presents significant challenges to the trustworthiness and transparency of research that use AI because some of the steps in the scientific process will not be fully open and understandable to humans, including AI experts. An important implication of the black box problem is that scientists who use AI are obligated to make their use of the technology explainable to their peers and the public. While precise details concerning what makes an AI system explainable may vary across disciplines and contexts, some baseline requirements for transparency may include:
The type, name, and version of AI system used.
What task(s) the system was used for.
How, when and by which contributor a system was used.
Why a certain system was used instead of alternatives (if available).
What aspects of a system are not explainable (e.g., weightings).
Technical details related to model’s architecture, training data and optimization procedures, influential features involved in model’s decisions, the reliability and accuracy of the system (if known).
Whether inferences drawn by the AI system are supported by currently accepted scientific theories, principles, or concepts.
This information should be expressed in plain language to allow non-experts to understand the whos, whats, hows, and whys related to the AI system. Ideally, this information would become a standard part of reported research that used AI. The information could be reported in the materials and methods section or in supplemental material, much that same way that information about statistical methods and software is currently reported.
As mentioned previously, making AI explainable does not completely solve the black box problem but it can play a key role in promoting transparency, accountability, and trust [ 7 , 9 ]. While there seems to be an emerging consensus on the utility and importance of making AI explainable, there is very little agreement about what explainability means in practice, because what makes AI explainable depends on the context of its use [ 58 ]. Clearly, this is a topic where more empirical research and ethical/policy analysis is needed.
7.5 AI and confidentiality
Using AI in research, especially generative AI models, raises ethical issues related to data privacy and confidentiality. ChatGPT, for example, stores the information submitted by users, including data submitted in initial prompts and subsequent interactions. Unless users opt out, this information could be used for training and other purposes. The data could potentially include personal and confidential information, such as information contained in drafts of scientific papers, grant proposals, experimental protocols, or institutional policies; computer code; legal strategies; business plans; and private information about human research participants [ 67 , 85 ]. Due to concerns about breaches of confidentiality, the National Institutes of Health (NIH) recently prohibited the use of generative AI technologies, such as LLMs, in grant peer review [ 159 ]. Footnote 22 Some US courts now require lawyers to disclose their use of generative AI in preparing legal documents and make assurances that they have taken appropriate steps to protect confidentiality [ 146 ].
While we are not suggesting that concerns about confidentiality justify prohibiting generative AI use in science, we think that considerable caution is warranted. Researchers who use generative AI to edit or review a document should assume that the material contained in it will not be kept confidential, and therefore, should not use these systems to edit or review anything containing confidential or personal information.
It is worth noting that technological solutions to the confidentiality problem may be developed in due course. For example, if an organization operates a local application of an LLM and places the technology behind a secure firewall, its members can use the technology safely. Electronic medical records, for example, have this type of security [ 127 ]. Some universities have already begun experimenting with operating their own AI systems for use by students, faculty, and administrators [ 225 ]. Also, as mentioned in Sect. 7.3 , the use of synthetic data may help to protect confidentiality.
7.6 AI and moral agency
The next issue we will discuss is whether AI can be considered a moral agent that participates in an epistemic community, that is, as a partner in knowledge generation. This became a major issue for the ethical norms of science in the winter of 2022–2023, when some researchers listed ChatGPT as authors on papers [ 102 ]. These publications initiated a vigorous debate in the research community, and journals scrambled to develop policies to deal with LLMs’ use in research. On one end of the spectrum, Jenkins and Lin [ 116 ] argued that AI systems can be authors if they make a substantial contribution to the research, and on the other end, Thorp [ 218 ] argued that AI systems cannot be named as authors and should not be used at all in preparing manuscripts. Currently, there seems to be an emerging consensus that falls in between these two extremes position, namely, that AI systems can be used in preparing manuscripts but that their use should be appropriately disclosed and discussed, [ 4 , 102 ]. In 2023, the International Committee of Medical Journal Editors (ICMJE), a highly influential organization with over 4,500 member journals, released the following statement about AI and authorship:
At submission, the journal should require authors to disclose whether they used artificial intelligence (AI)assisted technologies (such as Large Language Models [LLMs], chatbots, or image creators) in the production of submitted work. Authors who use such technology should describe, in both the cover letter and the submitted work, how they used it. Chatbots (such as ChatGPT) should not be listed as authors because they cannot be responsible for the accuracy, integrity, and originality of the work, and these responsibilities are required for authorship (see Section II.A.1). Therefore, humans are responsible for any submitted material that included the use of AI-assisted technologies. Authors should carefully review and edit the result because AI can generate authoritative-sounding output that can be incorrect, incomplete, or biased. Authors should not list AI and AI assisted technologies as an author or co-author, nor cite AI as an author. Authors should be able to assert that there is no plagiarism in their paper, including in text and images produced by the AI. Humans must ensure there is appropriate attribution of all quoted material, including full citations [ 113 ].
We agree with the ICMJE’s position, which mirrors views we defended in print before the ICMJE released its guidance [ 101 , 102 ].
Authorship on scientific papers is based not only on making a substantial contribution, but also on being accountable for the work [ 207 ]. Because authorship implies significant epistemic and ethical responsibilities, one should not be named as an author on a work if one cannot be accountable for one’s contribution to the work. If questions arise about the work after publication, one needs to be able to answer those questions intelligibly and if deemed liable, face possible legal, financial, or social consequences for one’s actions.
AI systems cannot be held accountable for their actions for two reasons: (1) they cannot provide intelligible explanations for what they did, (2) they cannot be held morally responsible for their actions, (3) they cannot suffer consequences nor can be sanctioned. The first reason has to do with the previously discussed black box problem. Although current proposals for making AI explainable may help to deal with this issue, they still fall far short of humanlike accountability, because these proposals do not require that the AI system, itself , should provide an explanation. Regarding the second reason, when we hold humans accountable, we expect them to explain their behavior in clear and intelligible language. Footnote 23 If a principal investigator wonders why a graduate student did not report all the data related to experiment, the investigator expects the student to explain why they did what they did. Current AI systems cannot do this. In some cases, someone else may be able to provide an explanation of how they work and what they do, but this not the same as the AI providing the explanation, which is a prerequisite for accountability. The third reason has to do with the link between accountabilities and sanctions. If an AI system makes a mistake which harms others, it cannot be sanctioned. These systems do not have interests, values, reputation and feelings in the same way that humans do and cannot be punished by law enforcement.
Even if an AI can intelligibly explain itself in the future, this does not imply that it can be morally responsible. While the concept of moral agency, like the concept of consciousness, is controversial, there is general agreement that moral agency requires the capacity to perform intentional (or purposeful) actions, understand moral norms, and make decisions based on moral norms. These capacities also presuppose additional capacities, such as consciousness, self-awareness, personal memory, perception, general intelligence, and emotions [ 46 , 95 , 213 ]. While computer scientists are making some progress on developing AI systems that have quasi-moral agency, that is, AI systems that can make decisions based on moral norms [ 71 , 196 , 203 ], they are still a long way from developing AGI or AC (see definitions of these terms in Sect. 2 ), which would seem to be required for genuine moral agency.
Moreover, other important implications follow from current AI’s lack of moral agency. First, AI systems cannot be named as inventors on patents, because inventorship also implies moral agency [ 62 ]. Patents are granted to individuals, i.e., persons, but since AI systems lack moral agency, they do not qualify as persons under the patent laws adopted by most countries. Second, AI systems cannot be copyright holders, because to own a copyright, one must be a person [ 49 ]. Copyrights, under US law, are granted only to people [ 224 ].
Although AI systems should not be named as authors or inventors, it is still important to appropriately recognize their contributions. Recognition should be granted not only to promote honesty and transparency in research but also to prevent human authors from receiving undue credit. For example, although many scientists and engineers deserve considerable accolades for solving the protein folding problem [ 118 , 176 ], failing to mention the role of AlphaFold in this discovery would be giving human contributors more credit than they deserve.
7.7 AI and research ethics education
The last topic we will address in this section has to do with education and mentoring in responsible conduct of research (RCR), which is widely recognized as essential to promoting ethical judgment, reasoning, and behavior in science [ 207 ]. In the US, the NIH and National Science Foundation (NSF) require RCR education for funded students and trainees, and many academic institutions require some form of RCR training for all research faculty [ 190 ]. Topics typically covered in RCR courses, seminars, workshops, or training sessions include data fabrication and falsification, plagiarism, investigation of misconduct, scientific record keeping, data management, rigor and reproducibility, authorship, peer review, publication, conflict of interest, mentoring, safe research environment, protection and human and animal subjects, and social responsibility [ 207 ]. As demonstrated in this paper, the use of AI in research has a direct bearing on most of these topics, but especially on authorship, rigor and reproducibility, peer review, and social responsibility. We recommend, therefore, that RCR education and training incorporate discussion of the use of AI in research, wherever relevant.
8 Conclusion
Using AI in research benefits science and society but also creates some novel and complex ethical issues that affect accountability, responsibility, transparency, trustworthiness, reproducibility, fairness, and objectivity, and other important values in research. Although scientists do not need to radically revise their ethical norms to deal with these issues, they do need new guidance for the appropriate use of AI in research. Table 2 provides a summary of our recommendations for this guidance. Since AI continues to advance rapidly, scientists, academic institutions, funding agencies and publishers, should continue to discuss AI’s impact on research and update their knowledge, ethical guidelines and policies accordingly. Guidance should be periodically revised as AI becomes woven into the fabric of scientific practice (or normalized) and researchers learn about it, adapt to it, and use it in novel ways. Since science has significant impacts on society, public engagement in such discussions is crucial for responsible the use, development, and AI in research [ 234 ].
In closing, we will observe that many scholars, including ourselves, assume that today’s AI systems lack the capacities necessary for moral agency. This assumption has played a key role in our analysis of ethical uses of AI in research and has informed our recommendations. We realize that a day may arrive, possibly sooner than many would like to believe, when AI will advance to the point that this assumption will need to be revised, and that society will need to come to terms with the moral rights and responsibilities of some types of AI systems. Perhaps AI systems will one day participate in science as full partners in discovery and innovation [ 33 , 126 ]. Although we do not view this as a matter that now demands immediate attention, we remain open to further discussion of this issue in the future.
There is not sufficient space in this paper to conduct a thorough review of all the ways that AI is being used in scientific research. For a review of the information, see Wang et al. [ 231 ] and Krenn et al. [ 126 ].
However, the National Institutes of Health has prohibited the use of AI to review grants (see Sect. 7.5 ).
This a simplified taxonomy of AI that we have found useful to frame the research ethics issues. For more detailed taxonomy, see Graziani et al. [ 86 ].
See Krenn et al. [ 126 ] for a thoughtful discussion of the possible role of AGI in scientific research.
We will use the term ‘input’ in a very general sense to refer to data which are routed into the system, such as numbers, text, or image pixels.
It is important to note that the [ 167 ] paper was corrected to remove ChatGPT as an author because the tool did not meet the journal’s authorship criteria. See O’Connor [ 166 ].
There are important, philosophical issues at stake here concerning whether AI users should regard an output as ‘acceptable’ or ‘true’, but these questions are beyond the scope of our paper.
The question of whether true randomness exists in nature is metaphysically controversial because some physicists and philosophers argue that nothing happens by pure chance [ 64 ]. We do not need to delve into this issue here, since most people agree that the distinction can be viewed as epistemic and not metaphysical, that is, an error is systemic or random relative to our knowledge about the generation of the error.
Some of the most well-known cases of bias involved the use of AI systems by private companies. For example, Amazon stopped using an AI hiring tool in 2018 after it discovered that the tool was biased against women [ 57 ]. In 2021, Facebook faced public ridicule and shame for using image recognition software the labelled images of African American men as non-human primates [ 117 ]. In 2021, Zillow lost hundreds of millions of dollars because its algorithm systematically overestimated the market value of homes the company purchased [ 170 ].
Fake citations and factual errors made by LLMs are often referred to as ‘hallucinations.’ We prefer not to use this term because it ascribes mental states to AI.
An additional, and perhaps more concerning, issue is that using chatbots to review the literature contributes to the deskilling of humanity because it involves trusting an AI’s interpretation and synthesis of the literature instead of reading it and thinking about it for oneself. Since deskilling is a problem with many different applications of AI, we will not explore it in depth here. See Vallor [ 226 ].
We are assuming here that the engineer or scientist has access to the computer code and training data, which private companies may to be loath to provide. For example, developers at OpenAI and Google have not provided the public with access to their training data and code [ 130 ].
Although our discussion of the black box problem focuses on ML, in theory this problem could arise in any type of AI in which its workings cannot be understood by human beings.
Galileo had to convince his critics that his telescope could be trusted to convey reliable information about heavenly bodies, such as the moon and Jupiter. Explaining how the telescope works and comparing it to the human eye played an important role in his defense of the instrument [ 36 ].
This response may also conflate trust with verification. According to some theories of trust, if you trust something, you do not need to continually verify it. If I trust someone to tell me the truth, I do not need to continually verify that they are telling the trust. Indeed, it seems that we verify because we do not trust. For further discussion, see McLeod [ 145 ].
One could argue that deviation from honesty might be justified to protect human research subjects in some situations. For example, pseudonyms are often used in qualitative social/behavioral research to refer to participants or communities in order to protect their privacy [ 92 ].
Sanitizing LLMs is a form of censorship, which may be necessary in some cases, but also carries significant risks for freedom of expression [ 236 ].
While public, community, and stakeholder engagement is widely accepted as important for promoting trust in science and technology but can be difficult to implement, especially since publics, communities, and stakeholders can be difficult to identify and may have conflicting interests [ 157 ].
US federal policy defines research misconduct as data fabrication or falsification or plagiarism [ 168 ].
While the difference between recklessness and negligence can be difficult to ascertain, one way of thinking of recklessness is that it involves an indifference to or disregard for the veracity or integrity of research. Although almost all misconduct findings claim that the accused person (or respondent) acted intentionally, knowingly, or recklessly, there have been a few cases in which the respondent was found only to have acted recklessly [ 42 , 193 , 194 ].
The distinction between synthetic and real data raises some interesting and important philosophical and policy issues that we will examine in more depth in future work.
Some editors and publishers have been using AI to review and screen journal submissions [ 35 , 212 ]. For a discussion of issues raised by using AI in peer review, see Hosseini and Horbach [ 98 , 99 ].
This issue reminds us of the scene in 2001: A Space Odyssey in which the human astronauts ask the ship’s computer, HAL, to explain why it incorrectly diagnosed a problem with the AE-35 unit. HAL responds that HAL 9000 computers have never made an error so the misdiagnosis must be due to human error.
Aboumatar, H., Thompson, C., Garcia-Morales, E., Gurses, A.P., Naqibuddin, M., Saunders, J., Kim, S.W., Wise, R.: Perspective on reducing errors in research. Contemp. Clin. Trials Commun. 23 , 100838 (2021)
Article Google Scholar
Alberts, B., Johnson, A., Lewis, J., Raff, M., Roberts, K., Walters, P.: Molecular Biology of the Cell, 4th edn. Garland Science, New York and London (2002)
Google Scholar
Ali, R., Connolly, I.D., Tang, O.Y., Mirza, F.N., Johnston, B., Abdulrazeq, H.F., Galamaga, P.F., Libby, T.J., Sodha, N.R., Groff, M.W., Gokaslan, Z.L., Telfeian, A.E., Shin, J.H., Asaad, W.F., Zou, J., Doberstein, C.E.: Bridging the literacy gap for surgical consents: an AI-human expert collaborative approach. NPJ Digit. Med. 7 (1), 63 (2024)
All European Academies.: The European Code of Conduct for Research Integrity, Revised Edition 2023 (2023). https://allea.org/code-of-conduct/
Allyn, B.: The Google engineer who sees company's AI as 'sentient' thinks a chatbot has a soul. NPR (2022). https://www.npr.org/2022/06/16/1105552435/google-ai-sentient
Alvarado, R.: Should we replace radiologists with deep learning? Bioethics 36 (2), 121–133 (2022)
Alvarado, R.: What kind of trust does AI deserve, if any? AI Ethics (2022). https://doi.org/10.1007/s43681-022-00224-x
Alvarado, R.: Computer simulations as scientific instruments. Found. Sci. 27 (3), 1183–1205 (2022)
Article MathSciNet Google Scholar
Alvarado, R.: AI as an epistemic technology. Sci. Eng. Ethics 29 , 32 (2023)
American Society of Microbiology.: Code of Conduct (2021). https://asm.org/Articles/Ethics/COEs/ASM-Code-of-Ethics-and-Conduct
Ankarstad, A.: What is explainable AI (XAI)? Towards Data Science (2020). https://towardsdatascience.com/what-is-explainable-ai-xai-afc56938d513
Antun, V., Renna, F., Poon, C., Adcock, B., Hansen, A.C.: On instabilities of deep learning in image reconstruction and the potential costs of AI. Proc. Natl. Acad. Sci. U.S.A. 117 (48), 30088–30095 (2020)
Assael, Y., Sommerschield, T., Shillingford, B., Bordbar, M., Pavlopoulos, J., Chatzipanagiotou, M., Androutsopoulos, I., Prag, J., de Freitas, N.: Restoring and attributing ancient texts using deep neural networks. Nature 603 , 280–283 (2022)
Babu, N.V., Kanaga, E.G.M.: Sentiment analysis in social media data for depression detection using artificial intelligence: a review. SN Comput. Sci. 3 , 74 (2022)
Badini, S., Regondi, S., Pugliese, R.: Unleashing the power of artificial intelligence in materials design. Materials 16 (17), 5927 (2023). https://doi.org/10.3390/ma16175927
Ball, P.: Is AI leading to a reproducibility crisis in science? Nature 624 , 22–25 (2023)
Barrera, F.J., Brown, E.D.L., Rojo, A., Obeso, J., Plata, H., Lincango, E.P., Terry, N., Rodríguez-Gutiérrez, R., Hall, J.E., Shekhar, S.: Application of machine learning and artificial intelligence in the diagnosis and classification of polycystic ovarian syndrome: a systematic review. Front. Endocrinol. (2023). https://doi.org/10.3389/fendo.2023.1106625
Bartosz, B.B., Bartosz, J.: Can artificial intelligences be moral agents? New Ideas Psychol. 54 , 101–106 (2019)
Baum, J., Villasenor, J.: The politics of AI: ChatGPT and political biases. Brookings (2023). https://www.brookings.edu/articles/the-politics-of-ai-chatgpt-and-political-bias/
BBC News.: Alexa tells 10-year-old girl to touch live plug with penny. BBC News (2021). https://www.bbc.com/news/technology-59810383
Begus, G., Sprouse, R., Leban, A., Silva, M., Gero, S.: Vowels and diphthongs in sperm whales (2024). https://doi.org/10.31219/osf.io/285cs
Bevier, C.: ChatGPT broke the Turing test—the race is on for new ways to assess AI. Nature (2023). https://www.nature.com/articles/d41586-023-02361-7
Bevier, C.: The easy intelligence test that AI chatbots fail. Nature 619 , 686–689 (2023)
Bhattacharyya, M., Miller, V.M., Bhattacharyya, D., Miller, L.E.: High rates of fabricated and inaccurate references in ChatGPT-generated medical content. Cureus 15 (5), e39238 (2023)
Biddle, S.: The internet’s new favorite AI proposes torturing Iranians and surveilling mosques. The Intercept (2022). https://theintercept.com/2022/12/08/openai-chatgpt-ai-bias-ethics/
Bird, S.J., Housman, D.E.: Trust and the collection, selection, analysis and interpretation of data: a scientist’s view. Sci. Eng. Ethics 1 (4), 371–382 (1995)
Biology for Life.: n.d. https://www.biologyforlife.com/error-analysis.html
Blumauer, A.: How ChatGPT works and the problems with non-explainable AI. Pool Party (2023). https://www.poolparty.biz/blogposts/how-chat-gpt-works-non-explainable-ai#:~:text=ChatGPT%20is%20the%20antithesis%20of,and%20explainability%20are%20critical%20requirements
Bogost, I.: ChatGPT is dumber than you think. The Atlantic (2022). https://www.theatlantic.com/technology/archive/2022/12/chatgpt-openai-artificial-intelligencewriting-ethics/672386/
Bolanos, F., Salatino, A., Osborne, F., Motta, E.: Artificial intelligence for literature reviews: opportunities and challenges (2024). arXiv:2402.08565
Bordukova, M., Makarov, N., Rodriguez-Esteban, P., Schmich, F., Menden, M.P.: Generative artificial intelligence empowers digital twins in drug discovery and clinical trials. Expert Opin. Drug Discov. 19 (1), 33–42 (2024)
Borowiec, M.L., Dikow, R.B., Frandsen, P.B., McKeeken, A., Valentini, G., White, A.E.: Deep learning as a tool for ecology and evolution. Methods Ecol. Evol. 13 (8), 1640–1660 (2022)
Bostrom, N.: Superintelligence: Paths, Dangers, Strategies. Oxford University Press, Oxford (2014)
Bothra, A., Cao, Y., Černý, J., Arora, G.: The epidemiology of infectious diseases meets AI: a match made in heaven. Pathogens 12 (2), 317 (2023)
Brainard, J.: As scientists face a flood of papers, AI developers aim to help. Science (2023). https://www.science.org/content/article/scientists-face-flood-papers-ai-developers-aim-help
Brown, H.I.: Galileo on the telescope and the eye. J. Hist. Ideas 46 (4), 487–501 (1985)
Brumfiel, G.: New proteins, better batteries: Scientists are using AI to speed up discoveries. NPR (2023). https://www.npr.org/sections/health-shots/2023/10/12/1205201928/artificial-intelligence-ai-scientific-discoveries-proteins-drugs-solar
Brunello, N.: Example of a deep neural network (2021). https://commons.wikimedia.org/wiki/File:Example_of_a_deep_neural_network.png
Burrell, J.: How the machine ‘thinks’: understanding opacity in machine learning algorithms. Big Data Soc. 3 (1), 2053951715622512 (2016)
Calder, T.: The concept of evil. Stanford Encyclopedia of Philosophy (2022). https://plato.stanford.edu/entries/concept-evil/#KanTheEvi
Callaway, A.: ‘The entire protein universe’: AI predicts shape of nearly every known protein. Nature 608 , 14–16 (2022)
Caron, M.M., Dohan, S.B., Barnes, M., Bierer, B.E.: Defining "recklessness" in research misconduct proceedings. Accountability in Research, pp. 1–23 (2023)
Castelvecchi, D.: AI chatbot shows surprising talent for predicting chemical properties and reactions. Nature (2024). https://www.nature.com/articles/d41586-024-00347-7
CBS News.: ChatGPT and large language model bias. CBS News (2023). https://www.cbsnews.com/news/chatgpt-large-language-model-bias-60-minutes-2023-03-05/
CC BY-SA 4.0 DEED.: Amino-acid chains, known as polypeptides, fold to form a protein (2020). https://en.wikipedia.org/wiki/AlphaFold#/media/File:Protein_folding_figure.png
Cervantes, J.A., López, S., Rodríguez, L.F., Cervantes, S., Cervantes, F., Ramos, F.: Artificial moral agents: a survey of the current status. Sci. Eng. Ethics 26 (2), 501–532 (2020)
Chan, B.: Black-box assisted medical decisions: AI power vs. ethical physician care. Med. Health Care Philos. 26 , 285–292 (2023)
ChatGPT, Zhavoronkov, A.: Rapamycin in the context of Pascal’s Wager: generative pre-trained transformer perspective. Oncoscience 9 , 82–84 (2022)
Chatterjee, M.: AI cannot hold copyright, federal judge rules. Politico (2023). https://www.politico.com/news/2023/08/21/ai-cannot-hold-copyright-federal-judge-rules-00111865#:~:text=Friday's%20ruling%20will%20be%20a%20critical%20component%20in%20future%20legal%20fights.&text=Artificial%20intelligence%20cannot%20hold%20a,a%20federal%20judge%20ruled%20Friday
Chen, R.J., Lu, M.Y., Chen, T.Y., Williamson, D.F., Mahmood, F.: Synthetic data in machine learning for medicine and healthcare. Nat. Biomed. Eng. 5 , 493–497 (2021)
Chen, S., Kann, B.H., Foote, M.B., Aerts, H.J.W.L., Savova, G.K., Mak, R.H., Bitterman, D.S.: Use of artificial intelligence chatbots for cancer treatment information. JAMA Oncol. 9 (10), 1459–1462 (2023)
Cyrus, L.: How to fold graciously. In: Mossbauer Spectroscopy in Biological Systems: Proceedings of a Meeting Held at Allerton House, Monticello, Illinois, pp. 22–24 (1969)
Conroy, G.: Scientists used ChatGPT to generate an entire paper from scratch—but is it any good? Nature 619 , 443–444 (2023)
Conroy, G.: How ChatGPT and other AI tools could disrupt scientific publishing. Nature (2023). https://www.nature.com/articles/d41586-023-03144-w
Dai, B., Xu, Z., Li, H., Wang, B., Cai, J., Liu, X.: Racial bias can confuse AI for genomic studies. Oncologie 24 (1), 113–130 (2022)
Daneshjou, R., Smith, M.P., Sun, M.D., Rotemberg, V., Zou, J.: Lack of transparency and potential bias in artificial intelligence data sets and algorithms: a scoping review. JAMA Dermatol. 157 (11), 1362–1369 (2021)
Dastin, J.: Amazon scraps secret AI recruiting tool that showed bias against women. Reuters (2018). https://www.reuters.com/article/us-amazon-com-jobs-automation-insight-idUSKCN1MK08G
de Bruijn, H., Warnier, M., Janssen, M.: The perils and pitfalls of explainable AI: strategies for explaining algorithmic decision-making. Gov. Inf. Q. 39 (2), 101666 (2022)
Delua, J.: Supervised vs. unsupervised learning: What’s the difference? IBM (2021). https://www.ibm.com/blog/supervised-vs-unsupervised-learning/
Dhinakaran, A.: Overcoming AI’s transparency paradox. Forbes (2021). https://www.forbes.com/sites/aparnadhinakaran/2021/09/10/overcoming-ais-transparency-paradox/?sh=6c6b18834b77
Dickson, B.: LLMs can’t self-correct in reasoning tasks, DeepMind study finds. Tech Talks (2023). https://bdtechtalks.com/2023/10/09/llm-self-correction-reasoning-failures
Dunlap, T.: Artificial intelligence (AI) as an inventor? Dunlap, Bennett and Ludwig (2023). https://www.dbllawyers.com/artificial-intelligence-as-an-inventor/
Durán, J.M., Jongsma, K.R.: Who is afraid of black box algorithms? On the epistemological and ethical basis of trust in medical AI. J. Med. Ethics 47 (5), 329–335 (2021)
Einstein, A.: Letter to Max Born. Walker and Company, New York (1926). Published in: Irene Born (translator), The Born-Einstein Letters (1971)
Eisenstein, M.: Teasing images apart, cell by cell. Nature 623 , 1095–1097 (2023)
Eliot, L.: Nobody can explain for sure why ChatGPT is so good at what it does, troubling AI ethics and AI Law. Forbes (2023). https://www.forbes.com/sites/lanceeliot/2023/04/17/nobody-can-explain-for-sure-why-chatgpt-is-so-good-at-what-it-does-troubling-ai-ethics-and-ai-law/?sh=334c95685041
Eliot, L.: Generative AI ChatGPT can disturbingly gobble up your private and confidential data, forewarns AI ethics and AI law. Forbes (2023). https://www.forbes.com/sites/lanceeliot/2023/01/27/generative-ai-chatgpt-can-disturbingly-gobble-up-your-private-and-confidential-data-forewarns-ai-ethics-and-ai-law/?sh=592b16547fdb
Elliott, K.C., Resnik, D.B.: Making open science work for science and society. Environ. Health Perspect. 127 (7), 75002 (2019)
Euro News.: Man ends his life after an AI chatbot 'encouraged' him to sacrifice himself to stop climate change. Euro News (2023). https://www.euronews.com/next/2023/03/31/man-ends-his-life-after-an-ai-chatbot-encouraged-him-to-sacrifice-himself-to-stop-climate
European Agency for Fundamental Rights.: Data quality and Artificial Intelligence—Mitigating Bias and Error to Protect Fundamental Rights (2019). https://fra.europa.eu/sites/default/files/fra_uploads/fra-2019-data-quality-and-ai_en.pdf
Evans, K., de Moura, N., Chauvier, S., Chatila, R., Dogan, E.: Ethical decision making in autonomous vehicles: the AV ethics project. Sci. Eng. Ethics 26 , 3285–3312 (2020)
Extance, A.: How AI technology can tame the scientific literature. Nature (2018). https://www.nature.com/articles/d41586-018-06617-5
Fanelli, D.: How many scientists fabricate and falsify research? A systematic review and meta-analysis of survey data. PLoS ONE 4 (5), e5738 (2009)
Food and Drug Administration.: Artificial intelligence (AI) and machine learning (ML) in medical devices (2020). https://www.fda.gov/media/142998/download
Food and Drug Administration.: Development and approval process: drugs (2023). https://www.fda.gov/drugs/development-approval-process-drugs
Fraenkel, A.S.: Complexity of protein folding. Bull. Math. Biol. 55 (6), 1199–1210 (1993)
Fuhrman, J.D., Gorre, N., Hu, Q., Li, H., El Naqa, I., Giger, M.L.: A review of explainable and interpretable AI with applications in COVID-19 imaging. Med. Phys. 49 (1), 1–14 (2022)
Garin, S.P., Parekh, V.S., Sulam, J., Yi, P.H.: Medical imaging data science competitions should report dataset demographics and evaluate for bias. Nat. Med. 29 (5), 1038–1039 (2023)
Giere, R., Bickle, J., Maudlin, R.F.: Understanding Scientific Reasoning, 5th edn. Wadsworth, Belmont (2005)
Gillispie, C.C.: The Edge of Objectivity. Princeton University Press, Princeton (1960)
Giuffrè, M., Shung, D.L.: Harnessing the power of synthetic data in healthcare: innovation, application, and privacy. NPJ Digit. Med. 6 , 186 (2023)
Godwin, R.C., Bryant, A.S., Wagener, B.M., Ness, T.J., DeBerryJJ, H.L.L., Graves, S.H., Archer, A.C., Melvin, R.L.: IRB-draft-generator: a generative AI tool to streamline the creation of institutional review board applications. SoftwareX 25 , 101601 (2024)
Google.: Responsible AI practices (2023). https://ai.google/responsibility/responsible-ai-practices/
Goldman, A.I.: Liaisons: philosophy meets the cognitive and social sciences. MIT Press, Cambridge (2003)
Grad, P.: Trick prompts ChatGPT to leak private data. TechXplore (2023). https://techxplore.com/news/2023-12-prompts-chatgpt-leak-private.html
Graziani, M., Dutkiewicz, L., Calvaresi, D., Amorim, J.P., Yordanova, K., Vered, M., Nair, R., Abreu, P.H., Blanke, T., Pulignano, V., Prior, J.O., Lauwaert, L., Reijers, W., Depeursinge, A., Andrearczyk, V., Müller, H.: A global taxonomy of interpretable AI: unifying the terminology for the technical and social sciences. Artif. Intell. Rev. 56 , 3473–3504 (2023)
Guinness, H.: The best AI image generators in 2023. Zappier (2023). https://zapier.com/blog/best-ai-image-generator/
Gulshan, V., Peng, L., Coram, M., Stumpe, M.C., Wu, D., Narayanaswamy, A., Venugopalan, S., Widner, K., Madams, T., Cuadros, J., Kim, R., Raman, R., Nelson, P.C., Mega, J.L., Webster, D.R.: Development and validation of a deep learning algorithm for detection of diabetic retinopathy in retinal fundus photographs. JAMA 316 (22), 2402–2410 (2016)
Haack, S.: Defending Science within Reason. Prometheus Books, New York (2007)
Hackernoon.: (2024). https://hackernoon.com/the-times-v-microsoftopenai-unauthorized-reproduction-of-times-works-in-gpt-model-training-10
Hagendorff, T., Fabi, S., Kosinski, M.: Human-like intuitive behavior and reasoning biases emerged in large language models but disappeared in ChatGPT. Nat. Comput. Sci. (2023). https://doi.org/10.1038/s43588-023-00527-x
Heaton, J.: “*Pseudonyms are used throughout”: a footnote, unpacked. Qual. Inq. 1 , 123–132 (2022)
Heikkilä, M.: How OpenAI is trying to make ChatGPT safer and less biased. The Atlantic (2023). https://www.technologyreview.com/2023/02/21/1068893/how-openai-is-trying-to-make-chatgpt-safer-and-less-biased/
Helmenstine, A.: Systematic vs random error—differences and examples. Science Notes (2021). https://sciencenotes.org/systematic-vs-random-error-differences-and-examples/
Himma, K.E.: Artificial agency, consciousness, and the criteria for moral agency: what properties must an artificial agent have to be a moral agent? Ethics Inf. Technol. 11 , 19–29 (2009)
Holzinger, A., Langs, G., Denk, H., Zatloukal, K., Müller, H.: Causability and explainability of artificial intelligence in medicine. Wires (2019). https://doi.org/10.1002/widm.1312
Hosseini, M., Holmes, K.: Is it ethical to use generative AI if you can’t tell whether it is right or wrong? [Blog Post]. Impact of Social Sciences(2024). https://blogs.lse.ac.uk/impactofsocialsciences/2024/03/15/is-it-ethical-to-use-generative-ai-if-you-cant-tell-whether-it-is-right-or-wrong/
Hosseini, M., Horbach, S.P.J.M.: Fighting reviewer fatigue or amplifying bias? Considerations and recommendations for use of ChatGPT and other large language models in scholarly peer review. Res. Integr. Peer Rev. 8 (1), 4 (2023)
Hosseini, M., Horbach, S.P.J.M.: Can generative AI add anything to academic peer review? [Blog Post] Impact of Social Sciences(2023). https://blogs.lse.ac.uk/impactofsocialsciences/2023/09/26/can-generative-ai-add-anything-to-academic-peer-review/
Hosseini, M., Senabre Hidalgo, E., Horbach, S.P.J.M., Güttinger, S., Penders, B.: Messing with Merton: the intersection between open science practices and Mertonian values. Accountability in Research, pp. 1–28 (2022)
Hosseini, M., Rasmussen, L.M., Resnik, D.B.: Using AI to write scholarly publications. Accountability in Research, pp. 1–9 (2023)
Hosseini, M., Resnik, D.B., Holmes, K.: The ethics of disclosing the use of artificial intelligence in tools writing scholarly manuscripts. Res. Ethics (2023). https://doi.org/10.1177/17470161231180449
Hosny, A., Parmar, C., Quackenbush, J., Schwartz, L.H., Aerts, H.J.W.L.: Artificial intelligence in radiology. Nat. Rev. Cancer 18 (8), 500–510 (2018)
Howson, C., Urbach, P.: Scientific Reasoning: A Bayesian Approach, 3rd edn. Open Court, New York (2005)
Humphreys, P.: Extending Ourselves: Computational Science, Empiricism, and Scientific Method. Oxford University Press, New York (2004)
Book Google Scholar
Huo, T., Li, L., Chen, X., Wang, Z., Zhang, X., Liu, S., Huang, J., Zhang, J., Yang, Q., Wu, W., Xie, Y., Wang, H., Ye, Z., Deng, K.: Artificial intelligence-aided method to detect uterine fibroids in ultrasound images: a retrospective study. Sci. Rep. 13 (1), 3714 (2023)
Hutson. M.: Hypotheses devised by AI could find ‘blind spots’ in research. Nature (2023). https://www.nature.com/articles/d41586-023-03596
IBM.: What is AI? (2023). https://www.ibm.com/topics/artificial-intelligence
IBM.: What is a Captcha? (2023). https://www.ibm.com/topics/captcha
IBM.: Explainable AI (2023). https://www.ibm.com/topics/explainable-ai
IBM.: What is generative AI? (2023). https://research.ibm.com/blog/what-is-generative-AI
IBM.: What is ML? (2024). https://www.ibm.com/topics/machine-learning
International Committee of Medical Journal Editors.: Recommendations for the Conduct, Reporting, Editing, and Publication of Scholarly work in Medical Journals (2023). https://www.icmje.org/icmje-recommendations.pdf
International Organization for Standardization.: What is AI? (2024). https://www.iso.org/artificial-intelligence/what-is-ai#:~:text=Artificial%20intelligence%20is%20%E2%80%9Ca%20technical,%2FIEC%2022989%3A2022%5D
Janowicz, K., Gao, S., McKenzie, G., Hu, Y., Bhaduri, B.: GeoAI: spatially explicit artificial intelligence techniques for geographic knowledge discovery and beyond. Int. J. Geogr. Inf. Sci. 34 (4), 625–636 (2020)
Jenkins, R., Lin, P.:. AI-assisted authorship: How to assign credit in synthetic scholarship. SSRN Scholarly Paper No. 4342909 (2023). https://doi.org/10.2139/ssrn.4342909
Jones, D.: Facebook apologizes after its AI labels black men as 'primates'. NPR (2021). https://www.npr.org/2021/09/04/1034368231/facebook-apologizes-ai-labels-black-men-primates-racial-bias
Jumper, J., Evans, R., Pritzel, A., Green, T., Figurnov, M., Ronneberger, O., Tunyasuvunakool, K., Bates, R., Žídek, A., Potapenko, A., Bridgland, A., Meyer, C., Kohl, S.A.A., Ballard, A.J., Cowie, A., Romera-Paredes, B., Nikolov, S., Jain, R., Adler, J., Back, T., Petersen, S., Reiman, D., Clancy, E., Zielinski, M., Steinegger, M., Pacholska, M., Berghammer, T., Bodenstein, S., Silver, D., Vinyals, O., Senior, A.W., Kavukcuoglu, K., Kohli, P., Hassabis, D.: Highly accurate protein structure prediction with AlphaFold. Nature 596 (7873), 583–589 (2021)
Junction AI.: What is ChatGPT not good at? Junction AI (2023). https://junction.ai/what-is-chatgpt-not-good-at/
Kahn, J.: What wrong with “explainable A.I.” Fortune (2022). https://fortune.com/2022/03/22/ai-explainable-radiology-medicine-crisis-eye-on-ai/
Kahneman, D.: Thinking, Fast and Slow. Farrar, Straus, Giroux, New York (2011)
Kembhavi, A., Pattnaik, R.: Machine learning in astronomy. J. Astrophys. Astron. 43 , 76 (2022)
Kennedy, B., Tyson, A., Funk, C.: Americans’ trust in scientists, other groups declines. Pew Research Center (2022). https://www.pewresearch.org/science/2022/02/15/americans-trust-in-scientists-other-groups-declines/
Kim, I., Kang, K., Song, Y., Kim, T.J.: Application of artificial intelligence in pathology: trends and challenges. Diagnostics (Basel) 12 (11), 2794 (2022)
Kitcher, P.: The Advancement of Knowledge. Oxford University Press, New York (1993)
Krenn, M., Pollice, R., Guo, S.Y., Aldeghi, M., Cervera-Lierta, A., Friederich, P., Gomes, G.P., Häse, F., Jinich, A., Nigam, A., Yao, Z., Aspuru-Guzik, A.: On scientific understanding with artificial intelligence. Nat. Rev. Phys. 4 , 761–769 (2022)
Kruse, C.S., Smith, B., Vanderlinden, H., Nealand, A.: Security techniques for the electronic health records. J. Med. Syst. 41 (8), 127 (2017)
Kuhn, T.S.: The Essential Tension. University of Chicago Press, Chicago (1977)
Lal, A., Pinevich, Y., Gajic, O., Herasevich, V., Pickering, B.: Artificial intelligence and computer simulation models in critical illness. World Journal of Critical Care Medicine 9 (2), 13–19 (2020)
La Malfa, E., Petrov, A., Frieder, S., Weinhuber, C., Burnell, R., Cohn, A.G., Shadbolt, N., Woolridge, M.: The ARRT of language-models-as-a-service: overview of a new paradigm and its challenges (2023). arXiv: 2309.16573
Larkin, Z.: AI bias—what Is it and how to avoid it? Levity (2022). https://levity.ai/blog/ai-bias-how-to-avoid
Lee, N.T., Resnick, P., Barton, G.: Algorithmic Bias Detection and Mitigation: Best Practices and Policies to Reduce Consumer Harms. Brookings Institute, Washington, DC (2019)
Leswing, K.: OpenAI announces GPT-4, claims it can beat 90% of humans on the SAT. CNBC (2023). https://www.cnbc.com/2023/03/14/openai-announces-gpt-4-says-beats-90percent-of-humans-on-sat.html
Licht, K., Licht, J.: Artificial intelligence, transparency, and public decision-making: Why explanations are key when trying to produce perceived legitimacy. AI Soc. 35 , 917–926 (2020)
Lipenkova, J.: Overcoming the limitations of large language models: how to enhance LLMs with human-like cognitive skills. Towards Data Science (2023). https://towardsdatascience.com/overcoming-the-limitations-of-large-language-models-9d4e92ad9823
London, A.J.: Artificial intelligence and black-box medical decisions: accuracy versus explainability. Hastings Cent. Rep. 49 (1), 15–21 (2019)
Longino, H.: Science as Social Knowledge. Princeton University Press, Princeton (1990)
Lubell, J.: ChatGPT passed the USMLE. What does it mean for med ed? AMA (2023). https://www.ama-assn.org/practice-management/digital/chatgpt-passed-usmle-what-does-it-mean-med-ed
Martinho, A., Poulsen, A., Kroesen, M., Chorus, C.: Perspectives about artificial moral agents. AI Ethics 1 , 477–490 (2021)
Martinson, B.C., Anderson, M.S., de Vries, R.: Scientists behaving badly. Nature 435 (7043), 737–738 (2005)
Martins, C., Padovan, P., Reed, C.: The role of explainable AI (XAI) in addressing AI liability. SSRN (2020). https://ssrn.com/abstract=3751740
Matta, V., Bansal, G., Akakpo, F., Christian, S., Jain, S., Poggemann, D., Rousseau, J., Ward, E.: Diverse perspectives on bias in AI. J. Inf. Technol. Case Appl. Res. 24 (2), 135–143 (2022)
Matthewson, J.: Trade-offs in model-building: a more target-oriented approach. Stud. Hist. Philos. Sci. Part A 42 (2), 324–333 (2011)
McCarthy, J.: What is artificial intelligence? (2007). https://www-formal.stanford.edu/jmc/whatisai.pdf
McLeod, C.: Trust. Stanford Encyclopedia of Philosophy (2020). https://plato.stanford.edu/entries/trust/
Merken, S.: Another US judge says lawyers must disclose AI use. Reuters (2023). https://www.reuters.com/legal/transactional/another-us-judge-says-lawyers-must-disclose-ai-use-2023-06-08/
Merton, R.: The Sociology of Science. University of Chicago Press, Chicago (1973)
Messeri, L., Crockett, M.J.: Artificial intelligence and illusions of understanding in scientific research. Nature (2024). https://doi.org/10.1038/s41586-024-07146-0
Mieth, B., Rozier, A., Rodriguez, J.A., Höhne, M.M., Görnitz, N., Müller, R.K.: DeepCOMBI: explainable artificial intelligence for the analysis and discovery in genome-wide association studies. NAR Genom. Bioinform. 3 (3), lqab065 (2021)
Milmo, D.: Two US lawyers fined for submitting fake court citations from ChatGPT. The Guardian (2023). https://www.theguardian.com/technology/2023/jun/23/two-us-lawyers-fined-submitting-fake-court-citations-chatgpt
Mitchell, M.: Artificial Intelligence. Picador, New York (2019)
Mitchell, M.: What does it mean for AI to understand? Quanta Magazine (2021). https://www.quantamagazine.org/what-does-it-mean-for-ai-to-understand-20211216/
Mitchell, M.: AI’s challenge of understanding the world. Science 382 (6671), eadm8175 (2023)
Mittermaier, M., Raza, M.M., Kvedar, J.C.: Bias in AI-based models for medical applications: challenges and mitigation strategies. NPJ Digit. Med. 6 , 113 (2023)
Naddaf, M.: ChatGPT generates fake data set to support scientific hypothesis. Nature (2023). https://www.nature.com/articles/d41586-023-03635-w#:~:text=Researchers%20say%20that%20the%20model,doesn't%20pass%20for%20authentic
Nahas, K.: Now AI can be used to generate proteins. The Scientist (2023). https://www.the-scientist.com/news-opinion/now-ai-can-be-used-to-design-new-proteins-70997
National Academies of Sciences, Engineering, and Medicine: Gene Drives on the Horizon: Advancing Science, Navigating Uncertainty, and Aligning Research with Public Values. National Academies Press, Washington, DC (2016)
National Institutes of Health.: Guidelines for the Conduct of Research in the Intramural Program of the NIH (2023). https://oir.nih.gov/system/files/media/file/2023-11/guidelines-conduct_research.pdf
National Institutes of Health.: The use of generative artificial intelligence technologies is prohibited for the NIH peer review process. NOT-OD-23-149 (2023). https://grants.nih.gov/grants/guide/notice-files/NOT-OD-23-149.html
National Transportation and Safety Board.: Investigations (2023). https://www.ntsb.gov/investigations/Pages/Investigations.aspx
Nawaz, M.S., Fournier-Viger, P., Shojaee, A., Fujita, H.: Using artificial intelligence techniques for COVID-19 genome analysis. Appl. Intell. (Dordrecht) 51 (5), 3086–3103 (2021)
Ng, G.W., Leung, W.C.: Strong artificial intelligence and consciousness. J. Artif. Intell. Conscious. 7 (1), 63–72 (2020)
Nordling, L.: How ChatGPT is transforming the postdoc experience. Nature 622 , 655–657 (2023)
Nost, E., Colven, E.: Earth for AI: a political ecology of data-driven climate initiatives. Geoforum 130 , 23–34 (2022)
Ntoutsi, E., Fafalios, P., Gadiraju, U., Iosifidis, V., Nejdl, W., Vidal, M.E., Ruggieri, S., Turini, F., Papadopoulos, S., Krasanakis, E., Kompatsiaris, I., Kinder-Kurlanda, K., Wagner, C., Karimi, F., Fernandez, M., Alani, H., Berendt, B., Kruegel, T., Heinze, C., Broelemann, K., Kasneci, K., Tiropanis, T., Staab, S.: Bias in data-driven artificial intelligence systems—an introductory survey. Wires (2020). https://doi.org/10.1002/widm
O’Connor, S.: Corrigendum to “Open artificial intelligence platforms in nursing education: tools for academic progress or abuse?” [Nurse Educ. Pract. 66 (2023) 103537]. Nurse Educ. Pract. 67 , 103572 (2023)
O’Connor, S., ChatGPT: Open artificial intelligence platforms in nursing education: tools for academic progress or abuse? Nurse Educ. Pract. 66 , 103537 (2023)
Office of Science and Technology Policy: Federal research misconduct policy. Fed. Reg. 65 (235), 76260–76264 (2000)
Office and Science and Technology Policy.: Blueprint for an AI Bill of Rights (2022). https://www.whitehouse.gov/ostp/ai-bill-of-rights/
Olavsrud, T.: 9 famous analytics and AI disasters. CIO (2023). https://www.cio.com/article/190888/5-famous-analytics-and-ai-disasters.html
Omiye, J.A., Lester, J.C., Spichak, S., Rotemberg, V., Daneshjou, R.: Large language models propagate race-based medicine. NPJ Digit. Med. 6 , 195 (2023)
Oncology Medical Physics.: Accuracy, precision, and error (2024). https://oncologymedicalphysics.com/quantifying-accuracy-precision-and-error/
OpenAI.: (2023). https://openai.com/chatgpt
Osoba, O., Welser, W.: An Intelligence in Our Image: The Risks of Bias and Errors in Artificial Intelligence. Rand Corporation (2017). https://www.rand.org/content/dam/rand/pubs/research_reports/RR1700/RR1744/RAND_RR1744.pdf
Othman, K.: Public acceptance and perception of autonomous vehicles: a comprehensive review. AI Ethics 1 , 355–387 (2021)
Ovchinnikov, S., Park, H., Varghese, N., Huang, P.S., Pavlopoulos, G.A., Kim, D.E., Kamisetty, H., Kyrpides, N.C., Baker, D.: Protein structure determination using metagenome sequence data. Science 355 (6322), 294–298 (2017)
Parikh, R.B., Teeple, S., Navathe, A.S.: Addressing bias in artificial intelligence in health care. J. Am. Med. Assoc. 322 (24), 2377–2378 (2019)
Parrilla, J.M.: ChatGPT use shows that the grant-application system is broken. Nature (2023). https://www.nature.com/articles/d41586-023-03238-5
Pearson, J.: Scientific Journal Publishes AI-Generated Rat with Gigantic Penis In Worrying Incident [Internet]. Vice (2024). https://www.vice.com/en/article/dy3jbz/scientific-journal-frontiers-publishes-ai-generated-rat-with-gigantic-penis-in-worrying-incident
Pennock, R.T.: An Instinct for Truth: Curiosity and the Moral Character of Science. MIT Press, Cambridge (2019)
Perni, S., Lehmann, L.S., Bitterman, D.S.: Patients should be informed when AI systems are used in clinical trials. Nat. Med. 29 (8), 1890–1891 (2023)
Perrigo, B.: OpenAI used Kenyan workers on less than $2 per hour to make ChatGPT less toxic. Time Magazine (2023). https://time.com/6247678/openai-chatgpt-kenya-workers/
Pew Charitable Trust.: How FDA regulates artificial intelligence in medical products. Issue brief (2021). https://www.pewtrusts.org/en/research-and-analysis/issue-briefs/2021/08/how-fda-regulates-artificial-intelligence-in-medical-products
Raeburn, A.: What’s the difference between accuracy and precision? Asana (2023). https://asana.com/resources/accuracy-vs-precision
Rasmussen, L.: Why and how to incorporate issues of race/ethnicity and gender in research integrity education. Accountability in Research (2023)
Ratti, E., Graves, M.: Explainable machine learning practices: opening another black box for reliable medical AI. AI Ethics 2 , 801–814 (2022)
Resnik, D.B.: Social epistemology and the ethics of research. Stud. Hist. Philos. Sci. 27 , 566–586 (1996)
Resnik, D.B.: The Price of Truth: How Money Affects the Norms of Science. Oxford University Press, New York (2007)
Resnik, D.B.: Playing Politics with Science: Balancing Scientific Independence and Government Oversight. Oxford University Press, New York (2009)
Resnik, D.B., Dinse, G.E.: Do U.S. research institutions meet or exceed federal mandates for instruction in responsible conduct of research? A national survey. Acad. Med. 87 , 1237–1242 (2012)
Resnik, D.B., Elliott, K.C.: Value-entanglement and the integrity of scientific research. Stud. Hist. Philos. Sci. 75 , 1–11 (2019)
Resnik, D.B., Elliott, K.C.: Science, values, and the new demarcation problem. J. Gen. Philos. Sci. 54 , 259–286 (2023)
Resnik, D.B., Elliott, K.C., Soranno, P.A., Smith, E.M.: Data-intensive science and research integrity. Account. Res. 24 (6), 344–358 (2017)
Resnik, D.B., Smith, E.M., Chen, S.H., Goller, C.: What is recklessness in scientific research? The Frank Sauer case. Account. Res. 24 (8), 497–502 (2017)
Roberts, M., Driggs, D., Thorpe, M., Gilbey, J., Yeung, M., Ursprung, S., Aviles-Rivero, A.I., Etmann, C., McCague, C., Beer, L., Weir-McCall, J.R., Teng, Z., Gkrania-Klotsas, E., AIX-COVNET, Rudd, J.H.F., Sala, E., Schönlieb, C.B.: Common pitfalls and recommendations for using machine learning to detect and prognosticate for COVID-19 using chest radiographs and CT scans. Nat. Mach. Intell. 3 , 199–217 (2021)
Rodgers, W., Murray, J.M., Stefanidis, A., Degbey, W.Y., Tarba, S.: An artificial intelligence algorithmic approach to ethical decision-making in human resource management processes. Hum. Resour. Manag. Rev. 33 (1), 100925 (2023)
Romero, A.: AI won’t master human language anytime soon. Towards Data Science (2021). https://towardsdatascience.com/ai-wont-master-human-language-anytime-soon-3e7e3561f943
Röösli, E., Rice, B., Hernandez-Boussard, T.: Bias at warp speed: how AI may contribute to the disparities gap in the time of COVID-19. J. Am. Med. Inform. Assoc. 28 (1), 190–192 (2021)
Savage, N.: Breaking into the black box of artificial intelligence. Nature (2022). https://www.nature.com/articles/d41586-022-00858-1
Savage, N.: Synthetic data could be better than real data. Nature (2023). https://www.nature.com/articles/d41586-023-01445-8
Schmidt, E.: This is how AI will transform the way science gets done. MIT Technology Review (2023). https://www.technologyreview.com/2023/07/05/1075865/eric-schmidt-ai-will-transform-science/#:~:text=AI%20can%20also%20spread%20the,promising%20candidates%20for%20new%20drugs
Schwartz, R., Vassilev, A., Greene, K., Perine, L., Burt, A., Hal, P.: Towards a standard for identifying and managing bias in artificial intelligence. National Institute of Standards and Technology (2022). https://view.ckcest.cn/AllFiles/ZKBG/Pages/264/c914336ac0e68a6e3e34187adf9dd83bb3b7c09f.pdf
Semler, J.: Artificial quasi moral agency. In: AIES '22: Proceedings of the 2022 AAAI/ACM Conference on AI, Ethics, and Society (2022). https://doi.org/10.1145/3514094.3539549
Service RF: The game has changed. AI trumphs at protein folding. Science 370 (6521), 1144–1145 (2022)
Service R.: Materials-predicting AI from DeepMind could revolutionize electronics, batteries, and solar cells. Science (2023). https://www.science.org/content/article/materials-predicting-ai-deepmind-could-revolutionize-electronics-batteries-and-solar
Seth, A.: Being You: A New Science of Consciousness. Faber and Faber, London (2021)
Shamoo, A.E., Resnik, D.B.: Responsible Conduct of Research, 4th edn. Oxford University Press, New York (2022)
Shapin, S.: Here and everywhere: sociology of scientific knowledge. Ann. Rev. Sociol. 21 , 289–321 (1995)
Solomon, M.: Social Empiricism. MIT Press, Cambridge (2007)
Southern, M.G.: ChatGPT update: Improved math capabilities. Search Engine Journal (2023). https://www.searchenginejournal.com/chatgpt-update-improved-math-capabilities/478057/
Straw, I., Callison-Burch, C.: Artificial Intelligence in mental health and the biases of language based models. PLoS ONE 15 (12), e0240376 (2020)
Swaak, T.: ‘We’re all using it’: Publishing decisions are increasingly aided by AI. That’s not always obvious. The Chronicle of Higher Education (2023). https://deal.town/the-chronicle-of-higher-education/academe-today-publishing-decisions-are-increasingly-aided-by-ai-but-thats-not-always-obvious-PK2J5KUC4
Talbert, M.: Moral responsibility. Stanford Encyclopedia of Philosophy (2019). https://plato.stanford.edu/entries/moral-responsibility/
Taloni, A., Scorcia, V., Giannaccre, G.: Large language model advanced data analysis abuse to create a fake data set in medical research. JAMA Ophthalmol. (2023). https://jamanetwork.com/journals/jamaophthalmology/fullarticle/2811505
Tambornino, L., Lanzerath, D., Rodrigues, R., Wright, D.: SIENNA D4.3: survey of REC approaches and codes for Artificial Intelligence & Robotics (2019). https://zenodo.org/records/4067990
Terwilliger, T.C., Liebschner, D., Croll, T.I., Williams, C.J., McCoy, A.J., Poon, B.K., Afonine, P.V., Oeffner, R.D., Richardson, J.S., Read, R.J., Adams, P.D.: AlphaFold predictions are valuable hypotheses and accelerate but do not replace experimental structure determination. Nat. Methods (2023). https://doi.org/10.1038/s41592-023-02087-4
The White House.: Biden-Harris administration secures voluntary commitments from leading artificial intelligence companies to manage the risks posed by AI (2023). https://www.whitehouse.gov/briefing-room/statements-releases/2023/07/21/fact-sheet-biden-harris-administration-secures-voluntary-commitments-from-leading-artificial-intelligence-companies-to-manage-the-risks-posed-by-ai/#:~:text=President%20Biden%20signed%20an%20Executive,the%20public%20from%20algorithmic%20discrimination
Thorp, H.H.: ChatGPT is fun, but not an author. Science 379 (6630), 313 (2023)
Turing.: Complete analysis of artificial intelligence vs artificial consciousness (2023). https://www.turing.com/kb/complete-analysis-of-artificial-intelligence-vs-artificial-consciousness
Turing, A.: Computing machinery and intelligence. Mind 59 (236), 433–460 (1950)
UK Statistic Authority.: Ethical considerations relating to the creation and use of synthetic data (2022). https://uksa.statisticsauthority.gov.uk/publication/ethical-considerations-relating-to-the-creation-and-use-of-synthetic-data/pages/2/
Unbable.: Why AI fails in the wild. Unbable (2019). https://resources.unbabel.com/blog/artificial-intelligence-fails
UNESCO.: Ethics of Artificial Intelligence (2024). https://www.unesco.org/en/artificial-intelligence/recommendation-ethics
US Copyright Office: Copyright registration guidance: works containing material generated by artificial intelligence. Fed. Reg. 88 (51), 16190–16194 (2023)
University of Michigan.: Generative artificial intelligence (2023). https://genai.umich.edu/
Vallor, S.: Moral deskilling and upskilling in a new machine age: reflections on the ambiguous future of character. Philos. Technol. 28 , 107–124 (2015)
Van Gulick, R.: Consciousness. Stanford Encyclopedia of Philosophy (2018). https://plato.stanford.edu/entries/consciousness/
Varoquaux, G., Cheplygina, V.: Machine learning for medical imaging: methodological failures and recommendations for the future. NPJ Digit. Med. 5 , 48 (2022)
Vanian, J., Leswing, K.: ChatGPT and generative AI are booming, but the costs can be extraordinary. CNBC (2023). https://www.cnbc.com/2023/03/13/chatgpt-and-generative-ai-are-booming-but-at-a-very-expensive-price.html
Walters, W.H., Wilder, E.I.: Fabrication and errors in the bibliographic citations generated by ChatGPT. Sci. Rep. 13 , 14045 (2023)
Wang, H., Fu, T., Du, Y., Gao, W., Huang, K., Liu, Z., Chandak, P., Liu, S., Van Katwyk, P., Deac, A., Anandkumar, A., Bergen, K., Gomes, C.P., Ho, S., Kohli, P., Lasenby, J., Leskovec, J., Liu, T.Y., Manrai, A., Marks, D., Ramsundar, B., Song, L., Sun, J., Tang, J., Veličković, P., Welling, M., Zhang, L., Coley, C.W., Bengio, Y., Zitnik, M.: Scientific discovery in the age of artificial intelligence. Nature 620 (7972), 47–60 (2023)
Weiss, D.C.: Latest version of ChatGPT aces bar exam with score nearing 90th percentile. ABA J. (2023). https://www.abajournal.com/web/article/latest-version-of-chatgpt-aces-the-bar-exam-with-score-in-90th-percentile
Whitbeck, C.: Truth and trustworthiness in research. Sci. Eng. Ethics 1 (4), 403–416 (1995)
Wilson, C.: Public engagement and AI: a values analysis of national strategies. Gov. Inf. Q. 39 (1), 101652 (2022)
World Conference on Research Integrity.: Singapore Statement (2010). http://www.singaporestatement.org/statement.html
Zheng, S.: China’s answers to ChatGPT have a censorship problem. Bloomberg (2023). https://www.bloomberg.com/news/newsletters/2023-05-02/china-s-chatgpt-answers-raise-questions-about-censoring-generative-ai
Ziman, J.: Real Science. Cambridge University Press, Cambridge (2000)
Download references
Open access funding provided by the National Institutes of Health. Funding was provided by Foundation for the National Institutes of Health (Grant number: ziaes102646-10).
Author information
Authors and affiliations.
National Institute of Environmental Health Sciences, Durham, USA
David B. Resnik
Department of Preventive Medicine, Northwestern University Feinberg School of Medicine, Chicago, IL, USA
Mohammad Hosseini
Galter Health Sciences Library and Learning Center, Northwestern University Feinberg School of Medicine, Chicago, IL, USA
You can also search for this author in PubMed Google Scholar
Corresponding author
Correspondence to David B. Resnik .
Ethics declarations
Conflict of interest.
On behalf of all authors, the corresponding author states that there is no conflict of interest.
Additional information
Publisher's note.
Springer Nature remains neutral with regard to jurisdictional claims in published maps and institutional affiliations.
Rights and permissions
Open Access This article is licensed under a Creative Commons Attribution 4.0 International License, which permits use, sharing, adaptation, distribution and reproduction in any medium or format, as long as you give appropriate credit to the original author(s) and the source, provide a link to the Creative Commons licence, and indicate if changes were made. The images or other third party material in this article are included in the article's Creative Commons licence, unless indicated otherwise in a credit line to the material. If material is not included in the article's Creative Commons licence and your intended use is not permitted by statutory regulation or exceeds the permitted use, you will need to obtain permission directly from the copyright holder. To view a copy of this licence, visit http://creativecommons.org/licenses/by/4.0/ .
Reprints and permissions
About this article
Resnik, D.B., Hosseini, M. The ethics of using artificial intelligence in scientific research: new guidance needed for a new tool. AI Ethics (2024). https://doi.org/10.1007/s43681-024-00493-8
Download citation
Received : 14 December 2023
Accepted : 07 May 2024
Published : 27 May 2024
DOI : https://doi.org/10.1007/s43681-024-00493-8
Share this article
Anyone you share the following link with will be able to read this content:
Sorry, a shareable link is not currently available for this article.
Provided by the Springer Nature SharedIt content-sharing initiative
- Artificial intelligence
- Transparency
- Accountability
- Explainability
- Social responsibility
- Find a journal
- Publish with us
- Track your research
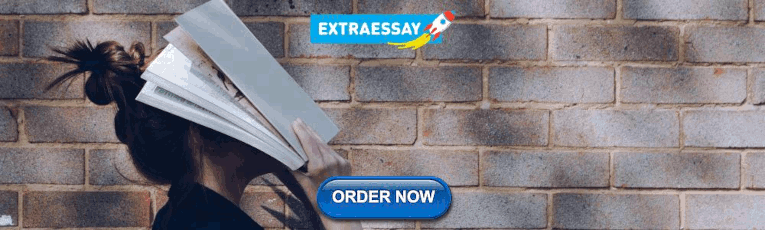
COMMENTS
Getting ethical approval for your study. Before you start any study involving data collection with people, you'll submit your research proposal to an institutional review board (IRB).. An IRB is a committee that checks whether your research aims and research design are ethically acceptable and follow your institution's code of conduct. They check that your research materials and procedures ...
Principles that come to mind might include autonomy, respect, dignity, privacy, informed consent and confidentiality. You may also have identified principles such as competence, integrity, wellbeing, justice and non-discrimination. Key ethical issues that you will address as an insider researcher include: Gaining trust.
Ethical Considerations. Ethical considerations in research refer to the principles and guidelines that researchers must follow to ensure that their studies are conducted in an ethical and responsible manner. These considerations are designed to protect the rights, safety, and well-being of research participants, as well as the integrity and credibility of the research itself
Underpayment is a common ethical issue that shouldn't be overlooked. Properly rewarding participants' time is critical for boosting engagement and obtaining high-quality data. While Prolific requires a minimum payment of £6.00 / $8.00 per hour, there are other factors you need to consider when deciding on a fair payment.
The inclusion of validating findings, ethical considerations (to be addressed shortly), the need for preliminary results, and early evidence of practical significance focus a reader's attention on key elements often overlooked in discussions about proposed projects. Format for a Qualitative Proposal
Ethical research therefore considers systemic and institutional factors that. are influencing the success with which goals and outcomes can be achieved. In the case of Nhung, there was a need for ...
Ethical considerations in research framework for evaluating research is outlined by Emanuel et al.7 Steps suggested in the process of eval-uating ethical research include: 1.Value in terms of the knowledge extracted and applied from the research 2.Scientific validity reflecting the methodology 3.Selection of subjects 4.Risk-benefit ratio
The third ethics principle of the Economic and Social Research Council (ESRC) states that: "The confidentiality of the information supplied by research subjects and the anonymity of respondents must be respected.". However, sometimes confidentiality is limited. For example, if a participant is at risk of harm, we must protect them.
Ethical considerations in research have always been challenging, including ethical concerns vis-a-vis time, funding, accessibility, and proper implementation of these concerns. ... In experimental research, especially involving human subjects, the researcher needs to submit an appropriate ethics proposal, although that process can slow down the ...
Ethical considerations in research are a set of principles that guide your research designs and practices. These principles include voluntary participation, informed consent, anonymity, confidentiality, potential for harm, and results communication. Scientists and researchers must always adhere to a certain code of conduct when collecting data ...
Ethical Considerations T he consideration of ethics in research, and in general business for that matter, is of growing importance. It is, therefore, critical that you understand the basics of ethical research and how this might affect your research project. This is especially important if your research involves inter-
In order to address ethical considerations aspect of your dissertation in an effective manner, you will need to expand discussions of each of the following points to at least one paragraph: 1. Voluntary participation of respondents in the research is important. Moreover, participants have rights to withdraw from the study at any stage if they ...
Education in research ethics is can help people get a better understanding of ethical standards, policies, and issues and improve ethical judgment and decision making. Many of the deviations that occur in research may occur because researchers simply do not know or have never thought seriously about some of the ethical norms of research.
NIH Clinical Center researchers published seven main principles to guide the conduct of ethical research: Social and clinical value. Scientific validity. Fair subject selection. Favorable risk-benefit ratio. Independent review. Informed consent. Respect for potential and enrolled subjects.
Researchers designing and conducting studies using human data should consider the values and principles of ethical conduct. Research ethics committees (RECs) typically evaluate the ethical acceptability of research proposals. Sometimes, differences arise between how researchers and RECs interpret et …
the research proposal to ensure inves tigator compliance with ethical aspects of the research. Some studies which have no apparent risk can be exempted from com mittee review and those with minimal risk may receive an expedited review. In these cases the committee chairperson judges the status of the proposal. Criteria for
INTRODUCTION. A clean, well-thought-out proposal forms the backbone for the research itself and hence becomes the most important step in the process of conduct of research.[] The objective of preparing a research proposal would be to obtain approvals from various committees including ethics committee [details under 'Research methodology II' section [Table 1] in this issue of IJA) and to ...
The research proposal should discuss potential ethical issues pertaining to the research. The researchers should pay special attention to vulnerable subjects to avoid breech of ethical codes (e.g. children, prisoners, pregnant women, mentally challenged, educationally and economically disadvantaged).
Getting ethical approval for your study. Before you start any study involving data collection with people, you'll submit your research proposal to an institutional review board (IRB).. An IRB is a committee that checks whether your research aims and research design are ethically acceptable and follow your institution's code of conduct. They check that your research materials and procedures ...
Abstract. Legal and ethical issues form an important component of modern research, related to the subject and researcher. This article seeks to briefly review the various international guidelines and regulations that exist on issues related to informed consent, confidentiality, providing incentives and various forms of research misconduct.
Factors contributing to proposal rejections included weak research questions or hypotheses, poor questionnaires/interview schedule design and inadequate research ethics consideration in the proposal.
Ethical Considerations in Research. Research is an important component of expanding knowledge and understanding the. world, and the people that inhabit the it. As Fiske (2018) notes, how people ...
Using artificial intelligence (AI) in research offers many important benefits for science and society but also creates novel and complex ethical issues. While these ethical issues do not necessitate changing established ethical norms of science, they require the scientific community to develop new guidance for the appropriate use of AI. In this article, we briefly introduce AI and explain how ...
The protectio n of human subjects through the. application of appropriat e ethical princi ples is. important in any research study (1). In a. qualitative study, et hical considerations have a ...