- Open access
- Published: 03 September 2022
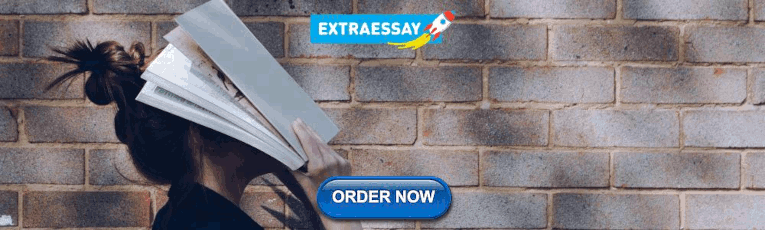
A literature review of risk, regulation, and profitability of banks using a scientometric study
- Shailesh Rastogi 1 ,
- Arpita Sharma 1 ,
- Geetanjali Pinto 2 &
- Venkata Mrudula Bhimavarapu ORCID: orcid.org/0000-0002-9757-1904 1 , 3
Future Business Journal volume 8 , Article number: 28 ( 2022 ) Cite this article
12k Accesses
1 Citations
Metrics details
This study presents a systematic literature review of regulation, profitability, and risk in the banking industry and explores the relationship between them. It proposes a policy initiative using a model that offers guidelines to establish the right mix among these variables. This is a systematic literature review study. Firstly, the necessary data are extracted using the relevant keywords from the Scopus database. The initial search results are then narrowed down, and the refined results are stored in a file. This file is finally used for data analysis. Data analysis is done using scientometrics tools, such as Table2net and Sciences cape software, and Gephi to conduct network, citation analysis, and page rank analysis. Additionally, content analysis of the relevant literature is done to construct a theoretical framework. The study identifies the prominent authors, keywords, and journals that researchers can use to understand the publication pattern in banking and the link between bank regulation, performance, and risk. It also finds that concentration banking, market power, large banks, and less competition significantly affect banks’ financial stability, profitability, and risk. Ownership structure and its impact on the performance of banks need to be investigated but have been inadequately explored in this study. This is an organized literature review exploring the relationship between regulation and bank performance. The limitations of the regulations and the importance of concentration banking are part of the findings.
Introduction
Globally, banks are under extreme pressure to enhance their performance and risk management. The financial industry still recalls the ignoble 2008 World Financial Crisis (WFC) as the worst economic disaster after the Great Depression of 1929. The regulatory mechanism before 2008 (mainly Basel II) was strongly criticized for its failure to address banks’ risks [ 47 , 87 ]. Thus, it is essential to investigate the regulation of banks [ 75 ]. This study systematically reviews the relevant literature on banks’ performance and risk management and proposes a probable solution.
Issues of performance and risk management of banks
Banks have always been hailed as engines of economic growth and have been the axis of the development of financial systems [ 70 , 85 ]. A vital parameter of a bank’s financial health is the volume of its non-performing assets (NPAs) on its balance sheet. NPAs are advances that delay in payment of interest or principal beyond a few quarters [ 108 , 118 ]. According to Ghosh [ 51 ], NPAs negatively affect the liquidity and profitability of banks, thus affecting credit growth and leading to financial instability in the economy. Hence, healthy banks translate into a healthy economy.
Despite regulations, such as high capital buffers and liquidity ratio requirements, during the second decade of the twenty-first century, the Indian banking sector still witnessed a substantial increase in NPAs. A recent report by the Indian central bank indicates that the gross NPA ratio reached an all-time peak of 11% in March 2018 and 12.2% in March 2019 [ 49 ]. Basel II has been criticized for several reasons [ 98 ]. Schwerter [ 116 ] and Pakravan [ 98 ] highlighted the systemic risk and gaps in Basel II, which could not address the systemic risk of WFC 2008. Basel III was designed to close the gaps in Basel II. However, Schwerter [ 116 ] criticized Basel III and suggested that more focus should have been on active risk management practices to avoid any impending financial crisis. Basel III was proposed to solve these issues, but it could not [ 3 , 116 ]. Samitas and Polyzos [ 113 ] found that Basel III had made banking challenging since it had reduced liquidity and failed to shield the contagion effect. Therefore, exploring some solutions to establish the right balance between regulation, performance, and risk management of banks is vital.
Keeley [ 67 ] introduced the idea of a balance among banks’ profitability, regulation, and NPA (risk-taking). This study presents the balancing act of profitability, regulation, and NPA (risk-taking) of banks as a probable solution to the issues of bank performance and risk management and calls it a triad . Figure 1 illustrates the concept of a triad. Several authors have discussed the triad in parts [ 32 , 96 , 110 , 112 ]. Triad was empirically tested in different countries by Agoraki et al. [ 1 ]. Though the idea of a triad is quite old, it is relevant in the current scenario. The spirit of the triad strongly and collectively admonishes the Basel Accord and exhibits new and exhaustive measures to take up and solve the issue of performance and risk management in banks [ 16 , 98 ]. The 2008 WFC may have caused an imbalance among profitability, regulation, and risk-taking of banks [ 57 ]. Less regulation , more competition (less profitability ), and incentive to take the risk were the cornerstones of the 2008 WFC [ 56 ]. Achieving a balance among the three elements of a triad is a real challenge for banks’ performance and risk management, which this study addresses.
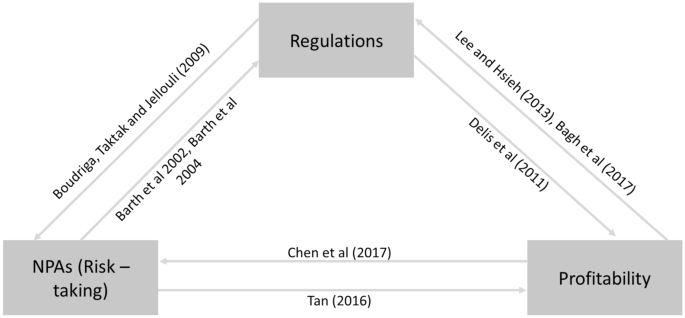
Triad of Profitability, regulation, and NPA (risk-taking). Note The triad [ 131 ] of profitability, regulation, and NPA (risk-taking) is shown in Fig. 1
Triki et al. [ 130 ] revealed that a bank’s performance is a trade-off between the elements of the triad. Reduction in competition increases the profitability of banks. However, in the long run, reduction in competition leads to either the success or failure of banks. Flexible but well-expressed regulation and less competition add value to a bank’s performance. The current review paper is an attempt to explore the literature on this triad of bank performance, regulation, and risk management. This paper has the following objectives:
To systematically explore the existing literature on the triad: performance, regulation, and risk management of banks; and
To propose a model for effective bank performance and risk management of banks.
Literature is replete with discussion across the world on the triad. However, there is a lack of acceptance of the triad as a solution to the woes of bank performance and risk management. Therefore, the findings of the current papers significantly contribute to this regard. This paper collates all the previous studies on the triad systematically and presents a curated view to facilitate the policy makers and stakeholders to make more informed decisions on the issue of bank performance and risk management. This paper also contributes significantly by proposing a DBS (differential banking system) model to solve the problem of banks (Fig. 7 ). This paper examines studies worldwide and therefore ensures the wider applicability of its findings. Applicability of the DBS model is not only limited to one nation but can also be implemented worldwide. To the best of the authors’ knowledge, this is the first study to systematically evaluate the publication pattern in banking using a blend of scientometrics analysis tools, network analysis tools, and content analysis to understand the link between bank regulation, performance, and risk.
This paper is divided into five sections. “ Data and research methods ” section discusses the research methodology used for the study. The data analysis for this study is presented in two parts. “ Bibliometric and network analysis ” section presents the results obtained using bibliometric and network analysis tools, followed by “ Content Analysis ” section, which presents the content analysis of the selected literature. “ Discussion of the findings ” section discusses the results and explains the study’s conclusion, followed by limitations and scope for further research.
Data and research methods
A literature review is a systematic, reproducible, and explicit way of identifying, evaluating, and synthesizing relevant research produced and published by researchers [ 50 , 100 ]. Analyzing existing literature helps researchers generate new themes and ideas to justify the contribution made to literature. The knowledge obtained through evidence-based research also improves decision-making leading to better practical implementation in the real corporate world [ 100 , 129 ].
As Kumar et al. [ 77 , 78 ] and Rowley and Slack [ 111 ] recommended conducting an SLR, this study also employs a three-step approach to understand the publication pattern in the banking area and establish a link between bank performance, regulation, and risk.
Determining the appropriate keywords for exploring the data
Many databases such as Google Scholar, Web of Science, and Scopus are available to extract the relevant data. The quality of a publication is associated with listing a journal in a database. Scopus is a quality database as it has a wider coverage of data [ 100 , 137 ]. Hence, this study uses the Scopus database to extract the relevant data.
For conducting an SLR, there is a need to determine the most appropriate keywords to be used in the database search engine [ 26 ]. Since this study seeks to explore a link between regulation, performance, and risk management of banks, the keywords used were “risk,” “regulation,” “profitability,” “bank,” and “banking.”
Initial search results and limiting criteria
Using the keywords identified in step 1, the search for relevant literature was conducted in December 2020 in the Scopus database. This resulted in the search of 4525 documents from inception till December 2020. Further, we limited our search to include “article” publications only and included subject areas: “Economics, Econometrics and Finance,” “Business, Management and Accounting,” and “Social sciences” only. This resulted in a final search result of 3457 articles. These results were stored in a.csv file which is then used as an input to conduct the SLR.
Data analysis tools and techniques
This study uses bibliometric and network analysis tools to understand the publication pattern in the area of research [ 13 , 48 , 100 , 122 , 129 , 134 ]. Some sub-analyses of network analysis are keyword word, author, citation, and page rank analysis. Author analysis explains the author’s contribution to literature or research collaboration, national and international [ 59 , 99 ]. Citation analysis focuses on many researchers’ most cited research articles [ 100 , 102 , 131 ].
The.csv file consists of all bibliometric data for 3457 articles. Gephi and other scientometrics tools, such as Table2net and ScienceScape software, were used for the network analysis. This.csv file is directly used as an input for this software to obtain network diagrams for better data visualization [ 77 ]. To ensure the study’s quality, the articles with 50 or more citations (216 in number) are selected for content analysis [ 53 , 102 ]. The contents of these 216 articles are analyzed to develop a conceptual model of banks’ triad of risk, regulation, and profitability. Figure 2 explains the data retrieval process for SLR.
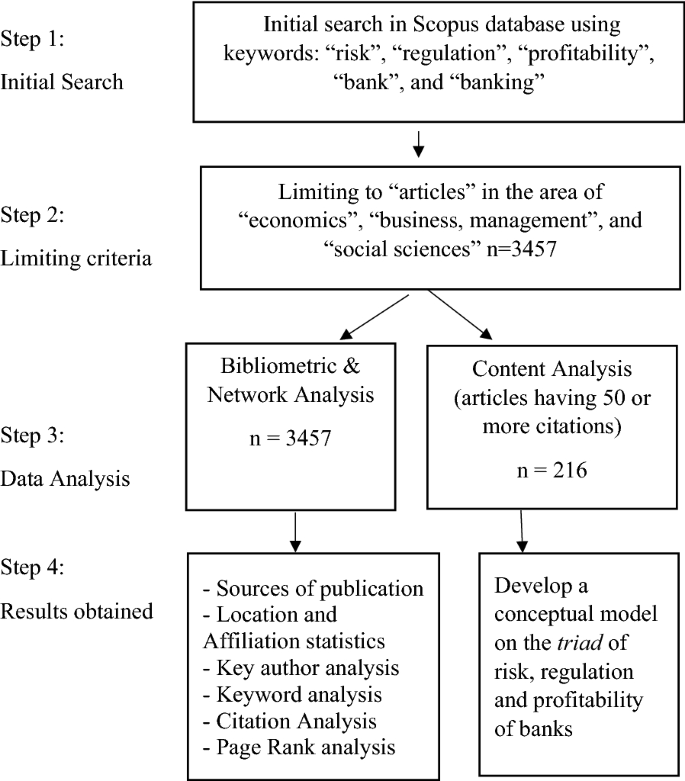
Data retrieval process for SLR. Note Stepwise SLR process and corresponding results obtained
Bibliometric and network analysis
Figure 3 [ 58 ] depicts the total number of studies that have been published on “risk,” “regulation,” “profitability,” “bank,” and “banking.” Figure 3 also depicts the pattern of the quality of the publications from the beginning till 2020. It undoubtedly shows an increasing trend in the number of articles published in the area of the triad: “risk” regulation” and “profitability.” Moreover, out of the 3457 articles published in the said area, 2098 were published recently in the last five years and contribute to 61% of total publications in this area.
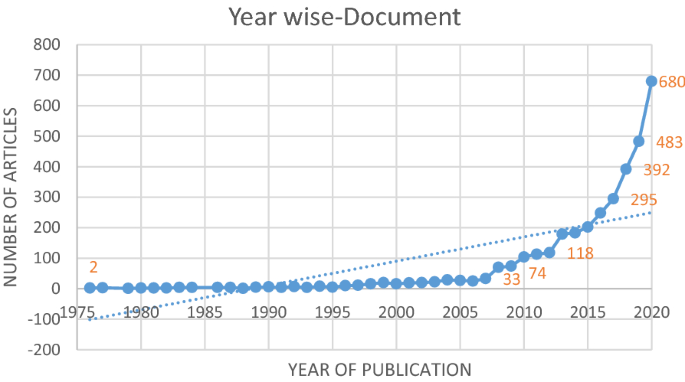
Articles published from 1976 till 2020 . Note The graph shows the number of documents published from 1976 till 2020 obtained from the Scopus database
Source of publications
A total of 160 journals have contributed to the publication of 3457 articles extracted from Scopus on the triad of risk, regulation, and profitability. Table 1 shows the top 10 sources of the publications based on the citation measure. Table 1 considers two sets of data. One data set is the universe of 3457 articles, and another is the set of 216 articles used for content analysis along with their corresponding citations. The global citations are considered for the study from the Scopus dataset, and the local citations are considered for the articles in the nodes [ 53 , 135 ]. The top 10 journals with 50 or more citations resulted in 96 articles. This is almost 45% of the literature used for content analysis ( n = 216). Table 1 also shows that the Journal of Banking and Finance is the most prominent in terms of the number of publications and citations. It has 46 articles published, which is about 21% of the literature used for content analysis. Table 1 also shows these core journals’ SCImago Journal Rank indicator and H index. SCImago Journal Rank indicator reflects the impact and prestige of the Journal. This indicator is calculated as the previous three years’ weighted average of the number of citations in the Journal since the year that the article was published. The h index is the number of articles (h) published in a journal and received at least h. The number explains the scientific impact and the scientific productivity of the Journal. Table 1 also explains the time span of the journals covering articles in the area of the triad of risk, regulation, and profitability [ 7 ].
Figure 4 depicts the network analysis, where the connections between the authors and source title (journals) are made. The network has 674 nodes and 911 edges. The network between the author and Journal is classified into 36 modularities. Sections of the graph with dense connections indicate high modularity. A modularity algorithm is a design that measures how strong the divided networks are grouped into modules; this means how well the nodes are connected through a denser route relative to other networks.
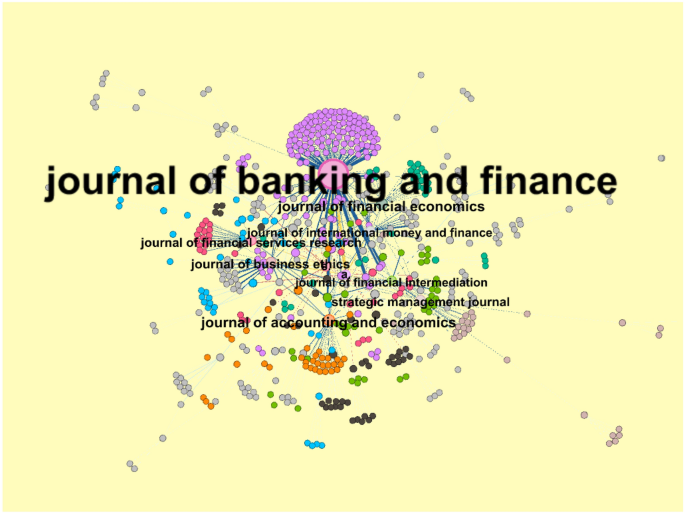
Network analysis between authors and journals. Note A node size explains the more linked authors to a journal
The size of the nodes is based on the rank of the degree. The degree explains the number of connections or edges linked to a node. In the current graph, a node represents the name of the Journal and authors; they are connected through the edges. Therefore, the more the authors are associated with the Journal, the higher the degree. The algorithm used for the layout is Yifan Hu’s.
Many authors are associated with the Journal of Banking and Finance, Journal of Accounting and Economics, Journal of Financial Economics, Journal of Financial Services Research, and Journal of Business Ethics. Therefore, they are the most relevant journals on banks’ risk, regulation, and profitability.
Location and affiliation analysis
Affiliation analysis helps to identify the top contributing countries and universities. Figure 5 shows the countries across the globe where articles have been published in the triad. The size of the circle in the map indicates the number of articles published in that country. Table 2 provides the details of the top contributing organizations.
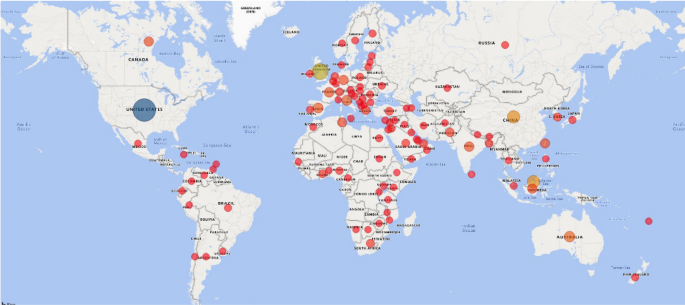
Location of articles published on Triad of profitability, regulation, and risk
Figure 5 shows that the most significant number of articles is published in the USA, followed by the UK. Malaysia and China have also contributed many articles in this area. Table 2 shows that the top contributing universities are also from Malaysia, the UK, and the USA.
Key author analysis
Table 3 shows the number of articles written by the authors out of the 3457 articles. The table also shows the top 10 authors of bank risk, regulation, and profitability.
Fadzlan Sufian, affiliated with the Universiti Islam Malaysia, has the maximum number, with 33 articles. Philip Molyneux and M. Kabir Hassan are from the University of Sharjah and the University of New Orleans, respectively; they contributed significantly, with 20 and 18 articles, respectively.
However, when the quality of the article is selected based on 50 or more citations, Fadzlan Sufian has only 3 articles with more than 50 citations. At the same time, Philip Molyneux and Allen Berger contributed more quality articles, with 8 and 11 articles, respectively.
Keyword analysis
Table 4 shows the keyword analysis (times they appeared in the articles). The top 10 keywords are listed in Table 4 . Banking and banks appeared 324 and 194 times, respectively, which forms the scope of this study, covering articles from the beginning till 2020. The keyword analysis helps to determine the factors affecting banks, such as profitability (244), efficiency (129), performance (107, corporate governance (153), risk (90), and regulation (89).
The keywords also show that efficiency through data envelopment analysis is a determinant of the performance of banks. The other significant determinants that appeared as keywords are credit risk (73), competition (70), financial stability (69), ownership structure (57), capital (56), corporate social responsibility (56), liquidity (46), diversification (45), sustainability (44), credit provision (41), economic growth (41), capital structure (39), microfinance (39), Basel III (37), non-performing assets (37), cost efficiency (30), lending behavior (30), interest rate (29), mergers and acquisition (28), capital adequacy (26), developing countries (23), net interest margin (23), board of directors (21), disclosure (21), leverage (21), productivity (20), innovation (18), firm size (16), and firm value (16).
Keyword analysis also shows the theories of banking and their determinants. Some of the theories are agency theory (23), information asymmetry (21), moral hazard (17), and market efficiency (16), which can be used by researchers when building a theory. The analysis also helps to determine the methodology that was used in the published articles; some of them are data envelopment analysis (89), which measures technical efficiency, panel data analysis (61), DEA (32), Z scores (27), regression analysis (23), stochastic frontier analysis (20), event study (15), and literature review (15). The count for literature review is only 15, which confirms that very few studies have conducted an SLR on bank risk, regulation, and profitability.
Citation analysis
One of the parameters used in judging the quality of the article is its “citation.” Table 5 shows the top 10 published articles with the highest number of citations. Ding and Cronin [ 44 ] indicated that the popularity of an article depends on the number of times it has been cited.
Tahamtan et al. [ 126 ] explained that the journal’s quality also affects its published articles’ citations. A quality journal will have a high impact factor and, therefore, more citations. The citation analysis helps researchers to identify seminal articles. The title of an article with 5900 citations is “A survey of corporate governance.”
Page Rank analysis
Goyal and Kumar [ 53 ] explain that the citation analysis indicates the ‘popularity’ and ‘prestige’ of the published research article. Apart from the citation analysis, one more analysis is essential: Page rank analysis. PageRank is given by Page et al. [ 97 ]. The impact of an article can be measured with one indicator called PageRank [ 135 ]. Page rank analysis indicates how many times an article is cited by other highly cited articles. The method helps analyze the web pages, which get the priority during any search done on google. The analysis helps in understanding the citation networks. Equation 1 explains the page rank (PR) of a published paper, N refers to the number of articles.
T 1,… T n indicates the paper, which refers paper P . C ( Ti ) indicates the number of citations. The damping factor is denoted by a “ d ” which varies in the range of 0 and 1. The page rank of all the papers is equal to 1. Table 6 shows the top papers based on page rank. Tables 5 and 6 together show a contrast in the top ranked articles based on citations and page rank, respectively. Only one article “A survey of corporate governance” falls under the prestigious articles based on the page rank.
Content analysis
Content Analysis is a research technique for conducting qualitative and quantitative analyses [ 124 ]. The content analysis is a helpful technique that provides the required information in classifying the articles depending on their nature (empirical or conceptual) [ 76 ]. By adopting the content analysis method [ 53 , 102 ], the selected articles are examined to determine their content. The classification of available content from the selected set of sample articles that are categorized under different subheads. The themes identified in the relationship between banking regulation, risk, and profitability are as follows.
Regulation and profitability of banks
The performance indicators of the banking industry have always been a topic of interest to researchers and practitioners. This area of research has assumed a special interest after the 2008 WFC [ 25 , 51 , 86 , 114 , 127 , 132 ]. According to research, the causes of poor performance and risk management are lousy banking practices, ineffective monitoring, inadequate supervision, and weak regulatory mechanisms [ 94 ]. Increased competition, deregulation, and complex financial instruments have made banks, including Indian banks, more vulnerable to risks [ 18 , 93 , 119 , 123 ]. Hence, it is essential to investigate the present regulatory machinery for the performance of banks.
There are two schools of thought on regulation and its possible impact on profitability. The first asserts that regulation does not affect profitability. The second asserts that regulation adds significant value to banks’ profitability and other performance indicators. This supports the concept that Delis et al. [ 41 ] advocated that the capital adequacy requirement and supervisory power do not affect productivity or profitability unless there is a financial crisis. Laeven and Majnoni [ 81 ] insisted that provision for loan loss should be part of capital requirements. This will significantly improve active risk management practices and ensure banks’ profitability.
Lee and Hsieh [ 83 ] proposed ambiguous findings that do not support either school of thought. According to Nguyen and Nghiem [ 95 ], while regulation is beneficial, it has a negative impact on bank profitability. As a result, when proposing regulations, it is critical to consider bank performance and risk management. According to Erfani and Vasigh [ 46 ], Islamic banks maintained their efficiency between 2006 and 2013, while most commercial banks lost, furthermore claimed that the financial crisis had no significant impact on Islamic bank profitability.
Regulation and NPA (risk-taking of banks)
The regulatory mechanism of banks in any country must address the following issues: capital adequacy ratio, prudent provisioning, concentration banking, the ownership structure of banks, market discipline, regulatory devices, presence of foreign capital, bank competition, official supervisory power, independence of supervisory bodies, private monitoring, and NPAs [ 25 ].
Kanoujiya et al. [ 64 ] revealed through empirical evidence that Indian bank regulations lack a proper understanding of what banks require and propose reforming and transforming regulation in Indian banks so that responsive governance and regulation can occur to make banks safer, supported by Rastogi et al. [ 105 ]. The positive impact of regulation on NPAs is widely discussed in the literature. [ 94 ] argue that regulation has multiple effects on banks, including reducing NPAs. The influence is more powerful if the country’s banking system is fragile. Regulation, particularly capital regulation, is extremely effective in reducing risk-taking in banks [ 103 ].
Rastogi and Kanoujiya [ 106 ] discovered evidence that disclosure regulations do not affect the profitability of Indian banks, supported by Karyani et al. [ 65 ] for the banks located in Asia. Furthermore, Rastogi and Kanoujiya [ 106 ] explain that disclosure is a difficult task as a regulatory requirement. It is less sustainable due to the nature of the imposed regulations in banks and may thus be perceived as a burden and may be overcome by realizing the benefits associated with disclosure regulation [ 31 , 54 , 101 ]. Zheng et al. [ 138 ] empirically discovered that regulation has no impact on the banks’ profitability in Bangladesh.
Governments enforce banking regulations to achieve a stable and efficient financial system [ 20 , 94 ]. The existing literature is inconclusive on the effects of regulatory compliance on banks’ risks or the reduction of NPAs [ 10 , 11 ]. Boudriga et al. [ 25 ] concluded that the regulatory mechanism plays an insignificant role in reducing NPAs. This is especially true in weak institutions, which are susceptible to corruption. Gonzalez [ 52 ] reported that firm regulations have a positive relationship with banks’ risk-taking, increasing the probability of NPAs. However, Boudriga et al. [ 25 ], Samitas and Polyzos [ 113 ], and Allen et al. [ 3 ] strongly oppose the use of regulation as a tool to reduce banks’ risk-taking.
Kwan and Laderman [ 79 ] proposed three levels in regulating banks, which are lax, liberal, and strict. The liberal regulatory framework leads to more diversification in banks. By contrast, the strict regulatory framework forces the banks to take inappropriate risks to compensate for the loss of business; this is a global problem [ 73 ].
Capital regulation reduces banks’ risk-taking [ 103 , 110 ]. Capital regulation leads to cost escalation, but the benefits outweigh the cost [ 103 ]. The trade-off is worth striking. Altman Z score is used to predict banks’ bankruptcy, and it found that the regulation increased the Altman’s Z-score [ 4 , 46 , 63 , 68 , 72 , 120 ]. Jin et al. [ 62 ] report a negative relationship between regulation and banks’ risk-taking. Capital requirements empowered regulators, and competition significantly reduced banks’ risk-taking [ 1 , 122 ]. Capital regulation has a limited impact on banks’ risk-taking [ 90 , 103 ].
Maji and De [ 90 ] suggested that human capital is more effective in managing banks’ credit risks. Besanko and Kanatas [ 21 ] highlighted that regulation on capital requirements might not mitigate risks in all scenarios, especially when recapitalization has been enforced. Klomp and De Haan [ 72 ] proposed that capital requirements and supervision substantially reduce banks’ risks.
A third-party audit may impart more legitimacy to the banking system [ 23 ]. The absence of third-party intervention is conspicuous, and this may raise a doubt about the reliability and effectiveness of the impact of regulation on bank’s risk-taking.
NPA (risk-taking) in banks and profitability
Profitability affects NPAs, and NPAs, in turn, affect profitability. According to the bad management hypothesis [ 17 ], higher profits would negatively affect NPAs. By contrast, higher profits may lead management to resort to a liberal credit policy (high earnings), which may eventually lead to higher NPAs [ 104 ].
Balasubramaniam [ 8 ] demonstrated that NPA has double negative effects on banks. NPAs increase stressed assets, reducing banks’ productive assets [ 92 , 117 , 136 ]. This phenomenon is relatively underexplored and therefore renders itself for future research.
Triad and the performance of banks
Regulation and triad.
Regulations and their impact on banks have been a matter of debate for a long time. Barth et al. [ 12 ] demonstrated that countries with a central bank as the sole regulatory body are prone to high NPAs. Although countries with multiple regulatory bodies have high liquidity risks, they have low capital requirements [ 40 ]. Barth et al. [ 12 ] supported the following steps to rationalize the existing regulatory mechanism on banks: (1) mandatory information [ 22 ], (2) empowered management of banks, and (3) increased incentive for private agents to exert corporate control. They show that profitability has an inverse relationship with banks’ risk-taking [ 114 ]. Therefore, standard regulatory practices, such as capital requirements, are not beneficial. However, small domestic banks benefit from capital restrictions.
DeYoung and Jang [ 43 ] showed that Basel III-based policies of liquidity convergence ratio (LCR) and net stable funding ratio (NSFR) are not fully executed across the globe, including the US. Dahir et al. [ 39 ] found that a decrease in liquidity and funding increases banks’ risk-taking, making banks vulnerable and reducing stability. Therefore, any regulation on liquidity risk is more likely to create problems for banks.
Concentration banking and triad
Kiran and Jones [ 71 ] asserted that large banks are marginally affected by NPAs, whereas small banks are significantly affected by high NPAs. They added a new dimension to NPAs and their impact on profitability: concentration banking or banks’ market power. Market power leads to less cost and more profitability, which can easily counter the adverse impact of NPAs on profitability [ 6 , 15 ].
The connection between the huge volume of research on the performance of banks and competition is the underlying concept of market power. Competition reduces market power, whereas concentration banking increases market power [ 25 ]. Concentration banking reduces competition, increases market power, rationalizes the banks’ risk-taking, and ensures profitability.
Tabak et al. [ 125 ] advocated that market power incentivizes banks to become risk-averse, leading to lower costs and high profits. They explained that an increase in market power reduces the risk-taking requirement of banks. Reducing banks’ risks due to market power significantly increases when capital regulation is executed objectively. Ariss [ 6 ] suggested that increased market power decreases competition, and thus, NPAs reduce, leading to increased banks’ stability.
Competition, the performance of banks, and triad
Boyd and De Nicolo [ 27 ] supported that competition and concentration banking are inversely related, whereas competition increases risk, and concentration banking decreases risk. A mere shift toward concentration banking can lead to risk rationalization. This finding has significant policy implications. Risk reduction can also be achieved through stringent regulations. Bolt and Tieman [ 24 ] explained that stringent regulation coupled with intense competition does more harm than good, especially concerning banks’ risk-taking.
Market deregulation, as well as intensifying competition, would reduce the market power of large banks. Thus, the entire banking system might take inappropriate and irrational risks [ 112 ]. Maji and Hazarika [ 91 ] added more confusion to the existing policy by proposing that, often, there is no relationship between capital regulation and banks’ risk-taking. However, some cases have reported a positive relationship. This implies that banks’ risk-taking is neutral to regulation or leads to increased risk. Furthermore, Maji and Hazarika [ 91 ] revealed that competition reduces banks’ risk-taking, contrary to popular belief.
Claessens and Laeven [ 36 ] posited that concentration banking influences competition. However, this competition exists only within the restricted circle of banks, which are part of concentration banking. Kasman and Kasman [ 66 ] found that low concentration banking increases banks’ stability. However, they were silent on the impact of low concentration banking on banks’ risk-taking. Baselga-Pascual et al. [ 14 ] endorsed the earlier findings that concentration banking reduces banks’ risk-taking.
Concentration banking and competition are inversely related because of the inherent design of concentration banking. Market power increases when only a few large banks are operating; thus, reduced competition is an obvious outcome. Barra and Zotti [ 9 ] supported the idea that market power, coupled with competition between the given players, injects financial stability into banks. Market power and concentration banking affect each other. Therefore, concentration banking with a moderate level of regulation, instead of indiscriminate regulation, would serve the purpose better. Baselga-Pascual et al. [ 14 ] also showed that concentration banking addresses banks’ risk-taking.
Schaeck et al. [ 115 ], in a landmark study, presented that concentration banking and competition reduce banks’ risk-taking. However, they did not address the relationship between concentration banking and competition, which are usually inversely related. This could be a subject for future research. Research on the relationship between concentration banking and competition is scant, identified as a research gap (“ Research Implications of the study ” section).
Transparency, corporate governance, and triad
One of the big problems with NPAs is the lack of transparency in both the regulatory bodies and banks [ 25 ]. Boudriga et al. [ 25 ] preferred to view NPAs as a governance issue and thus, recommended viewing it from a governance perspective. Ahmad and Ariff [ 2 ] concluded that regulatory capital and top-management quality determine banks’ credit risk. Furthermore, they asserted that credit risk in emerging economies is higher than that of developed economies.
Bad management practices and moral vulnerabilities are the key determinants of insolvency risks of Indian banks [ 95 ]. Banks are an integral part of the economy and engines of social growth. Therefore, banks enjoy liberal insolvency protection in India, especially public sector banks, which is a critical issue. Such a benevolent insolvency cover encourages a bank to be indifferent to its capital requirements. This indifference takes its toll on insolvency risk and profit efficiency. Insolvency protection makes the bank operationally inefficient and complacent.
Foreign equity and corporate governance practices help manage the adverse impact of banks’ risk-taking to ensure the profitability and stability of banks [ 33 , 34 ]. Eastburn and Sharland [ 45 ] advocated that sound management and a risk management system that can anticipate any impending risk are essential. A pragmatic risk mechanism should replace the existing conceptual risk management system.
Lo [ 87 ] found and advocated that the existing legislation and regulations are outdated. He insisted on a new perspective and asserted that giving equal importance to behavioral aspects and the rational expectations of customers of banks is vital. Buston [ 29 ] critiqued the balance sheet risk management practices prevailing globally. He proposed active risk management practices that provided risk protection measures to contain banks’ liquidity and solvency risks.
Klomp and De Haan [ 72 ] championed the cause of giving more autonomy to central banks of countries to provide stability in the banking system. Louzis et al. [ 88 ] showed that macroeconomic variables and the quality of bank management determine banks’ level of NPAs. Regulatory authorities are striving hard to make regulatory frameworks more structured and stringent. However, the recent increase in loan defaults (NPAs), scams, frauds, and cyber-attacks raise concerns about the effectiveness [ 19 ] of the existing banking regulations in India as well as globally.
Discussion of the findings
The findings of this study are based on the bibliometric and content analysis of the sample published articles.
The bibliometric study concludes that there is a growing demand for researchers and good quality research
The keyword analysis suggests that risk regulation, competition, profitability, and performance are key elements in understanding the banking system. The main authors, keywords, and journals are grouped in a Sankey diagram in Fig. 6 . Researchers can use the following information to understand the publication pattern on banking and its determinants.
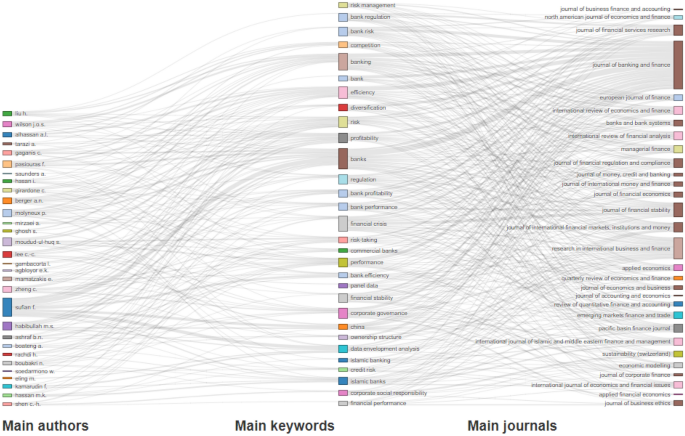
Sankey Diagram of main authors, keywords, and journals. Note Authors contribution using scientometrics tools
Research Implications of the study
The study also concludes that a balance among the three components of triad is the solution to the challenges of banks worldwide, including India. We propose the following recommendations and implications for banks:
This study found that “the lesser the better,” that is, less regulation enhances the performance and risk management of banks. However, less regulation does not imply the absence of regulation. Less regulation means the following:
Flexible but full enforcement of the regulations
Customization, instead of a one-size-fits-all regulatory system rooted in a nation’s indigenous requirements, is needed. Basel or generic regulation can never achieve what a customized compliance system can.
A third-party audit, which is above the country's central bank, should be mandatory, and this would ensure that all three aspects of audit (policy formulation, execution, and audit) are handled by different entities.
Competition
This study asserts that the existing literature is replete with poor performance and risk management due to excessive competition. Banking is an industry of a different genre, and it would be unfair to compare it with the fast-moving consumer goods (FMCG) or telecommunication industry, where competition injects efficiency into the system, leading to customer empowerment and satisfaction. By contrast, competition is a deterrent to the basic tenets of safe banking. Concentration banking is more effective in handling the multi-pronged balance between the elements of the triad. Concentration banking reduces competition to lower and manageable levels, reduces banks’ risk-taking, and enhances profitability.
No incentive to take risks
It is found that unless banks’ risk-taking is discouraged, the problem of high NPA (risk-taking) cannot be addressed. Concentration banking is a disincentive to risk-taking and can be a game-changer in handling banks’ performance and risk management.
Research on the risk and performance of banks reveals that the existing regulatory and policy arrangement is not a sustainable proposition, especially for a country where half of the people are unbanked [ 37 ]. Further, the triad presented by Keeley [ 67 ] is a formidable real challenge to bankers. The balance among profitability, risk-taking, and regulation is very subtle and becomes harder to strike, just as the banks globally have tried hard to achieve it. A pragmatic intervention is needed; hence, this study proposes a change in the banking structure by having two types of banks functioning simultaneously to solve the problems of risk and performance of banks. The proposed two-tier banking system explained in Fig. 7 can be a great solution. This arrangement will help achieve the much-needed balance among the elements of triad as presented by Keeley [ 67 ].
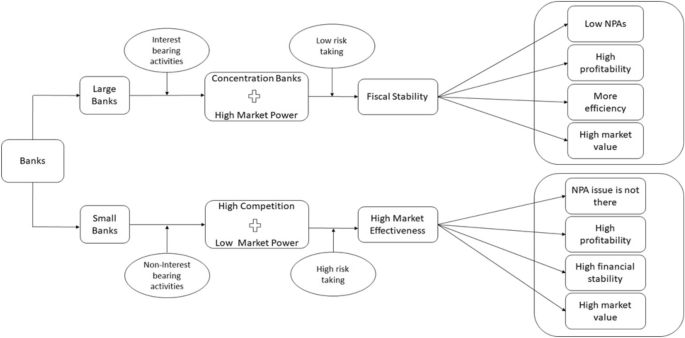
Conceptual Framework. Note Fig. 7 describes the conceptual framework of the study
The first set of banks could be conventional in terms of their structure and should primarily be large-sized. The number of such banks should be moderate. There is a logic in having only a few such banks to restrict competition; thus, reasonable market power could be assigned to them [ 55 ]. However, a reduction in competition cannot be over-assumed, and banks cannot become complacent. As customary, lending would be the main source of revenue and income for these banks (fund based activities) [ 82 ]. The proposed two-tier system can be successful only when regulation especially for risk is objectively executed [ 29 ]. The second set of banks could be smaller in size and more in number. Since they are more in number, they would encounter intense competition for survival and for generating more business. Small is beautiful, and thus, this set of banks would be more agile and adaptable and consequently more efficient and profitable. The main source of revenue for this set of banks would not be loans and advances. However, non-funding and non-interest-bearing activities would be the major revenue source. Unlike their traditional and large-sized counterparts, since these banks are smaller in size, they are less likely to face risk-taking and NPAs [ 74 ].
Sarmiento and Galán [ 114 ] presented the concerns of large and small banks and their relative ability and appetite for risk-taking. High risk could threaten the existence of small-sized banks; thus, they need robust risk shielding. Small size makes them prone to failure, and they cannot convert their risk into profitability. However, large banks benefit from their size and are thus less vulnerable and can convert risk into profitable opportunities.
India has experimented with this Differential Banking System (DBS) (two-tier system) only at the policy planning level. The execution is impending, and it highly depends on the political will, which does not appear to be strong now. The current agenda behind the DBS model is not to ensure the long-term sustainability of banks. However, it is currently being directed to support the agenda of financial inclusion by extending the formal credit system to the unbanked masses [ 107 ]. A shift in goal is needed to employ the DBS as a strategic decision, but not merely a tool for financial inclusion. Thus, the proposed two-tier banking system (DBS) can solve the issue of profitability through proper regulation and less risk-taking.
The findings of Triki et al. [ 130 ] support the proposed DBS model, in this study. Triki et al. [ 130 ] advocated that different component of regulations affect banks based on their size, risk-taking, and concentration banking (or market power). Large size, more concentration banking with high market power, and high risk-taking coupled with stringent regulation make the most efficient banks in African countries. Sharifi et al. [ 119 ] confirmed that size advantage offers better risk management to large banks than small banks. The banks should modify and work according to the economic environment in the country [ 69 ], and therefore, the proposed model could help in solving the current economic problems.
This is a fact that DBS is running across the world, including in India [ 60 ] and other countries [ 133 ]. India experimented with DBS in the form of not only regional rural banks (RRBs) but payments banks [ 109 ] and small finance banks as well [ 61 ]. However, the purpose of all the existing DBS models, whether RRBs [ 60 ], payment banks, or small finance banks, is financial inclusion, not bank performance and risk management. Hence, they are unable to sustain and are failing because their model is only social instead of a much-needed dual business-cum-social model. The two-tier model of DBS proposed in the current paper can help serve the dual purpose. It may not only be able to ensure bank performance and risk management but also serve the purpose of inclusive growth of the economy.
Conclusion of the study
The study’s conclusions have some significant ramifications. This study can assist researchers in determining their study plan on the current topic by using a scientific approach. Citation analysis has aided in the objective identification of essential papers and scholars. More collaboration between authors from various countries/universities may help countries/universities better understand risk regulation, competition, profitability, and performance, which are critical elements in understanding the banking system. The regulatory mechanism in place prior to 2008 failed to address the risk associated with banks [ 47 , 87 ]. There arises a necessity and motivates authors to investigate the current topic. The present study systematically explores the existing literature on banks’ triad: performance, regulation, and risk management and proposes a probable solution.
To conclude the bibliometric results obtained from the current study, from the number of articles published from 1976 to 2020, it is evident that most of the articles were published from the year 2010, and the highest number of articles were published in the last five years, i.e., is from 2015. The authors discovered that researchers evaluate articles based on the scope of critical journals within the subject area based on the detailed review. Most risk, regulation, and profitability articles are published in peer-reviewed journals like; “Journal of Banking and Finance,” “Journal of Accounting and Economics,” and “Journal of Financial Economics.” The rest of the journals are presented in Table 1 . From the affiliation statistics, it is clear that most of the research conducted was affiliated with developed countries such as Malaysia, the USA, and the UK. The researchers perform content analysis and Citation analysis to access the type of content where the research on the current field of knowledge is focused, and citation analysis helps the academicians understand the highest cited articles that have more impact in the current research area.
Practical implications of the study
The current study is unique in that it is the first to systematically evaluate the publication pattern in banking using a combination of scientometrics analysis tools, network analysis tools, and content analysis to understand the relationship between bank regulation, performance, and risk. The study’s practical implications are that analyzing existing literature helps researchers generate new themes and ideas to justify their contribution to literature. Evidence-based research knowledge also improves decision-making, resulting in better practical implementation in the real corporate world [ 100 , 129 ].
Limitations and scope for future research
The current study only considers a single database Scopus to conduct the study, and this is one of the limitations of the study spanning around the multiple databases can provide diverse results. The proposed DBS model is a conceptual framework that requires empirical testing, which is a limitation of this study. As a result, empirical testing of the proposed DBS model could be a future research topic.
Availability of data and materials
SCOPUS database.
Abbreviations
Systematic literature review
World Financial Crisis
Non-performing assets
Differential banking system
SCImago Journal Rank Indicator
Liquidity convergence ratio
Net stable funding ratio
Fast moving consumer goods
Regional rural banks
Agoraki M-EK, Delis MD, Pasiouras F (2011) Regulations, competition and bank risk-taking in transition countries. J Financ Stab 7(1):38–48
Google Scholar
Ahmad NH, Ariff M (2007) Multi-country study of bank credit risk determinants. Int J Bank Financ 5(1):35–62
Allen B, Chan KK, Milne A, Thomas S (2012) Basel III: Is the cure worse than the disease? Int Rev Financ Anal 25:159–166
Altman EI (2018) A fifty-year retrospective on credit risk models, the Altman Z-score family of models, and their applications to financial markets and managerial strategies. J Credit Risk 14(4):1–34
Alvarez F, Jermann UJ (2000) Efficiency, equilibrium, and asset pricing with risk of default. Econometrica 68(4):775–797
Ariss RT (2010) On the implications of market power in banking: evidence from developing countries. J Bank Financ 34(4):765–775
Aznar-Sánchez JA, Piquer-Rodríguez M, Velasco-Muñoz JF, Manzano-Agugliaro F (2019) Worldwide research trends on sustainable land use in agriculture. Land Use Policy 87:104069
Balasubramaniam C (2012) Non-performing assets and profitability of commercial banks in India: assessment and emerging issues. Nat Mon Refereed J Res Commer Manag 1(1):41–52
Barra C, Zotti R (2017) On the relationship between bank market concentration and stability of financial institutions: evidence from the Italian banking sector, MPRA working Paper No 79900. Last Accessed on Jan 2021 https://mpra.ub.uni-muenchen.de/79900/1/MPRA_paper_79900.pdf
Barth JR, Caprio G, Levine R (2004) Bank regulation and supervision: what works best? J Financ Intermed 2(13):205–248
Barth JR, Caprio G, Levine R (2008) Bank regulations are changing: For better or worse? Comp Econ Stud 50(4):537–563
Barth JR, Dopico LG, Nolle DE, Wilcox JA (2002) Bank safety and soundness and the structure of bank supervision: a cross-country analysis. Int Rev Financ 3(3–4):163–188
Bartolini M, Bottani E, Grosse EH (2019) Green warehousing: systematic literature review and bibliometric analysis. J Clean Prod 226:242–258
Baselga-Pascual L, Trujillo-Ponce A, Cardone-Riportella C (2015) Factors influencing bank risk in Europe: evidence from the financial crisis. N Am J Econ Financ 34(1):138–166
Beck T, Demirgüç-Kunt A, Levine R (2006) Bank concentration, competition, and crises: first results. J Bank Financ 30(5):1581–1603
Berger AN, Demsetz RS, Strahan PE (1999) The consolidation of the financial services industry: causes, consequences, and implications for the future. J Bank Financ 23(2–4):135–194
Berger AN, Deyoung R (1997) Problem loans and cost efficiency in commercial banks. J Bank Financ 21(6):849–870
Berger AN, Udell GF (1998) The economics of small business finance: the roles of private equity and debt markets in the financial growth cycle. J Bank Financ 22(6–8):613–673
Berger AN, Udell GF (2002) Small business credit availability and relationship lending: the importance of bank organisational structure. Econ J 112(477):F32–F53
Berger AN, Udell GF (2006) A more complete conceptual framework for SME finance. J Bank Financ 30(11):2945–2966
Besanko D, Kanatas G (1996) The regulation of bank capital: Do capital standards promote bank safety? J Financ Intermed 5(2):160–183
Beyer A, Cohen DA, Lys TZ, Walther BR (2010) The financial reporting environment: review of the recent literature. J Acc Econ 50(2–3):296–343
Bikker JA (2010) Measuring performance of banks: an assessment. J Appl Bus Econ 11(4):141–159
Bolt W, Tieman AF (2004) Banking competition, risk and regulation. Scand J Econ 106(4):783–804
Boudriga A, BoulilaTaktak N, Jellouli S (2009) Banking supervision and non-performing loans: a cross-country analysis. J Financ Econ Policy 1(4):286–318
Bouzon M, Miguel PAC, Rodriguez CMT (2014) Managing end of life products: a review of the literature on reverse logistics in Brazil. Manag Environ Qual Int J 25(5):564–584. https://doi.org/10.1108/MEQ-04-2013-0027
Article Google Scholar
Boyd JH, De Nicolo G (2005) The theory of bank risk taking and competition revisited. J Financ 60(3):1329–1343
Brealey RA, Myers SC, Allen F, Mohanty P (2012) Principles of corporate finance. Tata McGraw-Hill Education
Buston CS (2016) Active risk management and banking stability. J Bank Financ 72:S203–S215
Casu B, Girardone C (2006) Bank competition, concentration and efficiency in the single European market. Manch Sch 74(4):441–468
Charumathi B, Ramesh L (2020) Impact of voluntary disclosure on valuation of firms: evidence from Indian companies. Vision 24(2):194–203
Chen X (2007) Banking deregulation and credit risk: evidence from the EU. J Financ Stab 2(4):356–390
Chen H-J, Lin K-T (2016) How do banks make the trade-offs among risks? The role of corporate governance. J Bank Financ 72(1):S39–S69
Chen M, Wu J, Jeon BN, Wang R (2017) Do foreign banks take more risk? Evidence from emerging economies. J Bank Financ 82(1):20–39
Claessens S, Laeven L (2003) Financial development, property rights, and growth. J Financ 58(6):2401–2436. https://doi.org/10.1046/j.1540-6261.2003.00610.x
Claessens S, Laeven L (2004) What drives bank competition? Some international evidence. J Money Credit Bank 36(3):563–583
Cnaan RA, Moodithaya M, Handy F (2012) Financial inclusion: lessons from rural South India. J Soc Policy 41(1):183–205
Core JE, Holthausen RW, Larcker DF (1999) Corporate governance, chief executive officer compensation, and firm performance. J Financ Econ 51(3):371–406
Dahir AM, Mahat FB, Ali NAB (2018) Funding liquidity risk and bank risk-taking in BRICS countries: an application of system GMM approach. Int J Emerg Mark 13(1):231–248
Dechow P, Ge W, Schrand C (2010) Understanding earnings quality: a review of the proxies, their determinants, and their consequences. J Acc Econ 50(2–3):344–401
Delis MD, Molyneux P, Pasiouras F (2011) Regulations and productivity growth in banking: evidence from transition economies. J Money Credit Bank 43(4):735–764
Demirguc-Kunt A, Laeven L, Levine R (2003) Regulations, market structure, institutions, and the cost of financial intermediation (No. w9890). National Bureau of Economic Research.
Deyoung R, Jang KY (2016) Do banks actively manage their liquidity? J Bank Financ 66:143–161
Ding Y, Cronin B (2011) Popularand/orprestigious? Measures of scholarly esteem. Inf Process Manag 47(1):80–96
Eastburn RW, Sharland A (2017) Risk management and managerial mindset. J Risk Financ 18(1):21–47
Erfani GR, Vasigh B (2018) The impact of the global financial crisis on profitability of the banking industry: a comparative analysis. Economies 6(4):66
Erkens DH, Hung M, Matos P (2012) Corporate governance in the 2007–2008 financial crisis: evidence from financial institutions worldwide. J Corp Finan 18(2):389–411
Fahimnia B, Sarkis J, Davarzani H (2015) Green supply chain management: a review and bibliometric analysis. Int J Prod Econ 162:101–114
Financial Stability Report (2019) Financial stability report (20), December 2019. https://www.rbi.org.in/Scripts/PublicationReportDetails.aspx?UrlPage=&ID=946 Accesses on March 2020
Fink A (2005) Conducting Research Literature Reviews:From the Internet to Paper, 2nd edn. SAGE Publications
Ghosh A (2015) Banking-industry specific and regional economic determinants of non-performing loans: evidence from US states. J Financ Stab 20:93–104. https://doi.org/10.1016/j.jfs.2015.08.004
Gonzalez F (2005) Bank regulation and risk-taking incentives: an international comparison of bank risk. J Bank Financ 29(5):1153–1184
Goyal K, Kumar S (2021) Financial literacy: a systematic review and bibliometric analysis. Int J Consum Stud 45(1):80–105
Grassa R, Moumen N, Hussainey K (2020) Do ownership structures affect risk disclosure in Islamic banks? International evidence. J Financ Rep Acc 19(3):369–391
Haque F, Shahid R (2016) Ownership, risk-taking and performance of banks in emerging economies: evidence from India. J Financ Econ Policy 8(3):282–297
Hellmann TF, Murdock KC, Stiglitz JE (2000) Liberalization, moral hazard in banking, and prudential regulation: Are capital requirements enough? Am Econ Rev 90(1):147–165
Hirshleifer D (2001) Investor psychology and asset pricing. J Financ 56(4):1533–1597
Huang J, You JX, Liu HC, Song MS (2020) Failure mode and effect analysis improvement: a systematic literature review and future research agenda. Reliab Eng Syst Saf 199:106885
Ibáñez Zapata A (2017) Bibliometric analysis of the regulatory compliance function within the banking sector (Doctoral dissertation). Last Accessed on Jan 2021 https://riunet.upv.es/bitstream/handle/10251/85952/Bibliometric%20analysis_AIZ_v4.pdf?sequence=1
Ibrahim MS (2010) Performance evaluation of regional rural banks in India. Int Bus Res 3(4):203–211
Jayadev M, Singh H, Kumar P (2017) Small finance banks: challenges. IIMB Manag Rev 29(4):311–325
Jin JY, Kanagaretnam K, Lobo GJ, Mathieu R (2013) Impact of FDICIA internal controls on bank risk taking. J Bank Financ 37(2):614–624
Joshi MK (2020) Financial performance analysis of select Indian Public Sector Banks using Altman’s Z-Score model. SMART J Bus Manag Stud 16(2):74–87
Kanoujiya J, Bhimavarapu VM, Rastogi S (2021) Banks in India: a balancing act between profitability, regulation and NPA. Vision, 09722629211034417
Karyani E, Dewo SA, Santoso W, Frensidy B (2020) Risk governance and bank profitability in ASEAN-5: a comparative and empirical study. Int J Emerg Mark 15(5):949–969
Kasman S, Kasman A (2015) Bank competition, concentration and financial stability in the Turkish banking industry. Econ Syst 39(3):502–517
Keeley MC (1990) Deposit insurance, risk, and market power in banking. Am Econ Rev 1:1183–1200
Khaddafi M, Heikal M, Nandari A (2017) Analysis Z-score to predict bankruptcy in banks listed in indonesia stock exchange. Int J Econ Financ Issues 7(3):326–330
Khanna T, Yafeh Y (2007) Business groups in emerging markets: Paragons or parasites? J Econ Lit 45(2):331–372
King RG, Levine R (1993) Finance and growth: schumpeter might be right. Q J Econ 108(3):717–737
Kiran KP, Jones TM (2016) Effect of non performing assets on the profitability of banks–a selective study. Int J Bus Gen Manag 5(2):53–60
Klomp J, De Haan J (2015) Banking risk and regulation: Does one size fit all? J Bank Financ 36(12):3197–3212
Koehn M, Santomero AM (1980) Regulation of bank capital and portfolio risk. J Financ 35(5):1235–1244
Köhler M (2015) Which banks are more risky? The impact of business models on bank stability. J Financ Stab 16(1):195–212
Kothari SP (2001) Capital markets research in accounting. J Account Econ 31(1–3):105–231
Kumar S, Goyal N (2015) Behavioural biases in investment decision making – a systematic literature review. Qual Res Financ Mark 7(1):88–108
Kumar S, Kamble S, Roy MH (2020) Twenty-five years of Benchmarking: an International Journal (BIJ): a bibliometric overview. Benchmarking Int J 27(2):760–780. https://doi.org/10.1108/BIJ-07-2019-0314
Kumar S, Sureka R, Colombage S (2020) Capital structure of SMEs: a systematic literature review and bibliometric analysis. Manag Rev Q 70(4):535–565. https://doi.org/10.1007/s11301-019-00175-4
Kwan SH, Laderman ES (1999) On the portfolio effects of financial convergence-a review of the literature. Econ Rev 2:18–31
Lado AA, Boyd NG, Hanlon SC (1997) Competition, cooperation, and the search for economic rents: a syncretic model. Acad Manag Rev 22(1):110–141
Laeven L, Majnoni G (2003) Loan loss provisioning and economic slowdowns: Too much, too late? J Financ Intermed 12(2):178–197
Laeven L, Ratnovski L, Tong H (2016) Bank size, capital, and systemic risk: Some international evidence. J Bank Finance 69(1):S25–S34
Lee C-C, Hsieh M-F (2013) The impact of bank capital on profitability and risk in Asian banking. J Int Money Financ 32(1):251–281
Leech D, Leahy J (1991) Ownership structure, control type classifications and the performance of large British companies. Econ J 101(409):1418–1437
Levine R (1997) Financial development and economic growth: views and agenda. J Econ Lit 35(2):688–726
Lim CY, Woods M, Humphrey C, Seow JL (2017) The paradoxes of risk management in the banking sector. Br Acc Rev 49(1):75–90
Lo AW (2009) Regulatory reform in the wake of the financial crisis of 2007–2008. J Financ Econ Policy 1(1):4–43
Louzis DP, Vouldis AT, Metaxas VL (2012) Macroeconomic and bank-specific determinants of non-performing loans in Greece: a comparative study of mortgage, business and consumer loan portfolios. J Bank Financ 36(4):1012–1027
Maddaloni A, Peydró J-L (2011) Bank risk-taking, securitization, supervision, and low interest rates: evidence from the Euro-area and the U.S. lending standards. Rev Financ Stud 24(6):2121–2165. https://doi.org/10.1093/rfs/hhr015
Maji SG, De UK (2015) Regulatory capital and risk of Indian banks: a simultaneous equation approach. J Financ Econ Policy 7(2):140–156
Maji SG, Hazarika P (2018) Capital regulation, competition and risk-taking behavior of Indian banks in a simultaneous approach. Manag Financ 44(4):459–477
Messai AS, Jouini F (2013) Micro and macro determinants of non-performing loans. Int J Econ Financ Issues 3(4):852–860
Mitra S, Karathanasopoulos A, Sermpinis G, Dunis C, Hood J (2015) Operational risk: emerging markets, sectors and measurement. Eur J Oper Res 241(1):122–132
Mohsni S, Otchere I (2018) Does regulatory regime matter for bank risk-taking? A comparative analysis of US and Canada, d/Seas Working Papers-ISSN 2611-0172 1(1):28–28
Nguyen TPT, Nghiem SH (2015) The interrelationships among default risk, capital ratio and efficiency: evidence from Indian banks. Manag Financ 41(5):507–525
Niinimäki J-P (2004) The effects of competition on banks’ risk taking. J Econ 81(3):199–222
Page L, Brin S, Motwani R, Winograd T (1999) The PageRank citation ranking: bringing order to the web. Stanford InfoLab
Pakravan K (2014) Bank capital: the case against Basel. J Financ Regul Compl 22(3):208–218
Palacios-Callender M, Roberts SA, Roth-Berghofer T (2016) Evaluating patterns of national and international collaboration in Cuban science using bibliometric tools. J Doc 72(2):362–390. https://doi.org/10.1108/JD-11-2014-0164
Pinto G, Rastogi S, Kadam S, Sharma A (2019) Bibliometric study on dividend policy. Qual Res Financ Mark 12(1):72–95
Polizzi S, Scannella E (2020) An empirical investigation into market risk disclosure: Is there room to improve for Italian banks? J Financ Regul Compl 28(3):465–483
Prasad P, Narayanasamy S, Paul S, Chattopadhyay S, Saravanan P (2019) Review of literature on working capital management and future research agenda. J Econ Surv 33(3):827–861
Rahman MM, Zheng C, Ashraf BN, Rahman MM (2018) Capital requirements, the cost of financial intermediation and bank risk-taking: empirical evidence from Bangladesh. Res Int Bus Financ 44(1):488–503
Rajan RG (1994) Why bank credit policies fluctuate: a theory and some evidence. Q J Econ 109(2):399–441
Rastogi S, Gupte R, Meenakshi R (2021) A holistic perspective on bank performance using regulation, profitability, and risk-taking with a view on ownership concentration. J Risk Financ Manag 14(3):111
Rastogi S, Kanoujiya J (2022) Does transparency and disclosure (T&D) improve the performance of banks in India? Int J Product Perform Manag. https://doi.org/10.1108/IJPPM-10-2021-0613
Rastogi S, Ragabiruntha E (2018) Financial inclusion and socioeconomic development: gaps and solution. Int J Soc Econ 45(7):1122–1140
RBI (2001) Prudential Norms on income recognition, asset classification, and provisioning -pertaining to advances. Accessed on Apr 2020. https://rbidocs.rbi.org.in/rdocs/notification/PDFs/23068.pdf
Reddy S (2018) Announcement of payment banks and stock performance of commercial banks in India. J Internet Bank Commer 23(1):1–12
Repullo R (2004) Capital requirements, market power, and risk-taking in banking. J Financ Intermed 13(2):156–182
Rowley J, Slack F (2004) Conducting a literature review. Manag Res News 27(6):31–39. https://doi.org/10.1108/01409170410784185
Salas V, Saurina J (2003) Deregulation, market power and risk behaviour in Spanish banks. Eur Econ Rev 47(6):1061–1075
Samitas A, Polyzos S (2015) To Basel or not to Basel? Banking crises and contagion. Journal of Financial Regulation and Compliance 23(3):298–318
Sarmiento M, Galán JE (2017) The influence of risk-taking on bank efficiency: evidence from Colombia. Emerg Mark Rev 32:52–73. https://doi.org/10.1016/j.ememar.2017.05.007
Schaeck K, Cihak M, Wolfe S (2009) Are competitive banking systems more stable? J Money Credit Bank 41(4):711–734
Schwerter S (2011) Basel III’s ability to mitigate systemic risk. J Financ Regul Compl 19(4):337–354
Sen S, Sen RL (2014) Impact of NPAs on bank profitability: an empirical study. In: Ray N, Chakraborty K (eds) Handbook of research on strategic business infrastructure development and contemporary issues in finance. IGI Global, pp 124–134. https://doi.org/10.4018/978-1-4666-5154-8.ch010
Chapter Google Scholar
Shajahan K (1998) Non-performing assets of banks: Have they really declined? And on whose account? Econ Pol Wkly 33(12):671–674
Sharifi S, Haldar A, Rao SN (2016) Relationship between operational risk management, size, and ownership of Indian banks. Manag Financ 42(10):930–942
Sharma A, Theresa L, Mhatre J, Sajid M (2019) Application of altman Z-Score to RBI defaulters: Indian case. Asian J Res Bus Econ Manag 9(4):1–11
Shehzad CT, De Haan J (2015) Supervisory powers and bank risk taking. J Int Finan Markets Inst Money 39(1):15–24
Shen L, Xiong B, Hu J (2017) Research status, hotspotsandtrends forinformation behavior in China using bibliometric and co-word analysis. J Doc 73(4):618–633
Shleifer A, Vishny RW (1997) A survey of corporate governance. J Financ 52(2):737–783
Singh HP, Kumar S (2014) Working capital management: a literature review and research agenda. Qual Res Financ Mark 6(2):173–197
Tabak BM, Fazio DM, Cajueiro DO (2013) Systemically important banks and financial stability: the case of Latin America. J Bank Financ 37(10):3855–3866
Tahamtan I, SafipourAfshar A, Ahamdzadeh K (2016) Factors affecting number of citations: a comprehensive review of the literature. Scientometrics 107(3):1195–1225
Thakor AV (2018) Post-crisis regulatory reform in banking: Address insolvency risk, not illiquidity! J Financ Stab 37(1):107–111
Thomsen S, Pedersen T (2000) Ownership structure and economic performance in the largest European companies. Strategic Manag J 21(6):689–705
Tranfield D, Denyer D, Smart P (2003) Towards a methodology for developing evidence-informed management knowledge by means of systematic review. Br J Manag 14(3):207–222
Triki T, Kouki I, Dhaou MB, Calice P (2017) Bank regulation and efficiency: What works for Africa? Res Int Bus Financ 39(1):183–205
Tsay M, Shu Z (2011) Journal bibliometric analysis: a case study on the journal of documentation. J Doc 67(5):806–822
Vento GA, La Ganga P (2009) Bank liquidity risk management and supervision: which lessons from recent market turmoil. J Money Invest Bank 10(10):78–125
Wahid ANM (1994) The grameen bank and poverty alleviation in Bangladesh: theory, evidence and limitations. Am J Econ Sociol 53(1):1–15
Xiao Y, Watson M (2019) Guidance on conducting a systematic literature review. J Plan Educ Res 39(1):93–112
Xu X, Chen X, Jia F, Brown S, Gong Y, Xu Y (2018) Supply chain finance: a systematic literature review and bibliometric analysis. Int J Prod Econ 204:160–173
Yadav M (2011) Impact of non performing assets on profitability and productivity of public sector banks in India. AFBE J 4(1):232–239
Yong-Hak J (2013), Web of Science, Thomson Reuters
Zheng C, Rahman MM, Begum M, Ashraf BN (2017) Capital regulation, the cost of financial intermediation and bank profitability: evidence from Bangladesh. J Risk Financ Manag 10(2):9
Download references
Acknowledgements
Not Applicable.
Author information
Authors and affiliations.
Symbiosis Institute of Business Management, Symbiosis International (Deemed University), Pune, India
Shailesh Rastogi, Arpita Sharma & Venkata Mrudula Bhimavarapu
SIES School of Business Studies, Navi Mumbai, India
Geetanjali Pinto
School of Commerce and Management, D Y Patil International University, Akurdi, Pune, India
Venkata Mrudula Bhimavarapu
You can also search for this author in PubMed Google Scholar
Contributions
‘SR’ performed Abstract, Introduction, and Data methodology sections and was the major contributor; ‘AS’ performed Bibliometric and Network analysis and conceptual framework; ‘GP’ performed citation analysis and discussion section; ‘VMB’ collated data from the database and concluded the article. All authors read and approved the final manuscript.
Corresponding author
Correspondence to Venkata Mrudula Bhimavarapu .
Ethics declarations
Ethics approval and consent to participate, consent for publication, competing interests.
The authors declare that they have no competing interests.
Additional information
Publisher's note.
Springer Nature remains neutral with regard to jurisdictional claims in published maps and institutional affiliations.
Rights and permissions
Open Access This article is licensed under a Creative Commons Attribution 4.0 International License, which permits use, sharing, adaptation, distribution and reproduction in any medium or format, as long as you give appropriate credit to the original author(s) and the source, provide a link to the Creative Commons licence, and indicate if changes were made. The images or other third party material in this article are included in the article's Creative Commons licence, unless indicated otherwise in a credit line to the material. If material is not included in the article's Creative Commons licence and your intended use is not permitted by statutory regulation or exceeds the permitted use, you will need to obtain permission directly from the copyright holder. To view a copy of this licence, visit http://creativecommons.org/licenses/by/4.0/ .
Reprints and permissions
About this article
Cite this article.
Rastogi, S., Sharma, A., Pinto, G. et al. A literature review of risk, regulation, and profitability of banks using a scientometric study. Futur Bus J 8 , 28 (2022). https://doi.org/10.1186/s43093-022-00146-4
Download citation
Received : 11 March 2022
Accepted : 16 August 2022
Published : 03 September 2022
DOI : https://doi.org/10.1186/s43093-022-00146-4
Share this article
Anyone you share the following link with will be able to read this content:
Sorry, a shareable link is not currently available for this article.
Provided by the Springer Nature SharedIt content-sharing initiative
- Bank performance
- Profitability
- Bibliometric analysis
- Scientometric analysis
To read this content please select one of the options below:
Please note you do not have access to teaching notes, customer experience in digital banking: a review and future research directions.
International Journal of Quality and Service Sciences
ISSN : 1756-669X
Article publication date: 4 February 2022
Issue publication date: 3 May 2022
This study aims to demonstrate digital banking’s influence on customers’ evaluation of service experience and develop a framework identifying the most significant variables of digital banking that influence the financial performance of banks.
Design/methodology/approach
This structured review of literature, guided with the preferred reporting items for systematic reviews and meta-analyses framework, takes a digital banking perspective to identify 88 articles published between 2001 and 2021, examining distinct aspects of digital banking and their impact on financial performance.
Customer experience (CE) is determined by functional clues (functional quality, trust and convenience), mechanic clues (website attributes, website design, perceived usability) and humanic clues (customer complaint handling). The study is furthered to combine CE with the service profit chain model. This study also fills the gap to understand the use of “gamification” in technology-driven banking services to enhance CE. Finally, an integrative framework is proposed to link technology-related factors (digital banking clues and gamification), customer-related factors (CE, customer satisfaction and customer loyalty) and performance-related factors (financial performance).
Practical implications
The study conceptualises a “total” CE framework that banks can use to enhance their online presence. Banking service providers could also analyse their financial results based on digital banking’s impact on customers. Besides, banks can use this framework to strategically place “game-like features” in their digital platforms.
Originality/value
This study attempts to significantly contribute to the digital marketing literature related to CE with banks. It is one of the first studies to determine gamification explicitly in banking literature.
- Customer experience
- Digital banking
- Customer satisfaction
- Customer loyalty
- Financial performance
- Gamification
- Internet banking
Acknowledgements
Funding: Authors have not received any funding support to complete this work.
Conflict of interest statement: The authors declare that they have no conflict of interest.
Chauhan, S. , Akhtar, A. and Gupta, A. (2022), "Customer experience in digital banking: a review and future research directions", International Journal of Quality and Service Sciences , Vol. 14 No. 2, pp. 311-348. https://doi.org/10.1108/IJQSS-02-2021-0027
Emerald Publishing Limited
Copyright © 2022, Emerald Publishing Limited
Related articles
We’re listening — tell us what you think, something didn’t work….
Report bugs here
All feedback is valuable
Please share your general feedback
Join us on our journey
Platform update page.
Visit emeraldpublishing.com/platformupdate to discover the latest news and updates
Questions & More Information
Answers to the most commonly asked questions here
Academia.edu no longer supports Internet Explorer.
To browse Academia.edu and the wider internet faster and more securely, please take a few seconds to upgrade your browser .
Enter the email address you signed up with and we'll email you a reset link.
- We're Hiring!
- Help Center
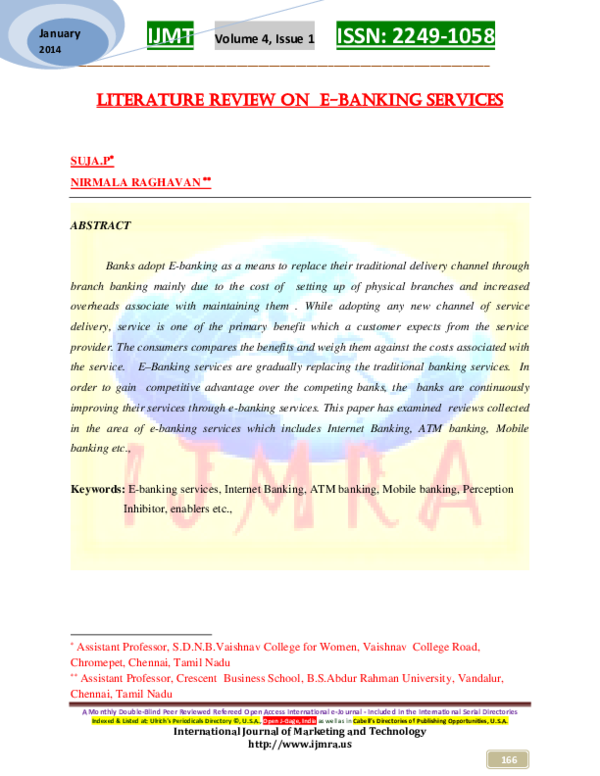
LITERATURE REVIEW ON E-BANKING SERVICES

2014, res publication
Banks adopt E-banking as a means to replace their traditional delivery channel through branch banking mainly due to the cost of setting up of physical branches and increased overheads associate with maintaining them. While adopting any new channel of service delivery, service is one of the primary benefit which a customer expects from the service provider. The consumers compares the benefits and weigh them against the costs associated with the service. E-Banking services are gradually replacing the traditional banking services. In order to gain competitive advantage over the competing banks, the banks are continuously improving their services through e-banking services. This paper has examined reviews collected in the area of e-banking services which includes Internet Banking, ATM banking, Mobile banking etc.
Related Papers
IOSR Journals
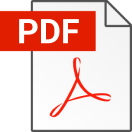
Md. Sazib Molla
shakir shaik
Kiran Kumar
Internet Banking becomes user friendly in today generation. Customer feels internet banking is safe and secure. Many application like online fund transfer, Payment of Income tax, mobile phone recharges, paying electricity bills, paying Dth recharge more. Customer doesn't have to go to the bank for transactions. Instead, customer can access your account any time and from any part of the world, and do so when you have the time, and not when the bank is open. For busy executives, students, and homemakers, e-banking is a virtual blessing. The research was conducted in Thiruvannamalai, Tamil Nadu. The research was based on consumer interested and perception about usage of electronic banking system and transaction.
In Today's scenario role of e-banking is very valuable. Without e-banking no banks can work. In this study we analyse, how much e-banking used in Public and Private sectors bank? (in reference to SBI and HDFC bank) Objective of the study is to find the consumer satisfaction in respect of e-banking and the perception of employees for using e-banking in Public and Private sectors banks. The method of the study is Primary and Secondary both. Study showed perception of customer regarding service quality and satisfaction of employee in internet banking services. As well as this study analyze the working style as a comparison between Public and Private sectors banks in respect of SBI and HDFC bank.
Mohammad Anisur Rahman
International Journal of Trend in Scientific Research and Development
Chandigarh University
isara solutions
International Research Journal Commerce arts science
Today traditional banking services, based on lending and accepting deposits related. The emergence of knowledge-based economy and the wider evolution of communication technology, banking services have undergone profound changes during the past decades. In order to provide the quality, customer service delivery and minimum transaction cost, banks have invested to a great extent in ICT and have adopted ICT networks for delivering a wide range of banking technologies and e-banking services in recent year. In this context, this study revealed that income, gender and age wise factors are placing an important role towards the usage of o electronic banking. The research confirmed the conceptual framework stating that if skills can be upgrade there will be a greater demand for E-Banking among customers.
reza seddighi
Electronic banking (e-banking) is a tool whose appropriate, accurate and timely utilization can lead to a successful performance in a competitive world. In other words, expanding e-banking should be considered for realizing customer orientation and customer satisfaction. However, when different types of e-banking services are provided but their quality is not acceptable, not only the customers would not be satisfied but they would be complaining about them. Considering the fact that all the organizations are seeking to attract new customers and improve their satisfaction, this issue is a critical one particularly in banks since they have a constant contact with the customers. On the other hand, the competition among banks and loan institutions as well as other financial supplies is increasing. Hence, realizing a competitive advantage seems essential for the survival of the banks. Modern banking services, which are closely related to information and communication technology, are among the most important factors in realizing competitive advantage for the banks and attracting new customers and increasing their satisfaction. In this study, using the field research method, we investigate the relationship between the usage of electronic services and satisfaction as well as attracting new customers in different branches of Eqtesade Novin Bank in Urmia. 1. INTRODUCTION With the increasing spread of information technology (IT), all the aspects of human life have fundamentally changed so that the modern world is in the course of a complete alteration. It can be said that the current industrial world have to embrace this change and being constant will cause interference in social, political and economic relationships of the individuals in a community or even in the international relations arena. Developing or lack thereof information technology in some societies has caused interference with the relations or the increase of relations among some countries. Modern communication technologies have conquered time and space dimensions and have changed the modern world into a global village so that it seems the modern human being has entered another world. During the two last decades of the twentieth century, three important innovations; namely fax, cellphone and the internet, have shown that how the expansion of communication can change the service industry and the daily life routines of people. Advancement in information and communication technologies (ICT) has both improved the supply of services and decreased the service costs. The impact of information and communication technology (ICT) in the field of trade and commerce has led to structural alterations in global trade as well as the emergence of a phenomenon called e-commerce, a process in which all the products will be exchanged through communicative or computerized connection networks or both of them. For instance, the internet, as a new channel for economic exchange, has provided the organizations with new resources for income generation and different opportunities and the volume of exchanges through internet is increasing on a daily basis and the companies avoiding this technology will soon be vanished from the face of the market arena. With the development of electronic systems, geographical distance has lost its meaning, which has led to an increasing competition among different companies and institutions including the banks. In order to reach the potential opportunities of the market and to overcome the different barriers and threats present in the complex business environment, the banks should possess competitive advantage. The electronic banking system is a context for reaching this competitive advantage. Nowadays, many banks in the global arena provide electronic services and an increasing majority of the customers tend to do their banking activities through electronic systems and without actually going to the bank branches. Using electronic banking services, the customers of the banks would be able to do their banking activities when and where they please and the banks will also enjoy lower operational costs due to the decrease in the number of staff and branches.
Dragos Paun
RELATED TOPICS
- We're Hiring!
- Help Center
- Find new research papers in:
- Health Sciences
- Earth Sciences
- Cognitive Science
- Mathematics
- Computer Science
- Academia ©2024
- Career Center
- Member Login
AcademyHealth Presents a Literature Review Summarizing the Research Findings of the Use of Internet Search Data in the Diagnosis of Diseases and Conditions
Sponsored by a grant from the Gordon and Betty Moore Foundation, and in partnership with Innovation Horizons, AcademyHealth conducted a literature review on the use of patient-generated data to assess the capability of the internet as a tool to inform clinical diagnosis practices.
As a component of an ongoing research project to address the gaps in medical care that contribute to delayed or missed diagnosis of serious disease and conditions, AcademyHealth has engaged in a study to examine how the use of a patient’s internet search data can be applied as a data source and tool to inform clinical diagnosis practices. Sponsored by a grant from the Gordon and Betty Moore Foundation, a literature review and comparative analyses were conducted utilizing data from internet searches by patients; the purpose of the review and analyses was to identify correlations (or a lack thereof) between each patient's searches and the corresponding patient's medical diagnoses. While many scientific reviews have previously summarized the utility of population-level internet search data for public health and health outcomes research, this is the first documented assessment of peer-reviewed publications focused on personalized medical diagnostic use. AcademyHealth conducted this study to help guide health services researchers, patient advocates, health care policy makers, and others to learn more about these data resources and their potential to inform new approaches to address diagnostic gaps and opportunities. This approach applies patient-generated data to assess the capability of the internet as a tool to improve understanding of symptoms, illuminate warning signs leading to a medical diagnostic workup, and empower caregivers to more effectively respond to health concerns.
The study design and findings represented in this report were reviewed by an Academy Health multidisciplinary steering committee that approved the report. The report details how researchers obtained and analyzed patient data from Microsoft and Google search engines to then link the data with the corresponding patient’s clinical information. All patient-generated data was provided by patients retrospectively with informed consent for research use. Of the 43 pee-reviewed publications identified and reviewed by AcademyHealth for inclusion in the report, all were retrospective analyses. The analyses included in the report represented a variety of medical and health considerations including cancer, mental health conditions, vulnerability, violence, and personal safety associated with neurodegenerative and aging disorders. Also included in the report were features published by researchers about tools that were developed to enable large data sets to be analyzed and integrated with other data, such as clinical trials and clinical care data. Cumulatively, these publications provide unique insights into the patient information needs, informed consent, and clinical research overview requirements needed to meet standards of conduct for research involving human subjects.
While many of these research publications identified signals of potential clinical use in diagnosis for specific diseases and conditions, none of the studies offered the type of confirmatory evidence that are required for medical use; at a minimum, evidence required would include prospective randomized studies. Therefore, this nascent type of diagnostic application research using large patient internet search data sets needs additional sponsorship to support infrastructure, analytic approaches, and importantly, innovative study design that enables prospective data collections to avoid bias and ultimately better understand the clinical efficacy and utility of this practice.
Further information can be found at the Exploring Consumer & Patient Internet Search Data to Improve Diagnosis Grant Program webpage . Innovation Horizons provided the research and analysis with support from the Gordon and Betty Moore Foundation.
Utilization of artificial intelligence in the banking sector: a systematic literature review
- Original Article
- Published: 11 August 2022
- Volume 28 , pages 835–852, ( 2023 )
Cite this article
- Omar H. Fares ORCID: orcid.org/0000-0003-0950-0661 1 ,
- Irfan Butt 1 &
- Seung Hwan Mark Lee 1
30k Accesses
22 Citations
56 Altmetric
Explore all metrics
This study provides a holistic and systematic review of the literature on the utilization of artificial intelligence (AI) in the banking sector since 2005. In this study, the authors examined 44 articles through a systematic literature review approach and conducted a thematic and content analysis on them. This review identifies research themes demonstrating the utilization of AI in banking, develops and classifies sub-themes of past research, and uses thematic findings coupled with prior research to propose an AI banking service framework that bridges the gap between academic research and industry knowledge. The findings demonstrate how the literature on AI and banking extends to three key areas of research: Strategy, Process, and Customer. These findings may benefit marketers and decision-makers in the banking sector to formulate strategic decisions regarding the utilization and optimization of value from AI technologies in the banking sector. This study also provides opportunities for future research.
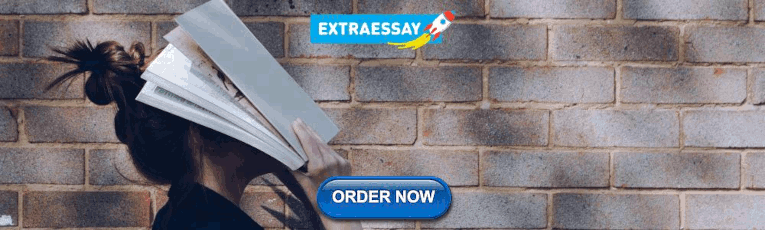
Similar content being viewed by others
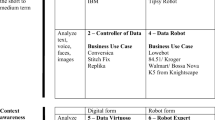
How artificial intelligence will change the future of marketing
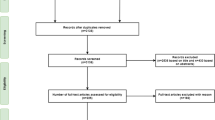
Artificial Intelligence and Business Value: a Literature Review
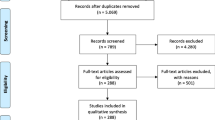
The role of artificial intelligence in healthcare: a structured literature review
Avoid common mistakes on your manuscript.
Introduction
Digital innovations in the modern banking landscape are no longer discretionary for financial institutions; instead, they are becoming necessary for financial institutions to cope with an increasingly competitive market and changing customer expectations (De Oliveira Santini, 2018 ; Eren, 2021 ; Hua et al., 2019 ; Rajaobelina and Ricard, 2021 ; Valsamidis et al., 2020 ; Yang, 2009 ). In the era of modern banking, many new digital technologies have been driven by artificial intelligence (AI) as the key engine (Dobrescu and Dobrescu, 2018 ), leading to innovative disruptions of banking channels (e.g., automated teller machines, online banking, mobile banking), services (e.g., imaging of checks, voice recognition, chatbots), and solutions (e.g., AI investment advisors and AI credit selectors).
The application of AI in banking is across the board, with uses in the front office (voice assistants and biometrics), middle office (anti-fraud risk monitoring and complex legal and compliance workflows), and back office (credit underwriting with smart contracts infrastructure). Banks are expected to save $447 billion by 2023, by employing AI applications. Almost 80% of the banks in the USA are cognizant of the potential benefits offered by AI (Digalaki, 2022 ). Indeed, the emergence of AI has generated a wealth of opportunities and challenges (Malali and Gopalakrishnan, 2020 ). In the banking context, the use of AI has led to more seamless sales and has guided the development of effective customer relationship management systems (Tarafdar et al., 2019 ). While the focus in the past was on the automation of credit scoring, analyses, and the grants process (Mehrotra, 2019 ), capabilities evolved to support internal systems and processes as well (Caron, 2019 ).
The term AI was first used in 1956 by John McCarthy (McCarthy et al., 1956 ); it refers to systems that act and think like humans in a rational way (Kok et al., 2009 ). In the aftermath of the dot com bubble in 2000, the field of AI shifted toward Web 2.0. era in 2005, and the growth of data and availability of information encouraged more research in AI and its potential (Larson, 2021 ). More recently, technological advancements have opened the doors for AI to facilitate enterprise cognitive computing, which involves embedding algorithms into applications to support organizational processes (Tarafdar et al., 2019 ). This includes improving the speed of information analysis, obtaining more accurate and reliable data outputs, and allowing employees to perform high-level tasks. In recent years, AI-based technologies have been shown to be effective and practical. However, many corporate executives still lack knowledge regarding the strategic utilization of AI in their organizations. For instance, Ransbotham et al. ( 2017 ) found that 85% of business executives viewed AI as a key tool for providing businesses with a sustainable competitive advantage; however, only 39% had a strategic plan for the use of AI, due to the lack of knowledge regarding implementation of AI for their organizations.
Here, we systematically analyze the past and current state of AI and banking literature to understand how it has been utilized within the banking sector historically, propose a service framework, and provide clear future research opportunities. In the past, a limited number of systematic literature reviews have studied AI within the management discipline (e.g., Bavaresco et al., 2020 ; Borges et al., 2020 ; Loureiro et al., 2020 ; Verma et al., 2021 ). However, the current literature lacks either research scope and depth, and/or industry focus. In response, we seek to differentiate our study from prior reviews by providing a specific focus on the banking sector and a more comprehensive analysis involving multiple modes of analysis.
In light of this, we aim to address the following research questions:
What are the themes and sub-themes that emerge from prior literature regarding the utilization of AI in the banking industry?
How does AI impact the customer's journey process in the banking sector, from customer acquisition to service delivery?
What are the current research deficits and future directions of research in this field?
Methodology
Selection of articles.
Adhering to the best practices for conducting a Systematic Literature Review (SLR) (see Khan et al., 2003 ; Tranfield et al, 2003 ; Xiao and Watson, 2019 ), we began by selecting the appropriate database and identifying keywords, based on an in-depth review of the literature. Research papers were extracted from Web of Science (WoS) and Scopus. These databases were selected to complement one another and provide access to scholarly articles (Mongeon and Paul-Hus, 2016 ); this was also the first step in ensuring the inclusion of high-quality articles (Harzing and Alakangas, 2016 ). The following query was used to search the title, abstract, and keywords: “Artificial intelligence OR machine learning OR deep learning OR neural networks OR Intelligent systems AND Bank AND consumer OR customer OR user.” The keywords were selected, based on prior literature review, with the goal of covering various business functions, especially focusing on the banking sector (Loureiro et al., 2020 ; Verma et al., 2021 ; Borges et al., 2020 ; Bavaresco et al., 2020 ). The initial search criteria yielded 11,684 papers. These papers were then filtered by “English,” “article only” publications, and using the subject area filter of “Management, Business Finance, accounting and Business,” which resulted in 626 papers.
In this study, we used the preferred reporting method for systematic reviews and meta-analyses (PRISMA) to ensure that we follow the systematic approach and track the flow of data across different stages of the SLR (Moher et al., 2009 ). After extracting the articles, each of the 626 papers was given a distinctive ID number to help differentiate the papers; the ID number was maintained throughout the analysis process. The data were then organized using the following columns: “ID number,” “database source,” “Author,” “title,” “Abstract,” “keywords,” “Year,” Australian Business Deans Council (ABDC) Journals, “and keyword validation columns.”
The exclusion of papers was done systematically in the following manner: a) All duplicate papers in the database were eliminated (105 duplicates); b) as a second quality check, papers not published in ABDC journals (163 papers) were omitted to ensure a quality standard for inclusion in the review,Query a practice consistent with other recent SLRs (Goyal and Kumar, 2021 ; Nusair et al., 2019 ; Pahlevan-Sharif et al., 2019 ); c) in order to ensure the relevance of articles included, and following our research objectives, we excluded non-consumer-related papers, searching for consumers (consumer, customer, user) in the title, abstract, and keywords; this resulted in the removal of 314 papers; d) for the remaining 48 papers, a relevance check was manually conducted to determine whether the papers were indeed related to AI and banking. Papers that specifically focused on the technical computational process of AI were removed (4 papers). This process resulted in the selection of 44 articles for subsequent analyses.
Thematic analysis
A thematic analysis classifies the topics and subtopics being researched. It is a method for identifying, analyzing, and reporting patterns within data (Boyatzis, 1998 ). We followed Chatha and Butt ( 2015 ) to classify the articles into themes and sub-themes using manual coding. Second, we employed the Leximancer software to supplement the manual classification process. The use of these two approaches provides additional validity and quality to the research findings.
Leximancer is a text-mining software that provides conceptual and relational information by identifying concept occurrences and co-occurrences (Leximancer, 2019 ). After uploading all the 44 papers onto Leximancer, we added “English” to the stoplist, which removed words such as “or/and/like” that are not relevant to developing themes. We manually removed irrelevant filler words, such as “pp.,” “Figure,” and “re.” Finally, our results consisted of two maps: a) a conceptual map wherein central themes and concepts are identified, and b) a relational cloud map where a network of connections and relationships are drawn among concepts.
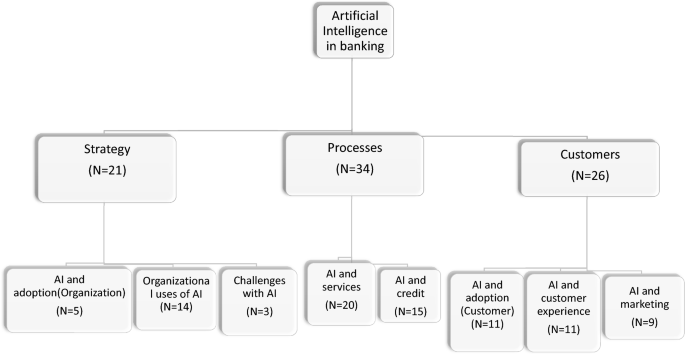
Thematic map
RQ 1: What are the themes and sub-themes that emerge from prior literature regarding the utilization of AI in the banking industry?
We began with a deductive approach to categorize articles into predetermined themes for the theme identification process. We then employed an inductive approach to identify the sub-themes and provide context for the primary themes (See Fig. 1 ). The procedure for determining the primary themes included, a) reviewing previous related systematic literature reviews (Bavaresco et al., 2020 ; Borges et al., 2020 ; Loureiro et al., 2020 ; Verma et al., 2021 ), b) identifying keywords and developing codes (themes) from selected papers; and c) reviewing titles, abstracts, and full papers, if needed, to identify appropriate allocation within these themes. Three primary themes were curated from the process: Strategy, Processes, and Customers (see Fig. 2 ).
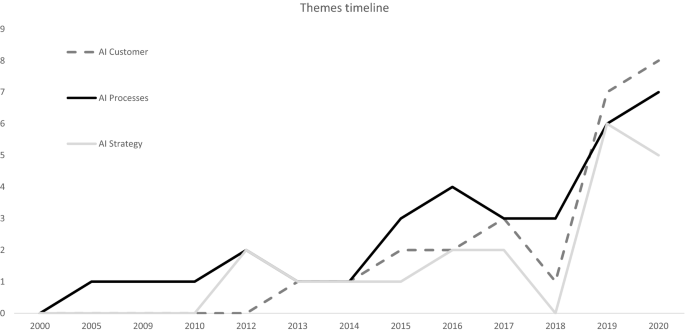
Themes by timeline
In the Strategy theme (21 papers), early research shows the potential uses and adoption of AI from an organizational perspective (e.g., Akkoç, 2012 ; Olson et al., 2012 ; Smeureanu et al., 2013 ). Data mining (an essential part of AI) has been used to predict bankruptcy (Olson et al., 2012 ) and to optimize risk models (Akkoç, 2012 ). The increasing use of AI-driven tools to drive organizational effectiveness creates greater business efficiency opportunities for financial institutions, as compared to traditional modes of strategizing and risk model development. The sub-theme Organizational use of AI (14 papers) covers a range of current activities wherein banks use AI to drive organizational value. These organizational uses include the use of AI to drive business strategies and internal business activities. Medhi and Mondal ( 2016 ) highlighted the use of an AI-driven model to predict outsourcing success. Our findings indicate the effectiveness of AI tools in driving efficient organizational strategies; however, there remain several challenges in implementing AI technologies, including the human resources aspect and the organizational culture to allow for such efficiencies (Fountain et al., 2019 ). More recently, there has been a noticeable focus on discussing some of the challenges associated with AI implementation in banking institutions (e.g., Jakšič and Marinč, 2019 ; Mohapatra, 2020 ). The sub-theme Challenges with AI (three papers) covers a range of challenges that organizations face, including the integration of AI in their organizations. Mohapatra ( 2020 ) characterizes some of the key challenges related to human–machine interactions to allow for the sustainable implementation of AI in banking. While much of the current research has focused on technology, our findings indicate that one of the main areas of opportunity in the future is related to adoption and integration. The sub-theme AI and adoption in financial institutions (six papers) covered a range of topics regarding motivation, and barriers to the adoption of AI technology from an organizational standpoint. Fountain et al. ( 2019 ) conceptually highlighted some barriers to organizational adoption, including workers’ fear, company culture, and budget constraints. Overall, in the Strategy theme, organizational uses of AI seemed to be the most prominent, which highlights the consistent focus on technology development compared with technology implementation. However, the literature remains limited in terms of discussions related to the organizational challenges associated with AI implementation.
In the Processes theme (34 papers), after the dot com bubble and with the emergence of Web 2.0, research on AI in the banking sector started to emerge. This could have been triggered by the suggested use of AI to predict stock market movements and stock selection (Kim and Lee, 2004 ; Tseng, 2003 ). At this stage, the literature on AI in the banking sector was related to its use in credit and loan analysis (Baesens et al., 2005 ; Ince and Aktan, 2009 ; Kao et al., 2012 ; Khandani et al., 2010 ). In the early stages of AI implementation, it is essential to develop fast and reliable AI infrastructure (Larson, 2021 ). Baesens et al. ( 2005 ) utilized a neural network approach to better predict loan defaults and early repayments. Ince and Aktan ( 2009 ) used a data mining technique to analyze credit scores and found that the AI-driven data mining approach was more effective than traditional methods. Similarly, Khandani et al. ( 2010 ) found machine-learning-driven models to be effective in analyzing consumer credit risk. The sub-theme, AI and credit (15 papers), covers the use of AI technology, such as machine learning and data mining, to improve credit scoring, analysis, and granting processes. For instance, Alborzi and Khanbabaei ( 2016 ) examined the use of data mining neural network techniques to develop a customer credit scoring model. Post-2013, there has been a noticeable increase in investigating how AI improves processes that go beyond credit analysis. The sub-theme AI and services (20 papers) covers the uses of AI for process improvement and enhancement. These process-related uses of technology include institutional uses of technology to improve internal service processes. For example, Soltani et al. ( 2019 ) examined the use of machine learning to optimize appointment scheduling time, and reduce service time. Overall, regarding the process theme, our findings highlight the usefulness of AI in improving banking processes; however, there remains a gap in practical research regarding the applied integration of technology in the banking system. In addition, while there is an abundance of research on credit risk, the exploration of other financial products remains limited.
In the Customer theme (26 papers), we uncovered the increasing use of AI as a methodological tool to better understand customer adoption of digital banking services. The sub-theme AI and Customer adoption (11 papers) covers the use of AI as a methodological tool to investigate customers’ adoption of digital banking technologies, including both barriers and motivational factors. For example, Arif et al. ( 2020 ) used a neural network approach to investigate barriers to internet-banking adoption by customers. Belanche et al. ( 2019 ) investigate factors related to AI-driven technology adoption in the banking sector. Payne et al. ( 2018 ) examine the drivers of the usage of AI-enabled mobile banking services. In addition, bank marketers have found an opportunity to use AI to better segment, target, and position their banking products and services. The sub-theme, AI and marketing (nine papers), covers the use of AI for different marketing activities, including customer segmentation, development of marketing models, and delivery of more effective marketing campaigns. For example, Smeureanu et al. ( 2013 ) proposed a machine learning technique to segment banking customers. Schwartz et al. ( 2017 ) utilized an AI-based method to examine the resource allocation in targeted advertisements. In recent years, there has been a noticeable trend in investigating how AI shapes customer experience (Soltani et al., 2019 ; Trivedi, 2019 ). The sub-theme of AI and customer experience (Papers 11) covers the use of AI to enhance banking experience and services for customers. For example, Trivedi ( 2019 ) investigated the use of chatbots in banking and their impact on customer experience.
Table 1 highlights the number of papers included in the themes and sub-themes. Overall, the papers related to Processes (77%) were the most frequently occurring, followed by Customer (59%) and Strategy-based (48%) papers. From 2013 onward, there was an increase in the inter-relation between all three areas of Strategy, Processes, and Customers. Since 2016, there has been a surge in research linking the themes of Processes and Customers. More recently, since 2017, papers combining Customers with Strategy have become more frequent.
Leximancer analysis
A Leximancer analysis was conducted on all the papers included in the study. This resulted in two major classifications and 56 distinct concepts. Here, a “concept” refers to a combination of closely related words. When referring to “concept co-occurrence,” we refer to the total number of times two concepts appear together. In comparison, the word association percentage refers to the conditional probability that two concepts will appear side-by-side.
Conceptual and relational analyses
Conceptual analysis refers to the analysis of data based on word frequency and word occurrence, whereas relational analysis refers to the analysis that draws connections between concepts and captures the co-occurrences between words (Leximancer, 2019 ). As Fig. 3 shows, the most prominent concept is “customer,” which provides additional credence to our customer theme. The concept “customer” appeared 2,231 times across all papers. For the concept “customer,” some of the key concept associations include satisfaction (324 co-occurrences and 64% word association), service (185 co-occurrences and 43% word association), and marketing (86 co-occurrences and 42% word association). This may imply the importance of utilizing AI in improving customer service and satisfaction, and in marketing to retain and grow the customer base. For instance, Trivedi ( 2019 ) examined the factors affecting chatbot satisfaction and found that information, system, and service quality, all have a significant positive association with it. Ekinci et al. ( 2014 ) proposed a customer lifetime value model, supported by a deep learning approach, to highlight key indicators in the banking sector. Xu et al. ( 2020 ) examined the effects of AI versus human customer service, and found that customers are more likely to use AI for low-complexity tasks, whereas a human agent is preferred for high-complexity tasks. It is worth noting that most of the research related to the customer theme has utilized a quantitative approach, with limited qualitative papers (i.e., four papers) in recent years.
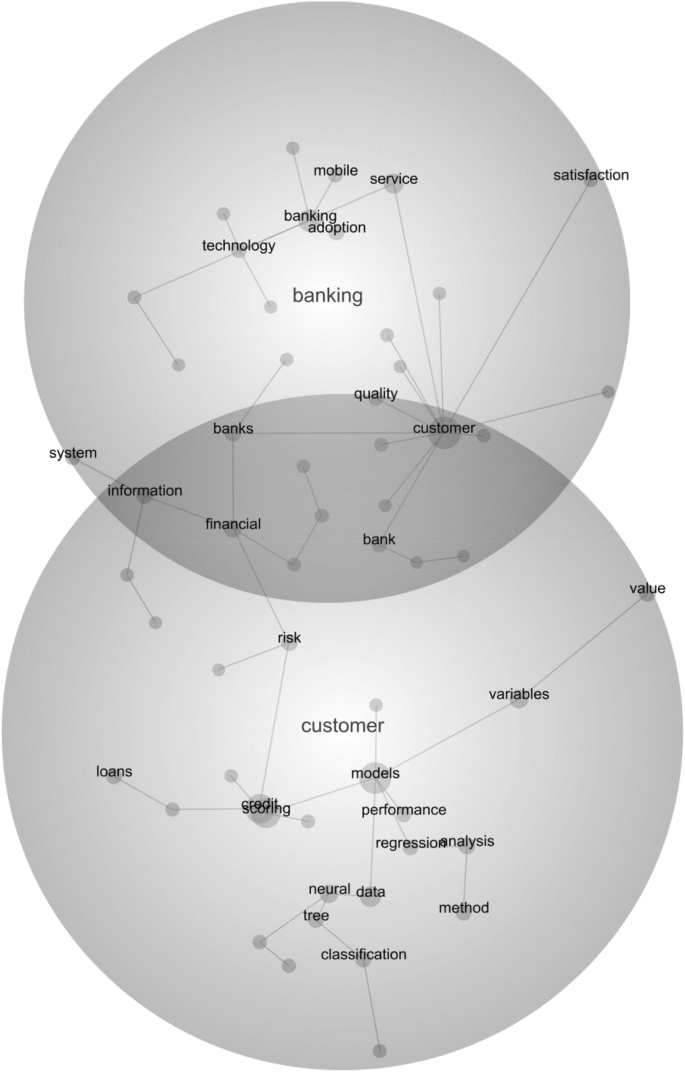
Concept map of content of all papers included in the study
Not surprisingly, the second most prominent concept is “banking,” which is expected as it is the sector that we are examining. The concept “banking” appeared 1,033 times across all the papers. In the “banking” concept, some of the key concept associations include mobile (248 co-occurrences and 88% word association), internet (152 co-occurrences and 82% word association), adoption (220 co-occurrences and 50% word association), and acceptance (71 co-occurrences and 42% word association). This implies the importance of utilizing AI in mobile- and internet-banking research, along with inquiries related to the adoption and acceptance of AI for such uses. Belanche et al. ( 2019 ) proposed a research framework to provide a deeper understanding of the factors driving AI-driven technology adoption in the banking sector. Payne et al. ( 2018 ) examined digital natives' comfort and attitudes toward AI-enabled mobile banking activities and found that the need for services, attitude toward AI, relative advantage, and trust had a significant positive association with the usage of AI-enabled mobile banking services.
Figure 4 highlights the concept associations and draws connections between concepts. The identification and classification of themes and sub-themes using the deductive method in thematic analysis, and the automated approach using Leximancer, provide a reliable and detailed overview of the prior literature.
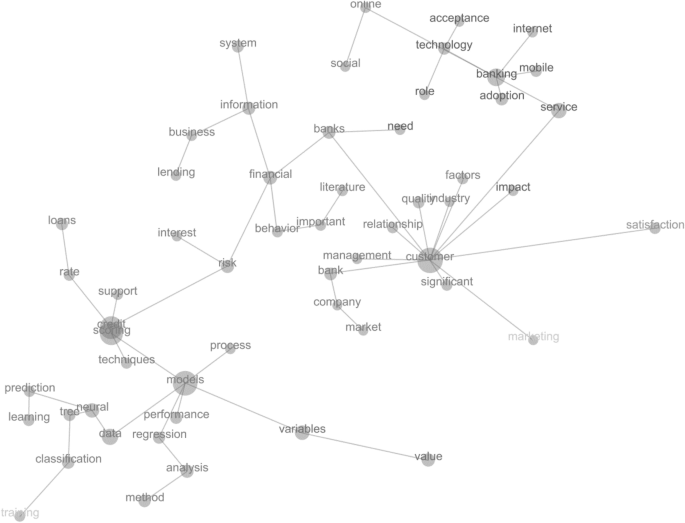
Cloud map of content of all papers included in the study
Customer credit solution application-service blueprint
RQ 2: How does AI impact the banking customer’s journey?
A service blueprint is a method that conceptualizes the customer journey while providing a framework for the front/back-end and support processes (Shostack, 1982 ). For a service blueprint to be effective, the core focus should be on the customer, and steps should be developed based on data and expertise (Bitner et al., 2008 ). As previously discussed, one of the key research areas, AI and banking, relates to credit applications and granting decisions; these are processes that directly impact customer accessibility and acquisition. Here, we develop and propose a Customer Credit Solution Application-Service Blueprint (CCSA) based on our earlier analyses.
Not only was the proposed design developed but the future research direction was also extracted from the articles included in this study. We also validated the framework through direct consultation with banking industry professionals. The CCSA model allows marketers, researchers, and banking professionals to gain a deeper understanding of the customer journey, understand the role of AI, provide an overview of future research directions, and highlight the potential for future growth in this field. As seen in Fig. 5 , we divided the service blueprint into four distinct segments: customer journey, front-stage, back-stage, and support processes. The customer journey is the first step in building a customer-centric blueprint, wherein we highlight the steps taken by customers to apply for a credit solution. The front-stage refers to how the customer interacts with a banking touchpoint (e.g., chatbots). Back-stage actions provide support to customer-facing front-stage actions. Support processes aid in internal organizational interactions and back-stage actions. This section lays out the steps for applying for credit solutions online and showcases the integration and use of AI in the process, with examples from the literature.
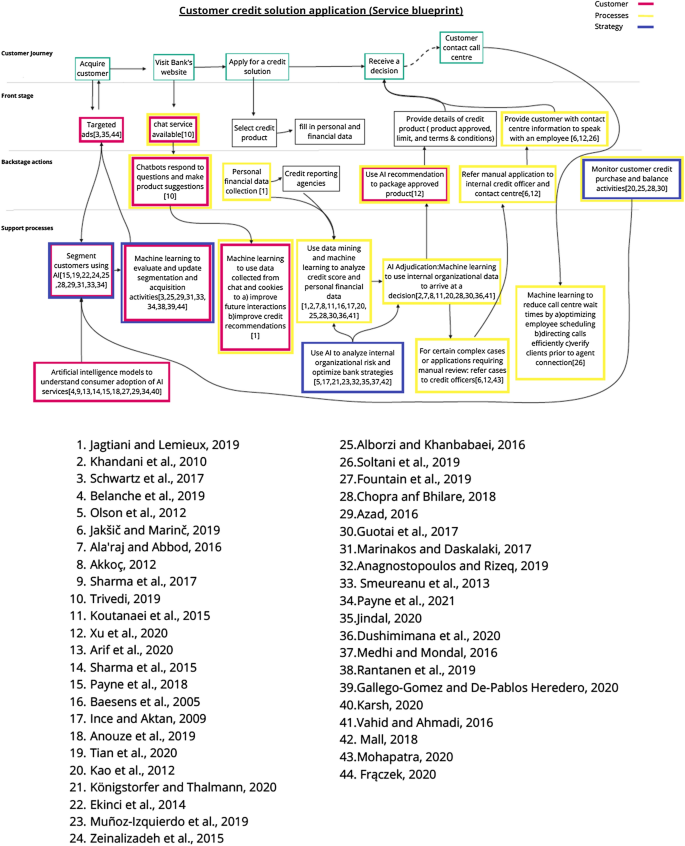
Customer credit solution application journey
Acquire customer
We begin from the initial step of customer acquisition, and proceed to credit decision, and post-decision (Broby, 2021 ). In the acquisition step, customers are targeted with the goal of landing them on the website and converting them to active customers. The front-stage includes targeted ads , where customers are exposed to ads that are tailored for them. For instance, Schwartz et al. ( 2017 ) utilized a multi-armed bandit approach for a large retail bank to improve customer acquisition, and proposed a method that allows bank marketers to maintain the balance between learning from advertisement data and optimizing advertisement investment. At this stage, the support processes focus on integrating AI as a methodological tool to better understand customers' banking adoption behaviors, in combination with utilizing machine learning to evaluate and update segmentation activities. The building block at this stage, is understanding the factors of online adoption. Sharma et al. ( 2017 ) used the neural network approach to investigate the factors influencing mobile banking adoption. Payne et al. ( 2018 ) examined digital natives' comfort and attitudes toward AI-enabled mobile banking activities. Markinos and Daskalaki ( 2017 ) used machine learning to classify bank customers based on their behavior toward advertisements.
Visit bank’s website & apply for a credit solution
At this stage, banking institutions aim to convert website traffic to credit solution applicants. The integration of robo-advisors will help customers select a credit solution that they can best qualify for, and which meets their banking needs. The availability of a robo-advisor can enhance the service offering, as it can help customers with the appropriate solution after gathering basic personal financial data and validating it instantly with credit reporting agencies. Trivedi ( 2019 ) found that information, system, and service quality are key to ensuring a seamless customer experience with the chatbot, with personalization moderating the constructs. Robo-advisors have task-oriented features (e.g., checking bank accounts) coupled with problem-solving features (e.g., processing credit applications). Following this, the data collected will be consistently examined through the use of machine learning to improve the offering and enhance customer experience. Jagtiani and Lemieux ( 2019 ) used machine learning to optimize data collected through different channels, which helps arrive at appropriate and inclusive credit recommendations. It is important to note that while the proposed process provides immense value to customers and banking institutions, many customers are hesitant to share their information; thus, trust in the banking institution is key to enhancing customer experience.
Receive a decision
After the data have been collected through the online channel, data mining and machine learning will aid in the analysis and provide optimal credit decisions. At this stage, the customer receives a credit decision through the robo-advisor. The traditional approaches for credit decisions usually take up to two weeks, as the application goes to the advisory network, then to the underwriting stage, and finally back to the customer. However, with the integration of AI, the customer can save time and be better informed by receiving an instant credit decision, allowing an increased sense of empowerment and control. The process of arriving at such decisions should provide a balance between managing organizational risk, maximizing profit, and increasing financial inclusion. For instance, Khandani et al. ( 2010 ) utilized machine learning techniques to build a model predicting customers' credit risk. Koutanaei et al. ( 2015 ) proposed a data mining model to provide more confidence in credit scoring systems. From an organizational risk standpoint, Mall ( 2018 ) used a neural network approach to examine the behavior of defaulting customers, so as to minimize credit risk, and increase profitability for credit-providing institutions.
Customer contact call center
At this stage, we outline the relationship between humans and AI. As Xu et al. ( 2020 ) found that customers prefer humans for high-complexity tasks, the integration of human employees for cases that require manual review is vital, as AI can make errors or misevaluate one of the C's of credit (Baiden, 2011 ). While AI provides a wealth of benefits for customers and organizations, we refer to Jakšič and Marinč's ( 2019 ) discussion that relationship banking still plays a key role in providing a competitive advantage for financial institutions. The integration of AI at this stage can be achieved by optimizing banking channels. For instance, banking institutions can optimize appointment scheduling time and reduce service time through the use of machine learning, as proposed by Soltani et al. ( 2019 ).
General discussion
Researchers have recognized the viable use of AI to provide enhanced customer service. As discussed in the CCSA service advice, facilities, such as robo-advisors, can aid in product selection, application for banking solutions, and time-saving in low-complexity tasks. As AI has been shown to be an effective tool for automating banking processes, improving customer satisfaction, and increasing profitability, the field has further evolved to examine issues pertaining to strategic insights. Recent research has been focused on investigating the use of AI to drive business strategies. For instance, researchers have examined the use of AI to simplify internal audit reports and evaluate strategic initiatives (Jindal, 2020 ; Muñoz-Izquierdo et al., 2019 ). The latest research also highlights the challenges associated with AI, whether from the perspective of implementation, culture, or organizational resistance (Fountain et al., 2019 ). Moreover, one of the key challenges uncovered in the CCSA is privacy and security concerns of customers in sharing their information. As AI technologies continue to grow in the banking sector, the privacy-personalization paradox has become a key research area that needs to be examined.
In addition, the COVID-19 pandemic has brought on a plethora of challenges in the implementation of AI in the banking sector. Although banks' interest in AI technologies remains high, the reduction in revenue has resulted in a decrease in short-term investment in AI technologies (Anderson et al., 2021 ). Wu and Olson ( 2020 ) highlight the need for banking institutions to continue investing in AI technologies to reduce future risks and enhance the integration between online and offline channels. From a customer perspective, COVID-19 has led to an uptick in the adoption of AI-driven services such as chatbots, E-KYC (Know your client), and robo-advisors (Agarwal et al., 2022 ).
Future research directions
RQ 3: What are the current research deficits and the future directions of research in this field?
Tables 2 , 3 , and 4 provide a complete list of recommendations for future research. These recommendations were developed by reviewing all the future research directions included in the 44 papers. We followed Watkins' ( 2017 ) rigorous and accelerated data reduction (RADaR) technique, which allows for an effective and systematic way to analyze and synthesize calls for future research (Watkins, 2017 ).
Regarding strategy, as AI continues to grow in the banking industry, financial institutions need to examine how internal stakeholders perceive the value of embracing AI, the role of leadership, and multiple other variables that impact the organizational adoption of AI. Therefore, we recommend that future research investigate the different factors (e.g., leadership role) that impact the organizational adoption of AI technologies. In addition, as more organizations use and accept AI, internal challenges emerge (Jöhnk et al., 2021 ). Thus, we recommend examining the different organizational challenges (e.g., organizational culture) associated with AI adoption.
Regarding processes, AI and credit is one of the areas that has been extensively explored since 2005 (Bhatore et al., 2020 ). We recommend expanding beyond the currently proposed models and challenging the underlying assumptions by exploring new aspects of risks presented with the introduction of AI technologies. In addition, we recommend the use of more practical case studies to validate new and existing models. Additionally, the growth of AI has evoked further exploration of how internal processes can be improved (Akerkar, 2019 ). For instance, we suggest investigating AI-driven models with other financial products/solutions (e.g., investments, deposit accounts, etc.).
Regarding customers, the key theories mentioned in the research papers included in the study are the Technology Acceptance Model (TAM) and diffusion of innovation theories (Anouze and Alamro, 2019 ; Azad, 2016 ; Belanche et al., 2019 ; Payne et al., 2018 ; Sharma et al., 2015 , 2017 ). However, as customers continue to become accustomed to AI, it may be imperative to develop theories that go beyond its acceptance and adoption. Thus, we recommend investigating different variables (e.g., social influence and user trends) and methods (e.g., cross-cultural studies) that impact customers' relationship with AI. The gradual shift toward its customer-centric utilization has prompted the exploration of new dimensions of AI that influence customer experience. Going forward, it is important to understand the impact of AI on customers and how it can be used to improve customer experience.
Limitations and implications
This study had several limitations. During our inclusion/exclusion criteria, it is plausible that some AI/banking papers may have been missed because of the specific keywords used to curate our dataset. In addition, articles may have been missed due to the time when the data were collected, such as Manrai and Gupta ( 2022 ), who examined investors' perceptions of robo-advisors. Second, regarding theme identification, there may be a potential bias toward selecting themes, which may lead to misclassification. In addition, we acknowledge that the papers were extracted only from the WoS and Scopus databases, which may limit our access to certain peer-reviewed outlets.
This research provides insights for practitioners and marketers in the North American banking sector. To assist in the implementation of AI-based decision-making, we encourage banking professionals to consider further refining their use of AI in the credit scoring, analysis, and granting processes to minimize risk, reduce costs, and improve customer experience. However, in doing so, we recommend using AI not only to improve internal processes but also as a tool (e.g., chatbots) to improve customer service for low-complexity tasks, thereby directing employees' efforts to other business-impacting activities. Moreover, we recommend using AI as a marketing segmentation tool to target customers for optimal solutions.
This study systematically reviewed the literature (44 papers) on AI and banking from 2005 to 2020. We believe that our findings may benefit industry professionals and decision-makers in formulating strategic decisions regarding the different uses of AI in the banking sector, and optimizing the value derived from AI technologies. We advance the field by providing a more comprehensive outlook specific to the area of AI and banking, reflecting the history and future opportunities for AI in shaping business strategies, improving logistics processes, and enhancing customer value.
Agarwal, P., Swami, S., & Malhotra, S. K. 2022. Artificial intelligence adoption in the post COVID-19 new-normal and role of smart technologies in transforming business: a review. Journal of Science and Technology Policy Management .
Akerkar, R. 2019. Employing AI in business. In artificial intelligence for business , 63–74. Cham: Springer.
Google Scholar
Akkoç, S. 2012. An empirical comparison of conventional techniques, neural networks and the three-stage hybrid adaptive neuro fuzzy inference system (ANFIS) model for credit scoring analysis: the case of Turkish credit card data. European Journal of Operational Research 222 (1): 168–178.
Article Google Scholar
Ala’raj, M., and M.F. Abbod. 2016. Classifiers consensus system approach for credit scoring. Knowledge-Based Systems 104: 89–105.
Alborzi, M., and M. Khanbabaei. 2016. Using data mining and neural networks techniques to propose a new hybrid customer behavior analysis and credit scoring model in banking services based on a developed RFM analysis method. International Journal of Business Information Systems 23 (1): 1–22.
Anagnostopoulos, I., and A. Rizeq. 2019. Confining value from neural networks: a sectoral study prediction of takeover targets in the US technology sector. Managerial Finance 45 (10–11): 1433–1457. https://doi.org/10.1108/MF-12-2017-0523 .
Anderson, J., Bholat, D., Gharbawi, M., & Thew, O. 2021. The impact of COVID-19 on artificial intelligence in banking. Bruegel-Blogs , NA-NA.
Anouze, A.L.M., and A.S. Alamro. 2019. Factors affecting intention to use e-banking in Jordan. International Journal of Bank Marketing 38: 86–112.
Arif, I., W. Aslam, and Y. Hwang. 2020. Barriers in adoption of internet banking: a structural equation modeling-neural network approach. Technology in Society 61: 101231.
Azad, M.A.K. 2016. Predicting mobile banking adoption in Bangladesh: a neural network approach. Transnational Corporations Review 8 (3): 207–214.
Baesens, B., T. Van Gestel, M. Stepanova, D. Van den Poel, and J. Vanthienen. 2005. Neural network survival analysis for personal loan data. Journal of the Operational Research Society 56 (9): 1089–1098.
Baiden, J.E. 2011. The 5 C's of Credit in the Lending Industry. Available at SSRN 1872804.
Bavaresco, R., D. Silveira, E. Reis, J. Barbosa, R. Righi, C. Costa, and C. Moreira. 2020. Conversational agents in business: a systematic literature review and future research directions. Computer Science Review 36: 100239.
Belanche, D., L.V. Casaló, and C. Flavián. 2019. Artificial intelligence in FinTech: understanding robo-advisors adoption among customers. Industrial Management Data Systems 119: 1411–1430.
Bhatore, S., L. Mohan, and Y.R. Reddy. 2020. Machine learning techniques for credit risk evaluation: A systematic literature review. Journal of Banking and Financial Technology 4 (1): 111–138.
Bitner, M.J., A.L. Ostrom, and F.N. Morgan. 2008. Service blueprinting: A practical technique for service innovation. California Management Review 50 (3): 66–94.
Borges, A.F., F.J. Laurindo, M.M. Spínola, R.F. Gonçalves, and C.A. Mattos. 2020. The strategic use of artificial intelligence in the digital era: Systematic literature review and future research directions. International Journal of Information Management 57: 102225.
Boyatzis, R.E. 1998. Transforming qualitative information: Thematic analysis and code development . Thousand Oaks, CA: Sage.
Broby, D. 2021. Financial technology and the future of banking. Financial Innovation 7 (1): 1–19.
Caron, M.S. 2019. The transformative effect of AI on the banking industry. Banking & Finance Law Review 34 (2): 169–214.
Chatha, K.A., and I. Butt. 2015. Themes of study in manufacturing strategy literature. International Journal of Operations & Production Management 35 (4): 604–698.
Chopra, A., and P. Bhilare. 2018. Application of ensemble models in credit scoring models. Business Perspectives and Research 6 (2): 129–141.
Daqar, M.A.A., and S. Arqawi. 2020. Fintech in the eyes of millennials and generation Z (the financial behavior and fintech perception). Banks and Bank Systems 15 (3): 20.
De Oliveira Santini, F., W.J. Ladeira, C.H. Sampaio, and M.G. Perin. 2018. Online banking services: A meta-analytic review and assessment of the impact of antecedents and consequents on satisfaction. Journal of Financial Services Marketing 23 (3): 168–178.
Digalaki, E. 2022. The impact of artificial intelligence in the banking sector & how AI is being used in 2022. https://www.businessinsider.com/ai-in-banking-report?r=US&IR=T
Dobrescu, E.M., and E.M. Dobrescu. 2018. Artificial intelligence (Ai)-the technology that shapes the world. Global Economic Observer 6 (2): 71–81.
Dushimimana, B., Y. Wambui, T. Lubega, and P.E. McSharry. 2020. Use of machine learning techniques to create a credit score model for airtime loans. Journal of Risk and Financial Management 13 (8): 180.
Ekinci, Y., N. Uray, and F. Ülengin. 2014. A customer lifetime value model for the banking industry: A guide to marketing actions. European Journal of Marketing 48 (3–4): 761–784.
Eren, B.A. 2021. Determinants of customer satisfaction in chatbot use: Evidence from a banking application in Turkey. International Journal of Bank Marketing 39 (2): 294–331.
Fountain, T., B. McCarthy, and T. Saleh. 2019. Building the AI-powered organization technology isn’t the biggest challenge, culture is. Harvard Business Review 97 (4): 62.
Frączek, B. 2020. A system to support the transparency of consumer credit offers. Journal of Risk and Financial Management 13 (12): 317.
Gallego-Gomez, C., and C. De-Pablos-Heredero. 2020. Artificial intelligence as an enabling tool for the development of dynamic capabilities in the banking industry. International Journal of Enterprise Information Systems (IJEIS) 16 (3): 20–33.
Goyal, K., and S. Kumar. 2021. Financial literacy: A systematic review and bibliometric analysis. International Journal of Consumer Studies 45 (1): 80–105.
Guotai, C., M.Z. Abedin, and F.E. Moula. 2017. Modeling credit approval data with neural networks: An experimental investigation and optimization. Journal of Business Economics and Management 18 (2): 224–240.
Harzing, A.W., and S. Alakangas. 2016. Google scholar, scopus and the web of science: A longitudinal and cross-disciplinary comparison. Scientometrics 106 (2): 787–804.
Hua, X., Y. Huang, and Y. Zheng. 2019. Current practices, new insights, and emerging trends of financial technologies. Industrial Management & Data Systems 119 (7): 1401–1410.
Ince, H., and B. Aktan. 2009. A comparison of data mining techniques for credit scoring in banking: A managerial perspective. Journal of Business Economics and Management 10 (3): 233–240.
Jagtiani, J., and C. Lemieux. 2019. The roles of alternative data and machine learning in fintech lending: Evidence from the LendingClub consumer platform. Financial Management 48 (4): 1009–1029.
Jakšič, M., and M. Marinč. 2019. Relationship banking and information technology: The role of artificial intelligence and FinTech. Risk Management 21 (1): 1–18.
Jindal, N. 2020. The impact of advertising and R&D on bankruptcy survival: A double-edged sword. Journal of Marketing 84 (5): 22–40.
Jöhnk, J., M. Weißert, and K. Wyrtki. 2021. Ready or not, AI comes—an interview study of organizational AI readiness factors. Business & Information Systems Engineering 63 (1): 5–20.
Kao, L.J., C.C. Chiu, and F.Y. Chiu. 2012. A Bayesian latent variable model with classification and regression tree approach for behavior and credit scoring. Knowledge-Based Systems 36: 245–252.
Khan, K.S., R. Kunz, J. Kleijnen, and G. Antes. 2003. Five steps to conducting a systematic review. Journal of the Royal Society of Medicine 96 (3): 118–121.
Khandani, A.E., A.J. Kim, and A.W. Lo. 2010. Consumer credit-risk models via machine-learning algorithms. Journal of Banking & Finance 34 (11): 2767–2787.
Kim, K.J., and W.B. Lee. 2004. Stock market prediction using artificial neural networks with optimal feature transformation. Neural Computing & Applications 13 (3): 255–260.
Kok, J.N., E.J. Boers, W.A. Kosters, P. Van der Putten, and M. Poel. 2009. Artificial intelligence: Definition, trends, techniques, and cases. Artificial Intelligence 1: 270–299.
Königstorfer, F., and S. Thalmann. 2020. Applications of artificial intelligence in commercial banks–a research agenda for behavioral finance. Journal of Behavioral and Experimental Finance 27: 100352.
Koutanaei, F.N., H. Sajedi, and M. Khanbabaei. 2015. A hybrid data mining model of feature selection algorithms and ensemble learning classifiers for credit scoring. Journal of Retailing and Consumer Services 27: 11–23.
Larson, E.J. 2021. The myth of artificial intelligence . In The Myth of Artificial Intelligence: Harvard University Press.
Book Google Scholar
Leximancer. (2019, November 25). Leximancer User Guide Release 5.0. Leximancer. https://static1.squarespace.com/static/539bebd7e4b045b6dc97e4f7/t/5e58d901137e3077d4409092/1582881372656/LeximancerUserGuide5.pdf .
Loureiro, S.M.C., J. Guerreiro, and I. Tussyadiah. 2020. Artificial intelligence in business: State of the art and future research agenda. Journal of Business Research . https://doi.org/10.1016/j.jbusres.2020.11.001 .
Malali, A.B., and S. Gopalakrishnan. 2020. Application of artificial intelligence and its powered technologies in the indian banking and financial industry: An overview. IOSR Journal of Humanities and Social Science 25 (4): 55–60.
Mall, S. 2018. An empirical study on credit risk management: The case of nonbanking financial companies. Journal of Credit Risk 14 (3): 49–66.
Manrai, R., and K.P. Gupta. 2022. Investor’s perceptions on artificial intelligence (AI) technology adoption in investment services in India. Journal of Financial Services Marketing . https://doi.org/10.1057/s41264-021-00134-9 .
Marinakos, G., and S. Daskalaki. 2017. Imbalanced customer classification for bank direct marketing. Journal of Marketing Analytics 5 (1): 14–30.
McCarthy, J., Minsky, M.L., & Rochester, N. 1956. The Dartmouth summer research project on artificial intelligence. Artificial intelligence: past, present, and future. Available at: http://www.dartmouth.edu/*vox/0607/ 0724/ai50.html
Medhi, P.K., and S. Mondal. 2016. A neural feature extraction model for classification of firms and prediction of outsourcing success: Advantage of using relational sources of information for new suppliers. International Journal of Production Research 54 (20): 6071–6081.
Mehrotra, A. (2019, April). Artificial Intelligence in Financial Services–Need to Blend Automation with Human Touch. In 2019 International Conference on Automation, Computational and Technology Management (ICACTM) (pp. 342–347). IEEE.
Mohapatra, S. 2020. Human and computer interaction in information system design for managing business. Information Systems and e-Business Management . https://doi.org/10.1007/s10257-020-00475-3 .
Moher, D., Liberati, A., Tetzlaff, J., Altman, D.G., & Prisma Group. 2009. Preferred reporting items for systematic reviews and meta-analyses: the PRISMA statement. PLoS medicine , 6(7), e1000097
Mongeon, P., and A. Paul-Hus. 2016. The journal coverage of Web of Science and Scopus: A comparative analysis. Scientometrics 106 (1): 213–228.
Muñoz-Izquierdo, N., M.D.M. Camacho-Miñano, M.J. Segovia-Vargas, and D. Pascual-Ezama. 2019. Is the external audit report useful for bankruptcy prediction? Evidence using artificial intelligence. International Journal of Financial Studies 7 (2): 20.
Nusair, K., I. Butt, and S.R. Nikhashemi. 2019. A bibliometric analysis of social media in hospitality and tourism research. International Journal of Contemporary Hospitality Management 31: 2691–2719.
Olson, D.L., D. Delen, and Y. Meng. 2012. Comparative analysis of data mining methods for bankruptcy prediction. Decision Support Systems 52 (2): 464–473.
Pahlevan-Sharif, S., P. Mura, and S.N. Wijesinghe. 2019. A systematic review of systematic reviews in tourism. Journal of Hospitality and Tourism Management 39: 158–165.
Payne, E.M., J.W. Peltier, and V.A. Barger. 2018. Mobile banking and AI-enabled mobile banking: The differential effects of technological and non-technological factors on digital natives’ perceptions and behavior. Journal of Research in Interactive Marketing 12 (3): 328–346.
Payne, E.H., J. Peltier, and V.A. Barger. 2021. Enhancing the value co-creation process: Artificial intelligence and mobile banking service platforms. Journal of Research in Interactive Marketing 15: 68–85.
Rajaobelina, L., and L. Ricard. 2021. Classifying potential users of live chat services and chatbots. Journal of Financial Services Marketing 26 (2): 81–94.
Ransbotham, S., Kiron, D., Gerbert, P., & Reeves, M. 2017. Reshaping business with artificial intelligence closing the gap between ambition and action. MIT Sloan Management Review , 59(1).
Rantanen, A., J. Salminen, F. Ginter, and B.J. Jansen. 2019. Classifying online corporate reputation with machine learning: A study in the banking domain. Internet Research 30: 45–66.
Schwartz, E.M., E.T. Bradlow, and P.S. Fader. 2017. Customer acquisition via display advertising using multi-armed bandit experiments. Marketing Science 36 (4): 500–522.
Sharma, S.K., S.M. Govindaluri, and S.M. Al Balushi. 2015. Predicting determinants of Internet banking adoption. Management Research Review 38 (7): 750–766.
Sharma, S.K., Govindaluri, S.M., Al-Muharrami, S., & Tarhini, A. 2017. A multi-analytical model for mobile banking adoption: a developing country perspective. Review of International Business and Strategy .
Shostack, G.L. 1982. How to design a service. European Journal of Marketing 16 (1): 49–63.
Smeureanu, I., G. Ruxanda, and L.M. Badea. 2013. Customer segmentation in private banking sector using machine learning techniques. Journal of Business Economics and Management 14 (5): 923–939.
Soltani, M., M. Samorani, and B. Kolfal. 2019. Appointment scheduling with multiple providers and stochastic service times. European Journal of Operational Research 277 (2): 667–683.
Tarafdar, M., C.M. Beath, and J.W. Ross. 2019. Using AI to enhance business operations. MIT Sloan Management Review 60 (4): 37–44.
Tian, Z., R.Y. Zhong, A. Vatankhah Barenji, Y.T. Wang, Z. Li, and Y. Rong. 2020. A blockchain-based evaluation approach for customer delivery satisfaction in sustainable urban logistics. International Journal of Production Research 59: 1–21.
Tranfield, D., D. Denyer, and P. Smart. 2003. Towards a methodology for developing evidence-informed management knowledge by means of systematic review. British Journal of Management 14 (3): 207–222.
Trivedi, J. 2019. Examining the customer experience of using banking Chatbots and its impact on brand love: The moderating role of perceived risk. Journal of Internet Commerce 18 (1): 91–111.
Tseng, C.C. (2003, July). Comparing artificial intelligence systems for stock portfolio selection. In The 9th international conference of computing in economics and finance (pp. 1–7).
Vahid, P.R., and A. Ahmadi. 2016. Modeling corporate customers’ credit risk considering the ensemble approaches in multiclass classification: Evidence from Iranian corporate credits. Journal of Credit Risk 12 (3): 71–95.
Valsamidis, S., Tsourgiannis, L., Pappas, D., & Mosxou, E. 2020. Digital banking in the new Era: Exploring customers' attitudes. In Business performance and financial institutions in Europe (pp. 91–104). Springer, Cham.
Verma, S., R. Sharma, S. Deb, and D. Maitra. 2021. Artificial intelligence in marketing: Systematic review and future research direction. International Journal of Information Management Data Insights 1: 100002.
Watkins, D.C. 2017. Rapid and rigorous qualitative data analysis: The “RADaR” technique for applied research. International Journal of Qualitative Methods 16 (1): 1609406917712131.
Wu, D.D., & Olson, D.L. 2020. The effect of COVID-19 on the banking sector. In Pandemic risk management in operations and finance (pp. 89–99). Springer, Cham.
Xiao, Y., and M. Watson. 2019. Guidance on conducting a systematic literature review. Journal of Planning Education and Research 39 (1): 93–112.
Xu, Y., C.H. Shieh, P. van Esch, and I.L. Ling. 2020. AI customer service: Task complexity, problem-solving ability, and usage intention. Australasian Marketing Journal 28 (4): 189–199.
Yang, A.S. 2009. Exploring adoption difficulties in mobile banking services. Canadian Journal of Administrative Sciences/revue Canadienne Des Sciences De L’administration 26 (2): 136–149.
Zeinalizadeh, N., A.A. Shojaie, and M. Shariatmadari. 2015. Modeling and analysis of bank customer satisfaction using neural networks approach. International Journal of Bank Marketing 33 (6): 717–732.
Download references
Author information
Authors and affiliations.
Ted Rogers School of Retail Management, Toronto Metropolitan University, 350 Victoria St, Toronto, ON, M5B 2K3, Canada
Omar H. Fares, Irfan Butt & Seung Hwan Mark Lee
You can also search for this author in PubMed Google Scholar
Corresponding author
Correspondence to Omar H. Fares .
Ethics declarations
Conflict of interest.
On behalf of all authors, the corresponding author states that there is no conflict of interest.
Additional information
Publisher's note.
Springer Nature remains neutral with regard to jurisdictional claims in published maps and institutional affiliations.
See Tables 2 , 3 and 4 .
Rights and permissions
Springer Nature or its licensor holds exclusive rights to this article under a publishing agreement with the author(s) or other rightsholder(s); author self-archiving of the accepted manuscript version of this article is solely governed by the terms of such publishing agreement and applicable law.
Reprints and permissions
About this article
Fares, O.H., Butt, I. & Lee, S.H.M. Utilization of artificial intelligence in the banking sector: a systematic literature review. J Financ Serv Mark 28 , 835–852 (2023). https://doi.org/10.1057/s41264-022-00176-7
Download citation
Received : 12 January 2022
Revised : 18 July 2022
Accepted : 27 July 2022
Published : 11 August 2022
Issue Date : December 2023
DOI : https://doi.org/10.1057/s41264-022-00176-7
Share this article
Anyone you share the following link with will be able to read this content:
Sorry, a shareable link is not currently available for this article.
Provided by the Springer Nature SharedIt content-sharing initiative
- Artificial intelligence
- Digital innovations
- Retail banking
- Customer journey map
- Systematic literature review
- Find a journal
- Publish with us
- Track your research
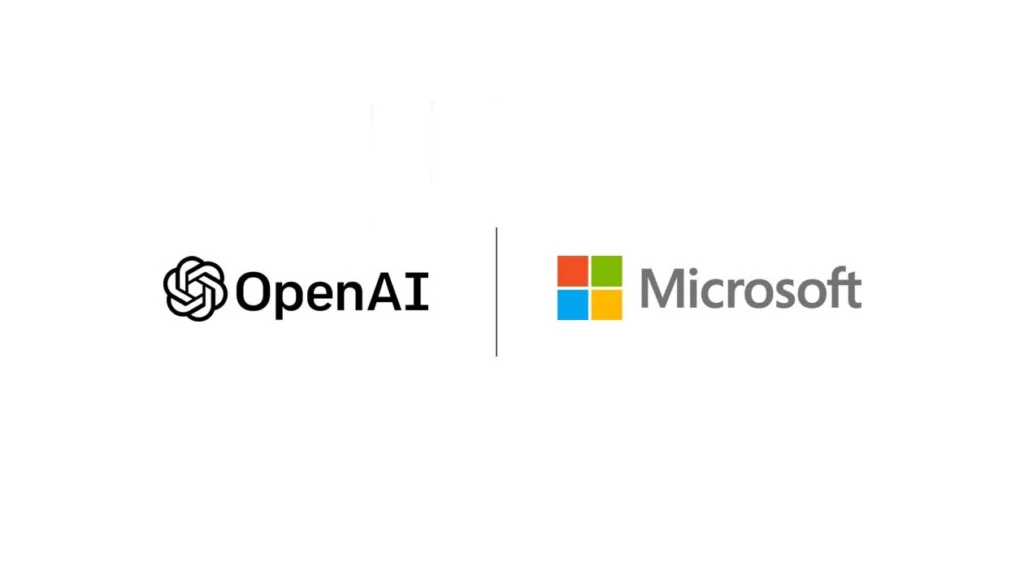
AI + Machine Learning , Announcements , Azure AI Content Safety , Azure AI Studio , Azure OpenAI Service , Partners
Introducing GPT-4o: OpenAI’s new flagship multimodal model now in preview on Azure
By Eric Boyd Corporate Vice President, Azure AI Platform, Microsoft
Posted on May 13, 2024 2 min read
- Tag: Copilot
- Tag: Generative AI
Microsoft is thrilled to announce the launch of GPT-4o, OpenAI’s new flagship model on Azure AI. This groundbreaking multimodal model integrates text, vision, and audio capabilities, setting a new standard for generative and conversational AI experiences. GPT-4o is available now in Azure OpenAI Service, to try in preview , with support for text and image.
Azure OpenAI Service
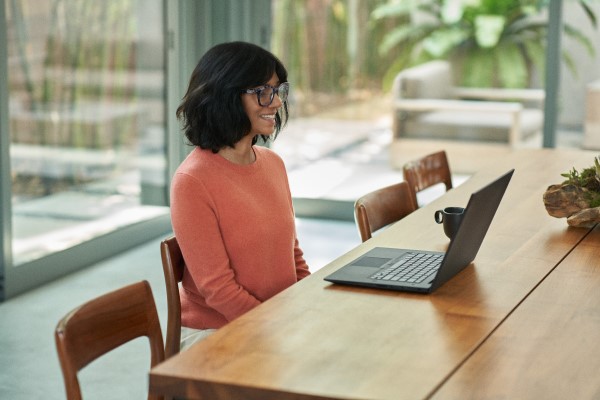
A step forward in generative AI for Azure OpenAI Service
GPT-4o offers a shift in how AI models interact with multimodal inputs. By seamlessly combining text, images, and audio, GPT-4o provides a richer, more engaging user experience.
Launch highlights: Immediate access and what you can expect
Azure OpenAI Service customers can explore GPT-4o’s extensive capabilities through a preview playground in Azure OpenAI Studio starting today in two regions in the US. This initial release focuses on text and vision inputs to provide a glimpse into the model’s potential, paving the way for further capabilities like audio and video.
Efficiency and cost-effectiveness
GPT-4o is engineered for speed and efficiency. Its advanced ability to handle complex queries with minimal resources can translate into cost savings and performance.
Potential use cases to explore with GPT-4o
The introduction of GPT-4o opens numerous possibilities for businesses in various sectors:
- Enhanced customer service : By integrating diverse data inputs, GPT-4o enables more dynamic and comprehensive customer support interactions.
- Advanced analytics : Leverage GPT-4o’s capability to process and analyze different types of data to enhance decision-making and uncover deeper insights.
- Content innovation : Use GPT-4o’s generative capabilities to create engaging and diverse content formats, catering to a broad range of consumer preferences.
Exciting future developments: GPT-4o at Microsoft Build 2024
We are eager to share more about GPT-4o and other Azure AI updates at Microsoft Build 2024 , to help developers further unlock the power of generative AI.
Get started with Azure OpenAI Service
Begin your journey with GPT-4o and Azure OpenAI Service by taking the following steps:
- Try out GPT-4o in Azure OpenAI Service Chat Playground (in preview).
- If you are not a current Azure OpenAI Service customer, apply for access by completing this form .
- Learn more about Azure OpenAI Service and the latest enhancements.
- Understand responsible AI tooling available in Azure with Azure AI Content Safety .
- Review the OpenAI blog on GPT-4o.
Let us know what you think of Azure and what you would like to see in the future.
Provide feedback
Build your cloud computing and Azure skills with free courses by Microsoft Learn.
Explore Azure learning
Related posts
AI + Machine Learning , Azure AI Studio , Customer stories
3 ways Microsoft Azure AI Studio helps accelerate the AI development journey chevron_right
AI + Machine Learning , Analyst Reports , Azure AI , Azure AI Content Safety , Azure AI Search , Azure AI Services , Azure AI Studio , Azure OpenAI Service , Partners
Microsoft is a Leader in the 2024 Gartner® Magic Quadrant™ for Cloud AI Developer Services chevron_right
AI + Machine Learning , Azure AI , Azure AI Content Safety , Azure Cognitive Search , Azure Kubernetes Service (AKS) , Azure OpenAI Service , Customer stories
AI-powered dialogues: Global telecommunications with Azure OpenAI Service chevron_right
AI + Machine Learning , Azure AI , Azure AI Content Safety , Azure OpenAI Service , Customer stories
Generative AI and the path to personalized medicine with Microsoft Azure chevron_right
Join the conversation, leave a reply cancel reply.
Your email address will not be published. Required fields are marked *
I understand by submitting this form Microsoft is collecting my name, email and comment as a means to track comments on this website. This information will also be processed by an outside service for Spam protection. For more information, please review our Privacy Policy and Terms of Use .
I agree to the above
Raisin (SaveBetter) Review 2024: High-Yield Accounts, but are They Safe and Reliable?
Find a Qualified Financial Advisor
Finding a financial advisor doesn't have to be hard. SmartAsset's free tool matches you with up to three fiduciary financial advisors that serve your area in minutes. Each advisor has been vetted by SmartAsset and is held to a fiduciary standard to act in your best interests. Start your search now.
The offers and details on this page may have updated or changed since the time of publication. See our article on Business Insider for current information.
Affiliate links for the products on this page are from partners that compensate us and terms apply to offers listed (see our advertiser disclosure with our list of partners for more details). However, our opinions are our own. See how we rate banking products to write unbiased product reviews.
Raisin is a great option if your ultimate goal is to earn the best savings account , money market account, and CD rates out there. Raisin offers APYs of up to 5.26% on savings and up to 5.31% on CDs. It's a marketplace that connects you with accounts you might not be able to access otherwise, including ones at community-driven and minority-owned institutions. You'll also earn higher rates than if you opened an account directly through one of its partner banks.
Raisin Interest Rates
- NexBank High Yield Savings Account: 5.26%
- Banc of California 3 Month CD: 5.28%
- Western Alliance Bank 6 Month CD: 5.23%
- Western Alliance Bank 1 Year CD: 5.05%
- Technology Credit Union 5 Month No Penalty CD: 5.15%
- Grand Bank Money Market Deposit Account: 5.25%
- Compare all Raisin offers
About Raisin
Raisin is a digital marketplace for finding high-yield accounts. It partners with banks and credit unions to offer you special interest rates if you open an account through Raisin rather than directly on the partner banks' websites.
Raisin has been providing a similar high-yield savings account marketplace in Europe. It works with over 400 banks and has more than 1 million direct customers. It started providing services in the U.S. through SaveBetter, and SaveBetter has now officially rebranded as Raisin.
Raisin is not a good choice if you want immediate access to your savings, though — it doesn't offer checking accounts, so you'll need to keep a checking account at another institution and make transfers between banks, which could take up to a few business days.
Raisin Pros and Cons
Raisin account pros.
One of the primary benefits of using Raisin is that you'll be able to find a variety of savings accounts, money market accounts, and CDs with high interest rates. Many of these rates are exclusive options, and the rates are higher than what you would find if you opened an account directly from a participating bank's website.
Raisin also stands out for its opening requirements. There's a $1 minimum opening deposit and no monthly service fee for all accounts. At traditional financial institutions, you may need to be mindful of monthly bank maintenance fees or higher minimum opening requirements.
Raisin account cons
Raisin offers several types of savings accounts , but it doesn't offer any checking or business bank accounts. As a result, if you transfer money to an external account, it may take one to three business days to process.
Because you're not directly banking with a financial institution, there are also a couple of unique downsides. For example, Raisin doesn't let you receive direct deposits or make cash deposits. You also can't connect your selected savings accounts to a third-party budgeting app like Rocket Money or YNAB App .
Raisin also has poor mobile app ratings on both the Apple Store and Google Play Store. That said, there are fewer than 60 reviews on each mobile app.
Raisin Savings Platform
Raisin partners with multiple banks and credit unions, including Sallie Mae , Mission Valley Bank, SkyOne Federal Credit Union, and more. Raisin can change partner companies at any time.
Many of the institutions are less well-known companies — but the good news is that your money is still safe with these less popular brands. You have FDIC insurance for banks (or NCUA insurance with credit unions) when you open accounts through Raisin. This means up to $250,000 is safe in an individual account, and $500,000 in a joint account.
Credit unions require you to become a member to open an account, and membership is usually only available to employees of certain companies or residents of specific counties. But when you open a credit union account through Raisin, you simply have to sign a membership agreement, and you'll qualify without having to pay any membership fees.
Some of the partner bank accounts are available nationwide, but you'll earn a higher rate through Raisin. Others are usually only available in certain areas, but they're available around the U.S. through Raisin.
The marketplace gives you the chance to earn high interest rates with the added bonus of working with institutions whose values you support. For example, Ponce Bank is certified as a community development financial institution (CDFI) , meaning it serves a local disadvantaged community. It's also a Hispanic-American owned bank . When you're searching for accounts, Raisin lets you filter by the type of institution you want, such as minority-led, family-owned, or one with a local focus.
Once you deposit money into your account through Raisin, it's held in an omnibus custodial account at the partner bank. The custodial account is managed by Lewis & Clark Bank , which is the custodian bank. When your funds are in a custodial account, the money is pooled with deposits from other Raisin customers.
Custodial accounts make it possible for Raisin to deposit money to separate banks, but they do come with drawbacks. The main downside is that it's a little difficult to access your savings. To deposit or withdraw money, you must link your Raisin account to an external account, then transfer money between the accounts. These transfers typically take one to three business days. So you'd always want to keep a checking account at a separate bank for these transfers.
Raisin Customer Service
To contact customer service, call Monday through Friday from 9 a.m. to 4 p.m. ET. You can also send an email or use automated chat on the website.
Some Redditor users have said they've experienced long wait times to receive customer support, though. Traditional banks and credit unions may offer more customer service availability. Some banks have 24/7 customer support through online chat or telephone.
Raisin is rated 2.0 out of 5 stars in the Apple store, and 3.1 out of 5 stars in the Google Play store. However, there are fewer than 60 reviews on each mobile app.
Is Raisin Trustworthy?
The Better Business Bureau grades companies based on responses to customer complaints, transparency about business practices, government actions against the businesses, and more. The BBB gives Raisin a B+ grade because there are several complaints against the company on the BBB website.
Raisin does not have any public controversies.
It can be good to read customer reviews or talk with friends and family who have used Raisin to get a better idea of what your experience could be like. You also may want to look into the BBB grade and customer reviews for a partner bank you're considering using through Raisin.
Raisin High-yield Accounts
Raisin high-yield savings and money market accounts.
The average savings account interest rate nationwide is just 0.46% APY. Many savings accounts at national banks charge monthly fees if you don't qualify to waive them. You'll earn significantly more through Raisin's partner savings and money market accounts, and you won't pay monthly fees.
Let's say you leave $10,000 in a savings account untouched. At a traditional bank that pays 0.05% APY compounded daily, you'd earn $5 in one year (which might be cancelled out by how much you pay in monthly fees). Maybe you open an account with Raisin that pays 5.00% APY. In one year, you'd earn $512.67 on that $10,000.
Raisin Fixed-Rate CDs
Savings and money market accounts have variable interest rates, so rates can fluctuate at any time. But CDs have fixed rates, meaning that if you open a 1-year CD with a 5% APY, that rate is set in stone and you'll earn 5% for the entire year.
There are other benefits to opening a fixed-rate CD through Raisin besides a higher rate. First, you may have access to a term length (say two months or nine months) that isn't available directly through the bank. Second, you only need $1 to open a CD through Raisin, regardless of which partner bank you choose. CDs typically require hundreds or even thousands to open an account. For example, you would normally need $2,500 to open a CD with Sallie Mae, but you only need $1 to open a Sallie Mae CD with Raisin.
Keep in mind that you will have to pay an early withdrawal penalty if you take out money before the term ends. When searching for fixed-rate CDs on the Raisin website, click on "product terms" next to the CD you're considering to see what that fee would be.
Raisin No-Penalty CDs
Unlike regular CDs, no-penalty CDs do not charge you for taking out money before a term ends. Most banks require you to take out all the money in one lump sum, though.
No-penalty CDs aren't super common, so it's nice that Raisin gives you even more options than you may find elsewhere.
Raisin Savings Alternatives
Raisin vs. ally.
If you're searching for the highest savings rates, Raisin will likely stand out more than Ally. Raisin has savings accounts, money market accounts, and CDs from a variety of banks and credit unions that pay more than any of Ally's bank accounts.
Although Raisin has partnerships with many banks, your overall banking options and services are still limited. Raisin doesn't offer checking accounts or access to other types of products like credit cards or loans.
If you're looking to do all your banking with one financial institution, Ally is a great option. Ally is one of the best online banks , and it has minimal fees and easy opening requirements for a variety of products.
Ally is also faster and makes it easier to access your money. You can simply transfer money between the bank's checking and savings accounts and see the change almost instantly.
Ally Bank Review
Raisin vs. Popular Direct
Raisin and the online bank Popular Direct both offer some of the best CD rates and savings account rates overall, so deciding between the two companies may ultimately depend on the account you want and how much you plan to deposit upfront.
Raisin may be a better choice if are looking for accounts with low minimum opening requirements – you only need $1 to get started. Meanwhile, Popular Direct has pretty steep opening requirements for an online bank. You'll need $100 to open a savings account and $10,000 to open a CD.
Popular Direct offers a checking account though, which Raisin doesn't offer. Hence, you may prefer Popular Direct if you want more direct access to your savings. Popular Direct also allows direct deposits, while Raisin does not.
Popular Direct Review
Why You Should Trust Us: How We Reviewed Raisin Accounts
At Personal Finance Insider, we rate products on a scale from zero to five stars. We use a bank account rating methodology that compares and contrasts the features and fine print of various products to help determine if the account may be right for you.
For example, we'll look at the minimum opening deposit, interest rate, monthly service fees, customer support availability, mobile app ratings, and ethics of a company to determine the rate for a savings account. For CDs, we'll look at similar criteria. However, we'll factor in early withdrawal penalties and CD variety, and we won't consider monthly service fees since that isn't applicable.
Raisin FAQs
Is raisin legitimate.
Yes, Raisin is a legitimate company. It connects you to bank accounts that are federally insured by the FDIC or NCUA.
Can I trust Raisin Bank?
Yes, Raisin is a trustworthy service overall. Raisin isn't a bank, it's an online marketplace that connects you with partner banks. Through these partner banks, your money is federally insured by the FDIC or NCUA. The American Institute of CPAs has given Raisin an SOC 2 certification, which means the AICPA has audited Raisin and found that the company has a high level of online security.
Does Raisin charge a fee?
No, Raisin doesn't charge a membership fee or a monthly bank maintenance fee. You'll only pay a fee if you withdraw money from a CD before the term ends — this is known as an early withdrawal penalty.
Is Raisin insured?
Yes, accounts you open through Raisin are insured. Accounts at partner banks are federally insured by the FDIC, and ones at partner credit unions are insured by the NCUA.
Who is Raisin owned by?
Raisin is owned by Raisin GmbH, a logistics firm founded in 2012. Raisin GmbH is based in Berlin, Germany, and owns both Raisin and Raisin UK.
How do I withdraw money from Raisin?
To withdraw money from Raisin, transfer funds from your Raisin-affiliated account to an external bank account. The transfer will probably take one to three business days.
If you enjoyed this story, be sure to follow Business Insider on Microsoft Start.
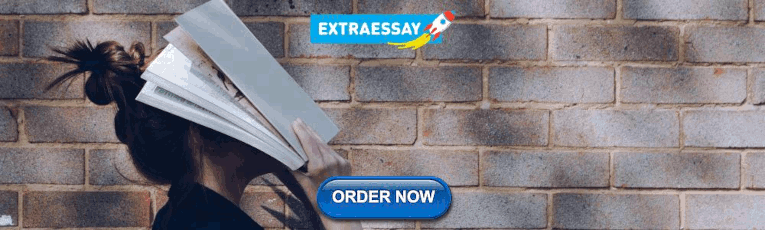
IMAGES
VIDEO
COMMENTS
This study presents a systematic literature review of regulation, profitability, and risk in the banking industry and explores the relationship between them. It proposes a policy initiative using a model that offers guidelines to establish the right mix among these variables. This is a systematic literature review study. Firstly, the necessary data are extracted using the relevant keywords ...
Published: September 01, 202 3. Abstract— The study aimed to present a systematic literature Review based on scientific research extracted for the Impact of e-banking. on Customer satisfaction ...
E-Banking has become one of the essential banking services that can, if properly implemented, increase customer satisfaction, and give banks a competitive advantage. ... The following section will present a thorough "Literature Review," followed by the "Method" and "Findings" of the study. An "Interpretation and Discussion ...
We review the burgeoning literature on FinTech and FinTech-enabled services, focusing on the opportunities and risks for banks. This paper is therefore timely because in most countries the traditional 'brick and mortar' model in banking has been combined with or replaced by an online model, where information technology and highly ...
This review article synthesizes the existing literature on the factors that influence the adoption and use of digital financial services, such as mobile money, online banking, and digital payment platforms. It identifies the main barriers and facilitators at the individual, social, and environmental levels, and provides implications for policy and practice.
The fast-growing trend of information technologies in banking and other businesses has led to computerizing banking transactions and other companies (Omotayo, 2020).This information technology-based development has given rise to new ways for business organizations to communicate with their customers, which supports improving banking and financial services (Raza et al., 2020).
Following a close review of the literature on cus-tomer satisfaction in general and specifically on customer satisfaction with banking services, as well as the related concepts of service quality and customer loyalty, we decided upon five main categories of determinants that impact customer satisfaction with banking services: convenience,
Objectives: This paper explains, synthesizes, reviews the main findings, and provides suggestions for future. research to deepen and enrich understanding of technology-based banking services ...
This paper provides a meta-analysis of the generalizations in the relationships between the antecedents and consequents of satisfaction with online banking services. In total, 118 observations were analysed, with a sample of 49,607 respondents in 39 published articles from studies indexed in ten databases (Jstor, Emerald, PsycINFO, Taylor & Francis, Elsevier Science Direct, Scopus, ProQuest ...
Structural equation modeling (SEM), followed by partial least squares SEM, is a majorly used method. There are three research streams in the literature: retail banking service quality, internet banking service quality and mobile banking service quality. Retail banking is the most studied stream, whereas mobile banking is the least studied stream.
review the literature to recognize the applicable advantages, the challenges which the Fintech firms could possess for the conventional system of banking. Financial services have altered significantly over the last few generations, owing pri-marily to advances in technology in telecommunications, information and technology, and banking policies.
The major contribution of this literature review is the identification of three major MFS domains, which are defined as a wide range of traditional and value-added services, retail transactions, banking activities and information accessible through portable devices and wearables (Dorfleitner et al., 2019). These three domains comprising the ...
LITERATURE REVIEW OF BANKING STUDIES 11 the Herfindahl-Hirschman index (HHI) 1 or an n-firm concentration ratio as exogenous indicators of market power and most of them were limited to analyzing the US banking markets, and used cross-section static panels or short-run time periods. 2
This study aims to demonstrate digital banking's influence on customers' evaluation of service experience and develop a framework identifying the most significant variables of digital banking that influence the financial performance of banks.,This structured review of literature, guided with the preferred reporting items for systematic ...
Banks also play an important role in diminishing informational asymmetries and risks in the financial system. Hence, the study of the banking industry and its impact on the economy is of the utmost importance. The effects of concentration and competition on bank performance are pertinent since they have important policy implications.
The framework integrates the rich literature on sustainable banking, maps the predominant research areas, and highlights the primary research gaps. Finally, the literature review demonstrates a progressive convergence of the instrumental and ethical approaches to sustainable banking. This convergence highlights the importance of both the vivid ...
Abstract. This study analyses existing literature review studies on banking sector performance. Specially, this research aim is to identify topics of interest and development niche for this vast ...
Mobile banking is the most popular and powerful mode of service delivery, which ensures the delivery of banking services anywhere and anytime. This article attempts to analyse the current status of research on mobile banking in order to identify the themes to be explored by future researchers.
IJMT January 2014 Volume 4, Issue 1 ISSN: 2249-1058 _____ LITERATURE REVIEW ON E-BANKING SERVICES SUJA.P NIRMALA RAGHAVAN ABSTRACT Banks adopt E-banking as a means to replace their traditional delivery channel through branch banking mainly due to the cost of setting up of physical branches and increased overheads associate with maintaining them .
E-BANKING: REVIEW OF LITERATURE. Anukool Manish, Hyde. Published 2017. Business, Computer Science, Economics. A feature of the banking industry across the globe has been that it is increasingly becoming turbulent and competitive, characterized by an increasing trend towards internationalization, mergers, takeovers and consolidation of the ...
system started in 2005 by the Reserve Bank of India (RBI), which enables bank customers in India to transfer funds between any two NEFT-enabled bank accounts on a one-to-one basis. Online innovation services and mobile banking have both grown in popularity in recent years. The Indian banking industry's transition to digitalization, which started
Online banking is one of the e-banking services relatively a new channel and is an umbrella term for the process by which a customer may perform banking transactions electronically without visiting a brick-and-mortar institution (Compeau & Higgins, 1995; Shah & Clarke, 2009).The fast-paced technology has affected almost all industries including banking industry.
Sponsored by a grant from the Gordon and Betty Moore Foundation, a literature review and comparative analyses were conducted utilizing data from internet searches by patients; the purpose of the review and analyses was to identify correlations (or a lack thereof) between each patient's searches and the corresponding patient's medical diagnoses ...
This study provides a holistic and systematic review of the literature on the utilization of artificial intelligence (AI) in the banking sector since 2005. In this study, the authors examined 44 articles through a systematic literature review approach and conducted a thematic and content analysis on them. This review identifies research themes demonstrating the utilization of AI in banking ...
Australian Banking Association chief executive Anna Bligh said ASIC's report found banks were doing a better job than their non-bank rivals in handling hardship. "There's always room for ...
Services for teams to share code, track work, and ship software. Azure Pipelines Continuously build, test, and deploy to any platform and cloud ... Review the OpenAI blog on GPT-4o. Explore . Let us know what you think of Azure and what you would like to see in the future. Provide feedback.
Raisin is a great option if your ultimate goal is to earn the best savings account, money market account, and CD rates out there. Raisin offers APYs of up to 5.26% on savings and up to 5.31% on ...