Using Data Mining Strategy in Qualitative Research
- Conference paper
- First Online: 29 October 2017
- Cite this conference paper
- Nadhirah Rasid 21 ,
- Puteri N. E. Nohuddin 21 ,
- Hamidah Alias 22 ,
- Irna Hamzah 21 &
- A. Imran Nordin 21
Part of the book series: Lecture Notes in Computer Science ((LNIP,volume 10645))
Included in the following conference series:
- International Visual Informatics Conference
2918 Accesses
2 Citations
Analyzing qualitative data can be tedious if it is done manually. There are several techniques available to conduct qualitative research such as thematic analysis, grounded theory and content analysis amongst other techniques. The data collected from these techniques are usually huge in amount. Little has been done to apply data mining strategy to analyzes data gathered using qualitative methodology. In this paper, we present a work done to apply text mining technique to analyzes data gathered from interviews – unstructured data. The aim of this study is to develop patterns of pediatric cancer patient’s activities in the ward. The result shows a pattern that suggests patients are mostly playing video games while receiving treatment and when they feel bored in the ward. This proposes that data mining techniques can be used to provide an initial insight of the information gathered qualitatively.
This is a preview of subscription content, log in via an institution to check access.
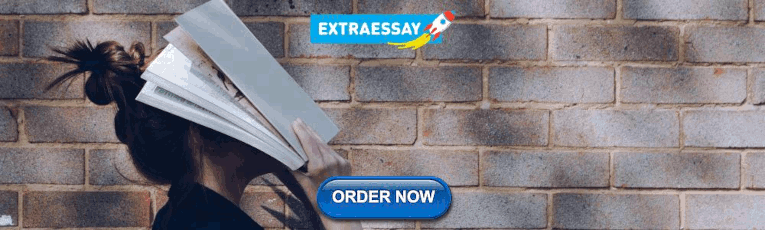
Access this chapter
- Available as PDF
- Read on any device
- Instant download
- Own it forever
- Available as EPUB and PDF
- Compact, lightweight edition
- Dispatched in 3 to 5 business days
- Free shipping worldwide - see info
Tax calculation will be finalised at checkout
Purchases are for personal use only
Institutional subscriptions
Woodgate, R.: Part I: an introduction to conducting qualitative research in children with cancer. J. Pediatr. Oncol. Nurs. 17 , 192–206 (2000)
Article Google Scholar
Rama Prasath, A., Ramya, M.M.: Automated drusen grading system in fundus image using fuzzy c-means clustering. Int. J. Eng. Technol. 6 , 833–841 (2014)
Google Scholar
Zhu, F., Patumcharoenpol, P., Zhang, C., Yang, Y., Chan, J., Meechai, A., Vongsangnak, W., Shen, B.: Biomedical text mining and its applications in cancer research. J. Biomed. Inform. 46 , 200–211 (2013)
Katal, A., Wazid, M., Goudar, R.H.: Big data: issues, challenges, tools and good practices. In: 2013 6th International Conference Contemporary Computing, IC3 2013, pp. 404–409 (2013)
Malterud, K.: Qualitative research: standards, challenges, and guidelines. Lancet 358 , 483–488 (2001)
Zainal, A.O., Nor Saleha, I.T.: National Cancer Registry Report (2011)
Lee, D.W., Kochenderfer, J.N., Stetler-Stevenson, M., Cui, Y.K., Delbrook, C., Feldman, S.A., Fry, T.J., Orentas, R., Sabatino, M., Shah, N.N., Steinberg, S.M., Stroncek, D., Tschernia, N., Yuan, C., Zhang, H., Zhang, L., Rosenberg, S.A., Wayne, A.S., Mackall, C.L.: T cells expressing CD19 chimeric antigen receptors for acute lymphoblastic leukaemia in children and young adults: a phase 1 dose-escalation trial. Lancet 385 , 517–528 (2015)
Taylor, R.M., Pearce, S., Gibson, F., Fern, L., Whelan, J.: Developing a conceptual model of teenage and young adult experiences of cancer through meta-synthesis. Int. J. Nurs. Stud. 50 , 832–846 (2013)
Tong, A., Palmer, S., Manns, B., Craig, J.C., Ruospo, M., Gargano, L., Johnson, D.W., Hegbrant, J., Olsson, M., Fishbane, S., Strippoli, G.F.M.: The beliefs and expectations of patients and caregivers about home haemodialysis: an interview study. BMJ Open 3 , e002148 (2013)
Wade, D.M., Brewin, C.R., Howell, D.C.J., White, E., Mythen, M.G., Weinman, J.A.: Intrusive memories of hallucinations and delusions in traumatized intensive care patients: an interview study. Br. J. Health. Psychol. 20 , 613–631 (2015)
Hrastinski, S., Aghaee, N.M.: How are campus students using social media to support their studies? An explorative interview study. Educ. Inf. Technol. 17 , 451–464 (2012)
Iosup, A., Epema, D.: An experience report on using gamification in technical higher education. In: Proceedings of 45th ACM Technical Symposium on Computer Science Education, SIGCSE 2014, pp. 27–32 (2014)
Ching, L.: A quantitative investigation of narratives: recycled drinking water. Water Policy 17 , 831–847 (2015)
Castellani, B., Castellani, J.: Data mining: qualitative analysis with health informatics data. Qual. Health Res. 13 , 1005–1018 (2003)
Alam, F., Pachauri, S.: Comparative study of J48, Naive Bayes and One-R classification technique for credit card fraud detection using WEKA. Adv. Comput. Sci. Technol. 10 , 1731–1743 (2017)
Fan, C., Xiao, F., Wang, S.: Development of prediction models for next-day building energy consumption and peak power demand using data mining techniques. Appl. Energy 127 , 1–10 (2014)
Slimani, T., Lazzez, A.: Efficient Analysis of Pattern and Association Rule Mining Approaches. arXiv preprint arXiv:1402.2892 (2014)
He, W.: Examining students’ online interaction in a live video streaming environment using data mining and text mining. Comput. Hum. Behav. 29 , 90–102 (2013)
Nohuddin, P.N.E., Coenen, F., Christley, R., Setzkorn, C.: Detecting temporal pattern and cluster changes in social networks: a study focusing UK cattle movement database. In: Shi, Z., Vadera, S., Aamodt, A., Leake, D. (eds.) Intelligent Information Processing V, IIP 2010. IFIP Advances in Information and Communication Technology, vol. 340, pp. 163–172. Springer, Heidelberg (2010). doi: 10.1007/978-3-642-16327-2_22
Vijiyarani, S., Sudha, S.: Disease prediction in data mining technique – a survey. Int. J. Comput. Appl. Inf. Technol. 2 , 17–21 (2013)
Ahmed, K., Emran, A.A., Jesmin, T., Mukti, R.F., Rahman, M.Z., Ahmed, F.: Early detection of lung cancer risk using data mining. Asian Pac. J. Cancer Prev. 14 , 595–598 (2013)
Eynon, R., Hjorth, I., Yasseri, T., Gillani, N.: Understanding Communication Patterns in MOOCs: Combining Data Mining and qualitative methods. arXiv preprint arXiv:1607.07495 (2016)
Dangare, C.S., Apte, S.S.: Improved study of heart disease prediction system using data mining classification techniques. Int. J. Comput. Appl. 0975–888 47 , 44–48 (2012)
Yukselturk, E., Ozekes, S., Türel, Y.K.: Predicting dropout student : an application of data mining methods in an online education program. Eur. J. Open Distance e‐Learning 17 , 118–133 (2014)
Khadjeh Nassirtoussi, A., Aghabozorgi, S., Ying Wah, T., Ngo, D.C.L.: Text mining of news-headlines for FOREX market prediction: a multi-layer dimension reduction algorithm with semantics and sentiment. Expert Syst. Appl. 42 , 306–324 (2015)
He, W., Zha, S., Li, L.: Social media competitive analysis and text mining: a case study in the pizza industry. Int. J. Inf. Manag. 33 , 464–472 (2013)
Bajaj, K., Pattabiraman, K., Mesbah, A.: Mining questions asked by web developers. In: Proceedings 11th Working Conference Mining Software Repositories - MSR 2014, pp. 112–121 (2014)
Berezina, K., Bilgihan, A., Cobanoglu, C., Okumus, F.: Understanding satisfied and dissatisfied hotel customers: text mining of online hotel reviews. J. Hosp. Mark. Manag. 25 , 1–24 (2015)
Khadjeh Nassirtoussi, A., Aghabozorgi, S., Ying Wah, T., Ngo, D.C.L.: Text mining for market prediction: a systematic review. Expert Syst. Appl. 41 , 7653–7670 (2014)
Rose, J., Lennerholt, C.: Low cost text mining as a strategy for qualitative researchers. Electron. J. Bus. Res. Method 15 , 2–16 (2017)
Yu, C.H., Jannasch-Pennell, A., Digangi, S., Yu, C.H.: Compatibility between text mining and qualitative research in the perspectives of grounded theory, content analysis, and reliability. Qual. Rep. 16 , 730–744 (2011)
Berglund, M., Westin, L., Svanstrom, R., Sundler, A.J.: Suffering caused by care patients’ experiences from hospital settings. Int. J. Qual. Stud. Health Well-Being 7 , 1–9 (2012)
Turnbull, M.: A qualitative investigation into the experiences, perceptions, beliefs and self-care management of people with type 2 diabetes (2015)
Morton, K., Balazinska, M., Grossman, D., Mackinlay, J.: Support the data enthusiast. Proc. VLDB Endow. 7 , 453–456 (2014)
Download references
Author information
Authors and affiliations.
Institute of Visual Informatics, Universiti Kebangsaan Malaysia, 43600, Bangi, Selangor, Malaysia
Nadhirah Rasid, Puteri N. E. Nohuddin, Irna Hamzah & A. Imran Nordin
Department of Pediatrics, Faculty of Medicine, Universiti Kebangsaan Malaysia, Cheras, 56000, Kuala Lumpur, Malaysia
Hamidah Alias
You can also search for this author in PubMed Google Scholar
Editor information
Editors and affiliations.
Universiti Kebangsaan Malaysia, Bangi, Selangor, Malaysia
Halimah Badioze Zaman
University of Cambridge, Cambridge, United Kingdom
Peter Robinson
Dublin City University, Dublin, Ireland
Alan F. Smeaton
National Central University, Jhongli, Taiwan
Timothy K. Shih
Carlos III University of Madrid, Madrid, Spain
Sergio Velastin
Toyo University, Kawagoe, Japan
Tada Terutoshi
Azizah Jaafar
Nazlena Mohamad Ali
Rights and permissions
Reprints and permissions
Copyright information
© 2017 Springer International Publishing AG
About this paper
Cite this paper.
Rasid, N., Nohuddin, P.N.E., Alias, H., Hamzah, I., Nordin, A.I. (2017). Using Data Mining Strategy in Qualitative Research. In: Badioze Zaman, H., et al. Advances in Visual Informatics. IVIC 2017. Lecture Notes in Computer Science(), vol 10645. Springer, Cham. https://doi.org/10.1007/978-3-319-70010-6_10
Download citation
DOI : https://doi.org/10.1007/978-3-319-70010-6_10
Published : 29 October 2017
Publisher Name : Springer, Cham
Print ISBN : 978-3-319-70009-0
Online ISBN : 978-3-319-70010-6
eBook Packages : Computer Science Computer Science (R0)
Share this paper
Anyone you share the following link with will be able to read this content:
Sorry, a shareable link is not currently available for this article.
Provided by the Springer Nature SharedIt content-sharing initiative
- Publish with us
Policies and ethics
- Find a journal
- Track your research
Analyzing Text Data
- Overview of Text Analysis and Text Mining
Choosing a Method
How much data do you need, word frequency analysis, machine learning/natural language processing, sentiment analysis.
- Library Databases
- Social Media
- Open Source
- Language Corpora
- Web Scraping
- Software for Text Analysis
- Text Data Citation
Library Data Services
Choosing the right text mining method is crucial because it significantly impacts the quality of insights and information you can extract from your text data. Each method provides different insights and requires different amounts of data, training, and iteration. Before you search for data, it is essential that you:
- identify the goals of your analysis
- determine the method you will use to meet those goals
- identify how much data you need for that method
- develop a sampling plan to build a data set that accurately represents your object of study.
Starting with this information in mind will make your project go more quickly and smoothly, and help you overcome a lot of hurdles such as incomplete data, too much or too little data, or problems with access to data.
More Resources:
- Content Analysis Method and Examples, from Mailman School of Public Health, Columbia University
- Qualitative Research Methods Overview, from Northeastern University
Before you start collecting data, think about how much data you really need. New researchers in text analysis often want to collect every source mentioning their topic, but this is usually not the best approach. Collecting so much data takes a lot of time, uses many computational resources, often goes against platform terms of service, and doesn't necessarily improve analysis.
In text analysis, an essential idea is saturation , where adding more data doesn't significantly improve performance. Saturation is when the model has learned as much as it can from the available data, and no new patterns are themes are emerging with additional data. Researchers often use experimentation and learning curves to determine when saturation occurs; you can start by analyzing a small or mid-sized dataset and see what happens if you add more data.
Once you know your research question, the next step is to create a sampling plan . In text analysis, sampling means selecting a representative subset of data from a larger dataset for analysis. This subset, called the sample, aims to capture the diversity of sentiments in the overall dataset. The goal is to analyze this smaller portion to draw conclusions about the information in the entire dataset.
For example, in a large collection of customer reviews, sampling may involve randomly selecting a subset for sentiment analysis instead of analyzing every single review. This approach saves computational resources and time while still providing insights into the overall sentiment distribution of the entire dataset. It's crucial to ensure that the sample accurately reflects the diversity of sentiments in the complete dataset for valid and reliable generalizations.
Example Sampling Plans
Sampling plans for text analysis involve selecting a subset of text data for analysis rather than analyzing the entire dataset. Here are two common sampling plans for text analysis:
Random Sampling:
- Description: Randomly select a subset of text documents from the entire dataset.
- Process: Assign each document a unique identifier and use a random number generator to choose documents for inclusion in the sample.
Stratified Sampling:
- Description: Divide the dataset into distinct strata or categories based on certain characteristics (e.g., product types, genres, age groups, race or ethnicity). Then, randomly sample from each stratum.
- Process: Divide the dataset into strata, and within each stratum, use random sampling to select a representative subset.
Remember, the choice of sampling plan depends on the specific goals of the analysis and the characteristics of the dataset. Random sampling is straightforward and commonly used when there's no need to account for specific characteristics in the dataset. Stratified sampling is useful when the dataset has distinct groups, and you want to ensure representation from each group in the sample.
Exactly How Many Sources do I need?
Determining the amount of data needed for text analysis involves a balance between having enough data to train a reliable model and avoiding unnecessary computational costs. The ideal dataset size depends on several factors, including the complexity of the task, the diversity of the data, and the specific algorithms or models being used.
- Task Complexity: If you are doing a simple task, like sentiment analysis or basic text classification, a few dozen articles might be enough. More complex tasks, like language translation or summarization, often require datasets on the scale of tens of thousands to millions.
- Model Complexity: Simple models like Naive Bayes often perform well with smaller datasets, whereas complex models, such as deep learning models with many parameters, will require larger datasets for effective training.
- Data Diversity: Ensure that the dataset is diverse and representative of the various scenarios the model will encounter. A more diverse dataset can lead to a more robust and generalizable model. A large dataset that is not diverse will yield worse results than a smaller, more diverse dataset.
- Domain-Specific Considerations: Sometimes there is not a lot of data available, and it is okay to make do with what you have!
Start by taking a look at articles in your field that have done a similar analysis. What approaches did they take? You can also schedule an appointment with a Data Services Librarian to get you started.
More Readings on Sampling Plans for Text Analysis:
- How to Choose a Sample Size in Qualitative Research, from LinkedIn Learning (members of the GW community have free access to LinkedIn Learning using their GW email account)
- Sampling in Qualitative Research , from Saylor Academy
- Lowe, A., Norris, A. C., Farris, A. J., & Babbage, D. R. (2018). Quantifying Thematic Saturation in Qualitative Data Analysis. Field Methods, 30(3), 191-207. https://doi.org/10.1177/1525822X17749386

Software for Word Frequency Analysis
- NVivo via GW's Virtual Computer Lab NVivo is a software package used for qualitative data analysis. It includes tools to support the analysis of textual data in a wide array of formats, as well as and audio, video, and image data. NVivo is available through the Virtual Computer Lab. Faculty and staff may find NVivo available for download from GW's Software Center.
- Analyzing Word and Document Frequency in R This chapter explains how to use tidy to analyze word and document frequency using Tidy Data in R.
- word clouds in R R programming functionality to create pretty word clouds, visualize differences and similarities between documents, and avoid over-plotting in scatter plots with text.
- ATLAS.ti Trial version of qualitative analysis workbench for processing text, image, audio, and video data. (Note: Health science students may have access to full version through Himmelfarb Library)
Related Tools Available Online
- Google ngram Viewer When you enter phrases into the Google Books Ngram Viewer, it displays a graph showing how those phrases have occurred in a corpus of books (e.g., "British English", "English Fiction", "French") over the selected years.
- HathiTrust This link opens in a new window HathiTrust is a partnership of academic and research institutions, offering a collection of millions of titles digitized from libraries around the world. To log in, select The George Washington University as your institution, then log in with your UserID and regular GW password.
- Voyant Voyant is an online point-and-click tool for text analysis. While the default graphics are impressive, it allows limited customizing of analysis and graphs and may be most useful for exploratory visualization.
Related Library Resources
- HathiTrust and Text Mining at GWU HathiTrust is an international community of research libraries committed to the long-term curation and availability of the cultural record. Through their common efforts and deep commitment to the public good, the libraries support the teaching and learning activities of the faculty, students or researchers at their home institutions, and the scholarly needs of the broader public as well.
- HathiTrust+Bookworn From the University of Illinois Library: HathiTrust+Bookworm is an online tool for visualizing trends in language over time. Developed by the HathiTrust Research Center using textual data from the HathiTrust Digital Library, it allows you to track changes in word use based on publication country, genre of works, and more.
- Python for Natural Language Processing A workshop offered through GW Libraries on natural language processing using Python.
- Text Mining Tutorials in R A collection of text mining course materials and tutorials developed for humanists and social scientists interested to learn R.
- Oxford English Dictionary This link opens in a new window The Oxford English Dictionary database will provide a word frequency analysis over time, drawing both from Google ngrams and the OED's own databases.
Example Projects Using Word Frequency Analysis
Robinson, J. S. and D. (n.d.). 3 Analyzing word and document frequency: Tf-idf | Text Mining with R . Retrieved November 21, 2023, from https://www.tidytextmining.com/tfidf.html
- Zhang, Z. (n.d.). Text Mining for Social and Behavioral Research Using R . Retrieved November 21, 2023, from https://books.psychstat.org/textmining/index.html
- Exploring Fascinating Insights with Word Frequency Analysis In the realm of data analysis, words hold immense power. They convey meaning, express ideas, and shape our understanding of the world. In this article, we’ll explore the fascinating world of textual data analysis by examining word frequencies. By counting the occurrence of words in a text, we can uncover interesting insights and gain a deeper understanding of the underlying themes and patterns. Join us on this word-centric journey as we dive into the realm of word frequency analysis using Python.
Machine learning for text analysis is a technology that teaches computers to understand and interpret written language by exposing them to examples. There are two types of machine learning for text analysis: supervised learning, in which a human helps to train the computer to detect patterns, and unsupervised learning, which enables computers to automatically categorize, analyze, and extract information from text without needing explicit programming.
One type of machine learning for text analysis is Natural Language Processing (NLP). NLP for text analysis is a field of artificial intelligence that involves the development and application of algorithms to automatically process, understand, and extract meaningful information from human language in textual form. NLP techniques are used to analyze and derive insights from large volumes of text data, enabling tasks such as sentiment analysis, named entity recognition, text classification, and language translation. The aim is to equip computers with the capability to comprehend and interpret written language, making it possible to automate various aspects of text-based information processing.
Software for Natural Language Processing
- NLTK for Python NLTK is a leading platform for building Python programs to work with human language data. It provides easy-to-use interfaces to over 50 corpora and lexical resources such as WordNet, along with a suite of text processing libraries for classification, tokenization, stemming, tagging, parsing, and semantic reasoning, wrappers for industrial-strength NLP libraries, and an active discussion forum.
- scikit Simple and efficient tools for predictive data analysis, using Python.
Related Resources Available Online
- [Large Language Models] LLMs in Scientific Research
- HathiTrust and Text Mining at GWU Information on text data mining using HathiTrust
- Social Feed Manager Social Feed Manager software was developed to support campus research about social media including Twitter, Tumblr, Flickr, and Sina Weibo platforms. It can be used to track mentions of you or your articles and other research products for the previous seven days and on into the future.. Email [email protected] to get started with Social Feed Manager or to schedule a consultation
Example Projects using Natural Language Processing
- Redd D, Workman TE, Shao Y, Cheng Y, Tekle S, Garvin JH, Brandt CA, Zeng-Treitler Q. Patient Dietary Supplements Use: Do Results from Natural Language Processing of Clinical Notes Agree with Survey Data? Medical Sciences . 2023; 11(2):37. https://doi.org/10.3390/medsci11020037
- Nguyen D, Liakata M, DeDeo S, Eisenstein J, Mimno D, Tromble R, Winters J. How We Do Things With Words: Analyzing Text as Social and Cultural Data. Front Artif Intell. 2020 Aug 25;3:62. doi: 10.3389/frai.2020.00062.
Sentiment analysis is a method of analyzing text to determine whether the emotional tone or sentiment expressed in a piece of text is positive, negative, or neutral. Sentiment analysis is commonly used in businesses to gauge customer feedback, social media monitoring, and market research.
Software for Sentiment Analysis
- Sentiment Analysis using NLTK for Python NLTK is a leading platform for building Python programs to work with human language data. It provides easy-to-use interfaces to over 50 corpora and lexical resources such as WordNet, along with a suite of text processing libraries for classification, tokenization, stemming, tagging, parsing, and semantic reasoning, wrappers for industrial-strength NLP libraries, and an active discussion forum.
- Sentiment Analysis with TidyData in R This chapter shows how to implement sentiment analysis using tidy data principles in R.
- Tableau Tableau works with numeric and categorical data to produce advanced graphics. Browse the Tableau public gallery to see examples of visuals and dashboards. Tableau offers free one-year Tableau licenses to students at accredited academic institutions, including GW. Visit https://www.tableau.com/academic/students for more about the program or to request a license.
- Qualtrics Text iQ Qualtrics is a powerful tool for collecting and analyzing survey data. Qualtrics Text iQ automatically performs sentiment analysis on collected data.
Related Resources Available Online
- finnstats. (2021, May 16). Sentiment analysis in R | R-bloggers. https://www.r-bloggers.com/2021/05/sentiment-analysis-in-r-3/
Example Projects Using Sentiment Analysis
- Duong, V., Luo, J., Pham, P., Yang, T., & Wang, Y. (2020). The Ivory Tower Lost: How College Students Respond Differently than the General Public to the COVID-19 Pandemic. 2020 IEEE/ACM International Conference on Advances in Social Networks Analysis and Mining (ASONAM), 126–130. https://doi.org/10.1109/ASONAM49781.2020.9381379
- Ali, R. H., Pinto, G., Lawrie, E., & Linstead, E. J. (2022). A large-scale sentiment analysis of tweets pertaining to the 2020 US presidential election. Journal of Big Data, 9(1). https://doi.org/10.1186/s40537-022-00633-z
- << Previous: Overview of Text Analysis and Text Mining
- Next: Finding Text Data >>
- Last Updated: Feb 7, 2024 10:26 AM
- URL: https://libguides.gwu.edu/textanalysis
Academia.edu no longer supports Internet Explorer.
To browse Academia.edu and the wider internet faster and more securely, please take a few seconds to upgrade your browser .
Enter the email address you signed up with and we'll email you a reset link.
- We're Hiring!
- Help Center
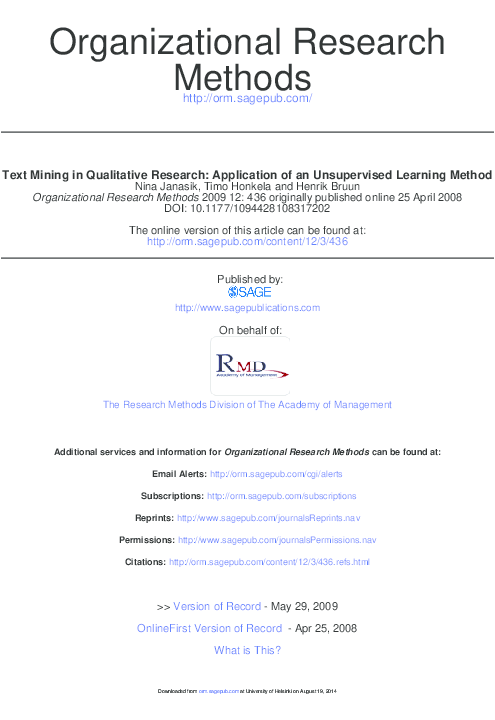
Text Mining in Qualitative Research: Application of an Unsupervised Learning Method

Related Papers
Organizational Research Methods
Despite the ubiquity of textual data, so far few researchers have applied text mining to answer organizational research questions. Text mining, which essentially entails a quantitative approach to the analysis of (usually) voluminous textual data, helps accelerate knowledge discovery by radically increasing the amount data that can be analyzed. This article aims to acquaint organizational researchers with the fundamental logic underpinning text mining, the analytical stages involved, and contemporary techniques that may be used to achieve different types of objectives. The specific analytical techniques reviewed are (a) dimensionality reduction, (b) distance and similarity computing, (c) clustering, (d) topic modeling, and (e) classification. We describe how text mining may extend contemporary organizational research by allowing the testing of existing or new research questions with data that are likely to be rich, contextualized, and ecologically valid. After an exploration of how ...
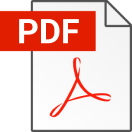
Expert Systems
Luiz Moutinho
Robab saadatdoost
This paper focuses on knowledge discovery among attributes of Iran Higher Education Institute using self organizing map (SOM); the key problem with massive volume of data is extracting knowledge and patterns that are hidden in data. Managerial needs to explore this data for the purpose of decision making and strategy making reveals its importance.. Furthermore it can be useful for researchers that study and research about higher education. Meanwhile planning for higher education has significant impact on developing of one society, successful planning needs to analysis some huge and historical data that is available in higher education institutes. SOM is a particular type of neural network used in clustering and helps discover patterns and relations without advanced knowledge about them. The steps of this approach can be discussed under five headings, which are (i) Data Preparation (ii) Data Loading, (iii) Initializing, (iv) Map training and (v) Interpretation of the results. The target dataset contains data of five universities located in Tehran, Iran affiliated to Medical Ministry of Iran and the most important attributes are program of study, learning style, study mode and degree. Results show that the number of enrolling students for Tehran medical university has decreased for the past 23 years from 1988 to 2005. This study also finds that Tehran University of Medical Science covers the majority of high degrees like MDdisplay(Doctor of Medicine) and PhD. The findings of this study can be used in improving of higher education decision making systems and the results of this study indicate SOM toolbox utility in similar institutes to knowledge discovery in a visualizing way.
Eleventh East-European Conference on Advances …
Leo Võhandu , Toomas Kirt
Chong Ho Yu
The objective of this article is to illustrate that text mining and qualitative research are epistemologically compatible. First, like many qualitative research approaches, such as grounded theory, text mining encourages open-mindedness and discourages preconceptions. Contrary to the popular belief that text mining is a linear and fully automated procedure, the text miner might add, delete, and revise the initial categories in an iterative fashion. Second, text mining is similar to content analysis, which also aims to extract common themes and threads by counting words. Although both of them utilize computer algorithms, text mining is characterized by its capability of processing natural languages. Last, the criteria of sound text mining adhere to those in qualitative research in terms of consistency and replicability.
Organization Development Review
Anton Shufutinsky
This award-winning paper deals with the need for bracketing and bridling in qualitative research for the purpose of limiting and abating biases. The paper discusses numerous methods of bracketing and epoch, including novel methodologies, for addressing the potential for biases in organization development, organization behavior, I/O psychology, human resources, and other organizational sciences.
The Qualitative …
Ann Russell
Dwi Santoso
RELATED PAPERS
WIDER Working Paper
Eva-Maria Egger
Antonio Liscidini
Progress In Electromagnetics Research Letters
fahmi hussin
Memorias del Instituto de Investigaciones en Ciencias de la Salud
Marta Inés Ferreira Gaona
Evaluation and Program Planning
Amber Summers
Critical Care
Alberto Tenorio-abreu
Physical Review Research
Ye-Hong Chen
volkan kahyalar
Trans-pasando Fronteras
Diana Salazar
Journal of Artificial Intelligence Research
Phan Thi Cam Tu B1911431
Cement and Concrete Research
Aditya Kumar
Arthroscopy: The Journal of Arthroscopic & Related Surgery
Stephen Snyder
Journal of South Carolina Water Resources
Lori Dickes
Technium Social Sciences Journal
M.nur Alamsyah
Journal of Healthcare Engineering
Miroslav Trajanovic
Landon Hillyard
Thaddeus Bartter
remo mombarg
Social Psychiatry and Psychiatric Epidemiology
Silvia Rizzi
Zenodo (CERN European Organization for Nuclear Research)
Jaume Targa
Sherryl Leone
Frontiers in Immunology
Roohi Rasool
American Journal of Infectious Diseases
Lalit Sharma
hjhjgfg freghrf
Jurnal Psikologi Teori dan Terapan
Muhammad Syafiq
- We're Hiring!
- Help Center
- Find new research papers in:
- Health Sciences
- Earth Sciences
- Cognitive Science
- Mathematics
- Computer Science
- Academia ©2024
Text mining & text analysis
- Why use text mining/analysis?
- What can you do with text mining/analysis?
- Text mining/analysis activities or tasks
- Examples of text mining/analysis
- Library databases
- Social media
- Open sources
- Web scraping
- Language Corpora
- Transcription of audio/video data
- Managing large data sets
- Tool directories
- Research methods
Machine learning
Natural language processing, topic modelling, network analysis, visualisations.
- Programming resources
- Considerations - Ethics, Copyright, Licencing, Etiquette
- Further help
Text analysis often relies on machine learning, a branch of computer science that trains computers to recognise patterns. There are two kinds of machine learning used in text analysis: supervised learning, where a human helps to train the pattern-detecting model, and unsupervised learning, where the computer finds patterns in text with little human intervention. An example of supervised learning is Naive Bayes Classification. See Natural Language Processing and Topic Modeling for examples of unsupervised machine learning
- Machine learning - reference entry - Encyclopedia of the Sciences of Learning more... less... UQ sign in required
- Naive Bayes Classification
Natural language processing, a kind of machine learning, is the attempt to use computational methods to extract meaning from free text. Among other things, natural language processing algorithms can derive: names of people and places, dates, sentiment, and parts of speech.
- Natural Language Processing more... less... UQ sign in required
- The Stanford Natural Language Processing Group Natural Language Processing software available to everyone.
Topic modeling, a form of machine learning, is a way of identifying patterns and themes in a body of text. Topic modeling is done by statistical algorithms, such as Latent Dirichlet Allocation, which groups words into "topics" based on which words frequently co-occur in a text
- Topic Modeling more... less... UQ sign in required
- Using Word Clouds for Topic Modeling Results
Network analysis is a method for finding connections between nodes representing people, concepts, sources, and more. These networks are usually visualised into graphs that show the interconnectedness of the nodes.
Social network analysis - the process of investigating social structures through the use of networks and graph theory. It characterises networked structures in terms of nodes (individual actors, people, or things within the network) and the edges, or links (relationships or interactions) that connect them.
Semantic network analysis - a network that represents semantic relations (meanings) between concepts. This is often used as a form of knowledge representation. It is a graph consisting of nodes, which represent concepts, and edges, which represent semantic relations between concepts.
- Social Network Analysis more... less... UQ sign in required
- Semantic Networks more... less... UQ sign in required
Text visualisation is a way to "see" your data. Text mining visualisation can help researchers see relationships between certain concepts. An example of a visualisation of data can be word clouds, graphs, maps, and other graphics that produce a visual depiction the data.
Various Text Analysis Projects with Visualisations
- With Criminal Intent - currently unavailable
- The state of our union is... dumber
- Novel Views: Les Miserables
- Tolkien's Books Analysed
Word Frequency Visualisations
- Google n-gram viewer - word frequencies over time
- Historical culturomics of pronoun frequencies - pronoun frequencies by gender over time
- The Words They Used - bubble cloud of words from national convention speeches, with size and color coding
- Ye Shall Know Them By Their Words - word frequencies by topic for presidential nomination speeches ( additional description )
- Mining Books to Map Emotions - frequencies of sentiment terms over time
- Text Visualisation more... less... UQ sign in required
- UQ Library - Data Visualisation Software
- << Previous: Tool directories
- Next: Programming resources >>
- Last Updated: Apr 3, 2024 10:15 AM
- URL: https://guides.library.uq.edu.au/research-techniques/text-mining-analysis
University Library, University of Illinois at Urbana-Champaign

Text Mining Tools and Methods
- Introduction
- Acquiring Text This link opens in a new window
- Text Analysis Methods
About NVivo
Nvivo basics, nvivo tips and troubleshooting, additional nvivo resources.
- Additional Downloadable Tools
- Web-Based Tools
- More resources
Scholarly Commons
CITL Data Analytics
What is NVivo?
NVivo is a software program used for qualitative and mixed-methods research. Specifically, it is used for the analysis of unstructured text, audio, video, and image data, including (but not limited to) interviews, focus groups, surveys, social media, and journal articles. It is produced by QSR International. As of October 2018, it is available for both Windows and Macintosh operating systems; however, the Macintosh version is missing some of the features that the Windows version has. Different editions of NVivo also have different features; see the comparison .
Why use NVivo?
- Analyze and organize unstructured text, audio, video, or image data.
- Playback ability for audio and video files, so that interviews can easily be transcribed in NVivo.
- Ability to capture social media data from Facebook, Twitter, and LinkedIn using the NCapture browser plug-in (NVivo 10 only).
- Import notes and captures from Evernote - great for field research.
- Import citations from EndNote, RefWorks, Mendeley, Zotero, or other bibliographic management software - great for literature reviews.
- User interface and text analysis available in English, French, German, Spanish, Portuguese, Japanese, and Simplified Chinese.
What versions of NVivo are there?
The newest version is NVivo 12 for Windows and Mac. There is a Pro and Plus edition for Windows. The Scholarly Commons hosts Windows version 11 Pro Edition.
The Mac version of NVivo lacks some features of the Windows version. See the comparison .
What file types are associated with NVivo?
- *.nvp - An NVivo for Windows project file.
- *.nvpx - An NVivo for Mac project file.
Is there a way to try NVivo for free?
At this time, QSR International offers free 14 day trials of NVivo 12 for Windows and Mac. Check out the free trial here .
Illinois affiliates can also use NVivo in the Scholarly Commons (version 11 Pro Edition).
Getting Sources into NVivo
To import a source, click the "Data" menu and choose the appropriate data option.
Click "Browse" to locate the file in your directory and select the desired file. This will now appear in your "Internals" folder in the Navigation Bar under "Sources." You may find it helpful to create folders under "Internals" to further specify what kind of internals are located there. These could include PDFs, audio files, video files, etc.
You can also get started testing NVivo's features and capabilities using the sample project .
Nodes are the containers for codes, themes in your project, memos you write to yourself, and more. They embody concepts and use your codes as evidence of the existence of that concept. You can create nodes as you analyze your data, or you can create them beforehand. This will largely depend on the kind of data you are analyzing as well as the chosen methodology of your project.
To create a new Node, click "Analyze" menu, then "New Node." You will need to name your new Node and provide a description. Your new Node will be stored in the "Nodes" folder automatically, but if you have existing nodes you can choose to nest your new Node under an existing Node. Check the "Aggregate coding from child nodes" box if you wish to nest Nodes.
Codes can be thought of as quotes you pull from your Sources that embody themes and share relationships with other Sources. You will always "Code at a Node." This simply means your highlighted portion of data (the Code) will be stored in a relevant container (the Node). Your Codes can be stored in multiple Nodes.
To Code, simply highlight a section of relevant text (or a segment of an audio or video file) and Code the Selection at a Node. This can be accomplished by right clicking and selecting at which Node to Code, or by going to the "Analyze" menu and selecting where to code. The former option is generally more cohesive to a steady workflow.
NCapture is a browser add-on that takes a snap-shot of the webpage you are currently on and saves it as a file-type specific to NVivo which then essentially works like a PDF. The NCapture button is located in different areas in each browser. You can import each NCapture into NVivo by selecting the "From Other Sources" option from the "External Data" tab in the "Ribbon." You can batch import NCaptures rather than one file at a time, streamlining the process. NCaptures make it easy to grab social media data, such as tweets or Facebook pages.
Tiny screen? Undock open source and node panes for ease of reading
You can undock the views of open sources, nodes, queries, or other project items by right-clicking on the item's tab and selecting "undock." this will open the item in its own viewer window. this is particularly useful if you have dual monitors: you can have a source document on one monitor, and have the node view on the other., choosing between word or pdf formats word and plain text files are easier to code in nvivo than pdfs., headers, footers, and page numbers in word docs are hidden when viewing the file in nvivo., need line numbers save your source as a pdf, to avoid corrupting your nvivo project files, do not store them on a network drive, external/removable drive, or cloud-synced directory..
- If you want to keep your NVivo project file on a network drive, change the "Update projects on networks drives" setting to On save. (See " How can I configure NVivo 10 to update the network file each time I save the project? ")
- If you are keeping your project file in a folder that is synced to a cloud storage service (such as Dropbox, Google Drive, Box, etc.), QSR recommends pausing syncing while you have the project open .
Switching between a Windows and a Mac computer?
See this tutorial on converting project files .
- Frequently Asked Questions QSR's official FAQ page.
- NVivo 11 for Windows Help QSR official help for NVivo 11 Windows version.
- NVivo for Mac Help QSR official help for NVivo Mac version.
- Official NVivo Video Tutorials For both Windows and Mac versions.
- NVivo Sample Project Download the sample project to get started working with NVivo.
- QSR Forum Discussion forum on QSR International's website, intended for peer discussion and information sharing.
- QSR on Twitter Follow QSR International on Twitter for the latest news and updates.
- The NVivo Blog QSR's official blog. In addition to NVivo news and tips, the blog also covers hot topics and current issues in qualitative research.
- << Previous: ATLAS.ti
- Next: R >>
- Last Updated: Nov 8, 2023 3:52 PM
- URL: https://guides.library.illinois.edu/textmining
A text mining and network analysis of topics and trends in major nursing research journals
Affiliations.
- 1 Department of Nursing, Faculty of Health Sciences, Lokman Hekim University, Ankara, Turkey.
- 2 School of Nursing and Health Sciences, Manhattanville College, Purchase, New York, USA.
- 3 Department of Health Services Administration, The University of Alabama at Birmingham, Birmingham, Alabama, USA.
- 4 Informatics Institute, The University of Alabama at Birmingham, Birmingham, Alabama, USA.
- 5 Electrical & Computer Engineering, The Center for Integrated Systems, The University of Alabama at Birmingham, Birmingham, Alabama, USA.
- PMID: 38268286
- PMCID: PMC10697125
- DOI: 10.1002/nop2.2050
Aim: This study is set to determine the main topics of the nursing field and to show the changing perspectives over time by analysing the abstracts of several major nursing research journals using text mining methodology.
Design: Text mining and network analysis.
Methods: Text analysis combines automatic and manual operations to identify patterns in unstructured data. Detailed searches covering 1998-2021 were conducted in PubMed archives to collect articles from six nursing journals: Journal of Advanced Nursing, International Journal of Nursing Studies, Western Journal of Nursing Research, Nursing Research, Journal of Nursing Scholarship and Research in Nursing and Health. This study uses a four-phase text mining and network approach, gathering text data and cleaning, preprocessing, text analysis and advanced analyses. Analyses and data visualization were performed using Endnote, JMP, Microsoft Excel, Tableau and VOSviewer versions. From six journals, 17,581 references in PubMed were combined into one EndNote file. Due to missing abstract information, 2496 references were excluded from the study. The remaining references (n = 15,085) were used for the text mining analyses.
Results: Eighteen subjects were determined into two main groups; research method topics and nursing research topics. The most striking topics are qualitative research, concept analysis, advanced practice in the downtrend, and literature search, statistical analysis, randomized control trials, quantitative research, nurse practice environment, risk assessment and nursing science. According to the network analysis results, nursing satisfaction and burnout and nursing practice environment are highly correlated and represent 10% of the total corpus. This study contributes in various ways to the field of nursing research enhanced by text mining. The study findings shed light on researchers becoming more aware of the latest research status, sub-fields and trends over the years, identifying gaps and planning future research agendas. No patient or public contribution.
Keywords: network analysis; nursing; research; text mining; topics; trends.
© 2023 The Authors. Nursing Open published by John Wiley & Sons Ltd.
- Data Mining
- Nursing Research*
- Periodicals as Topic*
- Open access
- Published: 30 April 2024
Explaining the barriers faced by veterinarians against preventing antimicrobial resistance: an innovative interdisciplinary qualitative study
- Razie Toghroli 1 ,
- Laleh Hassani 1 ,
- Teamur Aghamolaei 1 ,
- Manoj Sharma 2 ,
- Hamid Sharifi 3 , 5 &
- Maziar Jajarmi 4
BMC Infectious Diseases volume 24 , Article number: 455 ( 2024 ) Cite this article
Metrics details
Considering the significance of increased antimicrobial resistance (AMR) and its adverse effects on individual and social health and the important and effective role that veterinarians play in controlling this growing issue worldwide, it is essential to have effective preventive control programs. To this aim, the first step is to identify the factors behind the prevalence of AMR in Iran and the barriers veterinarians face to controlling this problem. Thus, the present study was conducted to explain the barriers veterinarians faced in the prevention of AMR from an Iranian veterinarian’s perspective.
The present research was done in three cities in Iran in 2021. The data were collected through in-depth interviews with 18 veterinarians selected through purposive and snowball sampling and analyzed using conventional qualitative content analysis.
The data analysis results were classified into 4 main categories and 44 subcategories. The former included: educational factors, administrative/legal factors, client-related factors, and veterinarian-related factors.
Conclusions
The increased AMR can be approached from multiple aspects. Considering the different factors that affect the increased AMR, it is necessary to consider them all through effective planning and policy-making at multi-level and multidisciplinary dimensions. There is special attention needed to scientific and practical interventions at the individual, interpersonal, social, and even political levels. At the same time, measures should be taken to rehabilitate and maintain the health of society to strengthen supervision and attract the full participation of interested organizations.
Peer Review reports
Introduction
Antimicrobial resistance (AMR) refers to the reduced effectiveness of antimicrobial agents, such as antibiotics, antivirals, antifungals, and antiparasitics, against infections caused by bacteria, viruses, fungi, and parasites [ 1 ]. This phenomenon makes infections harder to treat and increases the risk of disease spread, severe illness, and death. Misuse and overuse of these agents in humans, animals, and plants are key contributors to the development of AMR. AMR is a natural process that occurs gradually over time through genetic changes in microorganisms, but human activities, particularly the improper use of antimicrobials, significantly speed up this process. Veterinarians play a vital role in managing AMR, as they frequently prescribe antimicrobials to protect animal health. However, overprescription and misuse of antimicrobials in veterinary practice contribute to the development of AMR in humans. AMR presents a substantial challenge to global public health and economic stability. If left unchecked, AMR will lead to increased healthcare costs, decreased productivity, and potentially millions of avoidable deaths annually. To combat AMR, governments, healthcare providers, and researchers must collaborate to implement policies promoting judicious antimicrobial use, invest in innovative therapies, and foster educational initiatives to empower individuals to understand the importance of responsible antimicrobial stewardship [ 2 ].
AMR is an increasingly global issue that needs to be settled cooperatively. Resistant organisms exist in animals, humans, the environment, the food, and the main cause of this, is antimicrobial usage. AMR will become a leading cause of mortality in the world in the near future. As reported by some studies, by 2050, AMR will be the main cause of death on a global scale, which surpasses cancer deaths [ 3 , 4 ].
The mortality rate caused by microbial resistance is higher than the total number of deaths induced by cancer worldwide. Yet, the former has been largely neglected and, instead, issues such as cancer and how to treat it have been addressed more [ 5 , 6 , 7 ].
Unintentional antibiotic ingestion occurs frequently due to the widespread use of antibiotics in society. According to a report from 2010, approximately 10 pills, capsules, or teaspoons of antibiotics are taken annually by every person on Earth, which suggests a high degree of accidental consumption. Healthy individuals who consume significant amounts of antibiotics unintentionally may experience negative impacts on their health, particularly concerning the disturbance of the normal microbiome. This disturbance can lead to long-term complications, such as an increased risk of developing conditions like type 1 and 2 diabetes, inflammatory bowel diseases, celiac disease, allergies, and asthma [ 8 ]. Additionally, antibiotic exposure can contribute to the emergence of antibiotic-resistant strains, posing a challenge to public health. It is essential to note that antibiotics are necessary and lifesaving medicines when used appropriately under medical supervision. However, excessive or unnecessary use of antibiotics can pose risks to individual and population health [ 9 , 10 ].
According to the report of the World Health Organization, half of the antibiotics produced in the world are used in medicine and the other half in veterinary, agriculture and aquaculture [ 11 ]. In general, there is no difference between antibiotics used in veterinary medicine and antibiotics used in medicine. These drugs are used to prevent and treat diseases and promote growth in animal farms (pigs and poultry), unfortunately, the use of antibiotics in veterinary medicine leads to leaving residues in meat, milk and eggs [ 12 ]. Drug residues in food have adverse effects such as antibiotic resistance in humans, allergies, and inhibition of bacterial starter cultures used in dairy fermentation industries [ 13 ]. Despite the beneficial effects of antibiotics on the treatment of livestock infectious diseases, the presence of their residues in milk and animal meat, as well as their transfer to the human body have adverse effects on health, industry, and economics. As reported by the National Center for Rational Prescription of Antibiotics, consuming antibiotics in Iran is 16 times as high as the global standard. Some researchers believe that the spread of microbial resistance to antibiotics results not only from the unnecessary prescription and use of these compounds in humans but also from the widespread use of antimicrobial drugs in veterinary medicine. It has caused the transfer of such pathogenic bacteria from animals to human pathogens. The main difference between microbial resistance to antimicrobial drugs in humans and animals is that microbial resistance in humans affects the individual, whereas microbial resistance in livestock affects a large population due to the consumption of raw animal products by humans. Exposure to both resistant bacteria and antibiotic compounds prescribed for the treatment of infectious diseases for livestock through transmission causes the accumulation of drugs and drug residues in raw livestock products. It seems that attempts to prevent the occurrence of microbial resistance in livestock and its consequences for humans are effective and can be implemented efficiently by veterinarians and those active in this domain. What veterinarians can do with this respect is wide-ranging.
Most of the studies conducted in Iran in the field of antimicrobial resistance were in the medical and human fields, and the studies conducted in the veterinary field were mostly quantitative. A systematic review and meta-analysis showed a high level of antibiotic resistance in Staphylococcus aureus bovine mastitis in Iran. This pathogen is the common and main cause of bovine bacterial mastitis, which leads to high economic losses and can easily lead to the transmission of these treatment-resistant bacteria to humans [ 14 ]. In a qualitative study, which is one of the few qualitative and phenomenological studies conducted in Iran in the field of AMR, the lived experience of livestock breeders, their role and views in this field has been investigated. The results of this study have confirmed the importance of antibiotic resistance in Iran and the lack of existing research in this field, especially with a qualitative approach [ 15 ]. In another study that was conducted with semi-structured interviews with key stakeholders in Iran, including managers of the Ministry of Health, Iran Veterinary Organization, national professional associations and researchers through thematic analysis, the international enabling and predisposing factors related to It identified the control of AMR in Iran. The enabling factors that have been highlighted in this review were discussed in general, and more attention was paid to political factors such as formulation and implementation processes, and AMR surveillance, and challenges such as the smuggling of infected animals and antimicrobial drugs and livestock from neighboring countries and the impact of imposed sanctions. The review emphasizes the global nature of AMR as a challenge that requires consensus and international cooperation to effectively deal with this issue, but it does not specifically and specifically deal with why and analyze how AMR occurs and examine ways to prevent it, and only generally with the approaches Emphasizes political, including health diplomacy, to strengthen national efforts in the fight against AMR [ 16 ]. However, the present study, in an interdisciplinary manner, has specifically addressed one of the most important fields involved in the occurrence of AMR in human societies, and a field similar to it has received less attention before.
Yet, it is hard to make interventions with veterinarians directly involved because they are not easily available for research; therefore, veterinary students are the best and closest population for interventional studies. If this population adequately understand the principles of prescribing antibiotics, this successful learning will be productive in practice too [ 17 ].
Overcoming this problem will be possible with an One health approach, taking into account humans, animals, and environmental health altogether [ 18 , 19 , 20 ].
Today, AMR occurs in humans, wildlife, domestic animals, plants, and our environment directly by using antibiotics, and there is a risk on a much larger and more significant scale in animal-source foods consumed by humans indirectly. So it is logical to take a multidisciplinary health approach to solve this problem by eliminating the inappropriate use of antibiotics [ 21 , 22 ].
In the medical domain, extensive research has been done to examine physicians’ beliefs about prescribing antibiotics [ 23 ]. Many interventions have been made to reduce physicians’ over-prescription. The various aspects of antibiotic prescription have been extensively investigated so far in medical and clinical sciences. However, these interventions alone have not managed to prevent the occurrence of this important event. Thus, resolving this problem needs a multidimensional approach [ 24 ] .
Moreover, veterinarians prescribing drugs without using paraclinical services and selling over-the-counter (OTC) drugs are very common in several countries including Iran. In many stockbreeding industries, antibiotics are widely used not only for medical purposes but also as growth stimulants. There has been a serious lack of effective monitoring of these patterns of use. Similarly, there has scarcely been any strict preventive rule for this. Thus, it is likely that the AMR incidence rate is high in countries such as Iran [ 25 , 26 ].
A vast majority of research so far on the effect of AMR has only addressed this issue in human health [ 16 , 24 ]. In veterinary medicine, the body of existing literature has been limited to laboratory research and animal health. Veterinarians’ role in integrated health, especially AMR has not been adequately addressed. Therefore, there is no complete and clear understanding of veterinarians’ mental patterns and perceived social barriers to their decisions during diagnosis and treatment [ 27 ].
The over-prescribing of drugs is very common in animal products and animal-source foods (to be consumed by humans) in Iran. Moreover, each of these foods contains antimicrobial residues. Thus, it can be conjectured that people ingest significant amounts of antibiotics every day unintentionally without suffering from any infectious disease. Therefore, veterinarians must pay adequate attention to AMR in human health [ 28 ].
Overall, the world is faced with increasing availability and misuse of antibiotics in veterinary medicine, which threatens public health. There is a significant increase in AMR on a global scale, and there is a threat of increasing infections that do not respond well to treatment. It is essential to take appropriate measures and plan to prevent the over-prescription of antibiotics by veterinarians. There is an increasing need for education and empowerment policies, all deemed impossible unless the barriers facing veterinarians are recognized in appropriate prescribing. In other words, to deal with the AMR issue, the first step is to identify the causes and underlying contributing factors to this event in veterinary medicine and the disastrous conditions veterinarians face in Iran and the world. It is not possible to adequately approach what veterinarians go through and how they perceive the existing context only through quantitative research. A qualitative approach is needed to explore all aspects of this problem. Therefore, the present study employed this approach to explore the Iranian veterinarians’ perceptions of barriers to AMR prevention. We hope that the results generated from this study will help promote programs to curb slow down and the development of AMR in Iran and the world. The present findings can be used to make new social, economic, and even political decisions.
Materials and methods
Research design.
This research was conducted with a qualitative approach and qualitative content analysis method from three cities of Iran: Kerman (with a large population of large and small livestock), Bandar Abbas (a fishing and aquaculture hub), and Tehran (with a large population of pets and industrial poultry). The present qualitative study used semi-structured interviews with veterinarians who had experience in treating and prescribing antibiotics or office work in veterinary medicine, or those with sufficient experience and knowledge of issues in veterinary diagnosis and treatment. The interviews were held face-to-face from May 3, 2021 to August 13, 2021. In this study, theme analysis was used, which is a common type of qualitative content analysis. It seeks a deep understanding of the complexity, details, and embedded context of a given phenomenon. In this type of analysis, interviews with individuals provide a better understanding and richer information about participants’ experiences and perspectives. This research approach allows for an in-depth and rich exploration of participants’ experiences.
The panel of experts offering advice on research questions and reviewing the transcripts for reliability consisted of one epidemiologist, three health education and health promotion specialists, and two veterinary professors collaborating with three organizations: (Hormozgan University of Medical Sciences, Veterinary Department of Kerman University and Iranian Veterinary Organization). The present participants were selected through maximum variation and snowball sampling.
Participants
The research population consisted of veterinarians from three cities (Tehran, Kerman, and Bandar Abbas) in Iran, all dealing with a large population of livestock, poultry, and aquatic animals in 2021. The inclusion criteria were: veterinary work experience at least three years, the experience of therapeutic clinical work or working as a veterinary administrative and supervisory staff, willingness to participate (in the research), and ability to answer the questions. The exclusion criteria were unwillingness to participate and withdrawal from the interview. Purposive sampling was used with maximum variation (in terms of the province of work, age, sex, the field of work in a clinic or pharmacy, and affiliation with the public or private sector) at first steps and snowball sampling methods In the following. That means some veterinarians were concerned about expressing their opinions or reporting any illegal case they had dealt with. Therefore, they had to be selected through snowball sampling. After reaching the first participant and holding the interview, s/he was asked to suggest the next veterinarian who was aware of or was experienced in prescribing antibiotics against paraclinical rules. Therefore, each participant connected and introduced us to the next participant. Interviews were held in a public place at the interviewee’s convenience. In some cases, the interview was held in the clinic, and in others in the interviewee’s office. The data were collected and analyzed simultaneously. The interviews continued until the data were saturated (i.e. when no new information was obtained) and until all the extracted themes were sufficiently supported by the data. After the 17th interview, no new data were collected, but to be on the safe side, another interview was also conducted, and after the interview with the 18th participant, the sampling was stopped.
Data collection
Guided questions and semi-structured interviews were used for data collection. The interviews were held face-to-face and video calls. When required, a trained research assistant conducted a qualitative interview to increase the accuracy and speed of data collection. The interview questions were derived from a review of the existing literature on AMR with a focus on the underlying causes and also the comments made by a panel of experts. At the beginning of the interview, the purpose of the study was revealed to the participants and they were assured of the confidentiality of the information they provided and the anonymity of their responses. The interviewees were ensured they could withdraw from the study upon their will. Then, an informed letter of consent was signed. The required permission was gained to record all the conversations. The main focus of the questions included:
What are the barriers to veterinarians’ prevention of increased AMR? Explain.
What do you know about the causes and precursors of AMR occurrence in Iran?
What are the determinants of prescribing and using antibiotics in veterinary medicine in Iran in your opinion? Explain.
What are the determinants of the increased AMR in your opinion as a veterinarian?
Based on the participants’ previous answers, more exploratory questions were asked and, as a result, we extracted the main reasons why veterinarians over-prescribed drugs and why antibiotics were overconsumed in the animal source food industry. The sample size was determined by the theoretical data saturation criterion. In other words, during the data collection, when we concluded that more interviews and observations could not add any new information and only led to repeated findings, we stopped the data collection. Therefore, 18 active veterinarians in clinical, medical, educational and administrative fields were interviewed in Tehran, Kerman and Hormozgan (provinces). Individual interviews lasted between 42 and 57 min.
Data analysis
The process of data analysis was done using Granheim and Lundman method [ 29 , 30 ] and with the help of MAXQDA-2010 software by the first and second authors of the article. The first and second authors listened to recorded interviews and transcribed them into a written format in Word 2017 software immediately after every interview and on the same day with the help of other research colleagues. In the second step, the text of the interviews was read by the researchers very carefully to get a general view of their text. In the third stage, all the texts of the interviews were read line by line and very carefully, and the initial codes were started.
In the fourth step, the researchers placed the codes that were similar in terms of meaning and concept and were placed in a category in a subcategory and determined the relationship between them. In the fifth step, the codes and categories were placed in the main categories, which were conceptually more comprehensive and abstract [ 31 ]. Finally, in a joint meeting, the entire process of data analysis was shared and conflicting opinions on the content of a topic were discussed by a research team with two qualitative health researchers and two veterinarians.
Guba and Lincoln evaluation criteria [ 32 ] were used to check the trustworthiness of the findings. To substantiate the validity of the findings, the researcher’s self-review technique was used in data collection and analysis as well as a peer check during which the codes were provided to two participants to resolve misunderstandings. To substantiate the reliability of findings, intra- and inter-rater reliability tests were used. To this aim, the recorded and transcribed conversations were given to several experts for review. After analyzing the data, they were re-analyzed by colleagues. The next step was documentation to test the accuracy and comprehensibility of the procedures, and the underlying mechanisms of errors.
Ethical considerations
This research was approved by the Ethics Committee of Hormozgan University of Medical Sciences (IR.HUMS.REC.1400.207). In the interviews, the researcher, by introducing herself and also explaining the purposes of the study, tried to create an amicable atmosphere for the interview. The participants were also ensured of the confidentiality of the information they provided, the anonymity of recorded conversations, and also why they were selected. They consented to their voice being recorded. The participants were free to withdraw or leave the interview any time they requested.
The present study was conducted as interviews with 18 veterinarian participants in Tehran, Kerman, and Hormozgan provinces. Both sexes were included. There were 11 male and 7 female participants whose ages ranged between 27 and 58, with an average age of 42.5 years. The participants’ work experience ranged between 3 and 27 years, with an average of 15 years. The demographic information is summarized in Table 1 .
The data analysis led to the extraction of 4 main categories and 44 subcategories (see Table 2 ), each examined separately.
Educational factors
The first determinant of the increased AMR deals with academic issues in university. Among the most important issues are those concerning students, clients’ lacking awareness and knowledge of AMR in animals, and its transmission to humans (from animal source foods).
Unsystematic internship
During the summer holidays of the final 2–3 academic years of veterinary students, they are required to take the internship. Yet, some participants complained about the unsystematic and inefficiency of this internship.
“During our student days, we took up the internship, but we did not learn anything special at all”. (Participant #13)
Unadjusted curricula
The majority of participants agreed that during their studies, only in the bacteriology course, they learn about AMR (only superficially) and that in the university curriculum, this subject was not adequately included.
“All faculty members should teach something about AMR, not just the bacteriology professor. Also, do we not prescribe antibiotics once we diagnose viral diseases in clinical sciences and the like? If so, then why are we not taught what AMR actually is”? (Participant # 9)
Outdated education
As the participants described, it was essential to teach new things about AMR and to develop strict, principled, written instructions on this subject. The participants recommended following effective and efficient exemplar instructions (in foreign countries) to strengthen the educational system not also at university but in food and drug administrative organizations. It is essential to update basic and clinical sciences curricula and add AMR to all courses, as most participants agreed.
“We should keep up with the global community in this regard so that we can be fully aware of the new knowledge and instructions, and can create new instructions based on the preexisting ones”. (Participant #2) ”I think one thing that can definitely help is to see how successful projects in developed countries proceed. Let us follow their example”. (Participant #13) ”They still teach the way they did a hundred years ago. The subject matter should be changed. It seems as if discussing AMR does not matter at all”. (Participant #9)
Lack of specialized training courses
A key determinant of AMR prevention was the need for useful and effective training courses for all those somehow affected or affected by the AMR, including vets, the health staff, medics, therapists, as well as the livestock and poultry breeders, and the like.
“It is essential to hold relevant and useful training courses for ranchers, poultry farmers, pharmacists, as well as veterinarians, veterinary staff who perform inspection and monitoring work for others. So, everyone is expected to cooperate”. (Participant #2). “Farmers should know that adhering to the (medical) interval helps decrease antibiotic concentration in the animal being treated. Thus, the farmer or breeder needs to postpone the slaughter time. Or he is advised not to consume animal source foods while they are being medically treated”. (Participant #7).
Low specialized study index
As in many other sciences, gaining up-to-date knowledge requires studying the most recent research findings.
“We should not only encourage those who influence drug resistance to study about this subject, but universities should also encourage professors to study more about the specialized topic. If a professor fails to have updated knowledge, s/he cannot teach students well. Thus, how can we expect the students to act efficiently in near future”? (Participant #5)
Lack of empowering educational system
Some participants expressed concerns that the educational system did not adequately prepare students for accurate diagnosis and prescription in near future.
“At university, nothing matters more than studying and getting good marks. The educational system does not actually prepare students for the work market. In other words, it does not simulate real conditions before students leave academic life and enter the work market”. (Participant #9)
Lacking cooperation of all medical sectors affiliated with the university
In the present study, participants, all veterinary graduates or instructors, raised the question why discussing AMR was limited to the bacteriology course and not included in clinical and practical courses.
“Why is medical resistance only limited to bacteriology? All other basic and clinical sciences sectors at university are talking about diagnosis and treatment, and are prescribing drugs. But, when they come to medical resistance, they only refer to bacteriologists and the bacteriology labs”. (Participant #9)
Administrative and legal factors
There are issues about the rules/regulations and policies on veterinarians’ practice and that of all people somehow concerned with animal source foods, which can add to the existing problems. Here are the categories and the relevant excerpts:
Problems with rules and regulations
As the participants pinpointed, there is a strong need for food safety rules and regulations especially in terms of AMR. Adherence to these rules and regulations should be closely monitored too.
“Though there are rules, you can never be sure they are abided by fully. No one is afraid of not following the rules. Even I myself, who is doing clinical work, am not sure whether there is any prohibitory rule for this or not!” (Participant #8) . “Breeders who administer drugs themselves or those who slaughter animals being medically treated should be fined or prosecuted because they threaten public health. But, in reality there is no way to stop them”. (Participant #5)
Poor monitoring and administration
A number of participants acknowledged that even if there are rules and regulations, they are not fully observed. There has not been any efficient monitoring over how rules and regulations are followed. That is why rules have been ineffective.
“ All these are just instructions. In practice, there is no veterinary body monitoring how things are done. The rules are ineffective“(Participant #7) .
Selling over-the-counter (OTC) drugs or those without laboratory-based approval
Selling all kinds of drugs, including antibiotics without prescription, without laboratory approval and freely in Iran has caused serious trouble.
“ You can get any medicine you want from any pharmacy at any time. Actually, the main customers of pharmacies are those who buy drugs arbitrarily”. (Participant# 11) ” In my opinion, pharmacies should not sell every kind of drug especially antibiotics unless they receive a laboratory approval for the antibiogram test. Likewise, a vet should not prescribe antibiotics unless s/he receives the lab test result first”. (Participant #2) ” In my opinion, the sale of medicine, especially antibiotics, should be subject to laboratory approval. That is, a person should not be allowed to buy medicine until the laboratory has determined the type of disease or at least the effective antibiotic, even if the vet has prescribed it”. (Participant #9)
Inadequate advocacy
As some participants commented, gaining the full support of international, national and regional communities was a great issue.
“ We need the help of international and national organizations to solve this global issue. When a problem is global, the solution will definitely be achieved with the cooperation of international organizations”. (Participant #2)
Lack of interdisciplinary cooperative approach
According to some participants, to achieve an optimal solution to this problem, all administrative, supervisory, diagnostic, and medical sectors should cooperate.
“ Solving this problem is not what only one organization can do. Universities should teach students in the right way; veterinary administrative organizations should do their job efficiently; the private sector (e.g., clinics and pharmacies) should obey the rules. Most importantly, there should be strict rules made and abided by with all sectors cooperating”. (Participant #4) ” Our clients should be aware of the importance of AMR and also aware of how the drugs are cycled among the environment, animals, and humans. The Environment and Veterinary Organization, public health and agriculture, and the like should all take serious actions. If one ring is missing from this chain, the whole chain is broken. All efforts will end up fruitless”. (Participant #6).
Problems with the production and use of electronic health records (EHRs)
Developing systems such as the integrated prescription system and the use of EHRs can significantly help to prevent AMR occurrence.
“ If the EHR system was used, things would be better now. No pharmacy could then sell OTC drugs. Thus, no customer could buy antibiotics arbitrarily”. (Participant #14)
Slaughtering medically treated livestock
According to some participants, a stock not responding to an antibiotic treatment does not need any lab test. Neither does it need any abstinence interval. It can easily gain slaughter permission even in emergency cases.
“Here, an animal that is taking medicine and is not becoming well or is getting worse is sent for emergency slaughter. Is there any organization in charge here? Only if a buyer comes to know that an animal shows symptoms of a disease, he may buy it at a lower price”. “Before the slaughter, the antibiotic residues are controlled in poultry but not in macro-livestock (e.g., cattle, sheep and goat)”. (Participant #12)
Non-compulsory training courses before issuing a license for animal husbandry
Some participants insisted that the government should make it compulsory for applicants (for stockbreeding or husbandry) to complete AMR training courses before issuing a license for stock breeding. Here are some comments.
“ Certainly, people seeking for an establishment and operation license for livestock, poultry, fish ponds, and in short, any kind of livestock, must be obliged by the relevant governmental body to first pass a series of training courses and then get a license”. (Participant# 2)
Lacking coordination between medical and veterinary organizations
Due to the lack of the required infrastructure in veterinary medicine, this organization needs to cooperate with the Ministry of Health (for service provision), and medical and laboratory sectors especially to perform laboratory tests.
“ We can say that great concern is that veterinary medicine and medical sciences are affiliated with two different ministries. The former is deprived of the facilities provided by the ministry of health. Even for simple antibiogram tests, we should visit veterinary labs provincial centers, or big cities”. (Participant #11)
Lacking attention to micro-industries and micro-breeders
With the expansion and development of livestock, poultry, and aquaculture industries, the main attention has been focused on this group (of industries), and domestic and micro-breeders have been neglected.
“ If there are any rules and regulations, they are mostly about industries such as macro-level poultry breeding or husbandries. Yet, in practice, the national livestock is to a great extent bred by domestic and micro-level breeders that are largely neglected”. (Participant #3)
Limited facilities in small towns
The lack of diagnostic facilities such as laboratories equipped with antibiogram testing for cases sent from veterinary clinics have caused serious problems for clients and veterinarians.
“ For a simple antibiogram test, we have to refer to the provincial center, and this is both time-consuming and costly. More importantly, most of our livestock population is in small towns, not in provincial centers”! (Participant #1)
Client-related factors
Another determinant of the increased AMR as perceived by Iranian veterinarians is the factors related to livestock/poultry breeding and animal owners (termed here as “clients”). The clients’ choices, decisions, and behaviors will have significant effects on increasing AMR.
Quick response : Among the reasons for an emergent antibiotic prescription without any diagnostic test are: concerns about high mortality rate if the drug is not used immediately, the breeder’s referral at the onset or peak of a disease spread, or substantial losses in the herd, or the referral rush to improve conditions.
“ Mostly, livestock farmers especially poultry farmers or any other breeder with a significant number of livestock, poultry, or aquatic animals, insist on getting a strong antibiotic immediately so that the mortality rate does not rise any further. They cannot even wait for the antibiogram test result. If we do not prescribe antibiotics for them, they go get it from somewhere else, and even if they go for the antibiogram test, they may not be patient enough to receive the test result and, thus, arbitrarily begin other antibiotics”. (Participant #6)
Customer satisfaction
Some clients have used several specific drugs for years and found them effective. Thus, they have no faith in the lab diagnostic test result. Besides, some clinicians and especially vets are sometimes subject to too many demands, which can be tempting. They might occasionally be tempted to violate the existing rules and, upon a client’s persistence, they may neglect the protocols and easily give in.
“ For our clients, the drug manufacturing company even matters. Sometimes, they carry the former drug vial to show us and insist that the same drug be prescribed”. (Participant #3) ” Even when the required facilities were available, I faced too many suggestions. Some guys came to tell us to take it easy and let them get away with it (by granting or renewing their permit)”. (Participant #16)
Low purchasing power
As there is no drug and treatment insurance for animals in veterinary medicine in Iran, the cost of treatment or the price of drugs was found as another determinant of antibiotic prescription, as mentioned by the present veterinarian participants.
“ Sometimes prescriptions are written out according to the customer’s affordance. Sometimes, customers ask us to prescribe something they can afford to buy. As there is no insurance coverage for veterinary medicine, the price matters, and it significantly affects the act of prescription”. (Participant #7)
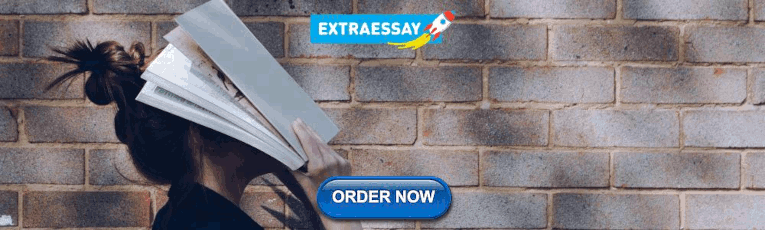
Social learning
An effective factor in antibiotic self-medication or arbitrary use of antibiotics is to learn about it. There are often others living in the same place (city or village) where the clients live, who used a certain drug and found it effective. Now the clients tend to follow their steps. Besides, self-medication cuts down on the diagnostic and therapeutic costs too.
“A farmer might come to us and insist on buying the same drug that his neighbor has already bought. He does not consider that the diseases might be different. Overall, clients are more influenced by neighbors than us”!
Pharmaceutical determinants
In some cases, what causes the clients to insist on our prescribing OTC drugs is the price and effectiveness of the drug (as perceived by the clients) and even the drug manufacturing company.
“ Some clients insist on buying a certain antibiotic because either they have already used and found it effective. Thus, they may ignore what the vet’s diagnosis is”. (Participant #11)
Unawareness of antibiotic residues and abstinence interval
While using antibiotics, the livestock, poultry, and aquaculture breeders should be aware of the animal source food abstinence interval. But in reality, they are mostly unaware of that.
“Many clients are not adequately aware of the abstinence interval after taking antibiotics, and this issue makes them send the animal products into the food cycle during the treatment period”. (Participant # 11)
Drug replacement or early cessation
When seemingly the symptoms of the disease are gone, some breeders ignore the medical instructions and cease the drug sooner than they should.
“For example, a drug should be taken for not shorter than a week. But when a client takes the drug for two days and feels the disease is gone, he stops administering the drug. He does not care about the medical resistance and how it occurs. He ignores them all”. (Participant # 5) ”A client may purchase an antibiotic (either prescribed or self-medicated) and begin the treatment. After one or two days, when there is no sign of recovery, he replaces the drug easily”. (Participant #8)
Antibiotics use as a growth stimulant
Antibiotics have long been used as growth stimulants on a large scale by breeders of raw animal products in Iran.
“ In large breeding industries such as livestock and poultry farming, antibiotics are used as a growth stimulant, and this is very common”. (Participant #4)
Self-medication or arbitrary use of drugs
As the participants mentioned, many clients take some therapeutic measures before visiting a veterinary diagnostic and medical center. They have already begun taking several antibiotics or have quit the treatment half in the way.
“ Sometimes a farmer arbitrarily buys and consumes several drugs before going to any veterinary, diagnostic or medical center”. (Participant #5) ” Some ranchers already take many antibiotics. When we ask them why they say they had it refrigerated since the last time they ever purchased and consumed the drug. They intended to use the remains of the drug and visit a clinic only if their self-medication did not prove effective”. (Participant #8)
The unconventionality of the antibiogram test
As perceived by the present participants, antibiogram testing is a new thing that has not been yet received well by many clients. Not many participants welcome or even prioritize this test. They think doing this test is not compulsory and, thus, they do not feel obliged to take it at all.
“Unless the customer is obliged to, he does not go for the test to a laboratory at all. Thus, it needs to be mandatory; yet in reality, it is not”! (Participant #15) ” When we tell a client that he should take a sample for an antibiogram test and wait until then, he is surprised. It seems as if he has never heard of such a thing. He wonders why none of his fellow breeders were already sent for such a test when they faced the same problem”. (Participant #18) ”Nobody cares about AMR in the future. They laugh at us and wonder what the consequences are”. (Participant #2)
Clients’ preference for over-prescribing vets
As perceived by the present participants, any veterinarian or clinician who prescribes more drugs to treat animals is more popular.
“ If you do not prescribe any drug, the client prefers to go to another vet. He will not wait at all for you to tell him about the importance of drug resistance. Now every doctor who prescribes more drugs becomes more popular and he is perceived as a better doctor”. (Participant #18)
Clients’ lacking foresight
Some participants acknowledged that the AMR problem is unthinkable in the future and far-fetched to many clients.
“ People do not really know what will happen in the future and people will suffer from drug resistance. No one can even imagine what will happen in the future. It is not tangible to them”. (Participant #2)
Rejection of paraclinical costs
Some clients, as the participants’ accounts, revealed, do not bear costs higher than those of the visit, including the cost of a laboratory.
“ Our clients are mostly reluctant to pay much, especially when the cost of the treatment is higher than that of, for example, a domestic chicken that they bring here for treatment”. (Participant #18)
Materialistic view
Many ranchers ignore many important things and are just concerned with more production and productivity, and gaining as much money as they can. So, they do things that are sometimes unethical and illegal but just cost-effective.
“I don’t think it matters how you make money. You just need to be smart and know when to do what. For example, I know a guy who drugs his chickens the day before slaughter but keeps some of them apart for his own family’s use. He sends one of the undrugged chickens to the laboratory so that he gets a negative lab test result. This way, if there is any loss, it will happen to the undrugged chickens and not the whole poultry”. (Participant #9) “Sometimes they breed a few chickens apart from others only to send them later on to the laboratory. The lab also cooperates with them and hides things in the actual report”. (Participant #13)
Veterinarian-related factors
In addition to the above-mentioned factors, veterinarians also sometimes cause an increase in AMR. Here we see how their characteristics affect their decisions on AMR development.
Inadequate job security
The current job market for veterinarians in Iran is not very prosperous and any factor that endangers the current position of activists in this field will fail.
“If you cannot keep the customer satisfied with yourself in the job market right now and put extra costs on the customer, he will quickly go to another clinic and another vet”. (Participant #14)
Lacking experience in the correct act of prescription
As perceived by the participants, many veterinarians who have just entered the work market lack any experience in prescribing drugs. Thus, they significantly account for the increased AMR.
“ As novice veterinarians do not have much experience in prescribing medicine, they prescribe several antibiotics together, with the hope that one of them works”. (Participant #13)
Prescription based on prior experience
Prescribing drugs based solely on diagnostic experience is common practice in more experienced veterinarians.
“ As soon as most colleagues see cases similar to what they have already faced and treated, they begin to write out the same prescription. It is generally well-established that certain drugs are always prescribed for respiratory infections, some for gastrointestinal infections, and so on”. (Participant #14)
The unconventionality of diagnostic tests among veterinarians
Many veterinarians have diagnosed diseases and prescribed them mainly based on their own experience. Antibiogram testing is a new therapeutic measure that has not been welcomed warmly by vets.
“There are very few vets who wait for the antibiogram test before writing out any prescription. Actually, antibiogram tests are still very uncommon”. (Participant #9)
Being labeled as inexperienced if dependent on laboratory diagnosis
As our participants described, a veterinarian who does not make a diagnosis or give treatment immediately and independently (from lab tests) and hinders it until the paraclinical test results are labeled as inexperienced.
“We have no problem sending the client to the lab, but unfortunately it seems as if we were unable to make a diagnosis ourselves and we were inexperienced and because of that we got help from the lab”. (Participant #12)
Fear of losing clients
Some veterinarians acknowledged if they delayed the diagnosis to a later time (to receive the lab test result), they could easily lose many customers.
“If you keep the client waiting or send him to a lab to fetch the test results, he will for sure prefer to visit another vet”. (Participant #5)
Diminishing ethical values
Another determining factor raised by the participants was the need to have a working conscience and commitment to livestock/poultry breeders, laboratories, and those having contracts with labs. In other words, the vets should rely on the lab test results.
“When I used to work on a poultry farm, I saw a separate hall for raising chickens with no antibiotics. The sample sent to the lab was taken from this hall. Or the chickens were slaughtered for the farmer’s own family. The other (drugged) chickens were sent to the slaughterhouse for public use”. (Participant #10) “Some colleagues are not committed enough to their job and do not feel it on their conscience. Similarly, the test results coming from some labs are not reliable either. So, the negative antibiotic results we receive from them might be false”. (Participant #5)
Lacking foresight
AMR is not familiar to many people in society. They do not adequately know what AMR is, which can affect their practice.
“Veterinarians cannot even imagine how dangerous AMR can be to human health in the future. When they have no idea what AMR is and can be, how can we expect them to be worried about it”? (Participant #8)
The insignificance of AMR
The AMR issue is not very important for some veterinarians in diagnosis, treatment, and monitoring.
“Rarely does veterinarian care about drug resistance. I do not think it is even their last priority to consider”! (Participant #10)
Lack of self-efficacy in overcoming barriers
A few interviewees admitted that they or some colleagues have a specific drug classification for most diseases according to which they act spontaneously. It means that they do not take different therapeutic measures when faced with different cases.
“Some clinicians do not consider that everyone can have his disease. I mean, they treat all patients the same way and prescribe strong broad-spectrum antibiotics for 90% of cases”. (Participant #5)
Competitive drug market
Many veterinarians are not required to sell drugs on a prescription, and selling without a prescription is a legal and common task. So active veterinarians in the field compete with each other for selling drugs and evidently for more income.
“Everyone likes to open up a pharmacy because he can easily earn money with no trouble with diagnostic and surgical measures. It is much better if you can persuade customers to buy more”. (Participant #8)
Apparent issues with prescriptions
The last subcategory of veterinarian-related factors was the appearance of prescriptions. The present prescriptions encourage vets to prescribe more drugs.
“The size and shape of prescriptions are such that the vet is encouraged to prescribe more drugs. The prescriptions should be refined in shape to allow for one or two drugs only and no more”. (Participant #5)
The present study aimed to explore the barriers faced by Irainian veterinarians against preventing Antimicrobial resistance. A few qualitative studies have been conducted on AMR, which dealt with the causes of progress and the obstacles faced by those involved in this problem, especially in the veterinary profession [ 33 , 34 ]. The results showed that different educational, legal/administrative and veterinarian-related factors account for the increased AMR in Iranian society. The first category included factors related to the educational system, such as the lack of any specialized training course for veterinary students, those in charge of monitoring veterinary practice, veterinary departments, and ranchers struggling with educational problems who may all be implicated in increasing AMR. In Iran, various educational initiatives have been implemented, such as the publication of a book on rational prescription principles, academic papers, and reports from the National Committee on Prescribing and Rational Drug Use. Despite these efforts, there are numerous educational obstacles in veterinary colleges in Iran when it comes to instructing students on prescription fundamentals and the rational utilization of medications.
The required material has been also developed; training and retraining programs have been planned based on eclectic drug use criteria; workshops, conferences, and seminars have been held too. A prescription can simply be representative of a whole nation’s sociocultural values and medical conditions. Many studies have been conducted worldwide to improve rational drug prescription and consumption [ 35 ]. The effects of educational interventions on the improved prescription pattern have been reported in Iranians and other studies too [ 36 , 37 ]. Continued training on rational drug prescription and pharmacy education has been recommended to doctors in the existing literature [ 38 , 39 ].
In Zareh’s study, the most commonly prescribed drugs were injections and antibiotics. The research findings showed that, after the training, there was an increase in the rational prescriptions for most prescribed drugs [ 40 ]. As for teaching strategies, the WHO has published The Guide to Good Prescribing for medical students. This guidebook contains six rational steps that can significantly reduce the irrational prescription of drugs: 1- defining the patient’s problem 2- defining the goals of treatment 3- ensuring that the treatment is appropriate for the patient. 4 – initiating the therapeutic measure 5 - providing information, instructions and warnings (if any) 6 - monitoring and ceasing the treatment [ 41 ]. Outdated education was a sub-category found in this study. Different studies showed that dentists often, due to a lack of knowledge about the side effects of improper prescribing of antibiotics, tend to over-prescribe them [ 42 , 43 ].
Veterinarians also are central to antimicrobial stewardship on farms, with their prescribing decisions significantly impacting AMR.A study on Canadian dairy cattle veterinarians’ revealed factors influencing their antimicrobial prescribing, attitudes towards reducing antimicrobial use, awareness of AMR, and perceived barriers to improving stewardship [ 44 ]. In addition educational resources have been developed to enhance veterinarians’ understanding of AMR and promote rational antimicrobial use. Online courses such as “Antimicrobial stewardship in veterinary practice” and “Farmed Animal Antimicrobial Stewardship Initiative” aim to educate veterinarians on responsible antimicrobial use [ 45 , 46 ].
What we need is a high-quality time management element added to the existing curricula so that students can be well-equipped with whatever they need to act professionally. Excessive imitation of medical sciences in specialized courses can only lower the efficiency of a vet’s profession. Rather, there is a need for incorporating courses on different animal species both at the general practitioner’s level and the specialized doctorate degrees [ 47 ].
There is also the issue of time management in the curriculum. Decreasing the quantity of content and increasing the quality (by adding more useful content) can better reform the veterinary curriculum. Goal-setting in veterinary sciences has already been revolutionized, and veterinary universities cannot ignore it. Thus, it is essential to consider the present and future needs in defining the required specialties to handle the existing national health issues, each of which can impose a loss of millions of dollars nationally. For many years, curricula have been developed in the European Union to achieve the necessary specializations by the existing needs, at least in the cattle breeding industry [ 48 ]. A deficient educational system is one factor that increases the overuse of antibiotics. Therefore, it is necessary to take basic measures based on the macro-planning of students’ knowledge and increase the quality of internships. In a study by Wushouer et al. in China, it was observed that an increasing awareness was followed by a decreasing rate of antibiotic administration [ 49 ]. Therefore, it is necessary to increase knowledge through a different approach in the educational system. Most experts believe that education in medical sciences should follow a different approach than other fields of study because knowledge construction in these fields of study (i.e., medicine, veterinary medicine) affects the content that students receive and the experiences they gain [ 50 ].
Failure to hold training courses for producers of raw animal products and unsystematic student internships can significantly lower the quality of education. Raising the study index in AMR and modeling on successful examples can be considered in curriculum design. Moreover, all departments of the veterinary faculties should cooperate and the heavy burden of teaching AMR should be removed from the bacteriology department only, and be shared by all basic sciences and clinical courses. Only then can we hope to see improved practice in students’ learning experiences and professional life in the near future.
The present findings showed that currently in our country, the educational system needs to be seriously reformed by appropriate training programs and pre-employment awareness-raising programs for veterinarians and ranchers [ 51 ]. People working in this field should be more empowered, better aware, and skilled enough at a correct diagnosis or proper functioning [ 52 ]. Only then we can hope that their self-efficacy is increased and they can learn to act more responsibly. These can help to prevent the occurrence of AMR and to begin to resolve it rather than worsening the issue.
The second category of the determinants of increased AMR was administrative and legal factors. Problems with the law, monitoring, and selling OTC drugs are important issues that can increase the costs of treatment too. This finding is consistent with several studies. For example, it is estimated that about 100,000 people in the United States die every year from the adverse effects of drugs [ 53 ]. In the United Kingdom, problems in 11% of prescriptions cost over € 400 million in loss, and about 16% of these problems harm patients [ 54 ]. Most of these errors are preventable, including drugs prescribed heedless of contraindications, those taken incorrectly, or those not having been properly monitored. The WHO, along with other relevant international organizations, proposed certain criteria to evaluate the quality of prescriptions to prevent the occurrence of problems and lower treatment costs [ 55 , 56 ]. A useful way of evaluating the prescription pattern in a country is to evaluate the doctors’ prescriptions. A simple prescription can represent the current state of medical education in a country, how laws and regulations affect the medical community, socio-cultural beliefs, and the medical condition [ 36 ]. Based on WHO guidelines use of medically important antimicrobials in food-producing animals, any level of restriction in antibiotic prescription should be considered, including a complete cessation of the use of one or more antibiotics. Examples of restrictions that WHO considered are: any prohibition on the use of antibiotics, such as but not limited to the prohibited use for specific indications (e.g., for prophylaxis of disease or growth promotion), the requirement of a prescription by a veterinarian for the use of antibiotics, voluntary restrictions on farms or organic interventions [ 55 ]. Drugs that need confirmation from a specially qualified person or organization should not be sold over the counter. Prescribed drugs are regulated by the US Food and Drug Administration (FDA). Having a federal license with a medical leaflet is a prerequisite for the packing of any drug. A medical leaflet usually consists of four parts: indications, contraindications, warnings, and dosage [ 57 ]. He who writes out a prescription decides who can consume the drug. A pharmacist can buy drugs, but he should sell them only to those authorized by a legally qualified person. Thus, a prescribed drug has 3 parts [ 58 ]: (1) The doctor’s prescription, (2) The pharmacist’s written prescription while delivering the drug, and (3) the drug package with a label on it. That is why officials are expected to always think about formulating new and public policies to implement correct and new strategies for the use of antibiotics [ 59 ]. Educational and political interventions, establishing and implementing laws regarding AMR stewardship may be effective and acceptable either before or during the livestock and poultry breeding programs, even for pet owners [ 60 ]. Success in the coordinated implementation of related laws is not possible without the advocacy of various stakeholders, including policymakers, veterinarians and ranchers, pet owners, public sector employees, farmers, and consumers [ 51 ]. It seems that the use of effective legislation, contractual requirements, professional obligations and the distribution of suitable facilities in more distant areas makes the implementation of this plan possible [ 21 , 22 ].
The third category was the client-related factors. Quick response and arbitrary drug use were among the sub-categories. With the expansion of public access to the internet system, people may want to refer less to vets and, instead, self-medicate or they may expect a quick response and begin to use antibiotics. In their research, Hofmeister et al. investigated veterinary visitors and found the internet connection speed as the third most important source of retrieving pet health information after GPs and specialized vets and before family and friends and other mass media [ 61 ]. Kogan et al. maintained that internet-based sources are considered an extra source of information about pet health for pet owners besides visiting vets for consultation [ 62 ]. Volk et al. reckoned that the internet and online health information could replace veterinarians and lead to fewer pet owners visiting veterinary clinics [ 63 ]. Thus, since some clients do not want to pay the visit and para clinic fees, by searching on the Internet and cyberspace, or based on their previous experience or else, they prescribe and take antibiotics arbitrarily before any visit to vets. If they do not find some proper treatment, they try other antibiotics, which leads to the problem of changing or stopping the antibiotics early before the end of the treatment period.
Some other poultry or aquatic breeders who have farms of several thousand pieces are very worried about the loss of their livestock, poultry and the aquatic population at the beginning of the disease. Since there may be a large population of their herd while waiting for the antibiogram test, they prefer to use a broad-spectrum and preferably cheaper antibiotic (for large-scale use for a large herd) to begin with and prevent their economic loss to a large extent [ 60 ]. Therefore, both in the producers and breeders of animal-origin food and in the owners of pets, the customer’s demand needs quick response and the customer’s demand should be prioritized [ 22 ].
In addition, a person who once used a broad-spectrum antibiotic without a prescription and got a response, suggests that to his/her colleagues or other breeders, and by promoting social learning, this behavior promotes the progress of antimicrobial resistance. In addition, many of these people are unaware of antibiotic residues and abstinence intervals, and currently do not feel threatened about the future of antimicrobial resistance. When they go to the vet, they prefer to go to a vet who prescribes some antibiotics to return home without any drug prescribed [ 33 ].
Another subcategory extracted from the present findings was the use of antibiotics as growth stimulants by poultry farmers. The use of antibiotics, both as a treatment in humans and as a therapeutic measure or growth stimulant in animals, has a great effect on the microbial flora of the intestine and also induces resistant strains in these animals [ 64 ]. When used as a growth stimulant, antibiotics can have adverse effects on humans and animals [ 65 ].
The fourth category was the veterinarian-related factors. The lack of an inter-sectoral approach was one subcategory extracted from the findings. Foreign studies mentioned a multi-sectoral approach and knowledge sharing in educational environments [ 66 ]. There seems to be a need for all institutions to have the required knowledge about the use of antibiotics through shared efforts between universities, the government, and the various professions. One subcategory was the insignificance of antimicrobial resistance to veterinarians. Antibiotic-containing products have harmful effects and there is a significant increase in the resistance of different types of infectious bacteria [ 67 ] besides the important role that antibiotic-containing animal products play in this process. Thus, global efforts are needed to reduce antibiotic use and attempt to control it. More control is needed over veterinary drugs and their use in livestock and poultry farms [ 68 ].
In line with the qualitative study in the UK, this study showed various behavioral and contextual factors involved in the participants’ beliefs about AMR stewardship and their responsibilities in the right direction [ 33 ]. One of these issues is the lack of experience in writing correct prescriptions among novice vets who prescribe several antibiotics at the same time in the hope that at least one works. This finding is in line with some studies that acknowledged that, when uncertain, most new clinicians tend to over-treat with antimicrobial drugs instead of refraining from treatment [ 1 , 69 , 70 , 71 ]. They prescribe several antibiotics in the hope that one works. The other extreme case is also possible when experienced veterinarians prescribe drugs based on their long-held experience. These clinicians have more faith in a series of antibiotics. On the other hand, the diagnosis of the disease and the prescription are dependent n each other. When they are told about the laboratory evidence, they react as if their credibility has been damaged. Therefore, they provide waves of unprincipled recommendations and increase antimicrobial resistance. If a veterinarian intends to prescribe antibiotics based on the principles and guidelines, s/he will face other problems, including the fear of losing clients because, as mentioned earlier, if the prescription is not in accordance with the client’s wishes or the urgency of responding to it, the client will prefer to go to another vet, and this issue will endanger the job security even more.
Another sub-category is lacking self-efficacy in dealing with different visits.In other words, the approach of veterinarians to prescribing antibiotics is to a great extent pre-established and classified. For example, oxytetracycline is the preferred antibiotic for most respiratory diseases. Any cause of disease that requires more attention to the self-efficacy of veterinarians and clinicians can be improved by training methods and participation in appropriate courses. Diminishing moral values becomes important in cases where full-time monitoring of antibiotic residues in animal products and their transfer to society and the environment is not possible, and where the government and regulatory agencies fail due to poor enforcement of laws. The regulatory forces cannot monitor and take care of the veterinary private sector employees and breeders. We can only hope that the vets will feel committed enough in their acts of diagnosis and prescription and the resultant effect on antimicrobial resistance. In the end, it is possible to recommend the modification of the appearance of prescriptions as a solution, because most of the headers of the veterinarians’ prescriptions in Iran are designed in a large way, which encourages the person to fill most of the prescription with writing the unnecessary drugs, so maybe it is recommended to design and implement a single protocol in limiting the written space of the prescriptions, we can take a step in reducing the obstacles facing the control of antimicrobial resistance.
Limitations, strengths and future directions
There were certain limitations in this study. As the interviews were face-to-face, participants might have been tempted to provide socially acceptable answers. Also, some veterinarians showed concerns about the illegal cases they were aware of and reported. So, they were selected through snowball sampling. In addition, selecting interviewees with work experience and an adequate understanding of the relevant problems and interviewing them in a private place were somehow difficult.
As in other qualitative studies, researchers’ beliefs may have influenced the study procedure from conceptualization to interaction with participants and data interpretation [ 72 ].There were chances that the interviewees’ comments did not cover all factors possibly because of the limited sample size. Sampling in qualitative studies continues until the saturation happens. Thus, in this study also the interviews continued until the data were saturated (i.e., when no new information was obtained) and until all the extracted themes were sufficiently supported by the data. No formula was included.
It is possible that besides the factors mentioned by the present participants, other experiences are gained in other parts of the country that cannot be subsumed under the present categories.
Despite the potential limitations, the present study has several strengths. The first is the sampling method with maximum variation (in terms of the province of work, age, sex, and work in the clinic or drug supply or employed in public and private sectors). The next strength is that during the interviews, some participants were dissatisfied with the current conditions, and this study provided an opportunity for them to find solutions. Moreover, there has been extensive research on AMR, but the vast majority of them are quantitative. Few have explored AMR determinants in society. The present study goes beyond the laboratory work, and with the One Health approach, using numerous interviews, it gains a deep understanding of work experience, and comprehensive and valid data to solve the AMR issue. The authors of this study intend to use the data from this study or at least part of the data for future educational interventions. A focus on the categories extracted from these studies helps to plan effective multidimensional interventions. This study can also guide future lines of research.
The results showed that AMR in veterinary medicine induced by veterinarians active in the clinical field occurs under the influence of different factors. To increase AMR stewardship, in the first step, the barriers facing all people involved should be deeply studied and identified. Appropriate plans and policies should be made to deal with the underlying factors. Educational, administrative and legal, client-related factors, and veterinarian-related factors should be considered as the determinants of the increased AMR. It is essential to reform the education system and strengthhen the interdisciplinary relationships, especially among universities and between the university and regulatory organizations. Removing the barriers these people face and reducing the consequent trouble can make the widespread emergence of AMR more evident. Its adverse effects on society will become a crisis which increases the causes of mortality due to the resistance produced to the antibiotics prescribed to patients.
Data availability
The original contributions presented in the study are included in the article/supplementary materials, further inquiries can be directed to the corresponding authors.
Abbreviations
Antimicrobial Resistance
over-the-counter
Prestinaci F et al. Antimicrobial resistance: a global multifaceted phenomenon 2015. 109(7): pp. 309–318.
Kovačević Z et al. Veterinary practitioners’ standpoints and comprehension towards antimicrobial use—are there opportunities for antimicrobial stewardship improvement? 2022. 11(7): p. 867.
Boucher HW. Bad bugs, no drugs 2002–2020: progress, challenges, and call to action. Trans Am Clin Climatol Assoc. 2020;131:65.
PubMed PubMed Central Google Scholar
Ghanbarpour R, et al. Domestic and game pigeons as reservoirs for Escherichia coli harbouring antimicrobial resistance genes. J Global Antimicrob Resist. 2020;22:571–7.
Article Google Scholar
Mahmoudi R, Golchin A, Farhoodi A. A Review on Antibiotic Residues in Animal-derived Foods in Iran over the Last Thirty Years 2014. 24(119): pp. 213–222.
Aidara-Kane A et al. World Health Organization (WHO) guidelines on use of medically important antimicrobials in food-producing animals 2018. 7: pp. 1–8.
Barlow JJ. Antimicrobial resistance and the use of antibiotics in the dairy industry: Facing consumer perceptions and producer realities 2011. 23: pp. 47–58.
Organization WH. Antimicrobial resistance: global report on surveillance. World Health Organization; 2014.
Kadri S. S.J.C.c.m., Key takeaways from the US CDC’s 2019 antibiotic resistance threats report for frontline providers 2020. 48(7): pp. 939–945.
Kuehn BJJ. Antibiotic Resist Threat Grows. 2019;322(24):2376–2376.
Google Scholar
Organization WHO. Use of antimicrobials outside human medicine 2001.
AL Wabel, N. The pharmacokinetics and milk residual behaviour of tylosin in lactating Najdi ewes 2008.
Nisha AJVw. Antibiotic residues-a Global Health Hazard. 2008;1(12):375.
Sharifi A, Sobhani K, Mahmoudi P. A systematic review and meta-analysis revealed a high-level antibiotic resistance of bovine mastitis Staphylococcus aureus in Iran 2023.
Toghroli R et al. Determinants of antimicrobial resistance occurrence in animal-based food, perceived by livestock farmers: a qualitative phenomenological study. 2023. 6(3): p. e1160.
Mehtarpour M et al. Control of antimicrobial resistance in Iran: The role of international factors 2020. 20: pp. 1–10.
Hassani L et al. An intervention to improve antibiotic prescription behavior in veterinary students: A protocol based on the multi-theory model to tackle antimicrobial resistance 2024. 7(2): p. e1886.
Laxminarayan R, et al. Antibiotic resistance—the need for global solutions. Lancet Infect Dis. 2013;13(12):1057–98.
Article PubMed Google Scholar
O’Neill J. Review on antimicrobial resistance: tackling drug-resistant infections globally: final report and recommendations Review on antimicrobial resistance: tackling drug-resistant infections globally: final report and recommendations., 2016.
Aenishaenslin C, et al. Evidence needed for antimicrobial resistance surveillance systems. Bull World Health Organ. 2019;97(4):283.
Article PubMed PubMed Central Google Scholar
Samuels R, Qekwana DN, Oguttu JW. Antibiotic prescription practices and attitudes towards the use of antimicrobials among veterinarians in the City of Tshwane, South Africa. PeerJ. 2021;9:e10144.
Van Boeckel TP, et al. Global trends in antimicrobial use in food animals. Proc Natl Acad Sci. 2015;112(18):p5649–5654.
Tonkin-Crine S, Yardley L, Little P. Antibiotic prescribing for acute respiratory tract infections in primary care: a systematic review and meta-ethnography. J Antimicrob Chemother. 2011;66(10):2215–23.
Article CAS PubMed Google Scholar
Drekonja DM, et al. Antimicrobial stewardship in outpatient settings: a systematic review. Infect Control Hosp Epidemiol. 2015;36(2):142–52.
van Dijk L, et al. Participatory policy making by dairy producers to reduce anti-microbial use on farms. Zoonoses Public Health. 2017;64(6):476–84.
Speksnijder DC, et al. Effect of structural animal health planning on antimicrobial use and animal health variables in conventional dairy farming in the Netherlands. J Dairy Sci. 2017;100(6):4903–13.
Sazmand A, et al. Assessment of knowledge, attitudes and practices relating to parasitic diseases and anthelmintic resistance among livestock farmers in Hamedan. Iran. 2020;7:584323.
Odetokun IA et al. Knowledge of antimicrobial resistance among veterinary students and their personal antibiotic use practices: a national cross-sectional survey. 2019. 8(4): p. 243.
Graneheim UH, Lundman B. Qualitative content analysis in nursing research: concepts, procedures and measures to achieve trustworthiness. Nurse Educ Today. 2004;24(2):105–12.
Toghroli R, et al. Explaining the determinants of Pediculosis Control and Prevention: a qualitative study in Southern Iran. INQUIRY: J Health Care Organ Provis Financing. 2022;59:00469580221086369.
Hsieh H-F, Shannon SE. Three approaches to qualitative content analysis. Qual Health Res. 2005;15(9):1277–88.
Lincoln YS, Lynham SA, Guba EG. Paradigmatic controversies, contradictions, and emerging confluences, revisited. Sage Handb Qualitative Res. 2011;4(2):97–128.
Golding SE, Ogden J, Higgins HM. Shared goals, different barriers: a qualitative study of UK veterinarians’ and farmers’ beliefs about antimicrobial resistance and stewardship. Front Veterinary Sci. 2019;6:132.
Doidge C, et al. Farmers’ perceptions of preventing antibiotic resistance on sheep and beef farms: risk, responsibility, and action. Front Veterinary Sci. 2020;7:524.
Irshaid YM, et al. A pharmacoepidemiological study of prescription pattern in outpatient clinics in Southwestern Saudi Arabia. Saudi Med J. 2004;25(12):1864–70.
PubMed Google Scholar
Mohagheghi M, et al. Community-based outpatient practice of antibiotics use in Tehran. Pharmacoepidemiol Drug Saf. 2005;14(2):135–8.
Madridejos-Mora R, Amado-Guirado E, Pérez-Rodríguez MT. Effectiveness of the combination of feedback and educational recommendations for improving drug prescription in general practice Medical care, 2004: pp. 643–648.
Chaturvedi V, Mathur A, Anand A. Rational drug use–As common as common sense? Med J Armed Forces India. 2012;68(3):206.
Article CAS PubMed PubMed Central Google Scholar
Rashmi S, Chaman L, Bhuvneshwar K. Antibacterial resistance: current problems and possible solutions. Indian J Med Sci. 2005;59:120–9.
Zare N et al. Effectiveness of the feedback and recalling education on quality of prescription by general practitioners in Shiraz 2008.
De Vries T, et al. Impact of a short course in pharmacotherapy for undergraduate medical students: an international randomised controlled study. Lancet. 1995;346(8988):1454–7.
Dar-Odeh NS et al. Antibiotic prescribing practices by dentists: a review Therapeutics and clinical risk management, 2010. 6: p. 301.
Öcek Z, et al. Development of a rational antibiotic usage course for dentists. Eur J Dent Educ. 2008;12(1):41–7.
Cobo-Angel C, Roche SM, LeBlanc SJJPo. Understanding the role of veterinarians in antimicrobial stewardship on Canadian dairy farms: a mixed-methods study. 2023. 18(7): p. e0289415.
Lloyd DH. S.W.J.A.r.i.b.f.l. Page, and c. animals, Antimicrobial stewardship in veterinary medicine 2018: pp. 675–697.
Allerton F, Russell JJJ-AR. Antimicrob Stewardship Veterinary Medicine: Rev Online Resour. 2023;5(3):dlad058.
Espinosa G-S, Román J et al. Veterinary Education and Training on Non-Traditional Companion Animals, Exotic, Zoo, and Wild Animals: Concepts Review and Challenging Perspective on Zoological Medicine 2023. 10(5): p. 357.
Barret D. Training and evaluation of the european cattle specialist of the future . in Proceeding of 24th World Buiatrics Congress . 2006.
Wushouer H et al. The impact of physicians’ knowledge on outpatient antibiotic use: Evidence from China’s county hospitals. Medicine, 2020. 99(3).
Holopainen A, Hakulinen-Viitanen T, Tossavainen K. Nurse teacherhood: systematic descriptive review and content analysis. Int J Nurs Stud. 2007;44(4):611–23.
Moradi G, et al. National action plan of the Islamic Republic of Iran for combating antimicrobial resistance during 2016–2021. Med J Islamic Repub Iran. 2018;32:82.
Organization WH. Strategic framework for collaboration on antimicrobial resistance: together for one health. Food & Agriculture Org; 2022.
Lazarou J, Pomeranz BH, Corey PN. Incidence of adverse drug reactions in hospitalized patients: a meta-analysis of prospective studies. JAMA. 1998;279(15):1200–5.
FitzGerald RJ. Medication errors: the importance of an accurate drug history. Br J Clin Pharmacol. 2009;67(6):671–5.
Organization WH. WHO guidelines on use of medically important antimicrobials in food-producing animals: web annex A: evidence base. World Health Organization; 2017.
Yimenu DK, et al. Assessment of antibiotic prescribing patterns at outpatient pharmacy using world health organization prescribing indicators. J Prim Care Community Health. 2019;10:2150132719886942.
Watson KT, Barash PG. The new Food and Drug Administration drug package insert: implications for patient safety and clinical care. Anesth Analgesia. 2009;108(1):211–8.
Wynn R, Meiller T, Crossley H. Drug information handbook for dentistry: oral medicine for medically-compromised patients & specific oral conditions. Lexi-Comp, US; 2000.
Ventola CL. The antibiotic resistance crisis: part 1: causes and threats. Pharm Ther. 2015;40(4):277.
Butcher A, Cañada JA, Sariola S. How to make noncoherent problems more productive: towards an AMR management plan for low resource livestock sectors. Humanit Social Sci Commun. 2021;8(1):1–10.
Hofmeister EH, et al. Validity and client use of information from the world wide web regarding veterinary anesthesia in dogs. J Am Vet Med Assoc. 2008;233(12):1860–4.
Kogan LR, Schoenfeld-Tacher R, Viera AR. The internet and health information: differences in pet owners based on age, gender, and education. J Med Libr Association: JMLA. 2012;100(3):197.
Volk JO, et al. Executive summary of the Bayer veterinary care usage study. J Am Vet Med Assoc. 2011;238(10):1275–82.
Price LB, et al. Elevated risk of carrying gentamicin-resistant Escherichia coli among US poultry workers. Environ Health Perspect. 2007;115(12):1738–42.
Awosanya EJ, Akande H. Animal health care seeking behavior of pets or livestock owners and knowledge and awareness on zoonoses in a university community. Veterinary World. 2015;8(7):841.
Santosh S, Panda S. Sharing of knowledge among faculty in a mega open university. Open Praxis. 2016;8(3):247–64.
Adib N, et al. Antibiotic resistance profile and virulence genes of uropathogenic Escherichia coli isolates in relation to phylogeny. Trop Biomed. 2014;31(1):17–25.
CAS PubMed Google Scholar
Kim H, Xie B. Health literacy in the eHealth era: a systematic review of the literature. Patient Educ Couns. 2017;100(6):1073–82.
Blaser MJJS. Antibiotic use and its consequences for the normal microbiome 2016. 352(6285): pp. 544–545.
Organization WH. World Health Organization Antimicrobial Resistance: Global Report on Surveillance. 2014 . 2014.
Weiner LM, et al. Vital signs: preventing antibiotic-resistant infections in hospitals—United States, 2014. Elsevier; 2016. pp. 2224–30.
Yeh CJ, Inman AG. Qualitative data analysis and interpretation in counseling psychology: strategies for best practices. Couns Psychol. 2007;35(3):369–403.
Download references
Acknowledgements
The authors would like to acknowledge all participants for their participation who patiently participated in this study.
This study received funding from Hormozgan University of Medical Sciences. The funder was not involved in the research design, collection, interpretation of data, analysis, the writing of the article or the decision to submit it for publication.
Author information
Authors and affiliations.
Social Determinants in Health Promotion Research Center, Hormozgan Health Institute, Hormozgan University of Medical Sciences, Bandar Abbas, Iran
Razie Toghroli, Laleh Hassani & Teamur Aghamolaei
Department of Social and Behavioral Health, School of Public Health, University of Nevada, Las Vegas (UNLV), Las Vegas, NV, 89119, USA
Manoj Sharma
HIV/STI Surveillance Research Center, and WHO Collaborating Center for HIV Surveillance, Institute for Futures Studies in Health, Kerman University of Medical Sciences, Kerman, Iran
Hamid Sharifi
Department of Pathobiology, Faculty of Veterinary Medicine, Shahid Bahonar University of Kerman, Kerman, Iran
Maziar Jajarmi
Institute for Global Health Sciences, University of California, San Francisco, CA, USA
You can also search for this author in PubMed Google Scholar
Contributions
RT, LH, TA and M-SH designed the study. MJ and RT conducted the laboratory analyses. RT, MJ collected the specimens. H-SH conducted the data analysis. RT, LH and M-SH wrote the main manuscript text. All authors reviewed and approved the manuscript.
Corresponding author
Correspondence to Laleh Hassani .
Ethics declarations
Ethics approval and consent to participate.
The study was approved by the Research Ethics Committee of Hormozgan University of Medical Sciences (IR.HUMS.REC.1400.207). A written informed consent was obtained from all the study participants. All methods were performed in accordance with the relevant guidelines and regulations by including a statement in the declarations.
Consent for publication
Not applicable.
Competing interests
All the authors declare that they have no competing interests.
Additional information
Publisher’s note.
Springer Nature remains neutral with regard to jurisdictional claims in published maps and institutional affiliations.
Note: Here, what we mean by “Livestock” is all animals, including cattle, sheep, goats, camels, poultry, and aquatic animals bred and consumed by humans and consumed as animal-origin food.
By “stockbreeder”, “breeder” and “client”, we mean all those who own livestock and pet. AMR represents antimicrobial resistance.
Rights and permissions
Open Access This article is licensed under a Creative Commons Attribution 4.0 International License, which permits use, sharing, adaptation, distribution and reproduction in any medium or format, as long as you give appropriate credit to the original author(s) and the source, provide a link to the Creative Commons licence, and indicate if changes were made. The images or other third party material in this article are included in the article’s Creative Commons licence, unless indicated otherwise in a credit line to the material. If material is not included in the article’s Creative Commons licence and your intended use is not permitted by statutory regulation or exceeds the permitted use, you will need to obtain permission directly from the copyright holder. To view a copy of this licence, visit http://creativecommons.org/licenses/by/4.0/ . The Creative Commons Public Domain Dedication waiver ( http://creativecommons.org/publicdomain/zero/1.0/ ) applies to the data made available in this article, unless otherwise stated in a credit line to the data.
Reprints and permissions
About this article
Cite this article.
Toghroli, R., Hassani, L., Aghamolaei, T. et al. Explaining the barriers faced by veterinarians against preventing antimicrobial resistance: an innovative interdisciplinary qualitative study. BMC Infect Dis 24 , 455 (2024). https://doi.org/10.1186/s12879-024-09352-7
Download citation
Received : 13 July 2023
Accepted : 25 April 2024
Published : 30 April 2024
DOI : https://doi.org/10.1186/s12879-024-09352-7
Share this article
Anyone you share the following link with will be able to read this content:
Sorry, a shareable link is not currently available for this article.
Provided by the Springer Nature SharedIt content-sharing initiative
- Antimicrobial resistance (AMR)
- Food Safety
- Veterinarian
- Qualitative research
BMC Infectious Diseases
ISSN: 1471-2334
- Submission enquiries: [email protected]
- General enquiries: [email protected]
- Open access
- Published: 01 May 2024
Hospital performance evaluation indicators: a scoping review
- Shirin Alsadat Hadian ORCID: orcid.org/0000-0002-1443-1990 1 ,
- Reza Rezayatmand ORCID: orcid.org/0000-0002-9907-3597 2 ,
- Nasrin Shaarbafchizadeh ORCID: orcid.org/0000-0001-7104-2214 3 ,
- Saeedeh Ketabi ORCID: orcid.org/0000-0002-6778-5645 4 &
- Ahmad Reza Pourghaderi ORCID: orcid.org/0000-0003-2682-2160 5
BMC Health Services Research volume 24 , Article number: 561 ( 2024 ) Cite this article
Metrics details
Hospitals are the biggest consumers of health system budgets and hence measuring hospital performance by quantitative or qualitative accessible and reliable indicators is crucial. This review aimed to categorize and present a set of indicators for evaluating overall hospital performance.
We conducted a literature search across three databases, i.e., PubMed, Scopus, and Web of Science, using possible keyword combinations. We included studies that explored hospital performance evaluation indicators from different dimensions.
We included 91 English language studies published in the past 10 years. In total, 1161 indicators were extracted from the included studies. We classified the extracted indicators into 3 categories, 14 subcategories, 21 performance dimensions, and 110 main indicators. Finally, we presented a comprehensive set of indicators with regard to different performance dimensions and classified them based on what they indicate in the production process, i.e., input, process, output, outcome and impact.
The findings provide a comprehensive set of indicators at different levels that can be used for hospital performance evaluation. Future studies can be conducted to validate and apply these indicators in different contexts. It seems that, depending on the specific conditions of each country, an appropriate set of indicators can be selected from this comprehensive list of indicators for use in the performance evaluation of hospitals in different settings.
Peer Review reports
Healthcare is complex [ 1 ] and a key sector [ 2 ] that is now globally faced with problems of rising costs, lack of service efficiency, competition, and equity as well as responsiveness to users [ 3 ]. One estimate by the WHO has shown a yearly waste of approximately 20–40% of total healthcare resources because of inefficiency [ 4 ]. European countries have spent on average 9.6% of their gross domestic product (GDP) on healthcare in 2017 and 9.92% in 2019. Germany, France, and Sweden reported the highest healthcare expenditures in Europe in 2018 (between 10.9% and 11.5% of GDP) [ 5 ]. In the U.S., healthcare spending consumes 18% of the GDP, which is likely to eclipse $6 trillion by 2027 [ 6 ].
Hospitals, as the biggest consumers of health system budgets [ 7 ], are the major part of the health system [ 8 ]. In many countries 50–80% of the health sector budget is dedicated to hospitals [ 8 , 9 ]. As a result, hospital performance analysis is becoming a routine task for every hospital manager. On the one hand, hospital managers worldwide are faced with difficult decisions regarding cost reduction, increasing service efficiency, and equity [ 10 ]. On the other hand, measuring hospital efficiency is an issue of interest among researchers because patients demand high-quality care at lower expenses [ 11 ].
To address the above mentioned need to measure hospital performance, implementing an appropriate hospital performance evaluation system is crucial in any hospital. In doing so, hospital administrators use various tools to analyse and monitor hospital activities [ 1 ], which need well-defined objectives, standards and quantitative indicators [ 12 ]. The latter are used to evaluate care provided to patients both quantitatively and qualitatively and are often related to input, output, processes, and outcomes. These indicators can be used for continuous quality improvement by monitoring, benchmarking, and prioritizing activities [ 13 ]. These parameters are developed to improve health outcomes and to provide comparative information for monitoring and managing and formulating policy objectives within and across health services [ 12 ]. Studies thus far have used their own set of indicators while evaluating hospital performance, which could be context dependent. In addition, those studies have mostly used a limited set of indicators that focus on few dimensions (2–6 dimensions) of hospital performance [ 14 , 15 , 16 , 17 , 18 ].
Therefore, comprehensive knowledge of potential indicators that can be used for hospital performance evaluation is necessary. It would help choose appropriate indicators when evaluating hospital performance in different contexts. It would also help researchers extend the range of analysis to evaluate performance from a wider perspective by considering more dimensions of performance. Although performance is a very commonly used term, it has several definitions [ 19 , 20 ], yet, it is often misunderstood [ 21 ]. Therefore, some researchers have expressed confusion about the related terms and considered them interchangeable. These terms are effectiveness, efficiency, productivity, quality, flexibility, creativity, sustainability, evaluation, and piloting [ 21 , 22 , 23 ]. Thus, this scoping review aimed to categorize and present a comprehensive set of indicators that can be used as a suitable set for hospital performance evaluation at any needed level of analysis, i.e., clinical, para-clinical, logistical, or departmental, and relate those indicators to the appropriate performance dimensions. The uniqueness of this paper is that it provides its readers with a comprehensive collection of indicators that have been used in different performance analysis studies.
Materials and methods
We conducted a scoping review of a body of literature. The scoping review can be of particular use when the topic has not yet been extensively reviewed or has a complex or heterogeneous nature. This type of review is commonly undertaken to examine the extent, range, and nature of research activity in a topic area; determine the value and potential scope and cost of undertaking a full systematic review; summarize and disseminate research findings; and identify research gaps in the existing literature. As a scoping review provides a rigorous and transparent method for mapping areas of research, it can be used as a standalone project or as a preliminary step to a systematic review [ 24 ]. While a systematic review (qualitative or quantitative) usually addresses a narrow topic/scope and is a method for integrating or comparing findings from previous studies [ 25 ].
In our study, we used the Preferred Reporting Items for Systematic reviews and Meta-Analyses extension for Scoping Reviews (PRISMA-ScR) Checklist following the methods outlined by Arksey and O’Malley [ 26 ] and Tricco [ 27 ]. A systematic search for published and English-language literature on hospital performance evaluation models was conducted, using three databases, i.e., PubMed, Scopus, and Web of Science, from 2013 to January 2023. Initially, the identified keywords were refined and validated by a team of experts. Then, a combination of vocabularies was identified by the authors through a brainstorming process. The search strategy was formulated using Boolean operators. The title and abstract of the formulas were searched in the online databases. The search query for each database is presented in Table 1 .
In the screening process, relevant references related to hospital performance evaluation were screened and abstracted into researcher-developed Microsoft® Excel forms by dual independent reviewers and conflicting information was provided by other reviewers.
The inclusion criteria were as follows: focused only on the hospital setting, available full text and written in English. We excluded studies that focused on health organization indicators, not specifically on hospital indicators; articles without appropriate data (only focused on models and not indicators; or qualitative checklist questionnaires); and articles that focused only on clinical or disease-related indicators, not hospital performance dimensions, and provided very general items as indicators, not the domains of the indicators themselves. Then, a PRISMA-ScR Checklist was used to improve transparency in our review [ 28 ].
To extract the data, researcher-developed Microsoft® Excel forms (data tables) were designed. The following data were subsequently extracted into Microsoft®Excel for synthesis and evaluation: title, author, article year, country, indicator category, study environment (number of hospitals studied), study time frame, indicator name, number of indicators, indicator level (hospital level, department level), evaluation perspective (performance, productivity, efficiency, effectiveness, quality, cost, safety, satisfaction, etc. ) , study type (quantitative or qualitative), indicator subtype (input (structure), process, output (result), outcome and impact), and other explanations. To create a descriptive summary of the results that address the objectives of this scoping review, numerical summarization was also used.
The purpose of creating the main category and the evaluation perspective section was to develop them and create new categories, which focused on the type of indicators related to the performance term. For example, in the “Category” section, the names of the departments or wards of the hospital (such as hospital laboratories, pharmacies, clinical departments, and warehouses) and in the “Evaluation perspective” section, various terms related to the evaluation of hospital performance were extracted. These two types were used after extracting their information under the title “performance dimension”.
The indicators’ levels were collected to determine the level of performance evaluation with the relevant index. Some indicators were used to evaluate the performance of the entire hospital, some were used to evaluate the performance of hospital departments, and some were used to evaluate the performance at the level of a specific project. For example, several indicators (such as bed occupancy ratio, length of stay, and waiting time) were used to evaluate the performance of the entire hospital, and other indicators (such as laboratory department indicators, energy consumption indicators, and neonatal department indicators) were used only to measure the performance of specific departments. This sections were used under the title “category”. The “category” and “indicator’s name” sections were defined according to the results of the “subcategory” section.
The subtypes of indicators (input (structure), process, output(result), outcome and impact) were defined based on the chain model, and each of the selected indicators was linked to it (Appendix 1 ). As a result of the chain model, inputs were used to carry out activities, activities led to the delivery of services or products (outputs). The outputs started to bring about change (outcomes), and eventually, this (hopefully) contributed to the impact [ 29 ]. The classification of the set of input, process, output, outcome and impact indicators was such that readers could access these categories if necessary according to their chosen evaluation models. The term was used under the title “Indicators by types”.
The type of study was considered quantitative or qualitative for determining whether an indicator was able to perform calculations. In this way, readers can choose articles that use quantitative or qualitative indicators to evaluate hospital performance.
We included 91 full-text studies (out of 7475) in English published between 2013 and January 2023 (Fig. 1 ), approximately 40% of which were published between 2020 and 2023. More than 20% of the retrieved studies were conducted in Iran and USA.
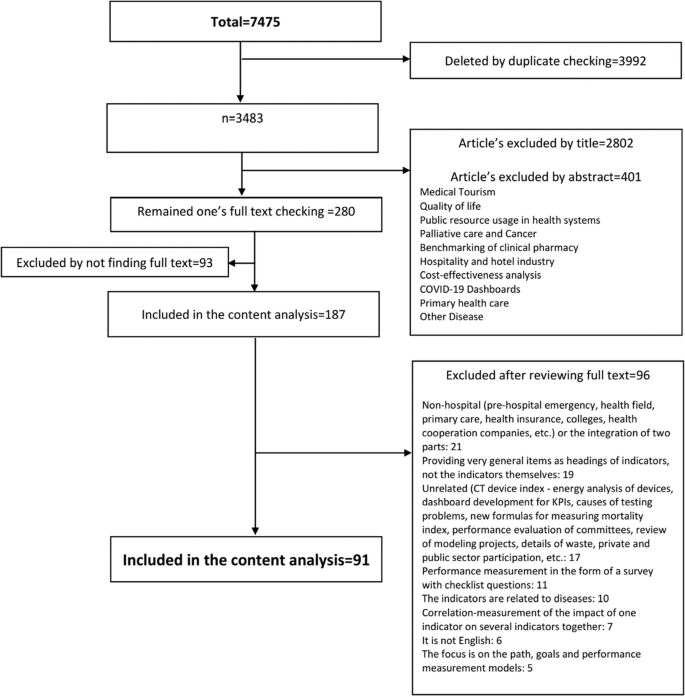
Study selection and data abstraction
Study characteristic
As shown in Table 2 , in 85% of the reviewed studies, a number of hospitals (1 to 3828 hospitals, 13,221 hospitals in total) were evaluated. More than 90% of the studies used a quantitative approach. In more than 70% of the studies, hospital evaluation occurred at the department level, which can also be divided into three levels: administrative, clinical ward, and paramedical department. In addition, the administrative departments consist of 13 departments, including financial management [ 48 , 55 , 61 , 67 , 68 , 80 , 83 , 109 , 113 ], supply chain management and warehouse [ 15 , 43 , 84 ], value-based purchasing [ 33 , 85 ], human resource management [ 97 , 101 ], medical equipment [ 32 , 87 ], health information management department [ 90 ], information systems [ 106 ], nutritional assessment [ 93 ], energy management [ 30 , 45 , 92 ], facility management [ 52 , 53 ], building sustainability and resilience [ 35 ], research activities [ 44 ], and education [ 107 ].
The clinical wards consisted of 8 wards, namely, emergency departments (EDs) [ 16 , 39 , 56 , 57 , 69 , 70 , 89 ], surgery departments [ 58 , 62 , 63 , 91 , 102 ], intensive care units (ICUs) [ 47 , 64 , 65 ], operating rooms (ORs) [ 38 , 88 , 108 ], surgical intensive care units (SICUs) [ 111 ], obstetrics and gynecology department [ 59 ], neonatal intensive care units (NICUs) [ 74 , 103 ] and quality of care [ 18 , 31 , 40 , 50 , 72 , 92 , 95 , 112 ] indicators. The paramedical departments consisted of 3 departments, pharmacy [ 60 , 76 , 98 ], laboratory and blood bank [ 37 , 42 , 43 , 49 ], and outpatient assessment [ 86 ] indicators.
With regard to data categorization, firstly, a total of 1204 indicators in 91 studies were extracted and after detailed examination, 43 indices (such as hospital ownership, level of care, admission process, and personal discipline) were removed due to their generality and impossibility of calculation in the hospital environment. Then, 1161 performance indicators were entered in this research and were categorized based on the performance criteria (more details about the indicators can be found in Appendix 1 ). Secondly, 145 functional dimensions, including divisions based on different departments and units of the hospital, were defined according to several focus group discussions with 5 health experts. Then, re-categorization and functional summarization were performed, after which 21 performance dimensions were finalized.
As shown in Table 4 , the 21 performance dimensions were divided into three parts: category, subcategory, and related indicators. Additionally, according to the hospital levels, there were three categories: ‘organizational management’, ‘clinical management’, and ‘administrative management’. Then, according to the type of indicators, fifteen subcategories were defined for the 110 selected main indicators.
Performance dimensions
The ‘productivity’ dimension focuses on indicators reflecting the macro-performance of the hospital, considering that this index is more effective and efficient. The ‘efficiency’ dimension focuses on general performance indicators for the optimal use of resources to create optimal output in the hospital. The ‘effectiveness’ dimension is a general performance indicator with an outcome view. The ‘speed’ dimension focuses on the indicators that show attention to the service delivery time and the speed of the procedures. The ‘development’ dimension focuses on matters related to employees’ and students’ training and related training courses. In terms of ‘safety’ dimension, there were issues related to patient safety, unwanted and harmful events, and hospital infections.
The “quality of work life” dimension emphasizes matters related to personnel volume and work conditions. The ‘quality’ dimension is related to the quality of service provided in different parts of the hospital and possible complications in improving the quality of services. The ‘satisfaction’ dimension focuses on the satisfaction of patients, employees, and their complaints. The ‘innovation’ dimension relates to the research process and its output. The ‘appropriateness’ dimension involves proper service from clinical departments, pharmaceutical services, and patient treatment. The ‘evaluation’ dimension focuses on the indicators related to the assessment scores of the para-clinical departments of the hospital.
The ‘profitability’ dimension focuses on the overall output indicators for income and profitability. The ‘cost’ dimension focuses on indicators related to general expenditures and the average cost per bed and patient and budgeting. The ‘economy’ dimension is related to financial rates and their indicators. The ‘coherence’ dimension emphasizes the indicators related to the continuity of the service delivery process. The ‘patient-centeredness’ dimension focuses on the indicators related to the patient’s experience of the facility, environment, treatment processes, communications, and relevant support for the patient. The ‘equity’ dimension studies indicators related to social and financial justice and life expectancy. The ‘relationship’ dimension evaluates the process of consultations and discussions required during the patients’ care provided by the treatment team. The ‘sustainability’ dimension focuses on indicators related to energy standards. The ‘flexibility’ dimension focuses on the hospital’s response to the crisis.
According to Table 4 , most studies focused on ‘efficiency’, ‘productivity’, ‘safety’ and ‘effectiveness’ as performance dimensions in 54, 53, 38 and 37 studies, respectively (40–70% of studies). In the ‘efficiency’ subcategory, resource management, supportive unit assessment, and human resource management indicators were the first to third most common indicators used in 26, 23 and 22 studies, respectively (approximately 25% of the studies).
In addition, for the ‘efficiency’ dimension, ‘medical staff numbers’, ‘emergency department bed numbers’, and ‘nonmedical staff numbers’ were reported in 16, 13, and 11 studies, respectively (between 20 and 30% of the studies). For the ‘productivity’ subcategory, ‘bed utilization rate’ and ‘service delivery and treatment’ were reported in 50% and 20% of the studies, respectively (46 and 19 out of 91).
Additionally, for the ‘productivity’ dimension, the ‘length of stay’ indicator was used more than others and reported in approximately 80% of the studies (43 out of 53), followed by the ‘bed occupancy rate’ in approximately 40% of the studies (21 out of 53). The ‘bed turnover ratio’ and ‘hospitalization rate’ were also reported in 12 studies. Furthermore, for ‘safety’ dimensions, all indicators were in the ‘patient safety’ subcategory, which has been reported in 38 studies, and ‘complications’, ‘accidents or adverse events’, and ‘incidents or errors rates’ were the most concentrated indicators by researchers in 13, 12, and 11 studies, respectively. The performance dimension of ‘effectiveness’ was presented in 37 studies (40%), with only two indicators, ‘mortality rate’ in 29 studies and ‘readmission rate’ in 23 studies.
Performance categories
Considering the three categories shown in Table 4 , ‘organizational management’ indicators were more commonly used among the other two categories (‘clinical’ and ‘administrative’) and were present in more than 85% of the studies (78 out of 91). Two categories, ‘clinical management’ and ‘administrative management’, were reported in 62 and 51 studies, respectively.
Performance subcategories
Considering the 14 subcategories shown in Table 4 , both the ‘bed utilization rate’ and ‘patient safety’ indicators were mentioned in 46 studies and were more common among the other subcategories. The second most common indicator of the ‘financial management’ subcategory was reported in 38 studies. At the third level, both the ‘human resource management’ and ‘time management’ indicators were presented in 31 studies. The ‘paramedical’ subcategory indicators were presented in less than 10% of the studies [ 60 , 96 , 97 , 98 , 106 , 113 ].
Performance indicators
According to the indicator columns in Table 3 , the most used indicators in reviewed studies were the length of stay, mortality rate, and readmission rate in 47%, 32%, and 25% of studies, respectively. Bed occupancy rate and non-personnel costs were reported in 23% of studies. Additionally, among the 110 indicators, 16 indicators, namely, the lab cancellation rate, exam-physician ratios, number of coded diagnoses, number of medical records, laboratory sample/report intervals, medical information request time, safety standards in the archives, nutritional risk screening, imaging quality control failures, errors in medical reports, average impact factor, nutritional measures, laboratory scoring, imaging inspection, discharge process and emergency response rate, were reported in less than 1% of the studies.
The classification of the indicators in Table 4 was performed based on the chain model, which included the input, process, output, outcome and impact. The assignment of the indicators to each category was performed according to the experts’ opinions. For instance, the number of publications by academic member of an academic hospital and the average impact factor of those publications were considered outcome indicators. As depicted in the Table 4 , most studies (80%) focused more on output indicators. Additionally, fifteen studies focused on introducing and extracting some of the input, process, output, outcome and impact indicators; among those, only one study [ 96 ] has examined the input, process, output and impact indicators simultaneously.
Additionally, in approximately 42% (36 out of 91) of the studies, the indicators’ definitions, formulas, or descriptions have been illustrated, while less than 10% of the studies have defined measuring units, standard or benchmark units for all studied indicators [ 15 , 43 , 45 , 51 , 52 , 57 , 67 ].
Overall, nine studies related to hospital performance evaluation were conducted using systematic review methodologies (five systematic reviews [ 16 , 29 , 30 , 56 , 113 ], two literature reviews [ 79 , 80 ], one narrative review [ 98 ] and one brief review [ 92 ]). Most of these studies focused on extracting performance indicators from one or more hospital departments (e.g., the emergency department) [ 16 , 56 ], hospital laboratory and radiology information systems [ 106 ], supply chain performance [ 29 ], resources and financial results and activity [ 113 ], hospital water consumption [ 30 ], and the pharmaceutical sector [ 98 ]. Other reviews included a three-step process to review, evaluate and rank these hospital indicators in a systematic approach [ 16 ], or to evaluate performance indicator models to create an interactive network and visualize the causal relationships between performance indicators [ 79 ]; moreover, some have focused on the importance of indicators to ensure adequate coverage of the relevant areas of health care services to be evaluated [ 92 ].
Only one scoping review aimed to identify current assessments of hospital performance and compared quality measures from each method in the context of the six qualitative domains of STEEEP (safety, timeliness, effectiveness, efficiency, equity, and patient-centeredness) of the Institute of Medicine (IOM) in accordance with Donabedian’s framework and formulating policy recommendations [ 115 ].
In addition, 21 studies divided performance indicators into 2 to 6 dimensions of performance. Also, the reviewed studies included 2–40 indicators in zero [ 29 , 30 , 98 ] to 6 domains [ 34 ]. Moreover, none of the studies have tried to comprehensively summarize and categorize the performance indicators in several categories, focusing on all the indicators reflecting the performance of the entire hospital organization, or the indicators of administrative units or clinical departments.
In this scoping review, a unique set of hospital performance evaluation indicators related to the various performance dimensions was categorized from 91 studies over the past ten years.
Similarly, in a study, 19 performance dimensions, 32 sub-dimensions, and 138 indicators were extracted from only six studies. Those dimensions were described by all studies included in the review, but only three studies specified the relevant indicators, and the list provided for all possible indicators was not comprehensive. Also, despite current review, there was no classification of indicators based on the hospital levels: managerial, clinical, or organizational levels [ 116 ]. Another study has similarly investigated the performance evaluation indicators of the hospital in such a way that among 42 studies, 111 indicators were presented in the four categories: input, output, outcome, and impact. But, there was no classification of indicators based on performance dimensions and hospital levels [ 117 ].
In this study, the importance of categorized indicators, for the first time to our knowledge, was determined based on their frequency of use in the published literature (Appendix 2 ). The ‘Organizational management’ indicators were the most common compared with the other two categories (‘clinical’ and ‘administrative’). It could be because of the fact that the indicators such as ‘bed occupancy rate’, ‘average length of stay’, ‘mortality rate’, ‘hospital infection rate’, and ‘patient safety’ are easier to be registered in hospital software compared to other indicators, and also they better reflect the overall performance of hospital. Thus, researchers are more interested in using these indicators.
Considering 14 subcategories, indicators related to three subcategories i.e. bed utilization, patient safety and financial management are the most frequent used indicators for hospital performance evaluation. It reflects the need of hospital managers to increase the profitability of hospital in one hand, and to control cost on the other hand. As a results, researchers have paid special attention to ‘cost income’, ‘profitability’, ‘economic’, etc., as indicators for evaluating hospital performance.
When considering indicators by type, more studies have focused on output indicators, while input indicators were the least common used. This might be because of the fact that at hospital level, it is difficult for managers to change those inputs such as ‘beds’, ‘human resources’, ‘equipment and facilities’. In addition, due to the complexity of interdepartmental relationships in hospitals, process indicators seemed to provide more variety for analysis than input indicators, so they were more often used. As mentioned above, output indicators were the most used indicators for hospital performance evaluation due to their ease of calculation and interpretation.
The main purpose of this paper was to identify a comprehensive set of indicators that can be used to evaluate hospital performance in various hospital settings by being distilled into a smaller and more related set of indicators for every hospital or department setting. future studies could be designed to validate each set of indicators in any specific context. In addition, they could investigate the relationship between the indicators and their outcomes of interest and the performance dimension each could address. This will enable hospital managers to build their own set of indicators for performance evaluation both at organization or at department level. Also it should be mentioned that.
Although some previous studies have provided definitions for each indicator and determined the standard criteria for them, this was not done in this study because the focus of this study was to provide a collection of all the indicators used in hospital performance evaluation, which resulted in the identification of more than a thousand indicators without limiting to specific country or context. So while preparing a smaller set of indicators, specific conditions of each country, such as the type of health system and its policy, the type of financing system, and the structure of services, should be taken into account to select appropriate indicators.
In addition, although it is important to examine the scope of each article to compare the list of indicators and the relationships between the dimensions of the hospital in terms of size and type and between the number and type of selected indicators, this was considered beyond the scope of this review due to the high number of indicators, which made the abovementioned investigations impossible. Future studies could do that while working with a smaller set of indicators.
This review aimed to categorize and present a comprehensive set of indicators for evaluating overall hospital performance in a systematic way. 1161 hospital performance indicators were drawn from 91 studies over the past ten years. They then were summarized into 110 main indicators, and categorized into three categories: 14 subcategories, and 21 performance dimensions This scoping review also highlighted the most frequent used indicators in performance evaluation studies which could reflect their importance for that purpose. The results of this review help hospital managers to build their own set of indicators for performance evaluation both at organization or at department level with regard to various performance dimensions.
As the results of this review was not limited to any specific country or context, specific conditions of each country, such as the type of health system and its policy, the type of financing system, and the structure of services, should be taken into account while selecting appropriate indicators as a smaller set of indicators for hospital performance evaluation in specific context.
Availability of data and materials
The datasets used and/or analyzed during the current study are available from the corresponding author on reasonable request.
Abbreviations
Gross domestic product
Preferred Reporting Items for Systematic reviews and Meta-Analyses extension for Scoping Reviews
Emergency departments
Intensive care unit
Operating room
Surgical intensive care unit
Neonatal intensive care unit
Readmission rate
Quality Control
Medication use evaluation
safety, timeliness, effectiveness, efficiency, equity, and patient-centeredness
Institute of Medicine
Abdullah A, Ahmad S, Athar MA, Rajpoot N, Talib F. Healthcare performance management using integrated FUCOM-MARCOS approach: the case of India. Int J Health Plann Manage. 2022;37(5):2635–68.
Article PubMed Google Scholar
Pestana M, Pereira R, Moro S. Improving health care management in hospitals through a productivity dashboard. J Med Syst. 2020;44(4):87.
Amos D. A practical framework for performance measurement of facilities management services in developing countries’ public hospitals. J Facil Manag. 2022;20(5):713–31.
Article Google Scholar
Ahmed S, Hasan MZ, MacLennan M, Dorin F, Ahmed MW, Hasan MM, et al. Measuring the efficiency of health systems in Asia: a data envelopment analysis. BMJ Open. 2019;9(3):e022155.
Article PubMed PubMed Central Google Scholar
Mitkova Z, Doneva M, Gerasimov N, Tachkov K, Dimitrova M, Kamusheva M, et al. Analysis of healthcare expenditures in Bulgaria. Healthc. 2022;10(2):274.
Patrinely JR, Walker SH, Glassman GE, Davis MJ, Abu-Ghname A, Khan U, et al. The importance of financial metrics in physician funding and performance evaluation. Plast Reconstr Surg. 2021;147:1213–8.
Article CAS PubMed Google Scholar
Buathong S, Bangchokdee S. The use of the performance measures in Thai public hospitals. ASIAN Rev Acc. 2017;25(4):472–85.
Google Scholar
Imani A, Alibabayee R, Golestani M, Dalal K. Key indicators affecting hospital efficiency: a systematic review. Front Public Heal. 2022;10:830102.
Mahdiyan S, Dehghani A, Tafti AD, Pakdaman M, Askari R. Hospitals’ efficiency in Iran: a systematic review and meta-analysis. J Educ Health Promot. 2019;8(1):126.
PubMed PubMed Central Google Scholar
Amos D, Musa ZN, Au-Yong CP. Performance measurement of facilities management services in Ghana’s public hospitals. Build Res Inf. 2020;48(2):218–38.
Feibert DC, Andersen B, Jacobsen P. Benchmarking healthcare logistics processes–a comparative case study of Danish and US hospitals. Total Qual Manag Bus Excell. 2019;30(1–2):108–34.
Gün I, Yilmaz F, Şenel IK. Efficiency analysis of health systems in world bank countries. Arch Heal Sci Res. 2021;8(2):147–52.
Breyer JZ, Giacomazzi J, Kuhmmer R, Lima KM, Hammes LS, Ribeiro RA, et al. Hospital quality indicators: a systematic review. Int J Health Care Qual Assur. 2019;32(2):474–87.
Regragui H, Sefiani N, Azzouzi H. Improving performance through measurement: the application of BSC and AHP in healthcare organization. In: Equipe De Recherche, Ingénierie, Innovation Et Management Des Systèmes Industriels, Université Abdelmalek Saadi. Tanger, Morocco: Institute of Electrical and Electronics Engineers Inc; 2018. p. 51–6.
Ghozali MT, Latifah DN, Darayani A. Analysis of Drug Supply Management of the Pharmacy Warehouse of Prof. Dr. Soerojo Mental Health Hospital, Magelang, Indonesia. Clin Schizophr Relat Psychoses. 2021;15:1–6.
Etu EE, Monplaisir L, Aguwa C, Arslanturk S, Masoud S, Markevych I, et al. Identifying indicators influencing emergency department performance during a medical surge: a consensus-based modified fuzzy Delphi approach. PLoS ONE. 2022;17(4 April):e0265101.
Article CAS PubMed PubMed Central Google Scholar
Lin C-Y, Shih F-C, Ho Y-H. Applying the balanced scorecard to build service performance measurements of medical institutions: An AHP-DEMATEL approach. Int J Environ Res Public Health. 2023;20(2):1022.
Backman C, Vanderloo S, Forster AJ. Measuring and improving quality in university hospitals in Canada: the collaborative for excellence in healthcare quality. Health Policy (New York). 2016;120(9):982–6.
Ghalem Â, Okar C, Chroqui R, Semma E. Performance: A concept to define. In: Performance: A concept to define. LOGISTIQUA 2016; 2016. p. 1–13.
Sonnentag S, Frese M. Performance Concepts and Performance Theory. In 2005. p. 1–25.
Tangen S. Demystifying productivity and performance. Int J Prod Perform Manag. 2005;54:34–46.
Elena-Iuliana I, Maria C. Organizational Performance – A Concept That Self-Seeks To Find Itself. Ann - Econ Ser Constantin Brancusi Univ Fac Econ. 2016;4(4):179–83.
Riratanaphong C, Van der Voordt T, Sarasoja A. Performance Measurement in the context of CREM and FM. In: Per Anker Jensen, Theo Van der Voordt CC, editor. The added value of facilities management: concepts, findings and perspectives. Lyngby Denmark: Polyteknisk Forlag; 2012. p. 1–21.
Pham M, Rajić A, Greig J, Sargeant J, Papadopoulos A, Mcewen S. A scoping review of scoping reviews: advancing the approach and enhancing the consistency. Res Synth Methods. 2014;5:371–85.
Chaney M. So you want to write a narrative review article? J Cardiothorac Vasc Anesth. 2021;35:3045–9.
Arksey H, O’Malley L. Scoping studies: towards a methodological framework. Int J Soc Res Methodol. 2005;8(1):19–32.
Tricco AC, Lillie E, Zarin W, O’Brien KK, Colquhoun H, Levac D, et al. PRISMA extension for scoping reviews (PRISMA-ScR): Checklist and explanation. Ann Intern Med. 2018;169(7):467–73.
Tricco A, Lillie E, Zarin W, O’Brien K, Colquhoun H, Levac D, et al. PRISMA extension for scoping reviews (PRISMA-ScR): Checklist and explanation. Ann Intern Med. 2018;169(7):467–73.
Dolatabad AH, Mahdiraji HA, Babgohari AZ, Garza-Reyes JA, Ai A. Analyzing the key performance indicators of circular supply chains by hybrid fuzzy cognitive mapping and Fuzzy DEMATEL: evidence from healthcare sector. Environ Dev Sustain. 2022;1–27.
Batista KJM, da Silva SR, Rabbani ERK, Zlatar T. Systematic review of indicators for the assessment of water consumption rates at hospitals. Water Supply. 2020;20(2):373–82.
Beta G, Role D, Berloviene D, Balkena Z. PATIENTS’ SATISFICATION AS THE QUALITY INDICATOR OF NURSING. In: Lubkina V, Kaupuzs A, Znotina D, editors. SOCIETY INTEGRATION EDUCATION, VOL VI: PUBLIC HEALTH AND SPORT, RESEARCHES IN ECONOMICS AND MANAGEMENT FOR SUSTAINABLE EDUCATION. 2020. p. 79–88.
Bhardwaj P, Joshi NK, Singh P, Suthar P, Joshi V, Jain YK, et al. Competence-based assessment of biomedical equipment management and maintenance system (e-Upkaran) using benefit evaluation framework. CUREUS J Med Sci. 2022;14(10):e30579.
Cheon O, Song M, Mccrea AM, Meier KJ. Health care in America: the relationship between subjective and objective assessments of hospitals. Int PUBLIC Manag J. 2021;24(5):596–622.
Craig KJT, McKillop MM, Huang HT, George J, Punwani ES, Rhee KB. US hospital performance methodologies: a scoping review to identify opportunities for crossing the quality chasm. BMC Health Serv Res. 2020;20(1):640.
Cristiano S, Ulgiati S, Gonella F. Systemic sustainability and resilience assessment of health systems, addressing global societal priorities: Learnings from a top nonprofit hospital in a bioclimatic building in Africa. Renew Sustain ENERGY Rev. 2021;141:110765.
Dadi D, Introna V, Santolamazza A, Salvio M, Martini C, Pastura T, et al. Private Hospital Energy Performance Benchmarking Using Energy Audit Data: An Italian Case Study. Energies. 2022;15(3):1–16.
Dawande PP, Wankhade RS, Akhtar FI, Noman O. Turnaround time: an efficacy measure for medical laboratories. CUREUS J Med Sci. 2022;14(9):e28824.
De Sousa LR, Mazzo A, De Almeida ACF, Tonello C, Lourençone LFM. Evaluation of quality indicators in the management of an operating room at a tertiary-level hospital. Med. 2022;55(1):1–8.
Drynda S, Schindler W, Slagman A, Pollmanns J, Horenkamp-Sonntag D, Schirrmeister W, et al. Evaluation of outcome relevance of quality indicators in the emergency department (ENQuIRE): study protocol for a prospective multicentre cohort study. BMJ Open. 2020;10(9):e038776.
Fekri O, Manukyan E, Klazinga N. Appropriateness, effectiveness and safety of care delivered in Canadian hospitals: a longitudinal assessment on the utility of publicly reported performance trend data between 2012–2013 and 2016–2017. BMJ Open. 2020;10(6):e035447.
Galloa AJO, Ramírez CA. Evaluating Colombian public hospitals productivity during 2004–2015. A luenberger-indicator approach. Rev Gerenc Y Polit Salud. 2020;19:1–23.
Gebreyes M, Sisay A, Tegen D, Asnake A, Wolde M. Evaluation of laboratory performance, associated factors and staff awareness towards achieving turnaround time in tertiary hospitals, Ethiopia. Ethiop J Health Sci. 2020;30(5):767–76.
Gnanaraj J, Kulkarni RG, Sahoo D, Abhishekh B. Assessment of the Key Performance Indicator Proposed by NABH in the Blood Centre of a Tertiary Health Care Hospital in Southern India. Indian J Hematol Blood Transfus. 2022;39:308–16.
Horenberg F, Lungu DA, Nuti S. Measuring research in the big data era: the evolution of performance measurement systems in the Italian teaching hospitals. Health Policy (New York). 2020;124(12):1387–94.
Hwang DK, Cho J, Moon J. Feasibility study on energy audit and data driven analysis procedure for building energy efficiency: bench-marking in Korean hospital buildings. Energies. 2019;14(15):3006.
Jaskova D. Efficiency of management, processes in a private hospital. Entrep Sustain Issues. 2021;9(1):436–46.
Jebraeily M, Valizadeh MA, Rahimi B, Saeidi S. The development of a clinical dashboard for monitoring of key performance indicators in ICU. J Iran Med Counc. 2022;5(2):308–17.
Kang Y, Kim M, Jung K. The equity of health care spending in South Korea: testing the impact of publicness. Int J Environ Res Public Health. 2020;17(5):1775.
Abou Tarieh RR, Zayyat R, Naoufal RN, Samaha HR. A case study exploring the impact of JCI standards implementation on staff productivity and motivation at the laboratory and blood bank. Heal Sci Rep. 2022;5(1):e497.
Kadoic N, Simic D, Mesaric J, Redep NB. Measuring quality of public hospitals in croatia using a multi-criteria Approach. Int J Environ Res Public Health. 2021;18:19.
Khalilabad T, Amir N, Asl P, Raeissi Shali M, Niknam N. Assessment of clinical and paraclinical departments of military hospitals based on the Pabon Lasso Model. J Educ Health Promot. 2020;9:1–6.
Lai JHK, Hou H, Edwards DJ, Yuen PL. An analytic network process model for hospital facilities management performance evaluation. Facilities. 2022;40(5–6):333–52.
Lai J, Yuen PL. Identification, classification and shortlisting of performance indicators for hospital facilities management. Facilities. 2021;39(1–2):4–18.
Lin CS, Chiu CM, Huang YC, Lang HC, Chen MS. Evaluating the operational efficiency and quality of Tertiary hospitals in Taiwan: the application of the EBITDA Indicator to the DEA Method and TOBIT Regression. Healthcare. 2022;10(1):58.
Matos R, Ferreira D, Pedro MI. Economic analysis of portuguese public hospitals through the construction of quality, efficiency, access, and financial related composite indicators. Soc Indic Res. 2021;157(1):361–92.
Morisod K, Luta X, Marti J, Spycher J, Malebranche M, Bodenmann P. Measuring health equity in emergency care using routinely collected data: a systematic review. Heal Equity. 2021;5(1):801–17.
Nik Hisamuddin R, Tuan Hairulnizam TK. Developing key performance indicators for emergency department of teaching hospitals: a mixed fuzzy Delphi and nominal group technique approach. Malays J Med Sci. 2022;29(2):114–25.
Ramírez Calazans A, Paredes Esteban RM, Grijalva Estrada OB, Ibarra Rodríguez MR. Assessment of quality indicators in pediatric major outpatient surgery. Influence of the COVID-19 pandemic. Cir Pediatr. 2023;36(1):17–21.
PubMed Google Scholar
Shaqura II, Gholami M, Akbari Sari A. Assessment of public hospitals performance in Gaza governorates using the Pabón Lasso Model. Int J Health Plann Manage. 2021;36(4):1223–35.
Al-Jazairi AS, Alnakhli AO. Quantifying clinical pharmacist activities in a tertiary care hospital using key performance indicators. Hosp Pharm. 2021;56(4):321–7.
Aloh HE, Onwujekwe OE, Aloh OG, Nweke CJ. Is bed turnover rate a good metric for hospital scale efficiency? A measure of resource utilization rate for hospitals in Southeast Nigeria. Cost Eff Resour Alloc. 2020;18(1):1–8.
Bari S, Incorvia J, Ahearn O, Dara L, Sharma S, Varallo J, et al. Building safe surgery knowledge and capacity in Cambodia: a mixed-methods evaluation of an innovative training and mentorship intervention. Glob Health Action. 2021;14(1):1998996.
Bari S, Incorvia J, Iverson KR, Bekele A, Garringer K, Ahearn O, et al. Surgical data strengthening in Ethiopia: results of a Kirkpatrick framework evaluation of a data quality intervention. Glob Health Action. 2021;14(1):1–11.
Bastos LSL, Hamacher S, Zampieri FG, Cavalcanti AB, Salluh JIF, Bozza FA. Structure and process associated with the efficiency of intensive care units in low-resource settings: an analysis of the CHECKLIST-ICU trial database. J Crit Care. 2020;59:118–23.
Bastos LSL, Wortel SA, de Keizer NF, Bakhshi-Raiez F, Salluh JIF, Dongelmans DA, et al. Comparing continuous versus categorical measures to assess and benchmark intensive care unit performance. J Crit Care. 2022;70:154063.
Kocisova K, Hass-Symotiuk M, Kludacz-Alessandri M. Use of the dea method to verify the performance model for hospitals. E M Ekon A Manag. 2018;21(4):125–40.
Lee D, Yu S, Yoon SN. Analysis of hospital management based on the characteristics of hospitals: focusing on financial indicators. Glob Bus Financ Rev. 2019;24(3):1–13.
Mirzaei A, Tabibi SJ, Nasiripour AA, Riahi L. Evaluating the feasibility of financial variables of health: A hospital administrator’s viewpoint. Galen Med J. 2016;5(1):25–30.
Middleton S, Gardner G, Gardner A, Considine J, FitzGerald G, Christofis L, et al. Are service and patient indicators different in the presence or absence of nurse practitioners? The EDPRAC cohort study of Australian emergency departments. BMJ Open. 2019;9(7):e024529.
Nobakht S, Jahangiri K, Hajinabi K. Correlation of performance indicators and productivity: A cross sectional study of emergency departments in Tehran, Iran during year 2016. Trauma Mon. 2018;23(5):1–6.
Nuti S, Grillo Ruggieri T, Podetti S. Do university hospitals perform better than general hospitals? A comparative analysis among Italian regions. BMJ Open. 2016;6(8):e011426.
Petrovic GM, Vukovic M, Vranes AJ. The impact of accreditation on health care quality in hospitals. Vojnosanit Pregl. 2018;75(8):803–8.
Pirani N, Zahiri M, Engali KA, Torabipour A. Hospital efficiency measurement before and after health sector evolution plan in Southwest of Iran: a DEA-panel data study. Acta Inf Med. 2018;26(2):106–10.
Profit J, Gould JB, Bennett M, Goldstein BA, Draper D, Phibbs CS, et al. The association of level of care with NICU quality. Pediatrics. 2016;137(3):44–51.
Rahimi H, Bahmaei J, Shojaei P, Kavosi Z, Khavasi M. Developing a strategy map to improve public hospitals performance with balanced scorecard and dematel approach. Shiraz E Med J. 2018;19(7):1–12.
Ahmed S, Hasan MZ, Laokri S, Jannat Z, Ahmed MW, Dorin F, et al. Technical efficiency of public district hospitals in Bangladesh: a data envelopment analysis. COST Eff Resour Alloc. 2019;17:17.
Rahman MH, Tumpa TJ, Ali SM, Paul SK. A grey approach to predicting healthcare performance. Meas J Int Meas Confed. 2019;134:307–25.
Sajadi HS, Sajadi ZS, Sajadi FA, Hadi M, Zahmatkesh M. The comparison of hospitals’ performance indicators before and after the Iran’s hospital care transformations plan. J Educ Health Promot. 2017;6:89.
Si S-L, You X-Y, Liu H-C, Huang J. Identifying key performance indicators for holistic hospital management with a modified DEMATEL approach. Int J Environ Res Public Health. 2017;14(8): 934.
Váchová L, Hajdíková T. Evaluation of Czech hospitals performance using MCDM methods. In: A SI, G WS, C D, editors. Department of exact methods, faculty of management, university of economics, Prague, Jarošovská 1117, Jindřichuv Hradec, vol. 37701. Czech Republic: Newswood Limited; 2017. p. 732–5.
Xenos P, Yfantopoulos J, Nektarios M, Polyzos N, Tinios P, Constantopoulos A. Efficiency and productivity assessment of public hospitals in Greece during the crisis period 2009–2012. Cost Eff Resour Alloc. 2017;15(1):6.
Zhang L, Liu R, Jiang S, Luo G, Liu H-C. Identification of key performance indicators for hospital management using an extended hesitant linguistic DEMATEL Approach. Healthc (Basel Switzerland). 2019;8(1):7.
Aksezer CS. A nonparametric approach for optimal reliability allocation in health services. Int J Qual Reliab Manag. 2016;33(2):284–94.
Cagliano AC, Grimaldi S, Rafele C. Assessing warehouse centralization and outsourcing in the healthcare sector: an Italian case study. In: Department of Management and Production Engineering, Politecnico Di Torino, Corso Duca Degli Abruzzi 24, Torino, 10129. Italy: AIDI - Italian Association of Industrial Operations Professors; 2017. p. 244–50.
Cefalu MS, Elliott MN, Setodji CM, Cleary PD, Hays RD. Hospital quality indicators are not unidimensional: a reanalysis of Lieberthal and Comer. Health Serv Res. 2019;54(2):502–8.
Gao H, Chen H, Feng J, Qin X, Wang X, Liang S, et al. Balanced scorecard-based performance evaluation of Chinese county hospitals in underdeveloped areas. J Int Med Res. 2018;46(5):1947–62.
Gonnelli V, Satta F, Frosini F, Iadanza E. Evidence-based approach to medical equipment maintenance monitoring. In: V HEO, V J, editors. University of Florence, Dept. of Information Engineering. Florence, Italy: Springer; 2017. p. 258–61.
Helkio P, Aantaa R, Virolainen P, Tuominen R. Productivity benchmarks for operative service units. ACTA Anaesthesiol Scand. 2016;60(4):450–6.
Khalifa M, Zabani I. Developing emergency room key performance indicators: What to measure and why should we measure it? J. M, A. H, P. G, A. K, M.S. H, editors. Vol. 226. King Faisal Specialist Hospital and Research Center, Jeddah, Saudi Arabia: IOS Press BV; 2016. p. 179–182.
Ajami S, Ebadsichani A, Tofighi S, Tavakoli N. Medical records department and balanced scorecard approach. J Educ Health Promot. 2013;2:7.
Bosse G, Mtatifikolo F, Abels W, Strosing C, Breuer J-P, Spies C. Immediate outcome indicators in perioperative care: a controlled intervention study on quality improvement in hospitals in Tanzania. PLoS One. 2013;8(6):e65428.
Hung K-Y, Jerng J-S. Time to have a paradigm shift in health care quality measurement. J Formos Med Assoc. 2014;113(10):673–9.
Jeejeebhoy KN, Keller H, Gramlich L, Allard JP, Laporte M, Duerksen DR, et al. Nutritional assessment: comparison of clinical assessment and objective variables for the prediction of length of hospital stay and readmission. Am J Clin Nutr. 2015;101(5):956–65.
Kittelsen SAC, Anthun KS, Goude F, Huitfeldt IMS, Häkkinen U, Kruse M, et al. Costs and quality at the hospital level in the nordic countries. Heal Econ (United Kingdom). 2015;24:140–63.
Koné Péfoyo AJ, Wodchis WP. Organizational performance impacting patient satisfaction in Ontario hospitals: a multilevel analysis. BMC Res Notes. 2013;6: 509.
Li CH, Yu CH. Performance evaluation of public non-profit hospitals using a BP Artificial neural network: the case of Hubei Province in China. Int J Environ Res Public Health. 2013;10(8):3619–33.
Liu K, Jain S, Shi J. Physician performance assessment using a composite quality index. Stat Med. 2013;32(15):2661–80.
Lloyd GF, Bajorek B, Barclay P, Goh S. Narrative review: Status of key performance indicators in contemporary hospital pharmacy practice. J Pharm Pract Res. 2015;45(4):396–403.
Mehrtak M, Yusefzadeh H, Jaafaripooyan E. Pabon Lasso and data envelopment analysis: a complementary approach to hospital performance measurement. Glob J Health Sci. 2014;6(4):107–16.
Mohammadi M, Ziapoor A, Mahboubi M, Faroukhi A, Amani N, Pour FH, et al. Performance evaluation of hospitals under supervision of Kermanshah medical sciences using pabonlasoty diagram of a five-year period (2008–2012). Life Sci J. 2014;11:77–81 ( 1 SPECL. ISSUE) ).
Niaksu O, Zaptorius J. Applying operational research and data mining to performance based medical personnel motivation system. In: Vilnius University, Institute of Mathematics and Informatics. Lithuania: IOS; 2014. p. 63–70.
Córdoba S, Caballero I, Navalón R, Martínez-Sánchez D, Martínez-Morán C, Borbujo J. Analysis of the surgical activity in the dermatology department of Fuenlabrada University Hospital, Madrid, Spain, between 2005 and 2010: determination of the standard operating times. Actas Dermosifiliogr. 2013;104(2):141–7.
Profit J, Kowalkowski MA, Zupancic JAF, Pietz K, Richardson P, Draper D, et al. Baby-MONITOR: a composite indicator of NICU Quality. Pediatrics. 2014;134(1):74–82.
Rabar D, Pap N. Evaluation of crotia’s regional hospital effiency: an application of data envelopment analysis . Bacher U, Barkovic D, Dernoscheg KH, LamzaMaronic M, Matic B, Runzheimer B, editors. Interdisciplinary Management Research IX. 2013;9:649–59.
Ramos MCA, da Cruz LP, Kishima VC, Pollara WM, de Lira ACO, Couttolenc BF. Performance evaluation of hospitals that provide care in the public health system, Brazil. Rev Saude Publica. 2015;49:1–9.
Schuers M, Joulakian MB, Griffon N, Pachéco J, Périgard C, Lepage E, et al. In: S IN, de PM AM, editors. Quality indicators from laboratory and radiology information systems. A. G. Volume 216. France: IOS; 2015. pp. 212–6. Department of Biomedical Informatics, Rouen University Hospital, Rouen Cedex, 76031,.
Tabrizi JS, Saadati M, Sadeghi-Bazargani H, Ebadi A, Golzari SEJ. Developing indicators to improve educational governance in hospitals. Clin Gov. 2014;19(2):117–25.
Costa A Jr, aS., Leão LE, Novais MA, Zucchi P. An assessment of the quality indicators of operative and non-operative times in a public university hospital. Einstein (Sao Paulo). 2015;13(4):594–9.
Coyne JS, Helton J. How prepared are US hospitals for the affordable care act? A financial condition analysis of US hospitals in 2011. J Health Care Finance. 2015;41(3).
Davis P, Milne B, Parker K, Hider P, Lay-Yee R, Cumming J, et al. Efficiency, effectiveness, equity (E-3). Evaluating hospital performance in three dimensions. Health Policy (New York). 2013;112(1–2):19–27.
Flatow VH, Ibragimova N, Divino CM, Eshak DSA, Twohig BC, Bassily-Marcus AM, et al. Quality outcomes in the surgical intensive care unit after electronic health record implementation. Appl Clin Inf. 2015;6(4):611–8.
Article CAS Google Scholar
Fonseca JRS, Ramos RMP, Santos AMP, Fonseca APSS. Policy effects on the quality of public health care: evaluating Portuguese public hospitals’ quality through customers’ views. Cent Eur J Public Policy. 2015;9(2):122–40.
Hadji B, Meyer R, Melikeche S, Escalon S, Degoulet P. Assessing the Relationships Between Hospital Resources and Activities: A Systematic Review. J Med Syst. 2014;38(10):1–21.
Hajduová Z, Herbrik G, Beslerová S. Application of DEA in the environment of Slovak hospitals. Invest Manag Financ Innov. 2015;12(4):148–53.
Thomas Craig KJ, McKillop MM, Huang HT, George J, Punwani ES, Rhee KB. U.S. hospital performance methodologies: a scoping review to identify opportunities for crossing the quality chasm. BMC Health Serv Res. 2020;20(1):640.
Carini E, Gabutti I, Frisicale EM, Di Pilla A, Pezzullo AM, de Waure C, et al. Assessing hospital performance indicators. What dimensions? Evidence from an umbrella review. BMC Health Serv Res. 2020;20(1):1038.
Rasi V, Delgoshaee B, Maleki M. Identification of common indicators of hospital performance evaluation models: a scoping review. J Educ Health Promot. 2020;9(1):63.
Xenos P, Yfantopoulos J, Nektarios M, Polyzos N, Tinios P, Constantopoulos A. Efficiency and productivity assessment of public hospitals in Greece during the crisis period 2009–2012. COST Eff Resour Alloc. 2017;15:15.
Shaqura II, Gholami M, Sari AA. Evaluation of performance at Palestinian public hospitals using Pabon Lasso model. Int J Health Plann Manage. 2021;36(3):896–910.
Li J, Seale H, Ray P, Wang Q, Yang P, Li S, et al. E-Health preparedness assessment in the context of an influenza pandemic: a qualitative study in China. BMJ Open. 2013;3(3):e002293.
Huang C-Y, Lai C-H. Effects of internal branding management in a hospital context. Serv Ind J. 2021;41(15–16):985–1006.
Download references
Acknowledgements
The authors are grateful for the support of the Vice Chancellor for Research of Isfahan University of Medical Sciences.
The present article is part of the result of a doctoral thesis approved by Isfahan University of Medical Sciences with code 55657 (IR.MUI.NUREMA.REC.1401.005), without financial source.
Author information
Authors and affiliations.
Student Research Committee, School of Management and Medical Information Sciences, Isfahan University of Medical Sciences, Isfahan, Iran
Shirin Alsadat Hadian
Health Management and Economics Research Center, Isfahan University of Medical Sciences, Isfahan, Iran
Reza Rezayatmand
Hospital Management Research Center, Health Management Research Institute, Iran University of Medical Sciences, Tehran, Iran
Nasrin Shaarbafchizadeh
Department of Management, Faculty of Administrative Sciences and Economics, University of Isfahan, Isfahan, Iran
Saeedeh Ketabi
School of Public Health and Preventive Medicine, Monash University, Victoria, Australia
Ahmad Reza Pourghaderi
You can also search for this author in PubMed Google Scholar
Contributions
Shirin Alsadat Hadian and Reza Rezayatmans and Saeedeh Ketabi: Study conceptualization and design. Acquisition of data: Shirin Alsadat Hadian, Reza Rezayatmand. Analysis and interpretation of data: Shirin Alsadat Hadian, Reza Rezayatmand, Nasrin Shaarbafchizadeh, Saeedeh Ketabi. Drafting of the manuscript: Shirin Alsadat Hadian, Reza Rezayatmand. Critical revision of the manuscript for important intellectual content: Reza Rezayatmand, Nasrin Shaarbafchizadeh, Saeedeh Ketabi, Ahmad Reza Pourghaderi.
Corresponding author
Correspondence to Reza Rezayatmand .
Ethics declarations
Ethics approval and consent to participate.
Not applicable.
Consent for publication
Competing interests.
The authors declare no competing interests.
Additional information
Publisher’s note.
Springer Nature remains neutral with regard to jurisdictional claims in published maps and institutional affiliations.
Supplementary Information
Supplementary material 1., supplementary material 2., supplementary material 3., rights and permissions.
Open Access This article is licensed under a Creative Commons Attribution 4.0 International License, which permits use, sharing, adaptation, distribution and reproduction in any medium or format, as long as you give appropriate credit to the original author(s) and the source, provide a link to the Creative Commons licence, and indicate if changes were made. The images or other third party material in this article are included in the article's Creative Commons licence, unless indicated otherwise in a credit line to the material. If material is not included in the article's Creative Commons licence and your intended use is not permitted by statutory regulation or exceeds the permitted use, you will need to obtain permission directly from the copyright holder. To view a copy of this licence, visit http://creativecommons.org/licenses/by/4.0/ . The Creative Commons Public Domain Dedication waiver ( http://creativecommons.org/publicdomain/zero/1.0/ ) applies to the data made available in this article, unless otherwise stated in a credit line to the data.
Reprints and permissions
About this article
Cite this article.
Hadian, S.A., Rezayatmand, R., Shaarbafchizadeh, N. et al. Hospital performance evaluation indicators: a scoping review. BMC Health Serv Res 24 , 561 (2024). https://doi.org/10.1186/s12913-024-10940-1
Download citation
Received : 03 January 2024
Accepted : 02 April 2024
Published : 01 May 2024
DOI : https://doi.org/10.1186/s12913-024-10940-1
Share this article
Anyone you share the following link with will be able to read this content:
Sorry, a shareable link is not currently available for this article.
Provided by the Springer Nature SharedIt content-sharing initiative
- Performance evaluation
BMC Health Services Research
ISSN: 1472-6963
- General enquiries: [email protected]
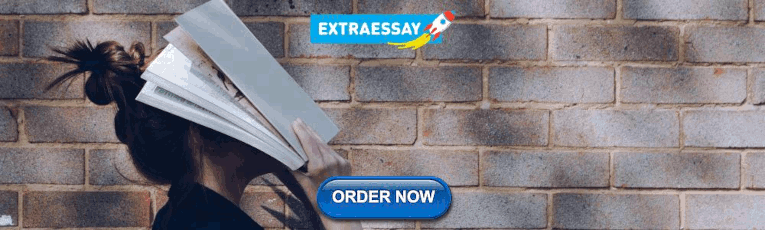
IMAGES
VIDEO
COMMENTS
Text mining somehow bridges qualitative and quantitative research traditions by structuring, analyzing, and understanding textual data at scale. ... we outline fields of research that are based both on our experience of using text mining in innovation research and are particularly salient or trending in the innovation research landscape (e.g ...
The authors' argument is that text mining using the SOM is particularly effective in improving inference quality within qualitative research. SOM creates multiple well-grounded perspectives on the data and thus improves the quality of the concepts and categories used in the analysis.
Qualitative text analysis emerged in the second half of the 20th century as a reply to what was seen as the superficiality of results of quantitative analyses ... the recent breakthrough in the use of text mining in sociological research mostly came from a more qualitative tradition (e.g., the 2013 issue of journal Poetics).
similarity. The authors' argument is that text mining using the SOM is particularly effective in improving inference quality within qualitative research. SOM creates multiple well-grounded perspectives on the data and thus improves the quality of the concepts and categories used in the analysis.
Text mining has been applied to improve reviewing efficiency in systematic reviews and used to identify, categorize, and summarize data for rapid evidence synthesis.2 However, the application of text mining software to qualitative social science research has been limited to date.15, 16 Applications have largely been directed at the task of ...
Text Mining in Qualitative Research: Application of an Unsupervised Learning Method. July 2009. Organizational Research Methods 12 (3):436-460. DOI: 10.1177/1094428108317202. Authors: Nina Janasik ...
Originality/value -This study demonstrates that combining content analysis with text mining techniques. improves the quality of the research output. Researchers may, therefore, better handle ...
The authors' argument is that text mining using the self-organizing map (SOM) is particularly effective in improving inference quality within qualitative research. The article provides an introduction to and a demonstration of the self-organizing map (SOM) method for organizational researchers interested in the use of qualitative data. The SOM is a versatile quantitative method very commonly ...
Text mining and text analytics (TM/TA) involve examining large volumes of unstructured text (corpus) aiming to obtain interpretable information, discover context, identify linguistic motifs, or transform the text and derive quantitative data that can be further analyzed. Natural language processing (NLP) is one example of a TM analytical technique.
Computer-aided analysis of qualitative data using Text Mining techniques or natural language processing is a kind of novelty in the field of quality sociology. ... DiGangi, S.: Compatibility between text mining and qualitative research in the perspectives of grounded theory, content analysis, and reliability. Qual. Rep. 16(3), 730-744 (2011 ...
The purpose of this article is to demonstrate that text mining and qualitative research are epistemologically compatible. First, like many qualitative research approaches, including grounded theory, text mining encourages open-mindedness and discourages preconceptions (Vilkinas, 2008). Second, text mining is similar to content analysis, which ...
The objective of this article is to illustrate that text mining and qualitative research are epistemologically compatible. First, like many qualitative research approaches, such as grounded theory, text mining encourages open-mindedness and discourages preconceptions. Contrary to the popular belief that text mining is a linear and fully automated procedure, the text miner might add, delete ...
Little has been done to apply data mining strategy to analyzes data gathered using qualitative methodology. In this paper, we present a work done to apply text mining technique to analyzes data gathered from interviews - unstructured data. The aim of this study is to develop patterns of pediatric cancer patient's activities in the ward.
4. DISCUSSION. In this study, we aimed to identify the most common research topics studied by researchers in the nursing field and analyse their trends between 1998 and 2021. First, we identified these topics using text mining strategies and grouped them according to their similarities.
VOSviewer tool is adopted to analyze and visualize the state-of-the-art efforts of existing research. Then, a qualitative analysis on the eight application fields discussed in depth. In line with this, current research gaps and promising research orientations about TM applications in AEC domains are identified. 2. Text mining
More Readings on Sampling Plans for Text Analysis: How to Choose a Sample Size in Qualitative Research, from LinkedIn Learning (members of the GW community have free access to LinkedIn Learning using their GW email account) Sampling in Qualitative Research, from Saylor Academy; Lowe, A., Norris, A. C., Farris, A. J., & Babbage, D. R. (2018).
Despite the difficulties, the combination of low cost. text mining with qualitative research is a promising methodological avenu e, and we specify some future. paths for this area of study ...
Despite the ubiquity of textual data, so far few researchers have applied text mining to answer organizational research questions. Text mining, which essentially entails a quantitative approach to the analysis of (usually) voluminous textual data, helps accelerate knowledge discovery by radically increasing the amount data that can be analyzed. This article aims to acquaint organizational ...
The objective of this article is to illustrate that text mining and qualitative research are epistemologically compatible. First, like many qualitative research approaches, such as grounded theory, text mining encourages open-mindedness and discourages preconceptions. Contrary to the popular belief that text mining is a linear and fully ...
Visualisations. Text visualisation is a way to "see" your data. Text mining visualisation can help researchers see relationships between certain concepts. An example of a visualisation of data can be word clouds, graphs, maps, and other graphics that produce a visual depiction the data. Various Text Analysis Projects with Visualisations.
NVivo is a software program used for qualitative and mixed-methods research. Specifically, it is used for the analysis of unstructured text, audio, video, and image data, including (but not limited to) interviews, focus groups, surveys, social media, and journal articles. It is produced by QSR International. As of October 2018, it is available ...
Aim: This study is set to determine the main topics of the nursing field and to show the changing perspectives over time by analysing the abstracts of several major nursing research journals using text mining methodology. Design: Text mining and network analysis. Methods: Text analysis combines automatic and manual operations to identify ...
The present research was done in three cities in Iran in 2021. The data were collected through in-depth interviews with 18 veterinarians selected through purposive and snowball sampling and analyzed using conventional qualitative content analysis. The data analysis results were classified into 4 main categories and 44 subcategories.
Hospitals are the biggest consumers of health system budgets and hence measuring hospital performance by quantitative or qualitative accessible and reliable indicators is crucial. This review aimed to categorize and present a set of indicators for evaluating overall hospital performance. We conducted a literature search across three databases, i.e., PubMed, Scopus, and Web of Science, using ...
The hydro-pneumatic suspension, known for enhancing vehicle ride comfort and stability, finds widespread use in engineering vehicles. Presently, the majority of mining trucks employ hydro-pneumatic suspension with a fixed damping hole, underscoring the critical importance of selecting appropriate damping hole parameters. Initially, an equilibrium mathematical model of the ¼ hydro-pneumatic ...