- Introduction to Genomics
- Educational Resources
- Policy Issues in Genomics
- The Human Genome Project
- Funding Opportunities
- Funded Programs & Projects
- Division and Program Directors
- Scientific Program Analysts
- Contact by Research Area
- News & Events
- Research Areas
- Research investigators
- Research Projects
- Clinical Research
- Data Tools & Resources
- Genomics & Medicine
- Family Health History
- For Patients & Families
- For Health Professionals
- Jobs at NHGRI
- Training at NHGRI
- Funding for Research Training
- Professional Development Programs
- NHGRI Culture
- Social Media
- Broadcast Media
- Image Gallery
- Press Resources
- Organization
- NHGRI Director
- Mission & Vision
- Policies & Guidance
- Institute Advisors
- Strategic Vision
- Leadership Initiatives
- Diversity, Equity, and Inclusion
- Partner with NHGRI
- Staff Search
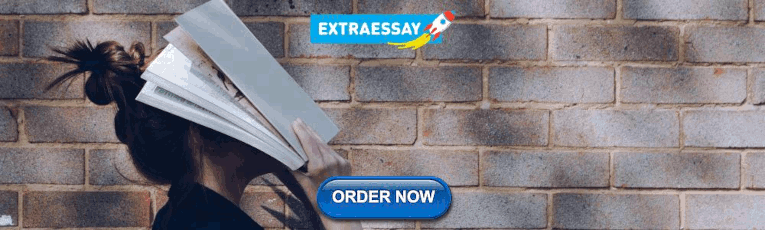
Genomic Data Science
As humans dig deeper into the genome, the analysis and interpretation of the genomic data collected are helping to better understand human health and disease, while also bringing up questions about privacy and ethics.
The Big Picture
- Genomic data science is a field of study that enables researchers to use powerful computational and statistical methods to decode the functional information hidden in DNA sequences.
- Estimates predict that genomics research will generate between 2 and 40 exabytes of data within the next decade.
- Our ability to sequence DNA has far outpaced our ability to decipher the information it contains, so genomic data science will be a vibrant field of research for many years to come.
- Performing genomic data science carries with it a set of ethical responsibilities, as each person's sequence data are associated with issues related to privacy and identity.
How it affects you
As biomedical research projects and large-scale collaborations grow rapidly, the amount of genomic data being generated is also increasing, with roughly 2 to 40 billion gigabytes of data now generated each year. Researchers are working to extract valuable information from such complicated and large datasets so they can better understand human health and disease.
What is genomic data science?
Genomic data science is a field of study that enables researchers to use powerful computational and statistical methods to decode the functional information hidden in DNA sequence. Applied in the context of genomic medicine, these data science tools help researchers and clinicians uncover how differences in DNA affect human health and disease.
Genomic data science emerged as a field in the 1990s to bring together two laboratory activities:
- Experimentation: Generating genomic information from studying the genomes of living organisms.
- Data analysis: Using statistical and computational tools to analyze and visualize genomic data, which includes processing and storing data and using algorithms and software to make predictions based on available genomic data.
Both activities help researchers acquire and gain insights from the vast amounts of genomic data.
Why does genomics involve so much data?
Human genomics gained mainstream attention in the early 2000s when the Human Genome Project successfully generated the first sequence of the chemical bases (“letters”) — As, Cs, Gs and Ts — in the human genome. Each of the trillions of cells in the human body contains a complete copy of the genome, i.e., our DNA blueprint). Most cells actually have two copies of the genome, which together reflect about 6 billion DNA letters.
Researchers are now generating more genomic data than ever before to understand how the genome functions and affects human health and disease. These data are coming from millions of people in various populations across the world. Data about a single human genome sequence alone would take up 200 gigabytes, or the space of about 200 copies of Jaws . We will need an estimated 40 exabytes to store the genome- sequence data generated worldwide by 2025. That’s almost one billion DVDs of Jaws ! In comparison, five exabytes could store all of the words ever spoken by human beings.
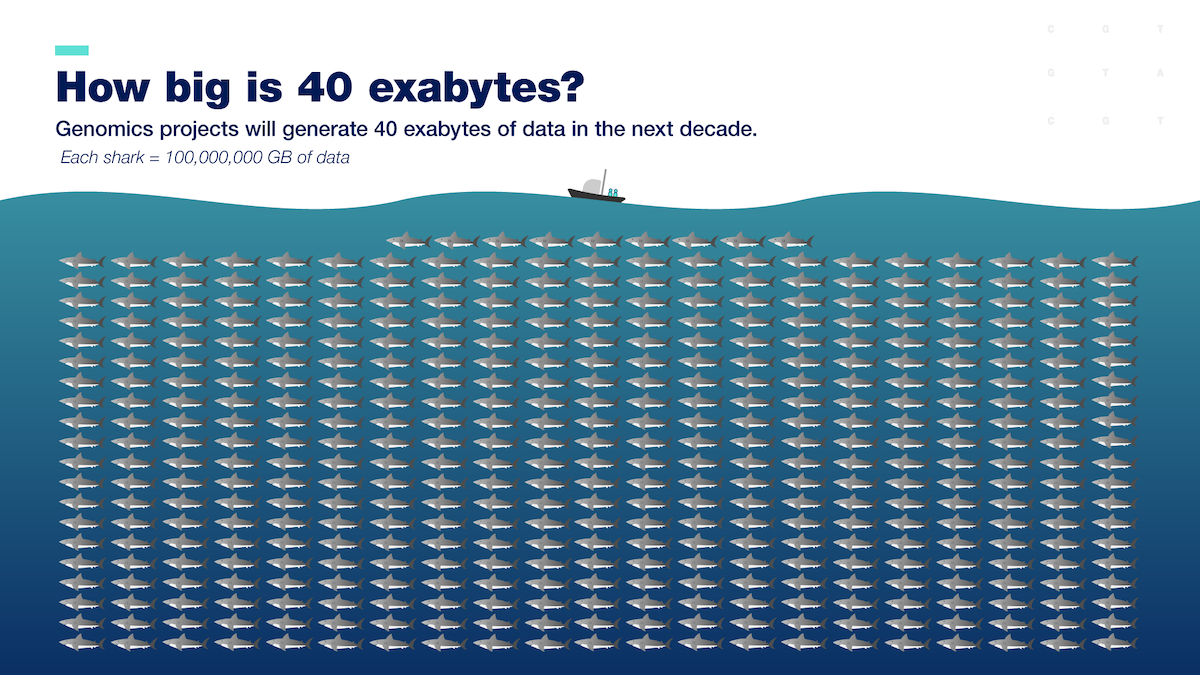
Because of the sizeable quantity of complex data associated with human genomes, genomics is now considered a "big data" field.
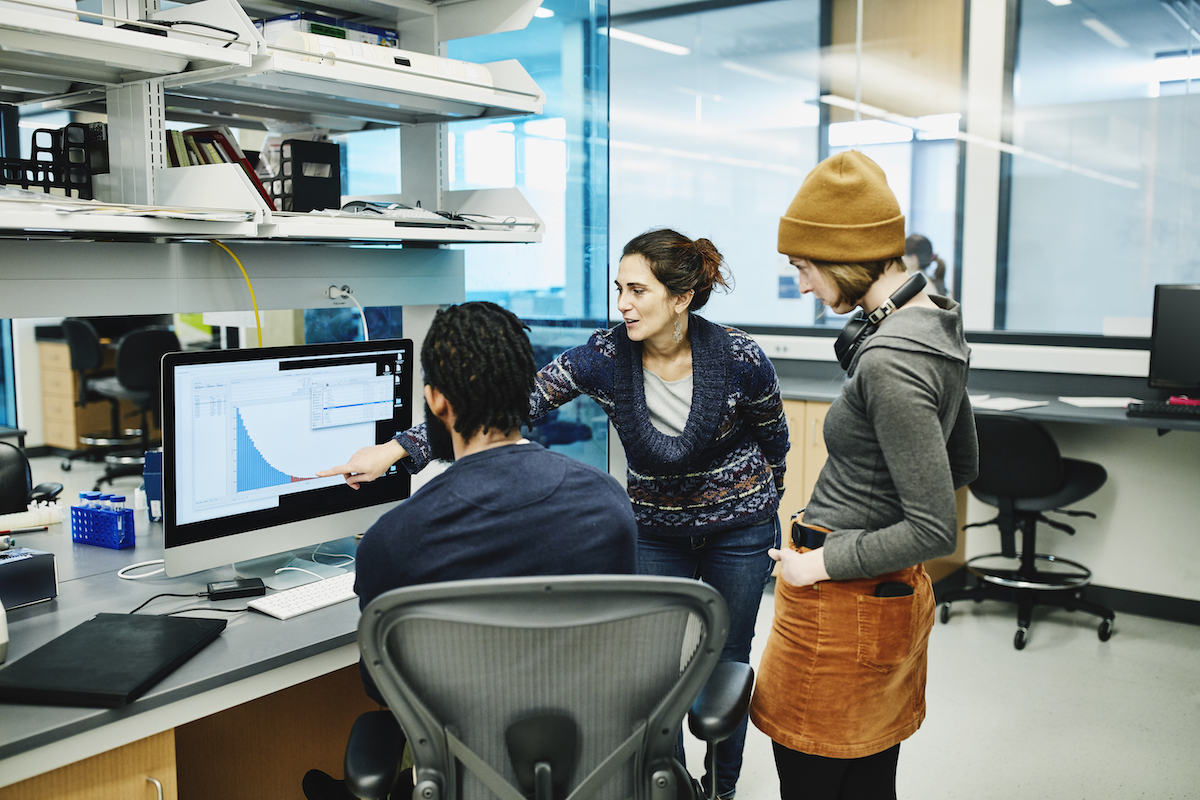
How do scientists study and use genomic data?
Researchers need special computational and analysis tools to find and interpret biological information hidden within the DNA of each person and also to manage the large volumes of data generated in genomics research projects.
Researchers use software tools called aligners to determine where individual pieces of DNA sequence lie on each part of a reference genome sequence.
Next, "variant callers" identify the places where a given human genome sequence differs from other human genome sequences. These genomic differences come in many sizes. The difference may be as small as one DNA letter (called a single-nucleotide polymorphism ), many letters long (called structural variants ) such as insertions or deletions , or substantially larger chromosomal abnormalities. These genomic differences may present no health risks, or they can directly cause inherited rare disorders, cancers or other more common diseases.
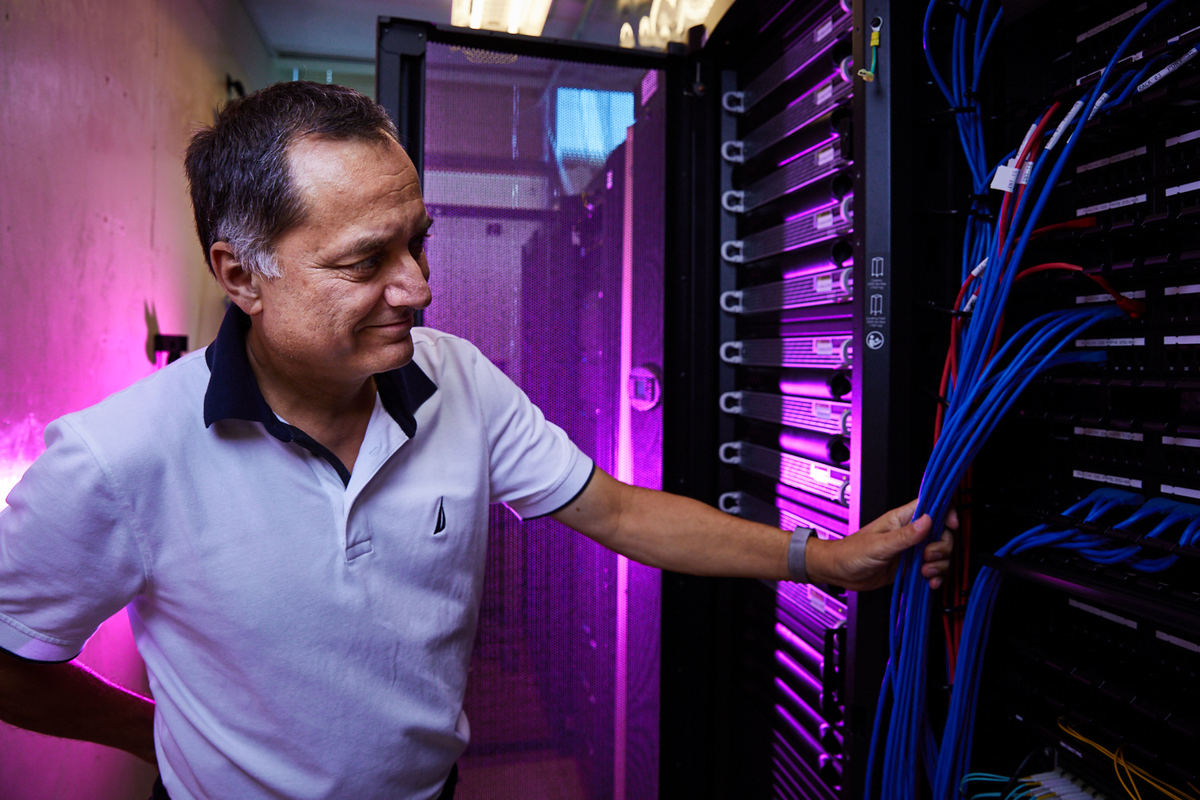
How do researchers manage and store such high volumes of genomic data?
Experts in both computer technologies and genomics manage and store genomic data by using various computer systems and software. Increasingly, data analysis and coordination centers are part of research networks and provide these services.
Generating genomic data requires significant financial support from institutions such as the National Human Genome Research Institute (NHGRI), which provides over $125 million each year to support various genomic data science endeavors.
The generated data resources are often made available to the broader scientific community to facilitate further data analyses. They organize and provide many types of information about the human genome, such as the locations of genes and variants in the DNA.
Many private and commercial cloud platforms work in collaboration with governmental and public entities, such as at the National Institutes of Health (NIH) through the STRIDES initiative. These initiatives provide storage and computing infrastructure for hosting genomic data and to provide the necessary security and privacy protections for human genomic data in particular.
What are some of the ethical, legal and societal implications of genomic data sharing?
Performing genomic research carries with it a set of ethical responsibilities, as information about a person's genome sequence is associated with complex issues related to privacy and identity.
- Informed Consent: Researchers usually ask for consent from individuals whose genomes are sequenced. But researchers must provide clear information about how they will use and share the resulting genome-sequence data in the process of gaining such informed consent.
- Privacy: Powerful computational tools can take sequence data from de-identified genomes and, under special circumstances, connect them back to the person whose DNA was sequenced. Investigators can use such tools for useful purposes, such as identifying criminals who left behind DNA at a crime scene. But the societal benefits must outweigh the potential risks of using genomic data in this way.
- Artificial Intelligence (AI): AI tools increasingly help researchers process vast quantities of genome-sequence data to look for hidden patterns in DNA. However, because AI algorithms often lack transparency, biases can creep in undetected when such algorithms are applied to DNA data. This area of genomic data science will need extensive ethics research to navigate the unique differences between current methods in genomic data science (which rely on human intelligence for interpretation of the results) and newer AI methods. While AI methods offer many promising advantages, they also draw conclusions in completely different ways than humans do, and hence need to be subject to careful ethics oversight.
With all these considerations, data scientists and genomics researchers must be educated about the implications of their studies and work closely with ethics researchers.
How do researchers share human genomic data?
Researchers are expected to share human genomic data according to the consent provided by the research participants. Genomic data are typically shared with the scientific community through data resources, which can be accessed in three ways:
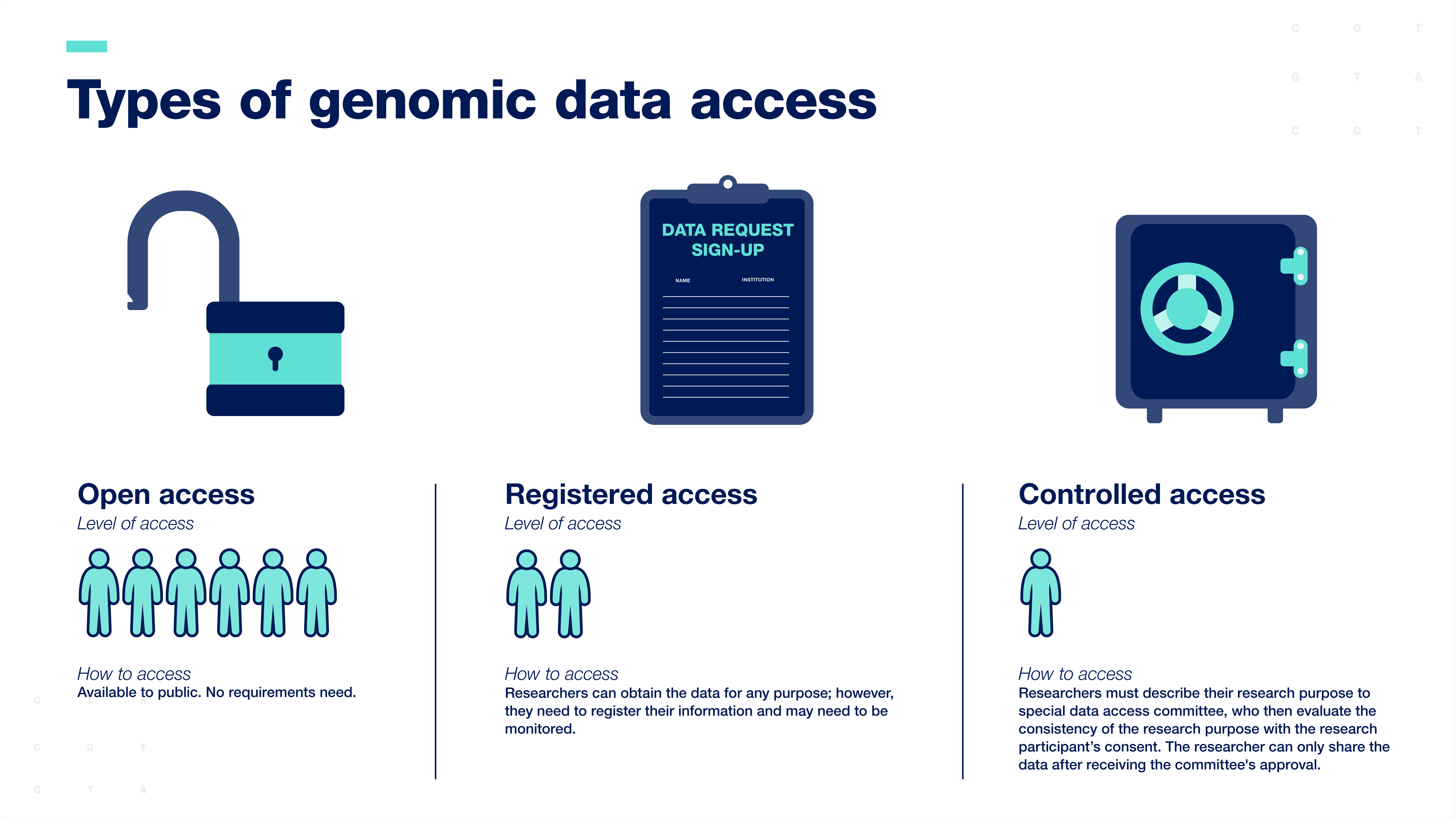
Open-access or unrestricted access is the broadest form of data sharing. Data are available to the public for any research purpose.
Registered access falls in between open-access and controlled-access. Researchers can obtain the data for any purpose; however, they must register their information, and their work with the data may need to be monitored.
Controlled-access data sharing requires researchers to describe their research purpose so that a special data access committee can evaluate the consistency of the research purpose with the participant’s consent. The researcher can only access the data after receiving the committee's approval.
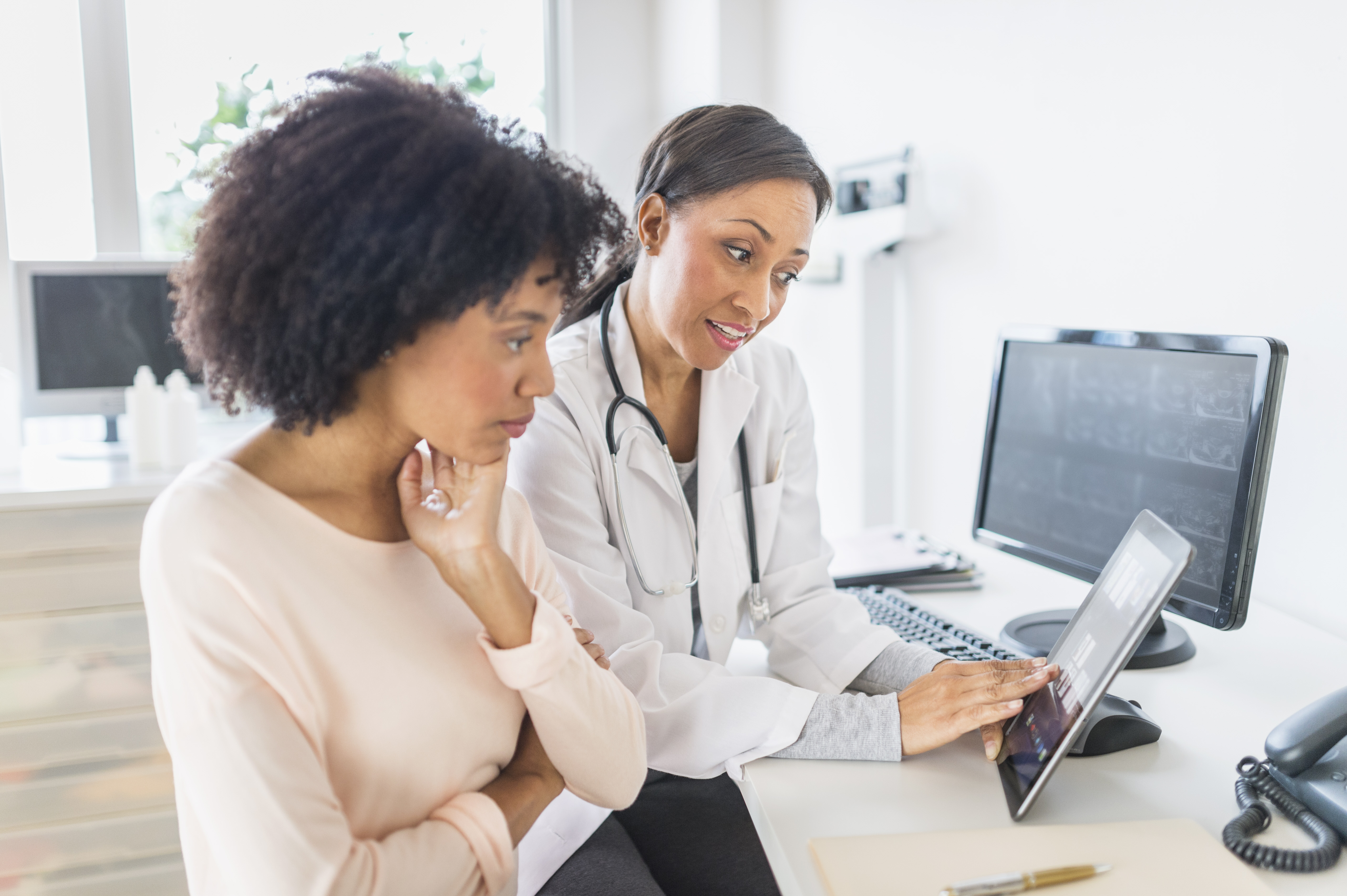
What are some emerging topics in genomic data science?
Human genomes contain many genomic variants (DNA letters that differ in particular places among individuals). Healthcare systems and researchers are building tools that identify these DNA differences and link them to medically relevant information, such as a risk for disease or an indication for a specific medication among several options. Researchers also use artificial intelligence systems to interpret genomic data for clinical purposes, such as diagnosing diseases at early stages or predicting risk for different diseases using genomic information .
In the last decade, cloud computing has become necessary for genomic data storage and analyses. Cloud computing decreases the need to duplicate large datasets, increases security and provides researchers more accessibility to genomic data science. Data scientists are creating tools to make data upload easier and to ensure privacy.
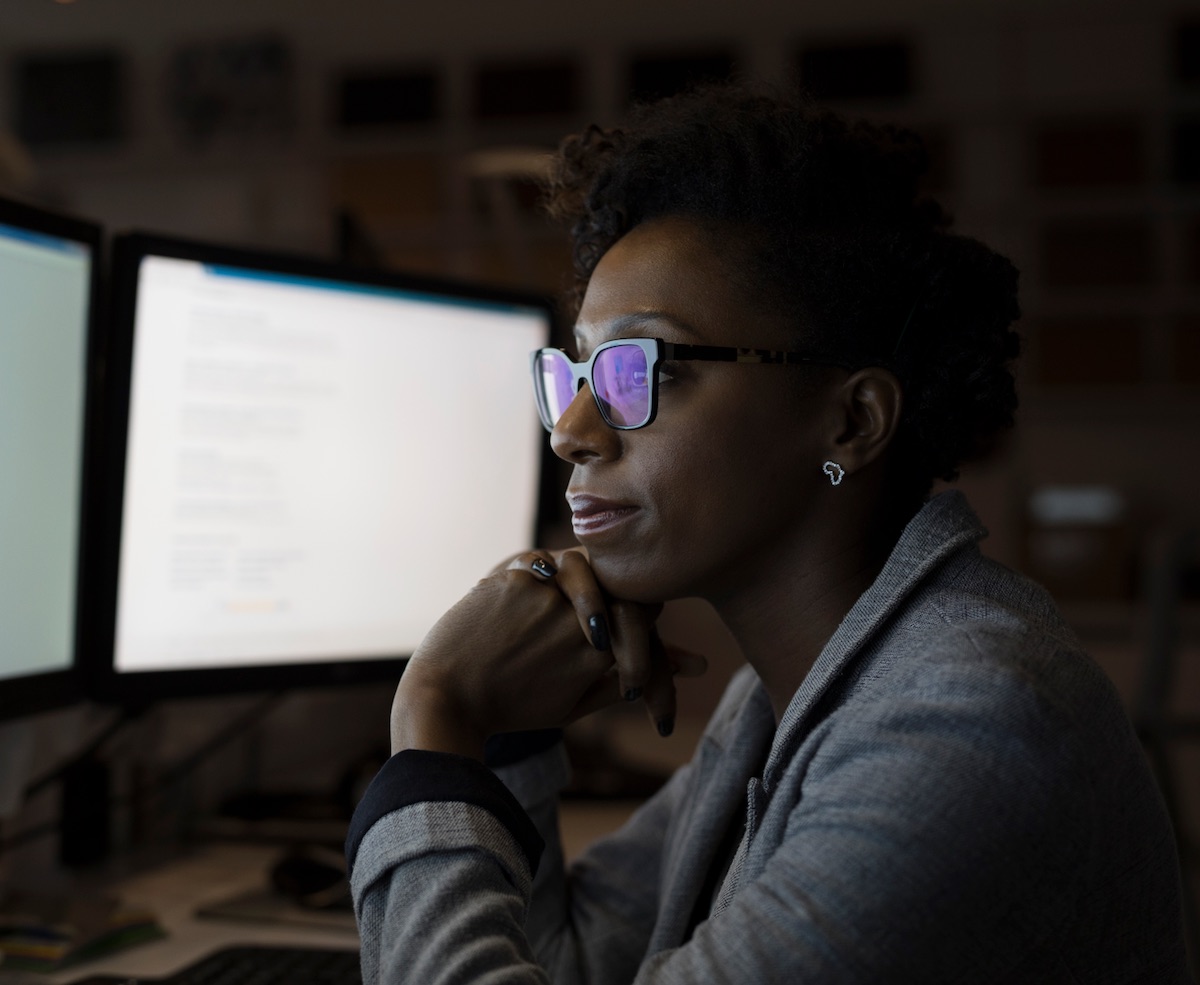
What is NHGRI’s role in working towards a more diverse and equitable workforce?
Women and various minorities groups have been largely underrepresented in genomic data science. NHGRI believes that it is critical to expand and enhance the diversity of the genomic data science workforce. Recent analyses show a significant lack of ethnic and gender diversity among data scientists, trainees and genomics researchers across US institutions.
NHGRI is making changes to enhance the presence of women and underrepresented group in the genomic data science workforce. Through NHGRI-funded training programs and by bringing larger numbers of people in the field of genomic data science, the Institute hopes to foster a more inclusive data science workforce in genomics and beyond.
Additional Resources
To learn more about NHGRI's involvement in genomic data science and data science activities at NIH, see the following resources:
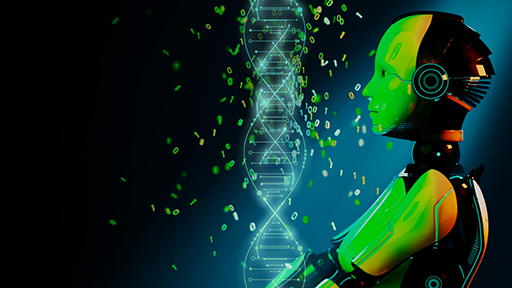
Last updated: April 5, 2022
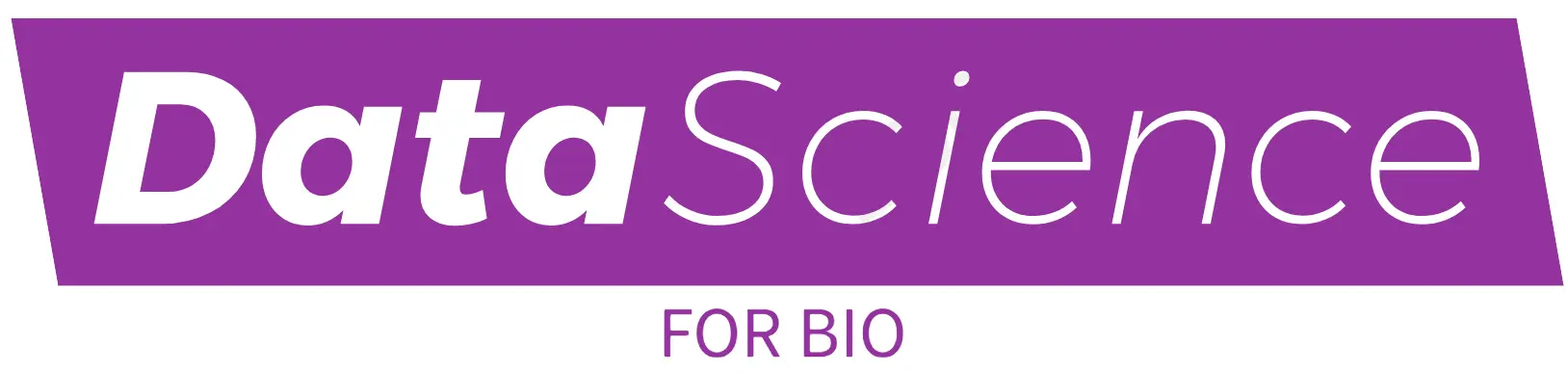
Scanpy vs Seurat: Two Powerhouses for Single Cell RNA-seq Data Analysis
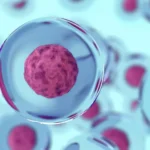
Visualizing Single-Cell Data with Scanpy UMAP, Dotplot & Heatmap: A Step-by-Step Guide
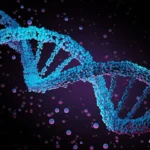
Python for Genomics: How to Simplify Complex Biological Data
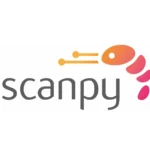
Single Cell RNA Sequencing: A Step by Step Scanpy Tutorial for Beginners

Type and hit Enter to search
Genomic data analysis: a beginner’s step-by-step guide with python and r examples.
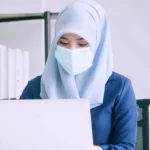
In the current biotechnology and medicine era, genomic data analysis is the compass guiding researchers through our genetic code.
This comprehensive guide aims to explain genomic data analysis along with Python and R coding , breaking down each step into digestible portions.
Before diving into the nitty-gritty of genomic data analysis, let’s grasp the concept of genomic data, its formats and significance of genomic data analysis.
Explore more about role of 20 Essential Python Codes for Bioinformatics Beginners.
What is Genomic Data?
Genomic data is the code embedded in DNA sequences of living organisms, serving as the fundamental blueprint of life. It contains the entire set of genetic instructions that govern the development, functioning, and characteristics of an organism. This data includes the sequence of nucleotide bases – adenine (A), thymine (T), cytosine (C), and guanine (G) – arranged in a specific order, forming genes that act as the building blocks of our biological existence.
Genomic data is the molecular language that scientists decode to understand the inherited traits, the origins of diseases, and the evolutionary paths of diverse species
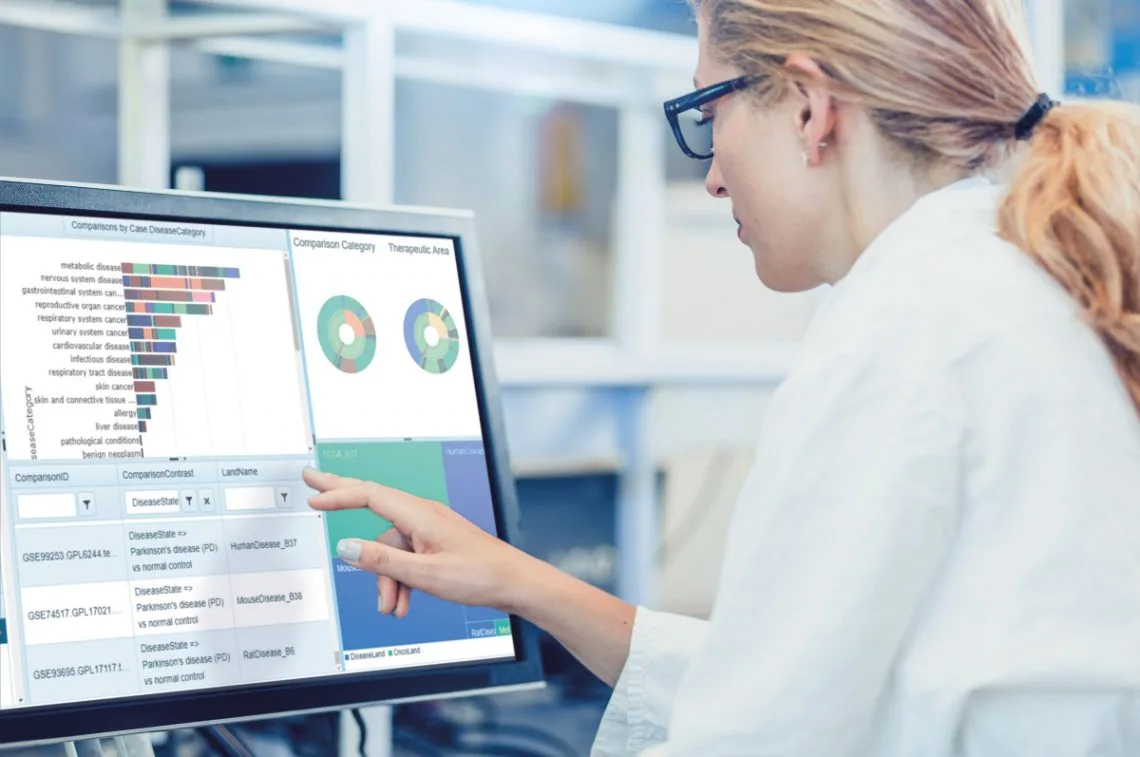
What are Common Genomic Data Formats?
Common genomic data formats include FASTQ, which holds raw sequence data, and BAM, a binary version of the Sequence Alignment/Map (SAM) format used for storing aligned sequence reads. Variant Call Format (VCF) is another vital format, specifically designed to represent genetic variations detected during genomic data analysis.
What is Genomic Data Analysis and Why it is Important?
Genomic data analysis is the systematic examination of the vast amount of genetic information contained within an organism’s DNA. It involves employing computational techniques and specialized tools to explore the genomic code.
The primary goal of genomic data analysis is to extract meaningful insights from the genetic data, understanding the functions of genes, identifying variations, and exploring the relationships between different elements within the genome. This process serves as a crucial bridge between the raw genetic information and actionable knowledge. It allows scientists, researchers, and healthcare professionals to comprehend the genetic basis of various phenomena, such as inherited traits, diseases, and evolutionary patterns. In essence, genomic data analysis provide a deeper understanding of life’s molecular intricacies and pave the way for advancements in medicine, biology, and genetics.
What Steps are Involved in Genomic Data Analysis?
Genomic data analysis involves a number of steps which are explained here in detail with python and R coding examples
Step 1: Gathering Genomic Data
Think of genomic data as the raw material for our genetic detective work. This data comes in various formats – FASTQ, BAM, or VCF. Imagine stepping into a vast library of genetic information, akin to NCBI’s Sequence Read Archive (SRA), where scientists worldwide deposit their genomic findings. Let’s say you’re interested in studying genetic variations in breast cancer. You’d download the relevant genomic data from SRA or collaborate with a renowned research institution specializing in cancer genomics.
Example: You access the SRA database and download genomic data from a study on breast cancer patients.
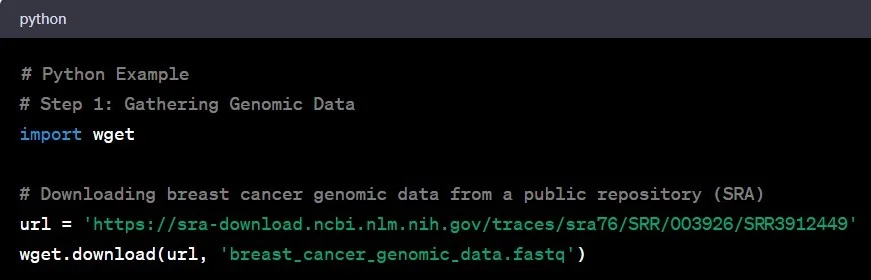
Step 2: Data Preprocessing
Now that we have our raw data, it’s time to clean it up – think of it as preparing a canvas before painting. This step, known as data preprocessing, involves removing noise, correcting errors, and ensuring the data’s overall quality.
Example: In our breast cancer study, you would eliminate low-quality reads, ensuring that the remaining data is reliable for subsequent analysis.
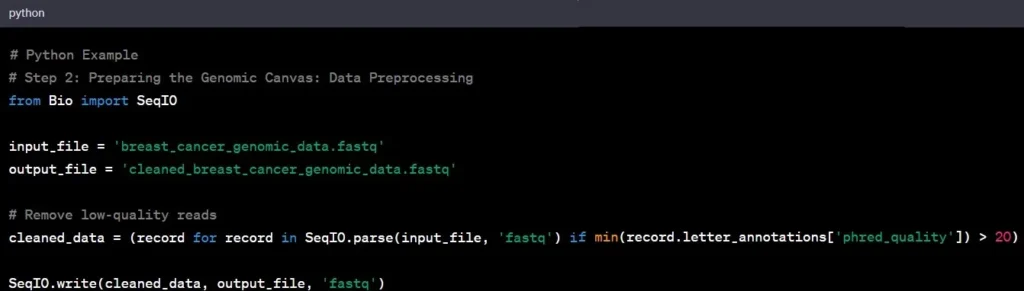
Step 3: Read Alignment
Reading the genomic sequence is the next step in genomic data analysis. Aligning the cleaned genomic data to a reference genome helps identify variations and understand the larger genomic landscape. It is like fitting puzzle pieces together to reveal the bigger picture.
Example: Aligning the genomic data of an individual with Asian ancestry to a reference genome based on individuals of European descent might unveil unique genetic variations specific to the Asian population.
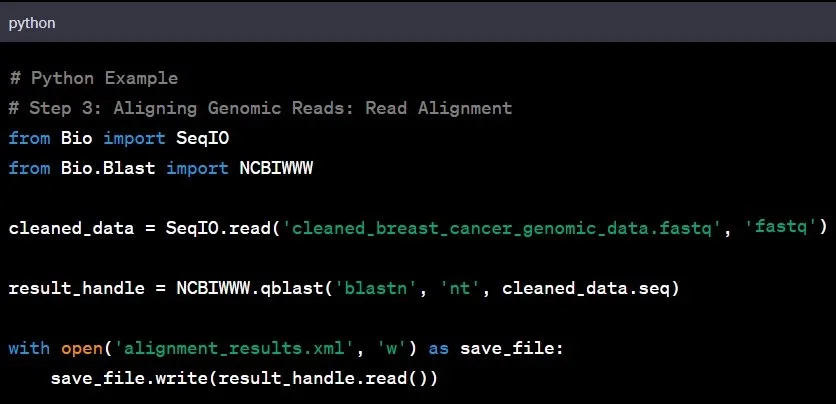
Step 4: Variant Calling
Variant calling involves identifying genetic variations – the differences between the aligned reads and the reference genome. Such as spotting single nucleotide polymorphisms (SNPs) and insertions/deletions (indels), by comparing aligned reads to the reference genome are common examples in genomic data analysis
Example: Identifying a single nucleotide polymorphism (SNP) in the BRCA1 gene can provide crucial information regarding breast cancer susceptibility.
Know more about R Bioinformatics Cheat Sheet for Beginners here.
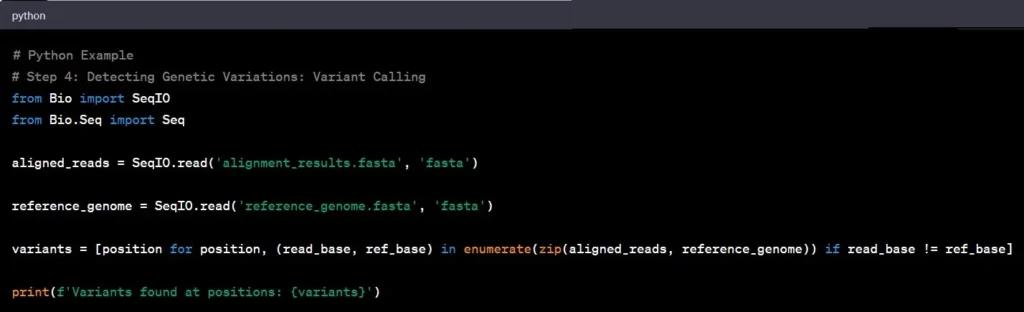
Step 5: Variant Annotation
Think of variant annotation as adding footnotes to the genomic text. It involves understanding the functional significance of identified variants and their potential impact on genes. It’s like deciphering the meaning behind the words in a book.
Example: Discovering a variant in a tumor suppressor gene may indicate a higher cancer risk, providing valuable insights for potential therapeutic strategies.
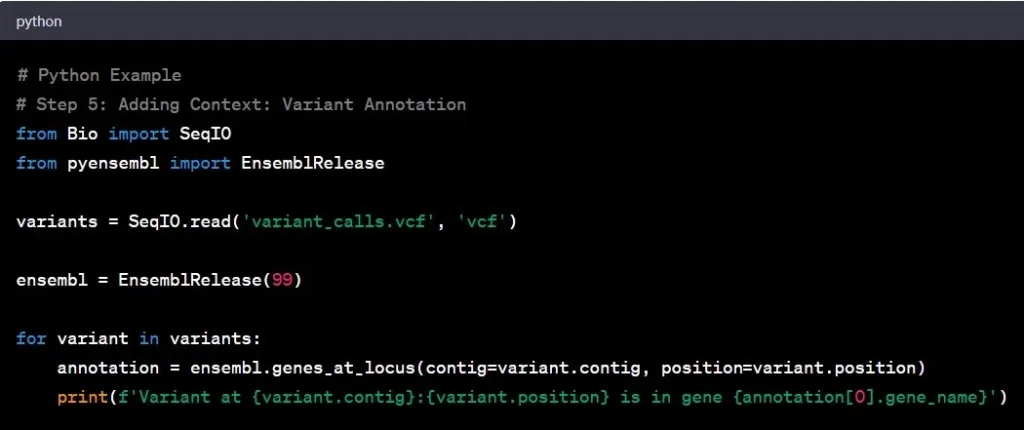
Step 6: Data Visualization
Now that we have our genomic data and insights, it’s time to visualize the findings. Visualization is the art of transforming raw data into meaningful stories. It is necessary step for genomic data analysis to gain insights and communicate findings effectively.
Example: Plotting the coverage across the genome could reveal regions with higher or lower sequencing depth, guiding further exploration.
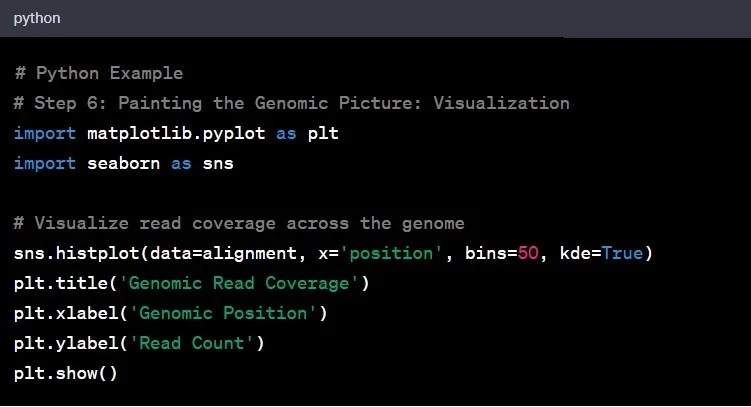
Conclusion:
Genomic data analysis is a multifaceted process that involves acquiring, preprocessing, aligning, calling variants, annotating, and visualizing data. The seamless integration of Python and R in this guide provides a versatile toolkit for researchers and data scientists in the field. Mastering these steps and techniques not only enhance understanding of genomics but also contribute to advancements in the ever-evolving field of biological data science genomic research.
Click here to explore 11 Python Packages for Biological Data and Cheat Sheet.
Share Article
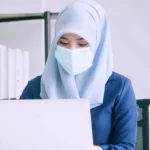
Tanzeela Arshad
Other articles.
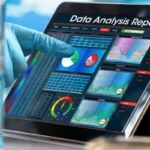
8 Essential Skills and Job Responsibilities of Clinical Data Analyst
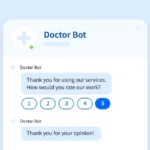
10 Future Applications of Conversational AI in Healthcare; Another Data Science Marvel
Data Analysis for Genomics
Drive your career forward.
This HarvardX professional certificate program gives learners the necessary skills and knowledge to tackle real-world data analysis challenges.
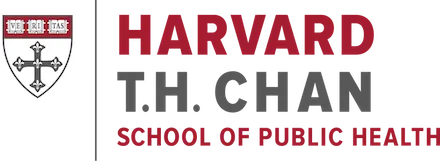
What You'll Learn
Advances in genomics have triggered fundamental changes in medicine and research. Genomic datasets are driving the next generation of discovery and treatment, and this series will enable you to analyze and interpret data generated by modern genomics technology.
Using open-source software, including R and Bioconductor, you will acquire skills to analyze and interpret genomic data. These courses are perfect for those who seek advanced training in high-throughput technology data. Problem sets will require coding in the R language to ensure mastery of key concepts. In the final course, you’ll investigate data analysis for several experimental protocols in genomics.
Enroll now to unlock the wealth of opportunities in modern genomics.
The course will be delivered via edX and connect learners around the world. After completing this series, you will understand how to:
- Bridge diverse genomic assay and annotation structures to data analysis and research presentations via innovative approaches to computing
- Use advanced techniques to analyze genomic data.
- Structure, annotate, normalize, and interpret genome-scale assays.
- Analyze data from several experimental protocols, using open-source software, including R and Bioconductor.
Courses in this Program
2–4 hours per week, for 4 weeks The structure, annotation, normalization, and interpretation of genome scale assays.
2–4 hours per week, for 5 weeks Perform RNA-Seq, ChIP-Seq, and DNA methylation data analyses, using open source software, including R and Bioconductor.
2–4 hours per week, for 4 weeks Learn advanced approaches to genomic visualization, reproducible analysis, data architecture, and exploration of cloud-scale consortium-generated genomic data.
Your Instructor
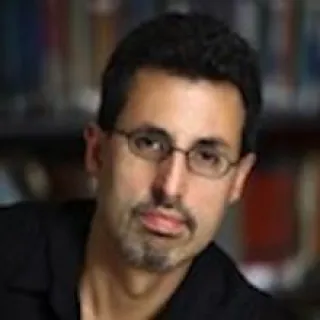
Rafael Irizarry
Professor of Biostatistics at Harvard University Read full bio.
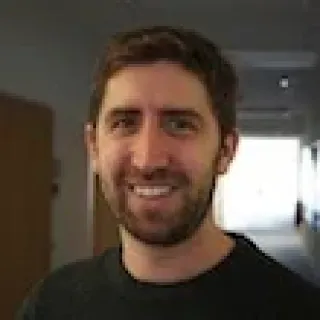
Michael Love
Assistant Professor, Departments of Biostatistics and Genetics at UNC Gillings School of Global Public Health Read full bio.
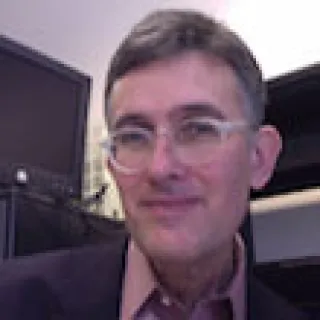
Vincent Carey
Professor, Medicine at Harvard Medical School Read full bio.
Job Outlook
- R is listed as a required skill in 64% of data science job postings and was Glassdoor’s Best Job in America in 2016 and 2017. (source: Glassdoor)
- Companies are leveraging the power of data analysis to drive innovation. Google data analysts use R to track trends in ad pricing and illuminate patterns in search data. Pfizer created customized packages for R so scientists can manipulate their own data.
- 32% of full-time data scientists started learning machine learning or data science through a MOOC, while 27% were self-taught. (source: Kaggle, 2017)
- Data Scientists are few in number and high in demand. (source: TechRepublic)
Ways to take this program
When you enroll in this program, you will register for a Verified Certificate for all 3 courses in the Professional Certificate Series.
Alternatively, learners can Audit the individual course for free and have access to select course material, activities, tests, and forums. Please note that Auditing the courses does not offer course or program certificates for learners who earn a passing grade.
Genomics Data Analysis
Learn advanced techniques to analyze genomics data

Associated Schools
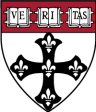
Harvard T.H. Chan School of Public Health
What you'll learn.
Advanced techniques to analyze genomic data
How to structure, annotate, normalize, and interpret genome-scale assays
How to bridge diverse genomic assay and annotation structures to data analysis and research presentations via innovative approaches to computing
How to analyze data from several experimental protocols, using open source software, including R and Bioconductor
About this series
The Genomics Data Analysis XSeries is an advanced series that will enable students to analyze and interpret data generated by modern genomics technology.
Using open-source software, including R and Bioconductor, you will acquire skills to analyze and interpret genomic data.
This XSeries is perfect for those who seek advanced training in high-throughput technology data. Problem sets will require coding in the R language to ensure learners fully grasp and master key concepts. The final course investigates data analysis for several experimental protocols in genomics.
This series includes
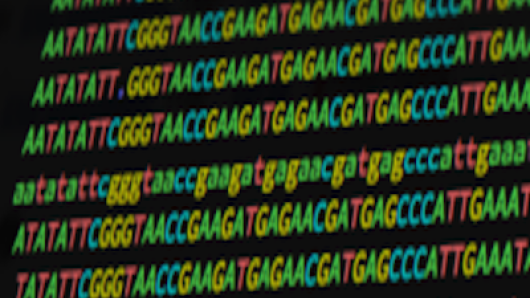
Case Studies in Functional Genomics
Perform RNA-Seq, ChIP-Seq, and DNA methylation data analyses, using open source software, including R and Bioconductor.

Introduction to Bioconductor
The structure, annotation, normalization, and interpretation of genome scale assays.
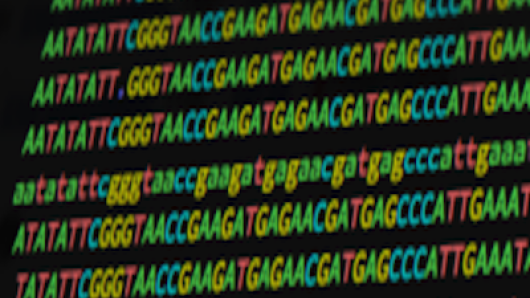
Advanced Bioconductor
Learn advanced approaches to genomic visualization, reproducible analysis, data architecture, and exploration of cloud-scale consortium-generated genomic data.
Instructors
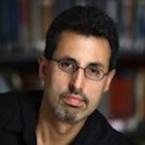
Rafael Irizarry
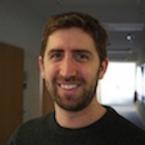
Michael Love
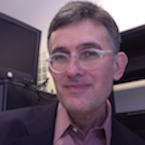
Vincent Carey
Join our list to learn more.
Genome Data Analysis
- © 2019
- Ju Han Kim 0
Division of Biomedical Informatics, Seoul National University College of Medicine, Seoul, Korea (Republic of)
You can also search for this author in PubMed Google Scholar
- Describes recent advances in genomics and bioinformatics
- Provides numerous examples of genome data analysis
- Meets the needs of life scientists, medical scientists, and others who are new to the field of bioinformatics
Part of the book series: Learning Materials in Biosciences (LMB)
64k Accesses
5 Citations
This is a preview of subscription content, log in via an institution to check access.
Access this book
- Available as EPUB and PDF
- Read on any device
- Instant download
- Own it forever
- Compact, lightweight edition
- Dispatched in 3 to 5 business days
- Free shipping worldwide - see info
Tax calculation will be finalised at checkout
Other ways to access
Licence this eBook for your library
Institutional subscriptions
Table of contents (21 chapters)
Front matter, bioinformatics for life and personal genome interpretation, bioinformatics for life, next-generation sequencing technology and personal genome data analysis, personal genome data analysis, personal genome interpretation and disease risk prediction, advanced microarray data analysis, gene expression data analysis, gene ontology and biological pathway-based analysis, gene set approaches and prognostic subgroup prediction, microrna data analysis, network biology, sequence, pathway and ontology informatics, motif and regulatory sequence analysis, molecular pathways and gene ontology, biological network analysis, snps, gwas and cnvs, informatics for genome variants, snps, gwas, cnvs: informatics for human genome variations, snp data analysis.
- Genome data analysis
- Bioinformatics
- Practice in data science
- Statistics using R
- Clinical informatics
About this book
This textbook describes recent advances in genomics and bioinformatics and provides numerous examples of genome data analysis that illustrate its relevance to real world problems and will improve the reader’s bioinformatics skills. Basic data preprocessing with normalization and filtering, primary pattern analysis, and machine learning algorithms using R and Python are demonstrated for gene-expression microarrays, genotyping microarrays, next-generation sequencing data, epigenomic data, and biological network and semantic analyses. In addition, detailed attention is devoted to integrative genomic data analysis, including multivariate data projection, gene-metabolic pathway mapping, automated biomolecular annotation, text mining of factual and literature databases, and integrated management of biomolecular databases.
The textbook is primarily intended for life scientists, medical scientists, statisticians, data processing researchers, engineers, and other beginners in bioinformatics who are experiencing difficulty in approaching the field. However, it will also serve as a simple guideline for experts unfamiliar with the new, developing subfield of genomic analysis within bioinformatics.
Authors and Affiliations
About the author.
Professor. Ju Han Kim, Division of Biomedical Informatics, Seoul National University College of Medicine, Seoul , South Korea.
Bibliographic Information
Book Title : Genome Data Analysis
Authors : Ju Han Kim
Series Title : Learning Materials in Biosciences
DOI : https://doi.org/10.1007/978-981-13-1942-6
Publisher : Springer Singapore
eBook Packages : Biomedical and Life Sciences , Biomedical and Life Sciences (R0)
Copyright Information : Springer Nature Singapore Pte Ltd. 2019
Softcover ISBN : 978-981-13-1941-9 Published: 10 May 2019
eBook ISBN : 978-981-13-1942-6 Published: 30 April 2019
Series ISSN : 2509-6125
Series E-ISSN : 2509-6133
Edition Number : 1
Number of Pages : XVI, 367
Number of Illustrations : 409 b/w illustrations, 236 illustrations in colour
Topics : Bioinformatics , Biomedicine general , Statistics for Life Sciences, Medicine, Health Sciences
- Publish with us
Policies and ethics
- Find a journal
- Track your research
Skip to Content
The value of genomic analysis
Genetic heritability is responsible for 30% of individual health outcomes , but is hardly used to guide disease prevention and care. Each individual carries 4-5 million genetic variants, each with varying influence on traits related to our health. The cost to sequence a genome has reduced drastically in recent years, and sequence data shows potential for ubiquitous use. However, the ability to read the sequence accurately and to meaningfully interpret it remain obstacles to broad adoption.
Improving the accuracy of genomic analysis
Sequencing genomes enables us to identify variants in a person’s DNA that indicate genetic disorders such as an elevated risk for breast cancer.
Highly accurate genomes with deep neural networks
Despite rapid advances in sequencing technologies, accurately calling genetic variants present in an individual genome from billions of short, errorful sequence reads remains challenging. As published in Nature Biotechnology , DeepVariant, an open-source variant caller that uses a deep neural network to call genetic variants from next-generation DNA sequencing data, significantly improves the accuracy in identifying variant locations, reducing the error rate by more than 50%. Learn more
Winner in PrecisionFDA V2 Truth Challenge
DeepVariant won awards for Best Overall accuracy in 3 of 4 instrument categories in the PrecisionFDA V2 Truth Challenge. Compared to previous state-of-the-art models, DeepVariant v1.0 significantly reduces the errors for widely-used sequencing data types, including Illumina and Pacific Biosciences. Read the article
Identifying disease-causing variants in cancer patients
Researchers wanted to understand if incorporating automated deep learning technology would improve the detection of disease-causing variants in patients with cancer. In a cross-sectional study published in JAMA of 2,367 prostate cancer and melanoma patients in the US and Europe, DeepVariant found disease-causing variants in 14% more individuals than prior state-of-the-art methods.
Building large-scale cohorts for genetic discovery research
Large cohorts of sequenced individuals are the foundations for discovery of novel genetic associations with disease. We developed best practices for generating cohorts that substantially improves over previous methods, which has been adopted by the UK Biobank for its large-scale sequencing efforts. Read the article
Improving genetic association discovery with machine learning
Discovering genetic variants associated with a trait of interest requires a large cohort of individuals with both genetic and trait information. As published in AJHG , we demonstrate that using a machine learning model to predict eye-disease-related traits from fundus images significantly improves discovery of genetic variants influencing those traits.
Our partners in genomics research
Because genomic data is highly personal, to the greatest extent possible we use datasets that are fully public or are broadly available to qualified researchers. We also partner with trusted organizations that contribute scientific and technology development to improve standards in genomic analysis and enhance the utility of sequencing data.
DeepVariant’s precisionFDA Truth Challenge V2 submission using PacBio HiFi reads achieved the highest single-technology accuracy, which has been featured on the PacBio blog and in a Nature Biotechnology retrospective . The collaboration also successfully launched DeepConsensus , which improves HiFi yield and read quality compared to existing consensus basecalling methods.
The Regeneron Genetics Center, one of the world’s largest human genomic research efforts, has adopted DeepVariant and re-trained specialized models for both internal projects and the delivery of 200,000 exomes to UKBiobank .
Benedict Paten ’s lab at UC Santa Cruz collaborated with Google on PEPPER-DeepVariant , which won best accuracy in the Oxford Nanopore Technologies category of the PrecisionFDA . The paper was also published in Nature Methods .
NVIDIA Clara Parabricks Pipelines software provides a suite of accelerated bioinformatic tools to support DNA and RNA applications, running on a GPU. Their implementation of DeepVariant processes a 30x whole human genome in less than 25 minutes from fastq to vcf using their latest A100 GPU.
GenapSys trained a custom DeepVariant model to provide a highly accurate variant caller for their new high accuracy, low cost, benchtop sequencing instrument.
ATGenomix builds a Spark framework which efficiently parallelizes DeepVariant , for their work with several clinical partners.
DNAnexus provides a secure and collaborative fit-for-purpose bioinformatics system that integrates cutting-edge tools like DeepVariant. They work with industry leaders like Google, the FDA, and UK Biobank to provide solutions to the scientific community.
DNAstack enables researchers to organize, share, and analyze genomics and biomedical data, using tools like DeepVariant, in an easy to use cloud environment. DNAstack's software products use open standards developed by the Global Alliance for Genomics & Health.

An official website of the United States government
The .gov means it’s official. Federal government websites often end in .gov or .mil. Before sharing sensitive information, make sure you’re on a federal government site.
The site is secure. The https:// ensures that you are connecting to the official website and that any information you provide is encrypted and transmitted securely.
- Publications
- Account settings
Preview improvements coming to the PMC website in October 2024. Learn More or Try it out now .
- Advanced Search
- Journal List
- Wiley Open Access Collection

Genome sequencing guide: An introductory toolbox to whole‐genome analysis methods
Alexis n. burian.
1 Department of Biology, Ithaca College, Ithaca New York, USA
2 Department of Biology, Davidson College, Davidson North Carolina, USA
Te‐Wen Lo
Deborah m. thurtle‐schmidt, associated data.
To fully appreciate genetics, one must understand the link between genotype (DNA sequence) and phenotype (observable characteristics). Advances in high‐throughput genomic sequencing technologies and applications, so‐called “‐omics,” have made genetic sequencing readily available across fields in biology from applications in non‐traditional study organisms to precision medicine. Thus, understanding these tools is critical for any biologist, especially those early in their career. This comprehensive review discusses the chronological development of different sequencing methods, the bioinformatics steps to analyzing this data, and social and ethical issues raised by these techniques that must be discussed and evaluated, including anticipatory guides and discussion questions for active engagement in the classroom. Additionally, the Supporting Information includes a case study to apply technical and ethical concepts from the text.
1. INTRODUCTION
Since DNA was established as the heritable material by Martha Chase and Alfred Hershey, scientists have sought to understand the structure and sequence of an organism's genome. 1 In 1953, the structure of DNA was determined, 2 , 3 , 4 yet it was not until 1996 that the first eukaryotic genome sequence—baking and brewing yeast Saccharomyces cerevisiae —was published. 5 Soon after, the first multicellular organism genome, C . elegans , 6 was completed, prompting the race to sequence the human genome, culminating in the draft human genome sequence in 2001. 7 Sequencing the human genome was a great achievement, but with the available technologies the effort was very labor and time‐intensive, prompting new advances in DNA sequencing. This second wave of sequencing technology—called next generation sequencing—drastically decreased sequencing costs, increasing the amount of genomic and genome‐scale information. 8 , 9 , 10 , 11 , 12 , 13
Genomic sequencing methods are now widely available, providing insight into basic molecular mechanisms from evolutionary analysis to personalized medicine. Additionally, genomic technologies can be applied to any methodology or organism in which nucleic acid can be extracted, making genomic methods widely accessible and “‐omic” techniques a staple across fields and organisms. Due to the ubiquity of these techniques, it is imperative for scientists early in their careers to understand both the power and the peril associated with genome sequencing techniques. This review introduces sequencing technologies, analysis methods, and socio‐ethical issues associated with genome sequencing to undergraduates. Through reading and engaging with the anticipatory guides and discussion questions with their peers and applying these concepts to the case study included in the Supporting Information , students should achieve the following learning outcomes:
- Explain the differences between Sanger and next‐generation sequencing methods.
- Compare and contrast chip‐based genotyping and whole‐genome sequencing methods.
- Identify advances in chemistry that enabled sequencing by synthesis.
- Outline the general pipeline for high‐throughput sequencing sample preparation and data analysis.
- Illustrate how various next‐generation sequencing techniques can be exploited to understand different aspects of gene expression.
- Discuss the social justice and ethical implications associated with genome sequencing techniques.
2. SEQUENCING AND WHOLE‐GENOME ANALYSIS METHODS
Nucleic acid sequencing techniques have evolved since their inception with each new technique building off of previous sequencing technology and addressing a prior shortcoming. In this section, we will review the development of various sequencing technologies.
2.1. Sanger sequencing
Anticipatory guides.
- What is necessary for DNA polymerase to add the next nucleotide?
- What is nucleic acid polarity and what implications does DNA polarity have on the double helix structure?
- How are DNA fragments separated during agarose gel electrophoresis?
- Review the steps of DNA amplification as in PCR .
Chain termination sequencing was the first nucleic acid sequencing method and revolutionized molecular biology, resulting in the 1980 Nobel Prize. Chain termination, also called Sanger sequencing as it was developed by Fred Sanger in 1977, uses the selective incorporation of dideoxynucleotides during an in vitro DNA replication reaction 14 (Figure 1 ). During DNA replication, DNA polymerase catalyzes the synthesis of DNA by forming a phosphodiester bond between the next complementary nucleotide and the hydroxyl group (─OH) of the 3′ end of the growing DNA strand. 15 Sanger sequencing exploits the requirement for an available 3′‐OH. The Sanger sequencing reaction contains both de oxynucleotides ( d NTPs) and dideoxynucleotides (ddNTPs). While dNTPs possess a 3′ carbon containing a hydroxyl group (Figure 1(b) ), ddNTPs lack the 3′‐OH (Figure 1(b) ) which prevents polymerase from adding the next base. Deoxynucleotides are present at high concentrations and will be incorporated by DNA polymerase in further synthesis most of the time. However, ddNTPs which are labeled with fluorescent dye are still incorporated albeit at a lower frequency, halting synthesis. This synthesis reaction results in numerous DNA fragments of varying lengths complementary to the sequenced template, each ending with the fluorescently labeled ddNTP (Figure 1(a) ). Each of the four different nucleotides are conjugated to a different dye, which emit a distinct wavelength when excited.

Chain termination sequencing. (a) Schematic of chain termination sequencing. DNA templates are amplified by DNA polymerase in a reaction containing a mixture of dNTPs and fluorescently labeled ddNTPs. Amplified fragments terminated at different lengths are separated by capillary gel electrophoresis followed by laser excitation and detection. Sequences are displayed in a chromatograph (as peaks) where each nucleotide is represented by a differently colored peak. The height of the peak indicates the confidence level at that nucleotide position. (b) Chemical structure of ddNTPs and dNTPs. The critical 3′ hydroxyl group in dNTPs is highlighted in red, which is not present in ddNTPs
The DNA molecule's sequence is determined by separating out all the newly synthesized DNA fragments by size, using capillary gel electrophoresis that separates DNA molecules by size with single base resolution, where smaller DNA molecules move faster through the capillary. At the end of the capillary, a laser excites the ddNTP at the end of the chain and the fluorescent dye color is detected, allowing the sequence to be recreated by the order of the laser excitations wavelengths observed (fluorescent dyes detected) (Figure 1(a) ).
2.2. Chip‐based detection methods
- Define heterozygous vs homozygous at a genetic locus .
- Write the complementary sequence for: 5 ′ ‐ATGCATCGTAT‐3 ′
- Describe the process of DNA denaturation and annealing (hybridization) during PCR .
- What is a single nucleotide polymorphism (SNP)? How are SNPs related to alleles?
- Why is determining more than one DNA sequence at a time beneficial?
Sanger sequencing can only sequence a single DNA fragment per reaction. Although powerful—Sanger sequencing was used to sequence the human genome 7 —sequencing a single fragment at a time has limitations. Chip‐based sequencing methods, called microarrays, sought to resolve this issue. 16 , 17 , 18 It is important to note that these methods do not actually sequence DNA but allow for the simultaneous detection of different DNA sequence variants and mRNAs at once. Generally, DNA microarray chips consist of a solid surface dotted with small wells that contain a collection of single‐stranded DNA specific to a gene, allele, or genomic region called the probe. Detection of different sequences is based on denatured, single‐stranded samples hybridizing (attaching through hydrogen bonding) to complementary probes on the surface of the chip. 19 Samples are prepared by extracting nucleic acid, fragmenting the nucleic acid into small pieces, denaturing the samples into single strands, and labeling the small fragments of nucleic acid with a fluorescent dye. These fragments are washed across the chip and hybridized to the DNA probes in wells that are complementary to the fluorescently labeled sample. The chip is then scanned and the quantity of each sample that anneals to each well is detected based on the amount of fluorescence present. The specific sequence and location on the chip for the DNA probe in each well is known, and the fluorescent signal correlates to the quantity of that sequence in the original sample.
To date, the main applications of DNA microarrays have been single nucleotide polymorphism (SNP) detection and relative mRNA quantification. For SNP detection, two adjacent wells contain probes specific to two common alleles in the population. The genotype of the sample is determined through detection of which wells the sample binds as the sample only binds to the well with the exact complementary sequence. These DNA microarray chips are still commonly used today to genotype people, as the human genome is costly to sequence. Additionally, microarrays can be used to determine relative gene expression through differential hybridization, correlating to gene expression, of differentially labeled control and experimental samples. Due to advances in sequencing by synthesis (see below), microarrays are not often used for RNA quantification anymore.
2.3. Sequencing by synthesis
- What challenges are there to sequencing an entire genome with Sanger sequencing?
- What types of questions could you address by sequencing all the DNA or RNA in an organism?
In the mid 1990s and early 2000s, two critical innovations brought on a fundamentally new sequencing methodology, still referred to as “next generation sequencing,” “second generation sequencing,” or more generally “sequencing by synthesis,” in which a single DNA molecule is continually sequenced. Continually sequencing the same molecule, as opposed to chain termination in Sanger sequencing, was made possible due to new chemistry termed “reversible terminator chemistry.” A nucleotide with a reversible terminator has a blocked 3′‐OH, similar to a ddNTP in Sanger sequencing, but after addition of another chemical solution the blocked 3′‐group is reversed to a 3′‐OH, again supporting sequencing (Figure 2 ). 13 , 20 Each of these modified dNTPs is labeled with a different fluorescent dye. After each base is added to the elongating DNA strand, synthesis is halted because of the blocked 3′‐OH, the dye is excited, and the color of the fluorescent nucleotide is recorded. Next, a chemical solution is added which both quenches the fluorescent dye (so that it no longer fluoresces) and reverses the blocked 3′‐OH, supporting the next round of sequencing. There are several reversible terminators used commercially. The most common of which are 3′ blocked reversible terminators with either a 3′‐ONH2, 3′‐O‐allyl, or 3′‐O‐azidomethyl. 20

Sequencing by synthesis. (a) Cycle of reversible terminator incorporation, identification of incorporated base by fluorescence imaging, followed by removal of the reversible terminator. (b) Chemical structure of a nucleotide with a reversible terminator attached. The 3′‐OH group is capped by a reversible terminator (black rectangle), with a fluorophore attached to the nitrogenous base (red circle). The fluorophore is then excited (red star), and the nucleotide is recorded. Finally, the fluorophore is cleaved from the nucleotide and the 3′‐OH (highlighted in red) is unblocked for the next round of sequencing
Another key innovation for sequencing by synthesis was simultaneous sequencing of multiple DNA sequences by attaching DNA strands to a flow cell, a two‐dimensional microfluidic device (which resembles a microscope slide)—very similar to a microarray used in chip‐based methods described above. First, DNA fragments are attached on one end to the flow cell. Next, each DNA molecule is amplified resulting in many copies of that DNA molecule in the same spot (or cluster) on the chip, amplifying the signal (sequence of the DNA molecule)—a step called “cluster generation”. 13 Spots on the chip have different initial DNA molecules and there can be millions of individual DNA molecules on each chip. After cluster generation, sequencing proceeds. For each base in the DNA strand, reversible terminator modified nucleotides are added and the attached fluorophores are excited and the chip imaged (Figure (Figure2). 2 ). The colored images are translated into a DNA sequence, resulting in a single sequence for each cluster on the chip. Sequencing occurs for a defined number of rounds (usually between 50–300 bases, but can be as long as 500 bases), creating what is termed a “short read” of DNA sequence. Identification of the nucleotide incorporated for each DNA fragment relies on the amplified signal from the many copies of that DNA fragment in the cluster. At times one of the DNA fragments in a cluster gets “off phase” from all other DNA fragments in the cluster by accidently incorporating more than one nucleotide at a time, resulting in an incorrect signal of the nucleotide incorporated for that DNA fragment. The longer the sequencing, the more likely it is that some of the DNA molecules in a cluster get “off phase,” limiting the length of the sequencing reads. 21 In addition, sequencing reads should be long enough to unambiguously map to the genome thus setting the limits of read lengths for sequencing by synthesis.
2.4. Third generation sequencing
- What are the advantages of being able to sequence longer fragments of DNA?
- What are the consequences if sequencing is not accurate?
- What is a processive enzyme and provide an example of a processive enzyme that uses a nucleotide substrate?
- What is a protein pore in membrane bilayers?
Third generation sequencing technologies, called single molecule sequencing (SMRT) and nanopore sequencing, rely on sequencing single nucleotide molecules. 22 Like Sanger sequencing and sequencing by synthesis, SMRT sequencing, developed by PacBio, also relies on synthesizing a new DNA strand by DNA polymerase. 23 However, the DNA polymerase is immobilized at the bottom of a tiny well in the sequencing chip. Each well has a single piece of DNA to be sequenced and each dNTP is given a fluorescent label with an unique emission spectrum. The immobilized DNA polymerase begins to replicate the DNA strand and as each dNTP is added, the fluorophores are excited. The sequence of the DNA can then be easily determined based on the emission spectra observed, which belong to the incorporated nucleotides that were detected.
A complementary third generation sequencing technology was developed that, like SMRT sequencing, relies on direct detection of a single nucleotide molecule but does not rely on DNA synthesis. Instead, nanopore sequencing (Figure 3 ) uses a membrane protein complex. This protein complex consists of two proteins: (a) an unwinding enzyme and (b) a pore protein which allows molecules to pass through a lipid bilayer. The unwinding enzyme, such as polymerase or helicase, unwinds the double helix so that a single nucleic acid strand (DNA or RNA) passes through the pore protein. 24 This pore protein is inserted in a synthetic lipid bilayer. A commonly used pore protein is MspA, which is a transmembrane protein found in Mycobacteria used to transport nutrients across the bacterial membrane. 25 , 27 , 28 The lipid bilayer has variable voltage on either side. As nucleotides pass through the unwinding enzyme and the pore, the mass of the nucleotide creates a distinct change in current. From the specific current signature detected, the sequence of the nucleotide strand is determined.

Nanopore sequencing. DNA double helix is unwound by unwinding enzyme and a single strand is fed through the pore inserted in a membrane. As the DNA moves through the protein nanopore, the nucleotides (colored circles) are identified by the change in ion current (yellow) across the membrane. Graph shows the identification of nucleotides in the DNA sequence based on the current measured over time
Third generation sequencing is characterized by its ability to sequence much longer reads. Both SMRT and nanopore technologies have reported reads of at least 8000 bp as compared to sequencing by synthesis in which the longest reads are 500 bp. 29 However, longer reads come at the expense of sequencing accuracy—both third generation technologies have much higher error rates than second generation sequencing. 29 , 30 To improve sequencing accuracy, in nanopore sequencing, the two strands of DNA are ligated with a hairpin structure, thus when the DNA is denatured and passed through the pore as a single stranded molecule, both complementary strands are sequenced (Figure 3 ). This provides twice the sequence for one strand, helping to resolve unclear base calls. Similarly, prior to SMRT sequencing, hairpins are ligated to both ends of DNA, resulting in a circular single stranded DNA. This DNA molecule can be continually sequenced by the immobilized polymerase, resulting in better base calling due to the multiple sequencing rounds.
2.5. General discussion questions
- How does a dideoxynucleotide prevent elongation by DNA polymerase?
- What aspects of Sanger sequencing gave way to sequencing by synthesis?
- What aspects of DNA microarray chips gave way to sequencing by synthesis?
- Draw a picture of the results on a DNA microarray for a sample homozygous at a locus and for a sample heterozygous at the locus .
- Why would adding all four nucleotides at the same time in sequencing by synthesis reaction result in more accurate sequencing?
- Pose a research question appropriate for each of the technologies discussed above .
- Compare and contrast the different sequencing/genome detection methods .
- For each of the sequencing methods above , enumerate the significance and limitations .
- If sequencing a new genome , why would using a combination of sequencing by synthesis and third generation sequencing be advantageous?
3. SEQUENCING PIPELINE
The sequencing pipeline is a three‐step process: sample and library preparation, sequencing, followed by data analysis and bioinformatics. Above described the second step—sequencing. This section will describe the process of steps one and three.
3.1. Sample and library preparation
- What are challenges to sequencing many different fragments of DNA at once using sequencing by synthesis?
- What are primers and why are they necessary for DNA replication?
- What is cDNA? How does cDNA sequence differ from the genomic DNA?
Before sequencing, the nucleic acid sample is isolated using traditional molecular biology techniques. Applications where determining the sequence or the amount of different specific sequences in a sample are typical applications of second and third generation sequencing technologies (reviewed in Reuter et al. 26 ). After nucleic acid isolation, one of the challenges to genomic sequencing methods is the preparation of many millions of different sequences for sequencing at the same time (Figure 4 ). For sequencing by synthesis and SMRT sequencing methods (which rely on DNA polymerase) all the sequences must have at least some common sequence to which a primer can anneal. In second generation sequencing, DNA sequences are adhered to the chip by hybridizing to a complementary single‐stranded DNA oligonucleotide and a primer also binds to this sequence supporting cluster generation. 13 Thus, the necessity of a common DNA sequence on each DNA fragment for chip hybridization, cluster generation, and sequencing is at odds with the innovation that many different DNA pieces of unknown sequences are sequenced simultaneously.

Sequencing by synthesis pipeline. (a) Genomic DNA is first fragmented into smaller templates which undergo modification, including 5′‐phosphorylation and addition of 3′‐a for adaptor ligation. Following size selection and PCR amplification, the library is denatured and amplified into clonal clusters that undergo linearization, blocking, and hybridization, preparing the flow cell for sequencing, using reversible terminators. (b) DNA fragment converted into library with adaptor and primer sequenced indicated
To overcome this problem, the nucleic acid sample is prepared into a “library”—a collection of DNA fragments each with common sequences (adaptors) on either end 13 (Figure 4(b) ). First, if the sample is RNA, it is converted to cDNA (complementary DNA) using reverse transcriptase as DNA is much more stable than RNA and DNA polymerase requires a DNA template. Since sequencing by synthesis requires short pieces of DNA, the DNA is sheared to less than 500 bps in length. Since each fragment of DNA is unique, the same adaptors (pieces of DNA) must be added to the ends of each fragment to replicate and sequence each unique fragment simultaneously. The first step in adaptor attachment is to add a single “A” base to the 5′ ends of each sequence. This off‐hanging “A” base allows the adaptors to attach through ligating to the complementary “T” overhang on the 3′ end of the adaptor. The ends of the adaptor are complementary to the end of the primer sequence, which through PCR both amplifies the library so that there is enough material for sequencing and extends to add the primer sequence. After this PCR step, the DNA can attach to the flow cell, and primers support cluster generation and subsequent sequencing by synthesis. Samples prepared for nanopore sequencing have a very similar library preparation step, adding adaptors to each of the fragments, however fragmentation is not necessary since these technologies support sequencing longer pieces. Even though nanopore sequencing relies on direct detection and not sequencing by synthesis, the adaptors are necessary to feed the nucleic acid through the pore by the ratcheting enzyme. 29
3.2. Data analysis and bioinformatics
- From the steps in sequencing by synthesis described above , identify what determines the length of the sequence returned to the user .
- What is the risk of only sequencing each base one time?
- What information would be useful from the sequencing reaction for analyzing the accuracy of the base call?
- What are intron/exon boundaries?
In high‐throughput sequencing, millions of reads are sequenced. A read is the sequence of each DNA fragment and in second generation sequencing the length of the sequence is defined by the number of sequencing cycles (the number of times modified dNTPs were added and imaged), typically from 50 to 300 base pairs in 50‐base‐pair increments. Thus, since the DNA is typically sheared to 200–500 base pairs during the library preparation, the entire DNA fragment is not sequenced. In a typical sequencing reaction, termed single‐end sequencing, the fragment is sequenced from just one end of the DNA fragment. A paired‐end sequencing reaction sequences each fragment from both of the DNA ends, providing twice as much sequencing information of the same piece of DNA. The reads are returned to the user in a plain text file termed a FASTQ file (Figure 5 ). 31 The FASTQ file format is a repeating unit of four lines: (a) the name of the read, which begins with an “@” symbol; (b) the sequence of the read; (c) a separator, a single “+” (plus) sign, to make the file easier to read; (d) the quality score line. Each base pair in the sequence receives a quality score termed the Q‐score ranging from 1 to 40 with 1 indicating the least confidence that the base call is correct and 40 being the most confident. 13 For example, “I” is a score of 40 which translates to 99.99% accuracy for that base call. The symbol code, which is an ASCII based code, ensures that each numerical score only takes up a single character space so that it lines up with the appropriate base. This repeating four lines continues for the millions of reads sequenced. For second generation sequencing, a single sequencing sample can produce over 150 million reads.

Whole genome sequencing analysis. (a) Example four lines of each read in a FASTQ file. Components in the FASTQ file are labeled with a text box of the same color, which include the sequence ID, nucleotide sequence, and quality score. (b) Example reads mapped to a reference genome (black). An example of 1x coverage (left) and 5x coverage (right) is shown. Reads common to both the 1X and 5X examples are shown in light gray, and reads only in the 5X example are shown in dark gray
Bioinformatic analysis consists of quality control of the reads and then mapping the reads to the genome of interest. For quality control, the fourth line for each sequence is read in the FASTQ file, to determine if there is sufficient confidence in each base call. Based on quality control results, some trimming of low‐quality bases may be required to ensure that only high‐quality bases are included in the analysis. Another common pre‐processing step is to remove the general adaptor sequence so that the reads map more reliably to the genome, which is the next step in bioinformatic analysis. Most often, second and third generation sequencing is not used to sequence a new genome from scratch but rather for analyzing and quantifying the sequences of a nucleic acid sample of interest from an organism with a sequenced reference genome by mapping the reads to this reference genome (Figure 5 ). For nucleic acid samples from DNA, mapping is straight‐forward (although computationally intensive), and reads are compared to the entire known genome to find the place that matches the read. After all reads are mapped to the genome, the amount of coverage is determined by approximating how many times each nucleotide is represented in all of the sequencing reads (Figure 5 ). For RNA‐seq, which sequences the mRNA of a sample and identifies gene expression and alternative splicing, more sophisticated mapping algorithms are used to map reads that span exon‐intron boundaries, which result in part of the read mapping in a different location than the other end of the read as compared to the genomic sequence. Once mapped, the coverage of the gene in RNA‐seq samples is used to determine that gene's expression in a sample. This expression can be compared across samples to determine differentially regulated genes between different conditions. More recently, new RNA‐seq mapping algorithms significantly decrease processing time by skipping over the labor‐intensive mapping portion and directly quantitating transcript levels. 32 , 33 , 34
3.3. General discussion questions
- Explain the purpose of the adaptor and primer sequences in a genomic library .
- On the flow‐chart diagram in Figure 4 , draw the library preparation steps for fragments of DNA .
- How can you determine how many reads were sequenced from the number of lines in a FASTQ file?
- Outline the different steps needed to go from RNA‐seq FASTQ files to gene expression quantitation .
- Explain how RNA‐seq could be used to detect alternative splicing .
- In what applications would paired‐end sequencing be desirable over single‐end sequencing?
- Why is “high coverage” important when trying to identify mutations in a sample?
4. SOCIAL IMPLICATION OF GENOME SEQUENCING
Power lies within the genomic tools discussed above. This power can be enormously beneficial to millions of people and change lives, but researchers must consider the long‐term consequences and contemplate the social and ethical ramifications. Below we illuminate some of the ethical and social consequences when genetic sequencing is used for medical advancements and placed directly in the hands of consumers.
4.1. Knowledge is power
- How will genetic testing change medical treatments?
- Is the application of genetic testing limited to human diseases?
The ease in sequencing and decrease in cost has led to numerous discoveries linking genes to diseases. 35 Genome‐wide association studies (GWAS) facilitate linking complex genetics to differential phenotypes. GWAS identifies specific SNPs associated with diseases by comparing common sequence variants and/or genomes between unaffected individuals to those individuals with a phenotype or interest. 36 Knowing what SNPs are associated with particular diseases, is the foundation of precision medicine. 37 Precision medicine allows medical professionals to choose the most effective treatments based on an individual's genetic sequence.
Sequencing technologies have led to numerous direct‐to‐consumer sequencing companies that allow individuals to learn about their own genetics without professional medical assistance. 38 In most cases, consumers simply mail a saliva sample which is genotyped, using a DNA microarray as described above with probes to common variants in the human population. Consumers learn the sequence of their genomic loci known to be associated with different phenotypes such as lactose intolerance, heart disease, or caffeine sensitivity. As a consumer, a person must decide what they hope to learn about their genetic make‐up to select the appropriate direct‐to‐consumer sequencing service.
4.2. Does everyone have equal access to this “power?”
- How do social inequalities affect access to genetic testing and relevant treatments?
- Who should be responsible for educating people about genetic testing and its implications?
Access to genomic technologies has traditionally been through clinical genetic testing. Sequencing by synthesis has revolutionized clinical genetic tests by allowing interrogation of multiple different genes or even the entire genome of a patient sample at once, significantly speeding up genetic testing. Results from these tests can be used both for diagnosis and to identify targeted therapies. However since clinical genetic testing is administered in a healthcare setting, social inequities which are well‐documented in healthcare also plague genetic testing. 39 Access to clinical genetic testing is not equivalent across all racial and socioeconomic communities 40 due to factors such as differences in comprehensive health insurance among racial groups 41 and mistrust of medical testing by individuals from groups historically excluded from healthcare. 40 These disparities put racial minorities at increased disadvantage to reaping the benefits of clinical genetic testing.
Even though direct‐to‐consumer tests can be more affordable ($60–$200 depending on the comprehensiveness of the test) the costs are out‐of‐pocket and still represent a significant barrier to access. Additionally, the health and lifestyle genetic risk factors that direct‐to‐consumer report on are from studies with inequitable racial representation. The majority of genetic research databases and GWAS include mostly European‐descent genomes, indicating a serious gap in “who” is being solicited to participate in genetic research. 42 This lack of representation decreases the applicability of results across populations and understanding of genetic diseases in non‐European populations. Thus, even if an individual has the means to access these tests, it is not a given if those results are applicable to them.
4.3. Can this “power” end up in the wrong hands?
- Who might you want to keep your genetic results from?
- How secure (private) are genetic testing results?
While there may be great benefits of having a better understanding of your genetic make‐up, before signing up with a direct‐to‐consumer genetic test, the consumers must consider who owns and has access to their data and fully understand the companies' privacy policies prior to submitting their sample. To help protect an individual's privacy, legislation has been enacted to protect the consumer's privacy. The 21st Century Cures Act seeks to protect an individual's confidentiality when genetic information is donated to federal research purposes by removing all identifiers (i.e., donor's name and contact information). 43 Any information obtained from the research cannot be released to law enforcement or government agencies. In addition, under the Health Insurance Portability and Accountability Act (HIPPA) one's genetic information is protected from employers, schools, and the public if it becomes a part of one's health record. 44 The entities that can access this information are law enforcement and health insurance.
With the rise of genetic testing came concern about genetic discrimination if health insurance companies had access to genetic testing results; companies could discriminate against those who tested positive for differing genetic predispositions and alter their healthcare coverage. The Genetic Nondiscrimination Act (GINA) was passed in 2008 45 to prevent health insurance companies from denying coverage and changing rates based on genetic predispositions. However, it only protects individuals who are not showing any symptoms of the predisposition. If symptoms of the genetic difference are present, then insurance companies could alter coverage and rates. GINA also prohibits employers from changing the employment status based on genetic testing results. Direct‐to‐consumer testing companies have their own privacy policies that should be considered before using the service. These considerations highlight how a scientist should also understand how a scientific tool is being used in addition to the development of such technologies.
4.4. Discussion questions
- What human diseases are ideal for genetic testing and precision medicine?
- What are the limitations of genetic testing?
- Is it always beneficial for someone to know genotype(s)? Are there genotypes that one might not want to know?
- What direct‐to‐consumer service(s) do you think provides the most interesting (relevant) information?
- Who should be responsible for genetic testing costs?
- Should restrictions be placed on genetic testing? Why or why not? If yes , what are appropriate restrictions?
- What are potential concerns regarding genetic testing? Would these concerns stop you from getting your DNA tested? Why or why not?
- Who should have access to genetic testing results?
- Is additional legislation necessary to regulate genetic testing and results? What issues should that legislation address?
Supporting information
Appendix S1 : Supporting Information.
ACKNOWLEDGMENT
Funding support provided by Davidson College to D. T‐S.
Burian AN, Zhao W, Lo T‐W, Thurtle‐Schmidt DM. Genome sequencing guide: An introductory toolbox to whole‐genome analysis methods . Biochem Mol Biol Educ . 2021; 49 :815–825. 10.1002/bmb.21561 [ PMC free article ] [ PubMed ] [ CrossRef ] [ Google Scholar ]
Funding information Davidson College
The Genomic Data Analysis Network
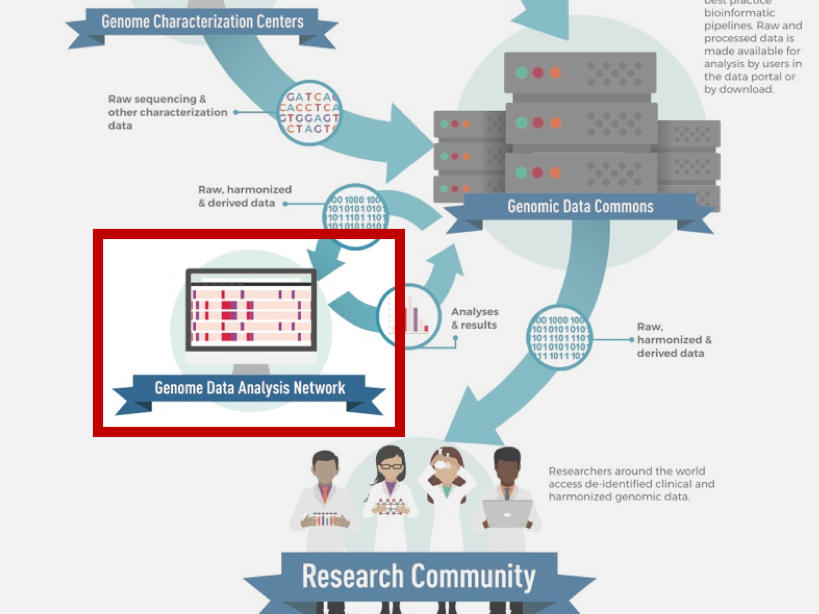
The Genomic Data Analysis Network (GDAN) serves to help the cancer research community leverage the genomic data and resources produced by CCG and other NCI programs
About the Genomic Data Analysis Network
While large genomic datasets are invaluable to the cancer research community, translating genomic data into biological insights into the development and treatment of cancer is not a straightforward task. Over a decade of experience from The Cancer Genome Atlas (TCGA) program demonstrated the power and necessity of “team science”—that successful analyses of large-scale genomic datasets require the coordination of a large body of researchers with a wide range of expertise in computational genomics, tumor biology, and clinical oncology.
CCG’s Genomic Data Analysis Network (GDAN) was formed from the need to harness TCGA data and a growing need at large for computational genomics. For TCGA, the network created standardized data formats and processing protocols, generated bioinformatics tools for the community, and performed a range of analyses on the data, notably generating clinically meaningful molecular subgroups of cancer and producing the PanCancer Atlas .
In the post-TCGA era, the GDAN continues to conduct key large-scale studies and generate genomic resources to support the genomic research community. The GDAN’s overall goal is to help the cancer research community leverage the genomic data and resources produced by CCG and other NCI programs for the benefit of cancer patients, largely by:
- developing and implementing new bioinformatic and computational tools to capture key biological insights about cancer (e.g., pathway analysis, data integration with visualization, and integrated cancer biology);
- developing data processing and quality control methods for working with large-scale genomic characterization data;
- processing and integrating a variety of analytical data types to generate disease-level findings and perform cross-disease analyses.
The GDAN is comprised of individual Genome Data Analysis Centers (GDACs), each specializing in a unique set of computational analyses, molecular platforms, data integration, or visualization techniques. The GDACs are tasked to cooperatively perform molecular analyses on new and existing data from CCG programs and work with the other components of CCG’s Genome Characterization Pipeline. Areas of expertise and examples of their utility include:
- DNA Mutations – Identifying mutations in coding and non-coding regions of the genome, classifying mutations as driver or passenger mutations, identifying chromosomal rearrangement events leading to fusion proteins, and determining potential enhancer or suppressor functionality of mutations.
- Gene Expression – Identifying mRNA expression patterns and correlating with relevant clinical parameters, identifying translocation or rearrangement events.
- Copy number and tumor purity - Clustering cases according to copy number alteration or loss-of-heterozygosity events, identifying candidate drivers of copy number alterations, estimating tumor purity of the samples.
- miRNA analysis - Analyzing miRNA expression to correlate with patterns of mRNA expression and identify expression regulation networks, correlating miRNA data with relevant clinical parameters.
- Long non coding RNA (lnRNA) – Analyzing lnRNA expression patterns and correlating with patterns of mRNA expression or expression regulation networks.
- Batch effects and data integration – Identifying batch effects that might have been accrued during processing of samples, devising bioinformatics methods to correct such effects, determining biologically relevant groups that can subsequently be analyzed in the context of clinical data.
- Methylation analysis – Identifying DNA methylation patterns of interest and correlating patterns with relevant clinical parameters, correlating patterns with mRNA expression data to propose gene regulation mechanisms.
- Pathway analysis – Identifying biological pathways that have been altered, performing multi-omic data analyses to identify altered pathways and potential clinical relevance.
- Single cell RNA sequencing – Identifying cell clusters according to gene expression patterns, extracting expression levels and correlating with relevant clinical parameters, identifying translocation/rearrangement events, and identifying cell clusters or subclones of interest.
- Circulating cell-free DNA (cfDNA) and circulating tumor DNA (ctDNA) – Analyzing “liquid biopsies,” or blood samples for cfDNA or ctDNA to establish correlations between mutations in tumor tissue and ctDNA, developing methods to utilize the technology as a diagnostic and prognostic tool, and creating models of disease burden and progression in cancer development.
- Long-read sequencing – Assembling genomes, identifying structural variants, sequencing through repetitive regions, phasing critical variants.
- Spatial genomics – Analyzing gene expression data with spatial information, produced from different emerging spatial genomics platforms.
- Digital Imaging – Mining histopathology images for elements that aid in diagnostic or prognostic efforts, applying machine learning to learn relevant features.
New Molecular Profiling Platforms to Explore New Facets of Cancer
CCG continues to expand and develop new genomic data and analysis resources for the cancer research community. Through the GDAN and other CCG programs, CCG is exploring new ways to mine the data and learn new things about cancer from the massive dataset.
Additionally, as new molecular platforms become available, CCG explores utilizing these platforms to complement existing datasets. New platforms may be utilized in new structural genomics projects or in some cases to further characterize existing samples. Existing or new Genome Characterization Centers may be sought out to provide these capabilities. These newer platforms include:
- Assay for transposase-accessible chromatin using sequencing (ATAC-seq)
- Single-cell RNA
- Single-cell DNA
- Spatial genomics
CCG considers how these new technologies may be applied to enhance what we can learn about cancer. For example, can single-cell or spatial technologies provide much needed insights into the tumor microenvironments of tumors that don’t respond to treatment? How can the technologies be used to further what we can learn from TCGA or other existing datasets?
For example, GDAN researchers applied the ATAC-seq chromatin accessibility assay to 410 TCGA tumor samples, getting an unprecedented systematic look at gene dysregulation in cancer. With this low-cost assay, the researchers were able to discover new DNA regulatory elements and a new class of mutations falling within these elements that may play a key role in cancer.
In addition to applying new molecular platforms to TCGA samples, CCG is also working to perform whole-genome sequencing for the complete set of TCGA samples. These rich datasets, along with analyses and methods developed by the GDAN, could help facilitate the discovery of new diagnostic and prognostic markers, new targets for pharmaceutical interventions, and new cancer prevention and treatment strategies.
Current GDAN Centers
The GDAN is comprised of individual Genome Data Analysis Centers (GDACs), each contributing distinct functions, capabilities, and analytical components. Each GDAC works collaboratively within the network and also with other components of CCG’s Genome Characterization Pipeline. The current GDACs and their area of expertise in computational genomics are described below.
Still accepting applications for online and hybrid programs!
- Skip to content
- Skip to search
- Accessibility Policy
- Report an Accessibility Issue

- New AI-powered statistics method has potential to improve tissue and disease research
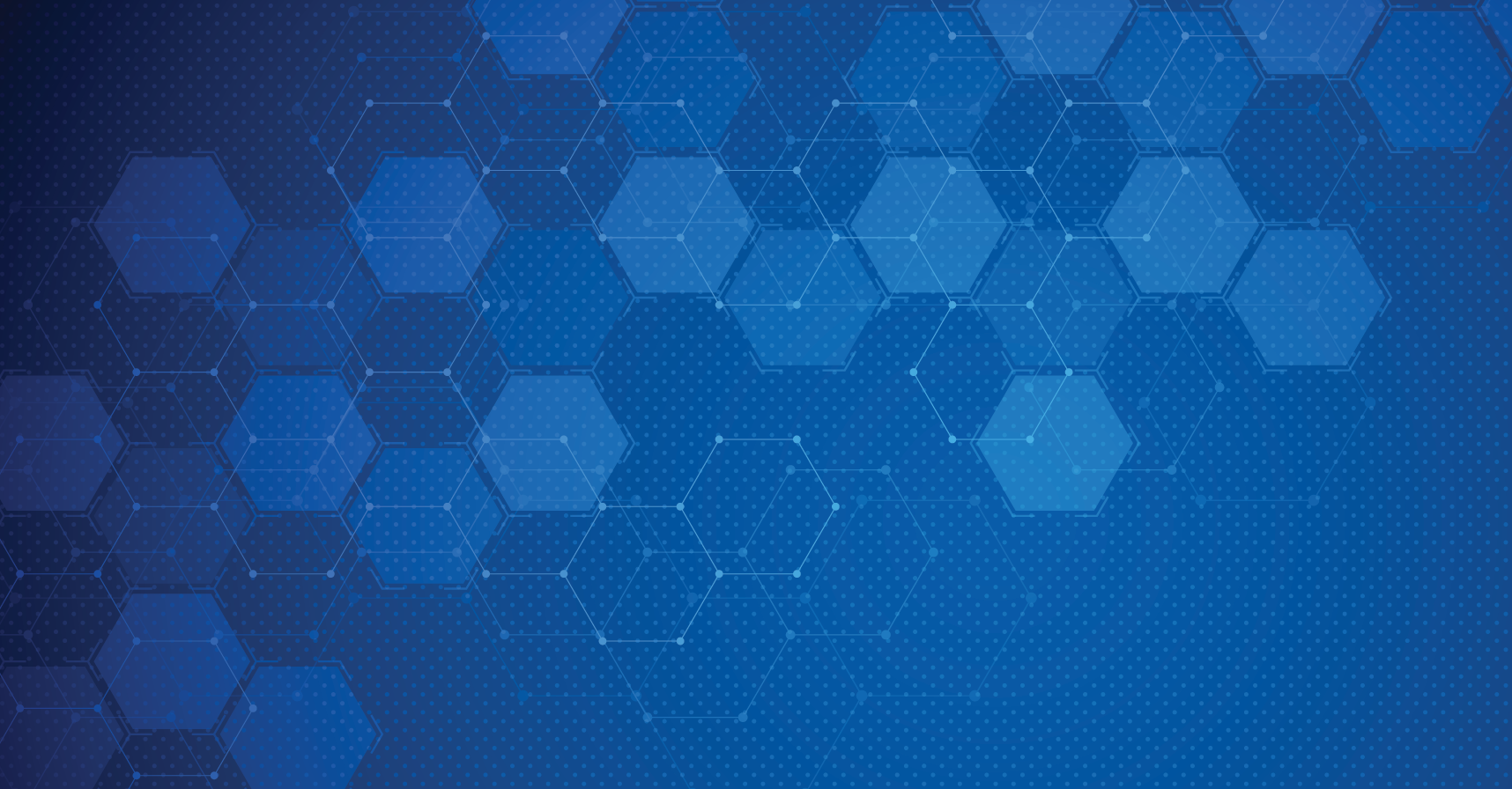
Research team hopeful that the method, called IRIS, can provide more detailed information for precision health treatment plans and health outcomes.
June 6, 2024
Researchers at the University of Michigan and Brown University have developed a new computational method, IRIS, to analyze complex tissue data which could transform our current understanding of diseases and how we treat them.
Integrative and Reference-Informed tissue Segmentation (IRIS) is a novel machine learning and artificial intelligence method that gives biomedical researchers the ability to view more precise information about tissue development, disease pathology and tumor organization.
The findings were published today in the journal Nature Methods .
IRIS draws from data generated by spatially resolved transcriptomics (SRT) and uniquely leverages single-cell RNA sequencing data as the reference to examine multiple layers of tissue simultaneously and distinguish various regions with unprecedented accuracy and computational speed.
Unlike traditional techniques that yield averaged data from tissue samples, SRT provides a much more granular view, pinpointing thousands of locations within a single tissue section. However, the challenge has always been to interpret this vast and detailed dataset, says Xiang Zhou , professor of Biostatistics at the University of Michigan School of Public Health and senior author of the paper. He worked with Ying Ma , assistant professor of Biostatistics at the Brown University School of Public Health , to develop IRIS.
Interpreting large and complex datasets is where IRIS becomes a helpful tool—its algorithms sort through the data to identify and segment various functional domains, such as tumor regions, and provide insights into cell interactions and disease progression mechanisms.
“Different from existing methods, IRIS directly characterizes the cellular landscape of the tissue and identifies biologically interpretable spatial domains, thus facilitating the understanding of the cellular mechanism underlying tissue function,” said Ma, who earned her Ph.D. in Biostatistics from the University of Michigan School of Public Health in 2023. “We anticipate that IRIS will serve as a powerful tool for large-scale multi-sample spatial transcriptomics data analysis across a wide range of biological systems.”
In the study, the researchers applied IRIS to six SRT datasets and compared its performance to other commonly used spatial domain methods. IRIS significantly outperformed other methods in accuracy. Ultimately as SRT technology continues to grow in popularity and use, the researchers hope to see methods like IRIS help to potentially develop targets for clinical interventions or drug targets, improving personalized treatment plans and patient health outcomes.
“The computational approach of IRIS pioneers a novel avenue for biologists to delve into the intricate architecture of complex tissues, offering unparalleled opportunities to explore the dynamic processes shaping tissue structure during development and disease progression. Through characterizing refined tissue structures and elucidating their alterations during disease states, IRIS holds the potential to unveil mechanistic insights crucial for understanding and combating various diseases.” said Zhou.
The study, “ Accurate and efficient integrative reference-informed spatial domain detection for spatial transcriptomics ,” was supported by grants from the National Institutes of Health.
Contact Destiny Cook Senior Public Relations Specialist University of Michigan School of Public Health [email protected] 734-647-8650
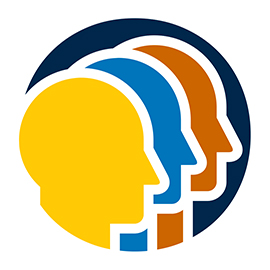
- Biostatistics
- Health Care
- Precision Health
Recent Posts
- Apple Hearing Study reveals prevalence of tinnitus
- Dolinoy, Peterson reappointed as department chairs at Michigan Public Health
- Housing crisis in Michigan: Report explores who owns, rents, has no home; examines racial gaps
What We’re Talking About
- Adolescent Health
- Air Quality
- Alternative Therapies
- Alumni News and Networking
- Child Health
- Chronic Disease
- Community Partnership
- Computational Epidemiology and Systems Modeling
- Disaster Relief
- Diversity Equity and Inclusion
- Engaged Learning
- Environmental Health
- Epidemiologic Science
- Epidemiology
- Epigenetics
- First Generation Students
- Food Policy
- Food Safety
- General Epidemiology
- Global Health Epidemiology
- Global Public Health
- Health Behavior and Health Education
- Health Care Access
- Health Care Management
- Health Care Policy
- Health Communication
- Health Disparities
- Health Informatics
- Health for Men
- Health for Women
- Heart Disease
- Hospital Administration
- Hospital and Molecular Epidemiology
- Immigration
- Industrial Hygiene
- Infectious Disease
- Internships
- LGBT Health
- Maternal Health
- Mental Health
- Mobile Health
- Occupational and Environmental Epidemiology
- Pain Management
- Pharmaceuticals
- Professional Development
- Reproductive Health
- Scholarships
- Sexual Health
- Social Epidemiology
- Social Media
- Student Organizations
- Urban Health
- Urban Planning
- Value-Based Care
- Water Quality
- What Is Public Health?
Information For
- Prospective Students
- Current Students
- Alumni and Donors
- Community Partners and Employers
- About Public Health
- How Do I Apply?
- Departments
- Findings magazine
Student Resources
- Career Development
- Certificates
- The Heights Intranet
- Update Contact Info
- Report Website Feedback
- History, Facts & Figures
- YSM Dean & Deputy Deans
- YSM Administration
- Department Chairs
- YSM Executive Group
- YSM Board of Permanent Officers
- FAC Documents
- Current FAC Members
- Appointments & Promotions Committees
- Ad Hoc Committees and Working Groups
- Chair Searches
- Leadership Searches
- Organization Charts
- Faculty Demographic Data
- Professionalism Reporting Data
- 2022 Diversity Engagement Survey
- State of the School Archive
- Faculty Climate Survey: YSM Results
- Strategic Planning
- Mission Statement & Process
- Beyond Sterling Hall
- COVID-19 Series Workshops
- Previous Workshops
- Departments & Centers
- Find People
- Biomedical Data Science
- Health Equity
- Inflammation
- Neuroscience
- Global Health
- Diabetes and Metabolism
- Policies & Procedures
- Media Relations
- A to Z YSM Lab Websites
- A-Z Faculty List
- A-Z Staff List
- A to Z Abbreviations
- Dept. Diversity Vice Chairs & Champions
- Dean’s Advisory Council on Lesbian, Gay, Bisexual, Transgender, Queer and Intersex Affairs Website
- Minority Organization for Retention and Expansion Website
- Office for Women in Medicine and Science
- Committee on the Status of Women in Medicine Website
- Director of Scientist Diversity and Inclusion
- Diversity Supplements
- Frequently Asked Questions
- Recruitment
- By Department & Program
- News & Events
- Executive Committee
- Aperture: Women in Medicine
- Self-Reflection
- Portraits of Strength
- Mindful: Mental Health Through Art
- Event Photo Galleries
- Additional Support
- MD-PhD Program
- PA Online Program
- Joint MD Programs
- How to Apply
- Advanced Health Sciences Research
- Clinical Informatics & Data Science
- Clinical Investigation
- Medical Education
- Visiting Student Programs
- Special Programs & Student Opportunities
- Residency & Fellowship Programs
- Center for Med Ed
- Organizational Chart
- Leadership & Staff
- Committee Procedural Info (Login Required)
- Faculty Affairs Department Teams
- Recent Appointments & Promotions
- Academic Clinician Track
- Clinician Educator-Scholar Track
- Clinican-Scientist Track
- Investigator Track
- Traditional Track
- Research Ranks
- Instructor/Lecturer
- Social Work Ranks
- Voluntary Ranks
- Adjunct Ranks
- Other Appt Types
- Appointments
- Reappointments
- Transfer of Track
- Term Extensions
- Timeline for A&P Processes
- Interfolio Faculty Search
- Interfolio A&P Processes
- Yale CV Part 1 (CV1)
- Yale CV Part 2 (CV2)
- Samples of Scholarship
- Teaching Evaluations
- Letters of Evaluation
- Dept A&P Narrative
- A&P Voting
- Faculty Affairs Staff Pages
- OAPD Faculty Workshops
- Leadership & Development Seminars
- List of Faculty Mentors
- Incoming Faculty Orientation
- Faculty Onboarding
- Past YSM Award Recipients
- Past PA Award Recipients
- Past YM Award Recipients
- International Award Recipients
- Nominations Calendar
- OAPD Newsletter
- Fostering a Shared Vision of Professionalism
- Academic Integrity
- Addressing Professionalism Concerns
- Consultation Support for Chairs & Section Chiefs
- Policies & Codes of Conduct
- First Fridays
- Fund for Physician-Scientist Mentorship
- Grant Library
- Grant Writing Course
- Mock Study Section
- Research Paper Writing
- Establishing a Thriving Research Program
- Funding Opportunities
- Join Our Voluntary Faculty
- Child Mental Health: Fostering Wellness in Children
- Faculty Resources
- Research by Keyword
- Research by Department
- Research by Global Location
- Translational Research
- Research Cores & Services
- Program for the Promotion of Interdisciplinary Team Science (POINTS)
- CEnR Steering Committee
- Experiential Learning Subcommittee
- Goals & Objectives
- Issues List
- Print Magazine PDFs
- Print Newsletter PDFs
- YSM Events Newsletter
- Social Media
- Patient Care
INFORMATION FOR
- Residents & Fellows
- Researchers
Single-Cell Genomics and Regulatory Networks for 388 Human Brains
Yale researchers who conducted the largest human brain analysis from a single-cell perspective hope their findings lead to better prediction of medicine that will target certain cells.
The study was led by co-corresponding authors Matthew Girgenti, PhD, assistant professor of psychiatry, and Mark Gerstein, PhD, Albert L. Williams Professor of Biomedical Informatics and professor of molecular biophysics & biochemistry, of computer science, and of statistics & data science at Yale School of Medicine. The findings were published in Science .
Single-cell genomics offers a powerful method to understand how genetic variants influence gene expression, especially across the numerous cell types in the human brain.
Moreover, it can potentially refine our understanding of the regulatory mechanisms underlying brain-related traits such as psychiatric disorders.
Girgenti and Gerstein participate in the PsychENCODE Consortium, which performed single-cell experiments (single-nucleus RNA-Seq, ATAC-Seq, and Multiome plus DNA sequencing) and computational analyses on samples from almost 400 human prefrontal-cortex samples of adults with a range of brain-related disorders such as schizophrenia, autism spectrum disorder, bipolar disorder, and Alzheimer’s disease, as well as controls.
These population-scale cohorts, with a wide range of brain phenotypes, are needed to infer significant associations among genetic variants and to develop models of regulation in specific cell types of the brain.
Integration of RNA expression and genotype data revealed >1.4M single-cell eQTLs (DNA positions that regulate gene expression), many of which were not seen in prior gene-expression datasets and a subset of which are involved in brain disorders. The researchers also found that expression patterns across cell types recapitulated the spatial architecture of neurons and enabled the identification of "dynamic eQTLs," with changes in regulatory effects across cortical layers.
The chromatin datasets in the resource allowed for identification of >550K single-cell cis regulatory elements, which were enriched at loci linked to brain-related traits. Combining gene expression, chromatin, and eQTL datasets, the researchers built cell-type-specific gene-regulatory networks and developed cell-to-cell communication networks, which highlighted differences in signaling pathways in the schizophrenic and bipolar disorder brain, including altered WNT and FGF signaling.
This integration allowed for accurate imputation of cell-type-specific expression and phenotype from genotype and allowed them to prioritize >250 risk genes and drug targets for brain-related disorders within specific cell types. Computationally simulated perturbation of individual genes led to predicted expression changes mirroring those for disease cases, increasing confidence in the predicted drug targets.
“This is the largest single cell multi-omic dataset of the human brain to date,” Girgenti said. “This population-scale resource for the human brain will help facilitate precision-medicine approaches for neuropsychiatric disorders, especially by prioritizing follow-up genes and drug targets linked to specific cell types.”
Funding was provided by the Simons Foundation and National Institute of Mental Health.
- Basic Science Research
Featured in this article
- Matthew Girgenti, PhD Assistant Professor of Psychiatry
- Mark Gerstein, PhD Albert L Williams Professor of Biomedical Informatics and Professor of Molecular Biophysics & Biochemistry, of Computer Science, and of Statistics & Data Science
- Open access
- Published: 03 June 2024
Genomic profiling informs therapies and prognosis for patients with hepatocellular carcinoma in clinical practice
- Mengqi Song 1 na1 ,
- Haoyue Cheng 2 na1 ,
- Hao Zou 1 na1 ,
- Kai Ma 1 na1 ,
- Lianfang Lu 1 na1 ,
- Qian Wei 1 na1 ,
- Zejiang Xu 1 na1 ,
- Zirui Tang 3 na1 ,
- Yuanzheng Zhang 4 na1 ,
- Yinan Wang 5 na1 &
- Chuandong Sun 1 na1
BMC Cancer volume 24 , Article number: 673 ( 2024 ) Cite this article
164 Accesses
1 Altmetric
Metrics details
Hepatocellular carcinoma (HCC) genomic research has discovered actionable genetic changes that might guide treatment decisions and clinical trials. Nonetheless, due to a lack of large-scale multicenter clinical validation, these putative targets have not been converted into patient survival advantages. So, it's crucial to ascertain whether genetic analysis is clinically feasible, useful, and whether it can be advantageous for patients. We sequenced tumour tissue and blood samples (as normal controls) from 111 Chinese HCC patients at Qingdao University Hospital using the 508-gene panel and the 688-gene panel, respectively. Approximately 95% of patients had gene variations related to targeted treatment, with 50% having clinically actionable mutations that offered significant information for targeted therapy. Immune cell infiltration was enhanced in individuals with TP53 mutations but decreased in patients with CTNNB1 and KMT2D mutations. More notably, we discovered that SPEN , EPPK1 , and BRCA2 mutations were related to decreased median overall survival, although MUC16 mutations were not. Furthermore, we found mutant MUC16 as an independent protective factor for the prognosis of HCC patients after curative hepatectomy. In conclusion, this study connects genetic abnormalities to clinical practice and potentially identifies individuals with poor prognoses who may benefit from targeted treatment or immunotherapy.
Peer Review reports
Introduction
Hepatocellular carcinoma (HCC) is a malignant and high heterogeneity tumour originating from the liver. It is the sixth most commonly diagnosed cancer and the third leading cause of cancer death worldwide in 2020 [ 1 ]. The incidence and mortality of HCC in China account for 45.3% and 47.1% of the world, respectively. The 5-year overall survival rate is currently only 14.1% [ 2 ]. The major risk factor for HCC is shifting from viral and alcoholic liver disease to obesity, type 2 diabetes, and nonalcoholic fatty liver disease [ 3 ]. The molecular pathogenesis of HCC involves the dysregulation of multiple signalling pathways, including Wnt/ß-Catenin, RAS/MAPK, PI3K/AKT/mTOR, TP53/cell cycle, IGFR, and MET, which is related to point mutations, copy number variations, epigenetic alternations, tumour suppressor inactivation and so on [ 4 ]. Hopefully, these discoveries will enable us to identify biomarkers for foretelling prognosis or responses to therapy.
The landscape of genetic alterations in HCC has a clear delineation, including the most prevalent mutations affecting TERT promoter (60%) [ 5 ], TP53 (12–48%) [ 6 , 7 , 8 ], and CTNNB1 (11–37%) [ 9 ]. The genetic alterations provide potential targets for treatment planning and prognostic assessment of HCC. About 25% of patients with HCC were detected potentially actionable mutations [ 10 ]. Sorafenib inhibits tumour growth and angiogenesis by targeting the RAF/MEK/ERK pathway and receptor tyrosine kinases [ 11 ]. PRI-724, a specific inhibitor targeting β-catenin, can be used to address HCC due to CTNNB1 mutation [ 12 ]. HCC caused by TERT promoter mutation can be intervened by using targeted drugs such as GX301, Imtelstat, and GV1001 [ 13 ]. Regarding prognostic markers, ARID1A , MLL , [ 14 ] LRP1B , and TP53 mutations, particularly the hotspot mutations R249S and V157F, are associated with poor prognosis for patients with HCC [ 15 , 16 ]. Song et al. found that TSC2 mutations were independently associated with early recurrence in HCC patients who underwent hepatectomy [ 17 ]. Nonetheless, these potential targets are yet to be translated into the actual survival benefits of patients due to the low mutation rates of most driver genes, no targeted drugs for oncogenic mutations, and the lack of large-scale multicenter clinical validation [ 13 ].
This study employed multigene sequencing panels targeting cancer driver genes involving key deregulated pathways in HCC, 175 drug-targeted genes, 23 immunotherapy-related genes, and 18 chemotherapy-related genes. Based on the real-world evidence from 111 patients with HCC, we aimed to determine the clinical viability and utility of genome analysis and whether patients can benefit from genomic profiling. Moreover, we identified mutations in four genes associated with survival, mutations in three other genes related to immune infiltration, and 292 novel potentially pathogenic mutations that could serve as potential targets for treatment decisions and prognostic assessment.
Materials and methods
Patient selection and clinical data collection.
This is a retrospective study. We screened 111 HCC patients with somatic mutations detected by targeted-capture sequencing. They were treated at the Affiliated Hospital of Qingdao University between October 2015 and November 2020. The follow-up was conducted up to January 15, 2022. Postoperative histopathological examinations confirmed clinical diagnoses. Clinicians gathered clinical data on the progression of the condition (Table S1). All patients were treated surgically. The extent of surgical resection is shown in Table S1. The study was authorized by the Ethics Committee of the Affiliated Hospital of Qingdao University (approval no. QYFYWZLL27327). The informed consent form was offered and signed by each patient. The experiment complied with the official key recommendations of the National Health and Family Planning Commission of China.
DNA extraction, library construction and sequencing
DNA was isolated from tumour tissue samples and whole blood samples (as normal controls) by QIAamp Fast DNA Tissue Kit and QIAamp DNA Blood mini Kit (QIAGEN), respectively. The concentration of DNA was determined using qubit fluorometry, and the integrity and purity were evaluated using agarose gel electrophoresis and the Qubit 2.0 fluorimeter (Thermo Fisher, USA). The targeted DNA sequence was then enriched and captured by two custom sequence capture probes (Nimblegen, USA) that targeted 7708 exons of 508 cancer-related genes and 10,176 exons of 688 cancer-related genes, respectively. Sequencing was performed on the MGISeq-2000 platform with a coverage depth of 1000 × for tumour tissue and 400 × for blood (MGI, Shenzhen, China).
The specific target gene list is in Table S2. There are 850 targeted genes captured by 688 and 508-gene panels, including 345 shared genes, 343 genes specific in the 688-gene panel, and 163 genes specific in the 508-gene panel. In the 508-gene panel, there were 135 genes involved in tumour signalling pathways, 89 associated with targeted therapy, and 16 associated with immunotherapy, 12 of which were associated with both targeted therapy and immunotherapy. The 688-gene panel included 452 genes involved in tumour signalling pathways, 11 associated with chemotherapy, 165 associated with targeted therapy, and 22 associated with immunotherapy, 15 of which involved both targeted therapy and immunotherapy.
Sequencing data analysis
SOAPnuke [ 18 ] was used to remove adapters and filter low-quality reads after obtaining raw sequencing data. Using bwa-mem2 ( https://github.com/bwa-mem2/bwa-mem2 ) [ 19 ], clean reads were mapped to the human reference genome (hg38). GATK (v 4.1.9.0) [ 20 ] was used to eliminate duplicates, identify somatic variants, and filter variants. The assessment of clinical importance and the prediction of the functional impact of sequence variants were done using ANNOVAR ( http://www.openbioinformatics.org/annovar/ ) [ 21 ]. Somatic variants were filtered based on the following criteria: i) variants with allele depth < 10 were excluded; ii) variants with allele frequencies < 0.1 were excluded; and iii) variants with population frequencies > 1% were excluded from the further investigation based on the Exome Aggregation Consortium dataset (ExAC http://exac.broadinstitute.org ), 1000 Genomes Project ( http://www.1000genomes.org/ ) [ 22 ]. ESP6500SI-V2 and avsnp150 databases. Additionally, actionable mutations were identified using OncoKB ( http://oncokb.org ) [ 23 ]. HCC driver genes were identified by IntOGen [ 24 ]. TIMER ( https://timer.comp-genomics.org/ ) [ 25 ] database offered tumour immune infiltration analysis.
Mutation statistics and visualization
Detailed information about mutations, including their features, distribution, and enrichment in oncogenic signalling pathways, was compiled and visualized using the R package maftools (version 2.8.05) [ 26 ]. We measured overall survival (OS) from the date of the first clinic visit to the last follow-up or death. Survival analysis was visualized using the R package survival (version 3.3.1) and survminer (version 0.4.9), using R package "jskm" to make landmark analysis. We used the Oviz-Bio platform to landscape the mutation type, mutated gene, mutation frequency, and clinical data about the patient [ 27 ]. Associations between driver genes and clinical features and the difference between the rates of affected cases in the TCGA cohort and this cohort were investigated using Fisher's exact test or the χ 2 test. P less than 0.05 was deemed significant.
Clinical characteristics of the patients with HCC
One hundred and eleven patients with HCC were included in this study (17 in female, 92 in male and two unknown). The median age was 53.5 years (range 33–78). According to TNM staging, the majority of patients (44.14%, 49/111) were in stage T1b. Over 40% of patients had small lesions with 2-5 cm tumour diameters, while 14.41% had large lesions with diameters greater than 10 cm. Lymph node metastasis was common in HCC and a key step in tumour metastasis. In our study, over one-quarter of patients developed lymph node metastasis. Furthermore, 30 patients (27.03%) experienced relapses, with the most common site of recurrence being intrahepatic (50%, 15/30). Over 85% of patients had one or more risk factors, including 88 patients with hepatitis B virus infection, 31 drinkers, and 17 with diabetes.
Concerning clinical indicators, the ASL/ALT ratios in serum were less than 0.8 in 29 patients and greater than 1.5 in 14 patients. The AFP level of 56 patients was above 25 ng/ml, and the CA19-9 level of 25 patients was more than or equal to 37 U/ml. In addition, most patients had normal CEA and CA125 levels (Table 1 and Table S1).
The spectrum of somatic mutations in genes
Of these 111 patients, 86 and 25 were detected mutations in 688 genes and 508 genes, respectively. In total, we detected 14,225 somatic mutations in all patients, including 1,125 SNVs, 1,789 insertions, and 1,017 deletions. Most mutations were located in the coding region, 32.1% in exonic regions, and 0.7% in splicing regions (Fig. 1 a). The two most common types of mutations were frameshift insertion and nonsynonymous SNV (Fig. 1 b). For SNV, C > T was the major mutant form (Fig. 1 c). In addition, there were 287 synonymous mutations, 9,148 variants in the intronic region, and 602 variants in the non-coding region, which were filtered out in the following analysis.
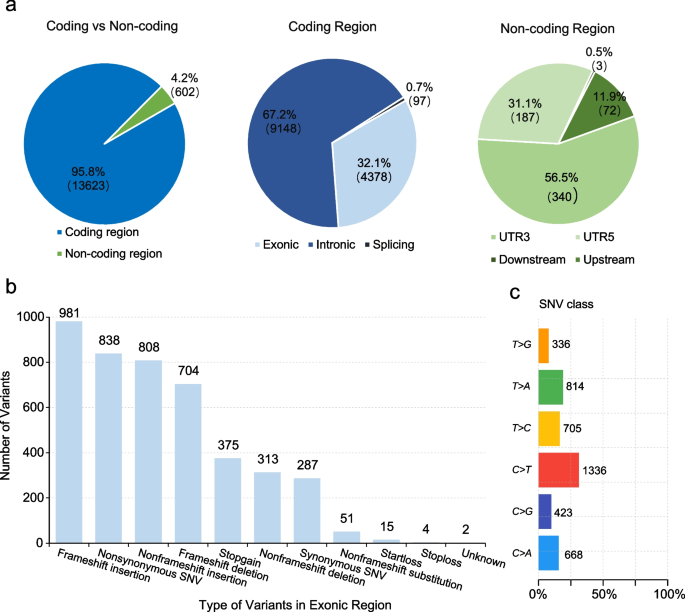
Results of variants calling in 111 patients with liver cancer. a Distribution of mutant sites in the coding and non-coding regions of DNA. b Types and numbers of variants. c Numbers of each SNV class
We identified 57 driver genes mutated in 111 patients in this study. About 34% (38/111) of patients harboured TERT promoter mutations. The frequently mutated driver genes were TP53 (50.45%, 56/111), KMT2D (36.05%, 31/86), FAT1 (30.23%, 26/86), FAT4 (29.07%, 25/86), and KMT2C (26.74%, 23/86; Fig. 2 ). The P53 structural domain was affected most frequently (Fig. 3 a). As driver genes, TP53 and CTNNB1 had no interactions with other genes, while Histone-lysine N-methyltransferase 2 ( KMT2 ) family genes had a synergistic effect with the FAT gene family, ARID1A/B , and GNAS (Fig. 3 b). Except for these driver genes, the top five high-frequency mutation genes were MUC16 (56.98%, 49/86), APOB (52.33%, 45/86), ZFHX4 (39.53%, 34/86), FAT3 (26.13%, 29/111), and EPPK1 (22.52%, 25/111; Figure S1). Epigenetic modifiers, such as ARID1A (21.62%, 24/111), ARID2 (17.12%, 19/111), and MLL genes, were also recurrently altered.
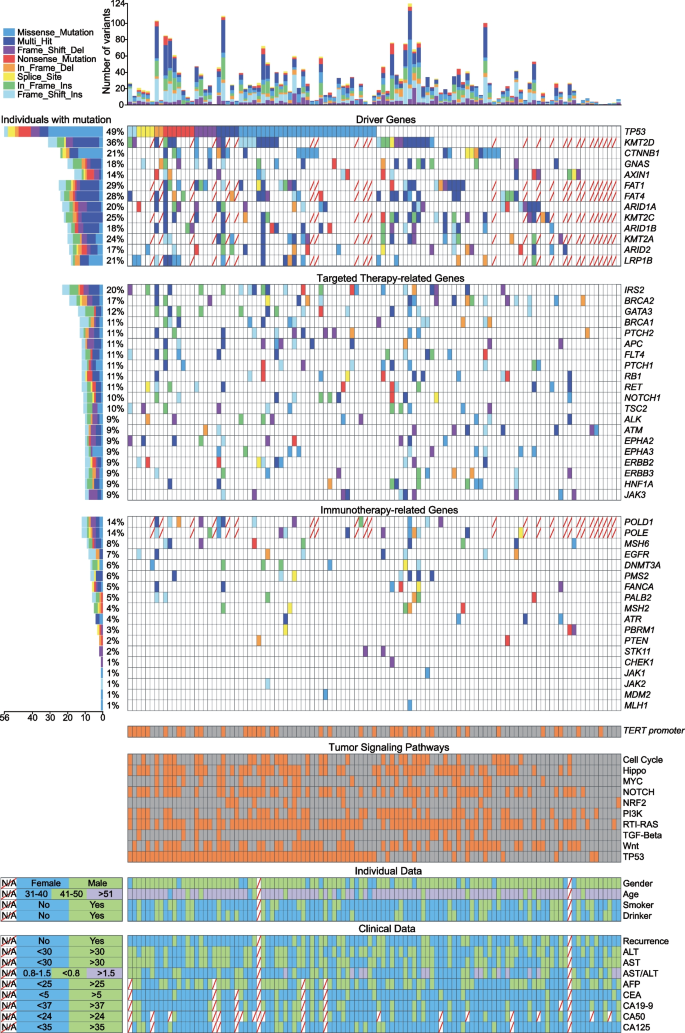
The landscape of frequently mutated genes of liver cancer. Significantly mutated genes in 111 patients. Above, the histogram shows the number of variants of each patient. Left, the percentages of patients with mutations. Diagonally indicated the information that was not available. Different colours correspond to different types of mutations. Variants annotated as Multhit_Hit are those genes that are mutated more than once in the same sample
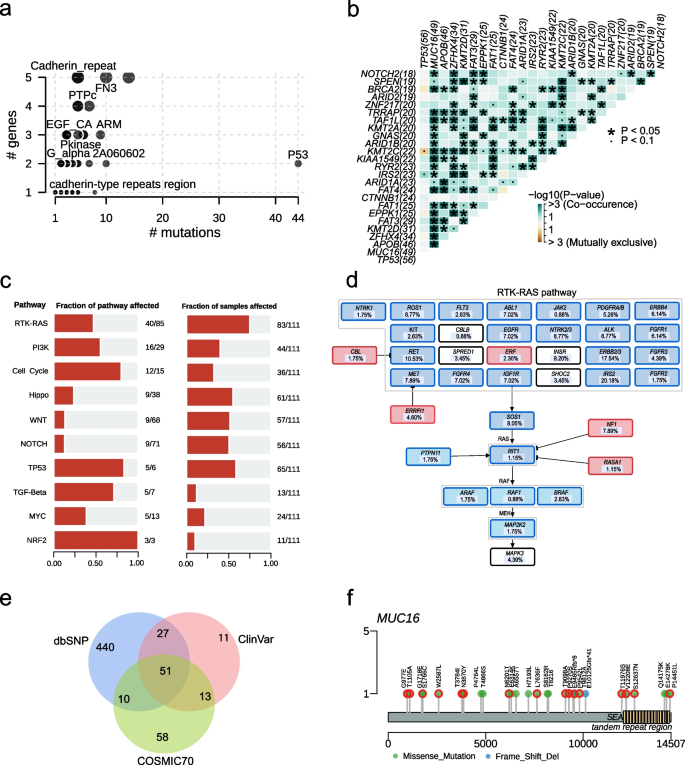
Clinical implications of mutations and domain and pathway enrichment analysis. a Frequently mutated Pfam protein domains in liver cancer. The bubble size is in proportion to the number of genes containing prominent display domains. b Somatic gene interactions. c Enrichment of known oncogenic signalling pathways. d Mutated genes in the RTK-RAS pathway. Tumor suppressor genes are in red, and oncogenes are in blue. e Venn plot of database-registered variants. f Location of the MUC16 mutations schematic. Red circles highlight novel missense mutations
The spectrum of somatic mutations in driver pathways
RTK-RAS (72.81%), TP53 (57.01%), Hippo (53.51%), Wnt/ß-Catenin (50%), NOTCH (49.12%), PI3K (38.60%), and Cell Cycle (31.58%) were activated frequently (Figs. 3 c and S1). We found 40 mutated genes involved in the RTK-RAS oncogenic signalling pathway, with five of the mutated genes being tumour suppressors and 35 being oncogenes. Oncogenes IRS2 (20.72%, 23/111), RET (10.81%, 12/111), and tumour suppressor genes NF1 (8.11%, 9/111) frequently mutated in the RTK-RAS pathway (Fig. 3 d). The mutations in KMT2D , CTNNB1 (21.62%, 24/111), GNAS (18.92%, 21/111), and AXIN1 (14.41%, 16/111) affected Wnt/ß-Catenin pathway and mutations in TP53 , ATM (9.01%, 10/111), RB1 (11.71%, 13/111), CDKN2A (8.11%, 9/111), and CDKN1A (5.41%, 6/111) altered cell cycle control (Figure S2). The oxidative stress pathway was altered in 9.65% of patients with mutations in NFE2L2 , KEAP1 , and CUL3 .
Clinical implications of mutations
We used the CLINVAR, dbSNP, COSMIC, and OncoKB databases to analyze the clinical significance of mutations. In our study, 528, 132, and 102 functional and meaningful variants have been registered in the dbSNP, CLINVAR, and COSMIC databases, respectively (Fig. 3 e). More importantly, 92.98% of patients had variants in targeted therapy-related genes (Fig. 2 ). Among them, 110 variants of 20 genes in 55 patients were reported as drug targets. In other words, 49.55% (55/111) of patients in this study had potentially actionable genomic alterations that required further clinical trials for HCC. For example, frameshift indels and stopain mutations in ARID1A and TSC1/2 were the targets of EZH2 inhibitors (Tazemetostat and GSK126) and mTOR inhibitors (ABI-009 and Everolimus), respectively. The nonsynonymous SNV, G3145C, in exon 21 of PIK3CA was the target of PIK3 inhibitors (Table S3). According to the follow-up results, 15 patients received targeted therapy, immunotherapy, and/or chemotherapy due to tumour recurrence (see Table S1 for treatment options). Three patients were found to carry TP53 mutations associated with sorafenib resistance. This provides evidence for the ultimate selection of lenvatinib. One patient who received sorafenib possessed CCND1 mutation that can result in sensitivity to sorafenib. Moreover, genotype CT of rs11598702 in NT5C2 suggested that one patient with this genotype may have a lower risk of toxic side effects with the use of gemcitabine. Ultimately, this patient also received chemotherapy with gemcitabine. The genetic testing results provided follow-up medication reference evidence for one-third of these 15 patients.
Three gene mutations have been found to be associated with immune infiltration. Based on the TIMER database, patients harbouring TP53 mutations had higher levels of B cells ( P = 0.039) and macrophages ( P = 0.023; Figure S3). In contrast, patients harboring CTNNB1 and KMT2D mutations had lower levels of CD8 + T cells ( P = 0.003 for CTNNB1 ; P = 0.004 for KMT2D ), macrophages ( P < 0.001; P = 0.004), neutrophils ( P < 0.001; P = 0.002) and dendritic cells ( P = 0.004; P = 0.007).
We performed pathogenic mutation prediction using 21 algorithms. For the 631 novel variants, at least five algorithms predicted that 292 variants were deleterious, of which we detected the most novel pathogenic mutations in MUC16 , following DNMT3A, UPF1, COL11A1 , and BIRC3 (Tables S4 and S5). The variants in MUC16 included 26 missense mutations and two frameshift deletions, of which 57.14% were novel pathogenic mutations. Most novel pathogenic mutations were located outside the SEA and tandem repeat region structural domains (Fig. 3 f).
Prognostic implications of genomic and clinical features
We compared survival between patients with or without mutations in genes. The median follow-up of 111 patients was 14.8 (IQR 0.1–84.0) months. We explored the relationship between genes with mutation frequencies greater than 15% and survival and found that alterations in four genes correlated with a poor or good prognosis. Patients harboring SPEN (18.3 vs. 15.0 months; Log-rank test, P = 0.024; Fig. 4 a), BRCA2 (20.3 vs. 15.1[months; P = 0.023; Fig. 4 b), and EPPK1 (18.3 vs.13.1 months; P = 0.044; Fig. 4 c) mutations had a shorter OS, while patients harboring MUC16 (18.7 vs. 15 months; P = 0.002, after landmark; Fig. 4 d) mutations had a longer OS. Mutations in BRCA2 (HR = 1.74), EPPK1 (HR = 1.59), and SPEN (HR = 2.02) were risk factors for patients with HCC, while MUC16 mutation (HR 0.32) was a protective factor.
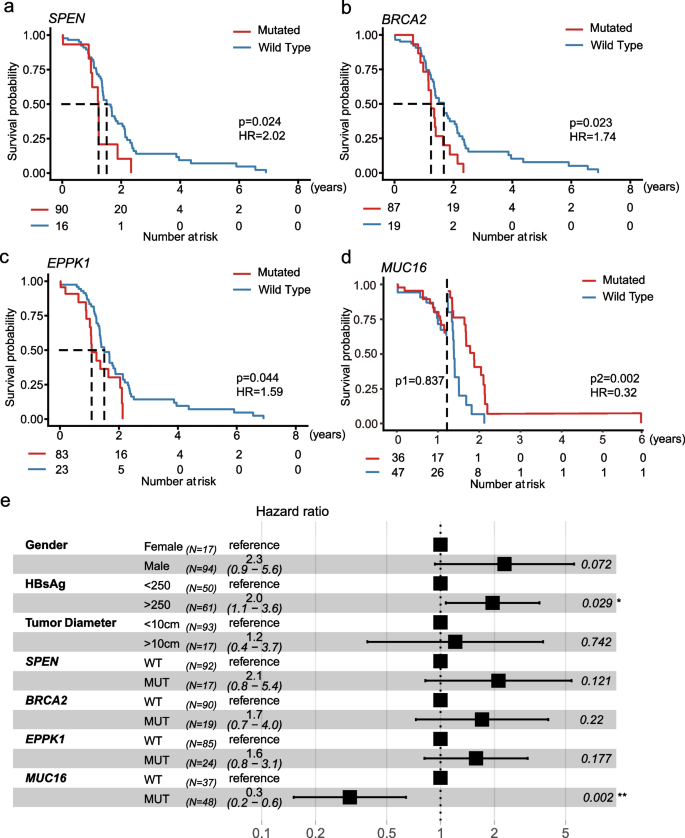
Survival analysis of mutated genes. a - d Survival analysis of SPEN , BRCA2 , EPPK1 , and MUC16 . The red line indicates the mutated group, and the blue line indicates the wild-type group. e Multivariate analysis of clinical prognostic factors of HCC. Log-rank test. HR: Hazard ratio
Moreover, we conducted univariate analysis considering clinical factors (e.g., gender, age, tumour size, HBsAg) and genes with mutation frequencies > 15%. Univariate analysis revealed that SPEN ( P = 0.028), BRCA2 ( P = 0.026), EPPK1 ( P = 0.047), and MUC16 ( P = 0.022) mutations were associated with the prognosis of HCC after hepatectomy. No clinical factor was found to be associated with prognosis. Next, we included the above four genes, as well as clinical factors (i.e., gender, HBsAg, and tumor size) in our multivariable regression analysis based on clinical significance and previous literature research findings. Positive HBsAg (HR = 2.3, 95% CI 1.2–4.6) was a risk factor for the prognosis of HCC patients after curative hepatectomy. Otherwise, mutant MUC16 (HR = 0.2, 95% CI 0.1–0.5) was a prognostic protective factor (Fig. 4 e).
Associations between driver genes and clinical characteristics
We explored the relationship between driver genes and clinical characteristics. LRP1B mutations were more common in smokers (55.88% vs 44.12%; χ 2 test, P = 0.03). Also, LRP1B mutations appeared to be associated with tumor diameter, which was more likely to be greater than 10 cm in patients with LRP1B mutations (68.75% vs. 31.25%; P = 0.01; Table S6).
Based on the real-world evidence from 111 Chinese patients, this study enhanced our comprehension of genome analysis's clinical viability and utility in HCC. Approximately 95% of patients had mutations in driver genes and/or pathways in HCC and 48.25% potentially actionable alterations, which yielded valuable information for targeted therapy or immunotherapy. TP53 , CTNNB1, and KMT2D mutations were related to immune cell infiltration. SPEN , EPPK1 , BRCA2 , and MUC16 mutations were associated with OS. More importantly, we identified mutant MUC16 as an independent protective factor for the prognosis of HCC patients after curative hepatectomy.
We revealed hotspot mutations in 111 Chinese patients with HCC, and the genomic mutation frequency of CTNNB1 (21.6% vs. 22.6%), AXIN1 (14.4% vs. 13.7%), RB1 (11.7% vs. 11.9%) in our cohort was not significantly different from previous reports [ 28 ]. However, the mutation frequency of TP53 (50.5% vs. 56.5%) and TERT (34% vs. 45.2%) in our cohort are lower to the cohort of Wang et al. [ 28 ]. We deduced that the difference in the number of patients with HBV infection may be one of the reasons. Some studies have reported that the mutation frequency of TP53 was higher in HCC caused by HBV infection than those without HBV infection [ 28 , 29 ]. In the cohort of Wang et al., 84.8% (140/165) of patients were positive for hepatitis B surface antigen (HBsAg), while in our cohort, only 54.1% (60/111) of patients were positive for HBsAg. Moreover, Wang et al. counted more mutation types than us, including gene amplification and fusion/rearrangement. Another reason might be that the criteria for patient enrollment are different. We screened HCC patients with somatic mutations and excluded patients without somatic mutations detected by targeted-capture sequencing. This might lead to a change in the frequency of gene mutations.
The immunological analysis revealed that mutations in TP53 were related to the level of immune infiltration. A report showed that HCC patients with mutant TP53 had significantly macrophage infiltration higher than those with wild-type TP53 [ 30 ], which coincided with our results. Loss or alteration of p53 caused by TP53 mutations can regulate the recruitment and activation of immune cells, resulting in the suppression or evasion of anti-tumor immune responses [ 31 , 32 ]. TP53 mutants can reprogram macrophages to tumour-associated macrophages (TAMs) [ 33 ] and were found to relate to the infiltration of TAMs into primary tumours [ 34 ]. One possible mechanism is that TP53 mutants lead to increased expression of the chemokine CCL2. CCL2, through the CCL2-CCR2 signalling axis, recruits TAMs to the tumour area [ 34 , 35 ]. Given the profound impact of the TP53 status of the cancer cell on the immune response, previous studies have found that TP53 mutations have the potential to serve as a predictive factor in guiding anti-PD-1/PD-L1 immunotherapy [ 36 , 37 ]. Because TP53 mutation significantly increased the expression of PD-1 and PD-L1. These studies focus on lung adenocarcinoma. High expression of PD-1 or PD-L1 has been consistently identified as a reliable predictor of a positive response to immunotherapy in various types of cancer. However, the association between TP53 and PD-L1 expression varies among cancer types [ 38 , 39 ]. Indeed, no definitive biomarkers have been identified to predict the efficacy of immunotherapy in HCC. Studies on PD-L1 expression in HCC are limited or have limited clinical value due to their low occurrence frequencies. The positive rate of PD-L1 expression in HCC tumour cells ranges from 10 to 20% [ 40 ], but objective responses have been observed in PD-1 monotherapy regardless of PD-L1 expression [ 41 , 42 ]. Therefore, more comprehensive and in-depth research is needed to determine whether TP53 mutants can serve as biomarkers for immune therapy in HCC.
Approximately 25% of potentially actionable mutations are found in HCC [ 6 ]. Unfortunately, the most prevalent drivers and trunk mutations, such as TERT promoter, AXIN1 , and TP53 mutations, are currently undruggable [ 43 ]. Nevertheless, recent studies have shown that there are already relevant, targeted drugs in Phase I to III trials [ 44 ]. For instance, CTNNB1 mutation-blocking drugs are expected to be useful for precision medicine [ 44 ]. A Japanese early clinical experience explored the effect of Atezolizumab plus Bevacizumab (ATZ/BV) in HCC patients harbouring CTNNB1 Mutation and found that ATZ/BV might improve the immunosuppressive tumour microenvironment caused by CTNNB1 mutation [ 45 ]. Patients harbouring CTNNB1 mutations are mainly manifested as immune rejection in the previous study [ 46 ] and our study. Thus, 25 patients harboring CTNNB1 mutations in our study might use ATZ/BV to improve immunosuppression. Additionally, Lim et al. conducted a phase II clinical study on treating RAS-mutant HCC using refametinib or refametinib plus sorafenib, which has shown promise [ 47 ]. This provided new potential treatment options for RAS-mutant HCC patients.
Notably, we found that mutant MUC16 was an independent protective factor for the prognosis of HCC patients after curative hepatectomy. MUC16 , encoding CA125, is the second most commonly mutated gene in HCC and has the most novel potential pathogenic variants in our study. Mutant MUC16 was also found to result in a better prognosis in gastric cancer and low-grade glioma [ 48 , 49 , 50 ]. The mechanisms underlying the favourable prognosis associated with MUC16 mutations remain unclear. In gastric cancer research, the group with MUC16 mutations showed increased infiltration of tumour-killing cells and decreased presence of immunosuppressive cells [ 48 ]. The infiltration of immune cells may significantly contribute to a better prognosis. However, we did not observe any differences in immune cell infiltration between the MUC16 mutation group and the wild-type group in our study on HCC. The mechanisms by which MUC16 mutation leads to a better prognosis may vary across different tumours. Therefore, MUC16 mutations may assist in HCC prognosis and should be further studied in this tumour type. Moreover, we observed that positive HBsAg was a risk factor for prognosis in multivariable analysis, but HBsAg did not show a significant association with prognosis in univariate analysis. A possible reason is that the effects of other factors are eliminated through multivariable analysis, revealing the independent effect of HBsAg on prognosis.
There are several limitations to our study. Firstly, targeted sequencing cannot detect changes in genes excluded from the assay, structure variation, and HBV/HCV integration. Secondly, sampling a single site cannot represent the whole tumour since HCC is highly heterogeneous. Thirdly, this study should be continued to collect more information on postoperative treatment and patient survival to link drug response and prognosis with molecular profiles. Fourthly, the average follow-up time of this study was not long enough (slightly over one year) to assess persistence of the impact of mutations. Despite these limitations, we identified novel potential immunotherapy efficacy and prognosis predictors.
Linking genomic alterations to clinical practice can identify patients who are likely to benefit from targeted therapies or immunotherapy and have a poor prognosis. We hope that our findings will make routine genetic testing more accessible in clinical practice and a research context.
Availability of data and materials
The dataset(s) supporting the conclusions of this article is(are) available in the China National Center for Bioinformation (CNCB) repository, [unique persistent identifier and hyperlink to dataset(s) in https://ngdc.cncb.ac.cn/gvm/getProjectDetail?project=GVM000754 ].
Abbreviations
- Hepatocellular carcinoma
Overall survival
Single nucleotide variants
Interquartile range
Hazard ratio
Atezolizumab plus Bevacizumab
Hepatitis B virus
Hepatitis C virus
Sung H, Ferlay J, Siegel RL, Laversanne M, Soerjomataram I, Jemal A, Bray F. Global cancer statistics 2020: GLOBOCAN estimates of incidence and mortality worldwide for 36 cancers in 185 countries. CA Cancer J Clin. 2021;71(3):209–49.
Article PubMed Google Scholar
McGlynn KA, Petrick JL, London WT. Global epidemiology of hepatocellular carcinoma: an emphasis on demographic and regional variability. Clin Liver Dis. 2015;19(2):223–38.
Article PubMed PubMed Central Google Scholar
Marengo A, Rosso C, Bugianesi E. Liver cancer: connections with obesity, fatty liver, and cirrhosis. Annu Rev Med. 2016;67:103–17.
Article CAS PubMed Google Scholar
Alqahtani A, Khan Z, Alloghbi A, Said Ahmed TS, Ashraf M, Hammouda DM: Hepatocellular Carcinoma: Molecular Mechanisms and Targeted Therapies. Medicina (Kaunas, Lithuania) 2019, 55(9):526.
Nault JC, Mallet M, Pilati C, Calderaro J, Bioulac-Sage P, Laurent C, Laurent A, Cherqui D, Balabaud C, Zucman-Rossi J. High frequency of telomerase reverse-transcriptase promoter somatic mutations in hepatocellular carcinoma and preneoplastic lesions. Nat Commun. 2013;4:2218.
Schulze K, Imbeaud S, Letouzé E, Alexandrov LB, Calderaro J, Rebouissou S, Couchy G, Meiller C, Shinde J, Soysouvanh F, et al. Exome sequencing of hepatocellular carcinomas identifies new mutational signatures and potential therapeutic targets. Nat Genet. 2015;47(5):505–11.
Article CAS PubMed PubMed Central Google Scholar
Fujimoto A, Furuta M, Shiraishi Y, Gotoh K, Kawakami Y, Arihiro K, Nakamura T, Ueno M, Ariizumi S, Nguyen HH, et al. Whole-genome mutational landscape of liver cancers displaying biliary phenotype reveals hepatitis impact and molecular diversity. Nat Commun. 2015;6:6120.
Guichard C, Amaddeo G, Imbeaud S, Ladeiro Y, Pelletier L, Maad IB, Calderaro J, Bioulac-Sage P, Letexier M, Degos F, et al. Integrated analysis of somatic mutations and focal copy-number changes identifies key genes and pathways in hepatocellular carcinoma. Nat Genet. 2012;44(6):694–8.
de La Coste A, Romagnolo B, Billuart P, Renard CA, Buendia MA, Soubrane O, Fabre M, Chelly J, Beldjord C, Kahn A, et al. Somatic mutations of the beta-catenin gene are frequent in mouse and human hepatocellular carcinomas. Proc Natl Acad Sci U S A. 1998;95(15):8847–51.
Llovet JM, Kelley RK, Villanueva A, Singal AG, Pikarsky E, Roayaie S, Lencioni R, Koike K, Zucman-Rossi J, Finn RS. Hepatocellular carcinoma. Nat Rev Dis Primers. 2021;7(1):6.
Abdelgalil AA, Alkahtani HM, Al-Jenoobi FI. Sorafenib. Profiles Drug Subst Excip Relat Methodol. 2019;44:239–66.
Lenz HJ, Kahn M. Safely targeting cancer stem cells via selective catenin coactivator antagonism. Cancer Sci. 2014;105(9):1087–92.
Zucman-Rossi J, Villanueva A, Nault JC, Llovet JM. Genetic landscape and biomarkers of hepatocellular carcinoma. Gastroenterology. 2015;149(5):1226–1239.e1224.
Li L, Rao X, Wen Z, Ding X, Wang X, Xu W, Meng C, Yi Y, Guan Y, Chen Y, et al. Implications of driver genes associated with a high tumor mutation burden identified using next-generation sequencing on immunotherapy in hepatocellular carcinoma. Oncol Lett. 2020;19(4):2739–48.
CAS PubMed PubMed Central Google Scholar
Woo HG, Wang XW, Budhu A, Kim YH, Kwon SM, Tang ZY, Sun Z, Harris CC, Thorgeirsson SS. Association of TP53 mutations with stem cell-like gene expression and survival of patients with hepatocellular carcinoma. Gastroenterology. 2011;140(3):1063–70.
Liu F, Hou W, Liang J, Zhu L, Luo C. LRP1B mutation: a novel independent prognostic factor and a predictive tumor mutation burden in hepatocellular carcinoma. J Cancer. 2021;12(13):4039–48.
Song K, He F, Xin Y, Guan G, Huo J, Zhu Q, Fan N, Guo Y, Zang Y, Wu L. TSC2 mutations were associated with the early recurrence of patients with HCC underwent hepatectomy. Pharmgenomics Pers Med. 2021;14:269–78.
PubMed PubMed Central Google Scholar
Chen Y, Chen Y, Shi C, Huang Z, Zhang Y, Li S, Li Y, Ye J, Yu C, Li Z, et al. SOAPnuke: a MapReduce acceleration-supported software for integrated quality control and preprocessing of high-throughput sequencing data. GigaScience. 2018;7(1):1–6.
Md V, Misra S, Li H, Aluru S. Efficient architecture-aware acceleration of BWA-MEM for multicore systems. In: 2019 IEEE International Parallel and Distributed Processing Symposium (IPDPS). 2019. p. 2314–24.
Google Scholar
McKenna A, Hanna M, Banks E, Sivachenko A, Cibulskis K, Kernytsky A, Garimella K, Altshuler D, Gabriel S, Daly M, et al. The Genome Analysis Toolkit: a MapReduce framework for analyzing next-generation DNA sequencing data. Genome Res. 2010;20(9):1297–303.
Wang K, Li M, Hakonarson H. ANNOVAR: functional annotation of genetic variants from high-throughput sequencing data. Nucleic Acids Res. 2010;38(16):e164.
Auton A, Brooks LD, Durbin RM, Garrison EP, Kang HM, Korbel JO, Marchini JL, McCarthy S, McVean GA, Abecasis GR. A global reference for human genetic variation. Nature. 2015;526(7571):68–74.
Chakravarty D, Gao J, Phillips SM, Kundra R, Zhang H, Wang J, Rudolph JE, Yaeger R, Soumerai T, Nissan MH, et al. OncoKB: a precision oncology knowledge base. JCO Precis Oncol. 2017;2017:PO.17.00011.
PubMed Google Scholar
Gonzalez-Perez A, Perez-Llamas C, Deu-Pons J, Tamborero D, Schroeder MP, Jene-Sanz A, Santos A, Lopez-Bigas N. IntOGen-mutations identifies cancer drivers across tumor types. Nat Methods. 2013;10(11):1081–2.
Li T, Fan J, Wang B, Traugh N, Chen Q, Liu JS, Li B, Liu XS. TIMER: a web server for comprehensive analysis of tumor-infiltrating immune cells. Can Res. 2017;77(21):e108–10.
Article CAS Google Scholar
Mayakonda A, Lin DC, Assenov Y, Plass C, Koeffler HP. Maftools: efficient and comprehensive analysis of somatic variants in cancer. Genome Res. 2018;28(11):1747–56.
Jia W, Li H, Li S, Chen L, Li SC. Oviz-Bio: a web-based platform for interactive cancer genomics data visualization. Nucleic Acids Res. 2020;48(W1):W415–w426.
Wang S, Shi H, Liu T, Li M, Zhou S, Qiu X, Wang Z, Hu W, Guo W, Chen X, et al. Mutation profile and its correlation with clinicopathology in Chinese hepatocellular carcinoma patients. Hepatobiliary Surg Nutr. 2021;10(2):172–9.
Amaddeo G, Cao Q, Ladeiro Y, Imbeaud S, Nault JC, Jaoui D, Gaston Mathe Y, Laurent C, Laurent A, Bioulac-Sage P, et al. Integration of tumour and viral genomic characterizations in HBV-related hepatocellular carcinomas. Gut. 2015;64(5):820–9.
El-Arabey AA, Abdalla M, Abd-Allah AR. SnapShot: TP53 status and macrophages infiltration in TCGA-analyzed tumors. Int Immunopharmacol. 2020;86:106758.
Carlsen L, Zhang S, Tian X, De La Cruz A, George A, Arnoff TE, El-Deiry WS. The role of p53 in anti-tumor immunity and response to immunotherapy. Front Mol Biosci. 2023;10:1148389.
Blagih J, Buck MD, Vousden KH. p53, cancer and the immune response. J Cell Sci. 2020;133(5):jcs237453.
Cooks T, Pateras IS, Jenkins LM, Patel KM, Robles AI, Morris J, Forshew T, Appella E, Gorgoulis VG, Harris CC. Mutant p53 cancers reprogram macrophages to tumor supporting macrophages via exosomal miR-1246. Nat Commun. 2018;9(1):771.
Walton J, Blagih J, Ennis D, Leung E, Dowson S, Farquharson M, Tookman LA, Orange C, Athineos D, Mason S, et al. CRISPR/Cas9-mediated Trp53 and Brca2 knockout to generate improved murine models of ovarian high-grade serous carcinoma. Can Res. 2016;76(20):6118–29.
Tesei A, Arienti C, Bossi G, Santi S, De Santis I, Bevilacqua A, Zanoni M, Pignatta S, Cortesi M, Zamagni A, et al. TP53 drives abscopal effect by secretion of senescence-associated molecular signals in non-small cell lung cancer. J Exp Clin Cancer Res. 2021;40(1):89.
Dong ZY, Zhong WZ, Zhang XC, Su J, Xie Z, Liu SY, Tu HY, Chen HJ, Sun YL, Zhou Q, et al. Potential Predictive Value of TP53 and KRAS Mutation Status for Response to PD-1 Blockade Immunotherapy in Lung Adenocarcinoma. Clin Cancer Res. 2017;23(12):3012–24.
Biton J, Mansuet-Lupo A, Pécuchet N, Alifano M, Ouakrim H, Arrondeau J, Boudou-Rouquette P, Goldwasser F, Leroy K, Goc J, et al. TP53, STK11, and EGFR mutations predict tumor immune profile and the response to anti-PD-1 in lung adenocarcinoma. Clin Cancer Res. 2018;24(22):5710–23.
Cortez MA, Ivan C, Valdecanas D, Wang X, Peltier HJ, Ye Y, Araujo L, Carbone DP, Shilo K, Giri DK, et al. PDL1 Regulation by p53 via miR-34. J Natl Cancer Inst 2016;108(1):djv303.
Yadollahi P, Jeon YK, Ng WL, Choi I. Current understanding of cancer-intrinsic PD-L1: regulation of expression and its protumoral activity. BMB Rep. 2021;54(1):12–20.
Pinato DJ, Mauri FA, Spina P, Cain O, Siddique A, Goldin R, Victor S, Pizio C, Akarca AU, Boldorini RL, et al. Clinical implications of heterogeneity in PD-L1 immunohistochemical detection in hepatocellular carcinoma: the Blueprint-HCC study. Br J Cancer. 2019;120(11):1033–6.
El-Khoueiry AB, Sangro B, Yau T, Crocenzi TS, Kudo M, Hsu C, Kim T-Y, Choo S-P, Trojan J, Welling TH 3rd, et al. Nivolumab in patients with advanced hepatocellular carcinoma (CheckMate 040): an open-label, non-comparative, phase 1/2 dose escalation and expansion trial. Lancet. 2017;389(10088):2492–502.
Zhu AX, Finn RS, Edeline J, Cattan S, Ogasawara S, Palmer D, Verslype C, Zagonel V, Fartoux L, Vogel A, et al. Pembrolizumab in patients with advanced hepatocellular carcinoma previously treated with sorafenib (KEYNOTE-224): a non-randomised, open-label phase 2 trial. Lancet Oncol. 2018;19(7):940–52.
Llovet JM, Pinyol R, Kelley RK, El-Khoueiry A, Reeves HL, Wang XW, Gores GJ, Villanueva A. Molecular pathogenesis and systemic therapies for hepatocellular carcinoma. Nat Cancer. 2022;3(4):386–401.
Llovet JM, Montal R, Sia D, Finn RS. Molecular therapies and precision medicine for hepatocellular carcinoma. Nat Rev Clin Oncol. 2018;15(10):599–616.
Ogawa K, Kanzaki H, Chiba T, Ao J, Qiang N, Ma Y, Zhang J, Yumita S, Ishino T, Unozawa H, et al. Effect of atezolizumab plus bevacizumab in patients with hepatocellular carcinoma harboring CTNNB1 mutation in early clinical experience. J Cancer. 2022;13(8):2656–61.
Shimada S, Mogushi K, Akiyama Y, Furuyama T, Watanabe S, Ogura T, Ogawa K, Ono H, Mitsunori Y, Ban D, et al. Comprehensive molecular and immunological characterization of hepatocellular carcinoma. EBioMedicine. 2019;40:457–70.
Lim HY, Merle P, Weiss KH, Yau T, Ross P, Mazzaferro V, Blanc J-F, Ma YT, Yen CJ, Kocsis J, et al. Phase II Studies with Refametinib or Refametinib plus Sorafenib in Patients with RAS-Mutated Hepatocellular Carcinoma. Clin Cancer Res. 2018;24(19):4650–61.
Huang YJ, Cao ZF, Wang J, Yang J, Wei YJ, Tang YC, Cheng YX, Zhou J, Zhang ZX. Why MUC16 mutations lead to a better prognosis: a study based on the cancer genome atlas gastric cancer cohort. World J Clin Cases. 2021;9(17):4143–58.
Zhang F, Li X, Chen H, Guo J, Xiong Z, Yin S, Jin L, Chen X, Luo D, Tang H et al. Mutation of MUC16 is associated with tumor mutational burden and lymph node metastasis in patients with gastric cancer. Front Med (Lausanne). 2022;9:836892.
Ferrer VP. MUC16 mutation is associated with tumor grade, clinical features, and prognosis in glioma patients. Cancer Genet. 2023;270–271:22–30.
Download references
This work was supported by the grant of Peking University Shenzhen Hospital Foundation (Grant No.KYQD2022132).
Author information
Mengqi Song and Haoyue Cheng contributed equally to this work.
Authors and Affiliations
Department of Hepatopancreatobiliary Surgery, The Affiliated Hospital of Qingdao University, Qingdao, Shandong, China
Mengqi Song, Hao Zou, Kai Ma, Lianfang Lu, Qian Wei, Zejiang Xu & Chuandong Sun
Department of Pathology, Beijing Chaoyang Hospital, Capital Medical University, Beijing, China
Haoyue Cheng
Software Engineering, Northeastern University, Shenyang, Liaoning, China
Collage of Medicine and Biological Information Engineering, Northeastern University, Shenyang, Liaoning, China
Yuanzheng Zhang
Department of Obstetrics and Gynecology, Peking University Shenzhen Hospital, Shenzhen, Guangdong, China
You can also search for this author in PubMed Google Scholar
Contributions
C.D.S and Y.N.W participated in the study conception and design. M.Q.S, H.Y.C and Z.R.T have performed analysis and interpretation of the data. M.Q.S, H.Z, K.M, L.F.L, Q.W and Z.J.X were involved in data analysis and interpretation. M.Q.S, H.Y.C, Z.R.T and Y.Z.Z prepared the manuscript and figures. H.Y.C conducted the statistical analysis. Y.N.W and C.D.S edited, critically read, and revised the manuscript. All authors contributed to the article and approved the submitted version.
Corresponding authors
Correspondence to Yinan Wang or Chuandong Sun .
Ethics declarations
Ethics approval and consent to participate.
The study was approved by the Ethics Committee of the Affiliated Hospital of Qingdao University (approval no. QYFYWZLL27327). The patients/participants provided their written informed consent to participate in this study.
Consent for publication
Not applicable.
Competing interests
The authors declare no competing interests.
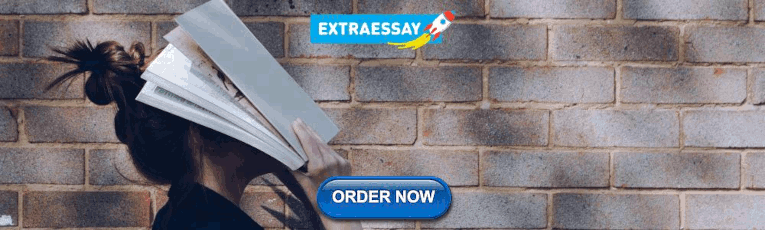
Additional information
Publisher’s note.
Springer Nature remains neutral with regard to jurisdictional claims in published maps and institutional affiliations.
Supplementary Information
12885_2024_12407_moesm1_esm.docx.
Additional file 1: Figure S1. Mutated genes in the TP53 pathway. Figure S2. The landscape of frequently mutated genes and chemotherapy-related genes in 111 patients. Figure S3. Association of immune infiltration with mutant genes.
12885_2024_12407_MOESM2_ESM.xlsx
Additional file 2: Table S1. Clinical information of 111 patients with Hepatocellular carcinoma. Table S2. List of the targeted genes. Table S3. Overview of the clinically actionable genomic alterations. Table S4. List of genes that have been detected by at least five software programs with new deleterious mutations. Table S5. The pathogenicity prediction results of the variants. Table S6. Thirteen mutation statuses stratified by clinical characteristics.
Rights and permissions
Open Access This article is licensed under a Creative Commons Attribution 4.0 International License, which permits use, sharing, adaptation, distribution and reproduction in any medium or format, as long as you give appropriate credit to the original author(s) and the source, provide a link to the Creative Commons licence, and indicate if changes were made. The images or other third party material in this article are included in the article's Creative Commons licence, unless indicated otherwise in a credit line to the material. If material is not included in the article's Creative Commons licence and your intended use is not permitted by statutory regulation or exceeds the permitted use, you will need to obtain permission directly from the copyright holder. To view a copy of this licence, visit http://creativecommons.org/licenses/by/4.0/ . The Creative Commons Public Domain Dedication waiver ( http://creativecommons.org/publicdomain/zero/1.0/ ) applies to the data made available in this article, unless otherwise stated in a credit line to the data.
Reprints and permissions
About this article
Cite this article.
Song, M., Cheng, H., Zou, H. et al. Genomic profiling informs therapies and prognosis for patients with hepatocellular carcinoma in clinical practice. BMC Cancer 24 , 673 (2024). https://doi.org/10.1186/s12885-024-12407-2
Download citation
Received : 31 August 2023
Accepted : 21 May 2024
Published : 03 June 2024
DOI : https://doi.org/10.1186/s12885-024-12407-2
Share this article
Anyone you share the following link with will be able to read this content:
Sorry, a shareable link is not currently available for this article.
Provided by the Springer Nature SharedIt content-sharing initiative
- Capture-based targeted sequencing
- Actionable genetic alterations
- Mutation landscape
ISSN: 1471-2407
- Submission enquiries: [email protected]
- General enquiries: [email protected]
- Search Menu
- Sign in through your institution
- Advance Articles
- Editor's Choice
- Information for authors
- Submission Site
- Open Access Options
- Why publish with the journal
- About DNA Research
- About the Kazusa DNA Research Institute
- Editorial Board
- Advertising and Corporate Services
- Journals Career Network
- Self-Archiving Policy
- Dispatch Dates
- Journals on Oxford Academic
- Books on Oxford Academic

Article Contents
Introduction, materials and methods, acknowledgements, conflict of interest, author contributions, data availability.
- < Previous
High-integrity Pueraria montana var. lobata genome and population analysis revealed the genetic diversity of Pueraria genus

Xuan-Zhao Huang, Shao-Da Gong and Xiao-hong Shang contributed equally to this work.
- Article contents
- Figures & tables
- Supplementary Data
Xuan-Zhao Huang, Shao-Da Gong, Xiao-hong Shang, Min Gao, Bo-Yuan Zhao, Liang Xiao, Ping-li Shi, Wen-dan Zeng, Sheng Cao, Zheng-dan Wu, Jia-Ming Song, Ling-Ling Chen, Hua-bing Yan, High-integrity Pueraria montana var. lobata genome and population analysis revealed the genetic diversity of Pueraria genus, DNA Research , Volume 31, Issue 3, June 2024, dsae017, https://doi.org/10.1093/dnares/dsae017
- Permissions Icon Permissions
Pueraria montana var. lobata ( P. lobata ) is a traditional medicinal plant belonging to the Pueraria genus of Fabaceae family. Pueraria montana var . thomsonii ( P. thomsonii ) and Pueraria montana var. montana ( P. montana ) are its related species. However, evolutionary history of the Pueraria genus is still largely unknown. Here, a high-integrity, chromosome-level genome of P. lobata and an improved genome of P. thomsonii were reported. It found evidence for an ancient whole-genome triplication and a recent whole-genome duplication shared with Fabaceae in three Pueraria species. Population genomics of 121 Pueraria accessions demonstrated that P. lobata populations had substantially higher genetic diversity, and P. thomsonii was probably derived from P. lobata by domestication as a subspecies. Selection sweep analysis identified candidate genes in P. thomsonii populations associated with the synthesis of auxin and gibberellin, which potentially play a role in the expansion and starch accumulation of tubers in P. thomsonii . Overall, the findings provide new insights into the evolutionary and domestication history of the Pueraria genome and offer a valuable genomic resource for the genetic improvement of these species.
Pueraria (2 n = 2 x = 22) is a genus of perennial vines in the Fabaceae family comprising more than 20 species native to Asia. Pueraria montana var. lobata ( P. lobata ) ( Fig. 1a ) with its related species, Pueraria montana var. thomsonii ( P. thomsonii ) and Pueraria montana var. montana ( P. montana ), are focal of the study in Pueraria. And their nutritional value, medicinal components, and morphological characteristics exhibited widely variations. 1 P. lobata and P. thomsonii are traditional Chinese medicinal materials with a long history of medicinal use in China, first recorded in the ‘Shen Nong’s Herbal Book’. Their roots are rich in puerarin, daidzein, genistein, and other flavonoids or isoflavone secondary metabolites. 2 , 3 The difference in morphological characteristics is that the roots of P. thomsonii are more expanded than those of P. lobata . However, P. montana is a variant with no expanded roots, exhibiting great difference with P. lobata and P. thomsonii ( Fig. 1b ). The root of P. montana does not contain puerarin, so it has low nutritional and medicinal value but strong resistance and can survive at low temperature. 4

Morphology and genome features of the P. lobata . (a) Morphological characteristics of P. lobata . (left, vines and leaves; middle, flower; right, root). (b) Evolutionary relationships of P. lobata , P. thomsonii , and P. montana with their divergence time (Mya, million years ago). (c) The landscape of genome features of P. lobata and P. thomsonii . (I) length of individual chromosomes; (II) GC contents; (III) repetitive sequences density; (IV) gene density; (V) DNA-Transposable elements density; (VI) long terminal repeat (LTR) density; (VII) inner lines indicate syntenic blocks.
P. montana could be differentiated from P. thomsonii and P. lobata based on significant differences in root morphology and nutrient content. However, it is challenging to distinguish P. thomsonii and P. lobata by traditional morphological characteristics or nutrient content. 5 , 6 The need for a precise classification of P. thomsonii and P. lobata significantly limits their potential value in medicine and nutrition. 7 In the past, DNA molecular marker technology and chloroplast genomic information have aided in classifying and utilizing Pueraria species, especially in distinguishing between P. thomsonii and P. lobata . 8–10 However, genome information has more significant advantages for addressing these issues, and high-quality genome assembly will provide the basis for detecting genomic variation and exploring evolutionary history. Advances in sequencing technology and reduced costs have enabled large-scale genome sequencing of plant populations, promoting plant research significantly. 11 , 12 Population genomic information can reveal populations’ genetic composition and diversity, effectively solve the challenging problem of classifying closely related species, better elucidate the evolutionary relationships among different subgroups and individuals, and provide critical evidence for species’ domestication and origin history. 13–15 Currently, research on the genomics and population genetics of Pueraria species needs to be developed more. Therefore, integrating these approaches to achieve a more precise classification of Pueraria species and exploit their potential genetic resources is of great significance.
The high-quality genomes of P. thomsonii and P. montana at the chromosome level were obtained in the previous study, and multi-omics analysis was used to comprehensively explore the biosynthetic pathways of critical secondary metabolites, such as isoflavones and puerarin in P. thomsonii . 4 , 16 Additionally, a comparison of gene families between P. thomsonii and P. montana was conducted to elucidate the underlying reasons behind distinct metabolic and cold-adaptation characteristics exhibited by these two species. 4 However, the more comprehensive comparative analysis of the genomes, phylogeny, and population genetics among the three Pueraria species has yet to be elucidated.
In this study, a high-quality chromosome-level genome of P. lobata was constructed using a combination of PacBio reads, paired-end reads, ONT reads, and Hi–C data. In addition, it generated an updated genome of P. thomsonii by removing the redundant haploid contigs. Whole-genome duplication (WGD) events, gene family expansion, and contraction occurring in Pueraria plants were explored through comparative genomics analysis. Whole-genome sequencing of 121 Pueraria accessions was used to address the evolutionary relationship among the species and identify selective signatures during domestication in P. thomsonii population. These results provide novel insights into the evolutionary history of the Pueraria genome and offer comprehensive information for breeding in the future.
Sample preparation and sequencing
The P. lobata accession ‘YG-19’ was selected for genome sequencing, having been grown in Luojiao Village, Guiping City, Guangxi Province. Genomic DNA was isolated from young leaves using a standard CTAB protocol and its integrity was verified by agarose gel electrophoresis. The genome sequencing was performed using Single-molecule real-time (SMRT) PacBio sequencing libraries, prepared using the SMRTbell Express Template Prep Kit 2.0 by Pacific Bioscience recommendation. The resulting libraries were sequenced on a PacBio Sequel II platform. Additionally, paired-end reads sequencing was performed using libraries selected for PE150 and sequenced using a BGIseq 500 sequencer. For ONT and Hi–C sequencing, the ONT and Hi–C libraries were prepared following the manufacturer’s instructions and the genome was sequenced on the Nanopore PromethION and BGI MGISEQ-2000 using standard protocols.
Genome assembly and quality assessment
For the P. lobata genome, a de novo assembly strategy was adopted. Short-read paired-end sequencing reads were used to count the total number and frequency of k-mer ( k = 17) using Jellyfish software (v2.2.9), 17 and then Genomescope (v1.0) 18 was used to predict genomic features. Pacbio long reads were preliminarily corrected and assembled with NextDenovo (v2.5.0, https://github.com/Nextomics/NextDenovo ), and both long reads and short reads were used to polish the resulting contig using NextPolish (v1.4.0). 19 We used 3D-DNA pipeline 20 to correct the resulting contigs with the Hi–C data, and then the PurgeHaplotigs software (v1.1.2) 21 was used to remove the redundant contigs. The final draft genome was assembled into scaffolds with Hi–C data using Juicer 22 and 3D-DNA pipeline. 20 These scaffolds were then manually curated using Juicebox. 23 Genomic sequence gaps were filled using TGS-gapcloser (v1.1.1) 24 and LR-gapcloser 25 in combination with ONT ultra-long reads (> 10 kb). Finally, Racon (v1.5.0) 26 with Pacbio reads and Pilon (v1.23) 27 with BGI short-reads were used to do a final error correction of the genomic gaps on the padding.
The quality of the P. thomsonii genome was improved by removing redundant sequences resulting from the high heterozygosity. The genome file was obtained from a previous study, 16 and genome size and heterozygosity were estimated by using the same process as applied to P. lobata genome. Redundant sequences were removed using Purge_Dups software (v1.2.5). 28 Finally, this updated version P. thomsonii genome was employed for subsequent analyses and evaluations.
The genome completeness was evaluated using BUSCO (v5.3.0) which contained 2,326 genes in the eudicots_odb10 database. 29 Short reads were mapped with BWA software (v0.7.17), 30 Pacbio long reads and ONT reads with minimap2 31 to assess the accuracy of the assembled genome. Picard software (v2.27.1) ( https://broadinstitute.github.io/picard ) was used to remove PCR duplicates in the short-read alignment, and BCFtools commands mpileup and call (v1.9) 32 were then used for SNV calling.
Genome annotation
The repetitive sequences in the P. lobata and P. thomsonii genomes were identified by using a combination of homologous similarity and de novo prediction. In the homology-based annotation step, repetitive sequences were predicted using RepeatMasker (v4.0.9) 33 and RepeatProteinMasker (v4.0.9) by searching against the RepBase library ( http://www.girinst.org/repbase ). During the de novo step, de novo repeat libraries were constructed based on the genome sequences using the de novo prediction program RepeatModeler (v1.0.11, http://www.repeatmasker.org/RepeatModeler/ ). Subsequently, the de novo repeat library was used to identify repetitive sequences using RepeatMasker (v4.0.9). We then combined all the repeat prediction results to remove redundancy and obtain the final set of the genome repeat sequences. Additionally, tandem repeats in the genome were identified using Tandem Repeats Finder ( http://tandem.bu.edu/trf/trf.html ). Three methods were used to predict protein-coding genes in the genomes of P. lobata and P. thomsonii , including homology-based prediction, de novo prediction, and transcriptomes data prediction. AUGUSTUS (v3.3.2), 34 Genscan (v1.0), 35 and GlimmmerHMM (v3.0.4) 36 were used to perform de novo gene prediction. The protein sequences of six species ( Medicago sativa , Oryza sativa , Melilotus albus , Medicago truncatula , Glycine max , and Arabidopsis thaliana ) were aligned to our genomes for homology-based gene prediction, and the structure of coding genes was predicted using Exonerate (v2.4.0). 37 The RNA sequencing reads were assembled using HISAT (v2.1.0) 38 and StringTie (v2.1.4), 39 and then TransDecoder ( https://github.com/TransDecoder ) were used for gene prediction. The results of the three methods are integrated and filtered to form a non-redundant, more complete gene set using MAKER (v2.31.10). 40
To predict gene functions, protein-coding genes to different protein databases, such as NR, UniProt, and Pfam, using BLASTP (with an E-value threshold of 1e-5) (v2.12.0+) 41 were mapped based on sequence similarity. We performed KEGG annotation using KOBAS (v3.0), 42 and extracted Gene Ontology information from the respective UniProt descriptions. Next, InterProScan (5.52-86.0) 43 was used to search the InterPro database and obtain the proteins’ conserved sequences, motifs, and structural domains.
Comparative genomics analysis
Orthofinder (v2.5.4, -S blast) 44 was used to detect gene families of P. lobata , P. thomsonii , P. montana , as well as the selected 12 species, including eight Fabaceae species outside the Pueraria genus ( A. duranensis , C. cajan , C. arietinum , G. max , M. sativa , P. vulgaris , M. truncatula , and P. sativum ), three non-Fabaceae dicotyledons ( V. vinifera , A. thaliana , and P. trichocarpa ) and one monocotyledon ( O. sativa ). Multiple sequence alignments were performed on the single-copy genes of 15 species using Muscle (v3.8.1). 45 The phylogenetic tree was then reconstructed using the maximum likelihood method with RAxML (v8.2.12). 46 The protein sequence alignment was converted to codon alignment with PAL2NAL ( http://www.bork.embl.de/pal2nal/ ). The MCMCTree program from the PAML package was used to estimate the species divergence times based on the TimeTree database. 47 Gene family expansions and contractions were analyzed using CAFE5 48 with default settings based on the identified phylogenetic tree and gene family statistics. Gene Ontology (GO) and Kyoto Encyclopedia of Genes and Genomes (KEGG) analyses of expanding gene families were performed using the R package clusterProfiler (v4.2.2, p < 0.05, q < 0.2). 49 We used the python version of MCScan (v20101014) 50 to identify syntenic blocks among P. lobata , P. thomsonii , and P. montana .
Analysis of WGD events
The paralogs and one-to-one orthologs from G. max , 51 V. vinifera , 51 P. thomsonii , P. lobata , and P. montana 4 were performed all-versus-all BLASTP (v2.12.0+) similarity searches with an E-value threshold of 1e−5. WGDI (v0.6.0) 52 was used to identify syntenic blocks and calculate synonymous substitutions rate (Ks) with default parameters based on the result of alignment within or between the selected species and corresponding genome annotation. The syntenic blocks of paralogs or one-to-one orthologs and the distribution of Ks values were used to determine WGD events.
Re-sequencing and variant calling
Young leaves of 110 accessions with P. thomsonii and P. lobata were individually harvested, and genomic DNA was extracted. DNA libraries construction and re-sequencing were performed with DNBSEQ workflow (BGI), generating 5.56 Tb reads with 150-bp paired-end. All Pueraria accessions re-sequenced in this study were identified, collected, and deposited in the Guangxi Academy of Agricultural Sciences, Nanning, China. Additionally, the genome sequencing data of 11 P. montana accessions were downloaded from our previous study. 4 Raw re-sequenced data was filtered by SOAPnuke software 53 with the following parameters: ‘-n 0.01 -l 20 -q 0.3 --adaMR 0.25 --ada_trim --polyX 50 --minReadLen 150’. The clean reads were mapped to the P. lobata genome by BWA with default parameters. Samtools (v1.15.1) was used to sort the alignment results and convert them into BAM files, and PCR duplicates were marked with Picard. Variant detection was performed by GATK (v3.8-0-ge9d8) 54 following the prescribed procedures for variation disclosure. GATK IndelRealigner locally realigned BAM files to eliminate mismatches around small-scale deletions and insertions. The genome Variant Call Formats (gVCFs) for each accession were generated by GATK HaplotypeCaller, and all gVCF files were merged to generate the VCF file of all accessions using GATK CombineGVCFs and GenotypeGVCFs. Furthermore, the hard filter was performed to raw variants using GATK VariantFiltration with following parameters: for SNPs, ‘QUAL < 30.0 || QD < 2.0 || MQ < 40.0 || FS > 60.0 || SOR > 3.0 || MQRankSum < -12.5 || ReadPosRankSum < -8.0’; for InDels, ‘QUAL < 30.0 || QD < 2.0 || ReadPosRankSum < -20.0 || InbreedingCoeff < -0.8 || FS > 200.0 || SOR > 10.0’. The SNPs and InDels of filtered variants were selected to screen further using parameters of ‘--maf 0.05 --max-missing 0.9 --max-alleles 2’ with VCFtools (v0.1.16), 55 generating a high-confidence SNPs and InDels set with biallelic, respectively. For InDels, only those with a length of less than 50 bp will be retained. The identified variants were annotated using SnpEff (v5.1d) 56 with P. lobata genome and its gene annotation file. SNPs and InDels were categorized based on their chromosome position and effects.
Population genetic analysis
Based on the high-confidence SNPs set, we implemented LD pruning with PLINK (v1.90b6.21) 57 option ‘--indep-pairwise 50 5 0.2’, generating a core set of 1,061,987 SNPs for population genetic analyses. The maximum-likelihood tree was constructed using IQ-TREE (v2.2.0.3), 58 based on the best model (TVM + F + I + R8) determined by the Bayesian information criterion, and the format conversion of the input file was performed using vcf2phlip (v2.8) ( https://github.com/edgardomortiz/vcf2phylip ). Bootstrap support values were calculated using the ultrafast bootstrap approach (UFboot) with 1,000 replicates. The closely related species G. max was used as an outgroup downloaded from a previous study. 59 The phylogenetic tree was visualized by iTOL ( https://itol.embl.de ). The genetic ancestry was examined using ADMIXTURE (v1.3.0) 60 with K values (the putative number of population) from 2 to 10, and the cross-validation (CV) was set to 5-fold. Even though the cross-validation (CV) error gradually decreased from K = 2 to 9, we chose K = 4 to divide groups or subgroups, rather than K = 9 with the minimum CV error. The reasons are as follows: (1) from K = 4 on, the CV error decreases (or increases) at a slower rate and falls within the narrow range of 0.31006 to 0.31749; (2) at K = 4, the corresponding ancestries are enough to categorize most P. montana , P. lobata , and P. thomsonii accessions into distinct groups or subgroups. Principal component analysis (PCA) was performed using GCTA (v1.93.2beta). 61 Considering the differences in genetic structure caused by the diversity of P. lobata accessions, the top three principal components were used to clearly distinguish the different groups or subgroups. Based on the population structure analyses mentioned above, 38 ungrouped accessions were removed from subsequent analyses. Additionally, the GMM93 ( P. montana ) accession within the subgroup G3-1 was suspected to be mis-identified and excluded from subsequent analysis. The squared correlation coefficient ( r 2 ) between pairwise SNPs in subgroups G3-1 and G3-2 was calculated to estimate the LD decay using PopLDdecay (v3.42). 62 Nucleotide diversity ( π ) and fixation index ( F ST ) of different populations were calculated by VCFtools with 50 kb windows sliding in 20 kb steps; Similarly, Tajima’s D value was calculated with 50 kb windows, and the statistical significance between subgroup G3-1 and G3-2 was compared using the two-sided Wilcoxon rank-sum test.
Identification of selective sweeps
Two statistical methods were employed to identify candidate genomic regions potentially affected by selection based on the high-confidence SNPs set. The cross-population composite likelihood ratio test (XP-CLR, https://github.com/hardingnj/xpclr ) was performed in subgroups G3-1 and G3-2 with 50 kb windows sliding in 20 kb steps. The windows with the top 5% XP-CLR score were taken as outliers. Based on the nucleotide diversity ( π ) of subgroups G3-1 and G3-2 calculated above, the statistic π (G3-2/G3-1) was then calculated concerning subgroups G3-1 and G3-2. An abnormally low value (5% outliers) suggests selection in subgroup G3-2, and the top value (5% outliers) indicates selection in subgroup G3-1. The overlapping regions with the top 5% XP-CLR score and low or top 5% π (G3-2/G3-1) value were assigned to candidate selective regions. Adjacent putative regions were merged into a single region to represent the effect of a single selective sweep. Haplotype differentiation patterns of gene loci were visualized by RectChr (v1.36, https://github.com/BGI-shenzhen/RectChr ).
Genome sequencing, assembly, and annotation
Multiple sequencing datasets were used to assemble the genome of P. lobata ( Fig. 1a ). First, k-mer analysis ( k = 17) revealed an estimated genome size of 1.06 Gb using 144.68 Gb (136 × coverage) of paired-end reads ( Supplementary Fig. S1 , Supplementary Table S1 ). Pacbio sequencing (182.63 Gb, 172 × coverage) was used for the initial contig assembly, followed by polishing and quality improvement of the resulting sequences ( Supplementary Table S1 ). Given the high heterozygosity in specific genomic regions, this may lead to the assembly of regional duplication. We further improved the assembly by removing redundant contigs, resulting in the removal of 193 Mb of sequences from the initial 1.21 Gb assembly ( Supplementary Table S2 ). The contigs were then grouped, sorted, and oriented using Hi–C interaction datasets (152.41 Gb, 144 × coverage) to achieve a chromosome-level assembly ( Supplementary Table S1 ). To enhance the continuity and completeness of the P. lobata genome, we used ONT ultra-long reads (82 Gb, 77 × coverage) with N50 of 36.56 kb to fill gaps in the genome ( Supplementary Table S1 ). As a result, we obtained a relatively complete genome of P. lobata , with a size of 1.05 Gb, and 91.69% of the sequences were anchored to 11 chromosomes, including 7 gap-free chromosomes, and the scaffold N50 was 86.86 Mb ( Fig. 1c , Supplementary Fig. S2,-S3 , Supplementary Table S3 ). Additionally, we performed a redundancy removal process on the previously published P. thomsonii genome, resulting in a new version of the genome with a size of 1.03 Gb and scaffold N50 was 98.03 Mb ( Fig. 1c , Supplementary Table S4 ).
The quality of de novo assembled P. lobata genome and the updated P. thomsonii genome were evaluated by using multiple methods. Accuracy of the two genomes was demonstrated by high mapping rates obtained from different sequencing data sets, including PacBio long reads, short paired-end reads, and ONT reads. The mapping rates for paired-end reads of P. lobata and P. thomsonii were 99.35% and 99.16%, respectively, and homozygous single-nucleotide variations (SNVs) accounted for approximately 0.0019% and 0.0057% of the two genomes ( Supplementary Table S5 ). Benchmarking Universal Single-Copy Orthologs (BUSCO) analysis estimated the completeness of the P. lobata and P. thomsonii genomes to be 99.0% (2,303 out of 2,326) and 98.5% (2,291 out of 2,326), respectively, indicating the high completeness of our genomes ( Supplementary Table S6 ).
Subsequently, repetitive sequence elements, protein-coding genes, and non-coding RNAs of both genomes were identified. It predicted 679.32 Mb (64.7%) and 651.91 Mb (62.9%) of repetitive sequences from P. lobata and P. thomsonii assemblies, respectively ( Supplementary Table S7 ), which is consistent with previous reports. 16 In P. lobata genome, Long Terminal Repeats (LTRs) were the most abundant repetitive elements (17.2%), followed by DNA transposable elements (3.67%) and LINEs (3.35%). Among the LTRs, Gypsy and Copia accounted for 9.91% and 6.84%, respectively. In P. thomsonii genome, LTRs (14.3%), LINEs (5.02%), and DNA transposable elements (3.42%) were the most abundant repetitive sequences, with Gypsy (6.37%) and Copia (7.41%) being the best represented among the LTRs.
Using homology analysis, de novo prediction, and transcriptome evidence, it annotated the protein-coding genes of P. lobata and P. thomsonii after masking repetitive sequences. It identified 38,386 genes in P. lobata genome, with an average gene length of 5,312 bp and average coding DNA sequences (CDS) length of 1,274 bp, and predicted 38,891 genes in P. thomsonii genome, with an average gene length of 4,477 bp and an average CDS length of 1,254 bp ( Supplementary Table S8 ). We further compared the number of genes, gene length, and CDS length among three Pueraria species ( P. lobata , P. thomsonii , and P. montana ) and Glycine max . The gene number of three Pueraria species was found to be similar, while P. lobata exhibited a more significant average gene length and average CDS length compared to the other three species ( Supplementary Table S8 ).
Compared to public databases, including Interpro, Pfam, GO, Uniprot, Pathway, and KEGG, approximately 97.27% (37,339) and 97.38% (37,873) of these genes were functionally annotated in P. lobata and P. thomsonii genome, respectively ( Supplementary Table S9 ). 3,432 and 3,072 non-coding RNAs were also identified, including microRNAs, transfer RNAs, ribosomal RNAs, and small nuclear RNAs ( Supplementary Table S10 ).
Comparative genomic and evolutionary analysis
To explore the genomic evolution of Pueraria , we clustered genes from the three Pueraria species ( P. lobata , P. thomsonii , and P. montana ), eight Fabaceae species outside the Pueraria genus ( A. duranensis , C. cajan , C. arietinum , G. max , M. sativa , P. vulgaris , M. truncatula , and P. sativum ), and other four representative species, including three non-Fabaceae dicotyledons ( V. vinifera , A. thaliana , and P. trichocarpa ) and one monocotyledon ( O. sativa ) ( Fig. 2a ). In total, we identified 35,121 gene families, with 17,416, 17,487, and 17,037 families detected in P. lobata , P. thomsonii , and P. montana , respectively ( Supplementary Table S11 ).

Genome comparison and evolution analysis. (a) Gene numbers of each category in 15 representative species. (b) Venn diagram showing the shared and unique gene families among P. lobata, P. thomsonii , P. montana , and G. max . (c) Phylogenomic analysis and expansion/contraction of the gene family. The numbers on the nodes represent the species divergence time with the confidence range list in brackets. (d) Genomic collinearity between three Pueraria crops. (e) Distribution of synonymous substitutions (Ks) values of syntenic paralogs in five selected species. Estimated whole-genome duplication (WGD) and whole-genome triplication (WGT) events occurring in Pueraria were highlighted.
The genes of three Pueraria species re-clustered by using G. max as the outgroup. These four species shared 18,203 gene families, while P. lobata , P. thomsonii , and P. montana shared 19,470 gene families, which can be considered a conservative gene set for the Pueraria species ( Fig. 2b ). It found 237 and 324 unique gene families in the genomes of P. lobata and P. thomsonii , including 1,074 genes and 1,286 genes respectively, which were significantly less than that (1,094 unique gene families; 3,954 genes) in P. montana genome ( Fig. 2b ). Importantly, P. lobata shared the majority gene families with P. thomsonii , indicating their closer evolutionary relationship.
Single-copy gene families obtained from 15 representative plant genomes were used to reconstruct a phylogenetic tree and estimate divergence times ( Fig. 2c ). The results supported a close evolutionary relationship among three Pueraria crops. Specifically, our phylogenetic analysis shows that P. lobata and P. thomsonii have the closest relationship, with a divergence time of 1.1 Mya. The common ancestor of P. lobata and P. thomsonii diverged from P. montana approximately 5.8 Mya. Moreover, the estimated divergence time between Pueraria species and G. max was 15.3 Mya. These findings provide valuable insights into the evolutionary history of Pueraria genus and its relationship with other plant species.
Gene family expansion and contraction were investigated in 15 representative plants ( Fig. 2c ). There were 800/624, 675/819, and 915/1133 expanded/contracted gene families in P. lobata , P. thomsonii , and P. montana genome, respectively. Subsequently, Gene Ontology (GO) and Kyoto Encyclopedia of Genes and Genomes (KEGG) analyses on the expanded gene families of each Pueraria species were conducted ( Supplementary Fig. S4 ). GO enrichment analysis indicated that the expanded gene families of P. lobata and P. thomsonii are related to stress responses, such as ‘response to oxidative stress’, ‘cellular response to salt stress’, and ‘response to herbicide’. This result suggests that P. lobata and P. thomsonii may be more capable of adapting to diverse environmental conditions. KEGG enrichment analysis shows that the expanded genes of P. lobata are involved in ‘Photosynthesis’, ‘Oxidative phosphorylation’, and ‘Ribosome’. Additionally, it was found that ‘Sesquiterpenoid and triterpenoid biosynthesis’ and ‘Quorum sensing’ are the most enriched terms of P. thomsonii and P. montana , respectively. Overall, the expansion of distinct gene families may have driven the observed differentiation among three Pueraria species. We also observed a significant expansion of gene families related to ‘Isoflavones biosynthesis’ and ‘Flavones and flavonols biosynthesis’ in the common ancestor of P. lobata and P. thomsonii ( Supplementary Fig. S5 ). In contrast, it does not exist in P. montana . This result indicates the potential role of these expanded gene families in enhancing the medicinal properties of Pueraria species.
Syntenic analysis and whole-genome duplication
We conducted genome comparisons among P. lobata , P. thomsonii , and P. montana , and identified syntenic blocks that are distributed across 11 chromosomes, indicating a strong interspecies synteny ( Fig. 2d ). A total of 500 syntenic blocks comprising 27,295 gene pairs were identified between P. lobata and P. thomsonii , of which 309 blocks containing 25,851 gene pairs were located on the same chromosome. Furthermore, it observed 361 syntenic blocks with 23,285 gene pairs shared between P. lobata and P. montana , with 231 blocks containing 18,083 gene pairs located on the same chromosome ( Supplementary Table S12 ). The largest block with the highest number of genes between P. lobata and P. thomsonii was located at the end of chromosome 9 and contained 951 pairs of orthologous genes, covering 26.7 Mb and 26.9 Mb of genomic regions on the P. lobata and P. thomsonii genomes, respectively. Meanwhile, 425 syntenic blocks containing 23,266 gene pairs were detected between P. thomsonii and P. montana , with 17,789 gene pairs located on the same chromosome ( Supplementary Table S12 ). High chromosomal relatedness was observed among the three Pueraria genomes, with an almost one-to-one synteny relationship between P. lobata and P. thomsonii , providing genomic evidence for the evolution of Pueraria species.
Two peaks of Ks values corresponding to two whole-genome duplication (WGD) events were detected in the Pueraria genus by analyzing synonymous substitutions (Ks). The first WGD event, represented by the ancient peak (Ks = 1.666–1.714), was consistent with the whole-genome triplication (γ event) in core eudicots, 63 as observed in V. vinifera (Ks = 1.240) and G. max (Ks = 1.738) ( Fig. 2e ). 64 , 65 The second WGD event, shared by Fabaceae, 65 is indicated by the recent peak (Ks = 0.563–0.572) ( Fig. 2e ). Syntenic blocks of paralogs with three Pueraria species indicate that the recent Ks peak was generated by a WGD event rather than segmental duplication ( Supplementary Fig. S6 ). Based on these results, we presume that the three Pueraria species did not undergo a lineage-specific WGD event.
To investigate the order of species differentiation, the syntenic blocks of orthologs and Ks values for two pairs of three Pueraria species were identified. We note that the Ks peak of P. thomsonii versus P. lobata (Ks = 0.004) is significantly lower than that in P. thomsonii versus P. montana (Ks = 0.043) or P. lobata versus P. montana (Ks = 0.041) ( Supplementary Fig. S7 ). According to the difference of Ks peak, it speculates that P. lobata and P. thomsonii are more closely related, which is also confirmed by phylogenetic tree ( Fig. 2c ).
Genomic polymorphism and population structure
To investigate genomic polymorphisms in three Pueraria species, a total of 121 Pueraria accessions were collected in this study, including 64 P. thomsonii and 46 P. lobata accessions with an average depth of 48×, 11 P. montana accessions from our previous study with an average depth of 28 × ( Supplementary Table S13 ). The P. thomsonii and P. lobata accessions sequenced in this study are mostly distributed in southern China, with over half of the accessions from Guangxi province and the remaining accessions mainly from Guangdong, Jiangxi, and Yunnan provinces ( Supplementary Fig. S8 ). All sequencing reads were mapped to P. lobata genome, and all mapping rates ranged from 96.07% to 99.20%, leading to the identification and annotation of 14,132,140 high-confidence single-nucleotide polymorphisms (SNPs) and 1,799,556 insertions/deletions (InDels) ( Supplementary Table S13 ). Of these, 577,166 SNPs (2.71%) and 26,355 InDels (0.90%) were in coding regions, with 308,061 nonsynonymous SNPs and 14,573 frameshift InDels that could potentially cause significant changes in protein sequences ( Supplementary Table S14–S15 ). Most of SNPs were found in intergenic regions (52.91%), followed by upstream (17.20%), downstream (16.25%), and intronic regions (9.36%).
We aimed to determine the evolutionary relationships among the three Pueraria species by examining their population genetics. To this end, we explored the phylogenetic relationships and population structure based on a core dataset of SNPs (Method). It conducted a phylogenetic tree and found that the 121 Pueraria accessions could be divided into three clades ( Fig. 3a ). Clade1 comprises nine P. montana accessions, representing the P. montana population. Clade2 and Clade3 harbor most P. lobata and P. thomsonii accessions, standing for the P. lobata and P. thomsonii populations ( Supplementary Table S13 ). Combining the results of estimated ancestral component and principal component analysis (PCA), G1 possesses a significant evolutionary distance from both G2 and G3 (Clade3) ( Fig. 3b , Supplementary Fig. S9 ). Notably, eight P. lobata accessions in G2 differ from those in G3 (Clade3), as revealed by principal components 2 (PC2) and 3 (PC3), suggesting an earlier divergence of G2 ( Fig. 3a–c , Supplementary Fig. S9 ). As the K value increased from 3 to 9, G3 (Clade3) could be further subdivided into two subgroups and 36 ungrouped accessions, which aligns with the optimal K value ( K = 4) we have chosen ( Fig. 3b , Supplementary Fig. S10 ). The classification criterion of the subgroup is as follows: (i) Any individual with a major ancestry component (> 70%) will be categorized into the corresponding subgroup; (ii) Subgroups must constitute a monophyletic branch in the phylogenetic tree. Subgroup G3-1 was predominantly composed of P. lobata accessions, with origins from Guangxi and Yunnan provinces ( Supplementary Table S13 ). The ungrouped accessions in G3 exhibit more admixture and relatively scattered PCs ( Fig. 3b–c , Supplementary Fig. S9 , S 11 ), likely resulting from wide crossing or wild introgression during domestication and cultivar development involving several related species. 66 Subgroup G3-2 includes most P. thomsonii accessions whose genetic structure demonstrates a remarkable similarity in all the analyses conducted, which is substantially different from the situation observed in other groups or subgroups ( Fig. 3a-c , Supplementary Fig. S9 , S 11 ). In summary, our results suggest that P. thomsonii (G3-2) was derived from P. lobata as a subspecies.

Phylogeny, population structure and genomic diversity of Pueraria accessions. (a) Maximum likelihood phylogenetic tree of 121 Pueraria accessions, using G. max as outgroup. The color of the branches represents different groups or subgroups. (b) Model-based clustering analysis with different numbers of groups or subgroups. Each vertical bar represents one individual, and colored segments represent the proportions of ancestral components. (c) PCA plot with the first two principal components. Colors of different groups or subgroups correspond to those in the phylogenetic tree. The more detailed principal components and quantities of G1 and subgroup G3-2 were shown in the zoomed-in view, respectively. (d) Nucleotide diversity (π) and fixation index ( F ST ) across the four groups and subgroups. Values in circles represent measures of π for the groups or subgroups, and values between pairs indicate F ST value.
Genomic diversity and selection signatures during domestication
Nucleotide diversity ( π ) and fixation indexes ( F ST ) were estimated to compare genome-wide genetic diversity among the above four major groups and subgroups. Despite having a larger accession size, subgroup G3-2 exhibited significantly lower nucleotide diversity ( π =1.79 × 10 −4 ) than subgroup G3-1 (π=2.45 × 10 −4 ) ( Fig. 3d ). This finding is likely due to that the accessions in subgroup G3-1 were mainly from wild populations, while those in subgroup G3-2 were mostly from landraces or cultivars ( Supplementary Table S13 ). The decrease in nucleotide diversity in subgroup G3-2 suggests that it may has undergone more artificial selection. The level of genetic differentiation between subgroup G3-1 and G2 is the lowest ( F ST = 0.2939), followed by that between subgroup G3-1 and G3-2 ( F ST = 0.3064), with the remaining levels of genetic differentiation among the other groups or subgroups being significantly higher ( Fig. 3d ). A significant proportion of SNPs (83.4%) identified in subgroup G3-2 are shared with subgroup G3-1, indicating that subgroup G3-1 has served as a primary source of genetic material for subgroup G3-2, potentially explaining the low level of genetic differentiation between them ( Supplementary Fig. S12a ). Linkage disequilibrium (LD), as indicated by r 2 , was calculated to compare LD decay between subgroups G3-2 and G3-1. The result showed that the LD decay reached half its maximum average correlation coefficient at a shorter distance in subgroup G3-2 (0.15 kb) than in subgroup G3-1 (2.61 kb) ( Supplementary Fig. S12b ). This finding is consistent with previous studies on G. max or V. vinifera , where wild populations and cultivars exhibited similar results. 14 , 67 The faster LD decay observed in subgroup G3-2 implies that it may have experienced stronger selection, as supported by the greater Tajima’s D value of subgroup G3-2 ( Supplementary Fig. S12c ).
The most significant phenotypic difference between P. thomsonii and P. lobata is that the root tuber of P. thomsonii is larger and accumulates a higher content of starch, traits that were likely selected during domestication. 68 , 69 To identify potential selective signals associated with P. thomsonii domestication, the cross-population composite likelihood ratio test (XP-CLR) and nucleotide diversity ratio were performed by comparing subgroup G3-2 versus G3-1. Combining these approaches, we identified only five selected regions in subgroup G3-1, consistent with our hypothesis that it underwent relatively weak selection. In contrast, 533 selected regions were found in subgroup G3-2, covering 3.85% (40.41 Mb) of the P. lobata genome and harboring 1,774 genes ( Fig. 4a–c , Supplementary Table S16 ). Previous studies conducted by our team have elucidated the puerarin synthesis pathway specific to P. lobata and P. thomsonii . 16 Within the selected region, we discovered the PlChr10.CHS (PlobChr10G00353750) is situated in the puerarin synthesis pathway, potentially contributing to variations in puerarin content between P. lobata and P. thomsonii ( Fig. 4a , Supplementary Table S17 ). In addition, several genes related to plant hormones were detected in selected regions, including PlChr01.IAA (PlobChr1G00059530), PlChr01.GA2ox (PlobChr1G00059170), PlChr07.GH3s (PlobChr7G00257580 and PlobChr7G00273940) and PlChr08.YUC (PlobChr8G00206820) ( Fig. 4a–c , Supplementary Table S17 ). Previous research has demonstrated that genes associated with auxin are significant contributors to starch synthesis. Specifically, the YUC gene, responsible for auxin synthesis, considerably influences the transcriptional levels of three key genes involved in starch granule synthesis and affects the accumulation of starch granules within the root apex of A. thaliana . 70 Gibberellin regulates the ratio of xylem to phloem in vascular tissue, 71 and the abnormal vascular tissue, consisting primarily of xylem and phloem, is responsible for the expansion of tubers. 72 In addition, the differentiation patterns of haplotypes for PlChr01.GA2ox and PlChr08.YUC were investigated, and it was determined that they carried unique haplotypes in subgroups G3-2 and G3-1, respectively ( Fig. 4d–e ). In summary, it suggests that genes associated with the synthesis of auxin and gibberellin, which have undergone strong selective pressure, may have played a significant role in the domestication process of root expansion and high starch accumulation in P. thomsonii .

Identification of selective sweeps associated with domestication traits in P. thomsonii . (a) Manhattan plot of the selective sweeps identified from the comparison of subgroup G3-2 and G3-1 by XP-CLR. Dashed lines represent the top 5% of the XP-CLR scores. Functionally characterized candidate genes associated with plant development were highlighted. (b–c) Distribution of nucleotide diversity (π) ratio with the upstream and downstream regions of PlChr01.GA2ox and PlChr08.YUC . Horizontal dashed lines represent the bottom 5% of the π ratio. Vertical dashed lines indicate the position of PlChr01.GA2ox and PlChr08.YUC . (d–e) Haplotype distributions were shown for SNPs within PlChr01.GA2ox and PlChr08.YUC . Each column represents the SNPs of PlChr01.GA2ox and PlChr08.YUC . Each row indicates the accessions of subgroups G3-2 or G3-1. Four colors represent different types of SNP allele respectively.
Pueraria belongs to the Fabaceae family and is a plant with significant economic value widely cultivated throughout Asia. Traditional Pueraria categories need to be clarified for evolutionary studies and exploitation of medicinal usage, mainly because of a lack of genomic and population genetic information. In this study, based on ONT reads and PacBio long reads, we constructed a high-quality genome of P. lobata with some chromosomes without gaps. The continuity of the P. lobata genome was improved by filling gaps with ONT ultra-long reads, although the specific regions corresponding to telomeres and centromeres remain unidentified. Completing these regions would necessitate the utilization of high-fidelity (HiFi) reads with low error rates. 73–75 Through a comparison of the sizes of P. lobata genome, P. montana genome, 4 and the previous P. thomsonii genome, 16 we observed that the genome size of P. lobata (~1.05 Gb) is more closely related to that of P. montana (~978 Mb), while exhibiting a substantial difference from that of previous P. thomsonii (~1.38 Gb). Based on k-mer analysis ( k = 17) with paired-end reads, the size and heterozygosity of the previous P. thomsonii genome were reevaluated to be 1.02 Gb and 1.25% ( Supplementary Fig. S13 ), respectively, which closely resemble those of P. lobata genome ( Supplementary Fig. S1 ). In addition, the result of BUSCO analysis shows that the completeness and duplication of the reference gene set in the previous P. thomsonii genome were 98.9% and 33.1% ( Supplementary Table S4 ), respectively. These results suggest that the previous P. thomsonii genome likely contains many redundant haploid contigs. This phenomenon could be attributed to the high levels of heterozygosity typical in the genomes of highly heterozygous species, such as lychee, 76 Medicago ruthenica , 77 and Cinnamomum camphora . 78 Therefore, we have excised the redundant regions from the previous P. thomsonii genome, leading to the generation of an updated genome. This improved genome provides a robust foundation for conducting more comprehensive investigations into P. thomsonii .
In the three Pueraria species, they all have a karyotype of 2 n = 2 x = 22. We observed significant genome syntenic relationships among P. lobata , P. thomsonii , and P. montana , indicating their high genetic relationship ( Fig. 2d ). This result suggests the possibility of extensive interspecific hybridization among Pueraria species. Enrichment analysis showed that gene families associated with stress responses were expanded in P. lobata and P. thomsonii . In contrast, changes in gene families associated with secondary metabolites such as isoflavonoids may have contributed to their different nutritional values. Numerous reports have been on the WGD events and evolutionary relationships of Fabaceae species; however, few researches have been conducted on Pueraria species. 65 , 79–81 In this study, we inferred that three Pueraria species collectively experienced two WGD events, with the most recent one being shared with Fabaceae plants. 82 Since then, none of these species has undergone separate WGD events. Previous studies have reported a recent WGD event in P. thomsonii (~4.8 Mya). 16 Nevertheless, such a recent WGD event could produce many collinearity blocks of paralogs, as seen in G. max , a closely related species to P. thomsonii . However, this was not observed in P. thomsonii genome ( Supplementary Fig. S14 ). 65 Therefore, it concludes that this event was likely due to segmental duplication rather than an authentic WGD event. A similar phenomenon has been observed in V. radiata . 79 Phylogenetic tree constructed using single-copy genes and Ks analysis based on orthologs also indicated that the three Pueraria species are closely related to G. max and belong to Papilionoideae ( Fig. 2c , Supplementary Fig. S7 ). 80 Among them, P. thomsonii and P. lobata exhibit a closer genetic relationship, consistent with their similar biological morphology and functions. Our results further contribute to the understanding of evolutionary relationships among Fabaceae species.
In addition, we first reported the characterization of genome-wide SNPs from 121 Pueraria accessions, which are mainly distributed in the south of China. Based on these SNPs, three Pueraria species populations were differentiated into three groups: G1, G2, and G3. Among them, G3 could be further divided into two subgroups. The subgroup G3-1 displayed greater population diversity than the subgroup G3-2, rendering it a valuable genetic resource for improving the P. thomsonii germplasm. Additionally, we found that the GMM93 ( P. montana ) accession was classified into subgroup G3-1 and exhibited a distant phylogenetic relationship with other P. montana accessions in both previous and current studies ( Fig. 3b–c ). 4 Given the current limitations of morphological-based identification of Pueraria species, we place greater confidence in the findings derived from genomics and population genetics analyses. Consequently, we infer that GMM93 is more likely to P. lobata rather than P. montana . Previous studies indicated that the three Pueraria species have a large mixed distribution in the southwestern of China, which may be the center of their origin. 83 Notably, most accessions in G1 and G2, located at the root of the phylogenetic tree, were collected from Yunnan and Guangxi provinces ( Supplementary Table S13 ). Thus, we speculate that the three Pueraria species may have originated from Yunnan and Guangxi provinces. The phylogenetic tree conducted with SNPs corroborated the close evolutionary relationship between P. thomsonii and P. lobata . Further combined with the differences in population diversity between subgroup G3-1 and G3-2, we suggest that P. thomsonii was likely domesticated from P. lobata as a subspecies. Even though our whole-genome SNP data could not able to display the whole scheme of P. thomsonii domestication history, it revealed some valuable details about the evolutionary relationship of three Pueraria species. This study offers a valuable genomic resource for the genetic improvement of Pueraria species.
This work was supported by the National Natural Science Foundation of China (32270712, 32100526, 82204563), Young Elite Scientists Sponsorship Program by CAST (2022QNRC001), the State Key Laboratory for Conservation and Utilization of Subtropical Agro-Bioresources (SKLCUSA-a202205), Chief Expert of Tuberous Crops Innovation Team in Guangxi Province (nycytxgxcxtd-2023-11-01), and Guangxi Science and Technology Major Program (GuikeAA23023035-6).
The authors declare no competing interests.
J.S., L.C., and H.Y. conceived and designed the study. X.S., L.X., P.S., W.Z., S.C., and Z.W. contributed to sample preparation. X.H., S.G., M.G., B.Z., and J.S. performed the bioinformatics analyses. X.H., S.G., X.S., J.S., H.Y., and L.C. wrote and revised the manuscript. All authors read and approved the final manuscript.
All data used in this study are publicly available. Whole genome sequencing data have been deposited in BioProject under accession numbers PRJCA016835 at https://ngdc.cncb.ac.cn/gwh .
Shang , X. , Huang , D. , Wang , Y. , et al. 2021 , Identification of nutritional ingredients and medicinal components of Pueraria lobata and its varieties using UPLC-MS/MS-based metabolomics , Molecules , 26 , 6587 .
Google Scholar
Zhou , Y.X. , Zhang , H. , and Peng , C. 2014 , Puerarin: a review of pharmacological effects , Phytother. Res. , 28 , 961 – 75 .
Ma , Y. , Shang , Y. , Zhong , Z. , et al. 2021 , A new isoflavone glycoside from flowers of Pueraria Montana var. lobata (Willd.) Sanjappa & Pradeep , Nat. Prod. Res. , 35 , 1459 – 64 .
Mo , C. , Wu , Z. , Shang , X. , et al. 2022 , Chromosome-level and graphic genomes provide insights into metabolism of bioactive metabolites and cold-adaption of Pueraria lobata var. montana , DNA Res. , 29 , dsac030 .
Huang , Z.Y. , Shen , Q.N. , Li , P. , et al. 2019 , [Quality research of Puerariae Lobatae Radix from different habitats with UPLC fingerprint and determination of multi-component content] , Zhongguo Zhong Yao Za Zhi , 44 , 2051 – 8 .
Zhang , G. , Liu , J. , Gao , M. , et al. 2020 , Tracing the edible and medicinal plant Pueraria montana and its products in the marketplace yields subspecies level distinction using DNA barcoding and DNA metabarcoding , Front. Pharmacol. , 11 , 336 .
Wong , K.H. , Razmovski-Naumovski , V. , Li , K.M. , Li , G.Q. , and Chan , K. 2015 , Comparing morphological, chemical and anti-diabetic characteristics of Puerariae lobatae Radix and Puerariae thomsonii Radix , J. Ethnopharmacol. , 164 , 53 – 63 .
Sun , Y. , Shaw , P.C. , and Fung , K.P. 2007 , Molecular authentication of Radix Puerariae lobatae and Radix Puerariae thomsonii by ITS and 5S rRNA spacer sequencing , Biol. Pharm. Bull. , 30 , 173 – 5 .
Adolfo , L.M. , Rao , X. , and Dixon , R.A. 2022 , Identification of Pueraria spp. through DNA barcoding and comparative transcriptomics , BMC Plant Biol. , 22 , 10 .
Li , J. , Yang , M. , Li , Y. , et al. 2022 , Chloroplast genomes of two Pueraria DC. species: sequencing, comparative analysis and molecular marker development , FEBS Open Bio , 12 , 349 – 61 .
Wang , W. , Mauleon , R. , Hu , Z. , et al. 2018 , Genomic variation in 3,010 diverse accessions of Asian cultivated rice , Nature , 557 , 43 – 9 .
Dong , Y. , Duan , S. , Xia , Q. , et al. 2023 , Dual domestications and origin of traits in grapevine evolution , Science , 379 , 892 – 901 .
Zhou , Y. , Massonnet , M. , Sanjak , J.S. , Cantu , D. , and Gaut , B.S. 2017 , Evolutionary genomics of grape ( Vitis vinifera ssp. vinifera ) domestication , Proc. Natl. Acad. Sci. USA , 114 , 11715 – 20 .
Liang , Z. , Duan , S. , Sheng , J. , et al. 2019 , Whole-genome resequencing of 472 Vitis accessions for grapevine diversity and demographic history analyses , Nat. Commun. , 10 , 1190 .
Low , Y.W. , Rajaraman , S. , Tomlin , C.M. , et al. 2022 , Genomic insights into rapid speciation within the world’s largest tree genus Syzygium , Nat. Commun. , 13 , 5031 .
Shang , X. , Yi , X. , Xiao , L. , et al. 2022 , Chromosomal-level genome and multi-omics dataset of Pueraria lobata var. thomsonii provide new insights into legume family and the isoflavone and puerarin biosynthesis pathways , Hortic. Res. , 9 , uhab035 .
Marçais , G. and Kingsford , C. 2011 , A fast, lock-free approach for efficient parallel counting of occurrences of k-mers , Bioinformatics , 27 , 764 – 70 .
Vurture , G.W. , Sedlazeck , F.J. , Nattestad , M. , et al. 2017 , GenomeScope: fast reference-free genome profiling from short reads , Bioinformatics , 33 , 2202 – 4 .
Hu , J. , Fan , J. , Sun , Z. , and Liu , S. 2020 , NextPolish: a fast and efficient genome polishing tool for long-read assembly , Bioinformatics , 36 , 2253 – 5 .
Dudchenko , O. , Batra , S.S. , Omer , A.D. , et al. 2017 , De novo assembly of the Aedes aegypti genome using Hi-C yields chromosome-length scaffolds , Science , 356 , 92 – 5 .
Roach , M.J. , Schmidt , S.A. , and Borneman , A.R. 2018 , Purge Haplotigs: allelic contig reassignment for third-gen diploid genome assemblies , BMC Bioinf. , 19 , 460 .
Durand , N.C. , Shamim , M.S. , Machol , I. , et al. 2016 , Juicer provides a one-click system for analyzing loop-resolution Hi-C experiments , Cell Syst , 3 , 95 – 8 .
Durand , N.C. , Robinson , J.T. , Shamim , M.S. , et al. 2016 , Juicebox provides a visualization system for Hi-C contact maps with unlimited zoom , Cell Syst , 3 , 99 – 101 .
Xu , M. , Guo , L. , Gu , S. , et al. 2020 , TGS-GapCloser: a fast and accurate gap closer for large genomes with low coverage of error-prone long reads , GigaScience , 9 , giaa094 .
Xu , G.-C. , Xu , T.-J. , Zhu , R. , et al. 2019 , LR_Gapcloser: a tiling path-based gap closer that uses long reads to complete genome assembly , GigaScience , 8 , giy157 .
Vaser , R. , Sović , I. , Nagarajan , N. , and Šikić , M. 2017 , Fast and accurate de novo genome assembly from long uncorrected reads , Genome Res. , 27 , 737 – 46 .
Walker , B.J. , Abeel , T. , Shea , T. , et al. 2014 , Pilon: an integrated tool for comprehensive microbial variant detection and genome assembly improvement , PLoS One , 9 , e112963 .
Guan , D. , McCarthy , S.A. , Wood , J. , Howe , K. , Wang , Y. , and Durbin , R. 2020 , Identifying and removing haplotypic duplication in primary genome assemblies , Bioinformatics , 36 , 2896 – 8 .
Simão , F.A. , Waterhouse , R.M. , Ioannidis , P. , Kriventseva , E.V. , and Zdobnov , E.M. 2015 , BUSCO: assessing genome assembly and annotation completeness with single-copy orthologs , Bioinformatics , 31 , 3210 – 2 .
Li , H. and Durbin , R. 2009 , Fast and accurate short read alignment with Burrows–Wheeler transform , Bioinformatics , 25 , 1754 – 60 .
Li , H. 2018 , Minimap2: pairwise alignment for nucleotide sequences , Bioinformatics , 34 , 3094 – 100 .
Danecek , P. , Bonfield , J.K. , Liddle , J. , et al. 2021 , Twelve years of SAMtools and BCFtools , GigaScience , 10 , giab008 .
Tarailo-Graovac , M. and Chen , N. 2009 , Using RepeatMasker to identify repetitive elements in genomic sequences , Curr. Protoc. Bioinformatics , Chapter 4 , 4.10.11 – 4 .
Stanke , M. and Waack , S. 2003 , Gene prediction with a hidden Markov model and a new intron submodel , Bioinformatics , 19 , ii215 – 25 .
Burge , C. and Karlin , S. 1997 , Prediction of complete gene structures in human genomic DNA , J. Mol. Biol. , 268 , 78 – 94 .
Majoros , W.H. , Pertea , M. , and Salzberg , S.L. 2004 , TigrScan and GlimmerHMM: two open source ab initio eukaryotic gene-finders , Bioinformatics , 20 , 2878 – 9 .
Slater , G.S.C. and Birney , E. 2005 , Automated generation of heuristics for biological sequence comparison , BMC Bioinf. , 6 , 31 .
Kim , D. , Langmead , B. , and Salzberg , S.L. 2015 , HISAT: a fast spliced aligner with low memory requirements , Nat. Methods , 12 , 357 – 60 .
Kovaka , S. , Zimin , A.V. , Pertea , G.M. , Razaghi , R. , Salzberg , S.L. , and Pertea , M. 2019 , Transcriptome assembly from long-read RNA-seq alignments with StringTie2 , Genome Biol. , 20 , 278 .
Holt , C. and Yandell , M. 2011 , MAKER2: an annotation pipeline and genome-database management tool for second-generation genome projects , BMC Bioinf. , 12 , 491 .
Altschul , S.F. , Gish , W. , Miller , W. , Myers , E.W. , and Lipman , D.J. 1990 , Basic local alignment search tool , J. Mol. Biol. , 215 , 403 – 10 .
Xie , C. , Mao , X. , Huang , J. , et al. 2011 , KOBAS 2.0: a web server for annotation and identification of enriched pathways and diseases , Nucleic Acids Res. , 39 , W316 – 22 .
Blum , M. , Chang , H.-Y. , Chuguransky , S. , et al. 2021 , The InterPro protein families and domains database: 20 years on , Nucleic Acids Res. , 49 , D344 – 54 .
Emms , D.M. and Kelly , S. 2019 , OrthoFinder: phylogenetic orthology inference for comparative genomics , Genome Biol. , 20 , 238 .
Edgar , R.C. 2004 , MUSCLE: multiple sequence alignment with high accuracy and high throughput , Nucleic Acids Res. , 32 , 1792 – 7 .
Stamatakis , A. 2014 , RAxML version 8: a tool for phylogenetic analysis and post-analysis of large phylogenies , Bioinformatics , 30 , 1312 – 3 .
Yang , Z. 2007 , PAML 4: phylogenetic analysis by maximum likelihood , Mol. Biol. Evol. , 24 , 1586 – 91 .
Mendes , F.K. , Vanderpool , D. , Fulton , B. , and Hahn , M.W. 2020 , CAFE 5 models variation in evolutionary rates among gene families , Bioinformatics , 36 , 5516 – 8 .
Yu , G. , Wang , L.-G. , Han , Y. , and He , Q.-Y. 2012 , clusterProfiler: an R package for comparing biological themes among gene clusters , OMICS , 16 , 284 – 7 .
Tang , H. , Bowers , J.E. , Wang , X. , Ming , R. , Alam , M. , and Paterson , A.H. 2008 , Synteny and collinearity in plant genomes , Science , 320 , 486 – 8 .
Goodstein , D.M. , Shu , S. , Howson , R. , et al. 2012 , Phytozome: a comparative platform for green plant genomics , Nucleic Acids Res. , 40 , D1178 – 86 .
Sun , P. , Jiao , B. , Yang , Y. , et al. 2022 , WGDI: a user-friendly toolkit for evolutionary analyses of whole-genome duplications and ancestral karyotypes , Mol. Plant , 15 , 1841 – 51 .
Chen , Y. , Chen , Y. , Shi , C. , et al. 2018 , SOAPnuke: a MapReduce acceleration-supported software for integrated quality control and preprocessing of high-throughput sequencing data , GigaScience , 7 , gix120 .
McKenna , A. , Hanna , M. , Banks , E. , et al. 2010 , The Genome Analysis Toolkit: a MapReduce framework for analyzing next-generation DNA sequencing data , Genome Res. , 20 , 1297 – 303 .
Danecek , P. , Auton , A. , Abecasis , G. , et al. ; 1000 Genomes Project Analysis Group . 2011 , The variant call format and VCFtools , Bioinformatics , 27 , 2156 – 8 .
Cingolani , P. , Platts , A. , Wang le , L. , et al. 2012 , A program for annotating and predicting the effects of single nucleotide polymorphisms, SnpEff: SNPs in the genome of Drosophila melanogaster strain w 1118 ; iso-2; iso-3 , Fly , 6 , 80 – 92 .
Purcell , S. , Neale , B. , Todd-Brown , K. , et al. 2007 , PLINK: a tool set for whole-genome association and population-based linkage analyses , Am. J. Hum. Genet. , 81 , 559 – 75 .
Minh , B.Q. , Schmidt , H.A. , Chernomor , O. , et al. 2020 , IQ-TREE 2: new models and efficient methods for phylogenetic inference in the genomic era , Mol. Biol. Evol. , 37 , 1530 – 4 .
Liu , Y. , Du , H. , Li , P. , et al. 2020 , Pan-genome of wild and cultivated soybeans , Cell , 182 , 162 – 76.e13 .
Alexander , D.H. , Novembre , J. , and Lange , K. 2009 , Fast model-based estimation of ancestry in unrelated individuals , Genome Res. , 19 , 1655 – 64 .
Yang , J. , Lee , S.H. , Goddard , M.E. , and Visscher , P.M. 2011 , GCTA: a tool for genome-wide complex trait analysis , Am. J. Hum. Genet. , 88 , 76 – 82 .
Zhang , C. , Dong , S.S. , Xu , J.Y. , He , W.M. , and Yang , T.L. 2019 , PopLDdecay: a fast and effective tool for linkage disequilibrium decay analysis based on variant call format files , Bioinformatics , 35 , 1786 – 8 .
Bowers , J.E. , Chapman , B.A. , Rong , J. , and Paterson , A.H. 2003 , Unravelling angiosperm genome evolution by phylogenetic analysis of chromosomal duplication events , Nature , 422 , 433 – 8 .
Jaillon , O. , Aury , J.M. , Noel , B. , et al. ; French-Italian Public Consortium for Grapevine Genome Characterization . 2007 , The grapevine genome sequence suggests ancestral hexaploidization in major angiosperm phyla , Nature , 449 , 463 – 7 .
Schmutz , J. , Cannon , S.B. , Schlueter , J. , et al. 2010 , Genome sequence of the palaeopolyploid soybean , Nature , 463 , 178 – 83 .
Yue , J. , VanBuren , R. , Liu , J. , et al. 2022 , SunUp and Sunset genomes revealed impact of particle bombardment mediated transformation and domestication history in papaya , Nat. Genet. , 54 , 715 – 24 .
Zhou , Z. , Jiang , Y. , Wang , Z. , et al. 2015 , Resequencing 302 wild and cultivated accessions identifies genes related to domestication and improvement in soybean , Nat. Biotechnol. , 33 , 408 – 14 .
Zeng , F. , Li , T. , Zhao , H. , Chen , H. , Yu , X. , and Liu , B. 2019 , Effect of debranching and temperature-cycled crystallization on the physicochemical properties of kudzu ( Pueraria lobata ) resistant starch , Int. J. Biol. Macromol. , 129 , 1148 – 54 .
Liu , D. , Ma , L. , Zhou , Z. , et al. 2021 , Starch and mineral element accumulation during root tuber expansion period of Pueraria thomsonii Benth , Food Chem. , 343 , 128445 .
Zhang , Y. , He , P. , Ma , X. , et al. 2019 , Auxin-mediated statolith production for root gravitropism , New Phytol. , 224 , 761 – 74 .
Mäkilä , R. , Wybouw , B. , Smetana , O. , et al. 2023 , Gibberellins promote polar auxin transport to regulate stem cell fate decisions in cambium , Nat. Plants , 9 , 631 – 44 .
Duan , H.Y. , Cheng , M.E. , Peng , H.S. , Zhang , H.T. , and Zhao , Y.J. 2015 , [Microscopic anatomy of abnormal structure in root tuber of Pueraria lobata ] , Zhongguo Zhong Yao Za Zhi , 40 , 4364 – 9 .
Song , J.M. , Xie , W.Z. , Wang , S. , et al. 2021 , Two gap-free reference genomes and a global view of the centromere architecture in rice , Mol. Plant , 14 , 1757 – 67 .
Han , X. , Zhang , Y. , Zhang , Q. , et al. 2023 , Two haplotype-resolved, gap-free genome assemblies for Actinidia latifolia and Actinidia chinensis shed light on the regulatory mechanisms of vitamin C and sucrose metabolism in kiwifruit , Mol. Plant , 16 , 452 – 70 .
Deng , Y. , Liu , S. , Zhang , Y. , et al. 2022 , A telomere-to-telomere gap-free reference genome of watermelon and its mutation library provide important resources for gene discovery and breeding , Mol. Plant , 15 , 1268 – 84 .
Hu , G. , Feng , J. , Xiang , X. , et al. 2022 , Two divergent haplotypes from a highly heterozygous lychee genome suggest independent domestication events for early and late-maturing cultivars , Nat. Genet. , 54 , 73 – 83 .
Wang , T. , Ren , L. , Li , C. , et al. 2021 , The genome of a wild Medicago species provides insights into the tolerant mechanisms of legume forage to environmental stress , BMC Biol. , 19 , 96 .
Wang , X.D. , Xu , C.Y. , Zheng , Y.J. , et al. 2022 , Chromosome-level genome assembly and resequencing of camphor tree ( Cinnamomum camphora ) provides insight into phylogeny and diversification of terpenoid and triglyceride biosynthesis of Cinnamomum , Hortic. Res. , 9 , uhac216 .
Kang , Y.J. , Kim , S.K. , Kim , M.Y. , et al. 2014 , Genome sequence of mungbean and insights into evolution within Vigna species , Nat. Commun. , 5 , 5443 .
Zhao , Y. , Zhang , R. , Jiang , K.W. , et al. 2021 , Nuclear phylotranscriptomics and phylogenomics support numerous polyploidization events and hypotheses for the evolution of rhizobial nitrogen-fixing symbiosis in Fabaceae , Mol. Plant , 14 , 748 – 73 .
Li , J. , Shen , J. , Wang , R. , et al. 2023 , The nearly complete assembly of the Cercis chinensis genome and Fabaceae phylogenomic studies provide insights into new gene evolution , Plant Commun , 4 , 100422 .
One Thousand Plant Transcriptomes Initiative . 2019 , One thousand plant transcriptomes and the phylogenomics of green plants , Nature , 574 , 679 – 85 .
Xie , L.-X. 2021 , A study on the Characteristics of Pharmacognosy of Three Varieties of Pueraria Montana (Lour.) Merr ., Jiangxi University of Chinese Medicine , Nanchang, Jiangxi Province, China .
Google Preview
Author notes
Supplementary data, email alerts, citing articles via.
- Author Guidelines
Affiliations
- Online ISSN 1756-1663
- Copyright © 2024 Kazusa DNA Research Institute
- About Oxford Academic
- Publish journals with us
- University press partners
- What we publish
- New features
- Open access
- Institutional account management
- Rights and permissions
- Get help with access
- Accessibility
- Advertising
- Media enquiries
- Oxford University Press
- Oxford Languages
- University of Oxford
Oxford University Press is a department of the University of Oxford. It furthers the University's objective of excellence in research, scholarship, and education by publishing worldwide
- Copyright © 2024 Oxford University Press
- Cookie settings
- Cookie policy
- Privacy policy
- Legal notice
This Feature Is Available To Subscribers Only
Sign In or Create an Account
This PDF is available to Subscribers Only
For full access to this pdf, sign in to an existing account, or purchase an annual subscription.
- Open access
- Published: 27 May 2024
Current status of community resources and priorities for weed genomics research
- Jacob Montgomery 1 ,
- Sarah Morran 1 ,
- Dana R. MacGregor ORCID: orcid.org/0000-0003-0543-0408 2 ,
- J. Scott McElroy ORCID: orcid.org/0000-0003-0331-3697 3 ,
- Paul Neve ORCID: orcid.org/0000-0002-3136-5286 4 ,
- Célia Neto ORCID: orcid.org/0000-0003-3256-5228 4 ,
- Martin M. Vila-Aiub ORCID: orcid.org/0000-0003-2118-290X 5 ,
- Maria Victoria Sandoval 5 ,
- Analia I. Menéndez ORCID: orcid.org/0000-0002-9681-0280 6 ,
- Julia M. Kreiner ORCID: orcid.org/0000-0002-8593-1394 7 ,
- Longjiang Fan ORCID: orcid.org/0000-0003-4846-0500 8 ,
- Ana L. Caicedo ORCID: orcid.org/0000-0002-0378-6374 9 ,
- Peter J. Maughan 10 ,
- Bianca Assis Barbosa Martins 11 ,
- Jagoda Mika 11 ,
- Alberto Collavo 11 ,
- Aldo Merotto Jr. ORCID: orcid.org/0000-0002-1581-0669 12 ,
- Nithya K. Subramanian ORCID: orcid.org/0000-0002-1659-7396 13 ,
- Muthukumar V. Bagavathiannan ORCID: orcid.org/0000-0002-1107-7148 13 ,
- Luan Cutti ORCID: orcid.org/0000-0002-2867-7158 14 ,
- Md. Mazharul Islam 15 ,
- Bikram S. Gill ORCID: orcid.org/0000-0003-4510-9459 16 ,
- Robert Cicchillo 17 ,
- Roger Gast 17 ,
- Neeta Soni ORCID: orcid.org/0000-0002-4647-8355 17 ,
- Terry R. Wright ORCID: orcid.org/0000-0002-3969-2812 18 ,
- Gina Zastrow-Hayes 18 ,
- Gregory May 18 ,
- Jenna M. Malone ORCID: orcid.org/0000-0002-9637-2073 19 ,
- Deepmala Sehgal ORCID: orcid.org/0000-0002-4141-1784 20 ,
- Shiv Shankhar Kaundun ORCID: orcid.org/0000-0002-7249-2046 20 ,
- Richard P. Dale 20 ,
- Barend Juan Vorster ORCID: orcid.org/0000-0003-3518-3508 21 ,
- Bodo Peters 11 ,
- Jens Lerchl ORCID: orcid.org/0000-0002-9633-2653 22 ,
- Patrick J. Tranel ORCID: orcid.org/0000-0003-0666-4564 23 ,
- Roland Beffa ORCID: orcid.org/0000-0003-3109-388X 24 ,
- Alexandre Fournier-Level ORCID: orcid.org/0000-0002-6047-7164 25 ,
- Mithila Jugulam ORCID: orcid.org/0000-0003-2065-9067 15 ,
- Kevin Fengler 18 ,
- Victor Llaca ORCID: orcid.org/0000-0003-4822-2924 18 ,
- Eric L. Patterson ORCID: orcid.org/0000-0001-7111-6287 14 &
- Todd A. Gaines ORCID: orcid.org/0000-0003-1485-7665 1
Genome Biology volume 25 , Article number: 139 ( 2024 ) Cite this article
886 Accesses
12 Altmetric
Metrics details
Weeds are attractive models for basic and applied research due to their impacts on agricultural systems and capacity to swiftly adapt in response to anthropogenic selection pressures. Currently, a lack of genomic information precludes research to elucidate the genetic basis of rapid adaptation for important traits like herbicide resistance and stress tolerance and the effect of evolutionary mechanisms on wild populations. The International Weed Genomics Consortium is a collaborative group of scientists focused on developing genomic resources to impact research into sustainable, effective weed control methods and to provide insights about stress tolerance and adaptation to assist crop breeding.
Each year globally, agricultural producers and landscape managers spend billions of US dollars [ 1 , 2 ] and countless hours attempting to control weedy plants and reduce their adverse effects. These management methods range from low-tech (e.g., pulling plants from the soil by hand) to extremely high-tech (e.g., computer vision-controlled spraying of herbicides). Regardless of technology level, effective control methods serve as strong selection pressures on weedy plants and often result in rapid evolution of weed populations resistant to such methods [ 3 , 4 , 5 , 6 , 7 ]. Thus, humans and weeds have been locked in an arms race, where humans develop new or improved control methods and weeds adapt and evolve to circumvent such methods.
Applying genomics to weed science offers a unique opportunity to study rapid adaptation, epigenetic responses, and examples of evolutionary rescue of diverse weedy species in the face of widespread and powerful selective pressures. Furthermore, lessons learned from these studies may also help to develop more sustainable control methods and to improve crop breeding efforts in the face of our ever-changing climate. While other research fields have used genetics and genomics to uncover the basis of many biological traits [ 8 , 9 , 10 , 11 ] and to understand how ecological factors affect evolution [ 12 , 13 ], the field of weed science has lagged behind in the development of genomic tools essential for such studies [ 14 ]. As research in human and crop genetics pushes into the era of pangenomics (i.e., multiple chromosome scale genome assemblies for a single species [ 15 , 16 ]), publicly available genomic information is still lacking or severely limited for the majority of weed species. Recent reviews of current weed genomes identified 26 [ 17 ] and 32 weed species with sequenced genomes [ 18 ]—many assembled to a sub-chromosome level.
Here, we summarize the current state of weed genomics, highlighting cases where genomics approaches have successfully provided insights on topics such as population genetic dynamics, genome evolution, and the genetic basis of herbicide resistance, rapid adaptation, and crop dedomestication. These highlighted investigations all relied upon genomic resources that are relatively rare for weedy species. Throughout, we identify additional resources that would advance the field of weed science and enable further progress in weed genomics. We then introduce the International Weed Genomics Consortium (IWGC), an open collaboration among researchers, and describe current efforts to generate these additional resources.
Evolution of weediness: potential research utilizing weed genomics tools
Weeds can evolve from non-weed progenitors through wild colonization, crop de-domestication, or crop-wild hybridization [ 19 ]. Because the time span in which weeds have evolved is necessarily limited by the origins of agriculture, these non-weed relatives often still exist and can be leveraged through population genomic and comparative genomic approaches to identify the adaptive changes that have driven the evolution of weediness. The ability to rapidly adapt, persist, and spread in agroecosystems are defining features of weedy plants, leading many to advocate agricultural weeds as ideal candidates for studying rapid plant adaptation [ 20 , 21 , 22 , 23 ]. The insights gained from applying plant ecological approaches to the study of rapid weed adaptation will move us towards the ultimate goals of mitigating such adaptation and increasing the efficacy of crop breeding and biotechnology [ 14 ].
Biology and ecological genomics of weeds
The impressive community effort to create and maintain resources for Arabidopsis thaliana ecological genomics provides a motivating example for the emerging study of weed genomics [ 24 , 25 , 26 , 27 ]. Arabidopsis thaliana was the first flowering plant species to have its genome fully sequenced [ 28 ] and rapidly became a model organism for plant molecular biology. As weedy genomes become available, collection, maintenance, and resequencing of globally distributed accessions of these species will help to replicate the success found in ecological studies of A. thaliana [ 29 , 30 , 31 , 32 , 33 , 34 , 35 ]. Evaluation of these accessions for traits of interest to produce large phenomics data sets (as in [ 36 , 37 , 38 , 39 , 40 ]) enables genome-wide association studies and population genomics analyses aimed at dissecting the genetic basis of variation in such traits [ 41 ]. Increasingly, these resources (e.g. the 1001 genomes project [ 29 ]) have enabled A. thaliana to be utilized as a model species to explore the eco-evolutionary basis of plant adaptation in a more realistic ecological context. Weedy species should supplement lessons in eco-evolutionary genomics learned from these experiments in A. thaliana .
Untargeted genomic approaches for understanding the evolutionary trajectories of populations and the genetic basis of traits as described above rely on the collection of genotypic information from across the genome of many individuals. While whole-genome resequencing accomplishes this requirement and requires no custom methodology, this approach provides more information than is necessary and is prohibitively expensive in species with large genomes. Development and optimization of genotype-by-sequencing methods for capturing reduced representations of newly sequence genomes like those described by [ 42 , 43 , 44 ] will reduce the cost and computational requirements of genetic mapping and population genetic experiments. Most major weed species do not currently have protocols for stable transformation, a key development in the popularity of A. thaliana as a model organism and a requirement for many functional genomic approaches. Functional validation of genes/variants believed to be responsible for traits of interest in weeds has thus far relied on transiently manipulating endogenous gene expression [ 45 , 46 ] or ectopic expression of a transgene in a model system [ 47 , 48 , 49 ]. While these methods have been successful, few weed species have well-studied viral vectors to adapt for use in virus induced gene silencing. Spray induced gene silencing is another potential option for functional investigation of candidate genes in weeds, but more research is needed to establish reliable delivery and gene knockdown [ 50 ]. Furthermore, traits with complex genetic architecture divergent between the researched and model species may not be amenable to functional genomic approaches using transgenesis techniques in model systems. Developing protocols for reduced representation sequencing, stable transformation, and gene editing/silencing in weeds will allow for more thorough characterization of candidate genetic variants underlying traits of interest.
Beyond rapid adaptation, some weedy species offer an opportunity to better understand co-evolution, like that between plants and pollinators and how their interaction leads to the spread of weedy alleles (Additional File 1 : Table S1). A suite of plant–insect traits has co-evolved to maximize the attraction of the insect pollinator community and the efficiency of pollen deposition between flowers ensuring fruit and seed production in many weeds [ 51 , 52 ]. Genetic mapping experiments have identified genes and genetic variants responsible for many floral traits affecting pollinator interaction including petal color [ 53 , 54 , 55 , 56 ], flower symmetry and size [ 57 , 58 , 59 ], and production of volatile organic compounds [ 60 , 61 , 62 ] and nectar [ 63 , 64 , 65 ]. While these studies reveal candidate genes for selection under co-evolution, herbicide resistance alleles may also have pleiotropic effects on the ecology of weeds [ 66 ], altering plant-pollinator interactions [ 67 ]. Discovery of genes and genetic variants involved in weed-pollinator interaction and their molecular and environmental control may create opportunities for better management of weeds with insect-mediated pollination. For example, if management can disrupt pollinator attraction/interaction with these weeds, the efficiency of reproduction may be reduced.
A more complete understanding of weed ecological genomics will undoubtedly elucidate many unresolved questions regarding the genetic basis of various aspects of weediness. For instance, when comparing populations of a species from agricultural and non-agricultural environments, is there evidence for contemporary evolution of weedy traits selected by agricultural management or were “natural” populations pre-adapted to agroecosystems? Where there is differentiation between weedy and natural populations, which traits are under selection and what is the genetic basis of variation in those traits? When comparing between weedy populations, is there evidence for parallel versus non-parallel evolution of weediness at the phenotypic and genotypic levels? Such studies may uncover fundamental truths about weediness. For example, is there a common phenotypic and/or genotypic basis for aspects of weediness among diverse weed species? The availability of characterized accessions and reference genomes for species of interest are required for such studies but only a few weedy species have these resources developed.
Population genomics
Weed species are certainly fierce competitors, able to outcompete crops and endemic species in their native environment, but they are also remarkable colonizers of perturbed habitats. Weeds achieve this through high fecundity, often producing tens of thousands of seeds per individual plant [ 68 , 69 , 70 ]. These large numbers in terms of demographic population size often combine with outcrossing reproduction to generate high levels of diversity with local effective population sizes in the hundreds of thousands [ 71 , 72 ]. This has two important consequences: weed populations retain standing genetic variation and generate many new mutations, supporting weed success in the face of harsh control. The generation of genomic tools to monitor weed populations at the molecular level is a game-changer to understanding weed dynamics and precisely testing the effect of artificial selection (i.e., management) and other evolutionary mechanisms on the genetic make-up of populations.
Population genomic data, without any environmental or phenotypic information, can be used to scan the genomes of weed and non-weed relatives to identify selective sweeps, pointing at loci supporting weed adaptation on micro- or macro-evolutionary scales. Two recent within-species examples include weedy rice, where population differentiation between weedy and domesticated populations was used to identify the genetic basis of weedy de-domestication [ 73 ], and common waterhemp, where consistent allelic differences among natural and agricultural collections resolved a complex set of agriculturally adaptive alleles [ 74 , 75 ]. A recent comparative population genomic study of weedy barnyardgrass and crop millet species has demonstrated how inter-specific investigations can resolve the signatures of crop and weed evolution [ 76 ] (also see [ 77 ] for a non-weed climate adaptation example). Multiple sequence alignments across numerous species provide complementary insight into adaptive convergence over deeper timescales, even with just one genomic sample per species (e.g., [ 78 , 79 ]). Thus, newly sequenced weed genomes combined with genomes available for closely related crops (outlined by [ 14 , 80 ]) and an effort to identify other non-weed wild relatives will be invaluable in characterizing the genetic architecture of weed adaptation and evolution across diverse species.
Weeds experience high levels of genetic selection, both artificial in response to agricultural practices and particularly herbicides, and natural in response to the environmental conditions they encounter [ 81 , 82 ]. Using genomic analysis to identify loci that are the targets of selection, whether natural or artificial, would point at vulnerabilities that could be leveraged against weeds to develop new and more sustainable management strategies [ 83 ]. This is a key motivation to develop genotype-by-environment association (GEA) and selective sweep scan approaches, which allow researchers to resolve the molecular basis of multi-dimensional adaptation [ 84 , 85 ]. GEA approaches, in particular, have been widely used on landscape-wide resequencing collections to determine the genetic basis of climate adaptation (e.g., [ 27 , 86 , 87 ]), but have yet to be fully exploited to diagnose the genetic basis of the various aspects of weediness [ 88 ]. Armed with data on environmental dimensions of agricultural settings, such as focal crop, soil quality, herbicide use, and climate, GEA approaches can help disentangle how discrete farming practices have influenced the evolution of weediness and resolve broader patterns of local adaptation across a weed’s range. Although non-weedy relatives are not technically required for GEA analyses, inclusion of environmental and genomic data from weed progenitors can further distinguish genetic variants underpinning weed origins from those involved in local adaptation.
New weeds emerge frequently [ 89 ], either through hybridization between species as documented for sea beet ( Beta vulgaris ssp. maritima) hybridizing with crop beet to produce progeny that are well adapted to agricultural conditions [ 90 , 91 , 92 ], or through the invasion of alien species that find a new range to colonize. Biosecurity measures are often in place to stop the introduction of new weeds; however, the vast scale of global agricultural commodity trade precludes the possibility of total control. Population genomic analysis is now able to measure gene flow between populations [ 74 , 93 , 94 , 95 ] and identify populations of origin for invasive species including weeds [ 96 , 97 , 98 ]. For example, the invasion route of the pest fruitfly Drosophila suzukii from Eastern Asia to North America and Europe through Hawaii was deciphered using Approximate Bayesian Computation on high-throughput sequencing data from a global sample of multiple populations [ 99 ]. Genomics can also be leveraged to predict invasion rather than explain it. The resequencing of a global sample of common ragweed ( Ambrosia artemisiifolia L.) elucidated a complex invasion route whereby Europe was invaded by multiple introductions of American ragweed that hybridized in Europe prior to a subsequent introduction to Australia [ 100 , 101 ]. In this context, the use of genomically informed species distribution models helps assess the risk associated with different source populations, which in the case of common ragweed, suggests that a source population from Florida would allow ragweed to invade most of northern Australia [ 102 ]. Globally coordinated research efforts to understand potential distribution models could support the transformation of biosecurity from perspective analysis towards predictive risk assessment.
Herbicide resistance and weed management
Herbicide resistance is among the numerous weedy traits that can evolve in plant populations exposed to agricultural selection pressures. Over-reliance on herbicides to control weeds, along with low diversity and lack of redundancy in weed management strategies, has resulted in globally widespread herbicide resistance [ 103 ]. To date, 272 herbicide-resistant weed species have been reported worldwide, and at least one resistance case exists for 21 of the 31 existing herbicide sites of action [ 104 ]—significantly limiting chemical weed control options available to agriculturalists. This limitation of control options is exacerbated by the recent lack of discovery of herbicides with new sites of action [ 105 ].
Herbicide resistance may result from several different physiological mechanisms. Such mechanisms have been classified into two main groups, target-site resistance (TSR) [ 4 , 106 ] and non-target-site resistance (NTSR) [ 4 , 107 ]. The first group encompasses changes that reduce binding affinity between a herbicide and its target [ 108 ]. These changes may provide resistance to multiple herbicides that have a common biochemical target [ 109 ] and can be effectively managed through mixture and/or rotation of herbicides targeting different sites of action [ 110 ]. The second group (NTSR), includes alterations in herbicide absorption, translocation, sequestration, and/or metabolism that may lead to unpredictable pleotropic cross-resistance profiles where structurally and functionally diverse herbicides are rendered ineffective by one or more genetic variant(s) [ 47 ]. This mechanism of resistance threatens not only the efficacy of existing herbicidal chemistries, but also ones yet to be discovered. While TSR is well understood because of the ease of identification and molecular characterization of target site variants, NTSR mechanisms are significantly more challenging to research because they are often polygenic, and the resistance causing element(s) are not well understood [ 111 ].
Improving the current understanding of metabolic NTSR mechanisms is not an easy task, since genes of diverse biochemical functions are involved, many of which exist as extensive gene families [ 109 , 112 ]. Expression changes of NTSR genes have been implicated in several resistance cases where the protein products of the genes are functionally equivalent across sensitive and resistant plants, but their relative abundance leads to resistance. Thus, regulatory elements of NTSR genes have been scrutinized to understand their role in NTSR mechanisms [ 113 ]. Similarly, epigenetic modifications have been hypothesized to play a role in NTSR, with much remaining to be explored [ 114 , 115 , 116 ]. Untargeted approaches such as genome-wide association, selective sweep scans, linkage mapping, RNA-sequencing, and metabolomic profiling have proven helpful to complement more specific biochemical- and chemo-characterization studies towards the elucidation of NTSR mechanisms as well as their regulation and evolution [ 47 , 117 , 118 , 119 , 120 , 121 , 122 , 123 , 124 ]. Even in cases where resistance has been attributed to TSR, genetic mapping approaches can detect other NTSR loci contributing to resistance (as shown by [ 123 ]) and provide further evidence for the role of TSR mutations across populations. Knowledge of the genetic basis of NTSR will aid the rational design of herbicides by screening new compounds for interaction with newly discovered NTSR proteins during early research phases and by identifying conserved chemical structures that interact with these proteins that should be avoided in small molecule design.
Genomic resources can also be used to predict the protein structure for novel herbicide target site and metabolism genes. This will allow for prediction of efficacy and selectivity for new candidate herbicides in silico to increase herbicide discovery throughput as well as aid in the design and development of next-generation technologies for sustainable weed management. Proteolysis targeting chimeras (PROTACs) have the potential to bind desired targets with great selectivity and degrade proteins by utilizing natural protein ubiquitination and degradation pathways within plants [ 125 ]. Spray-induced gene silencing in weeds using oligonucleotides has potential as a new, innovative, and sustainable method for weed management, but improved methods for design and delivery of oligonucleotides are needed to make this technique a viable management option [ 50 ]. Additionally, success in the field of pharmaceutical drug discovery in the development of molecules modulating protein–protein interactions offers another potential avenue towards the development of herbicides with novel targets [ 126 , 127 ]. High-quality reference genomes allow for the design of new weed management technologies like the ones listed here that are specific to—and effective across—weed species but have a null effect on non-target organisms.
Comparative genomics and genome biology
The genomes of weed species are as diverse as weed species themselves. Weeds are found across highly diverged plant families and often have no phylogenetically close model or crop species relatives for comparison. On all measurable metrics, weed genomes run the gamut. Some have smaller genomes like Cyperus spp. (~ 0.26 Gb) while others are larger, such as Avena fatua (~ 11.1 Gb) (Table 1 ). Some have high heterozygosity in terms of single-nucleotide polymorphisms, such as the Amaranthus spp., while others are primarily self-pollinated and quite homozygous, such as Poa annua [ 128 , 129 ]. Some are diploid such as Conyza canadensis and Echinochloa haploclada while others are polyploid such as C. sumetrensis , E. crus-galli , and E. colona [ 76 ]. The availability of genomic resources in these diverse, unexplored branches of the tree of life allows us to identify consistencies and anomalies in the field of genome biology.
The weed genomes published so far have focused mainly on weeds of agronomic crops, and studies have revolved around their ability to resist key herbicides. For example, genomic resources were vital in the elucidation of herbicide resistance cases involving target site gene copy number variants (CNVs). Gene CNVs of 5-enolpyruvylshikimate-3-phosphate synthase ( EPSPS ) have been found to confer resistance to the herbicide glyphosate in diverse weed species. To date, nine species have independently evolved EPSPS CNVs, and species achieve increased EPSPS copy number via different mechanisms [ 153 ]. For instance, the EPSPS CNV in Bassia scoparia is caused by tandem duplication, which is accredited to transposable element insertions flanking EPSPS and subsequent unequal crossing over events [ 154 , 155 ]. In Eleusine indica , a EPSPS CNV was caused by translocation of the EPSPS locus into the subtelomere followed by telomeric sequence exchange [ 156 ]. One of the most fascinating genome biology discoveries in weed science has been that of extra-chromosomal circular DNAs (eccDNAs) that harbor the EPSPS gene in the weed species Amaranthus palmeri [ 157 , 158 ]. In this case, the eccDNAs autonomously replicate separately from the nuclear genome and do not reintegrate into chromosomes, which has implications for inheritance, fitness, and genome structure [ 159 ]. These discoveries would not have been possible without reference assemblies of weed genomes, next-generation sequencing, and collaboration with experts in plant genomics and bioinformatics.
Another question that is often explored with weedy genomes is the nature and composition of gene families that are associated with NTSR. Gene families under consideration often include cytochrome P450s (CYPs), glutathione- S -transferases (GSTs), ABC transporters, etc. Some questions commonly considered with new weed genomes include how many genes are in each of these gene families, where are they located, and which weed accessions and species have an over-abundance of them that might explain their ability to evolve resistance so rapidly [ 76 , 146 , 160 , 161 ]? Weed genome resources are necessary to answer questions about gene family expansion or contraction during the evolution of weediness, including the role of polyploidy in NTSR gene family expansion as explored by [ 162 ].
Translational research and communication with weed management stakeholders
Whereas genomics of model plants is typically aimed at addressing fundamental questions in plant biology, and genomics of crop species has the obvious goal of crop improvement, goals of genomics of weedy plants also include the development of more effective and sustainable strategies for their management. Weed genomic resources assist with these objectives by providing novel molecular ecological and evolutionary insights from the context of intensive anthropogenic management (which is lacking in model plants), and offer knowledge and resources for trait discovery for crop improvement, especially given that many wild crop relatives are also important agronomic weeds (e.g., [ 163 ]). For instance, crop-wild relatives are valuable for improving crop breeding for marginal environments [ 164 ]. Thus, weed genomics presents unique opportunities and challenges relative to plant genomics more broadly. It should also be noted that although weed science at its core is an applied discipline, it draws broadly from many scientific disciplines such as, plant physiology, chemistry, ecology, and evolutionary biology, to name a few. The successful integration of weed-management strategies, therefore, requires extensive collaboration among individuals collectively possessing the necessary expertise [ 165 ].
With the growing complexity of herbicide resistance management, practitioners are beginning to recognize the importance of understanding resistance mechanisms to inform appropriate management tactics [ 14 ]. Although weed science practitioners do not need to understand the technical details of weed genomics, their appreciation of the power of weed genomics—together with their unique insights from field observations—will yield novel opportunities for applications of weed genomics to weed management. In particular, combining field management history with information on weed resistance mechanisms is expected to provide novel insights into evolutionary trajectories (e.g. [ 6 , 166 ]), which can be utilized for disrupting evolutionary adaptation. It can be difficult to obtain field history information from practitioners, but developing an understanding among them of the importance of such information can be invaluable.
Development of weed genomics resources by the IWGC
Weed genomics is a fast-growing field of research with many recent breakthroughs and many unexplored areas of study. The International Weed Genomics Consortium (IWGC) started in 2021 to address the roadblocks listed above and to promote the study of weedy plants. The IWGC is an open collaboration among academic, government, and industry researchers focused on producing genomic tools for weedy species from around the world. Through this collaboration, our initial aim is to provide chromosome-level reference genome assemblies for at least 50 important weedy species from across the globe that are chosen based on member input, economic impact, and global prevalence (Fig. 1 ). Each genome will include annotation of gene models and repetitive elements and will be freely available through public databases with no intellectual property restrictions. Additionally, future funding of the IWGC will focus on improving gene annotations and supplementing these reference genomes with tools that increase their utility.
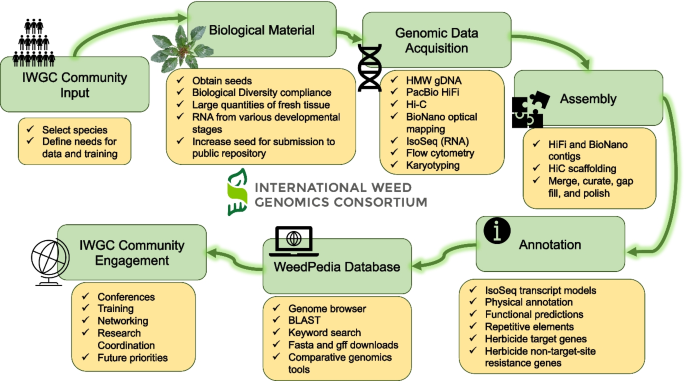
The International Weed Genomics Consortium (IWGC) collected input from the weed genomics community to develop plans for weed genome sequencing, annotation, user-friendly genome analysis tools, and community engagement
Reference genomes and data analysis tools
The first objective of the IWGC is to provide high-quality genomic resources for agriculturally important weeds. The IWGC therefore created two main resources for information about, access to, or analysis of weed genomic data (Fig. 1 ). The IWGC website (available at [ 167 ]) communicates the status and results of genome sequencing projects, information on training and funding opportunities, upcoming events, and news in weed genomics. It also contains details of all sequenced species including genome size, ploidy, chromosome number, herbicide resistance status, and reference genome assembly statistics. The IWGC either compiles existing data on genome size, ploidy, and chromosome number, or obtains the data using flow cytometry and cytogenetics (Fig. 1 ; Additional File 2 : Fig S1-S4). Through this website, users can request an account to access our second main resource, an online genome database called WeedPedia (accessible at [ 168 ]), with an account that is created within 3–5 working days of an account request submission. WeedPedia hosts IWGC-generated and other relevant publicly accessible genomic data as well as a suite of bioinformatic tools. Unlike what is available for other fields, weed science did not have a centralized hub for genomics information, data, and analysis prior to the IWGC. Our intention in creating WeedPedia is to encourage collaboration and equity of access to information across the research community. Importantly, all genome assemblies and annotations from the IWGC (Table 1 ), along with the raw data used to produce them, will be made available through NCBI GenBank. Upon completion of a 1-year sponsoring member data confidentiality period for each species (dates listed in Table 1 ), scientific teams within the IWGC produce the first genome-wide investigation to submit for publication including whole genome level analyses on genes, gene families, and repetitive sequences as well as comparative analysis with other species. Genome assemblies and data will be publicly available through NCBI as part of these initial publications for each species.
WeedPedia is a cloud-based omics database management platform built from the software “CropPedia” and licensed from KeyGene (Wageningen, The Netherlands). The interface allows users to access, visualize, and download genome assemblies along with structural and functional annotation. The platform includes a genome browser, comparative map viewer, pangenome tools, RNA-sequencing data visualization tools, genetic mapping and marker analysis tools, and alignment capabilities that allow searches by keyword or sequence. Additionally, genes encoding known target sites of herbicides have been specially annotated, allowing users to quickly identify and compare these genes of interest. The platform is flexible, making it compatible with future integration of other data types such as epigenetic or proteomic information. As an online platform with a graphical user interface, WeedPedia provides user-friendly, intuitive tools that encourage users to integrate genomics into their research while also allowing more advanced users to download genomic data to be used in custom analysis pipelines. We aspire for WeedPedia to mimic the success of other public genomic databases such as NCBI, CoGe, Phytozome, InsectBase, and Mycocosm to name a few. WeedPedia currently hosts reference genomes for 40 species (some of which are currently in their 1-year confidentiality period) with additional genomes in the pipeline to reach a currently planned total of 55 species (Table 1 ). These genomes include both de novo reference genomes generated or in progress by the IWGC (31 species; Table 1 ), and publicly available genome assemblies of 24 weedy or related species that were generated by independent research groups (Table 2 ). As of May 2024, WeedPedia has over 370 registered users from more than 27 countries spread across 6 continents.
The IWGC reference genomes are generated in partnership with the Corteva Agriscience Genome Center of Excellence (Johnston, Iowa) using a combination of single-molecule long-read sequencing, optical genome maps, and chromosome conformation mapping. This strategy has already yielded highly contiguous, phased, chromosome-level assemblies for 26 weed species, with additional assemblies currently in progress (Table 1 ). The IWGC assemblies have been completed as single or haplotype-resolved double-haplotype pseudomolecules in inbreeding and outbreeding species, respectively, with multiple genomes being near gapless. For example, the de novo assemblies of the allohexaploids Conyza sumatrensis and Chenopodium album have all chromosomes captured in single scaffolds and most chromosomes being gapless from telomere to telomere. Complementary full-length isoform (IsoSeq) sequencing of RNA collected from diverse tissue types and developmental stages assists in the development of gene models during annotation.
As with accessibility of data, a core objective of the IWGC is to facilitate open access to sequenced germplasm when possible for featured species. Historically, the weed science community has rarely shared or adopted standard germplasm (e.g., specific weed accessions). The IWGC has selected a specific accession of each species for reference genome assembly (typically susceptible to herbicides). In collaboration with a parallel effort by the Herbicide Resistant Plants committee of the Weed Science Society of America, seeds of the sequenced weed accessions will be deposited in the United States Department of Agriculture Germplasm Resources Information Network [ 186 ] for broad access by the scientific community and their accession numbers will be listed on the IWGC website. In some cases, it is not possible to generate enough seed to deposit into a public repository (e.g., plants that typically reproduce vegetatively, that are self-incompatible, or that produce very few seeds from a single individual). In these cases, the location of collection for sequenced accessions will at least inform the community where the sequenced individual came from and where they may expect to collect individuals with similar genotypes. The IWGC ensures that sequenced accessions are collected and documented to comply with the Nagoya Protocol on access to genetic resources and the fair and equitable sharing of benefits arising from their utilization under the Convention on Biological Diversity and related Access and Benefit Sharing Legislation [ 187 ]. As additional accessions of weed species are sequenced (e.g., pangenomes are obtained), the IWGC will facilitate germplasm sharing protocols to support collaboration. Further, to simplify the investigation of herbicide resistance, the IWGC will link WeedPedia with the International Herbicide-Resistant Weed Database [ 104 ], an already widely known and utilized database for weed scientists.
Training and collaboration in weed genomics
Beyond producing genomic tools and resources, a priority of the IWGC is to enable the utilization of these resources across a wide range of stakeholders. A holistic approach to training is required for weed science generally [ 188 ], and we would argue even more so for weed genomics. To accomplish our training goals, the IWGC is developing and delivering programs aimed at the full range of IWGC stakeholders and covering a breadth of relevant topics. We have taken care to ensure our approaches are diverse as to provide training to researchers with all levels of existing experience and differing reasons for engaging with these tools. Throughout, the focus is on ensuring that our training and outreach result in impacts that benefit a wide range of stakeholders.
Although recently developed tools are incredibly enabling and have great potential to replace antiquated methodology [ 189 ] and to solve pressing weed science problems [ 14 ], specialized computational skills are required to fully explore and unlock meaning from these highly complex datasets. Collaboration with, or training of, computational biologists equipped with these skills and resources developed by the IWGC will enable weed scientists to expand research programs and better understand the genetic underpinnings of weed evolution and herbicide resistance. To fill existing skill gaps, the IWGC is developing summer bootcamps and online modules directed specifically at weed scientists that will provide training on computational skills (Fig. 1 ). Because successful utilization of the IWGC resources requires more than general computational skills, we have created three targeted workshops that teach practical skills related to genomics databases, molecular biology, and population genomics (available at [ 190 ]). The IWGC has also hosted two official conference meetings, one in September of 2021 and one in January of 2023, with more conferences planned. These conferences have included invited speakers to present successful implementations of weed genomics, educational workshops to build computational skills, and networking opportunities for research to connect and collaborate.
Engagement opportunities during undergraduate degrees have been shown to improve academic outcomes [ 191 , 192 ]. As one activity to help achieve this goal, the IWGC has sponsored opportunities for US undergraduates to undertake a 10-week research experience, which includes an introduction to bioinformatics, a plant genomics research project that results in a presentation, and access to career building opportunities in diverse workplace environments. To increase equitable access to conferences and professional communities, we supported early career researchers to attend the first two IWGC conferences in the USA as well as workshops and bootcamps in Europe, South America, and Australia. These hybrid or in-person travel grants are intentionally designed to remove barriers and increase participation of individuals from backgrounds and experiences currently underrepresented within weed/plant science or genomics [ 193 ]. Recipients of these travel awards gave presentations and gained the measurable benefits that come from either virtual or in-person participation in conferences [ 194 ]. Moving forward, weed scientists must amass skills associated with genomic analyses and collaborate with other area experts to fully leverage resources developed by the IWGC.
The tools generated through the IWGC will enable many new research projects with diverse objectives like those listed above. In summary, contiguous genome assemblies and complete annotation information will allow weed scientists to join plant breeders in the use of genetic mapping for many traits including stress tolerance, plant architecture, and herbicide resistance (especially important for cases of NTSR). These assemblies will also allow for investigations of population structure, gene flow, and responses to evolutionary mechanisms like genetic bottlenecking and artificial selection. Understanding gene sequences across diverse weed species will be vital in modeling new herbicide target site proteins and designing novel effective herbicides with minimal off-target effects. The IWGC website will improve accessibility to weed genomics data by providing a single hub for reference genomes as well as phenotypic and genotypic information for accessions shared with the IWGC. Deposition of sequenced germplasm into public repositories will ensure that researchers are able to access and utilize these accessions in their own research to make the field more standardized and equitable. WeedPedia allows users of all backgrounds to quickly access information of interest such as herbicide target site gene sequence or subcellular localization of protein products for different genes. Users can also utilize server-based tools such as BLAST and genome browsing similar to other public genomic databases. Finally, the IWGC is committed to training and connecting weed genomicists through hosting trainings, workshops, and conferences.
Conclusions
Weeds are unique and fascinating plants, having significant impacts on agriculture and ecosystems; and yet, aspects of their biology, ecology, and genetics remain poorly understood. Weeds represent a unique area within plant biology, given their repeated rapid adaptation to sudden and severe shifts in the selective landscape of anthropogenic management practices. The production of a public genomics database with reference genomes and annotations for over 50 weed species represents a substantial step forward towards research goals that improve our understanding of the biology and evolution of weeds. Future work is needed to improve annotations, particularly for complex gene families involved in herbicide detoxification, structural variants, and mobile genetic elements. As reference genome assemblies become available; standard, affordable methods for gathering genotype information will allow for the identification of genetic variants underlying traits of interest. Further, methods for functional validation and hypothesis testing are needed in weeds to validate the effect of genetic variants detected through such experiments, including systems for transformation, gene editing, and transient gene silencing and expression. Future research should focus on utilizing weed genomes to investigate questions about evolutionary biology, ecology, genetics of weedy traits, and weed population dynamics. The IWGC plans to continue the public–private partnership model to host the WeedPedia database over time, integrate new datasets such as genome resequencing and transcriptomes, conduct trainings, and serve as a research coordination network to ensure that advances in weed science from around the world are shared across the research community (Fig. 1 ). Bridging basic plant genomics with translational applications in weeds is needed to deliver on the potential of weed genomics to improve weed management and crop breeding.
Availability of data and materials
All genome assemblies and related sequencing data produced by the IWGC will be available through NCBI as part of publications reporting the first genome-wide analysis for each species.
Gianessi LP, Nathan PR. The value of herbicides in U.S. crop production. Weed Technol. 2007;21(2):559–66.
Article Google Scholar
Pimentel D, Lach L, Zuniga R, Morrison D. Environmental and economic costs of nonindigenous species in the United States. Bioscience. 2000;50(1):53–65.
Barrett SH. Crop mimicry in weeds. Econ Bot. 1983;37(3):255–82.
Powles SB, Yu Q. Evolution in action: plants resistant to herbicides. Annu Rev Plant Biol. 2010;61:317–47.
Article CAS PubMed Google Scholar
Thurber CS, Reagon M, Gross BL, Olsen KM, Jia Y, Caicedo AL. Molecular evolution of shattering loci in U.S. weedy rice. Mol Ecol. 2010;19(16):3271–84.
Article PubMed PubMed Central Google Scholar
Comont D, Lowe C, Hull R, Crook L, Hicks HL, Onkokesung N, et al. Evolution of generalist resistance to herbicide mixtures reveals a trade-off in resistance management. Nat Commun. 2020;11(1):3086.
Article CAS PubMed PubMed Central Google Scholar
Ashworth MB, Walsh MJ, Flower KC, Vila-Aiub MM, Powles SB. Directional selection for flowering time leads to adaptive evolution in Raphanus raphanistrum (wild radish). Evol Appl. 2016;9(4):619–29.
Chan EK, Rowe HC, Kliebenstein DJ. Understanding the evolution of defense metabolites in Arabidopsis thaliana using genome-wide association mapping. Genetics. 2010;185(3):991–1007.
Frayling TM, Timpson NJ, Weedon MN, Zeggini E, Freathy RM, Lindgren CM, et al. A common variant in the FTO gene is associated with body mass index and predisposes to childhood and adult obesity. Science. 2007;316(5826):889–94.
Harkess A, Zhou J, Xu C, Bowers JE, Van der Hulst R, Ayyampalayam S, et al. The asparagus genome sheds light on the origin and evolution of a young Y chromosome. Nat Commun. 2017;8(1):1279.
Periyannan S, Moore J, Ayliffe M, Bansal U, Wang X, Huang L, et al. The gene Sr33 , an ortholog of barley Mla genes, encodes resistance to wheat stem rust race Ug99. Science. 2013;341(6147):786–8.
Ågren J, Oakley CG, McKay JK, Lovell JT, Schemske DW. Genetic mapping of adaptation reveals fitness tradeoffs in Arabidopsis thaliana . Proc Natl Acad Sci U S A. 2013;110(52):21077–82.
Article PubMed Central Google Scholar
Schartl M, Walter RB, Shen Y, Garcia T, Catchen J, Amores A, et al. The genome of the platyfish, Xiphophorus maculatus , provides insights into evolutionary adaptation and several complex traits. Nat Genet. 2013;45(5):567–72.
Ravet K, Patterson EL, Krähmer H, Hamouzová K, Fan L, Jasieniuk M, et al. The power and potential of genomics in weed biology and management. Pest Manag Sci. 2018;74(10):2216–25.
Hufford MB, Seetharam AS, Woodhouse MR, Chougule KM, Ou S, Liu J, et al. De novo assembly, annotation, and comparative analysis of 26 diverse maize genomes. Science. 2021;373(6555):655–62.
Liao W-W, Asri M, Ebler J, Doerr D, Haukness M, Hickey G, et al. A draft human pangenome reference. Nature. 2023;617(7960):312–24.
Huang Y, Wu D, Huang Z, Li X, Merotto A, Bai L, et al. Weed genomics: yielding insights into the genetics of weedy traits for crop improvement. aBIOTECH. 2023;4:20–30.
Chen K, Yang H, Wu D, Peng Y, Lian L, Bai L, et al. Weed biology and management in the multi-omics era: progress and perspectives. Plant Commun. 2024;5(4):100816.
De Wet JMJ, Harlan JR. Weeds and domesticates: evolution in the man-made habitat. Econ Bot. 1975;29(2):99–108.
Mahaut L, Cheptou PO, Fried G, Munoz F, Storkey J, Vasseur F, et al. Weeds: against the rules? Trends Plant Sci. 2020;25(11):1107–16.
Neve P, Vila-Aiub M, Roux F. Evolutionary-thinking in agricultural weed management. New Phytol. 2009;184(4):783–93.
Article PubMed Google Scholar
Sharma G, Barney JN, Westwood JH, Haak DC. Into the weeds: new insights in plant stress. Trends Plant Sci. 2021;26(10):1050–60.
Vigueira CC, Olsen KM, Caicedo AL. The red queen in the corn: agricultural weeds as models of rapid adaptive evolution. Heredity (Edinb). 2013;110(4):303–11.
Donohue K, Dorn L, Griffith C, Kim E, Aguilera A, Polisetty CR, et al. Niche construction through germination cueing: life-history responses to timing of germination in Arabidopsis thaliana . Evolution. 2005;59(4):771–85.
PubMed Google Scholar
Exposito-Alonso M. Seasonal timing adaptation across the geographic range of Arabidopsis thaliana . Proc Natl Acad Sci U S A. 2020;117(18):9665–7.
Fournier-Level A, Korte A, Cooper MD, Nordborg M, Schmitt J, Wilczek AM. A map of local adaptation in Arabidopsis thaliana . Science. 2011;334(6052):86–9.
Hancock AM, Brachi B, Faure N, Horton MW, Jarymowycz LB, Sperone FG, et al. Adaptation to climate across the Arabidopsis thaliana genome. Science. 2011;334(6052):83–6.
Initiative TAG. Analysis of the genome sequence of the flowering plant Arabidopsis thaliana . Nature. 2000;408(6814):796–815.
Alonso-Blanco C, Andrade J, Becker C, Bemm F, Bergelson J, Borgwardt KM, et al. 1,135 genomes reveal the global pattern of polymorphism in Arabidopsis thaliana . Cell. 2016;166(2):481–91.
Durvasula A, Fulgione A, Gutaker RM, Alacakaptan SI, Flood PJ, Neto C, et al. African genomes illuminate the early history and transition to selfing in Arabidopsis thaliana . Proc Natl Acad Sci U S A. 2017;114(20):5213–8.
Frachon L, Mayjonade B, Bartoli C, Hautekèete N-C, Roux F. Adaptation to plant communities across the genome of Arabidopsis thaliana . Mol Biol Evol. 2019;36(7):1442–56.
Fulgione A, Koornneef M, Roux F, Hermisson J, Hancock AM. Madeiran Arabidopsis thaliana reveals ancient long-range colonization and clarifies demography in Eurasia. Mol Biol Evol. 2018;35(3):564–74.
Fulgione A, Neto C, Elfarargi AF, Tergemina E, Ansari S, Göktay M, et al. Parallel reduction in flowering time from de novo mutations enable evolutionary rescue in colonizing lineages. Nat Commun. 2022;13(1):1461.
Kasulin L, Rowan BA, León RJC, Schuenemann VJ, Weigel D, Botto JF. A single haplotype hyposensitive to light and requiring strong vernalization dominates Arabidopsis thaliana populations in Patagonia. Argentina Mol Ecol. 2017;26(13):3389–404.
Picó FX, Méndez-Vigo B, Martínez-Zapater JM, Alonso-Blanco C. Natural genetic variation of Arabidopsis thaliana is geographically structured in the Iberian peninsula. Genetics. 2008;180(2):1009–21.
Atwell S, Huang YS, Vilhjálmsson BJ, Willems G, Horton M, Li Y, et al. Genome-wide association study of 107 phenotypes in Arabidopsis thaliana inbred lines. Nature. 2010;465(7298):627–31.
Flood PJ, Kruijer W, Schnabel SK, van der Schoor R, Jalink H, Snel JFH, et al. Phenomics for photosynthesis, growth and reflectance in Arabidopsis thaliana reveals circadian and long-term fluctuations in heritability. Plant Methods. 2016;12(1):14.
Marchadier E, Hanemian M, Tisné S, Bach L, Bazakos C, Gilbault E, et al. The complex genetic architecture of shoot growth natural variation in Arabidopsis thaliana . PLoS Genet. 2019;15(4):e1007954.
Tisné S, Serrand Y, Bach L, Gilbault E, Ben Ameur R, Balasse H, et al. Phenoscope: an automated large-scale phenotyping platform offering high spatial homogeneity. Plant J. 2013;74(3):534–44.
Tschiersch H, Junker A, Meyer RC, Altmann T. Establishment of integrated protocols for automated high throughput kinetic chlorophyll fluorescence analyses. Plant Methods. 2017;13:54.
Chen X, MacGregor DR, Stefanato FL, Zhang N, Barros-Galvão T, Penfield S. A VEL3 histone deacetylase complex establishes a maternal epigenetic state controlling progeny seed dormancy. Nat Commun. 2023;14(1):2220.
Choi M, Scholl UI, Ji W, Liu T, Tikhonova IR, Zumbo P, et al. Genetic diagnosis by whole exome capture and massively parallel DNA sequencing. Proc Natl Acad Sci U S A. 2009;106(45):19096–101.
Davey JW, Blaxter ML. RADSeq: next-generation population genetics. Brief Funct Genomics. 2010;9(5–6):416–23.
Elshire RJ, Glaubitz JC, Sun Q, Poland JA, Kawamoto K, Buckler ES, et al. A robust, simple genotyping-by-sequencing (GBS) approach for high diversity species. PLoS ONE. 2011;6(5):e19379.
MacGregor DR. What makes a weed a weed? How virus-mediated reverse genetics can help to explore the genetics of weediness. Outlooks Pest Manag. 2020;31(5):224–9.
Mellado-Sánchez M, McDiarmid F, Cardoso V, Kanyuka K, MacGregor DR. Virus-mediated transient expression techniques enable gene function studies in blackgrass. Plant Physiol. 2020;183(2):455–9.
Dimaano NG, Yamaguchi T, Fukunishi K, Tominaga T, Iwakami S. Functional characterization of Cytochrome P450 CYP81A subfamily to disclose the pattern of cross-resistance in Echinochloa phyllopogon . Plant Mol Biol. 2020;102(4–5):403–16.
de Figueiredo MRA, Küpper A, Malone JM, Petrovic T, de Figueiredo ABTB, Campagnola G, et al. An in-frame deletion mutation in the degron tail of auxin coreceptor IAA2 confers resistance to the herbicide 2,4-D in Sisymbrium orientale . Proc Natl Acad Sci U S A. 2022;119(9):e2105819119.
Patzoldt WL, Hager AG, McCormick JS, Tranel PJ. A codon deletion confers resistance to herbicides inhibiting protoporphyrinogen oxidase. Proc Natl Acad Sci U S A. 2006;103(33):12329–34.
Zabala-Pardo D, Gaines T, Lamego FP, Avila LA. RNAi as a tool for weed management: challenges and opportunities. Adv Weed Sci. 2022;40(spe1):e020220096.
Fattorini R, Glover BJ. Molecular mechanisms of pollination biology. Annu Rev Plant Biol. 2020;71:487–515.
Rollin O, Benelli G, Benvenuti S, Decourtye A, Wratten SD, Canale A, et al. Weed-insect pollinator networks as bio-indicators of ecological sustainability in agriculture. A review Agron Sustain Dev. 2016;36(1):8.
Irwin RE, Strauss SY. Flower color microevolution in wild radish: evolutionary response to pollinator-mediated selection. Am Nat. 2005;165(2):225–37.
Ma B, Wu J, Shi T-L, Yang Y-Y, Wang W-B, Zheng Y, et al. Lilac ( Syringa oblata ) genome provides insights into its evolution and molecular mechanism of petal color change. Commun Biol. 2022;5(1):686.
Xing A, Wang X, Nazir MF, Zhang X, Wang X, Yang R, et al. Transcriptomic and metabolomic profiling of flavonoid biosynthesis provides novel insights into petals coloration in Asian cotton ( Gossypium arboreum L.). BMC Plant Biol. 2022;22(1):416.
Zheng Y, Chen Y, Liu Z, Wu H, Jiao F, Xin H, et al. Important roles of key genes and transcription factors in flower color differences of Nicotiana alata . Genes (Basel). 2021;12(12):1976.
Krizek BA, Anderson JT. Control of flower size. J Exp Bot. 2013;64(6):1427–37.
Powell AE, Lenhard M. Control of organ size in plants. Curr Biol. 2012;22(9):R360–7.
Spencer V, Kim M. Re"CYC"ling molecular regulators in the evolution and development of flower symmetry. Semin Cell Dev Biol. 2018;79:16–26.
Amrad A, Moser M, Mandel T, de Vries M, Schuurink RC, Freitas L, et al. Gain and loss of floral scent production through changes in structural genes during pollinator-mediated speciation. Curr Biol. 2016;26(24):3303–12.
Delle-Vedove R, Schatz B, Dufay M. Understanding intraspecific variation of floral scent in light of evolutionary ecology. Ann Bot. 2017;120(1):1–20.
Pichersky E, Gershenzon J. The formation and function of plant volatiles: perfumes for pollinator attraction and defense. Curr Opin Plant Biol. 2002;5(3):237–43.
Ballerini ES, Kramer EM, Hodges SA. Comparative transcriptomics of early petal development across four diverse species of Aquilegia reveal few genes consistently associated with nectar spur development. BMC Genom. 2019;20(1):668.
Corbet SA, Willmer PG, Beament JWL, Unwin DM, Prys-Jones OE. Post-secretory determinants of sugar concentration in nectar. Plant Cell Environ. 1979;2(4):293–308.
Galliot C, Hoballah ME, Kuhlemeier C, Stuurman J. Genetics of flower size and nectar volume in Petunia pollination syndromes. Planta. 2006;225(1):203–12.
Vila-Aiub MM, Neve P, Powles SB. Fitness costs associated with evolved herbicide resistance alleles in plants. New Phytol. 2009;184(4):751–67.
Baucom RS. Evolutionary and ecological insights from herbicide-resistant weeds: what have we learned about plant adaptation, and what is left to uncover? New Phytol. 2019;223(1):68–82.
Bajwa AA, Latif S, Borger C, Iqbal N, Asaduzzaman M, Wu H, et al. The remarkable journey of a weed: biology and management of annual ryegrass ( Lolium rigidum ) in conservation cropping systems of Australia. Plants (Basel). 2021;10(8):1505.
Bitarafan Z, Andreasen C. Fecundity allocation in some european weed species competing with crops. Agronomy. 2022;12(5):1196.
Costea M, Weaver SE, Tardif FJ. The biology of Canadian weeds. 130. Amaranthus retroflexus L., A. powellii , A. powellii S. Watson, and A. hybridus L. Can J Plant Sci. 2004;84(2):631–68.
Dixon A, Comont D, Slavov GT, Neve P. Population genomics of selectively neutral genetic structure and herbicide resistance in UK populations of Alopecurus myosuroides . Pest Manag Sci. 2021;77(3):1520–9.
Kersten S, Chang J, Huber CD, Voichek Y, Lanz C, Hagmaier T, et al. Standing genetic variation fuels rapid evolution of herbicide resistance in blackgrass. Proc Natl Acad Sci U S A. 2023;120(16):e2206808120.
Qiu J, Zhou Y, Mao L, Ye C, Wang W, Zhang J, et al. Genomic variation associated with local adaptation of weedy rice during de-domestication. Nat Commun. 2017;8(1):15323.
Kreiner JM, Caballero A, Wright SI, Stinchcombe JR. Selective ancestral sorting and de novo evolution in the agricultural invasion of Amaranthus tuberculatus . Evolution. 2022;76(1):70–85.
Kreiner JM, Latorre SM, Burbano HA, Stinchcombe JR, Otto SP, Weigel D, et al. Rapid weed adaptation and range expansion in response to agriculture over the past two centuries. Science. 2022;378(6624):1079–85.
Wu D, Shen E, Jiang B, Feng Y, Tang W, Lao S, et al. Genomic insights into the evolution of Echinochloa species as weed and orphan crop. Nat Commun. 2022;13(1):689.
Yeaman S, Hodgins KA, Lotterhos KE, Suren H, Nadeau S, Degner JC, et al. Convergent local adaptation to climate in distantly related conifers. Science. 2016;353(6306):1431–3.
Haudry A, Platts AE, Vello E, Hoen DR, Leclercq M, Williamson RJ, et al. An atlas of over 90,000 conserved noncoding sequences provides insight into crucifer regulatory regions. Nat Genet. 2013;45(8):891–8.
Sackton TB, Grayson P, Cloutier A, Hu Z, Liu JS, Wheeler NE, et al. Convergent regulatory evolution and loss of flight in paleognathous birds. Science. 2019;364(6435):74–8.
Ye CY, Fan L. Orphan crops and their wild relatives in the genomic era. Mol Plant. 2021;14(1):27–39.
Clements DR, Jones VL. Ten ways that weed evolution defies human management efforts amidst a changing climate. Agronomy. 2021;11(2):284.
Article CAS Google Scholar
Weinig C. Rapid evolutionary responses to selection in heterogeneous environments among agricultural and nonagricultural weeds. Int J Plant Sci. 2005;166(4):641–7.
Cousens RD, Fournier-Level A. Herbicide resistance costs: what are we actually measuring and why? Pest Manag Sci. 2018;74(7):1539–46.
Lasky JR, Josephs EB, Morris GP. Genotype–environment associations to reveal the molecular basis of environmental adaptation. Plant Cell. 2023;35(1):125–38.
Lotterhos KE. The effect of neutral recombination variation on genome scans for selection. G3-Genes Genom Genet. 2019;9(6):1851–67.
Lovell JT, MacQueen AH, Mamidi S, Bonnette J, Jenkins J, Napier JD, et al. Genomic mechanisms of climate adaptation in polyploid bioenergy switchgrass. Nature. 2021;590(7846):438–44.
Todesco M, Owens GL, Bercovich N, Légaré J-S, Soudi S, Burge DO, et al. Massive haplotypes underlie ecotypic differentiation in sunflowers. Nature. 2020;584(7822):602–7.
Revolinski SR, Maughan PJ, Coleman CE, Burke IC. Preadapted to adapt: Underpinnings of adaptive plasticity revealed by the downy brome genome. Commun Biol. 2023;6(1):326.
Kuester A, Conner JK, Culley T, Baucom RS. How weeds emerge: a taxonomic and trait-based examination using United States data. New Phytol. 2014;202(3):1055–68.
Arnaud JF, Fénart S, Cordellier M, Cuguen J. Populations of weedy crop-wild hybrid beets show contrasting variation in mating system and population genetic structure. Evol Appl. 2010;3(3):305–18.
Ellstrand NC, Schierenbeck KA. Hybridization as a stimulus for the evolution of invasiveness in plants? Proc Natl Acad Sci U S A. 2000;97(13):7043–50.
Nakabayashi K, Leubner-Metzger G. Seed dormancy and weed emergence: from simulating environmental change to understanding trait plasticity, adaptive evolution, and population fitness. J Exp Bot. 2021;72(12):4181–5.
Busi R, Yu Q, Barrett-Lennard R, Powles S. Long distance pollen-mediated flow of herbicide resistance genes in Lolium rigidum . Theor Appl Genet. 2008;117(8):1281–90.
Délye C, Clément JAJ, Pernin F, Chauvel B, Le Corre V. High gene flow promotes the genetic homogeneity of arable weed populations at the landscape level. Basic Appl Ecol. 2010;11(6):504–12.
Roumet M, Noilhan C, Latreille M, David J, Muller MH. How to escape from crop-to-weed gene flow: phenological variation and isolation-by-time within weedy sunflower populations. New Phytol. 2013;197(2):642–54.
Moghadam SH, Alebrahim MT, Mohebodini M, MacGregor DR. Genetic variation of Amaranthus retroflexus L. and Chenopodium album L. (Amaranthaceae) suggests multiple independent introductions into Iran. Front Plant Sci. 2023;13:1024555.
Muller M-H, Latreille M, Tollon C. The origin and evolution of a recent agricultural weed: population genetic diversity of weedy populations of sunflower ( Helianthus annuus L.) in Spain and France. Evol Appl. 2011;4(3):499–514.
Wesse C, Welk E, Hurka H, Neuffer B. Geographical pattern of genetic diversity in Capsella bursa-pastoris (Brassicaceae) -A global perspective. Ecol Evol. 2021;11(1):199–213.
Fraimout A, Debat V, Fellous S, Hufbauer RA, Foucaud J, Pudlo P, et al. Deciphering the routes of invasion of Drosophila suzukii by means of ABC random forest. Mol Biol Evol. 2017;34(4):980–96.
CAS PubMed PubMed Central Google Scholar
Battlay P, Wilson J, Bieker VC, Lee C, Prapas D, Petersen B, et al. Large haploblocks underlie rapid adaptation in the invasive weed Ambrosia artemisiifolia . Nat Commun. 2023;14(1):1717.
van Boheemen LA, Hodgins KA. Rapid repeatable phenotypic and genomic adaptation following multiple introductions. Mol Ecol. 2020;29(21):4102–17.
Putra A, Hodgins K, Fournier-Level A. Assessing the invasive potential of different source populations of ragweed ( Ambrosia artemisiifolia L.) through genomically-informed species distribution modelling. Authorea. 2023;17(1):e13632.
Google Scholar
Bourguet D, Delmotte F, Franck P, Guillemaud T, Reboud X, Vacher C, et al. Heterogeneity of selection and the evolution of resistance. Trends Ecol Evol. 2013;28(2):110–8.
The International Herbicide-Resistant Weed Database. www.weedscience.org . Accessed 20 June 2023.
Powles S. Herbicide discovery through innovation and diversity. Adv Weed Sci. 2022;40(spe1):e020220074.
Murphy BP, Tranel PJ. Target-site mutations conferring herbicide resistance. Plants (Basel). 2019;8(10):382.
Gaines TA, Duke SO, Morran S, Rigon CAG, Tranel PJ, Küpper A, et al. Mechanisms of evolved herbicide resistance. J Biol Chem. 2020;295(30):10307–30.
Lonhienne T, Cheng Y, Garcia MD, Hu SH, Low YS, Schenk G, et al. Structural basis of resistance to herbicides that target acetohydroxyacid synthase. Nat Commun. 2022;13(1):3368.
Comont D, MacGregor DR, Crook L, Hull R, Nguyen L, Freckleton RP, et al. Dissecting weed adaptation: fitness and trait correlations in herbicide-resistant Alopecurus myosuroides . Pest Manag Sci. 2022;78(7):3039–50.
Neve P. Simulation modelling to understand the evolution and management of glyphosate resistance in weeds. Pest Manag Sci. 2008;64(4):392–401.
Torra J, Alcántara-de la Cruz R. Molecular mechanisms of herbicide resistance in weeds. Genes (Basel). 2022;13(11):2025.
Délye C, Gardin JAC, Boucansaud K, Chauvel B, Petit C. Non-target-site-based resistance should be the centre of attention for herbicide resistance research: Alopecurus myosuroides as an illustration. Weed Res. 2011;51(5):433–7.
Chandra S, Leon RG. Genome-wide evolutionary analysis of putative non-specific herbicide resistance genes and compilation of core promoters between monocots and dicots. Genes (Basel). 2022;13(7):1171.
Margaritopoulou T, Tani E, Chachalis D, Travlos I. Involvement of epigenetic mechanisms in herbicide resistance: the case of Conyza canadensis . Agriculture. 2018;8(1):17.
Pan L, Guo Q, Wang J, Shi L, Yang X, Zhou Y, et al. CYP81A68 confers metabolic resistance to ALS and ACCase-inhibiting herbicides and its epigenetic regulation in Echinochloa crus-galli . J Hazard Mater. 2022;428:128225.
Sen MK, Hamouzová K, Košnarová P, Roy A, Soukup J. Herbicide resistance in grass weeds: Epigenetic regulation matters too. Front Plant Sci. 2022;13:1040958.
Han H, Yu Q, Beffa R, González S, Maiwald F, Wang J, et al. Cytochrome P450 CYP81A10v7 in Lolium rigidum confers metabolic resistance to herbicides across at least five modes of action. Plant J. 2021;105(1):79–92.
Kubis GC, Marques RZ, Kitamura RS, Barroso AA, Juneau P, Gomes MP. Antioxidant enzyme and Cytochrome P450 activities are involved in horseweed ( Conyza sumatrensis ) resistance to glyphosate. Stress. 2023;3(1):47–57.
Qiao Y, Zhang N, Liu J, Yang H. Interpretation of ametryn biodegradation in rice based on joint analyses of transcriptome, metabolome and chemo-characterization. J Hazard Mater. 2023;445:130526.
Rouse CE, Roma-Burgos N, Barbosa Martins BA. Physiological assessment of non–target site restistance in multiple-resistant junglerice ( Echinochloa colona ). Weed Sci. 2019;67(6):622–32.
Abou-Khater L, Maalouf F, Jighly A, Alsamman AM, Rubiales D, Rispail N, et al. Genomic regions associated with herbicide tolerance in a worldwide faba bean ( Vicia faba L.) collection. Sci Rep. 2022;12(1):158.
Gupta S, Harkess A, Soble A, Van Etten M, Leebens-Mack J, Baucom RS. Interchromosomal linkage disequilibrium and linked fitness cost loci associated with selection for herbicide resistance. New Phytol. 2023;238(3):1263–77.
Kreiner JM, Tranel PJ, Weigel D, Stinchcombe JR, Wright SI. The genetic architecture and population genomic signatures of glyphosate resistance in Amaranthus tuberculatus . Mol Ecol. 2021;30(21):5373–89.
Parcharidou E, Dücker R, Zöllner P, Ries S, Orru R, Beffa R. Recombinant glutathione transferases from flufenacet-resistant black-grass ( Alopecurus myosuroides Huds.) form different flufenacet metabolites and differ in their interaction with pre- and post-emergence herbicides. Pest Manag Sci. 2023;79(9):3376–86.
Békés M, Langley DR, Crews CM. PROTAC targeted protein degraders: the past is prologue. Nat Rev Drug Discov. 2022;21(3):181–200.
Acuner Ozbabacan SE, Engin HB, Gursoy A, Keskin O. Transient protein-protein interactions. Protein Eng Des Sel. 2011;24(9):635–48.
Lu H, Zhou Q, He J, Jiang Z, Peng C, Tong R, et al. Recent advances in the development of protein–protein interactions modulators: mechanisms and clinical trials. Signal Transduct Target Ther. 2020;5(1):213.
Benson CW, Sheltra MR, Maughan PJ, Jellen EN, Robbins MD, Bushman BS, et al. Homoeologous evolution of the allotetraploid genome of Poa annua L. BMC Genom. 2023;24(1):350.
Robbins MD, Bushman BS, Huff DR, Benson CW, Warnke SE, Maughan CA, et al. Chromosome-scale genome assembly and annotation of allotetraploid annual bluegrass ( Poa annua L.). Genome Biol Evol. 2022;15(1):evac180.
Montgomery JS, Giacomini D, Waithaka B, Lanz C, Murphy BP, Campe R, et al. Draft genomes of Amaranthus tuberculatus , Amaranthus hybridus and Amaranthus palmeri . Genome Biol Evol. 2020;12(11):1988–93.
Jeschke MR, Tranel PJ, Rayburn AL. DNA content analysis of smooth pigweed ( Amaranthus hybridus ) and tall waterhemp ( A. tuberculatus ): implications for hybrid detection. Weed Sci. 2003;51(1):1–3.
Rayburn AL, McCloskey R, Tatum TC, Bollero GA, Jeschke MR, Tranel PJ. Genome size analysis of weedy Amaranthus species. Crop Sci. 2005;45(6):2557–62.
Laforest M, Martin SL, Bisaillon K, Soufiane B, Meloche S, Tardif FJ, et al. The ancestral karyotype of the Heliantheae Alliance, herbicide resistance, and human allergens: Insights from the genomes of common and giant ragweed. Plant Genome . 2024;e20442. https://doi.org/10.1002/tpg2.20442 .
Mulligan GA. Chromosome numbers of Canadian weeds. I Canad J Bot. 1957;35(5):779–89.
Meyer L, Causse R, Pernin F, Scalone R, Bailly G, Chauvel B, et al. New gSSR and EST-SSR markers reveal high genetic diversity in the invasive plant Ambrosia artemisiifolia L. and can be transferred to other invasive Ambrosia species. PLoS One. 2017;12(5):e0176197.
Pustahija F, Brown SC, Bogunić F, Bašić N, Muratović E, Ollier S, et al. Small genomes dominate in plants growing on serpentine soils in West Balkans, an exhaustive study of 8 habitats covering 308 taxa. Plant Soil. 2013;373(1):427–53.
Kubešová M, Moravcova L, Suda J, Jarošík V, Pyšek P. Naturalized plants have smaller genomes than their non-invading relatives: a flow cytometric analysis of the Czech alien flora. Preslia. 2010;82(1):81–96.
Thébaud C, Abbott RJ. Characterization of invasive Conyza species (Asteraceae) in Europe: quantitative trait and isozyme analysis. Am J Bot. 1995;82(3):360–8.
Garcia S, Hidalgo O, Jakovljević I, Siljak-Yakovlev S, Vigo J, Garnatje T, et al. New data on genome size in 128 Asteraceae species and subspecies, with first assessments for 40 genera, 3 tribes and 2 subfamilies. Plant Biosyst. 2013;147(4):1219–27.
Zhao X, Yi L, Ren Y, Li J, Ren W, Hou Z, et al. Chromosome-scale genome assembly of the yellow nutsedge ( Cyperus esculentus ). Genome Biol Evol. 2023;15(3):evad027.
Bennett MD, Leitch IJ, Hanson L. DNA amounts in two samples of angiosperm weeds. Ann Bot. 1998;82:121–34.
Schulz-Schaeffer J, Gerhardt S. Cytotaxonomic analysis of the Euphorbia spp. (leafy spurge) complex. II: Comparative study of the chromosome morphology. Biol Zentralbl. 1989;108(1):69–76.
Schaeffer JR, Gerhardt S. The impact of introgressive hybridization on the weediness of leafy spurge. Leafy Spurge Symposium. 1989;1989:97–105.
Bai C, Alverson WS, Follansbee A, Waller DM. New reports of nuclear DNA content for 407 vascular plant taxa from the United States. Ann Bot. 2012;110(8):1623–9.
Aarestrup JR, Karam D, Fernandes GW. Chromosome number and cytogenetics of Euphorbia heterophylla L. Genet Mol Res. 2008;7(1):217–22.
Wang L, Sun X, Peng Y, Chen K, Wu S, Guo Y, et al. Genomic insights into the origin, adaptive evolution, and herbicide resistance of Leptochloa chinensis , a devastating tetraploid weedy grass in rice fields. Mol Plant. 2022;15(6):1045–58.
Paril J, Pandey G, Barnett EM, Rane RV, Court L, Walsh T, et al. Rounding up the annual ryegrass genome: high-quality reference genome of Lolium rigidum . Front Genet. 2022;13:1012694.
Weiss-Schneeweiss H, Greilhuber J, Schneeweiss GM. Genome size evolution in holoparasitic Orobanche (Orobanchaceae) and related genera. Am J Bot. 2006;93(1):148–56.
Towers G, Mitchell J, Rodriguez E, Bennett F, Subba Rao P. Biology & chemistry of Parthenium hysterophorus L., a problem weed in India. Biol Rev. 1977;48:65–74.
CAS Google Scholar
Moghe GD, Hufnagel DE, Tang H, Xiao Y, Dworkin I, Town CD, et al. Consequences of whole-genome triplication as revealed by comparative genomic analyses of the wild radish ( Raphanus raphanistrum ) and three other Brassicaceae species. Plant Cell. 2014;26(5):1925–37.
Zhang X, Liu T, Wang J, Wang P, Qiu Y, Zhao W, et al. Pan-genome of Raphanus highlights genetic variation and introgression among domesticated, wild, and weedy radishes. Mol Plant. 2021;14(12):2032–55.
Chytrý M, Danihelka J, Kaplan Z, Wild J, Holubová D, Novotný P, et al. Pladias database of the Czech flora and vegetation. Preslia. 2021;93(1):1–87.
Patterson EL, Pettinga DJ, Ravet K, Neve P, Gaines TA. Glyphosate resistance and EPSPS gene duplication: Convergent evolution in multiple plant species. J Hered. 2018;109(2):117–25.
Jugulam M, Niehues K, Godar AS, Koo DH, Danilova T, Friebe B, et al. Tandem amplification of a chromosomal segment harboring 5-enolpyruvylshikimate-3-phosphate synthase locus confers glyphosate resistance in Kochia scoparia . Plant Physiol. 2014;166(3):1200–7.
Patterson EL, Saski CA, Sloan DB, Tranel PJ, Westra P, Gaines TA. The draft genome of Kochia scoparia and the mechanism of glyphosate resistance via transposon-mediated EPSPS tandem gene duplication. Genome Biol Evol. 2019;11(10):2927–40.
Zhang C, Johnson N, Hall N, Tian X, Yu Q, Patterson E. Subtelomeric 5-enolpyruvylshikimate-3-phosphate synthase ( EPSPS ) copy number variation confers glyphosate resistance in Eleusine indica . Nat Commun. 2023;14:4865.
Koo D-H, Molin WT, Saski CA, Jiang J, Putta K, Jugulam M, et al. Extrachromosomal circular DNA-based amplification and transmission of herbicide resistance in crop weed Amaranthus palmeri . Proc Natl Acad Sci U S A. 2018;115(13):3332–7.
Molin WT, Yaguchi A, Blenner M, Saski CA. The eccDNA Replicon: A heritable, extranuclear vehicle that enables gene amplification and glyphosate resistance in Amaranthus palmeri . Plant Cell. 2020;32(7):2132–40.
Jugulam M. Can non-Mendelian inheritance of extrachromosomal circular DNA-mediated EPSPS gene amplification provide an opportunity to reverse resistance to glyphosate? Weed Res. 2021;61(2):100–5.
Kreiner JM, Giacomini DA, Bemm F, Waithaka B, Regalado J, Lanz C, et al. Multiple modes of convergent adaptation in the spread of glyphosate-resistant Amaranthus tuberculatus . Proc Natl Acad Sci U S A. 2019;116(42):21076–84.
Cai L, Comont D, MacGregor D, Lowe C, Beffa R, Neve P, et al. The blackgrass genome reveals patterns of non-parallel evolution of polygenic herbicide resistance. New Phytol. 2023;237(5):1891–907.
Chen K, Yang H, Peng Y, Liu D, Zhang J, Zhao Z, et al. Genomic analyses provide insights into the polyploidization-driven herbicide adaptation in Leptochloa weeds. Plant Biotechnol J. 2023;21(8):1642–58.
Ohadi S, Hodnett G, Rooney W, Bagavathiannan M. Gene flow and its consequences in Sorghum spp. Crit Rev Plant Sci. 2017;36(5–6):367–85.
Renzi JP, Coyne CJ, Berger J, von Wettberg E, Nelson M, Ureta S, et al. How could the use of crop wild relatives in breeding increase the adaptation of crops to marginal environments? Front Plant Sci. 2022;13:886162.
Ward SM, Cousens RD, Bagavathiannan MV, Barney JN, Beckie HJ, Busi R, et al. Agricultural weed research: a critique and two proposals. Weed Sci. 2014;62(4):672–8.
Evans JA, Tranel PJ, Hager AG, Schutte B, Wu C, Chatham LA, et al. Managing the evolution of herbicide resistance. Pest Manag Sci. 2016;72(1):74–80.
International Weed Genomics Consortium Website. https://www.weedgenomics.org . Accessed 20 June 2023.
WeedPedia Database. https://weedpedia.weedgenomics.org/ . Accessed 20 June 2023.
Hall N, Chen J, Matzrafi M, Saski CA, Westra P, Gaines TA, et al. FHY3/FAR1 transposable elements generate adaptive genetic variation in the Bassia scoparia genome. bioRxiv . 2023; DOI: https://doi.org/10.1101/2023.05.26.542497 .
Jarvis DE, Sproul JS, Navarro-Domínguez B, Krak K, Jaggi K, Huang Y-F, et al. Chromosome-scale genome assembly of the hexaploid Taiwanese goosefoot “Djulis” ( Chenopodium formosanum ). Genome Biol Evol. 2022;14(8):evac120.
Ferreira LAI, de Oliveira RS, Jr., Constantin J, Brunharo C. Evolution of ACCase-inhibitor resistance in Chloris virgata is conferred by a Trp2027Cys mutation in the herbicide target site. Pest Manag Sci. 2023;79(12):5220–9.
Laforest M, Martin SL, Bisaillon K, Soufiane B, Meloche S, Page E. A chromosome-scale draft sequence of the Canada fleabane genome. Pest Manag Sci. 2020;76(6):2158–69.
Guo L, Qiu J, Ye C, Jin G, Mao L, Zhang H, et al. Echinochloa crus-galli genome analysis provides insight into its adaptation and invasiveness as a weed. Nat Commun. 2017;8(1):1031.
Sato MP, Iwakami S, Fukunishi K, Sugiura K, Yasuda K, Isobe S, et al. Telomere-to-telomere genome assembly of an allotetraploid pernicious weed, Echinochloa phyllopogon . DNA Res. 2023;30(5):dsad023.
Stein JC, Yu Y, Copetti D, Zwickl DJ, Zhang L, Zhang C, et al. Genomes of 13 domesticated and wild rice relatives highlight genetic conservation, turnover and innovation across the genus Oryza . Nat Genet. 2018;50(2):285–96.
Wu D, Xie L, Sun Y, Huang Y, Jia L, Dong C, et al. A syntelog-based pan-genome provides insights into rice domestication and de-domestication. Genome Biol. 2023;24(1):179.
Wang Z, Huang S, Yang Z, Lai J, Gao X, Shi J. A high-quality, phased genome assembly of broomcorn millet reveals the features of its subgenome evolution and 3D chromatin organization. Plant Commun. 2023;4(3):100557.
Mao Q, Huff DR. The evolutionary origin of Poa annua L. Crop Sci. 2012;52(4):1910–22.
Benson CW, Sheltra MR, Maughan JP, Jellen EN, Robbins MD, Bushman BS, et al. Homoeologous evolution of the allotetraploid genome of Poa annua L. Res Sq. 2023. https://doi.org/10.21203/rs.3.rs-2729084/v1 .
Brunharo C, Benson CW, Huff DR, Lasky JR. Chromosome-scale genome assembly of Poa trivialis and population genomics reveal widespread gene flow in a cool-season grass seed production system. Plant Direct. 2024;8(3):e575.
Mo C, Wu Z, Shang X, Shi P, Wei M, Wang H, et al. Chromosome-level and graphic genomes provide insights into metabolism of bioactive metabolites and cold-adaption of Pueraria lobata var. montana . DNA Research. 2022;29(5):dsac030.
Thielen PM, Pendleton AL, Player RA, Bowden KV, Lawton TJ, Wisecaver JH. Reference genome for the highly transformable Setaria viridis ME034V. G3 (Bethesda, Md). 2020;10(10):3467–78.
Yoshida S, Kim S, Wafula EK, Tanskanen J, Kim Y-M, Honaas L, et al. Genome sequence of Striga asiatica provides insight into the evolution of plant parasitism. Curr Biol. 2019;29(18):3041–52.
Qiu S, Bradley JM, Zhang P, Chaudhuri R, Blaxter M, Butlin RK, et al. Genome-enabled discovery of candidate virulence loci in Striga hermonthica , a devastating parasite of African cereal crops. New Phytol. 2022;236(2):622–38.
Nunn A, Rodríguez-Arévalo I, Tandukar Z, Frels K, Contreras-Garrido A, Carbonell-Bejerano P, et al. Chromosome-level Thlaspi arvense genome provides new tools for translational research and for a newly domesticated cash cover crop of the cooler climates. Plant Biotechnol J. 2022;20(5):944–63.
USDA-ARS Germplasm Resources Information Network (GRIN). https://www.ars-grin.gov/ . Accessed 20 June 2023.
Buck M, Hamilton C. The Nagoya Protocol on access to genetic resources and the fair and equitable sharing of benefits arising from their utilization to the convention on biological diversity. RECIEL. 2011;20(1):47–61.
Chauhan BS, Matloob A, Mahajan G, Aslam F, Florentine SK, Jha P. Emerging challenges and opportunities for education and research in weed science. Front Plant Sci. 2017;8:1537.
Shah S, Lonhienne T, Murray CE, Chen Y, Dougan KE, Low YS, et al. Genome-guided analysis of seven weed species reveals conserved sequence and structural features of key gene targets for herbicide development. Front Plant Sci. 2022;13:909073.
International Weed Genomics Consortium Training Resources. https://www.weedgenomics.org/training-resources/ . Accessed 20 June 2023.
Blackford S. Harnessing the power of communities: career networking strategies for bioscience PhD students and postdoctoral researchers. FEMS Microbiol Lett. 2018;365(8):fny033.
Pender M, Marcotte DE, Sto Domingo MR, Maton KI. The STEM pipeline: The role of summer research experience in minority students’ Ph.D. aspirations. Educ Policy Anal Arch. 2010;18(30):1–36.
PubMed PubMed Central Google Scholar
Burke A, Okrent A, Hale K. The state of U.S. science and engineering 2022. Foundation NS. https://ncses.nsf.gov/pubs/nsb20221 . 2022.
Wu J-Y, Liao C-H, Cheng T, Nian M-W. Using data analytics to investigate attendees’ behaviors and psychological states in a virtual academic conference. Educ Technol Soc. 2021;24(1):75–91.
Download references
Peer review information
Wenjing She was the primary editor of this article and managed its editorial process and peer review in collaboration with the rest of the editorial team.
The International Weed Genomics Consortium is supported by BASF SE, Bayer AG, Syngenta Ltd, Corteva Agriscience, CropLife International (Global Herbicide Resistance Action Committee), the Foundation for Food and Agriculture Research (Award DSnew-0000000024), and two conference grants from USDA-NIFA (Award numbers 2021–67013-33570 and 2023-67013-38785).
Author information
Authors and affiliations.
Department of Agricultural Biology, Colorado State University, 1177 Campus Delivery, Fort Collins, CO, 80523, USA
Jacob Montgomery, Sarah Morran & Todd A. Gaines
Protecting Crops and the Environment, Rothamsted Research, Harpenden, Hertfordshire, UK
Dana R. MacGregor
Department of Crop, Soil, and Environmental Sciences, Auburn University, Auburn, AL, USA
J. Scott McElroy
Department of Plant and Environmental Sciences, University of Copenhagen, Taastrup, Denmark
Paul Neve & Célia Neto
IFEVA-Conicet-Department of Ecology, University of Buenos Aires, Buenos Aires, Argentina
Martin M. Vila-Aiub & Maria Victoria Sandoval
Department of Ecology, Faculty of Agronomy, University of Buenos Aires, Buenos Aires, Argentina
Analia I. Menéndez
Department of Botany, The University of British Columbia, Vancouver, BC, Canada
Julia M. Kreiner
Institute of Crop Sciences, Zhejiang University, Hangzhou, China
Longjiang Fan
Department of Biology, University of Massachusetts Amherst, Amherst, MA, USA
Ana L. Caicedo
Department of Plant and Wildlife Sciences, Brigham Young University, Provo, UT, USA
Peter J. Maughan
Bayer AG, Weed Control Research, Frankfurt, Germany
Bianca Assis Barbosa Martins, Jagoda Mika, Alberto Collavo & Bodo Peters
Department of Crop Sciences, Federal University of Rio Grande Do Sul, Porto Alegre, Rio Grande Do Sul, Brazil
Aldo Merotto Jr.
Department of Soil and Crop Sciences, Texas A&M University, College Station, TX, USA
Nithya K. Subramanian & Muthukumar V. Bagavathiannan
Department of Plant, Soil and Microbial Sciences, Michigan State University, East Lansing, MI, USA
Luan Cutti & Eric L. Patterson
Department of Agronomy, Kansas State University, Manhattan, KS, USA
Md. Mazharul Islam & Mithila Jugulam
Department of Plant Pathology, Kansas State University, Manhattan, KS, USA
Bikram S. Gill
Crop Protection Discovery and Development, Corteva Agriscience, Indianapolis, IN, USA
Robert Cicchillo, Roger Gast & Neeta Soni
Genome Center of Excellence, Corteva Agriscience, Johnston, IA, USA
Terry R. Wright, Gina Zastrow-Hayes, Gregory May, Kevin Fengler & Victor Llaca
School of Agriculture, Food and Wine, University of Adelaide, Glen Osmond, South Australia, Australia
Jenna M. Malone
Jealott’s Hill International Research Centre, Syngenta Ltd, Bracknell, Berkshire, UK
Deepmala Sehgal, Shiv Shankhar Kaundun & Richard P. Dale
Department of Plant and Soil Sciences, University of Pretoria, Pretoria, South Africa
Barend Juan Vorster
BASF SE, Ludwigshafen Am Rhein, Germany
Jens Lerchl
Department of Crop Sciences, University of Illinois, Urbana, IL, USA
Patrick J. Tranel
Senior Scientist Consultant, Herbicide Resistance Action Committee / CropLife International, Liederbach, Germany
Roland Beffa
School of BioSciences, University of Melbourne, Parkville, VIC, Australia
Alexandre Fournier-Level
You can also search for this author in PubMed Google Scholar
Contributions
JMo and TG conceived and outlined the article. TG, DM, EP, RB, JSM, PJT, MJ wrote grants to obtain funding. MMI, BSG, and MJ performed mitotic chromosome visualization. VL performed sequencing. VL and KF assembled the genomes. LC and ELP annotated the genomes. JMo, SM, DRM, JSM, PN, CN, MV, MVS, AIM, JMK, LF, ALC, PJM, BABM, JMi, AC, MVB, LC, AFL, and ELP wrote the first draft of the article. All authors edited the article and improved the final version.
Corresponding author
Correspondence to Todd A. Gaines .
Ethics declarations
Ethics approval and consent to participate.
Ethical approval is not applicable for this article.
Competing interests
Some authors work for commercial agricultural companies (BASF, Bayer, Corteva Agriscience, or Syngenta) that develop and sell weed control products.
Additional information
Publisher’s note.
Springer Nature remains neutral with regard to jurisdictional claims in published maps and institutional affiliations.
Supplementary Information
13059_2024_3274_moesm1_esm.docx.
Additional file 1. List of completed and in-progress genome assemblies of weed species pollinated by insects (Table S1).
13059_2024_3274_MOESM2_ESM.docx
Additional file 2. Methods and results for visualizing and counting the metaphase chromosomes of hexaploid Avena fatua (Fig S1); diploid Lolium rigidum (Fig S2); tetraploid Phalaris minor (Fig S3); and tetraploid Salsola tragus (Fig S4).
Additional file 3. Review history.
Rights and permissions.
Open Access This article is licensed under a Creative Commons Attribution 4.0 International License, which permits use, sharing, adaptation, distribution and reproduction in any medium or format, as long as you give appropriate credit to the original author(s) and the source, provide a link to the Creative Commons licence, and indicate if changes were made. The images or other third party material in this article are included in the article's Creative Commons licence, unless indicated otherwise in a credit line to the material. If material is not included in the article's Creative Commons licence and your intended use is not permitted by statutory regulation or exceeds the permitted use, you will need to obtain permission directly from the copyright holder. To view a copy of this licence, visit http://creativecommons.org/licenses/by/4.0/ . The Creative Commons Public Domain Dedication waiver ( http://creativecommons.org/publicdomain/zero/1.0/ ) applies to the data made available in this article, unless otherwise stated in a credit line to the data.
Reprints and permissions
About this article
Cite this article.
Montgomery, J., Morran, S., MacGregor, D.R. et al. Current status of community resources and priorities for weed genomics research. Genome Biol 25 , 139 (2024). https://doi.org/10.1186/s13059-024-03274-y
Download citation
Received : 11 July 2023
Accepted : 13 May 2024
Published : 27 May 2024
DOI : https://doi.org/10.1186/s13059-024-03274-y
Share this article
Anyone you share the following link with will be able to read this content:
Sorry, a shareable link is not currently available for this article.
Provided by the Springer Nature SharedIt content-sharing initiative
- Weed science
- Reference genomes
- Rapid adaptation
- Herbicide resistance
- Public resources
Genome Biology
ISSN: 1474-760X
- Submission enquiries: [email protected]
- General enquiries: [email protected]
- Open access
- Published: 05 June 2024
Whole-genome sequencing reveals genomic diversity and selection signatures in Xia’nan cattle
- Xingya Song 1 na1 ,
- Zhi Yao 1 na1 ,
- Zijing Zhang 2 na1 ,
- Shijie Lyu 2 ,
- Ningbo Chen 1 ,
- Xingshan Qi 3 ,
- Xian Liu 4 ,
- Weidong Ma 5 ,
- Wusheng Wang 5 ,
- Chuzhao Lei 1 ,
- Yu Jiang 1 ,
- Eryao Wang 2 &
- Yongzhen Huang 1
BMC Genomics volume 25 , Article number: 559 ( 2024 ) Cite this article
Metrics details
The crossbreeding of specialized beef cattle breeds with Chinese indigenous cattle is a common method of genetic improvement. Xia’nan cattle, a crossbreed of Charolais and Nanyang cattle, is China’s first specialized beef cattle breed with independent intellectual property rights. After more than two decades of selective breeding, Xia’nan cattle exhibit a robust physique, good environmental adaptability, good tolerance to coarse feed, and high meat production rates. This study analyzed the population genetic structure, genetic diversity, and genomic variations of Xia’nan cattle using whole-genome sequencing data from 30 Xia’nan cattle and 178 published cattle genomic data.
The ancestry estimating composition analysis showed that the ancestry proportions for Xia’nan cattle were mainly Charolais with a small amount of Nanyang cattle. Through the genetic diversity studies (nucleotide diversity and linkage disequilibrium decay), we found that the genomic diversity of Xia’nan cattle is higher than that of specialized beef cattle breeds in Europe but lower than that of Chinese native cattle. Then, we used four methods to detect genome candidate regions influencing the excellent traits of Xia’nan cattle. Among the detected results, 42 genes (θπ and CLR) and 131 genes ( F ST and XP-EHH) were detected by two different detection strategies. In addition, we found a region in BTA8 with strong selection signals. Finally, we conducted functional annotation on the detected genes and found that these genes may influence body development ( NR6A1 ), meat quality traits ( MCCC1 ), growth traits ( WSCD1 , TMEM68 , MFN1 , NCKAP5 ), and immunity ( IL11RA , CNTFR , CCL27 , SLAMF1 , SLAMF7 , NAA35 , and GOLM1 ).
We elucidated the genomic features and population structure of Xia’nan cattle and detected some selection signals in genomic regions potentially associated with crucial economic traits in Xia’nan cattle. This research provided a basis for further breeding improvements in Xia’nan cattle and served as a reference for genetic enhancements in other crossbreed cattle.
Peer Review reports
Introduction
Domestic cattle include Bos taurus , Bos indicus , and their crossbreeds [ 1 ]. Previous research has divided domestic cattle around the world into five core populations based on geographic location: European taurine, Eurasian taurine, East Asian taurine, Chinese indicine, and Indian indicine [ 2 , 47 ]. Chinese native cattle, due to the development of agricultural civilization, have historically been bred for plowing, and it resulted in Chinese native cattle exhibiting better environmental adaptability and tolerance to coarse feed compared to specialized beef cattle breeds [ 3 , 4 ]. However, these local breeds notably lag behind specialized beef cattle in terms of meat production performance. In the 1980s, the demand for draft cattle gradually declined with the rapid advancement of agricultural mechanization. Concurrently, the market demand for beef cattle increased fast, necessitating improving both meat quantity and quality. To tap into the production potential of Chinese native cattle, breeders often introduced foreign bloodlines through hybridization to develop new breeds suitable for China. The Charolais, originating from France, are renowned as a large-sized beef cattle breed known for their rapid growth and high meat yield. Nanyang cattle, one of China’s five major native cattle breeds, are mainly distributed in Nanyang City, Henan Province. Previous study has shown that it belonged to the crossbreed of Bos taurus and Bos indicus . It has the advantages of the tall physique, fine meat quality, and more intramuscular fat, but also has the disadvantages of the slow growth rate and low slaughter rate [ 5 , 48 , 49 ]. Then, breeders in Henan province introduced Charolais cattle to crossbreed with the Nanyang cattle. After over two decades of selective breeding, they cultivated China’s first beef cattle breed with independent intellectual property rights. Xia’nan cattle possess several advantages, such as early maturity, rapid growth, good meat quality, and low dystocia rates, enhancing both beef production efficiency and the profitability of cattle farming for farmers.
In order to deeply study the genetic origin of excellent traits during Xia’nan cattle breeding, we sequenced 30 Xia’nan cattle’s genome and detected single nucleotide polymorphisms (SNPs) based on the reference genome of Bos taurus (ARS-UCD1.2). SNPs from Xia’nan cattle were compared with sites from beef cattle and Chinese native cattle previously collected.
Sequencing mapping and SNP detection
This study generated 6,574,344,629 clean reads from 30 Xia’nan cattle whole-genome sequencing data. The reads were mapped to the reference genome (ARS-UCD1.2) and the average coverage of these samples is 11.64×. Then, 20,546,726 biallelic SNPs discovered in these 30 Xia’nan cattle were annotated. Functional annotation of SNP sites showed that the majority of SNPs were located in intergenic regions (58.8%) or intronic regions (37.5%). Exonic SNPs comprised 0.7% of the total SNPs, including 54,180 non-synonymous SNPs and 74,085 synonymous SNPs (Table S3 ). The total number of detected SNPs in the breeds is shown in Table S3 . The count of SNPs in Xia’nan cattle is significantly lower than in Chinese indicine, Indian indicine, and Chinese native cattle (Nanyang cattle, Qinchuan cattle, and Jiaxian Red cattle) yet higher than in European taurine and Eurasian taurine. This distribution pattern of SNPs is similar to Pi’nan cattle, which is also a hybrid breed of Nanyang cattle in a previous study [ 6 ].
Population structure analysis and genetic diversity analysis
In order to delve deeper into the genetic background of Xia’nan cattle, this study conducted ancestry estimating analysis and Principal Component Analysis (PCA) and built the Neighbor-Joining (NJ) tree. The results from NJ tree and PCA exhibit similar patterns, revealing distinct geographical clustering among cattle populations. The first Principal Component (PC) explained 13.13% of the whole genome variation and the second PC explained 4.26%. In the NJ tree, these cattle are clearly divided into different clusters according to five “core” populations [ 2 ]. Xia’nan cattle was positioned between Chinese native cattle and Eurasian taurine, closer to the Eurasian taurine (Fig. 1 B and C). The result without Indian indicine is also shown in this study (Fig. S1 ), and it’s similar to Fig. 1 B. In the ancestry estimating analysis, we showed the cases which the CV error (Table S4 ) is small, and the rest were shown in Fig. S2 . When K = 2, There are clearly two components to the pedigree of these cattle: Bos taurus and Bos indicus . When K = 3, When K = 3, the Xia’nan cattle exhibited a higher resemblance to Charolais than Nanyang cattle, and it has more European taurine ancestry (Fig. 1 D). because these cattle were divided into six reference groups and one target group, we also plotted the case at K = 4–7 (Fig. S1 ).
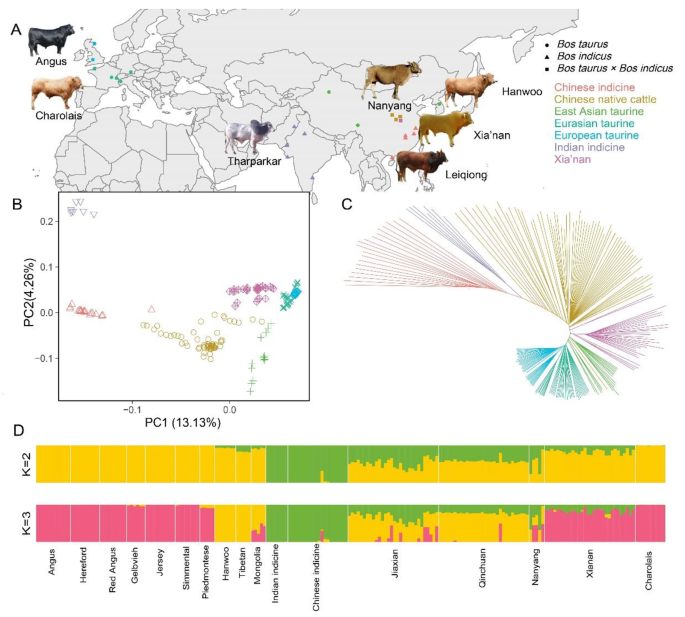
A Geographical distribution map of cattle breeds used in this study. B Principal component analysis of 17 cattle populations (208 individuals). C Neighbor-joining tree of the relationships in these populations. D Ancestry component analysis of these cattle breeds using ADMIXTURE with K = 2 and K = 3
To assess the genetic diversity of Xia’nan cattle, we calculated nucleotide diversity and linkage disequilibrium (LD) in Xia’nan cattle and other breeds. The results revealed that the highest nucleotide diversity was observed in Chinese indicine. Xia’nan cattle exhibited slightly higher nucleotide diversity compared to its paternal Charolais but lower than its maternal Nanyang cattle (Fig. 2 A). Linkage disequilibrium analysis at the distance of 100 kb indicated that Jiaxian Red cattle and Qinchuan cattle had the lowest LD values, while Tibetan cattle exhibited the highest LD value, followed by Mongolian cattle. Xia’nan cattle showed higher LD levels than Charolais but lower than Nanyang cattle (Fig. 2 B). In addition, we tested runs of homozygous (ROH) in these breeds and counted the distribution of ROHs of different lengths in different populations. We used the average of the number of ROHs in each breed to characterize the distribution pattern (Fig. S3 ). The results showed Chinese native cattle and Chinese indicine had more short ROHs (0.5-1 Mb and 1–2 Mb). Xia’nan cattle exhibited a pattern similar to that of the European taurine (Angus, Hereford and Red Angus).
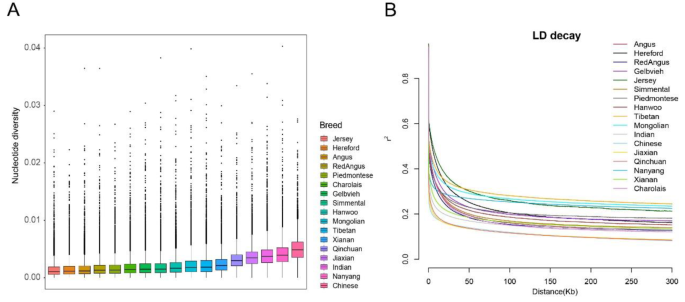
Genetic diversity analysis of these cattle. A Genome-wide distribution of nucleotide diversity of each breed in 50 kb windows with 50 kb steps. The horizontal line inside the box indicates the median of this distribution; box limits indicate the first and the thirds quartiles, points shows outliers. Data points outside the whiskers can be considered as outliers. B Genome-wide average LD decay estimated from each breed
Genome-wide selective sweep test
We employed nucleotide diversity (θπ) analysis and composite likelihood ratio (CLR) methods to detect and select genomic regions associated with genetic diversity. Regions that showed high signals (top 1%) by both methods were considered candidate selection regions (Fig. 3 A and B). In Xia’nan cattle, a total of 1184 genes (θπ) and 484 genes (CLR) exhibiting selection features were identified, with 40 genes overlapping (Tables S5 and S6 ). Functional enrichment analysis using Kyoto Encyclopedia of Genes and Genomes (KEGG) pathways and Gene Ontology (GO) was conducted for these overlapping genes. The top term enriched by KEGG is “Cytokine-cytokine receptor interaction” ( P -value = 0.006), Involving 3 genes ( IL11RA , CNTFR , CCL27 ). The GO terms with a significant ( P -value < 0.05) association in these overlapped genes include cytokine binding (GO:0019955), regulation of transcription, DNA-templated (GO:0006355), receptor complex (GO:0043235), and cytokine receptor activity (GO:0004896). Most terms are closely associated with the immune (Table S7 and S8 ).
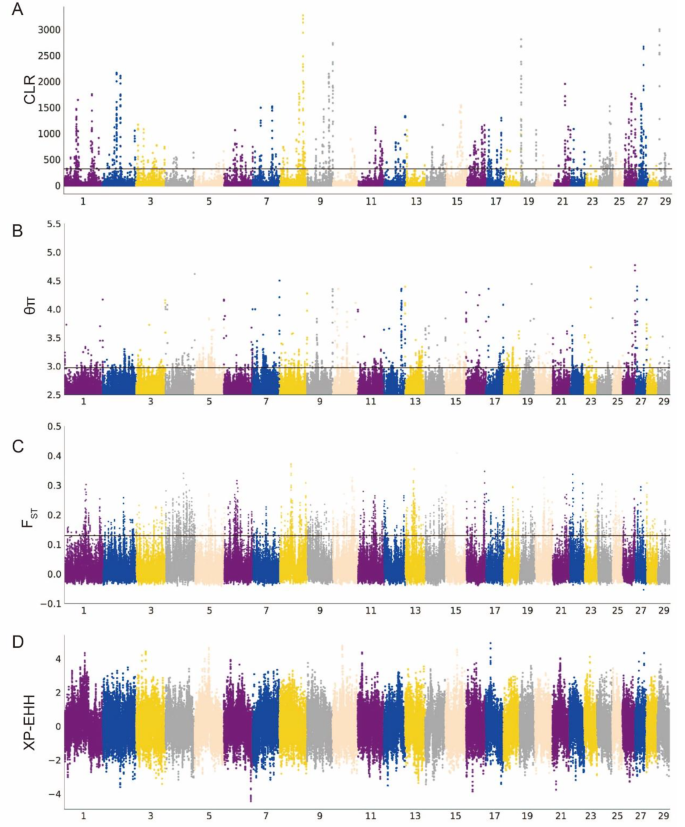
Manhattan plots of Analysis of the signatures of positive selection in the genome of Xia’nan cattle
Subsequently, we conducted fixation index ( F ST ) and cross-population extended homozygosity (XP-EHH) tests between the Xia’nan cattle and Nanyang cattle, and the top 1% of all sites are positive sites (Fig. 3 C and D). The F ST method detected 1344 genes, while the XP-EHH test identified 1355 genes (Tables S9 and S10 ). Among these, 131 genes were detected by both methods and confirmed as potential candidate genes specific to Xia’nan cattle. The KEGG and GO analysis results of these genes are shown in Table S12 . Additionally, some regions with strong signals of selection contained known genes associated with traits of interest, such as growth ( WSCD1 , TMEM68 , MFN1 , NCKAP5 ), environmental adaptation ( ITPR2 , GBA3 ), fat metabolism ( CRTC1 , HSPA12A ), and meat quality ( MCCC1 ).
In addition, we observed a significant peak on BTA8: 79.1–79.4 Mb, which was found by three methods (CLR, θπ, and F ST ) (Fig. 4 A and B). This region includes two immune-related genes: NAA35 and GOLM1 .
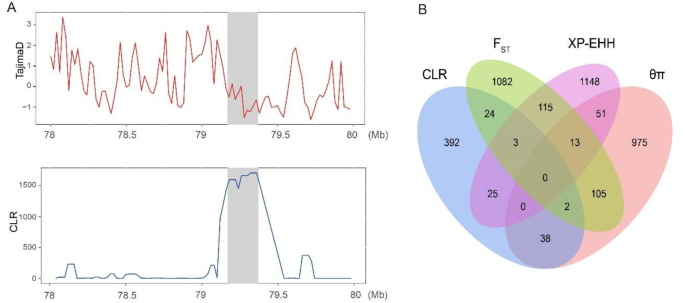
A Tajima’s D and CLR values on BTA8: 79.1–79.4 Mb region. B Venn diagram showing the overlapping gene counts among θπ, CLR, F ST and XP-EHH.
Population genetics and genetic diversity analyses can aid in assessing genetic resources and breed improvement in Xia’nan cattle. The results of ADMIXTURE software analysis show that the ancestry composition of Xia’nan cattle is more similar to that of Charolais cattle, with most European taurine ancestry. This tiny genetic contribution from Nanyang cattle results higher nucleotide diversity of the Xia’nan cattle genome than European taurine’s. However, the genomic diversity of Xia’nan cattle is lower than that of Chinese native cattle. It may be due to the founder effect or the recent intensive artificial selection in this population of Xia’nan cattle. The LD pattern of each variety was basically consistent with the results of nucleotide diversity. The higher LD level in Xia’nan cattle than in Charolais indicated that there may be more breeding in Xia’nan cattle. The differing LD imbalance intensity in Nanyang cattle compared to other Chinese local breeds might stem from a smaller sample size. The collected samples have undergone stronger artificial selection, emphasizing the rich variation sites and genetic resources inherent in Chinese native cattle breeds. The distribution pattern of ROHs can illustrate the breeding history of the cattle. The ROH distribution pattern of Xia’nan cattle is similar to that of European taurine, reflecting the recent intensive artificial selection in this breed. The pattern of Nanyang cattle and other Chinese native cattle breeds showed large differences, probably because the sample count of Nanyang cattle was too less.
Chinese native cattle are often better resistant and more adaptable than foreign beef breeds. In our selective sweep analysis of Xia’nan cattle, we found several genes associated with immune function, including IL11RA , CNTFR , and CCL27 , within the “cytokine-cytokine receptor interaction” pathway. Interleukin-11 receptor alpha (IL11RA) is the receptor of IL-11. In mice, the specific expression of IL-11 transgenes in fibroblasts or the injection of IL-11 leads to heart and kidney fibrosis, ultimately resulting in organ failure. Conversely, the deletion of IL11RA1 offered protection against these diseases [ 7 ]. Recent research has shown that inhibition of CNTFR could diminish the inhibitory effects of CLCF1 on mitochondrial biogenesis and thermogenesis [ 8 ]. The CC chemokine receptor 27 (CCL27), predominantly expressed by skin keratinocytes, plays a crucial role in the establishment of resident lymphocytes and maintaining immune balance in barrier tissues. It’s closely linked to normal skin and hair follicle development [ 9 ].
The candidate genes SLAMF1 and SLAMF7 are also related to immunity in cattle in previous research [ 10 ]. SLAMF1 and SLAMF7 both belong to the signaling lymphocytic activation molecule (SLAM) family, typically regarded as potential targets for inflammation and autoimmune diseases [ 11 , 12 ]. Previous studies have identified the SLAMF1 as a crucial negative regulator in humoral immune responses [ 13 ], initiating signal transduction networks across various immune cells [ 14 ]. Meanwhile, the SLAMF7 plays a significant role in macrophage hyperactivation and may have critical implications in modulating T-cell functionality within the tumor microenvironment [ 15 ]. Additionally, SLAMF7 is involved in regulating B-cell responses and adaptive immunity [ 16 ], potentially modulating susceptibility to autoimmune conditions in the central nervous system [ 17 ]. The strong selection region (BTA8: 79.1–79.4 Mb) that we found by three methods contains two genes: N-Alpha-Acetyltransferase 35 ( NAA35 ) and Golgi membrane protein 1 ( GOLM1 ). A previous study has shown that the cytokine quantitative trait locus (QTL), including the NAA35 - GOLM1 , significantly regulates the production of interleukin-6 in response to various pathogens [ 18 ].
These findings may be linked to the high disease resistance observed in Nanyang cattle and potentially contribute to Xia’nan cattle’s superior disease resistance compared to Charolais cattle [ 19 ]. Additionally, among the positively selected genes, we discovered NR6A1 , a gene associated with mammalian trunk development and vertebral count [ 20 , 21 ]. It may serve as a candidate locus influencing the body size of Xia’nan cattle.
We discovered lots of genes when comparing selection signals between Xia’nan and Charolais cattle. By searching the literature, we found that some of these genes influence important traits, for example, growth traits ( WSCD1 , TEME68 , MFN1 , NCKAP5 ), fat deposition ( CRTC1 , HSPA12A ), environmental adaptation ( ITPR2 , GBA3 ), and meat quality ( MCCC1 ). The WSC Domain Containing 1 ( WSCD1 ) gene encodes a protein exhibiting sulfotransferase activity and playing a role in glucose metabolism. This gene has been identified to be associated with daily feeding time and feeding rate in the White Duroc × Erhualian F population in a GWAS study [ 22 ], and it was linked to three reproductive periods’ body size in Simmental beef cattle in another GWAS study [ 8 ]. Transmembrane protein 68 ( TEME68 ) was found that it may be associated with feed intake and growth phenotypes in cattle [ 23 ]. Mitochondrial fusion protein 1 (MFN1) is a key regulator of mitochondrial fusion in mammalian cells, playing a pivotal role in maintaining the stability of mitochondrial morphology. The copy number of this gene was found to be related to the growth traits of beef cattle [ 24 ]. NCK-associated protein 5 ( NCKAP5 ) was found to be potentially associated with important phenotypes in Limousin cattle [ 25 ]. CREB-regulated transcription coactivator 1 ( CRTC1 ) and Heat shock protein 12 A ( HSPA12A ) can respectively regulate adipocyte differentiation and fat metabolism through the PPARγ pathway [ 26 , 27 ]. Inositol 1,4,5-Trisphosphate receptor type 2 ( ITPR2 ) and Glucosylceramidase Beta 3 ( GBA3 ) were reported as high-altitude adaptation genes [ 28 , 29 , 30 ]. Methylcrotonyl-CoA carboxylase 1 ( MCCC1 ) was identified as a candidate gene for pork meat quality through weighted gene co-expression network analysis [ 31 ].
These genes, which have been found to be related to immunity, fat deposition, meat quality, and adaptability, may be the components of the genetic basis of Nanyang cattle for good adaptability, excellent disease resistance, and tender meat quality. These screened genes may all play important roles in the formation of Xia’nan cattle’s excellent traits and can be used as candidate genes for breeding of beef cattle.
Conclusions
This study offers a thorough insight into genomic variations of Xia’nan cattle through Whole Genome Sequencing (WGS) data analysis. The exploration of population structure and genetic diversity in Xia’nan cattle will provide valuable guidance for developing informed and effective breeding strategies. Furthermore, we identified some candidate genes that may play crucial roles in growth traits, immune responses, and meat quality in beef cattle. Xia’nan cattle stand as a successful example of improvement among Chinese native cattle breeds, and unraveling the genetic factors behind their superior traits is pivotal for further enhancements within this breed and the development of novel breeds.
Sample and sequencing
We collected 30 blood samples of Xia’nan cattle from the Xia’nan breeding farm in Henan province (Table S1 ). The cattle were not treated harshly during sampling and were released after sampling. We employed the standard phenol-chloroform method for blood DNA extraction [ 32 ].
After assessing the DNA quality, gene libraries were assembled, each with an average fragment size of 300 bp per sample. The sequencing was carried out by BGI using the DNBSEQ-T7 sequencer.
To better elucidate the genetic variations in Xia’nan cattle, we expanded our sample collection by acquiring an additional 178 samples from five “core” cattle populations [ 2 ]. These samples encompass European taurine (Angus ( n = 11), Hereford ( n = 10), Red Angus ( n = 9)), Eurasian taurine (Chariolais ( n = 10), Gelbvieh ( n = 6), Jersey ( n = 10), Piedmontese ( n = 5), and Simmental ( n = 8)), East Asian taurine (Hanwoo ( n = 7), Mongolia ( n = 5), Tibetan ( n = 5)), Chinese indicine (Guangfeng cattle ( n = 4), Leiqiong cattle ( n = 3), Ji’an cattle ( n = 4), Jinjiang cattle ( n = 4), Wannan cattle ( n = 5)), Indian indicine (Gir ( n = 2), Haryana ( n = 1), Nelore ( n = 1), Sahiwal ( n = 1), Tharparkar ( n = 1), unidentified breed ( n = 1)) and Chinese native cattle ((Nanyang ( n = 5), Jiaxian ( n = 30), Qinchuan ( n = 30)) (Table S2 ).
Reads mapping, SNP calling, and annotation
The Trimmomatic software (version 0.38) [ 33 ]was utilized for trimming sequence reads, employing the following parameters: “LEADING:20, TRAILING:20, SLIDINGWINDWOE: 3:15, AVGQUAL:20, MINLEN:35, PHRED33”. The clean reads were aligned to the reference genome ARS-UCD1.2 by “bwa mem” software (version 0.7.13-r1126) with default parameters. Picard software ( http://broadinstitute.github.io/picard ) was used to remove PCR duplicates. After these, we used the “HaplotypeCaller”, “GenotypeGVCFs”, and “SelectVariants” modules in the Genome Analysis Toolkit (GATK, version 4.1.8.1) to detect SNPs and used the “VariantFiltration” module to filter SNPs with the parameters (QD < 2.0, QUAL < 30.0, SOR > 3.0, FS > 60.0, MQ < 40.0, MQRankSum < -12.5, and ReadPosRankSum < -8.0) [ 34 ]. Finally, BCFtools [ 35 ] was used to retain SNP sites with a genotype missing rate less than 0.1 and a minimum minor allele count greater than 2 (F_MISSING < 0.1 & MAC > 2). We employed ANNOVAR [ 30 ] software to annotate the functions of each SNP based on the Bos taurus reference genome annotation file ( https://ftp.ncbi.nlm.nih.gov/genomes/all/GCF/002/263/795/GCF_002263795.1_ARS-UCD1.2/GCF_002263795.1_ARS-UCD1.2_genomic.gff ).
Population genetic analysis
We used PLINK (version 1.90) [ 36 ] to remove the SNPs with high LD. The parameter is “--indep-pair-wise 50 5 0.2”. PopLDdecay [ 37 ] software was used to calculate and visualize Linkage disequilibrium (LD) decay with physical distance between SNPs. PCA was conducted using the “SmartPCA” module within Eigensoft (v6.1.4) [ 38 ]. For ancestry estimation analysis, ADMIXTURE (version 1.3) [ 39 ] was employed with the kinship (K-value) ranging from 2 to 7, and TBtools [ 40 ] was used for visualization. We utilized the matrix of pairwise genetic distances supplied by PLINK was used to construct an unrooted evolutionary tree. The visualization of the evolutionary tree was carried out using MEGA11 [ 41 ] and FigTree v1.4.4 ( http://tree.bio.ed.ac.uk/software/figtree/ ). We detected ROHs by PLINK with the following parameters: (1) the size of the window of 50 SNPs (--homozyg-window-snp 50); (2) required minimum density (--homozyg-density 50); (3) number of heterozygotes allowed in a window (--homozyg-window-het 3); (4) the number of missing calls allowed in window (--homozyg-window-missing 5). ROHs were divided into four categories based on length: 0.5-1 Mb, 1–2 Mb, 2–4 Mb, > 4 Mb.
Selective sweep identification
In order to reveal the signatures of selection influenced by artificial selection and genetic adaptation to the local environment, we employed various strategies to detect the genome selection signals of Xia’nan cattle. Within the Xia’nan cattle population, the composite likelihood ratio (CLR) method [ 42 ] and the nucleotide diversity (θπ) were employed. The value for nucleotide diversity in the plot is -log 10 (θπ). We used VCFtools [ 43 ] software to estimate the whole-genome nucleotide diversity using the sliding window approach utilizing window sizes of 50 kb and steps of 20 kb. SweepFinder2 [ 44 ] was employed to calculate the CLR for every 50 kb window. Empirical P -values of these windows were calculated. The regions with the empirical P -value in the top 1% are positive regions, and the genes detected by both methods are regarded as candidate genes.
Furthermore, fixation index ( F ST ) and cross-population extended haplotype homozygosity (XP-EHH) analyses were conducted to compare Xia’nan cattle with Nanyang cattle. F ST analysis was carried out by VCFtools with the same window size as CLR analysis. In the XP-EHH selection scans, the statistics were derived from the average of standardized XP-EHH scores calculated for every 50 kb region by SELSCAN V1.1 [ 45 ] software. The directional XP-EHH score indicated selection: a positive score suggested potential selection in Xia’nan cattle, while a negative score implied potential selection in the reference population. A significance threshold of P -value < 0.01 was applied to identify noteworthy genomic regions. Genes identified through both methods were considered as candidates for positive selection.
To further understand the functions and signaling pathways associated with these candidate genes, we used KOBAS (version 3.0) [ 46 ] to get enrichment pathways by GO and KEGG.
Data availability
Sequences are available from the Sequence Read Archive (SRA) database. Bioproject accession number is PRJNA1058368.
Decker JE, McKay SD, Rolf MM, Kim J, Molina Alcalá A, Sonstegard TS, Hanotte O, Götherström A, Seabury CM, Praharani L, et al. Worldwide patterns of ancestry, divergence, and admixture in domesticated cattle. PLoS Genet. 2014;10(3):e1004254.
Article PubMed PubMed Central Google Scholar
Chen N, Cai Y, Chen Q, Li R, Wang K, Huang Y, Hu S, Huang S, Zhang H, Zheng Z, et al. Whole-genome resequencing reveals world-wide ancestry and adaptive introgression events of domesticated cattle in East Asia. Nat Commun. 2018;9(1):2337.
Chen N, Xia X, Hanif Q, Zhang F, Dang R, Huang B, Lyu Y, Luo X, Zhang H, Yan H et al: Global genetic diversity, introgression, and evolutionary adaptation of indicine cattle revealed by whole genome sequencing. Nat Commun. 2023, 14(1):7803.
Article CAS PubMed PubMed Central Google Scholar
Gao Q, Liu H, Wang Z, Lan X, An J, Shen W, Wan F. Recent advances in feed and nutrition of beef cattle in China — a review. Anim Biosci. 2023;36(4):529–39.
Article CAS PubMed Google Scholar
Wei X, Zhu Y, Zhao X, Zhao Y, Jing Y, Liu G, Wang S, Li H, Ma Y. Transcriptome profiling of mRNAs in muscle tissue of Pinan cattle and Nanyang cattle. Gene. 2022;825:146435.
Zhang S, Yao Z, Li X, Zhang Z, Liu X, Yang P, Chen N, Xia X, Lyu S, Shi Q, et al. Assessing genomic diversity and signatures of selection in Pinan cattle using whole-genome sequencing data. BMC Genomics. 2022;23(1):460.
Schafer S, Viswanathan S, Widjaja AA, Lim WW, Moreno-Moral A, DeLaughter DM, Ng B, Patone G, Chow K, Khin E, et al. IL-11 is a crucial determinant of cardiovascular fibrosis. Nature. 2017;552(7683):110–5.
An B, Xu L, Xia J, Wang X, Miao J, Chang T, Song M, Ni J, Xu L, Zhang L, et al. Multiple association analysis of loci and candidate genes that regulate body size at three growth stages in Simmental beef cattle. BMC Genet. 2020;21(1):32.
Davila ML, Xu M, Huang C, Gaddes ER, Winter L, Cantorna MT, Wang Y, Xiong N. CCL27 is a crucial regulator of immune homeostasis of the skin and mucosal tissues. iScience. 2022;25(6):104426.
Xia X, Zhang S, Zhang H, Zhang Z, Chen N, Li Z, Sun H, Liu X, Lyu S, Wang X, et al. Assessing genomic diversity and signatures of selection in Jiaxian Red cattle using whole-genome sequencing data. BMC Genomics. 2021;22(1):43.
Dragovich MA, Mor A. The SLAM family receptors: potential therapeutic targets for inflammatory and autoimmune diseases. Autoimmun rev. 2018;17(7):674–82.
Farhangnia P, Ghomi SM, Mollazadehghomi S, Nickho H, Akbarpour M, Delbandi AA. SLAM-family receptors come of age as a potential molecular target in cancer immunotherapy. Front Immunol. 2023;14:1174138.
Wang N, Halibozek PJ, Yigit B, Zhao H, O’Keeffe MS, Sage P, Sharpe A, Terhorst C. Negative regulation of Humoral Immunity due to interplay between the SLAMF1, SLAMF5, and SLAMF6 receptors. Front Immunol. 2015;6:158.
Yurchenko M, Skjesol A, Ryan L, Richard GM, Kandasamy RK, Wang N, Terhorst C, Husebye H, Espevik T. SLAMF1 is required for TLR4-mediated TRAM-TRIF-dependent signaling in human macrophages. J Cell Biol. 2018;217(4):1411–29.
O’Connell P, Hyslop S, Blake MK, Godbehere S, Amalfitano A, Aldhamen YA. SLAMF7 signaling reprograms T cells toward exhaustion in the Tumor Microenvironment. J Immunol (Baltimore Md: 1950). 2021;206(1):193–205.
Article Google Scholar
O’Connell P, Blake MK, Godbehere S, Amalfitano A, Aldhamen YA. SLAMF7 modulates B cells and adaptive immunity to regulate susceptibility to CNS autoimmunity. J Neuroinflamm. 2022;19(1):241.
Simmons DP, Nguyen HN, Gomez-Rivas E, Jeong Y, Jonsson AH, Chen AF, Lange JK, Dyer GS, Blazar P, Earp BE, et al. SLAMF7 engagement superactivates macrophages in acute and chronic inflammation. Sci Immunol. 2022;7(68):eabf2846.
Li Y, Oosting M, Deelen P, Ricaño-Ponce I, Smeekens S, Jaeger M, Matzaraki V, Swertz MA, Xavier RJ, Franke L, et al. Inter-individual variability and genetic influences on cytokine responses to bacteria and fungi. Nat Med. 2016;22(8):952–60.
Mei C, Junjvlieke Z, Raza SHA, Wang H, Cheng G, Zhao C, Zhu W, Zan L. Copy number variation detection in Chinese indigenous cattle by whole genome sequencing. Genomics. 2020;112(1):831–6.
Chang YC, Manent J, Schroeder J, Wong SFL, Hauswirth GM, Shylo NA, Moore EL, Achilleos A, Garside V, Polo JM, et al. Nr6a1 controls hox expression dynamics and is a master regulator of vertebrate trunk development. Nat Commun. 2022;13(1):7766.
Zhang Y, Wang M, Yuan J, Zhou X, Xu S, Liu B. Association of polymorphisms in NR6A1, PLAG1 and VRTN with the number of vertebrae in Chinese tongcheng × large White crossbred pigs. Anim Genet. 2018;49(4):353–4.
Article PubMed Google Scholar
Guo YM, Zhang ZY, Ma JW, Ai HS, Ren J, Huang LS. A genomewide association study of feed efficiency and feeding behaviors at two fattening stages in a White Duroc × Erhualian F population. J Anim Sci. 2015;93(4):1481–9.
Lindholm-Perry AK, Kuehn LA, Smith TP, Ferrell CL, Jenkins TG, Freetly HC, Snelling WM. A region on BTA14 that includes the positional candidate genes LYPLA1, XKR4 and TMEM68 is associated with feed intake and growth phenotypes in cattle(1). Anim Genet. 2012;43(2):216–9.
Yao Z, Li J, Zhang Z, Chai Y, Liu X, Li J, Huang Y, Li L, Huang W, Yang G, et al. The relationship between MFN1 copy number variation and growth traits of beef cattle. Gene. 2022;811:146071.
Mariadassou M, Ramayo-Caldas Y, Charles M, Féménia M, Renand G, Rocha D. Detection of selection signatures in Limousin cattle using whole-genome resequencing. Anim Genet. 2020;51(5):815–9.
Hu Y, Lv J, Fang Y, Luo Q, He Y, Li L, Fan M, Wang Z. Crtc1 Deficiency causes obesity potentially via regulating PPARγ pathway in White Adipose. Front cell Dev Biology. 2021;9:602529.
Zhang X, Chen X, Qi T, Kong Q, Cheng H, Cao X, Li Y, Li C, Liu L, Ding Z. HSPA12A is required for adipocyte differentiation and diet-induced obesity through a positive feedback regulation with PPARγ. Cell Death Differ. 2019;26(11):2253–67.
Terefe E, Belay G, Han J, Hanotte O, Tijjani A. Genomic adaptation of Ethiopian indigenous cattle to high altitude. Front Genet. 2022;13:960234.
Huerta-Sánchez E, Degiorgio M, Pagani L, Tarekegn A, Ekong R, Antao T, Cardona A, Montgomery HE, Cavalleri GL, Robbins PA, et al. Genetic signatures reveal high-altitude adaptation in a set of Ethiopian populations. Mol Biol Evol. 2013;30(8):1877–88.
Sweet-Jones J, Lenis VP, Yurchenko AA, Yudin NS, Swain M, Larkin DM. Genotyping and whole-genome resequencing of Welsh Sheep Breeds Reveal Candidate Genes and variants for adaptation to local environment and socioeconomic traits. Front Genet. 2021;12:612492.
Zhao X, Wang C, Wang Y, Zhou L, Hu H, Bai L, Wang J. Weighted gene co-expression network analysis reveals potential candidate genes affecting drip loss in pork. Anim Genet. 2020;51(6):855–65.
Sambrook J, Fritsch EF, Maniatis T. Molecular cloning: a Laboratory Manual. 2nd ed. New York. CSH: Cold Spring Harbor; 1982.
Google Scholar
Bolger AM, Lohse M, Usadel B. Trimmomatic: a flexible trimmer for Illumina sequence data. Bioinf (Oxford England). 2014;30(15):2114–20.
CAS Google Scholar
Van der Auwera GA, Carneiro MO, Hartl C, Poplin R, Del Angel G, Levy-Moonshine A, Jordan T, Shakir K, Roazen D, Thibault J et al. From FastQ data to high confidence variant calls: the Genome Analysis Toolkit best practices pipeline. Current protocols in bioinformatics 2013, 43(1110):11.10.11–11.10.33.
Danecek P, Bonfield JK, Liddle J, Marshall J, Ohan V, Pollard MO, Whitwham A, Keane T, McCarthy SA, Davies RM et al. Twelve years of SAMtools and BCFtools. GigaScience 2021, 10(2).
Purcell S, Neale B, Todd-Brown K, Thomas L, Ferreira MA, Bender D, Maller J, Sklar P, de Bakker PI, Daly MJ, et al. PLINK: a tool set for whole-genome association and population-based linkage analyses. Am J Hum Genet. 2007;81(3):559–75.
Zhang C, Dong SS, Xu JY, He WM, Yang TL. PopLDdecay: a fast and effective tool for linkage disequilibrium decay analysis based on variant call format files. Bioinf (Oxford England). 2019;35(10):1786–8.
Patterson N, Price AL, Reich D. Population structure and eigenanalysis. PLoS Genet. 2006;2(12):e190.
Alexander DH, Lange K. Enhancements to the ADMIXTURE algorithm for individual ancestry estimation. BMC Bioinformatics. 2011;12:246.
Chen C, Chen H, Zhang Y, Thomas HR, Frank MH, He Y, Xia R. TBtools: an integrative Toolkit developed for interactive analyses of big Biological Data. Mol Plant. 2020;13(8):1194–202.
Tamura K, Stecher G, Kumar S. MEGA11: Molecular Evolutionary Genetics Analysis Version 11. Mol Biol Evol. 2021;38(7):3022–7.
Nielsen R, Williamson S, Kim Y, Hubisz MJ, Clark AG, Bustamante C. Genomic scans for selective sweeps using SNP data. Genome Res. 2005;15(11):1566–75.
Danecek P, Auton A, Abecasis G, Albers CA, Banks E, DePristo MA, Handsaker RE, Lunter G, Marth GT, Sherry ST, et al. The variant call format and VCFtools. Bioinf (Oxford England). 2011;27(15):2156–8.
DeGiorgio M, Huber CD, Hubisz MJ, Hellmann I, Nielsen R. SweepFinder2: increased sensitivity, robustness and flexibility. Bioinf (Oxford England). 2016;32(12):1895–7.
Szpiech ZA, Hernandez RD. Selscan: an efficient multithreaded program to perform EHH-based scans for positive selection. Mol Biol Evol. 2014;31(10):2824–7.
Bu D, Luo H, Huo P, Wang Z, Zhang S, He Z, Wu Y, Zhao L, Liu J, Guo J, et al. KOBAS-i: intelligent prioritization and exploratory visualization of biological functions for gene enrichment analysis. Nucleic Acids Res. 2021;49(W1):W317–25.
Xia X, Qu K, Wang Y, Sinding MS, Wang F, Hanif Q, Ahmed Z, Lenstra JA, Han J, Lei C et al: Global dispersal and adaptive evolution of domestic cattle: a genomic perspective. Stress Biol. 2023, 3(1):8.
Lyu Y, Wang F, Cheng H, Han J, Dang R, Xia X, Wang H, Zhong J, Lenstra JA, Zhang H et al: Recent selection and introgression facilitated high-altitude adaptation in cattle. Science Bulletin 2024.
Xia XT, Zhang FW, Li S, Luo XY, Peng LX, Dong Z, Pausch H, Leonard AS, Crysnanto D, Wang SK et al: Structural variation and introgression from wild populations in East Asian cattle genomes confer adaptation to local environment. Genome Biol 2023, 24(1):211.
Download references
Acknowledgements
We would like to thank Yu Jiang for his technical support.
This research has been supported by Technological Innovation 2030- Major Projects (2023ZD040480204); National Key R&D Plan (2022YFD1602310); 2022 Henan Province Central Leading Local Science and Technology Development Fund Project (Z20221343039); Major Science and Technology Projects in Henan Province (221100110200); China Agriculture Research System of MOF and MARA (CARS-37).
Author information
First Author: Xingya Song, Zhi Yao, Zijing Zhang and Shijie Lyu.
Authors and Affiliations
College of Animal Science and Technology, Northwest A&F University, No. 22 Xinong Road, Yangling Shaanxi, 712100, Shaanxi, People’s Republic of China
Xingya Song, Zhi Yao, Ningbo Chen, Chuzhao Lei, Yu Jiang & Yongzhen Huang
Institute of Animal Husbandry, Henan Academy of Agricultural Sciences, Zhengzhou, 450002, Henan, People’s Republic of China
Zijing Zhang, Shijie Lyu & Eryao Wang
Biyang County Xiananniu Technology Development Co., Ltd, Zhumadian, 463700, People’s Republic of China
Xingshan Qi
Henan Provincial Livestock Technology Promotion Station, Zhengzhou, 450008, Henan, People’s Republic of China
Shaanxi Agricultural and Animal Husbandry Seed Farm, Shaanxi Fufeng, 722203, People’s Republic of China
Weidong Ma & Wusheng Wang
You can also search for this author in PubMed Google Scholar
Contributions
YH conceived and designed the experiments. XS and ZY performed the statistical analysis and data upload. ZZ and SL performed the sample DNA extraction. EW provided suggestions for the revision of the manuscript. YJ, CL , and NC provided technical assistance. QX, XL, MW, WW, and EW contributed to the sample collections. YJ provides a data analysis platform. YH provided the laboratories for DNA extraction and statistical analysis. XS drafted the manuscript. All authors read and approved the final manuscript.
Corresponding authors
Correspondence to Eryao Wang or Yongzhen Huang .
Ethics declarations
Ethics approval and consent to participate.
All cattle were handled following the guidelines established by the Council for Animal Welfare of China. The protocols for sample collection and animal handling have been approved by the Faculty of Animal Policy and Welfare Committee of Northwest A&F University (FAPWCNWAFU, Protocol number, NWAFAC 1008).
Consent for publication
Not applicable.
Competing interests
The authors declare no competing interests.
Additional information
Publisher’s note.
Springer Nature remains neutral with regard to jurisdictional claims in published maps and institutional affiliations.
Electronic supplementary material
Below is the link to the electronic supplementary material.
Supplementary Material 1

Supplementary Material 2

Supplementary Material 3

Supplementary Material 4
Rights and permissions.
Open Access This article is licensed under a Creative Commons Attribution 4.0 International License, which permits use, sharing, adaptation, distribution and reproduction in any medium or format, as long as you give appropriate credit to the original author(s) and the source, provide a link to the Creative Commons licence, and indicate if changes were made. The images or other third party material in this article are included in the article’s Creative Commons licence, unless indicated otherwise in a credit line to the material. If material is not included in the article’s Creative Commons licence and your intended use is not permitted by statutory regulation or exceeds the permitted use, you will need to obtain permission directly from the copyright holder. To view a copy of this licence, visit http://creativecommons.org/licenses/by/4.0/ . The Creative Commons Public Domain Dedication waiver ( http://creativecommons.org/publicdomain/zero/1.0/ ) applies to the data made available in this article, unless otherwise stated in a credit line to the data.
Reprints and permissions
About this article
Cite this article.
Song, X., Yao, Z., Zhang, Z. et al. Whole-genome sequencing reveals genomic diversity and selection signatures in Xia’nan cattle. BMC Genomics 25 , 559 (2024). https://doi.org/10.1186/s12864-024-10463-3
Download citation
Received : 30 December 2023
Accepted : 28 May 2024
Published : 05 June 2024
DOI : https://doi.org/10.1186/s12864-024-10463-3
Share this article
Anyone you share the following link with will be able to read this content:
Sorry, a shareable link is not currently available for this article.
Provided by the Springer Nature SharedIt content-sharing initiative
- Xia’nan cattle
- Genetic diversity
- Population structure
- Genetic signatures
BMC Genomics
ISSN: 1471-2164
- Submission enquiries: [email protected]
- General enquiries: [email protected]
Thank you for visiting nature.com. You are using a browser version with limited support for CSS. To obtain the best experience, we recommend you use a more up to date browser (or turn off compatibility mode in Internet Explorer). In the meantime, to ensure continued support, we are displaying the site without styles and JavaScript.
- View all journals
- Explore content
- About the journal
- Publish with us
- Sign up for alerts
- Review Article
- Published: 30 January 2018
Cloud computing for genomic data analysis and collaboration
- Ben Langmead 1 &
- Abhinav Nellore 2
Nature Reviews Genetics volume 19 , pages 208–219 ( 2018 ) Cite this article
14k Accesses
169 Citations
99 Altmetric
Metrics details
- Computational biology and bioinformatics
- Genetic databases
- Next-generation sequencing
- Research data
A Corrigendum to this article was published on 12 February 2018
This article has been updated
Cloud computing is a paradigm whereby computational resources such as computers, storage and bandwidth can be rented on a pay-for-what-you-use basis.
The cloud's chief advantages are elasticity and convenience. Elasticity refers to the ability to rent and pay for the exact resources needed, and convenience refers to the fact that the user need not deal with the disadvantages of owning or maintaining the resources.
Archives of sequencing data are vast and rapidly growing. Cloud computing is an important enabler for recent efforts to reanalyse large cross-sections of archived sequencing data.
The cloud is becoming a popular venue for hosting large international collaborations, which benefit from the ability to hold data securely in a single location and proximate to the computational infrastructure that will be used to analyse it.
Funders of genomics research are increasingly aware of the cloud and its advantages and are beginning to allocate funds and create cloud-based resources accordingly.
Cloud clusters can be configured with security measures needed to adhere to privacy standards, such as those from the Database of Genotypes and Phenotypes (dbGaP).
Next-generation sequencing has made major strides in the past decade. Studies based on large sequencing data sets are growing in number, and public archives for raw sequencing data have been doubling in size every 18 months. Leveraging these data requires researchers to use large-scale computational resources. Cloud computing, a model whereby users rent computers and storage from large data centres, is a solution that is gaining traction in genomics research. Here, we describe how cloud computing is used in genomics for research and large-scale collaborations, and argue that its elasticity, reproducibility and privacy features make it ideally suited for the large-scale reanalysis of publicly available archived data, including privacy-protected data.
This is a preview of subscription content, access via your institution
Access options
Access Nature and 54 other Nature Portfolio journals
Get Nature+, our best-value online-access subscription
24,99 € / 30 days
cancel any time
Subscribe to this journal
Receive 12 print issues and online access
176,64 € per year
only 14,72 € per issue
Buy this article
- Purchase on Springer Link
- Instant access to full article PDF
Prices may be subject to local taxes which are calculated during checkout
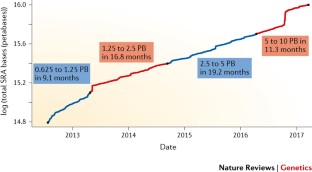
Similar content being viewed by others
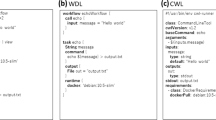
Practical guide for managing large-scale human genome data in research
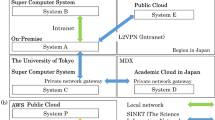
Design and implementation of a hybrid cloud system for large-scale human genomic research
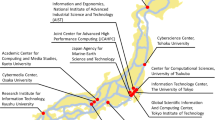
Building cloud computing environments for genome analysis in Japan
Change history, 12 february 2018.
The above article originally stated “FireCloud and CGC rely on AWS and the Google Cloud Platform for computing and data storage. In addition to charges for these commercial services, users pay convenience surcharges.” The second sentence was incorrect, as pointed out to and independently verified by the authors, and has been removed. Also, an incorrect citation was given for reference 66. The citation should have been: Bray, N. L., Pimentel, H., Melsted, P. & Pachter, L. Near-optimal probabilistic RNA-seq quantification. Nat. Biotech . 34 , 525–527 (2016). Finally, reference 67 referred to an older version of the CWL specification and has been updated. The article has been corrected online. The authors apologize for these errors.
Goodwin, S., McPherson, J. D. & McCombie, W. R. Coming of age: ten years of next-generation sequencing technologies. Nat. Rev. Genet. 17 , 333–351 (2016).
Article CAS PubMed Google Scholar
Stephens, Z. D. et al. Big data: astronomical or genomical? PLOS Biol. 13 , e1002195 (2015). This perspective puts the genomic data deluge in context with other sciences and shows how growth of archived genomics data is tracking improvements in technology.
Article CAS PubMed PubMed Central Google Scholar
Kodama, Y. et al. The sequence read archive: explosive growth of sequencing data. Nucleic Acids Res. 40 , D54–D56 (2012).
Leinonen, R. et al. The sequence read archive. Nucleic Acids Res. 39 , D19–D21 (2010).
Toribio, A. L. et al. European Nucleotide Archive in 2016. Nucleic Acids Res. 45 , D32–D36 (2017).
Denk, F. Don't let useful data go to waste. Nature 543 , 7 (2017).
Kuo, W. P., Jenssen, T.-K., Butte, A. J., Ohno-Machado, L. & Kohane, I. S. Analysis of matched mRNA measurements from two different microarray technologies. Bioinformatics 18 , 405–412 (2002).
Leek, J. T. et al. Tackling the widespread and critical impact of batch effects in high-throughput data. Nat. Rev. Genet. 11 , 733–739 (2010).
McCall, M. N., Bolstad, B. M. & Irizarry, R. A. Frozen robust multiarray analysis (fRMA). Biostatistics 11 , 242–253 (2010).
Article PubMed PubMed Central Google Scholar
Rhodes, D. R. et al. Large-scale meta-analysis of cancer microarray data identifies common transcriptional profiles of neoplastic transformation and progression. Proc. Natl Acad. Sci. USA 101 , 9309–9314 (2004).
Zeggini, E. et al. Meta-analysis of genome-wide association data and large-scale replication identifies additional susceptibility loci for type 2 diabetes. Nat. Genet. 40 , 638–645 (2008).
Marchionni, L., Afsari, B., Geman, D. & Leek, J. T. A simple and reproducible breast cancer prognostic test. BMC Genomics 14 , 336 (2013).
Lek, M. et al. Analysis of protein-coding genetic variation in 60,706 humans. Nature 536 , 285–291 (2016).
International Cancer Genome Consortium et al. International network of cancer genome projects. Nature 464 , 993–998 (2010).
GTEx Consortium. Genetic effects on gene expression across human tissues. Nature 550 , 204–213 (2017).
Melé, M. et al. Human genomics. The human transcriptome across tissues and individuals. Science 348 , 660–665 (2015).
Trans-Omics for Precision Medicine (TOPMed) Program. National Heart, Lung, and Blood Institute https://www.nhlbi.nih.gov/science/trans-omics-precision-medicine-topmed-program (2017).
Collins, F. S. & Varmus, H. A new initiative on precision medicine. N. Engl. J. Med. 372 , 793–795 (2015).
Gaziano, J. M. et al. Million Veteran Program: A mega-biobank to study genetic influences on health and disease. J. Clin. Epidemiol. 70 , 214–223 (2016).
Article PubMed Google Scholar
Foster, I. G. & Dennis, B. Cloud Computing for Science and Engineering (MIT Press, 2017). This book describes the public and private cloud offerings availabkle and how to use APIs for both commercial and OpenStack clouds to automate cloud tasks. It also describes Globus Auth and other important ideas related to identity federation, authentication and authorization.
Google Scholar
International Cancer Genes Consortium. PCAWG Data Portal and Visualizations. ICGC http://docs.icgc.org/pcawg/ (2017).
Birger, C. et al. FireCloud, a scalable cloud-based platform for collaborative genome analysis: strategies for reducing and controlling costs. bioRxiv , https://doi.org/10.1101/209494 (2017).
Lau, J. W. et al. The Cancer Genomics Cloud: collaborative, reproducible, and democratized – a new paradigm in large-scale computational research. Cancer Res. 77 , e3–e6 (2017).
Reynolds, S. M. et al. The ISB Cancer Genomics Cloud: a flexible cloud-based platform for cancer genomics research. Cancer Res. 77 , e7–e10 (2017).
Celniker, S. E. et al. Unlocking the secrets of the genome. Nature 459 , 927–930 (2009).
The ENCODE Project Consortium. An integrated encyclopedia of DNA elements in the human genome. Nature 489 , 57–74 (2012).
Mell, P. M. & Grance, T. SP 800–145. The NIST definition of cloud computing. National Institute of Standards and Technology http://csrc.nist.gov/publications/nistpubs/800-145/SP800-145.pdf (2011).
Wingfield, N., Streitfeld, D. & Lohr, S. Cloud produces sunny earnings at Amazon, Microsoft and Alphabet. New York Times https://www.nytimes.com/2017/04/27/technology/quarterly-earnings-cloud-computing-amazon-microsoft-alphabet.html (27 April 2017).
Mathews, L. Just how big is Amazon's AWS business? (hint: it's absolutely massive). Geek.com https://www.geek.com/chips/just-how-big-is-amazons-aws-business-hint-its-absolutely-massive-1610221/ (2014).
Sefraoui, O., Aissaoui, M. & Eleuldj, M. OpenStack: toward an open-source solution for cloud computing. Int. J. Comput. Appl. Technol. 55 , 38–42 (2012).
Moreno-Vozmediano, R., Montero, R. S. & Llorente, I. M. IaaS cloud architecture: from virtualized datacenters to federated cloud infrastructures. Computer 45 , 65–72 (2012).
Article Google Scholar
Das, S. et al. Next-generation genotype imputation service and methods. Nat. Genet. 48 , 1284–1287 (2016).
Stewart, C. A. et al. in Proc. 2015 XSEDE Conference: Scientific Advancements Enabled by Enhanced Cyberinfrastructure https://dl.acm.org/citation.cfm?id=2792745 (2015).
European Open Science Cloud [Editorial]. Nat. Genet. 48 , 821 (2016).
Madduri, R. K. et al. Experiences building Globus Genomics: a next-generation sequencing analysis service using Galaxy, Globus, and Amazon web services. Concurr. Comput. 26 , 2266–2279 (2014).
Yakneen, S., Waszak, S., Gertz, M. & Korbel, J. O. Enabling rapid cloud-based analysis of thousands of human genomes via Butler. bioRxiv https://doi.org/10.1101/185736 (2017).
Yung, C. K. et al. Large-scale uniform analysis of cancer whole genomes in multiple computing environments. bioRxiv https://doi.org/10.1101/161638 (2017).
Baggerly, K. A. & Coombes, K. R. Deriving chemosensitivity from cell lines: forensic bioinformatics and reproducible research in high-throughput biology. Ann. Appl. Statist. 3 , 1309–1334 (2009).
Dai, M. et al. Evolving gene/transcript definitions significantly alter the interpretation of GeneChip data. Nucleic Acids Res. 33 , e175 (2005).
Ioannidis, J. P. et al. Repeatability of published microarray gene expression analyses. Nat. Genet. 41 , 149–155 (2009).
Nekrutenko, A. & Taylor, J. Next-generation sequencing data interpretation: enhancing reproducibility and accessibility. Nat. Rev. Genet. 13 , 667–672 (2012).
Piccolo, S. R. & Frampton, M. B. Tools and techniques for computational reproducibility. Gigascience 5 , 30 (2016).
Angiuoli, S. V. et al. CloVR: a virtual machine for automated and portable sequence analysis from the desktop using cloud computing. BMC Bioinformatics 12 , 356 (2011).
Krampis, K. et al. Cloud BioLinux: pre-configured and on-demand bioinformatics computing for the genomics community. BMC Bioinformatics 13 , 42 (2012).
Merkel, D. Docker: lightweight Linux containers for consistent development and deployment. Linux J. 2014 , 2 (2014).
Kurtzer, G. M., Sochat, V. & Bauer, M. W. Singularity: scientific containers for mobility of compute. PLOS One 12 , e0177459 (2017).
The Clinical Cancer Genome Task Team of the Global Alliance for Genomics and Health. Sharing clinical and genomic data on cancer – the need for global solutions. N. Engl. J. Med. 376 , 2006–2009 (2017).
Bonazzi, V. R. & Bourne, P. E. Should biomedical research be like Airbnb? PLOS Biol. 15 , e2001818 (2017). The authors of this paper describe the NIH Data Commons and suggest cloud computing as a means for making large-scale genomics data sets available and associated analyses reproducible.
Bourne, P. E., Lorsch, J. R. & Green, E. D. Perspective: sustaining the big-data ecosystem. Nature 527 , S16–17 (2015).
Tryka, K. A. et al. NCBI's database of genotypes and phenotypes: dbGaP. Nucleic Acids Res. 42 , D975–D979 (2014).
Iyer, M. K. et al. The landscape of long noncoding RNAs in the human transcriptome. Nat. Genet. 47 , 199–208 (2015).
Brown, J. B. et al. Diversity and dynamics of the Drosophila transcriptome. Nature 512 , 393–399 (2014).
Graveley, B. The developmental transcriptome of Drosophila melanogaster . Genome Biol. 11 , I11 (2010).
Article PubMed Central Google Scholar
Gutzwiller, F. et al. Dynamics of Wolbachia pipientis gene expression across the Drosophila melanogaster life cycle. G3 5 , 2843–2856 (2015).
Bernstein, M. N., Doan, A. & Dewey, C. N. MetaSRA: normalized human sample-specific metadata for the sequence read archive. Bioinformatics 33 , 2914–2923 (2017).
Yung, C. K. et al. The Cancer Genome Collaboratory [abstract]. Cancer Res. 77 , 378 (2017).
Article CAS Google Scholar
Nellore, A. et al. Human splicing diversity and the extent of unannotated splice junctions across human RNA-seq samples on the sequence read archive. Genome Biol. 17 , 266 (2016).
Frazee, A. C., Langmead, B. & Leek, J. T. ReCount: a multi-experiment resource of analysis-ready RNA-seq gene count datasets. BMC Bioinformatics 12 , 449 (2011).
Langmead, B., Hansen, K. D. & Leek, J. T. Cloud-scale RNA-sequencing differential expression analysis with Myrna. Genome Biol. 11 , R83 (2010).
Nellore, A., Wilks, C., Hansen, K. D., Leek, J. T. & Langmead, B. Rail-dbGaP: analyzing dbGaP-protected data in the cloud with Amazon Elastic MapReduce. Bioinformatics 32 , 2551–2553 (2016). This work reports the use of cloud computing and MapReduce software to study tens of thousands of human RNA sequencing data sets, showing that many splice junctions that are well represented in public data are not present in popular gene annotations.
Collado-Torres, L. et al. Reproducible RNA-seq analysis using recount2. Nat. Biotechnol. 35 , 319–321 (2017).
Nellore, A. et al. Rail-RNA: scalable analysis of RNA-seq splicing and coverage. Bioinformatics 33 , 4003–4040 (2017).
Vivian, J. et al. Toil enables reproducible, open source, big biomedical data analyses. Nat. Biotechnol. 35 , 314–316 (2017).
Dobin, A. et al. STAR: ultrafast universal RNA-seq aligner. Bioinformatics 29 , 15–21 (2013).
Li, B. & Dewey, C. N. RSEM: accurate transcript quantification from RNA-Seq data with or without a reference genome. BMC Bioinformatics 12 , 323 (2011).
Bray, N. L., Pimentel, H., Melsted, P. & Pachter, L. Near-optimal probabilistic RNA-seq quantification. Nat. Biotech. 34 , 525–527 (2016).
Amstutz, P. et al. Common workflow language, v1.0. Figshare https://doi.org/10.6084/m9.figshare.3115156.v2 (2016).
Tatlow, P. J. & Piccolo, S. R. A cloud-based workflow to quantify transcript-expression levels in public cancer compendia. Sci. Rep. 6 , 39259 (2016). This study shows how cloud computing can be used to reanalyse over 12,000 human cancer RNA sequencing data sets for as little as US$0.09 per sample.
Foster, I. K., Carl. The Grid 2: Blueprint for a New Computing Infrastructure (Morgan Kaufmann, 2003).
Drew, K. et al. The Proteome Folding Project: proteome-scale prediction of structure and function. Genome Res. 21 , 1981–1994 (2011).
Rahman, M. et al. Alternative preprocessing of RNA-Sequencing data in The Cancer Genome Atlas leads to improved analysis results. Bioinformatics 31 , 3666–3672 (2015).
Stein, L. D. The case for cloud computing in genome informatics. Genome Biol. 11 , 207 (2010).
Bais, P., Namburi, S., Gatti, D. M., Zhang, X. & Chuang, J. H. CloudNeo: a cloud pipeline for identifying patient-specific tumor neoantigens. Bioinformatics 33 , 3110–3112 (2017).
Afgan, E. et al. The Galaxy platform for accessible, reproducible and collaborative biomedical analyses: 2016 update. Nucleic Acids Res. 44 , W3–W10 (2016).
Towns, J. et al. XSEDE: accelerating scientific discovery. Comput. Sci. Eng. 16 , 62–74 (2014).
Galaxy Community Hub. Publicly accessible Galaxy servers. Galaxy Project https://galaxyproject.org/public-galaxy-servers/ (2017).
Afgan, E. et al. Galaxy CloudMan: delivering cloud compute clusters. BMC Bioinformatics 11 (Suppl. 12), S4 (2010).
Liu, B. et al. Cloud-based bioinformatics workflow platform for large-scale next-generation sequencing analyses. J. Biomed. Inform. 49 , 119–133 (2014).
Foster, I. Globus Online: accelerating and democratizing science through cloud-based services. IEEE Internet Comput. 15 , 70–73 (2011).
Dana-Farber Cancer Institute. Dana-Farber Cancer Institute and Ontario Institute for Cancer Research join Collaborative Cancer Cloud http://www.dana-farber.org/newsroom/news-releases/2016/dana-farber-cancer-institute-and-ontario-institute-for-cancer-research-join-collaborative-cancer-cloud/ (2016).
Hawkins, T. The Collaborative Cancer Cloud: Intel and OHSU team up for cancer research. siliconANGLE http://siliconangle.com/blog/2016/12/16/collaborative-cancer-cloud-intel-ohsu-team-cancer-research-thecube/ (2016).
Global Alliance for Genomics and Health. A federated ecosystem for sharing genomic, clinical data. Science 352 , 1278–1280 (2016).
Amazon Web Services. AWS case study: DNAnexus. Amazon https://aws.amazon.com/solutions/case-studies/dnanexus/ (2017).
ICGC Data Coordination Center. About cloud partners. ICGC http://docs.icgc.org/cloud/about/ (2017).
modENCODE Project. modENCODE on the EC2 cloud. modENCODE http://data.modencode.org/modencode-cloud.html (2017).
Dean, J. & Ghemawat, S. MapReduce. Commun. ACM 51 , 107 (2008).
Kelly, B. J. et al. Churchill: an ultra-fast, deterministic, highly scalable and balanced parallelization strategy for the discovery of human genetic variation in clinical and population-scale genomics. Genome Biol. 16 , 6 (2015).
Langmead, B., Schatz, M. C., Lin, J., Pop, M. & Salzberg, S. L. Searching for SNPs with cloud computing. Genome Biol. 10 , R134 (2009).
Feng, X., Grossman, R. & Stein, L. PeakRanger: a cloud-enabled peak caller for ChIP-seq data. BMC Bioinformatics 12 , 139 (2011).
McKenna, A. et al. The Genome Analysis Toolkit: a MapReduce framework for analyzing next-generation DNA sequencing data. Genome Res. 20 , 1297–1303 (2010).
GA4GH-DREAM. GA4GH-DREAM Workflow Execution Challenge. Synapse https://www.synapse.org/WorkflowChallenge (2017).
Franke, A. et al. Genome-wide meta-analysis increases to 71 the number of confirmed Crohn's disease susceptibility loci. Nat. Genet. 42 , 1118–1125 (2010).
Petryszak, R. et al. The RNASeq-er API—a gateway to systematically updated analysis of public RNA-seq data. Bioinformatics 33 , 2218–2220 (2017).
Goldman, M., Craft, B., Zhu, J. & Haussler, D. The UCSC Xena system for cancer genomics data visualization and interpretation [Abstr. 2584]. Cancer Res. 77 , 2584 (2017).
Kolesnikov, N. et al. ArrayExpress update—simplifying data submissions. Nucleic Acids Res. 43 , D1113–D1116 (2015).
Google Compute Engine. Google Compute Engine pricing. Google Cloud Platform https://cloud.google.com/compute/pricing (2017).
Chard, R. et al. in 2015 IEEE 11th International Conference on e-Science , 136–144 (IEEE, 2015).
Book Google Scholar
Barr, J. Natural Language Processing at Clemson University – 1.1 Million vCPUs & EC2 Spot Instances. Amazon https://aws.amazon.com/blogs/aws/natural-language-processing-at-clemson-university-1-1-million-vcpus-ec2-spot-instances/ (2017).
NIH Commons. Commons Credits Pilot Portal. Commons Credits Pilot Portal https://www.commons-credit-portal.org/ (2017).
National Science Foundation. Amazon Web Services, Google Cloud, and Microsoft Azure join NSF's Big Data Program. National Science Foundation https://www.nsf.gov/news/news_summ.jsp?cntn_id=190830&WT.mc_ev=click (2017).
National Institute of Mental Health. Welcome to the NIMH Data Archive. NDA https://data-archive.nimh.nih.gov/ (2017).
Genomes Project Consortium. A global reference for human genetic variation. Nature 526 , 68–74 (2015).
Lappalainen, I. et al. The European Genome-Phenome Archive of human data consented for biomedical research. Nat. Genet. 47 , 692–695 (2015).
National Institutes of Health. NIH security best practices for controlled-access data subject to the NIH genomic data sharing (GDS) policy. NIH Office of Science Policy https://osp.od.nih.gov/wp-content/uploads/NIH_Best_Practices_for_Controlled-Access_Data_Subject_to_the_NIH_GDS_Policy.pdf (2015).
Stein, L. D., Knoppers, B. M., Campbell, P., Getz, G. & Korbel, J. O. Data analysis: Create a cloud commons. Nature 523 , 149–151 (2015). In this paper, the authors argue for the use of cloud computing in large consortia and describe plans for its use in the ICGC.
Deutsche Telekom. Deutsche Telekom launches highly secure public cloud based on Cisco platform. Deutsche Telekom https://www.telekom.com/en/media/media-information/archive/deutsche-telekom-launches-highly-secure-public-cloud-based-on-cisco-platform------362100 (2015).
Datta, S., Bettinger, K. & Snyder, M. Secure cloud computing for genomic data. Nat. Biotechnol. 34 , 588–591 (2016).
Dove, E. S. et al. Genomic cloud computing: legal and ethical points to consider. Eur. J. Hum. Genet. 23 , 1271–1278 (2015).
Francis, L. P. Genomic knowledge sharing: a review of the ethical and legal issues. Appl. Transl Genom. 3 , 111–115 (2014).
Seven Bridges Genomics. API Overview. Seven Bridges Genomics https://docs.sevenbridges.com/v1.0/docs/the-api (2017).
Ananthakrishnan, R., Chard, K., Foster, I. & Tuecke, S. Globus platform-as-a-service for collaborative science applications. Concurrency Comput. Pract. Exp. 27 , 290–305 (2015).
Chaterji, S. et al. Federation in genomics pipelines: techniques and challenges. Brief Bioinform. https://doi.org/10.1093/bib/bbx102 (2017).
Campbell, S. Teaching cloud computing. Computer 49 , 91–93 (2016).
Dudley, J. T. & Butte, A.J. In silico research in the era of cloud computing. Nat. Biotech. 28 , 1181–1185 (2010).
Barretina, J. et al. The Cancer Cell Line Encyclopedia enables predictive modelling of anticancer drug sensitivity. Nature 483 , 603–607 (2012).
Cancer Genome Atlas Research Network et al. The Cancer Genome Atlas Pan-Cancer analysis project. Nat. Genet. 45 , 1113–1120 (2013).
Heath, A. P. et al. Bionimbus: a cloud for managing, analyzing and sharing large genomics datasets. J. Am. Med. Inform. Assoc. 21 , 969–975 (2014).
Di Tommaso, P. et al. Nextflow enables reproducible computational workflows. Nat. Biotechnol. 35 , 316–319 (2017).
Fisch, K. M. et al. Omics Pipe: a community-based framework for reproducible multi-omics data analysis. Bioinformatics 31 , 1724–1728 (2015).
Allcock, W. et al. in Proceedings of the 2005 ACM/IEEE conference on Supercomputing 54 (Seattle, 2005).
Petryszak, R. et al. Expression Atlas update — a database of gene and transcript expression from microarray- and sequencing-based functional genomics experiments. Nucleic Acids Res. 42 , D926–D932 (2014).
Download references
Acknowledgements
The authors thank J. Taylor, E. Afgan, M. Schatz, J. Goecks and A. Margolin for reading through a draft of this work and providing helpful comments. B.L. was supported by the US National Institutes of Health/National Institute of General Medical Sciences grant 1R01GM118568.
Author information
Authors and affiliations.
Department of Computer Science, Center for Computational Biology, Johns Hopkins University, Baltimore, MD, USA
Ben Langmead
Department of Biomedical Engineering, Department of Surgery, Computational Biology Program, Oregon Health and Science University, Portland, OR, USA
Abhinav Nellore
You can also search for this author in PubMed Google Scholar
Contributions
The authors contributed equally to all aspects of this manuscript.
Corresponding authors
Correspondence to Ben Langmead or Abhinav Nellore .
Ethics declarations
Competing interests.
The authors declare no competing financial interests.
Related links
Further information.
European Genome–Phenome Archive
European Nucleotide Archive
Database of Genotypes and Phenotypes (dbGaP)
Sequence Read Archive
Galaxy cloud
PowerPoint slides
Powerpoint slide for fig. 1, powerpoint slide for fig. 2, powerpoint slide for fig. 3, powerpoint slide for fig. 4, powerpoint slide for table 1, powerpoint slide for table 2, powerpoint slide for table 3, powerpoint slide for table 4, powerpoint slide for table 5, supplementary information, supplementary information s1 (methods).
Supplementary Information for: Cloud computing for genomic data analysis and collaboration (PDF 228 kb)
Snippets of DNA sequence as reported by a DNA sequencer.
A component of a computer that stores data.
A central component of a computer in which the computation takes place.
A collection of connected computers that are able to work in a coordinated fashion to analyse data.
Information about a data set, often pertaining to how and from where it was collected. For example, for a human data set, metadata might include sex, age, cause of death and sequencing protocol used.
Similar to 'virtual machines', containers are 'virtual computers' that enable the use of multiple, isolated services on a single platform. They can run in the context of another computer, using a portion of the host computer's resources. Docker and Singularity are two container management systems.
Barriers that prevent unwanted, perhaps insecure network traffic from reaching a protected network.
(APIs). Formal specifications of the ways in which a user or program can interface with a system, for example, a cloud.
Rights and permissions
Reprints and permissions
About this article
Cite this article.
Langmead, B., Nellore, A. Cloud computing for genomic data analysis and collaboration. Nat Rev Genet 19 , 208–219 (2018). https://doi.org/10.1038/nrg.2017.113
Download citation
Published : 30 January 2018
Issue Date : April 2018
DOI : https://doi.org/10.1038/nrg.2017.113
Share this article
Anyone you share the following link with will be able to read this content:
Sorry, a shareable link is not currently available for this article.
Provided by the Springer Nature SharedIt content-sharing initiative
This article is cited by
Elasticblast: accelerating sequence search via cloud computing.
- Christiam Camacho
- Grzegorz M. Boratyn
- Thomas L. Madden
BMC Bioinformatics (2023)
Accelerating genomic workflows using NVIDIA Parabricks
- Kyle A. O’Connell
- Zelaikha B. Yosufzai
- Juergen A. Klenk
Application of deep learning technique in next generation sequence experiments
- Mehmet Orman
Journal of Big Data (2023)
BarleyExpDB: an integrative gene expression database for barley
- Tingting Li
BMC Plant Biology (2023)
Navigating bottlenecks and trade-offs in genomic data analysis
- Bonnie Berger
- Yun William Yu
Nature Reviews Genetics (2023)
Quick links
- Explore articles by subject
- Guide to authors
- Editorial policies
Sign up for the Nature Briefing: Translational Research newsletter — top stories in biotechnology, drug discovery and pharma.

- Open access
- Published: 06 June 2024
Genome-wide association study of BNT162b2 vaccine-related myocarditis identifies potential predisposing functional areas in Hong Kong adolescents
- Chun Hing She 1 na1 ,
- Hing Wai Tsang 1 na1 ,
- Xingtian Yang 1 ,
- Sabrina SL Tsao 1 ,
- Clara SM Tang 2 ,
- Sophelia HS Chan 1 ,
- Mike YW Kwan 1 ,
- Gilbert T Chua 1 ,
- Wanling Yang 1 &
- Patrick Ip 1
BMC Genomic Data volume 25 , Article number: 51 ( 2024 ) Cite this article
Metrics details
Vaccine-related myocarditis associated with the BNT162b2 vaccine is a rare complication, with a higher risk observed in male adolescents. However, the contribution of genetic factors to this condition remains uncertain. In this study, we conducted a comprehensive genetic association analysis in a cohort of 43 Hong Kong Chinese adolescents who were diagnosed with myocarditis shortly after receiving the BNT162b2 mRNA COVID-19 vaccine. A comparison of whole-genome sequencing data was performed between the confirmed myocarditis cases and a control group of 481 healthy individuals. To narrow down potential genomic regions of interest, we employed a novel clustering approach called ClusterAnalyzer, which prioritised 2,182 genomic regions overlapping with 1,499 genes for further investigation. Our pathway analysis revealed significant enrichment of these genes in functions related to cardiac conduction, ion channel activity, plasma membrane adhesion, and axonogenesis. These findings suggest a potential genetic predisposition in these specific functional areas that may contribute to the observed side effect of the vaccine. Nevertheless, further validation through larger-scale studies is imperative to confirm these findings. Given the increasing prominence of mRNA vaccines as a promising strategy for disease prevention and treatment, understanding the genetic factors associated with vaccine-related myocarditis assumes paramount importance. Our study provides valuable insights that significantly advance our understanding in this regard and serve as a valuable foundation for future research endeavours in this field.
Peer Review reports
Introduction
Since the COVID-19 outbreak, different types of vaccines have been developed and swiftly approved for emergency use worldwide by the US Food and Drug Administration (FDA) [ 1 , 2 , 3 ]. As early as April 2021, various platform-specific adverse effects have been reported following the administration of mRNA vaccines (Pfizer-BioNTech (BNT162b2) and Moderna (mRNA-1273) vaccines) through surveillance programs globally. These adverse effects include clotting disorders, Guillian-Barre syndrome, leukocytoclastic vasculitis [ 4 , 5 , 6 ], as well as acute myocarditis and pericarditis [ 7 , 8 ]. Comparing the incidence rates of vaccine-induced myocarditis in Hong Kong and the US, the incidence rate in Hong Kong was at 18.52 per 100,000 adolescents (age 12–17) [ 9 ] which is much higher than that of 6.28 per 100,000 in the US [ 10 ]. Furthermore, the incidence appeared to be higher in males than females, in adolescents (aged 12–29) compared to adults, and in individuals receiving the second dose compared to the first dose [ 9 , 11 , 12 , 13 , 14 , 15 , 16 ]. Most cases of BNT162b2-associated myocarditis presented with mild symptoms such as chest pain, which typically required either no treatment or the use of nonsteroidal anti-inflammatory drugs (NSAIDs) [ 9 ].
Messenger RNA (mRNA) vaccines introduce a small piece of genetic material called mRNA into host cells. In the case of BNT162b2 vaccines, the mRNA encoding the spike protein (S protein) of the virus is administered intramuscularly [ 17 ]. The mRNA delivered via lipid nanoparticles is taken up by host cells; it then escapes into host cell cytoplasm and instructs the production of the S protein [ 17 ]. S proteins produced by the host are expressed on the surface of host cells, and subsequently recognized, processed and presented by antigen-presenting cells to induce adaptive immune response via expansion of S protein-specific T cells (CD4 + and CD8 + ) [ 17 ]. Consequently, the generation of memory B cells and T cells enables a faster and stronger immune response on future insults.
While the etiology of vaccine-induced myocarditis remains unclear, molecular mimicry against S protein, where antibodies against SARS-CoV-2 cross-react with host tissue proteins, was believed to trigger the autoimmune response of vaccine-induced myocarditis [ 18 ]. The fact that subjects are more likely to develop myocarditis after second dose might be due to the fact that the first dose sensitized the immune system, and the second dose activates it [ 18 ]. High levels of testosterone were suspected to cause the higher incidence rate in males, possibly by promoting aggressive helper T cell response and downregulating anti-inflammatory immune cells [ 18 ]. The involvement of autoimmunity in mediating this complication motivated the hypothesis that genetic variations regulating the activities of autoimmune cells modulates disease susceptibility of vaccine-induced myocarditis. Recent identification of disease susceptibility loci in HLA (DRB1*14:01, DRB1*15:03, and motifs in HLA-A (Leu62 and Gln63)) and KIR ( KIR2DL5B(−)/KIR2DS3(+)/KIR2DS5(−)/KIR2DS4del(+) ) [ 19 , 20 ] suggested the involvement of T cells and NK cells in inducing myocarditis post vaccination, demonstrating the role of genetic predisposition in mediating susceptibility of vaccine-induced myocarditis. To our knowledge, most genetic studies in this field have taken a similar candidate gene approach, this however is biased towards immune-related genes. An unbiased genome-wide approach with the potential of uncovering disease susceptibility loci beyond immune-related genes could greatly impact personalized care in vaccine administration. This allows healthcare professionals to develop targeted screening strategies, prioritize monitoring for high-risk individuals, and implement early interventions. This personalized approach would enhance patient safety, improve outcomes, and contribute to the ongoing development of safer vaccines.
The important role of genetics can also be seen from a case of vaccine-induced myocarditis in 13-year-old monozygotic twins, which indicated a high degree of phenotypic similarity [ 21 ]. Studying rare variant associations would require a large cohort, necessitating ongoing recruitment and international collaboration. Common variants on the other hand, can be studied as clusters by linkage to enhance power in smaller cohorts. As a local effort to investigate the genetic role in vaccine-induced myocarditis, the current study aims to systematically analyze common variant associations of BNT162b2 vaccine-related myocarditis in a cohort of Hong Kong adolescent patients, leading to a hypothesis that disease susceptibility loci related to vaccine-induced myocarditis can be uncovered by using an unbiased genome-wide approach.
Methods and materials
Subject recruitment.
This study was conducted on a cohort of confirmed cases reported earlier by our team [ 9 , 22 ]. Individuals who received the mRNA COVID-19 vaccine consented to link their electronic health records from the Hospital Authority (HA) to their vaccination records through the COVID-19 vaccines Adverse events Response and Evaluation (CARE) programme. Participants aged 12–17 years with suspected post-vaccine acute myocarditis, having received the 1st, 2nd, or 3rd dose of the mRNA COVID-19 vaccine within 14 days before admission to a Hong Kong Hospital Authority (HA) hospital, were included in this study. A series of cardiological investigations were monitored for the admitted patients, including measuring serum cardiac troponin T, electrocardiograms (ECGs) and echocardiograms for the admitted patients. These cases were confirmed according to the criteria suggested by the Brighton Collaboration Case Definition of Myocarditis and Pericarditis and managed by the study team following the Hong Kong Paediatrics Investigation Protocol for Comirnaty-related Myocarditis/Pericarditis [ 23 ]. The exclusion criteria followed the epidemiological study published earlier by our group [ 9 ].
WGS data of 375 Hong Kong Chinese from a study of Hirschsprung’s disease conducted at The University of Hong Kong [ 24 ] and 106 healthy Hong Kong Chinese parents from another study on biliary atresia (unpublished) were used as controls for the association analysis. Allosomal data were not available for the dataset of 375 subjects. Importantly, since all control data were collected before the COVID-19 pandemic, none of the controls had a known diagnosis of vaccine-related myocarditis. To date, there have been no reported mechanistic or pathogenic crosstalk between the phenotypes of the controls (Hirschsprung’s disease and biliary atresia) and vaccine-related myocarditis, thus the sequencing data could be adopted as control dataset in conducting the association analysis in this study.
Whole genome sequencing, variant calling, and association analysis
Whole genome sequencing was performed on genomic DNA extracted from whole blood samples of 43 patients with vaccine-induced myocarditis. The sequencing was conducted using the DNBseq platform with paired-end reads (2 × 150 bp). However, 8 of the 43 samples had inconsistent FASTQ file formats and were excluded from downstream analysis. The raw reads from the remaining 35 samples were first trimmed using trimgalore v0.6.6 [ 25 ] to remove adapter sequences and low-quality bases. The trimmed reads were then aligned to the UCSC human reference genome build hg19 using bwa v0.7.17 [ 26 ]. The average depth of coverage was estimated to be 30X. Variant calling was performed using DeepVariant v1.4.0, and joint genotyping was conducted using GLnexus v1.4.1 [ 27 ] to generate the Variant Call Format (VCF) file for the cases. Variants from low coverage regions (DP < 10) were excluded to minimize variant calling errors.
The control dataset was obtained from other sources as previously described, and only genotype data in VCF format were available. The average depths of coverage were estimated to be approximately 30X for the control subjects from both the biliary atresia study and the Hirschsprung’s disease study. Three control subjects were found being related and therefore excluded from the analysis.
Quality control procedures were implemented using PLINK v1.90b5.3 [ 28 ] and an overview of these procedures was illustrated in Figure S1 . Non-autosomal SNPs and SNPs with genotype missingness greater than 10% or sample missingness greater than 10% were excluded. Additionally, based on the control VCF, SNPs with a minor allele frequency less than 1% or deviating from Hardy-Weinberg equilibrium ( p < 0.0001) were excluded. The association analysis was performed on the remaining 6,454,355 SNPs using an additive model to test their association with vaccine-induced myocarditis. Population stratification was adjusted using principal component analysis (PCA), and the first six principal components, along with sex, were included as covariates in the association analysis (Figure S2 and Figure S3 ).
ClusterAnalyzer to identify regions with consistent association P values among SNPs in intermediate to high LD
Based on the summary statistics of SNP associations for vaccine-related myocarditis, the ClusterAnalyzer was designed to prioritize genomic regions. (Fig. 1 ) Genomic regions are defined as non-overlapping sets of SNPs with a p < 0.05 (significant SNPs) located within 5 kb of one another. To ensure computational efficiency, only regions with a minimum of 10 significant SNPs were considered.
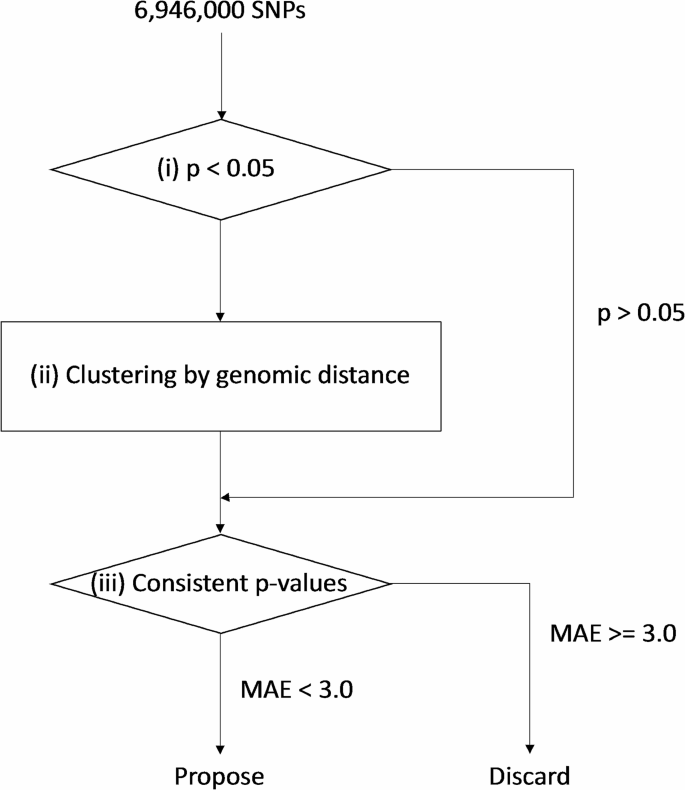
Schematics of ClusterAnalyzer. Based on summary statistics of SNPs tested for BNT162b2 vaccine-related myocarditis association, (i) SNPs with p < 0.05 were selected and (ii) clustered by genomic distance. P-value consistencies were evaluated for each signal within each cluster and quantified as Mean Absolute Error (MAEs). Clusters containing at least one signal with an MAE < 3.0 were prioritized
For each genomic region, SNPs in linkage equilibrium (LD; R 2 > 0.4) were categorized based on the direction of the effect (OR > 1 and OR < 1), enabling the separate evaluation of p-value consistencies for independent signals. Within each group, p-value consistency was determined by the deviation from the line shown in (A) and quantified as Mean Absolute Errors (MAEs, averaged residuals of K SNPs in the group) in (B). Here, s represents -log(P) of each SNPs, s max represents -log(P) of the top SNP, and r 2 represents LD(R 2 ) between the top SNP and other SNPs in the group. Larger MAE indicated poorer p-value consistencies and are thus more likely to be false signals.
Utilizing a user-defined threshold for MAE, a summary of prioritized genomic regions is presented in TSV format. Additionally, GWAS summary statistics of variants within the chosen regions are reported in PLINK format.
Gene set enrichment analysis
The prioritized genomic regions were extended by 5 kb on each end, and the genes within these regions were tabulated using hg19 gene regions obtained from the UCSC database ( http://api.genome.ucsc.edu/getData/track? genome=hg19;track=refGene ) and processed with pybedtools v0.9.1 [ 29 ]. The list of annotated genes in these regions was submitted to the g: Profiler Gene Ontology Statistics (g:GOSt) web server ( https://biit.cs.ut.ee/gprofiler/gost ) [ 30 ] for functional annotation. Gene set enrichment analysis was conducted based on Gene Ontology molecular function (GO:MF) and Gene Ontology biological process (GO:BP) manual annotations. The reference gene sets were derived from “gprofiler_full_hsapiens.name.gmt” and “gprofiler_full_hsapiens.ENSG.gmt.” Default statistical thresholds were used, except for “term size,” which refers to the number of genes enriched in a particular pathway. “Term size” was set within a range of 5-350 to exclude large pathways with limited interpretive value and small pathways with reduced statistical power [ 31 ]. Identified biological processes and molecular functions were clustered using the EnrichmentMap and AutoAnnotate applications (MCL Cluster) in Cytoscape v3.10.1 [ 32 ].
Statistical analysis and data visualization
Quality control procedures and LD computation were executed using PLINK v1.90b5.3 [ 28 ]. Manhattan plots and QQ-plots were generated with the Python package qmplot v0.3.2 ( https://github.com/ShujiaHuang/qmplot ), seaborn v0.12.1, and matplotlib v3.6.2. Regional association plots of selected regions were produced using LocusZoom v0.14.0 ( http://locuszoom.sph.umich.edu/ ). ClusterAnalyzer and other statistical analyses were implemented in Python.
Forty-three Hong Kong Chinese adolescents, aged between 12 and 17 years, with good past health were diagnosed with vaccine-related myocarditis between July 2021 to June 2022 after a median of 3 days after receiving the BNT162b2 vaccine (Comirnaty). The patient cohorts composed of 38 male patients (88.4%) and 5 female patients (12.6%). Majority (81.4%) presented with myocarditis after receiving the 2nd dose of the vaccine. Table 1 provides a summary of the demographic information of the recruited subjects. Detailed clinical background of the confirmed cases was shown in our earlier published studies [ 9 , 22 ]. The confirmed cases presented common cardiac symptoms including chest pain and palpitations. Abnormal ECGs and echocardiogram were observed with an elevated serum cTnT concentration in patients. Overall, these findings suggested that the confirmed cases exhibited an over-reacted host response to the BNT162b2 vaccine and experiencing the adverse cardiac events.
The effective 35 BNT162b2 vaccine-related myocarditis patients and 478 Hong Kong Chinese controls sequencing data were analyzed. Using an additive model, we tested the association of 6,454,355 SNPs related to vaccine-related myocarditis. Multiple SNPs reached genome-wide significance ( p < 5 × 10 − 8 ; Fig. 2 A), but only a small portion of SNPs formed stacks across the genome. In light of this, we designed a clustering approach to partially remove likely false positive signals due to the genotyping errors.
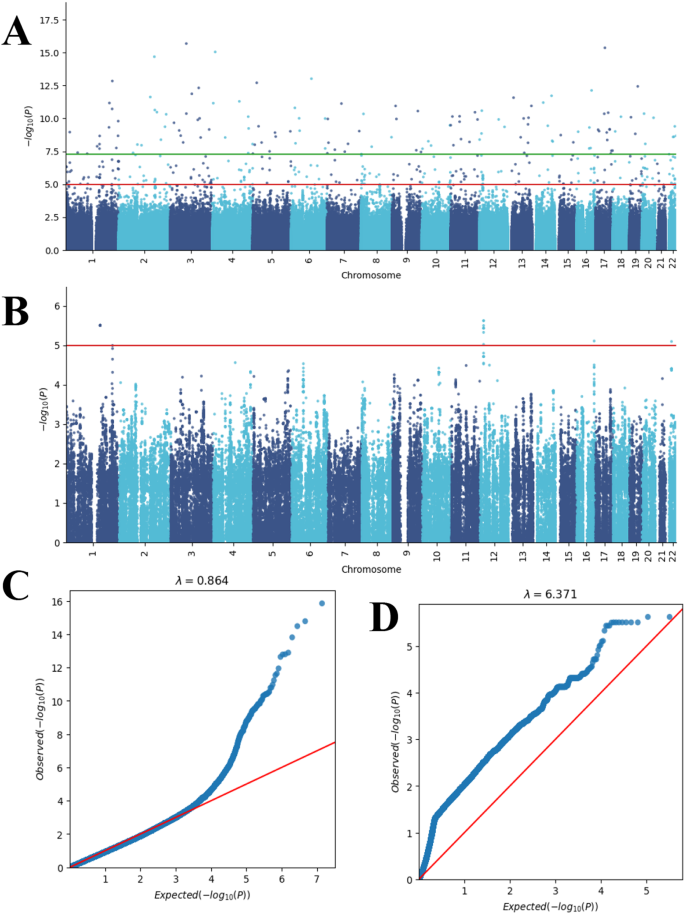
Manhattan and quantile-quantile (QQ) plots. Manhattan plot of (A) initial SNP associations and (B) prioritized SNP associations using ClusterAnalyzer. The horizontal axis in each plot represents the SNP location from chromosome 1 to chromosome 22, while the vertical axis represents significance level in negative logarithm. The red line corresponds to p = 1.0 × 10 − 5 . Green line corresponds to p = 5.0 × 10 − 8 . QQ plots are also provided for (C) initial SNP associations and (D) prioritized SNP associations
By setting a mean absolute error (MAE) threshold of 3.0 for the ClusterAnalyzer tool, we narrowed down the list of SNPs to 2,182 genomic regions, which contained 162,578 SNPs (Fig. 1 ). The average coverage of SNPs in these prioritized genomic regions was estimated at 27X, comparable to the genome average, indicating minimal bias towards sequence coverage. After applying the ClusterAnalyzer, the genomic SNPs appeared more organized as stacks (Fig. 2 A and B), and the genome inflation factor has increased significantly from 0.864 to 6.371 (Fig. 2 C and D). The tool effectively narrowed down the list of SNPs to a smaller subset, namely 2.5% of all SNPs. Two of the prioritized regions most enriched in significant SNPs were chr4:81,892,037–81,965,272 and chr11:40,473,809 − 49,585,162 (hg19; Fig. 3 ). Linkage-association plots showed linked SNPs have comparable log association values (within 3 logs units and adhered to the fitted line; Fig. 3 ), which is evidence of likely true signal. These regions localized to Bone Morphogenetic Protein 3 ( BMP3 ; Fig. 3 B) and Leucine-Rich Repeat Containing 4 C ( LRRC4C ; Fig. 3 D), suggesting a potential connection between these genomic regions and vaccine-related myocarditis.
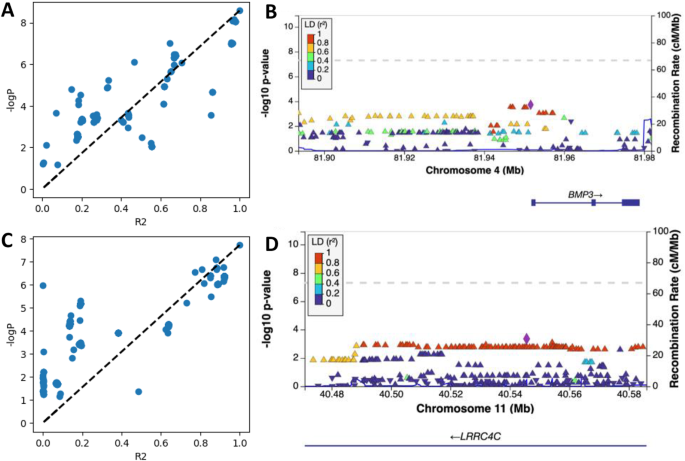
Linkage-association plots and regional association plots generated by LocusZoom for chr4:81,892,037–81,965,272 ( A , B ) and chr11:40,473,809 − 40,585,162 ( C , D ). SNPs with different direction of Odds Ratio (OR) compared to the top SNP are not shown. Dotted lines represent predicted log association P values based on Linkage Disequilibrium (LD, R 2 )
To functionally characterize the prioritized regions, a comprehensive analysis was conducted on 1,499 coding genes within those regions, including an additional 5 kb upstream and downstream for thorough examination. Gene set enrichment analysis was performed without automated electronic annotations to reveal biological insights. Among the 14 significantly enriched GO annotations with an FDR < 5% (Supplementary Table S2 ), “Axon Guidance” (GO:0007411) and “Ligand-gated channel activity” (GO:0022834) emerged as the most prominently enriched biological process and molecular function respectively. Clustering of the GO annotations revealed four distinct clusters related to ion channel activity, plasma membrane adhesion, cardiac conduction, and axonogenesis (Fig. 4 ). Taken together, these findings suggest a potential genetic predisposition in these specific functional areas that may contribute to BNT162b2 vaccine-induced myocarditis.
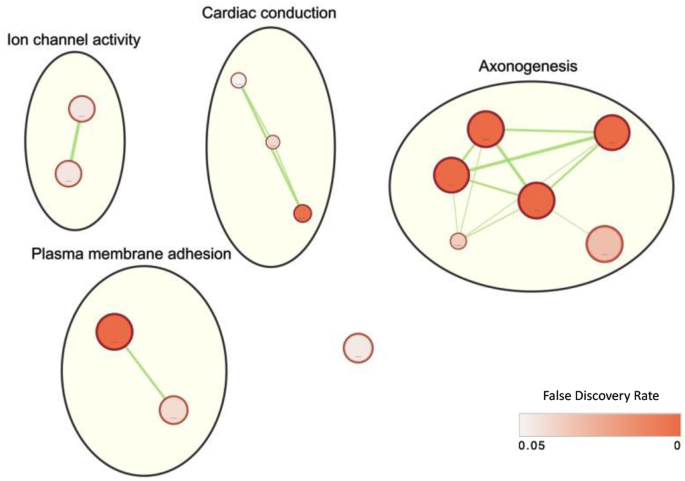
Visualization of gene set enrichment analysis results generated by Cytoscape, using g:Profiler for prioritized genes. Shades of red indicate false discovery rates, calculated based on g:SCS (Set Counts and Sizes, PMID: 17478515). Pathway clustering was carried out with AutoAnnotate, an app in Cytoscape, using the default settings
To the best of our knowledge, this is the first genomic study examining the genetic factors associated with the BNT162b2 vaccinated myocarditis in the largest patient cohort reported. Several epidemiological observations were widely accepted as risk factors associated with the vaccine-related myocarditis. Notable differences were observed in the incidence rate of vaccine-related myocarditis across various age, gender and ethnic group, the underlying reasons were also not fully understood [ 9 ]. In addition, long term complications of vaccine-related myocarditis are still not clearly defined. However, impairment of left ventricular and right ventricular myocardial deformation and persistence of late gadolinium enhancement was observed in certain subset of patients up to 1 year after diagnosed with BNT162b2 vaccine-related myocarditis, suggesting the potential long-term effects on the cardiac functions to the affected individuals [ 33 ]. By studying the largest representative Chinese vaccine-related myocarditis cohort, further analysis on the genetic background of the susceptible individuals may offer valuable information to identify vulnerable groups within the community and supplementing the explanations for current observed risk factors in the highest risk groups.
The present study offers a preliminary overview of the genetic factors contributing to BNT162b2 vaccine-related myocarditis in susceptible individuals. Specifically, the 2 prioritized clusters overlapped with BMP3 and LRRC4C which was found to be genes involved in inflammation and immunity. BMP3 codes for an inflammatory protein related to the regulation of the Smad signaling pathway and the release of pro-inflammatory cytokines including IL-6, IL-1β and IL-17A [ 34 ]. In the same functional study, BMP3 inhibition was associated with elevated levels of pro-inflammatory cytokines, suggesting that BMP3 expression may be involved in inflammation process [ 34 ]. Another region (chr11:40,473,809 − 40,585,162) was in the intronic region of Leucine-rich Repeat Containing 4C ( LRRC4C ) gene. LRRC4C functions are not directly involved in inflammation, but primarily in immune-related activity such as migration of monocytes, mast cells and M2 macrophages [ 35 ]. Moreover, the gene set enrichment analysis in this study provided a broader perspective on the genetic involvement in vaccine-related myocarditis. The identified gene sets in cardiac conduction and ion channel activity suggesting the pre-disposed cardiac functions were also a risk factor in developing the vaccine side effect. A dysfunction of cardiac ion channel and elevated high-sensitivity C-reactive proteins (CRP) was observed in a Brugada syndrome patient that presented with ventricular arrhythmias after receiving BNT162b2 mRNA vaccine, supporting the cardiac ion channel activity may be a potential target in developing the vaccine complications in the susceptible individuals [ 36 ]. Apart from that, gene sets related to plasma membrane adhesion revealed an aspect of molecular events that is rarely reported in BNT162b2 vaccine-related myocarditis. The RBD motif in the SARS-CoV-2 spike protein was found eliciting vascular inflammation by binding to the α5β1 integrin in the vascular endothelial cells, in which further demonstrated with systematic production of chemokines and cytokines including TNF-α, IL-1β and IL-6 in the mice model [ 37 ]. The molecular mimicry triggered by the spike protein encoded by the vaccine mRNA could elicit similar events but postulated with a possible stronger response to vascular endothelial cells in susceptible individuals. Further investigations are needed in elucidating the possible involvements of the identified genomic clusters and gene sets in BNT162b2 vaccine-related myocarditis. In addition, axonogensis, a biological process extends beyond the current knowledge of vaccine-related myocarditis, showed the most statistical significance in the enriched pathway analysis. Although the crosstalk between axonogensis and BNT162b2 vaccine-related myocarditis has not yet established, this finding may offer a new approach in investigating the vaccine effects and its associated complications.
Findings of this study need to be interpreted with the following caveats. First, due to the rarity of BNT162b2 vaccine-related myocarditis, the results should be interpreted cautiously as the number of patients included in this study was relatively small, although this study is among the largest cohort of BNT162b2 vaccine-related myocarditis reported among adolescents globally. The fast-response and efficient adverse drug reaction reporting system in COVID-19 vaccination and comprehensive clinical management network allowed us to recruit all the confirmed cases within the study period, establishing a BNT162b2 vaccine-related myocarditis patient cohort for further investigation. Second, the choice of controls may not be perfect for our study since the controls were not exposed to mRNA vaccines. We acknowledge this as a limitation of our study and justify that the use of this control cohort is valid as a population control of allele frequencies. Third, the limited analytical power may affect the accuracy of the associations to vaccine-related myocarditis. It is possible that false positives were included during the early analysis stage or due to sequencing errors, which could have influenced the subsequent interpretations. To address these issues, ClusterAnalyzer was utilized to maximize the sensitivity. The focus was primarily on clusters of signals with similar significance, rather than including single discrete signals, in order to minimize the inclusion of false positives. Last, the grouping of SNPs may be inaccurate due to uncertainties in p-value estimates and LD relationships estimates by ClusterAnalyzer. This could have limited the ability of the tool to exclude false positives or to include true positives. The current tool was unable to prioritize genetic variants in the HLA and KIR loci due to weak LD among variants intrinsic to the hypervariability of these loci. Further modifications should be implemented to enhance the precision and accuracy of the association signals.
In conclusion, this study has presented preliminary genetic factors associated with BNT162b2 vaccine-related myocarditis. The identified 2,182 genomic clusters potentially associated with the side effect. Further analysis in the genes located in the 2 selected clusters, as well as the enriched pathways analysis, not only align with the current understanding of the BNT162b2 vaccine side effect but also shed light on previously undocumented directions. The group of genes related to inflammation, cardiac conduction, and ion channel activity demonstrated the predisposed immunity and cardiac function differences among the susceptible individuals. Additionally, the highlighted enriched gene sets responsible for plasma membrane adhesion and axonogenesis provided new insights into the genetic factors associated with BNT-162b2 vaccine-related myocarditis. As the vaccine platform is poised to become the foundation for future development of immune protection against pathogens and even disease treatment, it is important to consider identifying individuals who may be more susceptible to major sole-effect of the mRNA platform. This would help mitigate any potential risks associated with mRNA COVID-19 vaccine-related myocarditis.
Data availability
Source code of ClusterAnalyzer is available on GitHub under BSD-3 license (http://github.com/snakesch/clusterAnalysis). Summary association statistics of raw association signals and prioritized signals were publicly available in the repository.
Pfizer-BioNTech. COVID-19 Vaccines . [cited 2023 16 Feb]; https://www.fda.gov/emergency-preparedness-and-response/coronavirus-disease-2019-covid-19/pfizer-biontech-covid-19-vaccines .
Baden LR, et al. Efficacy and safety of the mRNA-1273 SARS-CoV-2 vaccine. N Engl J Med. 2021;384(5):403–16.
Article CAS PubMed Google Scholar
Emary KRW, et al. Efficacy of ChAdOx1 nCoV-19 (AZD1222) vaccine against SARS-CoV-2 variant of concern 202012/01 (B.1.1.7): an exploratory analysis of a randomised controlled trial. Lancet. 2021;397(10282):1351–62.
Article CAS PubMed PubMed Central Google Scholar
Greinacher A, et al. Thrombotic Thrombocytopenia after ChAdOx1 nCov-19 vaccination. N Engl J Med. 2021;384(22):2092–101.
Liang I, Swaminathan S, Lee AYS. Emergence of de novo cutaneous vasculitis post coronavirus disease (COVID-19) vaccination. Clin Rheumatol. 2022;41(5):1611–2.
Article PubMed Google Scholar
Al Khames Aga QA, et al. Safety of COVID-19 vaccines. J Med Virol. 2021;93(12):6588–94.
Bautista Garcia J, et al. Acute myocarditis after administration of the BNT162b2 vaccine against COVID-19. Rev Esp Cardiol (Engl Ed). 2021;74(9):812–4.
Marshall M et al. Symptomatic Acute myocarditis in 7 adolescents after Pfizer-BioNTech COVID-19 vaccination. Pediatrics, 2021. 148(3).
Chua GT, et al. Epidemiology of Acute Myocarditis/Pericarditis in Hong Kong adolescents following Comirnaty Vaccination. Clin Infect Dis. 2022;75(4):673–81.
Gargano JW, et al. Use of mRNA COVID-19 vaccine after reports of Myocarditis among Vaccine recipients: update from the Advisory Committee on Immunization Practices - United States, June 2021. MMWR Morb Mortal Wkly Rep. 2021;70(27):977–82.
Pillay J, et al. Incidence, risk factors, natural history, and hypothesised mechanisms of myocarditis and pericarditis following covid-19 vaccination: living evidence syntheses and review. BMJ. 2022;378:e069445.
Oster ME, et al. Myocarditis cases reported after mRNA-Based COVID-19 vaccination in the US from December 2020 to August 2021. JAMA. 2022;327(4):331–40.
Mevorach D, et al. Myocarditis after BNT162b2 mRNA vaccine against Covid-19 in Israel. N Engl J Med. 2021;385(23):2140–9.
Tan JTC, et al. Adverse reactions and safety profile of the mRNA COVID-19 vaccines among Asian military personnel. Ann Acad Med Singap. 2021;50(11):827–37.
Diaz GA, et al. Myocarditis and Pericarditis after Vaccination for COVID-19. JAMA. 2021;326(12):1210–2.
Dickey JB, et al. A series of patients with myocarditis following SARS-CoV-2 Vaccination with mRNA-1279 and BNT162b2. JACC Cardiovasc Imaging. 2021;14(9):1862–3.
Article PubMed PubMed Central Google Scholar
Lamb YN. BNT162b2 mRNA COVID-19 vaccine: first approval. Drugs. 2021;81(4):495–501.
Munjal JS, et al. Covid- 19 vaccine-induced myocarditis. J Community Hosp Intern Med Perspect. 2023;13(5):44–9.
PubMed PubMed Central Google Scholar
Aharon A, et al. HLA binding-groove motifs are associated with myocarditis induction after Pfizer-BioNTech BNT162b2 vaccination. Eur J Clin Invest. 2024;54(4):e14142.
Tsang HW, et al. The central role of natural killer cells in mediating acute myocarditis after mRNA COVID-19 vaccination. Med. 2024;5(4):335–e3473.
Nishibayashi H, et al. Myocarditis in 13-Year-old Monochorionic Diamniotic Twins after COVID-19 vaccination. J Clin Immunol. 2022;42(7):1351–3.
Tsang HW et al. The central role of natural killer cells in mediating acute myocarditis after mRNA COVID-19 vaccination. Med, 2024.
Sexson Tejtel SK, et al. Myocarditis and pericarditis: case definition and guidelines for data collection, analysis, and presentation of immunization safety data. Vaccine. 2022;40(10):1499–511.
Cao Y, et al. NGS4THAL, a one-stop molecular diagnosis and Carrier Screening Tool for Thalassemia and other Hemoglobinopathies by Next-Generation sequencing. J Mol Diagn. 2022;24(10):1089–99.
Krueger F. Trim Galore! https://www.bioinformatics.babraham.ac.uk/projects/trim_galore/ .
Li H. Aligning sequence reads, clone sequences and assembly contigs with BWA-MEM . 2013, arXiv.
Yun T, et al. Accurate, scalable cohort variant calls using DeepVariant and GLnexus. Bioinformatics. 2021;36(24):5582–9.
Chang CC, et al. Second-generation PLINK: rising to the challenge of larger and richer datasets. Gigascience. 2015;4:7.
Dale RK, Pedersen BS, Quinlan AR. Pybedtools: a flexible Python library for manipulating genomic datasets and annotations. Bioinformatics. 2011;27(24):3423–4.
Kolberg L, et al. G:profiler—interoperable web service for functional enrichment analysis and gene identifier mapping (2023 update). Nucleic Acids Res. 2023;51(W1):W207–12.
Reimand J, et al. Pathway enrichment analysis and visualization of omics data using g:Profiler, GSEA, Cytoscape and EnrichmentMap. Nat Protoc. 2019;14(2):482–517.
Shannon P, et al. Cytoscape: a software environment for integrated models of biomolecular interaction networks. Genome Res. 2003;13(11):2498–504.
Yu CK, et al. Cardiovascular Assessment up to one year after COVID-19 Vaccine-Associated Myocarditis. Circulation. 2023;148(5):436–9.
Song B, et al. Inhibition of BMP3 increases the inflammatory response of fibroblast-like synoviocytes in rheumatoid arthritis. Aging. 2020;12(12):12305–23.
Yang X, et al. Prognostic value of LRRC4C in Colon and gastric cancers correlates with Tumour Microenvironment Immunity. Int J Biol Sci. 2021;17(5):1413–27.
Lim KH, Park J-S. COVID-19 Vaccination-Induced Ventricular Fibrillation in an Afebrile Patient with Brugada Syndrome. jkms. 2022;37(42):e306–0.
Robles JP, et al. The spike protein of SARS-CoV-2 induces endothelial inflammation through integrin α5β1 and NF-κB signaling. J Biol Chem. 2022;298(3):101695.
Download references
This work was supported by the Hong Kong Collaborative Research Fund (CRF) 2020/21 and the CRF Coronavirus and Novel Infectious Diseases Research Exercises (Reference Number: C7149-20G). The funding sources were not involved in the study design, data collection, analysis and interpretation, writing of the manuscripts, and the decision to submit the manuscript for publication.
Author information
Chun Hing She and Hing Wai Tsang contributed equally to this work.
Authors and Affiliations
Department of Paediatrics and Adolescent Medicine, Li Ka Shing Faculty of Medicine, The University of Hong Kong, Pokfulam, Hong Kong SAR, China
Chun Hing She, Hing Wai Tsang, Xingtian Yang, Sabrina SL Tsao, Sophelia HS Chan, Mike YW Kwan, Gilbert T Chua, Wanling Yang & Patrick Ip
Department of Surgery, Li Ka Shing Faculty of Medicine, The University of Hong Kong, Pokfulam, Hong Kong SAR, China
Clara SM Tang
You can also search for this author in PubMed Google Scholar
Contributions
The project was conceptualized by PI, GTC and HWT. The samples were contributed by GTC and prepared by HWT. CSMT contributed the control data. Design, execution and interpretation of genetic analysis results was conducted by CHS, WY and XY. Manuscript was drafted by CHS, HWT and GTC. PI and WY provided valuable comments to the study. ST acquired the relevant ethics approvals. SC and MK supported the analysis of the study.
Corresponding authors
Correspondence to Wanling Yang or Patrick Ip .
Ethics declarations
Ethical approval.
The study was approved by the Institutional Review Board of the University of Hong Kong/Hospital Authority Hong Kong West Cluster (Reference: UW20-292, UW21-149, UW21-157 and UW21-548), the Kowloon West Cluster Research Ethics Committee [Reference: KW/FR-20-086(148 − 10)] and the Department of Health Ethics Committee (Reference: LM21/2021). Written consent was obtained from parents or legal guardians of the participants.
Consent for publication
Not applicable.
Conflict of interest
The authors declare that the research was conducted in the absence of any commercial or financial relationships that could be construed as a potential conflict of interest.
Additional information
Publisher’s note.
Springer Nature remains neutral with regard to jurisdictional claims in published maps and institutional affiliations.
Electronic supplementary material
Below is the link to the electronic supplementary material.

Supplementary Material 1

Supplementary Material 2

Supplementary Material 3
Supplementary material 4, rights and permissions.
Open Access This article is licensed under a Creative Commons Attribution 4.0 International License, which permits use, sharing, adaptation, distribution and reproduction in any medium or format, as long as you give appropriate credit to the original author(s) and the source, provide a link to the Creative Commons licence, and indicate if changes were made. The images or other third party material in this article are included in the article’s Creative Commons licence, unless indicated otherwise in a credit line to the material. If material is not included in the article’s Creative Commons licence and your intended use is not permitted by statutory regulation or exceeds the permitted use, you will need to obtain permission directly from the copyright holder. To view a copy of this licence, visit http://creativecommons.org/licenses/by/4.0/ . The Creative Commons Public Domain Dedication waiver ( http://creativecommons.org/publicdomain/zero/1.0/ ) applies to the data made available in this article, unless otherwise stated in a credit line to the data.
Reprints and permissions
About this article
Cite this article.
She, C.H., Tsang, H.W., Yang, X. et al. Genome-wide association study of BNT162b2 vaccine-related myocarditis identifies potential predisposing functional areas in Hong Kong adolescents. BMC Genom Data 25 , 51 (2024). https://doi.org/10.1186/s12863-024-01238-6
Download citation
Received : 12 April 2024
Accepted : 27 May 2024
Published : 06 June 2024
DOI : https://doi.org/10.1186/s12863-024-01238-6
Share this article
Anyone you share the following link with will be able to read this content:
Sorry, a shareable link is not currently available for this article.
Provided by the Springer Nature SharedIt content-sharing initiative
- Vaccine-induced myocarditis
- BNT162b2 vaccine
- Genetic risk predisposition
- Vaccine side effect
BMC Genomic Data
ISSN: 2730-6844
- General enquiries: [email protected]
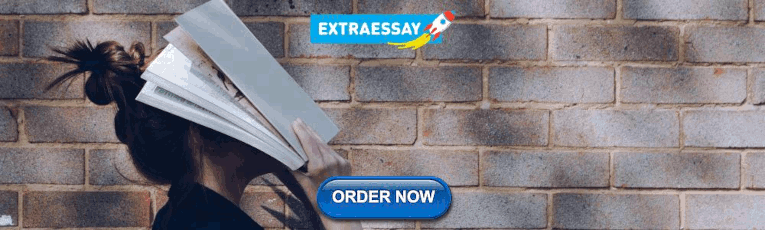
IMAGES
VIDEO
COMMENTS
Genomic data science emerged as a field in the 1990s to bring together two laboratory activities: Experimentation: Generating genomic information from studying the genomes of living organisms. Data analysis: Using statistical and computational tools to analyze and visualize genomic data, which includes processing and storing data and using ...
In the current biotechnology and medicine era, genomic data analysis is the compass guiding researchers through our genetic code. This comprehensive guide aims to explain genomic data analysis along with Python and R coding, breaking down each step into digestible portions.. Before diving into the nitty-gritty of genomic data analysis, let's grasp the concept of genomic data, its formats and ...
Advances in genomics have triggered fundamental changes in medicine and research. Genomic datasets are driving the next generation of discovery and treatment, and this series will enable you to analyze and interpret data generated by modern genomics technology. ... Bridge diverse genomic assay and annotation structures to data analysis and ...
This review aims to guide researchers in human genetics to process and analyze these large-scale genomic data to extract relevant information for improved downstream analyses in their specific ...
The Genomics Data Analysis XSeries is an advanced series that will enable students to analyze and interpret data generated by modern genomics technology. Using open-source software, including R and Bioconductor, you will acquire skills to analyze and interpret genomic data. This XSeries is perfect for those who seek advanced training in high ...
Given that the size of the project's phenotypic and genomic dataset is expected to reach 4.75 PB in 2023, the use of a central data store and cloud analysis tools will save funders an estimated ...
The Genomic Data Commons repository contains genomic, epigenomic, proteomic and clinical data from the TCGA and TARGET datasets. Here, the authors describe the analysis methods for how these ...
Transform Sequencing Data into Actionable Genetic Insights. The Computational Genome Analysis Platform (CGAP) is an intuitive, open-source analysis tool designed to support complex research & clinical genomics workflows.
The potential of transformer-based architectures and attention mechanisms in genome data analysis is vast and largely unexplored. They present a promising solution to tackle the massive scale and intricate nature of genomic data. The ability to capture long-range dependencies between genomic positions, consider multiple relevant genomic regions
Genomic Data Science is the field that applies statistics and data science to the genome. This Specialization covers the concepts and tools to understand, analyze, and interpret data from next generation sequencing experiments. It teaches the most common tools used in genomic data science including how to use the command line, along with a ...
Data science allows the extraction of practical insights from large-scale data. Here, we contextualize it as an umbrella term, encompassing several disparate subdomains. We focus on how genomics fits as a specific application subdomain, in terms of well-known 3 V data and 4 M process frameworks (volume-velocity-variety and measurement-mining-modeling-manipulation, respectively). We further ...
Softcover Book USD 99.99. Price excludes VAT (USA) Compact, lightweight edition. Dispatched in 3 to 5 business days. Free shipping worldwide - see info. Buy Softcover Book. Tax calculation will be finalised at checkout. This textbook describes recent advances in genomics and bioinformatics and provides numerous examples of genome data analysis ...
The value of genomic analysis. Genetic heritability is responsible for 30% of individual health outcomes, but is hardly used to guide disease prevention and care. Each individual carries 4-5 million genetic variants, each with varying influence on traits related to our health. The cost to sequence a genome has reduced drastically in recent ...
Objective. This study aims to provide an outline of the use of genomic data alongside routinely collected data in health research to date. As this field prepares to move forward, it is important to take stock of the current state of play in order to highlight new avenues for development, identify challenges, and ensure that adequate data governance models are in place for safe and socially ...
SEQUENCING PIPELINE. The sequencing pipeline is a three‐step process: sample and library preparation, sequencing, followed by data analysis and bioinformatics. Above described the second step—sequencing. This section will describe the process of steps one and three. 3.1. Sample and library preparation.
Bioinformatics and Big Data Analyt ics in Genomic Research. Qaiser asad. Department of health science, university of Public Health, Gujrat, India. Abstract: The field of genomics has witnessed a ...
The nature of genomic data. DNA has phenomenal storage potential by virtue of its compactness, stability, and quaternary code. Sequencing DNA to create computer files amenable to analysis therefore generates enormous volumes of data - one sequenced human genome takes around 200 gigabytes of storage (Citation 100, Citation 000 Genomes Project Citation 2018).
CCG's Genomic Data Analysis Network (GDAN) is a collaborative team that develops and applies computational analysis methods to large-scale datasets. The GDAN's goal is to help the research community leverage the genomic data produced by NCI and other programs.
Genomic analysis is the identification, measurement or comparison of genomic features such as DNA sequence, structural variation, gene expression, or regulatory and functional element annotation ...
Research team hopeful that the method, called IRIS, can provide more detailed information for precision health treatment plans and health outcomes. ... will serve as a powerful tool for large-scale multi-sample spatial transcriptomics data analysis across a wide range of biological systems." In the study, the researchers applied IRIS to six ...
Single-Cell Genomics and Regulatory Networks for 388 Human Brains. Yale researchers who conducted the largest human brain analysis from a single-cell perspective hope their findings lead to better prediction of medicine that will target certain cells. The study was led by co-corresponding authors Matthew Girgenti, PhD, assistant professor of ...
Hepatocellular carcinoma (HCC) genomic research has discovered actionable genetic changes that might guide treatment decisions and clinical trials. Nonetheless, due to a lack of large-scale multicenter clinical validation, these putative targets have not been converted into patient survival advantages. So, it's crucial to ascertain whether genetic analysis is clinically feasible, useful, and ...
Whole-genome duplication (WGD) events, gene family expansion, and contraction occurring in Pueraria plants were explored through comparative genomics analysis. Whole-genome sequencing of 121 Pueraria accessions was used to address the evolutionary relationship among the species and identify selective signatures during domestication in P ...
Weeds are attractive models for basic and applied research due to their impacts on agricultural systems and capacity to swiftly adapt in response to anthropogenic selection pressures. Currently, a lack of genomic information precludes research to elucidate the genetic basis of rapid adaptation for important traits like herbicide resistance and stress tolerance and the effect of evolutionary ...
Database of planctomycetotal genomes. In this study, we investigated the metabolic potential of Planctomycetota for polysaccharide degradation. We tried to identify the primary trends across Planctomycetota by concentrating the analysis on the class and order taxonomic levels (Fig. 1).We argue that lower than phylum taxonomic level genomic comparisons provide a more nuanced and detailed ...
Background Like all other species, fungi are susceptible to infection by viruses. The diversity of fungal viruses has been rapidly expanding in recent years due to the availability of advanced sequencing technologies. However, compared to other virome studies, the research on fungi-associated viruses remains limited. Results In this study, we downloaded and analyzed over 200 public datasets ...
This research provided a basis for further breeding improvements in Xia'nan cattle and served as a reference for genetic enhancements in other crossbreed cattle. ... This study offers a thorough insight into genomic variations of Xia'nan cattle through Whole Genome Sequencing (WGS) data analysis. The exploration of population structure and ...
This Review discusses the role of cloud computing in genomics research to facilitate data sharing and new analyses of archived sequencing data, as well as large-scale international collaborations ...
Vaccine-related myocarditis associated with the BNT162b2 vaccine is a rare complication, with a higher risk observed in male adolescents. However, the contribution of genetic factors to this condition remains uncertain. In this study, we conducted a comprehensive genetic association analysis in a cohort of 43 Hong Kong Chinese adolescents who were diagnosed with myocarditis shortly after ...