Accessibility Links
- Skip to content
- Skip to search IOPscience
- Skip to Journals list
- Accessibility help
- Accessibility Help
Click here to close this panel.
Purpose-led Publishing is a coalition of three not-for-profit publishers in the field of physical sciences: AIP Publishing, the American Physical Society and IOP Publishing.
Together, as publishers that will always put purpose above profit, we have defined a set of industry standards that underpin high-quality, ethical scholarly communications.
We are proudly declaring that science is our only shareholder.
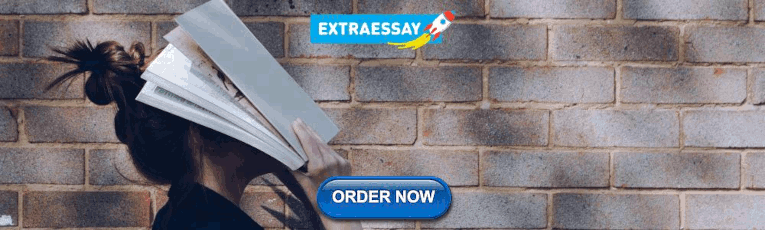
Factors Influencing the Effectiveness of Inventory Management in Manufacturing SMEs
Shiau Wei Chan 1 , R. Tasmin 1 , A. H. Nor Aziati 1 , Raja Zuraidah Rasi 1 , Fadillah Binti Ismail 1 and Li Ping Yaw 1
Published under licence by IOP Publishing Ltd IOP Conference Series: Materials Science and Engineering , Volume 226 , International Research and Innovation Summit (IRIS2017) 6–7 May 2017, Melaka, Malaysia Citation Shiau Wei Chan et al 2017 IOP Conf. Ser.: Mater. Sci. Eng. 226 012024 DOI 10.1088/1757-899X/226/1/012024
Article metrics
35324 Total downloads
Share this article
Author e-mails.
Author affiliations
1 Department of Production and Operation Management, Faculty of Technology Management and Business, Universiti Tun Hussein Onn Malaysia, Batu Pahat, Johor, Malaysia.
Buy this article in print
Effectiveness of inventory management is a vital part in the manufacturing organization to be more competitive. The previous studies have indicated that there are several factors influencing the effectiveness of inventory management in the organization but there is lack of researchers who carried out the research in the manufacturing small medium enterprise in Johor. Therefore, the purpose of this research is to identify the problem of inventory management faced by the manufacturing small medium enterprise and also to determine the factors that will influence the effectiveness of inventory management. In completing this research, 80 employees were selected randomly from the manufacturing small medium enterprise in Batu Pahat, Johor and they were requested to complete questionnaires. The result have shown that the problems of inventory management faced by manufacturing organization were underproduction, overproduction, stock out situation, delays in the delivery of raw materials and discrepancy of records. The factors, documentation/store records, planning, knowledge of employees/staff skill have shown to significantly influence the effectiveness of inventory management while the funds have shown slightly significant influence on the inventory management in manufacturing small medium enterprises. This quantitative study is important to the manufacturing organization in Malaysia because it provides the guidelines to the employers of manufacturing small medium enterprises in Batu Pahat, Johor.
Export citation and abstract BibTeX RIS
Content from this work may be used under the terms of the Creative Commons Attribution 3.0 licence . Any further distribution of this work must maintain attribution to the author(s) and the title of the work, journal citation and DOI.
A detailed Analysis of Use of AI in Inventory Management for technically better management
Ieee account.
- Change Username/Password
- Update Address
Purchase Details
- Payment Options
- Order History
- View Purchased Documents
Profile Information
- Communications Preferences
- Profession and Education
- Technical Interests
- US & Canada: +1 800 678 4333
- Worldwide: +1 732 981 0060
- Contact & Support
- About IEEE Xplore
- Accessibility
- Terms of Use
- Nondiscrimination Policy
- Privacy & Opting Out of Cookies
A not-for-profit organization, IEEE is the world's largest technical professional organization dedicated to advancing technology for the benefit of humanity. © Copyright 2024 IEEE - All rights reserved. Use of this web site signifies your agreement to the terms and conditions.
To read this content please select one of the options below:
Please note you do not have access to teaching notes, a review of inventory management research in major logistics journals: themes and future directions.
The International Journal of Logistics Management
ISSN : 0957-4093
Article publication date: 15 August 2008
The purpose of this paper is to provide a review of inventory management articles published in major logistics outlets, identify themes from the literature and provide future direction for inventory management research to be published in logistics journals.
Design/methodology/approach
Articles published in major logistics articles, beginning in 1976, which contribute to the inventory management literature are reviewed and cataloged. The articles are segmented based on major themes extracted from the literature as well as key assumptions made by the particular inventory management model.
Two major themes are found to emerge from logistics research focused on inventory management. First, logistics researchers have focused considerable attention on integrating traditional logistics decisions, such as transportation and warehousing, with inventory management decisions, using traditional inventory control models. Second, logistics researchers have more recently focused on examining inventory management through collaborative models.
Originality/value
This paper catalogs the inventory management articles published in the major logistics journals, facilitates the awareness and appreciation of such work, and stands to guide future inventory management research by highlighting gaps and unexplored topics in the extant literature.
- Inventory management
- Supply chain management
Williams, B.D. and Tokar, T. (2008), "A review of inventory management research in major logistics journals: Themes and future directions", The International Journal of Logistics Management , Vol. 19 No. 2, pp. 212-232. https://doi.org/10.1108/09574090810895960
Emerald Group Publishing Limited
Copyright © 2008, Emerald Group Publishing Limited
Related articles
We’re listening — tell us what you think, something didn’t work….
Report bugs here
All feedback is valuable
Please share your general feedback
Join us on our journey
Platform update page.
Visit emeraldpublishing.com/platformupdate to discover the latest news and updates
Questions & More Information
Answers to the most commonly asked questions here
Applications of Artificial Intelligence in Inventory Management: A Systematic Review of the Literature
- Review Article
- Published: 07 February 2023
- Volume 30 , pages 2605–2625, ( 2023 )
Cite this article
- Özge Albayrak Ünal ORCID: orcid.org/0000-0001-7798-8799 1 ,
- Burak Erkayman ORCID: orcid.org/0000-0002-9551-2679 1 &
- Bilal Usanmaz ORCID: orcid.org/0000-0003-0531-4618 2
5782 Accesses
8 Citations
Explore all metrics
Today, companies that want to keep up with technological development and globalization must be able to effectively manage their supply chains to achieve high quality, increased efficiency, and low costs. Diversified customer needs, global competitors, and market competition have led companies to pay more attention to inventory management. This article provides a comprehensive and up-to-date review of Artificial Intelligence (AI) applications used in inventory management through a systematic literature review. As a result of this analysis, which focused on research articles in two scientific databases published between 2012 and 2022 for detailed study, 59 articles were identified. Furthermore, the current situation is summarized and possible future aspects of inventory management are identified. The results show that the interest in AI methods has increased in recent years and machine learning algorithms are the most commonly used methods. This study is meticulously and comprehensively conducted so it will probably make significant contributions to the further studies in this field.
This is a preview of subscription content, log in via an institution to check access.
Access this article
Price includes VAT (Russian Federation)
Instant access to the full article PDF.
Rent this article via DeepDyve
Institutional subscriptions
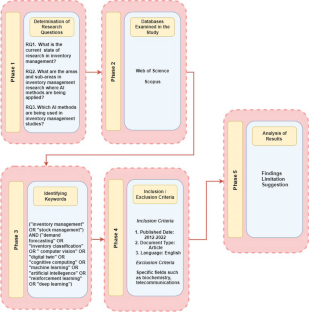
Similar content being viewed by others
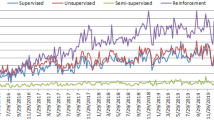
Machine Learning: Algorithms, Real-World Applications and Research Directions
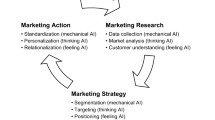
A strategic framework for artificial intelligence in marketing
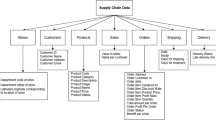
Predictive big data analytics for supply chain demand forecasting: methods, applications, and research opportunities
Perez HD et al (2021) Algorithmic approaches to inventory management optimization. Processes 9(1):102
Article Google Scholar
Singh D, Verma A (2018) Inventory management in supply chain. Mater Today 5(2):3867–3872
MathSciNet Google Scholar
Haberleitner H, Meyr H, Taudes A (2010) Implementation of a demand planning system using advance order information. Int J Prod Econ 128(2):518–526
Abbasimehr H, Shabani M, Yousefi M (2020) An optimized model using LSTM network for demand forecasting. Comput Ind Eng 143:106435
Beutel A-L, Minner S (2012) Safety stock planning under causal demand forecasting. Int J Prod Econ 140(2):637–645
Sridhar P, Vishnu C, Sridharan R (2021) Simulation of inventory management systems in retail stores: a case study. Mater Today 47:5130–5134
Google Scholar
Granillo-Macías R (2020) Inventory management and logistics optimization: a data mining practical approach. LogForum 16(4):535–547
Nallusamy S (2021) Performance measurement on inventory management and logistics through various forecasting techniques. Int J Perform Eng 17(2):216–228
Acosta ICG et al (2018) Design of an inventory management system in an agricultural supply chain considering the deterioration of the product: the case of small citrus producers in a developing country. J Appl Eng Sci 16(4):523–537
Varghese V et al (2012) Applying actual usage inventory management best practice in a health care supply chain. Int J Supply Chain Manage 1(2):1–10
Xie C, Wang L, Yang C (2021) Robust inventory management with multiple supply sources. Eur J Oper Res 295(2):463–474
Article MathSciNet MATH Google Scholar
Tranfield D, Denyer D, Smart P (2003) Towards a methodology for developing evidence-informed management knowledge by means of systematic review. Br J Manag 14(3):207–222
Evangelista P, Durst S (2015) Knowledge management in environmental sustainability practices of third-party logistics service providers. Vine 45(4):509–529
Bryman A (2007) The research question in social research: what is its role? Int J Soc Res Methodol 10(1):5–20
Nguyen KTP, Medjaher K (2019) A new dynamic predictive maintenance framework using deep learning for failure prognostics. Reliab Eng Syst Saf 188:251–262
Tabernik D, Skocaj D (2020) Deep learning for large-scale traffic-sign detection and recognition. IEEE Trans Intell Transp Syst 21(4):1427–1440
Mohammaditabar D, Ghodsypour SH, O’Brien C (2012) Inventory control system design by integrating inventory classification and policy selection. Int J Prod Econ 140(2):655–659
Liu JP et al (2016) A classification approach based on the outranking model for multiple criteria ABC analysis. Omega-International J Manage Sci 61:19–34
Kartal H et al (2016) An integrated decision analytic framework of machine learning with multi-criteria decision making for multi-attribute inventory classification. Comput Ind Eng 101:599–613
Aggarwal SC (1974) A review of current inventory theory and its applications. Int J Prod Res 12(4):443–482
Giannoccaro I, Pontrandolfo P (2002) Inventory management in supply chains: a reinforcement learning approach. Int J Prod Econ 78(2):153–161
Cachon GP, Fisher M (2000) Supply chain inventory management and the value of shared information. Manage Sci 46(8):1032–1048
Article MATH Google Scholar
Partovi FY, Anandarajan M (2002) Classifying inventory using an artificial neural network approach. Comput Ind Eng 41(4):389–404
Giannoccaro I, Pontrandolfo P, Scozzi B (2003) A fuzzy echelon approach for inventory management in supply chains. Eur J Oper Res 149(1):185–196
Yang L, Li H, Campbell JF (2020) Improving order fulfillment performance through integrated inventory management in a multi-item finished goods system. J Bus Logistics 41(1):54–66
Wang Z, Mersereau AJ (2017) Bayesian inventory management with potential change-points in demand. Prod Oper Manage 26(2):341–359
Calle M et al (2016) Integrated management of inventory and production systems based on floating decoupling point and real-time information: a simulation based analysis. Int J Prod Econ 181:48–57
KP ASR, Nayak N (2017) A study on the effectiveness of inventory management and control system in a milk producer organisation. Int J Logistics Syst Manage 28(2):253–266
Rana R, Oliveira FS (2015) Dynamic pricing policies for interdependent perishable products or services using reinforcement learning. Expert Syst Appl 42(1):426–436
Pirayesh Neghab D, Khayyati S, Karaesmen F (2022) An integrated data-driven method using deep learning for a newsvendor problem with unobservable features. Eur J Operational Res 302(2):482–496
Bandaru S et al (2015) Generalized higher-level automated innovization with application to inventory management. Eur J Oper Res 243(2):480–496
Kara A, Dogan I (2018) Reinforcement learning approaches for specifying ordering policies of perishable inventory systems. Expert Syst Appl 91:150–158
Zwaida TA, Pham C, Beauregard Y (2021) Optimization of inventory management to prevent drug shortages in the hospital supply chain. Appl Sci 11(6):2726
Katanyukul T (2014) Ruminative reinforcement learning: improve intelligent inventory control by ruminating on the past. J Comput 9(7):1530–1535
De Moor BJ, Gijsbrechts J, Boute RN (2021) Reward shaping to improve the performance of deep reinforcement learning in perishable inventory management. Eur J Operational Res 301(2):535–545
Boute RN et al (2021) Deep reinforcement learning for inventory control: a roadmap. Eur J Operational Res 298(2):401–412
Gijsbrechts J et al (2021) Can deep reinforcement learning improve inventory management? Performance on lost sales, dual-sourcing, and multi-echelon problems. M SOM-Manuf Serv Operations Manag. https://doi.org/10.1287/msom.2021.1064
Kegenbekov Z, Jackson I (2021) Adaptive supply chain: demand–supply synchronization using deep reinforcement learning. Algorithms 14(8):240
Meisheri H et al (2022) Scalable multi-product inventory control with lead time constraints using reinforcement learning. Neural Comput Appl 34(3):1735–1757
Priore P et al (2019) Applying machine learning to the dynamic selection of replenishment policies in fast-changing supply chain environments. Int J Prod Res 57(11):3663–3677
Demey YT, Wolff M (2017) SIMISS: a model-based searching strategy for inventory management systems. IEEE Internet Things J 4(1):172–182
Merrad Y et al (2020) A real-time mobile notification system for inventory stock out detection using SIFT and RANSAC. Int J Interactive Mobile Technol. https://doi.org/10.3991/ijim.v14i05.13315
Kalinov I et al (2020) WareVision: CNN barcode detection-based uav trajectory optimization for autonomous warehouse stocktaking. IEEE Rob Autom Lett 5(4):6647–6653
Giaconia C, Chamas A (2022) GAIA: great-distribution artificial intelligence-based algorithm for advanced large-scale commercial store management. Appl Sci-Basel 12(9):4798
Kosanoglu F, Atmis M, Turan HH (2022) A deep reinforcement learning assisted simulated annealing algorithm for a maintenance planning problem. Annals of Operations Research 15:1–32
Deutsch J, He D (2017) Using deep learning-based approach to predict remaining useful life of rotating components. IEEE Trans Syst Man Cybernetics: Syst 48(1):11–20
Tao F et al (2019) Digital twin-driven product design framework. Int J Prod Res 57(12):3935–3953
Fuller A et al (2020) Digital twin: enabling technologies, challenges and open research. IEEE Access 8:108952–108971
Abosuliman SS, Almagrabi AO (2021) Computer vision assisted human computer interaction for logistics management using deep learning. Comput Electr Eng 96:107555
Mao J et al (2020) The importance of public support in the implementation of green transportation in the smart cities. Computational Intell. https://doi.org/10.1111/coin.12326
Tian X, Wang H, Erjiang E (2021) Forecasting intermittent demand for inventory management by retailers: a new approach. J Retailing Consumer Serv 62:102662
do Rego JR, De Mesquita MA (2015) Demand forecasting and inventory control: a simulation study on automotive spare parts. Int J Prod Econ 161:1–16
Tangtisanon P (2018) Web service based food additive inventory management with forecasting system. in 2018 3rd International Conference on Computer and Communication Systems (ICCCS). IEEE
Yu Q et al (2017) Application of long short-term memory neural network to sales forecasting in retail—a case study. in International Workshop of Advanced Manufacturing and Automation. Springer
Kumar A, Shankar R, Aljohani NR (2020) A big data driven framework for demand-driven forecasting with effects of marketing-mix variables. Ind Mark Manage 90:493–507
Seyedan M, Mafakheri F, Wang C (2022) Cluster-based demand forecasting using bayesian model averaging: an ensemble learning approach. Decis Anal J 3:100033
Feizabadi J (2022) Machine learning demand forecasting and supply chain performance. Int J Logistics Res Appl 25(2):119–142
Deng CN, Liu YJ (2021) A deep learning-based inventory management and demand prediction optimization method for anomaly detection. Wirel Commun Mobile Comput. https://doi.org/10.1155/2021/9969357
Kack M, Freitag M (2021) Forecasting of customer demands for production planning by local k-nearest neighbor models. Int J Prod Econ 231:107837
Bala PK (2012) Improving inventory performance with clustering based demand forecasts. J Modelling Manage 7(1):23–37
Lee CY, Liang CL (2018) Manufacturer’s printing forecast, reprinting decision, and contract design in the educational publishing industry. Comput Ind Eng 125:678–687
Abbasi B et al (2020) Predicting solutions of large-scale optimization problems via machine learning: a case study in blood supply chain management. Comput Operations Res 119:104941
van Steenbergen RM, Mes MRK (2020) Forecasting demand profiles of new products. Decis Support Syst 139:113401
Zhu XD et al (2021) Demand forecasting with supply-chain information and machine learning: evidence in the Pharmaceutical Industry. Prod Oper Manage 30(9):3231–3252
Benhamida FZ et al (2021) Demand forecasting tool for inventory control smart systemsy. J Commun Softw Syst 17(2):185–196
Zhang P et al (2021) Pharmaceutical cold chain management based on blockchain and deep learning. J Internet Technol 22(7):1531–1542
Article MathSciNet Google Scholar
Ulrich M et al (2021) Distributional regression for demand forecasting in e-grocery. Eur J Oper Res 294(3):831–842
Li N et al (2021) A decision integration strategy for short-term demand forecasting and ordering for red blood cell components. Oper Res Health Care 29:100290
Ran H (2021) Construction and optimization of inventory management system via cloud-edge collaborative computing in supply chain environment in the internet of things era. PLoS ONE 16:e0259284
Sun X et al (2021) RBC inventory-management system based on XGBoost model. Indian J Hematol Blood Transfus 37(1):126–133
Aktepe A, Yanik E, Ersoz S (2021) Demand forecasting application with regression and artificial intelligence methods in a construction machinery company. J Intell Manuf 32(6):1587–1604
Galli L et al (2021) Prescriptive analytics for inventory management in health. J Oper Res Soc 72(10):2211–2224
Eljaouhari A et al (2022) Demand forecasting application with regression and iot based inventory management system: a case study of a semiconductor manufacturing company. Int J Eng Res Afr 60:189–210
Sucharitha RS, Lee S (2022) GMM clustering for in-depth food accessibility pattern exploration and prediction model of food demand behavior. Socio-Economic Plan Sci. https://doi.org/10.48550/arXiv.2202.01347
Wang Z et al (2022) Development and validation of a machine learning method to predict intraoperative red blood cell transfusions in cardiothoracic surgery. Sci Rep 12(1):1–9
Ulrich M et al (2022) Classification-based model selection in retail demand forecasting. Int J Forecast 38(1):209–223
Ji S et al (2019) An application of a three-stage XGboost-based model to sales forecasting of a cross-border e-commerce enterprise. Math Probl Eng. https://doi.org/10.1155/2019/8503252
Wang S, Yang Y (2021) M-GAN-XGBOOST model for sales prediction and precision marketing strategy making of each product in online stores. Data Technol Appl 55(5):749–770
Kim M et al (2022) Framework of 2D KDE and LSTM-based forecasting for cost-effective inventory management in smart manufacturing. Appl Sci-Basel 12(5):2380
Ntakolia C et al (2021) An explainable machine learning model for material backorder prediction in inventory management. Sensors 21(23):7926
Islam S, Amin SH (2020) Prediction of probable backorder scenarios in the supply chain using distributed random forest and gradient boosting machine learning techniques. J Big Data 7(1):1–22
O’Neil S et al (2016) Newsvendor problems with demand shocks and unknown demand distributions. Decis Sci 47(1):125–156
Lee CKM et al (2017) Design and development of inventory knowledge discovery system. Enterp Inform Syst 11(8):1262–1282
Van Belle J, Guns T, Verbeke W (2021) Using shared sell-through data to forecast wholesaler demand in multi-echelon supply chains. Eur J Oper Res 288(2):466–479
Tang Y-M et al (2022) Integrated smart warehouse and manufacturing management with demand forecasting in small-scale cyclical industries. Machines 10(6):472
Ecemiş O, Irmak S (2018) Paslanmaz çelik sektörü satış tahmininde veri madenciliği yöntemlerinin karşılaştırılması. Kilis 7 Aralık Üniversitesi Sosyal Bilimler Dergisi. 8:148–16915
Aktepe A et al (2018) An inventory classification approach combining expert systems, clustering, and fuzzy logic with the abc method, and an application. S Afr J Ind Eng 29(1):49–62
Huang B, Gan W, Li Z (2021) Application of medical material inventory model under deep learning in supply planning of public emergency. IEEE Access 9:44128–44138
Wang A, Gao XD (2021) A variable-scale dynamic clustering method. Comput Commun 171:163–172
Kaabi H, Jabeur K, Ladhari T (2018) A genetic algorithm-based classification approach for multicriteria ABC analysis. Int J Inform Technol Decis Mak 17(6):1805–1837
Maathavan KSK, Venkatraman S (2022) A secure encrypted classified electronic healthcare data for public cloud environment. Intell Autom Soft Comput 32(2):765–779
García-Barrios D et al (2021) A machine learning based method for managing multiple impulse purchase products: an inventory management approach. J Eng Sci Technol Rev 14(1):25–37
Yang K et al (2021) Multi-criteria spare parts classification using the deep convolutional neural network method. Appl Sci 11(15):7088
Zhang S et al (2020) Importance degree evaluation of spare parts based on clustering algorithm and back-propagation neural network. Math Problems Eng. https://doi.org/10.1155/2020/6161825
Balali V, Ashouri Rad A, Golparvar-Fard M (2015) Detection, classification, and mapping of U.S. traffic signs using google street view images for roadway inventory management. Vis Eng 3(1):1–18
Balali V, Golparvar-Fard M (2016) Evaluation of multiclass traffic sign detection and classification methods for us roadway asset inventory management. J Comput Civil Eng 30(2):04015022
Van Eck NJ et al (2010) A comparison of two techniques for bibliometric mapping: multidimensional scaling and VOS. J Am Soc Inform Sci Technol 61(12):2405–2416
Breiman L (2001) Random forests. Mach Learn 45(1):5–32
Bai J et al (2022) Multinomial random forest. Pattern Recogn 122:108331
Liu Y, Wang Y, Zhang J (2012) New machine learning algorithm: Random forest. International Conference on Information Computing and Applications. Springer, Berlin
Hong JS, Lie CH (1993) Joint reliability-importance of two edges in an undirected network. IEEE Trans Reliab 42(1):17–23
Ma M et al (2021) XGBoost-based method for flash flood risk assessment. J Hydrol 598:126382
Jawad J, Hawari AH, Zaidi SJ (2021) Artificial neural network modeling of wastewater treatment and desalination using membrane processes: a review. Chem Eng J 419:129540
Download references
This research did not receive any specific grant from funding agencies in the public, commercial, or not-for-profit sectors.
Author information
Authors and affiliations.
Department of Industrial Engineering, Engineering Faculty, Ataturk University, 25240, Erzurum, Turkey
Özge Albayrak Ünal & Burak Erkayman
Department of Computer Engineering, Engineering Faculty, Ataturk University, 25240, Erzurum, Turkey
Bilal Usanmaz
You can also search for this author in PubMed Google Scholar
Corresponding author
Correspondence to Burak Erkayman .
Additional information
Publisher’s note.
Springer Nature remains neutral with regard to jurisdictional claims in published maps and institutional affiliations.
Full names of the algorithms used in the study.
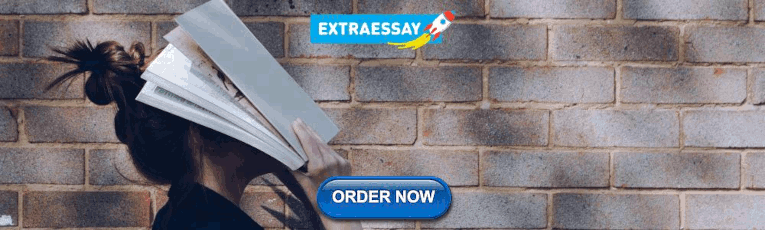
Rights and permissions
Springer Nature or its licensor (e.g. a society or other partner) holds exclusive rights to this article under a publishing agreement with the author(s) or other rightsholder(s); author self-archiving of the accepted manuscript version of this article is solely governed by the terms of such publishing agreement and applicable law.
Reprints and permissions
About this article
Albayrak Ünal, Ö., Erkayman, B. & Usanmaz, B. Applications of Artificial Intelligence in Inventory Management: A Systematic Review of the Literature. Arch Computat Methods Eng 30 , 2605–2625 (2023). https://doi.org/10.1007/s11831-022-09879-5
Download citation
Received : 13 August 2022
Accepted : 23 December 2022
Published : 07 February 2023
Issue Date : May 2023
DOI : https://doi.org/10.1007/s11831-022-09879-5
Share this article
Anyone you share the following link with will be able to read this content:
Sorry, a shareable link is not currently available for this article.
Provided by the Springer Nature SharedIt content-sharing initiative
- Inventory Management
- Artificial Intelligence
- Machine Learning
- Deep Learning
- Systematic Literature Review
- Find a journal
- Publish with us
- Track your research
- Search Menu
- Advance Articles
- Author Guidelines
- Submission Site
- Open Access
- Self-Archiving Policy
- Why Submit?
- About Journal of Forestry
- About Society of American Foresters
- Editorial Board
- Advertising & Corporate Services
- Journals Career Network
- Journals on Oxford Academic
- Books on Oxford Academic

Article Contents
Supplementary material, data availability, literature cited.
- < Previous
Characterizing Community Forests in the United States

Current affiliation: University of North Carolina Wilmington, Environmental Science, Wilmington, NC, USA
Current affiliation: US Department of the Interior, Office of Collaborative Action and Dispute Resolution Washington, DC, USA
- Article contents
- Figures & tables
- Supplementary Data
Reem Hajjar, Kathleen McGinley, Susan Charnley, Gregory E Frey, Meredith Hovis, Frederick W Cubbage, John Schelhas, Kailey Kornhauser, Characterizing Community Forests in the United States, Journal of Forestry , Volume 122, Issue 3, May 2024, Pages 273–284, https://doi.org/10.1093/jofore/fvad054
- Permissions Icon Permissions
Research on community forests (CFs), primarily governed and managed by local forest users in the United States, is limited, despite their growth in numbers over the past decade. We conducted a survey to inventory CFs in the United States and better understand their ownership and governance structures, management objectives, benefits, and financing. The ninety-eight CFs in our inventory are on private, public, and tribal lands. They had various ways of soliciting input from, or sharing decision-making authority with, local groups, organizations, and citizens. Recreation and environmental services were the most important management goals, but timber production occurred on more than two-thirds of CFs, contributing to income on many CFs, along with a diversity of other income sources to fund operations. We discuss the difficulties in creating a comprehensive CF inventory and typology given the diversity of models that exist, reflecting local social and environmental conditions and the bottom-up nature of community forestry in the United States.
Study Implications: Despite their small footprint in the United States, community forests are a rapidly developing model of forest ownership, governance, and management that helps protect forestlands and open space and demonstrates how market and nonmarket forest goods and services can be produced for broad and enduring community benefits. This study inventories and characterizes community forests in the United States to increase understanding of this model, its prevalence, and its potential. It provides a baseline of information that serves as a foundation for further exploration and research on the impacts and contributions of community forests.
Over the past few decades, many countries have increasingly promoted community forests (CFs) as a way to conserve forests, enhance rural livelihoods, and recognize the traditional and customary rights of local forest users to access, use, and manage forests ( Hajjar et al. 2021 ; Lund et al. 2018 ). CFs are delineated forest areas where community members have access to natural resources, are engaged in their governance, and receive indirect and direct benefits from their management ( Charnley and Poe 2007 ; McDermott and Schreckenberg 2009 ). Although CFs have existed in many forms across the globe for centuries, these more recent efforts are typically formal, government-sanctioned, and often government-sponsored. A total 14% of the world’s forests, and 28% of forests in low- and middle-income countries, are currently owned or managed by Indigenous peoples and local communities ( Rights and Resources Initiative 2018 ). Internationally, CF initiatives span a broad range of tenure regimes, institutional arrangements, relationships between communities and higher levels of government, activities, and outcomes that have evolved in line with local contexts, conditions, needs, and goals ( Charnley and Poe 2007 ; Hajjar and Molnar 2016 ).
In the United States, CFs have also existed in diverse forms for centuries ( Baker and Kusel 2003 ; McCullough 1995 ), although as elsewhere, formally designated community forests have been increasing in number since the 1990s. This relatively recent trend is likely driven by several factors. First, vertically integrated forest products companies nationwide have been divesting of their industrial timberlands since the late 1980s for economic reasons ( Zhang 2021 ), causing a large-scale shift in timberland ownership from industrial to institutional investors ( Zhang 2021 ). To prevent residential development, maintain access to local forests, conserve forest resources, and keep working forests working to provide economic opportunities for local residents, initiatives to acquire industrial timberland and manage it as CFs have proliferated ( Belsky 2008 ). Second, private family forest owners are aging; the average age of the primary decision-maker over family forestlands is 65 and, for about 20% of these ownerships, 75 or older ( Butler et al. 2021 ). Keeping their family forestland intact for future generations is a top concern for family forest owners ( Butler et al. 2021 ). If their descendants are uninterested or unable to keep this land in the family, community groups or municipalities may wish to acquire it as a CF to prevent its subdivision and fragmentation and provide community benefits.
Third, Indigenous peoples in the United States have regained greater control over ancestral lands, including forestlands, both on and off tribal trust lands over the past several decades ( McGinley et al. 2022 ). Some tribes have acquired forestland through fee simple purchase, including with funding designated for CF creation, and established CFs on those lands ( McGinley et al. 2022 ). Fourth, the 1990s saw a dramatic increase in citizen participation in decision-making about the management of public forestlands ( Baker and Kusel 2003 ; Charnley and Poe 2007 ). This trend has persisted, with community-based organizations, community members, forest collaborative groups, and other stakeholders playing a greater role in management decision-making and collaborative forest stewardship on federal lands ( Davis et al. 2020 ). In some cases, these arrangements may exhibit the characteristics of a CF.
Simultaneously, several programs providing funding for land acquisition to create CFs have arisen in the past two decades ( McGinley et al. 2022 ). Access to funding along with the emergence of supportive policies, organizations providing technical assistance, and practitioner networks have fostered a more favorable environment for CF creation since the 2000s ( Frey et al. forthcoming ). These trends have played out somewhat differently in different locations, but together they have contributed to a nationwide rise in CFs in the United States.
Unlike many other countries around the world with communal property systems, CFs in the United States do not exist as a distinct land tenure or ownership class. CFs have been established on a variety of public, private, and tribal lands and have diverse land tenure arrangements ( McGinley et al. 2022 ). Furthermore, there is no universally accepted definition of a CF in the United States ( Frey et al. forthcoming ). These two facts make studying CFs in the United States, as a distinct form of forest tenure, management, and governance, challenging. Literature on US CFs, most of it published since the 1990s, has primarily been descriptive in nature, relying on limited numbers of case studies to elaborate on the various motivations for creating CFs and the institutional and political context that pushed them forward ( Belsky 2015 ; McCarthy 2006 ); development of mechanisms and institutional arrangements for governance ( Abrams 2023 ; Abrams et al. 2015 ; Belsky and Barton 2018 ); and their potential benefits ( Christoffersen et al. 2008 ; Lyman et al. 2014 ). Belsky (2008) proposed a typology of CFs defined by who owns the CF—Indigenous groups, towns or municipalities, or community-based conservation organizations. A key message of the scientific literature is that a vast diversity of CFs exists in the United States, reflecting the diverse social, economic, and ecological contexts in which they occur.
To our knowledge, no prior research has attempted to document or characterize the full suite of CFs in the United States. Thus, the goals of this paper are to (1) identify, inventory, and characterize CFs in the United States; (2) enhance understanding of their ownership and governance structures, management objectives, and sources of income; (3) extend the discussion of the variability in forms of CFs and build on previous work to refine a CF typology; and (4) problematize how we recognize CFs in the United States (i.e., what is included, what is not, and why).
Defining CFs 1
A common but broad premise of CFs internationally is that place-based communities have some role in determining how local forests are to be managed for community benefit ( Hajjar et al. 2021 ). In the United States, communities associated with CFs are frequently not only place-based but also communities of interest and practice or some combination of these ( McGinley et al. 2022 ), complicating the notion of “community” and “local” (see Brosius et al. [2005 ] for a discussion). For purposes of deciding what to include in this study, we considered the following attributes of CFs, which are prevalent in the literature on US CFs (see Frey et al. forthcoming ): (1) ownership or tenure by a local governmental or nongovernmental organization (NGO) on behalf of the community; (2) communities are substantively involved in forest management and governance; (3) communities have secure rights to access and benefit from the forest; (4) social and economic benefits for local communities are a management priority; and (5) forest conservation values are permanently protected.
Creating a CF Inventory
To catalogue and characterize CFs in the United States, we first undertook an inventory of existing CFs, aiming to be as comprehensive as possible. Given the lack of a consistent definition or model of CFs, we used a hybrid approach to identify them ( Frey et al. forthcoming ). This meant first searching for entities that self-identify their property or initiative as a CF and for those that have participated in programs or policies related to CFs. Then we overlaid a series of inclusion criteria based on the attributes of CFs outlined above. Therefore, to be included in our study, local communities had to have rights of access and use and some form of management responsibility or decision-making authority (beyond consultation) over local forests. Additionally, these forests were managed to promote ecological sustainability and contribute to conservation while creating tangible local community benefits as a management priority.
We began by compiling a list of CFs and related information from a US Endowment for Forestry and Communities study ( Christoffersen et al. 2008 ) and a previous exploratory project ( Hovis et al. 2022 ). We then added to this list, drawing from CF lists provided by organizations that work with and support them, such as the Ford Foundation, the Northwest Community Forest Coalition, the Northern Forest Center, the Trust for Public Lands, the Open Space Institute, and the USDA Forest Service (Forest Service) Community Forest and Open Space Conservation Program. We also used Google Search Engine to identify any additional CFs not already included in our list. Search terms included: state name AND community forest OR community managed forest OR community-based forest OR town forest. We further consulted with various professionals in our networks involved with CFs (e.g., via the Northwest Community Forest Coalition annual meeting) to ensure the comprehensiveness of our list. Finally, we consulted with two project advisory committees that we set up at the start of the funded project under which this research was undertaken: one, a research advisory committee consisting of CF professionals across select government agencies, CF coalitions, and networks; the other, a tribal forestry advisory committee consisting of representatives of tribes with CFs and tribal natural resources networks.
We also used Google Search Engine to record any information on the identified CFs, usually landing on the websites of CF owners or their supporters. This information typically included the group name, forest location, acreage, landowner, governance, management objectives, history, URL, and contact information. Searches and consultations took place between 2019 and 2023, with more CFs identified and added continually as we heard of cases that were missed in our searches or that were being newly created. We examine the limitations of this approach in the Discussion section.
We initially located 136 possible CFs in the United States using these methods. Of these, thirty-two clearly did not meet our criteria, and we were unable to find additional information or contacts for eleven. To the remaining ninety-three CFs that met our inclusion criteria and for which we had contact information, we sent an internet-based survey using Qualtrics. We requested that a CF manager or other person familiar with the CF fill out the survey. The survey included questions about the CF, such as size, forest type, ownership, decision-making, who is involved in day-to-day management, management priorities, rules of access and use, and financing. Although most survey questions were designed to capture objective characteristics of the CF (i.e., size, ownership, etc.), we acknowledge that answers to a question asking about “management priorities” may not reflect the diversity of priorities a community may have for its forests. Rather, we expected that a CF manager responding to the survey would choose priorities that were being explicitly managed for, consistent with their management plan or mission statement.
To increase response rates ( Dillman et al. 2014 ), we followed up by sending reminder emails after 2 and 4 weeks and then through phone calls where phone numbers were available. Following this, for all nonresponses or cases where contact information could not be located, we filled out the survey ourselves to the extent possible using CF websites and other available resources. Not all survey questions had responses readily available from website sources, and so these surveys were not as complete. This resulted in some topic areas having smaller sample sizes, as displayed in the Results section. We also followed this protocol for newly identified CFs throughout the time period of the research (either newly created CFs or CFs discovered through our networks that met our criteria), for a combined total of ninety-eight CFs recorded up to April 2023. Survey responses were tabulated in SPSS, where descriptive statistics (frequencies and crosstabs) were used to show patterns across various CF characteristics.
We refer to three regions in discussing our results based on the Forest Service Resources Planning Act Assessment (RPA) regions: the West, combining the Pacific Coast and Rocky Mountain RPA regions, including CFs in Montana, Idaho, Washington, Oregon, California, and Arizona; the North, which includes CFs found in Maine, Vermont, New Hampshire, Massachusetts, New York, Connecticut, Michigan, and Wisconsin; and the South, which includes CFs in Georgia, North and South Carolina, Virginia, and Puerto Rico.
We collected data on ninety-eight CFs across the United States, constituting the sample used for this study ( SI Table 1 ). The survey response rate was 87% (eighty-five of ninety-eight); for the remaining thirteen survey nonresponses, we gathered information from internet sources. We expect the number of CFs to continue to grow in the coming years: after closing the survey in April 2023, we learned of at least four additional initiatives that were close to acquiring CF lands and nine that were seeking funds to purchase CF land. We believe that ninety-eight is close to the current total number of self-identifying CFs in the United States but acknowledge that it is likely an undercount of actual CFs that meet our inclusion criteria. We discuss the difficulties in accurately capturing all US CFs in the Discussion section. Rather than thinking of our sample as a complete inventory of all US CFs, we consider it sufficient for characterizing different types of CFs in the United States.
Location, Year Established, and Size
The greatest number of CFs per state were found in West Coast states ( figure 1 ; Washington, fourteen CFs; Oregon, twelve; California, nine); northeastern states (Maine, twelve CFs; Vermont, nine; New Hampshire, eight); and the upper midwestern states of Michigan and Wisconsin (five each). Fewer were located in southern states, with a handful spread across Georgia, North and South Carolina, and Virginia. The earliest recorded CFs in our sample were created in the 1930s and 1940s ( figure 2 ), mostly city and county forests in the northwestern United States (Montesano Community Forest, Hood River County Forest, Ashland Forestlands, Arcata Community Forest), and two town forests that self-describe as CFs in the Northeast (Gorham Town Forest, Mendon Town Forest). Most CFs in our sample were created after 2010 when there was a sharp increase in the number of CFs in all regions. This time period corresponded with new legislative support for CFs in some states (e.g., Washington State’s 2011 Community Forest Trust legislation) and at the federal level (e.g., the Forest Service's 2011 Community Forest Program), which have helped tribes, local governments, and nonprofit organizations acquire land at risk of development to establish CFs.

Location of CFs in our database. In this article, we refer to three regions in discussing our results: the West, which includes CFs found in Montana, Idaho, Washington, Oregon, California, and Arizona; the North, which includes Maine, Vermont, New Hampshire, Massachusetts, New York, Connecticut, Michigan, and Wisconsin; and the South, which includes Georgia, North and South Carolina, Virginia, and Puerto Rico.

Number of CFs in the United States since 1930.
The total area covered by CFs in our inventory is 436,411 acres (ac). Of this total, 87% of CFs were smaller than 5,000 ac ( figure 3 ), and 63% were less than 1,000 ac. By region, median sizes of CFs were: 1,360 ac in the West, 375 ac in the North, and 334 ac in the South. Nine CFs in the West were 5,000 ac or larger, compared to four in the North and none in the South. The majority of CFs less than 1,000 ac (thirty-nine of sixty-one CFs) were located in the North, with over half of those being between 100 and 500 ac (twenty-five of thirty-nine CFs). A total of 76% of reporting CFs said their forests were located on one contiguous parcel and 24% were on multiple unconnected parcels (varying from two to seventeen parcels).

Acreage of CFs across regions.
Ownership, Decision-Making Authority, and Management
As indicated in figure 4 , CFs in our sample were primarily owned by either a local government body (town, city, or county government) or by an NGO (e.g., a community-based organization, land trust, or other nonprofit). In the West, CFs were mostly purchased from private corporate owners (industrial timber companies, timber investment management organizations [TIMOs], or real estate investment trusts). In the North, CF lands were mostly acquired from private family forest owners. Ownership types included CFs of various sizes, although CFs larger than 1,000 ac tended to be held by a government body, whereas the majority of NGO-held CFs were smaller than 1,000 ac ( SI Table 2 ).

Ownership of CFs: (a) current landowner of forestlands designated as CFs and (b) previous landowners from whom the current landowner acquired the CF land. “Joint ownership” in (a) were parcels jointly owned by a local government body and a land trust ( n = 3), a private utilities firm ( n = 1), or a university ( n = 1); a tribe and a conservancy ( n = 1); and a land trust and private equity firm ( n = 1). “Other” in (b) were parcels that were pieced together from multiple ownerships.
Land ownership largely corresponded with the entity with ultimate decision-making authority over management, access, and use of the CF ( SI Figure 1 ). Government agencies largely had authority over government-owned CFs, tribes over tribally owned CFs, and NGOs over the land they owned. These entities had various ways of soliciting input from, or sharing decision-making authority with, local groups, organizations, and citizens. In some cases, this was institutionalized through formal joint decision-making processes. For example, there were eleven cases of local government ownership (town, city, or county-owned forests) where decision-making authority was jointly held by both that government body and formal citizen councils or committees established for this purpose. In other cases, although respondents did not describe decision-making as “joint,” they involved community members through mechanisms such as advisory committees and boards made up of local citizens, formal community and public consultation processes (mostly for city or town government ownerships), or various events, regular meetings, and other formal and informal mechanisms that sought community input (mostly for NGO ownerships). Local groups and volunteers contributed to day-to-day management of CFs across most ownerships ( SI Figure 2 ). In particular, various recreation-related volunteer groups helped to maintain trail systems. Otherwise, in many cases, forest consultants or forestry professionals from government agencies or NGOs contributed to forest planning and stewardship.
Management Goals and Allowed Activities
Survey respondents were asked to select the top four goals, from a list of options, that the CF was managed for ( figure 5 ). Across the country, the vast majority of CFs stated that recreation was a top management goal (82% of ninety-five reporting CFs). Collectively, conservation-oriented goals (watershed, habitat or open space protection, biodiversity conservation and restoration, and carbon sequestration, totaling 98% of reporting CFs), as well as other nonextractive goals (education, recreation, and cultural heritage protection, totaling 93% of reporting CFs) were much more prominent than extraction-oriented goals (timber production, nontimber forest products (NTFPs) management, agroforestry, and livestock grazing, totaling 47% of reporting CFs). However, timber production was among the top four management goals reported across the sample as a whole.

Primary management goals. Respondents were asked to list top four management goals for their CF.
There were few strong patterns between ownership type and management goals ( SI Table 3 ). Recreation was listed as one of the top management goals in over 75% of cases across CF ownership types, except for tribal (two of five CFs) and private corporate (one of two) owned CFs. All but two CFs owned by local governments listed a conservation-oriented goal. Half of local government-owned (twenty-two of forty-four CFs) and half of NGO-owned CFs (fifteen of thirty-two CFs) listed an extractive-oriented goal. Of the five tribal-owned CFs in our sample, only one listed an extractive-oriented goal (agroforestry) as a primary management goal and only one indicated that timber was produced but not as a primary goal. Local government and NGO-owned CFs reported slightly more often that producing timber was a primary goal (government: nineteen CFs listed it as a primary goal, twelve as a nonprimary goal, and twelve do not produce timber; NGO: thirteen, ten, and six, respectively).
Although timber production occurred on 70% of reporting CFs (sixty-five of ninety-three reporting CFs; figure 6A ), in almost half of those cases (twenty-eight of sixty-five cases) timber production was not one of the top four primary management goals of the CF. Geographically, no CFs in the southern region produced timber as a primary goal. In the North, slightly more CFs produced timber as a primary goal than not as a primary goal (twenty versus sixteen CFs), with only nine reporting no timber production. In the West, seventeen CFs reported producing timber as a primary goal, with ten producing but not primary and ten not producing. Timber production occurred across all acreages ( figure 6B ), including on almost two-thirds of the smallest CFs in our study (<1,000 ac) and on all CFs larger than 5,000 ac (although not always as a primary goal). Similarly, timber production occurred across all ownership types ( figure 6C ), whether as a primary goal or not. Of those engaged in timber production, a private consulting forester was used to oversee timber sales in 43% of fifty-one reporting cases, and internal staff from the CF owner in 26% of cases. Across ownerships, the entity that did the logging was most often a private contracting company (70% of fifty reporting cases). These entities were located at a place within 25 miles of the CF in 52% of forty-two reporting cases, or 26–50 miles in 33% of cases.

Status of timber production across CFs (a) by region, (b) CF size, and (c) ownership type.
Community forests had a variety of rules related to which activities were allowed and whether permits from CF owners were needed if allowed ( SI Figure 3 ). Motorized recreation, camping, and commercial uses of firewood or NTFPs were only allowed in a handful of CFs, often with the requirement of a free or paid permit. Hunting and fishing, in accordance with state regulations, were allowed in more than half of the reporting cases (69% of sixty-five reporting cases and 78% of sixty reporting cases, respectively) and rarely required a permit from CF owners. Personal use of firewood and other NTFPs were allowed in 22% and 40% of sixty-three reporting cases, respectively, although firewood use often required a permit. Altogether, 85% of sixty-six reporting cases allowed some nontimber extractive activities for personal use (either firewood, other NTFPs, hunting, or fishing). Only one CF did not allow recreation and four allowed it only with a free permit. In almost all cases, the same rules applied to the local community as to the general public, except for a few instances where NTFP and firewood use were limited to local community members.
Income Generation and Budgetary Support
A number of CFs across ownerships generated revenue from forest products and services (80% of forty-nine reporting CFs), mostly from timber sales ( figure 7 ; SI Table 4 ), although two-thirds of those reporting revenue generation stated that timber contributed to less than 30% of their budget. The few instances of revenue from hunting leases and payments for ecosystem services (mainly carbon offsets) were mostly reported in CFs owned by private nonprofits, whereas grazing permits or agriculture revenue were only reported in three state or local government-owned CFs ( SI Table 4 ). Timber revenue was reported across all ownerships where timber harvest occurred, except for the two cases of state government ownership, where it is anticipated in the future, once the forest regains commercial value following harvest by the previous owner.

Main sources of revenue generated from forest activities in 48 reporting CFs by region.
Grants from federal or state governments were the most frequently cited sources of annual budgetary support from 2018 to 2020, the period we asked about (70% of fifty-three reporting CFs; figure 8 ; SI Figure 4 ), although almost two-thirds of those CFs stated that grants contributed to less than 30% of their budget. Unsurprisingly, government-owned CFs more often reported (federal, state, or local) government sources for budgetary support. Local government-owned CFs were more reliant on local government funds: 71% of twenty-four reporting local government CFs stated they received funding from local governments (50% of them stating that they received more than 60% of their budget from this source), with only a handful of nongovernment owned CFs reporting support from this source. The NGO-owned CFs reported relying on donations from local community members and fundraiser events much more often than government-owned CFs (in three cases, community donations made up more than 60% of the budget). We did not track sources of funds used for acquiring forestlands in our survey.

Sources of budgetary support 2018–2020 by ownership type. Public ownership includes federal, state, and local governments, and private ownership includes both corporate and nonprofits. Polygons indicate largest differences between private and public ownerships.
As the results indicate, there are a variety of ownership and governance forms that CFs currently take in the United States, a variety of benefits that they provide, and a diversity of income sources that they rely on. As stated above, one goal of this study was to discuss the variability in CFs and develop a robust typology of them. Although Belsky (2008) proposed a CF typology based on ownership types, given the diversity of CFs we encountered in our survey (including within ownership types), we intended to develop a typology based on key characteristics, including ownership, decision-making, operational management, goals, size, and income sources. Two-step cluster analyses and Pearson’s χ 2 tests were performed to assess whether the CFs in our dataset could be empirically grouped according to various combinations of these characteristics. However, limited patterns emerged for creating definitive statistical typologies. Instead, we discuss here some emergent qualitative patterns based on the descriptive statistics reported in the Results section, reflect on the diversity of CFs in the United States, and propose a basic typology for practical purposes. Finally, we discuss the difficulties in creating a comprehensive CF inventory for the United States, given this diversity.
Ownership type emerged as a factor that seemed to shape some key functions of a CF—specifically, decision-making authority and sources of budgetary support. Publicly owned CFs (mostly by local city or town government) more often reported having either a government entity as ultimate decision-making authority or joint authority between local government and citizen councils or other local groups. They were also more reliant on government funding for budgetary support, either through federal or state grants, local government funds, or combinations of these. Privately owned CFs (mostly community-based organizations and local land trusts) more often reported having those same owners make decisions about the CF and less often reported that they formally engage in joint decision-making (although it is difficult to ascertain actual community participation in governance with our survey research design). They also more often reported relying on community donations and fundraiser events than local government funds. All five tribally owned CFs in our dataset were run by tribes themselves, including decision-making authority and operational management. Besides these basic characteristics, however, ownership type seems to have little influence on the size of CFs, management goals, allowed activities, timber production (equally present in public and private CFs), or earned income sources.
We saw moderate regional differences in ownership and size (more government ownership and larger sizes in the West), and who the CF owner bought their forestland from. Ownership history may help explain why the median size of CFs in the West was considerably larger than in the North. The majority of CF lands in the West were purchased from private corporate forest owners, whose holdings are often in the hundreds of thousands of acres ( Sass et al. 2021 ), and from TIMOs in particular, which typically sell land every 10 to 15 years ( Zhang 2021 ). In contrast, the majority of CF lands in the North were purchased from family forest owners; approximately 90% of these ownerships in the United States are under 50 ac ( Butler et al. 2021 ). Yet CFs larger than 5,000 ac occur in both the North and the West.
In both these regions, timber production often occurred across CFs of all sizes and was a primary management goal in roughly equal frequency, although not in our small sample of southern CFs. Timber production was not limited to any particular ownership type, or size class, of CF; rather, the potential to harvest timber as a management goal and source of revenue generation is likely influenced by the nature of the forest assets contained in a particular CF. Those with productive timberlands are presumably more likely than those lacking them to have timber production as a primary management goal. However, it may take years for this goal to be realized if the former owner recently harvested a substantial amount of commercial timber. All CFs across regions emphasized conservation goals, but forest restoration (phrased in the survey as “forest restoration, including wildfire management”) was cited more often in the West. Almost all CFs allowed public access for recreation and many for nontimber extractive activities for personal use. It is likely that some CFs regulate access more than others, but we could not capture this variation in our survey.
The difficulty in creating a typology of CFs is unsurprising given that, by definition, CFs reflect the values and priorities of the communities in which they are situated. Other historical, social, economic, and environmental factors also likely influence their characteristics. Additionally, policies and programs that provide funding opportunities to support CFs and their operations vary by state, influencing their sources of budgetary support. Investigating underlying factors that lead to the diversity in CF models and characteristics is a rich area for further research.
The second phase of our research (a larger project than reported here, aiming to better understand how CFs contribute to conservation and rural prosperity in the United States) uses a case-study sampling approach based on two characteristics that we postulated would be important distinguishing features of a typology: ownership of the CF and whether timber production is a primary management goal of the CF ( Table 1 ). We acknowledge that our survey results do not show that these two characteristics are statistically related to many other factors examined here but reasoned that ownership can influence CF governance and financing mechanisms, and that the role (or lack thereof) of timber production reflects the CF’s management goals, forest resources, financing mechanisms, and benefit streams. We recognize that CFs produce a host of benefits for communities beyond timber production. However, whether a CF prioritizes timber, harvests timber but does not prioritize it, or does not harvest timber emerged as an effective way to distinguish groups of CFs from each other in terms of their management priorities and resulting benefit streams. Otherwise, most CFs shared recreation and conservation-related goals.
A basic typology based on ownership and whether timber is a primary management goal of the CF. Percentages (in parentheses) reflect percentage of eighty-two CFs in our inventory that reported on timber status and ownership.
The diversity of CFs in the United States also reflects the grassroots nature of community forests across the country, making them somewhat unique relative to community forests globally. In many low- and middle-income countries, community forests are forests managed using a top-down model imposed and defined by national CF policies or land reforms and extensive financial and technical support from external donor organizations (e.g., national or international NGOs, multilateral/bilateral aid agencies), with communities receiving some rights and many responsibilities for forest management ( Charnley 2023 ; Hajjar et al. 2021 ; Ribot et al. 2006 ). In contrast, in the United States, CF establishment is typically driven from the bottom up, in most cases through local governments, locally based NGOs, or groups of citizens that come together to protect their local forests. There is no distinct CF tenure category at the national level and few national or state-level policies associated with community forests in the United States. Exceptions include Washington and New York states, where there are legislatively approved funding sources 2 to support CF acquisition and associated policy requirements once established, and the national-level Forest Service Community Forest and Open Space Conservation Program, which has supported the acquisition of numerous CFs in our inventory. This more grassroots approach results in a broad range of ownership, management, governance types, and rights and responsibilities among community members relative to many other countries. It also makes CFs somewhat hard to pinpoint in the United States, posing challenges for efforts to inventory them.
Stemming from this diversity in CFs, a key difficulty we faced in undertaking this inventory was determining what to include. Our approach to including CFs that self-identify as such or had participated in a program or policy related to CFs and met our criteria was naturally limiting. Although this approach was necessary to make an inventory possible, we acknowledge that many more CFs potentially exist than we included here, depending on how a CF is defined. In particular, our inventory captured many town forests and land trust forestlands, some tribal forests, and some state and federal forests. Yet these general ownership categories need further examination.
Town forests are local government-owned forests common across much of New England and the Northeast and in many cases may be considered CFs. They have long been established to generate income from timber and other resources for town budgets or specific projects and public services, to protect water, soil, and wildlife habitat, and to provide recreation and education opportunities for local community members and others ( Baker and Kusel 2003 ; Brown 1941 ; Hovis et al. 2022 ; McCullough 1995 ). The local ownership, management, and benefits of many town forests fulfill most of the criteria of CFs as laid out above. However, the acquisition and designation of a town forest does not guarantee its long-term protection from sale or development, and depending on how much the community participates in governance, it may or may not fulfil the governance criterion of CFs ( McGinley et al. 2022 ).
Similarly, many land trusts own forestlands that could be considered CFs, depending on how these forests are governed and managed, potentially increasing the number and extent of CFs in the United States. However, land trusts may not provide access for local communities or the general public to their forested land, may not provide for local community participation in decision-making, or may not manage their forests specifically for local benefits.
The extent to which tribal forests should be considered CFs is also complicated. Most tribal lands are trust lands, with about 56 million acres of land held in trust for tribes by the federal government (2.3% of US land area; DOI 2023 ). Although these lands are managed for the benefit of individual tribes, forest management activities take place under the direction of forest management and integrated resource management plans developed under the federal Bureau of Indian Affairs (BIA) guidelines and are subject to BIA approval. Since the passage of the Indian Self-Determination and Education Assistance Act of 1975 (Public Law 93-638), an increasing number of tribes have established contracts, known as 638 contracts, with the BIA by which tribal government forestry departments assume management responsibilities for forests on trust lands. These contracts are initiated by a formal request by a tribe to the BIA. By 2011, 112 tribes had taken advantage of these self-determination/self-governance opportunities for forest management, compared to 187 that relied on BIA to manage their lands directly ( Gordon et al. 2013 ). Given this complexity in governance, it is unclear to what extent the trust lands of individual tribes meet the criteria of CFs; such classification should be undertaken by tribes themselves. Tribes can also purchase and own fee lands to which they hold title. The five tribally owned CFs in our sample (they self-identify as such) were purchased this way from private landowners. Further research on tribal forests could explore the variations in ownership, benefits, and management of these forests on trust and fee lands.
Our inventory includes two CFs owned by Washington State and one that occurs on federal lands in California. These cases may appear to contradict our defining attributes of a CF, namely that they have local, long-term ownership or tenure, and that communities have significant decision-making authority. We included the state and federal CFs in our inventory primarily because they self-identified as CFs. However, they also display several attributes of a CF. The two state-owned CFs were acquired through Washington’s 2011 Community Forest Trust Program ( WA DNR, n.d. ). The legislation that created the program stipulated that CFs acquired with program funds (from state budget appropriations) be state-owned, and that state agencies have ultimate decision-making authority. But the legislation also stipulated that state-owned CFs have an advisory committee composed of roughly twenty members representing diverse stakeholder interests to inform those decisions and co-develop forest management plans with citizen input, and that CF management objectives should reflect the values of local communities ( WA Legislature 2011 ).
Regarding the federally owned case, Weaverville CF, the community manages the CF through a 10-year renewable cooperative stewardship agreement between the Forest Service and Bureau of Land Management (who own and administer different parts of the CF), and the local county resource conservation district (RCD) ( Frost 2014 ; Kelly 2018 ). The RCD is responsible for implementing forest management activities and is governed by a board of directors that oversees CF management, with input from a steering committee composed of ten to fifteen members, including local citizens and agency and RCD staff. Local residents have opportunities to provide input at community meetings that occur once or twice annually. The CF is managed to meet local community needs and priorities, such as wildfire risk reduction, habitat improvement for fish and wildlife, and recreation ( Frost 2014 ; Kelly 2018 ).
The question of whether CFs in the United States that self-identify as such should be considered CFs if they occur on land that is state- or federally owned—with the government retaining ultimate decision-making authority—deserves more attention and is a matter of debate among some practitioners and scholars (see Frey et al. forthcoming ). The international literature recognizes CFs that occur on national government-owned land where communities have concessions to manage the forests for a specified time period (e.g., several CFs in Canada [ Teitelbaum et al. 2006 ], Cameroon [ Piabuo et al. 2018 ], Guatemala [ Taylor 2010 ]); and CFs on national government land that are comanaged by the state and local communities (e.g., Tanzania; Blomley and Ramadhani, 2006 ). This highlights the importance of taking into account the governance criterion in defining CFs in the United States—the level of community involvement in decision-making—just as with town forests and land trusts, and opens the door for potential additional CFs on public lands that might fit the criteria but were not captured here.
The CFs we identified comprise less than 0.1% of all forests in the United States but are a rapidly developing model of forest ownership, governance, and management that provides local community benefits. They take a creative approach to funding and managing local forestlands through public, NGO, or tribal structures, generated income sources, and grant and donor fundraising. They have continued long-standing town and tribal forest ownership and management, helped protect forestlands and open space from imminent development, and offered innovative ways to form explicit community partnerships to manage existing public and private landscapes. As they solidify income sources and management capability, they also might serve as a new model of how market and nonmarket goods and services can be produced on forestlands for broad and enduring community benefits.
We have likely not included all individual CFs in the United States in this study and may have significant undercounts of certain types of CFs. Potential undercounts stem largely from ambiguity over which town, tribal, and private (e.g., land trust-held) forests meet our CF definition and criteria and lingering questions over whether CFs exist on federal lands. Nevertheless, the inventory will increase continually as communities develop proposals for CFs and obtain acquisition funding each year and new research is carried out. To help address this research limitation, we plan to create a centralized, publicly accessible repository that can serve as a living inventory to be updated as more CFs are either acknowledged as such or created. Although incomplete, our current inventory captures a fair representation of the variety of CF models in the United States, reflecting a diversity of ownerships, governance structures, management goals, benefit streams, and more.
This initial research to inventory and describe US CFs provides a sound base for further exploration. Future research could further explore levels of local participation in forest management and governance and when and how these variables would qualify a forest as a CF on public, private, or tribal lands. More in-depth research could also help refine our CF typology to include characteristics hard to ascertain from a survey instrument, such as level of community involvement or capacity and organizational development stage (e.g., incipient or mature). Furthermore, as more NGO-owned and town-owned forests self-identify with the label “community forest,” the consequences, advantages, and disadvantages of using that label will need further examination.
Future research could also compare CF models with traditional (noncommunity based) private and public forest ownerships to highlight their relative differences, advantages, and disadvantages. For example, some CF models share similarities but also have important differences with private family forest ownerships in terms of priority management objectives and timber production ( Butler et al. 2021 ; Shanafelt et al. 2023 ), warranting a systematic comparison of ownership types. Finally, we began this exercise of inventorying CFs in the United States to better understand their contributions to conservation and rural prosperity. Better understanding the ability of communities to capture CF monetary and nonmonetary benefits (and to do so equitably) can help inform the design of policies, programs, and actions to best support CFs.
Supplementary data are available at Journal of Forestry online.
This study was funded in part by the USDA National Institute of Food and Agriculture, Agriculture and Food Research Initiative (AFRI) Award number 2021-67023-34426. Partial funding was also provided by the USDA Forest Service’s Southern Research Station, Pacific Northwest Research Station, and International Institute of Tropical Forestry as well as Oregon State University and North Carolina State University.
The authors are developing a publicly accessible repository of community forests. In the meantime, the data used in this study will be made available upon reasonable request.
Abrams , Jesse. 2023 . Forest Policy and Governance in the United States . New York, NY : Routledge .
Google Scholar
Google Preview
Abrams , Jesse , E.J. Davis , and C. Moseley . 2015 . “Community-Based Organizations and Institutional Work in the Remote Rural West.” Review of Policy Research 32 ( 6 ): 675 – 698 . doi: 10.1111/ropr.12148 .
Baker , M. , and J. Kusel . 2003 . Community Forestry in the United States: Learning from the Past, Crafting the Future . Washington, DC : Island Press .
Belsky , Jill M. 2008 . “Creating Community Forests.” In Forest Community Connections: Implications for Research, Management, and Governance , edited by E.M. Donoghue and V.E. Sturtevant . New York, NY : Resources for the Future .
Belsky , Jill M. 2015 . “Community Forestry Engagement with Market Forces: A Comparative Perspective from Bhutan and Montana.” Forest Policy and Economics 58 ( 2015 ): 29 – 36 . doi: 10.1016/j.forpol.2014.11.004 .
Belsky , Jill M. , and Alexander Barton . 2018 . “Constitutionality in Montana: A Decade of Institution Building in the Blackfoot Community Conservation Area.” Human Ecology 46 ( 1 ): 91 – 92 . doi: 10.1007/s10745-018-9979-9 .
Blomley , T. , and H. Ramadhani . 2006 . “Going to Scale with Participatory Forest Management: Early Lessons from Tanzania.” International Forestry Review 8 ( 1 ): 93 – 100 . doi: 10.1505/ifor.8.1.93 .
Brosius , J. Peter , Anna Lowenhaupt Tsing , and Charles Zerner . 2005 . Communities and Conservation: Histories and Politics of Community-Based Natural Resource Management . Lanham, MD : Altamira Press .
Brown , N.C. 1941 . “Community Forests: Their Place in the American Forestry Program.” Journal of Forestry 39 ( 2 ): 171 – 179 .
Butler , Brett J. , Sarah M. Butler , Jesse Caputo , Jacqueline Dias , Amanda Robillard , and Emma M. Sass . 2021 . Family Forest Ownerships of the United States, 2018: Results from the USDA Forest Service, National Woodland Owner Survey. General Technical Report NRS-199 . Madison, WI : USDA Forest Service, Northern Research Station . https://www.fs.usda.gov/nrs/pubs/gtr/gtr_nrs199.pdf .
Charnley , Susan. 2023 . “Livelihood Investments as Incentives for Community Forestry in Africa.” World Development 168 ( August 2023 ): 106260 . doi: 10.1016/j.worlddev.2023.106260 .
Charnley , Susan , and Melissa R. Poe . 2007 . “Community Forestry in Theory and Practice: Where Are We Now?” Annual Review of Anthropology 36 ( 1 ): 301 – 336 . doi: 10.1146/annurev.anthro.36.081705.123143 .
Christoffersen , Nils , Don Harker , Martha West Lyman , and Barbara Wyckoff . 2008 . The Status of Community-Based Forestry in the United States: A Report to the US Endowment for Forestry and Communities . Greenville, SC : US Endowment for Forestry and Communities, Community Forest Consortium .
Davis , Emily Jane , Reem Hajjar , Susan Charnley , Cassandra Moseley , Kendra Wendel , and Meredith Jacobson . 2020 . “Community-Based Forestry on Federal Lands in the Western United States: A Synthesis and Call for Renewed Research.” Forest Policy and Economics 111 ( 2020 ): 102042 . doi: 10.1016/j.forpol.2019.102042 .
Dillman , D.A. , J.D. Smyth , and L.M. Christian . 2014 . Internet, Phone, Mail, and Mixed-Mode Surveys: The Tailored Design Method , fourth edition. Hoboken, NJ : Wiley Blackwell .
Frey , G.E. , R. Hajjar , S. Charnley , K. McGinley , J. Schelhas , N. Tarr , L. McCaskill , and F.W. Cubbage . Forthcoming . “‘Community Forests’ in the United States – How Do We Know One When We See One?” Submitted to Society and Natural Resources .
Frost , Pat. 2014 . Stitching the West Back Together: Conservation of Working Landscapes, edited by Susan Charnley , T.E. Sheridan , and G.P. Nabhan , 177 – 180 . Chicago, IL : University of Chicago Press .
Gordon , J.C. , J. Sessions , J. Bailey , D. Cleaves , V. Corrao , A. Leighton , L. Mason , M. Rasmussen , H. Salwasser , and M. Sterner . 2013 . Assessment of Indian Forests and Forest Management in the United States . Portland, OR : Indian Forest Management Assessment Team .
Hajjar , Reem , and Augusta Molnar . 2016 . “Decentralized and Community-Based Approaches.” In Forests, Business and Sustainability , edited by Rajat Panwar , Robert Kozak , and Eric Hansen . Abigdon, UK : Routledge .
Hajjar , Reem , Johan A. Oldekop , Peter Cronkleton , Peter Newton , Aaron J.M. Russell , and Wen Zhou . 2021 . “A Global Analysis of the Social and Environmental Outcomes of Community Forests.” Nature Sustainability 4 ( March ): 216 – 224 . doi: 10.1038/s41893-020-00633-y .
Hovis , Meredith , Gregory Frey , Kathleen McGinley , Frederick Cubbage , Xue Han , and Megan Lupek . 2022 . “Ownership, Governance, Uses, and Ecosystem Services of Community Forests in the Eastern United States.” Forests 13 ( 10 ): 1577 – 1523 . doi: 10.3390/f13101577 .
Kelly , Erin C. 2018 . “The Role of the Local Community on Federal Lands: The Weaverville Community Forest.” Humboldt Journal of Social Relations 1 ( 40 ): 163 – 177 . doi: 10.55671/0160-4341.1076 .
Lund , Jens Friis , Rebecca Leigh Rutt , Jesse Ribot . 2018 . “Trends in Research on Forestry Decentralization Policies.” Current Opinion in Environmental Sustainability 32 ( June 2018 ): 17 – 22 . doi: 10.1016/j.cosust.2018.02.003 .
Lyman , M.W. , C. Grimm , and J.R. Evans . 2014 . “Community Forests as a Wealth Creation Strategy for Rural Communities.” Community Development 45 ( 5 ): 474 – 489 .
McCarthy , James. 2006 . “Neoliberalism and the Politics of Alternatives: Community Forestry in British Columbia and the United States.” Annals of the Association of American Geographers 96 ( 1 ): 84 – 104 .
McCullough , R. 1995 . The Landscape of Community: A History of Communal Forests in New England . Hanover, NH : University Press of New England .
McDermott , M.H.K. , and K. Schreckenberg . 2009 . “Equity in Community Forestry: Insights from North and South.” International Forestry Review 11 ( 2 ): 157 – 170 . doi: 10.1505/ifor.11.2.155 .
McGinley , Kathleen , Susan Charnley , Frederick W. Cubbage , Reem Hajjar , Gregory E. Frey , John Schelhas , Meredith Hovis , and Kailey Kornahauser . 2022 . “Community Forest Ownership, Rights, and Governance Regimes in the United States.” In Routledge Handbook on Community Forestry , edited by J. Bulkan , J. Palmer , A.M. Larson , and M. Hobley , 11 – 28 . London : Routledge . doi: 10.4324/9780367488710-13 .
Piabuo , Serge Mandiefe , Divine Foundjem-Tita , and Peter A. Minang . 2018 . “Community Forest Governance in Cameroon: A Review.” Ecology and Society 23 ( 3 ): 15 . doi: 10.5751/ES-10330-230334 .
Ribot , Jesse C. , Arun Agrawal , and Anne M. Larson . 2006 . “Recentralizing While Decentralizing: How National Governments Reappropriate Forest Resources.” World Development 34 ( 11 ): 1864 – 1886 . doi: 10.1016/j.worlddev.2005.11.020 .
Rights and Resources Initiative . 2018 . At a Crossroads: Consequential Trends in Recognition of Community-Based Forest Tenure . Washington, DC : Rights and Resources Initiative .
Sass , Emma M. , Marla Markowski-Lindsay , Brett J. Butler , Jesse Caputo , Andrew Hartsell , Emily Huff , and Amanda Robillard . 2021 . “Dynamics of Large Corporate Forestland Ownerships in the United States.” Journal of Forestry 119 ( 4 ): 363 – 375 . doi: 10.1093/jofore/fvab013 .
Shanafelt , David W. , Jesse Caputo , Jens Abildtrup , and Brett J. Butler . 2023 . “If A Tree Falls in A Forest, Why Do People Care? An Analysis of Private Family Forest Owners’ Reasons for Owning Forest in the United States National Woodland Owner Survey.” Small-Scale Forestry 22 ( 2 ): 303 – 321 . doi: 10.1007/s11842-022-09530-y .
Taylor , Peter Leigh. 2010 . “Conservation, Community, and Culture? New Organizational Challenges of Community Forest Concessions in the Maya Biosphere Reserve of Guatemala.” Journal of Rural Studies 26 ( 2 ): 173 – 184 . doi: 10.1016/j.jrurstud.2009.09.006 .
Teitelbaum , S. , T.M. Beckely , and S. Nadeau . 2006 . “A National Portrait of Community Forestry in Canada.” The Forestry Chronicle 82 ( 3 ): 416 – 428 .
US Department of the Interior (DOI) . 2023 . “Native American Ownership and Governance of Natural Resources.” Natural Resources Revenue Data 2023 . https://revenuedata.doi.gov/how-revenue-works/native-american-ownership-governance/ .
Washington Department of Natural Resources (WA DNR) . n.d . “Washington Community Forest Trust Program.” https://www.dnr.wa.gov/managed-lands/washington-community-forest-trust-program .
Washington Legislature (WA Legislature) . 2011 . “Senate Bill Report ESHB 1421.” Washington State Legislature . https://lawfilesext.leg.wa.gov/biennium/2011-12/Pdf/Bill%20Reports/Senate/1421-S.E%20SBA%20NRMW%2011.pdf?q=20231016011547 .
Zhang , Daowei. 2021 . From Backwoods to Boardrooms: The Rise of Institutional Investment in Timberland . Corvallis, OR : Oregon State University Press .
Community forests, community forestry, and community-based forestry are terms that are often used interchangeably in the U.S. literature; however, see Frey et al. (forthcoming) and Belsky (2008) for a discussion of important differences.
The Washington State Community Forests Program was established by the state legislature in 2019 to provide grant funding for CF acquisition ( https://rco.wa.gov/grant/community-forests-program/ ). The New York Community Forest Conservation Grant program similarly funds municipal land acquisitions for community forests ( https://www.dec.ny.gov/lands/124345.html#:~:text=and%20contact%20information-,Program%20Overview,Leadership%20and%20Community%20Protection%20Act ).
Author notes
Supplementary data, email alerts, citing articles via.
- Recommend to Your Librarian
- Advertising and Corporate Services
Affiliations
- Online ISSN 1938-3746
- Print ISSN 0022-1201
- Copyright © 2024 Society of American Foresters
- About Oxford Academic
- Publish journals with us
- University press partners
- What we publish
- New features
- Open access
- Institutional account management
- Rights and permissions
- Get help with access
- Accessibility
- Advertising
- Media enquiries
- Oxford University Press
- Oxford Languages
- University of Oxford
Oxford University Press is a department of the University of Oxford. It furthers the University's objective of excellence in research, scholarship, and education by publishing worldwide
- Copyright © 2024 Oxford University Press
- Cookie settings
- Cookie policy
- Privacy policy
- Legal notice
This Feature Is Available To Subscribers Only
Sign In or Create an Account
This PDF is available to Subscribers Only
For full access to this pdf, sign in to an existing account, or purchase an annual subscription.
Article
- Volume 16, issue 5
- ESSD, 16, 2385–2405, 2024
- Peer review
- Related articles
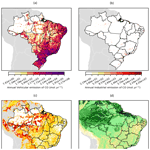
Brazilian Atmospheric Inventories – BRAIN: a comprehensive database of air quality in Brazil
Leonardo hoinaski, robson will, camilo bastos ribeiro.
Developing air quality management systems to control the impacts of air pollution requires reliable data. However, current initiatives do not provide datasets with large spatial and temporal resolutions for developing air pollution policies in Brazil. Here, we introduce the Brazilian Atmospheric Inventories (BRAIN), the first comprehensive database of air quality and its drivers in Brazil. BRAIN encompasses hourly datasets of meteorology, emissions, and air quality. The emissions dataset includes vehicular emissions derived from the Brazilian Vehicular Emissions Inventory Software (BRAVES), industrial emissions produced with local data from the Brazilian environmental agencies, biomass burning emissions from FINN – Fire INventory from the National Center for Atmospheric Research (NCAR), and biogenic emissions from the Model of Emissions of Gases and Aerosols from Nature (MEGAN) ( https://doi.org/10.57760/sciencedb.09858 , Hoinaski et al., 2023a; https://doi.org/10.57760/sciencedb.09886 , Hoinaski et al., 2023b). The meteorology dataset has been derived from the Weather Research and Forecasting Model (WRF) ( https://doi.org/10.57760/sciencedb.09857 , Hoinaski and Will, 2023a; https://doi.org/10.57760/sciencedb.09885 , Hoinaski and Will, 2023c). The air quality dataset contains the surface concentration of 216 air pollutants produced from coupling meteorological and emissions datasets with the Community Multiscale Air Quality Modeling System (CMAQ) ( https://doi.org/10.57760/sciencedb.09859 , Hoinaski and Will, 2023b; https://doi.org/10.57760/sciencedb.09884 , Hoinaski and Will, 2023d). We provide gridded data in two domains, one covering the Brazilian territory with 20×20 km spatial resolution and another covering southern Brazil with 4×4 km spatial resolution. This paper describes how the datasets were produced, their limitations, and their spatiotemporal features. To evaluate the quality of the database, we compare the air quality dataset with 244 air quality monitoring stations, providing the model's performance for each pollutant measured by the monitoring stations. We present a sample of the spatial variability of emissions, meteorology, and air quality in Brazil from 2019, revealing the hotspots of emissions and air pollution issues. By making BRAIN publicly available, we aim to provide the required data for developing air quality policies on municipal and state scales, especially for under-developed and data-scarce municipalities. We also envision that BRAIN has the potential to create new insights into and opportunities for air pollution research in Brazil.
- Article (PDF, 13528 KB)
- Supplement (215138 KB)
- Article (13528 KB)
- Full-text XML

Hoinaski, L., Will, R., and Ribeiro, C. B.: Brazilian Atmospheric Inventories – BRAIN: a comprehensive database of air quality in Brazil, Earth Syst. Sci. Data, 16, 2385–2405, https://doi.org/10.5194/essd-16-2385-2024, 2024.
It is consensus that air pollution threatens public health (OECD, 2024), economic progress (OECD, 2016), and climate (US EPA, 2023a). The negative outcomes associated with air pollution are not uniform within populations, and the impacts may be greater for more susceptible and exposed individuals (Makri and Stilianakis, 2008). Due to their social vulnerability and increasing emissions, developing countries urgently require reliable databases to provide information for designing air quality management systems to control air pollution (Sant'Anna et al., 2021).
Brazil has continental dimensions, is the seventh most populous country in the world, and has the 12th largest gross domestic product (IBGE, 2024). The combination of poorly planned development and the huge socioeconomic discrepancy has led to air quality impacts in Brazil. Air-pollution-related problems are not only restricted to great Brazilian cities and industrialized areas. Vehicular fleets and fuel consumption have also increased in small municipalities (CEIC, 2021; MME, 2023), posing a challenge to controlling vehicular emissions. In preserved and rural areas, large fire emissions have occurred due to illegal deforestation and poor soil management (Escobar, 2019; Rajão et al., 2020).
Following the practices of developed countries, Brazilian air quality policies have been enforced through legislative laws, using air quality standards as key components. However, the air quality management system remains incomplete in Brazil, with policies falling short of effectiveness due to a lack of air quality monitoring data across much of the country, primarily limited to well-developed areas (Sant'Anna et al., 2021). Moreover, Brazilian environmental agencies have not provided enough data and guidance to permit progress. Air quality consultants are still struggling to find mandatory inputs to understand and predict air quality for regulatory purposes. Efforts toward the permanent improvement of high-spatiotemporal-resolution emissions inventories and of meteorological and air quality data are needed.
An effective air quality management system must provide data to determine what emission reductions are needed to achieve the air quality standards (US EPA, 2023b). It requires air quality monitoring, a robust and detailed emissions inventory, reliable meteorological datasets, and methodologies to adapt the state-of-the-art air quality models to Brazil's reality. Moreover, it is crucial to undertake ongoing evaluation and to fully understand the air quality problem to design and implement the programs for pollution control. Currently, available initiatives including reanalysis and satellite products are still not providing datasets with large spatial and temporal resolutions for developing air pollution policies in Brazil. Global reanalyses such as the Copernicus Atmospheric Monitoring Service (CAMS) (Inness et al., 2019) and the second version of the Modern-Era Retrospective Analysis for Research and Applications (MERRA-2) (GMAO, 2015a, b) can provide estimates of air pollutants by combining chemical transport models (CTMs) with satellite and ground-based observations and physical information, assimilating data to constrain the results. However, the currently available reanalysis products do not provide data with high spatial resolution (up to 0.75° × 0.75° and 0.5° × 0.625°) and could be biased toward representing local and regional air quality (Arfan Ali et al., 2022). Moreover, they provide data for only a small list of air pollutants. Satellite-based products such as Sentinel-5P TROPOMI (Veefkind et al., 2012) and the Moderate-Resolution Imaging Spectroradiometer (MODIS) (Levy et al., 2015; Platnick et al., 2015) are still challenging due to their low temporal resolution, data gaps due to cloud coverage, and uncertainties (Shin et al., 2020). Besides, satellites rely on total tropospheric column measurements, which do not represent surface concentrations (Shin et al., 2019).
In this article, we present the Brazilian Atmospheric Inventories (BRAIN), the first comprehensive database to elaborate upon air quality management systems in Brazil. BRAIN combines local inventories, consolidated datasets, and the usage of internationally recommended models to provide hourly emissions and meteorological and air quality data covering the entire country.
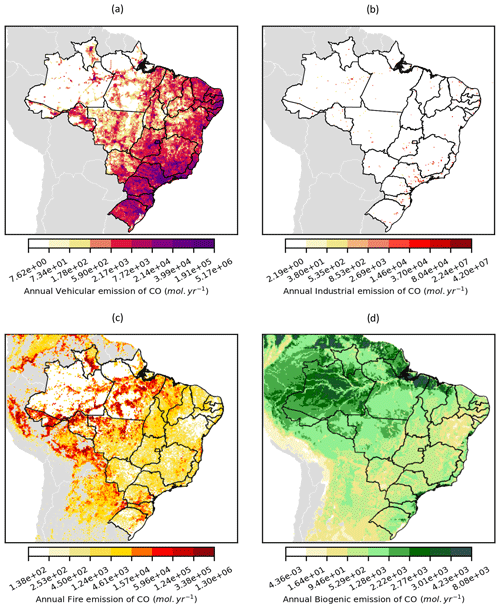
Figure 1 Spatial distribution of CO emissions from (a) vehicles, (b) industries, (c) biomass burning, and (d) biogenic sources, as provided by BRAIN.
BRAIN contains three types of hourly datasets: emissions, meteorology, and air quality. The emissions inventories include vehicular, industrial, biogenic, and biomass burning emissions. We provide meteorological data derived from the Weather Research and Forecasting (WRF) model. Coupling emissions, WRF, and the Community Multiscale Air Quality Modeling System (CMAQ) version 5.3.2, we provide air quality gridded data. All datasets are available on two spatial resolutions: the largest (Fig. S1 in the Supplement – d01) covers the entire country, while the smallest covers southern Brazil (Fig. S1 – d02). The BRAIN datasets in d01 are freely available at https://doi.org/10.57760/sciencedb.09858 (Hoinaski et al., 2023a), https://doi.org/10.57760/sciencedb.09857 (Hoinaski and Will, 2023a), and https://doi.org/10.57760/sciencedb.09859 (Hoinaski and Will, 2023b). The BRAIN datasets in d02 are available at https://doi.org/10.57760/sciencedb.09886 (Hoinaski et al., 2023b), https://doi.org/10.57760/sciencedb.09885 (Hoinaski and Will, 2023c), and https://doi.org/10.57760/sciencedb.09884 (Hoinaski and Will, 2023d). The Federal University of Santa Catarina (UFSC) institutional repository, https://brain.ens.ufsc.br/ (last access: 8 May 2024), and the web platform, https://hoinaski.prof.ufsc.br/BRAIN/ (last access: 8 May 2024), have served the BRAIN database since 2019. We envision making available more detailed datasets for other Brazilian regions, especially in the southeast, where the anthropogenic emission effects are more prominent. Future versions will also provide more detailed modeling outputs to properly cover medium- and small-sized cities.
BRAIN is intended to fill the gaps in those cases where adequately representative monitoring data to characterize the air quality are not available. BRAIN would be useful in providing background concentrations for a good procedure for licensing new sources of air pollution.
2.1 Emissions inventory
The BRAIN emissions inventory allows the spatiotemporal analysis of vehicular, industrial, biomass burning, and biogenic emissions in Brazil. The present version of this database does not account for other South American countries' emissions, apart from biomass burning and biogenic sources. We envision implementing other sources and a more detailed emissions inventory from other South American countries in a future version. Figure 1 presents a sample of the inventory, showing the annual carbon monoxide (CO) emissions by source. Section S2 in the Supplement (Table S1) summarizes the species in each emission source inventory. More information on each emissions dataset can be found in Sect. 2.1.1 to 2.1.5.
We observed higher vehicular emission rates of CO in urban areas with large population and vehicle fleet densities, mainly in the south and southeast (Fig. 1a). High industrial emission rates have been detected in the Brazilian regions, with large stationary sources such as refining units, thermoelectric power plants, and cement and paper industries (Fig. 1b) (Kawashima et al., 2020). In general, the north shows a higher concentration of biogenic and fire emissions. While the hotspots of biogenic emissions are predominately in the extreme north, the hotspots of fire emissions turn up in the midwestern, northern, and southern regions, as well as on the Brazilian western border (Fig. 1c–d).
2.1.1 Vehicular emissions
BRAIN uses the multispecies and high-spatiotemporal-resolution database of vehicular emissions from the Brazilian Vehicular Emission Inventory Software (BRAVES) (Hoinaski et al., 2022; Vasques and Hoinaski, 2021). The BRAVES database disaggregates municipality-aggregated emissions using the road density approach and temporal disaggregation based on vehicular flow profiles. SPECIATE 5.1 (Eyth et al., 2020) from the United States Environmental Protection Agency (USEPA, https://www.epa.gov/air-emissions-modeling/speciate , last access: 8 May 2024) speciates conventional pollutants in 41 species. BRAVES considers local studies (Nogueira et al., 2015) and data from Companhia Ambiental do Estado de São Paulo (CETESB) ( https://cetesb.sp.gov.br/veicular/relatorios-e-publicacoes/ , last access: 8 May 2024) to speciate acetaldehydes, formaldehyde, ethanol, and aldehydes in order to account for biofuel particularities in Brazil.
In BRAVES, vehicular activity is defined by fuel consumption in each municipality using data provided by the Brazilian National Agency for Oil, Natural Gas and Biofuel (ANP) ( https://www.gov.br/anp/pt-br/centrais-de-conteudo/dados-abertos/vendas-de-derivados-de-petroleo-e-biocombustiveis , last access: 8 May 2024). A fraction of fuel consumed by road transportation is based on data from the National Energy Balance (BEN) ( https://www.epe.gov.br/pt/publicacoes-dados-abertos/publicacoes/balanco-energetico-nacional-ben , last access: 8 May 2024), and MMA (2014). BRAVES calculates the weighted emission factor (EF) to address the effect of the fleet composition in terms of category, model year, and fuel utilization.
Vasques and Hoinaski (2021) compared BRAVES with different vehicular emission inventories from a local to national scale. On a national scale, vehicular emission rates from BRAVES underestimate the Emission Database for Global Atmospheric Research (EDGAR) and are slightly higher for CO (14 %) and non-methane volatile organic compounds (NMVOCs) (9 %) compared with the national inventory from Ministério do Meio Ambiente (MMA). The differences between estimates from BRAVES and from well-developed state inventories vary from −1 % to 35 % in São Paulo and from −2 % to 52 % in Minas Gerais. In addition, a relatively small bias between BRAVES and the Vehicular Emission Inventory (VEIN) was observed in São Paulo and Vale do Paraiba (Vasques and Hoinaski, 2021).
2.1.2 Industrial emissions
We derived the industrial emissions inventory by combining data from the state environmental agencies of Espírito Santo, Minas Gerais, and Santa Catarina. The emission rates of point sources from Espírito Santo and Minas Gerais are publicly provided by Instituto de Meio Ambiente e Recursos Hídricos do Espírito Santo (IEMA-ES) ( https://iema.es.gov.br/qualidadedoar/inventariodefontes , last access: 8 May 2024) and Fundação Estadual de Meio Ambiente (FEAM) ( http://www.feam.br/qualidade-do-ar/emissao-de-fontes-fixas , last access: 8 May 2024). Data from IEMA-ES contain emissions from the metropolitan region of Vitória from 2015, compiling measurements from regulatory procedures and emissions estimates. We did not convert the emissions inventory to the current modeling year since the data are not continuously updated. Therefore, we assumed that all emissions from these sources occurred in 2019.
In Santa Catarina, industrial emission data have been provided by Instituto de Meio Ambiente (IMA) ( https://www.ima.sc.gov.br/index.php , last access: 8 May 2024). These data are collected in the licensing process of potentially polluting industries. The base year of emission rates varies according to the availability. Summary information about the industrial sector types, the number of industries, and the respective emission rates in Santa Catarina can be found in Hoinaski et al. (2020) and at https://github.com/leohoinaski/IND_Inventory/blob/main/Inputs/BR_Ind.xlsx (last access: 8 May 2024). Emissions from large stationary sources (refining units, thermoelectric power plants, cement, and paper industries) provided by Kawashima et al. (2020) have been included when not encountered in the environmental agencies' inventories.
We chemically speciated the industrial emission rates by adopting the following steps: (i) grouping each point source using the same categories as in the Emission Database for Global Atmospheric Research (EDGAR) (Crippa et al., 2018) and the Intergovernmental Panel on Climate Change (IPCC) industrial segments, (ii) selecting compatible profiles in SPECIATE 5.1 for each group (Eyth et al., 2020), (iii) averaging the speciation factor by group and pollutant, and (iv) applying the speciation factor for the targeted pollutant (PM, NO x , VOCs). The SPECIATE 5.1 profiles used in this work are listed at https://github.com/leohoinaski/IND_Inventory/tree/main/IndustrialSpeciation (last access: 8 May 2024). The speciation factors by industrial group and pollutant are available at https://github.com/leohoinaski/IND_Inventory/blob/main/IndustrialSpeciation/IND_speciation.csv (last access: 8 May 2024).
We also vertically allocate the industrial emissions according to the plume's effective height, estimated by the sum of the geometric height and the superelevation of the plume. The plume superelevation was estimated by the Briggs method (Briggs, 1975, 1969). The initial vertical distribution of the plume has been estimated by disaggregating the emissions using a Gaussian approach, as proposed in the Sparse Matrix Operator Kernel Emissions (SMOKE) model (Bieser et al., 2011; Gordon et al., 2018; Guevara et al., 2014). Python code to estimate the plume's effective height and the initial vertical disaggregation of industrial emissions is available at https://doi.org/10.5281/zenodo.11167115 (Hoinaski, 2024a).
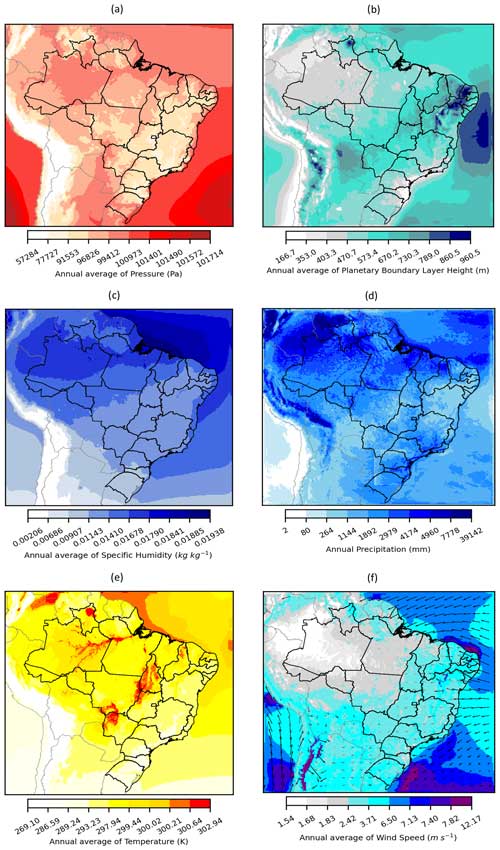
Figure 2 Annual average of meteorological variables in 2019, simulated by the WRF with 20 × 20 km resolution. (a) Atmospheric pressure, (b) planetary boundary layer height, (c) specific humidity, (d) annual accumulated precipitation, (e) temperature, (f) wind intensity and direction. All variables are annual averages except for precipitation, which represents the annual accumulated total.
2.1.3 Biomass burning emissions
The Fire INventory from NCAR (FINN) version 1.5 (Wiedinmyer et al., 2011) provides data on biomass burning emissions in BRAIN. FINN outputs contain daily emissions of trace gas and particle emissions from wildfires, agricultural fires, and prescribed burnings and do not include biofuel use and trash burning. Datasets have a 1 km spatial resolution and are available at https://www.acom.ucar.edu/Data/fire/ (last access: 8 May 2024).
Since CMAQ requires hourly emissions, a Python code ( https://github.com/barronh/finn2cmaq , last access: 8 May 2024) temporally disaggregates daily emissions into hourly emissions. The same code vertically splits the fire emissions to consider the plume rise effect and represents the vertical distribution (Henderson, 2022), converting text files into hourly 3D netCDF files.
Pereira et al. (2016) suggest that fire emissions estimated by FINN are strongly related to deforestation in many Brazilian regions. FINN estimates have a high correlation with both the Brazilian Biomass Burning Emission Model (3BEM) (0.86) and the Global Fire Assimilation System (GFAS) (0.84). The emissions estimated from FINN are commonly overestimated in comparison to other biomass burning emission inventories. An overestimation also occurs when FINN is used in air quality models and compared with observations. However, the use of FINN as input in air quality models can capture the temporal variability of pollutants emitted by biomass burning (Vongruang et al., 2017).
We have implemented the FINN v1.5 in this first version of BRAIN. However, FINN version 2.5 (Wiedinmyer et al., 2023) will be included in our emissions inventory in future work; this version uses an updated algorithm for determining fire size based on MODIS and Visible Infrared Imaging Radiometer Suite (VIIRS) satellite instruments. We also provide data from 2020 with the same modeling grid upgraded to FINN v2.5.
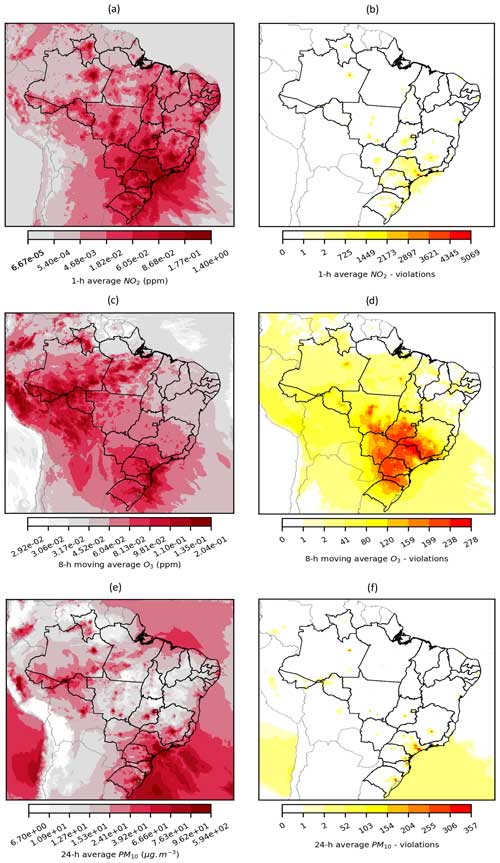
Figure 3 Spatial distribution of air pollutant concentration (a, c, e) and number of violations of air quality standards (b, d, f) for NO 2 (a–b) , O 3 (c–d) , and PM 10 (e–f) .
2.1.4 Biogenic emissions
We derived the biogenic emissions using the Model of Emissions of Gases and Aerosols from Nature (MEGAN) version 3.2 (Guenther et al., 2012; Silva et al., 2020). MEGAN is based on the leaf area index and plant functional groups. The model estimates emissions of gases and aerosols for different meteorological conditions and land cover types (Guenther et al., 2012). The leaf-level temperature and photosynthetically active radiation, as well as the vegetative stress conditions implemented in MEGAN, provide more physically realistic parameterizations for biosphere–atmosphere interactions (Silva et al., 2020). Input datasets, emission factor processors, and emission estimation modules are available at https://bai.ess.uci.edu/megan/data-and-code (last access: 8 May 2024). Data from WRF and the Meteorology-Chemistry Interface Processor (MCIP) have been used in MEGAN simulations.
MEGAN is commonly adopted to estimate emissions from biogenic fluxes, which constitute an important input for air quality modeling in many regions worldwide (Hogrefe et al., 2011; Kitagawa et al., 2022; Kota et al., 2015). Although MEGAN overestimates nighttime biogenic fluxes, the modeled emissions are correlated with measurements in the Amazon during both wet and dry seasons. The model is capable of capturing relatively well the seasonal variability of important organic pollutants in tropical forests (Sindelarova et al., 2014).
2.1.5 Sea spray aerosol emissions
Sea spray aerosol (SSA) is an important source of particles in the atmosphere. Due to its properties, SSA influences gas–particle partitioning in coastal environments (Gantt et al., 2015). SSA has been implemented in CMAQ as an inline source and requires the input of an ocean mask file (OCEAN) to identify the fractional coverage in each model grid cell allocated to the open ocean (OPEN) or surf zone (SURF). CMAQ uses this coverage information to calculate sea spray emission fluxes from the model's grid cells (US EPA, 2022). Detailed information on the mechanism of sea spray aerosol emissions and its implementation in CMAQ can be found in Gantt et al. (2015).
We provide a Python code ( https://github.com/leohoinaski/CMAQrunner/blob/master/hoinaskiSURFZONEv2.py , last access: 8 May 2024) to reproduce the OCEAN time-independent Input/Output Applications Programming Interface (I/O API) ( https://www.cmascenter.org/ioapi/ , last access: 8 May 2024) file so that it is ready to use in CMAQ. This code uses a shoreline Environmental Systems Research Institute (ESRI) shapefile from the National Oceanic and Atmospheric Administration (NOAA), available at https://www.ngdc.noaa.gov/mgg/shorelines/ (last access: 8 May 2024).
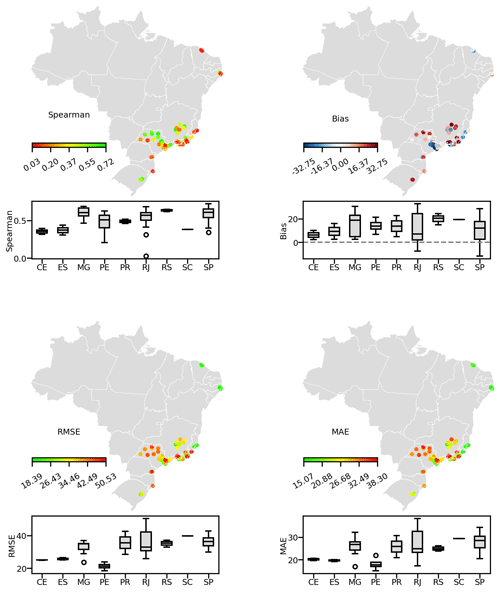
Figure 4 Spearman rank, bias, root mean squared error (RMSE), and mean absolute error (MAE) of O 3 dataset of BRAIN vs. observed values. Box plots of statistical metric by Brazilian state (considering only states with monitoring stations with representative data in 2019).
2.2 Meteorology
The WRF model has been used in this work to produce inputs for CMAQ and for meteorology characterization in Brazil. We provide hourly simulations in netCDF files. WRF has been set up to reproduce 36 h simulations, where the initial 12 h have been dedicated to model stabilization; these are excluded from the analysis. Thirty-three vertical levels have been employed, spaced at 50 hPa intervals. The parameterizations used in this work are described in Sect. S3. The remaining vertical levels followed a hybrid modeling scheme, accounting for terrain in the lower layers and gradually minimizing its influence at the higher levels. Details of WRF outputs can be found in Sect. S4 (Table S2).
The Global Forecast System (GFS) from the National Center for Atmospheric Research (NCAR) provided inputs with a spatial resolution of 0.25° × 0.25° and a temporal resolution of 6 h for the WRF simulations (Skamarock et al., 2008). Land use data and classification parameters are from the United States Geological Survey's (USGS) Moderate Resolution Imaging Spectroradiometer (MODIS).
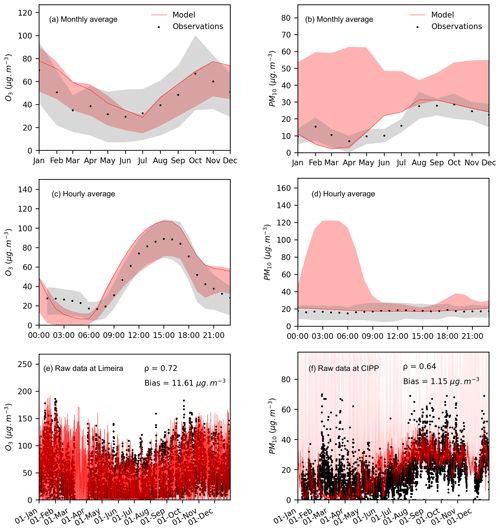
Figure 5 Time series of O 3 and PM 10 modeled and measured at Limeira (a, c, e) and CIPP (b, d, f) monitoring stations.
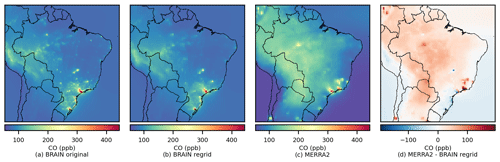
Figure 6 Annual average concentration of CO from BRAIN with its original resolution (a) , from BRAIN regridded to MERRA-2 resolution (b) , and from MERRA-2 (c) and the difference between MERRA-2 and BRAIN (d) .
Table 1 BRAIN datasets freely available.
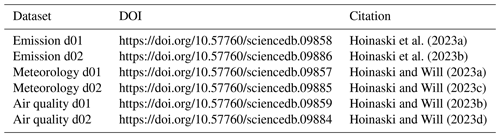
Download Print Version | Download XLSX
The Brazilian regions (north, northeast, midwest, southeast, and south) encompass three distinct climatic zones, namely the equatorial, tropical, and subtropical zones. The climatic diversity in Brazil is also shaped by topographical variations, landscape or vegetation, and the coastal areas. The temperature in Brazil follows a latitudinal pattern, increasing from south to north (Fig. 2e). The highest average temperatures are observed in the Amazon region, matching the historic data (Cavalcanti, 2016). The southern region exhibits the lowest average temperatures, which is also consistent with historical data (Cavalcanti, 2016).
The highest values of atmospheric pressure occurred in the northern region and in the extreme south of the country, and the lowest values were between the southeastern and southern regions (Fig. 2a). The planetary boundary layer height (PBLH) reaches the highest levels in the northeastern region and the lowest levels at the southern and southeastern coasts (Fig. 2b). The highest values of wind speed occurred in part of the northern and southern region. The Amazon region presented the lowest values of surface wind speed (Fig. 2f).
Humidity and precipitation exhibit similar patterns in the northern and northeastern regions (Fig. 2c, d) due to the trade winds that transport moisture from the tropical Atlantic (Mendonça and Danni-Oliveira, 2017). Except for the coast, the northeastern region is characterized by low humidity and drought during half of the year. The southern and southeastern regions have well-distributed rainfall throughout the year, as well as intermediate levels of humidity, except for the northern coast of the southern region, which has an elevated level of precipitation and humidity throughout the year.
The WRF model demonstrated the ability to reproduce diurnal and seasonal variability in winds in the Brazilian northeastern region (Souza et al., 2022a), although it underestimated the height of the planetary boundary layer (PBLH) by up to 20 %, as well as the temperature and humidity at 4 °C and 15 %, respectively. Pedruzzi et al. (2022) tested several model configurations, including an alternative land use scheme, and found a WRF tendency to overestimate temperature and humidity in the Brazilian southeastern region. Macedo et al. (2016) also evaluated the model's ability to predict extreme precipitation events. Although the WRF reasonably predicts the main meteorological aspects of the Brazilian southern region, the precipitation extremes were underestimated. A wind mapping study (Souza et al., 2022b) using WRF indicated that the average errors presented by the model in Brazil are minor, with an average bias of 2 m s −1 at 200 m in terms of wind intensity and errors at temperatures of 2 °C and humidity of approximately 10 %. Winds at lower levels tended to be overestimated, whereas PBLH was generally underestimated during the day.
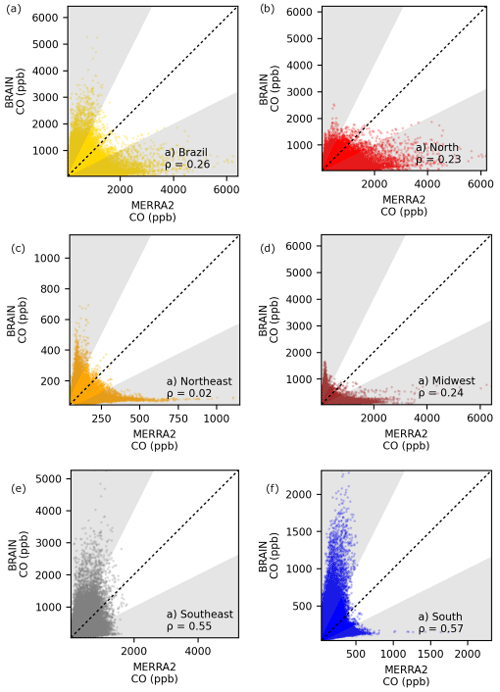
Figure 7 Concentration of CO from BRAIN vs. MERRA-2 in Brazil (a) , northern Brazil (b) , northeastern Brazil (c) , midwestern Brazil (d) , southeastern Brazil (e) , and southern Brazil (f) .
2.3 Air quality
We coupled emissions inventories, WRF, and CMAQ to produce the BRAIN air quality dataset for Brazil. CMAQ version 5.3.2 was set up using the third version of the Carbon Bond 6 chemical mechanism (cb6r3_ae7_aq) (Yarwood et al., 2010; Emery et al., 2015) with AERO7 treatment of secondary organic aerosol for standard cloud chemistry (Appel et al., 2021). The other model configurations used in this work can be found in Sect. S5 and at https://github.com/leohoinaski/CMAQrunner (last access: 8 May 2024). The pollutant list in CMAQ outputs, containing 216 species, can be found in Sect. S6 (Table S3).
The CMAQ standard profile of boundary conditions is used in the larger domain (d01), which provides the boundary conditions for the smaller one (d02). Further improvements to the database could include the boundary conditions derived from the GEOS-Chem model (Bey et al., 2001) ( https://geoschem.github.io/ , last access: 8 May 2024) or other better alternatives for the largest domain. The simulations have 24 h length and a time step interval of 1 h. The last hour of the previous simulation has been set up as the initial condition of the next one. We used the standard profile for the first hour of the first simulation (00:00:00 GMT on 1 January 2019). The figures with the spatial distribution and violations of criteria pollutants can be found in Sect. S7. Section S8 also presents the time series of criteria pollutants in Brazilian cities.
Using the BRAIN air quality dataset, we can observe the highest concentrations of NO 2 (Fig. 3a–b), O 3 (Fig. 3c–d), and PM 10 (Fig. 3e–f) in southeastern and southern Brazil. The concentration of O 3 violates the World Health Organization (WHO) air quality standards in multiple locations all over the country, while for NO 2 and PM 10 , this occurred mostly in southeastern and southern Brazil.
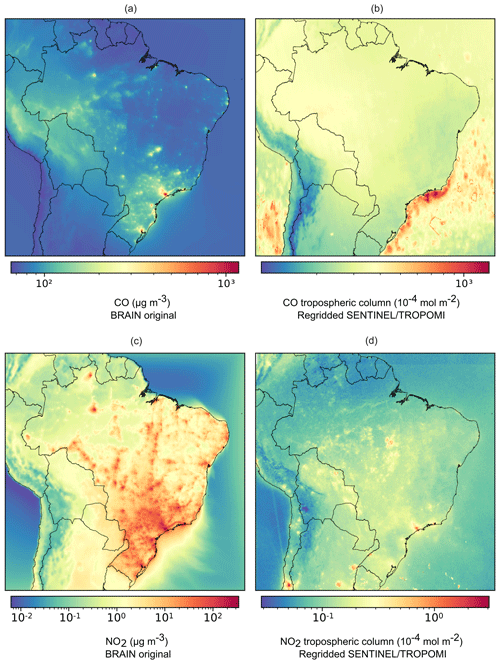
Figure 8 Annual average concentration of CO and NO 2 from BRAIN at its original resolution (a, c) and from Sentinel-5P TROPOMI spatially aligned to the BRAIN resolution (b, d) .
2.3.1 Models' performance
We sampled pixels around the monitoring station using a buffer of 0.5° to calculate the Spearman rank, bias, root mean squared error (RMSE), and mean absolute error (MAE) of the sampled pixels. We selected the highest Spearman rank of each pixel to demonstrate the model's performance in Figs. 4 and 5. Section S10 presents the box plots with overall statistical metrics for all stations. Section S11 presents statistical metrics by means of a monitoring station and pollutant, considering the pixel with the highest Spearman rank around each monitoring station. Section S12 presents the scatter plots comparing the BRAIN air quality dataset and the observations of each monitoring station. We used the simulations with domain d01 in the statistical analysis.
We observed the highest Spearman rank (0.72) in the state of São Paulo for O 3 concentration. Bias analysis revealed an underestimation in the São Paulo metropolitan area, while an overestimation occurred in Minas Gerais, Santa Catarina, Rio Grande do Sul, and the interior of São Paulo. In the northeast and in the state of Espírito Santo, bias is closer to zero. In Rio de Janeiro, the model over- and underestimated the observations. Regarding RMSE and MAE, the model performed better in coastal areas (maps in Fig. 4).
Comparing the states with air quality monitoring stations, the Spearman correlation of the O 3 dataset of BRAIN is higher in São Paulo, Minas Gerais, and Rio de Janeiro. However, these states also have a higher range of bias values, which could be negative and positive in São Paulo and Rio de Janeiro and are only positive in Minas Gerais (box plots in Fig. 4).
The heterogeneity in the stations' types and the insufficient spatial representativeness of observations in the Brazilian states must be considered while evaluating the model performance. According to the IEMA (2022), the strategic planning for the implementation of air quality monitoring stations, the financing and political efforts, and the technical characteristics (from installation to calibration and maintenance) vary significantly between Brazilian states. The lack of data quality assurance may compromise the credibility of the available air quality observations in Brazil.
BRAIN reproduced well the concentrations in moderately urbanized areas, such as Limeira and Piracicaba (Sect. S12). The database reached moderate performance in highly urbanized areas such as Copacabana and Rio de Janeiro (RJ) and at Marginal Tietê in the megacity of São Paulo (Sect. S12). Regarding the temporal profiles of O 3 and PM 10 , the seasonal and daily profiles are captured for both modeled pollutants, showing a suitable fit with the observations at the Limeira and Pecém Industrial and Port Complex (CIPP) air quality monitoring stations (Fig. 5). This reveals that the database can capture temporal patterns of air pollutant concentrations in urbanized and industrialized areas.
Figures with statistical metrics for other pollutants can be found in Sect. S13. Figures of modeled and observed time series for all monitoring stations can be found in Sect. S14.
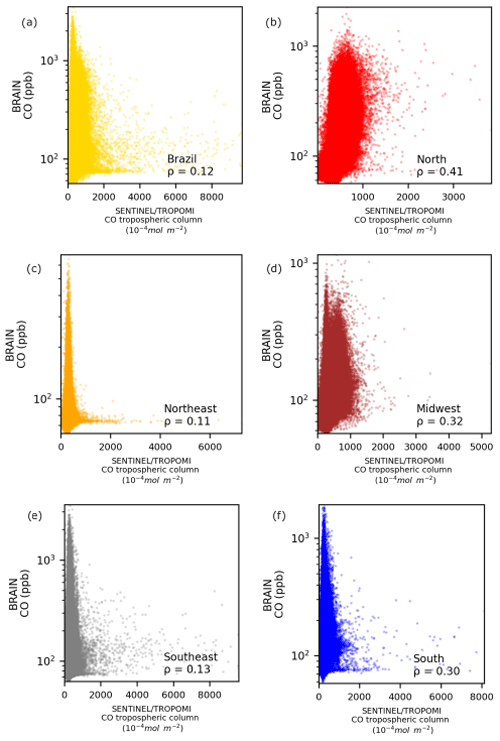
Figure 9 Concentration of CO from BRAIN vs. Sentinel-5P TROPOMI in Brazil (a) , northern Brazil (b) , northeastern Brazil (c) , midwestern Brazil (d) , southeastern Brazil (e) , and southern Brazil (f) .
Overall, the average concentrations are well simulated by CMAQ in BRAIN, with fair to good correlations (up to ∼ 0.7) between modeling and local measurements in São Paulo. Similar results have been reported by Albuquerque et al. (2018). Kitagawa et al. (2021) simulated PM 2.5 in a Brazilian coastal–urban area and showed that the CMAQ results commonly overestimated the observations, which agrees with the BRAIN air quality dataset. In another comparison between observations and CMAQ simulations (Kitagawa et al., 2022), the model overestimated the PM and NO 2 concentrations in the metropolitan region of Vitória (MRV) and underestimated O 3 . The authors suggest that the CMAQ simulations are suitable over the MRV, even though the model could not capture some local variabilities in air pollutant concentrations. It is already reported that the short-time abrupt variations are difficult to reproduce by means of air quality models (Albuquerque et al., 2018). The complex task of predicting air quality is associated with multiple error factors, including the lack of an emissions inventory, meteorology parameterizations, initial and boundary conditions, chemical mechanisms, numerical routines, etc. (Cheng et al., 2019; Albuquerque et al., 2018; Park et al., 2006; Pedruzzi et al., 2019).
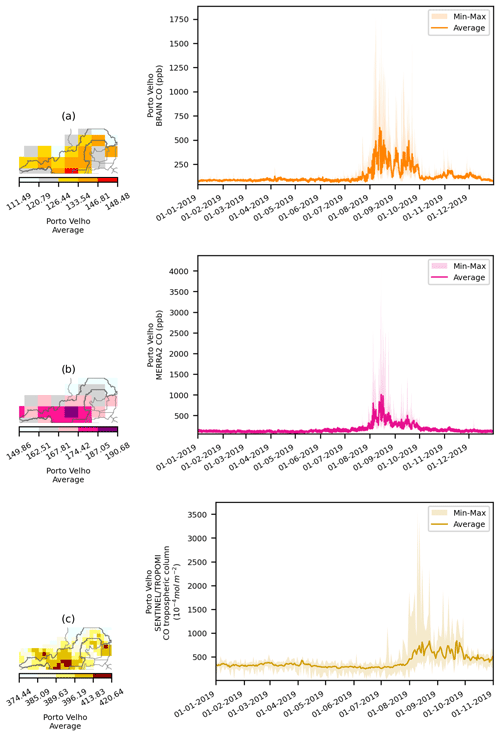
Figure 10 Annual average and hourly time series of CO from BRAIN (a) , MERRA-2 (b) , and Sentinel-5P TROPOMI (daily averages) (c) in Porto Velho, Brazil.
We analyzed the performances of 4×4 km simulations for CO, NO 2 , O 3 , and SO 2 , drawing a buffer of 0.5° degrees around monitoring station positions in southern Brazil. Our findings indicated higher Spearman values for the spatial resolution of 20×20 km for CO, O 3 , and SO 2 . Specifically, for O 3 , the best result at 20×20 km was 0.76, whereas the same point at 4×4 km resolution showed a correlation of 0.46. This pattern was also observed for CO, with the best result at 20×20 km being a Spearman value of 0.47 and 0.23 at the same point at 4×4 km resolution. The smallest differences in Spearman rank were observed for SO 2 (0.22: 20×20 , 0.19: 4×4 ). Even though improving the spatial resolution did not increase the correlation with measured data, we found the best results for bias, RMSE, and MAE for almost all pollutants at a 4×4 km resolution, except for CO. Please refer to Sect. S15 for the complete statistical analysis of 4×4 km simulations.
BRAIN captures seasonal patterns and the absolute magnitude of PM 2.5 in the northwest of the Amazonas state (near the Amazon Tall Tower Observatory – ATTO), as presented by Artaxo et al. (2013). This shows that our database can reproduce the concentrations in background areas (far from highly urbanized centers). Comparing BRAIN with observations at heavily biomass-burning-impacted sites in southwestern Amazonia (Porto Velho) (Artaxo et al., 2013) revealed that BRAIN can capture seasonal variations caused by wet and dry seasons, as well as the magnitude of average and peak concentrations. However, BRAIN PM 2.5 estimates are closer to the coarse mode of the time series rather than the fine mode shown in Artaxo et al. (2013). Even though BRAIN has captured the O 3 pattern observed by Artaxo et al. (2013), the estimates are around 2.7 times higher than the observations in the dry season and a factor of 2 higher for the wet season. It is worth mentioning that BRAIN uses 2019 data, while the study by Artaxo et al. (2013) consists of a sampling campaign from 2008 to 2012.
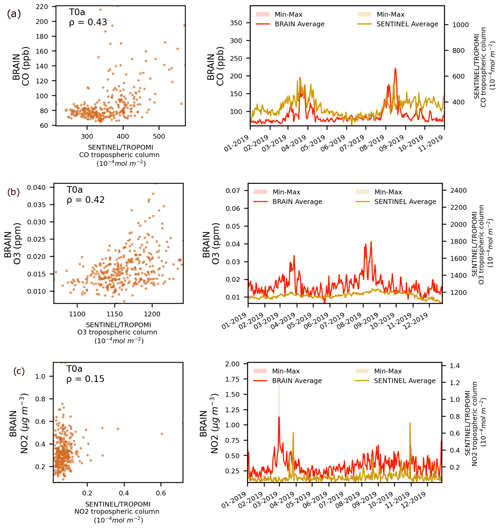
Figure 11 Scatter plot and daily time series of CO (a) , O 3 (b) , and NO 2 (c) from BRAIN and Sentinel-5P TROPOMI at T0a (GoAmazon reference). Values extracted using a buffer of 0.2° around the site.
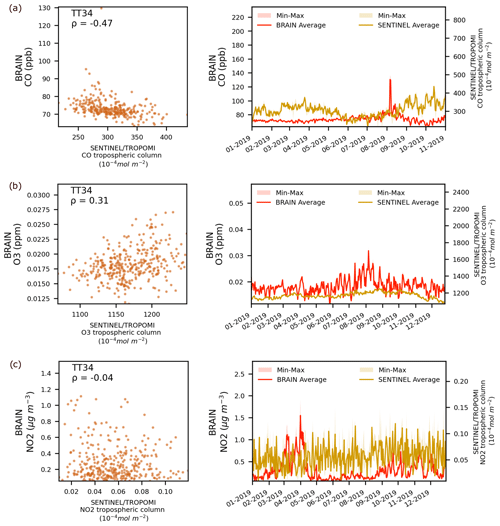
Figure 12 Scatterplot and daily time series of CO, O 3 , and NO 2 from BRAIN and Sentinel-5P TROPOMI at T0t/TT34 (GoAmazon reference). Values extracted using a buffer of 0.2° around the site.
BRAIN has a similar spatial pattern compared with MERRA-2 (GMAO, 2015a b), capturing hotspots in higher populated areas located in the southeast, south, and midwest. In the Amazon region, BRAIN can also capture hotspots similarly to MERRA-2 (Fig. 6). BRAIN estimates for carbon monoxide are lower than those of MERRA-2, except in the southern region and in some urban centers in the southeast and midwest (Fig. 6). Carbon monoxide concentrations estimated by BRAIN are moderately correlated with MERRA-2, mainly in the south (0.57) and southeast (0.55), while in the midwest, north, and northeast, the correlation is weaker (Fig. 7). Compared with the consolidated MERRA-2 database, BRAIN has the advantage since it uses local and more refined information and provides data at a higher spatial resolution for multiple species. We provide a detailed comparison between the MERRA-2 and BRAIN datasets for PM 2.5 , SO 2 , O 3 , and CO in Sect. S16.
We also compare our database with Sentinel-5P TROPOMI (Veefkind et al., 2012) data to demonstrate BRAIN's ability to capture the spatiotemporal variability of air pollutants in unmonitored areas (Fig. 8). We spatially realign Sentinel-5P TROPOMI products to the BRAIN resolution ( 20×20 km) using data from the NASA Goddard Earth Sciences Data and Information Services Center (GES-DISC) ( https://disc.gsfc.nasa.gov/ , last access: 8 May 2024). We merged all layers of the same day and interpolated them to match the BRAIN resolution. We computed the daily averages for both datasets. In this evaluation, we must consider the differences between the datasets since Sentinel-5P TROPOMI relies on tropospheric column measurements and BRAIN surface concentrations. BRAIN captured the hotspots of CO and NO 2 similarly to Sentinel-5P TROPOMI products, especially in southeastern Brazil. However, the hotspots of CO are dislocated towards the ocean in Sentinel-5P TROPOMI. NO 2 estimates from BRAIN present a higher number of hotspots. We emphasize that surface concentration data are more suitable than tropospheric column data in representing air quality. In this analysis, we removed negative values from Sentinel-5P TROPOMI products since they represent low-quality measurements (Eskes et al., 2022).
When we compared CO daily datasets from BRAIN and Sentinel-5P TROPOMI by Brazilian regions, we observed a moderate correlation in the north (0.41), midwest (0.32), and south (0.3). This analysis shows that BRAIN can reasonably detect temporal and spatial patterns of air pollutants. The complete comparison of CO and NO 2 from Sentinel-5P TROPOMI and BRAIN can be found in Sect. S17.
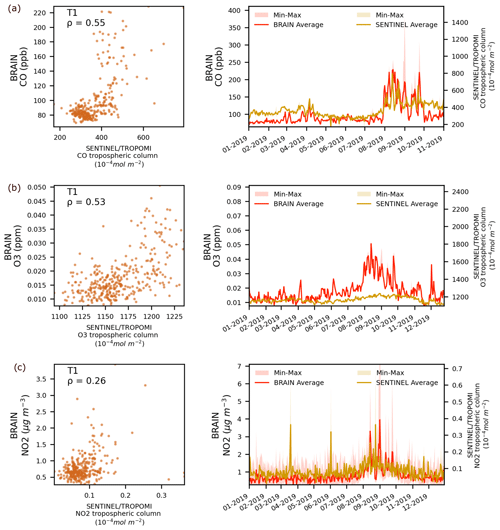
Figure 13 Scatter plot and daily time series of CO (a) , O 3 (b) , and NO 2 (c) from BRAIN and Sentinel-5P TROPOMI at T1 (GoAmazon reference). Values extracted using a buffer of 0.2° around the site.
We highlight that BRAIN, MERRA-2, and Sentinel-5P TROPOMI can capture similar temporal patterns of air pollutant concentrations in heavily biomass-burning-impacted sites such as Porto Velho in Rondônia (Fig. 10) and in urban areas such as São Paulo. We provide time series (minimum–maximum and average) of BRAIN, MERRA-2, and Sentinel-5P TROPOMI data spatially averaged within Brazilian capitals in Sect. S18. Section S19 contains time series (only average) of BRAIN data in Brazilian capitals.
To analyze BRAIN's performance in background regions (with low anthropogenic influence), we extracted data from two forested sites in the Brazilian northern region. As reference, we used the sampling sites of the GoAmazon experiment (Martin et al., 2016), named T0a (forested site situated 154.1 km from the Manaus urban area) and T0t/TT34 (a broken-canopy forested site situated 60.9 km from the city of Manaus). Sentinel-5P TROPOMI data spatially aligned to the BRAIN resolution were also extracted for comparison. A buffer of 0.2° around the sites selected the data of CO, O 3 , and NO 2 from both datasets. The results revealed that BRAIN captured the seasonal profile at T0a (Fig. 11), showing a moderate correlation with the tropospheric column measurements of Sentinel-5P TROPOMI, especially for CO and O 3 .
BRAIN estimates are slightly higher than the observed concentrations in background areas of CO, O 3 , and NO 2 in TT34 (Fig. 12) and T0a (Fig. 11). While O 3 concentrations simulated by BRAIN range around 18 ppb (average in 2019) at the TT34 site, observed concentrations in 2013 (Artaxo et al., 2013) were around 8.5 ppb ± 1.9 ppb. In T0a, BRAIN simulated concentrations around 16 ppb, overestimating the observations (7 ppb ± 2 ppb during the wet season from March to April 2013–2020) (Nascimento et al., 2022). Concerning CO, the concentrations simulated by BRAIN are slightly lower, ranging around 73 ppb (average) at TT34 compared to the 130 ppb observed during the GoAmazon experiment from 2010 to 2011 (Artaxo et al., 2013). We emphasize that the BRAIN and GoAmazon datasets are reported in different periods and, consequently, are influenced by different emission rates. For instance, fire emissions have changed significantly since 2011 in the Amazon (Copernicus, 2022; Naus et al., 2022).
We also analyzed BRAIN results in the Manaus urban area. We adopted the sampling site of the GoAmazon experiment (Martin et al., 2016) named T1 (INPA campus in Manaus). Compared with Sentinel-5P TROPOMI data, BRAIN reproduced fairly the temporal pattern of CO, O 3 , and NO 2 in the T1 site (Fig. 13). Abou Rafee et al. (2017) reported mean concentrations of 88.7 ppb for NO x and 382.6 pbb for CO in the Manaus urban area, while BRAIN reached 79 and 99 ppb (maximum of 383 ppb), revealing an underestimation in this area. Again, the sampling campaign presented by Abou Rafee et al. (2017) and the BRAIN simulations use different base years. Comparing BRAIN at T0a/TT34 (background sites) and T1 (urbanized), the database has reached consistent results, with lower concentration levels in preserved areas.
The inability to better predict the observations is mostly due to the quality of the emissions inventory. The lack of information on industrial emissions and their temporal variability is an important source of errors. Moreover, the vehicular emissions inventory also needs improvements to properly disaggregate the emissions in high-flow roads. Future versions of BRAIN could address these issues and incorporate other emission sources.
Emission data are available at https://doi.org/10.57760/sciencedb.09858 (Hoinaski et al., 2023a) and https://doi.org/10.57760/sciencedb.09886 (Hoinaski et al., 2023b). Meteorology data are available at https://doi.org/10.57760/sciencedb.09857 (Hoinaski and Will, 2023a) and https://doi.org/10.57760/sciencedb.09885 (Hoinaski and Will, 2023c). Air quality data are available at https://doi.org/10.57760/sciencedb.09859 (Hoinaski and Will, 2023b) and https://doi.org/10.57760/sciencedb.09884 (Hoinaski and Will, 2023d).
Code to generate the database, statistics, and figures is available at https://github.com/leohoinaski/CMAQrunner (last access: 8 May 2024) or https://doi.org/10.5281/zenodo.11166975 (Hoinaski, 2024b) and https://github.com/leohoinaski/IND_Inventory (last access: 8 May 2024) or https://doi.org/10.5281/zenodo.11167115 (Hoinaski, 2024a).
In this paper, we present BRAIN, the first comprehensive database for air quality management in Brazil. BRAIN provides emissions, meteorology, and air quality datasets for the entire country at a reliable spatiotemporal resolution. The BRAIN database covers a wide range of pollutant species (emissions and ambient concentrations) and atmospheric variables. So far, Brazil has lacked a comprehensive and easily accessible database for developing air quality management systems in urbanized and rural areas. This work contributes to overcoming this gap. BRAIN is a step forward toward a good procedure for licensing new sources of air pollution in Brazil.
Using a sample of BRAIN, we observed several violations of WHO air quality recommendations. The violations are not restricted to densely populated areas but also occur in rural ones. This reinforces the need for better air quality policies and a deep restructuring of the environmental agencies' procedures and data management in Brazil.
Compared with observations, the BRAIN air quality dataset has achieved good overall performance in predicting the criteria pollutants. However, there is plenty of room for improvement, mainly in relation to the quality of the emissions inventory. The lack of information on industrial emissions and their temporal variability is an important source of error. Moreover, the vehicular emissions inventory also needs improvements to properly disaggregate the emissions in high-flow roads. Improvements in boundary conditions and the inclusion of emission sources from other Latin American countries could also enhance the CMAQ performance. The influence of long-range transport will be addressed in a future version of the database by implementing boundary contributions from GEOSCHEM and other tools. Future versions of BRAIN could address these issues, incorporate other emission sources, and provide CMAQ outputs using different chemical mechanisms. We envision providing enough data to reproduce the historical pattern and future scenarios of air pollution in Brazil through a web platform to facilitate the access and usage of our database. We believe in an ongoing process that will improve the database.
The supplement related to this article is available online at: https://doi.org/10.5194/essd-16-2385-2024-supplement .
LH designed the methodology and developed the software. LH, RW, and CBR processed the data curation, conducted the formal analysis, and created the figures. LH, RW, and CBR prepared the original draft and revised the paper. LH is the project administrator and laboratory supervisor.
The contact author has declared that none of the authors has any competing interests.
Publisher’s note: Copernicus Publications remains neutral with regard to jurisdictional claims made in the text, published maps, institutional affiliations, or any other geographical representation in this paper. While Copernicus Publications makes every effort to include appropriate place names, the final responsibility lies with the authors.
The authors would like to thank the Secretaria de Estado do Desenvolvimento Econômico Sustentável do governo de Santa Catarina. The authors are grateful for the doctoral scholarships provided by the Coordenação de Aperfeiçoamento de Pessoal de Nível Superior – Brasil (CAPES).
This research has been supported by the Fundação de Amparo à Pesquisa e Inovação do Estado de Santa Catarina (grant no. 2018/TR/499; “Avaliação do impacto das emissões veiculares, queimadas, industriais e naturais na qualidade do ar em Santa Catarina”).
This paper was edited by Jing Wei and reviewed by two anonymous referees.
Abou Rafee, S. A., Martins, L. D., Kawashima, A. B., Almeida, D. S., Morais, M. V. B., Souza, R. V. A., Oliveira, M. B. L., Souza, R. A. F., Medeiros, A. S. S., Urbina, V., Freitas, E. D., Martin, S. T., and Martins, J. A.: Contributions of mobile, stationary and biogenic sources to air pollution in the Amazon rainforest: a numerical study with the WRF-Chem model, Atmos. Chem. Phys., 17, 7977–7995, https://doi.org/10.5194/acp-17-7977-2017 , 2017.
Albuquerque, T. T. A., de Fátima Andrade, M., Ynoue, R. Y., Moreira, D. M., Andreão, W. L., dos Santos, F. S., and Nascimento, E. G. S.: WRF-SMOKE-CMAQ modeling system for air quality evaluation in São Paulo megacity with a 2008 experimental campaign data, Environ. Sci. Pollut. Res., 25, 36555–36569, https://doi.org/10.1007/S11356-018-3583-9 , 2018.
Brazilian National Agency for Oil, Natural Gas and Biofuel (ANP): Vendas de derivados de petróleo e biocombustíveis, https://www.gov.br/anp/pt-br/centrais-de-conteudo/dados-abertos/vendas-de-derivados-de-petroleo-e-biocombustiveis (last access: 8 May 2024).
Appel, K. W., Bash, J. O., Fahey, K. M., Foley, K. M., Gilliam, R. C., Hogrefe, C., Hutzell, W. T., Kang, D., Mathur, R., Murphy, B. N., Napelenok, S. L., Nolte, C. G., Pleim, J. E., Pouliot, G. A., Pye, H. O. T., Ran, L., Roselle, S. J., Sarwar, G., Schwede, D. B., Sidi, F. I., Spero, T. L., and Wong, D. C.: The Community Multiscale Air Quality (CMAQ) model versions 5.3 and 5.3.1: system updates and evaluation, Geosci. Model Dev., 14, 2867–2897, https://doi.org/10.5194/gmd-14-2867-2021 , 2021.
Arfan Ali, Md., Bilal, M., Wang, Y., Nichol, J. E., Mhawish, A., Qiu, Z., de Leeuw, G., Zhang, Y., Zhan, Y., Liao, K., Almazroui, M., Dambul, R., Shahid, S., and Islam, M. N.: Accuracy assessment of CAMS and MERRA-2 reanalysis PM 2.5 and PM 10 concentrations over China, Atmos. Environ., 288, 119297, https://doi.org/10.1016/j.atmosenv.2022.119297 , 2022.
Artaxo, P., Rizzo, L. V., Brito, J. F., Barbosa, H. M., Arana, A., Sena, E. T., Cirino, G. G., Bastos, W., Martin, S. T., and Andreae, M. O.: Atmospheric aerosols in Amazonia and land use change: from natural biogenic to biomass burning conditions, Faraday Discuss., 165, 203–235, https://doi.org/10.1039/C3FD00052D , 2013.
Bey, I., Jacob, D. J., Yantosca, R. M., Logan, J. A., Field, B. D., Fiore, A. M., Li, Q., Liu, H. Y., Mickley, L. J., and Schultz, M. G.: Global modeling of tropospheric chemistry with assimilated meteorology: Model description and evaluation, J. Geophys. Res.-Atmos., 106, 23073–23095, https://doi.org/10.1029/2001JD000807 , 2001.
Bieser, J., Aulinger, A., Matthias, V., Quante, M., and Denier Van Der Gon, H. A. C.: Vertical emission profiles for Europe based on plume rise calculations, Environ. Pollut., 159, 2935–2946, https://doi.org/10.1016/J.ENVPOL.2011.04.030 , 2011.
Briggs, G. A.: Plume Rise: A Critical Survey, https://doi.org/10.2172/4743102 , 1969.
Briggs, G. A.: Plume rise predictions, TENNESSEE, U.S.A., Environ. Res. Labs., 59–111, https://doi.org/10.1007/978-1-935704-23-2_3 , 1975.
Cavalcanti, I. F.: Tempo e clima no Brasil, Oficina de textos, eISBN 978-85-7975-234-6, 2016.
CEIC: Brazil Vehicel Fleet: by Region, https://www.ceicdata.com/en/brazil/vehicle-fleet-by-region (last access: 8 May 2024) 2021.
Cheng, J., Su, J., Cui, T., Li, X., Dong, X., Sun, F., Yang, Y., Tong, D., Zheng, Y., Li, Y., Li, J., Zhang, Q., and He, K.: Dominant role of emission reduction in PM 2.5 air quality improvement in Beijing during 2013–2017: a model-based decomposition analysis, Atmos. Chem. Phys., 19, 6125–6146, https://doi.org/10.5194/acp-19-6125-2019 , 2019.
Copernicus: Wildfires: Amazonas records highest emissions in 20 years, https://atmosphere.copernicus.eu/wildfires-amazonas-records-highest-emissions-20-years (last access: 8 May 2024), 2022.
Crippa, M., Guizzardi, D., Muntean, M., Schaaf, E., Dentener, F., van Aardenne, J. A., Monni, S., Doering, U., Olivier, J. G. J., Pagliari, V., and Janssens-Maenhout, G.: Gridded emissions of air pollutants for the period 1970–2012 within EDGAR v4.3.2, Earth Syst. Sci. Data, 10, 1987–2013, https://doi.org/10.5194/essd-10-1987-2018 , 2018.
Eskes, H., Van Geffen, J., Boersma, F., Eichmann, K.U., Apituley, A., Pedergnana, M., Sneep, M., Veefkind, J.P., and Loyola, D.: Sentinel-5 precursor/TROPOMI Level 2 Product User Manual Nitrogendioxide document number: S5P-KNMI-L2-0021-MA, https://sentinels.copernicus.eu/documents/247904/2474726/Sentinel-5P-Level-2-Product-User-Manual-Nitrogen-Dioxide.pdf/ad25ea4c-3a9a-3067-0d1c-aaa56eb1746b?t=1658312035057 (last access: 8 May 2024), 2022.
Emery, C., Jung, J., Koo, B., and Yarwood, G.: Final Report, Improvements to CAMx Snow Cover Treatments and Carbon Bond Chemical Mechanism for Winter Ozone, Tech. rep., Ramboll Environ, Novato, CA, USA, https://www.camx.com/files/udaq_snowchem_final_6aug15.pdf (last access: 8 May 2024), 2015.
Escobar, H.: Amazon fires clearly linked to deforestation, scientists say, Science, 80, 853, https://doi.org/10.1126/science.365.6456.853 , 2019.
Eyth, A., Strum, M., Murphy, B., Epa, U.S., Shah, T., Shi, Y., Beardsley, R., Yarwood, G., and Houyoux, M.: Speciation Tool User's Guide Speciation Tool User's Guide Version 5.0 Ramboll-Speciation Tool User's Guide, https://www.cmascenter.org/speciation_tool/documentation/5.1/Ramboll_sptool_users_guide_V5.pdf (last access: 8 May 2024), 2020.
Gantt, B., Kelly, J. T., and Bash, J. O.: Updating sea spray aerosol emissions in the Community Multiscale Air Quality (CMAQ) model version 5.0.2, Geosci. Model Dev., 8, 3733–3746, https://doi.org/10.5194/gmd-8-3733-2015 , 2015.
Global Modeling and Assimilation Office (GMAO): MERRA-2 tavg1_2d_chm_Nx: 2d, 1-Hourly, Time-Averaged, Single-Level, Assimilation, Carbon Monoxide and Ozone Diagnostics V5.12.4, Greenbelt, MD, USA, Goddard Earth Sciences Data and Information Services Center (GES DISC) [data set], https://doi.org/10.5067/3RQ5YS674DGQ , 2015a.
Global Modeling and Assimilation Office (GMAO): MERRA-2 tavg1_2d_aer_Nx: 2d, 1-Hourly, Time-averaged, Single-Level, Assimilation, Aerosol Diagnostics V5.12.4, Greenbelt, MD, USA, Goddard Earth Sciences Data and Information Services Center (GES DISC) [data set], https://doi.org/10.5067/KLICLTZ8EM9D , 2015b.
Gordon, M., Makar, P. A., Staebler, R. M., Zhang, J., Akingunola, A., Gong, W., and Li, S.-M.: A comparison of plume rise algorithms to stack plume measurements in the Athabasca oil sands, Atmos. Chem. Phys., 18, 14695–14714, https://doi.org/10.5194/acp-18-14695-2018 , 2018.
Guenther, A. B., Jiang, X., Heald, C. L., Sakulyanontvittaya, T., Duhl, T., Emmons, L. K., and Wang, X.: The Model of Emissions of Gases and Aerosols from Nature version 2.1 (MEGAN2.1): an extended and updated framework for modeling biogenic emissions, Geosci. Model Dev., 5, 1471–1492, https://doi.org/10.5194/gmd-5-1471-2012 , 2012.
Guevara, M., Soret, A., Arévalo, G., Martínez, F., and Baldasano, J. M.: Implementation of plume rise and its impacts on emissions and air quality modelling, Atmos. Environ., 99, 618–629, https://doi.org/10.1016/J.ATMOSENV.2014.10.029 , 2014.
Henderson, B.: [Fire emission pre-processor for CMAQ], finntocmaq, GitHub [code], https://github.com/barronh/finn2cmaq (last access: 8 May 2024), 2022.
Hogrefe, C., Isukapalli, S. S., Tang, X., Georgopoulos, P. G., He, S., Zalewsky, E. E., Hao, W., Ku, J. Y., Key, T., and Sistla, G.: Impact of Biogenic Emission Uncertainties on the Simulated Response of Ozone and Fine Particulate Matter to Anthropogenic Emission Reductions, J. Air Waste Manage., 61, 92–108, https://doi.org/10.3155/1047-3289.61.1.92 , 2011.
Hoinaski, L.: leohoinaski/IND_Inventory: IND2CMAQ_v1.0 (IND2CMAQ_v1.0), Zenodo [code], https://doi.org/10.5281/zenodo.11167115 , 2024a.
Hoinaski, L.: CMAQrunner_v1.0, Zenodo [code], https://doi.org/10.5281/zenodo.11166975 , 2024b.
Hoinaski, L. and Will, R.: Brazilian Atmospheric Inventories – BRAIN version 1: meteorology dataset in Brazil, V1, Science Data Bank [data set], https://doi.org/10.57760/sciencedb.09857 , 2023a.
Hoinaski, L. and Will, R.: Brazilian Atmospheric Inventories – BRAIN version 1: air quality dataset in Brazil, V1, Science Data Bank [data set], https://doi.org/10.57760/sciencedb.09859 , 2023b.
Hoinaski, L., and Will, R.: Brazilian Atmospheric Inventories – BRAIN version 1: meteorology dataset in Southern Brazil, V1, Science Data Bank [data set], https://doi.org/10.57760/sciencedb.09885 , 2023c.
Hoinaski, L. and Will, R.: Brazilian Atmospheric Inventories – BRAIN version 1: air quality dataset in Southern Brazil, V1, Science Data Bank [data set], https://doi.org/10.57760/sciencedb.09884 , 2023d.
Hoinaski, L., Ribeiro, C. B., Santos, O. N., Vasques, T. V., Meotti, B., Will, R., and Rodella, F. H. C.: Avaliação do impacto das emissões veiculares, queimadas, industriais e naturais na qualidade do ar em Santa Catarina, 2020.
Hoinaski, L., Vasques, T. V., Ribeiro, C. B., and Meotti, B.: Multispecies and high-spatiotemporal-resolution database of vehicular emissions in Brazil, Earth Syst. Sci. Data, 14, 2939–2949, https://doi.org/10.5194/essd-14-2939-2022 , 2022.
Hoinaski, L., Will, R., and Ribeiro, C. B.: Brazilian Atmospheric Inventories – BRAIN version 1: emission dataset in Brazil, V1, Science Data Bank [data set], https://doi.org/10.57760/sciencedb.09858 , 2023a.
Hoinaski, L., Will, R., and Ribeiro, C. B.: Brazilian Atmospheric Inventories – BRAIN version 1: emission dataset in Southern Brazil, V1, Science Data Bank [data set], https://doi.org/10.57760/sciencedb.09886 , 2023b.
Inness, A., Ades, M., Agustí-Panareda, A., Barré, J., Benedictow, A., Blechschmidt, A.-M., Dominguez, J. J., Engelen, R., Eskes, H., Flemming, J., Huijnen, V., Jones, L., Kipling, Z., Massart, S., Parrington, M., Peuch, V.-H., Razinger, M., Remy, S., Schulz, M., and Suttie, M.: The CAMS reanalysis of atmospheric composition, Atmos. Chem. Phys., 19, 3515–3556, https://doi.org/10.5194/acp-19-3515-2019 , 2019.
Instituto Brasileiro de Geografia e Estatística (IBGE): Brasil em síntese, https://brasilemsintese.ibge.gov.br/territorio.html , last access: 8 May 2024.
Instituto de Energia e Meio Ambiente (IEMA): Recomendações para a expansão e a continuidade das redes de monitoramento da qualidade do ar no Brasil, https://energiaeambiente.org.br/wp-content/uploads/2022/07/IEMA_policypaper_qualidadedoar.pdf (last access: 8 May 2024), 2022.
Instituto de Energia e Meio Ambiente (IEMA): Plataforma de qualidade do ar, https://energiaeambiente.org.br/qualidadedoar/ , last access: 8 May 2024.
Kawashima, A. B., Martins, L. D., Abou Rafee, S. A., Rudke, A. P., de Morais, M. V., and Martins, J. A.: Development of a spatialized atmospheric emission inventory for the main industrial sources in Brazil, Environ. Sci. Pollut. Res., 27, 35941–35951, https://doi.org/10.1007/S11356-020-08281-7 , 2020.
Kitagawa, Y. K. L., Pedruzzi, R., Galvão, E. S., de Araújo, I. B., de Almeira Alburquerque, T. T., Kumar, P., Nascimento, E. G. S., and Moreira, D. M.: Source apportionment modelling of PM 2.5 using CMAQ-ISAM over a tropical coastal-urban area, Atmos. Pollut. Res., 12, 101250, https://doi.org/10.1016/J.APR.2021.101250 , 2021.
Kitagawa, Y. K. L., Kumar, P., Galvão, E. S., Santos, J. M., Reis, N. C., Nascimento, E. G. S., and Moreira, D. M.: Exposure and dose assessment of school children to air pollutants in a tropical coastal-urban area, Sci. Total Environ., 803, 149747, https://doi.org/10.1016/J.SCITOTENV.2021.149747 , 2022.
Kota, S. H., Schade, G., Estes, M., Boyer, D., and Ying, Q.: Evaluation of MEGAN predicted biogenic isoprene emissions at urban locations in Southeast Texas, Atmos. Environ., 110, 54–64, https://doi.org/10.1016/J.ATMOSENV.2015.03.027 , 2015.
Levy, R. and Hsu, C.: MODIS Atmosphere L2 Aerosol Product, NASA MODIS Adaptive Processing System, Goddard Space Flight Center, USA [data set], https://doi.org/10.5067/MODIS/MOD04_L2.006 , 2015.
Macedo, L. R., Basso, J. L. M., Yamasaki, Y., Macedo, L. R., Basso, J. L. M., and Yamasaki, Y.: Evaluation of the WRF Weather Forecasts over the Southern Region of Brazil, Am. J. Clim. Chang., 5, 103–115, https://doi.org/10.4236/AJCC.2016.51011 , 2016.
Makri, A. and Stilianakis, N. I.: Vulnerability to air pollution health effects, Int. J. Hyg. Environ. Health, 211, 326–336, https://doi.org/10.1016/J.IJHEH.2007.06.005 , 2008.
Martin, S. T., Artaxo, P., Machado, L. A. T., Manzi, A. O., Souza, R. A. F., Schumacher, C., Wang, J., Andreae, M. O., Barbosa, H. M. J., Fan, J., Fisch, G., Goldstein, A. H., Guenther, A., Jimenez, J. L., Pöschl, U., Silva Dias, M. A., Smith, J. N., and Wendisch, M.: Introduction: Observations and Modeling of the Green Ocean Amazon (GoAmazon2014/5), Atmos. Chem. Phys., 16, 4785–4797, https://doi.org/10.5194/acp-16-4785-2016 , 2016.
Mendonça, F. and Danni-Oliveira, I. M.: Climatologia: noções básicas e climas do Brasil, Oficina de textos, eISBN 978-85-7975-114-1, 2017.
Ministério de Minas e Energia (MME): Dados estatísticos, https://www.gov.br/anp/pt-br/centrais-de-conteudo/dados-estatisticos (last access: 8 May 2024).
Ministério do Meio Ambiente (MMA): Inventário Nacional de Emissões Atmosféricas por Veículos Automotores Rodoviários 2013, https://antigo.mma.gov.br/images/arquivo/80060/Inventario_de_Emissoes_por_Veiculos_Rodoviarios_2013.pdf (last access: 8 May 2024), 2014.
National Energy Balance (BEN): Balanço Energético Nacional, https://www.epe.gov.br/pt/publicacoes-dados-abertos/publicacoes/balanco-energetico-nacional-ben , last access: 8 May 2024.
Nascimento, J. P., Barbosa, H. M. J., Banducci, A. L., Rizzo, L. V., Vara-Vela, A. L., Meller, B. B., Gomes, H., Cezar, A., Franco, M. A., Ponczek, M., Wolff S., Bela, M. M., and Artaxo, P.: Major Regional-Scale Production of O 3 and Secondary Organic Aerosol in Remote Amazon Regions from the Dynamics and Photochemistry of Urban and Forest Emissions, Environ. Sci. Technol., 56, 9924–9935, https://doi.org/10.1021/acs.est.2c01358 , 2022.
Naus, S., Domingues, L. G., Krol, M., Luijkx, I. T., Gatti, L. V., Miller, J. B., Gloor, E., Basu, S., Correia, C., Koren, G., Worden, H. M., Flemming, J., Pétron, G., and Peters, W.: Sixteen years of MOPITT satellite data strongly constrain Amazon CO fire emissions, Atmos. Chem. Phys., 22, 14735–14750, https://doi.org/10.5194/acp-22-14735-2022 , 2022.
Nogueira, T., de Souza, K. F., Fornaro, A., de Fatima Andrade, M., and de Carvalho, L. R. F.: On-road emissions of carbonyls from vehicles powered by biofuel blends in traffic tunnels in the Metropolitan Area of Sao Paulo, Brazil, Atmos. Environ., 108, 88–97, https://doi.org/10.1016/J.ATMOSENV.2015.02.064 , 2015.
Organization of Economic Co-operation and Development (OECD): The economic consequences of outdoor air pollution, https://www.oecd.org/env/the-economic-consequences-of-outdoor-air-pollution-9789264257474-en.htm (last access: 8 May 2024), 2016.
Organization of Economic Co-operation and Development (OECD): Air pollution, https://www.oecd.org/environment/air-pollution/ , last access: 8 May 2024.
Park, S. K., Evan Cobb, C., Wade, K., Mulholland, J., Hu, Y., and Russell, A. G.: Uncertainty in air quality model evaluation for particulate matter due to spatial variations in pollutant concentrations, Atmos. Environ., 40, 563–573, https://doi.org/10.1016/J.ATMOSENV.2005.11.078 , 2006.
Pedruzzi, R., Baek, B. H., Henderson, B. H., Aravanis, N., Pinto, J. A., Araujo, I. B., Nascimento, E. G. S., Reis Junior, N. C., Moreira, D. M., and de Almeida Albuquerque, T. T.: Performance evaluation of a photochemical model using different boundary conditions over the urban and industrialized metropolitan area of Vitória, Brazil, Environ. Sci. Pollut. Res., 26, 16125–16144, https://doi.org/10.1007/S11356-019-04953-1 , 2019.
Pedruzzi, R., Andreão, W. L., Baek, B. H., Hudke, A. P., Glotfelty, T. W., Dias de Freitas, E., Martins, J. A., Bowden, J. H., Pinto, J. A., Alonso, M. F., and de Almeida Abuquerque, T. T.: Update of land use/land cover and soil texture for Brazil: Impact on WR F modeling results over São Paulo, Atmos. Environ., 268, 118760, https://doi.org/10.1016/J.ATMOSENV.2021.118760 , 2022.
Pereira, G., Siqueira, R., Rosário, N. E., Longo, K. L., Freitas, S. R., Cardozo, F. S., Kaiser, J. W., and Wooster, M. J.: Assessment of fire emission inventories during the South American Biomass Burning Analysis (SAMBBA) experiment, Atmos. Chem. Phys., 16, 6961–6975, https://doi.org/10.5194/acp-16-6961-2016 , 2016.
Platnick, S., Hubanks, P., Meyer, K., and King, M. D.: MODIS Atmosphere L3 Monthly Product (08_L3), NASA MODIS Adaptive Processing System, Goddard Space Flight Center [data set], https://doi.org/10.5067/MODIS/MOD08_M3.006 , 2015.
Rajão, R., Soares-Filho, B., Nunes, F., Börner, J., Machado, L., Assis, D., Oliveira, A., Pinto, L., Ribeiro, V., Rausch, L., Gibbs, H., and Figueira, D.: The rotten apples of Brazil's agribusiness, Science, 80, 369, 246–248, https://doi.org/10.1126/SCIENCE.ABA6646 , 2020.
Sant'Anna, A., Alencar, A., Araújo, C., Vormittag, E., Wicher, H., Cunha, K. B. da, Faria, M., de Fatima Andrade, M., Porto, P., Artaxo, P., Rocha, R., Simoni, W. De, Pinheiro, B., and Esturba, T.: O Estado da Qualidade do Ar no Brasil, https://www.wribrasil.org.br/sites/default/files/wri-o-estado-da-_qualidade-do-ar-no-brasil.pdf (last access: 8 May 2024), 2021.
Shin, M., Kang, Y., Park, S., Im, J., Yoo, C., and Quackenbush, L. J.: Estimating ground-level particulate matter concentrations using satellite-based data: a review, GISci. Remote Sens., 57, 174–189, https://doi.org/10.1080/15481603.2019.1703288 , 2020.
Skamarock, W. C., Klemp, J. B., Dudhia, J., Gill, D. O., Barker, D., Duda, M. G., and Powers, J. G.: A Description of the Advanced Research WRF Version 3 (No. NCAR/TN-475 + STR), University Corporation for Atmospheric Research, https://doi.org/10.5065/D68S4MVH , 2008.
Silva, S. J., Heald, C. L., and Guenther, A. B.: Development of a reduced-complexity plant canopy physics surrogate model for use in chemical transport models: a case study with GEOS-Chem v12.3.0, Geosci. Model Dev., 13, 2569–2585, https://doi.org/10.5194/gmd-13-2569-2020 , 2020.
Sindelarova, K., Granier, C., Bouarar, I., Guenther, A., Tilmes, S., Stavrakou, T., Müller, J.-F., Kuhn, U., Stefani, P., and Knorr, W.: Global data set of biogenic VOC emissions calculated by the MEGAN model over the last 30 years, Atmos. Chem. Phys., 14, 9317–9341, https://doi.org/10.5194/acp-14-9317-2014 , 2014.
Souza, N. B. P., Cardoso dos Santos, J. V., Sperandio Nascimento, E. G., Bandeira Santos, A. A., and Moreira, D. M.: Long-range correlations of the wind speed in a northeast region of Brazil, Energy, 243, 122742, https://doi.org/10.1016/J.ENERGY.2021.122742 , 2022a.
Souza, N. B. P., Sperandio, N. E. G., Santos, A. A. B., and Moreira, D. M.: Wind mapping using the mesoscale WRF model in a tropical region of Brazil, Energy, 240, 122491, https://doi.org/10.1016/J.ENERGY.2021.122491 , 2022b.
United States Environmental Protection Agency (U.S. EPA): Creating an OCEAN file for input to CMAQ, CMAQ, GitHub [code], https://github.com/U.S.EPA/CMAQ/blob/main/DOCS/Users_Guide/Tutorials/CMAQ_UG_tutorial_oceanfile.md (last access: 8 May 2024), 2022.
United States Environmental Protection Agency (U.S. EPA): Air Quality and Climate Change Research, https://www.epa.gov/air-research/air-quality-and-climate-change-research (last access: 8 May 2024), 2023a.
United States Environmental Protection Agency (U.S. EPA): Air Quality System (AQS), https://www.epa.gov/aqs (last access: 8 May 2024), 2023b.
Vasques, T. V., and Hoinaski, L.: Brazilian vehicular emission inventory software – BRAVES, Transp. Res. Part D Transp. Environ., 100, 103041, https://doi.org/10.1016/J.TRD.2021.103041 , 2021.
Veefkind, J. P., Aben, I., McMullan, K., Förster, H., de Vries, J., Otter, G., Claas, J., Eskes, H. J., de Haan, J. F., Kleipool, Q., van Weele, M., Hasekamp, O., Hoogeveen, R., Landgraf, J., Snel, R., Tol, P., Ingmann, P., Voors, R., Kruizinga, B., Vink, R., Visser, H., and Levelt, P. F.: TROPOMI on the ESA Sentinel-5 Precursor: A GMES mission for global observations of the atmospheric composition for climate, air quality and ozone layer applications, Remote Sens. Environ., 120, 70–83, https://doi.org/10.1016/j.rse.2011.09.027 , 2012.
Vongruang, P., Wongwises, P., and Pimonsree, S.: Assessment of fire emission inventories for simulating particulate matter in Upper Southeast Asia using WRF-CMAQ, Atmos. Pollut. Res., 8, 921–929, https://doi.org/10.1016/J.APR.2017.03.004 , 2017.
Wiedinmyer, C., Akagi, S. K., Yokelson, R. J., Emmons, L. K., Al-Saadi, J. A., Orlando, J. J., and Soja, A. J.: The Fire INventory from NCAR (FINN): a high resolution global model to estimate the emissions from open burning, Geosci. Model Dev., 4, 625–641, https://doi.org/10.5194/gmd-4-625-2011 , 2011.
Wiedinmyer, C., Kimura, Y., McDonald-Buller, E. C., Emmons, L. K., Buchholz, R. R., Tang, W., Seto, K., Joseph, M. B., Barsanti, K. C., Carlton, A. G., and Yokelson, R.: The Fire Inventory from NCAR version 2.5: an updated global fire emissions model for climate and chemistry applications, Geosci. Model Dev., 16, 3873–3891, https://doi.org/10.5194/gmd-16-3873-2023 , 2023.
Yarwood, G., Jung, J., Whitten, G., Heo, G., Mellberg, J., and Estes, M..: Updates to the Carbon Bond Mechanism for Version 6 (CB6), in: 9th Annual CMAS Conference, Chapel Hill, NC, 11–13, 1-4, https://www.cmascenter.org/conference/2010/abstracts/emery_updates_carbon_2010.pdf (last access: 8 May 2024), 2010.
- Introduction
- BRAIN database
- Data availability
- Code availability
- Author contributions
- Competing interests
- Acknowledgements
- Financial support
- Review statement
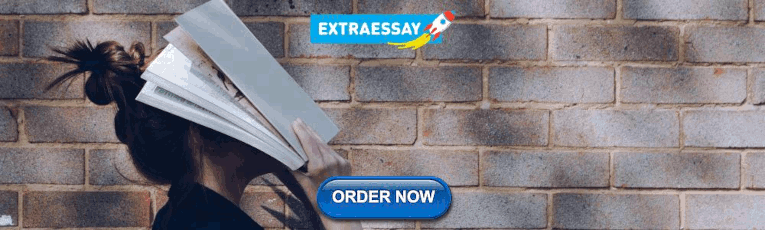
IMAGES
VIDEO
COMMENTS
2.4.2. Inventory management practices and organizational competitiveness. Inventory management is very crucial to any organization that is improving on its performance and attaining high levels of customer satisfaction. According to Nzuza (Citation 2015), the material held by an organization makes up for most of the organization assets. Most ...
Inventory is a central management function. It is a cornerstone of supply chain management and logistics in the material management system. Depending on the organisational objectives, inventories ...
PDF | On Mar 1, 2021, Cinthya Vanessa Munoz Macas and others published Inventory management for retail companies: A literature review and current trends | Find, read and cite all the research you ...
Inventory management (IM) is an essential component of the Supply Chain (SC). ... This paper reviews part of the work carried out, dealing with the optimization of the IM problem. ... B.D., Tokar, T.: A review of inventory management research in major logistics journals: themes and future directions. Int. J. Logist. Manage. 19, 212-232 (2008).
The aim of this article is to provide a comprehensive overview of the current and future research potential of inventory management through a systematic literature review of articles from 2012 to 2022. Inventory management and related AI techniques are categorized and presented in a way that facilitates orientation for researchers in the field.
This research covers five years, between 2015 and 2019, focusing specifically on the retail sector. The primary outcomes of this study are the leading inventory management systems and models, the Key Performance Indicators (KPIs) for their correct management, and the benefits and challenges for choosing or adopting an efficient inventory ...
The aim of this research is to examine the connection between inventory management practices (IMP) and their impact on the operational performance (OP) of SMEs. This investigation is conducted through a systematic literature review, with a specific focus on the perspective of supply chain and Industry 5.0 technology. This study uses bibliometric analysis and Scopus to extract and process ...
This paper delves into the multifaceted role of Artificial Intelligence (AI) in inventory management, encompassing its applications, challenges, and future opportunities. AI's integration into ...
Despite the widespread adoption of technology in inventory management, empirical research that examines the effect of these technologies is scarce. Does IT capability really have a positive effect on the inventory management? This paper empirically studies the usage of information technology by the definition of IT capability.
Inventory represents one of the most important assets of a business. The paper makes an attempt to understand the importance of inventory, Inventory issue and then to present a conceptual methodology for inventory issue. The objective of this paper are:- 1. To understand the concepts of inventory and inventory management in supply chain. 2.
In this research, the inventory management variable was measured using two aspects: inventory level control and demand forecasting. ... Hence, one of the significant contributions of this paper is related to the inventory management impacts on PSC performance in terms of quality, cost, and responsiveness. The theoretical contributions of this ...
Effectiveness of inventory management is a vital part in the manufacturing organization to be more competitive. The previous studies have indicated that there are several factors influencing the effectiveness of inventory management in the organization but there is lack of researchers who carried out the research in the manufacturing small medium enterprise in Johor.
Following that, the major findings of the reviewed research are compiled, with a focus on supply chain management, inventory control, and inventory optimization. The paper is concluded with a discussion of the potential possibilities for future research in this area, including the need for a stronger focus on real-world applications and the ...
This study investigated the effect of various inventory management factors on firm's efficiency. These factors included Capacity Utilization, Inventory Accuracy, Lean Inventory, and Stock Availability. Data was collected by the use of liker scale questionnaire from 250 individuals from different departmental stores in Karachi.
This research selects level 2 of the SCOR sourcing process "source make-to-order product (S2)" to modeling, evaluation, and analysis of the performance of our inventory management systems. The detailed codes of the SCOR sourcing processes source make-to-order product (S2) at level 3 are as follows:
Explore the latest full-text research PDFs, articles, conference papers, preprints and more on INVENTORY MANAGEMENT. Find methods information, sources, references or conduct a literature review on ...
An inventory management problem is addressed for a make-to-order supply chain that has inventory holding and/or manufacturing locations at each node. The lead times between nodes and production capacity limits are heterogeneous across the network. This study focuses on a single product, a multi-period centralized system in which a retailer is subject to an uncertain stationary consumer demand ...
The proposed research explores the potential impact of Artificial Intelligence (AI) on inventory management practices and their effect on organizational profitability. The study evaluates various AI techniques such as predictive analytics, machine learning, and computer vision and their role in reducing stock levels, improving demand forecasting accuracy, and reducing stock-out incidents. The ...
Second, logistics researchers have more recently focused on examining inventory management through collaborative models., - This paper catalogs the inventory management articles published in the major logistics journals, facilitates the awareness and appreciation of such work, and stands to guide future inventory management research by ...
monly used methods in inventory management are iden-tied. 4 Future research directions in the eld of inventory man-agement are identied. The article is organized as follows: After the Sect. 1, information regarding the research methodology is given in Sect. 2. The literature review and related work evalua-tion strategy are described in Sect. 3.
Search 218,418,368 papers from all fields of science. Search. Sign In Create Free ... , title={Effect of Healthcare Inventory Management Practices on the Performance of Pharmaceutical Companies' in Rwanda; A Case of Selected Retail Pharmacies in Gasabo District}, author={}, journal={Journal of Procurement \& Supply Chain}, year={2024 ...
Abstract. This research paper introduces an innovative Inventory Management System (IMS) designed to address common inventory challenges. The IMS leverages advanced technology, including barcode ...
Research on community forests (CFs), primarily governed and managed by local forest users in the United States, is limited, despite their growth in numbers over the past decade. We conducted a survey to inventory CFs in the United States and better understand their ownership and governance structures, management objectives, benefits, and financing.
Abstract. Developing air quality management systems to control the impacts of air pollution requires reliable data. However, current initiatives do not provide datasets with large spatial and temporal resolutions for developing air pollution policies in Brazil. Here, we introduce the Brazilian Atmospheric Inventories (BRAIN), the first comprehensive database of air quality and its drivers in ...
This paper provides a nontechnical survey of the status of inventory management systems. The central theme is an enumeration of practical problems that need research and analytic attention.
Access the portal of NASS, the official source of agricultural data and statistics in the US, and explore various reports and products.
Case Study. 1* Nazar Sohail, Krukshetra University. 2 Tariq Hussain Sheikh, Govt. Degree college Poonch, [email protected]. Abstract--- Inventory management is a challenging problem area ...