
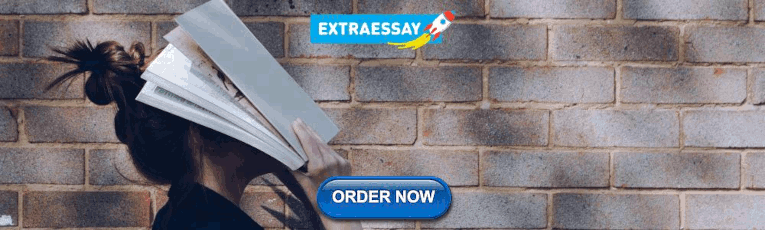
How to Use Creative Data Visualization Techniques for Easy Comprehension of Qualitative Research
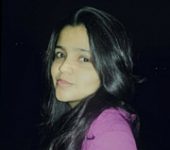
“A picture is worth a thousand words!”—an adage used so often stands true even whilst reporting your research data. Research studies with overwhelming data can perhaps be difficult to comprehend by some readers or can even be time-consuming. While presenting quantitative research data becomes easier with the help of graphs, pie charts, etc. researchers face an undeniable challenge whilst presenting qualitative research data. In this article, we will elaborate on effectively presenting qualitative research using data visualization techniques .
Table of Contents
What is Data Visualization?
Data visualization is the process of converting textual information into graphical and illustrative representations. It is imperative to think beyond numbers to get a holistic and comprehensive understanding of research data. Hence, this technique is adopted to help presenters communicate relevant research data in a way that’s easy for the viewer to interpret and draw conclusions.
What Is the Importance of Data Visualization in Qualitative Research?
According to the form in which the data is collected and expressed, it is broadly divided into qualitative data and quantitative data. Quantitative data expresses the size or quantity of data in a countable integer. Unlike quantitative data, qualitative data cannot be expressed in continuous integer values; it refers to data values described in the non-numeric form related to subjects, places, things, events, activities, or concepts.
What Are the Advantages of Good Data Visualization Techniques?
Excellent data visualization techniques have several benefits:
- Human eyes are often drawn to patterns and colors. Moreover, in this age of Big Data , visualization can be considered an asset to quickly and easily comprehend large amounts of data generated in a research study.
- Enables viewers to recognize emerging trends and accelerate their response time on the basis of what is seen and assimilated.
- Illustrations make it easier to identify correlated parameters.
- Allows the presenter to narrate a story whilst helping the viewer understand the data and draw conclusions from it.
- As humans can process visual images better than texts, data visualization techniques enable viewers to remember them for a longer time.
Different Types of Data Visualization Techniques in Qualitative Research
Here are several data visualization techniques for presenting qualitative data for better comprehension of research data.
1. Word Clouds
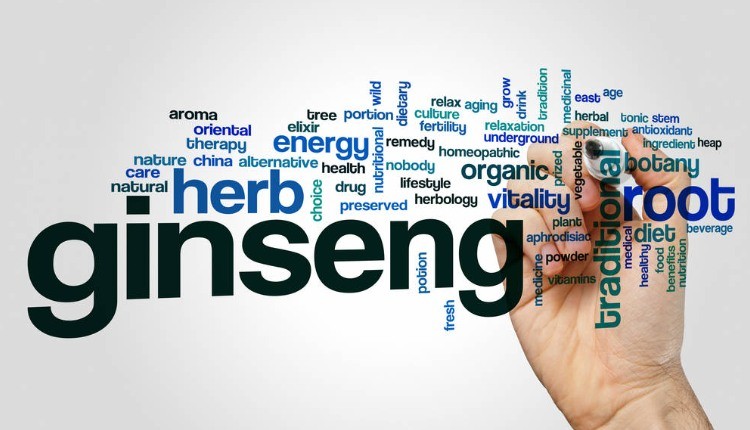
- Word Clouds is a type of data visualization technique which helps in visualizing one-word descriptions.
- It is a single image composing multiple words associated with a particular text or subject.
- The size of each word indicates its importance or frequency in the data.
- Wordle and Tagxedo are two majorly used tools to create word clouds.
2. Graphic Timelines
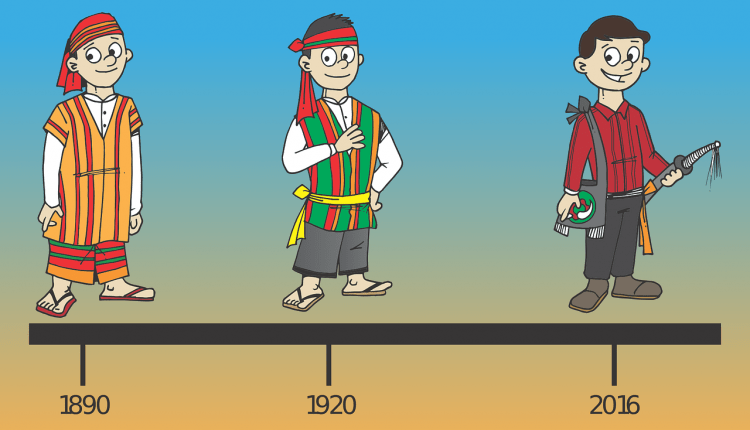
- Graphic timelines are created to present regular text-based timelines with pictorial illustrations or diagrams, photos, and other images.
- It visually displays a series of events in chronological order on a timescale.
- Furthermore, showcasing timelines in a graphical manner makes it easier to understand critical milestones in a study.
3. Icons Beside Descriptions
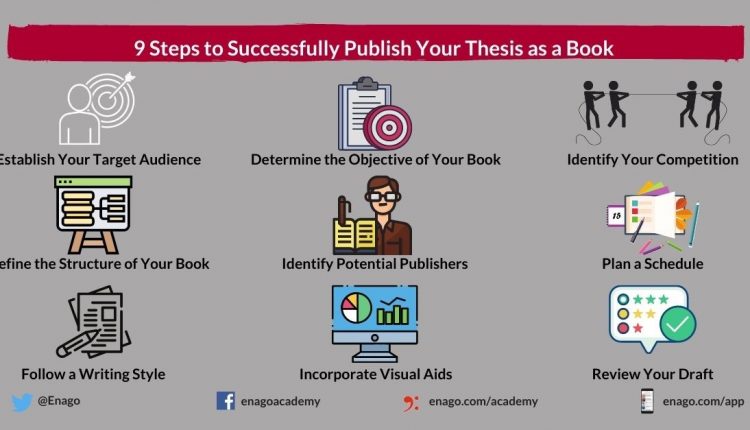
- Rather than writing long descriptive paragraphs, including resembling icons beside brief and concise points enable quick and easy comprehension.
4. Heat Map
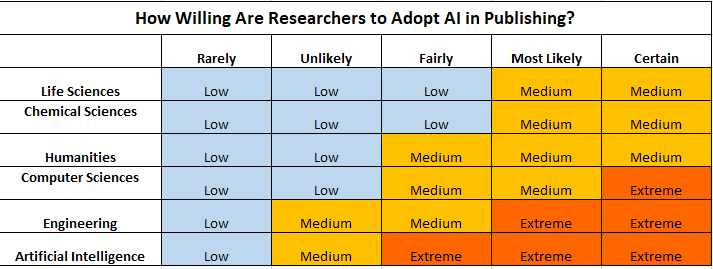
- Using a heat map as a data visualization technique better displays differences in data with color variations.
- The intensity and frequency of data is well addressed with the help of these color codes.
- However, a clear legend must be mentioned alongside the heat map to correctly interpret a heat map.
- Additionally, it also helps identify trends in data.
5. Mind Map
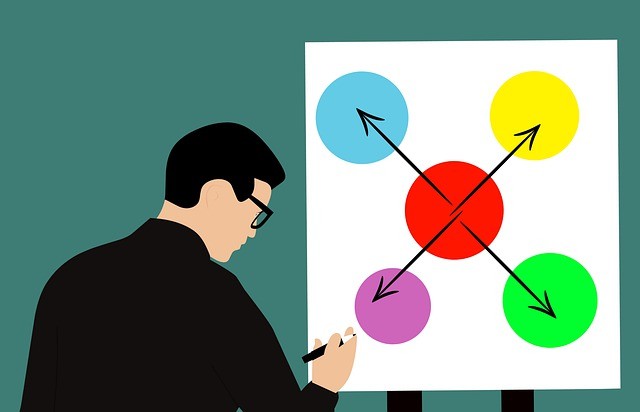
- A mind map helps explain concepts and ideas linked to a central idea.
- Allows visual structuring of ideas without overwhelming the viewer with large amounts of text.
- These can be used to present graphical abstracts
Do’s and Don’ts of Data Visualization Techniques
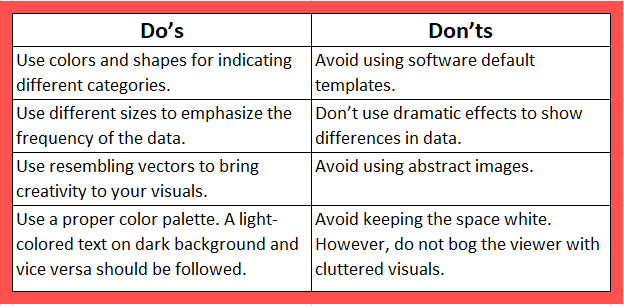
It perhaps is not easy to visualize qualitative data and make it recognizable and comprehensible to viewers at a glance. However, well-visualized qualitative data can be very useful in order to clearly convey the key points to readers and listeners in presentations.
Are you struggling with ways to display your qualitative data? Which data visualization techniques have you used before? Let us know about your experience in the comments section below!

nicely explained
None. And I want to use it from now.
Would it be ideal or suggested to use these techniques to display qualitative data in a thesis perhaps?
Using data visualization techniques in a qualitative research thesis can help convey your findings in a more engaging and comprehensible manner. Here’s a brief overview of how to incorporate data visualization in such a thesis:
Select Relevant Visualizations: Identify the types of data you have (e.g., textual, audio, visual) and the appropriate visualization techniques that can represent your qualitative data effectively. Common options include word clouds, charts, graphs, timelines, and thematic maps.
Data Preparation: Ensure your qualitative data is well-organized and coded appropriately. This might involve using qualitative analysis software like NVivo or Atlas.ti to tag and categorize data.
Create Visualizations: Generate visualizations that illustrate key themes, patterns, or trends within your qualitative data. For example: Word clouds can highlight frequently occurring terms or concepts. Bar charts or histograms can show the distribution of specific themes or categories. Timeline visualizations can help display chronological trends. Concept maps can illustrate the relationships between different concepts or ideas.
Integrate Visualizations into Your Thesis: Incorporate these visualizations within your thesis to complement your narrative. Place them strategically to support your arguments or findings. Include clear and concise captions and labels for each visualization, providing context and explaining their significance.
Interpretation: In the text of your thesis, interpret the visualizations. Explain what patterns or insights they reveal about your qualitative data. Offer meaningful insights and connections between the visuals and your research questions or hypotheses.
Maintain Consistency: Maintain a consistent style and formatting for your visualizations throughout the thesis. This ensures clarity and professionalism.
Ethical Considerations: If your qualitative research involves sensitive or personal data, consider ethical guidelines and privacy concerns when presenting visualizations. Anonymize or protect sensitive information as needed.
Review and Refinement: Before finalizing your thesis, review the visualizations for accuracy and clarity. Seek feedback from peers or advisors to ensure they effectively convey your qualitative findings.
Appendices: If you have a large number of visualizations or detailed data, consider placing some in appendices. This keeps the main body of your thesis uncluttered while providing interested readers with supplementary information.
Cite Sources: If you use specific software or tools to create your visualizations, acknowledge and cite them appropriately in your thesis.
Hope you find this helpful. Happy Learning!
Rate this article Cancel Reply
Your email address will not be published.
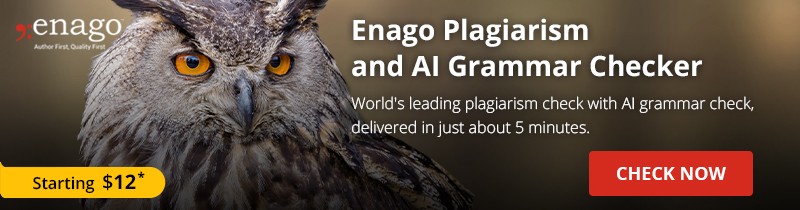
Enago Academy's Most Popular Articles
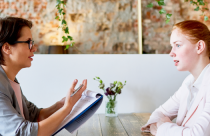
- Reporting Research
Research Interviews: An effective and insightful way of data collection
Research interviews play a pivotal role in collecting data for various academic, scientific, and professional…
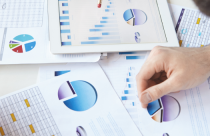
Planning Your Data Collection: Designing methods for effective research
Planning your research is very important to obtain desirable results. In research, the relevance of…
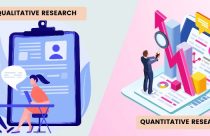
- Manuscript Preparation
- Publishing Research
Qualitative Vs. Quantitative Research — A step-wise guide to conduct research
A research study includes the collection and analysis of data. In quantitative research, the data…
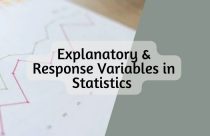
Explanatory & Response Variable in Statistics — A quick guide for early career researchers!
Often researchers have a difficult time choosing the parameters and variables (like explanatory and response…
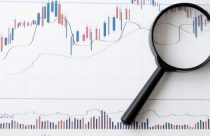
6 Steps to Evaluate the Effectiveness of Statistical Hypothesis Testing
You know what is tragic? Having the potential to complete the research study but not…

Sign-up to read more
Subscribe for free to get unrestricted access to all our resources on research writing and academic publishing including:
- 2000+ blog articles
- 50+ Webinars
- 10+ Expert podcasts
- 50+ Infographics
- 10+ Checklists
- Research Guides
We hate spam too. We promise to protect your privacy and never spam you.
Attachment Preview
0 \vgtccategory Research \authorfooter Ethan Kerzner and Miriah Meyer are with the University of Utah. E-mail: [email protected] and [email protected]. Sarah Goodwin is with the Royal Melbourne Institute of Technology and Monash University. E-mail: [email protected]. Jason Dykes and Sara Jones are with City, University of London. E-mail: [j.dykes,s.v.jones]@city.ac.uk. \shortauthortitle Kerzner et al. : A Framework for Creativity Workshops in Applied Visualization Research
A Framework for Creative Visualization-Opportunities Workshops
Applied visualization researchers often work closely with domain collaborators to explore new and useful applications of visualization. The early stages of collaborations are typically time consuming for all stakeholders as researchers piece together an understanding of domain challenges from disparate discussions and meetings. A number of recent projects, however, report on the use of creative visualization-opportunities (CVO) workshops to accelerate the early stages of applied work, eliciting a wealth of requirements in a few days of focused work. Yet, there is no established guidance for how to use such workshops effectively. In this paper, we present the results of a 2-year collaboration in which we analyzed the use of 17 workshops in 10 visualization contexts. Its primary contribution is a framework for CVO workshops that: 1) identifies a process model for using workshops; 2) describes a structure of what happens within effective workshops; 3) recommends \numberOfGuidelines actionable guidelines for future workshops; and 4) presents an example workshop and workshop methods. The creation of this framework exemplifies the use of critical reflection to learn about visualization in practice from diverse studies and experience.
K.6.1Management of Computing and Information SystemsProject and People ManagementLife Cycle; \CCScat K.7.mThe Computing ProfessionMiscellaneousEthics Introduction
Two key challenges in the early stages of applied visualization research are to find pressing domain problems and to translate them into interesting visualization opportunities. Researchers often discover such problems through a lengthy process of interviews and observations with domain collaborators that can sometimes take months [ 39 , 56 , 80 ] . A number of recent projects, however, report on the use of workshops to characterize domain problems in just a few days of focused work [ 16 , 17 , 18 , 35 , 64 , 88 ] . More specifically, these workshops are creative visualization-opportunities workshops (CVO workshops) , in which researchers and their collaborators explore opportunities for visualization in a domain [ 17 ] . When used effectively, such workshops reduce the time and effort needed for the early stages of applied visualization work, as noted by one participant: “The interpersonal leveling and intense revisiting of concepts made more progress in a day than we make in a year of lab meetings …[the workshop] created consensus by exposing shared user needs” [ 35 ] .
The CVO workshops reported in the literature were derived and adapted from software requirements workshops [ 33 ] and creative problem-solving workshops [ 1 ] to account for the specific needs of visualization design. These adaptations were necessary because existing workshop guidance does not appropriately emphasize three characteristics fundamental to applied visualization, which we term visualization specifics : the visualization mindset of researchers and collaborators characterized by a symbiotic collaboration [ 80 ] and a deep and changing understanding of domain challenges and relevant visualizations [ 54 ] ; the connection to visualization methodologies that include process and design decision models [ 62 , 80 ] ; and the use of visualization methods within workshops to focus on data analysis challenges and visualization opportunities [ 17 ] .
The successful use of CVO workshops resulted from an ad hoc process in which researchers modified existing workshop guidance to meet the needs of their specific projects and reported the results in varying levels of detail. For example, Goodwin et al. [ 17 ] provide rich details, but with a focus on their experience using a series of workshops in a collaboration with energy analysts. In contrast, Kerzner et al. [ 35 ] summarize their workshop with neuroscientists in one sentence even though it profoundly influenced their research. Thus, there is currently no structured guidance about how to design, run, and analyze CVO workshops. Researchers who are interested in using such workshops must adapt and refine disparate workshop descriptions.
In this paper, we — a group of visualization and creativity researchers who have been involved with every CVO workshop reported in the literature — reflect on our collective experience and offer guidance about how and why to use CVO workshops in applied visualization. More specifically, this paper results from a 2-year international collaboration in which we applied a methodology of critically reflective practice [ 7 ] to perform meta-analysis of our collective experience and research outputs from conducting 17 workshops in 10 visualization contexts [ 16 , 18 , 17 , 34 , 35 , 42 , 64 , 68 , 69 , 88 ] , combined with a review of the workshop literature from the domains of design [ 3 , 14 , 38 , 72 ] , software engineering [ 27 , 31 , 32 , 33 , 47 , 48 , 50 ] , and creative problem-solving [ 13 , 19 , 21 , 59 , 66 ] .
This paper’s primary contribution is a framework for CVO workshops. The framework consists of: 1) a process model that identifies actions before, during, and after workshops; 2) a structure that describes what happens in the beginning, in the middle, and at the end of effective workshops; 3) a set of 25 actionable guidelines for future workshops; and 4) an example workshop and example methods for future workshops. To further enhance the actionability of the framework, in Supplemental Materials 1 1 1 http://bit.ly/CVOWorkshops/ we provide documents with expanded details of the example workshop, additional example methods, and 25 pitfalls we have encountered when planning, running, and analyzing CVO workshops.
We tentatively offer a secondary contribution: this work exemplifies critically reflective practice that enables us to draw upon multiple diverse studies to generate new knowledge about visualization in practice. Towards this secondary contribution we include, in Supplemental Materials, an audit trail [ 10 , 41 ] of artifacts that shows how our thinking evolved over the 2-year collaboration.
In this paper, we first summarize the motivation for creating this framework and describe related work in Sec. 1 and 2 . Next, we describe our workshop experience and reflective analysis methods in Sec. 3 and 4 . Then, we introduce the framework in Sec. 5 – 9 . After that, we discuss implications and limitations of the work in Sec. 10 . We conclude with future work in Sec. 11 .
1 Motivation and Background
In our experience, CVO workshops provide tremendous value to applied visualization stakeholders — researchers and the domain specialists with whom they collaborate. CVO workshops provide time for focused thinking about a collaboration, which allows stakeholders to share expertise and explore visualization opportunities. In feedback, one participant reported the workshop was “a good way to stop thinking about technical issues and try to see the big picture” [ 18 ] .
CVO workshops can also help researchers understand analysis pipelines, work productively within organizational constraints, and efficiently use limited meeting time. As another participant said: “The structured format helped us to keep on topic and to use the short time wisely. It also helped us rapidly focus on what were the most critical needs going forward. At first I was a little hesitant, but it was spot-on and wise to implement” [ 42 ] .
Furthermore, CVO workshops can build trust, rapport, and a feeling of co-ownership among project stakeholders. Researchers and collaborators can leave workshops feeling inspired and excited to continue a project, as reported by one participant: “I enjoyed seeing all of the information visualization ideas …very stimulating for how these might be useful in my work” [ 18 ] .
Based on these reasons, our view is that CVO workshops have saved us significant amounts of time pursuing problem characterizations and task analysis when compared to traditional visualization design approaches that involve one-on-one interviews and observations. What may have taken several months, we accomplished with several days of workshop preparation, execution, and analysis. In this paper we draw upon 10 years of experience using and refining workshops to propose a framework that enables others to use CVO workshops in the future.
CVO workshops are based on workshops used for software requirements and creative problem-solving [ 17 ] . Software requirements workshops elicit specifications for large-scale systems [ 33 ] that can be used in requirements engineering [ 32 ] and agile development [ 26 ] . There are many documented uses of such workshops [ 31 , 48 , 49 , 50 ] , but they do not appropriately emphasize the mindset of visualization researchers or a focus on data and analysis.
More generally, creative problem-solving workshops are used to identify and solve problems in a number of domains [ 66 ] — many frameworks exist for such workshops [ 1 , 13 , 19 , 20 , 38 ] . Meta-analysis of these frameworks reveal common workshop characteristics that include: promoting trust and risk taking, exploring a broad space of ideas, providing time for focused work, emphasizing both problem finding and solving, and eliciting group creativity from the cross-pollination of ideas [ 63 ] .
Existing workshop guidance, however, does not completely describe CVO workshops. The key distinguishing feature of CVO workshops is the explicit focus on visualization, which implies three visualization specifics for effective workshops and workshop guidance:
Workshops should promote a visualization mindset — the set of beliefs and attitudes held by project stakeholders, including an evolving understanding about domain challenges and visualization [ 54 , 80 ] — that fosters and benefits an exploratory and visual approach to dealing with data while promoting trust and rapport among these stakeholders [ 82 ] ;
Workshops should contribute to visualization methodologies — the research practices of visualization, including process and decision models [ 56 , 62 ] — by creating artifacts and knowledge useful in the visualization design process; and
Workshops should use visualization methods that explicitly focus on data visualization and analysis by exploring visualization opportunities with the appropriate information location and task clarity [ 80 ] .
This paper is, in part, about adopting and adapting creative problem-solving workshops to account for these visualization specifics.
2 Related Work
Workshops are commonly used in a number of fields, such as business [ 20 , 21 , 83 ] and education [ 2 , 8 ] . Guidance from these fields, however, does not emphasize the role of workshops in a design process, which is central to applied visualization. Therefore, we focus this section on workshops as visualization design methods.
CVO workshops can be framed as a method for user-centered design [ 65 ] , participatory design [ 61 ] , or co-design [ 73 ] because they involve users directly in the design process — we draw on work from these fields that have characterized design methods. Sanders et al. [ 72 ] , for example, characterize methods by their role in the design process. Biskjaer et al. [ 3 ] analyze methods based on concrete, conceptual, and design space aspects. Vines et al. [ 86 ] propose ways of thinking about how users are involved in design. Dove [ 15 ] describes a framework for using data visualization in participatory workshops. A number of books also survey existing design methods [ 9 , 38 ] and practices [ 36 , 40 , 74 ] . These resources are valuable for understanding design methods but do not account for visualization specifics such as methodologies that emphasize the critical role of data early in the design process [ 43 ] .
CVO workshops can also be framed within existing visualization design process and decision models [ 51 , 56 , 62 , 80 , 85 ] . More specifically, CVO workshops focus on eliciting opportunities for visualization software from collaborators. They support the understand and ideate design activities [ 56 ] or fulfill the winnow , cast , and discover stages of the design study methodology’s nine-stage framework [ 80 ] .
A number of additional methods can be used in the early stages of applied work. Sakai and Aert [ 70 ] , for example, describe the use of card sorting for problem characterization. McKenna et al. [ 57 ] summarize the use of qualitative coding, personas, and data sketches in collaboration with security analysts. Koh et al. [ 37 ] describe workshops that demonstrate a wide range of visualizations to domain collaborators, a method that we have adapted for use in CVO workshops as described in Sec. 7.4 . Roberts et al. [ 67 ] describe a method for exploring and developing visualization ideas through structured sketching. This paper is about how to use these design methods, and others, within structured CVO workshops.
Visualization education workshops are also relevant to CVO workshops. Huron et al. [ 28 ] describe data physicalization workshops for constructive visualization with novices. He et al. [ 22 ] describe workshops for students to think about the relationships between domain problems and visualization designs. In contrast, we frame CVO workshops as a method for experienced researchers to pursue domain problem characterization. Nevertheless, we see opportunities for participatory methods, such as constructive visualization [ 29 ] and sketching [ 89 ] , to be integrated into CVO workshops.
3 Workshop Experience and Terminology
To develop the CVO workshop framework proposed in this paper, we gathered researchers who used workshops on 3 continents over the past 10 years. Our collective experience includes 17 workshops in 10 contexts: 15 workshops in 8 applied collaborations, summarized in Table 1 and Table 2 ; and 2 participatory workshops at IEEE VIS that focused on creating visualizations for domain specialists [ 68 , 69 ] .
The ways in which we use workshops have evolved over 10 years. In three of our projects, we used a series of workshops to explore opportunities, develop and iterate on prototypes, and evaluate the resulting visualizations in collaborations with cartographers [ 16 ] , energy analysts [ 17 ] , and defense analysts [ 88 ] . In three additional projects, we used a single workshop to jump-start applied collaborations with neuroscientists [ 35 ] , constraint programmers [ 18 ] , and psychiatrists [ 64 ] . Recently, we used two workshops to explore opportunities for funded collaboration with genealogists [ 34 ] and biologists [ 42 ] .
In our meta-analysis, we focused on the workshops used in the early stages of applied work or as the first in a series of workshops. To describe these workshops, we developed the term CVO workshops because they aim to deliberately and explicitly foster creativity while exploring opportunities for applied visualization collaborations.
Focused on CVO workshops, our experience includes the eight workshops in Table 2 . Since we analyzed more data than appeared in any resulting publications, including artifacts and experiential knowledge, we refer to workshops and their projects by identifiers, e.g., [ 1 ] refers to our collaboration with cartographers. In projects where we used more than one workshop [ 1 – 1 ], the identifier corresponds to the first workshop in the series, unless otherwise specified.
To describe our experience, we developed terminology for the role of researchers involved in each project. The primary researcher is responsible for deciding to use a CVO workshop, executing it, and integrating its results into a collaboration. Alternatively, supporting researchers provide guidance and support to the primary researcher. We have been involved with projects as both primary and supporting researchers (see Table 1 ).
We also adopt terminology to describe CVO workshops. Workshops are composed of methods — specific, repeatable and modular activities [ 12 ] . The methods are designed around a theme that identifies the workshop’s central topic or purpose [ 8 ] . The facilitators plan and guide the workshop, and the participants carry out the workshop methods. Typically the facilitators are visualization researchers and participants are domain collaborators, but, visualization researchers can participate [ 1 , 1 ], and collaborators can facilitate [ 1 , 1 ]. We adopted and refined this vocabulary during our reflective analysis.
4 Research Process
The contributions in this paper arise from reflection — the analysis of experiences to generate insights [ 5 , 78 ] . More specifically, we applied a methodology of critically reflective practice [ 7 ] , summarized by Thompson and Thompson [ 84 ] as “synthesizing experience, reflection, self-awareness and critical thinking to modify or change approaches to practice.”
We analyzed our collective experience and our CVO workshop data, which consisted of documentation, artifacts, participant feedback, and research outputs. The analysis methods that we used can be described through three metaphorical lenses of critically reflective practice:
The lens of our collective experience — we explored and articulated our experiential knowledge through interviews, discussions, card sorting, affinity diagramming, observation listing, and observations-to-insights [ 38 ] . We codified our experience, individually and collectively, in both written and diagram form. We iteratively and critically examined our ideas in light of workshop documentation and artifacts.
The lens of existing theory — we grounded our analysis and resulting framework in the literature of creativity and workshops [ 1 , 3 , 13 , 19 , 21 , 59 , 63 , 66 , 76 , 77 , 81 ] as well as visualization design theory [ 56 , 62 , 79 , 85 ] .
The lens of our learners (i.e., readers) — in addition to intertwining our analysis with additional workshops, we shared drafts of the framework with visualization researchers, and we used their feedback to make the framework more actionable and consistent.
Our reflective analysis, conducted over two years, was messy and iterative. It included periods of focused analysis and writing, followed by reflection on what we had written, which spurred additional analysis and rewriting. Throughout this time, we generated diverse artifacts, including models for thinking about how to use workshops, written reflections on which methods were valuable to workshop success, and collaborative writing about the value of workshops. This paper’s Supplemental Material contains a timeline of significant events in our reflective analysis and 30 supporting documents that show how our ideas evolved into the following framework.
5 Fundamentals of the Framework
The framework proposed in this paper describes how and why to use CVO workshops. We use the term framework because what we have created provides an interpretive understanding and approach to practice instead of causal or predictive knowledge [ 30 ] . The framework is a thinking tool to navigate the process of planning, running, and analyzing a workshop, but we note that it cannot resolve every question about workshops because the answers will vary with local experience, preference, and context. In this section, we describe a set of factors that contribute to workshop effectiveness, as well as introduce the workshop process model and structure. We intend for the framework to be complemented by existing workshop resources from outside of visualization [ 1 , 8 , 20 , 21 ] .
5.1 Tactics for Effective Workshops
Reflecting on our experience and reviewing the relevant literature [ 63 , 66 , 76 , 77 , 81 ] enabled us to identify several key factors that contribute to the effectiveness of workshops: focusing on the topic of visualization, data and analysis, while fostering, maintaining, and potentially varying the levels of agency, collegiality, trust, interest, and challenge associated with each. We term these factors TACTICs for effective workshops:
( T )opic — the space of ideas relevant to data, visualization, and domain challenges in the context of the workshop theme.
( A )gency — the sense of stakeholder ownership in the workshop, the workshop outcomes, and the research collaboration.
( C )ollegiality — the degree to which communication and collaboration occur among stakeholders.
( T )rust – the confidence that stakeholders have in each other, the workshop, the design process, and the researchers’ expertise.
( I )nterest — the amount of attention, energy, and engagement to workshop methods by the stakeholders.
( C )hallenge — the stakeholders’ barrier of entry to, and likelihood of success in, workshop methods.
The TACTICs are not independent, consistent, or measurable. The extent to which they are fostered depends upon the context in which they are used, including various characteristics of the workshop — often unknown in advance, although perhaps detectable by facilitators. Yet, selecting methods to maintain appropriate levels of agency, interest, and trust — while varying levels of challenge and approaching the topic from different perspectives — likely helps workshops to have a positive influence on the mindset of stakeholders and to generate ideas that move forward the methodology of the project. Hence, we refer to the TACTICs throughout this framework.
5.2 Process Model and Structure
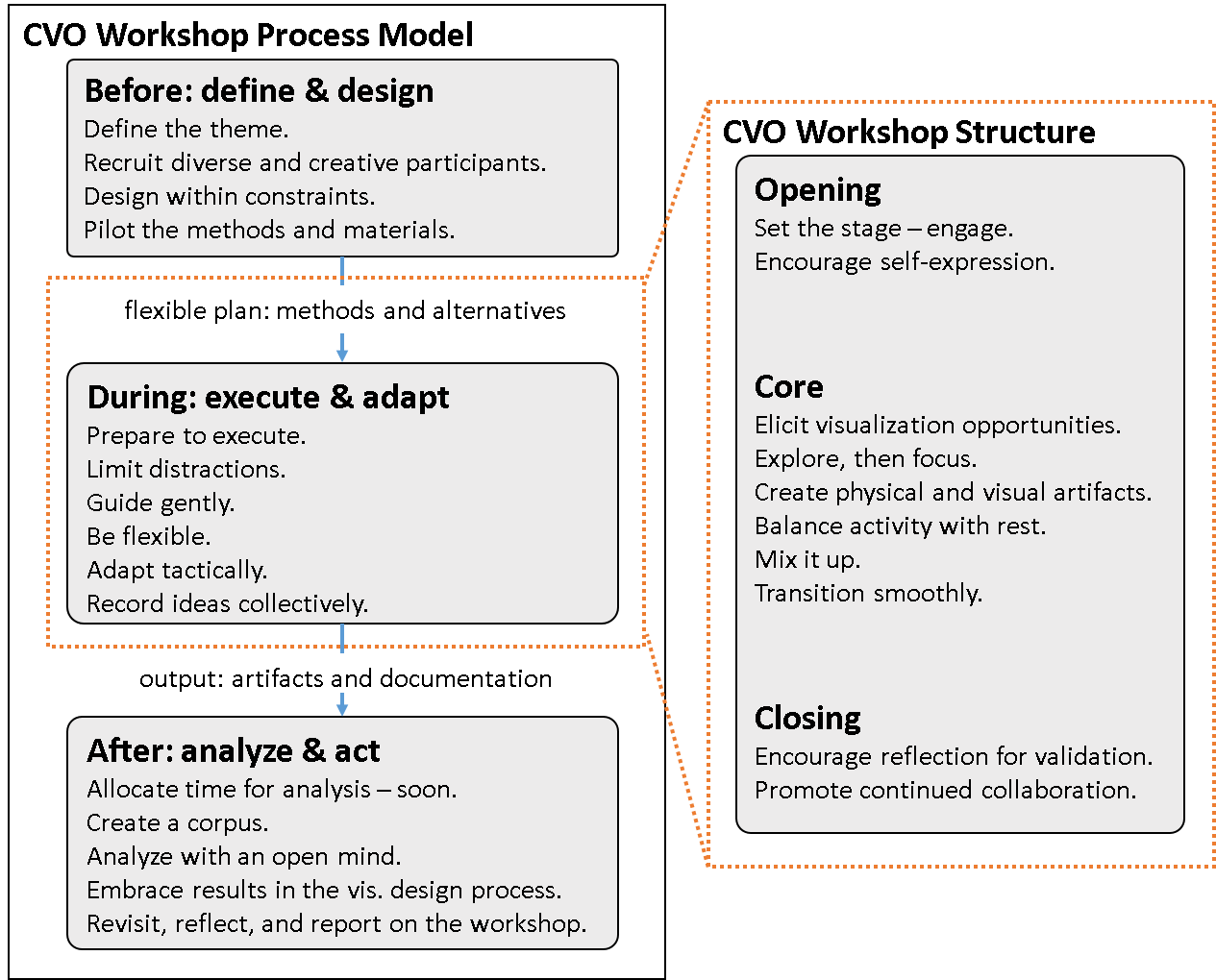
The framework proposes two models for describing how to use CVO workshops: a process model and a workshop structure. The models were adapted from the extensive literature that describes how to use workshops outside of visualization [ 1 , 8 , 13 , 15 , 20 , 21 , 66 ] .
The process model shown in Fig. 1 (left) consists of three stages that describe the actions of using CVO workshops:
Before: define & design. Define the workshop theme and design workshop methods, creating a flexible workshop plan.
During: execute & adapt. Perform the workshop plan, adapting it to participants’ reactions in light of the TACTICs, generating workshop output as a set of artifacts and documentation.
After: analyze & act. Make sense of the workshop output and use it in the downstream design process.
Nested within the process is the CVO workshop structure — Fig. 1 (right) — that identifies key aspects of the methods used in the beginning, middle, and end of workshops:
Opening. Establish shared context and interest while promoting trust, agency, and collegiality.
Core. Promote creative thinking about the topic, potentially varying challenge to maintain interest.
Closing. Provide time for reflection on the topic and promote continued collegiality in the collaboration.
The process model and structure are closely connected as shown by the orange box in Fig. 1 . As part of the workshop process, we design and execute a workshop plan. This plan follows the workshop structure because it organizes methods into the opening, core, and closing. In other words, the process is about how we use a workshop; the structure is about how methods are organized within a workshop.
We use the process model and structure to organize the following four sections of this paper. In these sections, we use paragraph-level headings to summarize 25 actionable workshop guidelines. Additionally, in Supplemental Materials we include a complementary set of 25 pitfalls that are positioned against these guidelines and the TACTICs to further enhance the actionability of the framework.
6 Before the Workshop: Define & Design
Creating an effective CVO workshop is a design problem: there is no single correct workshop, the ideal workshop depends on its intended outcomes, and the space of possible workshops is practically infinite. Accordingly, workshop design is an iterative process of defining a goal, testing solutions, evaluating their effectiveness, and improving ideas. The framework we have developed here is part of this process. In this section, we introduce four guidelines — summarized in paragraph-level headings — for workshop design.
Define the theme.
Just as design starts with defining a problem, creating a CVO workshop starts with defining its purpose, typically by articulating a concise theme. An effective theme piques interest in the workshop through a clear indication of the topic. It encourages a mindset of mutual learning among stakeholders. It also focuses on opportunities that exhibit the appropriate task clarity and information location of the design study methodology [ 80 ] . Examples from our work emphasize visualization opportunities (e.g., “enhancing legends with visualizations” [ 1 ]), domain challenges (e.g., “identify analysis and visualization opportunities for improved profiling of constraint programs” [ 1 ]), or broader areas of mutual interest (e.g., “explore opportunities for a funded collaboration with phylogenetic analysts” [ 1 ]).
Although we can improve the theme as our understanding of the domain evolves, posing a theme early can ground the design process and identify promising participants.
Recruit diverse and creative participants.
We recruit participants who have relevant knowledge and diverse perspectives about the topic. We also consider their openness to challenge and potential collegiality.
Examples of effective participants include a mix of frontline analysts, management, and support staff [ 1 ]; practitioners, teachers, and students [ 1 ]; or junior and senior analysts [ 1 ]. We recommend that participants attend the workshop in person because remote participation proved distracting in one workshop [ 1 ]. Recruiting fellow-tool builders [ 80 ] as participants should be approached with caution because their perspectives may distract from the topic — this happened in our workshop that did not result in active collaboration [ 1 ].
Design within constraints.
Identifying constraints can help winnow the possibilities for the workshop. Based on our experience, the following questions are particularly useful for workshop design:
Who will use the workshop results? Identifying the primary researcher early in the process is important because he or she will be responsible for the workshop and ultimately use its results. In a workshop where we did not clearly identify the primary researcher, the results went unused [ 1 ].
How many participants will be in the workshop? We typically recruit 5 to 15 participants — a majority domain collaborators, but sometimes designers and researchers [ 1 , 1 , 1 – 1 ].
Who will help to facilitate the workshop? We have facilitated our workshops as the primary researcher, with the assistance of supporting researchers or professional workshop facilitators. Domain collaborators can also be effective facilitators, especially if the domain vocabulary is complex and time is limited [ 1 , 1 ].
How long will the workshop be? Although we have run workshops that range from half a day [ 1 , 1 ] to two days [ 1 ], these extremes either feel rushed or require significant commitment from collaborators. We recommend that an effective workshop lasts about one working day.
Where will the workshop be run? Three factors are particularly important for determining the workshop venue: a mutually convenient location, a high quality projector for visualization examples, and ample space to complete the methods. We have had success with workshops at offsite locations [ 1 , 1 ], our workplaces, and our collaborators’ workplaces [ 1 – 1 ].
What are additional workshop constraints? Examples include the inability of collaborators to share sensitive data [ 1 , 1 ] and the available funding.
Pilot the methods and materials.
Piloting methods can ensure that the workshop will generate ideas relevant to the topic while maintaining appropriate levels of interest and challenge. We have piloted methods to evaluate how understandable they are [ 1 , 1 ], to test whether they create results that can be used to advance visualization design methodologies [ 1 , 1 ], to find mistakes in method prompts [ 1 , 1 , 1 , 1 ], and to ensure that the materials are effective — e.g., sticky notes are the correct size and visualizations are readable on the projector.
It is also useful to pilot workshops with proxy participants, such as researchers [ 1 ] or collaborators [ 1 ]. Feedback from collaborators during pilots has helped us revise the theme, identify promising participants, and refine the workshop methods.
7 Workshop Structure and Methods
This section describes guidelines for the methods used in the three phases of the CVO workshop structure (described in Sec. 5.2 ) — the opening, core, and closing. It concludes with a summary of an example workshop and resources for additional workshop methods.
7.1 Workshop Opening
The workshop opening communicates the goals and guidelines for participants, but it can be more than that. It can foster agency by encouraging self-expression and idea generation. It can encourage collegiality and trust by promoting open communication, acknowledging expertise, and establishing a safe co-owned environment. It can also garner interest by showing that the workshop will be useful and enjoyable. Two guidelines contribute to an effective opening.
Set the stage — engage.
CVO workshops typically open with a short introduction that reiterates the theme and establishes shared context for participants and facilitators. We have introduced workshops as “guided activities that are meant to help us understand: what would you like to do with visualization?” [ 1 ]. We have also used graphics that summarize the goals of our project, potentially priming participants to engage with the topic of visualization [ 1 ].
The opening can establish principles for creativity [ 1 , 66 ] , potentially fostering trust and collegiality. We used the following principles in one of our workshops [ 1 ]: 1) all ideas are valid, express and record them; 2) let everyone have their say; 3) be supportive of others; 4) instead of criticizing, create additional ideas; 5) think ‘possibility’ – not implementation; 6) speak in headlines and follow with detail; and 7) switch off all electronic devices.
Introduction presentations should be kept short to maintain interest. Passive methods, such as lectures and presentations, can discourage participation at the outset. For example, we started one workshop [ 1 ] with a presentation on the current state of analysis tools. This presentation encouraged participants to passively listen rather than actively explore, establishing a passive mindset that we had to overcome in subsequent methods. An effective opening engages participants.
Encourage self-expression.
We use methods that encourage self-expression to support interpersonal leveling and to act on the creativity principles — all ideas are valid and be supportive of others . Such interpersonal methods help to establish an atmosphere of trust and collegiality among participants and facilitators. They can also provide participants with a sense of agency [ 8 ] .
We have used interpersonal methods that ask participants to sketch ideas while suspending judgment [ 69 ] or to introduce themselves through analogies as a potential primer for creativity (see analogy introduction in Sec. 7.4 ). Overall, we use interpersonal methods in the opening to engage participants and facilitators, preparing them for the workshop core.
7.2 Workshop Core
In the workshop core, we harness the active and engaged mindset of participants by encouraging them to explore a wide ideaspace before selecting the more promising ideas. The methods in the core potentially generate hundreds of sticky notes, sketches, and other artifacts. Analysis of our experience and relevant literature leads us to suggest five guidelines for an effective core.
Elicit visualization opportunities.
We select workshop methods relevant to the topic, asking participants about their current analysis challenges, limitations of existing tools, characteristics of their data, or the ways in which they would like to use visualization. This can be achieved by adding a visualization twist to existing design and workshop methods.
In one workshop [ 1 ], for example, we used a method that “developed user stories, considered relevant datasets, discussed alternative scenarios and sketched solutions” with our domain collaborators. In retrospect, this method connected the topic into a more general workshop method, user stories [ 38 ] .
Explore, then focus.
We organize the core to first generate ideas using divergent methods that expand the ideaspace. Then, we evaluate ideas using convergent methods that winnow the ideaspace [ 66 ] . Using divergent methods early in the core allows us to consider many possibilities while also promoting agency and maintaining interest. Then, convergent methods can narrow the ideaspace to the more promising ideas.
Classifying methods as either divergent or convergent risks oversimplification as individual methods often include both divergent and convergent aspects. Consider our use of brainstorming [ 66 ] during one workshop [ 1 ]: we asked participants to record “problems and successes associated with the current clients on sticky notes” (divergent) and then to share the more interesting ideas (convergent). We classify this method as divergent because it creates ideas, despite the convergent discussion. In contrast, a convergent method may only involve grouping sticky notes from previous methods. Overall, in line with existing workshop guidance [ 1 , 13 , 21 , 66 ] , we judge methods by their intended impact on the ideaspace and organize the core with phases of divergent and convergent methods.
Create physical and visual artifacts.
We select methods by how they encourage participants to write, draw, or otherwise externalize their ideas. Externalizing ideas creates artifacts for us to analyze after the workshop. It aids creative thinking because expressing an idea forces the creator to elaborate it [ 77 ] , and promotes idea sharing that encourages collegiality.
We consider the artifact materials to be important. Sticky notes are particularly useful because they enable participants to group or rank ideas and potentially to discover emergent concepts in the ideaspace [ 15 ] . We have used sticky notes in almost all of our workshops, often using their color to encode information about which method generated an idea, and their positions to relate, differentiate, or rank ideas. This can help establish consensus. It can also aid post-workshop analysis by recording how ideas evolved and were valued throughout the workshop. Additional materials effective for externalizing ideas include handouts with structured prompts, butcher paper, and poster boards. Using whiteboards is tempting, but ideas are easily lost if the boards are erased.
We also consider the form of ideas to be important. Effective methods create artifacts relevant to the theme and topic of visualization. This can be achieved through the use of visual language (see wishful thinking in Sec. 7.4 ) and by encouraging participants to sketch or draw, such as in storyboarding [ 1 , 1 , 1 ]. We see many opportunities to create visual artifacts using existing methods, such as sketching with data [ 89 ] , constructive visualizations [ 29 ] , or parallel prototyping [ 67 ] approaches.
Balance activity with rest.
Because continuously generating or discussing ideas can be tiring for participants, we structure workshop methods to provide a balance between activity and rest. Specifically, we incorporate passive methods that provide time for incubation, the conscious and unconscious combination of ideas [ 77 ] .
Passive methods can include short breaks with food and coffee, informal discussions over meals, or methods where participants listen to presentations. When using methods that present ideas, asking participants to record their thoughts and reactions can promote interest and maintain a feeling of agency. We have typically used passive methods in full-day workshops [ 1 , 1 , 1 , 1 ], but we rely on breaks between methods for shorter workshops [ 1 ].
We consider the relationships among methods to be important as we strive to balance exploration with focus and activity with rest, while also using many materials for externalizing ideas. Considering methods that vary these factors can provide different levels of challenge because, for example, methods that require drawing ideas may be more difficult than discussing ideas. Using a variety of methods may also maintain interest because participants may become bored if too much time is spent on a specific idea.
Transition smoothly.
We avoid potentially jarring transitions between methods to preserve participant interest. Convergent discussions can be used to conclude individual methods by highlighting the interesting, exciting, or influential ideas. These discussions can promote collegiality by encouraging communication of ideas, agency by validating participants’ contributions, and interest in the ideas generated. Convergent discussions also highlight potentially important ideas for researchers to focus on after the workshop.
Convergent methods can also conclude the workshop core by grouping or ranking key ideas. We have used storyboarding to encourage the synthesis of ideas into a single narrative [ 1 , 1 , 1 ]. We have also asked participants to rank ideas, providing cues for analyzing the workshop results [ 1 ]. Convergent methods provide a sense of validation, potentially helping to build trust among researchers and collaborators as we transition to the closing.
7.3 Workshop Closing
The workshop closing sets the tone for continued collaboration. It is an opportunity to promote collegiality by reflecting on the shared creative experience. It allows for analysis that can potentially identify the more interesting visualization opportunities. The following two guidelines apply to effective closings.
Encourage reflection for validation.
We use discussions at the end of workshops to encourage reflection, potentially providing validation to participants and generating information valuable for workshop analysis. We encourage participants to reflect on how their ideas have evolved by asking, “What do you know now that you did not know this morning?” [ 1 ] or “What will you do differently tomorrow, given what you have learned today?” [ 1 ]. Responses to these questions can provide validation for the time committed to the workshop. One participant, for example, reported, “I was surprised by how much overlap there was with the challenges I face in my own work and those faced by others” [ 1 ].
Promote continued collaboration.
We conclude the workshop by identifying the next steps of action — continuing the methodology of the collaboration. We can explain how the ideas will be used to move the collaboration forward, often with design methods as we describe in Sec. 9 .
We can also ask participants for feedback about the workshop to learn more about their perceptions of visualization and to evaluate the effectiveness of workshop methods — encouraging the visualization mindset. E-mailing online surveys immediately after a workshop is effective for gathering feedback [ 1 , 1 ].
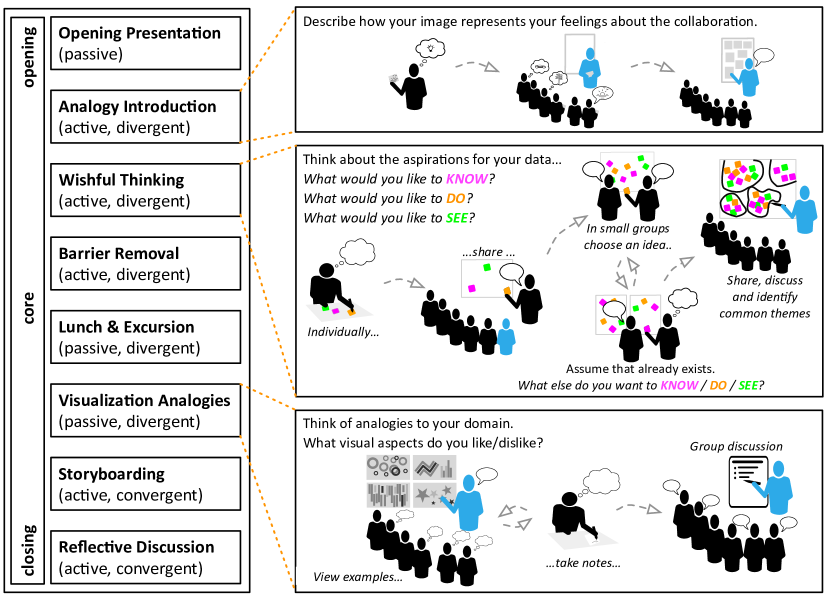
7.4 Example Workshop & Methods
To illustrate the workshop structure, we include an example workshop, shown in Fig. 2 . We selected this example because it has proven effective in three of our projects [ 1 , 1 , 1 ]. Here, we describe three methods of this workshop that we have also used successfully in additional workshops [ 1 , 1 ], and we refer to the Supplemental Material for descriptions of the remaining five methods. We emphasize that this is a starting place for thinking about workshops, and encourage that methods be adopted and adapted for local context.
To explain the workshop methods, we refer to their process — the steps of execution [ 3 ] . This process description abstracts and simplifies the methods because during their execution we adapt the process based on participant reactions and our own judgment of the TACTICs.
Analogy Introduction
We have used this active, interpersonal, and potentially divergent method in the workshop opening. A process of this method, shown in Fig. 2 (right, top), starts with a facilitator posing the analogy introduction prompt, e.g., “If you were to describe yourself as an animal, what would you be and why?” [ 1 ]. The facilitators and participants then respond to the prompt in turn — expressing themselves creatively.
Because everyone responds to the eccentric prompt, this method supports interpersonal leveling that helps to develop trust and collegiality among stakeholders. Using analogy can prime participants to think creatively [ 19 ] .
This method is simple to execute, and participants report that it has a profound impact on the workshop because of the leveling that occurs. The method helps to establish trust and that all ideas should be accepted and explored [ 1 ].
A more topical alternative requires more preparation. We have asked participants to come to the workshop with an image that represents their feelings about the project. Participants have created realistic images, clip-art, and sketches to present and discuss [ 1 ]. A visual analogy introduction can help establish the topic of visualization early in the workshop.
Wishful Thinking
We have used this divergent, active method early in the workshop core. It is based on creativity methods to generate aspirations [ 24 ] . We tailored these methods to visualization by prompting participants with a domain scenario and asking questions: “What would you like to know? What would you like to do? What would you like to see?”
One process of this method is shown in Fig. 2 (right, middle). First, we introduce the prompt and participants answer the know/do/see questions individually on sticky notes. Next, participants share ideas in a large group to encourage collegiality and cross-pollination of ideas. Then, participants form small groups and try to build on their responses by selecting interesting ideas, assuming that they have been completed, and responding to the know/do/see questions again — increasing the challenge. Finally, we lead a convergent discussion to highlight interesting ideas and to transition to the next method.
We encourage participants to record answers to the know/do/see questions on different color sticky notes because each prompt provides information that is useful at different points in the design process. Participants describe envisaged insights they would like to know and analysis tasks that they would like to do . Asking what participants would like to see is often more of a challenge, but ensures that a topic of visualization is established early.
We tailor the prompt to the workshop theme and project goals. For example, we asked energy analysts about long term goals for their project — “aspirations for the Smart Home programme…” They generated forward-thinking ideas, e.g., to better understand the value of the data [ 1 ]. In contrast, we asked neuroscientists about their current analysis needs — “suppose you are analyzing a connectome…” They created shorter term ideas, e.g., to see neuron connectivity [ 1 ].
Visualization Analogies
We have used this divergent, initially passive method later in the workshop core because it promotes incubation while allowing participants to specify visualization requirements by example. Similar to analogy-based creativity methods [ 19 ] and the visualization awareness method [ 37 ] , we present a curated collection of visualizations and ask participants to individually record analogies to their domain and to specify aspects of the visualizations that they like or dislike. We have used this method repeatedly, iteratively improving its process by reflecting on what worked in a number of our workshops [ 1 – 1 , 1 ].
One process of this method is shown in Fig. 2 (right, bottom). First, we provide participants with paper handouts that contain a representative image of each visualization — we have encouraged participants to annotate the handouts, externalizing their ideas [ 1 , 1 , 1 ]. Next, we present the curated visualizations on a projector and ask participants to think independently about how each visualization could apply to their domain and record their ideas. Then, we discuss these visualizations and analogies in a large group.
We curate the example visualizations to increase interest and establish participants’ trust in our visualization expertise. We have used visualizations that we created (to show authority and credibility); those that we did not create (for diversity and to show knowledge of the field); older examples (to show depth of knowledge); challenging examples (to stretch thinking); playful examples (to support engagement and creativity); closely related examples (to make analogies less of a challenge); and unrelated examples (to promote more challenging divergent thinking).
The discussions during this method have expanded the workshop ideaspace in surprising ways, including “What does it mean for legends to move?” [ 1 ], “What does it mean for energy to flow?” [ 1 ], and “What does it mean for neurons to rhyme?” [ 1 ]. Although this method is primarily passive, participants report that it is engaging and inspiring to see the possibilities of visualization and think about how such visualizations apply to their domain.
Additional Methods & Resources
We introduce the example workshop and methods as starting points for future workshops. Yet, the workshop design space is practically infinite and design should be approached with creativity in mind.
To help researchers navigate the design space, our Supplemental Material contains a list of 15 example methods that we have used or would consider using in future workshops. For these methods, we describe their process, their influence on the workshop ideaspace, their level of activity, and their potential impact on the TACTICs for effective workshops.
We have also found other resources particularly useful while designing workshops. These include books [ 1 , 20 , 21 , 25 , 38 , 58 ] and research papers [ 55 , 56 , 71 ] . Although these resources target a range of domains outside of visualization, we tailor the workshop methods such that they encourage a visualization mindset and focus on the topic of visualization opportunities.
8 During The Workshop: Execute & Adapt
Continuing the CVO workshop process model shown in Fig. 1 , we execute the workshop plan. This section proposes five guidelines for workshop execution.
Prepare to execute.
We prepare for the workshop in three ways: resolving details, reviewing how to facilitate effectively, and checking the venue. We encourage researchers to prepare for future workshops in the same ways.
We prepare by resolving many details, such as inviting participants, reserving the venue, ordering snacks for breaks, making arrangements for lunch, etc. Brooks-Harris and Stock-Ward [ 8 ] summarize many practical details that should be considered in preparing for execution. Our additional advice is to promote the visualization mindset in workshop preparation and execution.
We prepare by reviewing principles of effective facilitation, such as acting professionally, demonstrating acceptance, providing encouragement, and using humor [ 1 , 8 , 20 , 21 , 83 ] . We also assess our knowledge of the domain because, as facilitators, we will need to lead discussions. Effectively leading discussions can increase collegiality and trust between stakeholders as participants can feel that their ideas are valued and understood. In cases where we lacked domain knowledge, we recruited collaborators to serve as facilitators [ 1 , 1 ].
We also prepare by checking the venue for necessary supplies, such as a high quality projector, an Internet connection (if needed), and ample space for group activity. Within the venue, we arrange the furniture to promote a feeling of co-ownership and to encourage agency — a semi-circle seating arrangement works well for this [ 87 ] . A mistake in one of our workshops was to have a facilitator using a podium, which implied a hierarchy between facilitators and participants, hindering collegiality [ 68 ] .
Limit distractions.
Workshops provide a time to step away from normal responsibilities and to focus on the topic. Accordingly, participants and facilitators should be focused on the workshop without distractions, such as leaving for a meeting.
Communicating with people outside of the workshop — e.g., through e-mail — commonly distracts participants and facilitators. It should be discouraged in the workshop opening (e.g., switch off all electronic devices ). Principles in the workshop opening, however, should be justified to participants. Also, facilitators should lead by example at the risk of eroding trust and collegiality.
Guide gently.
While starting execution, the workshop opening can establish an atmosphere in which participants take initiative in completing methods. It is, however, sometimes necessary to redirect the participants in order to stay focused on the topic. Conversations that deviate from the workshop theme should be redirected. In one workshop [ 1 ], participants were allowed to discuss ideas more freely, and they reported in feedback that, “We had a tendency to get distracted [during discussions].” In a later workshop [ 1 ], we more confidently guided discussions, and participants reported “We were guided and kept from going too far off track …this was very effective.”
However, guiding participants requires judgment to determine whether a conversation is likely to be fruitful. It also requires us to be sensitive to the TACTICs — e.g., how would redirecting this conversation influence collegiality or agency? Redirection can be jolting and can contradict some of the guidelines (e.g., all ideas are valid ). We can prepare participants for redirection with another guideline during the workshop opening: Facilitators may keep you on track gently, so please be sensitive to their guidance.
Be flexible.
As we guide participants to stay on topic, it is important to be flexible in facilitation. For example, we may spend more time than initially planned on fruitful methods or cut short methods that bore participants.
Following this guideline can also blur the distinction between participants and facilitators. In one workshop [ 1 ], participants proposed a method that was more useful than what was planned. Thus, they became facilitators for this part of the workshop, which reinforced agency and maintained the interest of all stakeholders in the project. In the future, we may explore ways to plan this type of interaction, perhaps encouraging participants to create their own methods.
Adapt tactically.
As we guide the workshop, we interpret group dynamics and adapt methods to the changing situation. We can be forced to adapt for many reasons, such as a failing method ( nobody feels like an animal this morning ; sticky notes don’t stick ), a loss of interest ( there is no energy ; the room is too hot ; we had a tough away day yesterday ); a lack of agency ( some participants dominate some tasks ); or an equipment failure ( projector does not work ; no WiFi connection to present online demos [ 1 ]). Designing the workshop with alternative methods in mind — perhaps with varying degrees of challenge — can ensure that workshop time is used effectively.
Record ideas collectively.
Remember: conversations are ephemeral and anything not written down will likely be forgotten. We therefore encourage facilitators and participants to document ideas with context for later analysis. Selecting methods to create physical artifacts can help with recording ideas. As described in Sec. 7 , externalizing ideas on sticky notes and structured prompts has been effective in our workshops and addresses the visualization mindset.
We are uncertain about the use of audio recording to capture workshop ideas. Although it can be useful for shorter workshops [ 1 ], it can require tremendous time to transcribe before analysis [ 43 ] . Also, recording audio effectively can be challenging as participants move around during the workshop.
It can be useful to ensure that facilitators know that they are expected to help document ideas. A pilot workshop can help with this. In at least one of our projects [ 1 ], a pilot workshop may have reduced the note taking pressure on the primary researcher by setting clear expectations that all facilitators should help take notes.
9 After the Workshop: Analyze & Act
After the CVO workshop, we analyze its output and use the results of that analysis to influence the on-going collaboration. Here, we describe five guidelines for this analysis and action.
Allocate time for analysis — soon.
Effective CVO workshops generate rich and inspiring artifacts that can include hundreds of sticky notes, posters, sketches, and other documents. The exact output depends on the methods used in the workshop. Piloting methods can help prepare researchers for the analysis. Regardless, making sense of this output is labor intensive, often requiring more time than the workshop itself. Thus, it is important that we allocate time for analysis, particularly within a day of the workshop, so that we can analyze the workshop output while the experiences are still fresh in our memory.
Create a corpus.
We usually start analysis by creating a digital corpus of the CVO workshop output. We type or photograph the artifacts, organizing ideas into digital documents or spreadsheets. Through this process, we become familiar with key ideas contained in the artifacts. The corpus also preserves and organizes the artifacts, potentially allowing us to enlist diverse stakeholders — such as facilitators and collaborators — in analysis [ 1 ]. This can help in clarifying ambiguous ideas or adding context to seemingly incomplete ideas.
Analyze with an open mind.
Because the ideas in the workshop output will vary among projects, there are many ways to analyze this corpus of artifacts. We have used qualitative analysis methods — open coding, mindmapping, and other less formal processes — to group artifacts into common themes or tasks [ 1 , 1 – 1 ]. Quantitative analysis methods should be approached with caution as the frequency of an idea provides little information about its potential importance.
We have ranked the themes and tasks that we discovered in analysis according to various criteria, including novelty, ease of development, potential impact on the domain, and relevance to the project [ 1 , 1 – 1 ]. In other cases [ 1 , 1 ], workshop methods generated specific requirements, tasks, or scenarios that could be edited for clarity and directly integrated into the design process.
We encourage that analysis be approached with an open mind because of the many ways to make sense of the workshop data, including some approaches that we may not yet have considered.
Embrace results in the visualization design process.
Similarly, CVO workshop results can be integrated into visualization methodologies and processes in many ways. We have, for example, run additional workshops that explored the possibilities for visualization designs [ 1 , 1 ]. We have applied traditional user-centered design methods, such as interviews and contextual inquiry, to better understand collaborators’ tasks that emerged from the workshop [ 1 ]. We have created prototypes of varying fidelity, from sketches to functioning software [ 1 – 1 ], and we have identified key aims in proposals for funded collaboration [ 1 ].
In all of these cases, our actions were based on the reasons why we ran the workshops, and the workshop results profoundly influenced the direction of our collaboration. For example, in our collaboration with neuroscientists [ 1 ], the workshop helped us focus on graph connectivity, a topic that we were able to explore with technology probes and prototypes of increasing fidelity, ultimately resulting in new visualization tools and techniques.
Revisit, reflect, and report on the workshop.
The CVO workshop output is a trove of information that can be revisited throughout (and even beyond) the project. It can be used to document how ideas evolve throughout applied collaborations. It can also be used to evaluate and validate design decisions by demonstrating that any resulting software fulfills analysis needs that were identified in the workshop data [ 1 – 1 ]. Revisiting workshop output repeatedly throughout a project can continually inspire new ideas.
In our experience creating this paper, revisiting output from our own workshops allowed us to analyze how and why to use CVO workshops. We encourage researchers to reflect and report on their experiences using CVO workshops, the ways in which workshops influence collaborations, and ideas for future workshops. We hope that this framework provides a starting point for research into these topics.
10 Discussion
This section discusses implications and limitations of CVO workshops and the research methodology of critically reflective practice.
10.1 Limitations of CVO Workshops
Our experience across diverse domains — from cartography to neuroscience — provides evidence that CVO workshops are a valuable and general method for fostering the visualization mindset while creating artifacts that advance visualization methodologies. We argue that they achieve these goals through the use of methods that appropriately emphasize the topic of visualization opportunities while accounting for (inter)personal factors, including agency, collegiality, challenge, interest, and trust.
Yet, workshops may not be appropriate in some scenarios. Because using workshops requires researchers to ask interesting questions and potentially lead discussions about their collaborators’ domain, we caution the use of workshops as the first method in a project. Traditional user-centered approaches should be used to learn domain vocabulary and explore the feasibility of collaboration. In the project that did not result in ongoing collaboration [ 1 ], we lacked the domain knowledge needed to effectively design the workshop. Also, our collaborators were too busy to meet with us before the workshop, which should have been a warning about the nature of the project. Accordingly, we recommend researchers evaluate the preconditions of design studies [ 80 ] in projects where they are considering workshops.
We also recognize that workshops may not be well received by all of the stakeholders. In a full-day workshop [ 1 ], one participant reported that “Overall, it was good, but a bit long and slightly repetitive.” Similarly, after another full-day workshop [ 1 ], one participant said “There was too much time spent expanding and not enough focus …discussions were too shallow and nonspecific.” Nevertheless, both workshops were generally well received by stakeholders as they allowed us to explore a broad space of visualization opportunities. We can, however, improve future workshops by ensuring that the methods are closely related to the topic and that we facilitate workshops in a way that provides appropriate agency to all of the stakeholders.
More generally, whether workshops can enhance creativity is an open question [ 63 , 77 ] . Creativity is a complex phenomenon studied from many perspectives, including design [ 81 ] , psychology [ 77 ] , sociology [ 44 ] , and biology [ 52 ] . The results of several controlled experiments indicate that group-based methods can reduce creativity [ 4 , 60 ] . Yet, critics of these studies argue that they rely on contrived metrics and lack ecological validity [ 23 , 53 ] . Experimentally testing the relationship between workshops and creativity is beyond the scope of this paper. Instead, we focus on understanding and communicating how we use CVO workshops in applied collaborations.
10.2 Critically Reflective Practice
Throughout this project, we wrestled with a fundamental question: how can we rigorously learn from our diverse, collective experience? We first examined measurable attributes of workshops, such as their length, number of participants, and quantity of ideas generated. However, our workshops were conducted over 10 years in applied settings with no experimental controls. More importantly, it is difficult, if not impossible, to measure how ideas influence collaborations. Quantitative analysis, we decided, would not produce useful knowledge about how to use CVO workshops.
We also considered qualitative research methodologies and methods, such as grounded theory [ 11 ] and thematic analysis [ 6 ] . These approaches focus on extracting meaning from externalized data, but the the most meaningful and useful information about workshops resided in our collective, experiential knowledge. We therefore abandoned analysis methods that ignore (or seek to suppress) the role of experience in knowledge generation.
We found critically reflective practice to be an appropriate approach, providing a methodology to learn from the analysis of experience, documentation, and existing theory, while allowing for the use of additional analysis methods [ 7 , 84 ] . Due to the nature of reflection, however, the framework is not exhaustive, predictive, or objective. Nevertheless, it is consistent with our experience, grounded in existing theory, and, we argue, useful for future visualization research.
Yet, the use of reflective practice may raise questions about the validity of this work. After all, can the framework be validated without experimental data? We emphasize our choice of the term framework [ 30 ] because we intend for it to be evaluated by whether it provides an interpretive understanding of CVO workshops. Our position is that it achieves this goal because it enabled us to learn from our experience using workshops on 3 continents over the past 10 years. For example, we used the framework to identify and organize 25 pitfalls to avoid in future workshops — they are described in the Supplemental Material. This framework, however, is only a snapshot of our current understanding of CVO workshops, which will continue to evolve with additional research, practice, and reflection.
Given that this work results from the subjective analysis of our experience, we recognize that there could also be questions about its trustworthiness. Therefore, to increase the trustworthiness of our results, we provide an audit trail [ 10 , 41 ] of our work that contains a timeline of our analysis and our experience as well as diverse artifacts, including comparative analysis of our workshops, presentations outlining the framework, early written drafts of our framework, and structured written reflection to elicit ideas from all of this paper’s coauthors. This audit trail, in Supplemental Material, summarizes and includes 30 of the reflective artifacts, culled from the original set to protect the privacy of internal discussions and confidential materials from our domain collaborators.
In future reflective projects, we plan to establish guidelines that encourage transparency of reflective artifacts through mechanisms to flag documents as on- or off-the-record. Because our research and meta-analysis would have been impossible without well-preserved documentation, we hope that the audit trail inspires future thinking on how to document and preserve the decisions in visualization collaborations. We put forth both the audit trail and our documented use of critically reflective practice as secondary contributions.
11 Conclusion and Future Work
This paper contributes a framework for using workshops in the early stages of applied visualization research. The framework consists of two models for CVO workshops — a process model and a workshop structure. The framework also includes 25 actionable guidelines for future workshops and a validated example workshop.
We support the framework with Supplemental Material that includes extended details about the example workshop, 15 additional example workshop methods, 25 pitfalls to avoid in future workshops, and an analysis timeline and audit trail documenting how we developed the framework during a 2-year reflective collaboration. We hope that this framework inspires others to use and report on CVO workshops in applied visualization research.
Further thinking on the framework reveals opportunities for developing CVO workshop methods that emphasize the visualization mindset. For example, inspired by the Dear Data project [ 45 ] , we could ask participants to create graphics that reveal something about their daily life in the week before the workshop. The Dear Data Postcard Kit [ 46 ] offers guidance and materials for creating data visualizations about personal experiences, which could be adopted in CVO workshops.
We also hope to better understand the role of data in CVO workshops. Visualization methodologies stress the importance of using real data early in collaborative projects [ 43 , 80 ] . Our workshops, however, have focused participants on their perceptions of data rather than using real data because working with data is time consuming and unpredictable. In some projects, we incorporated data into the design process by using a series of workshops spaced over weeks or months, providing time for developers to design prototypes between workshops [ 1 – 1 ]. This development between workshops was expensive in terms of time and effort. But time moves on, and we may be able to reliably use data in workshops with new technologies and techniques, e.g., visualization design tools [ 90 ] , declarative visualization languages [ 75 ] , constructive visualization [ 29 ] , and sketching [ 89 ] .
Additionally, in this paper we focused on workshops to elicit visualization opportunities in the early stages of applied work. Exploring how the framework could be influenced by and extended for workshops that correspond to other stages of applied work — including the creation and analysis of prototypes, the exploration of data, or in the deployment, training and use of completed systems — may open up opportunities for using creativity in visualization design and research.
Acknowledgements.
- [1] Creative Problem-Solving Resource Guide . Creative Education Foundation, Scituate, MA, USA, 2015.
- [2] L. W. Anderson, D. R. Krathwohl, P. W. Airasian, K. A. Cruikshank, R. E. Mayer, P. R. Pintrich, J. Rathes, and M. C. Wittrock. A Taxonomy for Learning, Teaching, and Assessing: A Revision of Bloom’s Taxnomy of Educational Objectives, Abridged Edition . Pearson, 2000.
- [3] M. M. Biskjaer, P. Dalsgaard, and K. Halskov. Understanding creativity methods in design. In Proc. Conf. Designing Interactive Syst. , pages 839–851. ACM SIGCHI, 2017.
- [4] T. J. Bouchard. Personality, problem-solving procedure, and performance in small groups. J. Appl. Psychology , 53(1):1–29, 1969.
- [5] D. Boud, R. Keogh, and D. Walker. Reflection: Turning Experience into Learning . Routledge Taylor and Francis Group, London, UK, 1985.
- [6] V. Braun and V. Clarke. Using thematic analysis in psychology. Qualitative Res. Psychology , 3(2):77–101, 2006.
- [7] S. Brookfield. Critically reflective practice. J. of Continuing Edu. in the Health Professions , 18(4):197–205, 1998.
- [8] J. E. Brooks-Harris and S. R. Stock-Ward. Workshops: Designing and Facilitating Experiential Learning . SAGE Publications, Inc, Thousand Oaks, CA, USA, 1999.
- [9] B. Buxton. Sketching User Experiences: Getting the Design Right and the Right Desing . Morgan Kaufmann, San Francisco, CA, USA, 2010.
- [10] M. Carcary. The research audit trail-enhancing trustworthiness in qualitative inquiry. The Electron. J. of Bus. Res. Methods , 7(1), 2009.
- [11] J. Corbin and A. Strauss. Grounded theory research: Procedures, canons, and evaluative critera. Qualitative Sociology , 13(1):3–21, 1990.
- [12] M. Crotty. The Foundations of Social Research . SAGE Publications, Inc, London, UK, 1998.
- [13] E. de Bono. Lateral Thinking for Management . Pelican Books, Middlesex, UK, 1983.
- [14] G. Dove and S. Jones. Using data to stimulate creative thinking in the design of new products and services. In Proc. Conf. Designing Interactive Syst. , pages 443–452. ACM SIGCHI, 2014.
- [15] G. Dove, S. Julie, M. Mose, and N. Brodersen. Grouping notes through nodes: The functions of post-it notes in design team cognition. In Des. Thinking Res. Symp. , Copenhagen Business School, 2016.
- [16] J. Dykes, J. Wood, and A. Slingsby. Rethinking map legends with visualization. IEEE Trans. Vis. Comput. Graphics , 16(6):890–899, 2010.
- [17] S. Goodwin, J. Dykes, S. Jones, I. Dillingham, G. Dove, D. Allison, A. Kachkaev, A. Slingsby, and J. Wood. Creative user-centered design for energy analysts and modelers. IEEE Trans. Vis. Comput. Graphics , 19(12):2516–2525, 2013.
- [18] S. Goodwin, C. Mears, T. Dwyer, M. Garcia de la Banda, G. Tack, and M. Wallace. What do constraint programming users want to see? Eexploring the role of visualisation in profiling of models and search. IEEE Trans. Vis. Comput. Graphics , 23(1):281–290, 2016.
- [19] J. Gordon, William. Synectics - the Developmnent of Creative Capacity . Harper and Row, New York, NY, USA, 1961.
- [20] D. Gray, J. Macanufo, and S. Brown. Gamestorming: A Playbook for Innovators, Rulebreakers, and Changemakers . O’Reilly Media, Sebastopol, CA, USA, 2010.
- [21] P. Hamilton. The Workshop Book: How to Design and Lead Succesful Workshops . FT Press, Upper Saddle River, NJ, USA, 2016.
- [22] S. He and E. Adar. VizItCards: A card-based toolkit for infovis design education. IEEE Trans. Vis. Comput. Graphics , 23(1):561–570, 2017.
- [23] T. Hewett, M. Czerwinski, M. Terry, J. Nunamaker, L. Candy, B. Kules, and E. Sylvan. Creativity support tool evaluation methods and metrics. In NSF Workshop Report on Creativity Support Tools , 2005.
- [24] M. J. Hicks. Problem Solving and Decision Making: Hard, Soft, and Creative Approaches . Thomson Learning, London, UK, 2004.
- [25] L. Hohmann. Innovation Games: Creating Breakthrough Products Through Collaborative Play . Addison-Wesley, Boston, MA, USA, 2007.
- [26] B. Hollis and N. Maiden. Extending agile processes with creativity techniques. IEEE Software , 30(5):78–84, 2013.
- [27] J. Horkoff, N. Maiden, and J. Lockerbie. Creativity and goal modeling for software requirements engineering. In Proc. Conf. Creativity and Cognition , pages 165–168. ACM, 2015.
- [28] S. Huron, S. Carpendale, J. Boy, and J. D. Fekete. Using VisKit: A manual for running a constructive visualization workshop. In Pedagogy of Data Vis. Workshop at IEEE Vis , 2016.
- [29] S. Huron, S. Carpendale, A. Thudt, A. Tang, and M. Mauerer. Constructive visualization. In Proc. Conf. Designing Interactive Syst. , pages 433–442. ACM SIGCHI, 2014.
- [30] Y. Jabareen. Building a conceptual framework: Philosophy, definitions, and procedure. Intern. J. of Qualitative Methods , 8(4):49–62, 2008.
- [31] S. Jones, P. Lynch, N. Maiden, and S. Lindstaedt. Use and influence of creative ideas and requirements for a work-integrated learning system. In Int. Requirements Eng. Conf. , pages 289–294. IEEE, 2008.
- [32] S. Jones and N. Maiden. RESCUE: An integrated method for specifying requirements for complex socio-technical systems. In J. L. Mate and A. Silva, editors, Requirements Engineering for Sociotechnical Systems , pages 245–265. Information Resources Press, Arlington, VA, USA, 2005.
- [33] S. Jones, N. Maiden, and K. Karlsen. Creativity in the specification of large-scale socio-technical systems. In Conf. Creative Inventions, Innovations and Everyday Des. HCI , 2007.
- [34] E. Kerzner, A. Lex, and M. Meyer. Utah population database workshop (workshop, University of Utah). unpublished, 2017.
- [35] E. Kerzner, A. Lex, T. Urness, C. L. Sigulinsky, B. W. Jones, R. E. Marc, and M. Meyer. Graffinity: Visualizing connectivity in large graphs. Comput. Graph. Forum , 34(3):251–260, 2017.
- [36] J. Knapp, J. Zeratsky, and B. Kowitz. Sprint: How to Solve Big Problems and Test New Ideas in Just Five Days . Simon & Schuster, New York, NY, USA, 2016.
- [37] L. C. Koh, A. Slingsby, J. Dykes, and T. S. Kam. Developing and applying a user-centered model for the design and implementation of information visualization tools. In Proc. Int. Conf. Inform. Vis. , pages 90–95. IEEE, 2011.
- [38] V. Kumar and V. LaConte. 101 Design Methods: A Structured Approach to Driving Innovation in Your Organization . Wiley, San Francisco, CA, USA, 2012.
- [39] H. Lam, E. Bertini, P. Isenberg, and C. Plaisant. Empirical studies in information visualization: Seven scenarios. IEEE Trans. Vis. Comput. Graphics , 18(9):1520–1536, 2012.
- [40] B. Laural, editor. Design Research: Methods and Perspectives . MIT Press, Cambridge, MA, USA, 2003.
- [41] Y. S. Lincoln and E. Guba. Naturalistic Inquiry . SAGE Publications, Inc, Thousand Oaks, CA, USA, 1985.
- [42] C. Lisle, E. Kerzner, A. Lex, and M. Meyer. Arbor summit workshop (workshop, University of Utah). unpublished, 2017.
- [43] D. Lloyd and J. Dykes. Human-centered approaches in geovisualization design: Investigating multiple methods through a long-term case study. IEEE Trans. Vis. Comput. Graphics , 17(12):2498–2507, 2011.
- [44] T. I. Lubart. Creativity across cultures. In R. J. Sternberg, editor, Handbook of Creativity , pages 339–350. Cambridge University Press, Cambridge, UK, 1999.
- [45] G. Lupi and S. Posavec. Dear Data: The Story of a Friendship in Fifty-Two Postcards . Penguin, London, UK, 2016.
- [46] G. Lupi and S. Posavec. Dear Data Postcard Kit: For Two Friends to Draw and Share (Postcards) . Princeton Architectural Press, New York City, NY, USA, 2017.
- [47] N. Maiden, S. Jones, K. Karlsen, R. Neill, K. Zachos, and A. Milne. Requirements engineering as creative problem solving: A research agenda for idea finding. In Int. Requirements Eng. Conf. , pages 57–66. IEEE, 2010.
- [48] N. Maiden, S. Manning, S. Robertson, and J. Greenwood. Integrating creativity workshops into structured requirements processes. In Proc. Conf. Designing Interactive Syst. , pages 113–122. ACM SIGCHI, 2004.
- [49] N. Maiden, C. Ncube, and S. Robertson. Can requirements be creative? Experiences with an enhanced air space management system. In Int. Conf. Software Eng. , pages 632–641. IEEE, 2007.
- [50] N. Maiden and S. Robertson. Developing use cases and scenarios in the requirements process. In Proc. Intern. Conf. Software Eng. , pages 561–570. ACM, 2005.
- [51] G. E. Marai. Activity-centered domain characterization for problem-driven scientific visualization. IEEE Trans. Vis. Comput. Graphics , 24(1):913–922, 2018.
- [52] C. Martindale. Biological bases of creativity. In R. J. Sternberg, editor, Handbook of Creativity , pages 137–152. Cambridge University Press, Cambridge, UK, 1999.
- [53] R. Mayer. Fifty years of creativity research. In R. J. Sternberg, editor, Handbook of Creativity , pages 449–460. Cambridge University Press, Cambridge, UK, 1999.
- [54] N. McCurdy, J. Dykes, and M. Meyer. Action design research and visualization design. In Proc. Workshop on Beyond Time and Errors on Novel Evaluation Methods for Vis. (BELIV) , pages 10–18. ACM, 2016.
- [55] E. McFadzean. The creativity continuum:towards a classification of creative problem solving techniques. J. of Creativity and Innovation Manage. , 7(3):131–139, 1998.
- [56] S. McKenna, D. Mazur, J. Agutter, and M. Meyer. Design activity framework for visualization design. IEEE Trans. Vis. Comput. Graphics , 20(12):2191–2200, 2014.
- [57] S. McKenna, D. Staheli, and M. Meyer. Unlocking user-centered design methods for building cyber security visualizations. In IEEE Symp. Vis. for Cyber Security (VizSec) , 2015.
- [58] M. Michalko. Thinkertoys: A Handbook for Creative-Thinking Techniques . Ten Speed Press, Emeryville, CA, USA, 2006.
- [59] W. C. Miller. The Creative Edge: Fostering Innovation Where You Work . Basic Books, New York City, NY, USA, 1989.
- [60] B. Mullen, C. Salas, and E. Johnson. Productivity loss in brainstorming groups: A meta-analytical integration. Basic and Appl. Social Psychology , 12(1):3–23, 1991.
- [61] M. Muller and S. Kuhn. Participatory design. Commun. ACM , 36(6):24–28, 1993.
- [62] T. Munzner. A nested model for visualization design and validation. IEEE Trans. Vis. Comput. Graphics , 15(6):921–928, 2009.
- [63] R. S. Nickerson. Enhancing creativity. In R. J. Sternberg, editor, Handbook of Creativity , pages 392–430. Cambridge University Press, Cambridge, UK, 1999.
- [64] C. Nobre, N. Gehlenborg, H. Coon, and A. Lex. Lineage: Visualizing multivariate clinical data in genealogy graphs. IEEE Trans. Vis. Comput. Graphics, to be published. , 2018.
- [65] D. A. Norman and S. W. Draper. User Centered System Design; New Perspectives on Human-Computer Interaction . L. Erlbaum Associates Inc, Hillsdale, NJ, USA, 1986.
- [66] A. Osborn. Applied Immagination: Principles and Procedures of Creative Problem Solving . Charle Scribener’s Sons, New York, NY, USA, 1953.
- [67] J. C. Roberts, C. Headleand, and P. D. Ritsos. Sketching designs using the five design-sheet methodology. IEEE Trans. Vis. Comput. Graphics , 22(1):419–428, 2016.
- [68] D. H. Rogers, C. Aragon, D. Keefe, E. Kerzner, N. McCurdy, M. Meyer, and F. Samsel. Discovery Jam. In IEEE Vis (Workshops) , 2016.
- [69] D. H. Rogers, F. Samsel, C. Aragon, D. F. Keefe, N. McCurdy, E. Kerzner, and M. Meyer. Discovery Jam. In IEEE Vis (Workshops) , 2017.
- [70] R. Sakai and J. Aerts. Card sorting techniques for domain characterization in problem-driven visualization research. In Eurographics Conf. Vis. (Short Papers) . Eurographics, 2015.
- [71] E. B.-N. Sanders. Information, insipiration, and co-creation. In Conf. European Academy of Des. , 2005.
- [72] E. B.-N. Sanders, E. Brandt, and T. Binder. A framework for organizing the tools and techniques of participatory design. In Proc. Participatory Des. Conf. , pages 195–198, 2010.
- [73] E. B.-N. Sanders and P. J. Stappers. Co-creation and the new landscapes of design. CoDesign: Int. J. of CoCreation in Des. and the Arts , 4(1):5–18, 2008.
- [74] L. Sanders and P. J. Stappers. Convivial Toolbox: Generative Research for the Front End of Design . BIS Publishers, Amsterdam, The Netherlands, 2013.
- [75] A. Satyanarayan, D. Moritz, and K. Wongsuphasawat. Vega-Lite: A grammar of interactive graphics. IEEE Trans. Vis. Comput. Graphics , 23(1):341–350, 2017.
- [76] K. R. Sawyer. Group Creativity: Music, Theater, Collaboration . Lawrence Erlbaum Associates, Mahwah, NJ, USA, 2003.
- [77] K. R. Sawyer. Explaining Creativity - the Science of Human Innovation . Oxford University Press, New York, NY, USA, 2006.
- [78] D. A. Schon. The Reflective Practitioner . Basic Books, New York City, NY, USA, 1988.
- [79] M. Sedlmair, P. Isenberg, D. Baur, and A. Butz. Evaluating information visualization in large companies: Challenges, experiences and recommendations. In Proc. Workshop on Beyond Time and Errors on Novel Evaluation Methods for Vis. (BELIV) , pages 79–86. ACM, 2010.
- [80] M. Sedlmair, M. Meyer, and T. Munzner. Design study methodology: Reflections from the trenches and the stacks. IEEE Trans. Vis. Comput. Graphics , 18(12):2431–2440, 2012.
- [81] B. Shneiderman, G. Fischer, M. Czerwinski, and B. Myers. NSF Workshop Report on Creativity Support Tools . National Science Foundation, 2005.
- [82] B. Shneiderman and C. Plaisant. Strategies for evaluating information visualization tools. In Proc. Workshop on Beyond Time and Errors on Novel Evaluation Methods for Vis. (BELIV) , pages 1–7. ACM, 2006.
- [83] R. B. Stanfield. The Workshop Book: From Individual Creativity to Group Action . New Society Publishers, Gabriola Island, BC, Canada, 2002.
- [84] S. Thompson and N. Thompson. The Critically Reflective Practioner . Palgrave Macmillan, New York, NY, USA, 2008.
- [85] M. Tory and T. Moller. Human factors in visualization research. IEEE Trans. Vis. Comput. Graphics , 10(1):72–82, 2004.
- [86] J. Vines, R. Clarke, and P. Wright. Configuring participation: On how we involve people in design. In CHI ’13 Proceedings of the SIGCHI Conference on Human Factors in Computing Systems , volume 20, 2013.
- [87] R. S. Vosko. Where we learn shapes our learning. New Directions for Adult and Continuing Edu. , 50(Summer):23–32, 1991.
- [88] R. Walker, A. Slingsby, J. Dykes, K. Xu, J. Wood, P. H. Nguyen, D. Stephens, B. L. W. Wong, and Y. Zheng. An extensible framework for provenance in human terrain visual analytics. IEEE Trans. Vis. Comput. Graphics , 19(12):2139–2148, 2013.
- [89] J. Walny, S. Huron, and S. Carpendale. An exploratory study of data sketching for visual representation. Comput. Graph. Forum , 34(3):231–240, 2015.
- [90] K. Wongsuphasawat, D. Motitz, J. Mackinlay, B. Howe, and J. Heer. Voyager: Exploratory analysis via faceted browsing of visualization recommendations. IEEE Trans. Vis. Comput. Graphics , 22(1):649–658, 2016.
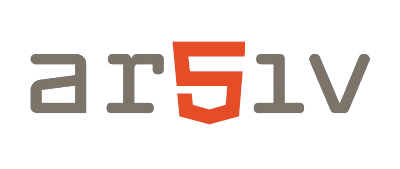
- Help & FAQ
A framework for creative visualization-opportunities workshops
Research output : Contribution to journal › Article › Research › peer-review
Applied visualization researchers often work closely with domain collaborators to explore new and useful applications of visualization. The early stages of collaborations are typically time consuming for all stakeholders as researchers piece together an understanding of domain challenges from disparate discussions and meetings. A number of recent projects, however, report on the use of creative visualization-opportunities (CVO) workshops to accelerate the early stages of applied work, eliciting a wealth of requirements in a few days of focused work. Yet, there is no established guidance for how to use such workshops effectively. In this paper, we present the results of a 2-year collaboration in which we analyzed the use of 17 workshops in 10 visualization contexts. Its primary contribution is a framework for CVO workshops that: 1) identifies a process model for using workshops; 2) describes a structure of what happens within effective workshops; 3) recommends 25 actionable guidelines for future workshops; and 4) presents an example workshop and workshop methods. The creation of this framework exemplifies the use of critical reflection to learn about visualization in practice from diverse studies and experience.
- Collaboration
- Conferences
- creativity workshops
- critically reflective practice
- Data visualization
- design studies
- Stakeholders
- User-centered visualization design
- Visualization
Access to Document
- 10.1109/TVCG.2018.2865241
Other files and links
- Link to publication in Scopus
T1 - A framework for creative visualization-opportunities workshops
AU - Kerzner, Ethan
AU - Goodwin, Sarah
AU - Dykes, Jason
AU - Jones, Sara
AU - Meyer, Miriah
PY - 2018/1
Y1 - 2018/1
N2 - Applied visualization researchers often work closely with domain collaborators to explore new and useful applications of visualization. The early stages of collaborations are typically time consuming for all stakeholders as researchers piece together an understanding of domain challenges from disparate discussions and meetings. A number of recent projects, however, report on the use of creative visualization-opportunities (CVO) workshops to accelerate the early stages of applied work, eliciting a wealth of requirements in a few days of focused work. Yet, there is no established guidance for how to use such workshops effectively. In this paper, we present the results of a 2-year collaboration in which we analyzed the use of 17 workshops in 10 visualization contexts. Its primary contribution is a framework for CVO workshops that: 1) identifies a process model for using workshops; 2) describes a structure of what happens within effective workshops; 3) recommends 25 actionable guidelines for future workshops; and 4) presents an example workshop and workshop methods. The creation of this framework exemplifies the use of critical reflection to learn about visualization in practice from diverse studies and experience.
AB - Applied visualization researchers often work closely with domain collaborators to explore new and useful applications of visualization. The early stages of collaborations are typically time consuming for all stakeholders as researchers piece together an understanding of domain challenges from disparate discussions and meetings. A number of recent projects, however, report on the use of creative visualization-opportunities (CVO) workshops to accelerate the early stages of applied work, eliciting a wealth of requirements in a few days of focused work. Yet, there is no established guidance for how to use such workshops effectively. In this paper, we present the results of a 2-year collaboration in which we analyzed the use of 17 workshops in 10 visualization contexts. Its primary contribution is a framework for CVO workshops that: 1) identifies a process model for using workshops; 2) describes a structure of what happens within effective workshops; 3) recommends 25 actionable guidelines for future workshops; and 4) presents an example workshop and workshop methods. The creation of this framework exemplifies the use of critical reflection to learn about visualization in practice from diverse studies and experience.
KW - Collaboration
KW - Conferences
KW - Creativity
KW - creativity workshops
KW - critically reflective practice
KW - Data visualization
KW - design studies
KW - Stakeholders
KW - User-centered visualization design
KW - Visualization
UR - http://www.scopus.com/inward/record.url?scp=85052625577&partnerID=8YFLogxK
U2 - 10.1109/TVCG.2018.2865241
DO - 10.1109/TVCG.2018.2865241
M3 - Article
C2 - 30137005
SN - 1077-2626
JO - IEEE Transactions on Visualization and Computer Graphics
JF - IEEE Transactions on Visualization and Computer Graphics
- Open access
- Published: 19 July 2015
The role of visual representations in scientific practices: from conceptual understanding and knowledge generation to ‘seeing’ how science works
- Maria Evagorou 1 ,
- Sibel Erduran 2 &
- Terhi Mäntylä 3
International Journal of STEM Education volume 2 , Article number: 11 ( 2015 ) Cite this article
74k Accesses
79 Citations
13 Altmetric
Metrics details
The use of visual representations (i.e., photographs, diagrams, models) has been part of science, and their use makes it possible for scientists to interact with and represent complex phenomena, not observable in other ways. Despite a wealth of research in science education on visual representations, the emphasis of such research has mainly been on the conceptual understanding when using visual representations and less on visual representations as epistemic objects. In this paper, we argue that by positioning visual representations as epistemic objects of scientific practices, science education can bring a renewed focus on how visualization contributes to knowledge formation in science from the learners’ perspective.
This is a theoretical paper, and in order to argue about the role of visualization, we first present a case study, that of the discovery of the structure of DNA that highlights the epistemic components of visual information in science. The second case study focuses on Faraday’s use of the lines of magnetic force. Faraday is known of his exploratory, creative, and yet systemic way of experimenting, and the visual reasoning leading to theoretical development was an inherent part of the experimentation. Third, we trace a contemporary account from science focusing on the experimental practices and how reproducibility of experimental procedures can be reinforced through video data.
Conclusions
Our conclusions suggest that in teaching science, the emphasis in visualization should shift from cognitive understanding—using the products of science to understand the content—to engaging in the processes of visualization. Furthermore, we suggest that is it essential to design curriculum materials and learning environments that create a social and epistemic context and invite students to engage in the practice of visualization as evidence, reasoning, experimental procedure, or a means of communication and reflect on these practices. Implications for teacher education include the need for teacher professional development programs to problematize the use of visual representations as epistemic objects that are part of scientific practices.
During the last decades, research and reform documents in science education across the world have been calling for an emphasis not only on the content but also on the processes of science (Bybee 2014 ; Eurydice 2012 ; Duschl and Bybee 2014 ; Osborne 2014 ; Schwartz et al. 2012 ), in order to make science accessible to the students and enable them to understand the epistemic foundation of science. Scientific practices, part of the process of science, are the cognitive and discursive activities that are targeted in science education to develop epistemic understanding and appreciation of the nature of science (Duschl et al. 2008 ) and have been the emphasis of recent reform documents in science education across the world (Achieve 2013 ; Eurydice 2012 ). With the term scientific practices, we refer to the processes that take place during scientific discoveries and include among others: asking questions, developing and using models, engaging in arguments, and constructing and communicating explanations (National Research Council 2012 ). The emphasis on scientific practices aims to move the teaching of science from knowledge to the understanding of the processes and the epistemic aspects of science. Additionally, by placing an emphasis on engaging students in scientific practices, we aim to help students acquire scientific knowledge in meaningful contexts that resemble the reality of scientific discoveries.
Despite a wealth of research in science education on visual representations, the emphasis of such research has mainly been on the conceptual understanding when using visual representations and less on visual representations as epistemic objects. In this paper, we argue that by positioning visual representations as epistemic objects, science education can bring a renewed focus on how visualization contributes to knowledge formation in science from the learners’ perspective. Specifically, the use of visual representations (i.e., photographs, diagrams, tables, charts) has been part of science and over the years has evolved with the new technologies (i.e., from drawings to advanced digital images and three dimensional models). Visualization makes it possible for scientists to interact with complex phenomena (Richards 2003 ), and they might convey important evidence not observable in other ways. Visual representations as a tool to support cognitive understanding in science have been studied extensively (i.e., Gilbert 2010 ; Wu and Shah 2004 ). Studies in science education have explored the use of images in science textbooks (i.e., Dimopoulos et al. 2003 ; Bungum 2008 ), students’ representations or models when doing science (i.e., Gilbert et al. 2008 ; Dori et al. 2003 ; Lehrer and Schauble 2012 ; Schwarz et al. 2009 ), and students’ images of science and scientists (i.e., Chambers 1983 ). Therefore, studies in the field of science education have been using the term visualization as “the formation of an internal representation from an external representation” (Gilbert et al. 2008 , p. 4) or as a tool for conceptual understanding for students.
In this paper, we do not refer to visualization as mental image, model, or presentation only (Gilbert et al. 2008 ; Philips et al. 2010 ) but instead focus on visual representations or visualization as epistemic objects. Specifically, we refer to visualization as a process for knowledge production and growth in science. In this respect, modeling is an aspect of visualization, but what we are focusing on with visualization is not on the use of model as a tool for cognitive understanding (Gilbert 2010 ; Wu and Shah 2004 ) but the on the process of modeling as a scientific practice which includes the construction and use of models, the use of other representations, the communication in the groups with the use of the visual representation, and the appreciation of the difficulties that the science phase in this process. Therefore, the purpose of this paper is to present through the history of science how visualization can be considered not only as a cognitive tool in science education but also as an epistemic object that can potentially support students to understand aspects of the nature of science.
Scientific practices and science education
According to the New Generation Science Standards (Achieve 2013 ), scientific practices refer to: asking questions and defining problems; developing and using models; planning and carrying out investigations; analyzing and interpreting data; using mathematical and computational thinking; constructing explanations and designing solutions; engaging in argument from evidence; and obtaining, evaluating, and communicating information. A significant aspect of scientific practices is that science learning is more than just about learning facts, concepts, theories, and laws. A fuller appreciation of science necessitates the understanding of the science relative to its epistemological grounding and the process that are involved in the production of knowledge (Hogan and Maglienti 2001 ; Wickman 2004 ).
The New Generation Science Standards is, among other changes, shifting away from science inquiry and towards the inclusion of scientific practices (Duschl and Bybee 2014 ; Osborne 2014 ). By comparing the abilities to do scientific inquiry (National Research Council 2000 ) with the set of scientific practices, it is evident that the latter is about engaging in the processes of doing science and experiencing in that way science in a more authentic way. Engaging in scientific practices according to Osborne ( 2014 ) “presents a more authentic picture of the endeavor that is science” (p.183) and also helps the students to develop a deeper understanding of the epistemic aspects of science. Furthermore, as Bybee ( 2014 ) argues, by engaging students in scientific practices, we involve them in an understanding of the nature of science and an understanding on the nature of scientific knowledge.
Science as a practice and scientific practices as a term emerged by the philosopher of science, Kuhn (Osborne 2014 ), refers to the processes in which the scientists engage during knowledge production and communication. The work that is followed by historians, philosophers, and sociologists of science (Latour 2011 ; Longino 2002 ; Nersessian 2008 ) revealed the scientific practices in which the scientists engage in and include among others theory development and specific ways of talking, modeling, and communicating the outcomes of science.
Visualization as an epistemic object
Schematic, pictorial symbols in the design of scientific instruments and analysis of the perceptual and functional information that is being stored in those images have been areas of investigation in philosophy of scientific experimentation (Gooding et al. 1993 ). The nature of visual perception, the relationship between thought and vision, and the role of reproducibility as a norm for experimental research form a central aspect of this domain of research in philosophy of science. For instance, Rothbart ( 1997 ) has argued that visualizations are commonplace in the theoretical sciences even if every scientific theory may not be defined by visualized models.
Visual representations (i.e., photographs, diagrams, tables, charts, models) have been used in science over the years to enable scientists to interact with complex phenomena (Richards 2003 ) and might convey important evidence not observable in other ways (Barber et al. 2006 ). Some authors (e.g., Ruivenkamp and Rip 2010 ) have argued that visualization is as a core activity of some scientific communities of practice (e.g., nanotechnology) while others (e.g., Lynch and Edgerton 1988 ) have differentiated the role of particular visualization techniques (e.g., of digital image processing in astronomy). Visualization in science includes the complex process through which scientists develop or produce imagery, schemes, and graphical representation, and therefore, what is of importance in this process is not only the result but also the methodology employed by the scientists, namely, how this result was produced. Visual representations in science may refer to objects that are believed to have some kind of material or physical existence but equally might refer to purely mental, conceptual, and abstract constructs (Pauwels 2006 ). More specifically, visual representations can be found for: (a) phenomena that are not observable with the eye (i.e., microscopic or macroscopic); (b) phenomena that do not exist as visual representations but can be translated as such (i.e., sound); and (c) in experimental settings to provide visual data representations (i.e., graphs presenting velocity of moving objects). Additionally, since science is not only about replicating reality but also about making it more understandable to people (either to the public or other scientists), visual representations are not only about reproducing the nature but also about: (a) functioning in helping solving a problem, (b) filling gaps in our knowledge, and (c) facilitating knowledge building or transfer (Lynch 2006 ).
Using or developing visual representations in the scientific practice can range from a straightforward to a complicated situation. More specifically, scientists can observe a phenomenon (i.e., mitosis) and represent it visually using a picture or diagram, which is quite straightforward. But they can also use a variety of complicated techniques (i.e., crystallography in the case of DNA studies) that are either available or need to be developed or refined in order to acquire the visual information that can be used in the process of theory development (i.e., Latour and Woolgar 1979 ). Furthermore, some visual representations need decoding, and the scientists need to learn how to read these images (i.e., radiologists); therefore, using visual representations in the process of science requires learning a new language that is specific to the medium/methods that is used (i.e., understanding an X-ray picture is different from understanding an MRI scan) and then communicating that language to other scientists and the public.
There are much intent and purposes of visual representations in scientific practices, as for example to make a diagnosis, compare, describe, and preserve for future study, verify and explore new territory, generate new data (Pauwels 2006 ), or present new methodologies. According to Latour and Woolgar ( 1979 ) and Knorr Cetina ( 1999 ), visual representations can be used either as primary data (i.e., image from a microscope). or can be used to help in concept development (i.e., models of DNA used by Watson and Crick), to uncover relationships and to make the abstract more concrete (graphs of sound waves). Therefore, visual representations and visual practices, in all forms, are an important aspect of the scientific practices in developing, clarifying, and transmitting scientific knowledge (Pauwels 2006 ).
Methods and Results: Merging Visualization and scientific practices in science
In this paper, we present three case studies that embody the working practices of scientists in an effort to present visualization as a scientific practice and present our argument about how visualization is a complex process that could include among others modeling and use of representation but is not only limited to that. The first case study explores the role of visualization in the construction of knowledge about the structure of DNA, using visuals as evidence. The second case study focuses on Faraday’s use of the lines of magnetic force and the visual reasoning leading to the theoretical development that was an inherent part of the experimentation. The third case study focuses on the current practices of scientists in the context of a peer-reviewed journal called the Journal of Visualized Experiments where the methodology is communicated through videotaped procedures. The three case studies represent the research interests of the three authors of this paper and were chosen to present how visualization as a practice can be involved in all stages of doing science, from hypothesizing and evaluating evidence (case study 1) to experimenting and reasoning (case study 2) to communicating the findings and methodology with the research community (case study 3), and represent in this way the three functions of visualization as presented by Lynch ( 2006 ). Furthermore, the last case study showcases how the development of visualization technologies has contributed to the communication of findings and methodologies in science and present in that way an aspect of current scientific practices. In all three cases, our approach is guided by the observation that the visual information is an integral part of scientific practices at the least and furthermore that they are particularly central in the scientific practices of science.
Case study 1: use visual representations as evidence in the discovery of DNA
The focus of the first case study is the discovery of the structure of DNA. The DNA was first isolated in 1869 by Friedrich Miescher, and by the late 1940s, it was known that it contained phosphate, sugar, and four nitrogen-containing chemical bases. However, no one had figured the structure of the DNA until Watson and Crick presented their model of DNA in 1953. Other than the social aspects of the discovery of the DNA, another important aspect was the role of visual evidence that led to knowledge development in the area. More specifically, by studying the personal accounts of Watson ( 1968 ) and Crick ( 1988 ) about the discovery of the structure of the DNA, the following main ideas regarding the role of visual representations in the production of knowledge can be identified: (a) The use of visual representations was an important part of knowledge growth and was often dependent upon the discovery of new technologies (i.e., better microscopes or better techniques in crystallography that would provide better visual representations as evidence of the helical structure of the DNA); and (b) Models (three-dimensional) were used as a way to represent the visual images (X-ray images) and connect them to the evidence provided by other sources to see whether the theory can be supported. Therefore, the model of DNA was built based on the combination of visual evidence and experimental data.
An example showcasing the importance of visual representations in the process of knowledge production in this case is provided by Watson, in his book The Double Helix (1968):
…since the middle of the summer Rosy [Rosalind Franklin] had had evidence for a new three-dimensional form of DNA. It occurred when the DNA 2molecules were surrounded by a large amount of water. When I asked what the pattern was like, Maurice went into the adjacent room to pick up a print of the new form they called the “B” structure. The instant I saw the picture, my mouth fell open and my pulse began to race. The pattern was unbelievably simpler than those previously obtained (A form). Moreover, the black cross of reflections which dominated the picture could arise only from a helical structure. With the A form the argument for the helix was never straightforward, and considerable ambiguity existed as to exactly which type of helical symmetry was present. With the B form however, mere inspection of its X-ray picture gave several of the vital helical parameters. (p. 167-169)
As suggested by Watson’s personal account of the discovery of the DNA, the photo taken by Rosalind Franklin (Fig. 1 ) convinced him that the DNA molecule must consist of two chains arranged in a paired helix, which resembles a spiral staircase or ladder, and on March 7, 1953, Watson and Crick finished and presented their model of the structure of DNA (Watson and Berry 2004 ; Watson 1968 ) which was based on the visual information provided by the X-ray image and their knowledge of chemistry.
X-ray chrystallography of DNA
In analyzing the visualization practice in this case study, we observe the following instances that highlight how the visual information played a role:
Asking questions and defining problems: The real world in the model of science can at some points only be observed through visual representations or representations, i.e., if we are using DNA as an example, the structure of DNA was only observable through the crystallography images produced by Rosalind Franklin in the laboratory. There was no other way to observe the structure of DNA, therefore the real world.
Analyzing and interpreting data: The images that resulted from crystallography as well as their interpretations served as the data for the scientists studying the structure of DNA.
Experimenting: The data in the form of visual information were used to predict the possible structure of the DNA.
Modeling: Based on the prediction, an actual three-dimensional model was prepared by Watson and Crick. The first model did not fit with the real world (refuted by Rosalind Franklin and her research group from King’s College) and Watson and Crick had to go through the same process again to find better visual evidence (better crystallography images) and create an improved visual model.
Example excerpts from Watson’s biography provide further evidence for how visualization practices were applied in the context of the discovery of DNA (Table 1 ).
In summary, by examining the history of the discovery of DNA, we showcased how visual data is used as scientific evidence in science, identifying in that way an aspect of the nature of science that is still unexplored in the history of science and an aspect that has been ignored in the teaching of science. Visual representations are used in many ways: as images, as models, as evidence to support or rebut a model, and as interpretations of reality.
Case study 2: applying visual reasoning in knowledge production, the example of the lines of magnetic force
The focus of this case study is on Faraday’s use of the lines of magnetic force. Faraday is known of his exploratory, creative, and yet systemic way of experimenting, and the visual reasoning leading to theoretical development was an inherent part of this experimentation (Gooding 2006 ). Faraday’s articles or notebooks do not include mathematical formulations; instead, they include images and illustrations from experimental devices and setups to the recapping of his theoretical ideas (Nersessian 2008 ). According to Gooding ( 2006 ), “Faraday’s visual method was designed not to copy apparent features of the world, but to analyse and replicate them” (2006, p. 46).
The lines of force played a central role in Faraday’s research on electricity and magnetism and in the development of his “field theory” (Faraday 1852a ; Nersessian 1984 ). Before Faraday, the experiments with iron filings around magnets were known and the term “magnetic curves” was used for the iron filing patterns and also for the geometrical constructs derived from the mathematical theory of magnetism (Gooding et al. 1993 ). However, Faraday used the lines of force for explaining his experimental observations and in constructing the theory of forces in magnetism and electricity. Examples of Faraday’s different illustrations of lines of magnetic force are given in Fig. 2 . Faraday gave the following experiment-based definition for the lines of magnetic forces:
a Iron filing pattern in case of bar magnet drawn by Faraday (Faraday 1852b , Plate IX, p. 158, Fig. 1), b Faraday’s drawing of lines of magnetic force in case of cylinder magnet, where the experimental procedure, knife blade showing the direction of lines, is combined into drawing (Faraday, 1855, vol. 1, plate 1)
A line of magnetic force may be defined as that line which is described by a very small magnetic needle, when it is so moved in either direction correspondent to its length, that the needle is constantly a tangent to the line of motion; or it is that line along which, if a transverse wire be moved in either direction, there is no tendency to the formation of any current in the wire, whilst if moved in any other direction there is such a tendency; or it is that line which coincides with the direction of the magnecrystallic axis of a crystal of bismuth, which is carried in either direction along it. The direction of these lines about and amongst magnets and electric currents, is easily represented and understood, in a general manner, by the ordinary use of iron filings. (Faraday 1852a , p. 25 (3071))
The definition describes the connection between the experiments and the visual representation of the results. Initially, the lines of force were just geometric representations, but later, Faraday treated them as physical objects (Nersessian 1984 ; Pocovi and Finlay 2002 ):
I have sometimes used the term lines of force so vaguely, as to leave the reader doubtful whether I intended it as a merely representative idea of the forces, or as the description of the path along which the power was continuously exerted. … wherever the expression line of force is taken simply to represent the disposition of forces, it shall have the fullness of that meaning; but that wherever it may seem to represent the idea of the physical mode of transmission of the force, it expresses in that respect the opinion to which I incline at present. The opinion may be erroneous, and yet all that relates or refers to the disposition of the force will remain the same. (Faraday, 1852a , p. 55-56 (3075))
He also felt that the lines of force had greater explanatory power than the dominant theory of action-at-a-distance:
Now it appears to me that these lines may be employed with great advantage to represent nature, condition, direction and comparative amount of the magnetic forces; and that in many cases they have, to the physical reasoned at least, a superiority over that method which represents the forces as concentrated in centres of action… (Faraday, 1852a , p. 26 (3074))
For giving some insight to Faraday’s visual reasoning as an epistemic practice, the following examples of Faraday’s studies of the lines of magnetic force (Faraday 1852a , 1852b ) are presented:
(a) Asking questions and defining problems: The iron filing patterns formed the empirical basis for the visual model: 2D visualization of lines of magnetic force as presented in Fig. 2 . According to Faraday, these iron filing patterns were suitable for illustrating the direction and form of the magnetic lines of force (emphasis added):
It must be well understood that these forms give no indication by their appearance of the relative strength of the magnetic force at different places, inasmuch as the appearance of the lines depends greatly upon the quantity of filings and the amount of tapping; but the direction and forms of these lines are well given, and these indicate, in a considerable degree, the direction in which the forces increase and diminish . (Faraday 1852b , p.158 (3237))
Despite being static and two dimensional on paper, the lines of magnetic force were dynamical (Nersessian 1992 , 2008 ) and three dimensional for Faraday (see Fig. 2 b). For instance, Faraday described the lines of force “expanding”, “bending,” and “being cut” (Nersessian 1992 ). In Fig. 2 b, Faraday has summarized his experiment (bar magnet and knife blade) and its results (lines of force) in one picture.
(b) Analyzing and interpreting data: The model was so powerful for Faraday that he ended up thinking them as physical objects (e.g., Nersessian 1984 ), i.e., making interpretations of the way forces act. Of course, he made a lot of experiments for showing the physical existence of the lines of force, but he did not succeed in it (Nersessian 1984 ). The following quote illuminates Faraday’s use of the lines of force in different situations:
The study of these lines has, at different times, been greatly influential in leading me to various results, which I think prove their utility as well as fertility. Thus, the law of magneto-electric induction; the earth’s inductive action; the relation of magnetism and light; diamagnetic action and its law, and magnetocrystallic action, are the cases of this kind… (Faraday 1852a , p. 55 (3174))
(c) Experimenting: In Faraday's case, he used a lot of exploratory experiments; in case of lines of magnetic force, he used, e.g., iron filings, magnetic needles, or current carrying wires (see the quote above). The magnetic field is not directly observable and the representation of lines of force was a visual model, which includes the direction, form, and magnitude of field.
(d) Modeling: There is no denying that the lines of magnetic force are visual by nature. Faraday’s views of lines of force developed gradually during the years, and he applied and developed them in different contexts such as electromagnetic, electrostatic, and magnetic induction (Nersessian 1984 ). An example of Faraday’s explanation of the effect of the wire b’s position to experiment is given in Fig. 3 . In Fig. 3 , few magnetic lines of force are drawn, and in the quote below, Faraday is explaining the effect using these magnetic lines of force (emphasis added):
Picture of an experiment with different arrangements of wires ( a , b’ , b” ), magnet, and galvanometer. Note the lines of force drawn around the magnet. (Faraday 1852a , p. 34)
It will be evident by inspection of Fig. 3 , that, however the wires are carried away, the general result will, according to the assumed principles of action, be the same; for if a be the axial wire, and b’, b”, b”’ the equatorial wire, represented in three different positions, whatever magnetic lines of force pass across the latter wire in one position, will also pass it in the other, or in any other position which can be given to it. The distance of the wire at the place of intersection with the lines of force, has been shown, by the experiments (3093.), to be unimportant. (Faraday 1852a , p. 34 (3099))
In summary, by examining the history of Faraday’s use of lines of force, we showed how visual imagery and reasoning played an important part in Faraday’s construction and representation of his “field theory”. As Gooding has stated, “many of Faraday’s sketches are far more that depictions of observation, they are tools for reasoning with and about phenomena” (2006, p. 59).
Case study 3: visualizing scientific methods, the case of a journal
The focus of the third case study is the Journal of Visualized Experiments (JoVE) , a peer-reviewed publication indexed in PubMed. The journal devoted to the publication of biological, medical, chemical, and physical research in a video format. The journal describes its history as follows:
JoVE was established as a new tool in life science publication and communication, with participation of scientists from leading research institutions. JoVE takes advantage of video technology to capture and transmit the multiple facets and intricacies of life science research. Visualization greatly facilitates the understanding and efficient reproduction of both basic and complex experimental techniques, thereby addressing two of the biggest challenges faced by today's life science research community: i) low transparency and poor reproducibility of biological experiments and ii) time and labor-intensive nature of learning new experimental techniques. ( http://www.jove.com/ )
By examining the journal content, we generate a set of categories that can be considered as indicators of relevance and significance in terms of epistemic practices of science that have relevance for science education. For example, the quote above illustrates how scientists view some norms of scientific practice including the norms of “transparency” and “reproducibility” of experimental methods and results, and how the visual format of the journal facilitates the implementation of these norms. “Reproducibility” can be considered as an epistemic criterion that sits at the heart of what counts as an experimental procedure in science:
Investigating what should be reproducible and by whom leads to different types of experimental reproducibility, which can be observed to play different roles in experimental practice. A successful application of the strategy of reproducing an experiment is an achievement that may depend on certain isiosyncratic aspects of a local situation. Yet a purely local experiment that cannot be carried out by other experimenters and in other experimental contexts will, in the end be unproductive in science. (Sarkar and Pfeifer 2006 , p.270)
We now turn to an article on “Elevated Plus Maze for Mice” that is available for free on the journal website ( http://www.jove.com/video/1088/elevated-plus-maze-for-mice ). The purpose of this experiment was to investigate anxiety levels in mice through behavioral analysis. The journal article consists of a 9-min video accompanied by text. The video illustrates the handling of the mice in soundproof location with less light, worksheets with characteristics of mice, computer software, apparatus, resources, setting up the computer software, and the video recording of mouse behavior on the computer. The authors describe the apparatus that is used in the experiment and state how procedural differences exist between research groups that lead to difficulties in the interpretation of results:
The apparatus consists of open arms and closed arms, crossed in the middle perpendicularly to each other, and a center area. Mice are given access to all of the arms and are allowed to move freely between them. The number of entries into the open arms and the time spent in the open arms are used as indices of open space-induced anxiety in mice. Unfortunately, the procedural differences that exist between laboratories make it difficult to duplicate and compare results among laboratories.
The authors’ emphasis on the particularity of procedural context echoes in the observations of some philosophers of science:
It is not just the knowledge of experimental objects and phenomena but also their actual existence and occurrence that prove to be dependent on specific, productive interventions by the experimenters” (Sarkar and Pfeifer 2006 , pp. 270-271)
The inclusion of a video of the experimental procedure specifies what the apparatus looks like (Fig. 4 ) and how the behavior of the mice is captured through video recording that feeds into a computer (Fig. 5 ). Subsequently, a computer software which captures different variables such as the distance traveled, the number of entries, and the time spent on each arm of the apparatus. Here, there is visual information at different levels of representation ranging from reconfiguration of raw video data to representations that analyze the data around the variables in question (Fig. 6 ). The practice of levels of visual representations is not particular to the biological sciences. For instance, they are commonplace in nanotechnological practices:
Visual illustration of apparatus
Video processing of experimental set-up
Computer software for video input and variable recording
In the visualization processes, instruments are needed that can register the nanoscale and provide raw data, which needs to be transformed into images. Some Imaging Techniques have software incorporated already where this transformation automatically takes place, providing raw images. Raw data must be translated through the use of Graphic Software and software is also used for the further manipulation of images to highlight what is of interest to capture the (inferred) phenomena -- and to capture the reader. There are two levels of choice: Scientists have to choose which imaging technique and embedded software to use for the job at hand, and they will then have to follow the structure of the software. Within such software, there are explicit choices for the scientists, e.g. about colour coding, and ways of sharpening images. (Ruivenkamp and Rip 2010 , pp.14–15)
On the text that accompanies the video, the authors highlight the role of visualization in their experiment:
Visualization of the protocol will promote better understanding of the details of the entire experimental procedure, allowing for standardization of the protocols used in different laboratories and comparisons of the behavioral phenotypes of various strains of mutant mice assessed using this test.
The software that takes the video data and transforms it into various representations allows the researchers to collect data on mouse behavior more reliably. For instance, the distance traveled across the arms of the apparatus or the time spent on each arm would have been difficult to observe and record precisely. A further aspect to note is how the visualization of the experiment facilitates control of bias. The authors illustrate how the olfactory bias between experimental procedures carried on mice in sequence is avoided by cleaning the equipment.
Our discussion highlights the role of visualization in science, particularly with respect to presenting visualization as part of the scientific practices. We have used case studies from the history of science highlighting a scientist’s account of how visualization played a role in the discovery of DNA and the magnetic field and from a contemporary illustration of a science journal’s practices in incorporating visualization as a way to communicate new findings and methodologies. Our implicit aim in drawing from these case studies was the need to align science education with scientific practices, particularly in terms of how visual representations, stable or dynamic, can engage students in the processes of science and not only to be used as tools for cognitive development in science. Our approach was guided by the notion of “knowledge-as-practice” as advanced by Knorr Cetina ( 1999 ) who studied scientists and characterized their knowledge as practice, a characterization which shifts focus away from ideas inside scientists’ minds to practices that are cultural and deeply contextualized within fields of science. She suggests that people working together can be examined as epistemic cultures whose collective knowledge exists as practice.
It is important to stress, however, that visual representations are not used in isolation, but are supported by other types of evidence as well, or other theories (i.e., in order to understand the helical form of DNA, or the structure, chemistry knowledge was needed). More importantly, this finding can also have implications when teaching science as argument (e.g., Erduran and Jimenez-Aleixandre 2008 ), since the verbal evidence used in the science classroom to maintain an argument could be supported by visual evidence (either a model, representation, image, graph, etc.). For example, in a group of students discussing the outcomes of an introduced species in an ecosystem, pictures of the species and the ecosystem over time, and videos showing the changes in the ecosystem, and the special characteristics of the different species could serve as visual evidence to help the students support their arguments (Evagorou et al. 2012 ). Therefore, an important implication for the teaching of science is the use of visual representations as evidence in the science curriculum as part of knowledge production. Even though studies in the area of science education have focused on the use of models and modeling as a way to support students in the learning of science (Dori et al. 2003 ; Lehrer and Schauble 2012 ; Mendonça and Justi 2013 ; Papaevripidou et al. 2007 ) or on the use of images (i.e., Korfiatis et al. 2003 ), with the term using visuals as evidence, we refer to the collection of all forms of visuals and the processes involved.
Another aspect that was identified through the case studies is that of the visual reasoning (an integral part of Faraday’s investigations). Both the verbalization and visualization were part of the process of generating new knowledge (Gooding 2006 ). Even today, most of the textbooks use the lines of force (or just field lines) as a geometrical representation of field, and the number of field lines is connected to the quantity of flux. Often, the textbooks use the same kind of visual imagery than in what is used by scientists. However, when using images, only certain aspects or features of the phenomena or data are captured or highlighted, and often in tacit ways. Especially in textbooks, the process of producing the image is not presented and instead only the product—image—is left. This could easily lead to an idea of images (i.e., photos, graphs, visual model) being just representations of knowledge and, in the worse case, misinterpreted representations of knowledge as the results of Pocovi and Finlay ( 2002 ) in case of electric field lines show. In order to avoid this, the teachers should be able to explain how the images are produced (what features of phenomena or data the images captures, on what ground the features are chosen to that image, and what features are omitted); in this way, the role of visualization in knowledge production can be made “visible” to students by engaging them in the process of visualization.
The implication of these norms for science teaching and learning is numerous. The classroom contexts can model the generation, sharing and evaluation of evidence, and experimental procedures carried out by students, thereby promoting not only some contemporary cultural norms in scientific practice but also enabling the learning of criteria, standards, and heuristics that scientists use in making decisions on scientific methods. As we have demonstrated with the three case studies, visual representations are part of the process of knowledge growth and communication in science, as demonstrated with two examples from the history of science and an example from current scientific practices. Additionally, visual information, especially with the use of technology is a part of students’ everyday lives. Therefore, we suggest making use of students’ knowledge and technological skills (i.e., how to produce their own videos showing their experimental method or how to identify or provide appropriate visual evidence for a given topic), in order to teach them the aspects of the nature of science that are often neglected both in the history of science and the design of curriculum. Specifically, what we suggest in this paper is that students should actively engage in visualization processes in order to appreciate the diverse nature of doing science and engage in authentic scientific practices.
However, as a word of caution, we need to distinguish the products and processes involved in visualization practices in science:
If one considers scientific representations and the ways in which they can foster or thwart our understanding, it is clear that a mere object approach, which would devote all attention to the representation as a free-standing product of scientific labor, is inadequate. What is needed is a process approach: each visual representation should be linked with its context of production (Pauwels 2006 , p.21).
The aforementioned suggests that the emphasis in visualization should shift from cognitive understanding—using the products of science to understand the content—to engaging in the processes of visualization. Therefore, an implication for the teaching of science includes designing curriculum materials and learning environments that create a social and epistemic context and invite students to engage in the practice of visualization as evidence, reasoning, experimental procedure, or a means of communication (as presented in the three case studies) and reflect on these practices (Ryu et al. 2015 ).
Finally, a question that arises from including visualization in science education, as well as from including scientific practices in science education is whether teachers themselves are prepared to include them as part of their teaching (Bybee 2014 ). Teacher preparation programs and teacher education have been critiqued, studied, and rethought since the time they emerged (Cochran-Smith 2004 ). Despite the years of history in teacher training and teacher education, the debate about initial teacher training and its content still pertains in our community and in policy circles (Cochran-Smith 2004 ; Conway et al. 2009 ). In the last decades, the debate has shifted from a behavioral view of learning and teaching to a learning problem—focusing on that way not only on teachers’ knowledge, skills, and beliefs but also on making the connection of the aforementioned with how and if pupils learn (Cochran-Smith 2004 ). The Science Education in Europe report recommended that “Good quality teachers, with up-to-date knowledge and skills, are the foundation of any system of formal science education” (Osborne and Dillon 2008 , p.9).
However, questions such as what should be the emphasis on pre-service and in-service science teacher training, especially with the new emphasis on scientific practices, still remain unanswered. As Bybee ( 2014 ) argues, starting from the new emphasis on scientific practices in the NGSS, we should consider teacher preparation programs “that would provide undergraduates opportunities to learn the science content and practices in contexts that would be aligned with their future work as teachers” (p.218). Therefore, engaging pre- and in-service teachers in visualization as a scientific practice should be one of the purposes of teacher preparation programs.
Achieve. (2013). The next generation science standards (pp. 1–3). Retrieved from http://www.nextgenscience.org/ .
Google Scholar
Barber, J, Pearson, D, & Cervetti, G. (2006). Seeds of science/roots of reading . California: The Regents of the University of California.
Bungum, B. (2008). Images of physics: an explorative study of the changing character of visual images in Norwegian physics textbooks. NorDiNa, 4 (2), 132–141.
Bybee, RW. (2014). NGSS and the next generation of science teachers. Journal of Science Teacher Education, 25 (2), 211–221. doi: 10.1007/s10972-014-9381-4 .
Article Google Scholar
Chambers, D. (1983). Stereotypic images of the scientist: the draw-a-scientist test. Science Education, 67 (2), 255–265.
Cochran-Smith, M. (2004). The problem of teacher education. Journal of Teacher Education, 55 (4), 295–299. doi: 10.1177/0022487104268057 .
Conway, PF, Murphy, R, & Rath, A. (2009). Learning to teach and its implications for the continuum of teacher education: a nine-country cross-national study .
Crick, F. (1988). What a mad pursuit . USA: Basic Books.
Dimopoulos, K, Koulaidis, V, & Sklaveniti, S. (2003). Towards an analysis of visual images in school science textbooks and press articles about science and technology. Research in Science Education, 33 , 189–216.
Dori, YJ, Tal, RT, & Tsaushu, M. (2003). Teaching biotechnology through case studies—can we improve higher order thinking skills of nonscience majors? Science Education, 87 (6), 767–793. doi: 10.1002/sce.10081 .
Duschl, RA, & Bybee, RW. (2014). Planning and carrying out investigations: an entry to learning and to teacher professional development around NGSS science and engineering practices. International Journal of STEM Education, 1 (1), 12. doi: 10.1186/s40594-014-0012-6 .
Duschl, R., Schweingruber, H. A., & Shouse, A. (2008). Taking science to school . Washington DC: National Academies Press.
Erduran, S, & Jimenez-Aleixandre, MP (Eds.). (2008). Argumentation in science education: perspectives from classroom-based research . Dordrecht: Springer.
Eurydice. (2012). Developing key competencies at school in Europe: challenges and opportunities for policy – 2011/12 (pp. 1–72).
Evagorou, M, Jimenez-Aleixandre, MP, & Osborne, J. (2012). “Should we kill the grey squirrels?” A study exploring students’ justifications and decision-making. International Journal of Science Education, 34 (3), 401–428. doi: 10.1080/09500693.2011.619211 .
Faraday, M. (1852a). Experimental researches in electricity. – Twenty-eighth series. Philosophical Transactions of the Royal Society of London, 142 , 25–56.
Faraday, M. (1852b). Experimental researches in electricity. – Twenty-ninth series. Philosophical Transactions of the Royal Society of London, 142 , 137–159.
Gilbert, JK. (2010). The role of visual representations in the learning and teaching of science: an introduction (pp. 1–19).
Gilbert, J., Reiner, M. & Nakhleh, M. (2008). Visualization: theory and practice in science education . Dordrecht, The Netherlands: Springer.
Gooding, D. (2006). From phenomenology to field theory: Faraday’s visual reasoning. Perspectives on Science, 14 (1), 40–65.
Gooding, D, Pinch, T, & Schaffer, S (Eds.). (1993). The uses of experiment: studies in the natural sciences . Cambridge: Cambridge University Press.
Hogan, K, & Maglienti, M. (2001). Comparing the epistemological underpinnings of students’ and scientists’ reasoning about conclusions. Journal of Research in Science Teaching, 38 (6), 663–687.
Knorr Cetina, K. (1999). Epistemic cultures: how the sciences make knowledge . Cambridge: Harvard University Press.
Korfiatis, KJ, Stamou, AG, & Paraskevopoulos, S. (2003). Images of nature in Greek primary school textbooks. Science Education, 88 (1), 72–89. doi: 10.1002/sce.10133 .
Latour, B. (2011). Visualisation and cognition: drawing things together (pp. 1–32).
Latour, B, & Woolgar, S. (1979). Laboratory life: the construction of scientific facts . Princeton: Princeton University Press.
Lehrer, R, & Schauble, L. (2012). Seeding evolutionary thinking by engaging children in modeling its foundations. Science Education, 96 (4), 701–724. doi: 10.1002/sce.20475 .
Longino, H. E. (2002). The fate of knowledge . Princeton: Princeton University Press.
Lynch, M. (2006). The production of scientific images: vision and re-vision in the history, philosophy, and sociology of science. In L Pauwels (Ed.), Visual cultures of science: rethinking representational practices in knowledge building and science communication (pp. 26–40). Lebanon, NH: Darthmouth College Press.
Lynch, M. & S. Y. Edgerton Jr. (1988). ‘Aesthetic and digital image processing representational craft in contemporary astronomy’, in G. Fyfe & J. Law (eds), Picturing Power; Visual Depictions and Social Relations (London, Routledge): 184 – 220.
Mendonça, PCC, & Justi, R. (2013). An instrument for analyzing arguments produced in modeling-based chemistry lessons. Journal of Research in Science Teaching, 51 (2), 192–218. doi: 10.1002/tea.21133 .
National Research Council (2000). Inquiry and the national science education standards . Washington DC: National Academies Press.
National Research Council (2012). A framework for K-12 science education . Washington DC: National Academies Press.
Nersessian, NJ. (1984). Faraday to Einstein: constructing meaning in scientific theories . Dordrecht: Martinus Nijhoff Publishers.
Book Google Scholar
Nersessian, NJ. (1992). How do scientists think? Capturing the dynamics of conceptual change in science. In RN Giere (Ed.), Cognitive Models of Science (pp. 3–45). Minneapolis: University of Minnesota Press.
Nersessian, NJ. (2008). Creating scientific concepts . Cambridge: The MIT Press.
Osborne, J. (2014). Teaching scientific practices: meeting the challenge of change. Journal of Science Teacher Education, 25 (2), 177–196. doi: 10.1007/s10972-014-9384-1 .
Osborne, J. & Dillon, J. (2008). Science education in Europe: critical reflections . London: Nuffield Foundation.
Papaevripidou, M, Constantinou, CP, & Zacharia, ZC. (2007). Modeling complex marine ecosystems: an investigation of two teaching approaches with fifth graders. Journal of Computer Assisted Learning, 23 (2), 145–157. doi: 10.1111/j.1365-2729.2006.00217.x .
Pauwels, L. (2006). A theoretical framework for assessing visual representational practices in knowledge building and science communications. In L Pauwels (Ed.), Visual cultures of science: rethinking representational practices in knowledge building and science communication (pp. 1–25). Lebanon, NH: Darthmouth College Press.
Philips, L., Norris, S. & McNab, J. (2010). Visualization in mathematics, reading and science education . Dordrecht, The Netherlands: Springer.
Pocovi, MC, & Finlay, F. (2002). Lines of force: Faraday’s and students’ views. Science & Education, 11 , 459–474.
Richards, A. (2003). Argument and authority in the visual representations of science. Technical Communication Quarterly, 12 (2), 183–206. doi: 10.1207/s15427625tcq1202_3 .
Rothbart, D. (1997). Explaining the growth of scientific knowledge: metaphors, models and meaning . Lewiston, NY: Mellen Press.
Ruivenkamp, M, & Rip, A. (2010). Visualizing the invisible nanoscale study: visualization practices in nanotechnology community of practice. Science Studies, 23 (1), 3–36.
Ryu, S, Han, Y, & Paik, S-H. (2015). Understanding co-development of conceptual and epistemic understanding through modeling practices with mobile internet. Journal of Science Education and Technology, 24 (2-3), 330–355. doi: 10.1007/s10956-014-9545-1 .
Sarkar, S, & Pfeifer, J. (2006). The philosophy of science, chapter on experimentation (Vol. 1, A-M). New York: Taylor & Francis.
Schwartz, RS, Lederman, NG, & Abd-el-Khalick, F. (2012). A series of misrepresentations: a response to Allchin’s whole approach to assessing nature of science understandings. Science Education, 96 (4), 685–692. doi: 10.1002/sce.21013 .
Schwarz, CV, Reiser, BJ, Davis, EA, Kenyon, L, Achér, A, Fortus, D, et al. (2009). Developing a learning progression for scientific modeling: making scientific modeling accessible and meaningful for learners. Journal of Research in Science Teaching, 46 (6), 632–654. doi: 10.1002/tea.20311 .
Watson, J. (1968). The Double Helix: a personal account of the discovery of the structure of DNA . New York: Scribner.
Watson, J, & Berry, A. (2004). DNA: the secret of life . New York: Alfred A. Knopf.
Wickman, PO. (2004). The practical epistemologies of the classroom: a study of laboratory work. Science Education, 88 , 325–344.
Wu, HK, & Shah, P. (2004). Exploring visuospatial thinking in chemistry learning. Science Education, 88 (3), 465–492. doi: 10.1002/sce.10126 .
Download references
Acknowledgements
The authors would like to acknowledge all reviewers for their valuable comments that have helped us improve the manuscript.
Author information
Authors and affiliations.
University of Nicosia, 46, Makedonitissa Avenue, Egkomi, 1700, Nicosia, Cyprus
Maria Evagorou
University of Limerick, Limerick, Ireland
Sibel Erduran
University of Tampere, Tampere, Finland
Terhi Mäntylä
You can also search for this author in PubMed Google Scholar
Corresponding author
Correspondence to Maria Evagorou .
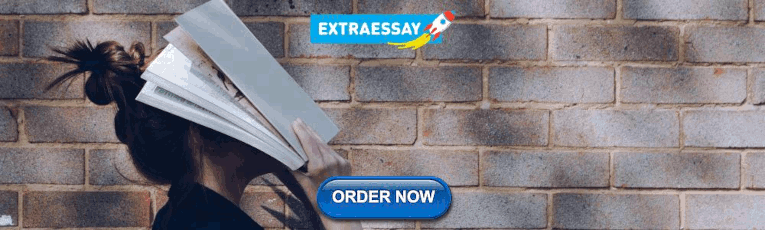
Additional information
Competing interests.
The authors declare that they have no competing interests.
Authors’ contributions
ME carried out the introductory literature review, the analysis of the first case study, and drafted the manuscript. SE carried out the analysis of the third case study and contributed towards the “Conclusions” section of the manuscript. TM carried out the second case study. All authors read and approved the final manuscript.
Rights and permissions
Open Access This article is distributed under the terms of the Creative Commons Attribution 4.0 International License ( https://creativecommons.org/licenses/by/4.0 ), which permits use, duplication, adaptation, distribution, and reproduction in any medium or format, as long as you give appropriate credit to the original author(s) and the source, provide a link to the Creative Commons license, and indicate if changes were made.
Reprints and permissions
About this article
Cite this article.
Evagorou, M., Erduran, S. & Mäntylä, T. The role of visual representations in scientific practices: from conceptual understanding and knowledge generation to ‘seeing’ how science works. IJ STEM Ed 2 , 11 (2015). https://doi.org/10.1186/s40594-015-0024-x
Download citation
Received : 29 September 2014
Accepted : 16 May 2015
Published : 19 July 2015
DOI : https://doi.org/10.1186/s40594-015-0024-x
Share this article
Anyone you share the following link with will be able to read this content:
Sorry, a shareable link is not currently available for this article.
Provided by the Springer Nature SharedIt content-sharing initiative
- Visual representations
- Epistemic practices
- Science learning
- Review article
- Open access
- Published: 11 July 2018
Decision making with visualizations: a cognitive framework across disciplines
- Lace M. Padilla ORCID: orcid.org/0000-0001-9251-5279 1 , 2 ,
- Sarah H. Creem-Regehr 2 ,
- Mary Hegarty 3 &
- Jeanine K. Stefanucci 2
Cognitive Research: Principles and Implications volume 3 , Article number: 29 ( 2018 ) Cite this article
34k Accesses
102 Citations
18 Altmetric
Metrics details
A Correction to this article was published on 02 September 2018
This article has been updated
Visualizations—visual representations of information, depicted in graphics—are studied by researchers in numerous ways, ranging from the study of the basic principles of creating visualizations, to the cognitive processes underlying their use, as well as how visualizations communicate complex information (such as in medical risk or spatial patterns). However, findings from different domains are rarely shared across domains though there may be domain-general principles underlying visualizations and their use. The limited cross-domain communication may be due to a lack of a unifying cognitive framework. This review aims to address this gap by proposing an integrative model that is grounded in models of visualization comprehension and a dual-process account of decision making. We review empirical studies of decision making with static two-dimensional visualizations motivated by a wide range of research goals and find significant direct and indirect support for a dual-process account of decision making with visualizations. Consistent with a dual-process model, the first type of visualization decision mechanism produces fast, easy, and computationally light decisions with visualizations. The second facilitates slower, more contemplative, and effortful decisions with visualizations. We illustrate the utility of a dual-process account of decision making with visualizations using four cross-domain findings that may constitute universal visualization principles. Further, we offer guidance for future research, including novel areas of exploration and practical recommendations for visualization designers based on cognitive theory and empirical findings.
Significance
People use visualizations to make large-scale decisions, such as whether to evacuate a town before a hurricane strike, and more personal decisions, such as which medical treatment to undergo. Given their widespread use and social impact, researchers in many domains, including cognitive psychology, information visualization, and medical decision making, study how we make decisions with visualizations. Even though researchers continue to develop a wealth of knowledge on decision making with visualizations, there are obstacles for scientists interested in integrating findings from other domains—including the lack of a cognitive model that accurately describes decision making with visualizations. Research that does not capitalize on all relevant findings progresses slower, lacks generalizability, and may miss novel solutions and insights. Considering the importance and impact of decisions made with visualizations, it is critical that researchers have the resources to utilize cross-domain findings on this topic. This review provides a cognitive model of decision making with visualizations that can be used to synthesize multiple approaches to visualization research. Further, it offers practical recommendations for visualization designers based on the reviewed studies while deepening our understanding of the cognitive processes involved when making decisions with visualizations.
Introduction
Every day we make numerous decisions with the aid of visualizations , including selecting a driving route, deciding whether to undergo a medical treatment, and comparing figures in a research paper. Visualizations are external visual representations that are systematically related to the information that they represent (Bertin, 1983 ; Stenning & Oberlander, 1995 ). The information represented might be about objects, events, or more abstract information (Hegarty, 2011 ). The scope of the previously mentioned examples illustrates the diversity of disciplines that have a vested interest in the influence of visualizations on decision making. While the term decision has a range of meanings in everyday language, here decision making is defined as a choice between two or more competing courses of action (Balleine, 2007 ).
We argue that for visualizations to be most effective, researchers need to integrate decision-making frameworks into visualization cognition research. Reviews of decision making with visual-spatial uncertainty also agree there has been a general lack of emphasis on mental processes within the visualization decision-making literature (Kinkeldey, MacEachren, Riveiro, & Schiewe, 2017 ; Kinkeldey, MacEachren, & Schiewe, 2014 ). The framework that has dominated applied decision-making research for the last 30 years is a dual-process account of decision making. Dual-process theories propose that we have two types of decision processes: one for automatic, easy decisions (Type 1); and another for more contemplative decisions (Type 2) (Kahneman & Frederick, 2002 ; Stanovich, 1999 ). Footnote 1 Even though many research areas involving higher-level cognition have made significant efforts to incorporate dual-process theories (Evans, 2008 ), visualization research has yet to directly test the application of current decision-making frameworks or develop an effective cognitive model for decision making with visualizations. The goal of this work is to integrate a dual-process account of decision making with established cognitive frameworks of visualization comprehension.
In this paper, we present an overview of current decision-making theories and existing visualization cognition frameworks, followed by a proposal for an integrated model of decision making with visualizations, and a selective review of visualization decision-making studies to determine if there is cross-domain support for a dual-process account of decision making with visualizations. As a preview, we will illustrate Type 1 and 2 processing in decision making with visualizations using four cross-domain findings that we observed in the literature review. Our focus here is on demonstrating how dual-processing can be a useful framework for examining visualization decision-making research. We selected the cross-domain findings as relevant demonstrations of Type 1 and 2 processing that were shared across the studies reviewed, but they do not represent all possible examples of dual-processing in visualization decision-making research. The review documents each of the cross-domain findings, in turn, using examples from studies in multiple domains. These cross-domain findings differ in their reliance on Type 1 and Type 2 processing. We conclude with recommendations for future work and implications for visualization designers.
Decision-making frameworks
Decision-making researchers have pursued two dominant research paths to study how humans make decisions under risk. The first assumes that humans make rational decisions, which are based on weighted and ordered probability functions and can be mathematically modeled (e.g. Kunz, 2004 ; Von Neumann, 1953 ). The second proposes that people often make intuitive decisions using heuristics (Gigerenzer, Todd, & ABC Research Group, 2000 ; Kahneman & Tversky, 1982 ). While there is fervent disagreement on the efficacy of heuristics and whether human behavior is rational (Vranas, 2000 ), there is more consensus that we can make both intuitive and strategic decisions (Epstein, Pacini, Denes-Raj, & Heier, 1996 ; Evans, 2008 ; Evans & Stanovich, 2013 ; cf. Keren & Schul, 2009 ). The capacity to make intuitive and strategic decisions is described by a dual-process account of decision making, which suggests that humans make fast, easy, and computationally light decisions (known as Type 1 processing) by default, but can also make slow, contemplative, and effortful decisions by employing Type 2 processing (Kahneman, 2011 ). Various versions of dual-processing theory exist, with the key distinctions being in the attributes associated with each type of process (for a more detailed review of dual-process theories, see Evans & Stanovich, 2013 ). For example, older dual-systems accounts of decision making suggest that each process is associated with specific cognitive or neurological systems. In contrast, dual-process (sometimes termed dual-type) theories propose that the processes are distinct but do not necessarily occur in separate cognitive or neurological systems (hence the use of process over system) (Evans & Stanovich, 2013 ).
Many applied domains have adapted a dual-processing model to explain task- and domain-specific decisions, with varying degrees of success (Evans, 2008 ). For example, when a physician is deciding if a patient should be assigned to a coronary care unit or a regular nursing bed, the doctor can use a heuristic or utilize heart disease predictive instruments to make the decision (Marewski & Gigerenzer, 2012 ). In the case of the heuristic, the doctor would employ a few simple rules (diagrammed in Fig. 1 ) that would guide her decision, such as considering the patient’s chief complaint being chest pain. Another approach is to apply deliberate mental effort to make a more time-consuming and effortful decision, which could include using heart disease predictive instruments (Marewski & Gigerenzer, 2012 ). In a review of how applied domains in higher-level cognition have implemented a dual-processing model for domain-specific decisions, Evans ( 2008 ) argues that prior work has conflicting accounts of Type 1 and 2 processing. Some studies suggest that the two types work in parallel while others reveal conflicts between the Types (Sloman, 2002 ). In the physician example proposed by Marewski and Gigerenzer ( 2012 ), the two types are not mutually exclusive, as doctors can utilize Type 2 to make a more thoughtful decision that is also influenced by some rules of thumb or Type 1. In sum, Evans ( 2008 ) argues that due to the inconsistency of classifying Type 1 and 2, the distinction between only two types is likely an oversimplification. Evans ( 2008 ) suggests that the literature only consistently supports the identification of processes that require a capacity-limited, working memory resource versus those that do not. Evans and Stanovich ( 2013 ) updated their definition based on new behavioral and neuroscience evidence stating, “the defining characteristic of Type 1 processes is their autonomy. They do not require ‘controlled attention,’ which is another way of saying that they make minimal demands on working memory resources” (p. 236). There is also debate on how to define the term working memory (Cowan, 2017 ). In line with prior work on decision making with visualizations (Patterson et al., 2014 ), we adopt the definition that working memory consists of multiple components that maintain a limited amount of information (their capacity) for a finite period (Cowan, 2017 ). Contemporary theories of working memory also stress the ability to engage attention in a controlled manner to suppress automatic responses and maintain the most task-relevant information with limited capacity (Engle, Kane, & Tuholski, 1999 ; Kane, Bleckley, Conway, & Engle, 2001 ; Shipstead, Harrison, & Engle, 2015 ).
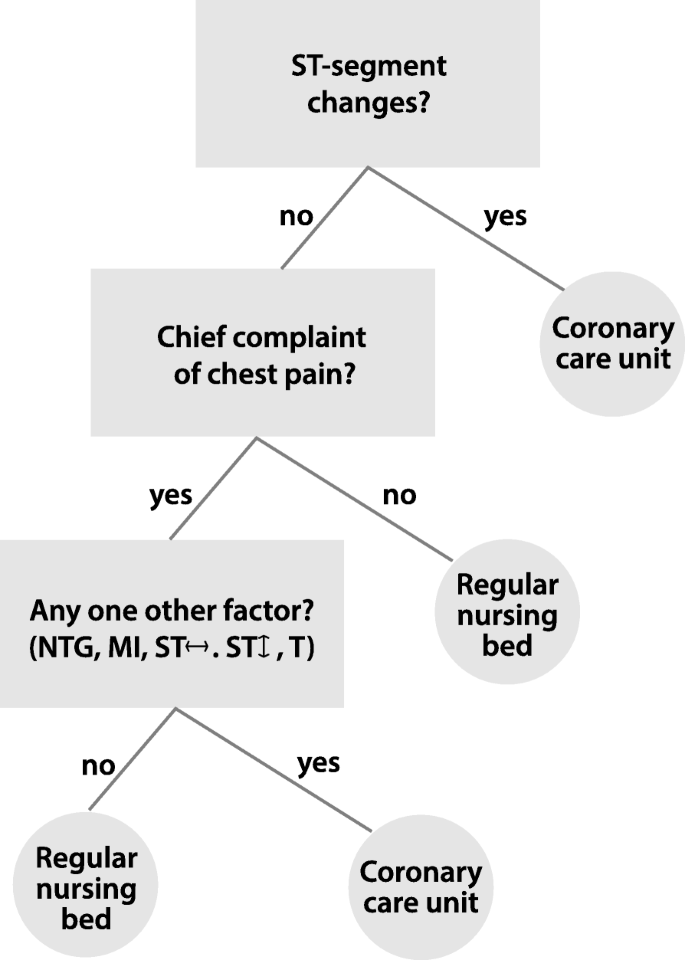
Coronary care unit decision tree, which illustrates a sequence of rules that a doctor could use to guide treatment decisions. Redrawn from “Heuristic decision making in medicine” by J. Marewski, and G. Gigerenzer 2012, Dialogues in clinical neuroscience, 14(1) , 77. ST-segment change refers to if certain anomaly appears in the patient’s electrocardiogram. NTG nitroglycerin, MI myocardial infarction, T T-waves with peaking or inversion
Identifying processes that require significant working memory provides a definition of Type 2 processing with observable neural correlates. Therefore, in line with Evans and Stanovich ( 2013 ), in the remainder of this manuscript, we will use significant working memory capacity demands and significant need for cognitive control, as defined above, as the criterion for Type 2 processing. In the context of visualization decision making, processes that require significant working memory are those that depend on the deliberate application of working memory to function. Type 1 processing occurs outside of users’ conscious awareness and may utilize small amounts of working memory but does not rely on conscious processing in working memory to drive the process. It should be noted that Type 1 and 2 processing are not mutually exclusive and many real-world decisions likely incorporate all processes. This review will attempt to identify tasks in visualization decision making that require significant working memory and capacity (Type 2 processing) and those that rely more heavily on Type 1 processing, as a first step to combining decision theory with visualization cognition.
Visualization cognition
Visualization cognition is a subset of visuospatial reasoning, which involves deriving meaning from external representations of visual information that maintain consistent spatial relations (Tversky, 2005 ). Broadly, two distinct approaches delineate visualization cognition models (Shah, Freedman, & Vekiri, 2005 ). The first approach refers to perceptually focused frameworks which attempt to specify the processes involved in perceiving visual information in displays and make predictions about the speed and efficiency of acquiring information from a visualization (e.g. Hollands & Spence, 1992 ; Lohse, 1993 ; Meyer, 2000 ; Simkin & Hastie, 1987 ). The second approach considers the influence of prior knowledge as well as perception. For example, Cognitive Fit Theory (Vessey, 1991), suggests that the user compares a learned graphic convention (mental schema) to the visual depiction. Visualizations that do not match the mental schema require cognitive transformations to make the visualization and mental representation align. For example, Fig. 2 illustrates a fictional relationship between the population growth of Species X and a predator species. At first glance, it may appear that when the predator species was introduced that the population of Species X dropped. However, after careful observation, you may notice that the higher population values are located lower on the Y-axis, which does not match our mental schema for graphs. With some effort, you can mentally reorder the values on the Y-axis to match your mental schema and then you may notice that the introduction of the predator species actually correlates with growth in the population of Species X. When the viewer is forced to mentally transform the visualization to match their mental schema, processing steps are increased, which may increase errors, time to complete a task, and demand on working memory (Vessey, 1991).
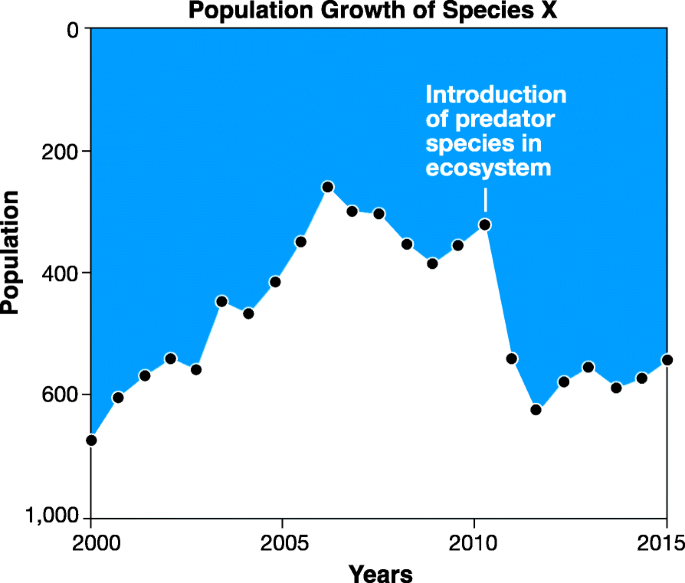
Fictional relationship between the population growth of Species X and a predator species, where the Y-axis ordering does not match standard graphic conventions. Notice that the y-axis is reverse ordered. This figure was inspired by a controversial graphic produced by Christine Chan of Reuters, which showed the relationship between Florida’s “Stand Your Ground” law and firearm murders with the Y-axis reversed ordered (Lallanilla, 2014 )
Pinker ( 1990 ) proposed a cognitive model (see Fig. 3 ), which provides an integrative structure that denotes the distinction between top-down and bottom-up encoding mechanisms in understanding data graphs. Researchers have generalized this model to propose theories of comprehension, learning, and memory with visual information (Hegarty, 2011 ; Kriz & Hegarty, 2007 ; Shah & Freedman, 2011 ). The Pinker ( 1990 ) model suggests that from the visual array , defined as the unprocessed neuronal firing in response to visualizations, bottom-up encoding mechanisms are utilized to construct a visual description , which is the mental encoding of the visual stimulus. Following encoding, viewers mentally search long-term memory for knowledge relevant for interpreting the visualization. This knowledge is proposed to be in the form of a graph schema.
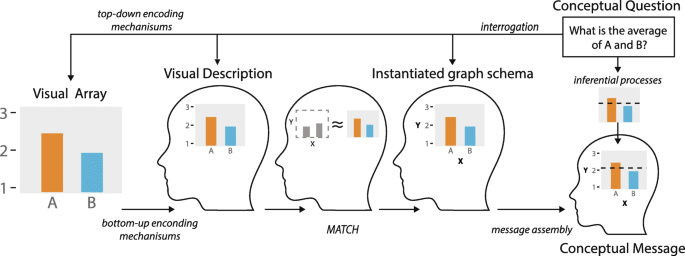
Adapted figure from the Pinker ( 1990 ) model of visualization comprehension, which illustrates each process
Then viewers use a match process, where the graph schema that is the most similar to the visual array is retrieved. When a matching graph schema is found, the schema becomes instantiated . The visualization conventions associated with the graph schema can then help the viewer interpret the visualization ( message assembly process). For example, Fig. 3 illustrates comprehension of a bar chart using the Pinker ( 1990 ) model. In this example, the matched graph schema for a bar graph specifies that the dependent variable is on the Y-axis and the independent variable is on the X-axis; the instantiated graph schema incorporates the visual description and this additional information. The conceptual message is the resulting mental representation of the visualization that includes all supplemental information from long-term memory and any mental transformations the viewer may perform on the visualization. Viewers may need to transform their mental representation of the visualization based on their task or conceptual question . In this example, the viewer’s task is to find the average of A and B. To do this, the viewer must interpolate information in the bar chart and update the conceptual message with this additional information. The conceptual question can guide the construction of the mental representation through interrogation , which is the process of seeking out information that is necessary to answer the conceptual question. Top-down encoding mechanisms can influence each of the processes.
The influences of top-down processes are also emphasized in a previous attempt by Patterson et al. ( 2014 ) to extend visualization cognition theories to decision making. The Patterson et al. ( 2014 ) model illustrates how top-down cognitive processing influences encoding, pattern recognition, and working memory, but not decision making or the response. Patterson et al. ( 2014 ) use the multicomponent definition of working memory, proposed by Baddeley and Hitch ( 1974 ) and summarized by Cowan ( 2017 ) as a “multicomponent system that holds information temporarily and mediates its use in ongoing mental activities” (p. 1160). In this conception of working memory, a central executive controls the functions of working memory. The central executive can, among other functions, control attention and hold information in a visuo-spatial temporary store , which is where information can be maintained temporally for decision making without being stored in long-term memory (Baddeley & Hitch, 1974 ).
While incorporating working memory into a visualization decision-making model is valuable, the Patterson et al. ( 2014 ) model leaves some open questions about relationships between components and processes. For example, their model lacks a pathway for working memory to influence decisions based on top-down processing, which is inconsistent with well-established research in decision science (e.g. Gigerenzer & Todd, 1999; Kahneman & Tversky, 1982 ). Additionally, the normal processing pathway, depicted in the Patterson model, is an oversimplification of the interaction between top-down and bottom-up processing that is documented in a large body of literature (e.g. Engel, Fries, & Singer, 2001 ; Mechelli, Price, Friston, & Ishai, 2004 ).
A proposed integrated model of decision making with visualizations
Our proposed model (Fig. 4 ) introduces a dual-process account of decision making (Evans & Stanovich, 2013 ; Gigerenzer & Gaissmaier, 2011 ; Kahneman, 2011 ) into the Pinker ( 1990 ) model of visualization comprehension. A primary addition of our model is the inclusion of working memory, which is utilized to answer the conceptual question and could have a subsequent impact on each stage of the decision-making process, except bottom-up attention. The final stage of our model includes a decision-making process that derives from the conceptual message and informs behavior. In line with a dual-process account (Evans & Stanovich, 2013 ; Gigerenzer & Gaissmaier, 2011 ; Kahneman, 2011 ), the decision step can either be completed with Type 1 processing, which only uses minimal working memory (Evans & Stanovich, 2013 ) or recruit significant working memory, constituting Type 2 processing. Also following Evans and Stanovich ( 2013 ), we argue that people can make a decision with a visualization while using minimal amounts of working memory. We classify this as Type 1 thinking. Lohse ( 1997 ) found that when participants made judgments about budget allocation using profit charts, individuals with less working memory capacity performed equally well compared to those with more working memory capacity, when they only made decisions about three regions (easier task). However, when participants made judgments about nine regions (harder task), individuals with more working memory capacity outperformed those with less working memory capacity. The results of the study reveal that individual differences in working memory capacity only influence performance on complex decision-making tasks (Lohse, 1997 ). Figure 5 (top) illustrates one way that a viewer could make a Type 1 decision about whether the average value of bars A and B is closer to 2 or 2.2. Figure 5 (top) illustrates how a viewer might make a fast and computationally light decision in which she decides that the middle point between the two bars is closer to the salient tick mark of 2 on the Y-axis and answers 2 (which is incorrect). In contrast, Fig. 5 (bottom) shows a second possible method of solving the same problem by utilizing significant working memory (Type 2 processing). In this example, the viewer has recently learned a strategy to address similar problems, uses working memory to guide a top-down attentional search of the visual array, and identifies the values of A and B. Next, she instantiates a different graph schema than in the prior example by utilizing working memory and completes an effortful mental computation of 2.4 + 1.9/2. Ultimately, the application of working memory leads to a different and more effortful decision than in Fig. 5 (top). This example illustrates how significant amounts of working memory can be used at early stages of the decision-making process and produce downstream effects and more considered responses. In the following sections, we provide a selective review of work on decision making with visualizations that demonstrates direct and indirect evidence for our proposed model.
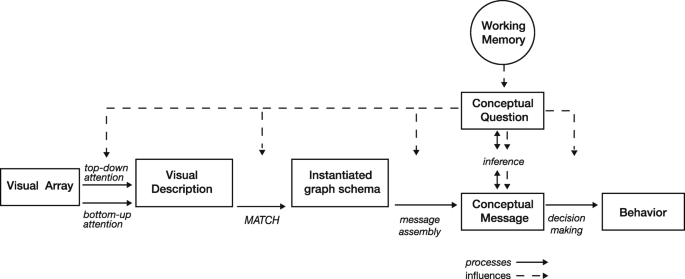
Model of visualization decision making, which emphasizes the influence of working memory. Long-term memory can influence all components and processes in the model either via pre-attentive processes or by conscious application of knowledge
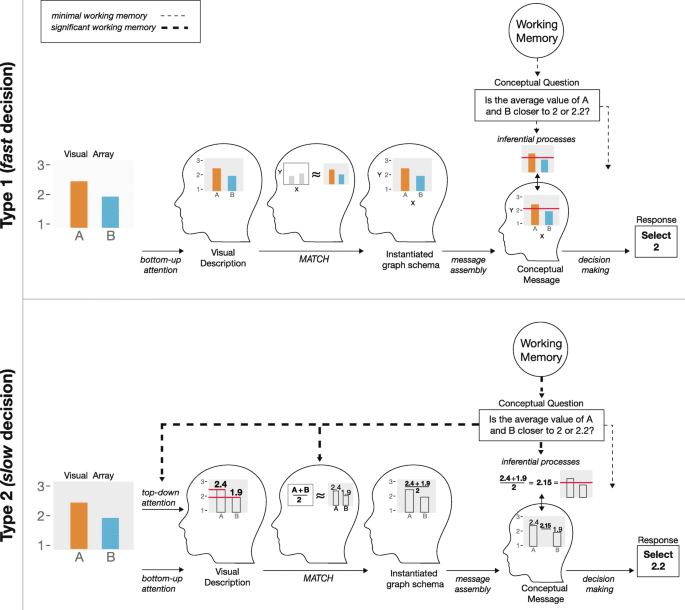
Examples of a fast Type 1 (top) and slow Type 2 (bottom) decision outlined in our proposed model of decision making with visualizations. In these examples, the viewer’s task is to decide if the average value of bars A and B are closer to 2 or 2.2. The thick dotted line denotes significant working memory and the thin dotted line negligible working memory
Empirical studies of visualization decision making
Review method.
To determine if there is cross-domain empirical support for a dual-process account of decision making with visualizations, we selectively reviewed studies of complex decision making with computer-generated two-dimensional (2D) static visualizations. To illustrate the application of a dual-process account of decision making to visualization research, this review highlights representative studies from diverse application areas. Interdisciplinary groups conducted many of these studies and, as such, it is not accurate to classify the studies in a single discipline. However, to help the reader evaluate the cross-domain nature of these findings, Table 1 includes the application area for the specific tasks used in each study.
In reviewing this work, we observed four key cross-domain findings that support a dual-process account of decision making (see Table 2 ). The first two support the inclusion of Type 1 processing, which is illustrated by the direct path for bottom-up attention to guide decision making with the minimal application of working memory (see Fig. 5 top). The first finding is that visualizations direct viewers’ bottom-up attention , which can both help and hinder decision making (see “ Bottom-up attention ”). The second finding is that visual-spatial biases comprise a unique category of bias that is a direct result of the visual encoding technique (see “ Visual-Spatial Biases ”). The third finding supports the inclusion of Type 2 processing in our proposed model and suggests that visualizations vary in cognitive fit between the visual description, graph schema, and conceptual question. If the fit is poor (i.e. there is a mismatch between the visualization and a decision-making component), working memory is used to perform corrective mental transformations (see “ Cognitive fit ”). The final cross-domain finding proposes that knowledge-driven processes may interact with the effects of the visual encoding technique (see “ Knowledge-driven processing ”) and could be a function of either Type 1 or 2 processes. Each of these findings will be detailed at length in the relevant sections. The four cross-domain findings do not represent an exhaustive list of all cross-domain findings that pertain to visualization cognition. However, these were selected as illustrative examples of Type 1 and 2 processing that include significant contributions from multiple domains. Further, some of the studies could fit into multiple sections and were included in a particular section as illustrative examples.
Bottom-up attention
The first cross-domain finding that characterizes Type 1 processing in visualization decision making is that visualizations direct participants’ bottom-up attention to specific visual features, which can be either beneficial or detrimental to decision making. Bottom-up attention consists of involuntary shifts in focus to salient features of a visualization and does not utilize working memory (Connor, Egeth, & Yantis, 2004 ), therefore it is a Type 1 process. The research reviewed in this section illustrates that bottom-up attention has a profound influence on decision making with visualizations. A summary of visual features that studies have used to attract bottom-up attention can be found in Table 3 .
Numerous studies show that salient information in a visualization draws viewers’ attention (Fabrikant, Hespanha, & Hegarty, 2010 ; Hegarty, Canham, & Fabrikant, 2010 ; Hegarty, Friedman, Boone, & Barrett, 2016 ; Padilla, Ruginski, & Creem-Regehr, 2017 ; Schirillo & Stone, 2005 ; Stone et al., 2003 ; Stone, Yates, & Parker, 1997 ). The most common methods for demonstrating that visualizations focus viewers’ attention is by showing that viewers miss non-salient but task-relevant information (Schirillo & Stone, 2005 ; Stone et al., 1997 ; Stone et al., 2003 ), viewers are biased by salient information (Hegarty et al., 2016 ; Padilla, Ruginski et al., 2017 ) or viewers spend more time looking at salient information in a visualization (Fabrikant et al., 2010 ; Hegarty et al., 2010 ). For example, Stone et al. ( 1997 ) demonstrated that when viewers are asked how much they would pay for an improved product using the visualizations in Fig. 6 , they focus on the number of icons while missing the base rate of 5,000,000. If a viewer simply totals the icons, the standard product appears to be twice as dangerous as the improved product, but because the base rate is large, the actual difference between the two products is insignificantly small (0.0000003; Stone et al., 1997 ). In one experiment, participants were willing to pay $125 more for improved tires when viewing the visualizations in Fig. 6 compared to a purely textual representation of the information. The authors also demonstrated the same effect for improved toothpaste, with participants paying $0.95 more when viewing a visual depiction compared to text. The authors’ term this heuristic of focusing on salient information and ignoring other data the foreground effect (Stone et al., 1997 ) (see also Schirillo & Stone, 2005 ; Stone et al., 2003 ).
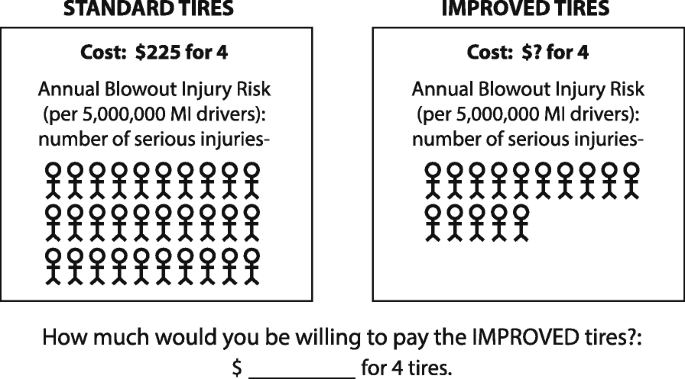
Icon arrays used to illustrate the risk of standard or improved tires. Participants were tasked with deciding how much they would pay for the improved tires. Note the base rate of 5 M drivers was represented in text. Redrawn from “Effects of numerical and graphical displays on professed risk-taking behavior” by E. R. Stone, J. F. Yates, & A. M. Parker. 1997, Journal of Experimental Psychology: Applied , 3 (4), 243
A more direct test of visualizations guiding bottom-up attention is to examine if salient information biases viewers’ judgments. One method involves identifying salient features using a behaviorally validated saliency model, which predicts the locations that will attract viewers’ bottom-up attention (Harel, 2015 ; Itti, Koch, & Niebur, 1998 ; Rosenholtz & Jin, 2005 ). In one study, researchers compared participants’ judgments with different hurricane forecast visualizations and then, using the Itti et al. ( 1998 ) saliency algorithm, found that the differences in what was salient in the two visualizations correlated with participants’ performance (Padilla, Ruginski et al., 2017 ). Specifically, they suggested that the salient borders of the Cone of Uncertainty (see Fig. 7 , left), which is used by the National Hurricane Center to display hurricane track forecasts, leads some people to incorrectly believe that the hurricane is growing in physical size, which is a misunderstanding of the probability distribution of hurricane paths that the cone is intended to represent (Padilla, Ruginski et al., 2017 ; see also Ruginski et al., 2016 ). Further, they found that when the same data were represented as individual hurricane paths, such that there was no salient boundary (see Fig. 7 , right), viewers intuited the probability of hurricane paths more effectively than the Cone of Uncertainty. However, an individual hurricane path biased viewers’ judgments if it intersected a point of interest. For example, in Fig. 7 (right), participants accurately judged that locations closer to the densely populated lines (highest likelihood of storm path) would receive more damage. This correct judgment changed when a location farther from the center of the storm was intersected by a path, but the closer location was not (see locations a and b in Fig. 7 right). With both visualizations, the researchers found that viewers were negatively biased by the salient features for some tasks (Padilla, Ruginski et al., 2017 ; Ruginski et al., 2016 ).
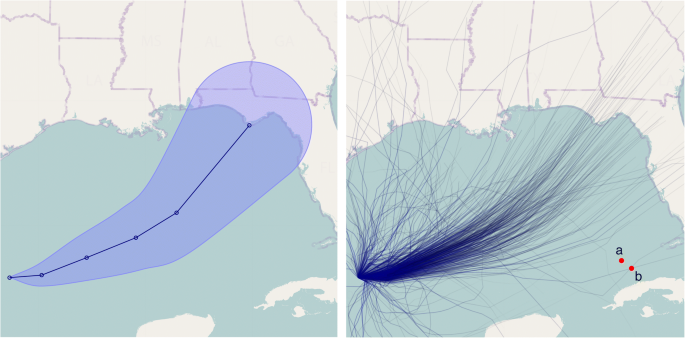
An example of the Cone of Uncertainty ( left ) and the same data represented as hurricane paths ( right ). Participants were tasked with evaluating the level of damage that would incur to offshore oil rigs at specific locations, based on the hurricane forecast visualization. Redrawn from “Effects of ensemble and summary displays on interpretations of geospatial uncertainty data” by L. M. Padilla, I. Ruginski, and S. H. Creem-Regehr. 2017, Cognitive Research: Principles and Implications , 2 (1), 40
That is not to say that saliency only negatively impacts decisions. When incorporated into visualization design, saliency can guide bottom-up attention to task-relevant information, thereby improving performance (e.g. Fabrikant et al., 2010 ; Fagerlin, Wang, & Ubel, 2005 ; Hegarty et al., 2010 ; Schirillo & Stone, 2005 ; Stone et al., 2003 ; Waters, Weinstein, Colditz, & Emmons, 2007 ). One compelling example using both eye-tracking measures and a saliency algorithm demonstrated that salient features of weather maps directed viewers’ attention to different variables that were visualized on the maps (Hegarty et al., 2010 ) (see also Fabrikant et al., 2010 ). Interestingly, when the researchers manipulated the relative salience of temperature versus pressure (see Fig. 8 ), the salient features captured viewers’ overt attention (as measured by eye fixations) but did not influence performance, until participants were trained on how to effectively interpret the features. Once viewers were trained, their judgments were facilitated when the relevant features were more salient (Hegarty et al., 2010 ). This is an instructive example of how saliency may direct viewers’ bottom-up attention but may not influence their performance until viewers have the relevant top-down knowledge to capitalize on the affordances of the visualization.
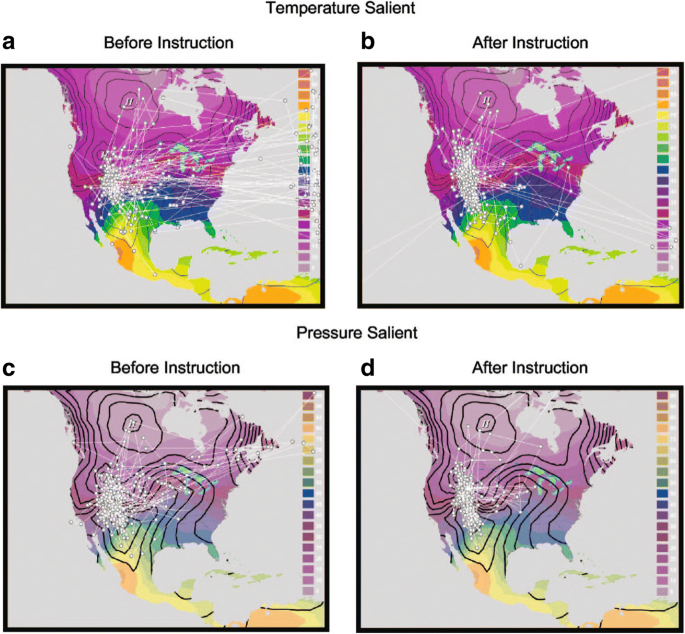
Eye-tracking data from Hegarty et al. ( 2010 ). Participants viewed an arrow located in Utah (obscured by eye-tracking data in the figure) and made judgments about whether the arrow correctly identified the wind direction. The black isobars were the task-relevant information. Notice that after instructions, viewers with the pressure-salient visualizations focused on the isobars surrounding Utah, rather than on the legend or in other regions. The panels correspond to the conditions in the original study
In sum, the reviewed studies suggest that bottom-up attention has a profound influence on decision making with visualizations. This is noteworthy because bottom-up attention is a Type 1 process. At a minimum, the work suggests that Type 1 processing influences the first stages of decision making with visualizations. Further, the studies cited in this section provide support for the inclusion of bottom-up attention in our proposed model.
- Visual-spatial biases
A second cross-domain finding that relates to Type 1 processing is that visualizations can give rise to visual-spatial biases that can be either beneficial or detrimental to decision making. We are proposing the new concept of visual-spatial biases and defining this term as a bias that elicits heuristics, which are a direct result of the visual encoding technique. Visual-spatial biases likely originate as a Type 1 process as we suspect they are connected to bottom-up attention, and if detrimental to decision making, have to be actively suppressed by top-down knowledge and cognitive control mechanisms (see Table 4 for summary of biases documented in this section). Visual-spatial biases can also improve decision-making performance. As Card, Mackinlay, and Shneiderman ( 1999 ) point out, we can use vision to think , meaning that visualizations can capitalize on visual perception to interpret a visualization without effort when the visual biases elucidated by the visualization are consistent with the correct interpretation.
Tversky ( 2011 ) presents a taxonomy of visual-spatial communications that are intrinsically related to thought, which are likely the bases for visual-spatial biases (see also Fabrikant & Skupin, 2005 ). One of the most commonly documented visual-spatial biases that we observed across domains is a containment conceptualization of boundary representations in visualizations. Tversky ( 2011 ) makes the analogy, “Framing a picture is a way of saying that what is inside the picture has a different status from what is outside the picture” (p. 522). Similarly, Fabrikant and Skupin ( 2005 ) describe how, “They [boundaries] help partition an information space into zones of relative semantic homogeneity” (p. 673). However, in visualization design, it is common to take continuous data and visually represent them with boundaries (i.e. summary statistics, error bars, isocontours, or regions of interest; Padilla et al., 2015 ; Padilla, Quinan, Meyer, & Creem-Regehr, 2017 ). Binning continuous data is a reasonable approach, particularly when intended to make the data simpler for viewers to understand (Padilla, Quinan, et al., 2017 ). However, it may have the unintended consequence of creating artificial boundaries that can bias users—leading them to respond as if data within a containment is more similar than data across boundaries. For example, McKenzie, Hegarty, Barrett, and Goodchild ( 2016 ) showed that participants were more likely to use a containment heuristic to make decisions about Google Map’s blue dot visualization when the positional uncertainty data were visualized as a bounded circle (Fig. 9 right) compared to a Gaussian fade (Fig. 9 left) (see also Newman & Scholl, 2012 ; Ruginski et al., 2016 ). Recent work by Grounds, Joslyn, and Otsuka ( 2017 ) found that viewers demonstrate a “deterministic construal error” or the belief that visualizations of temperature uncertainty represent a deterministic forecast. However, the deterministic construal error was not observed with textual representations of the same data (see also Joslyn & LeClerc, 2013 ).
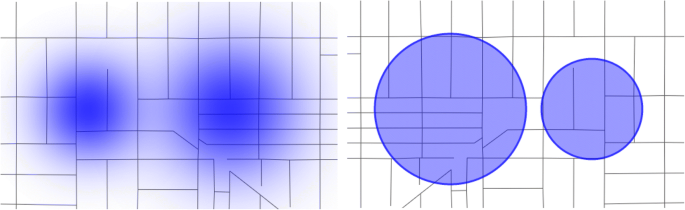
Example stimuli from McKenzie et al. ( 2016 ) showing circular semi-transparent overlays used by Google Maps to indicate the uncertainty of the users’ location. Participants compared two versions of these visualizations and determined which represented the most accurate positional location. Redrawn from “Assessing the effectiveness of different visualizations for judgments of positional uncertainty” by G. McKenzie, M. Hegarty, T. Barrett, and M. Goodchild. 2016, International Journal of Geographical Information Science , 30 (2), 221–239
Additionally, some visual-spatial biases follow the same principles as more well-known decision-making biases revealed by researchers in behavioral economics and decision science. In fact, some decision-making biases, such as anchoring , the tendency to use the first data point to make relative judgments, seem to have visual correlates (Belia, Fidler, Williams, & Cumming, 2005 ). For example, Belia et al. ( 2005 ) asked experts with experience in statistics to align two means (representing “Group 1” and “Group 2”) with error bars so that they represented data ranges that were just significantly different (see Fig. 10 for example of stimuli). They found that when the starting position of Group 2 was around 800 ms, participants placed Group 2 higher than when the starting position for Group 2 was at around 300 ms. This work demonstrates that participants used the starting mean of Group 2 as an anchor or starting point of reference, even though the starting position was arbitrary. Other work finds that visualizations can be used to reduce some decision-making biases including anecdotal evidence bias (Fagerlin et al., 2005 ), side effect aversion (Waters et al., 2007 ; Waters, Weinstein, Colditz, & Emmons, 2006 ), and risk aversion (Schirillo & Stone, 2005 ).
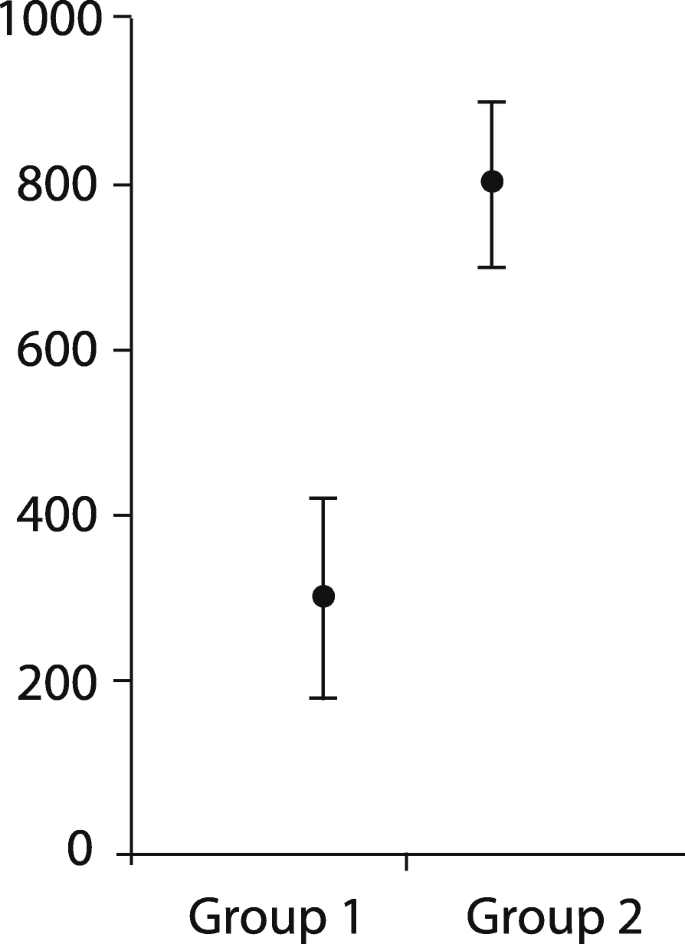
Example display and instructions from Belia et al. ( 2005 ). Redrawn from “Researchers misunderstand confidence intervals and standard error bars” by S. Belia, F. Fidler, J. Williams, and G. Cumming. 2005, Psychological Methods, 10 (4), 390. Copyright 2005 by “American Psychological Association”
Additionally, the mere presence of a visualization may inherently bias viewers. For example, viewers find scientific articles with high-quality neuroimaging figures to have greater scientific reasoning than the same article with a bar chart or without a figure (McCabe & Castel, 2008 ). People tend to unconsciously believe that high-quality scientific images reflect high-quality science—as illustrated by work from Keehner, Mayberry, and Fischer ( 2011 ) showing that viewers rate articles with three-dimensional brain images as more scientific than those with 2D images, schematic drawings, or diagrams (See Fig. 11 ). Unintuitively, however, high-quality complex images can be detrimental to performance compared to simpler visualizations (Hegarty, Smallman, & Stull, 2012 ; St. John, Cowen, Smallman, & Oonk, 2001 ; Wilkening & Fabrikant, 2011 ). Hegarty et al. ( 2012 ) demonstrated that novice users prefer realistically depicted maps (see Fig. 12 ), even though these maps increased the time taken to complete the task and focused participants’ attention on irrelevant information (Ancker, Senathirajah, Kukafka, & Starren, 2006 ; Brügger, Fabrikant, & Çöltekin, 2017 ; St. John et al., 2001 ; Wainer, Hambleton, & Meara, 1999 ; Wilkening & Fabrikant, 2011 ). Interestingly, professional meteorologists also demonstrated the same biases as novice viewers (Hegarty et al., 2012 ) (see also Nadav-Greenberg, Joslyn, & Taing, 2008 ).
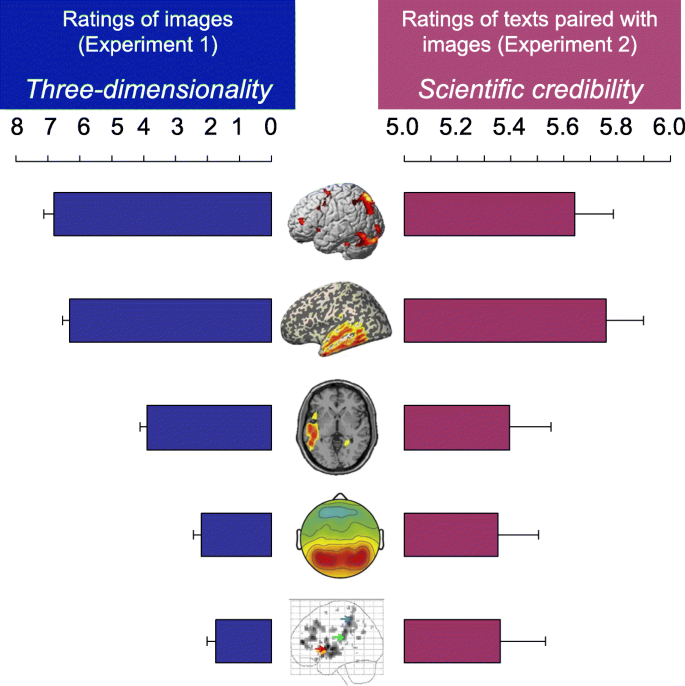
Image showing participants’ ratings of three-dimensionality and scientific credibility for a given neuroimaging visualization, originally published in grayscale (Keehner et al., 2011 )
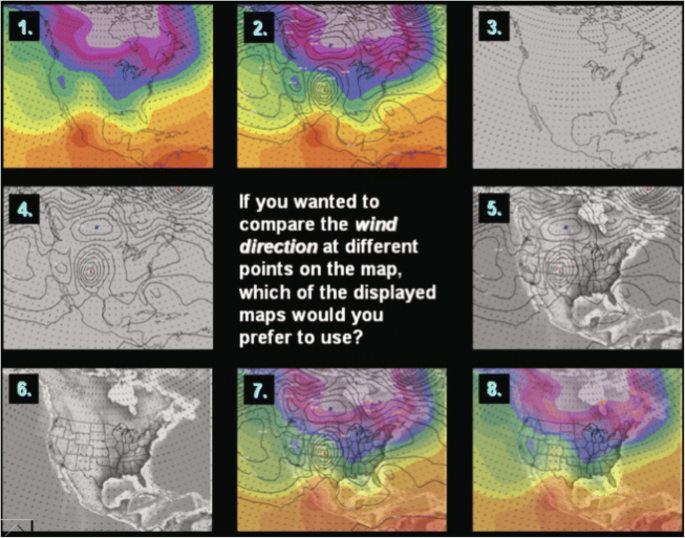
Example stimuli from Hegarty et al. ( 2012 ) showing maps with varying levels of realism. Both novice viewers and meteorologists were tasked with selecting a visualization to use and performing a geospatial task. The panels correspond to the conditions in the original study
We argue that visual-spatial biases reflect a Type 1 process, occurring automatically with minimal working memory. Work by Sanchez and Wiley ( 2006 ) provides direct evidence for this assertion using eye-tracking data to demonstrate that individuals with less working memory capacity attend to irrelevant images in a scientific article more than those with greater working memory capacity. The authors argue that we are naturally drawn to images (particularly high-quality depictions) and that significant working memory capacity is required to shift focus away from images that are task-irrelevant. The ease by which visualizations captivate our focus and direct our bottom-up attention to specific features likely increases the impact of these biases, which may be why some visual-spatial biases are notoriously difficult to override using working memory capacity (see Belia et al., 2005 ; Boone, Gunalp, & Hegarty, in press ; Joslyn & LeClerc, 2013 ; Newman & Scholl, 2012 ). We speculate that some visual-spatial biases are intertwined with bottom-up attention—occurring early in the decision-making process and influencing the down-stream processes (see our model in Fig. 4 for reference), making them particularly unremitting.
Cognitive fit
We also observe a cross-domain finding involving Type 2 processing, which suggests that if there is a mismatch between the visualization and a decision-making component, working memory is used to perform corrective mental transformations. Cognitive fit is a term used to describe the correspondence between the visualization and conceptual question or task (see our model for reference; for an overview of cognitive fit, see Vessey, Zhang, & Galletta, 2006 ). Those interested in examining cognitive fit generally attempt to identify and reduce mismatches between the visualization and one of the decision-making components (see Table 5 for a breakdown of the decision-making components that the reviewed studies evaluated). When there is a mismatch produced by the default Type 1 processing, it is argued that significant working memory (Type 2 processing) is required to resolve the discrepancy via mental transformations (Vessey et al., 2006 ). As working memory is capacity limited, the magnitude of mental transformation or amount of working memory required is one predictor of reaction times and errors.
Direct evidence for this claim comes from work demonstrating that cognitive fit differentially influenced the performance of individuals with more and less working memory capacity (Zhu & Watts, 2010 ). The task was to identify which two nodes in a social media network diagram should be removed to disconnect the maximal number of nodes. As predicted by cognitive fit theory, when the visualization did not facilitate the task (Fig. 13 left), participants with less working memory capacity were slower than those with more working memory capacity. However, when the visualization aligned with the task (Fig. 13 right), there was no difference in performance. This work suggests that when there is misalignment between the visualization and a decision-making process, people with more working memory capacity have the resources to resolve the conflict, while those with less resources show performance degradations. Footnote 2 Other work only found a modest relationship between working memory capacity and correct interpretations of high and low temperature forecast visualizations (Grounds et al., 2017 ), which suggests that, for some visualizations, viewers utilize little working memory.
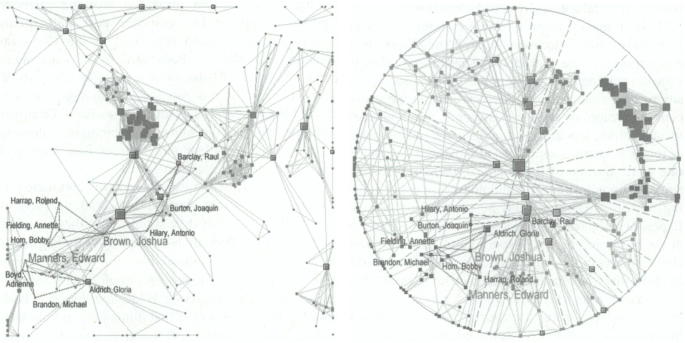
Examples of social media network diagrams from Zhu and Watts ( 2010 ). The authors argue that the figure on the right is more aligned with the task of identifying the most interconnected nodes than the figure on the left
As illustrated in our model, working memory can be recruited to aid all stages of the decision-making process except bottom-up attention. Work that examines cognitive fit theory provides indirect evidence that working memory is required to resolve conflicts in the schema matching and a decision-making component. For example, one way that a mismatch between a viewer’s mental schema and visualization can arise is when the viewer uses a schema that is not optimal for the task. Tversky, Corter, Yu, Mason, and Nickerson ( 2012 ) primed participants to use different schemas by describing the connections in Fig. 14 in terms of either transfer speed or security levels. Participants then decided on the most efficient or secure route for information to travel between computer nodes with either a visualization that encoded data using the thickness of connections, containment, or physical distance (see Fig. 14 ). Tversky et al. ( 2012 ) found that when the links were described based on their information transfer speed, thickness and distance visualizations were the most effective—suggesting that the speed mental schema was most closely matched to the thickness and distance visualizations, whereas the speed schema required mental transformations to align with the containment visualization. Similarly, the thickness and containment visualizations outperformed the distance visualization when the nodes were described as belonging to specific systems with different security levels. This work and others (Feeney, Hola, Liversedge, Findlay, & Metcalf, 2000 ; Gattis & Holyoak, 1996 ; Joslyn & LeClerc, 2013 ; Smelcer & Carmel, 1997 ) provides indirect evidence that gratuitous realignment between mental schema and the visualization can be error-prone and visualization designers should work to reduce the number of transformations required in the decision-making process.
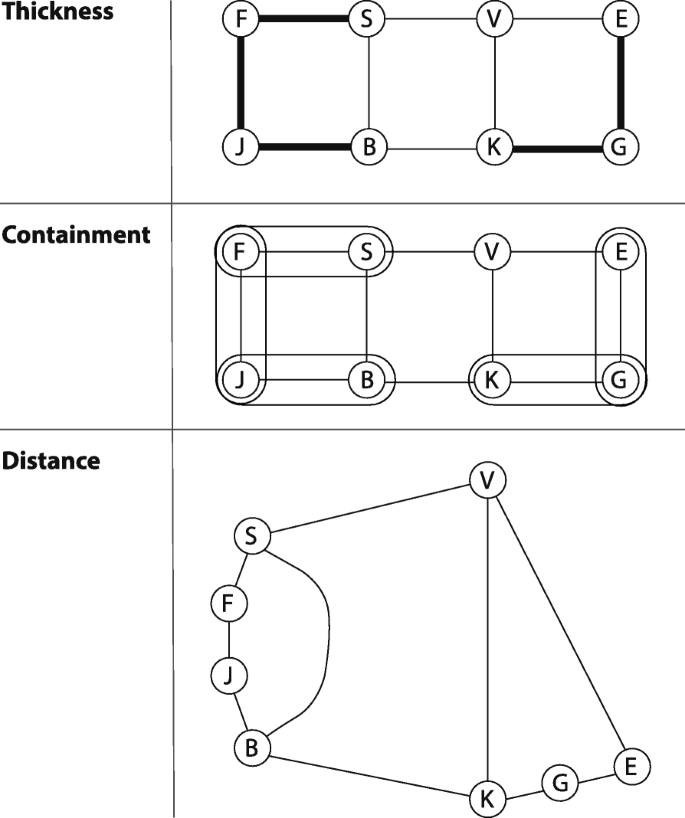
Example of stimuli from Tversky et al. ( 2012 ) showing three types of encoding techniques for connections between nodes (thickness, containment, and distance). Participants were asked to select routes between nodes with different descriptions of the visualizations. Redrawn from “Representing category and continuum: Visualizing thought” by B. Tversky, J. Corter, L. Yu, D. Mason, and J. Nickerson. In Diagrams 2012 (p. 27), P. Cox, P. Rodgers, and B. Plimmer (Eds.), 2012, Berlin Heidelberg: Springer-Verlag
Researchers from multiple domains have also documented cases of misalignment between the task, or conceptual question, and the visualization. For example, Vessey and Galletta ( 1991 ) found that participants completed a financial-based task faster when the visualization they chose (graph or table, see Fig. 15 ) matched the task (spatial or textual). For the spatial task, participants decided which month had the greatest difference between deposits and withdrawals. The textual or symbolic tasks involved reporting specific deposit and withdrawal amounts for various months. The authors argued that when there is a mismatch between the task and visualization, the additional transformation accounts for the increased time taken to complete the task (Vessey & Galletta, 1991 ) (see also Dennis & Carte, 1998 ; Huang et al., 2006 ), which likely takes place in the inference process of our proposed model.
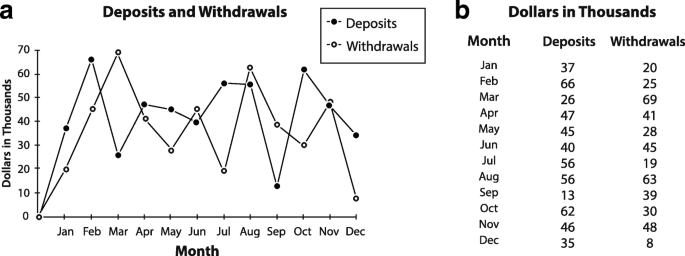
Examples of stimuli from Vessey and Galletta ( 1991 ) depicting deposits and withdraw amounts over the course of a year with a graph ( a ) and table ( b ). Participants completed either a spatial or textual task with the chart or table. Redrawn from “Cognitive fit: An empirical study of information acquisition” by I. Vessey, and D. Galletta. 1991, Information systems research, 2 (1), 72–73. Copyright 1991 by “INFORMS”
The aforementioned studies provide direct (Zhu & Watts, 2010 ) and indirect (Dennis & Carte, 1998 ; Feeney et al., 2000 ; Gattis & Holyoak, 1996 ; Huang et al., 2006 ; Joslyn & LeClerc, 2013 ; Smelcer & Carmel, 1997 ; Tversky et al., 2012 ; Vessey & Galletta, 1991 ) evidence that Type 2 processing recruits working memory to resolve misalignment between decision-making processes and the visualization that arise from default Type 1 processing. These examples of Type 2 processing using working memory to perform effortful mental computations are consistent with the assertions of Evans and Stanovich ( 2013 ) that Type 2 processes enact goal directed complex processing. However, it is not clear from the reviewed work how exactly the visualization and decision-making components are matched. Newman and Scholl ( 2012 ) propose that we match the schema and visualization based on the similarities between the salient visual features, although this proposal has not been tested. Further, work that assesses cognitive fit in terms of the visualization and task only examines the alignment of broad categories (i.e., spatial or semantic). Beyond these broad classifications, it is not clear how to predict if a task and visualization are aligned. In sum, there is not a sufficient cross-disciplinary theory for how mental schemas and tasks are matched to visualizations. However, it is apparent from the reviewed work that Type 2 processes (requiring working memory) can be recruited during the schema matching and inference processes.
Either type 1 and/or 2
Knowledge-driven processing.
In a review of map-reading cognition, Lobben ( 2004 ) states, “…research should focus not only on the needs of the map reader but also on their map-reading skills and abilities” (p. 271). In line with this statement, the final cross-domain finding is that the effects of knowledge can interact with the affordances or biases inherent in the visualization method. Knowledge may be held temporally in working memory (Type 2), held in long-term knowledge but effortfully used (Type 2), or held in long-term knowledge but automatically applied (Type 1). As a result, knowledge-driven processing can involve either Type 1 or Type 2 processes.
Both short- and long-term knowledge can influence visualization affordances and biases. However, it is difficult to distinguish whether Type 2 processing is using significant working memory capacity to temporarily hold knowledge or if participants have stored the relevant knowledge in long-term memory and processing is more automatic. Complicating the issue, knowledge stored in long-term memory can influence decision making with visualizations using both Type 1 and 2 processing. For example, if you try to remember Pythagorean’s Theorem, which you may have learned in high school or middle school, you may recall that a 2 + b 2 = c 2 , where c represents the length of the hypotenuse and a and b represent the lengths of the other two sides of a triangle. Unless you use geometry regularly, you likely had to strenuously search in long-term memory for the equation, which is a Type 2 process and requires significant working memory capacity. In contrast, if you are asked to recall your childhood phone number, the number might automatically come to mind with minimal working memory required (Type 1 processing).
In this section, we highlight cases where knowledge either influenced decision making with visualizations or was present but did not influence decisions (see Table 6 for the type of knowledge examined in each study). These studies are organized based on how much time the viewers had to incorporate the knowledge (i.e. short-term instructions and long-term individual differences in abilities and expertise), which may be indicative of where the knowledge is stored. However, many factors other than time influence the process of transferring knowledge by working memory capacity to long-term knowledge. Therefore, each of the studies cited in this section could be either Type 1, Type 2, or both types of processing.
One example of participants using short-term knowledge to override a familiarity bias comes from work by Bailey, Carswell, Grant, and Basham ( 2007 ) (see also Shen, Carswell, Santhanam, & Bailey, 2012 ). In a complex geospatial task for which participants made judgments about terrorism threats, participants were more likely to select familiar map-like visualizations rather than ones that would be optimal for the task (see Fig. 16 ) (Bailey et al., 2007 ). Using the same task and visualizations, Shen et al. ( 2012 ) showed that users were more likely to choose an efficacious visualization when given training concerning the importance of cognitive fit and effective visualization techniques. In this case, viewers were able to use knowledge-driven processing to improve their performance. However, Joslyn and LeClerc ( 2013 ) found that when participants viewed temperature uncertainty, visualized as error bars around a mean temperature prediction, they incorrectly believed that the error bars represented high and low temperatures. Surprisingly, participants maintained this belief despite a key, which detailed the correct way to interpret each temperature forecast (see also Boone et al., in press ). The authors speculated that the error bars might have matched viewers’ mental schema for high- and low-temperature forecasts (stored in long-term memory) and they incorrectly utilized the high-/low-temperature schema rather than incorporating new information from the key. Additionally, the authors propose that because the error bars were visually represented as discrete values, that viewers may have had difficulty reimagining the error bars as points on a distribution, which they term a deterministic construal error (Joslyn & LeClerc, 2013 ). Deterministic construal visual-spatial biases may also be one of the sources of misunderstanding of the Cone of Uncertainty (Padilla, Ruginski et al., 2017 ; Ruginski et al., 2016 ). A notable difference between these studies and the work of Shen et al. ( 2012 ) is that Shen et al. ( 2012 ) used instructions to correct a familiarity bias, which is a cognitive bias originally documented in the decision-making literature that is not based on the visual elements in the display. In contrast, the biases in Joslyn and LeClerc ( 2013 ) were visual-spatial biases. This provides further evidence that visual-spatial biases may be a unique category of biases that warrant dedicated exploration, as they are harder to influence with knowledge-driven processing.
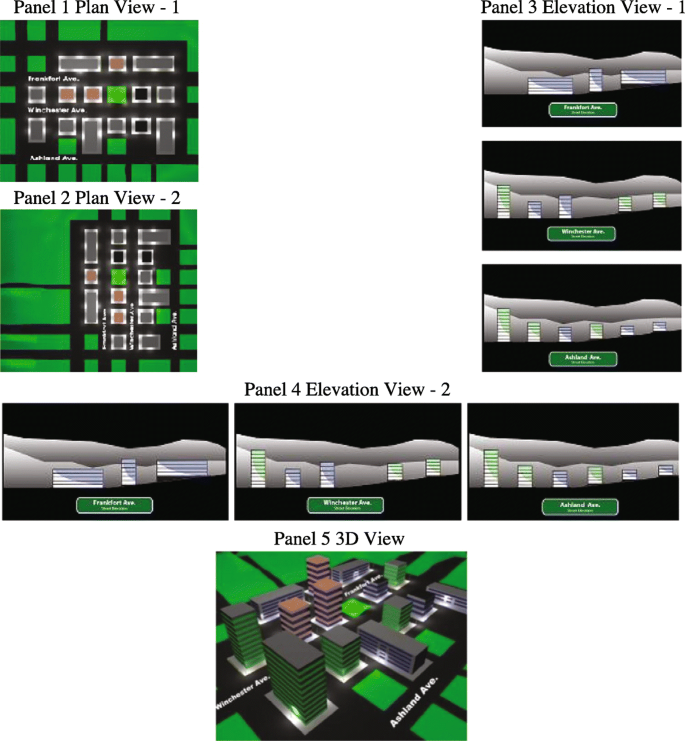
Example of different types of view orientations used by examined by Bailey et al. ( 2007 ). Participants selected one of these visualizations and then used their selection to make judgments including identifying safe passageways, determining appropriate locations for firefighters, and identifying suspicious locations based on the height of buildings. The panels correspond to the conditions in the original study
Regarding longer-term knowledge, there is substantial evidence that individual differences in knowledge impact decision making with visualizations. For example, numerous studies document the benefit of visualizations for individuals with less health literacy, graph literacy, and numeracy (Galesic & Garcia-Retamero, 2011 ; Galesic, Garcia-Retamero, & Gigerenzer, 2009 ; Keller, Siegrist, & Visschers, 2009 ; Okan, Galesic, & Garcia-Retamero, 2015 ; Okan, Garcia-Retamero, Cokely, & Maldonado, 2012 ; Okan, Garcia-Retamero, Galesic, & Cokely, 2012 ; Reyna, Nelson, Han, & Dieckmann, 2009 ; Rodríguez et al., 2013 ). Visual depictions of health data are particularly useful because health data often take the form of probabilities, which are unintuitive. Visualizations inherently illustrate probabilities (i.e. 10%) as natural frequencies (i.e. 10 out of 100), which are more intuitive (Hoffrage & Gigerenzer, 1998 ). Further, by depicting natural frequencies visually (see example in Fig. 17 ), viewers can make perceptual comparisons rather than mathematical calculations. This dual benefit is likely the reason visualizations produce facilitation for individuals with less health literacy, graph literacy, and numeracy.
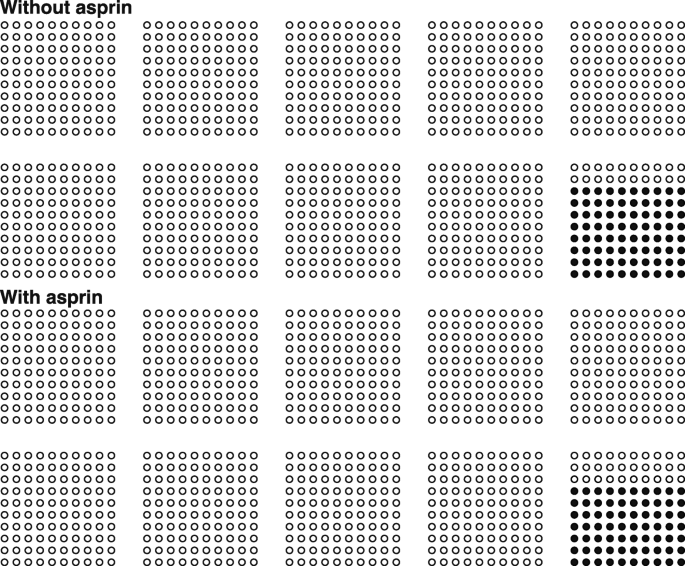
Example of stimuli used by Galesic et al. ( 2009 ) in a study demonstrating that natural frequency visualizations can help individuals overcome less numeracy. Participants completed three medical scenario tasks using similar visualizations as depicted here, in which they were asked about the effects of aspirin on risk of stroke or heart attack and about a hypothetical new drug. Redrawn from “Using icon arrays to communicate medical risks: overcoming less numeracy” by M. Galesic, R. Garcia-Retamero, and G. Gigerenzer. 2009, Health Psychology, 28 (2), 210
These studies are good examples of how designers can create visualizations that capitalize on Type 1 processing to help viewers accurately make decisions with complex data even when they lack relevant knowledge. Based on the reviewed work, we speculate that well-designed visualizations that utilize Type 1 processing to intuitively illustrate task-relevant relationships in the data may be particularly beneficial for individuals with less numeracy and graph literacy, even for simple tasks. However, poorly designed visualizations that require superfluous mental transformations may be detrimental to the same individuals. Further, individual differences in expertise, such as graph literacy, which have received more attention in healthcare communication (Galesic & Garcia-Retamero, 2011 ; Nayak et al., 2016 ; Okan et al., 2015 ; Okan, Garcia-Retamero, Cokely, & Maldonado, 2012 ; Okan, Garcia-Retamero, Galesic, & Cokely, 2012 ; Rodríguez et al., 2013 ), may play a large role in how viewers complete even simple tasks in other domains such as map-reading (Kinkeldey et al., 2017 ).
Less consistent are findings on how more experienced users incorporate knowledge acquired over longer periods of time to make decisions with visualizations. Some research finds that students’ decision-making and spatial abilities improved during a semester-long course on Geographic Information Science (GIS) (Lee & Bednarz, 2009 ). Other work finds that experts perform the same as novices (Riveiro, 2016 ), experts can exhibit visual-spatial biases (St. John et al., 2001 ) and experts perform more poorly than expected in their domain of visual expertise (Belia et al., 2005 ). This inconsistency may be due in part to the difficulty in identifying when and if more experienced viewers are automatically applying their knowledge or employing working memory. For example, it is unclear if the students in the GIS course documented by Lee and Bednarz ( 2009 ) developed automatic responses (Type 1) or if they learned the information and used working memory capacity to apply their training (Type 2).
Cheong et al. ( 2016 ) offer one way to gauge how performance may change when one is forced to use Type 1 processing, but then allowed to use Type 2 processing. In a wildfire task using multiple depictions of uncertainty (see Fig. 18 ), Cheong et al. ( 2016 ) found that the type of uncertainty visualization mattered when participants had to make fast Type 1 decisions (5 s) about evacuating from a wildfire. But when given sufficient time to make Type 2 decisions (30 s), participants were not influenced by the visualization technique (see also Wilkening & Fabrikant, 2011 ).
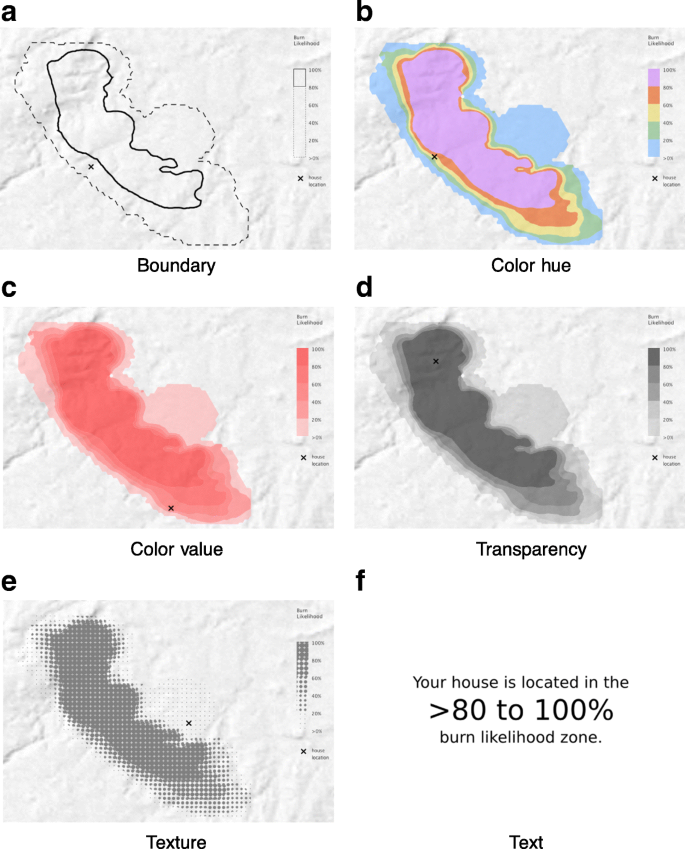
Example of multiple uncertainty visualization techniques for wildfire risk by Cheong et al. ( 2016 ). Participants were presented with a house location (indicated by an X), and asked if they would stay or leave based on one of the wildfire hazard communication techniques shown here. The panels correspond to the conditions in the original study
Interesting future work could limit experts’ time to complete a task (forcing Type 1 processing) and then determine if their judgments change when given more time to complete the task (allowing for Type 2 processing). To test this possibility further, a dual-task paradigm could be used such that experts’ working memory capacity is depleted by a difficult secondary task that also required working memory capacity. Some examples of secondary tasks in a dual-task paradigm include span tasks that require participants to remember or follow patterns of information, while completing the primary task, then report the remembered or relevant information from the pattern (for a full description of theoretical bases for a dual-task paradigm see Pashler, 1994 ). To our knowledge, only one study has used a dual-task paradigm to evaluate cognitive load of a visualization decision-making task (Bandlow et al., 2011 ). However, a growing body of research on other domains, such as wayfinding and spatial cognition, demonstrates the utility of using dual-task paradigms to understand the types of working memory that users employ for a task (Caffò, Picucci, Di Masi, & Bosco, 2011 ; Meilinger, Knauff, & Bülthoff, 2008 ; Ratliff & Newcombe, 2005 ; Trueswell & Papafragou, 2010 ).
Span tasks are examples of spatial or verbal secondary tasks, which include remembering the orientations of an arrow (taxes visual-spatial memory, (Shah & Miyake, 1996 ) or counting backward by 3 s (taxes verbal processing and short-term memory) (Castro, Strayer, Matzke, & Heathcote, 2018 ). One should expect more interference if the primary and secondary tasks recruit the same processes (i.e. visual-spatial primary task paired with a visual-spatial memory span task). An example of such an experimental design is illustrated in Fig. 19 . In the dual-task trial illustrated in Fig. 19 , if participants responses are as fast and accurate as the baseline trial then participants are likely not using significant amounts of working memory capacity for that task. If the task does require significant working memory capacity, then the inclusion of the secondary task should increase the time taken to complete the primary task and potentially produce errors in both the secondary and primary tasks. In visualization decision-making research, this is an open area of exploration for researchers and designers that are interested in understanding how working memory capacity and a dual-process account of decision making applies to their visualizations and application domains.
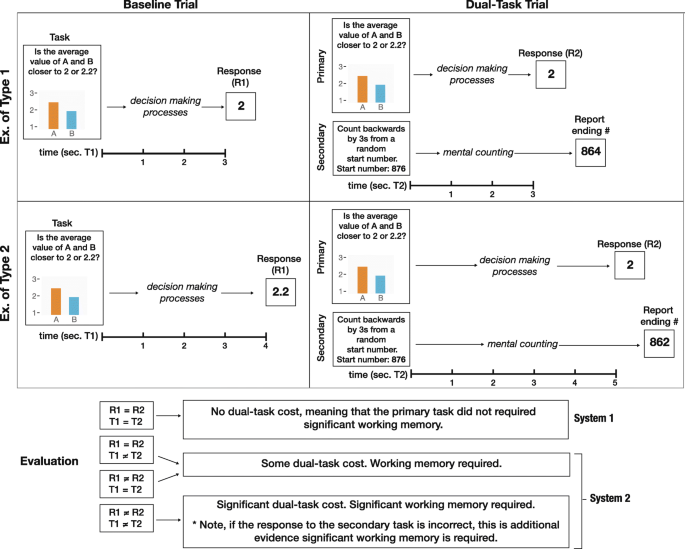
A diagram of a dual-tasking experiment is shown using the same task as in Fig. 5 . Responses resulting from Type 1 and 2 processing are illustrated. The dual-task trial illustrates how to place additional load on working memory capacity by having the participant perform a demanding secondary task. The impact of the secondary task is illustrated for both time and accuracy. Long-term memory can influence all components and processes in the model either via pre-attentive processes or by conscious application of knowledge
In sum, this section documents cases where knowledge-driven processing does and does not influence decision making with visualizations. Notably, we describe numerous studies where well-designed visualizations (capitalizing on Type 1 processing) focus viewers’ attention on task-relevant relationships in the data, which improves decision accuracy for individuals with less developed health literacy, graph literacy, and numeracy. However, the current work does not test how knowledge-driven processing maps on to the dual-process model of decision making. Knowledge may be held temporally by working memory capacity (Type 2), held in long-term knowledge but strenuously utilized (Type 2), or held in long-term knowledge but automatically applied (Type 1). More work is needed to understand if a dual-process account of decision making accurately describes the influence of knowledge-driven processing on decision making with visualizations. Finally, we detailed an example of a dual-task paradigm as one way to evaluate if viewers are employing Type 1 processing.
Review summary
Throughout this review, we have provided significant direct and indirect evidence that a dual-process account of decision making effectively describes prior findings from numerous domains interested in visualization decision making. The reviewed work provides support for specific processes in our proposed model including the influences of working memory, bottom-up attention, schema matching, inference processes, and decision making. Further, we identified key commonalities in the reviewed work relating to Type 1 and Type 2 processing, which we added to our proposed visualization decision-making model. The first is that utilizing Type 1 processing, visualizations serve to direct participants’ bottom-up attention to specific information, which can be either beneficial or detrimental for decision making (Fabrikant et al., 2010 ; Fagerlin et al., 2005 ; Hegarty et al., 2010 ; Hegarty et al., 2016 ; Padilla, Ruginski et al., 2017 ; Ruginski et al., 2016 ; Schirillo & Stone, 2005 ; Stone et al., 1997 ; Stone et al., 2003 ; Waters et al., 2007 ). Consistent with assertions from cognitive science and scientific visualization (Munzner, 2014 ), we propose that visualization designers should identify the critical information needed for a task and use a visual encoding technique that directs participants’ attention to this information. We encourage visualization designers who are interested in determining which elements in their visualizations will likely attract viewers’ bottom-up attention, to see the Itti et al. ( 1998 ) saliency model, which has been validated with eye-tracking measures (for implementation of this model along with Matlab code see Padilla, Ruginski et al., 2017 ). If deliberate effort is not made to capitalize on Type 1 processing by focusing the viewer’s attention on task-relevant information, then the viewer will likely focus on distractors via Type 1 processing, resulting in poor decision outcomes.
A second cross-domain finding is the introduction of a new concept, visual-spatial biases , which can also be both beneficial and detrimental to decision making. We define this term as a bias that elicits heuristics, which is a direct result of the visual encoding technique. We provide numerous examples of visual-spatial biases across domains (for implementation of this model along with Matlab code, see Padilla, Ruginski et al., 2017 ). The novel utility of identifying visual-spatial biases is that they potentially arise early in the decision-making process during bottom-up attention, thus influencing the entire downstream process, whereas standard heuristics do not exclusively occur at the first stage of decision making. This possibly accounts for the fact that visual-spatial biases have proven difficult to overcome (Belia et al., 2005 ; Grounds et al., 2017 ; Joslyn & LeClerc, 2013 ; Liu et al., 2016 ; McKenzie et al., 2016 ; Newman & Scholl, 2012 ; Padilla, Ruginski et al., 2017 ; Ruginski et al., 2016 ). Work by Tversky ( 2011 ) presents a taxonomy of visual-spatial communications that are intrinsically related to thought, which are likely the bases for visual-spatial biases.
We have also revealed cross-domain findings involving Type 2 processing, which suggest that if there is a mismatch between the visualization and a decision-making component, working memory is used to perform corrective mental transformations. In scenarios where the visualization is aligned with the mental schema and task, performance is fast and accurate (Joslyn & LeClerc, 2013 ). The types of mismatches observed in the reviewed literature are likely both domain-specific and domain-general. For example, situations where viewers employ the correct graph schema for the visualization, but the graph schema does not align with the task, are likely domain-specific (Dennis & Carte, 1998 ; Frownfelter-Lohrke, 1998 ; Gattis & Holyoak, 1996 ; Huang et al., 2006 ; Joslyn & LeClerc, 2013 ; Smelcer & Carmel, 1997 ; Tversky et al., 2012 ). However, other work demonstrates cases where viewers employ a graph schema that does not match the visualization, which is likely domain-general (e.g. Feeney et al., 2000 ; Gattis & Holyoak, 1996 ; Tversky et al., 2012 ). In these cases, viewers could accidentally use the wrong graph schema because it appears to match the visualization or they might not have learned a relevant schema. The likelihood of viewers making attribution errors because they do not know the corresponding schema increases when the visualization is less common, such as with uncertainty visualizations. When there is a mismatch, additional working memory is required resulting in increased time taken to complete the task and in some cases errors (e.g. Joslyn & LeClerc, 2013 ; McKenzie et al., 2016 ; Padilla, Ruginski et al., 2017 ). Based on these findings, we recommend that visualization designers should aim to create visualizations that most closely align with a viewer’s mental schema and task. However, additional empirical research is required to understand the nature of the alignment processes, including the exact method we use to mentally select a schema and the classifications of tasks that match visualizations.
The final cross-domain finding is that knowledge-driven processes can interact or override effects of visualization methods. We find that short-term (Dennis & Carte, 1998 ; Feeney et al., 2000 ; Gattis & Holyoak, 1996 ; Joslyn & LeClerc, 2013 ; Smelcer & Carmel, 1997 ; Tversky et al., 2012 ) and long-term knowledge acquisition (Shen et al., 2012 ) can influence decision making with visualizations. However, there are also examples of knowledge having little influence on decisions, even when prior knowledge could be used to improve performance (Galesic et al., 2009 ; Galesic & Garcia-Retamero, 2011 ; Keller et al., 2009 ; Lee & Bednarz, 2009 ; Okan et al., 2015 ; Okan, Garcia-Retamero, Cokely, & Maldonado, 2012 ; Okan, Garcia-Retamero, Galesic, & Cokely, 2012 ; Reyna et al., 2009 ; Rodríguez et al., 2013 ). We point out that prior knowledge seems to have more of an effect on non-visual-spatial biases, such as a familiarity bias (Belia et al., 2005 ; Joslyn & LeClerc, 2013 ; Riveiro, 2016 ; St. John et al., 2001 ), which suggests that visual-spatial biases may be closely related to bottom-up attention. Further, it is unclear from the reviewed work when knowledge switches from relying on working memory capacity for application to automatic application. We argue that Type 1 and 2 processing have unique advantages and disadvantages for visualization decision making. Therefore, it is valuable to understand which process users are applying for specific tasks in order to make visualizations that elicit optimal performance. In the case of experts and long-term knowledge, we propose that one interesting way to test if users are utilizing significant working memory capacity is to employ a dual-task paradigm (illustrated in Fig. 19 ). A dual-task paradigm can be used to evaluate the amount of working memory required and compare the relative working memory required between competing visualization techniques.
We have also proposed a variety of practical recommendations for visualization designers based on the empirical findings and our cognitive framework. Below is a summary list of our recommendations along with relevant section numbers for reference:
Identify the critical information needed for a task and use a visual encoding technique that directs participants’ attention to this information (“ Bottom-up attention ” section);
To determine which elements in a visualization will likely attract viewers’ bottom-up attention try employing a saliency algorithm (see Padilla, Quinan, et al., 2017 ) (see “ Bottom-up attention ”);
Aim to create visualizations that most closely align with a viewer’s mental schema and task demands (see “ Visual-Spatial Biases ”);
Work to reduce the number of transformations required in the decision-making process (see " Cognitive fit ");
To understand if a viewer is using Type 1 or 2 processing employ a dual-task paradigm (see Fig. 19 );
Consider evaluating the impact of individual differences such as graphic literacy and numeracy on visualization decision making.
Conclusions
We use visual information to inform many important decisions. To develop visualizations that account for real-life decision making, we must understand how and why we come to conclusions with visual information. We propose a dual-process cognitive framework expanding on visualization comprehension theory that is supported by empirical studies to describe the process of decision making with visualizations. We offer practical recommendations for visualization designers that take into account human decision-making processes. Finally, we propose a new avenue of research focused on the influence of visual-spatial biases on decision making.
Change history
02 september 2018.
The original article (Padilla et al., 2018) contained a formatting error in Table 2; this has now been corrected with the appropriate boxes marked clearly.
Dual-process theory will be described in greater detail in next section.
It should be noted that in some cases the activation of Type 2 processing should improve decision accuracy. More research is needed that examines cases where Type 2 could improve decision performance with visualizations.
Ancker, J. S., Senathirajah, Y., Kukafka, R., & Starren, J. B. (2006). Design features of graphs in health risk communication: A systematic review. Journal of the American Medical Informatics Association , 13 (6), 608–618.
Article Google Scholar
Baddeley, A. D., & Hitch, G. (1974). Working memory. Psychology of Learning and Motivation , 8 , 47–89.
Bailey, K., Carswell, C. M., Grant, R., & Basham, L. (2007). Geospatial perspective-taking: how well do decision makers choose their views? In Proceedings of the Human Factors and Ergonomics Society Annual Meeting (Vol. 51, No. 18, pp. 1246-1248). Los Angeles: SAGE Publications.
Balleine, B. W. (2007). The neural basis of choice and decision making. Journal of Neuroscience , 27 (31), 8159–8160.
Bandlow, A., Matzen, L. E., Cole, K. S., Dornburg, C. C., Geiseler, C. J., Greenfield, J. A., … Stevens-Adams, S. M. (2011). Evaluating Information Visualizations with Working Memory Metrics. In HCI International 2011–Posters’ Extended Abstracts , (pp. 265–269).
Chapter Google Scholar
Belia, S., Fidler, F., Williams, J., & Cumming, G. (2005). Researchers misunderstand confidence intervals and standard error bars. Psychological Methods , 10 (4), 389.
Bertin, J. (1983). Semiology of graphics: Diagrams, networks, maps . Madison: University of Wisconsin Press.
Boone, A., Gunalp, P., & Hegarty, M. (in press). Explicit versus Actionable Knowledge: The Influence of Explaining Graphical Conventions on Interpretation of Hurricane Forecast Visualizations. Journal of Experimental Psychology: Applied .
Brügger, A., Fabrikant, S. I., & Çöltekin, A. (2017). An empirical evaluation of three elevation change symbolization methods along routes in bicycle maps. Cartography and Geographic Information Science , 44 (5), 436–451.
Caffò, A. O., Picucci, L., Di Masi, M. N., & Bosco, A. (2011). Working memory components and virtual reorientation: A dual-task study. In Working memory: capacity, developments and improvement techniques , (pp. 249–266). Hauppage: Nova Science Publishers.
Google Scholar
Card, S. K., Mackinlay, J. D., & Shneiderman, B. (1999). Readings in information visualization: using vision to think . San Francisco: Morgan Kaufmann Publishers Inc.
Castro, S. C., Strayer, D. L., Matzke, D., & Heathcote, A. (2018). Cognitive Workload Measurement and Modeling Under Divided Attention. Journal of Experimental Psychology: General .
Cheong, L., Bleisch, S., Kealy, A., Tolhurst, K., Wilkening, T., & Duckham, M. (2016). Evaluating the impact of visualization of wildfire hazard upon decision-making under uncertainty. International Journal of Geographical Information Science , 30 (7), 1377–1404.
Connor, C. E., Egeth, H. E., & Yantis, S. (2004). Visual attention: Bottom-up versus top-down. Current Biology , 14 (19), R850–R852.
Cowan, N. (2017). The many faces of working memory and short-term storage. Psychonomic Bulletin & Review , 24 (4), 1158–1170.
Dennis, A. R., & Carte, T. A. (1998). Using geographical information systems for decision making: Extending cognitive fit theory to map-based presentations. Information Systems Research , 9 (2), 194–203.
Engel, A. K., Fries, P., & Singer, W. (2001). Dynamic predictions: Oscillations and synchrony in top–down processing. Nature Reviews Neuroscience , 2 (10), 704–716.
Engle, R. W., Kane, M. J., & Tuholski, S. W. (1999). Individual differences in working memory capacity and what they tell us about controlled attention, general fluid intelligence, and functions of the prefrontal cortex. In A. Miyake & P. Shah (Eds.), Models of working memory: Mechanisms of active maintenance and executive control (pp. 102-134). New York: Cambridge University Press.
Epstein, S., Pacini, R., Denes-Raj, V., & Heier, H. (1996). Individual differences in intuitive–experiential and analytical–rational thinking styles. Journal of Personality and Social Psychology , 71 (2), 390.
Evans, J. S. B. (2008). Dual-processing accounts of reasoning, judgment, and social cognition. Annual Review of Psychology , 59 , 255–278.
Evans, J. S. B., & Stanovich, K. E. (2013). Dual-process theories of higher cognition: Advancing the debate. Perspectives on Psychological Science , 8 (3), 223–241.
Fabrikant, S. I., Hespanha, S. R., & Hegarty, M. (2010). Cognitively inspired and perceptually salient graphic displays for efficient spatial inference making. Annals of the Association of American Geographers , 100 (1), 13–29.
Fabrikant, S. I., & Skupin, A. (2005). Cognitively plausible information visualization. In Exploring geovisualization , (pp. 667–690). Oxford: Elsevier.
Fagerlin, A., Wang, C., & Ubel, P. A. (2005). Reducing the influence of anecdotal reasoning on people’s health care decisions: Is a picture worth a thousand statistics? Medical Decision Making , 25 (4), 398–405.
Feeney, A., Hola, A. K. W., Liversedge, S. P., Findlay, J. M., & Metcalf, R. (2000). How people extract information from graphs: Evidence from a sentence-graph verification paradigm. In International Conference on Theory and Application of Diagrams (pp. 149-161). Berlin, Heidelberg: Springer.
Frownfelter-Lohrke, C. (1998). The effects of differing information presentations of general purpose financial statements on users’ decisions. Journal of Information Systems , 12 (2), 99–107.
Galesic, M., & Garcia-Retamero, R. (2011). Graph literacy: A cross-cultural comparison. Medical Decision Making , 31 (3), 444–457.
Galesic, M., Garcia-Retamero, R., & Gigerenzer, G. (2009). Using icon arrays to communicate medical risks: Overcoming low numeracy. Health Psychology , 28 (2), 210.
Garcia-Retamero, R., & Galesic, M. (2009). Trust in healthcare. In Kattan (Ed.), Encyclopedia of medical decision making , (pp. 1153–1155). Thousand Oaks: SAGE Publications.
Gattis, M., & Holyoak, K. J. (1996). Mapping conceptual to spatial relations in visual reasoning. Journal of Experimental Psychology: Learning, Memory, and Cognition , 22 (1), 231.
PubMed Google Scholar
Gigerenzer, G., & Gaissmaier, W. (2011). Heuristic decision making. Annual Review of Psychology , 62 , 451–482.
Gigerenzer, G., Todd, P. M., & ABC Research Group (2000). Simple Heuristics That Make Us Smart . Oxford: Oxford University Press.
Grounds, M. A., Joslyn, S., & Otsuka, K. (2017). Probabilistic interval forecasts: An individual differences approach to understanding forecast communication. Advances in Meteorology , 2017, 1-18.
Harel, J. (2015, July 24, 2012). A Saliency Implementation in MATLAB. Retrieved from http://www.vision.caltech.edu/~harel/share/gbvs.php
Hegarty, M. (2011). The cognitive science of visual-spatial displays: Implications for design. Topics in Cognitive Science , 3 (3), 446–474.
Hegarty, M., Canham, M. S., & Fabrikant, S. I. (2010). Thinking about the weather: How display salience and knowledge affect performance in a graphic inference task. Journal of Experimental Psychology: Learning, Memory, and Cognition , 36 (1), 37.
Hegarty, M., Friedman, A., Boone, A. P., & Barrett, T. J. (2016). Where are you? The effect of uncertainty and its visual representation on location judgments in GPS-like displays. Journal of Experimental Psychology: Applied , 22 (4), 381.
Hegarty, M., Smallman, H. S., & Stull, A. T. (2012). Choosing and using geospatial displays: Effects of design on performance and metacognition. Journal of Experimental Psychology: Applied , 18 (1), 1.
Hoffrage, U., & Gigerenzer, G. (1998). Using natural frequencies to improve diagnostic inferences. Academic Medicine , 73 (5), 538–540.
Hollands, J. G., & Spence, I. (1992). Judgments of change and proportion in graphical perception. Human Factors: The Journal of the Human Factors and Ergonomics Society , 34 (3), 313–334.
Huang, Z., Chen, H., Guo, F., Xu, J. J., Wu, S., & Chen, W.-H. (2006). Expertise visualization: An implementation and study based on cognitive fit theory. Decision Support Systems , 42 (3), 1539–1557.
Itti, L., Koch, C., & Niebur, E. (1998). A model of saliency-based visual attention for rapid scene analysis. IEEE Transactions on Pattern Analysis and Machine Intelligence , 20 (11), 1254–1259.
Joslyn, S., & LeClerc, J. (2013). Decisions with uncertainty: The glass half full. Current Directions in Psychological Science , 22 (4), 308–315.
Kahneman, D. (2011). Thinking, fast and slow . (Vol. 1). New York: Farrar, Straus and Giroux.
Kahneman, D., & Frederick, S. (2002). Representativeness revisited: Attribute substitution in intuitive judgment. In Heuristics and biases: The psychology of intuitive judgment , (p. 49).
Kahneman, D., & Tversky, A. (1982). Judgment under Uncertainty: Heuristics and Biases , (1st ed., ). Cambridge; NY: Cambridge University Press.
Book Google Scholar
Kane, M. J., Bleckley, M. K., Conway, A. R. A., & Engle, R. W. (2001). A controlled-attention view of working-memory capacity. Journal of Experimental Psychology: General , 130 (2), 169.
Keehner, M., Mayberry, L., & Fischer, M. H. (2011). Different clues from different views: The role of image format in public perceptions of neuroimaging results. Psychonomic Bulletin & Review , 18 (2), 422–428.
Keller, C., Siegrist, M., & Visschers, V. (2009). Effect of risk ladder format on risk perception in high-and low-numerate individuals. Risk Analysis , 29 (9), 1255–1264.
Keren, G., & Schul, Y. (2009). Two is not always better than one: A critical evaluation of two-system theories. Perspectives on Psychological Science , 4 (6), 533–550.
Kinkeldey, C., MacEachren, A. M., Riveiro, M., & Schiewe, J. (2017). Evaluating the effect of visually represented geodata uncertainty on decision-making: Systematic review, lessons learned, and recommendations. Cartography and Geographic Information Science , 44 (1), 1–21. https://doi.org/10.1080/15230406.2015.1089792 .
Kinkeldey, C., MacEachren, A. M., & Schiewe, J. (2014). How to assess visual communication of uncertainty? A systematic review of geospatial uncertainty visualisation user studies. The Cartographic Journal , 51 (4), 372–386.
Kriz, S., & Hegarty, M. (2007). Top-down and bottom-up influences on learning from animations. International Journal of Human-Computer Studies , 65 (11), 911–930.
Kunz, V. (2004). Rational choice . Frankfurt: Campus Verlag.
Lallanilla, M. (2014, April 24, 2014 10:15 am). Misleading Gun-Death Chart Draws Fire. https://www.livescience.com/45083-misleading-gun-death-chart.html
Lee, J., & Bednarz, R. (2009). Effect of GIS learning on spatial thinking. Journal of Geography in Higher Education , 33 (2), 183–198.
Liu, L., Boone, A., Ruginski, I., Padilla, L., Hegarty, M., Creem-Regehr, S. H., … House, D. H. (2016). Uncertainty Visualization by Representative Sampling from Prediction Ensembles. IEEE transactions on visualization and computer graphics, 23 (9), 2165-2178.
Lobben, A. K. (2004). Tasks, strategies, and cognitive processes associated with navigational map reading: A review perspective. The Professional Geographer , 56 (2), 270–281.
Lohse, G. L. (1993). A cognitive model for understanding graphical perception. Human Computer Interaction , 8 (4), 353–388.
Lohse, G. L. (1997). The role of working memory on graphical information processing. Behaviour & Information Technology , 16 (6), 297–308.
Marewski, J. N., & Gigerenzer, G. (2012). Heuristic decision making in medicine. Dialogues in Clinical Neuroscience , 14 (1), 77–89.
PubMed PubMed Central Google Scholar
McCabe, D. P., & Castel, A. D. (2008). Seeing is believing: The effect of brain images on judgments of scientific reasoning. Cognition , 107 (1), 343–352.
McKenzie, G., Hegarty, M., Barrett, T., & Goodchild, M. (2016). Assessing the effectiveness of different visualizations for judgments of positional uncertainty. International Journal of Geographical Information Science , 30 (2), 221–239.
Mechelli, A., Price, C. J., Friston, K. J., & Ishai, A. (2004). Where bottom-up meets top-down: Neuronal interactions during perception and imagery. Cerebral Cortex , 14 (11), 1256–1265.
Meilinger, T., Knauff, M., & Bülthoff, H. H. (2008). Working memory in wayfinding—A dual task experiment in a virtual city. Cognitive Science , 32 (4), 755–770.
Meyer, J. (2000). Performance with tables and graphs: Effects of training and a visual search model. Ergonomics , 43 (11), 1840–1865.
Munzner, T. (2014). Visualization analysis and design . Boca Raton, FL: CRC Press.
Nadav-Greenberg, L., Joslyn, S. L., & Taing, M. U. (2008). The effect of uncertainty visualizations on decision making in weather forecasting. Journal of Cognitive Engineering and Decision Making , 2 (1), 24–47.
Nayak, J. G., Hartzler, A. L., Macleod, L. C., Izard, J. P., Dalkin, B. M., & Gore, J. L. (2016). Relevance of graph literacy in the development of patient-centered communication tools. Patient Education and Counseling , 99 (3), 448–454.
Newman, G. E., & Scholl, B. J. (2012). Bar graphs depicting averages are perceptually misinterpreted: The within-the-bar bias. Psychonomic Bulletin & Review , 19 (4), 601–607. https://doi.org/10.3758/s13423-012-0247-5 .
Okan, Y., Galesic, M., & Garcia-Retamero, R. (2015). How people with low and high graph literacy process health graphs: Evidence from eye-tracking. Journal of Behavioral Decision Making .
Okan, Y., Garcia-Retamero, R., Cokely, E. T., & Maldonado, A. (2012). Individual differences in graph literacy: Overcoming denominator neglect in risk comprehension. Journal of Behavioral Decision Making , 25 (4), 390–401.
Okan, Y., Garcia-Retamero, R., Galesic, M., & Cokely, E. T. (2012). When higher bars are not larger quantities: On individual differences in the use of spatial information in graph comprehension. Spatial Cognition and Computation , 12 (2–3), 195–218.
Padilla, L., Hansen, G., Ruginski, I. T., Kramer, H. S., Thompson, W. B., & Creem-Regehr, S. H. (2015). The influence of different graphical displays on nonexpert decision making under uncertainty. Journal of Experimental Psychology: Applied , 21 (1), 37.
Padilla, L., Quinan, P. S., Meyer, M., & Creem-Regehr, S. H. (2017). Evaluating the impact of binning 2d scalar fields. IEEE Transactions on Visualization and Computer Graphics , 23 (1), 431–440.
Padilla, L., Ruginski, I. T., & Creem-Regehr, S. H. (2017). Effects of ensemble and summary displays on interpretations of geospatial uncertainty data. Cognitive Research: Principles and Implications , 2 (1), 40.
Pashler, H. (1994). Dual-task interference in simple tasks: Data and theory. Psychological Bulletin , 116 (2), 220.
Patterson, R. E., Blaha, L. M., Grinstein, G. G., Liggett, K. K., Kaveney, D. E., Sheldon, K. C., … Moore, J. A. (2014). A human cognition framework for information visualization. Computers & Graphics , 42 , 42–58.
Pinker, S. (1990). A theory of graph comprehension. In Artificial intelligence and the future of testing , (pp. 73–126).
Ratliff, K. R., & Newcombe, N. S. (2005). Human spatial reorientation using dual task paradigms . Paper presented at the Proceedings of the Annual Cognitive Science Society.
Reyna, V. F., Nelson, W. L., Han, P. K., & Dieckmann, N. F. (2009). How numeracy influences risk comprehension and medical decision making. Psychological Bulletin , 135 (6), 943.
Riveiro, M. (2016). Visually supported reasoning under uncertain conditions: Effects of domain expertise on air traffic risk assessment. Spatial Cognition and Computation , 16 (2), 133–153.
Rodríguez, V., Andrade, A. D., García-Retamero, R., Anam, R., Rodríguez, R., Lisigurski, M., … Ruiz, J. G. (2013). Health literacy, numeracy, and graphical literacy among veterans in primary care and their effect on shared decision making and trust in physicians. Journal of Health Communication , 18 (sup1), 273–289.
Rosenholtz, R., & Jin, Z. (2005). A computational form of the statistical saliency model for visual search. Journal of Vision , 5 (8), 777–777.
Ruginski, I. T., Boone, A. P., Padilla, L., Liu, L., Heydari, N., Kramer, H. S., … Creem-Regehr, S. H. (2016). Non-expert interpretations of hurricane forecast uncertainty visualizations. Spatial Cognition and Computation , 16 (2), 154–172.
Sanchez, C. A., & Wiley, J. (2006). An examination of the seductive details effect in terms of working memory capacity. Memory & Cognition , 34 (2), 344–355.
Schirillo, J. A., & Stone, E. R. (2005). The greater ability of graphical versus numerical displays to increase risk avoidance involves a common mechanism. Risk Analysis , 25 (3), 555–566.
Shah, P., & Freedman, E. G. (2011). Bar and line graph comprehension: An interaction of top-down and bottom-up processes. Topics in Cognitive Science , 3 (3), 560–578.
Shah, P., Freedman, E. G., & Vekiri, I. (2005). The Comprehension of Quantitative Information in Graphical Displays . In P. Shah (Ed.) & A. Miyake, The Cambridge Handbook of Visuospatial Thinking (pp. 426-476). New York: Cambridge University Press.
Shah, P., & Miyake, A. (1996). The separability of working memory resources for spatial thinking and language processing: An individual differences approach. Journal of Experimental Psychology: General , 125 (1), 4.
Shen, M., Carswell, M., Santhanam, R., & Bailey, K. (2012). Emergency management information systems: Could decision makers be supported in choosing display formats? Decision Support Systems , 52 (2), 318–330.
Shipstead, Z., Harrison, T. L., & Engle, R. W. (2015). Working memory capacity and the scope and control of attention. Attention, Perception, & Psychophysics , 77 (6), 1863–1880.
Simkin, D., & Hastie, R. (1987). An information-processing analysis of graph perception. Journal of the American Statistical Association , 82 (398), 454–465.
Sloman, S. A. (2002). Two systems of reasoning. In T. Gilovich, D. Griffin, & D. Kahneman (Eds.), Heuristics and biases : The psychology of intuitive judgment (pp. 379-396). New York: Cambridge University Press.
Smelcer, J. B., & Carmel, E. (1997). The effectiveness of different representations for managerial problem solving: Comparing tables and maps. Decision Sciences , 28 (2), 391.
St. John, M., Cowen, M. B., Smallman, H. S., & Oonk, H. M. (2001). The use of 2D and 3D displays for shape-understanding versus relative-position tasks. Human Factors , 43 (1), 79–98.
Stanovich, K. E. (1999). Who is rational? Studies of individual differences in reasoning . New York City: Psychology Press.
Stenning, K., & Oberlander, J. (1995). A cognitive theory of graphical and linguistic reasoning: Logic and implementation. Cognitive Science , 19 (1), 97–140.
Stone, E. R., Sieck, W. R., Bull, B. E., Yates, J. F., Parks, S. C., & Rush, C. J. (2003). Foreground: Background salience: Explaining the effects of graphical displays on risk avoidance. Organizational Behavior and Human Decision Processes , 90 (1), 19–36.
Stone, E. R., Yates, J. F., & Parker, A. M. (1997). Effects of numerical and graphical displays on professed risk-taking behavior. Journal of Experimental Psychology: Applied , 3 (4), 243.
Trueswell, J. C., & Papafragou, A. (2010). Perceiving and remembering events cross-linguistically: Evidence from dual-task paradigms. Journal of Memory and Language , 63 (1), 64–82.
Tversky, B. (2005). Visuospatial reasoning. In K. Holyoak and R. G. Morrison (eds.), The Cambridge Handbook of Thinking and Reasoning , (pp. 209-240). Cambridge: Cambridge University Press.
Tversky, B. (2011). Visualizing thought. Topics in Cognitive Science , 3 (3), 499–535.
Tversky, B., Corter, J. E., Yu, L., Mason, D. L., & Nickerson, J. V. (2012). Representing Category and Continuum: Visualizing Thought . Paper presented at the International Conference on Theory and Application of Diagrams, Berlin, Heidelberg.
Vessey, I., & Galletta, D. (1991). Cognitive fit: An empirical study of information acquisition. Information Systems Research , 2 (1), 63–84.
Vessey, I., Zhang, P., & Galletta, D. (2006). The theory of cognitive fit. In Human-computer interaction and management information systems: Foundations , (pp. 141–183).
Von Neumann, J. (1953). Morgenstern, 0.(1944) theory of games and economic behavior . Princeton, NJ: Princeton UP.
Vranas, P. B. M. (2000). Gigerenzer's normative critique of Kahneman and Tversky. Cognition , 76 (3), 179–193.
Wainer, H., Hambleton, R. K., & Meara, K. (1999). Alternative displays for communicating NAEP results: A redesign and validity study. Journal of Educational Measurement , 36 (4), 301–335.
Waters, E. A., Weinstein, N. D., Colditz, G. A., & Emmons, K. (2006). Formats for improving risk communication in medical tradeoff decisions. Journal of Health Communication , 11 (2), 167–182.
Waters, E. A., Weinstein, N. D., Colditz, G. A., & Emmons, K. M. (2007). Reducing aversion to side effects in preventive medical treatment decisions. Journal of Experimental Psychology: Applied , 13 (1), 11.
Wilkening, J., & Fabrikant, S. I. (2011). How do decision time and realism affect map-based decision making? Paper presented at the International Conference on Spatial Information Theory.
Zhu, B., & Watts, S. A. (2010). Visualization of network concepts: The impact of working memory capacity differences. Information Systems Research , 21 (2), 327–344.
Download references
This research is based upon work supported by the National Science Foundation under Grants 1212806, 1810498, and 1212577.
Availability of data and materials
No data were collected for this review.
Author information
Authors and affiliations.
Northwestern University, Evanston, USA
Lace M. Padilla
Department of Psychology, University of Utah, 380 S. 1530 E., Room 502, Salt Lake City, UT, 84112, USA
Lace M. Padilla, Sarah H. Creem-Regehr & Jeanine K. Stefanucci
Department of Psychology, University of California–Santa Barbara, Santa Barbara, USA
Mary Hegarty
You can also search for this author in PubMed Google Scholar
Contributions
LMP is the primary author of this study; she was central to the development, writing, and conclusions of this work. SHC, MH, and JS contributed to the theoretical development and manuscript preparation. All authors read and approved the final manuscript.
Corresponding author
Correspondence to Lace M. Padilla .
Ethics declarations
Authors’ information.
LMP is a Ph.D. student at the University of Utah in the Cognitive Neural Science department. LMP is a member of the Visual Perception and Spatial Cognition Research Group directed by Sarah Creem-Regehr, Ph.D., Jeanine Stefanucci, Ph.D., and William Thompson, Ph.D. Her work focuses on graphical cognition, decision making with visualizations, and visual perception. She works on large interdisciplinary projects with visualization scientists and anthropologists.
SHC is a Professor in the Psychology Department of the University of Utah. She received her MA and Ph.D. in Psychology from the University of Virginia. Her research serves joint goals of developing theories of perception-action processing mechanisms and applying these theories to relevant real-world problems in order to facilitate observers’ understanding of their spatial environments. In particular, her interests are in space perception, spatial cognition, embodied cognition, and virtual environments. She co-authored the book Visual Perception from a Computer Graphics Perspective ; previously, she was Associate Editor of Psychonomic Bulletin & Review and Experimental Psychology: Human Perception and Performance .
MH is a Professor in the Department of Psychological & Brain Sciences at the University of California, Santa Barbara. She received her Ph.D. in Psychology from Carnegie Mellon University. Her research is concerned with spatial cognition, broadly defined, and includes research on small-scale spatial abilities (e.g. mental rotation and perspective taking), large-scale spatial abilities involved in navigation, comprehension of graphics, and the role of spatial cognition in STEM learning. She served as chair of the governing board of the Cognitive Science Society and is associate editor of Topics in Cognitive Science and past Associate Editor of Journal of Experimental Psychology: Applied .
JS is an Associate Professor in the Psychology Department at the University of Utah. She received her M.A. and Ph.D. in Psychology from the University of Virginia. Her research focuses on better understanding if a person’s bodily states, whether emotional, physiological, or physical, affects their spatial perception and cognition. She conducts this research in natural settings (outdoor or indoor) and in virtual environments. This work is inherently interdisciplinary given it spans research on emotion, health, spatial perception and cognition, and virtual environments. She is on the editorial boards for the Journal of Experimental Psychology: General and Virtual Environments: Frontiers in Robotics and AI . She also co-authored the book Visual Perception from a Computer Graphics Perspective .
Ethics approval and consent to participate
The research reported in this paper was conducted in adherence to the Declaration of Helsinki and received IRB approval from the University of Utah, #IRB_00057678. No human subject data were collected for this work; therefore, no consent to participate was acquired.
Consent for publication
Consent to publish was not required for this review.
Competing interests
The authors declare that they have no competing interests.
Publisher’s Note
Springer Nature remains neutral with regard to jurisdictional claims in published maps and institutional affiliations.
Additional information
The original version of this article has been revised. Table 2 was corrected to be presented appropriately.
Rights and permissions
Open Access This article is distributed under the terms of the Creative Commons Attribution 4.0 International License ( http://creativecommons.org/licenses/by/4.0/ ), which permits unrestricted use, distribution, and reproduction in any medium, provided you give appropriate credit to the original author(s) and the source, provide a link to the Creative Commons license, and indicate if changes were made.
Reprints and permissions
About this article
Cite this article.
Padilla, L.M., Creem-Regehr, S.H., Hegarty, M. et al. Decision making with visualizations: a cognitive framework across disciplines. Cogn. Research 3 , 29 (2018). https://doi.org/10.1186/s41235-018-0120-9
Download citation
Received : 20 September 2017
Accepted : 05 June 2018
Published : 11 July 2018
DOI : https://doi.org/10.1186/s41235-018-0120-9
Share this article
Anyone you share the following link with will be able to read this content:
Sorry, a shareable link is not currently available for this article.
Provided by the Springer Nature SharedIt content-sharing initiative
- Decision making with visualizations review
- Cognitive model
- Geospatial visualizations
- Healthcare visualizations
- Weather forecast visualizations
- Uncertainty visualizations
- Graphical decision making
- Dual-process
- Help & FAQ
Reflections and Considerations on Running Creative Visualization Learning Activities
- School of Informatics
- Institute of Language, Cognition and Computation
- Design Informatics
- Language, Interaction, and Robotics
Research output : Chapter in Book/Report/Conference proceeding › Conference contribution
Abstract / Description of output
Keywords / materials (for non-textual outputs).
- Data visualization
- Information visualization
- Scientific visualization
- VisActivites
- Learning activities
Access to Document
- 10.1109/VisGuides57787.2022.00009 Licence: All Rights Reserved
- 2209.09807v1 Accepted author manuscript, 6.31 MB Licence: Creative Commons: Attribution (CC-BY)
- https://ieeexplore.ieee.org/document/9990915 Licence: All Rights Reserved
Fingerprint
- Visualization Computer Science 100%
- Learning Economics, Econometrics and Finance 100%
- Research Worker Computer Science 14%
- Learning Materials Computer Science 14%
- Best Practice Computer Science 14%
- Education Computer Science 14%
- Starting Point Computer Science 14%
- Learning Experience Economics, Econometrics and Finance 14%
T1 - Reflections and Considerations on Running Creative Visualization Learning Activities
AU - Roberts, Jonathan C.
AU - Bach, Benjamin
AU - Boucher, Magdalena
AU - Chevalier, Fanny
AU - Diehl, Alexandra
AU - Hinrichs, Uta
AU - Huron, Samuel
AU - Kirk, Andy
AU - Knudsen, Søren
AU - Meirelles, Isabel
AU - Noonan, Rebecca
AU - Pelchmann, Laura
AU - Rajabiyazdi, Fateme
AU - Stoiber, Christina
N1 - Conference code: 4
PY - 2022/12/22
Y1 - 2022/12/22
N2 - This paper draws together nine strategies for creative visualization activities. Teaching visualization often involves running learning activities where students perform tasks that directly support one or more topics that the teacher wishes to address in the lesson. As a group of educators and researchers in visualization, we reflect on our learning experiences. Our activities and experiences range from dividing the tasks into smaller parts, considering different learning materials, to encouraging debate. With this paper, our hope is that we can encourage, inspire, and guide other educators with visualization activities. Our reflections provide an initial starting point of methods and strategies to craft creative visualisation learning activities, and provide a foundation for developing best practices in visualization education.
AB - This paper draws together nine strategies for creative visualization activities. Teaching visualization often involves running learning activities where students perform tasks that directly support one or more topics that the teacher wishes to address in the lesson. As a group of educators and researchers in visualization, we reflect on our learning experiences. Our activities and experiences range from dividing the tasks into smaller parts, considering different learning materials, to encouraging debate. With this paper, our hope is that we can encourage, inspire, and guide other educators with visualization activities. Our reflections provide an initial starting point of methods and strategies to craft creative visualisation learning activities, and provide a foundation for developing best practices in visualization education.
KW - Data visualization
KW - Information visualization
KW - Scientific visualization
KW - VisActivites
KW - Learning activities
KW - Pedagogy
U2 - 10.1109/VisGuides57787.2022.00009
DO - 10.1109/VisGuides57787.2022.00009
M3 - Conference contribution
SN - 979-8-3503-9713-0
BT - Proceedings of the 4th IEEE Workshop on Visualization Guidelines in Research, Design, and Education
PB - Institute of Electrical and Electronics Engineers (IEEE)
T2 - 4th IEEE VIS Workshop on Visualization Guidelines – Visualization Guidelines in Research, Design, and Education, 2022
Y2 - 16 October 2022 through 16 October 2022
Advertisement
Visualizing qualitative data: unpacking the complexities and nuances of technology-supported learning processes
- Research Article
- Published: 28 July 2023
Cite this article
- Shiyan Jiang ORCID: orcid.org/0000-0003-4781-846X 1 , 3 ,
- Joey Huang 2 &
- Hollylynne S. Lee 1
399 Accesses
1 Altmetric
Explore all metrics
Analyzing qualitative data from learning processes is considered “messy” and time consuming (Chi in J Learn Sci 6(3):271–315, 1997). It is often challenging to summarize and synthesize such data in a manner that conveys the richness and complexity of learning processes in a clear and concise manner. Moreover, qualitative data often contains patterns that are not immediately apparent. Consequently, visualization can be an effective tool for representing and unpacking the complexities and multidimensions of learning processes. Additionally, visualizations provide a time-efficient approach to analyzing data and a high-level view of the learning process over time for researchers to zoom in on intriguing moments and patterns (Huang et al. in Comput Human Behav 87:480–492, 2018). In this conceptual paper, we provide a broad overview of research in the field of visualizing qualitative data and discuss two studies (1) visualizing role-changing patterns in an interdisciplinary learning environment and (2) operationalizing collaborative computational thinking practices via visualization. By leveraging these studies, we aim to demonstrate a visualization processing flow along with qualitative research and methods. Particularly, the processing flow includes three critical elements: research subjectivity, complexity of visual encoding, and purpose of visual encoding. The discussion highlights the iterative and creative nature of the visualization technique. Furthermore, we discuss the benefits, challenges, and limitations of using visualization in the context of qualitative studies.
This is a preview of subscription content, log in via an institution to check access.
Access this article
Price includes VAT (Russian Federation)
Instant access to the full article PDF.
Rent this article via DeepDyve
Institutional subscriptions
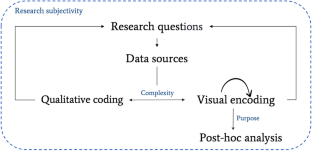
Similar content being viewed by others
Social Learning Theory—Albert Bandura
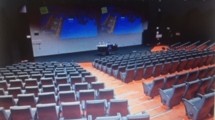
Learning environments’ influence on students’ learning experience in an Australian Faculty of Business and Economics
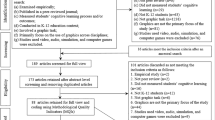
The Impact of Visual Displays on Learning Across the Disciplines: A Systematic Review
Alcalá, L., Rogoff, B., & Fraire, A. L. (2018). Sophisticated collaboration is common among Mexican-heritage US children. Proceedings of the National Academy of Sciences, 115 (45), 11377–11384.
Article Google Scholar
Archambault, S. G., Helouvry, J., Strohl, B., & Williams, G. (2015). Data visualization as a communication tool. Library Hi Tech News , 32 (2), 1–9.
Baker, R. S., Boser, U., & Snow, E. L. (2022). Learning engineering: A view on where the field is at, where it’s going, and the research needed. Technology, Mind, and Behavior . https://doi.org/10.1037/tmb0000058
Benbria (2022). A bubble-matrix chart based on d3.chart. GitHub. July 20 Retrieved from https://github.com/benbria/d3.chart.bubble-matrix
Börner, K., & Polley, D. E. (2014). Visual insights: A practical guide to making sense of data . MIT Press.
Google Scholar
Braun, V., & Clarke, V. (2021). One size fits all? what counts as quality practice in (reflexive) thematic analysis? Qualitative Research in Psychology, 18 (3), 328–352.
Brennan, K., Balch, C., & Chung, M. (2014). Creative computing . Harvard Graduate School of Education. CC BY-SA 4.0.
Cairo, A. (2012). The functional art: An introduction to information graphics and visualization . New Riders.
Calabrese Barton, A., Kang, H., Tan, E., O’Neill, T. B., Bautista-Guerra, J., & Brecklin, C. (2013). Crafting a future in science: Tracing middle school girls’ identity work over time and space. American Educational Research Journal, 50 (1), 37–75.
Campbell, T. G., & Hodges, T. S. (2020). Using positioning theory to examine how students collaborate in groups in mathematics. International Journal of Educational Research, 103 , 101632.
Chi, M. T. (1997). Quantifying qualitative analyses of verbal data: A practical guide. The Journal of the Learning Sciences, 6 (3), 271–315.
Cobb, P., Confrey, J., DiSessa, A., Lehrer, R., & Schauble, L. (2003). Design experiments in educational research. Educational Researcher , 32 (1), 9–13.
Csanadi, A., Eagan, B., Kollar, I., Shaffer, D. W., & Fischer, F. (2018). When coding-and-counting is not enough: Using epistemic network analysis (ENA) to analyze verbal data in CSCL research. International Journal of Computer-Supported Collaborative Learning , 13 , 419–438.
Derry, S. J., Pea, R. D., Barron, B., Engle, R. A., Erickson, F., Goldman, R., & Sherin, B. L. (2010). Conducting video research in the learning sciences: Guidance on selection, analysis, technology, and ethics. The Journal of the Learning Sciences, 19 (1), 3–53.
Fu, S., Zhao, J., Cheng, H. F., Zhu, H., & Marlow, J. (2018). T-cal: Understanding team conversational data with calendar-based visualization. Proceedings of the 2018 CHI conference on human factors in computing systems (pp. 1–13).
Gee, J. P. (2000). Identity as an analytic lens for research in education. Review of Research in Education , 25 , 99–125.
Gibbs, G. R. (2007). Thematic coding and categorizing. Analyzing Qualitative Data, 703 , 38–56.
Gisev, N., Bell, J. S., & Chen, T. F. (2013). Interrater agreement and interrater reliability: Key concepts, approaches, and applications. Research in Social and Administrative Pharmacy , 9 (3), 330–338.
Hao, J., Liu, L., von Davier, A. A., & Kyllonen, P. C. (2017). Initial steps towards a standardized assessment for collaborative problem solving (CPS): Practical challenges and strategies. In A. von Davier, M. Zhu, & P. Kyllonen (Eds.), Innovative assessment of collaboration (pp. 135–156). Springer. https://doi.org/10.1007/978-3-319-33261-1_9
Chapter Google Scholar
Hmelo-Silver, C. E. (2003). Analyzing collaborative knowledge construction: Multiple methods for integrated understanding. Computers & Education , 41 (4), 397–420.
Huang, J., & Parker, M. C. (2022). Developing computational thinking collaboratively: The nexus of computational practices within small groups. Computer Science Education . https://doi.org/10.1080/08993408.2022.2039488
Huang, J., Hmelo-Silver, C. E., Jordan, R., Gray, S., Frensley, T., Newman, G., & Stern, M. J. (2018). Scientific discourse of citizen scientists: Models as a boundary object for collaborative problem solving. Computers in Human Behavior , 87 , 480–492.
Jiang, S., Shen, J., & Smith, B. E. (2019). Designing discipline-specific roles for interdisciplinary learning: Two comparative cases in an afterschool STEM+L programme. International Journal of Science Education, 41 (6), 803–826.
Jiang, S., Smith, B. E., & Shen, J. (2021). Examining how different modes mediate adolescents’ interactions during their collaborative multimodal composing processes. Interactive Learning Environments , 29 (5), 807–820.
Kafai, Y. B., & Peppler, K. A. (2011). Youth, technology, and DIY: Developing participatory competencies in creative media production. Review of Research in Education, 35 (1), 89–119.
Kim, J. W., Ritter, F. E., & Koubek, R. J. (2013). An integrated theory for improved skill acquisition and retention in the three stages of learning. Theoretical Issues in Ergonomics Science , 14 (1), 22–37.
Koedinger, K. R., Brunskill, E., Baker, R. S., McLaughlin, E. A., & Stamper, J. (2013). New potentials for data-driven intelligent tutoring system development and optimization. AI Magazine , 34 (3), 27–41.
Lee, H. S., & Hollebrands, K. F. (2006). Students’ use of technological features while solving a mathematics problem. The Journal of Mathematical Behavior , 25 (3), 252–266.
Li, S., Chen, G., Xing, W., Zheng, J., & Xie, C. (2020). Longitudinal clustering of students’ self-regulated learning behaviors in engineering design. Computers & Education , 153 , 103899.
Marriott, K., Lee, B., Butler, M., Cutrell, E., Ellis, K., Goncu, C., & Szafir, D. A. (2021). Inclusive data visualization for people with disabilities: A call to action. Interactions , 28 (3), 47–51.
Munzner, T. (2018). Visualization. In S. Munzner & P. Shirley (Eds.), Fundamentals of computer graphics (pp. 665–699). CRC Press.
National Academies of Sciences, Engineering, and Medicine. (2018). How people learn II: Learners, contexts, and cultures . National Academies Press.
O’Dwyer, B. (2004). Qualitative data analysis: Illuminating a process for transforming a ‘messy’but ‘attractive’‘nuisance.’ In C. Humphrey & B. H. K. Lee (Eds.), The real life guide to accounting research (pp. 391–407). Oxford: Elsevier.
Papamitsiou, Z., & Economides, A. A. (2014). Learning analytics and educational data mining in practice: A systematic literature review of empirical evidence. Journal of Educational Technology & Society , 17 (4), 49–64.
Rabinovich, M., & Kacen, L. (2013). Qualitative coding methodology for interpersonal study. Psychoanalytic Psychology , 30 (2), 210.
Resnick, M., Maloney, J., Monroy-Hernández, A., Rusk, N., Eastmond, E., Brennan, K., & Kafai, Y. (2009). Scratch: Programming for all. Communications of the ACM , 52 (11), 60–67.
Riikonen, S., Seitamaa-Hakkarainen, P., & Hakkarainen, K. (2020). Bringing maker practices to school: Tracing discursive and materially mediated aspects of student teams’ collaborative making processes. International Journal of Computer-Supported Collaborative Learning , 15 (3), 319–349.
Rosé, C. (2018). Learning analytics in the learning sciences. In F. Fischer, C. E. Hmelo-Silver, S. R. Goldman, & P. Reimann (Eds.), International handbook of the learning sciences (pp. 511–519). Taylor & Francis.
Rosling, H., Ronnlund, A. R., & Rosling, O. (2005). New software brings statistics beyond the eye. In E. Giovannini (Ed.), Statistics, knowledge and policy: Key indicators to inform decision making (pp. 522–530). Organization for Economic Co-Operation and Development.
Sawyer, R. K. (2005). The cambridge handbook of the learning sciences . Cambridge University Press.
Book Google Scholar
Seufert, T. (2018). The interplay between self-regulation in learning and cognitive load. Educational Research Review , 24 , 116–129.
Smith, B. E. (2017). Composing across modes: A comparative analysis of adolescents’ multimodal composing processes. Learning Media and Technology , 42 (3), 259–278.
Stice, J. E. (1987). Using Kolb’s learning cycle to improve student learning. Engineering Education, 77 (5), 291–296.
Strauss, A., & Corbin, J. M. (1998). Basics of qualitative research: Techniques and procedures for developing grounded theory (2nd ed.). SAGE.
Stryker, S., & Burke, P. J. (2000). The past, present, and future of an identity theory. Social psychology quarterly, 63 , 284–297.
Sultana, S., Ahmed, S. I., & Rzeszotarski, J. M. (2021). Seeing in context: Traditional visual communication practices in rural bangladesh. Proceedings of the ACM on human-computer interaction , 4 (CSCW3), 1–31.
Teasley, S. D. (2011). Thinking about methods to capture effective collaborations. Analyzing interactions in CSCL (pp. 131–142). Springer.
Van Horne, K., & Bell, P. (2017). Youth disciplinary identification during participation in contemporary project-based science investigations in school. Journal of the Learning Sciences , 26 (3), 437–476.
Vieira, C., Parsons, P., & Byrd, V. (2018). Visual learning analytics of educational data: A systematic literature review and research agenda. Computers & Education , 122 , 119–135.
Weinberger, A., & Fischer, F. (2006). A framework to analyze argumentative knowledge construction in computer-supported collaborative learning. Computers & education , 46 (1), 71–95.
Wenger, E. (1998). C ommunities of practice: Learning, meaning, and identity. Cambridge University Press.
Wilkinson, L. (2012). The grammar of graphics (pp. 375–414). Springer.
Wise, A. F., Saghafian, M., & Padmanabhan, P. (2012). Towards more precise design guidance: Specifying and testing the functions of assigned student roles in online discussions. Educational Technology Research and Development , 60 , 55–82.
Download references
Author information
Authors and affiliations.
North Carolina State University, Raleigh, NC, USA
Shiyan Jiang & Hollylynne S. Lee
University of California Irvine, Irvine, CA, USA
Department of Teacher Education and Learning Sciences, North Carolina State University, Poe Hall, 208, 2310 Stinson Dr, Raleigh, NC, 27695, USA
Shiyan Jiang
You can also search for this author in PubMed Google Scholar
Corresponding author
Correspondence to Shiyan Jiang .
Ethics declarations
Conflict of interest.
The authors declare that they have no conflict of interest.
Informed consent
All consent processes and forms for the two studies were approved by the Solutions Institutional Review Board (IRB) ( https://www.solutionsirb.com/ ) prior to the study’s implementation. The parental consent forms were distributed and collected back before the study implementation. Classroom teachers asked students to bring home the parental consent forms for signature. Parents provided consent to allow researchers to use student-generated data and conduct interviews.
Research involving human and animal participants
The two studies were conducted with the university IRB (human subject protection) approval and adhered to ethical guidelines as the nature of study demanded.
Additional information
Publisher’s note.
Springer Nature remains neutral with regard to jurisdictional claims in published maps and institutional affiliations.
Rights and permissions
Springer Nature or its licensor (e.g. a society or other partner) holds exclusive rights to this article under a publishing agreement with the author(s) or other rightsholder(s); author self-archiving of the accepted manuscript version of this article is solely governed by the terms of such publishing agreement and applicable law.
Reprints and permissions
About this article
Jiang, S., Huang, J. & Lee, H.S. Visualizing qualitative data: unpacking the complexities and nuances of technology-supported learning processes. Education Tech Research Dev (2023). https://doi.org/10.1007/s11423-023-10272-7
Download citation
Accepted : 04 June 2023
Published : 28 July 2023
DOI : https://doi.org/10.1007/s11423-023-10272-7
Share this article
Anyone you share the following link with will be able to read this content:
Sorry, a shareable link is not currently available for this article.
Provided by the Springer Nature SharedIt content-sharing initiative
- Data visualization
- Qualitative data
- Learning processes
- Visual encoding
- Find a journal
- Publish with us
- Track your research
- Publisher Home
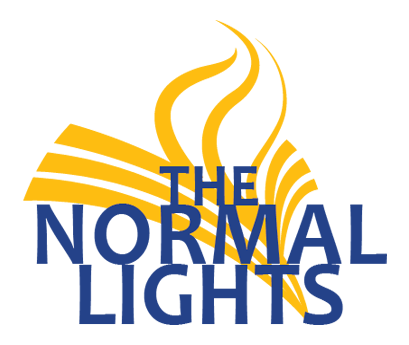
- Author Guidelines
- Editorial Team
- Quality Policy
- Submission Procedure
- Publication Ethics
- Announcements
Fostering Better Learning of Science Concepts through Creative Visualization
Main article content.
Creative visualization, learning of science concepts, creativity, education
Article Sidebar
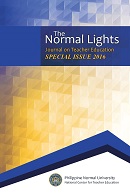
Article Details

This work is licensed under a Creative Commons Attribution-NonCommercial-NoDerivatives 4.0 International License .
Modal Header
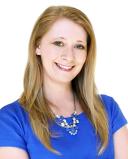
Seeing Is Believing: The Power of Visualization
Your best life, from the comfort of your armchair..
Posted December 3, 2009 | Reviewed by Jessica Schrader
Despite the great case for getting off our duffs, there are some amazingly cool and effective practices we can do from the comfort of our own recliners—without even budging a finger. For instance, you could practice your golf swing, work out your muscles, prepare to climb Mount Kilimanjaro, hone your chess skills, practice for tomorrow’s surgery, and you can even prepare for your best life!
Mental practice can get you closer to where you want to be in life, and it can prepare you for success! For instance, Natan Sharansky, a computer specialist who spent nine years in prison in the USSR after being accused of spying for U.S., has a lot of experience with mental practices. While in solitary confinement, he played himself in mental chess, saying: “I might as well use the opportunity to become the world champion!” Remarkably, in 1996, Sharansky beat world champion chess player Garry Kasparov!
A study looking at brain patterns in weightlifters found that the patterns activated when a weightlifter lifted hundreds of pounds were similarly activated when they only imagined lifting. In some cases, research has revealed that mental practices are almost effective as true physical practice . For instance, in his study on everyday people, Guang Yue , an exercise psychologist from Cleveland Clinic Foundation in Ohio, compared results of those who did physical exercises to the results of those who carried out virtual workouts in their heads. In the physical exercise group, finger abduction strength increased by 53%. In the group that did "mental contractions", their finger abduction strength increased by 35%. However, "the greatest gain (40%) was not achieved until 4 weeks after the training had ended" (Ranganathan et al., 2004). This demonstrates the mind's incredible power over the body and its muscles.
Noted as one form of mental rehearsal, visualization has been popular since the Soviets started using it back in the 1970s to compete in sports. Now, many athletes employ this technique, including Tiger Woods who has been using it since his pre- teen years. Seasoned athletes use vivid, highly detailed internal images and run-throughs of the entire performance, engaging all their senses in their mental rehearsal, and they combine their knowledge of the sports venue with mental rehearsal. World Champion Golfer, Jack Nicklaus has said: “I never hit a shot, not even in practice, without having a very sharp in-focus picture of it in my head." Even heavyweight champion Muhammad Ali used different mental practices to enhance his performance in the ring such as: “affirmation; visualization; mental rehearsal; self-confirmation; and perhaps the most powerful epigram of personal worth ever uttered: 'I am the greatest.'"
Brain studies now reveal that thoughts produce the same mental instructions as actions. Mental imagery impacts many cognitive processes in the brain: motor control, attention , perception, planning, and memory . So the brain is getting trained for actual performance during visualization. It’s been found that mental practices can enhance motivation , increase confidence and self-efficacy , improve motor performance, prime your brain for success, and increase states of flow—all relevant to achieving your best life!
For someone like Matthew Nagle who is paralyzed in all four limbs, mental practices have transformed his entire way of life . Matthew had a silicone chip implanted in brain. Astonishingly, after just four days of mental practice, he could move a computer cursor on a screen, open email, play a computer game, and control a robotic arm. While our circumstances may be less stringent than those that Matthew endures, it’s quite obvious that every person can benefit from mental practices.
So, if athletes and chess players use this technique to enhance performance, how can it enhance the lives of the "average Joe"? First, study results highlight the strength of the mind-body connection, or in other words the link between thoughts and behaviors—a very important connection for achieving your best life. While your future may not include achieving a great physique or becoming the heavyweight champ or winning the Masters Tournament, mental practice has a lot to offer you. Try it here!
Begin by establishing a highly specific goal. Imagine the future; you have already achieved your goal. Hold a mental "picture" of it as if it were occurring to you right at that moment. Imagine the scene in as much detail as possible. Engage as many of the five senses as you can in your visualization. Who are you with? Which emotions are you feeling right now? What are you wearing? Is there a smell in the air? What do you hear? What is your environment? Sit with a straight spine when you do this. Practice at night or in the morning (just before/after sleep). Eliminate any doubts, if they come to you. Repeat this practice often. Combine with meditation or an affirmation (e.g. “I am courageous; I am strong,” or to borrow from Ali, “I am the greatest!”).
Angie LeVan, MAPP , is a resilience coach, speaker, trainer, and writer, dedicated to helping individuals and organizations/businesses thrive! For more information, visit ajlevan.com .
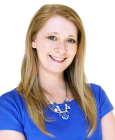
A.J. Adams, MAPP , specializes in workplace well-being, resilience, and burnout prevention.
- Find a Therapist
- Find a Treatment Center
- Find a Psychiatrist
- Find a Support Group
- Find Online Therapy
- United States
- Brooklyn, NY
- Chicago, IL
- Houston, TX
- Los Angeles, CA
- New York, NY
- Portland, OR
- San Diego, CA
- San Francisco, CA
- Seattle, WA
- Washington, DC
- Asperger's
- Bipolar Disorder
- Chronic Pain
- Eating Disorders
- Passive Aggression
- Personality
- Goal Setting
- Positive Psychology
- Stopping Smoking
- Low Sexual Desire
- Relationships
- Child Development
- Self Tests NEW
- Therapy Center
- Diagnosis Dictionary
- Types of Therapy
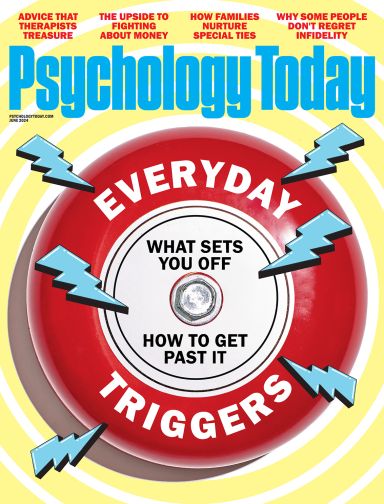
At any moment, someone’s aggravating behavior or our own bad luck can set us off on an emotional spiral that threatens to derail our entire day. Here’s how we can face our triggers with less reactivity so that we can get on with our lives.
- Emotional Intelligence
- Gaslighting
- Affective Forecasting
- Neuroscience

An official website of the United States government
The .gov means it’s official. Federal government websites often end in .gov or .mil. Before sharing sensitive information, make sure you’re on a federal government site.
The site is secure. The https:// ensures that you are connecting to the official website and that any information you provide is encrypted and transmitted securely.
- Publications
- Account settings
Preview improvements coming to the PMC website in October 2024. Learn More or Try it out now .
- Advanced Search
- Journal List
- Cogn Res Princ Implic
- v.3; 2018 Dec
Decision making with visualizations: a cognitive framework across disciplines
Lace m. padilla.
1 Northwestern University, Evanston, USA
2 Department of Psychology, University of Utah, 380 S. 1530 E., Room 502, Salt Lake City, UT 84112 USA
Sarah H. Creem-Regehr
Mary hegarty.
3 Department of Psychology, University of California–Santa Barbara, Santa Barbara, USA
Jeanine K. Stefanucci
Associated data.
No data were collected for this review.
Visualizations—visual representations of information, depicted in graphics—are studied by researchers in numerous ways, ranging from the study of the basic principles of creating visualizations, to the cognitive processes underlying their use, as well as how visualizations communicate complex information (such as in medical risk or spatial patterns). However, findings from different domains are rarely shared across domains though there may be domain-general principles underlying visualizations and their use. The limited cross-domain communication may be due to a lack of a unifying cognitive framework. This review aims to address this gap by proposing an integrative model that is grounded in models of visualization comprehension and a dual-process account of decision making. We review empirical studies of decision making with static two-dimensional visualizations motivated by a wide range of research goals and find significant direct and indirect support for a dual-process account of decision making with visualizations. Consistent with a dual-process model, the first type of visualization decision mechanism produces fast, easy, and computationally light decisions with visualizations. The second facilitates slower, more contemplative, and effortful decisions with visualizations. We illustrate the utility of a dual-process account of decision making with visualizations using four cross-domain findings that may constitute universal visualization principles. Further, we offer guidance for future research, including novel areas of exploration and practical recommendations for visualization designers based on cognitive theory and empirical findings.
Significance
People use visualizations to make large-scale decisions, such as whether to evacuate a town before a hurricane strike, and more personal decisions, such as which medical treatment to undergo. Given their widespread use and social impact, researchers in many domains, including cognitive psychology, information visualization, and medical decision making, study how we make decisions with visualizations. Even though researchers continue to develop a wealth of knowledge on decision making with visualizations, there are obstacles for scientists interested in integrating findings from other domains—including the lack of a cognitive model that accurately describes decision making with visualizations. Research that does not capitalize on all relevant findings progresses slower, lacks generalizability, and may miss novel solutions and insights. Considering the importance and impact of decisions made with visualizations, it is critical that researchers have the resources to utilize cross-domain findings on this topic. This review provides a cognitive model of decision making with visualizations that can be used to synthesize multiple approaches to visualization research. Further, it offers practical recommendations for visualization designers based on the reviewed studies while deepening our understanding of the cognitive processes involved when making decisions with visualizations.
Introduction
Every day we make numerous decisions with the aid of visualizations , including selecting a driving route, deciding whether to undergo a medical treatment, and comparing figures in a research paper. Visualizations are external visual representations that are systematically related to the information that they represent (Bertin, 1983 ; Stenning & Oberlander, 1995 ). The information represented might be about objects, events, or more abstract information (Hegarty, 2011 ). The scope of the previously mentioned examples illustrates the diversity of disciplines that have a vested interest in the influence of visualizations on decision making. While the term decision has a range of meanings in everyday language, here decision making is defined as a choice between two or more competing courses of action (Balleine, 2007 ).
We argue that for visualizations to be most effective, researchers need to integrate decision-making frameworks into visualization cognition research. Reviews of decision making with visual-spatial uncertainty also agree there has been a general lack of emphasis on mental processes within the visualization decision-making literature (Kinkeldey, MacEachren, Riveiro, & Schiewe, 2017 ; Kinkeldey, MacEachren, & Schiewe, 2014 ). The framework that has dominated applied decision-making research for the last 30 years is a dual-process account of decision making. Dual-process theories propose that we have two types of decision processes: one for automatic, easy decisions (Type 1); and another for more contemplative decisions (Type 2) (Kahneman & Frederick, 2002 ; Stanovich, 1999 ). 1 Even though many research areas involving higher-level cognition have made significant efforts to incorporate dual-process theories (Evans, 2008 ), visualization research has yet to directly test the application of current decision-making frameworks or develop an effective cognitive model for decision making with visualizations. The goal of this work is to integrate a dual-process account of decision making with established cognitive frameworks of visualization comprehension.
In this paper, we present an overview of current decision-making theories and existing visualization cognition frameworks, followed by a proposal for an integrated model of decision making with visualizations, and a selective review of visualization decision-making studies to determine if there is cross-domain support for a dual-process account of decision making with visualizations. As a preview, we will illustrate Type 1 and 2 processing in decision making with visualizations using four cross-domain findings that we observed in the literature review. Our focus here is on demonstrating how dual-processing can be a useful framework for examining visualization decision-making research. We selected the cross-domain findings as relevant demonstrations of Type 1 and 2 processing that were shared across the studies reviewed, but they do not represent all possible examples of dual-processing in visualization decision-making research. The review documents each of the cross-domain findings, in turn, using examples from studies in multiple domains. These cross-domain findings differ in their reliance on Type 1 and Type 2 processing. We conclude with recommendations for future work and implications for visualization designers.
Decision-making frameworks
Decision-making researchers have pursued two dominant research paths to study how humans make decisions under risk. The first assumes that humans make rational decisions, which are based on weighted and ordered probability functions and can be mathematically modeled (e.g. Kunz, 2004 ; Von Neumann, 1953 ). The second proposes that people often make intuitive decisions using heuristics (Gigerenzer, Todd, & ABC Research Group, 2000 ; Kahneman & Tversky, 1982 ). While there is fervent disagreement on the efficacy of heuristics and whether human behavior is rational (Vranas, 2000 ), there is more consensus that we can make both intuitive and strategic decisions (Epstein, Pacini, Denes-Raj, & Heier, 1996 ; Evans, 2008 ; Evans & Stanovich, 2013 ; cf. Keren & Schul, 2009 ). The capacity to make intuitive and strategic decisions is described by a dual-process account of decision making, which suggests that humans make fast, easy, and computationally light decisions (known as Type 1 processing) by default, but can also make slow, contemplative, and effortful decisions by employing Type 2 processing (Kahneman, 2011 ). Various versions of dual-processing theory exist, with the key distinctions being in the attributes associated with each type of process (for a more detailed review of dual-process theories, see Evans & Stanovich, 2013 ). For example, older dual-systems accounts of decision making suggest that each process is associated with specific cognitive or neurological systems. In contrast, dual-process (sometimes termed dual-type) theories propose that the processes are distinct but do not necessarily occur in separate cognitive or neurological systems (hence the use of process over system) (Evans & Stanovich, 2013 ).
Many applied domains have adapted a dual-processing model to explain task- and domain-specific decisions, with varying degrees of success (Evans, 2008 ). For example, when a physician is deciding if a patient should be assigned to a coronary care unit or a regular nursing bed, the doctor can use a heuristic or utilize heart disease predictive instruments to make the decision (Marewski & Gigerenzer, 2012 ). In the case of the heuristic, the doctor would employ a few simple rules (diagrammed in Fig. 1 ) that would guide her decision, such as considering the patient’s chief complaint being chest pain. Another approach is to apply deliberate mental effort to make a more time-consuming and effortful decision, which could include using heart disease predictive instruments (Marewski & Gigerenzer, 2012 ). In a review of how applied domains in higher-level cognition have implemented a dual-processing model for domain-specific decisions, Evans ( 2008 ) argues that prior work has conflicting accounts of Type 1 and 2 processing. Some studies suggest that the two types work in parallel while others reveal conflicts between the Types (Sloman, 2002 ). In the physician example proposed by Marewski and Gigerenzer ( 2012 ), the two types are not mutually exclusive, as doctors can utilize Type 2 to make a more thoughtful decision that is also influenced by some rules of thumb or Type 1. In sum, Evans ( 2008 ) argues that due to the inconsistency of classifying Type 1 and 2, the distinction between only two types is likely an oversimplification. Evans ( 2008 ) suggests that the literature only consistently supports the identification of processes that require a capacity-limited, working memory resource versus those that do not. Evans and Stanovich ( 2013 ) updated their definition based on new behavioral and neuroscience evidence stating, “the defining characteristic of Type 1 processes is their autonomy. They do not require ‘controlled attention,’ which is another way of saying that they make minimal demands on working memory resources” (p. 236). There is also debate on how to define the term working memory (Cowan, 2017 ). In line with prior work on decision making with visualizations (Patterson et al., 2014 ), we adopt the definition that working memory consists of multiple components that maintain a limited amount of information (their capacity) for a finite period (Cowan, 2017 ). Contemporary theories of working memory also stress the ability to engage attention in a controlled manner to suppress automatic responses and maintain the most task-relevant information with limited capacity (Engle, Kane, & Tuholski, 1999 ; Kane, Bleckley, Conway, & Engle, 2001 ; Shipstead, Harrison, & Engle, 2015 ).

Coronary care unit decision tree, which illustrates a sequence of rules that a doctor could use to guide treatment decisions. Redrawn from “Heuristic decision making in medicine” by J. Marewski, and G. Gigerenzer 2012, Dialogues in clinical neuroscience, 14(1) , 77. ST-segment change refers to if certain anomaly appears in the patient’s electrocardiogram. NTG nitroglycerin, MI myocardial infarction, T T-waves with peaking or inversion
Identifying processes that require significant working memory provides a definition of Type 2 processing with observable neural correlates. Therefore, in line with Evans and Stanovich ( 2013 ), in the remainder of this manuscript, we will use significant working memory capacity demands and significant need for cognitive control, as defined above, as the criterion for Type 2 processing. In the context of visualization decision making, processes that require significant working memory are those that depend on the deliberate application of working memory to function. Type 1 processing occurs outside of users’ conscious awareness and may utilize small amounts of working memory but does not rely on conscious processing in working memory to drive the process. It should be noted that Type 1 and 2 processing are not mutually exclusive and many real-world decisions likely incorporate all processes. This review will attempt to identify tasks in visualization decision making that require significant working memory and capacity (Type 2 processing) and those that rely more heavily on Type 1 processing, as a first step to combining decision theory with visualization cognition.
Visualization cognition
Visualization cognition is a subset of visuospatial reasoning, which involves deriving meaning from external representations of visual information that maintain consistent spatial relations (Tversky, 2005 ). Broadly, two distinct approaches delineate visualization cognition models (Shah, Freedman, & Vekiri, 2005 ). The first approach refers to perceptually focused frameworks which attempt to specify the processes involved in perceiving visual information in displays and make predictions about the speed and efficiency of acquiring information from a visualization (e.g. Hollands & Spence, 1992 ; Lohse, 1993 ; Meyer, 2000 ; Simkin & Hastie, 1987 ). The second approach considers the influence of prior knowledge as well as perception. For example, Cognitive Fit Theory (Vessey, 1991), suggests that the user compares a learned graphic convention (mental schema) to the visual depiction. Visualizations that do not match the mental schema require cognitive transformations to make the visualization and mental representation align. For example, Fig. 2 illustrates a fictional relationship between the population growth of Species X and a predator species. At first glance, it may appear that when the predator species was introduced that the population of Species X dropped. However, after careful observation, you may notice that the higher population values are located lower on the Y-axis, which does not match our mental schema for graphs. With some effort, you can mentally reorder the values on the Y-axis to match your mental schema and then you may notice that the introduction of the predator species actually correlates with growth in the population of Species X. When the viewer is forced to mentally transform the visualization to match their mental schema, processing steps are increased, which may increase errors, time to complete a task, and demand on working memory (Vessey, 1991).

Fictional relationship between the population growth of Species X and a predator species, where the Y-axis ordering does not match standard graphic conventions. Notice that the y-axis is reverse ordered. This figure was inspired by a controversial graphic produced by Christine Chan of Reuters, which showed the relationship between Florida’s “Stand Your Ground” law and firearm murders with the Y-axis reversed ordered (Lallanilla, 2014 )
Pinker ( 1990 ) proposed a cognitive model (see Fig. 3 ), which provides an integrative structure that denotes the distinction between top-down and bottom-up encoding mechanisms in understanding data graphs. Researchers have generalized this model to propose theories of comprehension, learning, and memory with visual information (Hegarty, 2011 ; Kriz & Hegarty, 2007 ; Shah & Freedman, 2011 ). The Pinker ( 1990 ) model suggests that from the visual array , defined as the unprocessed neuronal firing in response to visualizations, bottom-up encoding mechanisms are utilized to construct a visual description , which is the mental encoding of the visual stimulus. Following encoding, viewers mentally search long-term memory for knowledge relevant for interpreting the visualization. This knowledge is proposed to be in the form of a graph schema.

Adapted figure from the Pinker ( 1990 ) model of visualization comprehension, which illustrates each process
Then viewers use a match process, where the graph schema that is the most similar to the visual array is retrieved. When a matching graph schema is found, the schema becomes instantiated . The visualization conventions associated with the graph schema can then help the viewer interpret the visualization ( message assembly process). For example, Fig. Fig.3 3 illustrates comprehension of a bar chart using the Pinker ( 1990 ) model. In this example, the matched graph schema for a bar graph specifies that the dependent variable is on the Y-axis and the independent variable is on the X-axis; the instantiated graph schema incorporates the visual description and this additional information. The conceptual message is the resulting mental representation of the visualization that includes all supplemental information from long-term memory and any mental transformations the viewer may perform on the visualization. Viewers may need to transform their mental representation of the visualization based on their task or conceptual question . In this example, the viewer’s task is to find the average of A and B. To do this, the viewer must interpolate information in the bar chart and update the conceptual message with this additional information. The conceptual question can guide the construction of the mental representation through interrogation , which is the process of seeking out information that is necessary to answer the conceptual question. Top-down encoding mechanisms can influence each of the processes.
The influences of top-down processes are also emphasized in a previous attempt by Patterson et al. ( 2014 ) to extend visualization cognition theories to decision making. The Patterson et al. ( 2014 ) model illustrates how top-down cognitive processing influences encoding, pattern recognition, and working memory, but not decision making or the response. Patterson et al. ( 2014 ) use the multicomponent definition of working memory, proposed by Baddeley and Hitch ( 1974 ) and summarized by Cowan ( 2017 ) as a “multicomponent system that holds information temporarily and mediates its use in ongoing mental activities” (p. 1160). In this conception of working memory, a central executive controls the functions of working memory. The central executive can, among other functions, control attention and hold information in a visuo-spatial temporary store , which is where information can be maintained temporally for decision making without being stored in long-term memory (Baddeley & Hitch, 1974 ).
While incorporating working memory into a visualization decision-making model is valuable, the Patterson et al. ( 2014 ) model leaves some open questions about relationships between components and processes. For example, their model lacks a pathway for working memory to influence decisions based on top-down processing, which is inconsistent with well-established research in decision science (e.g. Gigerenzer & Todd, 1999; Kahneman & Tversky, 1982 ). Additionally, the normal processing pathway, depicted in the Patterson model, is an oversimplification of the interaction between top-down and bottom-up processing that is documented in a large body of literature (e.g. Engel, Fries, & Singer, 2001 ; Mechelli, Price, Friston, & Ishai, 2004 ).
A proposed integrated model of decision making with visualizations
Our proposed model (Fig. 4 ) introduces a dual-process account of decision making (Evans & Stanovich, 2013 ; Gigerenzer & Gaissmaier, 2011 ; Kahneman, 2011 ) into the Pinker ( 1990 ) model of visualization comprehension. A primary addition of our model is the inclusion of working memory, which is utilized to answer the conceptual question and could have a subsequent impact on each stage of the decision-making process, except bottom-up attention. The final stage of our model includes a decision-making process that derives from the conceptual message and informs behavior. In line with a dual-process account (Evans & Stanovich, 2013 ; Gigerenzer & Gaissmaier, 2011 ; Kahneman, 2011 ), the decision step can either be completed with Type 1 processing, which only uses minimal working memory (Evans & Stanovich, 2013 ) or recruit significant working memory, constituting Type 2 processing. Also following Evans and Stanovich ( 2013 ), we argue that people can make a decision with a visualization while using minimal amounts of working memory. We classify this as Type 1 thinking. Lohse ( 1997 ) found that when participants made judgments about budget allocation using profit charts, individuals with less working memory capacity performed equally well compared to those with more working memory capacity, when they only made decisions about three regions (easier task). However, when participants made judgments about nine regions (harder task), individuals with more working memory capacity outperformed those with less working memory capacity. The results of the study reveal that individual differences in working memory capacity only influence performance on complex decision-making tasks (Lohse, 1997 ). Figure 5 (top) illustrates one way that a viewer could make a Type 1 decision about whether the average value of bars A and B is closer to 2 or 2.2. Figure Figure5 5 (top) illustrates how a viewer might make a fast and computationally light decision in which she decides that the middle point between the two bars is closer to the salient tick mark of 2 on the Y-axis and answers 2 (which is incorrect). In contrast, Fig. 5 (bottom) shows a second possible method of solving the same problem by utilizing significant working memory (Type 2 processing). In this example, the viewer has recently learned a strategy to address similar problems, uses working memory to guide a top-down attentional search of the visual array, and identifies the values of A and B. Next, she instantiates a different graph schema than in the prior example by utilizing working memory and completes an effortful mental computation of 2.4 + 1.9/2. Ultimately, the application of working memory leads to a different and more effortful decision than in Fig. Fig.5 5 (top). This example illustrates how significant amounts of working memory can be used at early stages of the decision-making process and produce downstream effects and more considered responses. In the following sections, we provide a selective review of work on decision making with visualizations that demonstrates direct and indirect evidence for our proposed model.

Model of visualization decision making, which emphasizes the influence of working memory. Long-term memory can influence all components and processes in the model either via pre-attentive processes or by conscious application of knowledge

Examples of a fast Type 1 (top) and slow Type 2 (bottom) decision outlined in our proposed model of decision making with visualizations. In these examples, the viewer’s task is to decide if the average value of bars A and B are closer to 2 or 2.2. The thick dotted line denotes significant working memory and the thin dotted line negligible working memory
Empirical studies of visualization decision making
Review method.
To determine if there is cross-domain empirical support for a dual-process account of decision making with visualizations, we selectively reviewed studies of complex decision making with computer-generated two-dimensional (2D) static visualizations. To illustrate the application of a dual-process account of decision making to visualization research, this review highlights representative studies from diverse application areas. Interdisciplinary groups conducted many of these studies and, as such, it is not accurate to classify the studies in a single discipline. However, to help the reader evaluate the cross-domain nature of these findings, Table 1 includes the application area for the specific tasks used in each study.
Application area for the tasks used in the reviewed studies
In reviewing this work, we observed four key cross-domain findings that support a dual-process account of decision making (see Table 2 ). The first two support the inclusion of Type 1 processing, which is illustrated by the direct path for bottom-up attention to guide decision making with the minimal application of working memory (see Fig. Fig.5 5 top). The first finding is that visualizations direct viewers’ bottom-up attention , which can both help and hinder decision making (see “ Bottom-up attention ”). The second finding is that visual-spatial biases comprise a unique category of bias that is a direct result of the visual encoding technique (see “ Visual-Spatial Biases ”). The third finding supports the inclusion of Type 2 processing in our proposed model and suggests that visualizations vary in cognitive fit between the visual description, graph schema, and conceptual question. If the fit is poor (i.e. there is a mismatch between the visualization and a decision-making component), working memory is used to perform corrective mental transformations (see “ Cognitive fit ”). The final cross-domain finding proposes that knowledge-driven processes may interact with the effects of the visual encoding technique (see “ Knowledge-driven processing ”) and could be a function of either Type 1 or 2 processes. Each of these findings will be detailed at length in the relevant sections. The four cross-domain findings do not represent an exhaustive list of all cross-domain findings that pertain to visualization cognition. However, these were selected as illustrative examples of Type 1 and 2 processing that include significant contributions from multiple domains. Further, some of the studies could fit into multiple sections and were included in a particular section as illustrative examples.
Overview of the four cross-domain findings along with the type of processing that they reflect
The italicised words correspond to section titles
Bottom-up attention
The first cross-domain finding that characterizes Type 1 processing in visualization decision making is that visualizations direct participants’ bottom-up attention to specific visual features, which can be either beneficial or detrimental to decision making. Bottom-up attention consists of involuntary shifts in focus to salient features of a visualization and does not utilize working memory (Connor, Egeth, & Yantis, 2004 ), therefore it is a Type 1 process. The research reviewed in this section illustrates that bottom-up attention has a profound influence on decision making with visualizations. A summary of visual features that studies have used to attract bottom-up attention can be found in Table 3 .
Visual features used in the reviewed studies to attract bottom-up attention
Numerous studies show that salient information in a visualization draws viewers’ attention (Fabrikant, Hespanha, & Hegarty, 2010 ; Hegarty, Canham, & Fabrikant, 2010 ; Hegarty, Friedman, Boone, & Barrett, 2016 ; Padilla, Ruginski, & Creem-Regehr, 2017 ; Schirillo & Stone, 2005 ; Stone et al., 2003 ; Stone, Yates, & Parker, 1997 ). The most common methods for demonstrating that visualizations focus viewers’ attention is by showing that viewers miss non-salient but task-relevant information (Schirillo & Stone, 2005 ; Stone et al., 1997 ; Stone et al., 2003 ), viewers are biased by salient information (Hegarty et al., 2016 ; Padilla, Ruginski et al., 2017 ) or viewers spend more time looking at salient information in a visualization (Fabrikant et al., 2010 ; Hegarty et al., 2010 ). For example, Stone et al. ( 1997 ) demonstrated that when viewers are asked how much they would pay for an improved product using the visualizations in Fig. 6 , they focus on the number of icons while missing the base rate of 5,000,000. If a viewer simply totals the icons, the standard product appears to be twice as dangerous as the improved product, but because the base rate is large, the actual difference between the two products is insignificantly small (0.0000003; Stone et al., 1997 ). In one experiment, participants were willing to pay $125 more for improved tires when viewing the visualizations in Fig. Fig.6 6 compared to a purely textual representation of the information. The authors also demonstrated the same effect for improved toothpaste, with participants paying $0.95 more when viewing a visual depiction compared to text. The authors’ term this heuristic of focusing on salient information and ignoring other data the foreground effect (Stone et al., 1997 ) (see also Schirillo & Stone, 2005 ; Stone et al., 2003 ).

Icon arrays used to illustrate the risk of standard or improved tires. Participants were tasked with deciding how much they would pay for the improved tires. Note the base rate of 5 M drivers was represented in text. Redrawn from “Effects of numerical and graphical displays on professed risk-taking behavior” by E. R. Stone, J. F. Yates, & A. M. Parker. 1997, Journal of Experimental Psychology: Applied , 3 (4), 243
A more direct test of visualizations guiding bottom-up attention is to examine if salient information biases viewers’ judgments. One method involves identifying salient features using a behaviorally validated saliency model, which predicts the locations that will attract viewers’ bottom-up attention (Harel, 2015 ; Itti, Koch, & Niebur, 1998 ; Rosenholtz & Jin, 2005 ). In one study, researchers compared participants’ judgments with different hurricane forecast visualizations and then, using the Itti et al. ( 1998 ) saliency algorithm, found that the differences in what was salient in the two visualizations correlated with participants’ performance (Padilla, Ruginski et al., 2017 ). Specifically, they suggested that the salient borders of the Cone of Uncertainty (see Fig. 7 , left), which is used by the National Hurricane Center to display hurricane track forecasts, leads some people to incorrectly believe that the hurricane is growing in physical size, which is a misunderstanding of the probability distribution of hurricane paths that the cone is intended to represent (Padilla, Ruginski et al., 2017 ; see also Ruginski et al., 2016 ). Further, they found that when the same data were represented as individual hurricane paths, such that there was no salient boundary (see Fig. Fig.7, 7 , right), viewers intuited the probability of hurricane paths more effectively than the Cone of Uncertainty. However, an individual hurricane path biased viewers’ judgments if it intersected a point of interest. For example, in Fig. Fig.7 7 (right), participants accurately judged that locations closer to the densely populated lines (highest likelihood of storm path) would receive more damage. This correct judgment changed when a location farther from the center of the storm was intersected by a path, but the closer location was not (see locations a and b in Fig. Fig.7 7 right). With both visualizations, the researchers found that viewers were negatively biased by the salient features for some tasks (Padilla, Ruginski et al., 2017 ; Ruginski et al., 2016 ).

An example of the Cone of Uncertainty ( left ) and the same data represented as hurricane paths ( right ). Participants were tasked with evaluating the level of damage that would incur to offshore oil rigs at specific locations, based on the hurricane forecast visualization. Redrawn from “Effects of ensemble and summary displays on interpretations of geospatial uncertainty data” by L. M. Padilla, I. Ruginski, and S. H. Creem-Regehr. 2017, Cognitive Research: Principles and Implications , 2 (1), 40
That is not to say that saliency only negatively impacts decisions. When incorporated into visualization design, saliency can guide bottom-up attention to task-relevant information, thereby improving performance (e.g. Fabrikant et al., 2010 ; Fagerlin, Wang, & Ubel, 2005 ; Hegarty et al., 2010 ; Schirillo & Stone, 2005 ; Stone et al., 2003 ; Waters, Weinstein, Colditz, & Emmons, 2007 ). One compelling example using both eye-tracking measures and a saliency algorithm demonstrated that salient features of weather maps directed viewers’ attention to different variables that were visualized on the maps (Hegarty et al., 2010 ) (see also Fabrikant et al., 2010 ). Interestingly, when the researchers manipulated the relative salience of temperature versus pressure (see Fig. 8 ), the salient features captured viewers’ overt attention (as measured by eye fixations) but did not influence performance, until participants were trained on how to effectively interpret the features. Once viewers were trained, their judgments were facilitated when the relevant features were more salient (Hegarty et al., 2010 ). This is an instructive example of how saliency may direct viewers’ bottom-up attention but may not influence their performance until viewers have the relevant top-down knowledge to capitalize on the affordances of the visualization.

Eye-tracking data from Hegarty et al. ( 2010 ). Participants viewed an arrow located in Utah (obscured by eye-tracking data in the figure) and made judgments about whether the arrow correctly identified the wind direction. The black isobars were the task-relevant information. Notice that after instructions, viewers with the pressure-salient visualizations focused on the isobars surrounding Utah, rather than on the legend or in other regions. The panels correspond to the conditions in the original study
In sum, the reviewed studies suggest that bottom-up attention has a profound influence on decision making with visualizations. This is noteworthy because bottom-up attention is a Type 1 process. At a minimum, the work suggests that Type 1 processing influences the first stages of decision making with visualizations. Further, the studies cited in this section provide support for the inclusion of bottom-up attention in our proposed model.
Visual-spatial biases
A second cross-domain finding that relates to Type 1 processing is that visualizations can give rise to visual-spatial biases that can be either beneficial or detrimental to decision making. We are proposing the new concept of visual-spatial biases and defining this term as a bias that elicits heuristics, which are a direct result of the visual encoding technique. Visual-spatial biases likely originate as a Type 1 process as we suspect they are connected to bottom-up attention, and if detrimental to decision making, have to be actively suppressed by top-down knowledge and cognitive control mechanisms (see Table 4 for summary of biases documented in this section). Visual-spatial biases can also improve decision-making performance. As Card, Mackinlay, and Shneiderman ( 1999 ) point out, we can use vision to think , meaning that visualizations can capitalize on visual perception to interpret a visualization without effort when the visual biases elucidated by the visualization are consistent with the correct interpretation.
Biases documented in the reviewed studies
Tversky ( 2011 ) presents a taxonomy of visual-spatial communications that are intrinsically related to thought, which are likely the bases for visual-spatial biases (see also Fabrikant & Skupin, 2005 ). One of the most commonly documented visual-spatial biases that we observed across domains is a containment conceptualization of boundary representations in visualizations. Tversky ( 2011 ) makes the analogy, “Framing a picture is a way of saying that what is inside the picture has a different status from what is outside the picture” (p. 522). Similarly, Fabrikant and Skupin ( 2005 ) describe how, “They [boundaries] help partition an information space into zones of relative semantic homogeneity” (p. 673). However, in visualization design, it is common to take continuous data and visually represent them with boundaries (i.e. summary statistics, error bars, isocontours, or regions of interest; Padilla et al., 2015 ; Padilla, Quinan, Meyer, & Creem-Regehr, 2017 ). Binning continuous data is a reasonable approach, particularly when intended to make the data simpler for viewers to understand (Padilla, Quinan, et al., 2017 ). However, it may have the unintended consequence of creating artificial boundaries that can bias users—leading them to respond as if data within a containment is more similar than data across boundaries. For example, McKenzie, Hegarty, Barrett, and Goodchild ( 2016 ) showed that participants were more likely to use a containment heuristic to make decisions about Google Map’s blue dot visualization when the positional uncertainty data were visualized as a bounded circle (Fig. 9 right) compared to a Gaussian fade (Fig. (Fig.9 9 left) (see also Newman & Scholl, 2012 ; Ruginski et al., 2016 ). Recent work by Grounds, Joslyn, and Otsuka ( 2017 ) found that viewers demonstrate a “deterministic construal error” or the belief that visualizations of temperature uncertainty represent a deterministic forecast. However, the deterministic construal error was not observed with textual representations of the same data (see also Joslyn & LeClerc, 2013 ).

Example stimuli from McKenzie et al. ( 2016 ) showing circular semi-transparent overlays used by Google Maps to indicate the uncertainty of the users’ location. Participants compared two versions of these visualizations and determined which represented the most accurate positional location. Redrawn from “Assessing the effectiveness of different visualizations for judgments of positional uncertainty” by G. McKenzie, M. Hegarty, T. Barrett, and M. Goodchild. 2016, International Journal of Geographical Information Science , 30 (2), 221–239
Additionally, some visual-spatial biases follow the same principles as more well-known decision-making biases revealed by researchers in behavioral economics and decision science. In fact, some decision-making biases, such as anchoring , the tendency to use the first data point to make relative judgments, seem to have visual correlates (Belia, Fidler, Williams, & Cumming, 2005 ). For example, Belia et al. ( 2005 ) asked experts with experience in statistics to align two means (representing “Group 1” and “Group 2”) with error bars so that they represented data ranges that were just significantly different (see Fig. 10 for example of stimuli). They found that when the starting position of Group 2 was around 800 ms, participants placed Group 2 higher than when the starting position for Group 2 was at around 300 ms. This work demonstrates that participants used the starting mean of Group 2 as an anchor or starting point of reference, even though the starting position was arbitrary. Other work finds that visualizations can be used to reduce some decision-making biases including anecdotal evidence bias (Fagerlin et al., 2005 ), side effect aversion (Waters et al., 2007 ; Waters, Weinstein, Colditz, & Emmons, 2006 ), and risk aversion (Schirillo & Stone, 2005 ).

Example display and instructions from Belia et al. ( 2005 ). Redrawn from “Researchers misunderstand confidence intervals and standard error bars” by S. Belia, F. Fidler, J. Williams, and G. Cumming. 2005, Psychological Methods, 10 (4), 390. Copyright 2005 by “American Psychological Association”
Additionally, the mere presence of a visualization may inherently bias viewers. For example, viewers find scientific articles with high-quality neuroimaging figures to have greater scientific reasoning than the same article with a bar chart or without a figure (McCabe & Castel, 2008 ). People tend to unconsciously believe that high-quality scientific images reflect high-quality science—as illustrated by work from Keehner, Mayberry, and Fischer ( 2011 ) showing that viewers rate articles with three-dimensional brain images as more scientific than those with 2D images, schematic drawings, or diagrams (See Fig. 11 ). Unintuitively, however, high-quality complex images can be detrimental to performance compared to simpler visualizations (Hegarty, Smallman, & Stull, 2012 ; St. John, Cowen, Smallman, & Oonk, 2001 ; Wilkening & Fabrikant, 2011 ). Hegarty et al. ( 2012 ) demonstrated that novice users prefer realistically depicted maps (see Fig. 12 ), even though these maps increased the time taken to complete the task and focused participants’ attention on irrelevant information (Ancker, Senathirajah, Kukafka, & Starren, 2006 ; Brügger, Fabrikant, & Çöltekin, 2017 ; St. John et al., 2001 ; Wainer, Hambleton, & Meara, 1999 ; Wilkening & Fabrikant, 2011 ). Interestingly, professional meteorologists also demonstrated the same biases as novice viewers (Hegarty et al., 2012 ) (see also Nadav-Greenberg, Joslyn, & Taing, 2008 ).

Image showing participants’ ratings of three-dimensionality and scientific credibility for a given neuroimaging visualization, originally published in grayscale (Keehner et al., 2011 )

Example stimuli from Hegarty et al. ( 2012 ) showing maps with varying levels of realism. Both novice viewers and meteorologists were tasked with selecting a visualization to use and performing a geospatial task. The panels correspond to the conditions in the original study
We argue that visual-spatial biases reflect a Type 1 process, occurring automatically with minimal working memory. Work by Sanchez and Wiley ( 2006 ) provides direct evidence for this assertion using eye-tracking data to demonstrate that individuals with less working memory capacity attend to irrelevant images in a scientific article more than those with greater working memory capacity. The authors argue that we are naturally drawn to images (particularly high-quality depictions) and that significant working memory capacity is required to shift focus away from images that are task-irrelevant. The ease by which visualizations captivate our focus and direct our bottom-up attention to specific features likely increases the impact of these biases, which may be why some visual-spatial biases are notoriously difficult to override using working memory capacity (see Belia et al., 2005 ; Boone, Gunalp, & Hegarty, in press ; Joslyn & LeClerc, 2013 ; Newman & Scholl, 2012 ). We speculate that some visual-spatial biases are intertwined with bottom-up attention—occurring early in the decision-making process and influencing the down-stream processes (see our model in Fig. Fig.4 4 for reference), making them particularly unremitting.
Cognitive fit
We also observe a cross-domain finding involving Type 2 processing, which suggests that if there is a mismatch between the visualization and a decision-making component, working memory is used to perform corrective mental transformations. Cognitive fit is a term used to describe the correspondence between the visualization and conceptual question or task (see our model for reference; for an overview of cognitive fit, see Vessey, Zhang, & Galletta, 2006 ). Those interested in examining cognitive fit generally attempt to identify and reduce mismatches between the visualization and one of the decision-making components (see Table 5 for a breakdown of the decision-making components that the reviewed studies evaluated). When there is a mismatch produced by the default Type 1 processing, it is argued that significant working memory (Type 2 processing) is required to resolve the discrepancy via mental transformations (Vessey et al., 2006 ). As working memory is capacity limited, the magnitude of mental transformation or amount of working memory required is one predictor of reaction times and errors.
Decision-making components that the reviewed studies evaluated the cognitive fit of
Direct evidence for this claim comes from work demonstrating that cognitive fit differentially influenced the performance of individuals with more and less working memory capacity (Zhu & Watts, 2010 ). The task was to identify which two nodes in a social media network diagram should be removed to disconnect the maximal number of nodes. As predicted by cognitive fit theory, when the visualization did not facilitate the task (Fig. 13 left), participants with less working memory capacity were slower than those with more working memory capacity. However, when the visualization aligned with the task (Fig. 13 right), there was no difference in performance. This work suggests that when there is misalignment between the visualization and a decision-making process, people with more working memory capacity have the resources to resolve the conflict, while those with less resources show performance degradations. 2 Other work only found a modest relationship between working memory capacity and correct interpretations of high and low temperature forecast visualizations (Grounds et al., 2017 ), which suggests that, for some visualizations, viewers utilize little working memory.

Examples of social media network diagrams from Zhu and Watts ( 2010 ). The authors argue that the figure on the right is more aligned with the task of identifying the most interconnected nodes than the figure on the left
As illustrated in our model, working memory can be recruited to aid all stages of the decision-making process except bottom-up attention. Work that examines cognitive fit theory provides indirect evidence that working memory is required to resolve conflicts in the schema matching and a decision-making component. For example, one way that a mismatch between a viewer’s mental schema and visualization can arise is when the viewer uses a schema that is not optimal for the task. Tversky, Corter, Yu, Mason, and Nickerson ( 2012 ) primed participants to use different schemas by describing the connections in Fig. 14 in terms of either transfer speed or security levels. Participants then decided on the most efficient or secure route for information to travel between computer nodes with either a visualization that encoded data using the thickness of connections, containment, or physical distance (see Fig. 14 ). Tversky et al. ( 2012 ) found that when the links were described based on their information transfer speed, thickness and distance visualizations were the most effective—suggesting that the speed mental schema was most closely matched to the thickness and distance visualizations, whereas the speed schema required mental transformations to align with the containment visualization. Similarly, the thickness and containment visualizations outperformed the distance visualization when the nodes were described as belonging to specific systems with different security levels. This work and others (Feeney, Hola, Liversedge, Findlay, & Metcalf, 2000 ; Gattis & Holyoak, 1996 ; Joslyn & LeClerc, 2013 ; Smelcer & Carmel, 1997 ) provides indirect evidence that gratuitous realignment between mental schema and the visualization can be error-prone and visualization designers should work to reduce the number of transformations required in the decision-making process.

Example of stimuli from Tversky et al. ( 2012 ) showing three types of encoding techniques for connections between nodes (thickness, containment, and distance). Participants were asked to select routes between nodes with different descriptions of the visualizations. Redrawn from “Representing category and continuum: Visualizing thought” by B. Tversky, J. Corter, L. Yu, D. Mason, and J. Nickerson. In Diagrams 2012 (p. 27), P. Cox, P. Rodgers, and B. Plimmer (Eds.), 2012, Berlin Heidelberg: Springer-Verlag
Researchers from multiple domains have also documented cases of misalignment between the task, or conceptual question, and the visualization. For example, Vessey and Galletta ( 1991 ) found that participants completed a financial-based task faster when the visualization they chose (graph or table, see Fig. 15 ) matched the task (spatial or textual). For the spatial task, participants decided which month had the greatest difference between deposits and withdrawals. The textual or symbolic tasks involved reporting specific deposit and withdrawal amounts for various months. The authors argued that when there is a mismatch between the task and visualization, the additional transformation accounts for the increased time taken to complete the task (Vessey & Galletta, 1991 ) (see also Dennis & Carte, 1998 ; Huang et al., 2006 ), which likely takes place in the inference process of our proposed model.

Examples of stimuli from Vessey and Galletta ( 1991 ) depicting deposits and withdraw amounts over the course of a year with a graph ( a ) and table ( b ). Participants completed either a spatial or textual task with the chart or table. Redrawn from “Cognitive fit: An empirical study of information acquisition” by I. Vessey, and D. Galletta. 1991, Information systems research, 2 (1), 72–73. Copyright 1991 by “INFORMS”
The aforementioned studies provide direct (Zhu & Watts, 2010 ) and indirect (Dennis & Carte, 1998 ; Feeney et al., 2000 ; Gattis & Holyoak, 1996 ; Huang et al., 2006 ; Joslyn & LeClerc, 2013 ; Smelcer & Carmel, 1997 ; Tversky et al., 2012 ; Vessey & Galletta, 1991 ) evidence that Type 2 processing recruits working memory to resolve misalignment between decision-making processes and the visualization that arise from default Type 1 processing. These examples of Type 2 processing using working memory to perform effortful mental computations are consistent with the assertions of Evans and Stanovich ( 2013 ) that Type 2 processes enact goal directed complex processing. However, it is not clear from the reviewed work how exactly the visualization and decision-making components are matched. Newman and Scholl ( 2012 ) propose that we match the schema and visualization based on the similarities between the salient visual features, although this proposal has not been tested. Further, work that assesses cognitive fit in terms of the visualization and task only examines the alignment of broad categories (i.e., spatial or semantic). Beyond these broad classifications, it is not clear how to predict if a task and visualization are aligned. In sum, there is not a sufficient cross-disciplinary theory for how mental schemas and tasks are matched to visualizations. However, it is apparent from the reviewed work that Type 2 processes (requiring working memory) can be recruited during the schema matching and inference processes.
Either type 1 and/or 2
Knowledge-driven processing.
In a review of map-reading cognition, Lobben ( 2004 ) states, “…research should focus not only on the needs of the map reader but also on their map-reading skills and abilities” (p. 271). In line with this statement, the final cross-domain finding is that the effects of knowledge can interact with the affordances or biases inherent in the visualization method. Knowledge may be held temporally in working memory (Type 2), held in long-term knowledge but effortfully used (Type 2), or held in long-term knowledge but automatically applied (Type 1). As a result, knowledge-driven processing can involve either Type 1 or Type 2 processes.
Both short- and long-term knowledge can influence visualization affordances and biases. However, it is difficult to distinguish whether Type 2 processing is using significant working memory capacity to temporarily hold knowledge or if participants have stored the relevant knowledge in long-term memory and processing is more automatic. Complicating the issue, knowledge stored in long-term memory can influence decision making with visualizations using both Type 1 and 2 processing. For example, if you try to remember Pythagorean’s Theorem, which you may have learned in high school or middle school, you may recall that a 2 + b 2 = c 2 , where c represents the length of the hypotenuse and a and b represent the lengths of the other two sides of a triangle. Unless you use geometry regularly, you likely had to strenuously search in long-term memory for the equation, which is a Type 2 process and requires significant working memory capacity. In contrast, if you are asked to recall your childhood phone number, the number might automatically come to mind with minimal working memory required (Type 1 processing).
In this section, we highlight cases where knowledge either influenced decision making with visualizations or was present but did not influence decisions (see Table 6 for the type of knowledge examined in each study). These studies are organized based on how much time the viewers had to incorporate the knowledge (i.e. short-term instructions and long-term individual differences in abilities and expertise), which may be indicative of where the knowledge is stored. However, many factors other than time influence the process of transferring knowledge by working memory capacity to long-term knowledge. Therefore, each of the studies cited in this section could be either Type 1, Type 2, or both types of processing.
Type of knowledge examined in each study
One example of participants using short-term knowledge to override a familiarity bias comes from work by Bailey, Carswell, Grant, and Basham ( 2007 ) (see also Shen, Carswell, Santhanam, & Bailey, 2012 ). In a complex geospatial task for which participants made judgments about terrorism threats, participants were more likely to select familiar map-like visualizations rather than ones that would be optimal for the task (see Fig. 16 ) (Bailey et al., 2007 ). Using the same task and visualizations, Shen et al. ( 2012 ) showed that users were more likely to choose an efficacious visualization when given training concerning the importance of cognitive fit and effective visualization techniques. In this case, viewers were able to use knowledge-driven processing to improve their performance. However, Joslyn and LeClerc ( 2013 ) found that when participants viewed temperature uncertainty, visualized as error bars around a mean temperature prediction, they incorrectly believed that the error bars represented high and low temperatures. Surprisingly, participants maintained this belief despite a key, which detailed the correct way to interpret each temperature forecast (see also Boone et al., in press ). The authors speculated that the error bars might have matched viewers’ mental schema for high- and low-temperature forecasts (stored in long-term memory) and they incorrectly utilized the high-/low-temperature schema rather than incorporating new information from the key. Additionally, the authors propose that because the error bars were visually represented as discrete values, that viewers may have had difficulty reimagining the error bars as points on a distribution, which they term a deterministic construal error (Joslyn & LeClerc, 2013 ). Deterministic construal visual-spatial biases may also be one of the sources of misunderstanding of the Cone of Uncertainty (Padilla, Ruginski et al., 2017 ; Ruginski et al., 2016 ). A notable difference between these studies and the work of Shen et al. ( 2012 ) is that Shen et al. ( 2012 ) used instructions to correct a familiarity bias, which is a cognitive bias originally documented in the decision-making literature that is not based on the visual elements in the display. In contrast, the biases in Joslyn and LeClerc ( 2013 ) were visual-spatial biases. This provides further evidence that visual-spatial biases may be a unique category of biases that warrant dedicated exploration, as they are harder to influence with knowledge-driven processing.

Example of different types of view orientations used by examined by Bailey et al. ( 2007 ). Participants selected one of these visualizations and then used their selection to make judgments including identifying safe passageways, determining appropriate locations for firefighters, and identifying suspicious locations based on the height of buildings. The panels correspond to the conditions in the original study
Regarding longer-term knowledge, there is substantial evidence that individual differences in knowledge impact decision making with visualizations. For example, numerous studies document the benefit of visualizations for individuals with less health literacy, graph literacy, and numeracy (Galesic & Garcia-Retamero, 2011 ; Galesic, Garcia-Retamero, & Gigerenzer, 2009 ; Keller, Siegrist, & Visschers, 2009 ; Okan, Galesic, & Garcia-Retamero, 2015 ; Okan, Garcia-Retamero, Cokely, & Maldonado, 2012 ; Okan, Garcia-Retamero, Galesic, & Cokely, 2012 ; Reyna, Nelson, Han, & Dieckmann, 2009 ; Rodríguez et al., 2013 ). Visual depictions of health data are particularly useful because health data often take the form of probabilities, which are unintuitive. Visualizations inherently illustrate probabilities (i.e. 10%) as natural frequencies (i.e. 10 out of 100), which are more intuitive (Hoffrage & Gigerenzer, 1998 ). Further, by depicting natural frequencies visually (see example in Fig. 17 ), viewers can make perceptual comparisons rather than mathematical calculations. This dual benefit is likely the reason visualizations produce facilitation for individuals with less health literacy, graph literacy, and numeracy.

Example of stimuli used by Galesic et al. ( 2009 ) in a study demonstrating that natural frequency visualizations can help individuals overcome less numeracy. Participants completed three medical scenario tasks using similar visualizations as depicted here, in which they were asked about the effects of aspirin on risk of stroke or heart attack and about a hypothetical new drug. Redrawn from “Using icon arrays to communicate medical risks: overcoming less numeracy” by M. Galesic, R. Garcia-Retamero, and G. Gigerenzer. 2009, Health Psychology, 28 (2), 210
These studies are good examples of how designers can create visualizations that capitalize on Type 1 processing to help viewers accurately make decisions with complex data even when they lack relevant knowledge. Based on the reviewed work, we speculate that well-designed visualizations that utilize Type 1 processing to intuitively illustrate task-relevant relationships in the data may be particularly beneficial for individuals with less numeracy and graph literacy, even for simple tasks. However, poorly designed visualizations that require superfluous mental transformations may be detrimental to the same individuals. Further, individual differences in expertise, such as graph literacy, which have received more attention in healthcare communication (Galesic & Garcia-Retamero, 2011 ; Nayak et al., 2016 ; Okan et al., 2015 ; Okan, Garcia-Retamero, Cokely, & Maldonado, 2012 ; Okan, Garcia-Retamero, Galesic, & Cokely, 2012 ; Rodríguez et al., 2013 ), may play a large role in how viewers complete even simple tasks in other domains such as map-reading (Kinkeldey et al., 2017 ).
Less consistent are findings on how more experienced users incorporate knowledge acquired over longer periods of time to make decisions with visualizations. Some research finds that students’ decision-making and spatial abilities improved during a semester-long course on Geographic Information Science (GIS) (Lee & Bednarz, 2009 ). Other work finds that experts perform the same as novices (Riveiro, 2016 ), experts can exhibit visual-spatial biases (St. John et al., 2001 ) and experts perform more poorly than expected in their domain of visual expertise (Belia et al., 2005 ). This inconsistency may be due in part to the difficulty in identifying when and if more experienced viewers are automatically applying their knowledge or employing working memory. For example, it is unclear if the students in the GIS course documented by Lee and Bednarz ( 2009 ) developed automatic responses (Type 1) or if they learned the information and used working memory capacity to apply their training (Type 2).
Cheong et al. ( 2016 ) offer one way to gauge how performance may change when one is forced to use Type 1 processing, but then allowed to use Type 2 processing. In a wildfire task using multiple depictions of uncertainty (see Fig. 18 ), Cheong et al. ( 2016 ) found that the type of uncertainty visualization mattered when participants had to make fast Type 1 decisions (5 s) about evacuating from a wildfire. But when given sufficient time to make Type 2 decisions (30 s), participants were not influenced by the visualization technique (see also Wilkening & Fabrikant, 2011 ).

Example of multiple uncertainty visualization techniques for wildfire risk by Cheong et al. ( 2016 ). Participants were presented with a house location (indicated by an X), and asked if they would stay or leave based on one of the wildfire hazard communication techniques shown here. The panels correspond to the conditions in the original study
Interesting future work could limit experts’ time to complete a task (forcing Type 1 processing) and then determine if their judgments change when given more time to complete the task (allowing for Type 2 processing). To test this possibility further, a dual-task paradigm could be used such that experts’ working memory capacity is depleted by a difficult secondary task that also required working memory capacity. Some examples of secondary tasks in a dual-task paradigm include span tasks that require participants to remember or follow patterns of information, while completing the primary task, then report the remembered or relevant information from the pattern (for a full description of theoretical bases for a dual-task paradigm see Pashler, 1994 ). To our knowledge, only one study has used a dual-task paradigm to evaluate cognitive load of a visualization decision-making task (Bandlow et al., 2011 ). However, a growing body of research on other domains, such as wayfinding and spatial cognition, demonstrates the utility of using dual-task paradigms to understand the types of working memory that users employ for a task (Caffò, Picucci, Di Masi, & Bosco, 2011 ; Meilinger, Knauff, & Bülthoff, 2008 ; Ratliff & Newcombe, 2005 ; Trueswell & Papafragou, 2010 ).
Span tasks are examples of spatial or verbal secondary tasks, which include remembering the orientations of an arrow (taxes visual-spatial memory, (Shah & Miyake, 1996 ) or counting backward by 3 s (taxes verbal processing and short-term memory) (Castro, Strayer, Matzke, & Heathcote, 2018 ). One should expect more interference if the primary and secondary tasks recruit the same processes (i.e. visual-spatial primary task paired with a visual-spatial memory span task). An example of such an experimental design is illustrated in Fig. 19 . In the dual-task trial illustrated in Fig. 19 , if participants responses are as fast and accurate as the baseline trial then participants are likely not using significant amounts of working memory capacity for that task. If the task does require significant working memory capacity, then the inclusion of the secondary task should increase the time taken to complete the primary task and potentially produce errors in both the secondary and primary tasks. In visualization decision-making research, this is an open area of exploration for researchers and designers that are interested in understanding how working memory capacity and a dual-process account of decision making applies to their visualizations and application domains.

A diagram of a dual-tasking experiment is shown using the same task as in Fig. Fig.5. 5 . Responses resulting from Type 1 and 2 processing are illustrated. The dual-task trial illustrates how to place additional load on working memory capacity by having the participant perform a demanding secondary task. The impact of the secondary task is illustrated for both time and accuracy. Long-term memory can influence all components and processes in the model either via pre-attentive processes or by conscious application of knowledge
In sum, this section documents cases where knowledge-driven processing does and does not influence decision making with visualizations. Notably, we describe numerous studies where well-designed visualizations (capitalizing on Type 1 processing) focus viewers’ attention on task-relevant relationships in the data, which improves decision accuracy for individuals with less developed health literacy, graph literacy, and numeracy. However, the current work does not test how knowledge-driven processing maps on to the dual-process model of decision making. Knowledge may be held temporally by working memory capacity (Type 2), held in long-term knowledge but strenuously utilized (Type 2), or held in long-term knowledge but automatically applied (Type 1). More work is needed to understand if a dual-process account of decision making accurately describes the influence of knowledge-driven processing on decision making with visualizations. Finally, we detailed an example of a dual-task paradigm as one way to evaluate if viewers are employing Type 1 processing.
Review summary
Throughout this review, we have provided significant direct and indirect evidence that a dual-process account of decision making effectively describes prior findings from numerous domains interested in visualization decision making. The reviewed work provides support for specific processes in our proposed model including the influences of working memory, bottom-up attention, schema matching, inference processes, and decision making. Further, we identified key commonalities in the reviewed work relating to Type 1 and Type 2 processing, which we added to our proposed visualization decision-making model. The first is that utilizing Type 1 processing, visualizations serve to direct participants’ bottom-up attention to specific information, which can be either beneficial or detrimental for decision making (Fabrikant et al., 2010 ; Fagerlin et al., 2005 ; Hegarty et al., 2010 ; Hegarty et al., 2016 ; Padilla, Ruginski et al., 2017 ; Ruginski et al., 2016 ; Schirillo & Stone, 2005 ; Stone et al., 1997 ; Stone et al., 2003 ; Waters et al., 2007 ). Consistent with assertions from cognitive science and scientific visualization (Munzner, 2014 ), we propose that visualization designers should identify the critical information needed for a task and use a visual encoding technique that directs participants’ attention to this information. We encourage visualization designers who are interested in determining which elements in their visualizations will likely attract viewers’ bottom-up attention, to see the Itti et al. ( 1998 ) saliency model, which has been validated with eye-tracking measures (for implementation of this model along with Matlab code see Padilla, Ruginski et al., 2017 ). If deliberate effort is not made to capitalize on Type 1 processing by focusing the viewer’s attention on task-relevant information, then the viewer will likely focus on distractors via Type 1 processing, resulting in poor decision outcomes.
A second cross-domain finding is the introduction of a new concept, visual-spatial biases , which can also be both beneficial and detrimental to decision making. We define this term as a bias that elicits heuristics, which is a direct result of the visual encoding technique. We provide numerous examples of visual-spatial biases across domains (for implementation of this model along with Matlab code, see Padilla, Ruginski et al., 2017 ). The novel utility of identifying visual-spatial biases is that they potentially arise early in the decision-making process during bottom-up attention, thus influencing the entire downstream process, whereas standard heuristics do not exclusively occur at the first stage of decision making. This possibly accounts for the fact that visual-spatial biases have proven difficult to overcome (Belia et al., 2005 ; Grounds et al., 2017 ; Joslyn & LeClerc, 2013 ; Liu et al., 2016 ; McKenzie et al., 2016 ; Newman & Scholl, 2012 ; Padilla, Ruginski et al., 2017 ; Ruginski et al., 2016 ). Work by Tversky ( 2011 ) presents a taxonomy of visual-spatial communications that are intrinsically related to thought, which are likely the bases for visual-spatial biases.
We have also revealed cross-domain findings involving Type 2 processing, which suggest that if there is a mismatch between the visualization and a decision-making component, working memory is used to perform corrective mental transformations. In scenarios where the visualization is aligned with the mental schema and task, performance is fast and accurate (Joslyn & LeClerc, 2013 ). The types of mismatches observed in the reviewed literature are likely both domain-specific and domain-general. For example, situations where viewers employ the correct graph schema for the visualization, but the graph schema does not align with the task, are likely domain-specific (Dennis & Carte, 1998 ; Frownfelter-Lohrke, 1998 ; Gattis & Holyoak, 1996 ; Huang et al., 2006 ; Joslyn & LeClerc, 2013 ; Smelcer & Carmel, 1997 ; Tversky et al., 2012 ). However, other work demonstrates cases where viewers employ a graph schema that does not match the visualization, which is likely domain-general (e.g. Feeney et al., 2000 ; Gattis & Holyoak, 1996 ; Tversky et al., 2012 ). In these cases, viewers could accidentally use the wrong graph schema because it appears to match the visualization or they might not have learned a relevant schema. The likelihood of viewers making attribution errors because they do not know the corresponding schema increases when the visualization is less common, such as with uncertainty visualizations. When there is a mismatch, additional working memory is required resulting in increased time taken to complete the task and in some cases errors (e.g. Joslyn & LeClerc, 2013 ; McKenzie et al., 2016 ; Padilla, Ruginski et al., 2017 ). Based on these findings, we recommend that visualization designers should aim to create visualizations that most closely align with a viewer’s mental schema and task. However, additional empirical research is required to understand the nature of the alignment processes, including the exact method we use to mentally select a schema and the classifications of tasks that match visualizations.
The final cross-domain finding is that knowledge-driven processes can interact or override effects of visualization methods. We find that short-term (Dennis & Carte, 1998 ; Feeney et al., 2000 ; Gattis & Holyoak, 1996 ; Joslyn & LeClerc, 2013 ; Smelcer & Carmel, 1997 ; Tversky et al., 2012 ) and long-term knowledge acquisition (Shen et al., 2012 ) can influence decision making with visualizations. However, there are also examples of knowledge having little influence on decisions, even when prior knowledge could be used to improve performance (Galesic et al., 2009 ; Galesic & Garcia-Retamero, 2011 ; Keller et al., 2009 ; Lee & Bednarz, 2009 ; Okan et al., 2015 ; Okan, Garcia-Retamero, Cokely, & Maldonado, 2012 ; Okan, Garcia-Retamero, Galesic, & Cokely, 2012 ; Reyna et al., 2009 ; Rodríguez et al., 2013 ). We point out that prior knowledge seems to have more of an effect on non-visual-spatial biases, such as a familiarity bias (Belia et al., 2005 ; Joslyn & LeClerc, 2013 ; Riveiro, 2016 ; St. John et al., 2001 ), which suggests that visual-spatial biases may be closely related to bottom-up attention. Further, it is unclear from the reviewed work when knowledge switches from relying on working memory capacity for application to automatic application. We argue that Type 1 and 2 processing have unique advantages and disadvantages for visualization decision making. Therefore, it is valuable to understand which process users are applying for specific tasks in order to make visualizations that elicit optimal performance. In the case of experts and long-term knowledge, we propose that one interesting way to test if users are utilizing significant working memory capacity is to employ a dual-task paradigm (illustrated in Fig. 19 ). A dual-task paradigm can be used to evaluate the amount of working memory required and compare the relative working memory required between competing visualization techniques.
We have also proposed a variety of practical recommendations for visualization designers based on the empirical findings and our cognitive framework. Below is a summary list of our recommendations along with relevant section numbers for reference:
- Identify the critical information needed for a task and use a visual encoding technique that directs participants’ attention to this information (“ Bottom-up attention ” section);
- To determine which elements in a visualization will likely attract viewers’ bottom-up attention try employing a saliency algorithm (see Padilla, Quinan, et al., 2017 ) (see “ Bottom-up attention ”);
- Aim to create visualizations that most closely align with a viewer’s mental schema and task demands (see “ Visual-Spatial Biases ”);
- Work to reduce the number of transformations required in the decision-making process (see " Cognitive fit ");
- To understand if a viewer is using Type 1 or 2 processing employ a dual-task paradigm (see Fig. 19 );
- Consider evaluating the impact of individual differences such as graphic literacy and numeracy on visualization decision making.
Conclusions
We use visual information to inform many important decisions. To develop visualizations that account for real-life decision making, we must understand how and why we come to conclusions with visual information. We propose a dual-process cognitive framework expanding on visualization comprehension theory that is supported by empirical studies to describe the process of decision making with visualizations. We offer practical recommendations for visualization designers that take into account human decision-making processes. Finally, we propose a new avenue of research focused on the influence of visual-spatial biases on decision making.
This research is based upon work supported by the National Science Foundation under Grants 1212806, 1810498, and 1212577.
Availability of data and materials
Authors’ contributions.
LMP is the primary author of this study; she was central to the development, writing, and conclusions of this work. SHC, MH, and JS contributed to the theoretical development and manuscript preparation. All authors read and approved the final manuscript.
Authors’ information
LMP is a Ph.D. student at the University of Utah in the Cognitive Neural Science department. LMP is a member of the Visual Perception and Spatial Cognition Research Group directed by Sarah Creem-Regehr, Ph.D., Jeanine Stefanucci, Ph.D., and William Thompson, Ph.D. Her work focuses on graphical cognition, decision making with visualizations, and visual perception. She works on large interdisciplinary projects with visualization scientists and anthropologists.
SHC is a Professor in the Psychology Department of the University of Utah. She received her MA and Ph.D. in Psychology from the University of Virginia. Her research serves joint goals of developing theories of perception-action processing mechanisms and applying these theories to relevant real-world problems in order to facilitate observers’ understanding of their spatial environments. In particular, her interests are in space perception, spatial cognition, embodied cognition, and virtual environments. She co-authored the book Visual Perception from a Computer Graphics Perspective ; previously, she was Associate Editor of Psychonomic Bulletin & Review and Experimental Psychology: Human Perception and Performance .
MH is a Professor in the Department of Psychological & Brain Sciences at the University of California, Santa Barbara. She received her Ph.D. in Psychology from Carnegie Mellon University. Her research is concerned with spatial cognition, broadly defined, and includes research on small-scale spatial abilities (e.g. mental rotation and perspective taking), large-scale spatial abilities involved in navigation, comprehension of graphics, and the role of spatial cognition in STEM learning. She served as chair of the governing board of the Cognitive Science Society and is associate editor of Topics in Cognitive Science and past Associate Editor of Journal of Experimental Psychology: Applied .
JS is an Associate Professor in the Psychology Department at the University of Utah. She received her M.A. and Ph.D. in Psychology from the University of Virginia. Her research focuses on better understanding if a person’s bodily states, whether emotional, physiological, or physical, affects their spatial perception and cognition. She conducts this research in natural settings (outdoor or indoor) and in virtual environments. This work is inherently interdisciplinary given it spans research on emotion, health, spatial perception and cognition, and virtual environments. She is on the editorial boards for the Journal of Experimental Psychology: General and Virtual Environments: Frontiers in Robotics and AI . She also co-authored the book Visual Perception from a Computer Graphics Perspective .
Ethics approval and consent to participate
The research reported in this paper was conducted in adherence to the Declaration of Helsinki and received IRB approval from the University of Utah, #IRB_00057678. No human subject data were collected for this work; therefore, no consent to participate was acquired.
Consent for publication
Consent to publish was not required for this review.
Competing interests
The authors declare that they have no competing interests.
Publisher’s Note
Springer Nature remains neutral with regard to jurisdictional claims in published maps and institutional affiliations.
1 Dual-process theory will be described in greater detail in next section.
2 It should be noted that in some cases the activation of Type 2 processing should improve decision accuracy. More research is needed that examines cases where Type 2 could improve decision performance with visualizations.
The original version of this article has been revised. Table 2 was corrected to be presented appropriately.
Change history
The original article (Padilla et al., 2018) contained a formatting error in Table 2; this has now been corrected with the appropriate boxes marked clearly.
Contributor Information
Lace M. Padilla, Email: [email protected] .
Sarah H. Creem-Regehr, Email: [email protected] .
Mary Hegarty, Email: [email protected] .
Jeanine K. Stefanucci, Email: [email protected] .
- Ancker JS, Senathirajah Y, Kukafka R, Starren JB. Design features of graphs in health risk communication: A systematic review. Journal of the American Medical Informatics Association. 2006; 13 (6):608–618. doi: 10.1197/jamia.M2115. [ PMC free article ] [ PubMed ] [ CrossRef ] [ Google Scholar ]
- Baddeley AD, Hitch G. Working memory. Psychology of Learning and Motivation. 1974; 8 :47–89. doi: 10.1016/S0079-7421(08)60452-1. [ CrossRef ] [ Google Scholar ]
- Bailey, K., Carswell, C. M., Grant, R., & Basham, L. (2007). Geospatial perspective-taking: how well do decision makers choose their views? In Proceedings of the Human Factors and Ergonomics Society Annual Meeting (Vol. 51, No. 18, pp. 1246-1248). Los Angeles: SAGE Publications.
- Balleine BW. The neural basis of choice and decision making. Journal of Neuroscience. 2007; 27 (31):8159–8160. doi: 10.1523/JNEUROSCI.1939-07.2007. [ PMC free article ] [ PubMed ] [ CrossRef ] [ Google Scholar ]
- Bandlow A, Matzen LE, Cole KS, Dornburg CC, Geiseler CJ, Greenfield JA, et al. HCI International 2011–Posters’ Extended Abstracts. 2011. Evaluating Information Visualizations with Working Memory Metrics; pp. 265–269. [ Google Scholar ]
- Belia S, Fidler F, Williams J, Cumming G. Researchers misunderstand confidence intervals and standard error bars. Psychological Methods. 2005; 10 (4):389. doi: 10.1037/1082-989X.10.4.389. [ PubMed ] [ CrossRef ] [ Google Scholar ]
- Bertin, J. (1983). Semiology of graphics: Diagrams, networks, maps . Madison: University of Wisconsin Press.
- Boone, A., Gunalp, P., & Hegarty, M. (in press). Explicit versus Actionable Knowledge: The Influence of Explaining Graphical Conventions on Interpretation of Hurricane Forecast Visualizations. Journal of Experimental Psychology: Applied . [ PubMed ]
- Brügger A, Fabrikant SI, Çöltekin A. An empirical evaluation of three elevation change symbolization methods along routes in bicycle maps. Cartography and Geographic Information Science. 2017; 44 (5):436–451. doi: 10.1080/15230406.2016.1193766. [ CrossRef ] [ Google Scholar ]
- Caffò AO, Picucci L, Di Masi MN, Bosco A. Working memory: capacity, developments and improvement techniques. Hauppage: Nova Science Publishers; 2011. Working memory components and virtual reorientation: A dual-task study; pp. 249–266. [ Google Scholar ]
- Card, S. K., Mackinlay, J. D., & Shneiderman, B. (1999). Readings in information visualization: using vision to think . San Francisco: Morgan Kaufmann Publishers Inc.
- Castro, S. C., Strayer, D. L., Matzke, D., & Heathcote, A. (2018). Cognitive Workload Measurement and Modeling Under Divided Attention. Journal of Experimental Psychology: General . [ PubMed ]
- Cheong L, Bleisch S, Kealy A, Tolhurst K, Wilkening T, Duckham M. Evaluating the impact of visualization of wildfire hazard upon decision-making under uncertainty. International Journal of Geographical Information Science. 2016; 30 (7):1377–1404. doi: 10.1080/13658816.2015.1131829. [ CrossRef ] [ Google Scholar ]
- Connor CE, Egeth HE, Yantis S. Visual attention: Bottom-up versus top-down. Current Biology. 2004; 14 (19):R850–R852. doi: 10.1016/j.cub.2004.09.041. [ PubMed ] [ CrossRef ] [ Google Scholar ]
- Cowan N. The many faces of working memory and short-term storage. Psychonomic Bulletin & Review. 2017; 24 (4):1158–1170. doi: 10.3758/s13423-016-1191-6. [ PubMed ] [ CrossRef ] [ Google Scholar ]
- Dennis AR, Carte TA. Using geographical information systems for decision making: Extending cognitive fit theory to map-based presentations. Information Systems Research. 1998; 9 (2):194–203. doi: 10.1287/isre.9.2.194. [ CrossRef ] [ Google Scholar ]
- Engel AK, Fries P, Singer W. Dynamic predictions: Oscillations and synchrony in top–down processing. Nature Reviews Neuroscience. 2001; 2 (10):704–716. doi: 10.1038/35094565. [ PubMed ] [ CrossRef ] [ Google Scholar ]
- Engle, R. W., Kane, M. J., & Tuholski, S. W. (1999). Individual differences in working memory capacity and what they tell us about controlled attention, general fluid intelligence, and functions of the prefrontal cortex. In A. Miyake & P. Shah (Eds.), Models of working memory: Mechanisms of active maintenance and executive control (pp. 102-134). New York: Cambridge University Press.
- Epstein S, Pacini R, Denes-Raj V, Heier H. Individual differences in intuitive–experiential and analytical–rational thinking styles. Journal of Personality and Social Psychology. 1996; 71 (2):390. doi: 10.1037/0022-3514.71.2.390. [ PubMed ] [ CrossRef ] [ Google Scholar ]
- Evans JSB. Dual-processing accounts of reasoning, judgment, and social cognition. Annual Review of Psychology. 2008; 59 :255–278. doi: 10.1146/annurev.psych.59.103006.093629. [ PubMed ] [ CrossRef ] [ Google Scholar ]
- Evans JSB, Stanovich KE. Dual-process theories of higher cognition: Advancing the debate. Perspectives on Psychological Science. 2013; 8 (3):223–241. doi: 10.1177/1745691612460685. [ PubMed ] [ CrossRef ] [ Google Scholar ]
- Fabrikant SI, Hespanha SR, Hegarty M. Cognitively inspired and perceptually salient graphic displays for efficient spatial inference making. Annals of the Association of American Geographers. 2010; 100 (1):13–29. doi: 10.1080/00045600903362378. [ CrossRef ] [ Google Scholar ]
- Fabrikant Sara Irina, Skupin André. Exploring Geovisualization. 2005. Cognitively Plausible Information Visualization; pp. 667–690. [ Google Scholar ]
- Fagerlin A, Wang C, Ubel PA. Reducing the influence of anecdotal reasoning on people’s health care decisions: Is a picture worth a thousand statistics? Medical Decision Making. 2005; 25 (4):398–405. doi: 10.1177/0272989X05278931. [ PubMed ] [ CrossRef ] [ Google Scholar ]
- Feeney Aidan, Hola Ala K. W., Liversedge Simon P., Findlay John M., Metcalf Robert. Theory and Application of Diagrams. Berlin, Heidelberg: Springer Berlin Heidelberg; 2000. How People Extract Information from Graphs: Evidence from a Sentence-Graph Verification Paradigm; pp. 149–161. [ Google Scholar ]
- Frownfelter-Lohrke C. The effects of differing information presentations of general purpose financial statements on users’ decisions. Journal of Information Systems. 1998; 12 (2):99–107. [ Google Scholar ]
- Galesic M, Garcia-Retamero R. Graph literacy: A cross-cultural comparison. Medical Decision Making. 2011; 31 (3):444–457. doi: 10.1177/0272989X10373805. [ PubMed ] [ CrossRef ] [ Google Scholar ]
- Galesic M, Garcia-Retamero R, Gigerenzer G. Using icon arrays to communicate medical risks: Overcoming low numeracy. Health Psychology. 2009; 28 (2):210. doi: 10.1037/a0014474. [ PubMed ] [ CrossRef ] [ Google Scholar ]
- Garcia-Retamero, R., & Galesic, M. (2009). Trust in healthcare. In Kattan (Ed.), Encyclopedia of medical decision making , (pp. 1153–1155). Thousand Oaks: SAGE Publications.
- Gattis M, Holyoak KJ. Mapping conceptual to spatial relations in visual reasoning. Journal of Experimental Psychology: Learning, Memory, and Cognition. 1996; 22 (1):231. [ PubMed ] [ Google Scholar ]
- Gigerenzer G, Gaissmaier W. Heuristic decision making. Annual Review of Psychology. 2011; 62 :451–482. doi: 10.1146/annurev-psych-120709-145346. [ PubMed ] [ CrossRef ] [ Google Scholar ]
- Gigerenzer, G., Todd, P. M., & ABC Research Group (2000). Simple Heuristics That Make Us Smart . Oxford: Oxford University Press.
- Grounds Margaret A., Joslyn Susan, Otsuka Kyoko. Probabilistic Interval Forecasts: An Individual Differences Approach to Understanding Forecast Communication. Advances in Meteorology. 2017; 2017 :1–18. doi: 10.1155/2017/3932565. [ CrossRef ] [ Google Scholar ]
- Harel, J. (2015, July 24, 2012). A Saliency Implementation in MATLAB. Retrieved from http://www.vision.caltech.edu/~harel/share/gbvs.php
- Hegarty M. The cognitive science of visual-spatial displays: Implications for design. Topics in Cognitive Science. 2011; 3 (3):446–474. doi: 10.1111/j.1756-8765.2011.01150.x. [ PubMed ] [ CrossRef ] [ Google Scholar ]
- Hegarty M, Canham MS, Fabrikant SI. Thinking about the weather: How display salience and knowledge affect performance in a graphic inference task. Journal of Experimental Psychology: Learning, Memory, and Cognition. 2010; 36 (1):37. [ PubMed ] [ Google Scholar ]
- Hegarty M, Friedman A, Boone AP, Barrett TJ. Where are you? The effect of uncertainty and its visual representation on location judgments in GPS-like displays. Journal of Experimental Psychology: Applied. 2016; 22 (4):381. [ PubMed ] [ Google Scholar ]
- Hegarty M, Smallman HS, Stull AT. Choosing and using geospatial displays: Effects of design on performance and metacognition. Journal of Experimental Psychology: Applied. 2012; 18 (1):1. [ PubMed ] [ Google Scholar ]
- Hoffrage U, Gigerenzer G. Using natural frequencies to improve diagnostic inferences. Academic Medicine. 1998; 73 (5):538–540. doi: 10.1097/00001888-199805000-00024. [ PubMed ] [ CrossRef ] [ Google Scholar ]
- Hollands JG, Spence I. Judgments of change and proportion in graphical perception. Human Factors: The Journal of the Human Factors and Ergonomics Society. 1992; 34 (3):313–334. doi: 10.1177/001872089203400306. [ PubMed ] [ CrossRef ] [ Google Scholar ]
- Huang Z, Chen H, Guo F, Xu JJ, Wu S, Chen W-H. Expertise visualization: An implementation and study based on cognitive fit theory. Decision Support Systems. 2006; 42 (3):1539–1557. doi: 10.1016/j.dss.2006.01.006. [ CrossRef ] [ Google Scholar ]
- Itti L, Koch C, Niebur E. A model of saliency-based visual attention for rapid scene analysis. IEEE Transactions on Pattern Analysis and Machine Intelligence. 1998; 20 (11):1254–1259. doi: 10.1109/34.730558. [ CrossRef ] [ Google Scholar ]
- Joslyn S, LeClerc J. Decisions with uncertainty: The glass half full. Current Directions in Psychological Science. 2013; 22 (4):308–315. doi: 10.1177/0963721413481473. [ CrossRef ] [ Google Scholar ]
- Kahneman, D. (2011). Thinking, fast and slow . (Vol. 1). New York: Farrar, Straus and Giroux.
- Kahneman D, Frederick S. Heuristics and biases: The psychology of intuitive judgment. 2002. Representativeness revisited: Attribute substitution in intuitive judgment; p. 49. [ Google Scholar ]
- Kahneman D, Tversky A. Judgment under Uncertainty: Heuristics and Biases. 1. Cambridge; NY: Cambridge University Press; 1982. [ PubMed ] [ Google Scholar ]
- Kane MJ, Bleckley MK, Conway ARA, Engle RW. A controlled-attention view of working-memory capacity. Journal of Experimental Psychology: General. 2001; 130 (2):169. doi: 10.1037/0096-3445.130.2.169. [ PubMed ] [ CrossRef ] [ Google Scholar ]
- Keehner M, Mayberry L, Fischer MH. Different clues from different views: The role of image format in public perceptions of neuroimaging results. Psychonomic Bulletin & Review. 2011; 18 (2):422–428. doi: 10.3758/s13423-010-0048-7. [ PubMed ] [ CrossRef ] [ Google Scholar ]
- Keller C, Siegrist M, Visschers V. Effect of risk ladder format on risk perception in high-and low-numerate individuals. Risk Analysis. 2009; 29 (9):1255–1264. doi: 10.1111/j.1539-6924.2009.01261.x. [ PubMed ] [ CrossRef ] [ Google Scholar ]
- Keren G, Schul Y. Two is not always better than one: A critical evaluation of two-system theories. Perspectives on Psychological Science. 2009; 4 (6):533–550. doi: 10.1111/j.1745-6924.2009.01164.x. [ PubMed ] [ CrossRef ] [ Google Scholar ]
- Kinkeldey C, MacEachren AM, Riveiro M, Schiewe J. Evaluating the effect of visually represented geodata uncertainty on decision-making: Systematic review, lessons learned, and recommendations. Cartography and Geographic Information Science. 2017; 44 (1):1–21. doi: 10.1080/15230406.2015.1089792. [ CrossRef ] [ Google Scholar ]
- Kinkeldey C, MacEachren AM, Schiewe J. How to assess visual communication of uncertainty? A systematic review of geospatial uncertainty visualisation user studies. The Cartographic Journal. 2014; 51 (4):372–386. doi: 10.1179/1743277414Y.0000000099. [ CrossRef ] [ Google Scholar ]
- Kriz S, Hegarty M. Top-down and bottom-up influences on learning from animations. International Journal of Human-Computer Studies. 2007; 65 (11):911–930. doi: 10.1016/j.ijhcs.2007.06.005. [ CrossRef ] [ Google Scholar ]
- Kunz, V. (2004). Rational choice . Frankfurt: Campus Verlag.
- Lallanilla, M. (2014, April 24, 2014 10:15 am). Misleading Gun-Death Chart Draws Fire. https://www.livescience.com/45083-misleading-gun-death-chart.html
- Lee J, Bednarz R. Effect of GIS learning on spatial thinking. Journal of Geography in Higher Education. 2009; 33 (2):183–198. doi: 10.1080/03098260802276714. [ CrossRef ] [ Google Scholar ]
- Liu Le, Boone Alexander P., Ruginski Ian T., Padilla Lace, Hegarty Mary, Creem-Regehr Sarah H., Thompson William B., Yuksel Cem, House Donald H. Uncertainty Visualization by Representative Sampling from Prediction Ensembles. IEEE Transactions on Visualization and Computer Graphics. 2017; 23 (9):2165–2178. doi: 10.1109/TVCG.2016.2607204. [ PubMed ] [ CrossRef ] [ Google Scholar ]
- Lobben AK. Tasks, strategies, and cognitive processes associated with navigational map reading: A review perspective. The Professional Geographer. 2004; 56 (2):270–281. [ Google Scholar ]
- Lohse GL. A cognitive model for understanding graphical perception. Human Computer Interaction. 1993; 8 (4):353–388. doi: 10.1207/s15327051hci0804_3. [ CrossRef ] [ Google Scholar ]
- Lohse GL. The role of working memory on graphical information processing. Behaviour & Information Technology. 1997; 16 (6):297–308. doi: 10.1080/014492997119707. [ CrossRef ] [ Google Scholar ]
- Marewski JN, Gigerenzer G. Heuristic decision making in medicine. Dialogues in Clinical Neuroscience. 2012; 14 (1):77–89. [ PMC free article ] [ PubMed ] [ Google Scholar ]
- McCabe DP, Castel AD. Seeing is believing: The effect of brain images on judgments of scientific reasoning. Cognition. 2008; 107 (1):343–352. doi: 10.1016/j.cognition.2007.07.017. [ PubMed ] [ CrossRef ] [ Google Scholar ]
- McKenzie G, Hegarty M, Barrett T, Goodchild M. Assessing the effectiveness of different visualizations for judgments of positional uncertainty. International Journal of Geographical Information Science. 2016; 30 (2):221–239. doi: 10.1080/13658816.2015.1082566. [ CrossRef ] [ Google Scholar ]
- Mechelli A, Price CJ, Friston KJ, Ishai A. Where bottom-up meets top-down: Neuronal interactions during perception and imagery. Cerebral Cortex. 2004; 14 (11):1256–1265. doi: 10.1093/cercor/bhh087. [ PubMed ] [ CrossRef ] [ Google Scholar ]
- Meilinger T, Knauff M, Bülthoff HH. Working memory in wayfinding—A dual task experiment in a virtual city. Cognitive Science. 2008; 32 (4):755–770. doi: 10.1080/03640210802067004. [ PubMed ] [ CrossRef ] [ Google Scholar ]
- Meyer J. Performance with tables and graphs: Effects of training and a visual search model. Ergonomics. 2000; 43 (11):1840–1865. doi: 10.1080/00140130050174509. [ PubMed ] [ CrossRef ] [ Google Scholar ]
- Munzner T. Visualization analysis and design. Boca Raton, FL: CRC Press; 2014. [ Google Scholar ]
- Nadav-Greenberg L, Joslyn SL, Taing MU. The effect of uncertainty visualizations on decision making in weather forecasting. Journal of Cognitive Engineering and Decision Making. 2008; 2 (1):24–47. doi: 10.1518/155534308X284354. [ CrossRef ] [ Google Scholar ]
- Nayak JG, Hartzler AL, Macleod LC, Izard JP, Dalkin BM, Gore JL. Relevance of graph literacy in the development of patient-centered communication tools. Patient Education and Counseling. 2016; 99 (3):448–454. doi: 10.1016/j.pec.2015.09.009. [ PubMed ] [ CrossRef ] [ Google Scholar ]
- Newman GE, Scholl BJ. Bar graphs depicting averages are perceptually misinterpreted: The within-the-bar bias. Psychonomic Bulletin & Review. 2012; 19 (4):601–607. doi: 10.3758/s13423-012-0247-5. [ PubMed ] [ CrossRef ] [ Google Scholar ]
- Okan, Y., Galesic, M., & Garcia-Retamero, R. (2015). How people with low and high graph literacy process health graphs: Evidence from eye-tracking. Journal of Behavioral Decision Making .
- Okan Y, Garcia-Retamero R, Cokely ET, Maldonado A. Individual differences in graph literacy: Overcoming denominator neglect in risk comprehension. Journal of Behavioral Decision Making. 2012; 25 (4):390–401. doi: 10.1002/bdm.751. [ CrossRef ] [ Google Scholar ]
- Okan Y, Garcia-Retamero R, Galesic M, Cokely ET. When higher bars are not larger quantities: On individual differences in the use of spatial information in graph comprehension. Spatial Cognition and Computation. 2012; 12 (2–3):195–218. doi: 10.1080/13875868.2012.659302. [ CrossRef ] [ Google Scholar ]
- Padilla L, Hansen G, Ruginski IT, Kramer HS, Thompson WB, Creem-Regehr SH. The influence of different graphical displays on nonexpert decision making under uncertainty. Journal of Experimental Psychology: Applied. 2015; 21 (1):37. [ PubMed ] [ Google Scholar ]
- Padilla L, Quinan PS, Meyer M, Creem-Regehr SH. Evaluating the impact of binning 2d scalar fields. IEEE Transactions on Visualization and Computer Graphics. 2017; 23 (1):431–440. doi: 10.1109/TVCG.2016.2599106. [ PubMed ] [ CrossRef ] [ Google Scholar ]
- Padilla L, Ruginski IT, Creem-Regehr SH. Effects of ensemble and summary displays on interpretations of geospatial uncertainty data. Cognitive Research: Principles and Implications. 2017; 2 (1):40. [ PMC free article ] [ PubMed ] [ Google Scholar ]
- Pashler H. Dual-task interference in simple tasks: Data and theory. Psychological Bulletin. 1994; 116 (2):220. doi: 10.1037/0033-2909.116.2.220. [ PubMed ] [ CrossRef ] [ Google Scholar ]
- Patterson RE, Blaha LM, Grinstein GG, Liggett KK, Kaveney DE, Sheldon KC, et al. A human cognition framework for information visualization. Computers & Graphics. 2014; 42 :42–58. doi: 10.1016/j.cag.2014.03.002. [ CrossRef ] [ Google Scholar ]
- Pinker S. Artificial intelligence and the future of testing. 1990. A theory of graph comprehension; pp. 73–126. [ Google Scholar ]
- Ratliff, K. R., & Newcombe, N. S. (2005). Human spatial reorientation using dual task paradigms . Paper presented at the Proceedings of the Annual Cognitive Science Society.
- Reyna VF, Nelson WL, Han PK, Dieckmann NF. How numeracy influences risk comprehension and medical decision making. Psychological Bulletin. 2009; 135 (6):943. doi: 10.1037/a0017327. [ PMC free article ] [ PubMed ] [ CrossRef ] [ Google Scholar ]
- Riveiro M. Visually supported reasoning under uncertain conditions: Effects of domain expertise on air traffic risk assessment. Spatial Cognition and Computation. 2016; 16 (2):133–153. doi: 10.1080/13875868.2015.1137576. [ CrossRef ] [ Google Scholar ]
- Rodríguez V, Andrade AD, García-Retamero R, Anam R, Rodríguez R, Lisigurski M, et al. Health literacy, numeracy, and graphical literacy among veterans in primary care and their effect on shared decision making and trust in physicians. Journal of Health Communication. 2013; 18 (sup1):273–289. doi: 10.1080/10810730.2013.829137. [ PMC free article ] [ PubMed ] [ CrossRef ] [ Google Scholar ]
- Rosenholtz R, Jin Z. A computational form of the statistical saliency model for visual search. Journal of Vision. 2005; 5 (8):777–777. doi: 10.1167/5.8.777. [ CrossRef ] [ Google Scholar ]
- Ruginski IT, Boone AP, Padilla L, Liu L, Heydari N, Kramer HS, et al. Non-expert interpretations of hurricane forecast uncertainty visualizations. Spatial Cognition and Computation. 2016; 16 (2):154–172. doi: 10.1080/13875868.2015.1137577. [ CrossRef ] [ Google Scholar ]
- Sanchez CA, Wiley J. An examination of the seductive details effect in terms of working memory capacity. Memory & Cognition. 2006; 34 (2):344–355. doi: 10.3758/BF03193412. [ PubMed ] [ CrossRef ] [ Google Scholar ]
- Schirillo JA, Stone ER. The greater ability of graphical versus numerical displays to increase risk avoidance involves a common mechanism. Risk Analysis. 2005; 25 (3):555–566. doi: 10.1111/j.1539-6924.2005.00624.x. [ PubMed ] [ CrossRef ] [ Google Scholar ]
- Shah P, Freedman EG. Bar and line graph comprehension: An interaction of top-down and bottom-up processes. Topics in Cognitive Science. 2011; 3 (3):560–578. doi: 10.1111/j.1756-8765.2009.01066.x. [ PubMed ] [ CrossRef ] [ Google Scholar ]
- Shah, P., Freedman, E. G., & Vekiri, I. (2005). The Comprehension of Quantitative Information in Graphical Displays . In P. Shah (Ed.) & A. Miyake, The Cambridge Handbook of Visuospatial Thinking (pp. 426-476). New York: Cambridge University Press.
- Shah P, Miyake A. The separability of working memory resources for spatial thinking and language processing: An individual differences approach. Journal of Experimental Psychology: General. 1996; 125 (1):4. doi: 10.1037/0096-3445.125.1.4. [ PubMed ] [ CrossRef ] [ Google Scholar ]
- Shen M, Carswell M, Santhanam R, Bailey K. Emergency management information systems: Could decision makers be supported in choosing display formats? Decision Support Systems. 2012; 52 (2):318–330. doi: 10.1016/j.dss.2011.08.008. [ CrossRef ] [ Google Scholar ]
- Shipstead Z, Harrison TL, Engle RW. Working memory capacity and the scope and control of attention. Attention, Perception, & Psychophysics. 2015; 77 (6):1863–1880. doi: 10.3758/s13414-015-0899-0. [ PubMed ] [ CrossRef ] [ Google Scholar ]
- Simkin D, Hastie R. An information-processing analysis of graph perception. Journal of the American Statistical Association. 1987; 82 (398):454–465. doi: 10.1080/01621459.1987.10478448. [ CrossRef ] [ Google Scholar ]
- Sloman, S. A. (2002). Two systems of reasoning. In T. Gilovich, D. Griffin, & D. Kahneman (Eds.), Heuristics and biases : The psychology of intuitive judgment (pp. 379-396). New York: Cambridge University Press.
- Smelcer JB, Carmel E. The effectiveness of different representations for managerial problem solving: Comparing tables and maps. Decision Sciences. 1997; 28 (2):391. doi: 10.1111/j.1540-5915.1997.tb01316.x. [ CrossRef ] [ Google Scholar ]
- St. John M, Cowen MB, Smallman HS, Oonk HM. The use of 2D and 3D displays for shape-understanding versus relative-position tasks. Human Factors. 2001; 43 (1):79–98. doi: 10.1518/001872001775992534. [ PubMed ] [ CrossRef ] [ Google Scholar ]
- Stanovich Keith E. Who Is Rational? 1999. [ Google Scholar ]
- Stenning K, Oberlander J. A cognitive theory of graphical and linguistic reasoning: Logic and implementation. Cognitive Science. 1995; 19 (1):97–140. doi: 10.1207/s15516709cog1901_3. [ CrossRef ] [ Google Scholar ]
- Stone ER, Sieck WR, Bull BE, Yates JF, Parks SC, Rush CJ. Foreground: Background salience: Explaining the effects of graphical displays on risk avoidance. Organizational Behavior and Human Decision Processes. 2003; 90 (1):19–36. doi: 10.1016/S0749-5978(03)00003-7. [ CrossRef ] [ Google Scholar ]
- Stone ER, Yates JF, Parker AM. Effects of numerical and graphical displays on professed risk-taking behavior. Journal of Experimental Psychology: Applied. 1997; 3 (4):243. [ Google Scholar ]
- Trueswell JC, Papafragou A. Perceiving and remembering events cross-linguistically: Evidence from dual-task paradigms. Journal of Memory and Language. 2010; 63 (1):64–82. doi: 10.1016/j.jml.2010.02.006. [ CrossRef ] [ Google Scholar ]
- Tversky, B. (2005). Visuospatial reasoning. In K. Holyoak and R. G. Morrison (eds.), The Cambridge Handbook of Thinking and Reasoning , (pp. 209-240). Cambridge: Cambridge University Press.
- Tversky B. Visualizing thought. Topics in Cognitive Science. 2011; 3 (3):499–535. doi: 10.1111/j.1756-8765.2010.01113.x. [ PubMed ] [ CrossRef ] [ Google Scholar ]
- Tversky Barbara, Corter James E., Yu Lixiu, Mason David L., Nickerson Jeffrey V. Diagrammatic Representation and Inference. Berlin, Heidelberg: Springer Berlin Heidelberg; 2012. Representing Category and Continuum: Visualizing Thought; pp. 23–34. [ Google Scholar ]
- Vessey I, Galletta D. Cognitive fit: An empirical study of information acquisition. Information Systems Research. 1991; 2 (1):63–84. doi: 10.1287/isre.2.1.63. [ CrossRef ] [ Google Scholar ]
- Vessey I, Zhang P, Galletta D. Human-computer interaction and management information systems: Foundations. 2006. The theory of cognitive fit; pp. 141–183. [ Google Scholar ]
- Von Neumann J. Morgenstern, 0.(1944) theory of games and economic behavior. Princeton, NJ: Princeton UP; 1953. [ Google Scholar ]
- Vranas PBM. Gigerenzer's normative critique of Kahneman and Tversky. Cognition. 2000; 76 (3):179–193. doi: 10.1016/S0010-0277(99)00084-0. [ PubMed ] [ CrossRef ] [ Google Scholar ]
- Wainer H, Hambleton RK, Meara K. Alternative displays for communicating NAEP results: A redesign and validity study. Journal of Educational Measurement. 1999; 36 (4):301–335. doi: 10.1111/j.1745-3984.1999.tb00559.x. [ CrossRef ] [ Google Scholar ]
- Waters EA, Weinstein ND, Colditz GA, Emmons K. Formats for improving risk communication in medical tradeoff decisions. Journal of Health Communication. 2006; 11 (2):167–182. doi: 10.1080/10810730500526695. [ PubMed ] [ CrossRef ] [ Google Scholar ]
- Waters EA, Weinstein ND, Colditz GA, Emmons KM. Reducing aversion to side effects in preventive medical treatment decisions. Journal of Experimental Psychology: Applied. 2007; 13 (1):11. [ PubMed ] [ Google Scholar ]
- Wilkening Jan, Fabrikant Sara Irina. Spatial Information Theory. Berlin, Heidelberg: Springer Berlin Heidelberg; 2011. How Do Decision Time and Realism Affect Map-Based Decision Making? pp. 1–19. [ Google Scholar ]
- Zhu B, Watts SA. Visualization of network concepts: The impact of working memory capacity differences. Information Systems Research. 2010; 21 (2):327–344. doi: 10.1287/isre.1080.0215. [ CrossRef ] [ Google Scholar ]
How Creative Visualization Works: Achieving Success
Creative visualization.
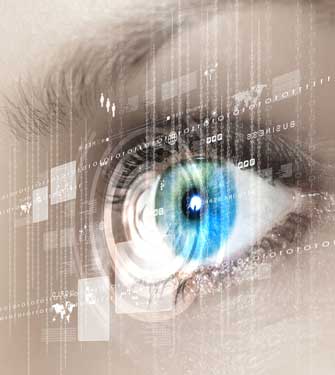
Did you know that some of the world’s most successful people, including professional athletes, actors, actresses, CEOs, artists, and spiritual leaders — attribute their success to one highly powerful mind technique?
It's called "creative visualization." Used by those who understand how the subconscious mind functions within the framework of our quantum reality, today more and more people are using this powerful law of attraction technique to manifest abundance and achieve personal success in their lives.
How does creative visualization work?
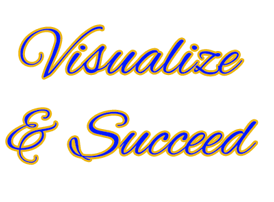
Creative visualization, put simply, involves the use of mental imagery to achieve a desired outcome. In other words, you must imagine yourself doing (or being) the thing that you want to do or be, successfully — with repetition.
For example, a tennis player wishing to improve their backhand would imagine themselves hitting it over and over in their mind (with proper form of course!). A person wishing to have financial success would imagine themselves as already living the life of someone with abundant wealth (nice house, nice car, etc.).
You know how famous people often claim to have "visualized" their success long before it ever happened for them in real life? It's the power of creative visualization that they are harnessing.
And once you see yourself as already having "achieved" your goal (whatever it may be), then your intuition and sense of "inner knowing" will light the proper path for you to follow (taking action is part of making this powerful technique work).
What's the secret to maximizing the law of attraction?
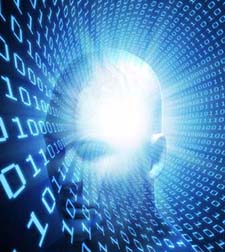
A few famous people who attribute their success to creative visualization are Oprah Winfrey, Jim Carrey, Richard Branson, Arnold Schwarzenegger, Michael Jordan, among many others.
Meditation. In order to have the best state of consciousness to manifest abundance — the mind must be still, and the mind must be clear.
Once meditation melts away whatever subconscious blockages we're dealing with (we all have them), and once meditation quiets down the mind chatter that blocks our intuition (a.k.a. "success GPS") — that's when our ability to visualize & manifest truly unlocks.
When you can finally see the "correct path" to your intended goal, whether it be love , wealth, more friends, or great success — then you start to believe.
"If you want to be successful, it's just this simple. Know what you are doing. Love what you are doing. And believe in what you are doing." — Will Rogers
Belief in what you are doing bends the quantum nature of our reality to your will. When you truly believe in something then nothing will stop you from achieving your dreams . How will you change the world? Meditation is the way.
Suggested Articles: How Meditation Makes Us Successful | How Meditation Awakens Intuition | The Law of Attraction: How To Manifest Abundance
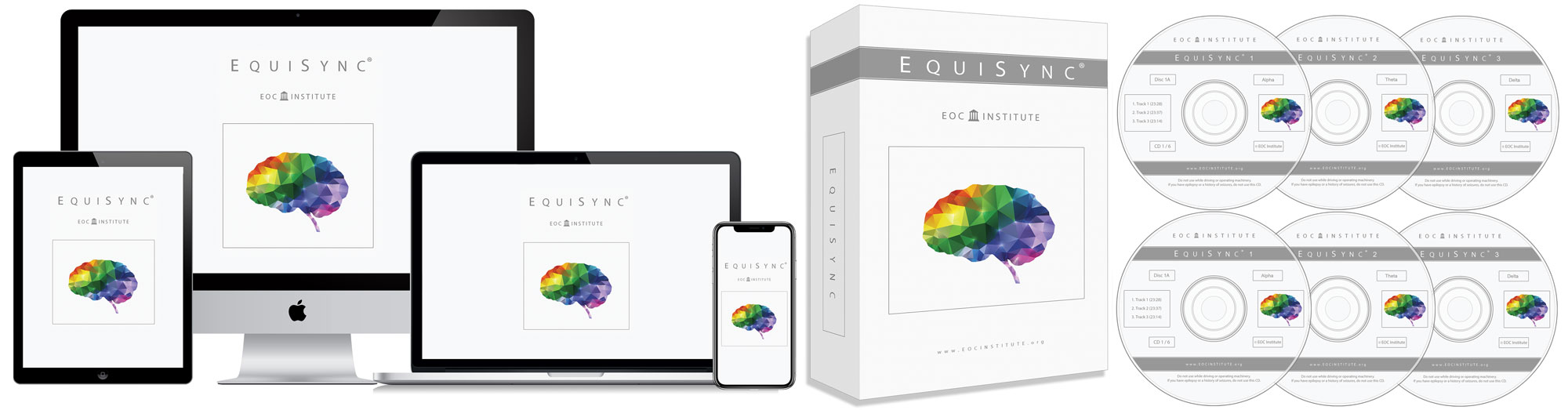
Click the buttons to play or pause the audio.