- Research article
- Open access
- Published: 30 June 2020
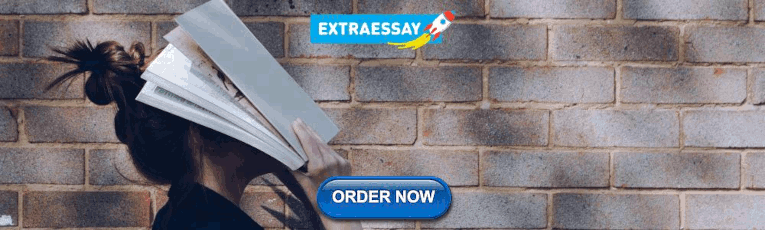
A meta-review of literature reviews assessing the capacity of patients with severe mental disorders to make decisions about their healthcare
- A. Calcedo-Barba 1 ,
- A. Fructuoso 2 ,
- J. Martinez-Raga 3 ,
- M. Sánchez de Carmona 5 &
- E. Vicens 6
BMC Psychiatry volume 20 , Article number: 339 ( 2020 ) Cite this article
7213 Accesses
29 Citations
18 Altmetric
Metrics details
Determining the mental capacity of psychiatric patients for making healthcare related decisions is crucial in clinical practice. This meta-review of review articles comprehensively examines the current evidence on the capacity of patients with a mental illness to make medical care decisions.
Systematic review of review articles following PRISMA recommendations. PubMed, Scopus, CINAHL and PsycInfo were electronically searched up to 31 January 2020. Free text searches and medical subject headings were combined to identify literature reviews and meta-analyses published in English, and summarising studies on the capacity of patients with serious mental illnesses to make healthcare and treatment related decisions, conducted in any clinical setting and with a quantitative synthesis of results. Publications were selected as per inclusion and exclusion criteria. The AMSTAR II tool was used to assess the quality of reviews.
Eleven publications were reviewed. Variability on methods across studies makes it difficult to precisely estimate the prevalence of decision-making capacity in patients with mental disorders. Nonetheless, up to three-quarters of psychiatric patients, including individuals with serious illnesses such as schizophrenia or bipolar disorder may have capacity to make medical decisions in the context of their illness. Most evidence comes from studies conducted in the hospital setting; much less information exists on the healthcare decision making capacity of mental disorder patients while in the community. Stable psychiatric and non-psychiatric patients may have a similar capacity to make healthcare related decisions. Patients with a mental illness have capacity to judge risk-reward situations and to adequately decide about the important treatment outcomes. Different symptoms may impair different domains of the decisional capacity of psychotic patients. Decisional capacity impairments in psychotic patients are temporal, identifiable, and responsive to interventions directed towards simplifying information, encouraging training and shared decision making. The publications complied satisfactorily with the AMSTAR II critical domains.
Conclusions
Whilst impairments in decision-making capacity may exist, most patients with a severe mental disorder, such as schizophrenia or bipolar disorder are able to make rational decisions about their healthcare. Best practice strategies should incorporate interventions to help mentally ill patients grow into the voluntary and safe use of medications.
Peer Review reports
In 1995, Appelbaum and Grisso stated that competence to consent to treatment relied on four legal standards: the ability to communicate a choice; the ability to understand relevant information; the ability to appreciate the situation and its likely consequences; and the ability to manipulate information rationally [ 1 ]. In healthcare, the capacity to make decisions regarding treatment is closely related to the autonomy, the exercise of self-governance, and the ability of an individual to take intentional actions [ 2 ]. The capacity to consent to treatment is often used in the clinical assessment of the ability to engage in authentic autonomous decision-making, a fundamental element of a person’s dignity and rights [ 3 ].
Assessment of mental capacity has become a key component of daily clinical practice [ 4 , 5 ]. Mental health legislation and medical ethics increasingly require physicians to empower patients to make decisions, and to respect the patient’s wishes with regard to accepting or refusing therapy [ 4 , 6 ]. However, it has been reported that coercive treatment, involuntary hospitalisations and medications are currently overused [ 7 ]; this has a direct negative impact on patients’ adherence to treatment and on their engagement and participation in shared decision-making with their healthcare professionals [ 8 ].
An increasing number of publications are assessing decision-making capacity in mental health. However, comparisons and contrasts of the findings of these articles are lacking and it becomes difficult to draw clear conclusions on what is the actual capacity of individuals with serious mental illnesses to make decisions about their healthcare and treatments [ 9 ]. This meta-review of review articles was designed as a comprehensive synthesis of the current state of knowledge in the field, with the aim of assessing the available evidence on the decision-making capacity of patients with various mental illnesses (especially schizophrenia, psychosis and bipolar disorder) with regard to the management of their disease and their treatment. The review compares the conclusions of various comprehensive publications, discusses the strength of these conclusions, and identifies existing gaps in the evidence.
The review of the literature was conducted in accordance with the Preferred Reporting Items for Systematic Review and Meta-Analysis (PRISMA) guidelines [ 10 ]. A series of steps, including the definition of the search strategy, identification and selection of publications, data extraction and synthesis, and quality assessment was followed.
Search strategy for identification and selection of publications
The aim of the search strategy was to provide a comprehensive list of published literature reviews assessing decision making capacity in patients with mental disorders. Four electronic databases (the Cumulative Index to Nursing and Allied Health Literature [CINAHL], PsycInfo, PubMed and Scopus) were searched up to 31 January 2020. The search strategy is described in Additional file 1 . Free text searches and medical subject headings were combined to identify literature reviews published in English, summarising studies conducted in any clinical setting and with a quantitative synthesis of results. Selection of publications was carried out as per inclusion and exclusion criteria (Table 1 ). Lists of references in the key papers retrieved were further checked to identify other relevant articles.
Potentially relevant abstracts were assessed by two expert reviewers to identify all papers suitable for inclusion. Full text copies were requested. Reviews which were identified after mutual agreement were included and data were extracted. A third reviewer was involved in the process to resolve any disagreements on the selection of publications.
Data extraction and quality assessment
Data extraction was carried out by one researcher. A data extraction form that covered citation, country, population, interventions, comparators, outcomes, settings, review type, aims, literature review size, strengths and limitations and key findings of the review as stated by authors was used to extract data (Tables 2 and 3 ). The AMSTAR II (A MeaSurement Tool to Assess systematic Reviews) [ 22 ] assessment tool was used to assess the quality of reviews.
A total of 1973 hits were initially identified; 1938 were either duplicated or deemed not relevant for the review based on the assessment of titles and abstracts; 22 full text publications were initially considered valid and retrieved for closer examination; 11 were excluded because they referred to diseases excluded from the review or did not assess decision making capacity. Data was finally extracted from 11 publications (Fig. 1 ).
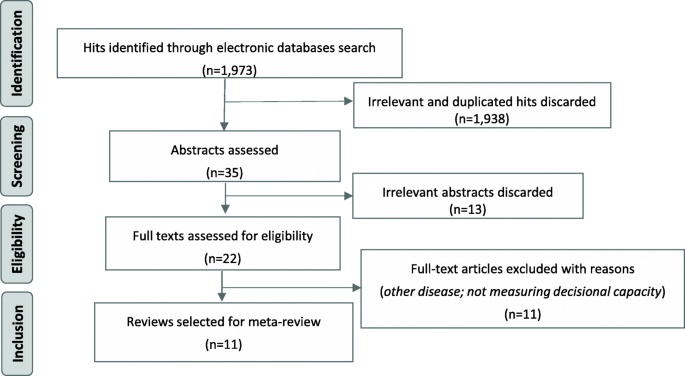
PRISMA diagram
The number of studies included in each review apprised varied between 7 [ 18 , 20 ] and 63 [ 16 ], and the number of patients with a mental disorder ranged from 6 [ 13 ] to 2483 [ 15 ]. Schizophrenia or schizoaffective disorders [ 12 , 13 , 19 , 20 ] and psychosis [ 14 , 18 , 21 ] were the most frequently explored mental illnesses. The general healthy population or patients with a non-mental disorder were the usual study comparators. Therefore, decisional capacity variance among mental illnesses of different nature has been little explored (Tables 2 and 3 ).
Prevalence of decision-making capacity
Information on the prevalence of capacity for making healthcare decisions among psychiatry patients can be derived from two systematic reviews. One systematic review of 37 empirical, quantitative studies of mental capacity in a mixed population of psychiatric patients reported that up to 67% of participants had the capacity to decide whether to be admitted to a psychiatric unit while a median of 71% had capacity for making treatment decisions (a median of 29%, interquartile range (IQR) 22–44, lacked capacity) [ 17 ]. Another systematic review (40 articles) found that 26% (95% confidence interval (CI): 18 to 36) to 67% (95% CI: 35 to 88) of people with schizophrenia or other non-affective disorders were able to make medical decisions related or unrelated to the management of their condition [(median: 48% (95% CI: 29 to 66)] [ 19 ]. In both reviews, up to three quarters of severe mental disorder patients, including individuals with schizophrenia would have capacity to make medical decisions in the context of their illness, in particular specific decisions related with their treatments [ 17 , 19 ]. Both reviews are also coincident in that heterogeneity between studies was high, with considerable variation in study design and measurements [ 17 , 19 ].
Decisional capacity in different clinical settings and patient groups
Four reviews assessed patients in diverse settings and explored the degree of impairment in each dimension of decision-making capacity. Lepping et al. [ 15 ] reported that 55% of patients in psychiatric and 66% of patients in non-psychiatric settings had the capacity to make medical decisions. Appreciation of the problem and necessity for treatment were more frequently compromised in psychiatric patients, while non-psychiatric patients struggled primarily with reasoning. The authors found a significant variation between studies due to heterogeneity in designs and methods that reached 86% in psychiatric settings and 90% in non-psychiatric settings. Jeste et al. [ 13 ] reported a 48 to 79% overlap between people with schizophrenia and non-psychiatric patients on the MacArthur subscales, which indicated that most patients with schizophrenia had comparably adequate decision-making capacity [ 13 ]. Psychotic inpatients had several characteristics which temporarily limited their capacity and distinguished them from outpatients. Greater severity of positive and negative symptoms, experiencing a stressful life event (e.g., hospitalisation), and often receiving higher doses of medication adversely impacted cognition among psychiatric inpatients [ 15 ]. Community-dwelling or clinically stable outpatients were much closer to non-psychiatric subjects in terms of the capacity for decision-making. The authors concluded that similar proportions of non-psychiatric and psychiatric outpatients either had or lacked capacity to consent to treatment or to hospital admission, and that impairment in the capacity to make decisions was not a distinguishing feature of schizophrenia patients [ 13 , 15 ].
Another meta-analysis of ten studies showed that compared to healthy controls, patients with schizophrenia or schizoaffective disorder were significantly more likely to have impaired decision-making capacity in terms of understanding, reasoning, appreciation and expression of a choice in clinical research and treatment, as measured by the MacArthur Competence Assessment Tool (MacCAT) instruments [ 20 ]. The standardised mean differences were more significant in older than in younger age subgroups, suggesting that, compared to their healthy counterparts, the impairment of decision-making capacity could be more obvious in older patients than in younger patients. In some of the studies included in this meta-analysis, decisional capacity improved in patients with schizophrenia following intensive educational interventions.
Another systematic review explored the degree of impairment in each dimension of decision-making capacity in schizophrenia patients compared to non-psychiatric controls, as assessed by the MacCAT [ 12 ]. The odds for a decreased understanding and a decreased appreciation were some five times higher in individuals with schizophrenia than in non-mentally ill controls, those for decreased reasoning almost four times higher, and those for a decreased aptitude to express a choice was over six times higher. The use of an enhanced informed consent form contributed to significant improvements in decision-making capacity compared to the use of standard forms. The authors concluded that even if patients with schizophrenia have a significantly decreased decision-making capacity, they should be considered to be as competent as non-mentally ill controls unless very severe changes were identifiable during the clinical examination [ 12 ].
In these four systematic reviews, the decisional capacity of patients with a psychiatric disease was compared with that of patients with a non-psychiatric clinical condition or with that of healthy individuals. All reviews are concurrent in the fact that impairments in decisional capacity can be found in both psychiatric and non-psychiatric patients, and therefore the diagnosis of a psychiatric condition should not be the upfront reason of incapacity. Despite most research being conducted in the hospital setting, those fewer addressing decisional capacity in psychiatric outpatients showed that their capacity to make medical decisions can be much alike to that of the non-psychiatric individuals. Likewise, studies coincidently acknowledge that decisional impairments amongst psychiatric inpatients are temporal and responsive to information-enhancing interventions.
Determining factors of decisional capacity in psychosis patients
In a systematic review and meta-analysis of factors that help or hinder treatment decision-making capacity in psychosis (23 studies, n = 1823) a moderate to large negative association between total psychotic symptom severity and the capacity of participants to understand information relevant to treatment decisions was found [ 14 ]. Poor insight was also associated with patients’ poor capacity to make treatment related decisions. Verbal cognitive function, metacognitive ability and years spent in education were positively associated with the ability of psychiatric individuals to understand information relating to treatment decision making. Decision-making capacity responded favourably to interventions, such as the simplification of the information, shared decision-making, and metacognitive training [ 14 ].
Likewise, Ruissen et al. [ 18 ] reported the findings of seven articles that assessed the relationship between competence to decide and insight of psychiatric inpatients and outpatients and of psychotic and non-psychotic patients. A large overlap between insight and competence to decide was reported among psychotic (schizophrenia, schizoaffective disorder, and psychotic episodes) and bipolar disorder (comprising both manic and depressive episodes) patients implying that a strong correlation existed between insight and capacity for making decisions, including decisions related to medical treatments and hospital admission. Psychotic patients with adequate insight were generally competent in making medical decisions.
Both reviews report findings on the capacity of psychotic patients to make treatment and other disease-related decisions. They are coincident in the relevance of insight as a determining factor of psychiatric patients’ decisional capacity. As expected, the burden and severity of psychotic symptoms can seriously compromise patients’ ability to make decisions.
Capacity of people with mental illness to make risk-reward decisions and to choose treatments
The capacity of mental illness patients for making value-based decisions was explored in literature reviews of studies based on gambling tasks and on preferences for medication-associated outcomes methods. A systematic review and meta-analysis explored the factors which may help or hinder the ability to make risk-reward decision making in a pooled sample of 4264 individuals with psychosis, based on their performance on the Iowa Gambling Tasks (IGT) and the Cambridge Gambling Tasks (CGT) [ 21 , 23 , 24 ]. Compared with healthy individuals, people with psychosis had moderately impaired risk-reward decision-making ability (g = − 0.57, 95% CI − 0.66 to − 0.48; I 2 45%; moderate quality) [ 21 ]. They were also more likely to value rewards over losses (k = 6, N = 516, g = 0.38, 95% CI: 0.05 to 0.70, I 2 64%), and to base decisions on recent rather than past outcomes (k = 6, N = 516, g = 0.30, 95% CI: − 0.04 to 0.65, I 2 68%). Analysis of the positive or negative influence of the type and dose of antipsychotics on decision-making capacity was inconclusive. The authors suggested that, although people with non-affective psychosis may make less effective decisions than healthy individuals in the IGT and CGT, their difficulties were moderate and comparable with those observed in other clinical groups.
Mukherjee and Kable [ 16 ] calculated that around 27% of patients with various mental disorders were similar to healthy individuals when deciding about losses and rewards on the IGT. Furthermore, individuals with mental illnesses had fewer deficits than individuals with frontal lobe lesions, for instance. The assessment of the severity of impairment across types of mental illnesses did not demonstrate any significant differences according to specific psychiatric diagnosis.
Eiring et al. [ 11 ] investigated the relative value adults with a mental illness place on treatment outcomes, including the attributes of particular medications or medication classes and the consequences and health states associated with their use. It reported that patients were able to provide valid preference measures with the different methods applied, generally understood the tasks, and gave sufficiently consistent answers. Among patients with schizophrenia, positive, acute or psychotic symptoms appeared consistently among the least desirable outcomes. Negative symptoms, such as reduced capacity for emotion, were found more desirable or less important than positive symptoms. Independence received high ratings and inpatient status low ratings. Overall, patients with schizophrenia tended to value disease states higher and side effects lower than other groups and perceived side effects more negatively than their therapists. Patients with bipolar disorder gave low values to mania and severe depression and reported weight gain to be important.
These reviews provide consistent evidence on the fact that patients with a serious mental disease, such as schizophrenia or bipolar disorder can make risk-reward decisions in the context of their illness and treatments. Furthermore, the studies summarised in the reviews show that these patients may achieve a level of ability for making value-based decisions equal to non-psychiatric patients. They can reliably decide about the important treatment outcomes and their most desirable treatment attributes.
Quality assessment
Reviews presented well-framed research questions based on the evidence based PICOS model [ 25 ] (Table 2 ) and were high quality according to the AMSTAR II assessment tool (Table 3 ) [ 22 ]. AMSTAR II was developed to evaluate systematic reviews of randomised trials or non-randomised studies of healthcare interventions, or both. Publications included in this review complied satisfactorily the AMSTAR II critical domains. No critical weaknesses were identified in the assessment. Therefore, the reviews provided an accurate and comprehensive summary of the results of studies of decision-making capacity in mental disorder patients.
This meta-review review brings together a set of high-quality reviews on the capacity of individuals with a severe mental illness to make decisions about their healthcare. It presents a thorough synthesis of current systematic review literature concerning the decision-making capacity of patients with mental disorders, including psychotic, schizophrenia and bipolar disorder individuals. It provides a picture of the state of the field in the complex task of assessing patients’ decisional capacity in psychiatry. It comprehensively summarizes a body of evidence supporting the idea that the decision-making capacity of psychiatric patients with serious mental illness is preserved in most circumstances and challenges the understanding that people with severe mental illnesses are unable to make their own choices [ 8 ].
Authors across studies are coincident in emphasising that most patients with a severe mental disorder are able to make rational decisions about their medical care and to participate in decision-making regarding treatments despite temporal impairments. Thus, most often the degree of impairment that may be inherent to the mental disorder does not constitute incapacity to make decisions. The findings also reveal that patients with psychotic disorders or other severe mental illnesses can make complex risk-reward decisions in usual clinical practice. Small deviations from optimal performance may arise due to deficits in the ability to fully represent the value of different choices and response options, a finding that aligns with results from experimental research in patients with schizophrenia [ 26 ].
Most of the reviews addressed the capacity to make decisions in people with severe mental disorders either already hospitalised or requiring hospital admission. This means that most studies included patients with more severe symptoms less responsive to usual therapies [ 27 ]. Even in these more ill psychiatric populations, between 60 and 70% had capacity to make some treatment decisions [ 1 , 17 , 19 ]. Hospitalised patients usually have greater care needs, even when their psychiatric symptoms are controlled, exhibit significantly more severe negative, positive, and manic symptoms, and have lower global functioning than outpatients [ 28 ]. Therefore, despite the scarcity of studies measuring decisional capacity in routine ambulatory practice in psychiatry, it can be expected a high level of health-related decision-making capacity in patients in everyday life in the community. Rigorous studies investigating this question as a primary outcome would be much welcome.
This meta- review also shows that people with schizophrenia have the capacity to make other difficult decisions related, for instance, to giving consent for hospital admission or to the type of treatment they prefer to receive. Likewise, other studies have reported that patients with schizophrenia or bipolar disorder are able to describe prodromal symptoms of relapse and to suggest a treatment and the need for hospitalisation in advance; that they can request or refuse medications and state their preferences for pre-emergency interventions, non-hospital alternatives and non-medical personal care [ 29 , 30 , 31 ]. In such circumstances, shared decision making and advancing crisis management plans may help reduce insecurity and improve healthcare outcomes for the patient with a mental illness. Studies have found that being involved in decision-making, whenever decisional capacity exists, renders more positive treatment results, better medication adherence, higher self-efficacy and autonomy, and lower decisional uncertainty among patients with mental disorders [ 32 , 33 ].
Patients’ awareness of decisional capacity and having the opportunity for sharing decisions on future care (crisis planning) for psychosis reduces the use of compulsory inpatient treatment by approximately 40% over 15 to 18 months [ 33 ]. In this context, advance directives are fundamental to ensure the timely provision of medical treatments, thus minimising decisional impairments in the acute stages of psychosis [ 34 ]. Psychosocial interventions are also important to address the complex health needs of people with serious mental illnesses. Combined with anti-psychotics, psychosocial interventions highly contribute to reduce the severity of symptoms, to benefit functioning, to encourage decision making and to decrease hospital readmissions [ 35 ].
Nevertheless, clinicians play the crucial role of judging the capacity of patients with severe mental disorder to decide about their treatments and healthcare, and tools exist to guide their assessment [ 36 ]. The final decision depends entirely on clinical judgement, based on the practitioner’s knowledge of the patient and of the course of the disease.
Beyond acute episodes, the findings also support the notion that continued training and learning, simplification and enhancement of the information improve the capacity of patients with severe mental disorders for decision-making both in hospital and in everyday life [ 37 ]. The results of various studies demonstrate that brief interventions aimed at recovering capacity for understanding can help schizophrenia patients to perform very much like healthy people in the four dimensions of decisional capacity (understanding, appreciation, reasoning and expression of a choice) [ 38 ]. Regular information reinforcement, strengthening neurocognitive functioning and training are important to maintain long-term levels of competence and to maximise decision making capacities of patients [ 39 , 40 ].
In sum, people with severe mental illness can benefit greatly from anticipation, prevention, gradual learning, enhanced information and enriched shared decision-making in order to strengthen their autonomous decision-making capacity, to increase their autonomy and to ultimately contribute to reducing the stigma of mental illness. Being able to make decisions in anticipation of, for instance, agitation or other acute symptoms should help patients to gain a sense of control over their own lives, and to enhance their health-related quality of life [ 41 ]. This review contributes to the growing body of evidence suggesting that the best medical practice should help severe mentally ill patients to grow into voluntary healthcare, safe users of medications.
Small sample size, heterogeneity, language and selection bias of participants were among the limitations frequently reported by the authors of the studies reviewed. However, since the publications included in the meta- review were systematic reviews and meta-analyses, the risk of bias was minimised. Nonetheless, it was limited to publications appeared in English. Although the search was comprehensive, papers in many other languages including French, Germany. Italian or Spanish may not have been identified. Several frequent mental illnesses were excluded for reasons of study feasibility. Important mental health conditions, such as dementia, depression and other disorders have not been addressed which may have limited the scope of the meta-review.
This meta-review of review articles provides a comprehensive synthesis of the current state of knowledge on the capacity of patients with mental illnesses to make decisions about their healthcare and medical treatments. It provides clinicians and other healthcare practitioners a summary of the evidence on the topic, contrasting key findings. It shows that whilst impairments in decision-making capacity may exist, most patients with a severe mental disorder are able to make adequate decisions about the care of their health. It denotes that best practice strategies should help mentally ill patients to exercise their decisional capacity to develop into autonomous and reliable users of medications. Keeping a sense of control over their illness and life may help them to improve the outcomes of their treatments and their health-related quality of life.
Availability of data and materials
The datasets used and/or analysed during the current study are available from the corresponding author on reasonable request.
Abbreviations
Description
A MeaSurement Tool to Assess systematic Reviews
Cambridge Gambling Tasks
Confidence Interval
Cumulative Index to Nursing and Allied Health Literature
Iowa Gambling Tasks
InterQuartile Range
MacArthur Competence Assessment Tool
MacArthur Competence Assessment Tool for Clinical Research
MacArthur Competence Assessment Tool for Treatment
Number Needed to Treat
Odds Ratios
Preferred Reporting Items for Systematic Review and Meta-Analysis
Standard Deviation
Standard Error
Standardized Mean Difference
Appelbaum P, Grisso T. The MacArthur treatment competence study. I: mental illness and competence to consent to treatment. Law Hum Behav. 1995;19(2):105–26.
PubMed Google Scholar
Owen GS, Freyenhagen F, Richardson G, Hotopf M. Mental capacity and decisional autonomy: an interdisciplinary challenge. Inquiry. 2009;52(1):79–107.
Google Scholar
Jeste D, Eglit G, PPalmer B, Martinis J, Blanck P, Saks E. Supported decision making in serious mental illness. Psychiatry. 2018;81(1):28–40.
United Nations. Convention on the rights of persons with disabilities. Report of the Convention. 2006; Available from: https://www.un.org/disabilities/documents/convention/convention_accessible_pdf.pdf .
Harding R, Taşcıoğlu E. Supported decision-making from theory to practice: implementing the right to enjoy legal capacity. Societies. 2018;8(2):25.
Morrissey F. The united nations convention on the rights of persons with disabilities: a new approach to decision-making in mental health law. Eur J Health Law. 2012;19(5):423–40.
Mahomed F, Stein MA, Patel V. Involuntary mental health treatment in the era of the United Nations convention on the rights of persons with disabilities. PLoS Med. 2018;15(10):e1002679.
PubMed PubMed Central Google Scholar
Danzer G, Rieger SM. Improving medication adherence for severely mentally ill adults by decreasing coercion and increasing cooperation. Bull Menn Clin. 2016;80(1):30–48.
Smith V, Devane D, Begley C, Clarke M. Methodology in conducting a systematic review of systematic reviews of healthcare interventions. BMC Med Res Methodol. 2011;11(1):15 Available from: http://www.biomedcentral.com/1471-2288/11/15 .
Moher D, Liberati A, Tetzlaff J, Altman D, Group. P. Preferred reporting items for systematic reviews and meta-analyses: the PRISMA statement. PLoS Med. 2009;6(7):e1000097 Available from: https://www.ncbi.nlm.nih.gov/pmc/articles/PMC2707599/pdf/pmed.1000097.pdf .
Eiring Ø, Landmark BF, Aas E, Salkeld G, Nylenna M, Nytrøen K. What matters to patients? A systematic review of preferences for medication-associated outcomes in mental disorders. BMJ Open. 2015;5(4):1–13.
Hostiuc S, Rusu MC, Negoi I, Drima E. Testing decision-making competency of schizophrenia participants in clinical trials. A meta-analysis and meta-regression. BMC Psychiatry. 2018;18(1):2.
Jeste DV, Depp CA, Palmer BW. Magnitude of impairment in decisional capacity in people with schizophrenia compared to normal subjects: an overview. Schizophr Bull. 2006;32(1):121–8.
Larkin A, Hutton P. Systematic review and meta-analysis of factors that help or hinder treatment decision-making capacity in psychosis. Br J Psychiatry. 2017;211(4):205–15.
Lepping P, Stanly T, Turner J. Systematic review on the prevalence of lack of capacity in medical and psychiatric settings. Clin Med J R Coll Physicians London. 2015;15(4):337–43.
Mukherjee D, Kable JW. Value-based decision making in mental illness: a meta-analysis. Clin Psychol Sci. 2014;2(6):767–82.
Okai D, Owen G, McGuire H, Singh S, Churchil R, Hotopf M. Mental capacity in psychiatric patients systematic review. Br J Psychiatry. 2007;191:291–7.
Ruissen AM, Widdershoven GAM, Meynen G, Abma TA, van Balkom AJLM. A systematic review of the literature about competence and poor insight. Acta Psychiatr Scand. 2012;125(2):103–13.
CAS PubMed Google Scholar
Spencer BWJ, Shields G, Gergel T, Hotopf M, Owen GS. Diversity or disarray? A systematic review of decision-making capacity for treatment and research in schizophrenia and other non-affective psychoses. Psychol Med. 2017;47(11):1906–22.
Bin WS, Wang YY, Ungvari GS, Ng CH, Wu RR, Wang J, et al. The MacArthur competence assessment tools for assessing decision-making capacity in schizophrenia: a meta-analysis. Schizophr Res. 2017;183:56–63. Available from. https://doi.org/10.1016/j.schres.2016.11.020 .
Article Google Scholar
Woodrow A, Sparks S, Bobrovskaia V, Paterson C, Murphy P, Hutton P. Decision-making ability in psychosis: a systematic review and meta-analysis of the magnitude, specificity and correlates of impaired performance on the Iowa and Cambridge Gambling Tasks. Psychol Med. 2019;49(1):32–38.
Shea BJ, Reeves BC, Wells G, Thuku M, Hamel C, Moran J, et al. AMSTAR 2: a critical appraisal tool for systematic reviews that include randomised or non-randomised studies of healthcare interventions, or both. BMJ. 2017;358:1–9.
Heerey E, Bell-Warren K, Gold J. Decision-making impairments in the context of intact reward sensitivity in schizophrenia. Biol Psychiatry. 2008;64(1):62–9.
Nestor P, Choate V, Niznikiewicz M, Levitt J, Shenton M, McCarley R. Neuropsychology of reward learning and negative symptoms in schizophrenia. Schizophr Res. 2014;159(0):506–8.
Cooke A, Smith D, Booth A. Beyond PICO. Qual Health Res. 2012;22(10):1435–43 Available from: http://journals.sagepub.com/doi/10.1177/1049732312452938 .
Gold JM, Waltz JA, Prentice KJ, Morris SE, Heerey EA. Reward processing in schizophrenia: a deficit in the representation of value. Schizophr Bull. 2008;34(5):835–47.
Maguire S, Rea SM. Convery. Electroconvulsive therapy - what do patients think of their treatment? Ulster Med J. 2016;85(3):182–6.
CAS PubMed PubMed Central Google Scholar
Nakanishi M, Setoya Y, Kodaka M, Makino H, Nishimura A, Yamauchi K, et al. Symptom dimensions and needs of care among patients with schizophrenia in hospital and the community. Psychiatry Clin Neurosci. 2007;61(5):495–501.
Maitre E, Debien C, Nicaise P, Wyngaerden F, LeGadulec M, et al. Advanced directives in psychiatry: a review of the qualitative literature, a state-of-the-art and viewpoints. Encephale. 2013;39(4):244–51.
Wilder C, Elbogen E, Moser L, Swanson J, Swartz M. Medication preferences and adherence among individuals with severe mental illness who completed psychiatric advance directives. Psychiatr Serv. 2010;61(4):380–5.
Gergel T, Owen GS. Fluctuating capacity and advance decision-making in bipolar affective disorder - self-binding directives and self-determination. Int J Law Psychiatry [Internet]. 2015;40:92–101. Available from:. https://doi.org/10.1016/j.ijlp.2015.04.004 .
Fisher A, Manicavasagar V, Kiln F, Juraskova I. Communication and decision-making in mental health: a systematic review focusing on bipolar disorder. Patient Educ Couns. 2016;99(7):1106–20. Available from:. https://doi.org/10.1016/j.pec.2016.02.011 .
Article PubMed Google Scholar
Stovell D, Morrison AP, Panayiotou M, Hutton P. Shared treatment decision-making and empowerment-related outcomes in psychosis: systematic review and meta-analysis. Br J Psychiatry. 2016;209(1):23–8.
Dornan J, Kennedy M, Garland J, Rutledge E, Kennedy HG. Functional mental capacity, treatment as usual and time: magnitude of change in secure hospital patients with major mental illness psychiatry. BMC Res Notes. 2015;8(1):1–9.
Asher L, Patel V, De Silva M. Community-based psychosocial interventions for people with schizophrenia in low and middle-income countries: systematic review and meta-analysis. BMC Psychiatry. 2017;17:355. https://doi.org/10.1186/s12888-017-1516-7 .
Schaefer LA. MacArthur competence assessment tools. In: Kreutzer J.S., DeLuca J., Caplan B. (eds) Encyclopedia of Clinical Neuropsychology. New York: Springer; 2011.
Owen G, David A, Hayward P, et al. Retrospective views of psychiatric in-patients regaining mental capacity. Br J Psychiatry. 2009;195(5):403–7.
Moser DJ, Reese RL, Hey CT, Schultz SK, Arndt S, Beglinger LJ, et al. Using a brief intervention to improve decisional capacity in schizophrenia research. Schizophr Bull. 2006;32(1):116–20.
Wang X, Yu X, Appelbaum S, Tang H, Yao G, Si T, et al. Longitudinal informed consent competency in stable community patients with schizophrenia: a one-week training and one-year follow-up study. Schizophr Res. 2016;170(1):162–7.
Sugawara N, Yasui-Furukori N, Sumiyoshi T. Competence to consent and its relationship with cognitive function in patients with schizophrenia. Front Psychiatry. 2019;10:195. Published 2019 Apr 12. https://doi.org/10.3389/fpsyt.2019.00195 .
Article PubMed PubMed Central Google Scholar
Jacob K. Recovery model of mental illness: a complementary approach to psychiatric care. Indian J Psychol Med. 2015;37(2):117–9.
Download references
Acknowledgements
Not applicable.
Ferrer funded the development of the study and the writing of the manuscript.
Author information
Authors and affiliations.
Department of Psychiatry, Hospital Gregorio Marañón; Medical School, Universidad Complutense de Madrid, Doctor Esquerdo 46, 28007, Madrid, Spain
A. Calcedo-Barba
Adult Psychiatry Service and Geneva Penal Medicine Division, Geneva University Hospitals, Puplinge, Switzerland
A. Fructuoso
Psychiatry Service, University Hospital Doctor Peset, University of Valencia, Valencia, Spain
J. Martinez-Raga
SmartWriting4U, Valencia, Spain
Medical School, Universidad Anáhuac, Mexico City, Mexico
M. Sánchez de Carmona
Department of Psychiatry, Parc Sanitari Sant Joan de Déu, Barcelona, Spain
You can also search for this author in PubMed Google Scholar
Contributions
SP, ACB, EV designed the study. SP developed the review and produced drafts of the manuscript. SP, ACB, EV, JMR, AF, MSC interpreted the data and were major contributors in writing the manuscript. SP, ACB, EV, JMR, AF, MSC substantially revised, and approved the final manuscript. SP, ACB, EV, JMR, AF, MSC agreed to be personally accountable for the author’s own contributions and to ensure that questions related to the accuracy or integrity of any part of the work are appropriately investigated, resolved, and the resolution documented in the literature.
Corresponding author
Correspondence to A. Calcedo-Barba .
Ethics declarations
Ethics approval and consent to participate, consent for publication, competing interests.
The authors declare that they have no competing interests.
Additional information
Publisher’s note.
Springer Nature remains neutral with regard to jurisdictional claims in published maps and institutional affiliations.
Supplementary information
Additional file 1..
Meta- review search strategy.
Rights and permissions
Open Access This article is licensed under a Creative Commons Attribution 4.0 International License, which permits use, sharing, adaptation, distribution and reproduction in any medium or format, as long as you give appropriate credit to the original author(s) and the source, provide a link to the Creative Commons licence, and indicate if changes were made. The images or other third party material in this article are included in the article's Creative Commons licence, unless indicated otherwise in a credit line to the material. If material is not included in the article's Creative Commons licence and your intended use is not permitted by statutory regulation or exceeds the permitted use, you will need to obtain permission directly from the copyright holder. To view a copy of this licence, visit http://creativecommons.org/licenses/by/4.0/ . The Creative Commons Public Domain Dedication waiver ( http://creativecommons.org/publicdomain/zero/1.0/ ) applies to the data made available in this article, unless otherwise stated in a credit line to the data.
Reprints and permissions
About this article
Cite this article.
Calcedo-Barba, A., Fructuoso, A., Martinez-Raga, J. et al. A meta-review of literature reviews assessing the capacity of patients with severe mental disorders to make decisions about their healthcare. BMC Psychiatry 20 , 339 (2020). https://doi.org/10.1186/s12888-020-02756-0
Download citation
Received : 23 December 2019
Accepted : 23 June 2020
Published : 30 June 2020
DOI : https://doi.org/10.1186/s12888-020-02756-0
Share this article
Anyone you share the following link with will be able to read this content:
Sorry, a shareable link is not currently available for this article.
Provided by the Springer Nature SharedIt content-sharing initiative
- Decision making capacity
- Mental disorder
- Schizophrenia
- Bipolar disorder
- Literature review
- Meta-review
BMC Psychiatry
ISSN: 1471-244X
- Submission enquiries: [email protected]
- General enquiries: [email protected]
Thank you for visiting nature.com. You are using a browser version with limited support for CSS. To obtain the best experience, we recommend you use a more up to date browser (or turn off compatibility mode in Internet Explorer). In the meantime, to ensure continued support, we are displaying the site without styles and JavaScript.
- View all journals
- My Account Login
- Explore content
- About the journal
- Publish with us
- Sign up for alerts
- Review Article
- Open access
- Published: 22 April 2020
Deep learning in mental health outcome research: a scoping review
- Chang Su 1 ,
- Zhenxing Xu 1 ,
- Jyotishman Pathak 1 &
- Fei Wang 1
Translational Psychiatry volume 10 , Article number: 116 ( 2020 ) Cite this article
49k Accesses
137 Citations
20 Altmetric
Metrics details
- Psychiatric disorders
Mental illnesses, such as depression, are highly prevalent and have been shown to impact an individual’s physical health. Recently, artificial intelligence (AI) methods have been introduced to assist mental health providers, including psychiatrists and psychologists, for decision-making based on patients’ historical data (e.g., medical records, behavioral data, social media usage, etc.). Deep learning (DL), as one of the most recent generation of AI technologies, has demonstrated superior performance in many real-world applications ranging from computer vision to healthcare. The goal of this study is to review existing research on applications of DL algorithms in mental health outcome research. Specifically, we first briefly overview the state-of-the-art DL techniques. Then we review the literature relevant to DL applications in mental health outcomes. According to the application scenarios, we categorize these relevant articles into four groups: diagnosis and prognosis based on clinical data, analysis of genetics and genomics data for understanding mental health conditions, vocal and visual expression data analysis for disease detection, and estimation of risk of mental illness using social media data. Finally, we discuss challenges in using DL algorithms to improve our understanding of mental health conditions and suggest several promising directions for their applications in improving mental health diagnosis and treatment.
Similar content being viewed by others
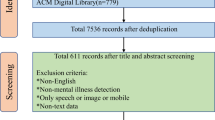
Natural language processing applied to mental illness detection: a narrative review
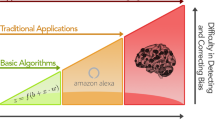
Predicting the future of neuroimaging predictive models in mental health
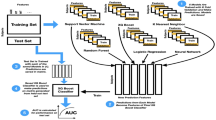
Predictive modeling of depression and anxiety using electronic health records and a novel machine learning approach with artificial intelligence
Introduction.
Mental illness is a type of health condition that changes a person’s mind, emotions, or behavior (or all three), and has been shown to impact an individual’s physical health 1 , 2 . Mental health issues including depression, schizophrenia, attention-deficit hyperactivity disorder (ADHD), and autism spectrum disorder (ASD), etc., are highly prevalent today and it is estimated that around 450 million people worldwide suffer from such problems 1 . In addition to adults, children and adolescents under the age of 18 years also face the risk of mental health disorders. Moreover, mental health illnesses have also been one of the most serious and prevalent public health problems. For example, depression is a leading cause of disability and can lead to an increased risk for suicidal ideation and suicide attempts 2 .
To better understand the mental health conditions and provide better patient care, early detection of mental health problems is an essential step. Different from the diagnosis of other chronic conditions that rely on laboratory tests and measurements, mental illnesses are typically diagnosed based on an individual’s self-report to specific questionnaires designed for the detection of specific patterns of feelings or social interactions 3 . Due to the increasing availability of data pertaining to an individual’s mental health status, artificial intelligence (AI) and machine learning (ML) technologies are being applied to improve our understanding of mental health conditions and have been engaged to assist mental health providers for improved clinical decision-making 4 , 5 , 6 . As one of the latest advances in AI and ML, deep learning (DL), which transforms the data through layers of nonlinear computational processing units, provides a new paradigm to effectively gain knowledge from complex data 7 . In recent years, DL algorithms have demonstrated superior performance in many data-rich application scenarios, including healthcare 8 , 9 , 10 .
In a previous study, Shatte et al. 11 explored the application of ML techniques in mental health. They reviewed literature by grouping them into four main application domains: diagnosis, prognosis, and treatment, public health, as well as research and clinical administration. In another study, Durstewitz et al. 9 explored the emerging area of application of DL techniques in psychiatry. They focused on DL in the studies of brain dynamics and subjects’ behaviors, and presented the insights of embedding the interpretable computational models into statistical context. In contrast, this study aims to provide a scoping review of the existing research applying DL methodologies on the analysis of different types of data related to mental health conditions. The reviewed articles are organized into four main groups according to the type of the data analyzed, including the following: (1) clinical data, (2) genetic and genomics data, (3) vocal and visual expression data, and (4) social media data. Finally, the challenges the current studies faced with, as well as future research directions towards bridging the gap between the application of DL algorithms and patient care, are discussed.
Deep learning overview
ML aims at developing computational algorithms or statistical models that can automatically infer hidden patterns from data 12 , 13 . Recent years have witnessed an increasing number of ML models being developed to analyze healthcare data 4 . However, conventional ML approaches require a significant amount of feature engineering for optimal performance—a step that is necessary for most application scenarios to obtain good performance, which is usually resource- and time-consuming.
As the newest wave of ML and AI technologies, DL approaches aim at the development of an end-to-end mechanism that maps the input raw features directly into the outputs through a multi-layer network structure that is able to capture the hidden patterns within the data. In this section, we will review several popular DL model architectures, including deep feedforward neural network (DFNN), recurrent neural network (RNN) 14 , convolutional neural network (CNN) 15 , and autoencoder 16 . Figure 1 provides an overview of these architectures.
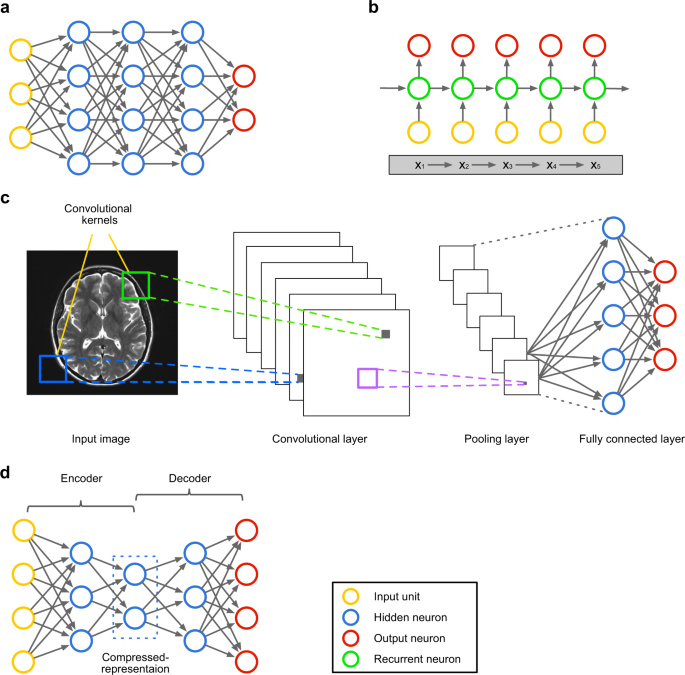
a Deep feedforward neural network (DFNN). It is the basic design of DL models. Commonly, a DFNN contains multiple hidden layers. b A recurrent neural network (RNN) is presented to process sequence data. To encode history information, each recurrent neuron receives the input element and the state vector of the predecessor neuron, and yields a hidden state fed to the successor neuron. For example, not only the individual information but also the dependence of the elements of the sequence x 1 → x 2 → x 3 → x 4 → x 5 is encoded by the RNN architecture. c Convolutional neural network (CNN). Between input layer (e.g., input neuroimage) and output layer, a CNN commonly contains three types of layers: the convolutional layer that is to generate feature maps by sliding convolutional kernels in the previous layer; the pooling layer is used to reduce dimensionality of previous convolutional layer; and the fully connected layer is to make prediction. For the illustrative purpose, this example only has one layer of each type; yet, a real-world CNN would have multiple convolutional and pooling layers (usually in an interpolated manner) and one fully connected layer. d Autoencoder consists of two components: the encoder, which learns to compress the input data into a latent representation layer by layer, whereas the decoder, inverse to the encoder, learns to reconstruct the data at the output layer. The learned compressed representations can be fed to the downstream predictive model.
Deep feedforward neural network
Artificial neural network (ANN) is proposed with the intention of mimicking how human brain works, where the basic element is an artificial neuron depicted in Fig. 2a . Mathematically, an artificial neuron is a nonlinear transformation unit, which takes the weighted summation of all inputs and feeds the result to an activation function, such as sigmoid, rectifier (i.e., rectified linear unit [ReLU]), or hyperbolic tangent (Fig. 2b ). An ANN is composed of multiple artificial neurons with different connection architectures. The simplest ANN architecture is the feedforward neural network (FNN), which stacks the neurons layer by layer in a feedforward manner (Fig. 1a ), where the neurons across adjacent layers are fully connected to each other. The first layer of the FNN is the input layer that each unit receives one dimension of the data vector. The last layer is the output layer that outputs the probabilities that a subject belonging to different classes (in classification). The layers between the input and output layers are the hidden layers. A DFNN usually contains multiple hidden layers. As shown in Fig. 2a , there is a weight parameter associated with each edge in the DFNN, which needs to be optimized by minimizing some training loss measured on a specific training dataset (usually through backpropagation 17 ). After the optimal set of parameters are learned, the DFNN can be used to predict the target value (e.g., class) of any testing data vectors. Therefore, a DFNN can be viewed as an end-to-end process that transforms a specific raw data vector to its target layer by layer. Compared with the traditional ML models, DFNN has shown superior performance in many data mining tasks and have been introduced to the analysis of clinical data and genetic data to predict mental health conditions. We will discuss the applications of these methods further in the Results section.
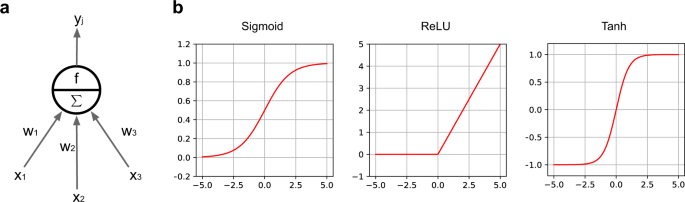
a An illustration of basic unit of neural networks, i.e., artificial neuron. Each input x i is associated with a weight w i . The weighted sum of all inputs Σ w i x i is fed to a nonlinear activation function f to generate the output y j of the j -th neuron, i.e., y j = f (Σ w i x i ). b Illustrations of the widely used nonlinear activation function.
Recurrent neural network
RNNs were designed to analyze sequential data such as natural language, speech, and video. Given an input sequence, the RNN processes one element of the sequence at a time by feeding to a recurrent neuron. To encode the historical information along the sequence, each recurrent neuron receives the input element at the corresponding time point and the output of the neuron at previous time stamp, and the output will also be provided to the neuron at next time stamp (this is also where the term “recurrent” comes from). An example RNN architecture is shown in Fig. 1b where the input is a sequence of words (a sentence). The recurrence link (i.e., the edge linking different neurons) enables RNN to capture the latent semantic dependencies among words and the syntax of the sentence. In recent years, different variants of RNN, such as long short-term memory (LSTM) 18 and gated recurrent unit 19 have been proposed, and the main difference among these models is how the input is mapped to the output for the recurrent neuron. RNN models have demonstrated state-of-the-art performance in various applications, especially natural language processing (NLP; e.g., machine translation and text-based classification); hence, they hold great premise in processing clinical notes and social media posts to detect mental health conditions as discussed below.
Convolutional neural network
CNN is a specific type of deep neural network originally designed for image analysis 15 , where each pixel corresponds to a specific input dimension describing the image. Similar to a DFNN, CNN also maps these input image pixels to the corresponding target (e.g., image class) through layers of nonlinear transformations. Different from DFNN, where only fully connected layers are considered, there are typically three types of layers in a CNN: a convolution–activation layer, a pooling layer, and a fully connected layer (Fig. 1c ). The convolution–activation layer first convolves the entire feature map obtained from previous layer with small two-dimensional convolution filters. The results from each convolution filter are activated through a nonlinear activation function in the same way as a DFNN. A pooling layer reduces the size of the feature map through sub-sampling. The fully connected layer is analogous to the hidden layer in a DFNN, where each neuron is connected to all neurons of the previous layer. The convolution–activation layer extracts locally invariant patterns from the feature maps. The pooling layer effectively reduces the feature dimensionality to avoid model overfitting. The fully connected layer explores the global feature interactions as in DFNNs. Different combinations of these three types of layers constitute different CNN architectures. Because of the various characteristics of images such as local self-similarity, compositionality, and translational and deformation invariance, CNN has demonstrated state-of-the-art performance in many computer vision tasks 7 . Hence, the CNN models are promising in processing clinical images and expression data (e.g., facial expression images) to detect mental health conditions. We will discuss the application of these methods in the Results section.
Autoencoder
Autoencoder is a special variant of the DFNN aimed at learning new (usually more compact) data representations that can optimally reconstruct the original data vectors 16 , 20 . An autoencoder typically consists of two components (Fig. 1d ) as follows: (1) the encoder, which learns new representations (usually with reduced dimensionality) from the input data through a multi-layer FNN; and (2) the decoder, which is exactly the reverse of the encoder, reconstructs the data in their original space from the representations derived from the encoder. The parameters in the autoencoder are learned through minimizing the reconstruction loss. Autoencoder has demonstrated the capacity of extracting meaningful features from raw data without any supervision information. In the studies of mental health outcomes, the use of autoencoder has resulted in desirable improvement in analyzing clinical and expression image data, which will be detailed in the Results section.
The processing and reporting of the results of this review were guided by the Preferred Reporting Items for Systematic Reviews and Meta-Analyses guidelines 21 . To thoroughly review the literature, a two-step method was used to retrieve all the studies on relevant topics. First, we conducted a search of the computerized bibliographic databases including PubMed and Web of Science. The search strategy is detailed in Supplementary Appendix 1 . The literature search comprised articles published until April 2019. Next, a snowball technique was applied to identify additional studies. Furthermore, we manually searched other resources, including Google Scholar, and Institute of Electrical and Electronics Engineers (IEEE Xplore), to find additional relevant articles.
Figure 3 presents the study selection process. All articles were evaluated carefully and studies were excluded if: (1) the main outcome is not a mental health condition; (2) the model involved is not a DL algorithm; (3) full-text of the article is not accessible; and (4) the article is written not in English.
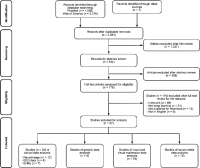
In total, 57 studies, in terms of clinical data analysis, genetic data analysis, vocal and visual expression data analysis, and social media data analysis, which met our eligibility criteria, were included in this review.
A total of 57 articles met our eligibility criteria. Most of the reviewed articles were published between 2014 and 2019. To clearly summarize these articles, we grouped them into four categories according to the types of data analyzed, including (1) clinical data, (2) genetic and genomics data, (3) vocal and visual expression data, and (4) social media data. Table 1 summarizes the characteristics of these selected studies.
Clinical data
Neuroimages.
Previous studies have shown that neuroimages can record evidence of neuropsychiatric disorders 22 , 23 . Two common types of neuroimage data analyzed in mental health studies are functional magnetic resonance imaging (fMRI) and structural MRI (sMRI) data. In fMRI data, the brain activity is measured by identification of the changes associated with blood flow, based on the fact that cerebral blood flow and neuronal activation are coupled 24 . In sMRI data, the neurological aspect of a brain is described based on the structural textures, which show some information in terms of the spatial arrangements of voxel intensities in 3D. Recently, DL technologies have been demonstrated in analyzing both fMRI and sMRI data.
One application of DL in fMRI and sMRI data is the identification of ADHD 25 , 26 , 27 , 28 , 29 , 30 , 31 . To learn meaningful information from the neuroimages, CNN and deep belief network (DBN) models were used. In particular, the CNN models were mainly used to identify local spatial patterns and DBN models were to obtain a deep hierarchical representation of the neuroimages. Different patterns were discovered between ADHDs and controls in the prefrontal cortex and cingulated cortex. Also, several studies analyzed sMRIs to investigate schizophrenia 32 , 33 , 34 , 35 , 36 , where DFNN, DBN, and autoencoder were utilized. These studies reported abnormal patterns of cortical regions and cortical–striatal–cerebellar circuit in the brain of schizophrenia patients, especially in the frontal, temporal, parietal, and insular cortices, and in some subcortical regions, including the corpus callosum, putamen, and cerebellum. Moreover, the use of DL in neuroimages also targeted at addressing other mental health disorders. Geng et al. 37 proposed to use CNN and autoencoder to acquire meaningful features from the original time series of fMRI data for predicting depression. Two studies 31 , 38 integrated the fMRI and sMRI data modalities to develop predictive models for ASDs. Significant relationships between fMRI and sMRI data were observed with regard to ASD prediction.
Challenges and opportunities
The aforementioned studies have demonstrated that the use of DL techniques in analyzing neuroimages can provide evidence in terms of mental health problems, which can be translated into clinical practice and facilitate the diagnosis of mental health illness. However, multiple challenges need to be addressed to achieve this objective. First, DL architectures generally require large data samples to train the models, which may pose a difficulty in neuroimaging analysis because of the lack of such data 39 . Second, typically the imaging data lie in a high-dimensional space, e.g., even a 64 × 64 2D neuroimage can result in 4096 features. This leads to the risk of overfitting by the DL models. To address this, most existing studies reported to utilize MRI data preprocessing tools such as Statistical Parametric Mapping ( https://www.fil.ion.ucl.ac.uk/spm/ ), Data Processing Assistant for Resting-State fMRI 40 , and fMRI Preprocessing Pipeline 41 to extract useful features before feeding to the DL models. Even though an intuitive attribute of DL is the capacity to learn meaningful features from raw data, feature engineering tools are needed especially in the case of small sample size and high-dimensionality, e.g., the neuroimage analysis. The use of such tools mitigates the overfitting risk of DL models. As reported in some selected studies 28 , 31 , 35 , 37 , the DL models can benefit from feature engineering techniques and have been shown to outperform the traditional ML models in the prediction of multiple conditions such as depression, schizophrenia, and ADHD. However, such tools extract features relying on prior knowledge; hence may omit some information that is meaningful for mental outcome research but unknown yet. An alternative way is to use CNN to automatically extract information from the raw data. As reported in the previous study 10 , CNNs perform well in processing raw neuroimage data. Among the studies reviewed in this study, three 29 , 30 , 37 reported to involve CNN layers and achieved desirable performances.
Electroencephalogram data
As a low-cost, small-size, and high temporal resolution signal containing up to several hundred channels, analysis of electroencephalogram (EEG) data has gained significant attention to study brain disorders 42 . As the EEG signal is one kind of streaming data that presents a high density and continuous characteristics, it challenges traditional feature engineering-based methods to obtain sufficient information from the raw EEG data to make accurate predictions. To address this, recently the DL models have been employed to analyze raw EEG signal data.
Four articles reviewed proposed to use DL in understanding mental health conditions based on the analysis of EEG signals. Acharya et al. 43 used CNN to extract features from the input EEG signals. They found that the EEG signals from the right hemisphere of the human brain are more distinctive in terms of the detection of depression than those from the left hemisphere. The findings provided shreds of evidence that depression is associated with a hyperactive right hemisphere. Mohan et al. 44 modeled the raw EEG signals by DFNN to obtain information about the human brain waves. They found that the signals collected from the central (C3 and C4) regions are marginally higher compared with other brain regions, which can be used to distinguish the depressed and normal subjects from the brain wave signals. Zhang et al. 45 proposed a concatenated structure of deep recurrent and 3D CNN to obtain EEG features across different tasks. They reported that the DL model can capture the spectral changes of EEG hemispheric asymmetry to distinguish different mental workload effectively. Li et al. 46 presented a computer-aided detection system by extracting multiple types of information (e.g., spectral, spatial, and temporal information) to recognize mild depression based on CNN architecture. The authors found that both spectral and temporal information of EEG are crucial for prediction of depression.
EEG data are usually classified as streaming data that are continuous and are of high density. Despite the initial success in applying DL algorithms to analyze EEG data for studying multiple mental health conditions, there exist several challenges. One major challenge is that raw EEG data gathered from sensors have a certain degree of erroneous, noisy, and redundant information caused by discharged batteries, failures in sensor readings, and intermittent communication loss in wireless sensor networks 47 . This may challenge the model in extracting meaningful information from noise. Multiple preprocessing steps (e.g., data denoising, data interpolation, data transformation, and data segmentation) are necessary for dealing with the raw EEG signal before feeding to the DL models. Besides, due to the dense characteristics in the raw EEG data, analysis of the streaming data is computationally more expensive, which poses a challenge for the model architecture selection. A proper model should be designed relatively with less training parameters. This is one reason why the reviewed studies are mainly based on the CNN architecture.
Electronic health records
Electronic health records (EHRs) are systematic collections of longitudinal, patient-centered records. Patients’ EHRs consist of both structured and unstructured data: the structured data include information about a patient’s diagnosis, medications, and laboratory test results, and the unstructured data include information in clinical notes. Recently, DL models have been applied to analyze EHR data to study mental health disorders 48 .
The first and foremost issue for analyzing the structured EHR data is how to appropriately handle the longitudinal records. Traditional ML models address this by collapsing patients’ records within a certain time window into vectors, which comprised the summary of statistics of the features in different dimensions 49 . For instance, to estimate the probability of suicide deaths, Choi et al. 50 leveraged a DFNN to model the baseline characteristics. One major limitation of these studies is the omittance of temporality among the clinical events within EHRs. To overcome this issue, RNNs are more commonly used for EHR data analysis as an RNN intuitively handles time-series data. DeepCare 51 , a long short-term memory network (LSTM)-based DL model, encodes patient’s long-term health state trajectories to predict the future outcomes of depressive episodes. As the LSTM architecture appropriately captures disease progression by modeling the illness history and the medical interventions, DeepCare achieved over 15% improvement in prediction, compared with the conventional ML methods. In addition, Lin et al. 52 designed two DFNN models for the prediction of antidepressant treatment response and remission. The authors reported that the proposed DFNN can achieve an area under the receiver operating characteristic curve (AUC) of 0.823 in predicting antidepressant response.
Analyzing the unstructured clinical notes in EHRs refers to the long-standing topic of NLP. To extract meaningful knowledge from the text, conventional NLP approaches mostly define rules or regular expressions before the analysis. However, it is challenging to enumerate all possible rules or regular expressions. Due to the recent advance of DL in NLP tasks, DL models have been developed to mine clinical text data from EHRs to study mental health conditions. Geraci et al. 53 utilized term frequency-inverse document frequency to represent the clinical documents by words and developed a DFNN model to identify individuals with depression. One major limitation of such an approach is that the semantics and syntax of sentences are lost. In this context, CNN 54 and RNN 55 have shown superiority in modeling syntax for text-based prediction. In particular, CNN has been used to mine the neuropsychiatric notes for predicting psychiatric symptom severity 56 , 57 . Tran and Kavuluru 58 used an RNN to analyze the history of present illness in neuropsychiatric notes for predicting mental health conditions. The model engaged an attention mechanism 55 , which can specify the importance of the words in prediction, making the model more interpretable than their previous CNN model 56 .
Although DL has achieved promising results in EHR analysis, several challenges remain unsolved. On one hand, different from diagnosing physical health condition such as diabetes, the diagnosis of mental health conditions lacks direct quantitative tests, such as a blood chemistry test, a buccal swab, or urinalysis. Instead, the clinicians evaluate signs and symptoms through patient interviews and questionnaires during which they gather information based on patient’s self-report. Collection and deriving inferences from such data deeply relies on the experience and subjectivity of the clinician. This may account for signals buried in noise and affect the robustness of the DL model. To address this challenge, a potential way is to comprehensively integrate multimodal clinical information, including structured and unstructured EHR information, as well as neuroimaging and EEG data. Another way is to incorporate existing medical knowledge, which can guide model being trained in the right direction. For instance, the biomedical knowledge bases contain massive verified interactions between biomedical entities, e.g., diseases, genes, and drugs 59 . Incorporating such information brings in meaningful medical constraints and may help to reduce the effects of noise on model training process. On the other hand, implementing a DL model trained from one EHR system into another system is challenging, because EHR data collection and representation is rarely standardized across hospitals and clinics. To address this issue, national/international collaborative efforts such as Observational Health Data Sciences and Informatics ( https://ohdsi.org ) have developed common data models, such as OMOP, to standardize EHR data representation for conducting observational data analysis 60 .
Genetic data
Multiple studies have found that mental disorders, e.g., depression, can be associated with genetic factors 61 , 62 . Conventional statistical studies in genetics and genomics, such as genome-wide association studies, have identified many common and rare genetic variants, such as single-nucleotide polymorphisms (SNPs), associated with mental health disorders 63 , 64 . Yet, the effect of the genetic factors is small and many more have not been discovered. With the recent developments in next-generation sequencing techniques, a massive volume of high-throughput genome or exome sequencing data are being generated, enabling researchers to study patients with mental health disorders by examining all types of genetic variations across an individual’s genome. In recent years, DL 65 , 66 has been applied to identify genetic risk factors associated with mental illness, by borrowing the capacity of DL in identifying highly complex patterns in large datasets. Khan and Wang 67 integrated genetic annotations, known brain expression quantitative trait locus, and enhancer/promoter peaks to generate feature vectors of variants, and developed a DFNN, named ncDeepBrain, to prioritized non-coding variants associated with mental disorders. To further prioritize susceptibility genes, they designed another deep model, iMEGES 68 , which integrates the ncDeepBrain score, general gene scores, and disease-specific scores for estimating gene risk. Wang et al. 69 developed a novel deep architecture that combines deep Boltzmann machine architecture 70 with conditional and lateral connections derived from the gene regulatory network. The model provided insights about intermediate phenotypes and their connections to high-level phenotypes (disease traits). Laksshman et al. 71 used exome sequencing data to predict bipolar disorder outcomes of patients. They developed a CNN and used the convolution mechanism to capture correlations of the neighboring loci within the chromosome.
Although the use of genetic data in DL in studying mental health conditions shows promise, multiple challenges need to be addressed. For DL-based risk c/gene prioritization efforts, one major challenge is the limitation of labeled data. On one hand, the positive samples are limited, as known risk SNPs or genes associated with mental health conditions are limited. For example, there are about 108 risk loci that were genome-wide significant in ASD. On the other hand, the negative samples (i.e., SNPs, variants, or genes) may not be the “true” negative, as it is unclear whether they are associated with the mental illness yet. Moreover, it is also challenging to develop DL models for analyzing patient’s sequencing data for mental illness prediction, as the sequencing data are extremely high-dimensional (over five million SNPs in the human genome). More prior domain knowledge is needed to guide the DL model extracting patterns from the high-dimensional genomic space.
Vocal and visual expression data
The use of vocal (voice or speech) and visual (video or image of facial or body behaviors) expression data has gained the attention of many studies in mental health disorders. Modeling the evolution of people’s emotional states from these modalities has been used to identify mental health status. In essence, the voice data are continuous and dense signals, whereas the video data are sequences of frames, i.e., images. Conventional ML models for analyzing such types of data suffer from the sophisticated feature extraction process. Due to the recent success of applying DL in computer vision and sequence data modeling, such models have been introduced to analyze the vocal and/or visual expression data. In this work, most articles reviewed are to predict mental health disorders based on two public datasets: (i) the Chi-Mei corpus, collected by using six emotional videos to elicit facial expressions and speech responses of the subjects of bipolar disorder, unipolar depression, and healthy controls; 72 and (ii) the International Audio/Visual Emotion Recognition Challenges (AVEC) depression dataset 73 , 74 , 75 , collected within human–computer interaction scenario. The proposed models include CNNs, RNNs, autoencoders, as well as hybrid models based on the above ones. In particular, CNNs were leveraged to encode the temporal and spectral features from the voice signals 76 , 77 , 78 , 79 , 80 and static facial or physical expression features from the video frames 79 , 81 , 82 , 83 , 84 . Autoencoders were used to learn low-dimensional representations for people’s vocal 85 , 86 and visual expression 87 , 88 , and RNNs were engaged to characterize the temporal evolution of emotion based on the CNN-learned features and/or other handcraft features 76 , 81 , 84 , 85 , 86 , 87 , 88 , 89 , 90 . Few studies focused on analyzing static images using a CNN architecture to predict mental health status. Prasetio et al. 91 identified the stress types (e.g., neutral, low stress, and high stress) from facial frontal images. Their proposed CNN model outperforms the conventional ML models by 7% in terms of prediction accuracy. Jaiswal et al. 92 investigated the relationship between facial expression/gestures and neurodevelopmental conditions. They reported accuracy over 0.93 in the diagnostic prediction of ADHD and ASD by using the CNN architecture. In addition, thermal images that track persons’ breathing patterns were also fed to a deep model to estimate psychological stress level (mental overload) 93 .
From the above summary, we can observe that analyzing vocal and visual expression data can capture the pattern of subjects’ emotion evolution to predict mental health conditions. Despite the promising initial results, there remain challenges for developing DL models in this field. One major challenge is to link vocal and visual expression data with the clinical data of patients, given the difficulties involved in collecting such expression data during clinical practice. Current studies analyzed vocal and visual expression over individual datasets. Without clinical guidance, the developed prediction models have limited clinical meanings. Linking patients’ expression information with clinical variables may help to improve both the interpretability and robustness of the model. For example, Gupta et al. 94 designed a DFNN for affective prediction from audio and video modalities. The model incorporated depression severity as the parameter, linking the effects of depression on subjects’ affective expressions. Another challenge is the limitation of the samples. For example, the Chi-Mei dataset contains vocal–visual data from only 45 individuals (15 with bipolar disorder, 15 with unipolar disorder, and 15 healthy controls). Also, there is a lack of “emotion labels” for people’s vocal and visual expression. Apart from improving the datasets, an alternative way to solve this challenge is to use transfer learning, which transfers knowledge gained with one dataset (usually more general) to the target dataset. For example, some studies trained autoencoder in public emotion database such as eNTERFACE 95 to generate emotion profiles (EPs). Other studies 83 , 84 pre-trained CNN over general facial expression datasets 96 , 97 for extracting face appearance features.
Social media data
With the widespread proliferation of social media platforms, such as Twitter and Reddit, individuals are increasingly and publicly sharing information about their mood, behavior, and any ailments one might be suffering. Such social media data have been used to identify users’ mental health state (e.g., psychological stress and suicidal ideation) 6 .
In this study, the articles that used DL to analyze social media data mainly focused on stress detection 98 , 99 , 100 , 101 , depression identification 102 , 103 , 104 , 105 , 106 , and estimation of suicide risk 103 , 105 , 107 , 108 , 109 . In general, the core concept across these work is to mine the textual, and where applicable graphical, content of users’ social media posts to discover cues for mental health disorders. In this context, the RNN and CNN were largely used by the researchers. Especially, RNN usually introduces an attention mechanism to specify the importance of the input elements in the classification process 55 . This provides some interpretability for the predictive results. For example, Ive et al. 103 proposed a hierarchical RNN architecture with an attention mechanism to predict the classes of the posts (including depression, autism, suicidewatch, anxiety, etc.). The authors observed that, benefitting from the attention mechanism, the model can predict risk text efficiently and extract text elements crucial for making decisions. Coppersmith et al. 107 used LSTM to discover quantifiable signals about suicide attempts based on social media posts. The proposed model can capture contextual information between words and obtain nuances of language related to suicide.
Apart from text, users also post images on social media. The properties of the images (e.g., color theme, saturation, and brightness) provide some cues reflecting users’ mental health status. In addition, millions of interactions and relationships among users can reflect the social environment of individuals that is also a kind of risk factors for mental illness. An increasing number of studies attempted to combine these two types of information with text content for predictive modeling. For example, Lin et al. 99 leveraged the autoencoder to extract low-level and middle-level representations from texts, images, and comments based on psychological and art theories. They further extended their work with a hybrid model based on CNN by integrating post content and social interactions 101 . The results provided an implication that the social structure of the stressed users’ friends tended to be less connected than that of the users without stress.
The aforementioned studies have demonstrated that using social media data has the potential to detect users with mental health problems. However, there are multiple challenges towards the analysis of social media data. First, given that social media data are typically de-identified, there is no straightforward way to confirm the “true positives” and “true negatives” for a given mental health condition. Enabling the linkage of user’s social media data with their EHR data—with appropriate consent and privacy protection—is challenging to scale, but has been done in a few settings 110 . In addition, most of the previous studies mainly analyzed textual and image data from social media platforms, and did not consider analyzing the social network of users. In one study, Rosenquist et al. 111 reported that the symptoms of depression are highly correlated inside the circle of friends, indicating that social network analysis is likely to be a potential way to study the prevalence of mental health problems. However, comprehensively modeling text information and network structure remains challenging. In this context, graph convolutional networks 112 have been developed to address networked data mining. Moreover, although it is possible to discover online users with mental illness by social media analysis, translation of this innovation into practical applications and offer aid to users, such as providing real-time interventions, are largely needed 113 .
Discussion: findings, open issues, and future directions
Principle findings.
The purpose of this study is to investigate the current state of applications of DL techniques in studying mental health outcomes. Out of 2261 articles identified based on our search terms, 57 studies met our inclusion criteria and were reviewed. Some studies that involved DL models but did not highlight the DL algorithms’ features on analysis were excluded. From the above results, we observed that there are a growing number of studies using DL models for studying mental health outcomes. Particularly, multiple studies have developed disease risk prediction models using both clinical and non-clinical data, and have achieved promising initial results.
DL models “think to learn” like a human brain relying on their multiple layers of interconnected computing neurons. Therefore, to train a deep neural network, there are multiple parameters (i.e., weights associated links between neurons within the network) being required to learn. This is one reason why DL has achieved great success in the fields where a massive volume of data can be easily collected, such as computer vision and text mining. Yet, in the health domain, the availability of large-scale data is very limited. For most selected studies in this review, the sample sizes are under a scale of 10 4 . Data availability is even more scarce in the fields of neuroimaging, EEG, and gene expression data, as such data reside in a very high-dimensional space. This then leads to the problem of “curse of dimensionality” 114 , which challenges the optimization of the model parameters.
One potential way to address this challenge is to reduce the dimensionality of the data by feature engineering before feeding information to the DL models. On one hand, feature extraction approaches can be used to obtain different types of features from the raw data. For example, several studies reported in this review have attempted to use preprocessing tools to extract features from neuroimaging data. On the other hand, feature selection that is commonly used in conventional ML models is also an option to reduce data dimensionality. However, the feature selection approaches are not often used in the DL application scenario, as one of the intuitive attributes of DL is the capacity to learn meaningful features from “all” available data. The alternative way to address the issue of data bias is to use transfer learning where the objective is to improve learning a new task through the transfer of knowledge from a related task that has already been learned 115 . The basic idea is that data representations learned in the earlier layers are more general, whereas those learned in the latter layers are more specific to the prediction task 116 . In particular, one can first pre-train a deep neural network in a large-scale “source” dataset, then stack fully connected layers on the top of the network and fine-tune it in the small “target” dataset in a standard backpropagation manner. Usually, samples in the “source” dataset are more general (e.g., general image data), whereas those in the “target” dataset are specific to the task (e.g., medical image data). A popular example of the success of transfer learning in the health domain is the dermatologist-level classification of skin cancer 117 . The authors introduced Google’s Inception v3 CNN architecture pre-trained over 1.28 million general images and fine-tuned in the clinical image dataset. The model achieved very high-performance results of skin cancer classification in epidermal (AUC = 0.96), melanocytic (AUC = 0.96), and melanocytic–dermoscopic images (AUC = 0.94). In facial expression-based depression prediction, Zhu et al. 83 pre-trained CNN on the public face recognition dataset to model the static facial appearance, which overcomes the issue that there is no facial expression label information. Chao et al. 84 also pre-trained CNN to encode facial expression information. The transfer scheme of both of the two studies has been demonstrated to be able to improve the prediction performance.
Diagnosis and prediction issues
Unlike the diagnosis of physical conditions that can be based on lab tests, diagnoses of the mental illness typically rely on mental health professionals’ judgment and patient self-report data. As a result, such a diagnostic system may not accurately capture the psychological deficits and symptom progression to provide appropriate therapeutic interventions 118 , 119 . This issue accordingly accounts for the limitation of the prediction models to assist clinicians to make decisions. Except for several studies using the unsupervised autoencoder for learning low-dimensional representations, most studies reviewed in this study reported using supervised DL models, which need the training set containing “true” (i.e., expert provided) labels to optimize the model parameters before the model being used to predict labels of new subjects. Inevitably, the quality of the expert-provided diagnostic labels used for training sets the upper-bound for the prediction performance of the model.
One intuitive route to address this issue is to use an unsupervised learning scheme that, instead of learning to predict clinical outcomes, aims at learning compacted yet informative representations of the raw data. A typical example is the autoencoder (as shown in Fig. 1d ), which encodes the raw data into a low-dimensional space, from which the raw data can be reconstructed. Some studies reviewed have proposed to leverage autoencoder to improve our understanding of mental health outcomes. A constraint of the autoencoder is that the input data should be preprocessed to vectors, which may lead to information loss for image and sequence data. To address this, recently convolutional-autoencoder 120 and LSTM-autoencoder 121 have been developed, which integrate the convolution layers and recurrent layers with the autoencoder architecture and enable us to learn informative low-dimensional representations from the raw image data and sequence data, respectively. For instance, Baytas et al. 122 developed a variation of LSTM-autoencoder on patient EHRs and grouped Parkinson’s disease patients into meaningful subtypes. Another potential way is to predict other clinical outcomes instead of the diagnostic labels. For example, several selected studies proposed to predict symptom severity scores 56 , 57 , 77 , 82 , 84 , 87 , 89 . In addition, Du et al. 108 attempted to identify suicide-related psychiatric stressors from users’ posts on Twitter, which plays an important role in the early prevention of suicidal behaviors. Furthermore, training model to predict future outcomes such as treatment response, emotion assessments, and relapse time is also a promising future direction.
Multimodal modeling
The field of mental health is heterogeneous. On one hand, mental illness refers to a variety of disorders that affect people’s emotions and behaviors. On the other hand, though the exact causes of most mental illnesses are unknown to date, it is becoming increasingly clear that the risk factors for these diseases are multifactorial as multiple genetic, environmental, and social factors interact to influence an individual’s mental health 123 , 124 . As a result of domain heterogeneity, researchers have the chance to study the mental health problems from different perspectives, from molecular, genomic, clinical, medical imaging, physiological signal to facial, and body expressive and online behavioral. Integrative modeling of such multimodal data means comprehensively considering different aspects of the disease, thus likely obtaining deep insight into mental health. In this context, DL models have been developed for multimodal modeling. As shown in Fig. 4 , the hierarchical structure of DL makes it easily compatible with multimodal integration. In particular, one can model each modality with a specific network and combine them by the final fully connected layers, such that parameters can be jointly learned by a typical backpropagation manner. In this review, we found an increasing number of studies have attempted to use multimodal modeling. For example, Zou et al. 28 developed a multimodal model composed of two CNNs for modeling fMRI and sMRI modalities, respectively. The model achieved 69.15% accuracy in predicting ADHD, which outperformed the unimodal models (66.04% for fMRI modal-based and 65.86% for sMRI modal-based). Yang et al. 79 proposed a multimodal model to combine vocal and visual expression for depression cognition. The model results in 39% lower prediction error than the unimodal models.
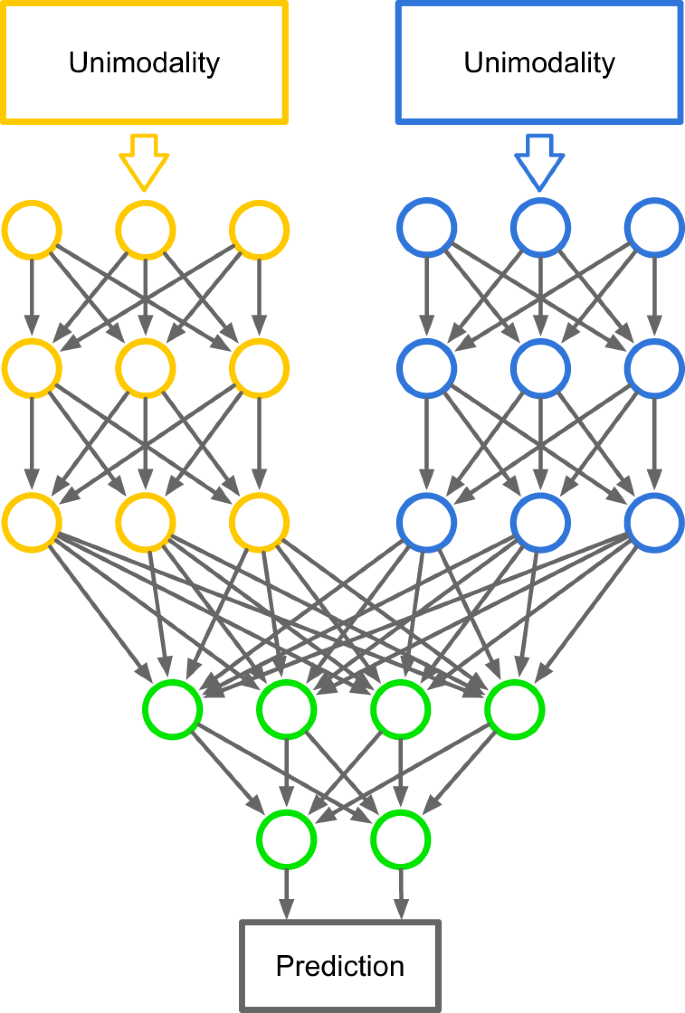
One can model each modality with a specific network and combine them using the final fully-connected layers. In this way, parameters of the entire neural network can be jointly learned in a typical backpropagation manner.
Model interpretability
Due to the end-to-end design, the DL models usually appear to be “black boxes”: they take raw data (e.g., MRI images, free-text of clinical notes, and EEG signals) as input, and yield output to reach a conclusion (e.g., the risk of a mental health disorder) without clear explanations of their inner working. Although this might not be an issue in other application domains such as identifying animals from images, in health not only the model’s prediction performance but also the clues for making the decision are important. For example in the neuroimage-based depression identification, despite estimation of the probability that a patient suffers from mental health deficits, the clinicians would focus more on recognizing abnormal regions or patterns of the brain associated with the disease. This is really important for convincing the clinical experts about the actions recommended from the predictive model, as well as for guiding appropriate interventions. In addition, as discussed above, the introduction of multimodal modeling leads to an increased challenge in making the models more interpretable. Attempts have been made to open the “black box” of DL 59 , 125 , 126 , 127 . Currently, there are two general directions for interpretable modeling: one is to involve the systematic modification of the input and the measure of any resulting changes in the output, as well as in the activation of the artificial neurons in the hidden layers. Such a strategy is usually used in CNN in identifying specific regions of an image being captured by a convolutional layer 128 . Another way is to derive tools to determine the contribution of one or more features of the input data to the output. In this case, the widely used tools include Shapley Additive Explanation 129 , LIME 127 , DeepLIFT 130 , etc., which are able to assign each feature an importance score for the specific prediction task.
Connection to therapeutic interventions
According to the studies reviewed, it is now possible to detect patients with mental illness based on different types of data. Compared with the traditional ML techniques, most of the reviewed DL models reported higher prediction accuracy. The findings suggested that the DL models are likely to assist clinicians in improved diagnosis of mental health conditions. However, to associate diagnosis of a condition with evidence-based interventions and treatment, including identification of appropriate medication 131 , prediction of treatment response 52 , and estimation of relapse risk 132 still remains a challenge. Among the reviewed studies, only one 52 proposed to target at addressing these issues. Thus, further efforts are needed to link the DL techniques with the therapeutic intervention of mental illness.
Domain knowledge
Another important direction is to incorporate domain knowledge. The existing biomedical knowledge bases are invaluable sources for solving healthcare problems 133 , 134 . Incorporating domain knowledge could address the limitation of data volume, problems of data quality, as well as model generalizability. For example, the unified medical language system 135 can help to identify medical entities from the text and gene–gene interaction databases 136 could help to identify meaningful patterns from genomic profiles.
Recent years have witnessed the increasing use of DL algorithms in healthcare and medicine. In this study, we reviewed existing studies on DL applications to study mental health outcomes. All the results available in the literature reviewed in this work illustrate the applicability and promise of DL in improving the diagnosis and treatment of patients with mental health conditions. Also, this review highlights multiple existing challenges in making DL algorithms clinically actionable for routine care, as well as promising future directions in this field.
World Health Organization. The World Health Report 2001: Mental Health: New Understanding, New Hope (World Health Organization, Switzerland, 2001).
Google Scholar
Marcus, M., Yasamy, M. T., van Ommeren, M., Chisholm, D. & Saxena, S. Depression: A Global Public Health Concern (World Federation of Mental Health, World Health Organisation, Perth, 2012).
Hamilton, M. Development of a rating scale for primary depressive illness. Br. J. Soc. Clin. Psychol. 6 , 278–296 (1967).
CAS PubMed Google Scholar
Dwyer, D. B., Falkai, P. & Koutsouleris, N. Machine learning approaches for clinical psychology and psychiatry. Annu. Rev. Clin. Psychol. 14 , 91–118 (2018).
PubMed Google Scholar
Lovejoy, C. A., Buch, V. & Maruthappu, M. Technology and mental health: the role of artificial intelligence. Eur. Psychiatry 55 , 1–3 (2019).
Wongkoblap, A., Vadillo, M. A. & Curcin, V. Researching mental health disorders in the era of social media: systematic review. J. Med. Internet Res. 19 , e228 (2017).
PubMed PubMed Central Google Scholar
LeCun, Y., Bengio, Y. & Hinton, G. Deep learning. Nature 521 , 436 (2015).
Miotto, R., Wang, F., Wang, S., Jiang, X. & Dudley, J. T. Deep learning for healthcare: review, opportunities and challenges. Brief. Bioinformatics 19 , 1236–1246 (2017).
Durstewitz, D., Koppe, G. & Meyer-Lindenberg, A. Deep neural networks in psychiatry. Mol. Psychiatry 24 , 1583–1598 (2019).
Vieira, S., Pinaya, W. H. & Mechelli, A. Using deep learning to investigate the neuroimaging correlates of psychiatric and neurological disorders: methods and applications. Neurosci. Biobehav. Rev. 74 , 58–75 (2017).
Shatte, A. B., Hutchinson, D. M. & Teague, S. J. Machine learning in mental health: a scoping review of methods and applications. Psychol. Med. 49 , 1426–1448 (2019).
Murphy, K. P. Machine Learning: A Probabilistic Perspective (MIT Press, Cambridge, 2012).
Biship, C. M. Pattern Recognition and Machine Learning (Information Science and Statistics) (Springer-Verlag, Berlin, 2007).
Bengio, Y., Simard, P. & Frasconi, P. Learning long-term dependencies with gradient descent is difficult. IEEE Trans. Neural Netw. Learn. Syst. 5 , 157–166 (1994).
CAS Google Scholar
LeCun, Y., Bottou, L., Bengio, Y. & Haffner, P. Gradient-based learning applied to document recognition. Proc. IEEE 86 , 2278–2324 (1998).
Vincent, P., Larochelle, H., Lajoie, I., Bengio, Y. & Manzagol, P. A. Stacked denoising autoencoders: learning useful representations in a deep network with a local denoising criterion. J. Mach. Learn. Res. 11 , 3371–3408 (2010).
Rumelhart, D. E., Hinton, G. E. & Williams, R. J. Learning representations by back-propagating errors. Cogn. modeling. 5 , 1 (1988).
Hochreiter, S. & Schmidhuber, J. Long short-term memory. Neural Comput. 9 , 1735–1780 (1997).
Cho, K., Van Merriënboer, B., Bahdanau, D. & Bengio, Y. On the properties of neural machine translation: encoder-decoder approaches. In Proc . SSST-8, Eighth Workshop on Syntax, Semantics and Structure in Statistical Translation 103–111 (Doha, Qatar, 2014).
Liou, C., Cheng, W., Liou, J. & Liou, D. Autoencoder for words. Neurocomputing 139 , 84–96 (2014).
Moher, D., Liberati, A., Tetzlaff, J. & Altman, D. G. Preferred reporting items for systematic reviews and meta-analyses: the PRISMA statement. Ann. Intern. Med. 151 , 264–269 (2009).
Schnack, H. G. et al. Can structural MRI aid in clinical classification? A machine learning study in two independent samples of patients with schizophrenia, bipolar disorder and healthy subjects. Neuroimage 84 , 299–306 (2014).
O’Toole, A. J. et al. Theoretical, statistical, and practical perspectives on pattern-based classification approaches to the analysis of functional neuroimaging data. J. Cogn. Neurosci. 19 , 1735–1752 (2007).
Logothetis, N. K., Pauls, J., Augath, M., Trinath, T. & Oeltermann, A. Neurophysiological investigation of the basis of the fMRI signal. Nature 412 , 150 (2001).
Kuang, D. & He, L. Classification on ADHD with deep learning. In Proc . Int. Conference on Cloud Computing and Big Data 27–32 (Wuhan, China, 2014).
Kuang, D., Guo, X., An, X., Zhao, Y. & He, L. Discrimination of ADHD based on fMRI data with deep belief network. In Proc . Int. Conference on Intelligent Computing 225–232 (Taiyuan, China, 2014).
Farzi, S., Kianian, S. & Rastkhadive, I. Diagnosis of attention deficit hyperactivity disorder using deep belief network based on greedy approach. In Proc . 5th Int. Symposium on Computational and Business Intelligence 96–99 (Dubai, United Arab Emirates, 2017).
Zou, L., Zheng, J. & McKeown, M. J. Deep learning based automatic diagnoses of attention deficit hyperactive disorder. In Proc . 2017 IEEE Global Conference on Signal and Information Processing (GlobalSIP) 962–966 (Montreal, Canada, 2017).
Riaz A. et al. Deep fMRI: an end-to-end deep network for classification of fMRI data. In Proc . 2018 IEEE 15th Int. Symposium on Biomedical Imaging . 1419–1422 (Washington, DC, USA, 2018).
Zou, L., Zheng, J., Miao, C., Mckeown, M. J. & Wang, Z. J. 3D CNN based automatic diagnosis of attention deficit hyperactivity disorder using functional and structural MRI. IEEE Access. 5 , 23626–23636 (2017).
Sen, B., Borle, N. C., Greiner, R. & Brown, M. R. A general prediction model for the detection of ADHD and Autism using structural and functional MRI. PLoS ONE 13 , e0194856 (2018).
Zeng, L. et al. Multi-site diagnostic classification of schizophrenia using discriminant deep learning with functional connectivity MRI. EBioMedicine 30 , 74–85 (2018).
Pinaya, W. H. et al. Using deep belief network modelling to characterize differences in brain morphometry in schizophrenia. Sci. Rep. 6 , 38897 (2016).
CAS PubMed PubMed Central Google Scholar
Pinaya, W. H., Mechelli, A. & Sato, J. R. Using deep autoencoders to identify abnormal brain structural patterns in neuropsychiatric disorders: a large-scale multi-sample study. Hum. Brain Mapp. 40 , 944–954 (2019).
Ulloa, A., Plis, S., Erhardt, E. & Calhoun, V. Synthetic structural magnetic resonance image generator improves deep learning prediction of schizophrenia. In Proc . 25th IEEE Int. Workshop on Machine Learning for Signal Processing (MLSP) 1–6 (Boston, MA, USA, 2015).
Matsubara, T., Tashiro, T. & Uehara, K. Deep neural generative model of functional MRI images for psychiatric disorder diagnosis. IEEE Trans. Biomed. Eng . 99 (2019).
Geng, X. & Xu, J. Application of autoencoder in depression diagnosis. In 2017 3rd Int. Conference on Computer Science and Mechanical Automation (Wuhan, China, 2017).
Aghdam, M. A., Sharifi, A. & Pedram, M. M. Combination of rs-fMRI and sMRI data to discriminate autism spectrum disorders in young children using deep belief network. J. Digit. Imaging 31 , 895–903 (2018).
Shen, D., Wu, G. & Suk, H. -I. Deep learning in medical image analysis. Annu. Rev. Biomed. Eng. 19 , 221–248 (2017).
Yan, C. & Zang, Y. DPARSF: a MATLAB toolbox for “pipeline” data analysis of resting-state fMRI. Front. Syst. Neurosci. 4 , 13 (2010).
Esteban, O. et al. fMRIPrep: a robust preprocessing pipeline for functional MRI. Nat. Methods 16 , 111–116 (2019).
Herrmann, C. & Demiralp, T. Human EEG gamma oscillations in neuropsychiatric disorders. Clin. Neurophysiol. 116 , 2719–2733 (2005).
Acharya, U. R. et al. Automated EEG-based screening of depression using deep convolutional neural network. Comput. Meth. Prog. Biol. 161 , 103–113 (2018).
Mohan, Y., Chee, S. S., Xin, D. K. P. & Foong, L. P. Artificial neural network for classification of depressive and normal. In EEG Proc . 2016 IEEE EMBS Conference on Biomedical Engineering and Sciences 286–290 (Kuala Lumpur, Malaysia, 2016).
Zhang, P., Wang, X., Zhang, W. & Chen, J. Learning spatial–spectral–temporal EEG features with recurrent 3D convolutional neural networks for cross-task mental workload assessment. IEEE Trans. Neural Syst. Rehabil. Eng. 27 , 31–42 (2018).
Li, X. et al. EEG-based mild depression recognition using convolutional neural network. Med. Biol. Eng. Comput . 47 , 1341–1352 (2019).
Patel, S., Park, H., Bonato, P., Chan, L. & Rodgers, M. A review of wearable sensors and systems with application in rehabilitation. J. Neuroeng. Rehabil. 9 , 21 (2012).
Smoller, J. W. The use of electronic health records for psychiatric phenotyping and genomics. Am. J. Med. Genet. B Neuropsychiatr. Genet. 177 , 601–612 (2018).
Wu, J., Roy, J. & Stewart, W. F. Prediction modeling using EHR data: challenges, strategies, and a comparison of machine learning approaches. Med. Care. 48 , S106–S113 (2010).
Choi, S. B., Lee, W., Yoon, J. H., Won, J. U. & Kim, D. W. Ten-year prediction of suicide death using Cox regression and machine learning in a nationwide retrospective cohort study in South Korea. J. Affect. Disord. 231 , 8–14 (2018).
Pham, T., Tran, T., Phung, D. & Venkatesh, S. Predicting healthcare trajectories from medical records: a deep learning approach. J. Biomed. Inform. 69 , 218–229 (2017).
Lin, E. et al. A deep learning approach for predicting antidepressant response in major depression using clinical and genetic biomarkers. Front. Psychiatry 9 , 290 (2018).
Geraci, J. et al. Applying deep neural networks to unstructured text notes in electronic medical records for phenotyping youth depression. Evid. Based Ment. Health 20 , 83–87 (2017).
Kim, Y. Convolutional neural networks for sentence classification. arXiv Prepr. arXiv 1408 , 5882 (2014).
Yang, Z. et al. Hierarchical attention networks for document classification. In Proc . 2016 Conference of the North American Chapter of the Association for Computational Linguistics: Human Language Technologies 1480–1489 (San Diego, California, USA, 2016).
Rios, A. & Kavuluru, R. Ordinal convolutional neural networks for predicting RDoC positive valence psychiatric symptom severity scores. J. Biomed. Inform. 75 , S85–S93 (2017).
Dai, H. & Jonnagaddala, J. Assessing the severity of positive valence symptoms in initial psychiatric evaluation records: Should we use convolutional neural networks? PLoS ONE 13 , e0204493 (2018).
Tran, T. & Kavuluru, R. Predicting mental conditions based on “history of present illness” in psychiatric notes with deep neural networks. J. Biomed. Inform. 75 , S138–S148 (2017).
Samek, W., Binder, A., Montavon, G., Lapuschkin, S. & Müller, K.-R. Evaluating the visualization of what a deep neural network has learned. IEEE Trans. Neural Netw. Learn. Syst. 28 , 2660–2673 (2016).
Hripcsak, G. et al. Characterizing treatment pathways at scale using the OHDSI network. Proc. Natl. Acad. Sci . USA 113 , 7329–7336 (2016).
McGuffin, P., Owen, M. J. & Gottesman, I. I. Psychiatric Genetics and Genomics (Oxford Univ. Press, New York, 2004).
Levinson, D. F. The genetics of depression: a review. Biol. Psychiatry 60 , 84–92 (2006).
Wray, N. R. et al. Genome-wide association analyses identify 44 risk variants and refine the genetic architecture of major depression. Nat. Genet. 50 , 668 (2018).
Mullins, N. & Lewis, C. M. Genetics of depression: progress at last. Curr. Psychiatry Rep. 19 , 43 (2017).
Zou, J. et al. A primer on deep learning in genomics. Nat. Genet. 51 , 12–18 (2019).
Yue, T. & Wang, H. Deep learning for genomics: a concise overview. Preprint at arXiv:1802.00810 (2018).
Khan, A. & Wang, K. A deep learning based scoring system for prioritizing susceptibility variants for mental disorders. In Proc . 2017 IEEE Int. Conference on Bioinformatics and Biomedicine (BIBM) 1698–1705 (Kansas City, USA, 2017).
Khan, A., Liu, Q. & Wang, K. iMEGES: integrated mental-disorder genome score by deep neural network for prioritizing the susceptibility genes for mental disorders in personal genomes. BMC Bioinformatics 19 , 501 (2018).
Wang, D. et al. Comprehensive functional genomic resource and integrative model for the human brain. Science 362 , eaat8464 (2018).
Salakhutdinov, R. & Hinton, G. Deep Boltzmann machines. In Proc . 12th Int. Conference on Artificial Intelligence and Statistics 448–455 (Clearwater, Florida, USA, 2009).
Laksshman, S., Bhat, R. R., Viswanath, V. & Li, X. DeepBipolar: Identifying genomic mutations for bipolar disorder via deep learning. Hum. Mutat. 38 , 1217–1224 (2017).
CAS PubMed Central Google Scholar
Huang, K.-Y. et al. Data collection of elicited facial expressions and speech responses for mood disorder detection. In Proc . 2015 Int. Conference on Orange Technologies (ICOT) 42–45 (Hong Kong, China, 2015).
Valstar, M. et al. AVEC 2013: the continuous audio/visual emotion and depression recognition challenge. In Proc . 3rd ACM Int. Workshop on Audio/Visual Emotion Challenge 3–10 (Barcelona, Spain, 2013).
Valstar, M. et al. Avec 2014: 3d dimensional affect and depression recognition challenge. In Proc . 4th Int. Workshop on Audio/Visual Emotion Challenge 3–10 (Orlando, Florida, USA, 2014).
Valstar, M. et al. Avec 2016: depression, mood, and emotion recognition workshop and challenge. In Proc . 6th Int. Workshop on Audio/Visual Emotion Challenge 3–10 (Amsterdam, The Netherlands, 2016).
Ma, X., Yang, H., Chen, Q., Huang, D. & Wang, Y. Depaudionet: an efficient deep model for audio based depression classification. In Proc . 6th Int. Workshop on Audio/Visual Emotion Challenge 35–42 (Amsterdam, The Netherlands, 2016).
He, L. & Cao, C. Automated depression analysis using convolutional neural networks from speech. J. Biomed. Inform. 83 , 103–111 (2018).
Li, J., Fu, X., Shao, Z. & Shang, Y. Improvement on speech depression recognition based on deep networks. In Proc . 2018 Chinese Automation Congress (CAC) 2705–2709 (Xi’an, China, 2018).
Yang, L., Jiang, D., Han, W. & Sahli, H. DCNN and DNN based multi-modal depression recognition. In Proc . 2017 7th Int. Conference on Affective Computing and Intelligent Interaction 484–489 (San Antonio, Texas, USA, 2017).
Huang, K. Y., Wu, C. H. & Su, M. H. Attention-based convolutional neural network and long short-term memory for short-term detection of mood disorders based on elicited speech responses. Pattern Recogn. 88 , 668–678 (2019).
Dawood, A., Turner, S. & Perepa, P. Affective computational model to extract natural affective states of students with Asperger syndrome (AS) in computer-based learning environment. IEEE Access. 6 , 67026–67034 (2018).
Song, S., Shen, L. & Valstar, M. Human behaviour-based automatic depression analysis using hand-crafted statistics and deep learned spectral features. In Proc . 13th IEEE Int. Conference on Automatic Face & Gesture Recognition 158–165 (Xi’an, China, 2018).
Zhu, Y., Shang, Y., Shao, Z. & Guo, G. Automated depression diagnosis based on deep networks to encode facial appearance and dynamics. IEEE Trans. Affect. Comput. 9 , 578–584 (2018).
Chao, L., Tao, J., Yang, M. & Li, Y. Multi task sequence learning for depression scale prediction from video. In Proc . 2015 Int. Conference on Affective Computing and Intelligent Interaction (ACII) 526–531 (Xi’an, China, 2015).
Yang, T. H., Wu, C. H., Huang, K. Y. & Su, M. H. Detection of mood disorder using speech emotion profiles and LSTM. In Proc . 10th Int. Symposium on Chinese Spoken Language Processing (ISCSLP) 1–5 (Tianjin, China, 2016).
Huang, K. Y., Wu, C. H., Su, M. H. & Chou, C. H. Mood disorder identification using deep bottleneck features of elicited speech. In Proc . 2017 Asia-Pacific Signal and Information Processing Association Annual Summit and Conference (APSIPA ASC) 1648–1652 (Kuala Lumpur, Malaysia, 2017).
Jan, A., Meng, H., Gaus, Y. F. B. A. & Zhang, F. Artificial intelligent system for automatic depression level analysis through visual and vocal expressions. IEEE Trans. Cogn. Dev. Syst. 10 , 668–680 (2017).
Su, M. H., Wu, C. H., Huang, K. Y. & Yang, T. H. Cell-coupled long short-term memory with l-skip fusion mechanism for mood disorder detection through elicited audiovisual features. IEEE Trans. Neural Netw. Learn. Syst . 31 (2019).
Harati, S., Crowell, A., Mayberg, H. & Nemati, S. Depression severity classification from speech emotion. In Proc . 40th Annual International Conference of the IEEE Engineering in Medicine and Biology Society (EMBC) 5763–5766 (Honolulu, HI, USA, 2018).
Su, M. H., Wu, C. H., Huang, K. Y., Hong, Q. B. & Wang, H. M. Exploring microscopic fluctuation of facial expression for mood disorder classification. In Proc . 2017 Int. Conference on Orange Technologies (ICOT) 65–69 (Singapore, 2017).
Prasetio, B. H., Tamura, H. & Tanno, K. The facial stress recognition based on multi-histogram features and convolutional neural network. In Proc . 2018 IEEE Int. Conference on Systems, Man, and Cybernetics (SMC) 881–887 (Miyazaki, Japan, 2018).
Jaiswal, S., Valstar, M. F., Gillott, A. & Daley, D. Automatic detection of ADHD and ASD from expressive behaviour in RGBD data. In Proc . 12th IEEE Int. Conference on Automatic Face & Gesture Recognition 762–769 (Washington, DC, USA, 2017).
Cho, Y., Bianchi-Berthouze, N. & Julier, S. J. DeepBreath: deep learning of breathing patterns for automatic stress recognition using low-cost thermal imaging in unconstrained settings. In Proc . 2017 7th Int. Conference on Affective Computing and Intelligent Interaction (ACII) 456–463 (San Antonio, Texas, USA, 2017).
Gupta, R., Sahu, S., Espy-Wilson, C. Y. & Narayanan, S. S. An affect prediction approach through depression severity parameter incorporation in neural networks. In Proc . 2017 Int. Conference on INTERSPEECH 3122–3126 (Stockholm, Sweden, 2017).
Martin, O., Kotsia, I., Macq, B. & Pitas, I. The eNTERFACE'05 audio-visual emotion database. In Proc . 22nd Int. Conference on Data Engineering Workshops 8–8 (Atlanta, GA, USA, 2006).
Goodfellow, I. J. et al. Challenges in representation learning: A report on three machine learning contests. In Proc . Int. Conference on Neural Information Processing 117–124 (Daegu, Korea, 2013).
Yi, D., Lei, Z., Liao, S. & Li, S. Z.. Learning face representation from scratch. Preprint at arXiv 1411.7923 (2014).
Lin, H. et al. User-level psychological stress detection from social media using deep neural network. In Proc . 22nd ACM Int. Conference on Multimedia 507–516 (Orlando, Florida, USA, 2014).
Lin, H. et al. Psychological stress detection from cross-media microblog data using deep sparse neural network. In Proc . 2014 IEEE Int. Conference on Multimedia and Expo 1–6 (Chengdu, China, 2014).
Li, Q. et al. Correlating stressor events for social network based adolescent stress prediction. In Proc . Int. Conference on Database Systems for Advanced Applications 642–658 (Suzhou, China, 2017).
Lin, H. et al. Detecting stress based on social interactions in social networks. IEEE Trans. Knowl. Data En. 29 , 1820–1833 (2017).
Cong, Q. et al. X-A-BiLSTM: a deep learning approach for depression detection in imbalanced data. In Proc . 2018 IEEE Int. Conference on Bioinformatics and Biomedicine (BIBM) 1624–1627 (Madrid, Spain, 2018).
Ive, J., Gkotsis, G., Dutta, R., Stewart, R. & Velupillai, S. Hierarchical neural model with attention mechanisms for the classification of social media text related to mental health. In Proc . Fifth Workshop on Computational Linguistics and Clinical Psychology: From Keyboard to Clinic 69–77 (New Orleans, Los Angeles, USA, 2018).
Sadeque, F., Xu, D. & Bethard, S. UArizona at the CLEF eRisk 2017 pilot task: linear and recurrent models for early depression detection. CEUR Workshop Proc . 1866 (2017).
Fraga, B. S., da Silva, A. P. C. & Murai, F. Online social networks in health care: a study of mental disorders on Reddit. In Proc . 2018 IEEE/WIC/ACM Int. Conference on Web Intelligence (WI) 568–573 (Santiago, Chile, 2018).
Gkotsis, G. et al. Characterisation of mental health conditions in social media using Informed Deep Learning. Sci. Rep. 7 , 45141 (2017).
Coppersmith, G., Leary, R., Crutchley, P. & Fine, A. Natural language processing of social media as screening for suicide risk. Biomed. Inform. Insights 10 , 1178222618792860 (2018).
Du, J. et al. Extracting psychiatric stressors for suicide from social media using deep learning. BMC Med. Inform. Decis. Mak. 18 , 43 (2018).
Alambo, A. et al. Question answering for suicide risk assessment using Reddit. In Proc . IEEE 13th Int. Conference on Semantic Computing 468–473 (Newport Beach, California, USA, 2019).
Eichstaedt, J. C. et al. Facebook language predicts depression in medical records. Proc. Natl Acad. Sci. USA 115 , 11203–11208 (2018).
Rosenquist, J. N., Fowler, J. H. & Christakis, N. A. Social network determinants of depression. Mol. Psychiatry 16 , 273 (2011).
Kipf, T. N. & Welling, M. Semi-supervised classification with graph convolutional networks. In Proc. 2017 Int. Conference on Learning Representations (Toulon, France, 2017).
Rice, S. M. et al. Online and social networking interventions for the treatment of depression in young people: a systematic review. J. Med. Internet Res. 16 , e206 (2014).
Hastie, T., Tibshirani, R. & Friedman, J. The elements of statistical learning: data mining, inference, and prediction. Springer Series in Statistics. Math. Intell. 27 , 83–85 (2009).
Torrey, L. & Shavlik, J. in Handbook of Research on Machine Learning Applications and Trends: Algorithms, Methods, and Techniques 242–264 (IGI Global, 2010).
Yosinski, J., Clune, J., Bengio, Y. & Lipson, H. How transferable are features in deep neural networks? In Proc . Advances in Neural Information Processing Systems 3320–3328 (Montreal, Canada, 2014).
Esteva, A. et al. Dermatologist-level classification of skin cancer with deep neural networks. Nature 542 , 115 (2017).
Insel, T. et al. Research domain criteria (RDoC): toward a new classification framework for research on mental disorders. Am. Psychiatr. Assoc. 167 , 748–751 (2010).
Nelson, B., McGorry, P. D., Wichers, M., Wigman, J. T. & Hartmann, J. A. Moving from static to dynamic models of the onset of mental disorder: a review. JAMA Psychiatry 74 , 528–534 (2017).
Guo, X., Liu, X., Zhu, E. & Yin, J. Deep clustering with convolutional autoencoders. In Proc . Int. Conference on Neural Information Processing 373–382 (Guangzhou, China, 2017).
Srivastava, N., Mansimov, E. & Salakhudinov, R. Unsupervised learning of video representations using LSTMs. In Proc . Int. Conference on Machine Learning 843–852 (Lille, France, 2015).
Baytas, I. M. et al. Patient subtyping via time-aware LSTM networks. In Proc . 23rd ACM SIGKDD Int. Conference on Knowledge Discovery and Data Mining 65–74 (Halifax, Canada, 2017).
American Psychiatric Association. Diagnostic and Statistical Manual of Mental Disorders (DSM-5®) (American Psychiatric Pub, Washington, DC, 2013).
Biological Sciences Curriculum Study. In: NIH Curriculum Supplement Series (Internet) (National Institutes of Health, USA, 2007).
Noh, H., Hong, S. & Han, B. Learning deconvolution network for semantic segmentation. In Proc . IEEE Int. Conference on Computer Vision 1520–1528 (Santiago, Chile, 2015).
Grün, F., Rupprecht, C., Navab, N. & Tombari, F. A taxonomy and library for visualizing learned features in convolutional neural networks. In Proc. 33rd Int. Conference on Machine Learning (ICML) Workshop on Visualization for Deep Learning (New York, USA, 2016).
Ribeiro, M. T., Singh, S. & Guestrin, C. Why should I trust you?: Explaining the predictions of any classifier. In Proc . 22nd ACM SIGKDD Int. Conference on Knowledge Discovery and Data Mining 1135–1144 (San Francisco, CA, 2016).
Zhang, Q. S. & Zhu, S. C. Visual interpretability for deep learning: a survey. Front. Inf. Technol. Electron. Eng. 19 , 27–39 (2018).
Lundberg, S. M. & Lee, S. I. A unified approach to interpreting model predictions. In Proc . 31st Conference on Neural Information Processing Systems 4765–4774 (Long Beach, CA, 2017).
Shrikumar, A., Greenside, P., Shcherbina, A. & Kundaje, A. Not just a black box: learning important features through propagating activation differences. In Proc . 33rd Int. Conference on Machine Learning (New York, NY, 2016).
Gawehn, E., Hiss, J. A. & Schneider, G. Deep learning in drug discovery. Mol. Inform. 35 , 3–14 (2016).
Jerez-Aragonés, J. M., Gómez-Ruiz, J. A., Ramos-Jiménez, G., Muñoz-Pérez, J. & Alba-Conejo, E. A combined neural network and decision trees model for prognosis of breast cancer relapse. Artif. Intell. Med. 27 , 45–63 (2003).
Zhu, Y., Elemento, O., Pathak, J. & Wang, F. Drug knowledge bases and their applications in biomedical informatics research. Brief. Bioinformatics 20 , 1308–1321 (2018).
Su, C., Tong, J., Zhu, Y., Cui, P. & Wang, F. Network embedding in biomedical data science. Brief. Bioinform . https://doi.org/10.1093/bib/bby117 (2018).
Bodenreider, O. The unified medical language system (UMLS): integrating biomedical terminology. Nucleic Acids Res. 32 (suppl_1), D267–D270 (2004).
Szklarczyk, D. et al. STRING v10: protein–protein interaction networks, integrated over the tree of life. Nucleic Acids Res. 43 , D447–D452 (2014).
Download references
Acknowledgements
The work is supported by NSF 1750326, R01 MH112148, R01 MH105384, R01 MH119177, R01 MH121922, and P50 MH113838.
Author information
Authors and affiliations.
Department of Healthcare Policy and Research, Weill Cornell Medicine, New York, NY, USA
Chang Su, Zhenxing Xu, Jyotishman Pathak & Fei Wang
You can also search for this author in PubMed Google Scholar
Contributions
C.S., Z.X. and F.W. planned and structured the whole paper. C.S. and Z.X. conducted the literature review and drafted the manuscript. J.P. and F.W. reviewed and edited the manuscript.
Corresponding author
Correspondence to Fei Wang .
Ethics declarations
Competing interests.
The authors declare no competing interests.
Additional information
Publisher’s note Springer Nature remains neutral with regard to jurisdictional claims in published maps and institutional affiliations.
Supplementary information
Supplemental material, rights and permissions.
Open Access This article is licensed under a Creative Commons Attribution 4.0 International License, which permits use, sharing, adaptation, distribution and reproduction in any medium or format, as long as you give appropriate credit to the original author(s) and the source, provide a link to the Creative Commons license, and indicate if changes were made. The images or other third party material in this article are included in the article’s Creative Commons license, unless indicated otherwise in a credit line to the material. If material is not included in the article’s Creative Commons license and your intended use is not permitted by statutory regulation or exceeds the permitted use, you will need to obtain permission directly from the copyright holder. To view a copy of this license, visit http://creativecommons.org/licenses/by/4.0/ .
Reprints and permissions
About this article
Cite this article.
Su, C., Xu, Z., Pathak, J. et al. Deep learning in mental health outcome research: a scoping review. Transl Psychiatry 10 , 116 (2020). https://doi.org/10.1038/s41398-020-0780-3
Download citation
Received : 31 August 2019
Revised : 17 February 2020
Accepted : 26 February 2020
Published : 22 April 2020
DOI : https://doi.org/10.1038/s41398-020-0780-3
Share this article
Anyone you share the following link with will be able to read this content:
Sorry, a shareable link is not currently available for this article.
Provided by the Springer Nature SharedIt content-sharing initiative
This article is cited by
Automated mood disorder symptoms monitoring from multivariate time-series sensory data: getting the full picture beyond a single number.
- Filippo Corponi
- Bryan M. Li
- Antonio Vergari
Translational Psychiatry (2024)
Detecting your depression with your smartphone? – An ethical analysis of epistemic injustice in passive self-tracking apps
- Mirjam Faissner
- Sebastian Laacke
Ethics and Information Technology (2024)
Development of intelligent system based on synthesis of affective signals and deep neural networks to foster mental health of the Indian virtual community
- Mandeep Kaur Arora
- Jaspreet Singh
Social Network Analysis and Mining (2024)
Prevalence and predictors of self-rated mental health among farm and non-farm adult rural residents of Saskatchewan
- Md Saiful Alam
- Bonnie Janzen
- Punam Pahwa
Current Psychology (2024)
Unraveling minds in the digital era: a review on mapping mental health disorders through machine learning techniques using online social media
Quick links.
- Explore articles by subject
- Guide to authors
- Editorial policies

- Open access
- Published: 02 May 2022
Rethinking mental wellness among adolescents: an integrative review protocol of mental health components
- Zaida Orth ORCID: orcid.org/0000-0002-2895-0417 1 &
- Brian van Wyk ORCID: orcid.org/0000-0003-1032-1847 1
Systematic Reviews volume 11 , Article number: 83 ( 2022 ) Cite this article
6483 Accesses
4 Citations
1 Altmetric
Metrics details
Adolescents have been overlooked in global public health initiatives as this period is generally considered to be the healthiest in an individual’s life course. However, the growth of the global adolescent population and their changing health profiles have called attention to the diverse health needs of adolescents. The increased attention toward adolescent health has accentuated existing gaps as global health reports have emphasised that there is a continued need for valid and reliable health data. In this context, evidence has shown that mental health issues constitute one of the greatest burdens of disease for adolescents. This integrative review aims to unpack the meaning of mental wellness among adolescents and its associated constructs by analysing and synthesising empirical and theoretical research on adolescent mental wellness. In doing this, we will develop a working definition of adolescent mental wellness that can be used to develop an instrument aimed at measuring adolescent mental wellness.
The integrative review is guided by the five steps described by Whittemore and Knafl. A comprehensive search strategy which will include carefully selected terms that correspond to the domains of interest (positive mental health/mental wellness) will be used to search for relevant literature on electronic databases, grey literature and government or non-governmental organisations (NGO) websites. Studies will be included if they describe and/or define general mental wellness in adolescent populations aged 10–19. The screening and reporting of the review will be conducted following the Preferred Reporting Items for Systematic Reviews and Meta-Analyses (PRISMA) guidelines. Data from the integrative review will be analysed using narrative framework synthesis for qualitative and quantitative studies.
This integrative review aims to search for and synthesise current research regarding adolescent mental wellness to identify how wellness is being described and conceptualised. We aim to identify gaps and to contribute to a more comprehensive definition of mental wellness which can aid in the development of an age- and culturally appropriate measure of adolescent mental wellness.
Peer Review reports
In 2016, adolescents (10–19 years) were estimated at 1.2 billion (18%) of the world population, making them the largest population of adolescents in history [ 1 , 2 ]. Adolescents have been largely overlooked in global health and social policies, because this period is generally considered to be the healthiest in an individual’s life-course [ 2 ], and the unique health problems associated with adolescence have been misconstrued or ignored in favour of more pressing public health concerns [ 3 ]. However, changing health profiles of adolescents in both developed and developing countries have called attention to the diverse health needs of adolescents [ 1 ]. According to the World Health Organization (WHO), more than 1.1 million adolescents died in 2016—mostly from preventable or treatable causes [ 4 ]. Therefore, the considerable gains from global investments in child and maternal health programmes would yield fewer long term benefits without simultaneous investments in adolescent health [ 5 , 6 ].
According to the life course approach, all stages of an individual’s life are intricately intertwined and interconnected with each other, and other people in society including past, present and future generations of their families [ 7 ]. In other words, evidence has shown that early life experiences, including events experienced in the pre-conception phase, play a role in determining the developmental origins and trajectories of health and wellness or disease across an individual’s life course [ 7 ]. From this perspective, it is understood that the health and wellness of individuals, as well as communities, depend on interactions oscillating between multiple risk and protective factors throughout one’s life. Based on this, early and appropriate interventions during child and adolescent years are shown to be the most effective prevention strategies to promote optimal public health and human development [ 5 , 6 , 8 ]. Additionally, following the life course approach, it is argued that these early investments in child and adolescent health will yield a triple dividend as they will grow into healthier adults who can contribute positively to society, as well as the health and development of the next generation [ 2 , 9 ].
Globally, mental health issues constitute one of the greatest burdens of disease for adolescents. According to the WHO, in 2016 mental health conditions accounted for 16% of the global burden of disease and injury for adolescents, with depression being identified as one of the leading causes of illness and disability, followed by suicide as the second leading cause of death in adolescents and self-harm the third [ 4 , 9 ]. UNICEF propagates that half of all lifetime mental disorders have onset during adolescence [ 10 ]. The recent inclusion of adolescents on the global health agenda as a target group for intervention represents a key step toward reducing the global burden of disease attributed to mental health disorders and reducing preventable deaths [ 2 , 10 , 11 , 12 , 13 ]. However, due to the previous neglect of mental health as a public health issue, efforts to address adolescent mental health are met with various challenges.
Currently, there is a lack of data concerning mental health conditions among adolescents, especially those living in low- and middle-income countries (LMICs) [ 11 ]. The lack of a body of quality evidence can affect the way adolescents are represented in national policies, as well as the ways in which government and healthcare officials respond to treatment and prevention [ 2 , 14 ]. According to WHO, a 2014 review of health policy documents from 109 countries showed that 84% have given some attention to adolescents, with three-quarters of them addressing sexual and reproductive health; one-third addressing tobacco and alcohol use, and one-quarter focusing on mental health [ 1 ]. In LMICs, efforts to improve child and adolescent mental health services (CAMHS) are hindered by a lack of specific CAMHS policies, resources and, fewer child and adolescent psychiatrists and other mental health professionals [ 12 , 13 ]. Furthermore, studies from developed countries have suggested that while CAMHS and policies are in place, there is a lack of mental health service uptake among children and adolescents due to various attitudinal, stigma-related, and structural barriers to accessing mental health services [ 14 ]. These challenges and barriers to CAMHS in both LMICs and higher-income countries are particularly apparent among adolescents living with a chronic disorder or disease [ 15 , 16 ]. Mental health conditions are increasingly recognised in children and adolescents with chronic disorders. Studies have shown that living with a chronic health condition is associated with increased risk of developing comorbid physical and mental health problems, which in turn influence treatment adherence and quality of life [ 16 , 17 , 18 ].
Another recurring obstacle for integrating mental health into global public health initiatives and frameworks is the lack of consensus of a definition of mental health [ 19 ]. Despite the growth of mental health and wellness research in recent decades, the question of how mental wellness should be defined remains largely unresolved [ 20 ]. This has given rise to broad and ambiguous definitions which, consequently, result in concepts such as mental health, mental wellness and mental wellbeing being used interchangeably. Currently, the term ‘mental health’ is often used as a euphemism to refer to mental illness, referring to conditions that adversely affect cognition, emotion and behaviour (i.e. depression and anxiety) [ 21 ]. This use reflects in the literature as the majority of adolescent mental health research adopts the dominant pathological view of health by focusing on mental health disorders such as psychiatric disorders, general mental health disorders, emotional and behavioural problems and psychological distress [ 22 , 23 ]. Similarly, global health initiatives such as AAH-HA! focus majorly on the burden of disease of mental disorders by reporting on self-harm, depressive disorders, childhood behavioural disorders and anxiety [ 3 ]. This dominant pathological view of mental health persists despite the contributions of positive health and wellbeing research which argues that wellness and illness are not two ends of the same continuum as previously thought, rather these constructs represent two independent continua [ 19 , 23 ]. In other words, the absence of mental illness does not necessarily indicate a state of mental health/wellness [ 1 , 23 , 24 ]. Therefore, it is imperative to consider both mental wellness and mental illness in research, and to move away from the previous ‘absence of disease’ model to one that emphasises positive psychological functioning for mental health [ 23 , 24 ]. In this model, wellness refers to the degree one feels positive about life, and one’s capacity to manage one’s feelings, behaviours, and limitations [ 23 ]. From this model, addressing adolescent mental wellness is seen as more than treating and mitigating the burden of disease of mental illness, rather it is also useful in maintaining lifelong mental and physical wellness and preventing the development of mental disorders [ 23 ]. Adolescents in particular experience multiple physical, social, and emotional changes, which can positively or negatively impact on their mental wellness. Therefore, interventions at this stage are crucial as research shows that providing psychosocial support and mental health promotion, such as psycho-education and community empowerment, facilitates the development of mental wellness (positive mental health) which is protective against psychopathology (mental illness) [ 9 , 24 ].
There is a need to develop accurate and culturally appropriate measures of mental wellness to support research endeavours that aim to improve adolescent mental health. Therefore, there is a greater necessity to clarify what is being measured, and how the resulting data from the measure should be interpreted to undertake fair and valid assessments. As such, developing a definition of mental wellness should encompass more than the description of wellness itself (as is the case with current definitions) to a clear and definite statement of the exact meaning of the construct.
To this end, this integrative review forms part of a larger study which aims to unpack the meaning of mental wellness among adolescents and its associated constructs by analysing and synthesising relevant literature and empirical and theoretical research on adolescent mental wellness. In doing this, we aim to use this information to develop and conceptualise adolescent mental wellness as a construct. Additionally, by focusing on conceptualising mental wellness, we hope to provide clarity regarding the way concepts such as mental wellness are used in the literature by clearly distinguishing between mental health (as a euphemism for mental illness) and mental wellness as a positive state of mental health. We aim to develop an instrument which can measure mental wellness as an indicator of general mental health and wellness among adolescents.
Methodology
The integrative review has been identified as a unique tool in healthcare for synthesising investigations available on a given topic or phenomena and for directing practise based on scientific knowledge [ 25 ]. The existing body of literature on mental health among adolescents is varied and complex as there are many concepts associated with mental health research ranging from positive aspects such as ‘resilience’ and ‘self-efficacy’ to negative aspects such as ‘depression’ and ‘anxiety’. As such, it is not possible for one study to capture all the dimensions associated with mental health. However, by adopting the integrative review method, we will be able to include the various sources and methodologies used in research to summarise existing empirical and theoretical literature associated with [positive] mental wellness concepts to better understand and conceptualise mental wellness among adolescents. The integrative review method proposed by Whittemore and Knafl [ 26 ] will be used: (1) problem identification, (2) literature search, (3) data evaluation, (4) data analysis, and (5) presentation of the integrative review.
Problem identification
The problem identification stage is a crucial first step in an integrative review. Therefore, we aim to approach this as a phase in itself. This means, going beyond the initial research questions to fully develop a framework of the problem and all its related variables. In this section, we describe some approaches we will use to identify the problem which the integrative review will address. As previously mentioned, our interest lies in understanding how mental wellness is conceptualised among adolescents, to aid in the conceptualisation and development of a mental wellness instrument for adolescent populations. Based on our initial reading of the literature, we have identified two recurring issues in this regard: firstly, there is a lack of validated mental wellness instruments for adolescents; and secondly, despite a growing body of research, the question of how mental wellness should be defined remains largely unresolved. Based on this, we have proposed to follow two research questions to aid us in identifying the problem.
How is the concept of mental wellness defined in research involving adolescents?
What indicators of mental wellness are being explored/investigated in research?
These two questions allow us to investigate how research has approached the study of mental wellness, what variables were of interest and how these were defined. To answer these questions, we will follow an iterative approach to gather and assess the available information to present a clear identification of the problem and all the variables of interest. To this end, we are currently conducting a systematic review of mental health instruments used in research with adolescent populations [ 21 ].
Understanding how mental wellness has been defined in research is an important part of our problem identification, as it will show us what theories and/or definitions of mental wellness are dominant, and which are missing. As Dodge et al. [ 20 ] argued, current definitions of wellness are more descriptive in the sense that they describe aspects of wellness rather than the construct itself. This lack of definition poses a problem in measurement development as the definition of a construct ultimately influences how it is being measured and how the resulting data should be interpreted. Therefore, to further aid our problem identification, we will compare the data from the systematic review with data from qualitative interviews exploring mental wellness among adolescents living with HIV (ALHIV). As previously mentioned, this review forms part of a larger study aimed at developing an instrument to measure mental wellness among adolescents. We have chosen to include the interviews with ALHIV for the problem identification stage as we want to develop an instrument that can measure mental wellness among healthy populations and those living with a chronic illness such as HIV. This is necessary as Manderscheid et al. [ 23 ] argue that a dual emphasis on mental and physical health is essential as studies have shown that positive health may influence biological functioning. This information will be used to identify the problem of the integrative review (Fig. 1 ). Using the information from the problem identification phase, we will move on to the second phase to conduct a literature search of mental health concepts used in research with adolescent populations.
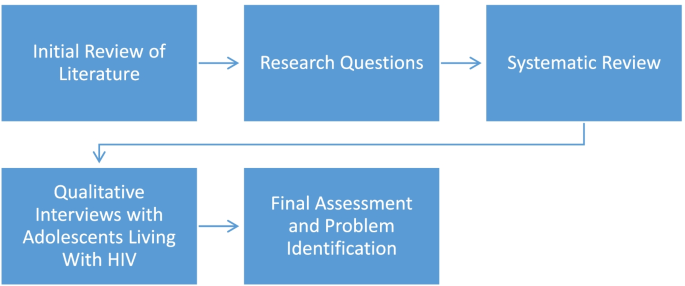
Steps followed to identify the problem for an integrative review
Literature search
A comprehensive search strategy which will include carefully selected terms that correspond to the domains of interest (mental health/mental wellness) will be used to search for relevant literature on electronic databases, grey literature and government or non-governmental organisations (NGO) websites. A systematic database search will be performed using Ebscohost (Psycharticles, Academic Search Premier, SocIndex), Educational Resource Information Center (ERIC), Medical Literature Analysis Retrieval System Online (MEDLINE) and Sabinet. A list of initial keywords has been identified for the search strategy: ((adolescen* OR teenage* OR young people OR youth) [AND] (“psychological wellbeing” OR “mental health wellbeing” OR “mental wellness” OR “mental health”). As the integrative review allows for a more iterative process, the list of keywords will be modified as the initial search reveals more relevant and refined search terms.
Inclusion and exclusion criteria
Studies will be included if they describe and/or define mental wellness in adolescent populations. As the interest lies in conceptualising mental wellness for adolescents, only studies dealing with general mental health, wellbeing and wellness will be included. In other words, studies focused on mental disorders or mental illnesses among adolescents will be excluded. For this review, studies will be included for all adolescents aged 10–19 who have not been diagnosed with a mental illness or disorder. Eligible studies will include qualitative, quantitative, and mixed-method studies published from 2000 to 2022. The time period of the search strategy was chosen due to the paucity of research in this area [ 3 , 22 , 27 ]. Furthermore, the prioritization of adolescent health and the focus on adolescent-friendly services occurred after 2000 [ 28 ].
Screening and selection process
Study selection.
The PICOT mnemonics (Table 1 ) for reviews will be used to guide study selection.
The above-mentioned criteria and search strategy will be used to search the databases. The screening and reporting of the review will be conducted following the Preferred Reporting Items for Systematic Reviews and Meta-Analyses (PRISMA) guideline and checklist [ 29 ]. The number of hits for each database will be recorded and the citations will be exported to Mendeley citation software. Following this, two reviewers will screen all the titles and abstracts to assess which articles are appropriate for inclusion. The full-text articles of the included abstracts will be downloaded and reviewed again to determine which articles should be included for the final assessment [ 30 ]. Any discrepancies between the two reviewers will be resolved by a third party. Additionally, based on the information retrieved from the screening, the researcher may modify the search to include other relevant sources.
Data evaluation
In this integrative review, the primary sources will include both empirical and theoretical literature - which increases the complexity of evaluating the quality of the included sources [ 26 ]. According to Whittmore and Knafl [ 26 ], integrative reviews using diverse sampling frames may adopt an approach to data evaluation that is similarly used in historical research. In this case, the authenticity, methodological quality, informational value, and representativeness of the available sources should be discussed in the final report. To minimise bias, the two reviewers will utilise two existing quality criteria instruments to evaluate the different types of data [ 26 ]. Firstly, the Mixed Methods Appraisal Tool (MMAT) (Additional file 1 : Appendix A) will be used to assess the methodological quality of the studies as it allows for summarising the overall quality across a range of study designs [ 31 ]. Secondly, the SFS scoring system version E (Additional file 1 : Appendix B) will be used to assess the quality of the methodologies of the included articles [ 32 ]. The SFS scoring system version E is appropriate as it allows for screening of both quantitative and qualitative research and allows for the appraisal of the definitions of constructs being investigated [ 32 ].
Data analysis
Once the selection of included articles has been finalised, we will extract the relevant data into a Microsoft Excel document to organise the information and prepare for the data synthesis. The Excel sheet will include information regarding the purpose of the study, study characteristics, results, and appraisal of the study as well as any other supporting information. All data will be cross-checked for quality purposes.
Data from the integrative review will be analysed using narrative framework synthesis for qualitative and quantitative studies. Framework synthesis begins with a tentative framework that can either be borrowed from previous studies or can be developed from key concepts [ 26 , 33 ]. With framework synthesis, the included studies are coded according to the developing framework in an iterative process until the body of evidence can be presented coherently.
In the final stage, the findings from the review will be discussed and presented in either tabular or diagrammatic form. Additionally, the limitations of the review will be discussed as well as recommendations for future research.
This integrative review aims to synthesise current literature on adolescent mental wellness to identify the ways in which this is being described and applied in research. The purpose of this is to identify gaps and to contribute to the conceptualisation of a more comprehensive definition of mental wellness which can aid in the development of an age- and culturally appropriate measure of adolescent mental wellness. Such measures are much needed in adolescent health research as it may be used to better understand the mental wellness needs of adolescents and contribute to the development of interventions and programmes aimed at improving psychological wellbeing and/or mental wellness of adolescents.
Strengths and limitations
According to our knowledge, this protocol describes the first integrative review to investigate and describe how mental wellness is defined in research among adolescents. Understanding how mental wellness among adolescents has been conceptualised is necessary to identify what are the strengths and limitations of such definitions. This will allow researchers to rethink what mental wellness means to adolescents and how this can and should be measured in research. A limitation of this study is related to the search strategy, notably around the time span (2000–2022) and the identification of grey literature, as not all possible sources of literature may be accessed.
Availability of data and materials
Not applicable.
Abbreviations
Adolescents living with HIV
Antiretroviral therapy
Child and Adolescent Mental Health
Low- and middle-income countries
Mixed Methods Appraisal Tool
SFS Scoring System
United Nations International Children’s Emergency Fund
World Health Organization
World Health Organization. Health for the world’s adolescents: a second chance in the second decade. 2014. Available from: https://www.who.int/maternal_child_adolescent/documents/second-decade/en/
Google Scholar
Patton GC, Sawyer SM, Santelli JS, Ross DA, Afifi R, Allen NB, et al. Our future: a Lancet commission on adolescent health and wellbeing. Lancet. 2016;387:2423–78. https://doi.org/10.1016/S0140-6736(16)00579-1 .
Article PubMed PubMed Central Google Scholar
World Health Organization. Global accelerated action for the health of adolescents (AA-HA!) guidance to support country implementation. 2017. Available from: http://www.who.int/maternal_child_adolescent/topics/adolescence/framework-accelerated-action/en/ .
World Health Organization. Adolescents: health risks and solutions. 2014. Available from: http://www.who.int/mediacentre/factsheets/fs345/en/ .
James S, Pisa PT, Imrie J, Beery MP, Martin C, Skosana C, et al. Assessment of adolescent and youth friendly services in primary healthcare facilities in two provinces in South Africa. BMC Health Serv Res. 2018;18:1–10. https://doi.org/10.1186/s12913-018-3623-7 .
Article CAS Google Scholar
Sawyer SM, Afifi RA, Bearinger LH, Blakemore SJ, Dick B, Ezeh AC, et al. Adolescence: a foundation for future health. Lancet. 2012;379:1630–40. https://doi.org/10.1016/S0140-6736(12)60072-5 .
Article PubMed Google Scholar
World Health Organization. The life-course approach: from theory to practice. Case stories from two small countries in Europe. 2018. Available from: http://www.euro.who.int/__data/assets/pdf_file/0004/374359/life-course-iceland-malta-eng.pdf?ua=1 .
Armstrong A, Nagata JM, Vicari M, Irvine C, Cluver L, Sohn AH, et al. A global research agenda for adolescents living with HIV. J Acquir Immune Defic Syndr. 2018;78:S16–21. https://doi.org/10.1097/QAI.0000000000001744 .
World Health Organization. Adolescent mental health, 2018. Available from: https://www.who.int/news-room/fact-sheets/detail/adolescent-mental-health .
UNICEF. Measurement of mental health among adolescents at the population level (MMAP): conceptual framework and the roadmap to the measurement of mental health, 2018. Available from: https://data.unicef.org/wp-content/uploads/2018/11/Conceptual-framework-and-Roadmap-19-july.pdf .
Guthold R, Moller AB, Azzopardi P, Ba MG, Fagan L, Baltag V, et al. The Global Action for Measurement of Adolescent health (GAMA) initiative—rethinking adolescent metrics. J Adolesc Health. 2019;64:697–9. https://doi.org/10.1016/j.jadohealth.2019.03.008 .
Mokitimi S, Schneider M, de Vries PJ. Child and adolescent mental health policy in South Africa: history, current policy development and implementation, and policy analysis. Int J Ment Health Syst. 2008;12(36):1–15. https://doi.org/10.1186/s13033-018-0213-3 .
Article Google Scholar
Juengsiragulwit D. Opportunities and obstacles in child and adolescent mental health services in low- and middle-income countries: a review of the literature. WHO South-East Asia J Public Health. 2015;4:110. https://doi.org/10.4103/2224-3151.206680 .
Campo JV, Bridge JA, Fontanella CA. Access to mental health services implementing an integrated solution. JAMA Pediatr. 2015;169:299–300. https://doi.org/10.1001/jamapediatrics.2014.3558 .
Sawyer SM, Drew S, Yeo MS, Britto MT. Adolescents with a chronic condition: challenges living, challenges treating. Lancet. 2007;369:1481–9. https://doi.org/10.1016/S0140-6736(07)60370-5 .
Delamater AM, Guzman A, Aparicio K. Mental health issues in children and adolescents with chronic illness. Int J Hum Rights Healthc. 2017;10:163–73. https://doi.org/10.1108/IJHRH-05-2017-0020 .
Duffus SH, Cooper KL, Agans RP, Jain N. Mental health and behavioral screening in pediatric type 1 diabetes. Diabetes Spectr. 2019;32:171–5. https://doi.org/10.2337/ds18-0053.22 .
Lee S, Chung NG, Choi JY. Comparison of resilience and quality of life between adolescent blood cancer survivors and those with congenital heart disease: a cross sectional study. Health Qual Life Outcomes. 2020;18:1–7. https://doi.org/10.1186/s12955-020-01487-w .
Manwell LA, Barbic SP, Roberts K, Durisko Z, Lee C, Ware E, et al. What is mental health? Evidence towards a new definition from a mixed methods multidisciplinary international survey. BMJ Open. 2015;5:1–11. https://doi.org/10.1136/bmjopen-2014-007079 .
Dodge R, Daly AP, Huyton J, Sanders LD. The challenge of defining wellbeing. Int J Wellbeing. 2012;2:222–35. https://doi.org/10.5502/ijw.v2i3.4 .
Orth Z, Van Wyk B. Adolescent mental wellness: a systematic review protocol of instruments measuring general mental health and well-being. BMJ Open. 2020;10:37237. https://doi.org/10.1136/bmjopen-2020-037237 .
Vreeman RC, Mccoy BM, Lee S. Review article mental health challenges among adolescents living with HIV. J Int AIDS Soc. 2017;20:100–9. https://doi.org/10.7448/IAS.20.4.21497 .
Manderscheid RW, Ryff CD, Freeman EJ, Mcknight-eily LR, Dhingra S, Strine TW. Evolving definitions of mental illness and wellness. Prev Chronic Dis. 2010;7:5–10 http://www.cdc.gov/pcd/issues/2010/jan/09_0124.htm .
Keyes CLM. Promoting and protecting mental health as flourishing. Am Psychol. 2005:95–108. https://doi.org/10.1037/0003-066X.62.2.95 .
De Souza MT, De Carvalho R. Integrative review: what is it? How to do it? Einstein. 2010;8:102–7. https://doi.org/10.1590/S1679-45082010RW1134 .
Whittemore R, Knafl K. The integrative review: updated methodology. J Adv Nurs. 2005;52(5):546–53. https://doi.org/10.1111/j.1365-2648.2005.03621 .
Sherr L, Cluver LD, Toska E, He E. Differing psychological vulnerabilities among behaviourally and perinatally HIV infected adolescents in South Africa–implications for targeted health service provision. AIDS Care. 2018;30:92–101. https://doi.org/10.1080/09540121.2018.1476664 .
Article CAS PubMed Google Scholar
World Health Organization. Promoting mental health: concepts, emerging evidence, practice (summary report). Geneva: World Health Organization; 2004.
Page MJ, McKenzie JE, Bossuyt PM, Boutron I, Hoffmann TC, Mulrow CD, et al. The PRISMA 2020 statement: an updated guideline for reporting systematic reviews. BMJ. 2021;372:n71. https://doi.org/10.1136/bmj.n71 .
Eggar M, Smith GD, Altman DG. Systematic reviews in health care: meta analysis in context. 2nd ed. London: BMJ Publishing Group; 2001.
Book Google Scholar
Hong QN, Fàbregues S, Bartlett G, Boardman F, Cargo M, Dagenais P, et al. The mixed methods appraisal tool (MMAT) version 2018 for information professionals and researchers. Educ Inf. 2018;34:285–91. https://doi.org/10.3233/EFI-180221 .
Smith MR, Franciscus G, Swartbooi C, Munnik E, Jacobs W. The SFS scoring system. In: Smith MR (Ed., Chair). Symposium on methodological rigour and coherence: deconstructing the quality appraisal tool in systematic review methodology, conducted at the 21 st National Conference of the Psychological Association of South Africa. South Africa; 2015.
Snilstveit B, Oliver S, Vojtkova M. Narrative approaches to systematic review and synthesis of evidence for international development policy and practice. J Dev Eff. 2012;4:409–29. https://doi.org/10.1080/19439342.2012.710641 .
Download references
Acknowledgements
ZO is supported by the National Research Foundation, grant number (118160). Additionally, the authors would like to acknowledge funding form the Belgian Development Cooperation, through the Institute of Tropical Medicine Antwerp. Any opinion, finding and conclusion or recommendation expressed in this material is that of the authors and not the funders.
Author information
Authors and affiliations.
School of Public Health, University of the Western Cape, Bellville, 7535, South Africa
Zaida Orth & Brian van Wyk
You can also search for this author in PubMed Google Scholar
Contributions
ZO conceived the idea, developed the research question and study methods, and contributed meaningfully to the drafting and editing; she also approved the final manuscript. BvW aided in developing the research question and study methods, contributed meaningfully to the drafting and editing, and approved the final manuscript.
Corresponding author
Correspondence to Zaida Orth .
Ethics declarations
Ethics approval and consent to participate.
This integrative review forms part of the corresponding author’s PhD work and has been approved by the University of the Western Capes’ Biomedical Science Research Ethics Committee.
Ethics Reference Number: BM19/9/18
Consent for publication
Competing interests.
The authors declare that they have no competing interests.
Additional information
Publisher’s note.
Springer Nature remains neutral with regard to jurisdictional claims in published maps and institutional affiliations.
Supplementary Information
Additional file 1: appendix a..
Mixed Methods Appraisal Tool (MMAT). Appendix B. SFS Scoring System (Version E). Appendix C. PRISMA-P Checklist.
Rights and permissions
Open Access This article is licensed under a Creative Commons Attribution 4.0 International License, which permits use, sharing, adaptation, distribution and reproduction in any medium or format, as long as you give appropriate credit to the original author(s) and the source, provide a link to the Creative Commons licence, and indicate if changes were made. The images or other third party material in this article are included in the article's Creative Commons licence, unless indicated otherwise in a credit line to the material. If material is not included in the article's Creative Commons licence and your intended use is not permitted by statutory regulation or exceeds the permitted use, you will need to obtain permission directly from the copyright holder. To view a copy of this licence, visit http://creativecommons.org/licenses/by/4.0/ . The Creative Commons Public Domain Dedication waiver ( http://creativecommons.org/publicdomain/zero/1.0/ ) applies to the data made available in this article, unless otherwise stated in a credit line to the data.
Reprints and permissions
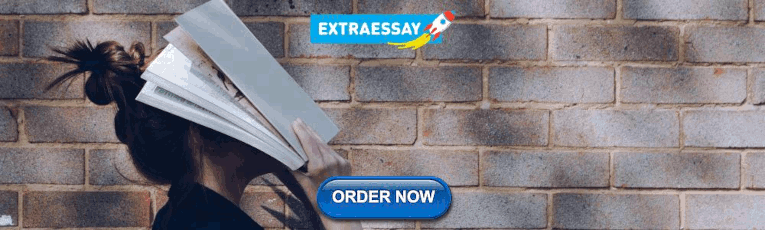
About this article
Cite this article.
Orth, Z., van Wyk, B. Rethinking mental wellness among adolescents: an integrative review protocol of mental health components. Syst Rev 11 , 83 (2022). https://doi.org/10.1186/s13643-022-01961-0
Download citation
Received : 05 October 2020
Accepted : 15 April 2022
Published : 02 May 2022
DOI : https://doi.org/10.1186/s13643-022-01961-0
Share this article
Anyone you share the following link with will be able to read this content:
Sorry, a shareable link is not currently available for this article.
Provided by the Springer Nature SharedIt content-sharing initiative
- Adolescent mental health
- Mental wellness
- Integrative review
- Positive psychology
Systematic Reviews
ISSN: 2046-4053
- Submission enquiries: Access here and click Contact Us
- General enquiries: [email protected]
- Research article
- Open access
- Published: 11 September 2013
A systematic review of the effectiveness of mental health promotion interventions for young people in low and middle income countries
- Margaret M Barry 1 ,
- Aleisha M Clarke 1 ,
- Rachel Jenkins 2 &
- Vikram Patel 3 , 4
BMC Public Health volume 13 , Article number: 835 ( 2013 ) Cite this article
64k Accesses
279 Citations
94 Altmetric
Metrics details
This systematic review provides a narrative synthesis of the evidence on the effectiveness of mental health promotion interventions for young people in low and middle-income countries (LMICs). Commissioned by the WHO, a review of the evidence for mental health promotion interventions across the lifespan from early years to adulthood was conducted. This paper reports on the findings for interventions promoting the positive mental health of young people (aged 6–18 years) in school and community-based settings.
Searching a range of electronic databases, 22 studies employing RCTs (N = 11) and quasi-experimental designs conducted in LMICs since 2000 were identified. Fourteen studies of school-based interventions implemented in eight LMICs were reviewed; seven of which included interventions for children living in areas of armed conflict and six interventions of multicomponent lifeskills and resilience training. Eight studies evaluating out-of-school community interventions for adolescents were identified in five countries. Using the Effective Public Health Practice Project (EPHPP) criteria, two reviewers independently assessed the quality of the evidence.
The findings from the majority of the school-based interventions are strong. Structured universal interventions for children living in conflict areas indicate generally significant positive effects on students’ emotional and behavioural wellbeing, including improved self-esteem and coping skills. However, mixed results were also reported, including differential effects for gender and age groups, and two studies reported nonsignficant findings. The majority of the school-based lifeskills and resilience programmes received a moderate quality rating, with findings indicating positive effects on students’ self-esteem, motivation and self-efficacy. The quality of evidence from the community-based interventions for adolescents was moderate to strong with promising findings concerning the potential of multicomponent interventions to impact on youth mental health and social wellbeing.
Conclusions
The review findings indicate that interventions promoting the mental health of young people can be implemented effectively in LMIC school and community settings with moderate to strong evidence of their impact on both positive and negative mental health outcomes. There is a paucity of evidence relating to interventions for younger children in LMIC primary schools. Evidence for the scaling up and sustainability of mental health promotion interventions in LMICs needs to be strengthened.
Peer Review reports
Mental health is fundamental to good health and wellbeing and influences social and economic outcomes across the lifespan [ 1 – 3 ]. Childhood and adolescence are crucial periods for laying the foundations for healthy development and good mental health. It is estimated that 10-20% of young people worldwide experience mental health problems [ 4 ]. Poor mental health in childhood is associated with health and social problems such as school failure, delinquency and substance misuse, and increases the risk of poverty and other adverse outcomes in adulthood [ 3 ]. Interventions that promote positive mental health equip young people with the necessary life skills, supports and resources to fulfill their potential and overcome adversity. Systematic reviews of the international evidence, which come predominantly from high income countries (HICs), show that comprehensive mental health promotion interventions carried out in collaboration with families, schools and communities, lead to improvements not only in mental health but also improved social functioning, academic and work performance, and general health behaviours [ 5 – 13 ].
Despite the recognition of the importance of mental health promotion for children and adolescents, mental health remains a neglected public health issue, especially in low and middle-income countries (LMICs). Mental health is inequitably distributed as people living in poverty and other forms of social disadvantage bear a disproportionate burden of mental disorders and their adverse consequences [ 14 – 17 ]. There is increasing recognition of the relevance of mental health to global development strategies, and in particular to the achievement of the Millennium Development Goals (MDGs), including improving child and maternal health, universal education, combating HIV/AIDS and other diseases, and eradicating poverty [ 18 , 19 ]. As 90% of the world’s children and adolescents live in LMICs, where they constitute up to 50% of the population [ 20 ], there is an urgent need to address the mental health of young people as part of the wider health promotion and development agenda.
Schools are one of the most important community settings for promoting the mental health of young people [ 21 ]. The school setting provides a forum for promoting emotional and social competence as well as academic learning and offers a means of reaching the significant number of young people who experience mental health problems [ 22 – 25 ]. Educational opportunities throughout life are associated with improved mental health outcomes. The promotion of emotional health and wellbeing is a core feature of the WHO’s Health Promoting Schools initiative [ 26 ]. There is good evidence that mental health promotion programmes in schools, especially those adopting a whole school approach, lead to positive mental health, social and educational outcomes [ 13 , 27 – 29 ]. Programmes incorporating life skills, social and emotional learning and early interventions to address emotional and behavioural problems, produce long-term benefits for young people, including improved emotional and social functioning, positive health behaviours, and improved academic performance [ 5 , 13 , 25 , 27 – 31 ]. To date there has been comparatively little research on school and community-based mental health promotion interventions for young people in LMIC settings and no systematic attempt to synthesize the evidence from such settings. This is the goal of this paper. The work described here was undertaken in 2011–2012 as part of the World Health Organization Task Force on Mainstreaming Health Promotion. Established on foot of the WHO 7 th Global Conference on Health Promotion [ 32 ], the Task Force sought to develop a package of evidence-based health promotion actions addressing priority public health conditions in LMICs.
The objectives of the review were:
To synthesize evidence on the effectiveness of mental health promotion interventions for young people that have been implemented in LMICs.
To identify gaps in the existing evidence and highlight areas where further research is needed.
Study selection
This systematic review conforms to the guidelines outlined by the PRISMA 2009 checklist. A research protocol for the original review was agreed with the Members of the WHO Task Force and the Cochrane Public Health Group (CPHG). Studies were eligible for inclusion if the intervention was designed to promote positive mental health for young people in LMIC settings. For the purpose of this review, mental health promotion interventions were defined as any planned action, programme or policy, which was undertaken with the aim of improving mental health or modifying its determinants. Evidence in relation to the studies for young people aged 6–18 years across all school and community settings was included, with no exclusions based on gender or ethnicity. Academic and grey literature published from 2000 onwards in printed or electronic format was deemed eligible for inclusion. In order to include studies of comparable quality, we considered study designs including randomized controlled trials, cluster randomized controlled trials, and quasi-experimental study designs. The primary outcomes of interest were mental health and wellbeing benefits including; indicators of positive mental health such as self-esteem, self-efficacy, coping skills, resilience, emotional wellbeing; negative mental health such as depression, anxiety, psychological distress, suicidal behaviour; and wellbeing indicators such as social participation, empowerment, communication and social support. Secondary health related outcomes were also noted. Studies with the following characteristics were excluded from the review; (i) selective and indicated prevention interventions, as defined by Mrazek and Haggerty [ 33 ], (ii) studies with no control/comparison group, and (iii) qualitative only studies.
Search strategy
Academic databases including PubMed, PsychInfo, Scopus, ISI Web of Knowledge, Cochrane database of systematic reviews were searched. Health Promotion and Public Health Review databases were also searched including Evidence for Policy and Practice information and Coordinating (EPPI) Centre; University of York National Health Service Centre for reviews and dissemination; National Institute of Clinical Excellence (NICE); Effective Public Health Practice, Health Evidence Canada; WHO programmes and projects. Additional sources included Google Scholar and reference list of relevant articles, book chapters and reviews. Key individuals and organizations identified through the search process were contacted to identify further details on publications. The electronic search strategy used across all databases is provided in Table 1 . The last search for the original systematic review of mental health promotion interventions was completed on 11 th March 2011 and included articles published between January 2000 - December 2010. A repeated search was conducted on 7th September 2012 to update results and included articles published between January 2011 – June 2012.
Study selection and data collection
Using the search strategy described above, all titles and abstracts retrieved were scanned for relevance. Duplicates, articles not relevant, and articles that did not meet the inclusion criteria were removed. Full text papers were obtained for studies that were selected for inclusion. Studies were subsequently selected relating to young people and were classified according to (i) school-based programmes (ii) community-based programmes for adolescents. Two reviewers assessed the studies in order to ensure that they met the inclusion criteria set out for this review.
Data analysis
As the interventions and outcomes evaluated in the included studies were too diverse to allow a quantitative synthesis of the study findings, a narrative synthesis was undertaken. Following the guidelines of the Cochrane Public Health Group, the methodological quality of the intervention evaluations was assessed using the Quality Assessment Tool for Quantitative Studies developed by the Effective Public Health Practice Project [ 34 ]. Studies were assessed for selection bias, study design, confounders, blinding, data collection and withdrawals and drop-outs. Each study was rated independently by two reviewers (MB and AC). The quality assessments were compared and disagreements were resolved through discussion. Based on the ratings of each of the six components, each study received an overall global rating of strong, moderate or weak. Following the quality assessment stage, the inclusion of studies and extraction of key findings was finalized. Extracted data were entered into a table of study characteristics (Table 2 ) including the quality assessment ratings for each study.
The results of the search and study selection are shown in Figure 1 . The original search process carried out in 2011 produced 10,471 articles, 188 articles of which were selected for full review and exported to Endnote. Of these, 146 were either contextual articles related to mental health promotion in LMICs or studies that did not meet one of our inclusion criteria. Seven articles were systematic/summary reviews of the evidence base in LMICs, five of which were reviews of interventions for young people. A total of 35 primary studies were selected for review. Of these, 14 studies evaluated school or community-based interventions for young people in LMICs. During the repeated search performed in September 2012, a further eight studies evaluating school-based interventions were identified. The combined searches resulted in a total of 22 studies (14 school and eight community-based studies) undergoing quality assessment. No studies in non-English language specific to school and community based-interventions were identified in the review process.
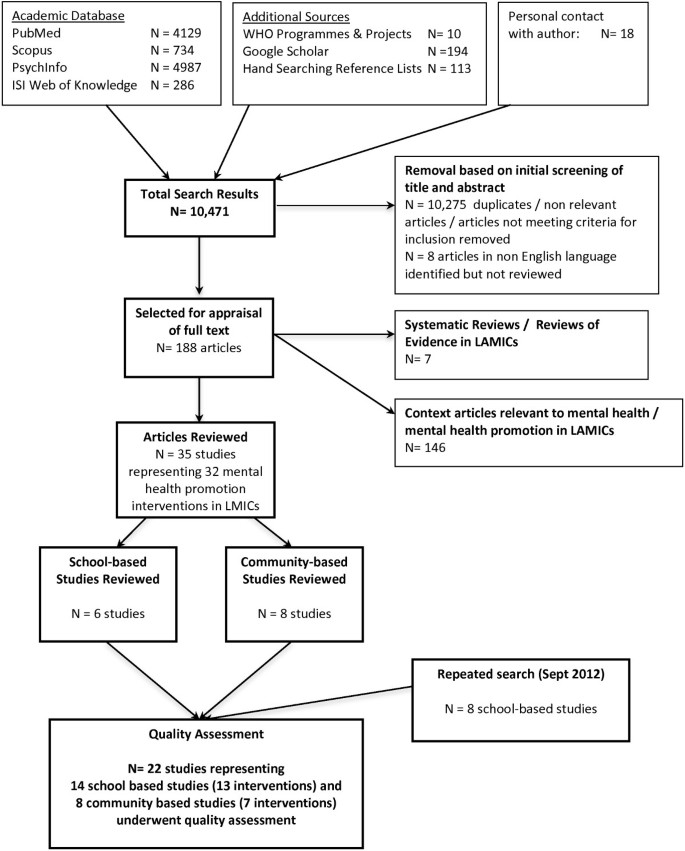
Search results from original search of mental health promotion interventions in LMICs.
The five systematic review articles from LMICs that were identified examined the effectiveness of HIV related lifeskills interventions [ 69 , 70 ] and psychosocial interventions for children and adolescents affected by armed conflict in LMICs [ 71 – 73 ]. All relevant interventions across the reviews were identified and cross-referenced with the primary articles retrieved through the electronic search. Given the specific focus of this systematic review on mental health promotion and primary prevention, several studies from these systematic reviews did not meet the inclusion criteria for this review.
Regarding the number and percentage of evaluation studies carried out across LMICs, 18.2% (N = 4) of the interventions were carried out in low income countries, 36.4% (N = 8) were carried out in lower middle income countries and 45.4% (N = 10) were carried out in upper middle income countries. Just under one third of the interventions (N = 7) were carried out in South Africa alone.
School-based programmes
Fourteen studies describing thirteen interventions implemented in school settings in eight LMIC countries were identified. Four studies were carried out in Gaza/Palestine [ 48 , 50 , 55 , 56 ], three were carried out in South Africa [ 37 , 38 , 41 , 44 ], two in Uganda [ 45 , 49 ] and one intervention was carried out in India [ 35 ], Chile [ 36 ], Mauritius [ 42 ], Nepal [ 47 ], and the Lebanon [ 54 ]. The majority of studies (>60%) were published between 2010–2012. The quality of evidence from the majority of studies was strong. A total of eight studies received a strong quality rating [ 42 , 45 , 47 , 48 , 50 , 54 – 56 ], five studies received a moderate quality assessment rating as a result of selection bias [ 36 , 44 ] and not reporting the percentage of withdrawals/dropouts [ 35 , 38 , 49 ]. One study received a weak quality assessment rating due to selection bias, not reporting confounders and not reporting level of withdrawals [ 41 ].
The programmes were mental health promotion and universal prevention interventions designed for all children and adolescents of school going age. Interventions varied slightly in their focus from the development of social, emotional, problem solving and coping skills [ 35 , 41 ] to a combined mental health promotion with physical fitness programme [ 36 ], combined mental health promotion and sexuality education [ 37 , 38 ] and a universal depression prevention intervention [ 42 ]. Two interventions were designed specifically to support AIDS orphaned children, one was an art intervention [ 44 ], another was a peer support intervention led by teachers [ 45 ]. Seven interventions (eight studies) were school-based psychosocial interventions implemented in countries affected by armed conflict [ 47 – 50 , 54 – 56 ]. These interventions were designed to reduce distress, enhance resilience and coping skills. Four of these interventions incorporated cognitive behavioural techniques (CBT) and trauma related psychoeducation modules [ 47 – 50 , 54 ]. One intervention consisted of short writing sessions [ 55 ], another provided structured recreational activities [ 56 ].
Seven of the school-based interventions were designed for post-primary school students (>12 years of age). Four interventions were implemented with a broad age range from 6 – 18 years [ 44 , 48 , 54 , 56 ]. Three interventions were implemented with children in the senior end of primary school (>10 years of age) [ 45 , 49 , 50 ]. Eight interventions were implemented by the class teacher [ 35 – 38 , 42 , 44 , 49 , 54 , 55 ], with the remaining interventions implemented by mental health professionals [ 41 , 48 , 50 ], locally trained paraprofessionals [ 47 ] and local youth workers [ 44 , 56 ]. The majority of session ranged in length from 11 – 16 sessions implemented weekly. One intervention provided six booster sessions at 12 months following completion of the programme [ 37 , 38 ]. Eight school interventions were developed in the implementing country. Five interventions were adapted versions of evidence-based interventions from high income countries [ 37 , 38 , 42 , 47 – 49 ].
Regarding intervention outcomes, in terms of the seven universal programmes implemented with children affected by armed conflict, the findings are generally positive but with some studies reporting mixed effects. Loughry et al. [ 56 ] reported that the after-school recreational activities implemented over one year had a significant positive impact on children and adolescents’ externalising and internalising problem scores and also improved parental support as a result of parental involvement in the structured activities. Khamis et al. [ 48 ] reported that the Classroom-Based Intervention (CBI) had a significant positive effect on children (age 6–11) and adolescents (age 13–16) in terms of improved social and emotional wellbeing, communication skills and reduced conduct and peer problems and hyperactivity levels. Ager et al. [ 49 ] reported similar findings for the school-based Psychosocial Structures Activities intervention (PSSA) which is based on principles of the CBI, with the intervention having a significant positive effect on primary school children’s (mean age 10 years) wellbeing. Interestingly, the CBI study carried out in Nepal reported specific gender effects, with significant reductions in psychological difficulties and aggression among males only and improved prosocial behaviour among females only [ 47 ]. Two studies reported less positive findings. Karam et al. [ 54 ] found that the cognitive behavioural therapy intervention (CBT) implemented over 12 consecutive days had no significant effect on participant rates of depression, separation anxiety and post-traumatic stress disorder (PTSD). Lange-Nielsen et al. [ 55 ] reported that the three day short-term writing intervention had no effect on participants’ PTSD symptoms and anxiety scores. This study also reported that the writing intervention lead initially to significantly increased depression symptoms for participants between pre and post-intervention but that symptoms significantly declined at five months follow up. Contrasting findings in terms of gender effects were reported across three studies; two studies reported that the interventions have a more positive effect on girls [ 48 , 49 ] while another intervention reported no programme effect for girls with PTSD scores improving only in male participants [ 50 ].
Regarding the universal lifeskills and resilience school-based interventions, all six studies reported significant positive effects on students’ mental health and wellbeing in terms of improved self-esteem [ 35 , 36 , 42 ], motivation [ 38 ] and self-efficacy [ 44 ]. The peer-group support intervention implemented with AIDS orphan children resulted in significant improvements in participants’ depression, anger and anxiety scores but not for self-concept [ 45 ]. The combined fitness lifeskills education intervention reported improvements in anxiety symptoms, however, there was no change in participants’ depression scores [ 36 ]. The depression prevention intervention on the other hand reported a significant reduction in depressive symptoms (medium effect size) and hopelessness (medium effect size) and a significant increase in coping skills (medium effect size) amongst participants in the intervention group [ 42 ]. Long-term findings from this depression prevention intervention included improved self-esteem and coping skills (medium effect size) at six months follow up. In addition, the resilience intervention in South Africa also reported long-term findings with improved self-appraisal scores maintained at three months follow up [ 41 ]. Additional outcomes from these studies include improved behaviour [ 35 ], school adjustment [ 35 ], fitness [ 36 ], attitudes about reproductive and sexual health [ 37 ] and a reduction in the level of substance misuse [ 37 ]. The art intervention for AIDS orphan children reported the least positive findings with a significant improvement reported in the intervention groups’ self-efficacy score but no change in participants’ depression, self-esteem and emotional and behavioural scores [ 44 ]. While the results from the resiliency intervention indicated significant improvements in participants’ emotional reactivity, self appraisal and interpersonal strength, the weak quality of this study must be considered when interpreting these findings.
Community-based interventions
This review identified eight studies evaluating seven out-of-school community interventions for adolescents in five countries. Four studies were carried out in South Africa [ 60 , 61 , 63 , 64 ], one study was carried out in India [ 57 ], Honduras [ 58 ], Egypt [ 59 ] and Uganda [ 65 – 68 ]. All eight studies were published between 2006 and 2010. The quality of evidence from these studies was moderate to strong. Four studies received a strong quality assessment rating [ 57 , 60 , 61 , 65 ] and four studies received a moderate quality assessment rating due to small sample size [ 58 ] and failure to report validity and reliability of measures used in three studies [ 59 , 63 , 64 ].
Interventions included a multi-component school and community-based intervention for youth aged 16–24 years [ 57 ]; a family-based strengthening programme ( Familias Fuertas ) for parents and their adolescent children [ 58 ]; a multidimensional programme ( Ishraq ) aimed at improving the life skills, literacy, recreational activities and health knowledge of 13–15 year old girls in Egypt [ 59 ] and combined HIV prevention and lifeskills interventions ( Stepping Stones and CHAMPSA ) for adolescents in South Africa [ 60 , 61 ]. Two studies evaluated the Intervention with Microfinance for AIDS and Gender Equity ( IMAGE ), a poverty-focused microfinance initiative for women that is combined with a 12–15 month gender and HIV education curriculum [ 63 , 64 ]. One study examined the effects of small individual loans and mentorship on health and mental health functioning of primary school children [ 65 – 68 ]. Five of the seven interventions were designed for young people aged 13+. The Familias Fuertas intervention was designed for children age 10–14 and one of the evaluations of the IMAGE microfinance intervention was implemented with females aged 18 and over. Two interventions provided parent training [ 58 , 61 ] and two interventions were designed specifically for females [ 59 , 63 , 64 ]. Five of the interventions were implemented by local trained community caregivers [ 59 – 61 , 63 – 68 ]. The Familias Fuertas intervention was implemented by a local nurse [ 58 ] and the multi-component school and community intervention in India was implemented by a team of social workers, psychologists and peer educators [ 57 ]. Five of the interventions were developed in the implementing country. Two interventions were adapted versions of evidence-based interventions that were developed in the United States [ 58 , 61 ].
Collectively, the results from these studies indicate the significant positive effect of community-based mental health promotion interventions on young people’s mental health and social wellbeing. Five interventions provided strong evidence of their positive impact on mental health. Balaji et al. [ 57 ] reported that the community-based youth health intervention in India resulted in significant improvements in participants’ depression scores, reported levels of suicidal behaviour, and knowledge and attitudes about mental health. South Africa’s IMAGE intervention resulted in significant improvements in empowerment, social participation and levels of openness among women in the combined IMAGE-microfinance intervention, with no change evident the microfinance only intervention [ 64 ]. In addition, Pronijk et al. [ 64 ] reported that participants in the IMAGE intervention were significantly more likely to participate in training, and had greater participation in social and community groups. Ssewamala et al. [ 65 , 66 ] reported that the SUUBI economic empowerment intervention for AIDS orphaned children had a significant positive impact on participants’ self-esteem and levels of depression. Results from the parent-youth interventions indicate the significant effect of the programmes on positive parenting communication and behaviours, parental self-esteem and family relations [ 58 , 61 ].
Other reported outcomes also included significantly improved: peer relations [ 59 ]; academic performance [ 59 , 68 ]; student-teacher relations [ 57 ]; communication [ 64 ]; improved gender roles [ 64 ] and significantly reduced sexual risk behaviour [ 60 , 63 ] one year follow up; physical and sexual partner violence [ 57 , 60 , 63 , 64 ]; and substance abuse [ 57 , 60 ]. Bell et al., [ 61 ] reported medium effect sizes for improved caregiver communication comfort. Long-term findings from the Stepping Stones intervention include reduced physical and sexual partner violence at two years follow- up, and reduced substance abuse at one year follow up [ 60 ].
This review sought to determine the effectiveness of mental health promotion interventions designed for young people (aged 6–18 years) in LMICs. A total of 22 studies evaluating 20 interventions were identified. The majority of interventions were implemented in upper and lower middle income countries, thus highlighting the paucity of evidence from low income countries. Four interventions were carried out in low income countries, three of which were conducted in Uganda. It is encouraging to note, however, the significant increase in publications from LMICs in the last four years, with the majority of interventions identified in this review published since 2008.
With regard to the school-based interventions, the quality of evidence from the 14 studies is moderate to strong. Findings from these studies indicate that there is reasonably robust evidence that school-based programmes implemented across diverse LMICs can have significant positive effects on students’ emotional and behavioural wellbeing, including reduced depression and anxiety and improved coping skills. Promising interventions include the Resourceful Adolescent Program (RAP-A), which was implemented by teachers in Mauritius [ 42 ]. This study is an example of an evidence-based intervention adapted from a HIC for implementation in a LMIC and points to the potential of such interventions when adapted to meet the cultural needs of young people in LMICs. Another promising intervention is the teacher led peer-group support intervention for AIDs orphaned children which was implemented in a low-income country [ 45 ]. The findings from this study suggest the potential of peer support mental health promotion interventions in optimizing adjustment and decreasing the psychological distress associated with AIDS orphanhood in the adolescent age group. Such interventions may have great potential in addressing the increased risk of depression, peer relationship problems, post-traumatic stress and conduct problems among AIDS orphans [ 74 – 76 ]. There is also some encouraging evidence that interventions which combine lifeskills with reproductive and sexual health education [ 37 , 38 ] and physical health and fitness [ 36 ] can have a significant positive effect on pupils’ risk-taking and prosocial behaviour. These findings are consistent with the substantive evidence from multiple reviews of school-based interventions in HICs which report the greater effectiveness of multi-component interventions (i.e. interventions that adopt a social competence approach and develop supportive environments), when compared with interventions that focus on specific problem behaviours [ 8 , 28 , 77 – 79 ]. The integration of multicomponent programmes within a whole school approach [ 13 ] based on generic social and emotional skills training addressing comon risk and protective factors, delivered within a supportive school environment in partnership with parents and the local community, has the potential to reach larger population groups with fewer resources.
The evidence for universal interventions implemented with young people affected by war attests to the important role of the school as an accessible setting for such interventions. Similar to previous reviews [ 72 , 73 ], the heterogeneity across the studies in terms of programme content, delivery, duration, and study sample makes it difficult to draw general conclusions about the effectiveness of these interventions as a whole. However, there is evidence that the more structured interventions of longer duration can have a significant positive effect on mental health and wellbeing. The results from the Classroom-Based Intervention (CBI) and the school-based Psychosocial Structured Activities intervention (PSSA), which is based on CBI principles, highlight the positive effect of these interventions on young people’s social, emotional and behavioural wellbeing. The differential effects according to gender reported across these interventions, however, calls for further investigation into possible gender specific components. The optimum age for programme implementation also needs further examination. There is evidence from Khamis et al. [ 48 ] that CBI did not yield the same significant positive changes with older males (12–16 years) as with the younger group (aged 6–11). This finding is in line with substantive evidence from HICs regarding the need to reach children when they are young in order to sustain their existing resilience and strengthen their coping capabilities [ 4 , 12 , 80 – 82 ].
Non-significant findings were also found for a writing intervention implemented with young people aged 12–17 [ 55 ] and a CBT intervention implemented with children and adolescents aged 5–16 [ 54 ]. It is important to note the initial negative impact of the writing intervention on participants’ depression symptoms, which then subsequently declined at follow-up. Common characteristics of these interventions were their short duration and the broad age range of the intervention participants. This is in contrast to the year long after-school intervention implemented with children and adolescents and their parents living in Gaza and the West Bank, which resulted in significant improvement in participants’ social and emotional wellbeing and parenting behaviours [ 56 ]. The results from these studies underscore the importance of understanding optimum programme components in terms of content, duration, and target age range in order to ensure the development of effective school-based interventions in conflict areas. This is in line with recommendations from previous reviews of school-based interventions implemented in war exposed countries [ 71 , 72 ] including those from secondary prevention interventions, not covered in this review, which also point to the need for more rigorous research on the differential intervention effects related to age, gender and war-related experiences [ 73 , 83 ]. The studies in this review support previous findings concerning the role of universal school programmes for children living in conflict areas as an effective, accessible and efficient means of enhancing and protecting good mental health alongside more targeted approaches for students at higher risk [ 84 ]. The exploration of a whole school approach to interventions in this area carries potential for reaching the wider community through the school setting.
The majority of the school-based interventions included in this review were implemented with young people age 12–16 years. In view of the paucity of evidence of mental health promotion interventions for young children in primary schools in LMICs (age 5–10 years), there is an urgent need for high quality studies with longitudinal designs to assess the impact of school-based intervention for younger primary school children in order to strengthen the evidence base in this area. Schools are arguably one of the most important settings for reaching out to young children and their families and early intervention is recognised as one of the key principles of effective mental health promotion and prevention interventions [ 4 , 8 , 12 , 80 ]. In addition, eight of the interventions were implemented by trained class teachers, with the remaining interventions implemented by psychologists, paraprofessionals and youth workers. As Srikala & Kumar [ 35 ] argue, any programme incorporated into the education system in LMICs has to be feasible and cost-effective. The findings from this review suggest that trained teachers can effectively deliver mental health promotion interventions. Similar to findings from HICs, several of the studies reviewed highlighted the importance of teacher training and the provision of ongoing support during programme implementation. Harnessing the skills of teachers and providing support in the school setting offers a sustainable and low cost method of improving children’s emotional and behavioural wellbeing, developing positive coping strategies and promoting school performance. As the Millennium Development Goals have set out as a target that all boys and girls will be able to complete a full course of primary schools by 2015, the integration of social and emotional learning and lifeskills development in the primary school curriculum and the development of a whole school approach to health promotion is an important component of this development agenda.
In terms of the evidence for community-based interventions in LMICs, there are a limited number of very promising youth interventions addressing sexual and emotional health, HIV prevention, substance misuse, violence prevention, functional literacy, economic empowerment and social participation among excluded groups. The results from these multicomponent interventions are impressive given the improvements that were shown across a broad range of adolescent health outcomes. Although limited in number, the three microfinance interventions for young adults and primary school children included in this review, provide encouraging evidence that combined microfinance and training interventions promoting essential lifeskills, asset building and reourcefulness, can result in significant mental health and wellbeing benefits. Further evaluations of such multicomponent community-based interventions are needed to determine the long-term impact on more specific mental health outcomes.
Study limitations
This systematic review has a number of important limitations, which impact on its validity. Firstly, there are limitations relating to the scope of the systematic search, which impact on the validity of the findings. Due to the timescale and resources available, a systematic search for studies published in the grey literature was not included, and neither was effort made to find well-designed studies that had not been reported at all due to non-significant findings. Furthermore, a search in languages other than English was not undertaken and, therefore, key studies in the other former colonial languages of French, Spanish, Portuguese and Dutch were not included.
Secondly, there are limitations relating to the selection criteria, which also impact on the validity of the findings. Studies not employing traditional experimental or quasi-experimental designs were excluded from the search and therefore, qualitative and other such study designs were discarded in the search process. Of the studies that were included, justification of sample size and validation of the outcome measures employed were not reported in a small number of the papers. It could be argued that such studies should also have been excluded from the review, but in our methodology they were included but received lower quality assessment ratings due to the absence of information on these issues. Finally, as a narrative synthesis the review is not designed to generate summary statistics derived from meta-analyses. Despite these limitations, the studies included in this review clearly demonstrate that high quality and effective mental health promotion interventions, and their evaluation through well-designed research studies, are feasible in LMIC settings.
The review findings indicate that mental health promotion interventions for young people can be implemented effectively in LMIC settings. There is good quality evidence regarding the impact of school-based programmes and promising evidence from multicomponent community-based studies that such interventions offer a viable means of promoting the mental health and wellbeing of young people. Notably, the studies reviewed demonstrate the feasibility and effectiveness of integrating mental health promotion interventions into education and community programmes such as community empowerment, poverty reduction, HIV/AIDS prevention, reproductive and sexual health. While the mental health promotion interventions identified in this review have achieved success across a diverse range of countries, relatively few have been systematically scaled up to serve the needs of young people at a regional or national level. Thus, evidence for their sustainability and effectiveness when scaled up through the educational system and community settings in LMICs needs to be strengthened, especially in low-income countries. In addition, the short-term follow-up periods of many of the studies point to the need for future research to evaluate long-term outcomes. Research is also needed to strengthen the evidence-base on the interrelationship between mental health and other health, educational and social wellbeing outcomes. Such research would strengthen the case for mainstreaming the integration of mental health into key health, education and development priorities for young people in LMICs.
The studies reviewed demonstrate the feasibility and potential sustainability of implementing mental health promotion interventions in LMICs through employing existing infrastructures and resources, working with local teachers, community workers, young people and their families. Further research is needed on the contextual factors influencing the adoption and adaptation in LMICs of well-validated interventions that have been developed in low resource settings in HIC countries. In particular, implementation research is needed to ensure the successful adaptation and transfer of school-based interventions for younger primary school children across educational, cultural and socio-economic settings. The development of culturally valid measures of mental health, that will support the evaluation of culturally appropriate interventions in LMICs, is also identified as an area for methodological development. Existing standardized mental health measures from HICs need to be locally validated and the development of culturally sensitive indicators of positive mental health and wellbeing will be particularly important in determining the benefits of mental health promotion interventions delivered in diverse cultural contexts. Developing capacity in LMICs for the implementation and evaluation of mental health promotion policies and practices is fundamental to promoting and sustaining action for positive youth mental health development.
Herrman H, Jané-Llopis E: The status of mental health promotion. Publ Health Rev. 2012, 34 (2): 1-21.
Google Scholar
Barry MM, Friedli L: The influence of social, demographic and physical factors on positive mental health in children, adults and older people. In Foresight Mental Capital and Wellbeing Project. State-of-Science Review: SR-B3. 2008, London: Government Office of Science and Innovation
Jenkins R, Baingana F, Ahmad R, McDaid D, Atun R: Social, economic, human rights and political challenges to global mental health. Mental Health Fam Med. 2011, 8: 87-96.
Kieling C, Baker-Henningham H, Belfer M, Conti G, Ertem I, Omigbodun O, Rohde LA, Srinath S: Child and adolescent mental health worldwide: evidence for action. Lancet. 2011, 378: 1515-1525. 10.1016/S0140-6736(11)60827-1.
Article PubMed Google Scholar
Durlak JA, Wells AM: Primary prevention mental health programs for children and adolescents: a meta-analytic review. Am J Community Psychol. 1997, 25 (2): 115-152. 10.1023/A:1024654026646.
Article CAS PubMed Google Scholar
Hosman C, Jané-Llopis E: The Evidence of Health Promotion Effectiveness: Shaping Public Health in a new Europe , A Report for the European Commission. Political challenge 2: mental health. 1999, Paris: International Union for Health Promotion and Education
Jané-Llopis E, Barry MM, Hosman C, Patel V: The Evidence of Mental Health Promotion Effectiveness Strategies for Action. Edited by: Jane-Llopis E, Barry MM, Hosman C, Patel V. 2005, 2: 9-25. Mental health promotion works: a review, Promotion and Education Supplement
Herrman H, Saxena S, Moodie R: Promoting Mental Health: Concepts, Emerging Evidence, Practice. 2005, Geneva: World Health Organization: A report of the World Health Organization, Department of Mental Health and Substance Abuse in collaboration with the Victorian Health Promotion Foundation and Univeristy of Melbourne
Barry MM, Jenkins R: Implementing Mental Health Promotion. 2007, Oxford: Churchill Livingstone Elsevier
Nores M, Barnett WS: Benefits of early childhood interventions across the world: (Under) Investing in the very young. Econ Educ Rev. 2010, 29 (2): 271-282. 10.1016/j.econedurev.2009.09.001.
Article Google Scholar
Baker-Henningham H, Lopez Boo F: Early Childhood Stimulation Interventions in Developing Countries: A comprehensive literature review. 2010, Washington, DC: Inter-American Development Bank
Stewart-Brown SL, Schrader-McMillan A: Parenting for mental health: What does the evidence say we need to do? Report of Workpackage 2 of the DataPrev project. Health Promot Int. 2011, 26 (SUPPL. 1): i10-i28.
Weare K, Nind M: Mental health promotion and problem prevention in schools: what does the evidence say?. Health Promot Int. 2011, 26 (SUPPL. 1): i29-i69.
Melzer D, Fryers T, Jenkins R: Maudsley Monographs. Social Inequalities and the Distribution of Common Mental Disorders. 2004, Hove: Psychology Press
Jenkins R, Bhugra D, Bebbington P, Brugha T, Farrell M, Coid J, Fryers T, Weich S, Singleton N, Meltzer H: Debt, income and mental disorder in the general population. Psychol Med. 2008, 38 (10): 1485-1493.
Lund C, Breen A, Flisher AJ, Kakuma R, Corrigall J, Joska JA, Swartz L, Patel V: Poverty and common mental disorders in low and middle income countries: a systematic review. Soc Sci Med. 2010, 71 (3): 517-528. 10.1016/j.socscimed.2010.04.027.
Patel V, Lund C, Hatherill S, Plagerson S, Corrigall J, Fundl M, Flisher AJ: Mental disorders: equity and social determinants. Equity, Social Determinants and Public Health Programmes. Edited by: Blas E, Sivasankara Kurup A. 2010, Geneva: World Health Organization, 115-134.
Miranda JJ, Patel V: Achieving the millennium development goals: does mental health play a role?. PLoS Med. 2005, 2 (10): 0962-0965.
United Nations General Assembly, 65th Session: Global Health and Foreign Policy Resolution. A/65/L.27.New York, 9 th December 2010. Accessible at: http://www.un.org/ga/search/view_doc.asp?symbol=A/65/95
Patel V, Flisher AJ, Nikapota A, Malhotra S: Promoting child and adolescent mental health in low and middle income countries. J Child Psychol Psychiatr. 2008, 49 (3): 313-334. 10.1111/j.1469-7610.2007.01824.x.
World Health Organization: The World Health Report. Mental Health: New Understanding, New Hope. 2001, Geneva: World Health Organization
Weare K: Promoting mental, emotional and social health: A whole school approach. 2000, London: Routledge
Book Google Scholar
Rowling L: Mental health promotion. Mental health promotion and young people: concepts and practice. Edited by: Rowling L. 2002, Australia: McGraw-Hill, 10-23.
Zins J, Weissberg R, Wang M, Walberg H: Building academic success on social and emotional learning: What does the research say?. 2004, Columbia University New York and London: Teachers College Press
Payton J, Weissberg RP, Durlak JA, Dymnicki AB, Taylor RD, Schellinger KB, Pachan M: The positive impact of social and emotional learning for kindergarten to eight-grade students: Findings from three scientific reviews. 2008, Chicago, IL: Collaborative for Academic, Social, and Emotional Learning
World Health Organization: WHO’s Global School Health Initiative: Health Promoting Schools. 1998, Geneva: World Health Organization
Lister-Sharp D, Chapman S, Stewart-Brown S, Sowden A: Health promoting schools and health promotion in schools: two systematic reviews. Health Technol Assess. 1999, 3 (22): 1-207.
CAS PubMed Google Scholar
Wells J, Barlow J, Stewart-Brown S: A systematic review of universal approaches to mental health promotion in schools. Health Educ. 2003, 103 (4): 197-220. 10.1108/09654280310485546.
Tennant R, Goens C, Barlow J, Day C, Stewart-Brown S: A systematic review of reviews of interventions to promote mental health and prevent mental health problems in children and young people. J Publ Mental Health. 2007, 6 (1): 25-32. 10.1108/17465729200700005.
Harden A, Rees R, Shepherd J, Brunton G, Oliver S, Oakley A: Young people and mental health: a systematic review of research on barriers and facilitators. 2001, London: EPPI Centre
Wells J, Barlow J, Stewart-Brown S: A systematic review of universal approaches to mental health promotion in schools. 2001, Oxford: Health Service Research Unit
World Health Organization: Seventh Global Conference on Health Promotion: Promoting Health and Development: closing the Implementation Gap. 26th-30th October 2009, Nairobi, Kenya: , Accessible at: http://www.who.int/healthpromotion/conferences/7gchp/en/index.html
Mrazek CJ, Haggerty RJ: Reducing risks for mental disorders: frontiers for prevention intervention research. 1994, Washington DC: National Academic Press
Jackson N, Waters E, Anderson L, Bailie R, Hawe P, Naccarella L, Norris S, Oliver S, Petticrew M, Pienaar E, Popay J, Roberts H, Rogers W, Shepherd J, Sowden A, Thomas H: The challenges of systematically reviewing public health interventions. J Publ Health. 2004, 26 (3): 303-307. 10.1093/pubmed/fdh164.
Article CAS Google Scholar
Srikala B, Kumar KV: Empowering adolescents with life skills education in schools-School mental health program: Does it work. Indian J Psychiatr. 2010, 52 (4): 344-349. 10.4103/0019-5545.74310.
Bonhauser M, Fernandez G, Püschel K, Yaňez F, Montero J, Thompson B, Coronado G: Improving physical fitness and emotional well-being in adolescents of low socioeconomic status in Chile: results of a school-based controlled trial. Health Promot Int. 2005, 20 (2): 113-122. 10.1093/heapro/dah603.
Smith EA, Palen LA, Caldwell LL, Flisher AJ, Graham JW, Mathews C, Wegner L, Vergnani T: Substance use and sexual risk prevention in Cape Town, South Africa: an evaluation of the HealthWise program. Prev Sci. 2008, 9 (4): 311-321. 10.1007/s11121-008-0103-z.
Caldwell LL, Patrick ME, Smith EA, Palen LA, Wegner L: Influencing Adolescent Leisure Motivation: Intervention Effects of HealthWise South Africa. J Leis Res. 2010, 42 (2): 203-220.
PubMed PubMed Central Google Scholar
Caldwell LL, Smith EA, Wegner L, Vernani T, Mpofu E, Flisher AJ, Matthews C: HealthWise South Africa: development of a life skills curriculum for young adults. World Leisure. 2004, 3: 4-17.
Botvin GJ, Schinke S, Orlandi MA: Drug abuse prevention with multiethnic youth. 1995, Thousand Oaks, CA: Sage
De Villiers M, van den Berg H: The implementation and evaluation of a resiliency programme for children. South Afr J Psychol. 2012, 42 (1): 93-102. 10.1177/008124631204200110.
Rivet-Duval E, Heriot S, Hunt C: Preventing Adolescent Depression in Mauritius: A Universal School-Based Program. Child Adolesc Mental Health. 2011, 16 (2): 86-91. 10.1111/j.1475-3588.2010.00584.x.
Shochet IM, Ham D: Universal school-based approaches to preventing adolescent depression: Past findings and future directions of the Resourceful Adolescent Program. Int J Ment Heal Promot. 2004, 6: 17-25.
Mueller J, Alie C, Jonas B, Brown E, Sherr L: A quasi-experimental evaluation of a community-based art therapy intervention exploring the psychosocial health of children affected by HIV in South Africa. Trop Med Int Health. 2011, 16 (1): 57-66. 10.1111/j.1365-3156.2010.02682.x.
Kumakech E, Cantor-Graae E, Maling S, Bajunirwe F: Peer-group support intervention improves the psychosocial well-being of AIDS orphans: Cluster randomized trial. Soc Sci Med. 2009, 68 (6): 1038-1043. 10.1016/j.socscimed.2008.10.033.
Hope A, Trimmel S: Training for transformation, a handbook for community workers. Vols. 1–3. 1995, Nairobi: Gweru Mambo Press
Jordans MJD, Komproe IH, Tol WA, Kohrt BA, Luitel NP, Macy RD, De Jong JT: Evaluation of a classroom-based psychosocial intervention in conflict-affected Nepal: a cluster randomized controlled trial. J Child Psychol Psychiatr. 2010, 51 (7): 818-826. 10.1111/j.1469-7610.2010.02209.x.
Khamis V, Macy R, Coignez V: The Impact of the Classroom/Community/Camp-Based Intervention (CBI) Program on Palestinian Children. 2004, USA: Save the Children
Ager A, Akesson B, Stark L, Flouri E, Okot B, McCollister F, Boothby N: The impact of the school-based Psychosocial Structured Activities (PSSA) program on conflict-affected children in northern Uganda. J Child Psychol Psychiatr. 2011, 52 (11): 1124-1133. 10.1111/j.1469-7610.2011.02407.x.
Qouta SR, Palosaari E, Diab M, Punamaki RL: Intervention effectiveness among war-affected children: a cluster randomized controlled trial on improving mental health. J Trauma Stress 2012. 2012, 25 (3): 288-298.
Smith P, Dyregrov A, Yule W: Children and war: Teaching recovery techniques. 2000, Bergen, Norway: Foundation for Children and War
Ehntholt KA, Smith PA, Yule W: School-based cognitive-behavioral therapy group intervention for refugee children who have experienced war related trauma. Clin Child Psychol Psychiatr. 2005, 10: 235-250. 10.1177/1359104505051214.
Giannopoulo J, Dikaiakou A, Yule W: Cognitive-behavioural group intervention for PTSD symptoms in children following the Athens 1999 earthquake: a pilot study. Clin Child Psychol Psychiatr. 2006, 11: 543-553. 10.1177/1359104506067876.
Karam EG, Fayyad J, Karam AN, Tabet CC, Melhem N, Mneimneh Z, Dimassi H: Effectiveness and specificity of a classroom-based group intervention in children and adolescents exposed to war in Lebanon. World Psychiatr. 2008, 7 (2): 103-109.
Lange-Nielsen II, Kolltveit S, Thabet AAM, Dyregrov A, Pallesen S, Johnsen TB, Christian J: Short-Term Effects of a Writing Intervention Among Adolescents in Gaza. J Loss Trauma. 2012, 17 (5): 403-422. 10.1080/15325024.2011.650128.
Loughry M, Ager A, Flouri E, Khamis V, Afana AH, Qouta S: The impact of structured activities among Palestinian children in a time of conflict. J Child Psychol Psychiatr. 2006, 47 (12): 1211-1218.
Balaji M, Andrews T, Andrew G, Patel V: The acceptability, feasibility, and effectiveness of a population-based intervention to promote youth health: an exploratory study in Goa, India. J Adolesc Heal. 2011, 48 (5): 453-460. 10.1016/j.jadohealth.2010.07.029.
Vasquez M, Meza L, Almandarez O, Santos A, Matute RC, Canaca LD, Cruz A, Cacosta A, Bacilla MEG, Wilson L: Evaluation of a Strengthening Families (Familias Fuertes) Intervention for Parents and Adolescents in Hondurus. Nurs Res South Online J. 2010, 10 (3):
Brady M, Assaad R, Ibrahim B, Salem A, Salem R, Zibani N: Providing new opportunities to adolescent girls in socially conservative settings: the Ishraq program in rural upper Egypt. 2007, New York: Population Council
Jewkes R, Nduna M, Levin J, Jama N, Dunkle K, Puren A, Duvvury N: Impact of stepping stones on incidence of HIV and HSV-2 and sexual behaviour in rural South Africa: cluster randomised controlled trial. BMJ. 2008, 337: a507-
Bell CC, Bhana A, Petersen I, McKay MM, Gibbons R, Bannon W, Amatya A: Building protective factors to offset sexually risky behaviors among black youths: a randomized control trial. J Natl Med Assoc. 2008, 100 (8): 936-944.
Article PubMed PubMed Central Google Scholar
McKay MM, Chasse KT, Paikoff R, McKinney LD, Baptiste D, Coleman D, Madison S, Bell CC: Family-level impact of the CHAMP Family Program: a community collaborative effort to support urban families and reduce youth HIV risk exposure. Fam Process. 2004, 43 (1): 79-93. 10.1111/j.1545-5300.2004.04301007.x.
Kim J, Ferrari G, Abramsky T, Watts C, Hargreaves J, Morison L, Phetla G, Porter J, Pronyk P: Assessing the incremental effects of combining economic and health interventions: The IMAGE study in South Africa. Bull World Health Organ. 2009, 87 (11): 824-832. 10.2471/BLT.08.056580.
Pronyk PM, Hargreaves JR, Kim JC, Morison LA, Phetla G, Watts C, Busza J, Porter JD: Effect of a structural intervention for the prevention of intimate-partner violence and HIV in rural South Africa: a cluster randomised trial. Lancet. 2006, 368 (9551): 1973-1983. 10.1016/S0140-6736(06)69744-4.
Ssewamala FM, Neilands TB, Waldfogel J, Ismayilova L: The impact of a comprehensive microfinance intervention on depression levels of AIDS-orphaned children in Uganda. J Adolesc Heal. 2012, 50 (4): 346-352. 10.1016/j.jadohealth.2011.08.008.
Ssewamala FM, Han CK, Neilands TB: Asset ownership and health and mental health functioning among AIDS-orphaned adolescents: Findings from a randomized clinical trial in rural Uganda. Soc Sci Med. 2009, 69 (2): 191-198. 10.1016/j.socscimed.2009.05.019.
Ssewamala FM, Karimli L, Han CK, Ismayilova L: Social capital, savings, and educational performance of orphaned adolescents in Sub-Saharan Africa. Child Youth Serv Rev. 2010, 32 (12): 1704-1710. 10.1016/j.childyouth.2010.07.013.
Ssewamala FM, Ismayilova L: Integrating children’s savings accounts in the care and support of orphaned adolescents in rural Uganda. Soc Serv Rev. 2009, 83 (3): 453-472. 10.1086/605941.
Paul-Ebhohimhen VA, Poobalan A, Van Teijlingen ER: A systematic review of school-based sexual health interventions to prevent STI/HIV in sub-Saharan Africa. BMC Publ Health. 2008, 8: 4-10.1186/1471-2458-8-4.
Harrison A, Newell ML, Imrie J, Hoddinott G: HIV prevention for South African youth: which interventions work? A systematic review of current evidence. BMC Publ Health. 2010, 10: 102-10.1186/1471-2458-10-102.
Persson TJ, Rousseau C: School-based interventions for minors in war-exposed countries: a review of targeted and general programmes. Torture Q J Rehabil Torture Victims Prev Torture. 2009, 19 (2): 88-101.
CAS Google Scholar
Jordans MJD, Tol WA, Komproe IH, De Jong JVTM: Systematic review of evidence and treatment approaches: psychosocial and mental health care for children in war. Child Adolesc Mental Health. 2009, 14 (1): 2-14. 10.1111/j.1475-3588.2008.00515.x.
Tol WA, Barbui C, Galappatti A, Silove D, Betancourt TS, Souza R, Golaz A, Van Ommeren M: Mental health and psychosocial support in humanitarian settings: linking practice and research. Lancet. 2011, 378 (9802): 1581-1591. 10.1016/S0140-6736(11)61094-5.
Cluver L, Gardner F, Operario D: Psychological distress amongst AIDS-orphaned children in urban South Africa. J Child Psychol Psychiatr. 2007, 48 (8): 755-763. 10.1111/j.1469-7610.2007.01757.x.
Nyamukapa CA, Gregson S, Lopman B, Saito S, Watts HJ, Monasch R, Jukes MCH: HIV-associated orphanhood and children’s psychosocial distress: theoretical framework tested with data from Zimbabwe. Am J Publ Health. 2008, 98 (1): 133-141. 10.2105/AJPH.2007.116038.
Atwine B, Cantor-Graae E, Bajunirwe F: Psychological distress among AIDS orphans in rural Uganda. Soc Sci Med. 2005, 61 (3): 555-564. 10.1016/j.socscimed.2004.12.018.
Green J, Howes F, Waters E, Maher E, Oberklaid F: Promoting the social and emotional health of primary school-aged children: reviewing the evidence base for school-based interventions. Int J Ment Heal Promot. 2005, 7 (3): 30-36. 10.1080/14623730.2005.9721872.
Adi Y: Systematic review of the effectiveness of interventions to promote mental wellbeing in primary schools Report 1: Universal approaches which do not focus on violence or bullying. 2007, London: National Institute of Clinical Excellence
Stewart-Brown S: What is the evidence on school health promotion in improving health or preventing disease and, specifically, what is the effectiveness of the health promoting schools approach. 2006, Copenhagen: WHO Regional Office for Europe
Greenberg M, Domitrovich C, Bumbarger B: The prevention of mental disorders in school-aged children: current state of the field. Prev Treatment. 2001, 4 (1): 1-52.
Browne G, Gafni A, Roberts J, Byrne C, Majumdar B: Effective/efficient mental health programs for school-age children: a synthesis of reviews. Soc Sci Med. 2004, 58 (7): 1367-1384. 10.1016/S0277-9536(03)00332-0.
Shucksmith J, Summerbell C, Jones S, Whittaker V: Mental wellbeing of children in primary education (targeted/indicated activities). 2007, London: National Institute of Clinical Excellence
Tol WA, Komproe IH, Jordans MJD, Vallipuram A, Sipsma H, Sivayokan S, Macy RD, deJong JT: Outcomes and moderators of a preventive school-based mental health intervention for children affected by war in Sri Lanka: a cluster randomized trial. World Psychiatr. 2012, 11 (2): 114-122. 10.1016/j.wpsyc.2012.05.008.
Layne CM, Saltzman WR, Poppleton L, Burlingame GM, Pasalic A, Durakovic E, Music M, Campara N, Dapo N, Arslanagic B, Steinberg AM, Pynoos RS: Effectiveness of a school-based group psychotherapy program for war-exposed adolescents: a randomized controlled trial. J Am Acad Child Adolesc Psychiatr. 2008, 47 (9): 1048-1062. 10.1097/CHI.0b013e31817eecae.
Pre-publication history
The pre-publication history for this paper can be accessed here: http://www.biomedcentral.com/1471-2458/13/835/prepub
Download references
Acknowledgements
The authors wish to acknowledge the support of the WHO Task Force on Mainstreaming Health Promotion Evidence Project led by Dr Gauden Galea at WHO Geneva, who commisioned the original evidence review on which this paper is based. We are grateful to Dr Taghi Mohammad Yasamy, WHO Department of Mental Health and Substance Abuse, who acted as WHO focal point, and Professor Elizabeth Waters, Coordinating Editor of the Cochrane Public Health Group and Consulting Editor for the Mainstreaming Health Promotion Evidence Project, for their technical guidance and comments on the original review. We also acknowledge the assistance of a number of study authors globally who supplied us with additional information on the interventions and their evaluation. The views expressed in this paper are solely those of the authors and do not necessarily reflect the views of WHO. The authors have declared that no competing interests exist. VP is supported by a Wellcome Trust Senior Research Fellowship in Tropical Medicine. His work with young people is additionally supported by the MacArthur Foundation.
Author information
Authors and affiliations.
WHO Collaborating Centre for Health Promotion Research, National University of Ireland Galway, University Road, Galway, Ireland
Margaret M Barry & Aleisha M Clarke
WHO Collaborating Centre for Research and Training for Mental Health, Institute of Psychiatry, King’s College London, 16 De Crespigny Park, London, SE5 8AF, UK
Rachel Jenkins
Centre for Global Mental Health, London School of Hygiene & Tropical Medicine, Keppel Street, London, WC1E 7HT, UK
Vikram Patel
Sangath, Goa, India
You can also search for this author in PubMed Google Scholar
Corresponding author
Correspondence to Margaret M Barry .
Additional information
Competing interests.
The authors declare that they have no competing interests.
Authors’ contributions
MB designed the study and AC performed the data search; MB and AC reviewed the studies and carried out the quality assessment ratings; RJ and VP contributed to the interpretation of the data and the drafting of the manuscript. All authors read and approved the final manuscript.
Authors’ original submitted files for images
Below are the links to the authors’ original submitted files for images.
Authors’ original file for figure 1
Rights and permissions.
This article is published under license to BioMed Central Ltd. This is an Open Access article distributed under the terms of the Creative Commons Attribution License ( http://creativecommons.org/licenses/by/2.0 ), which permits unrestricted use, distribution, and reproduction in any medium, provided the original work is properly cited.
Reprints and permissions
About this article
Cite this article.
Barry, M.M., Clarke, A.M., Jenkins, R. et al. A systematic review of the effectiveness of mental health promotion interventions for young people in low and middle income countries. BMC Public Health 13 , 835 (2013). https://doi.org/10.1186/1471-2458-13-835
Download citation
Received : 17 January 2013
Accepted : 20 August 2013
Published : 11 September 2013
DOI : https://doi.org/10.1186/1471-2458-13-835
Share this article
Anyone you share the following link with will be able to read this content:
Sorry, a shareable link is not currently available for this article.
Provided by the Springer Nature SharedIt content-sharing initiative
- Mental health promotion
- Young people
- Low and middle income countries
- Systematic review
BMC Public Health
ISSN: 1471-2458
- General enquiries: [email protected]
Mosaic Brain Aneuploidy in Mental Illnesses: An Association of Low-level Post-zygotic Aneuploidy with Schizophrenia and Comorbid Psychiatric Disorders
Affiliations.
- 1 Mental Health Research Center, Moscow, Russian Federation.
- 2 Separated Structural Unit "Clinical Research Institute of Pediatrics named after Y.E Veltishev", Pirogov Russian National Research Medical University, Moscow, Russian Federation.
- 3 Moscow State University of Psychology and Education, Moscow, Russian Federation.
- 4 Department of Medical Genetics, Russian Medical Academy of Postgraduate Education, Ministry of Health, Moscow, Russian Federation.
- PMID: 29606903
- PMCID: PMC5850504
- DOI: 10.2174/1389202918666170717154340
Background: Postzygotic chromosomal variation in neuronal cells is hypothesized to make a substantial contribution to the etiology and pathogenesis of neuropsychiatric disorders. However, the role of somatic genome instability and mosaic genome variations in common mental illnesses is a matter of conjecture.
Materials and methods: To estimate the pathogenic burden of somatic chromosomal mutations, we determined the frequency of mosaic aneuploidy in autopsy brain tissues of subjects with schizophrenia and other psychiatric disorders (intellectual disability comorbid with autism spectrum disorders). Recently, post-mortem brain tissues of subjects with schizophrenia, intellectual disability and unaffected controls were analyzed by Interphase Multicolor FISH (MFISH), Quantitative Fluorescent in situ Hybridization (QFISH) specially designed to register rare mosaic chromosomal mutations such as lowlevel aneuploidy (whole chromosome mosaic deletion/duplication). The low-level mosaic aneuploidy in the diseased brain demonstrated significant 2-3-fold frequency increase in schizophrenia (p=0.0028) and 4-fold increase in intellectual disability comorbid with autism (p=0.0037) compared to unaffected controls. Strong associations of low-level autosomal/sex chromosome aneuploidy (p=0.001, OR=19.0) and sex chromosome-specific mosaic aneuploidy (p=0.006, OR=9.6) with schizophrenia were revealed.
Conclusion: Reviewing these data and literature supports the hypothesis suggesting that an association of low-level mosaic aneuploidy with common and, probably, overlapping psychiatric disorders does exist. Accordingly, we propose a pathway for common neuropsychiatric disorders involving increased burden of rare de novo somatic chromosomal mutations manifesting as low-level mosaic aneuploidy mediating local and general brain dysfunction.
Keywords: Aging; Aneuploidy; Chromosomal instability; Genome instability; Human brain; Ontogeny; Psychiatric disorders; Somatic genome variations.
Publication types
Advertisement
Maslow and Mental Health Recovery: A Comparative Study of Homeless Programs for Adults with Serious Mental Illness
- Original Article
- Published: 12 February 2014
- Volume 42 , pages 220–228, ( 2015 )
Cite this article
- Benjamin F. Henwood 1 ,
- Katie-Sue Derejko 2 ,
- Julie Couture 1 &
- Deborah K. Padgett 2
12k Accesses
65 Citations
33 Altmetric
Explore all metrics
This mixed-methods study uses Maslow’s hierarchy as a theoretical lens to investigate the experiences of 63 newly enrolled clients of housing first and traditional programs for adults with serious mental illness who have experienced homelessness. Quantitative findings suggests that identifying self-actualization goals is associated with not having one’s basic needs met rather than from the fulfillment of basic needs. Qualitative findings suggest a more complex relationship between basic needs, goal setting, and the meaning of self-actualization. Transforming mental health care into a recovery-oriented system will require further consideration of person-centered care planning as well as the impact of limited resources especially for those living in poverty.
This is a preview of subscription content, log in via an institution to check access.
Access this article
Price includes VAT (Russian Federation)
Instant access to the full article PDF.
Rent this article via DeepDyve
Institutional subscriptions
Similar content being viewed by others
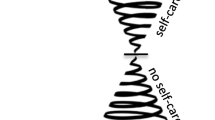
Dear Mental Health Practitioners, Take Care of Yourselves: a Literature Review on Self-Care
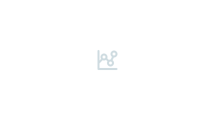
The Impact of Financial Hardship on Single Parents: An Exploration of the Journey From Social Distress to Seeking Help
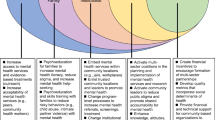
Community Interventions to Promote Mental Health and Social Equity
Charmaz, K. (2006). Constructing grounded theory: a practical guide through qualitative analysis . Los Angeles: Sage.
Google Scholar
Clarke, S., Oades, L. G., & Crowe, T. P. (2012). Recovery in mental health: A movement towards well-being and meaning in contrast to an avoidance of symptoms. Psychiatric Rehabilitation Journal, 35 , 297–304.
Article PubMed Google Scholar
Collins, S. E., Malone, D. K., & Clifasefi, S. L. (2013). Housing retention in single-site housing first for chronically homeless individuals with severe alcohol problems. American Journal of Public Health, 103 (S2), S269–S274.
Article PubMed Central PubMed Google Scholar
Culhane, D. (2008). The costs of homelessness: A perspective from the United States. European Journal of Homelessness, 2 , 97–114.
Dordick, G. A. (2002). Recovering from homelessness: Determining the “quality of sobriety” in a transitional housing program. Qualitative Sociology, 25 (1), 7–32.
Article Google Scholar
Draine, J., Salzer, M., Culhane, D., & Hadley, T. (2002a). Poverty, social problems, and serious mental illness. Psychiatric Services, 53 , 899.
Draine, J., Salzer, M. S., Culhane, D. P., & Hadley, T. R. (2002b). Role of social disadvantage in crime, joblessness, and homelessness among persons with serious mental illness. Psychiatric Services, 53 , 565–573.
Freund, K. S., & Lous, J. (2012). The effect of preventive consultations on young adults with psychosocial problems: a randomized trial. Health Education Research, 27 (5), 927–945.
Fukui, S., Starnino, V. R., Susana, M., Davidson, L. J., Cook, K., Rapp, C. A., et al. (2011). Effect of Wellness Recovery Action Plan (WRAP) participation on psychiatric symptoms, sense of hope, and recovery. Psychiatric Rehabilitation Journal, 34 (3), 214.
Gorman, D. (2010). Maslow’s hierarchy and social and emotional wellbeing. Aboriginal and Islander Health Worker Journal, 34 , 27–29.
Greenwood, R. M., Stefancic, A., & Tsemberis, S. (2013). Pathways housing first for homeless persons with psychiatric disabilities: Program innovation, research, and advocacy. Journal of Social Issues, 69 (4), 645–663.
Henwood, B. F., Stanhope, V., & Padgett, D. K. (2011). The role of housing: a comparison of front-line provider views in housing first and traditional programs. Administration and Policy in Mental Health, 38 , 77–85.
Hopper, K. (2007). Rethinking social recovery in schizophrenia: what a capabilities approach might offer. Social Science and Medicine, 65 (5), 868–879.
Hopper, K. (2012). Commentary: the counter-reformation that failed? A commentary on the mixed legacy of supported housing. Psychiatric Services, 63 (5), 461–463.
Hopper, K., Jost, J., Hay, T., & Welber, S. (1997). Homelessness, severe mental illness, and the institutional circuit. Psychiatric Services, 48 (5), 659–665.
Article CAS PubMed Google Scholar
Jacobson, N., & Greenley, D. (2001). What is recovery? A conceptual model and explication. Psychiatric Services, 52 , 482–485.
Kenrick, D. T., Griskevicius, V., Neuberg, S. L., & Schaller, M. (2010). Renovating the pyramid of needs. Perspectives on Psychological Science, 5 , 292–314.
Kertesz, S. G., Crouch, K., Milby, J. B., Cusimano, R. E., & Schumacher, J. E. (2009). Housing first for homeless persons with active addiction: are we overreaching? Milbank Quarterly, 87 (2), 495–534.
Kiel, J. M. (1999). Reshaping Maslow’s hierarchy of needs to reflect today’s educational and managerial philosophies. Journal of Instructional Psychology, 26 , 167.
Maslow, A. H. (1943). A theory of human motivation. Psychological Review, 50 , 370–396.
Maslow, A. H. (1970). Motivation and personality (2nd ed.). New York: Harper & Row.
Miles, M. B., & Huberman, A. M. (1994). Qualitative data analysis: An expanded sourcebook . Los Angeles: Sage.
Nelson, G., Lord, J., & Ochocka, J. (2001). Empowerment and mental health in community: Narratives of psychiatric consumer/survivors. Journal of Community & Applied Social Psychology, 11 , 125–142.
Nussbaum, M. C. (2006). Frontiers of justice: disability, nationality, species membership . Cambridge: Harvard University Press.
Ochocka, J., Nelson, G., & Janzen, R. (2005). Moving forward: Negotiating self and external circumstances in recovery. Psychiatric Rehabilitation Journal, 28 , 315–322.
Onken, S. J., Craig, C. M., Ridgway, P., Ralph, R. O., & Cook, J. A. (2007). An analysis of the definitions and elements of recovery: a review of the literature. Psychiatric Rehabilitation Journal, 31 , 9–22.
Padgett, D. K. (2007). There’s no place like (a) home: Ontological security among persons with serious mental illness in the United States. Social Science and Medicine, 64 (9), 1925–1936.
Padgett, D. K. (2008). Qualitative methods in social work research . Los Angeles: Sage.
Padgett, D. K., Smith, B., Henwood, B. F., & Tiderington, E. (2012). Life course adversity in the lives of formerly homeless persons with serious mental illness: context and meaning. American Journal of Orthopsychiatry, 82 , 421–430.
Palinkas, L. A., Horwitz, S. M., Chamberlain, P., Hurlburt, M. S., & Landsverk, J. (2011). Mixed-methods designs in mental health services research: A review. Psychiatric Services, 62 (3), 255–263.
Pearson, C., Montgomery, A. E., & Locke, G. (2009). Housing stability among homeless individuals with serious mental illness participating in Housing First programs. Journal of Community Psychology, 37 (3), 404–417.
Rawls, J. (1971). A theory of justice . Cambridge: Harvard University Press.
Ridgway, P. (2001). Restorying psychiatric disability: Learning from first person recovery narratives. Psychiatric Rehabilitation Journal, 24 , 335–343.
Roychowdhury, A. (2011). Bridging the gap between risk and recovery: a human needs approach. The Psychiatrist, 35 , 68–73.
SAMHSA (2011). SAMHSA announces a working definition of “recovery” from mental disorders and substance use disorders. Retrieved July 11, 2012, from http://www.samhsa.gov/newsroom/advisories/1112223420.aspx .
Sandelowski, M. (2001). Real qualitative researchers do not count: The use of numbers in qualitative research. Research in Nursing & Health, 24 , 230–240.
Article CAS Google Scholar
Stake, R. E. (1995). The art of case study research . Thousand Oaks: Sage.
Stefancic, A., & Tsemberis, S. (2007). Housing First for long-term shelter dwellers with psychiatric disabilities in a suburban county: A four-year study of housing access and retention. J Prim Prev, 28 (3–4), 265–279.
Tondora, J., Miller, R., & Davidson, L. (2012). The Top Ten Concerns about Person-Centered Care Planning in Mental Health Systems. International Journal of Person Centered Medicine, 2 (3), 410–420.
Trattner, W. I. (1998). From poor law to welfare state: A history of social welfare in America (6th ed.). New York: Free.
Tsemberis, S., & Eisenberg, R. F. (2000). Pathways to housing: Supported housing for street- dwelling homeless individuals with psychiatric disabilities. Psychiatric Services (Washington, D. C.), 51 (4), 487–493.
Tsemberis, S., Gulcur, L., & Nakae, M. (2004). Housing First, consumer choice, and harm reduction for homeless individuals with a dual diagnosis. American Journal of Public Health, 94 , 651–656.
Viron, M., Bello, I., Freudenreich, O., & Shtasel, D. (2013). Characteristics of homeless adults with serious mental illness served by a state mental health transitional shelter. Community Mental Health Journal, 1–6. doi: 10.1007/s10597-013-9607-5 .
Wahba, M. A., & Bridwell, L. G. (1976). Maslow reconsidered: A review of research on the need hierarchy theory. Organizational Behavior and Human Performance, 15 , 212–240.
Download references
Acknowledgments
This research was supported by the National Institute of Mental Health (R01 69865).
Author information
Authors and affiliations.
School of Social Work, University of Southern California, 1150 S. Olive Street, #1429, Los Angeles, CA, 90015-2211, USA
Benjamin F. Henwood & Julie Couture
Silver School of Social Work, 838 Broadway, 3rd Floor, New York Recovery Study, New York, NY, 10003, USA
Katie-Sue Derejko & Deborah K. Padgett
You can also search for this author in PubMed Google Scholar
Corresponding author
Correspondence to Benjamin F. Henwood .
Rights and permissions
Reprints and permissions
About this article
Henwood, B.F., Derejko, KS., Couture, J. et al. Maslow and Mental Health Recovery: A Comparative Study of Homeless Programs for Adults with Serious Mental Illness. Adm Policy Ment Health 42 , 220–228 (2015). https://doi.org/10.1007/s10488-014-0542-8
Download citation
Published : 12 February 2014
Issue Date : March 2015
DOI : https://doi.org/10.1007/s10488-014-0542-8
Share this article
Anyone you share the following link with will be able to read this content:
Sorry, a shareable link is not currently available for this article.
Provided by the Springer Nature SharedIt content-sharing initiative
- Mental health recovery
- Housing first
- Homelessness
- Serious mental illness
- Find a journal
- Publish with us
- Track your research

An official website of the United States government
The .gov means it’s official. Federal government websites often end in .gov or .mil. Before sharing sensitive information, make sure you’re on a federal government site.
The site is secure. The https:// ensures that you are connecting to the official website and that any information you provide is encrypted and transmitted securely.
- Publications
- Account settings
Preview improvements coming to the PMC website in October 2024. Learn More or Try it out now .
- Advanced Search
- Journal List
- JMIR Ment Health
- v.7(6); 2020 Jun
Peer Support in Mental Health: Literature Review
Reham a hameed shalaby.
1 Department of Psychiatry, University of Alberta, Edmonton, AB, Canada
Vincent I O Agyapong
A growing gap has emerged between people with mental illness and health care professionals, which in recent years has been successfully closed through the adoption of peer support services (PSSs). Peer support in mental health has been variously defined in the literature and is simply known as the help and support that people with lived experience of mental illness or a learning disability can give to one another. Although PSSs date back to several centuries, it is only in the last few decades that these services have formally evolved, grown, and become an integral part of the health care system. Debates around peer support in mental health have been raised frequently in the literature. Although many authors have emphasized the utmost importance of incorporating peer support into the health care system to instill hope; to improve engagement, quality of life, self-confidence, and integrity; and to reduce the burden on the health care system, other studies suggest that there are neutral effects from integrating PSSs into health care systems, with a probable waste of resources.
In this general review, we aimed to examine the literature, exploring the evolution, growth, types, function, generating tools, evaluation, challenges, and the effect of PSSs in the field of mental health and addiction. In addition, we aimed to describe PSSs in different, nonexhaustive contexts, as shown in the literature, that aims to draw attention to the proposed values of PSSs in such fields.
The review was conducted through a general search of the literature on MEDLINE, Google Scholar, EMBASE, Scopus, Chemical Abstracts, and PsycINFO. Search terms included peer support, peer support in mental health, social support, peer, family support, and integrated care.
There is abundant literature defining and describing PSSs in different contexts as well as tracking their origins. Two main transformational concepts have been described, namely, intentional peer support and transformation from patients to peer support providers. The effects of PSSs are extensive and integrated into different fields, such as forensic PSSs, addiction, and mental health, and in different age groups and mental health condition severity. Satisfaction of and challenges to PSS integration have been clearly dependent on a number of factors and consequently impact the future prospect of this workforce.
Conclusions
There is an internationally growing trend to adopt PSSs within addiction and mental health services, and despite the ongoing challenges, large sections of the current literature support the inclusion of peer support workers in the mental health care workforce. The feasibility and maintenance of a robust PSS in health care would only be possible through collaborative efforts and ongoing support and engagement from all health care practitioners, managers, and other stakeholders.
Introduction
Peer support services (PSSs) are novel interventions recently adopted in mental health systems worldwide. It is believed, however, that PSSs date back to more than three centuries to the moral treatment era [ 1 ], albeit on an informal basis. Diverse definitions and classifications for PSSs have been provided in the literature [ 2 - 4 ], and numerous reports have praised and supported the service provided by peer support workers (PSWs) [ 5 - 8 ]. However, other literature suggests the neutral effects of PSSs, with weak associated evidence to support such services [ 9 , 10 ]. The potential impact of PSWs on their peers [ 11 - 14 ] has received considerable attention in the literature.
PSSs have been introduced in different contexts, such as family PSWs [ 15 - 19 ], the forensic field [ 20 , 21 ], and online PSSs. A considerable number of strategies were proposed to generate an effective PSS in the mental health field amid a number of associated concerns and challenges [ 22 - 25 ].
This general review sheds light on PSWs’ experiences, benefits, challenges, opportunities to expand access to quality addiction, and mental health care using PSSs. The review was conducted through a general search of the literature on MEDLINE, Google Scholar, EMBASE, Scopus, Chemical Abstracts, and PsycINFO. Search terms included peer support, peer support in mental health, social support, peer, family support, and integrated care. We began the review with an examination of the definitions, origins, and types of peer support contributions and within different clinical contexts, aiming at deepening the view to the diverse effects of such a workforce. We then continued with examining the transition from a patient role to a PSW role and their incorporation into mental health systems. Thereafter, we provided a conceptual framework for the effects of peer support and stigma in relation to PSWs. We concluded the review by examining the benefits and challenges associated with PSSs and provided a commentary on future directions for PSSs in mental health.
Definitions
Peer support has diverse meanings in the literature. For example, it is a system of giving and receiving help founded on key principles of respect, shared responsibility, and an agreement of what is helpful [ 26 ]. A peer is defined as an equal , someone with whom one shares demographic or social similarities, whereas support refers to “the kind of deeply felt empathy, encouragement, and assistance that people with shared experiences can offer one another within a reciprocal relationship” [ 3 ]. The Mental Health Foundation in the United Kingdom defined peer support in mental health as “the help and support that people with lived experience of a mental illness or a learning disability can give to one another” [ 27 ]. Peer employees were also defined as “individuals who fill designated unique peer positions as well as peers who are hired into traditional MH positions” [ 28 ]. In 1976, authors defined self-help groups as “voluntary small group structures for mutual aid in the accomplishment of a specific purpose...usually formed by peers who have come together for mutual assistance in satisfying a common need, overcoming a common handicap or life-disrupting problem, and bringing about desired social and/or personal change” [ 28 ]. Although the mutual relationship was sometimes overlooked and rather described as an asymmetric or nearly one-directional relationship [ 29 ], it is emphasized upon as 1 of the 4 main tasks for peer support accomplishments, which are mutuality, connection, worldwide, and moving toward rather than moving away [ 30 ].
Origin and Growth of Peer Support
Davidson et al [ 11 ] have expressed the paradigm that calls for new models of community-based practice, which turned away from case management and from conceptualizing old practices under new terms. In the 1990s, peer support was formally introduced as a service in community mental health care. However, there is evidence of its practice throughout history, including during the moral treatment era in France at the end of the 18th century [ 1 ]. Recently, peer support has been rapidly growing in many countries and could attract a considerable amount of research [ 22 ]. Although Lunatic Friends’ Society is known as the earliest peer support group in mental health, which was founded in England in the middle of the 19th century [ 31 ], self-help groups were described as the oldest and most pervasive of peer support types [ 28 ]. Some peer-run groups also formed in Germany in the late 19th century, which protested on involuntary confinement laws. In addition to this, several individuals in the 18th and 19th centuries publicized their protests about their treatment in autobiographies and petitions [ 32 ]. The origin of peer support even reaches further back than the earliest asylums [ 33 ]. Some authors suggest that peer support is not based on psychiatric models and diagnostic criteria [ 3 ]; however, it is about “understanding another’s situation empathically through the shared experience of emotional and psychological pain” [ 34 ]. In the United States, the start of legitimacy for peer support was ignited in 2007 by considering the conditions under which PSSs could be reimbursed by Medicaid [ 35 ]. Although this reform was entailing a recovery model, which has been adopted by health care providers and stakeholders in many “English-speaking” countries, it was not the case in many other countries, in which this reform was yet to be well formulated [ 36 ].
Transformational Concepts in Peer Support Service
Intentional peer support: informal to formal peer support evolution.
Intentional peer support (IPS) is described as a philosophical descendant of the informal peer support of the ex-patients’ movement in the 1970s [ 3 ]. It depends on a way of communication that immerses the provider into the recipient experience by stepping back from one’s story and being eagerly open to others’ stories [ 30 ]. In the field of psychiatry, trauma is blamed for playing a pivotal role in the experience, diagnosis, and treatment, and peer support is described as the logical environment for disseminating trauma-informed care (TIC) or service, which enables building relationships based on mutuality, shared power, and respect [ 37 ]. In the same context, trauma-informed peer support usually begins with the main question, “What happened to you?” instead of “What is wrong with you?” [ 30 ]. TIC is an explanatory model that identifies PSWs sharing lived experiences, ensuring safety and functioning as an advocate, and a liaison to patient management plans, where empowerment and intervention models are strongly emphasized upon [ 38 , 39 ]. The shift from a traditional biomedical model to recovery-oriented practice is meant to perceive trauma as a coping mechanism rather than a pathology [ 38 , 40 ]. This clearly entails training of all service providers for better acknowledgment and comfort in dealing with trauma survivors , with an understanding of trauma as an expectation rather than an exception [ 41 ]. Although the TIC concept has evolved over the years, it still lacks guidance, training, staff knowledge, and governmental support, which are necessary to ensure successful policy implementation [ 40 ]. The role of PSWs also extended to support those at risk of trauma events because of the nature of their work, including child protection workers, who are at risk of posttraumatic stress disorder or anxiety disorder [ 42 ]. Although IPS grew from the informal practices of grassroots-initiated peer support, it differs from earlier approaches because it is a theoretically based, manualized approach with clear goals and a fidelity tool for practitioners [ 14 ]. It instead focuses on the nature and purpose of the peer support relationship and its attention to skill building to purposefully engage in peer support relationships that promote mutual healing and growth [ 3 ]. Transitioning from informal to formal roles provides not only well-formulated expectations of the role but also a better chance to identify the potential conflict of the PSWs’ mixed identity [ 43 ].
Research conducted on PSWs has been conceptualized throughout history [ 22 ]. Starting with feasibility studies, at the initial stage, it is followed by studies comparing peer staff with nonpeer staff and, finally, the studies that answer questions such as the following:
- Do interventions provided by peers differ from those provided by nonpeers?
- What makes peer support a unique form of service delivery?
If so, to the previous question, what are the active ingredients of these aspects of peer support, and what outcomes can they produce?
Studies that provide answers to the latter set of questions are expected to provide a deeper understanding of the philosophical underpinnings of the IPS concept for PSSs.
The Transformation From Patient to Peer Support Providers
The shift from being a service recipient to a service provider has been contributing as a driving force to restore fundamental human rights, especially among those with serious mental illnesses (SMIs) [ 22 ]. Telling the personal lived experience leads to a profound shift, from telling an “illness story” to a “recovery story” [ 4 ]. This involved an identity transformation from being perceived as a victim or a patient to a person fully engaged in life with various opportunities ahead [ 4 ]. This transition is seen as a gradual process and one that is supported by several other personal changes with expected challenges [ 44 ]. Moving a full circle to include PSWs as the service provider has been undertaken by mental health services to further exceed the transformational role, which was primarily the main aim of providing such a service [ 45 ]. A liminal identity was given for PSWs as laying between several roles, being service users, friends, and staff. Thus, the professionalism of the PSW role might not be a successful way to ensure individual well-being or to promote the peer support initiative [ 46 ]. Thus, successful transitioning from the patient to PSW role involves fundamental functional shifts achieved through overcoming multiple barriers at the personal, health system, and societal levels.
Effects of Peer Support Service in Different Contexts
Trained PSWs or mentors can use communication behaviors useful to different client groups. Many studies showed the effectiveness and feasibility of applying for peer support as follows:
Severe or Serious Mental Illness
Generally, the evidence for peer support interventions for people with SMIs has been described as moderate to limited with mixed intervention effects [ 2 , 47 ]. On the one hand, adding PSSs to intensive case management teams proved to improve activation in terms of knowledge, skills, confidence, and attitudes for managing health and treatment. Hence, patients become healthier, report better quality of life (QOL), engage in more health care practices, and report more treatment satisfaction [ 48 , 49 ]. On the other hand, a systematic review of randomized controlled trials (RCTs) involving adults with SMIs, while showing some evidence of positive effects on measures of hope, recovery, and empowerment at and beyond the end of the PSS intervention in this review, did not show any positive effects on hospitalization, satisfaction, or overall symptoms [ 10 ]. Similarly, a Cochrane systematic review of PSSs for people with schizophrenia found inconclusive results, with a high risk of bias in most of the studies and insufficient data to support or refute the PSS for this group [ 50 ].
Addiction and Drug Users
In recent years, peer recovery support services have become an accepted part of the treatment for substance use disorders, providing a more extensive array of services that are typically associated with the mutual supportive intervention [ 51 ]. This is in contrast to the use of peer support for SMIs where evidence is still developing. The Substance Abuse and Mental Health Services Administration (SAMHSA) defined peer recovery support for substance use disorders as “a set of nonclinical, peer-based activities that engage, educate, and support individuals so that they can make life changes that are necessary to recover from substance use disorders” [ 51 ]. Despite the long-term nature of substance abuse, immersion in peer support groups and activities and active engagement in the community are considered the 2 critical predictors of recovery for more than half the dependent substance users [ 52 ].
A number of trials studied the peer support effect on drug users, especially in the emergency department [ 53 , 54 ]. Another randomized trial found that a socially focused treatment can affect change in the patient’s social network and hence increase support for abstinence, for example, an increase of one nondrinking friend in the social network is translated into a 27% increase in the probability of reporting abstinence on 90% of days or more at all follow-up visits, which extended to 15 months [ 55 ].
Forensic Peer Support Service
The forensic peer system refers to the engagement of peer specialists who have histories of mental illness as well as criminal justice involvement and who are trained to help other patients sharing similar accounts [ 20 ]. As referred to by Davidson and Rowe [ 20 ], “Forensic Peer Specialists embody the potential for recovery for people who confront the dual stigmas associated with SMI and criminal justice system involvement.”
They offer day-to-day support for those released early from jail by accompanying them to initial probation meetings or treatment appointments and referring them to potential employers and landlords, helping people to negotiate and minimize continuing criminal sanctions and training professional staff on engaging consumers with criminal justice history [ 20 , 21 ]. PSWs with incarceration histories could successfully identify the liminal space in being supportive rather than providing support for the criminal offense, in contrast with the conventional methods that directly confront criminality [ 56 ]. In fact, having criminal history is the “critical component” for achieving recovery [ 56 ]. Multiple initiatives have been introduced to facilitate a reentry process for people recently released from incarceration, including Forensic Assertive Community Treatment, Assertive Community Treatment, Critical Time Intervention, and Women’s Initiative Supporting Health Transitions Clinic, through diverse community support groups involving PSWs [ 57 , 58 ].
A peer support program undertaken by older community volunteers was effective in improving general and physical health, social functioning, depression parameters, and social support satisfaction, especially in socially isolated, low-income older adults [ 59 ]. The Reclaiming Joy Peer Support intervention (a mental health intervention that pairs an older adult volunteer with a participant) has the potential for decreasing depression symptoms and improving QOL indicators for both anxiety and depression [ 60 ]. Engaging the community in health research could be of a high value in acknowledging their own health needs [ 61 ].
Youth and Adolescents
Peer support programs are mostly needed for university students, where challenges with loneliness and isolation are well recognized [ 62 ]. Hence, a need emerged for training peers to support their peer adolescents with the prospective challenges at this age [ 63 ]. Trained peer support students without necessarily having a lived experience were also examined in England [ 64 ]. The study included university students measuring the acceptability and impact of the volunteer peer support program through 6 weekly sessions. Students with lower mental well-being were more likely to complete the course, and an improvement in mental well-being was recorded for those who attended more frequently. Overall, peers remain to be an essential source of support for young people experiencing mental health and substance use problems [ 65 ].
Medically and Socially Disadvantaged Subgroups
A peer-led, medical self-management program intervention has been beneficial for medically and socially disadvantaged subgroups [ 60 ]. The Reclaiming Joy Peer Support intervention has the potential for increasing QOL and reducing depression in low-income older adults who have physical health conditions [ 60 ]. Similarly, for those who are “hardly reached,” it was indicated that the PSS provided is even more effective in these marginalized populations [ 66 ]. A Health and Recovery Peer program was delivered by mental health peer leaders for people with SMIs, resulting in an improvement in the physical health–related QOL parameters such as physical activity and medication adherence [ 49 ]. Peer-delivered and technology-supported interventions are feasible and acceptable and are associated with improvements in psychiatric, medical self-management skills, QOL, and empowerment of older adults with SMIs and or chronic health conditions [ 67 , 68 ].
Persons With Disabilities
The United Nations’ Convention on the Rights of Persons with Disabilities (CRPD) was adopted in 2007 and stated that “persons with disabilities should have equal recognition before the law and the right to exercise their legal capacity” [ 69 - 71 ]. Therefore, a positive emphasis upon the supported decision making and the fight against discrimination is evident through the convention. Nevertheless, these initiatives have been perceived as incomplete considering many challenges such as the community social status and ongoing perceived stigma of people with disabilities (PWDs) [ 70 , 72 ]. “Circle of support” is an elaborate example of an applicable peer support model for PWDs that has helped in decision making and facilitating communication [ 70 , 73 , 74 ]. This is clearly aligned with the paradigm shift from the biomedical to the socially supportive model of disability, which was provided by CRPD [ 70 ].
Peer Support for Families
Families may act either as sources of understanding and support or stigmatization through ignorance, prejudice, and discrimination, with subsequent negative impact [ 19 ]. In addition, the distress and burden associated with caring for a family member with mental illness are evident, where 29% to 60% endure significant psychological distress [ 17 ]. Family support can be financial or emotional; however, moral support was perceived as the substantial motivating factor for relatives who are ill [ 19 ]. In the last few decades, consistent and growing evidence that supports the inclusion of family members in the treatment and care of their misfortunate relatives has been developed. This has been mainly evident in the youth mental health system that urged the transformation change, which incorporates family members in the health care service provided to their youth [ 18 , 75 ]. Many PSWs have been engaged in family psychoeducation as family peers or parent partners, especially for those with the first episode of psychosis [ 76 ]. Although familial education is crucial and needs to be provided through different scales [ 19 ], an extensive matching of PSWs and the caregivers has not been perceived as a necessity to create a successful volunteer mentoring relationship [ 77 ]. Multiple initiatives have taken place all over the world. In India, a program titled “Saathi” was established for family members of residential and outpatient mental health service users that had dual goals of offering information and developing a peer support mechanism for family members of people with different mental health conditions [ 19 ]. In Melbourne, Australia, “Families Helping Families” was developed, where family PSWs are positioned in the service assessment area and in the inpatient unit to ensure early involvement [ 18 ]. An impressive peer support guide for parents of children or youth with mental health problems is provided by the Canadian Mental Health Association, British Colombia Division [ 15 ]. In Ontario, family matters programs are provided through provincial peer support programs [ 16 ].
The term “transforming mental health care” entails active involvement of families in orienting the mental health system toward recovery [ 78 ]. Family members are to have access to timely and accurate information that promotes learning, self-monitoring, and accountability [ 79 ]. The inclusion of family members as partners of the medical service is the new philosophy, with a subsequent shift from the concept of clinic-based practice to a community-based service approach [ 78 ].
Peer Support Service in Low- and Middle-Income Countries
Several initiatives took place in low- and middle-income countries, such as in rural Uganda, where a trained peer-led team provided 12 successful training sessions of perinatal service for a group of parents over a 6-month period, which resulted in better maternal well-being and child development, compared with another control group [ 80 ]. Similarly, successful community peer groups were conducted in rural India and Nepal, with high feasibility and effectiveness rates, and perceived as “potential alternative to health-worker-led interventions” [ 81 - 83 ]. In addition, adding counseling and social support groups entailing PSWs to the conventional medication treatment for patients with psychotic disorders was tried in a cohort study in Uganda; however, the results were not significantly different from those who received only medications [ 84 ]. This might be because of the underpowering of community services offered, compared with the robust medication regimens [ 85 ].
It is evident from the aforementioned information that there is mixed evidence on the effectiveness of PSW interventions in different contexts. For example, for patients with SMIs, systematic reviews suggest that there is some evidence of positive effects on measures of hope, recovery, and empowerment but no positive effects on hospitalization, satisfaction, or overall symptoms [ 10 ]. Similarly, for patients with addiction issues, although being involved in a peer network did not reduce social assistance for alcohol, they somewhat increased behavioral and attitudinal support for abstinence as well as involvement with Alcoholics Anonymous [ 55 ]. Furthermore, although many observational studies support the PSW role in the other contexts described above, there is a current dearth of literature involving RCTs and systematic reviews reporting on the effectiveness of PSWs in these specific contexts. Thus, there exist opportunities for conducting RCTs in the described contexts.
The Conceptual Framework for the Effects of Peer Support Service
The conceptual framework is based on empirical evidence, suggesting that the impact of PSWs reflects upon the recipients of such a service [ 4 , 76 , 86 - 90 ], the global health system [ 22 , 47 , 76 , 86 , 91 , 92 ], and the PSWs themselves [ 13 , 28 , 76 , 93 ], as shown in Figure 1 . The framework has, therefore, been developed by authors through a general review of the literature that examines the effects of PSSs on patients, health care systems, and also PSWs themselves so as to provide evidence-based material supporting all possible effects of PSW roles.

The conceptual framework for the impact of peer support workers in mental health. MH: mental health; PSS: peer support service; PSW: peer support worker.
Supportive social relationships can have a dual opposing effect on individuals’ lives, either as a family member or as social and professional networks through sharing their disappointments and pains or their joy and successes [ 11 ]. Useful roles for PSSs are identified in many studies. For example, adding 3 peer specialists to a team of 10 intensive case managers provided better QOL with greater satisfaction [ 12 ], stigma reduction, and less health service utilization [ 89 , 91 ]. The economic impact of PSSs has been extensively studied in the literature, concluding cost containment for the health care system in terms of reduction of readmission rates, emergency visits, and fewer hospital stays, which altogether substantially exceed the cost of running a peer support program [ 92 ]. Moreover, PSWs are looked at as providers of a service at a cheaper cost compared with other health care providers [ 94 , 95 ]. For example, about US $23,000 is paid to PSWs in the United States compared with around US $100,000 for a nurse practitioner [ 96 ]. However, a PSS is not posited as a substitute for clinical services, rather it is perceived as an intrapersonal and social service that provides a dual role of effective service and with humanizing care and support [ 14 , 26 , 97 ]. This role extends to cover PSWs themselves, in terms of improved overall well-being and self-confidence, reframing identity, and enhancing responsibility either toward themselves or their peers [ 13 , 93 ].
Although PSWs can play a variety of tasks, managers who hire them may want to ensure that improving patient activation is included in their range of duties [ 48 ]. In 2 concurrent studies, a significant increase in QOL satisfaction, reduction of rehospitalization rates, and reduction in the number of hospital days were recorded when adding PSSs to usual care [ 22 , 98 ]. In another study engaging 31 peer providers in diverse mental health, agencies identified 5 broad domains of wellness, including foundational, emotional, growth and spiritual, social, and occupational wellness [ 4 ]. In a systematic literature review for people with SMIs, peer-navigator interventions and self-management were the most promising interventions [ 47 ]. PSWs’ effects are diversified through sharing in different contexts. For example, positive impacts on the physical health of their peers have been recorded [ 49 ]. Peer-based approaches have been used to deliver behavioral weight loss interventions [ 90 ]. For young students, structured peer support for depression may have benefits in improving students’ mental well-being [ 64 ]. In the case of crisis houses, greater satisfaction was achieved through a provided informal PSS [ 99 ]. Robust studies, therefore, recommend implementing peer support programs [ 10 , 18 ].
On the other hand, authors found that PSSs met moderate levels of evidence and that effectiveness varied across service types, for example, with “peers in existing clinical roles” was described as being less effective than the “peer staff added to traditional services” and “peer staff delivering structured curricula” [ 3 ]. Other reviews suggested that current evidence does not support recommendations or mandatory requirements from policy makers to offer programs for peer support [ 9 , 10 ].
Peer Support Workers’ Satisfaction and Challenges
PSWs experience different problems alongside their diverse job roles, including low pay, stigma, unclear work roles, alienation, struggling with skill deficits, lack of training opportunities, emotional stress in helping others, and, on top of that, maintaining their personal physical and mental health wellness [ 100 , 101 ]. Researchers found that PSWs experience discrimination and prejudice from nonpeer workers, in addition to the encountered difficulties of how to manage the transition from being a patient to a PSW. As a result, high attrition rates were noted among PSWs in mental health settings [ 102 , 103 ]. Peer job satisfaction is strongly dependent on several factors [ 100 , 104 , 105 ]. Role clarity and psychological empowerment, organizational culture, and working partnership with peers were the most significant predictors of PSW job satisfaction, while professional prejudice was not perceived as a significant predictor [ 106 , 107 ]. Other studies noted that the main problems were experiencing marginalization, lack of understanding, and a sense of exclusion [ 108 - 110 ]. Payment could also contribute to the amount of satisfaction of PSWs [ 76 ], as compensation helps through facilitation and engagement motivation [ 109 ]. Nevertheless, it seems that not the payment, which ranged from US $10 to US $20 per hour, but the lack of recognition and acknowledgment are the causes for job nonsatisfaction [ 104 ].
An interesting literature review grouped these challenges and barriers facing PSWs during fulfilling their assigned roles into 6 main categories: nature of the innovation, individual professional, service user, social context, organizational context, and economic and political contexts [ 111 ].
It is evident from the abovementioned information that the PSW role is challenged at multiple levels, including at the personal, societal, and organizational levels. These challenges have a direct bearing on PSW satisfaction, and the successful integration of the PSW role into the health care system depends to a great extent on how these challenges are overcome.
Novel Technology in Peer Support Service (Online and Telephone)
Online support groups are usually conducted through bulletin boards, emails, or live chatting software [ 28 ]. Online groups are familiar with people whose illnesses are similar to SMIs or affecting the body shape that have forced them to experience embarrassment and social stigmatization [ 23 , 24 ]. Therefore, they split from the social contexts and redirect toward novel ways of help, such as PSWs and online support groups, and web-based communities provided a suitable medium for people with SMIs by following and learning from their peers on the web, which positively helped them to fight against stigma, instilling hope and gaining insight and empowerment for better health control [ 25 ]. Increasingly, social media grew as a target for individuals with SMIs, such as schizophrenia, schizoaffective disorder, or bipolar disorder, seeking advice and supporting each other [ 112 - 114 ]. For someone with SMIs, the decision to reach out and connect with others typically occurs at a time of increased instability or when facing significant life challenges [ 115 ]. In a qualitative study, popular social media, such as YouTube, appeared useful for allowing people with SMIs to feel less alone, find hope, support each other, and share personal experiences and coping strategies with day-to-day challenges of living with mental illness through listening and posting comments [ 114 ]. Mobile phone–based peer support was found to be a feasible and acceptable way to the youngsters during their pregnancy as well as in the postpartum period [ 116 ]. In addition, when coupled with frequent face-to-face meetings with PSWs and with “text for support,” it could be of high value for patients with different mental illnesses [ 117 ]. Although online peer networks actively fight against discrimination and stigma, their accessibility to diverse patients’ sectors regarding their income and ethnicity is still questionable [ 25 ].
Future of Peer Support Services
Potential new roles, such as community health workers, peer whole health coaches, peer wellness coaches, and peer navigators, have been suggested for such a workforce [ 76 ]. They are described as an “ill-defined potential new layer of professionals” [ 118 ]. Through an initiative undertaken by SAMHSA via its “Bringing Recovery Supports to Scale Technical Assistance Center Strategy,” a successful identification of abilities and critical knowledge necessarily required for PSWs who provide help and support for those recovering from mental health and substance abuse was noted [ 76 ]. At present, peer support is seen as a growing paradigm in many countries, including the United Kingdom, Canada, New Zealand, France, and the Netherlands [ 103 , 119 ]. As an evolving culture, peer support has the opportunity to forge not just mental health system change but social change as well [ 37 ]. A novel peer support system termed “Edmonton peer support system” (EPSS) is currently being tested in a randomized controlled pilot trial [ 117 ]. In this study, investigators are evaluating the effectiveness of an innovative peer support program that incorporates leadership training, mentorship, recognition, and reward systems for PSWs, coupled with automated daily supportive text messaging, which has proven effectiveness in feasibility trials involving patients with depression and alcohol use disorders [ 120 , 121 ]. Previous studies have examined the effect of PSSs in different contexts, including outpatient departments [ 122 ], emergency departments [ 53 , 54 ], community mental health clinics [ 123 , 124 ], and inpatient sites [ 125 ]. On the contrary, the EPSS study focuses on patients who have been discharged from acute care hospitals. These patients are being randomized into 1 of the 4 main groups: enrollment in a peer support system, enrollment in a peer support system plus automated daily supportive and reminder text messages, enrollment in automated daily supportive and reminder text messages alone, or treatment as usual follow-up care. The research team hypothesizes that patients who are assigned to a peer support system plus automated daily supportive and reminder text messages will show the best outcome.
Organizations may facilitate peer support through their values, actions, and oversight [ 119 ] and through a robust supervision system with available educational access, which could be the adequate path for creating a positive and risk-free environment for PSWs throughout their complex workloads [ 126 ]. On the other hand, ethics committees play essential roles in the inclusion of PSWs in applied research studies by avoiding repetition of the work of other trusted agencies and considering the ethical validity of consent procedures for peer support interventions [ 127 ].
There is an internationally growing trend to adopt PSSs within addiction and mental health services, and despite the ongoing challenges, large sections of the current literature support the inclusion of the PSWs into the mental health care workforce. The literature suggests that the benefits of PSSs impact not only the recipients of mental health services but also extend to the PSWs and the whole health care system. Although the expected benefits of PSSs might be directly measured in terms of service utilization or patient improvement indicators, this could also extend to include wellness and empowerment for PSWs, who may still be fragile, vulnerable, and in need of ongoing acknowledgment and recognition. Thus, the potential for PSSs to be embedded into routine care and the opportunities for the development of innovative models of care for addiction and mental health patients such as the EPSS, which incorporates PSSs and supportive text messaging [ 117 ], are evidently a high valued priority. However, the feasibility and maintenance of a robust PSS in health care would only be possible through collaborative efforts and ongoing support and engagement from all health care practitioners, managers, and other stakeholders.
This literature review has several limitations. First, the review is not a systematic review or meta-analysis, and as such, there were no well-defined inclusion or exclusion criteria of studies, which potentially could lead to the exclusion of some essential related studies. Second, the search was conducted in English publications only. Consequently, there is a high probability of missing critical related publications published in non-English languages. Finally, as the review depended mainly on the available literature from the aforementioned sources, which showed marked variability in their design and covered diverse ideas under the central theme, the different weights for each idea throughout the review could be noted.
Acknowledgments
This work was supported by Douglas Harding Trust Fund and Alberta Health Services.
Abbreviations
Conflicts of Interest: None declared.
REVIEW article
Depression and anxiety disorders in patients with inflammatory bowel disease.

- 1 Department of Gastroenterology, The Second Affiliated Hospital, Zhejiang University School of Medicine, Hangzhou, China
- 2 Department of Psychiatry, The Second Affiliated Hospital, Zhejiang University School of Medicine, Hangzhou, China
Mental health is a significant yet overlooked aspect of inflammatory bowel disease (IBD) patient care, with challenges in determining optimal treatments and psychological health resources. The most common psychological conditions in patients with IBD are anxiety and depression. The increased prevalence of these mental disorders appeals to mental screening of each person diagnosed with IBD at initial consultation. There are simple and clinically viable methods available to screen for mental problems. Psychological methods may be as or even more significant as a therapeutic modality. Herein we discuss the three major areas of psychological co-morbidity in IBD: (1) the prevalence and risk factors associated with anxiety and depression disorders for patients with IBD; (2) diagnosis of psychological disorders for patients with IBD; (3) treatment with patients with IBD and mental disorders. The gastroenterologists are encouraged to screen and treat these patients with IBD and mental disorders, which may improve outcomes.
Introduction
Inflammatory Bowel Diseases (IBD), including Crohn's disease (CD) and ulcerative colitis (UC), are characterized as chronic and relapsing inflammatory disorders with the peak incidence around 20 years of age ( 1 ). The incidence and prevalence of IBD are increasing worldwide. In China, the incidence rate of IBD was 1.74 per 100,000 person-years (UC: 1.18 per 100,000; CD: 0.4 per 100,000) ( 1 ). The patients with IBD had significant physical, psychosocial, and economic burden related to high risk of adverse health outcomes, including morbidity, hospitalization, surgery, work disability and even mortality ( 2 ). Health-related quality of life (HRQoL) is a concept that includes domains related to physical factors (such as natural history of disease and medications), mental factors (such as anxiety and depression), and social factors (such as job status and occupations) ( 3 ). Anxiety and depression are the most common psychological disorders for patients with IBD. One recent study showed that patients with IBD and mental health disease generated significantly higher costs compared with patients without mental disorders ( 4 ). Psychological disorders in patients with IBD may lead to high risk of relapse and poor treatment compliance. Moreover, systematic literature review suggested that psychiatric treatment may be effective in reducing anxiety and depression disorders, gastrointestinal symptoms and disease activity ( 5 ).
It has long been believed that patients with IBD might have psychological illness, including symptoms of common mental disorders and somatization, which may be related to the complex bidirectional interaction via the gut-brain axis ( 6 ). In a study of 405 patients with IBD following for 2 years, the authors observed that patients with normal anxiety scores but who were active at baseline had a nearly 6-fold increase in the risk of anxiety symptoms ( 7 ). Therefore, the occurrence of anxiety and depression may be related to the manifestations of active intestinal inflammation related to brain-gut axis imbalance ( 6 , 7 ).
The identified articles for this review used three different electronic databases: MEDLINE, PsychINFO, and EMBASE. The search terms were used: “inflammatory bowel diseases”, “ulcerative colitis”, “Crohn's disease”. The set operator AND was used to combine these terms with the following terms: “anxiety”, “depression”, and “mood disorder” or “mental disorder”. Herein we discuss the three major areas of psychological co-morbidity in IBD: (1) the prevalence and risk factors associated with anxiety and depression disorders for patients with IBD; (2) diagnosis of psychological disorders for patients with IBD; (3) treatment with patients with IBD and mental disorders. The clinicians are encouraged to detect and treat these patients with IBD and mental disorders, which may improve outcomes.
Prevalence and Risk factors Associated With Anxiety and Depression Disorders
Compared with the general population, patients with IBD had a higher lifetime rate of psychiatric disorders such as anxiety and depression, which were known to have negative impact on the quality of their lives and the severity of disease ( 8 , 9 ). Recently, in a systemic review and meta-analysis, the authors performed subgroup analyses by gender, disease location and disease activity, country and method used to define anxiety and depression ( 10 ). The meta-analysis showed that patients with IBD were exposed to high prevalence of symptoms of anxiety and depression, with approximately one in three patients affected by anxiety symptoms and one in four patients affected by depression symptoms ( 10 ). Furthermore, the prevalence of symptoms of anxiety or depression were higher inpatients with active IBD than in patients with inactive disease ( 10 ). Another study reported that patients with IBD had 3–5 times more likely to develop anxiety disorders and 2–4 times to develop depression disorders in the lifetime compared with general population ( 11 ).There was a Korea nationwide study with 46,707 non-IBD as control and 15,569 patients with IBD who were followed for 6 years, patients with IBD experienced significantly more anxiety (12.2 vs. 8.7%) and depression (8.0 vs. 4.7%) ( 12 ). One systematic review showed that patients with IBD had ~15 and 20%prevalence rate of depression and anxiety respectively ( 13 ). It was worth mentioning that one population-based study demonstrated that depression and anxiety disorders might precede IBD diagnosis by at least 5 years ( 14 ). In another population-based cohort study in Canada, nearly 80% of patients with IBD and anxiety were diagnosed with anxiety more than 2 years before the diagnosis of IBD ( 11 ).
By identifying factors related to the development of mental disorders in patients with IBD, it is possible to reverse anxiety and depressive disorders, not only to improve the quality of life, but also to improve the course of the disease. Based on data from the ISSEO cohort studies, factors associated with anxiety and depression disorders were severe disease, disease flares and socioeconomic deprivation ( 15 ). The Korea national study demonstrated a higher risk of new onset anxiety among individuals with CD (hazard ratio, 1.63) or UC (hazard ratio, 1.60) compared with matched controls. The risk ratios of depression after diagnosis of CD and UC are 2.09 and 2.00, respectively ( 12 ). This phenomenon was also observed in a case-control study in southern England, indicating that the risk of anxiety and depression is highest within 1 year after IBD diagnosis ( 16 ). Another prospective longitudinal follow-up conducted in Ontario, Canada showed that patients with abnormal anxiety HADS (Hospital Anxiety and Depression Scale, HADS) scores had higher IBD-related outcomes compared with patients whose anxiety scores did not increase (odds ratio, 3.36) ( 17 ). However, no difference was observed in those with abnormal depression HADS scores compared with those without elevated depression scores (odds ratio, 0.43) in IBD-related outcomes ( 17 ). Moreover, the results suggested that elevated anxiety HADS scores at baseline was an independent predictor of adverse IBD-related outcomes ( 17 ). A study of 204 patients with IBD (103 with UC and 101 with CD) showed that perceived stress was associated with mood disorders in IBD ( 18 ). However, in UC, other factors like a new diagnosis of IBD were related to anxiety and stress, while inpatient status and active diseases were related to depression. In CD, the factors such as abdominal pain, lower socioeconomic status were related to anxiety, while increasing age was related to depression ( 18 ).
Furthermore, large longitudinal studies had consistently shown more frequent IBD flares and worse disease activity in those with symptoms of anxiety and depression disorders ( 19 ). In a cohort of 2,007 patients followed over 9 years from the Swiss, the results showed an association between depressive symptoms and clinical recurrence of IBD ( 20 ). In a large longitudinal study of patients with IBD (1,516 with UC and 2,798 with CD) from USA with almost 2 years follow-up, Bharati Kochar et al. found that baseline depression patients with CD have an increased risk of recurrence (risk ratio, RR: 2.3), hospitalization (RR:1.3) or IBD-related surgery (RR:1.3). UC patients with baseline depression also have an increased risk of relapse (RR: 1.6), hospitalization (RR: 1.3), or surgery (RR: 1.8) at follow-up ( 21 ).
In conclusion, new onset of disease, disease activity, side effects of medications, stressful life events, inpatient status and lower socioeconomic status can easily affect the mood of one or two types of IBD ( 22 ). The increased prevalence of anxiety and depression disorders required a mental screening of each patient with IBD at initial consultation.
Diagnosis of Psychological Disorders
Identifying the psychological disorders of patients with IBD at the time of diagnosis or during the course of the disease is highly related to the patient's care, timely initiation of appropriate treatment, or improvement of the outcome of the disease ( 23 , 24 ). Screening and monitoring the psychological disorders in patients with IBD play significant role in both primary care and specialist settings. Unfortunately, no IBD specific instruments to measure anxiety or depression disorders have been validated to date. Tools developed for common illnesses of depression and anxiety include self-reported or clinician-reported approaches, and structured clinician psychiatric interviews ( 25 ). One approach to determine anxiety and depression is to use existing screening measures that have established reliability and effectiveness, which are easy to score and can be coded into electronic medical records. No measure can replace the doctor's judgment, and all positive screenings should be linked to an action plan involving further patient evaluation and treatment ( 26 ). It was important that clinicians and researchers understood the strengths and weaknesses of the various screening tools, and were informed about the interpretation of the resultant scores. The questionnaire scales and cutoff used to define presence of patients with symptoms of anxiety or depression were different ( 10 ). The detailed characteristics of depression and anxiety symptom scales were described as follows (also listed in Table 1 ).
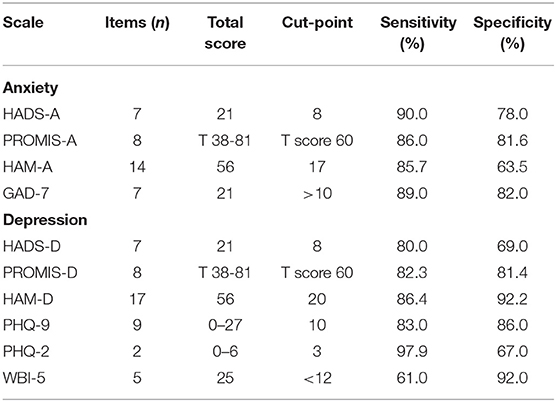
Table 1 . A summary of the characteristics of the scales.
Structured Clinical Interview for DSM Disorders (SCID)
The SCID is a semi-structured clinical interview that uses DSM-IV (Diagnostic and Statistical Manual of Mental Disorders IV criteria) to identify mental illness. SCID-based diagnosis can be used as a reference standard for standard validity analysis ( 14 ). “SCID depression” refers to any current or lifetime SCID diagnosis of major depression or thymic disorder; “SCID anxiety disorder” refers to any current or lifetime SCID diagnosis of panic disorder ( 27 ).
Hospital Anxiety and Depression Scale (HADS)
The HADS is a 14-item self-report questionnaire used to assess anxiety (A) and depression (D) levels in the past week ( 28 ). Each question is assessed using a 4-point like rt scale and the total score is 42 (21 with depression and 21 with anxiety). And the higher scores, the larger degree of anxiety and depression. It has been extensively studied to describe the prevalence of anxiety and depression in IBD developed for outpatients. The items on the scale are less directly influenced by disease-related symptoms which are different from other scales ( 18 , 29 ). The cut-point of 8 is used to identify possible anxiety or depression ( 18 , 29 ). The detection sensitivity and specificity of HADS are 80 and 69% for depression, respectively, and 90 and 78% for anxiety ( 28 ). However, HADS-D and HADS-A are the lowest sensitivity relative to other measures.
Patient-Reported Outcomes Measurement Information System (PROMIS)
The PROMIS, including Depression Short-Form 8a (PROMIS Depression) and Anxiety Short-Form 8a (PROMIS Anxiety), is an 8-item self-report questionnaire used to assess the level of anxiety and depression in the past week ( 30 ). The American Psychiatric Association has provided guidance on the use of a native scale for PROMIS Anxiety and Depression to identify the severity of psychological distress ( 31 , 32 ). Among other outcome variables, the PROMIS instrument generates anxiety and depression scores ( 33 ). The raw scores for anxiety and depression are translated to standardized T-scores with a population mean of 50 ± 10 ( 30 , 34 ). Unlike other measures, the PROMIS instruments are based on the item response theory framework to develop item banks, which allow delivery by computer-adaptive testing ( 33 ). PROMIS anxiety and depression can detect generalized anxiety screening positive (sensitivity 86.0%; specificity 81.6%) and possible major depression (sensitivity 82.3%; specificity 81.4%) ( 35 ). However, scoring PROMIS response patterns for research purposes can be very time-consuming.
Hamilton Rating Scale (HAM)
Hamilton Anxiety Rating Scale (HAM-A) and Hamilton Depression Rating Scale (HAM-D) are the two oldest tools for assessing anxiety and depression ( 36 , 37 ). These are scales assessed by clinicians and consist of 14 and 17 items respectively. They are used to assess the severity of patient anxiety and depression, which are the most used anxiety and depression scale assessed by clinicians in the world. Interestingly, the main value of HAM-A and HAM-D is to assess the patient's response to a course of treatment, not a diagnostic or screening tool (39). Through continuous use of the scale, doctors can evaluate the results of medication or psychotherapy ( 38 ). The detection sensitivity and specificity of HAM-A are 85.7 and 63.5% for anxiety, and 86.4 and 92.2% for depression, respectively.
Patient Health Questionnaire-9 (PHQ-9)
The PHQ-9 is a self-administered scale developed by Spitzer et al. for screening depression which is freely available in more than 60 languages ( 39 ). The shorter versions without the suicidal item are PHQ-4 and PHQ-2. The total score is 27 and the cut off score for PHQ-9 is 10 ( 40 ). The management time of the questionnaire is <5 min. The PHQ scale is a multi-purpose instrument that can also be used to diagnose, monitor and measure the severity of depression ( 39 ). The sensitivity and specificity of this scale are 83 and 86%, respectively ( 41 ). While the detection sensitivity and specificity of PHQ-2 are 97 and 67% for depression, respectively ( 42 ).
World Health Organization Well-Being index (WBI-5)
The WBI-5 is a short questionnaire consisting of five simple non-invasive questions.
It has sufficient effectiveness in screening depression and measuring the results of clinical trials, and has been successfully applied in a wide range of research fields ( 43 ). Currently, WBI-5 has been translated into more than 30 languages and has been used in research projects around the world. The total score is 25, and score <12 is consistent with clinical depression ( 44 ). Moreover, the WBI-5 detects depression with 61% insensitivity and 92% in specificity ( 43 ).
Symptom Checklist Anxiety Scale (SCL-A20)
SCL-A20 is a subscale of Symptom Checklist 90 Revised (SCL90-R) designed by Derogatis LR, which composed of 20 items and is used as a screening tool for anxiety ( 45 ). SCL90-R is composed of 90 items and assesses a wide range of mental symptoms, which have been widely used and proven to be reliable. Compared to SCL90-R, SCL-A20 takes a shorter time to administer. The cut off score of SCL-A20 for clinical anxiety is 30.
Generalized Anxiety Disorder-7 (GAD-7)
The GAD-7 is a 7-item self-report questionnaire assessing levels of anxiety over the last 2 weeks, which has demonstrated promise as a scale with good clinical applicability and powerful psychometric characteristics ( 46 ). It has demonstrated good reliability and structural validity ( 47 , 48 ). The total score is 21, and score >10 is consistent with moderate anxiety. Furthermore, GAD-7 has a sensitivity of 89% for GAD and a specificity of 82%, which moderately good at screening panic disorder (sensitivity 74%, specificity 81%), social anxiety disorder (sensitivity 72%, specificity 80%) and post-traumatic stress disorder (sensitivity 66%, specificity 81%), etc., ( 47 , 49 ). However, GAD-7 may not be able to capture the anxiety associated with anxiety disorders other than generalized anxiety disorder.
Moreover, a study systematically assessed the reliability and validity of multiple symptom rating scales for anxiety and depression in patients with IBD ( 14 ). The study showed that the symptom rating scales for anxiety and depression were similar in psychometric attributes. However, the anxiety scale was not as good as the depression scale ( 14 ). To be mentioned, most studies on self-reported symptom scales that assess anxiety and depression are conducted in primary care settings, but not in people with chronic diseases (such as IBD) who suffer from depression. The risk of illness and anxiety is particularly high, and the result may be higher ( 50 ). However, the accurate screening rate for mental disorders in primary care is estimated to be <50%, and the recognition rate for depression in primary care may be only 3.4% ( 51 – 53 ). Future research will need to determine to what extent the consistent use of the symptom scale in the IBD population will improve the detection of depression and anxiety. Clinicians evaluating patients with IBD should incorporate symptom scales into their practice, provided that when the score exceeds a certain cut-off point for the population, appropriate follow-up actions are available to clarify the background and manage symptoms. Unless we have more precise and standardized tools to quantify the psychological and inflammatory aspects of IBD, the dispute on the link between common mental disorders and IBD may continue. Therefore, clinicians may improve their detection of depression and anxiety through carrying out a symptom scale.
Supporting care that includes medical care and psychosocial, environmental, and behavioral interventions to improve health, which may achieve the highest health value ( 54 ). Both anxiety disorders and depression can be treated effectively by pharmacotherapy as well as psychotherapy ( Table 2 ). Psychological therapies should be considered in patients with IBD and functional symptoms ( 55 ). Most cases of mental disorders can be successfully treated with behavioral interventions, such as cognitive behavioral therapy (CBT), hypnosis, and mindfulness techniques for patients with and without IBD ( 56 – 60 ). In CBT, CBT has not been studied to continuously change the outcome of the disease, but it can effectively improve medical compliance and underlying symptoms of anxiety or depression ( 61 , 62 ). CBT is better than usual controls and treatments, but not better than other positive behavioral interventions. Mindfulness meditation programs can regulate pain, anxiety and depression of various physical health diseases ( 63 ). Hypnotherapy is a promising adjunctive treatment for IBD and has further proved beneficial for pain and anxiety in non-IBD populations ( 64 , 65 ). One study demonstrated the effect of 12 hypnotherapy on patients with IBD taking steroids for 5 years, the result showed that 60% patients stopped using steroids after hypnotherapy ( 66 ). Although hypnosis is efficacious, there are few behavioral professionals trained in medical hypnosis.
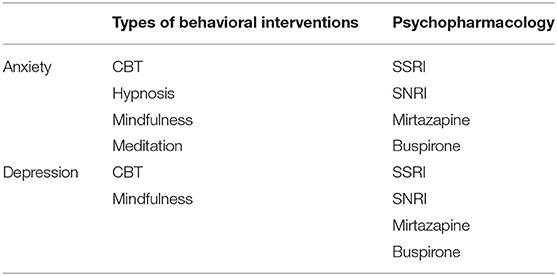
Table 2 . The behavioral interventions and psychopharmacology treatment.
Along with psychotherapy, antidepressants have been recognized as treatments for gut–brain disorders that might benefit both psychological and gastrointestinal health ( 67 ). Antidepressants have been found to be effective for the treatment of depression, anxiety, and chronic pain syndromes, but the overall support for their efficacy is modest at best. Although there are no large randomized trials for patients with IBD, there is evidence that selective serotonin reuptake inhibitors (SSRIs), serotonin and norepinephrine reuptake inhibitors (SNRIs), and tricyclic antidepressants (TCAs) can reduce anxiety and depression psychotherapy when used in combination with the following drugs ( 68 – 70 ).
Several clinical trials have addressed the impact of SSRI treatment on gastrointestinal dysfunction ( 71 ). The impact of SSRI on IBD is controversial. Most studies have shown that SSRI treatment has some benefits for patients with IBD, while other studies have failed to prove superior to placebo, especially if patients with depression are excluded from the trial ( 72 , 73 ). SSRI antidepressants can help relieve symptoms of depression, such as depression, irritability, feelings of worthlessness, irritability, anxiety, and trouble sleeping ( 74 ). SSRIs are generally well tolerated. However, like most antidepressants, SSRIs are associated with activation and increased suicidal ideation at higher doses.
SNRIs are a newer category that can block presynaptic serotonin and norepinephrine transporters, thereby increasing the post-synaptic stimulation of these receptors, which may be useful for refractory depression, anxiety, and chronic pain. The symptom is effective, and its side effects are similar to SSRIs and TCAs, with minor side effects ( 75 ).
Although TCA may have many serious side effects, TCA has shown some efficacy on functional gastrointestinal symptoms. In the Australian IBD treatment survey, approximately one-third of patients used antidepressants including TCA ( 69 ). The most common reason for this prescription is depression or anxiety, but TCA is rarely used for physical illness. A second retrospective study found that high-dose TCA prescribed for depression can reduce disease episodes, endoscopy and steroid use ( 76 ).
Mirtazapine
Mirtazapine is a noradrenergic and specific serotonergic antidepressant (NaSSA) with a variety of unique receptor activities, including blocking postsynaptic 5-HT2 and 5-HT3 receptors, Inhibition of presynaptic α2-adrenergic receptors, potent histamine H1 antagonism and moderate muscarinic receptor antagonism. The patients who did not tolerate SSRI therapy had efficacyfrom50 to 73% when treated with Mirtazapine ( 77 ). A study has shown that it is effective in the treatment of IBS, which is dominated by diarrhea and accompanied by depression and anxiety ( 78 ). If the side effects of sedation and weight gain can be tolerated, mirtazapine has a moderate effect on depression, and there is some support for the use of nausea and vomiting ( 68 ).
Compared with SSRIs, bupropion seems to be more effective in treating drowsiness and fatigue, but has less benefit in depression-related anxiety ( 73 , 79 ). Bupropion targets the reuptake of dopamine and norepinephrine, has a moderate effect on depression, and has a certain supportive effect on fatigue and smoking cessation ( 68 , 80 ). Buspirone has indications for generalized anxiety disorder, but studies have shown only small benefits. There is currently more and more evidence to treat functional dyspepsia ( 68 ). Unlike benzodiazepines, it has no side effects of physical dependence and cognitive impairment ( 81 , 82 ).
Most of these drugs have physiological effects on the brain, immune system and gastroenterology (except bupropion), so their treatment and side effects are represented in these systems. In a national study in Denmark, it was found that the use of antidepressants is beneficial to the course of patients with UC and CD, especially those who did not use antidepressants before the onset of IBD ( 83 ). A systematic review showed that antidepressant treatment may have a beneficial effect on IBD activity, disease recurrence, IBD-related surgery, quality of life, and treatment compliance ( 5 , 83 – 85 ). In the comparison of IBD depression patients who insisted and did not adhere to antidepressant treatment, the IBD activity, depression and anxiety of the patients who insisted on treatment decreased, and the quality of life improved ( 8 ). Some studies have shown that patients with IBD are more prone to depression, which can worsen the prognosis of IBD ( 86 ). Recently, an analysis of a large retrospective cohort study showed that patients with depression have a significantly higher risk of CD (adjusted HR: 2.11) and UC (adjusted HR: 2.23) ( 85 ). In addition, this study showed that SSRIs and TCAs have a protective effect on CD, while mirtazapine, SNRIs, SSRIs, serotonin modulators and TCAs have a protective effect on UC ( 85 ). The study showed that depression increases the risk of IBD, and the use of antidepressants during the treatment of depression may reduce this risk.
Conclusions and Perspectives
Psychological conditions like anxiety and depression are common and can affect the quality of life and the course of IBD. The increasing prevalence of these mental illnesses requires that every person diagnosed with IBD be mentally screened during the initial consultation. Therefore, it is necessary and feasible to call for patients with IBD to see a psychologist once a year. There are simple and clinically feasible tools that can be used to screen for psychological problems. Psychological factors are essential for proper IBD management, as there are complex interactions between the physical and emotional health of patients with IBD. Psychological methods may be as important as treatment methods, or even more important. Behavioral interventions can improve outcomes in part by changing relationships, compliance, and preventing recurrence. Antidepressants may have a beneficial effect on IBD activity, disease recurrence, IBD-related surgery, quality of life, and treatment compliance. The comprehensive management method of patients with IBD composed of gastroenterologists and psychiatrists should be included as the standard of care.
Author Contributions
CW and YaC: conceptualization. SH: writing - original draft. YiC and CW: writing - review and editing. All authors contributed to the article and approved the submitted version.
Conflict of Interest
The authors declare that the research was conducted in the absence of any commercial or financial relationships that could be construed as a potential conflict of interest.
Publisher's Note
All claims expressed in this article are solely those of the authors and do not necessarily represent those of their affiliated organizations, or those of the publisher, the editors and the reviewers. Any product that may be evaluated in this article, or claim that may be made by its manufacturer, is not guaranteed or endorsed by the publisher.
1. Li X, Song P, Li J, Tao Y, Li G, Li X, et al. The disease burden and clinical characteristics of inflammatory bowel disease in the Chinese population: A systematic review and meta-analysis. Int J Environ Res Public Health. (2017) 14:238. doi: 10.3390/ijerph14030238
PubMed Abstract | CrossRef Full Text | Google Scholar
2. Burisch J, Jess T, Martinato M, Lakatos PL. The burden of inflammatory bowel disease in Europe. J Crohn's Colitis. (2013) 7:322–37. doi: 10.1016/j.crohns.2013.01.010
3. Ishak WW, Pan D, Steiner AJ, Feldman E, Mann A, Mirocha J, et al. Patient-reported outcomes of quality of life, functioning, and GI/psychiatric symptom severity in patients with inflammatory bowel disease (IBD). Inflamm Bowel Dis. (2017) 23:798–803. doi: 10.1097/MIB.0000000000001060
4. Szigethy E, Murphy SM, Ehrlich OG, Engel-Nitz NM, Heller CA, Henrichsen K, et al. Mental health costs of inflammatory bowel diseases. Inflamm Bowel Di s. (2021) 27:40–8. doi: 10.1093/ibd/izaa030
5. Tarricone I, Giulia M, Bonucci G, Rizzello F, Carini G, Muratori R, et al. Prevalence and effectiveness of psychiatric treatments for patients with IBD : A systematic literature review. J Psychosom Res. (2017) 101:68–95. doi: 10.1016/j.jpsychores.2017.07.001
6. Gracie DJ, Hamlin PJ, Ford AC. The influence of the brain-gut axis in inflammatory bowel disease and possible implications for treatment. Lancet Gastroenterol Hepatol. (2019) 4:632–42. doi: 10.1016/S2468-1253(19)30089-5
7. Gracie DJ, Guthrie EA, Hamlin PJ, Ford AC. Bi-directionality of brain–gut interactions in patients with inflammatory bowel disease. Gastroenterology. (2018) 54:1635–46.e3. doi: 10.1053/j.gastro.2018.01.027
8. Yanartas O, Kani HT, Bicakci E, Kilic I, Banzragch M, Acikel C, et al. The effects of psychiatric treatment on depression, anxiety, quality of life, and sexual dysfunction in patients with inflammatory bowel disease. Neuropsychiatr Dis Treat. (2016) 12:673–83. doi: 10.2147/NDT.S106039
9. van den Brink G, Stapersma L, Marroun H El, Henrichs J, Szigethy EM, Utens EM, et al. Effectiveness of disease-specific cognitive-behavioural therapy on depression, anxiety, quality of life and the clinical course of disease in adolescents with inflammatory bowel disease: study protocol of a multicentre randomised controlled trial (HAPPY-IBD). BMJ Open Gastroenterol. (2016) 3: e000071. doi: 10.1136/bmjgast-2015-000071
10. Barberio B, Zamani M, Black CJ, Savarino E V, Ford AC. Prevalence of symptoms of anxiety and depression in patients with inflammatory bowel disease: a systematic review and meta-analysis. Lancet Gastroenterol Hepatol. (2021) 6:359–70. doi: 10.1016/S2468-1253(21)00014-5
11. Walker JR, Ediger JP, Graff LA, Greenfeld JM, Clara I, Lix L, et al. The Manitoba IBD cohort study: A population-based study of the prevalence of lifetime and 12-month anxiety and mood disorders. Am J Gastroenterol. (2008) 103:1989–97. doi: 10.1111/j.1572-0241.2008.01980.x
12. Choi K, Chun J, Han K, Park S, Soh H, Kim J, et al. Risk of anxiety and depression in patients with inflammatory bowel disease : a nationwide, population-based study. J Clin Med. (2019) 8:654. doi: 10.3390/jcm8050654
13. Neuendorf R, Harding A, Stello N, Hanes D, Wahbeh H. Depression and anxiety in patients with Inflammatory Bowel Disease: A systematic review. J Psychosom Res. (2016) 87:70–80. doi: 10.1016/j.jpsychores.2016.06.001
14. Bernstein CN, Zhang L, Lix LM, Graff LA, Walker JR, Fisk JD, et al. The validity and reliability of screening measures for depression and anxiety disorders in inflammatory bowel disease. Inflamm Bowel Dis. (2018) 24:1867–75. doi: 10.1093/ibd/izy068
15. Nahon S, Lahmek P, Durance C, Olympie A, Lesgourgues B, Colombel JF, et al. Risk factors of anxiety and depression in inflammatory bowel disease. Inflamm Bowel Dis. (2012) 18:2086–91. doi: 10.1002/ibd.22888
16. Kurina LM, Goldacre MJ, Yeates D, Gill LE. Depression and anxiety in people with inflammatory bowel disease. J Epidemiol Community Health. (2001) 55:716–20. doi: 10.1136/jech.55.10.716
17. Narula N, Pinto-Sanchez MI, Calo NC, Ford AC, Bercik P, Reinisch W, et al. Anxiety but not depression predicts poor outcomes in inflammatory bowel disease. Inflamm Bowel Dis. (2019) 25:1255–61. doi: 10.1093/ibd/izy385
18. Goodhand JR, Wahed M, Mawdsley JE, Farmer AD, Aziz Q, Rampton DS. Mood disorders in inflammatory bowel disease: Relation to diagnosis, disease activity, perceived stress, and other factors. Inflamm Bowel Dis. (2012) 18:2301–9. doi: 10.1002/ibd.22916
19. Mikocka-Walus A, Andrews JM. It is high time to examine the psyche while treating IBD. Nat Rev Gastroenterol Hepatol. (2018) 15:329–30. doi: 10.1038/s41575-018-0004-y
20. Mikocka-Walus A, Pittet V, Rossel JB, von Känel R. Symptoms of Depression and Anxiety Are Independently Associated With Clinical Recurrence of Inflammatory Bowel Disease. Clin Gastroenterol Hepatol. (2016) 14:829–35.e1. doi: 10.1016/j.cgh.2015.12.045
21. Kochar B, Barnes EL, Long MD, Cushing KC, Galanko J, Martin CF, et al. Depression Is associated with more aggressive inflammatory bowel disease. Am J Gastroenterol. (2018) 113:80–5. doi: 10.1038/ajg.2017.423
22. Krishnan V, Nestler EJ. The molecular neurobiology of depression. Nature. (2008) 455:894–902. doi: 10.1038/nature07455
23. Weaver E, Szigethy E. Managing pain and psychosocial care in IBD: a primer for the practicing gastroenterologist. Curr Gastroenterol Rep. (2020) 22:20. doi: 10.1007/s11894-020-0757-7
24. Rozich JJ, Holmer A, Singh S. Effect of lifestyle factors on outcomes in patients with inflammatory bowel diseases. Am J Gastroenterol. (2020) 115:832–40. doi: 10.14309/ajg.0000000000000608
25. Ali GC, Ryan G, De Silva MJ. Validated screening tools for common mental disorders in low and middle income countries: a systematic review. PLoS ONE. (2016) 11:e0156939. doi: 10.1371/journal.pone.0156939
26. Regueiro M, Greer JB, Szigethy E. Etiology and treatment of pain and psychosocial issues. Gastroenterology. (2017) 152:430–9.e4. doi: 10.1053/j.gastro.2016.10.036
27. Lewis K, Marrie RA, Bernstein CN, Graff LA, Patten SB, Sareen J, et al. The prevalence and risk factors of undiagnosed depression and anxiety disorders among patients with inflammatory bowel disease. Inflamm Bowel Dis. (2019) 25:1674–80. doi: 10.1093/ibd/izz045
28. Bjelland I, Dahl AA, Haug TT, Neckelmann D. The validity of the hospital anxiety and depression scale: an updated literature review. J Psychosom Res. (2002) 52:69–77. doi: 10.1016/S0022-3999(01)00296-3
29. Zigmond AS, Snaith RP. The hospital anxiety and depression scale. Acta Psychiatr Scand. (1983) 67:361–70. doi: 10.1111/j.1600-0447.1983.tb09716.x
30. Cella D, Riley W, Stone A, Rothrock N, Reeve B, Yount S, et al. The patient-reported outcomes measurement information system (PROMIS) developed and tested its first wave of adult self-reported health outcome item banks: 2005-2008. J Clin Epidemiol. (2010) 63:1179–94. doi: 10.1016/j.jclinepi.2010.04.011
31. Tarescavage AM, Forner EH, Ben-Porath Y. Construct validity of DSM-5 level 2 assessments (PROMIS depression, anxiety, and anger): evidence from the MMPI-2-RF. Assessment. (2021) 28:788–95. doi: 10.1177/1073191120911092
32. McGuire FH, Carl A, Woodcock L, Frey L, Dake E, Matthews DD, et al. Differences in patient and parent informant reports of depression and anxiety symptoms in a clinical sample of transgender and gender diverse youth. LGBT Health. (2021) 8:404–11. doi: 10.1089/lgbt.2020.0478
33. Kroenke K, Yu Z, Wu J, Kean J, Monahan PO. Operating characteristics of PROMIS four-item depression and anxiety scales in primary care patients with chronic pain. Pain Med. (2014) 15:1892–901. doi: 10.1111/pme.12537
34. Pilkonis PA, Choi SW, Reise SP, Stover AM, Riley WT, Cella D, et al. Item banks for measuring emotional distress from the patient-reported outcomes measurement information system (PROMIS®): Depression, anxiety, and anger. Assessment. (2011) 18:263–83. doi: 10.1177/1073191111411667
35. Purvis TE, Neuman BJ, Riley LH, Skolasky RL. Comparison of PROMIS Anxiety and Depression, PHQ-8, and GAD-7 to screen for anxiety and depression among patients presenting for spine surgery. J Neurosurg Spine. (2019) 18:1–8. doi: 10.3171/2018.9.SPINE18521
36. Bech P. Measuring states of anxiety with clinician-rated and patient-rated scales. In: Selek S, editor. Different Views of Anxiety Disorders . IntechOpen (2011). p. 169–84.
Google Scholar
37. Zimmerman M, Martinez JH, Young D, Chelminski I, Dalrymple K. Severity classification on the Hamilton depression rating scale. J Affect Disord. (2013) 150:384–8. doi: 10.1016/j.jad.2013.04.028
38. Kummer A, Cardoso F, Teixeira AL. Generalized anxiety disorder and the Hamilton Anxiety Rating Scale in Parkinson's disease. Arq Neuropsiquiatr. (2010) 68:495–501. doi: 10.1590/s0004-282x2010000400005
39. Kroenke K, Spitzer RL, Williams JBW. The PHQ-9: Validity of a brief depression severity measure. J Gen Intern Med. (2001) 16:606–13. doi: 10.1046/j.1525-1497.2001.016009606.x
40. Manea L, Gilbody S, McMillan D. Optimal cut-off score for diagnosing depression with the Patient Health Questionnaire (PHQ-9): A meta-analysis. CMAJ. (2012) 184:E191–6. doi: 10.1503/cmaj.110829
41. Marc LG, Henderson WR, Desrosiers A, Testa MA, Jean SE, Akom EE. Reliability and validity of the haitian creole PHQ-9. J Gen Intern Med. (2014) 29:1679–86. doi: 10.1007/s11606-014-2951-5
42. Kroenke K, Spitzer RL, Williams JBW. The patient health questionnaire-2: Validity of a two-item depression screener. Med Care. (2003) 41:1284–92. doi: 10.1097/01.MLR.0000093487.78664.3C
43. Topp CW, Østergaard SD, Søndergaard S, Bech P. The WHO-5 well-being index: A systematic review of the literature. Psychother Psychosom. (2015) 84:167–76. doi: 10.1159/000376585
44. Blom EH, Bech P, Högberg G, Larsson JO, Serlachius E. Screening for depressed mood in an adolescent psychiatric context by brief self-assessment scales - testing psychometric validity of WHO-5 and BDI-6 indices by latent trait analyses. Health Qual Life Outcomes. (2012) 10:149. doi: 10.1186/1477-7525-10-149
45. Derogatis LR, Unger R. Symptom Checklist-90-Revised (2010). In: Weiner IB, Craighead WE. The Corsini Encyclopedia of Psychology. Available online at: https://doi.org/10.1002/9780470479216.corpsy0970
46. Spitzer RL, Kroenke K, Williams JBW, Löwe B. A brief measure for assessing generalized anxiety disorder: The GAD-7. Arch Intern Med. (2006) 166:1092–7. doi: 10.1001/archinte.166.10.1092
47. Kroenke K, Spitzer RL, Williams JBW, Monahan PO, Löwe B. Anxiety disorders in primary care: Prevalence, impairment, comorbidity, and detection. Ann Intern Med. (2007) 146:317–25. doi: 10.7326/0003-4819-146-5-200703060-00004
48. Löwe B, Decker O, Müller S, Brähler E, Schellberg D, Herzog W, et al. Validation and standardization of the generalized anxiety disorder screener (GAD-7) in the general population. Med Care. (2008) 46:266–74. doi: 10.1097/MLR.0b013e318160d093
49. Swinson RP. The GAD-7 scale was accurate for diagnosing generalised anxiety disorder. Evid Based Med. (2006) 11:184. doi: 10.1136/ebm.11.6.184
50. Mitchell AJ. Clinical utility of screening for clinical depression and bipolar disorder. Curr Opin Psychiatry. (2012) 25:24–31. doi: 10.1097/YCO.0b013e32834de45b
51. Sareen J, Stein MB, Campbell DW, Hassard T, Menec V. The relation between perceived need for mental health treatment, DSM diagnosis, and quality of life: A Canadian population-based survey. Can J Psychiatry. (2005) 50:87–94. doi: 10.1177/070674370505000203
52. Weiller E, Bisserbe JC, Maier W, Lecrubier Y. Prevalence and recognition of anxiety syndromes in five European primary care settings. A report from the WHO study on Psychological Problems in General Health Care. Br J Psychiatry. (1998) 34:18–23. doi: 10.1192/S0007125000293471
53. Wittchen HU, Mühlig S, Beesdo K. Mental disorders in primary care. Dialogues Clin Neurosci. (2003) 5:115–28. doi: 10.1159/000079760
54. Szigethy EM, Allen JI, Reiss M, Cohen W, Perera LP, Brillstein L, et al. White paper aga: the impact of mental and psychosocial factors on the care of patients with inflammatory bowel disease. Clin Gastroenterol Hepatol. (2017) 15:986–97. doi: 10.1016/j.cgh.2017.02.037
55. Colombel JF, Shin A, Gibson PR, AGA. Clinical practice update on functional gastrointestinal symptoms in patients with inflammatory bowel disease: expert review. Clin Gastroenterol Hepatol. (2019) 17:380–90. doi: 10.1016/j.cgh.2018.08.001
56. McCombie AM, Mulder RT, Gearry RB. Psychotherapy for inflammatory bowel disease: A review and update. J Crohn's Colitis. (2013) 7:935–49. doi: 10.1016/j.crohns.2013.02.004
57. Szigethy E, Bujoreanu SI, Youk AO, Weisz J, Benhayon D, Fairclough D, et al. Randomized efficacy trial of two psychotherapies for depression in youth with inflammatory bowel disease. J Am Acad Child Adolesc Psychiatry. (2014) 53:726–35. doi: 10.1016/j.jaac.2014.04.014
58. Neilson K, Ftanou M, Monshat K, Salzberg M, Bell S, Kamm MA, et al. A Controlled study of a group mindfulness intervention for individuals living with inflammatory bowel disease. Inflamm Bowel Dis. (2016) 22:694–701. doi: 10.1097/MIB.0000000000000629
59. Gerbarg PL, Jacob VE, Stevens L, Bosworth BP, Chabouni F, Defilippis EM, et al. The effect of breathing, movement, and meditation on psychological and physical symptoms and inflammatory biomarkers in inflammatory bowel disease: a randomized controlled trial. Inflamm Bowel Dis. (2015) 21:2886–96. doi: 10.1097/MIB.0000000000000568
60. Shikatani B, Antony MM, Kuo JR, Cassin SE. The impact of cognitive restructuring and mindfulness strategies on postevent processing and affect in social anxiety disorder. J Anxiety Disord. (2014) 28:570–9. doi: 10.1016/j.janxdis.2014.05.012
61. Mikocka-Walus A, Bampton P, Hetzel D, Hughes P, Esterman A, Andrews JM. Cognitive-behavioural therapy for inflammatory bowel disease: 24-month data from a randomised controlled trial. Int J Behav Med. (2017) 24:127–35. doi: 10.1007/s12529-016-9580-9
62. Mikocka-Walus A, Bampton P, Hetzel D, Hughes P, Esterman A, Andrews JM. Cognitive-behavioural therapy has no effect on disease activity but improves quality of life in subgroups of patients with inflammatory bowel disease: A pilot randomised controlled trial. BMC Gastroenterol. (2015) 15:54. doi: 10.1186/s12876-015-0278-2
63. Goyal M, Singh S, Sibinga EMS, Gould NF, Rowland-Seymour A, Sharma R, et al. Meditation programs for psychological stress and well-being: A systematic review and meta-analysis. JAMA Intern Med. (2014) 174:357–68. doi: 10.1001/jamainternmed.2013.13018
64. Keefer L, Keshavarzian A. Feasibility and acceptability of gut-directed hypnosis on inflammatory bowel disease: A brief communication. Int J Clin Exp Hypn. (2007) 55:457–66. doi: 10.1080/00207140701506565
65. Mawdsley JE, Jenkins DG, Macey MG, Langmead L, Rampton DS. The effect of hypnosis on systemic and rectal mucosal measures of inflammation in ulcerative colitis. Am J Gastroenterol. (2008) 103:1460–9. doi: 10.1111/j.1572-0241.2008.01845.x
66. Miller V, Whorwell PJ. Treatment of inflammatory bowel disease: A role for hypnotherapy? Int J Clin Exp Hypn. (2008) 56:306–17. doi: 10.1080/00207140802041884
67. Mikocka-Walus A, Ford AC, Drossman DA. Antidepressants in inflammatory bowel disease. Nat Rev Gastroenterol Hepatol. (2020) 17:184–92. doi: 10.1038/s41575-019-0259-y
68. Thorkelson G, Bielefeldt K, Szigethy E. Empirically supported use of psychiatric medications in adolescents and adults with IBD. Inflamm Bowel Dis. (2016) 22:1509–22. doi: 10.1097/MIB.0000000000000734
69. Goodhand JR, Greig FIS, Koodun Y, McDermott A, Wahed M, Langmead L, et al. Do antidepressants influence the disease course in inflammatory bowel disease? A retrospective case-matched observational study. Inflamm Bowel Dis. (2012) 18:1232–9. doi: 10.1002/ibd.21846
70. Cámara RJA, Ziegler R, Begré S, Schoepfer AM, Von Känel R. The role of psychological stress in inflammatory bowel disease: Quality assessment of methods of 18 prospective studies and suggestions for future research. Digestion. (2009) 80:129–39. doi: 10.1159/000226087
71. Ostovaneh MR, Saeidi B, Hajifathalian K, Farrokhi-Khajeh-Pasha Y, Fotouhi A, Mirbagheri SS, et al. Comparing omeprazole with fluoxetine for treatment of patients with heartburn and normal endoscopy who failed once daily proton pump inhibitors: Double-blind placebo-controlled trial. Neurogastroenterol Motil. (2014) 26:670–8. doi: 10.1111/nmo.12313
72. Tabas G, Beaves M, Wang J, Friday P, Mardini H, Arnold G. Paroxetine to treat irritable bowel syndrome not responding to high-fiber diet: A double-blind, placebo-controlled trial. Am J Gastroenterol. (2004) 99:914–20. doi: 10.1111/j.1572-0241.2004.04127.x
73. Papakostas GI, Stahl SM, Krishen A, Seifert CA, Tucker VL, Goodale EP, et al. Efficacy of bupropion and the selective serotonin reuptake inhibitors in the treatment of major depressive disorder with high levels of anxiety (anxious depression): A pooled analysis of 10 studies. J Clin Psychiatry. (2008) 69:1287–92. doi: 10.4088/JCP.v69n0812
74. Fujitsuka N, Asakawa A, Hayashi M, Sameshima M, Amitani H, Kojima S, et al. Selective serotonin reuptake inhibitors modify physiological gastrointestinal motor activities via 5-HT2c receptor and acyl ghrelin. Biol Psychiatry. (2009) 65:748–59. doi: 10.1016/j.biopsych.2008.10.031
75. Thase ME. Are SNRIs more effective than SSRIs? A review of the current state of the controversy. Psychopharmacol Bull. (2008) 41:58–85.
PubMed Abstract | Google Scholar
76. Iskandar HN, Cassell B, Kanuri N, Gyawali CP, Gutierrez A, Dassopoulos T, et al. Tricyclic antidepressants for management of residual symptoms in inflammatory bowel disease. J Clin Gastroenterol. (2014) 48:423–9. doi: 10.1097/MCG.0000000000000049
77. Croom KF, Perry CM, Plosker GL. Mirtazapine: A review of its use in major depression and other psychiatric disorders. CNS Drugs. (2009) 23:427–52. doi: 10.2165/00023210-200923050-00006
78. Akama F, Mikami K, Watanabe N, Kimoto K, Yamamoto K, Matsumoto H. Efficacy of mirtazapine on irritable bowel syndrome with anxiety and depression: A case study. J Nippon Med Sch. (2018) 85:330–3. doi: 10.1272/jnms.JNMS.2018_85-53
79. Papakostas GI, Nutt DJ, Hallett LA, Tucker VL, Krishen A, Fava M. Resolution of sleepiness and fatigue in major depressive disorder: a comparison of bupropion and the selective serotonin reuptake inhibitors. Biol Psychiatry. (2006) 60:1350–5. doi: 10.1016/j.biopsych.2006.06.015
80. Jefferson JW, Rush AJ, Nelson JC, VanMeter SA, Krishen A, Hampton KD, et al. Extended-release bupropion for patients with major depressive disorder presenting with symptoms of reduced energy, pleasure, and interest: Findings from a randomized, double-blind, placebo-controlled study. J Clin Psychiatry. (2006) 67:865–73. doi: 10.4088/JCP.v67n0602
81. Lader M, Farr I, Morton S. A comparison of alpidem and placebo in relieving benzodiazepine withdrawal symptoms. Int Clin Psychopharmacol. (1993) 8:31–6. doi: 10.1097/00004850-199300810-00005
82. Lader M, Olajide D. A comparison of buspirone and placebo in relieving benzodiazepine withdrawal symptoms. J Clin Psychopharmacol. (1987) 7:11–5. doi: 10.1097/00004714-198702000-00003
83. Kristensen MS, Kjærulff TM, Ersbøll AK, Green A, Hallas J, Thygesen LC. The influence of antidepressants on the disease course among patients with Crohn's Disease and Ulcerative Colitis - A Danish Nationwide Register-Based Cohort Study. Inflamm Bowel Dis. (2019) 25:886–93. doi: 10.1093/ibd/izy367
84. Macer BJD, Prady SL, Mikocka-Walus A. Antidepressants in Inflammatory Bowel Disease: A Systematic Review. Inflamm Bowel Dis. (2017) 23:534–50. doi: 10.1097/MIB.0000000000001059
85. Frolkis AD, Vallerand IA, Shaheen AA, Lowerison MW, Swain MG, Barnabe C, et al. Depression increases the risk of inflammatory bowel disease, which may be mitigated by the use of antidepressants in the treatment of depression. Gut. (2019) 68:1606–12. doi: 10.1136/gutjnl-2018-317182
86. Mittermaier C, Dejaco C, Waldhoer T, Oefferlbauer-Ernst A, Miehsler W, Beier M, et al. Impact of Depressive mood on relapse in patients with inflammatory bowel disease: a prospective 18-month follow-up study. Psychosom Med. (2004) 66:79–84. doi: 10.1097/01.PSY.0000106907.24881.F2
Keywords: depression disorders, anxiety disorders, inflammatory bowel disease, psychological treatment, diagnose of psychological disorders
Citation: Hu S, Chen Y, Chen Y and Wang C (2021) Depression and Anxiety Disorders in Patients With Inflammatory Bowel Disease. Front. Psychiatry 12:714057. doi: 10.3389/fpsyt.2021.714057
Received: 17 June 2021; Accepted: 30 August 2021; Published: 08 October 2021.
Reviewed by:
Copyright © 2021 Hu, Chen, Chen and Wang. This is an open-access article distributed under the terms of the Creative Commons Attribution License (CC BY) . The use, distribution or reproduction in other forums is permitted, provided the original author(s) and the copyright owner(s) are credited and that the original publication in this journal is cited, in accordance with accepted academic practice. No use, distribution or reproduction is permitted which does not comply with these terms.
*Correspondence: Caihua Wang, wangcaihua@zju.edu.cn
Disclaimer: All claims expressed in this article are solely those of the authors and do not necessarily represent those of their affiliated organizations, or those of the publisher, the editors and the reviewers. Any product that may be evaluated in this article or claim that may be made by its manufacturer is not guaranteed or endorsed by the publisher.
2 hr 26 min
Does Lifting Really Improve Mental Health? The Stronger By Science Podcast
Greg, Pak, and Milo review the literature on lifting for mental health. Does exercising improve your mood? How long does this last? What about lifting? Can we improve our long-term mental health through physical activity and lifting weights?
- Episode Website
- More Episodes
- 2024 Stronger By Science LLC • The Stronger By Science Podcast
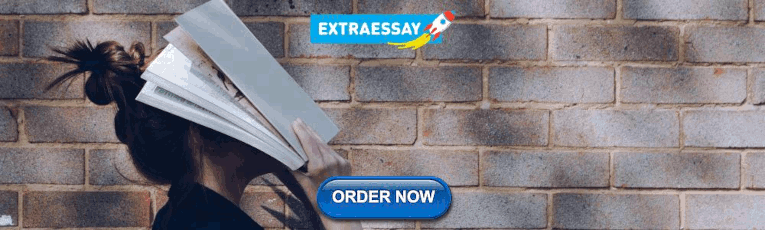
IMAGES
VIDEO
COMMENTS
A scoping review of the academic literature on the mental health of physicians and physicians-in-training in North America was conducted using Arksey and O'Malley's [] methodological framework.Our review objectives and broad focus, including the general questions posed to conduct the review, lend themselves to a scoping review approach, which is suitable for the analysis of a broader range ...
Scope of Mental Health Promotion and Prevention in the Current Situation. Literature provides considerable evidence on the effectiveness of various preventive mental health interventions targeting risk and protective factors for various mental illnesses (18, 36-42).There is also modest evidence of the effectiveness of programs focusing on early identification and intervention for severe ...
With an increased political interest in school-based mental health education, the dominant understanding and measurement of mental health literacy (MHL) in adolescent research should be critically appraised. This systematic literature review aimed to investigate the conceptualisation and measurement of MHL in adolescent research and the extent of methodological homogeneity in the field for ...
Reviews the extant literature on mental health and well-being. • Identifies the antecedents and consequences of employees' mental health and well-being. • Highlights the gaps in the existing literature and under researched areas. • Presents the findings as well as the future research directions at three interconnected levels.
Introduction. Childhood and adolescence are critical periods to promote mental health as more than half of mental health problems start at these stages, and many of these persist throughout adult life (Kessler et al., 2005).Currently, this has become a priority as worldwide data shows an increase in the prevalence of mental health issues in childhood and adolescence (de la Barra M, 2009) and ...
Background Determining the mental capacity of psychiatric patients for making healthcare related decisions is crucial in clinical practice. This meta-review of review articles comprehensively examines the current evidence on the capacity of patients with a mental illness to make medical care decisions. Methods Systematic review of review articles following PRISMA recommendations. PubMed ...
The COVID-19 pandemic is a major health crisis affecting several nations, with over 720,000 cases and 33,000 confirmed deaths reported to date. Such widespread outbreaks are associated with adverse mental health consequences. Keeping this in mind, existing literature on the COVID-19 outbreak pertinent to mental health was retrieved via a ...
Mental illness is a type of health condition that changes a person's mind, emotions, or behavior (or all three), and has been shown to impact an individual's physical health 1,2.Mental health ...
Objective: A scoping review of studies published in the first year of the COVID-19 pandemic focused on individuals with pre-existing symptoms of depression, anxiety, and specified stressor-related disorders, with the objective of mapping the research conducted. Eligibility criteria: (1) direct study of individuals with pre-existing depressive, anxiety, and/or specified stressor-related (i.e ...
The integrative review has been identified as a unique tool in healthcare for synthesising investigations available on a given topic or phenomena and for directing practise based on scientific knowledge [].The existing body of literature on mental health among adolescents is varied and complex as there are many concepts associated with mental health research ranging from positive aspects such ...
This review of the literature included empirical studies (qualitative and quantitative), literature reviews, and meta-analyses that assessed mental health in Black American adolescents. We focused on adolescents (ages 10-17) to inform prevention and intervention efforts.
Children and adolescent mental health. The World Health Organization (WHO, Citation 2017) reported that 10-20% of children and adolescents worldwide experience mental health problems.It is estimated that 50% of all mental disorders are established by the age of 14 and 75% by the age of 18 (Kessler et al., Citation 2007; Kim-Cohen et al., Citation 2003).
Mental health is a state of complete physical, psychological, and social well-being. 1,2 According to WHO's "Comprehensive Mental Health Action Plan 2013-2030," it is vital that each member state meets children's and adolescents' needs. 3 Mental health conditions are among the most common clinical challenges affecting approximately 20% of children and adolescents. 4,5 Mental disorders ...
Property cluster models are an approach that proposes that networks of mechanistic clusters cause mental health problems. For example, Kendler et al. ( 2011) argue that this model is the best way of understanding mental health problems as it acknowledges that mental health problems are multi-factorial or "fuzzy".
A Systematic Literature Review that was conducted by Fernandez et al. focused on evaluating the effect of setting-based interventions that stimulated and improved the mental health and well-being of university students and employees . That review constitutes an asset for universities seeking to adopt setting-based strategies that were proven ...
Background This systematic review provides a narrative synthesis of the evidence on the effectiveness of mental health promotion interventions for young people in low and middle-income countries (LMICs). Commissioned by the WHO, a review of the evidence for mental health promotion interventions across the lifespan from early years to adulthood was conducted. This paper reports on the findings ...
Running head: MENT AL HEAL TH LITERACY. Mental health literacy: A review of what it is and why it matters. Abstract. An increasing amount of scholarly work has attempted to understand the reasons ...
Problem-solving therapy (PST) is a potential psychological intervention aimed at preventing and treating psychological issues in stroke patients, although its efficacy is not clearly established. This systematic review assessed the effectiveness of PST in improving mental health, functioning, quality of life, and mortality in this population. Six databases were searched for literature indexed ...
This scoping review aimed to examine and summarize current knowledge on adolescents' voices regarding self-engagement in mental health treatment. Method: The review followed the scoping methodology of Arksey and O'Malley from 2005, updated by Levac and colleagues in 2010, involving five stages: (1) identifying the research question, (2 ...
Family involvement in mental health care has received increased attention, and there is a growing consensus in policies and clinical guidelines that families should be involved. ... Addressing the support needs of families during the acute hospitalization of a parent with mental illness: A narrative literature review. International Journal of ...
A review of 11 randomized, controlled trials (RCTs) concluded that R. rosea may have beneficial effects on physical performance, mental performance, and mental illness. A pharmacological review noted that R. rosea extracts and one of the constituents, salidroside, demonstrated anti-aging, anti-inflammatory, immunostimulant, DNA reparative, and ...
This literature review focused on the mental health and coping strategies of healthcare workers amidst pandemic. Coronavirus disease 2019 (COVID-19) has produced a worldwide health catastrophe ...
Conclusion: Reviewing these data and literature supports the hypothesis suggesting that an association of low-level mosaic aneuploidy with common and, probably, overlapping psychiatric disorders does exist. Accordingly, we propose a pathway for common neuropsychiatric disorders involving increased burden of rare de novo somatic chromosomal ...
Transforming mental health care into a recovery-oriented system will require further consideration of person-centered care planning as well as the impact of limited resources especially for those living in poverty. ... Ralph, R. O., & Cook, J. A. (2007). An analysis of the definitions and elements of recovery: a review of the literature ...
Peer Support in Mental Health: Literature Review. Reham A Hameed Shalaby, Department of Psychiatry, University of Alberta, 1E1 Walter Mackenzie Health Sciences Centre, 8440 112 St NW, Edmonton, AB, T6G 2B7, Canada, Phone: 1 4034702050, Email: ac.atrebalau@ybalahsr.
Page | 1 Literature Review: Effectiveness of Mental Health Awareness Campaigns Authors: Amir Chapel Institute for Social Research, UNM Date: 11/28/16 Definition: The World Health Organization (WHO) defines health as "a state of complete physical, mental, and social well- being and not merely the absence of disease or infirmity (WHO, 2016)."
Mental health conditions included articles related to suicide, mental health overall, depression and self-harm. Nurse suicide was the focus of three articles which were all published between 2019 and 2020, possibly signifying a new focus in research on nurse health. ... A Narrative Literature Review. Show details Hide details. Marco Grech ...
The HADS is a 14-item self-report questionnaire used to assess anxiety (A) and depression (D) levels in the past week ( 28 ). Each question is assessed using a 4-point like rt scale and the total score is 42 (21 with depression and 21 with anxiety). And the higher scores, the larger degree of anxiety and depression.
Greg, Pak, and Milo review the literature on lifting for mental health. Does exercising improve your mood? How long does this last? What about lifting? Can we improve our long-term mental health through physical activity and lifting weights?
The portrayal of mental health in contemporary literature has become an increasingly prevalent and important topic in recent years. As society's understanding and awareness of mental health issues have evolved, so too have the ways in which these issues are depicted in literature. In this essay, we will explore the historical context of mental health in literature, key figures who have ...