
An official website of the United States government
The .gov means it’s official. Federal government websites often end in .gov or .mil. Before sharing sensitive information, make sure you’re on a federal government site.
The site is secure. The https:// ensures that you are connecting to the official website and that any information you provide is encrypted and transmitted securely.
- Publications
- Account settings
Preview improvements coming to the PMC website in October 2024. Learn More or Try it out now .
- Advanced Search
- Journal List
- Front Sports Act Living

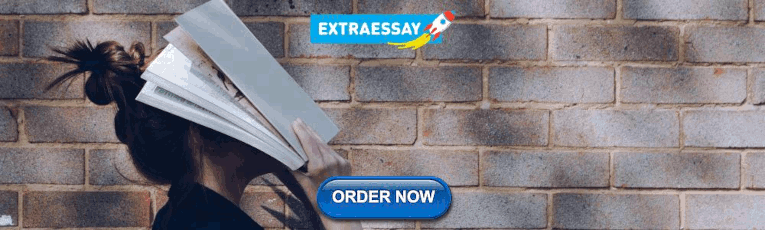
Stress in Academic and Athletic Performance in Collegiate Athletes: A Narrative Review of Sources and Monitoring Strategies
Marcel lopes dos santos.
1 School of Kinesiology, Applied Health and Recreation, Oklahoma State University, Stillwater, OK, United States
Melissa Uftring
Cody a. stahl, robert g. lockie.
2 Department of Kinesiology, California State University, Fullerton, CA, United States
Brent Alvar
3 Department of Kinesiology, Point Loma Nazarene University, San Diego, CA, United States
J. Bryan Mann
4 Department of Kinesiology and Sport Sciences, University of Miami, Miami, FL, United States
J. Jay Dawes
College students are required to manage a variety of stressors related to academic, social, and financial commitments. In addition to the burdens facing most college students, collegiate athletes must devote a substantial amount of time to improving their sporting abilities. The strength and conditioning professional sees the athlete on nearly a daily basis and is able to recognize the changes in performance and behavior an athlete may exhibit as a result of these stressors. As such, the strength and conditioning professional may serve an integral role in the monitoring of these stressors and may be able to alter training programs to improve both performance and wellness. The purpose of this paper is to discuss stressors experienced by collegiate athletes, developing an early detection system through monitoring techniques that identify the detrimental effects of stress, and discuss appropriate stress management strategies for this population.
Introduction
The college years are a period of time when young adults experience a significant amount of change and a variety of novel challenges. Academic performance, social demands, adjusting to life away from home, and financial challenges are just a few of the burdens college students must confront (Humphrey et al., 2000 ; Paule and Gilson, 2010 ; Aquilina, 2013 ). In addition to these stressors, collegiate athletes are required to spend a substantial amount of time participating in activities related to their sport, such as attending practices and training sessions, team meetings, travel, and competitions (Humphrey et al., 2000 ; López de Subijana et al., 2015 ; Davis et al., 2019 ; Hyatt and Kavazis, 2019 ). These commitments, in addition to the normal stress associated with college life, may increase a collegiate-athlete's risk of experiencing both physical and mental issues (Li et al., 2017 ; Moreland et al., 2018 ) that may affect their overall health and wellness. For these reasons, it is essential that coaches understand the types of stressors collegiate athletes face in order to help them manage the potentially deleterious effects stress may have on athletic and academic performance.
Strength and conditioning coaches are allied health care professionals whose primary job is to enhance fitness of individuals for the purpose of improving athletic performance (Massey et al., 2002 , 2004 , 2009 ). As such, many universities and colleges hire strength and conditioning coaches as part of their athletic staff to help athletes maximize their physical potential (Massey et al., 2002 , 2004 , 2009 ). Strength and conditioning coaches strive to increase athletic performance by the systematic application of physical stress to the body via resistance training, and other forms of exercise, to yield a positive adaptation response (Massey et al., 2002 , 2004 , 2009 ). For this reason, they need to understand and to learn how to manage athletes' stress. Additionally, based on the cumulative nature of stress, it is important that both mental and emotional stressors are also considered in programming. It is imperative that strength and conditioning coaches are aware of the multitude of stressors collegiate athletes encounter, in order to incorporate illness and injury risk management education into their training programs (Radcliffe et al., 2015 ; Ivarsson et al., 2017 ).
Based on the large number of contact hours strength and conditioning coaches spend with their athletes, they are in an optimal position to assist athletes with developing effective coping strategies to manage stress. By doing so, strength and conditioning coaches may be able to help reach the overarching goal of improving the health, wellness, fitness, and performance of the athletes they coach. The purpose of this review article is to provide the strength and conditioning professional with a foundational understanding of the types of stressors collegiate athletes may experience, and how these stressors may impact mental health and athletic performance. Suggestions for assisting athletes with developing effective coping strategies to reduce potential physiological and psychological impacts of stress will also be provided.
Stress and the Stress Response
In its most simplistic definition, stress can be described as a state of physical and psychological activation in response to external demands that exceed one's ability to cope and requires a person to adapt or change behavior. As such, both cognitive or environmental events that trigger stress are called stressors (Statler and DuBois, 2016 ). Stressors can be acute or chronic based on the duration of activation. Acute stressors may be defined as a stressful situation that occurs suddenly and results in physiological arousal (e.g., increase in hormonal levels, blood flow, cardiac output, blood sugar levels, pupil and airway dilation, etc.) (Selye, 1976 ). Once the situation is normalized, a cascade of hormonal reactions occurs to help the body return to a resting state (i.e., homeostasis). However, when acute stressors become chronic in nature, they may increase an individual's risk of developing anxiety, depression, or metabolic disorders (Selye, 1976 ). Moreover, the literature has shown that cumulative stress is correlated with an increased susceptibility to illness and injury (Szivak and Kraemer, 2015 ; Mann et al., 2016 ; Hamlin et al., 2019 ). The impact of stress is individualistic and subjective by nature (Williams and Andersen, 1998 ; Ivarsson et al., 2017 ). Additionally, the manner in which athletes respond to a situational or environmental stressor is often determined by their individual perception of the event (Gould and Udry, 1994 ; Williams and Andersen, 1998 ; Ivarsson et al., 2017 ). In this regard, the athlete's perception can either be positive (eustress) or negative (distress). Even though they both cause physiological arousal, eustress also generates positive mental energy whereas distress generates anxiety (Statler and DuBois, 2016 ). Therefore, it is essential that an athlete has the tools and ability to cope with these stressors in order to have the capacity to manage both acute and chronic stress. As such, it is important to understand the types of stressors collegiate athletes are confronted with and how these stressors impact an athlete's performance, both athletically and academically.
Literature Search/Data Collection
The articles included in this review were identified via online databases PubMed, MEDLINE, and ISI Web of Knowledge from October 15th 2019 through January 15th 2020. The search strategy combined the keywords “academic stress,” “athletic stress,” “stress,” “stressor,” “college athletes,” “student athletes,” “collegiate athletes,” “injury,” “training,” “monitoring.” Duplicated articles were then removed. After reading the titles and abstracts, all articles that met the inclusion criteria were considered eligible for inclusion in the review. Subsequently, all eligible articles were read in their entirety and were either included or removed from the present review.
Inclusion Criteria
The studies included met all the following criteria: (i) published in English-language journals; (ii) targeted college athletes; (iii) publication was either an original research paper or a literature review; (iv) allowed the extraction of data for analysis.
Data Analysis
Relevant data regarding participant characteristics (i.e., gender, academic status, sports) and study characteristics were extracted. Articles were analyzed and divided into two separate sections based on their specific topics: Academic Stress and Athletic Stress. Then, strategies for monitoring and workload management are discussed in the final section.
Academic Stress
Fundamentally, collegiate athletes have two major roles they must balance as part of their commitment to a university: being a college student and an athlete. Academic performance is a significant source of stress for most college students (Aquilina, 2013 ; López de Subijana et al., 2015 ; de Brandt et al., 2018 ; Davis et al., 2019 ). This stress may be further compounded among collegiate athletes based on their need to be successful in the classroom, while simultaneously excelling in their respective sport (Aquilina, 2013 ; López de Subijana et al., 2015 ; Huml et al., 2016 ; Hamlin et al., 2019 ). Davis et al. ( 2019 ) conducted surveys on 173 elite junior alpine skiers and reported significant moderate to strong correlations between perceived stress and several variables including depressed mood ( r = 0.591), sleep disturbance ( r = 0.459), fatigue ( r = 0.457), performance demands ( r = 0.523), and goals and development ( r = 0.544). Academic requirements were the highest scoring source of stress of all variables and was most strongly correlated with perceived stress ( r = 0.467). Interestingly, it was not academic rigor that was viewed by the athletes as the largest source of direct stress; rather, the athletes surveyed reported time management as being their biggest challenge related to academic performance (Davis et al., 2019 ). This further corroborates the findings of Hamlin et al. ( 2019 ). The investigators reported that during periods of the academic year in which levels of perceived academic stress were at their highest, students had trouble managing sport practices and studying. These stressors were also associated with a decrease in energy levels and overall sleep quality. These factors may significantly increase the collegiate athlete's susceptibility to illness and injury (Hamlin et al., 2019 ). For this reason, coaches should be aware of and sensitive to the stressors athletes experience as part of the cyclical nature of the academic year and attempt to help athletes find solutions to balancing athletic and academic demands.
According to Aquilina ( 2013 ), collegiate athletes tend to be more committed to sports development and may view their academic career as a contingency plan to their athletic career, rather than a source of personal development. As a result, collegiate athletes often, but certainly not always, prioritize athletic participation over their academic responsibilities (Miller and Kerr, 2002 ; Cosh and Tully, 2014 , 2015 ). Nonetheless, scholarships are usually predicated on both athletic and academic performance. For instance, the National Collegiate Athletic Association (NCAA) requires collegiate athletes to achieve and maintain a certain grade point average (GPA). Furthermore, they are also often required to also uphold a certain GPA to maintain an athletic scholarship. The pressure to maintain both high levels of academic and athletic performance may increase the likelihood of triggering mental health issues (i.e., anxiety and depression) (Li et al., 2017 ; Moreland et al., 2018 ).
Mental health issues are a significant concern among college students. There has been an increased emphasis placed on the mental health of collegiate athletes in recent years (Petrie et al., 2014 ; Li et al., 2017 , 2019 ; Reardon et al., 2019 ). Based on the 2019 National College Health Assessment survey from the American College Health Association (ACHA) consisting of 67,972 participants, 27.8% of college students reported anxiety, and 20.2% reported experiencing depression which negatively affected their academic performance (American College Health Association American College Health Association-National College Health Assessment II, 2019 ). Approximately 65.7% (50.7% males and 71.8% females) reported feeling overwhelming anxiety in the past 12 months, and 45.1% (37.1% males and 47.6% females) reported feeling so depressed that it was difficult for them to function. However, only 24.3% (13% males and 28.4% females) reported being diagnosed and treated by a professional in the past 12 months. Collegiate athletes are not immune to these types of issues. According to information presented by the NCAA, many certified athletic trainers anecdotally state that anxiety is an issue affecting the collegiate-athlete population (NCAA, 2014 ). However, despite the fact that collegiate athletes are exposed to numerous stressors, they are less likely to seek help at a university counseling center than non-athletes (NCAA, 2014 ), which could be related to stigmas that surround mental health services (NCAA, 2014 ; Kaier et al., 2015 ; Egan, 2019 ). This not only has significant implications related to their psychological well-being, but also their physiological health, and consequently their performance. For instance, in a study by Li et al. ( 2017 ) it was found that NCAA Division I athletes who reported preseason anxiety symptoms had a 2.3 times greater injury incidence rate compared to athletes who did not report. This same study discovered that male athletes who reported preseason anxiety and depression had a 2.1 times greater injury incidence, compared to male athletes who did not report symptoms of anxiety and depression. (Lavallée and Flint, 1996 ) also reported a correlation between anxiety and both injury frequency and severity among college football players ( r = 0.43 and r = 0.44, respectively). In their study, athletes reporting high tension/anxiety had a higher rate of injury. It has been suggested that the occurrence of stress and anxiety may cause physiological responses, such as an increase in muscle tension, physical fatigue, and a decrease in neurocognitive and perception processes that can lead to physical injuries (Ivarsson et al., 2017 ). For this reason, it is reasonable to consider that academic stressors may potentiate effects of stress and result in injury and illness in collegiate athletes.
Periods of more intense academic stress increase the susceptibility to illness or injury (Mann et al., 2016 ; Hamlin et al., 2019 ; Li et al., 2019 ). For example, Hamlin et al. ( 2019 ) investigated levels of perceived stress, training loads, injury, and illness incidence in 182 collegiate athletes for the period of one academic year. The highest levels of stress and incidence of illness arise during the examination weeks occurring within the competitive season. In addition, the authors also reported the odds ratio, which is the occurrence of the outcome of interest (i.e., injury), based off the given exposure to the variables of interest (i.e., perceived mood, sleep duration, increased academic stress, and energy levels). Based on a logistic regression, they found that each of the four variables (i.e., mood, energy, sleep duration, and academic stress) was related to the collegiate athletes' likelihood to incur injuries. In summary, decreased levels of perceived mood (odds ratio of 0.89, 0.85–0.0.94 CI) and sleep duration (odds ratio of 0.94, 0.91–0.97 CI), and increased academic stress (odds ratio of 0.91, 0.88–0.94 CI) and energy levels (odds ratio of 1.07, 1.01–1.14 CI), were able to predict injury in these athletes. This corroborates Mann et al. ( 2016 ) who found NCAA Division I football athletes at a Bowl Championship Subdivision university were more likely to become ill or injured during an academically stressful period (i.e., midterm exams or other common test weeks) than during a non-testing week (odds ratio of 1.78 for high academic stress). The athletes were also less likely to get injured during training camp (odds ratio of 3.65 for training camp). Freshmen collegiate athletes may be especially more susceptible to mental health issues than older students. Their transition includes not only the academic environment with its requirements and expectations, but also the adaptation to working with a new coach and teammates. In this regard, Yang et al. ( 2007 ) found an increase in the likelihood of depression that freshmen athletes experienced, as these freshmen were 3.27 times more likely to experience depression than their older teammates. While some stressors are recurrent and inherent in academic life (e.g., attending classes, homework, etc.), others are more situational (e.g., exams, midterms, projects) and may be anticipated by the strength and conditioning coach.
Athletic Stress
The domain of athletics can expose collegiate athletes to additional stressors that are specific to their cohort (e.g., sport-specific, team vs. individual sport) (Aquilina, 2013 ). Time spent training (e.g., physical conditioning and sports practice), competition schedules (e.g., travel time, missing class), dealing with injuries (e.g., physical therapy/rehabilitation, etc.), sport-specific social support (e.g., teammates, coaches) and playing status (e.g., starting, non-starter, being benched, etc.) are just a few of the additional challenges collegiate athletes must confront relative to their dual role of being a student and an athlete (Maloney and McCormick, 1993 ; Scott et al., 2008 ; Etzel, 2009 ; Fogaca, 2019 ). Collegiate athletes who view the demands of stressors from academics and sports as a positive challenge (i.e., an individual's self-confidence or belief in oneself to accomplish the task outweighs any anxiety or emotional worry that is felt) may potentially increase learning capacity and competency (NCAA, 2014 ). However, when these demands are perceived as exceeding the athlete's capacity, this stress can be detrimental to the student's mental and physical health as well as to sport performance (Ivarsson et al., 2017 ; Li et al., 2017 ).
As previously stated, time management has been shown to be a challenge to collegiate athletes. The NCAA rules state that collegiate athletes may only engage in required athletic activities for 4 h per day and 20 h/week during in-season and 8 h/week during off-season throughout the academic year. Although these rules have been clearly outlined, the most recent NCAA GOALS (2016) study reported alarming numbers regarding time commitment to athletic-related activities. Data from over 21,000 collegiate athletes from 600 schools across Divisions I, II, and III were included in this study. Although a breakdown of time commitments was not provided, collegiate athletes reported dedicating up to 34 h per week to athletics (e.g., practices, weight training, meetings with coaches, tactical training, competitions, etc.), in addition to spending between 38.5 and 40 h per week working on academic-related tasks. This report also showed a notable trend related to athletes spending an increase of ~2 more athletics-related hours per week compared to the 2010 GOALS study, along with a decrease of 2 h of personal time (from 19.5 h per week in 2010 to 17.1 in 2015). Furthermore, ~66% of Division I and II and 50% of Division III athletes reported spending as much or more time in their practices during the off-season as during the competitive season (DTHOMAS, 2013 ). These numbers show how important it is for collegiate athletes to develop time management skills to be successful in both academics and athletics. Overall, most collegiate athletes have expressed a need to find time to enjoy their college experience outside of athletic obligations (Paule and Gilson, 2010 ). Despite that, because of the increasing demand for excellence in academics and athletics, collegiate athletes' free time with family and friends is often scarce (Paule and Gilson, 2010 ). Consequently, trainers, coaches, and teammates will likely be the primary source of their weekly social interactivity.
Social interactions within their sport have also been found to relate to factors that may impact an athlete's perceived stress. Interactions with coaches and trainers can be effective or deleterious to an athlete. Effective coaching includes a coaching style that allows for a boost of the athlete's motivation, self-esteem, and efficacy in addition to mitigating the effects of anxiety. On the other hand, poor coaching (i.e., the opposite of effective coaching) can have detrimental psychological effects on an athlete (Gearity and Murray, 2011 ). In a closer examination of the concept of poor coaching practices, Gearity and Murray ( 2011 ) interviewed athletes about their experiences of receiving poor coaching. Following analysis of the interviews, the authors identified the main themes of the “coach being uncaring and unfair,” “practicing poor teaching inhibiting athlete's mental skills,” and “athlete coping.” They stated that inhibition of an athlete's mental skills and coping are associated with the psychological well-being of an athlete. Also, poor coaching may result in mental skills inhibition, distraction, insecurity, and ultimately team division (Gearity and Murray, 2011 ). This combination of factors may compound the negative impacts of stress in athletes and might be especially important for in injured athletes.
Injured athletes have previously been reported to have elevated stress as a result of heightened worry about returning to pre-competition status (Crossman, 1997 ), isolation from teammates if the injury is over a long period of time (Podlog and Eklund, 2007 ) and/or reduced mood or depressive symptoms (Daly et al., 1995 ). In addition, athletes who experience prolonged negative thoughts may be more likely to have decreased rehabilitation attendance or adherence, worse functional outcomes from rehabilitation (e.g., on measures of proprioception, muscular endurance, and agility), and worse post-injury performance (Brewer, 2012 ).
Monitoring Considerations
In addition to poor coaching, insufficient workload management can hinder an athlete's ability to recover and adapt to training, leading to fatigue accumulation (Gabbett et al., 2017 ). Excessive fatigue can impair decision-making ability, coordination and neuromuscular control, and ultimately result in overtraining and injury (Soligard et al., 2016 ). For instance, central fatigue was found to be a direct contributor to anterior cruciate ligament injuries in soccer players (Mclean and Samorezov, 2009 ). Introducing monitoring tools may serve as a means to reduce the detrimental effects of stress in collegiate athletes. Recent research on relationships between athlete workloads, injury, and performance has highlighted the benefits of athlete monitoring (Drew and Finch, 2016 ; Jaspers et al., 2017 ).
Athlete monitoring is often assessed with the measuring and management of workload associated with a combination of sport-related and non-sport-related stressors (Soligard et al., 2016 ). An effective workload management program should aim to detect excessive fatigue, identify its causes, and constantly adapt rest, recovery, training, and competition loads respectively (Soligard et al., 2016 ). The workload for each athlete is based off their current levels of physical and psychological fatigue, wellness, fitness, health, and recovery (Soligard et al., 2016 ). Accumulation of situational or physical stressors will likely result in day-to-day fluctuations in the ability to move external loads and strength train effectively (Fry and Kraemer, 1997 ). Periods of increased academic stress may cause increased levels of fatigue, which can be identified by using these monitoring tools, thereby assisting the coaches with modulating the workload during these specific periods. Coaches who plan to incorporate monitoring and management strategies must have a clear understanding of what they want to achieve from athlete monitoring (Gabbett et al., 2017 ; Thornton et al., 2019 ).
Monitoring External Loads
External load refers to the physical work (e.g., number of sprints, weight lifted, distance traveled, etc.) completed by the athlete during competition, training, and activities of daily living (Soligard et al., 2016 ). This type of load is independent of the athlete's individual characteristics (Wallace et al., 2009 ). Monitoring external loading can aid in the designing of training programs which mimic the external load demands of an athlete's sport, guide rehabilitation programs, and aid in the detection of spikes in external load that may increase the risk of injury (Clubb and McGuigan, 2018 ).
The means of quantifying external load can involve metrics as simple as pitch counts in baseball and softball (Fleisig and Andrews, 2012 ; Shanley et al., 2012 ) or quantifying lifting session training loads (e.g., sum value of weight lifted during an exercise x number of repetitions × the number of sets). Neuromuscular function testing is another more common way of analyzing external load. This is typically done using such measures such as the counter movement jump, squat jump, or drop jump. A force platform can be used to measure a myriad of outcomes (e.g., peak power, ground contact time, time to take-off, reactive strength index, and jump height), or simply measure jump height in a more traditional manner. Jumping protocols, such as the countermovement jump, have been adopted to examine the recovery of neuromuscular function after athletic competition with significant decreases for up to 72 h commonly reported (Andersson et al., 2008 ; Magalhães et al., 2010 ; Twist and Highton, 2013 ). (Gathercole et al., 2015 ) found reductions in 18 different neuromuscular variables in collegiate athletes following a fatiguing protocol. The variables of eccentric duration, concentric duration, total duration, time to peak force/power, and flight time:contraction time ratio, derived from a countermovement jump were deemed suitable for detecting neuromuscular fatigue with the rise in the use of technology for monitoring, certain sports have adopted specific software that can aid in the monitoring of stress. For example, power output can be measured using devices such as SRM™ or PowerTap™ in cycling (Jobson et al., 2009 ). This data can be analyzed to provide information such as average power or normalized power. The power output can then be converted into a Training Stress Score™ via commercially available software (Marino, 2011 ). More sophisticated measures of external load may involve the use of wearable technology devices such as Global Positioning System (GPS) devices, accelerometers, magnetometer, and gyroscope inertial sensors (Akenhead and Nassis, 2016 ). These devices can quantify external load in several ways, such as duration of movement, total distance covered, speed of movement, acceleration, and decelerations, as well as sport specific movement such as number and height of jumps, number of tackles, or breakaways, etc. (Akenhead and Nassis, 2016 ). The expansion of marketing of wearable devices has been substantial; however, there are questions of validity and reliability related to external load tracking limitations related to proprietary metrics, as well as the overall cost that should be considered when considering the adoption of such devices (Aughey et al., 2016 ; Torres-Ronda and Schelling, 2017 ).
Monitoring Internal Loads
While external load may provide information about an athlete's performance capacity and work completed, it does not provide clear evidence of how athletes are coping with and adapting to the external load (Halson, 2014 ). This type of information comes from the monitoring of internal loads. The term internal load refers to the individual physiological and psychological response to the external stress or load imposed (Wallace et al., 2009 ). Internal load is influenced by a number of factors such as daily life stressors, the environment around the athlete, and coping ability (Soligard et al., 2016 ). Indirect measures, such as the use of heart rate (HR) monitoring, and subjective measurements, such as perceived effort (i.e., ratings of perceived exertion), are examples of internal load monitoring. Using subjective measurement systems is a simple and practical method when dealing with large numbers of athletes (Saw et al., 2016 ; Nässi et al., 2017 ). Subjective reporting of training load (Rating of Perceived Exertion—RPE) (Coyne et al., 2018 ), Session Rating of Perceived Exertion—sRPE) (Coyne et al., 2018 ), perceived stress and recovery (Recovery Stress Questionnaire for Athletes—RESTQ-S), and psychological mood states (Profile of Mood States—POMS) have all been found to be a reliable indicator of training load (Robson-Ansley et al., 2009 ; Saw et al., 2016 ) and only take a few moments to complete. In addition, subjective measures can be more responsive to tracking changes or training responses in athletes than objective measures (Saw et al., 2016 ).
Heart rate (HR) monitoring is a common intrinsic measure of how the body is responding to stress. With training, the reduction of resting HR is typically a clear indication of the heart becoming more efficient and not having to beat as frequently. Alternately, increases of resting HR over time with a continuation of training may be an indicator of too much stress. Improper nutrition, such as regular or ongoing suboptimal intakes of vitamins or minerals, may result in increased ventilation and/or increased heart rate (Lukaski, 2004 ). It has been suggested that the additional stress may lead to parasympathetic hyperactivity, leading to an increase in resting HR (Statler and DuBois, 2016 ). This largely stems from research examining the sensitivity of various HR derived metrics, such as resting HR, HR variability (HRV), and HR recovery (HRR) to fluctuations in training load (Borresen and Ian Lambert, 2009 ). HRR in athlete monitoring is the rate of HR decline after the cessation of exercise. A common measure of HHR is the use of a 2 min step test followed by a 60 s HR measurement. The combination of the exercise (stress) on the cardiovascular system and then its subsequent return toward baseline has been used as an indicator of autonomic function and training status in athletes (Daanen et al., 2012 ). In collegiate athletes it was found that hydration status impacted HRR following moderate to hard straining sessions (Ayotte and Corcoran, 2018 ). Athletes who followed a prescription hydration plan performed better in the standing long jump, tracked objects faster, and showed faster HRR vs. athletes who followed their normal self-selected hydration plan (Ayotte and Corcoran, 2018 ). To date, HR monitoring and the various derivatives have mainly been successful in detecting changes in training load and performance in endurance athletes (Borresen and Ian Lambert, 2009 ; Lamberts et al., 2009 ; Thorpe et al., 2017 ). Although heart rate monitoring can provide additional physiological insight for aerobic sessions or events, it thus far has not been found to be an accurate measurement for quantifying internal load during many explosive, short duration anaerobic activities (Bosquet et al., 2008 ).
A multitude of studies have reported the reliability and validity of using RPE and sRPE across a range of training modalities (Foster, 1998 ; Impellizzeri et al., 2004 ; Sweet et al., 2004 ). This measure can be used to create a number of metrics such as session load (sRPE × duration in minutes), daily load (sum of all session loads for that day), weekly training load (sum of all daily training loads for entire week), monotony (standard deviation of weekly training load), and strain (daily or weekly training load × monotony) (Foster, 1998 ). Qualitative questionnaires that monitor stress and fatigue have been well-established as tools to use with athletes (see Table 1 for examples of commonly used questionnaires in research). Using short daily wellness questionnaires may allow coaches to generate a wellness score which then can be adjusted based off of the stress the athlete may be feeling to meet the daily load target (Foster, 1998 ; Robson-Ansley et al., 2009 ). However, strength and conditioning coaches need to be mindful that these questionnaires may require sports psychologist or other licensed professional to examine and provide the results. An alternative that may be better suited for strength and conditioning professionals to use could be to incorporate some of the themes of those questionnaires into programing.
Overview of common tool/measures used by researchers to monitor training load.
A Multifaceted Approach
Dissociation between external and internal load units may be indicative of the state of fatigue of an athlete. Utilizing a monitoring system in which the athlete is able to make adjustments to their training loads in accordance with how they are feeling in that moment can be a useful tool for assisting the athlete in managing stress. Auto-regulation is a method of programming that allows for adjustments based on the results of one or more readiness tests. When implemented properly, auto regulation enables the coach or athlete to optimize training based on the athlete's given readiness for training on a particular day, thereby aiming to avoid potential overtraining (Kraemer and Fleck, 2018 ). Several studies have found that using movement velocity to designate resistance training intensities can result in significant improvements in maximal strength and athletic performance (Pareja-Blanco et al., 2014 , 2017 ; Mann et al., 2015 ). Velocity based training allows the coach and athlete to view real time feedback for the given lifts, thereby allowing them to observe how the athlete is performing in that moment. If the athlete is failing to meet the prescribed velocity or the velocity drops greater than a predetermined amount between sets, then this should signal the coach to investigate. If there is a higher than normal amount of stress on that athlete for the day, that could be a potential reason. This type of combination style program of using a quantitative or objective measurement (s) and a subjective measure of wellness (qualitative questionnaire) has recently been reported to be an effective tool in monitoring individuals apart of a team (Starling et al., 2019 ). The subjective measure in this study was the readiness to train questionnaire (RTT-Q) and the objective measures were the HRR 6min test (specifically the HRR 60s = recorded as decrease in HR in the 60 s after termination of the test) to assess autonomic function and the standing long jump (SLJ) to measure neuromuscular function. The findings found that, based on the absolute typical error of measurement, the HRR 60s and SLJ could detect medium and large changes in fatigue and readiness. The test took roughly 8 min for the entire team, which included a group consisting of 24 college-age athletes. There are many other combinations of monitoring variables and strategies that coaches and athletes may utilize.
Data Analysis – How to Utilize the Measures
Regardless of what type of monitoring tool a coach or athlete may incorporate, it is essential to understand how to analyze this data. There are excellent resources available which discuss this topic in great detail (Gabbett et al., 2017 ; Clubb and McGuigan, 2018 ; Thornton et al., 2019 ). This section will highlight two main conclusions from these sources and briefly describe two of the main statistical practices and concepts discussed. The use of z-scores or modified z-scores has been proposed as a method of detecting meaningful change in athlete data (Clubb and McGuigan, 2018 ; Thornton et al., 2019 ). For different monitoring tools listed in Table 1 , the following formula would be an example of how to assess changes: (Athlete daily score—Baseline score)/Standard deviation of baseline. The baseline would likely be based off an appropriate period such as the scores across 2 weeks during the preseason.
In sports and sports science, the use of a magnitude-based inference (MBI) has been suggested as more appropriate and easier to understand when examining meaningful changes in athletic data, than null-hypothesis significance testing (NHST) (Buchheit, 2014 ). Additional methods to assess meaningful change that are similar to MBI are using standard deviation, typical error, effect sizes, smallest worthwhile change (SWC), and coefficient of variation (Thornton et al., 2019 ). It should be noted that all of these methods have faced criticism from sources such as statisticians. It is important to understand that the testing methods, measurements, and analysis should be based on the resources and intended goals from use, which will differ from every group and individual. Once identified, it is up to the practitioner to keep this system the same, in order to collect data that can then be examined to understand meaningful information for each setting (Thornton et al., 2019 ).
Managing and Coping Strategies
Once the collegiate-athlete has been able to identify the need to balance their stress levels, the athlete may then need to seek out options for managing their stress. Coaches are be able to assist them by sharing information on health and wellness resources available for the students, both on and off campus. Another way a coach can potentially support their athletes is by establishing an open-door policy, wherein the team members feel comfortable approaching a member of the strength and conditioning staff in order to seek out resources for coping with challenges related to stress.
There are some basic skills that strength and conditioning coaches can teach (while staying within their scope of practice). Coaches can introduce their athletes to basic lifestyle concepts, such as practicing deep breathing techniques, positive self-talk, and developing healthy sleep habits (i.e., turning off their mobile devices 1 h before bed and aiming for 8 h of sleep each night, etc.). A survey of strength and conditioning practitioners by Radcliffe et al. ( 2015 ) found that strategies used by practitioners included a mix of cognitive and behavioral strategies, which was used as justification for recommending practitioners find opportunities to guide professional development toward awareness strategies. Practitioners reported using a wide variety of psychological skills and strategies, which following survey analysis, highlighted a significant emphasis on strategies that may influence athlete self-confidence and goal setting. Themes identified by Radcliffe et al. ( 2015 ) included confidence building, arousal management, and skill acquisition. Additionally, similar lower level themes that are connected (i.e., goal setting, increasing, or decreasing arousal intensities, self-talk, mental imagery) are all discussed in the 4th edition of the NSCA Essentials of Strength and Conditioning book (Haff et al., 2016 ). When the interventions aiming to improve mental health expand from basic concepts to mental training beyond a coach's scope, it would be pertinent for the coach to refer the collegiate-athlete to a sport psychology or other mental health consultant (Fogaca, 2019 ). Moreover, strength and conditioning coaches may find themselves in a position to become key players in facilitating management strategies for collegiate athletes, thereby guiding the athlete in their quest to learn how to best manage the mental and physical energy levels required in the quest for overall optimal performance (Statler and DuBois, 2016 ).
Conclusion and Future Directions
This review article has summarized some of the ways that strength and conditioning professionals may be able to gain a better understanding of the types of stressors encountered by collegiate athletes, the impact these stressors may have on athletic performance, and suggestions for assisting athletes with developing effective coping strategies to reduce the potential negative physiological and psychological impacts of stress. It has been suggested that strategies learned in the context of training may have a carry-over effect into other areas such as competition. More education is needed in order for strength and conditioning professionals to gain a greater understanding of how to support their athletes with stress-management techniques and resources. Some ways to disseminate further education on stress-management tools for coaches to share with their athletes may include professional development events, such as conferences and clinics.
Author Contributions
All of the authors have contributed to the development of the manuscript both in writing and conceptual development.
Conflict of Interest
The authors declare that the research was conducted in the absence of any commercial or financial relationships that could be construed as a potential conflict of interest. The handling editor declared a past collaboration with one of the authors RL.
- Akenhead R., Nassis G. P. (2016). Training load and player monitoring in high-level football: current practice and perceptions . Int. J. Sports Physiol. Perform. 11 , 587–593. 10.1123/ijspp.2015-0331 [ PubMed ] [ CrossRef ] [ Google Scholar ]
- American College Health Association and American College Health Association-National College Health Assessment II (2019). Reference Group Executive Summary Spring 2019 . Silver Spring, MD: American College Health Association. [ Google Scholar ]
- Andersson H., Raastad T., Nilsson J., Paulsen G., Garthe I., Kadi F. (2008). Neuromuscular fatigue and recovery in Elite female soccer: effects of active recovery . Med. Sci. Sports Exerc. 40 , 372–380. 10.1249/mss.0b013e31815b8497 [ PubMed ] [ CrossRef ] [ Google Scholar ]
- Aquilina D. (2013). A study of the relationship between elite athletes' educational development and sporting performance . Int. J. Hist. Sport 30 , 374–392. 10.1080/09523367.2013.765723 [ CrossRef ] [ Google Scholar ]
- Aughey R. J. (2011). Applications of GPS technologies to field sports . Int. J. Sports Physiol. Perform. 6 , 295–310. 10.1123/ijspp.6.3.295 [ PubMed ] [ CrossRef ] [ Google Scholar ]
- Aughey R. J., Elias G. P., Esmaeili A., Lazarus B., Stewart A. M. (2016). Does the recent internal load and strain on players affect match outcome in elite Australian football? J. Sci. Med. Sport. 19 , 182–186. 10.1016/j.jsams.2015.02.005 [ PubMed ] [ CrossRef ] [ Google Scholar ]
- Ayotte D., Corcoran M. P. (2018). Individualized hydration plans improve performance outcomes for collegiate athletes engaging in in-season training . J. Int. Soc. Sports Nutr. 15 :27. 10.1186/s12970-018-0230-2 [ PMC free article ] [ PubMed ] [ CrossRef ] [ Google Scholar ]
- Borresen J., Ian Lambert M. (2009). The quantification of training load, the training response and the effect on performance . Sports Med. 39 , 779–795. 10.2165/11317780-000000000-00000 [ PubMed ] [ CrossRef ] [ Google Scholar ]
- Bosquet L., Merkari S., Arvisais D., Aubert A. E. (2008). Is heart rate a convenient tool to monitor over-reaching? a systematic review of the literature. Br. J. Sports Med. 42 , 709–714. 10.1136/bjsm.2007.042200 [ PubMed ] [ CrossRef ] [ Google Scholar ]
- Brewer B. W. (2012). Psychology of sport injury rehabilitation, in Handbook of Sport Psychology , eds G. Tenenbaum, and R. C. Eklund (Hoboken, NJ: John Wiley & Sons, Inc; ), 404–424. 10.1002/9781118270011.ch18 [ CrossRef ] [ Google Scholar ]
- Buchheit M. (2014). Monitoring training status with HR measures: do all roads lead to Rome? Front. Physiol. 5 :73. 10.3389/fphys.2014.00073 [ PMC free article ] [ PubMed ] [ CrossRef ] [ Google Scholar ]
- Clubb J., McGuigan M. (2018). Developing cost-effective, evidence-based load monitoring systems in strength and conditioning practice . Strength Cond. J. 40 , 75–81. 10.1519/SSC.0000000000000396 [ CrossRef ] [ Google Scholar ]
- Cosh S., Tully P. J. (2014). All I have to do is pass: a discursive analysis of student athletes' talk about prioritising sport to the detriment of education to overcome stressors encountered in combining elite sport and tertiary education . Psychol. Sport Exerc. 15 , 180–189. 10.1016/j.psychsport.2013.10.015 [ CrossRef ] [ Google Scholar ]
- Cosh S., Tully P. J. (2015). Stressors, coping, and support mechanisms for student athletes combining elite sport and tertiary education: implications for practice . Sport Psychol. 29 , 120–133. 10.1123/tsp.2014-0102 [ CrossRef ] [ Google Scholar ]
- Coyne J., Haff G., Coutts A., Newton R., Nimphius S. (2018). The current state of subjective training load monitoring—a practical perspective and call to action . Sports Med. 4 :58. 10.1186/s40798-018-0172-x [ PMC free article ] [ PubMed ] [ CrossRef ] [ Google Scholar ]
- Crossman J. (1997). Psychological rehabilitation from sports injuries . Sports Med. 23 , 333–339. 10.2165/00007256-199723050-00005 [ PubMed ] [ CrossRef ] [ Google Scholar ]
- Daanen H. A. M., Lamberts R. P., Kallen V. L., Jin A., van Meeteren N. L. U. (2012). A systematic review on heart-rate recovery to monitor changes in training status in athletes . Int. J. Sports Physiol. Perform. 7 , 251–260. 10.1123/ijspp.7.3.251 [ PubMed ] [ CrossRef ] [ Google Scholar ]
- Daly J. M., Brewer B. W., van Raalte J. L., Petitpas A. J., Sklar J. H. (1995). Cognitive appraisal, emotional adjustment, and adherence to rehabilitation following knee surgery . J. Sport Rehabil. 4 , 23–30. 10.1123/jsr.4.1.23 [ CrossRef ] [ Google Scholar ]
- Davis P., Halvarsson A., Lundström W., Lundqvist C. (2019). Alpine ski coaches' and athletes' perceptions of factors influencing adaptation to stress in the classroom and on the slopes . Front. Psychol. 10 :1641. 10.3389/fpsyg.2019.01641 [ PMC free article ] [ PubMed ] [ CrossRef ] [ Google Scholar ]
- de Brandt K., Wylleman P., Torregrossa M., Schipper-van Veldhoven N., Minelli D., Defruyt S., et al. (2018). Exploring the factor structure of the dual career competency questionnaire for Athletes in European pupil- and student-athletes . J. Sport. Exercise. Psy. 1–18. 10.1080/1612197X.2018.1511619 [ CrossRef ] [ Google Scholar ]
- Drew M. K., Finch C. F. (2016). The relationship between training load and injury, illness and soreness: a systematic and literature review . Sports Med. 46 , 861–883. 10.1007/s40279-015-0459-8 [ PubMed ] [ CrossRef ] [ Google Scholar ]
- DTHOMAS (2013). NCAA GOALS Study. NCAA.Org - The Official Site of the NCAA . Available online at: http://www.ncaa.org/about/resources/research/ncaa-goals-study (accessed October 5, 2019).
- Egan K. P. (2019). Supporting mental health and well-being among student-athletes . Clin. Sports Med. 38 , 537–544. 10.1016/j.csm.2019.05.003 [ PubMed ] [ CrossRef ] [ Google Scholar ]
- Etzel E. F. ed. (2009). Counseling and Psychological Services for College Student-Athletes . Morgantown, WV: Fitness Information Technology. [ Google Scholar ]
- Fleisig G. S., Andrews J. R. (2012). Prevention of elbow injuries in youth baseball pitchers . Sports Health 4 , 419–424. 10.1177/1941738112454828 [ PMC free article ] [ PubMed ] [ CrossRef ] [ Google Scholar ]
- Fogaca J. L. (2019). Combining mental health and performance interventions: coping and social support for student-athletes . J. Appl. Sport Psychol. 1–16. 10.1080/10413200.2019.1648326 [ CrossRef ] [ Google Scholar ]
- Foster C. (1998). Monitoring training in athletes with reference to overtraining syndrome . Med. Sci. Sports Exerc. 30 , 1164–1168. 10.1097/00005768-199807000-00023 [ PubMed ] [ CrossRef ] [ Google Scholar ]
- Fry A. C., Kraemer W. J. (1997). Resistance exercise overtraining and overreaching: neuroendocrine responses . Sports Med. 23 , 106–129. 10.2165/00007256-199723020-00004 [ PubMed ] [ CrossRef ] [ Google Scholar ]
- Gabbett T. J., Nassis G. P., Oetter E., Pretorius J., Johnston N., Medina D., et al.. (2017). The athlete monitoring cycle: a practical guide to interpreting and applying training monitoring data . Br. J. Sports Med. 51 , 1451–1452. 10.1136/bjsports-2016-097298 [ PubMed ] [ CrossRef ] [ Google Scholar ]
- Gathercole R. J., Sporer B. C., Stellingwerff T., Sleivert G. G. (2015). Comparison of the capacity of different jump and sprint field tests to detect neuromuscular fatigue . J. Strength Cond. Res. 29 , 2522–2531. 10.1519/JSC.0000000000000912 [ PubMed ] [ CrossRef ] [ Google Scholar ]
- Gearity B. T., Murray M. A. (2011). Athletes' experiences of the psychological effects of poor coaching . Psychol. Sport Exerc. 12 , 213–221. 10.1016/j.psychsport.2010.11.004 [ CrossRef ] [ Google Scholar ]
- Gould D., Udry E. (1994). Psychological skills for enhancing performance: arousal regulation strategies . Med. Sci. Sports Exerc. 26 , 478–485. [ PubMed ] [ Google Scholar ]
- Haff G., Triplett N. T., National Strength Conditioning Association (U.S.) eds. (2016). Essentials of Strength Training and Conditioning . 4th Edn Champaign, IL: Human Kinetics. [ Google Scholar ]
- Halson S. L. (2014). Monitoring training load to understand fatigue in athletes . Sports Med. 44 , 139–147. 10.1007/s40279-014-0253-z [ PMC free article ] [ PubMed ] [ CrossRef ] [ Google Scholar ]
- Hamlin M. J., Wilkes D., Elliot C. A., Lizamore C. A., Kathiravel Y. (2019). Monitoring training loads and perceived stress in young elite university athletes . Front. Physiol. 10 :34. 10.3389/fphys.2019.00034 [ PMC free article ] [ PubMed ] [ CrossRef ] [ Google Scholar ]
- Hopkins W. G. (1991). Quantification of training in competitive sports: methods and applications . Sports Med. 12 , 161–183. 10.2165/00007256-199112030-00003 [ PubMed ] [ CrossRef ] [ Google Scholar ]
- Huml M. R., Hambrick M. E., Hums M. A. (2016). Coaches' perceptions of the reduction of athletic commitment for division II student-athletes: development and validation of a measure of athletic/academic balance . J. Intercoll. Sport 9 , 303–325. 10.1123/jis.2015-0055 [ CrossRef ] [ Google Scholar ]
- Humphrey J. H., Yow D. A., Bowden W. W. (2000). Stress in College Athletics: Causes, Consequences, Coping . New York, NY: Haworth Half-Court Press. [ Google Scholar ]
- Hyatt H. W., Kavazis A. N. (2019). Body composition and perceived stress through a calendar year in NCAA I female volleyball players . Int. J. Exerc. Sci. 12 , 433–443. [ PMC free article ] [ PubMed ] [ Google Scholar ]
- Impellizzeri F. M., Rampinini E., Coutts A. J., Sassi A., Marcora S. M. (2004). Use of RPE-based training load in Soccer . Med. Sci. Sports Exerc. 36 , 1042–1047. 10.1249/01.MSS.0000128199.23901.2F [ PubMed ] [ CrossRef ] [ Google Scholar ]
- Ivarsson A., Johnson U., Andersen M. B., Tranaeus U., Stenling A., Lindwall M. (2017). Psychosocial factors and sport injuries: meta-analyses for prediction and prevention . Sports Med. 47 , 353–365. 10.1007/s40279-016-0578-x [ PubMed ] [ CrossRef ] [ Google Scholar ]
- Jaspers A., Brink M. S., Probst S. G. M., Frencken W. G. P., Helsen W. F. (2017). Relationships between training load indicators and training outcomes in professional Soccer . Sports Med. 47 , 533–544. 10.1007/s40279-016-0591-0 [ PubMed ] [ CrossRef ] [ Google Scholar ]
- Jobson S. A., Passfield L., Atkinson G., Barton G., Scarf P. (2009). The analysis and utilization of cycling training data . Sports Med. 39 , 833–844. 10.2165/11317840-000000000-00000 [ PubMed ] [ CrossRef ] [ Google Scholar ]
- Kaier E., Cromer L. D., Johnson M. D., Strunk K., Davis J. L. (2015). Perceptions of mental illness stigma: comparisons of athletes to nonathlete peers . J. Coll. Stud. Dev. 56 , 735–739. 10.1353/csd.2015.0079 [ CrossRef ] [ Google Scholar ]
- Kallus W., Kellmann M. (2016). The Recovery-Stress . Questionnaires: User Manual . Frankfurt: Pearson Assessment & Information GmbH. [ Google Scholar ]
- Kenttä G., Hassmén P. (1998). Overtraining and recovery: a conceptual model . Sports Med. 26 , 1–16. 10.2165/00007256-199826010-00001 [ PubMed ] [ CrossRef ] [ Google Scholar ]
- Kraemer W. J., Fleck S. J. (2018). Optimizing Strength Training: Designing Nonlinear Periodization Workouts . Champaign: Human Kinetics; Available online at: https://public.ebookcentral.proquest.com/choice/publicfullrecord.aspx?p=5730619 (accessed January 30, 2020). [ Google Scholar ]
- Lamberts R. P., Swart J., Capostagno B., Noakes T. D., Lambert M. I. (2009). Heart rate recovery as a guide to monitor fatigue and predict changes in performance parameters: heart rate recovery to monitor of performance . Scand. J. Med. Sci. Sports 20 , 449–457. 10.1111/j.1600-0838.2009.00977.x [ PubMed ] [ CrossRef ] [ Google Scholar ]
- Lavallée L., Flint F. (1996). The relationship of stress, competitive anxiety, mood state, and social support to athletic injury . J. Athl. Train. 31 , 296–299. [ PMC free article ] [ PubMed ] [ Google Scholar ]
- Li C., Ivarsson A., Lam L. T., Sun J. (2019). Basic psychological needs satisfaction and frustration, stress, and sports injury among university athletes: a four-wave prospective survey . Front. Psychol. 10 :665. 10.3389/fpsyg.2019.00665 [ PMC free article ] [ PubMed ] [ CrossRef ] [ Google Scholar ]
- Li H., Moreland J. J., Peek-Asa C., Yang J. (2017). Preseason anxiety and depressive symptoms and prospective injury risk in collegiate athletes . Am. J. Sports Med. 45 , 2148–2155. 10.1177/0363546517702847 [ PubMed ] [ CrossRef ] [ Google Scholar ]
- López de Subijana C., Barriopedro M., Conde E. (2015). Supporting dual career in Spain: Elite athletes' barriers to study . Psychol. Sport Exerc. 21 , 57–64. 10.1016/j.psychsport.2015.04.012 [ CrossRef ] [ Google Scholar ]
- Lukaski H. C. (2004). Vitamin and mineral status: effects on physical performance . Nutrition 20 , 632–644. 10.1016/j.nut.2004.04.001 [ PubMed ] [ CrossRef ] [ Google Scholar ]
- Magalhães J., Rebelo A., Oliveira E., Silva J. R., Marques F., Ascensão A. (2010). Impact of loughborough intermittent shuttle test versus soccer match on physiological, biochemical and neuromuscular parameters . Eur. J. Appl. Physiol. 108 , 39–48. 10.1007/s00421-009-1161-z [ PubMed ] [ CrossRef ] [ Google Scholar ]
- Maloney M. T., McCormick R. E. (1993). An examination of the role that intercollegiate athletic participation plays in academic achievement: athletes' feats in the classroom . J. Hum. Resour. 28 :555 10.2307/146160 [ CrossRef ] [ Google Scholar ]
- Mann J. B., Bryant K. R., Johnstone B., Ivey P. A., Sayers S. P. (2016). Effect of physical and academic stress on illness and injury in division 1 college football players . J. Strength Cond. Res. 30 , 20–25. 10.1519/JSC.0000000000001055 [ PubMed ] [ CrossRef ] [ Google Scholar ]
- Mann J. B., Ivey P. A., Sayers S. P. (2015). Velocity-based training in football . Strength Cond. J. 37 , 52–57. 10.1519/SSC.0000000000000177 [ CrossRef ] [ Google Scholar ]
- Marino F. E. (ed.). (2011). Regulation of Fatigue in Exercise . Hauppauge, NY: Nova Science Publishers. [ Google Scholar ]
- Martin D. T., Andersen M. B. (2000). Heart rate-perceived exertion relationship during training and taper . J. Sports Med. Phys. Fitness 40 , 201–208. [ PubMed ] [ Google Scholar ]
- Massey C. D., Maneval M. W., Phillips J., Vincent J., White G., Zoeller B. (2002). An analysis of teaching and coaching behaviors of elite strength and conditioning coaches . J. Strength Cond. Res. 16 , 456–460. 10.1519/00124278-200208000-00019 [ PubMed ] [ CrossRef ] [ Google Scholar ]
- Massey C. D., Schwind J. J., Andrews D. C., Maneval M. W. (2009). An analysis of the job of strength and conditioning coach for football at the division II Level . J. Strength Cond. Res. 23 , 2493–2499. 10.1519/JSC.0b013e3181bbe9b6 [ PubMed ] [ CrossRef ] [ Google Scholar ]
- Massey C. D., Vincent J., Maneval M. (2004). Job analysis of college division I-A football strength and conditioning coaches . J. Strength Cond. Res. 18 , 19–25. 10.1519/1533-4287(2004)018<0019:jaocdi>2.0.co;2 [ PubMed ] [ CrossRef ] [ Google Scholar ]
- Mclean S. G., Samorezov J. E. (2009). Fatigue-induced ACL injury risk stems from a degradation in central control . Med. Sci. Sport Exerc. 41 , 1662–1673. 10.1249/MSS.0b013e31819ca07b [ PubMed ] [ CrossRef ] [ Google Scholar ]
- Miller P. S., Kerr G. (2002). The athletic, academic and social experiences of intercollegiate student athletes . J. Sport Behav. 25 , 346–367. [ Google Scholar ]
- Moreland J. J., Coxe K. A., Yang J. (2018). Collegiate athletes' mental health services utilization: A systematic review of conceptualizations, operationalizations, facilitators, and barriers . J. Sport Health Sci. 7 , 58–69. 10.1016/j.jshs.2017.04.009 [ PMC free article ] [ PubMed ] [ CrossRef ] [ Google Scholar ]
- Morgan W. P., Brown D. R., Raglin J. S., O'Connor P. J., Ellickson K. A. (1987). Psychological monitoring of overtraining and staleness . Br. J. Sports Med. 21 , 107–114. 10.1136/bjsm.21.3.107 [ PMC free article ] [ PubMed ] [ CrossRef ] [ Google Scholar ]
- Morton R. H., Fitz-Clarke J. R., Banister E. W. (1990). Modeling human performance in running . J. Appl. Physiol. 69 , 1171–1177. 10.1152/jappl.1990.69.3.1171 [ PubMed ] [ CrossRef ] [ Google Scholar ]
- Nässi A., Ferrauti A., Meyer T., Pfeiffer M., Kellmann M. (2017). Psychological tools used for monitoring training responses of athletes . Perform. Enhanc. Health 5 , 125–133. 10.1016/j.peh.2017.05.001 [ CrossRef ] [ Google Scholar ]
- NCAA (2014). Mind Body and Sport: Understanding and Supporting Student-Athlete Mental Wellness. Independent Publisher . Available online at: https://books.google.com/books?id=JA-5rQEACAAJ (accessed October 01, 2019).
- Pareja-Blanco F., Rodríguez-Rosell D., Sánchez-Medina L., Gorostiaga E., González-Badillo J. (2014). Effect of movement velocity during resistance training on neuromuscular performance . Int. J. Sports Med. 35 , 916–924. 10.1055/s-0033-1363985 [ PubMed ] [ CrossRef ] [ Google Scholar ]
- Pareja-Blanco F., Rodríguez-Rosell D., Sánchez-Medina L., Sanchis-Moysi J., Dorado C., Mora-Custodio R., et al.. (2017). Effects of velocity loss during resistance training on athletic performance, strength gains and muscle adaptations . Scand. J. Med. Sci. Sports 27 , 724–735. 10.1111/sms.12678 [ PubMed ] [ CrossRef ] [ Google Scholar ]
- Paule A. L., Gilson T. A. (2010). Current collegiate experiences of big-time, non-revenue, NCAA athletes . J. Intercoll. Sport 3 , 333–347. 10.1123/jis.3.2.333 [ CrossRef ] [ Google Scholar ]
- Petrie T. A., Deiters J., Harmison R. J. (2014). Mental toughness, social support, and athletic identity: Moderators of the life stress–injury relationship in collegiate football players . Sport Exerc. Perform. Psychol. 3 , 13–27. 10.1037/a0032698 [ CrossRef ] [ Google Scholar ]
- Plews D. J., Laursen P. B., Stanley J., Kilding A. E., Buchheit M. (2013). Training adaptation and heart rate variability in elite endurance athletes: opening the door to effective monitoring . Sports Med. 43 , 773–781. 10.1007/s40279-013-0071-8 [ PubMed ] [ CrossRef ] [ Google Scholar ]
- Podlog L., Eklund R. C. (2007). Professional coaches' perspectives on the return to sport following serious injury . J. Appl. Sport Psychol. 19 , 207–225. 10.1080/10413200701188951 [ CrossRef ] [ Google Scholar ]
- Pyne D. B., Martin D. T. (2011). Fatigue – insights from individual and team sports. in Regulation of Fatigue in Exercise , ed F. E. Marino, (New York, NY: Nova Publishers; ), 177–186. [ Google Scholar ]
- Radcliffe J. N., Comfort P., Fawcett T. (2015). Psychological strategies included by strength and conditioning coaches in applied strength and conditioning: J. Strength . Cond. Res. 29 , 2641–2654. 10.1519/JSC.0000000000000919 [ PubMed ] [ CrossRef ] [ Google Scholar ]
- Reardon C. L., Hainline B., Aron C. M., Baron D., Baum A. L., Bindra A., et al.. (2019). Mental health in elite athletes: international olympic committee consensus statement 2019 . Br. J. Sports Med. 53 , 667–699. 10.1136/bjsports-2019-100715 [ PubMed ] [ CrossRef ] [ Google Scholar ]
- Robson-Ansley P. J., Gleeson M., Ansley L. (2009). Fatigue management in the preparation of Olympic athletes . J. Sports Sci. 27 , 1409–1420. 10.1080/02640410802702186 [ PubMed ] [ CrossRef ] [ Google Scholar ]
- Rushall B. S. (1990). A tool for measuring stress tolerance in elite athletes . J. Appl. Sport Psychol. 2 , 51–66. 10.1080/10413209008406420 [ CrossRef ] [ Google Scholar ]
- Saw A. E., Main L. C., Gastin P. B. (2016). Monitoring the athlete training response: subjective self-reported measures trump commonly used objective measures: a systematic review . Br. J. Sports Med. 50 :281. 10.1136/bjsports-2015-094758 [ PMC free article ] [ PubMed ] [ CrossRef ] [ Google Scholar ]
- Scott B. M., Paskus T. S., Miranda M., Petr T. A., McArdle J. J. (2008). In-season vs. out-of-season academic performance of college student-athletes . J. Intercoll. Sport 1 , 202–226. 10.1123/jis.1.2.202 [ CrossRef ] [ Google Scholar ]
- Selye H. (ed.). (1976). The Stress of Life . New York, NY : McGraw-Hill. [ Google Scholar ]
- Shanley E., Michener L., Ellenbecker T., Rauh M. (2012). Shoulder range of motion, pitch count, and injuries among interscholastic female softball pitchers: a descriptive study . Int. J. Sports Phys. Ther. 7 , 548–557. [ PMC free article ] [ PubMed ] [ Google Scholar ]
- Soligard T., Schwellnus M., Alonso J.-M., Bahr R., Clarsen B., Dijkstra H. P., et al.. (2016). How much is too much? (Part 1) International Olympic Committee consensus statement on load in sport and risk of injury . Br. J. Sports Med. 50 , 1030–1041. 10.1136/bjsports-2016-096581 [ PubMed ] [ CrossRef ] [ Google Scholar ]
- Starling L. T., Nellemann S., Parkes A., Lambert M. I. (2019). The Fatigue and Fitness Test for Teams (FFITT): a practical option for monitoring athletes in a team as individuals . Eur. J. Sport Sci . 20 , 1–9. 10.1080/17461391.2019.1612951 [ PubMed ] [ CrossRef ] [ Google Scholar ]
- Statler T., DuBois A. (2016). Psychology of athletic preparation and performance, in Essentials of Strength Training and Conditioning , eds. G. Haff, and N. T. Triplett (Champaign, IL: Human Kinetics; ), 155–172. [ Google Scholar ]
- Sweet T. W., Foster C., McGuigan M. R., Brice G. (2004). Quantitation of resistance training using the session rating of perceived exertion method . J. Strength Cond. Res. 18 :796. 10.1519/14153.1 [ PubMed ] [ CrossRef ] [ Google Scholar ]
- Szivak T. K., Kraemer W. J. (2015). Physiological readiness and resilience: pillars of military preparedness . J. Strength Cond. Res. 29 , S34–S39. 10.1519/JSC.0000000000001073 [ PubMed ] [ CrossRef ] [ Google Scholar ]
- Taylor K.-L., Chapman D., Cronin J., Newton M., Gill N. (2012). Fatigue monitoring in high performance sport: a survey of current trends . J. Aust. Strength Cond. 20 , 12–23. [ Google Scholar ]
- Thornton H. R., Delaney J. A., Duthie G. M., Dascombe B. J. (2019). Developing athlete monitoring systems in team sports: data analysis and visualization . Int. J. Sports Physiol. Perform. 14 , 698–705. 10.1123/ijspp.2018-0169 [ PubMed ] [ CrossRef ] [ Google Scholar ]
- Thorpe R. T., Atkinson G., Drust B., Gregson W. (2017). Monitoring fatigue status in elite team-sport athletes: implications for practice . Int. J. Sports Physiol. Perfrom. 12 , S2-27-S2–34. 10.1123/ijspp.2016-0434 [ PubMed ] [ CrossRef ] [ Google Scholar ]
- Torres-Ronda L., Schelling X. (2017). Critical process for the implementation of technology in sport organizations . Strength Cond. J. 39 , 54–59. 10.1519/SSC.0000000000000339 [ CrossRef ] [ Google Scholar ]
- Twist C., Highton J. (2013). Monitoring fatigue and recovery in rugby league players . Int. J. Sports Physiol. Perform. 8 , 467–474. 10.1123/ijspp.8.5.467 [ PubMed ] [ CrossRef ] [ Google Scholar ]
- Wallace L. K., Slattery K. M., Coutts A. J. (2009). The ecological validity and application of the session-rpe method for quantifying training loads in swimming . J. Strength Cond. Res. 23 , 33–38. 10.1519/JSC.0b013e3181874512 [ PubMed ] [ CrossRef ] [ Google Scholar ]
- Williams J. M., Andersen M. B. (1998). Psychosocial antecedents of sport injury: review and critique of the stress and injury model . J. Appl. Sport Psychol. 10 , 5–25. 10.1080/10413209808406375 [ CrossRef ] [ Google Scholar ]
- Yang J., Peek-Asa C., Corlette J. D., Cheng G., Foster D. T., Albright J. (2007). Prevalence of and risk factors associated with symptoms of depression in competitive collegiate student athletes . Clin. J. Sport Med. 17 , 481–487. 10.1097/JSM.0b013e31815aed6b [ PubMed ] [ CrossRef ] [ Google Scholar ]
Collegiate athletes' mental health services utilization: A systematic review of conceptualizations, operationalizations, facilitators, and barriers
Affiliations.
- 1 The Research Institute at Nationwide Children's Hospital, Columbus, OH 43205, USA.
- 2 Ohio Department of Mental Health & Addiction Services Office of Quality, Planning & Research, Columbus, OH 43215, USA.
- 3 Department of Pediatrics, College of Medicine, The Ohio State University, Columbus, OH 43210, USA.
- PMID: 30356496
- PMCID: PMC6180550
- DOI: 10.1016/j.jshs.2017.04.009
Background: While mental health among collegiate athletes is receiving increased attention, research on factors surrounding collegiate athletes' decision to seek mental health services is limited. The goal of the present review was to analyze and synthesize the current literature concerning collegiate athletes' utilization of mental health services, including the facilitators of and barriers to use of these services.
Methods: The analysis was guided and organized using a socio-ecological framework, which considered the unique context in which collegiate athletes study and perform. A total of 21 articles, published between 2005 and 2016, which concern U.S. collegiate athletes' mental health services utilization (MHSU) were selected and included for the final analysis. Conceptualizations and operationalizations of MHSU were compared and contrasted. Facilitators of and barriers to athletes MHSU were examined and summarized while appropriately considering the proximity of each factor (facilitator or barrier) to the athletes.
Results: Results showed variations in conceptualizations and operationalizations of MHSU in the articles analyzed, which made interpretation and cross comparison difficult. Collegiate athletes are willing to utilize mental health services, but gender, perceived stigma, peer norms-for athletes and coaches-plus service availability impact their MHSU.
Conclusion: Key stakeholders, administrators, and public health officials should partner to eliminate MHSU barriers, support facilitators, and generally empower collegiate athletes to actively manage their mental health.
Keywords: College athlete; Mental health; Mental health services; NCAA; Psychology; Sport psychology; Systematic review.
Publication types
The NCAA is committed to making policy decisions based on quality research data. The NCAA research staff conducts national research for its members on a wide variety of topics including academic performance, student-athlete well-being, finances of intercollegiate athletics programs, gender-equity and diversity issues and many others. Meet the Research Staff
Undergraduate Experiences and Post-College Outcomes of NCAA Student-Athletes
Gallup interviewed nearly 5,000 former NCAA student-athletes about their college experiences and current well-being.
Graduation Rates
The NCAA has devoted attention to researching student-athlete graduation rates for more than two decades.
Research Resources
- NCAA Graduate Student Research Grant
- NCAA Innovations in Research and Practice Grant
- Data Sharing: Download Data Files for Sharing
- Interactive Databases
- Register for the NCAA Alumni Research Panel
- Journal Articles and Media
- NCAA Research Committee
Probability of Going Pro
Of the nearly 8 million students participating in high school athletics in the United States only a fraction will become a professional athlete.
NCAA Demographics Database
Search for specific race/ethnicity data regarding NCAA student-athletes, coaches and administrators by gender, division and sport.
NCAA GOALS Study
What did 21,000 NCAA student-athletes have to say about their college experiences?
Have questions for the NCAA research or library staff?
- Email us at [email protected]
- Send historical sport questions to [email protected]
Financial Research
- Finances of Intercollegiate Athletics
Academics Research
- Graduation Rates
- Division I Academic Progress Rate (APR)
- Division I and II Diploma Dashboards
- Research on Student-Athlete Transfers
Well-Being Research
- NCAA Student-Athlete Health and Wellness Study
- NCAA Student-Athlete Well-Being Study
- NCAA Coach Well-Being Study
- NCAA Student-Athlete Activism and Racial Justice Engagement Study
- NCAA National Study on Collegiate Wagering
- NCAA Social Environments Study
- NCAA Student-Athlete Substance Use Study
- NCAA GOALS Study
- Gallup Study: Undergraduate Experiences and Post-College Outcomes of NCAA Student-Athletes
Participation, Demographics and Institutional Personnel Research
- Institutional Characteristics of NCAA Member Schools
- International Student-Athlete Participation
- Diversity Research
- Sports Sponsorship and Participation Research
- Probability of Competing Beyond High School
- NCAA Faculty Athletics Representatives Survey
- NCAA Historical Membership Dashboard
Divisional Research
- Division I Research
- Division II Research
- Division III Research

Thanks for visiting !
The use of software that blocks ads hinders our ability to serve you the content you came here to enjoy.
We ask that you consider turning off your ad blocker so we can deliver you the best experience possible while you are here.
Thank you for your support!
- Follow us on Facebook
- Follow us on Twitter
- Criminal Justice
- Environment
- Politics & Government
- Race & Gender
Expert Commentary
Performance-enhancing drugs in athletics: Research roundup
2015 roundup of research on the use of performance-enhancing drugs in athletics and academics as well as their potential health effects.
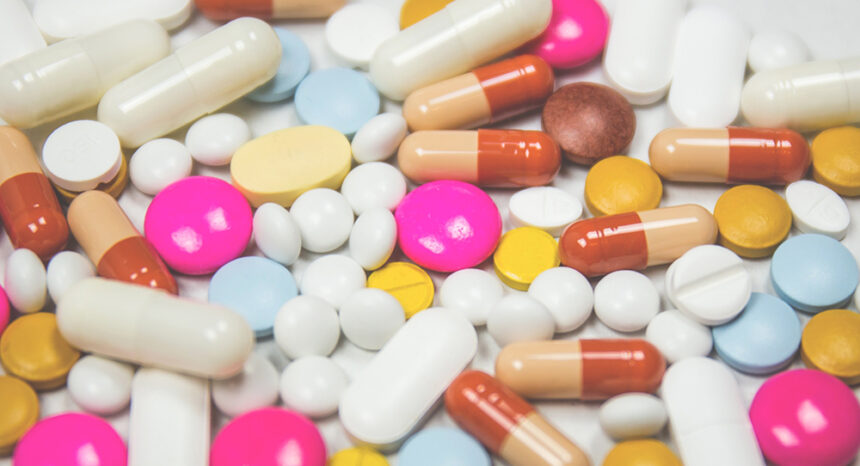
Republish this article

This work is licensed under a Creative Commons Attribution-NoDerivatives 4.0 International License .
by Leighton Walter Kille, The Journalist's Resource June 9, 2015
This <a target="_blank" href="https://journalistsresource.org/health/athletic-academic-performance-enhancing-drugs-research-roundup/">article</a> first appeared on <a target="_blank" href="https://journalistsresource.org">The Journalist's Resource</a> and is republished here under a Creative Commons license.<img src="https://journalistsresource.org/wp-content/uploads/2020/11/cropped-jr-favicon-150x150.png" style="width:1em;height:1em;margin-left:10px;">
Performance-enhancing drugs have a long history in sports, of course, but pharmacological research has led to a surge in the number of substances available, each with its own potential for misuse.
Given the potential financial rewards of athletic success, it’s no surprise that we’ve been witness to a seemingly endless procession of allegations and scandals. Sluggers Barry Bonds (steroids) and Alex Rodriguez (human growth hormone); cyclists Lance Armstrong (EPO), Floyd Landis (testosterone) and Alberto Contador (clenbuterol); runners Tyson Gay (steroids) and Justin Gatlin (testosterone); and golfer Vijay Singh (IGF-1) are only some of the more prominent professionals implicated in such behavior. The complicity of medical professionals and shadowy labs is often involved, and a 2015 report from the International Cycling Union (UCI) found the sport’s own governing body bore significant responsibility.
Not surprisingly, hard numbers on rates of usage are difficult to come by, but anecdotal evidence isn’t lacking and anonymous surveys have provided some insight. Questionable use of medications and supplements have also been reported in the U.S. armed forces , fire and police departments , amateur athletics , and even high schools .
Below is a selection of studies on a range of issues related to performance-enhancing drugs. It has sections on their potential economic impacts, prevalence , health effects and athletes’ attitudes . For additional studies on these topics, you can search PubMed , which is the federal clearinghouse for all medical research. At bottom, we have also included some studies relating to cognitive-enhancing drugs and the related academic dimensions of this issue.
—————————-
“The Economics of Corruption in Sports: The Special Case of Doping” Dimant, Eugen; Deutscher, Christian. Edmond J. Safra Working Papers, No. 55, January 2015.
Abstract: “Corruption in general and doping in particular are ubiquitous in both amateur and professional sports and have taken the character of a systemic threat. In creating unfair advantages, doping distorts the level playing field in sporting competition. With higher stakes involved, such distortions create negative externalities not only on the individual level (lasting health damages, for example) but also frictions on the aggregate level (such as loss of media interest) and erode the principle of sports. In this paper, we provide a comprehensive literature overview of the individual’s incentive to dope, the concomitant detrimental effects and respective countermeasures. In explaining the athlete’s motivation to use performance enhancing drugs, we enrich the discussion by adapting insights from behavioral economics. These insights help to understand such an athlete’s decision beyond a clear-cut rationale but rather as a product of the interaction with the underlying environment. We stress that in order to ensure clean sports and fair competition, more sophisticated measurement methods have to be formulated, and the respective data made publicly available in order to facilitate more extensive studies in the future. So far, the lack of data is alarming, especially in the area of elite sports where the stakes are high and doping has a substantial influence.”
“The Frequency of Doping in Elite Sport: Results of a Replication Study” Pitsch, Werner; Emrich, Eike. International Review for the Sociology of Sport , October 2012, Vol. 47, No. 5, 559-580. doi: 10.1177/1012690211413969.
Abstract: “The difficulty of measuring the prevalence of doping in elite sport is a recurring topic in the scientific literature on doping. The Randomized Response Technique is a method for asking such embarrassing or even threatening questions while allowing the respondents to answer honestly. It was used to measure the prevalence of doping among German squad athletes by Pitsch et al. (2005, 2007). In a replication study with better sampling control, it was possible to replicate the general trend of the data from the 2005 study…. The paper-based survey resulted in a rate of 10.2% ‘honest dopers,’ irrespective of the disciplines, obtained with the question: ‘Have you ever knowingly used illicit drugs or methods in order to enhance your performance?’ By adding the rate of cheaters (24.7%), whose behaviour the researchers know nothing about, one can calculate the interval (10.2%, 34.9%), which should include the true rate of dopers throughout their career among German elite athletes. In contrast, this means that the larger proportion of athletes, namely, 65.2%, represents ‘honest non-dopers.’ In the 2008 season, this figure was 65%.”
“Growth Hormone Doping in Sports: A Critical Review of Use and Detection Strategies” Baumann, Gerhard P. Endocrine Reviews , April 2012, Vol. 33, No. 2 155-186. doi: 10.1210/er.2011-1035.
Abstract: “[Growth hormone] is believed to be widely employed in sports as a performance-enhancing substance. Its use in athletic competition is banned by the World Anti-Doping Agency, and athletes are required to submit to testing for GH exposure…. The scientific evidence for the [performance-enhancing characteristics] of GH is weak, a fact that is not widely appreciated in athletic circles or by the general public. Also insufficiently appreciated is the risk of serious health consequences associated with high-dose, prolonged GH use. This review discusses the GH biology relevant to GH doping; the virtues and limitations of detection tests in blood, urine, and saliva; secretagogue efficacy; IGF-I doping; and information about the effectiveness of GH as a performance-enhancing agent.”
“Supplements in Top-Level Track and Field Athletes” Tscholl, Philippe; Alonso, Juan M.; Dollé, Gabriel; Junge, Astrid; Dvorak, Jiri. American Journal of Sports Medicine , January 2010, Vol. 38, No. 1, 133-140. doi: 10.1177/0363546509344071.
Abstract: “Analysis of 3,887 doping control forms undertaken during 12 International Association of Athletics Federations World Championships and one out-of-competitions season in track and field. Results: There were 6,523 nutritional supplements (1.7 per athlete) and 3,237 medications (0.8 per athlete) reported. Nonsteroidal anti-inflammatory drugs (NSAIDs; 0.27 per athlete, n = 884), respiratory drugs (0.21 per athlete, n = 682), and alternative analgesics (0.13, n = 423) were used most frequently. Medication use increased with age (0.33 to 0.87 per athlete) and decreased with increasing duration of the event (from sprints to endurance events; 1.0 to 0.63 per athlete). African and Asian track and field athletes reported using significantly fewer supplements (0.85 vs. 1.93 per athlete) and medications (0.41 vs. 0.96 per athlete) than athletes from other continents. The final ranking in the championships was unrelated to the quantity of reported medications or supplements taken. Compared with middle-distance and long-distance runners, athletes in power and sprint disciplines reported using more NSAIDs, creatine, and amino acids, and fewer antimicrobial agents. Conclusion: The use of NSAIDs in track and field is less than that reported for team-sport events. However, nutritional supplements are used more than twice as often as they are in soccer and other multisport events; this inadvertently increases the risk of positive results of doping tests.”
“Alcohol, Tobacco, Illicit Drugs and Performance Enhancers: A Comparison of Use by College Student Athletes and Nonathletes” Yusko, David A.; et al. American Journal of Sports Medicine, August 2010. doi: 10.3200/JACH.57.3.281-290.
Abstract: Compares the prevalence and pattern of substance use in undergraduate student athletes and nonathletes from 2005-2006. Data was collected using questionnaires from male (n = 418) and female (n = 475) student athletes and nonathletes from 2005-2006 to assess prevalence, quantity, and frequency of alcohol and drug use, and to determine patterns of student athletes’ alcohol and drug use during their athletic season versus out of season. Male student athletes were found to be at high risk for heavy drinking and performance-enhancing drug use. Considerable in-season versus out-of-season substance use fluctuations were identified in male and female student athletes. Additional, and possibly alternative, factors are involved in a student athlete’s decision-making process regarding drug and alcohol use, which suggests that the development of prevention programs that are specifically designed to meet the unique needs of the college student athlete may be beneficial.”
Health effects
“Performance Enhancing Drug Abuse and Cardiovascular Risk in Athletes” Angell, Peter J.; Chester, Neil; Sculthorpe, Nick; Whyte, Greg; George, Keith; Somauroo, John. British Journal of Sports Medicine , July 2012. doi:10.1136/bjsports-2012-091186.
Abstract: “Despite continuing methodological developments to detect drug use and associated punishments for positive dope tests, there are still many athletes who choose to use performance- and image-enhancing drugs. Of primary concern to this review are the health consequences of drug use by athletes…. We will address current knowledge, controversies and emerging evidence in relation to cardiovascular (CV) health of athletes taking drugs. Further, we delimit our discussion to the CV consequences of anabolic steroids and stimulant (including amphetamines and cocaine) use. These drugs are reported in the majority of adverse findings in athlete drug screenings and thus are more likely to be relevant to the healthcare professionals responsible for the well-being of athletes.”
“Illicit Anabolic-Androgenic Steroid Use” Kanayama, Gen; Hudson, James I.; Pope Jr., Harrison G. Hormones and Behavior , Volume 58, Issue 1, June 2010, Pages 111-121. doi: 10.1016/j.yhbeh.2009.09.006.
Abstract: “The anabolic-androgenic steroids (AAS) are a family of hormones that includes testosterone and its derivatives. These substances have been used by elite athletes since the 1950s, but they did not become widespread drugs of abuse in the general population until the 1980s. Thus, knowledge of the medical and behavioral effects of illicit AAS use is still evolving. Surveys suggest that many millions of boys and men, primarily in Western countries, have abused AAS to enhance athletic performance or personal appearance. AAS use among girls and women is much less common. Taken in supraphysiologic doses, AAS show various long-term adverse medical effects, especially cardiovascular toxicity. Behavioral effects of AAS include hypomanic or manic symptoms, sometimes accompanied by aggression or violence, which usually occur while taking AAS, and depressive symptoms occurring during AAS withdrawal. However, these symptoms are idiosyncratic and afflict only a minority of illicit users; the mechanism of these idiosyncratic responses remains unclear. AAS users may also ingest a range of other illicit drugs, including both “body image” drugs to enhance physical appearance or performance, and classical drugs of abuse. In particular, AAS users appear particularly prone to opioid use. There may well be a biological basis for this association, since both human and animal data suggest that AAS and opioids may share similar brain mechanisms. Finally, AAS may cause a dependence syndrome in a substantial minority of users. AAS dependence may pose a growing public health problem in future years but remains little studied.”
“Adverse Health Effects of Anabolic-Androgenic Steroids” Van Amsterdama, Jan; Opperhuizena, Antoon; Hartgensb, Fred. Regulatory Toxicology and Pharmacology , Volume 57, Issue 1, June 2010, Pages 117-123. doi: 10.1016/j.yrtph.2010.02.001.
Abstract: “Anabolic-androgenic steroids (AAS) are synthetic drugs derived from testosterone. Illegally, these drugs are regularly self-administered by body builders and power lifters to enhance their sportive performance. Adverse side effects of AAS include sexual dysfunction, alterations of the cardiovascular system, psyche and behavior, and liver toxicity. However, severe side effects appear only following prolonged use of AAS at high dose and their occurrence is limited…. The overwhelming stereotype about AAS is that these compounds cause aggressive behavior in males. However, the underlying personality traits of a specific subgroup of the AAS abusers, who show aggression and hostility, may be relevant, as well. Use of AAS in combination with alcohol largely increases the risk of violence and aggression. The dependence liability of AAS is very low, and withdrawal effects are relatively mild. Based on the scores for acute and chronic adverse health effects, the prevalence of use, social harm and criminality, AAS were ranked among 19 illicit drugs as a group of drugs with a relatively low harm.”
“Effects of Growth Hormone Therapy on Exercise Performance in Men” Triay, Jessica M.; Ahmad, Bushra N. Trends in Urology & Men’s Health , July/August 2012, Vol. 3, Issue 4, 23-26. doi: 10.1002/tre.274.
Conclusions: “In the athletic arena, [growth hormone] doping is considered to be widespread and used in combination with other agents, and regimens vary depending on individual preferences and cost implications…. It must be recognised that the effects of GH administration in adults with a normal GH/IGF-1 axis are not comparable to those in GH deficiency and that the complexity of processes influencing GH release and peripheral actions means that overall performance should be considered as opposed to isolated effects. Although studies to date have been small in both subject numbers and treatment times, they have demonstrated measurable changes in GH and IGF-1 levels, as well as possible deleterious effects on exercise performance that should be taken seriously.”
“Performance-Enhancing Drugs on the Web: A Growing Public-Health Issue” Brennan, Brian P.; Kanayama, Gen; Pope Jr., Harrison G. American Journal on Addictions , March-April 2013, Vol. 22, Issue 2, 158-161. doi: 10.1111/j.1521-0391.2013.00311.x.
Abstract: “Today’s Internet provides extensive “underground” guidelines for obtaining and using illicit substances, including especially anabolic-androgenic steroids (AAS) and other appearance- and performance-enhancing drugs (APEDs). We attempted to qualitatively characterize APED-related Internet sites. We used relevant Internet search terms [and] found thousands of sites involving AAS and other APEDs. Most sites presented an unabashedly pro-drug position, often openly questioning the qualifications and motivations of mainstream medical practitioners. Offers of AAS and other APEDs for sale, together with medical advice of varying legitimacy, was widespread across sites. Importantly, many sites provided detailed guidelines for exotic forms of APED use, some likely associated with serious health risks, which are probably unknown to most practicing clinicians.”
“Doping in Sport: A Review of Elite Athletes’ Attitudes, Beliefs and Knowledge” Morente-Sánchez, Jaime; Zabala, Mikel. Sports Medicine , March 2013. doi: 10.1007/s40279-013-0037-x.
Abstract: “Although most athletes acknowledge that doping is cheating, unhealthy and risky because of sanctions, its effectiveness is also widely recognized. There is a general belief about the inefficacy of anti-doping programmes, and athletes criticise the way tests are carried out. Most athletes consider the severity of punishment is appropriate or not severe enough. There are some differences between sports, as team-based sports and sports requiring motor skills could be less influenced by doping practices than individual self-paced sports. However, anti-doping controls are less exhaustive in team sports. The use of banned substance also differs according to the demand of the specific sport. Coaches appear to be the main influence and source of information for athletes, whereas doctors and other specialists do not seem to act as principal advisors. Athletes are becoming increasingly familiar with anti-doping rules, but there is still a lack of knowledge that should be remedied using appropriate educational programmes. There is also a lack of information on dietary supplements and the side effects of [performance-enhancing substances].”
“Age and Gender Specific Variations in Attitudes to Performance Enhancing Drugs and Methods” Singhammer, John. Sport Science Review , December 2012. doi: 10.2478/v10237-012-0017-3.
Abstract: “Using a population-based cross-sectional sample of 1,703 Danish men and women aged 15-60 years, the present study examined age and gender variation in attitudes to performance enhancing drugs and methods…. Overall, participants held negative attitudes to drugs and methods enhancing predominantly cognitive-abilities-enhancing performance drugs and to appearance-modifying methods, but were positive to drugs for restoring physical functioning conditions. However, attitudes varied nonlinearly across age. Lenient attitudes peaked at around age 25 and subsequently decreased. Lenient attitudes to use of drugs against common disorders decreased in a linear fashion. No gender differences were observed and attitude did not vary with level of education, self-reported health or weekly hours of physical activity.”
“Drugs, Sweat and Gears: An Organizational Analysis of Performance Enhancing Drug Use in the 2010 Tour De France” Palmer, Donald; Yenkey, Christopher. University of California, Davis; University of Chicago. March 2013.
Abstract: “This paper seeks a more comprehensive explanation of wrongdoing in organizations by theorizing two under-explored causes: the criticality of a person’s role in their organization’s strategy-based structure, and social ties to known deviants within their organization and industry. We investigate how these factors might have influenced wrongdoing in the context of professional cyclists’ use of banned performance enhancing drugs (PEDs) in advance of the 2010 Tour de France….. We find substantial support for our prediction that actors who are more critical to the organization’s strategy-based structure are more likely to engage in wrongdoing. Further, we find that while undifferentiated social ties to known wrongdoers did not increase the likelihood of wrongdoing, ties to unpunished offenders increased the probability of wrongdoing and ties to severely punished offenders decreased it. These effects were robust to consideration of other known causes of wrongdoing: weak governance regimes and permissive cultural contexts, performance strain, and individual propensities to engage in wrongdoing.”
“Elite Athletes’ Estimates of the Prevalence of Illicit Drug Use: Evidence for the False Consensus Effect” Dunn, Matthew; Thomas, Johanna O.; Swift, Wendy; Burns, Lucinda. Drug and Alcohol Review , January 2012, Vol. 31, Issue 1, 27-32. doi: 10.1111/j.1465-3362.2011.00307.x.
Abstract: “The false consensus effect (FCE) is the tendency for people to assume that others share their attitudes and behaviours to a greater extent than they actually do…. The FCE was investigated among 974 elite Australian athletes who were classified according to their drug use history. Participants tended to report that there was a higher prevalence of drug use among athletes in general compared with athletes in their sport, and these estimates appeared to be influenced by participants’ drug-use history. While overestimation of drug use by participants was not common, this overestimation also appeared to be influenced by athletes’ drug use history.”
“The Role of Sports Physicians in Doping: A Note on Incentives” Korn, Evelyn; Robeck, Volker. Philipps-Universitat, Marburg, March 2013.
Abstract: “How to ban the fraudulent use of performance-enhancing drugs is an issue in all professional — and increasingly in amateur — sports. The main effort in enforcing a ‘clean sport’ has concentrated on proving an abuse of performance-enhancing drugs and on imposing sanctions on teams and athletes. An investigation started by Freiburg university hospital against two of its employees who had been working as physicians for a professional cycling team has drawn attention to another group of actors: physicians. It reveals a multi-layered contractual relations between sports teams, physicians, hospitals, and sports associations that provided string incentives for the two doctors to support the use performance-enhancing drugs. This paper argues that these misled incentives are not singular but a structural part of modern sports caused by cross effects between the labor market for sports medicine specialists (especially if they are researchers) and for professional athletes.”
“Socio-economic Determinants of Adolescent Use of Performance Enhancing Drugs” Humphreys, Brad R.; Ruseski, Jane E. Journal of Socio-Economics , April 2011, Vol. 40, Issue 2, 208-216. doi: 10.1016/j.socec.2011.01.008.
Abstract: “Evidence indicates that adolescents (athletes and non-athletes use performance enhancing drugs. We posit that adolescent athletes have different socio-economic incentives to use steroids than non-athletes. We examine adolescent steroid use using data from the Youth Risk Behavior Surveillance System. Multi-sport upperclassmen and black males have a higher probability of steroid use. Steroid use is associated with motivations to change physical appearance and experimentation with illicit substances. These results suggest there are different socio-economic motivations for adolescent steroid use and that steroid use is an important component of overall adolescent drug use.”
Cognitive-enhancing drugs
“Randomized Response Estimates for the 12-Month Prevalence of Cognitive-Enhancing Drug Use in University Students” Dietz, Pavel; et al. Pharmacotherapy , January 2013, Vol. 33, Issue 1, 44-50. doi: 10.1002/phar.1166.
Results: “An anonymous, specialized questionnaire that used the randomized response technique was distributed to students at the beginning of classes and was collected afterward. From the responses, we calculated the prevalence of students taking drugs only to improve their cognitive performance and not to treat underlying mental disorders such as attention-deficit-hyperactivity disorder, depression, and sleep disorders. The estimated 12-month prevalence of using cognitive-enhancing drugs was 20%. Prevalence varied by sex (male 23.7%, female 17.0%), field of study (highest in students studying sports-related fields, 25.4%), and semester (first semester 24.3%, beyond first semester 16.7%).”
“The Diversion and Misuse of Pharmaceutical Stimulants: What Do We Know and Why Should We Care?” Kaye, Sharlene; Darke, Shane. Addiction , February 2012, Vol. 107, Issue 3, 467-477. doi: 10.1111/j.1360-0443.2011.03720.x.
Results: “The evidence to date suggests that the prevalence of diversion and misuse of pharmaceutical stimulants varies across adolescent and young adult student populations, but is higher than that among the general population, with the highest prevalence found among adults with attention deficit-hyperactive disorder (ADHD) and users of other illicit drugs. Concerns that these practices have become more prevalent as a result of increased prescribing are not supported by large-scale population surveys…. Despite recognition of the abuse liability of these medications, there is a paucity of data on the prevalence, patterns and harms of diversion and misuse among populations where problematic use and abuse may be most likely to occur (e.g. adolescents, young adults, illicit drug users). Comprehensive investigations of diversion and misuse among these populations should be a major research priority, as should the assessment of abuse and dependence criteria among those identified as regular users.”
“Adderall Abuse on College Campuses: A Comprehensive Literature Review” Varga, Matthew D. Journal of Evidence-Based Social Work , 2012, Vol. 9, Issue 3. doi: 10.1080/15433714.2010.525402.
Abstract: “Prescription stimulant abuse has dramatically increased over the past 10 years, but the amount of research regarding college students and illicit prescription stimulant use is still very limited. This has important implications for college mental health professionals and higher education administrators. In this comprehensive literature review the author explores factors contributing to illicit use, self-medication, and recreational use of controlled prescription stimulants; discusses the potential consequences for those students abusing stimulants; and provides recommendations for educating, combating, and assisting students who illicitly use prescription stimulants on college campuses.”
“A Comparison of Attitudes Toward Cognitive Enhancement and Legalized Doping in Sport in a Community Sample of Australian Adults” Partridge, Brad; Lucke, Jayne; Hall, Wayne. AJOB Primary Research , November 2012. doi: 10.1080/21507716.2012.720639.
Abstract: “This article compares public attitudes toward the use of prescription drugs for cognitive enhancement with the use of performance enhancing drugs in sport. We explore attitudes toward the acceptability of both practices; the extent to which familiarity with cognitive enhancement is related to its perceived acceptability; and relationships between the acceptability of cognitive enhancement and legalized doping in sport. Of 1,265 [survey] participants, 7% agreed that cognitive enhancement is acceptable; 2.4% of the total sample said they had taken prescription drugs to enhance their concentration or alertness in the absence of a diagnosed disorder, and a further 8% said they knew someone who had done so. These participants were twice as likely to think cognitive enhancement was acceptable. Only 3.6% of participants agreed that people who play professional sport should be allowed to use performance-enhancing drugs if they wanted to. Participants who found cognitive enhancement acceptable were 9.5 times more likely to agree with legalized doping.”
Keywords: drugs, youth, sports, cheating, higher education, corruption, ADHD, research roundup
About The Author
Leighton Walter Kille
- Reference Manager
- Simple TEXT file
People also looked at
Review article, stress in academic and athletic performance in collegiate athletes: a narrative review of sources and monitoring strategies.
- 1 School of Kinesiology, Applied Health and Recreation, Oklahoma State University, Stillwater, OK, United States
- 2 Department of Kinesiology, California State University, Fullerton, CA, United States
- 3 Department of Kinesiology, Point Loma Nazarene University, San Diego, CA, United States
- 4 Department of Kinesiology and Sport Sciences, University of Miami, Miami, FL, United States
College students are required to manage a variety of stressors related to academic, social, and financial commitments. In addition to the burdens facing most college students, collegiate athletes must devote a substantial amount of time to improving their sporting abilities. The strength and conditioning professional sees the athlete on nearly a daily basis and is able to recognize the changes in performance and behavior an athlete may exhibit as a result of these stressors. As such, the strength and conditioning professional may serve an integral role in the monitoring of these stressors and may be able to alter training programs to improve both performance and wellness. The purpose of this paper is to discuss stressors experienced by collegiate athletes, developing an early detection system through monitoring techniques that identify the detrimental effects of stress, and discuss appropriate stress management strategies for this population.
Introduction
The college years are a period of time when young adults experience a significant amount of change and a variety of novel challenges. Academic performance, social demands, adjusting to life away from home, and financial challenges are just a few of the burdens college students must confront ( Humphrey et al., 2000 ; Paule and Gilson, 2010 ; Aquilina, 2013 ). In addition to these stressors, collegiate athletes are required to spend a substantial amount of time participating in activities related to their sport, such as attending practices and training sessions, team meetings, travel, and competitions ( Humphrey et al., 2000 ; López de Subijana et al., 2015 ; Davis et al., 2019 ; Hyatt and Kavazis, 2019 ). These commitments, in addition to the normal stress associated with college life, may increase a collegiate-athlete's risk of experiencing both physical and mental issues ( Li et al., 2017 ; Moreland et al., 2018 ) that may affect their overall health and wellness. For these reasons, it is essential that coaches understand the types of stressors collegiate athletes face in order to help them manage the potentially deleterious effects stress may have on athletic and academic performance.
Strength and conditioning coaches are allied health care professionals whose primary job is to enhance fitness of individuals for the purpose of improving athletic performance ( Massey et al., 2002 , 2004 , 2009 ). As such, many universities and colleges hire strength and conditioning coaches as part of their athletic staff to help athletes maximize their physical potential ( Massey et al., 2002 , 2004 , 2009 ). Strength and conditioning coaches strive to increase athletic performance by the systematic application of physical stress to the body via resistance training, and other forms of exercise, to yield a positive adaptation response ( Massey et al., 2002 , 2004 , 2009 ). For this reason, they need to understand and to learn how to manage athletes' stress. Additionally, based on the cumulative nature of stress, it is important that both mental and emotional stressors are also considered in programming. It is imperative that strength and conditioning coaches are aware of the multitude of stressors collegiate athletes encounter, in order to incorporate illness and injury risk management education into their training programs ( Radcliffe et al., 2015 ; Ivarsson et al., 2017 ).
Based on the large number of contact hours strength and conditioning coaches spend with their athletes, they are in an optimal position to assist athletes with developing effective coping strategies to manage stress. By doing so, strength and conditioning coaches may be able to help reach the overarching goal of improving the health, wellness, fitness, and performance of the athletes they coach. The purpose of this review article is to provide the strength and conditioning professional with a foundational understanding of the types of stressors collegiate athletes may experience, and how these stressors may impact mental health and athletic performance. Suggestions for assisting athletes with developing effective coping strategies to reduce potential physiological and psychological impacts of stress will also be provided.
Stress and the Stress Response
In its most simplistic definition, stress can be described as a state of physical and psychological activation in response to external demands that exceed one's ability to cope and requires a person to adapt or change behavior. As such, both cognitive or environmental events that trigger stress are called stressors ( Statler and DuBois, 2016 ). Stressors can be acute or chronic based on the duration of activation. Acute stressors may be defined as a stressful situation that occurs suddenly and results in physiological arousal (e.g., increase in hormonal levels, blood flow, cardiac output, blood sugar levels, pupil and airway dilation, etc.) ( Selye, 1976 ). Once the situation is normalized, a cascade of hormonal reactions occurs to help the body return to a resting state (i.e., homeostasis). However, when acute stressors become chronic in nature, they may increase an individual's risk of developing anxiety, depression, or metabolic disorders ( Selye, 1976 ). Moreover, the literature has shown that cumulative stress is correlated with an increased susceptibility to illness and injury ( Szivak and Kraemer, 2015 ; Mann et al., 2016 ; Hamlin et al., 2019 ). The impact of stress is individualistic and subjective by nature ( Williams and Andersen, 1998 ; Ivarsson et al., 2017 ). Additionally, the manner in which athletes respond to a situational or environmental stressor is often determined by their individual perception of the event ( Gould and Udry, 1994 ; Williams and Andersen, 1998 ; Ivarsson et al., 2017 ). In this regard, the athlete's perception can either be positive (eustress) or negative (distress). Even though they both cause physiological arousal, eustress also generates positive mental energy whereas distress generates anxiety ( Statler and DuBois, 2016 ). Therefore, it is essential that an athlete has the tools and ability to cope with these stressors in order to have the capacity to manage both acute and chronic stress. As such, it is important to understand the types of stressors collegiate athletes are confronted with and how these stressors impact an athlete's performance, both athletically and academically.
Literature Search/Data Collection
The articles included in this review were identified via online databases PubMed, MEDLINE, and ISI Web of Knowledge from October 15th 2019 through January 15th 2020. The search strategy combined the keywords “academic stress,” “athletic stress,” “stress,” “stressor,” “college athletes,” “student athletes,” “collegiate athletes,” “injury,” “training,” “monitoring.” Duplicated articles were then removed. After reading the titles and abstracts, all articles that met the inclusion criteria were considered eligible for inclusion in the review. Subsequently, all eligible articles were read in their entirety and were either included or removed from the present review.
Inclusion Criteria
The studies included met all the following criteria: (i) published in English-language journals; (ii) targeted college athletes; (iii) publication was either an original research paper or a literature review; (iv) allowed the extraction of data for analysis.
Data Analysis
Relevant data regarding participant characteristics (i.e., gender, academic status, sports) and study characteristics were extracted. Articles were analyzed and divided into two separate sections based on their specific topics: Academic Stress and Athletic Stress. Then, strategies for monitoring and workload management are discussed in the final section.
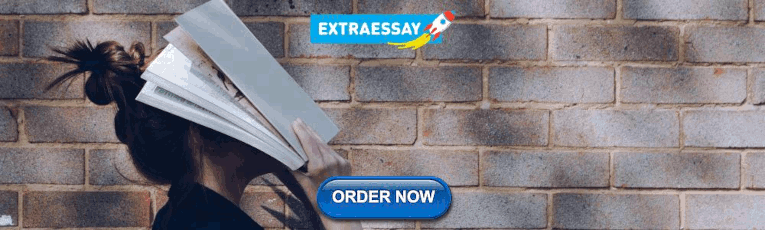
Academic Stress
Fundamentally, collegiate athletes have two major roles they must balance as part of their commitment to a university: being a college student and an athlete. Academic performance is a significant source of stress for most college students ( Aquilina, 2013 ; López de Subijana et al., 2015 ; de Brandt et al., 2018 ; Davis et al., 2019 ). This stress may be further compounded among collegiate athletes based on their need to be successful in the classroom, while simultaneously excelling in their respective sport ( Aquilina, 2013 ; López de Subijana et al., 2015 ; Huml et al., 2016 ; Hamlin et al., 2019 ). Davis et al. (2019) conducted surveys on 173 elite junior alpine skiers and reported significant moderate to strong correlations between perceived stress and several variables including depressed mood ( r = 0.591), sleep disturbance ( r = 0.459), fatigue ( r = 0.457), performance demands ( r = 0.523), and goals and development ( r = 0.544). Academic requirements were the highest scoring source of stress of all variables and was most strongly correlated with perceived stress ( r = 0.467). Interestingly, it was not academic rigor that was viewed by the athletes as the largest source of direct stress; rather, the athletes surveyed reported time management as being their biggest challenge related to academic performance ( Davis et al., 2019 ). This further corroborates the findings of Hamlin et al. (2019) . The investigators reported that during periods of the academic year in which levels of perceived academic stress were at their highest, students had trouble managing sport practices and studying. These stressors were also associated with a decrease in energy levels and overall sleep quality. These factors may significantly increase the collegiate athlete's susceptibility to illness and injury ( Hamlin et al., 2019 ). For this reason, coaches should be aware of and sensitive to the stressors athletes experience as part of the cyclical nature of the academic year and attempt to help athletes find solutions to balancing athletic and academic demands.
According to Aquilina (2013) , collegiate athletes tend to be more committed to sports development and may view their academic career as a contingency plan to their athletic career, rather than a source of personal development. As a result, collegiate athletes often, but certainly not always, prioritize athletic participation over their academic responsibilities ( Miller and Kerr, 2002 ; Cosh and Tully, 2014 , 2015 ). Nonetheless, scholarships are usually predicated on both athletic and academic performance. For instance, the National Collegiate Athletic Association (NCAA) requires collegiate athletes to achieve and maintain a certain grade point average (GPA). Furthermore, they are also often required to also uphold a certain GPA to maintain an athletic scholarship. The pressure to maintain both high levels of academic and athletic performance may increase the likelihood of triggering mental health issues (i.e., anxiety and depression) ( Li et al., 2017 ; Moreland et al., 2018 ).
Mental health issues are a significant concern among college students. There has been an increased emphasis placed on the mental health of collegiate athletes in recent years ( Petrie et al., 2014 ; Li et al., 2017 , 2019 ; Reardon et al., 2019 ). Based on the 2019 National College Health Assessment survey from the American College Health Association (ACHA) consisting of 67,972 participants, 27.8% of college students reported anxiety, and 20.2% reported experiencing depression which negatively affected their academic performance ( American College Health Association American College Health Association-National College Health Assessment II, 2019 ). Approximately 65.7% (50.7% males and 71.8% females) reported feeling overwhelming anxiety in the past 12 months, and 45.1% (37.1% males and 47.6% females) reported feeling so depressed that it was difficult for them to function. However, only 24.3% (13% males and 28.4% females) reported being diagnosed and treated by a professional in the past 12 months. Collegiate athletes are not immune to these types of issues. According to information presented by the NCAA, many certified athletic trainers anecdotally state that anxiety is an issue affecting the collegiate-athlete population ( NCAA, 2014 ). However, despite the fact that collegiate athletes are exposed to numerous stressors, they are less likely to seek help at a university counseling center than non-athletes ( NCAA, 2014 ), which could be related to stigmas that surround mental health services ( NCAA, 2014 ; Kaier et al., 2015 ; Egan, 2019 ). This not only has significant implications related to their psychological well-being, but also their physiological health, and consequently their performance. For instance, in a study by Li et al. (2017) it was found that NCAA Division I athletes who reported preseason anxiety symptoms had a 2.3 times greater injury incidence rate compared to athletes who did not report. This same study discovered that male athletes who reported preseason anxiety and depression had a 2.1 times greater injury incidence, compared to male athletes who did not report symptoms of anxiety and depression. ( Lavallée and Flint, 1996 ) also reported a correlation between anxiety and both injury frequency and severity among college football players ( r = 0.43 and r = 0.44, respectively). In their study, athletes reporting high tension/anxiety had a higher rate of injury. It has been suggested that the occurrence of stress and anxiety may cause physiological responses, such as an increase in muscle tension, physical fatigue, and a decrease in neurocognitive and perception processes that can lead to physical injuries ( Ivarsson et al., 2017 ). For this reason, it is reasonable to consider that academic stressors may potentiate effects of stress and result in injury and illness in collegiate athletes.
Periods of more intense academic stress increase the susceptibility to illness or injury ( Mann et al., 2016 ; Hamlin et al., 2019 ; Li et al., 2019 ). For example, Hamlin et al. (2019) investigated levels of perceived stress, training loads, injury, and illness incidence in 182 collegiate athletes for the period of one academic year. The highest levels of stress and incidence of illness arise during the examination weeks occurring within the competitive season. In addition, the authors also reported the odds ratio, which is the occurrence of the outcome of interest (i.e., injury), based off the given exposure to the variables of interest (i.e., perceived mood, sleep duration, increased academic stress, and energy levels). Based on a logistic regression, they found that each of the four variables (i.e., mood, energy, sleep duration, and academic stress) was related to the collegiate athletes' likelihood to incur injuries. In summary, decreased levels of perceived mood (odds ratio of 0.89, 0.85–0.0.94 CI) and sleep duration (odds ratio of 0.94, 0.91–0.97 CI), and increased academic stress (odds ratio of 0.91, 0.88–0.94 CI) and energy levels (odds ratio of 1.07, 1.01–1.14 CI), were able to predict injury in these athletes. This corroborates Mann et al. (2016) who found NCAA Division I football athletes at a Bowl Championship Subdivision university were more likely to become ill or injured during an academically stressful period (i.e., midterm exams or other common test weeks) than during a non-testing week (odds ratio of 1.78 for high academic stress). The athletes were also less likely to get injured during training camp (odds ratio of 3.65 for training camp). Freshmen collegiate athletes may be especially more susceptible to mental health issues than older students. Their transition includes not only the academic environment with its requirements and expectations, but also the adaptation to working with a new coach and teammates. In this regard, Yang et al. (2007) found an increase in the likelihood of depression that freshmen athletes experienced, as these freshmen were 3.27 times more likely to experience depression than their older teammates. While some stressors are recurrent and inherent in academic life (e.g., attending classes, homework, etc.), others are more situational (e.g., exams, midterms, projects) and may be anticipated by the strength and conditioning coach.
Athletic Stress
The domain of athletics can expose collegiate athletes to additional stressors that are specific to their cohort (e.g., sport-specific, team vs. individual sport) ( Aquilina, 2013 ). Time spent training (e.g., physical conditioning and sports practice), competition schedules (e.g., travel time, missing class), dealing with injuries (e.g., physical therapy/rehabilitation, etc.), sport-specific social support (e.g., teammates, coaches) and playing status (e.g., starting, non-starter, being benched, etc.) are just a few of the additional challenges collegiate athletes must confront relative to their dual role of being a student and an athlete ( Maloney and McCormick, 1993 ; Scott et al., 2008 ; Etzel, 2009 ; Fogaca, 2019 ). Collegiate athletes who view the demands of stressors from academics and sports as a positive challenge (i.e., an individual's self-confidence or belief in oneself to accomplish the task outweighs any anxiety or emotional worry that is felt) may potentially increase learning capacity and competency ( NCAA, 2014 ). However, when these demands are perceived as exceeding the athlete's capacity, this stress can be detrimental to the student's mental and physical health as well as to sport performance ( Ivarsson et al., 2017 ; Li et al., 2017 ).
As previously stated, time management has been shown to be a challenge to collegiate athletes. The NCAA rules state that collegiate athletes may only engage in required athletic activities for 4 h per day and 20 h/week during in-season and 8 h/week during off-season throughout the academic year. Although these rules have been clearly outlined, the most recent NCAA GOALS (2016) study reported alarming numbers regarding time commitment to athletic-related activities. Data from over 21,000 collegiate athletes from 600 schools across Divisions I, II, and III were included in this study. Although a breakdown of time commitments was not provided, collegiate athletes reported dedicating up to 34 h per week to athletics (e.g., practices, weight training, meetings with coaches, tactical training, competitions, etc.), in addition to spending between 38.5 and 40 h per week working on academic-related tasks. This report also showed a notable trend related to athletes spending an increase of ~2 more athletics-related hours per week compared to the 2010 GOALS study, along with a decrease of 2 h of personal time (from 19.5 h per week in 2010 to 17.1 in 2015). Furthermore, ~66% of Division I and II and 50% of Division III athletes reported spending as much or more time in their practices during the off-season as during the competitive season ( DTHOMAS, 2013 ). These numbers show how important it is for collegiate athletes to develop time management skills to be successful in both academics and athletics. Overall, most collegiate athletes have expressed a need to find time to enjoy their college experience outside of athletic obligations ( Paule and Gilson, 2010 ). Despite that, because of the increasing demand for excellence in academics and athletics, collegiate athletes' free time with family and friends is often scarce ( Paule and Gilson, 2010 ). Consequently, trainers, coaches, and teammates will likely be the primary source of their weekly social interactivity.
Social interactions within their sport have also been found to relate to factors that may impact an athlete's perceived stress. Interactions with coaches and trainers can be effective or deleterious to an athlete. Effective coaching includes a coaching style that allows for a boost of the athlete's motivation, self-esteem, and efficacy in addition to mitigating the effects of anxiety. On the other hand, poor coaching (i.e., the opposite of effective coaching) can have detrimental psychological effects on an athlete ( Gearity and Murray, 2011 ). In a closer examination of the concept of poor coaching practices, Gearity and Murray (2011) interviewed athletes about their experiences of receiving poor coaching. Following analysis of the interviews, the authors identified the main themes of the “coach being uncaring and unfair,” “practicing poor teaching inhibiting athlete's mental skills,” and “athlete coping.” They stated that inhibition of an athlete's mental skills and coping are associated with the psychological well-being of an athlete. Also, poor coaching may result in mental skills inhibition, distraction, insecurity, and ultimately team division ( Gearity and Murray, 2011 ). This combination of factors may compound the negative impacts of stress in athletes and might be especially important for in injured athletes.
Injured athletes have previously been reported to have elevated stress as a result of heightened worry about returning to pre-competition status ( Crossman, 1997 ), isolation from teammates if the injury is over a long period of time ( Podlog and Eklund, 2007 ) and/or reduced mood or depressive symptoms ( Daly et al., 1995 ). In addition, athletes who experience prolonged negative thoughts may be more likely to have decreased rehabilitation attendance or adherence, worse functional outcomes from rehabilitation (e.g., on measures of proprioception, muscular endurance, and agility), and worse post-injury performance ( Brewer, 2012 ).
Monitoring Considerations
In addition to poor coaching, insufficient workload management can hinder an athlete's ability to recover and adapt to training, leading to fatigue accumulation ( Gabbett et al., 2017 ). Excessive fatigue can impair decision-making ability, coordination and neuromuscular control, and ultimately result in overtraining and injury ( Soligard et al., 2016 ). For instance, central fatigue was found to be a direct contributor to anterior cruciate ligament injuries in soccer players ( Mclean and Samorezov, 2009 ). Introducing monitoring tools may serve as a means to reduce the detrimental effects of stress in collegiate athletes. Recent research on relationships between athlete workloads, injury, and performance has highlighted the benefits of athlete monitoring ( Drew and Finch, 2016 ; Jaspers et al., 2017 ).
Athlete monitoring is often assessed with the measuring and management of workload associated with a combination of sport-related and non-sport-related stressors ( Soligard et al., 2016 ). An effective workload management program should aim to detect excessive fatigue, identify its causes, and constantly adapt rest, recovery, training, and competition loads respectively ( Soligard et al., 2016 ). The workload for each athlete is based off their current levels of physical and psychological fatigue, wellness, fitness, health, and recovery ( Soligard et al., 2016 ). Accumulation of situational or physical stressors will likely result in day-to-day fluctuations in the ability to move external loads and strength train effectively ( Fry and Kraemer, 1997 ). Periods of increased academic stress may cause increased levels of fatigue, which can be identified by using these monitoring tools, thereby assisting the coaches with modulating the workload during these specific periods. Coaches who plan to incorporate monitoring and management strategies must have a clear understanding of what they want to achieve from athlete monitoring ( Gabbett et al., 2017 ; Thornton et al., 2019 ).
Monitoring External Loads
External load refers to the physical work (e.g., number of sprints, weight lifted, distance traveled, etc.) completed by the athlete during competition, training, and activities of daily living ( Soligard et al., 2016 ). This type of load is independent of the athlete's individual characteristics ( Wallace et al., 2009 ). Monitoring external loading can aid in the designing of training programs which mimic the external load demands of an athlete's sport, guide rehabilitation programs, and aid in the detection of spikes in external load that may increase the risk of injury ( Clubb and McGuigan, 2018 ).
The means of quantifying external load can involve metrics as simple as pitch counts in baseball and softball ( Fleisig and Andrews, 2012 ; Shanley et al., 2012 ) or quantifying lifting session training loads (e.g., sum value of weight lifted during an exercise x number of repetitions × the number of sets). Neuromuscular function testing is another more common way of analyzing external load. This is typically done using such measures such as the counter movement jump, squat jump, or drop jump. A force platform can be used to measure a myriad of outcomes (e.g., peak power, ground contact time, time to take-off, reactive strength index, and jump height), or simply measure jump height in a more traditional manner. Jumping protocols, such as the countermovement jump, have been adopted to examine the recovery of neuromuscular function after athletic competition with significant decreases for up to 72 h commonly reported ( Andersson et al., 2008 ; Magalhães et al., 2010 ; Twist and Highton, 2013 ). ( Gathercole et al., 2015 ) found reductions in 18 different neuromuscular variables in collegiate athletes following a fatiguing protocol. The variables of eccentric duration, concentric duration, total duration, time to peak force/power, and flight time:contraction time ratio, derived from a countermovement jump were deemed suitable for detecting neuromuscular fatigue with the rise in the use of technology for monitoring, certain sports have adopted specific software that can aid in the monitoring of stress. For example, power output can be measured using devices such as SRM™ or PowerTap™ in cycling ( Jobson et al., 2009 ). This data can be analyzed to provide information such as average power or normalized power. The power output can then be converted into a Training Stress Score™ via commercially available software ( Marino, 2011 ). More sophisticated measures of external load may involve the use of wearable technology devices such as Global Positioning System (GPS) devices, accelerometers, magnetometer, and gyroscope inertial sensors ( Akenhead and Nassis, 2016 ). These devices can quantify external load in several ways, such as duration of movement, total distance covered, speed of movement, acceleration, and decelerations, as well as sport specific movement such as number and height of jumps, number of tackles, or breakaways, etc. ( Akenhead and Nassis, 2016 ). The expansion of marketing of wearable devices has been substantial; however, there are questions of validity and reliability related to external load tracking limitations related to proprietary metrics, as well as the overall cost that should be considered when considering the adoption of such devices ( Aughey et al., 2016 ; Torres-Ronda and Schelling, 2017 ).
Monitoring Internal Loads
While external load may provide information about an athlete's performance capacity and work completed, it does not provide clear evidence of how athletes are coping with and adapting to the external load ( Halson, 2014 ). This type of information comes from the monitoring of internal loads. The term internal load refers to the individual physiological and psychological response to the external stress or load imposed ( Wallace et al., 2009 ). Internal load is influenced by a number of factors such as daily life stressors, the environment around the athlete, and coping ability ( Soligard et al., 2016 ). Indirect measures, such as the use of heart rate (HR) monitoring, and subjective measurements, such as perceived effort (i.e., ratings of perceived exertion), are examples of internal load monitoring. Using subjective measurement systems is a simple and practical method when dealing with large numbers of athletes ( Saw et al., 2016 ; Nässi et al., 2017 ). Subjective reporting of training load (Rating of Perceived Exertion—RPE) ( Coyne et al., 2018 ), Session Rating of Perceived Exertion—sRPE) ( Coyne et al., 2018 ), perceived stress and recovery (Recovery Stress Questionnaire for Athletes—RESTQ-S), and psychological mood states (Profile of Mood States—POMS) have all been found to be a reliable indicator of training load ( Robson-Ansley et al., 2009 ; Saw et al., 2016 ) and only take a few moments to complete. In addition, subjective measures can be more responsive to tracking changes or training responses in athletes than objective measures ( Saw et al., 2016 ).
Heart rate (HR) monitoring is a common intrinsic measure of how the body is responding to stress. With training, the reduction of resting HR is typically a clear indication of the heart becoming more efficient and not having to beat as frequently. Alternately, increases of resting HR over time with a continuation of training may be an indicator of too much stress. Improper nutrition, such as regular or ongoing suboptimal intakes of vitamins or minerals, may result in increased ventilation and/or increased heart rate ( Lukaski, 2004 ). It has been suggested that the additional stress may lead to parasympathetic hyperactivity, leading to an increase in resting HR ( Statler and DuBois, 2016 ). This largely stems from research examining the sensitivity of various HR derived metrics, such as resting HR, HR variability (HRV), and HR recovery (HRR) to fluctuations in training load ( Borresen and Ian Lambert, 2009 ). HRR in athlete monitoring is the rate of HR decline after the cessation of exercise. A common measure of HHR is the use of a 2 min step test followed by a 60 s HR measurement. The combination of the exercise (stress) on the cardiovascular system and then its subsequent return toward baseline has been used as an indicator of autonomic function and training status in athletes ( Daanen et al., 2012 ). In collegiate athletes it was found that hydration status impacted HRR following moderate to hard straining sessions ( Ayotte and Corcoran, 2018 ). Athletes who followed a prescription hydration plan performed better in the standing long jump, tracked objects faster, and showed faster HRR vs. athletes who followed their normal self-selected hydration plan ( Ayotte and Corcoran, 2018 ). To date, HR monitoring and the various derivatives have mainly been successful in detecting changes in training load and performance in endurance athletes ( Borresen and Ian Lambert, 2009 ; Lamberts et al., 2009 ; Thorpe et al., 2017 ). Although heart rate monitoring can provide additional physiological insight for aerobic sessions or events, it thus far has not been found to be an accurate measurement for quantifying internal load during many explosive, short duration anaerobic activities ( Bosquet et al., 2008 ).
A multitude of studies have reported the reliability and validity of using RPE and sRPE across a range of training modalities ( Foster, 1998 ; Impellizzeri et al., 2004 ; Sweet et al., 2004 ). This measure can be used to create a number of metrics such as session load (sRPE × duration in minutes), daily load (sum of all session loads for that day), weekly training load (sum of all daily training loads for entire week), monotony (standard deviation of weekly training load), and strain (daily or weekly training load × monotony) ( Foster, 1998 ). Qualitative questionnaires that monitor stress and fatigue have been well-established as tools to use with athletes (see Table 1 for examples of commonly used questionnaires in research). Using short daily wellness questionnaires may allow coaches to generate a wellness score which then can be adjusted based off of the stress the athlete may be feeling to meet the daily load target ( Foster, 1998 ; Robson-Ansley et al., 2009 ). However, strength and conditioning coaches need to be mindful that these questionnaires may require sports psychologist or other licensed professional to examine and provide the results. An alternative that may be better suited for strength and conditioning professionals to use could be to incorporate some of the themes of those questionnaires into programing.
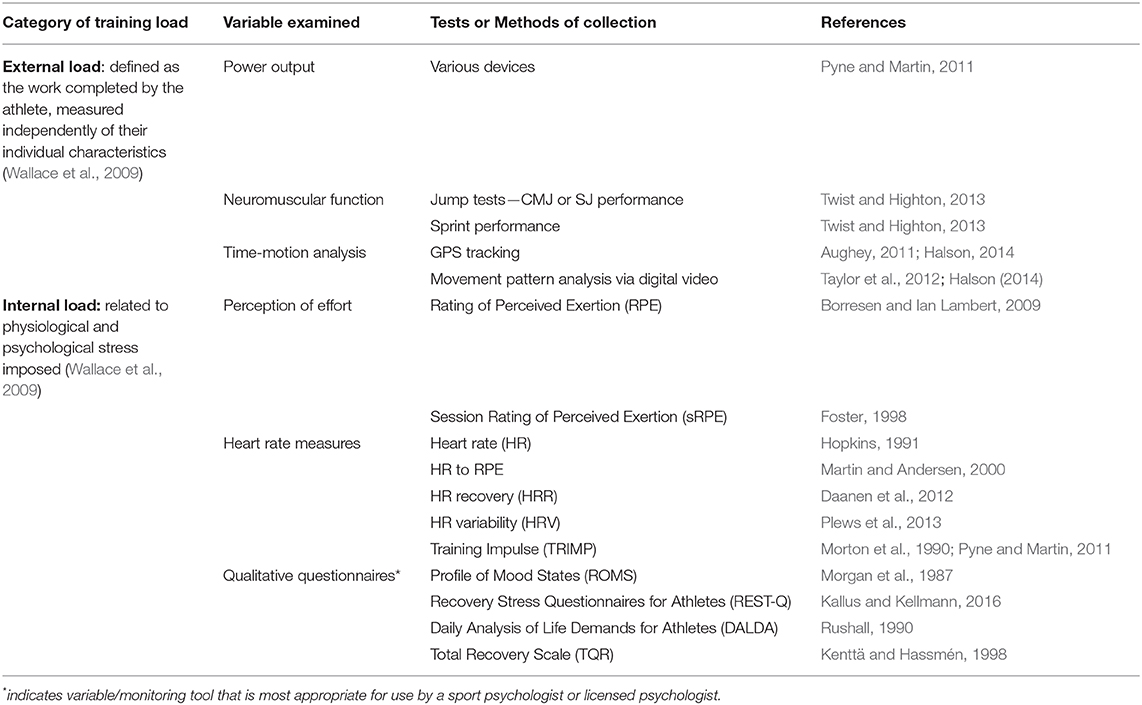
Table 1 . Overview of common tool/measures used by researchers to monitor training load.
A Multifaceted Approach
Dissociation between external and internal load units may be indicative of the state of fatigue of an athlete. Utilizing a monitoring system in which the athlete is able to make adjustments to their training loads in accordance with how they are feeling in that moment can be a useful tool for assisting the athlete in managing stress. Auto-regulation is a method of programming that allows for adjustments based on the results of one or more readiness tests. When implemented properly, auto regulation enables the coach or athlete to optimize training based on the athlete's given readiness for training on a particular day, thereby aiming to avoid potential overtraining ( Kraemer and Fleck, 2018 ). Several studies have found that using movement velocity to designate resistance training intensities can result in significant improvements in maximal strength and athletic performance ( Pareja-Blanco et al., 2014 , 2017 ; Mann et al., 2015 ). Velocity based training allows the coach and athlete to view real time feedback for the given lifts, thereby allowing them to observe how the athlete is performing in that moment. If the athlete is failing to meet the prescribed velocity or the velocity drops greater than a predetermined amount between sets, then this should signal the coach to investigate. If there is a higher than normal amount of stress on that athlete for the day, that could be a potential reason. This type of combination style program of using a quantitative or objective measurement (s) and a subjective measure of wellness (qualitative questionnaire) has recently been reported to be an effective tool in monitoring individuals apart of a team ( Starling et al., 2019 ). The subjective measure in this study was the readiness to train questionnaire (RTT-Q) and the objective measures were the HRR 6min test (specifically the HRR 60s = recorded as decrease in HR in the 60 s after termination of the test) to assess autonomic function and the standing long jump (SLJ) to measure neuromuscular function. The findings found that, based on the absolute typical error of measurement, the HRR 60s and SLJ could detect medium and large changes in fatigue and readiness. The test took roughly 8 min for the entire team, which included a group consisting of 24 college-age athletes. There are many other combinations of monitoring variables and strategies that coaches and athletes may utilize.
Data Analysis – How to Utilize the Measures
Regardless of what type of monitoring tool a coach or athlete may incorporate, it is essential to understand how to analyze this data. There are excellent resources available which discuss this topic in great detail ( Gabbett et al., 2017 ; Clubb and McGuigan, 2018 ; Thornton et al., 2019 ). This section will highlight two main conclusions from these sources and briefly describe two of the main statistical practices and concepts discussed. The use of z-scores or modified z-scores has been proposed as a method of detecting meaningful change in athlete data ( Clubb and McGuigan, 2018 ; Thornton et al., 2019 ). For different monitoring tools listed in Table 1 , the following formula would be an example of how to assess changes: (Athlete daily score—Baseline score)/Standard deviation of baseline. The baseline would likely be based off an appropriate period such as the scores across 2 weeks during the preseason.
In sports and sports science, the use of a magnitude-based inference (MBI) has been suggested as more appropriate and easier to understand when examining meaningful changes in athletic data, than null-hypothesis significance testing (NHST) ( Buchheit, 2014 ). Additional methods to assess meaningful change that are similar to MBI are using standard deviation, typical error, effect sizes, smallest worthwhile change (SWC), and coefficient of variation ( Thornton et al., 2019 ). It should be noted that all of these methods have faced criticism from sources such as statisticians. It is important to understand that the testing methods, measurements, and analysis should be based on the resources and intended goals from use, which will differ from every group and individual. Once identified, it is up to the practitioner to keep this system the same, in order to collect data that can then be examined to understand meaningful information for each setting ( Thornton et al., 2019 ).
Managing and Coping Strategies
Once the collegiate-athlete has been able to identify the need to balance their stress levels, the athlete may then need to seek out options for managing their stress. Coaches are be able to assist them by sharing information on health and wellness resources available for the students, both on and off campus. Another way a coach can potentially support their athletes is by establishing an open-door policy, wherein the team members feel comfortable approaching a member of the strength and conditioning staff in order to seek out resources for coping with challenges related to stress.
There are some basic skills that strength and conditioning coaches can teach (while staying within their scope of practice). Coaches can introduce their athletes to basic lifestyle concepts, such as practicing deep breathing techniques, positive self-talk, and developing healthy sleep habits (i.e., turning off their mobile devices 1 h before bed and aiming for 8 h of sleep each night, etc.). A survey of strength and conditioning practitioners by Radcliffe et al. (2015) found that strategies used by practitioners included a mix of cognitive and behavioral strategies, which was used as justification for recommending practitioners find opportunities to guide professional development toward awareness strategies. Practitioners reported using a wide variety of psychological skills and strategies, which following survey analysis, highlighted a significant emphasis on strategies that may influence athlete self-confidence and goal setting. Themes identified by Radcliffe et al. (2015) included confidence building, arousal management, and skill acquisition. Additionally, similar lower level themes that are connected (i.e., goal setting, increasing, or decreasing arousal intensities, self-talk, mental imagery) are all discussed in the 4th edition of the NSCA Essentials of Strength and Conditioning book ( Haff et al., 2016 ). When the interventions aiming to improve mental health expand from basic concepts to mental training beyond a coach's scope, it would be pertinent for the coach to refer the collegiate-athlete to a sport psychology or other mental health consultant ( Fogaca, 2019 ). Moreover, strength and conditioning coaches may find themselves in a position to become key players in facilitating management strategies for collegiate athletes, thereby guiding the athlete in their quest to learn how to best manage the mental and physical energy levels required in the quest for overall optimal performance ( Statler and DuBois, 2016 ).
Conclusion and Future Directions
This review article has summarized some of the ways that strength and conditioning professionals may be able to gain a better understanding of the types of stressors encountered by collegiate athletes, the impact these stressors may have on athletic performance, and suggestions for assisting athletes with developing effective coping strategies to reduce the potential negative physiological and psychological impacts of stress. It has been suggested that strategies learned in the context of training may have a carry-over effect into other areas such as competition. More education is needed in order for strength and conditioning professionals to gain a greater understanding of how to support their athletes with stress-management techniques and resources. Some ways to disseminate further education on stress-management tools for coaches to share with their athletes may include professional development events, such as conferences and clinics.
Author Contributions
All of the authors have contributed to the development of the manuscript both in writing and conceptual development.
Conflict of Interest
The authors declare that the research was conducted in the absence of any commercial or financial relationships that could be construed as a potential conflict of interest.
The handling editor declared a past collaboration with one of the authors RL.
Akenhead, R., and Nassis, G. P. (2016). Training load and player monitoring in high-level football: current practice and perceptions. Int. J. Sports Physiol. Perform. 11, 587–593. doi: 10.1123/ijspp.2015-0331
PubMed Abstract | CrossRef Full Text | Google Scholar
American College Health Association and American College Health Association-National College Health Assessment II (2019). Reference Group Executive Summary Spring 2019 . Silver Spring, MD: American College Health Association.
Google Scholar
Andersson, H., Raastad, T., Nilsson, J., Paulsen, G., Garthe, I., and Kadi, F. (2008). Neuromuscular fatigue and recovery in Elite female soccer: effects of active recovery. Med. Sci. Sports Exerc. 40, 372–380. doi: 10.1249/mss.0b013e31815b8497
Aquilina, D. (2013). A study of the relationship between elite athletes' educational development and sporting performance. Int. J. Hist. Sport 30, 374–392. doi: 10.1080/09523367.2013.765723
CrossRef Full Text | Google Scholar
Aughey, R. J. (2011). Applications of GPS technologies to field sports. Int. J. Sports Physiol. Perform. 6, 295–310. doi: 10.1123/ijspp.6.3.295
Aughey, R. J., Elias, G. P., Esmaeili, A., Lazarus, B., and Stewart, A. M. (2016). Does the recent internal load and strain on players affect match outcome in elite Australian football? J. Sci. Med. Sport. 19, 182–186. doi: 10.1016/j.jsams.2015.02.005
Ayotte, D., and Corcoran, M. P. (2018). Individualized hydration plans improve performance outcomes for collegiate athletes engaging in in-season training. J. Int. Soc. Sports Nutr. 15:27. doi: 10.1186/s12970-018-0230-2
Borresen, J., and Ian Lambert, M. (2009). The quantification of training load, the training response and the effect on performance. Sports Med. 39, 779–795. doi: 10.2165/11317780-000000000-00000
Bosquet, L., Merkari, S., Arvisais, D., and Aubert, A. E. (2008). Is heart rate a convenient tool to monitor over-reaching? a systematic review of the literature. Br. J. Sports Med. 42, 709–714. doi: 10.1136/bjsm.2007.042200
Brewer, B. W. (2012). “Psychology of sport injury rehabilitation,” in Handbook of Sport Psychology , eds G. Tenenbaum, and R. C. Eklund (Hoboken, NJ: John Wiley & Sons, Inc.), 404–424. doi: 10.1002/9781118270011.ch18
Buchheit, M. (2014). Monitoring training status with HR measures: do all roads lead to Rome? Front. Physiol. 5:73. doi: 10.3389/fphys.2014.00073
Clubb, J., and McGuigan, M. (2018). Developing cost-effective, evidence-based load monitoring systems in strength and conditioning practice. Strength Cond. J. 40, 75–81. doi: 10.1519/SSC.0000000000000396
Cosh, S., and Tully, P. J. (2014). “All I have to do is pass”: a discursive analysis of student athletes' talk about prioritising sport to the detriment of education to overcome stressors encountered in combining elite sport and tertiary education. Psychol. Sport Exerc. 15, 180–189. doi: 10.1016/j.psychsport.2013.10.015
Cosh, S., and Tully, P. J. (2015). Stressors, coping, and support mechanisms for student athletes combining elite sport and tertiary education: implications for practice. Sport Psychol. 29, 120–133. doi: 10.1123/tsp.2014-0102
Coyne, J., Haff, G., Coutts, A., Newton, R., and Nimphius, S. (2018). The current state of subjective training load monitoring—a practical perspective and call to action. Sports Med. 4:58. doi: 10.1186/s40798-018-0172-x
Crossman, J. (1997). Psychological rehabilitation from sports injuries. Sports Med. 23, 333–339. doi: 10.2165/00007256-199723050-00005
Daanen, H. A. M., Lamberts, R. P., Kallen, V. L., Jin, A., and van Meeteren, N. L. U. (2012). A systematic review on heart-rate recovery to monitor changes in training status in athletes. Int. J. Sports Physiol. Perform. 7, 251–260. doi: 10.1123/ijspp.7.3.251
Daly, J. M., Brewer, B. W., van Raalte, J. L., Petitpas, A. J., and Sklar, J. H. (1995). Cognitive appraisal, emotional adjustment, and adherence to rehabilitation following knee surgery. J. Sport Rehabil. 4, 23–30. doi: 10.1123/jsr.4.1.23
Davis, P., Halvarsson, A., Lundström, W., and Lundqvist, C. (2019). Alpine ski coaches' and athletes' perceptions of factors influencing adaptation to stress in the classroom and on the slopes. Front. Psychol. 10:1641. doi: 10.3389/fpsyg.2019.01641
de Brandt, K., Wylleman, P., Torregrossa, M., Schipper-van Veldhoven, N., Minelli, D., Defruyt, S., et al. (2018). Exploring the factor structure of the dual career competency questionnaire for Athletes in European pupil- and student-athletes. J. Sport. Exercise. Psy. 1–18. doi: 10.1080/1612197X.2018.1511619
Drew, M. K., and Finch, C. F. (2016). The relationship between training load and injury, illness and soreness: a systematic and literature review. Sports Med. 46, 861–883. doi: 10.1007/s40279-015-0459-8
DTHOMAS (2013). NCAA GOALS Study. NCAA.Org - The Official Site of the NCAA . Available online at: http://www.ncaa.org/about/resources/research/ncaa-goals-study (accessed October 5, 2019).
Egan, K. P. (2019). Supporting mental health and well-being among student-athletes. Clin. Sports Med. 38, 537–544. doi: 10.1016/j.csm.2019.05.003
Etzel, E. F. ed. (2009). Counseling and Psychological Services for College Student-Athletes. Morgantown, WV: Fitness Information Technology.
Fleisig, G. S., and Andrews, J. R. (2012). Prevention of elbow injuries in youth baseball pitchers. Sports Health 4, 419–424. doi: 10.1177/1941738112454828
Fogaca, J. L. (2019). Combining mental health and performance interventions: coping and social support for student-athletes. J. Appl. Sport Psychol. 1–16. doi: 10.1080/10413200.2019.1648326
Foster, C. (1998). Monitoring training in athletes with reference to overtraining syndrome. Med. Sci. Sports Exerc. 30, 1164–1168. doi: 10.1097/00005768-199807000-00023
Fry, A. C., and Kraemer, W. J. (1997). Resistance exercise overtraining and overreaching: neuroendocrine responses. Sports Med. 23, 106–129. doi: 10.2165/00007256-199723020-00004
Gabbett, T. J., Nassis, G. P., Oetter, E., Pretorius, J., Johnston, N., Medina, D., et al. (2017). The athlete monitoring cycle: a practical guide to interpreting and applying training monitoring data. Br. J. Sports Med. 51, 1451–1452. doi: 10.1136/bjsports-2016-097298
Gathercole, R. J., Sporer, B. C., Stellingwerff, T., and Sleivert, G. G. (2015). Comparison of the capacity of different jump and sprint field tests to detect neuromuscular fatigue. J. Strength Cond. Res. 29, 2522–2531. doi: 10.1519/JSC.0000000000000912
Gearity, B. T., and Murray, M. A. (2011). Athletes' experiences of the psychological effects of poor coaching. Psychol. Sport Exerc. 12, 213–221. doi: 10.1016/j.psychsport.2010.11.004
Gould, D., and Udry, E. (1994). Psychological skills for enhancing performance: arousal regulation strategies. Med. Sci. Sports Exerc. 26, 478–485.
PubMed Abstract | Google Scholar
Haff, G., Triplett, N. T., and National Strength Conditioning Association (U.S.) eds. (2016). Essentials of Strength Training and Conditioning . 4th Edn. Champaign, IL: Human Kinetics.
Halson, S. L. (2014). Monitoring training load to understand fatigue in athletes. Sports Med. 44, 139–147. doi: 10.1007/s40279-014-0253-z
Hamlin, M. J., Wilkes, D., Elliot, C. A., Lizamore, C. A., and Kathiravel, Y. (2019). Monitoring training loads and perceived stress in young elite university athletes. Front. Physiol. 10:34. doi: 10.3389/fphys.2019.00034
Hopkins, W. G. (1991). Quantification of training in competitive sports: methods and applications. Sports Med. 12, 161–183. doi: 10.2165/00007256-199112030-00003
Huml, M. R., Hambrick, M. E., and Hums, M. A. (2016). Coaches' perceptions of the reduction of athletic commitment for division II student-athletes: development and validation of a measure of athletic/academic balance. J. Intercoll. Sport 9, 303–325. doi: 10.1123/jis.2015-0055
Humphrey, J. H., Yow, D. A., and Bowden, W. W. (2000). Stress in College Athletics: Causes, Consequences, Coping . New York, NY: Haworth Half-Court Press.
Hyatt, H. W., and Kavazis, A. N. (2019). Body composition and perceived stress through a calendar year in NCAA I female volleyball players. Int. J. Exerc. Sci. 12, 433–443.
Impellizzeri, F. M., Rampinini, E., Coutts, A. J., Sassi, A., and Marcora, S. M. (2004). Use of RPE-based training load in Soccer. Med. Sci. Sports Exerc. 36, 1042–1047. doi: 10.1249/01.MSS.0000128199.23901.2F
Ivarsson, A., Johnson, U., Andersen, M. B., Tranaeus, U., Stenling, A., and Lindwall, M. (2017). Psychosocial factors and sport injuries: meta-analyses for prediction and prevention. Sports Med. 47, 353–365. doi: 10.1007/s40279-016-0578-x
Jaspers, A., Brink, M. S., Probst, S. G. M., Frencken, W. G. P., and Helsen, W. F. (2017). Relationships between training load indicators and training outcomes in professional Soccer. Sports Med. 47, 533–544. doi: 10.1007/s40279-016-0591-0
Jobson, S. A., Passfield, L., Atkinson, G., Barton, G., and Scarf, P. (2009). The analysis and utilization of cycling training data. Sports Med. 39, 833–844. doi: 10.2165/11317840-000000000-00000
Kaier, E., Cromer, L. D., Johnson, M. D., Strunk, K., and Davis, J. L. (2015). Perceptions of mental illness stigma: comparisons of athletes to nonathlete peers. J. Coll. Stud. Dev. 56, 735–739. doi: 10.1353/csd.2015.0079
Kallus, W., and Kellmann, M. (2016). The Recovery-Stress. Questionnaires: User Manual . Frankfurt: Pearson Assessment & Information GmbH.
Kenttä, G., and Hassmén, P. (1998). Overtraining and recovery: a conceptual model. Sports Med. 26, 1–16. doi: 10.2165/00007256-199826010-00001
Kraemer, W. J., and Fleck, S. J. (2018). Optimizing Strength Training: Designing Nonlinear Periodization Workouts . Champaign: Human Kinetics. Available online at: https://public.ebookcentral.proquest.com/choice/publicfullrecord.aspx?p=5730619 (accessed January 30, 2020).
Lamberts, R. P., Swart, J., Capostagno, B., Noakes, T. D., and Lambert, M. I. (2009). Heart rate recovery as a guide to monitor fatigue and predict changes in performance parameters: heart rate recovery to monitor of performance. Scand. J. Med. Sci. Sports 20, 449–457. doi: 10.1111/j.1600-0838.2009.00977.x
Lavallée, L., and Flint, F. (1996). The relationship of stress, competitive anxiety, mood state, and social support to athletic injury. J. Athl. Train. 31, 296–299.
Li, C., Ivarsson, A., Lam, L. T., and Sun, J. (2019). Basic psychological needs satisfaction and frustration, stress, and sports injury among university athletes: a four-wave prospective survey. Front. Psychol. 10:665. doi: 10.3389/fpsyg.2019.00665
Li, H., Moreland, J. J., Peek-Asa, C., and Yang, J. (2017). Preseason anxiety and depressive symptoms and prospective injury risk in collegiate athletes. Am. J. Sports Med. 45, 2148–2155. doi: 10.1177/0363546517702847
López de Subijana, C., Barriopedro, M., and Conde, E. (2015). Supporting dual career in Spain: Elite athletes' barriers to study. Psychol. Sport Exerc. 21, 57–64. doi: 10.1016/j.psychsport.2015.04.012
Lukaski, H. C. (2004). Vitamin and mineral status: effects on physical performance. Nutrition 20, 632–644. doi: 10.1016/j.nut.2004.04.001
Magalhães, J., Rebelo, A., Oliveira, E., Silva, J. R., Marques, F., and Ascensão, A. (2010). Impact of loughborough intermittent shuttle test versus soccer match on physiological, biochemical and neuromuscular parameters. Eur. J. Appl. Physiol. 108, 39–48. doi: 10.1007/s00421-009-1161-z
Maloney, M. T., and McCormick, R. E. (1993). An examination of the role that intercollegiate athletic participation plays in academic achievement: athletes' feats in the classroom. J. Hum. Resour. 28:555. doi: 10.2307/146160
Mann, J. B., Bryant, K. R., Johnstone, B., Ivey, P. A., and Sayers, S. P. (2016). Effect of physical and academic stress on illness and injury in division 1 college football players. J. Strength Cond. Res. 30, 20–25. doi: 10.1519/JSC.0000000000001055
Mann, J. B., Ivey, P. A., and Sayers, S. P. (2015). Velocity-based training in football. Strength Cond. J. 37, 52–57. doi: 10.1519/SSC.0000000000000177
Marino, F. E., (ed.). (2011). Regulation of Fatigue in Exercise . Hauppauge, NY: Nova Science Publishers.
Martin, D. T., and Andersen, M. B. (2000). Heart rate-perceived exertion relationship during training and taper. J. Sports Med. Phys. Fitness 40, 201–208.
Massey, C. D., Maneval, M. W., Phillips, J., Vincent, J., White, G., and Zoeller, B. (2002). An analysis of teaching and coaching behaviors of elite strength and conditioning coaches. J. Strength Cond. Res. 16, 456–460. doi: 10.1519/00124278-200208000-00019
Massey, C. D., Schwind, J. J., Andrews, D. C., and Maneval, M. W. (2009). An analysis of the job of strength and conditioning coach for football at the division II Level. J. Strength Cond. Res. 23, 2493–2499. doi: 10.1519/JSC.0b013e3181bbe9b6
Massey, C. D., Vincent, J., and Maneval, M. (2004). Job analysis of college division I-A football strength and conditioning coaches. J. Strength Cond. Res. 18, 19–25. doi: 10.1519/1533-4287(2004)018<0019:jaocdi>2.0.co;2
Mclean, S. G., and Samorezov, J. E. (2009). Fatigue-induced ACL injury risk stems from a degradation in central control. Med. Sci. Sport Exerc. 41, 1662–1673. doi: 10.1249/MSS.0b013e31819ca07b
Miller, P. S., and Kerr, G. (2002). The athletic, academic and social experiences of intercollegiate student athletes. J. Sport Behav. 25, 346–367.
Moreland, J. J., Coxe, K. A., and Yang, J. (2018). Collegiate athletes' mental health services utilization: A systematic review of conceptualizations, operationalizations, facilitators, and barriers. J. Sport Health Sci. 7, 58–69. doi: 10.1016/j.jshs.2017.04.009
Morgan, W. P., Brown, D. R., Raglin, J. S., O'Connor, P. J., and Ellickson, K. A. (1987). Psychological monitoring of overtraining and staleness. Br. J. Sports Med. 21, 107–114. doi: 10.1136/bjsm.21.3.107
Morton, R. H., Fitz-Clarke, J. R., and Banister, E. W. (1990). Modeling human performance in running. J. Appl. Physiol. 69, 1171–1177. doi: 10.1152/jappl.1990.69.3.1171
Nässi, A., Ferrauti, A., Meyer, T., Pfeiffer, M., and Kellmann, M. (2017). Psychological tools used for monitoring training responses of athletes. Perform. Enhanc. Health 5, 125–133. doi: 10.1016/j.peh.2017.05.001
NCAA (2014). Mind Body and Sport: Understanding and Supporting Student-Athlete Mental Wellness. Independent Publisher . Available online at: https://books.google.com/books?id=JA-5rQEACAAJ (accessed October 01, 2019).
Pareja-Blanco, F., Rodríguez-Rosell, D., Sánchez-Medina, L., Gorostiaga, E., and González-Badillo, J. (2014). Effect of movement velocity during resistance training on neuromuscular performance. Int. J. Sports Med. 35, 916–924. doi: 10.1055/s-0033-1363985
Pareja-Blanco, F., Rodríguez-Rosell, D., Sánchez-Medina, L., Sanchis-Moysi, J., Dorado, C., Mora-Custodio, R., et al. (2017). Effects of velocity loss during resistance training on athletic performance, strength gains and muscle adaptations. Scand. J. Med. Sci. Sports 27, 724–735. doi: 10.1111/sms.12678
Paule, A. L., and Gilson, T. A. (2010). Current collegiate experiences of big-time, non-revenue, NCAA athletes. J. Intercoll. Sport 3, 333–347. doi: 10.1123/jis.3.2.333
Petrie, T. A., Deiters, J., and Harmison, R. J. (2014). Mental toughness, social support, and athletic identity: Moderators of the life stress–injury relationship in collegiate football players. Sport Exerc. Perform. Psychol. 3, 13–27. doi: 10.1037/a0032698
Plews, D. J., Laursen, P. B., Stanley, J., Kilding, A. E., and Buchheit, M. (2013). Training adaptation and heart rate variability in elite endurance athletes: opening the door to effective monitoring. Sports Med. 43, 773–781. doi: 10.1007/s40279-013-0071-8
Podlog, L., and Eklund, R. C. (2007). Professional coaches' perspectives on the return to sport following serious injury. J. Appl. Sport Psychol. 19, 207–225. doi: 10.1080/10413200701188951
Pyne, D. B., and Martin, D. T. (2011). “Fatigue – insights from individual and team sports.” in Regulation of Fatigue in Exercise , ed F. E. Marino, (New York, NY: Nova Publishers), 177–186.
Radcliffe, J. N., Comfort, P., and Fawcett, T. (2015). Psychological strategies included by strength and conditioning coaches in applied strength and conditioning: J. Strength . Cond. Res. 29, 2641–2654. doi: 10.1519/JSC.0000000000000919
Reardon, C. L., Hainline, B., Aron, C. M., Baron, D., Baum, A. L., Bindra, A., et al. (2019). Mental health in elite athletes: international olympic committee consensus statement 2019. Br. J. Sports Med. 53, 667–699. doi: 10.1136/bjsports-2019-100715
Robson-Ansley, P. J., Gleeson, M., and Ansley, L. (2009). Fatigue management in the preparation of Olympic athletes. J. Sports Sci. 27, 1409–1420. doi: 10.1080/02640410802702186
Rushall, B. S. (1990). A tool for measuring stress tolerance in elite athletes. J. Appl. Sport Psychol. 2, 51–66. doi: 10.1080/10413209008406420
Saw, A. E., Main, L. C., and Gastin, P. B. (2016). Monitoring the athlete training response: subjective self-reported measures trump commonly used objective measures: a systematic review. Br. J. Sports Med. 50:281. doi: 10.1136/bjsports-2015-094758
Scott, B. M., Paskus, T. S., Miranda, M., Petr, T. A., and McArdle, J. J. (2008). In-season vs. out-of-season academic performance of college student-athletes . J. Intercoll. Sport 1, 202–226. doi: 10.1123/jis.1.2.202
Selye, H., (ed.). (1976). The Stress of Life . New York, NY : McGraw-Hill.
Shanley, E., Michener, L., Ellenbecker, T., and Rauh, M. (2012). Shoulder range of motion, pitch count, and injuries among interscholastic female softball pitchers: a descriptive study. Int. J. Sports Phys. Ther. 7, 548–557.
Soligard, T., Schwellnus, M., Alonso, J.-M., Bahr, R., Clarsen, B., Dijkstra, H. P., et al. (2016). How much is too much? (Part 1) International Olympic Committee consensus statement on load in sport and risk of injury. Br. J. Sports Med. 50, 1030–1041. doi: 10.1136/bjsports-2016-096581
Starling, L. T., Nellemann, S., Parkes, A., and Lambert, M. I. (2019). The Fatigue and Fitness Test for Teams (FFITT): a practical option for monitoring athletes in a team as individuals. Eur. J. Sport Sci . 20, 1–9. doi: 10.1080/17461391.2019.1612951
Statler, T., and DuBois, A. (2016). “Psychology of athletic preparation and performance,” in Essentials of Strength Training and Conditioning , eds. G. Haff, and N. T. Triplett (Champaign, IL: Human Kinetics), 155–172.
Sweet, T. W., Foster, C., McGuigan, M. R., and Brice, G. (2004). Quantitation of resistance training using the session rating of perceived exertion method. J. Strength Cond. Res. 18:796. doi: 10.1519/14153.1
Szivak, T. K., and Kraemer, W. J. (2015). Physiological readiness and resilience: pillars of military preparedness. J. Strength Cond. Res. 29, S34–S39. doi: 10.1519/JSC.0000000000001073
Taylor, K.-L., Chapman, D., Cronin, J., Newton, M., and Gill, N. (2012). Fatigue monitoring in high performance sport: a survey of current trends. J. Aust. Strength Cond. 20, 12–23.
Thornton, H. R., Delaney, J. A., Duthie, G. M., and Dascombe, B. J. (2019). Developing athlete monitoring systems in team sports: data analysis and visualization. Int. J. Sports Physiol. Perform. 14, 698–705. doi: 10.1123/ijspp.2018-0169
Thorpe, R. T., Atkinson, G., Drust, B., and Gregson, W. (2017). Monitoring fatigue status in elite team-sport athletes: implications for practice. Int. J. Sports Physiol. Perfrom. 12, S2-27-S2–34. doi: 10.1123/ijspp.2016-0434
Torres-Ronda, L., and Schelling, X. (2017). Critical process for the implementation of technology in sport organizations. Strength Cond. J. 39, 54–59. doi: 10.1519/SSC.0000000000000339
Twist, C., and Highton, J. (2013). Monitoring fatigue and recovery in rugby league players. Int. J. Sports Physiol. Perform. 8, 467–474. doi: 10.1123/ijspp.8.5.467
Wallace, L. K., Slattery, K. M., and Coutts, A. J. (2009). The ecological validity and application of the session-rpe method for quantifying training loads in swimming. J. Strength Cond. Res. 23, 33–38. doi: 10.1519/JSC.0b013e3181874512
Williams, J. M., and Andersen, M. B. (1998). Psychosocial antecedents of sport injury: review and critique of the stress and injury model. J. Appl. Sport Psychol. 10, 5–25. doi: 10.1080/10413209808406375
Yang, J., Peek-Asa, C., Corlette, J. D., Cheng, G., Foster, D. T., and Albright, J. (2007). Prevalence of and risk factors associated with symptoms of depression in competitive collegiate student athletes. Clin. J. Sport Med. 17, 481–487. doi: 10.1097/JSM.0b013e31815aed6b
Keywords: stress, load management, academic stress, stress management, injury
Citation: Lopes Dos Santos M, Uftring M, Stahl CA, Lockie RG, Alvar B, Mann JB and Dawes JJ (2020) Stress in Academic and Athletic Performance in Collegiate Athletes: A Narrative Review of Sources and Monitoring Strategies. Front. Sports Act. Living 2:42. doi: 10.3389/fspor.2020.00042
Received: 05 October 2019; Accepted: 30 March 2020; Published: 08 May 2020.
Reviewed by:
Copyright © 2020 Lopes Dos Santos, Uftring, Stahl, Lockie, Alvar, Mann and Dawes. This is an open-access article distributed under the terms of the Creative Commons Attribution License (CC BY) . The use, distribution or reproduction in other forums is permitted, provided the original author(s) and the copyright owner(s) are credited and that the original publication in this journal is cited, in accordance with accepted academic practice. No use, distribution or reproduction is permitted which does not comply with these terms.
*Correspondence: J. Bryan Mann, Bmann@miami.edu
Log in using your username and password
- Search More Search for this keyword Advanced search
- Latest content
- Current issue
- For authors
- New editors
- BMJ Journals More You are viewing from: Google Indexer
You are here
- Volume 58, Issue 11
- Strength, power and aerobic capacity of transgender athletes: a cross-sectional study
- Article Text
- Article info
- Citation Tools
- Rapid Responses
- Article metrics

- http://orcid.org/0000-0001-7412-1188 Blair Hamilton 1 , 2 ,
- http://orcid.org/0009-0005-9553-3081 Andrew Brown 2 ,
- http://orcid.org/0009-0007-0957-5002 Stephanie Montagner-Moraes 2 ,
- http://orcid.org/0000-0001-9483-3262 Cristina Comeras-Chueca 3 ,
- http://orcid.org/0000-0001-8609-2812 Peter G Bush 2 ,
- http://orcid.org/0000-0002-8526-9169 Fergus M Guppy 4 ,
- http://orcid.org/0000-0001-6210-2449 Yannis P Pitsiladis 5 , 6
- 1 School of Sport and Health Sciences , University of Brighton , Brighton , UK
- 2 School of Applied Sciences University , Brighton , UK
- 3 Health Sciences Faculty , Universidad San Jorge , Zaragoza , Spain
- 4 Heriot-Watt University , Edinburgh , UK
- 5 Department of Movement, Human and Health Sciences , University of Rome ‘Foro Italico’ , Rome , Italy
- 6 Department of Sport, Physical Education and Health , Hong Kong Baptist University , Hong Kong , Hong Kong SAR
- Correspondence to Professor Yannis P Pitsiladis, Department of Sport, Physical Education and Health, Hong Kong Baptist University, Hong Kong, Hong Kong SAR; ypitsiladis{at}hkbu.edu.hk
Objective The primary objective of this cross-sectional study was to compare standard laboratory performance metrics of transgender athletes to cisgender athletes.
Methods 19 cisgender men (CM) (mean±SD, age: 37±9 years), 12 transgender men (TM) (age: 34±7 years), 23 transgender women (TW) (age: 34±10 years) and 21 cisgender women (CW) (age: 30±9 years) underwent a series of standard laboratory performance tests, including body composition, lung function, cardiopulmonary exercise testing, strength and lower body power. Haemoglobin concentration in capillary blood and testosterone and oestradiol in serum were also measured.
Results In this cohort of athletes, TW had similar testosterone concentration (TW 0.7±0.5 nmol/L, CW 0.9±0.4 nmol/), higher oestrogen (TW 742.4±801.9 pmol/L, CW 336.0±266.3 pmol/L, p=0.045), higher absolute handgrip strength (TW 40.7±6.8 kg, CW 34.2±3.7 kg, p=0.01), lower forced expiratory volume in 1 s:forced vital capacity ratio (TW 0.83±0.07, CW 0.88±0.04, p=0.04), lower relative jump height (TW 0.7±0.2 cm/kg; CW 1.0±0.2 cm/kg, p<0.001) and lower relative V̇O 2 max (TW 45.1±13.3 mL/kg/min/, CW 54.1±6.0 mL/kg/min, p<0.001) compared with CW athletes. TM had similar testosterone concentration (TM 20.5±5.8 nmol/L, CM 24.8±12.3 nmol/L), lower absolute hand grip strength (TM 38.8±7.5 kg, CM 45.7±6.9 kg, p = 0.03) and lower absolute V̇O 2 max (TM 3635±644 mL/min, CM 4467±641 mL/min p = 0.002) than CM.
Conclusion While longitudinal transitioning studies of transgender athletes are urgently needed, these results should caution against precautionary bans and sport eligibility exclusions that are not based on sport-specific (or sport-relevant) research.
Data availability statement
Data are available on reasonable request.
This is an open access article distributed in accordance with the Creative Commons Attribution Non Commercial (CC BY-NC 4.0) license, which permits others to distribute, remix, adapt, build upon this work non-commercially, and license their derivative works on different terms, provided the original work is properly cited, appropriate credit is given, any changes made indicated, and the use is non-commercial. See: http://creativecommons.org/licenses/by-nc/4.0/ .
https://doi.org/10.1136/bjsports-2023-108029
Statistics from Altmetric.com
Request permissions.
If you wish to reuse any or all of this article please use the link below which will take you to the Copyright Clearance Center’s RightsLink service. You will be able to get a quick price and instant permission to reuse the content in many different ways.
WHAT IS ALREADY KNOWN ON THIS TOPIC
There is currently a lack of laboratory data on strength, power and V̇O 2 max from transgender athlete populations.
WHAT THIS STUDY ADDS
This research compares laboratory measures of strength, power and V̇O 2 max of transgender male and female athletes to their cisgender counterparts.
Transgender women athletes demonstrated lower performance than cisgender women in the metrics of forced expiratory volume in 1 s:forced vital capacity ratio, jump height and relative V̇O 2 max.
Transgender women athletes demonstrated higher absolute handgrip strength than cisgender women, with no difference found relative to fat-free mass or hand size.
HOW THIS STUDY MIGHT AFFECT RESEARCH, PRACTICE, OR POLICY
This study provides sport governing bodies with laboratory-based performance-related data from transgender athletes.
Longitudinal studies are needed to confirm if these results are a direct result of gender affirmation hormone therapy.
Sports-specific studies are necessary to inform policy-making.
Introduction
Transgender athletes can experience conflict between the gender that they were assigned and their experienced gender. 1 The question of integrating transgender athletes into their affirmed gender categories is becoming more prominent, with sports’ governing bodies using varied approaches, from bans on transgender women in the female category 2 requiring the reduction of testosterone in the female category for some time 3 to self-identification into the athletes chosen category. 4
As part of gender affirmation hormone therapy (GAHT), some transgender women undergo testosterone suppression (target ≤1.8 nmol/L 5 ) coupled with oestrogen supplementation (target 400–600 pmol/L 5 ), while some transgender men undergo testosterone supplementation (National Health Service (NHS, UK) target 15–20 nmol/L, 6 Endocrine Society Target 11–34.7 nmol/L 7 ). Testosterone is known to impact sporting performances, with differences in circulating testosterone concentration between cisgender men (CM) and women proposed to explain most of the laboratory-measured differences in sports performance. 8 9 GAHT of transgender men and women alters the body composition of transgender athletes via testosterone-mediated effects on fat-free mass 8 and oestrogens on subcutaneous fat distribution 9 and maintenance of muscle mass. 10 An often-held assumption against transgender women athletes competing in the female category of sport is that transgender women have benefited from a high testosterone concentration from assigned male-at-birth puberty until the administration of GAHT that cannot be mitigated 11 and that cisgender women competitors are unable to achieve similar benefits naturally. 12 To date, this assumption has yet to be tested and confirmed in transgender athlete cohorts. The low serum testosterone concentrations from an assigned female-at-birth puberty would hypothetically not give transgender men the competitive advantages of higher testosterone concentrations over CM, and this viewpoint is reflected in the current inclusion sports policies for transgender men. 2
Lab-derived data on a cohort of transgender athletes, as requested in article 6.1b of the International Olympic Committee Framework On Fairness, Inclusion And Non-Discrimination based on Gender Identity and Sex Variations, 4 must be generated to better inform a decision-making process. 13 Therefore, the primary aim of this study was to compare cardiorespiratory fitness, strength and body composition of transgender women and men athletes to that of matched cisgender cohorts.
Study design
This cross-sectional study involved a single visit to the laboratory at the School of Applied Sciences, University of Brighton, UK. Each participant arrived at ~9:00 hours. after an overnight fast and departed from testing at ~15:00 hours. The complete study design can be found in the study protocol, available as a preprint. 14
Recruitment
Following ethical approval (ref: 9496), 75 (19 CM, 12 transgender men, 23 transgender women and 21 cisgender women) participants were recruited through social media advertising on Meta Platforms (Facebook and Instagram, Meta Platforms, California, USA) and X (Twitter, California, USA). Following the initial response, all participants were provided with the participant information sheet by email at least 7 days before being invited to travel to the laboratory, with further oral information about the study procedures and written informed consent provided on their visit to the laboratory.
Participants and eligibility criteria
Participants were required to participate in competitive sports or undergo physical training at least three times per week. Following written consent, participants were asked to record their last four training sessions and self-rate their training intensity for each session on a scale of 1–10 (10=maximum intensity). The mean of the four sessions was recorded to represent the athletes’ training intensity. The transgender athletes must have completed ≥1 year of GAHT, voluntarily disclosed during consent and verified during blood test analysis. The full inclusion/exclusion criteria can be found in the study protocol, available as a preprint. 14 Two cisgender women and one transgender man could not provide blood samples and were consequently excluded from all analyses as their endocrine profiles could not be verified. Furthermore, two transgender women and one cisgender woman were excluded from all analyses due to testosterone concentrations exceeding recommended female testosterone concentrations (2.7 nmol/L 15 ).
Laboratory assessments
Blood sampling and analysis.
Prior to venous blood sampling, haemoglobin concentration ((Hb)) was sampled via the third drop of a Unistik 3 Comfort lancet (Owen Mumford, Woodstock, UK) finger prick capillary blood sample analysed immediately using a HemoCue 201+ (HemoCue AB, Ängelholm, Sweden). Capillary blood was used for (Hb) analysis for practical reasons such as ease of use. It is important to note that the HemoCue 201+used in the present study is expected to yield higher (Hb) values than venous blood. 16 After capillary sampling, one 10 mL whole venous blood sample was collected from an antecubital vein into a BD serum tube (Becton, Dickinson and Company, Wokingham, Berkshire, UK) for serum extraction. Once collected, the tubes were left at room temperature (18°C±5°C) for 1 hour and then stored in a fridge (3°C±2°C) for up to 4 hours before being centrifuged (PK 120 centrifuge, ALC, Winchester, Virginia, USA) using a T515 rotor at 1300G for 10 min at 4°C, before storage at −80°C until analysis. Before analysis, the samples were stored between −25°C and −15°C, thawed at room temp until liquid, vortexing to remix samples, centrifuged at 2876G for 8 min to remove any precipitant and then analysed for participant’s testosterone and oestradiol concentrations on an immunoassay analyser (Roche Cobas 8000 e801, Roche Diagnostics, Burgess Hill, UK).
Body composition and bone mass
Participants’ body mass was measured (OMRON Healthcare, Kyoto, Japan) while participants were lightly dressed, representing clothed body mass. Body composition and bone mass were measured by DXA (Horizon W, Hologic, Massachusetts, USA). Each participant underwent a whole-body, a proximal-femur and a lumbar spine scan. The participant was asked to lie on the scan bed, and the first author (BH) performed all participant placement and scanning for the three scans. Due to inbuilt assumptions of body fat percentage for the head and scanning bed area imitations, whole-body less head data are reported for the whole-body scan. Body mass index (BMI), Fat Mass Index (FMI) and Fat-Free Mass Index (FFMI) were calculated by taking the appropriate mass value and dividing it by height (m 2 ).
Lung function
Lung function was measured using a Vitalograph Alpha spirometer (Vitalograph, Kansas, USA) with an antibacterial filter and a nose clip on the bridge of the participant’s nose. Each participant was asked to perform the flow-volume-loop spirometry to test forced vital capacity (FVC), forced expiratory volume in 1 s (FEV 1 ) and peak expiratory flow. The test was repeated until a trend of declining performance occurred. The highest numeric value for each metric obtained during a test with the correct procedure was then recorded. The FEV1:FVC ratio was used to assess the presence of obstructed lung function.
Strength was measured using a handgrip dynamometer (TAKEI 5401, TAKEI Scientific Instruments, Japan). The participants’ hand sizes were also measured around the metacarpophalangeal joints of both hands prior to testing. Each hand was tested three times in sequential order of left-right to allow each hand to rest; the mean scores were taken from the three attempts for each hand.
Lower body power
Lower body power was measured with the countermovement jump on a JUM001 Jump Mat (Probotics, Alabama, USA). During the test, if the participant went beyond 45° of countermovement or the hands came off the hips, the test would be declared void for that attempt. After recording three legitimate attempts, the mean scores were recorded.
Cardiopulmonary exercise testing
Cardiopulmonary exercise testing was performed using a 95T Engage Treadmill ergometer (Life Fitness, Illinois, USA) and a COSMED QUARK (COSMED, Rome, Italy). All V̇O 2 max tests were conducted and analysed by the first author (BH) to avoid interinvestigator variability. 17 The ramp protocol of Badawy and Muaidi treadmill V̇O 2 max testing 18 was used for each V̇O 2 max test, involving gradual increases in speed every 3 min at a 1% incline. One cisgender man and two cisgender women were excluded from the analysis as they did not meet the required respiratory exchange ratio of ≥1.1 to classify the test as maximal (cisgender men (CM), n=18, transgender men (TM), n=11; cisgender women (CW) n=16; transgender women (TW), n=21).
Statistical analysis
Data meeting the assumptions of normality and homogeneity of variance were analysed using a one-way analysis of variance along with Bonferroni post hoc corrections for pairwise comparisons. Data not meeting the parametric assumptions were compared using a Kruskal-Wallis ANOVA with Dwass-Steel-Critchlow-Fligner post hoc test for multiple comparisons, with an alpha level of 0.05 for both types of analysis. Statistical analysis and presentation are consistent with the checklist for statistical assessment of medical papers statement 19 found in online supplemental files 1–3 at Hamilton et al , The Strength, Power and Aerobic Capacity of Transgender Athletes: A Cross-Sectional Study (Internet). OSF; 2023. Available from: osf.io/a684b.
Supplemental material
Equity, diversity and inclusion statement.
The author group consists of early (n=3) and senior researchers (n=3) from different disciplines and universities (n=3). Two authors are members of a marginalised community; the lead early-career author is a transgender woman, and one of the junior authors is a woman from the global south. Our study population included male and female transgender athletes from within the UK participating in competitive sports in comparison with cisgender male and female athletes participating in competitive sports; thus, findings may not be generalisable to global athlete populations.
Participant characteristics
Our investigation encompassed a diverse cohort of athletes, with endurance sports representing 36% of the athlete cohort, team sports representing 26% and power sports representing 38%. No cisgender or transgender athletes were competing at the national or international level. No significant differences were found in age (F (3–66) =1.9, p=0.14), training intensity score (χ 2 (3) =1.2, p=0.76) or length of GAHT between transgender men and transgender women (F (1–32) =0.5, p=0.48, table 1 ).
- View inline
Significant differences were found in height (F (3–66) =21.3, p<0.001), with CM being taller than transgender men (t (66) =3.8, p=0.002, table 1 ). Transgender women were also taller than transgender men (t (66) =3.3, p=0.01) and cisgender women (t (66) =6.5, p<0.001, table 1 ).
Significant differences were found in clothed mass (F (3–66) =10.6, p<0.001), with transgender women found to be heavier than cisgender women (t (66) =5.6, p<0.001, table 1 ).
BMI was also significantly different between the groups in this Study (F (3–66) =3.6, p=0.02). Transgender women athletes demonstrated higher BMI than cisgender women (t (66) =2.9, p=0.03, table 1 ), with no further differences observed.
Blood measures
There was a significant gender effect on testosterone concentration (F (3–66) =80.6, p<0.001). CM (20.5±5.8 nmol/L) exhibited significantly higher total testosterone concentration than transgender women (0.7±0.5 nmol/L, t (66) = 11.1, p<0.001, figure 1A ). Transgender men (24.8±12.3 nmol/L) had elevated total testosterone concentration compared with transgender women (t (66) =11.3) and cisgender women (0.9±0.4 nmol/L, t (66) =10.9, both p<0.001, figure 1A ). There was also a significant gender effect on oestradiol concentration (F (3−66) =7.6, p<0.001), with transgender women (742.4±801.9 pmol/L) showing higher oestradiol concentration than CM (104.3±24.8 pmol/L, t (66) =4.4 p<0.001), cisgender women (336.0±266.3 pmol/L, t (66) =2.7, p=0.045) and transgender men (150.2±59.4 pmol/L, t (66) =3.4, p=0.01, figure 1B ).
- Download figure
- Open in new tab
- Download powerpoint
Blood measures. (A) testosterone; (B) oestradiol; (C) haemoglobin. *p<0.05, **p<0.01, ***p<0.001, ****p<0.0001. CM, cisgender men; CW, cisgender women; TM, transgender men; TW, transgender women.
Transgender women’s total testosterone concentration (0.7±0.5 nmol/L) falls within the recommendations for GAHT of ≤1.8 nmol/L, 5 and oestradiol concentrations (742.4±801.9 pmol/L) exceed the target of 400–600 pmol/L 5 for GAHT. Transgender men’s testosterone concentration (24.8±12.3 nmol/L) exceeds the NHS target of 15–20 nmol/L 6 for GAHT, although not the Endocrine Society target of 11–34.7 nmol/L. 7
Differences were reported in (Hb) concentration (F (3–66) =3.3, p=0.03), although a post hoc Bonferroni analysis showed no differences between the various groups (CM 142.8±12.5 g/L; transgender men, 143.3±19.5 g/L; transgender women, 131.3±14.2 g/L; cisgender women, 133.3±12.7 g/L; figure 1C ).
DXA assessment
There was a significant gender effect on percentage fat mass (F (3–66) =6.6, p<0.001), with CM having a lower percentage fat mass than transgender women (t (66) =−4.4, p<0.001, table 2 ), with no other differences observed. A significant gender effect was also found on absolute fat mass (F (3–66) =6.6, p<0.001), with transgender women having more absolute fat mass than CM (t (66) =3.8, p=0.002, table 2 ) and cisgender women (t (66) =3.9, p=0.002, table 2 ). FMI measures revealed a gender effect (F (3–66) =5.2, p=0.003), with transgender women found to have a higher FMI than CM (t (66) =3.7, p=0.002, table 2 ) and cisgender women (t (66) =2.8, p=0.04, table 2 ). Android to gynoid ratio analysis (F (3–66) =10.7, p<0.001) revealed cisgender women had a lower ratio than transgender men (t (66) =−2.9, p=0.03, table 2 ), and transgender women (t (66) =−4.0, p=0.001, table 2 ).
Body composition, BMD data, handgrip strength, lower anaerobic power and cardiopulmonary exercise testing
Fat-free mass
There was a significant gender effect on absolute fat-free mass (F (3–66) =24.6, p<0.001), with CM having significantly more absolute fat-free mass than transgender men (t (66) =3.5, p=0.01, table 2 ). Cisgender women had less absolute fat-free mass than transgender men (t (66) =−3.5, p=0.01, table 2 ) and transgender women (t (66) =−6.6, p<0.001, table 2 ). No gender-based effects were found when comparing transgender women athletes to cisgender women athletes, or transgender men athletes to CM athletes in the measures of FFMI (F (3–66) =3.7, p=0.02, table 2 ), percentage of fat-free mass (F (3–66) =2.4, p=0.08, table 2 ) or appendicular FFMI (F (3–66) =5.1, p=0.003, table 2 ).
Bone mineral density
No differences in whole-body bone mineral density (BMD) (F (3–66) =4.6, p=0.01), femoral neck BMD (F (3–66) =1.0, p=0.39, table 2 ), total proximal femur BMD (F (3–66) =1.5, p=0.22, table 2 ) or total lumbar spine BMD (F (3–66) =0.4, p=0.78, table 2 ) were found between transgender athletes and cisgender athletes ( table 2 ).
Lung function data for all groups can be found in table 2 . FEV 1 had an effect of gender (F (3–66) =14.7, p<0.001), with CM having greater FEV 1 than transgender men (t (66) = 4.5, p<0.001, figure 2A ). Transgender women also had greater FEV 1 than cisgender women (t (66) =4.2, p<0.001, figure 2A ) and transgender men (t (66) =2.9, p=0.03, figure 2A ). There was a similar effect of gender on FVC (F (3–66) =21.6, p<0.001, figure 2B ), with CM having greater FVC than transgender men (t (66) =5.2, p<0.001, figure 2B ). Transgender women also had greater FVC than cisgender women (t (66) =5.6, p<0.001, figure 2B ) and transgender men (t (66) =4.0, p=0.001, figure 2B ). A significant effect of gender was also seen on the FEV 1 :FVC ratio (F (3–66) =3.3, p=0.03 figure 2C ), with transgender women showing a reduced FEV 1 :FVC ratio compared with cisgender women (t (66) =−2.8, p=0.04, figure 2C ) with no differences observed in transgender or CM. Peak expiratory flow (F (3–66) =5.5, p=0.002) had a minor gender-based effect, with cisgender women having lower peak expiratory flow than transgender women (t (66) −3.0, p=0.02, figure 2D ).
Lung function measures. (A) Forced rxpiratory volume in 1 s (FEV 1 ); (B) forced vital capacity (FVC) (C) modified Tiffeneau-Pinelli Index (FEV 1 :FVC); (D) peak expiratory flow (PEF). *p<0.05, **p<0.01, ***p<0.001, ****p<0.0001. CM, cisgender men; CW, cisgender women; TM, transgender men; TW, transgender women.
Handgrip strength
Handgrip strength data can be found in table 2 . Absolute right handgrip strength was significantly different between the groups (F (3–66) =10.5, p<0.001), with CM having greater absolute right handgrip strength than transgender men (t (66) =2.9, p=0.03, figure 3B ). Transgender women also had greater absolute right handgrip strength than cisgender women (t (66) =3.2, p=0.01, figure 3B ). Absolute left handgrip was significantly different between the groups (F (3–66) =8.6, p<0.001). However, no differences were found between transgender and cisgender athletes ( figure 3A ). There was no effect on the right (F (3–66) =0.8, p=0.53, figure 3F ) or left-hand grip strength (F (3–66) =1.0, p=0.39, figure 3E ) relative to fat-free mass, nor was there any gender effect on the right (F (3–66) =1.6, p=0.20, figure 3D ) or left-hand grip-strength (F (3–66) =2.1, p=0.11) relative to hand size.
Absolute and relative handgrip strength (GS) measures. (A) Absolute strength (right hand); B) Absolute strength (left hand) (C) relative strength to hand size (right hand); (D) relative strength to hand size (left hand); (E) relative strength to fat-free mass (FFM) (right hand); (F) relative strength to fat-free mass (left hand). *p<0.05, ***p<0.001, ****p<0.0001. CM, cisgender men; CW, cisgender women; TM, transgender men; TW, transgender women.
Lower body anaerobic power
Lower body anaerobic power data are shown in table 2 . Gender had a significant effect on absolute countermovement jump height (F (3–66) =7.2, p<0.001), with CM having greater absolute jump height than transgender women (t (66) =4.5, p<0.001, figure 4A ). A significant effect of gender was found in countermovement jump height relative to fat-free mass (F (3–66) =10.1, p<0.001, figure 4B ), with transgender women found to have lower countermovement jump height relative to fat-free mass than both cisgender women (t (66) =−5.3, p<0.001) and transgender men (t (66) =–3.2, p=0.01, figure 4B ).
Absolute and relative anaerobic power measures. (A) Absolute CMJ height; B) Relative CMJ height to fat-free mass (FFM); (C) absolute peak power; (D) relative peak power to FFM; (E) absolute average power; (F) relative average power to FFM. *p<0.05, **p<0.01, ***p<0.001, ****p<0.0001. CM, cisgender men; CMJ, Counter Movement Jump; CW, cisgender women; TM, transgender men; TW, transgender women.
There was a significant difference in absolute peak power (F (3–66) =8.7, p<0.001), with cisgender women having reduced peak power compared with transgender men (t (66) =−3.3, p=0.01) and transgender women (t (66) =−3.6, p=0.004, figure 4C ). Peak power relative to fat-free mass had a more negligible gender effect (F (3–66) =4.2, p=0.01), with no difference in peak power relative to fat-free mass found between transgender and cisgender athletes ( figure 4D ).
There was a significant gender effect of absolute average power (F (3–66) =5.9, p=0.001), with cisgender women having reduced absolute average power compared with transgender men (t (66) =–3.1, p=0.02, figure 4E ). There was no effect of gender on average power relative to fat-free mass (F (3–66) =2.6, p=0.06, figure 4F ).
Cardiopulmonary exercise testing data are shown in table 2 . A significant effect of gender was found on absolute V̇O 2 max (F (3–62) =14.1, p<0.001) with CM having greater absolute V̇O 2 max than transgender men (t (66) =3.8, p=0.002, figure 5A ) and transgender women (t (66) =4.3, p<0.001, figure 5A ). Relative V̇O 2 max to body mass also showed a significant gender effect (F (3–62) =9.8, p<0.001) with transgender women having lower relative V̇O 2 max than CM (t (66) =–5.3, p<0.001, figure 5B ) and cisgender women (t (66) =−3.3, p=0.01, figure 5B ). No significant gender effect was found on the measure of V̇O 2 max relative to fat-free mass (F (3–62) =2.0, p=0.12).
Absolute and relative cardiopulmonary exercise testing measures. (A) Absolute V̇O 2 max; (B) relative V̇O 2 max to body weight; (C) absolute anaerobic threshold (AT); (D) anaerobic threshold (%V̇O 2 max); (E) relative anaerobic threshold relative to body mass; (F) AT relative to at-free mass (FFM). *p<0.05, **p<0.01, ***p<0.001, ****p<0.0001. CM, cisgender men; CW, cisgender women; TM, transgender men; TW, transgender women.
Gender affected the absolute anaerobic threshold (F (3–62) =14.1, p<0.001), with cisgender (3924±628 mL/min) men having a higher absolute anaerobic threshold than transgender men (3089±546 mL/min, t (66) =4.2, p<0.001, figure 5C ), and transgender women (3122±438 mL/min, t (66) =4.8, p<0.001, figure 5C ). No significant gender effect was found on the measure of anaerobic threshold as a percentage of V̇O 2 max (F (3–62) =0.8, p=0.51, figure 5D ). A gender effect was also seen on the anaerobic threshold relative to body mass (F (3–62) =10.7, p<0.001), with transgender women (38.3±6.6 mL/kg/min) showing a lower relative anaerobic threshold than both cisgender women (47.2±6.1 mL/kg/min, t (66) =–3.3, p=0.01, figure 5E ) and CM (52.2±9.5 mL/kg/min, t (66) =−5.4, p<0.001, figure 5E ). CM also showed a higher relative anaerobic threshold than transgender men (42.1±9.9 mL/kg/min, t (66) =3.3, p=0.01, figure 5E ). Anaerobic threshold relative to fat-free mass also had a small gender effect (F (3–62) =3.2, p=0.03), with transgender women (60.8±12.2 mL/kg FFM /min) having a lower anaerobic threshold relative to fat-free mass than CM (71.2±13.3 mL/kg FFM /min, t (66) =−2.8, p=0.045, figure 5F ).
The results presented in this study provide valuable insights into laboratory-based performance-related metrics of gender-diverse athletes participating in competitive sports. Given the primary aim of GAHT, 20 it is noteworthy that although this study is cross-sectional in design, transgender women’s oestradiol was higher than that of cisgender women ( figure 1B ). The presence of outliers affecting transgender women’s oestrogen concentration ( figure 1B ) is evident. This underscores that transgender women in this cohort of athletes exhibit a distinct endocrine profile from CM and share a similar endocrine profile with cisgender women, whom many transgender women aim to integrate into a sporting category. One of the most noticeable disparities between gender groups was in height and mass ( table 1 ), with CM and transgender women being taller and heavier than their cisgender and transgender counterparts ( table 1 ). Body composition measures (fat mass % and fat-free mass %, table 2 ) between transgender women and cisgender women found no difference. However, transgender women are, on average as a cohort taller and heavier.
In this cohort, the average difference in haemoglobin (Hb) between cisgender women and CM athletes was 7% ( figure 1C ), lower than previously described (12% 8 ). Notably, the (Hb) profiles of all the athlete groups were not significantly different, concurring with earlier research 21 and contradicting research in sedentary populations. 22 (Hb) is crucial in O 2 transport 23 and vital for endurance sports performance, 24 with O 2 delivery to the tissues a limiting factor in V̇O 2 max attainment. 25 The lack of differences in (Hb) is consistent with the lack of observed difference in absolute V̇O 2 max between transgender women, transgender men and cisgender women in this cohort. However, as cardiac output, the most crucial variable influencing V̇O 2 max 25 was not assessed in the present study, a more comprehensive mechanistic explanation for the similar maximal aerobic capacity between groups cannot be provided.
No differences in BMD were observed between transgender and cisgender women athletes in this study ( table 2 ), despite prior research hypothesising that transgender women athletes have a significant BMD advantage over cisgender women. 11 The sample size for each gender was n<30 participants and may be insufficient to characterise BMD differences reliably. Exercise has been shown to have a protective effect on BMD in CM 26 and CW, 27 and our results suggest a protective effect of exercise in transgender women, given that there is evidence of low BMD in transgender women with low weekly sports activity. 28 Nevertheless, the results suggest the complexity of bone health in athlete populations and the need for a more comprehensive assessment to understand the long-term impact of GAHT on transgender athletes’ BMD.
The differences observed in body composition in this population ( table 2 ) indirectly show the potential role of androgens in body composition, owing to the role of oestradiol in fat accumulation 29 and transgender women’s oestradiol concentrations ( figure 1B ) and fat mass ( table 2 ) being greater than all other groups. Body composition differences may have implications for sports that prioritise exercise economy, 30 defined as the average V̇O 2 relative to body mass between submaximal intensities, 31 as athletes with a higher fat mass percentage will present with a lower exercise economy owing to the increased O 2 cost of exercise. 32 The android-to-gynoid ratio analysis ( table 2 ) suggests that hormone therapy ( figure 1A,B ) influences differences in fat distribution patterns. However, fat distribution patterns of the present transgender female athlete cohort ( table 2 ) do not reach ratios previously reported in cisgender female populations (0.8). 33 Understanding these variations is essential for evaluating performance in sports where body composition is a determining factor, for example, weightlifting or boxing.
Cisgender women had lower absolute fat-free mass than transgender men and transgender women ( table 2 ). When analysing absolute fat and fat-free mass data ( table 2 ), these results can be affected by sample size and/or athlete diversity limitations. A purposefully designed future study with height-matched and sport-matched cisgender and transgender female athletes is crucial to understanding differences in these parameters, as they are influenced by height disparities ( table 1 ) and variations in sampled sports.
FVC, FEV 1 and FEV 1 :FVC ratio are higher in athletes than in the normal sedentary control individuals, 34 and there is no difference in all three metrics between aerobic athletes and anaerobic athletes. 35 Therefore, the lung function differences observed in figure 2A,B may be attributed to factors such as skeletal size benefiting lung capacity and function, 36 with transgender women’s FVC results ( figure 2B ) suggesting gender-affirming hormone care did not impact changing lung volumes owing to the GAHTs lack of effect on skeletal stature. 11 Transgender women showed a significantly reduced FEV 1 :FVC ratio compared with cisgender women ( figure 2C ). The FEV 1 :FVC ratio has been used as a screening index for identifying obstructive lung conditions globally, 37 as a lower FEV 1 owing to obstruction of air escaping from the lungs will reduce the FEV 1 :FVC ratio. Transgender women’s results ( figure 2C ) suggest obstructed airflow in the lungs 38 when compared with cisgender women. However, this observation of transgender women is unlikely to be pathological (<0.70), 39 as seen in chronic obstructive pulmonary disease.
Nevertheless, this reduced airflow could potentially lead to exercise-induced dyspnoea, resulting in performance limitations 40 in comparison to cisgender women. When comparing both the CM and transgender women athletes’ groups with identical heights (1.8 m, table 1 ), while both groups exhibit similar FVC, transgender women demonstrate a lower FEV 1 , leading to a reduced FEV 1 :FVC ratio compared with CM, although not significant. If there were a significant difference between CM and transgender women, our preliminary hypothesis would have attributed this divergence to testosterone suppression in transgender women. However, comparing transgender women to cisgender women who do not share similar height and or exhibit a comparable FVC, the observed differences become more complex to interpret. The possibility arises that factors beyond hormonal influences, such as varying levels of aerobic training, may contribute to the significant difference found in the FEV 1 :FVC ratio between transgender women and cisgender women. Further longitudinal investigation is required to elucidate if the causation underlying these pulmonary function disparities is indeed testosterone suppression.
Strength results in figure 3 disagree with previous literature in a non-athlete transgender cohort using the same methodology that showed transgender women and CM had significantly different absolute and relative hand grip strength. 41 Our results showed no differences in absolute strength between transgender women and CM and no difference in relative handgrip between any of the groups in this study ( figure 3 ). These results highlight the differences between athlete and sedentary populations. However, the results relative to hand size also concur with the notion that greater handgrip strength is caused by greater hand size, 42 as there were no differences in results between the four groups when normalised for hand size ( figure 3C,D ). Therefore, investigations with more accurate measures of strength are warranted in transgender athletes.
Transgender women presented lower absolute jump height than CM and lower relative jump height, normalised for fat-free mass, than transgender men and cisgender women ( figure 4 ). These results in this study cohort suggest that transgender women lack lower body anaerobic power compared with the other groups. Transgender women’s higher absolute peak power than cisgender women ( figure 4C ), coupled with higher fat mass potentially driven by higher oestradiol concentrations ( figure 1B ), suggest that transgender women had more inertia to overcome during the explosive phase of the countermovement jump, which may lead to decreased performance. However, when normalised for fat-free mass ( figure 4D ), transgender women’s peak power was lower than that of cisgender women, showing that this cohort also lacks peak power relatively, indicating that the higher fat mass may not be the primary contributing factor. Further investigations are warranted to find the causation of this poor lower anaerobic power performance in transgender women.
The lack of differences in anaerobic threshold (%V̇O 2 max, figure 5D ) suggests that the athletes in this study had a similar fitness status, which is an essential underlying finding given that CM showed greater absolute V̇O 2 max than all groups ( figure 5A ), with no differences between transgender women and cisgender women found, and transgender women exhibited lower relative V̇O 2 max compared with both CM and women ( figure 5B ). In this cohort, the finding of no statistical difference in absolute V̇O 2 max between transgender women and cisgender women contrasts the idea that transgender women’s bigger lung size ( table 2 ) is an inherent respiratory function advantage over cisgender women. 11 Both the absolute and relative V̇O 2 max differences between groups contradict one previous study in non-athlete transgender populations that found transgender women had higher absolute V̇O 2 peak and no difference in relative V̇O 2 peak compared with cisgender women. 41 This contradictory finding further highlights population differences between non-athlete and athlete cohorts while also contradicting literature hypothesising that there would be a baseline gap in aerobic capacity between transgender women and cisgender women. 11 The results in this athlete cohort warrant further research to elucidate the mechanisms behind this deviation, as they may be metabolic, as transgender women also exhibited a lower relative anaerobic threshold ( figure 5E ). The findings in table 2 reveal notable disparities in fat mass, fat-free mass, laboratory sports performance measures and hand-grip strength measures between cisgender male and transgender female athletes. These differences underscore the inadequacy of using cisgender male athletes as proxies for transgender women athletes. Therefore, based on these limited findings, we recommend that transgender women athletes be evaluated as their own demographic group, in accordance with the principles outlined in Article 6.1b of the International Olympic Committee Framework on Fairness, Inclusion and Non-Discrimination based on Gender Identity and Sex Variations. 4
Study limitations
The limitations of this study primarily relate to its cross-sectional design, making it challenging to establish causation or examine if the performance of athletes changes as a result of undergoing GAHT. Longitudinal studies are needed to examine how GAHT, and other factors impact athletes’ physiology and performance over time. Additionally, the composition of the study cohort may not fully represent the diversity of athletes in elite sports from worldwide populations. Athletes from various sporting disciplines and performance levels were included, and the athlete training intensity was self-reported. Therefore, the results may suffer from selection and recall bias. 43 The results may not apply to all levels or ages of athletes, specifically as this research did not include any adolescent athletes competing at the national or international level. The athletes participating in the present study represented a variety of different sports, and this would have undoubtedly impacted the results of the study as different sports stress different training and sports modalities. Exercise type, intensity and duration all have an impact on physiological responses and overall laboratory performance metrics. 44 The subgroups of sports that emerged were also too dissimilar to allow meaningful subgroup analysis. The complexity and difficulty of this area of activity means that while this study provides a starting point for understanding the complex physiology in GAHT and athletic performance, this study does not provide evidence that is sufficient to influence policy for either inclusion or exclusion. However, this is the first study to assess laboratory-based measures of performance in transgender athletes, and this opens up interesting avenues for replication and extension into the longitudinal effects of GAHT on athletic performance.
Future research should include more extensive and diverse samples to enhance the generalisability of findings or smaller, more specific cohorts to hone in on a particular sports discipline. However, such studies may be complex due to the low numbers of transgender athletes. The recruitment method of this study also provided a limitation as social media advertising was used rather than recruitment from a clinical provider. Social media recruitment leaves this study open to sample bias as social media advertising, although great for recruiting hard-to-reach participants for observational studies, 44 45 does not represent a clinical population in 86% of comparisons. 44 As the participants were not recruited from a clinic, this also means that the gender-affirming treatment of the transgender athletes was not controlled. For example, different testosterone suppression methods have different efficacies, 46 and future studies should consider differences in the prescribed GAHT to participants. Lastly, the participants were not screened by a clinician before participation, and any medical conditions were self-reported in the physical activity readiness questionnaire (PAR-Q). This method of medical reporting leaves the data open to self-reporting bias, which can mislead descriptive statistics and causal inferences 47 as participants’ cognitive processes, such as social desirability, can alter participants’ responses. 48 Therefore, it is recommended to use a clinic to screen and recruit participants to avoid such bias in a longitudinal study of transgender athlete sports performance.
Conclusions
This research compares transgender male and transgender female athletes to their cisgender counterparts. Compared with cisgender women, transgender women have decreased lung function, increasing their work in breathing. Regardless of fat-free mass distribution, transgender women performed worse on the countermovement jump than cisgender women and CM. Although transgender women have comparable absolute V̇O 2 max values to cisgender women, when normalised for body weight, transgender women’s cardiovascular fitness is lower than CM and women. Therefore, this research shows the potential complexity of transgender athlete physiology and its effects on the laboratory measures of physical performance. A long-term longitudinal study is needed to confirm whether these findings are directly related to gender-affirming hormone therapy owing to the study’s shortcomings, particularly its cross-sectional design and limited sample size, which make confirming the causal effect of gender-affirmative care on sports performance problematic.
Ethics statements
Patient consent for publication.
Not applicable.
Ethics approval
This study involves human participants and ethical approval for this study has been granted by the School of Applied Sciences Research Ethics Committee of the University of Brighton, Brighton, UK (Ref: 9496). Participants gave informed consent to participate in the study before taking part.
Acknowledgments
We thank Associate Professor Ada Cheung of the Department of Medicine (Austin Health) at the University of Melbourne, Australia for her valuable review of this work prior to publication.
- Arcelus J ,
- Bouman WP , et al
- World Aquatics
- USA Gymnastics
- International Olympic Committee
- National Health Service
- Hembree WC ,
- Cohen-Kettenis PT ,
- Gooren L , et al
- Handelsman DJ ,
- Hirschberg AL ,
- Alvero-Cruz JR ,
- Chilibeck P , et al
- Gharahdaghi N ,
- Phillips BE ,
- Szewczyk NJ , et al
- Hilton EN ,
- Lundberg TR
- Hamilton BR ,
- Barrett J , et al
- Hamilton B ,
- Comeras-Chueca C ,
- Bush P , et al
- Leitman SF , et al
- Popović ZB ,
- Badawy MM ,
- Mansournia MA ,
- Collins GS ,
- Nielsen RO , et al
- Malczewska-Lenczowska J ,
- Sitkowski D ,
- Orysiak J , et al
- Roberts TK ,
- French D , et al
- Schmidt W ,
- Bassett DR ,
- Staines KA ,
- Kelley GA , et al
- Hofmeister M
- Rothman MS ,
- Mareschal J ,
- Karsegard VL , et al
- Losnegard T ,
- Schäfer D ,
- V Mendonca G ,
- Imboden MT ,
- Swartz AM , et al
- Rajaure YS ,
- Budhathoki L , et al
- van der Plaat D ,
- Dharmage S , et al
- Min HK , et al
- Schiöler L ,
- Lindberg A , et al
- Anzueto A , et al
- Alvares LAM ,
- Santos MR ,
- Souza FR , et al
- Alahmari KA ,
- Kakaraparthi VN ,
- Reddy RS , et al
- Tripepi G ,
- Dekker FW , et al
- Topolovec-Vranic J ,
- Natarajan K
- Whitaker C ,
- Stevelink S ,
- Leemaqz S ,
- Ooi O , et al
- Mathiowetz N
Supplementary materials
Supplementary data.
This web only file has been produced by the BMJ Publishing Group from an electronic file supplied by the author(s) and has not been edited for content.
- Data supplement 1
- Data supplement 2
- Data supplement 3
X @BlairH_PhD
Contributors BH, FMG and YPP designed the study. Material preparation, reporting and critical revision of the work were performed by BH, PGB, FMG and YPP. Data collection was performed by CC-C, AB, SM-M and BH. BH wrote the first draft of the manuscript, and all authors critically revised subsequent versions until all authors could approve the final manuscript. YPP is the guarantor.
Funding The study has been funded by a research grant awarded by the International Olympic Committee, Lausanne, Switzerland.
Competing interests YPP is a member of the IOC Medical and Scientific Commission, which recently published articles and framework documents on the topic. BH and FMG have recently published articles on the topic on behalf of the International Federation of Sports Medicine (FIMS). All authors declare no further conflict of interest or competing interests.
Patient and public involvement Patients and/or the public were not involved in the design, or conduct, or reporting, or dissemination plans of this research.
Provenance and peer review Not commissioned; externally peer reviewed.
Supplemental material This content has been supplied by the author(s). It has not been vetted by BMJ Publishing Group Limited (BMJ) and may not have been peer-reviewed. Any opinions or recommendations discussed are solely those of the author(s) and are not endorsed by BMJ. BMJ disclaims all liability and responsibility arising from any reliance placed on the content. Where the content includes any translated material, BMJ does not warrant the accuracy and reliability of the translations (including but not limited to local regulations, clinical guidelines, terminology, drug names and drug dosages), and is not responsible for any error and/or omissions arising from translation and adaptation or otherwise.
Read the full text or download the PDF:
- Share full article
Advertisement
Supported by
N.C.A.A. Athletes’ Pay Deal Raises Questions About Future of College Sports
The landmark settlement made many wonder what the reality — and impact — of revenue-sharing plans with college athletes would look like.

By David W. Chen , Jacey Fortin and Anna Betts
Brent Jacquette knows a thing or two about college sports. A former collegiate soccer player and coach in Pennsylvania who is now an executive at a consulting firm for athletic recruiting, he’s well aware of issues surrounding pay for college athletes.
But even for an industry veteran like Mr. Jacquette, the news of the N.C.A.A.’s staggering settlement in a class-action antitrust lawsuit on Thursday came as a surprise, with more than a little anxiety. The first words that came to mind, he said, were “trepidation” and “confusion.”
And he was not alone in feeling unsettled. Interviews, statements and social media posts mere hours after the settlement was announced showed that many were uncertain and concerned about what the future of collegiate sports holds.
“These are unprecedented times, and these decisions will have a seismic effect on the permanent landscape of collegiate athletics,” Phil DiStefano, chancellor of the University of Colorado Boulder, and Rick George, the school’s athletic director, said in a statement .
If the $2.8 billion settlement is approved by a judge, it would allow for a revenue-sharing plan through which Division I athletes can be paid directly by their schools for playing sports — a first in the nearly 120-year history of the N.C.A.A. Division 1 schools would be allowed to use about $20 million a year to pay their athletes as soon as the 2025 football season.
Mr. Jacquette thought of the word “trepidation” because of the impact that the settlement, shaped by the biggest and wealthiest universities with robust football programs, could have on coaches and athletes at smaller institutions and in low-profile sports.
And “confusion," because families of college athletes might soon be overwhelmed by all the possible ways for a student to get paid. In past years, the only form of compensation that students could get was athletic scholarships. But now, with the possibility of a revenue-sharing plan and pay arrangements involving students’ name, image and likeness , they have a lot more to consider when planning their collegiate sports careers.
“As this makes big news, people see this pot of gold at the end of the tunnel,” Mr. Jacquette said.
Many questioned what the financial burdens stemming from a revenue-sharing plan would look like.
Smaller conferences, such as the Big East — which includes Georgetown, Villanova and the University of Connecticut — have voiced “strong objections ” to the settlement, worried about shouldering an unfair burden of costs involved in revenue sharing. They said schools that have higher-profile sports teams and are part of bigger conferences, which often have TV contracts and much higher revenues, should be responsible for covering more of the costs.
Even coaches at powerful athletic programs, such as the University of Florida, which is part of the Southeastern Conference, had qualms. Tim Walton, the school’s softball coach, wondered in an interview with The Associated Press what this would mean for smaller sports programs on campus, and how the university would handle sharing revenues with athletes.
Another concern from critics of the settlement was whether female athletes would be compensated fairly. The settlement did not address how they would be paid compared to men, but Title IX prohibits sex-based discrimination in educational settings.
“Oftentimes, we put all of our eggs in the revenue-generating sports, which is comprised of mostly male sports,” said Jasher Cox, athletic director of Allen University, a historically Black and Division II school in South Carolina.
But many still questioned how the settlement would pan out in practice. Terry Connor, the athletic director at Thomas More University in Kentucky, said in an interview that the reality of revenue sharing felt somewhat distant. Although his school is Division II and would not necessarily be part of a revenue-sharing plan, Mr. Connor said that this still affects college sports as a whole.
The settlement is only the latest in a series of big changes at the N.C.A.A. in recent years, Mr. Connor said, and “how we’ll have to adapt to that is going to be the big question.”
Mr. Jacquette cautioned that it was still “premature” to assess the full impact of the settlement, since the details had not been released. And Sam C. Ehrlich, a professor at Boise State University who has written extensively about college athletes, said that while the settlement was a milestone, it should not be seen as the be-all and end-all of college sports.
Pointing to the Supreme Court’s unanimous ruling in 2021 that the N.C.A.A. could not bar payments to college athletes, Mr. Ehrlich said the college sports landscape has been ever-changing. That decision paved the way for N.I.L. arrangements, or payments based on an athlete’s name, image and likeness. He also cited Johnson v. N.C.A.A. , a pending case over whether college athletes should be classified as employees, which could have profound labor and tax implications.
“This is a big moment, for sure, but it’s not like we have reached the finish line,” Mr. Ehrlich said. “I wouldn’t even necessarily go as far to say, ‘this is the climax here.’ This is still going to be ongoing for several years.”
Billy Witz contributed reporting.
David W. Chen reports on state legislatures, state level policymaking and the political forces behind them. More about David W. Chen
Jacey Fortin covers a wide range of subjects for the National desk of The Times, including extreme weather, court cases and state politics all across the country. More about Jacey Fortin
Anna Betts reports on national events, including politics, education, and natural or man-made disasters, among other things. More about Anna Betts
Inside the World of Sports
Dive deeper into the people, issues and trends shaping professional, collegiate and amateur athletics..
The N.C.A.A.’s New Era: If a judge approves, a $2.8 billion settlement would let colleges and universities pay athletes directly for the first time. How would it work ?
Bringing Back Reebok: Shaquille O’Neal pushed to be in charge of the company’s return to the hypercompetitive world of basketball sneakers. The Hall of Famer understands the stakes .
The Capital of Women’s Soccer: The success of Barcelona Femení has made the Spanish city, and the broader region of Catalonia, a laboratory for finding out what happens when the women’s game has prominence similar to the men’s .
A Minnesota Rallying Cry: Fans of the Minnesota Timberwolves have picked up on a phrase uttered by the team’s star, Anthony Edwards, and are hardly put off by its mild vulgarity.
A Beloved Manager: A coach’s soccer legacy is often reduced to titles and trophies. In Liverpool, Jürgen Klopp will endure in murals, music and shared memories .
AI image misinformation has surged, Google researchers find
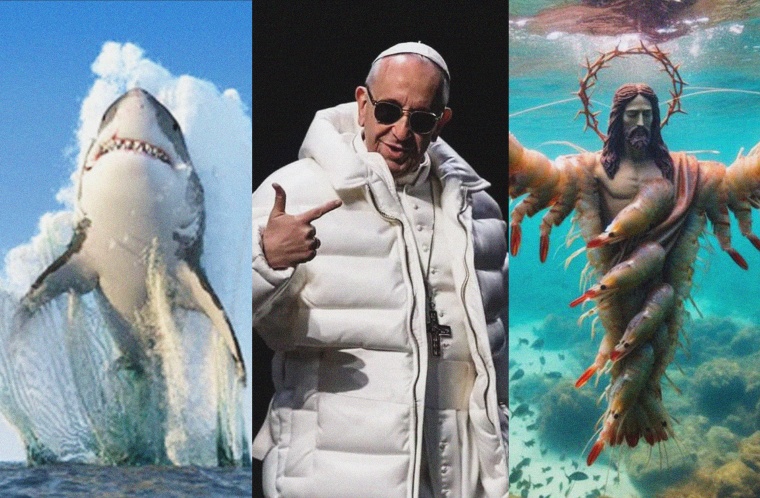
Fake images generated by artificial intelligence have proliferated so quickly that they’re now nearly as common as those manipulated by text or traditional editing tools like Photoshop, according to researchers at Google and several fact-checking organizations.
The findings offer an indication of just how quickly the technology has been embraced by people seeking to spread false information. But researchers warned that AI is still just one way in which pictures are used to mislead the public — the most common continues to be real images taken out of context.
In a paper released online this month but not yet peer-reviewed, the researchers tracked misinformation trends by analyzing nearly 136,000 fact-checks dating back to 1995, with the majority of them published after 2016 and ending in November 2023. They found that AI accounted for very little image-based misinformation until spring of 2023, right around when fake photos of Pope Francis in a puffer coat went viral.
“The sudden prominence of AI-generated content in fact checked misinformation claims suggests a rapidly changing landscape,” the researchers wrote.
The lead researchers and representatives for Google did not comment in time for publication.
Alexios Mantzarlis, who first flagged and reviewed the latest research in his newsletter, Faked Up , said the democratization of generative AI tools has made it easy for almost anyone to spread false information online.
“We go through waves of technological advancements that shock us in their capacity to manipulate and alter reality, and we are going through one now,” said Mantzarlis, who is the director of the Security, Trust, and Safety Initiative at Cornell Tech, Cornell University’s graduate campus in New York City. “The question is, how quickly can we adapt? And then, what safeguards can we put in place to avoid their harms?”
We go through waves of technological advancements that shock us in their capacity to manipulate and alter reality, and we are going through one now.
-Alexios Mantzarlis, director of the Security, Trust, and Safety Initiative at Cornell Tech
The researchers found that about 80% of fact-checked misinformation claims involve media such as images and video, with video increasingly dominating those claims since 2022.
Even with AI, the study found that real images paired with false claims about what they depict or imply continue to spread without the need for AI or even photo-editing.
“While AI-generated images did not cause content manipulations to overtake context manipulations, our data collection ended in late 2023 and this may have changed since,” the researchers wrote. “Regardless, generative-AI images are now a sizable fraction of all misinformation-associated images.”
Text is also a component in about 80% of all image-based misinformation, most commonly seen in screenshots.
“We were surprised to note that such cases comprise the majority of context manipulations,” the paper stated. “These images are highly shareable on social media platforms, as they don’t require that the individual sharing them replicate the false context claim themselves: they’re embedded in the image.”
Cayce Myers, a public relations professor and graduate studies director at Virginia Tech’s School of Communication, said context manipulations can be even harder to detect than AI-generated images because they already look authentic.
“In that sense, that’s a much more insidious problem,” Myers, who reviewed the recent findings prior to being interviewed, said. “Because if you have, let’s say, a totally AI-generated image that someone can look at and say, ‘That doesn’t look quite right,’ that’s a lot different than seeing an actual image that is captioned in a way that is misrepresenting what the image is of.”
Even AI-based misinformation, however, is quickly growing harder to detect as technology advances. Myers said traditional hallmarks of an AI-generated image — abnormalities such as misshapen hands, garbled text or a dog with five legs — have diminished “tremendously” since these tools first became widespread.
Earlier this month, during the Met Gala, two viral AI-generated images of Katy Perry (who wasn’t at the event) looked so realistic at first glance that even her mom mistakenly thought the singer was in attendance.
And while the study stated AI models aren’t typically trained to generate images like screenshots and memes, it’s possible they will quickly learn to reliably produce those types of images as new iterations of advanced language models continue to roll out.
To reliably distinguish misinformation as AI tools grow more sophisticated, Mantzarlis said people will have to learn to question the content’s source or distributor rather than the visuals themselves.
“The content alone is no longer going to be sufficient for us to make an assessment of truthfulness of trustworthiness veracity,” Mantzarlis said. “I think you need to have the full context: Who shared it with you? How was it shared? How do you know it was them?”
But the study noted that relying solely on fact-checked claims doesn’t capture the whole scope of misinformation out there, as it’s often the images that go viral that end up being fact checked. It also relied only on misinformation claims made in English. This leaves out many lesser-viewed or non-English pieces of misinformation that float unchecked in the wild.
Still, Mantzarlis said he believes the study reflects a “good sample” of English-language misinformation cases online, particularly those that have reached a substantial enough audience for fact-checkers to take notice.
For Myers, the bigger limitation affecting any study on disinformation — especially in the age of AI — will be the fast-changing nature of the disinformation itself.
“The problem for people who are looking at how to get a handle on disinformation is that it’s an evolving technological reality,” Myers said. “And capturing that is difficult, because what you study in May of 2024 may already be a very different reality in June of 2024.”

Angela Yang is a culture and trends reporter for NBC News.
Purdue Hospitality and Tourism Management graduate student wins best paper award for innovative technology research
Written By: Rebecca Hoffa, [email protected]
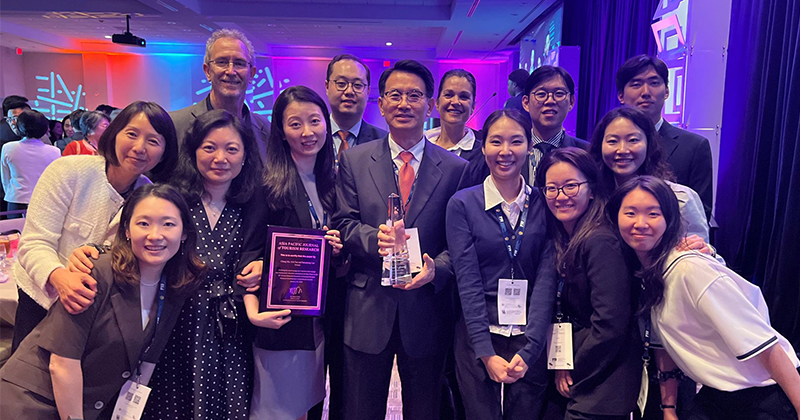
Evita Ma poses with the fellow Purdue HTM attendees at the 29th Annual Graduate Education and Graduate Student Research Conference in Hospitality and Tourism in January. (Photo provided)
As Starship robots deliver food to hungry Boilermakers across Purdue University’s West Lafayette campus, their presence is often welcomed like that of a neighborhood pet — students are often seen helping them when they get stuck or smiling and moving out of their way when they meet them on the sidewalk. When Alei (Aileen) Fan , associate professor in the White Lodging-J.W. Marriott, Jr. School of Hospitality and Tourism Management (HTM) and an expert in service innovation and experience design, helped one along its way one day, she was met with a low-tone, male voice saying, “Thank you.” This contradicted the “cute” vision Fan had in her mind of the robots being like her dog at home.
When Fan relayed the experience to her PhD student Chang (Evita) Ma , the College of Health and Human Sciences graduate student was inspired to investigate deeper how the combination of appearance and voice impacted the consumer’s overall experience in the service encounter.
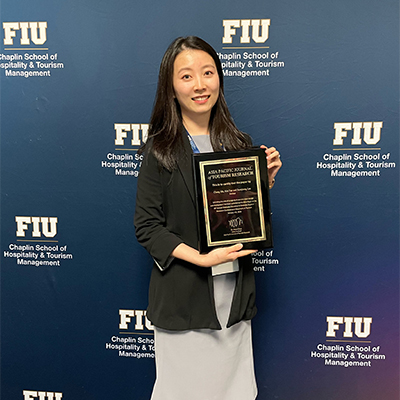
Evita Ma poses with her best paper award at the 29th Annual Graduate Education and Graduate Student Research Conference in Hospitality and Tourism. (Photo provided)
These efforts culminated in winning a best paper award at the 29th Annual Graduate Education and Graduate Student Research Conference in Hospitality and Tourism in January.
“I’m really honored,” Ma said. “As far as I know, for the past three years or so, no one from our school has gotten the award, so it was a pleasure to have that. It’s very competitive — over the 130-some presentations, I was selected as one of the four winners.”
The study looked at how people’s reactions differed when comparing matched, or congruent, voice and appearance and mismatched, or incongruent, voice and appearance. The researchers found that depending on the robot’s function, people tended to be either more or less accepting of when a robot’s voice does not match its appearance.
In a utilitarian scenario where the consumer really only desires the robot to perform a job function and doesn’t care as much about having an emotional connection, people often prefer the congruent voice and appearance because they feel the incongruent one is not well-designed or not capable of performing their desired outcome. When consumers are in a hedonic scenario where they don’t care as much about the functions the robot is performing but simply wish to have a connection or engage with the robot, the congruency doesn’t matter as much, and some of the surprising elements, such as the mismatched voice and appearance, may attract people to engage with it.
“We as human beings actually view things holistically,” Ma said. “We don’t separate the different parts.”
Considering how these distinct factors work together to shape consumer perceptions could ultimately shape the characteristics of future service robots to improve service encounters across the industry.
“It’s a timely topic,” Fan said. “Whether you like it or not, technology takes up a lot of our lives. This research provided practical guidelines for industries and companies on how to design different robots to better serve our customer.”
This study resulted as a product of Ma’s study “Decoding the Shared Pathways of Consumer Technology Experience in Hospitality and Tourism: A Meta-Analysis,” which examined the literature currently available to investigate the different types of technology and how humans interact with them. Ma ultimately noticed a gap in papers that combined elements — many simply looked at appearance or voice independently.
“Very few of the papers actually combine all the different subtle elements together; they just focus on one single perspective like appearance or voice,” Ma said. “So, I began looking at: What is the combination between those? Our initial idea was we wanted to know how the combination of design elements of service robots impacts the customer’s reactions and feelings of the service in different scenarios.”
Prior to coming to Purdue, Ma spent four years gaining industry experience at the Hospitality Financial and Technology Professionals nonprofit in Hong Kong, where she made many connections on the technology side of the industry and solidified her interest in coming to a very technology-focused university to earn her PhD.
“Purdue has such a strong background in terms of technology and engineering, so that was also one of the reasons I wanted to keep focusing on that for my research area,” Ma said. “After taking a class with Dr. Fan, I decided to join her team.”
Beyond research, Ma has fully immersed herself in the teaching and engagement areas of graduate student life as well. Ma teaches two 200-level marketing courses to undergraduate HTM students, where she’s maximized opportunities for the students to engage in experiential education. She’s also working on curriculum development with HTM faculty and has become involved in several graduate student organizations, including the Purdue Graduate Student Government, where she is a senator.
After graduating from the program, Ma plans to pursue a faculty position that allows her to combine her passion for research with her love for teaching and service.
“She’s really the star student,” Fan said. “When we evaluate a PhD student, there are three aspects: research, teaching and service. Evita is excellent in all of these.”
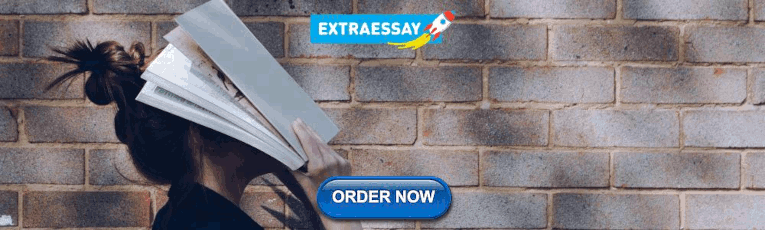
IMAGES
COMMENTS
Fundamentally, collegiate athletes have two major roles they must balance as part of their commitment to a university: being a college student and an athlete. Academic performance is a significant source of stress for most college students (Aquilina, 2013 ; López de Subijana et al., 2015 ; de Brandt et al., 2018 ; Davis et al., 2019 ).
These students attended one of two schools in this area and the study consisted of 284 non-athletes and 95 athletes. The study's results showed that 70% of people agreed that being an athlete motivated them to attend school regularly. This study also found that 71% said participation led to better time management.
Athletes may need lawyers, agents, accountants, and financial advisers who can help them in this process; 40% of NCAA athletes have expressed a desire for more resources navigating NIL. 3 The fact that such a high number of college athletes feel that they are lacking in terms of this resource indicates a potential source of stress, as well as ...
Student athletes on average spend over twenty hours per. week in practice or play, sustain bodily injury and fatigue, and miss a fair. number of classes when their sport is in season (W att and ...
This paper presents a. systematic literature review examining empirical research into the academic and. athletic identities of student-athletes in dual (education and sport) careers. The 42 ...
In these senses, nonrevenue college athletes are doubly advantaged—i.e., in background and positive sport impact—something that we hope future quantitative and qualitative research will interrogate. CONCLUSIONS. Our study's main aim is to investigate the academic achievement and attainment of student athletes in higher education.
A Qualitative Study of College Athletes' Experiences of the COVID-19 Pandemic Carra Johnson . The novel coronavirus (COVID-19) pandemic took a toll on collegiate athletics, as all in-person ... bringing the joy, challenge, and humor to my research papers the last few years—fingers crossed this is the one that gets us published! And, a big ...
Kegelaers et al. ( 2022) conducted a systematic scoping review on studies of the mental health of student-athletes, which also included intervention studies. The results from the intervention studies were, however, merely described and not examined or discussed in depth. Moreover, the review provided no future directions of research or ...
Researching college athletes can be difficult due to the many different levels of ... There is also research on athletes attitudes towards seeking help, but few papers offer an approach that focuses on specific mental illnesses as well as the help seeking behaviors associated with them. This paper serves to evaluate the extent to which
Background: While mental health among collegiate athletes is receiving increased attention, research on factors surrounding collegiate athletes' decision to seek mental health services is limited. The goal of the present review was to analyze and synthesize the current literature concerning collegiate athletes' utilization of mental health services, including the facilitators of and barriers ...
surrounding mental health issues in college athletes. This chapter will discuss the methods and procedures used for data collection. All data was collected through the Drake Memorial Library Database at The College of Brockport, State University of New York. Data Collection For this synthesis paper all data was collected through the Drake Memorial
PDF | On Dec 1, 2014, Timothy L Neal published Mental Health and the College Student Athlete: Developing a Plan to Recognize and Refer Student Athletes with Psychological Concerns at the ...
The NCAA is committed to making policy decisions based on quality research data. The NCAA research staff conducts national research for its members on a wide variety of topics including academic performance, student-athlete well-being, finances of intercollegiate athletics programs, gender-equity and diversity issues and many others.
Abstract. After fifty years of Title IX, the gap in participation rates between men and women in college athletics has closed significantly. In 1982, women comprised only 28% of all NCAA college athletes. In 2020, they made up 44%. Despite the progress in participation rates, a substantial gap in resources allocated to men's and women's ...
Examples of schools with athletic department budgets near the median include Maryland, Connecticut, Mississippi State, Iowa State, Georgia Tech, and Colorado. For an institution with 20,000 undergraduates, like Georgia Tech or Mississippi. from football was $20.3 million and from men's basketball $5.6 million.
Results: There were 6,523 nutritional supplements (1.7 per athlete) and 3,237 medications (0.8 per athlete) reported. Nonsteroidal anti-inflammatory drugs (NSAIDs; 0.27 per athlete, n = 884), respiratory drugs (0.21 per athlete, n = 682), and alternative analgesics (0.13, n = 423) were used most frequently.
The agreement calls for the NCAA and the conferences to pay $2.77 billion over 10 years to more than 14,000 former and current college athletes who say now-defunct rules prevented them from ...
A 2022 analysis by USA Today found that 49 of 107 public schools in the Football Bowl Subdivision, the highest classification of college football, had underfunded women's programs by a combined ...
College students are required to manage a variety of stressors related to academic, social, and financial commitments. In addition to the burdens facing most college students, collegiate athletes must devote a substantial amount of time to improving their sporting abilities. The strength and conditioning professional sees the athlete on nearly a daily basis and is able to recognize the changes ...
Objective The primary objective of this cross-sectional study was to compare standard laboratory performance metrics of transgender athletes to cisgender athletes. Methods 19 cisgender men (CM) (mean±SD, age: 37±9 years), 12 transgender men (TM) (age: 34±7 years), 23 transgender women (TW) (age: 34±10 years) and 21 cisgender women (CW) (age: 30±9 years) underwent a series of standard ...
This study uses new data from the National Sports. and Society Survey ( N = 3,993) to assess recent public opinions about allowing college athletes. to be paid more than it costs them to go to ...
If the agreement in House v. N.C.A.A. is approved, Division I schools could set aside as much as about $20 million each to pay their athletes as soon as the 2025 football season. Vasha Hunt ...
This study explored the effects of partial sleep deprivation (PSD) on Sanda athletes' athletic performance, an area with limited research. Using a randomized crossover controlled trial with 18 male athletes, the PSD group had 4 hours of sleep while the NSN group had 8 hours.
The settlement is only the latest in a series of big changes at the N.C.A.A. in recent years, Mr. Connor said, and "how we'll have to adapt to that is going to be the big question.". Mr ...
In a paper released online this month but not yet peer-reviewed, the researchers tracked misinformation trends by analyzing nearly 136,000 fact-checks dating back to 1995, with the majority of ...
These efforts culminated in winning a best paper award at the 29th Annual Graduate Education and Graduate Student Research Conference in Hospitality and Tourism in January. "I'm really honored," Ma said. "As far as I know, for the past three years or so, no one from our school has gotten the award, so it was a pleasure to have that.