EEG seizure detection: concepts, techniques, challenges, and future trends
- Published: 04 April 2023
- Volume 82 , pages 42021–42051, ( 2023 )
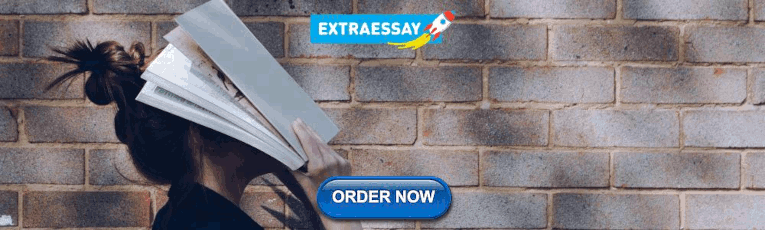
Cite this article
- Athar A. Ein Shoka 1 ,
- Mohamed M. Dessouky 1 , 2 ,
- Ayman El-Sayed ORCID: orcid.org/0000-0002-4437-259X 1 &
- Ezz El-Din Hemdan ORCID: orcid.org/0000-0001-9910-144X 1
5670 Accesses
12 Citations
1 Altmetric
Explore all metrics
A central nervous system disorder is usually referred to as epilepsy. In epilepsy brain activity becomes abnormal, leading to times of abnormal behavior or seizures, and at times loss of awareness. Consequently, epilepsy patients face problems in daily life due to precautions they must take to adapt to this condition, particularly when they use heavy equipment, e.g., vehicle derivation. Epilepsy studies rely primarily on electroencephalography (EEG) signals to evaluate brain activity during seizures. It is troublesome and time-consuming to manually decide the location of seizures in EEG signals. The automatic detection framework is one of the principal tools to help doctors and patients take appropriate precautions. This paper reviews the epilepsy mentality disorder and the types of seizure, preprocessing operations that are performed on EEG data, a generally extracted feature from the signal, and a detailed view on classification procedures used in this problem and provide insights on the difficulties and future research directions in this innovative theme. Therefore, this paper presents a review of work on recent methods for the epileptic seizure process along with providing perspectives and concepts to researchers to present an automated EEG-based epileptic seizure detection system using IoT and machine learning classifiers for remote patient monitoring in the context of smart healthcare systems. Finally, challenges and open research points in EEG seizure detection are investigated.
Similar content being viewed by others
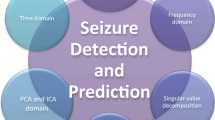
EEG seizure detection and prediction algorithms: a survey
A Comparative Study on Epileptic Seizure Detection Methods
Epileptic Seizure Detection Contribution in Healthcare Sustainability
Avoid common mistakes on your manuscript.
1 Introduction
Epilepsy, a neurological issue, has been considered a worldwide problem and is one of the principal dangers to human lives. As demonstrated by the reports of the World Health Organization (WHO), around 50 million individuals around the world are suffering from epilepsy, which makes it quite possibly the most broadly recognized global neurological disease [ 13 , 27 ]. Epilepsy influences both females, males, and even children. The seizure’s symptoms can vary broadly [ 20 , 41 ].
A few groups of individuals with epilepsy just stare blankly for a couple of moments during a seizure, while others repeatedly jerk their arms or legs. Having one seizure attack doesn’t mean you have epilepsy. At least two seizure attacks without stimulus are generally required for an epilepsy diagnosis. To detect brain abnormalities, EEG is one of the widely common techniques utilized to measure electrical disturbances in the human brain for the diagnosis of epileptic seizures [ 88 , 142 ].
The normal shape of EEG signals gets modified during an epileptic seizure. Thus, based on the variety of EEG signal characteristics, epileptic patients’ states can be grouped into three phases, normal, preictal, and ictal. Many electrical disturbances start happening in the cerebrum of epileptic patients before a seizure’s actual onset, which is termed a preictal stage. For recognition of seizures at this stage, such electrical disturbances in the patient’s brain need to be recorded during the transition from normal to the ictal stage [ 109 ]. Consequently, this process of early recognition of epileptic seizures at the preictal stage could save the existence of patients by enabling them to take precautionary measures to prevent injurious and life-threatening accidents. In EEG tests, electrodes are associated with your scalp using a paste-like substance or cap. The electrodes register the electrical activity of your brain [ 47 , 67 , 107 ].
As previously stated, there is an urgent need for an automatic efficient method for early recognition of epileptic seizures to save the lives of thousands of epileptic patients every year, capable of alerting the patients, their families, and nearby hospitals before the actual occurrence of epileptic seizures. so, this system could help epileptic patients in the case of an emergency to save their lives and for improving their quality of life [ 3 , 39 ].
Recently, research is being attempted for the detection of epileptic seizures to support for automatic diagnosis system to help clinicians from burdensome work. In this respect, an enormous number of research papers is published for the identification of epileptic seizures. Automatic seizure detection can prove efficient by making the process reliable and faster. Therefore, this domain attracts the researchers to investigate several types of techniques and domains such as the frequency domain, time domain, time-frequency domain, Empirical mode decomposition, and nonlinear methods. Nevertheless, experiments showed significant performance improvements when two or more conventional methods are combined. U. Rajendra discusses various entropies utilized for an automated diagnosis of epilepsy using EEG signals, and also the applications of entropies with their advantages and disadvantages [ 4 ]. In [ 124 ] automated epileptic seizure detection techniques based on multi-domain approaches were reviewed. The authors in [ 102 ] review pattern recognition techniques to detect seizures from EEG data, and also study the performance of DWT features with different classifiers. Focal and non-focal characterization are identified to determine areas affected by seizures [ 5 ]. Md Shafiqul Islam suggested a dynamic approach utilizing a deep learning model (Epileptic-Net) to detect an epileptic seizure. This approach included dense convolutional blocks, feature attention modules, residual blocks, and the hypercolumn technique [ 49 ]. Gaetano Zazzaro and Luigi Pavone evaluate the performance of a seizure detection system by studying its performance in correctly identifying seizures and in minimizing false alarms and to decide if it is generalizable to several patients [ 146 ]. In [ 74 ] explore the possibilities of wearable multi-modal monitoring in epilepsy and identify effective strategies for seizure-detection.
Nowadays, the Internet of Things (IoT) is playing a dynamic vital role in medical care by providing significant solutions for many medical and healthcare applications. The IoT technologies provide continuous and real-time observation of patients’ health using wearable devices. These technologies are also applied for the acquisition and transmission of EEG signals of epileptic patients. Along with such technologies, machine learning algorithms provide promising solutions for the effective detection of seizure stages from received EEG signals. Also, IoT utilized in combination with AI procedures and cloud computing services has arisen as a powerful technology to resolve many problems in the medical care area. The need to present an automatic epileptic seizure recognition framework for early identification of epileptic seizures utilizing existing communication technologies in collaboration with machine learning, IoT, and cloud computing [ 3 , 93 ].
This work will bring researchers up to date on the significant feature extraction techniques, statistical and machine learning classifiers, and recent deep learning algorithms. Another contribution of this review is to help researchers to identify publicly available databases of recorded epileptic seizure signals. Finally, based on this current review, suggestions on future research directions are provided. In conclusion, the primary contributions of this work can condense as follows:
Provide an overview of EEG signals and explore the seizure detection process along with providing information on available EEG datasets.
Reviewing works done using various deep learning models for automated detection of epileptic seizures by various modalities.
Explore challenges in the detection of epileptic seizures as well as analyze the best performing model for various modalities of data.
Explore and present AI-based seizure detection and IoT-based automated seizure detection.
Provide opportunities and future research directions for this cutting-edge research subject.
This paper is organized as follows, Section 2 defines preliminaries for EEG data, available datasets, and the seizure detection process, which is separated into three steps: preprocessing step, feature extraction techniques, and classification algorithms. Section 3 presents the AI-based Seizure detection. The IoT-based automated seizure detection is provided in Section 4 . Section 5 shows the challenges and the future research direction. Finally, the paper ended with the conclusion and references.
2 EEG signal acquisition
This section explores the basic knowledge of EEG signals and the available datasets with understanding the methodology of EEG signal acquisition.
2.1 EEG data
Epilepsy is a neurological issue described by the erratic intrusion of typical cerebrum exercises. In epilepsy, certain zones or all zones of the cerebrum are overactive, this prompts seizures. Epileptic seizures fluctuate from one person to another one, they may last for a few seconds and unnoticed, they might influence just a single arm or one leg or the entire body, and sometimes individuals end up oblivious. Thusly, there are two primary classes of epileptic seizure as shown in Fig. 1 :
Generalized seizures
Partial seizures
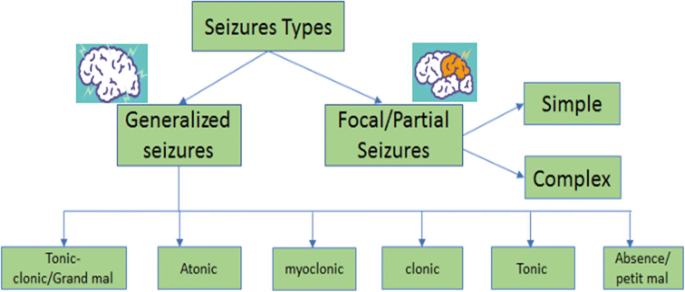
Types of seizures and sub-types
Generalized seizures affect the whole brain. It results in a lack of consciousness and makes the whole frame convulse. Focal or partial epileptic seizures include just a specific piece of the cerebrum. As in Fig. 1 , generalized epileptic seizures can be subdivided into absence (petit mal), tonic-clonic (grand mal), atonic, myoclonic, clonic, and tonic seizures [ 92 ]. To diagnose epileptic seizures, Various screening techniques have been developed. This is accomplished by using various methods like Electro Encephalography (EEG), Magneto Encephalography (MEG), functional Magnetic Resonance Imaging (fMRI), and Near Infra-Red Spectroscopy (NIRS). Table 1 presents a comparison between the various kinds of brain signal acquisition.
EEG is the most widely preferred signal acquisition method. This is due to EEG signals being economical, portable, and obtaining clear rhythms in the frequency domain. The EEG provides the voltage variations produced by the ionic current of neurons in the brain, which indicate the brain’s bioelectric activity. Diagnosing epilepsy with EEG signals is time-consuming and strenuous, as the epileptologist or neurologist needs to screen the EEG signals minutely. Also, there is a possibility of human error, and hence, developing a computer-based diagnosis may alleviate these problems.
Electroencephalography (EEG) is a simple test that estimates electrical activity in the cerebrum. It is generally utilized for the recognition and examination of epileptic seizures. EEG signals can be partitioned into four stages, which are marked by epilepsy patients. These stages are an ictal, preictal, postictal, and interictal state as in Fig. 2 [ 17 , 61 ].
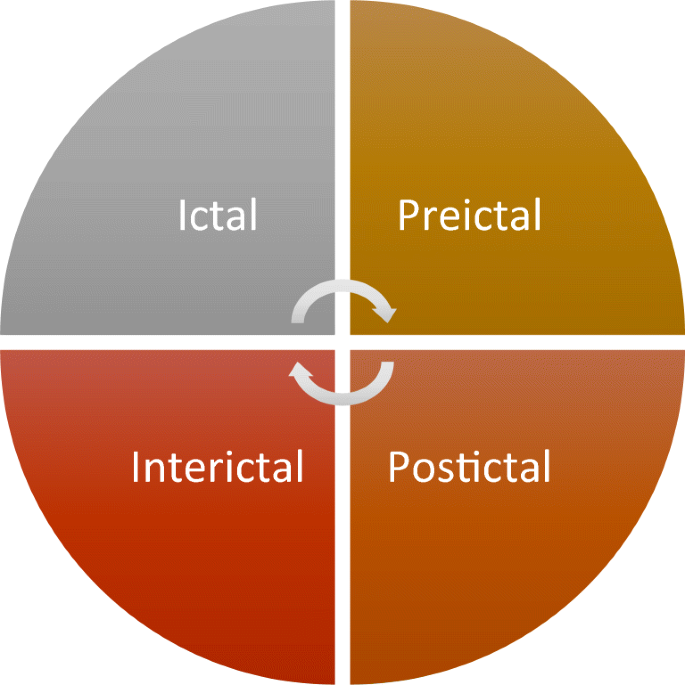
Different stages of EEG signals
EEG signals divided into five main rhythms are perceived from an EEG recording: Alpha 0.5 − 4 Hz, Beta 4 − 8 Hz, Gamma 8 − 12 Hz, Delta 12 − 30 Hz, and Theta over 30 Hz. The measure of activity in various EEG frequency bands can be quantified by employing spectral analysis techniques as in Table 2 [ 32 , 128 ].
EEG signals are normally obtained using superficial scalp electrodes, placed using a 10–20 international system presented in Fig. 3 . Electrode Placement using a 10–20 System is a strategy used to portray the area of electrodes on the scalp. It is relying upon the relationship between the area of an electrode and the layer of the cerebral cortex. Each site has a letter (to recognize the lobe) and a letter or number to recognize the area. The letters T, F, C, O, and P stand for Temporal, Frontal, Central, Occipital, and Parietal. The left hemisphere is directed by (1,3,5,7) while (2,4,6,8) numbers refer to the right hemisphere. Electrodes put on the midline are referred to using the letter z. Smaller numbers refer to a closed position to the midline [ 32 ].
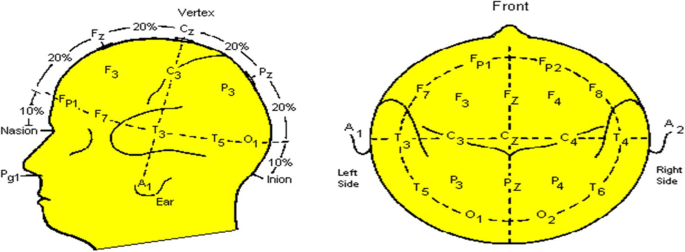
10–20 International system for Electrode Placement
2.2 EEG datasets
A dataset plays an important role for researchers and scientists in developing accurate and robust Computer-aided designs to evaluate the performance of their models. EEG recording is a broadly common tool for observing brain activity. These recordings play a vital role in machine learning classifiers to explore the novel methods for seizure detection in different ways such as onset seizure detection, quick seizure detection, patient seizure detection, and seizure localization. The significance of openly accessible datasets is to give a benchmark to dissect and contrast the outcomes with others.
To develop and evaluate the automated epileptic seizure detection systems, several publicly available datasets: CHB-MIT [ 43 , 127 , 144 , 150 ], ECoG Dataset [ 72 , 108 ], Freiburg epilepsy dataset [ 112 , 150 ], Bonn seizure dataset [ 82 , 119 , 128 , 136 ], BERN- Barcelona dataset [ 44 , 97 ], Kaggle dataset, Flint-Hills eplipsiae, Hauz Khas and Zenodo dataset. The signals obtained from these datasets are recorded either intracranially or from the scalp of humans or animals. Table 3 summarizes the supplementary information for this public dataset.
3 Epileptic seizure detection system
Artificial Intelligence (AI) plays a significant role in making support and assisting in the medical field. Especially, in EEG-based Seizure detection for early diagnosis of epilepsy. The seizure detection process can be separated into three main phases: preprocessing, feature extraction, and classification, as in Fig. 4 . These phases would be presented in detail.
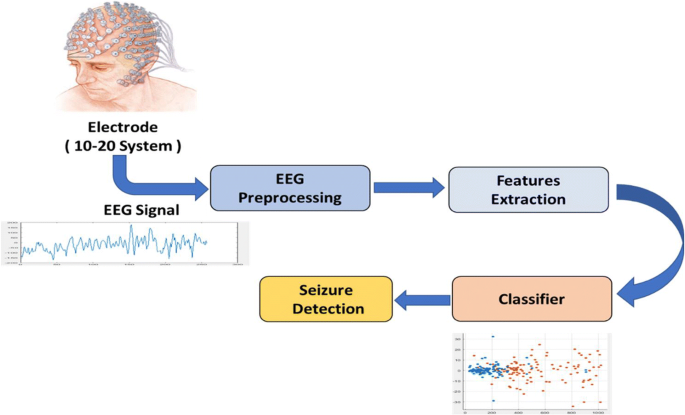
A seizure discovery framework
3.1 EEG preprocessing
Raw EEG signal is non-stationary and has a low spatial resolution. EEG signals are susceptible and highly affected by artifacts and noise. These artifacts may affect information and analysis of the recorded signals. Therefore, the necessity of artifact identification and removal, either in clinical diagnosis or practical applications, is the most significant preprocessing step before being utilized to reduce their impact on the feature extraction stage. In this step also, it is crucial to decide on frequency and channel from EEG as it is produced from numerous electrodes. Signal pre-processing sometimes is named Signal Enhancement. In general, the acquired cerebrum signals are contaminated by noise and artifacts.
The artifacts are divided into two types physiologic and extra-physiologic. Extra-physiologic or Exterior Artifacts are created from outside the body like equipment, faulty electrodes, Power lines, ventilation, and digital artifacts (loose wiring, etc.). Physiological artifacts also called (Interior Artifacts) created from the body such as eye blinks, eye movements (EOG), heartbeat (ECG), muscular movement (EMG), Skin resistance, and power line interferences are also merged with brain signals, these types of artifacts are more muddled to eliminate. Figure 5 illustrate the EEG signal having physiological artifacts [ 48 , 53 , 61 ]. Types of artifacts in EEG signals are illustrated in Table 4 .
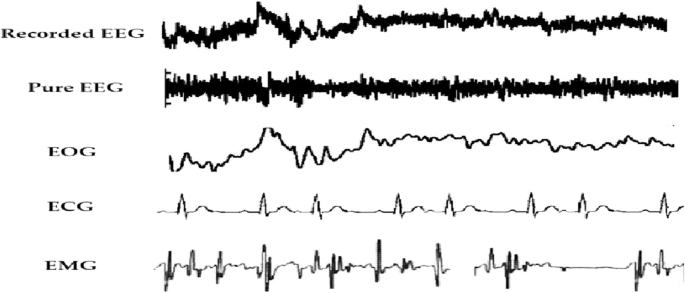
EEG signal with physiological artifacts
Several efficient techniques are used for artifact removal particularly for physiological artifacts, as demonstrated in Fig. 6 . It demonstrates that the most generally use algorithms are BSS-based methods, particularly ICA (34%) [ 54 ]. Also, it is worthy to take note that because of the constraints of single strategies like single Regression and BSS, a lot of researchers incline toward the hybrid method (20%) to upgrade the exhibition of methods in recent years. Filtering (13%) and wavelet transform (9%) are also artifact removal algorithms. Despite the extensive research on artifact removal from EEG signals, there is no agreement ideal answer for all types of artifacts [ 53 , 57 , 104 ].
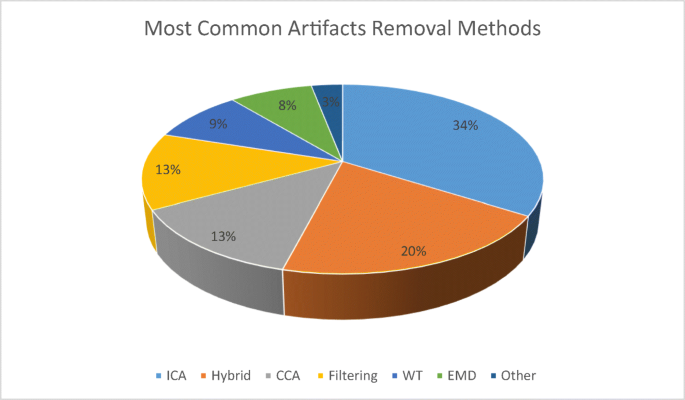
The percentages for the most common artifacts removal methods [ 53 ]
The constraints mean working on single methods sometimes not achieved acceptable results so the choice of using hybrid methods is good to achieve good performance on EEG seizure detection
Artifact removal takes place by using Common Average Referencing (CAR), Common Spatial Patterns (CSP), Principal Component Analysis (PCA), Surface Laplacian (SL), Independent Component Analysis (ICA), Frequency Normalization (Freq-Norm), Single Value Decomposition (SVD), Common Spatio-Spatial Patterns (CSSP), Common Spatial Subspace Decomposition (CSSD), Differential Window (DW), Local Averaging Technique (LAT), Robust Kalman Filtering, Butter worth filter, and simple classifier, etc. The most frequently used techniques are ICA, PCA, CAR, SL, CSP, and Adaptive Filtering [ 19 , 80 ].
Artifact removal can be divided into two different categories. The first one is single removal techniques, and the other is the hybrid one as displayed in Figs. 7 , 8 , and 9 .
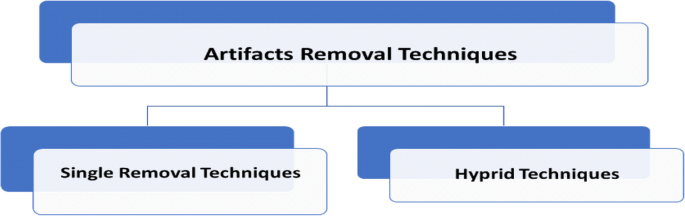
Techniques of artifacts removal
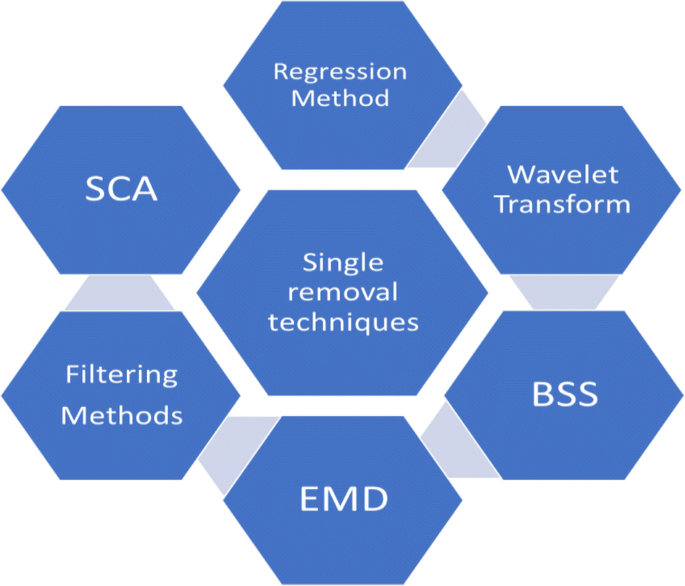
Single removal techniques
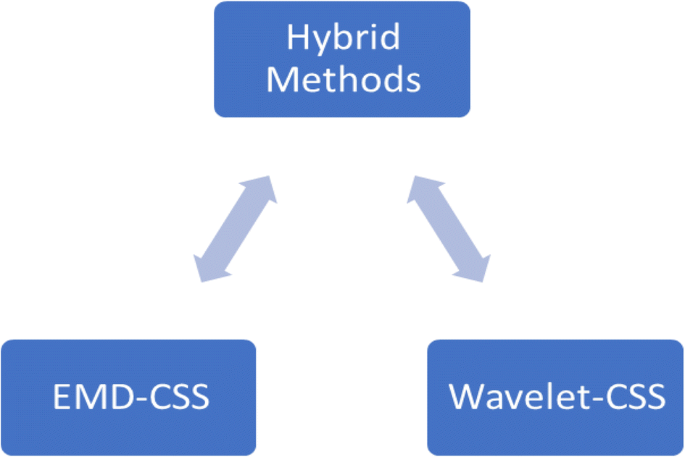
Basic hybrid methods
Tables 5 and 6 explore the techniques of artifact removal by providing their advantages and disadvantages [ 53 , 104 ].
Most of the practical applications of EEG are often needed real-time signal processing to be robust to artifacts. The artifact removal methods are required to be automatic and have a low computational cost. The automatic process indicates the chosen method can automatically identify and eliminate the artifact with no manual intervention. The regression and filtering approach can execute automatically. Furthermore, BSS methods will be automatic when there is a subsequent procedure, as cited in Table 5 , above, like SVM.
Another factor is the number of channels used. Specifically for the home healthcare environment, less channel is often required. BBS algorithms cannot be applied in such conditions because the assumption of BSS is that more channels will lead to better accuracy. However, wavelet transform and EMD-based methods can be executed with a single channel.
ICA-based algorithms can deal with artifacts that appeared in EEG recordings. Regression and adaptive filters are more achievable choices when the reference channels for specific artifacts are available. Apart from ICA, CCA and its combinations of other methods appear to be a good choice for the removal of muscle artifacts. For application to a few channels, EMD, and its hybrid methods with BSS or WT could be an ideal choice. Wavelet transform fails to identify completely artifacts that overlap with spectral properties. EMD also suffers from the drawback of mode-mixing. Therefore, it is quite difficult to find a single method that is both efficient and accurate enough to satisfy all the conditions perfectly.
3.2 Feature extraction
After obtaining the (noise and artifacts)-free signals from the preprocessing phase, effective and meaningful features need to be extracted from the brain EEG signals. Different techniques for feature extraction from EEG signals such as Genetic Algorithms (GA), Wavelet Transformations (WT), Wavelet Packet Decomposition (WPD), Empirical Mode Decomposition (EMD), Fast Fourier Transformations (FFT), PCA, ICA, Adaptive Auto-Regressive parameters (AAR), bilinear AAR, and multivariate AAR. The most common techniques are ICA, PCA, WT, AR, WPD, and FFT. A comparison between these techniques is presented in Table 7 [ 48 , 61 , 149 ].
3.3 Classification techniques
Once the preprocessing and features extraction phases of the detection framework are discussed, this is the time to separate between seizure and seizure-free. The quality of classification algorithms is largely dependent on the feature extracted that is fed to the classifier. The classifier is used to classify the signal into various classes so, the classifier is considered a decision-making system. A classifier can be separated into three types; linear classifiers, nonlinear classifiers, and nearest neighbor classifiers. The most used in BCI is linear and nonlinear classifiers. Table 5 , above, shows a comparison among different types of classifiers [ 48 , 61 ].
Two main steps can be carried out in the classification stage, that is, the training and testing phases. The extracted features are divided into those phases, and after training the classifier, the new data can be classified with the trained network this is called the testing phase. Clustering, machine learning, or, recently, deep neural networks are the classifier used for epileptic seizure detection systems.
Machine Learning Techniques
Artificial Intelligent (AI) algorithms are the most generally utilized classifiers in the automatic epilepsy detection framework. The conventional feature extraction methods are used to extract features and statistically analyze rank and select data that are used as input to AI classifiers. Several classification techniques have been proposed in the literature, such as k-nearest neighbor (k-NN), logistic regression, random forest, artificial neural networks (ANNs), fuzzy logic, and SVMs with various kernel functions. A list of studies utilizing machine learning algorithms with different feature extraction techniques is displayed in Table 8 . And the comparison is applied to the most popular and commonly used classifier. This comparison takes into their consideration the advantages and disadvantages of each classifier.
From Table 8 , it can be noticed that the genetic algorithm, Bayesian net, and fuzzy clustering are not popular classifiers in EEG signal processing [ 51 , 62 , 84 ]. LDA, ANN, and KNN are quite promising classifiers with great accuracy [ 9 , 55 , 64 ]. But, SVM is the most commonly applied classifier [ 22 , 101 , 105 ].
The performance of various EEG detection algorithms from 2014 to 2021 is illustrated in Table 9 . This table shows the performance of the classifier and the feature extraction technique. Upadhyay et al. [ 131 ] recorded an accuracy of 100% with the DWT and LS-SVM classifier. Sriraam et al. [ 106 ] achieve 96.66% accuracy using a new feature, Teager energy with supervised backpropagation. Some studies like the work of Saminu et al. [ 111 ] have employed multiple features with an ML technique. This study employed SVM with a feedforward neural network (FFNN) to detect and classify ictal and interictal signals. It was computationally less complex with high accuracy of 99.6%.
Mahjoub et al. [ 23 ] was a mix of linear and non-linear parameters and multiple features as its edge. It utilized feature extraction of epileptic EEGs with tunable-Q wavelet transform (TQWT) and intrinsic mode functions (IMFs) of multivariate empirical mode decomposition (MEMD) and directly from the EEG raw data. This approach provides an accuracy of 98.7% with SVM.
From Table 9 , it is noticed that there are several algorithms that give high accuracy (equal to 100%) such as the algorithm in reference [ 65 , 83 , 100 , 101 , 131 ].
Deep Learning Techniques
Shallow networks are a second name to conventional neural networks, CNN consists of many hidden layers (more than 2 layers to hundreds of layers). Due to the massive number of these hidden layers, the parameters of the network are increased. The feature extraction step in CNN architecture is made automatically in the process of classifying the EEG signal. While the max-pooling layer carries forward the significant feature decided/chosen by the convolutional layer. The fully connected layer simply compiles the extracted data for the SoftMax layer that conducts the binary classification, i.e., converting the data into probabilities between 0 and 1.
Nowadays, seizure detection systems employed deep learning algorithms to overcome the limitations associated with machine learning techniques. DL uses multilayer architecture so; features do not need to be extracted manually. it can manage huge datasets. Several models are proposed using DL techniques: long short-term memory (LSTM) and Gated-Recurrent-Units (GRU) are recurrent neural networks, convolutional neural networks (CNNs), AE, and DBN are employed as unsupervised learning. Table 9 , above, shows the automated epilepsy detection works and analysis that utilized the deep learning method.
Table 10 summarized the performance of previously EEG detection and classification algorithms employed by researchers.
From Table 10 , it is noticed that the algorithm in reference [ 77 ] gives high accuracy (equal to 100%).
In the last years, there are several techniques for seizure classification. It is essential to review the statistical evaluation of the performance of these techniques. A summary of the different techniques, the database used, and their performance in terms of accuracy sensitivity, and specificity, is shown in Table 11 .
4 IoT-based automated epileptic seizure detection
Seizures are a very diverse group of disorders, with such a variety of epilepsy smart devices available which are not easily managed. it is difficult for a clinician to realize how to locate the best device for the seizure patient’s needs. Also, seizures can be varying for every individual; simply realizing that somebody has epilepsy doesn’t mention to you what their epilepsy is like, or what seizures they have. So, there is a need to develop an accurate and refined way of remotely monitoring patients at home.
Remote monitoring allows patients to use mobile medical devices to do routine tests at home and send the test information to a specialist in real-time. A challenge in this research is to blend the devices into the web environment and make them accessible, discoverable, and secure. A ‘seamless’ way of integrating these physical objects or things from the physical world to the cyber world is The Internet of Things [ 70 ].
Internet of Things (IoT) means the practice of designing and molding Internet-connected Things through computer networks. The term ‘IoT’ signifies that instead of using smaller effective gadgets like laptops, Tablets, and smartphones, it is better to use several efficient devices. The traditional methods used for epilepsy detection based on wired hospital monitoring systems are not appropriate for the recognition of long-term monitoring outdoors. Consequently, there must be a framework that can identify seizures in patients to alarm the members of the family or clinical staff for help, based on a wearable device, or a portable application in a framework dependent on the utilization of IoT and cloud computing.
There are many existing epilepsy devices smart and non-smart as in Table 12 . it is difficult to know the best device to implement. For instance, the patient could be in the bath, in bed, or watching TV when the seizure occurs. For instance, a Smartwatch used for monitoring shaking may not be comfortable to wear in bed, so a Smart Mattress would take its place. Likewise, a Companion Monitor which monitors bed movement and sound but would not be fitting in the shower. Another variant is that various kinds of seizures will present themselves and there are not always solutions at the clinician’s fingertips; devices are just not reasonable for all the different environments we live in.
Epilepsy patients often preferred the use of smart devices in their daily routines, due to the recent advances in sensor development, processing, and displays that have enabled devices. Depending on the patient, some wearable devices can be placed on almost any part of the body: wrist, ankle, waist, chest, arm, legs, etc. Product design is another factor that affects patient-device suitability. A good design can reduce mental and physical stress, reduce the learning curve, improve user device operability in using the device and thus improve overall product quality. Recently, there are epilepsy devices and apps that track medication and send reminders to take medication, some are for an emergency with buttons to press before losing consciousness with a seizure and some detect a seizure based on the pattern of jerks. Therefore, it is vital to choose the most appropriate device to find evidence on device reliability for a seizure type, and specifically to avoid “false alarms which can be disruptive for the family.” Patients with epilepsy must “discuss with their doctors the pros and cons of each device. Table 13 presents a comparative study for different IoT methods. Finally, Table 14 is added to clarify the general difference among ML/DL in addition IoT system.
The basic building blocks of the automatic epileptic seizure detection system are shown in Fig. 10 . The suggested framework consists of three key phases that collaborate to achieve the system objective. Each phase provides and delivers a specific task and operation in harmonizing with the other phases. The three phases can be as the following:
Stage 1: In this phase, IoT-based wearable medical sensors and smart-phone can be used for data acquisition in real-time. These will be connected to the patient’s heat to collect EEG data.
Stage 2: In this phase, the cloud will be used to provide a pool of processing and storage resources for receiving patients’ data from their smart phone over the internet to be sorted, and then it became available for doctor’s inspections. Besides, the data exploration and handling will be held in the cloud for any disorder detection in patient’s data, therefore, the abnormal changes in patient’s data will be classified based on patient status. All results in acquired data and extracted knowledge will be reported either to health-givers such as friends, family, and medical staff reliably and efficiently. In summary, the cloud enables collaboration and information sharing through its infrastructure which allows medical staff and experts to host information, analytics, and diagnostics. This discloses in earlier medicaments and real-time updates to the patient’s data and status.
Stage 3: In this phase, the medical staff uses a cloud-based web monitoring system to monitor a patient’s records and sensory EEG data. The staff will be able to inspect reports provided by the cloud-based analytical system and they able to take appropriate actions.
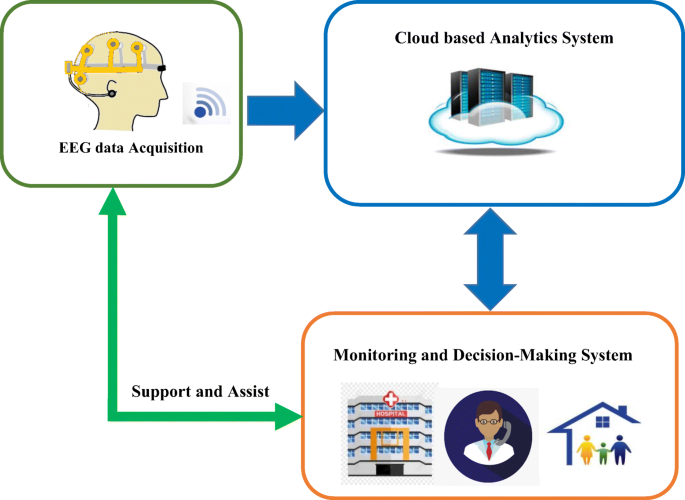
IoT-based cloud system for automatic epileptic seizure detection
5 Challenges and open research points
Working on the EEG based Seizure detection poses several challenges as the following:
The automated seizure registration techniques for improving the quality of seizure data.
An accurate professional database, because seizure monitoring is crucial for therapeutic decisions for patients or caretakers. Inaccurate seizure documentation has a bad effect on the patient’s treatment.
A real-time seizure detection system and subsequent evaluation by experts are still required.
The issue of obtaining longer recordings is due to technical reasons.
emergency call systems that require actively pushing an alarm button are inappropriate for most epilepsy patients and alternative approaches are needed.
Diagnostic accuracy is still missing so multimodal approaches which combine the measurement of autonomous parameters (e.g., heart rate, or muscular activity) will be required to detect these seizures.
The trade-off between seizure detection algorithm (SDA) accuracy vs. detection speed, such improvements may come at the expense of increased invasiveness of monitoring.
Dealing with non-stationarity and noise. EEG is prone to many different artifacts which obstruct the view of underlying brain activity.
Minimizing computational cost.
SDA Performance Assessment. Extensive prospective validation of any SDA is required to properly assess its performance.
Regarding this interesting topic, several open research points can lead the researcher in working with future work as the following:
Study the effect of the Corona Virus on epilepsy patients and how it affects the EEG brain signals.
Develop an efficient seizure detection model using spectrogram images for the EEG signals.
Using the Internet of Things for remotely monitoring patients who suffer from epilepsy.
Deep learning structures must be carefully chosen based on the problem’s peculiarities and include relevant datasets for real-time epilepsy detection. Likewise, hybrid deep learning techniques should be broadly investigated.
AI-based classifiers must be chosen carefully so that will not miss or skip all the relevant EEG channels and electrodes.
Develop mobile applications for remotely observing epilepsy patients by doctors and family members.
The epileptic seizure datasets that are with a large size and high dimension, it is necessary to utilize dimensional reduction methods to reduce the dataset dimension and still retain the important signal information need to be further explored. So, appropriate features that reduce the classifier’s computational complexity and time should be believed.
6 Conclusion
In recent years, using manual monitoring and studying EEG to diagnose epilepsy is a very difficult and challenging task of observing long recordings and decision-making through experience. With the growth of epilepsy patients, accurate identification becomes increasingly significant. Likewise, recognizing seizures accurately from an enormous amount of information becomes challenging. Besides, the machine and deep learning algorithms classifiers as a useful and suitable tool for accurate seizure identification due to the complexity of EEG signals in such datasets. Henceforward, there is a prerequisite for providing an automated seizure detection system that is a promising tool for neurologists in making epilepsy diagnoses. Further investigation must be thoroughly conducted on seizure detection techniques to improve the outcome. Therefore, this study investigated and reviewed various automated EEG epileptic seizure detection and classification techniques. Additionally. It highlighted both traditional feature extraction techniques and statistical and machine learning classifiers. Moreover, this work focused on the trends in the IoT framework for epilepsy detection. Finally, the challenges and open research points in EEG seizure detection are inspected.
Data availability
The data is available up to a request from the author.
Aayesha M, Qureshi B, Afzaal M, Qureshi MS, Fayaz M (2021) Machine learning-based EEG signals classification model for epileptic seizure detection. Multimed Tools Appl 80(12):17849–17877. https://doi.org/10.1007/s11042-021-10597-6
Article Google Scholar
Abdelhameed A, Bayoumi M (2021) A deep learning approach for automatic seizure detection in children with epilepsy. Front Comput Neurosci 15:29. https://doi.org/10.3389/fncom.2021.650050
Abualsaud K, Mohamed A, Khattab T, Yaacoub E, Hasna M, Guizani M (2018) Classification for imperfect EEG epileptic seizure in IoT applications: a comparative study. 2018 14th Int. Wirel. Commun. Mob. Comput. Conf. IWCMC 2018, pp 364–369. https://doi.org/10.1109/IWCMC.2018.8450279
Acharya UR, Fujita H, Sudarshan VK, Bhat S, Koh JEW (2015) Application of entropies for automated diagnosis of epilepsy using EEG signals: a review. Knowl-Based Syst 88:85–96. https://doi.org/10.1016/j.knosys.2015.08.004
Acharya UR et al (2019) Characterization of focal EEG signals: a review. Futur Gener Comput Syst 91:290–299. https://doi.org/10.1016/j.future.2018.08.044
Achilles F, Tombari F, Belagiannis V, Loesch-Biffar A, Noachtar S, Navab N (2016) Convolutional neural networks for real-time epileptic seizure detection. Comput Methods Biomech Biomed Eng Imaging Vis 6:1–6. https://doi.org/10.1080/21681163.2016.1141062
Ahammad N, Fathima T, Joseph P (2014) Detection of epileptic seizure event and onset using EEG. Biomed Res Int 2014:450573. https://doi.org/10.1155/2014/450573
Ahmad I et al (2022) EEG-based epileptic seizure detection via machine/deep learning approaches: a systematic review. Comput Intell Neurosci 2022:6486570. https://doi.org/10.1155/2022/6486570
Aileni RM, Pasca S, Florescu A (2020) EEG-brain activity monitoring and predictive analysis of signals using artificial neural networks. Sensors (Basel) 20(12):3346. https://doi.org/10.3390/s20123346
Akareddy S, Kulkarni P (2013) EEG signal classification for epilepsy seizure detection using improved approximate entropy. Int J Public Health Sci 2:23–32. https://doi.org/10.11591/ijphs.v2i1.1836
Akut R (2019) Wavelet based deep learning approach for epilepsy detection. Heal Inf Sci Syst 7:1–9. https://doi.org/10.1007/s13755-019-0069-1
Al Ghayab HR, Li Y, Abdulla S, Diykh M, Wan X (2016) Classification of epileptic EEG signals based on simple random sampling and sequential feature selection. Brain Inform 3(2):85–91. https://doi.org/10.1007/s40708-016-0039-1
Alam SM, Bhuiyan M (2013) Detection of seizure and epilepsy using higher order statistics in the EMD domain. IEEE J Biomed Health Inform 17:312–318. https://doi.org/10.1109/JBHI.2012.2237409
Alhussein M, Muhammad G, Hossain MS (2019) EEG pathology detection based on deep learning. IEEE Access 7:27781–27788. https://doi.org/10.1109/ACCESS.2019.2901672
Almustafa KM (2020) Classification of epileptic seizure dataset using different machine learning algorithms. Inform Med Unlocked 21:100444. https://doi.org/10.1016/j.imu.2020.100444
Alotaibi SM, Atta-ur-Rahman, Basheer MI, Khan MA (2021) Ensemble machine learning based identification of pediatric epilepsy. Comput Mater Contin 68(1):149–165. https://doi.org/10.32604/cmc.2021.015976
Alotaiby TN, Alshebeili SA, Alshawi T, Ahmad I, Abd El-Samie FE (2014) EEG seizure detection and prediction algorithms: a survey. EURASIP J Adv Signal Process 2014(1):183. https://doi.org/10.1186/1687-6180-2014-183
Antoniades A, Spyrou L, Took CC, Sanei S (2016) Deep learning for epileptic intracranial EEG data. In: 2016 IEEE 26th International Workshop on Machine Learning for Signal Processing (MLSP), pp 1–6. https://doi.org/10.1109/MLSP.2016.7738824
Bashashati A, Fatourechi M, Ward R, Birch G (2007) A survey of signal processing algorithms in brain-computer interfaces based on electrical brain signals. J Neural Eng 4:R32–R57. https://doi.org/10.1088/1741-2560/4/2/R03
Ben Slimen I, Boubchir L, Seddik H (2020) Epileptic seizure prediction based on EEG spikes detection of ictal-preictal states. J Biomed Res 34(3):162–169. https://doi.org/10.7555/JBR.34.20190097
Bhattacharyya A, Sharma M, Pachori RB, Sircar P, Acharya UR (2018) A novel approach for automated detection of focal EEG signals using empirical wavelet transform. Neural Comput Appl 29(8):47–57. https://doi.org/10.1007/s00521-016-2646-4
Bose R, Pratiher S, Chatterjee S (2019) Detection of epileptic seizure employing a novel set of features extracted from multifractal spectrum of electroencephalogram signals. IET Signal Process 13(2):157–164
Chahira M, Le Bouquin Jeannès R, Lajnef T, Kachouri A (2020) Epileptic seizure detection on EEG signals using machine learning techniques and advanced preprocessing methods. Biomedical Engineering/Biomedizinische Technik 65(1):33–50
Chen G, Xie W, Bui TD, Krzyżak A (2017) Automatic epileptic seizure detection in EEG using nonsubsampled wavelet–Fourier features. J Med Biol Eng 37(1):123–131. https://doi.org/10.1007/s40846-016-0214-0
Cho K-O, Jang H-J (2020) Comparison of different input modalities and network structures for deep learning-based seizure detection. Sci Rep 10:122. https://doi.org/10.1038/s41598-019-56958-y
Choudhury NR, Roy SS, Pal A, Chatterjee S, Bose R (2018) Epileptic seizure detection employing cross-hyperbolic stockwell transform. In: 2018 Fourth International Conference on Research in Computational Intelligence and Communication Networks (ICRCICN), pp 70–74. https://doi.org/10.1109/ICRCICN.2018.8718687
Chapter Google Scholar
Daoud H, Williams P, Bayoumi M (2020) IoT based efficient epileptic seizure prediction system using deep learning. In: 2020 IEEE 6th World Forum on Internet of Things (WF-IoT), pp 1–6. https://doi.org/10.1109/WF-IoT48130.2020.9221169
De Cooman T et al (2018) Adaptive nocturnal seizure detection using heart rate and low-complexity novelty detection. Seizure 59:48–53. https://doi.org/10.1016/j.seizure.2018.04.020
Dhif I, Hachicha K, Pinna A, Hochberg S, Garda P (2017) Epileptic seizure detection based on expected activity measurement and neural network classification, pp 2814–2817
Diykh M, Li Y, Wen P (2017) Classify epileptic EEG signals using weighted complex networks based community structure detection. Expert Syst Appl 90:87–100. https://doi.org/10.1016/j.eswa.2017.08.012
Djoufack Nkengfack LC, Tchiotsop D, Atangana R, Louis-Door V, Wolf D (2021) Classification of EEG signals for epileptic seizures detection and eye states identification using Jacobi polynomial transforms-based measures of complexity and least-square support vector machine. Inform Med Unlocked 23:100536. https://doi.org/10.1016/j.imu.2021.100536
Durai S, Vanathi P (2017) EEG signal separation using improved EEMD - fast IVA algorithm. Asian J Res Soc Sci Humanit 7:1230. https://doi.org/10.5958/2249-7315.2017.00239.8
Ein Shoka AA, Alkinani MH, El-Sherbeny AS, El-Sayed A, Dessouky MM (2021) Automated seizure diagnosis system based on feature extraction and channel selection using EEG signals. Brain Inform 8(1):1. https://doi.org/10.1186/s40708-021-00123-7
Elgohary S, Eldawlatly S, Khalil MI (2016) Epileptic seizure prediction using zero-crossings analysis of EEG wavelet detail coefficients. In: 2016 IEEE Conference on Computational Intelligence in Bioinformatics and Computational Biology (CIBCB), pp 1–6. https://doi.org/10.1109/CIBCB.2016.7758115
Fasil OK, Rajesh R (2019) Time-domain exponential energy for epileptic EEG signal classification. Neurosci Lett 694:1–8. https://doi.org/10.1016/j.neulet.2018.10.062
Feng B, Zhao J, Fu W (2018) Automated classification of epileptic eeg signals based on multi-feature extraction. In: 2018 IEEE 9th International Conference on Software Engineering and Service Science (ICSESS), pp 382–386. https://doi.org/10.1109/ICSESS.2018.8663773
Gajic D, Djurovic Z, Di Gennaro S, Gustafsson F (2014) Classification of EEG signals for detection of epileptic seizures based on wavelets and statistical pattern recognition. Biomed Eng Appl Basis Commun 26:1450021. https://doi.org/10.4015/S1016237214500215
Gao Y, Gao B, Chen Q, Liu J, Zhang Y (2020) Deep convolutional neural network-based epileptic electroencephalogram (EEG) signal classification. Front Neurol 11:375. https://doi.org/10.3389/fneur.2020.00375
Garcés Correa A, Orosco LL, Diez P, Laciar Leber E (2019) Adaptive filtering for epileptic event detection in the EEG. J Med Biol Eng 39:1–7. https://doi.org/10.1007/s40846-019-00467-w
Gill AF et al (2014) Time domain analysis of EEG signals for detection of epileptic seizure. In: 2014 IEEE Symposium on Industrial Electronics & Applications (ISIEA), pp 32–35. https://doi.org/10.1109/ISIEA.2014.8049867
Giourou E, Stavropoulou-Deli A, Giannakopouou A, Kostopoulos G, Koutroumanidis M (2015) Introduction to epilepsy and related brain disorders, pp 11–38
Gogna A, Majumdar A, Ward R (2017) Semi-supervised stacked label consistent autoencoder for reconstruction and analysis of biomedical signals. IEEE Trans Biomed Eng 64(9):2196–2205. https://doi.org/10.1109/TBME.2016.2631620
Gómez C, Arbeláez P, Navarrete M, Alvarado-Rojas C, Le Van Quyen M, Valderrama M (2020) Automatic seizure detection based on imaged-EEG signals through fully convolutional networks. Sci Rep 10(1):21833. https://doi.org/10.1038/s41598-020-78784-3
Gupta V, Nishad A, Pachori RB (2018) Focal EEG signal detection based on constant-bandwidth TQWT filter-banks. In: 2018 IEEE International Conference on Bioinformatics and Biomedicine (BIBM), pp 2597–2604. https://doi.org/10.1109/BIBM.2018.8621311
Hussain W, Sadiq MT, Siuly S, Rehman AU (2021) Epileptic seizure detection using 1 D-convolutional long short-term memory neural networks. Appl Acoust 177:107941. https://doi.org/10.1016/j.apacoust.2021.107941
Hussein R, Palangi H, Ward RK, Wang ZJ (2019) Optimized deep neural network architecture for robust detection of epileptic seizures using EEG signals. Clin Neurophysiol 130(1):25–37. https://doi.org/10.1016/j.clinph.2018.10.010
Ibrahim F, Abd-Elateif el-Gindy S, el-Dolil SM, el-Fishawy AS, el-Rabaie ESM, Dessouky MI, Eldokany IM, Alotaiby TN, Alshebeili SA, Abd el-Samie FE (2019) A statistical framework for EEG channel selection and seizure prediction on mobile. Int J Speech Technol 22(1):191–203. https://doi.org/10.1007/s10772-018-09565-7
Iftikhar M, Khan SA, Hassan A (2018) A survey of deep learning and traditional approaches for EEG signal processing and classification. In: 2018 IEEE 9th annual information technology, electronics and mobile communication conference (IEMCON), pp 395–400. https://doi.org/10.1109/IEMCON.2018.8614893
Islam MS, Thapa K, Yang S-H (2022) Epileptic-net: an improved epileptic seizure detection system using dense convolutional block with attention network from EEG. Sensors 22(3):728
Jaiswal AK, Banka H (2018) Epileptic seizure detection in EEG signal using machine learning techniques. Australas Phys Eng Sci Med 41(1):81–94. https://doi.org/10.1007/s13246-017-0610-y
Jaiswal P, Koushal R (2015) EEG signal classification using Modified Fuzzy Clustering algorithm. Int J Comput Sci Inform Technol 6(3):2031–2034
Jang H-J, Cho K-O (2019) Dual deep neural network-based classifiers to detect experimental seizures. Korean J Physiol Pharmacol 23:131–139. https://doi.org/10.4196/kjpp.2019.23.2.131
Jiang X, Bian G-B, Tian Z (2019) Removal of artifacts from EEG signals: a review. Sensors 19:987. https://doi.org/10.3390/s19050987
Jindal K, Upadhyay R, Singh HS (2018) Eeg artifact removal and noise suppression using hybrid Glct -Ica technique. In: 2018 10th International Congress on Ultra Modern Telecommunications and Control Systems and Workshops (ICUMT), pp 1–5. https://doi.org/10.1109/ICUMT.2018.8631219
Karabiber Cura O, Kocaaslan Atli S, Türe HS, Akan A (2020) Epileptic seizure classifications using empirical mode decomposition and its derivative. Biomed Eng Online 19(1):10. https://doi.org/10.1186/s12938-020-0754-y
Khalid MI, Alotaiby T, Aldosari SA, Alshebeili SA, Al-hameed MH, Almohammed FSY (2016) Epileptic MEG spikes detection using common spatial patterns and linear discriminant analysis. IEEE Access 4:4629–4634
Kim M, Kim S (2018) A comparsion of artifact rejection methods for a BCI using event related potentials. In: 2018 6th International Conference on Brain-Computer Interface (BCI), pp 1–4. https://doi.org/10.1109/IWW-BCI.2018.8311530
Kocadagli O, Langari R (2017) Classification of EEG signals for epileptic seizures using hybrid artificial neural networks based wavelet transforms and fuzzy relations. Expert Syst Appl 88:419–434. https://doi.org/10.1016/j.eswa.2017.07.020
Kumar Y, Dewal ML, Anand RS (2014) Epileptic seizure detection using DWT based fuzzy approximate entropy and support vector machine. Neurocomputing 133:271–279. https://doi.org/10.1016/j.neucom.2013.11.009
Lahmiri S, Shmuel A (2019) Accurate classification of seizure and seizure-free intervals of intracranial EEG signals from epileptic patients. IEEE Trans Instrum Meas 68(3):791–796. https://doi.org/10.1109/TIM.2018.2855518
Lakshmi MR, Prasad TV, Dr Prakash VC (2014) "Survey on EEG signal processing methods." International journal of advanced research in computer science and software engineering 4.1.
Li P, Karmakar C, Yan C, Palaniswami M, Liu C (2016) Classification of 5-S epileptic EEG recordings using distribution entropy and sample entropy. Front Physiol 7:136. https://doi.org/10.3389/fphys.2016.00136
Li M, Chen W, Zhang T (2016) Automatic epilepsy detection using wavelet-based nonlinear analysis and optimized SVM. Biocybern Biomed Eng 36:708–718. https://doi.org/10.1016/j.bbe.2016.07.004
Li M, Chen W, Zhang T (2017) Application of MODWT and log-normal distribution model for automatic epilepsy identification. Biocybern. Biomed. Eng. 37(4):679–689. https://doi.org/10.1016/j.bbe.2017.08.003
Li Y, Cui W, Luo M, Li K, Wang L (2018) Epileptic seizure detection based on time-frequency images of EEG signals using gaussian mixture model and gray level co-occurrence matrix features. Int J Neural Syst 28(7):1850003. https://doi.org/10.1142/S012906571850003X
Li M, Chen W, Zhang T (2018) A novel seizure diagnostic model based on kernel density estimation and least squares support vector machine. Biomed Signal Process Control 41:233–241. https://doi.org/10.1016/j.bspc.2017.12.005
Li F, Liang Y, Zhang L, Yi C, Liao Y, Jiang Y, Si Y, Zhang Y, Yao D, Yu L, Xu P (2019) Transition of brain networks from an interictal to a preictal state preceding a seizure revealed by scalp EEG network analysis. Cogn Neurodyn 13(2):175–181. https://doi.org/10.1007/s11571-018-09517-6
Liu H, Xi L, Zhao Y, Li Z (2019) Using deep learning and machine learning to detect epileptic seizure with electroencephalography (EEG) data. Mach Learn Res 4:39. https://doi.org/10.11648/j.mlr.20190403.11
M NR, Prabu V, Kumar R, Ahamed MY (2019) Iot based epilepsy monitoring and detection using ambulatory system. Int J Innov Res Adv Eng 6(03):123–125
Google Scholar
McHale SA, Pereira E, Weishmann U, Hall M, Fang H (2017) An IoT approach to personalised remote monitoring and management of epilepsy. In: 2017 14th International Symposium on Pervasive Systems, Algorithms and Networks & 2017 11th International Conference on Frontier of Computer Science and Technology & 2017 Third International Symposium of Creative Computing (ISPAN-FCST-ISCC), pp 414–418. https://doi.org/10.1109/ISPAN-FCST-ISCC.2017.34
Mohammadpoory Z, Nasrolahzadeh M, Haddadnia J (2017) Epileptic seizure detection in EEGs signals based on the weighted visibility graph entropy. Seizure 50:202–208. https://doi.org/10.1016/j.seizure.2017.07.001
Mohammadpoory Z, Nasrolahzadeh M, Mahmoodian N, Sayyah M, Haddadnia J (2019) Complex network based models of ECoG signals for detection of induced epileptic seizures in rats. Cogn Neurodyn 13(4):325–339. https://doi.org/10.1007/s11571-019-09527-y
Muhammad G, Masud M, Amin SU, Alrobaea R, Alhamid MF (2018) Automatic seizure detection in a Mobile multimedia framework. IEEE Access 6:45372–45383. https://doi.org/10.1109/ACCESS.2018.2859267
Munch Nielsen J, Zibrandtsen IC, Masulli P, Lykke Sørensen T, Andersen TS, Wesenberg Kjær T (2022) Towards a wearable multi-modal seizure detection system in epilepsy: a pilot study. Clin Neurophysiol 136:40–48. https://doi.org/10.1016/j.clinph.2022.01.005
Mursalin M, Zhang Y, Chen Y, Chawla NV (2017) Automated epileptic seizure detection using improved correlation-based feature selection with random forest classifier. Neurocomputing 241:204–214. https://doi.org/10.1016/j.neucom.2017.02.053
Nagappan I, Khan N, Shahina A (2020) Deep learning approach to detect seizure using reconstructed phase space images. J Biomed Res 34:238. https://doi.org/10.7555/JBR.34.20190043
Nogay HS, Adeli H (2020) Detection of epileptic seizure using Pretrained deep convolutional neural network and transfer learning. Eur Neurol 83(6):602–614. https://doi.org/10.1159/000512985
Osman AH, Alzahrani AA (2019) New approach for automated epileptic disease diagnosis using an integrated self-organization map and radial basis function neural network algorithm. IEEE Access 7:4741–4747. https://doi.org/10.1109/ACCESS.2018.2886608
Park C et al (2018) Epileptic seizure detection for multi-channel EEG with deep convolutional neural network. In: 2018 International Conference on Electronics, Information, and Communication (ICEIC), pp 1–5. https://doi.org/10.23919/ELINFOCOM.2018.8330671
Parvez MZ, Paul M (2017) Seizure prediction using undulated global and local features. IEEE Trans Biomed Eng 64(1):208–217. https://doi.org/10.1109/TBME.2016.2553131
Patidar S, Panigrahi T (2017) Detection of epileptic seizure using Kraskov entropy applied on tunable-Q wavelet transform of EEG signals. Biomed Signal Process Control 34:74–80. https://doi.org/10.1016/j.bspc.2017.01.001
Peachap AB, Tchiotsop D (2019) Epileptic seizures detection based on some new Laguerre polynomial wavelets, artificial neural networks and support vector machines. Informatics Med. Unlocked 16:100209. https://doi.org/10.1016/j.imu.2019.100209
Peker M, Sen B, Delen D (2016) A novel method for automated diagnosis of epilepsy using complex-valued classifiers. IEEE J Biomed Health Inform 20(1):108–118. https://doi.org/10.1109/JBHI.2014.2387795
Pippa E et al (2016) Improving classification of epileptic and non-epileptic EEG events by feature selection. Neurocomputing 171:576–585. https://doi.org/10.1016/j.neucom.2015.06.071
Pisano F, Sias G, Fanni A, Cannas B, Dourado A, Pisano B, Teixeira CA (2020) Convolutional neural network for seizure detection of nocturnal frontal lobe epilepsy. Complexity 2020:4825767–4825710. https://doi.org/10.1155/2020/4825767
Prabhakar SK, Rajaguru H (2017) Conceptual analysis of epilepsy classification using probabilistic mixture models. 2017 5th int. winter conf. brain-computer interface, pp 81–84. https://doi.org/10.1109/IWW-BCI.2017.7858166 .
Qi Y, Wang Y, Zhang J, Zhu J, Zheng X (2014) Robust deep network with maximum Correntropy criterion for seizure detection. Biomed Res Int 2014:703816. https://doi.org/10.1155/2014/703816
Rajaei H, Cabrerizo M, Janwattanapong P, Pinzon-Ardila A, Gonzalez-Arias S, Adjouadi M (2016) Connectivity maps of different types of epileptogenic patterns. In: 2016 38th Annual International Conference of the IEEE Engineering in Medicine and Biology Society (EMBC), pp 1018–1021. https://doi.org/10.1109/EMBC.2016.7590875
Rajaguru H (2017) Non linear ICA and logistic regression for classification of epilepsy from eeg signals. Int. Conf. Electron. Commun. Aerosp. Technol., pp 577–580
Rajaguru H (2017) Sparse PCA and soft decision tree classifiers for epilepsy classification from EEG signals. Int. Conf. Electron. Commun. Aerosp. Technol. ICECA, pp 581–584
Rajaguru H, Thangavel V (2014) Wavelets and morphological operators based classification of epilepsy risk levels. Math Probl Eng 2014:813197–813113. https://doi.org/10.1155/2014/813197
Rizzo C (2019) In: Mecarelli O (ed) EEG signal acquisition BT - clinical electroencephalography. Springer International Publishing, Cham, pp 53–73
Rukasha T, Woolley SI, Kyriacou T, Collins T (2020) Evaluation of wearable electronics for epilepsy: A systematic review. Electronics 9(6):968
Sai CY, Mokhtar N, Arof H, Cumming P, Iwahashi M (2018) Automated classification and removal of EEG artifacts with SVM and wavelet-ICA. IEEE J Biomed Health Inform 22(3):664–670. https://doi.org/10.1109/JBHI.2017.2723420
Samie F, Paul S, Bauer L, Henkel J (2018) Highly efficient and accurate seizure prediction on constrained IoT devices. In: 2018 Design, Automation & Test in Europe Conference & Exhibition (DATE), pp 955–960. https://doi.org/10.23919/DATE.2018.8342147
Saminu S, Xu G, Zhang S, Isselmou AEK, Zakariyya RS, Jabire AH (2019) Epilepsy detection and classification for smart iot devices using hybrid technique. In: 2019 15th International Conference on Electronics, Computer and Computation (ICECCO), pp 1–6. https://doi.org/10.1109/ICECCO48375.2019.9043241
San-Segundo R, Gil-Martín M, D’Haro-Enríquez LF, Pardo JM (2019) Classification of epileptic EEG recordings using signal transforms and convolutional neural networks. Comput Biol Med 109:148–158. https://doi.org/10.1016/j.compbiomed.2019.04.031
Sareen S, Sood S, Gupta S (2016) An automatic prediction of epileptic seizures using cloud computing and wireless sensor networks. J Med Syst 40:226. https://doi.org/10.1007/s10916-016-0579-1
Sayeed A, Mohanty SP, Kougianos E, Yanambaka VP, Zaveri H (2018) A robust and fast seizure detector for IoT edge. In: 2018 IEEE International Symposium on Smart Electronic Systems (iSES) (Formerly iNiS), pp 156–160. https://doi.org/10.1109/iSES.2018.00042
Sharma M, Pachori R (2017) A novel approach to detect epileptic seizures using a combination of tunable-Q wavelet transform and fractal dimension. J Mech Med Biol 17:1740003 (20 pages). https://doi.org/10.1142/S0219519417400036
Sharma M, Bhurane AA, Rajendra Acharya U (2018) MMSFL-OWFB: a novel class of orthogonal wavelet filters for epileptic seizure detection. Knowl-Based Syst 160:265–277. https://doi.org/10.1016/j.knosys.2018.07.019
Sharmila A (2018) Epilepsy detection from EEG signals: a review. J Med Eng Technol 42(5):368–380. https://doi.org/10.1080/03091902.2018.1513576
Sharmila A, Geethanjali P (2016) DWT based detection of epileptic seizure from EEG signals using naive Bayes and k-NN classifiers. IEEE Access 4:7716–7727. https://doi.org/10.1109/ACCESS.2016.2585661
Sheela P, Puthankattil SD (2020) A hybrid method for artifact removal of visual evoked EEG. J Neurosci Methods 336:108638. https://doi.org/10.1016/j.jneumeth.2020.108638
Shivarudhrappa R, Sriraam N, Temel Y, Rao S, Hegde A, Kubben P (2019) Performance evaluation of DWT based sigmoid entropy in time and frequency domains for automated detection of epileptic seizures using SVM classifier. Comput Biol Med 110:127–143. https://doi.org/10.1016/j.compbiomed.2019.05.016
Shoeibi A et al (2021) Epileptic seizures detection using deep learning techniques: A review. Int J Environ Res Public Health 18(11):5780
Shoka A, Dessouky M, El-Sherbeny A, El-Sayed A (2019) Literature review on EEG preprocessing, feature extraction, and classifications techniques. Menoufia J Electron Eng Res 28(ICEEM2019-Special Issue):292–299. https://doi.org/10.21608/mjeer.2019.64927
Siddiqui MK, Islam M, Kabir A (2019) A novel quick seizure detection and localization through brain data mining on ECoG dataset. Neural Comput Appl 31:1–14. https://doi.org/10.1007/s00521-018-3381-9
Singh K, Malhotra J (2019) IoT and cloud computing based automatic epileptic seizure detection using HOS features based random forest classification. J Ambient Intell Humaniz Comput. https://doi.org/10.1007/s12652-019-01613-7
Singh G, Kaur M, Singh D (2015) Detection of epileptic seizure using wavelet transformation and spike based features. In: 2015 2nd International Conference on Recent Advances in Engineering & Computational Sciences (RAECS), pp 1–4. https://doi.org/10.1109/RAECS.2015.7453376
Sriraam N et al (2018) Multichannel EEG based inter-ictal seizures detection using teager energy with backpropagation neural network classifier. Australas Phys Eng Sci Med 41:1047–1055. https://doi.org/10.1007/s13246-018-0694-z
Stevenson NJ, Tapani K, Lauronen L, Vanhatalo S (2019) A dataset of neonatal EEG recordings with seizure annotations. Sci. Data 6(1):190039. https://doi.org/10.1038/sdata.2019.39
Subasi A (2005) Epileptic seizure detection using dynamic wavelet network. Expert Syst Appl 29(2):343–355. https://doi.org/10.1016/j.eswa.2005.04.007
Sudalaimani C, Sivakumaran N, Elizabeth T, Rominus V (2018) Automated detection of the preseizure state in EEG signal using neural networks. Biocybern Biomed Eng 39. https://doi.org/10.1016/j.bbe.2018.11.007
Supriya S, Siuly S, Zhang Y (2016) Automatic epilepsy detection from EEG introducing a new edge weight method in the complex network. Electron Lett 52(17):1430–1432. https://doi.org/10.1049/el.2016.1992
Tanveer M, Pachori RB (eds.) (2018) Machine intelligence and signal analysis. vol 748. Springer
Tanveer M, Pachori RB, Angami NV (2018) Entropy based features in FAWT framework for automated detection of epileptic seizure EEG signals. In: 2018 IEEE Symposium Series on Computational Intelligence (SSCI), pp 1946–1952. https://doi.org/10.1109/SSCI.2018.8628733
Thara DK, PremaSudha BG, Xiong F (2019) Auto-detection of epileptic seizure events using deep neural network with different feature scaling techniques. Pattern Recognit Lett 128:544–550. https://doi.org/10.1016/j.patrec.2019.10.029
Thara DK, Premasudha BG, Nayak RS, Murthy TV, Ananth Prabhu G, Hanoon N (2020) Electroencephalogram for epileptic seizure detection using stacked bidirectional LSTM_GAP neural network. Evol Intell. https://doi.org/10.1007/s12065-020-00459-9
Thodoroff P, Pineau J, Lim A (2016) Learning robust features using deep learning for automatic seizure detection. CoRR, vol abs/1608.0, [Online]. Available: http://arxiv.org/abs/1608.00220
Thomas J, Comoretto L, Jing J, Dauwels J, Cash S, Westover MB (2018) EEG classification via convolutional neural network-based interictal epileptiform event detection. In: 2018 40th Annual International Conference of the IEEE Engineering in Medicine and Biology Society (EMBC). IEEE
Tiwari AK, Pachori RB, Kanhangad V, Panigrahi BK (2017) Automated diagnosis of epilepsy using key-point-based local binary pattern of EEG signals. IEEE J Biomed Health Inform 21(4):888–896. https://doi.org/10.1109/JBHI.2016.2589971
Tjepkema-Cloostermans M, Carvalho R, van Putten M (2018) Deep learning for detection of focal epileptiform discharges from scalp EEG recordings. Clin Neurophysiol 129:2191–2196. https://doi.org/10.1016/j.clinph.2018.06.024
Torse D, Desai V, Khanai R (2017) A review on seizure detection systems with Emphasis on multi-domain feature extraction and classification using machine learning. Brain-Broad Res Artif Intell Neurosci 8(4):109–129
Torse D, Desai V, Khanai R (2018) A review on seizure detection systems with emphasis on multi-domain 752feature extraction and classification using machine learning
Torse D, Desai V, Khanai R (2019) Classification of EEG signals in seizure detection system using ellipse area features and support vector machine: ICDECT 2017, pp 87–96
Tsiouris KM, Konitsiotis S, Koutsouris DD, Fotiadis DI (2019) Unsupervised seizure detection based on rhythmical activity and spike detection in EEG signals. In: 2019 IEEE EMBS International Conference on Biomedical & Health Informatics (BHI), pp 1–4. https://doi.org/10.1109/BHI.2019.8834644
Tsipouras MG (2019) Spectral information of EEG signals with respect to epilepsy classification. EURASIP J Adv Signal Process 2019(1):10. https://doi.org/10.1186/s13634-019-0606-8
Tzimourta KD, Tzallas AT, Giannakeas N, Astrakas LG, Tsalikakis DG, Angelidis P, Tsipouras MG (2019) A robust methodology for classification of epileptic seizures in EEG signals. Health Technol (Berl) 9(2):135–142. https://doi.org/10.1007/s12553-018-0265-z
Ullah I, Hussain M, Qazi E-H, Aboalsamh H (2018) An automated system for epilepsy detection using EEG brain signals based on deep learning approach. Expert Syst Appl 107:61–71. https://doi.org/10.1016/j.eswa.2018.04.021
Upadhyay R, Padhy PK, Kankar PK (2016) A comparative study of feature ranking techniques for epileptic seizure detection using wavelet transform. Comput Electr Eng 53:163–176. https://doi.org/10.1016/j.compeleceng.2016.05.016
Vandecasteele K et al (2017) Automated epileptic seizure detection based on wearable ECG and PPG in a hospital environment. Sensors (Basel) 17(10):2338. https://doi.org/10.3390/s17102338
Vergara PM, de la Cal E, Villar JR, González VM, Sedano J (2017) An IoT platform for epilepsy monitoring and supervising. J Sensors 2017:6043069–6043018. https://doi.org/10.1155/2017/6043069
Wang S, Li Y, Wen P, Zhu G (2015) Analyzing EEG signals using graph entropy based principle component analysis and J48 decision tree. In: Proceedings of the 6th International Conference on Signal Processing Systems (ICSPS 2014). International Journal of Signal Processing Systems
Wang G, Ren D, Li K, Wang D, Wang M, Yan X (2018) EEG-based detection of epileptic seizures through the use of a directed transfer function method. IEEE Access 6:47189–47198. https://doi.org/10.1109/ACCESS.2018.2867008
Wang X, Gong G, Li N (2019) Automated recognition of epileptic EEG states using a combination of symlet wavelet processing, gradient boosting machine, and grid search optimizer. Sensors (Basel) 19(2):219. https://doi.org/10.3390/s19020219
Article MathSciNet Google Scholar
Wei X, Zhou L, Chen Z, Zhang L, Zhou Y (2018) Automatic seizure detection using three-dimensional CNN based on multi-channel EEG. BMC Med Inform Decis Mak 18(Suppl 5):111. https://doi.org/10.1186/s12911-018-0693-8
Yayik A, Yildirim E, Kutlu Y, Yildirim S (2015) Epileptic state detection: pre-ictal, Inter-ictal, ictal. Int J Intell Syst Appl Eng 3(1):14. https://doi.org/10.18201/ijisae.14531
Yildiz M, Bergil E, Oral C (2017) Comparison of different classification methods for the preictal stage detection in EEG signals. Biomed Res 28(2):858–865
Yol S, Ozdemir MA, Akan A, Chaparro LF (2018) Detection of epileptic seizures by the analysis of eeg signals using empirical mode decomposition. In: 2018 Medical Technologies National Congress (TIPTEKNO), pp 1–4. https://doi.org/10.1109/TIPTEKNO.2018.8596780
Yuan Y, Xun G, Jia K, Zhang A (2017) A multi-view deep learning method for epileptic seizure detection using short-time fourier transform. In: Proceedings of the 8th ACM International Conference on Bioinformatics, Computational Biology, and Health Informatics
Yuan Y, Xun G, Jia K, Zhang A (2019) A multi-view deep learning framework for EEG seizure detection. IEEE J Biomed Health Inform 23(1):83–94. https://doi.org/10.1109/JBHI.2018.2871678
Yuvaraj R, Thomas J, Kluge T, Dauwels J (2018) A deep learning scheme for automatic seizure detection from long-term scalp EEG. In: 2018 52nd Asilomar conference on signals, systems, and computers, pp 368–372. https://doi.org/10.1109/ACSSC.2018.8645301
Zabihi M, Kiranyaz S, Ince T, Gabbouj M (2013) Patient-specific epileptic seizure detection in long-term EEG recording in paediatric patients with intractable seizures. In: IET Intelligent Signal Processing Conference 2013 (ISP 2013), pp 1–7. https://doi.org/10.1049/cp.2013.2060
Zahra A, Kanwal N, ur Rehman N, Ehsan S, McDonald-Maier KD (2017) Seizure detection from EEG signals using multivariate empirical mode decomposition. Comput Biol Med 88:132–141. https://doi.org/10.1016/j.compbiomed.2017.07.010
Zazzaro G, Pavone L (2022) Machine learning characterization of ictal and interictal states in EEG aimed at automated seizure detection. Biomedicines 10(7):1491
Zhang G et al (2020) MNL-Network: a multi-scale non-local network for epilepsy detection from EEG signals. Front Neurosci 14:870. https://doi.org/10.3389/fnins.2020.00870
Zhao W, Zhao W, Wang W, Jiang X, Zhang X, Peng Y, Zhang B, Zhang G (2020) A novel deep neural network for robust detection of seizures using EEG signals. Comput Math Methods Med 2020:9689821. https://doi.org/10.1155/2020/9689821
Zhou D, Li X (2020) Epilepsy EEG signal classification algorithm based on improved RBF. Front Neurosci 14:606. [Online]. Available: https://www.frontiersin.org/article/10.3389/fnins.2020.00606
Zhou M, Tian C, Cao R, Wang B, Niu Y, Hu T, Guo H, Xiang J (2018) Epileptic seizure detection based on EEG signals and CNN. Front Neuroinform 12:95. https://doi.org/10.3389/fninf.2018.00095
Download references
Author information
Authors and affiliations.
Faculty of Electronic Engineering, Computer Science and Engineering Department, Menoufia University, Menouf, Egypt
Athar A. Ein Shoka, Mohamed M. Dessouky, Ayman El-Sayed & Ezz El-Din Hemdan
Department of Computer Science & Artificial Intelligence, College of Computer Science and Engineering, University of Jeddah, Jeddah, Saudi Arabia
Mohamed M. Dessouky
You can also search for this author in PubMed Google Scholar
Corresponding author
Correspondence to Athar A. Ein Shoka .
Ethics declarations
Competing interests.
The author(s) declare(s) that they have no competing interests.
Additional information
Publisher’s note.
Springer Nature remains neutral with regard to jurisdictional claims in published maps and institutional affiliations.
Rights and permissions
Springer Nature or its licensor (e.g. a society or other partner) holds exclusive rights to this article under a publishing agreement with the author(s) or other rightsholder(s); author self-archiving of the accepted manuscript version of this article is solely governed by the terms of such publishing agreement and applicable law.
Reprints and permissions
About this article
Ein Shoka, A.A., Dessouky, M.M., El-Sayed, A. et al. EEG seizure detection: concepts, techniques, challenges, and future trends. Multimed Tools Appl 82 , 42021–42051 (2023). https://doi.org/10.1007/s11042-023-15052-2
Download citation
Received : 25 April 2022
Revised : 07 August 2022
Accepted : 27 February 2023
Published : 04 April 2023
Issue Date : November 2023
DOI : https://doi.org/10.1007/s11042-023-15052-2
Share this article
Anyone you share the following link with will be able to read this content:
Sorry, a shareable link is not currently available for this article.
Provided by the Springer Nature SharedIt content-sharing initiative
- Electroencephalography (EEG)
- Features extraction
- Classification
- Artificial intelligence
- Find a journal
- Publish with us
- Track your research
- Open access
- Published: 25 May 2020
A review of epileptic seizure detection using machine learning classifiers
- Mohammad Khubeb Siddiqui ORCID: orcid.org/0000-0001-6699-6216 1 na1 ,
- Ruben Morales-Menendez 1 ,
- Xiaodi Huang 2 na1 &
- Nasir Hussain 3
Brain Informatics volume 7 , Article number: 5 ( 2020 ) Cite this article
21k Accesses
200 Citations
1 Altmetric
Metrics details
Epilepsy is a serious chronic neurological disorder, can be detected by analyzing the brain signals produced by brain neurons. Neurons are connected to each other in a complex way to communicate with human organs and generate signals. The monitoring of these brain signals is commonly done using Electroencephalogram (EEG) and Electrocorticography (ECoG) media. These signals are complex, noisy, non-linear, non-stationary and produce a high volume of data. Hence, the detection of seizures and discovery of the brain-related knowledge is a challenging task. Machine learning classifiers are able to classify EEG data and detect seizures along with revealing relevant sensible patterns without compromising performance. As such, various researchers have developed number of approaches to seizure detection using machine learning classifiers and statistical features. The main challenges are selecting appropriate classifiers and features. The aim of this paper is to present an overview of the wide varieties of these techniques over the last few years based on the taxonomy of statistical features and machine learning classifiers—‘black-box’ and ‘non-black-box’. The presented state-of-the-art methods and ideas will give a detailed understanding about seizure detection and classification, and research directions in the future.
1 Introduction
The word epilepsy originates from the Latin and Greek word ‘epilepsia’ which means ‘seizure’ or ‘to seize upon’. It is a serious neurological disorder with unique characteristics, tending of recurrent seizures [ 1 ]. The context of epilepsy, found in the Babylonian text on medicine, was written over 3000 years ago [ 2 , 3 ]. This disease is not limited to human beings, but extends to cover all species of mammals such as dogs, cats and rats. However, the word epilepsy does not give any types of clues about the cause or severity of the seizures; it is unremarkable and uniformly distributed around the world [ 1 , 4 ].
Several theories about the cause are already available. The main cause is electrical activity disturbance inside a brain [ 1 , 5 , 6 ], which could be originated by several reasons [ 7 ] such as malformations, shortage of oxygen during childbirth, and low sugar level in blood [ 8 , 9 ]. Globally, epilepsy affects approximately 50 million people, with 100 million being affected at least once in their lifetime [ 5 , 10 ]. Overall, it accounts for 1% of the world’s burden of diseases, and the prevalence rate is reported at 0.5–1% [ 4 , 11 ]. The main symptom of epilepsy is to experience more than one seizure by a patient. It causes a sudden breakdown or unusual activity in the brain that impulses an involuntary alteration in a patient’s behaviour, sensation, and loss of momentary consciousness. Typically, seizures last from seconds to a few minute(s), and can happen at any time without any aura. This leads to serious injuries including fractures, burns, and sometimes death [ 12 ].
1.1 Seizure type
Based on the symptoms, seizures are categorized by neuro-experts into two main categories—partial and generalized [ 7 , 13 ]—as shown in Fig. 1 . Partial seizure, also called ‘focal seizure’, causes only a section of the cerebral hemisphere to be affected. There are two types of Partial seizure: simple-partial and complex-partial. In the simple-partial, a patient does not lose consciousness but cannot communicate properly. In the complex-partial, a person gets confused about the surroundings and starts behaving abnormally like chewing and mumbling; this is known as ‘focal impaired awareness seizure’. On the contrary, in the generalized seizures, all regions of the brain suffer and entire brain networks get affected quickly [ 14 ]. Generalized seizures are of many types, but they are broadly divided into two categories: convulsive and non-convulsive.
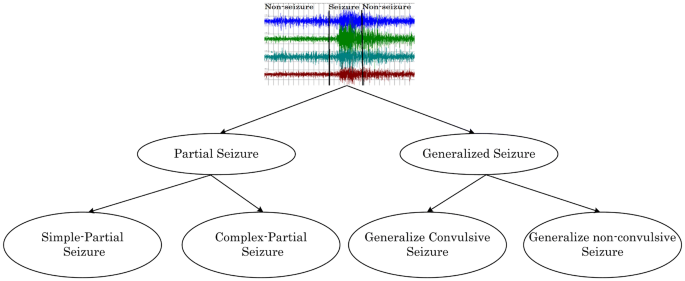
Types of seizure. Showing types of seizure and its sub-types
1.2 Main contributions of the paper
In brief, the contributions of this paper are as follows:
We have done the review according to five main dimensions. First, researchers who adopted the EEG, ECoG or both for seizure detection; second, significant features; third, machine learning classifiers; fourth, the performance of the classifier during a seizure, and last, knowledge discovery (e.g., seizure localization).
Through study, it has been explored that an ensemble of decision trees (i.e., decision forest–random forest) classifier outperforms other classifiers (ANN, KNN, SVM, single Decision Tree).
We also suggest, how decision forest algorithms could be more effective for other knowledge discovery tasks besides seizure detection.
This study will help the researchers with their data science backgrounds to identify which statistical and machine learning classifiers are more relevant for further improvement to the existing methods for seizure detection.
The study will also help the readers for understanding about the publicly available epilepsy datasets.
In the end, we have provided our observations by the current review and suggestions for future research in this area.
The structure of the paper is organized as follows. “ Role of data scientists in epileptic seizure detection ” section gives the overview of machine learning experts in EEG datasets. The preliminaries requirements are provided in “ A framework for seizure detection ” section; it presents a general model of seizure detection and explains each step in a subsequent manner. “ Publicly available datasets ” section provides the details of benchmark datasets with their description. “ Seizure detection based on statistical features and machine learning classifiers ” section explains the review of literature work done on seizure detection using different machine learning classifiers, with a detailed comparison. “ Seizure localization ” section reviews the work done in identifying the affected lobes of the brain using machine learning classifiers. In “ Problems identified in existing literature ” section, we have explored the issues in the previous work and highlighted the gap. Overall, “ observation about capable classifiers and statistical features ” section reports our observations from the review about a suitable classifier and feature. “ Research directions in seizure detection ” section emphasizes the future directions in this research area, followed by “ Conclusion ” section on the summary of the paper.
2 Role of data scientists in epileptic seizure detection
Applications of machine learning are significantly seen on health and biological data sets for better outcomes [ 15 , 16 ]. Researchers/scientists on different areas, specifically, data mining and machine learning, are actively involved in proposing solutions for better seizure detection. Machine learning has been significantly applied to discover sensible and meaningful patterns from different domain datasets [ 17 , 18 ]. It plays a significant and potential role in solving the problems of various disciplines like healthcare [ 17 , 19 , 20 , 21 , 22 , 23 , 24 , 25 ]. Applications of machine learning can also be seen on brain datasets for seizure detection, epilepsy lateralization, differentiating seizure sates, and localization [ 26 , 27 , 28 , 29 ]. This has been done by various machine learning classifiers such as ANN, SVM, decision tree, decision forest, and random forest [ 26 , 28 ].
Certainly, in the past, numerous reviews have been carried out on seizure detection along with applied features, classifiers, and claimed accuracy [ 27 , 30 , 31 , 32 , 33 ] without focusing on the challenges faced by the data scientists whilst doing research on datasets of neurological disorders. Therefore, this article provides a detailed study of machine learning applications on epileptic seizure detection and other related knowledge discovery tasks. In this review, the collected articles are from well-known journals of their relevant field. These references are either indexed by SCOPUS or Web of Science (WOS) . Besides, we also considered some good ranked conference papers. Extensive literature is available covering the deep analysis of different features and classifiers applied on EEG datasets for seizure detection [ 31 , 34 , 35 ]. Both, feature extraction and applying classification techniques are challenging tasks. Previous literature reveals that for the past few years, interest has been increased in the application of machine learning classifiers for extracting meaningful patterns from EEG signals, which helps for detecting seizures, its location in the brain, and other impressive related knowledge discoveries [ 28 , 36 , 37 ]. Three decades ago, Jean Gotman [ 6 , 38 , 39 , 40 ], analyzed and proposed the model for effective usage of EEG signals by applying different computational and statistical techniques for automatic seizure detection. Furthermore, the research has been carried out by different signal processing methods and data science methods to provide better outcomes [ 27 , 34 , 41 , 42 , 43 , 44 , 45 , 46 , 47 ].
3 A framework for seizure detection
In this section, we present a pictorial framework of the model used for seizure detection from an EEG/ECoG seizure dataset, illustrated in Fig. 2 . The process comprises four steps: Data Collection, Data Preparation, Applying Machine Learning Classifiers and Performance Evaluation.
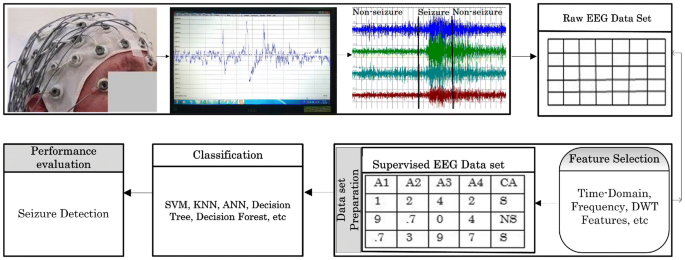
Basic model of epileptic seizure detection. This explains the basic steps to collect the dataset by EEG medium, display of raw EEG signals, transform EEG signals to two-dimensional table, feature selection, prepare the dataset with seizure (S) and non-seizure (NS) , apply machine learning classifier(s) and seizure detection, or other related tasks
3.1 Data collection
The initial requirement is to collect the dataset of brain signals. For this, different monitoring tools are used. Typically, the mostly used devices are EEG and ECoG, because their channels or electrodes are implanted by glue on the surface of the scalp as per 10–20 International system [ 48 ] at different lobes. Each of them has a wire connection to the EEG device, providing timely information about the variations in voltage, along with temporal and spatial information [ 49 ]. As highlighted in Fig. 2 , the EEG channels are placed on the subject’s scalp, and the electrical signals are read by the EEG monitoring tool and it displays these raw signals over the screen. Further, these raw signals have been carefully monitored by the analyst and classified into ‘seizure’ and ‘non-seizure’ states.
3.2 Data transformation
After data collection, the next crucial step is to transform the signal data into a 2-D Table format. The reason for this is to make it easier for analysis and provide necessary knowledge like seizure detection. This datum is raw because it has not been processed yet. Therefore, it will not be suitable to give relevant information. To do the processing, different feature selection modalities have been applied. This step also presents the dataset as supervised, which means that it provides the class attribute with possible class-values.
3.3 Dataset preparation
For data transformation, data processing is a decisive step to extract meaningful information from the collected raw dataset. As such, different feature extraction techniques have been used; as shown in Table 1 . These methods are generally applied to the extracted EEG signal dataset [ 31 , 34 ]. The raw dataset becomes rich in terms of different statistical measure values.
After feature extraction processing, the dataset becomes more informative that it ultimately helps the classifier for retrieving better knowledge.
3.4 Applying machine learning classifiers and performance evaluation
To achieve a high accuracy of seizure detection rate and explore relevant knowledge from the EEG processed dataset, different supervised and unsupervised machine learning have been used.
3.4.1 Classification
In classification, a dataset D has a set of ‘non-class attributes’, and a ‘class attribute’. They are the principal components and their pertinent knowledge is very important, as both have a strong association for potential classification. The target attribute is defined as the ‘class attribute’ C , and it comprises more than one class values, e.g., seizure and non-seizure . On the contrary, attributes \(A=\{A_1,A_2.A_3 \ldots A_n\}\) are known as ‘non-class attributes’ or predictors [ 50 , 51 ]. The following classifiers have been popularly used in seizure detection. Common classifiers such as SVM [ 52 ], decision tree [ 53 ] and decision forest [ 54 ] are applied to the processed EEG dataset for seizure detection.
3.4.2 Performance evaluation
The accuracy of the obtained results is used to evaluate different methods. The most popular training approach is tenfold cross-validation [ 55 ], where each fold, i.e., one horizontal segment of the dataset is considered to be the testing dataset and the remaining nine segments are used as the training dataset [ 56 , 57 ].
Except for the accuracy, the performance of the classifiers is commonly measured by the following metrics such as precision, recall, and f-measure [ 58 ]. These are based on four possible classification outcomes—True-Positive (TP), True-Negative (TN), False-Positive (FP), and False-Negative (FN) as presented in Table 2 .
Precision is the ratio of true-positives to the total number of cases that are detected as positive (TP+FP). It is the percentage of selected cases that are correct, as shown in Eq. 1 . High precision means the low false-positive rate.
Recall is the ratio of true-positive cases to the cases that are actually positive. Equation 2 shows the percentage of corrected cases that are selected.
Despite getting the high Recall results of the classifier, it does not indicate that the classifier performs well in terms of precision. As a result, it is mandatory to calculate the weighted harmonic mean of Precision and Recall; this measure is known as F-measure score, shown in Eq. 3 . The false-positives and the false-negatives are taken into account. Generally, it is more useful than accuracy, especially when the dataset is imbalanced.
4 Publicly available datasets
For data scientists and researchers, a dataset used is important for evaluating the performance of their proposed models. Similarly, in epileptic seizure detection, we need to capture the brain signals. EEG recording is the most used method for monitoring brain activity. These recordings play a vital role in machine learning classifiers to explore the novel methods for seizure detection in different ways such as onset seizure detection, quick seizure detection, patient seizure detection, and seizure localization. The significance of publicly available datasets is that they provide a benchmark to analyze and compare the results to others. In the following section, we will describe the popular datasets that are widely used on epilepsy.
4.1 Children Hospital Boston, Massachusetts Institute of Technology—EEG dataset
This dataset is publicly available on a physionet server and prepared at Children Hospital Boston, Massachusetts Institute of Technology (CHB-MIT) [ 59 , 60 ]. It can be collected easily via Cygwin tool which interacts with the physionet server. It contains the number of seizure and non-seizure EEG recordings for each patient of the CHB [ 61 ]. The dataset comprises 23 patients; 5 males, aged 3–22 years, and 17 females aged 1.5–19. Each patient contains multiple seizure and non-seizure recording files in European data format (.edf), representing the spikes with seizure start and end time, which is easily visible at a browser called an ‘EDFbrowser’. The primary datasets are in the 1-D format, containing EEG signals that are obtained through the different types of channels that were placed on the surface of the brain as per 10-20 International System. All these signals of the dataset were sampled at the frequency of 256Hz.
4.2 ECoG Dataset, Epilepsy Centre, University of California
This is a publicly available dataset of electrocorticogram (ECoG) signals from an epileptic patient, which was collected from the Epilepsy Center, University of California, San Francisco (UCSF) [ 62 ]. It was originally collected by implanting 76 electrodes on the scalp in both invasive (12-electrodes) and non-invasive manner (64-electrodes). It comprises 16 files altogether. Out of these, eight files ( \(F1, F2, \cdots F8\) ) are classified as ‘pre-ictal’ meaning the stage before the seizure. The rest of the files ( \(F9, F10, F11, \cdots F16\) ) represent the ‘ictal’ stage data. The collected data are sampled at the frequency of 400 Hz (i.e., 400 cycles/s) and the total duration is 10 s. As a result, there are (400 cycles/s \(\times\) 10 s) 4000 cycles in each file [ 63 ].
4.3 The Freiburg—EEG dataset
This dataset was collected from the invasive EEG recordings of 21 patients (8 males aged 13–47 years, 13 females aged 10–50 years) suffering from medically intractable focal epilepsy. It was recorded during an invasive pre-surgical epilepsy monitoring at the Epilepsy Centre of the University Hospital of Freiburg, Germany [ 64 ]. Out of 21 patients, 13 patients had 24 h of recordings, and 8 patients had less than 24 h. These recordings are inter-ictal, and together they provide 88 seizures.
4.4 Bonn University—EEG dataset
The dataset comprises five subsets, where each one denoted as (A–E) contains 100 single-channels recording, and each of them has a 23.6 s duration, captured by the international 10–20 electrode placement scheme. All the signals are recorded with the same 128-channel amplifier system channel [ 65 ].
4.5 BERN-BARCELONA—EEG dataset
This dataset comprised EEG recordings derived from five pharmacoresistant temporal lobe epilepsy patients with 3750 focal and 3750 non-focal bivariate EEG files. Three patients were seizure-free, with two patients only having auras but no other seizures following surgery. The multichannel EEG signals were recorded with an intracranial strip and depth electrodes. The 10–20 positioning was used for the electrodes’ implantation. EEG signals were either sampled at 512 or 1024 Hz, depending on whether they were recorded with more or less than 64 channels. According to the intracranial EEG recordings, they were able to localize the brain areas where seizures started for all five patients [ 66 ]. This dataset is good for the seizure localization purpose.
5 Seizure detection based on statistical features and machine learning classifiers
This section explains the comprehensive detail of work on seizure detection using statistical features, classifiers—‘black-box’ and ‘non-black-box’. They are illustrated in Table 3 . In brief, the ‘black-box’ classifiers are those which provide the accuracy without mentioning the reasons behind the results such as ANN and SVM [ 67 ]. They are unable to explain their classification steps. Whereas, ‘non-black-box’ classifiers such as decision forest and random forest can able to explain each step of the processing, which is human-understandable. As a result, it helps in human-interpretable knowledge with high accuracy [ 68 ].
5.1 Seizure detection based on statistical features
If we apply machine learning classifier(s) directly to raw EEG/ECoG datasets, it may not produce enough sensible patterns. Therefore, selecting significant and capable statistical features from EEG and ECoG raw datasets is one of the challenges and a crucial task. The nature of EEG and ECoG signals is very complex, non-stationary and time-dependent [ 105 , 106 , 107 ]. As such, we can apply the machine learning classifier(s) to the processed datasets, which will ultimately assist to solve various neurological problems; for example, identifying seizure’s stages, accurate seizure detection, fast detection, etc. In Table 3 , we summarize a review of several studies.
The significant statistical features were extracted by different types of transformation techniques; discrete wavelet transformations (DWT), continuous wavelet transformation (CWT), Fourier transformation (FT), discrete cosine transformation (DCT), singular value decomposition (SVD), intrinsic mode function (IMF), and time–frequency domain from EEG datasets [ 34 , 71 , 79 , 108 ]. Logesparan et al. [ 34 ] used different types of feature extraction methods for seizure detection, but they reported that two features—‘line length’ and ‘relative power’—are the good performers for seizure detection. Guerrero-Mosquera [ 109 ] applied three time-domain features—line length, frequency, and energy on the raw EEG dataset. These features claim to be suitable for seizure detection and other brain-related applications such as computer interface (BCI). The claimed performance was evaluated using the following metrics such as sensitivity, specificity, F-score, receiver operating characteristics (ROC) curve, and percentile bootstrap measures. Duo Chen [ 84 ] used DWT with the SVM classifier on two benchmark datasets—CHB-MIT and Bonn University, achieved seizure detection accuracies of 92.30% and 99.33%, respectively. Ramy Hussein et al. [ 100 ] proposed a new featured L1-penalized robust regression (L1PRR) for seizure detection, the issue with their approach is computational complexity. Zavid and Paul [ 99 ] focused on classifying the ‘ictal’ and ‘inter-ictal’ states, where they used four features DCT, DCT-DWT, SVD, and IMF; the obtained signals are further classified by LS-SVM due to less computational cost.
Several researchers have contributed to seizure detection using a single feature [ 108 , 110 ]. The feature ‘line length’ [ 108 , 110 ] was applied to an EEG dataset; approximately 4.1 s of mean detection latency is recorded at a false alarm rate of 0.051 Fp/h. Further, Guo et al. [ 69 ] also used ‘line length’ but with the ANN for classifying the records obtained by EEG signals. Their automated seizure detection accuracy is 99.6%. A system was proposed by Koolen et al. [ 70 ] to detect seizures from EEG recordings. This detection system uses a single feature—‘line length’. The performance of this system shows 84.27% accuracy, 84.00% sensitivity and 85.70% specificity, which are comparatively lower than the results of Guo et al. [ 69 ].
After 3 years of study on several of statistical features [ 34 ], Logesparan et al. [ 71 ] proposed the ‘line length’ feature for normalization and discrimination of class values from EEG datasets. It is noted that ‘line length’ could be taken as the strongest feature and provides considerable output. Based on previous studies, the ‘line length’ can be taken with other features, and the result would be more promising, specifically in machine learning. This is because the dataset dimension would also increase with meaningful statistical information in the attributes.
Some other studies on seizure detection based on a single feature, i.e., entropy and its sub-types such as approximate entropy (AE) and sample entropy (SE), have also been done [ 45 , 72 , 73 , 111 ]. The entropy feature helps to find the random behaviour of EEG signals and takes depth benefits in measuring the impurity of the signals [ 112 , 113 ]. The entropy feature has been used widely where data are in the form of signals such as ECG, [ 114 ], EEG, and ECoG [ 36 ]. This helps in further steps of the detection model.
Acharya et al. [ 111 ] used four different types of entropy-based features: sample entropy, approximate entropy, phase entropy (S1), and phase entropy (S2) of the EEG datasets. The processed dataset from these entropy features was used for seizure detection. In another study, Chen et al. [ 90 ] used eight different kinds of entropy feature—approximate, sample, spectral, fuzzy, permutation, Shannon, conditional and correction conditional on a raw EEG dataset; further, the processed data were classified into three class values: ‘ictal’, ‘inter-ictal’ and ‘normal stage’, and their accuracy is 99.50%. A tool was proposed by Selvakumari et al. [ 89 ] using four features—entropy, root mean square (RMS), variance, and energy. Based on these features, the detection was done using SVM and naïve Bayesian classifiers with a reported accuracy of 95.63%. The tool is also able to find the seizure region in the brain; however, they did not mention the exact percentage of seizure location. Song and Li [ 72 ] built classification models by two classifiers—Extreme Learner Machine (ELM) and the back-propagation neural network (BPNN). Overall, their findings show 95.6% of classification accuracy with less execution time. Yong Zhang et al. [ 73 ] applied two entropy features—AE and SE on two different classifiers—ELM and SVM for processing EEG dataset. The SE features with ELM provide good classification accuracy compared to the AE feature whilst detecting the seizure.
The energy feature has been significantly used in seizure detection [ 115 ]. It plays a vital role particularly when the seizure is detected by the epoch- or windows-based method. This means that the EEG signals are divided into various segments [ 79 , 94 ]. An exponential energy feature has been introduced by Fasil and Rajesh [ 97 ], which helps in identifying the irregularities in amplitude EEG signals.
Observations This section has provided an overview of the contributions of statistical features to seizure detection and their importance. Some researchers detect seizures using multiple sets of features, whilst others select a single feature such as ‘line length’. We recommend the ‘line length’ feature to be in the list of the set of suitable features for seizure detection because it is helpful in measuring the EEG signals complexity. It plays a sensitive role in the changes at the frequency and amplitude of signals. As a result, it helps to discriminate against the ‘seizure’ and ‘non-seizure’ cases. However, from the data science point of view, it is very important to see the various perspectives of each brain signals by observing other statistical features. Furthermore, we also suggest not to use the irrelevant feature(s) as they will unnecessarily increase the dataset size which results in an increase in computational time and gives insensible patterns too. As a result, it becomes a hassle to machine learning classifiers and users rather than providing the benefit. Some researchers [ 95 , 98 , 101 ] used a large number of features, which increases the attribute size, and results in more computational time and less accuracy. So, if we take the fewer features as previous researchers have done [ 71 , 73 , 79 ] this will give the low-dimensional dataset, which will not be fruitful for the knowledge discovery process. The next section illustrates the seizure detection by ‘black-box’ classifiers. As far as the classification purpose is concerned, it would be better to take more relevant statistical features, which can be integrated into knowledge discovery and a good performance rate.
5.2 Seizure detection based on black-box classifiers
The classifiers such as SVM, ANN, and KNN are considered as prominent ones due to their remarkable performances in different domains [ 67 , 116 ]. Each technique has its pros and cons, and ‘black-box’ methods are not an exception to this [ 104 ]. Even though these classifiers contribute well to brain datasets, some of the relevant works on seizure detection using these classifiers are reported here.
The study of Satapathy et al. [ 85 ] was based on two ‘black-box’ approaches—SVM and Neural networks using different kernel methods for seizure detection against a large EEG dataset. The performance of each classifier is measured independently by the majority voting system, and it was found that SVM was more capable than other neural networks. Subasi et al. [ 87 ] proposed the solution to detect seizure using a hybrid approach of SVM, genetic algorithm (GA), and particle swarm optimization (PSO). The method achieved impressive accuracy, i.e., 99.38%, but the problem is that the classifier trains the dataset twice, one for SVM-GA and another for SVM-PSO. This could be a time-consuming.
Shoeb and Guttag [ 41 ] performed seizure detection on their arranged dataset of Child Hospital Bostan, MIT (CHB-MIT) [ 60 ] using SVM with the vector feature and achieved the estimated accuracy of 96%. Dorai and Ponnambalam [ 42 ] came with an idea of the epoch, which means dividing the dataset into smaller time frames. Further, they applied an ensemble of four ‘black-box’ approaches—LDA, KNN, CVE, and SVM on these epoch EEG datasets. This approach provides the prediction of onset seizures 65 s earlier. Classifying the EEG data into two class ‘ ‘seizure” and ‘non-seizure’, Birjandtalab et al. [ 117 ] used a Gaussian mixture model (GMM) before detecting the seizure, and obtained 90% accuracy with 85.1% F-measure. They also raised the issue of class imbalance in their dataset. Tzallas et al. [ 103 ] used time–frequency-domain features with ANN for the EEG dataset and obtained 100% accuracy for the ‘seizure’ and ‘non-seizure’ classification problem; with epochs’ datasets the accuracy is 97.7% from (A, B, C, and D) for ‘non-seizure’ and set E for ‘seizure’ epoch classes. Amin et al. [ 79 ] extracted relative energy features from the DWT method, and four classifiers—SVM, MLP, KNN, and Naïve Bayes—were applied for the classification purpose, the result shows 98% of SVM accuracy, which outperforms remaining classifiers. A framework had been proposed by K. Abualsaud et al. [ 118 ] using the ensemble of ‘black-box’ classifiers for automated seizure detection on noisy EEG signals, and the reported classification accuracy is 95%. However, the ensemble approach did not provide good accuracy as desired because all four classifiers were ‘black-box’.
In 2018, Lahmiri et al. [ 92 ] used generalized Hurst exponent (GHE) and KNN, to propose a system for identifying the ‘seizure’ and ‘non-seizure’ classes from intracranial EEG recordings, detection rate, with 100% accuracy rate. Further, Lahmiri et al. [ 43 ] exploited GHE with SVM, to classify the ‘seizure’ and ‘non-seizure’, and also they found 100% accuracy in less time. Here, the good indication is that authors claim the good accuracy in less time for seizure detection. But, the authors did not clearly define how many times the seizure can be detected. In another study by Al Ghayab et al. [ 88 ], the obtained accuracy is 100% as a result of using the concept of Information gain theory, to extract and rank the meaningful features from EEG signal dataset. The least square-support vector machine (LS-SVM) is then applied to classify the seizure cases. Moreover, due to the ‘black-box’’s nature of applied classifiers, the authors could not explore any other related aspects in terms of Knowledge discovery. Zabihi et al. [ 81 ] did patient-specific seizure detection using SVM classifier on the processed dataset with a good set of features, comprising time-domain, frequency-domain, time–frequency domain, and non-linear feature. The performance of their model has achieved an average of 93.78% sensitivity and a specificity of 99.05%. Here, it is noteworthy that they skip an important feature—‘line length’, from the available literature, which is prominently used in seizure detection. We also argue that CHB-MIT dataset [ 60 ] is imbalanced because, in an hour(s) of recording, a seizure time span is for a few seconds.
Observations
The main issue with ‘black-box’ classifiers is that they only make prediction without providing logic rules or patterns. That is why, they are not recommended for extracting sensible knowledge. For example, for class imbalance issues in EEG datasets, insufficient related literature is found, and the researchers who attempted to work on this problem did not provide a conceivable solution as to how to solve the class imbalance issue whilst detecting the seizure.
5.3 Seizure detection based on non-black-box classifiers
‘Black-box’ classifiers are unable to express their classification procedure for human interpretation [ 67 , 104 , 116 ]. Consequently, there are fewer chances for knowledge discovery and better accuracy performance. Therefore, the concept of ‘non-black-box’ classifiers such as decision trees, and decision forests came into practice.
Chen et al. [ 119 ] first introduced the decision tree to the EEG dataset for seizure detection. Kemal and Saleh [ 120 ] used a C5.0 decision tree [ 121 ] algorithm to explore the logic rules for seizure detection, with an average accuracy of 75%. When the same C5.0 was applied to the same dataset processed by Fourier transformation the obtained accuracy with cross-validation was, however, 98.62%. A few related works are been available, where only a decision tree method is applied seizure detection because of less accuracy and a limited number of patterns obtained from the logic rules of a decision tree [ 122 ]. As a result, both the knowledge discovery and accuracy suffer. However, this gap can be filled by applying decision forest approaches instead [ 51 , 57 , 123 ].
Through the literature, it is found that the decision forest approaches are more effective than the single decision tree [ 57 , 124 ], because the decision tree often gives a confined set of rules and overfitting issue is also raised [ 68 ]. The rules are extracted from training data by a decision tree that generates either limited or a single set of logic rules (Say, wherever C2_Entropy value \(\le 101.01\) then \(Class\_value=seizure\) ) and stops growing the tree further records in the training dataset once the rule is accepted. However, if we generate a decision forest on the training data, we can achieve multiple sets of decision trees with the combination of sensible logic rules and a higher accuracy rate due to the majority voting method [ 57 ]. Decision forest classifiers [ 54 , 68 ] are the type of ensemble methods that are used frequently. These are also used in seizure detection as they provide a high accuracy rate which depends on the majority voting method from the ensemble of decision trees. Moreover, they produce more logic rules as multiple decision trees from the training data ( D ) [ 123 ]. These logic rules are humanly interpretable, and data scientists can easily interrelate them with other seizure-related information from EEG datasets.
Siddiqui and Islam [ 125 ] used Systematic Forest (SySFor) to detect the seizure on ECoG without epoch reduction. Further, Siddiqui et al. [ 63 ] applied two decision forests—Systematic Forest (SysFor) [ 123 ] and Forest CERN [ 51 ] on nine statistical features for quick seizure detection using the concept of epoch length reduction. It is based on dividing the size of training dataset D into \(D_1, D_2\) , ... \(D_n\) and testing the accuracy at every epoch of the dataset. These sub-datasets are in descending order in terms of time duration. If the seizure can be detected in a shorter epoch length without a decline in accuracy, then we can use the same one, which results in fast seizure detection. They achieved 100% accuracy. The limitation of this work is that authors have taken the dataset of a single patient, this could be tested for more patients. Several researchers have taken the advantages of random forest classifier for detecting the seizures [ 76 , 78 , 82 , 126 ]. Because researchers/data scientists are able to see the logic rules and interpret them correspondingly. Moreover, it also provides good accuracy [ 44 , 76 , 77 , 78 , 80 , 82 ]. Donos et al. [ 44 ] applied decision forest classifier—random forest, on time and frequency domains’ feature, which was extracted from an IEEG (Intra-cranial EEG) dataset. It helped in selecting the intra-cranial channels for early seizure detection in a closed-loop circuit. The results claimed that the system can detect the seizure with 93.8% sensitivity. Wang et al. [ 94 ] developed the greedy approach of random forest, i.e., forest-grid search optimization (RF-GSO), with this method and they found 96.7% accuracy. The shortcoming of this technique is that the performance could decline if EEG signals are too noisy. Tzimourta et al. [ 93 ] applied random forest to monitor seizure activities on the two benchmark epilepsy datasets [ 64 , 65 ], the reported performance is 99.74%. Pinto-Orellana and Fábio R. Cerqueira [ 76 ] also used the random forest on the processed CHB-MIT dataset by a Spectro-temporal feature, and 70s, and the accuracy of each block is 98.30%.
Truong ND et al. [ 82 ] had carried out novel work of channel selection whilst detecting the seizure. Their key contribution is that they also focus on channels contributing mostly to automatic seizure detection. They used the random forest to solve channel selection and seizure detection, and which achieving 96.94% area under the curve (AUC). In another work, Mursalin et al. [ 80 ] proposed a method for seizure detection by selecting features with an Improved Correlation-based Feature Selection(ICFS). Basically it is a fusion of time and frequency domain. Then, a random forest classifier was applied for the seizure detection model. The obtained average classification accuracy by this approach was 98.75%.
Some other works have used an ensemble of ‘non-black-box’ classifiers such as boosting, bagging and random subspace [ 78 , 127 ]. Yan et al. [ 78 ] applied a boosting classifier achieving 94.26% of accuracy, although the results were not as impressive as the ones obtained by [ 44 ], which used a random forest classifier. Hosseini [ 128 ] used Random subspace classifier along with an SVM classifier, to classify and detect seizures. Here, the benefit of applying a subspace on big datasets is to divide them into sub-datasets based on the random subspace concept, and then the SVM classifier was applied to each sub-dataset. Ensemble accuracy (EA) was calculated by the majority voting method, which was 95%. Apart from this study, the same authors of Hosseini et al. [ 126 ] recently did another research using an ensemble of classifiers. First, they created bootstrap samples using a random subspace method, and then applied classifiers such as SVM, KNN, extended nearest neighbor (ENN), and multilayer perceptron (MLP) obtaining 97% accuracy. Hussein et al. [ 100 ], proposed a novel feature extraction method, i.e., L1-penalized robust regression (L1PRR), which uses three common symptoms during seizures—muscles artifacts, eyes movement, and white noise. Inputting these features help the random forest classifier to obtain 100% accuracy.
Observations In comparison to decision trees, decision forest classifiers are tremendously used on brain datasets for exploring different research goals. It is difficult to suggest a particular classifier whilst dealing with a high-dimensional dataset, but a random forest classifier can be a capable classifier. However, it also criticizes that not all the ‘non-black-box’ classifiers are peculiar to detect seizures and have also pointed out the objection on the drawback of using a single decision tree classifier.
5.4 Seizure detection based on black-box and non-black-box machine learning classifiers
From the literature, it is found that just a single machine learning classifier is not sufficient. Therefore, to take advantage of both ‘black-box’ and ‘non-black-box’ classifiers, some researchers utilized them in their experiments. This section provides a comprehensive review of classifiers applied together to detect the seizure.
Acharya et al. [ 111 ] used the ensemble of seven different classifiers—Fuzzy surgeon classifier (FSC), SVM, KNN, Probabilistic neural network, GMM, decision tree and Naïve Bayes for distinguishing the three states of a patient as ‘normal, ‘pre-ictal’ and ‘ictal’. The overall accuracy is 98.1%. Fergus et al. [ 83 ] also used distinct classifiers such as linear discriminant analysis (LDA), quadratic discriminant classifier (QDC), logistic classifier, uncorrelated normal density-based classifier (UDC), polynomial classifier, KNN, PARZEN, SVM, and decision tree on the processed data with seven features such as entropy, RMS, skewness, and variance. They contributed that the detected patient is suffering from a ‘Generalize seizure’ (means affecting whole brain region) across different patients without prior information about the seizure focal points. Mursalin et al. [ 101 ] proposed a method to reduce the data size, statistical sampling technique called optimum sample allocation technique, and to reduce the features they develop a feature selection algorithm. The analysis was done on the combination of five classifiers—SVM, KNN, NB, Logistic Model Trees (LMT) and Random forest.
Rand and Sriram [ 95 ] used four classifiers such as SVM, KNN, random forest, and Adaboost on a high-dimensional dataset prepared by 28 features. Their result shows that SVM outperforms on the cubic kernel. In another study, Manzouri et al. [ 98 ] used SVM and random forest on the dataset produced by 10-time and frequency features. In comparison to SVM-based detector, random forest classifier outperforms. Subasi et al. [ 96 ] achieved 100% of accuracy using four machine learning classifiers such as ANN, KNN, SVM, and random forest on two popular datasets—Freiburg and CHB-MIT to classify the three different states of seizures ‘pre-ictal’, ‘ictal’, and ‘inter-ictal’. Sharma et al. [ 102 ] proposed an automated system using iterative filtering and random forest for classifying the EEG signals. This work achieved classification accuracies of 99.5% on BONN dataset (A-E), for A versus E subsets, 96% for D versus E subsets, and 98.4% for ABCD versus E classes of EEG signals. Birjandtalab et al. [ 77 ] used two classifiers for different purposes; KNN is used to discriminate the ‘seizure’ and ‘non-seizure’ classes, whereas random forest is used to explore the significant channels. Here, the random forest also helps in the dimension reduction problem. The main benefit of selecting suitable channels is that it helps in providing relevant required information from the chosen channels, and reduces the computational cost of a classifier too. However, the authors did not mention here the important information from channel selection like finding the seizure location from the brain scalp. The main critic in [ 95 , 98 , 101 ] is that because of a large number of features, the attribute size of dataset will increases, and as a result the accuracy and computation time suffer.
5.4.1 Observations
We observe that some work used an ensemble of distinguished classifiers to take the benefits separately. For example, influential channel selection can be independently done using decision forest classifiers like a random forest. But authors used other classifiers such as SVM and KNN for classifying the seizure records with good accuracy.
6 Seizure localization
After a successful seizure detection, localization is an essential task for epileptic surgery [ 129 , 130 , 131 ]. Typically, localized seizures can be cured by surgery which arises either from the left or right region of the brain. The seizure monitoring tools such as ECoG and EEG are prominently helpful to identify the seizure location. The electrodes/channels are implanted in a non-invasive (for EEG) and an invasive manner (for ECoG). Their positioning is based on the 10/20 (10–20) International system, which helps in identifying the seizure location [ 132 ]. The concept of seizure localization means identifying the region of the brain affected by a seizure. Though some types of seizures such as ‘tonic-clonic’ are cured by anti-epileptic drugs (AED), patients with partial seizures in some cases might go for surgery [ 13 ]. To solve this problem, finding the seizure location is an essential and challenging task for neurologists and neurosurgeon [ 129 , 130 ]. The surgical target is to find a point/location/focal area from where a seizure is originating. The 10–20 positioning system gives some clues for identifying the location of a seizure. Recently, computational and machine learning methods have been applied to identify a seizure location [ 130 , 133 ].
Acar et al. [ 133 ] used trucker and non-linear multi-way Trucker kernels, and claimed that other classifiers such as SVD and principal component analysis (PCA) were unable to localize a seizure. Ghannad-Rezaie [ 134 ] applied an advanced swarm intelligence algorithm to seizure data for finding seizure location. Their study produced some appreciable results, and explored whether the patient’s temporal lobe was affected by a seizure or not. They also suggested that SVM might be able to detect the seizure location. Moreover, they also focused on the reduction of ECoG electrodes. Mansouri et al. [ 135 ] proposed an algorithm for Seizure localization, which was tested on 10 sec of EEG dataset from Karuniya University. Here, they have taken the small-size dataset, because recording usually takes several hours. If they had tested on a big dataset, it would have been much better. Fakhraei et al. [ 130 ] calculated the sensitivity of each region of the brain. The confident prediction rate (CPR) was compared with the AUC of ROC plots obtained by six classifiers from the dataset of 79 patients (31 males, 48 females) with 197 medical features. The study found that CPR was more suitable than ROC. They also explored that 43 patients had the temporal lobe epilepsy (TLE) on their left sides whilst 36 patients had it on the right sides of their brains. Likewise, Rai et al. [ 136 ] proposed a method for identifying the focal points of the seizure by applying two entropy-based features—‘renyi entropy’ and ‘negentropy’ with the neural network classifier. Siddiqui et al. [ 63 ] localize the seizure using two decision forest classifiers, and their results showed that the left hemisphere of a brain was more affected by the seizures.
Observation
It is found that compared to seizure detection, machine learning classifiers have not been extensively applied for seizure localization. But some literature exist on this problem. In these reported works, authors did not mention the percentage of the affected region of the brain by a seizure, and they were not able to identify the exact location at the lobes such as occipital, frontal, parietal left and parietal right. Although, it is not our primary objective in this review paper, whilst discussing the related published research, we found some interesting clues for seizure localization.
7 Problems identified in existing literature
One of the most significant and decisive steps is to select suitable statistical features because each channel or electrode implanted on the brain provides different statistical measures. Undoubtedly, earlier researchers made their consistent efforts to find the best features. Whilst some researchers used many features [ 34 , 79 ], the others applied a few features [ 31 , 36 , 108 , 112 , 137 ] for detecting the seizure. As a data scientist, it is very important to see the different statistical perspectives of each brain signal by analyzing the statistical properties of the features such as entropy, energy, and skewness. And we must not focus on taking irrelevant feature(s) as such since it will unnecessarily increase the dataset size. Consequently, it will be more a burden to machine learning classifiers than a benefit, and if we take few features as previous researchers did [ 71 , 73 , 79 ], this will give the low-dimensional dataset and it will not be beneficial for an effective knowledge discovery process. Therefore, we should select those potential features that can to provide logical results. Hence, it is advisable to select a group of features to avoid a burden to the machine learning classifiers and to get help in related knowledge discovery.
Each classifier has its own merits and demerits, depending on the dataset attributes and requirements [ 138 ]. In general, it is very difficult to point out which classifier was the most effective for brain datasets. To identify the capable classifier, several classifiers have been tested on EEG datasets and their performance has been evaluated, and the one which performs well is to be considered in solving seizure detection and imparting knowledge discovery. The literature reveals that previous researchers had applied different approaches, most of which were from ‘black-box’ such as ANN, KNN and SVM. The biggest shortcoming in them is that they are unable to provide the appropriate explanations for patterns and the logic rules hidden inside the models. That is why, they are not suggested for remarkable knowledge discovery process. Data scientists may not explore the internal processing of patterns [ 51 , 104 ]. However, from the literature, it is noted that the ‘non-black-box’ approach, especially, random forest, is widely used for seizure detection [ 44 , 76 , 77 ], because of its nature of generating bootstrap samples [ 124 , 139 ] whilst building a decision forest. An analysis has been done to estimate the performance of machine learning classifiers on EEG datasets and has been found that ensemble non-black-classifiers performs effectively [ 104 ]. We argue that the random forest is based on bootstrap samples and it misses some influential attributes, because it randomly selects the attribute and sometimes generates the same set of logic rules also. As a result, sometimes, it creates irrelevant information too. To overcome this issue, we also suggest some other decision forest algorithms such as SysFor [ 123 ] and Forest CERN [ 51 ] methods in seizure detection.
All these findings on seizure detection raise few interesting research questions such as selecting suitable statistical features and machine learning classifiers to take less computation time as dataset has a high volume with high dimension, and the most significant missing information from machine learning classifiers is locating the accurate point of seizure at the brain lobe(s).
7.1 Class imbalance issue in seizure detection
Class imbalance is one of the serious problems [ 140 ] in machine learning and the majority is seen in medical datasets [ 141 ], particularly in EEG signals. This is because the duration of EEG recording is long, time-consuming and seizure duration is for a few seconds, which results in being prone to errors [ 91 ]. As a result, the dataset becomes highly imbalanced. Previous researchers have focused on seizure detection. Over the last few years, researchers have been focusing on the class imbalance challenge whilst detecting the seizures, and attempting to solve it by applying different conventional approaches with some novelties. Javad Birjandtalab et al. [ 91 ] used ANN with a weighted cost function to imbalanced EEG dataset, by achieving 86% F-measure. El Saadi et al. [ 142 ] obtained 97.3% accuracy using the under-sampling method with the SVM classifier. In another work by Saadullah and Awais [ 143 ], they used a combination of SMOTE and RUSTBOST techniques for detecting seizure to imbalance seizure data with 97% accuracy. However, the research done by Yuan Qi et al. [ 86 ] was very close to the satisfactory result as they assigned the heavy weights to a minority class of the data to maintain the effective balance and solved the biasing issue. The main critique of this work is that the authors did not mentioned what weights were assigned and what was their threshold level? Here, we argue that despite of EEG data are highly imbalanced as a result of their long-hour EEG recordings, the recordings continue until the seizure is detected. The seizure(s) time spans from only seconds to minute(s). Although researchers [ 76 , 86 , 117 , 143 ] made their efforts in addressing this issue using both ‘black-box’ and ‘non-black-box’ classifiers, they did not propose any justifiable solutions, in terms of how big weights should be assigned to the minority (seizure) classes.
8 Overall observation about capable classifiers and statistical features
It is challenging to suggest that a specific classifier should be capable for seizure detection. If we discuss classifiers, three constraints are very important whilst selecting a classifier—able to handle the high-dimensional dataset, high accuracy of the model, and able to retrieve the sensible knowledge. Not all machine learning classifiers are suitable for seizure detection and knowledge discovery tasks, mainly because of their black-box nature. This means that the logic rules/patterns are not visible and understandable to data scientists. In ‘non-black-box’ classifiers amongst decision trees [ 53 ] and decision forests [ 54 ], only decision forest algorithms are more capable, because the logic rules and knowledge discovered by a single decision tree are often limited and insufficient. For example, if we build a decision tree on a training dataset—it provides a limited or single set of logic rules and stops growing the tree further as all the data points in the training set accept that rule. On the other hand, if we build a decision forest on the same training set, we get multiple decision trees with more sensible logic rules. Siddiqui et al. [ 104 ] have done the analysis on CHB-MIT dataset to know which classifier performs better. For this, they applied two black-box (SVM and KNN) and two non-black-box (decision tree and ensemble of trees i.e., bagging, random subspace, boosting); they found non-black box classifier (ensemble) outperforms compared to other classifiers of black-box. Even ensemble also performs better than a single decision tree which is a non-black box classifier. Siddiqui et al. [ 63 ] applied two decision forests—Systematic Forest (SysFor) and Forest CERN for quick seizure detection using the concept of epoch length reduction. They achieved 100% of accuracy. Similarly, Hussein et al. [ 100 ] also achieved 100% accuracy using decision forest–random forest approach.
The literature reveals that in the last few years, ‘non-black-box’ classifiers, particularly decision forest approach, were widely used on brain datasets of EEG and ECoG for different research goals [ 76 , 82 , 94 , 144 ]. The reasons for using the decision forest for seizure detection are as follows:
A decision forest overcomes some of the disadvantages of a decision tree. A decision tree discovers only a single set of logic rules from an input dataset. The logic rules that are discovered by a single decision tree may fail to correctly predict and classify the class values;
A decision forest can produce more set of logic rules/patterns compared to a single decision tree and there is a high chance of good prediction/classification compared to a single decision tree;
Able to handle high-dimensional sets;
Due to its ensemble nature a decision forest mostly produces a high accuracy compared to a single tree and other classifiers [ 54 ];
Less computational time (specifically for Random forest);
Logic rules are clear and humanly interpretable such as analysts/domain experts can easily understand and suggest best opinions. For example, affected brain lobe by seizure, identifying suitable statistical features, etc.
Furthermore, many statistical features have been used for seizure detection. However, a comparison between them is difficult because of their heterogeneous nature. Some researchers used a single feature such as energy and entropy. On the other hand, a combination of statistical features such as energy, kurtosis, line length, entropy, skewness, max, standard deviation, and min may produce promising outcomes. Most research [ 34 , 46 , 92 , 100 , 109 , 145 ] have achieved better results using these features. The novelty of [ 29 , 63 , 104 , 125 ] is the selected nine statistical features are able to assist in seizure detection with high accuracy, i.e., 100%. This also provides the clue about seizure localization with the help of sensible logical rules. Hence, the selected group of features will not be a burden to the machine learning classifier but it will assist in related knowledge discovery.
9 Research directions in seizure detection
In this research analysis, we surveyed different machine learning classifiers used for seizure detection. No doubt, the progress of the persistent attempt has been found in this topic but few interesting research questions are also raised. In this section, we identify significant challenges which can uplift the future research in this area.
Selecting suitable statistical features and machine learning classifiers to take less computation time as the dataset has a high volume with a high dimension.
Accurate seizure detection on imbalanced datasets of long duration EEG recording datasets.
Quick seizure detection on long-hour EEG recording.
Whilst selecting the machine classifier it should be kept in mind that the classifier does not miss any necessary EEG channel/electrode.
Knowledge discovery from machine learning classifiers such as seizure localization which exactly points affected brain lobe(s), channel importance, and based on participating channels in seizure a knowledge could be provided to neurologist or neurosurgeon for suggesting epilepsy category.
10 Conclusion
With the increase of epilepsy, its accurate detection becomes increasingly important. A major challenge is to detect seizures correctly from a large volume of data. Due to the complexity of EEG signals in such datasets, machine learning classifiers are suitable for accurate seizure detection. Selecting suitable classifiers and features are, however, crucial.
As such, this paper has comprehensively reviewed machine learning approaches for seizure detection. As a result, we conclude that ‘non-black-box’ classifiers—decision forest (ensemble of decision trees)—is most effective. This is because it can produce multiple sensible, explanatory logic rules with high accuracy of prediction. Further, it can help discover some relevant information such as seizure localization and exploring seizure types. On the contrary, ‘black-box’ classifiers cannot generate logic rules, although they can achieve high predictive accuracy. As for selecting suitable features, we should select those that can provide logical results. By the review of the literature, the use of the features such as entropy, line length, energy, skewness, kurtosis, and standard deviation can achieve 100% accuracy in the classifiers. We suggest not to use the irrelevant features as the dimension of the data increases. This is because the computation cost of a classifier will grow high, and it may also produce insensible patterns. If we use just one or two features such as line length and energy, the low-dimensional dataset will be generated. However, this dataset will not be fruitful for the knowledge discovery process.
This review paper has provided new perspectives to data scientists who are working on epileptic seizure detection using EEG signals. In summary, this paper focuses on the review of selecting machine learning classifiers and suitable features.
Availability of data and materials
Not applicable.
Organization WH (2006) Neurological disorders: public health challenges. World Health Organization, New York
Google Scholar
Chaudhary UJ, Duncan JS, Lemieux L (2011) A dialogue with historical concepts of epilepsy from the babylonians to hughlings jackson: persistent beliefs. Epilepsy Behav 21(2):109–114
Article Google Scholar
Reynolds EH (2009) Milestones in epilepsy*. Epilepsia 50(3):338–342. https://doi.org/10.1111/j.1528-1167.2009.02050.x
WHO (2005) Atlas: Epilepsy care in the world. World Health Organization, Geneva
Alarcón G, Valentín A (2012) Introduction to Epilepsy. Cambridge University Press, Cambridge
Book Google Scholar
Qu H, Gotman J (1993) Improvement in seizure detection performance by automatic adaptation to the eeg of each patient. Electroencephalogr Clin Neurophysiol 86(2):79–87
Sazgar M, Young MG (2019) Seizures and Epilepsy. In: Absolute Epilepsy and EEG rotation review, pp. 9–46. Springer, New York
Chapter Google Scholar
Schachter S, Shafer P, Sirven J (2013) What causes epilepsy and seizures. Epilepsy Foundation
Delanty N, Vaughan CJ, French JA (1998) Medical causes of seizures. Lancet 352(9125):383–390
WHO: Media Center Epilepsy, (Fact sheet N999). http://www.who.int/mediacentre/factsheets/fs999/en/ (2015) Accessed 15 July 2019
Shafer PO, Sirven JI (2014) Epilepsy statistics. Epilepsy Foundation
Hannah JA, Brodie MJ (1998) Epilepsy and learning disabilities—a challenge for the next millennium? Seizure 7(1):3–13
Thurman DJ, Beghi E, Begley CE, Berg AT, Buchhalter JR, Ding D, Hesdorffer DC, Hauser WA, Kazis L, Kobau R et al (2011) Standards for epidemiologic studies and surveillance of epilepsy. Epilepsia 52(s7):2–26
Fisher RS (2017) The new classification of seizures by the international league against epilepsy 2017. Curr Neurol Neurosci Rep 17(6):48
Mahmud M, Kaiser MS, Hussain A, Vassanelli S (2018) Applications of deep learning and reinforcement learning to biological data. IEEE Trans Neural Networks Learn Syst 29(6):2063–2079
Article MathSciNet Google Scholar
Mahmud M, Kaiser MS, Hussain A (2020) Deep learning in mining biological data. arXiv:2003.00108
Fayyad UM, Piatetsky-Shapiro G, Smyth P, Uthurusamy R (eds.) (1996) Advances in Knowledge Discovery and Data Mining. American Association for Artificial Intelligence, Menlo Park, CA, USA
Yin Y, Kaku I, Tang J, Zhu J (2011) Data mining: concepts, methods and applications in management and engineering design. Springer, New York
Islam MZ, D’Alessandro S, Furner M, Johnson L, Gray D, Carter L (2016) Brand switching pattern discovery by data mining techniques for the telecommunication industry in australia. Aust J Inform Syst. https://doi.org/10.3127/ajis.v20i0.1420
Aljumah AA, Ahamad MG, Siddiqui MK (2013) Application of data mining: diabetes health care in young and old patients. J King Saud Univ Comput Inform Sci 25(2):127–136
Aljumah A, Siddiqui M (2016) Data mining perspective: prognosis of life style on hypertension and diabetes. Int Arab J Inf Technol 13(1)
Siddiqui MK, Morales-Menendez R, Gupta PK, Iqbal HM, Hussain F, Khatoon K, Ahmad S (2020) Correlation between temperature and COVID-19 (suspected, confirmed and death) cases based on machine learning analysis. J Pure Appl Microbiol 14(Spl Edn.)
Aljumah AA, Siddiqui MK (2014) Hypertension interventions using classification based data mining. Res J Appl Sci Eng Technol 7(17):3593–3602
Almazyad AS, Ahamad MG, Siddiqui MK, Almazyad AS (2010) Effective hypertensive treatment using data mining in Saudi Arabia. J Clin Monit Comput 24(6):391–401
Singh GA, Gupta PK (2019) Performance analysis of various machine learning-based approaches for detection and classification of lung cancer in humans. Neural Comput Appl 31(10):6863–6877
Fu T-c (2011) A review on time series data mining. Eng Appl Artif Intell 24(1):164–181
Tzallas AT, Tsipouras MG, Tsalikakis DG, Karvounis EC, Astrakas L, Konitsiotis S, Tzaphlidou M (2012) Automated epileptic seizure detection methods: a review study. In: Epilepsy-histological, electroencephalographic and psychological aspects. InTech
Abbasi B, Goldenholz DM (2019) Machine learning applications in epilepsy. Epilepsia
Siddiqui MK. Brain data mining for epileptic seizure-detection. Doctoral Dissertation, Charles Sturt University, Australia
Paul Y (2018) Various epileptic seizure detection techniques using biomedical signals: a review. Brain Inform 5(2):6
Boonyakitanont P, Lek-uthai A, Chomtho K, Songsiri J (2020) A review of feature extraction and performance evaluation in epileptic seizure detection using eeg. Biomed Signal Process Control 57:101702
Sharmila A, Geethanjali P (2019) A review on the pattern detection methods for epilepsy seizure detection from EEG signals. Biomed Eng /Biomedizinische Technik. 64(5):507–17
van Westrhenen A, De Cooman T, Lazeron RH, Van Huffel S, Thijs RD (2019) Ictal autonomic changes as a tool for seizure detection: a systematic review. Clin Auton Res 29(2):161–181
Logesparan AJC Lojini, Rodriguez-Villegas E (2012) Optimal features for online seizure detection. Med Biol Eng Comput 50(7):659–669
Ramgopal S, Thome-Souza S, Jackson M, Kadish NE, Fernández IS, Klehm J, Bosl W, Reinsberger C, Schachter S, Loddenkemper T (2014) Seizure detection, seizure prediction, and closed-loop warning systems in epilepsy. Epilepsy Behav 37:291–307
Quintero-Rincón A, D’Giano C, Batatia H (2019) Seizure onset detection in eeg signals based on entropy from generalized gaussian pdf modeling and ensemble bagging classifier. In: Digital Health Approach for Predictive, Preventive, Personalised and Participatory Medicine, pp. 1–10. Springer, New York
Greene B, Faul S, Marnane W, Lightbody G, Korotchikova I, Boylan G (2008) A comparison of quantitative eeg features for neonatal seizure detection. Clin Neurophysiol 119(6):1248–1261
Gotman J, Ives J, Gloor P (1979) Automatic recognition of inter-ictal epileptic activity in prolonged eeg recordings. Electroencephalogr Clin Neurophysiol 46(5):510–520
Gotman J (1982) Automatic recognition of epileptic seizures in the eeg. Electroencephalogr Clin Neurophysiol 54(5):530–540
Koffler D, Gotman J (1985) Automatic detection of spike-and-wave bursts in ambulatory eeg recordings. Electroencephalogr Clin Neurophysiol 61(2):165–180
Shoeb A, Guttag J (2010) Application of machine learning to epileptic seizure detection. In: 2010 the 27th International Conference on Machinelearning, Haifa, Israel
Dorai A, Ponnambalam K (2010) Automated epileptic seizure onset detection. In: Autonomous and Intelligent Systems (AIS), 2010 International Conference On, pp. 1–4 . IEEE
Lahmiri S (2018) An accurate system to distinguish between normal and abnormal electroencephalogram records with epileptic seizure free intervals. Biomed Sign Process Control 40:312–317
Donos C, Dümpelmann M, Schulze-Bonhage A (2015) Early seizure detection algorithm based on intracranial EEG and random forest classification. Int J Neur Syst 25(05):1550023
Ocak H (2009) Automatic detection of epileptic seizures in eeg using discrete wavelet transform and approximate entropy. Exp Syst Appl 36(2):2027–2036
Thomas E, Temko A, Lightbody G, Marnane W, Boylan G (2011) Advances in automated neonatal seizure detection. New Adv Intell Sign Process 1:93–113
Al Ghayab HR, Li Y, Abdulla S, Diykh M, Wan X (2016) Classification of epileptic EEG signals based on simple random sampling and sequential feature selection. Brain Inform 3(2):85–91
Herwig U, Satrapi P, Schönfeldt-Lecuona C (2003) Using the international 10–20 eeg system for positioning of transcranial magnetic stimulation. Brain Topogr 16(2):95–99
Misulis KE (2013) Atlas of EEG, seizure semiology, and management. Oxford University Press, Oxford
Sammut C, Webb GI (2017) Encyclopedia of machine learning and data mining. Springer, New York
Book MATH Google Scholar
Adnan MN, Islam MZ (2016) Forest CERN: A new decision forest building technique. In: Pacific-Asia Conference on Knowledge Discovery and Data Mining, pp. 304–315. Springer, New York
Cortes C, Vapnik V (1995) Support-vector networks. Mach Learn 20(3):273–297
MATH Google Scholar
Quinlan JR (1993) C4.5: Programs for machine learning. Morgan Kaufmann Publishers Inc., San Francisco
Breiman L (2001) Random forests. Mach Learn 45(1):5–32. https://doi.org/10.1023/A:1010933404324
Article MATH Google Scholar
Arlot S, Celisse A et al (2010) A survey of cross-validation procedures for model selection. Statistics surveys 4:40–79
Article MathSciNet MATH Google Scholar
Kurgan LA, Cios KJ (2004) CAIM discretization algorithm. IEEE Trans Knowl Data Eng 16(2):145–153
Li J, Liu H (2003) Ensembles of cascading trees. In: Data Mining, 2003. ICDM 2003. Third IEEE International Conference On, pp. 585–588. IEEE
Powers DM (2011) Evaluation: from precision, recall and f-measure to roc, informedness, markedness and correlation
Goldberger A, Amaral L, Glass L, Hausdorff J, Ivanov PC, Mark R, Mietus J, Moody G, Peng C, Stanley H (2000) PhysioBank, PhysioToolkit, and PhysioNet: components of a new research resource for complex physiologic signals. Circulation 101(23):e215–e220
CHB-MIT Scalp EEG Database. https://physionet.org/pn6/chbmit/ . Accessed 20 Jun 2015
Shoeb A, Edwards H, Connolly J, Bourgeois B, Treves ST, Guttag J (2004) Patient-specific seizure onset detection. Epilepsy Behav 5(4):483–498
Kramer MA, Kolaczyk ED, Kirsch HE (2008) Emergent network topology at seizure onset in humans. Epilepsy Res 79(2):173–186
Siddiqui MK, Islam MZ, Kabir MA (2018) A novel quick seizure detection and localization through brain data mining on ecog dataset. Neural Computing and Applications 1–14
Freiburg seizure prediction project. Freiburg, Germany. http://epilepsy.uni-freiburg.de/freiburg-seizure-prediction-project/eeg-database (2003)
Andrzejak RG, Lehnertz K, Mormann F, Rieke C, David P, Elger CE (2001) Indications of nonlinear deterministic and finite-dimensional structures in time series of brain electrical activity: Dependence on recording region and brain state. Phys Rev E 64(6):061907
Andrzejak RG, Schindler K, Rummel C (2012) Nonrandomness, nonlinear dependence, and nonstationarity of electroencephalographic recordings from epilepsy patients. Phys Rev E 86(4):046206
Lee H, Kim S (2016) Black-box classifier interpretation using decision tree and fuzzy logic-based classifier implementation. Int J Fuzzy Logic Intell Syst 16(1):27–35
Adnan MN, Islam MZ (2017) Forex++: A new framework for knowledge discovery from decision forests. Aust J Inform Syst. https://doi.org/10.3127/ajis.v21i0.1539
Guo L, Rivero D, Dorado J, Rabunal JR, Pazos A (2010) Automatic epileptic seizure detection in eegs based on line length feature and artificial neural networks. J Neurosci Methods 191(1):101–109
Koolen N, Jansen K, Vervisch J, Matic V, De Vos M, Naulaers G, Van Huffel S (2014) Line length as a robust method to detect high-activity events: automated burst detection in premature eeg recordings. Clin Neurophysiol 125(10):1985–1994
Logesparan L, Rodriguez-Villegas E, Casson AJ (2015) The impact of signal normalization on seizure detection using line length features. Med Biol Eng Comput 53(10):929–942
Song Y, Liò P (2010) A new approach for epileptic seizure detection: sample entropy based feature extraction and extreme learning machine. J Biomed Sci Eng 3(06):556
Zhang Y, Zhang Y, Wang J, Zheng X (2014) Comparison of classification methods on EEG signals based on wavelet packet decomposition. Neural Comput Appl 26(5):1217–1225. https://doi.org/10.1007/s00521-014-1786-7
Ahmad MA, Khan NA, Majeed W (2014) Computer assisted analysis system of electroencephalogram for diagnosing epilepsy. In: Pattern Recognition (ICPR), 2014 22nd International Conference On, pp. 3386–3391 . IEEE
Gill AF, Fatima SA, Akram MU, Khawaja SG, Awan SE (2015) Analysis of eeg signals for detection of epileptic seizure using hybrid feature set. In: Theory and Applications of Applied Electromagnetics, pp. 49–57. Springer
Orellana MP, Cerqueira F (2016) Personalized epilepsy seizure detection using random forest classification over one-dimension transformed EEG data. bioRxiv, 070300
Birjandtalab J, Pouyan MB, Cogan D, Nourani M, Harvey J (2017) Automated seizure detection using limited-channel eeg and non-linear dimension reduction. Comput Biol Med 82:49–58
Yan A, Zhou W, Yuan Q, Yuan S, Wu Q, Zhao X, Wang J (2015) Automatic seizure detection using stockwell transform and boosting algorithm for long-term eeg. Epilepsy Behav 45:8–14
Amin HU, Malik AS, Ahmad RF, Badruddin N, Kamel N, Hussain M, Chooi W-T (2015) Feature extraction and classification for eeg signals using wavelet transform and machine learning techniques. Austr Phys Eng Sci Med 38(1):139–149
Mursalin M, Zhang Y, Chen Y, Chawla NV (2017) Automated epileptic seizure detection using improved correlation-based feature selection with random forest classifier. Neurocomputing 241:204–214
Zabihi M, Kiranyaz S, Ince T, Gabbouj M (2013) Patient-specific epileptic seizure detection in long-term eeg recording in paediatric patients with intractable seizures
Truong ND, Kuhlmann L, Bonyadi MR, Yang J, Faulks A, Kavehei O (2017) Supervised learning in automatic channel selection for epileptic seizure detection. Exp Syst Appl
Fergus P, Hussain A, Hignett D, Al-Jumeily D, Abdel-Aziz K, Hamdan H (2016) A machine learning system for automated whole-brain seizure detection. Appl Comput Inform 12(1):70–89
Chen D, Wan S, Xiang J, Bao FS (2017) A high-performance seizure detection algorithm based on discrete wavelet transform (dwt) and eeg. PLoS ONE 12(3):0173138
Satapathy SK, Jagadev AK, Dehuri S (2017) Weighted majority voting based ensemble of classifiers using different machine learning techniques for classification of eeg signal to detect epileptic seizure. Informatica 41(1):99
MathSciNet Google Scholar
Yuan Q, Zhou W, Zhang L, Zhang F, Xu F, Leng Y, Wei D, Chen M (2017) Epileptic seizure detection based on imbalanced classification and wavelet packet transform. Seizure
Subasi A, Kevric J, Canbaz MA (2019) Epileptic seizure detection using hybrid machine learning methods. Neural Comput Appl 31(1):317–325
Al Ghayab HR, Li Y, Siuly S, Abdulla S (2019) Epileptic seizures detection in eegs blending frequency domain with information gain technique. Soft Comput 23(1):227–239
Selvakumari RS, Mahalakshmi M, Prashalee P (2019) Patient-specific seizure detection method using hybrid classifier with optimized electrodes. J Med Syst 43(5):121
Chen S, Zhang X, Chen L, Yang Z (2019) Automatic diagnosis of epileptic seizure in electroencephalography signals using nonlinear dynamics features. IEEE Access 7:61046–61056
Birjandtalab J, Jarmale VN, Nourani M, Harvey J (2018) Imbalance learning using neural networks for seizure detection. In: 2018 IEEE Biomedical Circuits and Systems Conference (BioCAS), pp. 1–4 . IEEE
Lahmiri S, Shmuel A (2018) Accurate classification of seizure and seizure-free intervals of intracranial eeg signals from epileptic patients. IEEE Trans Instrum Meas 68(3):791–796
Tzimourta KD, Tzallas AT, Giannakeas N, Astrakas LG, Tsalikakis DG, Angelidis P, Tsipouras MG (2019) A robust methodology for classification of epileptic seizures in eeg signals. Health Technol 9(2):135–142
Wang X, Gong G, Li N, Qiu S (2019) Detection analysis of epileptic EEG using a novel random forest model combined with grid search optimization. Front Human Neurosci 13:12
Raghu S, Sriraam N (2018) Classification of focal and non-focal eeg signals using neighborhood component analysis and machine learning algorithms. Exp Syst Appl 113:18–32
Alickovic E, Kevric J, Subasi A (2018) Performance evaluation of empirical mode decomposition, discrete wavelet transform, and wavelet packed decomposition for automated epileptic seizure detection and prediction. Biomed Sign Process Contr 39:94–102
Fasil O, Rajesh R (2019) Time-domain exponential energy for epileptic eeg signal classification. Neurosci Lett 694:1–8
Manzouri F, Heller S, Dümpelmann M, Woias P, Schulze-Bonhage A (2018) A comparison of machine learning classifiers for energy-efficient implementation of seizure detection. Front Syst Neurosci 12: 43
Parvez MZ, Paul M (2014) Epileptic seizure detection by analyzing eeg signals using different transformation techniques. Neurocomputing 145:190–200
Hussein R, Elgendi M, Wang ZJ, Ward RK (2018) Robust detection of epileptic seizures based on l1-penalized robust regression of EEG signals. Exp Syst Appl 104:153–167
Mursalin M, Islam SS, Noman MK (2019) Epileptic seizure classification using statistical sampling and a novel feature selection algorithm. arXiv preprint arXiv:1902.09962
Sharma RR, Varshney P, Pachori RB, Vishvakarma SK (2018) Automated system for epileptic EEG detection using iterative filtering. IEEE Sens Lett 2(4):1–4
Tzallas AT, Tsipouras MG (2007) Fotiadis DI (2007) Automatic seizure detection based on time-frequency analysis and artificial neural networks. Comput Intell Neurosci 2007:80510
Siddiqui MK, Islam MZ, Kabir MA (2017) Analyzing performance of classification techniques in detecting epileptic seizure. In: International Conference on Advanced Data Mining and Applications, pp. 386–398. Springer, New York
Sanei S, Chambers JA (2013) EEG signal processing. Wiley, New York
Chaovalitwongse WA, Prokopyev OA, Pardalos PM (2006) Electroencephalogram (eeg) time series classification: applications in epilepsy. Ann Ope Res 148(1):227–250
Moselhy HF (2011) Psychosocial and cultural aspects of epilepsy. In: Novel Aspects on Epilepsy. InTech
Esteller R, Echauz J, Tcheng T, Litt B, Pless B (2001) Line length: an efficient feature for seizure onset detection. In: Engineering in Medicine and Biology Society, 2001. Proceedings of the 23rd Annual International Conference of the IEEE, vol. 2, pp. 1707–1710 . IEEE
Guerrero-Mosquera C, Trigueros AM, Franco JI, Navia-Vázquez Á (2010) New feature extraction approach for epileptic eeg signal detection using time-frequency distributions. Med Biol Eng Comput 48(4):321–330
Olsen DE, Lesser RP, Harris JC, Webber WRS, Cristion JA (1994) Automatic detection of seizures using electroencephalographic signals. Google Patents. US Patent 5,311, 876
Acharya UR, Molinari F, Sree SV, Chattopadhyay S, Ng K-H, Suri JS (2012) Automated diagnosis of epileptic EEG using entropies. Biomed Sign Process Contr 7(4):401–408
Kannathal N, Choo ML, Acharya UR, Sadasivan P (2005) Entropies for detection of epilepsy in eeg. Comput Meth Progr Biomed 80(3):187–194
Kumar Y, Dewal M (2011) Complexity measures for normal and epileptic eeg signals using apen, sampen and sen. IJCCT 2(7):6–12
Shimizu M, Iiya M, Fujii H, Kimura S, Suzuki M, Nishizaki M (2019) Left ventricular end-systolic contractile entropy can predict cardiac prognosis in patients with complete left bundle branch block. Journal of Nuclear Cardiology 1–10
Pal PR, Panda R (2010) Classification of eeg signals for epileptic seizure evaluation. In: Students’ Technology Symposium (TechSym), 2010 IEEE, pp. 72–76. IEEE
Cepukenas J, Lin C, Sleeman D (2015) Applying rule extraction and rule refinement techniques to (blackbox) classifiers. In: Proceedings of the 8th international conference on knowledge capture, p. 27. ACM
Birjandtalab J, Pouyan MB, Nourani M (2016) Unsupervised eeg analysis for automated epileptic seizure detection. In: Proceedings of the first international workshop on pattern recognition, international society for optics and photonics, pp. 100110–100110
Abualsaud K, Mahmuddin M, Saleh M, Mohamed A (2015) Ensemble classifier for epileptic seizure detection for imperfect eeg data. Sci World J 2015:945689
Chen C, Liu J, Syu J (2012) Application of chaos theory and data mining to seizure detection of epilepsy. Proc Conf. IPCSIT/Hong Kong 25:23–28
Polat K, Güneş S (2007) Classification of epileptiform eeg using a hybrid system based on decision tree classifier and fast fourier transform. Appl Math Comput 187(2):1017–1026
MathSciNet MATH Google Scholar
Quinlan R (2004) Data mining tools see5 and c5. 0
Cios KJ, Pedrycz W, Swiniarski RW, Kurgan LA (2007) Data mining: a knowledge discovery approach. Springer, New York
Islam MZ, Giggins H (2011) Knowledge discovery through sysfor: a systematically developed forest of multiple decision trees. In: Proceedings of the Ninth Australasian Data Mining Conference-Volume 121, pp. 195–204. Australian Computer Society, Inc
Ho TK (1998) The random subspace method for constructing decision forests. IEEE Trans Patt Analy Mach Intell 20(8):832–844
Siddiqui MK, Islam MZ (2016) Data mining approach in seizure detection. In: 2016 IEEE Region 10 Conference (TENCON), Singapore, pp. 3579–3583. Institute of Electrical and Electronics Engineers (IEEE), https://doi.org/10.1109/tencon.2016.7848724
Hosseini M-P, Pompili D, Elisevich K, Soltanian-Zadeh H (2018) Random ensemble learning for eeg classification. Artif Intell Med 84:146–158
Hassan AR, Siuly S, Zhang Y (2016) Epileptic seizure detection in eeg signals using tunable-q factor wavelet transform and bootstrap aggregating. Comput Meth Prog Biomed 137:247–259
Hosseini M-P, Hajisami A, Pompili D (2016) Real-time epileptic seizure detection from eeg signals via random subspace ensemble learning. In: Autonomic Computing (ICAC), 2016 IEEE International Conference On, pp. 209–218 . IEEE
Jurcak V, Tsuzuki D, Dan I (2007) 10/20, 10/10, and 10/5 systems revisited: Their validity as relative head-surface-based positioning systems. NeuroImage 34(4):1600–1611. https://doi.org/10.1016/j.neuroimage.2006.09.024
Fakhraei S, Soltanian-Zadeh H, Fotouhi F, Elisevich K (2011) Confidence in medical decision making: Application in temporal lobe epilepsy data mining. In: Proceedings of the 2011 Workshop on Data Mining for Medicine and Healthcare. DMMH ’11, pp. 60–63. ACM, New York, NY, USA . https://doi.org/10.1145/2023582.2023593
Keraudren K, Kainz B, Oktay O, Kyriakopoulou V, Rutherford M, Hajnal JV, Rueckert D (2015) Automated localization of fetal organs in mri using random forests with steerable features. In: International conference on medical image computing and computer-assisted Intervention, pp. 620–627. Springer, New York
Trans-Cranial-Technologies: 10/20 System Positioning. https://www.trans-cranial.com/local/manuals/10_20_pos_man_v1_0_pdf.pdf . Accessed 7 Oct 2015
Acar E, Bingöl CA, Bingöl H, Yener B (2006) Computational analysis of epileptic focus localization. In: Proceedings of the 24th IASTED International Conference on Biomedical Engineering. BioMed’06, pp. 317–322. ACTA Press, Anaheim, CA, USA. http://dl.acm.org/citation.cfm?id=1166506.1166562
Ghannad-Rezaie M, Soltanain-Zadeh H, Siadat MR, Elisevich KV (2006) Medical data mining using particle swarm optimization for temporal lobe epilepsy. In: 2006 IEEE International conference on evolutionary computation, pp. 761–768. https://doi.org/10.1109/CEC.2006.1688388
Mansouri A, Singh SP, Sayood K (2019) Online eeg seizure detection and localization. Algorithms 12(9):176
Rai K, Bajaj V, Kumar A (2015) Features extraction for classification of focal and non-focal eeg signals. Inform Sci Appl Lect Notes Electr Eng 339:599–605
Hadoush H, Alafeef M, Abdulhay E (2019) Brain complexity in children with mild and severe autism spectrum disorders: Analysis of multiscale entropy in eeg. Brain topography 1–8
Fernández-Delgado M, Cernadas E, Barro S, Amorim D (2014) Do we need hundreds of classifiers to solve real world classification problems? J Mach Learn Res 15(1):3133–3181
Breiman L (1996) Bagging predictors. Mach Learn 24(2):123–140
Galar M, Fernandez A, Barrenechea E, Bustince H, Herrera F (2012) A review on ensembles for the class imbalance problem: bagging-, boosting-, and hybrid-based approaches. IEEE Trans Syst Man Cybern C 42(4):463–484
Yang F, Wang H-Z, Mi H, Cai W-W et al (2009) Using random forest for reliable classification and cost-sensitive learning for medical diagnosis. BMC Bioinform 10(1):22
El Saadi H, Al-Sadek AF, Fakhr MW (2012) Informed under-sampling for enhancing patient specific epileptic seizure detection. Int J Comput Appl 57:16
Amin S, Kamboh AM (2016) A robust approach towards epileptic seizure detection. In: Machine Learning for Signal Processing (MLSP), 2016 IEEE 26th International Workshop On, pp. 1–6 . IEEE
Yang Z, Choupan J, Reutens D, Hocking J (2015) Lateralization of temporal lobe epilepsy based on resting-state functional magnetic resonance imaging and machine learning. Front Neurol 6:184
Tito M, Cabrerizo M, Ayala M, Jayakar P, Adjouadi M (2009) Seizure detection: an assessment of time-and frequency-based features in a unified two-dimensional decisional space using nonlinear decision functions. J Clin Neurophysiol 26(6):381–391
Download references
Acknowledgements
We acknowledge the contribution of Dr. Khudeja Khatoon, MD, Faculty member of the Hayat Unani Medical College & Research Centre, India for carefully looking the medical terminologies in the paper. Also, thankful to Mr. Mohammad Arshad, English language expert, Shoumou Investment and Trading Company, KSA for proofreading the paper.
Author information
Mohammad Khubeb Siddiqui and Xiaodi Huang contributed equally to this work
Authors and Affiliations
School of Engineering and Sciences, Tecnologico de Monterrey, Av. E. Garza Sada 2501, Monterrey, Nuevo Leon, Mexico
Mohammad Khubeb Siddiqui & Ruben Morales-Menendez
School of Computing and Mathematics, Charles Sturt University, 2640, Albury, NSW, Australia
Xiaodi Huang
College of Applied Studies and Community Service, King Saud University, Riyadh, Kingdom of Saudi Arabia
Nasir Hussain
You can also search for this author in PubMed Google Scholar
Contributions
MKS: acquisition of related works, machine learning study, comparative study, arguments, writeup. RMM reviewed the overall paper and provided comments. XH: review the article on Machine Learning perspective, main contributions and direction of research. NH: discussion and table analysis. All authors read and approved the final manuscript.
Corresponding author
Correspondence to Ruben Morales-Menendez .
Ethics declarations
Competing interests.
The authors declare that they have no competing interests.
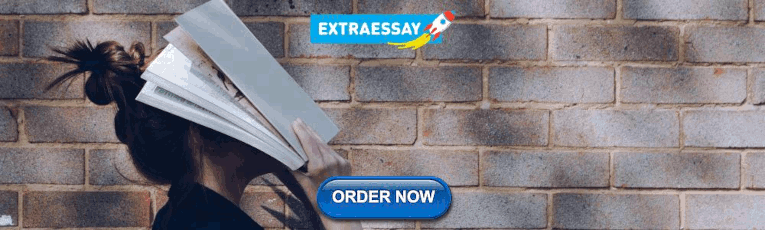
Additional information
Publisher's note.
Springer Nature remains neutral with regard to jurisdictional claims in published maps and institutional affiliations.
Rights and permissions
Open Access This article is licensed under a Creative Commons Attribution 4.0 International License, which permits use, sharing, adaptation, distribution and reproduction in any medium or format, as long as you give appropriate credit to the original author(s) and the source, provide a link to the Creative Commons licence, and indicate if changes were made. The images or other third party material in this article are included in the article's Creative Commons licence, unless indicated otherwise in a credit line to the material. If material is not included in the article's Creative Commons licence and your intended use is not permitted by statutory regulation or exceeds the permitted use, you will need to obtain permission directly from the copyright holder. To view a copy of this licence, visit http://creativecommons.org/licenses/by/4.0/ .
Reprints and permissions
About this article
Cite this article.
Siddiqui, M.K., Morales-Menendez, R., Huang, X. et al. A review of epileptic seizure detection using machine learning classifiers. Brain Inf. 7 , 5 (2020). https://doi.org/10.1186/s40708-020-00105-1
Download citation
Received : 24 December 2019
Accepted : 09 May 2020
Published : 25 May 2020
DOI : https://doi.org/10.1186/s40708-020-00105-1
Share this article
Anyone you share the following link with will be able to read this content:
Sorry, a shareable link is not currently available for this article.
Provided by the Springer Nature SharedIt content-sharing initiative
- Applications of machine learning on epilepsy
- Statistical features
- Seizure detection
- Seizure localization
- Black-box and non-black-box classifiers
- EEG signals
Seizure Forecasting: Patient and Caregiver Perspectives
Affiliations.
- 1 Epilepsy Foundation of America, Greater Landover, MD, United States.
- 2 Coalition for Aligning Science, Chevy Chase, MD, United States.
- PMID: 34616352
- PMCID: PMC8488220
- DOI: 10.3389/fneur.2021.717428
Accurate seizure forecasting is emerging as a near-term possibility due to recent advancements in machine learning and EEG technology improvements. Large-scale data curation and new data element collection through consumer wearables and digital health tools such as longitudinal seizure diary data has uncovered new possibilities for personalized algorithm development that may be used to predict the likelihood of future seizures. The Epilepsy Foundation recognized the unmet need for development in seizure forecasting following a 2016 survey where an overwhelming majority of respondents across all seizure types and frequencies reported that unpredictability of seizures had the strongest impact on their life while living with or caring for someone living with epilepsy. In early 2021, the Epilepsy Foundation conducted an updated survey among those living with epilepsies and/or their caregivers to better understand the use-cases that best suit the needs of our community as seizure forecast research advances. These results will provide researchers with insight into user-acceptance of using a forecasting tool and incorporation into their daily life. Ultimately, this input from people living with epilepsy and caregivers will provide timely feedback on what the community needs are and ensure researchers and companies first and foremost consider these needs in seizure forecasting tools/product development.
Keywords: community survey; epilepsy; patient perception; seizure forecasting; seizure forecasting devices; wearable sensors.
Copyright © 2021 Grzeskowiak and Dumanis.
- Open access
- Published: 19 January 2024
Detection of epileptic seizure in EEG signals using machine learning and deep learning techniques
- Pankaj Kunekar ORCID: orcid.org/0000-0001-6729-5315 1 ,
- Mukesh Kumar Gupta 1 &
- Pramod Gaur 2
Journal of Engineering and Applied Science volume 71 , Article number: 21 ( 2024 ) Cite this article
1700 Accesses
1 Citations
Metrics details
Around 50 million individuals worldwide suffer from epilepsy, a chronic, non-communicable brain disorder. Several screening methods, including electroencephalography, have been proposed to identify epileptic episodes. EEG data, which are frequently utilised to enhance epilepsy analysis, offer essential information on the electrical processes of the brain. Prior to the emergence of deep learning (DL), feature extraction was accomplished by standard machine learning techniques. As a result, they were only as good as the people who made the features by hand. But with DL, both feature extraction and classification are fully automated. These methods have significantly advanced several fields of medicine, including the diagnosis of epilepsy. In this paper, the works focused on automated epileptic seizure detection using ML and DL techniques are presented as well as their comparative analysis is done. The UCI-Epileptic Seizure Recognition dataset is used for training and validation. Some of the conventional ML and DL algorithms are used with a proposed model which uses long short-term memory (LSTM) to find the best approach. Post that comparative analysis is performed on these algorithms to find the best approach for epileptic seizure detection. As a result, the proposed model LSTM gives a validation accuracy of 97% giving the most appropriate and precise result as compared to other mentioned algorithms used in this study.
Introduction
Epilepsy is a persistent, non-communicable brain condition. A hereditary condition or an acquired brain disorder, such as a trauma or stroke, may cause epilepsy. A person who is having a seizure exhibits strange behaviour, symptoms and sensations, sometimes even losing consciousness. According to the most recent assessment by the World Health Organization (WHO), around 50 million people worldwide experience epileptic seizures, and the majority of them are unaware of their illness.
Most of the time seizure attacks are the cause of accidents. Seizures are a result of excessive electrical discharges in a group of brain cells. A patient who has a seizure attack for more than 5 min needs to be medicated as soon as possible, but due to the lack of knowledge about this condition, it is not treated at early stages. It is not possible to treat any brain-related condition just by observing the patient. The ionic current passing through the brain neurons is observed using electroencephalogram (EEG) which provides a graph of temporal and spatial information about the brain. However, it is challenging to obtain comprehensive information about these dynamic biological signals due to the non-linear and non-stationary nature of EEG signals.
Depending on the seizure characteristics, epilepsy has various types of epilepsy seizures [ 1 ]. In recent years, treatment of epileptic seizures is possible due to advancements in the medical field, but still, detection and classification of epileptic seizures are tedious tasks without using automation that can be done using various machine learning and deep learning techniques. Also, there are different times to observe patient brain signals. Using various ML and DL techniques on EEG signal data to detect seizure helps to get insights into a patient’s brain condition more preciously.
There is a lot of work done in this domain already, but most algorithm fails to get validation accuracy and other model performance parameters. Also, the implemented model sometimes fails because of a lack of dataset.
The proposed system is intended to solve the problem of automation in epileptic seizure detection. The proposed model uses LSTM to detect seizure patients or normal persons by considering observations from the UCI-Epileptic Seizure detection dataset. Also, the designed system matches the research gaps in this area by providing a more accurate classification of the signal into two categories.
The proposed model is implemented by considering various ML algorithms and deep learning algorithms and their performance on the UCI dataset, so that the validation accuracy can be increased by observing other models’ performance. The detailed comparison of machine learning and deep learning models is done with a proposed model to solve many problems.
Related work
Various papers were studied to know about the research done in the area. Some of the important surveys and techniques are explained below:
In [ 2 ], a survey on scalable technologies assisting in early screening and predictive analysis for lifestyle diseases is done. Study [ 3 ] explores various forms of artificial intelligence on large volumes of data in medical technology. In [ 4 , 5 , 6 ], various studies explored that machine learning and deep learning techniques have been efficiently used for enhanced medical innovations.
In the paper [ 7 ], the authors proposed a system which uses empirical mode decomposition (EMD) and extracted time and frequency domain features for extracting features from EEG signals. The CHB-MIT dataset is used here. The model has given a higher true-positive rate of 92.23%, and the average prediction time is 23.60 min on the scalp for all subjects in the dataset.
In the paper [ 8 ], emphasis is placed on computational complexity, and other models are also compared by systems with multiple classifications. The three main basic parts are as follows: the first one is feature extraction, the second one is hierarchical attention layer and the last is classification layer. Three scenarios were used to evaluate this system: based on combinations of interictal, preictal and ictal.
In the paper [ 9 ], the author proposed a system focused on feature extraction and classification. The model used Taylor Fourier, rhythm-specific and filter bank for pre-processing and at the end feature extraction and classification SVM used. The model achieved an accuracy of 94.88%. The Bonn University Database is used here.
In the paper [ 10 ], using a corpus of EEG data from Temple University Hospital, the authors applied CNN and transfer learning to categorise seven variations of seizures with non-seizure EEG. This study’s goal is to carry out a multiclass categorisation. Before being fed as input to CNN, the signal was transformed into a spectrogram. To choose the best network for the given study, many DL pre-trained networks were employed. 82.85% (Googlenet) and 88.30% (Inceptionv3) are the best categorisation accuracy models used in this study.
In this system [ 11 ], to examine alternative model assessment parameters, two fusion methods were considered: the first one is ensemble, and the second one is Choquet fuzzy integral used with the deep neural network used in the system.
In the paper [ 12 ], a singular value decomposition fuzzy k -nearest neighbour classifier methodology based on discrete wavelet transform offers about 100% accuracy and nearly about 93.33% on two and three classes using the Bonn University Dataset.
In [ 13 ], the authors categorise EEG data into ictal and interictal types. The primary problem here is the non-stationary and non-linear character of the EEG signal while attempting to understand brain output. The main emphasis is on feature extraction from seizure EEG recordings to create a method for epileptic seizure identification on the CHB-MIT dataset that uses both fuzzy-based and conventional machine learning techniques.
In [ 14 ], the authors suggest using deep learning to identify seizures in paediatric patients. To implement the supervised classifier, the CHB-MIT dataset is used to classify ictal and interictal brain state signals. Two-dimensional deep convolution auto-encoder connected to a neural network.
The paper [ 15 ] intended to use the principal components analysis used for the feature reduction approach to the signals in order to obtain the optimum classification algorithm for epileptic seizures. Using the dataset to predict epilepsy, KNN, RF, SVM, ANN and DT algorithms are used, and the performance of classifiers is examined both with and without PCA.
This paper [ 16 ] reviews different deep learning algorithms with CNN for 1D CNN, 2D CNN, CNN using transfer learning and LSTM. The author reviewed different approaches for each technique in which the CNN and LSTM were showing significant accuracy of around 99%. The different dataset was considered CHB-MIT, BON, Flint Hills and Bern Barcelona.
The paper [ 17 ] presents a model which solves the issues of data imbalance, low accuracy and classification model with sampling techniques including downsampling, random sampling and the synthetic minority oversampling technique. The authors proposed the heterogeneous deep ensemble model which gives an accuracy score of 0.93) and an F -measure value of 0.91.
The paper’s [ 18 ] authors say manual observation of EEG is performed to detect epilepsy which makes it difficult and easy to switch over automated diagnosis system. The Bonn EEG Dataset is used. The authors proposed a least squares support vector machine (LSSVM) as a better approach to deal with linear equations with an accuracy of 94.7%.
In the paper [ 19 ], the authors worked on EEG signal noise removal. The EEG signal gets compromised by background noise or any muscle movement which makes it difficult to detect in automatic mode. After taking this limitation, the paper reviews different automatic approaches which state feature selection and classification are the tedious and error-prone area in epilepsy.
In the paper [ 20 ], the authors proposed ResNET-50 as an automated system which will define the EEG data into non-ictal, ictal and pre-ictal classes. The CHB-MIT, Freiburg, BONN Dataset and BERN Dataset use CNN by transforming the 2D EEG images from 1D EEG images which give 94.88% accuracy.
In the paper [ 21 ], the authors proposed CNN for the classification of an epileptic seizure. The proposed model contains four models: the CNN model, fusion of two CNN; fusion of 3 CNN, fusion of 4 CNN model and transfer learning using ResNet 50. The fusion of 3 and 4 CNN models gave significantly best results with 95% accuracy. The two convolution layers with 32 filter and 3 × 3 kernel size are used as a single CNN model which after concatenated for fusion of 2, 3 and 4 CNN models.
In the paper [ 22 ], the authors proposed a deep neural network with hierarchical attention mechanisms. The system starting layer was of two separate CNN for extracting the feature and connected to the hierarchical attention layer and fully connected layers for classification. The computation time of the proposed model was 0.23 s for classification and 0.014 for feature extraction.
In the paper [ 23 ], a novel seizure prediction model called TASM ResNet was proposed by the authors. It is based on an intracranial EEG signal-based pre-trained ResNet and a temporal attention simulation module. The simulation module was created to take raw EEG data, transform it into data that resembles images and then extract temporal characteristics. ResNet was utilised in this case to decrease the amount of training data. Additionally, the final outcomes demonstrated that an image network that has been pre-trained on a sizable dataset using a simulation module can migrate EEG signals.
Proposed methods
The proposed system follows the traditional way of model training and testing as shown in Fig. 1 . The UCI-Epileptic Seizure Dataset is firstly pre-processed to feed to the model. After dataset pre-processing done, the dataset split into training and testing data. After that model is selected, there are logistic regression, KNN, SVM, ANN and LSTM. Next, the selected model is trained based on UCI training dataset. The testing data is feed to model to get results. Finally, the model was evaluated based on various parameters such as accuracy, confusion matrix, precision, recall and F1 score.
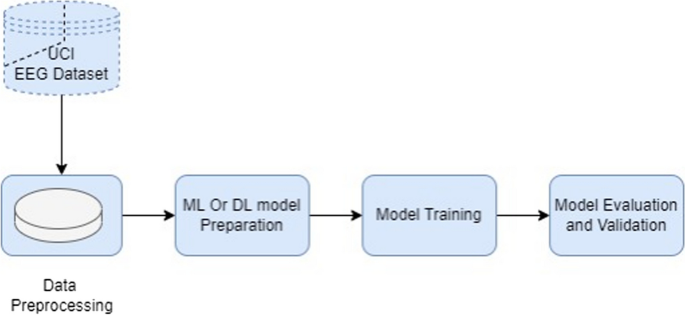
General system architecture where model is replaced
The UCI-Epileptic Seizure Dataset is the set of data that is utilised for model performance. The original dataset consists of 100 files in 5 separate folders, each of which corresponds to a particular patient. 23.6-s recording of brain activity is stored in each file. A total of 4097 information/data points from the associated time series are sampled. Each data point represents the EEG recording’s value at a particular time point. There are 500 people in all, each having 4097 data points. Every 4097 information points, it is split and jumbled into 23 sets, each of which has 178 information points for 1 s and represents the value of the EEG recording at a particular time. As a result, there are now 23 (sets) × 500 (people) = 11,500 informational pieces, each of which comprises 178 information points for 1 s (a column), the last column represents the class as y {1,2,3,4,5}. The dataset therefore has 179 total columns, the first 178 of which are input vectors, and the 179th of which is a categorisation for patients (Table 1 ).
Dataset preprocessing
The dataset consists of 5 different classes mentioned as follows:
5—Keeping patient eyes open while taking a brain EEG graph
4—Keeping patient eyes closed while taking a brain EEG graph
3—EEG activity from a healthy part of the brain
2—EEG activity in the vicinity of the tumour
1—Seizure activity recording
All patients in classes 5, 4, 3 and 2 are those where there is no experience of seizures, according to an analysis of the dataset. The dataset is then encoded using the One-Hot method for binary classification, with all classes except 1 being transformed to 0 to indicate no seizures and 1 to indicate seizure sufferers.
ML and DL model selection
As the part of comparative study, there are a total of five models compared based on model evaluation parameters. Logistic regression, SVM classifier and KNN are traditional machine learning algorithm; as there is binary classification, these models were selected. The artificial neural network was used for complex analysis, and the last model was the LSTM-based neural network; with this model, other models were compared.
Results and discussion
Traditional machine learning model, model 1: logistic regression.
The regression algorithm for binary classification is used for detecting seizure activity. The model used test spilt as 33% of the dataset for validation. The training accuracy of the model is 66.92%, and the validation accuracy is 63.9%. Using validation data, as shown in Fig. 2 , the true-negative value percentage was 67.17%, true-positive 0.0%, false-positive rate 32.83% and false negative 0.0%. As the confusion matrix is shown in Fig. 2 , the classes are not perfectly classified, and it is not useful for this classification task.
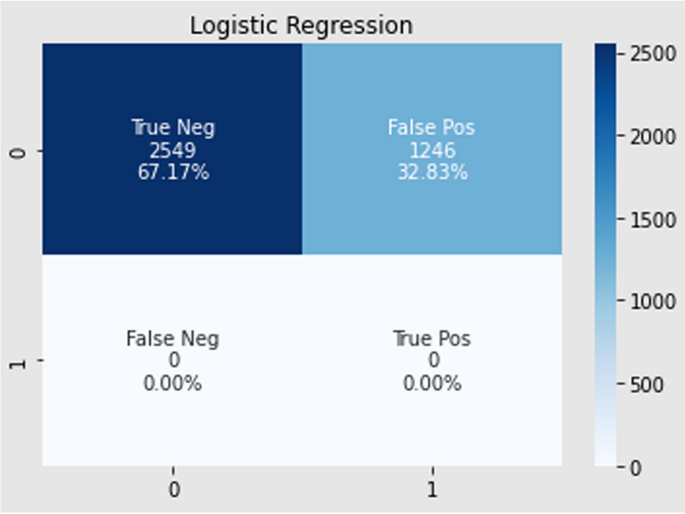
Logistic regression confusion matrix
Model 2: SVM
For binary classification, SVM is used. The model is trained on 67% of the dataset. The validation accuracy is 97.2%, and the training accuracy is 98.09%. The model has a 0.0% true-positive classification on validation data. As shown in Fig. 3 , false positive 19.10%. The true negative was 80.90%, and the false negative was 0%. The validation data is 720 rows as positive class and 3025 as negative class. As the confusion matrix is shown in Fig. 3 , the classes are not perfectly classified, and it is not useful for this classification task.
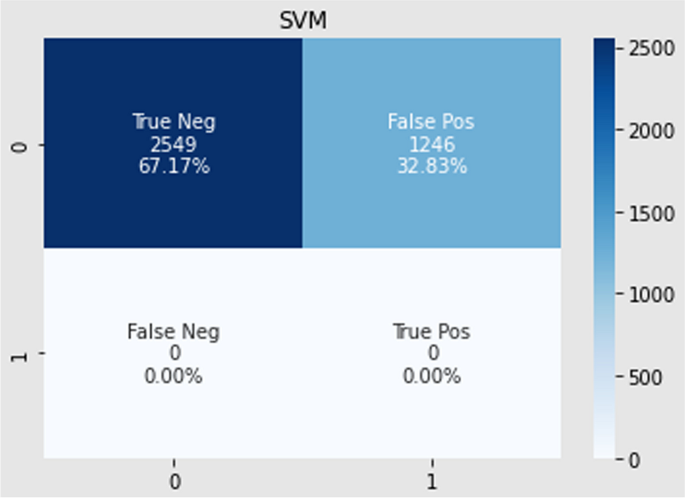
SVM confusion matrix
Model 3: K-nearest neighbour
The KNN is used for the classification of seizure activity. The neighbour value is 5, and the distance formula is Euclidean distance. The training accuracy of the model is 93.61%, and the validation accuracy is 91.96% based on 33% data of the dataset. Using validation data, the true-negative percentage was 87.59% and the true positive as 0.0%. The false-negative value is 0.0%, and the false-positive value is 12.41% as shown in Fig. 4 . As the confusion matrix shown in Fig. 4 , the classes are not perfectly classified, and it is not useful for this classification task.
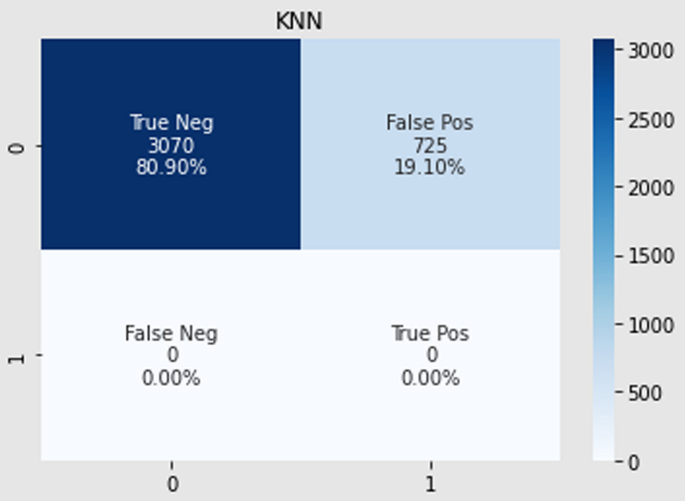
KNN confusion matrix
Deep neural network
Model 1: artificial neural network (ann).
The multiple-layer ANN model is implemented with four layers. The input shape for the model was 178.1 that is 178 data points. The first, second and third, layers with 32 neurons and Relu is the activation function, and the fourth layer with 2 neurons with softmax is the activation function. Adam optimiser was used as a model optimiser. Binary cross entropy is used as loss as there is binary classification. The total trainable parameters are 7906. The model is trained on 67% data. The model training accuracy was 98.9%, and the validation accuracy was 97% which is tested on 33% of testing dataset. It was observed a sudden increase in training accuracy while training the model after the first epoch from 0.91 to 0. 96% and a slow increase in accuracy shown in Fig. 5 .
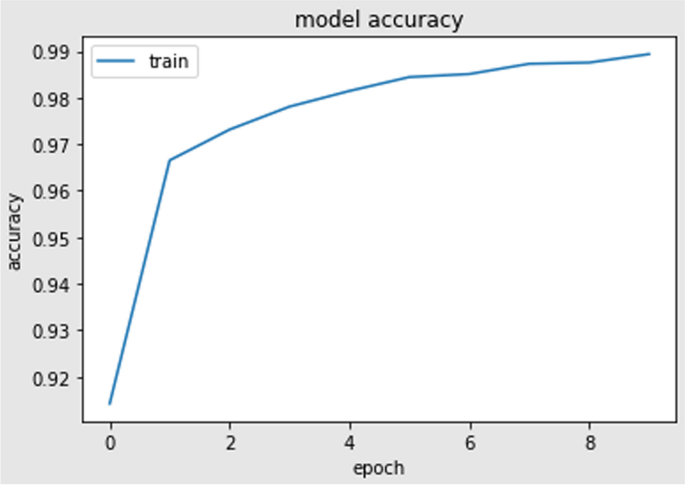
ANN accuracy
The training loss is 0.03, and the validation loss is 0.08 as shown in Fig. 6 . Based on validation data, the confusion matrix is shown in Fig. 7 .
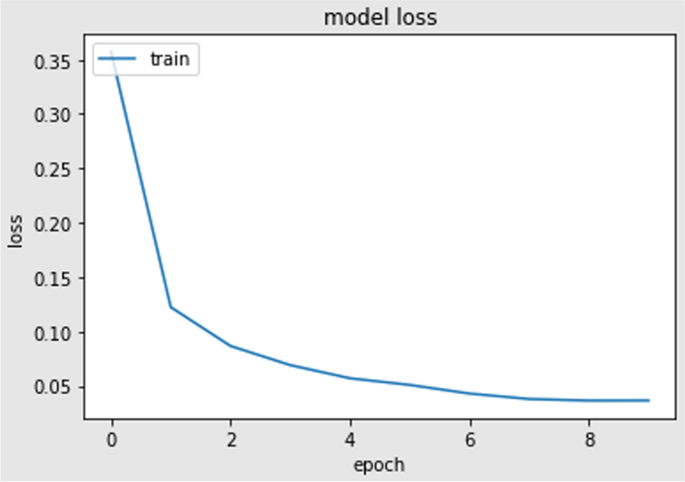
Confusion matrix
True negative (TN) is 78.89%, true positive (TP) is 18.16%, false positive is 0.81% and false negative is 2.13%. The model validation values for negative class (no seizure) precision were 0.97%, recall 0.99% and F1 score 0.98% and positive class (seizure activity) precision 0.96%, recall 0.89% and F1 score 0.92%. The total number of negative class data rows is 3025 and the positive class 770. As the system works for the medical domain, there is importance to the false-negative value which looks effective in ANN, so using the ANN model for this task is not that much good choice.
Proposed model
Model 1: proposed model—long short-term memory.
The multiple-layer LSTM model is implemented with three layers. The input shape for the model was 1178 that is 178 data points. The first LSTM layer with 64 neurons and Relu as activation function. The second LSTM layer with 32 neurons and Relu as an activation function. The last layer with 2 neurons with softmax as an activation function converts the output to a weighted sum to probability which sums to 1. Adam optimiser is used as an optimiser. Binary cross entropy is used as loss as there is binary classification. Total trainable parameters as 74,690. The model is trained on 67% data. The model training accuracy is 99.9%, and the validation accuracy is 97% which is tested on 33% of the dataset. It was observed a sudden increase in training accuracy while training the model after the first epoch from 0.91 to 0.96%, and a slow increase in accuracy is shown in Fig. 8 .
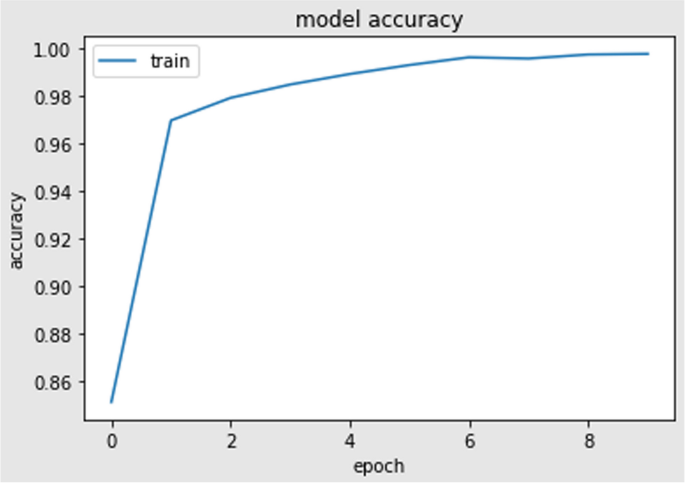
LSTM accuracy
The training loss is 0.006, and the validation loss is 0.106 as shown in Fig. 9 . Based on validation data, the confusion matrix is shown in Fig. 10 .
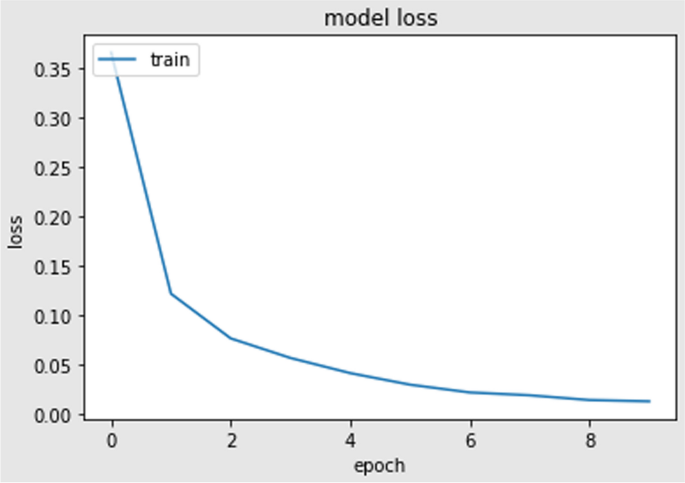
LSTM confusion matrix
True negative (TN) is 79.05%, true positive (TP) 18.23%, false-positive percentage 0.66% and false negative 2.06%. The model validation values for negative class (no seizure) precision 0.97%, recall 0.99%, and F1 score 0.98% and positive class (seizure activity) precision 0.97%, recall 0.90% and F1 score 0.93%. The total number of negative class data rows is 3025 and positive class 770.
Comparitive analysis
There are a total of five models trained and tested on the UCI-Epileptic Seizure Dataset. Each model was compared with other models based on several model evaluation parameters. In this study, precision, recall, training accuracy, validation accuracy, F1-Score and confusion matrix are the parameters considered.
The matrix below shows how much actual values as the same as predicted values by model, based on that true negative, false negatives, false positive and true positive are calculated.
Actual values
It tells how much the per cent model gives accurate values.
It reveals how many of the positive data points that the model identified as positive are truly positive.
The recall measures how accurately the model has identified real data items.
Table 2 gives a detailed comparison of all 5 models used in this study. All the models were trained and validated on the same dataset with validation data as 33% UCI dataset and 67% for training models. As shown in Table 2 , the positive class (seizure activity) have 770 rows for validation and 3025 as a negative class (no seizure). The logistic regression achieves training and validation accuracy of 66.92% and 63.9%, respectively, comparatively less than the SVM used on the same dataset. Similarly, with precision, the F1 score and recall value in the logistic regression algorithm show very less effect in seizure classification. Refer to the confusion matrix of SVM shown in Fig. 3 great training accuracy of 98.09% and validation accuracy of 97.23% but not able to predict classes as shown as true positive. The KNN showed training accuracy and validation accuracy of 93.61% and 91.96%, respectively, but not able to detect classes as the true positive rate is 0.0%.
The ANN model was able to classify true-positive rate (sensitivity) value with minimal false positive compared to SVM. ANN shown in Table 2 gives a precision value of 0.96, recall 0.89 and F1 score 0.92 for seizure signals and precision value 0.97, recall 0.99 and F1 score 0.98 for healthy signals. The proposed model LSTM was able to classify more accurately than ANN with very minimal difference in training and validation accuracy. The proposed model was able to achieve 99.88% accuracy on training data and a validation accuracy of 97.1% as compared to ANN validation accuracy of 97.0%. LSTM shown in Table 2 gives a precision value of 0.96, recall 0.90 and F1 score 0.93 for seizure signals and precision value 0.97, recall 0.99 and F1 score 0.98 for healthy signals. Based on the overall comparison, LSTM-based model performs better.
Conclusions
As the proposed system intended to classify the healthy person’s brain EEG signal and seizure patient brain EEG signal, the system classifies the signal data with the LSTM model with a validation accuracy of 97% and false negative 2.06%. As shown in Table 2 , the conventional machine learning algorithms logistic regression, SVM and KNN achieve good accuracy but not work fine in classification. Also, considering the ANN achieves good model evaluation parameters but somewhat less precise in false negative area. In conclusion, the proposed model works better as compared to other models used in this study. The system can be useful in epileptic seizure detection.
The proposed system currently works good in binary classification. There are also types of epileptic seizure that can be detected precisely as it deals with the medical domain. The dataset used in this study is somewhat insufficient to train model, and also dataset is unbalanced as the other categories are converted using one-hot encoding for binary classification. Also, in the proposed model, there is a scope of improvement in the false-negative section. The limitation of the proposed system is that it needs to test for multiclass classification of epilepsy seizures. The similar work has been carried out in [ 24 ]. Also, work needs to be tested with other datasets like state-of-the-art work [ 25 , 26 , 27 ]. The other alternatives to test the results are to use EEG datasets like CHB-MIT, TUH EEG Corpus and Bonn University with methods like CNN, SofMax and Bi-LSTM to improve false negatives.
Availability of data and materials
Data is publicly available on Kaggle and UCI Repository.
Abbreviations
Machine learning
Deep learning
University of California Irvine
Long short-term memory
World Health Organization
- Electroencephalogram
Empirical mode decomposition
Convolutional neural network
k -Nearest neighbours
Random forest
Support vector machine
Artificial neural networks
Decision tree
Principal component analysis
Least squares support vector machine
Kunekar PR, Gupta M, Agarwal B (2020) Deep learning with multi modal ensemble fusion for epilepsy diagnosis. 2020 3rd International Conference on Emerging Technologies in Computer Engineering: Machine Learning and Internet of Things (ICETCE). pp 80–84. https://doi.org/10.1109/ICETCE48199.2020.9091742
Chapter Google Scholar
Kunekar PR, Gupta M, Agarwal B (2019) Detection and analysis of life style based diseases in early phase of life: a survey. In: Somani A, Ramakrishna S, Chaudhary A, Choudhary C, Agarwal B (eds) Emerging technologies in computer engineering: microservices in big data analytics. ICETCE 2019. Communications in Computer and Information Science, vol 985. Springer, Singapore. https://doi.org/10.1007/978-981-13-8300-7_6
Kumar Boddu RS, Chakravarthi DS, Venkateswararao N, Chakravarthy DSK, Devarajan A, Kunekar PR (2021) The effects of artificial intelligence and medical technology on the life of human. J Pharm Res Int 33(50A):25–32. Article no.JPRI.76516, ISSN: 2456-9119
Article Google Scholar
Arora B, Jadhav P, Sulaiman RB, Kareem A, Kunekar P, Pant B (2022) Integrating artificial intelligence and deep learning for enhanced medical innovation. 2022 5th International Conference on Contemporary Computing and Informatics (IC3I). pp 327–331. https://doi.org/10.1109/IC3I56241.2022.10073054
Kunekar P, Vaishnav R, Kalaivani E, Gangodkar D, Kaur C, Dhanraj JA (2022) Applications of machine learning techniques in detecting skin cancer. 2022 5th International Conference on Contemporary Computing and Informatics (IC3I). pp 265–270. https://doi.org/10.1109/IC3I56241.2022.10072834
Chakrapani IS, Tyagi N, Tyagi S, Kunekar P, Padmaja DL, Pant K (2022) Applications of Deep Learning (DL) techniques in detecting breast cancer and malignant cells. 2022 5th International Conference on Contemporary Computing and Informatics (IC3I). pp 217–222. https://doi.org/10.1109/IC3I56241.2022.10072720
Usman SM, Usman M, Fong S (2017) Epileptic seizures prediction using machine learning methods. Comput Math Methods Med 2017:9074759
Article MathSciNet Google Scholar
Zhou M, Tian C, Cao R, Wang B, Niu Y, Hu T, Guo H, Xiang J (2018) Epileptic seizure detection based on EEG signals and CNN. Front Neuroinform 12:95
Serna J, Paternina MRA, Zamora-Méndez ARK. Tripathy R, Pachori R (2020) EEG-Rhythm Specific Taylor-Fourier Filter Bank Implemented With O-Splines for the Detection of Epilepsy Using EEG Signals. IEEE Sens J. https://doi.org/10.1109/JSEN.2020.2976519
Raghu S, Sriraam N, Temel Y, Rao SV, Kubben PL (2020) EEG based multi-class seizure type classification using convolutional neural network and transfer learning. Neural Netw 124:202–212
Ludwig SA (2020) Multi-label classification for epileptic seizure recognition: deep neural network ensemble versus Choquet fuzzy integral fusion. 2020 IEEE Symposium Series on Computational Intelligence (SSCI). pp 836–841
Singh N, Dehuri S (2020) Multiclass classification of EEG signal for epilepsy detection using DWT based SVD and fuzzy kNN classifier. Intell Decis Technol. 14:1–14. https://doi.org/10.3233/IDT-190043
Aayesha Z, Qureshi M, Afzaal M, Qureshi M, Fayaz M (2021) Machine learning-based EEG signals classification model for epileptic seizure detection. Multimed Tools Appl. 80:1–29. https://doi.org/10.1007/s11042-021-10597-6
Abdelhameed A, Bayoumi M (2021) A deep learning approach for automatic seizure detection in children with epilepsy. Front Comput Neurosci 15:650050. United States
Nahzat S, Yaganoglu M (2021) Classification of epileptic seizure dataset using different machine learning algorithms and PCA feature reduction technique. J Invest Eng Technol 4(2):47–60
Google Scholar
Shoeibi A, Khodatars M, Ghassemi N, Jafari M, Moridian P, Alizadehsani R et al (2021) Epileptic Seizures Detection Using Deep Learning Techniques: A Review. Int J Environ Res Public Health. 18(11):5780. https://doi.org/10.3390/ijerph18115780
Raibag MAA, Franklin JV, Sarkar R (2022) Multi-feature learning model for epilepsy classification supervised by a highly robust heterogeneous deep ensemble. Turk J Comput Math Educ 13:273–284
Torse DA, Khanai R (2022) Classification of epileptic seizures using ensemble empirical mode decomposition and least squares support vector machine. 2021 International Conference on Computer Communication and Informatics (ICCCI). pp 1–5
Natu M, Bachute M, Gite S, Kotecha K, Vidyarthi A (2022) Review on epileptic seizure prediction: machine learning and deep learning approaches. Comput Math Methods Med 2022:7751263
George F et al (2022) Epileptic seizure prediction using EEG images. 2020 International Conference on Communication and Signal Processing (ICCSP). pp 1595–1598
Ouichka O, Echtioui A, Hamam H (2022) Deep Learning Models for Predicting Epileptic Seizures Using iEEG Signals. Electronics. 11(4):605. https://doi.org/10.3390/electronics11040605
Chirasani SKR, Manikandan S (2022) A deep neural network for the classification of epileptic seizures using hierarchical attention mechanism. Appl Soft Comput 26:5389–5397
Jiang Y, Yao Lu, Yang L (2022) An epileptic seizure prediction model based on a time-wise attention simulation module and a pretrained ResNet. Methods 202:117–126
Oliva JT, Rosa JLG (2021) Binary and multiclass classifiers based on multitaper spectral features for epilepsy detection. Biomed Signal Process Control 66:102469
Raghu S, Sriraam N, Temel Y, Rao SV, Kubben PL (2020) EEG based multi-class seizure type classification using convolutional neural network and transfer learning. Neural Netw. 124:202–212. https://doi.org/10.1016/j.neunet.2020.01.017 . Epub 2020 Jan 25
Ilias L, Askounis D, Psarras J (2022) Multimodal detection of epilepsy with deep neural networks. Expert Syst Appl. 213:119010. https://doi.org/10.1016/j.eswa.2022.119010
Yao X, Cheng Q, Zhang GQ (2019) A novel independent RNN approach to classification of seizures against non-seizures. https://arxiv.org/abs/1903.09326
Download references
Acknowledgements
Not applicable.
Author information
Authors and affiliations.
Department of CSE, Swami Keshvanand Institute of Technology, Management & Gramothan, Jaipur, Rajasthan, India
Pankaj Kunekar & Mukesh Kumar Gupta
BITS Pilani, Dubai Campus, Dubai, UAE
Pramod Gaur
You can also search for this author in PubMed Google Scholar
Contributions
The original idea of the research work is of PK. Literature work and implementation work are done by PK. Reviews, suggestions and inputs to research work are done by MG and PG. Also, MG and PG have contributed to the implementation work. Results are validated and verified by PK, MG and PG. All authors have read and approved the manuscript.
Corresponding author
Correspondence to Pankaj Kunekar .
Ethics declarations
Competing interests.
The authors declare that they have no competing interests.
Additional information
Publisher’s note.
Springer Nature remains neutral with regard to jurisdictional claims in published maps and institutional affiliations.
Rights and permissions
Open Access This article is licensed under a Creative Commons Attribution 4.0 International License, which permits use, sharing, adaptation, distribution and reproduction in any medium or format, as long as you give appropriate credit to the original author(s) and the source, provide a link to the Creative Commons licence, and indicate if changes were made. The images or other third party material in this article are included in the article's Creative Commons licence, unless indicated otherwise in a credit line to the material. If material is not included in the article's Creative Commons licence and your intended use is not permitted by statutory regulation or exceeds the permitted use, you will need to obtain permission directly from the copyright holder. To view a copy of this licence, visit http://creativecommons.org/licenses/by/4.0/ . The Creative Commons Public Domain Dedication waiver ( http://creativecommons.org/publicdomain/zero/1.0/ ) applies to the data made available in this article, unless otherwise stated in a credit line to the data.
Reprints and permissions
About this article
Cite this article.
Kunekar, P., Gupta, M.K. & Gaur, P. Detection of epileptic seizure in EEG signals using machine learning and deep learning techniques. J. Eng. Appl. Sci. 71 , 21 (2024). https://doi.org/10.1186/s44147-023-00353-y
Download citation
Received : 09 May 2023
Accepted : 23 December 2023
Published : 19 January 2024
DOI : https://doi.org/10.1186/s44147-023-00353-y
Share this article
Anyone you share the following link with will be able to read this content:
Sorry, a shareable link is not currently available for this article.
Provided by the Springer Nature SharedIt content-sharing initiative
- Epilepsy analysis
- Epileptic seizure detection
- Comparative analysis
- UCI dataset
MINI REVIEW article
Personality disorders in people with epilepsy: a review.

- 1 Department of Biomedical and NeuroMotor Sciences, University of Bologna, Bologna, Italy
- 2 IRCCS Istituto delle Scienze Neurologiche di Bologna, Epilepsy Center (full member of the European Reference Network EpiCARE), Bologna, Italy
- 3 Department of Medicine and Surgery, University of Milano Bicocca, Milan, Italy
Epileptologists and psychiatrists have long observed a correlation between epilepsy and personality disorders (PDs) in their clinical practice. We conducted a comprehensive PubMed search looking for evidence on PDs in people with epilepsy (PwE). Out of over 600 results obtained without applying any time restriction, we selected only relevant studies (both analytical and descriptive) limited to English, Italian, French and Spanish languages, with a specific focus on PDs, rather than traits or symptoms, thus narrowing our search down to 23 eligible studies. PDs have been investigated in focal epilepsy (predominantly temporal lobe epilepsy - TLE), juvenile myoclonic epilepsy (JME) and psychogenic non-epileptic seizures (PNES), with heterogeneous methodology. Prevalence rates of PDs in focal epilepsy ranged from 18 to 42% in surgical candidates or post-surgical individuals, with Cluster C personality disorders or related traits and symptoms being most common. In JME, prevalence rates ranged from 8 to 23%, with no strong correlation with any specific PDs subtype. In PNES, prevalence rates ranged from 30 to 60%, with a notable association with Cluster B personality disorders, particularly borderline personality disorder. The presence of a PD in PwE, irrespective of subtype, complicates treatment management. However, substantial gaps of knowledge exist concerning the neurobiological substrate, effects of antiseizure medications and epilepsy surgery on concomitant PDs, all of which are indeed potential paths for future research.
Introduction
Epilepsy is a chronic disease of the brain defined as “an enduring predisposition to generate epileptic seizures, and by the neurobiologic, cognitive, psychological, and social consequences of this condition” ( 1 ). It is a common neurological disease, with a calculated incidence rate of 61.4 per 100,000 person-years and a prevalence of 7.60 per 1,000 population ( 2 ), it affects approximately 46 million people, therefore representing a significant fraction of the worldwide disease burden ( 3 ). Remarkably, nearly half of people with epilepsy (PwE) experience comorbidities, with certain conditions exhibiting a higher prevalence among PwE compared to the general population, often impacting on epilepsy prognosis itself ( 4 ). Psychiatric disorders have garnered particular attention, due to their association with poor seizure outcome, drug-resistance, heightened suicide risk ( 5 – 7 ). While extensive research has been devoted to psychotic, anxiety and mood disorders, limited attention has been directed to the relationship between epilepsy and personality disorders (PDs). This neglect is somewhat surprising given historical observations dating back to the last century, originating from studies involving institutionalized PwE. Several clinical conditions have been described, such as Geschwind syndrome ( 8 ), gliscroid personality or Blumer syndrome ( 9 ). These data were not universally supported or agreed upon ( 10 ) and were related, in particular, to the presence of temporal lobe epilepsies (TLE) with drug-resistant seizures, social isolation, and the use of certain medications, such as phenobarbital or bromine. Subsequent studies showed that other types of epilepsy, such as the Idiopathic Generalized Epilepsies (IGE), showed very different personological characteristics from those described for TLE ( 11 ). Features such as superficiality, tendency to elation, poor compliance and critical traits were noted, often attributed to frontal lobe involvement ( 11 ). However the debate about the existence of personological alterations during epilepsy has waned in subsequent years, partly in connection with the use of medications with less impact on cognitive function and with the improved social integration of PwE, so much so that the Commission on Epilepsy, Risks and Insurance of the International Bureau for Epilepsy regarded the risk for psychological disorders in epilepsy to be negligible, at least when considered as such ( 12 ). Furthermore, the emergence of a revised classification system both for epilepsies and for personality disorders (DSM-5) has provided more rigorous and precise definitions, warranting a reevaluation of the association between epilepsy and PDs. In light of these developments, it is imperative to revisit the nexus between epilepsy and PDs, incorporating contemporary scientific evidence and examining diverse populations.
Our review focuses specifically on PDs in PwE, with the aim to collect and synthesize existing evidence, identify gaps of knowledge and propose potential avenues for future research.
Material and methods
In November 2023 we performed a search on PubMed database using the following terms: “Epilepsy”[Mesh] AND [“personality disorder”(All Fields) OR “paranoid personality disorder” (MeSH) OR “schizoid personality disorder” (Mesh) OR “schizotypal personality disorder” (MeSH) OR “antisocial personality disorder” [MeSH] OR “borderline personality disorder “(MeSH) OR “histrionic personality disorder” (MeSH) OR “narcissistic personality disorder” (All Fields) OR “avoidant personality disorder” (All Fields) OR “dependent personality disorder” (Mesh) OR “obsessive compulsive disorder” (MeSH)].
We selected studies (both descriptive and analytical) written in English, Italian, French and Spanish investigating the prevalence of personality disorders in PwE. Exclusion criteria were reviews, case reports, case series with small populations (i.e. less than 10 patients) and editorial comments. Additionally, studies emphasizing personality traits or symptoms without a formal diagnosis of personality disorder were excluded. Studies focusing on personality traits or symptoms without a diagnosis of personality disorder were excluded. No time restrictions were applied, and the entire selection process was carried out manually without the use of any automated tool.
The identified references were screened and provisionally selected for inclusion on the basis of title and abstract, when available. The full texts of articles meeting the inclusion criteria were then assessed.
The initial PubMed search yielded a total of 638 articles. Following a multi-step selection process, 23 studies met the inclusion criteria and were considered eligible for analysis (see Figure 1 for further details). The findings of these studies are presented in distinct subchapters, according to the main themes investigated (see Table 1 ).
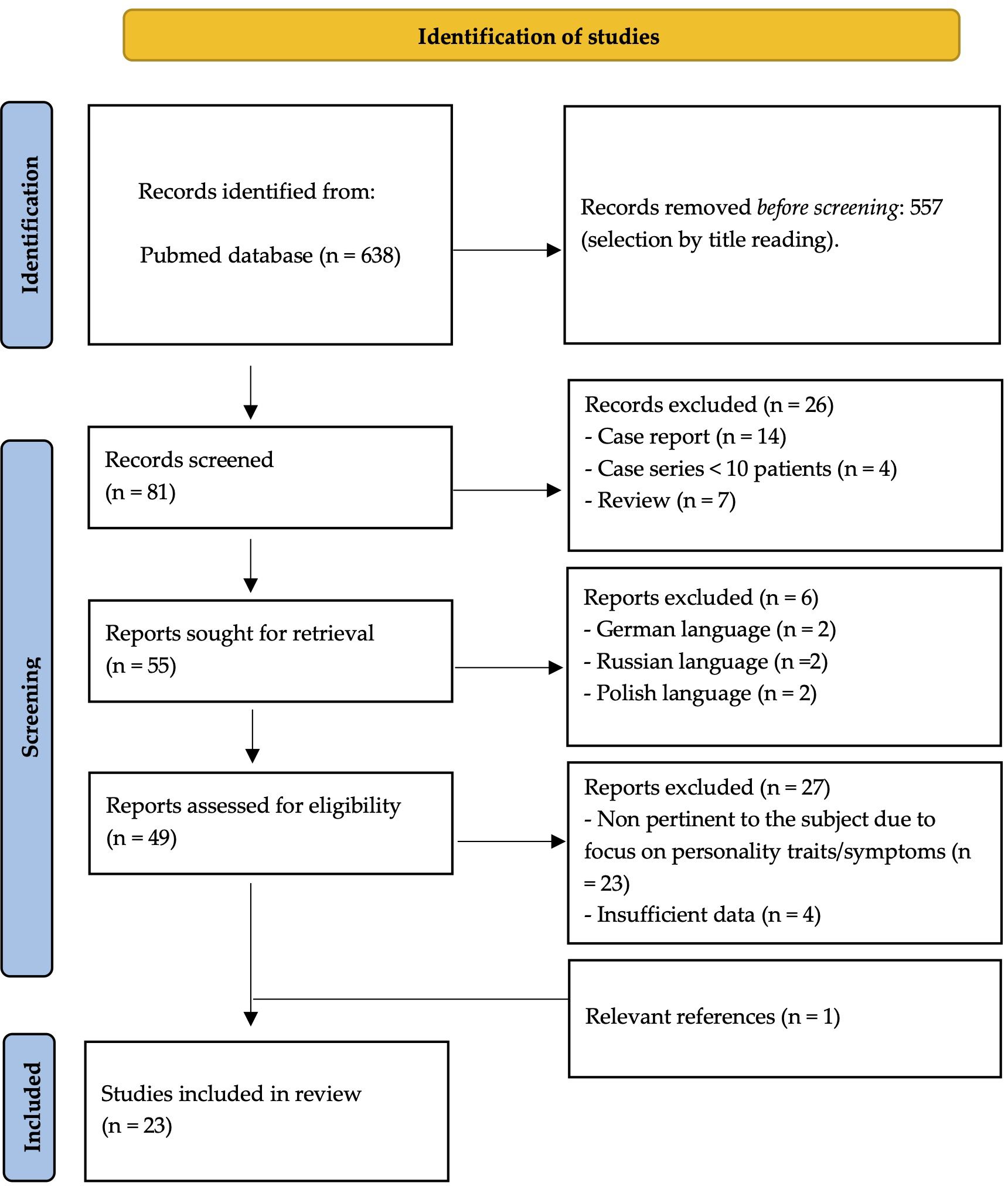
Figure 1 Detailed multi-step process for source identification.
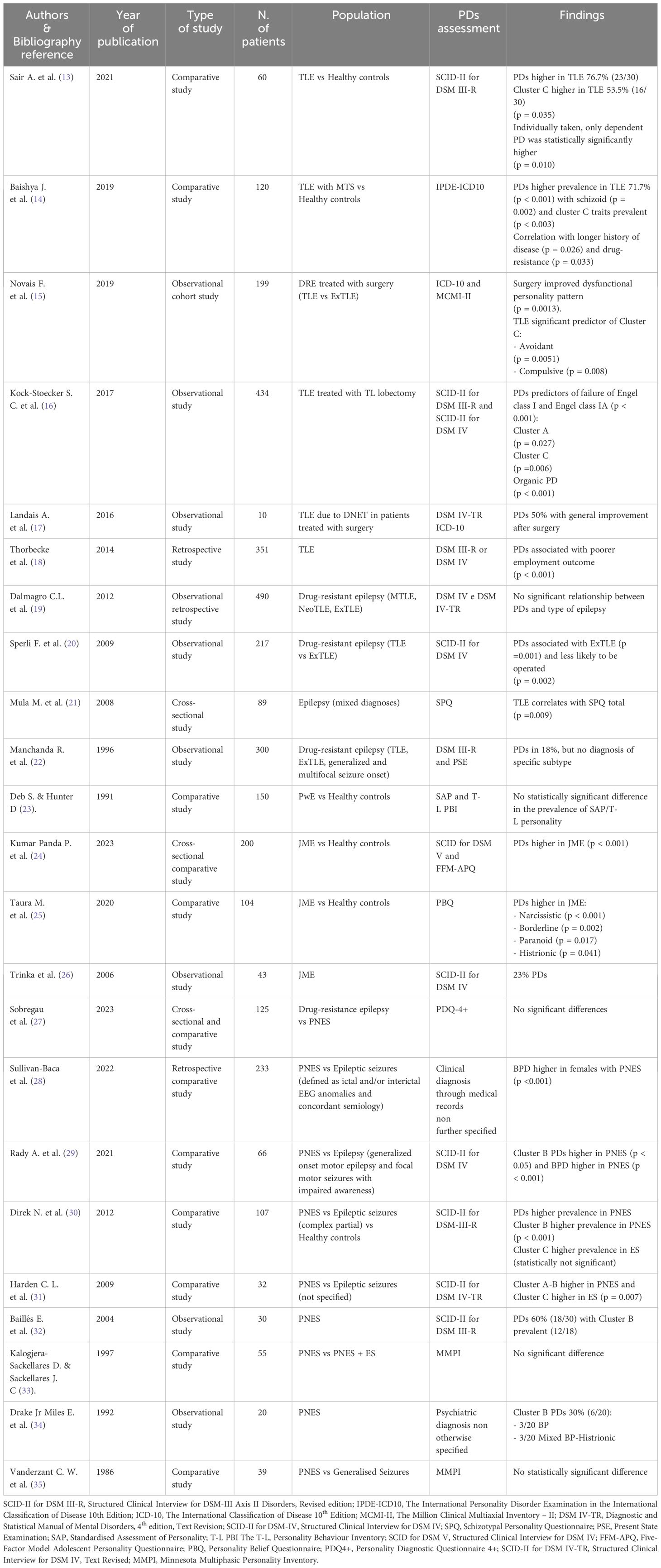
Table 1 Results.
Personality disorders and focal epilepsy
Among the included studies, eleven investigated the relationship between PDs and focal epilepsy. These studies showed a significant prevalence of PDs within the studied population, with the strongest association observed between TLE and Cluster C PDs ( 13 – 15 ) and, to a lesser extent, between TLE and schizotypal personality ( 21 ).
Several studies investigated PDs in people with focal epilepsy who underwent epilepsy surgery, investigating its impact on both medical conditions. Notably, one study reported a statistically significant improvement in pre-surgical PD symptoms, although details on seizure outcomes were not provided ( 15 ). In another work, surgical resection of the epileptogenic zone led to remarkable improvements in all five patients diagnosed with concomitant PD, with two even being able to discontinue antipsychotic medications. Post-surgical seizure outcomes, assessed using the Engel Surgical Outcome Scale, were also highly favorable, with patients either becoming completely seizure-free or experiencing only rare seizures ( 12 ). Interestingly, a large population- based study in people with TLE revealed that PDs were predictive of surgery failure, defined as significantly lower rates of Engel class I and IA ( 16 ). The authors speculated that complex brain microstructural abnormalities in people with TLE and psychiatric comorbidities might underlie this association, emphasizing the need for further research.
In a separate comparative study, PDs were more commonly associated with extratemporal lobe epilepsy (ExTLE) rather than TLE, leading to lower rates of surgical intervention for individuals with this comorbidity. Nevertheless, those who did undergo surgery exhibited similarly successful outcomes ( 20 ). Moreover, people with epilepsy and PDs seem to suffer from an even bleaker stigma, as shown by lower employment rates two years after surgery ( 18 ).
Conversely, three studies did not detect significant differences in PD prevalence among PwE. One uncontrolled study focused only on people with intellectual disability and epilepsy, thus this finding could be attributed to a population selection bias ( 23 ). In the other two studies, discrepancies in results might be attributed to the specific assessment scales utilized – as questioned by the Authors themselves ( 17 ) - or the comparison of PD prevalence across different subtypes of focal epilepsy ( 19 ) that did not yield statistically significant differences.
Personality disorders and juvenile myoclonic epilepsy
Three studies on PDs and juvenile myoclonic epilepsy (JME) were identified. Two recent comparative studies showed that PDs were significantly more prevalent in JME compared to healthy controls. While one study did not identify specific correlation between PD subtypes and JME ( 24 ), the other reported strong associations between narcissistic, borderline, paranoid and histrionic PDs and JME ( 25 ). These discrepancies may stem from variations in assessment scales used, as the populations examined exhibited otherwise similar characteristics. A third older and purely descriptive study focused on assessing the prevalence of PDs in a medium-size sample of patients with JME, without providing statistical analyses ( 23 ).
Personality disorders and psychogenic non-epileptic seizures
Among the 9 studies investing the association between PDs and psychogenic non-epileptic seizures (PNES), seven were comparative studies comparing people with PNES to various control groups, including those with epilepsy/epileptic seizures alone ( 27 – 31 , 35 ), PwE and PNES ( 33 ), or healthy controls ( 30 ). The remaining two studies were observational uncontrolled studies that gathered data on people with PNES through medical history and hospital charts ( 32 , 34 ). While the evidence from these latter two studies was undoubtedly of lower quality due to the lack of proper statistical analysis and, in one case, insufficient details on the methods used to diagnose PD ( 34 ), we deemed it important to include them as seminal works that highlighted a higher prevalence of Cluster B PDs in people with PNES, a finding largely confirmed by subsequent literature and deemed statistically significant in the majority of our results ( 28 – 31 ). However, three studies presented discordant findings in this regard, potentially influenced by factors such as the assessments tools used. For instance, one study ( 27 ) reported statistically significant high scores in the “extraversion” section of the NEO-Personality Inventory-Revised among patients with PNES. The other two studies assessed PDs by the Minnesota Multiphasic Personality Inventory (MMPI), despite criticisms regarding its limitations and inconsistency of results obtained using this tool ( 36 , 37 ).
Regarding gender differences, two studies show a statistically significant higher prevalence of PDs in women with PNES ( 28 , 29 ).
Despite the known higher prevalence of psychiatric comorbidities in PwE, the relationship between epilepsy and PDs has been inadequately investigated in the most recent scientific literature ( 38 ).
Our review demonstrates that the literature available on this topic is extremely heterogeneous in terms of methodology, population size, type of epilepsy investigated and assessment tools used for evaluating the disorder, with many works even failing to differentiate between psychiatric disorders in general and PDs ( 38 – 43 ). This last issue seems particularly relevant as the vast majority of the examined studies tend to combine and investigate various psychiatric conditions together ( 44 ). Conversely, when studies do focus on personality comorbidities, they often center on traits or symptoms rather than formally diagnosed PDs ( 39 – 41 ). Such methodological inconsistencies present a significant barrier to the comprehensive inclusion of pertinent evidence in our analysis, consequently constraining our capacity to derive meaningful insights regarding the relationship between PDs and epilepsy.
Another issue encountered in reviewing the existing literature on this subject is the lack of strong evidence due to the limited scope of studies carried out thus far, as, in spite of the higher prevalence of psychiatric comorbidities in PwE and the impact they seem to have on epilepsy prognosis itself, the number of well-designed case-control studies investigating their correlation remains insufficient ( 24 ). Ideally, to ensure statistical validity and clinical relevance, systematic comparisons should be made among different types of epilepsy and between PWE and healthy controls. Moreover, it seems very interesting to observe that there is relatively little standardized work on the issue of diagnostic tools used to identify PDs in epilepsy. Many studies relied on the MMPI, despite concerns raised by some researchers ( 36 , 37 ). The consequence of it, however, is the vast array of diagnostic tools used subsequently, such as the Bear-Fedio Inventory (BFI), the DSM-IV axis I and axis II, the Structured Clinical Interview for DSM-III-R PDs (SCID-II), the Clinical Interview Schedule (CIS) and many others, making the different results obtained arduous to analyse in a comprehensive fashion ( 38 ). Moreover, as reiterated by Trimble ( 36 ), the diagnosis made by scales cannot be considered valid tout court for a clinical diagnosis.
On the other hand, the change in the classification of the same disorders in relation to different editions of the DSM, the same different classification of epilepsies, the change in the treatment of them in recent years and, in particular, the increased social integration of individuals with epilepsy do not allow the historically acquired data to be considered valid.
Furthermore, the literature revision is complicated by its heterogeneity, as specific different types of epilepsy were investigated in individual studies, while, on the contrary, some neglected to differentiate between epilepsy and isolated seizures. There is a long-standing and established interest in exploring the relationship between PDs and PNES ( 35 ) and, more recently, a rapidly growing body of evidence on PDs in people with focal epilepsy has emerged ( 19 – 21 ), even applying the recently proposed dimensional approach to personality profile ( 45 ). However, there are only scattered studies on other subtypes of epilepsy, such as JME ( 24 ), and, to our best knowledge, very few works on the correlation between PDs and epilepsy as a whole, most of which also happen to be quite dated ( 36 , 46 – 48 ).
Given these limitations, our study aimed to aggregate and summarize available findings to offer a current perspective on the state of research in this area. By highlighting these constraints, we hope to identify potential research directions for future exploration. The relationship between PDs and focal epilepsy, particularly TLE, is well-established, with prevalence rates ranging from 18 to 42% in surgical candidates or post-surgical individuals ( 49 ). While there is no clear agreement as to what type of PD is the most prevalent, cluster C personality disorders or related traits and symptoms seem to be the most frequent ( 13 – 15 , 31 , 40 , 50 ). However, the correlation between TLE, PDs and surgical treatment remains inconclusive, highlighting the need for future works to delve in this complex relationship, due to its profound impact on patients’ outcome.
In the context of JME, the prevalence and specific types of PDs remain contentious among the limited available studies ( 51 ). Nevertheless, the significance of exploring this relationship is undeniable, given the prevalence of JME. Analysing the correlation between JME and PDs within the broader framework of the idiopathic generalized epilepsies, ideally comparing JME with the other subtypes, could yield robust evidence on their psychiatric and personality profiles.
Our research underscores a strong connection PDs and PNES, particularly in females, with a strong association with cluster B personality disorders, notably borderline personality disorder ( 52 ). Delayed diagnosis of PNES, averaging 7-9 years from the initial clinical episode, leads to unnecessary hospitalizations and inappropriate treatment with antiseizure medications (ASMs) ( 53 ). Regarding the association with PNES, it must, in any case, be considered, that since PNES is a psychiatric disorder, many authors wonder whether we are really facing an association of different pathologies or a single one ( 11 ).
Regarding ASMs in general, our review highlights that the presence of a PD in PwE, regardless of the subtype, complicates treatment management. Clinicians face challenges in maintaining a delicate balance due to the potential for ASMs to worsen or exacerbate underlying PDs, as observed with drugs like perampanel and levetiracetam ( 7 , 54 – 57 ).
This review has methodological limitations. We conducted our research through only one database, potentially excluding relevant evidence available from other online sources. Moreover, few studies were not included for language reasons ( 58 – 61 ). Lastly, we did not offer an analysis of the evidence strength provided by each work as the grand variety of statistical methodology used, population size, type of epilepsy and parameters considered made us opt for a purely descriptive presentation of our findings. However, we do not think that these limits significantly reduce the strength of our results, which allowed us to identify research paths worthy of further investigation.
Conclusions and directions for future research
While the relationship between psychiatric comorbidities and epilepsy has been discussed since time immemorial ( 47 ), our study reveals a notable lack of definitive data on various aspects concerning PDs in PwE. The literature in this domain is limited and methodologically diverse, yet our findings suggest that PDs are a prevalent comorbidity in PwE.
Future research should prioritize the identification of significant correlations between PDs and specific types of epilepsy, as well as elucidate how their co-occurrence influence patients’ prognosis. Additionally, it is crucial to investigate the effects of current ASMs on concomitant PDs.
Given the increasing number of patients being considered for epilepsy surgery, it is of the utmost importance to deepen our understanding of the neurological basis and pathological mechanisms that intertwine PDs and epilepsy, given the current scarcity of evidence that may inadvertently hinder optimal treatment decisions for some patients.
Based on our findings and with the aim of enhancing patient care and wellbeing, we advocate for a collaborative, multidisciplinary approach involving neurologists and psychiatrists, which should begin from the early stages of diagnosis and extend to the selection of personalized therapeutic strategies for each patient.
Author contributions
VV: Writing – original draft, Conceptualization, Investigation, Methodology. FB: Writing – review & editing. CC: Writing – review & editing. LF: Writing – review & editing. LL: Writing – review & editing. LM: Writing – review & editing. BM: Supervision, Writing – review & editing, Conceptualization, Funding acquisition, Methodology.
The author(s) declare financial support was received for the research, authorship, and/or publication of this article. The publication of this article was supported by the “Ricerca Corrente” funding from the Italian Ministry of Health.
Acknowledgments
The authors would like to thank Loretta Giuliano for her help in creating our search string for PubMed.
Conflict of interest
The authors declare that the research was conducted in the absence of any commercial or financial relationships that could be construed as a potential conflict of interest.
The author(s) declared that they were an editorial board member of Frontiers, at the time of submission. This had no impact on the peer review process and the final decision.
Publisher’s note
All claims expressed in this article are solely those of the authors and do not necessarily represent those of their affiliated organizations, or those of the publisher, the editors and the reviewers. Any product that may be evaluated in this article, or claim that may be made by its manufacturer, is not guaranteed or endorsed by the publisher.
1. Fisher RS, Acevedo C, Arzimanoglou A, Bogacz A, Cross JH, Elger CE, et al. ILAE official report: a practical clinical definition of epilepsy. Epilepsia . (2014) 55:475–82. doi: 10.1111/epi.12550
PubMed Abstract | CrossRef Full Text | Google Scholar
2. Beghi E. The epidemiology of epilepsy. Neuroepidemiology . (2020) 54:185–91. doi: 10.1159/000503831
3. Feigin VL, Nichols E, Alam T, Bannick MS, Beghi E, Blake N, et al. Global, regional, and national burden of neurological disorders, 1990-2016: a systematic analysis for the Global Burden of Disease Study 2016. Lancet Neurol . (2019) 18:459–80. doi: 10.1016/S1474-4422(18)30499-X
4. Keezer MR, Sisodiya SM, Sander JW. Comorbidities of epilepsy: current concepts and future perspectives. Lancet Neurol . (2016) 15:106–15. doi: 10.1016/S1474-4422(15)00225-2
5. Taylor RS, Sander JW, Taylor RJ, Baker GA. Predictors of health-related quality of life and costs in adults with epilepsy: a systematic review. Epilepsia . (2011) 52:2168–80. doi: 10.1111/j.1528-1167.2011.03213.x
6. Ioannou P, Foster DL, Sander JW, Dupont S, Gil-Nagel A, Drogon O’Flaherty E, et al. The burden of epilepsy and unmet need in people with focal seizures. Brain Behav . (2022) 12:e2589. doi: 10.1002/brb3.2589
7. Memon AM, Katwala J, Douille C, Kelley C, Monga V. Exploring the complex relationship between antiepileptic drugs and suicidality: A systematic literature review. Innov Clin Neurosci . (2023) 20:47–51.
PubMed Abstract | Google Scholar
8. Benson DF. The geschwind syndrome. Adv Neurol . (1991) 55:411–21.
9. Blumer D. Evidence supporting the temporal lobe epilepsy personality syndrome. Neurology . (1999) 53:S9–12.
10. Devinsky O, Najjar S. Evidence against the existence of a temporal lobe epilepsy personality syndrome. Neurology . (1999) 53:S13–25.
11. Beghi M, Beghi E, Cornaggia CM, Gobbi G. Idiopathic generalized epilepsies of adolescence. Epilepsia . (2006) 47 Suppl 2:107–10. doi: 10.1111/j.1528-1167.2006.00706.x
12. Cornaggia CM. Epilepsy and risks: a first-step evaluation . Milano: Ghedini editore (1993).
Google Scholar
13. Sair A, Şair YB, Saracoğlu İ, Sevincok L, Akyol A. The relation of major depression, OCD, personality disorders and affective temperaments with Temporal lobe epilepsy. Epilepsy Res . (2021) 171:106565. doi: 10.1016/j.eplepsyres.2021.106565
14. Baishya J, Ravish Rajiv K, Chandran A, Unnithan G, Menon RN, Thomas SV, et al. Personality disorders in temporal lobe epilepsy: What do they signify? Acta Neurol Scand . (2020) 142:210–5. doi: 10.1111/ane.13259
15. Novais F, Franco A, Loureiro S, Andrea M, Figueira ML, Pimentel J, et al. Personality patterns of people with medically refractory epilepsy - Does the epileptogenic zone matter? Epilepsy Behav EB . (2019) 97:130–4. doi: 10.1016/j.yebeh.2019.05.049
CrossRef Full Text | Google Scholar
16. Koch-Stoecker SC, Bien CG, Schulz R, May TW. Psychiatric lifetime diagnoses are associated with a reduced chance of seizure freedom after temporal lobe surgery. Epilepsia . (2017) 58:983–93. doi: 10.1111/epi.13736
17. Landais A, Crespel A, Moulis JL, Coubes P, Gelisse P. Psychiatric comorbidity in temporal DNET and improvement after surgery. Neurochirurgie . (2016) 62:165–70. doi: 10.1016/j.neuchi.2016.02.002
18. Thorbecke R, May TW, Koch-Stoecker S, Ebner A, Bien CG, Specht U. Effects of an inpatient rehabilitation program after temporal lobe epilepsy surgery and other factors on employment 2 years after epilepsy surgery. Epilepsia . (2014) 55:725–33. doi: 10.1111/epi.12573
19. Dalmagro CL, Velasco TR, Bianchin MM, Martins APP, Guarnieri R, Cescato MP, et al. Psychiatric comorbidity in refractory focal epilepsy: a study of 490 patients. Epilepsy Behav EB . (2012) 25:593–7. doi: 10.1016/j.yebeh.2012.09.026
20. Sperli F, Rentsch D, Despland PA, Foletti G, Jallon P, Picard F, et al. Psychiatric comorbidity in patients evaluated for chronic epilepsy: a differential role of the right hemisphere? Eur Neurol . (2009) 61:350–7. doi: 10.1159/000210547
21. Mula M, Cavanna A, Collimedaglia L, Viana M, Barbagli D, Tota G, et al. Clinical correlates of schizotypy in patients with epilepsy. J Neuropsychiatry Clin Neurosci . (2008) 20:441–6. doi: 10.1176/appi.neuropsych.20.4.441
22. Manchanda R, Schaefer B, McLachlan RS, Blume WT, Wiebe S, Girvin JP, et al. Psychiatric disorders in candidates for surgery for epilepsy. J Neurol Neurosurg Psychiatry . (1996) 61:82–9. doi: 10.1136/jnnp.61.1.82
23. Deb S, Hunter D. Psychopathology of people with mental handicap and epilepsy. III: Personality disorder. Br J Psychiatry J Ment Sci . (1991) 159:830–4. doi: 10.1192/bjp.159.6.830
24. Panda PK, Ramachandran A, Tomar A, Elwadhi A, Kumar V, Sharawat IK. Prevalence, nature, and severity of the psychiatric comorbidities and their impact on quality of life in adolescents with Juvenile myoclonic epilepsy. Epilepsy Behav EB . (2023) 142:109216. doi: 10.1016/j.yebeh.2023.109216
25. Taura M, Gama AP, Sousa AVM, Noffs MHS, Alonso NB, Yacubian EM, et al. Dysfunctional personality beliefs and executive performance in patients with juvenile myoclonic epilepsy. Epilepsy Behav EB . (2020) 105:106958. doi: 10.1016/j.yebeh.2020.106958
26. Trinka E, Kienpointner G, Unterberger I, Luef G, Bauer G, Doering LB, et al. Psychiatric comorbidity in juvenile myoclonic epilepsy. Epilepsia . (2006) 47:2086–91. doi: 10.1111/j.1528-1167.2006.00828.x
27. Sobregrau P, Baillès E, Carreño M, Donaire A, Boget T, Setoain X, et al. Psychiatric and psychological assessment of Spanish patients with drug-resistant epilepsy and psychogenic nonepileptic seizures (PNES) with no response to previous treatments. Epilepsy Behav EB . (2023) 145:109329. doi: 10.1016/j.yebeh.2023.109329
28. Sullivan-Baca E, Weitzner DS, Choudhury TK, Fadipe M, Miller BI, Haneef Z. Characterizing differences in psychiatric profiles between male and female veterans with epilepsy and psychogenic non-epileptic seizures. Epilepsy Res . (2022) 186:106995. doi: 10.1016/j.eplepsyres.2022.106995
29. Rady A, Elfatatry A, Molokhia T, Radwan A. Psychiatric comorbidities in patients with psychogenic nonepileptic seizures. Epilepsy Behav EB . (2021) 118:107918. doi: 10.1016/j.yebeh.2021.107918
30. Direk N, Kulaksizoglu IB, Alpay K, Gurses C. Using personality disorders to distinguish between patients with psychogenic nonepileptic seizures and those with epileptic seizures. Epilepsy Behav EB . (2012) 23:138–41. doi: 10.1016/j.yebeh.2011.11.013
31. Harden CL, Jovine L, Burgut FT, Carey BT, Nikolov BG, Ferrando SJ. A comparison of personality disorder characteristics of patients with nonepileptic psychogenic pseudoseizures with those of patients with epilepsy. Epilepsy Behav EB . (2009) 14:481–3. doi: 10.1016/j.yebeh.2008.12.012
32. Baillés E, Pintor L, Fernandez-Egea E, Torres X, Matrai S, De Pablo J, et al. Psychiatric disorders, trauma, and MMPI profile in a Spanish sample of nonepileptic seizure patients. Gen Hosp Psychiatry . (2004) 26:310–5. doi: 10.1016/j.genhosppsych.2004.04.003
33. Kalogjera-Sackellares D, Sackellares JC. Personality profiles of patients with pseudoseizures. Seizure . (1997) 6:1–7. doi: 10.1016/S1059-1311(97)80045-3
34. Drake MEJ, Pakalnis A, Phillips BB. Neuropsychological and psychiatric correlates of intractable pseudoseizures. Seizure . (1992) 1:11–3. doi: 10.1016/1059-1311(92)90048-6
35. Vanderzant CW, Giordani B, Berent S, Dreifuss FE, Sackellares JC. Personality of patients with pseudoseizures. Neurology . (1986) 36:664–8. doi: 10.1212/WNL.36.5.664
36. Trimble MR. Personality disturbances in epilepsy. Neurology . (1983) 33:1332–4. doi: 10.1212/WNL.33.10.1332-a
37. Perini GI, Tosin C, Carraro C, Bernasconi G, Canevini MP, Canger R, et al. Interictal mood and personality disorders in temporal lobe epilepsy and juvenile myoclonic epilepsy. J Neurol Neurosurg Psychiatry . (1996) 61:601–5. doi: 10.1136/jnnp.61.6.601
38. Swinkels WAM, Duijsens IJ, Spinhoven P. Personality disorder traits in patients with epilepsy. Seizure . (2003) 12:587–94. doi: 10.1016/S1059-1311(03)00098-0
39. Guo X, Lin W, Zhong R, Han Y, Yu J, Yan K, et al. Factors related to the severity of obsessive-compulsive symptoms and their impact on suicide risk in epileptic patients. Epilepsy Behav EB . (2023), 146:109362. doi: 10.1016/j.yebeh.2023.109362
40. Kilicaslan EE, Türe HS, Kasal Mİ, Çavuş NN, Akyüz DA, Akhan G, et al. Differences in obsessive-compulsive symptom dimensions between patients with epilepsy with obsessive-compulsive symptoms and patients with OCD. Epilepsy Behav EB . (2020) 102:106640. doi: 10.1016/j.yebeh.2019.106640
41. Kim SJ, Lee SA, Ryu HU, Han SH, Lee GH, Jo KD, et al. Factors associated with obsessive-compulsive symptoms in people with epilepsy. Epilepsy Behav EB . (2020) 102:106723. doi: 10.1016/j.yebeh.2019.106723
42. Ertekin BA, Kulaksizoğlu IB, Ertekin E, Gürses C, Bebek N, Gökyiğit A, et al. A comparative study of obsessive-compulsive disorder and other psychiatric comorbidities in patients with temporal lobe epilepsy and idiopathic generalized epilepsy. Epilepsy Behav EB . (2009) 14:634–9. doi: 10.1016/j.yebeh.2009.01.016
43. Isaacs KL, Philbeck JW, Barr WB, Devinsky O, Alper K. Obsessive-compulsive symptoms in patients with temporal lobe epilepsy. Epilepsy Behav EB . (2004) 5:569–74. doi: 10.1016/j.yebeh.2004.04.009
44. de Oliveira GNM, Kummer A, Salgado JV, Portela EJ, Sousa-Pereira SR, David AS, et al. Psychiatric disorders in temporal lobe epilepsy: an overview from a tertiary service in Brazil. Seizure . (2010) 19:479–84. doi: 10.1016/j.seizure.2010.07.004
45. Kustov G, Zhuravlev D, Zinchuk M, Popova S, Tikhonova O, Yakovlev A, et al. Maladaptive personality traits in patients with epilepsy and psychogenic non-epileptic seizures. Seizure . (2024) 117:77–82. doi: 10.1016/j.seizure.2024.02.005
46. Jeżowska-Jurczyk K, Kotas R, Jurczyk P, Nowakowska-Kotas M, Budrewicz S, Pokryszko-Dragan A. Mental disorders in patients with epilepsy. Psychiatr Pol . (2020) 54:51–68. doi: 10.12740/PP/93886
47. Trimble MR. Personality disorders and epilepsy. Acta Neurochir Suppl (Wien) . (1988) 44:98–101. doi: 10.1007/978-3-7091-9005-0_20
48. Trimble MR. Psychiatric aspects of epilepsy. Psychiatr Dev . (1987) 5:285–300.
49. Gaitatzis A, Trimble MR, Sander JW. The psychiatric comorbidity of epilepsy. Acta Neurol Scand . (2004) 110:207–20. doi: 10.1111/j.1600-0404.2004.00324.x
50. Hamed SA, Elserogy YM, Abd-Elhafeez HA. Psychopathological and peripheral levels of neurobiological correlates of obsessive-compulsive symptoms in patients with epilepsy: a hospital-based study. Epilepsy Behav EB . (2013) 27:409–15. doi: 10.1016/j.yebeh.2013.01.022
51. Gélisse P, Thomas P, Samuelian JC, Gentin P. Psychiatric disorders in juvenile myoclonic epilepsy. Epilepsia . (2007) 48:1032–3. doi: 10.1111/j.1528-1167.2007.01009_4.x
52. Lacey C, Cook M, Salzberg M. The neurologist, psychogenic nonepileptic seizures, and borderline personality disorder. Epilepsy Behav EB . (2007) 11:492–8. doi: 10.1016/j.yebeh.2007.09.010
53. Oto MM. The misdiagnosis of epilepsy: Appraising risks and managing uncertainty. Seizure . (2017) 44:143–6. doi: 10.1016/j.seizure.2016.11.029
54. Villanueva V, Garcés M, López-González FJ, Rodriguez-Osorio X, Toledo M, Salas-Puig J, et al. Safety, efficacy and outcome-related factors of perampanel over 12 months in a real-world setting: The FYDATA study. Epilepsy Res . (2016) 126:201–10. doi: 10.1016/j.eplepsyres.2016.08.001
55. Yumnam S, Bhagwat C, Saini L, Sharma A, Shah R. Levetiracetam-associated obsessive-compulsive symptoms in a preschooler. J Clin Psychopharmacol . (2021) 41:495–6. doi: 10.1097/JCP.0000000000001402
56. Fujikawa M, Kishimoto Y, Kakisaka Y, Jin K, Kato K, Iwasaki M, et al. Obsessive-compulsive behavior induced by levetiracetam. J Child Neurol . (2015) 30:942–4. doi: 10.1177/0883073814541471
57. Mbizvo GK, Dixon P, Hutton JL, Marson AG. The adverse effects profile of levetiracetam in epilepsy: a more detailed look. Int J Neurosci . (2014) 124:627–34. doi: 10.3109/00207454.2013.866951
58. Usykina MV, Kornilova SV, Lavrushchik MV. [Cognitive impairment and social functioning in organic personality disorder due to epilepsy]. Zh Nevrol Psikhiatr Im S Korsakova . (2021) 121:21–6. doi: 10.17116/jnevro202112106121
59. Dvirskiĭ AE, Shevtsov AG. [Clinical manifestations of epilepsy in hereditary schizophrenia]. Zhurnal Nevropatol Psikhiatrii Im SS Korsakova Mosc Russ 1952 . (1991) 91:28–30.
60. Ekiert H, Jarzebowska E, Nurowska K, Welbel L. [Personality of epileptic patients and their relatives with special regard to traits and symptoms not included in the epileptic character]. Psychiatr Pol . (1967) 1:15–21.
61. Bilikiewicz A, Gromska J. Mental symptoms and character disorders in epilepsy with the “Temporal Syndrome”. Neurol Neurochir Psychiatr Pol . (1964) 14:879–82.
Keywords: epilepsy, personality disorders (PDs), PNES, juvenile myoclonic epilepsy (JME), temporal lobe epilepsy, epilepsy surgery
Citation: Viola V, Bisulli F, Cornaggia CM, Ferri L, Licchetta L, Muccioli L and Mostacci B (2024) Personality disorders in people with epilepsy: a review. Front. Psychiatry 15:1404856. doi: 10.3389/fpsyt.2024.1404856
Received: 21 March 2024; Accepted: 24 April 2024; Published: 10 May 2024.
Reviewed by:
Copyright © 2024 Viola, Bisulli, Cornaggia, Ferri, Licchetta, Muccioli and Mostacci. This is an open-access article distributed under the terms of the Creative Commons Attribution License (CC BY) . The use, distribution or reproduction in other forums is permitted, provided the original author(s) and the copyright owner(s) are credited and that the original publication in this journal is cited, in accordance with accepted academic practice. No use, distribution or reproduction is permitted which does not comply with these terms.
*Correspondence: Barbara Mostacci, [email protected]
Disclaimer: All claims expressed in this article are solely those of the authors and do not necessarily represent those of their affiliated organizations, or those of the publisher, the editors and the reviewers. Any product that may be evaluated in this article or claim that may be made by its manufacturer is not guaranteed or endorsed by the publisher.
Thank you for visiting nature.com. You are using a browser version with limited support for CSS. To obtain the best experience, we recommend you use a more up to date browser (or turn off compatibility mode in Internet Explorer). In the meantime, to ensure continued support, we are displaying the site without styles and JavaScript.
- View all journals
- Explore content
- About the journal
- Publish with us
- Sign up for alerts
- 09 May 2024
Cubic millimetre of brain mapped in spectacular detail
- Carissa Wong
You can also search for this author in PubMed Google Scholar
Rendering based on electron-microscope data, showing the positions of neurons in a fragment of the brain cortex. Neurons are coloured according to size. Credit: Google Research & Lichtman Lab (Harvard University). Renderings by D. Berger (Harvard University)
Researchers have mapped a tiny piece of the human brain in astonishing detail. The resulting cell atlas, which was described today in Science 1 and is available online , reveals new patterns of connections between brain cells called neurons, as well as cells that wrap around themselves to form knots, and pairs of neurons that are almost mirror images of each other.
The 3D map covers a volume of about one cubic millimetre, one-millionth of a whole brain, and contains roughly 57,000 cells and 150 million synapses — the connections between neurons. It incorporates a colossal 1.4 petabytes of data. “It’s a little bit humbling,” says Viren Jain, a neuroscientist at Google in Mountain View, California, and a co-author of the paper. “How are we ever going to really come to terms with all this complexity?”
Slivers of brain
The brain fragment was taken from a 45-year-old woman when she underwent surgery to treat her epilepsy. It came from the cortex, a part of the brain involved in learning, problem-solving and processing sensory signals. The sample was immersed in preservatives and stained with heavy metals to make the cells easier to see. Neuroscientist Jeff Lichtman at Harvard University in Cambridge, Massachusetts, and his colleagues then cut the sample into around 5,000 slices — each just 34 nanometres thick — that could be imaged using electron microscopes.
Jain’s team then built artificial-intelligence models that were able to stitch the microscope images together to reconstruct the whole sample in 3D. “I remember this moment, going into the map and looking at one individual synapse from this woman’s brain, and then zooming out into these other millions of pixels,” says Jain. “It felt sort of spiritual.”
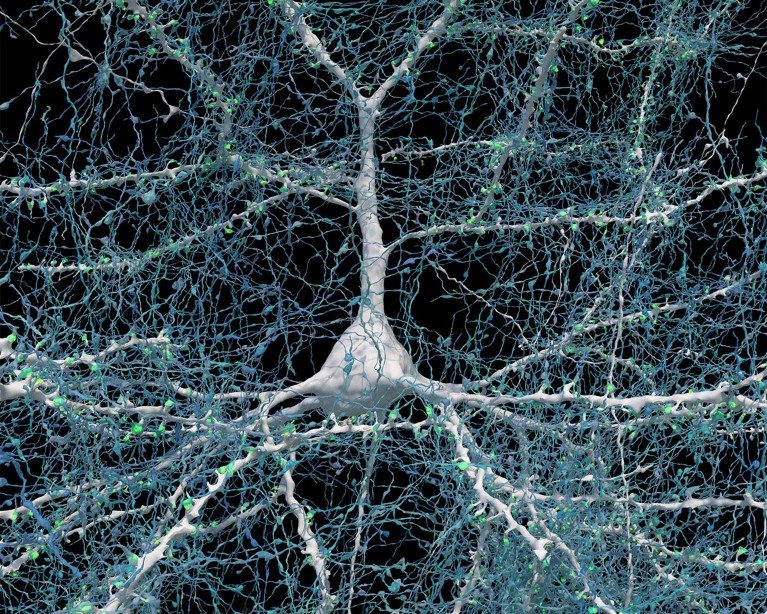
A single neuron (white) shown with 5,600 of the axons (blue) that connect to it. The synapses that make these connections are shown in green. Credit: Google Research & Lichtman Lab (Harvard University). Renderings by D. Berger (Harvard University)
When examining the model in detail, the researchers discovered unconventional neurons, including some that made up to 50 connections with each other. “In general, you would find a couple of connections at most between two neurons,” says Jain. Elsewhere, the model showed neurons with tendrils that formed knots around themselves. “Nobody had seen anything like this before,” Jain adds.
The team also found pairs of neurons that were near-perfect mirror images of each other. “We found two groups that would send their dendrites in two different directions, and sometimes there was a kind of mirror symmetry,” Jain says. It is unclear what role these features have in the brain.
Proofreaders needed
The map is so large that most of it has yet to be manually checked, and it could still contain errors created by the process of stitching so many images together. “Hundreds of cells have been ‘proofread’, but that’s obviously a few per cent of the 50,000 cells in there,” says Jain. He hopes that others will help to proofread parts of the map they are interested in. The team plans to produce similar maps of brain samples from other people — but a map of the entire brain is unlikely in the next few decades, he says.
“This paper is really the tour de force creation of a human cortex data set,” says Hongkui Zeng, director of the Allen Institute for Brain Science in Seattle. The vast amount of data that has been made freely accessible will “allow the community to look deeper into the micro-circuitry in the human cortex”, she adds.
Gaining a deeper understanding of how the cortex works could offer clues about how to treat some psychiatric and neurodegenerative diseases. “This map provides unprecedented details that can unveil new rules of neural connections and help to decipher the inner working of the human brain,” says Yongsoo Kim, a neuroscientist at Pennsylvania State University in Hershey.
doi: https://doi.org/10.1038/d41586-024-01387-9
Shapson-Coe, A. et al. Science 384 , eadk4858 (2024).
Article Google Scholar
Download references
Reprints and permissions
Related Articles
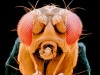
- Neuroscience
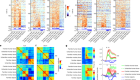
Temporal multiplexing of perception and memory codes in IT cortex
Article 15 MAY 24
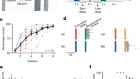
Volatile working memory representations crystallize with practice
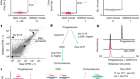
Evolution of a novel adrenal cell type that promotes parental care
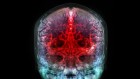
Organoids merge to model the blood–brain barrier
Research Highlight 15 MAY 24
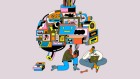
How does ChatGPT ‘think’? Psychology and neuroscience crack open AI large language models
News Feature 14 MAY 24
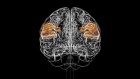
Brain-reading device is best yet at decoding ‘internal speech’
News 13 MAY 24
Postdoc Fellowships
Train with world-renowned cancer researchers at NIH? Consider joining the Center for Cancer Research (CCR) at the National Cancer Institute
Bethesda, Maryland
NIH National Cancer Institute (NCI)
Faculty Recruitment, Westlake University School of Medicine
Faculty positions are open at four distinct ranks: Assistant Professor, Associate Professor, Full Professor, and Chair Professor.
Hangzhou, Zhejiang, China
Westlake University
PhD/master's Candidate
PhD/master's Candidate Graduate School of Frontier Science Initiative, Kanazawa University is seeking candidates for PhD and master's students i...
Kanazawa University
Senior Research Assistant in Human Immunology (wet lab)
Senior Research Scientist in Human Immunology, high-dimensional (40+) cytometry, ICS and automated robotic platforms.
Boston, Massachusetts (US)
Boston University Atomic Lab
Postdoctoral Fellow in Systems Immunology (dry lab)
Postdoc in systems immunology with expertise in AI and data-driven approaches for deciphering human immune responses to vaccines and diseases.
Sign up for the Nature Briefing newsletter — what matters in science, free to your inbox daily.
Quick links
- Explore articles by subject
- Guide to authors
- Editorial policies

An official website of the United States government
The .gov means it’s official. Federal government websites often end in .gov or .mil. Before sharing sensitive information, make sure you’re on a federal government site.
The site is secure. The https:// ensures that you are connecting to the official website and that any information you provide is encrypted and transmitted securely.
- Publications
- Account settings
Preview improvements coming to the PMC website in October 2024. Learn More or Try it out now .
- Advanced Search
- Journal List
- Wolters Kluwer - PMC COVID-19 Collection
- PMC10171097

The Association Between COVID-19 and Febrile Seizure
Sean m. hanlon.
From the ∗ Department of Emergency Medicine
† Department of Internal Medicine and Pediatrics
Jack G. Schneider
‡ Department of Pediatrics, The Ryan White Center for Pediatric Infectious Disease and Global Health, Indiana University School of Medicine
§ Department of Biostatistics, Indiana University-Purdue University Indianapolis
Sean M. Thompson
∥ Department of Emergency Medicine, Indiana University School of Medicine, Indianapolis, IN
Background/Objective
Throughout the pandemic, febrile seizures have resulted from infection secondary to severe acute respiratory syndrome coronavirus-2 (SARS-CoV-2). The objective of this study is to determine if there is an increased association between COVID-19 and febrile seizures as compared with other causes of febrile seizures.
This was a retrospective case control study. Data were collected from the National Institute of Health (NIH) supported National COVID Cohort Collaborative (N3C). Patients from 6 to 60 months who were tested for COVID-19 were included; cases were defined as COVID-19–positive patients whereas controls were defined as COVID-19–negative patients. Febrile seizures diagnosed within 48 hours of the COVID-19 test were considered to be associated with the test result. Patients were subjected to a stratified gender and date matching design followed by a logistic regression controlling for age and race.
During the study period, 27,692 patients were included. Of those, 6923 patients were COVID-19–positive, among which 189 had febrile seizures (2.7%). After logistic regression, the likelihood of having febrile seizures concurrently with COVID-19 as compared with other causes was 0.96 ( P = 0.949; confidence interval, 0.81, 1.14).
Conclusions
There were 2.7% of the patients with COVID-19 that were diagnosed with a febrile seizure. However, when subjected to a matched case control design with logistic regression controlling for confounding variables, there does not appear to be an increased risk of febrile seizures secondary to COVID-19 as compared with other causes.
Febrile seizures have long been a common presentation to emergency departments and are the most common form of childhood seizures, occurring in 2% to 5% of the population. Generally, these are defined as seizures that occur in a pediatric patient 6 months to 60 months of age in the setting of febrile illness and the absence of central nervous system infection. Febrile seizures are generally categorized into either simple or complex seizures. Simple febrile seizures are generalized or non-focal seizures, last less than 15 minutes, and do not recur in the proceeding 24 hours. Complex febrile seizures are any seizures that do not meet the above criteria. 1
The most common cause of febrile seizures includes viral illnesses, bacterial infections, and vaccinations, notably the combined MMR-V vaccine. One of the largest studies to date, the EFES study, was a prospective multicenter study that enrolled patients with febrile seizures and performed a respiratory multiplex array to determine which respiratory viruses were most associated with the condition. Results showed at least one respiratory virus was detected in nearly 83% of patients with febrile seizures, with adenovirus being the highest at 55%. Coronaviruses OC43 and 229 were also tested in the study and found to be the ninth and twelfth most common viruses (7% and 3.5%, respectively). These results, however, were published before the discovery of Severe Acute Respiratory Syndrome Coronavirus-2 (SARS-CoV-2). 2
Early in the SARS-CoV-2 pandemic, case reports documented the association between COVID-19 infection and febrile seizures. 3 , 4 In addition, studies have examined the general frequency of febrile seizures in COVID-19 patients. 5 Currently, there are no published studies determining whether there is an increased risk of febrile seizures with COVID-19 infections compared with other causes. The primary aim of this study was to determine whether there is an increased association between COVID-19 infection and febrile seizures as compared with patients who are COVID-19–negative.
Study Design
We conducted a retrospective case-control evaluation of COVID-19 infected patients to assess the risk of febrile seizures compared with other causes. Secondary data were collected using the National Institute of Health (NIH) supported National COVID Cohort Collaborative (N3C) database, which is powered by the Palantir platform. The N3C is a partnership among 70 different healthcare institutions across the United States of America that have agreed to share and disseminate clinical data related to COVID-19. Data are obtained from outpatient, emergency department, and inpatient settings. Currently, there are 6.1 million COVID-19–positive patients registered within the database with over 18 billion rows of data available, which include, but are not limited to, laboratory data, vital signs, diagnoses, and mortality. 6 The project protocol was submitted to our institutional review board. It was deemed not to be human subject research and did not require further review as the data set was deidentified. Our funding was only used to expedite our statistical analysis.
Study Setting, Population, and Protocol
The study population was designed within the enclave, the N3C's individualized secure platform, using SQL coding language. All pediatric patients between the ages of 6 and 60 months with a COVID-19 test performed (both rapid and PCR) from July 1, 2020, to December 31, 2021, were initially included in the study. If they had a past medical history of any of the following conditions they were excluded: epilepsy, cerebral palsy, CNS infection, ventricular shunt, intracranial mass. Concept sets to standardize working definitions and diagnoses were created within ATLAS. These were created for all the exclusion criteria and the diagnosis of febrile seizures (includes both simple and complex). 7
A stratified matching design using gender and date was employed before statistical analysis. Any patients without a gender listed were excluded. After patients were divided into gender groups, those who tested positive for COVID-19 were cases and the date of their first positive test was designated as the case index date. Only the initial positive test was used in the analysis as each patient may have had multiple tests over the study period. Similarly, patients who tested negative for COVID-19 within the same timeframe (ie, same month and year) were controls, and the date of their first negative test was designated as the control index date. Only the initial index date was used to produce one data point per patient.
Outcome Measures
Diagnosis of febrile seizure was considered to be associated with either the case or control if it occurred 2 days before or 2 days after the index date. This was the primary outcome and was included in the final analysis.
Data Analysis
Within the above strata, each case was randomly matched to three controls (1:3 ratio). After gender and date matching were completed, the data were subjected to a multivariate logistic regression accounting for age and race. Age was viewed as a continuous variable. We did examine age separately as a categorical value, but it did not change the outcome. Thus, it was not included. The regression resulted in an odds ratio for febrile seizures and COVID-19. Furthermore, baseline characteristics were recorded. Febrile seizure types were also compared between COVID-19–positive and –negative patients.
Between July 1, 2020 and December 31 st 2021, 74,052 patients met both inclusion and exclusion criteria. It is important to note that these are not unique patients as some had multiple COVID test results over the time period. 133 patients were excluded as no gender was identified. Patients were then split into month-year groups for matching based on case and control index dates. Ultimately, there were 39 separate month-year and gender groupings.
After matching based on gender and index dates was performed in a 1:3 ratio between COVID-positive and -negative patients and subsequent encounters were removed, 27,692 unique patients were identified and included in the final analysis (Fig. (Fig.1). 1 ). Of these, 12,632 (45.6%) were female and 15,060 (54.4%) were male. The average age of the patients in the study population was 28.4 months (Table (Table1 1 ).

Stratified matching design by gender and COVID test result (month-year).
Baseline Demographic Information About the Population
Of the study population, 759 unique patients were diagnosed with febrile seizures. Notably, while a patient could have had multiple febrile seizure ICD-10 codes during the study period, only the initial index date was accounted for in the analysis. In some cases, there were multiple codes for febrile seizures during the 48-hour window around the initial index date. The 759 unique patients accounted for 836 febrile seizure diagnosis codes. One hundred eighty-nine of the 6932 COVID-19–positive patients had a febrile seizure within the window (2.7%), compared with 570 of the 20,769 COVID-19–negative patients diagnosed with febrile seizure (2.7%). Among COVID-19–positive patients, 83.2% were diagnosed with simple febrile seizure and 16.8% with complex febrile seizure. In comparison, 80% of COVID-19–negative patients were diagnosed with simple febrile seizure and 20% with complex febrile seizure.
For the logistic regression, age was viewed as a continuous variable. The odds ratio for febrile seizures based on age in one-month increments was 0.984 ( P -value <0.001; CI 0.979, 0.989). African Americans and patients with race identified as “other” were both more likely to be diagnosed with febrile seizures than their White counterparts (OR 1.31, P -value 0.004 [CI 1.09, 1.55] and OR 1.47, P -value 0.003 [CI 1.14, 1.90] respectively). This is independent of COVID test result. The odds ratio for febrile seizures in patients testing positive for COVID was 0.96 ( P -value 0.949 [CI 0.81, 1.14]) (Table (Table2 2 ).
Logistic Regression Using Age as a Continuous Variable
The main objective of this multicenter retrospective case control study was to determine if there is an increased association between COVID-19 and diagnosis of febrile seizures versus other common causes of febrile seizures. Based on the logistic regression model, there was no statistically significant association. Thus, it can be inferred that patients with COVID-19 are as likely to be diagnosed with febrile seizures as patients diagnosed with other common respiratory illnesses. It is important to note that the controls in this study are patients that tested negative for COVID-19; based on this design, control patients could have had one of many diagnoses, both viral and bacterial, as the underlying etiology of their febrile seizures. In addition, the controls patients could have simply been screened for COVID-19 and not had active febrile illness. Interestingly, it was found that 189 of the 6,932 COVID-19–positive patients (approximately 2.7%) had febrile seizure within the 48-hour window. In addition, 83% of COVID-19–positive patients had simple febrile seizures.
Both percentages are higher compared with a similar retrospective study, which reported only 0.5% of COVID-19–positive patients being diagnosed with febrile seizures within a three-day window; 68% of those cases were simple febrile seizures. The sample size of COVID-19–positive patients is similar in both studies. 5 We were unable to evaluate co-infection in our study. For that reason, the true incidence of febrile seizures solely secondary to COVID-19 patients may be somewhat lower. Referring to the prospective EFES study from 2016 in Turkey, coronavirus OC43 and 229 both were found to be associated with a higher proportion of complex febrile seizures. 2 It is important to mention that, compared with both of the previous studies, selection bias was a key limitation in our study design as only patients tested for COVID-19 were included as cases or controls. This is directly related to the design of the N3C database. However, as expected because of the pandemic, there was a high prevalence of COVID-19 testing during the study, which in turn, reduces the impact of selection bias. As for the distribution of simple versus complex febrile seizures in our study, it is possible that simple febrile seizure cases are overrepresented. Since the 48-hour window is based on COVID-19 test date and time, if a seizure occurs near the end of the window, it may only be recorded as simple. However, it is possible that another seizure occurred within 24 hours of the initial occurrence, which would then qualify as a complex febrile seizure and not have been appropriately accounted for.
Even though there was no increased association seen between COVID-19 and febrile seizure diagnoses, there are some other findings worth noting. Referring to Table Table2, 2 , African Americans and patients categorized in the “other” racial group are more likely to be diagnosed with febrile seizure compared with their White counterparts, with odds ratios of 1.31 and 1.47, respectively. This contradicts findings from previous studies, where there was no difference among racial groups in hospitalized patients diagnosed with febrile seizures. 8 , 9 Though the reason for this disparity is unclear, it can be speculated that race may play an underlying role in affecting already established risk factors for febrile seizures, such as genetic predisposition. Alternatively, patients within this cohort may have been incorrectly diagnosed due to implicit bias.
Limitations
There are several limitations to the study, most of which are attributable to the inherent aspects of a retrospective study. Mainly, there are multiple confounders that cannot be controlled for. In addition, the N3C is a novel and constantly updating database. First, medical histories were only included in the Enclave if they were updated after January 2018. Thus, if a patient had a diagnosis before that date that had not been updated in their electronic medical record, it potentially could have been omitted. In addition, we were unable to consider the timing of a patient's medical condition in relation to COVID-19 testing or diagnosis of febrile seizures. For example, if a patient was diagnosed with a brain malignancy months or years after having COVID-19 with a febrile seizure diagnosis, he/she would have been erroneously excluded from the analysis. Second, due to confidentiality purposes, the ages of patients within the N3C database were randomly shifted by up to 6 months at the time of data collection. We chose patients aged 6 months to 5.5 years, which would have theoretically captured any patient from age 0 months to 6 years of age. Lastly, the Palantir platform did not recognize any medical terminology from the ICD03 classification system at the time of data analysis; due to this limitation, 1700+ concepts were dropped from the intracranial mass exclusion criteria concept set created on ATLAS when transferred to Palantir. Some examples of supported classification systems include SNOMED and Nebraska Lexicon. N3C support staff acknowledged the two previous limitations and are expected to remedy these soon.
CONCLUSIONS
The results of this study demonstrate that there is an association between COVID-19 and febrile seizures; 2.7% of COVID-19–positive patients had febrile seizures within a 48-hour window. However, when subjected to a gender-matched case control design with logistic regression to control for confounding variables, there does not appear to be an increased risk of febrile seizures secondary to COVID-19 when compared with other common causes of febrile seizures. The data gathered from this study can assist emergency department providers with evaluation of febrile seizures and the guidance given to families of COVID-19–positive patients. To further assess the risk of COVID-19 causing febrile seizures as compared with other causes a multicenter and prospective study is needed.
Disclosure: The authors declare no conflict of interest.
Author Contributions: S.H. and D.S. were involved in acquisition of the data. S.H., D.S., J.S., and S.T. were involved in study design, interpreting data, and drafting the article. J.S. and S.T. were involved in critical revision of the article. Z.Y. was involved in design and statistical expertise. Z.Y. approves the methodology and provided a statistical review.
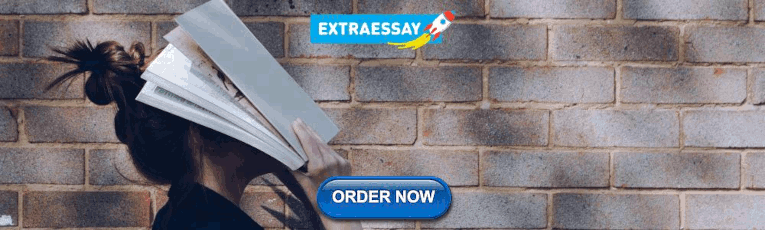
IMAGES
VIDEO
COMMENTS
Many clinical conundrums in need of attention by researchers were discussed in a recent opinion paper in which the following topics were deemed ripe for neuroscientific investigation: the roles of genes versus acquired factors in seizure predisposition; how epilepsy develops in an otherwise normal brain and how to prevent the consequences of ...
Seizures affect the lives of 10% of the global population and result in epilepsy in 1 to 2% of people around the world. Current knowledge about etiology, diagnosis, and treatments for epilepsy is constantly evolving. ... It is also essential for research purposes to have proper definitions, so that appropriate populations can be identified and ...
Clinical and experimental research papers adopting fresh conceptual approaches to the study of epilepsy and its treatment are encouraged. The overriding criteria for publication are novelty, significant clinical or experimental relevance, and interest to a multidisciplinary audience in the broad arena of epilepsy.
The content published in Cureus is the result of clinical experience and/or research by independent individuals or organizations. ... Operational classification of seizure types by the International League Against Epilepsy: position paper of the ILAE Commission for Classification and Terminology. Fisher RS, Cross JH, French JA, et al. Epilepsia ...
In recent years, a lot of research has been done in the epileptic seizures detection field using artificial intelligence methods [167,168,169,170,171,172,173,174,175]. In this paper, a comprehensive review of works done in the field of epileptic seizure detection using various DL techniques such as CNNs, RNNs, and AEs is presented.
This paper discusses state-of-the-art techniques for (1) capturing the physiology signals of seizures, (2) detecting and classifying types of seizures, (3) seizures therapy, and (4) the challenges and potential seizure-related research directions.
In 2022, epilepsy research has made advances across a range of clinically important areas, from self-management, genetics, imaging, and surgical planning to understanding febrile seizures and coma-related periodic patterns. Most notably, in May 2022, the World Health Assembly adopted the Intersectoral Global Action Plan on Epilepsy and Other Neurological Disorders, which aims to address gaps ...
In this paper, we will review the research progress of seizure prediction methods based on scalp EEG and iEEG respectively. By observing clinical symptoms and analyzing the EEG signals of epilepsy patients, the EEG signals can be classified into four periods: ictal, pre-ictal, inter-ictal, and post-ictal (Usman et al. 2019c). The ictal period ...
This paper reviews the epilepsy mentality disorder and the types of seizure, preprocessing operations that are performed on EEG data, a generally extracted feature from the signal, and a detailed view on classification procedures used in this problem and provide insights on the difficulties and future research directions in this innovative theme.
2005 — Volumes 63-67. Page 1 of 2. Incorporating Journal of Epilepsy; Read the latest articles of Epilepsy Research at ScienceDirect.com, Elsevier's leading platform of peer-reviewed scholarly literature.
Fisher, R. S. et al. Operational classification of seizure types by the International League Against Epilepsy: position paper of the ILAE Commission for Classification and Terminology. Epilepsia ...
Epilepsia. Epilepsia is the leading, authoritative journal for innovative clinical and basic science research for all aspects of epilepsy and seizures. In addition, Epilepsia publishes critical reviews, opinion pieces, and guidelines that foster understanding and aim to improve the diagnosis and treatment of people with seizures and epilepsy.
1.2 Main contributions of the paper. In brief, the contributions of this paper are as follows: 1. We have done the review according to five main dimensions. First, researchers who adopted the EEG, ECoG or both for seizure detection; second, significant features; third, machine learning classifiers; fourth, the performance of the classifier during a seizure, and last, knowledge discovery (e.g ...
Epilepsy is a chronic disease of the brain characterized by an enduring (i.e., persisting) predisposition to generate seizures, unprovoked by any immediate central nervous system insult, and by the neurobiologic, cognitive, psychological, and social consequences of seizure recurrences. Epilepsy affects both sexes and all ages with worldwide ...
RSS Feed. Epilepsy refers to a group of neurological disorders of varying aetiology, characterized by recurrent brain dysfunction that result from sudden excessive and disordered neuronal ...
In early 2021, the Epilepsy Foundation conducted an updated survey among those living with epilepsies and/or their caregivers to better understand the use-cases that best suit the needs of our community as seizure forecast research advances. These results will provide researchers with insight into user-acceptance of using a forecasting tool and ...
Seizure - European Journal of Epilepsy is an international journal owned by Epilepsy Action (the largest member led epilepsy organisation in the UK). It provides a forum for papers on all topics related to epilepsy and seizure disorders. Seizure focuses especially on clinical and psychosocial aspects, but will publish papers on the basic sciences related to the condition itself, the ...
Epilepsy is a life-threatening neurological brain disorder that gives rise to recurrent unprovoked seizures. It occurs due to abnormal chemical changes in our brains. For many years, studies have been conducted to support the automatic diagnosis of epileptic seizures for clinicians' ease. For that, several studies entail machine learning methods for early predicting epileptic seizures.
This paper reviews advances in epilepsy in recent years with an emphasis on therapeutics and underlying mechanisms, including status epilepticus, drug and surgical treatments. Lessons from rarer ...
Abstract. Epilepsy is commonly known as seizure-associated medical conditions either morbid or comorbid altogether. Although many different procedures were determined for diagnosing and treating ...
These methods have significantly advanced several fields of medicine, including the diagnosis of epilepsy. In this paper, the works focused on automated epileptic seizure detection using ML and DL techniques are presented as well as their comparative analysis is done. ... Also, the designed system matches the research gaps in this area by ...
Introduction. Epilepsy is the enduring predisposition of the brain to generate seizures, a condition that carries neurobiological, cognitive, psychological, and social consequences ().Over 50 million people worldwide are affected by epilepsy and its causes remain partially elusive, leaving physicians, and patients an unclear insight into the etiology of the disease and the best treatment ...
Introduction. Epilepsy is a chronic disease of the brain defined as "an enduring predisposition to generate epileptic seizures, and by the neurobiologic, cognitive, psychological, and social consequences of this condition" ().It is a common neurological disease, with a calculated incidence rate of 61.4 per 100,000 person-years and a prevalence of 7.60 per 1,000 population (), it affects ...
Credit: Google Research & Lichtman Lab (Harvard University). Renderings by D. Berger (Harvard University) Researchers have mapped a tiny piece of the human brain in astonishing detail.
Neonatal seizures are a frequent clinical manifestation in the neonatal period and they can present within the framework of four clinical scenarios, each with a distinct post-neonatal evolution (Fig. 1).In scenarios A, C, and D, neonatal seizures correspond to acute symptomatic seizures that may be self-limiting (A), initially stop but eventually evolve after a latent period in post-neonatal ...
One hundred eighty-nine of the 6932 COVID-19-positive patients had a febrile seizure within the window (2.7%), compared with 570 of the 20,769 COVID-19-negative patients diagnosed with febrile seizure (2.7%). Among COVID-19-positive patients, 83.2% were diagnosed with simple febrile seizure and 16.8% with complex febrile seizure.