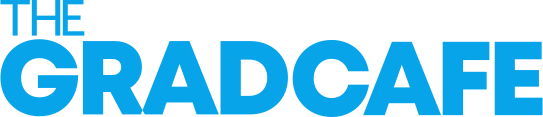
- Remember me Not recommended on shared computers
Forgot your password?
- Mathematics and Statistics
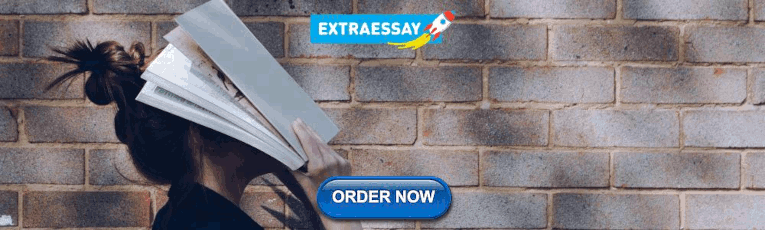
2023 Applicant Profiles and Admission Results for Statistics/Biostatistics
By abm495 April 1, 2023 in Mathematics and Statistics
Recommended Posts
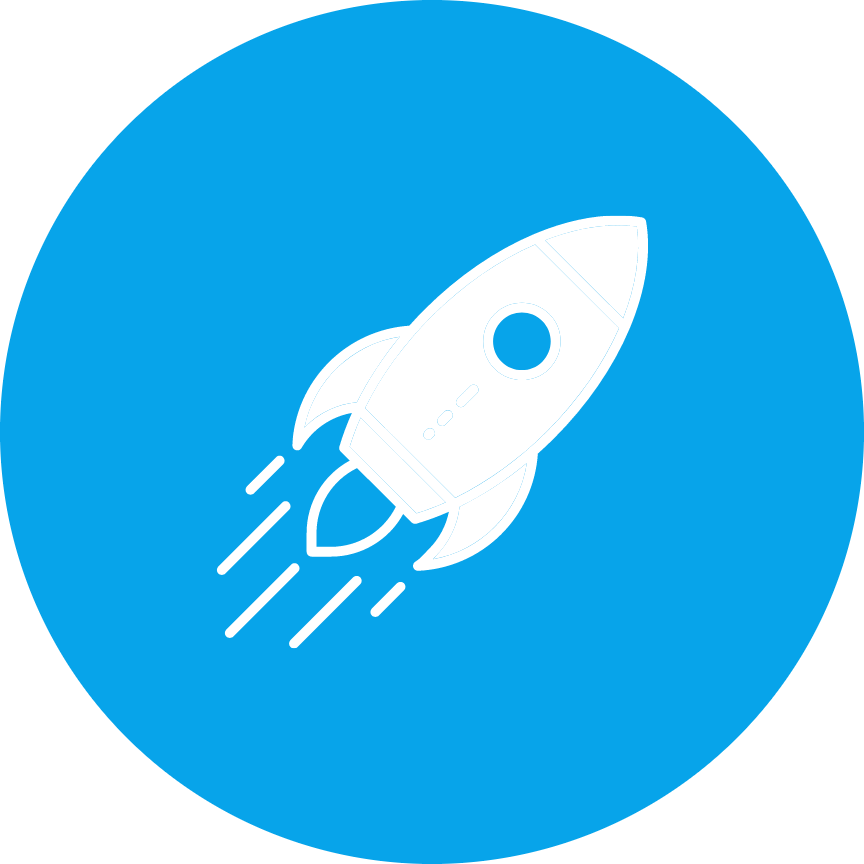
- prgp , Counterfactual , FunctionalAnalysis and 2 others
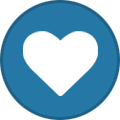
Link to comment
Share on other sites.
- 2 years of University Sponsored REU in Bayesian Statistics.
- 1 year of research during gap year with well known organization in Spatial Statistics.
- Two University Sponsored projects involving regression analysis
- Published one first-author paper at a relatively unknown conference. Second paper in writing.
- Did a poster presentation at local conference.
- Valedictorian + Summa Cum Laude
- Outstanding Research Award + Extra Funding
- Math Honor Society
- 1 Very Strong - From my research advisor. Known very well in the engineering field. 3+ years
- 1 Good - Professor I took two classes with and organized study groups for.
- 1 Decent - Professor I took Mathematical Statistics II with and received a 100% in the course. Also was aware of my extenuating circumstances.
- dirichletprior
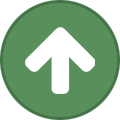
aspiringsadistician
Undergrad Institution: Medium State School, Top 200 US News Major(s): Math Minor(s): GPA: ~3.87
Type of Student: Domestic White Male GRE General Test: Q: 166 (84%) V: 158 (78%) W: 4.0 (54%)
Programs Applying: Stats PhD Programs
Research Experience: Undergrad thesis in Pure Math, along with a research experience in algebra with a professor at my school. No published papers
Letters of Recommendation:
LOR 1: Thesis Advisor, took some grad courses with them as well.
LOR 2: Did well in a graduate course with them.
LOR 3: I took the algebra research experience with them.
Overall these Professors could speak strongly about my mathematical abilities.
Math/Statistics Grades: Mostly A’s with some A-‘s and a single B. The most relevant are:
7 Grad courses including Analysis I/II and Functional Analysis.
8 independent study courses in pure math.
Probability Theory, Quantum Mechanics, Calc I – III, Linear Optimization, Adv calc I/II.
Any Miscellaneous Points that Might Help: My school’s Honors program allowed me to take basically only math courses. So that's what I did. Applying to Where: (Color use here is welcome) Iowa State - Admitted + Fellowship - Accepted!
University of Florida - Admitted
Florida State University – Admitted
University of Connecticut - Admitted
University of Pittsburgh - Admitted
University of South Carolina - Admitted + Fellowship
Virginia Tech – Admitted
North Carolina State – Waitlist
University of Virginia - Waitlist
Texas A&M – No response
Ohio State – No response
Rutgers – No response
George Washington University – No response
University of Iowa – No response
Colorado State University – No response
Reflection:
I applied late in the cycle (~Jan 10) and missed the deadline for most top 20 programs. I suspect I would have had good results if I had applied to these programs. That said, I’m very happy with Iowa State.
On 4/4/2023 at 1:31 PM, prgp said: Undergrad Institution: Unknown State School (100% admittance rate) Major(s): Applied Mathematics (Essentially Applied Statistics) Minor(s): GPA: 3.25/4 😰 Type of Student: Domestic Hispanic Male GRE General Test: Taken: 08/2019 (Refused to retake for Ph.D. applications) Q: 149 (38%) V: 158 (61%) W: 3.0 (13%) GRE Subject Test in Mathematics: M : NA Grad Institution: Large State School USWN (50-100) Concentration: Statistics GPA: 3.95/4 😏 Programs Applying: Statistics Ph.D. (Wide variety of interests) Research Experience: Undergraduate Research and presented at 3 conferences/symposiums. Graduate thesis research in soft statistics for machine learning(publishable) and will develop an R package for it (Summer), presented at 2 conferences. Graduate research in biology mathematics for stochastic simulation presented at local conference. (Technically forgot to add this to resume, but in my math stat 1 course, we developed a workflow for the 4-parameter uniform (location-scale family) that should be getting published eventually) Awards/Honors/Recognitions: Fully funded MS student. Pertinent Activities or Jobs: x2 TA for high level undergraduate probability course (rice textbook), Recitation leader for Intro to Stats with R course. Graduate Instructor for College Prep, Trigonometry, and Intro to Stats. Letters of Recommendation: Recommendations should be good, they are from Advisor (Assistant Department Head), Faculty who I did the bioMath research with (Associate Department Head), Math Stat I Instructor (A in class). Math/Statistics Grades: Undergraduate: Typical core 5 for undergraduate (C's and B's), Stats and Probability courses (B's and A's). Graduate : Applied Spatial, Data Vis, Intro to Mathematical Statistics, R Computation, Bioinformatics, Mathematical Statistics I (Casella and Berger) (A), Geostatistics (A-) Any Miscellaneous Points that Might Help: I did everything that I could to rectify my poor undergraduate gpa, and GRE scores. I believe I accomplished that. UC Berkeley - Statistics Ph.D. Rejected Washington - Statistics Ph.D. Rejected Duke - Statistical Science Ph.D. Rejected North Carolina CH - Statistics and OR Ph.D. Rejected NCSU - Statistics Ph.D. Rejected Rejected UC Davis - Statistics Ph.D. Ghosted/Waitlisted Texas A&M - Statistics Ph.D. Ghosted/Waitlisted UCLA - Statistics Ph.D. Ghosted/Waitlisted Iowa State - Statistics Ph.D. Ghosted/Waitlisted UC Irvine - Statistics Ph.D. Interview 1/20 - unofficial admittance 1/26 - Official Admittance + UC Funding 2/1 - Matriculated 3/31 UT Austin - Statistics and DS Ph.D. Rejected Colorado State - Statistics Ph.D. Rejected Virginia Tech - Statistics Ph.D. Rejected Oregon State - Statistics Ph.D. Ghosted/Waitlisted Home University - Statistics Ph.D. Withdrawn Reflection: I knew going into this that my low undergrad GPA and bad GRE, will potentially overshadow all of the great diverse research that I have done. It was clear on my rejection from Virginia Tech, since my reason for rejection was "non-competitive". Other programs have me waitlisted, officially and unofficially, personally those programs took too long in making a decision. Regardless of the outcomes from the remaining programs, I plan on attending UCI. They have something really special cooking out there, and I am excited to be apart of that. Update: Rejects from CSU and UNC, It appears that UG GPA is weighted importantly.
Very happy with these results! I got an early acceptance to Pitt because of rolling admissions so I cut down my application list and only applied to schools that I would choose over that scholarship. I applied to Duke partially because I knew that they gave half tuition scholarships but I didn't expect a 75% scholarship! My math background isn’t the strongest because I decided on biostatistics after finishing undergrad. I took Calc 1-3 and linear algebra through community college night classes. This might have been a negative for some schools but it worked out fine for me in the end.
- 3 weeks later...
Counterfactual
Undergrad Institution: University locally known for business & social science in Taiwan (ranked around 200 globally in business in USNR) Major(s): Double Major in Finance and Statistics Minor(s): N/A GPA: 4.29/4.3 (4.0/4.0 after WES evaluation) Relevant courses: Calculus (A+/A+), Linear Algebra I/II (A+/A+), Mathematical Statistics I/II (A+/A+), Regression Analysis I (A+), Multivariate Analysis (A+), Programming 101 (A+, in Python), Programming and Statistical Software (A+, in R)
Exchange program institute: UC Berkeley (one-year international exchange program) GPA: 3.95/4.0 Relevant courses: Analysis I/II (A/A-), Statistical Prediction and ML (A), Causal Inference (A), Grad Experimental Design (A), Grad Intro to Stat Computing (A)
Type of Student: International (Asian) GRE General Test: Q: 166 (84%) V: 157 (74%) W: 4.0 (54%) GRE Subject Test in Mathematics: N/A TOEFL Score: R30/L28/S25/W28
Programs Applying: A mix of Statistics/Biostatistics Masters and PhD programs
Research Interests: Causal Inference, Network Analysis
Research Experience: 1 conference paper in community detection. I worked as a student researcher at a statistical institute in Taiwan, main theme of the research is community detection algorithms. Awards/Honors/Recognitions: Presidential Award x6, Study abroad scholarship, Research Scholarship Pertinent Activities or Jobs: N/A, nothing beyond my undergraduate research
Letters of Recommendation: 4 letters from instructors at Berkeley (Likely the typical did-well in class letter), 1 from my undergraduate research supervisor (strong) Any Miscellaneous Points that Might Help: The letter from my undergraduate research supervisor should be very strong, he said he would love to have me as his PhD student, and I think that definitely helped tremendously!! Applying to Where: (Color use here is welcome) Ohio State University Biostatistics PhD - admitted 1/26 with official letter received 3/1 Iowa State University Statistics PhD - admitted 1/31 University of Michigan, Ann Arbor Biostatistics PhD - admitted 2/9 - Attending!! University of Florida Statistics PhD - admitted 3/7 UC Davis Statistics PhD - interviewed on 2/15, admitted 2/25 University of Minnesota, Twin City - MS Biostatistics - admitted w/o funding 2/4 (rejected from PhD) Harvard University SM 80 Biostatistics - admitted w/o funding 2/10 UW - Seattle Biostatistics MS Capstone - admitted w/o funding 2/14 UC Berkeley Statistics MA - rejected 3/11 UW Seattle Statistics MS - rejected 3/27 UT Austin Statistics PhD - rejected 3/27 University of Michigan, Ann Arbor Statistics PhD - rejected 3/28 UW Madison Statistics PhD - rejected 3/29 UC Davis Biostatistics PhD - rejected 4/6 Penn State University Statistics PhD - rejected 4/11 Purdue University Statistics PhD - rejected 4/11 University of Minnesota, Twin City Statistics PhD - rejected 4/14 Ohio State University Statistics PhD - rejected 4/18 Texas A&M Statistics PhD - rejected 4/29 NC State Statistics PhD - rejected 4/20 UCLA Statistics PhD - rejected 5/19
Thoughts and Thanks: I feel super blessed this applications cycle and am so very grateful for the admission results. I know for a fact that none of the above are safety schools, and though out of the 21 schools I applied to, most resulted in a straight rejection (didn't even make it to the edge of the waitlist). At the end of the day, I got into many schools I never thought I would've gotten into. I didn't have a strong math background compared to most of the international applicants, nor do I have fabulous GRE scores (my quant section was a mess). I didn't have a 3-stack strong letter that are all from reputed professors (my letters were a mix of did well in class letters from Berkeley and one strong letter from the professor whom I did research with in Taiwan). Other than a great undergrad GPA, I think the SOP carried more weight than I thought it would. And submitting a large number of applications to schools with matching interests was also definitely helpful, though hindsight 20/20. I've been following the forum ever since I was a freshmen in college, and I'm glad I finally made it to a PhD program!! Special thanks to @bayessays @Stat Assistant Professor @cyberwulf @trynagetby and many others! Your career and application advice helped me tremendously in terms of career planning and in the application process!! I know there's a long way to go, but I am glad I can be making contributions to the scientific community through developing better estimation methods and help create better decision criteria via math and data! Can't wait to start the Fall semester!
- bayessays and Ryuk
- 2 weeks later...
dominatedconvergence

explodingpineapple
- 1 month later...
gradcafereader103
Create an account or sign in to comment.
You need to be a member in order to leave a comment
Create an account
Sign up for a new account in our community. It's easy!
Already have an account? Sign in here.
- Existing user? Sign In
- Online Users
- All Activity
- My Activity Streams
- Unread Content
- Content I Started
- Results Search
- Post Results
- Leaderboard
- Create New...
Important Information
This website uses cookies to ensure you get the best experience on our website. See our Privacy Policy and Terms of Use
- Skip to Content
- Berkeley Academic Guide Home
- Institution Home
Berkeley Berkeley Academic Guide: Academic Guide 2023-24

The Department of Statistics grants BA, MA, and PhD degrees in Statistics. The undergraduate and graduate programs allow students to participate in a field that is growing in importance and breadth of application. Understanding the natural and human worlds in the Information Age increasingly requires statistical reasoning and methods, and stochastic models are essential components of research and applications across a vast spectrum of fields. The Department of Statistics provides students with world-class resources for study and research, including access to the extensive computational facilities maintained by the Statistical Computing Facility.
Facilities and Resources
The Statistical Computing Facility (SCF) is a unit of the Department of Statistics. Its mission is to provide undergraduate students, graduate students, postdocs, and faculty in the Statistics Department at Berkeley with state-of-the-art computing resources, services, and technical knowledge; supporting them in carrying out cutting-edge research activities, innovative instructional programs, and efficient day-to-day computing activities. The SCF also supports the students and faculty of the Econometrics Laboratory of the Department of Economics.
The Department of Statistics operates a consulting service in which advanced graduate students, under faculty supervision, are available as consultants during specified hours. The service is associated with the course, which may be taken for credit. Consulting is free to members of the campus community. Statistical advice can be sought at any stage of the research process. Those seeking statistical advice are encouraged to contact consultants early in the research process. Refer to the Department of Statistics website to find out which faculty member is currently coordinating this service.
Three seminars regularly take place in the department: the Neyman seminar , the probability seminar , and the statistics and genomics seminar . Each year, the department also has two joint seminars with Stanford and a joint seminar with UC Davis.
Undergraduate Programs
Statistics : BA, Minor
Graduate Programs
Statistics : MA, PhD
Visit Department Website
STAT 0PX Preparatory Statistics 1 Unit
Terms offered: Summer 2016 10 Week Session, Summer 2015 10 Week Session, Summer 2014 10 Week Session This course assists entering Freshman students with basic statistical concepts and problem solving. Designed for students who do not meet the prerequisites for 2. Offered through the Student Learning Center. Preparatory Statistics: Read More [+]
Rules & Requirements
Prerequisites: Consent of instructor
Hours & Format
Summer: 6 weeks - 5 hours of lecture and 4.5 hours of workshop per week 8 weeks - 5 hours of lecture and 4.5 hours of workshop per week
Additional Format: Five hours of Lecture and Four and one-half hours of Workshop per week for 8 weeks. Five hours of Lecture and Four and one-half hours of Workshop per week for 6 weeks.
Additional Details
Subject/Course Level: Statistics/Undergraduate
Grading/Final exam status: Offered for pass/not pass grade only. Final exam required.
Instructor: Purves
Preparatory Statistics: Read Less [-]
STAT 2 Introduction to Statistics 4 Units
Terms offered: Fall 2024, Summer 2024 8 Week Session, Spring 2024 Population and variables. Standard measures of location, spread and association. Normal approximation. Regression. Probability and sampling. Interval estimation. Some standard significance tests. Introduction to Statistics: Read More [+]
Credit Restrictions: Students will receive no credit for STAT 2 after completing STAT W21 , STAT 20 , STAT 21 , STAT 25, STAT S2, STAT 21X, STAT N21, STAT 5, or STAT 2X.
Fall and/or spring: 15 weeks - 3 hours of lecture and 2 hours of laboratory per week
Summer: 6 weeks - 7.5 hours of lecture and 5 hours of laboratory per week 8 weeks - 5 hours of lecture and 4 hours of laboratory per week
Additional Format: Three hours of lecture and two hours of laboratory per week. Five hours of lecture and four hours of laboratory per week for 8 weeks. Seven and one-half hours of lecture and five hours of laboratory per week for 6 weeks.
Grading/Final exam status: Letter grade. Final exam required.
Introduction to Statistics: Read Less [-]
STAT C8 Foundations of Data Science 4 Units
Terms offered: Fall 2024, Summer 2024 8 Week Session, Spring 2024, Fall 2023, Spring 2023, Fall 2022, Spring 2022, Fall 2021, Summer 2021 8 Week Session, Fall 2020 Foundations of data science from three perspectives: inferential thinking, computational thinking, and real-world relevance. Given data arising from some real-world phenomenon, how does one analyze that data so as to understand that phenomenon? The course teaches critical concepts and skills in computer programming and statistical inference, in conjunction with hands-on analysis of real-world datasets, including economic data, document collections, geographical data, and social networks. It delves into social and legal issues surrounding data analysis, including issues of privacy and data ownership. Foundations of Data Science: Read More [+]
Prerequisites: This course may be taken on its own, but students are encouraged to take it concurrently with a data science connector course (numbered 88 in a range of departments)
Credit Restrictions: Students will receive no credit for DATA C8 \ COMPSCI C8 \ INFO C8 \ STAT C8 after completing COMPSCI 8, or DATA 8. A deficient grade in DATA C8 \ COMPSCI C8 \ INFO C8 \ STAT C8 may be removed by taking COMPSCI 8, COMPSCI 8, or DATA 8.
Summer: 8 weeks - 6 hours of lecture and 4 hours of laboratory per week
Additional Format: Three hours of lecture and two hours of laboratory per week. Six hours of lecture and four hours of laboratory per week for 8 weeks.
Formerly known as: Computer Science C8/Statistics C8/Information C8
Also listed as: COMPSCI C8/DATA C8/INFO C8
Foundations of Data Science: Read Less [-]
STAT 20 Introduction to Probability and Statistics 4 Units
Terms offered: Fall 2024, Summer 2024 8 Week Session, Spring 2024 For students with mathematical background who wish to acquire basic concepts. Relative frequencies, discrete probability, random variables, expectation. Testing hypotheses. Estimation. Illustrations from various fields. Introduction to Probability and Statistics: Read More [+]
Prerequisites: Mathematics 1A, Mathematics 16A, Mathematics 10A/10B, or consent of instructor.,One semester of calculus
Credit Restrictions: Students will receive no credit for STAT 20 after completing STAT W21 , STAT 2 , STAT 5, STAT 21 , STAT N21, STAT 2X, STAT S20, STAT 21X, or STAT 25. A deficient grade in STAT 20 may be removed by taking STAT W21 , STAT 21 , or STAT N21.,Students who have taken 2, 2X, 5, 21, 21X, or 25 will receive no credit for 20.
Summer: 8 weeks - 6 hours of lecture and 3 hours of laboratory per week
Additional Format: Three hours of lecture and two hours of laboratory per week. Six hours of lecture and three hours of laboratory per week for 8 weeks.,Three hours of Lecture and Two hours of Laboratory per week for 15 weeks. Six hours of Lecture and Three hours of Laboratory per week for 8 weeks.
Introduction to Probability and Statistics: Read Less [-]
STAT 21 Introductory Probability and Statistics for Business 4 Units
Terms offered: Summer 2024 8 Week Session, Summer 2023 8 Week Session, Summer 2022 8 Week Session Descriptive statistics, probability models and related concepts, sample surveys, estimates, confidence intervals, tests of significance, controlled experiments vs. observational studies, correlation and regression. Introductory Probability and Statistics for Business: Read More [+]
Prerequisites: One semester of calculus
Credit Restrictions: Students will receive no credit for STAT 21 after completing STAT 20 , STAT W21 , STAT 25, STAT 2X, STAT 21X, STAT S21, STAT 5, STAT 2 , or STAT N21. A deficient grade in STAT 21 may be removed by taking STAT 20 , STAT W21 , or STAT N21.
Fall and/or spring: 15 weeks - 3 hours of lecture per week
Summer: 8 weeks - 7.5 hours of lecture per week
Additional Format: Three hours of lecture per week. Seven and one-half hours of lecture per week for 8 weeks.
Introductory Probability and Statistics for Business: Read Less [-]
STAT W21 Introductory Probability and Statistics for Business 4 Units
Terms offered: Summer 2021 8 Week Session, Summer 2020 8 Week Session, Summer 2019 8 Week Session Reasoning and fallacies, descriptive statistics, probability models and related concepts, combinatorics, sample surveys, estimates, confidence intervals, tests of significance, controlled experiments vs. observational studies, correlation and regression. Introductory Probability and Statistics for Business: Read More [+]
Credit Restrictions: Students will receive no credit for Statistics W21 after completing Statistics 2, 20, 21, N21 or 25. A deficient grade in Statistics 21, N21 maybe removed by taking Statistics W21.
Fall and/or spring: 15 weeks - 3 hours of web-based lecture per week
Summer: 8 weeks - 7.5 hours of web-based lecture per week
Additional Format: Three hours of Web-based lecture per week for 15 weeks. Seven and one-half hours of Web-based lecture per week for 8 weeks.
Online: This is an online course.
Formerly known as: N21
STAT 24 Freshman Seminars 1 Unit
Terms offered: Spring 2021, Fall 2016, Fall 2003 The Berkeley Seminar Program has been designed to provide new students with the opportunity to explore an intellectual topic with a faculty member in a small-seminar setting. Berkeley seminars are offered in all campus departments, and topics vary from department to department and semester to semester. Enrollment limited to 15 freshmen. Freshman Seminars: Read More [+]
Repeat rules: Course may be repeated for credit when topic changes.
Fall and/or spring: 15 weeks - 1 hour of seminar per week
Additional Format: One hour of Seminar per week for 15 weeks.
Grading/Final exam status: The grading option will be decided by the instructor when the class is offered. Final exam required.
Freshman Seminars: Read Less [-]
STAT 33A Introduction to Programming in R 1 Unit
Terms offered: Spring 2024, Fall 2023, Spring 2023 An introduction to the R statistical software for students with minimal prior experience with programming. This course prepares students for data analysis with R. The focus is on the computational model that underlies the R language with the goal of providing a foundation for coding. Topics include data types and structures, such as vectors, data frames and lists; the REPL evaluation model; function calls, argument matching, and environments; writing simple functions and control flow. Tools for reading, analyzing, and plotting data are covered, such as data input/output, reshaping data, the formula language, and graphics models. Introduction to Programming in R: Read More [+]
Credit Restrictions: Students will receive no credit for STAT 33A after completing STAT 33B , or STAT 133 . A deficient grade in STAT 33A may be removed by taking STAT 33B , or STAT 133 .
Fall and/or spring: 15 weeks - 1 hour of lecture and 1 hour of laboratory per week
Summer: 6 weeks - 2 hours of lecture and 3 hours of laboratory per week
Additional Format: One hour of lecture and one hour of laboratory per week. Two hours of lecture and three hours of laboratory per week for 6 weeks.
Introduction to Programming in R: Read Less [-]
STAT 33B Introduction to Advanced Programming in R 1 Unit
Terms offered: Spring 2024, Fall 2023, Spring 2023 The course is designed primarily for those who are already familiar with programming in another language, such as python, and want to understand how R works, and for those who already know the basics of R programming and want to gain a more in-depth understanding of the language in order to improve their coding. The focus is on the underlying paradigms in R, such as functional programming, atomic vectors, complex data structures, environments , and object systems. The goal of this course is to better understand programming principles in general and to write better R code that capitalizes on the language's design. Introduction to Advanced Programming in R: Read More [+]
Prerequisites: Compsci 61A or equivalent programming background
Credit Restrictions: Students will receive no credit for STAT 33B after completing STAT 133 . A deficient grade in STAT 33B may be removed by taking STAT 133 .
Introduction to Advanced Programming in R: Read Less [-]
STAT 39D Freshman/Sophomore Seminar 2 - 4 Units
Terms offered: Fall 2008, Fall 2007 Freshman and sophomore seminars offer lower division students the opportunity to explore an intellectual topic with a faculty member and a group of peers in a small-seminar setting. These seminars are offered in all campus departments; topics vary from department to department and from semester to semester. Freshman/Sophomore Seminar: Read More [+]
Prerequisites: Priority given to freshmen and sophomores
Repeat rules: Course may be repeated for credit without restriction.
Fall and/or spring: 15 weeks - 2-4 hours of seminar per week
Additional Format: Seminar format.
Freshman/Sophomore Seminar: Read Less [-]
STAT C79 Societal Risks and the Law 3 Units
Terms offered: Spring 2013 Defining, perceiving, quantifying and measuring risk; identifying risks and estimating their importance; determining whether laws and regulations can protect us from these risks; examining how well existing laws work and how they could be improved; evaluting costs and benefits. Applications may vary by term. This course cannot be used to complete engineering unit or technical elective requirements for students in the College of Engineering. Societal Risks and the Law: Read More [+]
Fall and/or spring: 15 weeks - 3 hours of lecture and 1 hour of discussion per week
Additional Format: Three hours of Lecture and One hour of Discussion per week for 15 weeks.
Grading/Final exam status: Letter grade. Final exam not required.
Also listed as: COMPSCI C79/POL SCI C79
Societal Risks and the Law: Read Less [-]
STAT C88S Probability and Mathematical Statistics in Data Science 3 Units
Terms offered: Spring 2024, Summer 2023 8 Week Session, Spring 2023, Fall 2022 In this connector course we will state precisely and prove results discovered while exploring data in Data C8. Topics include: probability, conditioning, and independence; random variables; distributions and joint distributions; expectation, variance, tail bounds; Central Limit Theorem; symmetries in random permutations; prior and posterior distributions; probabilistic models; bias-variance tradeoff; testing hypotheses; correlation and the regression model. Probability and Mathematical Statistics in Data Science: Read More [+]
Prerequisites: Prerequisite: one semester of calculus at the level of Math 16A, Math 10A, or Math 1A. Corequisite or Prerequisite: Foundations of Data Science ( COMPSCI C8 / DATA C8 / INFO C8 / STAT C8 )
Credit Restrictions: Students will receive no credit for DATA C88S after completing STAT 134 , STAT 140, STAT 135 , or DATA C102 .
Fall and/or spring: 15 weeks - 3 hours of lecture and 2 hours of discussion per week
Summer: 8 weeks - 6 hours of lecture and 4 hours of discussion per week
Additional Format: Three hours of lecture and two hours of discussion per week. Six hours of lecture and four hours of discussion per week for 8 weeks.
Formerly known as: Statistics 88
Also listed as: DATA C88S
Probability and Mathematical Statistics in Data Science: Read Less [-]
STAT 89A Linear Algebra for Data Science 4 Units
Terms offered: Spring 2022, Spring 2021, Spring 2020 An introduction to linear algebra for data science. The course will cover introductory topics in linear algebra, starting with the basics; discrete probability and how prob- ability can be used to understand high-dimensional vector spaces; matrices and graphs as popular mathematical structures with which to model data (e.g., as models for term-document corpora, high-dimensional regression problems, ranking/classification of web data, adjacency properties of social network data, etc.); and geometric approaches to eigendecompositions, least-squares, principal components analysis, etc. Linear Algebra for Data Science: Read More [+]
Prerequisites: One year of calculus. Prerequisite or corequisite: Foundations of Data Science ( COMPSCI C8 / INFO C8 / STAT C8 )
Additional Format: Three hours of lecture and two hours of laboratory per week.
Linear Algebra for Data Science: Read Less [-]
STAT 94 Special Topics in Probability and Statistics 1 - 4 Units
Terms offered: Fall 2015 Topics will vary semester to semester. Special Topics in Probability and Statistics: Read More [+]
Fall and/or spring: 15 weeks - 1-3 hours of lecture and 0-2 hours of discussion per week
Additional Format: One to three hours of lecture and zero to two hours of discussion per week.
Special Topics in Probability and Statistics: Read Less [-]
STAT 97 Field Study in Statistics 1 - 3 Units
Terms offered: Fall 2015, Spring 2012 Supervised experience relevant to specific aspects of statistics in off-campus settings. Individual and/or group meetings with faculty. Field Study in Statistics: Read More [+]
Fall and/or spring: 15 weeks - 1-3 hours of fieldwork per week
Summer: 6 weeks - 2.5-7.5 hours of fieldwork per week 8 weeks - 1.5-5.5 hours of fieldwork per week
Additional Format: One to three hours of fieldwork per week. One and one-half to five and one-half hours of fieldwork per week for 8 weeks. Two and one-half to seven and one-half hours of fieldwork per week for 6 weeks.
Grading/Final exam status: Offered for pass/not pass grade only. Final exam not required.
Field Study in Statistics: Read Less [-]
STAT 98 Directed Group Study 1 - 3 Units
Terms offered: Fall 2023, Spring 2023, Fall 2022 Must be taken at the same time as either Statistics 2 or 21. This course assists lower division statistics students with structured problem solving, interpretation and making conclusions. Directed Group Study: Read More [+]
Fall and/or spring: 15 weeks - 2-3 hours of directed group study per week
Summer: 8 weeks - 4-6 hours of directed group study per week
Additional Format: Two to three hours of directed group study per week. Four to six hours of directed group study per week for 8 weeks.
Directed Group Study: Read Less [-]
STAT C100 Principles & Techniques of Data Science 4 Units
Terms offered: Fall 2024, Summer 2024 8 Week Session, Spring 2024, Summer 2023 8 Week Session, Fall 2022, Fall 2021, Fall 2020 In this course, students will explore the data science lifecycle, including question formulation, data collection and cleaning, exploratory data analysis and visualization, statistical inference and prediction, and decision-making. This class will focus on quantitative critical thinking and key principles and techniques needed to carry out this cycle. These include languages for transforming, querying and analyzing data; algorithms for machine learning methods including regression, classification and clustering; principles behind creating informative data visualizations; statistical concepts of measurement error and prediction; and techniques for scalable data processing. Principles & Techniques of Data Science: Read More [+]
Prerequisites: COMPSCI C8 / DATA C8 / INFO C8 / STAT C8 with a C- or better, or Pass; and COMPSCI 61A , COMPSCI/DATA C88C, or ENGIN 7 with a C- or better, or Pass; Corequisite: MATH 54 , 56 or EECS 16A (C- or better, or Pass, required if completed prior to Data C100)
Credit Restrictions: Students will receive no credit for DATA C100 \ STAT C100 \ COMPSCI C100 after completing DATA 100. A deficient grade in DATA C100 \ STAT C100 \ COMPSCI C100 may be removed by taking DATA 100.
Fall and/or spring: 15 weeks - 3-3 hours of lecture, 1-1 hours of discussion, and 0-1 hours of laboratory per week
Summer: 8 weeks - 6-6 hours of lecture, 2-2 hours of discussion, and 0-2 hours of laboratory per week
Additional Format: Three hours of lecture and one hour of discussion and zero to one hours of laboratory per week. Six hours of lecture and two hours of discussion and zero to two hours of laboratory per week for 8 weeks.
Instructors: Gonzalez, Nourozi, Perez, Yan
Formerly known as: Statistics C100/Computer Science C100
Also listed as: COMPSCI C100/DATA C100
Principles & Techniques of Data Science: Read Less [-]
STAT C102 Data, Inference, and Decisions 4 Units
Terms offered: Fall 2024, Spring 2024, Fall 2023 This course develops the probabilistic foundations of inference in data science, and builds a comprehensive view of the modeling and decision-making life cycle in data science including its human, social, and ethical implications. Topics include: frequentist and Bayesian decision-making, permutation testing, false discovery rate, probabilistic interpretations of models, Bayesian hierarchical models, basics of experimental design, confidence intervals , causal inference, Thompson sampling, optimal control, Q-learning, differential privacy, clustering algorithms, recommendation systems and an introduction to machine learning tools including decision trees, neural networks and ensemble methods. Data, Inference, and Decisions: Read More [+]
Prerequisites: Math 54 or 56 or 110 or Stat 89A or Physics 89 or both of EECS 16A and 16B with a C- or better, or Pass; Data/Stat/CompSci C100 with a C- or better, or Pass; and any of EECS 126 , Data/Stat C140, Stat 134, IndEng 172, Math 106 with a C- or better, or Pass. Data/Stat C140 or EECS 126 are preferred
Credit Restrictions: Students will receive no credit for DATA C102 after completing STAT 102, or DATA 102. A deficient grade in DATA C102 may be removed by taking STAT 102, STAT 102, or DATA 102.
Fall and/or spring: 15 weeks - 3 hours of lecture, 1 hour of discussion, and 1 hour of laboratory per week
Additional Format: Three hours of lecture and one hour of discussion and one hour of laboratory per week.
Formerly known as: Statistics 102
Also listed as: DATA C102
Data, Inference, and Decisions: Read Less [-]
STAT C131A Statistical Methods for Data Science 4 Units
Terms offered: Fall 2024, Fall 2023, Spring 2023 This course teaches a broad range of statistical methods that are used to solve data problems. Topics include group comparisons and ANOVA, standard parametric statistical models, multivariate data visualization, multiple linear regression, logistic regression and classification, regression trees and random forests. An important focus of the course is on statistical computing and reproducible statistical analysis. The course and lab include hands-on experience in analyzing real world data from the social, life, and physical sciences. The R statistical language is used. Statistical Methods for Data Science: Read More [+]
Prerequisites: Statistics/Computer Science/Information C8 or Statistics 20; and Mathematics 1A, Mathematics 16A, or Mathematics 10A/10B. Strongly recommended corequisite: Statistics 33A or Statistics 133
Formerly known as: Statistics 131A
Also listed as: DATA C131A
Statistical Methods for Data Science: Read Less [-]
STAT 133 Concepts in Computing with Data 3 Units
Terms offered: Fall 2024, Spring 2024, Fall 2023 An introduction to computationally intensive applied statistics. Topics will include organization and use of databases, visualization and graphics, statistical learning and data mining, model validation procedures, and the presentation of results. This course uses R as its primary computing language; details are determined by the instructor. Concepts in Computing with Data: Read More [+]
Summer: 10 weeks - 4 hours of lecture and 3 hours of laboratory per week
Additional Format: Three hours of lecture and two hours of computer laboratory per week. Four hours of lecture and three hours of computer laboratory per week for ten weeks.
Concepts in Computing with Data: Read Less [-]
STAT 134 Concepts of Probability 4 Units
Terms offered: Fall 2024, Summer 2024 8 Week Session, Spring 2024 An introduction to probability, emphasizing concepts and applications. Conditional expectation, independence, laws of large numbers. Discrete and continuous random variables. Central limit theorem. Selected topics such as the Poisson process, Markov chains, characteristic functions. Concepts of Probability: Read More [+]
Prerequisites: One year of calculus
Credit Restrictions: Students will not receive credit for 134 after taking 140 or 201A.
Concepts of Probability: Read Less [-]
STAT 135 Concepts of Statistics 4 Units
Terms offered: Fall 2024, Summer 2024 8 Week Session, Spring 2024 A comprehensive survey course in statistical theory and methodology. Topics include descriptive statistics, maximum likelihood estimation, non-parametric methods, introduction to optimality, goodness-of-fit tests, analysis of variance, bootstrap and computer-intensive methods and least squares estimation. The laboratory includes computer-based data-analytic applications to science and engineering. Concepts of Statistics: Read More [+]
Prerequisites: STAT 134 or STAT 140; and MATH 54 , EL ENG 16A, STAT 89A , MATH 110 or equivalent linear algebra. Strongly recommended corerequisite: STAT 133
Additional Format: Three hours of Lecture and Two hours of Laboratory per week for 15 weeks. Six hours of Lecture and Four hours of Laboratory per week for 8 weeks.
Concepts of Statistics: Read Less [-]
STAT C140 Probability for Data Science 4 Units
Terms offered: Fall 2024, Spring 2024, Fall 2023, Spring 2023 An introduction to probability, emphasizing the combined use of mathematics and programming. Discrete and continuous families of distributions. Bounds and approximations. Transforms and convergence. Markov chains and Markov Chain Monte Carlo. Dependence, conditioning, Bayesian methods. Maximum likelihood, least squares prediction, the multivariate normal, and multiple regression. Random permutations, symmetry, and order statistics. Use of numerical computation, graphics, simulation, and computer algebra. Probability for Data Science: Read More [+]
Objectives & Outcomes
Course Objectives: Data/Stat C140 is a probability course for Data C8 graduates who have taken more mathematics and wish to go deeper into data science. The emphasis on simulation and the bootstrap in Data C8 gives students a concrete sense of randomness and sampling variability. Data/Stat C140 capitalizes on this, abstraction and computation complementing each other throughout. Topics in statistical theory are included to allow students to proceed to modeling and statistical learning classes without taking a further semester of mathematical statistics.
Student Learning Outcomes: Understand the difference between math and simulation, and appreciate the power of both Use a variety of approaches to problem solving Work with probability concepts algebraically, numerically, and graphically
Prerequisites: DATA/COMPSCI/INFO/STAT C8, or both STAT 20 and one of COMPSCI 61A or COMPSCI/DATA C88C with C- or better, or Pass; and one year of calculus at the level of MATH 1A -1B or higher, with C- or better, or Pass. Corequisite: MATH 54 , MATH 56 , EECS 16B , MATH 110 or equivalent linear algebra (C- or better, or Pass, required if completed prior to enrollment in Data/Stat C140)
Credit Restrictions: Students will receive no credit for STAT C140 after completing STAT 134 , or EECS 126 .
Fall and/or spring: 15 weeks - 3-3 hours of lecture, 1-1 hours of discussion, 1-1 hours of supplement, and 0-1 hours of voluntary per week 15 weeks - 3-3 hours of lecture, 2-2 hours of discussion, 0-0 hours of supplement, and 0-1 hours of voluntary per week
Additional Format: Three hours of lecture and two hours of discussion and zero hour of supplement and zero to one hours of voluntary per week. Three hours of lecture and one hour of discussion and one hour of supplement and zero to one hours of voluntary per week.
Formerly known as: Statistics 140
Also listed as: DATA C140
Probability for Data Science: Read Less [-]
STAT 150 Stochastic Processes 3 Units
Terms offered: Fall 2024, Spring 2024, Fall 2023 Random walks, discrete time Markov chains, Poisson processes. Further topics such as: continuous time Markov chains, queueing theory, point processes, branching processes, renewal theory, stationary processes, Gaussian processes. Stochastic Processes: Read More [+]
Prerequisites: 101 or 103A or 134
Additional Format: Three hours of Lecture per week for 15 weeks.
Stochastic Processes: Read Less [-]
STAT 151A Linear Modelling: Theory and Applications 4 Units
Terms offered: Fall 2024, Spring 2024, Fall 2023 A coordinated treatment of linear and generalized linear models and their application. Linear regression, analysis of variance and covariance, random effects, design and analysis of experiments, quality improvement, log-linear models for discrete multivariate data, model selection, robustness, graphical techniques, productive use of computers, in-depth case studies. This course uses either R or Python as its primary computing language, as determined by the instructor. Linear Modelling: Theory and Applications: Read More [+]
Prerequisites: STAT 135 . STAT 133 recommended
Additional Format: Three hours of Lecture and Two hours of Laboratory per week for 15 weeks.
Linear Modelling: Theory and Applications: Read Less [-]
STAT 152 Sampling Surveys 4 Units
Terms offered: Spring 2020, Spring 2019, Spring 2018 Theory and practice of sampling from finite populations. Simple random, stratified, cluster, and double sampling. Sampling with unequal probabilities. Properties of various estimators including ratio, regression, and difference estimators. Error estimation for complex samples. Sampling Surveys: Read More [+]
Prerequisites: 101 or 134. 133 and 135 recommended
Sampling Surveys: Read Less [-]
STAT 153 Introduction to Time Series 4 Units
Terms offered: Fall 2024, Spring 2024, Fall 2023 An introduction to time series analysis in the time domain and spectral domain. Topics will include: estimation of trends and seasonal effects, autoregressive moving average models, forecasting, indicators, harmonic analysis, spectra. This course uses either R or Python as its primary computing language, as determined by the instructor. Introduction to Time Series: Read More [+]
Prerequisites: 134 or consent of instructor. 133 or 135 recommended
Introduction to Time Series: Read Less [-]
STAT 154 Modern Statistical Prediction and Machine Learning 4 Units
Terms offered: Fall 2024, Spring 2024, Fall 2023 Theory and practice of statistical prediction. Contemporary methods as extensions of classical methods. Topics: optimal prediction rules, the curse of dimensionality, empirical risk, linear regression and classification, basis expansions, regularization, splines, the bootstrap, model selection, classification and regression trees, boosting, support vector machines. Computational efficiency versus predictive performance. Emphasis on experience with real data and assessing statistical assumptions. This course uses Python as its primary computing language; details are determined by the instructor. Modern Statistical Prediction and Machine Learning: Read More [+]
Prerequisites: Mathematics 53 or equivalent; Mathematics 54, Electrical Engineering 16A, Statistics 89A, Mathematics 110 or equivalent linear algebra; Statistics 135, the combination of Data/Stat C140 and Data/Stat/Compsci C100, or equivalent; experience with some programming language. Recommended prerequisite: Mathematics 55 or equivalent exposure to counting arguments
Summer: 10 weeks - 4.5 hours of lecture and 3 hours of laboratory per week
Additional Format: Three hours of lecture and two hours of laboratory per week. Four and one-half hours of lecture and three hours of laboratory per week for 10 weeks.
Modern Statistical Prediction and Machine Learning: Read Less [-]
STAT 155 Game Theory 3 Units
Terms offered: Fall 2024, Summer 2024 8 Week Session, Spring 2024 General theory of zero-sum, two-person games, including games in extensive form and continuous games, and illustrated by detailed study of examples. Game Theory: Read More [+]
Prerequisites: 101 or 134
Summer: 8 weeks - 6 hours of lecture per week
Additional Format: Three hours of Lecture per week for 15 weeks. Six hours of Lecture per week for 8 weeks.
Game Theory: Read Less [-]
STAT 156 Causal Inference 4 Units
Terms offered: Fall 2024, Fall 2023, Fall 2022 This course will focus on approaches to causal inference using the potential outcomes framework. It will also use causal diagrams at an intuitive level. The main topics are classical randomized experiments, observational studies, instrumental variables, principal stratification and mediation analysis. Applications are drawn from a variety of fields including political science, economics, sociology, public health, and medicine. This course is a mix of statistical theory and data analysis. Students will be exposed to statistical questions that are relevant to decision and policy making. This course uses R as its primary computing language; details are determined by the instructor. Causal Inference: Read More [+]
Prerequisites: Statistics 135
Causal Inference: Read Less [-]
STAT 157 Seminar on Topics in Probability and Statistics 3 Units
Terms offered: Spring 2024, Fall 2023, Spring 2023 Substantial student participation required. The topics to be covered each semester that the course may be offered will be announced by the middle of the preceding semester; see departmental bulletins. Recent topics include: Bayesian statistics, statistics and finance, random matrix theory, high-dimensional statistics. Seminar on Topics in Probability and Statistics: Read More [+]
Prerequisites: Mathematics 53-54, Statistics 134, 135. Knowledge of scientific computing environment (R or Matlab) often required. Prerequisites might vary with instructor and topics
Repeat rules: Course may be repeated for credit with instructor consent.
Fall and/or spring: 15 weeks - 3 hours of seminar per week
Additional Format: Three hours of Seminar per week for 15 weeks.
Seminar on Topics in Probability and Statistics: Read Less [-]
STAT 158 Experimental Design 4 Units
Terms offered: Fall 2023, Spring 2023, Spring 2021 This course will review the statistical foundations of randomized experiments and study principles for addressing common setbacks in experimental design and analysis in practice. We will cover the notion of potential outcomes for causal inference and the Fisherian principles for experimentation (randomization, blocking, and replications). We will also cover experiments with complex structures (clustering in units, factorial design, hierarchy in treatments, sequential assignment, etc). We will also address practical complications in experiments, including noncompliance, missing data, and measurement error. This course uses R as its primary computing language; details are determined by the instructor. Experimental Design: Read More [+]
Prerequisites: Statistics 134 and Statistics 135 and experience with Software R, or consent of instructor
Experimental Design: Read Less [-]
STAT 159 Reproducible and Collaborative Statistical Data Science 4 Units
Terms offered: Spring 2023, Spring 2022, Spring 2021 A project-based introduction to statistical data analysis. Through case studies, computer laboratories, and a term project, students will learn practical techniques and tools for producing statistically sound and appropriate, reproducible, and verifiable computational answers to scientific questions. Course emphasizes version control, testing, process automation, code review, and collaborative programming. Software tools may include Bash , Git, Python, and LaTeX. Reproducible and Collaborative Statistical Data Science: Read More [+]
Prerequisites: Statistics 133, Statistics 134, and Statistics 135 (or equivalent)
Grading/Final exam status: Letter grade. Alternative to final exam.
Reproducible and Collaborative Statistical Data Science: Read Less [-]
STAT 165 Forecasting 3 Units
Terms offered: Spring 2024 Forecasting has been used to predict elections, climate change, and the spread of COVID-19. Poor forecasts led to the 2008 financial crisis. In our daily lives, good forecasting ability can help us plan our work, be on time to events, and make informed career decisions. This practically-oriented class will provide students with tools to make good forecasts, including Fermi estimates, calibration training, base rates, scope sensitivity, and power laws. This course uses Python as its primary computing language; details are determined by the instructor. Forecasting: Read More [+]
Course Objectives: Discuss several historical instances of successful and unsuccessful forecasts. Practice making forecasts about our own lives, about current events, and about scientific progress
Student Learning Outcomes: Formulate questions that are relevant to their own life or work. Identify well-defined versus poorly-defined forecasting questions. Provide forecasts that are well-calibrated. Understand common forecasting pitfalls, such as improper independence assumptions, and how to identify and guard against them. Understand how forecasts evolve across time in response to new information. Use forecasts to inform decisions. Utilize a variety of forecasting tools, such as base rates, to improve their forecasts. Utilize and filter data across a variety of sources to inform their forecasts. Work in teams to improve forecasts.
Prerequisites: Stat 134, Data/Stat C140, EECS 126 , Math 106, IND ENG 172 , or equivalent; and familiarity with Python; or consent of instructor. Strongly Recommended: Compsci 61A, Data/Compsci C88C, or equivalent
Additional Format: Three hours of lecture per week.
Forecasting: Read Less [-]
STAT H195 Special Study for Honors Candidates 1 - 4 Units
Terms offered: Spring 2015, Fall 2014, Fall 2010 Special Study for Honors Candidates: Read More [+]
Fall and/or spring: 15 weeks - 0 hours of independent study per week
Summer: 6 weeks - 1-5 hours of independent study per week 8 weeks - 1-4 hours of independent study per week
Additional Format: Zero hours of Independent study per week for 15 weeks. One to Four hour of Independent study per week for 8 weeks. One to Five hour of Independent study per week for 6 weeks.
Special Study for Honors Candidates: Read Less [-]
STAT 197 Field Study in Statistics 0.5 - 3 Units
Terms offered: Fall 2021, Fall 2020, Spring 2017 Supervised experience relevant to specific aspects of statistics in on-campus or off-campus settings. Individual and/or group meetings with faculty. Field Study in Statistics: Read More [+]
Credit Restrictions: Enrollment is restricted; see the Introduction to Courses and Curricula section of this catalog.
Fall and/or spring: 15 weeks - 2-9 hours of fieldwork per week
Summer: 6 weeks - 3-22 hours of fieldwork per week 8 weeks - 2-16 hours of fieldwork per week 10 weeks - 2-12 hours of fieldwork per week
Additional Format: Two to nine hours of fieldwork per week. Two to twelve hours of fieldwork per week for 10 weeks. Two to sixteen hours of fieldwork per week for 8 weeks. Three to twenty two hours of fieldwork per week for 6 weeks.
STAT 198 Directed Study for Undergraduates 1 - 3 Units
Terms offered: Spring 2024, Fall 2023, Spring 2023 Special tutorial or seminar on selected topics. Directed Study for Undergraduates: Read More [+]
Fall and/or spring: 15 weeks - 1-3 hours of directed group study per week
Summer: 6 weeks - 2.5-7.5 hours of directed group study per week 8 weeks - 1.5-5.5 hours of directed group study per week
Additional Format: One to Three hour of Directed group study per week for 15 weeks. One and one-half to Five and one-half hours of Directed group study per week for 8 weeks. Two and one-half to Seven and one-half hours of Directed group study per week for 6 weeks.
Directed Study for Undergraduates: Read Less [-]
STAT 199 Supervised Independent Study and Research 1 - 3 Units
Terms offered: Fall 2019, Fall 2018, Spring 2017 Supervised Independent Study and Research: Read More [+]
Fall and/or spring: 15 weeks - 1-3 hours of independent study per week
Summer: 6 weeks - 1-4 hours of independent study per week 8 weeks - 1-3 hours of independent study per week 10 weeks - 1-3 hours of independent study per week
Additional Format: One to three hours of independent study per week. One to three hours of independent study per week for 10 weeks. One to three hours of independent study per week for 8 weeks. One to four hours of independent study per week for 6 weeks.
Supervised Independent Study and Research: Read Less [-]
STAT 200A Introduction to Probability and Statistics at an Advanced Level 4 Units
Terms offered: Fall 2018, Fall 2011, Fall 2010 Probability spaces, random variables, distributions in probability and statistics, central limit theorem, Poisson processes, transformations involving random variables, estimation, confidence intervals, hypothesis testing, linear models, large sample theory, categorical models, decision theory. Introduction to Probability and Statistics at an Advanced Level: Read More [+]
Prerequisites: Multivariable calculus and one semester of linear algebra
Credit Restrictions: Students will receive no credit for Statistics 200A after completing Statistics 201A-201B.
Subject/Course Level: Statistics/Graduate
Grading: Letter grade.
Introduction to Probability and Statistics at an Advanced Level: Read Less [-]
STAT 200B Introduction to Probability and Statistics at an Advanced Level 4 Units
Terms offered: Spring 2019, Spring 2012, Spring 2011 Probability spaces, random variables, distributions in probability and statistics, central limit theorem, Poisson processes, transformations involving random variables, estimation, confidence intervals, hypothesis testing, linear models, large sample theory, categorical models, decision theory. Introduction to Probability and Statistics at an Advanced Level: Read More [+]
Credit Restrictions: Students will receive no credit for Statistics 200A-200B after completing Statistics 201A-201B.
STAT C200C Principles and Techniques of Data Science 4 Units
Terms offered: Fall 2024, Spring 2024, Fall 2023, Spring 2023, Spring 2022, Spring 2021, Spring 2020 Explores the data science lifecycle: question formulation, data collection and cleaning, exploratory, analysis, visualization, statistical inference, prediction, and decision-making. Focuses on quantitative critical thinking and key principles and techniques: languages for transforming, querying and analyzing data; algorithms for machine learning methods: regression, classification and clustering; principles of informative visualization; measurement error and prediction; and techniques for scalable data processing. Research term project. Principles and Techniques of Data Science: Read More [+]
Prerequisites: COMPSCI C8 / INFO C8 / STAT C8 or ENGIN 7 ; and either COMPSCI 61A or COMPSCI 88. Corequisites: MATH 54 or EECS 16A
Credit Restrictions: Students will receive no credit for DATA C200 \ COMPSCI C200A \ STAT C200C after completing DATA C100 .
Fall and/or spring: 8 weeks - 6-6 hours of lecture, 2-2 hours of discussion, and 0-2 hours of laboratory per week 15 weeks - 3-3 hours of lecture, 1-1 hours of discussion, and 0-1 hours of laboratory per week
Additional Format: Three hours of lecture and one hour of discussion and zero to one hours of laboratory per week. Six hours of lecture and two hours of discussion and zero to two hours of laboratory per week for 8 weeks. Six hours of lecture and two hours of discussion and zero to two hours of laboratory per week for 8 weeks.
Formerly known as: Statistics C200C/Computer Science C200A
Also listed as: COMPSCI C200A/DATA C200
Principles and Techniques of Data Science: Read Less [-]
STAT 201A Introduction to Probability at an Advanced Level 4 Units
Terms offered: Fall 2024, Fall 2023, Fall 2022 Distributions in probability and statistics, central limit theorem, Poisson processes, modes of convergence, transformations involving random variables. Introduction to Probability at an Advanced Level: Read More [+]
Prerequisites: Undergraduate probability at the level of Statistics 134, multivariable calculus (at the level of Berkeley’s Mathematics 53) and linear algebra (at the level of Berkeley’s Mathematics 54)
Credit Restrictions: Students will receive no credit for STAT 201A after completing STAT 200A .
Introduction to Probability at an Advanced Level: Read Less [-]
STAT 201B Introduction to Statistics at an Advanced Level 4 Units
Terms offered: Fall 2024, Fall 2023, Fall 2022 Estimation, confidence intervals, hypothesis testing, linear models, large sample theory, categorical models, decision theory. Introduction to Statistics at an Advanced Level: Read More [+]
Credit Restrictions: Students will receive no credit for Statistics 201B after completing Statistics 200B.
Introduction to Statistics at an Advanced Level: Read Less [-]
STAT 204 Probability for Applications 4 Units
Terms offered: Fall 2023, Fall 2019, Spring 2017 A treatment of ideas and techniques most commonly found in the applications of probability: Gaussian and Poisson processes, limit theorems, large deviation principles, information, Markov chains and Markov chain Monte Carlo, martingales, Brownian motion and diffusion. Probability for Applications: Read More [+]
Credit Restrictions: Students will receive no credit for Statistics 204 after completing Statistics 205A-205B.
Instructor: Evans
Probability for Applications: Read Less [-]
STAT C205A Probability Theory 4 Units
Terms offered: Fall 2024, Fall 2023, Fall 2022 The course is designed as a sequence with Statistics C205B/Mathematics C218B with the following combined syllabus. Measure theory concepts needed for probability. Expection, distributions. Laws of large numbers and central limit theorems for independent random variables. Characteristic function methods. Conditional expectations, martingales and martingale convergence theorems. Markov chains. Stationary processes. Brownian motion. Probability Theory: Read More [+]
Also listed as: MATH C218A
Probability Theory: Read Less [-]
STAT C205B Probability Theory 4 Units
Terms offered: Spring 2024, Spring 2023, Spring 2022 The course is designed as a sequence with with Statistics C205A/Mathematics C218A with the following combined syllabus. Measure theory concepts needed for probability. Expection, distributions. Laws of large numbers and central limit theorems for independent random variables. Characteristic function methods. Conditional expectations, martingales and martingale convergence theorems. Markov chains. Stationary processes. Brownian motion. Probability Theory: Read More [+]
Also listed as: MATH C218B
STAT C206A Advanced Topics in Probability and Stochastic Process 3 Units
Terms offered: Fall 2024, Fall 2020, Fall 2016, Fall 2014 The topics of this course change each semester, and multiple sections may be offered. Advanced topics in probability offered according to students demand and faculty availability. Advanced Topics in Probability and Stochastic Process: Read More [+]
Prerequisites: Statistics C205A-C205B or consent of instructor
Also listed as: MATH C223A
Advanced Topics in Probability and Stochastic Process: Read Less [-]
STAT C206B Advanced Topics in Probability and Stochastic Processes 3 Units
Terms offered: Spring 2024, Spring 2023, Spring 2022 The topics of this course change each semester, and multiple sections may be offered. Advanced topics in probability offered according to students demand and faculty availability. Advanced Topics in Probability and Stochastic Processes: Read More [+]
Also listed as: MATH C223B
Advanced Topics in Probability and Stochastic Processes: Read Less [-]
STAT 210A Theoretical Statistics 4 Units
Terms offered: Fall 2024, Fall 2023, Fall 2022 An introduction to mathematical statistics, covering both frequentist and Bayesian aspects of modeling, inference, and decision-making. Topics include statistical decision theory; point estimation; minimax and admissibility; Bayesian methods; exponential families; hypothesis testing; confidence intervals; small and large sample theory; and M-estimation. Theoretical Statistics: Read More [+]
Prerequisites: Linear algebra, real analysis, and a year of upper division probability and statistics
Theoretical Statistics: Read Less [-]
STAT 210B Theoretical Statistics 4 Units
Terms offered: Spring 2024, Spring 2023, Spring 2022 Introduction to modern theory of statistics; empirical processes, influence functions, M-estimation, U and V statistics and associated stochastic decompositions; non-parametric function estimation and associated minimax theory; semiparametric models; Monte Carlo methods and bootstrap methods; distributionfree and equivariant procedures; topics in machine learning. Topics covered may vary with instructor. Theoretical Statistics: Read More [+]
Prerequisites: Statistics 210A and a graduate level probability course; a good understanding of various notions of stochastic convergence
STAT 212A Topics in Theoretical Statistics 3 Units
Terms offered: Spring 2021, Fall 2015, Fall 2012 This course introduces the student to topics of current research interest in theoretical statistics. Recent topics include information theory, multivariate analysis and random matrix theory, high-dimensional inference. Typical topics have been model selection; empirical and point processes; the bootstrap, stochastic search, and Monte Carlo integration; information theory and statistics; semi- and non-parametric modeling; time series and survival analysis. Topics in Theoretical Statistics: Read More [+]
Prerequisites: 210 or 205 and 215
Formerly known as: 216A-216B and 217A-217B
Topics in Theoretical Statistics: Read Less [-]
STAT 212B Topics in Theoretical Statistics 3 Units
Terms offered: Spring 2016 This course introduces the student to topics of current research interest in theoretical statistics. Recent topics include information theory, multivariate analysis and random matrix theory, high-dimensional inference. Typical topics have been model selection; empirical and point processes; the bootstrap, stochastic search, and Monte Carlo integration; information theory and statistics; semi- and non-parametric modeling; time series and survival analysis. Topics in Theoretical Statistics: Read More [+]
STAT 215A Applied Statistics and Machine Learning 4 Units
Terms offered: Fall 2024, Fall 2023, Fall 2022 Applied statistics and machine learning, focusing on answering scientific questions using data, the data science life cycle, critical thinking, reasoning, methodology, and trustworthy and reproducible computational practice. Hands-on-experience in open-ended data labs, using programming languages such as R and Python. Emphasis on understanding and examining the assumptions behind standard statistical models and methods and the match between the assumptions and the scientific question. Exploratory data analysis. Model formulation, fitting, model testing and validation, interpretation, and communication of results. Methods, including linear regression and generalizations, decision trees, random forests, simulation, and randomization methods. Applied Statistics and Machine Learning: Read More [+]
Prerequisites: Linear algebra, calculus, upper division probability and statistics, and familiarity with high-level programming languages. Statistics 133, 134, and 135 recommended
Applied Statistics and Machine Learning: Read Less [-]
STAT 215B Statistical Models: Theory and Application 4 Units
Terms offered: Spring 2024, Spring 2023, Spring 2022 Course builds on 215A in developing critical thinking skills and the techniques of advanced applied statistics. Particular topics vary with instructor. Examples of possible topics include planning and design of experiments, ANOVA and random effects models, splines, classification, spatial statistics, categorical data analysis, survival analysis, and multivariate analysis. Statistical Models: Theory and Application: Read More [+]
Prerequisites: Statistics 215A or consent of instructor
Statistical Models: Theory and Application: Read Less [-]
STAT 222 Masters of Statistics Capstone Project 4 Units
Terms offered: Spring 2024, Spring 2023, Spring 2022 The capstone project is part of the masters degree program in statistics. Students engage in professionally-oriented group research under the supervision of a research advisor. The research synthesizes the statistical, computational, economic, and social issues involved in solving complex real-world problems. Masters of Statistics Capstone Project: Read More [+]
Prerequisites: Statistics 201A-201B, 243. Restricted to students who have been admitted to the one-year Masters Program in Statistics beginning fall 2012 or later
Fall and/or spring: 15 weeks - 3 hours of seminar and 1 hour of laboratory per week
Additional Format: One hour of laboratory and three hours of seminar per week.
Masters of Statistics Capstone Project: Read Less [-]
STAT 230A Linear Models 4 Units
Terms offered: Spring 2024, Spring 2023, Spring 2022 Theory of least squares estimation, interval estimation, and tests under the general linear fixed effects model with normally distributed errors. Large sample theory for non-normal linear models. Two and higher way layouts, residual analysis. Effects of departures from the underlying assumptions. Robust alternatives to least squares. Linear Models: Read More [+]
Prerequisites: Matrix algebra, a year of calculus, two semesters of upper division or graduate probability and statistics
Linear Models: Read Less [-]
STAT 232 Experimental Design 4 Units
Terms offered: Spring 2023, Spring 2022, Fall 2018 This course will review the statistical foundations of randomized experiments and study principles for addressing common setbacks in experimental design and analysis in practice. We will cover the notion of potential outcomes for causal inference and the Fisherian principles for experimentation (randomization, blocking, and replications). We will also cover experiments with complex structures (clustering in units, factorial design, hierarchy in treatments, sequential assignment, etc). We will also address practical complications in experiments, including noncompliance, missing data, and measurement error. Experimental Design: Read More [+]
STAT 238 Bayesian Statistics 3 Units
Terms offered: Fall 2016 Bayesian methods and concepts: conditional probability, one-parameter and multiparameter models, prior distributions, hierarchical and multi-level models, predictive checking and sensitivity analysis, model selection, linear and generalized linear models, multiple testing and high-dimensional data, mixtures, non-parametric methods. Case studies of applied modeling. In-depth computational implementation using Markov chain Monte Carlo and other techniques. Basic theory for Bayesian methods and decision theory. The selection of topics may vary from year to year. Bayesian Statistics: Read More [+]
Course Objectives: develop Bayesian models for new types of data implement Bayesian models and interpret the results read and discuss Bayesian methods in the literature select and build appropriate Bayesian models for data to answer research questions understand and describe the Bayesian perspective and its advantages and disadvantages compared to classical methods
Prerequisites: Probability and mathematical statistics at the level of Stat 134 and Stat 135 or, ideally, Stat 201A and Stat 201B
Fall and/or spring: 15 weeks - 3 hours of lecture and 1 hour of laboratory per week
Additional Format: Three hours of lecture and one hour of laboratory per week.
Bayesian Statistics: Read Less [-]
STAT 239A The Statistics of Causal Inference in the Social Science 4 Units
Terms offered: Fall 2015, Fall 2014 Approaches to causal inference using the potential outcomes framework. Covers observational studies with and without ignorable treatment assignment, randomized experiments with and without noncompliance, instrumental variables, regression discontinuity, sensitivity analysis and randomization inference. Applications are drawn from a variety of fields including political science, economics, sociology, public health and medicine. The Statistics of Causal Inference in the Social Science: Read More [+]
Prerequisites: At least one graduate matrix based multivariate regression course in addition to introductory statistics and probability
Fall and/or spring: 15 weeks - 3-3 hours of lecture and 1-2 hours of discussion per week
Additional Format: Three hours of lecture and one to two hours of discussion per week.
Grading: Letter grade. This is part one of a year long series course. A provisional grade of IP (in progress) will be applied and later replaced with the final grade after completing part two of the series.
Instructor: Sekhon
The Statistics of Causal Inference in the Social Science: Read Less [-]
STAT 239B Quantitative Methodology in the Social Sciences Seminar 4 Units
Terms offered: Spring 2016, Spring 2015 A seminar on successful research designs and a forum for students to discuss the research methods needed in their own work, supplemented by lectures on relevant statistical and computational topics such as matching methods, instrumental variables, regression discontinuity, and Bayesian, maximum likelihood and robust estimation. Applications are drawn from political science, economics, sociology, and public health. Experience with R is assumed. Quantitative Methodology in the Social Sciences Seminar: Read More [+]
Prerequisites: Statistics 239A or equivalent
Grading: Letter grade. This is part two of a year long series course. Upon completion, the final grade will be applied to both parts of the series.
Quantitative Methodology in the Social Sciences Seminar: Read Less [-]
STAT C239A The Statistics of Causal Inference in the Social Science 4 Units
Terms offered: Fall 2018, Fall 2017, Fall 2016 Approaches to causal inference using the potential outcomes framework. Covers observational studies with and without ignorable treatment assignment, randomized experiments with and without noncompliance, instrumental variables, regression discontinuity, sensitivity analysis and randomization inference. Applications are drawn from a variety of fields including political science, economics, sociology, public health and medicine. The Statistics of Causal Inference in the Social Science: Read More [+]
Additional Format: Three hours of Lecture and Two hours of Discussion per week for 15 weeks.
Also listed as: POL SCI C236A
STAT C239B Quantitative Methodology in the Social Sciences Seminar 4 Units
Terms offered: Spring 2018, Spring 2017 A seminar on successful research designs and a forum for students to discuss the research methods needed in their own work, supplemented by lectures on relevant statistical and computational topics such as matching methods, instrumental variables, regression discontinuity, and Bayesian, maximum likelihood and robust estimation. Applications are drawn from political science, economics, sociology, and public health. Experience with R is assumed. Quantitative Methodology in the Social Sciences Seminar: Read More [+]
Additional Format: Three hours of lecture and two hours of discussion per week.
Also listed as: POL SCI C236B
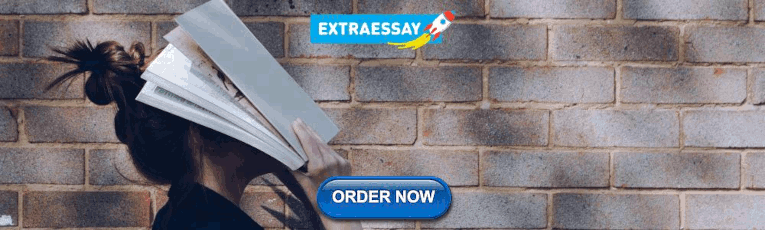
STAT 240 Nonparametric and Robust Methods 4 Units
Terms offered: Spring 2023, Spring 2021, Fall 2017 Standard nonparametric tests and confidence intervals for continuous and categorical data; nonparametric estimation of quantiles; robust estimation of location and scale parameters. Efficiency comparison with the classical procedures. Nonparametric and Robust Methods: Read More [+]
Prerequisites: A year of upper division probability and statistics
Nonparametric and Robust Methods: Read Less [-]
STAT C241A Statistical Learning Theory 3 Units
Terms offered: Fall 2023, Fall 2021, Fall 2020 Classification regression, clustering, dimensionality, reduction, and density estimation. Mixture models, hierarchical models, factorial models, hidden Markov, and state space models, Markov properties, and recursive algorithms for general probabilistic inference nonparametric methods including decision trees, kernal methods, neural networks, and wavelets. Ensemble methods. Statistical Learning Theory: Read More [+]
Instructors: Bartlett, Jordan, Wainwright
Also listed as: COMPSCI C281A
Statistical Learning Theory: Read Less [-]
STAT C241B Advanced Topics in Learning and Decision Making 3 Units
Terms offered: Spring 2024, Spring 2023, Spring 2022 Recent topics include: Graphical models and approximate inference algorithms. Markov chain Monte Carlo, mean field and probability propagation methods. Model selection and stochastic realization. Bayesian information theoretic and structural risk minimization approaches. Markov decision processes and partially observable Markov decision processes. Reinforcement learning. Advanced Topics in Learning and Decision Making: Read More [+]
Also listed as: COMPSCI C281B
Advanced Topics in Learning and Decision Making: Read Less [-]
STAT 243 Introduction to Statistical Computing 4 Units
Terms offered: Fall 2024, Fall 2023, Fall 2022 Concepts in statistical programming and statistical computation, including programming principles, data and text manipulation, parallel processing, simulation, numerical linear algebra, and optimization. Introduction to Statistical Computing: Read More [+]
Student Learning Outcomes: Become familiar with concepts and tools for reproducible research and good scientific computing practices. Operate effectively in a UNIX environment and on remote servers. Program effectively in languages including R and Python with an advanced knowledge of language functionality and an understanding of general programming concepts. Understand in depth and make use of principles of numerical linear algebra, optimization, and simulation for statistics-related research.
Prerequisites: Graduate standing
Introduction to Statistical Computing: Read Less [-]
STAT 244 Statistical Computing 4 Units
Terms offered: Spring 2011, Spring 2010, Spring 2009 Algorithms in statistical computing: random number generation, generating other distributions, random sampling and permutations. Matrix computations in linear models. Non-linear optimization with applications to statistical procedures. Other topics of current interest, such as issues of efficiency, and use of graphics. Statistical Computing: Read More [+]
Prerequisites: Knowledge of a higher level programming language
Statistical Computing: Read Less [-]
STAT C245A Introduction to Modern Biostatistical Theory and Practice 4 Units
Terms offered: Spring 2024, Spring 2023, Spring 2022 Course covers major topics in general statistical theory, with a focus on statistical methods in epidemiology. The course provides a broad theoretical framework for understanding the properties of commonly-used and more advanced methods. Emphasis is on estimation in nonparametric models in the context of contingency tables, regression (e.g., linear, logistic), density estimation and more. Topics include maximum likelihood and loss-based estimation , asymptotic linearity/normality, the delta method, bootstrapping, machine learning, targeted maximum likelihood estimation. Comprehension of broad concepts is the main goal, but practical implementation in R is also emphasized. Basic knowledge of probability/statistics and calculus are assume Introduction to Modern Biostatistical Theory and Practice: Read More [+]
Prerequisites: Statistics 200A (may be taken concurrently)
Instructor: Hubbard
Also listed as: PB HLTH C240A
Introduction to Modern Biostatistical Theory and Practice: Read Less [-]
STAT C245B Biostatistical Methods: Survival Analysis and Causality 4 Units
Terms offered: Fall 2024, Fall 2023, Fall 2022 Analysis of survival time data using parametric and non-parametric models, hypothesis testing, and methods for analyzing censored (partially observed) data with covariates. Topics include marginal estimation of a survival function, estimation of a generalized multivariate linear regression model (allowing missing covariates and/or outcomes), estimation of a multiplicative intensity model (such as Cox proportional hazards model) and estimation of causal parameters assuming marginal structural models. General theory for developing locally efficient estimators of the parameters of interest in censored data models. Computing techniques, numerical methods, simulation and general implementation of biostatistical analysis techniques with emphasis on data applications. Biostatistical Methods: Survival Analysis and Causality: Read More [+]
Prerequisites: Statistics 200B (may be taken concurrently)
Instructor: van der Laan
Also listed as: PB HLTH C240B
Biostatistical Methods: Survival Analysis and Causality: Read Less [-]
STAT C245C Biostatistical Methods: Computational Statistics with Applications in Biology and Medicine 4 Units
Terms offered: Fall 2023, Fall 2022, Fall 2021 This course provides an introduction to computational statistics, with emphasis on statistical methods and software for addressing high-dimensional inference problems in biology and medicine. Topics include numerical and graphical data summaries, loss-based estimation (regression, classification, density estimation), smoothing, EM algorithm, Markov chain Monte-Carlo, clustering, multiple testing, resampling, hidden Markov models, in silico exp eriments. Biostatistical Methods: Computational Statistics with Applications in Biology and Medicine: Read More [+]
Prerequisites: Statistics 200A or equivalent (may be taken concurrently)
Instructor: Dudoit
Also listed as: PB HLTH C240C
Biostatistical Methods: Computational Statistics with Applications in Biology and Medicine: Read Less [-]
STAT C245D Biostatistical Methods: Computational Statistics with Applications in Biology and Medicine II 4 Units
Terms offered: Fall 2017, Fall 2015, Fall 2013 This course and Pb Hlth C240C/Stat C245C provide an introduction to computational statistics with emphasis on statistical methods and software for addressing high-dimensional inference problems that arise in current biological and medical research. The courses also discusses statistical computing resources, with emphasis on the R language and environment (www.r-project.org). Programming topics to be discussed include: data structures, functions , statistical models, graphical procedures, designing an R package, object-oriented programming, inter-system interfaces. The statistical and computational methods are motivated by and illustrated on data structures that arise in current high-dimensional inference problems in biology and medicine. Biostatistical Methods: Computational Statistics with Applications in Biology and Medicine II: Read More [+]
Prerequisites: Statistics 200A-200B or Statistics 201A-201B (may be taken concurrently) or consent of instructor
Also listed as: PB HLTH C240D
Biostatistical Methods: Computational Statistics with Applications in Biology and Medicine II: Read Less [-]
STAT C245F Statistical Genomics 4 Units
Terms offered: Spring 2022, Spring 2021, Spring 2020, Spring 2018, Spring 2017 Genomics is one of the fundamental areas of research in the biological sciences and is rapidly becoming one of the most important application areas in statistics. The first course in this two-semester sequence is Public Health C240E/Statistics C245E. This is the second course, which focuses on sequence analysis, phylogenetics, and high-throughput microarray and sequencing gene expression experiments. The courses are primarily intended for graduate students and advanced undergraduate students from the mathematical sciences. Statistical Genomics: Read More [+]
Additional Format: Three hours of lecture and one hour of discussion per week.
Instructors: Dudoit, Huang, Nielsen, Song
Also listed as: PB HLTH C240F
Statistical Genomics: Read Less [-]
STAT C247C Longitudinal Data Analysis 4 Units
Terms offered: Fall 2024, Fall 2023, Fall 2021 Course covers statistical issues surrounding estimation of effects using data on units followed through time. Course emphasizes a regression model approach for estimating associations of disease incidence modeling, continuous outcome data/linear models & longitudinal extensions to nonlinear models forms (e.g., logistic). Course emphasizes complexities that repeated measures has on the estimation process & opportunities it provides if data is modeled appropriately. Most time is spent on 2 approaches: mixed models based upon explicit (latent variable) maximum likelihood estimation of the sources of the dependence, versus empirical estimating equation approaches (generalized estimating equations). Primary focus is from the analysis side. Longitudinal Data Analysis: Read More [+]
Course Objectives: After successfully completing the course, you will be able to: • frame data science questions relevant to longitudinal studies as the estimation of statistical parameters generated from regression, • derive consistent statistical inference in the presence of correlated, repeated measures data using likelihood-based mixed models and estimating equation approaches (generalized estimating equations; GEE), • implement the relevant methods using R. • interpret the regression output, including both coefficients and variance components and
Prerequisites: 142, 145, 241 or equivalent courses in basic statistics, linear and logistic regression
Also listed as: PB HLTH C242C
Longitudinal Data Analysis: Read Less [-]
STAT 248 Analysis of Time Series 4 Units
Terms offered: Spring 2022, Spring 2021, Spring 2020 Frequency-based techniques of time series analysis, spectral theory, linear filters, estimation of spectra, estimation of transfer functions, design, system identification, vector-valued stationary processes, model building. Analysis of Time Series: Read More [+]
Prerequisites: 102 or equivalent
Analysis of Time Series: Read Less [-]
STAT 251 Stochastic Analysis with Applications to Mathematical Finance 3 Units
Terms offered: Spring 2008, Spring 2006, Spring 2005 The essentials of stochastic analysis, particularly those most relevant to financial engineering, will be surveyed: Brownian motion, stochastic integrals, Ito's formula, representation of martingales, Girsanov's theorem, stochastic differential equations, and diffusion processes. Examples will be taken from the Black-Scholes-Merton theory of pricing and hedging contingent claims such as options, foreign market derivatives, and interest rate related contracts. Stochastic Analysis with Applications to Mathematical Finance: Read More [+]
Prerequisites: 205A or consent of instructor
Stochastic Analysis with Applications to Mathematical Finance: Read Less [-]
STAT 254 Modern Statistical Prediction and Machine Learning 4 Units
Terms offered: Fall 2024, Spring 2024, Fall 2023 This course is about statistical learning methods and their use for data analysis. Upon completion, students will be able to build baseline models for real world data analysis problems, implement models using programming languages and draw conclusions from models. The course will cover principled statistical methodology for basic machine learning tasks such as regression, classification, dimension reduction and clustering. Methods discussed will include linear regression, subset selection, ridge regression, LASSO, logistic regression, kernel smoothing methods, tree based methods, bagging and boosting, neural networks, Bayesian methods, as well as inference techniques based on resampling, cross validation and sample splitting. Modern Statistical Prediction and Machine Learning: Read More [+]
Prerequisites: STAT 135 , the combination of DATA/STAT/COMPSCI C100 and DATA/STAT C140, or equivalent
STAT 256 Causal Inference 4 Units
Terms offered: Fall 2024, Fall 2023, Fall 2022 This course will focus on approaches to causal inference using the potential outcomes framework. It will also use causal diagrams at an intuitive level. The main topics are classical randomized experiments, observational studies, instrumental variables, principal stratification and mediation analysis. Applications are drawn from a variety of fields including political science, economics, sociology, public health, and medicine. This course is a mix of statistical theory and data analysis. Students will be exposed to statistical questions that are relevant to decision and policy making. Causal Inference: Read More [+]
Prerequisites: Statistics 201B or Statistics 210A
STAT 259 Reproducible and Collaborative Statistical Data Science 4 Units
Credit Restrictions: Students will receive no credit for Statistics 259 after taking Statistics 159.
STAT 260 Topics in Probability and Statistics 3 Units
Terms offered: Fall 2024, Spring 2024, Spring 2023 Special topics in probability and statistics offered according to student demand and faculty availability. Topics in Probability and Statistics: Read More [+]
Topics in Probability and Statistics: Read Less [-]
STAT C261 Quantitative/Statistical Research Methods in Social Sciences 3 Units
Terms offered: Spring 2016, Spring 2015, Spring 2014 Selected topics in quantitative/statistical methods of research in the social sciences and particularly in sociology. Possible topics include: analysis of qualitative/categorical data; loglinear models and latent-structure analysis; the analysis of cross-classified data having ordered and unordered categories; measure, models, and graphical displays in the analysis of cross-classified data; correspondence analysis, association analysis, and related methods of data analysis. Quantitative/Statistical Research Methods in Social Sciences: Read More [+]
Fall and/or spring: 15 weeks - 2 hours of lecture per week
Additional Format: Two hours of Lecture per week for 15 weeks.
Also listed as: SOCIOL C271D
Quantitative/Statistical Research Methods in Social Sciences: Read Less [-]
STAT 265 Forecasting 3 Units
Terms offered: Spring 2024 Forecasting has been used to predict elections, climate change, and the spread of COVID-19. Poor forecasts led to the 2008 financial crisis. In our daily lives, good forecasting ability can help us plan our work, be on time to events, and make informed career decisions. This practically-oriented class will provide students with tools to make good forecasts, including Fermi estimates, calibration training, base rates, scope sensitivity, and power laws. Forecasting: Read More [+]
Course Objectives: We’ll discuss several historical instances of successful and unsuccessful forecasts, and practice making forecasts about our own lives, about current events, and about scientific progress.
STAT 272 Statistical Consulting 3 Units
Terms offered: Fall 2024, Spring 2024, Fall 2023 To be taken concurrently with service as a consultant in the department's drop-in consulting service. Participants will work on problems arising in the service and will discuss general ways of handling such problems. There will be working sessions with researchers in substantive fields and occasional lectures on consulting. Statistical Consulting: Read More [+]
Prerequisites: Some course work in applied statistics and permission of instructor
Fall and/or spring: 15 weeks - 2 hours of session per week
Additional Format: Two hours of session per week and individual meetings as necessary.
Grading: Offered for satisfactory/unsatisfactory grade only.
Statistical Consulting: Read Less [-]
STAT 278B Statistics Research Seminar 1 - 4 Units
Terms offered: Fall 2024, Spring 2024, Fall 2023 Special topics, by means of lectures and informational conferences. Statistics Research Seminar: Read More [+]
Fall and/or spring: 15 weeks - 0 hours of seminar per week
Additional Format: Two or more hours of seminar per week.
Statistics Research Seminar: Read Less [-]
STAT 298 Directed Study for Graduate Students 1 - 12 Units
Terms offered: Fall 2024, Spring 2024, Fall 2023 Special tutorial or seminar on selected topics. Directed Study for Graduate Students: Read More [+]
Summer: 6 weeks - 1-16 hours of independent study per week 8 weeks - 1-12 hours of independent study per week
Additional Format: Zero hours of Independent study per week for 15 weeks. One to Twelve hour of Independent study per week for 8 weeks. One to Sixteen hour of Independent study per week for 6 weeks.
Directed Study for Graduate Students: Read Less [-]
STAT 299 Individual Study Leading to Higher Degrees 0.5 - 12 Units
Terms offered: Fall 2024, Spring 2024, Fall 2023 Individual study Individual Study Leading to Higher Degrees: Read More [+]
Fall and/or spring: 15 weeks - 2-36 hours of independent study per week
Summer: 6 weeks - 4-45 hours of independent study per week 8 weeks - 3-36 hours of independent study per week 10 weeks - 2.5-27 hours of independent study per week
Additional Format: Work hours to be arrange based on unit value. Offered for 1-6 units during Summer Session.
Individual Study Leading to Higher Degrees: Read Less [-]
STAT 375 Professional Preparation: Teaching of Probability and Statistics 2 - 4 Units
Terms offered: Fall 2024, Spring 2024, Fall 2023 Discussion, problem review and development, guidance of laboratory classes, course development, supervised practice teaching. Professional Preparation: Teaching of Probability and Statistics: Read More [+]
Prerequisites: Graduate standing and appointment as a graduate student instructor
Fall and/or spring: 15 weeks - 2 hours of lecture and 4 hours of laboratory per week
Additional Format: One or two hours of lecture and two to four of laboratory per week.
Subject/Course Level: Statistics/Professional course for teachers or prospective teachers
Formerly known as: Statistics 300
Professional Preparation: Teaching of Probability and Statistics: Read Less [-]
STAT 601 Individual Study for Master's Candidates 0.5 - 8 Units
Terms offered: Fall 2024, Spring 2024, Fall 2023 Individual study in consultation with the graduate adviser, intended to provide an opportunity for qualified students to prepare themselves for the master's comprehensive examinations. Units may not be used to meet either unit or residence requirements for a master's degree. Individual Study for Master's Candidates: Read More [+]
Repeat rules: Course may be repeated for credit up to a total of 16 units.
Fall and/or spring: 15 weeks - 0.5-8 hours of independent study per week
Summer: 6 weeks - 1.5-20 hours of independent study per week 8 weeks - 1-15 hours of independent study per week 10 weeks - 1-12 hours of independent study per week
Additional Format: One-half to eight hours of independent study per week. One to twelve hours of independent study per week for 10 weeks. One to fifteen hours of independent study per week for 8 weeks. One and one-half to twenty hours of independent study per week for 6 weeks.
Subject/Course Level: Statistics/Graduate examination preparation
Individual Study for Master's Candidates: Read Less [-]
STAT 602 Individual Study for Doctoral Candidates 0.5 - 8 Units
Terms offered: Fall 2024, Spring 2024, Fall 2023 Individual study in consultation with the graduate adviser, intended to provide an opportunity for qualified students to prepare themselves for certain examinations required of candidates for the Ph.D. degree. Individual Study for Doctoral Candidates: Read More [+]
Prerequisites: One year of full-time graduate study and permission of the graduate adviser
Credit Restrictions: Course does not satisfy unit or residence requirements for doctoral degree.
Individual Study for Doctoral Candidates: Read Less [-]
STAT 700 Statistics Colloquium 0.0 Units
Terms offered: Prior to 2007 The Statistics Colloquium is a forum for talks on the theory and applications of Statistics to be given to the faculty and graduate students of the Statistics Department and other interested parties. Statistics Colloquium: Read More [+]
Fall and/or spring: 15 weeks - 1-2 hours of colloquium per week
Additional Format: One to two hours of colloquium per week.
Grading: The grading option will be decided by the instructor when the class is offered.
Formerly known as: Statistics 999
Statistics Colloquium: Read Less [-]
Contact Information
Department of statistics.
367 Evans Hall
Phone: 510-642-2781
Fax: 510-641-7892
Department Chair
Prof. Haiyan Huang
PhD Program Coordinator
La Shana Porlaris
373 Evans Hall
Phone: 510-642-5361
Master's Program Coordinator
David Apilado Jr.
375 Evans Hall
Phone: 510-643-0589
Print Options
When you print this page, you are actually printing everything within the tabs on the page you are on: this may include all the Related Courses and Faculty, in addition to the Requirements or Overview. If you just want to print information on specific tabs, you're better off downloading a PDF of the page, opening it, and then selecting the pages you really want to print.
The PDF will include all information unique to this page.
You are using an outdated browser. Please upgrade your browser to improve your experience.
Updating Live
Most active programs.

Department of Demography
University of California Berkeley
- Graduate Program
UC Berkeley Demography offers three graduate degree tracks independently and in conjunction with the department of Sociology.
Ph.D. in Demography
The doctoral program is intended to provide advanced training in demography, with particular attention to research and theoretical issues.
Graduate Group in Sociology and Demography (GGSD)
The purpose of this Ph.D. program, administered by the Graduate Group in Sociology and Demography (GGSD), is to foster intellectual exchange between graduate students and faculty in the two disciplines, and to improve the competitiveness of graduate students with interests in both sociology and demography on the academic job market.
Master of Arts in Demography
The terminal Master’s program is intended to provide training for those interested exclusively in the M.A. in Demography and for those who will acquire the degree in addition to a doctoral degree in another subject. Applicants interested in earning a Ph.D. in Demography should apply directly to the Ph.D. program, whether or not they already hold a Master’s degree.
Occasionally students holding or earning a Master’s degree in another subject may wish to earn an M.A. in Demography as well. They may do so provided that there is no overlap between the courses used to satisfy the requirements for the two degrees. Individuals holding a Master’s degree in Demography from another institution (even if under a different title, like Population Studies) cannot earn an M.A. in Demography at UC Berkeley.
Common First-Year Curriculum
The first-year curriculum is designed to introduce all graduate students to the essentials of demographic theory and methods. Therefore, students on all three degree tracks begin by completing a set of required courses in the first year as outlined below. Students in the doctoral program take the same curriculum in the first year as Master’s students and sit for the same written examinations, which they must pass at a level commensurate with the requirements of the doctoral program. All first-year courses, with the exception of DEMOG 213, must be taken on a letter-grade basis.
Fall semester
Spring semester.
Toward the end of the Spring semester of the first year, students take an M.A. Qualifying/Ph.D. Preliminary Exam. This M.A. exam is a written test of fluency in demographic theory, methods, and substance. The exam consists of two 3-hour long sessions, one devoted to methods and the other to substance and theory. Although all of the content included on the M.A. exam is covered in the required courses, the exam is not a simple repetition of course exams. Instead, students are required to demonstrate the ability to synthesize forms of knowledge acquired in different courses and to think critically about the assumptions and implications of demographic models. Non-circulating copies of old exams are available in the Sharlin Library and are now also available on-line to Demography students.
Upon the successful completion of the first-year coursework and the M.A. exam, students earn their Master’s degrees in Demography. Students in the doctoral programs continue on their respective tracks, fulfilling the requirements for advancement to candidacy and submitting their dissertations.
Demography Ph.D. Degree Requirements
Those working for a doctoral degree are expected to enter with, or obtain concurrently at Berkeley, a Master’s degree in a different but related subject (e.g., Sociology , Economics , Anthropology , Statistics , Public Policy , Public Health , Biostatistics , Regional Planning , Geography ). This ancillary Master’s degree must be earned before the dissertation stage. We recognize that some students may enter the doctoral program with a Master’s degree in Demography, or with a one in a subject not clearly related to their demographic work. The applicability of such degrees to the doctoral program is determined on an individual basis.
Students in the Ph.D. program must pass an oral qualifying examination before they can be advanced to candidacy. Students are responsible for making all arrangements for the exam including: 1) filling out the necessary paperwork with help from the Graduate Services Advisor at least 3 weeks in advance of your expected exam date, 2) forming an examination committee (see below) with the advice and consent of the Graduate Advisor and/or other faculty members, 3) scheduling a date and time for the exam that is agreeable to all committee members, and 4) preparing reading lists for the two specialized sections of the exam (see below), which must be approved by the Graduate Advisor; 5) providing copies of the final reading lists, plus the two designated “showpiece” articles, to all committee members at least two weeks before the exam.
COMMITTEE MEMBERS
The examination committee must have four members. Students should choose committee members to achieve an appropriate balance in light of their chosen areas of specialization (see below). Two members of the examination committee must be chosen from the regular faculty and/or emeriti of the Department of Demography (Feehan, Goldstein, Hammel, Johnson-Hanks, Lee, Wachter, and Wilmoth). One of these individuals should be designated as chair of the orals committee, although note that the chair of the orals committee cannot also serve as chair of the student´s dissertation committee.
The third “inside member” can be either a regular or an affiliated faculty of the Department. (For a current list of affiliated faculty, please refer to the list of all Faculty members.) Professors from other departments on the Berkeley campus who are not formally designated as affiliates of the Department may serve as the third inside member with approval from the Graduate Advisor. Visiting professors or faculty from other campuses may serve as an inside member with an approval of the Graduate Dean.
One member of the committee must be from outside the Department. Graduate Division guidelines require that the designated “outside member” must belong to the Berkeley Division of the Academic Senate. In other words, the outside member must be regular faculty in another department on the Berkeley campus. Exceptions to this rule are not possible.
Affiliated faculty of the Department of Demography may serve as either the third inside member, or as the sole outside member of the examination committee. Sociology/Demography students should refer to the program description for further details on selection of oral exam committees specific to their program.
AREAS OF SPECIALIZATION
Students are tested in three subject areas, which must include General Demography plus two specialized fields chosen by the student in consultation with the Graduate Advisor. There can be considerable flexibility in the choice of the areas of specialization, but they must not duplicate field exams or other requirements for degrees being earned in other departments. Typical choices include: Fertility, Mortality, Mathematical Demography, Economic Demography, Anthropological Demography, Migration, Historical Demography, Family/Kinship, etc.
READING LISTS
Reading lists should be prepared by the student for the specialized areas. These lists should be comparable in scope and length to a one-semester course syllabus on the topic. If the student has attended a specialized course on the topic, s/he may wish to use the syllabus for that course as the basis for assembling the reading list, though usually with some modifications to reflect his/her own interests. Each reading list should be organized into sections based on thematic content. Students should consult with the exam committee members who are most knowledgeable in each specialized area and then obtain final approval of their reading lists from the Graduate Advisor at least one month prior to the exam.
Each of the two specialized reading lists must contain one article (or chapter, if appropriate) that is designated as a “showpiece.” The article chosen for this purpose should have analytical depth and be regarded as a major contribution to the broader subject area. Students should be familiar with all aspects (theory, data, methods, policy implications, etc.) of these articles and be prepared to discuss them in detail.
PREPARATION
Students should think about three aspects of preparation for this examination. First, the General Demography section is a test of “what every young demographer should know and be able to explain clearly.” Sample questions include: “What is a synthetic cohort?,” “What is the range of TFRs observed for national populations in the world today?,” “What is the demographic transition?,” “What are stable and stationary populations?,” etc. Clarity of presentation is especially important in this section of the exam.
Second, the two specialized sections of the exam are a test of whether the student could prepare to teach a graduate-level semester course on either of these topics. Students will be asked to demonstrate a broad and detailed knowledge of their chosen areas, although they are not expected to prepare full lectures on specific subjects for sake of the orals exam. Students may also be asked to comment on possibilities for further research in their specialized areas; for example, they could be asked to respond to a question like, “What open questions remain in this area?”
Third, the two showpiece articles are a test of whether the student can give an in-depth evaluation of a specific piece of scholarly research. The student should demonstrate not only a thorough knowledge of the author´s methods, arguments, and findings, but also an ability to analyze the general problem considered in the article and to propose alternative approaches.
The dissertation prospectus is an important step toward completing a doctoral degree in Demography. It is generally the final requirement that a student must complete before being approved for “advancement to candidacy” for the Ph.D. (see also “Guidelines for Advancement to Candidacy, Normative Time, and Filing Fee”). This document describes procedures and suggests a format for the prospectus.
The prospectus must be approved by the faculty member who will serve as chair of the student’s dissertation committee. Signed approval of the prospectus by the dissertation chair is needed before the Graduate Advisor can sign the forms for advancement to candidacy. All decisions about the appropriate form, length, and content of a dissertation prospectus lie with the dissertation chair, not the Graduate Advisor. Thus, the guidelines provided here are merely suggestions, which may serve as a starting point for discussion with the dissertation chair about directions and requirements for a successful prospectus. In making plans for the dissertation prospectus, the student and her/his chair should keep in mind the deadline for advancement to candidacy that will qualify the student for the normative time fee reduction.
As a suggestion, the form and content of the dissertation prospectus should follow (roughly) the specifications for a standard NIH grant application (i.e., an R01 award). The Research Plan in such an application has four essential parts, which are intended to answer the following questions: (1) What do you intend to do? (2) Why is this work important? (3) What has already been done? (4) How are you going to do the work? Thus, the four sections of an NIH grant application, modified as appropriate for a dissertation prospectus, are as follows:
a) Specific Aims. List the research objectives and specific goals of the dissertation research project. State the hypotheses to be tested (1 page is recommended) .
b) Background and Significance. Briefly sketch the background leading to the proposed dissertation, critically evaluate existing knowledge, and specifically identify the gaps which the project is intended to fill (2-3 pages are recommended).
c) Preliminary Studies. Describe previous studies by the student that are relevant to the proposed project. This could include a summary of a class paper, other preliminary data analyses, results of a pilot survey, etc. (6-8 pages are recommended) .
d) Research design and methods. Describe the research design and procedures to be used to accomplish the specific aims of the project. Include how the data will be collected, analyzed, and interpreted. Describe any new methodology and its advantage over existing methodologies. Discuss the potential difficulties and limitations of the proposed procedures and alternative approaches to achieve the aims. Provide also a tentative sequence or time-table for completing the research and writing the dissertation (14-16 pages are recommended) .
The research plan for an NIH grant application may not exceed 25 single-spaced pages, although the distribution of pages within these four section may vary. A dissertation prospectus should be no longer than this, although it could be somewhat shorter (25 double-spaced pages, for example). In any event, the proportional distribution between the four sections should be maintained at least approximately. In particular, it is very important for the fourth section to be the most prominent.
Upon successful completion of the foreign language requirement, the oral qualifying exam, and the prospectus, students enter into the final phase of the program, doctoral candidacy. During this period, students are expected to take another research seminar, Demography 296, every semester until the completion of the dissertation. This phase should last two or three academic years.
Sociology and Demography Ph.D. (GGSD) Requirements
The Demography preliminary examination must be passed at a level commensurate with the usual requirements for the doctoral program in the Department of Demography in order for a student to continue toward the Ph.D. in Sociology and Demography.
Consistent with the standard practice in the Department of Sociology, students in the joint program are required to write an M.A. research paper. This paper is written with the supervision and approval of a personal M.A. committee comprising two Sociology faculty members. The student’s M.A. committee must approve in advance all details regarding the design, research, content, length, and timetable for the research paper project. Each candidate for the M.A. selects a faculty member as the Chair of her/his M.A. committee (with that professor’s approval) and a second reader. The student may ask the Graduate Advisor of the GGSD to assist in finding a second reader. The M.A. research paper requirement is fulfilled when both members of the M.A. committee have signed and dated the cover page.
After all the coursework, methods, and paper requirements in Sociology are met, each student in the program is required to have an M.A. Advising Conference, organized by GGSD in conjunction with the Department of Sociology (and following the rules prevailing in that department), where it is decided whether the student satisfies doctoral requirements or not. Thus, in addition to the aforementioned requirements in the Department of Demography a student must satisfy the usual doctoral requirements in the Department of Sociology in order to continue toward the Ph.D. in Sociology and Demography.
This program includes one oral Qualifying Examination, to be taken when all pre-qualifying requirements have been met, including the language examination. The orals committee consists of five members, all of whom must be members of the Berkeley Division of the Academic Senate. At least three but not more than four of the committee members must be core faculty in the GGSD, with at least one committee member representing the Department of Demography and another representing the Department of Sociology. One of these “inside” members will serve as chair of the Orals Committee. That person may not also serve as Chair of the student’s dissertation committee. At least one and not more than two members must be outside of the core faculty of the GGSD. The Graduate Adviser of the GGSD recommends the membership of the Qualifying Exam committee and the topics of examination to the Graduate Dean.
The student prepares four fields of study for the oral Qualifying Examination, including Sociological Theory, General Demography, and two specialized fields in sociology and/or demography. Preparation for the exam is supervised by the Chair of the orals committee, who may separate and delegate the task of supervision to other committee members with expertise in one of the student’s fields of examination. The oral Qualifying Examination itself consists of questions on each of the four topics, in an order chosen by the student. For a successful outcome, the committee must vote unanimously for a “pass.” The jurisdiction for ruling on split votes rests with the Administrative Committee.
After passing the oral Qualifying Examination, the student assembles a provisional dissertation committee. This provisional committee will become the permanent dissertation committee once the student is advanced to candidacy for the Ph.D. This committee will consist of three members, including two core faculty member in the GGSD and one outside member who does not belong to core faculty of the GGSD. All committee members must be members of the Berkeley Division of the Academic Senate.
With the guidance of the Chair of the provisional committee, the student prepares a dissertation prospectus. Once the provisional committee approves the dissertation prospectus, the student will be advanced to candidacy for the Ph.D. Once the dissertation committee members have accepted the dissertation, they sign and date the cover page. A public dissertation defense is not required although students are strongly encouraged to give a final presentation to the Graduate Group.
As with the oral Qualifying Examination, dissertation committee members are chosen by the Graduate Adviser of the GGSD in consultation with the student. The dissertation committee is approved formally by the Graduate Dean.
The graduate program in Sociology and Demography does not allow students to earn a joint M.A. degree since the goal of the program is doctoral studies only. If there is no overlap between required courses and other requirements, students can earn both an M.A. in Sociology and an M.A. in Demography at the discretion of the two Departments. These degrees are not the end goal, but rather milestones en route towards the Ph.D. However, in those cases where a student’s progress is not deemed sufficient for advancement to candidacy for the Ph.D. in Sociology and Demography, terminal M.A. degrees may be granted by one or both departments.
Graduate Group in Sociology and Demography Core Faculty
Daniel Aldana Cohen, Assistant Professor of Sociology
Irene Bloemraad, Professor of Sociology
William Dow, Professor of Public Health
Dennis Feehan, Assistant Professor of Demography
Neil Fligstein, Professor of Sociology
Marion Fourcade, Professor of Sociology
Joshua Goldstein, Professor of Demography
David Harding, Associate Professor of Sociology
Heather Haveman, Professor of Sociology
Jennifer Johnson-Hanks, Professor of Demography and Sociology
Rucker Johnson, Associate Professor of Public Policy
Danya Lagos, Assistant Professor of Sociology
Mara Loveman, Professor of Sociology
Samuel Lucas, Professor of Sociology
Ayesha Mahmud, Assistant Professor of Demography
Jane Mauldon, Associate Teaching Professor of Public Policy
Trond Petersen, Professor of Sociology
Sandra Smith, Professor of Sociology
Comprehensive list of Sociology and Demography Ph.D. Program requirements 202 3
- About Berkeley Demography
- About Our People
- Graduate Students
- Students & Alumni
- Visitors, Post-Docs & Trainees
- Graduate Admission Information
- Undergraduate Minor
- The Demography Lab
- Our Publications
- Berkeley Interdisciplinary Migration Initiative
- Berkeley Opportunity Lab
- Berkeley Population Center
- Bixby Center
- California Policy Lab
- Human Mortality Database
- Events Calendar
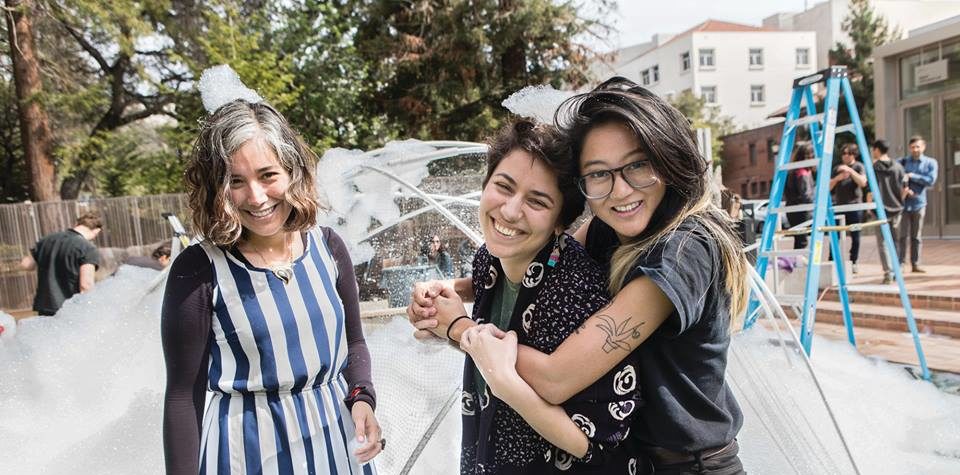
Berkeley Graduate Student Profile
Berkeley graduate student profile 2022-23.
UC Berkeley is famed for the breadth, depth, and reach of more than 120 graduate programs in 15 schools and colleges. Our passion for critical inquiry, debate, discovery and innovation is informed by a deep commitment to contributing to a better world. Nearly all first-year students cite the outstanding reputation and quality of their graduate program as important in their decision to enroll at Berkeley. Typically, half of admitted applicants choose Berkeley over other offers. View our Graduate Student Profile brochure as a PDF .
Career Outcomes
Student funding, rankings and other measures of excellence.
Overall UC Berkeley rankings can be found here .
U.S. News and World Report
Yet again U.S. News & World Report ranked UC Berkeley as the number 1 public university in the nation, tied with UCLA.
The new “Best Global Universities Rankings” of U.S. News & World Report placed UC Berkeley fourth in the world . Only Harvard, MIT, and Stanford ranked higher.
In the new rankings, Berkeley’s graduate programs placed first in the world in English, history, psychology, and sociology; second in chemistry, computer science, earth sciences, physics and statistics. Nine other subject areas ranked in the top 10.
- 1st – English, History, Psychology, Sociology
- 2nd – Chemistry, Computer Science, Earth Sciences, Physics, Statistics
- 3rd – Mathematics, Biological Sciences, Clinical Psychology, Engineering, Social Welfare, Public Affairs
- 4th – Economics, Political Science
- 7th – Biostatistics
- 8th – Business (#2 in Part-time MBA), Public Health
- 9th – Law (#1 for business corporate law)
Academic Rankings of World Universities
New global rankings place UC Berkeley at the pinnacle of public universities on the U.S. and fifth overall in the world . The 2022 Academic Rankings of World Universities is an assessment of 500 top institutions around the globe by the ShanghaiRanking Consultancy.
Among 54 subject areas, Berkeley ranked first in the world in chemistry and electrical and electronic engineering, and third in the world in computer science and engineering and statistics.
In addition, Berkeley ranked fifth in economics and sociology.
Berkeley Stands Tall in World Reputation Rankings
For the sixth year in a row, UC Berkeley ranks sixth in the world when it comes to its reputation among its peers, according to new rankings from Times Higher Education .
The six “most powerful global brands” in the U.K. publication’s annual World Reputation Rankings vary little from year to year, with Harvard at the top, then MIT, Stanford, Oxford, Cambridge, and then Berkeley.
Berkeley Again Shines as Top U.S. Public University in Forbes Rankings
New rankings from the financial publication Forbes , again place Berkeley at the very top of the list of public universities in the United States.
On the new Forbes Top Colleges 2021 list, Berkeley is the only public university among the top five schools overall.
Graduate Alumni Accomplishments
UC Berkeley alumni are among the most accomplished in their fields. Below is just a snapshot of graduate alumni accolades:
- 26 faculty Nobel Prize winners,including 10 current faculty members; Cal Facts 2022
- 32 alumni Nobel Prize winners; Cal Facts 2022
- 75 Fellows from 1981 to 2022; MacArthur Fellows
- 30 National Medal of Science Recipients; The President’s National Medal of Science: Recipient Search
The Berkeley Graduate Profile is produced by the Institutional Research and Communications units in the Graduate Division .
Additional Resources
- Faculty Excellence
- Research Centers
2022-23 Graduate Student Enrollment
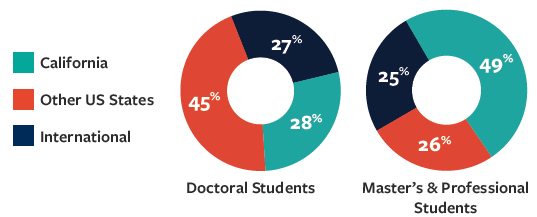
Among the 45,307 students at Berkeley, nearly 28% are pursuing doctoral and master’s degrees in more than 100 disciplines.
The fall 2022 incoming graduate class of 4,041 students was selected from more than 40,000 applicants .
Graduate majors (Fall 2022 Enrollment)
Berkeley offers more than 100 graduate majors (master’s and doctoral), 93 of which grant doctoral degrees.
Degrees Granted
Top 5 academic institutions that hire berkeley ph.d.s.
- University of California System
Long-term employment trends
(Based on a survey of Ph.D. recipients who graduated between 2013 to 2017)
- 54.1% of Berkeley doctoral alumni (and 78% arts and humanities Ph.D.s) work in 4-year academic institutions compared to 41.4% nation-wide
- 31.4% work in the private for-profit sector
- 3.4% work in the non-profit sector or for the state, local or national government
- 3.9% are self-employed or have other employment
Career placement of doctoral degree recipients
First positions of students who graduated between 2011 – 2020 (based on a survey conducted 12-18 months after graduation).
*First positions taken by Berkeley Ph.D.s who went into academia:
Sources of financial support for graduate students (2022 – 2023)
Most admitted doctoral students are funded in full for the majority of their study. In some cases that funding includes teaching and research appointments which are covered by collective bargaining agreements and come with many benefits of employment.
Funding packages
Berkeley offers multi-year funding packages to most entering doctoral students, including those who will enhance the diversity of the graduate student population. Recipients are selected by their departments at the admissions stage.
Multi-year packages typically include:
- 2 years of fellowship funding
- and/or 1 year of Doctoral Completion Fellowship*
Departmental funding often includes funding from a variety of sources, including university fellowships, departmental fellowships, training grant awards, GSI (teaching) appointments, and GSR (research) positions. Note that GSI and GSR positions, and all terms and conditions of employment, are covered by a collective bargaining agreement.
External multi-year fellowships, such as the NSF GRFP, NDSEG (DoD), Hertz, and others, are integrated into departmental funding packages.
*The Doctoral Completion Fellowship provides one year of fellowship funding to students in certain Humanities and Social Science disciplines, for use after advancement to doctoral candidacy.
Other Campus Funding Opportunities
- Conference Travel Grants
- Parent Grants
- Research Grants/Fellowships (from Institutes/Centers and others)
- Training Grant Programs
Doctoral Program
Program summary.
Students are required to
- master the material in the prerequisite courses ;
- pass the first-year core program;
- attempt all three parts of the qualifying examinations and show acceptable performance in at least two of them (end of 1st year);
- satisfy the depth and breadth requirements (2nd/3rd/4th year);
- successfully complete the thesis proposal meeting (winter quarter of the 3rd year);
- present a draft of their dissertation and pass the university oral examination (4th/5th year).
The PhD requires a minimum of 135 units. Students are required to take a minimum of nine units of advanced topics courses (for depth) offered by the department (not including literature, research, consulting or Year 1 coursework), and a minimum of nine units outside of the Statistics Department (for breadth). Courses for the depth and breadth requirements must equal a combined minimum of 24 units. In addition, students must enroll in STATS 390 Statistical Consulting, taking it at least twice.
All students who have passed the qualifying exams but have not yet passed the Thesis Proposal Meeting must take STATS 319 at least once each year. For example, a student taking the qualifying exams in the summer after Year 1 and having the dissertation proposal meeting in Year 3, would take 319 in Years 2 and 3. Students in their second year are strongly encouraged to take STATS 399 with at least one faculty member. All details of program requirements can be found in our PhD handbook (available to Stanford affiliates only, using Stanford authentication. Requests for access from non-affiliates will not be approved).
Statistics Department PhD Handbook
All students are expected to abide by the Honor Code and the Fundamental Standard .
Doctoral and Research Advisors
During the first two years of the program, students' academic progress is monitored by the department's Graduate Director. Each student should meet at least once a quarter with the Graduate Director to discuss their academic plans and their progress towards choosing a thesis advisor (before the final study list deadline of spring of the second year). From the third year onward students are advised by their selected advisor.
Qualifying Examinations
Qualifying examinations are part of most PhD programs in the United States. At Stanford these exams are intended to test the student's level of knowledge when the first-year program, common to all students, has been completed. There are separate examinations in the three core subjects of statistical theory and methods, applied statistics, and probability theory, which are typically taken during the summer at the end of the student's first year. Students are expected to attempt all three examinations and show acceptable performance in at least two of them. Letter grades are not given. Qualifying exams may be taken only once. After passing the qualifying exams, students must file for Ph.D. Candidacy, a university milestone, by the end of spring quarter of their second year.
While nearly all students pass the qualifying examinations, those who do not can arrange to have their financial support continued for up to three quarters while alternative plans are made. Usually students are able to complete the requirements for the M.S. degree in Statistics in two years or less, whether or not they have passed the PhD qualifying exams.
Thesis Proposal Meeting and Dissertation Reading Committee
The thesis proposal meeting is intended to demonstrate a student's depth in some areas of statistics, and to examine the general plan for their research. In the meeting the student gives a 60-minute presentation involving ideas developed to date and plans for completing a PhD dissertation, and for another 60 minutes answers questions posed by the committee. which consists of their advisor and two other members. The meeting must be successfully completed by the end of winter quarter of the third year. If a student does not pass, the exam must be repeated. Repeated failure can lead to a loss of financial support.
The Dissertation Reading Committee consists of the student’s advisor plus two faculty readers, all of whom are responsible for reading the full dissertation. Of these three, at least two must be members of the Statistics Department (faculty with a full or joint appointment in Statistics but excluding for this purpose those with only a courtesy or adjunct appointment). Normally, all committee members are members of the Stanford University Academic Council or are emeritus Academic Council members; the principal dissertation advisor must be an Academic Council member.
The Doctoral Dissertation Reading Committee form should be completed and signed at the Dissertation Proposal Meeting. The form must be submitted before approval of TGR status or before scheduling a University Oral Examination.
For further information on the Dissertation Reading Committee, please see the Graduate Academic Policies and Procedures (GAP) Handbook section 4.8.
University Oral Examinations
The oral examination consists of a public, approximately 60-minute, presentation on the thesis topic, followed by a 60 minute question and answer period attended only by members of the examining committee. The questions relate to the student's presentation and also explore the student's familiarity with broader statistical topics related to the thesis research. The oral examination is normally completed during the last few months of the student's PhD period. The examining committee typically consists of four faculty members from the Statistics Department and a fifth faculty member from outside the department serving as the committee chair. Four out of five passing votes are required and no grades are given. Nearly all students can expect to pass this examination, although it is common for specific recommendations to be made regarding completion of the thesis.
The Dissertation Reading Committee must also read and approve the thesis.
For further information on university oral examinations and committees, please see the Graduate Academic Policies and Procedures (GAP) Handbook section 4.7 .
Dissertation
The dissertation is the capstone of the PhD degree. It is expected to be an original piece of work of publishable quality. The research advisor and two additional faculty members constitute the student's dissertation reading committee.
Commencement 2024
The UC Berkeley Department of Statistics celebrated the graduates of the class of 2024 on Wednesday, May 15th, at Zellerbach Playhouse on the Berkeley campus. Department Chair Haiyan Huang led commencement activities, which featured an address by Professor Kate Crawford. After the ceremony, family and friends joined the graduates for a reception at the Alumni House.
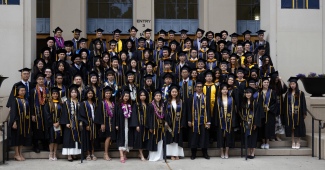
Department Citation in the Statistics Master's Program Given the recognition of excellence in the theory and application of statistics at the master's level and outstanding performance in the master's program, The 2024 winners are Nicolás Nuñez Sahr and Aarushi Somani.
Department Citation in Probability Given in recognition of an outstanding Ph.D. student in probability. The 2024 winner is Adam Jaffee, whose research focuses on the manner in which mathematical statistics develops rigorous foundations for problems at the intersection of statistics and geometry.
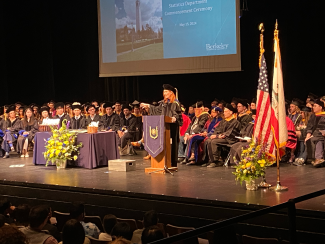
Eric L. Lehman Citation Established in 1999, this prize consists of a gift certificate for a scientific book and a citation. It is awarded for an outstanding Ph.D. dissertation in theoretical statistics (interpreted broadly). Nikhil Ghosh is the 2024 Lehman winner.
Outstanding Graduate Student Instructor Award The Outstanding Graduate Student Instructor (OGSI) Award honors UC Berkeley GSIs each year for their outstanding work in teaching on the Berkeley campus. These OGSI recipients are nominated from within their teaching departments. The GSI Teaching & Resource Center provides the award recipients certificates of distinction and hosts a celebratory ceremony in the spring. This award is sponsored by the GSI Teaching & Resource Center. The 2024 winners are:
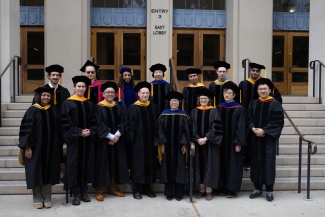
Tae Joo Ahn "Near-optimal multiple testing procedure in Bayesian linear models" Advised By: Professor Song Mei
Nikhil Ghosh "Theoretical Foundations of Deep Learning: Optimization, Generalization, and Scaling" Advised By: Professors Bin Yu & Song Mei
Amanda Glazer “From Bikers to Batters: Steering Statistics Through Real-World Problems” Advised By: Professor Philip Stark
Ella Hiesmayr “The spectral edge of sparse random graphs and mean-field interacting branching processes" Advised By: Professor Steven N. Evans & Shirshendu Ganguly
Adam Quinn Jaffe "Geometry, Analysis, and Optimization in Probability Theory" Advised By: Professor Steven N. Evans
Shuni Li "Statistical and Computational Methods for Applications in Materials Science and Biology" Advised By: Professor Haiyan Huang
Benjamin Yenji Lu "Essays on Causal Inference" Advised By: Professors Avi Feller & Peng Ding
Facundo Sapienza “Physics-Informed Machine Learning for the Earth Sciences: Applications to Glaciology and Paleomagnetism” Advised By: Professors Fernando Pérez & Jonathan Taylor Jacob Spertus "Stratified sequential nonparametrics: inferential validity by design, any way you slice it" Advised By: Professor Philip Stark Biostatistics:
James Duncan “Trustworthy Data Science: Scalable Software Frameworks and Interdisciplinary Geoscience Research” Advised By: Professor Bin Yu
Hao Wang "Statistical Analysis and Visualization of scRNA-Seq data at Population Scale" Advised By: Professor Elizabeth Purdom Yutong Wang "Statistical and Machine Learning Methods for Understanding Spatial Biology and Cellular Communication” Advised By: Professor Yun S. Song Commencement Address - Kate Crawford Kate Crawford is an internationally leading scholar of artificial intelligence and its impacts. She is a research professor at the University of Southern California in Los Angeles, a senior principal researcher at Microsoft Research in New York, and was the inaugural Visiting Chair in AI and Justice at the École Normale Supérieure in Paris. Her research has appeared in venues such as Nature and Science, Technology & Human Values, and she has advised policymakers in the United Nations, the White House, and the European Parliament. Her book Atlas of AI: Power, Politics, and the Planetary Costs of Artificial Intelligence has won multiple awards including the Sally Hacker Prize, is translated into more than ten languages, and was named one of the books of the year by both New Scientist and The Financial Times. Kate is a joint winner of the Ayrton Prize from the British Society for the History of Science.
In addition to her academic research, Kate produces art installations and visual investigations which have been shown in more than a hundred museums and biennales around the world. Her project Anatomy of an AI System with Vladan Joler is currently exhibited at the MoMA in New York (2022-2024) and is in the museum's permanent collection, as well as the permanent collections of the V&A in London, the Ars Electronica Center, and the Design Museum in London. It was also given the Design of the Year Award. Their new project, Calculating Empires: A Genealogy of Power and Technology premiered at Fondazione Prada in Milan, and was next exhibited at the KW Institute in Berlin in 2024.
Kate leads the Knowing Machines Project, a transatlantic research collaboration of scientists, artists, and legal scholars that investigates training data. She was named on the TIME100 list in 2023 as one of the most influential people in AI.
Chair Haiyan Huang on the class of 2024 “As graduates from the Statistics Department, you are poised to be at the forefront of creating a more just and secure world. Your expertise in statistics and data science is vital across numerous fields, including technology, medicine, finances, material sciences, criminal justice, politics, and even professional athletics. Use your skills to learn from data, promote ethical data usage, and shape a better future. We are counting on you to make a significant impact and drive positive change in the world!”
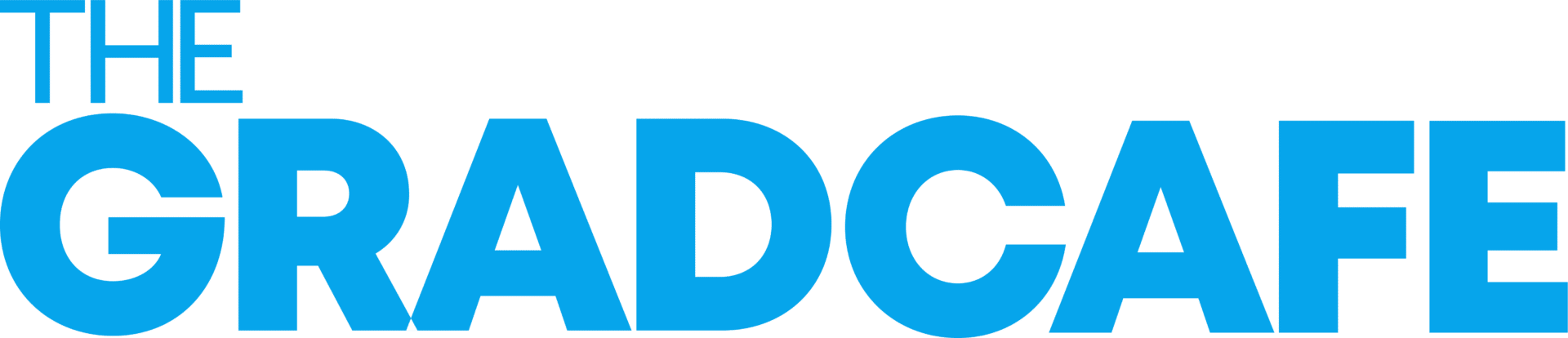
- Majors & Careers
- Online Grad School
- Preparing For Grad School
- Student Life
- Grad Trends: Interest in Artificial Intelligence Surges
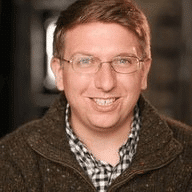
Ignore Artificial Intelligence at your peril. While expansive news coverage might make it seem like another tech fad, our graduate admissions data shows sustained interest from universities and graduate students.
Table of Contents
What The Data Says
Graduate admissions data from The GradCafe shows the percentage of graduate applications related to artificial intelligence makes up about 0.3 percent of all graduate applications on record. That’s more than double the rate we’ve seen in the past, where AI programs (including natural-language processing and machine learning) made up less than a tenth of a percent of applications.
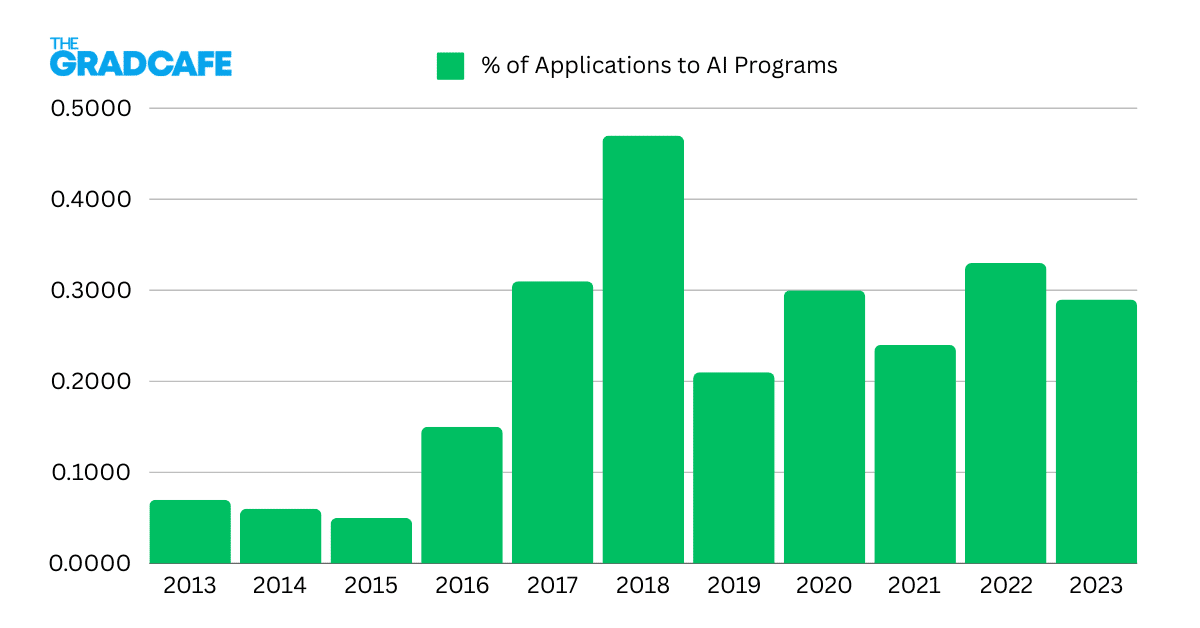
Here’s the full data from applicant surveys. Note the surge of applications in 2016, the spike in 2018, and the ongoing increase through 2023. This is the most recent data available as of May of 2024. The GradCafe will release new data as it becomes available.
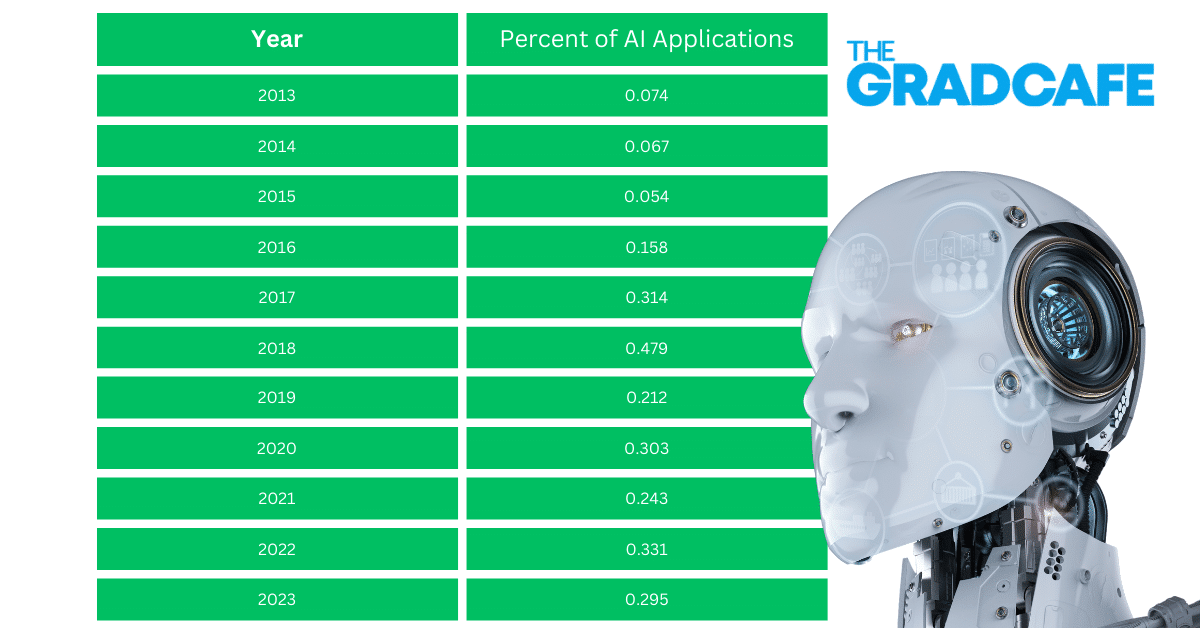
To put this in context, psychology grad programs make up between two and three percent of applications each year. Econ programs also have some of the highest average application rates, usually between 5 and 7.5 percent.
Here’s the data for economics grad programs.
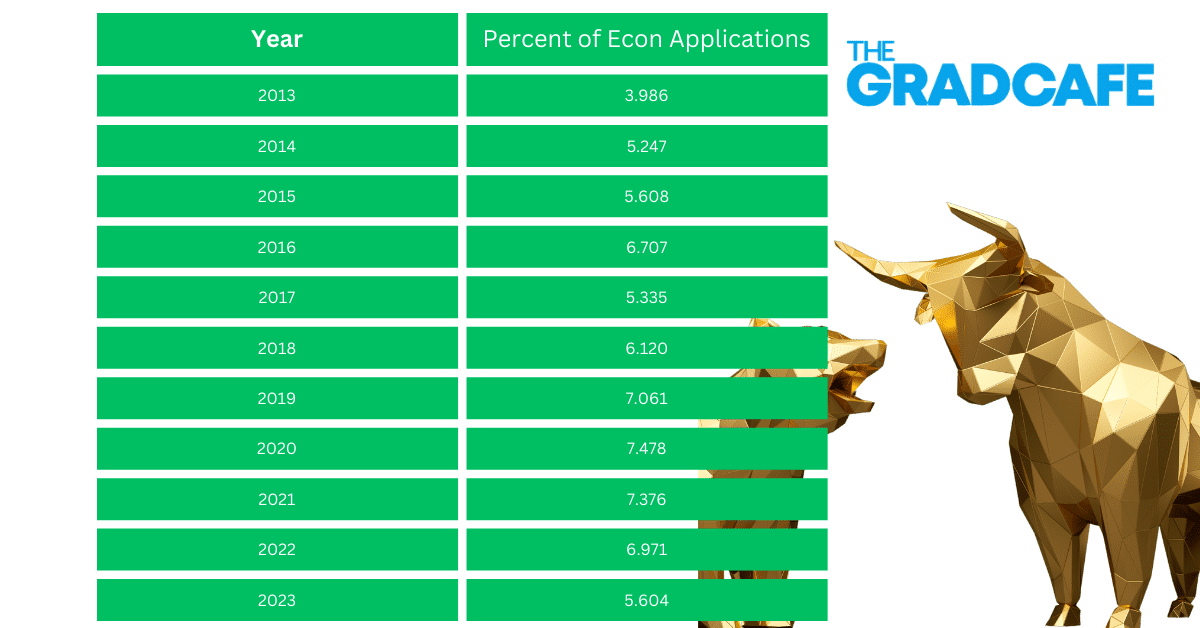
Artificial intelligence programs, often requiring a background in statistics and some programming knowledge like Python, are not included in the overarching computer science degree programs. This wide categorization usually makes up six to 10 percent of annual graduate applications.
As always, you can review live graduate admissions results at The GradCafe.
The Changing Landscape of AI Grad Programs
It’s also important to consider how these graduate programs have changed. Their names provide one insight into each program’s focus, and a trend in naming conventions shows how the industry has evolved.
Over the past ten years, the conversation shifted away from the popular “machine learning” label into the more general title of “artificial intelligence”. And note that computer vision spiked in 2015.
Here’s the data.
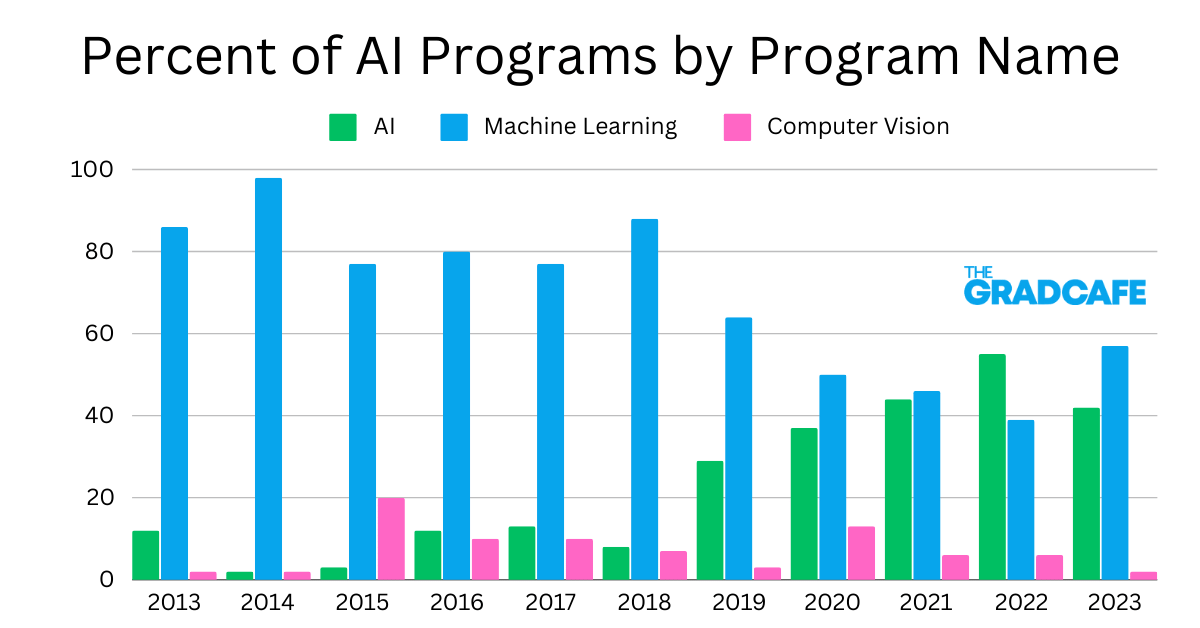
How Graduate Admissions Data Affects the Working World
When we evaluate graduate admissions data, we always consider the working world. Consider self-driving cars. In a 2016 report , Stanford cited transportation as one of the most salient industries for artificial intelligence. The GradCafe’s graduate admissions data reflects that.
Tesla’s first “autopilot” feature rolled out in late 2015 . (Note the spike of interest in “computer vision” grad programs that coincides with Tesla’s announcement.) And in 2016, graduate admissions for AI programs surged. That number continued to climb well before the release of chat-GPT, which launched in 2022.
The surge of applications for artificial intelligence graduate programs mirrors a real-world drive to enhance every industry. After all, artificial intelligence isn’t a monolith. It encompasses machine learning, natural language processing, computer vision, and many other intricacies.
As interest in artificial intelligence has grown, so too have graduate admissions in related programs. For years, universities have offered courses on natural language processing, machine learning, and computer vision. Now we’re seeing a trend where more applicants apply to graduate programs focused specifically on artificial intelligence.
Have you applied to a grad program this season? Please remember to submit your survey when you hear back with an acceptance, rejection, or waitlist.
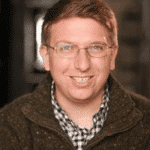
Jim Markus is the director at Venture Kite. He hosted Frugal Living, a popular finance podcast, and co-created The Ink & Blood Dueling Society, a theatrical writing event that appeared at conventions across the United States. He’s also an award-winning game designer and sales professional.
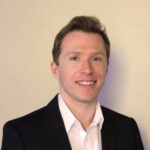
Dr. Robert Johns
Dr. Johns is the Technical Editor for Hackr.io. He has two PhDs (structural and blast engineering) and worked for years as a data scientist.
- Dr. Robert Johns https://blog.thegradcafe.com/author/dr_johns/ Grad Trends: Interest in Artificial Intelligence Surges
- Dr. Robert Johns https://blog.thegradcafe.com/author/dr_johns/ Breaking Records: Yale Sees Most Selective Grad Admissions Season Yet
- Breaking Records: Yale Sees Most Selective Grad Admissions Season Yet
Related Posts
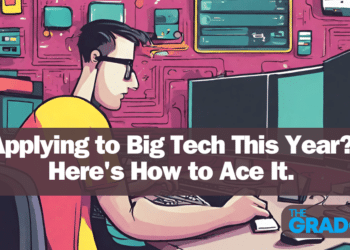
- Applying to Big Tech This Year? Here’s How to Ace It.
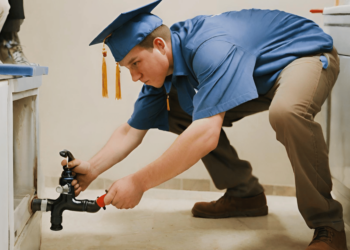
73% of job seekers believe a degree is needed for a well-paying role–but is it?
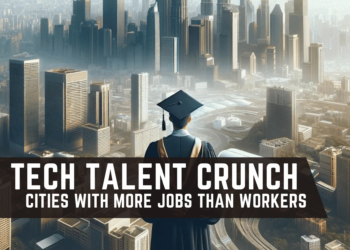
Tech Talent Crunch: Cities with More Jobs Than Workers
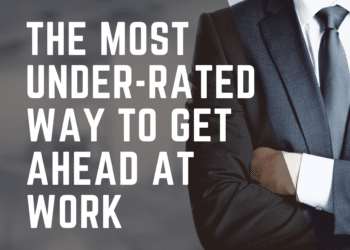
The Most Under-Rated Career Advancement Tip for 2024
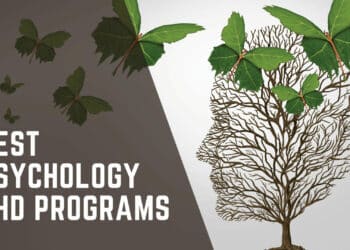
Top 5 Best Psychology PhD Programs in 2024
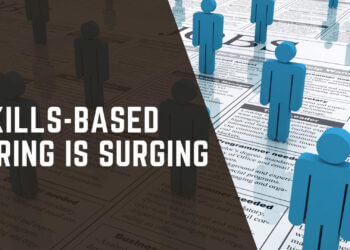
Good News For Early Careers: Skills-Based Hiring is Surging
Leave a reply cancel reply.
Your email address will not be published. Required fields are marked *
Save my name, email, and website in this browser for the next time I comment.
Recent Posts
- 12 Best Laptops for Computer Science Students
- Is a Master’s Degree Worth It? [2024 Guide]
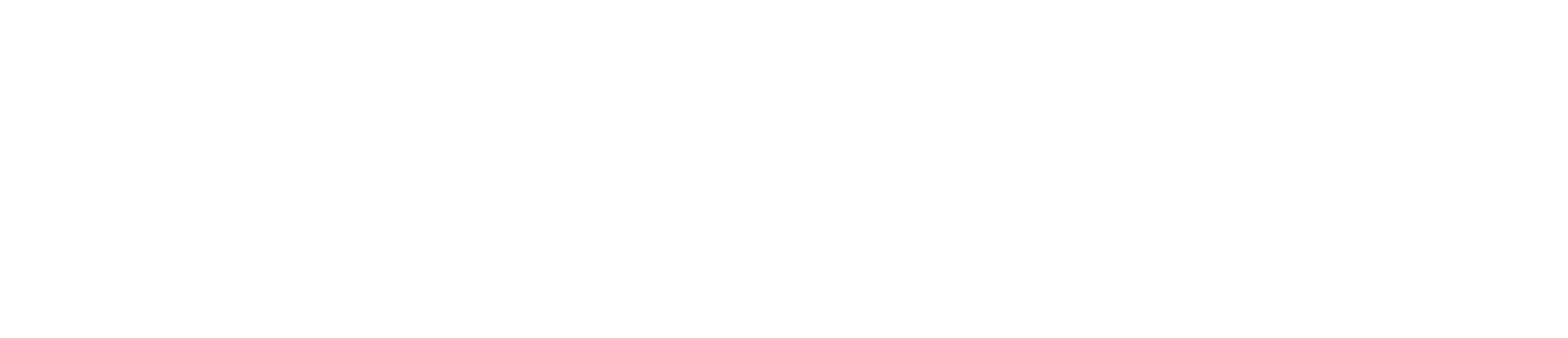
© 2023 TheGradCafe.com All rights reserved
- Partner With Us
- Results Search
- Submit Your Results
- Write For Us
Former Berkeley Law Dean and Prominent Domestic Policy Expert Christopher Edley Jr. Dies at 71

- Share article on Facebook
- Share article on Twitter
- Share article on LinkedIn
- Email article
By Andrew Cohen
Christopher Edley Jr., Berkeley Law’s dean from 2004 to 2013 and a renowned figure in administrative law, education policy, and civil rights, died unexpectedly on May 10 at age 71.
“As dean, he made an enormous positive difference in every aspect of the law school, from the hiring of many terrific faculty, to his initiative to build the South Addition, to dramatically increasing support for public interest grants for students, to the creation of many research centers,” says Berkeley Law Dean Erwin Chemerinsky. “Chris and I were law school classmates. He has been a dear friend and has provided me invaluable wisdom and support in my years as a dean. I know I speak for all of us in saying how terribly much we will miss him.”
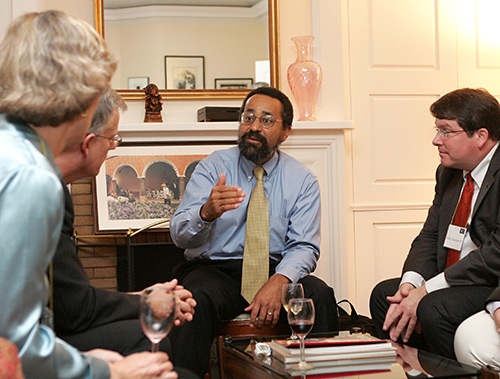
Edley forged a distinguished career in both academia and public service. He spent 23 years as a professor at Harvard Law School, where co-founded the Harvard Civil Rights Project, regularly taught Administrative Law and a Law & Governance seminar, and also taught Environmental Law, National Security Law, Poverty & the Law, Defense Department Procurement Law & Policy, and Taxation.
Born in Boston and raised in Philadelphia and New Rochelle, New York, Edley earned degrees from Swarthmore College, the Harvard School of Public Policy, and Harvard Law School. He served in White House policy and budget positions under Presidents Jimmy Carter and Bill Clinton; for Carter, his responsibilities included welfare reform, social security, food and nutrition programs, and several other anti-poverty agencies.
For Clinton, Edley oversaw budgets and legislative initiatives for five cabinet departments — Justice, Commerce, Housing and Urban Development, Transportation, and Treasury — spanning more than 40 independent agencies, with budget responsibility totaling hundreds of billions of dollars. As senior counsel to Clinton, he also directed a government-wide review of affirmative action programs.
“Chris Edley had a brilliant mind and a kind, good heart that he put to use to build a better, fairer, more just America,” Clinton said in a statement. “From his groundbreaking academic career to his service in multiple key roles in my administration, he always believed that law and policy are ultimately about people. He mastered the minute details but never lost sight of the big picture — giving more people the chance to live their best lives. He is gone far too soon, but his legacy will endure.”
A valued voice
Edley held senior positions in five presidential campaigns, including senior policy adviser for Barack Obama, his former student at Harvard Law. He then served on Obama’s Transition Board with responsibility for education, immigration, and health.
As co-chair of the congressionally chartered National Commission on Education Equity and Excellence from 2011 to 2013, Edley led its charge to recommend pathways for reform and steered a nationwide follow-up effort to advance those recommendations.
He also served as interim dean for UC Berkeley’s School of Education — a role he stepped into at the request of Chancellor Carol Christ — from 2021 to 2023. In that position, Edley helped formalize the school’s undergraduate major in education and the school officially became the Berkeley School of Education after having served only graduate students for more than 50 years.
“As our dean, Chris championed initiatives to expand online education, bolster early childhood education, and supported the school’s groundbreaking statewide effort for equity-based school leadership programs,” says current Dean Michelle D. Young. “Chris has left an indelible mark on the nation’s civil rights dialogue, the Berkeley School of Education, and most certainly on our hearts.”
Young’s full statement about his impact describes how he urged colleagues to embrace the mantra, “Educate like democracy depends on it.”
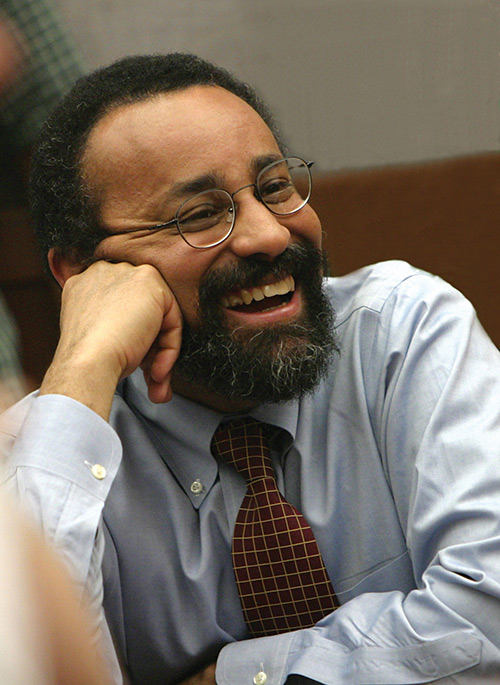
Involved in a wide range of civic endeavors, Edley served on the U.S. Commission on Civil Rights, was a board member with several social justice-focused nonprofits, and co-founded The Opportunity Institute, a charitable non-governmental organization that focuses on federal and state policy related to education and social mobility. He also served on the board of The Century Foundation, the Russell Sage Foundation, and the American Council on Germany.
Edley was a fellow of the American Academy of Arts & Sciences, the National Academy of Public Administration, the Gates Foundation’s National Programs Advisory Panel, the Council on Foreign Relations, and the American Law Institute, among other prominent organizations. He also served on many committees for the National Academies of Science’s National Research Council, a committee to evaluate performance standards and design a national system of education equity indicators.
“Chris Edley was a relentless force for good in the world — the good he accomplished directly and the good that he empowered other people to do,” says Molly Van Houweling, a Berkeley Law professor and faculty co-director of the school’s Berkeley Center for Law & Technology. “He set an example of how to make a complicated world better with grit and good cheer. As sad as I am, I can’t help but smile when I think of him.”
A tailor-made role
Chosen as Berkeley Law’s dean from a candidate pool of nearly 200 people, Edley embraced the school’s public mission.
“I have turned aside deanship inquiries in the past, but this opportunity is unique,” Edley said after accepting the job. “The mission of a great public law school is different from that of an elite private school because we must consider not only the excellence of our training and scholarship, but also the access to and inclusiveness of our enterprise. We must also provide intellectual capital and leadership to the state, nation, and world.”
In addition to expanding Berkeley Law’s faculty ranks, summer grants for students working in public interest positions, and research centers, Edley led a major campaign that provided much-needed physical space through renovations and the South Addition — a major project that added 55,000 square feet for new classrooms, a student center, library expansion, and more.
Faculty members recall his willingness to fight through bureaucratic red tape to pursue innovative, ambitious ideas he thought would enrich the school and its learning environment.
“He took on that kind of challenge with an infectious optimism that brought excitement, positivity, and school spirit to Berkeley Law,” Van Houweling says. “We have benefitted so much from the hard but important things he accomplished for the school … and even more so from the lessons he taught us about how to persevere, and how to serve the greater good.”
Relentless tenacity
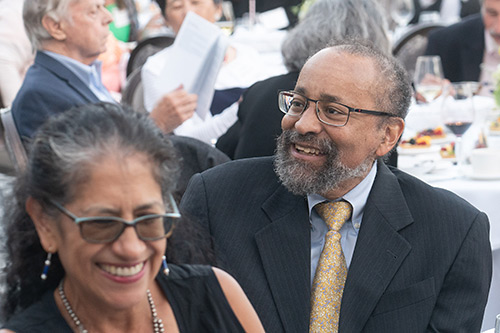
Professor Daniel Farber, faculty director of the school’s Center for Law, Energy & the Environment, says Berkeley Law was grappling with internal dissension and low faculty morale when Edley was hired.
“Chris re-energized the law school,” Farber says. “Because he believed deeply in the public service mission of law schools, he fostered the growth of new research centers to address issues facing society. All in all, it was one of the most eventful deanships in the history of the law school. He will be greatly missed.”
Edley, whose father Christopher Edley Sr. was a lawyer and longtime leader of the United Negro College Fund, continued as a professor at Berkeley Law after stepping down as dean. This past semester, he taught Truth, Proof, and Evidence: Comparing Courtroom, Politics, News, Lab, and Church, as well as The Struggle for Education Equity and Excellence with Chemerinsky and Edley’s wife, Berkeley Law lecturer Maria Echaveste ’80.
Many colleagues sharing reflections on social media conveyed deep appreciation for Edley’s determination and humanity.
“Chris Edley was a force,” Berkeley Law Professor Kenneth Bamberger tweeted. “He was a committed, curious, and supportive mentor and friend. He transformed Berkeley Law, both physically and programmatically. He devoted himself to the belief that a just society required access to excellent and affordable education.”
Former Berkeley Law professor and interim dean Melissa Murray, now a law professor at New York University, tweeted: “Chris Edley hired me and totally changed my life. He was a beloved mentor and friend who believed completely in the transformative power of education. This is an unimaginable loss — for Berkeley and all of us who loved and admired him.”
Edley is survived by his son Christopher Edley III, Echaveste, their children Zara and Elias, a grandson, and his sister Judith Edley. A memorial service will be announced at a later date.
Dean Edley was deeply passionate about supporting students who pursue public interest work during the summers before their second and third years of law school. These fellowships, affectionately named Edley Grants, reflect his commitment. Please consider contributing to the Summer Public Interest Fellowship Fund in his memory.
05/13/2024 Topics: Diversity , Faculty News , Social Justice and Public Interest
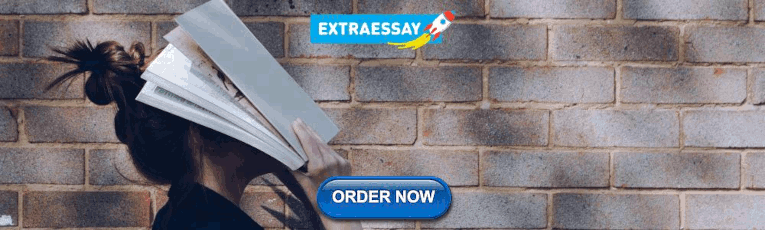
IMAGES
VIDEO
COMMENTS
4. 44032. 44033. Search and submit to the largest database of graduate school admission results. Find out who got in where and when from 2006 to 2024.
Posted February 19, 2021 (edited) Yeah, Harvard is really, really strong in the areas of causal inference and MCMC. For deep/maching learning and probability theory, I would say that Columbia, UC Berkeley, and UPenn Wharton have an edge over Harvard (e.g. you've got David Blei at Columbia, Michael Jordan and Martin Wainwright at Berkeley, Edgar ...
The Statistics PhD program is rigorous, yet welcoming to students with interdisciplinary interests and different levels of preparation. The standard PhD program in statistics provides a broad background in probability theory and applied and theoretical statistics. There are three designated emphasis (DE) tracks available to students in the PhD ...
The academic job market in Statistics/Biostatistics is crowded and competitive enough these days that many PhD holders/postdocs from the Ivy League, Stanford, Berkeley, etc. are taking jobs at schools whose programs are ranked much lower than their alma mater/postdoc institution.
The Statistics PhD program is rigorous, yet welcoming to students with interdisciplinary interests and different levels of preparation. Students in the PhD program take core courses on the theory and application of probability and statistics during their first year. The second year typically includes additional course work and a transition to ...
PhD Admissions. The Berkeley Statistics Department is a community of researchers and educators studying diverse topics within statistics, data science, and probability. We believe that individuals from diverse backgrounds offer unique perspectives that intellectually enrich our field. We are central to research life on campus and have forged ...
UC Davis Statistics PhD - interviewed on 2/15, admitted 2/25 University of Minnesota, Twin City - MS Biostatistics - admitted w/o funding 2/4 (rejected from PhD) ... UC Berkeley (Statistics PhD) Admitted. Harvard (Statistics PhD) Rejected. ... I got so stressed seeing people post their acceptances on GradCafe when I had not received anything ...
The Statistics MA program prepares students for careers that require statistical skills. It focuses on tackling statistical challenges encountered by industry rather than preparing for a PhD. The program is for full-time students and is designed to be completed in two semesters (fall and spring).
PhD Application Requirements. Thank you for your interest in our Ph.D Program. The application for admission for Fall 2025 will open in September. The application will close in early December. We do not offer spring admissions. The application process is entirely online. All supplemental materials such as your transcripts, the descriptive list ...
Search up-to-date admission results to more than 250 graduate schools. With over 840,000 admission results submitted, TheGradCafe helps millions of grad students each year with their admissions journey. ... Berkeley Computer Science. 10. ALL Economics. 2024 Most popular Master Programs By School. 1. University Of Southern California Computer ...
The Statistical Computing Facility (SCF) is a unit of the Department of Statistics. Its mission is to provide undergraduate students, graduate students, postdocs, and faculty in the Statistics Department at Berkeley with state-of-the-art computing resources, services, and technical knowledge; supporting them in carrying out cutting-edge ...
Submissions live results. Join the community. Register for our newsletter to get the inside scoop on graduate application trends, admissions acceptance data, and to receive activity alerts on your graduate school or program.
Statistics at UC Berkeley. We are a community engaged in research and education in probability and statistics. In addition to developing fundamental theory and methodology, we are actively involved in statistical problems that arise in such diverse fields as molecular biology, geophysics, astronomy, AIDS research, neurophysiology, sociology, political science, education, demography, and the U ...
Q18: How many students are admitted each year? Statistics receives between 150-200 applications for admission to the PhD program each year and approximately 400-600 for the MA program. We typically recommend for admission between 14-20 PhD students to achieve an incoming class of 8-10 PhD students.
Graduate-level courses in Demography or other disciplines relevant to the student's research interests. Min. 7 (taken for letter grades) TOTAL. Min. 24 units. Toward the end of the Spring semester of the first year, students take an M.A. Qualifying/Ph.D. Preliminary Exam. This M.A. exam is a written test of fluency in demographic theory ...
Berkeley Graduate Student Profile 2022-23. UC Berkeley is famed for the breadth, depth, and reach of more than 120 graduate programs in 15 schools and colleges. Our passion for critical inquiry, debate, discovery and innovation is informed by a deep commitment to contributing to a better world. Nearly all first-year students cite the ...
Statistics Department PhD Handbook. All students are expected to abide by the Honor Code and the Fundamental Standard. Doctoral and Research Advisors. During the first two years of the program, students' academic progress is monitored by the department's Graduate Director. Each student should meet at least once a quarter with the Graduate ...
The program is for full-time students and is designed to be completed in two semesters (fall and spring). In order to obtain the M.A. in Statistics, admitted M.A. students must complete a minimum of 24 units of courses and pass a comprehensive examination. In the first semester, all students will take intensive graduate courses in probability ...
The University of California Berkeley consistently tops US rankings in scientific fields, including biological sciences, ecology, and statistics, though the school's statistics master's program is in the arts. US News ranks UCLA Berkeley no. 2 on its list of best statistics master's programs. Unlike many statistics graduate programs, UCLA ...
May 16, 2024. The UC Berkeley Department of Statistics celebrated the graduates of the class of 2024 on Wednesday, May 15th, at Zellerbach Playhouse on the Berkeley campus. Department Chair Haiyan Huang led commencement activities, which featured an address by Professor Kate Crawford. After the ceremony, family and friends joined the graduates ...
What The Data Says. Graduate admissions data from The GradCafe shows the percentage of graduate applications related to artificial intelligence makes up about 0.3 percent of all graduate applications on record. That's more than double the rate we've seen in the past, where AI programs (including natural-language processing and machine learning) made up less than a tenth of a percent of ...
By Andrew Cohen. Christopher Edley Jr., Berkeley Law's dean from 2004 to 2013 and a renowned figure in administrative law, education policy, and civil rights, died unexpectedly on May 10 at age 71. "As dean, he made an enormous positive difference in every aspect of the law school, from the hiring of many terrific faculty, to his initiative ...