- Data Center
- Applications
- Open Source
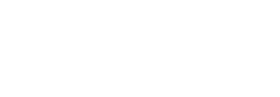
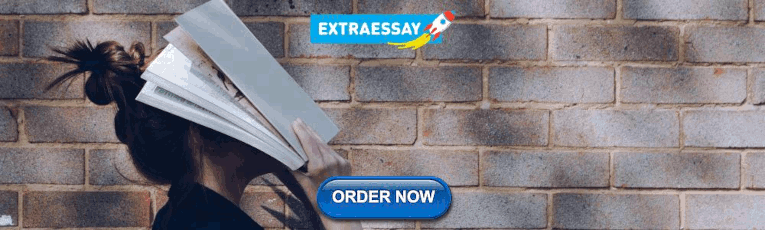
How Data Mining is Used by Nasdaq, DHL, Cerner, PBS, and The Pegasus Group: Case Studies
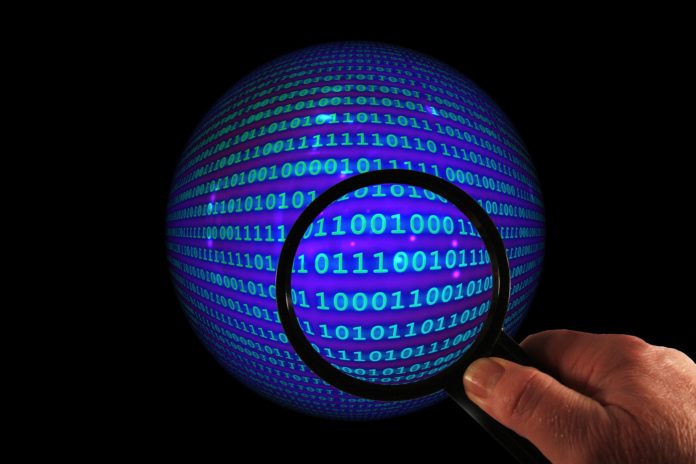
Datamation content and product recommendations are editorially independent. We may make money when you click on links to our partners. Learn More .
Companies understand that data mining can provide insights to improve the organization. Yet, many struggle with the right types of data to collect, where to start, or what project may benefit from data mining.
Examining the data mining success of others in a variety of circumstances illuminates how certain methods and software in the market can assist companies. See below how five organizations benefited from data mining in different industries: cybersecurity, finance, health care, logistics, and media.
See more: What is Data Mining? Types & Examples
1. Cerner Corporation
Over 14,000 hospitals, physician’s offices, and other medical facilities use Cerner Corporation’s software solutions.
Cerner’s access allows them to combine patient medical records and medical device data to create an integrated medical database and improve health care.
Using Cloudera’s data mining allows different devices to feed into a common database and predict medical conditions.
“In our first attempts to build this common platform, we immediately ran into roadblocks,” says Ryan Brush, senior director and distinguished engineer at Cerner.
“Our clients are reporting that the new system has actually saved hundreds of lives by being able to predict if a patient is septic more effectively than they could before.”
Industry: Health care
Data mining provider: Cloudera
- Collect data from unlimited and different sources
- Enhance operational and financial performance for health care facilities
- Improve patient diagnosis and save lives
Read the Cerner Corporation and Cloudera, Inc. case study.
DHL Temperature Management Solutions provides temperature controlled pharmaceutical logistics to ensure pharmaceutical and biological goods stay within required temperature ranges to retain potency.
Previously, DHL transferred data into spreadsheets that took a week to compile and would only contain a portion of the potential information.
Moving to DOMO’s data mining platform allows for real-time reporting of a broader set of data categories to improve insight.
“We’re able to pinpoint issues that we couldn’t see before. For example, a certain product, on a certain lane, at a certain station is experiencing an issue repeatedly,” says Dina Bunn, global head of central operations and IT for DHL Temperature Management Solutions.
Industry: Logistics
Data mining provider: DOMO
- Real-time versus week-old logistics information
- More insight into sources of delays or problems at both a high and a detailed level
- More customer engagement
Read the DHL and DOMO case study.
See more: Current Trends & Future Scope of Data Mining
The Nasdaq electronic stock exchange integrates Sisense’s data mining capabilities into their IR Insight software to help customers analyze huge data sets.
“Our customers rely on a range of content sets, including information that they license from others, as well as data that they input themselves,” says James Tickner, head of data analytics for Nasdaq Corporate Solutions.
“Being able to layer those together and attain a new level of value from content that they’ve been looking at for years but in another context.”
The combined application provides real-time analysis and clear reports easy for customers to understand and communicate internally.
Industry: Finance
Data mining provider: Sisense
- Meets rigorous data security regulations
- Quickly processes huge data sets from a variety of sources
- Provides clients with new ways to visualize and interpret data to extract new value
Read or watch the Nasdaq and Sisense case study.
The Public Broadcasting System (PBS) of the U.S. manages an online website to service 353 PBS member stations and their viewers. Their 330 million sessions, 800 million page views, and 17.5 million episode plays generate enormous data that the PBS team struggled to analyze.
PBS worked with LunaMetrics to perform data mining on the Google Analytics 360 platform to speed up insights into PBS customers.
Dan Haggerty, director of digital analytics for PBS, says “that was the coolest thing about it. A machine took our data without prior assumptions and reaffirmed and strengthened ideas that subject matter experts already suspected about our audiences based on our contextual knowledge.”
Industry: Media
Data mining provider: Google Analytics and LunaMetrics
- Identified seven key audience segments based on web behaviors
- Developed in-depth personas per segment through data mining
- Insights help direct future content and feature development
Read the PBS, LunaMetrics, and Google Analytics case study.
5. The Pegasus Group
Cyber attackers compromised and targeted the data mining system (DMS) of a major network client of The Pegasus Group and launched a distributed denial-of-service (DDoS) attack against 1,500 services.
Under extreme time pressure, The Pegasus Group needed to find a way to use data mining to analyze up to 35GB of data with no prior knowledge of the data contents.
“[I analyzed] the first three million lines and [used RapidMiner’s data mining to perform] a stratified sampling to see which ones [were] benign, which packets [were] really part of the network, and which packets were part of the attack,” says Rodrigo Fuentealba Cartes of The Pegasus Group.
“In just 15 minutes … I used this amazing simulator to see what kinds of parameters I could use to filter packets … and in another two hours, the attack was stopped.”
Industry: Cybersecurity
Data mining provider: RapidMinder
- Uploaded and analyzed three million lines of data
- Recommended analysis models provided answers within 15 minutes
- Data analysis suggested solutions that stopped the attack within two hours
Watch The Pegasus Group and RapidMiner case study.
See more: Top Data Mining Tools
Subscribe to Data Insider
Learn the latest news and best practices about data science, big data analytics, artificial intelligence, data security, and more.
Similar articles
Business intelligence vs. predictive analysis: how do they differ, 6 industry-leading predictive analytics examples & applications, top 7 data science tools: essentials for 2024, get the free newsletter.
Subscribe to Data Insider for top news, trends & analysis
Latest Articles
What is sentiment analysis..., business intelligence vs. predictive..., 6 industry-leading predictive analytics..., top 7 data science....
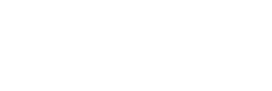
Data Mining Case Studies & Benefits

- Key Takeaways
Data mining has improved the decision-making process for over 80% of companies. (Source: Gartner).
Statista reports that global spending on robotic process automation (RPA) is projected to reach $98 billion by 2024, indicating a significant investment in automation technologies.
According to Grand View Research, the global data mining market will reach $16,9 billion in 2027.
Ethical Data Mining preserves individual rights and fosters trust.
A successful implementation requires defining clear goals, choosing data wisely, and constant adaptation.
Data mining case studies help businesses explore data for smart decision-making. It’s about finding valuable insights from big datasets. This is crucial for businesses in all industries as data guides strategic planning. By spotting patterns in data, businesses gain intelligence to innovate and stay competitive. Real examples show how data mining improves marketing and healthcare. Data mining isn’t just about analyzing data; it’s about using it wisely for meaningful changes.
The Importance of Data Mining for Modern Business:

Data mining has taken on a central role in the modern world of business. Data is a major issue for businesses today. Making informed decisions with this data can be crucial to staying competitive. This article explores the many aspects of data mining and its impact on decisions.
- Unraveling Data Landscape
Businesses generate a staggering amount of data, including customer interactions, market patterns, and internal operations. Decision-makers face an information overload without effective tools for sorting through all this data.
Data mining is a process which not only organizes, structures and extracts patterns and insights from this vast amount of data. It acts as a compass to guide decision makers through the complex landscape of data.
- Empowering Strategic Decision Making
Data mining is a powerful tool for strategic decision making. Businesses can predict future trends and market behavior by analyzing historical data. This insight allows businesses to better align their strategies with predicted shifts.
Data mining can provide the strategic insights required for successful decision making, whether it is launching a product, optimizing supply chain, or adjusting pricing strategies.
- Customer-Centric Determining
Understanding and meeting the needs of customers is paramount in an era where customer-centricity reigns. Data mining is crucial in determining customer preferences, behaviors, and feedback.
This information allows businesses to customize products and services in order to meet the expectations of customers, increase satisfaction and build lasting relationships. With customer-centric insights, decision-makers can make choices that resonate with their target audiences and foster loyalty and brand advocacy.
Data Mining: Applications across industries
Data mining is transforming the way companies operate and make business decisions. This article explores the various applications of data-mining, highlighting case studies that illuminate its impact in the healthcare, retail, and finance sectors.
- Healthcare Case Studies:

Data mining is a powerful tool in the healthcare industry. It can improve patient outcomes and treatment plans. Discover compelling case studies in which data mining played a crucial role in predicting patterns of disease, optimizing treatment and improving patient care. These examples, which range from early detection of health risks to personalized medicines, show the impact that data mining has had on the healthcare industry.
State of Technology 2024
Humanity's Quantum Leap Forward
Explore 'State of Technology 2024' for strategic insights into 7 emerging technologies reshaping 10 critical industries. Dive into sector-wide transformations and global tech dynamics, offering critical analysis for tech leaders and enthusiasts alike, on how to navigate the future's technology landscape.
- Retail Success stories:
Retail is at the forefront of leveraging data mining to enhance customer experiences and streamline operations. Discover success stories of how data mining empowered businesses to better understand consumer behavior, optimize their inventory management and create personalized marketing strategies.
These case studies, which range from e-commerce giants and brick-and-mortar shops, show how data mining can boost sales, improve customer satisfaction, transform the retail landscape, etc.
- Financial Sector Examples:
Data mining is a valuable tool in the finance industry, where precision and risk assessment are key. Explore case studies that demonstrate how data mining can be used for fraud detection and risk assessment. These examples demonstrate how financial institutions use data mining to make better decisions, protect against fraud, and customize services to their clients’ needs.
- Data Mining and Education:
Data mining has been used in the education sector to enhance learning beyond healthcare, retail and finance. Learn how educational institutions use data mining to optimize learning outcomes, analyze student performance and personalize materials. These examples, ranging from adaptive learning platforms and predictive analytics to predictive modeling, demonstrate the potential for data mining to revolutionize how we approach education.
- Manufacturing efficiency:

Data mining is a powerful tool for streamlining manufacturing processes. Examine case studies that demonstrate how data mining can be used to improve supply chain management, predict maintenance requirements, and increase overall operational efficiency. These examples show how data-driven insights can lead to cost savings, increased productivity, and a competitive advantage in manufacturing.
Data mining is a key component in each of these applications. It unlocks insights, streamlines operations, and shapes the future of decisions. Data mining is transforming the landscapes of many industries, including healthcare, retail, education, finance, and manufacturing.
Data Mining Techniques
Data mining techniques help businesses gain an edge by extracting valuable insights and information from large datasets. This exploration will provide an overview of the most popular data mining methods, and back each one with insightful case studies.
- Popular Data Mining Techniques
Clustering Analysis
The clustering technique involves grouping data points based on a set of criteria. This method is useful for detecting patterns in data sets and can be used to segment customers, detect anomalies, or recognize patterns. The case studies will show how clustering can be used to improve marketing strategies, streamline products, and increase overall operational efficiency.
Association Rule Mining
Association rule mining reveals relationships between variables within large datasets. Market basket analysis is a common application of association rule mining, which identifies patterns in co-occurring products in transactions. Real-world examples of how association rule mining is used in retail to improve product placements, increase sales, and enhance the customer experience.
Decision Tree Analysis
The decision tree is a visual representation of the process of making decisions. This technique is a powerful tool for classification tasks. It helps businesses make decisions using a set of criteria. Through case studies, you will learn how decision tree analyses have been used in the healthcare industry for disease diagnosis and fraud detection, as well as predictive maintenance in manufacturing.
Regression Analysis
Regression analysis is a way to explore the relationship between variables. This allows businesses to predict and understand how one variable affects another. Discover case studies that demonstrate how regression analysis is used to predict customer behavior, forecast sales trends, and optimize pricing strategies.
Benefits and ROI:
Businesses are increasingly realizing the benefits of data mining in the current dynamic environment. The benefits are numerous and tangible, ranging from improved decision-making to increased operational efficiency. We’ll explore these benefits, and how businesses can leverage data mining to achieve significant gains.
- Enhancing Decision Making
Data mining provides businesses with actionable insight derived from massive datasets. Analyzing patterns and trends allows organizations to make more informed decisions. This reduces uncertainty and increases the chances of success. There are many case studies that show how data mining has transformed the decision-making process of businesses in various sectors.
- Operational Efficiency
Data mining is essential to achieving efficiency, which is the cornerstone of any successful business. Organizations can improve their efficiency by optimizing processes, identifying bottlenecks, and streamlining operations. These real-world examples show how businesses have made remarkable improvements in their operations, leading to savings and resource optimization.
- Personalized Customer Experiences
Data mining has the ability to customize experiences for customers. Businesses can increase customer satisfaction and loyalty by analyzing the behavior and preferences of their customers. Discover case studies that show how data mining has been used to create engaging and personalized customer journeys.
- Competitive Advantage
Gaining a competitive advantage is essential in today’s highly competitive environment. Data mining gives businesses insights into the market, competitor strategies, and customer expectations. These insights can give organizations a competitive edge and help them achieve success. Look at case studies that show how companies have outperformed their competitors by using data mining.
Calculating ROI and Benefits
To justify investments, businesses must also quantify their return on investment. Calculating ROI for data mining initiatives requires a thorough analysis of the costs, benefits, and long-term impacts. Let’s examine the complexities of ROI within the context of data-mining.
- Cost-Benefit Analysis
Prior to focusing on ROI, companies must perform a cost-benefit assessment of their data mining projects. It involves comparing the costs associated with implementing data-mining tools, training staff, and maintaining infrastructure to the benefits anticipated, such as higher revenue, cost savings and better decision-making. Case studies from real-world situations provide insight into cost-benefit analysis.
- Quantifying Tangible and intangible benefits
Data mining initiatives can yield tangible and intangible benefits. Quantifying tangible benefits such as an increase in sales or a reduction in operational costs is easier. Intangible benefits such as improved brand reputation or customer satisfaction are also important, but they may require a nuanced measurement approach. Examine case studies that quantify both types.
- Long-term Impact Assessment
ROI calculations should not be restricted to immediate gains. Businesses need to assess the impact their data mining projects will have in the future. Consider factors like sustainability, scalability, and ongoing benefits. Case studies that demonstrate the success of data-mining strategies over time can provide valuable insight into long-term impact assessment.
- Key Performance Indicators for ROI
Businesses must establish KPIs that are aligned with their goals in order to measure ROI. KPIs can be used to evaluate the success of data-mining initiatives, whether it is tracking sales growth, customer satisfaction rates, or operational efficiency. Explore case studies to learn how to select and monitor KPIs strategically for ROI measurement.
Data Mining Ethics
Data mining is a field where ethical considerations are crucial to ensuring transparent and responsible practices. It is important to carefully navigate the ethical landscape as organizations use data to extract valuable insights. This section examines ethical issues in data mining and highlights cases that demonstrate ethical practices.
- Understanding Ethical Considerations
Data mining ethics revolves around privacy, consent, and responsible information use. Businesses are faced with the question of how they use and collect data. Ethics also includes the biases in data and the fairness of algorithms.
- Balance Innovation and Privacy
Finding the right balance between privacy and innovation is a major ethical issue in data mining. In order to gain an edge in the market through data insights and to innovate, organizations must walk a tightrope between innovation and privacy. Case studies will illuminate how companies have successfully balanced innovation and privacy.
- Transparency and informed consent
Transparency in the processes is another important aspect of ethical data mining. This is to ensure that individuals are informed and consented before their data is used. This subtopic will explore the importance of transparency in data collection and processing, with case studies that highlight instances where organizations have established exemplary standards to obtain informed consent.
Exploring Data Mining Ethics is crucial as data usage evolves. Businesses must balance innovation, privacy, and transparency while gaining informed consent. Real-world cases show how ethical data mining protects privacy and builds trust.
Implementing Data Mining is complex yet rewarding. This guide helps set goals, choose data sources, and use algorithms effectively. Challenges like data security and resistance to change are common but manageable.
Considering ethics while implementing data mining shows responsibility and opens new opportunities. Organizations prioritizing ethical practices become industry leaders, mitigating risks and achieving positive impacts on business, society, and technology. Ethics and implementation synergize in data mining, unlocking its true potential.
- Q. What ethical considerations are important in data mining?
Privacy and consent are important ethical considerations for data mining.
- Q. How can companies avoid common pitfalls when implementing data mining?
By ensuring the security of data, addressing cultural opposition, and encouraging continuous learning and adaptation.
- Q. Why is transparency important in data mining?
Transparency and consent to use collected data ethically are key elements of building trust.
- Q. What are the main steps to implement data mining in businesses?
Define your objectives, select data sources, select algorithms and monitor continuously.
- Q. How can successful organizations use data mining to gain a strategic advantage?
By taking informed decisions, improving operations and staying on top of the competition.
How useful was this post?
Click on a star to rate it!
Average rating 0 / 5. Vote count: 0
No votes so far! Be the first to rate this post.

Related Post
Understanding the basics of cluster analysis in data mining, exploring multivariate regression analysis in data mining: techniques and applications, the role of data visualization in exploratory data analysis, the power of data management platforms (dmps): your complete guide, understanding distributed file systems: a comprehensive guide, relational database management systems: a guide for modern businesses, table of contents.
Expand My Business is Asia's largest marketplace platform which helps you find various IT Services like Web and App Development, Digital Marketing Services and all others.
- IT Staff Augmentation
- Data & AI
- E-commerce Development
Article Categories
- Technology 610
- Business 306
- Digital Marketing 245
- Social Media Marketing 127
- E-Commerce 120
- Website Development 98
Copyright © 2024 Mantarav Private Limited. All Rights Reserved.

- Privacy Overview
- Strictly Necessary Cookies
This website uses cookies so that we can provide you with the best user experience possible. Cookie information is stored in your browser and performs functions such as recognising you when you return to our website and helping our team to understand which sections of the website you find most interesting and useful.
Strictly Necessary Cookie should be enabled at all times so that we can save your preferences for cookie settings.
If you disable this cookie, we will not be able to save your preferences. This means that every time you visit this website you will need to enable or disable cookies again.
Data mining tools -a case study for network intrusion detection
- Open access
- Published: 02 October 2020
- Volume 80 , pages 4999–5019, ( 2021 )
Cite this article
You have full access to this open access article
- Soodeh Hosseini 1 , 2 &
- Saman Rafiee Sardo 1
8534 Accesses
11 Citations
Explore all metrics
With the growth of data mining and machine learning approaches in recent years, many efforts have been made to generalize these sciences so that researchers from any field can easily utilize these sciences. One of the most important of these efforts is the development of data mining tools that try to hide the complexities from researchers so that they can achieve a professional output with any level of knowledge. This paper is focused on reviewing and comparing data mining and machine learning tools including WEKA, KNIME, Keel, Orange, Azure, IBM SPSS Modeler, R and Scikit-Learn to show what approach each of these methods has taken in the face of the complexities and problems of different scenarios of generalization of data mining and machine learning. In addition, for a more detailed review, this paper examines the challenge of network intrusion detection in two tools, Knime with graphical interface and Scikit-Learn with coding environment.
Similar content being viewed by others
A Practical Review on Intrusion Detection Systems by Known Data Mining Methods
Analysis of Machine Learning Classification Techniques for Anomaly Detection with NSL-KDD Data Set
Machine Learning Approaches on Intrusion Detection System: A Holistic Review
Avoid common mistakes on your manuscript.
1 Introduction
The growth and penetration of the Internet has led to the production of large amounts of data by companies. In addition, many software and databases have been developed to help companies maintain this data. There has also been a lot of research in recent years to extract useful information from these data. These researches are very valuable for companies and as a result, have led to the growth of data mining and machine learning technologies. For example, the Chinese Electric Company data review, examined in [ 36 ], can be analyzed to discover the peak hours of power consumption.
Data mining is an appropriate extraction of hidden predictive information totally stored or captured in massive data centers. Recently, many free and commercial data mining and data analysis tools have been developed for solving problems across fields such as life sciences, financial services, telecom, and insurance [ 17 ]. Data mining or Knowledge Discovery from Data (KDD) tools allows us to analyze large datasets to solve decision problems. The data mining tools use historical information to build a model to predict customer’s behavior e.g., which customers are likely to respond to a new product. Another example is intrusion detection in local systems or networks by analyzing the activity of system and network and processes them by the data mining algorithm in data mining tools. However, these tools are not all powerful enough and do not support all issues, like the research done by the researcher in [ 20 , 35 ], which can be out of range of many of these tools. However, the goal of all data mining tools is to provide a simple environment for us. In general, when we choose a data mining tool to use, there are many affective factors. Does it run natively on our computer? Does the KDD tool provide all the methods we use? If not, how extensible is it? Does that extensibility use its own language or another language (e.g. R, Python, and SQL) that is generally accessible from many packages? [ 28 ]. In the following, we introduce the tools that have been studied in this paper.
KEEL (Knowledge Extraction based on Evolutionary Learning) is an open source (GPLv3) Java software tool that supports data management and a designer of experiments. This tool pays special attention to the implementation of evolutionary learning and soft computing based techniques for Data Mining problems containing regression, classification, clustering, pattern mining and so on [ 2 ].
The open source analytics platform KNIME is a modular environment that allows interactive execution and simple visual assembly of workflows [ 5 ]. KNIME like most of data mining tools is a graphical tool contain over than 1000 nodes that connected to each other and perform the data mining algorithm. In KNIME you can do classification, clustering, image processing, using WEKA and many more algorithms with programming languages such as python and R [ 17 , 19 ].
Weka is the strong tool for data mining and machine learning that includes a perfect collection of preprocessing data tools and machine learning algorithms. Moreover, Weka can apply several learners to data and compare and evaluate their performance in order to choose the best learner for prediction [ 12 ].
Orange is a Python-based tool for data mining and machine learning suite, featuring a visual programming front-end for exploratory data analysis. It consists of a set of widgets for data preprocessing, features compute modeling, model comparison, and exploration methods. Components are called widgets and they range from simple data visualization, subset selection and preprocessing, to empirical evaluation of learning algorithms and predictive modeling [ 24 ].
Rapid Miner is a free open-source software for machine learning and data mining processes written in Java. RapidMiner has a flexible operator for a different format of input and output. It contains many learning schemes for regression, clustering and classification tasks. The graphical user interface of RapidMiner provides Plot View, Meta Data View, and a Data View in the result perspective when working with results.
The Azure Machine Learning service and its development environment are cloud-based and fully scalable which allow the user easily build an analytic model [ 7 ]. Another advantage of Azure is providing a circumstance for users that they can drag and drop analytic modules and data sets onto the experimental environment. In experimental environment users can connect two or more modules to each other, forming a model, editing and saving the model and use the model to learn and predict the new pattern.
IBM SPSS Modeler is one of the data mining software applications from IBM. It is a data mining and text analytics tool for building predictive models [ 1 ]. IBM SPSS Modeler has many types of modeling methods taken from artificial intelligence, machine learning, and statistics. The methods available on the Modeling menu allow you to deduce new information from your data and to create predictive models.
R is a statistical language that has a faster rate than code-centric software. One of the usages of programing language R is in data mining and text mining project, especially large-scale projects [ 33 ]. The R application includes statistical techniques (including linear and nonlinear modeling, statistical classical tests, time series analysis, classification, clustering, etc.) and graphical capabilities.
Scikit-learn is an open source machine learning software developed by David Cournapeau in 2007. Scikit-learn is released under the new BSD license. Scikit-learn offers a set of clustering and classification algorithms. It is offered as a package in Python language and is dependent on data science packages like Numpy and Pandas [ 26 ].
This paper is an extension of previous works on comparing introduced data mining tools. We want to compare a number of popular KDD tools, which are being used by organizations in taking proper business decisions and making optimal use of resources for business development. We present three of the most popular license tools and five open source tools. We provide comprehensive and simple tables for anyone who wants to compare tools with each other in short time in terms of important properties of data mining tools. Also, in the following, as a practical application, we present a case-study in which two of these tools challenged in terms of detecting attacks on computer networks to see how these can solve our problem.
The rest of paper is organized as follows. In Section 2 , we explain some related work on comparing data mining tools. In Section 3 , we provide examine and compare KDD tools ability to support different algorithms and scenarios, and present the results of this review in detail in several tables. In Section 4 , we present a case study and use two of the tools to examine the challenge presented in Section 3 . Finally, in Section 5 , we conclude the paper.
2 Related works
Nowadays, most of the people and commercial companies which deal with data mining for solving their problem, use some known data mining and machine learning tools and do not program data mining algorithms from the beginning. These tools have several learning algorithms and several other packages for analyze data sets. In this section, we talk about similar related work which concentrates on comparing data mining and machine learning tools.
John et al. [ 9 ] presented a paper and talk about 17 data mining tools which very famous at that time. The authors examined the tools in terms of comprehensiveness, project construction steps, cost, and support for various classification, standalone algorithms, and objectives. One of their major problems is the lack of full coverage of data mining and machine learning algorithms, especially clustering algorithms. Most of those tools do not exist in the market at this time or they simply lost popularity among users like WizWhy from WizSoft.
Goebel et al. [ 11 ] presented a survey of data mining tools in 1999. In this survey, they talked about common knowledge discovery tasks, suggestions to solve these tasks, and available tools which equipped with modules that can handle these tasks. The authors have studied 43 tools, which are well-researched in terms of operating system and support for various algorithms, but the tools under review are very weak compared to current tools.
In another survey, Mikut et al. [ 23 ] classified data mining tools into nine different types based on variant criteria such as user groups, data mining tasks and methods, data structures, visualization and interaction styles, import and export options for data and models, platforms, and license policies.
Abdulrahman et al. in [ 3 ] compared 19 open source data mining tools. The main aim of this paper was to present a comparative study, which prepares the features contained in each data mining tool for the user. The evaluation is carried out by scores provided by experts to produce a subjective judgment of each tool and objective analysis about which features are satisfied by each tool.
The report available on [ 29 ], like data miner survey in 2011, shows that decision tree and regression are two most popular data mining algorithm among data miners. This survey also shows that about 76% of analytic professionals use R for solving their data mining problem and most professional analytic select R as a primary tool since 2013. R has the first place in most used data mining tool since 2010.
However, KNIME and IBM SPSS Modeler are two tools which have won the highest satisfaction among users who work with these tools.
In [ 27 ], authors worked in three phase. In the first phase, they make a list of data mining tools from other papers which work on data mining tools. In the second phase, they delete out of dated tools from the list and in the last phase, the compared rest of tools with each other. They presented their results in the form of a table so that the reader can make the right decision with the support of each tool.
Alan et al. [ 2 ] described the properties of six most used tools for general data mining problem which available today: Rapid Miner, R, Weka, KNIME, Orange, and Scikit-learn. They compared these tools and concluded that there is no single best tool. Each tool has some advantage and disadvantage and Rapid Miner, R, Weka, and KNIME recommended for most of the data mining problems because of their user-friendly environment. In this paper, authors have not provided any exact reasons and documentation to support different algorithms and scenarios, and most of their efforts have been focused on studying different datasets.
Hong [ 15 ] introduced a prediction model which considers the recurrent support vector regression model with chaotic artificial bee colony algorithm to enhance the performance of forecasting. Fan et al. [ 10 ] presented a support vector regression model hybridized with the differential empirical mode decomposition (DEMD) method and auto regression (AR) to provide electric load forecasting with good accuracy. Hong et al. [ 16 ] introduced support vector regression based on a forecasting model with a new algorithm which is named chaotic genetic algorithm (CGA), to enhance the performance of forecasting. Hong et al. [ 36 ] hybridized several machine learning methods, such as the support vector regression model, the cuckoo search algorithm, the Tent chaotic mapping function, the out-bound-back mechanism, the VMD method, and the SR mechanism to improve the forecasting accuracy. Li et al. [ 20 ] implemented a periodogram estimation method (PEM) with considering the LSSVR model to improve the accuracy prediction. Hong et al. [ 35 ] proposed a novel electric load model of forecasting, which considers quantum computing mechanism with intelligent models such as the support vector regression model.
3 Data mining algorithms and scenarios supported by the tools
In this chapter, the tools of WEKA, KNIME, Keel, Orange, Azure, IBM SPSS Modeler, R and Scikit-Learn have been examined and then, they have been introduced and compared based on a number of basic criteria.
Machine learning algorithms can be divided into four categories [ 8 ]:
Supervised learning
In supervised learning, a set of samples with their labels is given to the machine and the machine should find a relationship between the samples and their labels. The goal in these algorithms is that the algorithm should be able to reduce the error in future examples. Classification and preference learning are some of its examples. Some examples of supervised learning algorithms include Decision tree, Random Forest, Artificial Neural Network, Support Vector Machine and Bayesian Network.
Unsupervised learning
Samples used in unsupervised learning are unlabeled. In these algorithms a cost function and a distance measure are defined that, algorithms must reduce the value of the cost function according to the distance measure. Predicting future inputs, decision making, clustering or grouping, dimensionality reduction, and so on are part of the unsupervised learning subcategories. Some examples of unsupervised learning algorithms include K-means clustering, Markov chain model, Expectation maximization algorithm, Density-based spatial clustering of applications with noise (DBSCAN) and Apriori algorithm.
Semi-supervised learning
The samples used in the semi-monitored approach are a combination of labeled and unlabeled samples. This approach requires less data than other methods, such as supervised learning and unsupervised learning, which reduces the cost of resources.
Reinforcement learning
In this scenario, the machine is depicted as an agent and the surrounding as its environment. Also, information is not given to the machine in reinforcement learning. But the machine can interact with the environment with some actions and receive information and rewards from it. When, the machine receives a reward, it can learn how to improve itself so that it can receive more rewards in the future by doing some actions.
3.1 Support: Data mining algorithms and scenarios
After introducing some of the data mining tools, we are now reviewing these tools based on performance criteria. Tables 1 , 2 , 3 and 4 shows the main characteristics of these data mining tools. In Table 5 , the supported machine learning algorithms for each data mining tool are summarized. Figure 1 also shows the different parts for creating a machine learning model. In this paper, we will look at how tools support these components.
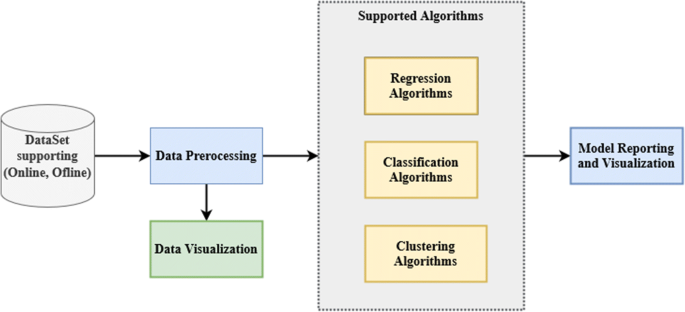
Different parts of a machine learning model
We recognize four levels of support in these characteristics: none (N), basic support (B), intermediate support (I) and advanced support (A). The notation Yes (Y) is used for supporting, and No (N) is used for no-supporting, if characteristics do not have intermediate levels of support. The (+) specifies that the tools implement the algorithm, apply an external add-on (A) to support it; (S) indicates some degree of support for the method, or do not (−). Since most tools are upgraded in a constant state, the data in Tables should be considered temporarily. However, summarizing their capabilities is important and useful so that interested users can choose the suitable environment to handle their problem.
3.1.1 Pre-processing variety
This part contains discretization [ 21 ], feature selection [ 25 ], instance selection [ 34 ] and missing values imputation [ 4 ]. The most of the suites try to offer a good feature selection and discretization set of methods, but they ignore specialized methods of missing values imputation and instance selection. Usually, the contributions included are basic modules of replacing or generating null values and methods for sampling the data sets by random (stratified or not) or by value-dependence.
3.1.2 Learning variety
This is support over main areas of data mining, such as predictive tasks (classification, regression, anomaly/deviation detection), and descriptive tasks (clustering, association rule discovery, sequential pattern discovery) [ 31 ]. In addition, we take several novel data mining scenarios such as multiple instance learning (MIL), Semi-Supervised Learning (SSL), and Imbalanced Classification.
3.1.3 Advanced features
This part includes some of the less common criteria incorporated such as post-processing techniques, meta-learning, statistical tests, evolutionary algorithms (EAs), fuzzy learning schemes, multi-classifiers for extending the functionality of the software tool.
4 Case study: Intrusion detection challenge in selected tools
In this section, we challenge one of the introduced tools to review its function. The proposed challenge is network intrusion detection system (NIDS), which we use for NSL-KDD data. This dataset requires proper preprocesses that the selected tool should be able to do. Also, the accuracy of the proposed model is of great importance to us. Finally, the tool should be able to give us a proper report. Figure 2 shows the proposed model for intrusion detection. We have implemented our model in two tools, Knime with graphical interface and Scikit-Learn with coding environment.

Proposed model for intrusion detection
4.1 Intrusion detection challenge
A NIDS monitors activities in the network and can detect malicious activities. In general, the main goal is to examine network activities and provide a report on whether an attack has occurred [ 18 ]. In most networks, data is vital, so a NIDS must have the proper accuracy in detecting attacks. The method for doing this in NIDS is one of two methods:
A) Anomaly Detection : Any activity with abnormal behavior is known as an attack. These methods can better detect unknown attacks, but the rate of missing report is low [ 14 ].
B) Misuse Detection : In these methods, a standard pattern for known attacks is created, and an activity is detected as an attack, if it is similar to one of the stored patterns. These methods can well detect known attacks, but they are unable to detect new attacks. Also, the rate of misplaced report is high [ 6 ].
When designing a NIDS, we need to consider the following steps: data collection, preprocessing, intrusion detection and reporting. In this paper, what we expect from a tool is to be able to pre-process our data well, then train the proposed model for intrusion detection and finally provide a proper report for the test data set.
4.2 NSL-KDD dataset
NSL-KDD is a dataset to fix the KDD Cup 99 dataset problem. Some of the KDD Cup 99 problems have been resolved in this dataset but still have some problems [ 22 , 32 ]. However, it is used as a standard data set. Each sample in the NSL-KDD has 41 features. This dataset contains 125,973 training data from 23 network attack types and a normal state and 18,794 test data that includes 38 attack types and normal mode. In our data, there are new types of attacks to check the compatibility of the model to new attacks. Table 6 provides the complete information of the NSL-KDD dataset.
As shown in Table 6 , NSL-KDD has 5 classes including 1 normal and 4 types of attacks such as DoS, Probe, R2L, and U2R.
Denial of Service (DoS) attack: is an attack in which the attacker makes some computing (or memory) resource too busy (respectively, too full) to handle legitimate requests, or denies legitimate users access to a resource/service.
User to Root (U2R) attack: is an attack in which the attacker starts out with access to a normal user account on a system and is able to exploit some vulnerability to gain root access to the system.
Remote to Local (R2L) attack: occurs when an attacker who has the ability to send packets to a machine over a network but who does not have an account on that machine exploits some vulnerability to gain local access as a user on that machine.
Prob attack: is an attack that tries to find knowledge about how the computers are connected to each other by bypassing security controls.
4.3 Selected tools
Our goal is to choose tools that solve NIDS challenges well. Here we try to solve this challenge with two approaches: The first approach is choosing a graphical interface tool that suits those who are not strong in programming and conversely, in the second approach, we choose a tool without a graphical interface to suit those who are strong in programming. For the first approach, we have more choices than the second approach. We are looking for a tool that can pre-process the NSL-KDD data, as well as classification algorithms that can evaluate the model proper performance. The solution presented here for the NIDS challenge is not so complex that the tools analyzed above are not able to solve it. As the aim is more to work on a tool than to find an optimal model for NIDS, so almost all tools are able to implement the model with a slight difference in the solution. Accordingly, we select the KNIME tool with the view that the other tools are also suitable. For the second approach, among the tools reviewed are two R and SCIKIT-LEARN tools with no user interface and both are robust enough to solve the NIDS challenge. We choose SCIKIT-LEARN, which does not mean that R is weak or unable to solve the challenge.
So, we use KNIME and Scikit-Learn tools in this challenge. These tools are powerful enough and giving us two different views, one with the graphical environment and one with the coding environment. Figure 3 shows an overview of work flow in the KNIME tool. This implementation has three parts. The first part is to read data and perform preprocessing on the data to become the proper data. The second part is the training of a model on pre-processed data. The third step is to evaluate the model on the test data. Also, we follow all these steps in the Scikit-Learn tool and execute them all with coding.
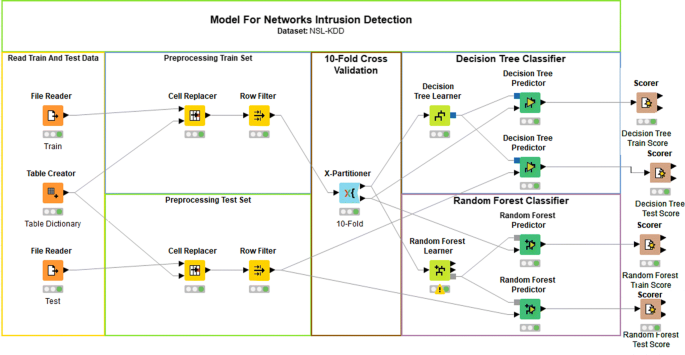
The KNIME Work Flow
4.3.1 Preprocessing
In the first implementation step, we perform the necessary preprocessing on the data to provide the proper data that we want to convert. In this step, we try to remove classes with fewer than 20 iterations because our model does not properly study them. Then, according to Table 6 , we convert the existing class into the five main classes of DoS, Probe, R2L, U2R, and Normal. Now we have a 5-class problem and implement the model according to it. According to Fig. 1 , the preprocessing process in the KNIME tool is accomplished with the help of a node of the type “Table Creator” and two “Cell Replacer” and “Row Filter” nodes. In “Table Creator” node, a dictionary is defined to change the unnecessary classes to null, and convert the required classes into a 5-class format. And the “Cell Replacer” node executes the dictionary defined in the previous step on the label column. In the following, “Row Filter” node removes samples that are not labeled.
Also in the Scikit-Learn tool, we first use the Numpy library to find the number of iterations of each class and delete it in a loop of classes with less than 20 iterations. Then, we define a dictionary specifying that each key value is converted to a 5-class format. Figure 4 shows the preprocessing in Scikit-Learn.
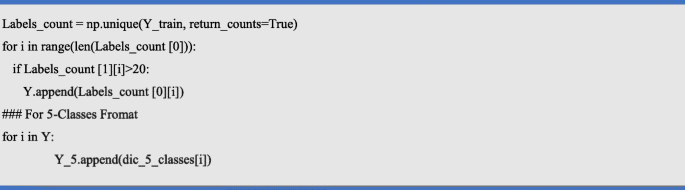
Pre-processing on Scikit-Learn
4.3.2 Results
After converting the data to the desired shape, we run the Random Forest and Decision Tree algorithms 10-fold. We use accuracy, precision, recall and F1-score criteria to evaluate the algorithms, the formula of which is given below [ 13 ].
Figure 5 shows the model definition and implementation steps in the Scikit-Learn tool. Table 7 demonstrates the results of Random Forest and Decision Tree algorithms for each data class in KNIME and Table 8 shows these results in Scikit-Learn. Table 9 also shows the accuracy of each algorithm in KNIME and Table 10 shows these results in Scikit-Learn. Figure 6 indicates Train and Validation Accuracy for Random Forest and Decision Tree in Scikit-Learn.
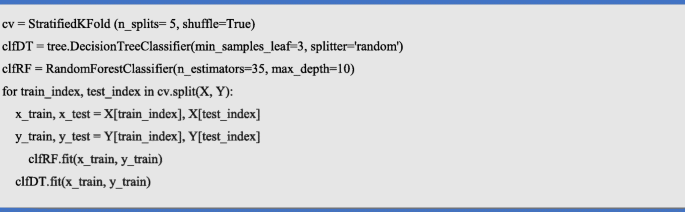
generating and training model in Scikit-Learn
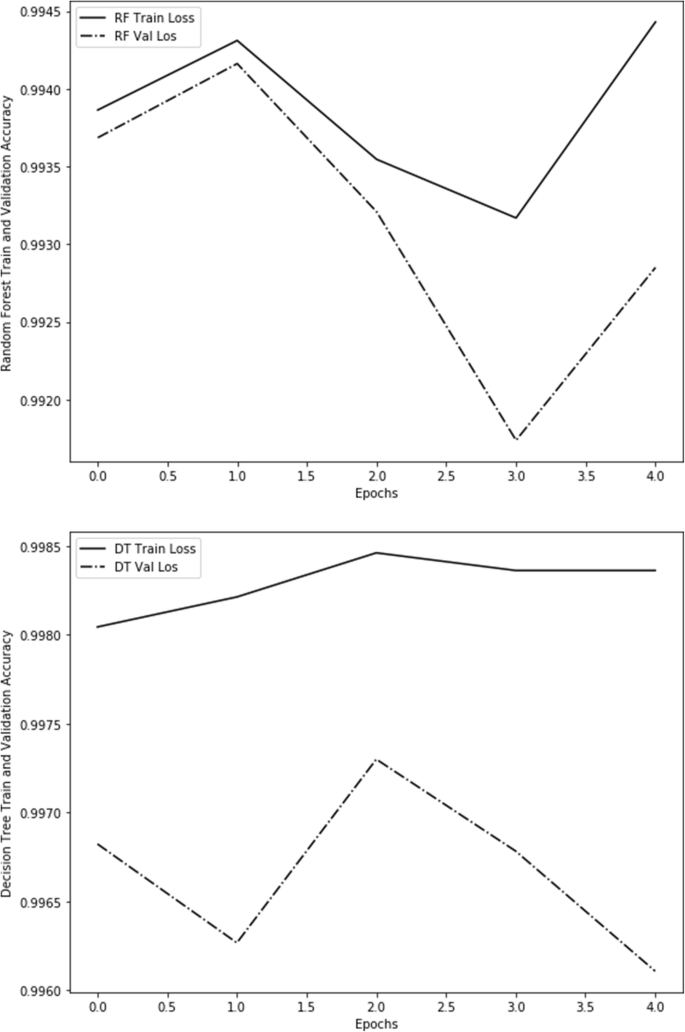
Train and Validation Accuracy for Random Forest and Decision Tree in Scikit-Learn
The results show that, the accuracy of the Random Forest algorithm in the KNIME tool was better than the Scikit-Learn tool. However, the results for the Decision tree algorithm on the two tools are opposite, which could indicate differences in implementation. These two algorithms are in these two tools. Therefore, it can be concluded that in addition to support of tools for different algorithms, it is possible that the results of those algorithms are different in two tools on the same data set, and researchers should pay attention to this point.
We then perform a statistical test using the STAC tool [ 30 ] to evaluate the superiority of each of the algorithms. Figure 7 shows how to choose a suitable statistical test. The results of this test are shown in Table 11 , STAC results show that although there are differences between the accuracy of the two algorithms in both tools, but both tools have the same rank in performance on this data set.
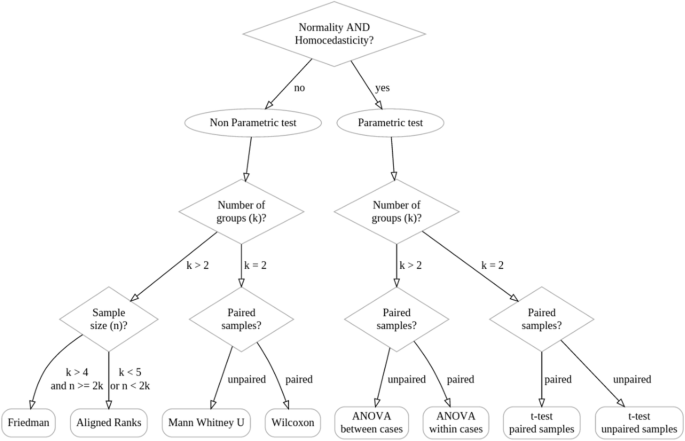
STAC assistant decision tree [ 30 ]
For comparing the examined algorithms, we apply a statistical test using STAC. Since the data distribution are not specified, we use a nonparametric test with considering two machine learning algorithms (Random Forest and Decision Tree) thus the number of algorithms are 2 (k = 2), based on STAC diagram in Fig. 7 , Mann Withney U test is chosen.
Table 12 shows the rank of algorithms in STAC. As shown in Table 12 , the Random Forest receives the maximum score.
5 Conclusions
In this paper, we compared a number of popular KDD tools such as WEKA, KNIME, KEEL, Orange, Azure, IBM SPSS Modeler, and R tools in terms of platforms, features, and algorithms. We presented comprehensive and simple tables to analyze and compare these tools with each other on important properties. Also, we reviewed the challenge of NIDS in the KNIME tool and, with the help of it, we created a model on the NSL-KDD dataset to examine how the KNIME and Scikit-Learn tools works.
Given the enormous growth of data in industry and science, the data analysis has become a significant problem. In recent years, tools for data mining and machine learning have grown enormously. In this paper, several data mining tools are considered together to helps us to select the appropriate software for extracting the useful data. We have examined them based on support for various algorithms and scenarios, operating systems, open source, and more. The authors believe that all the tools under review have been developed to make it easier to use data mining and machine learning; however, there are differences in how they are implemented and supported, and newer versions can change their superiority; for example, support for video data can be very impressive.
Abdar M (2015) A survey and compare the performance of IBM SPSS modeler and rapid miner software for predicting liver disease by using various data mining algorithms. J Sci (CSJ) 36:1–12
Google Scholar
Alcalá-Fdez J, Fernández A, Luengo J, Derrac J, García S, Sánchez L, Herrera F (2011) Keel data-mining software tool: data set repository, integration of algorithms and experimental analysis framework. J Multiple-Valued Logic Soft Comput 17:255–287
Altalhi AH, Luna JM, Vallejo M, Ventura S (2017) Evaluation and comparison of open source software suites for data mining and knowledge discovery. Wiley Interdisc Rev: Data Mining Knowl Discov 7(3):e1204
Batista GE, Monard MC (2003) An analysis of four missing data treatment methods for supervised learning. Appl Artif Intell 17(5–6):519–533
Article Google Scholar
Berthold MR, Cebron N, Dill F, Gabriel TR, Kötter T, Meinl T, Ohl P, Thiel K, Wiswedel B (2009) KNIME-the Konstanz information miner: version 2.0 and beyond. AcM SIGKDD Explorations Newslett 11(1):26–31
Casas P, Mazel J, Owezarski P (2012) Unsupervised network intrusion detection systems: detecting the unknown without knowledge. Comput Commun 35(7):772–783
Chappel, D. (2015) Introduction Azure machine learning: a guide for technical professionals. Chappel & Associates 2015: 1–17
Elavarasan D, Vincent DR, Sharma V, Zomaya AY, Srinivasan K (2018) Forecasting yield by integrating agrarian factors and machine learning models: a survey. Comput Electron Agric 155:257–282
Elder JF, Abbott DW (1998) A comparison of leading data mining tools. Fourth International Conference on Knowledge Discovery and Data Mining, pp. 1–68
Fan G-F, Peng L-L, Hong W-C, Sun F (2016) Electric load forecasting by the SVR model with differential empirical mode decomposition and auto regression. Neurocomputing 173:958–970
Goebel M, Gruenwald L (1999) A survey of data mining and knowledge discovery software tools. ACM SIGKDD Explorations Newslett 1(1):20–33
Graczyk M, Lasota T, Trawiński B (2009) Comparative analysis of premises valuation models using KEEL, RapidMiner, and WEKA. International conference on computational collective intelligence: Springer. pp. 800-812
Hassan MM, Gumaei A, Alsanad A, Alrubaian M, Fortino G (2020) A hybrid deep learning model for efficient intrusion detection in big data environment. Inf Sci 513:386–396
Hodo E, Bellekens X, Hamilton A, Tachtatzis C, Atkinson R (2017) Shallow and deep networks intrusion detection system: A taxonomy and survey. arXiv preprint arXiv:1701.02145
Hong W-C (2011) Electric load forecasting by seasonal recurrent SVR (support vector regression) with chaotic artificial bee colony algorithm. Energy 36(9):5568–5578
Hong W-C, Dong Y, Zhang WY, Chen L-Y, Panigrahi B (2013) Cyclic electric load forecasting by seasonal SVR with chaotic genetic algorithm. Int J Electr Power Energy Syst 44(1):604–614
Jovic A, Brkic K, Bogunovic N (2014) An overview of free software tools for general data mining. 2014 37th international Convention on Information and Communication Technology, Electronics and Microelectronics (MIPRO): IEEE. pp. 1112–1117
Juniper Networks (2015) Juniper Networks - How many Packets per Second per port are needed to achieve Wire-Speed?" https://www.kb.juniper.net/InfoCenter/index?page=contentfn&gid=KB14737 . Accessed 22 Feb 2020
Kadaru BB, UmaMaheswararao M (2017) An overview of general data mining tools. Int Res J Eng Technol 4(9):930–936
Li M-W, Geng J, Hong W-C, Zhang L-D (2019) Periodogram estimation based on LSSVR-CCPSO compensation for forecasting ship motion. Nonlinear Dyn 97(4):2579–2594
Liu H, Hussain F, Tan CL, Dash M (2002) Discretization: an enabling technique. Data Min Knowl Disc 6(4):393–423
Article MathSciNet Google Scholar
McHugh J (2000) Testing intrusion detection systems: a critique of the 1998 and 1999 darpa intrusion detection system evaluations as performed by Lincoln laboratory. ACM Trans Info System Secur (TISSEC) 3(4):262–294
Mikut R, Reischl M (2011) Data mining tools. Wiley Interdisc Rev: Data Mining Knowl Discov 1(5):431–443
Naik A, Samant L (2016) Correlation review of classification algorithm using data mining tool: WEKA, Rapidminer, Tanagra, Orange and Knime. Procedia Comp Sci 85:662–668
Oh I-S, Lee J-S, Moon B-R (2004) Hybrid genetic algorithms for feature selection. IEEE Trans Pattern Anal Mach Intell 26(11):1424–1437
Pedregosa F, Varoquaux G, Gramfort A, Michel V, Thirion B, Grisel O, Blondel M, Prettenhofer P, Weiss R, Dubourg V (2011) Scikit-learn: machine learning in Python. J Mach Learn Res 12:2825–2830
MathSciNet MATH Google Scholar
Pop, D., & Iuhasz, G. Overview of machine learning tools and libraries. Inst. e-Austria Timisoara.
Raut R, Nathe A (2015) Comparative study of commercial data mining tools. Int J Electron Commun Soft Comput Sci Eng (IJECSCSE) 2015:128–132
Rexer K, Gearan P, Allen H (2015) Data science survey. Rexer Analytics. Winchester, Massachusetts, 2015
Rodríguez-Fdez I, Canosa A, Mucientes M, Bugarín A (2015) STAC: a web platform for the comparison of algorithms using statistical tests. 2015 IEEE international conference on fuzzy systems (FUZZ-IEEE): IEEE. pp. 1-8
Tan, P.-N., Steinbach, M., & Kumar, V (2006) Introduction to data mining, Pearson education. Inc., New Delhi.
Tavallaee M, Bagheri E, Lu W, Ghorbani AA (2009) A detailed analysis of the KDD CUP 99 data set. IEEE symposium on computational intelligence for security and defense applications: IEEE. p. 1–6
Team RC (2000) R language definition. R foundation for statistical computing, Vienna
Wilson DR, Martinez TR (2000) Reduction techniques for instance-based learning algorithms. Mach Learn 38(3):257–286
Zhang Z, Hong W-C (2019) Electric load forecasting by complete ensemble empirical mode decomposition adaptive noise and support vector regression with quantum-based dragonfly algorithm. Nonlinear Dynamics 98(2):1107–1136
Zhang Z, Hong W-C, Li J (2020) Electric load forecasting by hybrid self-recurrent support vector regression model with variational mode decomposition and improved cuckoo search algorithm. IEEE Access 8:14642–14658
Download references
Author information
Authors and affiliations.
Department of Computer Science, Faculty of Mathematics and Computer, Shahid Bahonar University of Kerman, Kerman, Iran
Soodeh Hosseini & Saman Rafiee Sardo
Mahani Mathematical Research Center, Shahid Bahonar University of Kerman, Kerman, Iran
Soodeh Hosseini
You can also search for this author in PubMed Google Scholar
Corresponding author
Correspondence to Soodeh Hosseini .
Ethics declarations
Conflict of interest.
The authors declare that they have no conflict of interest.
Additional information
Publisher’s note.
Springer Nature remains neutral with regard to jurisdictional claims in published maps and institutional affiliations.
Rights and permissions
Open Access This article is licensed under a Creative Commons Attribution 4.0 International License, which permits use, sharing, adaptation, distribution and reproduction in any medium or format, as long as you give appropriate credit to the original author(s) and the source, provide a link to the Creative Commons licence, and indicate if changes were made. The images or other third party material in this article are included in the article's Creative Commons licence, unless indicated otherwise in a credit line to the material. If material is not included in the article's Creative Commons licence and your intended use is not permitted by statutory regulation or exceeds the permitted use, you will need to obtain permission directly from the copyright holder. To view a copy of this licence, visit http://creativecommons.org/licenses/by/4.0/ .
Reprints and permissions
About this article
Hosseini, S., Sardo, S.R. Data mining tools -a case study for network intrusion detection. Multimed Tools Appl 80 , 4999–5019 (2021). https://doi.org/10.1007/s11042-020-09916-0
Download citation
Received : 07 June 2020
Revised : 21 August 2020
Accepted : 16 September 2020
Published : 02 October 2020
Issue Date : February 2021
DOI : https://doi.org/10.1007/s11042-020-09916-0
Share this article
Anyone you share the following link with will be able to read this content:
Sorry, a shareable link is not currently available for this article.
Provided by the Springer Nature SharedIt content-sharing initiative
- Data mining tools
- Machine learning algorithms
- Intrusion detection
- Scikit-learn
- Find a journal
- Publish with us
- Track your research
Understanding Data Mining With the Help Of Case Studies On Data Mining In Market Analysis
Data | 0 comments
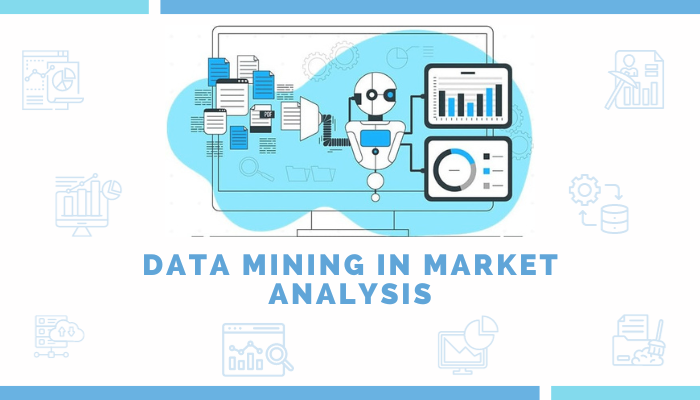
The modern generation of the 21st century witnessed a massive growth of businesses and companies around the globe. Every other day you would find a business lifting its head into existence. The arrival of so many businesses together leveled up the game of the competition. The competition is for the sense of existence in the market. One of the major technologies that gained immense popularity and is growing rapidly among businesses is Data mining.
Data mining technology assists the various companies to look into the compilation of huge quantities of data they brought together and use them to develop collaboration and improve relationships to boost its efficiency. It helps them gather accurate and detailed information about customers, which helps them strategize an influential plan and make better decisions.
Let us first understand what Data Mining is before we delve deep into the case studies in data mining applications or the various data mining case study examples.
What Is Data Mining?
Data mining is a mechanical tool used by companies that helps extract all the information from a compilation of data. Such information helps in making predictions and acting as per the same. Various statistical and mathematical calculations are used to remove the cover from relations and trends among the huge quantities of data stored in the company database. Data mining is a perfect combination of statistics, data warehousing, artificial intelligence technology, and machine learning.
Statistics resembled the starting point of data mining. Regression analysis, standard deviation, and variance are the statistical functions that act as tools in facilitating people’s study of relationships between data and their reliability. Statistics is one of the pillars of data mining technology, as most data mining actions function according to it.
Data warehouses saw their birth in the 1970s when they used large mainframe systems and the COBOL techniques of programming to store data. All these saw the arrival of big databases that we now know as data warehouses. These warehouses are for the management, retrieval, and storage of data. Megabytes and terabytes of data get data management systems reflecting sophistication. Such storage is an integral part of data mining as it helps the company to manipulate organized data.
Artificial intelligence is another basic pillar of data mining like data warehouses and statistics. The beginning of artificial intelligence in the 1980s gave a set of algorithms with a design to help the computer learn by itself. The passage of time helped massive development that led to algorithms becoming data manipulation tools, with applications in large sets of data.
Data mining collaborated with artificial intelligence instead of applying an early-defined hypothesis that helped generate relationships between the data. Artificial intelligence helped analyze the data and find collaborations between the data, thus developing models to help the developers assume diverse relationships.
Artificial intelligence paved the way for machine learning. Experts define machine learning as the machine’s capability to improve its performance after assessing its earlier results. Machine learning comes right after artificial intelligence as it works towards bringing together the study of trial and error with the help of statistical analysis. It provides an opportunity for the software to learn things by itself and makes all the data without any external help.
Tasks Of Data Mining

Classification
Classification refers to a procedure of finding out a model that explains the classes and concepts of data. The primary objective of classification is to predict the class objects whose identities are still behind the curtain. This derivation of this model works on the analytical results of the training sets of data.
You can understand it better with the following example;
- Political parties are assigning the voters in their known buckets.
- Including new customers into an existing customer group.
When we talk about statistical modeling, regression analysis comes first in discussion. It refers to a statistical process for estimating the relationship among the diverse variables. Regression comes with a lot of analyzing and modeling techniques for many variables. Here the spotlight is on the relationship between a dependent variable and one or more than one independent variable.
Following are some examples;
- Predictions concerning the unemployment rates for the following year.
- Making estimates about the insurance premium.
Detection Of Anomaly
Anomalies refer to the problems and issues within the software. This is a process of identifying the events, items, or observations that do not fall in line with the patterns or items expected in the dataset.
Example: Fraud transactions in your credit card account.
Time Series
A time series refers to a series of data points that are in a list, graph, or index, depending on the order of time. Commonly speaking, time-series signifies the action sequence taken along successive points of time, placed at regular intervals. Therefore, it is a discrete sequence of data.
Example: Production forecasting, forecasting of sales, etc.
Clustering refers to sorting the objects into diverse groups, where each group would consist of objects having similar characteristics. The features of the objects in one group will differ from the objects in another group. Following is a static example of clustering;
- Searching the customer segments in a company, depending on their transactions, customer calls, and web.
Analysis Of Association
Association is one of those data mining functions that finds out the possibility of an item recurring while in a collection. Association rules explain the rules of relationship between the items that co-occur.
Example: Search for the various opportunities for cross-selling for a retailer, as per his transaction history.
Expanding And Exploring Business
As we already know, data mining refers to a process in businesses where large chunks of data get explored to find meaningful rules and patterns. Companies can use data mining to gain that competitive edge over their fellow companies and push their business to better heights.
History Of Data Mining
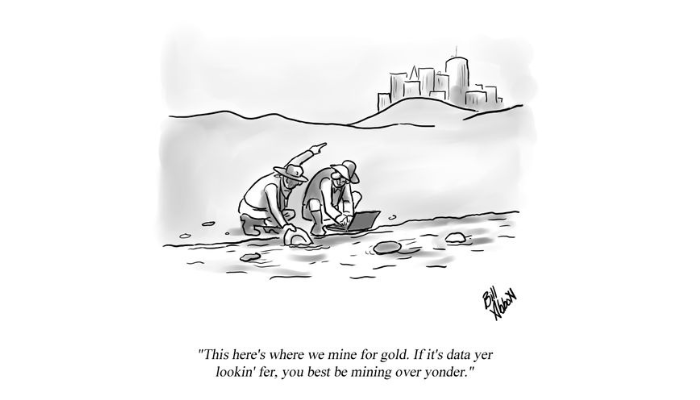
You might feel that data mining is a new concept. This term might be a new one, but the concept has been around for quite a few years. Classical statistics, artificial intelligence, and machine learning together led to the development of data mining . Everything began in the 1960s when the concept of data collection surfaced. It refers to the storage of data and information in computers. Tapes, disks, and computers were the technology available during that time.
Next, we saw the arrival of the concept of Data access in the 1980s. The concept of data access brought the introduction of relational databases and structured languages for the query. Both these helped in educating us humans more about data. Dynamic availability of data at a record level came with Data access.
Decision reports and Data warehousing came in the 1990s, which unveiled the procedure of management and retrieval of centralized data. This came with the following characteristics;
- Maintenance of a central address for keeping all the data concerning the organization.
- It will help you in analyzing the data and concentrating on the specific characteristics.
- Dynamic delivery of data at multiple levels.
The present data mining is all about making predictions and generalizing the patterns.
Influential Events And Personalities In Data Mining
Data mining saw the inception of its mention in the hands of John Herry Holland in 1975, who wrote the book “Adaptation in Natural and Artificial Systems.” This book was a thesis on genetic algorithms. However, the term “Data Mining” came into the limelight in the 1990s, with its mention in the database community for the first time. Moving further, William S . Cleveland brought forward Data mining as an independent concept in 2001. Data mining gained its epitome of prominence in February 2015, when The White House of the United States Of America hired D.J. Patil as their data scientist.
Methods Of Analysis In Data Mining

There are different methods of analytical observation in Data mining. They are as follows;
Artificial Neural Networks
These are the non-linear models of prediction that have a stark resemblance with the neural networks in biology when it comes to structuring.
Genetic Algorithms
Genetic algorithms refer to optimizing that utilizes the combination of genetics, natural selection, and mutation based on the concept of natural evolution.
Induction Of Rules
The application of all the rules found via extraction is the ones found from the significance of statistics.
Decision Trees
These are structures with the shape of trees that help in showing a set of decisions. These decisions help in generating rules for classifying a dataset.
- Chi-square Automatic Interaction Detection (CHAID), Classification and Regression Trees (CART).
- Classification and Regression tree works towards segmentation of the dataset by creating a spill that goes two ways.
- Chi-square Automatic Interaction Detection uses the chi-square tests to create splits in multiple directions.
Method Of Nearest Neighbour
The nearest neighbor technique works towards classifying each record within a dataset. It depends on combining the classes of the k-records that have the highest similarity with the dataset. (K=1)
Visualization Of Data
- Data visualization refers to the interpretation of complex relationships via visuals within data with multi-dimensions.
- One of the substantial examples is the usage of graphical tools for the illustration purposes of data relationships.
Also Read: Top 10 Data Mining Techniques for Business Success
Application Of Data Mining
You can establish data mining for your company via the feature of modeling. Modeling refers to the act of building a model that has an application to a particular situation, following which you can use that model in a different situation that does not have any existing model. Such models help you in making predictions regarding the patterns.
Data Mining In The Field Of Marketing
Companies garnered huge benefits and returns in their marketing area when considering data mining and working on its advancement. Companies use all the data gathered via data mining to push their efforts towards tailoring their discount coupons, and gift vouchers and working on their sales and advertisements to target their customers.
When you devise your marketing strategy through such mined data, you can devise a better marketing strategy and increase your sales effectiveness. Your company will also save a lot of money depending on such information.
Case Studies On Data Mining
Let us now go through the various case studies of data mining applications that will help us understand the importance of this procedure in various companies and businesses;
Case Study No.1: Target
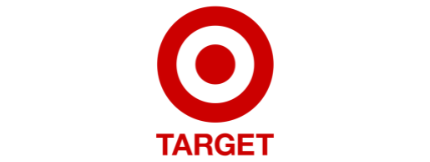
The first data mining case study example is that of the company named ‘ Target .’ Target vouchers use data mining for tailoring their discount coupons. They hope that sending such discount coupons to the customers will make them buy their products regularly. The strategists of Target assume this as an effective mechanism to prevent the customers from changing their loyalties to other brands or companies.
- When most customers are vulnerable to changing brand loyalties , due to varied choices and changing preferences. Like in tech sphere, innovative features like longer battery life and a more advanced camera system can be some factors.
- The stores like Target try to utilize such time to exploit the opportunity and lure customers into making purchases from their brand. Not just temporarily, but they try to engage them till the very end.
The company puts all its data into useful, which they collect while you are in its store for purchase or on its online website for buying something. They also collect such data by purchasing it from other companies. Duhigg states that Target has been collecting data for decades through the customers who walk into their stores regularly. They assign distinctive, unique codes to diverse customers from time to time.
This unique code is the Guest ID number within the working of Target, which keeps track of everything they purchase. Later, they use this very data to analyze the tastes and preferences of the customers and take effective steps to boost their marketing strategy.
Andrew Pole, an analyst, started his analysis of the “Pregnancy prediction model” by going through the history of the company’s baby shower registry. He tried to use this data to predict a woman’s changes regarding their shopping habits when they are expecting a baby. He formulated a list of 25 items based on this information to determine whether a woman is pregnant or not. This model successfully predicted whether a customer was pregnant or not but also assumed the date of delivery.
Case Study No.2: Amazon
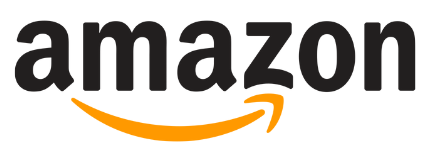
Here we are going to talk about Amazon . The case study of Amazon is one of the best case studies on data mining in market analysis. Amazon tries to use all the data they mined so that they can improve their customer service. The data mined consists of the customer’s name, home address, and personal details. Such data also consists of the customer’s preferences and the issues they are dealing with to find a solution.
They try to collect all the data regarding the customer from the various departments of the firm. Once they have all the necessary data, they synchronize and compile them for sending them to the human representative. The human representative then uses the data to have a great personalized conversation with the particular customer.
The employees of the customer service department of Amazon have all such needful information in their hands. This adds to making the conversation with the customer a lot more convenient. The employees of customer service have enough information about you which helps them make the conversation personal. However, there are no worries about the conversation being creepy.
Also check: Amazon AWS data partner marketplace.
Case Study No.3: Starbucks

Starbucks is one of the leading coffee shops with innumerable branches around the globe. Their case study will be a perfect example of the case studies on data mining in market analysis. Starbucks indulges in data mining to determine the perfect locations for setting up its stores. Tactics of Data mining and modeling assist the numerous Starbucks locations within proximity.
They try to analyze the data based on the locations, the population composition of the location, and traffic in the streets around to predict whether setting up a store there will be successful or not. Starbucks seeks assistance from a data platform named ArcGIS, developed by a company named Esri. They help them gather all the necessary information about the concerned location, demographic structure, the presence of Customer homes around, work, and other outings. All these data supplement the monitoring and boosting of their sales.
This particular company named Esri gathers a lot of data from Starbucks and, after ingrained analysis, positions them on platforms that are easily understandable for the employees there.
Case Study No.4: Usage Of Association Rule Mining In the Systems Of Recommendation
Recommender systems gained immense popularity among various fields of the industry at the current time. Music, movies, books, search queries, research books, social tags, etc., are widely-known fields. These recommendation systems assist enterprises by combining ideas from intelligent systems, information retrieval, and machine learning to make assumptions regarding the customer’s behavior. Recommender systems have two distinct approaches for their functioning;
Collaborative Filtering
The method of collaborative filtering indulges in collecting and analyzing a huge chunk of information regarding the user’s preferences, behavior, activities, etc. this will help them predict the like of a user in accordance with the other users. One of the approaches here is the usage of the Apriori algorithm.
Here you would know how the Apriori algorithm is used to squeeze out data concerning association rules from the user profiles. PVT is one of the prominent examples in this regard. PVT is one of those recommender systems that recommend various TV channels to the viewers, depending on their viewing experience. Channels with both positive and negative reviews work under the management of PVT. It treats TV viewers as transactions and the program ratings as itemsets.
One can use the Apriori algorithm to find out a set of rules and attached confidence levels between the programs. The confidence values resemble the similarity scores, and the system uses them to fill a program similarity matrix. There is an initiative to create a bridge between two TV shows. One who watches Splitsville or Roadies will not take an interest in shows like Kaun Banega Crorepati. However, if there is a line drawn between MTV Spitsvilla and Kaun Banega Crorepati, it will result in pattern watching.
Case Study No. 5: Model Of Classification For Selection of Targets In Direct Marketing
Historical data of purchase helped develop a prediction model of response along with data mining techniques. This development was to make predictions about whether a customer of the Ebedi Microfinance Bank of Nigeria would revert to a promotional offer or not. Data mining techniques helped develop a prediction model with the help of data regarding the customer’s purchase history. The data found its storage in a data warehouse to assist the decision of the management. The customer’s purchases in history and his demographic dataset helped in formulating a response model.
Then the development of the model took inputs from the following purchase variables;
Recent Purchases
It refers to the number of months from the time of the first purchase till the time the customer made the last purchase. It is one of the most powerful weapons to predict whether the promotional offer will succeed or fail. It is quite a logical observation. The primary statement through this point is that if you made some recent purchases, you are more likely to respond to the offer as against the fact that your last purchase was way back.
Frequency stands for the number of purchases the customer made. This data concerning the number of purchases can be within a definite period or all the purchases to date. This characteristic feature comes second to the factor of recent purchases when it comes to making predictions.
Monetary value
Monetary value resembles the total amount spent by the customer on making purchases from the company. You can draw some resemblance with frequency as such data can have a definite period or the total money spent to date. It is the least favorable tool to predict the steps of the customer.
However, when all three characteristic tools come together, it can sharpen the chances of the prediction being correct.
The customer’s demographic information includes his features and details concerning his sex, postal address, age, occupation, etc. The Bayesian algorithm, rather than the Naïve Bayesian algorithm, was a primary ingredient in constructing the classifier system. The selection techniques of both wrapper and filter features were in an application for selecting the inputs of this model.
The data results revealed that the Ebedi Microfinance Bank of Nigeria could plan effective strategies for marketing their goods and services. They can do it by making a detailed report on the status of customers that will guide their path to making correct decisions regarding the disbursement of funds. Therefore, they can use those funds on useful marketing tactics rather than waste them on failed strategies.
Read More: Case Study: Walmart
The Future Of Data Mining
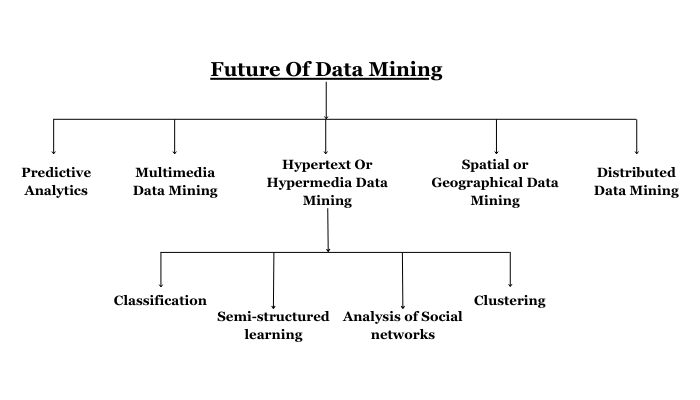
You can perceive the future of data mining through the following characteristics;
Predictive Analytics
Predictive analytics states that you can achieve “one-click data mining” through a simple and more efficient data mining process.
- You should allow the application of advanced analytics across various subjects.
- The area that will bring the highest revolution will be medicine. The researchers can analyze prediction to determine the factors associated with a particular disease and what medicine might work wonders on the affected patient.
Distributed Data Mining
Distributed data mining refers to the act of mining data spread across different locations. Combine the facilities of local data analysis and a global data model to get the best results out of data mining.
Hypertext Or Hypermedia Data Mining
This type of data mining includes hyperlinks, texts, marked texts, and any other form of information related to hypermedia. It has the following techniques;
- Classification.
- Clustering.
- Semi-structured learning.
- Analysis of Social Networks.
Multimedia Data Mining
The data from Multimedia data mining includes multimedia like videos, images, animation, audio, etc. This form of data requires a separate representation compared to traditional data.
Spatial or Geographical Data Mining
Data mining consisting of space or geography includes the analytical information concerning satellite images, natural resources, and all data from topography. All of the data comes from diverse locations, with most of them being pictures.
Things That Bother About Data Mining
There are a few drawbacks or rather concerns that experts have regarding data mining. They are as follows;
Assurance Of Privacy
Data mining is gaining immense popularity and momentum among various industries. This results in the collection of more information about every individual. When such accurate and personal information comes to the public domain, the chances of exploitation increase by manifolds. Some people or applications can use this information to meet their narrow desires. So you can very well understand that data mining allows easy accessibility of data, thus exposing it to the ills of exploitation. People can steal your identity and use it to fraud someone else.
Issues With User Interface
The experts dealing with data mining studies are skeptical about whether visualization tools can expose the true knowledge any data holds. The chances of people understanding any visual data and discovering the true meaning are difficult at times.
Problems Concerning The Performance Of Data Mining
When we talk about data mining tools, numerous statistics, and other analytical methods had a design for discovering smaller quantities of data. Such tools might fail to accommodate the rising gravity and depth of information.
There is no guarantee that the collection of data and its mining will mitigate the risks in the future. Therefore, data mining is still not a flawless procedure.
The above information can help you better understand data mining through the various case studies in data mining applications. Data mining can be a boon for various industries as that will help them secure more relevant information about the customers and boost all strategies for garnering better customer experiences.
When we talk about the few drawbacks of data mining, various companies’ advanced technology and software developments can mitigate the risks. Moreover, no company will sacrifice its brand name for the sake of some cheap benefits. You can get the best data solution services from http://bizprospex.com , which can help you with their AML Sanctions List , PEP List , Data Appending , and Skip Tracing services to gather the best and most authentic data available.
Submit a Comment Cancel reply
Your email address will not be published. Required fields are marked *
This site uses Akismet to reduce spam. Learn how your comment data is processed .
Don't miss updates from us!
Test us free, maybe.
We are very sure of our services and quality of work. How sure if you may ask?
We offer 100% money back with a 10-hour free trial.*
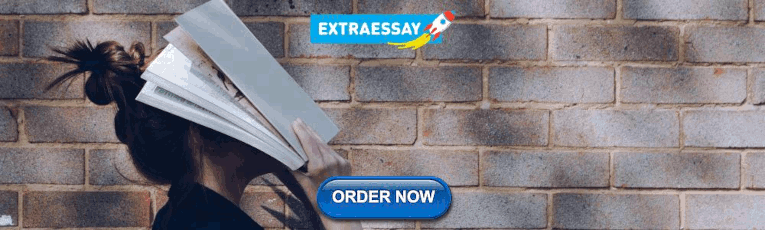
Recent Posts
- Exploring the role of data marketplace in your business’s success
- Leverage data to secure funding for your business
- Using data analytics solutions for business decision-making
- How jobs feed data can unlock market trends?
- Future of B2B Marketing: Insights and Statistics
- CASE STUDIES (6)
- Data mining (31)
- data solution (13)
- marketing (26)
Free sample data available
- Subject guides
- Text and data mining
- Case studies
Text and data mining: Case studies
- Licensed data sources
- Open data sources
- Researcher community developments
- Issues to consider
This page outlines different case studies and use cases. The librarian-researcher case studies highlight the interaction between library professionals, researchers, scholarly resources and tools, while the external case studies focus on the research impact from text and data mining activities.
Librarian-Researcher case studies
- Content Extraction from Web of Science This case study shows the interplay between researchers, library professionals and licensed databases to get the appropriate content and licensing sorted before the next part of the research project can begin.
- Digital Humanities Assessment Case Study This case study shows the interplay between lecturers/researchers, licensed databases, open data, students and librarians to get the appropriate content and licensing sorted before the student assessment can be tested, clarified and set.
Data Mining in Healthcare: Applying Strategic Intelligence Techniques to Depict 25 Years of Research Development
Maikel luis kolling.
1 Graduate Program of Industrial Systems and Processes, University of Santa Cruz do Sul, Santa Cruz do Sul 96816-501, Brazil; [email protected] (M.L.K.); [email protected] (M.K.S.)
Leonardo B. Furstenau
2 Department of Industrial Engineering, Federal University of Rio Grande do Sul, Porto Alegre 90035-190, Brazil; rb.csinu.2xm@uanetsrufodranoel
Michele Kremer Sott
Bruna rabaioli.
3 Department of Medicine, University of Santa Cruz do Sul, Santa Cruz do Sul 96816-501, Brazil; moc.liamg@iloiabbaranurb
Pedro Henrique Ulmi
4 Department of Computer Science, University of Santa Cruz do Sul, Santa Cruz do Sul 96816-501, Brazil; [email protected]
Nicola Luigi Bragazzi
5 Laboratory for Industrial and Applied Mathematics (LIAM), Department of Mathematics and Statistics, York University, Toronto, ON M3J 1P3, Canada
Leonel Pablo Carvalho Tedesco
Associated data.
Not applicable.
In order to identify the strategic topics and the thematic evolution structure of data mining applied to healthcare, in this paper, a bibliometric performance and network analysis (BPNA) was conducted. For this purpose, 6138 articles were sourced from the Web of Science covering the period from 1995 to July 2020 and the SciMAT software was used. Our results present a strategic diagram composed of 19 themes, of which the 8 motor themes (‘NEURAL-NETWORKS’, ‘CANCER’, ‘ELETRONIC-HEALTH-RECORDS’, ‘DIABETES-MELLITUS’, ‘ALZHEIMER’S-DISEASE’, ‘BREAST-CANCER’, ‘DEPRESSION’, and ‘RANDOM-FOREST’) are depicted in a thematic network. An in-depth analysis was carried out in order to find hidden patterns and to provide a general perspective of the field. The thematic network structure is arranged thusly that its subjects are organized into two different areas, (i) practices and techniques related to data mining in healthcare, and (ii) health concepts and disease supported by data mining, embodying, respectively, the hotspots related to the data mining and medical scopes, hence demonstrating the field’s evolution over time. Such results make it possible to form the basis for future research and facilitate decision-making by researchers and practitioners, institutions, and governments interested in data mining in healthcare.
1. Introduction
Deriving from Industry 4.0 that pursues the expansion of its autonomy and efficiency through data-driven automatization and artificial intelligence employing cyber-physical spaces, the Healthcare 4.0 portrays the overhaul of medical business models towards a data-driven management [ 1 ]. In akin environments, substantial amounts of information associated to organizational processes and patient care are generated. Furthermore, the maturation of state-of-the-art technologies, namely, wearable devices, which are likely to transform the whole industry through more personalized and proactive treatments, will lead to a noteworthy increase in user patient data. Moreover, the forecast for the annual global growth in healthcare data should exceed soon 1.2 exabytes a year [ 1 ]. Despite the massive and growing volume of health and patient care information [ 2 ], it is still, to a great extent, underused [ 3 ].
Data mining, a subfield of artificial intelligence that makes use of vast amounts of data in order to allow significant information to be extracted through previously unknown patterns, has been progressively applied in healthcare to assist clinical diagnoses and disease predictions [ 2 ]. This information has been known to be rather complex and difficult to analyze. Furthermore, data mining concepts can also perform the analysis and classification of colossal bulks of information, grouping variables with similar behaviors, foreseeing future events, amid other advantages for monitoring and managing health systems ceaselessly seeking to look after the patients’ privacy [ 4 ]. The knowledge resulting from the application of the aforesaid methods may potentially improve resource management and patient care systems, assist in infection control and risk stratification [ 5 ]. Several studies in healthcare have explored data mining techniques to predict incidence [ 6 ] and characteristics of patients in pandemic scenarios [ 7 ], identification of depressive symptoms [ 8 ], prediction of diabetes [ 9 ], cancer [ 10 ], scenarios in emergency departments [ 11 ], amidst others. Thus, the utilization of data mining in health organizations ameliorates the efficiency of service provision [ 12 ], quality of decision making, and reduces human subjectivity and errors [ 13 ].
The understanding of data mining in the healthcare sector is, in this context, vital and some researchers have executed bibliometric analyses in the field with the intention of investigating the challenges, limitations, novel opportunities, and trends [ 14 , 15 , 16 , 17 ]. However, at the time of this study, there were no published works that provided a complete analysis of the field using a bibliometric performance and network analysis (BPNA) (see Table 1 . In the light of this, we have defined three research questions:
- RQ1: What are the strategic themes of data mining in healthcare?
- RQ2: How is the thematic evolution structure of data mining in healthcare?
- RQ3: What are the trends and opportunities of data mining in healthcare for academics and practitioners?
Existing bibliometric analysis of data mining in healthcare in Web of Science (WoS).
Thus, with the objective to lay out a superior understanding of the data mining usage in the healthcare sector and to answer the defined research questions, we have performed a bibliometric performance and network analysis (BPNA) to set fourth an overview of the area. We used the Science Mapping Analysis Software Tool (SciMAT), a software developed by Cobo et al. [ 18 ] with the purpose of identifying strategic themes and the thematic evolution structure of a given field, which can be used as a strategic intelligence tool. The strategic intelligence, an approach that can enhance decision-making in terms of science and technology trends [ 19 , 20 , 21 , 22 , 23 , 24 , 25 , 26 , 27 ], can help researchers and practitioners to understand the area and devise new ideas for future works as well as to identify the trends and opportunities of data mining in healthcare.
This research is structured as follows: Section 2 highlights the methodology and the dataset. Section 3 presents the bibliometric performance of data mining in healthcare. In Section 4 , the strategic diagram presents the most relevant themes according to our bibliometric indicators as well as the thematic network structure of the motor themes and the thematic evolution structure, which provide a complete overview of data mining over time. Section 5 presents the conclusions, limitations, and suggestions for future works.
2. Methodology and Dataset
Attracting attention from companies, universities, and scientific journals, bibliometric analysis enhances decision-making by providing a reliable method to collect information from databases, to transform the aforementioned data into knowledge, and to stimulate wisdom development. Furthermore, the techniques of bibliometric analysis can provide higher and different perspectives of scientific production by using advanced measurement tools and methods to depict how authors, works, journals and institutions are advancing in a specific field of research through the hidden patterns that are embedded in large datasets.
The existing works on bibliometric analysis of data mining in health care in the Web of Science are shown in Table 1 , where it is depicted that only three studies have been performed and the differences between these approaches and this work are explained.
2.1. Methodology
For this study we have applied BPNA, a method that combines science mapping with performance analysis, to the field of data mining in healthcare with the support of the SciMAT software. This methodology has been chosen in view of the fact that such a combination, in addition to assisting decision-making for academics and practitioners, allows us to perform a deep investigation into the field of research by giving a new perspective of its intricacies. The BPNA conducted in this paper was composed of four steps outlined below.
2.1.1. Discovery of Research Themes
The themes were identified using a frequency and network reduction of keywords. In this process, the keywords were firstly normalized using the Salton’s Cosine, a correlation coefficient, and then clustered through the simple center algorithm. Finally, the thematic evolution structure co-word network was normalized using the equivalence index.
2.1.2. Depicting Research Themes
The previously identified themes were then plotted on a bi-dimensional diagram composed of four quadrants, in which the “vertical axis” characterizes the density (D) and the “horizontal axis” characterizes the centrality (C) of the theme [ 28 , 29 ] ( Figure 1 a) [ 18 , 20 , 25 , 30 , 31 , 32 , 33 ].

Strategic diagram ( a ). Thematic network structure ( b ). Thematic evolution structure ( c ).
- (a) First quadrant—motor themes: trending themes for the field of research with high development.
- (b) Second quadrant—basic and transversal themes: themes that are inclined to become motor themes in the future due to their high centrality.
- (c) Third quadrant—emerging or declining themes: themes that require a qualitative analysis to define whether they are emerging or declining.
- (d) Fourth quadrant—highly developed and isolated themes: themes that are no longer trending due to a new concept or technology.
2.1.3. Thematic Network Structure and Detection of Thematic Areas
The results were organized and structured in (a) a strategic diagram (b) a thematic network structure of motor themes, and (c) a thematic evolution structure. The thematic network structure ( Figure 1 b) represents the co-occurrence between the research themes and underlines the number of relationships (C) and internal strength among them (D). The thematic evolution structure ( Figure 1 c) provides a proper picture of how the themes preserve a conceptual nexus throughout the following sub-periods [ 23 , 34 ]. The size of the clusters is proportional to the number of core documents and the links indicate co-occurrence among the clusters. Solid lines indicate that clusters share the main theme, and dashed lines represent the shared cluster elements that are not the name of the themes [ 35 ]. The thickness of the lines is proportional to the inclusion index, which indicates that the themes have elements in common [ 35 ]. Furthermore, in the thematic network structure the themes were then manually classified between data mining techniques and medical research concepts.
2.1.4. Performance Analysis
The scientific contribution was measured by analyzing the most important research themes and thematic areas using the h-index, sum of citations, core documents centrality, density, and nexus among themes. The results can be used as a strategic intelligence approach to identify the most relevant topics in the research field.
2.2. Dataset
Composed of 6138 non-duplicated articles and reviews in English language, the dataset used in this work was sourced from the Web of Science (WoS) database utilizing the following query string (“data mining” and (“health*” OR “clinic*” OR “medic* OR “disease”)). The documents were then processed and had their keywords, both the author’s and the index controlled and uncontrolled terms, extracted and grouped in accordance with their meaning. In order to remove duplicates and terms which had less than two occurrences in the documents, a preprocessing step was applied to the authors, years, publication dates, and keywords. For instance, the preprocessing has reduced the total number of keywords from 21,838 to 5310, thus improving the bibliometric analysis clarity. With the exception of the strategic diagram that was plotted utilizing a single period (1995–July 2020), in this study, the timeline was divided into three sub-periods: 1995–2003, 2004–2012, and 2013–July 2020.
Subsequently, a network reduction was applied in order to exclude irrelevant words and co-occurrences. For the network extraction we wanted to identify co-occurrence among words. For the mapping process, we used a simple center algorithm. Finally, a core mapper was used, and the h-index and sum citations were selected. Figure 2 shows a good representation of the steps of the BPNA.

Workflow of the bibliometric performance and network analysis (BPNA).
3. Bibliometric Performance of Data Mining in Healthcare
In this section, we measured the performance of the field of data mining in healthcare in terms of publications and citations over time, the most productive and cited researchers, as well as productivity of scientific journals, institutions, countries, and most important research areas in the WoS. To do this, we used indicators such as: number of publications, sum of citations by year, journal impact factor (JIF), geographic distribution of publications, and research field. For this, we examined the complete period (1995 to July 2020).
3.1. Publications and Citations Overtime
Figure 3 shows the performance analysis of publications and citations of data mining in healthcare over time from 1995 to July 2020 in the WoS. The first sub-period (1995–2003) shows the beginning of the research field with 316 documents and a total of 13,483 citations. Besides, the first article in the WoS was published by Szolovits (1995) [ 36 ] who presented a tutorial for handling uncertainty in healthcare and highlighted the importance to develop data mining techniques in order to assist the healthcare sector. This sub-period shows a slightly increasing number of citations until 2003 and the year with the highest number of citations was 2002.

Number of publications over time (1995–July 2020).
The slightly increasing number continues from the first sub-period to the second subperiod (2004–2013) with a total of 1572 publications and 55,734 citations. The year 2006 presents the highest number of citations mainly due to the study of Fawcett [ 37 ] which attracted 7762 citations. The author introduced the concept of Receiver Operating Characteristics (ROC). This technique is widely used in data mining to assist medical decision-making.
From the second to the third sub-period, it is possible to observe a huge increase in the number of publications (4250 publications) and 41,821 citations. This elevated increase may have occurred due to the creation of strategies to implement emerging technologies in the healthcare sector in order to move forward with the third digital revolution in healthcare, the so-called Healthcare 4.0 [ 1 , 38 ]. Furthermore, although the citations are showing a positive trend, it is still possible to observe a downward trend from 2014 to 2020. This may happen, as Wang [ 39 ] highlights, due to the fact that a scientific document needs three to seven years to reach its peak point of citation [ 34 ]. Therefore, this is not a real trend.
3.2. Most Productive and Cited Authors
Table 2 displays the most productive and cited authors from 1995 to July 2020 of data mining in healthcare in the WoS. Leading as the most productive researcher in the field of data mining in healthcare is Li, Chien-Feng, a pathologist at Chi Mei Hospital which is sixth-ranked in publication numbers. He dedicates his studies to the molecular diagnosis of cancer with innovative technologies. In the sequence, Acharya, U. Rajendra, ranked in the top 1% of highly cited researchers in five consecutive years (2016, 2017, 2018, 2019, and 2020) in computer science according to Thomson’s essential science indicators, shares second place with Chung, Kyungyong from the Division of Engineering and Computer Science at the Kyonggi University in Su-won-si, South Korea. On the other hand, Bate, Andrew C., a member of the Food and Drug Administration (FDA) Science Council of Pharmacovigilance Subcommittee, which is the fourth-ranked institution in publication count as the most cited researcher with 945 citations. Subsequently, Lindquist, Marie, who monitors global pharmacovigilance and data management development at the World Health Organization (WHO), is ranked second with 943 citations. Last but not least, Edwards, E.R., an orthopedic surgeon at the Royal Australasian College of Surgeons is ranked third with 888 citations. Notably, this study does not demonstrate a direct correlation between the number of publications and the number of citations.
Most Cited/Productive authors from 1995 to July 2020.
3.3. Productivity of Scientific Journals, Universities, Countries and Most Important Research Fields
Table 3 shows the journals that publish studies related to data mining in healthcare. PLOS One is the first ranked with 124 publications, followed by Expert Systems with Applications with 105, and Artificial Intelligence in Medicine with 75. On the other hand, the journal Expert Systems with Applications is the journal that had the highest Journal Impact Factor (JIF) from 2019–2020.
Journals that publish studies to data mining in healthcare.
Table 4 shows the most productive institutions and the most productive countries. The first ranked is Columbia University followed by U.S. FDA Registration and Harvard University. In terms of country productivity, United States is the first in the rank, followed by China and England. In comparison with Table 2 , it is possible to notice that the most productive author is not related to the most productive institutions (Columbia University and U.S. FDA Registration). Besides, the institution with the highest number of publications is in the United States, which is found to be the most productive country.
Institutions and countries that publish studies to data mining in healthcare.
Regarding Columbia University, it is possible to verify its prominence in data mining in healthcare through its advanced data science programs, which are one of the best evaluated and advanced in the world. We highlight the Columbia Data Science Society, an interdisciplinary society that promotes data science at Columbia University and the New York City community.
The U.S. FDA Registration has a data mining council to promote the prioritization and governance of data mining initiatives within the Center for Biological Research and Evaluation to assess spontaneous reports of adverse events after the administration of regulated medical products. In addition, they created an Advanced and Standards-Based Network Analyzer for Clinical Assessment and Evaluation (PANACEA), which supports the application of standards recognition and network analysis for reporting these adverse events. It is noteworthy that the FDA Adverse Events Reporting System (FAERS) database is the main resource that identifies adverse reactions in medications marketed in the United States. A text mining system based on EHR that retrieves important clinical and temporal information is also highlighted along with support for the Cancer Prevention and Control Division at the Centers for Disease Control and Prevention in a big data project.
The Harvard University offers online data mining courses and has a Center for Healthcare Data Analytics created by the need to analyze data in large public or private data sets. Harvard research includes funding and providing healthcare, quality of care, studies on special and disadvantaged populations, and access to care.
Table 5 presents the most important WoS subject research fields of data mining in healthcare from 1995 to July 2020. Computer Science Artificial Intelligence is the first ranked with 768 documents, followed by Medical Informatics with 744 documents, and Computer Science Information Systems with 722 documents.
Most relevant WoS subject categories and research fields.
4. Science Mapping Analysis of Data Mining in Healthcare
In this section the science mapping analysis of data mining in healthcare is depicted. The strategic diagram shows the most relevant themes in terms of centrality and density. The thematic network structure uncovers the relationship (co-occurrence) between themes and hidden patterns. Lastly, the thematic evolution structure underlines the most important themes of each sub-period and shows how the field of study is evolving over time.
4.1. Strategic Diagram Analysis
Figure 4 presents 19 clusters, 8 of which are categorized as motor themes (‘NEURAL-NETWORKS’, ‘CANCER’, ‘ELETRONIC-HEALTH-RECORDS’, ‘DIABETES-MELLITUS’, ‘ADVERSE-DRUG-EVENTS’, ‘BREAST-CANCER’, ‘DEPRESSION’ and ‘RANDOM-FOREST’), 2 as basic and transversal themes (‘CORONARY-ARTERY-DISEASE’ and ‘PHOSPHORYLATION’), 7 as emerging or declining themes (‘PERSONALIZED-MEDICINE’, ‘DATA-INTEGRATION’, ‘INTENSIVE-CARE-UNIT’, ‘CLUSTER-ANALYSIS’, ‘INFORMATION-EXTRACTION’, ‘CLOUD-COMPUTING’ and ‘SENSORS’), and 2 as highly developed and isolated themes (‘ALZHEIMERS-DISEASE’, and ‘METABOLOMICS’).

Strategic diagram of data mining in healthcare (1995–July 2020).
Each cluster of themes was measured in terms of core documents, h-index, citations, centrality, and density. The cluster ‘NEURAL-NETWORKS’ has the highest number of core documents (336) and is ranked first in terms of centrality and density. On the other hand, the cluster ‘CANCER’ is the most widely cited with 5810 citations.
4.2. Thematic Network Structure Analysis of Motor Themes
The motor themes have an important role regarding the shape and future of the research field because they correspond to the key topics to everyone interested in the subject. Therefore, they can be considered as strategic themes in order to develop the field of data mining in healthcare. The eight motor themes are discussed below, and they are displayed below in Figure 5 together with the network structure of each theme.

Thematic network structure of mining in healthcare (1995–July 2020). ( a ) The cluster ‘NEURAL-NETWORKS’. ( b ) The cluster ‘CANCER’. ( c ) The cluster ‘ELECTRONIC-HEALTH-RECORDS’. ( d ) The cluster ‘DIABETES-MELLITUS’. ( e ) The cluster ‘BREAST-CANCER’. ( f ) The cluster ‘ALZHEIMER’S DISEASE’. ( g ) The cluster ‘DEPRESSION’. ( h ) The cluster ‘RANDOM-FOREST’.
4.2.1. Neural Network (a)
The cluster ‘NEURAL-NETWORKS’ ( Figure 5 a) is the first ranked in terms of core documents, h-index, centrality, and density. The ‘NEURAL-NETWORKS’ cluster is strongly influenced by subthemes related to data science algorithms, such as ‘SUPPORT-VECTOR-MACHINE’, ‘DECISION-TREE’, among others. This network represents the use of data mining techniques to detect patterns and find important information correlated to patient health and medical diagnosis. A reasonable explanation for this network might be related to the high number of studies which conducted benchmarking of neural networks with other techniques to evaluate performance (e.g., resource usage, efficiency, accuracy, scalability, etc.) [ 40 , 41 , 42 ]. Besides, the significant size of the cluster ‘MACHINE-LEARNING’ is expected since neural networks is a type of machine learning. On the other hand, the subtheme ‘HEART-DISEASE’ stands out as the single disease in this network, which can be justified by the high number of researches with the goal to apply data mining to support decision-making in heart disease treatment and diagnosis.
4.2.2. Cancer (b)
The cluster ‘CANCER’ ( Figure 5 b) is the second ranked in terms of core documents, h-index, and density. On the other hand, it is the first in terms of citations (5810). This cluster is highly influenced by the subthemes related to the studies of cancer genes mutations, such as ‘BIOMAKERS’, ‘GENE-EXPRESSION’, among others. The use of data mining techniques has been attracting attention and efforts from academics in order to help solve problems in the field of oncology. Cancer is known as the disease that kills the most people in the 21st century due to various environmental pollutions, food pesticides and additives [ 14 ], eating habits, mental health, among others. Thus, controlling any form of cancer is a global strategy and can be enhanced by applying data mining techniques. Furthermore, the subtheme ‘PROSTATE-CANCER’ highlights that the most efforts of data mining applications focused on prostate cancer’s studies. Prostate cancer is the most common cancer in men. Although the benefits of traditional clinical exams for screening (digital rectal examination, the prostate-specific antigen and blood test and transrectal ultrasound), there is still a lack in terms of efficacy to reduce mortality with the use of such tests [ 43 ]. In this sense, data mining may be a suitable solution since it has been used in bioinformatics analyses to understand prostate cancer mutation [ 44 , 45 ] and uncover useful information that can be used for diagnoses and future prognostic tests which enhance both patients and clinical decision-making [ 46 ].
4.2.3. Electronic Health Records (HER—c)
The cluster ‘ELECTRONIC-HEALTH-RECORDS’ ( Figure 5 c) represents the concept in which patient’s health data are stored. Such data are continuously increasing over time, thereby creating a large amount of data (big data) which has been used as input (EHR) for healthcare decision support systems to enhance clinical decision-making. The clusters ‘NATURAL-LANGUAGE-PROCESSING’ and ‘TEXT MINING’ highlight that these mining techniques are the most frequently used with data mining in healthcare. Another pattern that must be highlighted is the considerable density among the clusters ‘SIGNAL-DETECTION’ and ‘PHARMACOVIGILANCE’ which represents the use of data mining to depict a broad range of adverse drug effects and to identify signals almost in real-time by using EHR [ 47 , 48 ]. Besides, the cluster ‘MISSING-DATA’ is related to studies focused on the challenge regarding to incomplete EHR and missing data in healthcare centers, which compromise the performance of several prediction models [ 49 ]. In this sense, techniques to handle missing data have been under improvement in order to move forward with the accurate prediction based on medical data mining applications [ 50 ].
4.2.4. Diabetes Mellitus (DM—d)
Nowadays, DM is one of the most frequent endocrine disorders [ 51 ] and affected more than 450 million people worldwide in 2017 and is expected to grow to 693 million by the year 2045. The same applies for the 850 billion dollars spent just in 2017 by the health sector [ 52 ]. The cluster ‘DIABETES-MELLITUS’ ( Figure 5 d) has a strong association with the risk factor subtheme group (e.g., ‘INSULIN-RESISTENCE’, ‘OBESITY’, ‘BODY-MASS-INDEX’, ‘CARDIOVASCULAR-DISEASE’, and ‘HYPERTENSION’). However, the obesity (cluster ‘OBESITY’) is the major risk factor related to DM, particularly in Type 2 Diabetes (T2D) [ 51 ]. T2D shows a prevalence of 90% of worldwide diabetic patients when compared with T1D and T3D, mainly characterized by insulin resistance [ 51 ]. Thus, this might justify the presence of the clusters ‘TYPE-2-DIABETES’ and ‘INSULIN-RESISTANCE’ which seems to be highly developed by data mining academics and practitioners. The massive number of researches into all facets of DM has led to the formation of huge volumes of EHR, in which the mostly applied data mining technique is the association rules technique. It is used to identify associations among risk factors [ 51 ], thusly justifying the appearance of the cluster ‘ASSOCIATION-RULES’.
4.2.5. Breast Cancer (e)
The cluster ‘BREAST-CANCER’ ( Figure 5 e) presents the most prevalent type of cancer affecting approximately 12.5% of women worldwide [ 53 , 54 ]. The cluster ‘OVEREXPRESSION’ and ‘METASTASIS’ highlights the high number of studies using data mining to understand the association of overexpression of molecules (e.g., MUC1 [ 54 ], TRIM29 [ 55 ], FKBP4 [ 56 ], etc.) with breast cancer metastasis. Such overexpression of molecules also appears in other forms of cancers, justifying the group of subthemes: ‘LUNG CANCER’, ‘GASTRIC-CANCER’, ‘OVARIAN-CANCER’, and ‘COLORECTALCANCER’. Moreover, the cluster ‘IMPUTATION’ highlight efforts to develop imputation techniques (data missingness) for breast cancer record analysis [ 57 , 58 ]. Besides, the application of data mining to depict breast cancer characteristics and their causes and effects has been highly supported by ‘MICROARRAY-DATA’ [ 59 , 60 ], ‘PATHWAY’ [ 61 ], and ‘COMPUTER-AIDED-DIAGNOSIS’ [ 62 ].
4.2.6. Alzheimer’s Disease (AD—f)
The cluster ‘ALZHEIMER’S DISEASE’ ( Figure 5 f) is highly influenced by subthemes related to diseases, such as ‘DEMENTIA’ and ‘PARKINSON’S-DISEASE’. This co-occurrence happens because the AD is a neurodegenerative illness which leads to dementia and Parkinson’s disease. Studies show that the money spent on AD in 2015 was about $828 billion [ 63 ]. In this sense, data mining has been widely used with ‘GENOME-WIDE-ASSOCIATION’ techniques in order to identify genes related to the AD [ 64 , 65 ] and prediction of AD by using data mining in ‘MRI’ Brain images [ 66 , 67 ]. The cluster ‘NF-KAPPA-B’ highlights the efforts to identify associations of NF-κB (factor nuclear kappa B) with AD by using data mining techniques which can be used to advance anti-drug developments [ 68 ].
4.2.7. Depression (g)
The cluster ‘DEPRESSION’ ( Figure 5 g) presents a common disease which affects over 260 million people. In the worst case, it can lead to suicide which is the second leading cause of death in young adults. The cluster ‘DEPRESSION’ is a highly associated cluster. Its connections mostly represent the subthemes that have been the research focus of data mining applications [ 69 ]. The connection between both the sub theme ‘SOCIAL-MEDIA’ and ‘ADOLESCENTS’, especially in times of social isolation, are extremely relevant to help identify early symptoms and tendencies among the population [ 70 ]. Furthermore, the presence of the ‘COMORBIDITY’ and ‘SYMPTONS’ is not surprising given knowledge discovery properties of the data mining field could provide significant insights into the etiology of depression [ 71 ].
4.2.8. Random Forest (h)
An ensemble learning method that is used in this study is the last cluster approach, which, among other things, is used for classification. The presence of the ‘BAYESIAN-NETWORK’ subtheme, supported by the connection between both and the ‘INFERENCE’, might represent another alternative to which the applications in data mining using random forest are benchmarked against [ 72 ]. Since the ‘RANDOM-FOREST’ ( Figure 5 h) cluster has barely passed the threshold from a basic and transversal theme to a motor theme, the works developed under this cluster are not yet as interconnected as the previous one. Thus, the theme with the most representativeness is the ‘AIR-POLLUTION’ in conjunction with ‘POLLUTION’, where studies have been performed in order to obtain ‘RISK-ASSESSMENT’ through the exploration of the knowledge hidden in large databases [ 73 ].
4.3. Thematic Evolution Structure Analysis
The Computer Science’s themes related to data mining and the medical research concepts, depicted, respectively, in the grey and blue areas of the thematic evolution diagram ( Figure 6 ), demonstrates the evolution of the research field over the different sub-periods addressed in this study. In this way, each individual theme relevance is illustrated through its cluster size as well as with its relationships throughout the different sub-periods. Thus, in this section, an analysis of the different trends on themes will be presented to give a brief insight into the factors that might have influenced its evolution. Furthermore, the proceeding analysis will be split into two thematic areas where, firstly, the grey area (practices and techniques related to data mining in healthcare) will be discussed followed by the blue one (health concepts and disease supported by data mining).

Thematic evolution structure of mining in healthcare (1995–July 2020).
4.3.1. Practices and Techniques Related to Data Mining in Healthcare
The cluster ‘KNOWLEDGE-DISCOVERY’ ( Figure 6 , 1995–2012), often known as a synonym for data mining, provides a broader view of the field differing in this way from the algorithm focused theme, that is data mining, where its appearance and, later in the third period, its fading could provide a first insight into the overall evolution of the data mining papers applied to healthcare. The occurrence of the cluster knowledge discovery in the first two periods could demonstrate the focus of the application of the data mining techniques in order to classify and predict conditions in the medical field. This gives rise to a competition with early machine learning techniques that could be potentially evidenced through the presence of the cluster ‘NEURAL-NETWORK’, which the data mining techniques could probably be benchmarked against. The introduction of the ‘FEATURE-SELECTION’, ‘ARTIFICIAL-INTELLIGENCE’, and ‘MACHINE-LEARNING’ clusters together with the fading of ‘KNOWLEDGE-DISCOVERY’ could imply the occurrence of a disruption of the field in the third sub-period that has led to a change in the perspective on the studies.
One instance that could represent such a disruption could have been a well-known paper published by Alex Krizhevsky, Ilya Sutskever, and Geoffrey Hinton [ 74 ], where a novel technique in neural networks was firstly applied to a major image recognition competition. A vast advantage over the other algorithms that have been used was obtained. The connection between the work previously mentioned and its impact on the data mining on healthcare research could be majorly supported by the disappearance of the cluster ‘IMAGE- MINING’ of the second sub-period which has no connections further on. Furthermore, the presence of the clusters ‘MACHINE-LEARNING’, ‘ARTIFICIAL-INTELLIGENCE’, ‘SUPPORT-VECTOR-MACHINES’, and ‘LOGISTIC-REGRESSION’ may be the evidence of a shift of focus on the data mining community for health care where, besides attempting to compete with machine learning algorithms, they are now striving to further improve the results previously obtained with machine learning through data mining. Moreover, given the presence of the colossal feature selection cluster, which circumscribes algorithms that enhance classification accuracy through a better selection of parameters, this trend could be given credence in consequence of its presence since it may be encompassing publications from the formerly stated clusters.
Although still small, the presence of the cluster ‘SECURITY’ in the last sub-period ( Figure 6 , 2013–2020) is, at the very least, relevant given the sensitive data that is handled in the medical space, such as patient’s history and diseases. Above all, the recent leaks of personal information have devised an ever-increasing attention to this topic focusing on, among other things, the de-identification of the personal information [ 75 , 76 , 77 ]. These kind of security processes allow, among others, data mining researchers to make use of the vast sensitive information that is stored in hospitals without any linkage that could associate a person to the data. For instance, the MIMIC Critical Care Database [ 78 ], an example of a de-identified database, has been allowing further research into many diseases and conditions in a secure way that would otherwise have been extremely impaired due to data limitations.
4.3.2. Health Concepts and Disease Supported by Data Mining
The cluster ‘GENE-EXPRESSION’ stands out in the first period and second period ( Figure 6 , 1995–2012) of medical research concepts and establishes strong co-occurrence with the cluster ‘CANCER’ in the third sub-period. This link can be explained by research involving the microarray technology, which makes it possible to detect deletions and duplications in the human genome by analyzing the expression of thousands of genes in different tissues. It is also possible to confirm the importance of genetic screening not only for cancer, but for several diseases, such as ‘ALZHEIMER’ and other brain disorders, thereby assisting in preventive medicine and enabling more efficient treatment plans [ 79 ]. For example, a research was carried out to analyze complex brain disorders such as schizophrenia from expression gene microarrays [ 80 ].
Sequencing technologies have undergone major improvements in recent decades to determine evolutionary changes in genetic, epigenetic mechanisms, and in the ‘MOLECULAR-CLASSIFICATION’, a topic that gained prominence as a cluster in the first period. An example of this can be found in a study published in 2010 which combined a global optimization algorithm called Dongguang Li (DGL) with cancer diagnostic methods based on gene selection and microarray analysis. It performed the molecular classification of colon cancers and leukemia and demonstrated the importance of machine learning, data mining, and good optimization algorithms for analyzing microarray data in the presence of subsets of thousands of genes [ 81 ].
The cluster ‘PROSTATE-CANCER’ in the second period ( Figure 6 , 2004–2012) presents a higher conceptual nexus to ‘MOLECULAR-CLASSIFICATION’ in the first sub-period and the same happens with clusters, such as ‘METASTASIS’, ‘BREAST-CANCER’, and ‘ALZHEIMER’, which appear more recently in the third sub-period. The significant increase in the incidence of prostate cancer in recent years results in the need for greater understanding of the disease in order to increase patient survival, since prostate cancer with metastasis was not well explored, despite having a survival rate much smaller compared to the early stages. In this sense, the understanding of age-specific survival of patients with prostate cancer in a hospital in using machine learning started to gain attention by academics and highlighted the importance of knowing survival after diagnosis for decision making and better genetic counseling [ 82 ]. In addition, the relationship between prostate cancer and Alzheimer’s disease is explained by the fact that the use of androgen deprivation therapy, used to treat prostate cancer, is associated with an increased risk of Alzheimer’s disease and dementia [ 81 ]. Therefore, the risks and benefits of long-term exposure to this therapy must be weighed. Finally, the relationship between prostate cancer and breast cancer in the thematic evolution can be explained due to the fact that studies are showing that men with a family history of breast cancer have a 21% higher risk of developing prostate cancer, including lethal disease [ 83 ].
The cluster ‘PHARMACOVIGILANCE’ appears in the second sub-period ( Figure 6 , 2004–2012) showing a strong co-occurrence with clusters of the third sub-period: ‘ADVERSE-DRUGS-REACTIONS’ and ‘ELECTRONIC-HEALTH-RECORDS’. In recent years, data-mining algorithms have stood out for their usefulness in detecting and screening patients with potential adverse drug reactions and, consequently, they have become a central component of pharmacovigilance, important for reducing the morbidity and mortality associated with the use of medications [ 48 ]. The importance of electronic medical records for pharmacovigilance is evident, which act as a health database and enable drug safety assessors to collect information. In addition, such medical records are also essential to optimize processes within health institutions, ensure more safety of patient data, integrate information, and facilitate the promotion of science and research in the health field [ 84 ]. These characteristics explain the large number of studies of ‘ELECTRONIC-HEALTH-RECORDS’ in the third sub-period and the growth of this theme in recent years, since the world has started to introduce electronic medical records, although currently there are few institutions that still use physical medical records.
The ‘DEPRESSION’ appears in the second sub-period ( Figure 6 , 2004–2012) and remains as a trend in the third sub-period with a significant increase in publications on the topic. It is known that this disease is numerous and is increasing worldwide, but that it still has many stigmas in its treatment and diagnosis. Globalization and the contemporary work environment [ 85 ] can be explanatory factors for the increase in the theme from the 2000s onwards and the COVID-19 pandemic certainly contributed to the large number of articles on mental health published in 2020. In this context, improving the detection of mental disorders is essential for global health, which can be enhanced by applying data mining to quantitative electroencephalogram signals to classify between depressed and healthy people and can act as an adjuvant clinical decision support to identify depression [ 69 ].
5. Conclusions
In this research, we have performed a BPNA to depict the strategic themes, the thematic network structure, and the thematic evolution structure of the data mining applied in healthcare. Our results highlighted several significant pieces of information that can be used by decision-makers to advance the field of data mining in healthcare systems. For instance, our results could be used by editors from scientific journals to enhance decision-making regarding special issues and manuscript review. From the same perspective, healthcare institutions could use this research in the recruiting process to better align the position needs to the candidate’s qualifications based on the expanded clusters. Furthermore, Table 2 presents a series of authors whose collaboration network may be used as a reference to identify emerging talents in a specific research field and might become persons of interest to greatly expand a healthcare institution’s research division. Additionally, Table 3 and Table 4 could also be used by researchers to enhance the alignment of their research intentions and partner institutions to, for instance, encourage the development of data mining applications in healthcare and advance the field’s knowledge.
The strategic diagram ( Figure 4 ) depicted the most important themes in terms of centrality and density. Such results could be used by researchers to provide insights for a better comprehension of how diseases like ‘CANCER’, ‘DIABETES-MELLITUS’, ‘ALZHEIMER’S-DISEASE’, ‘BREAST-CANCER’, ‘DEPRESSION’, and ‘CORONARY-ARTERY-DISEASE’ have made use of the innovations in the data mining field. Interestingly, none of the clusters have highlighted studies related to infectious diseases, and, therefore, it is reasonable to suggest the exploration of data mining techniques in this domain, especially given the global impact that the coronavirus pandemic has had on the world.
The thematic network structure ( Figure 5 ) demonstrates the co-occurrences among clusters and may be used to identify hidden patterns in the field of research to expand the knowledge and promote the development of scientific insights. Even though exhaustive research of the motor themes and their subthemes has been performed in this article, future research must be conducted in order to depict themes from the other quadrants (Q2, Q3, and Q4), especially emerging and declining themes, to bring to light relations between the rise and decay of themes that might be hidden inside the clusters.
The thematic evolution structure showed how the field is evolving over time and presented future trends of data mining in healthcare. It is reasonable to predict that clusters such as ‘NEURAL-NETWORKS’, ‘FEATURE-SELECTION’, ‘EHR’ will not decay in the near future due to their prevalence in the field and, most likely, due to the exponential increase in the amount of patient health that is being generated and stored daily in large data lakes. This unprecedented increase in data volume, which is often of dubious quality, leads to great challenges in the search for hidden information through data mining. Moreover, as a consequence of the ever-increasing data sensitivity, the cluster ‘SECURITY’, which is related to the confidentiality of the patient’s information, is likely to remain growing during the next years as government and institutions further develop structures, algorithms, and laws that aim to assure the data’s security. In this context, blockchain technologies specifically designed to ensure integrity and publicity of de-identified, similarly as it is done by the MIMIC-III (Medical Information Mart for Intensive Care III) [ 78 ], may be crucial to accelerate the advancement of the field by providing reliable information for health researchers across the world. Furthermore, future researches should be conducted in order to understand how these themes will behave and evolve during the next years, and interpret the cluster changes to properly assess the trends here presented. These results could also be used as teaching material for classes, as it provides strategic intelligence applications and the field’s historical data.
In terms of limitations, we used the WoS database since it has index journals with high JIF. Therefore, we suggest to analyze other databases, such as Scopus, PubMed, among others in future works. Besides, we used the SciMAT to perform the analysis and other bibliometric software, such as VOS viewer, Cite Space, Sci2tool, etc., could be used to explore different points of view. Such information will support this study and future works to advance the field of data mining in healthcare.
Author Contributions
Conceptualization, M.L.K., L.B.F., L.P.C.T. and N.L.B.; Data curation, L.B.F.; Formal analysis, L.B.F., B.R., and P.H.U.; Funding acquisition, N.L.B.; Investigation, M.L.K., L.B.F., L.P.C.T. and M.K.S.; Methodology, L.B.F.; Project administration, L.B.F., N.L.B. and L.P.C.T.; Resources, N.L.B.; Supervision, L.B.F., N.L.B. and L.P.C.T.; Validation, N.L.B. and L.P.C.T.; Visualization, N.L.B.; Writing—original draft, L.B.F. and N.L.B.; Writing—review & editing, N.L.B. All authors have read and agreed to the published version of the manuscript.
This study was financed in part by the Coordenação de Aperfeiçoamento de Pessoal de Nível Superior—Brazil (CAPES)—Finance Code 001, and in part by the Brazilian Ministry of Health. N.L.B. is partially supported by the CIHR 2019 Novel Coronavirus (COVID-19) rapid research program.
Institutional Review Board Statement
Informed consent statement, data availability statement, conflicts of interest.
The authors declare no conflict of interest.
Publisher’s Note: MDPI stays neutral with regard to jurisdictional claims in published maps and institutional affiliations.

Tech Business Guide
Insights on how to realize the benefits of new technologies.

15 Examples of Data Mining in Real Life: Uncovering Hidden Insights
Data mining has become increasingly important for organizations seeking to uncover insights and make informed decisions. As a result, it is paramount for business leaders and entrepreneurs to study examples of data mining in real life.
By analyzing large amounts of data, mining algorithms can identify correlations and trends that might not appear at first glance.
In this blog post, we’ll take a look at ten examples of data mining in real life. Indeed, these examples demonstrate how data mining can drive innovation and make a tangible impact on people’s lives.
In this article:
What Are Examples of Data Mining?
Data mining involves using sophisticated methods such as machine learning processes to identify patterns from large datasets. Afterward, it uses them for various purposes, such as predicting customer behavior or market trends.
Some standard techniques include clustering, regression analysis and classification. Clustering identifies similar groups within a dataset; regression predicts future outcomes based on past events and classification determines to which category data points belong.
Organizations worldwide have adopted these methods to quickly process information with greater accuracy than manual analysis could ever achieve alone.
Examples for Data Mining in Healthcare
Healthcare providers and other stakeholders increasingly embrace data mining tools to analyze patient records quickly and accurately. Moreover, not only does it enable faster processing, but it also improves accuracy when compared to manual methods. Ultimately, they seek to make informed decisions regarding diagnosis and treatment options available.
Following are some examples of data mining in real life in the healthcare industry:
1. – Improving Patient Outcomes Study

Photo by Marcelo Leal .
A study from Stanford University used data mining to analyze electronic health records (EHRs) and identify factors that can predict hospital readmissions. Above all, by understanding these predictors, healthcare providers can take proactive steps to prevent readmissions and improve patient outcomes.
The study highlights the potential of big data and artificial intelligence to enhance healthcare decision-making, particularly in readmission prevention. Above all, early intervention can have a significant impact on preventing subsequent clinic readmissions. Nevertheless, data mining techniques in healthcare are still a relatively new field. Still, it holds promise for improving the quality and efficiency of healthcare delivery in the future.
2. – BCBSMA Reduces Readmissions With Preventive Care
A study from Blue Cross Blue Shield of Massachusetts (BCBSMA) used data mining to identify patients at risk for developing chronic conditions. In addition, by targeting these patients with preventative care, the health insurer reduced healthcare costs.
The BCBSMA case study exemplifies how big data analytics can help healthcare organizations to gain better decision-making and savings. Similarly, big data can be instrumental in reducing hospital readmissions, identifying high-risk patients, and improving patient outcomes.
The use of big data is likely to become more prevalent in healthcare practice and research. Subsequently, it will play an essential role in shaping the future of healthcare delivery.
3. – Managing Resources of a South Korean Hospital

Photo by Marcel Scholte .
A study from a hospital in South Korea used data mining to analyze patient data and identify the length of stay and resource use patterns. Consequently, they optimized the hospital’s resource allocation, reduced patient wait times and improved overall efficiency.
The study used electronic health records (EHRs) to analyze patient data and identify the length of stay predictors. For instance, the authors used a k-means clustering algorithm to identify patient clusters with distinct length-of-stay patterns. Then they used decision tree analysis to identify the factors influencing the length of stay. Above all, it highlights how data mining can identify patient subgroups, providing insights to improve resource allocation and patient outcomes.
Data Mining Examples in Businesses
Businesses rely heavily on accurate forecasting models when making important strategic decisions regarding investments and marketing campaigns. To this end, they utilize predictive analytics powered by machine learning algorithms, extracting relevant insights from vast amounts of historical data, including financial, operational, customer service, purchase order, inventory, sales performance and others. Therefore, managers comprehensively view all factors influencing the company’s bottom line.
Here are some data mining examples in real life in businesses:
4. – Walmart’s Big Data Mining in Real Life

Photo by Marques Thomas .
The world’s largest retailer, Walmart, has been making strides in using big data analytics. Certainly, with over 20,000, Walmart has access to vast data that offer an invaluable understanding of their customer’s behaviors and preferences.
Walmart’s use of big data analytics has been one of the driving forces behind its growth and success. Further, According to Bernard Marr , a prominent thought leader in business and technology, Walmart has built a state-of-the-art analytics system that runs on a petabyte data cloud, allowing them to make more informed decisions about everything from inventory management to marketing and customer engagement.
5. – Target’s Predictive Analysis Example of Data Mining in Real Life
This Forbes article highlights that Big Data’s power can be fascinating and alarming.
Target used the insights generated by their data mining system to send customized advertisements to expectant mothers. Also, the company used this information to provide them with helpful product recommendations.
Examples like this raise important questions about consumer privacy, targeted advertising, and ethical marketing practices.
Subsequently, Target later modified its advertising approach to be less intrusive.
6. – Amazon Recommender System

Photo by SOCIAL.CUT .
Amazon has become a household name due to its convenient online shopping platform. Still, behind the scenes, Amazon leverages sophisticated data mining tools to target customers with personalized offers and recommendations. Furthermore, association rule learning and market basket analysis are among the tools we covered in previous blog posts.
The company’s data-driven approach has increased customer loyalty, driven sales, and streamlined operations.
As businesses increasingly rely on big data, having a robust data strategy and the right tools is crucial.
For an example of market basket analysis, check out our article: How to Create a Market Basket Analysis in Tableau .
7. – American Apparel RFID System
Retailer American Apparel implemented an RFID system to improve inventory accuracy and streamline operations.
RFID stands for Radio-Frequency Identification , a technology that uses electromagnetic fields to identify and track tags attached to objects automatically.
The RFID system consists of a tiny radio transponder, a radio receiver and a transmitter. Moreover, it stores tag information in non-volatile memory. After that, it transmits the information when triggered by an electromagnetic interrogation pulse from a nearby RFID reader device back to the reader.
American Apparel used RFID tags to track inventory levels in real time, which helped the company identify sales trends and adjust inventory levels accordingly. As a result, the retailer increased its inventory accuracy and reduced the time and labor required for inventory management.
Beyond retail, industries such as automotive manufacturing, supply chain management, inventory control, animal tracking and libraries use RFID tags. However, there are concerns about the privacy implications of RFID technology since systems can potentially read personal data without consent. So, to address these concerns, the industry developed standards to protect privacy and security.
8. – JPMorgan Chase Data Mining Fraud Prevention System

Photo by David Jones .
In 2016, JPMorgan Chase launched a machine learning-enabled fraud detection system that uses predictive modeling to identify potential fraudulent activity in real time. Firstly, it combines transactional, customer behavior and demographic data to create a risk score for each transaction. Then, the system alerts human investigators when it detects unusual activity or high-risk transactions. Consequently, the system has reduced false alarms and improved detection rates for fraudulent activities.
Using sophisticated models based on customer demographic information and historical transactions is essential for banks to identify potential fraudulent activities and prevent financial losses.
9. – Zurich Claims Assessment Data Mining Example in Real Life
Zurich Insurance giant partnered with a drone services provider to survey properties and assess damage claims from natural disasters.
Using machine learning algorithms to analyze the drone footage, Zurich reduced the time and personnel required to assess claims. As a result, they improved customer experience.
This case study demonstrates how insurers leverage data mining and machine learning to transform business operations and provide more efficient and accurate customer services.
10. – Caterpillar’s Heavy Equipment Preventive Maintenance

Photo by Boom & Bucket .
Caterpillar has a strong presence in the mining industry and has innovated to bring cutting-edge technology to the market.
One of its offerings is the Cat MineStar System , which includes a suite of predictive analytics tools. Further, the system helps mining companies improve equipment performance, reduce downtime, and improve overall productivity.
The system uses advanced data analytics and machine learning to generate insights into equipment health and performance. Further, it offers various services, including equipment maintenance and component replacement schedules. As a result, mining companies can reduce the risk of equipment failure and keep their operations running at peak efficiency. In addition, the tools can help mining companies to prioritize maintenance activities, optimize equipment utilization, and plan for equipment upgrades or replacements.
11. – Netflix’s Real-Life Data Mining Example
Streaming giant Netflix built a highly successful business model by analyzing customer data to personalize its recommendations and predict what original content will be successful.
Netflix uses algorithms to recommend movies and TV shows to users based on their viewing history. Additionally, it uses analytics to predict content genres that will become popular, such as the hit series “House of Cards.”
By analyzing user preferences data, the company could determine that a political drama would succeed before they produced the show. As a result, Netflix has become a leader in the entertainment industry.
12. – Google Adwords Advertising Platform

Photo by Myriam Jessier .
Google AdWords is one of the most widely used online advertising platforms. Above all, one of the critical ways AdWords optimizes ad campaigns is by extracting insights and patterns from large data sets. Further, advanced analytics techniques like linear regression models allow AdWords to identify the most effective ad placements and targeting strategies. As a result, advertisers can focus on potential buyers more effectively.
AdWords can analyze demographic information, browsing history and search queries through data mining. Therefore, it can identify users more likely to click on ads, purchase goods or sign up for newsletters. Moreover, AdWords can also use data mining to determine the optimal times and locations for displaying ads, ensuring that ad placements are relevant and compelling.
13. – Microsoft’s Machine Learning for Analytics and Personalization (MLAP)
MLAP , developed by Microsoft Research, helps businesses and organizations better understand user behavior and preferences. Above all, it can analyze large data sets using machine learning algorithms to extract meaningful insights and provide tools for personalizing user experiences, thus improving the customer experience for products and services. Furthermore, it supports various data formats, visualization tools for presenting insights and results, and integrations with other Microsoft products and services.
14. – LinkedIn’s Talent Solutions Insights Toolkit

Photo by Georgia de Lotz .
LinkedIn’s Talent Solutions Insights Toolkit is another example of data mining in real life.
The toolkit gives businesses valuable insights into talent acquisition strategies . To this end, it provides access to real-time data and analytics. These include Job postings, applicant profiles, competitor insights, customized reporting, and the ability to share data with team members.
Further, additional features include candidate analysis, market analysis, and company insights, enabling recruiters to identify top talent, monitor industry trends, and analyze competitor strategies.
15. – Facebook’s Social Networking Insights
Facebook collects vast amounts of personal information from users, allowing advertisers to analyze and build detailed interest demographics profiles, helping them reach desired audience segments. For example, suppose a company wants to advertise shoes to women aged 25–35. In that case, Facebook uses mined insights to create custom audiences to match those criteria, allowing marketers to focus.
Additionally, Facebook provides resources for advertisers to create and target custom audiences based on various criteria, including demographics, interests, behaviors, and more. This information is accessible through the Facebook Ads Manager platform and in many articles and tutorials on Facebook’s website.
Harness the Power of Data Mining
Data mining is transforming the way we understand the world around us. Most importantly, unlocking insights that might otherwise remain hidden fosters more informed decisions and drives positive outcomes for businesses, industries, and society.
Throughout this post, we have explored 15 remarkable examples of how data mining is employed in real-life scenarios to uncover valuable insights. Additionally, these examples demonstrate the power of data mining in a diverse range of fields. Moreover, as organizations continue to generate ever-increasing amounts of data, data mining will undoubtedly become an even more important tool in unlocking the secrets of our complex world.
What are your thoughts about these examples of data mining in real life? How has your experience been implementing data mining? Leave a comment below.
Subscribe To Our List
Did you like our blog post? Would you like to get free updates directly in your email? Subscribe to our list in the form below.
Email address:
We use Mailchimp as our marketing platform. By clicking below to subscribe, you acknowledge that your information will be transferred to Mailchimp for processing. You also acknowledge that you would like to hear from Tech Business Guide via emails. You can unsubscribe at any time by clicking the link in the footer of our emails. For information about our privacy practices, please visit our website.
Related Articles

6 Machine Learning Steps Explained for the Business
Imagine where you could take your business if you knew the next product a customer will buy or if a transaction is fraudulent. This is what Machine Learning (ML) promises. Machine Learning allows businesses to challenge the status quo, creating tremendous disruption. Already, many companies are using Machine Learning to develop new business opportunities. Are […]

Machine Learning Engineer vs Data Scientist: How To Choose
As Artificial Intelligence business applications become commonplace, you may find yourself with the question of whether to pursue a Machine Learning Engineer vs Data Scientist hire or career decision. The industry tends to confuse between the two job roles. However, while they share many of the skills required to do both jobs, there are subtle […]

10 Data Mining Tools: Uncovering Insights for Business Success
Data mining tools have significantly changed the way businesses analyze their data. With the vast quantity of information available to organizations today, it can be challenging to extract valuable insights from it. Therefore, tools like data mining software come in handy, providing various features to help businesses sift through their data and gain valuable insights. […]
Leave a Reply Cancel reply
Your email address will not be published. Required fields are marked *
Data Mining: use cases & benefits
- April 27, 2021
- Data Science
Share This Post
Table of Contents
In the last decade, advances in processing power and speed have allowed us to move from tedious and time-consuming manual practices to fast and easy automated data analysis. The more complex the data sets collected, the greater the potential to uncover relevant information. Retailers, banks, manufacturers, healthcare companies, etc., are using data mining to uncover the relationships between everything from price optimisation, promotions and demographics to how economics, risk, competition and online presence affect their business models, revenues, operations and customer relationships. Today, data scientists have become indispensable to organisations around the world as companies seek to achieve bigger goals than ever before with data science. In this article, you will learn about the main use cases of data mining and how it has opened up a world of possibilities for businesses.
Today, organisations have access to more data than ever before. However, making sense of the huge volumes of structured and unstructured data to implement improvements across the organisation can be extremely difficult due to the sheer volume of information.
What is Data Mining
Data mining is the process of analyzing massive volumes of data to discover business intelligence that helps companies solve problems, mitigate risks, and seize new opportunities. Data mining, also called knowledge discovery in databases, in computer science, the process of discovering interesting and useful patterns and relationships in large volumes of data. The field combines tools from statistics and artificial intelligence with database management to analyze large digital collections, known as data sets. Data mining is widely used in business , science research, and government security. It is the process of finding anomalies, patterns and correlations within large data sets to predict outcomes. It is a process used by companies to turn raw data into useful information.
The data mining process breaks down into five steps:
1. Organizations collect data and load it into their data warehouses 2. They store and manage the data, either on in-house servers or the cloud 3. Business analysts, management teams and information technology professionals access the data and determine 4. how they want to organize it 5. Application software sorts the data based on the user’s results 6. The end-user presents the data in an easy-to-share format, such as a graph or table.
Data mining practitioners typically achieve timely, reliable results by following a structured, repeatable process that involves these six steps :
- Business understanding Developing a thorough understanding of the project parameters, including the current business situation, the primary business objective of the project, and the criteria for success.
- Data understanding Determining the data that will be needed to solve the problem and gathering it from all available sources.
- Data preparation Preparing the data in the appropriate format to answer the business question, fixing any data quality problems such as missing or duplicate data.
- Modeling Using algorithms to identify patterns within the data.
- Evaluation Determining whether and how well the results delivered by a given model will help achieve the business goal. There is often an iterative phase to find the best algorithm in order to achieve the best result.
- Deployment Making the results of the project available to decision-makers.
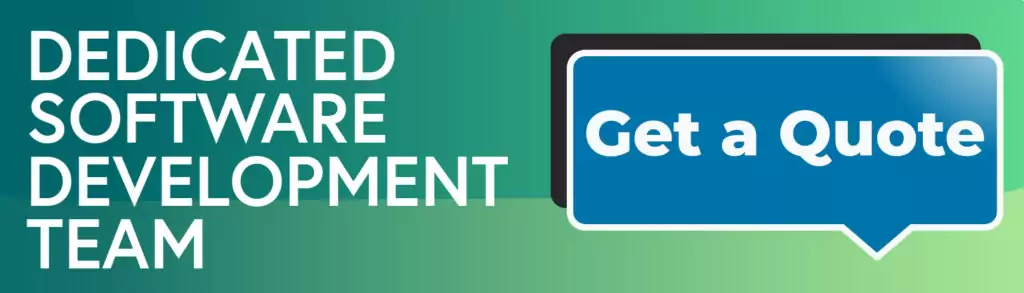
Data Mining Techniques
There are many data mining techniques that organisations can use to turn raw data into actionable insights. These techniques range from advanced AI to the fundamentals of data preparation, which are essential to maximising the value of data investments: 1. Pattern tracking Pattern tracking is a fundamental technique of data mining. It is about identifying and monitoring trends or patterns in data to make intelligent inferences about business outcomes. When an organisation identifies a trend in sales data, for example, it has a basis for taking action to leverage that information. If it is determined that a certain product sells better than others for a particular demographic, an organisation can use this knowledge to create similar products or services, or simply stock the original product better for that demographic.
2. Data cleaning and preparation Data cleaning and preparation is an essential part of the data mining process. Raw data must be cleaned and formatted to be useful for the various analysis methods. Data cleaning and preparation includes various elements of data modelling, transformation, migration, integration and aggregation. It is a necessary step in understanding the basic characteristics and attributes of the data to determine its best use.
3. Classification Classification-based data mining techniques involve analysing the various attributes associated with different types of data. Once organisations have identified the key characteristics of these data types, they can categorise or classify the corresponding data. This is essential for identifying, for example, personally identifiable information that organisations may wish to protect or delete from records.
4. Outlier detection Outlier detection identifies anomalies in data sets. Once organisations have found outliers in their data, it is easier to understand why these anomalies occur and to prepare for any future occurrences to better meet business objectives. For example, if there is a spike in the use of transactional credit card systems at a certain time of day, organisations can leverage this information by discovering the reason for the spike to optimise their sales for the rest of the day.
5. Association Association is a data mining technique related to statistics. It indicates that certain data is related to other data or data-driven events. It is similar to the notion of co-occurrence in machine learning, where the probability of one data-based event is indicated by the presence of another. This means that data analysis shows that there is a relationship between two data events: for example, the fact that the purchase of hamburgers is frequently accompanied by the purchase of Chips.
6. Clustering Clustering is an analysis technique that relies on visual approaches to understanding data. Clustering mechanisms use graphs to show where the distribution of data is with respect to different types of metrics. Clustering techniques also use different colours to show the distribution of data. Graphical approaches are ideal for using cluster analysis. With graphs and clustering, in particular, users can visually see how data is distributed to identify trends that are relevant to their business objectives. 7. Regression Regression techniques are useful for identifying the nature of the relationship between variables in a data set. These relationships may be causal in some cases, or simply correlated in others. Regression is a simple white box technique that clearly reveals the relationship between variables. Regression techniques are used in some aspects of forecasting and data modelling. 8. Sequential patterns This data mining technique focuses on finding a series of events that occur in sequence. It is particularly useful for transactional data mining. For example, this technique can reveal which items of clothing customers are most likely to buy after an initial purchase of, say, a pair of shoes. Understanding sequential patterns can help organisations to recommend additional items to customers to boost sales. 9. Prediction Prediction is a very powerful aspect of data mining and is one of the four branches of analytics. Predictive analytics uses patterns found in current or historical data to extend them into the future. In this way, it gives organisations insight into trends that will occur in their data in the future. There are several different approaches to using predictive analytics. Some of the more advanced ones involve aspects of machine learning and artificial intelligence. However, predictive analytics does not necessarily rely on these techniques, but can also be facilitated by simpler algorithms.
10. Decision trees Decision trees are a specific type of predictive model that allows organisations to efficiently extract data. Technically, a decision tree is part of machine learning, but it is better known as a “white box” machine learning technique due to its extremely simple nature. A decision tree allows users to clearly understand how data inputs affect outcomes. When multiple decision tree models are combined, they create predictive analytics models known as a random forest. Complicated random forest models are considered “black box” machine learning techniques because it is not always easy to understand their results based on their inputs. However, in most cases, this basic form of ensemble modelling is more accurate than using decision trees alone.
11. Neural networks A neural network is a specific type of machine learning model that is often used with AI and deep learning. So-called because they have different layers that resemble the functioning of neurons in the human brain, neural networks are one of the most accurate machine learning models used today. 12. Visualization Data visualisations are another important part of data mining. They offer users a view of data based on sensory perceptions that people can see. Today’s data visualisations are dynamic, useful for real-time data streaming, and are characterised by different colours that reveal different trends and patterns in the data. Dashboards are a powerful way to use data visualisations to uncover information about data operations. Organisations can base dashboards on different metrics and use visualisations to highlight patterns in the data, rather than simply using numerical results from statistical models.
13. Statistical techniques Statistical techniques are at the heart of most analyses involved in the data mining process. Different analysis models are based on statistical concepts, which produce numerical values applicable to specific business objectives. For example, neural networks use complex statistics based on different weights and measures to determine whether an image is a dog or a cat in image recognition systems. 14. Long-term memory processing Long-term memory processing refers to the ability to analyse data over long periods. Historical data stored in data warehouses are useful for this purpose. When an organisation can analyse over a long period of time, it is able to identify patterns that would otherwise be too subtle to detect. For example, by analysing attrition over a period of several years, an organisation can find subtle clues that could lead to a reduction in attrition in finance.
15. Data warehousing Data warehousing is an important part of the data mining process. Traditionally, data warehousing was about storing structured data in relational database management systems so that it could be analysed for business intelligence, reporting and basic dashboards. Today, there are cloud-based data warehouses and semi-structured and unstructured data warehouses such as Hadoop. While data warehouses were traditionally used for historical data, many modern approaches can provide deep analysis of data in real time.
16. Machine learning and artificial intelligence Machine learning and artificial intelligence (AI) represent some of the most advanced developments in the field of data mining. Advanced forms of machine learning, such as deep learning, offer highly accurate predictions when working with large-scale data. They are therefore useful for data processing in AI implementations such as computer vision, speech recognition or sophisticated text analysis using natural language processing. These data mining techniques help to determine the value of semi-structured and unstructured data.
Why is data mining important?
Data mining allows you to:
- Sift through all the chaotic and repetitive noise in your data.
- Understand what is relevant and then make good use of that information to assess likely outcomes.
- Accelerate the pace of making informed decisions.
Benefits of Data Mining
- Data mining helps companies to get knowledge-based information.
- It can be implemented in new systems as well as existing platforms
- Data mining helps organizations to make the profitable adjustments in operation and production.
- Facilitates automated prediction of trends and behaviors as well as automated discovery of hidden patterns.
- The data mining is a cost-effective and efficient solution compared to other statistical data applications.
- Data mining helps with the decision-making process.
- It is the speedy process which makes it easy for the users to analyze huge amount of data in less time.
Data Mining use cases and examples
The predictive capacity of data mining has changed the design of business strategies. Now, you can understand the present to anticipate the future. These are some uses cases and examples of data mining in current industry:
- Marketing Data mining is used to explore increasingly large databases and to improve market segmentation. By analysing the relationships between parameters such as customer age, gender, tastes, etc., it is possible to guess their behaviour in order to direct personalised loyalty campaigns. Data mining in marketing also predicts which users are likely to unsubscribe from a service, what interests them based on their searches, or what a mailing list should include to achieve a higher response rate.
- Banking Banks use data mining to better understand market risks. It is commonly applied to credit ratings and to intelligent anti-fraud systems to analyse transactions, card transactions, purchasing patterns and customer financial data. Data mining also allows banks to learn more about our online preferences or habits to optimise the return on their marketing campaigns, study the performance of sales channels or manage regulatory compliance obligations.
- Education Data mining benefits educators to access student data, predict achievement levels and find students or groups of students which need extra attention. For example, students who are weak in maths subject.
- E-Commerce E-commerce websites use Data Mining to offer cross-sells and up-sells through their websites. One of the most famous names is Amazon, who use Data mining techniques to get more customers into their eCommerce store.
- Retail Supermarkets, for example, use joint purchasing patterns to identify product associations and decide how to place them in the aisles and on the shelves. Data mining also detects which offers are most valued by customers or increase sales at the checkout queue.
- Service Providers Service providers like mobile phone and utility industries use Data Mining to predict the reasons when a customer leaves their company. They analyze billing details, customer service interactions, complaints made to the company to assign each customer a probability score and offer incentives.
- Medicine Data mining enables more accurate diagnostics. Having all of the patient’s information, such as medical records, physical examinations, and treatment patterns, allows more effective treatments to be prescribed. It also enables more effective, efficient and cost-effective management of health resources by identifying risks, predicting illnesses in certain segments of the population or forecasting the length of hospital admission. Detecting fraud and irregularities, and strengthening ties with patients with an enhanced knowledge of their needs are also advantages of using data mining in medicine.
- Insurance Data mining helps insurance companies to price their products profitable and promote new offers to their new or existing customers.
- Manufacturing With the help of Data Mining Manufacturers can predict wear and tear of production assets. They can anticipate maintenance which helps them reduce them to minimize downtime.
- Crime Investigation Data Mining helps crime investigation agencies to deploy police workforce (where is a crime most likely to happen and when?), who to search at a border crossing etc.
- Television and radio There are networks that apply real time data mining to measure their online television (IPTV) and radio audiences. These systems collect and analyse, on the fly, anonymous information from channel views, broadcasts and programming. Data mining allows networks to make personalised recommendations to radio listeners and TV viewers, as well as get to know their interests and activities in real time and better understand their behaviour. Networks also gain valuable knowledge for their advertisers, who use this data to target their potential customers more accurately.
Organizations across industries are achieving transformative results from data mining:
- Bayer helps farmers with sustainable food production Weeds that damage crops have been a problem for farmers since farming began. A proper solution is to apply a narrow spectrum herbicide that effectively kills the exact species of weed in the field while having as few undesirable side effects as possible. But to do that, farmers first need to accurately identify the weeds in their fields. Using Talend Real-time Big Data, Bayer Digital Farming developed WEEDSCOUT, a new application farmers can download free. The app uses machine learning and artificial intelligence to match photos of weeds in a Bayer database with weed photos farmers send in. It gives the grower the opportunity to more precisely predict the impact of his or her actions such as, choice of seed variety, application rate of crop protection products, or harvest timing.
- Air France KLM caters to customer travel preferences The airline uses data mining techniques to create a 360-degree customer view by integrating data from trip searches, bookings, and flight operations with web, social media, call center, and airport lounge interactions. They use this deep customer insight to create personalized travel experiences.
- Groupon aligns marketing activities One of Groupon’s key challenges is processing the massive volume of data it uses to provide its shopping service. Every day, the company processes more than a terabyte of raw data in real time and stores this information in various database systems. Data mining allows Groupon to align marketing activities more closely with customer preferences, analyzing 1 terabyte of customer data in real time and helping the company identify trends as they emerge.
- Domino’s helps customers build the perfect pizza The largest pizza company in the world collects 85,000 structured and unstructured data sources, including point of sales systems and 26 supply chain centers, and through all its channels, including text messages, social media, and Amazon Echo. This level of insight has improved business performance while enabling one-to-one buying experiences across touchpoints.
You can use data mining to solve almost any business problem that involves data, including:
- Increasing revenue.
- Understanding customer segments and preferences.
- Acquiring new customers.
- Improving cross-selling and up-selling.
- Retaining customers and increasing loyalty.
- Increasing ROI from marketing campaigns.
- Detecting fraud.
- Identifying credit risks.
- Monitoring operational performance.
Data mining tools
Organizations can get started with data mining by accessing the necessary tools. Because the data mining process starts right after data ingestion, it’s critical to find data preparation tools that support different data structures necessary for data mining analytics. Organizations will also want to classify data in order to explore it with the numerous techniques discussed above.
1. Oracle Data Mining Oracle Data Mining popularly knownn as ODM is a module of the Oracle Advanced Analytics Database. This Data mining tool allows data analysts to generate detailed insights and make predictions. It helps predict customer behavior, develops customer profiles, identifies cross-selling opportunities.
2. Rapid Miner Rapid Miner is one of the best predictive analysis systems, it is written in JAVA programming language. It provides an integrated environment for deep learning, text mining, machine learning & predictive analysis. It offers a range of products to build new data mining processes and predictive setup analysis.
3. Orange Data Mining It is a perfect software suite for machine learning & data mining. It best aids the data visualization and is a component based software. The components of Orange are called “widgets.” These widgets range from preprocessing and data visualization to the assessment of algorithms and predictive modeling. Widgets deliver significant functionalities such as: displaying data table and allowing to select features, data reading, training predictors and comparison of learning algorithms, data element visualization, etc.
4. Weka Weka has a GUI that facilitates easy access to all its features. It is written in JAVA programming language. Weka is an open-source machine learning software with a vast collection of algorithms for data mining. It supports different data mining tasks, like preprocessing, classification, regression, clustering, and visualization, in a graphical interface that makes it easy to use. For each of these tasks, Weka provides built-in machine learning algorithms which allow you to quickly test your ideas and deploy models without writing any code. 5. KNIME It is the best integration platform for data analytics and reporting developed by KNIME.com AG. It operates on the concept of the modular data pipeline. KNIME constitutes of various machine learning and data mining components embedded together. It is a free, open-source platform for data mining and machine learning. Its intuitive interface allows you to create end-to-end data science workflows, from modeling to production. And different pre-built components enable fast modeling without entering a single line of code. A set of powerful extensions and integrations make KNIME a versatile and scalable platform to process complex types of data and use advanced algorithms. With KNIME, data scientists can create applications and services for analytics or business intelligence. In the financial industry, for instance, common use cases include credit scoring, fraud detection, and credit risk assessment.
6. Sisense Sisense is another effective Data mining tool. Sisense is extremely useful and best suited BI software when it comes to reporting purposes within the organization. It has a brilliant capability to handle and process data for the small scale/large scale organizations. It instantly analyzes and visualizes both big and disparate datasets. It is an ideal tool for creating dashboards with a wide variety of visualizations. It allows combining data from various sources to build a common repository and further, refines data to generate rich reports that get shared across departments for reporting. Sisense generates reports which are highly visual. It is specially designed for users that are non-technical. It allows drag & drop facility as well as widgets. Different widgets can be selected to generate the reports in form of pie charts, line charts, bar graphs etc. based on the purpose of an organization. Reports can be further drilled down by simply clicking to check details and comprehensive data.
7. Dundas Dundas is another excellent dashboard, reporting & data analytics tool. Dundas is quite reliable with its rapid integrations & quick insights. It provides unlimited data transformation patterns with attractive tables, charts & graphs. Dundas BI puts data in well-defined structures in a specific manner in order to ease the processing for the user. It constitutes of relational methods that facilitate multi-dimensional analysis and focuses on business-critical matters. As it generates reliable reports, thus it reduces cost and eliminates the requirement of other additional software.
8. Intetsoft Intetsoft is analytics dashboard and reporting tool that provides iterative development of data reports/views & generates pixel perfect reports. It allows the quick and flexible transformation of data from various sources.
9. Qlik Qlik is Data mining and visualization tool. It also offers dashboards and supports multiple data sources and file types.It has the following features: drag-and-drop interfaces to create flexible, interactive data visualizations, instantly respond to interactions and changes, supports multiple data sources and file types, allows easy security for data and content across all devices, allows you to share relevant analyses, including apps and stories, using a centralized hub.
10. MonkeyLearn MonkeyLearn is a machine learning platform that specializes in text mining. Available in a user-friendly interface, you can easily integrate MonkeyLearn with your existing tools to perform data mining in real-time. Start immediately with pre-trained text mining models like this sentiment analyzer, below, or build a customized solution to cater to more specific business needs. MonkeyLearn supports various data mining tasks, from detecting topics, sentiment, and intent, to extracting keywords and named entities.MonkeyLearn’s text mining tools are already being used to automate ticket tagging and routing in customer support, automatically detect negative feedback in social media, and deliver fine-grained insights that lead to better decision making.
I hope you found this article useful, if you need any help with Data Mining or Data Science Project in general, contact us ! We have experts in this field.
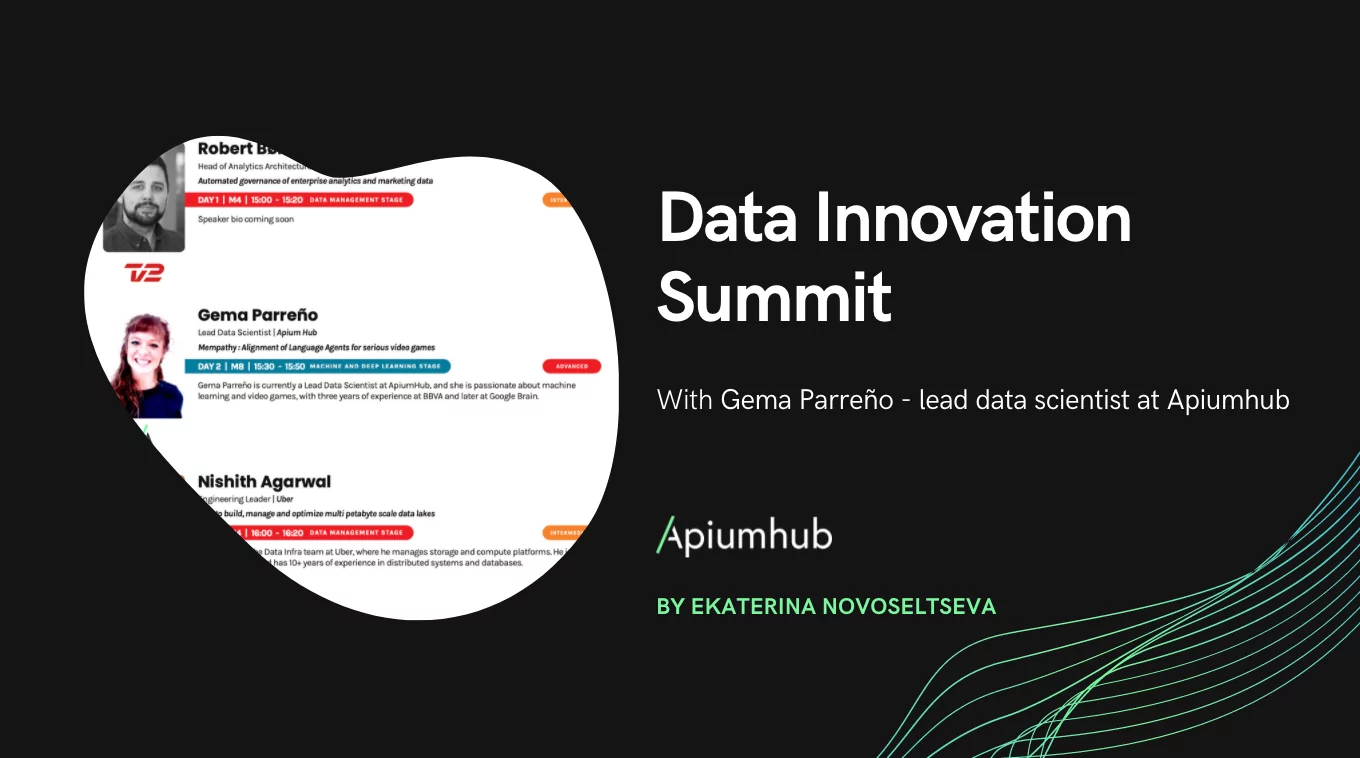
Leave a Reply Cancel Reply
Your email address will not be published. Required fields are marked *
You may use these HTML tags and attributes: <a href="" title=""> <abbr title=""> <acronym title=""> <b> <blockquote cite=""> <cite> <code> <del datetime=""> <em> <i> <q cite=""> <s> <strike> <strong>
Save my name, email, and website in this browser for the next time I comment.
Subscribe To Our Newsletter
Get updates from our latest tech findings
About Apiumhub
Apiumhub brings together a community of software developers & architects to help you transform your idea into a powerful and scalable product. Our Tech Hub specialises in Software Architecture , Web Development & Mobile App Development . Here we share with you industry tips & best practices, based on our experience.
Estimate Your Project
- Agile web and app development
- Offshoring and outsourcing
- Product Ownership
- Software architecture
- Software Architecture Sonar
- Software Quality Assurance Category
- Technology industry trends
- User Experience Design
Popular posts
- Custom Software Development Services & Modern Practices
- 5 Major Software Architecture Patterns
- Software Development Service Providers You Can Trust
- Software Outsourcing: Interesting Statistics, Predictions, Facts & Key Players
- Tech Of The Future: Technology Predictions For Our World In 2050
Get our Book : Software Architecture Metrics
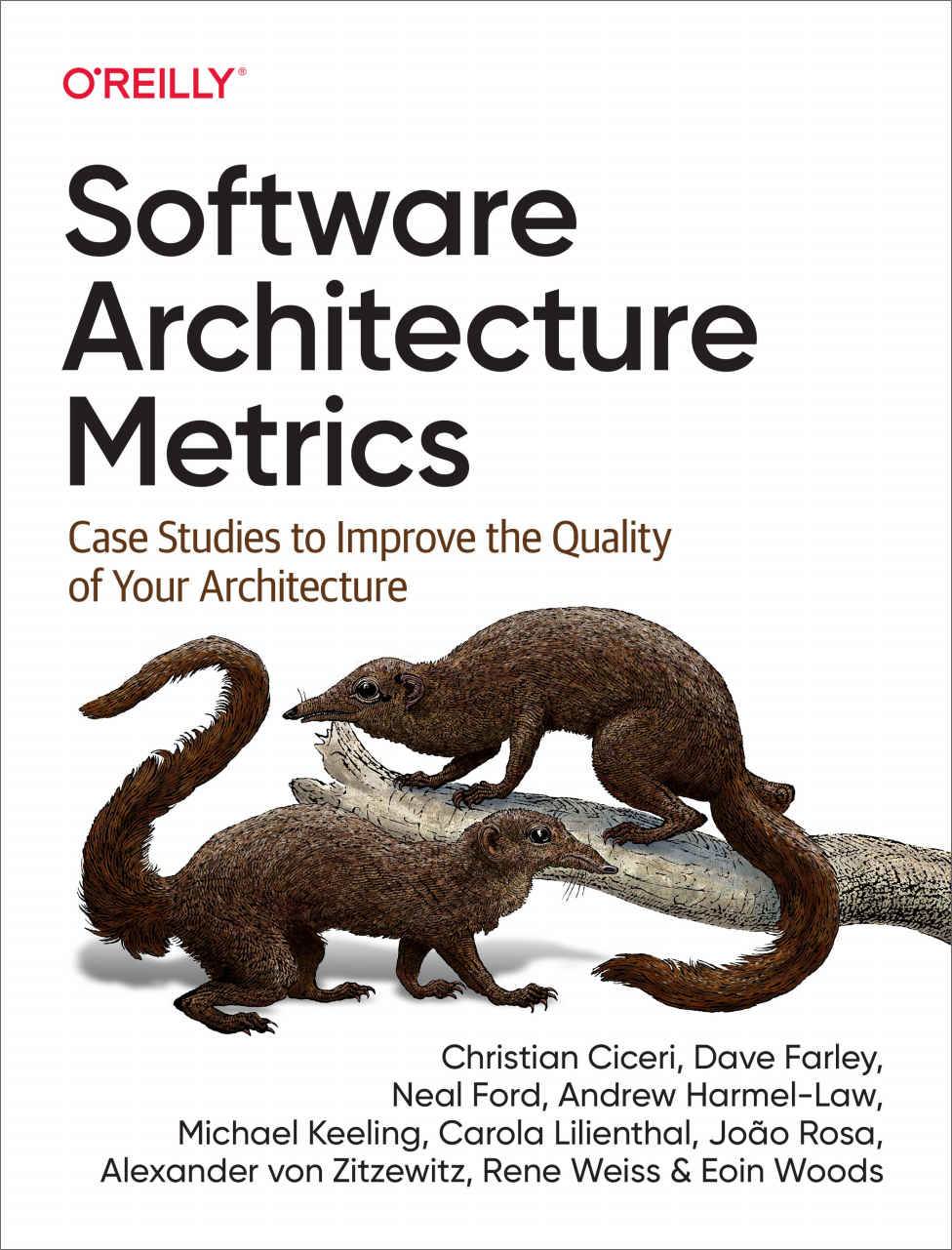
Have a challenging project?
We Can Work On It Together
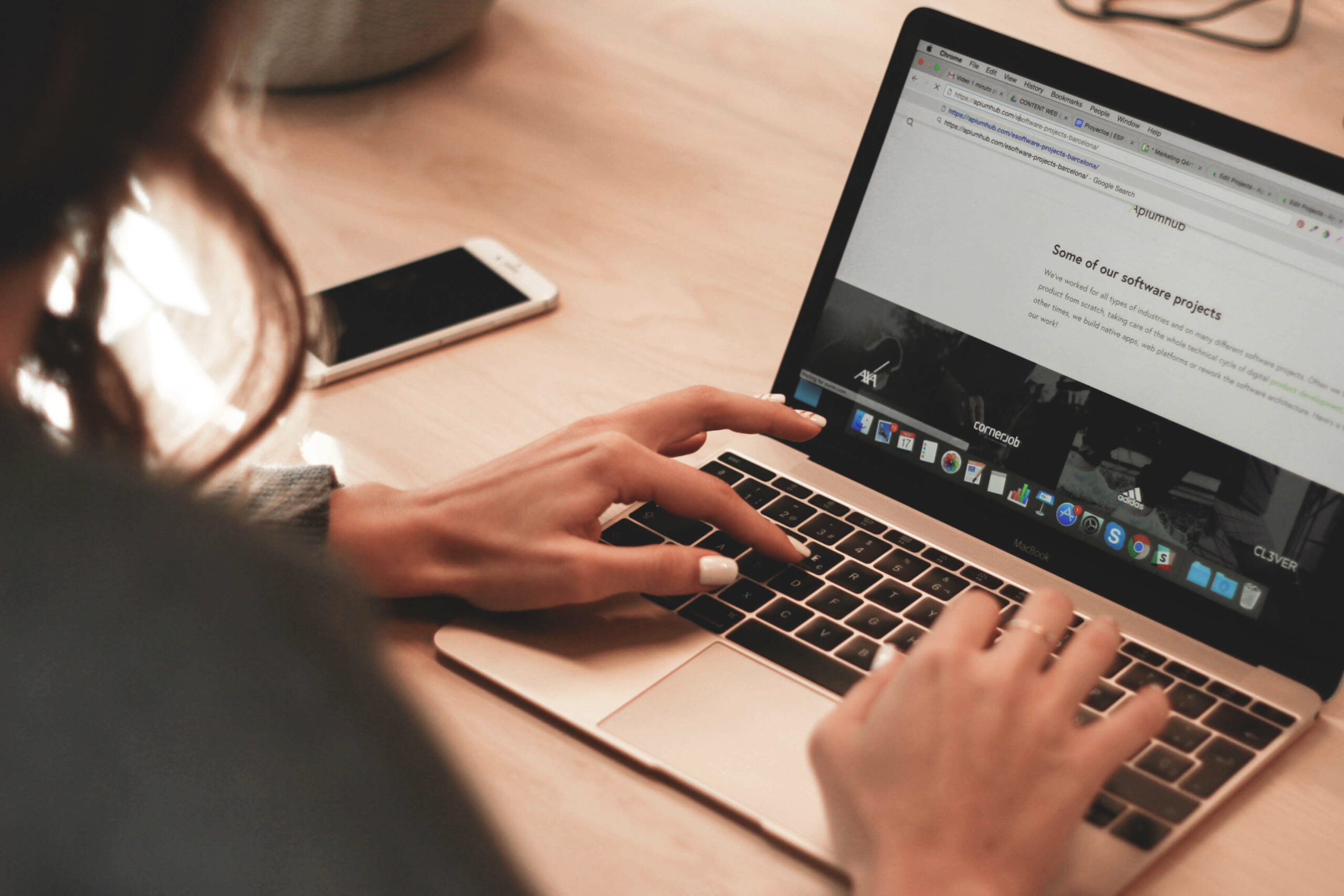
- (+34) 934 815 085
- [email protected]
- Consell de Cent, 333, 7o, 08007 Barcelona
OUR SERVICES
- Dedicated Team
- Team Extension
- Software Architecture
- Web Development
- Mobile App Development
- Agile Product Manager
- QA Automation
LATEST BLOG NEWS
7 Benefits of Using GitHub
HTMX vs React: Choosing the Right Frontend Approach for Your Project
5 Books for QA Engineers
Key Android and iOS Accessibility Features
USEFUL LINKS
- Free Discovery Session
- Green Software
- Become a Contributor
- Privacy Policy
- Whistleblower Channel
Receive the latest tech industry news and events


- Free Case Studies
- Business Essays
Write My Case Study
Buy Case Study
Case Study Help
- Case Study For Sale
- Case Study Service
- Hire Writer
Case Study on Data Mining
Data mining case study:.
Data mining is the complicated process which is characterized with the collection and analysis of the unknown data and its transformation into simpler algorithms which are known by the people who do not have mathematical education in order to use this data in various spheres of human life.
Data mining is closely connected with computer science and studies the methods of information management which includes the methods of classification, modelling, prediction, etc. which are based on the use of artificial intelligence, genetic algorithms, decision tree learning, evolutionary programming, content-addressable memory, fuzzy logic, etc. Among the methods of data mining there are many statistic methods (descriptive analysis, factor analysis, analysis of variance, component analysis, linear discriminant analysis and others).However, such methods presuppose certain knowledge about the data under analysis, that does not meet the aims of data mining (discovery of the earlier unknown data which can be useful on practice). The process of the solution of the problem with the help of the methods of data mining consists of several core components. First of all there is a great data base.
We Will Write a Custom Case Study Specifically For You For Only $13.90/page!
Then, there is a supposition that there is certain hidden knowledge in this data basis, which should be found.Artificial intelligence tries to find this information with the help of various methods worked out by the experts. Under the term of hidden knowledge one understands the knowledge which is not known by anyone, because it can not be seen (it can be discovered only with the help of profound calculations). Moreover, this information should have practical use and possibility for interpretation.In order to succeed in the process of data mining case study writing one should research the topic deeply to improve knowledge on the problem. If one is aware of the principles of the problem, he will be able to solve the puzzle of a case study suggested by the professor.
A good case study should possess a logical structure, brand new ideas, up-to-date content, etc. A student is supposed to use his critical thinking skills to define the cause of the problem and its effect. In the end, one should analyze the value of the problem and suggest the effective methods for its solution.The Internet and a free example case study on data mining tools can be the best solution of the problem connected with the process of case study writing, because every example paper is written by the professional writer. With the help of a free sample case study on data mining and data warehousing one can learn to analyze the problem correctly, format and construct the proper composition for the paper and draw the right conclusions.
Related posts:
- Case study: Jaeger uses data mining to reduce losses
- Data Mining
- Case Study on Data Communication and Networking
- Arctic Mining Case Study
- Case Study Qmont Mining
- Mining Service Case Study Analysis
- Case Study on Data Warehousing
Quick Links
Privacy Policy
Terms and Conditions
Testimonials
Our Services
Case Study Writing Service
Case Studies For Sale
Our Company
Welcome to the world of case studies that can bring you high grades! Here, at ACaseStudy.com, we deliver professionally written papers, and the best grades for you from your professors are guaranteed!
[email protected] 804-506-0782 350 5th Ave, New York, NY 10118, USA
Acasestudy.com © 2007-2019 All rights reserved.

Hi! I'm Anna
Would you like to get a custom case study? How about receiving a customized one?
Haven't Found The Case Study You Want?
For Only $13.90/page
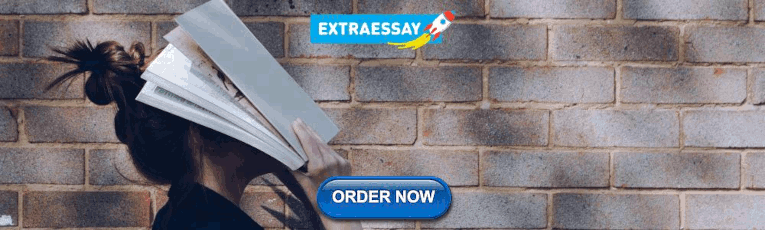
IMAGES
VIDEO
COMMENTS
Read the PBS, LunaMetrics, and Google Analytics case study. 5. The Pegasus Group. Cyber attackers compromised and targeted the data mining system (DMS) of a major network client of The Pegasus Group and launched a distributed denial-of-service (DDoS) attack against 1,500 services. Under extreme time pressure, The Pegasus Group needed to find a ...
A successful implementation requires defining clear goals, choosing data wisely, and constant adaptation. Data mining case studies help businesses explore data for smart decision-making. It's about finding valuable insights from big datasets. This is crucial for businesses in all industries as data guides strategic planning.
International Journal of Computer Sciences and Engin eering Open Access. Research Paper Vol-6, Special Issue-8, Oct 2018 E-ISSN: 2347 -2693. A CASE STUDY ON DATA MINING APPLICATIONS ON BANKING ...
Data Mining Case Studies and Practice Prize is an international peer-reviewed workshop highlighting successful real-world applications of data mining. DMCS applications are wide-ranging: (a) data mining systems that have uncovered massive tax fraud rings (MITRE) (b) identification of patients at risk of heart disease, and detection of breast ...
Data mining or Knowledge Discovery from Data (KDD) tools allows us to analyze large datasets to solve decision problems. The data mining tools use historical information to build a model to predict customer's behavior e.g., which customers are likely to respond to a new product.
Abstract. We report on the panel discussion held at the ICDM'10 conference on the top 10 data mining case studies in order to provide a snapshot of where and how data mining techniques have made significant real-world impact. The tasks covered by 10 case studies range from the detection of anomalies such as cancer, fraud, and system failures to ...
process and popular data mining techniques. It also presents R and its packages, functions and task views for data mining. At last, some datasets used in this book are described. 1.1 Data Mining Data mining is the process to discover interesting knowledge from large amounts of data [Han 1 R
The methodological purpose of this article is to demonstrate how data mining contributes to rapid complex case study descriptions. Our complexity-informed design draws on freely accessible datasets reporting the public health response surrounding the onset of the COVID-19 pandemic in Alberta (Canada) and involves the cross analysis of integrated findings across six periods of fluctuation ...
ABSTRACT. Data Mining with R: Learning with Case Studies, Second Edition uses practical examples to illustrate the power of R and data mining. Providing an extensive update to the best-selling first edition, this new edition is divided into two parts. The first part will feature introductory material, including a new chapter that provides an ...
The case studies uses data mining algorithm implementations from CRAN packages. It is devoted to the classification of individuals described by socioeconomic Census attributes into income categories. The primary objective of the case study is to predict the number of violent crimes (per population) in US communities based on attributes ...
Built on the state-of-the-art data mining techniques, Mo-bileMiner presents a real case study on how to integrate data mining techniques into a business solution. In a large mobile communication company like China Mo-bile Communication Corporation, there are many analytical tasks where data mining can help to address the business interests of ...
Web Data Mining: A Case Study. Samia Jones Galveston College, Galveston, TX 77550. Omprakash K. Gupta Prairie View A&M, Prairie View, TX 77446 [email protected]. Abstract. With an enormous amount of data stored in databases and data warehouses, it is increasingly important to develop powerful tools for analysis of such data and mining ...
Case Study No.3: Starbucks. Starbucks is one of the leading coffee shops with innumerable branches around the globe. Their case study will be a perfect example of the case studies on data mining in market analysis. Starbucks indulges in data mining to determine the perfect locations for setting up its stores.
Text and data mining: Case studies. This page outlines different case studies and use cases. The librarian-researcher case studies highlight the interaction between library professionals, researchers, scholarly resources and tools, while the external case studies focus on the research impact from text and data mining activities.
The field of data mining provides techniques for automated discovery of valuable information from the accumulated data of computerized operations of enterprises. This book offers a clear and comprehensive introduction to both data mining theory and practice. It is written primarily as a textbook for the students of computer science, management, computer applications, and information technology.
The Harvard University offers online data mining courses and has a Center for Healthcare Data Analytics created by the need to analyze data in large public or private data sets. Harvard research includes funding and providing healthcare, quality of care, studies on special and disadvantaged populations, and access to care.
As a result, it is paramount for business leaders and entrepreneurs to study examples of data mining in real life. ... This case study demonstrates how insurers leverage data mining and machine learning to transform business operations and provide more efficient and accurate customer services. 10. - Caterpillar's Heavy Equipment Preventive ...
It is the process of finding anomalies, patterns and correlations within large data sets to predict outcomes. It is a process used by companies to turn raw data into useful information. The data mining process breaks down into five steps: 1. Organizations collect data and load it into their data warehouses. 2.
Airline Data Mining Case Study. The airline industry is experiencing rapid evolution, driven by technological advancements, centralized planning, and the entry of new industry players. Air travel ...
This paper proposes an innovative use of data mining, visualization and OLAP techniques for decision support in services related to electricity connections and regional level of management of case ...
Case Study on Data Mining Application in Health Care Monitoring Systems 82 Data mining applications in healthcare sector: Healthcare sector nowadays creates a large amounts of complex data about patients, hospital resources, disease diagnosis, electronic patient records and various types of medical devices . Larger amounts of data are a
3. Network Analysis. When we talk about text mining, it is inevitable to talk also about social media. Indeed, together with the texts exchanged in conversations, the network of users — posting, commenting, influencing, and following — is often investigated to learn more about connections, groups, and upcoming trends.
Data Mining Case Study: Data mining is the complicated process which is characterized with the collection and analysis of the unknown data and its transformation into simpler algorithms which are known by the people who do not have mathematical education in order to use this data in various spheres of human life.. Data mining is closely connected with computer science and studies the methods ...