- Open access
- Published: 06 April 2020
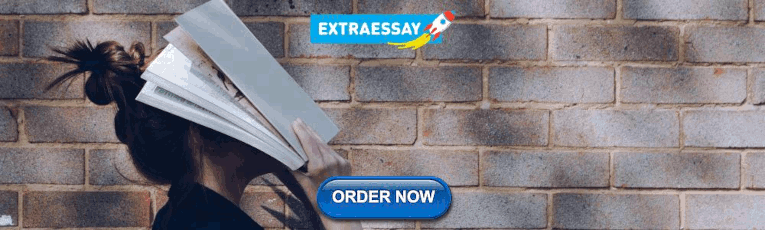
The influence of education on health: an empirical assessment of OECD countries for the period 1995–2015
- Viju Raghupathi 1 &
- Wullianallur Raghupathi 2
Archives of Public Health volume 78 , Article number: 20 ( 2020 ) Cite this article
217k Accesses
270 Citations
172 Altmetric
Metrics details
A clear understanding of the macro-level contexts in which education impacts health is integral to improving national health administration and policy. In this research, we use a visual analytic approach to explore the association between education and health over a 20-year period for countries around the world.
Using empirical data from the OECD and the World Bank for 26 OECD countries for the years 1995–2015, we identify patterns/associations between education and health indicators. By incorporating pre- and post-educational attainment indicators, we highlight the dual role of education as both a driver of opportunity as well as of inequality.
Adults with higher educational attainment have better health and lifespans compared to their less-educated peers. We highlight that tertiary education, particularly, is critical in influencing infant mortality, life expectancy, child vaccination, and enrollment rates. In addition, an economy needs to consider potential years of life lost (premature mortality) as a measure of health quality.
Conclusions
We bring to light the health disparities across countries and suggest implications for governments to target educational interventions that can reduce inequalities and improve health. Our country-level findings on NEET (Not in Employment, Education or Training) rates offer implications for economies to address a broad array of vulnerabilities ranging from unemployment, school life expectancy, and labor market discouragement. The health effects of education are at the grass roots-creating better overall self-awareness on personal health and making healthcare more accessible.
Peer Review reports
Introduction
Is education generally associated with good health? There is a growing body of research that has been exploring the influence of education on health. Even in highly developed countries like the United States, it has been observed that adults with lower educational attainment suffer from poor health when compared to other populations [ 36 ]. This pattern is attributed to the large health inequalities brought about by education. A clear understanding of the health benefits of education can therefore serve as the key to reducing health disparities and improving the well-being of future populations. Despite the growing attention, research in the education–health area does not offer definitive answers to some critical questions. Part of the reason is the fact that the two phenomena are interlinked through life spans within and across generations of populations [ 36 ], thereby involving a larger social context within which the association is embedded. To some extent, research has also not considered the variances in the education–health relationship through the course of life or across birth cohorts [ 20 ], or if there is causality in the same. There is therefore a growing need for new directions in education–health research.
The avenues through which education affects health are complex and interwoven. For one, at the very outset, the distribution and content of education changes over time [ 20 ]. Second, the relationship between the mediators and health may change over time, as healthcare becomes more expensive and/or industries become either more, or less hazardous. Third, some research has documented that even relative changes in socioeconomic status (SES) can affect health, and thus changes in the distribution of education implies potential changes in the relationship between education and health. The relative index of inequality summarizes the magnitude of SES as a source of inequalities in health [ 11 , 21 , 27 , 29 ]. Fourth, changes in the distribution of health and mortality imply that the paths to poor health may have changed, thereby affecting the association with education.
Research has proposed that the relationship between education and health is attributable to three general classes of mediators: economic; social, psychological, and interpersonal; and behavioral health [ 31 ]. Economic variables such as income and occupation mediate the relationship between education and health by controlling and determining access to acute and preventive medical care [ 1 , 2 , 19 ]. Social, psychological, and interpersonal resources allow people with different levels of education to access coping resources and strategies [ 10 , 34 ], social support [ 5 , 22 ], and problem-solving and cognitive abilities to handle ill-health consequences such as stress [ 16 ]. Healthy behaviors enable educated individuals to recognize symptoms of ill health in a timely manner and seek appropriate medical help [ 14 , 35 ].
While the positive association between education and health has been established, the explanations for this association are not [ 31 ]. People who are well educated experience better health as reflected in the high levels of self-reported health and low levels of morbidity, mortality, and disability. By extension, low educational attainment is associated with self-reported poor health, shorter life expectancy, and shorter survival when sick. Prior research has suggested that the association between education and health is a complicated one, with a range of potential indicators that include (but are not limited to) interrelationships between demographic and family background indicators [ 8 ] - effects of poor health in childhood, greater resources associated with higher levels of education, appreciation of good health behaviors, and access to social networks. Some evidence suggests that education is strongly linked to health determinants such as preventative care [ 9 ]. Education helps promote and sustain healthy lifestyles and positive choices, nurture relationships, and enhance personal, family, and community well-being. However, there are some adverse effects of education too [ 9 ]. Education may result in increased attention to preventive care, which, though beneficial in the long term, raises healthcare costs in the short term. Some studies have found a positive association between education and some forms of illicit drug and alcohol use. Finally, although education is said to be effective for depression, it has been found to have much less substantial impact in general happiness or well-being [ 9 ].
On a universal scale, it has been accepted that several social factors outside the realm of healthcare influence the health outcomes [ 37 ]. The differences in morbidity, mortality and risk factors in research, conducted within and between countries, are impacted by the characteristics of the physical and social environment, and the structural policies that shape them [ 37 ]. Among the developed countries, the United States reflects huge disparities in educational status over the last few decades [ 15 , 24 ]. Life expectancy, while increasing for all others, has decreased among white Americans without a high school diploma - particularly women [ 25 , 26 , 32 ]. The sources of inequality in educational opportunities for American youth include the neighborhood they live in, the color of their skin, the schools they attend, and the financial resources of their families. In addition, the adverse trends in mortality and morbidity brought on by opioids resulting in suicides and overdoses (referred to as deaths of despair) exacerbated the disparities [ 21 ]. Collectively, these trends have brought about large economic and social inequalities in society such that the people with more education are likely to have more health literacy, live longer, experience better health outcomes, practice health promoting behaviors, and obtain timely health checkups [ 21 , 17 ].
Education enables people to develop a broad range of skills and traits (including cognitive and problem-solving abilities, learned effectiveness, and personal control) that predispose them towards improved health outcomes [ 23 ], ultimately contributing to human capital. Over the years, education has paved the way for a country’s financial security, stable employment, and social success [ 3 ]. Countries that adopt policies for the improvement of education also reap the benefits of healthy behavior such as reducing the population rates of smoking and obesity. Reducing health disparities and improving citizen health can be accomplished only through a thorough understanding of the health benefits conferred by education.
There is an iterative relationship between education and health. While poor education is associated with poor health due to income, resources, healthy behaviors, healthy neighborhood, and other socioeconomic factors, poor health, in turn, is associated with educational setbacks and interference with schooling through difficulties with learning disabilities, absenteeism, or cognitive disorders [ 30 ]. Education is therefore considered an important social determinant of health. The influence of national education on health works through a variety of mechanisms. Generally, education shows a relationship with self-rated health, and thus those with the highest education may have the best health [ 30 ]. Also, health-risk behaviors seem to be reduced by higher expenditure into the publicly funded education system [ 18 ], and those with good education are likely to have better knowledge of diseases [ 33 ]. In general, the education–health gradients for individuals have been growing over time [ 38 ].
To inform future education and health policies effectively, one needs to observe and analyze the opportunities that education generates during the early life span of individuals. This necessitates the adoption of some fundamental premises in research. Research must go beyond pure educational attainment and consider the associated effects preceding and succeeding such attainment. Research should consider the variations brought about by the education–health association across place and time, including the drivers that influence such variations [ 36 ].
In the current research, we analyze the association between education and health indicators for various countries using empirical data from reliable sources such as the Organization for Economic Cooperation and Development (OECD) and World Bank. While many studies explore the relationship between education and health at a conceptual level, we deploy an empirical approach in investigating the patterns and relationships between the two sets of indicators. In addition, for the educational indicators, we not only incorporate the level of educational attainment, but also look at the potential socioeconomic benefits, such as enrollment rates (in each sector of educational level) and school life expectancy (at each educational level). We investigate the influences of educational indicators on national health indicators of infant mortality, child vaccinations, life expectancy at birth, premature mortality arising from lack of educational attainment, employment and training, and the level of national health expenditure. Our research question is:
What are some key influencers/drivers in the education-health relationship at a country level?
The current study is important because policy makers have an increasing concern on national health issues and on policies that support it. The effect of education is at the root level—creating better overall self-awareness on personal health and making healthcare more accessible. The paper is organized as follows: Section 2 discusses the background for the research. Section 3 discusses the research method; Section 4 offers the analysis and results; Section 5 provides a synthesis of the results and offers an integrated discussion; Section 6 contains the scope and limitations of the research; Section 7 offers conclusions with implications and directions for future research.
Research has traditionally drawn from three broad theoretical perspectives in conceptualizing the relationship between education and health. The majority of research over the past two decades has been grounded in the Fundamental Cause Theory (FCT) [ 28 ], which posits that factors such as education are fundamental social causes of health inequalities because they determine access to resources (such as income, safe neighborhoods, or healthier lifestyles) that can assist in protecting or enhancing health [ 36 ]. Some of the key social resources that contribute to socioeconomic status include education (knowledge), money, power, prestige, and social connections. As some of these undergo change, they will be associated with differentials in the health status of the population [ 12 ].
Education has also been conceptualized using the Human Capital Theory (HCT) that views it as a return on investment in the form of increased productivity [ 4 ]. Education improves knowledge, skills, reasoning, effectiveness, and a broad range of other abilities that can be applied to improving health. The third approach - the signaling or credentialing perspective [ 6 ] - is adopted to address the large discontinuities in health at 12 and 16 years of schooling, which are typically associated with the receipt of a high school diploma and a college degree, respectively. This perspective considers the earned credentials of a person as a potential source that warrants social and economic returns. All these theoretical perspectives postulate a strong association between education and health and identify mechanisms through which education influences health. While the HCT proposes the mechanisms as embodied skills and abilities, FCT emphasizes the dynamism and flexibility of mechanisms, and the credentialing perspective proposes educational attainment through social responses. It needs to be stated, however, that all these approaches focus on education solely in terms of attainment, without emphasizing other institutional factors such as quality or type of education that may independently influence health. Additionally, while these approaches highlight the individual factors (individual attainment, attainment effects, and mechanisms), they do not give much emphasis to the social context in which education and health processes are embedded.
In the current research while we acknowledge the tenets of these theoretical perspectives, we incorporate the social mechanisms in education such as level of education, skills and abilities brought about by enrollment, school life expectancy, and the potential loss brought about by premature mortality. In this manner, we highlight the relevance of the social context in which the education and health domains are situated. We also study the dynamism of the mechanisms over countries and over time and incorporate the influences that precede and succeed educational attainment.
We analyze country level education and health data from the OECD and World Bank for a period of 21 years (1995–2015). Our variables include the education indicators of adult education level; enrollment rates at various educational levels; NEET (Not in Employment, Education or Training) rates; school life expectancy; and the health indicators of infant mortality, child vaccination rates, deaths from cancer, life expectancy at birth, potential years of life lost and smoking rates (Table 1 ). The data was processed using the tools of Tableau for visualization, and SAS for correlation and descriptive statistics. Approaches for analysis include ranking, association, and data visualization of the health and education data.
Analyses and results
In this section we identify and analyze patterns and associations between education and health indicators and discuss the results. Since countries vary in population sizes and other criteria, we use the estimated averages in all our analyses.
Comparison of health outcomes for countries by GDP per capita
We first analyzed to see if our data reflected the expectation that countries with higher GDP per capita have better health status (Fig. 1 ). We compared the average life expectancy at birth, average infant mortality, average deaths from cancer and average potential year of life lost, for different levels of GDP per capita (Fig. 1 ).
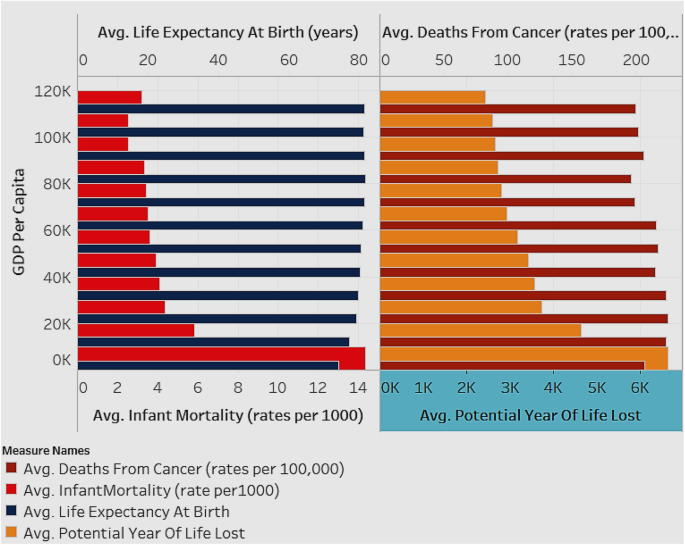
Associations between Average Life Expectancy (years) and Average Infant Mortality rate (per 1000), and between Deaths from Cancer (rates per 100,000) and Average Potential Years of Life Lost (years), by GDP per capita (for all countries for years 1995–2015)
Figure 1 depicts two charts with the estimated averages of variables for all countries in the sample. The X-axis of the first chart depicts average infant mortality rate (per 1000), while that of the second chart depicts average potential years of life lost (years). The Y-axis for both charts depicts the GDP per capita shown in intervals of 10 K ranging from 0 K–110 K (US Dollars). The analysis is shown as an average for all the countries in the sample and for all the years (1995–2015). As seen in Fig. 1 , countries with lower GDP per capita have higher infant mortality rate and increased potential year of life lost (which represents the average years a person would have lived if he or she had not died prematurely - a measure of premature mortality). Life expectancy and deaths from cancer are not affected by GDP level. When studying infant mortality and potential year lost, in order to avoid the influence of a control variable, it was necessary to group the samples by their GDP per capita level.
Association of Infant Mortality Rates with enrollment rates and education levels
We explored the association of infant mortality rates with the enrollment rates and adult educational levels for all countries (Fig. 2 ). The expectation is that with higher education and employment the infant mortality rate decreases.
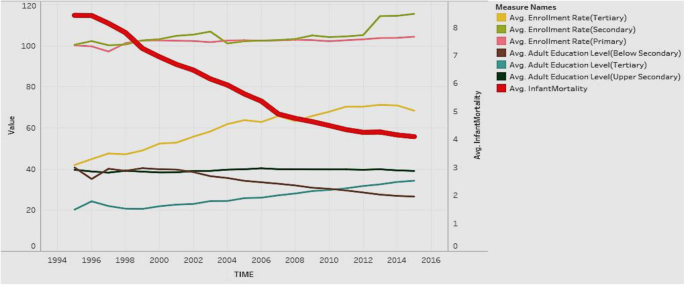
Association of Adult Education Levels (ratio) and Enrollment Rates (ratio) with Infant Mortality Rate (per 1000)
Figure 2 depicts the analysis for all countries in the sample. The figure shows the years from 1995 to 2015 on the X axis. It shows two Y-axes with one axis denoting average infant mortality rate (per 1000 live births), and the other showing the rates from 0 to 120 to depict enrollment rates (primary/secondary/tertiary) and education levels (below secondary/upper secondary/tertiary). Regarding the Y axis showing rates over 100, it is worth noting that the enrollment rates denote a ratio of the total enrollment (regardless of age) at a level of education to the official population of the age group in that education level. Therefore, it is possible for the number of children enrolled at a level to exceed the official population of students in the age group for that level (due to repetition or late entry). This can lead to ratios over 100%. The figure shows that in general, all education indicators tend to rise over time, except for adult education level below secondary, which decreases over time. Infant mortality shows a steep decreasing trend over time, which is favorable. In general, countries have increasing health status and education over time, along with decreasing infant mortality rates. This suggests a negative association of education and enrollment rates with mortality rates.
Association of Education Outcomes with life expectancy at birth
We explored if the education outcomes of adult education level (tertiary), school life expectancy (tertiary), and NEET (not in employment, education, or training) rates, affected life expectancy at birth (Fig. 3 ). Our expectation is that adult education and school life expectancy, particularly tertiary, have a positive influence, while NEET has an adverse influence, on life expectancy at birth.
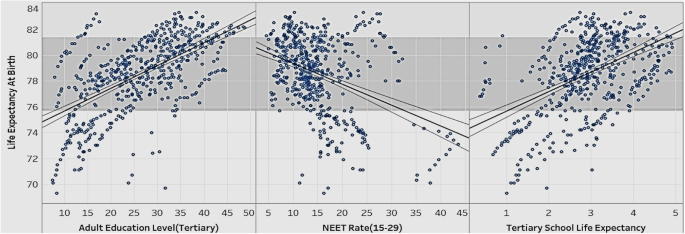
Association of Adult Education Level (Tertiary), NEET rate, School Life Expectancy (Tertiary), with Life Expectancy at Birth
Figure 3 show the relationships between various education indicators (adult education level-tertiary, NEET rate, school life expectancy-tertiary) and life expectancy at birth for all countries in the sample. The figure suggests that life expectancy at birth rises as adult education level (tertiary) and tertiary school life expectancy go up. Life expectancy at birth drops as the NEET rate goes up. In order to extend people’s life expectancy, governments should try to improve tertiary education, and control the number of youths dropping out of school and ending up unemployed (the NEET rate).
Association of Tertiary Enrollment and Education with potential years of life lost
We wanted to explore if the potential years of life lost rates are affected by tertiary enrollment rates and tertiary adult education levels (Fig. 4 ).
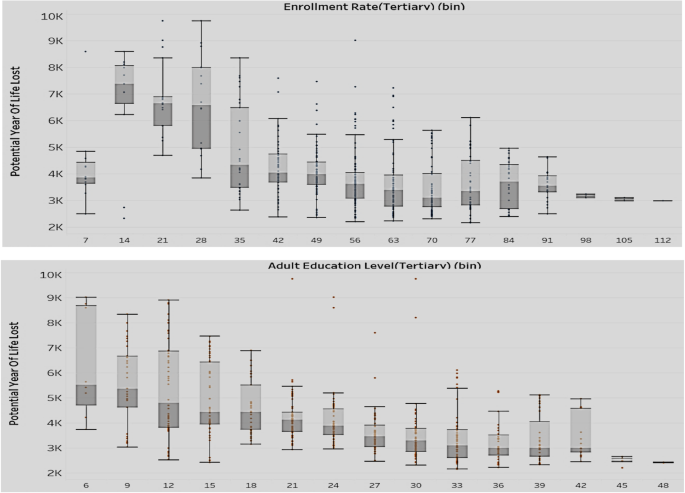
Association of Enrollment rate-tertiary (top) and Adult Education Level-Tertiary (bottom) with Potential Years of Life Lost (Y axis)
The two sets of box plots in Fig. 4 compare the enrollment rates with potential years of life lost (above set) and the education level with potential years of life lost (below set). The analysis is for all countries in the sample. As mentioned earlier, the enrollment rates are expressed as ratios and can exceed 100% if the number of children enrolled at a level (regardless of age) exceed the official population of students in the age group for that level. Potential years of life lost represents the average years a person would have lived, had he/she not died prematurely. The results show that with the rise of tertiary adult education level and tertiary enrollment rate, there is a decrease in both value and variation of the potential years of life lost. We can conclude that lower levels in tertiary education adversely affect a country’s health situation in terms of premature mortality.
Association of Tertiary Enrollment and Education with child vaccination rates
We compared the performance of tertiary education level and enrollment rates with the child vaccination rates (Fig. 5 ) to assess if there was a positive impact of education on preventive healthcare.
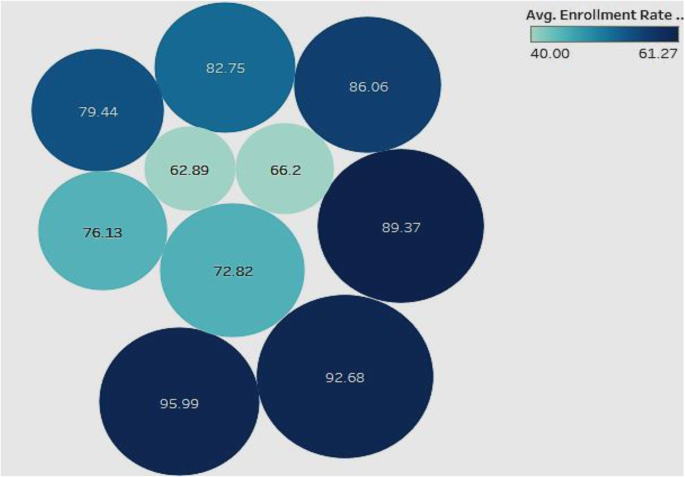
Association of Adult Education Level-Tertiary and Enrollment Rate-Tertiary with Child Vaccination Rates
In this analysis (Fig. 5 ), we looked for associations of child vaccination rates with tertiary enrollment and tertiary education. The analysis is for all countries in the sample. The color of the bubble represents the tertiary enrollment rate such that the darker the color, the higher the enrollment rate, and the size of the bubble represents the level of tertiary education. The labels inside the bubbles denote the child vaccination rates. The figure shows a general positive association of high child vaccination rate with tertiary enrollment and tertiary education levels. This indicates that countries that have high child vaccination rates tend to be better at tertiary enrollment and have more adults educated in tertiary institutions. Therefore, countries that focus more on tertiary education and enrollment may confer more health awareness in the population, which can be reflected in improved child vaccination rates.
Association of NEET rates (15–19; 20–24) with infant mortality rates and deaths from Cancer
In the realm of child health, we also looked at the infant mortality rates. We explored if infant mortality rates are associated with the NEET rates in different age groups (Fig. 6 ).
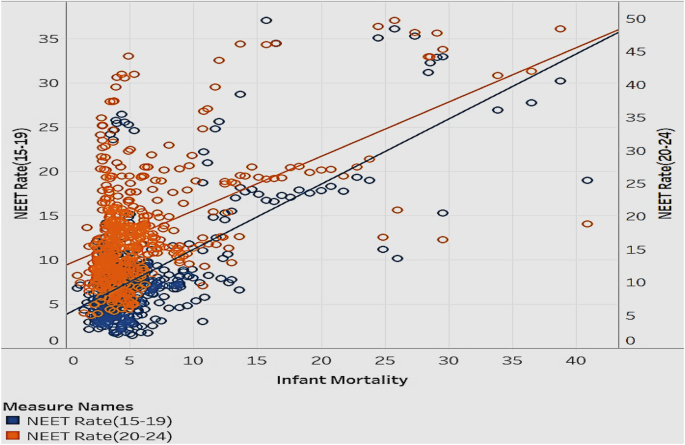
Association of Infant Mortality rates with NEET Rates (15–19) and NEET Rates (20–24)
Figure 6 is a scatterplot that explores the correlation between infant mortality and NEET rates in the age groups 15–19 and 20–24. The data is for all countries in the sample. Most data points are clustered in the lower infant mortality and lower NEET rate range. Infant mortality and NEET rates move in the same direction—as infant mortality increases/decrease, the NEET rate goes up/down. The NEET rate for the age group 20–24 has a slightly higher infant mortality rate than the NEET rate for the age group 15–19. This implies that when people in the age group 20–24 are uneducated or unemployed, the implications on infant mortality are higher than in other age groups. This is a reasonable association, since there is the potential to have more people with children in this age group than in the teenage group. To reduce the risk of infant mortality, governments should decrease NEET rates through promotional programs that disseminate the benefits of being educated, employed, and trained [ 7 ]. Additionally, they can offer financial aid to public schools and companies to offer more resources to raise general health awareness in people.
We looked to see if the distribution of population without employment, education, or training (NEET) in various categories of high, medium, and low impacted the rate of deaths from cancer (Fig. 7 ). Our expectation is that high rates of NEET will positively influence deaths from cancer.
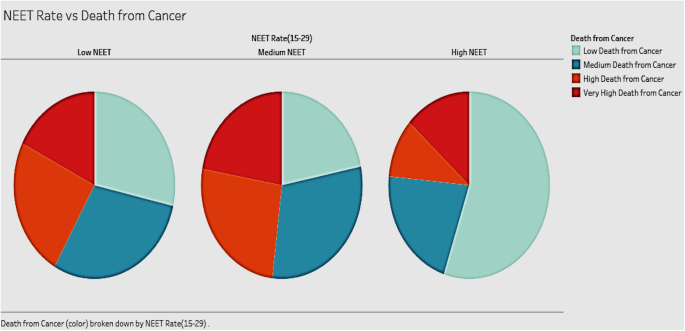
Association of Deaths from Cancer and different NEET Rates
The three pie charts in Fig. 7 show the distribution of deaths from cancer in groups of countries with different NEET rates (high, medium, and low). The analysis includes all countries in the sample. The expectation was that high rates of NEET would be associated with high rates of cancer deaths. Our results, however, show that countries with medium NEET rates tend to have the highest deaths from cancer. Countries with high NEET rates have the lowest deaths from cancer among the three groups. Contrary to expectations, countries with low NEET rates do not show the lowest death rates from cancer. A possible explanation for this can be attributed to the fact that in this group, the people in the labor force may be suffering from work-related hazards including stress, that endanger their health.
Association between adult education levels and health expenditure
It is interesting to note the relationship between health expenditure and adult education levels (Fig. 8 ). We expect them to be positively associated.
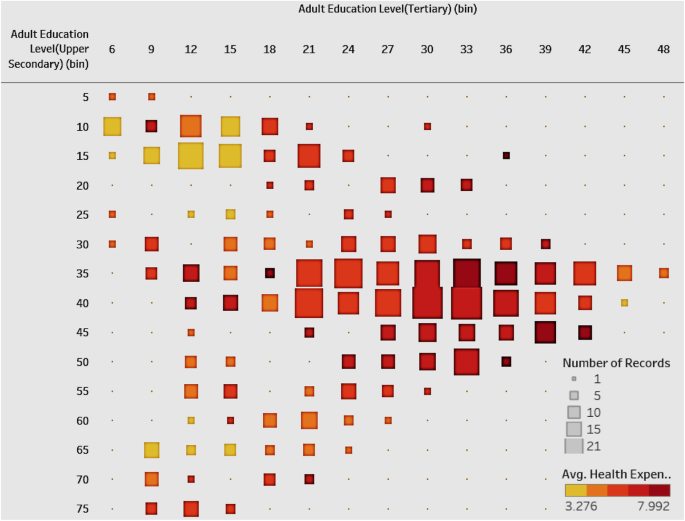
Association of Health Expenditure and Adult Education Level-Tertiary & Upper Secondary
Figure 8 shows a heat map with the number of countries in different combinations of groups between tertiary and upper-secondary adult education level. We emphasize the higher levels of adult education. The color of the square shows the average of health expenditure. The plot shows that most of the countries are divided into two clusters. One cluster has a high tertiary education level as well as a high upper-secondary education level and it has high average health expenditure. The other cluster has relatively low tertiary and upper secondary education level with low average health expenditure. Overall, the figure shows a positive correlation between adult education level and compulsory health expenditure. Governments of countries with low levels of education should allocate more health expenditure, which will have an influence on the educational levels. Alternatively, to improve public health, governments can frame educational policies to improve the overall national education level, which then produces more health awareness, contributing to national healthcare.
Association of Compulsory Health Expenditure with NEET rates by country and region
Having explored the relationship between health expenditure and adult education, we then explored the relationship between health expenditure and NEET rates of different countries (Fig. 9 ). We expect compulsory health expenditure to be negatively associated with NEET rates.
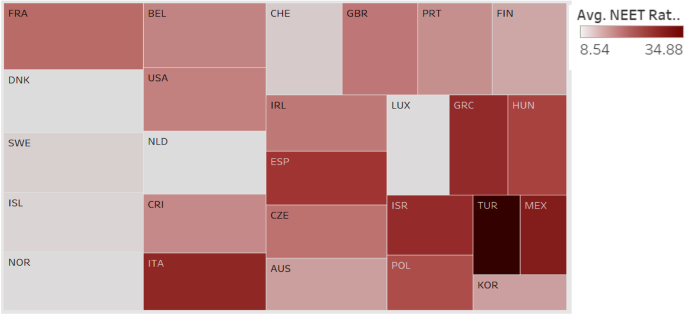
Association between Compulsory Health Expenditure and NEET Rate by Country and Region
In Fig. 9 , each box represents a country or region; the size of the box indicates the extent of compulsory health expenditure such that a larger box implies that the country has greater compulsory health expenditure. The intensity of the color of the box represents the NEET rate such that the darker color implies a higher NEET rate. Turkey has the highest NEET rate with low health expenditure. Most European countries such as France, Belgium, Sweden, and Norway have low NEET rates and high health expenditure. The chart shows a general association between low compulsory health expenditure and high NEET rates. The relationship, however, is not consistent, as there are countries with high NEET and high health expenditures. Our suggestion is for most countries to improve the social education for the youth through free training programs and other means to effectively improve the public health while they attempt to raise the compulsory expenditure.
Distribution of life expectancy at birth and tertiary enrollment rate
The distribution of enrollment rate (tertiary) and life expectancy of all the countries in the sample can give an idea of the current status of both education and health (Fig. 10 ). We expect these to be positively associated.
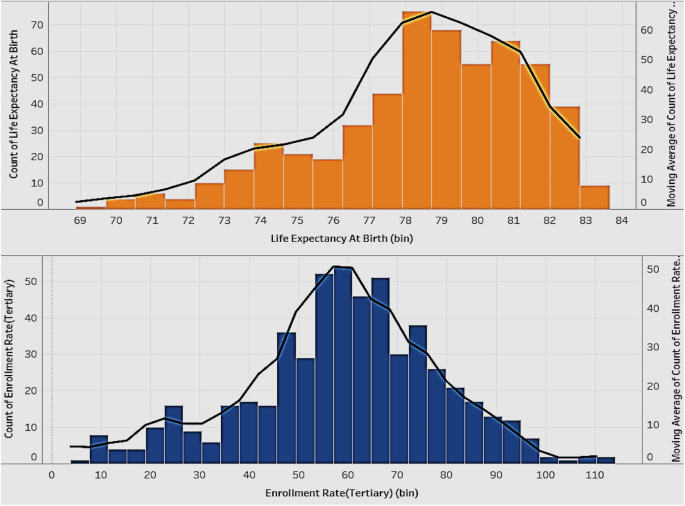
Distribution of Life Expectancy at Birth (years) and Tertiary Enrollment Rate
Figure 10 shows two histograms with the lines representing the distribution of life expectancy at birth and the tertiary enrollment rate of all the countries. The distribution of life expectancy at birth is skewed right, which means most of the countries have quite a high life expectancy and there are few countries with a very low life expectancy. The tertiary enrollment rate has a good distribution, which is closer to a normal distribution. Governments of countries with an extremely low life expectancy should try to identify the cause of this problem and take actions in time to improve the overall national health.
Comparison of adult education levels and deaths from Cancer at various levels of GDP per capita
We wanted to see if various levels of GDP per capita influence the levels of adult education and deaths from cancer in countries (Fig. 11 ).
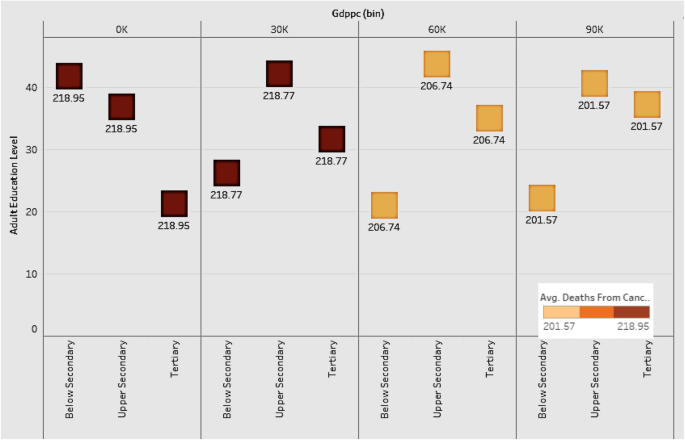
Comparison of Adult Education Levels and Deaths from Cancer at various levels of GDP per capita
Figure 11 shows the distribution of various adult education levels for countries by groups of GDP per capita. The plot shows that as GDP grows, the level of below-secondary adult education becomes lower, and the level of tertiary education gets higher. The upper-secondary education level is constant among all the groups. The implication is that tertiary education is the most important factor among all the education levels for a country to improve its economic power and health level. Countries should therefore focus on tertiary education as a driver of economic development. As for deaths from cancer, countries with lower GDP have higher death rates, indicating the negative association between economic development and deaths from cancer.
Distribution of infant mortality rates by continent
Infant mortality is an important indicator of a country’s health status. Figure 12 shows the distribution of infant mortality for the continents of Asia, Europe, Oceania, North and South America. We grouped the countries in each continent into high, medium, and low, based on infant mortality rates.
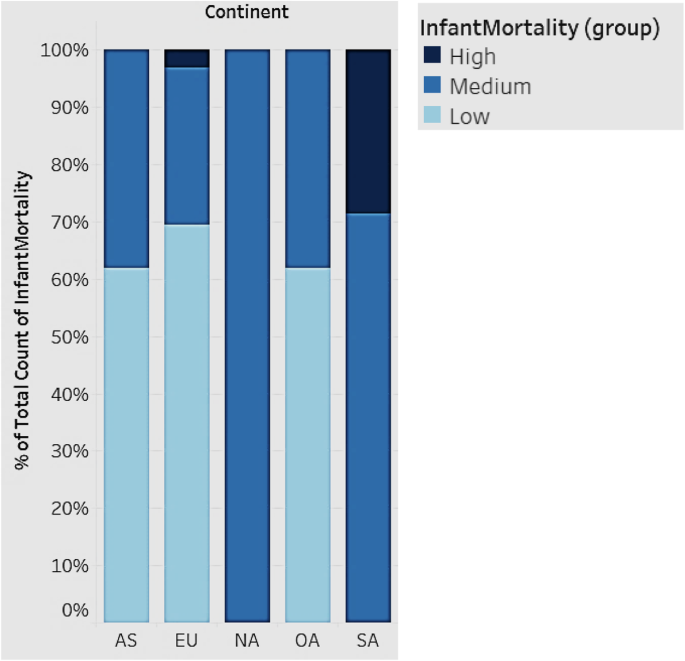
Distribution of Infant Mortality rates by Continent
In Fig. 12 , each bar represents a continent. All countries fall into three groups (high, medium, and low) based on infant mortality rates. South America has the highest infant mortality, followed by Asia, Europe, and Oceania. North America falls in the medium range of infant mortality. South American countries, in general, should strive to improve infant mortality. While Europe, in general, has the lowest infant mortality rates, there are some countries that have high rates as depicted.
Association between child vaccination rates and NEET rates
We looked at the association between child vaccination rates and NEET rates in various countries (Fig. 13 ). We expect countries that have high NEET rates to have low child vaccination rates.
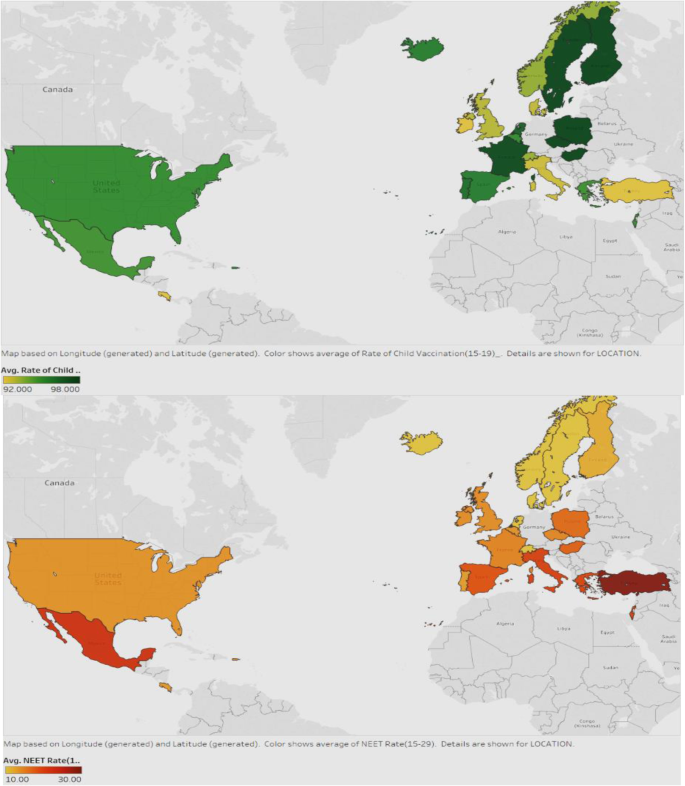
Association between Child Vaccination Rates and NEET rates
Figure 13 displays the child vaccination rates in the first map and the NEET rates in the second map, for all countries. The darker green color shows countries with higher rates of vaccination and the darker red represents those with higher NEET rates. It can be seen that in general, the countries with lower NEET also have better vaccination rates. Examples are USA, UK, Iceland, France, and North European countries. Countries should therefore strive to reduce NEET rates by enrolling a good proportion of the youth into initiatives or programs that will help them be more productive in the future, and be able to afford preventive healthcare for the families, particularly, the children.
Average smoking rate in different continents over time
We compared the trend of average smoking rate for the years 1995–201 for the continents in the sample (Fig. 14 ).
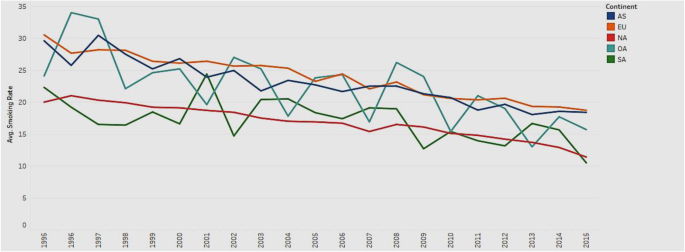
Trend of average smoking rate in different continents from 1995 to 2015
Figure 14 depicts the line charts of average smoking rates for the continents of Asia, Europe, Oceania, North and South America. All the lines show an overall downward trend, which indicates that the average smoking rate decreases with time. The trend illustrates that people have become more health conscious and realize the harmful effects of smoking over time. However, the smoking rate in Europe (EU) is consistently higher than that in other continents, while the smoking rate in North America (NA) is consistently lower over the years. Governments in Europe should pay attention to the usage of tobacco and increase health consciousness among the public.
Association between adult education levels and deaths from Cancer
We explored if adult education levels (below-secondary, upper-secondary, and tertiary) are associated with deaths from cancer (Fig. 15 ) such that higher levels of education will mitigate the rates of deaths from cancer, due to increased awareness and proactive health behavior.
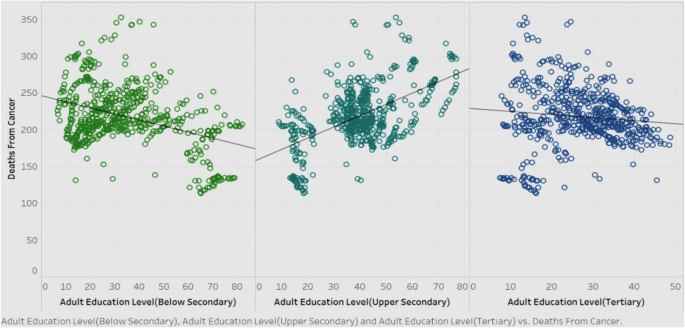
Association of deaths from cancer with adult education levels
Figure 15 shows the correlations of deaths from cancer among the three adult education levels, for all countries in the sample. It is obvious that below-secondary and tertiary adult education levels have a negative correlation with deaths from cancer, while the upper-secondary adult education level shows a positive correlation. Barring upper-secondary results, we can surmise that in general, as education level goes higher, the deaths from cancer will decrease. The rationale for this could be that education fosters more health awareness and encourages people to adopt healthy behavioral practices. Governments should therefore pay attention to frame policies that promote education. However, the counterintuitive result of the positive correlation between upper-secondary levels of adult education with the deaths from cancer warrants more investigation.
We drilled down further into the correlation between the upper-secondary education level and deaths from cancer. Figure 16 shows this correlation, along with a breakdown of the total number of records for each continent, to see if there is an explanation for the unique result.
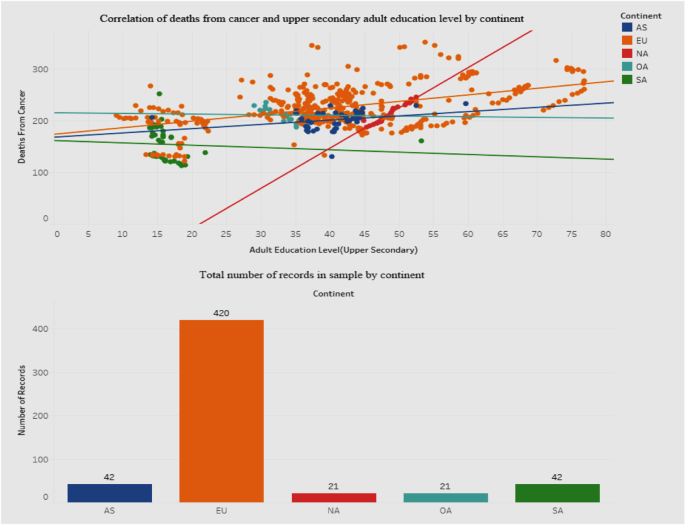
Association between deaths from cancer and adult education level-upper secondary
Figure 16 shows a dashboard containing two graphs - a scatterplot of the correlation between deaths from cancer and education level, and a bar graph showing the breakdown of the total sample by continent. We included a breakdown by continent in order to explore variances that may clarify or explain the positive association for deaths from cancer with the upper-secondary education level. The scatterplot shows that for the European Union (EU) the points are much more scattered than for the other continents. Also, the correlation between deaths and education level for the EU is positive. The bottom bar graph depicts how the sample contains a disproportionately high number of records for the EU than for other continents. It is possible that this may have influenced the results of the correlation. The governments in the EU should investigate the reasons behind this phenomenon. Also, we defer to future research to explore this in greater detail by incorporating other socioeconomic parameters that may have to be factored into the relationship.
Association between average tertiary school life expectancy and health expenditure
We moved our focus to the trends of tertiary school life expectancy and health expenditure from 1995 to 2015 (Fig. 17 ) to check for positive associations.
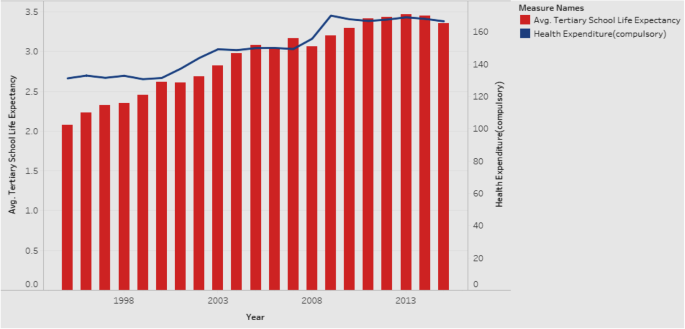
Association between Average Tertiary School Life Expectancy and Health Expenditure
Figure 17 is a combination chart explaining the trends of tertiary school life expectancy and health expenditure, for all countries in the sample. The rationale is that if there is a positive association between the two, it would be worthwhile for the government to allocate more resources towards health expenditure. Both tertiary school life expectancy and health expenditure show an increase over the years from 1995 to 2015. Our additional analysis shows that they continue to increase even after 2015. Hence, governments are encouraged to increase the health expenditure in order to see gains in tertiary school life expectancy, which will have positive implications for national health. Given that the measured effects of education are large, investments in education might prove to be a cost-effective means of achieving better health.
Our results reveal how interlinked education and health can be. We show how a country can improve its health scenario by focusing on appropriate indicators of education. Countries with higher education levels are more likely to have better national health conditions. Among the adult education levels, tertiary education is the most critical indicator influencing healthcare in terms of infant mortality, life expectancy, child vaccination rates, and enrollment rates. Our results emphasize the role that education plays in the potential years of life lost, which is a measure that represents the average years a person would have lived had he/she not died prematurely. In addition to mortality rate, an economy needs to consider this indicator as a measure of health quality.
Other educational indicators that are major drivers of health include school life expectancy, particularly at the tertiary level. In order to improve the school life expectancy of the population, governments should control the number of youths ending up unemployed, dropping out of school, and without skills or training (the NEET rate). Education allows people to gain skills/abilities and knowledge on general health, enhancing their awareness of healthy behaviors and preventive care. By targeting promotions and campaigns that emphasize the importance of skills and employment, governments can reduce the NEET rates. And, by reducing the NEET rates, governments have the potential to address a broad array of vulnerabilities among youth, ranging from unemployment, early school dropouts, and labor market discouragement, which are all social issues that warrant attention in a growing economy.
We also bring to light the health disparities across countries and suggest implications for governments to target educational interventions that can reduce inequalities and improve health, at a macro level. The health effects of education are at the grass roots level - creating better overall self-awareness on personal health and making healthcare more accessible.
Scope and limitations
Our research suffers from a few limitations. For one, the number of countries is limited, and being that the data are primarily drawn from OECD, they pertain to the continent of Europe. We also considered a limited set of variables. A more extensive study can encompass a larger range of variables drawn from heterogeneous sources. With the objective of acquiring a macro perspective on the education–health association, we incorporated some dependent variables that may not traditionally be viewed as pure health parameters. For example, the variable potential years of life lost is affected by premature deaths that may be caused by non-health related factors too. Also there may be some intervening variables in the education–health relationship that need to be considered. Lastly, while our study explores associations and relationships between variables, it does not investigate causality.
Conclusions and future research
Both education and health are at the center of individual and population health and well-being. Conceptualizations of both phenomena should go beyond the individual focus to incorporate and consider the social context and structure within which the education–health relationship is embedded. Such an approach calls for a combination of interdisciplinary research, novel conceptual models, and rich data sources. As health differences are widening across the world, there is need for new directions in research and policy on health returns on education and vice versa. In developing interventions and policies, governments would do well to keep in mind the dual role played by education—as a driver of opportunity as well as a reproducer of inequality [ 36 ]. Reducing these macro-level inequalities requires interventions directed at a macro level. Researchers and policy makers have mutual responsibilities in this endeavor, with researchers investigating and communicating the insights and recommendations to policy makers, and policy makers conveying the challenges and needs of health and educational practices to researchers. Researchers can leverage national differences in the political system to study the impact of various welfare systems on the education–health association. In terms of investment in education, we make a call for governments to focus on education in the early stages of life course so as to prevent the reproduction of social inequalities and change upcoming educational trajectories; we also urge governments to make efforts to mitigate the rising dropout rate in postsecondary enrollment that often leads to detrimental health (e.g., due to stress or rising student debt). There is a need to look into the circumstances that can modify the postsecondary experience of youth so as to improve their health.
Our study offers several prospects for future research. Future research can incorporate geographic and environmental variables—such as the quality of air level or latitude—for additional analysis. Also, we can incorporate data from other sources to include more countries and more variables, especially non-European ones, so as to increase the breadth of analysis. In terms of methodology, future studies can deploy meta-regression analysis to compare the relationships between health and some macro-level socioeconomic indicators [ 13 ]. Future research should also expand beyond the individual to the social context in which education and health are situated. Such an approach will help generate findings that will inform effective educational and health policies and interventions to reduce disparities.
Availability of data and materials
The dataset analyzed during the current study is available from the corresponding author on reasonable request.
Abbreviations
Fundamental Cause Theory
Human Capital Theory
Not in Employment, Education, or Training
Organization for Economic Cooperation and Development
Socio-economic status
Andersen RM, Newman JF. Societal and individual determinants of medical care utilization in the United States. Milbank Mem Fund Q Health Soc. 1973;51(1):95–124.
Article CAS Google Scholar
Andersen RM. Revisiting the behavioral model and access to medical care: does it matter? J Health Social Behav. 1995;36(1):1–10.
Baker DP. The Schooled Society: The Educational Transformation of Global Culture. Stanford, CA: Stanford Univ. Press: 2014; 360.
Becker GS. Human capital: a theoretical and empirical analysis, with special reference to education. Chicago: Univ. Chicago Press; 1964.
Google Scholar
Berkman LF, Syme SL. Social networks, host resistance, and mortality: a nine-year follow-up study of Alameda County residents. Oxford Acad J. 1979;109(2):186–204.
CAS Google Scholar
Crimmins EM, Kim JK, Vasunilashorn S. Biodemography: new approaches to understanding trends and differences in population health and mortality. Demography. 2010;47:S41–64.
Article Google Scholar
Spence M. Job market signalling. The Quarterly J Econ. 1973;87:355–79.
Cutler DM, Lleras-Muney A. Education and Health: Evaluating Theories and Evidence: NBER Working Papers; 2006. p. 12352.
Feinstein L. What are the effects of education on health? OECD Proceedings of the Copenhagen Symposium; 2006 . .
Folkman S, Lazarus RS. An analysis of coping in a middle-aged community sample. J Health Soc Behav. 1980;21(3):219–39.
Freedman VA, Martin LG. The role of education in explaining and forecasting trends in functional limitations among older Americans. Demography. 1999;36(4):461–73.
Freese J, Lutfey K. Fundamental causality: challenges of an animating concept for medical sociology. In: Pescosolido BA, Martin JK, McLeod JD, Rogers A, editors. Handbook of the Sociology of Health, Illness, and Healing: a blueprint for the 21st century. New York: Springer; 2011. p. 67–81.
Chapter Google Scholar
Fouweather T, Gillies C, Wohland P, Van Oyen H, Nusselde W, Robine J, Cambois E, Jagger C. Comparison of socio-economic indicators explaining inequalities in healthy life years at age 50 in Europe: 2005 and 2010. Eur J Pub Health. 2015;25:978–83.
George LK, Gwyther LP. Caregiver Weil-being: a multidimensional examination of family caregivers of demented adults. Gerontologist. 1986;26(3):253–9.
Goldman D, Smith JP. The increasing value of education to health. Soc Sc Med. 2011;72(10):1728–37.
Harper AC, Lambert LJ. The health of populations: an introduction. New York: Springer Publishing Company; 1994.
Health 2020: Education and health through the life-course. WHO Europe Sector Brief on Education Health; 2015. http://www.euro.who.int/__data/assets/pdf_file/0007/324619/Health-2020-Education-and-health-through-the-life-course-en.pdf?ua=1 .
Kino S, Bernabé E, Sabbah W. The role of healthcare and education systems in co-occurrence of health risk behaviours in 27 European countries. Eur J Public Health. 2018;28(1):186–92.
Landerman LR, Burns BJ, Swartz MS, Wagner HR, George LK. The relationship between insurance coverage and psychiatric disorder in predicting use of mental health services. Am J Psychol. 1994;151(12):1785.
Lynch SM. Cohort and life-course patterns in the relationship between education and health: a hierarchical approach. Demography. 2003;40(2):309–31.
Mackenbach J, Kunst A. Measuring the magnitude of socio-economic inequalities in health: an overview of available measures illustrated with two examples from Europe. Soc Sci Med. 1997;44(6):757–71. https://doi.org/10.1016/S0277-9536(96)00073-1 .
Article CAS PubMed Google Scholar
Manton KG, Corder L, Stallard E. Chronic disability trends in elderly United States populations: 1982-1994. Natl Acad Sci. 1997;94(6):2593–8.
Mirowski J, Ross CE. Education, learned effectiveness and health. London Rev Edu. 2005;3(3):205–20.
Montez JK, Berkman LF. Trends in the educational gradient of mortality among US adults aged 45 to 84 years: bringing regional context into the explanation. Am J Pub Health. 2014;104(1):e82–90.
Montez JK, Zajacova A. Trends in mortality risk by education level and cause of death among US white women from 1986 to 2006. Am J Pub Health. 2013;103:473–9.
Olshansky SJ, Antonucci T, Berkman L, Binstock RH, Boersch-Supan A, Cacioppo JT, Carnes BA, Carstensen LL, Fried LP, Goldman DP, Jackson J, Kohli M, Rother J, Zheng Y, Rowe J. Differences in life expectancy due to race and educational differences are widening, and many may not catch up. Health Aff. 2012;31(8):1803–13.
Pamuk ER. Social-class inequality in infant mortality in England and Wales from 1921 to 1980. Eur J Popul 1988; 4 , 1–21, https://doi.org/10.1007/BF01797104.
Phelan JC , Link BG , Tehranifar P . Social conditions as fundamental causes of health inequalities: Theory, evidence, and policy implications . J Health Soc Behav 2010 ; 51 : S28 S40 . doi : 10.1177/0022146510383498.
Renard F, Devleesschauwer B, Speybroeck N, Deboosere P. Monitoring health inequalities when the socio-economic composition changes: are the slope and relative indices of inequality appropriate? Results of a simulation study. BMC Public Health 2019; 19: 662. https://doi.org/10.1186/s12889-019-6980-1.
Ro A, Geronimus A, Bound J, Griffith D, Gee G. Educational gradients in five Asian immigrant populations: do country of origin, duration and generational status moderate the education-health relationship? Prev Med Rep. 2016;4:338–43.
Ross CE, Wu CL. The links between education and health. Am Soc Rev. 1995;60(5):719–45.
Shiels MS, Chernyavskiy P, Anderson WF, Best AF, Haozous EA. Diverging trends in premature mortality in the U.S. by sex, race, and ethnicity in the 21st century. Lancet. 2017;389:1043–54.
Tsou MT. Association of Education, health behaviors, concerns, and knowledge with metabolic syndrome among urban elderly in one medical Center in Taiwan. Int J Gerontology. 2017;11(3:138–43.
Wheaton B. Stress, personal coping resources, and psychiatric symptoms: an investigation of interactive models. J Health Soc Behav. 1983;24(3):208–29.
Williams DR, Collins C. US socioeconomic and racial differences in health: patterns and explanations. Ann Rev Soc. 1995;21:349–86.
Zajacova A, Lawrence EM. The relationship between education and health: reducing disparities through a contextual approach. Ann Rev Pub Health. 2018;39:273–89.
Zimmerman EB, Woolf SH. Understanding the relationship between education and health. Discussion Paper, Inst Med. Washington DC; 2014. https://nam.edu/wp-content/uploads/2015/06/BPH-UnderstandingTheRelationship1.pdf .
Zhong H. An over time analysis on the mechanisms behind the education–health gradients in China. China Econ Rev. 2015;34(C):135–49.
Download references
Acknowledgements
Not applicable.
Author information
Authors and affiliations.
Koppelman School of Business, Brooklyn College of the City University of New York, 2900 Bedford Ave, Brooklyn, NY, 11210, USA
Viju Raghupathi
Gabelli School of Business, Fordham University, 140 W. 62nd Street, New York, NY, 10023, USA
Wullianallur Raghupathi
You can also search for this author in PubMed Google Scholar
Contributions
Both authors contributed equally to all parts of the manuscript.
Corresponding author
Correspondence to Viju Raghupathi .
Ethics declarations
Ethics approval and consent to participate, consent for publication, competing interests.
The authors declare that they have no competing interests.
Additional information
Publisher’s note.
Springer Nature remains neutral with regard to jurisdictional claims in published maps and institutional affiliations.
Rights and permissions
Open Access This article is licensed under a Creative Commons Attribution 4.0 International License, which permits use, sharing, adaptation, distribution and reproduction in any medium or format, as long as you give appropriate credit to the original author(s) and the source, provide a link to the Creative Commons licence, and indicate if changes were made. The images or other third party material in this article are included in the article's Creative Commons licence, unless indicated otherwise in a credit line to the material. If material is not included in the article's Creative Commons licence and your intended use is not permitted by statutory regulation or exceeds the permitted use, you will need to obtain permission directly from the copyright holder. To view a copy of this licence, visit http://creativecommons.org/licenses/by/4.0/ . The Creative Commons Public Domain Dedication waiver ( http://creativecommons.org/publicdomain/zero/1.0/ ) applies to the data made available in this article, unless otherwise stated in a credit line to the data.
Reprints and permissions
About this article
Cite this article.
Raghupathi, V., Raghupathi, W. The influence of education on health: an empirical assessment of OECD countries for the period 1995–2015. Arch Public Health 78 , 20 (2020). https://doi.org/10.1186/s13690-020-00402-5
Download citation
Received : 16 October 2019
Accepted : 26 February 2020
Published : 06 April 2020
DOI : https://doi.org/10.1186/s13690-020-00402-5
Share this article
Anyone you share the following link with will be able to read this content:
Sorry, a shareable link is not currently available for this article.
Provided by the Springer Nature SharedIt content-sharing initiative
- Education level
- Enrollment rate
- Life expectancy
- Potential years of life lost
- Infant mortality
- Deaths from cancer
Archives of Public Health
ISSN: 2049-3258
- General enquiries: [email protected]
Journal of Population Sciences
- Original Article
- Open access
- Published: 07 March 2019
The impact of increasing education levels on rising life expectancy: a decomposition analysis for Italy, Denmark, and the USA
- Marc Luy 1 , 2 ,
- Marina Zannella 1 , 2 , 7 ,
- Christian Wegner-Siegmundt 1 , 2 ,
- Yuka Minagawa 5 ,
- Wolfgang Lutz 1 , 2 , 3 , 4 &
- Graziella Caselli 6
Genus volume 75 , Article number: 11 ( 2019 ) Cite this article
39k Accesses
46 Citations
138 Altmetric
Metrics details
Significant reductions in mortality are reflected in strong increases in life expectancy particularly in industrialized countries. Previous analyses relate these improvements primarily to medical innovations and advances in health-related behaviors. Mostly ignored, however, is the question to what extent the gains in life expectancy are related to structural changes in the populations due to increasing education levels. We decompose changes of the total populations’ life expectancy at age 30 in Italy, Denmark, and the USA, over the 20-year period between 1990 and 2010 into the effects of education-specific mortality changes (“M effect”) and changes in the populations’ educational structure (“P effect”). We use the “replacement decomposition technique” to further subdivide the M effect into the contributions by the individual education groups. While most of the increases in life expectancy are due to the effect of changing mortality, a large proportion of improvements in longevity can indeed be attributed to the changing structure of the population by level of education in all three countries. The estimated contribution of the P effect ranges from around 15% for men in the USA to approximately 40% for women in Denmark. This study demonstrates strong associations between education and overall population health, suggesting that education policies can also be seen as indirect health policies.
Introduction
Reductions in mortality which started in Europe in the middle of the eighteenth century have continued to spread globally and led to huge increases in life expectancy, with industrialized countries being most advanced in this process. Most of these changes were due to a shift in cause-specific mortality patterns from communicable diseases at younger ages, including neo-natal and childhood ages, to non-communicable conditions more prevalent at advanced ages, as described in Omran’s ( 1971 ) “epidemiologic transition theory.” Recently, the potentials of human longevity were further extended by the “cardiovascular revolution” which started in the 1970s and initiated a new period of decreasing mortality (Caselli 2015 ; Olshansky and Ault 1986 ; Omran 1983 ; Rogers and Hackenberg 1987 ). Studies have tended to relate these improvements to public health measures and new medical advancements, such as developments in screening, prevention, and treatment of cardiovascular diseases (Mackenbach et al. 1988 ; Vallin and Meslé 2004 ; Wilmoth 2000 ). Improvements in living conditions and health-related behaviors have also reduced mortality, thereby leading to further increase in survival chances (Denney et al. 2010 ; Janssen and Kunst 2005 ; Rogers et al. 1999 , 2010 ).
In tandem with the decrease of mortality, the populations have experienced significant increases in educational attainment (Lutz et al. 2014a ). Between 1970 and 2009, for instance, the average number of years of education rose by 3.6 years for people aged 25 and older in 175 countries (Gakidou et al. 2010 ). A great deal of research acknowledges the strong impact of socioeconomic resources on various health outcomes, suggesting socioeconomic conditions as a “fundamental cause” of inequalities in health and mortality (Link and Phelan 1995 ). Many studies have shown that highly educated people stand to benefit first and most from the prevailing drivers of decreasing mortality, particularly with regard to the risk factors of cardiovascular diseases (Hummer and Lariscy 2011 ; Mirowsky and Ross 2003 ). These inequalities translate into differences in life expectancy of several years between individuals with low and high educational attainment (Caselli et al. 2014 ). Lleras-Muney ( 2005 ) estimated—with data for the USA in 1960—that one more year of education increased average life expectancy at age 35 by as much as 1.7 years. Further, Woolf et al. ( 2007 ) estimated that eliminating education-specific inequalities in mortality would save even more lives than medical advances.
Naturally, higher education does not automatically lead to better health. It is also unclear to what extent education itself plays a direct role inside the complex network of various health-related socioeconomic factors (Kaplan et al. 2014 ). Although evidence infers that it is justified to assume some direct functional causality (Geyer and Peter 2000 ; Lleras-Muney 2005 ; Lutz and Skirbekk 2014 ; van Kippersluis et al. 2011 ), it appears that the association between education and mortality operates to a larger extent along an indirect pathway in which education acts as access criterion to other drivers of health and longevity (Arendt 2005 ; Clark and Royer 2013 ; Davey Smith et al. 1998 ; Kilpi et al. 2016 ; Kröger et al. 2015 ; Lager and Torssander 2012 ). As Hayward et al. ( 2015 : 16) put it, “…there is no inherent causal association between educational attainment and adult mortality; instead, the causal association is dependent upon time, place, and social environment under study.”
Here, we briefly summarize the major mechanisms linking education to health and mortality (an extensive compilation of recent studies can be found in Montez and Friedman ( 2015 )). Schooling develops basic cognitive functioning, such as reading, writing, and communicating, and teaches individuals how to think logically, critically analyze data, solve problems, and implement plans (Kingston et al. 2003 ). Higher education is the key to stable and well-paid jobs, and increased income helps to pay for nutritious food, better-quality housing, and high-quality medical care (Mirowsky and Ross 2003 ). In addition, education promotes healthy lifestyles through the development of effective human agency. Highly educated people use their knowledge, information, and past experiences to avoid health-related risk factors and engage in health-enhancing behaviors, such as smoking cessation, alcohol abstinence, and frequent physical exercise (Denney et al. 2010 ; Laaksonen et al. 2008 ). Moreover, education provides socio-psychological resources that can contribute to health and longevity through emotional and instrumental support (House et al. 1988 ). That well-educated people are more likely to be and remain married also contributes to the relationship between education and health (Qian and Preston 1993 ).
These examples indicate how higher education contributes to better health and longer lives on the individual level. Furthermore, investments in education also improve the health status on the population level. Baker et al.’s ( 2011 ) systematic review demonstrates the strong relationship between education and adult mortality across countries at different levels of development. These insights raise the question to what extent the increasing education levels constitute an additional factor behind populations’ rising life expectancy. If educational attainment improves in a population, it usually follows that the proportion of individuals with lower education levels decreases, while the proportion of those with higher education increases. Consequently, the health of the overall population should increase accordingly, even if the mortality rates of each education category remain unchanged. The question to what extent the gains in life expectancy were caused by such changes in the populations’ education structure, in addition to the effect of decreasing mortality within the education groups, has been addressed only rarely so far. Shkolnikov et al. ( 2006 ) and Jasilionis et al. ( 2007 ) provided such estimates for some northern, central, and eastern European countries between the late 1980s and 1990s. They found that the changes in the education-specific composition had in fact a remarkable positive effect on the populations’ life expectancy levels. Similar conclusions were derived in studies on specific national subpopulations (De Grande et al. 2014 ; Klein et al. 2006 ; Turra et al. 2016 ).
Guided by the works of Shkolnikov et al. ( 2006 ) and Jasilionis et al. ( 2007 ), we examine, on the basis of the same methodology, how changes in the educational attainment by age and sex contributed to increasing overall life expectancy at age 30 ( e 30 ) in Italy, Denmark, and the USA between around 1990 and 2010. Thus, our study extends the literature on the relationship between populations’ levels of education and life expectancy by adding insights from new populations and by analyzing a longer and more recent observation period. Also, the basic research question differs from the previous studies. Shkolnikov et al. ( 2006 ) and Jasilionis et al. ( 2007 ) examined primarily whether the stalls and decreases of life expectancy in central and eastern Europe during the 1980s were driven by certain education-specific subpopulations. The present study focuses, by contrast, on western populations and the specific question to what extent changes in the educational structure of the populations contributed to their steady increases in life expectancy.
The populations of Italy, Denmark, and the USA form an interesting sample to assess the relationship between populations’ levels of education and life expectancy because they differ in terms of life expectancy, magnitudes of education-specific differentials in mortality, and the education profile of the populations. In the USA, formal schooling lasts 13 years until around age 18 with some variations between the states. Notably, the US population experienced substantial changes over the years in terms of the levels, content, and importance of education (Hummer and Lariscy 2011 ). The proportion of the population at age 25 and older with at least high school education more than doubled since the early 1960s to almost 90% in 2015 (Ryan and Bauman 2016 ). In the two European states, compulsory education is shorter than in the USA (OECD 2016 ). Also, the process of education expansion started later. The proportion of adults with tertiary education is therefore still distinctly lower, above all in Italy (OECD 2016 ). More effective population policies in the past let Denmark move ahead in the process of education expansion (Arendt 2005 ), giving the country the intermediate position among the three study populations. All these characteristics of the national education systems refer exclusively to quantitative aspects. Certainly, education systems differ also in qualitative aspects. These are, however, not the topic of this study. We will come back to this issue briefly in the “ Discussion ” section of the paper.
In the following two sections, we describe the data and methods we used to construct the life tables by education and to decompose the changes in e 30 over the observed 20-year period into the effects of changing education-specific mortality (“M effect”) and changing educational structure of the populations (“P effect”). In the “ Results ” section, we summarize the outcomes of our analyses which confirm that increasing education levels are strongly related to the rising levels of life expectancy. This finding has policy implications, particularly for newly industrializing countries and other populations of the global south where education levels are projected to increase even more rapidly than in the industrialized world (Lutz et al. 2014a ). Because further rises of average education levels are bound to occur by the fact that in most countries the younger cohorts are better educated than the older ones, our results also fuel the expectation of continuing increases in life expectancy—an issue that is highly controversial among demographers and split the community into “optimists” and “pessimists”. All these issues and the limitations of the study are discussed in the final section of the paper.
The analysis requires age- and education-specific data for the populations at risk and the deaths for the start and end years of our observation period. Our study starts at age 30 because this is the age when education is usually completed. We defined educational attainment on the basis of the ISCED-97 scale (UNESCO 1996 ) with the three levels low (ISCED 0–2), medium (ISCED 3–4), and high education (ISCED 5–6). Obtaining this data for our study populations was a challenging task, in particular for the early 1990s. Because the aim of this study is to estimate the impact of changes in populations’ educational structures on overall life expectancy, we gave priority to the most similar derivation of life tables for the studied populations rather than the most accurate estimate of life expectancy by education for each population. A cross-country comparison of differentials in life expectancy by education level would be a different research question which is not only affected by the characteristics of the data sources. The same formal education levels can include significant differences in the quality of education. Finally, the number of considered education levels plays a role with regard to both, the extent of differentials between the levels and the estimation of the effects of corresponding structural changes. The following paragraphs summarize the data sources and how we constructed our database as homogeneous as possible.
Information about the population by age, sex, and education level was taken from Italian census data which is available online at the data warehouse of the National Statistical Institute of Italy (ISTAT). Footnote 1 Age-, sex-, and education-specific numbers of death stem from the Italian national vital statistics registry on causes of death and are based on death certificates. Data for 2011 were downloaded from the ISTAT data warehouse. Data for 1991 are not available online and were manually collected and digitalized by the authors from the printed reports “Nascite e decessi” at the ISTAT archive in Rome, Italy. In all data sources, education refers to the highest educational attainment which allowed us to translate the numbers directly into the ISCED-97 classification. The data for the population at risk and the number of deaths cover the ages 30 to 85+ in 5-year age groups. Cases with unknown education level (4.3% of deaths in 1991) have been distributed according to the education-specific proportions for valid cases for each age and sex group. Because of the introduction of new data-check plans in 2004, there were no cases with missing information in the data for 2011.
Information about the population by age, sex, and education level comes from nationwide population registers, covering the ages 30 to 100. Information about age-, sex-, and education-specific mortality was available in form of probabilities of dying for ages 30 to 64. These were derived by register linkage at the individual level by use of the unique personal identification number assigned to all Danish citizens (Thygesen et al. 2011 ). For ages 65 and older, age- and sex-specific probabilities of dying were only available for the total population without separation by education level. All data were available for single ages and represent averages of two subsequent calendar years (i.e., the data for 1990 refers to the average of 1989 and 1990, and the data for 2010 to the average of 2009 and 2010). The databases did not include any cases with missing information. Education level was defined according to ISCED-97 and categorized into the levels 1–2, 3A, 3C & 4A, and 5–6. We merged the data for ISCED levels 3A, 3C, and 4A (ISCED 3–4) and derived the age-, sex-, and education-specific numbers of death from the data for the population and the corresponding probabilities of dying. Education-specific probabilities of dying above age 64 were derived by extrapolating the available data for ages 30 to 64 with the Brass logit life table model (Brass 1971 ). We used the age-specific mortality schedules of the total Danish population by sex (for 1989 respective to 2009) from the Human Mortality Database ( 2017 ) as standard life tables. Footnote 2 The Brass parameters were estimated from the age- and education-specific probabilities of dying at ages 30 to 64 (see the “ Life tables by education level ” section for more details on our estimations with the Brass model).
Information about the population by age, sex, and education level stems from the data collection IPUMS-USA (Ruggles et al. 2015 ). Footnote 3 Age-, sex-, and education-specific numbers of death stem from the Division of Vital Statistics of the National Center for Health Statistics and are based on death certificates. The data were downloaded from the website of the Centers for Disease Control and Prevention (CDC). Footnote 4 Both datasets are available in micro-data form, and we merged them into 5-year age groups from age 30 to 90+. In the data for the population at risk, education refers to the highest educational attainment, which enabled us to translate the numbers directly into the ISCED-97 classification. In the mortality data for 1990, education is classified by the number of actual years of schooling. In the mortality data for 2010, education refers to the highest educational attainment as defined in the Revision of the Standard Certificate of Death of the year 2003. Footnote 5 Given that not all states have adopted the new classification, the mortality data for 2010 includes both classifications: number of years in school and the highest educational attainment. For the states which used the new regulation, we were able to translate the numbers directly into the ISCED-97 classification. To obtain corresponding education classifications for the deaths of 1990 and for those of 2010 which were still classified by the number of school years, we performed an approximate reclassification into the ISCED-97 system on the basis of the EDATTAIN International Recode of Educational Attainment. Footnote 6 Cases with unknown education levels (23.6% of deaths in 1990 and 2.2% of deaths in 2010) were distributed according to the education-specific proportions for valid cases for each age and sex group.
As described in the previous section, the characteristics of the underlying data differ between our study populations, e.g., with regard to the construction of the three ISCED education groups. Another important difference concerns the data sources for the education-specific populations at risk and the corresponding number of deaths. Whereas for Denmark both stem from linked population registers, we have different and unlinked data sources for Italy and the USA. Consequently, the estimated education-specific differentials in life expectancy cannot be directly compared between the populations. However, as outlined at the beginning of the “ Data ” section and later in more detail in the “ Discussion ” section of this paper, the purpose of this study lies exclusively in the estimation of the relationship between increasing education levels and overall life expectancy. In order to compare results across our study populations, we constructed life tables as basis for the decomposition analysis. Life tables were constructed as consistently as possible in regards to the available data for each study population.
Life tables by education level
We constructed abridged period life tables from age 30 for 5-year age groups for the populations of Italy (1991 and 2011), Denmark (1990 and 2010), and the USA (1990 and 2010) on the basis of age-, sex-, and education-specific death rates derived from the data described in the “ Data ” section. The age-specific fractions of last age interval of life were estimated with the Keyfitz method (Preston et al. 2001 ). In order to get a homogeneous basis for our analysis, we extrapolated all life tables with the Brass logit model (Brass 1971 ) to age 110. The Brass parameters were estimated from the age- and education-specific probabilities of dying at ages 30 to 64 (because of the restriction set by the data for Denmark, see the “ Denmark ” section). Country- and period-specific life tables from the Human Mortality Database ( 2017 ) were used as standard. Footnote 7 The extrapolated life tables for each sex- and education-specific subpopulation were then used to derive the death rates for the country-specific last open age intervals. These start at different ages as determined by the country-specific availability of data for the living population (see the “ Data ” section). The basic data on deaths and populations at risk by education levels and the life tables by educational attainment for each country and observation period are available in the Additional file 1 .
Decomposition of changes in life expectancy
The central aim of this study is to decompose the changes in life expectancy at age 30 ( e 30 ) for the total populations of Italy, Denmark, and the USA between around 1990 (time t 1) and around 2010 (time t 2) into the contributions of changes in education-specific mortality (M effect) and changes in the populations’ educational structure (P effect). The terms are adopted from Shkolnikov et al. ( 2006 ) who introduced this specific decomposition approach. Isolating the M and P effects is difficult because they are complexly interrelated. On the one hand, changes in education-specific mortality cause changes in the education-specific structure of the population through increasing or decreasing survival chances. On the other hand, changes in the educational structure of the population affect the corresponding mortality levels because the risk compositions of the education-specific subpopulations are changing, e.g., when a larger proportion of formerly less-educated individuals with higher mortality risks move to higher education levels or vice versa. Between t 1 and t 2, both changes occur simultaneously with different and varying magnitudes what makes the interrelation even more complex.
Although several approaches have been proposed to decompose the change in the death rates, they all follow the same basic idea of standardization, that is to isolate the effect of changing mortality under constant population composition, or vice versa, the effect of changing composition of the population under constant mortality. The challenging issue is to decide the condition for which mortality and population compositions are standardized for. Three different standards are possible: (1) the conditions prevailing at t 1, (2) the conditions prevailing at t 2, and (3) the average of conditions prevailing at t 1 and t 2. We used the replacement decomposition technique (Andreev et al. 2002 ; Shkolnikov et al. 2003 ) which estimates the M and P effects on the basis of standard (3). The basic approach of this method is to apply a “[…] stepwise replacement of elements from one vector of age-specific mortality rates by respective elements of another vector” (Andreev et al. 2002 : 500).
In a nutshell, the replacement decomposition technique works as follows. Starting with the original vector of education-specific death rates at t 1, we estimate e 30 . In the first replacement step, the education-specific death rates of the age group 30–34 of t 1 are substituted by the corresponding death rates of t 2, while the educational structure of the population is kept constant at the level of t 1. The difference between the resulting modeled values for e 30 at t 2 and those of the start year t 1 provides an estimate for the M effect of the age group 30–34 on the basis of standard (1). In the second step, the P effect originating from education-specific structural changes in this age group is estimated accordingly by substituting the population’s educational structure in age group 30–34 of t 1 by those of t 2, while the education-specific death rates are kept constant at the level of t 1. These steps are repeated for all possible combinations of age- and education-specific death rates and the population’s educational structure until the conditions prevailing at t 1 are completely replaced by the corresponding conditions at t 2. Then, the procedure is performed equivalently on the basis of standardization procedure (2) for the transition from t 2 to t 1 through substituting the education- and age-specific death rates and the educational structure at t 2 stepwise by those prevalent at t 1.
The different directions across the replacement paths, the subpopulations, and the age schedule cause different combinations of vectors which should be fully considered in an ideal decomposition procedure. To avoid the corresponding bulk of calculations, the usually applied strategy is to perform the replacement procedure only from the youngest to the oldest age group. The resulting set of age-specific M and P effects is then summed up to the overall effects and averaged for the results obtained by standards (1) and (2). Consequently, the yielded estimates can be regarded as approximate overall M and P effects, i.e., estimates for the contributions of changes in education-specific mortality (respective contributions of changes in the educational structure of the population) to changing death rates between t 1 and t 2, when the population’s educational structure (respective mortality conditions) remain constant. The particular advantage of the replacement decomposition technique is that the replacement can be performed separately for each education subgroup. This allows a subdivision of the M effect into the individual contributions of education group-specific changes in mortality (more and specific details about the method can be found in Shkolnikov et al. 2006 ). For our analyses, we used the MS Excel macro provided by Andreev and Shkolnikov ( 2012 ). Footnote 8
Table 1 presents the distribution of and changes in educational attainment at ages 30 and above for Italy, Denmark, and the USA during the study period. Substantial changes have been made in educational attainment levels in these countries between 1990 and 2010. The proportion of the population with low education strongly declined, and consequently, there were large increases of the proportions in the medium and high education categories. Italy had massive shifts particularly between the low and medium levels, while the proportion of highly educated people increased somewhat less. Denmark experienced similar decreases at the low education level but with more distinct differences between women and men. In contrast to Italy, the high education category increased more than the medium category in both sexes. Partly due to starting from a higher level, the USA saw considerably smaller increases in the education levels over the same period. Nonetheless, the share of those with low educational attainment also declined during the study period, while the proportion of medium education level changed only slightly. Hence, the shift in education level occurred primarily at the extreme ends of low and high education levels. Despite these increases in education levels in all three countries, large proportions of the populations still remained in the low education groups, mostly concentrated in the older ages. In the most recent observation years, 55.4% of the Italian population had less than upper secondary education, and the respective figures are 32.3% in Denmark and 12.8% in the USA.
Table 2 summarizes how life expectancy at age 30 ( e 30 ) increased during the 20 years of observation and how it varied by education group in the three countries. For the total populations, e 30 increased by 4.4 years in Italy, 3.6 years in Denmark, and 2.8 years in the USA. In all populations, there was a clear gradient in e 30 by level of education. Those with high education had the highest life expectancy, followed by medium and low education groups (except for Italian women in 1991 and the total Italian population in 2011). Two points are worth emphasizing. First, the pattern of impact of education on life expectancy was different among countries. In Italy, the largest gap in e 30 existed between the low education level and the two others, suggesting that completion of upper secondary education was a mortality threshold in the Italian population during the 1990s and 2000s. By contrast, tertiary education seems to have been the key to life expectancy improvements in the USA: differences between medium and high education were larger than those between medium and low education. Denmark shows the smallest inequalities across education groups, albeit with more notable sex differences in the highest education category. Second, educational differences in e 30 have widened over time in most cases. The differentials between high and low education groups were consistently larger in more recent years for both sexes in all three countries. Increases can be observed between medium and low education as well, though the extent of changes compared to those between low and high education differed between populations and sexes. The only exceptions are the decreasing differences between high and medium education among men and the total population in Italy, and among women in Denmark.
Results from the decomposition analyses are summarized in Table 3 and in Figs. 1 and 2 . It becomes apparent that improvements in e 30 were driven primarily by the M effect caused by decreasing education-specific death rates. The M effect was responsible for 80.9% of the total gain in e 30 in the USA, for 80.1% in Italy, and for 75.6% in Denmark. As expected, the pattern of the M effect broken down into specific education categories was not uniform across countries. In Italy, most of the M effect was caused by the low education group, resulting in a 61.5% increase in e 30 of the total population between 1991 and 2011 (57.9% for men; 67.7% for women). In the USA, the mortality reductions in the medium education category made the largest contribution to the increase in e 30 (51.5% for the total population; 48.4% for men; 62.5% for women). The low education group contributed least to increasing life expectancy in the USA. Among women, the contribution was even negative due to the decrease of e 30 between 1990 and 2010 (see Table 2 ). In Denmark, no specific education group dominated the mortality decline. The highest contribution originated from the low education level (33.4% for the total population; 34.6% for men; 26.4% for women), but the contribution of the medium education level was not much smaller (27.1% for the total population; 28.3% for men; 23.1% for women). The contribution of mortality declines in the high education level ranged from 3.8% among Italian women to 28.1% in the total population of the USA.
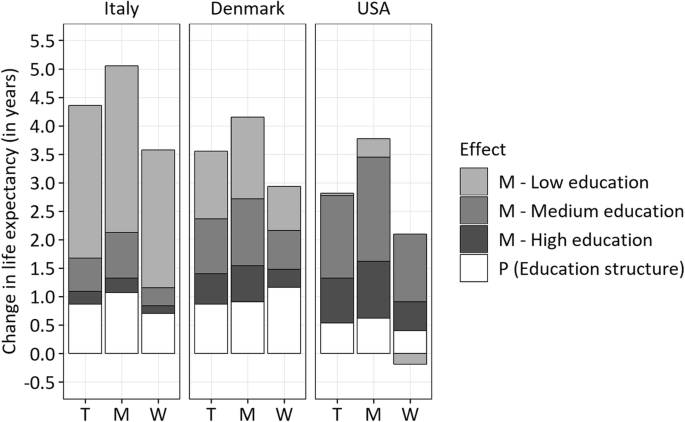
Absolute contributions of changes in mortality (M effect) and populations’ educational structure (P effect) to differences in life expectancy at age 30 (in years) for Italy (1991–2011), Denmark (1990–2010), and the USA (1990–2010), stratified by gender. Source: authors’ own calculations; the data sources for the three populations can be found in the “ Data ” section. Note: T refers to total population, M is men, and W is women
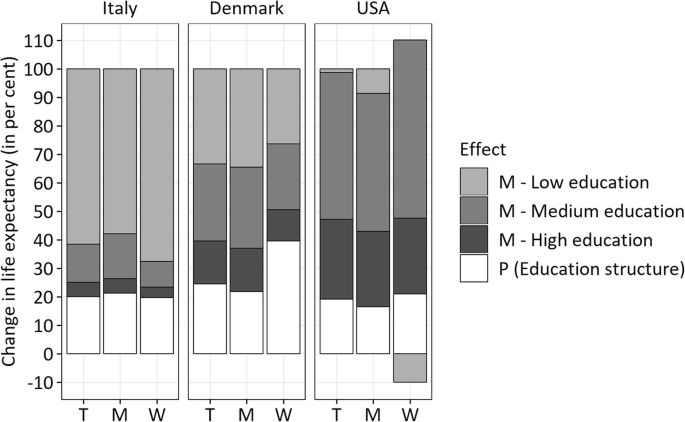
Relative contributions of changes in mortality (M effect) and populations’ educational structure (P effect) to differences in life expectancy at age 30 (in percent) for Italy (1991–2011), Denmark (1990–2010), and the USA (1990–2010), stratified by gender. Source: authors’ own calculations; the data sources for the three populations can be found in the “ Data ” section. Note: T refers to total population, M is men, and W is women
While the most powerful contributor to increasing life expectancy was the effect of decreasing mortality within education groups, the changing educational structure of the populations also contributed substantially to the increase in e 30 in all three countries (see Fig. 1 ). In Italy and Denmark, the structural change in the populations accounted for approximately 1 year of the increases in e 30 between around 1990 and 2010, and in the USA for about half a year. The smaller absolute increase attributable to the P effect in the USA can be primarily explained by the smaller overall increase in life expectancy (see Table 2 ). When looking at the relative contributions, the effect of changes in the educational structure on changes in e 30 was of similar magnitude in all three populations: 19.1% in the USA, 19.9% in Italy, and 24.4% in Denmark.
As shown in Table 3 and Fig. 2 , the relative effects are also similar across the male and female populations in all three countries. The only outlier is Danish women where the effect of changing educational composition on increasing life expectancy was particularly high, accounting for almost 40% of the change in e 30 between 1990 and 2010. This reflects the fact that of all analyzed populations, Danish women experienced the greatest shifts in educational structure during our study period, with the largest decrease in the proportion of less educated and the largest increase in the proportion of highly educated individuals (see Table 1 ). Overall, these results illustrate the substantial contributions of progress in educational attainment to the improvements in life expectancy between 1990 and 2010 in all three countries, in addition to all the other forces leading to decreasing overall mortality.
Methodological and conceptual issues
Several technical notes are in order. We performed a series of sensitivity analyses to test whether, and to what extent, our results are affected by the definition used for education levels, the restriction of the decomposition to changes between a start and an end year of a defined observation period, the chosen observation period itself, and the decomposition technique applied. With regard to the latter issue, we tested an alternative approach developed by Das Gupta ( 1993 ) which combines the classic age decomposition of a change in life expectancy (Andreev 1982 ; Pressat 1985 ) with the general method for decomposing differences between rates (Das Gupta 1978 ). We compared the results of this method with those derived with the replacement decomposition technique on the basis of the correlation coefficients for the estimated age-specific M and P effects. All coefficients were positive and close to 1.0, the minimum value being 0.9883 for the M effect of medium-educated women in Denmark. Thus, both methods do not differ with regard to the direction of the estimated effects and they vary only marginally with regard to their magnitudes.
Further, we tested to what extent different definitions of education levels affect the results of our study. We did this with the data for Italy by performing the analysis on the basis of different classifications of education, including a narrower definition of low education (ISCED 0–1) and a broader definition of medium education (ISCED 2–4) as well as the use of four education classes (ISCED 0–1, 2–3, 4, 5–6). In all cases, the estimated P effects went in the same direction but were larger than in the presented data based on the conventional three education classes (ISCED 0–2, 3–4, 5–6). Note that if a continuous underlying relationship between length of education and level of life expectancy is assumed, then any categorization into discrete education categories will necessarily result in an underestimation of the P effect. This underestimation gets the stronger the smaller the number of categories is and the broader the education ranges they cover. If over time the average length of education within, e.g., the middle category of education increases, this would also lead to an increase in the life expectancy of the people belonging to this category. Under the three-category-framework, this increase in life expectancy would, however, not be classified as an education effect but rather as an exogenous mortality decline effect. If, on the other hand, the middle education category would be split into two, part of this improvement in both education and life expectancy would result in more people moving from the lower middle to the upper middle education category and the resulting life expectancy increase would result in a stronger P effect. The above mentioned results of the sensitivity analysis with four education categories clearly confirm this pattern. More generally, it can be said that the larger the number of education categories—i.e., the more closely it reflects the likely continuous underlying relationship—the larger will be the effect attributed to P.
The data for Italy also allowed us to carry out the decomposition for the 30-year period from 1981 to 2011. This analysis yielded basically identical outcomes in terms of the relative contribution of the increasing education level to rising life expectancy. Finally, a year-by-year decomposition with annual data for Denmark revealed that the P effect builds up gradually and shows less fluctuations than the M effect, indicating that our conclusions with regard to the effect of populations’ changing educational structure on changing life expectancy are not affected by the direct decomposition of changes between the start and the end year of the study period.
Life tables derived from different data sources for deaths and population at risk as done in the present study for Italy and the USA always entail the risk of a numerator-denominator bias (Sorlie and Johnson 1996 ). Note that our study focuses on the estimation of the association of increasing education levels with overall life expectancy. Our data are period life tables which model what would happen to a population if a given set of mortality conditions were experienced: the empirical data are an approximate reflection of actual conditions. Therefore, it is most important to estimate for each population education-specific death rates for the start and end years with the same methodological approach, what was only possible in the performed manner. Yet, it seems unlikely that our conclusions are influenced by this issue as well as by possible inaccuracies in the reporting of education levels and our imputation strategy for missing data. Regarding the latter, it is likely that our proportional redistribution of deaths with unknown education level on the basis of the deaths with information about education leads to a slight underestimation of the P effect. Footnote 9 There might be further sources for bias connected to some other technical and conceptual issues (see Jasilionis and Shkolnikov 2016 ), but we expect these to be of only minor relevance for the studied populations and the quality of the underlying data.
In sum, the sensitivity analyses suggest that none of these methodological and technical issues affected the conclusions of this study, and that the presented magnitude of the P effect is rather conservative. It is important to note, however, that the estimated life expectancy by education level cannot be compared across the studied populations because of differences in the underlying data characteristics as described in the “ Data ” section. For the same reason, our levels of education-specific life expectancy can differ from other studies which use different data for the same populations. In fact, our estimates for life expectancy at age 30 by education level provide larger inequalities between the highest and lowest education levels than comparable data from EUROSTAT or OECD (Corsini 2010 ; Murtin et al. 2017 ). These deviations are strongest for the populations from Italy and the USA, what indicates that our use of unlinked data leads to an overestimation of differences in life expectancy compared to linked data. However, linked data were only available for population subsamples for our earlier observation period. Given that we gave priority to use data that covers the total populations, we could not avoid the use of unlinked data for Italy and the USA. For estimating maximum precise levels of education-specific differences in mortality, such a linkage is preferable to our analysis based on independent data sources for the education-specific risk populations and numbers of death by age (see also Valkonen 2006 ).
Summary of findings
This study examined the extent to which changes in the educational structure are related to changes in life expectancy at age 30 in Italy (1991–2011), Denmark (1990–2010), and the USA (1990–2010). We used the same analytical strategy as Shkolnikov et al. ( 2006 ) and Jasilionis et al. ( 2007 ) to decompose changes in life expectancy at age 30 into the effects of education-specific mortality changes (M effect) and the effects of changes in the populations’ educational structure (P effect). The present study improves upon prior research by (1) testing the association between changes in the educational structure of the populations and changes in life expectancy at adult ages, (2) utilizing data from three different countries which markedly differ in terms of populations’ health status and education profile, and (3) including more recent years in the analyses. Our findings contribute to the debate about the role of educational attainment in changing population health status.
Three main findings emerge from our analyses. First, there were considerable changes in the educational composition in all three countries during the study period. The proportion of those with low educational attainment decreased, while the proportion of medium and highly educated individuals increased. There were particularly large increases in Italy and Denmark. Second, life expectancy was distributed in a graded fashion across education groups. Life expectancy was highest for those who had more than high school education, followed by those with medium and low educational attainment levels. Importantly, the gap in e 30 between the highest and lowest educational levels increased over the two decades of our study period. Third, the results from decomposition analysis show that the structural change in education accounted for a substantial proportion of improvements in overall life expectancy in all three countries. Specifically, we found that populations’ changes in educational attainment explained between around 15% (men in the USA) and approximately 40% (women in Denmark) of the increases in life expectancy at age 30 between 1990 and 2010.
Interpretation of findings
The present study provides some extension of our understanding of the mechanisms behind recent improvements in life expectancy. Prior research has tended to focus on medical advancements, in particular developments in the screening, treatment, and prevention of cardiovascular diseases, as major contributors to improved population health (Mackenbach et al. 1988 ; Vallin and Meslé 2004 ; Wilmoth 2000 ). Our results demonstrate the additional importance of education in this process. As described in the fundamental social causes of health inequalities theory, education helps individuals to develop health-related resources, allowing highly educated people to enjoy longer and healthier lives (Link and Phelan 1995 ). When new health technologies are developed, it is likely that people with higher education use resources they own, namely money, knowledge, prestige, power and beneficial social connections, to gain access to life-saving innovations.
The presented findings contain also some policy implications. For instance, they provide support for previous studies which suggested to consider education policy within the framework of health policy (e.g., Lleras-Muney 2005 ; Schoeni and Ofstedal 2010 ; Stringhini et al. 2017 ). The Millennium Development Goals (MDGs) sought to achieve universal primary education, with a special focus on less-developed countries. The post-MDGs international development agenda, Sustainable Development Goals (SDGs), also includes goals related to education, such as ensuring inclusive and equitable quality of education and lifelong learning for all. The present findings suggest that these efforts not only contribute to the expansion of mass education but are likely to lead also to reductions in mortality and an increase in populations’ life expectancy.
Building on this conclusion, the findings of our study add also insights into the discussion about the future of human longevity. Two major lines of thought have developed in recent years, which are referred to as “optimistic” and “pessimistic” (see Caselli et al. 2014 for details). In principle, both perspectives agree that mortality will continue to decrease. They differ, however, in their opinions on the time course and magnitude of these improvements. According to the pessimists, future progress will be smaller than in the past because the eradication of infectious diseases in industrialized societies will slow down the potential and the pace of mortality declines (Olshansky et al. 1990 ). In addition, new risk factors, such as obesity and global pandemic outbreaks, are thought to moderate improvements in human longevity (Olshansky 2005 ). In contrast, the optimists claim that mortality rates will further decline particularly at older ages, leading to un-damped gains in life expectancy (Christensen et al. 2009 ; Vaupel 2010 ). Although debate continues regarding future scenarios of human longevity, the current study provides a so far neglected factor in support of the optimistic perspective. The average years of education in the world are projected to increase from 7.9 years in 2010 to 11.0 years by 2060 (Lutz et al. 2014a ). It seems likely that these further increases of the average education level will continue to contribute to the process of mortality reduction (see also Deeg et al. 2013 ).
Nonetheless, measures and programs to improve the health of less-educated individuals are needed. Despite impressive changes in the distribution of educational attainment during the past 20 years, a large proportion of the studied populations still had less than upper secondary education. Moreover, educational gradients in life expectancy widened during the last decades in many industrialized populations as reflected in the presented estimations (see also Caselli et al. 2014 ; Shkolnikov et al. 2012 ). Hendi ( 2015 ) and Goldring et al. ( 2016 ) have shown that much of these changes in education-specific life expectancy are due to the shifts in the populations’ educational structure. Note that, the mortality benefits of education appear to be particularly large for better-educated people, while those with low education continue to be left behind. In this context, it is important to note that the improvements in educational attainment might cause the decreasing proportion of people with low education to become increasingly selected towards adverse health conditions (Deboosere et al. 2009 ; Hendi 2017 ).
Some issues should be noted which could provide the ground for fruitful follow-up research. First, our study includes only three countries, though their populations cover a broad spectrum of education levels and transitions across modern western nations. Shkolnikov et al. ( 2006 ) and Jasilionis et al. ( 2007 ) performed a comparable analysis for some northern, central, and eastern European countries between the late 1980s and 1990s. For Finland, they found a similar range of (annual) contributions of the P effect to changes in life expectancy as we did for Italy, Denmark, and the USA. The Czech Republic shows smaller effects of structural changes. For Russia, Estonia, and Lithuania, the P effects were even larger than in our study but overlaid by the drastic negative M effects prevalent at that time. Sweden shows the largest effects of education-specific changes in the population structure. Larger effects of the structural changes compared to our estimates were also presented by Klein et al. ( 2006 ) for the increase in life expectancy between the female cohorts 1925 and 1955 of Germany, by Turra et al. ( 2016 ) for changes in life expectancy among Brazilian women between 1960 and 2010, and by De Grande et al. ( 2014 ) for changes between the periods 1991/1996 and 2001/2006 among specific urban and non-urban subpopulations from Belgium. The latter study restricted the analysis, however, to young adults aged 19–34 and decomposed changes in the standardized mortality rates instead of life expectancy. In any case, all estimates available to date refer to industrialized societies. As improvements in educational attainment have also been (and will further be) made in the less-developed countries of the world (Gakidou et al. 2010 ; Lutz et al. 2014a ), including more countries into the analyses—both more-developed and less-developed nations—should be a topic of future investigations.
Second, we partitioned changes in life expectancy only into changes in mortality and educational structure. Although evidence shows that education itself is in part directly related to mortality (Geyer and Peter 1999 ; Lleras-Muney 2005 ; van Kippersluis et al. 2011 ), there is also a strong correlation with other dimensions of socioeconomic status, such as income, occupation, and wealth (Ross and Wu 1995 ). It would be challenging if it were possible to include these factors into the decomposition. Moreover, the macro-level effect of education on mortality might be moderated through other characteristics of the populations, such as ethnicity or country of birth. For instance, a large literature suggests substantial black-white mortality differences in the USA (Williams 2005 ), which are associated with mortality differences by education level. Montez et al. ( 2012 ) have shown that high school education is a threshold for mortality reduction for blacks in the USA, while whites enjoy mortality benefits from continued education after high school. Such heterogeneity effects are manifold and can arise from different causal pathways.
Finally, we assumed the comparability of education levels across countries during the observation time. Although we used a standardized measurement of education (ISCED-97), educational systems as well as the quality of education may differ significantly. Moreover, the studied populations experienced differences in the timing and dynamics of the process of the educational transition. This point has a notable relevance for the described between-country differences in the education-specific origins of the M effects. Lower secondary education became mandatory in Italy only after the 1950s and until age 16 only after 2007. Therefore, most of the people in the low education group around 1990 had no educational attainment at all, especially among the old and oldest old. By the early 2010s, a large proportion of these cohorts had died and the majority of the less-educated subpopulation had a lower secondary degree. In Denmark and the USA, these shifts occurred already earlier. This explains why enrollment into secondary education yielded larger M effects in Italy than in the other two countries. Thus, our understanding of the education-mortality association on the population level would also be deepened by research that accounts for the context and quality of populations’ education and related cohort effects.
To conclude, this study demonstrates that progress in education has made important contributions to increasing life expectancy in Italy, Denmark, and the USA over the past two decades. These findings are in line with the theoretical heterogeneity approach, which states that mortality levels and differences in mortality are strongly influenced by the specific risk group composition of populations (Vaupel et al. 1979 ; Vaupel and Yashin 1985 ). In addition to all the other important benefits of education (Lutz 2009 ; Lutz et al. 2014b ), it can also be viewed as a powerful health policy which allows more people to enjoy both better and longer lives. As aptly summarized by Kaplan et al. ( 2014 : 193), “Enhancing health outcomes through improved educational attainment is an attractive alternative, although we still need better evidence that interventions to improve educational attainment will increase life expectancy.” Our contribution tried to provide a piece of this evidence.
Web address: http://dati.istat.it/
Web address: http://www.mortality.org
Web address: https://usa.ipums.org/
Web address: http://www.cdc.gov/nchs/nvss.htm
See: http://www.cdc.gov/nchs/nvss/vital_certificate_revisions.htm
See: https://international.ipums.org/international-action/variables/EDATTAIN#description_section
We used the Brass model instead of the more commonly used models of Gompertz or Kannisto because of the comparatively young age 65 as starting point for the extrapolation. The Brass model adjusts the survival function of a complete standard life table (in our case life tables for the total populations with single age data from 30 to 110) to fit the available but truncated information about the mortality of the analyzed population (in our case the subpopulations by education level from ages 30 to 64). The Brass model uses two parameters for this adjustment: the alpha parameter reflecting the level of the subpopulations’ overall mortality and the beta parameter for the subpopulations’ specific age pattern of mortality, both in relation to the standard life table. Luy et al. ( 2015 ) have demonstrated the functionality of this approach in their study of life expectancy by education, income, and occupation for the German population. Details about the extrapolation of age-specific probabilities of dying with the Brass model can be found there. Descriptions of the derivation of the Brass parameters alpha and beta from empirical data can be found in Ngom and Bawah ( 2004 ) and Stewart ( 2004 ).
MS Excel File: Decomposition_replacement_from_young_to_old_ages(4).xls
In an alternative analysis, we assigned all deaths with unknown education to the lowest education level (based on the assumption that the majority of deaths with unknown education level—albeit not all—are likely to belong to this group). This scenario led to huge differences compared to our old model for the population of the USA because the life expectancy of the lowest education decreased strongly for the low education group in period 1 (from 41.7 to 34.4 years among men and from 49.5 to 42.9 years among women). Consequently, the low education group experienced by far the strongest increase in life expectancy between period 1 and period 2. This led (1) to an extreme shift of the education-specific M effects from the high to the low education level and (2) regarding the total effects to a shift from the total M effect to the P effect. Among men of the USA, the P effect increased from 0.6 years in our old model to 2.1 years, and among women of the USA from 0.4 to 1.7 years. For the Italian population, these shifts were considerably smaller (because of the much lower number of deaths with unknown education level in period 1), with the P effects increasing from 1.1 to 1.5 years among men and from 0.7 to 1.2 years among women.
Andreev, E. M. (1982). Metod komponent v analize prodoljitelnosty zjizni [the method of components in the analysis of length of life]. Vestnik Statistiki, 9 (3), 42–47.
Google Scholar
Andreev, E. M., & Shkolnikov, V. M. (2012). An Excel spreadsheet for the decomposition of a difference between two values of an aggregate demographic measure by stepwise replacement running from young to old ages. MPIDR technical report TR-2012-002 . Rostock: Max Planck Institute for Demographic Research.
Andreev, E. M., Shkolnikov, V. M., & Begun, A. Z. (2002). Algorithm for decomposition of differences between aggregate demographic measures and its application to life expectancies, healthy life expectancies, parity-progression ratios and total fertility rates. Demographic Research, 7 (14), 499–522.
Arendt, J. N. (2005). Does education cause better health? A panel data analysis using school reforms for identification. Economics of Education Review, 24 (2), 149–160.
Baker, D. P., Leon, J., Smith Greenaway, E. G., Collins, J., & Movit, M. (2011). The education effect on population health: a reassessment. Population and Development Review, 37 (2), 307–332.
Brass, W. (1971). On the scale of mortality. In W. Brass (Ed.), Biological aspects of demography (pp. 69–110). London: Taylor and Francis.
Caselli, G. (2015). Mortality, epidemiological, and health transitions. In J. D. Wright (Ed.), International encyclopedia of the social & behavioral Sciences (2nd ed., pp. 857–862). Oxford: Elsevier.
Caselli, G., Drefahl, S., Wegner-Siegmundt, C., & Luy, M. (2014). Future mortality in low mortality countries. In W. Lutz, W. P. Butz, & S. KC (Eds.), World population and human capital in the twenty-first century (pp. 226–272). Oxford: Oxford University Press.
Christensen, K., Doblhammer, G., Rau, R., & Vaupel, J. W. (2009). Ageing populations: the challenges ahead. Lancet, 374 (9696), 1196–1208.
Clark, D., & Royer, H. (2013). The effect of education on adult mortality and health: evidence from Britain. American Economic Review, 103 (6), 2087–2120.
Corsini, V. (2010). Highly educated men and women likely to live longer. Life expectancy by educational attainment. Statistics in focus 24/2010 . Brussels: Eurostat.
Das Gupta, P. (1978). A general method of decomposing a difference between two rates into several components. Demography, 15 (1), 99–112.
Das Gupta, P. (1993). Standardisation and decomposition of rates: a user’s manual. Current population reports P23–186 . Wasghington: U.S. Government Printing Office.
Davey Smith, G., Hart, C., Hole, D., MacKinnon, P., Gillis, C., Watt, G., Blane, D., & Hawthorne, V. (1998). Education and occupational social class: which is the more important indicator of mortality risk? Journal of Epidemiology and Community Health, 52 (3), 153–160.
De Grande, H., Vandenheede, H., & Deboosere, P. (2014). Trends in young-adult mortality between the 1990s and the 2000s in urban and non-urban areas in Belgium: the role of a changing educational composition in overall mortality decline. Health & Place, 30 , 61–69.
Deboosere, P., Gadeyne, S., & Van Oyen, H. (2009). The 1991-2004 evolution in life expectancy by educational level in Belgium based on linked census and population register data. European Journal of Population, 25 (2), 175–196.
Deeg, D. J. H., van Vliet, M. J. G., Kardaun, J. W. P. F., & Huisman, M. (2013). Understanding the mortality decline at older ages. Improved life course or improved present period? Annual Review of Gerontology and Geriatrics, 33 (1), 261–291.
Denney, J. T., Rogers, R. G., Hummer, R. A., & Pampel, F. C. (2010). Education inequality in mortality: the age and gender specific mediating effects of cigarette smoking. Social Science Research, 39 (4), 662–673.
Gakidou, E., Cowling, K., Lozano, R., & Murray, C. J. L. (2010). Increased educational attainment and its effect on child mortality in 175 countries between 1970 and 2009: a systematic analysis. The Lancet, 376 (9745), 959–974.
Geyer, S., & Peter, R. (1999). Occupational status and all-cause mortality. A study with health insurance data from Nordrhein-Westfalen, Germany. European Journal of Public Health, 9 (2), 114–118.
Geyer, S., & Peter, R. (2000). Income, occupational position, qualification and health inequalities—competing risks? (comparing indicators of social status). Journal of Epidemiology and Community Health, 54 (4), 299–305.
Goldring, T., Lange, F., & Richards-Shubik, S. (2016). Testing for changes in the SES-mortality gradient when the distribution of education changes too. Journal of Health Economics, 46 , 120–130.
Hayward, M. D., Hummer, R. A., & Sasson, I. (2015). Trends and group differences in the association between educational attainment and U.S. adult mortality: implications for understanding education's causal influence. Social Science & Medicine, 127 , 8–18.
Hendi, A. S. (2015). Trends in U.S. life expectancy gradients: the role of changing educational composition. International Journal of Epidemiology, 44 (3), 946–955.
Hendi, A. S. (2017). Trends in education-specific life expectancy, data quality, and shifting education distributions: a note on recent research. Demography, 54 (3), 1203–1213.
House, J. S., Landis, K. R., & Umberson, D. (1988). Social relationships and health. Science, 241 (4865), 540–545.
Human Mortality Database. 2017. University of California, Berkeley (USA), and Max Planck Institute for Demographic Research (Germany). Available at www.mortality.org or www.humanmortality.de (data downloaded on 25 Sept 2017).
Hummer, R. A., & Lariscy, J. T. (2011). Educational attainment and adult mortality. In R. G. Rogers & E. M. Crimmins (Eds.), International handbook of adult mortality (pp. 241–261). Dordrecht, Heidelberg, London, New York: Springer.
Janssen, F., & Kunst, A. E. (2005). Cohort patterns in mortality trends among the elderly in seven European countries, 1950-99. International Journal of Epidemiology, 34 (5), 1149–1159.
Jasilionis, D., & Shkolnikov, V. M. (2016). Longevity and education: a demographic perspective. Gerontology, 62 (3), 253–262.
Jasilionis, D., Jdanov, D., & Leinsalu, M. (2007). Der Zusammenhang von Bildung und Lebenserwartung in Mittel-und Osteuropa. Jahrbuch der Max-Planck-Gesellschaft, 2006-2007 , 103–108.
Kaplan, R. M., Spittel, M. L., & Zeno, T. L. (2014). Educational attainment and life expectancy. Policy Insights From the Behavioral and Brain Sciences, 1 (1), 189–194.
Kilpi, F., Silventoinen, K., Konttinen, H., & Martikainen, P. (2016). Disentangling the relative importance of different socioeconomic resources for myocardial infarction incidence and survival: a longitudinal study of over 300 000 Finnish adults. The European Journal of Public Health, 26 (2), 260–266.
Kingston, P. W., Hubbard, R., Lapp, B., Schroeder, P., & Wilson, J. (2003). Why education matters. Sociology of Education, 76 (1), 53–70.
van Kippersluis, H., O’Donnell, O., & van Doorslaer, E. (2011). Long-run returns to education: does schooling lead to an extended old age? Journal of Human Resources, 46 (4), 695–721.
Klein, T., Unger, R., & Schulze, A. (2006). Bildungsexpansion und Lebenserwartung. In A. Hadjar & R. Becker (Eds.), Die Bildungsexpansion. Erwartete und unerwartete Folgen (pp. 311–331). Wiesbaden: VS Verlag für Sozialwissenschaften.
Kröger, H., Pakpahan, E., & Hoffmann, R. (2015). What causes health inequality? A systematic review on the relative importance of social causation and health selection. The European Journal of Public Health, 25 (6), 951–960.
Laaksonen, M., Talala, K., Martelin, T., Rahkonen, O., Roos, E., Helakorpi, S., Laatikainen, T., & Prättälä, R. (2008). Health behaviours as explanations for educational level differences in cardiovascular and all-cause mortality: a follow-up of 60 000 men and women over 23 years. The European Journal of Public Health, 18 (1), 38–43.
Lager, A. C. J., & Torssander, J. (2012). Causal effect of education on mortality in a quasi-experiment on 1.2 million Swedes. Proceedings of the National Academy of Sciences, 109 (22), 8461–8466.
Link, B. G., & Phelan, J. (1995). Social conditions as fundamental causes of disease. Journal of Health and Social Behavior, 35 (extra issue), 80–94.
Lleras-Muney, A. (2005). The relationship between education and adult mortality in the United States. Review of Economic Studies, 72 (1), 189–221.
Lutz, W. (2009). Sola schola et sanitate: human capital as the root cause and priority for international development? Philosophical Transactions of the Royal Society of London B: Biological Sciences, 364 (1532), 3031–3047.
Lutz, W., & Skirbekk, V. (2014). How education drives demography and knowledge informs projection. In W. Lutz, W. P. Butz, & S. KC (Eds.), World population and human capital in the twenty-first century (pp. 14–38). Oxford: Oxford University Press.
Lutz, W., Butz, W. P., & S. KC. (2014a). World population and human capital in the twenty-first century . Oxford: Oxford University Press.
Lutz, W., Muttarak, R., & Striessnig, E. (2014b). Universal education is key to enhanced climate adaptation. Science, 346 (6213), 1061–1062.
Luy, M., Wegner-Siegmundt, C., Wiedemann, A., & Spijker, J. (2015). Life expectancy by education, income and occupation in Germany: estimations using the longitudinal survival method. Comparative Population Studies, 40 (4), 399–436.
Mackenbach, J. P., Looman, C. W. N., Kunst, A. E., Habbema, J. D. F., & van der Maas, P. J. (1988). Post-1950 mortality trends and medical care: gains in life expectancy due to declines in mortality from conditions amenable to medical intervention in the Netherlands. Social Science & Medicine, 27 (9), 889–894.
Mirowsky, J., & Ross, C. E. (2003). Education, social status, and health . New York: Aldine de Gruyter.
Montez, J. K., & Friedman, E. M. (2015). Educational attainment and adult health: contextualizing causality . Social Science & Medicine Special Issue. Elsevier.
Montez, J. K., Hummer, R. A., & Hayward, M. D. (2012). Educational attainment and adult mortality in the United States: a systematic analysis of functional form. Demography, 49 (1), 315–336.
Murtin, F., Mackenbach, J., Jasilionis, D., & Mira d'Ercole, M. (2017). Inequalities in longevity by education in OECD countries. Insights from new OECD estimates. OECD statistics working papers 2017/02 . Paris: OECD Publishing.
Ngom, P., & Bawah, A. A. (2004). INDEPTH model life tables for sub-Saharan Africa . Aldershot, Burlington: Ashgate.
OECD. (2016). Education at a glance 2016: OECD indicators . Paris: OECD Publishing.
Olshansky, S. J. (2005). Projecting the future of U.S. health and longevity. Health Affairs, 24 (supplement 2), W5R86–W85R89.
Olshansky, S. J., & Ault, A. B. (1986). The fourth stage of the epidemiologic transition: the age at delayed degenerative diseases. The Milbank Quarterly, 64 (3), 355–391.
Olshansky, S. J., Carnes, B. A., & Cassel, C. (1990). In search of Methuselah: estimating the upper limits to human longevity. Science, 250 (4981), 634–640.
Omran, A. R. (1971). The epidemiologic transition. A theory of the epidemiology of population change. The Milbank Memorial Fund Quarterly, 49 (4), 509–537.
Omran, A. R. (1983). The epidemiologic transition theory. A preliminary update. Journal of Tropical Pediatrics, 29 (6), 305–316.
Pressat, R. (1985). Contribution des écarts de mortalité par âge á la différence des vies moyennes. Population, 4 (5), 766–770.
Preston, S. H., Heuveline, P., & Guillot, M. (2001). Demography: Measuring and modeling population processes . Oxford: Blackwell.
Qian, Z., & Preston, S. H. (1993). Changes in American marriage, 1972 to 1987: availability and forces of attraction by age and education. American Sociological Review, 58 (4), 482–495.
Rogers, R. G., & Hackenberg, R. (1987). Extending epidemiologic transition theory: a new stage. Social Biology, 34 (3–4), 234–243.
Rogers, R. G., Hummer, R. A., & Nam, C. B. (1999). Living and dying in the USA: behavioral, health, and social differentials of adult mortality . San Diego: Academic Press.
Rogers, R. G., Everett, B. G., Zajacova, A., & Hummer, R. A. (2010). Educational degrees and adult mortality risk in the United States. Biodemography and Social Biology, 56 (1), 80–99.
Ross, C. E., & Wu, C.-l. (1995). The links between education and health. American Sociological Review, 60 (5), 719–745.
Ruggles, S., Genadek, K., Goeken, R., Grover, J., & Sobek, M. (2015). Integrated public use microdata series: Version 6.0 [machine-readable database] . Minneapolis: University of Minnesota.
Ryan, C. L., & Bauman, K. (2016). Educational attainment in the United States: 2015 . Current Population Reports Washington: United States Census Bureau.
Schoeni, R. F., & Ofstedal, M. B. (2010). Key themes in research on the demography of aging. Demography, 47 (supplement), S5–S15.
Shkolnikov, V. M., Andreev, E. M., & Begun, A. Z. (2003). Gini coefficient as a life table function: computation from discrete data, decomposition of differences and empirical examples. Demographic Research, 8 (11), 305–358.
Shkolnikov, V. M., Andreev, E. M., Jasilionis, D., Leinsalu, M., Antonova, O. I., & McKee, M. (2006). The changing relation between education and life expectancy in central and eastern Europe in the 1990s. Journal of Epidemiology and Community Health, 60 (10), 875–881.
Shkolnikov, V. M., Andreev, E. M., Jdanov, D. A., Jasilionis, D., Kravdal, Ø., Vågerö, D., & Valkonen, T. (2012). Increasing absolute mortality disparities by education in Finland, Norway and Sweden, 1971–2000. Journal of Epidemiology and Community Health, 66 (4), 372–378.
Sorlie, P. D., & Johnson, N. J. (1996). Validity of education information on the death certificate. Epidemiology, 7 (4), 437–439.
Stewart, Q. T. (2004). Brass' relational model: a statistical analysis. Mathematical Population Studies, 11 (1), 51–72.
Stringhini, S., Carmeli, C., Jokela, M., Avendaño, M., Muennig, P., Guida, F., Ricceri, F., d'Errico, A., Barros, H., Bochud, M., Chadeau-Hyam, M., Clavel-Chapelon, F., Costa, G., Delpierre, C., Fraga, S., Goldberg, M., Giles, G. G., Krogh, V., Kelly-Irving, M., Layte, R., Lasserre, A. M., Marmot, M. G., Preisig, M., Shipley, M. J., Vollenweider, P., Zins, M., Kawachi, I., Steptoe, A., Mackenbach, J. P., Vineis, P., & Kivimäki, M. (2017). Socioeconomic status and the 25x25 risk factors as determinants of premature mortality: a multicohort study and meta-analysis of 1.7 million men and women. The Lancet . https://doi.org/10.1016/S0140-6736(1016)32380-32387 .
Thygesen, L. C., Daasnes, C., Thaulow, I., & Brønnum-Hansen, H. (2011). Introduction to Danish (nationwide) registers on health and social issues: structure, access, legislation, and archiving. Scandinavian Journal of Public Health, 39 (7 suppl), 12–16.
Turra, C. M., Renteria, E., & Guimarães, R. (2016). The effect of changes in educational composition on adult female mortality in Brazil. Research on Aging, 38 (3), 283–298.
UNESCO. (1996). International standard classification of education ISCED 1997 . Montreal: UNESCO-UIS.
Valkonen, T. (2006). Social inequalities in mortality. In G. Caselli, J. Vallin, & G. Wunsch (Eds.), Demography: analysis and synthesis (Vol. 2, pp. 195–206). London: Academic Press.
Vallin, J., & Meslé, F. (2004). Convergences and divergences in mortality. A new approach to health transition. Demographic Research, 2 (2), 11–44.
Vaupel, J. W. (2010). Biodemography of human ageing. Nature, 465 (25 march), 536–542.
Vaupel, J. W., & Yashin, A. I. (1985). Heterogeneity's ruses: some surprising effects of selection on population dynamics. The American Statistician, 39 (3), 176–185.
Vaupel, J. W., Manton, K. G., & Stallard, E. (1979). The impact of heterogeneity in individual frailty on the dynamics of mortality. Demography, 16 (3), 439–454.
Williams, D. R. (2005). The health of U.S. racial and ethnic populations. The Journals of Gerontology Series B: Psychological Sciences and Social Sciences, 60 (special issue 2), S53–S62.
Wilmoth, J. R. (2000). Demography of longevity: past, present, and future trends. Experimental Gerontology, 35 , 1111–1129.
Woolf, S. H., Johnson, R. E., Phillips, R. L., & Philipsen, M. (2007). Giving everyone the health of the educated: an examination of whether social change would save more lives than medical advances. American Journal of Public Health, 97 (4), 679–683.
Download references
Acknowledgements
We thank Henrik Brønnum-Hansen (University of Copenhagen) for preparing and providing us the data for Denmark, two anonymous reviewers of this manuscript, and Werner Richter for language editing.
This research was supported by the European Research Council, within the European Community’s Seventh Framework Programme (FP7/2007–2013), ERC Grant Agreement No. 262663 (HEMOX) and within the EU Framework Programme for Research and Innovation Horizon 2020, ERC Grant Agreement No. 725187 (LETHE).
Availability of data and materials
All data are available online or in printed form as described in the " Data " section. The life table calculations have been made with MS Excel and the decomposition analyses with the MS Excel macro “Decomposition_replacement_from_young_to_old_ages(4).xls” provided by Andreev and Shkolnikov ( 2012 ) (see " Methods " section). All data and results are online available in the Additional file.
Author information
Authors and affiliations.
Wittgenstein Centre for Demography and Global Human Capital (IIASA, VID/ÖAW, WU), Welthandelsplatz 2 / Level 2, 1020, Vienna, Austria
Marc Luy, Marina Zannella, Christian Wegner-Siegmundt & Wolfgang Lutz
Vienna Institute of Demography of the Austrian Academy of Sciences, Welthandelsplatz 2 / Level 2, 1020, Vienna, Austria
International Institute for Applied Systems Analysis, Laxenburg, Austria
Wolfgang Lutz
Vienna University of Economics and Business, Welthandelsplatz 2 / Level 3, 1020, Vienna, Austria
Faculty of Liberal Art, Sophia University, 7-1 Kioicho Chiyodaku, Tokyo, 102-8554, Japan
Yuka Minagawa
Department of Statistical Sciences, Sapienza University of Rome, Viale Regina Elena 295, 00161, Rome, Italy
Graziella Caselli
Department of Methods and Models for Economics, Territory and Finance, Sapienza University of Rome, Via Del Castro Laurenziano 9, 00161, Rome, Italy
Marina Zannella
You can also search for this author in PubMed Google Scholar
Contributions
ML and GC developed the research idea and designed the study. ML directed and supervised the analyses and wrote large parts of the paper. CW-S performed the analyses, directed a systematic literature review with PubMed, and gave inputs to the paper, in particular the “ Methods ” section. MZ collected and prepared the data for Italy and the USA, supported the analyses, and gave inputs to the paper. YM reviewed the literature and prepared large parts of the paper. WL and GC contributed to the interpretation of the data and commented on all parts of the paper with corresponding inputs to the text. All authors read and approved the final manuscript.
Corresponding author
Correspondence to Marc Luy .
Ethics declarations
Competing interests.
The authors declare that they have no competing interests.
Publisher’s Note
Springer Nature remains neutral with regard to jurisdictional claims in published maps and institutional affiliations.
Additional file:
Additional file 1:.
Basic data and life tables by educational attainment. (CSV 54 kb)
Rights and permissions
Open Access This article is distributed under the terms of the Creative Commons Attribution 4.0 International License ( http://creativecommons.org/licenses/by/4.0/ ), which permits unrestricted use, distribution, and reproduction in any medium, provided you give appropriate credit to the original author(s) and the source, provide a link to the Creative Commons license, and indicate if changes were made.
Reprints and permissions
About this article
Cite this article.
Luy, M., Zannella, M., Wegner-Siegmundt, C. et al. The impact of increasing education levels on rising life expectancy: a decomposition analysis for Italy, Denmark, and the USA. Genus 75 , 11 (2019). https://doi.org/10.1186/s41118-019-0055-0
Download citation
Received : 10 October 2018
Accepted : 15 January 2019
Published : 07 March 2019
DOI : https://doi.org/10.1186/s41118-019-0055-0
Share this article
Anyone you share the following link with will be able to read this content:
Sorry, a shareable link is not currently available for this article.
Provided by the Springer Nature SharedIt content-sharing initiative
- Life expectancy
- Human capital
- Population structure
- Decomposition; Health policy
Thank you for visiting nature.com. You are using a browser version with limited support for CSS. To obtain the best experience, we recommend you use a more up to date browser (or turn off compatibility mode in Internet Explorer). In the meantime, to ensure continued support, we are displaying the site without styles and JavaScript.
- View all journals
- Explore content
- About the journal
- Publish with us
- Sign up for alerts
- Published: 13 April 2020
The effect of education on determinants of climate change risks
- Brian C. O’Neill ORCID: orcid.org/0000-0001-7505-8897 1 ,
- Leiwen Jiang ORCID: orcid.org/0000-0002-2073-6440 2 , 3 ,
- Samir KC 2 , 4 ,
- Regina Fuchs 5 ,
- Shonali Pachauri ORCID: orcid.org/0000-0001-8138-3178 4 ,
- Emily K. Laidlaw 4 ,
- Tiantian Zhang 6 ,
- Wei Zhou 6 &
- Xiaolin Ren 7
Nature Sustainability volume 3 , pages 520–528 ( 2020 ) Cite this article
3258 Accesses
41 Citations
94 Altmetric
Metrics details
- Socioeconomic scenarios
- Sustainability
Increased educational attainment is a sustainable development priority and has been posited to have benefits for other social and environmental issues, including climate change. However, links between education and climate change risks can involve both synergies and trade-offs, and the balance of these effects remains ambiguous. Increases in educational attainment could lead to faster economic growth and therefore higher emissions, more climate change and higher risks. At the same time, improved attainment would be associated with faster fertility decline in many countries, slower population growth and therefore lower emissions, and would also be likely to reduce vulnerability to climate impacts. We employ a multiregion, multisector model of the world economy, driven with country-specific projections of future population by level of education, to test the net effect of education on emissions and on the Human Development Index (HDI), an indicator that correlates with adaptive capacity to climate impacts. We find that improved educational attainment is associated with a modest net increase in emissions but substantial improvement in the HDI values in developing country regions. Avoiding stalled progress in educational attainment and achieving gains at least consistent with historical trends is especially important in reducing future vulnerability.
This is a preview of subscription content, access via your institution
Access options
Access Nature and 54 other Nature Portfolio journals
Get Nature+, our best-value online-access subscription
24,99 € / 30 days
cancel any time
Subscribe to this journal
Receive 12 digital issues and online access to articles
111,21 € per year
only 9,27 € per issue
Buy this article
- Purchase on Springer Link
- Instant access to full article PDF
Prices may be subject to local taxes which are calculated during checkout
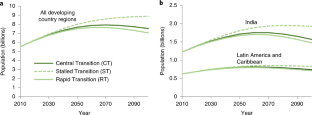
Similar content being viewed by others
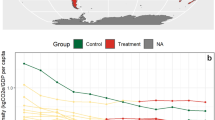
Effectiveness of carbon dioxide emission target is linked to country ambition and education level
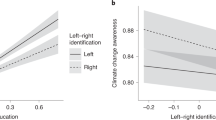
Right-wing ideology reduces the effects of education on climate change beliefs in more developed countries
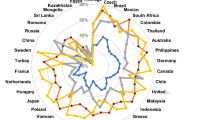
The international role of education in sustainable lifestyles and economic development
Data availability.
The samples of census datasets analysed during the current study are publicly available from IPUMS International at https://international.ipums.org/international/ . The national sample household survey data analysed for this study are publicly available for Brazil ( https://www.ibge.gov.br/estatisticas/sociais/habitacao/9050-pesquisa-de-orcamentos-familiares.html?=&t=downloads ), China ( https://opendata.pku.edu.cn/dataverse/CFPS?language=en ), India (Human Development Survey, https://www.icpsr.umich.edu/icpsrweb/DSDR/studies/36151 ), Mexico ( http://en.www.inegi.org.mx/programas/enigh/tradicional/2005/ ), South Africa ( https://www.datafirst.uct.ac.za/dataportal/index.php/catalog/316 ) and Uganda ( http://microdata.worldbank.org/index.php/catalog/2059 ), after registering and submitting requests. The national survey data for some countries are available but restrictions apply to the availability of these data, which were used under licence for the current study and so are not publicly available. These include India (National Sample Survey 2004–2005 and 2011–2012, http://www.icssrdataservice.in/datarepository/index.php/ ) and Indonesia ( https://microdata.bps.go.id/mikrodata/index.php/catalog/SUSENAS ). While these original full datasets have restrictions on availability, tables of derived results from the original datasets can be provided upon request.
Code availability
The code for the version of the iPETS model used to produce economic and emissions projections for this analysis is available upon request. It will eventually be publicly available at ipetsmodel.com.
Smith, W. C., Anderson, E., Salinas, D., Horvatek, R. & Baker, D. P. A meta-analysis of education effects on chronic disease: the causal dynamics of the population education transition curve. Soc. Sci. Med. 127 , 29–40 (2015).
Article Google Scholar
Coady, D. & Dizioli, A. Income inequality and education revisited: persistence, endogeneity and heterogeneity. Appl. Econ. 50 , 2747–2761 (2018).
Hanmer, L. & Klugman, J. Exploring women’s agency and empowerment in developing countries: where do we stand? Fem. Econ. 22 , 237–263 (2016).
Transforming Our World: The 2030 Agenda for Sustainable Development (United Nations, 2015).
A Guide to SDG Interactions: From Science to Implementation (International Council for Science, 2017).
McCollum, D. L. et al. Connecting the sustainable development goals by their energy inter-linkages. Environ. Res. Lett. 13 , 033006 (2018).
Pradhan, P., Costa, L., Rybski, D., Lucht, W. & Kropp, J. P. A systematic study of sustainable development goal (SDG) interactions. Earth’s Future 5 , 1169–1179 (2017).
Moyer, J. D. & Bohl, D. K. Alternative pathways to human development: assessing trade-offs and synergies in achieving the Sustainable Development Goals. Futures 105 , 199–210 (2019).
Gomez-Echeverri, L. Climate and development: enhancing impact through stronger linkages in the implementation of the Paris Agreement and the Sustainable Development Goals (SDGs). Phil. Trans. R. Soc. A 376 , 20160444 (2018).
Oppenheimer, M. et al. in IPCC Climate Change 2014: Impacts, Adaptation and Vulnerability (eds Field, C. B. et al.) 1039–1100 (Cambridge Univ. Press, 2014) .
O’Neill, B. C. et al. Global demographic trends and future carbon emissions. Proc. Natl Acad. Sci. USA 107 , 17521–17526 (2010).
Crespo Cuaresma, J., Lutz, W. & Sanderson, W. Is the demographic dividend an education dividend? Demography 51 , 299–315 (2014).
Lutz, W., Muttarak, R. & Striessnig, E. Universal education is key to enhanced climate adaptation. Science 346 , 1061–1062 (2014).
Article CAS Google Scholar
Gall, M. Indices of Social Vulnerability to Natural Hazards: A Comparative Evaluation (Univ. South Carolina, 2007).
Füssel, H.-M. Review and Quantitative Analysis of Indices of Climate Change Exposure, Adaptive Capacity, Sensitivity, and Impacts (World Bank, 2010); https://openknowledge.worldbank.org/handle/10986/9193
KC, S. & Lutz, W. The human core of the shared socioeconomic pathways: population scenarios by age, sex and level of education for all countries to 2100. Glob. Environ. Change 42 , 181–192 (2017).
O’Neill, B. C. et al. The roads ahead: narratives for shared socioeconomic pathways describing world futures in the 21st century. Glob. Environ. Change 42 , 169–180 (2017).
Riahi, K. et al. The shared socioeconomic pathways and their energy, land use, and greenhouse gas emissions implications: an overview. Glob. Environ. Change 42 , 153–168 (2017).
Ren, X. et al. Avoided economic impacts of climate change on agriculture: integrating a land surface model (CLM) with a global economic model (iPETS). Clim. Change 146 , 517–531 (2018).
Ren, X., Lu, Y., O'Neill, B. C. & Weitzel, M. Economic and biophysical impacts on agriculture under 1.5 °C and 2 °C warming. Environ. Res. Lett. 13 , 115006 (2018).
Böhringer, C. & Löschel, A. Computable general equilibrium models for sustainability impact assessment: status quo and prospects. Ecol. Econ. 60 , 49–64 (2006).
Scrieciu, S. S. The inherent dangers of using computable general equilibrium models as a single integrated modelling framework for sustainability impact assessment. A critical note on Böhringer and Löschel (2006). Ecol. Econ. 60 , 678–684 (2007).
Lutz, W. & Skirbekk, V. in World Population & Human Capital in the Twenty-First Century: An Overview (eds Lutz, W. et al.) 14–38 (Oxford Univ. Press, 2014).
IPCC: Summary for Policymakers. In Climate Change 2013: The Physical Science Basis (eds Stocker, T. F. et al.) (Cambridge Univ. Press, 2013).
Fuso Nerini, F. et al. Mapping synergies and trade-offs between energy and the sustainable development goals. Nat. Energy 3 , 10–15 (2018).
Wiedenhofer, D., Smetschka, B., Akenji, L., Jalas, M. & Haberl, H. Household time use, carbon footprints, and urban form: a review of the potential contributions of everyday living to the 1.5 °C climate target. Curr. Opin. Environ. Sustain. 30 , 7–17 (2018).
Duarte, R. et al. Modeling the carbon consequences of pro-environmental consumer behavior. Appl. Energy 184 , 1207–1216 (2016).
Dickson, J. R., Hughes, B. B. & Irfan, M. T. Advancing Global Education (Routledge, 2010).
Burke, M., Davis, W. M. & Diffenbaugh, N. S. Large potential reduction in economic damages under UN mitigation targets. Nature 557 , 549–553 (2018).
IPCC Climate Change 2014: Synthesis Report (eds Core Writing Team, Pachauri, R. K. & Meyer, L. A.) (IPCC, 2014).
Casey, G. & Galor, O. Is faster economic growth compatible with reductions in carbon emissions? The role of diminished population growth. Environ. Res. Lett. 12 , 014003 (2017).
Bongaarts, J. & O’Neill, B. C. Global warming policy: is population left out in the cold? Science 361 , 650–652 (2018).
Jiang, L. & O’Neill, B. C. Global urbanization projections for the shared socioeconomic pathways. Glob. Environ. Change 42 , 193–199 (2017).
Dellink, R., Chateau, J., Lanzi, E. & Magné, B. Long-term economic growth projections in the shared socioeconomic pathways. Glob. Environ. Change 42 , 200–214 (2017).
Lutz, W. & Kc, S. Global human capital: integrating education and population. Science 333 , 587–592 (2011).
KC, S. et al. Projection of populations by level of educational attainment, age, and sex for 120 countries for 2005–2050. Demogr. Res. 22 , 383–472 (2010).
KC, S., Potancokova, M., Bauer, R., Goujon, A. & Striessnig, E. in World Population and Human Capital in the Twenty-First Century (eds Lutz, W. et al.) 434–518 (Oxford Univ. Press, 2014).
Cutler, D. & Lleras-Muney, A. in Encyclopedia of Health Economics (ed. Culyer, A. J.) 232–245 (Elsevier, 2014).
Baker, D. P., Leon, J., Smith Greenaway, E. G., Collins, J. & Movit, M. The education effect on population health: a reassessment. Popul. Dev. Rev. 37 , 307–332 (2011).
KC, S. & Lentzner, H. The effect of education on adult mortality and disability: a global perspective. Vienna Yearb. Popul. Res. 8 , 201–235 (2010).
Rindfuss, R. R., St. John, C. & Bumpass, L. L. Education and the timing of motherhood: disentangling causation. J. Marriage Fam. 46 , 981–984 (1984).
Gerster, M., Ejrnæs, M. & Keiding, N. The causal effect of educational attainment on completed fertility for a cohort of Danish women—does feedback play a role? Stat. Biosci. 6 , 204–222 (2014).
Kravdal, Ø. Effects of current education on second- and third-birth rates among Norwegian women and men born in 1964: substantive interpretations and methodological issues. Demogr. Res. 17 , 211–246 (2007).
Forced Out: Mandatory Pregnancy Testing and the Expulsion of Pregnant Students in Tanzanian Schools (CRR, 2013); https://go.nature.com/2WJOg9J
Bongaarts, J. The causes of educational differences in fertility in Sub-Saharan Africa. Vienna Yearb. Popul. Res. 8 , 31–50 (2010).
Clarke, D. Children and their parents: a review of fertility and causality. J. Econ. Surv. 32 , 518–540 (2018).
Hoem, J. M. & Kreyenfeld, M. Anticipatory analysis and its alternatives in life-course research. Part 1: the role of education in the study of first childbearing. Demogr. Res. 15 , 461–484 (2006).
Calvin, K. et al. The SSP4: a world of deepening inequality. Glob. Environ. Change 42 , 284–296 (2017).
Rogelj, J. et al. Scenarios towards limiting global mean temperature increase below 1.5 °C. Nat. Clim. Change 8 , 325–332 (2018).
Download references
Acknowledgements
We thank the Asian Demographic Research Institute at Shanghai University for hosting research stays for B.C.O. during which parts of this work were carried out. A substantial amount of the work for this study was completed while B.C.O., L.J., E.K.L. and X.R. were at the National Center for Atmospheric Research, Boulder, CO.
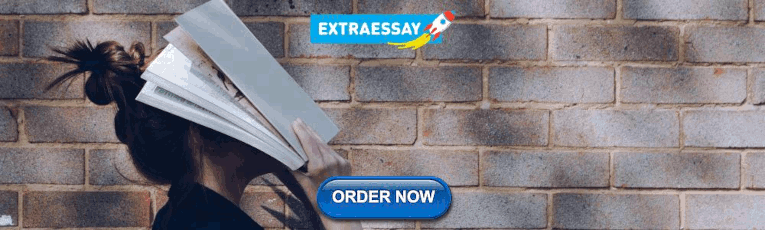
Author information
Authors and affiliations.
Josef Korbel School of International Studies and Pardee Center for International Futures, University of Denver, Denver, CO, USA
Brian C. O’Neill
Asian Demographic Research Institute, Shanghai University, Shanghai, China
Leiwen Jiang & Samir KC
Population Council, New York, NY, USA
- Leiwen Jiang
International Institute for Applied Systems Analysis, Laxenburg, Austria
Samir KC, Shonali Pachauri & Emily K. Laidlaw
Statistics Austria, Vienna, Austria
Regina Fuchs
Institute of Population and Development Studies, Zhejiang University, Hangzhou, China
Tiantian Zhang & Wei Zhou
National Center for Atmospheric Research, Boulder, CO, USA
Xiaolin Ren
You can also search for this author in PubMed Google Scholar
Contributions
B.C.O. led, and L.J., S.KC and S.P. contributed to, the design of the study. B.C.O. coordinated the paper and led the writing, with contributions from L.J., S.KC, S.P. and E.K.L. L.J., R.F., S.P. and E.K.L. led the analysis of household survey data, with contributions from T.Z. and W.Z. S.KC carried out the population–education projections. L.J. carried out the household projections. X.R. carried out the iPETS model projections, with contributions from B.C.O. B.C.O., L.J., S.KC, S.P., E.K.L. and X.R. interpreted results.
Corresponding author
Correspondence to Brian C. O’Neill .
Ethics declarations
Competing interests.
The authors declare no competing interests.
Additional information
Publisher’s note Springer Nature remains neutral with regard to jurisdictional claims in published maps and institutional affiliations.
Supplementary information
Supplementary information.
Supplementary Methods 1–3, Tables 1–4, Figs. 1–4 and references.
Reporting Summary
Rights and permissions.
Reprints and permissions
About this article
Cite this article.
O’Neill, B.C., Jiang, L., KC, S. et al. The effect of education on determinants of climate change risks. Nat Sustain 3 , 520–528 (2020). https://doi.org/10.1038/s41893-020-0512-y
Download citation
Received : 03 August 2018
Accepted : 11 March 2020
Published : 13 April 2020
Issue Date : July 2020
DOI : https://doi.org/10.1038/s41893-020-0512-y
Share this article
Anyone you share the following link with will be able to read this content:
Sorry, a shareable link is not currently available for this article.
Provided by the Springer Nature SharedIt content-sharing initiative
This article is cited by
Spatial and temporal changes in social vulnerability to natural hazards: a case study for china counties.
Natural Hazards (2024)
Achieving carbon neutrality in Africa is possible: the impact of education, employment, and renewable energy consumption on carbon emissions
- Chinyere Ori Elom
- Robert Ugochukwu Onyeneke
- Chidebe Chijioke Uwaleke
Carbon Research (2024)
Climate change unequally affects nitrogen use and losses in global croplands
- Chenchen Ren
- Xiuming Zhang
Nature Food (2023)
Assessing populations exposed to climate change: a focus on Africa in a global context
- Daniela Ghio
- Anne Goujon
- Thomas Petroliagkis
Population and Environment (2023)
Digitalization and its impact on labour market and education. Selected aspects
- Piotr Hetmańczyk
Education and Information Technologies (2023)
Quick links
- Explore articles by subject
- Guide to authors
- Editorial policies
Sign up for the Nature Briefing newsletter — what matters in science, free to your inbox daily.

Among nurses, how does education level impact professional values? A systematic review
Affiliation.
- 1 School of Nursing, College of Human and Health Services, George Mason University, Fairfax, VA, USA.
- PMID: 28657173
- DOI: 10.1111/inr.12390
Background: Professional nursing values have been acknowledged globally as the foundation of daily nursing care practice. Understanding how nurses identify, comprehend and apply their professional nursing values is an important step towards improving nursing practice and patient care quality. Research has demonstrated that nurses' professional values are cultivated during prelicensure academic education.
Aim: The aim of this systematic review was to determine how level of education affects professional nursing values of clinical practising nurses.
Methods: A systematic search of quantitative research published through December 2015 was performed in the following five electronic databases: CINAHL, Cochrane Library, MEDLINE, Web of Science and Religion and Philosophy Collection. The search was not limited to country of origin. The studies were assessed for methodological quality using established criteria.
Results: Of 1501 articles identified through the literature search, only seven studies met the inclusion criteria with the majority being of good to high quality. Most of the studies found registered nurses pursuing a bachelor of science in nursing or higher had a greater awareness and application of professional values than nurses with lower levels of academic or non-academic education. Nurses with higher education also embraced professional values as fundamental for quality nursing care practice.
Implications for nursing and health policy: Health and academic institutions should support nurses through quality continuing and higher education that reinforces professional values, thus improving the quality of patient care.
Conclusions: The level of nurses' education appears to play an important role in developing both an awareness and an integration of professional values into practice. More research is needed to discover methods that may be used to promote nurses' professional values among nurses already practising clinically.
Keywords: Educational Level; Educational Status; Ethics; Morals; Nurse; Nursing Education; Nursing Staff; Professional Values; Values.
© 2017 International Council of Nurses.
Publication types
- Systematic Review
- Attitude of Health Personnel*
- Attitude to Health
- Educational Status*
- Middle Aged
- Nursing Care / psychology*
- Nursing Staff / psychology*
- Nursing Staff / standards*
- Professional Competence*

An official website of the United States government
The .gov means it’s official. Federal government websites often end in .gov or .mil. Before sharing sensitive information, make sure you’re on a federal government site.
The site is secure. The https:// ensures that you are connecting to the official website and that any information you provide is encrypted and transmitted securely.
- Publications
- Account settings
Preview improvements coming to the PMC website in October 2024. Learn More or Try it out now .
- Advanced Search
- Journal List
- HHS Author Manuscripts

The Impact of Education on Depression Assessment: Differential Item Functioning Analysis
Level of education can impact access to healthcare and health outcomes. Increasing rates of depression are a major public health concern, and vulnerability to depression is compounded for individuals with a lower level of education. Assessment of depression is integral to many domains of healthcare including primary care and mental health specialty care. This investigation examined the degree to which education influences the psychometric properties of self-report items that measure depressive symptoms. This study was a secondary data analysis of three large internet panel studies. Together, the studies included the BDI-II, CES-D, PHQ-9, and PROMIS measures of depression. Using a differential item functioning (DIF) approach, we found evidence of DIF such that some items on each questionnaire were flagged for DIF (i.e., different psychometric properties across education groups); effect sizes ranged, McFadden’s Pseudo R 2 = .005 to .022. For example, education influenced psychometric properties for several double-barreled questions (i.e., questions that ask about more than one concept). Depression questionnaires vary in complexity, interacting with the respondent’s level of education. Measurement of depression should include consideration of possible educational disparities, to identify people who may struggle with a written questionnaire, or may be subject to subtle psychometric biases associated with education.
Depression is a common and increasing public health concern ( Vos et al., 2016 ). The lifetime risk for depression in US adults has previously been reported as 29.9% ( Kessler, Petukhova, Sampson, Zaslavsky, & Wittchen, 2012 ). Individuals with lower levels of education face socioeconomic challenges, including poorer access to healthcare ( Goldman & Smith, 2011 ). Therefore, individuals with lower educational attainment and depression are vulnerable on multiple levels— people with lower levels of education may have less access to screening and treatment for depression. Effective treatment begins with accurate and appropriate assessment. Thus, assessment should be precise and unbiased across levels of education. We investigated the influence of educational attainment on the psychometric properties of commonly used measures of depression. Simply stated, it is important to know how education affects individuals’ responses to questions about depression.
The literature suggests many associations between depression and education. Unsurprisingly, higher rates of depression are observed among individuals with lower levels of education, relative to people with higher levels of education ( Bauldry, 2015 ). Relatedly, several studies have demonstrated that a higher level of education is associated with lower levels of depression ( Fletcher, 2010 ; Lorant et al., 2003 ). The connection between depression and education is likely bidirectional. Fletcher (2008) found that females with depressive symptoms are more likely to drop out of high school, not enroll in college, and are less likely to enroll in a 4-year college. Bjelland et al. (2008) found that a higher level of education was associated with lower levels of anxiety and depression over time. Moreover, having a psychiatric diagnosis in general has been associated with school termination from primary school through college ( Breslau et al., 2009 ). It is important to note that the relationship between level of education and depression may be unique to the United States, as this association was not found in 11 other countries studied; this finding may be related to other sociodemographic factors such as gender ( Andrade et al., 2003 ).
Level of education has been associated with overall health and well-being, including health conditions such as diabetes and hypertension. Interestingly, Mezuk, Eaton, Golden, and Ding (2008) found that education moderated the association between type II diabetes and major depressive disorder. Level of education is related to level of literacy, consistent with findings that both lower educational attainment and limited literacy significantly predict poorer knowledge about hypertension and poorer control of symptoms, with literacy having mediated the relationship between education and knowledge about one’s hypertension ( Pandit et al., 2009 ). Thus, literacy and education have the potential to impact health outcomes, with education being a distal variable that influences factors that are more proximal to health, including literacy (and perhaps more specifically health literacy; Paasche-Orlow & Wolf, 2007 ). Importantly, education is negatively associated with higher mortality risk ( Baker, Leon, Smith Greenaway, Collins, & Movit, 2011 ). For example, individuals with lower education have higher mortality risk related to worse kidney health, dialysis complications, and poorer kidney transplant outcomes ( Green & Cavanaugh, 2015 ). Given the complex relationships among depression, health outcomes, and education, depression assessments should be accurate across levels of education.
Level of Education and Assessment
Educational attainment influences psychological assessment and self-reporting on questionnaires. This is widely known, such that standard cognitive assessments such as the Wechsler Adult Intelligence Scale IV (WAIS IV) provide supplemental scoring norms based on demographics such as level of education ( Brooks, Holdnack, & Iverson, 2011 ). Among healthy adults, education moderated the association between depressive symptoms and performance on tests of immediate and delayed verbal memory. Individuals with greater educational attainment performed well regardless of depressive symptomology, but those with lower education and depression demonstrated poorer performance ( McLaren, Szymkowicz, Kirton, & Dotson, 2015 ). Thus, accurate and equitable assessment in research and the clinic should consider the effect of educational attainment on individuals’ understanding of questionnaires.
Differential Item Functioning (DIF) exists when there is a difference in the relationship between a questionnaire item and a construct (e.g., depression) across groups (e.g., more vs. less education). Previous studies that have examined DIF by education have illuminated potentially biased or problematic items in the questionnaire development process. For example, the Mini-Mental State Exam was found to include significant DIF by education for 4–5 items, which led to overestimation of cognitive errors for individuals with a lower level of education ( Crane et al., 2006 ; Jones & Gallo, 2002 ; Murden, McRae, Kaner, & Bucknam, 1991 ; Ramirez, Teresi, Holmes, Gurland, & Lantigua, 2006 ). The Mattis Dementia Rating Scale was found to contain four items with DIF by education; one item demonstrated a large effect of DIF (digit span backwards; Teresi, Kleinman, & Ocepek-Welikson, 2000 ). Teresi et al. (2009) investigated DIF by several demographic factors including education for 32 PROMIS Depression items; one item demonstrated DIF by education such that individuals with higher education had a larger item slope. We built upon this prior work by examining PROMIS Depression and other items used to assess depression in the current study.
This study is unique because we had the opportunity to examine DIF in PROMIS measures as well as three other measures of depression. We hypothesized that DIF would be observed across levels of educational attainment for complex items on legacy measures of depression: the Beck Depression Inventory II (BDI-II), the Center for Epidemiologic Studies Depression Scale (CES-D), and the Patient Health Questionnaire (PHQ-9). When an item is flagged for DIF, it means that one or more of its item response theory (IRT) parameters are not equivalent across groups, which may suggest the possibility of a problematic and/or biased item. In the context of education, we hypothesized that different levels of education might lead to non-equivalent understanding of the items, potentially undermining the precision of depression assessment across the full range of education. Legacy measures of depression often include complicated wording and long items, which may be difficult for people with lower education to comprehend, which in turn could make it difficult for them to choose a response option that matches their experience. Taple, Griffith, and Wolf (2019) found DIF by health literacy on a PROMIS Depression short form, such that individuals with limited health literacy had lower item slopes. Similarly, we hypothesized that individuals with lower educational attainment would have lower item slopes on items with DIF, meaning that the item is not as informative. To our knowledge, this is the first study to investigate the broad impact of educational attainment on the PHQ-9, CES-D, BDI-II, and PROMIS Depression items using DIF analysis.
Participants
The present study included data from three internet panel samples (for details see Choi, Schalet, Cook, & Cella, 2014 ). All three samples were recruited by independent companies. The first sample, PROMIS 1 Wave 1 ( N = 744; ages 18–88) was recruited by Polimetrix online. The second sample, was originally recruited for the calibration of NIH Toolbox ( N = 748; ages 18–92) by Greenfield Online. Note that Greenfield Online is now called Toluna. Finally, the company Op4G recruited the third sample, PROsetta Stone ( N = 1,104, ages 18–88).
This study is a secondary data analysis of data from a study linking PROMIS to legacy measures of depression (see Choi et al., 2014 for details). This study was approved by the university’s Institutional Review Board. Participants completed a battery of measures online, mostly for the purpose of calibrating IRT parameters and examining other psychometric properties; the other measures are beyond the scope of the current investigation. The three samples included in the present study were recruited online from the general US population.
This study investigates the effect of education on four separate measures of depression – three questionnaires commonly used to assess depressive symptoms in the clinical setting, as well as items measuring depression from subsets of the NIH Patient Reported Outcomes Measurement Information System (PROMIS) item bank. Subsets from the PROMIS Depression item bank were administered to each sample, and included 28, 20, and 15 items, with fewer items selected to minimize participant burden. Fourteen PROMIS items were consistent across all three samples. Collectively we refer to the BDI-II, CES-D, and PHQ-9 as “legacy measures” of depression ( Choi et al., 2014 ). Cronbach’s alpha for all four measures of depression have been previously reported as exceeding 0.9 ( Choi et al., 2014 ). Table 1 presents a detailed breakdown of which measures were administered to each sample.
Measures Administered by Sample
Beck Depression Inventory II (BDI-II).
The BDI-II is a questionnaire that assesses symptoms of depression. It contains 21 items ( Beck, Steer, & Brown, 1996 ), each with response options ranging from 0 to 3; each response option contains the full item stem, in addition to the severity or frequency of the symptom. For example, the response options for an item assessing sadness include: “ 0: I do not feel sad; 1: I feel sad much of the time; 2: I am sad all the time; 3: I am so sad or unhappy that I can’t stand it ” ( Beck et al., 1996 ). Respondents are instructed to endorse the option that describes their experiences over the past two weeks. Internal consistency for the BDI-II has been previously described as Cronbach’s alpha ranging from .73 to .92 for non-clinical populations ( Dozois, Dobson, & Ahnberg, 1998 ).
Center for Epidemiologic Studies Depression Scale (CES-D).
The CES-D was designed for depression assessment in the general population ( Choi et al., 2014 ; Zimmerman & Coryell, 1994 ). The CES-D is comprised of 20 items ( Radloff, 1977 ). Possible responses range from 0 ( Rarely or none of the time ) to 3 ( Most or all of the time ). Internal consistency has been reported at Cronbach’s alpha = 0.85 in the general population, and other studies have supported its construct validity ( Radloff, 1977 ; Zimmerman & Coryell, 1994 ).
Patient Health Questionnaire (PHQ-9).
The PHQ-9 is used frequently in mental health settings as well as in primary care. The PHQ-9 consists of nine items based on the DSM-IV diagnostic criteria for a major depressive episode. Each item has four possible response options, 0 ( Not at all ) to 3 ( Nearly every day ). Internal consistency of the PHQ-9 has been previously reported with Cronbach’s alpha ranging from .86 to .89 ( Kroenke, Spitzer, & Williams, 2001 ). The PHQ-9 has demonstrated high criterion validity when compared to clinical interviews by mental health professionals ( Kroenke et al., 2001 ).
PROMIS Depression.
The National Institutes of Health (NIH) supported the development of the PROMIS system of standardized assessments of patient-reported outcomes (PROs; Cella et al., 2007, 2010; Fries, Bruce, & Cella, 2005; Hays, Bjorner, Revicki, Spritzer, & Cella, 2009; Liu et al., 2010). PROMIS has item banks for computer adaptive testing, as well as static short forms created from the item banks. For depression the items are rated on a five-point frequency scale with response options from 1 ( never ) to 5 ( always ; Pilkonis et al., 2011). The depression item bank includes items such as, “ In the past 7 days…I felt worthless ” and “ In the past 7 days…I felt I had nothing to look forward to .” Scores are expressed as T scores. Internal consistency of PROMIS Depression scales has been reported, Cronbach’s alpha = .95. Moreover, convergent validity with the CES-D has been previously reported as r = .83 (Pilkonis et al., 2011).
Differential Item Functioning (DIF)
DIF analysis is a method used to statistically quantify whether group membership (e.g., level of education) affects the relationship that a questionnaire item has with the construct it measures (e.g., depression). One method for detecting DIF is Item Response Theory (IRT; Cho, Suh, & Lee, 2016 ). If DIF is present, an item has different IRT properties across groups, even at the same level of the latent construct ( Reeve & Fayers, 2005 ; Shealy & Stout, 1993 ). In the current analyses, if an item shows DIF, then the level of depression may be under- or overestimated in individuals with a lower level of education. It is important to note that DIF with small effect size for individual items can cumulatively affect the entire test or measure ( Shealy & Stout, 1993 ). Discrepancies at the test level can influence construct validity, such that interpretations across groups may be inaccurate ( Taple et al., 2019 ).
Analytical Strategy
Descriptive statistics were conducted with the pastecs package (Grosjean & Ibanez, 2014) in R (R Core Team, 2013). DIF analyses were carried out for each item from the measures described above, in each sample separately, using the lordif package in R (Choi, Gibbons, & Crane, 2011). A similar methodology was used and recommended by Taple et al. (2019) . lordif employs a graded response model (GRM), a type of IRT model, which was well suited to the measures in this study because each item had several ordinal response options ( Reeve & Fayers, 2005 ; Samejima, 2016 ). Within lordif , items were flagged for DIF based on McFadden’s pseudo R 2 . Given concerns about Type 1 errors with chi-square detection tests (Choi et al., 2011), we opted for McFadden’s pseudo R 2 , an indicator of effect size.
DIF analyses were conducted using three levels of education: high school and below, some college or technical school, and college graduate and above. Educational attainment was split into these three groups so that each education group comprised roughly one-third of each sample. We used effect sizes rather than statistical significance to determine the presence and magnitude of DIF. McFadden’s pseudo R 2 was used to indicate the cutoff for flagging items for DIF. We chose to analyze DIF with cutoffs specifically chosen for each sample and measure. To determine the final cutoffs, we ran DIF across a wide range of effect sizes then inspected the output for where the number of items flagged for DIF stabilized. We then chose the largest (i.e., the most stringent) effect size where the number of items flagged remained consistent. That chosen effect size was used to determine how many items were flagged for each scale in each sample. The cutoffs used in the present study are larger than cutoffs determined by Monte Carlo simulations, which create a distribution of observed effect sizes based on a no-DIF null hypothesis. Thus, our observed effect sizes are more conservative than the results of the Monte Carlo simulations, because the simulations determine the minimum effect size that would be considered indicative of DIF.
Using the procedure described above, the cutoffs used to determine if an item would be flagged for DIF were selected for each measure in each sample. Effect size cutoffs by sample and measures are detailed in Table 2 . If an item had an effect size larger than the appropriate cutoff, it was flagged for DIF. It is important to distinguish the McFadden Pseudo R 2 cutoffs listed here from the observed pseudo R 2 values from the DIF analyses (see results below); the latter pseudo R 2 values are the observed impact of DIF (i.e., the observed impact of education on IRT parameters for depression items).
McFadden Pseudo R 2 Cutoffs by Sample and Measure
We examined IRT parameters across groups for all items flagged for DIF. Looking at the IRT parameters visually with item characteristic curves (ICCs) provides additional information about the nature of the DIF for that item. ICCs are determined by two types of IRT parameters – item slopes and item thresholds. Item slopes indicated how strongly an item relates to the construct (depression in this case) for each group. Item thresholds demonstrate the level of the construct (i.e., depression) for someone from an educational group to choose a particular response to the item. We examined ICCs for all items flagged for DIF.
As described above, we hypothesized that people with a lower level of education would have lower item slopes. We anticipated that the particular items flagged in this way would require a higher level of vocabulary. Therefore, we conducted several post-hoc readability analyses, using the readability package in R ( Rinker, 2017 ), to gain more information about the various measures of depression. These commonly used readability statistics demonstrate the average grade level required to read a passage ( Coleman & Liau, 1975 ; Flesch, 1948 ; Gunning, 1952 ; McLaughlin, 1969 ; Smith & Senter, 1967 ).
Descriptive Statistics
Descriptive statistics of demographics for each sample are displayed in Table 3 . Level of education was evenly dispersed across all samples. In the NIH Toolbox sample, age ranged from 18 to 92 years ( Mean ± SD = 47.2 ± 15.2). Fifty six percent of the Toolbox participants identified as female. PROMIS 1 Wave 1 participants ranged in age from 18 to 88 years ( Mean ± SD = 51.0 ± 18.8). Roughly half of the sample (52%) was female. Lastly, the PROsetta Stone sample comprised individuals from ages 18 to 88 years ( Mean ± SD = 46.3 ± 17.5). Fifty two percent of the PROsetta Stone participants were female.
Sample Characteristics
At least one item on each questionnaire in each sample showed DIF. Effect sizes for all items flagged for DIF are displayed in Table 4 . Content of item stems for all items flagged for DIF is presented in Table 5 . Of the measures administered in the NIH Toolbox sample, PROMIS Depression had one item with DIF, two items on the PHQ-9 were flagged, and one item on the CES-D (item 17; “ I had crying spells ”) demonstrated DIF with the largest effect size of items flagged in this sample ( R 2 = .017). In the PROMIS 1 Wave 1 sample, PROMIS Depression demonstrated four items with DIF, and the CES-D showed five items with DIF. Again, one of the items flagged on the CES-D (item 10; “ I felt fearful ”) had the largest effect size ( R 2 = .022). One item from the BDI-II was flagged for DIF in the third sample, PROsetta Stone, and four items from PROMIS Depression. One of the items flagged from PROMIS Depression (item 17; “In the past 7 days, I felt sad” ) had the largest effect size in this sample ( R 2 = .02). ICCs for these items with the largest effect sizes in each sample are displayed in Figures 1a – 1c .

Item characteristic curves (ICCs) for PROMIS Depression Item 17. Theta, displayed on the X axis, is the depression metric transformed to a standardized scale ( Mean = 0, SD = 1). The Y axis shows the probability of response type. As shown the curves are non-overlapping across groups.

Item characteristic curves (ICCs) for CES-D Item 10. Theta, displayed on the X axis, is the depression metric transformed to a standardized scale ( Mean = 0, SD = 1). The Y axis shows the probability of response type. Thresholds are collapsed when fewer than five participants use certain response categories.
Differential Item Functioning (DIF) Results
Note . Numbers displayed are the McFadden Pseudo R 2 effect sizes for each item flagged for DIF. – indicates that the measure was not administered in that sample. Item # refers to which items from each questionnaire were flagged for DIF.
Item Stems of Items Flagged for DIF
Note . – indicates that the measure was not administered in that sample. Item # refers to which items from each questionnaire were flagged for DIF. Item content is included in the response options for the BDI-II.
DIF findings were as follows. In the Toolbox Calibration sample, the ICCs for PROMIS Depression Item 46 (EDDEP46) and PHQ-9 items 5 and 8 showed that individuals with the highest level of education (college graduate or above) had steeper item slopes than the other two education groups, and thresholds varied. Similarly, BDI-II Item 9 in the PROsetta Stone sample showed that individuals with the highest level of education had steeper item slopes. In contrast, the ICCs for the items flagged for DIF in the PROMIS 1 Wave 1 sample, with the exception of PROMIS Depression Item 9 (EDDEP9) and CES-D Item 2, showed that individuals with the lowest level of education had the steepest item slopes. Within PROsetta Stone, slopes of PROMIS items flagged for DIF showed mixed results, varying in strength among the groups. Three of the four PROMIS items flagged demonstrated that individuals with a high school education or below had higher response thresholds than the other two educational groups.
Post-hoc Readability Analyses
We found that the PHQ-9, as shown by readability indices, requires the highest reading level of the questionnaires studied. The BDI-II also required a high reading level across most indices. High readability scores indicate that a higher reading level is needed to understand the item. Readability analyses are presented in detail in Table 6 . The CES-D demonstrated moderate difficulty based on readability statistics. Readability analyses revealed low to moderate difficulty of PROMIS Depression items.
Readability Statistics Findings
Note . Numbers indicate estimated grade level required to read and comprehend the text
Across all samples, on several items flagged for DIF, individuals with high school education or below (the lowest educational attainment category) had higher item slopes than the other two education groups. Teresi et al. (2009) found a similar item slope pattern on one PROMIS Depression item that demonstrated DIF by education. For individuals with higher levels of education, some items with DIF had lower item slopes (e.g., EDDEP 14: “ I felt that I was not as good as other people ”). It is possible that individuals with high education spent more time thinking about the content of the items leading to more variability in interpretation of the items. In contrast, perhaps individuals with lower education took a more literal interpretation. The pattern of DIF was not always in a consistent direction, however. Some items were flagged for DIF with individuals with the highest level of education (college or above) having the steepest item slopes. For example, PHQ-9 item 5 asks about “ poor appetite or overeating ”– opposite responses within one item. For these items, it is likely that responses for people with higher education are more closely related to their level of depression because they were able to understand these items more clearly than people with less educational attainment.
In the present study, items with DIF had small effect sizes. These small effect size can have a cumulative effect, however, on overall survey scores (Crane et al., 2007). If one looks at the proportion of items flagged, PROMIS does as well or better than legacy measures of depression in terms of equitable assessment across levels of education. In other words, PROMIS items were flagged for DIF at the lowest rate. Moreover, in the PROsetta Stone sample, the IRT parameters (i.e., slope and thresholds) of the PROMIS items flagged for DIF were large in an absolute sense, and relative to BDI-II item 9. Despite being flagged for DIF, this finding indicates that these PROMIS items were closely related to the latent construct of depression.
At the item level, educational attainment impacted participant responses on at least one item for each measure in all three samples. Given that DIF was found across all measures and that there is wide variability within levels of education, health literacy may have a stronger influence on IRT parameters than education for items measuring depression (e.g., Paasche-Orlow & Wolf, 2007 ; Taple et al., 2019 ). For instance, all 4-year college programs do not consist of equivalent content – there is likely different coverage of depression, for example, in a psychology major versus an engineering or fine-arts major. Thus, people within the college graduate and above education stratum could vary in their exposure to health information including depression. Nonetheless, these findings demonstrate that examining questionnaires for DIF can be a valuable tool to illuminate items that are challenging for some respondents, as well as items that are subject to variable interpretation. Researchers and clinicians should be cognizant of the effects of level of education on questionnaire responses, especially when new questionnaires are being developed and psychometric analyses are being used to select and remove items.
Of note, items 5 and 8 on the PHQ-9 were flagged for DIF in the NIH Toolbox Calibration sample; both items are double-barreled, meaning that the item asks about two separate concepts within a single item. In the field of test development there is wide consensus that double-barreled items are problematic, and should be avoided, because they can evoke confusion ( Dillman, Smyth, & Christian, 2014 ). Moreover, different responders may focus on different aspects of the item ( Gehlbach, 2015 ; Gideon, 2012 ; Sinkowitz-Cochran, 2013 ). In addition to DIF, we also examined the difficulty of items with readability analyses. It is worth noting that readability analyses are intended to evaluate larger blocks of texts, rather than single statements like a survey item, and do not consider the context of reading or individual differences in reading. Nonetheless, it was interesting to compare these findings with the DIF analyses. PROMIS Depression items in the NIH Toolbox sample were flagged for DIF at the lowest rate (in terms of total number of items on the questionnaire), which was consistent with PROMIS items being easier to read than the other questionnaires according to readability indices. Moreover, because readability analyses may fail to detect certain problems, it is helpful to use psychometric tools (e.g., DIF analysis) as a context and person-sensitive adjunctive tool for evaluating questionnaires items used in healthcare.
Our findings have implications for clinical practice. The legacy measures studied are widely used in clinical settings. If questionnaires have several items with DIF, this sets the stage for inaccurate assessment of depressive symptoms in patients with lower educational attainment. Downstream, depression questionnaires have the potential to influence referrals to specialty care, diagnostic decisions, treatment planning, and the assessment of change during treatment. DIF methodology is informative for any clinical assessment, given that respondents (e.g., patients) are likely to have a range of education. Questionnaires should be equally applicable across levels of education, so that all individuals may be accurately assessed. The fact that DIF by education is observed for all depression measures in this study suggests that the overall experience of completing the questionnaire may also be different (e.g., perhaps people with low levels of education find the questionnaires more intimidating); this should be assessed in future studies so that comfort as well as accuracy during questionnaire testing is maintained.
Limitations and Future Directions
We acknowledge that this study had limitations. First, the three samples were Internet based, and thus, were self-selecting and subject to the sampling procedures used by the panel companies. Second, the number of PROMIS Depression items, and thereby the content of some items, was not consistent across the three samples. Third, although several racial groups were represented in this research, the samples were predominantly White. Efforts should be made to recruit more diverse samples in future research to ensure that effects are generalizable. Fourth and finally, the Internet panels were individuals from the general US population. Effects of DIF may be even greater in a clinical sample (e.g., patients with major depression), and different results may be obtained in other countries. Future studies that investigate the impact of education on questionnaires assessing symptoms of depression in a sample with more moderate to severe symptomatology would be informative.
Future research can explore other methods for analyzing DIF. It may be useful to examine and compare the IRT parameters of different measures (e.g., PROMIS Depression compared to PHQ-9). It is possible, for example, that even if more PROMIS Depression items were flagged for DIF, PROMIS Depression as a questionnaire may be more strongly related to the construct of depression (e.g., larger item slopes). Ideally, questionnaire items would be free of DIF with large item slopes.
All measures displayed DIF by education for at least one item. Overall, findings were mixed such that item slopes were sometimes higher for individuals with high school education or below; for other items slopes were lower for people with less educational attainment. Therefore, depending on the item content, some items are less informative of a person’s actual symptoms depending on their level of educational attainment. Level of education needs to be considered during development and administration of instruments measuring depression. DIF is a useful tool to indicate which items may be more difficult for individuals with lower educational attainment.

Item characteristic curves (ICCs) for CES-D Item 17. Theta, displayed on the X axis, is the depression metric transformed to a standardized scale ( Mean = 0, SD = 1). The Y axis shows the probability of response type. Thresholds are collapsed when fewer than five participants use certain response categories.
- Andrade L, Caraveo-Anduaga JJ, Berglund P, Bijl RV, De Graaf R, Vollebergh W, … Wittchen HU (2003). The epidemiology of major depressive episodes: Results from the International Consortium of Psychiatric Epidemiology (ICPE) Surveys . International Journal of Methods in Psychiatric Research , 12 ( 1 ), 3–21. Retrieved from https://www.ncbi.nlm.nih.gov/pubmed/12830306 [ PMC free article ] [ PubMed ] [ Google Scholar ]
- Baker DP, Leon J, Smith Greenaway EG, Collins J, & Movit M. (2011). The education effect on population health: A reassessment . Population and Development Review , 37 ( 2 ), 307–332. doi: 10.1111/j.1728-4457.2011.00412.x [ PMC free article ] [ PubMed ] [ CrossRef ] [ Google Scholar ]
- Bauldry S. (2015). Variation in the protective effect of higher education against depression . Society and Ment Health , 5 ( 2 ), 145–161. doi: 10.1177/2156869314564399 [ PMC free article ] [ PubMed ] [ CrossRef ] [ Google Scholar ]
- Beck AT, Steer RA, & Brown GK (1996). Manual for the Beck Depression Inventory-II . Psychological Corporation. [ Google Scholar ]
- Bjelland I, Krokstad S, Mykletun A, Dahl AA, Tell GS, & Tambs K. (2008). Does a higher educational level protect against anxiety and depression? The HUNT study . Social Science & Medicine , 66 ( 6 ), 1334–1345. doi: 10.1016/j.socscimed.2007.12.019 [ PubMed ] [ CrossRef ] [ Google Scholar ]
- Breslau J, Miller E, Breslau N, Bohnert K, Lucia V, & Schweitzer J. (2009). The impact of early behavior disturbances on academic achievement in high school . Pediatrics , 123 ( 6 ), 1472–1476. doi: 10.1542/peds.2008-1406 [ PMC free article ] [ PubMed ] [ CrossRef ] [ Google Scholar ]
- Brooks BL, Holdnack JA, & Iverson GL (2011). Advanced clinical interpretation of the WAIS-IV and WMS-IV: Prevalence of low scores varies by level of intelligence and years of education . Assessment , 18 ( 2 ), 156–167. doi: 10.1177/1073191110385316 [ PubMed ] [ CrossRef ] [ Google Scholar ]
- Cho SJ, Suh Y, & Lee WY (2016). After differential item functioning is detected: IRT item calibration and scoring in the presence of DIF . Applied Psychological Measurement , 40 ( 8 ), 573–591. 10.1177/0146621616664304 [ PMC free article ] [ PubMed ] [ CrossRef ] [ Google Scholar ]
- Choi SW, Schalet B, Cook KF, & Cella D. (2014). Establishing a common metric for depressive symptoms: Linking the BDI-II, CES-D, and PHQ-9 to PROMIS depression . Psychological Assessment , 26 ( 2 ), 513–527. doi: 10.1037/a0035768 [ PMC free article ] [ PubMed ] [ CrossRef ] [ Google Scholar ]
- Coleman M, & Liau TL (1975). A computer readability formula designed for machine scoring . Journal of Applied Psychology , 60 ( 2 ), 283–284. doi: 10.1037/h0076540 [ CrossRef ] [ Google Scholar ]
- Crane PK, Gibbons LE, Jolley L, van Belle G, Selleri R, Dalmonte E, & De Ronchi D. (2006). Differential item functioning related to education and age in the Italian version of the Mini-Mental State Examination . International Psychogeriatrics , 18 ( 3 ), 505–515. doi: 10.1017/S1041610205002978 [ PubMed ] [ CrossRef ] [ Google Scholar ]
- Dillman DA, Smyth JD, & Christian LM (2014). Internet, phone, mail, and mixed-mode surveys: The tailored design method . John Wiley & Sons. [ Google Scholar ]
- Dozois DJ, Dobson KS, & Ahnberg JL (1998). A psychometric evaluation of the Beck Depression Inventory–II . Psychological Assessment , 10 ( 2 ), 83–89. 10.1037/1040-3590.10.2.83 [ CrossRef ] [ Google Scholar ]
- Flesch R. (1948). A new readability yardstick . Journal of Applied Psychology , 32 ( 3 ), 221–233. Retrieved from https://www.ncbi.nlm.nih.gov/pubmed/18867058 . [ PubMed ] [ Google Scholar ]
- Fletcher JM (2010). Adolescent depression and educational attainment: Results using sibling fixed effects . Health Economics , 19 ( 7 ), 855–871. doi: 10.1002/hec.1526 [ PubMed ] [ CrossRef ] [ Google Scholar ]
- Gehlbach H. (2015). Seven survey sins . The Journal of Early Adolescence , 35 ( 5–6 ), 883–897. doi: 10.1177/0272431615578276 [ CrossRef ] [ Google Scholar ]
- Gideon L. (2012). The art of question phrasing. In Handbook of survey methodology for the social sciences (pp. 91–107). Springer. [ Google Scholar ]
- Goldman D, & Smith JP (2011). The increasing value of education to health . Social Science & Medicine , 72 ( 10 ), 1728–1737. doi: 10.1016/j.socscimed.2011.02.047 [ PMC free article ] [ PubMed ] [ CrossRef ] [ Google Scholar ]
- Green JA, & Cavanaugh KL (2015). Understanding the influence of educational attainment on kidney health and opportunities for improved care . Advances in Chronic Kidney Disease , 22 ( 1 ), 24–30. doi: 10.1053/j.ackd.2014.07.004 [ PubMed ] [ CrossRef ] [ Google Scholar ]
- Gunning R. (1952). The technique of clear writing . New York, NY: McGraw-Hill. [ Google Scholar ]
- Jones RN, & Gallo JJ (2002). Education and sex differences in the Mini-Mental State Examination: Effects of differential item functioning . The Journals of Gerontology: Series B: Psychological Sciences and Social Sciences , 57 ( 6 ), P548–P558. doi: 10.1093/geronb/57.6.P548 [ PubMed ] [ CrossRef ] [ Google Scholar ]
- Kessler RC, Petukhova M, Sampson NA, Zaslavsky AM, & Wittchen H-U (2012). Twelve-month and lifetime prevalence and lifetime morbid risk of anxiety and mood disorders in the United States . International Journal of Methods in Psychiatric Research , 21 ( 3 ), 169–184. doi: 10.1002/mpr.1359 [ PMC free article ] [ PubMed ] [ CrossRef ] [ Google Scholar ]
- Kroenke K, Spitzer RL, & Williams JBW (2001). The PHQ-9: Validity of a brief depression severity measure . Journal of General Internal Medicine , 16 ( 9 ), 606–613. doi: 10.1046/j.1525-1497.2001.016009606.x [ PMC free article ] [ PubMed ] [ CrossRef ] [ Google Scholar ]
- Lorant V, Deliege D, Eaton W, Robert A, Philippot P, & Ansseau M. (2003). Socioeconomic inequalities in depression: A meta-analysis . American Journal of Epidemiology , 157 ( 2 ), 98–112. Retrieved from https://www.ncbi.nlm.nih.gov/pubmed/12522017 . [ PubMed ] [ Google Scholar ]
- McLaren ME, Szymkowicz SM, Kirton JW, & Dotson VM (2015). Impact of education on memory deficits in subclinical depression . Archives of Clinical Neuropsychology , 30 ( 5 ), 387–393. doi: 10.1093/arclin/acv038 [ PMC free article ] [ PubMed ] [ CrossRef ] [ Google Scholar ]
- McLaughlin GH (1969). SMOG grading: A new readability formula . Journal of Reading , 12 ( 8 ), 639–646. Retrieved from https://www.jstor.org/stable/40011226 [ Google Scholar ]
- Murden RA, McRae TD, Kaner S, & Bucknam ME (1991). Mini-Mental State exam scores vary with education in Blacks and Whites . Journal of the American Geriatrics Society , 39 ( 2 ), 149–155. doi: 10.1111/j.1532-5415.1991.tb01617.x [ PubMed ] [ CrossRef ] [ Google Scholar ]
- Paasche-Orlow MK, & Wolf MS (2007). The causal pathways linking health literacy to health outcomes . American Journal of Health Behavior , 31 Suppl 1 , S19–26. doi: 10.5555/ajhb.2007.31.supp.S19 [ PubMed ] [ CrossRef ] [ Google Scholar ]
- Pandit AU, Tang JW, Bailey SC, Davis TC, Bocchini MV, Persell SD, … Wolf MS (2009). Education, literacy, and health: Mediating effects on hypertension knowledge and control . Patient Education and Counseling , 75 ( 3 ), 381–385. doi: 10.1016/j.pec.2009.04.006 [ PubMed ] [ CrossRef ] [ Google Scholar ]
- Radloff LS (1977). The CES-D Scale: A self-report depression scale for research in the general population . Applied Psychological Measurement , 1 ( 3 ), 385–401. doi: 10.1177/014662167700100306 [ CrossRef ] [ Google Scholar ]
- Ramirez M, Teresi JA, Holmes D, Gurland B, & Lantigua R. (2006). Differential item functioning (DIF) and the Mini-Mental State Examination (MMSE): Overview, sample, and issues of translation . Medical Care , 44 ( 11 , Suppl 3). doi: 10.1097/01.mlr.0000245181.96133.db [ PubMed ] [ CrossRef ] [ Google Scholar ]
- Reeve BB, & Fayers P. (2005). Applying item response theory modeling for evaluating questionnaire item and scale properties. In Assessing quality of life in clinical trials: Methods of Practice (pp. 55–73). Oxford University Press. [ Google Scholar ]
- Rinker T. (2017). Readability: Tools to calculate readability scores (Version 0.1.0) [Software]. doi: 10.5281/zenodo.31950 [ CrossRef ] [ Google Scholar ]
- Samejima F. (2016). Graded Response Models. In Handbook of Item Response Theory (Vol. 1: Models, pp. 95–107). CRC Press. [ Google Scholar ]
- Shealy R, & Stout W. (1993). A model-based standardization approach that separates true bias/DIF from group ability differences and detects test bias/DTF as well as item bias/DIF . Psychometrika , 58 ( 2 ), 159–194. doi: 10.1007/bf02294572 [ CrossRef ] [ Google Scholar ]
- Sinkowitz-Cochran RL (2013). Survey design: To ask or not to ask? That is the question …. Clinical Infectious Diseases , 56 ( 8 ), 1159–1164. doi: 10.1093/cid/cit005 [ PubMed ] [ CrossRef ] [ Google Scholar ]
- Smith EA, & Senter RJ (1967). Automated readability index . AMRL TR, Aerospace Medical Research Laboratories (US) , 1–14. Retrieved from https://www.ncbi.nlm.nih.gov/pubmed/5302480 . [ PubMed ] [ Google Scholar ]
- Taple BJ, Griffith JW, & Wolf MS (2019). Interview administration of PROMIS depression and anxiety short forms . Health Literacy Research and Practice , 3 ( 3 ), e196–e204. 10.3928/24748307-20190626-01 [ PMC free article ] [ PubMed ] [ CrossRef ] [ Google Scholar ]
- Teresi JA, Kleinman M, & Ocepek-Welikson K. (2000). Modern psychometric methods for detection of differential item functioning: Application to cognitive assessment measures . Statistics in Medicine , 19 ( 11–12 ), 1651–1683. Retrieved from https://www.ncbi.nlm.nih.gov/pubmed/10844726 . [ PubMed ] [ Google Scholar ]
- Vos T, Allen C, Arora M, Barber RM, Bhutta ZA, Brown A, … Incidence, G. D. I. (2016). Global, regional, and national incidence, prevalence, and years lived with disability for 310 diseases and injuries, 1990–2015: A systematic analysis for the Global Burden of Disease Study 2015 . Lancet , 388 (10053), 1545–1602. doi: 10.1016/S0140-6736(16)31678-6 [ PMC free article ] [ PubMed ] [ CrossRef ] [ Google Scholar ]
- Zimmerman M, & Coryell W. (1994). Screening for major depressive disorder in the community: A comparison of measures . Psychological Assessment , 6 ( 1 ), 71–74. doi: 10.1037/1040-3590.6.1.71 [ CrossRef ] [ Google Scholar ]
Numbers, Facts and Trends Shaping Your World
Read our research on:
Full Topic List
Regions & Countries
Publications
- Our Methods
- Short Reads
- Tools & Resources
Read Our Research On:
Internet & Technology
6 facts about americans and tiktok.
62% of U.S. adults under 30 say they use TikTok, compared with 39% of those ages 30 to 49, 24% of those 50 to 64, and 10% of those 65 and older.
Many Americans think generative AI programs should credit the sources they rely on
Americans’ use of chatgpt is ticking up, but few trust its election information, whatsapp and facebook dominate the social media landscape in middle-income nations, sign up for our internet, science, and tech newsletter.
New findings, delivered monthly
Electric Vehicle Charging Infrastructure in the U.S.
64% of Americans live within 2 miles of a public electric vehicle charging station, and those who live closest to chargers view EVs more positively.
When Online Content Disappears
A quarter of all webpages that existed at one point between 2013 and 2023 are no longer accessible.
A quarter of U.S. teachers say AI tools do more harm than good in K-12 education
High school teachers are more likely than elementary and middle school teachers to hold negative views about AI tools in education.
Teens and Video Games Today
85% of U.S. teens say they play video games. They see both positive and negative sides, from making friends to harassment and sleep loss.
Americans’ Views of Technology Companies
Most Americans are wary of social media’s role in politics and its overall impact on the country, and these concerns are ticking up among Democrats. Still, Republicans stand out on several measures, with a majority believing major technology companies are biased toward liberals.
22% of Americans say they interact with artificial intelligence almost constantly or several times a day. 27% say they do this about once a day or several times a week.
About one-in-five U.S. adults have used ChatGPT to learn something new (17%) or for entertainment (17%).
Across eight countries surveyed in Latin America, Africa and South Asia, a median of 73% of adults say they use WhatsApp and 62% say they use Facebook.
5 facts about Americans and sports
About half of Americans (48%) say they took part in organized, competitive sports in high school or college.
REFINE YOUR SELECTION
Research teams, signature reports.
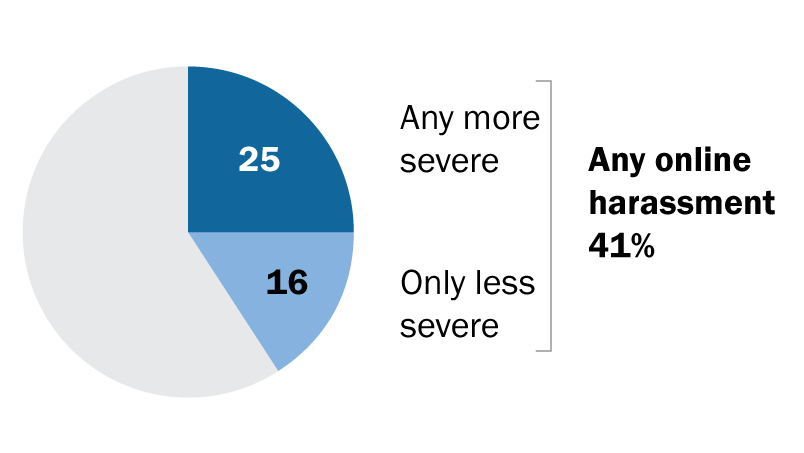
The State of Online Harassment
Roughly four-in-ten Americans have experienced online harassment, with half of this group citing politics as the reason they think they were targeted. Growing shares face more severe online abuse such as sexual harassment or stalking
Parenting Children in the Age of Screens
Two-thirds of parents in the U.S. say parenting is harder today than it was 20 years ago, with many citing technologies – like social media or smartphones – as a reason.
Dating and Relationships in the Digital Age
From distractions to jealousy, how Americans navigate cellphones and social media in their romantic relationships.
Americans and Privacy: Concerned, Confused and Feeling Lack of Control Over Their Personal Information
Majorities of U.S. adults believe their personal data is less secure now, that data collection poses more risks than benefits, and that it is not possible to go through daily life without being tracked.
Americans and ‘Cancel Culture’: Where Some See Calls for Accountability, Others See Censorship, Punishment
Social media fact sheet, digital knowledge quiz, video: how do americans define online harassment.
1615 L St. NW, Suite 800 Washington, DC 20036 USA (+1) 202-419-4300 | Main (+1) 202-857-8562 | Fax (+1) 202-419-4372 | Media Inquiries
Research Topics
- Email Newsletters
ABOUT PEW RESEARCH CENTER Pew Research Center is a nonpartisan fact tank that informs the public about the issues, attitudes and trends shaping the world. It conducts public opinion polling, demographic research, media content analysis and other empirical social science research. Pew Research Center does not take policy positions. It is a subsidiary of The Pew Charitable Trusts .
© 2024 Pew Research Center
Medication Literacy Test for Older Adults: psychometric analysis and standardization of the new instrument
- Research Article
- Published: 01 June 2024
Cite this article
- Laís Lessa Neiva Pantuzza 1 ,
- Adriano Max Moreira Reis ORCID: orcid.org/0000-0002-0017-7338 2 ,
- Stephanie Ferreira Botelho 1 ,
- Ana Luiza Pereira da Rocha 2 ,
- Maria Auxiliadora Parreiras Martins 2 ,
- Mariana Martins Gonzaga do Nascimento 2 ,
- Liliana Batista Vieira 3 ,
- Ronara Camila de Souza Groia Veloso 4 &
- Elizabeth do Nascimento 5
Explore all metrics
Low medication literacy is prevalent among older adults and is associated with adverse drug events. The Medication Literacy Test for Older Adults (TELUMI) was developed and content validated in a previously published study.
To evaluate the psychometric properties and provide norms for TELUMI scores.
This was a cross-sectional methodological study with older adults selected from the community and from two outpatient services. Descriptive item-analysis, exploratory factor analysis (EFA), item response theory (IRT), reliability, and validity analysis with schooling and health literacy were performed to test the psychometric properties of the TELUMI. The classification of the TELUMI scores was performed using percentile norms.
A total of 344 participants, with a mean age of 68.7 years (standard deviation = 6.7), were included; most were female (66.6%), black/brown (61.8%), had low schooling level (60.2%) and low income (55.2%). The EFA pointed to the one-dimensional structure of TELUMI. A three-parameter logistic model was adopted for IRT. All items had an adequate difficulty index. One item had discrimination < 0.65, and three items had an unacceptable guessing index (< 0.35) and were excluded. The 29-item version of TELUMI had excellent internal consistency (KR20 = 0.89). There was a positive and strong association between TELUMI scores and health literacy and education level. The scores were classified as inadequate medication literacy (≤ 10.0 points), medium medication literacy (11–20 points), and adequate medication literacy (≥ 21 points).
The results suggest that the 29-item version of TELUMI is psychometrically adequate for measuring medication literacy in older adults.
This is a preview of subscription content, log in via an institution to check access.
Access this article
Price includes VAT (Russian Federation)
Instant access to the full article PDF.
Rent this article via DeepDyve
Institutional subscriptions
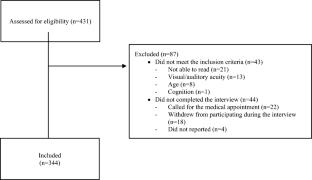
Similar content being viewed by others
Mediation effects of medication information processing and adherence on association between health literacy and quality of life.
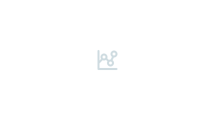
The impact of health literacy on beliefs about medication in a Dutch medication-using population
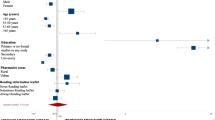
Age and education as factors associated with medication literacy: a community pharmacy perspective
Pouliot A, Vaillancourt R, Stacey D, et al. Defining and identifying concepts of medication literacy: an international perspective. Res Soc Adm Pharm. 2018;14:797–804. https://doi.org/10.1016/j.sapharm.2017.11.005 .
Article Google Scholar
Pouliot A, Vaillancourt R. Medication literacy: why pharmacists should pay attention. Can J Hosp Pharm. 2016;69:335–6. https://doi.org/10.4212/cjhp.v69i4.1576 .
Article PubMed PubMed Central Google Scholar
Gentizon J, Bovet E, Rapp E, et al. Medication literacy in hospitalized older adults: concept development. Health Lit Res Pract. 2022;6:e79–83. https://doi.org/10.3928/24748307-20220309-02 .
Neiva Pantuzza LL, Nascimento E, Crepalde-Ribeiro K, et al. Medication literacy: a conceptual model. Res Soc Adm Pharm. 2022;18:2675–82. https://doi.org/10.1016/j.sapharm.2021.06.003 .
Cutilli CC. Health literacy in geriatric patients: an integrative review of the literature. Orthop Nurs. 2007;26:43–8. https://doi.org/10.1097/00006416-200701000-00014 .
Article PubMed Google Scholar
Chesser AK, Keene Woods N, Smothers K, et al. Health literacy and older adults. Gerontol Geriatr Med. 2016;2:233372141663049. https://doi.org/10.1177/2333721416630492 .
Horvat N, Kos M. Development, validation and performance of a newly designed tool to evaluate functional medication literacy in Slovenia. Int J Clin Pharm. 2020;42:1490–8. https://doi.org/10.1007/s11096-020-01138-6 .
Pantuzza LLN, Nascimento E, Botelho SF, et al. Mapping the construct and measurement of medication literacy: a scoping review. Br J Clin Pharmacol. 2021;87:754–75. https://doi.org/10.1111/bcp.14490 .
Gentizon J, Fleury M, Pilet E, et al. Conceptualization and content validation of the MEDication literacy assessment of geriatric patients and informal caregivers (MED-fLAG). J Patient Rep Outcomes. 2022;6:87. https://doi.org/10.1186/s41687-022-00495-2 .
Mei C, Xu B, Cai X, et al. Factors affecting the medication literacy of older adults and targeted initiatives for improvement: a cross-sectional study in central China. Front Public Health. 2024;11:1249022. https://doi.org/10.3389/fpubh.2023.1249022 .
Pantuzza LLN, Nascimento E, Botelho SF, et al. Development and content validation of the medication literacy test for older adults (TELUMI). Arch Gerontol Geriatr. 2023;12:105027. https://doi.org/10.1016/j.archger.2023.105027 .
United Nations. World Population Prospects—Data Booklet [Internet]. Department of Economic and Social Affairs. 2015. Available from: www.unpopulation.org . Accessed 01 Oct 2022
DeVellis RF. Scale development theory and applications. SAGE Publications, Newbury Park (Applied Social Research Methods Series, 26); 2003. ISBN-10 1412980445/ISBN-13 978-1412980449
Moraes EN, Carmo JA, Moraes FL, et al. Clinical-functional vulnerability Index-20 (IVCF-20): rapid recognition of frail older adults. Rev Saúde Publica. 2016;50:81. https://doi.org/10.1590/S1518-8787.2016050006963 .
Saliba D, Elliott M, Rubenstein LZ, et al. The vulnerable elders survey: a tool for identifying vulnerable older people in the community. J Am Geriatr Soc. 2001;49:1691–9. https://doi.org/10.1046/j.1532-5415.2001.49281.x .
Article CAS PubMed Google Scholar
Gnjidic D, Hilmer SN, Blyth FM, et al. Polypharmacy cutoff and outcomes: five or more medicines were used to identify community-dwelling older men at risk of different adverse outcomes. J Clin Epidemiol. 2012;65:989–95. https://doi.org/10.1016/j.jclinepi.2012.02.018 .
Damascene A, Delicio AM, Mazo DF, et al. Validation of the Brazilian version of mini-test CASI-S. Arq Neuropsiquiatr. 2005;63:416–21. https://doi.org/10.1590/s0004-282x2005000300010 .
Apolinario D, Braga RCOP, Magaldi RM, et al. Short assessment of health literacy for portuguese-speaking adults. Rev Saude Publica. 2012;46:702–11. https://doi.org/10.1590/s0034-89102012005000047 .
Crawford JR, Garthwaite PH, Slick DJ. On percentile norms in neuropsychology: proposed reporting standards and methods for quantifying the uncertainty over the percentile ranks of test scores. Clin Neuropsychol. 2009;23:1173–95. https://doi.org/10.1080/13854040902795018 .
Muzaffar B. The development and validation of a scale to measure training culture: the TC scale. J Cult Soc Dev. 2016;23:49–58.
Google Scholar
Almeida-Brasil CC, Nascimento ED, Silveira MR, et al. New patient-reported outcome measure to assess perceived barriers to antiretroviral therapy adherence: the PEDIA scale. Cad Saude Publica. 2019;35:e00184218. https://doi.org/10.1590/0102-311x00184218 .
Birnbaum A. Some latent trait models and their use in inferring an examinee’s Ability. In. Statistical theories of mental test scores, Addison-Wesley, Reading; 1968. ISBN 10: 0201043106 ISBN 13: 9780201043105
Maydeu-Olivares AJH. Limited information goodness-of-fit testing in multidimensional contingency tables. Psychometrika. 2006;71:713–32. https://doi.org/10.1007/s11336-005-1295-9 .
Baker FB. The basics of item response theory, 2nd edn. ERIC Clearinghouse on Assessment and Evaluation; 2001. ISBN-1-886047-03-0
Kuder GF, Richardson MW. The theory of the estimation of test reliability. Psychometrika. 1937;2:151–60. https://doi.org/10.1007/BF02288391 .
Plaza-Zamora J, Legaz I, Osuna E, et al. Age and education as factors associated with medication literacy: a community pharmacy perspective. BMC Geriatr. 2020;20:501. https://doi.org/10.1186/s12877-020-01881-5 .
Nutbeam D. Defining, measuring and improving health literacy. Health Eval Promot. 2015;42:450–5.
Ping W, Cao W, Tan H, et al. Health protective behavior scale: development and psychometric evaluation. PLoS ONE. 2018;13:e0190390. https://doi.org/10.1371/journal.pone.0190390 .
Article CAS PubMed PubMed Central Google Scholar
Tennant R, Hiller L, Fishwick R, et al. The Warwick-Dinburgh mental well-being scale (WEMWBS): development and UK validation. Health Qual Life Outcomes. 2007;5:63. https://doi.org/10.1186/1477-7525-5-63 .
Lin HW, Chang EH, Ko Y, et al. Conceptualization, development and psychometric evaluations of a new medication-related health literacy instrument: the Chinese medication literacy measurement. Int J Environ Res Public Health. 2020;17:6951. https://doi.org/10.3390/ijerph17196951 .
Vervloet M, van Dijk L, Rademakers JJDJM, et al. Recognizing and addressing limited pharmaceutical literacy: development of the RALPH interview guide. Res Social Adm Pharm. 2018;14:805–11. https://doi.org/10.1016/j.sapharm.2018.04.031 .
Gentizon J, Hirt J, Jaques C, et al. Instruments assessing medication literacy in adult recipients of care: a systematic review of measurement properties. Int J Nurs Stud. 2021;113:103785. https://doi.org/10.1016/j.ijnurstu.2020.103785 .
Sauceda JA, Loya AM, Sias JJ, et al. Medication literacy in Spanish and English: psychometric evaluation of a new assessment tool. J Am Pharm Assoc. 2012;52:e231–40. https://doi.org/10.1331/JAPhA.2012.11264 .
Download references
This work was supported by the Conselho Nacional de Desenvolvimento Científico e Tecnológico—CNPq [Grant Number 409435/2018–0] and the Coordenação de Aperfeiçoamento de Pessoal de Nível Superior—Brazil (CAPES) [Finance Code 001]. The sponsors were not involved in the study design; in the collection, analysis, and interpretation of the data; in the writing of the report; and in the decision to submit the article for publication.
Author information
Authors and affiliations.
Programa de Pós-Graduação em Medicamentos e Assistência Farmacêutica, Faculdade de Farmácia, Universidade Federal de Minas Gerais, 6627 Presidente Antônio Carlos Ave., Pampulha, Belo Horizonte, Minas Gerais, Brazil
Laís Lessa Neiva Pantuzza & Stephanie Ferreira Botelho
Faculdade de Farmácia, Universidade Federal de Minas Gerais, 6627 Presidente Antônio Carlos Ave., Pampulha, Belo Horizonte, Minas Gerais, Brazil
Adriano Max Moreira Reis, Ana Luiza Pereira da Rocha, Maria Auxiliadora Parreiras Martins & Mariana Martins Gonzaga do Nascimento
Faculdade de Ciências Farmacêuticas, Universidade Federal de Alfenas, 700 Gabriel Monteiro da Silva St., Centro, Alfenas, Minas Gerais, Brazil
Liliana Batista Vieira
Hospital das Clínicas, Universidade Federal de Minas Gerais, 110 Professor Alfredo Balena Ave., Santa Efigênia, Belo Horizonte, Minas Gerais, Brazil
Ronara Camila de Souza Groia Veloso
Departamento de Psicologia, Faculdade de Filosofia e Ciências Humanas, Universidade Federal de Minas Gerais, 6627 Presidente Antônio Carlos Ave., Pampulha, Belo Horizonte, Minas Gerais, Brazil
Elizabeth do Nascimento
You can also search for this author in PubMed Google Scholar
Corresponding author
Correspondence to Adriano Max Moreira Reis .
Ethics declarations
Conflicts of interest.
The authors have no conflicts of interest to declare.
Additional information
Publisher's note.
Springer Nature remains neutral with regard to jurisdictional claims in published maps and institutional affiliations.
Supplementary Information
Below is the link to the electronic supplementary material.
Supplementary file1 (DOCX 246 kb)
Supplementary file2 (docx 381 kb), supplementary file3 (docx 390 kb), rights and permissions.
Springer Nature or its licensor (e.g. a society or other partner) holds exclusive rights to this article under a publishing agreement with the author(s) or other rightsholder(s); author self-archiving of the accepted manuscript version of this article is solely governed by the terms of such publishing agreement and applicable law.
Reprints and permissions
About this article
Neiva Pantuzza, L.L., Reis, A.M.M., Botelho, S.F. et al. Medication Literacy Test for Older Adults: psychometric analysis and standardization of the new instrument. Int J Clin Pharm (2024). https://doi.org/10.1007/s11096-024-01744-8
Download citation
Received : 06 February 2024
Accepted : 25 April 2024
Published : 01 June 2024
DOI : https://doi.org/10.1007/s11096-024-01744-8
Share this article
Anyone you share the following link with will be able to read this content:
Sorry, a shareable link is not currently available for this article.
Provided by the Springer Nature SharedIt content-sharing initiative
- Health literacy
- Medication literacy
- Older adults
- Validation study
- Find a journal
- Publish with us
- Track your research
- Election 2024
- Entertainment
- Newsletters
- Photography
- Personal Finance
- AP Investigations
- AP Buyline Personal Finance
- AP Buyline Shopping
- Press Releases
- Israel-Hamas War
- Russia-Ukraine War
- Global elections
- Asia Pacific
- Latin America
- Middle East
- Election Results
- Delegate Tracker
- AP & Elections
- Auto Racing
- 2024 Paris Olympic Games
- Movie reviews
- Book reviews
- Personal finance
- Financial Markets
- Business Highlights
- Financial wellness
- Artificial Intelligence
- Social Media
Nearly 3 out of 10 children in Afghanistan face crisis or emergency level of hunger in 2024
FILE - Afghan children eat in a makeshift shelter after an earthquake in Gayan district in Paktika province, Afghanistan, Saturday, June 25, 2022. About 6.5 million children in Afghanistan were forecast to experience crisis levels of hunger in 2024, a nongovernmental organization said Tuesday, May 29, 2024.(AP Photo/Ebrahim Nooroozi, File)
This is a locator map for Afghanistan with its capital, Kabul. (AP Photo)
- Copy Link copied
ISLAMABAD (AP) — About 6.5 million children in Afghanistan were forecast to experience crisis levels of hunger in 2024, a nongovernmental organization said.
Nearly three out of 10 Afghan children will face crisis or emergency levels of hunger this year as the country feels the immediate impacts of floods, the long-term effects of drought, and the return of Afghans from neighboring Pakistan and Iran, according to a report released late Tuesday by Save The Children.
New figures from global hunger monitoring body Integrated Food Security Phase Classification forecast that 28% of Afghanistan’s population, about 12.4 million people, will face acute food insecurity before October. Of those, nearly 2.4 million are predicted to experience emergency levels of hunger, which is one level above famine, according to Save the Children.
The figures show a slight improvement from the last report, released in October 2023, but underline the continuing need for assistance, with poverty affecting half of the population.
Torrential rain and flash floods hit northern Afghanistan in May , killing more than 400 people. Thousands of homes were destroyed or damaged and farmland was turned into mud.
Save the Children is operating a “clinic on wheels” in Baghlan province, which was hit the worst by floods, as part of its emergency response program. The organization added that an estimated 2.9 million children under the age of 5 are projected to suffer from acute malnutrition in 2024.
Arshad Malik, country director for Save the Children in Afghanistan, said that the NGO has treated more than 7,000 children for severe or acute malnutrition so far this year.
“Those numbers are a sign of the massive need for continuing support for families as they experience shock after shock,” Malik said. Children are feeling the devastating impacts of three years of drought, high levels of unemployment , and the return of more than 1.4 million Afghans from Pakistan and Iran, he added.
“We need long-term, community-based solutions to help families rebuild their lives,” Malik said.
More than 557,000 Afghans have returned from Pakistan since September 2023, after Pakistan began cracking down on foreigners it alleges are in the country illegally, including 1.7 million Afghans. It insists the campaign isn’t directed against Afghans specifically, but they make up most of the foreigners in the South Asian country.
In April, Save the Children said that a quarter-million Afghan children need education, food and homes after being forcibly returned from Pakistan.
Malik added that only 16% of funding for the 2024 humanitarian response plan has been met so far, but nearly half the population needs assistance.
“This is not the time for the world to look away,” he said.
Meanwhile, the European Union is allocating an additional 10 million euros (nearly $10.9 million) to the U.N. food agency for school feeding activities in Afghanistan. These latest funds from the EU follow an earlier contribution of 20.9 million euros ($22.7 million) towards the World Food Program’s school meal program in Afghanistan for 2022 and 2023.
The funding comes at a timely moment and averts WFP having to downsize its school meal program this year because of a lack of funding, the WFP said in a statement.
“Hunger can be a barrier to education. The additional EU funding to our long-standing partner WFP ensures that more children in Afghanistan receive nutritious food,” said Raffaella Iodice, chargé d’affaires of the EU’s delegation to Afghanistan.
The WFP’s statement said that the agency will be able to use the funding to distribute fortified biscuits or locally produced nutritious school snacks to pupils in more than 10,000 schools in the eight provinces of Farah, Ghor, Jawzjan, Nangarhar, Nuristan, Paktika, Uruzgan and Zabul.
Last year, WFP supported 1.5 million school-age children through this program.
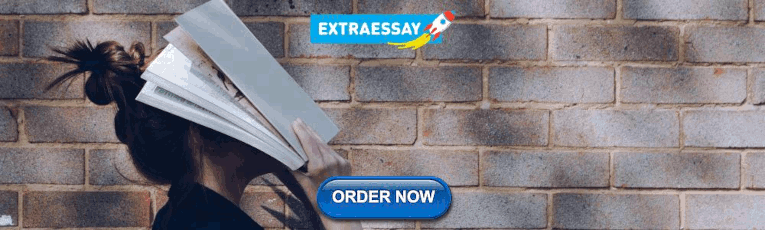
IMAGES
VIDEO
COMMENTS
URGENT NEED FOR NEW DIRECTIONS IN EDUCATION-HEALTH RESEARCH. Americans have worse health than people in other high-income countries, and have been falling further behind in recent decades ().This is partially due to the large health inequalities and poor health of adults with low education ().Understanding the health benefits of education is thus integral to reducing health disparities and ...
Education and health and wellbeing are intrinsically linked. The evidence behind the importance of education as a determinant of health is amongst the most compelling. Education is strongly associated with life expectancy, morbidity, health behaviours, and educational attainment plays an important role in health by shaping opportunities, employment, and income. In this issue of The Lancet ...
The American Educational Research Journal (AERJ) is the flagship journal of AERA, with articles that advance the empirical, theoretical, and methodological understanding of education and learning. It publishes original peer-reviewed analyses spanning the field of education research across all subfields and disciplines and all levels of analysis, all levels of education throughout the life span ...
Additionally, Perdima et al. [12] analysed journal articles in the Scopus database related to educational technology in physical education. However, none of these reviews addressed studies on educational technology at the primary or elementary education level.
A clear understanding of the macro-level contexts in which education impacts health is integral to improving national health administration and policy. In this research, we use a visual analytic approach to explore the association between education and health over a 20-year period for countries around the world. Using empirical data from the OECD and the World Bank for 26 OECD countries for ...
In Denmark, no specific education group dominated the mortality decline. The highest contribution originated from the low education level (33.4% for the total population; 34.6% for men; 26.4% for women), but the contribution of the medium education level was not much smaller (27.1% for the total population; 28.3% for men; 23.1% for women).
Education is a process and a product.From a societal perspective, the process of education (from the Latin, ducere, "to lead," and e, "out from," yield education, "a leading out") intentionally engages the receptive capacities of children and others to imbue them with knowledge, skills of reasoning, values, socio-emotional awareness and control, and social interaction, so they can ...
Education is strongly associated with life expectancy, morbidity, health behaviours, and educational attainment plays an important role in health by shaping opportunities, employment, and income. In this issue of The Lancet Public Health, two research Articles emphasise the lifelong impact of education on health. The study by Yu-Tzu Wu and ...
Level of education is a characteristic of individuals and populations that plays a key role in determining a number of aspects of well-being, including health, income and empowerment 1,2,3 ...
Journal of Education. The oldest educational publication in the country, the Journal of Education's mission is to disseminate knowledge that informs practice in PK-12, higher, and professional education. A refereed publication, the Journal offers … | View full journal description. This journal is a member of the Committee on Publication ...
A further 11 studies reported findings from interventions completed with students enrolled in pre-service teacher education programmes. Second level students were also represented in the final review, with five studies reporting across middle and high school levels. ... Journal of Education for Sustainable Development 2 : 141-156.10.1177 ...
Adults with higher educational attainment live healthier and longer lives compared with their less educated peers. The disparities are large and widening. We posit that understanding the educational and macrolevel contexts in which this association occurs is key to reducing health disparities and improving population health. In this article, we briefly review and critically assess the current ...
4 issues/year. 0195-6744. 1549-6511. 2.5. Ranked #123 out of 269 "Education & Educational Research" journals. 3.3. Ranked #414 out of 1,469 "Education" journals. The American Journal of Education seeks to bridge and integrate the intellectual, methodological, and substantive diversity of educational scholarship and to encourage a ...
In education this might mean, for example, that where a family has no history of education beyond primary or secondary level, the individual concerned may have a disposition that predisposes them to leave school early in line with familial traditions. ... European Journal of Education 53(3): 293-309. Crossref.
175 Journals in JSTOR. Date Range. Academe. 1979 - 2020. AAUP Bulletin. 1956 - 1978. Bulletin of the American Association of University Professors (1915-1955) 1915 - 1955. Academy of Management Learning & Education.
Introduction. Girls' education and gender inequalities associated with education were areas of major policy attention before the COVID-19 pandemic, and remain central to the agendas of governments, multilateral organisations and international NGOs in thinking about agendas to build back better, more equal or to build forward (Save the Children Citation 2020; UN Women Citation 2021; UNESCO ...
The analysis of the field and suggestions for future research in this positional article is primarily based on (a) a review of influential articles in inclusive education research (Nilholm and Göransson Citation 2017); (b) an overview (Nilholm Citation submitted) of influential reviews in five leading special needs journals (European Journal ...
Research has demonstrated that nurses' professional values are cultivated during prelicensure academic education. Aim: The aim of this systematic review was to determine how level of education affects professional nursing values of clinical practising nurses. Methods: A systematic search of quantitative research published through December 2015 ...
Level of education can impact access to healthcare and health outcomes. Increasing rates of depression are a major public health concern, and vulnerability to depression is compounded for individuals with a lower level of education. ... Mini-Mental State exam scores vary with education in Blacks and Whites. Journal of the American Geriatrics ...
Health and academic institutions should support nurses through quality continuing and higher education that reinforces professional values, thus improving the quality of patient care. Conclusions. The level of nurses' education appears to play an important role in developing both an awareness and an integration of professional values into practice.
Selectively indexed journals contain an average of 50-79% education-related articles and are critical to topic area coverage; ERIC applies a manual article-by-article selection process and indexes only the articles that conform to the standard and criteria outlined in the ERIC selection policy.
Digital technologies strive to decrease or eliminate pollution and waste while increasing production and efficiency. These technologies have shown a powerful impact on the education system. The recent COVID-19 Pandemic has further institutionalised the applications of digital technologies in education.
rson correlation, and the coefficient of determination to analyze the citations reported by ARC-PA during January 2021 to February 2023. Concurrent first-time taker Physician Assistant National Certifying Exam (PANCE) results were used to determine whether a correlation exists between pass rates and citations. In addition, a survey was sent to each institution's current program director to ...
The impact of school leadership on student outcomes is an important aspect of educational research, policy and practice. The assumption that high-quality leadership contributes significantly to enhanced school and student outcomes is well supported by research. Leithwood et al.'s (2006) widely cited study shows that total leadership explains up ...
Americans' Views of Technology Companies. Most Americans are wary of social media's role in politics and its overall impact on the country, and these concerns are ticking up among Democrats. Still, Republicans stand out on several measures, with a majority believing major technology companies are biased toward liberals. short readsApr 3, 2024.
Medication literacy refers to cognitive and social skills to access, understand, critically evaluate, communicate, and calculate medication information to make informed decisions about drug therapy [1,2,3,4].Low medication literacy is highly prevalent among older adults due to different age-related changes, including deteriorated cognition, loss of visual and hearing acuity, and decreased ...
Submit an article Journal homepage. Free access. 20 Views 0 ... Vet in higher education is one of the themes discussed having as a scenario the digital storytelling and photography to enhance the level of English of tourism students. Still addressing students' online realities, one of the studies in this volume looked at how a new digital ...
Of those, nearly 2.4 million are predicted to experience emergency levels of hunger, which is one level above famine, according to Save the Children. The figures show a slight improvement from the last report, released in October 2023, but underline the continuing need for assistance, with poverty affecting half of the population.
Calorimetry is one of the best experiments to do in an introductory-level chemistry laboratory course, as it has high accuracy and requires relatively inexpensive materials. Like other traditional general chemistry laboratory experiments, calorimetry is not easily converted to at-home or remote experimentation. The in-lab experiment typically requires relatively inaccessible equipment, such as ...
At a broader level, these articles illustrate how education can be related to important marketing frameworks. The 4Ps framework can be related to education-relevant decisions about product (Sen and Tucker 2022), pricing (Yoon, Yang, and Morewedge 2022), communication (Bollinger et al. 2022), and delivery mode (Lu, Bradlow, and Hutchinson 2022).