- Tools and Resources
- Customer Services
- Affective Science
- Biological Foundations of Psychology
- Clinical Psychology: Disorders and Therapies
- Cognitive Psychology/Neuroscience
- Developmental Psychology
- Educational/School Psychology
- Forensic Psychology
- Health Psychology
- History and Systems of Psychology
- Individual Differences
- Methods and Approaches in Psychology
- Neuropsychology
- Organizational and Institutional Psychology
- Personality
- Psychology and Other Disciplines
- Social Psychology
- Sports Psychology
- Share This Facebook LinkedIn Twitter
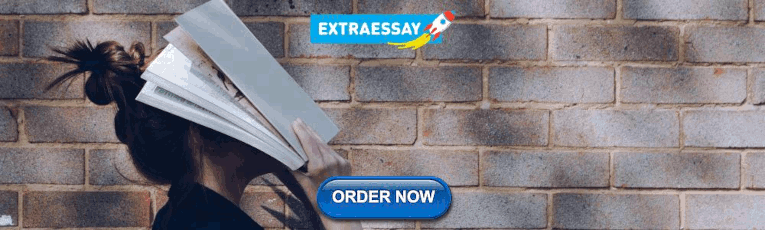
Article contents
Job and work design.
- Anja Van den Broeck Anja Van den Broeck KU Leuven
- and Sharon K. Parker Sharon K. Parker University of Western Australia
- https://doi.org/10.1093/acrefore/9780190236557.013.15
- Published online: 24 May 2017
Job design or work design refers to the content, structure, and organization of tasks and activities. It is mostly studied in terms of job characteristics, such as autonomy, workload, role problems, and feedback. Throughout history, job design has moved away from a sole focus on efficiency and productivity to more motivational job designs, including the social approach toward work, Herzberg’s two-factor model, Hackman and Oldham’s job characteristics model, the job demand control model of Karasek, Warr’s vitamin model, and the job demands resources model of Bakker and Demerouti. The models make it clear that a variety of job characteristics make up the quality of job design that benefits employees and employers alike. Job design is crucial for a whole range of outcomes, including (a) employee health and well-being, (b) attitudes like job satisfaction and commitment, (c) employee cognitions and learning, and (d) behaviors like productivity, absenteeism, proactivity, and innovation. Employee personal characteristics play an important role in job design. They influence how employees themselves perceive and seek out particular job characteristics, help in understanding how job design exerts its influence, and have the potential to change the impact of job design.
- work design
- job characteristics
- satisfaction
- performance
- proactivity
“It is about a search, too, for daily meaning as well as daily bread, for recognition as well as cash, for astonishment rather than torpor; in short, for a sort of life rather than a Monday through Friday sort of dying” (Terkel, 1974 , p. xi).
Billions of people spend most of their waking lives at work, so it is fortunate that work can be a positive feature of living. Obviously, the associated salary helps to pay the bills and provides a means for a certain standard of living. But good work also structures one’s time, builds identity, allows for social contact, and enables engagement in meaningful activities (Jahoda, 1982 ). Nevertheless, although work can serve these important functions, it can also be a threat to people’s well-being, cause alienation, and result in burnout. As an extreme example of its negative effects, Chinese and French telecom workers have been reported committing suicide because of work-related issues.
Whether work is beneficial or detrimental is largely dependent upon how it is designed. Work design is defined as the content, structure, and organization of one’s task and activities (Parker, 2014 ). It is mostly studied in terms of job characteristics, such as job autonomy and workload, which are like the building blocks of work design. Meta-analytical results show that these job characteristics predict employees’ health and well-being, their cognitions and learning, and their attitudes and behavior (Humphrey, Nahrgang, & Morgeson, 2007 ; Nahrgang, Morgeson, & Hofmann, 2011 ). There is no doubt that work design is important, so it is not surprising that it has received considerable research attention (Parker, Morgeson, & Johns, 2017 ).
Throughout the 20th century , several authors developed different job-design models, which have been expanded into various contemporary perspectives. The net effect is that the literature on work design is somewhat fragmented. Rather than providing one overall framework to study the design of jobs—similar to the Big 5 framework in personality, for example—job-design models consider the topic from different angles. This diversity may be an advantage in understanding the complexity of job design, but an overview—let alone on overarching model—is lacking, which inhibits the sharing of knowledge and ultimately our understanding of job design.
Against this background, it is necessary to review the approaches that have dominated the literature around the world and the contemporary models that have emerged from them. This overview reveals the basic principles that guide views on job design: how work is conceptualized, how job characteristics relate to important outcomes, and the roles personal aspects play in job design–outcome relationships.
This article makes several contributions to the literature. First, by providing an overview of important job-design models that have dominated the work-design literature around the globe, the article introduces job-design scholars working in one research tradition to other traditions. Second, the article explicates the most important assumptions about the impact of job design across the different models and brings the assumptions together in one integrative work design (IWD) model. Finally, the article supplies an overview of fruitful avenues for future research that might stimulate future research on the important topic of job design. Although previously it had been argued that “we know all there is to know” about job design (Ambrose & Kulik, 1999 ), one in three employees in Europe still has a job of poor intrinsic quality (Lorenz & Valeyre, 2005 ) and different influences put pressure on the quality of jobs (Parker, Holman, & Van den Broeck, 2017 ) . Building on this overall model, different pathways emerge for the rejuvenation of the literature (Parker et al., 2017 ) and for fostering knowledge on how jobs can be designed so that work brings out the best in people.
Historical Overview of Models Around the Globe
Job design has a long history. Ever since people organized themselves to hunt and gather for food, or even to build the Aztec temples, people identified activities, tasks, and roles and distributed them among collaborators. The scientific study of work design, however, started with the work of Adam Smith, who described in his book The Wealth of Nations how the division of labor could increase productivity. Previously, the timing and location of work fitted seamlessly in with everyday activities, and many industries were characterized by craftsmen, who developed a product from the beginning to the end (Barley & Kunda, 2001 ). A blacksmith would craft pins starting from iron ore, while a carpenter made cupboards out of trees. But Adam Smith advocated the dissection of labor into different tasks, and the division of these task among employees, so that each would repetitively execute small tasks: One employee would cut the metal plate, while another one would polish the pins.
Scientific Management
The principle of the division of labor was further developed into scientific management (Taylor, 2004 ). Adopting a scientific approach to work in order to increase efficiency, Taylor argued that ideal jobs included single, highly simplified, and specialized activities that were repeated throughout the working day, with little time to waste in between (Campion & Thayer, 1988 ). Taylor developed his ideas in the realm of the Industrial Revolution, which made it possible to automate many of the activities in people’s jobs. Employees were essentially considered parts of the machinery, with the idea they could easily be replaced. While previously employees inherited or chose their trades and learned on the job by trial and error, with Taylorism, employees were selected and trained to execute specific tasks according to prescribed procedures and standards. Supervisors were tasked with monitoring employees’ actions, leading to the division between unskilled manual labor and skilled managerial tasks, and to the rewarding of employees according to their performance (e.g., via piecework) so that the goals of the employees (i.e., making money) would be aligned with the goal of the company (i.e., making profit).
Essential principles of Taylorism thus include simplification and specialization, but also the selection and training of employees to achieve a fit between demands of the job and employees’ abilities. Because of these principles, efficiency rocketed, and Taylorism was soon also adopted for office jobs. Today, Taylorism still inspires the design of both manufacturing and service jobs in many organizations (Parker et al., 2017 ).
Despite its positive consequences in terms of productivity, one downside of this mechanical approach to job design was that employee morale dropped. For instance, in the Midvale Steel plant, where Taylorism was implemented, employees experienced mental and physical fatigue and boredom, resulting in sabotage and absenteeism (Walker & Guest, 1952 ). The negative effects of Taylorism eventually led to the development of several less mechanistic and more motivational work designs, including social and psychological approaches.
Social Approaches to Work
In further exploring the effects of Taylorism, Mayo and his colleagues uncovered the importance of individual attitudes toward work and teams. In the famous “Hawthorne studies,” focusing on a team of Western Electric Company workers, Mayo and colleagues aimed to improve employees’ working conditions, but they failed to find strong effects of interventions like increasing or decreasing illumination, or shortening or lengthening the working day, on individual employee performance, even though such effects would be expected based on Taylorism. Rather, production went up over the course of the period in which the employees were involved and consulted in the experiment. The free expression of ideas and feelings to the management, and sustained cooperation in teams, increased employee morale and ultimately efficiency. Group norms were shown to have a strong effect on employee attitudes and behavior and were more effective in generating employee productivity than individual rewards, potentially because being part of a group increased feelings of security. In a Taylorism model, people were seen as a part of a machine, but according to Mayo, employees should be regarded as part of a social group.
The focus on groups was further developed into sociotechnical systems theory by human relations scholars at the Tavistock Institute in the United Kingdom (Pasmore, 1995 ). The scholars aimed to optimize the alignment of technical systems and employees. To make optimal use of the available technology, the scholars were convinced that teams of employees should have the autonomy to organize themselves (without too much supervision) and to manage technological problems and to suggest improvements, thereby breaking with the previous division between manual labor and managerial tasks. Furthermore, rather than advocating specialization, human relations scholars argued that, within the teams, employees should work on a meaningful and relatively broad set of tasks and that team members should be allowed to rotate, so that they would have some variety and become multi-skilled (Pasmore, 1995 ).
Sociotechnical systems theory gave rise to the use of autonomous working groups, later labeled self-managing teams . Several studies provided evidence for the positive effects of autonomous working groups on job satisfaction and performance, but the positive effects were not always found Some have therefore argued that autonomous work teams need to be implemented with care and may be most effective in uncertain contexts, where individuals can make a difference (Wageman, 1997 ; Wright & Cordery, 1999 ).
Herzberg’s Two-Factor Theory
Building on the importance of employees’ attitudes, the first major model that made an explicit link between job design and employee motivation is the two-factor theory of Herzberg ( 1968 ). Herzberg started from Maslow’s need pyramid ( 1954 ) and argued that, while some job aspects caused job satisfaction, other were responsible for employee dissatisfaction. Satisfaction and dissatisfaction were thus considered independent states, with different antecedents. Dissatisfaction was said to occur when employees feel deprived of their physical, animal needs, due to a lack of “hygiene factors,” such as a decent salary, security, safe working conditions, status, good relationships at work, and attention to one’s personal life. Satisfaction, in contrast, was said to be intertwined with growth-oriented human needs and is influenced by the availability of motivators like achievement, recognition, responsibility, and growth.
Although Herzberg’s model has been criticized and has received little empirical support (Wall & Stephenson, 1970 ), it has had a vast impact on the literature on job design. First, Herzberg provided the building blocks for the meta-theory underlying job design. In building on the differentiation between basic animal needs and more human, higher order growth needs, Herzberg inspired McGregor ( 1960 ) to develop Theory X and Theory Y, two views on humankind that managers may hold that have implications for how jobs should be designed. Theory X assumes that employees are passive and lazy and need to be pushed (i.e., the stick approach to motivation) or pulled (i.e., the carrot approach to motivation) using the principles of Taylorism. In contrast, managers who hold Theory Y see employees as active and growth-oriented human beings who like to interact with their environment. Adopting this theory likely stimulates managers to design highly satisfying and motivational jobs that make optimal use of the interest and energy of employees.
Second, Herzberg was the first to develop a well-defined job-design model and to advocate the empirical study of people’s jobs, thereby paving the way for the tradition of job-design research that we know today. Moreover, Herzberg pointed out the importance of a fair wage and good working conditions, similar to Taylorism and social relations at work, as did the human relations movement. In addition, he called for attention to opportunities to learn and develop oneself, which were to be found in the content of the job. As such, Herzberg was arguably the first to advance that the true motivational potential of work is linked to the content of one’s job. He further advanced that jobs could become more motivating by job enlargement (i.e., adding additional tasks of similar difficulty) and—most importantly—job enrichment (i.e., by adding more complex task and decision authority). As in the case of autonomous teams, these practices can lead to beneficial outcomes, although the effects ultimately depend on the context and manner in which they are implemented (Axtell & Parker, 2003 ; Campion, Mumford, Morgeson, & Nahrgang, 2005 )
Hackman and Oldham’s Job Characteristics Model
Hackman and Oldham followed through on the idea of motivational jobs and exclusively focused on job content in their job characteristics model (JCM; Hackman & Oldham, 1976 ). Specifically, they argued that the motivating potential of jobs could be determined by assessing the degree of task significance, task identity, and variety, as well as the autonomy and feedback directly from the job. Moving beyond mere job satisfaction, these job characteristics were argued to lead to an expanded set of outcomes of job design, including internal motivation, performance, absenteeism, and turnover. Furthermore, where previous models were silent about the psychological process through which job design may have its impact, Hackman and Oldham proposed three psychological states as mediating mechanism: having knowledge of results, feelings responsible, and experiencing meaningfulness in the job. These states were expected to be influenced by feedback, autonomy, and the combination of task identity, task significance, and variety, respectively. While both autonomy and feedback are essential for jobs to be motivating, as each of the latter aspects relate to the same critical psychological state, task identity, task significance, and variety were considered to be interchangeable, which introduced the possibility that particular job aspects can compensate for each other, so that low task identity wouldn’t be problematic when employees experience high levels of variety.
An important contribution from Hackman and Oldham is that they acknowledged the importance of individual differences. They assumed that peoples’ skills, knowledge, and ability, as well as general satisfaction with the work context, may impact the strength of the relations between the job characteristics and the critical psychological states, and between the latter and the work outcomes (Oldham, Hackman, & Pearce, 1976 ). Perhaps the most important moderator in the JCM is peoples’ growth-need strength, which is defined as the degree to which employees want to develop in the context of work. Highly growth-oriented employees may benefit more from job enrichment.
In addition to developing the JCM, Hackman and Oldham also contributed to the job-design literature by presenting a measure (Hackman & Oldham, 1976 ), which spurred empirical research. Meta-analysis supported the basic tenets of the model, showing that motivational characteristics lead to favorable attitudinal and behavioral outcomes, via some of the critical psychological states (Fried & Ferris, 1987 ; Humphrey et al., 2007 ; Johns, Xie, & Gang, 1992 ). However, criticism has been directed at the inclusion of only a limited set of job characteristics, mediating mechanisms, and behavioral outcomes, as well as at the model’s focus only on the motivational aspects of work, while ignoring the stressful aspects (Parker, Wall, & Cordery, 2001 ) proposed an elaborated version of the JCM that identified an expanded set of work characteristics (including those more important in contemporary work, such as emotional demands and performance-monitoring demands), elaborated moderators (including, for example, operational uncertainty), and outcomes (including creativity, proactivity, and safety). This model also proposed antecedents of work design.
Karasek’s Job Demand Control Model
Karasek ( 1979 ) built on the criticisms of the JCM. In his job demand control model, he synthesized the traditions on detrimental aspects of work design (i.e., demands, including workload and role stressors) and the beneficial aspects (i.e., job control, including autonomy and skill variety) mentioned in the literature following the development of the Michigan Model (Caplan, Cobb, French, Harrison, & Pinneau, 1975 ) and the JCM, respectively. Rather than considering both aspects separately, seeing all structural work aspects as a demand, Karasek argued that job demands and job control have to be examined in combination, as the effects of each may be fundamentally different depending on the level of the other.
Specifically, Karasek built his theory on four types of jobs: Passive jobs are characterized by low demands and low control, while high-strain jobs include high job demands and low job control. Low-strain jobs are characterized by low demands and high job control, while active jobs include both high demands and high job control. These four types of jobs fall along two continua. Low- and high-strain jobs are modeled on a continuum from low strain to high strain, which over time may result in stress and health problems, while passive and active jobs are modeled on a growth-related continuum ranging from low to high activation, fostering motivation, learning, and development. Following up on the assumptions of the Michigan Model, Karasek proposed that job design not only may have short-term effects, but also in the long run, may affect employee personality: Continuous exposure to stressful jobs leads to accumulated strain, which then causes long-term anxiety that inhibits learning. Continuous exposure to active jobs, in contrast, builds experiences of mastery, which then buffers the perception of strain (Theorell & Karasek, 1996 ).
Apart from an expanded focus on the content of work in terms of job demands and job control, Karasek expanded his model by reintroducing social support, as a beneficial aspect of job design, and more specifically as an antidote to job demands. The role of social relations at work was acknowledged by Herzberg (although only as a hygiene factor) but was not included in the JCM, which continued to dominate the job-design literature in the United States.
Karasek’s model spurred research on job stress, as well as on health-related outcomes, such mortality and cardiovascular diseases (Van der Doef & Maes, 1998 ). The additive effects of job demands and job control are often found, but more cross-sectionally than over time, which suggests that reciprocal or reversed effects may also occur, with well-being, motivation, and learning also predicting job design (Hausser, Mojzisch, Niesel, & Schulz-Hardt, 2010 ). Results for the interaction between job demands and job control are limited (Van der Doef & Maes, 1999 ), even among high-quality studies (de Lange, Taris, Kompier, Houtman, & Bongers, 2003 ).
Warr’s Vitamin Model
Warr ( 1987 ) further expanded on the number of job characteristics that may influence people’s well-being. Going beyond the design of jobs, per se, Warr examined environmental aspects that may serve as vitamins for people’s well-being, in or outside the context of work. Well-being is herein broadly defined, including affective well-being, which is arranged around three axes—pleasure and displeasure, anxiety versus comfort, and depression versus enthusiasm—as well as competence, aspiration, autonomy, and integrated functioning of feeling harmonious. In total, Warr discerned nine different broad environmental factors that affect aspects of well-being, including:
Availability of money or a decent salary.
Physical security (good working conditions and working material).
Environmental clarity (low job insecurity, high role clarity, predictable outcomes, and task feedback).
A valued social position associated with, for example, task significance and the possibility to contribute to society.
Contact with others or the possibility of having (good) social relations at work, being able to depend on others, and working on a nice team.
Variety or having changes in one’s task context and social relations.
Externally generated goals or a challenging workload, with low levels of role conflict and conflict or competition with others.
Opportunity for skill use and acquisition or the potential to apply and extend one’s skills.
Opportunities for personal control or having autonomy, discretion, and opportunities to participate (Warr, 1987 ).
Intriguingly, Warr was the first to recognize that these job characteristics are not necessarily linearly related to employee well-being. Some job characteristics, and more specifically money, safety, and a valued social position, are the vitamins C and E. First, they affect employee well-being linearly, but only a certain amount, with their effects plateaus maintaining a constant effect (CE). The other job characteristics, however, are vitamins A and D and affect employee well-being in a curvilinear way: both low and high levels are detrimental, with any addition beyond a certain level leading to decrease in well-being (AD). In assuming these relations, Warr captured the widely held assumption that there can be too much of a good thing (Pierce & Aguinis, 2011 ). For example, while some amount of workload can be beneficial, too much workload may be detrimental for employees’ well-being. Similarly, too much job stimulation may contribute to negative health outcomes (Fried et al., 2013 ).
Furthermore, in line with Hackman and Oldham, Warr proposed that some employees are more susceptible to the impact of particular job characteristics than others, because their personal values or abilities fit better with particular job characteristics. For example, employees with low preference for independence benefit less from autonomy, while employees having a high tolerance for ambiguity suffer less when their environment provides less clear guidelines (Warr, 1987 ).
Research has provided some support for the vitamin model, showing that externally generated goals, autonomy, and social support may indeed have curvilinear relations with employee well-being (De Jonge & Schaufeli, 1998 ; Xie & Johns, 1995 ), but these results are not always replicated, especially not longitudinally (Mäkikangas, Feldt, & Kinnunen, 2007 ) or when general, rather than job-related, well-being is assessed (Rydstedt, Ferrie, & Head, 2006 ). One of the merits of the vitamin model is, however, that it broadened researchers’ horizons in terms of which job characteristics could influence employee well-being.
The Job Demands Resources Model of Bakker, Demerouti, and Schaufeli
The job demands resources model (JD-R model; Bakker, Demerouti, & Sanz-Vergel, 2014 ; Demerouti, Bakker, Nachreiner, & Schaufeli, 2001 ) aimed to provide an integrative view of job characteristics. At the core of the model lies the various job characteristics that may impact employees, which can be meaningfully classified as job demands and job resources. Job demands are defined as “those physical, psychological, social, or organizational aspects of the job that require sustained physical and/or psychological (cognitive and emotional) effort or skills and are therefore associated with certain physiological and/or psychological costs” (Bakker & Demerouti, 2007 , p. 312). They are not necessarily negative, but turn into job stressors when they exceed workers’ capacities, which makes it hard for them to recover. Job resources are defined as the “physical, psychological, social, or organizational aspects of the job that … (1) [are] functional in achieving work goals, (2) reduce job demands and the associated physiological and psychological costs, [or] (3) stimulate personal growth, learning, and development” (Bakker & Demerouti, 2007 , p. 312).
Just like the vitamin model, the JD-R model focuses on employee well-being as a crucial outcome. Following the positive psychology movement advocating the balanced study of the bright side of employees’ functioning (Seligman & Csikszentmihalyi, 2000 ) along with the dark side, both negative (i.e., burnout) and positive (i.e., work engagement) aspects of well-being are considered as the crucial pathways through which job demands and job resources relate to a host of other outcomes, including employee physical health and well-being, job satisfaction, organizational commitment, and different types of behaviors, including in-role and extra-role performance, as well as counterproductive behavior (for an overview, see Van den Broeck, Van Ruysseveldt, Vanbelle, & De Witte, 2013 ).
Job demands are considered the main cause of burnout. In being continuously confronted with job demands, employees can become emotionally exhausted because they put all their energy into the job. Under particular situations, such as when all their effort is in vain, they likely start withdrawing from their job as a means to protect themselves and become cynical, which is part of the burnout response. Job resources can also have a (limited) direct negative relationship with burnout (Schaufeli & Bakker, 2004 ), but they are most crucial for the development of vigor and dedication, the main components of work engagement. Job demands and job resources are also assumed to interact, so that high levels of resources may attenuate (i.e., buffer) the association between job demands and burnout, while job demands are said to strengthen (i.e., boost) the association between job resources and work engagement.
Within the JD-R model, individual factors are modeled as personal resources, which are defined as malleable lower-order, cognitive-affective personal aspects reflecting a positive belief in oneself or the world (van den Heuvel, Demerouti, Bakker, & Schaufeli, 2010 ). As in the job characteristics model, personal resources can represent the underlying process through which job resources prevent burnout and foster work engagement (Xanthopoulou, Bakker, Demerouti, & Schaufeli, 2007 ), moderate, and—more specifically—buffer the health-impairing impact of job demands, as job resources do, and they may serve as antecedents of the job characteristics, preventing the occurrence of job demands and increasing the (perceived) availability of job resources.
Evidence supporting the JD-R model is abundant, but the model is used mostly in the European literature. Job demands and job resources are convincingly shown to relate to burnout and work engagement (Nahrgang et al., 2011 ), while some evidence is provided for their interactions and the role of personal resources (Van den Broeck et al., 2013 ).
Contemporary Job-Design Models
Over the years, various other models have been developed. They may range from slightly different perspectives on job characteristics and their roles in the prediction of employee functioning to more fundamental changes in how we could perceive job design.
For example, some scholars suggested that not all job demands are equal, but need to be differentiated into challenging and hindering job demands. While challenges are obstacles that can be overcome and hold the potential for learning, hindrances are threatening obstacles that drain people’s energy and prevent goal achievement (Lepine, Podsakoff, & Lepine, 2005 ). Some authors suggested that job demands can be either challenging, or hindering, or both, depending on the appraisal of the individual employee (Rodríguez, Kozusnik, & Peiro, 2013 ; Webster, Beehr, & Christiansen, 2010 ). Others, in contrast, argued that employees generally categorize particular job demands as challenging (e.g., workload and time pressure) or hindering (e.g., red tape and role conflict), in relatively clear-cut categorizations (Cavanaugh, Boswell, Roehling, & Boudreau, 2000 ; Van den Broeck, De Cuyper, De Witte, & Vansteenkiste, 2010 ).
Morgeson and Humphrey ( 2006 ) aimed to integrate the various job characteristics that have been examined in the literature and encouraged job-design scholars not only to focus on task characteristics, such as autonomy and variety, and social characteristics, such as social support and interdependence, but also to pick up on the work context and consider ergonomics, equipment use, and work conditions. This call aligns with the observations of Campion and Thayer ( 1985 ). They noticed that job-design scholars seem to have specialized in either the biological (e.g., concern with noise and lifting), the ergonomic (e.g., lighting, information input), the motivational (e.g., autonomy, variety), or the mechanistic (e.g., specialization, simplification) job characteristics, while Taylor, for example, considered each of these aspects in designing jobs. The more integrative view may be more beneficial, because motivational job characteristics may also have an impact on biological functioning (e.g., heart disease), and the best results may be achieved when ergonomic and motivational factors are jointly considered. For example, Das, Shikdar, and Winters ( 2007 ) found that drill press operators who had the most ergonomic tools and received training were more satisfied and performed better than their counterparts who also could use the ergonomic tools but didn’t receive any training.
New developments in the job-design literature also focused on the relations between the job characteristics and outcomes. The Demand-Induced Strain Compensation model (DISC model; de Jonge & Dormann, 2003 ), for example, further refined job-design theory by qualifying the interaction between job demands and job resources. Specifically, the DISC model assumes that job resources have more potential to buffer the negative effect of job demands on employee well-being when the demands, resources, and outcomes are all physical, cognitive, or emotional. That is, emotional resources, such as social support, may best buffer the impact of emotional demands on emotional stability (Van de Ven, De Jonge, & Vlerick, 2014 ).
More profound changes in job-design theory have been launched. For example, building on the notions of role conflict and role ambiguity (Kahn et al., 1964 ), Ilgen and Hollenbeck ( 1992 ) argued for the study of work roles, which are generally broader than people’s prescribed jobs because they also include emergent and self-imitated tasks. The focus on work roles led to a flourishing literature on role breadth self-efficacy (Parker, 1998 ), personal initiative (Frese, Garst, & Fay, 2007 ), and proactive work behavior (Parker, Williams, & Turner, 2006 ), with the argument being that work design is an especially important facilitator of these outcomes.
The relational perspective of Grant and colleagues is also a novel extension (e.g., Grant, 2007 ). In this approach, a powerful way to design work is to ensure that employees are connected with those that benefit from the work. Such an approach enhances task significance, and thereby promotes greater prosocial motivation amongst employees, which in turn benefits employee performance (for a review, see Grant & Parker, 2009 ).
Another development has been to recognize the role of work design in promoting learning. As Parker ( 2014 , p. 671) argued: “Motivational theories of work design have dominated psychological approaches to work design. However, we need to expand the criterion space beyond motivation, not just by adding extra dependent variables to empirical studies but by exploring when, why, and how work design can help to achieve different purposes.” Parker outlined existing theory and research that suggest work design might be a powerful—yet currently rather neglected—intervention for promoting learning outcomes, such as the accelerated acquisition of expert knowledge, as well as for promoting developmental outcomes over the lifespan (such as the development of cognitive complexity, or even moral development).
Nevertheless, despite, or perhaps because of, the different perspectives, the current job-design literature can be fragmented, with different job-design models offering insights to different parts of the puzzle but not necessarily the whole puzzle. To move the job-design literature forward, a more synthesized mental model of the literature has been developed that describes what can already be considered established knowledge and that highlights fruitful ways forward.
The Integrative Work Design Model
Figure 1. The Integrated Work Design (IWD) Model.
Building from the job-design models featured in the literature and the principles they put forward, an integrative work design model can be developed. The model may stimulate scholars to think broadly when studying job design and to develop new areas for research. It may equally assist managers to consider various aspects of people’s jobs when assessing the adequateness of the jobs they design. The model includes job characteristics as antecedents, and their possible relations with employee outcomes, including employee well-being, cognitions, attitudes, and behaviors. Finally, personal characteristics are taken into account as intervening variables in these relationships. The core aspects of the integrative work design (IWD) model are outlined in Figure 1 .
Job Characteristics as Antecedents
In keeping with the approach of the JD-R, the differentiation between job resources and job demands is maintained as a valuable framework for grouping job characteristics. This approach is not without criticism (Van den Broeck et al., 2013 ). For example, not all job characteristics can be easily classified as either a job demand or a job resource (e.g., job security could be a resource, while job insecurity could be a demand). However, within the IWD model it is maintained that various positive and negative events are not simply opposite ends of the spectrum (e.g., the absence of aggressive or troublesome patients doesn’t necessarily turn patient contacts into positive experiences; Hakanen, Bakker, & Demerouti, 2005 ). The difference between positive and negative reflects the universal differentiation between the positive and the negative, which is rooted in our neurophysiology and how we appraise each encounter with the environment (Barrett, Mesquita, Ochsner, & Gross, 2007 ). Negatives typically loom larger than positives and have a stronger impact on negative aspects of employee functioning, while positive aspects are more predictive of positive outcomes (Baumeister, Bratslavsky, Finkenauer, & Vohs, 2001 ). This suggests that having a mindset that looks at both job demands and job resources allows scholars and managers alike to take a balanced perspective on the beneficial and detrimental characteristics of a job.
Although Warr, as well as the JD-R model, start from the assumption that several job characteristics may have an impact on employee functioning, by far the most empirical attention has been paid to the restricted list of job characteristics proposed by Karasek: autonomy, workload, and social support (Humphrey et al., 2007 ), and/or the five job characteristics covered in the JCM. To overcome this issue, a broader view on job design seems necessary. People may be inspired by new developments in the job-design literature differentiating between job challenges (e.g., responsibility) and job hindrances (e.g., red tape), as was done in the development of a model including job hindrances, challenges, and resources (Crawford, Lepine, & Rich, 2010 ; Van den Broeck et al., 2010 ). Second, the classification by Campion and Thayer ( 1985 , 1988 ) may be a source of inspiration to also include job characteristics related to human factors (e.g., equipment) and biological factors (e.g., noise, temperature), along with the mechanical (e.g., repetition) and motivational (e.g., promotion, task significance) job characteristics. Job-design scholars may consider the inclusion of context-specific job-specific hindrances, challenges, and resources, such as student aggression for teachers, number and duration of interventions for firefighters, or having contact with the patients’ families for nurses (for an overview, see Van den Broeck et al., 2013 ).
The study of specific and general job characteristics may also take into account recent developments in the labor market, such as the digital revolution, and the changes in demographics. Few studies have included the consequences of these changes in the study of contemporary jobs, although they have caused dramatic changes in job design (Cordery & Parker, 2012 ). For example, due to technological advances, jobs have undergone profound changes. While some jobs are disappearing due to automation and digitalization, the remaining jobs—for example, in supporting, maintaining, and repairing technology—have become more analytical and problem-solving in nature. Recent job-design scales therefore include concentration and precision as cognitive or mental demands (Van Veldhoven, Prins, Van der Laken, & Dijkstra, 2016 ), which may become extremely relevant for older workers who experience a decline in fluid intelligence (Krings, Sczesny, & Kluge, 2011 ). Due to the technological revolution, employees also become increasingly dependent on technology, leading to techno-stress (Tarafdar, D’Arcy, Turel, & Gupta, 2015 ). The growing body of research on this issue, however, developed outside of I/O psychology, with the leading publications in information and computer sciences. Similarly, the use of digital technology has made it possible to work from home. This increased the degree to which work-related activities intruded into private life and had implications for job design in terms of autonomy and social support (Allen, Golden, & Shockley, 2015 ; Gajendran & Harrison, 2007 ). Research interest on the impact of telework is growing, but—again—mostly as a separate field, rather than as an aspect of job design (Bailey & Kurland, 2002 ).
Finally, and again in line with the JD-R model and the recent work of Morgeson and Humphrey ( 2006 ), job demands and job resources can be found at the level of one’s task and job in general—relating to the content of work—but also at the level of the social relations at work (e.g., conflict vs. social support), which includes the social support component of the Karasek model and the call for more research on the role of social influences at work (Grant & Parker, 2009 ). Apart from the social aspects, attention could also be paid to job characteristics at the team level. In 2012 , Hollenbeck, Beersma, and Schouten noted that up to 80% of all Fortune organizations rely on teamwork to achieve their goals. Team characteristics, such as interdependence and team autonomy, have an impact on employee task characteristics, such as autonomy and well-being and performance (Langfred, 2007 ; Van Mierlo, Rutte, Vermunt, Kompier, & Doorewaard, 2007 ) and could therefore be taken into account.
Furthermore, scholars may want to go one step further and incorporate aspects at the level of the organization, such as HR-related demands and resources (e.g., strategic impact; De Cooman, Stynen, Van den Broeck, Sels, & De Witte, 2013 ) and organizational climate (e.g., safety climate; Dollard & Bakker, 2010 ). Apart from examining the direct impact of these characteristics on employee functioning, job-design scholars could also examine their interplay, in terms of how organizational and team-level variables influence social and task characteristics, as well as how the different levels may buffer, amplify, or boost each other’s impact (Parker, Van den Broeck, & Holman, 2017 ). Furthermore, scholars could examine the interplay between job characteristics through profile analyses (Van den Broeck, De Cuyper, Luyckx, & De Witte, 2012 ).
The Relations of Job Characteristics with Outcomes
The previous models have outlined that job characteristics may influence employee outcomes in many different ways. While most models assume linear relations, Warr argued for curvilinear relations, where both too little and too much of a job characteristic, such as workload, would lead to lower levels of employee well-being, an assumption that also seems to be implicit in the definition of job demands in the JD-R model. Others have argued that such curvilinear relationships are nothing less than “urban myths,” as they are difficult to establish empirically (Taris, 2006 ). Although the lack of empirical support for curvilinear relations may also be attributable to methodological shortcomings, this challenging statement has encouraged other scholars to argue that not the amount, but the type, of job demands matters for how they relate to employee functioning (Lepine et al., 2005 ; Van den Broeck et al., 2010 ). This approach ties in with the appraisal theory (Folkman & Lazarus, 1985 ), which states that the interpretation of an event as challenging or threatening determines how people react to it. Future studies may follow through on these potential curvilinear or differentiated results.
Moreover, it would be interesting to see more research on the differentiated results of particular job characteristics. While Hackman and Oldham argued that all motivational job characteristics would have an impact on employee motivation, performance, and turnover, other frameworks (e.g., Herzberg’s two-factor theory, Karasek’s job demand control model, the JD-R, and the DISC model) propose that some job characteristics would be more strongly related to particular outcomes. This is in line with the meta-analysis findings that motivational characteristics may, for example, explain more variance in performance than social characteristics, but the latter seemed to be most important in the prediction of turnover intentions (Humphrey et al., 2007 ). Furthermore, this meta-analysis showed that work-scheduling autonomy is less predictive of job satisfaction than decision-making autonomy, which shows that it is worthwhile to examine the different effects of different job characteristics.
Different Outcomes of Job Design
Within the job-design literature, different outcomes of job design have been put to the fore. Whereas Taylor mostly focused on performance, most of the motivational and health-oriented job-design models focused on aspects of employee well-being and attitudes. But none of the existing job-design models does full justice to the rich amount of consequences that have been empirically studied. The immediate, i.e., individual-level, outcomes of job design are here grouped in terms of health and well-being, cognitions and learning, attitudes, and behaviors (Cordery & Parker, 2012 ; Humphrey et al., 2007 ). The IWD model thus goes beyond mere well-being, core task performance, absenteeism, and turnover.
Health and Well-being
First, following through on their importance in job-design models, employee health and well-being have arguably been the most studied outcomes of job design. Meta-analytic results convincingly show that job characteristics like autonomy, feedback, and social support increase employee engagement and prevent employees from feeling anxious, stressed, or burned out (Humphrey et al., 2007 ; Nahrgang et al., 2011 ). In line with these results, many countries developed policies that urge employers to take care of the psychosocial risk factors—including mostly quantitative and qualitative demands, job control, and opportunities for skill development—to prevent these outcomes (Formazin et al., 2014 ).
A policy-oriented focus on the improvement of job design also has the potential to prevent more injuries and somatic health problems related to job design. For example, job characteristics are also important precursors of accidents, injuries, and unsafe behavior (Nahrgang et al., 2011 ), because high job demands and low job resources might cause employees to routinely violate safety rules (Hansez & Chmiel, 2010 ). People working in jobs of low quality also have higher risk of stroke and the development of heart disease (Backé, Seidler, Latza, Rossnagel, & Schumann, 2012 ; Eller et al., 2009 ). Similar results have been found for the experience of low back pain, pain in the shoulders or knees (Bernal et al., 2015 ), or obesity (Fried et al., 2013 ; Kim & Han, 2015 ). Interestingly, these results are mostly reported in journals featuring biomedical and human factors research (Parker et al., 2017 ), leaving these far-reaching consequences of job design relatively unnoticed in I/O psychology.
A considerable body of research established the importance of job design for employee attitudes toward work, such as organizational commitment, job involvement, and job satisfaction (Humphrey et al., 2007 ). Meta-analytic results, for example, show that job demands explain 28% of the variance in job design, while job resources can explain no less than 62% to 85% (Humphrey et al., 2007 ; Nahrgang et al., 2011 ). Results are inconclusive whether high levels of job satisfaction should be attributed primarily to good social relations at work or to the motivational characteristics defined by Hackman and Oldham.
Cognitions and Learning
While much attention has been devoted to health and well-being, research interest in cognitions as outcomes of job design has only been emerging recently and is a promising avenue for the future (Parker, 2014 ). Although more complex jobs may be challenging (Van Veldhoven et al., 2016 ), in their systematic review, Then et al. ( 2014 ) demonstrated that this might also have positive consequences, as high work complexity—together with high job control—has a protective effect against the decline of cognitive functions later in life and dementia. Increasing cognitive demands may thus start a process in which cognitive processes are maintained, if not increased. Similarly, work pressure may reduce daytime intuitive decision making, but enhance analytical thinking (Gordon, Demerouti, Bipp, & Le Blanc, 2015 ) and foster learning (De Witte, Verhofstadt, & Omey, 2007 ), as could be expected based on Karasek’s model and German action theory (Frese & Zapf, 1994 ). For example, Holman et al. ( 2012 ) showed that blue collar workers in a vehicle manufacturer improved their learning strategies when being allotted job control, while solving complex problems. Similar results were found in a diary study (Niessen, Sonnentag, & Friederike, 2012 ), where job resources, such as having meaning on one’s job, allowed employees to maintain focus and explore new information, which then led to employees’ thriving, defined as a combination of learning and high levels of energy.
In the work context, different behaviors are valued, ranging from performance and adaptivity to proactivity (Griffin, Neal, & Parker, 2007 ). Taylor’s primary aim was to design jobs to increase job performance according to the scientific standards he established. Although performance has received less attention throughout the different classic job-design models, it is still considered crucial in job design research. Results are somewhat mixed. While meta-analyses show that a range of job resources (e.g., autonomy, skill or task variety, task significance, feedback) all relate positively to self-rated performance, only autonomy seems to relate to objective performance (Humphrey et al., 2007 ). Motivational and social factors like autonomy, task identity, feedback from the job, and social support are also important predictor of behaviors like absenteeism, while social aspects of work design, such as social support, feedback from others, and interdependence, prove to be most important for turnover intentions (Humphrey et al., 2007 ). Job resources like feedback and intrinsically motivating tasks also predict extra role behaviors, such as altruism, courtesy, conscientiousness, and civic virtue, while job demands like role ambiguity, role conflict, and task routinization are negatively related to these behaviors (Podskaoff, Mackenzie, Paine, & Bachrach, 2000 ).
Job design also affects adaptivity, or the degree employees cope well with the ongoing change and adversities in organizations (Griffin et al., 2007 ). For example, sportsmanship, or tolerating work-related inconveniences without complaining, is fostered when employees find their jobs inherently satisfying and receive feedback, while role problems likely forestall sportsmanship (Podskaoff et al., 2000 ).
More than just adapting to the rapid changes and the insecurity characterizing the contemporary labor market, employees are also required to proactively anticipate and act upon potential future opportunities (Griffin et al., 2007 ). Job design had not always been considered essential for employee proactivity (Anderson, De Dreu, & Nijstad, 2004 ), but recent views see job design—and most importantly autonomy and social support—as an important antecedent (Parker et al., 2006 ), which is confirmed by meta-analytic results (Tornau & Frese, 2013 ). Employees in enriched jobs are more inclined to be proactive than employees in jobs characterized by routinization and formalization (Marinova, Peng, Lorinkova, Van Dyne, & Chiaburu, 2015 ).
Job design is also an important moderator for proactivity, allowing proactive motivations to materialize in proactive behavior (Parker, Bindl, & Strauss, 2010 ). Meta-analyses, for example, suggest that employees don’t need autonomy to generate ideas, but require autonomy for idea implementation (Hammond, Neff, Farr, Schwall, & Zhao, 2011 ). As for job demands, the relations may be complex: while uncertainty relates negatively to feedback seeking (Anseel, Beatty, Shen, Lievens, & Sackett, 2015 ), job demands like complexity associate positively with proactive innovation (Hammond et al., 2011 ). Similar results are found at the within-person level. For example, civil servants are more likely to proactively try to improve procedures and introduce new ways of working when they are challenged by time pressure and situational constraints (Fritz & Sonnentag, 2007 ). Proactive behavior may also become a challenge in itself, because one has to plan his actions and invest additional hours or effort in the proactive behavior (Podsakoff, Podsakoff, MacKenzie, Maynes, & Spoelma, 2014 ). Job characteristics like job insecurity may also lead to counterproductive behaviors toward the organization (Van den Broeck et al., 2014 ) and also toward other employees. For example, Van den Broeck, Baillien, and De Witte ( 2011 ) found that job demands like workload are a risk factor for bullying behavior, while job resources like autonomy and supervisory support seem to reduce this risk. However, employees having both high job demands and high job resources were most at risk of becoming bullies at work.
Employee health and well-being, cognitions, attitudes, and behavior are treated as independent elements in the IWD model. They are, however, most likely to influence each other. High levels of well-being have, for example, been shown to relate to commitment and behavioral outcomes (Nahrgang et al., 2011 ). Moreover, thus far, mostly short-term outcomes at the level of the employee were mentioned, leaving outcomes that evolve only over time or develop at the organizational unexplored. Job design is, however, also related to several such outcomes, potentially through its impact on well-being, cognitions, attitudes, and behaviors. For example, job design also has an effect on one’s self-definition (Parker, Wall, & Jackson, 2016 ) and careers (Fried, Grant, Levi, Hadani, & Slowik, 2007 ). Longitudinal studies among more than 2,000 employees show that having high job demands and few opportunities for skill development or social support cause employees to retire early, even above and beyond their impact on mental and physical health (de Wind et al., 2014 ; de Wind, Geuskens, Ybema, Bongers, & van der Beek, 2015 ). Job design also leads to the financial success of the organization. High levels of job resources during one’s shift, for example, increase the financial returns in the fast food industry, as they contributed to the work engagement of the employees (Xanthopoulou, Bakker, Demerouti, & Schaufeli, 2009 ). High job resources equally increase the service climate within the hospitality sector, which then associates with customer loyalty (Salanova, Agut, & Peiró, 2005 ). These outcomes not only may be caused by job characteristics, but also feed into job characteristics (i.e., they have reciprocal relationships), and they may be dependent on employees’ personal characteristics.
Personal Characteristics
Several of the job-characteristics models have considered the role of personal characteristics within job design. Rightly so, as employee functioning is likely to be a function of both situation (i.e., to be job related) and person factors. Most attention with regard to the role of person factors has been paid to personal resources, which are defined as highly valued aspects, relating to resilience and contributing to individuals’ potential to successfully control and influence the environment (Hobfoll, Johnson, Ennis, & Jackson, 2003 ).
Several personal characteristics have been considered personal resources. Within the JCM, for example, growth-need strength can be seen as a personal resource, as are the critical psychological states of meaning, knowledge of results, and responsibility. Within Warr’s vitamin model, employees’ values are considered essential to how employees respond to certain contexts, while a host of personal resources have been studied in the realm of the JD-R model, ranging from hope and optimism to the core self-evaluations of self-esteem, generalized self-efficacy, emotional stability, and locus of control (Van den Broeck et al., 2013 ).
In our view, personal resources may play at least three different roles in the job-design literature. First, they may moderate the impact of job characteristics on employee functioning (i.e., job resources as moderators). Conservation of resources Theory (COR), for example, assumes that having resources allows people to cope with demanding circumstances, so that personal resources may buffer the negative consequences (Hobfoll, 1989 ). In line with this view, employees endowed with self-esteem and optimism were found to experience less psychological distress when confronted with job demands like time pressure (Mäkangas & Kinnunen, 2003 ), while customer orientation buffers the association between job demands and burnout (Babakus, Yavas, & Ashill, 2009 ).
In addition to the buffering effect, job resources can also amplify the positive effects of resourceful job characteristics, as was also mentioned by Hackman and Oldham, as well as by Warr. Again following COR, employees holding high levels of personal resources in a resourceful environment may build resource caravans, which may then lead to low levels of stress and high performance (Hobfoll, 2002 ). More specifically, personal resources may boost the impact of job resources, because a fit between the personal resources and the job characteristics causes employees to pay more attention to the availability of the job characteristics, but also because they have more adaptive ways to act upon the job characteristics (Kristof-brown, Zimmerman, & Johnson, 2005 ). For example, employees who aim to develop themselves see more opportunities for development and may make better use of such opportunities, which then increases their well-being (Van den Broeck, Schreurs, Guenter, & van Emmerik, 2015 ; Van den Broeck, Van Ruysseveldt, Smulders, & De Witte, 2011 ).
Apart from the relatively straightforward moderating effects, personal resources may also influence the impact of job characteristics in a more complex way. Because of their boosting effect on job resources, personal resources may also enable employees to use their job resources better to offset the negative effects of job demands, leading to a three-way interaction between personal and job resources and job demands. For example, employees having an internal locus of control may make optimal use of the job control at their disposal to attenuate the effects of daily stressors, while for employees having an external locus of control, job control may not be put in practice and in some research actually predicted poorer well-being and health (Meier, Semmer, Elfering, & Jacobshagen, 2008 ; for a similar study, see Parker & Sprigg, 1999 ). Considering the curvilinear effects, personal resources may affect the tipping point at which increases in job characteristics stop being positive or even start to have negative consequences, so that employees with a high need for security may appreciate higher levels of role clarity than employees who are more adventurous (Warr, 1987 ). Overall, personal resources may allow employees to make better use of job resources, while dealing better with job demands, leading to different outcomes for employees working in the same jobs.
A second role of personal resources may be in explaining the relationships between job characteristics and their outcomes (i.e., personal resources as mediators). Hackman and Oldham suggested that the environment could influence employees’ psychological states, which then explains why job characteristics affect, for example, employee motivation and performance. According to JD-R scholars, this is true not only for the critical psychological states, but also for various personal resources. An important addition to the assumption is that not only may job resources add to psychological states, but also job demands can be assumed to take away employees’ energy and hinder their goal orientation, thereby decreasing employees’ personal resources (Hobfoll, 1989 ). In support of this, research shows that the same psychological resources may indeed explain the effects of both motivational and demanding job characteristics: While job resources lead to high levels of engagement and low levels of burnout through increased satisfaction of SDT’s basic needs for autonomy, competence, and relatedness, job demands hinder the experience of basic need satisfaction and therefore lead to higher levels of burnout and more counterproductive behavior (Van den Broeck, Suela, Vander Elst, Fischmann, Iliescu, & De Witte, 2014 ; Van den Broeck, Vansteenkiste, De Witte, & Lens, 2008 ). In exploring the mediating role of personal resources, future research may answer the call for more attention to the processes underpinning the relations between job characteristics and outcomes (Parker et al., 2001 ).
Finally, personal resources may serve as antecedents of job demands and job resources. This may be because managers provide more favorable job conditions to highly motivated employees (Rousseau, 2001 ), because such employees craft their job to include more motivational and less demanding characteristics (Wrzesniewski & Dutton, 2001 ), or because resourceful employees appraise their job situation as more benign or challenging and less threatening (Folkman & Lazarus, 1985 ).
Notably, thus far, the job-design literature focuses on relatively changeable and positive personal characteristics. However, more stable personal characteristics may also play a role, as they may shape employees’ directedness to particular goals and thereby equally serve as antecedents and moderators of job characteristics (Barrick, Mount, & Li, 2013 ). The personality trait of neuroticism may, for example, cause employees to report higher job demands, while extroverted employees experience more job resources (Bakker et al., 2010 ). Moreover, recently, it was also shown that job characteristics may change employees’ personality (Wu, 2016 ). A final consideration is that particular personal aspects may also make employees more vulnerable to the negative impact of job demands or make it more difficult to benefit from positive aspects.
The job-design literature has a long history and continues to grow. Although some job-design scholars have argued there is nothing left to know about job design, new aspects are still unraveling and many aspects of the nature of job characteristics and their relationship with various outcomes, as well as the role of personal characteristics, remain underexplored. Because job design strongly associates with a host of outcomes and various jobs are still of low quality, job design still deserves scholarly and managerial attention. The integrative work design (IWD) model may assist in the process.
Further Reading
- Grant, A. M. (2008). The significance of task significance: Job performance effects, relational mechanisms, and boundary conditions . Journal of Applied Psychology , 93 (1), 108–124.
- Oldham, G. R. , & Fried, Y. (2016). Job design research and theory: Past, present and future . Organizational Behavior and Human Decision Processes , 136 , 20–35.
- Oldham, G. R. , & Hackman, J. R. (2010). Not what it was and not what it will be: The future of job design research . Journal of Organizational Behavior , 31 (2–3), 463–479.
- Allen, T. D. , Golden, T. D. , & Shockley, K. M. (2015). How effective is telecommuting? Assessing the status of our scientific findings . Psychological Science in the Public Interest , 16 (2), 40–68.
- Ambrose, M. L. , & Kulik, C. (1999). Old friends, new faces: Motivation research in the 1990. Journal of Management , 25 (3), 231–292.
- Anderson, N. , De Dreu, C. K. W. , & Nijstad, B. A. (2004). The routinization of innovation research: A constructively critical review of the state-of-the-science . Journal of Organizational Behavior , 25 (2), 147–173.
- Anseel, F. , Beatty, A. S. , Shen, W. , Lievens, F. , & Sackett, P. R. (2015). How are we doing after 30 years? A meta-analytic review of the antecedents and outcomes of feedback-seeking behavior . Journal of Management , 41 (1), 318–348.
- Axtell, C. M. , & Parker, S. K. (2003). Promoting role breadth self-efficacy through involvement, work redesign and training . Human Relations , 56 (1), 113–131.
- Babakus, E. , Yavas, U. , & Ashill, N. J. (2009). The role of customer orientation as a moderator of the job demand–burnout–performance relationship: A surface-level trait perspective . Journal of Retailing , 85 (4), 480–492.
- Backé, E.-M. , Seidler, A. , Latza, U. , Rossnagel, K. , & Schumann, B. (2012). The role of psychosocial stress at work for the development of cardiovascular diseases: A systematic review . International Archives of Occupational and Environmental Health , 85 (1), 67–79.
- Bailey, D. E. , & Kurland, N. B. (2002). A review of telework research: Findings, new directions, and lessons for the study of modern work . Journal of Organizational Behavior , 23 , 383–400.
- Bakker, A. B. , Boyd, C. M. , Dollard, M. , Gillespie, N. , Winefield, A. H. , & Stough, C. (2010). The role of personality in the job demands-resources model: A study of Australian academic staff . Career Development International , 15 (7), 622–636.
- Bakker, A. B. , & Demerouti, E. (2007). The job demands-resources model: State of the art . Journal of Managerial Psychology , 22 (3), 309–328.
- Bakker, A. B. , Demerouti, E. , & Sanz-Vergel, A. I. (2014). Burnout and work engagement: The JD–R approach . Annual Review of Organizational Psychology and Organizational Behavior , 1 (1), 389–411.
- Barley, S. R. , & Kunda, G. (2001). Bringing work back in . Organization Science , 12 (1), 76–95.
- Barrett, L. F. , Mesquita, B. , Ochsner, K. N. , & Gross, J. J. (2007). The experience of emotion . Annual Review of Psychology , 58 , 373–403.
- Barrick, M. R. , Mount, M. K. , & Li, N. (2013). The theory of purposeful work behavior: The role of personality, higher-order goals and job characteristics. Academy of Management Review , 38 (1), 132–153.
- Baumeister, R. F. , Bratslavsky, E. , Finkenauer, C. , & Vohs, K. D. (2001). Bad is stronger than good . Review of General Psychology , 5 (4), 323–370.
- Bernal, D. , Campos-Serna, J. , Tobias, A. , Vargas-Prada, S. , Benavides, F. G. , & Serra, C. (2015). Work-related psychosocial risk factors and musculoskeletal disorders in hospital nurses and nursing aides: A systematic review and meta-analysis . International Journal of Nursing Studies , 52 (2), 635–648.
- Campion, M. A. , Mumford, T. V. , Morgeson, F. P. , & Nahrgang, J. D. (2005). Work redesign: Eight obstacles and opportunities . Human Resource Management , 44 (4), 367–390.
- Campion, M. A. , & Thayer, P. W. (1985). Development and field evaluation of an interdisciplinary measure of job design . Journal of Applied Psychology , 70 (1), 29–43.
- Campion, M. A. , & Thayer, P. W. (1988). Job design: Approaches, outcomes and trade-offs. Organizational Dynamics , 15 (3), 66–79.
- Caplan, R. D. , Cobb, S. , French, J. R. R, Jr. , Harrison, R. V. , & Pinneau, S. R., Jr. (1975). Demands and worker health: Main effects and occupational differences . Washington, DC.: US Government Printing Office
- Cavanaugh, M. A. , Boswell, W. R. , Roehling, M. V. , & Boudreau, J. W. (2000). An empirical examination of self-reported work stress among U.S. managers . Journal of Applied Psychology , 85 (1), 65–74.
- Cordery, J. , & Parker, S. K. (2007). Organization of work. In P. F. Boxall , J. Purcell , & P. M. Wright (Eds.), Oxford Handbook of Human Resource management (Vol. 1, pp. 12–13). Oxford: Oxford University Press.
- Cordery, J. L. , & Parker, S. K. (2012). Job and role design. In S. Kozlowski (Ed.), Oxford handbook of industrial and organizational psychology (Vol 1, pp. 247–284). Oxford: Oxford University Press.
- Crawford, E. R. , Lepine, J. A. , & Rich, B. L. (2010). Linking job demands and resources to employee engagement and burnout: A theoretical extension and meta-analytic test . The Journal of Applied Psychology , 95 (5), 834–848.
- Das, B. , Shikdar, A. A. , & Winters, T. (2007). Workstation redesign for a repetitive drill press operation: A combined work design and ergonomics approach . Human Factors and Ergonomics in Manufacturing , 17 (4), 395–410.
- De Cooman, R. , Stynen, D. , Van den Broeck, A. , Sels, L. , & De Witte, H. (2013). How job characteristics relate to need satisfaction and autonomous motivation: Implications for work effort . Journal of Applied Social Psychology , 43 (6), 1342–1352.
- De Jonge, J. , & Dormann, C. (2003). The DISC model: Demand-induced strain compensation mechanisms in job stress. In M. F. Dollard , H. R. Winefield , & A. H. Winefield (Eds.), Occupational stress in the service professions (pp. 43–74). London: Taylor & Francis.
- De Jonge, J. , & Schaufeli, W. B. (1998). Job characteristics and employee well-being: A test of Warr’s vitamin model in health care workers using structural equation modelling . Journal of Organizational Behavior , 19 (4), 387–407.
- de Lange, A. H. , Taris, T. W. , Kompier, M. A. J. , Houtman, I. L. D. , & Bongers, P. M. (2003). “The very best of the millennium”: Longitudinal research and the demand-control (-support) model . Journal of Occupational Health Psychology , 8 (4), 282–305.
- de Wind, A. , Geuskens, G. A. , Ybema, J. F. , Blatter, B. M. , Burdorf, A. , Bongers, P. M. , et al. (2014). Health, job characteristics, skills, and social and financial factors in relation to early retirement—Results from a longitudinal study in the Netherlands . Scandinavian Journal of Work, Environment & Health , 40 (2), 186–194.
- de Wind, A. , Geuskens, G. A. , Ybema, J. F. , Bongers, P. M. , & van der Beek, A. J. (2015). The role of ability, motivation, and opportunity to work in the transition from work to early retirement—Testing and optimizing the Early Retirement Model . Scandinavian Journal of Work, Environment & Health , 41 (1), 24–35.
- De Witte, H. , Verhofstadt, E. , & Omey, E. (2007). Testing Karasek’s learning and strain hypotheses on young workers in their first job . Work & Stress , 21 (2), 131–141.
- Demerouti, E. , Bakker, A. B. , Nachreiner, F. , & Schaufeli, W. B. (2001). The job demands-resources model of burnout . Journal of Applied Psychology , 86 (3), 499–512.
- Dollard, M. F. , & Bakker, A. B. (2010). Psychosocial safety climate as a precursor to conducive work environments, psychological health problems, and employee engagement . Journal of Occupational and Organizational Psychology , 83 (3), 579–599.
- Eller, N. H. , Netterstrøm, B. , Gyntelberg, F. , Kristensen, T. S. , Nielsen, F. , Steptoe, A. , et al. (2009). Work-related psychosocial factors and the development of ischemic heart disease . Cardiology in Review , 17 (2), 83–97.
- Folkman, S. , & Lazarus, R. S. (1985). If it changes it must be a process: Study of emotion and coping during three stages of a college examination . Journal of Personality and Social Psychology , 48 (1), 150–170.
- Formazin, M. , Burr, H. , Aagestad, C. , Tynes, T. , Thorsen, S. V. , Perkio-Makela, M. , et al. (2014). Dimensional comparability of psychosocial working conditions as covered in European monitoring questionnaires . BMC Public Health , 14 , 1251.
- Frese, M. , Garst, H. , & Fay, D. (2007). Making things happen: Reciprocal relationships between work characteristics and personal initiative in a four-wave longitudinal structural equation model . The Journal of Applied Psychology , 92 (4), 1084–1102.
- Frese, M. , & Zapf, D. (1994). Action as the core of work psychology: A German approach. In H. C. Triandis , M. D. Dunnette , & L. M. Hough (Eds.), Handbook of industrial and organizational psychology (Vol. 4, pp. 271–340). Palo Alto, CA: Consulting Psychologists Press.
- Fried, Y. , & Ferris, G. (1987). The validity of the job characteristics model: A review and meta-analysis . Personnel Psychology , 40 (2), 287–322.
- Fried, Y. , Grant, A. M. , Levi, A. S. , Hadani, M. , & Slowik, L. H. (2007). Job design in temporal context: A career dynamics perspective . Journal of Organizational Behavior , 28 (7), 911–927.
- Fried, Y. , Laurence, G. A. , Shirom, A. , Melamed, S. , Toker, S. , Berliner, S. , et al. (2013). The relationship between job enrichment and abdominal obesity: A longitudinal field study of apparently healthy individuals . Journal of Occupational Health Psychology , 18 (4), 458–468.
- Fritz, C. , & Sonnentag, S. (2007). Antecedents of day-level proactive behavior: A look at job stressors and positive affect during the workday . Journal of Management , 35 (1), 94–111.
- Gajendran, R. S. , & Harrison, D. A. (2007). The good, the bad, and the unknown about telecommuting: Meta-analysis of psychological mediators and individual consequences . The Journal of Applied Psychology , 92 (6), 1524–1541.
- Gordon, H. J. , Demerouti, E. , Bipp, T. , & Le Blanc, P. M. (2015). The Job Demands and Resources Decision Making (JD-R-DM) model . European Journal of Work and Organizational Psychology , 24 (1), 44–58.
- Grant, A. M. (2007). Relational job design and the motivation to make a prosocial difference. Academy of Management Review , 32 (2), 393–417.
- Grant, A. M. , & Parker, S. K. (2009). Redesigning work design theories: The rise of relational and proactive perspectives . Academy of Management Annals , 3 (1), 317–375.
- Griffin, M. A. , Neal, A. , & Parker, S. K. (2007). A new model of work role performance: Positive behavior in uncertain and interdependent contexts. Academy of Management Journal , 50 (2), 327–347.
- Hackman, J. R. , & Oldham, G. R. (1976). Motivation through the design of work: Test of a theory. Organizational Behavior and Human Performance , 16 , 250–279.
- Hakanen, J. J. , Bakker, A. B. , & Demerouti, E. (2005). How dentists cope with their job demands and stay engaged: The moderating role of job resources . European Journal of Oral Sciences , 113 (6), 479–487.
- Hammond, M. M. , Neff, N. L. , Farr, J. L. , Schwall, A. R. , & Zhao, X. (2011). Predictors of individual-level innovation at work: A meta-analysis . Psychology of Aesthetics, Creativity, and the Arts , 5 (1), 90–105.
- Hansez, I. , & Chmiel, N. (2010). Safety behavior: Job demands, job resources, and perceived management commitment to safety . Journal of Occupational Health Psychology , 15 (3), 267–278.
- Hausser, J. A. , Mojzisch, A. , Niesel, M. , & Schulz-Hardt, S. (2010). Ten years on: A review of recent research on the Job Demand-Control (-Support) model and psychological well-being . Work and Stress , 24 (1), 1–35.
- Herzberg, F. (1968). One more time: How do you motivate employees? Harvard Business Review , 48 , 53–62.
- Hobfoll, S. E. (1989). Conservation of resources: A new attempt at conceptualizing stress. American Psychologist , 44 (3), 513–524.
- Hobfoll, S. E. (2002). Social and psychological resources and adaptation . Review of General Psychology , 6 (4), 307–324.
- Hobfoll, S. E. , Johnson, R. J. , Ennis, N. , & Jackson, A. P. (2003). Resource loss, resource gain, and emotional outcomes among inner city women . Journal of Personality and Social Psychology , 84 (3), 632–643.
- Holman, D. , Totterdell, P. , Axtell, C. , Stride, C. , Port, R. , Svensson, R. , & Zibarras, L. (2012). Job design and the employee innovation process: The mediating role of learning strategies . Journal of Business and Psychology , 27 , 177–191.
- Huang, Y. , Xu, S. , Hua, J. , Zhu, D. , Liu, C. , Hu, Y. , et al. (2015). Association between job strain and risk of incident stroke . Neurology , 85 , 1–7.
- Humphrey, S. E. , Nahrgang, J. D. , & Morgeson, F. P. (2007). Integrating motivational, social, and contextual work design features: A meta-analytic summary and theoretical extension of the work design literature . Journal of Applied Psychology , 92 (5), 1332–1356.
- Ilgen, D. R. , & Hollenbeck, J. R. (1992). The structure ofwork: Job design and roles. In M. D. Dunnette & L. M. Hough (Eds.), Handbook of industrial and organizational psychology (2d ed., Vol. 2, pp. 165–207). Palo Alto, CA: Consulting Psychologists Press.
- Jahoda, M. (1982). Employment and unemployment: A social-psychological analysis . Cambridge, England: Cambridge University Press.
- Johns, G. , Xie, J. L. , & Gang, Y. (1992). Mediating and moderating effects in job design. Journal of Management , 18 (4), 657–676.
- Kahn, R. L. , Wolfe, D. M. , Quinn, R. P. , Snoek, J. D. , & Rosenthal, R. A. (1964). Occupational stress: Studies in role conflict and ambiguity . New York: John Wiley and Sons.
- Karasek, R. A. (1979). Job demands, job decision latitude, and mental strain: Implications for job redesign. Administrative Science Quarterly , 24 , 285–308.
- Kim, T. H. , & Han, E. (2015). Impact of body mass on job quality . Economics and Human Biology , 17 , 75–85.
- Krings, F. , Sczesny, S. , & Kluge, A. (2011). Stereotypical inferences as mediators of age discrimination: The role of competence and warmth . British Journal of Management , 22 (2), 187–201.
- Kristof-brown, A. M. Y. L. , Zimmerman, R. D. , & Johnson, E. C. (2005). Consequences of individuals’ fit at work: A meta-analysis of person-job, person-organization, person-group and person-supervisor fit. Personnel Psychology , 58 , 281–342.
- Langfred, C. W. (2007). The downside of self-management: A longitudinal study of the effects of conflict on trust, autonomy and task interdependence in self-managing teams. The Academy of Management Journal , 50 (4), 885–900.
- Lepine, J. A. , Podsakoff, N. P. , & Lepine, M. A. (2005). A meta-analytic test of the challenge stressor–hindrance stressor framework: An explanation for inconsistent relationships among stressors and performance. Academy of Management Journal , 48 (5), 764–775.
- Lorenz, E. , & Valeyre, A. (2005). Organisational innovation, human resource management and labour market structure: A comparison of the EU-15 . The Journal of Industrial Relations , 47 (4), 424–442.
- Mäkikangas, A. , Feldt, T. , & Kinnunen, U. (2007). Warr’s scale of job-related affective well-being: A longitudinal examination of its structure and relationships with work characteristics . Work & Stress , 21 (3), 197–219.
- Mäkangas, A. , & Kinnunen, U. (2003). Psychosocial work stressors and well-being: Selfesteem and optimism as moderators in a one-year longitudinal sample. Personality and Individual Differences , 35 , 537–557.
- Marinova, S. V. , Peng, C. , Lorinkova, N. , Van Dyne, L. , & Chiaburu, D. (2015). Change-oriented behavior: A meta-analysis of individual and job design predictors . Journal of Vocational Behavior , 88 , 104–120.
- Maslow, A. H. (1954). Motivation and personality . New York: Harper & Row.
- McGregor, D. , (1960). The human side of enterprise . New York: McGraw-Hill.
- Meier, L. L. , Semmer, N. K. , Elfering, A. , & Jacobshagen, N. (2008). The double meaning of control: Three-way interactions between internal resources, job control, and stressors at work . Journal of Occupational Health Psychology , 13 (3), 244–258.
- Morgeson, F. P. , & Humphrey, S. E. (2006). The Work Design Questionnaire (WDQ): Developing and validating a comprehensive measure for assessing job design and the nature of work . Journal of Applied Psychology , 91 (6), 1321–1339.
- Nahrgang, J. D. , Morgeson, F. P. , & Hofmann, D. A. (2011). Safety at work: A meta-analytic investigation of the link between job demands, job resources, burnout, engagement, and safety outcomes . The Journal of Applied Psychology , 96 (1), 71–94.
- Niessen, C. , Sonnentag, S. , & Friederike, S. (2012). Thriving at work—A diary study . Journal of Organizational Behavior , 33 , 468–487.
- Oldham, G. R. , Hackman, J. R. , & Pearce, J. L. (1976). Conditions under which employees respond positively to enriched work . Journal of Applied Psychology , 61 (4), 395–403.
- Parker, S. K. (1998). Enhancing role breadth self-efficacy: The roles of job enrichment and other organizational interventions. The Journal of Applied Psychology , 83 (6), 835–852. Retrieved from http://www.ncbi.nlm.nih.gov/pubmed/9885197 .
- Parker, S. K. (2014). Beyond motivation: Job and work design for development, health, ambidexterity, and more . Annual Review of Psychology , 65 , 661–691.
- Parker, S. K. , Bindl, U. K. , & Strauss, K. (2010). Making things happen: A model of proactive motivation . Journal of Management , 36 (4), 827–856.
- Parker, S. K. , Holman, D. , & Van den Broeck, A. (2017). Work design influences: A synthesis of multi-level factors that affect the design of work . Academy of Management Annals , 11 , 267–308.
- Parker, S. K. , Morgeson, F. P. , & Johns, G. (2017). One hundred years of work design research: Looking back and looking forward. Journal of Applied Psychology , 102 , 403.
- Parker, S. K. , & Sprigg, C. A. (1999). Minimizing strain and maximizing learning: The role of job demands, job conrol, and proactive personality. Journal of Applied Psychology , 84 (6), 925–939.
- Parker, S. K. , Wall, T. D. , & Cordery, J. L. (2001). Future work design research and practice: Towards an elaborated model of work design. Journal of Occupational and Organizational Psychology , 74 , 413–440.
- Parker, S. K. , Wall, T. D. , & Jackson, P. R. (2016). “That’s Not My Job”: Developing flexible employee work orientations. Academy of Management Journal , 40 (4), 899–929.
- Parker, S. K. , Williams, H. M. , & Turner, N. (2006). Modeling the antecedents of proactive behavior at work . Journal of Applied Psychology , 91 (3), 636–652.
- Pasmore, W. A. (1995). Social science transformed: The socio-technical perspective. Human Relations , 48 (1), 1–21.
- Pierce, J. R. , & Aguinis, H. (2011). The too-much-of-a-good-thing effect in management . Journal of Management , 39 (2), 313–338.
- Podsakoff, N. P. , Podsakoff, P. M. , MacKenzie, S. B. , Maynes, T. D. , & Spoelma, T. M. (2014). Consequences of unit-level organizational citizenship behaviors: A review and recommendations for future research. Journal of Organizational Behavior , 35 , 87–119.
- Podskaoff, P. M. , Mackenzie, S. B. , Paine, J. B. , & Bachrach, D. G. (2000). Organizational citizenship behaviors: A critical review of the theoretical and future research. Journal of Management , 26 (3), 513–563.
- Rodríguez, I. , Kozusnik, M. W. , & Peiro, J. M. (2013). Development and validation of the Valencia Eustress-Distress Appraisal Scale . International. Journal of Stress Management , 20 (4), 279–308.
- Rousseau, D. M. (2001). Flexibility versus fairness? Organizational Dynamics , 29 (4), 260–273.
- Rydstedt, L. W. , Ferrie, J. , & Head, J. (2006). Is there support for curvilinear relationships between psychosocial work characteristics and mental well-being? Cross-sectional and long-term data from the Whitehall II study . Work and Stress , 20 (1), 6–20.
- Salanova, M. , Agut, S. , & Peiró, J. M. (2005). Linking organizational resources and work engagement to employee performance and customer loyalty: The mediation of service climate . The Journal of Applied Psychology , 90 (6), 1217–1227.
- Schaufeli, W. B. , & Bakker, A. B. (2004). Job demands, job resources, and their relationship with burnout and engagement: A multi-sample study. Journal of Organizational Behavior , 25 , 293–315.
- Seligman, M. E. P. , & Csikszentmihalyi, M. (2000). Positive psychology: An introduction . American Psychologist , 55 (1), 5–14.
- Tarafdar, M. , D’Arcy, J. , Turel, O. , & Gupta, A. (2015). The dark side of information technology. MIT Sloan Management Review , 56 (2), 61–70. Retrieved from http://mitsmr.com/1wD3N7E.
- Taris, T. W. (2006). Bricks without clay: On urban myths in occupational health psychology . Work & Stress , 20 (2), 99–104.
- Taylor, F. W. (2004). Scientific management . London. Routledge.
- Terkel, S. , (Ed.). (1974). Working: People talk about what they do all day and how they feel about what they do . The New Press.
- Then, F. S. , Luck, T. , Luppa, M. , Thinschmidt, M. , Deckert, S. , Nieuwenhuijsen, K. , et al. (2014). Systematic review of the effect of the psychosocial working environment on cognition and dementia . Occupational and Environmental Medicine , 71 (5), 358–365.
- Theorell, T. , & Karasek, R. A. (1996). Current issues relating to psychosocial job strain and cardiovascular disease research . Journal of Occupational Health Psychology , 1 (1), 9–26.
- Tornau, K. , & Frese, M. (2013). Construct clean-up in proactivity research: A meta-analysis on the nomological net of work-related proactivity concepts and their incremental validities . Applied Psychology: An International Review , 62 (1), 44–96.
- Van de Ven, B. , De Jonge, J. , & Vlerick, P. (2014). Testing the triple-match principle in the technology sector: A two-wave longitudinal panel study . Applied Psychology , 63 (2), 300–325.
- Van den Broeck, A. , Baillien, E. , & De Witte, H. (2011). Workplace bullying: A perspective from the Job Demands-Resources model . South African Journal of Industrial Psychology , 37 (2), 1–12.
- Van den Broeck, A. , De Cuyper, N. , De Witte, H. , & Vansteenkiste, M. (2010). Not all job demands are equal: Differentiating job hindrances and job challenges in the Job Demands–Resources model . European Journal of Work and Organizational Psychology , 19 (6), 735–759.
- Van den Broeck, A. , De Cuyper, N. , Luyckx, K. , & De Witte, H. (2012). Employees’ job demands–resources profiles, burnout and work engagement: A person-centred examination. Economic Industrial Democracy , 33 (4), 691–706.
- Van den Broeck, A. , Schreurs, B. , Guenter, H. , & van Emmerik, I. H. (2015). Skill utilization and well-being: A cross-level story of day-to-day fluctuations and personal intrinsic values . Work & Stress , 29 (3), 306–323.
- Van den Broeck, A. , Suela, C. , Vander Elst, T. , Fischmann, G. , Iliescu, D. , & De Witte, H. (2014). The mediating role of psychological needs in the relation between qualitative job insecurity and counterproductive work behavior . Career Development International , 19 (5), 526–547.
- Van den Broeck, A. , Van Ruysseveldt, J. , Smulders, P. , & De Witte, H. (2011). Does an intrinsic work value orientation strengthen the impact of job resources? A perspective from the Job Demands–Resources model . European Journal of Work and Organizational Psychology , 20 (5), 581–609.
- Van den Broeck, A. , Van Ruysseveldt, J. , Vanbelle, E. , & De Witte, H. (2013). The Job Demands–Resources model: Overview and suggestions for future research. In A. B. Bakker (Ed.), Advances in positive organizational psychology (Vol. 1, pp. 83–105). Emerald, Bingley, U.K.: Emerald Group Publishing Limited.
- Van den Broeck, A. , Vansteenkiste, M. , De Witte, H. , & Lens, W. (2008). Explaining the relationships between job characteristics, burnout, and engagement: The role of basic psychological need satisfaction . Work & Stress , 22 (3), 277–294.
- van den Heuvel, M. , Demerouti, E. , Bakker, A. B. , & Schaufeli, W. B. (2010). Personal resources and work engagement in the face of change. Contemporary occupational health psychology: Global Perspectives on Research and Practice , 1 , 124–150.
- van den Heuvel, M. , Demerouti, E. , Bakker, A. B. , & Schaufeli, W. B. (2013). Adapting to change: The value of change information and meaning-making . Journal of Vocational Behavior , 83 (1), 11–21.
- Van der Doef, M. , & Maes, S. (1998). The job demand-control (-support) model and physical health outcomes: A review of the strain and buffer hypotheses . Psychology & Health , 13 (5), 909–936.
- Van der Doef, M. , & Maes, S. (1999). The job demand-control (-support) model and psychological well-being: A review of 20 years of empirical research . Work & Stress , 13 (2), 87–114.
- Van Mierlo, H. , Rutte, C. G. , Vermunt, J. K. , Kompier, M. A. J. , & Doorewaard, J. A. C. M. (2007). A multi-level mediation model of the relationships between team autonomy, individual task design and psychological well-being . Journal of Occupational and Organizational Psychology , 80 (4), 647–664.
- Van Veldhoven, M. , Prins, J. , Van der Laken, P. , & Dijkstra, L. (2016). Manual QEEW2.0: 42 short scales for survey research on work, well-being and performance . Amsterdam: SKB.
- Warr, P. (1987). Work, unemployment, and mental health . Oxford: Clarendon Press.
- Wageman, R. (1997). Critical success factors for creating superb teams . Organizational Dynamics , 26 , 49–61.
- Walker, C. R. , & Guest, R. H. (1952). The man on the assembly line . Cambridge, Mass. Harvard University Press.
- Wall, T. D. , & Stephenson, G. M. (1970). Herzberg’s two-factor theory of job attitudes: A critical evaluation and some fresh evidence . Industrial Relations Journal , 1 (3), 41–65.
- Webster, J. R. , Beehr, T. a. , & Christiansen, N. D. (2010). Toward a better understanding of the effects of hindrance and challenge stressors on work behavior . Journal of Vocational Behavior , 76 (1), 68–77.
- Wright, B. M. , & Cordery, J. L. (1999). Production uncertainty as a contextual moderator of employee reactions to job design . The Journal of Applied Psychology , 84 (3), 456–463.
- Wrzesniewski, A. , & Dutton, J. E. (2001). Crafting a job: Revisioning employees as active crafters of their work . The Academy of Management Review , 26 (2), 179–201.
- Wu, C. H. (2016). Personality change via work: A job demand-control model of Big-Five personality changes . Journal of Vocational Behavior , 92 , 157–166.
- Xanthopoulou, D. , Bakker, A. B. , Demerouti, E. , & Schaufeli, W. B. (2007). The role of personal resources in the job demands-resources model . International Journal of Stress Management , 14 (2), 121–141.
- Xanthopoulou, D. , Bakker, A. B. , Demerouti, E. , & Schaufeli, W. B. (2009). Work engagement and financial returns: A diary study on the role of job and personal resources . Journal of Occupational and Organizational Psychology , 82 (1), 183–200.
- Xie, J. L. , & Johns, G. (1995). Job scope and stress: Can job scope be too high? Academy of Management Journal , 38 (5), 1288–1309.
Related Articles
- Action Regulation Theory
- Work and Family
- Occupational Health Psychology
- The Psychology of Work Engagement
- Corporate Social Responsibility: An Overview from an Organizational and Psychological Perspective
Printed from Oxford Research Encyclopedias, Psychology. Under the terms of the licence agreement, an individual user may print out a single article for personal use (for details see Privacy Policy and Legal Notice).
date: 27 May 2024
- Cookie Policy
- Privacy Policy
- Legal Notice
- Accessibility
- [66.249.64.20|81.177.180.204]
- 81.177.180.204
Character limit 500 /500

Research Design 101
Everything You Need To Get Started (With Examples)
By: Derek Jansen (MBA) | Reviewers: Eunice Rautenbach (DTech) & Kerryn Warren (PhD) | April 2023
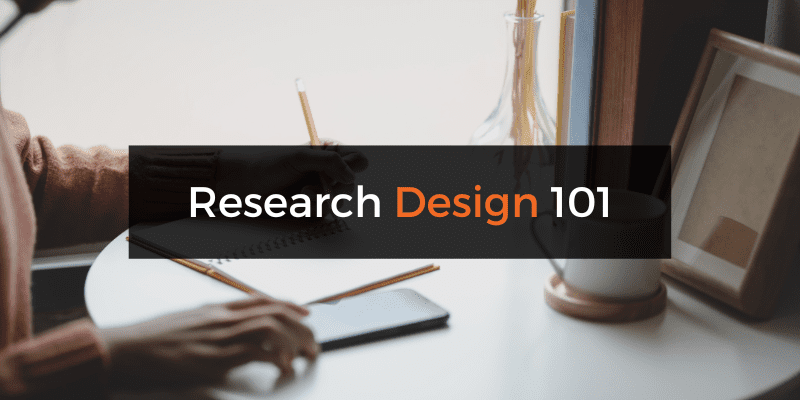
Navigating the world of research can be daunting, especially if you’re a first-time researcher. One concept you’re bound to run into fairly early in your research journey is that of “ research design ”. Here, we’ll guide you through the basics using practical examples , so that you can approach your research with confidence.
Overview: Research Design 101
What is research design.
- Research design types for quantitative studies
- Video explainer : quantitative research design
- Research design types for qualitative studies
- Video explainer : qualitative research design
- How to choose a research design
- Key takeaways
Research design refers to the overall plan, structure or strategy that guides a research project , from its conception to the final data analysis. A good research design serves as the blueprint for how you, as the researcher, will collect and analyse data while ensuring consistency, reliability and validity throughout your study.
Understanding different types of research designs is essential as helps ensure that your approach is suitable given your research aims, objectives and questions , as well as the resources you have available to you. Without a clear big-picture view of how you’ll design your research, you run the risk of potentially making misaligned choices in terms of your methodology – especially your sampling , data collection and data analysis decisions.
The problem with defining research design…
One of the reasons students struggle with a clear definition of research design is because the term is used very loosely across the internet, and even within academia.
Some sources claim that the three research design types are qualitative, quantitative and mixed methods , which isn’t quite accurate (these just refer to the type of data that you’ll collect and analyse). Other sources state that research design refers to the sum of all your design choices, suggesting it’s more like a research methodology . Others run off on other less common tangents. No wonder there’s confusion!
In this article, we’ll clear up the confusion. We’ll explain the most common research design types for both qualitative and quantitative research projects, whether that is for a full dissertation or thesis, or a smaller research paper or article.
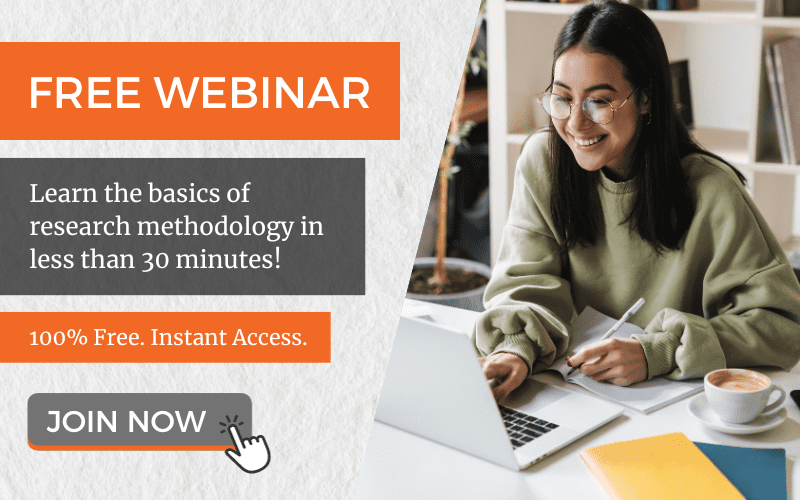
Research Design: Quantitative Studies
Quantitative research involves collecting and analysing data in a numerical form. Broadly speaking, there are four types of quantitative research designs: descriptive , correlational , experimental , and quasi-experimental .
Descriptive Research Design
As the name suggests, descriptive research design focuses on describing existing conditions, behaviours, or characteristics by systematically gathering information without manipulating any variables. In other words, there is no intervention on the researcher’s part – only data collection.
For example, if you’re studying smartphone addiction among adolescents in your community, you could deploy a survey to a sample of teens asking them to rate their agreement with certain statements that relate to smartphone addiction. The collected data would then provide insight regarding how widespread the issue may be – in other words, it would describe the situation.
The key defining attribute of this type of research design is that it purely describes the situation . In other words, descriptive research design does not explore potential relationships between different variables or the causes that may underlie those relationships. Therefore, descriptive research is useful for generating insight into a research problem by describing its characteristics . By doing so, it can provide valuable insights and is often used as a precursor to other research design types.
Correlational Research Design
Correlational design is a popular choice for researchers aiming to identify and measure the relationship between two or more variables without manipulating them . In other words, this type of research design is useful when you want to know whether a change in one thing tends to be accompanied by a change in another thing.
For example, if you wanted to explore the relationship between exercise frequency and overall health, you could use a correlational design to help you achieve this. In this case, you might gather data on participants’ exercise habits, as well as records of their health indicators like blood pressure, heart rate, or body mass index. Thereafter, you’d use a statistical test to assess whether there’s a relationship between the two variables (exercise frequency and health).
As you can see, correlational research design is useful when you want to explore potential relationships between variables that cannot be manipulated or controlled for ethical, practical, or logistical reasons. It is particularly helpful in terms of developing predictions , and given that it doesn’t involve the manipulation of variables, it can be implemented at a large scale more easily than experimental designs (which will look at next).
That said, it’s important to keep in mind that correlational research design has limitations – most notably that it cannot be used to establish causality . In other words, correlation does not equal causation . To establish causality, you’ll need to move into the realm of experimental design, coming up next…
Need a helping hand?
Experimental Research Design
Experimental research design is used to determine if there is a causal relationship between two or more variables . With this type of research design, you, as the researcher, manipulate one variable (the independent variable) while controlling others (dependent variables). Doing so allows you to observe the effect of the former on the latter and draw conclusions about potential causality.
For example, if you wanted to measure if/how different types of fertiliser affect plant growth, you could set up several groups of plants, with each group receiving a different type of fertiliser, as well as one with no fertiliser at all. You could then measure how much each plant group grew (on average) over time and compare the results from the different groups to see which fertiliser was most effective.
Overall, experimental research design provides researchers with a powerful way to identify and measure causal relationships (and the direction of causality) between variables. However, developing a rigorous experimental design can be challenging as it’s not always easy to control all the variables in a study. This often results in smaller sample sizes , which can reduce the statistical power and generalisability of the results.
Moreover, experimental research design requires random assignment . This means that the researcher needs to assign participants to different groups or conditions in a way that each participant has an equal chance of being assigned to any group (note that this is not the same as random sampling ). Doing so helps reduce the potential for bias and confounding variables . This need for random assignment can lead to ethics-related issues . For example, withholding a potentially beneficial medical treatment from a control group may be considered unethical in certain situations.
Quasi-Experimental Research Design
Quasi-experimental research design is used when the research aims involve identifying causal relations , but one cannot (or doesn’t want to) randomly assign participants to different groups (for practical or ethical reasons). Instead, with a quasi-experimental research design, the researcher relies on existing groups or pre-existing conditions to form groups for comparison.
For example, if you were studying the effects of a new teaching method on student achievement in a particular school district, you may be unable to randomly assign students to either group and instead have to choose classes or schools that already use different teaching methods. This way, you still achieve separate groups, without having to assign participants to specific groups yourself.
Naturally, quasi-experimental research designs have limitations when compared to experimental designs. Given that participant assignment is not random, it’s more difficult to confidently establish causality between variables, and, as a researcher, you have less control over other variables that may impact findings.
All that said, quasi-experimental designs can still be valuable in research contexts where random assignment is not possible and can often be undertaken on a much larger scale than experimental research, thus increasing the statistical power of the results. What’s important is that you, as the researcher, understand the limitations of the design and conduct your quasi-experiment as rigorously as possible, paying careful attention to any potential confounding variables .
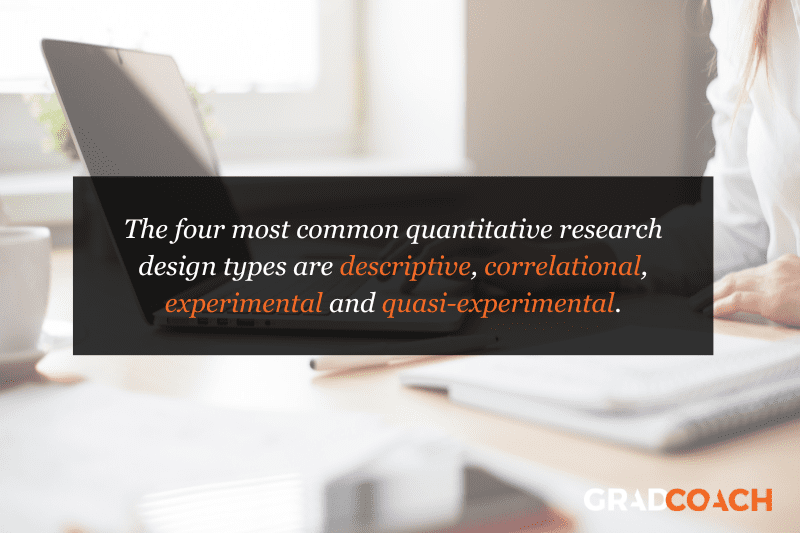
Research Design: Qualitative Studies
There are many different research design types when it comes to qualitative studies, but here we’ll narrow our focus to explore the “Big 4”. Specifically, we’ll look at phenomenological design, grounded theory design, ethnographic design, and case study design.
Phenomenological Research Design
Phenomenological design involves exploring the meaning of lived experiences and how they are perceived by individuals. This type of research design seeks to understand people’s perspectives , emotions, and behaviours in specific situations. Here, the aim for researchers is to uncover the essence of human experience without making any assumptions or imposing preconceived ideas on their subjects.
For example, you could adopt a phenomenological design to study why cancer survivors have such varied perceptions of their lives after overcoming their disease. This could be achieved by interviewing survivors and then analysing the data using a qualitative analysis method such as thematic analysis to identify commonalities and differences.
Phenomenological research design typically involves in-depth interviews or open-ended questionnaires to collect rich, detailed data about participants’ subjective experiences. This richness is one of the key strengths of phenomenological research design but, naturally, it also has limitations. These include potential biases in data collection and interpretation and the lack of generalisability of findings to broader populations.
Grounded Theory Research Design
Grounded theory (also referred to as “GT”) aims to develop theories by continuously and iteratively analysing and comparing data collected from a relatively large number of participants in a study. It takes an inductive (bottom-up) approach, with a focus on letting the data “speak for itself”, without being influenced by preexisting theories or the researcher’s preconceptions.
As an example, let’s assume your research aims involved understanding how people cope with chronic pain from a specific medical condition, with a view to developing a theory around this. In this case, grounded theory design would allow you to explore this concept thoroughly without preconceptions about what coping mechanisms might exist. You may find that some patients prefer cognitive-behavioural therapy (CBT) while others prefer to rely on herbal remedies. Based on multiple, iterative rounds of analysis, you could then develop a theory in this regard, derived directly from the data (as opposed to other preexisting theories and models).
Grounded theory typically involves collecting data through interviews or observations and then analysing it to identify patterns and themes that emerge from the data. These emerging ideas are then validated by collecting more data until a saturation point is reached (i.e., no new information can be squeezed from the data). From that base, a theory can then be developed .
As you can see, grounded theory is ideally suited to studies where the research aims involve theory generation , especially in under-researched areas. Keep in mind though that this type of research design can be quite time-intensive , given the need for multiple rounds of data collection and analysis.
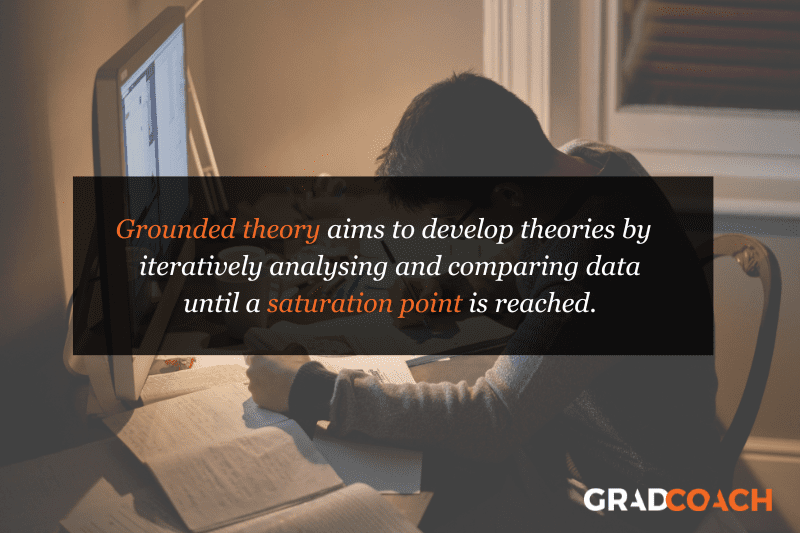
Ethnographic Research Design
Ethnographic design involves observing and studying a culture-sharing group of people in their natural setting to gain insight into their behaviours, beliefs, and values. The focus here is on observing participants in their natural environment (as opposed to a controlled environment). This typically involves the researcher spending an extended period of time with the participants in their environment, carefully observing and taking field notes .
All of this is not to say that ethnographic research design relies purely on observation. On the contrary, this design typically also involves in-depth interviews to explore participants’ views, beliefs, etc. However, unobtrusive observation is a core component of the ethnographic approach.
As an example, an ethnographer may study how different communities celebrate traditional festivals or how individuals from different generations interact with technology differently. This may involve a lengthy period of observation, combined with in-depth interviews to further explore specific areas of interest that emerge as a result of the observations that the researcher has made.
As you can probably imagine, ethnographic research design has the ability to provide rich, contextually embedded insights into the socio-cultural dynamics of human behaviour within a natural, uncontrived setting. Naturally, however, it does come with its own set of challenges, including researcher bias (since the researcher can become quite immersed in the group), participant confidentiality and, predictably, ethical complexities . All of these need to be carefully managed if you choose to adopt this type of research design.
Case Study Design
With case study research design, you, as the researcher, investigate a single individual (or a single group of individuals) to gain an in-depth understanding of their experiences, behaviours or outcomes. Unlike other research designs that are aimed at larger sample sizes, case studies offer a deep dive into the specific circumstances surrounding a person, group of people, event or phenomenon, generally within a bounded setting or context .
As an example, a case study design could be used to explore the factors influencing the success of a specific small business. This would involve diving deeply into the organisation to explore and understand what makes it tick – from marketing to HR to finance. In terms of data collection, this could include interviews with staff and management, review of policy documents and financial statements, surveying customers, etc.
While the above example is focused squarely on one organisation, it’s worth noting that case study research designs can have different variation s, including single-case, multiple-case and longitudinal designs. As you can see in the example, a single-case design involves intensely examining a single entity to understand its unique characteristics and complexities. Conversely, in a multiple-case design , multiple cases are compared and contrasted to identify patterns and commonalities. Lastly, in a longitudinal case design , a single case or multiple cases are studied over an extended period of time to understand how factors develop over time.
As you can see, a case study research design is particularly useful where a deep and contextualised understanding of a specific phenomenon or issue is desired. However, this strength is also its weakness. In other words, you can’t generalise the findings from a case study to the broader population. So, keep this in mind if you’re considering going the case study route.
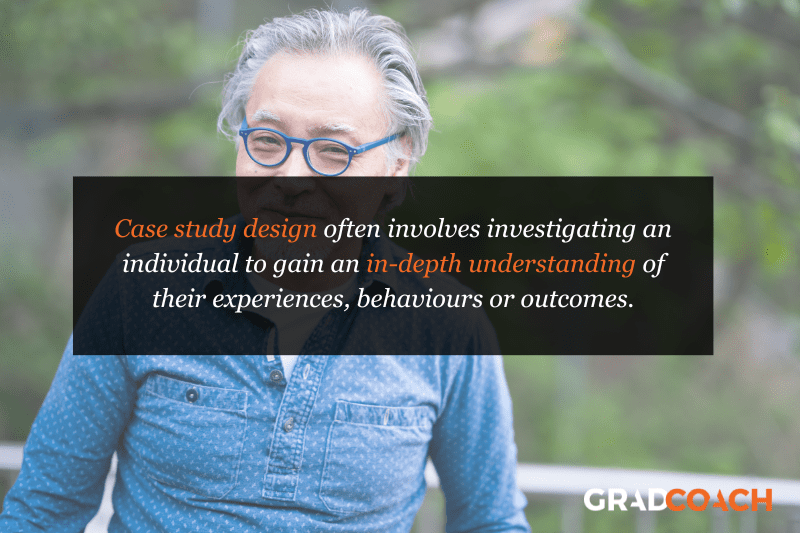
How To Choose A Research Design
Having worked through all of these potential research designs, you’d be forgiven for feeling a little overwhelmed and wondering, “ But how do I decide which research design to use? ”. While we could write an entire post covering that alone, here are a few factors to consider that will help you choose a suitable research design for your study.
Data type: The first determining factor is naturally the type of data you plan to be collecting – i.e., qualitative or quantitative. This may sound obvious, but we have to be clear about this – don’t try to use a quantitative research design on qualitative data (or vice versa)!
Research aim(s) and question(s): As with all methodological decisions, your research aim and research questions will heavily influence your research design. For example, if your research aims involve developing a theory from qualitative data, grounded theory would be a strong option. Similarly, if your research aims involve identifying and measuring relationships between variables, one of the experimental designs would likely be a better option.
Time: It’s essential that you consider any time constraints you have, as this will impact the type of research design you can choose. For example, if you’ve only got a month to complete your project, a lengthy design such as ethnography wouldn’t be a good fit.
Resources: Take into account the resources realistically available to you, as these need to factor into your research design choice. For example, if you require highly specialised lab equipment to execute an experimental design, you need to be sure that you’ll have access to that before you make a decision.
Keep in mind that when it comes to research, it’s important to manage your risks and play as conservatively as possible. If your entire project relies on you achieving a huge sample, having access to niche equipment or holding interviews with very difficult-to-reach participants, you’re creating risks that could kill your project. So, be sure to think through your choices carefully and make sure that you have backup plans for any existential risks. Remember that a relatively simple methodology executed well generally will typically earn better marks than a highly-complex methodology executed poorly.
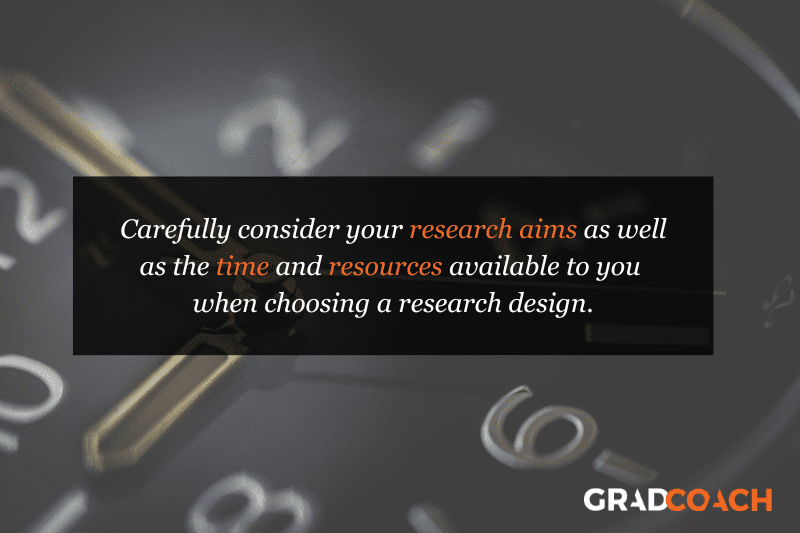
Recap: Key Takeaways
We’ve covered a lot of ground here. Let’s recap by looking at the key takeaways:
- Research design refers to the overall plan, structure or strategy that guides a research project, from its conception to the final analysis of data.
- Research designs for quantitative studies include descriptive , correlational , experimental and quasi-experimenta l designs.
- Research designs for qualitative studies include phenomenological , grounded theory , ethnographic and case study designs.
- When choosing a research design, you need to consider a variety of factors, including the type of data you’ll be working with, your research aims and questions, your time and the resources available to you.
If you need a helping hand with your research design (or any other aspect of your research), check out our private coaching services .
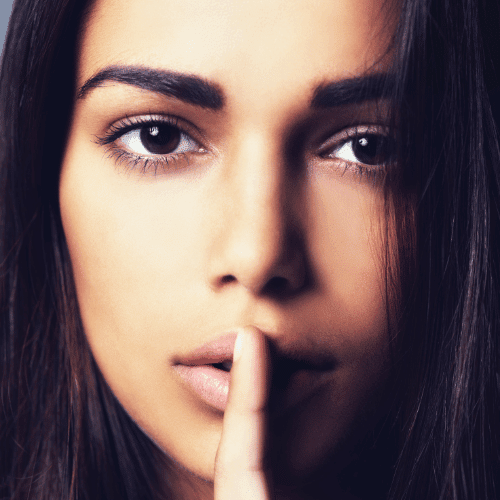
Psst... there’s more!
This post was based on one of our popular Research Bootcamps . If you're working on a research project, you'll definitely want to check this out ...
You Might Also Like:
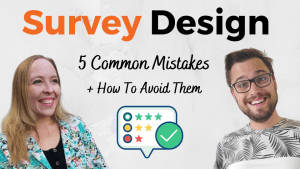
10 Comments
Is there any blog article explaining more on Case study research design? Is there a Case study write-up template? Thank you.
Thanks this was quite valuable to clarify such an important concept.
Thanks for this simplified explanations. it is quite very helpful.
This was really helpful. thanks
Thank you for your explanation. I think case study research design and the use of secondary data in researches needs to be talked about more in your videos and articles because there a lot of case studies research design tailored projects out there.
Please is there any template for a case study research design whose data type is a secondary data on your repository?
This post is very clear, comprehensive and has been very helpful to me. It has cleared the confusion I had in regard to research design and methodology.
This post is helpful, easy to understand, and deconstructs what a research design is. Thanks
how to cite this page
Thank you very much for the post. It is wonderful and has cleared many worries in my mind regarding research designs. I really appreciate .
how can I put this blog as my reference(APA style) in bibliography part?
Submit a Comment Cancel reply
Your email address will not be published. Required fields are marked *
Save my name, email, and website in this browser for the next time I comment.
- Print Friendly
Have a language expert improve your writing
Run a free plagiarism check in 10 minutes, automatically generate references for free.
- Knowledge Base
- Methodology
Research Design | Step-by-Step Guide with Examples
Published on 5 May 2022 by Shona McCombes . Revised on 20 March 2023.
A research design is a strategy for answering your research question using empirical data. Creating a research design means making decisions about:
- Your overall aims and approach
- The type of research design you’ll use
- Your sampling methods or criteria for selecting subjects
- Your data collection methods
- The procedures you’ll follow to collect data
- Your data analysis methods
A well-planned research design helps ensure that your methods match your research aims and that you use the right kind of analysis for your data.
Table of contents
Step 1: consider your aims and approach, step 2: choose a type of research design, step 3: identify your population and sampling method, step 4: choose your data collection methods, step 5: plan your data collection procedures, step 6: decide on your data analysis strategies, frequently asked questions.
- Introduction
Before you can start designing your research, you should already have a clear idea of the research question you want to investigate.
There are many different ways you could go about answering this question. Your research design choices should be driven by your aims and priorities – start by thinking carefully about what you want to achieve.
The first choice you need to make is whether you’ll take a qualitative or quantitative approach.
Qualitative research designs tend to be more flexible and inductive , allowing you to adjust your approach based on what you find throughout the research process.
Quantitative research designs tend to be more fixed and deductive , with variables and hypotheses clearly defined in advance of data collection.
It’s also possible to use a mixed methods design that integrates aspects of both approaches. By combining qualitative and quantitative insights, you can gain a more complete picture of the problem you’re studying and strengthen the credibility of your conclusions.
Practical and ethical considerations when designing research
As well as scientific considerations, you need to think practically when designing your research. If your research involves people or animals, you also need to consider research ethics .
- How much time do you have to collect data and write up the research?
- Will you be able to gain access to the data you need (e.g., by travelling to a specific location or contacting specific people)?
- Do you have the necessary research skills (e.g., statistical analysis or interview techniques)?
- Will you need ethical approval ?
At each stage of the research design process, make sure that your choices are practically feasible.
Prevent plagiarism, run a free check.
Within both qualitative and quantitative approaches, there are several types of research design to choose from. Each type provides a framework for the overall shape of your research.
Types of quantitative research designs
Quantitative designs can be split into four main types. Experimental and quasi-experimental designs allow you to test cause-and-effect relationships, while descriptive and correlational designs allow you to measure variables and describe relationships between them.
With descriptive and correlational designs, you can get a clear picture of characteristics, trends, and relationships as they exist in the real world. However, you can’t draw conclusions about cause and effect (because correlation doesn’t imply causation ).
Experiments are the strongest way to test cause-and-effect relationships without the risk of other variables influencing the results. However, their controlled conditions may not always reflect how things work in the real world. They’re often also more difficult and expensive to implement.
Types of qualitative research designs
Qualitative designs are less strictly defined. This approach is about gaining a rich, detailed understanding of a specific context or phenomenon, and you can often be more creative and flexible in designing your research.
The table below shows some common types of qualitative design. They often have similar approaches in terms of data collection, but focus on different aspects when analysing the data.
Your research design should clearly define who or what your research will focus on, and how you’ll go about choosing your participants or subjects.
In research, a population is the entire group that you want to draw conclusions about, while a sample is the smaller group of individuals you’ll actually collect data from.
Defining the population
A population can be made up of anything you want to study – plants, animals, organisations, texts, countries, etc. In the social sciences, it most often refers to a group of people.
For example, will you focus on people from a specific demographic, region, or background? Are you interested in people with a certain job or medical condition, or users of a particular product?
The more precisely you define your population, the easier it will be to gather a representative sample.
Sampling methods
Even with a narrowly defined population, it’s rarely possible to collect data from every individual. Instead, you’ll collect data from a sample.
To select a sample, there are two main approaches: probability sampling and non-probability sampling . The sampling method you use affects how confidently you can generalise your results to the population as a whole.
Probability sampling is the most statistically valid option, but it’s often difficult to achieve unless you’re dealing with a very small and accessible population.
For practical reasons, many studies use non-probability sampling, but it’s important to be aware of the limitations and carefully consider potential biases. You should always make an effort to gather a sample that’s as representative as possible of the population.
Case selection in qualitative research
In some types of qualitative designs, sampling may not be relevant.
For example, in an ethnography or a case study, your aim is to deeply understand a specific context, not to generalise to a population. Instead of sampling, you may simply aim to collect as much data as possible about the context you are studying.
In these types of design, you still have to carefully consider your choice of case or community. You should have a clear rationale for why this particular case is suitable for answering your research question.
For example, you might choose a case study that reveals an unusual or neglected aspect of your research problem, or you might choose several very similar or very different cases in order to compare them.
Data collection methods are ways of directly measuring variables and gathering information. They allow you to gain first-hand knowledge and original insights into your research problem.
You can choose just one data collection method, or use several methods in the same study.
Survey methods
Surveys allow you to collect data about opinions, behaviours, experiences, and characteristics by asking people directly. There are two main survey methods to choose from: questionnaires and interviews.
Observation methods
Observations allow you to collect data unobtrusively, observing characteristics, behaviours, or social interactions without relying on self-reporting.
Observations may be conducted in real time, taking notes as you observe, or you might make audiovisual recordings for later analysis. They can be qualitative or quantitative.
Other methods of data collection
There are many other ways you might collect data depending on your field and topic.
If you’re not sure which methods will work best for your research design, try reading some papers in your field to see what data collection methods they used.
Secondary data
If you don’t have the time or resources to collect data from the population you’re interested in, you can also choose to use secondary data that other researchers already collected – for example, datasets from government surveys or previous studies on your topic.
With this raw data, you can do your own analysis to answer new research questions that weren’t addressed by the original study.
Using secondary data can expand the scope of your research, as you may be able to access much larger and more varied samples than you could collect yourself.
However, it also means you don’t have any control over which variables to measure or how to measure them, so the conclusions you can draw may be limited.
As well as deciding on your methods, you need to plan exactly how you’ll use these methods to collect data that’s consistent, accurate, and unbiased.
Planning systematic procedures is especially important in quantitative research, where you need to precisely define your variables and ensure your measurements are reliable and valid.
Operationalisation
Some variables, like height or age, are easily measured. But often you’ll be dealing with more abstract concepts, like satisfaction, anxiety, or competence. Operationalisation means turning these fuzzy ideas into measurable indicators.
If you’re using observations , which events or actions will you count?
If you’re using surveys , which questions will you ask and what range of responses will be offered?
You may also choose to use or adapt existing materials designed to measure the concept you’re interested in – for example, questionnaires or inventories whose reliability and validity has already been established.
Reliability and validity
Reliability means your results can be consistently reproduced , while validity means that you’re actually measuring the concept you’re interested in.
For valid and reliable results, your measurement materials should be thoroughly researched and carefully designed. Plan your procedures to make sure you carry out the same steps in the same way for each participant.
If you’re developing a new questionnaire or other instrument to measure a specific concept, running a pilot study allows you to check its validity and reliability in advance.
Sampling procedures
As well as choosing an appropriate sampling method, you need a concrete plan for how you’ll actually contact and recruit your selected sample.
That means making decisions about things like:
- How many participants do you need for an adequate sample size?
- What inclusion and exclusion criteria will you use to identify eligible participants?
- How will you contact your sample – by mail, online, by phone, or in person?
If you’re using a probability sampling method, it’s important that everyone who is randomly selected actually participates in the study. How will you ensure a high response rate?
If you’re using a non-probability method, how will you avoid bias and ensure a representative sample?
Data management
It’s also important to create a data management plan for organising and storing your data.
Will you need to transcribe interviews or perform data entry for observations? You should anonymise and safeguard any sensitive data, and make sure it’s backed up regularly.
Keeping your data well organised will save time when it comes to analysing them. It can also help other researchers validate and add to your findings.
On their own, raw data can’t answer your research question. The last step of designing your research is planning how you’ll analyse the data.
Quantitative data analysis
In quantitative research, you’ll most likely use some form of statistical analysis . With statistics, you can summarise your sample data, make estimates, and test hypotheses.
Using descriptive statistics , you can summarise your sample data in terms of:
- The distribution of the data (e.g., the frequency of each score on a test)
- The central tendency of the data (e.g., the mean to describe the average score)
- The variability of the data (e.g., the standard deviation to describe how spread out the scores are)
The specific calculations you can do depend on the level of measurement of your variables.
Using inferential statistics , you can:
- Make estimates about the population based on your sample data.
- Test hypotheses about a relationship between variables.
Regression and correlation tests look for associations between two or more variables, while comparison tests (such as t tests and ANOVAs ) look for differences in the outcomes of different groups.
Your choice of statistical test depends on various aspects of your research design, including the types of variables you’re dealing with and the distribution of your data.
Qualitative data analysis
In qualitative research, your data will usually be very dense with information and ideas. Instead of summing it up in numbers, you’ll need to comb through the data in detail, interpret its meanings, identify patterns, and extract the parts that are most relevant to your research question.
Two of the most common approaches to doing this are thematic analysis and discourse analysis .
There are many other ways of analysing qualitative data depending on the aims of your research. To get a sense of potential approaches, try reading some qualitative research papers in your field.
A sample is a subset of individuals from a larger population. Sampling means selecting the group that you will actually collect data from in your research.
For example, if you are researching the opinions of students in your university, you could survey a sample of 100 students.
Statistical sampling allows you to test a hypothesis about the characteristics of a population. There are various sampling methods you can use to ensure that your sample is representative of the population as a whole.
Operationalisation means turning abstract conceptual ideas into measurable observations.
For example, the concept of social anxiety isn’t directly observable, but it can be operationally defined in terms of self-rating scores, behavioural avoidance of crowded places, or physical anxiety symptoms in social situations.
Before collecting data , it’s important to consider how you will operationalise the variables that you want to measure.
The research methods you use depend on the type of data you need to answer your research question .
- If you want to measure something or test a hypothesis , use quantitative methods . If you want to explore ideas, thoughts, and meanings, use qualitative methods .
- If you want to analyse a large amount of readily available data, use secondary data. If you want data specific to your purposes with control over how they are generated, collect primary data.
- If you want to establish cause-and-effect relationships between variables , use experimental methods. If you want to understand the characteristics of a research subject, use descriptive methods.
Cite this Scribbr article
If you want to cite this source, you can copy and paste the citation or click the ‘Cite this Scribbr article’ button to automatically add the citation to our free Reference Generator.
McCombes, S. (2023, March 20). Research Design | Step-by-Step Guide with Examples. Scribbr. Retrieved 26 May 2024, from https://www.scribbr.co.uk/research-methods/research-design/
Is this article helpful?
Shona McCombes
- USC Libraries
- Research Guides
Organizing Your Social Sciences Research Paper
- Types of Research Designs
- Purpose of Guide
- Design Flaws to Avoid
- Independent and Dependent Variables
- Glossary of Research Terms
- Reading Research Effectively
- Narrowing a Topic Idea
- Broadening a Topic Idea
- Extending the Timeliness of a Topic Idea
- Academic Writing Style
- Applying Critical Thinking
- Choosing a Title
- Making an Outline
- Paragraph Development
- Research Process Video Series
- Executive Summary
- The C.A.R.S. Model
- Background Information
- The Research Problem/Question
- Theoretical Framework
- Citation Tracking
- Content Alert Services
- Evaluating Sources
- Primary Sources
- Secondary Sources
- Tiertiary Sources
- Scholarly vs. Popular Publications
- Qualitative Methods
- Quantitative Methods
- Insiderness
- Using Non-Textual Elements
- Limitations of the Study
- Common Grammar Mistakes
- Writing Concisely
- Avoiding Plagiarism
- Footnotes or Endnotes?
- Further Readings
- Generative AI and Writing
- USC Libraries Tutorials and Other Guides
- Bibliography
Introduction
Before beginning your paper, you need to decide how you plan to design the study .
The research design refers to the overall strategy and analytical approach that you have chosen in order to integrate, in a coherent and logical way, the different components of the study, thus ensuring that the research problem will be thoroughly investigated. It constitutes the blueprint for the collection, measurement, and interpretation of information and data. Note that the research problem determines the type of design you choose, not the other way around!
De Vaus, D. A. Research Design in Social Research . London: SAGE, 2001; Trochim, William M.K. Research Methods Knowledge Base. 2006.
General Structure and Writing Style
The function of a research design is to ensure that the evidence obtained enables you to effectively address the research problem logically and as unambiguously as possible . In social sciences research, obtaining information relevant to the research problem generally entails specifying the type of evidence needed to test the underlying assumptions of a theory, to evaluate a program, or to accurately describe and assess meaning related to an observable phenomenon.
With this in mind, a common mistake made by researchers is that they begin their investigations before they have thought critically about what information is required to address the research problem. Without attending to these design issues beforehand, the overall research problem will not be adequately addressed and any conclusions drawn will run the risk of being weak and unconvincing. As a consequence, the overall validity of the study will be undermined.
The length and complexity of describing the research design in your paper can vary considerably, but any well-developed description will achieve the following :
- Identify the research problem clearly and justify its selection, particularly in relation to any valid alternative designs that could have been used,
- Review and synthesize previously published literature associated with the research problem,
- Clearly and explicitly specify hypotheses [i.e., research questions] central to the problem,
- Effectively describe the information and/or data which will be necessary for an adequate testing of the hypotheses and explain how such information and/or data will be obtained, and
- Describe the methods of analysis to be applied to the data in determining whether or not the hypotheses are true or false.
The research design is usually incorporated into the introduction of your paper . You can obtain an overall sense of what to do by reviewing studies that have utilized the same research design [e.g., using a case study approach]. This can help you develop an outline to follow for your own paper.
NOTE: Use the SAGE Research Methods Online and Cases and the SAGE Research Methods Videos databases to search for scholarly resources on how to apply specific research designs and methods . The Research Methods Online database contains links to more than 175,000 pages of SAGE publisher's book, journal, and reference content on quantitative, qualitative, and mixed research methodologies. Also included is a collection of case studies of social research projects that can be used to help you better understand abstract or complex methodological concepts. The Research Methods Videos database contains hours of tutorials, interviews, video case studies, and mini-documentaries covering the entire research process.
Creswell, John W. and J. David Creswell. Research Design: Qualitative, Quantitative, and Mixed Methods Approaches . 5th edition. Thousand Oaks, CA: Sage, 2018; De Vaus, D. A. Research Design in Social Research . London: SAGE, 2001; Gorard, Stephen. Research Design: Creating Robust Approaches for the Social Sciences . Thousand Oaks, CA: Sage, 2013; Leedy, Paul D. and Jeanne Ellis Ormrod. Practical Research: Planning and Design . Tenth edition. Boston, MA: Pearson, 2013; Vogt, W. Paul, Dianna C. Gardner, and Lynne M. Haeffele. When to Use What Research Design . New York: Guilford, 2012.
Action Research Design
Definition and Purpose
The essentials of action research design follow a characteristic cycle whereby initially an exploratory stance is adopted, where an understanding of a problem is developed and plans are made for some form of interventionary strategy. Then the intervention is carried out [the "action" in action research] during which time, pertinent observations are collected in various forms. The new interventional strategies are carried out, and this cyclic process repeats, continuing until a sufficient understanding of [or a valid implementation solution for] the problem is achieved. The protocol is iterative or cyclical in nature and is intended to foster deeper understanding of a given situation, starting with conceptualizing and particularizing the problem and moving through several interventions and evaluations.
What do these studies tell you ?
- This is a collaborative and adaptive research design that lends itself to use in work or community situations.
- Design focuses on pragmatic and solution-driven research outcomes rather than testing theories.
- When practitioners use action research, it has the potential to increase the amount they learn consciously from their experience; the action research cycle can be regarded as a learning cycle.
- Action research studies often have direct and obvious relevance to improving practice and advocating for change.
- There are no hidden controls or preemption of direction by the researcher.
What these studies don't tell you ?
- It is harder to do than conducting conventional research because the researcher takes on responsibilities of advocating for change as well as for researching the topic.
- Action research is much harder to write up because it is less likely that you can use a standard format to report your findings effectively [i.e., data is often in the form of stories or observation].
- Personal over-involvement of the researcher may bias research results.
- The cyclic nature of action research to achieve its twin outcomes of action [e.g. change] and research [e.g. understanding] is time-consuming and complex to conduct.
- Advocating for change usually requires buy-in from study participants.
Coghlan, David and Mary Brydon-Miller. The Sage Encyclopedia of Action Research . Thousand Oaks, CA: Sage, 2014; Efron, Sara Efrat and Ruth Ravid. Action Research in Education: A Practical Guide . New York: Guilford, 2013; Gall, Meredith. Educational Research: An Introduction . Chapter 18, Action Research. 8th ed. Boston, MA: Pearson/Allyn and Bacon, 2007; Gorard, Stephen. Research Design: Creating Robust Approaches for the Social Sciences . Thousand Oaks, CA: Sage, 2013; Kemmis, Stephen and Robin McTaggart. “Participatory Action Research.” In Handbook of Qualitative Research . Norman Denzin and Yvonna S. Lincoln, eds. 2nd ed. (Thousand Oaks, CA: SAGE, 2000), pp. 567-605; McNiff, Jean. Writing and Doing Action Research . London: Sage, 2014; Reason, Peter and Hilary Bradbury. Handbook of Action Research: Participative Inquiry and Practice . Thousand Oaks, CA: SAGE, 2001.
Case Study Design
A case study is an in-depth study of a particular research problem rather than a sweeping statistical survey or comprehensive comparative inquiry. It is often used to narrow down a very broad field of research into one or a few easily researchable examples. The case study research design is also useful for testing whether a specific theory and model actually applies to phenomena in the real world. It is a useful design when not much is known about an issue or phenomenon.
- Approach excels at bringing us to an understanding of a complex issue through detailed contextual analysis of a limited number of events or conditions and their relationships.
- A researcher using a case study design can apply a variety of methodologies and rely on a variety of sources to investigate a research problem.
- Design can extend experience or add strength to what is already known through previous research.
- Social scientists, in particular, make wide use of this research design to examine contemporary real-life situations and provide the basis for the application of concepts and theories and the extension of methodologies.
- The design can provide detailed descriptions of specific and rare cases.
- A single or small number of cases offers little basis for establishing reliability or to generalize the findings to a wider population of people, places, or things.
- Intense exposure to the study of a case may bias a researcher's interpretation of the findings.
- Design does not facilitate assessment of cause and effect relationships.
- Vital information may be missing, making the case hard to interpret.
- The case may not be representative or typical of the larger problem being investigated.
- If the criteria for selecting a case is because it represents a very unusual or unique phenomenon or problem for study, then your interpretation of the findings can only apply to that particular case.
Case Studies. Writing@CSU. Colorado State University; Anastas, Jeane W. Research Design for Social Work and the Human Services . Chapter 4, Flexible Methods: Case Study Design. 2nd ed. New York: Columbia University Press, 1999; Gerring, John. “What Is a Case Study and What Is It Good for?” American Political Science Review 98 (May 2004): 341-354; Greenhalgh, Trisha, editor. Case Study Evaluation: Past, Present and Future Challenges . Bingley, UK: Emerald Group Publishing, 2015; Mills, Albert J. , Gabrielle Durepos, and Eiden Wiebe, editors. Encyclopedia of Case Study Research . Thousand Oaks, CA: SAGE Publications, 2010; Stake, Robert E. The Art of Case Study Research . Thousand Oaks, CA: SAGE, 1995; Yin, Robert K. Case Study Research: Design and Theory . Applied Social Research Methods Series, no. 5. 3rd ed. Thousand Oaks, CA: SAGE, 2003.
Causal Design
Causality studies may be thought of as understanding a phenomenon in terms of conditional statements in the form, “If X, then Y.” This type of research is used to measure what impact a specific change will have on existing norms and assumptions. Most social scientists seek causal explanations that reflect tests of hypotheses. Causal effect (nomothetic perspective) occurs when variation in one phenomenon, an independent variable, leads to or results, on average, in variation in another phenomenon, the dependent variable.
Conditions necessary for determining causality:
- Empirical association -- a valid conclusion is based on finding an association between the independent variable and the dependent variable.
- Appropriate time order -- to conclude that causation was involved, one must see that cases were exposed to variation in the independent variable before variation in the dependent variable.
- Nonspuriousness -- a relationship between two variables that is not due to variation in a third variable.
- Causality research designs assist researchers in understanding why the world works the way it does through the process of proving a causal link between variables and by the process of eliminating other possibilities.
- Replication is possible.
- There is greater confidence the study has internal validity due to the systematic subject selection and equity of groups being compared.
- Not all relationships are causal! The possibility always exists that, by sheer coincidence, two unrelated events appear to be related [e.g., Punxatawney Phil could accurately predict the duration of Winter for five consecutive years but, the fact remains, he's just a big, furry rodent].
- Conclusions about causal relationships are difficult to determine due to a variety of extraneous and confounding variables that exist in a social environment. This means causality can only be inferred, never proven.
- If two variables are correlated, the cause must come before the effect. However, even though two variables might be causally related, it can sometimes be difficult to determine which variable comes first and, therefore, to establish which variable is the actual cause and which is the actual effect.
Beach, Derek and Rasmus Brun Pedersen. Causal Case Study Methods: Foundations and Guidelines for Comparing, Matching, and Tracing . Ann Arbor, MI: University of Michigan Press, 2016; Bachman, Ronet. The Practice of Research in Criminology and Criminal Justice . Chapter 5, Causation and Research Designs. 3rd ed. Thousand Oaks, CA: Pine Forge Press, 2007; Brewer, Ernest W. and Jennifer Kubn. “Causal-Comparative Design.” In Encyclopedia of Research Design . Neil J. Salkind, editor. (Thousand Oaks, CA: Sage, 2010), pp. 125-132; Causal Research Design: Experimentation. Anonymous SlideShare Presentation; Gall, Meredith. Educational Research: An Introduction . Chapter 11, Nonexperimental Research: Correlational Designs. 8th ed. Boston, MA: Pearson/Allyn and Bacon, 2007; Trochim, William M.K. Research Methods Knowledge Base. 2006.
Cohort Design
Often used in the medical sciences, but also found in the applied social sciences, a cohort study generally refers to a study conducted over a period of time involving members of a population which the subject or representative member comes from, and who are united by some commonality or similarity. Using a quantitative framework, a cohort study makes note of statistical occurrence within a specialized subgroup, united by same or similar characteristics that are relevant to the research problem being investigated, rather than studying statistical occurrence within the general population. Using a qualitative framework, cohort studies generally gather data using methods of observation. Cohorts can be either "open" or "closed."
- Open Cohort Studies [dynamic populations, such as the population of Los Angeles] involve a population that is defined just by the state of being a part of the study in question (and being monitored for the outcome). Date of entry and exit from the study is individually defined, therefore, the size of the study population is not constant. In open cohort studies, researchers can only calculate rate based data, such as, incidence rates and variants thereof.
- Closed Cohort Studies [static populations, such as patients entered into a clinical trial] involve participants who enter into the study at one defining point in time and where it is presumed that no new participants can enter the cohort. Given this, the number of study participants remains constant (or can only decrease).
- The use of cohorts is often mandatory because a randomized control study may be unethical. For example, you cannot deliberately expose people to asbestos, you can only study its effects on those who have already been exposed. Research that measures risk factors often relies upon cohort designs.
- Because cohort studies measure potential causes before the outcome has occurred, they can demonstrate that these “causes” preceded the outcome, thereby avoiding the debate as to which is the cause and which is the effect.
- Cohort analysis is highly flexible and can provide insight into effects over time and related to a variety of different types of changes [e.g., social, cultural, political, economic, etc.].
- Either original data or secondary data can be used in this design.
- In cases where a comparative analysis of two cohorts is made [e.g., studying the effects of one group exposed to asbestos and one that has not], a researcher cannot control for all other factors that might differ between the two groups. These factors are known as confounding variables.
- Cohort studies can end up taking a long time to complete if the researcher must wait for the conditions of interest to develop within the group. This also increases the chance that key variables change during the course of the study, potentially impacting the validity of the findings.
- Due to the lack of randominization in the cohort design, its external validity is lower than that of study designs where the researcher randomly assigns participants.
Healy P, Devane D. “Methodological Considerations in Cohort Study Designs.” Nurse Researcher 18 (2011): 32-36; Glenn, Norval D, editor. Cohort Analysis . 2nd edition. Thousand Oaks, CA: Sage, 2005; Levin, Kate Ann. Study Design IV: Cohort Studies. Evidence-Based Dentistry 7 (2003): 51–52; Payne, Geoff. “Cohort Study.” In The SAGE Dictionary of Social Research Methods . Victor Jupp, editor. (Thousand Oaks, CA: Sage, 2006), pp. 31-33; Study Design 101. Himmelfarb Health Sciences Library. George Washington University, November 2011; Cohort Study. Wikipedia.
Cross-Sectional Design
Cross-sectional research designs have three distinctive features: no time dimension; a reliance on existing differences rather than change following intervention; and, groups are selected based on existing differences rather than random allocation. The cross-sectional design can only measure differences between or from among a variety of people, subjects, or phenomena rather than a process of change. As such, researchers using this design can only employ a relatively passive approach to making causal inferences based on findings.
- Cross-sectional studies provide a clear 'snapshot' of the outcome and the characteristics associated with it, at a specific point in time.
- Unlike an experimental design, where there is an active intervention by the researcher to produce and measure change or to create differences, cross-sectional designs focus on studying and drawing inferences from existing differences between people, subjects, or phenomena.
- Entails collecting data at and concerning one point in time. While longitudinal studies involve taking multiple measures over an extended period of time, cross-sectional research is focused on finding relationships between variables at one moment in time.
- Groups identified for study are purposely selected based upon existing differences in the sample rather than seeking random sampling.
- Cross-section studies are capable of using data from a large number of subjects and, unlike observational studies, is not geographically bound.
- Can estimate prevalence of an outcome of interest because the sample is usually taken from the whole population.
- Because cross-sectional designs generally use survey techniques to gather data, they are relatively inexpensive and take up little time to conduct.
- Finding people, subjects, or phenomena to study that are very similar except in one specific variable can be difficult.
- Results are static and time bound and, therefore, give no indication of a sequence of events or reveal historical or temporal contexts.
- Studies cannot be utilized to establish cause and effect relationships.
- This design only provides a snapshot of analysis so there is always the possibility that a study could have differing results if another time-frame had been chosen.
- There is no follow up to the findings.
Bethlehem, Jelke. "7: Cross-sectional Research." In Research Methodology in the Social, Behavioural and Life Sciences . Herman J Adèr and Gideon J Mellenbergh, editors. (London, England: Sage, 1999), pp. 110-43; Bourque, Linda B. “Cross-Sectional Design.” In The SAGE Encyclopedia of Social Science Research Methods . Michael S. Lewis-Beck, Alan Bryman, and Tim Futing Liao. (Thousand Oaks, CA: 2004), pp. 230-231; Hall, John. “Cross-Sectional Survey Design.” In Encyclopedia of Survey Research Methods . Paul J. Lavrakas, ed. (Thousand Oaks, CA: Sage, 2008), pp. 173-174; Helen Barratt, Maria Kirwan. Cross-Sectional Studies: Design Application, Strengths and Weaknesses of Cross-Sectional Studies. Healthknowledge, 2009. Cross-Sectional Study. Wikipedia.
Descriptive Design
Descriptive research designs help provide answers to the questions of who, what, when, where, and how associated with a particular research problem; a descriptive study cannot conclusively ascertain answers to why. Descriptive research is used to obtain information concerning the current status of the phenomena and to describe "what exists" with respect to variables or conditions in a situation.
- The subject is being observed in a completely natural and unchanged natural environment. True experiments, whilst giving analyzable data, often adversely influence the normal behavior of the subject [a.k.a., the Heisenberg effect whereby measurements of certain systems cannot be made without affecting the systems].
- Descriptive research is often used as a pre-cursor to more quantitative research designs with the general overview giving some valuable pointers as to what variables are worth testing quantitatively.
- If the limitations are understood, they can be a useful tool in developing a more focused study.
- Descriptive studies can yield rich data that lead to important recommendations in practice.
- Appoach collects a large amount of data for detailed analysis.
- The results from a descriptive research cannot be used to discover a definitive answer or to disprove a hypothesis.
- Because descriptive designs often utilize observational methods [as opposed to quantitative methods], the results cannot be replicated.
- The descriptive function of research is heavily dependent on instrumentation for measurement and observation.
Anastas, Jeane W. Research Design for Social Work and the Human Services . Chapter 5, Flexible Methods: Descriptive Research. 2nd ed. New York: Columbia University Press, 1999; Given, Lisa M. "Descriptive Research." In Encyclopedia of Measurement and Statistics . Neil J. Salkind and Kristin Rasmussen, editors. (Thousand Oaks, CA: Sage, 2007), pp. 251-254; McNabb, Connie. Descriptive Research Methodologies. Powerpoint Presentation; Shuttleworth, Martyn. Descriptive Research Design, September 26, 2008; Erickson, G. Scott. "Descriptive Research Design." In New Methods of Market Research and Analysis . (Northampton, MA: Edward Elgar Publishing, 2017), pp. 51-77; Sahin, Sagufta, and Jayanta Mete. "A Brief Study on Descriptive Research: Its Nature and Application in Social Science." International Journal of Research and Analysis in Humanities 1 (2021): 11; K. Swatzell and P. Jennings. “Descriptive Research: The Nuts and Bolts.” Journal of the American Academy of Physician Assistants 20 (2007), pp. 55-56; Kane, E. Doing Your Own Research: Basic Descriptive Research in the Social Sciences and Humanities . London: Marion Boyars, 1985.
Experimental Design
A blueprint of the procedure that enables the researcher to maintain control over all factors that may affect the result of an experiment. In doing this, the researcher attempts to determine or predict what may occur. Experimental research is often used where there is time priority in a causal relationship (cause precedes effect), there is consistency in a causal relationship (a cause will always lead to the same effect), and the magnitude of the correlation is great. The classic experimental design specifies an experimental group and a control group. The independent variable is administered to the experimental group and not to the control group, and both groups are measured on the same dependent variable. Subsequent experimental designs have used more groups and more measurements over longer periods. True experiments must have control, randomization, and manipulation.
- Experimental research allows the researcher to control the situation. In so doing, it allows researchers to answer the question, “What causes something to occur?”
- Permits the researcher to identify cause and effect relationships between variables and to distinguish placebo effects from treatment effects.
- Experimental research designs support the ability to limit alternative explanations and to infer direct causal relationships in the study.
- Approach provides the highest level of evidence for single studies.
- The design is artificial, and results may not generalize well to the real world.
- The artificial settings of experiments may alter the behaviors or responses of participants.
- Experimental designs can be costly if special equipment or facilities are needed.
- Some research problems cannot be studied using an experiment because of ethical or technical reasons.
- Difficult to apply ethnographic and other qualitative methods to experimentally designed studies.
Anastas, Jeane W. Research Design for Social Work and the Human Services . Chapter 7, Flexible Methods: Experimental Research. 2nd ed. New York: Columbia University Press, 1999; Chapter 2: Research Design, Experimental Designs. School of Psychology, University of New England, 2000; Chow, Siu L. "Experimental Design." In Encyclopedia of Research Design . Neil J. Salkind, editor. (Thousand Oaks, CA: Sage, 2010), pp. 448-453; "Experimental Design." In Social Research Methods . Nicholas Walliman, editor. (London, England: Sage, 2006), pp, 101-110; Experimental Research. Research Methods by Dummies. Department of Psychology. California State University, Fresno, 2006; Kirk, Roger E. Experimental Design: Procedures for the Behavioral Sciences . 4th edition. Thousand Oaks, CA: Sage, 2013; Trochim, William M.K. Experimental Design. Research Methods Knowledge Base. 2006; Rasool, Shafqat. Experimental Research. Slideshare presentation.
Exploratory Design
An exploratory design is conducted about a research problem when there are few or no earlier studies to refer to or rely upon to predict an outcome . The focus is on gaining insights and familiarity for later investigation or undertaken when research problems are in a preliminary stage of investigation. Exploratory designs are often used to establish an understanding of how best to proceed in studying an issue or what methodology would effectively apply to gathering information about the issue.
The goals of exploratory research are intended to produce the following possible insights:
- Familiarity with basic details, settings, and concerns.
- Well grounded picture of the situation being developed.
- Generation of new ideas and assumptions.
- Development of tentative theories or hypotheses.
- Determination about whether a study is feasible in the future.
- Issues get refined for more systematic investigation and formulation of new research questions.
- Direction for future research and techniques get developed.
- Design is a useful approach for gaining background information on a particular topic.
- Exploratory research is flexible and can address research questions of all types (what, why, how).
- Provides an opportunity to define new terms and clarify existing concepts.
- Exploratory research is often used to generate formal hypotheses and develop more precise research problems.
- In the policy arena or applied to practice, exploratory studies help establish research priorities and where resources should be allocated.
- Exploratory research generally utilizes small sample sizes and, thus, findings are typically not generalizable to the population at large.
- The exploratory nature of the research inhibits an ability to make definitive conclusions about the findings. They provide insight but not definitive conclusions.
- The research process underpinning exploratory studies is flexible but often unstructured, leading to only tentative results that have limited value to decision-makers.
- Design lacks rigorous standards applied to methods of data gathering and analysis because one of the areas for exploration could be to determine what method or methodologies could best fit the research problem.
Cuthill, Michael. “Exploratory Research: Citizen Participation, Local Government, and Sustainable Development in Australia.” Sustainable Development 10 (2002): 79-89; Streb, Christoph K. "Exploratory Case Study." In Encyclopedia of Case Study Research . Albert J. Mills, Gabrielle Durepos and Eiden Wiebe, editors. (Thousand Oaks, CA: Sage, 2010), pp. 372-374; Taylor, P. J., G. Catalano, and D.R.F. Walker. “Exploratory Analysis of the World City Network.” Urban Studies 39 (December 2002): 2377-2394; Exploratory Research. Wikipedia.
Field Research Design
Sometimes referred to as ethnography or participant observation, designs around field research encompass a variety of interpretative procedures [e.g., observation and interviews] rooted in qualitative approaches to studying people individually or in groups while inhabiting their natural environment as opposed to using survey instruments or other forms of impersonal methods of data gathering. Information acquired from observational research takes the form of “ field notes ” that involves documenting what the researcher actually sees and hears while in the field. Findings do not consist of conclusive statements derived from numbers and statistics because field research involves analysis of words and observations of behavior. Conclusions, therefore, are developed from an interpretation of findings that reveal overriding themes, concepts, and ideas. More information can be found HERE .
- Field research is often necessary to fill gaps in understanding the research problem applied to local conditions or to specific groups of people that cannot be ascertained from existing data.
- The research helps contextualize already known information about a research problem, thereby facilitating ways to assess the origins, scope, and scale of a problem and to gage the causes, consequences, and means to resolve an issue based on deliberate interaction with people in their natural inhabited spaces.
- Enables the researcher to corroborate or confirm data by gathering additional information that supports or refutes findings reported in prior studies of the topic.
- Because the researcher in embedded in the field, they are better able to make observations or ask questions that reflect the specific cultural context of the setting being investigated.
- Observing the local reality offers the opportunity to gain new perspectives or obtain unique data that challenges existing theoretical propositions or long-standing assumptions found in the literature.
What these studies don't tell you
- A field research study requires extensive time and resources to carry out the multiple steps involved with preparing for the gathering of information, including for example, examining background information about the study site, obtaining permission to access the study site, and building trust and rapport with subjects.
- Requires a commitment to staying engaged in the field to ensure that you can adequately document events and behaviors as they unfold.
- The unpredictable nature of fieldwork means that researchers can never fully control the process of data gathering. They must maintain a flexible approach to studying the setting because events and circumstances can change quickly or unexpectedly.
- Findings can be difficult to interpret and verify without access to documents and other source materials that help to enhance the credibility of information obtained from the field [i.e., the act of triangulating the data].
- Linking the research problem to the selection of study participants inhabiting their natural environment is critical. However, this specificity limits the ability to generalize findings to different situations or in other contexts or to infer courses of action applied to other settings or groups of people.
- The reporting of findings must take into account how the researcher themselves may have inadvertently affected respondents and their behaviors.
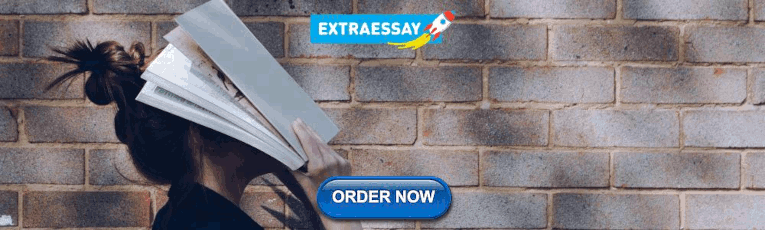
Historical Design
The purpose of a historical research design is to collect, verify, and synthesize evidence from the past to establish facts that defend or refute a hypothesis. It uses secondary sources and a variety of primary documentary evidence, such as, diaries, official records, reports, archives, and non-textual information [maps, pictures, audio and visual recordings]. The limitation is that the sources must be both authentic and valid.
- The historical research design is unobtrusive; the act of research does not affect the results of the study.
- The historical approach is well suited for trend analysis.
- Historical records can add important contextual background required to more fully understand and interpret a research problem.
- There is often no possibility of researcher-subject interaction that could affect the findings.
- Historical sources can be used over and over to study different research problems or to replicate a previous study.
- The ability to fulfill the aims of your research are directly related to the amount and quality of documentation available to understand the research problem.
- Since historical research relies on data from the past, there is no way to manipulate it to control for contemporary contexts.
- Interpreting historical sources can be very time consuming.
- The sources of historical materials must be archived consistently to ensure access. This may especially challenging for digital or online-only sources.
- Original authors bring their own perspectives and biases to the interpretation of past events and these biases are more difficult to ascertain in historical resources.
- Due to the lack of control over external variables, historical research is very weak with regard to the demands of internal validity.
- It is rare that the entirety of historical documentation needed to fully address a research problem is available for interpretation, therefore, gaps need to be acknowledged.
Howell, Martha C. and Walter Prevenier. From Reliable Sources: An Introduction to Historical Methods . Ithaca, NY: Cornell University Press, 2001; Lundy, Karen Saucier. "Historical Research." In The Sage Encyclopedia of Qualitative Research Methods . Lisa M. Given, editor. (Thousand Oaks, CA: Sage, 2008), pp. 396-400; Marius, Richard. and Melvin E. Page. A Short Guide to Writing about History . 9th edition. Boston, MA: Pearson, 2015; Savitt, Ronald. “Historical Research in Marketing.” Journal of Marketing 44 (Autumn, 1980): 52-58; Gall, Meredith. Educational Research: An Introduction . Chapter 16, Historical Research. 8th ed. Boston, MA: Pearson/Allyn and Bacon, 2007.
Longitudinal Design
A longitudinal study follows the same sample over time and makes repeated observations. For example, with longitudinal surveys, the same group of people is interviewed at regular intervals, enabling researchers to track changes over time and to relate them to variables that might explain why the changes occur. Longitudinal research designs describe patterns of change and help establish the direction and magnitude of causal relationships. Measurements are taken on each variable over two or more distinct time periods. This allows the researcher to measure change in variables over time. It is a type of observational study sometimes referred to as a panel study.
- Longitudinal data facilitate the analysis of the duration of a particular phenomenon.
- Enables survey researchers to get close to the kinds of causal explanations usually attainable only with experiments.
- The design permits the measurement of differences or change in a variable from one period to another [i.e., the description of patterns of change over time].
- Longitudinal studies facilitate the prediction of future outcomes based upon earlier factors.
- The data collection method may change over time.
- Maintaining the integrity of the original sample can be difficult over an extended period of time.
- It can be difficult to show more than one variable at a time.
- This design often needs qualitative research data to explain fluctuations in the results.
- A longitudinal research design assumes present trends will continue unchanged.
- It can take a long period of time to gather results.
- There is a need to have a large sample size and accurate sampling to reach representativness.
Anastas, Jeane W. Research Design for Social Work and the Human Services . Chapter 6, Flexible Methods: Relational and Longitudinal Research. 2nd ed. New York: Columbia University Press, 1999; Forgues, Bernard, and Isabelle Vandangeon-Derumez. "Longitudinal Analyses." In Doing Management Research . Raymond-Alain Thiétart and Samantha Wauchope, editors. (London, England: Sage, 2001), pp. 332-351; Kalaian, Sema A. and Rafa M. Kasim. "Longitudinal Studies." In Encyclopedia of Survey Research Methods . Paul J. Lavrakas, ed. (Thousand Oaks, CA: Sage, 2008), pp. 440-441; Menard, Scott, editor. Longitudinal Research . Thousand Oaks, CA: Sage, 2002; Ployhart, Robert E. and Robert J. Vandenberg. "Longitudinal Research: The Theory, Design, and Analysis of Change.” Journal of Management 36 (January 2010): 94-120; Longitudinal Study. Wikipedia.
Meta-Analysis Design
Meta-analysis is an analytical methodology designed to systematically evaluate and summarize the results from a number of individual studies, thereby, increasing the overall sample size and the ability of the researcher to study effects of interest. The purpose is to not simply summarize existing knowledge, but to develop a new understanding of a research problem using synoptic reasoning. The main objectives of meta-analysis include analyzing differences in the results among studies and increasing the precision by which effects are estimated. A well-designed meta-analysis depends upon strict adherence to the criteria used for selecting studies and the availability of information in each study to properly analyze their findings. Lack of information can severely limit the type of analyzes and conclusions that can be reached. In addition, the more dissimilarity there is in the results among individual studies [heterogeneity], the more difficult it is to justify interpretations that govern a valid synopsis of results. A meta-analysis needs to fulfill the following requirements to ensure the validity of your findings:
- Clearly defined description of objectives, including precise definitions of the variables and outcomes that are being evaluated;
- A well-reasoned and well-documented justification for identification and selection of the studies;
- Assessment and explicit acknowledgment of any researcher bias in the identification and selection of those studies;
- Description and evaluation of the degree of heterogeneity among the sample size of studies reviewed; and,
- Justification of the techniques used to evaluate the studies.
- Can be an effective strategy for determining gaps in the literature.
- Provides a means of reviewing research published about a particular topic over an extended period of time and from a variety of sources.
- Is useful in clarifying what policy or programmatic actions can be justified on the basis of analyzing research results from multiple studies.
- Provides a method for overcoming small sample sizes in individual studies that previously may have had little relationship to each other.
- Can be used to generate new hypotheses or highlight research problems for future studies.
- Small violations in defining the criteria used for content analysis can lead to difficult to interpret and/or meaningless findings.
- A large sample size can yield reliable, but not necessarily valid, results.
- A lack of uniformity regarding, for example, the type of literature reviewed, how methods are applied, and how findings are measured within the sample of studies you are analyzing, can make the process of synthesis difficult to perform.
- Depending on the sample size, the process of reviewing and synthesizing multiple studies can be very time consuming.
Beck, Lewis W. "The Synoptic Method." The Journal of Philosophy 36 (1939): 337-345; Cooper, Harris, Larry V. Hedges, and Jeffrey C. Valentine, eds. The Handbook of Research Synthesis and Meta-Analysis . 2nd edition. New York: Russell Sage Foundation, 2009; Guzzo, Richard A., Susan E. Jackson and Raymond A. Katzell. “Meta-Analysis Analysis.” In Research in Organizational Behavior , Volume 9. (Greenwich, CT: JAI Press, 1987), pp 407-442; Lipsey, Mark W. and David B. Wilson. Practical Meta-Analysis . Thousand Oaks, CA: Sage Publications, 2001; Study Design 101. Meta-Analysis. The Himmelfarb Health Sciences Library, George Washington University; Timulak, Ladislav. “Qualitative Meta-Analysis.” In The SAGE Handbook of Qualitative Data Analysis . Uwe Flick, editor. (Los Angeles, CA: Sage, 2013), pp. 481-495; Walker, Esteban, Adrian V. Hernandez, and Micheal W. Kattan. "Meta-Analysis: It's Strengths and Limitations." Cleveland Clinic Journal of Medicine 75 (June 2008): 431-439.
Mixed-Method Design
- Narrative and non-textual information can add meaning to numeric data, while numeric data can add precision to narrative and non-textual information.
- Can utilize existing data while at the same time generating and testing a grounded theory approach to describe and explain the phenomenon under study.
- A broader, more complex research problem can be investigated because the researcher is not constrained by using only one method.
- The strengths of one method can be used to overcome the inherent weaknesses of another method.
- Can provide stronger, more robust evidence to support a conclusion or set of recommendations.
- May generate new knowledge new insights or uncover hidden insights, patterns, or relationships that a single methodological approach might not reveal.
- Produces more complete knowledge and understanding of the research problem that can be used to increase the generalizability of findings applied to theory or practice.
- A researcher must be proficient in understanding how to apply multiple methods to investigating a research problem as well as be proficient in optimizing how to design a study that coherently melds them together.
- Can increase the likelihood of conflicting results or ambiguous findings that inhibit drawing a valid conclusion or setting forth a recommended course of action [e.g., sample interview responses do not support existing statistical data].
- Because the research design can be very complex, reporting the findings requires a well-organized narrative, clear writing style, and precise word choice.
- Design invites collaboration among experts. However, merging different investigative approaches and writing styles requires more attention to the overall research process than studies conducted using only one methodological paradigm.
- Concurrent merging of quantitative and qualitative research requires greater attention to having adequate sample sizes, using comparable samples, and applying a consistent unit of analysis. For sequential designs where one phase of qualitative research builds on the quantitative phase or vice versa, decisions about what results from the first phase to use in the next phase, the choice of samples and estimating reasonable sample sizes for both phases, and the interpretation of results from both phases can be difficult.
- Due to multiple forms of data being collected and analyzed, this design requires extensive time and resources to carry out the multiple steps involved in data gathering and interpretation.
Burch, Patricia and Carolyn J. Heinrich. Mixed Methods for Policy Research and Program Evaluation . Thousand Oaks, CA: Sage, 2016; Creswell, John w. et al. Best Practices for Mixed Methods Research in the Health Sciences . Bethesda, MD: Office of Behavioral and Social Sciences Research, National Institutes of Health, 2010Creswell, John W. Research Design: Qualitative, Quantitative, and Mixed Methods Approaches . 4th edition. Thousand Oaks, CA: Sage Publications, 2014; Domínguez, Silvia, editor. Mixed Methods Social Networks Research . Cambridge, UK: Cambridge University Press, 2014; Hesse-Biber, Sharlene Nagy. Mixed Methods Research: Merging Theory with Practice . New York: Guilford Press, 2010; Niglas, Katrin. “How the Novice Researcher Can Make Sense of Mixed Methods Designs.” International Journal of Multiple Research Approaches 3 (2009): 34-46; Onwuegbuzie, Anthony J. and Nancy L. Leech. “Linking Research Questions to Mixed Methods Data Analysis Procedures.” The Qualitative Report 11 (September 2006): 474-498; Tashakorri, Abbas and John W. Creswell. “The New Era of Mixed Methods.” Journal of Mixed Methods Research 1 (January 2007): 3-7; Zhanga, Wanqing. “Mixed Methods Application in Health Intervention Research: A Multiple Case Study.” International Journal of Multiple Research Approaches 8 (2014): 24-35 .
Observational Design
This type of research design draws a conclusion by comparing subjects against a control group, in cases where the researcher has no control over the experiment. There are two general types of observational designs. In direct observations, people know that you are watching them. Unobtrusive measures involve any method for studying behavior where individuals do not know they are being observed. An observational study allows a useful insight into a phenomenon and avoids the ethical and practical difficulties of setting up a large and cumbersome research project.
- Observational studies are usually flexible and do not necessarily need to be structured around a hypothesis about what you expect to observe [data is emergent rather than pre-existing].
- The researcher is able to collect in-depth information about a particular behavior.
- Can reveal interrelationships among multifaceted dimensions of group interactions.
- You can generalize your results to real life situations.
- Observational research is useful for discovering what variables may be important before applying other methods like experiments.
- Observation research designs account for the complexity of group behaviors.
- Reliability of data is low because seeing behaviors occur over and over again may be a time consuming task and are difficult to replicate.
- In observational research, findings may only reflect a unique sample population and, thus, cannot be generalized to other groups.
- There can be problems with bias as the researcher may only "see what they want to see."
- There is no possibility to determine "cause and effect" relationships since nothing is manipulated.
- Sources or subjects may not all be equally credible.
- Any group that is knowingly studied is altered to some degree by the presence of the researcher, therefore, potentially skewing any data collected.
Atkinson, Paul and Martyn Hammersley. “Ethnography and Participant Observation.” In Handbook of Qualitative Research . Norman K. Denzin and Yvonna S. Lincoln, eds. (Thousand Oaks, CA: Sage, 1994), pp. 248-261; Observational Research. Research Methods by Dummies. Department of Psychology. California State University, Fresno, 2006; Patton Michael Quinn. Qualitiative Research and Evaluation Methods . Chapter 6, Fieldwork Strategies and Observational Methods. 3rd ed. Thousand Oaks, CA: Sage, 2002; Payne, Geoff and Judy Payne. "Observation." In Key Concepts in Social Research . The SAGE Key Concepts series. (London, England: Sage, 2004), pp. 158-162; Rosenbaum, Paul R. Design of Observational Studies . New York: Springer, 2010;Williams, J. Patrick. "Nonparticipant Observation." In The Sage Encyclopedia of Qualitative Research Methods . Lisa M. Given, editor.(Thousand Oaks, CA: Sage, 2008), pp. 562-563.
Philosophical Design
Understood more as an broad approach to examining a research problem than a methodological design, philosophical analysis and argumentation is intended to challenge deeply embedded, often intractable, assumptions underpinning an area of study. This approach uses the tools of argumentation derived from philosophical traditions, concepts, models, and theories to critically explore and challenge, for example, the relevance of logic and evidence in academic debates, to analyze arguments about fundamental issues, or to discuss the root of existing discourse about a research problem. These overarching tools of analysis can be framed in three ways:
- Ontology -- the study that describes the nature of reality; for example, what is real and what is not, what is fundamental and what is derivative?
- Epistemology -- the study that explores the nature of knowledge; for example, by what means does knowledge and understanding depend upon and how can we be certain of what we know?
- Axiology -- the study of values; for example, what values does an individual or group hold and why? How are values related to interest, desire, will, experience, and means-to-end? And, what is the difference between a matter of fact and a matter of value?
- Can provide a basis for applying ethical decision-making to practice.
- Functions as a means of gaining greater self-understanding and self-knowledge about the purposes of research.
- Brings clarity to general guiding practices and principles of an individual or group.
- Philosophy informs methodology.
- Refine concepts and theories that are invoked in relatively unreflective modes of thought and discourse.
- Beyond methodology, philosophy also informs critical thinking about epistemology and the structure of reality (metaphysics).
- Offers clarity and definition to the practical and theoretical uses of terms, concepts, and ideas.
- Limited application to specific research problems [answering the "So What?" question in social science research].
- Analysis can be abstract, argumentative, and limited in its practical application to real-life issues.
- While a philosophical analysis may render problematic that which was once simple or taken-for-granted, the writing can be dense and subject to unnecessary jargon, overstatement, and/or excessive quotation and documentation.
- There are limitations in the use of metaphor as a vehicle of philosophical analysis.
- There can be analytical difficulties in moving from philosophy to advocacy and between abstract thought and application to the phenomenal world.
Burton, Dawn. "Part I, Philosophy of the Social Sciences." In Research Training for Social Scientists . (London, England: Sage, 2000), pp. 1-5; Chapter 4, Research Methodology and Design. Unisa Institutional Repository (UnisaIR), University of South Africa; Jarvie, Ian C., and Jesús Zamora-Bonilla, editors. The SAGE Handbook of the Philosophy of Social Sciences . London: Sage, 2011; Labaree, Robert V. and Ross Scimeca. “The Philosophical Problem of Truth in Librarianship.” The Library Quarterly 78 (January 2008): 43-70; Maykut, Pamela S. Beginning Qualitative Research: A Philosophic and Practical Guide . Washington, DC: Falmer Press, 1994; McLaughlin, Hugh. "The Philosophy of Social Research." In Understanding Social Work Research . 2nd edition. (London: SAGE Publications Ltd., 2012), pp. 24-47; Stanford Encyclopedia of Philosophy . Metaphysics Research Lab, CSLI, Stanford University, 2013.
Sequential Design
- The researcher has a limitless option when it comes to sample size and the sampling schedule.
- Due to the repetitive nature of this research design, minor changes and adjustments can be done during the initial parts of the study to correct and hone the research method.
- This is a useful design for exploratory studies.
- There is very little effort on the part of the researcher when performing this technique. It is generally not expensive, time consuming, or workforce intensive.
- Because the study is conducted serially, the results of one sample are known before the next sample is taken and analyzed. This provides opportunities for continuous improvement of sampling and methods of analysis.
- The sampling method is not representative of the entire population. The only possibility of approaching representativeness is when the researcher chooses to use a very large sample size significant enough to represent a significant portion of the entire population. In this case, moving on to study a second or more specific sample can be difficult.
- The design cannot be used to create conclusions and interpretations that pertain to an entire population because the sampling technique is not randomized. Generalizability from findings is, therefore, limited.
- Difficult to account for and interpret variation from one sample to another over time, particularly when using qualitative methods of data collection.
Betensky, Rebecca. Harvard University, Course Lecture Note slides; Bovaird, James A. and Kevin A. Kupzyk. "Sequential Design." In Encyclopedia of Research Design . Neil J. Salkind, editor. (Thousand Oaks, CA: Sage, 2010), pp. 1347-1352; Cresswell, John W. Et al. “Advanced Mixed-Methods Research Designs.” In Handbook of Mixed Methods in Social and Behavioral Research . Abbas Tashakkori and Charles Teddle, eds. (Thousand Oaks, CA: Sage, 2003), pp. 209-240; Henry, Gary T. "Sequential Sampling." In The SAGE Encyclopedia of Social Science Research Methods . Michael S. Lewis-Beck, Alan Bryman and Tim Futing Liao, editors. (Thousand Oaks, CA: Sage, 2004), pp. 1027-1028; Nataliya V. Ivankova. “Using Mixed-Methods Sequential Explanatory Design: From Theory to Practice.” Field Methods 18 (February 2006): 3-20; Bovaird, James A. and Kevin A. Kupzyk. “Sequential Design.” In Encyclopedia of Research Design . Neil J. Salkind, ed. Thousand Oaks, CA: Sage, 2010; Sequential Analysis. Wikipedia.
Systematic Review
- A systematic review synthesizes the findings of multiple studies related to each other by incorporating strategies of analysis and interpretation intended to reduce biases and random errors.
- The application of critical exploration, evaluation, and synthesis methods separates insignificant, unsound, or redundant research from the most salient and relevant studies worthy of reflection.
- They can be use to identify, justify, and refine hypotheses, recognize and avoid hidden problems in prior studies, and explain data inconsistencies and conflicts in data.
- Systematic reviews can be used to help policy makers formulate evidence-based guidelines and regulations.
- The use of strict, explicit, and pre-determined methods of synthesis, when applied appropriately, provide reliable estimates about the effects of interventions, evaluations, and effects related to the overarching research problem investigated by each study under review.
- Systematic reviews illuminate where knowledge or thorough understanding of a research problem is lacking and, therefore, can then be used to guide future research.
- The accepted inclusion of unpublished studies [i.e., grey literature] ensures the broadest possible way to analyze and interpret research on a topic.
- Results of the synthesis can be generalized and the findings extrapolated into the general population with more validity than most other types of studies .
- Systematic reviews do not create new knowledge per se; they are a method for synthesizing existing studies about a research problem in order to gain new insights and determine gaps in the literature.
- The way researchers have carried out their investigations [e.g., the period of time covered, number of participants, sources of data analyzed, etc.] can make it difficult to effectively synthesize studies.
- The inclusion of unpublished studies can introduce bias into the review because they may not have undergone a rigorous peer-review process prior to publication. Examples may include conference presentations or proceedings, publications from government agencies, white papers, working papers, and internal documents from organizations, and doctoral dissertations and Master's theses.
Denyer, David and David Tranfield. "Producing a Systematic Review." In The Sage Handbook of Organizational Research Methods . David A. Buchanan and Alan Bryman, editors. ( Thousand Oaks, CA: Sage Publications, 2009), pp. 671-689; Foster, Margaret J. and Sarah T. Jewell, editors. Assembling the Pieces of a Systematic Review: A Guide for Librarians . Lanham, MD: Rowman and Littlefield, 2017; Gough, David, Sandy Oliver, James Thomas, editors. Introduction to Systematic Reviews . 2nd edition. Los Angeles, CA: Sage Publications, 2017; Gopalakrishnan, S. and P. Ganeshkumar. “Systematic Reviews and Meta-analysis: Understanding the Best Evidence in Primary Healthcare.” Journal of Family Medicine and Primary Care 2 (2013): 9-14; Gough, David, James Thomas, and Sandy Oliver. "Clarifying Differences between Review Designs and Methods." Systematic Reviews 1 (2012): 1-9; Khan, Khalid S., Regina Kunz, Jos Kleijnen, and Gerd Antes. “Five Steps to Conducting a Systematic Review.” Journal of the Royal Society of Medicine 96 (2003): 118-121; Mulrow, C. D. “Systematic Reviews: Rationale for Systematic Reviews.” BMJ 309:597 (September 1994); O'Dwyer, Linda C., and Q. Eileen Wafford. "Addressing Challenges with Systematic Review Teams through Effective Communication: A Case Report." Journal of the Medical Library Association 109 (October 2021): 643-647; Okoli, Chitu, and Kira Schabram. "A Guide to Conducting a Systematic Literature Review of Information Systems Research." Sprouts: Working Papers on Information Systems 10 (2010); Siddaway, Andy P., Alex M. Wood, and Larry V. Hedges. "How to Do a Systematic Review: A Best Practice Guide for Conducting and Reporting Narrative Reviews, Meta-analyses, and Meta-syntheses." Annual Review of Psychology 70 (2019): 747-770; Torgerson, Carole J. “Publication Bias: The Achilles’ Heel of Systematic Reviews?” British Journal of Educational Studies 54 (March 2006): 89-102; Torgerson, Carole. Systematic Reviews . New York: Continuum, 2003.
- << Previous: Purpose of Guide
- Next: Design Flaws to Avoid >>
- Last Updated: May 25, 2024 4:09 PM
- URL: https://libguides.usc.edu/writingguide
- Privacy Policy

Home » Research Design – Types, Methods and Examples
Research Design – Types, Methods and Examples
Table of Contents

Research Design
Definition:
Research design refers to the overall strategy or plan for conducting a research study. It outlines the methods and procedures that will be used to collect and analyze data, as well as the goals and objectives of the study. Research design is important because it guides the entire research process and ensures that the study is conducted in a systematic and rigorous manner.
Types of Research Design
Types of Research Design are as follows:
Descriptive Research Design
This type of research design is used to describe a phenomenon or situation. It involves collecting data through surveys, questionnaires, interviews, and observations. The aim of descriptive research is to provide an accurate and detailed portrayal of a particular group, event, or situation. It can be useful in identifying patterns, trends, and relationships in the data.
Correlational Research Design
Correlational research design is used to determine if there is a relationship between two or more variables. This type of research design involves collecting data from participants and analyzing the relationship between the variables using statistical methods. The aim of correlational research is to identify the strength and direction of the relationship between the variables.
Experimental Research Design
Experimental research design is used to investigate cause-and-effect relationships between variables. This type of research design involves manipulating one variable and measuring the effect on another variable. It usually involves randomly assigning participants to groups and manipulating an independent variable to determine its effect on a dependent variable. The aim of experimental research is to establish causality.
Quasi-experimental Research Design
Quasi-experimental research design is similar to experimental research design, but it lacks one or more of the features of a true experiment. For example, there may not be random assignment to groups or a control group. This type of research design is used when it is not feasible or ethical to conduct a true experiment.
Case Study Research Design
Case study research design is used to investigate a single case or a small number of cases in depth. It involves collecting data through various methods, such as interviews, observations, and document analysis. The aim of case study research is to provide an in-depth understanding of a particular case or situation.
Longitudinal Research Design
Longitudinal research design is used to study changes in a particular phenomenon over time. It involves collecting data at multiple time points and analyzing the changes that occur. The aim of longitudinal research is to provide insights into the development, growth, or decline of a particular phenomenon over time.
Structure of Research Design
The format of a research design typically includes the following sections:
- Introduction : This section provides an overview of the research problem, the research questions, and the importance of the study. It also includes a brief literature review that summarizes previous research on the topic and identifies gaps in the existing knowledge.
- Research Questions or Hypotheses: This section identifies the specific research questions or hypotheses that the study will address. These questions should be clear, specific, and testable.
- Research Methods : This section describes the methods that will be used to collect and analyze data. It includes details about the study design, the sampling strategy, the data collection instruments, and the data analysis techniques.
- Data Collection: This section describes how the data will be collected, including the sample size, data collection procedures, and any ethical considerations.
- Data Analysis: This section describes how the data will be analyzed, including the statistical techniques that will be used to test the research questions or hypotheses.
- Results : This section presents the findings of the study, including descriptive statistics and statistical tests.
- Discussion and Conclusion : This section summarizes the key findings of the study, interprets the results, and discusses the implications of the findings. It also includes recommendations for future research.
- References : This section lists the sources cited in the research design.
Example of Research Design
An Example of Research Design could be:
Research question: Does the use of social media affect the academic performance of high school students?
Research design:
- Research approach : The research approach will be quantitative as it involves collecting numerical data to test the hypothesis.
- Research design : The research design will be a quasi-experimental design, with a pretest-posttest control group design.
- Sample : The sample will be 200 high school students from two schools, with 100 students in the experimental group and 100 students in the control group.
- Data collection : The data will be collected through surveys administered to the students at the beginning and end of the academic year. The surveys will include questions about their social media usage and academic performance.
- Data analysis : The data collected will be analyzed using statistical software. The mean scores of the experimental and control groups will be compared to determine whether there is a significant difference in academic performance between the two groups.
- Limitations : The limitations of the study will be acknowledged, including the fact that social media usage can vary greatly among individuals, and the study only focuses on two schools, which may not be representative of the entire population.
- Ethical considerations: Ethical considerations will be taken into account, such as obtaining informed consent from the participants and ensuring their anonymity and confidentiality.
How to Write Research Design
Writing a research design involves planning and outlining the methodology and approach that will be used to answer a research question or hypothesis. Here are some steps to help you write a research design:
- Define the research question or hypothesis : Before beginning your research design, you should clearly define your research question or hypothesis. This will guide your research design and help you select appropriate methods.
- Select a research design: There are many different research designs to choose from, including experimental, survey, case study, and qualitative designs. Choose a design that best fits your research question and objectives.
- Develop a sampling plan : If your research involves collecting data from a sample, you will need to develop a sampling plan. This should outline how you will select participants and how many participants you will include.
- Define variables: Clearly define the variables you will be measuring or manipulating in your study. This will help ensure that your results are meaningful and relevant to your research question.
- Choose data collection methods : Decide on the data collection methods you will use to gather information. This may include surveys, interviews, observations, experiments, or secondary data sources.
- Create a data analysis plan: Develop a plan for analyzing your data, including the statistical or qualitative techniques you will use.
- Consider ethical concerns : Finally, be sure to consider any ethical concerns related to your research, such as participant confidentiality or potential harm.
When to Write Research Design
Research design should be written before conducting any research study. It is an important planning phase that outlines the research methodology, data collection methods, and data analysis techniques that will be used to investigate a research question or problem. The research design helps to ensure that the research is conducted in a systematic and logical manner, and that the data collected is relevant and reliable.
Ideally, the research design should be developed as early as possible in the research process, before any data is collected. This allows the researcher to carefully consider the research question, identify the most appropriate research methodology, and plan the data collection and analysis procedures in advance. By doing so, the research can be conducted in a more efficient and effective manner, and the results are more likely to be valid and reliable.
Purpose of Research Design
The purpose of research design is to plan and structure a research study in a way that enables the researcher to achieve the desired research goals with accuracy, validity, and reliability. Research design is the blueprint or the framework for conducting a study that outlines the methods, procedures, techniques, and tools for data collection and analysis.
Some of the key purposes of research design include:
- Providing a clear and concise plan of action for the research study.
- Ensuring that the research is conducted ethically and with rigor.
- Maximizing the accuracy and reliability of the research findings.
- Minimizing the possibility of errors, biases, or confounding variables.
- Ensuring that the research is feasible, practical, and cost-effective.
- Determining the appropriate research methodology to answer the research question(s).
- Identifying the sample size, sampling method, and data collection techniques.
- Determining the data analysis method and statistical tests to be used.
- Facilitating the replication of the study by other researchers.
- Enhancing the validity and generalizability of the research findings.
Applications of Research Design
There are numerous applications of research design in various fields, some of which are:
- Social sciences: In fields such as psychology, sociology, and anthropology, research design is used to investigate human behavior and social phenomena. Researchers use various research designs, such as experimental, quasi-experimental, and correlational designs, to study different aspects of social behavior.
- Education : Research design is essential in the field of education to investigate the effectiveness of different teaching methods and learning strategies. Researchers use various designs such as experimental, quasi-experimental, and case study designs to understand how students learn and how to improve teaching practices.
- Health sciences : In the health sciences, research design is used to investigate the causes, prevention, and treatment of diseases. Researchers use various designs, such as randomized controlled trials, cohort studies, and case-control studies, to study different aspects of health and healthcare.
- Business : Research design is used in the field of business to investigate consumer behavior, marketing strategies, and the impact of different business practices. Researchers use various designs, such as survey research, experimental research, and case studies, to study different aspects of the business world.
- Engineering : In the field of engineering, research design is used to investigate the development and implementation of new technologies. Researchers use various designs, such as experimental research and case studies, to study the effectiveness of new technologies and to identify areas for improvement.
Advantages of Research Design
Here are some advantages of research design:
- Systematic and organized approach : A well-designed research plan ensures that the research is conducted in a systematic and organized manner, which makes it easier to manage and analyze the data.
- Clear objectives: The research design helps to clarify the objectives of the study, which makes it easier to identify the variables that need to be measured, and the methods that need to be used to collect and analyze data.
- Minimizes bias: A well-designed research plan minimizes the chances of bias, by ensuring that the data is collected and analyzed objectively, and that the results are not influenced by the researcher’s personal biases or preferences.
- Efficient use of resources: A well-designed research plan helps to ensure that the resources (time, money, and personnel) are used efficiently and effectively, by focusing on the most important variables and methods.
- Replicability: A well-designed research plan makes it easier for other researchers to replicate the study, which enhances the credibility and reliability of the findings.
- Validity: A well-designed research plan helps to ensure that the findings are valid, by ensuring that the methods used to collect and analyze data are appropriate for the research question.
- Generalizability : A well-designed research plan helps to ensure that the findings can be generalized to other populations, settings, or situations, which increases the external validity of the study.
Research Design Vs Research Methodology
About the author.
Muhammad Hassan
Researcher, Academic Writer, Web developer
You may also like

How to Cite Research Paper – All Formats and...

Data Collection – Methods Types and Examples

Delimitations in Research – Types, Examples and...

Research Paper Format – Types, Examples and...

Research Process – Steps, Examples and Tips

Institutional Review Board – Application Sample...
Leave a comment x.
Save my name, email, and website in this browser for the next time I comment.

An official website of the United States government
The .gov means it’s official. Federal government websites often end in .gov or .mil. Before sharing sensitive information, make sure you’re on a federal government site.
The site is secure. The https:// ensures that you are connecting to the official website and that any information you provide is encrypted and transmitted securely.
- Publications
- Account settings
Preview improvements coming to the PMC website in October 2024. Learn More or Try it out now .
- Advanced Search
- Journal List
- Indian J Crit Care Med
- v.23(Suppl 4); 2019 Dec
Understanding Research Study Designs
Priya ranganathan.
Department of Anesthesiology, Critical Care and Pain, Tata Memorial Hospital, Mumbai, Maharashtra, India
In this article, we will look at the important features of various types of research study designs used commonly in biomedical research.
How to cite this article
Ranganathan P. Understanding Research Study Designs. Indian J Crit Care Med 2019;23(Suppl 4):S305–S307.
We use a variety of research study designs in biomedical research. In this article, the main features of each of these designs are summarized.
TERMS USED IN RESEARCH DESIGNS
Exposure vs outcome.
Exposure refers to any factor that may be associated with the outcome of interest. It is also called the predictor variable or independent variable or risk factor. Outcome refers to the variable that is studied to assess the impact of the exposure on the population. It is also known as the predicted variable or the dependent variable. For example, in a study looking at nerve damage after organophosphate (OPC) poisoning, the exposure would be OPC and the outcome would be nerve damage.
Longitudinal vs Transversal Studies
In longitudinal studies, participants are followed over time to determine the association between exposure and outcome (or outcome and exposure). On the other hand, in transversal studies, observations about exposure and outcome are made at a single point in time.
Forward vs Backward Directed Studies
In forward-directed studies, the direction of enquiry moves from exposure to outcome. In backward-directed studies, the line of enquiry starts with outcome and then determines exposure.
Prospective vs Retrospective Studies
In prospective studies, the outcome has not occurred at the time of initiation of the study. The researcher determines exposure and follows participants into the future to assess outcomes. In retrospective studies, the outcome of interest has already occurred when the study commences.
CLASSIFICATION OF STUDY DESIGNS
Broadly, study designs can be classified as descriptive or analytical (inferential) studies.
Descriptive Studies
Descriptive studies describe the characteristics of interest in the study population (also referred to as sample, to differentiate it from the entire population in the universe). These studies do not have a comparison group. The simplest type of descriptive study is the case report. In a case report, the researcher describes his/her experience with symptoms, signs, diagnosis, or treatment of a patient. Sometimes, a group of patients having a similar experience may be grouped to form a case series.
Case reports and case series form the lowest level of evidence in biomedical research and, as such, are considered hypothesis-generating studies. However, they are easy to write and may be a good starting point for the budding researcher. The recognition of some important associations in the field of medicine—such as that of thalidomide with phocomelia and Kaposi's sarcoma with HIV infection—resulted from case reports and case series. The reader can look up several published case reports and case series related to complications after OPC poisoning. 1 , 2
Analytical (Inferential) Studies
Analytical or inferential studies try to prove a hypothesis and establish an association between an exposure and an outcome. These studies usually have a comparator group. Analytical studies are further classified as observational or interventional studies.
In observational studies, there is no intervention by the researcher. The researcher merely observes outcomes in different groups of participants who, for natural reasons, have or have not been exposed to a particular risk factor. Examples of observational studies include cross-sectional, case–control, and cohort studies.
Cross-sectional Studies
These are transversal studies where data are collected from the study population at a single point in time. Exposure and outcome are determined simultaneously. Cross-sectional studies are easy to conduct, involve no follow-up, and need limited resources. They offer useful information on prevalence of health conditions and possible associations between risk factors and outcomes. However, there are two major limitations of cross-sectional studies. First, it may not be possible to establish a clear cause–benefit relationship. For example, in a study of association between colon cancer and dietary fiber intake, it may be difficult to establish whether the low fiber intake preceded the symptoms of colon cancer or whether the symptoms of colon cancer resulted in a change in dietary fiber intake. Another important limitation of cross-sectional studies is survival bias. For example, in a study looking at alcohol intake vs mortality due to chronic liver disease, among the participants with the highest alcohol intake, several may have died of liver disease; this will not be picked up by the study and will give biased results. An example of a cross-sectional study is a survey on nurses’ knowledge and practices of initial management of acute poisoning. 3
Case–control Studies
Case–control studies are backward-directed studies. Here, the direction of enquiry begins with the outcome and then proceeds to exposure. Case–control studies are always retrospective, i.e., the outcome of interest has occurred when the study begins. The researcher identifies participants who have developed the outcome of interest (cases) and chooses matching participants who do not have the outcome (controls). Matching is done based on factors that are likely to influence the exposure or outcome (e.g., age, gender, socioeconomic status). The researcher then proceeds to determine exposure in cases and controls. If cases have a higher incidence of exposure than controls, it suggests an association between exposure and outcome. Case–control studies are relatively quick to conduct, need limited resources, and are useful when the outcome is rare. They also allow the researcher to study multiple exposures for a particular outcome. However, they have several limitations. First, matching of cases with controls may not be easy since many unknown confounders may affect exposure and outcome. Second, there may be biased in the way the history of exposure is determined in cases vs controls; one way to overcome this is to have a blinded assessor determining the exposure using a standard technique (e.g., a standardized questionnaire). However, despite this, it has been shown that cases are far more likely than controls to recall history of exposure—the “recall bias.” For example, mothers of babies born with congenital anomalies may provide a more detailed history of drugs ingested during their pregnancy than those with normal babies. Also, since case-control studies do not begin with a population at risk, it is not possible to determine the true risk of outcome. Instead, one can only calculate the odds of association between exposure and outcome.
Kendrick and colleagues designed a case–control study to look at the association between domestic poison prevention practices and medically attended poisoning in children. They identified children presenting with unintentional poisoning at home (cases with the outcome), matched them with community participants (controls without the outcome), and then elicited data from parents and caregivers on home safety practices (exposure). 4
Cohort Studies
Cohort studies resemble clinical trials except that the exposure is naturally determined instead of being decided by the investigator. Here, the direction of enquiry begins with the exposure and then proceeds to outcome. The researcher begins with a group of individuals who are free of outcome at baseline; of these, some have the exposure (study cohort) while others do not (control group). The groups are followed up over a period of time to determine occurrence of outcome. Cohort studies may be prospective (involving a period of follow-up after the start of the study) or retrospective (e.g., using medical records or registry data). Cohort studies are considered the strongest among the observational study designs. They provide proof of temporal relationship (exposure occurred before outcome), allow determination of risk, and permit multiple outcomes to be studied for a single exposure. However, they are expensive to conduct and time-consuming, there may be several losses to follow-up, and they are not suitable for studying rare outcomes. Also, there may be unknown confounders other than the exposure affecting the occurrence of the outcome.
Jayasinghe conducted a cohort study to look at the effect of acute organophosphorus poisoning on nerve function. They recruited 70 patients with OPC poisoning (exposed group) and 70 matched controls without history of pesticide exposure (unexposed controls). Participants were followed up or 6 weeks for neurophysiological assessments to determine nerve damage (outcome). Hung carried out a retrospective cohort study using a nationwide research database to look at the long-term effects of OPC poisoning on cardiovascular disease. From the database, he identified an OPC-exposed cohort and an unexposed control cohort (matched for gender and age) from several years back and then examined later records to look at the development of cardiovascular diseases in both groups. 5
Interventional Studies
In interventional studies (also known as experimental studies or clinical trials), the researcher deliberately allots participants to receive one of several interventions; of these, some may be experimental while others may be controls (either standard of care or placebo). Allotment of participants to a particular treatment arm is carried out through the process of randomization, which ensures that every participant has a similar chance of being in any of the arms, eliminating bias in selection. There are several other aspects crucial to the validity of the results of a clinical trial such as allocation concealment, blinding, choice of control, and statistical analysis plan. These will be discussed in a separate article.
The randomized controlled clinical trial is considered the gold standard for evaluating the efficacy of a treatment. Randomization leads to equal distribution of known and unknown confounders between treatment arms; therefore, we can be reasonably certain that any difference in outcome is a treatment effect and not due to other factors. The temporal sequence of cause and effect is established. It is possible to determine risk of the outcome in each treatment arm accurately. However, randomized controlled trials have their limitations and may not be possible in every situation. For example, it is unethical to randomize participants to an intervention that is likely to cause harm—e.g., smoking. In such cases, well-designed observational studies are the only option. Also, these trials are expensive to conduct and resource-intensive.
In a randomized controlled trial, Li et al. randomly allocated patients of paraquat poisoning to receive either conventional therapy (control group) or continuous veno-venous hemofiltration (intervention). Patients were followed up to look for mortality or other adverse events (outcome). 6
Researchers need to understand the features of different study designs, with their advantages and limitations so that the most appropriate design can be chosen for a particular research question. The Centre for Evidence Based Medicine offers an useful tool to determine the type of research design used in a particular study. 7
Source of support: Nil
Conflict of interest: None

- SUGGESTED TOPICS
- The Magazine
- Newsletters
- Managing Yourself
- Managing Teams
- Work-life Balance
- The Big Idea
- Data & Visuals
- Reading Lists
- Case Selections
- HBR Learning
- Topic Feeds
- Account Settings
- Email Preferences
What Job Crafting Looks Like
- Jane E. Dutton
- Amy Wrzesniewski

Stories of three people who changed their jobs to find more meaning.
Twenty years ago, the authors started studying job crafting — the act of altering your job to make it more meaningful. Since then, they’ve identified different forms this concept can take. They include: task crafting , which involves changing the type, scope, sequence, and number of tasks that make up your job; relational crafting, where you alter who you interact with in your work; and cognitive crafting , where you modify the way you interpret the tasks and/or work you’re doing. The authors share stories of three individuals that illustrate what each of these types look like and how employees were able to make their jobs more meaningful and engaging.
Job crafting — changing your job to make it more engaging and meaningful — can take many forms. We’ve been studying job crafting for 20 years and our research among hospital cleaners , employees in a manufacturing firm, a women’s advocacy nonprofit , and tech workers identified three main forms these changes can take.

- JD Jane E. Dutton is the Robert L. Kahn Distinguished University Professor of Business Administration and Psychology at the University of Michigan’s Ross School of Business. She is co-founder of the Center for Positive Organizations at Ross.
- AW Amy Wrzesniewski ( [email protected] ) is a professor of organizational behavior at the Yale School of Management.
Partner Center
- This transformative approach breaks away from the single chip per package model, improving performance and time to market while reducing manufacturing costs and power consumption.
- Advanced multichip packaging substantially minimizes the semiconductor form factor, making it well suited for mobile devices, automotive computing, and generative artificial intelligence (GenAI).
- Primary changes in chip industry structure will be evident through elevating the role of system design, shifting focus from the front end to the back end, and adapting to greater complexity.
- In this new environment, each segment of the chipmaking ecosystem—including fabless chipmakers, foundries, integrated device manufacturers and outsourced semiconductor assembly and test vendors—will have to alter their business models to remain profitable.
Subscribe to receive the latest insights on Technology, Media, and Telecommunications.
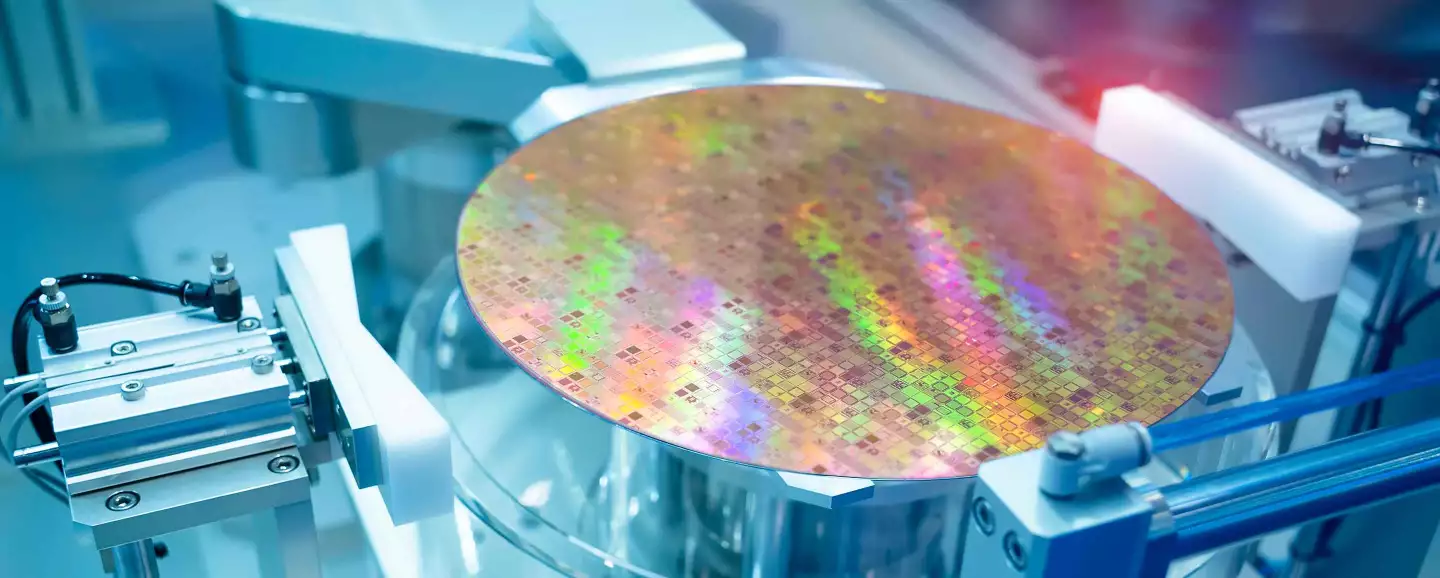
Semiconductors
/ article, advanced packaging is radically reshaping the chip ecosystem.
By Joseph Fitzgerald , Jan-Hinnerk Mohr , Nadim Sah , Jörg Jeschke , Karl Breidenbach , Nicola Kerschbaumer , Sohini Kar , and Ramiro Palma
Key Takeaways
Periodically, analysts commenting on the chip industry will pronounce that Moore’s Law is obsolete. Yet while the speed of progress is slowing down, chipmakers are still doubling the number of transistors on an integrated circuit (IC) approximately every 2.5 years. In the past, fundamentally new approaches for chip design never seemed to catch on. That’s no longer the case as fresh ideas that are essential for running some of today’s most important applications are rapidly gaining favor.
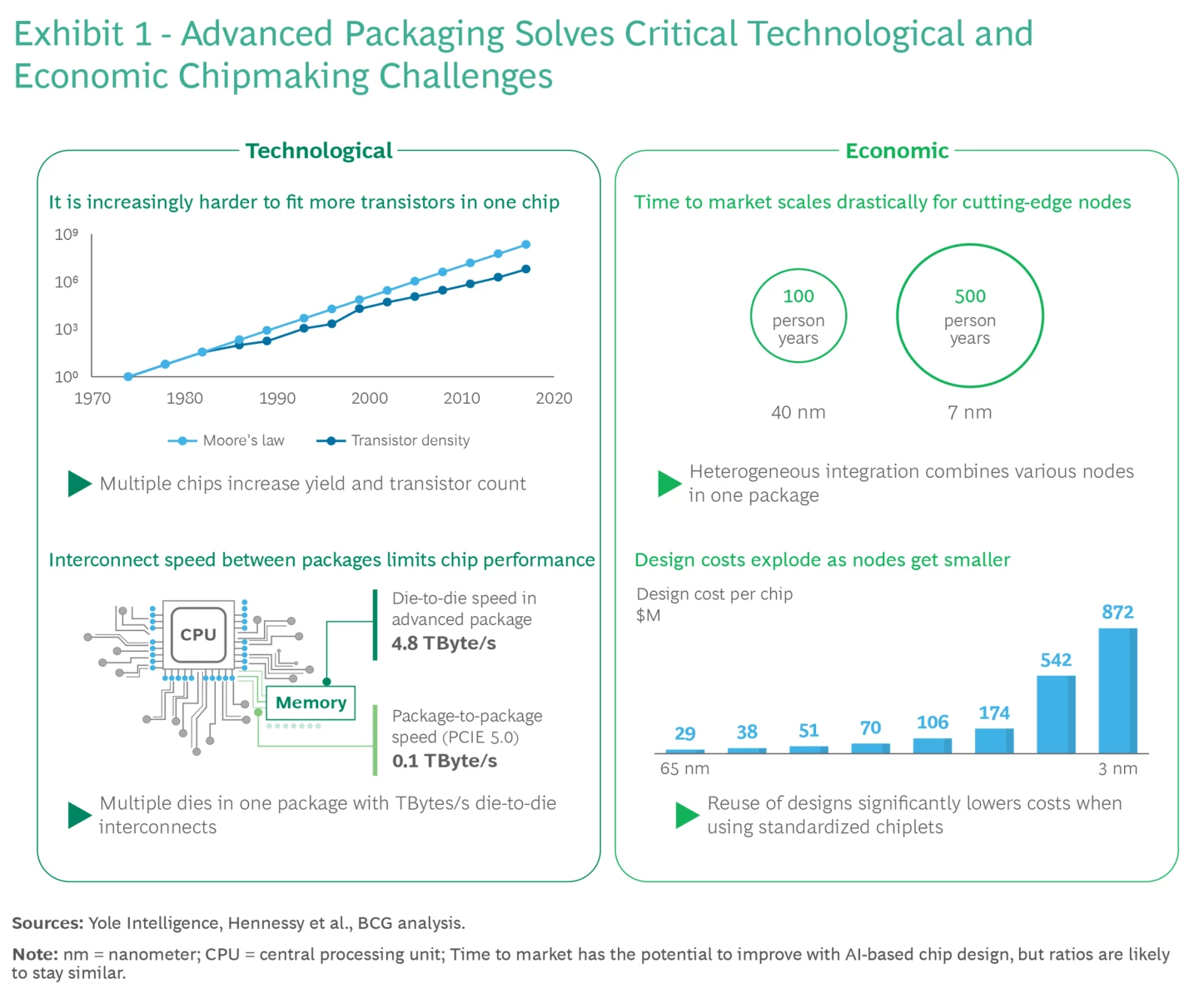
One of the most significant of these new concepts is advanced packaging, which essentially accommodates an ever-increasing number of transistors by decreasing the size of electrical contacts. Traditionally, a semiconductor chip focuses on performing one particular action or process. By comparison, an advanced multichip package incorporates multiple chips and processes into one component. This transformative approach integrates a multitude of semiconductor components into a single package, directly addressing the most critical semiconductor technical and commercial constraints. (See Exhibit 1.)
Advanced multichip packaging improves performance and time to market while reducing chip manufacturing costs and power consumption. Moreover, because it is such a radical change in chip integration, unlocking greater functionality and a reduced form factor, advanced packaging of chips is perfectly suited for key applications such as mobile devices and, in the coming years, automotive computing and generative artificial intelligence (GenAI).
For chipmakers, investors, and computing device and equipment makers, the emergence of advanced packaging represents a radical shift in the semiconductor industry landscape. The next generation of industry-leading organizations will be those that realize value creation is migrating towards companies that can design and integrate complex, system-level chip solutions using concepts like advanced packaging. Merely making individual components will rapidly lose luster, replaced by collaborative efforts that bring together the best in design, packaging, and system integration to meet the demands of the market.
Package or Perish
We are now in the “More than Moore” era. As predicted by Gordon Moore himself, it was inevitable that his law would eventually meet a threshold of diminishing economic returns. As the industry reaches the physical limitations of transistor density and chip size, the costs to cram more into a small space are exploding. But rather than try to squeeze additional transistors onto a single chip, advanced multichip packaging enables the combining of smaller, cost- and performance-optimized dies—the unpackaged semiconductor chips comprised of functional integrated circuits. It does so by using high-bandwidth interconnects and the latest front-end or wafer fabrication technologies to unleash the combined power of the packaged components. Perhaps most importantly, each of these components can have a different purpose, with computing and memory running on smaller technology nodes than components that have already reached their scaling limits, such as input/output blocks.
These components can be placed in closer proximity on advanced substrates, driving data transfer rates up to 35 times faster than the latest motherboard. This also allows for the reuse of die designs across multiple systems because the modular components can be combined in any number of ways to drive various and varied applications. By contrast, more widely used systems on a chip (SoC) are only designed for a single application.
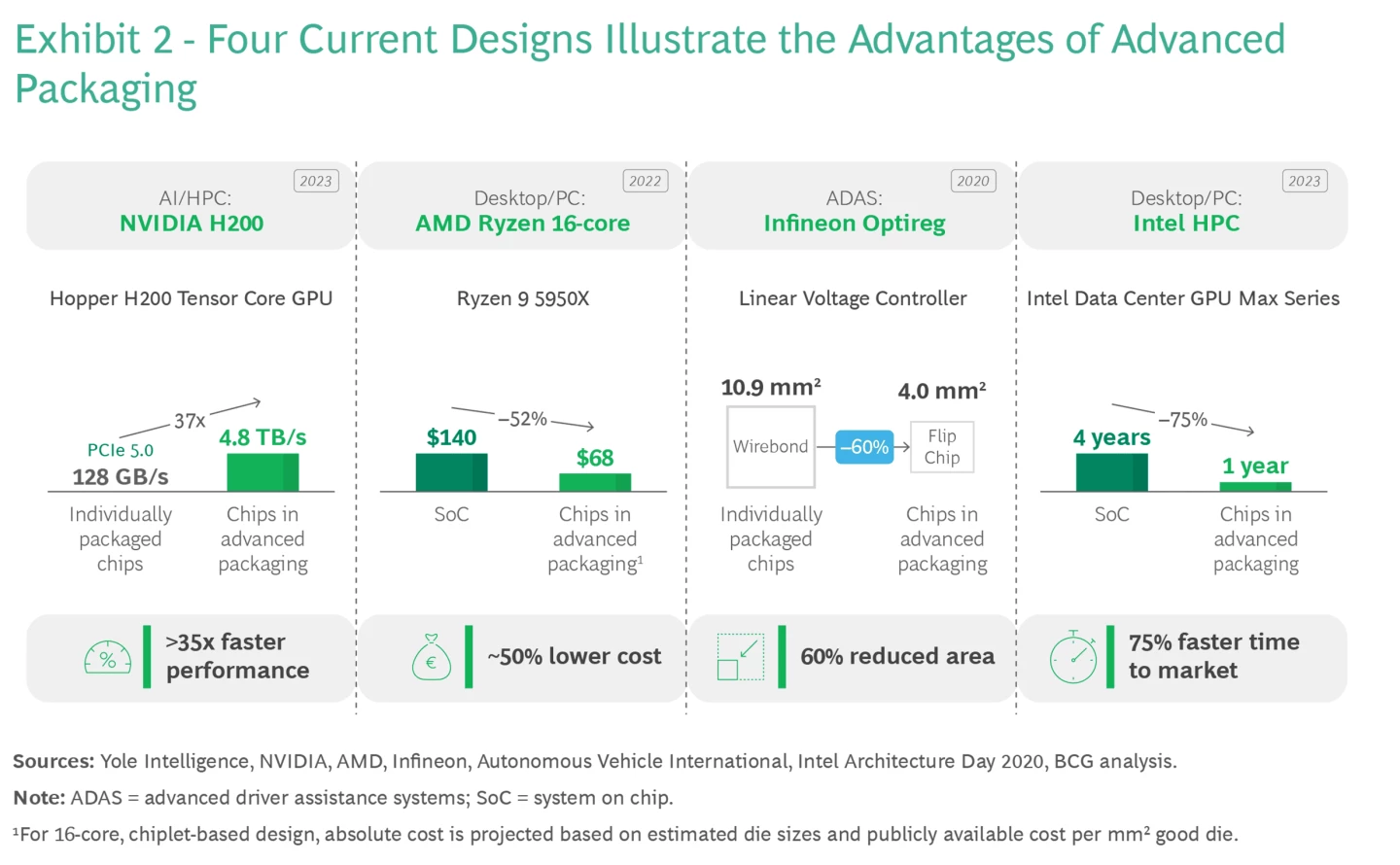
Four examples illustrate the advantages of advanced packaging. (See Exhibit 2.) The NVIDIA Hopper H200 is one of the most innovative AI core graphics processing units (GPUs) today. Six high-bandwidth memory (HBM) stacks are packaged with the central IC chips, allowing for interconnection speeds of up to 4.8 TB/s. Conventional systems, where chips are connected via a printed circuit board, are typically limited to less than 200 GB/s. Close interconnect distances also drastically decrease the power consumption of these chips, making the data centers that power large language models (LLMs) economically feasible.
The second example is the AMD Ryzen series. By opting for multiple smaller chips rather than a single large SoC, designers were able to select from a range of node sizes for each of the heterogeneously integrated dies and choose chips that were optimized for each of the package’s functions. This approach cut manufacturing costs by as much as 50%. The smaller die sizes contribute to this cost reduction by increasing fabrication yield rates because the same number of defects on a wafer is distributed across more dies.
The third illustration involves chip area size. By integrating the individual chips used in Optireg linear voltage controllers into an advanced package, Infineon was able to reduce the part’s footprint by 60%. Condensing the space required for complex systems enables a new generation of compact devices without compromising computational power or functionality. This miniaturization is key in industries where space is at a premium, such as in mobile and Internet of Things (IoT) devices, small medical equipment like hearing aids and pacemakers, and automotive computing systems.
Finally, Intel, among other integrated design manufacturers (IDMs), has greatly expanded investment in the manufacturing sides of its business to develop advanced multichip packaging capabilities. Intel has already shown that switching from a single large SoC to multiple dies in its Data Center GPU Max Series can minimize die complexity and allow existing die designs to be reused in multiple packages. In the process, Intel found that this can reduce time to market by up to 75%.
Advanced Packaging Shakes Up the Value Chain
Advanced packaging accounts for about 8% of the total semiconductor market today and is projected to double by 2030 to more than $96 billion, outpacing the rest of the chip industry. Currently, consumer electronics like smartphones dominate advanced packaging applications, but the burst of activity in the AI segment will propel future growth. AI requires rapid data exchange between computing and memory elements, which is enabled by 2.5D and 3D packaging. These approaches place more than two chips next to each other to generate high interconnect speeds at a relatively low cost. AI applications account for 25% of the total advanced packaging market already, and this is poised to grow at around 20% per year through the next decade.
As this growth trend picks up speed, advanced packaging will change the semiconductor ecosystem significantly. (See Exhibit 3.) Traditionally, the value chain of chipmaking was rather simple: one chip designer, one front-end manufacturer, and a back-end company for packaging and testing. Sometimes all these roles are handled by the same integrated device manufacturer. In this scenario, the lion’s share of value capture is concentrated in chip design and the front end—where most of the innovation lies—while packaging is relegated to a lower-margin role.
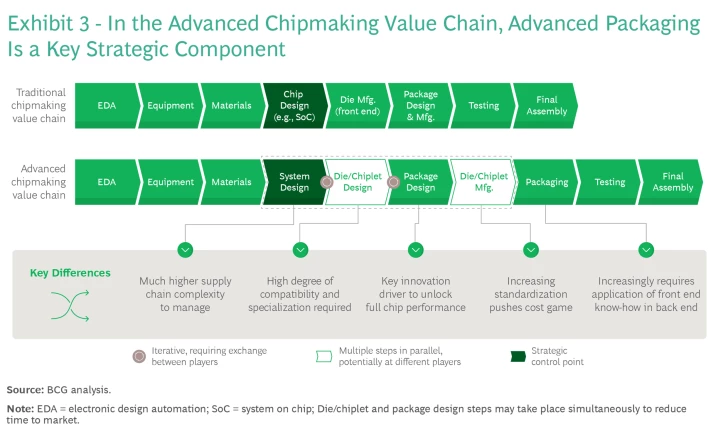
But advanced multichip packaging demands a profound rethinking of package design and how to improve it efficiently and continuously. It calls for elevated capabilities in each segment of the chip value chain. As the package becomes a core determinant of system performance, multiple semiconductor dies—often designed and manufactured by different companies—will have to be integrated in the same package, which itself is potentially produced by yet another company. As a result, system designers will need to orchestrate this new and more complex supply chain and drive close collaboration and alignment among all players.
This imminent radical alteration of the traditional chip manufacturing cycle is already prompting companies to reallocate capital expenditures and refocus R&D efforts to get out ahead of the emerging trend. In our view, the primary changes in chip industry structure will be evident in three ways:
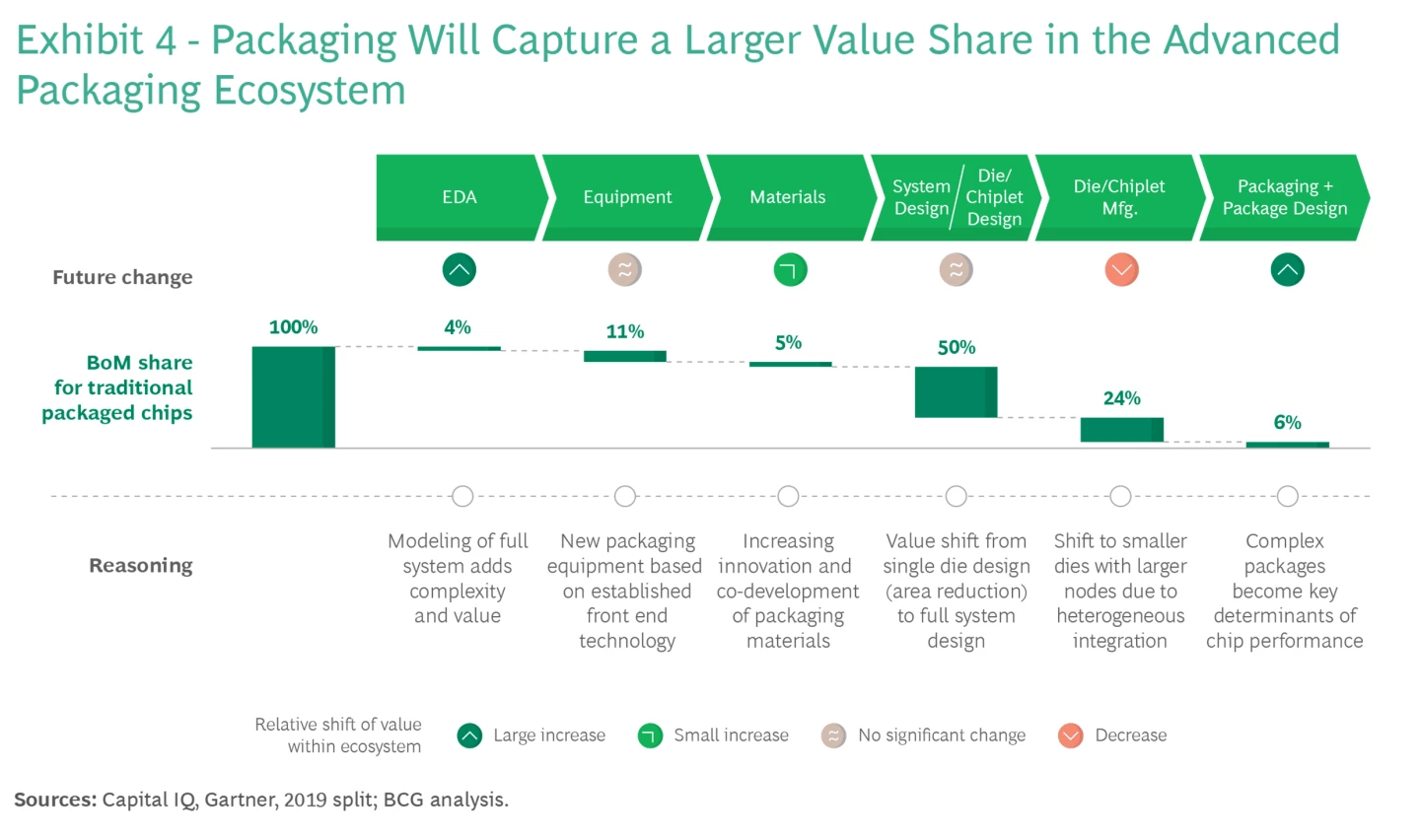
- Elevating the Role of System Design. The value share contributed by advanced package design will rise significantly, underscoring its strategic importance. (See Exhibit 4.) In response, chip designers are solidifying their grasp on this crucial segment by extending design from the single chip to the whole system, including integrating multiple dies into an advanced package.
- Shifting from Front End to Back End. The package will become a point of innovation, a differentiation driver pivotal to system performance. While front-end manufacturing will continue to command a high share of value generation, back-end design and packaging will gain in importance and profit value.
- Adapting to Complexity. Making advanced semiconductor packages is a complex process. To manage it, electronic design automation (EDA) software must be programmed to design and simulate multiple chips in a package and how their interplay may impact operating conditions, such as thermal dissipation and warpage. Similarly, materials suppliers must develop new, innovative materials to address, among other things, thermal expansion and heat transfer at the numerous interfaces in advanced packages. And packaging equipment must be modified to meet the decreasing feature sizes and increasing precision requirements of advanced packaging.
The Altered Face of the Chip Industry
As these technology changes take hold, the face of the semiconductor industry will be altered in significant ways. Chiefly, there will be a much greater degree of collaboration across chip design, package engineering, and system architecture, as each will directly impact the other. One die’s power profile will affect the next die’s thermal load and may require customized materials to optimize system performance.
Like today’s front-end landscape in which chip design and manufacturing co-evolve, strategic partnerships will increasingly extend from multiple front-end players to back-end package manufacturing. A good example of this, NVIDIA’s Hopper H100, uses a TSMC CoWoS-S package, which combines chips designed by NVIDIA and manufactured by TSMC with HBM designed and manufactured by SK Hynix. Another illustration: chip designers today work with a specific foundry’s fabrication process development kit in their electronic design automation software when building a chip. Tomorrow, this kit may contain the specific package solution in a similar manner.
Another potential area of greater collaboration will involve GenAI and machine intelligence. Integrated in EDA software, artificial intelligence capabilities can automate IC layout and floor planning; optimize power, performance, area (PPA), the essential component of semiconductor design; and simplify and expedite chip production to an unparalleled degree. But as the success of AI platforms is strongly dependent on the size and accuracy of its learning dataset, EDA software vendors and chip designers will have to balance pooling internal blueprints and frameworks to enhance the AI knowledge base without revealing their design secrets to competitors.
And even as companies jockey for position, geopolitical and regulatory constraints and opportunities will arise to affect supply chains and market access. Subsidies for advanced multichip packaging are increasingly in vogue as governments try to attract, retain, and support technology innovation in chipmaking. At the same time, since semiconductors are considered critical for national and economic security, trade barriers are being erected in various regions to protect domestic manufacturing. However, these measures could impact the availability of supplies, partners, and customers for semiconductor companies.
Strategic Solutions for Semiconductor Players
With so much change in the offing due to advanced packaging, different players in the semiconductor industry will need to adopt distinct strategic imperatives to position themselves for sustained differentiation and value capture. Here’s a breakdown of how segments of the industry can position themselves best. (See Exhibit 5.)
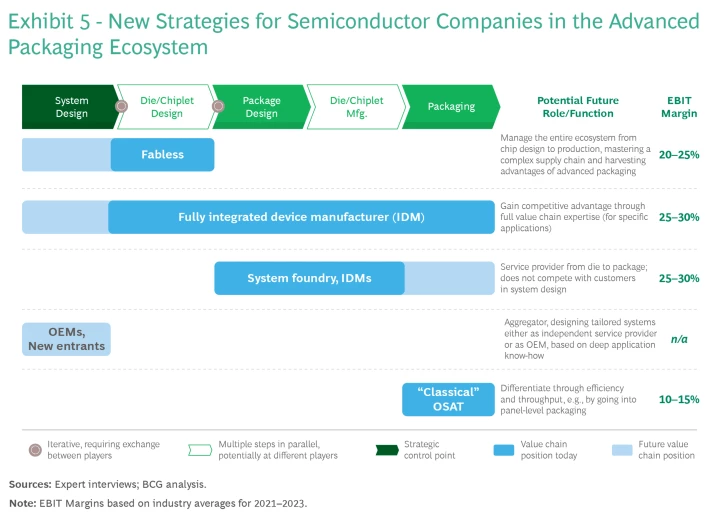
Fabless Chipmakers
Fabless chipmakers, which focus on designing chips and partner with foundries for manufacturing, will have to expand their business models quite a bit to address the challenges of advanced packaging. As the single chip becomes part of an advanced multichip package, fabless chip designers that successfully integrate the whole system development and production will capture the highest value share. However, this comes with the massive challenge of orchestrating and managing a complex supply chain across other chip designers, multiple foundries, and even material suppliers. Beyond practical execution, system designers will also be accountable to customers for the complex product’s performance, and a package manufacturer that has trusting relationships with its collaborators in this venture may be willing to take the overall liability for the system. Alternately, all involved parties in the supply chain may agree to share liabilities for a certain period after product launch when they have had enough samples to determine the most common points of failure.
Advanced packaging will threaten revenues of leading-node foundries as it reduces demand for large monolithic SoCs, replacing them with smaller, more standardized dies. To maintain their profit levels, leading-node foundries should extend their offering into advanced packaging, positioning themselves as system foundries.
However, foundries focused on mature nodes will struggle to develop advanced packaging solutions that can directly compete with those of their leading-node peers, who are more comfortable with innovation and less hesitant to enter new markets. Those foundries can still make inroads into advanced packaging by adding to their portfolios through-silicon vias (TSVs) for silicon interposers—key ingredients that facilitate connections and communications in many advanced packages. This would allow them to increase fab utilization in times of low front-end demand for their mature chips.
Integrated Device Manufacturers
Traditionally, IDMs design, manufacture, and package their own chips, even if parts of the value chain may be outsourced. However, the economics of this business model may be challenged in an advanced packaging environment where system designers increasingly demand unpackaged dies for integration into other suppliers’ packages, limiting demand for the IDM’s packaged end product. Leading IDMs may be able to capture the system designer role themselves, particularly for lucrative, specialized applications.
Another alternative is for the IDM to offer their front- and back-end facilities to fabless customers, thereby providing systems foundry services. While that would certainly improve asset utilization, it would require strong firewalls between the IDM’s own products and those manufactured for fabless competitors that become customers. These firewalls should ensure strict separation of production lines or teams. This significantly increases complexity, but it may be imperative to create a strategy that overcomes this challenge if an IDM hopes to avoid losing its role in the chip ecosystem as advanced packaging takes hold.
Outsourced Semiconductor Assembly and Test (OSAT) Vendors
With around 30% of advanced packaging wafers already produced by IDMs and foundries, traditional OSATs must carefully assess where they best fit in a market that—even as it develops—is to a degree shifting towards other parts of the value chain. In the new packaging world, OSATs need to determine their most advantageous position between simple wire bonds and the most advanced 2.5D/3D packages.
OSATs should build on their traditional strengths of high-volume, cost-efficient manufacturing, focusing on essential packaging activities that will still be required for some time, such as bumping, in which bumps or balls of solder are spread across an entire wafer before it is diced into individual chips. And taking a more innovative approach, OSATs can develop capabilities in panel-level packaging. Dealing with large panel sizes increases the number of dies processed simultaneously and is less expensive than wafer-level packaging typically used by foundries.
In the dawn of the advanced packaging era, the landscape offers a lot of promise but also much to be wary about. Companies that recognize and invest in the strategic value of advanced packaging are setting themselves up for success. They are not only expanding their own competitive edge but are also shaping the future direction of the semiconductor industry. The winners will be companies that have successfully innovated, navigated global government policies, forged deep connections with customers and their application ecosystems, and leveraged the latest in AI and design processes.
These industry front-runners are poised to capitalize on the shift in value creation from the front end to more nuanced, complex, and value-rich back-end processes. And they are in a position to be in the sweet spot when GenAI applications—virtually all of which rely on advanced packaging components—transform from an intriguing, novel idea today to a starkly dominant platform in the coming years.
For investors, strategic partners, and semiconductor companies, the message is clear: the time to invest in and prioritize advanced packaging is now. Those that do will thrive, steering the semiconductor industry towards its next performance frontier and into a future marked by ingenuity, speed, and sustained growth.
The authors would like to thank Changwook Kim, Shreya Dwarakanath, and Jack Tu for their contributions to this article.

Managing Director & Partner
San Francisco - Bay Area

Project Leader

Associate Director

Knowledge Director
ACC – Bengaluru

ABOUT BOSTON CONSULTING GROUP
Boston Consulting Group partners with leaders in business and society to tackle their most important challenges and capture their greatest opportunities. BCG was the pioneer in business strategy when it was founded in 1963. Today, we work closely with clients to embrace a transformational approach aimed at benefiting all stakeholders—empowering organizations to grow, build sustainable competitive advantage, and drive positive societal impact.
Our diverse, global teams bring deep industry and functional expertise and a range of perspectives that question the status quo and spark change. BCG delivers solutions through leading-edge management consulting, technology and design, and corporate and digital ventures. We work in a uniquely collaborative model across the firm and throughout all levels of the client organization, fueled by the goal of helping our clients thrive and enabling them to make the world a better place.
© Boston Consulting Group 2024. All rights reserved.
For information or permission to reprint, please contact BCG at [email protected] . To find the latest BCG content and register to receive e-alerts on this topic or others, please visit bcg.com . Follow Boston Consulting Group on Facebook and X (formerly Twitter) .
Pica code: 2024-adrj145 - Design and characterization of Environmental Barrier Coatings (EBC) for high-T applications omissis

Job Information
Offer description.
The research will contribute to the design, manufacturing and characterization of Environmental Barrier Coatings (EBCs) for high temperature applications deposited by thermal spraying as planned in the research activities of PRIN 2022 “GENESIS” project. The design step will focus on rare earth mono- and di-silicates. omissis
Requirements
Additional information.
Eligible destination country/ies for fellows:
Eligibility of fellows: country/ies of residence:
Eligibility of fellows: nationality/ies:
Selective public procedure by qualifications and interview
- Contact email: [email protected]
- Contact phone: 059/2056512-6445-6075-7078-6450
- Annual budget: 27204
- Frequency of calls: 12
- International mobility required: no
Work Location(s)
Where to apply.
- Skip to main content
- Keyboard shortcuts for audio player
Benedictine College nuns denounce Harrison Butker's speech at their school
John Helton
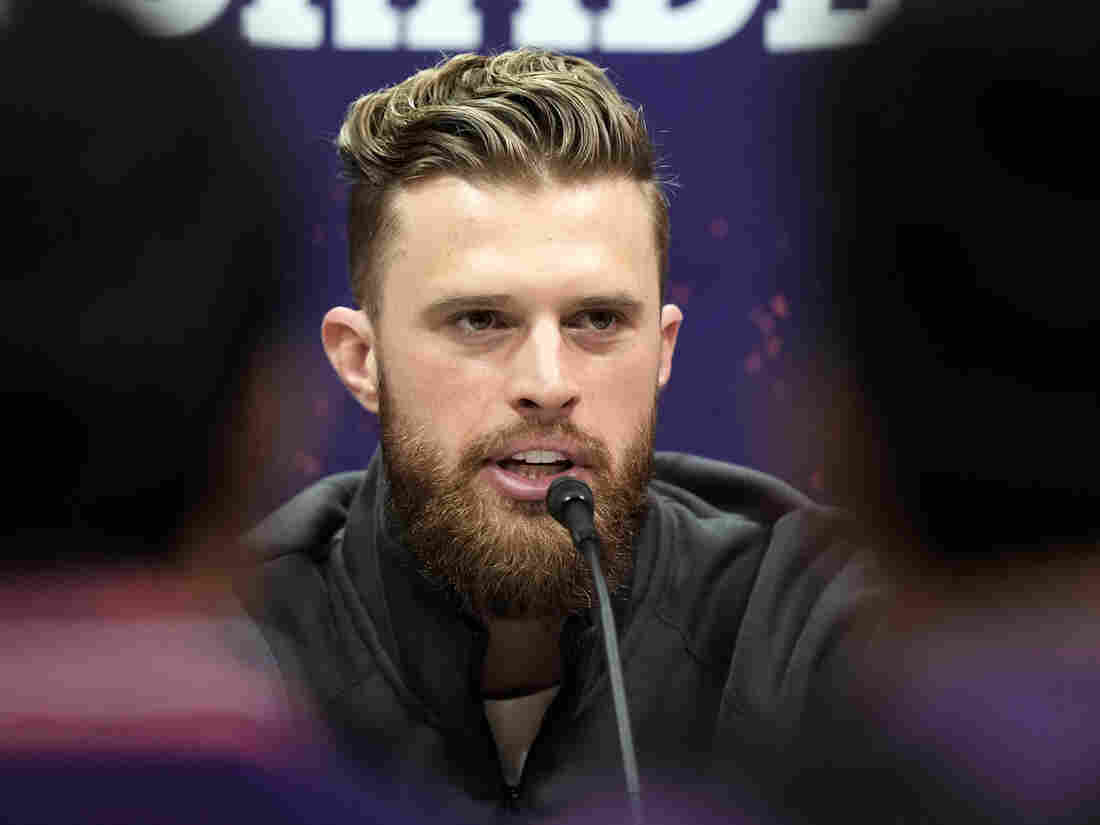
Kansas City Chiefs kicker Harrison Butker speaks to the media during NFL football Super Bowl 58 opening night on Feb. 5, 2024, in Las Vegas. Butker railed against Pride month along with President Biden's leadership during the COVID-19 pandemic and his stance on abortion during a commencement address at Benedictine College last weekend. Charlie Riedel/AP hide caption
Kansas City Chiefs kicker Harrison Butker speaks to the media during NFL football Super Bowl 58 opening night on Feb. 5, 2024, in Las Vegas. Butker railed against Pride month along with President Biden's leadership during the COVID-19 pandemic and his stance on abortion during a commencement address at Benedictine College last weekend.
An order of nuns affiliated with Benedictine College rejected Kansas City Chiefs kicker Harrison's Butker's comments in a commencement speech there last weekend that stirred up a culture war skirmish.
"The sisters of Mount St. Scholastica do not believe that Harrison Butker's comments in his 2024 Benedictine College commencement address represent the Catholic, Benedictine, liberal arts college that our founders envisioned and in which we have been so invested," the nuns wrote in a statement posted on Facebook .
In his 20-minute address , Butker denounced abortion rights, Pride Month, COVID-19 lockdowns and "the tyranny of diversity, equity and inclusion" at the Catholic liberal arts college in Atchison, Kan.
He also told women in the audience to embrace the "vocation" of homemaker.
"I want to speak directly to you briefly because I think it is you, the women, who have had the most diabolical lies told to you. How many of you are sitting here now about to cross the stage, and are thinking about all the promotions and titles you're going to get in your career?" he asked. "Some of you may go on to lead successful careers in the world. But I would venture to guess that the majority of you are most excited about your marriage and the children you will bring into this world."

For many Missouri Catholics, abortion rights means choosing between faith, politics
That was one of the themes that the sisters of Mount St. Scholastica took issue with.
"Instead of promoting unity in our church, our nation, and the world, his comments seem to have fostered division," they wrote. "One of our concerns was the assertion that being a homemaker is the highest calling for a woman. We sisters have dedicated our lives to God and God's people, including the many women whom we have taught and influenced during the past 160 years. These women have made a tremendous difference in the world in their roles as wives and mothers and through their God-given gifts in leadership, scholarship, and their careers."
The Benedictine sisters of Mount St. Scholastica founded a school for girls in Atchinson in the 1860s. It merged with St. Benedict's College in 1971 to form Benedictine College.
Neither Butker nor the Chiefs have commented on the controversy. An online petition calling for the Chiefs to release the kicker had nearly 215,000 signatures as of Sunday morning.

6 in 10 U.S. Catholics are in favor of abortion rights, Pew Research report finds
The NFL, for its part, has distanced itself from Butker's remarks.
"Harrison Butker gave a speech in his personal capacity," Jonathan Beane, the NFL's senior VP and chief diversity and inclusion officer told NPR on Thursday. "His views are not those of the NFL as an organization."
Meanwhile, Butker's No. 7 jersey is one of the league's top-sellers , rivaling those of better-known teammates Patrick Mahomes and Travis Kelce.
Butker has been open about his faith. The 28-year-old father of two told the Eternal Word Television Network in 2019 that he grew up Catholic but practiced less in high school and college before rediscovering his belief later in life.
His comments have gotten some support from football fan social media accounts and Christian and conservative media personalities .
A video of his speech posted on Benedictine College's YouTube channel has 1.5 million views.
Rachel Treisman contributed to this story.
- Harrison Butker
- benedictine college
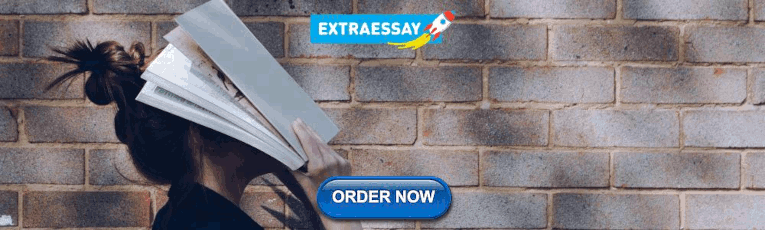
IMAGES
VIDEO
COMMENTS
A research design is a strategy for answering your research question using empirical data. Creating a research design means making decisions about: Your overall research objectives and approach. Whether you'll rely on primary research or secondary research. Your sampling methods or criteria for selecting subjects. Your data collection methods.
A research design is defined as the overall plan or structure that guides the process of conducting research. It is a critical component of the research process and serves as a blueprint for how a study will be carried out, including the methods and techniques that will be used to collect and analyze data.
Cohort design involves examining research subjects who have already been exposed to a research topic, making it especially effective for conducting ethical research on medical topics or risk factors. This design type is very flexible, and it applies to both primary and secondary data. 13. Historical research design.
Job Design "It is about a search, too, for daily meaning as well as daily bread, for recognition as well as cash, for astonishment rather than torpor; in short, for a sort of life rather than a Monday through Friday sort of dying" (Terkel, 1974, p. xi). Billions of people spend most of their waking lives at work, so it is fortunate that work can be a positive feature of living.
Research design refers to the overall plan, structure or strategy that guides a research project, from its conception to the final analysis of data. Research designs for quantitative studies include descriptive, correlational, experimental and quasi-experimenta l designs. Research designs for qualitative studies include phenomenological ...
We begin by defining job design and discussing the early work that was instrumental in shaping the direction of research on the topic. We then move to a discussion of the state of current research and theory on job design. Finally, we conclude with some ideas for future research. 2. Early work on job design.
Step 2: Choose a type of research design. Step 3: Identify your population and sampling method. Step 4: Choose your data collection methods. Step 5: Plan your data collection procedures. Step 6: Decide on your data analysis strategies. Frequently asked questions. Introduction. Step 1. Step 2.
Research design is a researcher's method of conducting a research project or study. Typically, the research problem determines the design, which guides researchers to collect, analyse and measure data. The design incorporates the research problem and identifies the specific topic it's addressing. This information usually forms part of the study ...
A research method is a strategy for implementing your research design. It includes ways to collect and analyze data. For example, surveys, interviews, and experiments are research methods you might consider using to gather information. Deciding on a suitable method typically requires you to understand your research goal.
Job design is a topic that continues to receive substantial attention from researchers and practitioners alike. In this article we have reviewed much of the early and current research and theory on the topic, including discussing work on Motivation-Hygiene Theory (Herzberg, 1966), JCT (Hackman & Oldham, 1976), and the Interdisciplinary ...
While many books and articles guide various qualitative research methods and analyses, there is currently no concise resource that explains and differentiates among the most common qualitative approaches. We believe novice qualitative researchers, students planning the design of a qualitative study or taking an introductory qualitative research course, and faculty teaching such courses can ...
Before beginning your paper, you need to decide how you plan to design the study.. The research design refers to the overall strategy and analytical approach that you have chosen in order to integrate, in a coherent and logical way, the different components of the study, thus ensuring that the research problem will be thoroughly investigated. It constitutes the blueprint for the collection ...
Research design is a critical topic that is central to research studies in science, social science, and many other disciplines. After identifying the research topic and formulating questions, selecting the appropriate design is perhaps the most important decision a researcher makes.
Job design and well-being. Job design is concerned with the activities of workers, their duties, the tasks required to perform their work, and how those tasks and duties are structured and scheduled (Morgeson and Humphrey Citation 2008; Parker and Ohly Citation 2008).Modern typologies of job design include factors such as: job demands, job control, skill use, task variety, role clarity, use of ...
Research Design. Definition: Research design refers to the overall strategy or plan for conducting a research study. It outlines the methods and procedures that will be used to collect and analyze data, as well as the goals and objectives of the study. Research design is important because it guides the entire research process and ensures that ...
Ranganathan P. Understanding Research Study Designs. Indian J Crit Care Med 2019;23 (Suppl 4):S305-S307. Keywords: Clinical trials as topic, Observational studies as topic, Research designs. We use a variety of research study designs in biomedical research. In this article, the main features of each of these designs are summarized. Go to:
for validity and reliability. Design is basically concerned with the aims, uses, purposes, intentions and plans within the. pr actical constraint of location, time, money and the researcher's ...
Job design is a process that companies use to create a new job or add duties to an existing job. This allows a company to more easily reach its goals by having more employees perform more tasks within the organization. Job design may involve developing a new position or simply adjusting the set of tasks that a current position encompasses.
Research design is the plan, structure and strategy and investigation concaved so as to obtain search question and control variance" (Borwankar, 1995). ... describe and assess meaning related to ...
Job design is the division of work tasks assigned to an individual in an organization that specifies what the worker does, how, and why. ... employees manage meaning will help to increase emplo ...
What Job Crafting Looks Like. Stories of three people who changed their jobs to find more meaning. Twenty years ago, the authors started studying job crafting — the act of altering your job to ...
Download the 2024 Gen Z and Millennial Report. 5 MB PDF. To learn more about the mental health findings, read the Mental Health Deep Dive. The 13th edition of Deloitte's Gen Z and Millennial Survey connected with nearly 23,000 respondents across 44 countries to track their experiences and expectations at work and in the world more broadly.
One of the most significant of these new concepts is advanced packaging, which essentially accommodates an ever-increasing number of transistors by decreasing the size of electrical contacts. Traditionally, a semiconductor chip focuses on performing one particular action or process. By comparison, an advanced multichip package incorporates ...
Research design is a logical and systematic plan prepared for directing a research study. It specifies the objectives of the study, the methodology, and the techniques to be adopted for achieving ...
The research will contribute to the design, manufacturing and characterization of Environmental Barrier Coatings (EBCs) for high temperature applications deposited by thermal spraying as planned in the research activities of PRIN 2022 "GENESIS" project. The design step will focus on rare earth mono- and di-silicates. omissis
"Instead of promoting unity in our church, our nation, and the world, his comments seem to have fostered division," the sisters wrote of the NFL kicker's controversial commencement address.