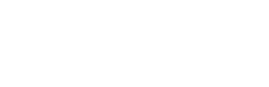
- About UTAS-Muscat
- Quality Assurance
- Mail and Documentation
- Partnership and Entrepreneurship
- Research and Consultation
- Innovation and Technology Transfer
- Academic Affairs
- Training and Career Guidance
- Admission and Registration
- Information Systems and Educational Technologies
- Student Affairs
- Administrative Affairs
- Financial Affairs
- Human Resources
- Applied Sciences
- Business Studies
- Engineering
- Fashion Design
- Information Technology
- Photography
- Preparatory Studies Centre
- It Symposium

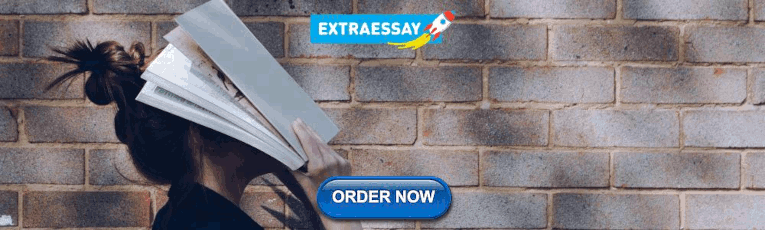
Events-Details
Business studies department - matrix literature review.
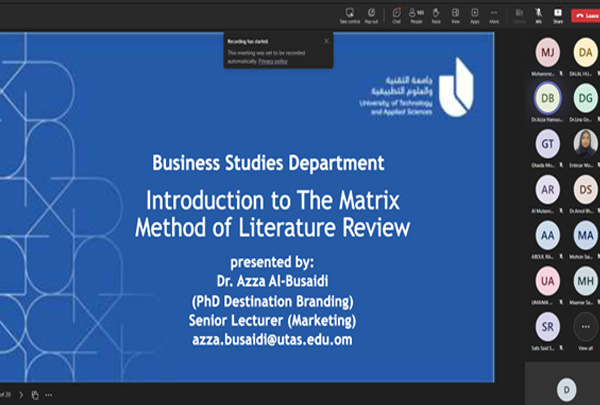
The CEBA Muscat branch organized a workshop on “Matrix Literature Review”. This workshop was presented by Dr Azza Al Busaidi, Senior Lecturer, CEBA Muscat. The main theme of this workshop is to gain a perfect knowledge on literature review. The Venue, Date and Time of the workshop is on the 12st of October 2023 at 12 PM to 2 PM through MS Teams.
Dr Azza started the session with the brief introduction about the literature review, scaffolding to dig ideas. And she focused on the following points:
- Process of constructing the matrix
- To search key words / search strings in Google.
- Google scholar search Hacks
- Combining articles in groups
- Creating Matrix, organize it and code it with colors.
- Sample Matrices
- Summary of Article analysis.
- Using “Gamification “in literature review
The session was informative and effective. Questions were raised by the faculties and the doubts are clarified. To conclude, Dr Azza presented the session in an effective way, this will help the faculties and students to write the literature review in a creative way.
University of Tasmania, Australia
Literature reviews.
- What is a literature review?
- How to develop a researchable question
- How to find the literature
- How to manage the reading and take notes that make sense
- Organising your Literature Review
Advice, other sites and examples
- Advice and examples for nursing students
Organising your literature review
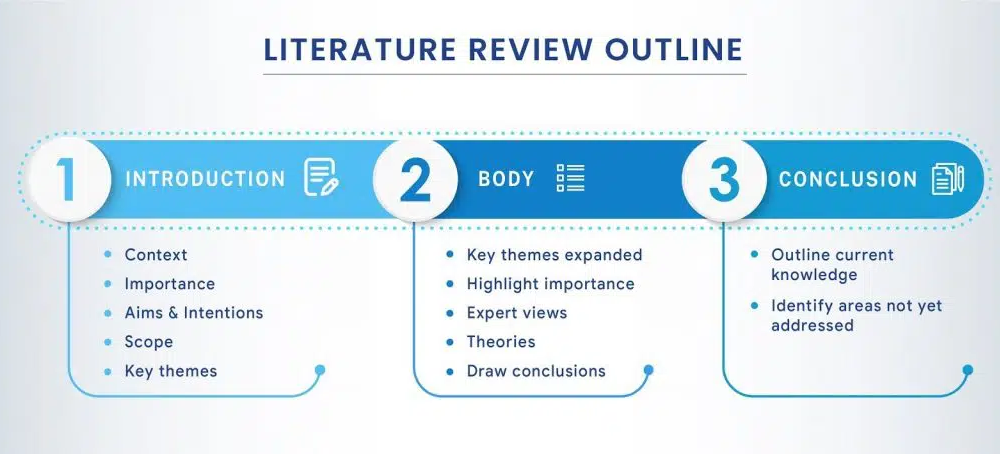
https://www.helpforassessment.com/blog/how-to-outline-a-literature-review/
Using your notes from the matrix, it is now time to plan before writing.
The usual structure of Introduction, Body and Conclusion apply here but after that, you need to make decisions about how the information will be organised:
- Sequentially/Chronological?
- Topical - subtopics?
- Theoretical?
- Methodology?
- Importance?
This will sometimes depend on your discipline. For example, in Science disciplines it might be better to organise by the methods of research used if you are going to find gaps in those methods, while in Education or the Social Sciences, it might be better to organise by synthesising theory.
Some literature reviews will require a description of the search strategy. For more information, you may want to look at the advice on this page: Steps of Building Search Strategies
This video from Western Sydney University explains further and has examples for geographical and thematic organisation.
Language and structure
Once you have written a draft, think carefully about the language you have used to signpost the structure of your review in order to build a convincing and logical synthesis.
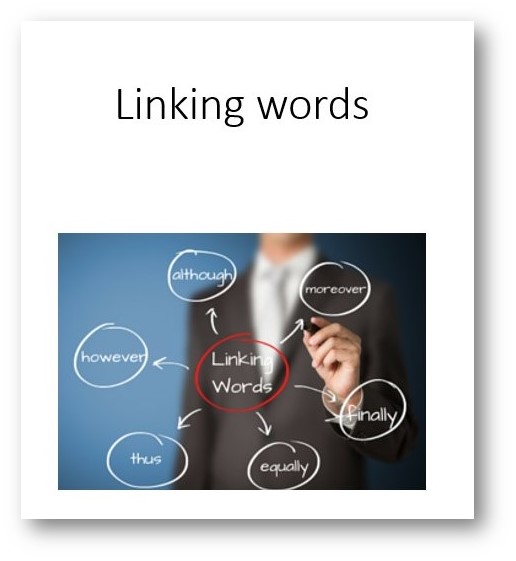
Carefully choosing transition/linking words and reporting verbs will strengthen your writing.
- Transition and Linking Language
- Reporting verbs
- Academic Phrasebank: referring to sources This academic phrasebank has many more examples of using language to build the structure of your argument.
- 1. Guidance notes for Lit Review
- 2. Literature Review purpose
- Cite/Write: Writing a literature Review, QUT
- RMIT Learning Lab: Literature Review overview
Advice and examples for nursing students (may be useful for other Health Sciences)
- Postgrad Literature Review Good example
- Postgrad Literature Review Poor Example
- Southern Cross University Literature Review Examples
- Literature review outline Boswell, C & Cannon, S (eds) 2014, Introduction to nursing research: incorporating evidence-based practice, Jones and Bartlett Learning, Sudbury, viewed 5 October 2017, Ovid, http://ovidsp.ovid.com.ezproxy.utas.edu.au/ovidweb.cgi?T=JS&CSC=Y&NEWS=N&PAGE=booktext&D=books1&AN=01965234/4th_Edition/2&EPUB=Y
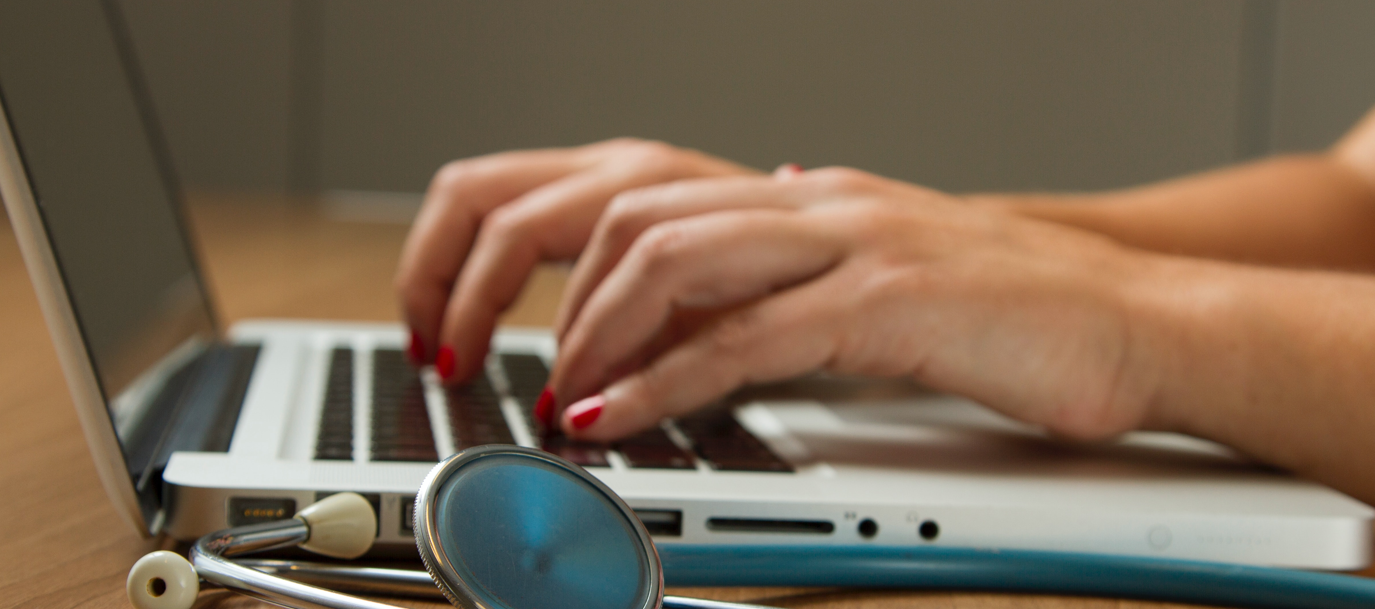
- << Previous: How to manage the reading and take notes that make sense
- Last Updated: Apr 10, 2024 11:56 AM
- URL: https://utas.libguides.com/literaturereviews

- Search entire site
- Search for a course
- Browse study areas
Analytics and Data Science
- Data Science and Innovation
- Postgraduate Research Courses
- Business Research Programs
- Undergraduate Business Programs
- Entrepreneurship
- MBA Programs
- Postgraduate Business Programs
Communication
- Animation Production
- Business Consulting and Technology Implementation
- Digital and Social Media
- Media Arts and Production
- Media Business
- Media Practice and Industry
- Music and Sound Design
- Social and Political Sciences
- Strategic Communication
- Writing and Publishing
- Postgraduate Communication Research Degrees
Design, Architecture and Building
- Architecture
- Built Environment
- DAB Research
- Public Policy and Governance
- Secondary Education
- Education (Learning and Leadership)
- Learning Design
- Postgraduate Education Research Degrees
- Primary Education
Engineering
- Civil and Environmental
- Computer Systems and Software
- Engineering Management
- Mechanical and Mechatronic
- Systems and Operations
- Telecommunications
- Postgraduate Engineering courses
- Undergraduate Engineering courses
- Sport and Exercise
- Palliative Care
- Public Health
- Nursing (Undergraduate)
- Nursing (Postgraduate)
- Health (Postgraduate)
- Research and Honours
- Health Services Management
- Child and Family Health
- Women's and Children's Health
Health (GEM)
- Coursework Degrees
- Clinical Psychology
- Genetic Counselling
- Good Manufacturing Practice
- Physiotherapy
- Speech Pathology
- Research Degrees
Information Technology
- Business Analysis and Information Systems
- Computer Science, Data Analytics/Mining
- Games, Graphics and Multimedia
- IT Management and Leadership
- Networking and Security
- Software Development and Programming
- Systems Design and Analysis
- Web and Cloud Computing
- Postgraduate IT courses
- Postgraduate IT online courses
- Undergraduate Information Technology courses
- International Studies
- Criminology
- International Relations
- Postgraduate International Studies Research Degrees
- Sustainability and Environment
- Practical Legal Training
- Commercial and Business Law
- Juris Doctor
- Legal Studies
- Master of Laws
- Intellectual Property
- Migration Law and Practice
- Overseas Qualified Lawyers
- Postgraduate Law Programs
- Postgraduate Law Research
- Undergraduate Law Programs
- Life Sciences
- Mathematical and Physical Sciences
- Postgraduate Science Programs
- Science Research Programs
- Undergraduate Science Programs
Transdisciplinary Innovation
- Creative Intelligence and Innovation
- Diploma in Innovation
- Transdisciplinary Learning
- Postgraduate Research Degree
Literature reviews

A literature review may form an assignment by itself, in which case the aim is to summarise the key research relating to your topic. Alternatively, it may form part of a larger paper, such as a thesis or a research report, in which case the aim is to explain why more research needs to be done on your chosen topic.
Process of a literature review
click on each process for more information
1. Deciding on a research question or topic
Whether you’ve been given a topic by your teacher, or you’ve been asked to decide upon your own topic or research question, it’s best to rephrase the topic as a specific question that you’re attempting to answer.
If your purpose is to summarise the existing research on the topic, it may be quite appropriate to have a very broad question, such as the example below.
If your purpose is to justify the need to conduct further primary research, you will need a more specific research question which takes into account how the existing research has failed to adequately answer the question you’re planning to address.
This question allows the writer to narrow the focus of their literature review and, hopefully, find gaps in the amount or type of research conducted into this very specific topic. Sometimes you may start with a broader topic or question, then conduct some initial research into the existing literature, and then narrow the focus of your research question based on what you find.
2. Searching the literature
When searching for relevant sources, it’s important to first decide the criteria you will use when trying to find existing research. This includes setting the scope of your research to decide what is important and why.
- your discipline
- the purpose of your research
- the amount of research that has been conducted on that topic
- the speed with which findings are considered no longer valid
- Which databases will you use? What types of journals are considered acceptable? Speaking with your teacher, and with a Librarian from UTS Library, can help you make these decisions.
- Are you focusing on research relating to a particular country, and if so, why?
- Are you focusing on particular research methods or specific theoretical approaches? If so, you need to explain to the reader what they are and why you have made these decisions.
3. Taking notes
What notes you take depends on your research question. Knowing what you’re trying to achieve or what question you’re trying to answer will help you choose what to focus on when reading the literature. Common aspects to look out for when reading include:
- the main research findings
- the researchers’ claims (usually based on their interpretation of the findings)
- where, when and how the research was conducted
- the scope and/or limitations of the research being reviewed
Remember to also take notes about your own response to the literature. If you see weaknesses in a particular study, or assumptions being made when interpreting the findings of a study, make a note of it, as your critical analysis of the literature is a key aspect of a literature review.
4. Grouping your materials
Before drafting your literature review, it’s useful to group together your sources according to theme. A good literature review is not structured based on having one paragraph for each paper that you review. Instead, paragraphs are based on topics or themes that have been identified when conducting your research, with various sources synthesised within each paragraph.
How you decide on your groupings will depend on the purpose of your literature review. You may be clear on this before you begin researching, or your themes may emerge during the research process. Examples can include:
- the methodology used to conduct the research
- the theoretical perspective used by the researchers
- themes identified when analysing the various research findings
- opinions presented by the researchers based on their analysis of the findings
5. Writing the review
Your literature review should tell some sort of story. After reading the various studies published on your topic, are you able to clearly answer your initial research question? If so, was it an answer you were expecting, and what evidence was most useful in helping you answer the question. If there is no clear answer to your question, is that because:
- not enough research has been conducted on the topic?
- the findings of the various studies are contradictory or inconclusive?
- the results are too dependent on a specific context?
- there are too many variables to consider?
Whatever you decide, you need to explain this clearly to your reader, guiding them through how you got to your answer by explaining what you were looking for and what connections you found when looking through the existing literature.
Structure of a literature review

Introduction
The introduction is usually one paragraph in a short literature review, or series of paragraphs in a longer review, outlining:
- the content being covered,
- the structure (or how the review is organised), and
- the scope of what will be covered.
- Literature reviews are usually organised so that each paragraph or section covers one theme or sub-topic.
- Each section ends with a brief summary which relates this theme to the main focus of the research area.
The conclusion summarises the main themes that were identified when reviewing the literature.
Click on each component for more information and some examples
- the limits of what will be covered.
Example introduction to a literature review
[1] Education is one aspect of society that everyone has experienced, and that everyone therefore has an opinion about. However, despite decades of research into pedagogical approaches to education, there is still surprisingly little consensus regarding how learning and teaching should be conducted in order to be most effective. This is especially true with regards to critical pedagogy, which can be defined as the theory and practice of helping students achieve critical consciousness about what they are learning, how they are learning it, and the context which shapes both of these aspects (Windsor, 2018). [2] The purpose of this literature review is to demonstrate the lack of analytical approaches to teaching in the higher education sphere, and the importance of critical pedagogy in enhancing curriculum development, teacher training and classroom practice. When reviewing the literature, it soon becomes clear that research studies in the ESL field are dominated by language instruction techniques, with less attention given to ways teachers can adopt a more critical stance with their learners (Pennycook 1999; Saroub & Quadros 2015). [3] For the purposes of this review , respected ESL and Adult Education journals, online publications, unpublished theses and academic books from the late 1980s to 2016 will be examined, with a coverage of sources from Australia, the US, Asia, the Middle East and South America. Pennycook (1994, 1999), Giroux (1988) and Freire (1970) are commonly cited in these research studies. It is rare to find authors or research questioning the value of critical pedagogy; Ellsworth (1989) and Johnson (1999) are notable exceptions. [4] This literature review covers two main areas. Firstly, research into what pre-service and existing teachers know and think about critical pedagogy is examined. The second area investigates teacher and student resistance to some critical teaching practices.
Rather than writing one paragraph for each piece of research being discussed, literature reviews are usually organised so that each paragraph (or section) covers one theme or sub-topic. These themes will differ depending on your topic and your purpose, but may relate to:
- different theoretical perspectives on your topic
- different ways of conducting research on the topic
- different sub-topics within the broader topic
Each section ends with a brief summary which relates this theme to the main focus of the research area. It may do this by focusing on parts of the topic where the literature agrees or disagrees. The body paragraphs should be well organised and structured. See our resources on effective paragraph writing .
Example body paragraph from a literature review
[1] Resistance to change is another area that was found to restrict the adoption of critical teaching practices in the ESL area. [2] Canh and Barnard’s small case study (2009) of Vietnamese teachers’ capacity to take on a national curriculum change directed by the Vietnamese government found implementation was different from the ‘idealised world of innovation designers’ (p. 30). While also recommending better teacher training, they cited the need for an adjustment of teachers’ belief systems to make change happen, since an individual’s practice ‘behind the closed doors of their classroom’ (p. 21) is a largely unobserved space, despite mandated curriculum changes. [3] Similarly, resistance and avoidance among EFL teachers was noted by Cox & De Assis–Peterson’s Brazilian study (1999). They found that teachers often avoided political language questions from students, for example, ‘Why should we learn English if we’re Brazilian?’ [4] This suggests that any uptake of critical practices may be more dependent on teacher attitudes, reflecting their internal reality, than on external factors.
Example conclusion from a literature review
[1] This review of relevant literature has quite clearly shown a lack of understanding of critical pedagogy among a range of teachers, despite the strong likelihood of it being included in their training. [2] It also demonstrated that many teachers used avoidance when faced with difficult topics or situations related to critical language education. [3] Calls for curriculum changes, better training and more teaching materials were common in research recommendations, and it may be that teachers’ personal attitudes also play an important role in changing classroom practice.
If the literature review is part of a larger research project, the conclusion should also summarise any gaps in the existing literature, and use this to justify the need for your own proposed research project. The types of gaps in the existing literature may relate to:
- a lack of research into a particular aspect of the topic, or
- the fact that existing evidence is conflicting or inconclusive, and therefore more evidence is required to help provide conclusive evidence, or
- problems in the methodology used in previous research, meaning that a different research method is required.
Literature review as an individual assignment
If the purpose of your literature review is to summarise the existing literature on a topic, you will be expected to:
- summarise the most important (or most recent) literature on your specified topic
- discuss any common themes that emerge in the literature, such as similar types of research that have been conducted, similar findings from the research, or similar interpretations of the findings
- discuss any differences in the research findings or interpretation of evidence from the literature
- Does the author make any assumptions that weaken their claims?
- Are the author’s claims supported by adequate evidence?
- Was the research conducted in a way that is valid and credible?
- Is there anything missing from their discussion of the topic?
Literature review as part of a thesis or research report
If your literature review is part of a thesis or research report, as well as doing everything listed above, you will also need to:
- discuss ‘gaps’ in the current literature, which means finding important areas of research that have not yet been adequately covered, or for which further evidence is still required
- explain the significance of your research
- show how your work builds on previous research
- show how your work can be differentiated from previous research (i.e. what makes your research different from previous studies that have been done on this topic?)
Verb tense in literature Reviews
Always consider the verb tense when presenting a review of previously published work. There are three main verb tenses used in literature reviews. Please click on each occasion to check which verb tense is appropriate.
1. Describing a particular study
When describing a particular study or piece of research (or the researchers who conducted it), it is common to use past tense .
For example:
After conducting a meta-review of studies on effective exam preparation techniques, Wang & Li (2016) concluded that ….
2. Giving opinions about a study
If you are sharing your own views about a previous study, or conveying the views of other experts, then present tense is more common.
Although the research conducted by Lopez et al. (2017) was an important contribution to the field, their claims are too strong given the lack of supporting evidence.
3. Making generalisations
If you are making generalisations about past research, present perfect tense is used.
Several researchers have studied the effects of stress on very young children (Baggio, 2014; Suarez, 2017; Van Djik et al., 2020).

Back to top
UTS acknowledges the Gadigal people of the Eora Nation, the Boorooberongal people of the Dharug Nation, the Bidiagal people and the Gamaygal people, upon whose ancestral lands our university stands. We would also like to pay respect to the Elders both past and present, acknowledging them as the traditional custodians of knowledge for these lands.

Advertisement
Publics’ views on ethical challenges of artificial intelligence: a scoping review
- Open access
- Published: 19 December 2023
Cite this article
You have full access to this open access article
- Helena Machado ORCID: orcid.org/0000-0001-8554-7619 1 ,
- Susana Silva ORCID: orcid.org/0000-0002-1335-8648 2 &
- Laura Neiva ORCID: orcid.org/0000-0002-1954-7597 3
2555 Accesses
9 Altmetric
Explore all metrics
This scoping review examines the research landscape about publics’ views on the ethical challenges of AI. To elucidate how the concerns voiced by the publics are translated within the research domain, this study scrutinizes 64 publications sourced from PubMed ® and Web of Science™. The central inquiry revolves around discerning the motivations, stakeholders, and ethical quandaries that emerge in research on this topic. The analysis reveals that innovation and legitimation stand out as the primary impetuses for engaging the public in deliberations concerning the ethical dilemmas associated with AI technologies. Supplementary motives are rooted in educational endeavors, democratization initiatives, and inspirational pursuits, whereas politicization emerges as a comparatively infrequent incentive. The study participants predominantly comprise the general public and professional groups, followed by AI system developers, industry and business managers, students, scholars, consumers, and policymakers. The ethical dimensions most commonly explored in the literature encompass human agency and oversight, followed by issues centered on privacy and data governance. Conversely, topics related to diversity, nondiscrimination, fairness, societal and environmental well-being, technical robustness, safety, transparency, and accountability receive comparatively less attention. This paper delineates the concrete operationalization of calls for public involvement in AI governance within the research sphere. It underscores the intricate interplay between ethical concerns, public involvement, and societal structures, including political and economic agendas, which serve to bolster technical proficiency and affirm the legitimacy of AI development in accordance with the institutional norms that underlie responsible research practices.
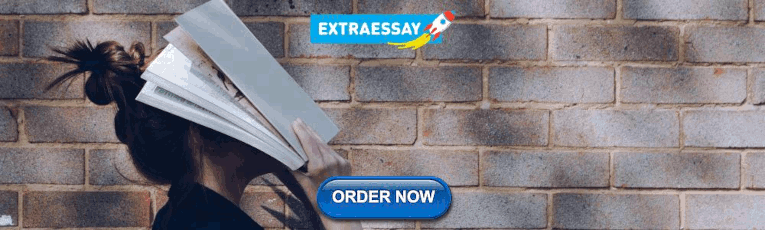
Similar content being viewed by others
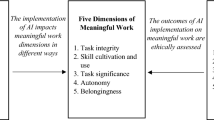
The Ethical Implications of Artificial Intelligence (AI) For Meaningful Work
Sarah Bankins & Paul Formosa
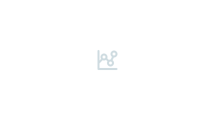
The Ethics of AI Ethics: An Evaluation of Guidelines
Thilo Hagendorff
Research Methodology: An Introduction
Avoid common mistakes on your manuscript.
1 Introduction
Current advances in the research, development, and application of artificial intelligence (AI) systems have yielded a far-reaching discourse on AI ethics that is accompanied by calls for AI technology to be democratically accountable and trustworthy from the publics’ Footnote 1 perspective [ 1 , 2 , 3 , 4 , 5 ]. Consequently, several ethics guidelines for AI have been released in recent years. As of early 2020, there were 167 AI ethics guidelines documents around the world [ 6 ]. Organizations such as the European Commission (EC), the Organization for Economic Co-operation and Development (OECD), and the United Nations Educational, Scientific and Cultural Organization (UNESCO) recognize that public participation is crucial for ensuring the responsible development and deployment of AI technologies, Footnote 2 emphasizing the importance of inclusivity, transparency, and democratic processes to effectively address the societal implications of AI [ 11 , 12 ]. These efforts were publicly announced as aiming to create a common understanding of ethical AI development and foster responsible practices that address societal concerns while maximizing AI’s potential benefits [ 13 , 14 ]. The concept of human-centric AI has emerged as a key principle in many of these regulatory initiatives, with the purposes of ensuring that human values are incorporated into the design of algorithms, that humans do not lose control over automated systems, and that AI is used in the service of humanity and the common good to improve human welfare and human rights [ 15 ]. Using the same rationale, the opacity and rapid diffusion of AI have prompted debate about how such technologies ought to be governed and which actors and values should be involved in shaping governance regimes [ 1 , 2 ].
While industry and business have traditionally tended to be seen as having no or little incentive to engage with ethics or in dialogue, AI leaders currently sponsor AI ethics [ 6 , 16 , 17 ]. However, some concerns call for ethics, public participation, and human-centric approaches in areas such as AI with high economic and political importance to be used within an instrumental rationale by the AI industry. A growing corpus of critical literature has conceived the development of AI ethics as efforts to reduce ethics to another form of industrial capital or to coopt and capture researchers as part of efforts to control public narratives [ 12 , 18 ]. According to some authors, one of the reasons why ethics is so appealing to many AI companies is to calm critical voices from the publics; therefore, AI ethics is seen as a way of gaining or restoring trust, credibility and support, as well as legitimation, while criticized practices are calmed down to maintain the agenda of industry and science [ 12 , 17 , 19 , 20 ].
Critical approaches also point out that despite regulatory initiatives explicitly invoking the need to incorporate human values into AI systems, they have the main objective of setting rules and standards to enable AI-based products and services to circulate in markets [ 20 , 21 , 22 ] and might serve to avoid or delay binding regulation [ 12 , 23 ]. Other critical studies argue that AI ethics fails to mitigate the racial, social, and environmental damage of AI technologies in any meaningful sense [ 24 ] and excludes alternative ethical practices [ 25 , 26 ]. As explained by Su [ 13 ], in a paper that considers the promise and perils of international human rights in AI governance, while human rights can serve as an authoritative source for holding AI developers accountable, its application to AI governance in practice shows a lack of effectiveness, an inability to effect structural change, and the problem of cooptation.
In a value analysis of AI national strategies, Wilson [ 5 ] concludes that the publics are primarily cast as recipients of AI’s abstract benefits, users of AI-driven services and products, a workforce in need of training and upskilling, or an important element for thriving democratic society that unlocks AI's potential. According to the author, when AI strategies articulate a governance role for the publics, it is more like an afterthought or rhetorical gesture than a clear commitment to putting “society-in-the-loop” into AI design and implementation [ 5 , pp. 7–8]. Another study of how public participation is framed in AI policy documents [ 4 ] shows that high expectations are assigned to public participation as a solution to address concerns about the concentration of power, increases in inequality, lack of diversity, and bias. However, in practice, this framing thus far gives little consideration to some of the challenges well known for researchers and practitioners of public participation with science and technology, such as the difficulty of achieving consensus among diverse societal views, the high resource requirements for public participation exercises, and the risks of capture by vested interests [ 4 , pp. 170–171]. These studies consistently reveal a noteworthy pattern: while references to public participation in AI governance are prevalent in the majority of AI national strategies, they tend to remain abstract and are often overshadowed by other roles, values, and policy concerns.
Some authors thus contended that the increasing demand to involve multiple stakeholders in AI governance, including the publics, signifies a discernible transformation within the sphere of science and technology policy. This transformation frequently embraces the framework of “responsible innovation”, Footnote 3 which emphasizes alignment with societal imperatives, responsiveness to evolving ethical, social, and environmental considerations, and the participation of the publics as well as traditionally defined stakeholders [ 3 , 28 ]. When investigating how the conception and promotion of public participation in European science and technology policies have evolved, Macq, Tancoine, and Strasser [ 29 ] distinguish between “participation in decision-making” (pertaining to science policy decisions or decisions on research topics) and “participation in knowledge and innovation-making”. They find that “while public participation had initially been conceived and promoted as a way to build legitimacy of research policy decisions by involving publics into decision-making processes, it is now also promoted as a way to produce better or more knowledge and innovation by involving publics into knowledge and innovation-making processes, and thus building legitimacy for science and technology as a whole” [ 29 , p. 508]. Although this shift in science and technology research policies has been noted, there exists a noticeable void in the literature in regard to understanding how concrete research practices incorporate public perspectives and embrace multistakeholder approaches, inclusion, and dialogue.
While several studies have delved into the framing of the publics’ role within AI governance in several instances (from Big Tech initiatives to hiring ethics teams and guidelines issued from multiple institutions to governments’ national policies related to AI development), discussing the underlying motivations driving the publics’ participation and the ethical considerations resulting from such involvement, there remains a notable scarcity of knowledge concerning how publicly voiced concerns are concretely translated into research efforts [ 30 , pp. 3–4, 31 , p. 8, 6]. To address this crucial gap, our scoping review endeavors to analyse the research landscape about the publics’ views on the ethical challenges of AI. Our primary objective is to uncover the motivations behind involving the publics in research initiatives, identify the segments of the publics that are considered in these studies, and illuminate the ethical concerns that warrant specific attention. Through this scoping review, we aim to enhance the understanding of the political and social backdrop within which debates and prior commitments regarding values and conditions for publics’ participation in matters related to science and technology are formulated and expressed [ 29 , 32 , 33 ] and which specific normative social commitments are projected and performed by institutional science [ 34 , p. 108, [ 35 , p. 856].
We followed the guidance for descriptive systematic scoping reviews by Levac et al. [ 36 ], based on the methodological framework developed by Arksey and O’Malley [ 37 ]. The steps of the review are listed below:
2.1 Stage 1: identifying the research question
The central question guiding this scoping review is the following: What motivations, publics and ethical issues emerge in research addressing the publics’ views on the ethical challenges of AI? We ask:
What motivations for engaging the publics with AI technologies are articulated?
Who are the publics invited?
Which ethical issues concerning AI technologies are perceived as needing the participation of the publics?
2.2 Stage 2: identifying relevant studies
A search of the publications on PubMed® and Web of Science™ was conducted on 19 May 2023, with no restriction set for language or time of publication, using the following search expression: (“AI” OR “artificial intelligence”) AND (“public” OR “citizen”) AND “ethics”. The search was followed by backwards reference tracking, examining the references of the selected publications based on full-text assessment.
2.3 Stage 3: study selection
The inclusion criteria allowed only empirical, peer-reviewed, original full-length studies written in English to explore publics’ views on the ethical challenges of AI as their main outcome. The exclusion criteria disallowed studies focusing on media discourses and texts. The titles of 1612 records were retrieved. After the removal of duplicates, 1485 records were examined. Two authors (HM and SS) independently screened all the papers retrieved initially, based on the title and abstract, and afterward, based on the full text. This was crosschecked and discussed in both phases, and perfect agreement was achieved.
The screening process is summarized in Fig. 1 . Based on title and abstract assessments, 1265 records were excluded because they were neither original full-length peer-reviewed empirical studies nor focused on the publics’ views on the ethical challenges of AI. Of the 220 fully read papers, 54 met the inclusion criteria. After backwards reference tracking, 10 papers were included, and the final review was composed of 64 papers.
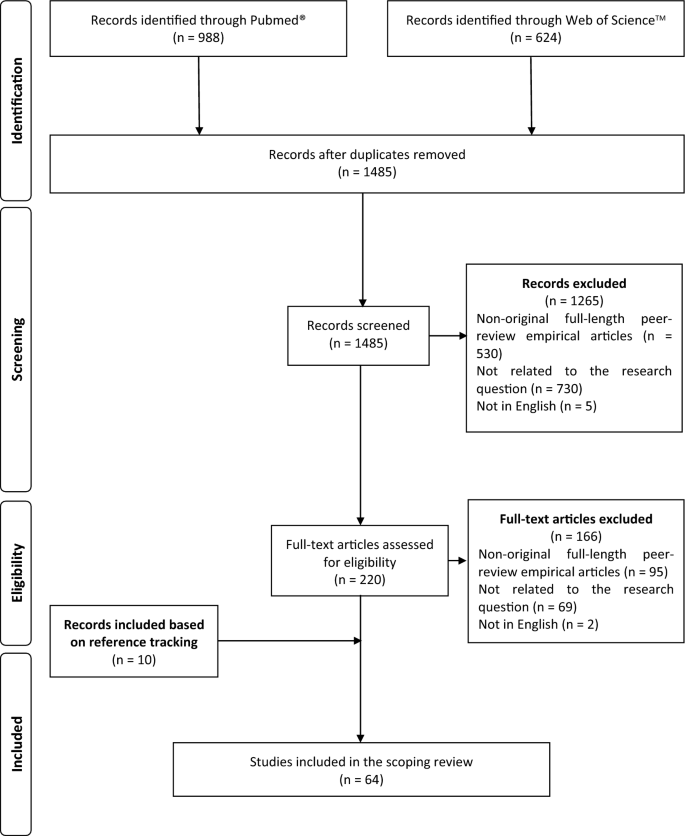
Flowchart showing the search results and screening process for the scoping review of publics’ views on ethical challenges of AI
2.4 Stage 4: charting the data
A standardized data extraction sheet was initially developed by two authors (HM and SS) and completed by two coders (SS and LN), including both quantitative and qualitative data (Supplemental Table “Data Extraction”). We used MS Excel to chart the data from the studies.
The two coders independently charted the first 10 records, with any disagreements or uncertainties in abstractions being discussed and resolved by consensus. The forms were further refined and finalized upon consensus before completing the data charting process. Each of the remaining records was charted by one coder. Two meetings were held to ensure consistency in data charting and to verify accuracy. The first author (HM) reviewed the results.
Descriptive data for the characterization of studies included information about the authors and publication year, the country where the study was developed, study aims, type of research (quantitative, qualitative, or other), assessment of the publics’ views, and sample. The types of research participants recruited as publics were coded into 11 categories: developers of AI systems; managers from industry and business; representatives of governance bodies; policymakers; academics and researchers; students; professional groups; general public; local communities; patients/consumers; and other (specify).
Data on the main motivations for researching the publics’ views on the ethical challenges of AI were also gathered. Authors’ accounts of their motivations were synthesized into eight categories according to the coding framework proposed by Weingart and colleagues [ 33 ] concerning public engagement with science and technology-related issues: education (to inform and educate the public about AI, improving public access to scientific knowledge); innovation (to promote innovation, the publics are considered to be a valuable source of knowledge and are called upon to contribute to knowledge production, bridge building and including knowledge outside ‘formal’ ethics); legitimation (to promote public trust in and acceptance of AI, as well as of policies supporting AI); inspiration (to inspire and raise interest in AI, to secure a STEM-educated labor force); politicization (to address past political injustices and historical exclusion); democratization (to empower citizens to participate competently in society and/or to participate in AI); other (specify); and not clearly evident.
Based on the content analysis technique [ 38 ], ethical issues perceived as needing the participation of the publics were identified through quotations stated in the studies. These were then summarized in seven key ethical principles, according to the proposal outlined by the EC's Ethics Guidelines for Trustworthy AI [ 39 ]: human agency and oversight; technical robustness and safety; privacy and data governance; transparency; diversity, nondiscrimination and fairness; societal and environmental well-being; and accountability.
2.5 Stage 5: collating, summarizing, and reporting the results
The main characteristics of the 64 studies included can be found in Table 1 . Studies were grouped by type of research and ordered by the year of publication. The findings regarding the publics invited to participate are presented in Fig. 2 . The main motivations for engaging the publics with AI technologies and the ethical issues perceived as needing the participation of the publics are summarized in Tables 2 and 3 , respectively. The results are presented below in a narrative format, with complimentary tables and figures to provide a visual representation of key findings.
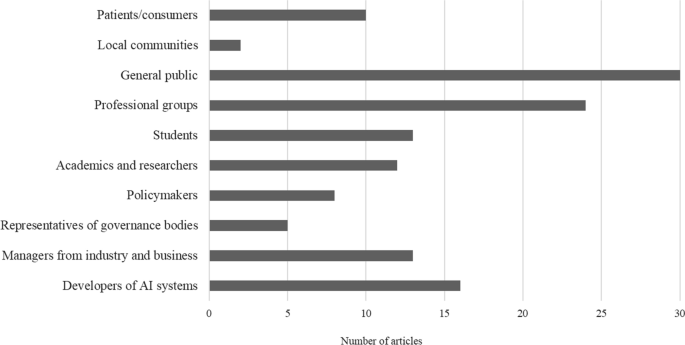
Publics invited to engage with issues framed as ethical challenges of AI
There are some methodological limitations in this scoping review that should be taken into account when interpreting the results. The use of only two search engines may preclude the inclusion of relevant studies, although supplemented by scanning the reference list of eligible studies. An in-depth analysis of the topics explored within each of the seven key ethical principles outlined by the EC's Ethics Guidelines for Trustworthy AI was not conducted. This assessment would lead to a detailed understanding of the publics’ views on ethical challenges of AI.
3.1 Study characteristics
Most of the studies were in recent years, with 35 of the 64 studies being published in 2022 and 2023. Journals were listed either on the databases related to Science Citation Index Expanded (n = 25) or the Social Science Citation Index (n = 23), with fewer journals indexed in the Emerging Sources Citation Index (n = 7) and the Arts and Humanities Citation Index (n = 2). Works covered a wide range of fields, including health and medicine (services, policy, medical informatics, medical ethics, public and environmental health); education; business, management and public administration; computer science; information sciences; engineering; robotics; communication; psychology; political science; and transportation. Beyond the general assessment of publics’ attitudes toward, preferences for, and expectations and concerns about AI, the publics’ views on ethical challenges of AI technologies have been studied mainly concerning healthcare and public services and less frequently regarding autonomous vehicles (AV), education, robotic technologies, and smart homes. Most of the studies (n = 47) were funded by research agencies, with 7 papers reporting conflicts of interest.
Quantitative research approaches have assessed the publics’ views on the ethical challenges of AI mainly through online or web-based surveys and experimental platforms, relying on Delphi studies, moral judgment studies, hypothetical vignettes, and choice-based/comparative conjoint surveys. The 25 qualitative studies collected data mainly by semistructured or in-depth interviews. Analysis of publicly available material reporting on AI-use cases, focus groups, a post hoc self-assessment, World Café, participatory research, and practice-based design research were used once or twice. Multi or mixed-methods studies relied on surveys with open-ended and closed questions, frequently combined with focus groups, in-depth interviews, literature reviews, expert opinions, examinations of relevant curriculum examples, tests, and reflexive writings.
The studies were performed (where stated) in a wide variety of countries, including the USA and Australia. More than half of the studies (n = 38) were conducted in a single country. Almost all studies used nonprobability sampling techniques. In quantitative studies, sample sizes varied from 2.3 M internet users in an online experimental platform study [ 40 ] to 20 participants in a Delphi study [ 41 ]. In qualitative studies, the samples varied from 123 participants in 21 focus groups [ 42 ] to six expert interviews [ 43 ]. In multi or mixed-methods studies, samples varied from 2036 participants [ 44 ] to 21 participants [ 45 ].
3.2 Motivations for engaging the publics
The qualitative synthesis of the motivations for researching the publics’ views on the ethical challenges of AI is presented in Table 2 and ordered by the number of studies referencing them in the scoping review. More than half of the studies (n = 37) addressed a single motivation. Innovation (n = 33) and legitimation (n = 29) proved to have the highest relevance as motivations for engaging the publics in the ethical challenges of AI technologies, as articulated in 15 studies. Additional motivations are rooted in education (n = 13), democratization (n = 11), and inspiration (n = 9). Politicization was mentioned in five studies. Although they were not authors’ motivations, few studies were found to have educational [ 46 , 47 ], democratization [ 48 , 49 ], and legitimation or inspirations effects [ 50 ].
To consider the publics as a valuable source of knowledge that can add real value to innovation processes in both the private and public sectors was the most frequent motivation mentioned in the literature. The call for public participation is rooted in the aspiration to add knowledge outside “formal” ethics at three interrelated levels. First, at a societal level, by asking what kind of AI we want as a society based on novel experiments on public policy preferences [ 51 ] and on the study of public perceptions, values, and concerns regarding AI design, development, and implementation in domains such as health care [ 46 , 52 , 53 , 54 , 55 ], public and social services [ 49 , 56 , 57 , 58 ], AV [ 59 , 60 ] and journalism [ 61 ]. Second, at a practical level, the literature provides insights into the perceived usefulness of AI applications [ 62 , 63 ] and choices between boosting developers’ voluntary adoption of ethical standards or imposing ethical standards via regulation and oversight [ 64 ], as well as suggesting specific guidance for the development and use of AI systems [ 65 , 66 , 67 ]. Finally, at a theoretical level, literature expands the social-technical perspective [ 68 ] and motivated-reasoning theory [ 69 ].
Legitimation was also a frequent motivation for engaging the publics. It was underpinned by the need for public trust in and social licences for implementing AI technologies. To ensure the long-term social acceptability of AI as a trustworthy technology [ 70 , 71 ] was perceived as essential to support its use and to justify its implementation. In one study [ 72 ], the authors developed an AI ethics scale to quantify how AI research is accepted in society and which area of ethical, legal, and social issues (ELSI) people are most concerned with. Public trust in and acceptance of AI is claimed by social institutions such as governments, private sectors, industry bodies, and the science community, behaving in a trustworthy manner, respecting public concerns, aligning with societal values, and involving members of the publics in decision-making and public policy [ 46 , 48 , 73 , 74 , 75 ], as well as in the responsible design and integration of AI technologies [ 52 , 76 , 77 ].
Education, democratization, and inspiration had a more modest presence as motivations to explore the publics’ views on the ethical challenges of AI. Considering the emergence of new roles and tasks related to AI, the literature has pointed to the public need to ensure the safe use of AI technologies by incorporating ethics and career futures into the education, preparation, and training of both middle school and university students and the current and future health workforce. Improvements in education and guidance for developers and older adults were also noticed. The views of the publics on what needs to be learned or how this learning may be supported or assessed were perceived as crucial. In one study [ 78 ], the authors developed strategies that promote learning related to AI through collaborative media production, connecting computational thinking to civic issues and creative expression. In another study [ 79 ], real-world scenarios were successfully used as a novel approach to teaching AI ethics. Rhim et al. [ 76 ] provided AV moral behavior design guidelines for policymakers, developers, and the publics by reducing the abstractness of AV morality.
Studies motivated by democratization promoted broader public participation in AI, aiming to empower citizens both to express their understandings, apprehensions, and concerns about AI [ 43 , 78 , 80 , 81 ] and to address ethical issues in AI as critical consumers, (potential future) developers of AI technologies or would-be participants in codesign processes [ 40 , 43 , 45 , 78 , 82 , 83 ]. Understanding the publics’ views on the ethical challenges of AI is expected to influence companies and policymakers [ 40 ]. In one study [ 45 ], the authors explored how a digital app might support citizens’ engagement in AI governance by informing them, raising public awareness, measuring publics’ attitudes and supporting collective decision-making.
Inspiration revolved around three main motivations: to raise public interest in AI [ 46 , 48 ]; to guide future empirical and design studies [ 79 ]; and to promote developers’ moral awareness through close collaboration between all those involved in the implementation, use, and design of AI technologies [ 46 , 61 , 78 , 84 , 85 ].
Politicization was the less frequent motivation reported in the literature for engaging the publics. Recognizing the need for mitigation of social biases [ 86 ], public participation to address historically marginalized populations [ 78 , 87 ], and promoting social equity [ 79 ] were the highlighted motives.
3.3 The invited publics
Study participants were mostly the general public and professional groups, followed by developers of AI systems, managers from industry and business, students, academics and researchers, patients/consumers, and policymakers (Fig. 2 ). The views of local communities and representatives of governance bodies were rarely assessed.
Representative samples of the general public were used in five papers related to studies conducted in the USA [ 88 ], Denmark [ 73 ], Germany [ 48 ], and Austria [ 49 , 63 ]. The remaining random or purposive samples from the general public comprised mainly adults and current and potential users of AI products and services, with few studies involving informal caregivers or family members of patients (n = 3), older people (n = 2), and university staff (n = 2).
Samples of professional groups included mainly healthcare professionals (19 out of 24 studies). Educators, law enforcement, media practitioners, and GLAM professionals (galleries, libraries, archives, and museums) were invited once.
3.4 Ethical issues
The ethical issues concerning AI technologies perceived as needing the participation of the publics are depicted in Table 3 . They were mapped by measuring the number of studies referencing them in the scoping review. Human agency and oversight (n = 55) was the most frequent ethical aspect that was studied in the literature, followed by those centered on privacy and data governance (n = 43). Diversity, nondiscrimination and fairness (n = 39), societal and environmental well-being (n = 39), technical robustness and safety (n = 38), transparency (n = 35), and accountability (n = 31) were less frequently discussed.
The concerns regarding human agency and oversight were the replacement of human beings by AI technologies and deskilling [ 47 , 55 , 67 , 74 , 75 , 89 , 90 ]; the loss of autonomy, critical thinking, and innovative capacities [ 50 , 58 , 61 , 77 , 78 , 83 , 85 , 90 ]; the erosion of human judgment and oversight [ 41 , 70 , 91 ]; and the potential for (over)dependence on technology and “oversimplified” decisions [ 90 ] due to the lack of publics’ expertise in judging and controlling AI technologies [ 68 ]. Beyond these ethical challenges, the following contributions of AI systems to empower human beings were noted: more fruitful and empathetic social relationships [ 47 , 68 , 90 ]; enhancing human capabilities and quality of life [ 68 , 70 , 74 , 83 , 92 ]; improving efficiency and productivity at work [ 50 , 53 , 62 , 65 , 83 ] by reducing errors [ 77 ], relieving the burden of professionals and/or increasing accuracy in decisions [ 47 , 55 , 90 ]; and facilitating and expanding access to safe and fair healthcare [ 42 , 53 , 54 ] through earlier diagnosis, increased screening and monitoring, and personalized prescriptions [ 47 , 90 ]. To foster human rights, allowing people to make informed decisions, the last say was up to the person themselves [ 42 , 43 , 46 , 55 , 64 , 67 , 73 , 76 ]. People should determine where and when to use automated functions and which functions to use [ 44 , 54 ], developing “job sharing” arrangements with machines and humans complementing and enriching each other [ 56 , 65 , 90 ]. The literature highlights the need to build AI systems that are under human control [ 48 , 70 ] whether to confirm or to correct the AI system’s outputs and recommendations [ 66 , 90 ]. Proper oversight mechanisms were seen as crucial to ensure accuracy and completeness, with divergent views about who should be involved in public participation approaches [ 86 , 87 ].
Data sharing and/or data misuse were considered the major roadblocks regarding privacy and data governance, with some studies pointing out distrust of participants related to commercial interests in health data [ 55 , 90 , 93 , 94 , 95 ] and concerns regarding risks of information getting into the hands of hackers, banks, employers, insurance companies, or governments [ 66 ]. As data are the backbone of AI, secure methods of data storage and protection are understood as needing to be provided from the input to the output data. Recognizing that in contemporary societies, people are aware of the consequences of smartphone use resulting in the minimization of privacy concerns [ 93 ], some studies have focused on the impacts of data breaches and loss of privacy and confidentiality [ 43 , 45 , 46 , 60 , 62 , 80 ] in relation to health-sensitive personal data [ 46 , 93 ], potentially affecting more vulnerable populations, such as senior citizens and mentally ill patients [ 82 , 90 ] as well as those at young ages [ 50 ], and when journalistic organizations collect user data to provide personalized news suggestions [ 61 ]. The need to find a balance between widening access to data and ensuring confidentiality and respect for privacy [ 53 ] was often expressed in three interrelated terms: first, the ability of data subjects to be fully informed about how data will be used and given the option of providing informed consent [ 46 , 58 , 78 ] and controlling personal information about oneself [ 57 ]; second, the need for regulation [ 52 , 65 , 87 ], with one study reporting that AI developers complain about the complexity, slowness, and obstacles created by regulation [ 64 ]; and last, the testing and certification of AI-enabled products and services [ 71 ]. The study by De Graaf et al. [ 91 ] discussed the robots’ right to store and process the data they collect, while Jenkins and Draper [ 42 ] explored less intrusive ways in which the robot could use information to report back to carers about the patient’s adherence to healthcare.
Studies discussing diversity, nondiscrimination, and fairness have pointed to the development of AI systems that reflect and reify social inequalities [ 45 , 78 ] through nonrepresentative datasets [ 55 , 58 , 96 , 97 ] and algorithmic bias [ 41 , 45 , 85 , 98 ] that might benefit some more than others. This could have multiple negative consequences for different groups based on ethnicity, disease, physical disability, age, gender, culture, or socioeconomic status [ 43 , 55 , 58 , 78 , 82 , 87 ], from the dissemination of hate speech [ 79 ] to the exacerbation of discrimination, which negatively impacts peace and harmony within society [ 58 ]. As there were cross-country differences and issue variations in the publics’ views of discriminatory bias [ 51 , 72 , 73 ], fostering diversity, inclusiveness, and cultural plurality [ 61 ] was perceived as crucial to ensure the transferability/effectiveness of AI systems in all social groups [ 60 , 94 ]. Diversity, nondiscrimination, and fairness were also discussed as a means to help reduce health inequalities [ 41 , 67 , 90 ], to compensate for human preconceptions about certain individuals [ 66 ], and to promote equitable distribution of benefits and burdens [ 57 , 71 , 80 , 93 ], namely, supporting access by all to the same updated and high-quality AI systems [ 50 ]. In one study [ 83 ], students provided constructive solutions to build an unbiased AI system, such as using a dataset that includes a diverse dataset engaging people of different ages, genders, ethnicities, and cultures. In another study [ 86 ], participants recommended diverse approaches to mitigate algorithmic bias, from open disclosure of limitations to consumer and patient engagement, representation of marginalized groups, incorporation of equity considerations into sampling methods and legal recourse, and identification of a wide range of stakeholders who may be responsible for addressing AI bias: developers, healthcare workers, manufacturers and vendors, policymakers and regulators, AI researchers and consumers.
Impacts on employment and social relationships were considered two major ethical challenges regarding societal and environmental well-being. The literature has discussed tensions between job creation [ 51 ] and job displacement [ 42 , 90 ], efficiency [ 90 ], and deskilling [ 57 ]. The concerns regarding future social relationships were the loss of empathy, humanity, and/or sensitivity [ 52 , 66 , 90 , 99 ]; isolation and fewer social connections [ 42 , 47 , 90 ]; laziness [ 50 , 83 ]; anxious counterreactions [ 83 , 99 ]; communication problems [ 90 ]; technology dependence [ 60 ]; plagiarism and cheating in education [ 50 ]; and becoming too emotionally attached to a robot [ 65 ]. To overcome social unawareness [ 56 ] and lack of acceptance [ 65 ] due to financial costs [ 56 , 90 ], ecological burden [ 45 ], fear of the unknown [ 65 , 83 ] and/or moral issues [ 44 , 59 , 100 ], AI systems need to provide public benefit sharing [ 55 ], consider discrepancies between public discourse about AI and the utility of the tools in real-world settings and practices [ 53 ], conform to the best standards of sustainability and address climate change and environmental justice [ 60 , 71 ]. Successful strategies in promoting the acceptability of robots across contexts included an approachable and friendly looking as possible, but not too human-like [ 49 , 65 ], and working with, rather than in competition, with humans [ 42 ].
The publics were invited to participate in the following ethical issues related to technical robustness and safety: usability, reliability, liability, and quality assurance checks of AI tools [ 44 , 45 , 55 , 62 , 99 ]; validity of big data analytic tools [ 87 ]; the degree to which an AI system can perform tasks without errors or mistakes [ 50 , 57 , 66 , 84 , 90 , 93 ]; and needed resources to perform appropriate (cyber)security [ 62 , 101 ]. Other studies approached the need to consider both material and normative concerns of AI applications [ 51 ], namely, assuring that AI systems are developed responsibly with proper consideration of risks [ 71 ] and sufficient proof of benefits [ 96 ]. One study [ 64 ] highlighted that AI developers tend to be reluctant to recognize safety issues, bias, errors, and failures, and when they do so, they do so in a selective manner and in their terms by adopting positive-sounding professional jargon as AI robustness.
Some studies recognized the need for greater transparency that reduces the mystery and opaqueness of AI systems [ 71 , 82 , 101 ] and opens its “black box” [ 64 , 71 , 98 ]. Clear insights about “what AI is/is not” and “how AI technology works” (definition, applications, implications, consequences, risks, limitations, weaknesses, threats, rewards, strengths, opportunities) were considered as needed to debunk the myth about AI as an independent entity [ 53 ] and for providing sufficient information and understandable explanations of “what’s happening” to society and individuals [ 43 , 48 , 72 , 73 , 78 , 102 ]. Other studies considered that people, when using AI tools, should be made fully aware that these AI devices are capturing and using their data [ 46 ] and how data are collected [ 58 ] and used [ 41 , 46 , 93 ]. Other transparency issues reported in the literature included the need for more information about the composition of data training sets [ 55 ], how algorithms work [ 51 , 55 , 84 , 94 , 97 ], how AI makes a decision [ 57 ] and the motivations for that decision [ 98 ]. Transparency requirements were also addressed as needing the involvement of multiple stakeholders: one study reported that transparency requirements should be seen as a mediator of debate between experts, citizens, communities, and stakeholders [ 87 ] and cannot be reduced to a product feature, avoiding experiences where people feel overwhelmed by explanations [ 98 ] or “too much information” [ 66 ].
Accountability was perceived by the publics as an important ethical issue [ 48 ], while developers expressed mixed attitudes, from moral disengagement to a sense of responsibility and moral conflict and uncertainty [ 85 ]. The literature has revealed public skepticism regarding accountability mechanisms [ 93 ] and criticism about the shift of responsibility away from tech industries that develop and own AI technologies [ 53 , 68 ], as it opens space for users to assume their own individual responsibility [ 78 ]. This was the case in studies that explored accountability concerns regarding the assignment of fault and responsibility for car accidents using self-driving technology [ 60 , 76 , 77 , 88 ]. Other studies considered that more attention is needed to scrutinize each application across the AI life cycle [ 41 , 71 , 94 ], to explainability of AI algorithms that provide to the publics the cause of AI outcomes [ 58 ], and to regulations that assign clear responsibility concerning litigation and liability [ 52 , 89 , 101 , 103 ].
4 Discussion
Within the realm of research studies encompassed in the scoping review, the contemporary impetus for engaging the publics in ethical considerations related to AI predominantly revolves around two key motivations: innovation and legitimation. This might be explained by the current emphasis on responsible innovation, which values the publics’ participation in knowledge and innovation-making [ 29 ] within a prioritization of the instrumental role of science for innovation and economic return [ 33 ]. Considering the publics as a valuable source of knowledge that should be called upon to contribute to knowledge innovation production is underpinned by the desire for legitimacy, specifically centered around securing the publics’ endorsement of scientific and technological advancements [ 33 , 104 ]. Approaching the publics’ views on the ethical challenges of AI can also be used as a form of risk prevention to reduce conflict and close vital debates in contention areas [ 5 , 34 , 105 ].
A second aspect that stood out in this finding is a shift in the motivations frequently reported as central for engaging the publics with AI technologies. Previous studies analysing AI national policies and international guidelines addressing AI governance [ 3 , 4 , 5 ] and a study analysing science communication journals [ 33 ] highlighted education, inspiration and democratization as the most prominent motivations. Our scoping review did not yield similar findings, which might signal a departure, in science policy related to public participation, from the past emphasis on education associated with the deficit model of public understanding of science and democratization of the model of public engagement with science [ 106 , 107 ].
The underlying motives for the publics’ engagement raise the question of the kinds of publics it addresses, i.e., who are the publics that are supposed to be recruited as research participants [ 32 ]. Our findings show a prevalence of the general public followed by professional groups and developers of AI systems. The wider presence of the general public indicates not only what Hagendijk and Irwin [ 32 , p. 167] describe as a fashionable tendency in policy circles since the late 1990s, and especially in Europe, focused on engaging 'the public' in scientific and technological change but also the avoidance of the issues of democratic representation [ 12 , 18 ]. Additionally, the unspecificity of the “public” does not stipulate any particular action [ 24 ] that allows for securing legitimacy for and protecting the interests of a wide range of stakeholders [ 19 , 108 ] while bringing the risk of silencing the voices of the very publics with whom engagement is sought [ 33 ]. The focus on approaching the publics’ views on the ethical challenges of AI through the general public also demonstrates how seeking to “lay” people’s opinions may be driven by a desire to promote public trust and acceptance of AI developments, showing how science negotiates challenges and reinstates its authority [ 109 ].
While this strategy is based on nonscientific audiences or individuals who are not associated with any scientific discipline or area of inquiry as part of their professional activities, the converse strategy—i.e., involving professional groups and AI developers—is also noticeable in our findings. This suggests that technocratic expert-dominated approaches coexist with a call for more inclusive multistakeholder approaches [ 3 ]. This coexistence is reinforced by the normative principles of the “responsible innovation” framework, in particular the prescription that innovation should include the publics as well as traditionally defined stakeholders [ 3 , 110 ], whose input has become so commonplace that seeking the input of laypeople on emerging technologies is sometimes described as a “standard procedure” [ 111 , p. 153].
In the body of literature included in the scoping review, human agency and oversight emerged as the predominant ethical dimension under investigation. This finding underscores the pervasive significance attributed to human centricity, which is progressively integrated into public discourses concerning AI, innovation initiatives, and market-driven endeavours [ 15 , 112 ]. In our perspective, the importance given to human-centric AI is emblematic of the “techno-regulatory imaginary” suggested by Rommetveit and van Dijk [ 35 ] in their study about privacy engineering applied in the European Union’s General Data Protection Regulation. This term encapsulates the evolving collective vision and conceptualization of the role of technology in regulatory and oversight contexts. At least two aspects stand out in the techno-regulatory imaginary, as they are meant to embed technoscience in societally acceptable ways. First, it reinstates pivotal demarcations between humans and nonhumans while concurrently producing intensified blurring between these two realms. Second, the potential resolutions offered relate to embedding fundamental rights within the structural underpinnings of technological architectures [ 35 ].
Following human agency and oversight, the most frequent ethical issue discussed in the studies contained in our scoping review was privacy and data governance. Our findings evidence additional central aspects of the “techno-regulatory imaginary” in the sense that instead of the traditional regulatory sites, modes of protecting privacy and data are increasingly located within more privatized and business-oriented institutions [ 6 , 35 ] and crafted according to a human-centric view of rights. The focus on secure ways of data storage and protection as in need to be provided from the input to the output data, the testing and certification of AI-enabled products and services, the risks of data breaches, and calls for finding a balance between widening access to data and ensuring confidentiality and respect for privacy, exhibited by many studies in this scoping review, portray an increasing framing of privacy and data protection within technological and standardization sites. This tendency shows how forms of expertise for privacy and data protection are shifting away from traditional regulatory and legal professionals towards privacy engineers and risk assessors in information security and software development. Another salient element to highlight pertains to the distribution of responsibility for privacy and data governance [ 6 , 113 ] within the realm of AI development through engagement with external stakeholders, including users, governmental bodies, and regulatory authorities. It extends from an emphasis on issues derived from data sharing and data misuse to facilitating individuals to exercise control over their data and privacy preferences and to advocating for regulatory frameworks that do not impede the pace of innovation. This distribution of responsibility shared among the contributions and expectations of different actors is usually convoked when the operationalization of ethics principles conflicts with AI deployment [ 6 ]. In this sense, privacy and data governance are reconstituted as a “normative transversal” [ 113 , p. 20], both of which work to stabilize or close controversies, while their operationalization does not modify any underlying operations in AI development.
Diversity, nondiscrimination and fairness, societal and environmental well-being, technical robustness and safety, transparency, and accountability were the ethical issues less frequently discussed in the studies included in this scoping review. In contrast, ethical issues of technical robustness and safety, transparency, and accountability “are those for which technical fixes can be or have already been developed” and “implemented in terms of technical solutions” [ 12 , p. 103]. The recognition of issues related to technical robustness and safety expresses explicit admissions of expert ignorance, error, or lack of control, which opens space for politics of “optimization of algorithms” [ 114 , p. 17] while reinforcing “strategic ignorance” [ 114 , p. 89]. In the words of the sociologist Linsey McGoey, strategic ignorance refers to “any actions which mobilize, manufacture or exploit unknowns in a wider environment to avoid liability for earlier actions” [ 115 , p. 3].
According to the analysis of Jobin et al. [ 11 ] of the global landscape of existing ethics guidelines for AI, transparency comprising efforts to increase explainability, interpretability, or other acts of communication and disclosure is the most prevalent principle in the current literature. Transparency gains high relevance in ethics guidelines because this principle has become a pro-ethical condition “enabling or impairing other ethical practices or principles” [Turilli and Floridi 2009, [ 11 ], p. 14]. Our findings highlight transparency as a crucial ethical concern for explainability and disclosure. However, as emphasized by Ananny and Crawford [ 116 , p. 973], there are serious limitations to the transparency ideal in making black boxes visible (i.e., disclosing and explaining algorithms), since “being able to see a system is sometimes equated with being able to know how it works and governs it—a pattern that recurs in recent work about transparency and computational systems”. The emphasis on transparency mirrors Aradau and Blanke’s [ 114 ] observation that Big Tech firms are creating their version of transparency. They are prompting discussions about their data usage, whether it is for “explaining algorithms” or addressing bias and discrimination openly.
The framing of ethical issues related to accountability, as elucidated by the studies within this scoping review, manifests as a commitment to ethical conduct and the transparent allocation of responsibility and legal obligations in instances where the publics encounters algorithmic deficiencies, glitches, or other imperfections. Within this framework, accountability becomes intricately intertwined with the notion of distributed responsibility, as expounded upon in our examination of how the literature addresses challenges in privacy and data governance. Simultaneously, it converges with our discussion on optimizing algorithms concerning ethical concerns on technical robustness and safety by which AI systems are portrayed as fallible yet eternally evolving towards optimization. As astutely observed by Aradau and Blanke [ 114 , p. 171], “forms of accountability through error enact algorithmic systems as fallible but ultimately correctable and therefore always desirable. Errors become temporary malfunctions, while the future of algorithms is that of indefinite optimization”.
5 Conclusion
This scoping review of how publics' views on ethical challenges of AI are framed, articulated, and concretely operationalized in the research sector shows that ethical issues and publics formation are closely entangled with symbolic and social orders, including political and economic agendas and visions. While Steinhoff [ 6 ] highlights the subordinated nature of AI ethics within an innovation network, drawing on insights from diverse sources beyond Big Tech, we assert that this network is dynamically evolving towards greater hybridity and boundary fusion. In this regard, we extend Steinhoff's argument by emphasizing the imperative for a more nuanced understanding of how this network operates within diverse contexts. Specifically, within the research sector, it operates through a convergence of boundaries, engaging human and nonhuman entities and various disciplines and stakeholders. Concurrently, the advocacy for diversity and inclusivity, along with the acknowledgement of errors and flaws, serves to bolster technical expertise and reaffirm the establishment of order and legitimacy in alignment with the institutional norms underpinning responsible research practices.
Our analysis underscores the growing importance of involving the publics in AI knowledge creation and innovation, both to secure public endorsement and as a tool for risk prevention and conflict mitigation. We observe two distinct approaches: one engaging nonscientific audiences and the other involving professional groups and AI developers, emphasizing the need for inclusivity while safeguarding expert knowledge. Human-centred approaches are gaining prominence, emphasizing the distinction and blending of human and nonhuman entities and embedding fundamental rights in technological systems. Privacy and data governance emerge as the second most prevalent ethical concern, shifting expertise away from traditional regulatory experts to privacy engineers and risk assessors. The distribution of responsibility for privacy and data governance is a recurring theme, especially in cases of ethical conflicts with AI deployment. However, there is a notable imbalance in attention, with less focus on diversity, nondiscrimination, fairness, societal, and environmental well-being, compared to human-centric AI, privacy, and data governance being managed through technical fixes. Last, acknowledging technical robustness and safety, transparency, and accountability as foundational ethics principles reveals an openness to expert limitations, allowing room for the politics of algorithm optimization, framing AI systems as correctable and perpetually evolving.
Data availability
This manuscript has data included as electronic supplementary material. The dataset constructed by the authors, resulting from a search of publications on PubMed ® and Web of Science™, analysed in the current study, is not publicly available. But it can be available from the corresponding author on reasonable request.
In this article, we will employ the term "publics" rather than the singular "public" to delineate our viewpoint concerning public participation in AI. Our option is meant to acknowledge that there are no uniform, monolithic viewpoints or interests. From our perspective, the term "publics" allows for a more nuanced understanding of the various groups, communities, and individuals who may have different attitudes, beliefs, and concerns regarding AI. This choice may differ from the terminology employed in the referenced literature.
The following examples are particularly illustrative of the multiplicity of organizations emphasizing the need for public participation in AI. The OECD Recommendations of the Council on AI specifically emphasizes the importance of empowering stakeholders considering essential their engagement to adoption of trustworthy [ 7 , p. 6]. The UNESCO Recommendation on the Ethics of AI emphasizes that public awareness and understanding of AI technologies should be promoted (recommendation 44) and it encourages governments and other stakeholders to involve the publics in AI decision-making processes (recommendation 47) [ 8 , p. 23]. The European Union (EU) White Paper on AI [ 9 , p. 259] outlines the EU’s approach to AI, including the need for public consultation and engagement. The Ethics Guidelines for Trustworthy AI [ 10 , pp. 19, 239], developed by the High-Level Expert Group on AI (HLEG) appointed by the EC, emphasize the importance of public participation and consultation in the design, development, and deployment of AI systems.
“Responsible Innovation” (RI) and “Responsible Research and Innovation” (RRI) have emerged in parallel and are often used interchangeably, but they are not the same thing [ 27 , 28 ]. RRI is a policy-driven discourse that emerged from the EC in the early 2010s, while RI emerged largely from academic roots. For this paper, we will not consider the distinctive features of each discourse, but instead focus on the common features they share.
Cath, C., Wachter, S., Mittelstadt, B., Taddeo, M., Floridi, L.: Artificial intelligence and the ‘good society’: the US, EU, and UK approach. Sci. Eng. Ethics 24 , 505–528 (2017). https://doi.org/10.1007/s11948-017-9901-7
Article Google Scholar
Cussins, J.N.: Decision points in AI governance. CLTC white paper series. Center for Long-term Cybersecurity. https://cltc.berkeley.edu/publication/decision-points-in-ai-governance/ (2020). Accessed 8 July 2023
Ulnicane, I., Okaibedi Eke, D., Knight, W., Ogoh, G., Stahl, B.: Good governance as a response to discontents? Déjà vu, or lessons for AI from other emerging technologies. Interdiscip. Sci. Rev. 46 (1–2), 71–93 (2021). https://doi.org/10.1080/03080188.2020.1840220
Ulnicane, I., Knight, W., Leach, T., Stahl, B., Wanjiku, W.: Framing governance for a contested emerging technology: insights from AI policy. Policy Soc. 40 (2), 158–177 (2021). https://doi.org/10.1080/14494035.2020.1855800
Wilson, C.: Public engagement and AI: a values analysis of national strategies. Gov. Inf. Q. 39 (1), 101652 (2022). https://doi.org/10.1016/j.giq.2021.101652
Steinhoff, J.: AI ethics as subordinated innovation network. AI Soc. (2023). https://doi.org/10.1007/s00146-023-01658-5
Organization for Economic Co-operation and Development. Recommendation of the Council on Artificial Intelligence. https://legalinstruments.oecd.org/en/instruments/oecd-legal-0449 (2019). Accessed 8 July 2023
United Nations Educational, Scientific and Cultural Organization. Recommendation on the Ethics of Artificial Intelligence. https://unesdoc.unesco.org/ark:/48223/pf0000381137 (2021). Accessed 28 June 2023
European Commission. On artificial intelligence – a European approach to excellence and trust. White paper. COM(2020) 65 final. https://commission.europa.eu/publications/white-paper-artificial-intelligence-european-approach-excellence-and-trust_en (2020). Accessed 28 June 2023
European Commission. The ethics guidelines for trustworthy AI. Directorate-General for Communications Networks, Content and Technology, EC Publications Office. https://digital-strategy.ec.europa.eu/en/library/ethics-guidelines-trustworthy-ai (2019). Accessed 10 July 2023
Jobin, A., Ienca, M., Vayena, E.: The global landscape of AI ethics guidelines. Nat. Mach. Intell. 1 , 389–399 (2019). https://doi.org/10.1038/s42256-019-0088-2
Hagendorff, T.: The ethics of AI ethics: an evaluation of guidelines. Minds Mach. 30 , 99–120 (2020). https://doi.org/10.1007/s11023-020-09517-8
Su, A.: The promise and perils of international human rights law for AI governance. Law Technol. Hum. 4 (2), 166–182 (2022). https://doi.org/10.5204/lthj.2332
Article MathSciNet Google Scholar
Ulnicane, I.: Emerging technology for economic competitiveness or societal challenges? Framing purpose in artificial intelligence policy. GPPG. 2 , 326–345 (2022). https://doi.org/10.1007/s43508-022-00049-8
Sigfrids, A., Leikas, J., Salo-Pöntinen, H., Koskimies, E.: Human-centricity in AI governance: a systemic approach. Front Artif. Intell. 6 , 976887 (2023). https://doi.org/10.3389/frai.2023.976887
Benkler, Y.: Don’t let industry write the rules for AI. Nature 569 (7755), 161 (2019). https://doi.org/10.1038/d41586-019-01413-1
Phan, T., Goldenfein, J., Mann, M., Kuch, D.: Economies of virtue: the circulation of ‘ethics’ in Big Tech. Sci. Cult. 31 (1), 121–135 (2022). https://doi.org/10.1080/09505431.2021.1990875
Ochigame, R.: The invention of “ethical AI”: how big tech manipulates academia to avoid regulation. Intercept. https://theintercept.com/2019/12/20/mit-ethical-ai-artificial-intelligence/ (2019). Accessed 10 July 2023
Ferretti, T.: An institutionalist approach to ai ethics: justifying the priority of government regulation over self-regulation. MOPP 9 (2), 239–265 (2022). https://doi.org/10.1515/mopp-2020-0056
van Maanen, G.: AI ethics, ethics washing, and the need to politicize data ethics. DISO 1 (9), 1–23 (2022). https://doi.org/10.1007/s44206-022-00013-3
Gerdes, A.: The tech industry hijacking of the AI ethics research agenda and why we should reclaim it. Discov. Artif. Intell. 2 (25), 1–8 (2022). https://doi.org/10.1007/s44163-022-00043-3
Amariles, D.R., Baquero, P.M.: Promises and limits of law for a human-centric artificial intelligence. Comput. Law Secur. Rev. 48 (105795), 1–10 (2023). https://doi.org/10.1016/j.clsr.2023.105795
Mittelstadt, B.: Principles alone cannot guarantee ethical AI. Nat. Mach. Intell. 1 (11), 501–507 (2019). https://doi.org/10.1038/s42256-019-0114-4
Munn, L.: The uselessness of AI ethics. AI Ethics 3 , 869–877 (2022). https://doi.org/10.1007/s43681-022-00209-w
Heilinger, J.C.: The ethics of AI ethics. A constructive critique. Philos. Technol. 35 (61), 1–20 (2022). https://doi.org/10.1007/s13347-022-00557-9
Roche, C., Wall, P.J., Lewis, D.: Ethics and diversity in artificial intelligence policies, strategies and initiatives. AI Ethics (2022). https://doi.org/10.1007/s43681-022-00218-9
Diercks, G., Larsen, H., Steward, F.: Transformative innovation policy: addressing variety in an emerging policy paradigm. Res. Policy 48 (4), 880–894 (2019). https://doi.org/10.1016/j.respol.2018.10.028
Owen, R., Pansera, M.: Responsible innovation and responsible research and innovation. In: Dagmar, S., Kuhlmann, S., Stamm, J., Canzler, W. (eds.) Handbook on Science and Public Policy, pp. 26–48. Edward Elgar, Cheltenham (2019)
Google Scholar
Macq, H., Tancoigne, E., Strasser, B.J.: From deliberation to production: public participation in science and technology policies of the European Commission (1998–2019). Minerva 58 (4), 489–512 (2020). https://doi.org/10.1007/s11024-020-09405-6
Cath, C.: Governing artificial intelligence: ethical, legal and technical opportunities and challenges. Philos. Trans. Royal Soc. A. 376 , 20180080 (2018). https://doi.org/10.1098/rsta.2018.0080
Wilson, C.: The socialization of civic participation norms in government?: Assessing the effect of the Open Government Partnership on countries’e-participation. Gov. Inf. Q. 37 (4), 101476 (2020). https://doi.org/10.1016/j.giq.2020.101476
Hagendijk, R., Irwin, A.: Public deliberation and governance: engaging with science and technology in contemporary Europe. Minerva 44 (2), 167–184 (2006). https://doi.org/10.1007/s11024-006-0012-x
Weingart, P., Joubert, M., Connoway, K.: Public engagement with science - origins, motives and impact in academic literature and science policy. PLoS One 16 (7), e0254201 (2021). https://doi.org/10.1371/journal.pone.0254201
Wynne, B.: Public participation in science and technology: performing and obscuring a political–conceptual category mistake. East Asian Sci. 1 (1), 99–110 (2007). https://doi.org/10.1215/s12280-007-9004-7
Rommetveit, K., Van Dijk, N.: Privacy engineering and the techno-regulatory imaginary. Soc. Stud. Sci. 52 (6), 853–877 (2022). https://doi.org/10.1177/03063127221119424
Levac, D., Colquhoun, H., O’Brien, K.: Scoping studies: advancing the methodology. Implement. Sci. 5 (69), 1–9 (2010). https://doi.org/10.1186/1748-5908-5-69
Arksey, H., O’Malley, L.: Scoping studies: towards a methodological framework. Int. J. Soc. Res. Methodol. 8 (1), 19–32 (2005). https://doi.org/10.1080/1364557032000119616
Stemler, S.: An overview of content analysis. Pract. Asses. Res. Eval. 7 (17), 1–9 (2001). https://doi.org/10.7275/z6fm-2e34
European Commission. European Commission's ethics guidelines for trustworthy AI. https://digital-strategy.ec.europa.eu/en/library/ethics-guidelines-trustworthy-ai (2021). Accessed 8 July 2023
Awad, E., Dsouza, S., Kim, R., Schulz, J., Henrich, J., Shariff, A., et al.: The moral machine experiment. Nature 563 (7729), 59–64 (2018). https://doi.org/10.1038/s41586-018-0637-6
Liyanage, H., Liaw, S.T., Jonnagaddala, J., Schreiber, R., Kuziemsky, C., Terry, A.L., de Lusignan, S.: Artificial intelligence in primary health care: perceptions, issues, and challenges. Yearb. Med. Inform. 28 (1), 41–46 (2019). https://doi.org/10.1055/s-0039-1677901
Jenkins, S., Draper, H.: Care, monitoring, and companionship: views on care robots from older people and their carers. Int. J. Soc. Robot. 7 (5), 673–683 (2015). https://doi.org/10.1007/s12369-015-0322-y
Tzouganatou, A.: Openness and privacy in born-digital archives: reflecting the role of AI development. AI Soc. 37 (3), 991–999 (2022). https://doi.org/10.1007/s00146-021-01361-3
Liljamo, T., Liimatainen, H., Pollanen, M.: Attitudes and concerns on automated vehicles. Transp. Res. Part F Traffic Psychol. Behav. 59 , 24–44 (2018). https://doi.org/10.1016/j.trf.2018.08.010
Couture, V., Roy, M.C., Dez, E., Laperle, S., Belisle-Pipon, J.C.: Ethical implications of artificial intelligence in population health and the public’s role in its governance: perspectives from a citizen and expert panel. J. Med. Internet Res. 25 , e44357 (2023). https://doi.org/10.2196/44357
McCradden, M.D., Sarker, T., Paprica, P.A.: Conditionally positive: a qualitative study of public perceptions about using health data for artificial intelligence research. BMJ Open 10 (10), e039798 (2020). https://doi.org/10.1136/bmjopen-2020-039798
Blease, C., Kharko, A., Annoni, M., Gaab, J., Locher, C.: Machine learning in clinical psychology and psychotherapy education: a mixed methods pilot survey of postgraduate students at a Swiss University. Front. Public Health 9 (623088), 1–8 (2021). https://doi.org/10.3389/fpubh.2021.623088
Kieslich, K., Keller, B., Starke, C.: Artificial intelligence ethics by design. Evaluating public perception on the importance of ethical design principles of artificial intelligence. Big Data Soc. 9 (1), 1–15 (2022). https://doi.org/10.1177/20539517221092956
Willems, J., Schmidthuber, L., Vogel, D., Ebinger, F., Vanderelst, D.: Ethics of robotized public services: the role of robot design and its actions. Gov. Inf. Q. 39 (101683), 1–11 (2022). https://doi.org/10.1016/J.Giq.2022.101683
Tlili, A., Shehata, B., Adarkwah, M.A., Bozkurt, A., Hickey, D.T., Huang, R.H., Agyemang, B.: What if the devil is my guardian angel: ChatGPT as a case study of using chatbots in education. Smart Learn Environ. 10 (15), 1–24 (2023). https://doi.org/10.1186/S40561-023-00237-X
Ehret, S.: Public preferences for governing AI technology: comparative evidence. J. Eur. Public Policy 29 (11), 1779–1798 (2022). https://doi.org/10.1080/13501763.2022.2094988
Esmaeilzadeh, P.: Use of AI-based tools for healthcare purposes: a survey study from consumers’ perspectives. BMC Med. Inform. Decis. Mak. 20 (170), 1–19 (2020). https://doi.org/10.1186/s12911-020-01191-1
Laïï, M.C., Brian, M., Mamzer, M.F.: Perceptions of artificial intelligence in healthcare: findings from a qualitative survey study among actors in France. J. Transl. Med. 18 (14), 1–13 (2020). https://doi.org/10.1186/S12967-019-02204-Y
Valles-Peris, N., Barat-Auleda, O., Domenech, M.: Robots in healthcare? What patients say. Int. J. Environ. Res. Public Health 18 (9933), 1–18 (2021). https://doi.org/10.3390/ijerph18189933
Hallowell, N., Badger, S., Sauerbrei, A., Nellaker, C., Kerasidou, A.: “I don’t think people are ready to trust these algorithms at face value”: trust and the use of machine learning algorithms in the diagnosis of rare disease. BMC Med. Ethics 23 (112), 1–14 (2022). https://doi.org/10.1186/s12910-022-00842-4
Criado, J.I., de Zarate-Alcarazo, L.O.: Technological frames, CIOs, and artificial intelligence in public administration: a socio-cognitive exploratory study in spanish local governments. Gov. Inf. Q. 39 (3), 1–13 (2022). https://doi.org/10.1016/J.Giq.2022.101688
Isbanner, S., O’Shaughnessy, P.: The adoption of artificial intelligence in health care and social services in Australia: findings from a methodologically innovative national survey of values and attitudes (the AVA-AI Study). J. Med. Internet Res. 24 (8), e37611 (2022). https://doi.org/10.2196/37611
Kuberkar, S., Singhal, T.K., Singh, S.: Fate of AI for smart city services in India: a qualitative study. Int. J. Electron. Gov. Res. 18 (2), 1–21 (2022). https://doi.org/10.4018/Ijegr.298216
Kallioinen, N., Pershina, M., Zeiser, J., Nezami, F., Pipa, G., Stephan, A., Konig, P.: Moral judgements on the actions of self-driving cars and human drivers in dilemma situations from different perspectives. Front. Psychol. 10 (2415), 1–15 (2019). https://doi.org/10.3389/fpsyg.2019.02415
Vrščaj, D., Nyholm, S., Verbong, G.P.J.: Is tomorrow’s car appealing today? Ethical issues and user attitudes beyond automation. AI Soc. 35 (4), 1033–1046 (2020). https://doi.org/10.1007/s00146-020-00941-z
Bastian, M., Helberger, N., Makhortykh, M.: Safeguarding the journalistic DNA: attitudes towards the role of professional values in algorithmic news recommender designs. Digit. Journal. 9 (6), 835–863 (2021). https://doi.org/10.1080/21670811.2021.1912622
Kaur, K., Rampersad, G.: Trust in driverless cars: investigating key factors influencing the adoption of driverless cars. J. Eng. Technol. Manag. 48 , 87–96 (2018). https://doi.org/10.1016/j.jengtecman.2018.04.006
Willems, J., Schmid, M.J., Vanderelst, D., Vogel, D., Ebinger, F.: AI-driven public services and the privacy paradox: do citizens really care about their privacy? Public Manag. Rev. (2022). https://doi.org/10.1080/14719037.2022.2063934
Duke, S.A.: Deny, dismiss and downplay: developers’ attitudes towards risk and their role in risk creation in the field of healthcare-AI. Ethics Inf. Technol. 24 (1), 1–15 (2022). https://doi.org/10.1007/s10676-022-09627-0
Cresswell, K., Cunningham-Burley, S., Sheikh, A.: Health care robotics: qualitative exploration of key challenges and future directions. J. Med. Internet Res. 20 (7), e10410 (2018). https://doi.org/10.2196/10410
Amann, J., Vayena, E., Ormond, K.E., Frey, D., Madai, V.I., Blasimme, A.: Expectations and attitudes towards medical artificial intelligence: a qualitative study in the field of stroke. PLoS One 18 (1), e0279088 (2023). https://doi.org/10.1371/journal.pone.0279088
Aquino, Y.S.J., Rogers, W.A., Braunack-Mayer, A., Frazer, H., Win, K.T., Houssami, N., et al.: Utopia versus dystopia: professional perspectives on the impact of healthcare artificial intelligence on clinical roles and skills. Int. J. Med. Inform. 169 (104903), 1–10 (2023). https://doi.org/10.1016/j.ijmedinf.2022.104903
Sartori, L., Bocca, G.: Minding the gap(s): public perceptions of AI and socio-technical imaginaries. AI Soc. 38 (2), 443–458 (2022). https://doi.org/10.1007/s00146-022-01422-1
Chen, Y.-N.K., Wen, C.-H.R.: Impacts of attitudes toward government and corporations on public trust in artificial intelligence. Commun. Stud. 72 (1), 115–131 (2021). https://doi.org/10.1080/10510974.2020.1807380
Aitken, M., Ng, M., Horsfall, D., Coopamootoo, K.P.L., van Moorsel, A., Elliott, K.: In pursuit of socially ly-minded data-intensive innovation in banking: a focus group study of public expectations of digital innovation in banking. Technol. Soc. 66 (101666), 1–10 (2021). https://doi.org/10.1016/j.techsoc.2021.101666
Choung, H., David, P., Ross, A.: Trust and ethics in AI. AI Soc. 38 (2), 733–745 (2023). https://doi.org/10.1007/s00146-022-01473-4
Hartwig, T., Ikkatai, Y., Takanashi, N., Yokoyama, H.M.: Artificial intelligence ELSI score for science and technology: a comparison between Japan and the US. AI Soc. 38 (4), 1609–1626 (2023). https://doi.org/10.1007/s00146-021-01323-9
Ploug, T., Sundby, A., Moeslund, T.B., Holm, S.: Population preferences for performance and explainability of artificial intelligence in health care: choice-based conjoint survey. J. Med. Internet Res. 23 (12), e26611 (2021). https://doi.org/10.2196/26611
Zheng, B., Wu, M.N., Zhu, S.J., Zhou, H.X., Hao, X.L., Fei, F.Q., et al.: Attitudes of medical workers in China toward artificial intelligence in ophthalmology: a comparative survey. BMC Health Serv. Res. 21 (1067), 1–13 (2021). https://doi.org/10.1186/S12913-021-07044-5
Ma, J., Tojib, D., Tsarenko, Y.: Sex robots: are we ready for them? An exploration of the psychological mechanisms underlying people’s receptiveness of sex robots. J. Bus. Ethics 178 (4), 1091–1107 (2022). https://doi.org/10.1007/s10551-022-05059-4
Rhim, J., Lee, G.B., Lee, J.H.: Human moral reasoning types in autonomous vehicle moral dilemma: a cross-cultural comparison of Korea and Canada. Comput. Hum. Behav. 102 , 39–56 (2020). https://doi.org/10.1016/j.chb.2019.08.010
Dempsey, R.P., Brunet, J.R., Dubljevic, V.: Exploring and understanding law enforcement’s relationship with technology: a qualitative interview study of police officers in North Carolina. Appl. Sci-Basel 13 (6), 1–17 (2023). https://doi.org/10.3390/App13063887
Lee, C.H., Gobir, N., Gurn, A., Soep, E.: In the black mirror: youth investigations into artificial intelligence. ACM Trans. Comput. Educ. 22 (3), 1–25 (2022). https://doi.org/10.1145/3484495
Kong, S.C., Cheung, W.M.Y., Zhang, G.: Evaluating an artificial intelligence literacy programme for developing university students? Conceptual understanding, literacy, empowerment and ethical awareness. Educ. Technol. Soc. 26 (1), 16–30 (2023). https://doi.org/10.30191/Ets.202301_26(1).0002
Street, J., Barrie, H., Eliott, J., Carolan, L., McCorry, F., Cebulla, A., et al.: Older adults’ perspectives of smart technologies to support aging at home: insights from five world cafe forums. Int. J. Environ. Res. Public Health 19 (7817), 1–22 (2022). https://doi.org/10.3390/Ijerph19137817
Ikkatai, Y., Hartwig, T., Takanashi, N., Yokoyama, H.M.: Octagon measurement: public attitudes toward AI ethics. Int J Hum-Comput Int. 38 (17), 1589–1606 (2022). https://doi.org/10.1080/10447318.2021.2009669
Wang, S., Bolling, K., Mao, W., Reichstadt, J., Jeste, D., Kim, H.C., Nebeker, C.: Technology to support aging in place: older adults’ perspectives. Healthcare (Basel) 7 (60), 1–18 (2019). https://doi.org/10.3390/healthcare7020060
Zhang, H., Lee, I., Ali, S., DiPaola, D., Cheng, Y.H., Breazeal, C.: Integrating ethics and career futures with technical learning to promote AI literacy for middle school students: an exploratory study. Int. J. Artif. Intell. Educ. 33 , 290–324 (2022). https://doi.org/10.1007/s40593-022-00293-3
Henriksen, A., Blond, L.: Executive-centered AI? Designing predictive systems for the public sector. Soc. Stud. Sci. (2023). https://doi.org/10.1177/03063127231163756
Nichol, A.A., Halley, M.C., Federico, C.A., Cho, M.K., Sankar, P.L.: Not in my AI: moral engagement and disengagement in health care AI development. Pac. Symp. Biocomput. 28 , 496–506 (2023)
Aquino, Y.S.J., Carter, S.M., Houssami, N., Braunack-Mayer, A., Win, K.T., Degeling, C., et al.: Practical, epistemic and normative implications of algorithmic bias in healthcare artificial intelligence: a qualitative study of multidisciplinary expert perspectives. J. Med. Ethics (2023). https://doi.org/10.1136/jme-2022-108850
Nichol, A.A., Bendavid, E., Mutenherwa, F., Patel, C., Cho, M.K.: Diverse experts’ perspectives on ethical issues of using machine learning to predict HIV/AIDS risk in sub-Saharan Africa: a modified Delphi study. BMJ Open 11 (7), e052287 (2021). https://doi.org/10.1136/bmjopen-2021-052287
Awad, E., Levine, S., Kleiman-Weiner, M., Dsouza, S., Tenenbaum, J.B., Shariff, A., et al.: Drivers are blamed more than their automated cars when both make mistakes. Nat. Hum. Behav. 4 (2), 134–143 (2020). https://doi.org/10.1038/s41562-019-0762-8
Blease, C., Kaptchuk, T.J., Bernstein, M.H., Mandl, K.D., Halamka, J.D., DesRoches, C.M.: Artificial intelligence and the future of primary care: exploratory qualitative study of UK general practitioners’ views. J. Med. Internet Res. 21 (3), e12802 (2019). https://doi.org/10.2196/12802
Blease, C., Locher, C., Leon-Carlyle, M., Doraiswamy, M.: Artificial intelligence and the future of psychiatry: qualitative findings from a global physician survey. Digit. Health 6 , 1–18 (2020). https://doi.org/10.1177/2055207620968355
De Graaf, M.M.A., Hindriks, F.A., Hindriks, K.V.: Who wants to grant robots rights? Front Robot AI 8 , 781985 (2022). https://doi.org/10.3389/frobt.2021.781985
Guerouaou, N., Vaiva, G., Aucouturier, J.-J.: The shallow of your smile: the ethics of expressive vocal deep-fakes. Philos. Trans. R Soc. B Biol. Sci. 377 (1841), 1–11 (2022). https://doi.org/10.1098/rstb.2021.0083
McCradden, M.D., Baba, A., Saha, A., Ahmad, S., Boparai, K., Fadaiefard, P., Cusimano, M.D.: Ethical concerns around use of artificial intelligence in health care research from the perspective of patients with meningioma, caregivers and health care providers: a qualitative study. CMAJ Open 8 (1), E90–E95 (2020). https://doi.org/10.9778/cmajo.20190151
Rogers, W.A., Draper, H., Carter, S.M.: Evaluation of artificial intelligence clinical applications: Detailed case analyses show value of healthcare ethics approach in identifying patient care issues. Bioethics 36 (4), 624–633 (2021). https://doi.org/10.1111/bioe.12885
Tosoni, S., Voruganti, I., Lajkosz, K., Habal, F., Murphy, P., Wong, R.K.S., et al.: The use of personal health information outside the circle of care: consent preferences of patients from an academic health care institution. BMC Med. Ethics 22 (29), 1–14 (2021). https://doi.org/10.1186/S12910-021-00598-3
Allahabadi, H., Amann, J., Balot, I., Beretta, A., Binkley, C., Bozenhard, J., et al.: Assessing trustworthy AI in times of COVID-19: deep learning for predicting a multiregional score conveying the degree of lung compromise in COVID-19 patients. IEEE Trans. Technol. Soc. 3 (4), 272–289 (2022). https://doi.org/10.1109/TTS.2022.3195114
Gray, K., Slavotinek, J., Dimaguila, G.L., Choo, D.: Artificial intelligence education for the health workforce: expert survey of approaches and needs. JMIR Med. Educ. 8 (2), e35223 (2022). https://doi.org/10.2196/35223
Alfrink, K., Keller, I., Doorn, N., Kortuem, G.: Tensions in transparent urban AI: designing a smart electric vehicle charge point. AI Soc. 38 (3), 1049–1065 (2022). https://doi.org/10.1007/s00146-022-01436-9
Bourla, A., Ferreri, F., Ogorzelec, L., Peretti, C.S., Guinchard, C., Mouchabac, S.: Psychiatrists’ attitudes toward disruptive new technologies: mixed-methods study. JMIR Ment. Health 5 (4), e10240 (2018). https://doi.org/10.2196/10240
Kopecky, R., Kosova, M.J., Novotny, D.D., Flegr, J., Cerny, D.: How virtue signalling makes us better: moral preferences with respect to autonomous vehicle type choices. AI Soc. 38 , 937–946 (2022). https://doi.org/10.1007/s00146-022-01461-8
Lam, K., Abramoff, M.D., Balibrea, J.M., Bishop, S.M., Brady, R.R., Callcut, R.A., et al.: A Delphi consensus statement for digital surgery. NPJ Digit. Med. 5 (100), 1–9 (2022). https://doi.org/10.1038/s41746-022-00641-6
Karaca, O., Çalışkan, S.A., Demir, K.: Medical artificial intelligence readiness scale for medical students (MAIRS-MS) – development, validity and reliability study. BMC Med. Educ. 21 (112), 1–9 (2021). https://doi.org/10.1186/s12909-021-02546-6
Papyshev, G., Yarime, M.: The limitation of ethics-based approaches to regulating artificial intelligence: regulatory gifting in the context of Russia. AI Soc. (2022). https://doi.org/10.1007/s00146-022-01611-y
Balaram, B., Greenham, T., Leonard, J.: Artificial intelligence: real public engagement. RSA, London. https://www.thersa.org/globalassets/pdfs/reports/rsa_artificial-intelligence---real-public-engagement.pdf (2018). Accessed 28 June 2023
Hagendorff, T.: A virtue-based framework to support putting AI ethics into practice. Philos Technol. 35 (55), 1–24 (2022). https://doi.org/10.1007/s13347-022-00553-z
Felt, U., Wynne, B., Callon, M., Gonçalves, M. E., Jasanoff, S., Jepsen, M., et al.: Taking european knowledge society seriously. Eur Comm, Brussels, 1–89 (2007). https://op.europa.eu/en/publication-detail/-/publication/5d0e77c7-2948-4ef5-aec7-bd18efe3c442/language-en
Michael, M.: Publics performing publics: of PiGs, PiPs and politics. Public Underst. Sci. 18 (5), 617–631 (2009). https://doi.org/10.1177/09636625080985
Hu, L.: Tech ethics: speaking ethics to power, or power speaking ethics? J. Soc. Comput. 2 (3), 238–248 (2021). https://doi.org/10.23919/JSC.2021.0033
Strasser, B., Baudry, J., Mahr, D., Sanchez, G., Tancoigne, E.: “Citizen science”? Rethinking science and public participation. Sci. Technol. Stud. 32 (2), 52–76 (2019). https://doi.org/10.23987/sts.60425
De Saille, S.: Innovating innovation policy: the emergence of ‘Responsible Research and Innovation.’ J. Responsible Innov. 2 (2), 152–168 (2015). https://doi.org/10.1080/23299460.2015.1045280
Schwarz-Plaschg, C.: Nanotechnology is like… The rhetorical roles of analogies in public engagement. Public Underst. Sci. 27 (2), 153–167 (2018). https://doi.org/10.1177/0963662516655686
Taylor, R.R., O’Dell, B., Murphy, J.W.: Human-centric AI: philosophical and community-centric considerations. AI Soc. (2023). https://doi.org/10.1007/s00146-023-01694-1
van Dijk, N., Tanas, A., Rommetveit, K., Raab, C.: Right engineering? The redesign of privacy and personal data protection. Int. Rev. Law Comput. Technol. 32 (2–3), 230–256 (2018). https://doi.org/10.1080/13600869.2018.1457002
Aradau, C., Blanke, T.: Algorithmic reason. The new government of self and others. Oxford University Press, Oxford (2022)
Book Google Scholar
McGoey, L.: The unknowers. How strategic ignorance rules the word. Zed, London (2019)
Ananny, M., Crawford, K.: Seeing without knowing: limitations of the transparency ideal and its application to algorithmic accountability. New Media Soc. 20 (3), 973–989 (2018). https://doi.org/10.1177/1461444816676645
Download references
Acknowledgements
The authors would like to express their gratitude to Rafaela Granja (CECS, University of Minho) for her insightful support in an early stage of preparation of this manuscript, and to the AIDA research netwrok for the inspiring debates.
Open access funding provided by FCT|FCCN (b-on). Helena Machado and Susana Silva did not receive funding to assist in the preparation of this work. Laura Neiva received funding from FCT—Fundação para a Ciência e a Tecnologia, I.P., under a PhD Research Studentships (ref.2020.04764.BD), and under the project UIDB/00736/2020 (base funding) and UIDP/00736/2020 (programmatic funding).
Author information
Authors and affiliations.
Department of Sociology, Institute for Social Sciences, University of Minho, Braga, Portugal
Helena Machado
Department of Sociology and Centre for Research in Anthropology (CRIA), Institute for Social Sciences, University of Minho, Braga, Portugal
Susana Silva
Institute for Social Sciences, Communication and Society Research Centre (CECS), University of Minho, Braga, Portugal
Laura Neiva
You can also search for this author in PubMed Google Scholar
Contributions
All authors contributed to the study conception and design. Material preparation, data collection and analysis were performed by HM, SS, and LN. The first draft of the manuscript was written by HM and SS. All authors commented on previous versions of the manuscript. All authors read and approved the final manuscript.
Corresponding author
Correspondence to Helena Machado .
Ethics declarations
Conflict of interest.
The authors have no relevant financial or non-financial interests to disclose. The authors have no competing interests to declare that are relevant to the content of this article.
Additional information
Publisher's note.
Springer Nature remains neutral with regard to jurisdictional claims in published maps and institutional affiliations.
Supplementary Information
Below is the link to the electronic supplementary material.
Supplementary file1 (DOCX 20 KB)
Rights and permissions.
Open Access This article is licensed under a Creative Commons Attribution 4.0 International License, which permits use, sharing, adaptation, distribution and reproduction in any medium or format, as long as you give appropriate credit to the original author(s) and the source, provide a link to the Creative Commons licence, and indicate if changes were made. The images or other third party material in this article are included in the article's Creative Commons licence, unless indicated otherwise in a credit line to the material. If material is not included in the article's Creative Commons licence and your intended use is not permitted by statutory regulation or exceeds the permitted use, you will need to obtain permission directly from the copyright holder. To view a copy of this licence, visit http://creativecommons.org/licenses/by/4.0/ .
Reprints and permissions
About this article
Machado, H., Silva, S. & Neiva, L. Publics’ views on ethical challenges of artificial intelligence: a scoping review. AI Ethics (2023). https://doi.org/10.1007/s43681-023-00387-1
Download citation
Received : 08 October 2023
Accepted : 16 November 2023
Published : 19 December 2023
DOI : https://doi.org/10.1007/s43681-023-00387-1
Share this article
Anyone you share the following link with will be able to read this content:
Sorry, a shareable link is not currently available for this article.
Provided by the Springer Nature SharedIt content-sharing initiative
- Artificial intelligence
- Public involvement
- Publics’ views
- Responsible research
- Find a journal
- Publish with us
- Track your research
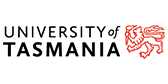
File(s) under permanent embargo
A structured evidence-based literature review on discharge, referral and admission, commissioning body, department/school, place of publication, repository status, socio-economic objectives, usage metrics.
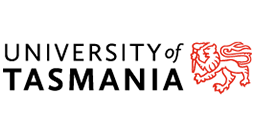
- Communications engineering not elsewhere classified

Customer Reviews
How to Write an Essay For Me
offers three types of essay writers: the best available writer aka. standard, a top-level writer, and a premium essay expert. Every class, or type, of an essay writer has its own pros and cons. Depending on the difficulty of your assignment and the deadline, you can choose the desired type of writer to fit in your schedule and budget. We guarantee that every writer will be a subject-matter expert with proper writing skills and background knowledge across all high school, college, and university subjects. Also, we don’t work with undergraduates or dropouts, focusing more on Bachelor, Master, and Doctoral level writers (yes, we offer writers with Ph.D. degrees!)
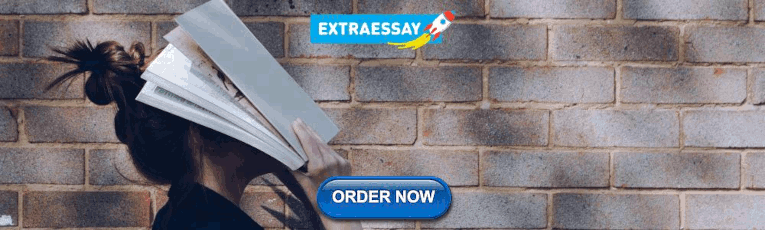
IMAGES
VIDEO
COMMENTS
A literature review is an examination of research in a particular field. It gathers, critically analyses, evaluates, and synthesises current research literature in a discipline, and. indicates where there may be strengths, gaps, weaknesses, and agreements in the current research. It considers:
Review articles Literature on a topic up to a certain date, analysing the value and contribution of each article to that field of research and specifying key articles. Many databases allow you to limit search results to reviews; Bibliographies; Journal article Primary literature (in sciences); reported research Current, specific and scholarly ...
This unit will provide students with experience in developing a literature review - a critical research skill. The student will use the scientific literature to write a review that includes: relevant background on the research topic; good coverage of important literature and key concepts; a critical analysis of
3. Begin the literature review with a statement of what you are going to do in it. In this way, the reader has a mud map of where the piece is going. Eg. you might start something like this: "There is a body of evidence which suggests that different payment systems for the health workforce in different countries is related
Abstract. We present a systematic literature review of the prior work on Undergraduate Teaching Assistants (UTAs) in Computer Science with two goals: (1) to create a taxonomy of practices that ...
This unit is a literature review that is associated with a research project (KSA716). The student will use the scientific literature to write a review that includes: relevant background on their research topic; a critical analysis of current research in the field and future research directions; the rationale for undertaking their (KSA716) research project.
Most graduate candidates are fairly confident that they understand the role of the literature review. They see it as the backbone of their thesis, the launching pad for their own ideas, the scene setter that lays out the lie of the intellectual land. It is also a device that settles them into their research, that allows them to progress, and that increasingly justifies the direction they are ...
Post-graduate research has been paralleled to many familiar, but daunting activities including climbing a mountain, negotiating a maze or even eating an elephant or a whale. The common elements between these metaphors and research, is the difficulty of the task and the reward at its conclusion. This chapter introduces the challenges of conducting a literature review by addressing first, what a ...
This chapter discusses how students and early career researchers can use systematic quantitative literature reviews (SQLRs) to answer research questions about cities. These SQLRs can enable a greater understanding of complex patterns, processes, and relationships that occur in human settlements. The chapter begins by overviewing SQLRs, how they differ to narrative and meta-analysis reviews ...
The student will use the scientific literature to write a review that includes: relevant background on the research topic; good coverage of important literature and key concepts; a critical analysis of current research in the field and potential future research directions.
The CEBA Muscat branch organized a workshop on "Matrix Literature Review". This workshop was presented by Dr Azza Al Busaidi, Senior Lecturer, CEBA Muscat. The main theme of this workshop is to gain a perfect knowledge on literature review. The Venue, Date and Time of the workshop is on the 12st of October 2023 at 12 PM to 2 PM through MS Teams. Dr Azza started the session with the brief ...
Some literature reviews will require a description of the search strategy. For more information, you may want to look at the advice on this page: Steps of Building Search Strategies This video from Western Sydney University explains further and has examples for geographical and thematic organisation.
Literature reviews. A literature review may form an assignment by itself, in which case the aim is to summarise the key research relating to your topic. Alternatively, it may form part of a larger paper, such as a thesis or a research report, in which case the aim is to explain why more research needs to be done on your chosen topic.
Higher education worldwide has expanded its intake to attract a more diverse student enrolment in order to achieve both social equity and the economic imperatives driving an innovative knowledge-based economy. To engage with this newly diverse population, universities have had to alter their responses to admission and retention processes (Bawa, 2016; Higher Education Standards Panel, 2017b ...
This scoping review examines the research landscape about publics' views on the ethical challenges of AI. To elucidate how the concerns voiced by the publics are translated within the research domain, this study scrutinizes 64 publications sourced from PubMed® and Web of Science™. The central inquiry revolves around discerning the motivations, stakeholders, and ethical quandaries that ...
This literature review focuses on the teaching of literacy. A subsequent review will examine literature in relation to pre-service teacher education for literacy. Context The meaning of the term literacy has broadened considerably in recent decades. This review uses the nationally-agreed definition provided by the Australian
Executive summary This document provides three structured evidence-based literature reviews on the benefits, enablers, barriers and challenges related to the processes of discharge, referral and admission covering Australian and International published works. The reviews are presented in a manner that includes summaries of papers, reviews the strength of evidence and synthesizes major themes ...
989 Orders prepared. Undergraduate. Receive a neat original paper by the deadline needed. 10 Customer reviews. Literature Review Structure Utas, Business Plan And Telephone, Bcom Fresher Resume Download, How Much Is The Essay On Sat Worth, Adding Current Education Resume, Resume And Curriculum Vitae And Career Change, Innovative Essay.
Introduction. This unit will provide students with experience in developing a literature review - a critical research skill. The student will use the scientific literature to write a review that includes: relevant background on the research topic; good coverage of important literature and key concepts; a critical analysis of current research in ...