Covering a story? Visit our page for journalists or call (773) 702-8360.
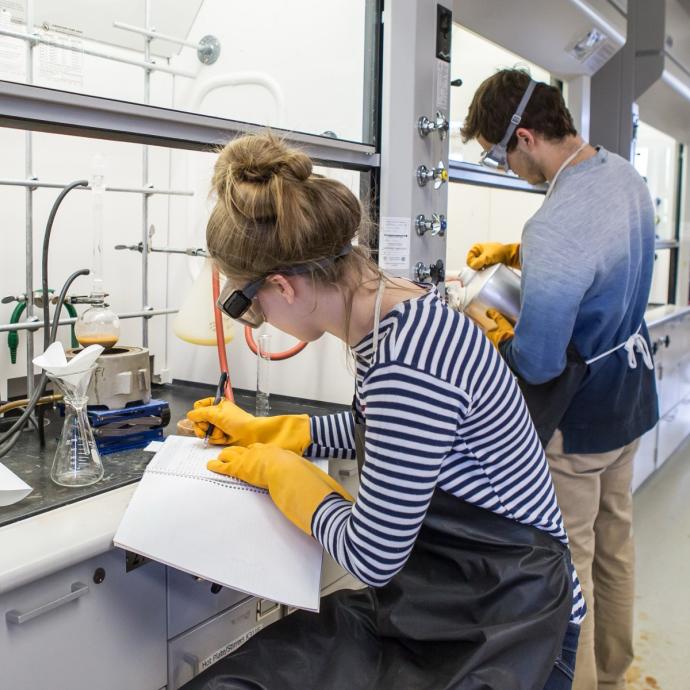
New Certificate Program
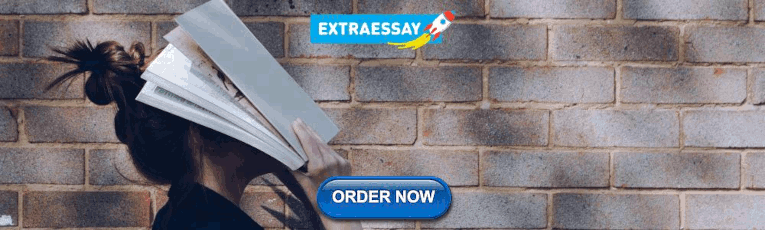
Top Stories
- Scientists may have found the spot where our supermassive black hole “vents”
- UChicago scientist seeks to make plastic more recyclable
- UChicago announces 2024 winners of Quantrell and PhD Teaching Awards
Field experiments, explained
Editor’s note: This is part of a series called “The Day Tomorrow Began,” which explores the history of breakthroughs at UChicago. Learn more here.
A field experiment is a research method that uses some controlled elements of traditional lab experiments, but takes place in natural, real-world settings. This type of experiment can help scientists explore questions like: Why do people vote the way they do? Why do schools fail? Why are certain people hired less often or paid less money?
University of Chicago economists were early pioneers in the modern use of field experiments and conducted innovative research that impacts our everyday lives—from policymaking to marketing to farming and agriculture.
Jump to a section:
What is a field experiment, why do a field experiment, what are examples of field experiments, when did field experiments become popular in modern economics, what are criticisms of field experiments.
Field experiments bridge the highly controlled lab environment and the messy real world. Social scientists have taken inspiration from traditional medical or physical science lab experiments. In a typical drug trial, for instance, participants are randomly assigned into two groups. The control group gets the placebo—a pill that has no effect. The treatment group will receive the new pill. The scientist can then compare the outcomes for each group.
A field experiment works similarly, just in the setting of real life.
It can be difficult to understand why a person chooses to buy one product over another or how effective a policy is when dozens of variables affect the choices we make each day. “That type of thinking, for centuries, caused economists to believe you can't do field experimentation in economics because the market is really messy,” said Prof. John List, a UChicago economist who has used field experiments to study everything from how people use Uber and Lyft to how to close the achievement gap in Chicago-area schools . “There are a lot of things that are simultaneously moving.”
The key to cleaning up the mess is randomization —or assigning participants randomly to either the control group or the treatment group. “The beauty of randomization is that each group has the same amount of bad stuff, or noise or dirt,” List said. “That gets differenced out if you have large enough samples.”
Though lab experiments are still common in the social sciences, field experiments are now often used by psychologists, sociologists and political scientists. They’ve also become an essential tool in the economist’s toolbox.
Some issues are too big and too complex to study in a lab or on paper—that’s where field experiments come in.
In a laboratory setting, a researcher wants to control as many variables as possible. These experiments are excellent for testing new medications or measuring brain functions, but they aren’t always great for answering complex questions about attitudes or behavior.
Labs are highly artificial with relatively small sample sizes—it’s difficult to know if results will still apply in the real world. Also, people are aware they are being observed in a lab, which can alter their behavior. This phenomenon, sometimes called the Hawthorne effect, can affect results.
Traditional economics often uses theories or existing data to analyze problems. But, when a researcher wants to study if a policy will be effective or not, field experiments are a useful way to look at how results may play out in real life.
In 2019, UChicago economist Michael Kremer (then at Harvard) was awarded the Nobel Prize alongside Abhijit Banerjee and Esther Duflo of MIT for their groundbreaking work using field experiments to help reduce poverty . In the 1990s and 2000s, Kremer conducted several randomized controlled trials in Kenyan schools testing potential interventions to improve student performance.
In the 1990s, Kremer worked alongside an NGO to figure out if buying students new textbooks made a difference in academic performance. Half the schools got new textbooks; the other half didn’t. The results were unexpected—textbooks had no impact.
“Things we think are common sense, sometimes they turn out to be right, sometimes they turn out to be wrong,” said Kremer on an episode of the Big Brains podcast. “And things that we thought would have minimal impact or no impact turn out to have a big impact.”
In the early 2000s, Kremer returned to Kenya to study a school-based deworming program. He and a colleague found that providing deworming pills to all students reduced absenteeism by more than 25%. After the study, the program was scaled nationwide by the Kenyan government. From there it was picked up by multiple Indian states—and then by the Indian national government.
“Experiments are a way to get at causal impact, but they’re also much more than that,” Kremer said in his Nobel Prize lecture . “They give the researcher a richer sense of context, promote broader collaboration and address specific practical problems.”
Among many other things, field experiments can be used to:
Study bias and discrimination
A 2004 study published by UChicago economists Marianne Bertrand and Sendhil Mullainathan (then at MIT) examined racial discrimination in the labor market. They sent over 5,000 resumes to real job ads in Chicago and Boston. The resumes were exactly the same in all ways but one—the name at the top. Half the resumes bore white-sounding names like Emily Walsh or Greg Baker. The other half sported African American names like Lakisha Washington or Jamal Jones. The study found that applications with white-sounding names were 50% more likely to receive a callback.
Examine voting behavior
Political scientist Harold Gosnell , PhD 1922, pioneered the use of field experiments to examine voting behavior while at UChicago in the 1920s and ‘30s. In his study “Getting out the vote,” Gosnell sorted 6,000 Chicagoans across 12 districts into groups. One group received voter registration info for the 1924 presidential election and the control group did not. Voter registration jumped substantially among those who received the informational notices. Not only did the study prove that get-out-the-vote mailings could have a substantial effect on voter turnout, but also that field experiments were an effective tool in political science.
Test ways to reduce crime and shape public policy
Researchers at UChicago’s Crime Lab use field experiments to gather data on crime as well as policies and programs meant to reduce it. For example, Crime Lab director and economist Jens Ludwig co-authored a 2015 study on the effectiveness of the school mentoring program Becoming a Man . Developed by the non-profit Youth Guidance, Becoming a Man focuses on guiding male students between 7th and 12th grade to help boost school engagement and reduce arrests. In two field experiments, the Crime Lab found that while students participated in the program, total arrests were reduced by 28–35%, violent-crime arrests went down by 45–50% and graduation rates increased by 12–19%.
The earliest field experiments took place—literally—in fields. Starting in the 1800s, European farmers began experimenting with fertilizers to see how they affected crop yields. In the 1920s, two statisticians, Jerzy Neyman and Ronald Fisher, were tasked with assisting with these agricultural experiments. They are credited with identifying randomization as a key element of the method—making sure each plot had the same chance of being treated as the next.
The earliest large-scale field experiments in the U.S. took place in the late 1960s to help evaluate various government programs. Typically, these experiments were used to test minor changes to things like electricity pricing or unemployment programs.
Though field experiments were used in some capacity throughout the 20th century, this method didn’t truly gain popularity in economics until the 2000s. Kremer and List were early pioneers and first began experimenting with the method in the 1990s.
In 2004, List co-authored a seminal paper defining field experiments and arguing for the importance of the method. In 2008, he and UChicago economist Steven Levitt published another study tracing the history of field experiments and their impact on economics.
In the past few decades, the use of field experiments has exploded. Today, economists often work alongside NGOs or nonprofit organizations to study the efficacy of programs or policies. They also partner with companies to test products and understand how people use services.
There are several ethical discussions happening among scholars as field experiments grow in popularity. Chief among them is the issue of informed consent. All studies that involve human test subjects must be approved by an institutional review board (IRB) to ensure that people are protected.
However, participants in field experiments often don’t know they are in an experiment. While an experiment may be given the stamp of approval in the research community, some argue that taking away peoples’ ability to opt out is inherently unethical. Others advocate for stricter review processes as field experiments continue to evolve.
According to List, another major issue in field experiments is the issue of scale . Many experiments only test small groups—say, dozens to hundreds of people. This may mean the results are not applicable to broader situations. For example, if a scientist runs an experiment at one school and finds their method works there, does that mean it will also work for an entire city? Or an entire country?
List believes that in addition to testing option A and option B, researchers need a third option that accounts for the limitations that come with a larger scale. “Option C is what I call critical scale features. I want you to bring in all of the warts, all of the constraints, whether they're regulatory constraints, or constraints by law,” List said. “Option C is like your reality test, or what I call policy-based evidence.”
This problem isn’t unique to field experiments, but List believes tackling the issue of scale is the next major frontier for a new generation of economists.
Hero photo copyright Shutterstock.com
More Explainers
Improv, Explained
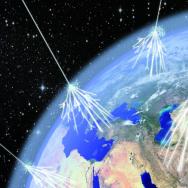
Cosmic rays, explained
Get more with UChicago News delivered to your inbox.
Recommended Stories
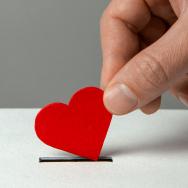
An economist illuminates our giving habits—during the pandemic and…
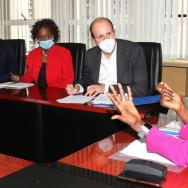
Collaborating with Kenyan government on development innovations is…
Related Topics
Latest news, big brains podcast: storm warning: why hurricanes are growing beyond measure.
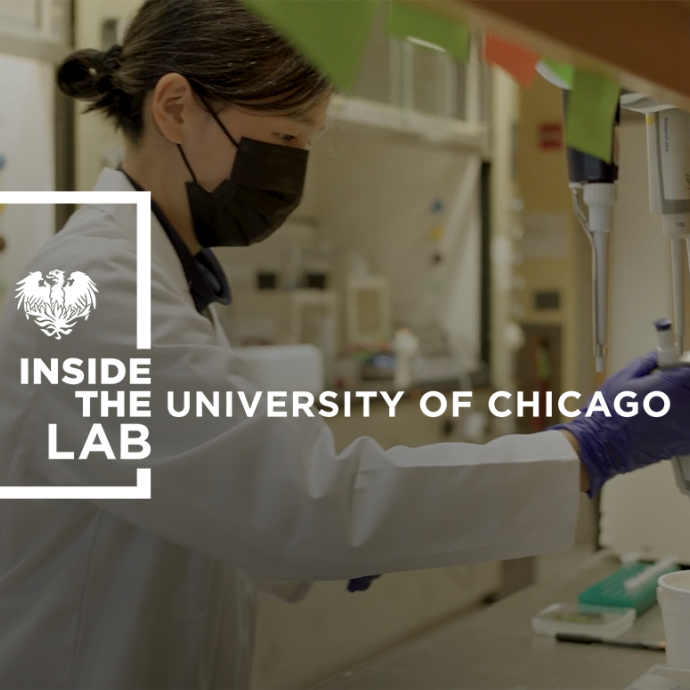
‘Inside the Lab’ series provides a unique look at UChicago research
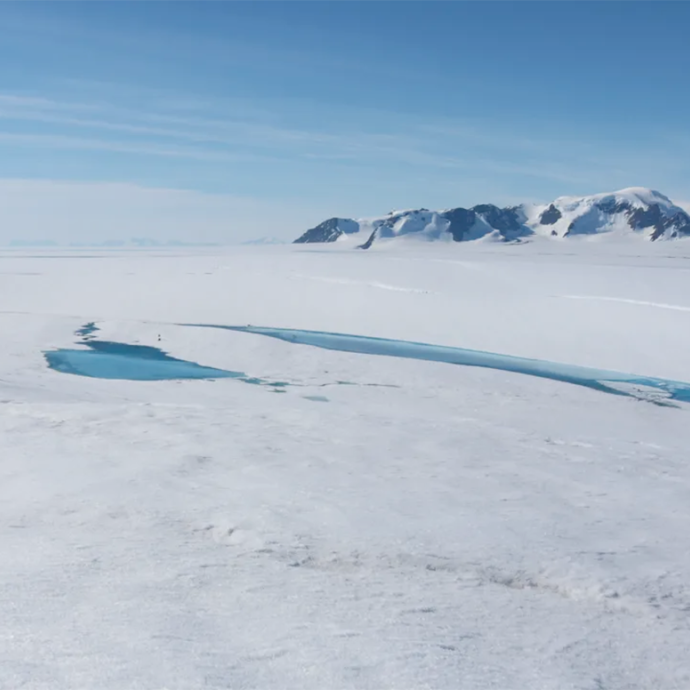
Geophysical Sciences
Scientists find evidence that meltwater is fracturing ice shelves in Antarctica
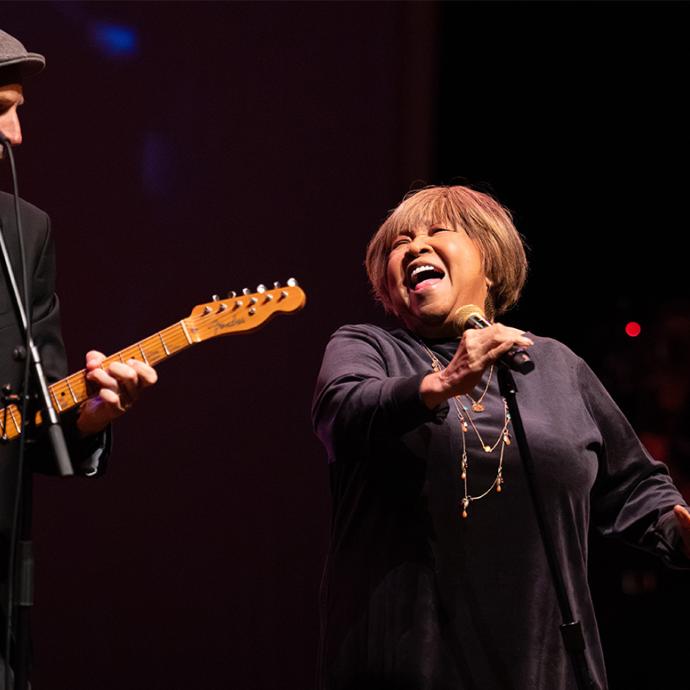
Mavis Staples, legendary singer and activist, returns to UChicago to inspire next generation

Where do breakthrough discoveries and ideas come from?
Explore The Day Tomorrow Began
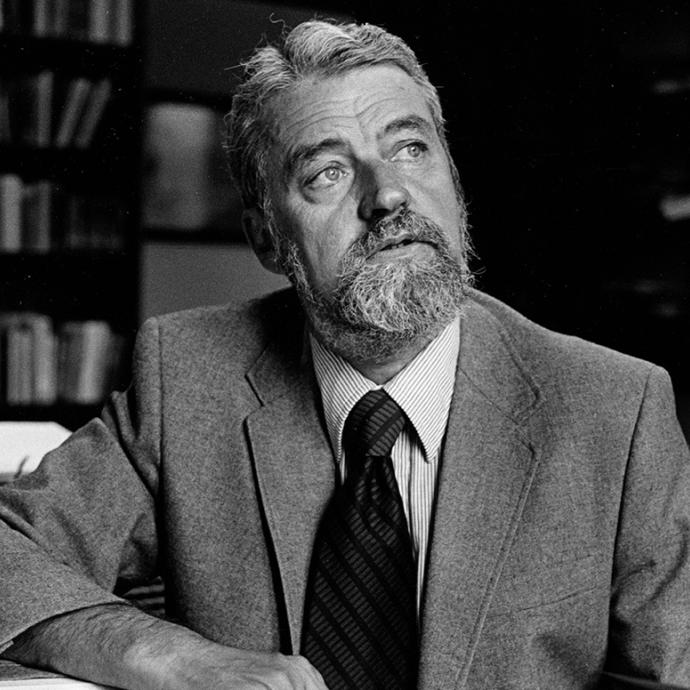
W. Ralph Johnson, pre-eminent UChicago critic of Latin poetry, 1933‒2024
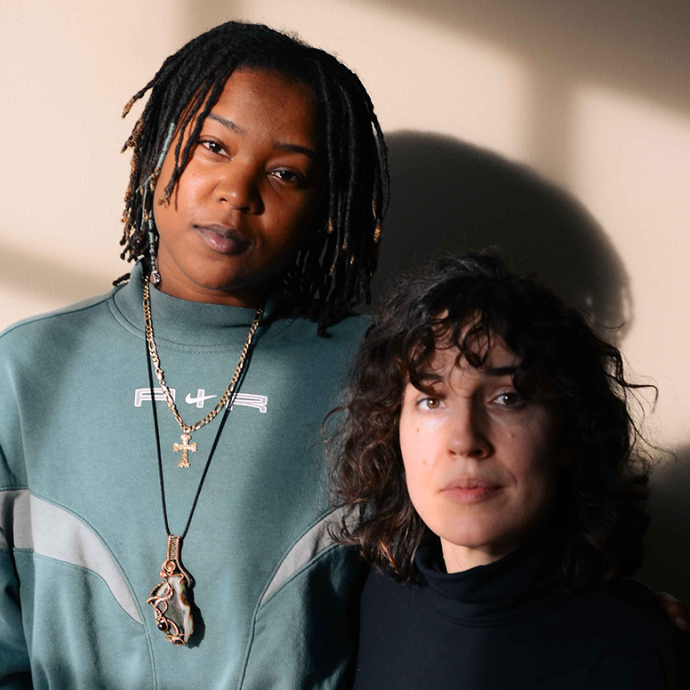
Pulitzer Prize
Trina Reynolds-Tyler, MPP'20, wins Pulitzer Prize in Local Reporting
Around uchicago, uchicago to offer new postbaccalaureate premedical certificate program.
Carnegie Fellow
UChicago political scientist Molly Offer-Westort named Carnegie Fellow
National Academy of Sciences
Five UChicago faculty elected to National Academy of Sciences in 2024
Laing Award
UChicago Press awards top honor to Margareta Ingrid Christian for ‘Objects in A…
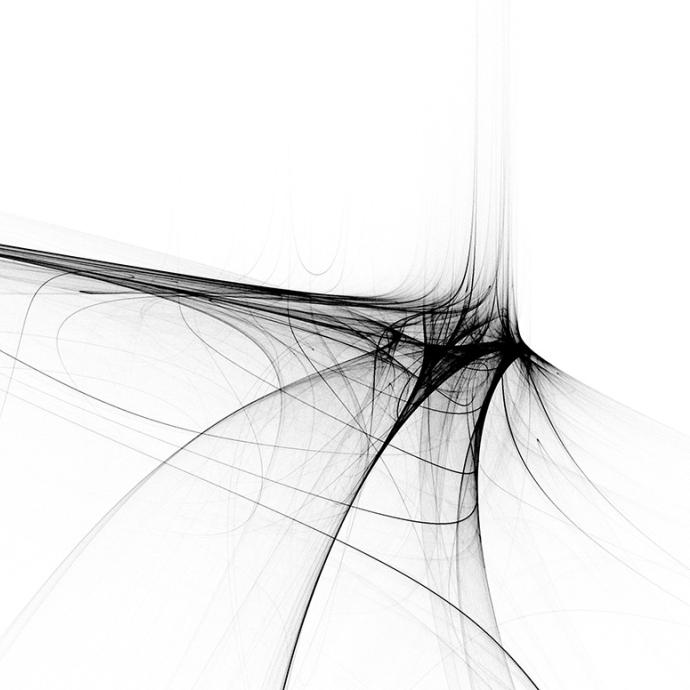
Winners of the 2024 UChicago Science as Art competition announced
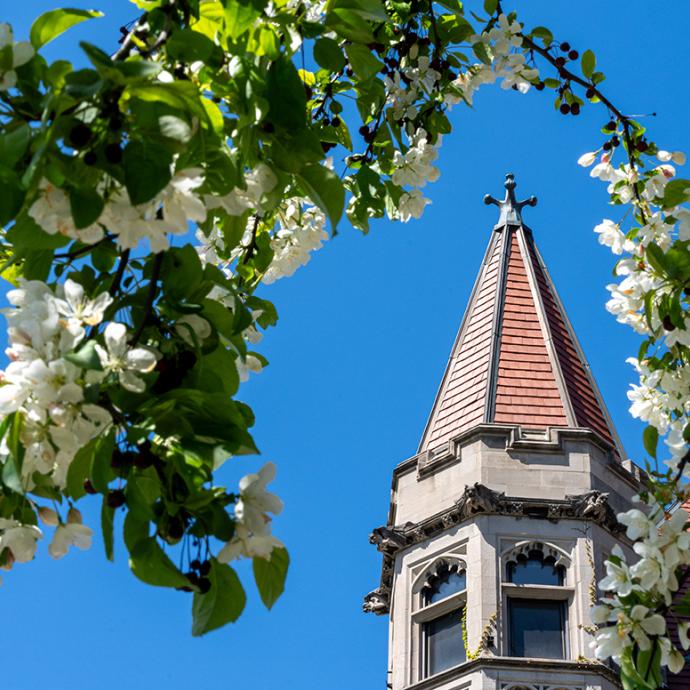
Six UChicago scholars elected to American Academy of Arts and Sciences in 2024
Biological Sciences Division
“You have to be open minded, planning to reinvent yourself every five to seven years.”
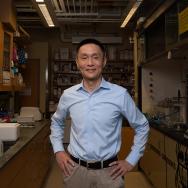
UChicago paleontologist Paul Sereno’s fossil lab moves to Washington Park
D-Lab's workshops and consulting services are paused for the summer. Our core staff will be focusing on special projects and other endeavors. We look forward to seeing you in the fall and hope you have a great summer.
Introduction to Field Experiments and Randomized Controlled Trials

Have you ever been curious about the methods researchers employ to determine causal relationships among various factors, ultimately leading to significant breakthroughs and progress in numerous fields? In this article, we offer an overview of field experimentation and its importance in discerning cause and effect relationships. We outline how randomized experiments represent an unbiased method for determining what works. Furthermore, we discuss key aspects of experiments, such as intervention, excludability, and non-interference. To illustrate these concepts, we present a hypothetical example of a randomized controlled trial evaluating the efficacy of an experimental drug called Covi-Mapp.
Why experiments?
Every day, we find ourselves faced with questions of cause and effect. Understanding the driving forces behind outcomes is crucial, ranging from personal decisions like parenting strategies to organizational challenges such as effective advertising. This blog aims to provide a systematic introduction to experimentation, igniting enthusiasm for primary research and highlighting the myriad of experimental applications and opportunities available.
The challenge for those who seek to answer causal questions convincingly is to develop a research methodology that doesn't require identifying or measuring all potential confounders. Since no planned design can eliminate every possible systematic difference between treatment and control groups, random assignment emerges as a powerful tool for minimizing bias. In the contentious world of causal claims, randomized experiments represent an unbiased method for determining what works. Random assignment means participants are assigned to different groups or conditions in a study purely by chance. Basically, each participant has an equal chance to be assigned to a control group or a treatment group.
Field experiments, or randomized studies conducted in real-world settings, can take many forms. While experiments on college campuses are often considered lab studies, certain experiments on campus – such as those examining club participation – may be regarded as field experiments, depending on the experimental design. Ultimately, whether a study is considered a field experiment hinges on the definition of "the field."
Researchers may employ two main scenarios for randomization. The first involves gathering study participants and randomizing them at the time of the experiment. The second capitalizes on naturally occurring randomizations, such as the Vietnam draft lottery.
Intervention, Excludability, and Non-Interference
Three essential features of any experiment are intervention, excludability, and non-interference. In a general sense, the intervention refers to the treatment or action being tested in an experiment. The excludability principle is satisfied when the only difference between the experimental and control groups is the presence or absence of the intervention. The non-interference principle holds when the outcome of one participant in the study does not influence the outcomes of other participants. Together, these principles ensure that the experiment is designed to provide unbiased and reliable results, isolating the causal effect of the intervention under study.
Omitted Variables and Non-Compliance
To ensure unbiased results, researchers must randomize as much as possible to minimize omitted variable bias. Omitted variables are factors that influence the outcome but are not measured or are difficult to measure. These unmeasured attributes, sometimes called confounding variables or unobserved heterogeneity, must be accounted for to guarantee accurate findings.
Non-compliance can also complicate experiments. One-sided non-compliance occurs when individuals assigned to a treatment group don't receive the treatment (failure to treat), while two-sided non-compliance occurs when some subjects assigned to the treatment group go untreated or individuals assigned to the control group receive the treatment. Addressing these issues at the design level by implementing a blind or double-blind study can help mitigate potential biases.
Achieving Precision through Covariate Balance
To ensure the control and treatment groups are comparatively similar in all relevant aspects, particularly when the sample size (n) is small, it is essential to achieve covariate balance. Covariance measures the association between two variables, while a covariate is a factor that influences the outcome variable. By balancing covariates, we can more accurately isolate the effects of the treatment, leading to improved precision in our findings.
Fictional Example of Randomized Controlled Trial of Covi-Mapp for COVID-19 Management
Let's explore a fictional example to better understand experiments: a one-week randomized controlled trial of the experimental drug Covi-Mapp for managing Covid. In this case, the control group receives the standard care for Covid patients, while the treatment group receives the standard care plus Covi-Mapp. The outcome of interest is whether patients have cough symptoms on day 7, as subsidizing cough symptoms is an encouraging sign in Covid recovery. We'll measure the presence of cough on day 0 and day 7, as well as temperature on day 0 and day 7. Gender is also tracked. The control represents the standard care for COVID-19 patients, while the treatment includes standard care plus the experimental drug.
In this Covi-Mapp example, the intervention is the Covi-Mapp drug, the excludability principle is satisfied if the only difference in patient care between the groups is the drug administration, and the non-interference principle holds if one patient's outcome doesn't affect another's.
First, let's assume we have a dataset containing the relevant information for each patient, including cough status on day 0 and day 7, temperature on day 0 and day 7, treatment assignment, and gender. We'll read the data and explore the dataset:
Simple treatment effect of the experimental drug
Without any covariates, let's first look at the estimated effect of the treatment on the presence of cough on day 7. The estimated proportion of patients with a cough on day 7 for the control group (not receiving the experimental drug) is 0.847458. In other words, about 84.7% of patients in the control group are expected to have a cough on day 7, all else being equal. The estimated effect of the experimental drug on the presence of cough on day 7 is -0.23. This means that, on average, receiving the experimental drug reduces the proportion of patients with a cough on day 7 by 23.8% compared to the control group.
We know that a patient's initial condition would affect the final outcome. If the patient has a cough and a fever on day 0, they might not fare well with the treatment. To better understand the treatment's effect, let's add these covariates:
The output shows the results of a linear regression model, estimating the effect of the experimental drug (treat_covid_mapp) on the presence of cough on day 7, adjusting for cough on day 0 and temperature on day 0. The experimental drug significantly reduces the presence of cough on day 7 by approximately 16.6% compared to the control group (p-value = 0.046242). The presence of cough on day 0 does not significantly predict the presence of cough on day 7 (p-value = 0.717689). A one-unit increase in temperature on day 0 is associated with a 20.6% increase in the presence of cough on day 7, and this effect is statistically significant (p-value = 0.009859).
Should we add day 7 temperature as a covariate? By including it, we might find that the treatment is no longer statistically significant since the temperature on day 7 could be affected by the treatment itself. It is a post-treatment variable, and by including it, the experiment loses value as we used something that was affected by intervention as our covariate.
However, we'd like to investigate if the treatment affects men or women differently. Since we collected gender as part of the study, we could check for Heterogeneous Treatment Effect (HTE) for male vs. female. The experimental drug has a marginally significant effect on the outcome variable for females, reducing it by approximately 23.1% (p-value = 0.05391).
Which group, those coded as male == 0 or male == 1, have better health outcomes (cough) in control? What about in treatment? How does this help to contextualize any heterogeneous treatment effect that might have been estimated?
Stargazer is a popular R package that enables users to create well-formatted tables and reports for statistical analysis results.
Looking at this regression report, we see that males in control have a temperature of 102; females in control have a temperature of 98.6 (which is very nearly a normal temperature). So, in control, males are worse off. In treatment, males have a temperature of 102 - 2.59 = 99.41. While this is closer to a normal temperature, this is still elevated. Females in treatment have a temperature of 98.5 - .32 = 98.18, which is slightly lower than a normal temperature, and is better than an elevated temperature. It appears that the treatment is able to have a stronger effect among male participants than females because males are *more sick* at baseline.
In conclusion, experimentation offers a fascinating and valuable avenue for primary research, allowing us to address causal questions and enhance our understanding of the world around us. Covariate control helps to isolate the causal effect of the treatment on the outcome variable, ensuring that the observed effect is not driven by confounding factors. Proper control of covariates enhances the internal validity of the study and ensures that the estimated treatment effect is an accurate representation of the true causal relationship. By exploring and accounting for sub groups in data, researchers can identify whether the treatment has different effects on different groups, such as men and women or younger and older individuals. This information can be critical for making informed policy decisions and developing targeted interventions that maximize the benefits for specific groups. The ongoing investigation of experimental methodologies and their potential applications represents a compelling and significant area of inquiry.
Gerber, A. S., & Green, D. P. (2012). Field Experiments: Design, Analysis, and Interpretation . W. W. Norton.
“DALL·E 2.” OpenAI , https://openai.com/product/dall-e-2
“Data Science 241. Experiments and Causal Inference.” UC Berkeley School of Information , https://www.ischool.berkeley.edu/courses/datasci/241

An official website of the United States government
The .gov means it’s official. Federal government websites often end in .gov or .mil. Before sharing sensitive information, make sure you’re on a federal government site.
The site is secure. The https:// ensures that you are connecting to the official website and that any information you provide is encrypted and transmitted securely.
- Publications
- Account settings
Preview improvements coming to the PMC website in October 2024. Learn More or Try it out now .
- Advanced Search
- Journal List
- Perspect Clin Res
- v.9(4); Oct-Dec 2018
Study designs: Part 1 – An overview and classification
Priya ranganathan.
Department of Anaesthesiology, Tata Memorial Centre, Mumbai, Maharashtra, India
Rakesh Aggarwal
1 Department of Gastroenterology, Sanjay Gandhi Postgraduate Institute of Medical Sciences, Lucknow, Uttar Pradesh, India
There are several types of research study designs, each with its inherent strengths and flaws. The study design used to answer a particular research question depends on the nature of the question and the availability of resources. In this article, which is the first part of a series on “study designs,” we provide an overview of research study designs and their classification. The subsequent articles will focus on individual designs.
INTRODUCTION
Research study design is a framework, or the set of methods and procedures used to collect and analyze data on variables specified in a particular research problem.
Research study designs are of many types, each with its advantages and limitations. The type of study design used to answer a particular research question is determined by the nature of question, the goal of research, and the availability of resources. Since the design of a study can affect the validity of its results, it is important to understand the different types of study designs and their strengths and limitations.
There are some terms that are used frequently while classifying study designs which are described in the following sections.
A variable represents a measurable attribute that varies across study units, for example, individual participants in a study, or at times even when measured in an individual person over time. Some examples of variables include age, sex, weight, height, health status, alive/dead, diseased/healthy, annual income, smoking yes/no, and treated/untreated.
Exposure (or intervention) and outcome variables
A large proportion of research studies assess the relationship between two variables. Here, the question is whether one variable is associated with or responsible for change in the value of the other variable. Exposure (or intervention) refers to the risk factor whose effect is being studied. It is also referred to as the independent or the predictor variable. The outcome (or predicted or dependent) variable develops as a consequence of the exposure (or intervention). Typically, the term “exposure” is used when the “causative” variable is naturally determined (as in observational studies – examples include age, sex, smoking, and educational status), and the term “intervention” is preferred where the researcher assigns some or all participants to receive a particular treatment for the purpose of the study (experimental studies – e.g., administration of a drug). If a drug had been started in some individuals but not in the others, before the study started, this counts as exposure, and not as intervention – since the drug was not started specifically for the study.
Observational versus interventional (or experimental) studies
Observational studies are those where the researcher is documenting a naturally occurring relationship between the exposure and the outcome that he/she is studying. The researcher does not do any active intervention in any individual, and the exposure has already been decided naturally or by some other factor. For example, looking at the incidence of lung cancer in smokers versus nonsmokers, or comparing the antenatal dietary habits of mothers with normal and low-birth babies. In these studies, the investigator did not play any role in determining the smoking or dietary habit in individuals.
For an exposure to determine the outcome, it must precede the latter. Any variable that occurs simultaneously with or following the outcome cannot be causative, and hence is not considered as an “exposure.”
Observational studies can be either descriptive (nonanalytical) or analytical (inferential) – this is discussed later in this article.
Interventional studies are experiments where the researcher actively performs an intervention in some or all members of a group of participants. This intervention could take many forms – for example, administration of a drug or vaccine, performance of a diagnostic or therapeutic procedure, and introduction of an educational tool. For example, a study could randomly assign persons to receive aspirin or placebo for a specific duration and assess the effect on the risk of developing cerebrovascular events.
Descriptive versus analytical studies
Descriptive (or nonanalytical) studies, as the name suggests, merely try to describe the data on one or more characteristics of a group of individuals. These do not try to answer questions or establish relationships between variables. Examples of descriptive studies include case reports, case series, and cross-sectional surveys (please note that cross-sectional surveys may be analytical studies as well – this will be discussed in the next article in this series). Examples of descriptive studies include a survey of dietary habits among pregnant women or a case series of patients with an unusual reaction to a drug.
Analytical studies attempt to test a hypothesis and establish causal relationships between variables. In these studies, the researcher assesses the effect of an exposure (or intervention) on an outcome. As described earlier, analytical studies can be observational (if the exposure is naturally determined) or interventional (if the researcher actively administers the intervention).
Directionality of study designs
Based on the direction of inquiry, study designs may be classified as forward-direction or backward-direction. In forward-direction studies, the researcher starts with determining the exposure to a risk factor and then assesses whether the outcome occurs at a future time point. This design is known as a cohort study. For example, a researcher can follow a group of smokers and a group of nonsmokers to determine the incidence of lung cancer in each. In backward-direction studies, the researcher begins by determining whether the outcome is present (cases vs. noncases [also called controls]) and then traces the presence of prior exposure to a risk factor. These are known as case–control studies. For example, a researcher identifies a group of normal-weight babies and a group of low-birth weight babies and then asks the mothers about their dietary habits during the index pregnancy.
Prospective versus retrospective study designs
The terms “prospective” and “retrospective” refer to the timing of the research in relation to the development of the outcome. In retrospective studies, the outcome of interest has already occurred (or not occurred – e.g., in controls) in each individual by the time s/he is enrolled, and the data are collected either from records or by asking participants to recall exposures. There is no follow-up of participants. By contrast, in prospective studies, the outcome (and sometimes even the exposure or intervention) has not occurred when the study starts and participants are followed up over a period of time to determine the occurrence of outcomes. Typically, most cohort studies are prospective studies (though there may be retrospective cohorts), whereas case–control studies are retrospective studies. An interventional study has to be, by definition, a prospective study since the investigator determines the exposure for each study participant and then follows them to observe outcomes.
The terms “prospective” versus “retrospective” studies can be confusing. Let us think of an investigator who starts a case–control study. To him/her, the process of enrolling cases and controls over a period of several months appears prospective. Hence, the use of these terms is best avoided. Or, at the very least, one must be clear that the terms relate to work flow for each individual study participant, and not to the study as a whole.
Classification of study designs
Figure 1 depicts a simple classification of research study designs. The Centre for Evidence-based Medicine has put forward a useful three-point algorithm which can help determine the design of a research study from its methods section:[ 1 ]

Classification of research study designs
- Does the study describe the characteristics of a sample or does it attempt to analyze (or draw inferences about) the relationship between two variables? – If no, then it is a descriptive study, and if yes, it is an analytical (inferential) study
- If analytical, did the investigator determine the exposure? – If no, it is an observational study, and if yes, it is an experimental study
- If observational, when was the outcome determined? – at the start of the study (case–control study), at the end of a period of follow-up (cohort study), or simultaneously (cross sectional).
In the next few pieces in the series, we will discuss various study designs in greater detail.
Financial support and sponsorship
Conflicts of interest.
There are no conflicts of interest.
Thank you for visiting nature.com. You are using a browser version with limited support for CSS. To obtain the best experience, we recommend you use a more up to date browser (or turn off compatibility mode in Internet Explorer). In the meantime, to ensure continued support, we are displaying the site without styles and JavaScript.
- View all journals
- Explore content
- About the journal
- Publish with us
- Sign up for alerts
- Published: 23 March 2022
Embracing field studies as a tool for learning
- Jon M. Jachimowicz ORCID: orcid.org/0000-0002-1197-8958 1
Nature Reviews Psychology volume 1 , pages 249–250 ( 2022 ) Cite this article
233 Accesses
3 Citations
28 Altmetric
Metrics details
- Human behaviour
- Social sciences
Field studies in social psychology tend to focus on validating existing insights. In addition to learning from the laboratory and bringing those insights to the field — which researchers currently favour — we should also conduct field studies that aim to learn in the field first.
This is a preview of subscription content, access via your institution
Relevant articles
Open Access articles citing this article.
What Can Educational Psychology Learn From, and Contribute to, Theory Development Scholarship?
- Jeffrey A. Greene
Educational Psychology Review Open Access 23 May 2022
Access options
Subscribe to this journal
Receive 12 digital issues and online access to articles
55,14 € per year
only 4,60 € per issue
Buy this article
- Purchase on Springer Link
- Instant access to full article PDF
Prices may be subject to local taxes which are calculated during checkout
Premachandra, B. & Lewis, N. A. Jr. Do we report the information that is necessary to give psychology away? a scoping review of the psychological intervention literature 2000–2018. Perspect. Psychol. Sci. 17 , 226–238 (2022).
Article Google Scholar
Milkman, K. L. et al. Megastudies improve the impact of applied behavioural science. Nature 600 , 478–483 (2021).
IJzerman, H. et al. Use caution when applying behavioural science to policy. Nat. Hum. Behav. 4 , 1092–1094 (2020).
Vazire, S. Implications of the credibility revolution for productivity, creativity, and progress. Perspect. Psychol. Sci. 13 , 411–417 (2018).
Chatman, J. A. & Flynn, F. J. Full-cycle micro-organizational behavior research. Organ. Sci. 16 , 434–447 (2005).
Eisenhardt, K. M. Building theories from case study research. Acad. Manage. Rev. 14 , 532–550 (1989).
Ranganathan, A. The artisan and his audience: identification with work and price setting in a handicraft cluster in southern india. Adm. Sci. Q. 63 , 637–667 (2018).
Jachimowicz, J. The study premortem: why publishing null results is only the first step. Behavioral Scientist , https://go.nature.com/3KONKNt (16 October 2018).
Whillans, A. & West, C. Alleviating time poverty among the working poor: a pre-registered longitudinal field experiment. Sci. Rep. 12 , 719 (2022).
Perlow, L. A. The time famine: toward a sociology of work time. Adm. Sci. Q. 44 , 57–81 (1999).
Download references
Acknowledgements
The author thanks Z. Berry, M. Gorges, O. Hauser, K. Krautter, J. Murray, C. Vinluan and A. Whillans for their excellent comments on an earlier version of this Comment
Author information
Authors and affiliations.
Harvard Business School, Harvard University, Boston, MA, USA
Jon M. Jachimowicz
You can also search for this author in PubMed Google Scholar
Corresponding author
Correspondence to Jon M. Jachimowicz .
Ethics declarations
Competing interests.
The author declares no competing interests.
Rights and permissions
Reprints and permissions
About this article
Cite this article.
Jachimowicz, J.M. Embracing field studies as a tool for learning. Nat Rev Psychol 1 , 249–250 (2022). https://doi.org/10.1038/s44159-022-00047-x
Download citation
Published : 23 March 2022
Issue Date : May 2022
DOI : https://doi.org/10.1038/s44159-022-00047-x
Share this article
Anyone you share the following link with will be able to read this content:
Sorry, a shareable link is not currently available for this article.
Provided by the Springer Nature SharedIt content-sharing initiative
This article is cited by
Educational Psychology Review (2022)
Quick links
- Explore articles by subject
- Guide to authors
- Editorial policies
Sign up for the Nature Briefing newsletter — what matters in science, free to your inbox daily.

- Search Menu
- Author Guidelines
- Submission Site
- Open Access
- About International Studies Review
- About the International Studies Association
- Editorial Board
- Advertising and Corporate Services
- Journals Career Network
- Self-Archiving Policy
- Dispatch Dates
- Journals on Oxford Academic
- Books on Oxford Academic

Article Contents
Introduction, what is fieldwork, purpose of fieldwork, physical safety, mental wellbeing and affect, ethical considerations, remote fieldwork, concluding thoughts, acknowledgments, funder information.
- < Previous
Field Research: A Graduate Student's Guide

- Article contents
- Figures & tables
- Supplementary Data
Ezgi Irgil, Anne-Kathrin Kreft, Myunghee Lee, Charmaine N Willis, Kelebogile Zvobgo, Field Research: A Graduate Student's Guide, International Studies Review , Volume 23, Issue 4, December 2021, Pages 1495–1517, https://doi.org/10.1093/isr/viab023
- Permissions Icon Permissions
What is field research? Is it just for qualitative scholars? Must it be done in a foreign country? How much time in the field is “enough”? A lack of disciplinary consensus on what constitutes “field research” or “fieldwork” has left graduate students in political science underinformed and thus underequipped to leverage site-intensive research to address issues of interest and urgency across the subfields. Uneven training in Ph.D. programs has also left early-career researchers underprepared for the logistics of fieldwork, from developing networks and effective sampling strategies to building respondents’ trust, and related issues of funding, physical safety, mental health, research ethics, and crisis response. Based on the experience of five junior scholars, this paper offers answers to questions that graduate students puzzle over, often without the benefit of others’ “lessons learned.” This practical guide engages theory and praxis, in support of an epistemologically and methodologically pluralistic discipline.
¿Qué es la investigación de campo? ¿Es solo para académicos cualitativos? ¿Debe realizarse en un país extranjero? ¿Cuánto tiempo en el terreno es “suficiente”? La falta de consenso disciplinario con respecto a qué constituye la “investigación de campo” o el “trabajo de campo” ha causado que los estudiantes de posgrado en ciencias políticas estén poco informados y, por lo tanto, capacitados de manera insuficiente para aprovechar la investigación exhaustiva en el sitio con el objetivo de abordar los asuntos urgentes y de interés en los subcampos. La capacitación desigual en los programas de doctorado también ha provocado que los investigadores en las primeras etapas de su carrera estén poco preparados para la logística del trabajo de campo, desde desarrollar redes y estrategias de muestreo efectivas hasta generar la confianza de las personas que facilitan la información, y las cuestiones relacionadas con la financiación, la seguridad física, la salud mental, la ética de la investigación y la respuesta a las situaciones de crisis. Con base en la experiencia de cinco académicos novatos, este artículo ofrece respuestas a las preguntas que desconciertan a los estudiantes de posgrado, a menudo, sin el beneficio de las “lecciones aprendidas” de otras personas. Esta guía práctica incluye teoría y praxis, en apoyo de una disciplina pluralista desde el punto de vista epistemológico y metodológico.
En quoi consiste la recherche de terain ? Est-elle uniquement réservée aux chercheurs qualitatifs ? Doit-elle être effectuée dans un pays étranger ? Combien de temps faut-il passer sur le terrain pour que ce soit « suffisant » ? Le manque de consensus disciplinaire sur ce qui constitue une « recherche de terrain » ou un « travail de terrain » a laissé les étudiants diplômés en sciences politiques sous-informés et donc sous-équipés pour tirer parti des recherches de terrain intensives afin d'aborder les questions d'intérêt et d'urgence dans les sous-domaines. L'inégalité de formation des programmes de doctorat a mené à une préparation insuffisante des chercheurs en début de carrière à la logistique du travail de terrain, qu'il s'agisse du développement de réseaux et de stratégies d’échantillonnage efficaces, de l'acquisition de la confiance des personnes interrogées ou des questions de financement, de sécurité physique, de santé mentale, d’éthique de recherche et de réponse aux crises qui y sont associées. Cet article s'appuie sur l'expérience de cinq jeunes chercheurs pour proposer des réponses aux questions que les étudiants diplômés se posent, souvent sans bénéficier des « enseignements tirés » par les autres. Ce guide pratique engage théorie et pratique en soutien à une discipline épistémologiquement et méthodologiquement pluraliste.
Days before embarking on her first field research trip, a Ph.D. student worries about whether she will be able to collect the qualitative data that she needs for her dissertation. Despite sending dozens of emails, she has received only a handful of responses to her interview requests. She wonders if she will be able to gain more traction in-country. Meanwhile, in the midst of drafting her thesis proposal, an M.A. student speculates about the feasibility of his project, given a modest budget. Thousands of miles away from home, a postdoc is concerned about their safety, as protests erupt outside their window and state security forces descend into the streets.
These anecdotes provide a small glimpse into the concerns of early-career researchers undertaking significant projects with a field research component. Many of these fieldwork-related concerns arise from an unfortunate shortage in curricular offerings for qualitative and mixed-method research in political science graduate programs ( Emmons and Moravcsik 2020 ), 1 as well as the scarcity of instructional materials for qualitative and mixed-method research, relative to those available for quantitative research ( Elman, Kapiszewski, and Kirilova 2015 ; Kapiszewski, MacLean, and Read 2015 ; Mosley 2013 ). A recent survey among the leading United States Political Science programs in Comparative Politics and International Relations found that among graduate students who have carried out international fieldwork, 62 percent had not received any formal fieldwork training and only 20 percent felt very or mostly prepared for their fieldwork ( Schwartz and Cronin-Furman 2020 , 7–8). This shortfall in training and instruction means that many young researchers are underprepared for the logistics of fieldwork, from developing networks and effective sampling strategies to building respondents’ trust. In addition, there is a notable lack of preparation around issues of funding, physical safety, mental health, research ethics, and crisis response. This is troubling, as field research is highly valued and, in some parts of the field, it is all but expected, for instance in comparative politics.
Beyond subfield-specific expectations, research that leverages multiple types of data and methods, including fieldwork, is one of the ways that scholars throughout the discipline can more fully answer questions of interest and urgency. Indeed, multimethod work, a critical means by which scholars can parse and evaluate causal pathways, is on the rise ( Weller and Barnes 2016 ). The growing appearance of multimethod research in leading journals and university presses makes adequate training and preparation all the more significant ( Seawright 2016 ; Nexon 2019 ).
We are five political scientists interested in providing graduate students and other early-career researchers helpful resources for field research that we lacked when we first began our work. Each of us has recently completed or will soon complete a Ph.D. at a United States or Swedish university, though we come from many different national backgrounds. We have conducted field research in our home countries and abroad. From Colombia and Guatemala to the United States, from Europe to Turkey, and throughout East and Southeast Asia, we have spanned the globe to investigate civil society activism and transitional justice in post-violence societies, conflict-related sexual violence, social movements, authoritarianism and contentious politics, and the everyday politics and interactions between refugees and host-country citizens.
While some of us have studied in departments that offer strong training in field research methods, most of us have had to self-teach, learning through trial and error. Some of us have also been fortunate to participate in short courses and workshops hosted by universities such as the Consortium for Qualitative Research Methods and interdisciplinary institutions such as the Peace Research Institute Oslo. Recognizing that these opportunities are not available to or feasible for all, and hoping to ease the concerns of our more junior colleagues, we decided to compile our experiences and recommendations for first-time field researchers.
Our experiences in the field differ in several key respects, from the time we spent in the field to the locations we visited, and how we conducted our research. The diversity of our experiences, we hope, will help us reach and assist the broadest possible swath of graduate students interested in field research. Some of us have spent as little as ten days in a given country or as much as several months, in some instances visiting a given field site location just once and in other instances returning several times. At times, we have been able to plan weeks and months in advance. Other times, we have quickly arranged focus groups and impromptu interviews. Other times still, we have completed interviews virtually, when research participants were in remote locations or when we ourselves were unable to travel, of note during the coronavirus pandemic. We have worked in countries where we are fluent or have professional proficiency in the language, and in countries where we have relied on interpreters. We have worked in settings with precarious security as well as in locations that feel as comfortable as home. Our guide is not intended to be prescriptive or exhaustive. What we offer is a set of experience-based suggestions to be implemented as deemed relevant and appropriate by the researcher and their advisor(s).
In terms of the types of research and data sources and collection, we have conducted archival research, interviews, focus groups, and ethnographies with diplomats, bureaucrats, military personnel, ex-combatants, civil society advocates, survivors of political violence, refugees, and ordinary citizens. We have grappled with ethical dilemmas, chief among them how to get useful data for our research projects in ways that exceed the minimal standards of human subjects’ research evaluation panels. Relatedly, we have contemplated how to use our platforms to give back to the individuals and communities who have so generously lent us their time and knowledge, and shared with us their personal and sometimes harrowing stories.
Our target audience is first and foremost graduate students and early-career researchers who are interested in possibly conducting fieldwork but who either (1) do not know the full potential or value of fieldwork, (2) know the potential and value of fieldwork but think that it is excessively cost-prohibitive or otherwise infeasible, or (3) who have the interest, the will, and the means but not necessarily the know-how. We also hope that this resource will be of value to graduate programs, as they endeavor to better support students interested in or already conducting field research. Further, we target instructional faculty and graduate advisors (and other institutional gatekeepers like journal and book reviewers), to show that fieldwork does not have to be year-long, to give just one example. Instead, the length of time spent in the field is a function of the aims and scope of a given project. We also seek to formalize and normalize the idea of remote field research, whether conducted because of security concerns in conflict zones, for instance, or because of health and safety concerns, like the Covid-19 pandemic. Accordingly, researchers in the field for shorter stints or who conduct fieldwork remotely should not be penalized.
We note that several excellent resources on fieldwork such as the bibliography compiled by Advancing Conflict Research (2020) catalogue an impressive list of articles addressing questions such as ethics, safety, mental health, reflexivity, and methods. Further resources can be found about the positionality of the researcher in the field while engaging vulnerable communities, such as in the research field of migration ( Jacobsen and Landau 2003 ; Carling, Bivand Erdal, and Ezzati 2014 ; Nowicka and Cieslik 2014 ; Zapata-Barrero and Yalaz 2019 ). However, little has been written beyond conflict-affected contexts, fragile settings, and vulnerable communities. Moreover, as we consulted different texts and resources, we found no comprehensive guide to fieldwork explicitly written with graduate students in mind. It is this gap that we aim to fill.
In this paper, we address five general categories of questions that graduate students puzzle over, often without the benefit of others’ “lessons learned.” First, What is field research? Is it just for qualitative scholars? Must it be conducted in a foreign country? How much time in the field is “enough”? Second, What is the purpose of fieldwork? When does it make sense to travel to a field site to collect data? How can fieldwork data be used? Third, What are the nuts and bolts? How does one get ready and how can one optimize limited time and financial resources? Fourth, How does one conduct fieldwork safely? What should a researcher do to keep themselves, research assistants, and research subjects safe? What measures should they take to protect their mental health? Fifth, How does one conduct ethical, beneficent field research?
Finally, the Covid-19 pandemic has impressed upon the discipline the volatility of research projects centered around in-person fieldwork. Lockdowns and closed borders left researchers sequestered at home and unable to travel, forced others to cut short any trips already begun, and unexpectedly confined others still to their fieldwork sites. Other factors that may necessitate a (spontaneous) readjustment of planned field research include natural disasters, a deteriorating security situation in the field site, researcher illness, and unexpected changes in personal circumstances. We, therefore, conclude with a section on the promise and potential pitfalls of remote (or virtual) fieldwork. Throughout this guide, we engage theory and praxis to support an epistemologically and methodologically pluralistic discipline.
The concept of “fieldwork” is not well defined in political science. While several symposia discuss the “nuts and bolts” of conducting research in the field within the pages of political science journals, few ever define it ( Ortbals and Rincker 2009 ; Hsueh, Jensenius, and Newsome 2014 ). Defining the concept of fieldwork is important because assumptions about what it is and what it is not underpin any suggestions for conducting it. A lack of disciplinary consensus about what constitutes “fieldwork,” we believe, explains the lack of a unified definition. Below, we discuss three areas of current disagreement about what “fieldwork” is, including the purpose of fieldwork, where it occurs, and how long it should be. We follow this by offering our definition of fieldwork.
First, we find that many in the discipline view fieldwork as squarely in the domain of qualitative research, whether interpretivist or positivist. However, field research can also serve quantitative projects—for example, by providing crucial context, supporting triangulation, or illustrating causal mechanisms. For instance, Kreft (2019) elaborated her theory of women's civil society mobilization in response to conflict-related sexual violence based on interviews she carried out in Colombia. She then examined cross-national patterns through statistical analysis. Conversely, Willis's research on the United States military in East Asia began with quantitative data collection and analysis of protest events before turning to fieldwork to understand why protests occurred in some instances but not others. Researchers can also find quantifiable data in the field that is otherwise unavailable to them at home ( Read 2006 ; Chambers-Ju 2014 ; Jensenius 2014 ). Accordingly, fieldwork is not in the domain of any particular epistemology or methodology as its purpose is to acquire data for further information.
Second, comparative politics and international relations scholars often opine that fieldwork requires leaving the country in which one's institution is based. Instead, we propose that what matters most is the nature of the research project, not the locale. For instance, some of us in the international relations subfield have interviewed representatives of intergovernmental organizations (IGOs) and international nongovernmental organizations (INGOs), whose headquarters are generally located in Global North countries. For someone pursuing a Ph.D. in the United States and writing on transnational advocacy networks, interviews with INGO representatives in New York certainly count as fieldwork ( Zvobgo 2020 ). Similarly, a graduate student who returns to her home country to interview refugees and native citizens is conducting a field study as much as a researcher for whom the context is wholly foreign. Such interviews can provide necessary insights and information that would not have been gained otherwise—one of the key reasons researchers conduct fieldwork in the first place. In other instances, conducting any in-person research is simply not possible, due to financial constraints, safety concerns, or other reasons. For example, the Covid-19 pandemic has forced many researchers to shift their face-to-face research plans to remote data collection, either over the phone or virtually ( Howlett 2021 , 2). For some research projects, gathering data through remote methods may yield the same if not similar information than in-person research ( Howlett 2021 , 3–4). As Howlett (2021 , 11) notes, digital platforms may offer researchers the ability to “embed ourselves in other contexts from a distance” and glimpse into our subjects’ lives in ways similar to in-person research. By adopting a broader definition of fieldwork, researchers can be more flexible in getting access to data sources and interacting with research subjects.
Third, there is a tendency, especially among comparativists, to only count fieldwork that spans the better part of a year; even “surgical strike” field research entails one to three months, according to some scholars ( Ortbals and Rincker 2009 ; Weiss, Hicken, and Kuhonta 2017 ). The emphasis on spending as much time as possible in the field is likely due to ethnographic research traditions, reflected in classics such as James Scott's Weapons of the Weak , which entail year-long stints of research. However, we suggest that the appropriate amount of time in the field should be assessed on a project-by-project basis. Some studies require the researcher to be in the field for long periods; others do not. For example, Willis's research on the discourse around the United States’ military presence in overseas host communities has required months in the field. By contrast, Kreft only needed ten days in New York to carry out interviews with diplomats and United Nations staff, in a context with which she already had some familiarity from a prior internship. Likewise, Zvobgo spent a couple of weeks in her field research sites, conducting interviews with directors and managers of prominent human rights nongovernmental organizations. This population is not so large as to require a whole month or even a few months. This has also been the case for Irgil, as she had spent one month in the field site conducting interviews with ordinary citizens. The goal of the project was to acquire information on citizens’ perceptions of refugees. As we discuss in the next section, when deciding how long to spend in the field, scholars must consider the information their project requires and consider the practicalities of fieldwork, notably cost.
Thus, we highlight three essential points in fieldwork and offer a definition accordingly: fieldwork involves acquiring information, using any set of appropriate data collection techniques, for qualitative, quantitative, or experimental analysis through embedded research whose location and duration is dependent on the project. We argue that adopting such a definition of “fieldwork” is necessary to include the multitude of forms fieldwork can take, including remote methods, whose value and challenges the Covid-19 pandemic has impressed upon the discipline.
When does a researcher need to conduct fieldwork? Fieldwork can be effective for (1) data collection, (2) theory building, and (3) theory testing. First, when a researcher is interested in a research topic, yet they could not find an available and/or reliable data source for the topic, fieldwork could provide the researcher with plenty of options. Some research agendas can require researchers to visit archives to review historical documents. For example, Greitens (2016) visited national archives in the Philippines, South Korea, Taiwan, and the United States to find historical documents about the development of coercive institutions in past authoritarian governments for her book, Dictators and Their Secret Police . Also, newly declassified archival documents can open new possibilities for researchers to examine restricted topics. To illustrate, thanks to the newly released archival records of the Chinese Communist Party's communications, and exchange of visits with the European communist world, Sarotte (2012) was able to study the Party's decision to crack down on Tiananmen protesters, which had previously been deemed as an unstudiable topic due to the limited data.
Other research agendas can require researchers to conduct (semistructured) in-depth interviews to understand human behavior or a situation more closely, for example, by revealing the meanings of concepts for people and showing how people perceive the world. For example, O'Brien and Li (2005) conducted in-depth interviews with activists, elites, and villagers to understand how these actors interact with each other and what are the outcomes of the interaction in contentious movements in rural China. Through research, they revealed that protests have deeply influenced all these actors’ minds, a fact not directly observable without in-depth interviews.
Finally, data collection through fieldwork should not be confined to qualitative data ( Jensenius 2014 ). While some quantitative datasets can be easily compiled or accessed through use of the internet or contact with data-collection agencies, other datasets can only be built or obtained through relationships with “gatekeepers” such as government officials, and thus require researchers to visit the field ( Jensenius 2014 ). Researchers can even collect their own quantitative datasets by launching surveys or quantifying data contained in archives. In a nutshell, fieldwork will allow researchers to use different techniques to collect and access original/primary data sources, whether these are qualitative, quantitative, or experimental in nature, and regardless of the intended method of analysis. 2
But fieldwork is not just for data collection as such. Researchers can accomplish two other fundamental elements of the research process: theory building and theory testing. When a researcher finds a case where existing theories about a phenomenon do not provide plausible explanations, they can build a theory through fieldwork ( Geddes 2003 ). Lee's experience provides a good example. When studying the rise of a protest movement in South Korea for her dissertation, Lee applied commonly discussed social movement theories, grievances, political opportunity, resource mobilization, and repression, to explain the movement's eruption and found that these theories do not offer a convincing explanation for the protest movement. She then moved on to fieldwork and conducted interviews with the movement participants to understand their motivations. Finally, through those interviews, she offered an alternative theory that the protest participants’ collective identity shaped during the authoritarian past played a unifying factor and eventually led them to participate in the movement. Her example shows that theorization can take place through careful review and rigorous inference during fieldwork.
Moreover, researchers can test their theory through fieldwork. Quantitative observational data has limitations in revealing causal mechanisms ( Esarey 2017 ). Therefore, many political scientists turn their attention to conducting field experiments or lab-in-the-field experiments to reveal causality ( Druckman et al. 2006 ; Beath, Christia, and Enikolopov 2013 ; Finseraas and Kotsadam 2017 ), or to leveraging in-depth insights or historical records gained through qualitative or archival research in process-tracing ( Collier 2011 ; Ricks and Liu 2018 ). Surveys and survey experiments may also be useful tools to substantiate a theoretical story or test a theory ( Marston 2020 ). Of course, for most Ph.D. students, especially those not affiliated with more extensive research projects, some of these options will be financially prohibitive.
A central concern for graduate students, especially those working with a small budget and limited time, is optimizing time in the field and integrating remote work. We offer three pieces of advice: have a plan, build in flexibility, and be strategic, focusing on collecting data that are unavailable at home. We also discuss working with local translators or research assistants. Before we turn to these more practical issues arising during fieldwork, we address a no less important issue: funding.
The challenge of securing funds is often overlooked in discussions of what constitutes field research. Months- or year-long in-person research can be cost-prohibitive, something academic gatekeepers must consider when evaluating “what counts” and “what is enough.” Unlike their predecessors, many graduate students today have a significant amount of debt and little savings. 3 Additionally, if researchers are not able to procure funding, they have to pay out of pocket and possibly take on more debt. Not only is in-person fieldwork costly, but researchers may also have to forego working while they are in the field, making long stretches in the field infeasible for some.
For researchers whose fieldwork involves travelling to another location, procuring funding via grants, fellowships, or other sources is a necessity, regardless of how long one plans to be in the field. A good mantra for applying for research funding is “apply early and often” ( Kelsky 2015 , 110). Funding applications take a considerable amount of time to prepare, from writing research statements to requesting letters of recommendation. Even adapting one's materials for different applications takes time. Not only is the application process itself time-consuming, but the time between applying for and receiving funds, if successful, can be quite long, from several months to a year. For example, after defending her prospectus in May 2019, Willis began applying to funding sources for her dissertation, all of which had deadlines between June and September. She received notifications between November and January; however, funds from her successful applications were not available until March and April, almost a year later. 4 Accordingly, we recommend applying for funding as early as possible; this not only increases one's chances of hitting the ground running in the field, but the application process can also help clarify the goals and parameters of one's research.
Graduate students should also apply often for funding opportunities. There are different types of funding for fieldwork: some are larger, more competitive grants such as the National Science Foundation Political Science Doctoral Dissertation Improvement Grant in the United States, others, including sources through one's own institution, are smaller. Some countries, like Sweden, boast a plethora of smaller funding agencies that disburse grants of 20,000–30,0000 Swedish Kronor (approx. 2,500–3,500 U.S. dollars) to Ph.D. students in the social sciences. Listings of potential funding sources are often found on various websites including those belonging to universities, professional organizations (such as the American Political Science Association or the European Consortium for Political Research), and governmental institutions dealing with foreign affairs. Once you have identified fellowships and grants for which you and your project are a good match, we highly recommend soliciting information and advice from colleagues who have successfully applied for them. This can include asking them to share their applications with you, and if possible, to have them, another colleague or set of colleagues read through your project description and research plan (especially for bigger awards) to ensure that you have made the best possible case for why you should be selected. While both large and small pots of funding are worth applying for, many researchers end up funding their fieldwork through several small grants or fellowships. One small award may not be sufficient to fund the entirety of one's fieldwork, but several may. For example, Willis's fieldwork in Japan and South Korea was supported through fellowships within each country. Similarly, Irgil was able to conduct her fieldwork abroad through two different and relatively smaller grants by applying to them each year.
Of course, situations vary in different countries with respect to what kinds of grants from what kinds of funders are available. An essential part of preparing for fieldwork is researching the funding landscape well in advance, even as early as the start of the Ph.D. We encourage first-time field researchers to be aware that universities and departments may themselves not be aware of the full range of possible funds available, so it is always a good idea to do your own research and watch research-related social media channels. The amount of funding needed thereby depends on the nature of one's project and how long one intends to be in the field. As we elaborate in the next section, scholars should think carefully about their project goals, the data required to meet those goals, and the requisite time to attain them. For some projects, even a couple of weeks in the field is sufficient to get the needed information.
Preparing to Enter “the field”
It is important to prepare for the field as much as possible. What kind of preparations do researchers need? For someone conducting interviews with NGO representatives, this might involve identifying the largest possible pool of potential respondents, securing their contact information, sending them study invitation letters, finding a mutually agreeable time to meet, and pulling together short biographies for each interviewee in order to use your time together most effectively. If you plan to travel to conduct interviews, you should reach out to potential respondents roughly four to six weeks prior to your arrival. For individuals who do not respond, you can follow up one to two weeks before you arrive and, if needed, once more when you are there. This is still no guarantee for success, of course. For Kreft, contacting potential interviewees in Colombia initially proved more challenging than anticipated, as many of the people she targeted did not respond to her emails. It turned out that many Colombians have a preference for communicating via phone or, in particular, WhatsApp. Some of those who responded to her emails sent in advance of her field trip asked her to simply be in touch once she was in the country, to set up appointments on short notice. This made planning and arranging her interview schedule more complicated. Therefore, a general piece of advice is to research your target population's preferred communication channels and mediums in the field site if email requests yield no or few responses.
In general, we note for the reader that contacting potential research participants should come after one has designed an interview questionnaire (plus an informed consent protocol) and sought and received, where applicable, approval from institutional review boards (IRBs) or other ethical review procedures in place (both at one's home institution/in the country of the home institution as well as in the country where one plans to conduct research if travelling abroad). The most obvious advantage of having the interview questionnaire in place and having secured all necessary institutional approvals before you start contacting potential interviewees is that you have a clearer idea of the universe of individuals you would like to interview, and for what purpose. Therefore, it is better to start sooner rather than later and be mindful of “high seasons,” when institutional and ethical review boards are receiving, processing, and making decisions on numerous proposals. It may take a few months for them to issue approvals.
On the subject of ethics and review panels, we encourage you to consider talking openly and honestly with your supervisors and/or funders about the situations where a written consent form may not be suitable and might need to be replaced with “verbal consent.” For instance, doing fieldwork in politically unstable contexts, highly scrutinized environments, or vulnerable communities, like refugees, might create obstacles for the interviewees as well as the researcher. The literature discusses the dilemma in offering the interviewees anonymity and requesting signed written consent in addition to the emphasis on total confidentiality ( Jacobsen and Landau 2003 ; Mackenzie, McDowell, and Pittaway 2007 ; Saunders, Kitzinger, and Kitzinger 2015 ). Therefore, in those situations, the researcher might need to take the initiative on how to act while doing the interviews as rigorously as possible. In her fieldwork, Irgil faced this situation as the political context of Turkey did not guarantee that there would not be any adverse consequences for interviewees on both sides of her story: citizens of Turkey and Syrian refugees. Consequently, she took hand-written notes and asked interviewees for their verbal consent in a safe interview atmosphere. This is something respondents greatly appreciated ( Irgil 2020 ).
Ethical considerations, of course, also affect the research design itself, with ramifications for fieldwork. When Kreft began developing her Ph.D. proposal to study women's political and civil society mobilization in response to conflict-related sexual violence, she initially aimed to recruit interviewees from the universe of victims of this violence, to examine variation among those who did and those who did not mobilize politically. As a result of deeper engagement with the literature on researching conflict-related sexual violence, conversations with senior colleagues who had interviewed victims, and critical self-reflection of her status as a researcher (with no background in psychology or social work), she decided to change focus and shift toward representatives of civil society organizations and victims’ associations. This constituted a major reconfiguration of her research design, from one geared toward identifying the factors that drive mobilization of victims toward using insights from interviews to understand better how those mobilize perceive and “make sense” of conflict-related sexual violence. Needless to say, this required alterations to research strategies and interview guides, including reassessing her planned fieldwork. Kreft's primary consideration was not to cause harm to her research participants, particularly in the form of re-traumatization. She opted to speak only with those women who on account of their work are used to speaking about conflict-related sexual violence. In no instance did she inquire about interviewees’ personal experiences with sexual violence, although several brought this up on their own during the interviews.
Finally, if you are conducting research in another country where you have less-than-professional fluency in the language, pre-fieldwork planning should include hiring a translator or research assistant, for example, through an online hiring platform like Upwork, or a local university. Your national embassy or consulate is another option; many diplomatic offices have lists of individuals who they have previously contracted. More generally, establishing contact with a local university can be beneficial, either in the form of a visiting researcher arrangement, which grants access to research groups and facilities like libraries or informally contacting individual researchers. The latter may have valuable insights into the local context, contacts to potential research participants, and they may even be able to recommend translators or research assistants. Kreft, for example, hired local research assistants recommended by researchers at a Bogotá-based university and remunerated them equivalent to the salary they would have received as graduate research assistants at the university, while also covering necessary travel expenses. Irgil, on the other hand, established contacts with native citizens and Syrian gatekeepers, who are shop owners in the area where she conducted her research because she had the opportunity to visit the fieldwork site multiple times.
Depending on the research agenda, researchers may visit national archives, local government offices, etc. Before visiting, researchers should contact these facilities and make sure the materials that they need are accessible. For example, Lee visited the Ronald Reagan Presidential Library Archives to find the United States’ strategic evaluations on South Korea's dictator in the 1980s. Before her visit, she contacted librarians in the archives, telling them her visit plans and her research purpose. Librarians made suggestions on which categories she should start to review based on her research goal, and thus she was able to make a list of categories of the materials she needed, saving her a lot of her time.
Accessibility of and access to certain facilities/libraries can differ depending on locations/countries and types of facilities. Facilities in authoritarian countries might not be easily accessible to foreign researchers. Within democratic countries, some facilities are more restrictive than others. Situations like the pandemic or national holidays can also restrict accessibility. Therefore, researchers are well advised to do preliminary research on whether a certain facility opens during the time they visit and is accessible to researchers regardless of their citizenship status. Moreover, researchers must contact the staff of facilities to know whether identity verification is needed and if so, what kind of documents (photo I.D. or passport) should be exhibited.
Adapting to the Reality of the Field
Researchers need to be flexible because you may meet people you did not make appointments with, come across opportunities you did not expect, or stumble upon new ideas about collecting data in the field. These happenings will enrich your field experience and will ultimately be beneficial for your research. Similarly, researchers should not be discouraged by interviews that do not go according to plan; they present an opportunity to pursue relevant people who can provide an alternative path to your work. Note that planning ahead does not preclude fortuitous encounters or epiphanies. Rather, it provides a structure for them to happen.
If your fieldwork entails travelling abroad, you will also be able to recruit more interviewees once you arrive at your research site. In fact, you may have greater success in-country; not everyone is willing to respond to a cold email from an unknown researcher in a foreign country. In Irgil's fieldwork, she contacted store owners that are known in the area and who know the community. This eased her process of introduction into the community and recruiting interviewees. For Zvobgo, she had fewer than a dozen interviews scheduled when she travelled to Guatemala to study civil society activism and transitional justice since the internal armed conflict. But she was able to recruit additional participants in-country. Interviewees with whom she built a rapport connected her to other NGOs, government offices, and the United Nations country office, sometimes even making the call and scheduling interviews for her. Through snowball sampling, she was able to triple the number of participants. Likewise, snowball sampling was central to Kreft's recruitment of interview partners. Several of her interviewees connected her to highly relevant individuals she would never have been able to identify and contact based on web searches alone.
While in the field, you may nonetheless encounter obstacles that necessitate adjustments to your original plans. Once Kreft had arrived in Colombia, for example, it transpired quickly that carrying out in-person interviews in more remote/rural areas was near impossible given her means, as these were not easily accessible by bus/coach, further complicated by a complex security situation. Instead, she adjusted her research design and shifted her focus to the big cities, where most of the major civil society organizations are based. She complemented the in-person interviews carried out there with a smaller number of phone interviews with civil society activists in rural areas, and she was also able to meet a few activists operating in rural or otherwise inaccessible areas as they were visiting the major cities. The resulting focus on urban settings changed the kinds of generalizations she was able to make based on her fieldwork data and produced a somewhat different study than initially anticipated.
This also has been the case for Irgil, despite her prior arrangements with the Syrian gatekeepers, which required adjustments as in the case of Kreft. Irgil acquired research clearance one year before, during the interviews with native citizens, conducting the interviews with Syrian refugees. She also had her questionnaire ready based on the previously collected data and the media search she had conducted for over a year before travelling to the field site. As she was able to visit the field site multiple times, two months before conducting interviews with Syrian refugees, she developed a schedule with the Syrian gatekeepers and informants. Yet, once she was in the field, influenced by Turkey's recent political events and the policy of increasing control over Syrian refugees, half of the previously agreed informants changed their minds or did not want to participate in interviews. As Irgil was following the policies and the news related to Syrian refugees in Turkey closely, this did not come as that big of a surprise but challenged the previously developed strategy to recruit interviewees. Thus, she changed the strategy of finding interviewees in the field site, such as asking people, almost one by one, whether they would like to participate in the interview. Eventually, she could not find willing Syrian women refugees as she had planned, which resulted in a male-dominant sample. As researchers encounter such situations, it is essential to remind oneself that not everything can go according to plan, that “different” does not equate to “worse,” but that it is important to consider what changes to fieldwork data collection and sampling imply for the study's overall findings and the contribution it makes to the literature.
We should note that conducting interviews is very taxing—especially when opportunities multiply, as in Zvobgo's case. Depending on the project, each interview can take an hour, if not two or more. Hence, you should make a reasonable schedule: we recommend no more than two interviews per day. You do not want to have to cut off an interview because you need to rush to another one, whether the interviews are in-person or remote. And you do not want to be too exhausted to have a robust engagement with your respondent who is generously lending you their time. Limiting the number of interviews per day is also important to ensure that you can write comprehensive and meaningful fieldnotes, which becomes even more essential where it is not possible to audio-record your interviews. Also, be sure to remember to eat, stay hydrated, and try to get enough sleep.
Finally, whether to provide gifts or payments to the subject also requires adapting to the reality of the field. You must think about payments beforehand when you apply for IRB approval (or whatever other ethical review processes may be in place) since these applications usually contain questions about payments. Obviously, the first step is to carefully evaluate whether the gifts and payments provided can harm the subject or are likely to unduly affect the responses they will give in response to your questions. If that is not the case, you have to make payment decisions based on your budget, field situation, and difficulties in recruitment. Usually, payment of respondents is more common in survey research, whereas it is less common in interviews and focus groups.
Nevertheless, payment practices vary depending on the field and the target group. In some cases, it may become a custom to provide small gifts or payments when interviewing a certain group. In other cases, interviewees might be offended if they are provided with money. Therefore, knowing past practices and field situations is important. For example, Lee provided small coffee gift cards to one group while she did not to the other based on previous practices of other researchers. That is, for a particular group, it has become a custom for interviewers to pay interviewees. Sometimes, you may want to reimburse your subject's interview costs such as travel expenses and provide beverages and snacks during the conduct of research, as Kreft did when conducting focus groups in Colombia. To express your gratitude to your respondents, you can prepare small gifts such as your university memorabilia (e.g., notebooks and pens). Since past practices about payments can affect your interactions and interviews with a target group, you want to seek advice from your colleagues and other researchers who had experiences interacting with the target group. If you cannot find researchers who have this knowledge, you can search for published works on the target population to find if the authors share their interview experiences. You may also consider contacting the authors for advice before your interviews.
Researching Strategically
Distinguishing between things that can only be done in person at a particular site and things that can be accomplished later at home is vital. Prioritize the former over the latter. Lee's fieldwork experience serves as a good example. She studied a conservative protest movement called the Taegeukgi Rally in South Korea. She planned to conduct interviews with the rally participants to examine their motivations for participating. But she only had one month in South Korea. So, she focused on things that could only be done in the field: she went to the rally sites, she observed how protests proceeded, which tactics and chants were used, and she met participants and had some casual conversations with them. Then, she used the contacts she made while attending the rallies to create a social network to solicit interviews from ordinary protesters, her target population. She was able to recruit twenty-five interviewees through good rapport with the people she met. The actual interviews proceeded via phone after she returned to the United States. In a nutshell, we advise you not to be obsessed with finishing interviews in the field. Sometimes, it is more beneficial to use your time in the field to build relationships and networks.
Working With Assistants and Translators
A final consideration on logistics is working with research assistants or translators; it affects how you can carry out interviews, focus groups, etc. To what extent constant back-and-forth translation is necessary or advisable depends on the researcher's skills in the interview language and considerations about time and efficiency. For example, Kreft soon realized that she was generally able to follow along quite well during her interviews in Colombia. In order to avoid precious time being lost to translation, she had her research assistant follow the interview guide Kreft had developed, and interjected follow-up questions in Spanish or English (then to be translated) as they arose.
Irgil's and Zvobgo's interviews went a little differently. Irgil's Syrian refugee interviewees in Turkey were native Arabic speakers, and Zvobgo's interviewees in Guatemala were native Spanish speakers. Both Irgil and Zvobgo worked with research assistants. In Irgil's case, her assistant was a Syrian man, who was outside of the area. Meanwhile, Zvobgo's assistant was an undergraduate from her home institution with a Spanish language background. Irgil and Zvobgo began preparing their assistants a couple of months before entering the field, over Skype for Irgil and in-person for Zvobgo. They offered their assistants readings and other resources to provide them with the necessary background to work well. Both Irgil and Zvobgo's research assistants joined them in the interviews and actually did most of the speaking, introducing the principal investigator, explaining the research, and then asking the questions. In Zvobgo's case, interviewee responses were relayed via a professional interpreter whom she had also hired. After every interview, Irgil and Zvobgo and their respective assistants discussed the answers of the interviewees, potential improvements in phrasing, and elaborated on their hand-written interview notes. As a backup, Zvobgo, with the consent of her respondents, had accompanying audio recordings.
Researchers may carry out fieldwork in a country that is considerably less safe than what they are used to, a setting affected by conflict violence or high crime rates, for instance. Feelings of insecurity can be compounded by linguistic barriers, cultural particularities, and being far away from friends and family. Insecurity is also often gendered, differentially affecting women and raising the specter of unwanted sexual advances, street harassment, or even sexual assault ( Gifford and Hall-Clifford 2008 ; Mügge 2013 ). In a recent survey of Political Science graduate students in the United States, about half of those who had done fieldwork internationally reported having encountered safety issues in the field, (54 percent female, 47 percent male), and only 21 percent agreed that their Ph.D. programs had prepared them to carry out their fieldwork safely ( Schwartz and Cronin-Furman 2020 , 8–9).
Preventative measures scholars may adopt in an unsafe context may involve, at their most fundamental, adjustments to everyday routines and habits, restricting one's movements temporally and spatially. Reliance on gatekeepers may also necessitate adopting new strategies, such as a less vehement and cold rejection of unwanted sexual advances than one ordinarily would exhibit, as Mügge (2013) illustratively discusses. At the same time, a competitive academic job market, imperatives to collect novel and useful data, and harmful discourses surrounding dangerous fieldwork also, problematically, shape incentives for junior researchers to relax their own standards of what constitutes acceptable risk ( Gallien 2021 ).
Others have carefully collected a range of safety precautions that field researchers in fragile or conflict-affected settings may take before and during fieldwork ( Hilhorst et al. 2016 ). Therefore, we are more concise in our discussion of recommendations, focusing on the specific situations of graduate students. Apart from ensuring that supervisors and university administrators have the researcher's contact information in the field (and possibly also that of a local contact person), researchers can register with their country's embassy or foreign office and any crisis monitoring and prevention systems it has in place. That way, they will be informed of any possible unfolding emergencies and the authorities have a record of them being in the country.
It may also be advisable to set up more individualized safety protocols with one or two trusted individuals, such as friends, supervisors, or colleagues at home or in the fieldwork setting itself. The latter option makes sense in particular if one has an official affiliation with a local institution for the duration of the fieldwork, which is often advisable. Still, we would also recommend establishing relationships with local researchers in the absence of a formal affiliation. To keep others informed of her whereabouts, Kreft, for instance, made arrangements with her supervisors to be in touch via email at regular intervals to report on progress and wellbeing. This kept her supervisors in the loop, while an interruption in communication would have alerted them early if something were wrong. In addition, she announced planned trips to other parts of the country and granted her supervisors and a colleague at her home institution emergency reading access to her digital calendar. To most of her interviews, she was moreover accompanied by her local research assistant/translator. If the nature of the research, ethical considerations, and the safety situation allow, it might also be possible to bring a local friend along to interviews as an “assistant,” purely for safety reasons. This option needs to be carefully considered already in the planning stage and should, particularly in settings of fragility or if carrying out research on politically exposed individuals, be noted in any ethical and institutional review processes where these are required. Adequate compensation for such an assistant should be ensured. It may also be advisable to put in place an emergency plan, that is, choose emergency contacts back home and “in the field,” know whom to contact if something happens, and know how to get to the nearest hospital or clinic.
We would be remiss if we did not mention that, when in an unfamiliar context, one's safety radar may be misguided, so it is essential to listen to people who know the context. For example, locals can give advice on which means of transport are safe and which are not, a question that is of the utmost importance when traveling to appointments. For example, Kreft was warned that in Colombia regular taxis are often unsafe, especially if waved down in the streets, and that to get to her interviews safely, she should rely on a ride-share service. In one instance, a Colombian friend suggested that when there was no alternative to a regular taxi, Kreft should book through the app and share the order details, including the taxi registration number or license plate, with a friend. Likewise, sharing one's cell phone location with a trusted friend while traveling or when one feels unsafe may be a viable option. Finally, it is prudent to heed the safety recommendations and travel advisories provided by state authorities and embassies to determine when and where it is safe to travel. Especially if researchers have a responsibility not only for themselves but also for research assistants and research participants, safety must be a top priority.
This does not mean that a researcher should be careless in a context they know either. Of course, conducting fieldwork in a context that is known to the researcher offers many advantages. However, one should be prepared to encounter unwanted events too. For instance, Irgil has conducted fieldwork in her country of origin in a city she knows very well. Therefore, access to the site, moving around the site, and blending in has not been a problem; she also has the advantage of speaking the native language. Yet, she took notes of the streets she walked in, as she often returned from the field site after dark and thought she might get confused after a tiring day. She also established a closer relationship with two or three store owners in different parts of the field site if she needed something urgent, like running out of battery. Above all, one should always be aware of one's surroundings and use common sense. If something feels unsafe, chances are it is.
Fieldwork may negatively affect the researcher's mental health and mental wellbeing regardless of where one's “field” is, whether related to concerns about crime and insecurity, linguistic barriers, social isolation, or the practicalities of identifying, contacting and interviewing research participants. Coping with these different sources of stress can be both mentally and physically exhausting. Then there are the things you may hear, see and learn during the research itself, such as gruesome accounts of violence and suffering conveyed in interviews or archival documents one peruses. Kreft and Zvobgo have spoken with women victims of conflict-related sexual violence, who sometimes displayed strong emotions of pain and anger during the interviews. Likewise, Irgil and Willis have spoken with members of other vulnerable populations such as refugees and former sex workers ( Willis 2020 ).
Prior accounts ( Wood 2006 ; Loyle and Simoni 2017 ; Skjelsbæk 2018 ; Hummel and El Kurd 2020 ; Williamson et al. 2020 ; Schulz and Kreft 2021 ) show that it is natural for sensitive research and fieldwork challenges to affect or even (vicariously) traumatize the researcher. By removing researchers from their regular routines and support networks, fieldwork may also exacerbate existing mental health conditions ( Hummel and El Kurd 2020 ). Nonetheless, mental wellbeing is rarely incorporated into fieldwork courses and guidelines, where these exist at all. But even if you know to anticipate some sort of reaction, you rarely know what that reaction will be until you experience it. When researching sensitive or difficult topics, for example, reactions can include sadness, frustration, anger, fear, helplessness, and flashbacks to personal experiences of violence ( Williamson et al. 2020 ). For example, Kreft responded with episodic feelings of depression and both mental and physical exhaustion. But curiously, these reactions emerged most strongly after she had returned from fieldwork and in particular as she spent extended periods analyzing her interview data, reliving some of the more emotional scenes during the interviews and being confronted with accounts of (sexual) violence against women in a concentrated fashion. This is a crucial reminder that fieldwork does not end when one returns home; the after-effects may linger. Likewise, Zvobgo was physically and mentally drained upon her return from the field. Both Kreft and Zvobgo were unable to concentrate for long periods of time and experienced lower-than-normal levels of productivity for weeks afterward, patterns that formal and informal conversations with other scholars confirm to be common ( Schulz and Kreft 2021 ). Furthermore, the boundaries between “field” and “home” are blurred when conducting remote fieldwork ( Howlett 2021 , 11).
Nor are these adverse reactions limited to cases where the researcher has carried out the interviews themselves. Accounts of violence, pain, and suffering transported in reports, secondary literature, or other sources can evoke similar emotional stress, as Kreft experienced when engaging in a concentrated fashion with additional accounts of conflict-related sexual violence in Colombia and with the feminist literature on sexual and gender-based violence in the comfort of her Swedish office. This could also be applicable to Irgil's fieldwork as she interviewed refugees whose traumas have come out during the interviews or recall specific events triggered by the questions. Likewise, Lee has reviewed primary and secondary materials on North Korean defectors in the national archives and these materials contain violent, intense, emotional narratives.
Fortunately, there are several strategies to cope with and manage such adverse consequences. In a candid and insightful piece, other researchers have discussed the usefulness of distractions, sharing with colleagues, counseling, exercise, and, probably less advisable in the long term, comfort eating and drinking ( Williamson et al. 2020 ; see also Loyle and Simoni 2017 ; Hummel and El Kurd 2020 ). Our experiences largely tally with their observations. In this section, we explore some of these in more detail.
First, in the face of adverse consequences on your mental wellbeing, whether in the field or after your return, it is essential to be patient and generous with yourself. Negative effects on the researcher's mental wellbeing can hit in unexpected ways and at unexpected times. Even if you think that certain reactions are disproportionate or unwarranted at that specific moment, they may simply have been building up over a long time. They are legitimate. Second, the importance of taking breaks and finding distractions, whether that is exercise, socializing with friends, reading a good book, or watching a new series, cannot be overstated. It is easy to fall into a mode of thinking that you constantly have to be productive while you are “in the field,” to maximize your time. But as with all other areas in life, balance is key and rest is necessary. Taking your mind off your research and the research questions you puzzle over is also a good way to more fully soak up and appreciate the context in which you find yourself, in the case of in-person fieldwork, and about which you ultimately write.
Third, we cannot stress enough the importance of investing in social relations. Before going on fieldwork, researchers may want to consult others who have done it before them. Try to find (junior) scholars who have done fieldwork on similar kinds of topics or in the same country or countries you are planning to visit. Utilizing colleagues’ contacts and forging connections using social media are valuable strategies to expand your networks (in fact, this very paper is the result of a social media conversation and several of the authors have never met in person). Having been in the same situation before, most field researchers are, in our experience, generous with their time and advice. Before embarking on her first trip to Colombia, Kreft contacted other researchers in her immediate and extended network and received useful advice on questions such as how to move around Bogotá, whom to speak to, and how to find a research assistant. After completing her fieldwork, she has passed on her experiences to others who contacted her before their first fieldwork trip. Informal networks are, in the absence of more formalized fieldwork preparation, your best friend.
In the field, seeking the company of locals and of other researchers who are also doing fieldwork alleviates anxiety and makes fieldwork more enjoyable. Exchanging experiences, advice and potential interviewee contacts with peers can be extremely beneficial and make the many challenges inherent in fieldwork (on difficult topics) seem more manageable. While researchers conducting remote fieldwork may be physically isolated from other researchers, even connecting with others doing remote fieldwork may be comforting. And even when there are no precise solutions to be found, it is heartening or even cathartic to meet others who are in the same boat and with whom you can talk through your experiences. When Kreft shared some of her fieldwork-related struggles with another researcher she had just met in Bogotá and realized that they were encountering very similar challenges, it was like a weight was lifted off her shoulders. Similarly, peer support can help with readjustment after the fieldwork trip, even if it serves only to reassure you that a post-fieldwork dip in productivity and mental wellbeing is entirely natural. Bear in mind that certain challenges are part of the fieldwork experience and that they do not result from inadequacy on the part of the researcher.
Finally, we would like to stress a point made by Inger Skjelsbæk (2018 , 509) and which has not received sufficient attention: as a discipline, we need to take the question of researcher mental wellbeing more seriously—not only in graduate education, fieldwork preparation, and at conferences, but also in reflecting on how it affects the research process itself: “When strong emotions arise, through reading about, coding, or talking to people who have been impacted by [conflict-related sexual violence] (as victims or perpetrators), it may create a feeling of being unprofessional, nonscientific, and too subjective.”
We contend that this is a challenge not only for research on sensitive issues but also for fieldwork more generally. To what extent is it possible, and desirable, to uphold the image of the objective researcher during fieldwork, when we are at our foundation human beings? And going even further, how do the (anticipated) effects of our research on our wellbeing, and the safety precautions we take ( Gifford and Hall-Clifford 2008 ), affect the kinds of questions we ask, the kinds of places we visit and with whom we speak? How do they affect the methods we use and how we interpret our findings? An honest discussion of affective responses to our research in methods sections seems utopian, as emotionality in the research process continues to be silenced and relegated to the personal, often in gendered ways, which in turn is considered unconnected to the objective and scientific research process ( Jamar and Chappuis 2016 ). But as Gifford and Hall-Clifford (2008 , 26) aptly put it: “Graduate education should acknowledge the reality that fieldwork is scholarly but also intimately personal,” and we contend that the two shape each other. Therefore, we encourage political science as a discipline to reflect on researcher wellbeing and affective responses to fieldwork more carefully, and we see the need for methods courses that embrace a more holistic notion of the subjectivity of the researcher.
Interacting with people in the field is one of the most challenging yet rewarding parts of the work that we do, especially in comparison to impersonal, often tedious wrangling and analysis of quantitative data. Field researchers often make personal connections with their interviewees. Consequently, maintaining boundaries can be a bit tricky. Here, we recommend being honest with everyone with whom you interact without overstating the abilities of a researcher. This appears as a challenge in the field, particularly when you empathize with people and when they share profound parts of their lives with you for your research in addition to being “human subjects” ( Fujii 2012 ). For instance, when Irgil interviewed native citizens about the changes in their neighborhood following the arrival of Syrian refugees, many interviewees questioned what she would offer them in return for their participation. Irgil responded that her primary contribution would be her published work. She also noted, however, that academic papers can take a year, sometimes longer, to go through the peer-reviewed process and, once published, many studies have a limited audience. The Syrian refugees posed similar questions. Irgil responded not only with honesty but also, given this population's vulnerable status, she provided them contact information for NGOs with which they could connect if they needed help or answers to specific questions.
For her part, Zvobgo was very upfront with her interviewees about her role as a researcher: she recognized that she is not someone who is on the frontlines of the fight for human rights and transitional justice like they are. All she could/can do is use her platform to amplify their stories, bringing attention to their vital work through her future peer-reviewed publications. She also committed to sending them copies of the work, as electronic journal articles are often inaccessible due to paywalls and university press books are very expensive, especially for nonprofits. Interviewees were very receptive; some were even moved by the degree of self-awareness and the commitment to do right by them. In some cases, this prompted them to share even more, because they knew that the researcher was really there to listen and learn. This is something that junior scholars, and all scholars really, should always remember. We enter the field to be taught. Likewise, Kreft circulated among her interviewees Spanish-language versions of an academic article and a policy brief based on the fieldwork she had carried out in Colombia.
As researchers from the Global North, we recognize a possible power differential between us and our research subjects, and certainly an imbalance in power between the countries where we have been trained and some of the countries where we have done and continue to do field research, particularly in politically dynamic contexts ( Knott 2019 ). This is why we are so concerned with being open and transparent with everyone with whom we come into contact in the field and why we are committed to giving back to those who so generously lend us their time and knowledge. Knott (2019 , 148) summarizes this as “Reflexive openness is a form of transparency that is methodologically and ethically superior to providing access to data in its raw form, at least for qualitative data.”
We also recognize that academics, including in the social sciences and especially those hailing from countries in the Global North, have a long and troubled history of exploiting their power over others for the sake of their research—including failing to be upfront about their research goals, misrepresenting the on-the-ground realities of their field research sites (including remote fieldwork), and publishing essentializing, paternalistic, and damaging views and analyses of the people there. No one should build their career on the backs of others, least of all in a field concerned with the possession and exercise of power. Thus, it is highly crucial to acknowledge the power hierarchies between the researcher and the interviewees, and to reflect on them both in the field and beyond the field upon return.
A major challenge to conducting fieldwork is when researchers’ carefully planned designs do not go as planned due to unforeseen events outside of our control, such as pandemics, natural disasters, deteriorating security situations in the field, or even the researcher falling ill. As the Covid-19 pandemic has made painfully clear, researchers may face situations where in-person research is simply not possible. In some cases, researchers may be barred entry to their fieldwork site; in others, the ethical implications of entering the field greatly outweigh the importance of fieldwork. Such barriers to conducting in-person research require us to reconsider conventional notions of what constitutes fieldwork. Researchers may need to shift their data collection methods, for example, conducting interviews remotely instead of in person. Even while researchers are in the field, they may still need to carry out part of their interviews or surveys virtually or by phone. For example, Kreft (2020) carried out a small number of interviews remotely while she was based in Bogotá, because some of the women's civil society activists with whom she intended to speak were based in parts of the country that were difficult and/or dangerous to access.
Remote field research, which we define as the collection of data over the internet or over the phone where in-person fieldwork is not possible due to security, health or other risks, comes with its own sets of challenges. For one, there may be certain populations that researchers cannot reach remotely due to a lack of internet connectivity or technology such as cellphones and computers. In such instances, there will be a sampling bias toward individuals and groups that do have these resources, a point worth noting when scholars interpret their research findings. In the case of virtual research, the risk of online surveillance, hacking, or wiretapping may also produce reluctance on the part of interviewees to discuss sensitive issues that may compromise their safety. Researchers need to carefully consider how the use of digital technology may increase the risk to research participants and what changes to the research design and any interview guides this necessitates. In general, it is imperative that researchers reflect on how they can ethically use digital technology in their fieldwork ( Van Baalen 2018 ). Remote interviews may also be challenging to arrange for researchers who have not made connections in person with people in their community of interest.
Some of the serendipitous happenings we discussed earlier may also be less likely and snowball sampling more difficult. For example, in phone or virtual interviews, it is harder to build good rapport and trust with interviewees as compared to face-to-face interviews. Accordingly, researchers should be more careful in communicating with interviewees and creating a comfortable interview environment. Especially when dealing with sensitive topics, researchers may have to make several phone calls and sometimes have to open themselves to establishing trust with interviewees. Also, researchers must be careful in protecting interviewees in phone or virtual interviews when they deal with sensitive topics of countries interviewees reside in.
The inability to physically visit one's community of interest may also encourage scholars to critically reflect on how much time in the field is essential to completing their research and to consider creative, alternative means for accessing information to complete their projects. While data collection techniques such as face-to-face interviews and archival work in the field may be ideal in normal times, there exist other data sources that can provide comparably useful information. For example, in her research on the role of framing in the United States base politics, Willis found that social media accounts and websites yielded information useful to her project. Many archives across the world have also been digitized. Researchers may also consider crowdsourcing data from the field among their networks, as fellow academics tend to collect much more data in the field than they ever use in their published works. They may also elect to hire someone, perhaps a graduate student, in a city or a country where they cannot travel and have the individual access, scan, and send archival materials. This final suggestion may prove generally useful to researchers with limited time and financial resources.
Remote qualitative data collection techniques, while they will likely never be “the gold-standard,” also pose several advantages. These techniques may help researchers avoid some of the issues mentioned previously. Remote interviews, for example, are less time-consuming in terms of travel to the interview site ( Archibald et al. 2019 ). The implication is that researchers may have less fatigue from conducting interviews and/or may be able to conduct more interviews. For example, while Willis had little energy to do anything else after an in-person interview (or two) in a given day, she had much more energy after completing remote interviews. Second, remote fieldwork also helps researchers avoid potentially dangerous situations in the field mentioned previously. Lastly, remote fieldwork generally presents fewer financial barriers than in-person research ( Archibald et al. 2019 ). In that sense, considering remote qualitative data collection, a type of “fieldwork” may make fieldwork more accessible to a greater number of scholars.
Many of the substantive, methodological and practical challenges that arise during fieldwork can be anticipated. Proper preparation can help you hit the ground running once you enter your fieldwork destination, whether in-person or virtually. Nonetheless, there is no such thing as being perfectly prepared for the field. Some things will simply be beyond your control, and especially as a newcomer to field research, and you should be prepared for things to not go as planned. New questions will arise, interview participants may cancel appointments, and you might not get the answers you expected. Be ready to make adjustments to research plans, interview guides, or questionnaires. And, be mindful of your affective reactions to the overall fieldwork situation and be gentle with yourself.
We recommend approaching fieldwork as a learning experience as much as, or perhaps even more than, a data collection effort. This also applies to your research topic. While it is prudent always to exercise a healthy amount of skepticism about what people tell you and why, the participants in your research will likely have unique perspectives and knowledge that will challenge yours. Be an attentive listener and remember that they are experts of their own experiences.
We encourage more institutions to offer courses that cover field research preparation and planning, practical advice on safety and wellbeing, and discussion of ethics. Specifically, we align with Schwartz and Cronin-Furman's (2020 , 3) contention “that treating fieldwork preparation as the methodology will improve individual scholars’ experiences and research.” In this article, we outline a set of issue areas in which we think formal preparation is necessary, but we note that our discussion is by no means exhaustive. Formal fieldwork preparation should also extend beyond what we have covered in this article, such as issues of data security and preparing for nonqualitative fieldwork methods. We also note that field research is one area that has yet to be comprehensively addressed in conversations on diversity and equity in the political science discipline and the broader academic profession. In a recent article, Brielle Harbin (2021) begins to fill this gap by sharing her experiences conducting in-person election surveys as a Black woman in a conservative and predominantly white region of the United States and the challenges that she encountered. Beyond race and gender, citizenship, immigration status, one's Ph.D. institution and distance to the field also affect who is able to do what type of field research, where, and for how long. Future research should explore these and related questions in greater detail because limits on who is able to conduct field research constrict the sociological imagination of our field.
While Emmons and Moravcsik (2020) focus on leading Political Science Ph.D. programs in the United States, these trends likely obtain, both in lower ranked institutions in the broader United States as well as in graduate education throughout North America and Europe.
As all the authors have carried out qualitative fieldwork, this is the primary focus of this guide. This does not, however, mean that we exclude quantitative or experimental data collection from our definition of fieldwork.
There is great variation in graduate students’ financial situations, even in the Global North. For example, while higher education is tax-funded in most countries in Europe and Ph.D. students in countries such as Sweden, Norway, Denmark, the Netherlands, and Switzerland receive a comparatively generous full-time salary, healthcare and contributions to pension schemes, Ph.D. programs in other contexts like the United States and the United Kingdom have (high) enrollment fees and rely on scholarships, stipends, or departmental duties like teaching to (partially) offset these, while again others, such as Germany, are commonly financed by part-time (50 percent) employment at the university with tasks substantively unrelated to the dissertation. These different preconditions leave many Ph.D. students struggling financially and even incurring debt, while others are in a more comfortable financial position. Likewise, Ph.D. programs around the globe differ in structure, such as required coursework, duration and supervision relationships. Naturally, all of these factors have a bearing on the extent to which fieldwork is feasible. We acknowledge unequal preconditions across institutions and contexts, and trust that those Ph.D. students interested in pursuing fieldwork are best able to assess the structural and institutional context in which they operate and what this implies for how, when, and how long to carry out fieldwork.
In our experience, this is not only the general cycle for graduate students in North America, but also in Europe and likely elsewhere.
For helpful advice and feedback on earlier drafts, we wish to thank the editors and reviewers at International Studies Review , and Cassandra Emmons. We are also grateful to our interlocuters in Argentina, Canada, Colombia, Germany, Guatemala, Japan, Kenya, Norway, the Philippines, Sierra Leone, South Korea, Spain, Sweden, Turkey, the United Kingdom, and the United States, without whom this reflection on fieldwork would not have been possible. All authors contributed equally to this manuscript.
This material is based upon work supported by the Forskraftstiftelsen Theodor Adelswärds Minne, Knut and Alice Wallenberg Foundation(KAW 2013.0178), National Science Foundation Graduate Research Fellowship Program(DGE-1418060), Southeast Asia Research Group (Pre-Dissertation Fellowship), University at Albany (Initiatives for Women and the Benevolent Association), University of Missouri (John D. Bies International Travel Award Program and Kinder Institute on Constitutional Democracy), University of Southern California (Provost Fellowship in the Social Sciences), Vetenskapsrådet(Diarienummer 2019-06298), Wilhelm och Martina Lundgrens Vetenskapsfond(2016-1102; 2018-2272), and William & Mary (Global Research Institute Pre-doctoral Fellowship).
Advancing Conflict Research . 2020 . The ARC Bibliography . Accessed September 6, 2020, https://advancingconflictresearch.com/resources-1 .
Google Scholar
Google Preview
Archibald Mandy M. , Ambagtsheer Rachel C. , Casey Mavourneen G. , Lawless Michael . 2019 . “ Using Zoom Videoconferencing for Qualitative Data Collection: Perceptions and Experiences of Researchers and Participants .” International Journal of Qualitative Methods 18 : 1 – 18 .
Beath Andrew , Christia Fotini , Enikolopov Ruben . 2013 . “ Empowering Women Through Development Aid: Evidence from a Field Experiment in Afghanistan .” American Political Science Review 107 ( 3 ): 540 – 57 .
Carling Jorgen , Erdal Marta Bivand , Ezzati Rojan . 2014 . “ Beyond the Insider–Outsider Divide in Migration Research .” Migration Studies 2 ( 1 ): 36 – 54 .
Chambers-Ju Christopher . 2014 . “ Data Collection, Opportunity Costs, and Problem Solving: Lessons from Field Research on Teachers’ Unions in Latin America .” P.S.: Political Science & Politics 47 ( 2 ): 405 – 9 .
Collier David . 2011 . “ Understanding Process Tracing .” P.S.: Political Science and Politics 44 ( 4 ): 823 – 30 .
Druckman James N. , Green Donald P. , Kuklinski James H. , Lupia Arthur . 2006 . “ The Growth and Development of Experimental Research in Political Science .” American Political Science Review 100 ( 4 ): 627 – 35 .
Elman Colin , Kapiszewski Diana , Kirilova Dessislava . 2015 . “ Learning Through Research: Using Data to Train Undergraduates in Qualitative Methods .” P.S.: Political Science & Politics 48 ( 1 ): 39 – 43 .
Emmons Cassandra V. , Moravcsik Andrew M. . 2020 . “ Graduate Qualitative Methods Training in Political Science: A Disciplinary Crisis .” P.S.: Political Science & Politics 53 ( 2 ): 258 – 64 .
Esarey Justin. 2017 . “ Causal Inference with Observational Data .” In Analytics, Policy, and Governance , edited by Bachner Jennifer , Hill Kathryn Wagner , Ginsberg Benjamin , 40 – 66 . New Haven : Yale University Press .
Finseraas Henning , Kotsadam Andreas . 2017 . “ Does Personal Contact with Ethnic Minorities Affect anti-immigrant Sentiments? Evidence from a Field Experiment .” European Journal of Political Research 56 : 703 – 22 .
Fujii Lee Ann . 2012 . “ Research Ethics 101: Dilemmas and Responsibilities .” P.S.: Political Science & Politics 45 ( 4 ): 717 – 23 .
Gallien Max . 2021 . “ Solitary Decision-Making and Fieldwork Safety .” In The Companion to Peace and Conflict Fieldwork , edited by Ginty Roger Mac , Brett Roddy , Vogel Birte , 163 – 74 . Cham, Switzerland : Palgrave Macmillan .
Geddes Barbara . 2003 . Paradigms and Sand Castles: Theory Building and Research Design in Comparative Politics . Ann Arbor : University of Michigan Press .
Gifford Lindsay , Hall-Clifford Rachel . 2008 . “ From Catcalls to Kidnapping: Towards an Open Dialogue on the Fieldwork Experiences of Graduate Women .” Anthropology News 49 ( 6 ): 26 – 7 .
Greitens Sheena C. 2016 . Dictators and Their Secret Police: Coercive Institutions and State Violence . Cambridge : Cambridge University Press .
Harbin Brielle M. 2021 . “ Who's Able to Do Political Science Work? My Experience with Exit Polling and What It Reveals about Issues of Race and Equity .” PS: Political Science & Politics 54 ( 1 ): 144 – 6 .
Hilhorst Dorothea , Hogson Lucy , Jansen Bram , Mena Rodrigo Fluhmann . 2016 . Security Guidelines for Field Research in Complex, Remote and Hazardous Places . Accessed August 25, 2020, http://hdl.handle.net/1765/93256 .
Howlett Marnie. 2021 . “ Looking At the ‘Field’ Through a Zoom Lens: Methodological Reflections on Conducting Online Research During a Global Pandemic .” Qualitative Research . Online first .
Hsueh Roselyn , Jensenius Francesca Refsum , Newsome Akasemi . 2014 . “ Fieldwork in Political Science: Encountering Challenges and Crafting Solutions: Introduction .” PS: Political Science & Politics 47 ( 2 ): 391 – 3 .
Hummel Calla , El Kurd Dana . 2020 . “ Mental Health and Fieldwork .” P.S.: Political Science & Politics 54 ( 1 ): 121 – 5 .
Irgil Ezgi. 2020 . “ Broadening the Positionality in Migration Studies: Assigned Insider Category .” Migration Studies . Online first .
Jacobsen Karen , Landau Lauren B. . 2003 . “ The Dual Imperative in Refugee Research: Some Methodological and Ethical Considerations in Social Science Research on Forced Migration .” Disasters 27 ( 3 ): 185 – 206 .
Jamar Astrid , Chappuis Fairlie . 2016 . “ Conventions of Silence: Emotions and Knowledge Production in War-Affected Research Environments .” Parcours Anthropologiques 11 : 95 – 117 .
Jensenius Francesca R. 2014 . “ The Fieldwork of Quantitative Data Collection .” P.S.: Political Science & Politics 47 ( 2 ): 402 – 4 .
Kapiszewski Diana , MacLean Lauren M. , Read Benjamin L. . 2015 . Field Research in Political Science: Practices and Principles . Cambridge : Cambridge University Press .
Kelsky Karen . 2015 . The Professor Is In: The Essential Guide to Turning Your Ph.D. Into a Job . New York : Three Rivers Press .
Knott Eleanor . 2019 . “ Beyond the Field: Ethics After Fieldwork in Politically Dynamic Contexts .” Perspectives on Politics 17 ( 1 ): 140 – 53 .
Kreft Anne-Kathrin . 2019 . “ Responding to Sexual Violence: Women's Mobilization in War .” Journal of Peace Research 56 ( 2 ): 220 – 33 .
Kreft Anne-Kathrin . 2020 . “ Civil Society Perspectives on Sexual Violence in Conflict: Patriarchy and War Strategy in Colombia .” International Affairs 96 ( 2 ): 457 – 78 .
Loyle Cyanne E. , Simoni Alicia . 2017 . “ Researching Under Fire: Political Science and Researcher Trauma .” P.S.: Political Science & Politics 50 ( 1 ): 141 – 5 .
Mackenzie Catriona , McDowell Christopher , Pittaway Eileen . 2007 . “ Beyond ‘do No Harm’: The Challenge of Constructing Ethical Relationships in Refugee Research .” Journal of Refugee Studies 20 ( 2 ): 299 – 319 .
Marston Jerome F. 2020 . “ Resisting Displacement: Leveraging Interpersonal Ties to Remain Despite Criminal Violence in Medellín, Colombia .” Comparative Political Studies 53 ( 13 ): 1995 – 2028 .
Mosley Layna , ed. 2013 . Interview Research in Political Science . Ithaca : Cornell University Press .
Mügge Liza M. 2013 . “ Sexually Harassed by Gatekeepers: Reflections on Fieldwork in Surinam and Turkey .” International Journal of Social Research Methodology 16 ( 6 ): 541 – 6 .
Nexon Daniel. 2019 . International Studies Quarterly (ISQ) 2019 Annual Editorial Report . Accessed August 25, 2020, https://www.isanet.org/Portals/0/Documents/ISQ/2019_ISQ%20Report.pdf?ver = 2019-11-06-103524-300 .
Nowicka Magdalena , Cieslik Anna . 2014 . “ Beyond Methodological Nationalism in Insider Research with Migrants .” Migration Studies 2 ( 1 ): 1 – 15 .
O'Brien Kevin J. , Li Lianjiang . 2005 . “ Popular Contention and Its Impact in Rural China .” Comparative Political Studies 38 ( 3 ): 235 – 59 .
Ortbals Candice D. , Rincker Meg E. . 2009 . “ Fieldwork, Identities, and Intersectionality: Negotiating Gender, Race, Class, Religion, Nationality, and Age in the Research Field Abroad: Editors’ Introduction .” P.S.: Political Science & Politics 42 ( 2 ): 287 – 90 .
Read Benjamin. 2006 . “ Site-intensive Methods: Fenno and Scott in Search of Coalition .” Qualitative & Multi-method Research 4 ( 2 ): 10 – 3 .
Ricks Jacob I. , Liu Amy H. . 2018 . “ Process-Tracing Research Designs: A Practical Guide .” P.S.: Political Science & Politics 51 ( 4 ): 842 – 6 .
Sarotte Mary E. 2012 . “ China's Fear of Contagion: Tiananmen Square and the Power of the European Example .” International Security 37 ( 2 ): 156 – 82 .
Saunders Benjamin , Kitzinger Jenny , Kitzinger Celia . 2015 . “ Anonymizing Interview Data: Challenges and Compromise in Practice .” Qualitative Research 15 ( 5 ): 616 – 32 .
Schulz Philipp , Kreft Anne-Kathrin . 2021 . “ Researching Conflict-Related Sexual Violence: A Conversation Between Early Career Researchers .” International Feminist Journal of Politics . Advance online access .
Schwartz Stephanie , Cronin-Furman Kate . 2020 . “ Ill-Prepared: International Fieldwork Methods Training in Political Science .” Working Paper .
Seawright Jason . 2016 . “ Better Multimethod Design: The Promise of Integrative Multimethod Research .” Security Studies 25 ( 1 ): 42 – 9 .
Skjelsbæk Inger . 2018 . “ Silence Breakers in War and Peace: Research on Gender and Violence with an Ethics of Engagement .” Social Politics: International Studies in Gender , State & Society 25 ( 4 ): 496 – 520 .
Van Baalen Sebastian . 2018 . “ ‘Google Wants to Know Your Location’: The Ethical Challenges of Fieldwork in the Digital Age .” Research Ethics 14 ( 4 ): 1 – 17 .
Weiss Meredith L. , Hicken Allen , Kuhonta Eric Martinez . 2017 . “ Political Science Field Research & Ethics: Introduction .” The American Political Science Association—Comparative Democratization Newsletter 15 ( 3 ): 3 – 5 .
Weller Nicholas , Barnes Jeb . 2016 . “ Pathway Analysis and the Search for Causal Mechanisms .” Sociological Methods & Research 45 ( 3 ): 424 – 57 .
Williamson Emma , Gregory Alison , Abrahams Hilary , Aghtaie Nadia , Walker Sarah-Jane , Hester Marianne . 2020 . “ Secondary Trauma: Emotional Safety in Sensitive Research .” Journal of Academic Ethics 18 ( 1 ): 55 – 70 .
Willis Charmaine . 2020 . “ Revealing Hidden Injustices: The Filipino Struggle Against U.S. Military Presence .” Minds of the Movement (blog). October 27, 2020, https://www.nonviolent-conflict.org/blog_post/revealing-hidden-injustices-the-filipino-struggle-against-u-s-military-presence/ .
Wood Elizabeth Jean . 2006 . “ The Ethical Challenges of Field Research in Conflict Zones .” Qualitative Sociology 29 ( 3 ): 373 – 86 .
Zapata-Barrero Ricard , Yalaz Evren . 2019 . “ Qualitative Migration Research Ethics: Mapping the Core Challenges .” GRITIM-UPF Working Paper Series No. 42 .
Zvobgo Kelebogile . 2020 . “ Demanding Truth: The Global Transitional Justice Network and the Creation of Truth Commissions .” International Studies Quarterly 64 ( 3 ): 609 – 25 .
Email alerts
Citing articles via.
- Recommend to your Library
Affiliations
- Online ISSN 1468-2486
- Print ISSN 1521-9488
- Copyright © 2024 International Studies Association
- About Oxford Academic
- Publish journals with us
- University press partners
- What we publish
- New features
- Open access
- Institutional account management
- Rights and permissions
- Get help with access
- Accessibility
- Advertising
- Media enquiries
- Oxford University Press
- Oxford Languages
- University of Oxford
Oxford University Press is a department of the University of Oxford. It furthers the University's objective of excellence in research, scholarship, and education by publishing worldwide
- Copyright © 2024 Oxford University Press
- Cookie settings
- Cookie policy
- Privacy policy
- Legal notice
This Feature Is Available To Subscribers Only
Sign In or Create an Account
This PDF is available to Subscribers Only
For full access to this pdf, sign in to an existing account, or purchase an annual subscription.

Want to create or adapt books like this? Learn more about how Pressbooks supports open publishing practices.
6.3 Conducting Experiments
Learning objectives.
- Describe several strategies for recruiting participants for an experiment.
- Explain why it is important to standardize the procedure of an experiment and several ways to do this.
- Explain what pilot testing is and why it is important.
The information presented so far in this chapter is enough to design a basic experiment. When it comes time to conduct that experiment, however, several additional practical issues arise. In this section, we consider some of these issues and how to deal with them. Much of this information applies to nonexperimental studies as well as experimental ones.
Recruiting Participants
Of course, you should be thinking about how you will obtain your participants from the beginning of any research project. Unless you have access to people with schizophrenia or incarcerated juvenile offenders, for example, then there is no point designing a study that focuses on these populations. But even if you plan to use a convenience sample, you will have to recruit participants for your study.
There are several approaches to recruiting participants. One is to use participants from a formal subject pool —an established group of people who have agreed to be contacted about participating in research studies. For example, at many colleges and universities, there is a subject pool consisting of students enrolled in introductory psychology courses who must participate in a certain number of studies to meet a course requirement. Researchers post descriptions of their studies and students sign up to participate, usually via an online system. Participants who are not in subject pools can also be recruited by posting or publishing advertisements or making personal appeals to groups that represent the population of interest. For example, a researcher interested in studying older adults could arrange to speak at a meeting of the residents at a retirement community to explain the study and ask for volunteers.
The Volunteer Subject
Even if the participants in a study receive compensation in the form of course credit, a small amount of money, or a chance at being treated for a psychological problem, they are still essentially volunteers. This is worth considering because people who volunteer to participate in psychological research have been shown to differ in predictable ways from those who do not volunteer. Specifically, there is good evidence that on average, volunteers have the following characteristics compared with nonvolunteers (Rosenthal & Rosnow, 1976):
- They are more interested in the topic of the research.
- They are more educated.
- They have a greater need for approval.
- They have higher intelligence quotients (IQs).
- They are more sociable.
- They are higher in social class.
This can be an issue of external validity if there is reason to believe that participants with these characteristics are likely to behave differently than the general population. For example, in testing different methods of persuading people, a rational argument might work better on volunteers than it does on the general population because of their generally higher educational level and IQ.
In many field experiments, the task is not recruiting participants but selecting them. For example, researchers Nicolas Guéguen and Marie-Agnès de Gail conducted a field experiment on the effect of being smiled at on helping, in which the participants were shoppers at a supermarket. A confederate walking down a stairway gazed directly at a shopper walking up the stairway and either smiled or did not smile. Shortly afterward, the shopper encountered another confederate, who dropped some computer diskettes on the ground. The dependent variable was whether or not the shopper stopped to help pick up the diskettes (Guéguen & de Gail, 2003). Notice that these participants were not “recruited,” but the researchers still had to select them from among all the shoppers taking the stairs that day. It is extremely important that this kind of selection be done according to a well-defined set of rules that is established before the data collection begins and can be explained clearly afterward. In this case, with each trip down the stairs, the confederate was instructed to gaze at the first person he encountered who appeared to be between the ages of 20 and 50. Only if the person gazed back did he or she become a participant in the study. The point of having a well-defined selection rule is to avoid bias in the selection of participants. For example, if the confederate was free to choose which shoppers he would gaze at, he might choose friendly-looking shoppers when he was set to smile and unfriendly-looking ones when he was not set to smile. As we will see shortly, such biases can be entirely unintentional.
Standardizing the Procedure
It is surprisingly easy to introduce extraneous variables during the procedure. For example, the same experimenter might give clear instructions to one participant but vague instructions to another. Or one experimenter might greet participants warmly while another barely makes eye contact with them. To the extent that such variables affect participants’ behavior, they add noise to the data and make the effect of the independent variable more difficult to detect. If they vary across conditions, they become confounding variables and provide alternative explanations for the results. For example, if participants in a treatment group are tested by a warm and friendly experimenter and participants in a control group are tested by a cold and unfriendly one, then what appears to be an effect of the treatment might actually be an effect of experimenter demeanor.
Experimenter’s Sex as an Extraneous Variable
It is well known that whether research participants are male or female can affect the results of a study. But what about whether the experimenter is male or female? There is plenty of evidence that this matters too. Male and female experimenters have slightly different ways of interacting with their participants, and of course participants also respond differently to male and female experimenters (Rosenthal, 1976). For example, in a recent study on pain perception, participants immersed their hands in icy water for as long as they could (Ibolya, Brake, & Voss, 2004). Male participants tolerated the pain longer when the experimenter was a woman, and female participants tolerated it longer when the experimenter was a man.
Researcher Robert Rosenthal has spent much of his career showing that this kind of unintended variation in the procedure does, in fact, affect participants’ behavior. Furthermore, one important source of such variation is the experimenter’s expectations about how participants “should” behave in the experiment. This is referred to as an experimenter expectancy effect (Rosenthal, 1976). For example, if an experimenter expects participants in a treatment group to perform better on a task than participants in a control group, then he or she might unintentionally give the treatment group participants clearer instructions or more encouragement or allow them more time to complete the task. In a striking example, Rosenthal and Kermit Fode had several students in a laboratory course in psychology train rats to run through a maze. Although the rats were genetically similar, some of the students were told that they were working with “maze-bright” rats that had been bred to be good learners, and other students were told that they were working with “maze-dull” rats that had been bred to be poor learners. Sure enough, over five days of training, the “maze-bright” rats made more correct responses, made the correct response more quickly, and improved more steadily than the “maze-dull” rats (Rosenthal & Fode, 1963). Clearly it had to have been the students’ expectations about how the rats would perform that made the difference. But how? Some clues come from data gathered at the end of the study, which showed that students who expected their rats to learn quickly felt more positively about their animals and reported behaving toward them in a more friendly manner (e.g., handling them more).
The way to minimize unintended variation in the procedure is to standardize it as much as possible so that it is carried out in the same way for all participants regardless of the condition they are in. Here are several ways to do this:
- Create a written protocol that specifies everything that the experimenters are to do and say from the time they greet participants to the time they dismiss them.
- Create standard instructions that participants read themselves or that are read to them word for word by the experimenter.
- Automate the rest of the procedure as much as possible by using software packages for this purpose or even simple computer slide shows.
- Anticipate participants’ questions and either raise and answer them in the instructions or develop standard answers for them.
- Train multiple experimenters on the protocol together and have them practice on each other.
- Be sure that each experimenter tests participants in all conditions.
Another good practice is to arrange for the experimenters to be “blind” to the research question or to the condition that each participant is tested in. The idea is to minimize experimenter expectancy effects by minimizing the experimenters’ expectations. For example, in a drug study in which each participant receives the drug or a placebo, it is often the case that neither the participants nor the experimenter who interacts with the participants know which condition he or she has been assigned to. Because both the participants and the experimenters are blind to the condition, this is referred to as a double-blind study. (A single-blind study is one in which the participant, but not the experimenter, is blind to the condition.) Of course, there are many times this is not possible. For example, if you are both the investigator and the only experimenter, it is not possible for you to remain blind to the research question. Also, in many studies the experimenter must know the condition because he or she must carry out the procedure in a different way in the different conditions.
Record Keeping
It is essential to keep good records when you conduct an experiment. As discussed earlier, it is typical for experimenters to generate a written sequence of conditions before the study begins and then to test each new participant in the next condition in the sequence. As you test them, it is a good idea to add to this list basic demographic information; the date, time, and place of testing; and the name of the experimenter who did the testing. It is also a good idea to have a place for the experimenter to write down comments about unusual occurrences (e.g., a confused or uncooperative participant) or questions that come up. This kind of information can be useful later if you decide to analyze sex differences or effects of different experimenters, or if a question arises about a particular participant or testing session.
It can also be useful to assign an identification number to each participant as you test them. Simply numbering them consecutively beginning with 1 is usually sufficient. This number can then also be written on any response sheets or questionnaires that participants generate, making it easier to keep them together.
Pilot Testing
It is always a good idea to conduct a pilot test of your experiment. A pilot test is a small-scale study conducted to make sure that a new procedure works as planned. In a pilot test, you can recruit participants formally (e.g., from an established participant pool) or you can recruit them informally from among family, friends, classmates, and so on. The number of participants can be small, but it should be enough to give you confidence that your procedure works as planned. There are several important questions that you can answer by conducting a pilot test:
- Do participants understand the instructions?
- What kind of misunderstandings do participants have, what kind of mistakes do they make, and what kind of questions do they ask?
- Do participants become bored or frustrated?
- Is an indirect manipulation effective? (You will need to include a manipulation check.)
- Can participants guess the research question or hypothesis?
- How long does the procedure take?
- Are computer programs or other automated procedures working properly?
- Are data being recorded correctly?
Of course, to answer some of these questions you will need to observe participants carefully during the procedure and talk with them about it afterward. Participants are often hesitant to criticize a study in front of the researcher, so be sure they understand that this is a pilot test and you are genuinely interested in feedback that will help you improve the procedure. If the procedure works as planned, then you can proceed with the actual study. If there are problems to be solved, you can solve them, pilot test the new procedure, and continue with this process until you are ready to proceed.
Key Takeaways
- There are several effective methods you can use to recruit research participants for your experiment, including through formal subject pools, advertisements, and personal appeals. Field experiments require well-defined participant selection procedures.
- It is important to standardize experimental procedures to minimize extraneous variables, including experimenter expectancy effects.
- It is important to conduct one or more small-scale pilot tests of an experiment to be sure that the procedure works as planned.
- Practice: List two ways that you might recruit participants from each of the following populations: (a) elderly adults, (b) unemployed people, (c) regular exercisers, and (d) math majors.
- Discussion: Imagine a study in which you will visually present participants with a list of 20 words, one at a time, wait for a short time, and then ask them to recall as many of the words as they can. In the stressed condition, they are told that they might also be chosen to give a short speech in front of a small audience. In the unstressed condition, they are not told that they might have to give a speech. What are several specific things that you could do to standardize the procedure?
Guéguen, N., & de Gail, Marie-Agnès. (2003). The effect of smiling on helping behavior: Smiling and good Samaritan behavior. Communication Reports, 16 , 133–140.
Ibolya, K., Brake, A., & Voss, U. (2004). The effect of experimenter characteristics on pain reports in women and men. Pain, 112 , 142–147.
Rosenthal, R. (1976). Experimenter effects in behavioral research (enlarged ed.). New York, NY: Wiley.
Rosenthal, R., & Fode, K. (1963). The effect of experimenter bias on performance of the albino rat. Behavioral Science, 8 , 183-189.
Rosenthal, R., & Rosnow, R. L. (1976). The volunteer subject . New York, NY: Wiley.
Research Methods in Psychology Copyright © 2016 by University of Minnesota is licensed under a Creative Commons Attribution-NonCommercial-ShareAlike 4.0 International License , except where otherwise noted.
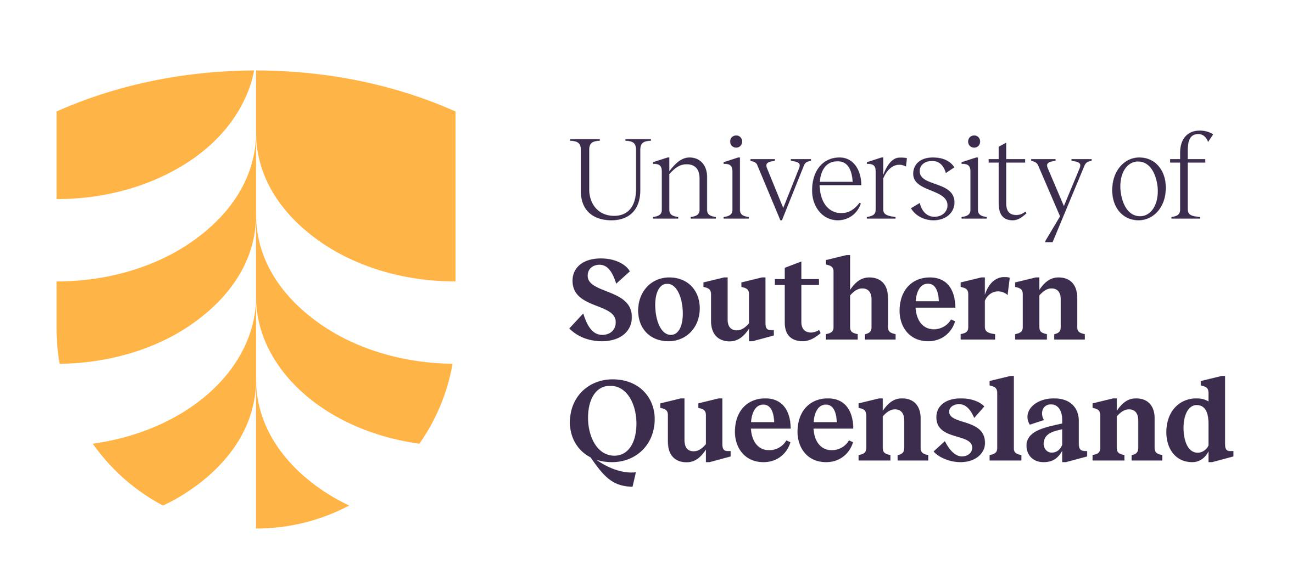
Want to create or adapt books like this? Learn more about how Pressbooks supports open publishing practices.
10 Experimental research
Experimental research—often considered to be the ‘gold standard’ in research designs—is one of the most rigorous of all research designs. In this design, one or more independent variables are manipulated by the researcher (as treatments), subjects are randomly assigned to different treatment levels (random assignment), and the results of the treatments on outcomes (dependent variables) are observed. The unique strength of experimental research is its internal validity (causality) due to its ability to link cause and effect through treatment manipulation, while controlling for the spurious effect of extraneous variable.
Experimental research is best suited for explanatory research—rather than for descriptive or exploratory research—where the goal of the study is to examine cause-effect relationships. It also works well for research that involves a relatively limited and well-defined set of independent variables that can either be manipulated or controlled. Experimental research can be conducted in laboratory or field settings. Laboratory experiments , conducted in laboratory (artificial) settings, tend to be high in internal validity, but this comes at the cost of low external validity (generalisability), because the artificial (laboratory) setting in which the study is conducted may not reflect the real world. Field experiments are conducted in field settings such as in a real organisation, and are high in both internal and external validity. But such experiments are relatively rare, because of the difficulties associated with manipulating treatments and controlling for extraneous effects in a field setting.
Experimental research can be grouped into two broad categories: true experimental designs and quasi-experimental designs. Both designs require treatment manipulation, but while true experiments also require random assignment, quasi-experiments do not. Sometimes, we also refer to non-experimental research, which is not really a research design, but an all-inclusive term that includes all types of research that do not employ treatment manipulation or random assignment, such as survey research, observational research, and correlational studies.
Basic concepts
Treatment and control groups. In experimental research, some subjects are administered one or more experimental stimulus called a treatment (the treatment group ) while other subjects are not given such a stimulus (the control group ). The treatment may be considered successful if subjects in the treatment group rate more favourably on outcome variables than control group subjects. Multiple levels of experimental stimulus may be administered, in which case, there may be more than one treatment group. For example, in order to test the effects of a new drug intended to treat a certain medical condition like dementia, if a sample of dementia patients is randomly divided into three groups, with the first group receiving a high dosage of the drug, the second group receiving a low dosage, and the third group receiving a placebo such as a sugar pill (control group), then the first two groups are experimental groups and the third group is a control group. After administering the drug for a period of time, if the condition of the experimental group subjects improved significantly more than the control group subjects, we can say that the drug is effective. We can also compare the conditions of the high and low dosage experimental groups to determine if the high dose is more effective than the low dose.
Treatment manipulation. Treatments are the unique feature of experimental research that sets this design apart from all other research methods. Treatment manipulation helps control for the ‘cause’ in cause-effect relationships. Naturally, the validity of experimental research depends on how well the treatment was manipulated. Treatment manipulation must be checked using pretests and pilot tests prior to the experimental study. Any measurements conducted before the treatment is administered are called pretest measures , while those conducted after the treatment are posttest measures .
Random selection and assignment. Random selection is the process of randomly drawing a sample from a population or a sampling frame. This approach is typically employed in survey research, and ensures that each unit in the population has a positive chance of being selected into the sample. Random assignment, however, is a process of randomly assigning subjects to experimental or control groups. This is a standard practice in true experimental research to ensure that treatment groups are similar (equivalent) to each other and to the control group prior to treatment administration. Random selection is related to sampling, and is therefore more closely related to the external validity (generalisability) of findings. However, random assignment is related to design, and is therefore most related to internal validity. It is possible to have both random selection and random assignment in well-designed experimental research, but quasi-experimental research involves neither random selection nor random assignment.
Threats to internal validity. Although experimental designs are considered more rigorous than other research methods in terms of the internal validity of their inferences (by virtue of their ability to control causes through treatment manipulation), they are not immune to internal validity threats. Some of these threats to internal validity are described below, within the context of a study of the impact of a special remedial math tutoring program for improving the math abilities of high school students.
History threat is the possibility that the observed effects (dependent variables) are caused by extraneous or historical events rather than by the experimental treatment. For instance, students’ post-remedial math score improvement may have been caused by their preparation for a math exam at their school, rather than the remedial math program.
Maturation threat refers to the possibility that observed effects are caused by natural maturation of subjects (e.g., a general improvement in their intellectual ability to understand complex concepts) rather than the experimental treatment.
Testing threat is a threat in pre-post designs where subjects’ posttest responses are conditioned by their pretest responses. For instance, if students remember their answers from the pretest evaluation, they may tend to repeat them in the posttest exam.
Not conducting a pretest can help avoid this threat.
Instrumentation threat , which also occurs in pre-post designs, refers to the possibility that the difference between pretest and posttest scores is not due to the remedial math program, but due to changes in the administered test, such as the posttest having a higher or lower degree of difficulty than the pretest.
Mortality threat refers to the possibility that subjects may be dropping out of the study at differential rates between the treatment and control groups due to a systematic reason, such that the dropouts were mostly students who scored low on the pretest. If the low-performing students drop out, the results of the posttest will be artificially inflated by the preponderance of high-performing students.
Regression threat —also called a regression to the mean—refers to the statistical tendency of a group’s overall performance to regress toward the mean during a posttest rather than in the anticipated direction. For instance, if subjects scored high on a pretest, they will have a tendency to score lower on the posttest (closer to the mean) because their high scores (away from the mean) during the pretest were possibly a statistical aberration. This problem tends to be more prevalent in non-random samples and when the two measures are imperfectly correlated.
Two-group experimental designs

Pretest-posttest control group design . In this design, subjects are randomly assigned to treatment and control groups, subjected to an initial (pretest) measurement of the dependent variables of interest, the treatment group is administered a treatment (representing the independent variable of interest), and the dependent variables measured again (posttest). The notation of this design is shown in Figure 10.1.

Statistical analysis of this design involves a simple analysis of variance (ANOVA) between the treatment and control groups. The pretest-posttest design handles several threats to internal validity, such as maturation, testing, and regression, since these threats can be expected to influence both treatment and control groups in a similar (random) manner. The selection threat is controlled via random assignment. However, additional threats to internal validity may exist. For instance, mortality can be a problem if there are differential dropout rates between the two groups, and the pretest measurement may bias the posttest measurement—especially if the pretest introduces unusual topics or content.
Posttest -only control group design . This design is a simpler version of the pretest-posttest design where pretest measurements are omitted. The design notation is shown in Figure 10.2.

The treatment effect is measured simply as the difference in the posttest scores between the two groups:
![Rendered by QuickLaTeX.com \[E = (O_{1} - O_{2})\,.\]](https://usq.pressbooks.pub/app/uploads/quicklatex/quicklatex.com-90f2ce47275e7b35def5c5b52b7d7d45_l3.png)
The appropriate statistical analysis of this design is also a two-group analysis of variance (ANOVA). The simplicity of this design makes it more attractive than the pretest-posttest design in terms of internal validity. This design controls for maturation, testing, regression, selection, and pretest-posttest interaction, though the mortality threat may continue to exist.

Because the pretest measure is not a measurement of the dependent variable, but rather a covariate, the treatment effect is measured as the difference in the posttest scores between the treatment and control groups as:
Due to the presence of covariates, the right statistical analysis of this design is a two-group analysis of covariance (ANCOVA). This design has all the advantages of posttest-only design, but with internal validity due to the controlling of covariates. Covariance designs can also be extended to pretest-posttest control group design.
Factorial designs
Two-group designs are inadequate if your research requires manipulation of two or more independent variables (treatments). In such cases, you would need four or higher-group designs. Such designs, quite popular in experimental research, are commonly called factorial designs. Each independent variable in this design is called a factor , and each subdivision of a factor is called a level . Factorial designs enable the researcher to examine not only the individual effect of each treatment on the dependent variables (called main effects), but also their joint effect (called interaction effects).

In a factorial design, a main effect is said to exist if the dependent variable shows a significant difference between multiple levels of one factor, at all levels of other factors. No change in the dependent variable across factor levels is the null case (baseline), from which main effects are evaluated. In the above example, you may see a main effect of instructional type, instructional time, or both on learning outcomes. An interaction effect exists when the effect of differences in one factor depends upon the level of a second factor. In our example, if the effect of instructional type on learning outcomes is greater for three hours/week of instructional time than for one and a half hours/week, then we can say that there is an interaction effect between instructional type and instructional time on learning outcomes. Note that the presence of interaction effects dominate and make main effects irrelevant, and it is not meaningful to interpret main effects if interaction effects are significant.
Hybrid experimental designs
Hybrid designs are those that are formed by combining features of more established designs. Three such hybrid designs are randomised bocks design, Solomon four-group design, and switched replications design.
Randomised block design. This is a variation of the posttest-only or pretest-posttest control group design where the subject population can be grouped into relatively homogeneous subgroups (called blocks ) within which the experiment is replicated. For instance, if you want to replicate the same posttest-only design among university students and full-time working professionals (two homogeneous blocks), subjects in both blocks are randomly split between the treatment group (receiving the same treatment) and the control group (see Figure 10.5). The purpose of this design is to reduce the ‘noise’ or variance in data that may be attributable to differences between the blocks so that the actual effect of interest can be detected more accurately.
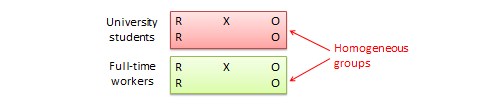
Solomon four-group design . In this design, the sample is divided into two treatment groups and two control groups. One treatment group and one control group receive the pretest, and the other two groups do not. This design represents a combination of posttest-only and pretest-posttest control group design, and is intended to test for the potential biasing effect of pretest measurement on posttest measures that tends to occur in pretest-posttest designs, but not in posttest-only designs. The design notation is shown in Figure 10.6.
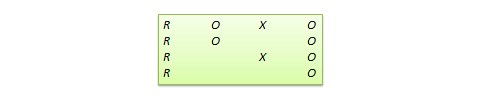
Switched replication design . This is a two-group design implemented in two phases with three waves of measurement. The treatment group in the first phase serves as the control group in the second phase, and the control group in the first phase becomes the treatment group in the second phase, as illustrated in Figure 10.7. In other words, the original design is repeated or replicated temporally with treatment/control roles switched between the two groups. By the end of the study, all participants will have received the treatment either during the first or the second phase. This design is most feasible in organisational contexts where organisational programs (e.g., employee training) are implemented in a phased manner or are repeated at regular intervals.

Quasi-experimental designs
Quasi-experimental designs are almost identical to true experimental designs, but lacking one key ingredient: random assignment. For instance, one entire class section or one organisation is used as the treatment group, while another section of the same class or a different organisation in the same industry is used as the control group. This lack of random assignment potentially results in groups that are non-equivalent, such as one group possessing greater mastery of certain content than the other group, say by virtue of having a better teacher in a previous semester, which introduces the possibility of selection bias . Quasi-experimental designs are therefore inferior to true experimental designs in interval validity due to the presence of a variety of selection related threats such as selection-maturation threat (the treatment and control groups maturing at different rates), selection-history threat (the treatment and control groups being differentially impacted by extraneous or historical events), selection-regression threat (the treatment and control groups regressing toward the mean between pretest and posttest at different rates), selection-instrumentation threat (the treatment and control groups responding differently to the measurement), selection-testing (the treatment and control groups responding differently to the pretest), and selection-mortality (the treatment and control groups demonstrating differential dropout rates). Given these selection threats, it is generally preferable to avoid quasi-experimental designs to the greatest extent possible.

In addition, there are quite a few unique non-equivalent designs without corresponding true experimental design cousins. Some of the more useful of these designs are discussed next.
Regression discontinuity (RD) design . This is a non-equivalent pretest-posttest design where subjects are assigned to the treatment or control group based on a cut-off score on a preprogram measure. For instance, patients who are severely ill may be assigned to a treatment group to test the efficacy of a new drug or treatment protocol and those who are mildly ill are assigned to the control group. In another example, students who are lagging behind on standardised test scores may be selected for a remedial curriculum program intended to improve their performance, while those who score high on such tests are not selected from the remedial program.

Because of the use of a cut-off score, it is possible that the observed results may be a function of the cut-off score rather than the treatment, which introduces a new threat to internal validity. However, using the cut-off score also ensures that limited or costly resources are distributed to people who need them the most, rather than randomly across a population, while simultaneously allowing a quasi-experimental treatment. The control group scores in the RD design do not serve as a benchmark for comparing treatment group scores, given the systematic non-equivalence between the two groups. Rather, if there is no discontinuity between pretest and posttest scores in the control group, but such a discontinuity persists in the treatment group, then this discontinuity is viewed as evidence of the treatment effect.
Proxy pretest design . This design, shown in Figure 10.11, looks very similar to the standard NEGD (pretest-posttest) design, with one critical difference: the pretest score is collected after the treatment is administered. A typical application of this design is when a researcher is brought in to test the efficacy of a program (e.g., an educational program) after the program has already started and pretest data is not available. Under such circumstances, the best option for the researcher is often to use a different prerecorded measure, such as students’ grade point average before the start of the program, as a proxy for pretest data. A variation of the proxy pretest design is to use subjects’ posttest recollection of pretest data, which may be subject to recall bias, but nevertheless may provide a measure of perceived gain or change in the dependent variable.

Separate pretest-posttest samples design . This design is useful if it is not possible to collect pretest and posttest data from the same subjects for some reason. As shown in Figure 10.12, there are four groups in this design, but two groups come from a single non-equivalent group, while the other two groups come from a different non-equivalent group. For instance, say you want to test customer satisfaction with a new online service that is implemented in one city but not in another. In this case, customers in the first city serve as the treatment group and those in the second city constitute the control group. If it is not possible to obtain pretest and posttest measures from the same customers, you can measure customer satisfaction at one point in time, implement the new service program, and measure customer satisfaction (with a different set of customers) after the program is implemented. Customer satisfaction is also measured in the control group at the same times as in the treatment group, but without the new program implementation. The design is not particularly strong, because you cannot examine the changes in any specific customer’s satisfaction score before and after the implementation, but you can only examine average customer satisfaction scores. Despite the lower internal validity, this design may still be a useful way of collecting quasi-experimental data when pretest and posttest data is not available from the same subjects.
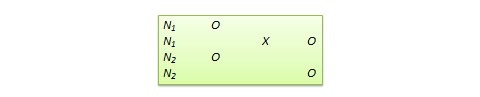
An interesting variation of the NEDV design is a pattern-matching NEDV design , which employs multiple outcome variables and a theory that explains how much each variable will be affected by the treatment. The researcher can then examine if the theoretical prediction is matched in actual observations. This pattern-matching technique—based on the degree of correspondence between theoretical and observed patterns—is a powerful way of alleviating internal validity concerns in the original NEDV design.

Perils of experimental research
Experimental research is one of the most difficult of research designs, and should not be taken lightly. This type of research is often best with a multitude of methodological problems. First, though experimental research requires theories for framing hypotheses for testing, much of current experimental research is atheoretical. Without theories, the hypotheses being tested tend to be ad hoc, possibly illogical, and meaningless. Second, many of the measurement instruments used in experimental research are not tested for reliability and validity, and are incomparable across studies. Consequently, results generated using such instruments are also incomparable. Third, often experimental research uses inappropriate research designs, such as irrelevant dependent variables, no interaction effects, no experimental controls, and non-equivalent stimulus across treatment groups. Findings from such studies tend to lack internal validity and are highly suspect. Fourth, the treatments (tasks) used in experimental research may be diverse, incomparable, and inconsistent across studies, and sometimes inappropriate for the subject population. For instance, undergraduate student subjects are often asked to pretend that they are marketing managers and asked to perform a complex budget allocation task in which they have no experience or expertise. The use of such inappropriate tasks, introduces new threats to internal validity (i.e., subject’s performance may be an artefact of the content or difficulty of the task setting), generates findings that are non-interpretable and meaningless, and makes integration of findings across studies impossible.
The design of proper experimental treatments is a very important task in experimental design, because the treatment is the raison d’etre of the experimental method, and must never be rushed or neglected. To design an adequate and appropriate task, researchers should use prevalidated tasks if available, conduct treatment manipulation checks to check for the adequacy of such tasks (by debriefing subjects after performing the assigned task), conduct pilot tests (repeatedly, if necessary), and if in doubt, use tasks that are simple and familiar for the respondent sample rather than tasks that are complex or unfamiliar.
In summary, this chapter introduced key concepts in the experimental design research method and introduced a variety of true experimental and quasi-experimental designs. Although these designs vary widely in internal validity, designs with less internal validity should not be overlooked and may sometimes be useful under specific circumstances and empirical contingencies.
Social Science Research: Principles, Methods and Practices (Revised edition) Copyright © 2019 by Anol Bhattacherjee is licensed under a Creative Commons Attribution-NonCommercial-ShareAlike 4.0 International License , except where otherwise noted.
Share This Book
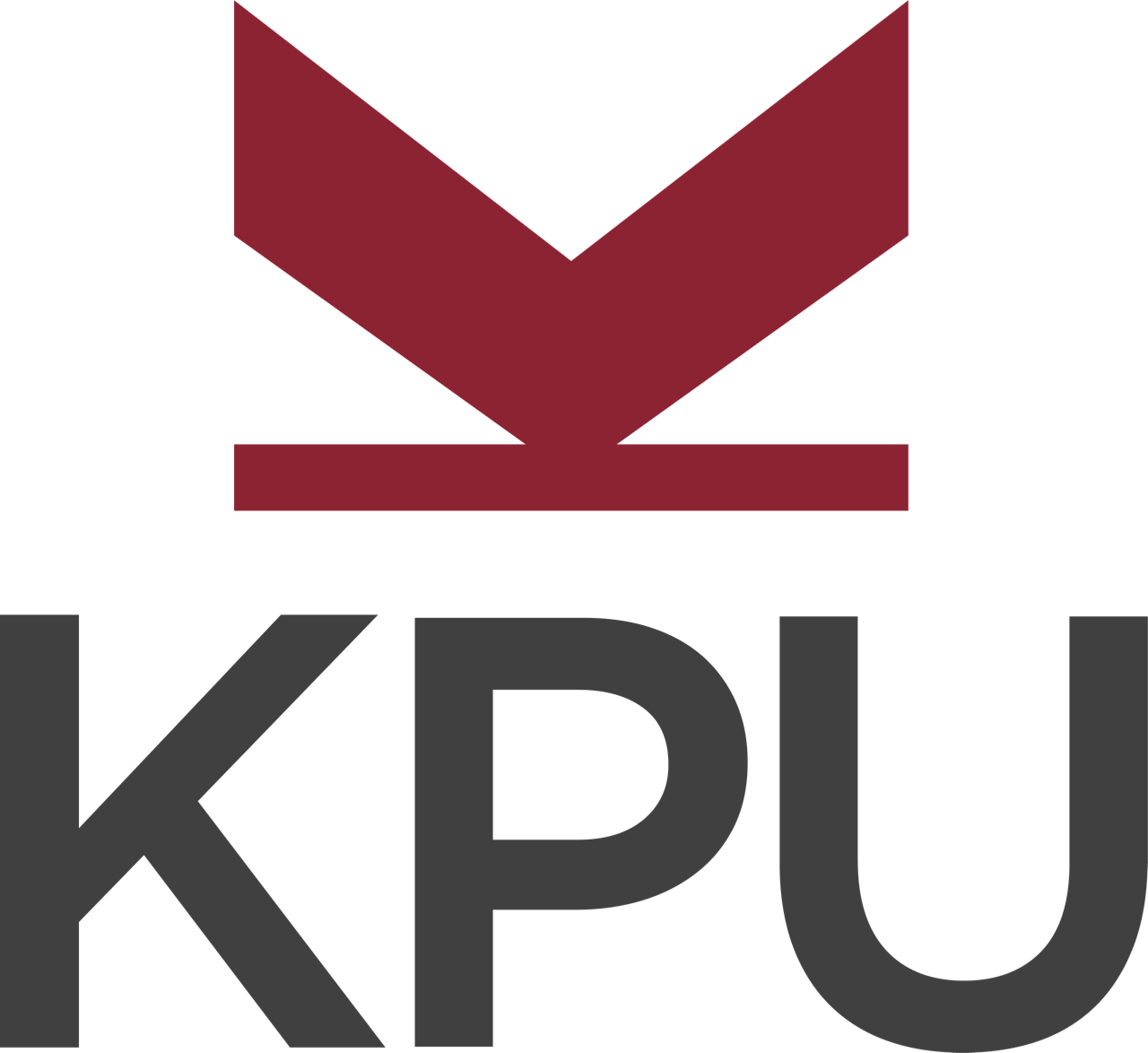
Want to create or adapt books like this? Learn more about how Pressbooks supports open publishing practices.
Overview of the Scientific Method
11 Designing a Research Study
Learning objectives.
- Define the concept of a variable, distinguish quantitative from categorical variables, and give examples of variables that might be of interest to psychologists.
- Explain the difference between a population and a sample.
- Distinguish between experimental and non-experimental research.
- Distinguish between lab studies, field studies, and field experiments.
Identifying and Defining the Variables and Population
Variables and operational definitions.
Part of generating a hypothesis involves identifying the variables that you want to study and operationally defining those variables so that they can be measured. Research questions in psychology are about variables. A variable is a quantity or quality that varies across people or situations. For example, the height of the students enrolled in a university course is a variable because it varies from student to student. The chosen major of the students is also a variable as long as not everyone in the class has declared the same major. Almost everything in our world varies and as such thinking of examples of constants (things that don’t vary) is far more difficult. A rare example of a constant is the speed of light. Variables can be either quantitative or categorical. A quantitative variable is a quantity, such as height, that is typically measured by assigning a number to each individual. Other examples of quantitative variables include people’s level of talkativeness, how depressed they are, and the number of siblings they have. A categorical variable is a quality, such as chosen major, and is typically measured by assigning a category label to each individual (e.g., Psychology, English, Nursing, etc.). Other examples include people’s nationality, their occupation, and whether they are receiving psychotherapy.
After the researcher generates their hypothesis and selects the variables they want to manipulate and measure, the researcher needs to find ways to actually measure the variables of interest. This requires an operational definition —a definition of the variable in terms of precisely how it is to be measured. Most variables that researchers are interested in studying cannot be directly observed or measured and this poses a problem because empiricism (observation) is at the heart of the scientific method. Operationally defining a variable involves taking an abstract construct like depression that cannot be directly observed and transforming it into something that can be directly observed and measured. Most variables can be operationally defined in many different ways. For example, depression can be operationally defined as people’s scores on a paper-and-pencil depression scale such as the Beck Depression Inventory, the number of depressive symptoms they are experiencing, or whether they have been diagnosed with major depressive disorder. Researchers are wise to choose an operational definition that has been used extensively in the research literature.
Sampling and Measurement
In addition to identifying which variables to manipulate and measure, and operationally defining those variables, researchers need to identify the population of interest. Researchers in psychology are usually interested in drawing conclusions about some very large group of people. This is called the population . It could be all American teenagers, children with autism, professional athletes, or even just human beings—depending on the interests and goals of the researcher. But they usually study only a small subset or sample of the population. For example, a researcher might measure the talkativeness of a few hundred university students with the intention of drawing conclusions about the talkativeness of men and women in general. It is important, therefore, for researchers to use a representative sample—one that is similar to the population in important respects.
One method of obtaining a sample is simple random sampling , in which every member of the population has an equal chance of being selected for the sample. For example, a pollster could start with a list of all the registered voters in a city (the population), randomly select 100 of them from the list (the sample), and ask those 100 whom they intend to vote for. Unfortunately, random sampling is difficult or impossible in most psychological research because the populations are less clearly defined than the registered voters in a city. How could a researcher give all American teenagers or all children with autism an equal chance of being selected for a sample? The most common alternative to random sampling is convenience sampling , in which the sample consists of individuals who happen to be nearby and willing to participate (such as introductory psychology students). Of course, the obvious problem with convenience sampling is that the sample might not be representative of the population and therefore it may be less appropriate to generalize the results from the sample to that population.
Experimental vs. Non-Experimental Research
The next step a researcher must take is to decide which type of approach they will use to collect the data. As you will learn in your research methods course there are many different approaches to research that can be divided in many different ways. One of the most fundamental distinctions is between experimental and non-experimental research.
Experimental Research
Researchers who want to test hypotheses about causal relationships between variables (i.e., their goal is to explain) need to use an experimental method. This is because the experimental method is the only method that allows us to determine causal relationships. Using the experimental approach, researchers first manipulate one or more variables while attempting to control extraneous variables, and then they measure how the manipulated variables affect participants’ responses.
The terms independent variable and dependent variable are used in the context of experimental research. The independent variable is the variable the experimenter manipulates (it is the presumed cause) and the dependent variable is the variable the experimenter measures (it is the presumed effect).
Extraneous variables are any variable other than the dependent variable. Confounds are a specific type of extraneous variable that systematically varies along with the variables under investigation and therefore provides an alternative explanation for the results. When researchers design an experiment they need to ensure that they control for confounds; they need to ensure that extraneous variables don’t become confounding variables because in order to make a causal conclusion they need to make sure alternative explanations for the results have been ruled out.
As an example, if we manipulate the lighting in the room and examine the effects of that manipulation on workers’ productivity, then the lighting conditions (bright lights vs. dim lights) would be considered the independent variable and the workers’ productivity would be considered the dependent variable. If the bright lights are noisy then that noise would be a confound since the noise would be present whenever the lights are bright and the noise would be absent when the lights are dim. If noise is varying systematically with light then we wouldn’t know if a difference in worker productivity across the two lighting conditions is due to noise or light. So confounds are bad, they disrupt our ability to make causal conclusions about the nature of the relationship between variables. However, if there is noise in the room both when the lights are on and when the lights are off then noise is merely an extraneous variable (it is a variable other than the independent or dependent variable) and we don’t worry much about extraneous variables. This is because unless a variable varies systematically with the manipulated independent variable it cannot be a competing explanation for the results.
Non-Experimental Research
Researchers who are simply interested in describing characteristics of people, describing relationships between variables, and using those relationships to make predictions can use non-experimental research. Using the non-experimental approach, the researcher simply measures variables as they naturally occur, but they do not manipulate them. For instance, if I just measured the number of traffic fatalities in America last year that involved the use of a cell phone but I did not actually manipulate cell phone use then this would be categorized as non-experimental research. Alternatively, if I stood at a busy intersection and recorded drivers’ genders and whether or not they were using a cell phone when they passed through the intersection to see whether men or women are more likely to use a cell phone when driving, then this would be non-experimental research. It is important to point out that non-experimental does not mean nonscientific. Non-experimental research is scientific in nature. It can be used to fulfill two of the three goals of science (to describe and to predict). However, unlike with experimental research, we cannot make causal conclusions using this method; we cannot say that one variable causes another variable using this method.
Laboratory vs. Field Research
The next major distinction between research methods is between laboratory and field studies. A laboratory study is a study that is conducted in the laboratory environment. In contrast, a field study is a study that is conducted in the real-world, in a natural environment.
Laboratory experiments typically have high internal validity . Internal validity refers to the degree to which we can confidently infer a causal relationship between variables. When we conduct an experimental study in a laboratory environment we have very high internal validity because we manipulate one variable while controlling all other outside extraneous variables. When we manipulate an independent variable and observe an effect on a dependent variable and we control for everything else so that the only difference between our experimental groups or conditions is the one manipulated variable then we can be quite confident that it is the independent variable that is causing the change in the dependent variable. In contrast, because field studies are conducted in the real-world, the experimenter typically has less control over the environment and potential extraneous variables, and this decreases internal validity, making it less appropriate to arrive at causal conclusions.
But there is typically a trade-off between internal and external validity. External validity simply refers to the degree to which we can generalize the findings to other circumstances or settings, like the real-world environment. When internal validity is high, external validity tends to be low; and when internal validity is low, external validity tends to be high. So laboratory studies are typically low in external validity, while field studies are typically high in external validity. Since field studies are conducted in the real-world environment it is far more appropriate to generalize the findings to that real-world environment than when the research is conducted in the more artificial sterile laboratory.
Finally, there are field studies which are non-experimental in nature because nothing is manipulated. But there are also field experiment s where an independent variable is manipulated in a natural setting and extraneous variables are controlled. Depending on their overall quality and the level of control of extraneous variables, such field experiments can have high external and high internal validity.
A quantity or quality that varies across people or situations.
A quantity, such as height, that is typically measured by assigning a number to each individual.
A variable that represents a characteristic of an individual, such as chosen major, and is typically measured by assigning each individual's response to one of several categories (e.g., Psychology, English, Nursing, Engineering, etc.).
A definition of the variable in terms of precisely how it is to be measured.
A large group of people about whom researchers in psychology are usually interested in drawing conclusions, and from whom the sample is drawn.
A smaller portion of the population the researcher would like to study.
A common method of non-probability sampling in which the sample consists of individuals who happen to be easily available and willing to participate (such as introductory psychology students).
The variable the experimenter manipulates.
The variable the experimenter measures (it is the presumed effect).
Any variable other than the dependent and independent variable.
A specific type of extraneous variable that systematically varies along with the variables under investigation and therefore provides an alternative explanation for the results.
A study that is conducted in the laboratory environment.
A study that is conducted in a "real world" environment outside the laboratory.
Refers to the degree to which we can confidently infer a causal relationship between variables.
Refers to the degree to which we can generalize the findings to other circumstances or settings, like the real-world environment.
A type of field study where an independent variable is manipulated in a natural setting and extraneous variables are controlled as much as possible.
Research Methods in Psychology Copyright © 2019 by Rajiv S. Jhangiani, I-Chant A. Chiang, Carrie Cuttler, & Dana C. Leighton is licensed under a Creative Commons Attribution-NonCommercial-ShareAlike 4.0 International License , except where otherwise noted.
Share This Book
- Bipolar Disorder
- Therapy Center
- When To See a Therapist
- Types of Therapy
- Best Online Therapy
- Best Couples Therapy
- Best Family Therapy
- Managing Stress
- Sleep and Dreaming
- Understanding Emotions
- Self-Improvement
- Healthy Relationships
- Student Resources
- Personality Types
- Guided Meditations
- Verywell Mind Insights
- 2024 Verywell Mind 25
- Mental Health in the Classroom
- Editorial Process
- Meet Our Review Board
- Crisis Support
How the Experimental Method Works in Psychology
Kendra Cherry, MS, is a psychosocial rehabilitation specialist, psychology educator, and author of the "Everything Psychology Book."
:max_bytes(150000):strip_icc():format(webp)/IMG_9791-89504ab694d54b66bbd72cb84ffb860e.jpg)
Amanda Tust is a fact-checker, researcher, and writer with a Master of Science in Journalism from Northwestern University's Medill School of Journalism.
:max_bytes(150000):strip_icc():format(webp)/Amanda-Tust-1000-ffe096be0137462fbfba1f0759e07eb9.jpg)
sturti/Getty Images
The Experimental Process
Types of experiments, potential pitfalls of the experimental method.
The experimental method is a type of research procedure that involves manipulating variables to determine if there is a cause-and-effect relationship. The results obtained through the experimental method are useful but do not prove with 100% certainty that a singular cause always creates a specific effect. Instead, they show the probability that a cause will or will not lead to a particular effect.
At a Glance
While there are many different research techniques available, the experimental method allows researchers to look at cause-and-effect relationships. Using the experimental method, researchers randomly assign participants to a control or experimental group and manipulate levels of an independent variable. If changes in the independent variable lead to changes in the dependent variable, it indicates there is likely a causal relationship between them.
What Is the Experimental Method in Psychology?
The experimental method involves manipulating one variable to determine if this causes changes in another variable. This method relies on controlled research methods and random assignment of study subjects to test a hypothesis.
For example, researchers may want to learn how different visual patterns may impact our perception. Or they might wonder whether certain actions can improve memory . Experiments are conducted on many behavioral topics, including:
The scientific method forms the basis of the experimental method. This is a process used to determine the relationship between two variables—in this case, to explain human behavior .
Positivism is also important in the experimental method. It refers to factual knowledge that is obtained through observation, which is considered to be trustworthy.
When using the experimental method, researchers first identify and define key variables. Then they formulate a hypothesis, manipulate the variables, and collect data on the results. Unrelated or irrelevant variables are carefully controlled to minimize the potential impact on the experiment outcome.
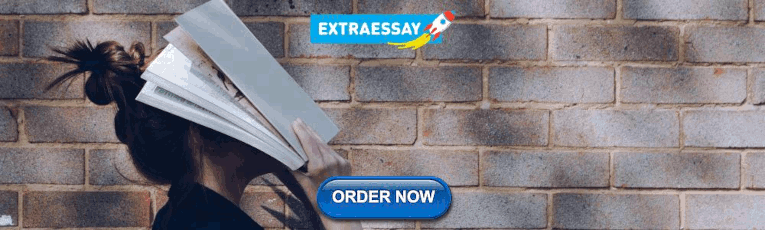
History of the Experimental Method
The idea of using experiments to better understand human psychology began toward the end of the nineteenth century. Wilhelm Wundt established the first formal laboratory in 1879.
Wundt is often called the father of experimental psychology. He believed that experiments could help explain how psychology works, and used this approach to study consciousness .
Wundt coined the term "physiological psychology." This is a hybrid of physiology and psychology, or how the body affects the brain.
Other early contributors to the development and evolution of experimental psychology as we know it today include:
- Gustav Fechner (1801-1887), who helped develop procedures for measuring sensations according to the size of the stimulus
- Hermann von Helmholtz (1821-1894), who analyzed philosophical assumptions through research in an attempt to arrive at scientific conclusions
- Franz Brentano (1838-1917), who called for a combination of first-person and third-person research methods when studying psychology
- Georg Elias Müller (1850-1934), who performed an early experiment on attitude which involved the sensory discrimination of weights and revealed how anticipation can affect this discrimination
Key Terms to Know
To understand how the experimental method works, it is important to know some key terms.
Dependent Variable
The dependent variable is the effect that the experimenter is measuring. If a researcher was investigating how sleep influences test scores, for example, the test scores would be the dependent variable.
Independent Variable
The independent variable is the variable that the experimenter manipulates. In the previous example, the amount of sleep an individual gets would be the independent variable.
A hypothesis is a tentative statement or a guess about the possible relationship between two or more variables. In looking at how sleep influences test scores, the researcher might hypothesize that people who get more sleep will perform better on a math test the following day. The purpose of the experiment, then, is to either support or reject this hypothesis.
Operational definitions are necessary when performing an experiment. When we say that something is an independent or dependent variable, we must have a very clear and specific definition of the meaning and scope of that variable.
Extraneous Variables
Extraneous variables are other variables that may also affect the outcome of an experiment. Types of extraneous variables include participant variables, situational variables, demand characteristics, and experimenter effects. In some cases, researchers can take steps to control for extraneous variables.
Demand Characteristics
Demand characteristics are subtle hints that indicate what an experimenter is hoping to find in a psychology experiment. This can sometimes cause participants to alter their behavior, which can affect the results of the experiment.
Intervening Variables
Intervening variables are factors that can affect the relationship between two other variables.
Confounding Variables
Confounding variables are variables that can affect the dependent variable, but that experimenters cannot control for. Confounding variables can make it difficult to determine if the effect was due to changes in the independent variable or if the confounding variable may have played a role.
Psychologists, like other scientists, use the scientific method when conducting an experiment. The scientific method is a set of procedures and principles that guide how scientists develop research questions, collect data, and come to conclusions.
The five basic steps of the experimental process are:
- Identifying a problem to study
- Devising the research protocol
- Conducting the experiment
- Analyzing the data collected
- Sharing the findings (usually in writing or via presentation)
Most psychology students are expected to use the experimental method at some point in their academic careers. Learning how to conduct an experiment is important to understanding how psychologists prove and disprove theories in this field.
There are a few different types of experiments that researchers might use when studying psychology. Each has pros and cons depending on the participants being studied, the hypothesis, and the resources available to conduct the research.
Lab Experiments
Lab experiments are common in psychology because they allow experimenters more control over the variables. These experiments can also be easier for other researchers to replicate. The drawback of this research type is that what takes place in a lab is not always what takes place in the real world.
Field Experiments
Sometimes researchers opt to conduct their experiments in the field. For example, a social psychologist interested in researching prosocial behavior might have a person pretend to faint and observe how long it takes onlookers to respond.
This type of experiment can be a great way to see behavioral responses in realistic settings. But it is more difficult for researchers to control the many variables existing in these settings that could potentially influence the experiment's results.
Quasi-Experiments
While lab experiments are known as true experiments, researchers can also utilize a quasi-experiment. Quasi-experiments are often referred to as natural experiments because the researchers do not have true control over the independent variable.
A researcher looking at personality differences and birth order, for example, is not able to manipulate the independent variable in the situation (personality traits). Participants also cannot be randomly assigned because they naturally fall into pre-existing groups based on their birth order.
So why would a researcher use a quasi-experiment? This is a good choice in situations where scientists are interested in studying phenomena in natural, real-world settings. It's also beneficial if there are limits on research funds or time.
Field experiments can be either quasi-experiments or true experiments.
Examples of the Experimental Method in Use
The experimental method can provide insight into human thoughts and behaviors, Researchers use experiments to study many aspects of psychology.
A 2019 study investigated whether splitting attention between electronic devices and classroom lectures had an effect on college students' learning abilities. It found that dividing attention between these two mediums did not affect lecture comprehension. However, it did impact long-term retention of the lecture information, which affected students' exam performance.
An experiment used participants' eye movements and electroencephalogram (EEG) data to better understand cognitive processing differences between experts and novices. It found that experts had higher power in their theta brain waves than novices, suggesting that they also had a higher cognitive load.
A study looked at whether chatting online with a computer via a chatbot changed the positive effects of emotional disclosure often received when talking with an actual human. It found that the effects were the same in both cases.
One experimental study evaluated whether exercise timing impacts information recall. It found that engaging in exercise prior to performing a memory task helped improve participants' short-term memory abilities.
Sometimes researchers use the experimental method to get a bigger-picture view of psychological behaviors and impacts. For example, one 2018 study examined several lab experiments to learn more about the impact of various environmental factors on building occupant perceptions.
A 2020 study set out to determine the role that sensation-seeking plays in political violence. This research found that sensation-seeking individuals have a higher propensity for engaging in political violence. It also found that providing access to a more peaceful, yet still exciting political group helps reduce this effect.
While the experimental method can be a valuable tool for learning more about psychology and its impacts, it also comes with a few pitfalls.
Experiments may produce artificial results, which are difficult to apply to real-world situations. Similarly, researcher bias can impact the data collected. Results may not be able to be reproduced, meaning the results have low reliability .
Since humans are unpredictable and their behavior can be subjective, it can be hard to measure responses in an experiment. In addition, political pressure may alter the results. The subjects may not be a good representation of the population, or groups used may not be comparable.
And finally, since researchers are human too, results may be degraded due to human error.
What This Means For You
Every psychological research method has its pros and cons. The experimental method can help establish cause and effect, and it's also beneficial when research funds are limited or time is of the essence.
At the same time, it's essential to be aware of this method's pitfalls, such as how biases can affect the results or the potential for low reliability. Keeping these in mind can help you review and assess research studies more accurately, giving you a better idea of whether the results can be trusted or have limitations.
Colorado State University. Experimental and quasi-experimental research .
American Psychological Association. Experimental psychology studies human and animals .
Mayrhofer R, Kuhbandner C, Lindner C. The practice of experimental psychology: An inevitably postmodern endeavor . Front Psychol . 2021;11:612805. doi:10.3389/fpsyg.2020.612805
Mandler G. A History of Modern Experimental Psychology .
Stanford University. Wilhelm Maximilian Wundt . Stanford Encyclopedia of Philosophy.
Britannica. Gustav Fechner .
Britannica. Hermann von Helmholtz .
Meyer A, Hackert B, Weger U. Franz Brentano and the beginning of experimental psychology: implications for the study of psychological phenomena today . Psychol Res . 2018;82:245-254. doi:10.1007/s00426-016-0825-7
Britannica. Georg Elias Müller .
McCambridge J, de Bruin M, Witton J. The effects of demand characteristics on research participant behaviours in non-laboratory settings: A systematic review . PLoS ONE . 2012;7(6):e39116. doi:10.1371/journal.pone.0039116
Laboratory experiments . In: The Sage Encyclopedia of Communication Research Methods. Allen M, ed. SAGE Publications, Inc. doi:10.4135/9781483381411.n287
Schweizer M, Braun B, Milstone A. Research methods in healthcare epidemiology and antimicrobial stewardship — quasi-experimental designs . Infect Control Hosp Epidemiol . 2016;37(10):1135-1140. doi:10.1017/ice.2016.117
Glass A, Kang M. Dividing attention in the classroom reduces exam performance . Educ Psychol . 2019;39(3):395-408. doi:10.1080/01443410.2018.1489046
Keskin M, Ooms K, Dogru AO, De Maeyer P. Exploring the cognitive load of expert and novice map users using EEG and eye tracking . ISPRS Int J Geo-Inf . 2020;9(7):429. doi:10.3390.ijgi9070429
Ho A, Hancock J, Miner A. Psychological, relational, and emotional effects of self-disclosure after conversations with a chatbot . J Commun . 2018;68(4):712-733. doi:10.1093/joc/jqy026
Haynes IV J, Frith E, Sng E, Loprinzi P. Experimental effects of acute exercise on episodic memory function: Considerations for the timing of exercise . Psychol Rep . 2018;122(5):1744-1754. doi:10.1177/0033294118786688
Torresin S, Pernigotto G, Cappelletti F, Gasparella A. Combined effects of environmental factors on human perception and objective performance: A review of experimental laboratory works . Indoor Air . 2018;28(4):525-538. doi:10.1111/ina.12457
Schumpe BM, Belanger JJ, Moyano M, Nisa CF. The role of sensation seeking in political violence: An extension of the significance quest theory . J Personal Social Psychol . 2020;118(4):743-761. doi:10.1037/pspp0000223
By Kendra Cherry, MSEd Kendra Cherry, MS, is a psychosocial rehabilitation specialist, psychology educator, and author of the "Everything Psychology Book."
Research Methods In Psychology
Saul Mcleod, PhD
Editor-in-Chief for Simply Psychology
BSc (Hons) Psychology, MRes, PhD, University of Manchester
Saul Mcleod, PhD., is a qualified psychology teacher with over 18 years of experience in further and higher education. He has been published in peer-reviewed journals, including the Journal of Clinical Psychology.
Learn about our Editorial Process
Olivia Guy-Evans, MSc
Associate Editor for Simply Psychology
BSc (Hons) Psychology, MSc Psychology of Education
Olivia Guy-Evans is a writer and associate editor for Simply Psychology. She has previously worked in healthcare and educational sectors.
Research methods in psychology are systematic procedures used to observe, describe, predict, and explain behavior and mental processes. They include experiments, surveys, case studies, and naturalistic observations, ensuring data collection is objective and reliable to understand and explain psychological phenomena.
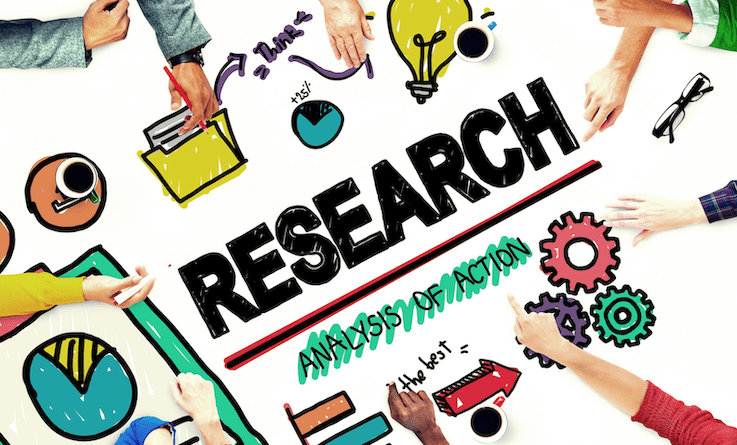
Hypotheses are statements about the prediction of the results, that can be verified or disproved by some investigation.
There are four types of hypotheses :
- Null Hypotheses (H0 ) – these predict that no difference will be found in the results between the conditions. Typically these are written ‘There will be no difference…’
- Alternative Hypotheses (Ha or H1) – these predict that there will be a significant difference in the results between the two conditions. This is also known as the experimental hypothesis.
- One-tailed (directional) hypotheses – these state the specific direction the researcher expects the results to move in, e.g. higher, lower, more, less. In a correlation study, the predicted direction of the correlation can be either positive or negative.
- Two-tailed (non-directional) hypotheses – these state that a difference will be found between the conditions of the independent variable but does not state the direction of a difference or relationship. Typically these are always written ‘There will be a difference ….’
All research has an alternative hypothesis (either a one-tailed or two-tailed) and a corresponding null hypothesis.
Once the research is conducted and results are found, psychologists must accept one hypothesis and reject the other.
So, if a difference is found, the Psychologist would accept the alternative hypothesis and reject the null. The opposite applies if no difference is found.
Sampling techniques
Sampling is the process of selecting a representative group from the population under study.
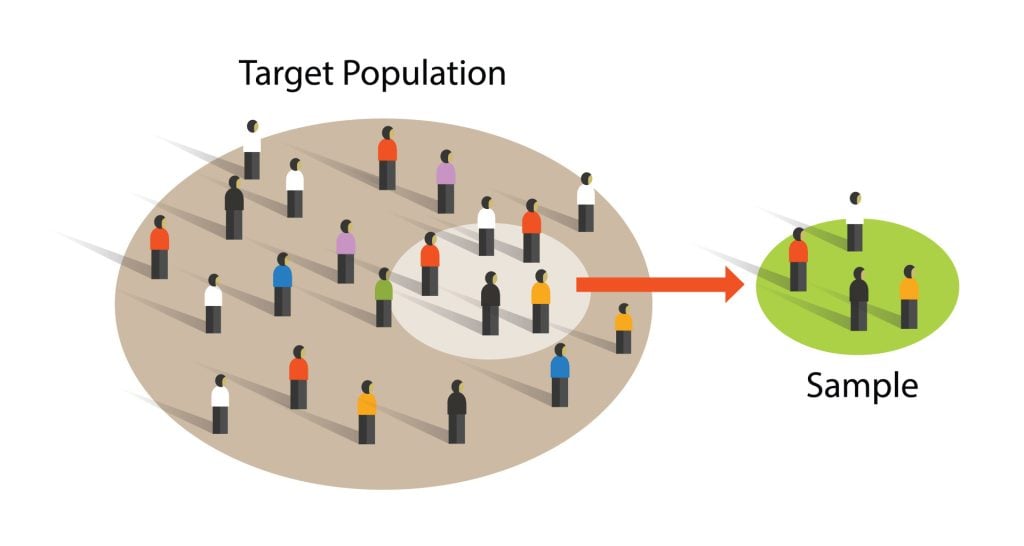
A sample is the participants you select from a target population (the group you are interested in) to make generalizations about.
Representative means the extent to which a sample mirrors a researcher’s target population and reflects its characteristics.
Generalisability means the extent to which their findings can be applied to the larger population of which their sample was a part.
- Volunteer sample : where participants pick themselves through newspaper adverts, noticeboards or online.
- Opportunity sampling : also known as convenience sampling , uses people who are available at the time the study is carried out and willing to take part. It is based on convenience.
- Random sampling : when every person in the target population has an equal chance of being selected. An example of random sampling would be picking names out of a hat.
- Systematic sampling : when a system is used to select participants. Picking every Nth person from all possible participants. N = the number of people in the research population / the number of people needed for the sample.
- Stratified sampling : when you identify the subgroups and select participants in proportion to their occurrences.
- Snowball sampling : when researchers find a few participants, and then ask them to find participants themselves and so on.
- Quota sampling : when researchers will be told to ensure the sample fits certain quotas, for example they might be told to find 90 participants, with 30 of them being unemployed.
Experiments always have an independent and dependent variable .
- The independent variable is the one the experimenter manipulates (the thing that changes between the conditions the participants are placed into). It is assumed to have a direct effect on the dependent variable.
- The dependent variable is the thing being measured, or the results of the experiment.
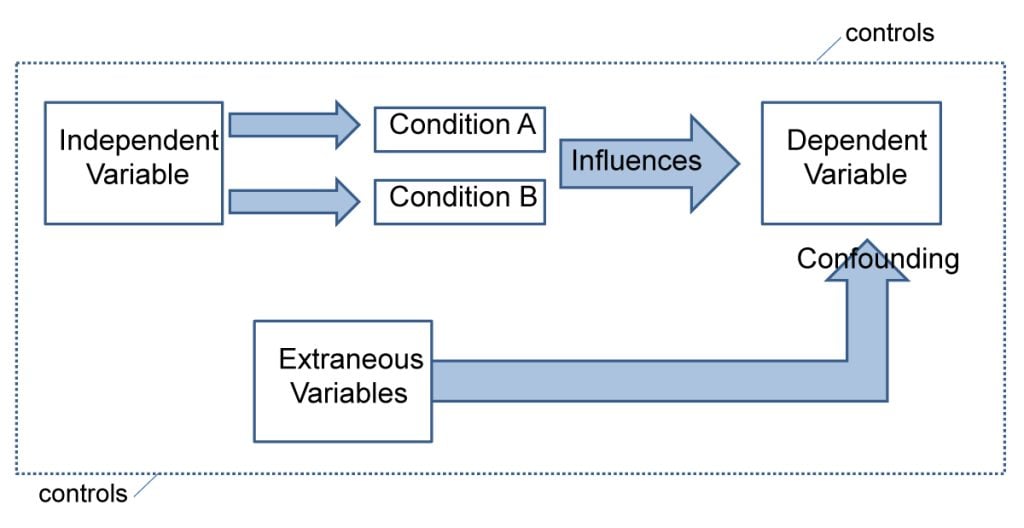
Operationalization of variables means making them measurable/quantifiable. We must use operationalization to ensure that variables are in a form that can be easily tested.
For instance, we can’t really measure ‘happiness’, but we can measure how many times a person smiles within a two-hour period.
By operationalizing variables, we make it easy for someone else to replicate our research. Remember, this is important because we can check if our findings are reliable.
Extraneous variables are all variables which are not independent variable but could affect the results of the experiment.
It can be a natural characteristic of the participant, such as intelligence levels, gender, or age for example, or it could be a situational feature of the environment such as lighting or noise.
Demand characteristics are a type of extraneous variable that occurs if the participants work out the aims of the research study, they may begin to behave in a certain way.
For example, in Milgram’s research , critics argued that participants worked out that the shocks were not real and they administered them as they thought this was what was required of them.
Extraneous variables must be controlled so that they do not affect (confound) the results.
Randomly allocating participants to their conditions or using a matched pairs experimental design can help to reduce participant variables.
Situational variables are controlled by using standardized procedures, ensuring every participant in a given condition is treated in the same way
Experimental Design
Experimental design refers to how participants are allocated to each condition of the independent variable, such as a control or experimental group.
- Independent design ( between-groups design ): each participant is selected for only one group. With the independent design, the most common way of deciding which participants go into which group is by means of randomization.
- Matched participants design : each participant is selected for only one group, but the participants in the two groups are matched for some relevant factor or factors (e.g. ability; sex; age).
- Repeated measures design ( within groups) : each participant appears in both groups, so that there are exactly the same participants in each group.
- The main problem with the repeated measures design is that there may well be order effects. Their experiences during the experiment may change the participants in various ways.
- They may perform better when they appear in the second group because they have gained useful information about the experiment or about the task. On the other hand, they may perform less well on the second occasion because of tiredness or boredom.
- Counterbalancing is the best way of preventing order effects from disrupting the findings of an experiment, and involves ensuring that each condition is equally likely to be used first and second by the participants.
If we wish to compare two groups with respect to a given independent variable, it is essential to make sure that the two groups do not differ in any other important way.
Experimental Methods
All experimental methods involve an iv (independent variable) and dv (dependent variable)..
- Field experiments are conducted in the everyday (natural) environment of the participants. The experimenter still manipulates the IV, but in a real-life setting. It may be possible to control extraneous variables, though such control is more difficult than in a lab experiment.
- Natural experiments are when a naturally occurring IV is investigated that isn’t deliberately manipulated, it exists anyway. Participants are not randomly allocated, and the natural event may only occur rarely.
Case studies are in-depth investigations of a person, group, event, or community. It uses information from a range of sources, such as from the person concerned and also from their family and friends.
Many techniques may be used such as interviews, psychological tests, observations and experiments. Case studies are generally longitudinal: in other words, they follow the individual or group over an extended period of time.
Case studies are widely used in psychology and among the best-known ones carried out were by Sigmund Freud . He conducted very detailed investigations into the private lives of his patients in an attempt to both understand and help them overcome their illnesses.
Case studies provide rich qualitative data and have high levels of ecological validity. However, it is difficult to generalize from individual cases as each one has unique characteristics.
Correlational Studies
Correlation means association; it is a measure of the extent to which two variables are related. One of the variables can be regarded as the predictor variable with the other one as the outcome variable.
Correlational studies typically involve obtaining two different measures from a group of participants, and then assessing the degree of association between the measures.
The predictor variable can be seen as occurring before the outcome variable in some sense. It is called the predictor variable, because it forms the basis for predicting the value of the outcome variable.
Relationships between variables can be displayed on a graph or as a numerical score called a correlation coefficient.
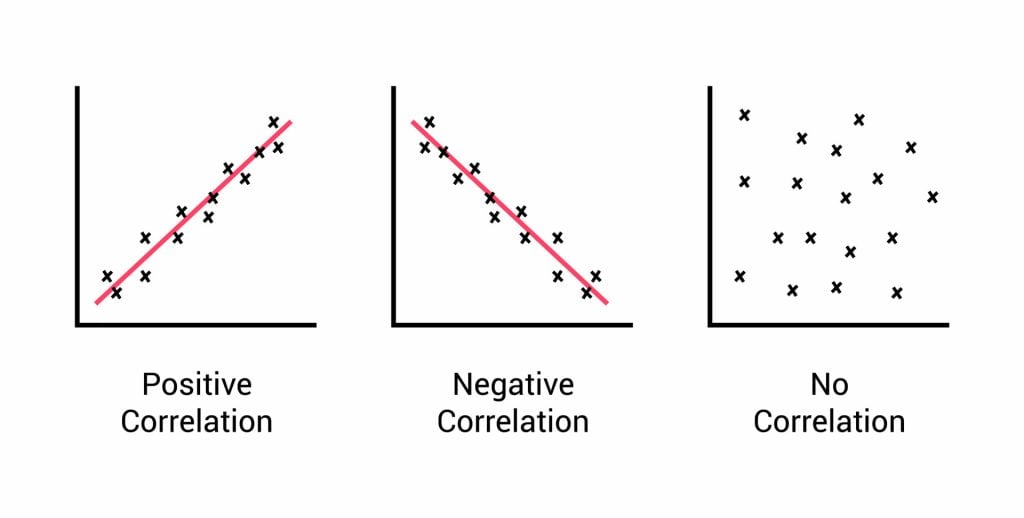
- If an increase in one variable tends to be associated with an increase in the other, then this is known as a positive correlation .
- If an increase in one variable tends to be associated with a decrease in the other, then this is known as a negative correlation .
- A zero correlation occurs when there is no relationship between variables.
After looking at the scattergraph, if we want to be sure that a significant relationship does exist between the two variables, a statistical test of correlation can be conducted, such as Spearman’s rho.
The test will give us a score, called a correlation coefficient . This is a value between 0 and 1, and the closer to 1 the score is, the stronger the relationship between the variables. This value can be both positive e.g. 0.63, or negative -0.63.
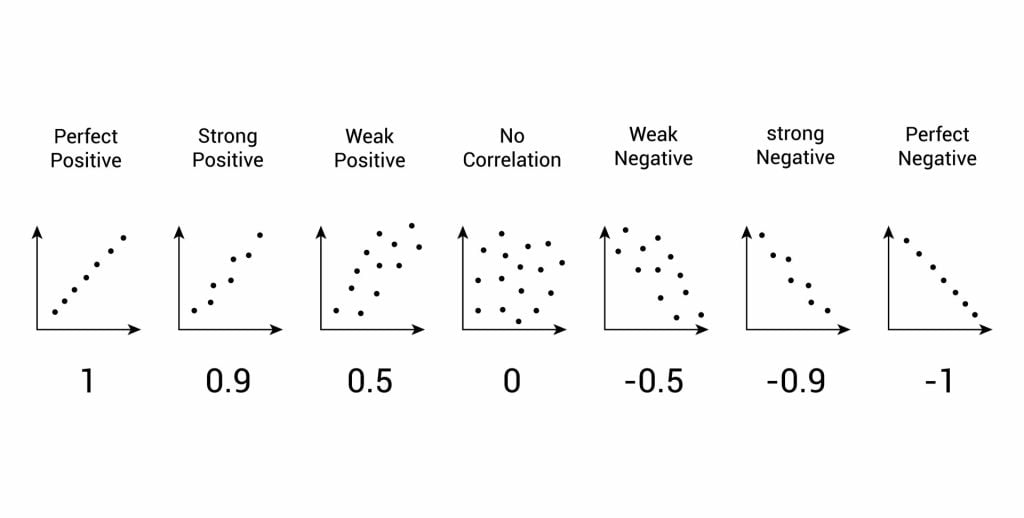
A correlation between variables, however, does not automatically mean that the change in one variable is the cause of the change in the values of the other variable. A correlation only shows if there is a relationship between variables.
Correlation does not always prove causation, as a third variable may be involved.
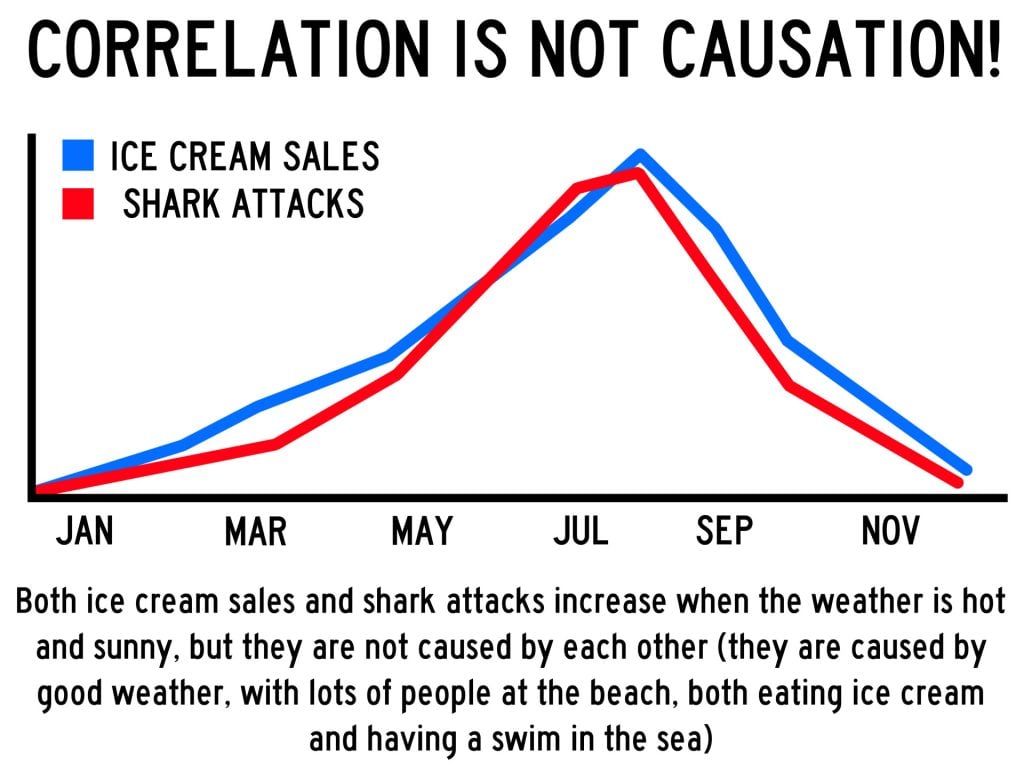
Interview Methods
Interviews are commonly divided into two types: structured and unstructured.
A fixed, predetermined set of questions is put to every participant in the same order and in the same way.
Responses are recorded on a questionnaire, and the researcher presets the order and wording of questions, and sometimes the range of alternative answers.
The interviewer stays within their role and maintains social distance from the interviewee.
There are no set questions, and the participant can raise whatever topics he/she feels are relevant and ask them in their own way. Questions are posed about participants’ answers to the subject
Unstructured interviews are most useful in qualitative research to analyze attitudes and values.
Though they rarely provide a valid basis for generalization, their main advantage is that they enable the researcher to probe social actors’ subjective point of view.
Questionnaire Method
Questionnaires can be thought of as a kind of written interview. They can be carried out face to face, by telephone, or post.
The choice of questions is important because of the need to avoid bias or ambiguity in the questions, ‘leading’ the respondent or causing offense.
- Open questions are designed to encourage a full, meaningful answer using the subject’s own knowledge and feelings. They provide insights into feelings, opinions, and understanding. Example: “How do you feel about that situation?”
- Closed questions can be answered with a simple “yes” or “no” or specific information, limiting the depth of response. They are useful for gathering specific facts or confirming details. Example: “Do you feel anxious in crowds?”
Its other practical advantages are that it is cheaper than face-to-face interviews and can be used to contact many respondents scattered over a wide area relatively quickly.
Observations
There are different types of observation methods :
- Covert observation is where the researcher doesn’t tell the participants they are being observed until after the study is complete. There could be ethical problems or deception and consent with this particular observation method.
- Overt observation is where a researcher tells the participants they are being observed and what they are being observed for.
- Controlled : behavior is observed under controlled laboratory conditions (e.g., Bandura’s Bobo doll study).
- Natural : Here, spontaneous behavior is recorded in a natural setting.
- Participant : Here, the observer has direct contact with the group of people they are observing. The researcher becomes a member of the group they are researching.
- Non-participant (aka “fly on the wall): The researcher does not have direct contact with the people being observed. The observation of participants’ behavior is from a distance
Pilot Study
A pilot study is a small scale preliminary study conducted in order to evaluate the feasibility of the key s teps in a future, full-scale project.
A pilot study is an initial run-through of the procedures to be used in an investigation; it involves selecting a few people and trying out the study on them. It is possible to save time, and in some cases, money, by identifying any flaws in the procedures designed by the researcher.
A pilot study can help the researcher spot any ambiguities (i.e. unusual things) or confusion in the information given to participants or problems with the task devised.
Sometimes the task is too hard, and the researcher may get a floor effect, because none of the participants can score at all or can complete the task – all performances are low.
The opposite effect is a ceiling effect, when the task is so easy that all achieve virtually full marks or top performances and are “hitting the ceiling”.
Research Design
In cross-sectional research , a researcher compares multiple segments of the population at the same time
Sometimes, we want to see how people change over time, as in studies of human development and lifespan. Longitudinal research is a research design in which data-gathering is administered repeatedly over an extended period of time.
In cohort studies , the participants must share a common factor or characteristic such as age, demographic, or occupation. A cohort study is a type of longitudinal study in which researchers monitor and observe a chosen population over an extended period.
Triangulation means using more than one research method to improve the study’s validity.
Reliability
Reliability is a measure of consistency, if a particular measurement is repeated and the same result is obtained then it is described as being reliable.
- Test-retest reliability : assessing the same person on two different occasions which shows the extent to which the test produces the same answers.
- Inter-observer reliability : the extent to which there is an agreement between two or more observers.
Meta-Analysis
A meta-analysis is a systematic review that involves identifying an aim and then searching for research studies that have addressed similar aims/hypotheses.
This is done by looking through various databases, and then decisions are made about what studies are to be included/excluded.
Strengths: Increases the conclusions’ validity as they’re based on a wider range.
Weaknesses: Research designs in studies can vary, so they are not truly comparable.
Peer Review
A researcher submits an article to a journal. The choice of the journal may be determined by the journal’s audience or prestige.
The journal selects two or more appropriate experts (psychologists working in a similar field) to peer review the article without payment. The peer reviewers assess: the methods and designs used, originality of the findings, the validity of the original research findings and its content, structure and language.
Feedback from the reviewer determines whether the article is accepted. The article may be: Accepted as it is, accepted with revisions, sent back to the author to revise and re-submit or rejected without the possibility of submission.
The editor makes the final decision whether to accept or reject the research report based on the reviewers comments/ recommendations.
Peer review is important because it prevent faulty data from entering the public domain, it provides a way of checking the validity of findings and the quality of the methodology and is used to assess the research rating of university departments.
Peer reviews may be an ideal, whereas in practice there are lots of problems. For example, it slows publication down and may prevent unusual, new work being published. Some reviewers might use it as an opportunity to prevent competing researchers from publishing work.
Some people doubt whether peer review can really prevent the publication of fraudulent research.
The advent of the internet means that a lot of research and academic comment is being published without official peer reviews than before, though systems are evolving on the internet where everyone really has a chance to offer their opinions and police the quality of research.
Types of Data
- Quantitative data is numerical data e.g. reaction time or number of mistakes. It represents how much or how long, how many there are of something. A tally of behavioral categories and closed questions in a questionnaire collect quantitative data.
- Qualitative data is virtually any type of information that can be observed and recorded that is not numerical in nature and can be in the form of written or verbal communication. Open questions in questionnaires and accounts from observational studies collect qualitative data.
- Primary data is first-hand data collected for the purpose of the investigation.
- Secondary data is information that has been collected by someone other than the person who is conducting the research e.g. taken from journals, books or articles.
Validity means how well a piece of research actually measures what it sets out to, or how well it reflects the reality it claims to represent.
Validity is whether the observed effect is genuine and represents what is actually out there in the world.
- Concurrent validity is the extent to which a psychological measure relates to an existing similar measure and obtains close results. For example, a new intelligence test compared to an established test.
- Face validity : does the test measure what it’s supposed to measure ‘on the face of it’. This is done by ‘eyeballing’ the measuring or by passing it to an expert to check.
- Ecological validit y is the extent to which findings from a research study can be generalized to other settings / real life.
- Temporal validity is the extent to which findings from a research study can be generalized to other historical times.
Features of Science
- Paradigm – A set of shared assumptions and agreed methods within a scientific discipline.
- Paradigm shift – The result of the scientific revolution: a significant change in the dominant unifying theory within a scientific discipline.
- Objectivity – When all sources of personal bias are minimised so not to distort or influence the research process.
- Empirical method – Scientific approaches that are based on the gathering of evidence through direct observation and experience.
- Replicability – The extent to which scientific procedures and findings can be repeated by other researchers.
- Falsifiability – The principle that a theory cannot be considered scientific unless it admits the possibility of being proved untrue.
Statistical Testing
A significant result is one where there is a low probability that chance factors were responsible for any observed difference, correlation, or association in the variables tested.
If our test is significant, we can reject our null hypothesis and accept our alternative hypothesis.
If our test is not significant, we can accept our null hypothesis and reject our alternative hypothesis. A null hypothesis is a statement of no effect.
In Psychology, we use p < 0.05 (as it strikes a balance between making a type I and II error) but p < 0.01 is used in tests that could cause harm like introducing a new drug.
A type I error is when the null hypothesis is rejected when it should have been accepted (happens when a lenient significance level is used, an error of optimism).
A type II error is when the null hypothesis is accepted when it should have been rejected (happens when a stringent significance level is used, an error of pessimism).
Ethical Issues
- Informed consent is when participants are able to make an informed judgment about whether to take part. It causes them to guess the aims of the study and change their behavior.
- To deal with it, we can gain presumptive consent or ask them to formally indicate their agreement to participate but it may invalidate the purpose of the study and it is not guaranteed that the participants would understand.
- Deception should only be used when it is approved by an ethics committee, as it involves deliberately misleading or withholding information. Participants should be fully debriefed after the study but debriefing can’t turn the clock back.
- All participants should be informed at the beginning that they have the right to withdraw if they ever feel distressed or uncomfortable.
- It causes bias as the ones that stayed are obedient and some may not withdraw as they may have been given incentives or feel like they’re spoiling the study. Researchers can offer the right to withdraw data after participation.
- Participants should all have protection from harm . The researcher should avoid risks greater than those experienced in everyday life and they should stop the study if any harm is suspected. However, the harm may not be apparent at the time of the study.
- Confidentiality concerns the communication of personal information. The researchers should not record any names but use numbers or false names though it may not be possible as it is sometimes possible to work out who the researchers were.

Related Articles
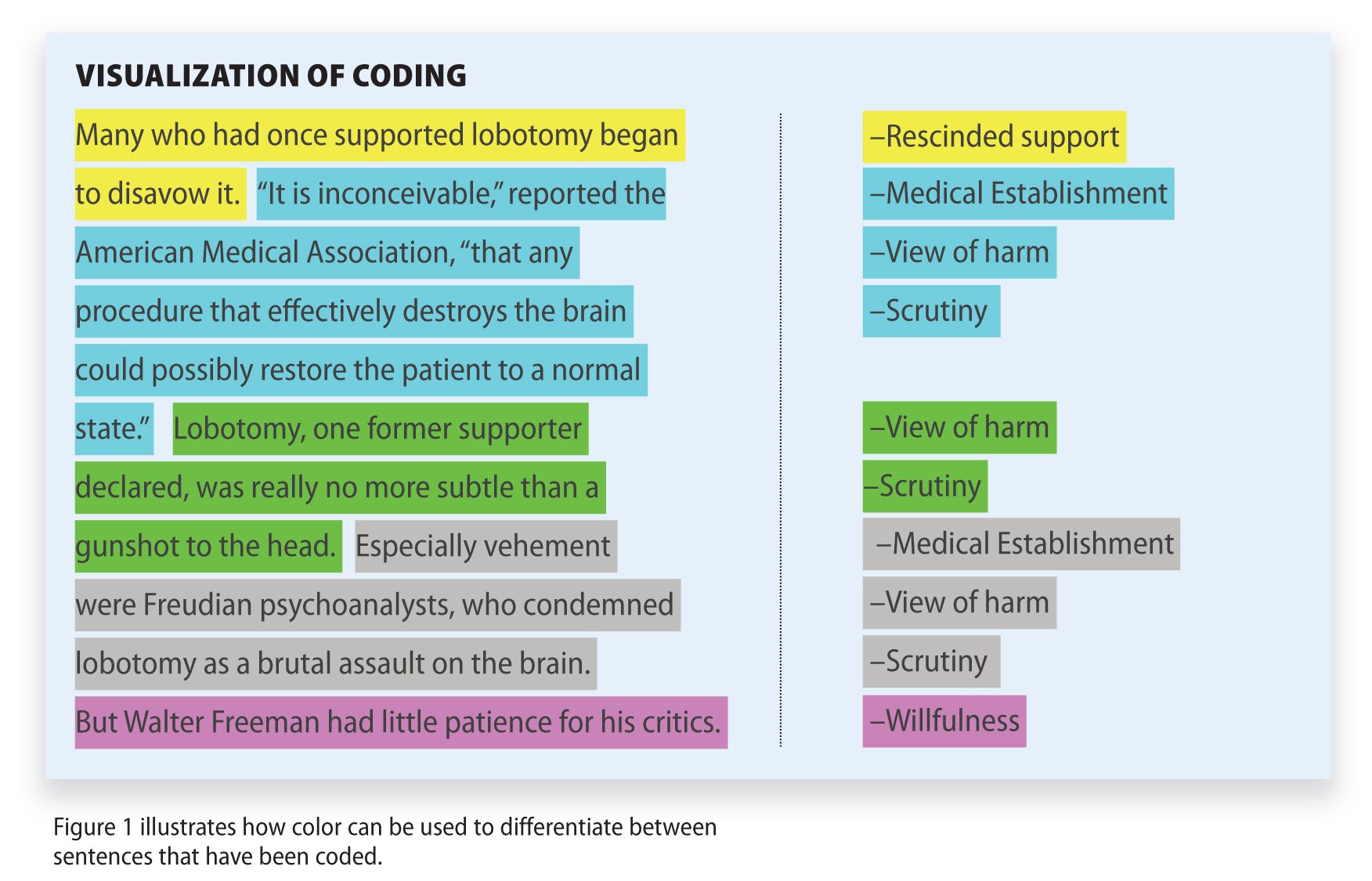
Research Methodology
Qualitative Data Coding
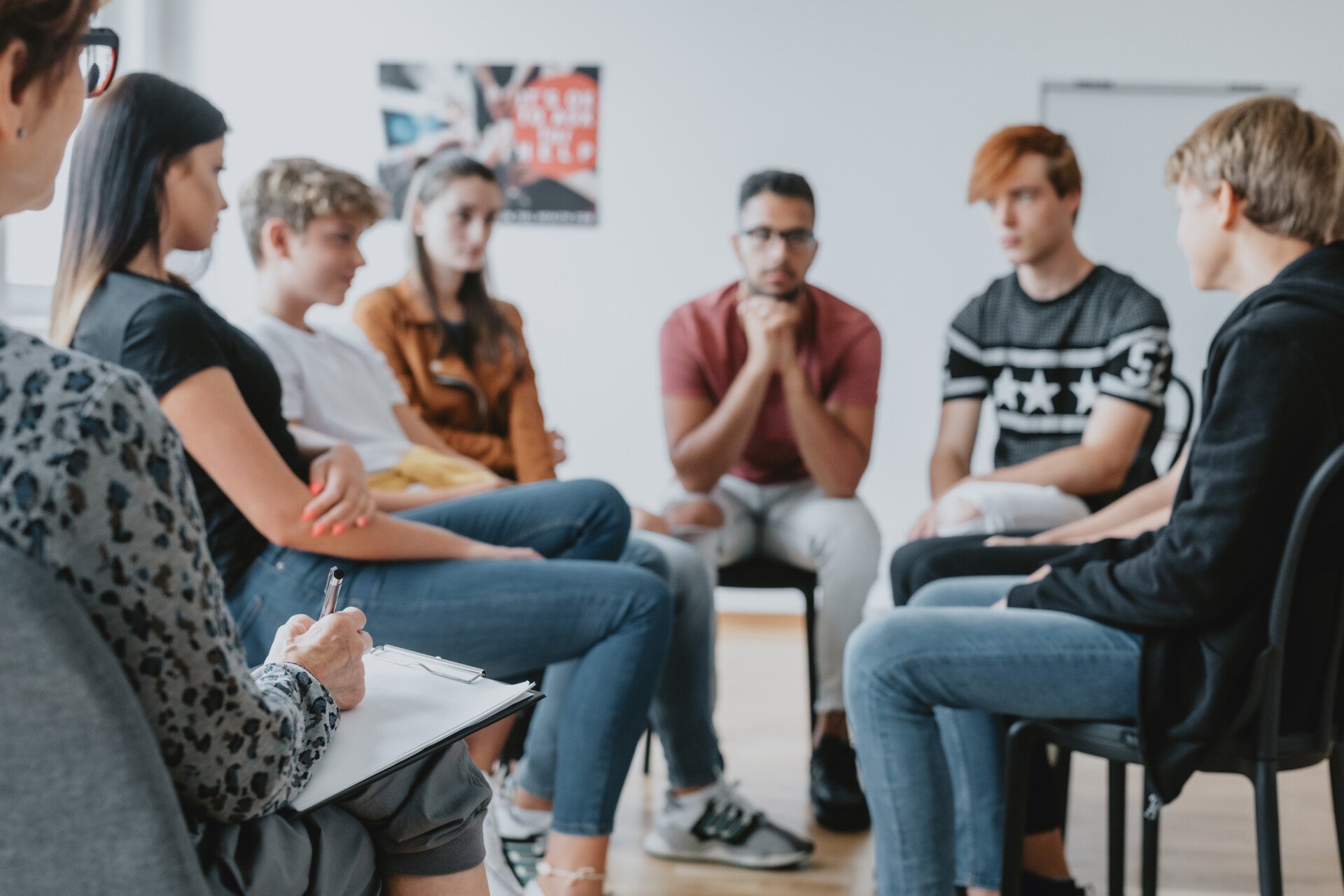
What Is a Focus Group?
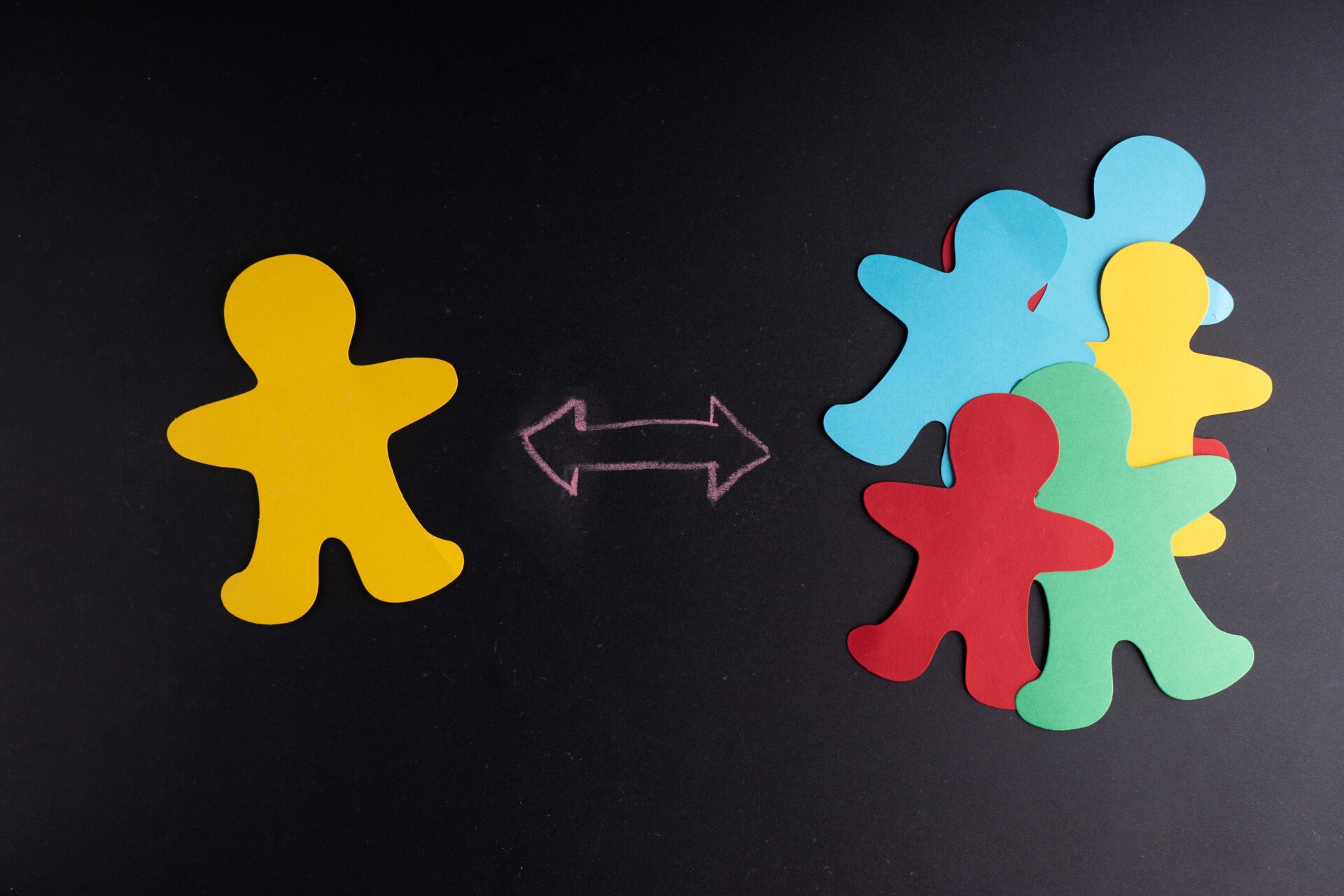
Cross-Cultural Research Methodology In Psychology
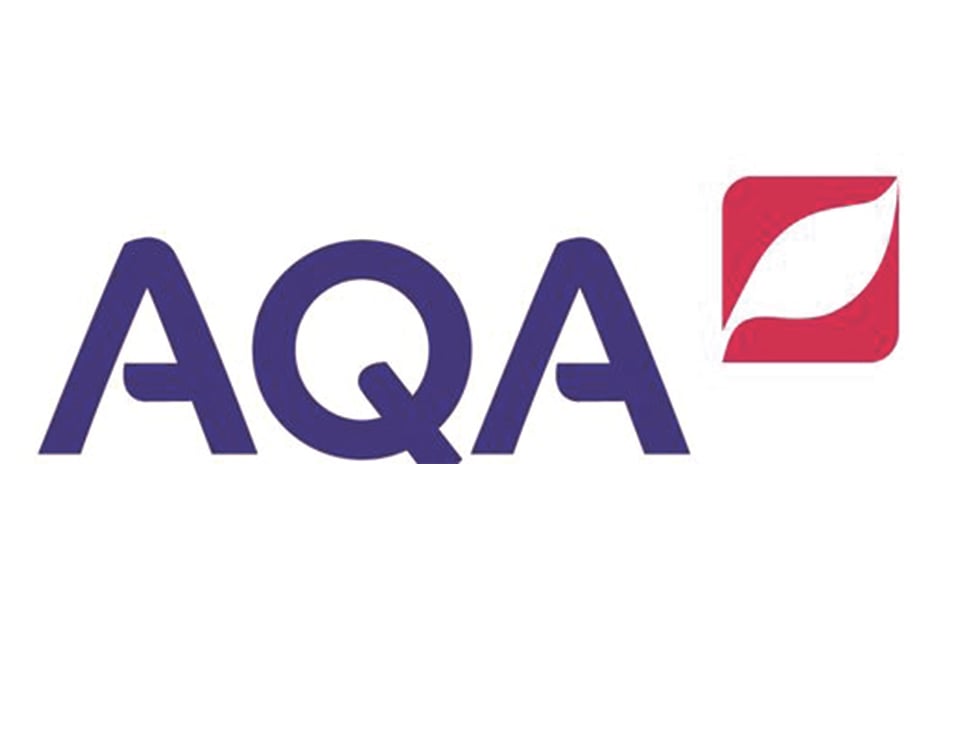
A-Level Psychology
A-level Psychology AQA Revision Notes
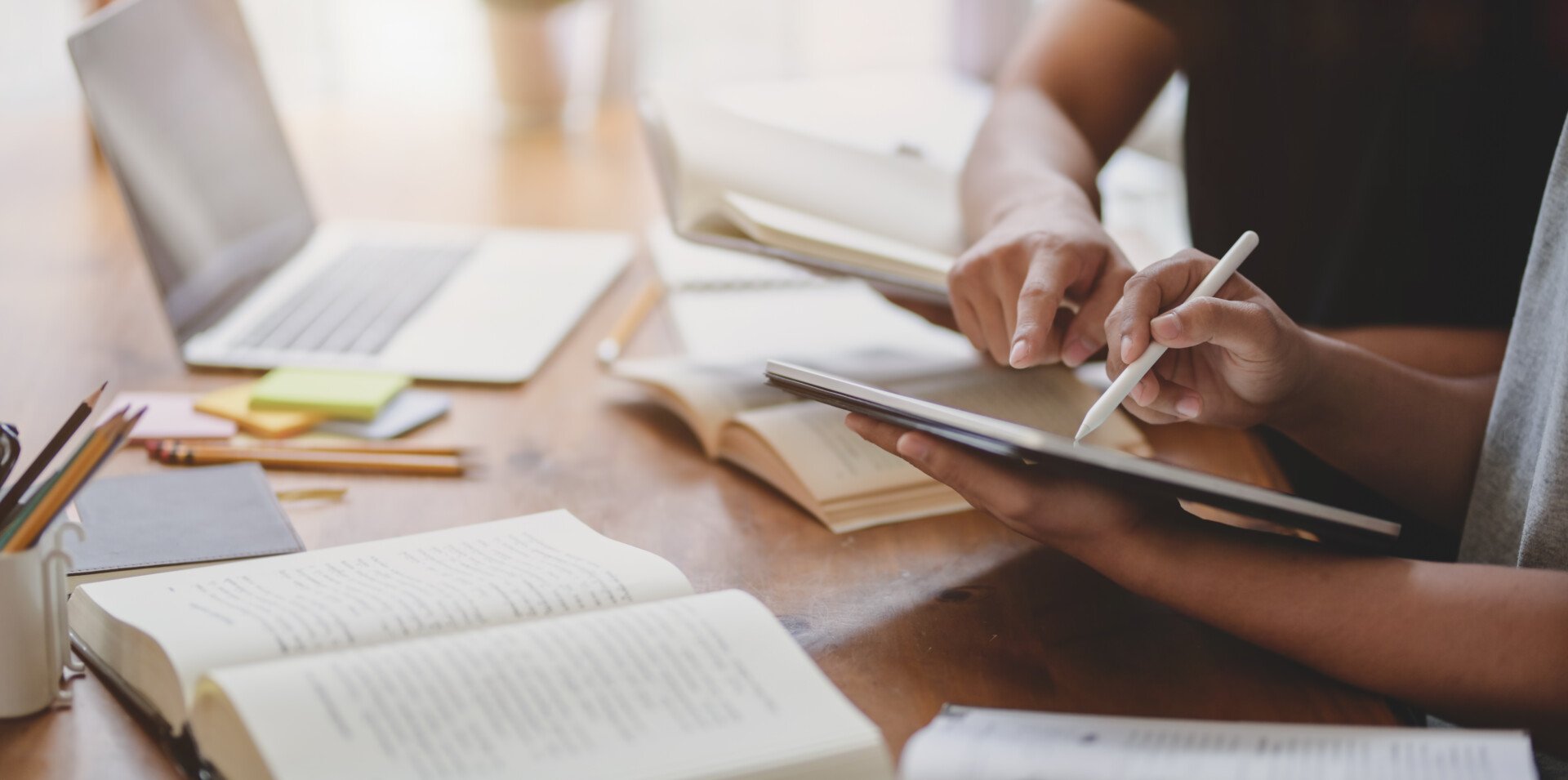
What Is Internal Validity In Research?
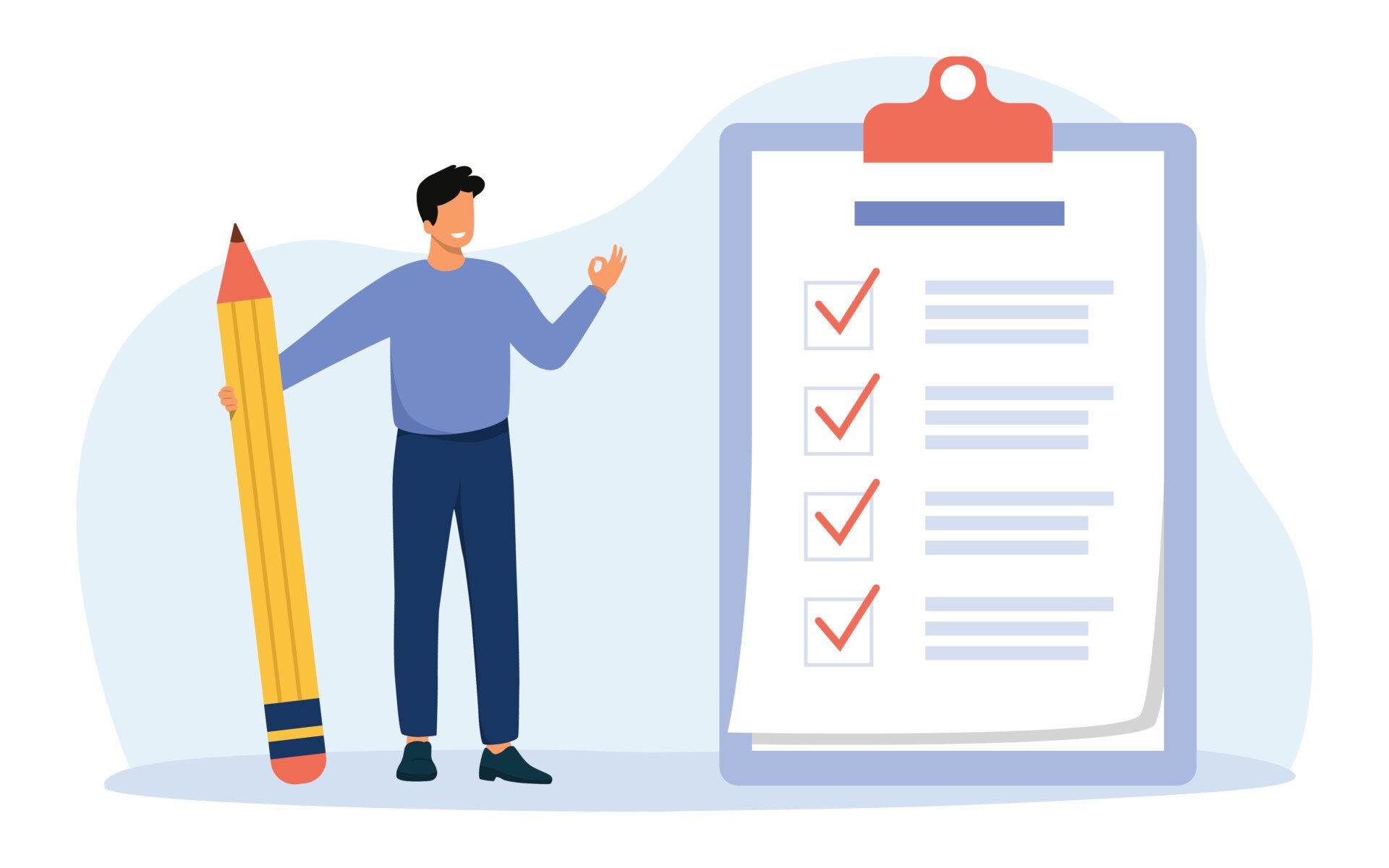
Research Methodology , Statistics
What Is Face Validity In Research? Importance & How To Measure
Live revision! Join us for our free exam revision livestreams Watch now →
Reference Library
Collections
- See what's new
- All Resources
- Student Resources
- Assessment Resources
- Teaching Resources
- CPD Courses
- Livestreams
Study notes, videos, interactive activities and more!
Psychology news, insights and enrichment
Currated collections of free resources
Browse resources by topic
- All Psychology Resources
Resource Selections
Currated lists of resources
Study Notes
Field Experiments
Last updated 22 Mar 2021
- Share on Facebook
- Share on Twitter
- Share by Email
Experiments look for the effect that manipulated variables (independent variables) have on measured variables (dependent variables), i.e. causal effects.
Field experiments are conducted in a natural setting (e.g. at a sports event or on public transport), as opposed to the artificial environment created in laboratory experiments. Some variables cannot be controlled due to the unpredictability of these real-life settings (e.g. the public interacting with participants), but an independent variable will still be altered for a dependent variable to be measured against.
Evaluation of field experiments:
- Field experiments generally yield results with higher ecological validity than laboratory experiments, as the natural settings will relate to real life.
- Demand characteristics are less of an issue with field experiments than laboratory experiments (i.e. participants are less likely to adjust their natural behaviour according to their interpretation of the study’s purpose, as they might not know they are in a study).
- Extraneous variables could confound results due to the reduced control experimenters have over them in non-artificial environments, which makes it difficult to find truly causal effects between independent and dependent variables.
- Ethical principles have to be considered, such as the lack of informed consent; if participants are not made aware of their participation in an experiment, privacy must be respected during observations and participants must be debriefed appropriately when observations come to an end.
- Precise replication of the natural environment of field experiments is understandably difficult, so they have poor reliability, unlike laboratory experiments where the exact conditions can be recreated.
- Field experiments are more susceptible to sample bias, as participants are often not randomly allocated to experimental conditions (i.e. participants’ groups are already pre-set rather than randomly assigned).
- Field experiments
You might also like
Types of experiment: overview, our subjects.
- › Criminology
- › Economics
- › Geography
- › Health & Social Care
- › Psychology
- › Sociology
- › Teaching & learning resources
- › Student revision workshops
- › Online student courses
- › CPD for teachers
- › Livestreams
- › Teaching jobs
Boston House, 214 High Street, Boston Spa, West Yorkshire, LS23 6AD Tel: 01937 848885
- › Contact us
- › Terms of use
- › Privacy & cookies
© 2002-2024 Tutor2u Limited. Company Reg no: 04489574. VAT reg no 816865400.
ReviseSociology
A level sociology revision – education, families, research methods, crime and deviance and more!
Field Experiments in sociology
The practical, ethical and theoretical strengths and limitations of field experiments in comparison to lab experiments, relevant to sociology.
Table of Contents
Last Updated on February 24, 2023 by Karl Thompson
Field Experiments take place in real-life settings such as a classroom, the work place or even the high street. Field experiments are much more common in sociology than laboratory experiments. In fact sociologists hardly ever use lab experiments because the artificial environment of the laboratory is so far removed from real life that most sociologists believe that the results gained from such experiments tell us very little about how respondents would actually act in real life.
It is actually quite easy to set up a field experiment. If you wanted to measure the effectiveness of different teaching methods on educational performance in a school for example, all you would need to do is to get teachers to administer a short test to measure current performance levels, and then get them to change one aspect of their teaching for one class, or for a sample of some pupils, but not for the others, for a period of time (say one term) and then measure and compare the results of all pupils at the end.
You need to know about field experiments for the research methods component of A-level sociology and the AQA exam board does seem to like setting exam questions on experiments!

The advantages of Field Experiments over Lab Experiments
Better external validity – The big advantage which field experiments obviously have better external validity than lab experiments, because they take place in normally occurring social settings.
Larger Scale Settings – Practically it is possible to do field experiments in large institutions – in schools or workplaces in which thousands of people interact for example, which isn’t possible in laboratory experiments.
The disadvantages of Field Experiments
It is not possible to control variables as closely as with laboratory experiments – With the Rosenthal and Jacobson experiment, for example we simply don’t know what else might have influenced the ‘spurting group’ besides ‘higher teacher expectations’.
The Hawthorne Effect (or Experimental Effect) may reduce the validity of results. The Hawthorne effect is where respondents may act differently just because they know they are part of an experiment. The Hawthorne Effect was a phrase coined by Elton Mayo (1927) who did research into workers’ productivity at the Western Electric Company’s Hawthorne plant. With the workers agreement (they knew that an experiment was taking place, and the purpose of the experiment), Mayo set about varying things such as lighting levels, the speed of conveyor belts and toilet breaks. However, whatever he did, the worker’s productivity always increased from the norm, even when conditions were worsened. He concluded that the respondents were simply trying to please the researcher. NB – The Hawthorne effect can also apply to laboratory experiments.
Practical Problems – Access is likely to be more of a problem with lab experiments. Schools and workplaces might be reluctant to allow researchers in.
Ethical Problems – Just as with lab experiments – it is often possible to not inform people that an experiment is taking place in order for them to act naturally, so the issues of deception and lack of informed consent apply here too, as does the issue of harm.
Rosenthal and Jacobson’s 1968 Field Experiment
Rosenthal and Jacobson’s classic 1968 field experiment on the effects of teacher expectations (aka Pygmalion in the Classroom) illustrates some of the strengths and limitation of this method
The aim of this research was to measure the effect of high teacher expectation on the educational performance of pupils.
Rosenthal and Jacobson carried out their research in a California primary school they called ‘Oak School’. Pupils were given an IQ test and on the basis of this R and J informed teachers that 20% of the pupils were likely ‘spurt’ academically in the next year. In reality, however, the 20% were randomly selected.
All of the pupils were re-tested 8 months later and he spurters had gained 12 IQ points compared to an average of 8.
Rosenthal and Jacobsen concluded that higher teacher expectations were responsible for this difference in achievement.
Limitations of the Experiment
Deception/ Lack of Informed Consent is an issue : In order for the experiment to work, R and J had to deceive the teachers about the real nature of the experiment, and the pupils had no idea what was going on.
Ethical problems : while the spurters seem to have benefited from this study, the other 80% of pupils did not, in fact it is possible that they were harmed because of the teachers giving disproportionate amounts of attention to the spurting group. Given that child rights and child welfare are more central to education today it is unlikely that such an experiment would be allowed to take place.
Reliability is a problem : while the research design was relatively simple and thus easy to repeat (in fact within five years of the original study this was repeated 242 times) the exact conditions are not possible to repeat – given differences between schools and the type and mixture of pupils who attend different schools.
Finally, it’s not possible to rule out the role of extraneous variables . Rosenthal and Jacobson claim that higher teacher expectation led to the higher achievement of the ‘spurters’ but they did not conduct any observations of this taking place. It may have been other factors.
Signposting and Related Posts
Seven Examples of Field Experiments in Sociology
An Introduction to Experiments in Sociology
Laboratory Experiments in Sociology
Are Chinese Teaching Methods the Best? – A Field Experiment in ‘tough teaching methods’ in the UK conducted in 2015.
Theory and Methods A Level Sociology Revision Bundle

If you like this sort of thing, then you might like my Theory and Methods Revision Bundle – specifically designed to get students through the theory and methods sections of A level sociology papers 1 and 3.
Contents include:
- 74 pages of revision notes
- 15 mind maps on various topics within theory and methods
- Five theory and methods essays
- ‘How to write methods in context essays’.
Share this:
- Share on Tumblr
7 thoughts on “Field Experiments in sociology”
- Pingback: Non-Participant Observation – ReviseSociology
- Pingback: Hoe passen we neurowetenschap toe in het klaslokaal? – Donders Wonders
- Pingback: How can we apply neuroscience findings in the classroom? – Donders Wonders
- Pingback: Experiments in Sociology – Revision Notes | ReviseSociology
- Pingback: Experiments in Sociology | ReviseSociology
- Pingback: Seven Examples of Field Experiments for Sociology | ReviseSociology
- Pingback: Laboratory Experiments: Definition, Explanation, Advantages and Disadvantages | ReviseSociology
Leave a Reply Cancel reply
This site uses Akismet to reduce spam. Learn how your comment data is processed .
Discover more from ReviseSociology
Subscribe now to keep reading and get access to the full archive.
Continue reading

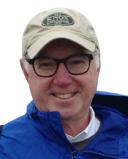
How to Get Started on Your First Psychology Experiment
Acquiring even a little expertise in advance makes science research easier..
Updated May 16, 2024 | Reviewed by Ray Parker
- Why Education Is Important
- Find a Child Therapist
- Students often struggle at the beginning of research projects—knowing how to begin.
- Research projects can sometimes be inspired by everyday life or personal concerns.
- Becoming something of an "expert" on a topic in advance makes designing a study go more smoothly.
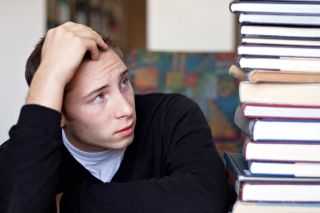
One of the most rewarding and frustrating parts of my long career as a psychology professor at a small liberal arts college has been guiding students through the senior capstone research experience required near the end of their college years. Each psychology major must conduct an independent experiment in which they collect data to test a hypothesis, analyze the data, write a research paper, and present their results at a college poster session or at a professional conference.
The rewarding part of the process is clear: The students' pride at seeing their poster on display and maybe even getting their name on an article in a professional journal allows us professors to get a glimpse of students being happy and excited—for a change. I also derive great satisfaction from watching a student discover that he or she has an aptitude for research and perhaps start shifting their career plans accordingly.
The frustrating part comes at the beginning of the research process when students are attempting to find a topic to work on. There is a lot of floundering around as students get stuck by doing something that seems to make sense: They begin by trying to “think up a study.”
The problem is that even if the student's research interest is driven by some very personal topic that is deeply relevant to their own life, they simply do not yet know enough to know where to begin. They do not know what has already been done by others, nor do they know how researchers typically attack that topic.
Students also tend to think in terms of mission statements (I want to cure eating disorders) rather than in terms of research questions (Why are people of some ages or genders more susceptible to eating disorders than others?).
Needless to say, attempting to solve a serious, long-standing societal problem in a few weeks while conducting one’s first psychology experiment can be a showstopper.
Even a Little Bit of Expertise Can Go a Long Way
My usual approach to helping students get past this floundering stage is to tell them to try to avoid thinking up a study altogether. Instead, I tell them to conceive of their mission as becoming an “expert” on some topic that they find interesting. They begin by reading journal articles, writing summaries of these articles, and talking to me about them. As the student learns more about the topic, our conversations become more sophisticated and interesting. Researchable questions begin to emerge, and soon, the student is ready to start writing a literature review that will sharpen the focus of their research question.
In short, even a little bit of expertise on a subject makes it infinitely easier to craft an experiment on that topic because the research done by others provides a framework into which the student can fit his or her own work.
This was a lesson I learned early in my career when I was working on my own undergraduate capstone experience. Faced with the necessity of coming up with a research topic and lacking any urgent personal issues that I was trying to resolve, I fell back on what little psychological expertise I had already accumulated.
In a previous psychology course, I had written a literature review on why some information fails to move from short-term memory into long-term memory. The journal articles that I had read for this paper relied primarily on laboratory studies with mice, and the debate that was going on between researchers who had produced different results in their labs revolved around subtle differences in the way that mice were released into the experimental apparatus in the studies.
Because I already had done some homework on this, I had a ready-made research question available: What if the experimental task was set up so that the researcher had no influence on how the mouse entered the apparatus at all? I was able to design a simple animal memory experiment that fit very nicely into the psychological literature that was already out there, and this prevented a lot of angst.
Please note that my undergraduate research project was guided by the “expertise” that I had already acquired rather than by a burning desire to solve some sort of personal or social problem. I guarantee that I had not been walking around as an undergraduate student worrying about why mice forget things, but I was nonetheless able to complete a fun and interesting study.

My first experiment may not have changed the world, but it successfully launched my research career, and I fondly remember it as I work with my students 50 years later.
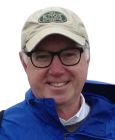
Frank McAndrew, Ph.D., is the Cornelia H. Dudley Professor of Psychology at Knox College.
- Find a Therapist
- Find a Treatment Center
- Find a Psychiatrist
- Find a Support Group
- Find Online Therapy
- United States
- Brooklyn, NY
- Chicago, IL
- Houston, TX
- Los Angeles, CA
- New York, NY
- Portland, OR
- San Diego, CA
- San Francisco, CA
- Seattle, WA
- Washington, DC
- Asperger's
- Bipolar Disorder
- Chronic Pain
- Eating Disorders
- Passive Aggression
- Personality
- Goal Setting
- Positive Psychology
- Stopping Smoking
- Low Sexual Desire
- Relationships
- Child Development
- Self Tests NEW
- Therapy Center
- Diagnosis Dictionary
- Types of Therapy
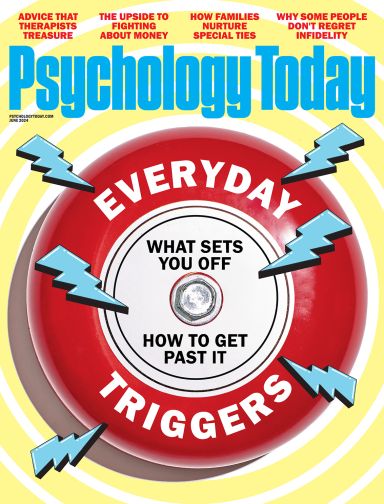
At any moment, someone’s aggravating behavior or our own bad luck can set us off on an emotional spiral that threatens to derail our entire day. Here’s how we can face our triggers with less reactivity so that we can get on with our lives.
- Emotional Intelligence
- Gaslighting
- Affective Forecasting
- Neuroscience
Risk–return preferences, gender inequalities and the moderating role of a counselling intervention on choice of major: evidence from a field and survey experiment
- Open access
- Published: 14 May 2024
Cite this article
You have full access to this open access article
- Lukas Fervers ORCID: orcid.org/0000-0002-7850-700X 1 , 2 ,
- Marita Jacob 2 ,
- Janina Beckmann 3 &
- Joachim G. Piepenburg 1 , 4
38 Accesses
Explore all metrics
In this study, we examine gender inequalities in educational decision-making. Specifically, we consider high school students selecting a higher education study programme and examine gender-specific risk and return preferences regarding monetary returns and the risk of failure in the programme. Moreover, we assess whether a counselling intervention can mitigate these gender inequalities. We employ a research design that combines a factorial survey and a field experiment to test our hypotheses. Consistent with our theoretical expectations, the results of the factorial survey confirm that girls are disproportionally deterred by the higher failure rates of possible study programmes, whereas boys are attracted more strongly by higher expected returns after graduation. Overall, the counselling intervention reduces the dissuasive effect of higher failure rates. Contrary to our expectations, the moderating effect is not stronger for girls but (if at all) is stronger for boys.
Similar content being viewed by others
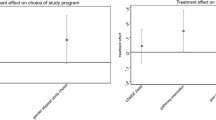
Do students need more information to leave the beaten paths? The impact of a counseling intervention on high school students’ choice of major
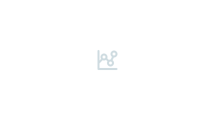
Gender, motivation and labour market beliefs in higher education choices
Missing men: determinants of the gender gap in education majors.
Avoid common mistakes on your manuscript.
Introduction
Gender differences in the choice of major occur in almost all industrialized countries (Barone, 2011 ). One frequently observed pattern is the overrepresentation of women in fields such as humanities and pedagogics, whereas men are overrepresented in science, technology, engineering and mathematics, with the proportion of one gender reaching more than 90% in some study programmes (Destatis, 2019 ; Liu & Zuo, 2019 ). These gender inequalities in the choice of major have consequences that transcend the educational system itself, as educational choices are important determinants of future employment and earnings (Gerber & Cheung, 2008 ; Horowitz, 2018 ; Jacob & Klein, 2019 ; Smyth, 2005 ). Consequently, a large body of literature on higher education has investigated the link between gender and field of study intention and choice (Bieri et al., 2016 ; Jonsson, 1999 ; Mann & Diprete, 2013 ; Morgan et al., 2013 ; Ochsenfeld, 2016 ; Reimer & Steinmetz, 2009 ; Silander et al., 2022 ; Xie et al., 2015 ), as well as the degree to which interventions can mitigate these gender differences (Barone et al., 2019 ; Finger et al., 2020 ; Scheeren et al., 2018 ).
This paper adds to this discussion in two ways. First, we build on psychological research that consistently documents gendered differences in risk aversion and return preferences (Niederle & Vesterlund, 2007 ; Niederle & Vesterlund, 2011 ; Paola & Gioia, 2012 ; Sutter et al., 2016 ; Sutter & Glätzle-Rützler, 2014 ) and argue that these differences may partly explain gendered differences in higher education choices. This argument is motivated by descriptive evidence showing a strong correlation between the risk–return profile of a certain study programme and the proportion of men to women in the programme. Study programmes with a high share of male students, such as engineering or computer science, offer the most favourable employment prospects after graduation but are also characterized by very high failure rates, while study programmes with a high share of female students often show the exact opposite pattern (for data on Germany, see Neugebauer et al., 2019 ).
Second, we assess whether the role of risk and return preferences permits policy interventions to mitigate gender differences. If a high (perceived) risk of failure deters female students from pursuing study programmes that yield higher employment prospects, counselling that strengthens self-confidence and fosters problem-solving skills for study-related difficulties could increase enrolment in these types of study programmes, thus reducing gender disparities in the choice of major. As girls tend to underestimate their skills, particularly regarding ambitious educational paths, counselling could indirectly mitigate gender differences by correcting these gender-biased ability beliefs, which have been documented by several studies (Marshman et al., 2018 ; mathematics, Perez-Felkner et al., 2017 ).
Methodologically, neither question is trivial to answer. First, study programmes differ in many respects beyond their risk–return profiles. Therefore, the observed correlation between risk–return profile and the share of male and female students may be spurious. Second, drawing causal inferences on the moderating effects of counselling may be complicated by endogenous self-selection into counselling programmes, which undermines the comparability between participants and non-participants (Imbens & Rubin, 2015 ). Therefore, we conducted a survey experiment (factorial survey) embedded in a field experiment. In the first step, we recruited high school students and divided them into treatment and control group. The treatment group was invited to participate in a counselling workshop, while the control group was compensated through participation in a prize draw. In the second step, we conducted a factorial survey a few months after the workshop and prize draw. We asked participants to rate the attractiveness of study programmes, experimentally varying the failure rates and expected income after graduation while holding all other parameters constant. This research design enabled us to assess whether risk–return profiles exert different influences on boys versus girls and identify the possible mitigating role of the counselling workshop.
Theory and related work
Risk, return and the role of counselling.
Rational choice theory suggests that individuals will select the educational pathways promising the highest utility. Two major determinants of utility are the (perceived) benefits of a certain choice ( return ) and the probability of success ( risk ) . Individuals will, therefore, opt for an educational pathway that maximizes utility by offering the most favourable risk–benefit ratio (Breen et al., 2014 ; Breen & Goldthorpe, 1997 ; Gabay-Egozi et al., 2010 ; Tutić, 2017 ). Building on and extending this framework, we argue that individual utility depends not only on objectively measured risk and returns but also on individual risk and return preferences. For example, risk-averse individuals are more likely to refrain from pursuing challenging educational paths (such as those with high failure rates), even with accurate information. As laboratory experiments in economics and psychology indicate substantially higher risk aversion among women (e.g. Niederle & Vesterlund, 2007 ), women may be more strongly deterred by fields of study that present a higher risk of failure. Similarly, men could be disproportionately attracted by higher returns as they tend to emphasize income and career (Busch-Heizmann, 2015 ; Jurczyk et al., 2019 ; Wolter et al., 2019 ).
This raises the role of counselling. We suggest that interventions could (indirectly) mitigate perceived risks by fostering students’ confidence in their abilities and discussing problem-solving strategies to address study-related difficulties. This could lower the perceived risk of failure in a certain study programme, thereby mitigating the deterring effect of higher failure rates and encouraging riskier and, possibly, more rewarding choices (we provide a detailed description of the content of the intervention in the “ The intervention ” section). While interventions to lower perceived risk could be expected to increase the probability of making risky choices for both boys and girls, there are reasons to expect that the effect will be larger for girls. First, as girls display lower average levels of risk affinity than boys, they may profit more from counselling due to ceiling effects at the top of the risk-affinity distribution. Second, girls might be more responsive to feedback regarding their risk evaluations, irrespective of their starting level, because they generally underestimate their competencies in various ambitious study programmes, such as those in STEM fields, compared to boys (see, e.g. physics, Marshman et al., 2018 ; mathematics, Perez-Felkner et al., 2017 ), leaving more room to correct their downward-biased risk perceptions. Therefore, boys and girls are expected to become more similar in their subjective evaluations of a given choice, which would contribute to less gendered choices of major. In this study, we focus on risk, as our counselling approach targets risk preferences and perceptions rather than return preferences. In sum, we hypothesize that:
(H1) Girls are more strongly guided by risk attributes while boys emphasize return characteristics when making educational choices.
(H2) Participation in counselling interventions mitigates the negative impact of higher risk on individual utility.
(H3) The effect of a counselling intervention on taking riskier choices will be stronger for girls.
Related work
At a general level, this paper contributes to several fields, including gender inequalities in higher education (Buchmann et al., 2008 ; Cech et al., 2011 ; Herd et al., 2019 ; Mann & Diprete, 2013 ; Morgan et al., 2013 ; Schwerter & Ilg, 2021 ), as well as the role of risk and return preferences or personality traits for study and career choices (Breen et al., 2014 ; Buser et al., 2014 ; Chen & Simpson, 2015 ; Daniel & Watermann, 2018 ; Finger, 2016 ; Sanabria & Penner, 2017 ; Sax et al., 2017 ). More specifically, we add to the policy-oriented literature that assesses the effects of interventions on gendered differences in higher education choices. Previous research in this field has mostly provided short info-treatments on actual risks and returns, seeking to correct social or gender-specific misperceptions about the risks and returns of certain educational pathways (Barone et al., 2016 , 2019 ; Bleemer & Zafar, 2018 ; Callender & Melis, 2022 ; Ehlert et al., 2017 ; Evans & Boatman, 2018 ; Finger et al., 2020 ; French & Oreopoulos, 2017 ; Herbaut & Geven, 2020 ; Ruder & van Noy, 2017 ). While these interventions appear to reduce social inequalities with respect to socioeconomic background (for a review, see French & Oreopoulos, 2017 ), there is no evidence that they reduce gender inequalities. If at all, the effect appears to be stronger for boys. One reason for this finding could be that perceptions of actual risk and returns do not differ between genders, even without counselling workshops (as Barone et al., 2019 , reported). Consequently, the approach of correcting gender-specific concepts may be of limited utility. At the same time, our theoretical reasoning suggests that men and women may differ more strongly in their risk and return preferences rather than their knowledge of actual risks and returns. Therefore, we extend the previous research by analysing the impact of an intervention that (indirectly) targets the perceived risk of failure.
Research design
Essentially, our research design combines a survey and field experiment. The field experiment consists of a counselling workshop, with participants randomly assigned into treatment and control group (randomized controlled trial, RCT), while the survey experiment consists of a factorial survey conducted after the counselling workshops. For the RCT, we recruit high school students from the area surrounding two large German cities who are between 6 and 18 months from graduating high school (i.e. attaining the German higher education entrance diploma, Abitur ). During recruitment, we point out that participation would consist of multiple online surveys with monetary compensation (10 euros each). Moreover, we indicate that a randomly allocated subset of study participants would be invited to participate in a university guidance and counselling workshop offered by the Department for Student Services. Those who were not offered the workshop would take part in a raffle where five prizes of 100 euros could be won in addition to the monetary compensation for the surveys. The recruiting strategy for our study was similar to the approach typically employed for such workshops, e.g. providing flyers to high school students or contacting schools to relay information on guidance workshops to their students. Therefore, the resulting sample closely mirrors the target group that would usually participate in such interventions.
After registering for the study, participants complete an online questionnaire (first wave of the survey) that mostly consists of pre-treatment covariates. At the end of the first survey, participants are randomly assigned to either the treatment or control group, and only members of the treatment group were offered places in a 1-day workshop. Between 3 and 6 months after the end of the first survey, participants are invited to complete a second online survey, which contains a survey experiment assessing the importance of risk and return characteristics for study programme choice (see Fig. 1 ). This setup enables us to analyse whether the importance of risk and return varies between genders, whether participation in the workshops has a moderating effect on the importance of risk, and whether this moderating effect is stronger for girls. We summarize the research design in Fig. 1 and outline its components in more detail in the following sections.
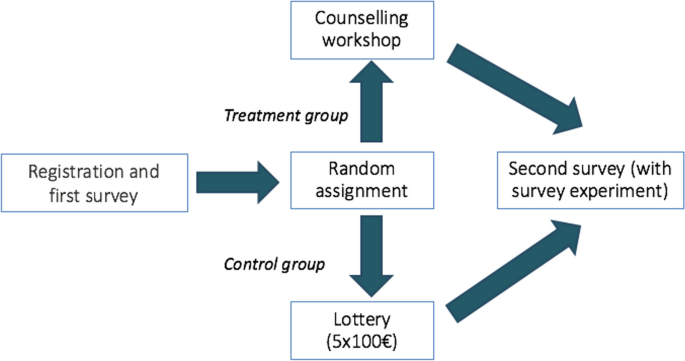
Visualization of research design
The intervention
The intervention consists of a 1-day counselling workshop and takes place between the two survey waves. The workshop was conducted by professional counsellors from two universities and consists of two modules offering general information as well as psychological counselling. First, students complete three exercises designed to increase their awareness of their cognitive abilities, occupational interests and personal values. For example, they receive individual feedback on a self-assessment of their cognitive abilities that they completed before the workshop. These exercises constituted the basis of the discussion about suitable majors for the students. Second, students receive general information about study opportunities, such as the differences between universities and universities of applied sciences or access to reliable sources of information. More importantly in our context, the third part of the workshop consists of psychological counselling dedicated to strategies and resources for handling problems in possible study programmes; this stage includes an already enrolled student. The student provided examples of their experiences along with the difficulties they encountered during the study programme and described strategies to handle those issues. Additional individual exercises and group work encouraged participants to think about how to handle difficulties by discussing their past experiences and future applications. The general notion conveyed by the intervention is that while studying may present several challenges and demands, students should not be deterred from pursuing their preferred study programmes, as resources and problem-solving strategies are available to help overcome difficulties. The workshop did not target a specific study programme but emphasized that participants should choose based on their preferences.
Consequently, workshop participation can alleviate the deterrent effect of a higher average failure rate because participants become aware of the resources and strategies available to them in case of difficulties. In addition, they will have encountered an experienced student who has overcome difficulties encountered during their studies, increasing the credibility of the information they receive.
The factorial survey
The factorial survey was conducted in the second survey wave, after the intervention. It was designed to vary the risk–return pattern of potential study programmes while keeping all other factors fixed. This allows us to disentangle the impact of risk and return characteristics on the attractiveness of a certain choice from possible confounders. To increase the robustness of our findings, we ask two types of questions to observe participants’ risk and return preferences. We use a factorial survey offering different vignettes of risk and return profiles and asking participants to rate the different programmes, as well as a choice question that asks participants to decide between specific study programmes. We adopt these two approaches to confirm whether our results could be sensitive to different survey designs.
In the vignette study, we present participants with the following scenario and ask the following question:
"Imagine that you already know which major you wish to study. The major is offered at three universities nearby. The study programs differ with respect to dropout rates and expected income after graduation. Assume you can study your preferred study program at University (A/ B/ C). The average income after graduation is (32,000 / 43,000 / 54,000€), the failure rate is (22 / 31 / 40%). On a scale from 0 to 10, how likely will you apply for this study program?"
By stating that the participants can study their preferred major at some university not further specified, we reassure that systematic variations in programme attractiveness reflect only the differences in risk and return outlined in the description of the study programme. This, therefore, fulfils our goal of creating exogenous variation in the risk–return pattern of a certain study programme that is not confounded by other characteristics, such as gender-specific vocational interests. Importantly, the experiment is also not confounded by the participants’ actual study intentions because the experimental design rules out factors such as students with more ambitious study intentions or different return preferences receiving higher values in their vignettes. While the effects may vary between students depending on their study intentions, the estimation of the average effect across the sample remains unaffected. At the same time, the intervention may affect study intentions; therefore, participants from the treatment group might have a slightly different set of study programmes in mind. However, the analysis relies on within-respondent variation (the fixed-effects analyses exclusively assess within-respondent variation), implying that survey participants have the same major in mind when responding to the vignettes.
Every participant responded to three vignettes. As Table 1 shows, we use three levels for the two dimensions, “risk” and “return”, generating a vignette universe of nine. We follow an algorithm suggested by Nguyen ( 2001 ) to assign the vignettes to three blocks. The exact blocking used in this study is presented in Table A.5 . Each participant is randomly assigned to one block. As we are particularly interested in the interaction between the vignette dimensions and individual-level variables (treatment status and gender), we could limit the vignette dimensions to two options with three levels each.
As a second measure of participants’ risk and return preferences, we asked participants a simple choice question:
“Assume you can study your preferred study program at University A and University B. At University A, the failure rate is 27%, the average income after completion is 34,000€. At University B, the failure rate is 39%, the average income after completion 47,000€.”
Which option do you prefer?
The choice question also fulfils our main purpose of creating exogenous variation in the risk–return profile. Varying risk and return simultaneously prevents the disentangling of the dimensions and tests them jointly. As this question focuses on both dimensions simultaneously, it is less relevant to the analysis of the treatment, which was not intended to affect return preferences. Nevertheless, the choice question is a reasonable method to assess the role of gender in choosing high-risk high-return vs. low-risk low-return programmes.
Data and variables
Our approaches produce different data structures. The vignette study produces a clustered dataset with vignettes clustered by person, with each observation including the corresponding rating and vignette variables (risk/return). The choice question results in a cross-section dataset including a variable of choosing University A or B (covariates are measured before the start of the treatment, and the survey experiment was conducted afterwards. As most of the covariates measured before the treatment are time-invariant, we are de facto dealing with a cross-section dataset including a variable of choosing University A or B). Both datasets contain an exogenous treatment indicator, an indicator for actual treatment status (not identical due to non-compliance) and the covariates measured in survey wave one, i.e. before participation in the counselling workshop. These include participants’ age in months, gender, school grade in maths and German and parents’ educational background (a dummy variable indicating whether both parents hold university degrees). Moreover, we coded two dummy variables for household composition (living with both parents and having siblings) and the share of schoolmates who planned to enter university. Finally, we included an indicator for the self-reported start of gathering information on study opportunities. As this is an experiment, controlling for confounders is less relevant for the treatment effect estimations, but it may be helpful to assess how sensitive the gender effect is to the inclusion of covariates.
In total, 725 high school students registered for our study and completed the initial survey before being assigned to experimental groups. Of those, 608 students participated in the second wave, which is a response rate of more than 80%. Panel attrition was strongly reduced by inviting participants via e-mail, text message and up to 20 telephone calls. The remaining panel attrition was mostly limited to participants with invalid phone numbers or who never answered the phone. Data cleaning (e.g. due to missing information on important covariates) reduced the sample to 580 cases. Due to randomization, no selection bias towards treatment (i.e. covariate imbalance) is apparent. A summary of the participants’ descriptive statistics is provided in Table A.1 . The two most remarkable findings are a strong overrepresentation of girls (about 75%) and, on average, a certain preference for high-risk high-return choices (also about 75%). The overrepresentation of girls is consistent with experiences from similar workshops, reinforcing that we are utilizing a sample that is similar to the real-world conditions of such workshops but not representative of all students. Moreover, we must account for two-sided non-compliance in the estimations (see the “ Estimation, inference and robustness checks ” section), although the compliance rates are rather high (almost 80% in the treatment group and more than 95% in the control group).
Estimation, inference and robustness checks
We made our estimates in two steps. First, we relied on the experimental vignette study and the choice question to describe gendered differences in risk and return preferences. In the second step, we jointly considered the results from the survey and the field experiment to assess the counselling workshop’s potential to reduce the impact of risk preferences on students’ study programme choices. This allows our estimation strategy to account for the peculiarities of the two experiments’ respective data structures.
Gender and risk/return
To analyse gendered differences in risk–return preferences, we start by analysing the data from the vignette study. To do so, we run random effects multilevel regressions to account for the nested data structure of ratings on vignette variables (risk/return) and covariates. To assess the differences in the importance of risk/return between genders, we additionally insert cross-level interaction terms between gender and risk/return.
As stated in the previous section, we confirmed the robustness of our approach with the choice question. Due to the cross-sectional data structure, we simply regressed the dummy variable indicating the high-risk high-return option on gender using linear probability models (LPM) with robust standard errors. To assess the sensitivity of the effect of gender, we run additional regressions with different sets of control variables.
The moderating role of the counselling workshop
In the second part of the analysis, we focus on the moderating role that treatment participation plays in students’ risk preferences. To do so, we focus on the factorial survey, as the workshop is expected to affect risk preferences but not return preferences. Therefore, we do not expect an impact on the binary choice question, although we briefly report the results of that analysis as well. Generally speaking, the approach for analysing the moderating role is very similar to the first part, except we exchange the risk/return × gender interaction for the risk × treatment interaction (see Eq. 2 ).
Here, we have to address an endogeneity issue caused by two-sided non-compliance; that is, a certain proportion of the invited participants did not attend the workshop (about 20% of those who were assigned to the treatment group), while ten participants who were assigned to the control group achieved placement in the workshops by registering for the study multiple times (non-compliance rate 3%). Excluding these participants completely would have biased the results, as (apparently) particularly motivated participants from the control group would have been excluded, generating a correlation between treatment assignment and unobserved confounders (per protocol analysis; Imbens & Rubin, 2015 ). However, actual treatment status is not randomized anymore and therefore possibly endogenous. Therefore, we use treatment assignment and the treatment assignment × risk interaction as instruments for actual treatment status and the treatment status × risk interaction, and estimate Eq. 2 by generalized least squares instrumental variable (GLS-IV) estimation (Imbens & Rubin, 2015 ). We estimated this equation for the whole sample as well as for both genders separately to analyse gendered differences in the moderating role of the treatment.
Robustness checks
To further substantiate the robustness of our results, we conduct two additional robustness checks. First, we conduct an intent-to-treat (ITT) analysis instead of our IV approach. This involves estimating the moderating effect of treatment assignment rather than actual treatment status to circumvent the problem of non-compliance. Second, we replace the GLS-IV regressions with FE-IV regressions to re-confirm the reliability of our research design.
Most importantly, we check whether endogenous panel attrition induces a correlation between treatment assignment and confounders among participants who participated in the second survey wave. To this end, we first run a selection regression (among all participants from wave 1) of participation in wave 2 on treatment assignment on all covariates. To further confirm whether attrition leads to covariate imbalance, we conduct a multivariate balancing test among second-wave participants by regressing treatment assignment on all covariates. Finally, we include a full set of treatment × covariate interactions in the selection regression to explicitly test for different selection patterns between groups.
Results and discussion
Risk, return and gender.
We start by presenting the first part of our analysis, the description of gendered differences in risk and return preferences. First, we look at descriptive statistics on the relationship between the vignette dimensions and the outcome. As Fig. 2 shows, the relationship follows the expected pattern. The left panel shows a decreasing average rating for higher failure rates. Similarly, the average rating strongly increases for higher expected income.
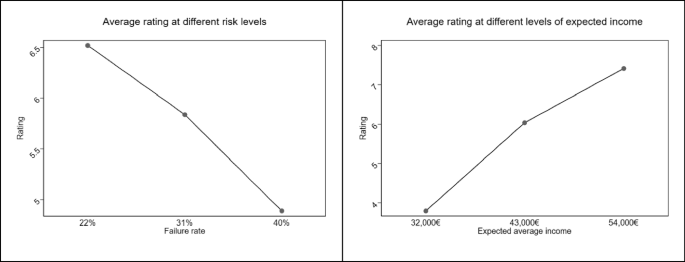
Descriptive results for the relation of vignette variables and the outcome
We proceed with the results of the regression analyses. Table 2 summarizes the results of the factorial survey. Models 1 and 2 present regressions on the rating of the vignette variables with (Model 2) and without (Model 1) covariates, while Model 3 includes the interaction terms. In all models, the vignette variables enter as dummy variables with the lowest category as the reference category.
Models 1 and 2 confirm that the effect of the vignette variables is significant at the 1% level. Theoretically speaking, the most interesting results are those from the interaction terms model. To facilitate interpretation, we present the coefficients in Fig. 3 .
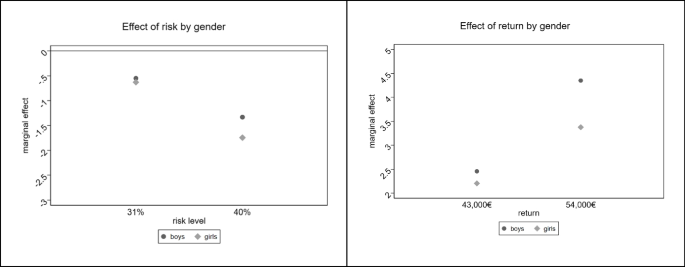
Visualization of gender × vignette interactions. The corresponding regression is displayed in Table 2 , model 3
The displayed coefficients show the estimated effect of moving from the lowest to the medium or highest category separately for both genders. For both dimensions, the observed gender differences are consistent with our theoretical expectations but differ in magnitude as well as statistical significance. As the left panel shows, boys and girls assign (ceteris paribus) lower ratings to study programmes with higher failure rates, but not to the same degree. While there is no difference in the medium category, the difference in the highest category confirms that girls react more strongly than boys to higher failure rates. The point estimates (see Table 2 ) imply a negative effect for boys of − 1.3, whereas the effect for girls is − 1.7. This amounts to a relative difference of about 30%, meaning the negative effect is 30% stronger for girls than boys. The interaction effect barely misses statistical significance ( t -value, − 1.49). The opposite picture is revealed for returns, as shown in the right panel. Once again, both genders assign higher ratings to study programmes with higher expected income, with larger gender differences in the highest category. The positive effect of going from the lowest to the highest category is 4.4 for boys, but only 3.4 for girls, which means that the effect is, again, about 30% greater for boys. While the gender difference revealed here is comparable to that for risk, the estimated difference is significant at the 1% level. Altogether, these results confirm the hypotheses that girls are deterred more strongly by higher failure rates, whereas boys are disproportionately attracted by higher income potential in their selection of specific study programmes.
To reaffirm the robustness of our results, we proceed with the results from the LPMs that regress the choice of the high-risk high-return option on gender plus different sets of covariates. The results are shown in Table 3 . The coefficient of female expresses the difference in the probability of choosing the high-risk high-return option, compared to boys, in percentage points. In all models, the effect is significant at the 1% level and the effect size ranges between 12 and 15% points. Notably, the effect does not consistently diminish when covariates are included, suggesting that gender differences are not driven by omitted (unobservable) variables. As expected, treatment status does not affect the chosen programme as it constitutes a combined risk and return measure and there is no theoretical reason to believe that the treatment affects return preferences. Taken together, both parts of our experiment confirm our first hypothesis, that risk and return preferences vary along gender lines and may play an important role in students’ choice of major.
The moderating effect of the treatment
We now examine whether the counselling intervention can mitigate the dissuasive effect of higher failure rates among high school students in general and girls in particular. We proceed by presenting the results from the risk-treatment interactions as outlined in Eq. ( 2 ). Table 4 displays the results for the entire sample (Model 1) as well as boys alone (Model 2) and girls alone (Model 3). We display the coefficients of the interaction term for Model 1, including both genders, in Fig. 4 .
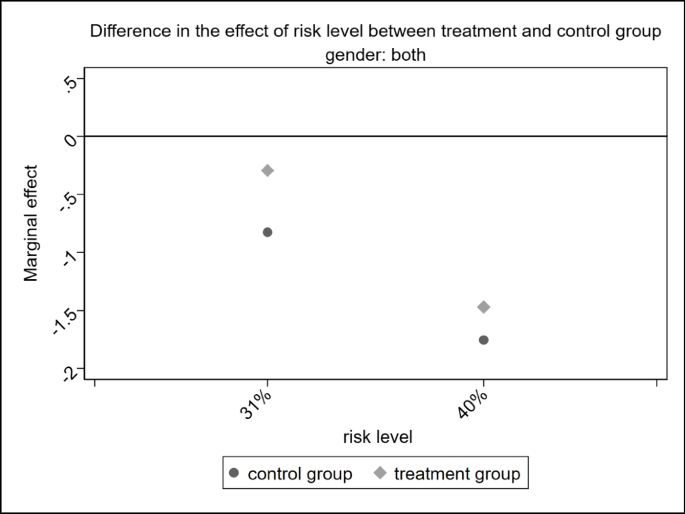
Mitigating role of counselling for the effect of risk aversion on rating (whole sample). Corresponding regression is given in Table 4 (model 1)
Participation in the counselling workshop appears to considerably mitigate the negative effect of higher failure rates, especially in the medium category. The negative risk effect of − 0.83 shrinks to − 0.29 with treatment participation, implying that the deterrent effect decreases to almost one-third of its original size (significant at the 10% level). This confirms our second hypothesis that the counselling workshop made participants less sensitive to higher failure rates for medium- vs. low-risk options. When interpreting the moderator, it should be noted that its maximum positive effect is limited by design, as higher failure rates can (ceteris paribus) rarely be regarded as positive. This demonstrates the volume of the estimated decrease.
We further display the coefficients from the regressions for both genders separately in Fig. 5 . As the left (girls) and right (boys) panels indicate, the intervention alleviated the negative effect of higher failure rates for both genders, once again especially in the medium category. However, the difference between the two groups belies our theoretical expectations. Contrary to our hypothesis, the difference in point estimates between the treatment and control groups is larger for boys for both risk categories. For example, for girls without treatment, the rating of a study programme decreases by 1.796 scale points when the risk increases from the lowest to the highest category. This deterrent effect slightly decreases to − 1.660 with treatment. In contrast, for boys, the deterrent effect decreases from 1.637 in the control group to 0.824 in the treatment group. This corresponds to a decrease of 0.28 standard deviations (SD = 2.91) for boys compared to a decrease of 0.05 standard deviations for girls (SD = 2.74). While this difference is non-negligible, it does not reach statistical significance due to the small number of cases in each subgroup and the overall small number of male students in our sample. It remains subject to future research whether significant differences can be found in larger samples.
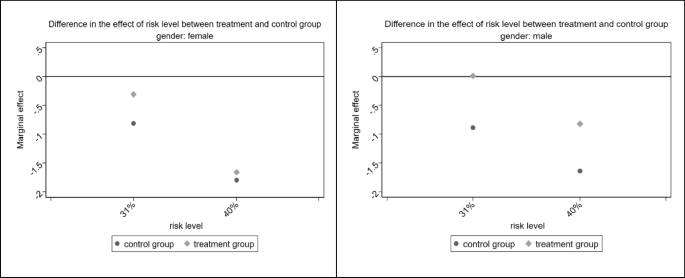
Moderating effect of treatment, separately by gender. Corresponding regressions are displayed in Table 4 (model 2 and 3)
Our robustness checks (ITT analysis and FE estimations, summarized in Tables A.2 and A.3) show that methodological choices play a minor role in our results. While the FE estimates rarely diverge from the results presented in this section, the ITT analyses vary in the expected way as the coefficients are smaller, although the significance levels are more or less unchanged. Moreover, panel attrition does not seem to induce confounding. While response rates are slightly higher in the treatment group (though not significant at the 5% level; Table A.4 , Model 1), a multivariate check on covariate imbalance among individuals who participated in the second survey wave shows that none of the covariates is related to treatment assignment (Model 2; the p -value of joint significance is 0.87). This is substantiated by selection regressions including interaction terms (Models 3 and 4), which show that the treatment and control groups follow very similar selection patterns. In sum, this implies that while we observed panel attrition, it did not induce confounding and does not, therefore, indicate biased treatment effect estimates. Unobserved confounding cannot be ruled out completely, but this seems unlikely in light of the results concerning the observed covariates.
Discussion and conclusion
This paper been inspired by consistent gendered differences in the choice of major in higher education, as well as previous research into interventions to mitigate these differences. We focused on seeking a theoretical explanation for gendered differences that would highlight the role of risk/return preferences. Subsequently, we have assessed the moderating impact of an intervention that does not focus on providing objective information about risks and returns but instead aims to strengthen students’ self-confidence in their abilities to mitigate the deterrent effect of higher failure rates. To answer these research questions, we employed a survey experiment combined with a field experiment.
Our results partly confirm and partly subvert our theoretical expectations. On one hand, female participants are indeed disproportionately deterred by higher failure rates, whereas male participants are disproportionately attracted by higher returns. Moreover, participation in the counselling workshop mitigate the deterrent effect of higher failure rates. On the other hand, and contrary to our expectations, the mitigating effect of the workshop is not stronger for girls, but, if at all, stronger for boys (but these differences are not statistically significant).
These results inform higher education research and indicate new directions for future research in three ways. First, and at the most abstract level, our results confirm the general notion outlined in rational choice approaches that utility considerations matter for educational choices. Moreover, the apparent gendered differences reveal that the evaluation of options depends heavily on the subjective evaluation of their risk–return patterns, implying that individual risk–return preferences matter even in cases of perfect information.
Second, our results add to the understanding of gender inequalities in educational decision-making in general, and particularly in higher education. As outlined in the introduction, the correlation between risk–return patterns and the proportion of male to female students in college majors is quite apparent at the aggregate level. However, causal claims about this relationship are hampered because the relevant study programmes differ in numerous other ways that may confound the relationship between risk–return patterns and student gender proportions. Our survey experiment, therefore, substantiates the argument that risk/return preferences might drive this relationship.
Finally, our results show that the importance of perceived risk and returns could be exploited by interventions designed to support students’ decisions. However, the results from the gender-specific analyses counter our theoretical expectations, as the counselling intervention exerted a stronger effect on boys than girls. There are multiple explanations for this unexpected result. On one hand, this may have resulted from a classical Matthew effect, in which people at the higher rather than the lower end of the risk-affinity distribution are pushed further upwards even heavier. On the other hand, these results also raise the question of whether we still lack a sufficient understanding of the mechanisms behind gender differences, as well as the heterogeneous effects of educational interventions on both genders. This argument is reinforced by the fact that we are not the first to observe effect heterogeneity that favours boys when stronger effects on girls were expected. For example, see other researchers’ results on the provision of objective information about risk and returns (Barone et al., 2019 ; Finger et al., 2020 ; Peters et al. 2023 ), curricular demands (Görlitz & Gravert, 2018 ; Jacob et al., 2020 ) or gendered responses to failure in “weed out courses” (Sanabria & Penner, 2017 ). In this regard, pursuing a deeper understanding of the gender-specific mechanisms of study choice, as well as the effect of educational interventions, should remain an important part of the higher education research agenda.
Despite its contributions to the field, our research design is not without limitations. First, offering the counselling workshop under real conditions is both an advantage and a disadvantage, as the findings concerning the importance of risk–return preferences do not necessarily generalize to the population of all students but rather to those who participate in similar interventions. (For further considerations of participation rates, see Pietrzyk & Erdmann, 2020 .) This reaffirms the external validity of the policy conclusions drawn concerning the moderating effect of the intervention. Moreover, our results align with existing research into gendered differences in risk and return preferences that relied on representative samples (Sanabria & Penner, 2017 ), suggesting that the observed differences in risk–return preferences are not merely due to sample selection. Second, we investigated intended rather than actual study choice. While the results presented by Buser et al. ( 2014 ) imply that intended choice may translate into actual study choice, future research must determine whether similar interventions actually lead to different study choices. Third, and relatedly, as we wanted students to evaluate different study programmes for their preferred major, it remains to be seen whether students would change their preferred major if their risk and return preferences changed. While we asked students to compare the same majors at different universities, it seems reasonable to assume that the effect would translate to comparisons of different majors. Finally, the notion of encouraging students to engage in more challenging and rewarding educational paths is often regarded positively in education research (Lent et al., 2018 ) as it may guide pupils to pursue study choices based on interest rather than anxiety. However, this does not guarantee their mastery of the resulting challenges. Therefore, future research should investigate whether the possible effect on study choice correlates with higher success and satisfaction during tertiary education.
In sum, our results reinforce the importance of both actual risk–return patterns and subjective perceptions, while also highlighting potential avenues for future research regarding long-term consequences and possible policy options.
Barone, C. (2011). Some things never change: Gender segregation in Higher Education across eight nations and three decades. Sociology of Education , 84 (2), 157–176. https://doi.org/10.1177/0038040711402099 .
Barone, C., Schizzerotto, A., Abbiati, G., & Argentin, G. (2016). Information barriers, social inequality, and plans for higher education: Evidence from a field experiment. European Sociological Review, 33 (1), 84–96. https://doi.org/10.1093/esr/jcw050
Article Google Scholar
Barone, C., Schizzerotto, A., Assirelli, G., & Abbiati, G. (2019). Nudging gender desegregation: A field experiment on the causal effect of information barriers on gender inequalities in higher education. European Societies, 21 (3), 356–377. https://doi.org/10.1080/14616696.2018.1442929
Bieri, F., Imdorf, C., Stoilova, R., & Boyadijeva, P. (2016). The Bulgarian Educational system and gender segregation in the labour market. European Societies, 18 (2), 158–179. https://doi.org/10.1080/14616696.2016.1141305
Bleemer, Z., & Zafar, B. (2018). Intended College Attendance: Evidence from an experiment on College returns and costs. Journal of Public Economics, 157 (C), 184–211. https://doi.org/10.1016/j.jpubeco.2017.11.002
Breen, R., & Goldthorpe, J. H. (1997). Explaining Educational differentials. Rationality and Society , 9 (3), 275–305. https://doi.org/10.1177/104346397009003002 .
Breen, R., van de Werfhorst, H. G., & Mads Meier, J. (2014). Deciding under doubt: A theory of risk aversion, Time Discounting preferences, and Educational decision-making. European Sociological Review , 30 (2), 258–270. https://doi.org/10.1093/esr/jcu039 .
Buchmann, C., DiPrete, T. A., & McDaniel, A. (2008). Gender inequalities in education. Annual Review of Sociology, 34 (1), 319–337. https://doi.org/10.1146/annurev.soc.34.040507.134719
Busch-Heizmann, A. (2015). Supply-side explanations for occupational gender segregation: Adolescents’ work values and Gender-(A)typical occupational aspirations. European Sociological Review , 31 (1), 48–64. https://doi.org/10.1093/esr/jcu081 .
Buser, T., Niederle, M., & Oosterbeek, H. (2014). Gender, competitiveness, and Career choices *. The Quarterly Journal of Economics, 129 (3), 1409–1447. https://doi.org/10.1093/qje/qju009
Callender, C., & Melis, G. (2022). The privilege of choice: How prospective College students’ financial concerns influence their choice of Higher Education Institution and subject of study in England. The Journal of Higher Education, 93 (3), 477–501. https://doi.org/10.1080/00221546.2021.1996169
Cech, E., Rubineau, B., Silbey, S., & Seron, C. (2011). Professional Role confidence and gendered persistence in Engineering. American Sociological Review, 76 (5), 641–666. https://doi.org/10.1177/0003122411420815
Chen, P., Daniel, & Simpson, P. A. (2015). Does Personality Matter? Applying Holland’s typology to Analyze Students’ Self-Selection into Science, Technology, Engineering, and Mathematics Majors. The Journal of Higher Education , 86 (5), 725–750. https://doi.org/10.1080/00221546.2015.11777381 .
Daniel, A., & Watermann, R. (2018). The role of Perceived benefits, costs, and probability of success in students’ plans for higher education. A quasi-experimental test of rational choice theory. European Sociological Review, 34 (5), 539–553. https://doi.org/10.1093/esr/jcy022
de Paola, M., & Gioia, F. (2012). Risk aversion and field of study choice: The role of individual ability. Bulletin of Economic Research, 64 (3), s193-209. https://doi.org/10.1111/j.1467-8586.2012.00445.x
Destatis (2019). GENESIS-Online Datenbank: Studierende: Deutschland, Semester, Nationalität, Geschlecht, Studienfach . https://www-genesis.destatis.de/genesis/online/data;sid=4E99294813B9C28601324B33819E6535.GO_2_1?operation=ergebnistabelleUmfang&levelindex=3&levelid=1569830665884&downloadname=21311-0003
Ehlert, M., Finger, C., Rusconi, A., & Solga, H. (2017). Applying to College: Do Information Deficits Lower the Likelihood of College-Eligible Students from Less-Privileged Families to Pursue Their College Intentions? Evidence from a Field Experiment. Social Science Research, 67 , 193–212. https://doi.org/10.1016/j.ssresearch.2017.04.005
Evans, B. J., & Boatman, A. (2018). Understanding how Information affects loan aversion: A Randomized Control Trial of Providing Federal Loan Information to High School seniors. The Journal of Higher Education, 90 (5), 800–832. https://doi.org/10.1080/00221546.2019.1574542
Finger, C. (2016). Institutional Constraints and the Translation of College Aspirations into Intentions—Evidence from a Factorial Survey. Research in Social Stratification and Mobility, 46 , 112–128. https://doi.org/10.1016/j.rssm.2016.08.001
Finger, C., Solga, H., Ehlert, M., & Rusconi, A. (2020). Gender differences in the choice of field of study and the relevance of income information. Insights from a field experiment. Research in Social Stratification and Mobility, 65 , 100457. https://doi.org/10.1016/j.rssm.2019.100457.
Peter, F., Schober, P., & Spiess, C. K. (2023). Information intervention on long-term earnings prospects and the gender gap in major choice. European Sociological Review, jcad055. https://doi.org/10.1093/esr/jcad055.
French, R., & Oreopoulos, P. (2017). Behavioral Barriers Transitioning to College. Labour Economics, 47 , 48–63. https://doi.org/10.1016/j.labeco.2017.05.005
Gabay-Egozi, L., Shavit, Y., & Yaish, M. (2010). Curricular choice: A test of a rational choice model of education. European Sociological Review, 26 (4), 447–463. https://doi.org/10.1093/esr/jcp031
Gerber, T. P., & Cheung, S. Y. (2008). Horizontal stratification in postsecondary education: Forms, explanations, and implications. Annual Review of Sociology, 34 (1), 299–318. https://doi.org/10.1146/annurev.soc.34.040507.134604
Görlitz, K., & Gravert, C. (2018). The effects of a High School Curriculum Reform on University Enrollment and the choice of College Major. Education Economics, 26 (3), 321–336. https://doi.org/10.1080/09645292.2018.1426731
Herbaut, E., & Geven, K. (2020). What works to reduce inequalities in higher education? A systematic review of the (quasi-) experimental literature on outreach and financial aid. Research in Social Stratification and Mobility, 65 , 100442. https://doi.org/10.1016/j.rssm.2019.100442.
Herd, P., Freese, J., Sicinski, K., Domingue, B. W., Harris, K. M., Wei, C., & Hauser, R. M. (2019). Genes, gender inequality, and Educational Attainment. American Sociological Review, 84 (6), 1069–1098. https://doi.org/10.1177/0003122419886550
Horowitz, J. (2018). Relative education and the advantage of a College Degree. American Sociological Review , 83 (4), 771–801. https://doi.org/10.1177/0003122418785371 .
Imbens, G. W., & Rubin, D. B. (2015). Causal inference for statistics, Social, and Biomedical sciences: An introduction . Cambridge University Press. https://doi.org/10.1017/CBO9781139025751 .
Jacob, M., & Klein, M. (2019). Social origin, field of study and graduates’ career progression: Does social inequality vary across fields? The British Journal of Sociology, 70 (5), 1850–1873. https://doi.org/10.1111/1468-4446.12696
Jacob, M., Iannelli, C., Duta, A., & Smyth, E. (2020). Secondary school subjects and gendered STEM enrollment in Higher Education in Germany, Ireland, and Scotland. International Journal of Comparative Sociology, 61 (1), 59–78. https://doi.org/10.1177/0020715220913043
Jonsson, J. O. (1999). Explaining sex differences in Educational Choice an Empirical Assessment of a rational choice model. European Sociological Review , 15 (4), 391–404. https://doi.org/10.1093/oxfordjournals.esr.a018272 .
Jurczyk, K., Jentsch, B., Sailer, J., & Schier, M. (2019). Female-breadwinner families in Germany: New gender roles? Journal of Family Issues , 40 (13), 1731–1754. https://doi.org/10.1177/0192513X19843149 .
Lent, R. W., Sheu, H. B., Miller, M. J., Cusick, M. E., Lee, T., Penn, & Truong, N. N. (2018). Predictors of Science, Technology, Engineering, and Mathematics Choice options: A Meta-Analytic path analysis of the Social-Cognitive choice model by gender and Race/Ethnicity. Journal of Counseling Psychology , 65 (1), 17–35. https://doi.org/10.1037/cou0000243 .
Liu, E. M., & Zuo, S. X. (2019). Measuring the impact of interaction between children of a matrilineal and a patriarchal culture on gender differences in risk aversion. Proceedings of the National Academy of Sciences, 116 (14), 6713–6719. https://doi.org/10.1073/pnas.1808336116
Mann, A., & DiPrete, T. A. (2013). Trends in gender segregation in the choice of Science and Engineering Majors. Social Science Research , 42 (6), 1519–1541. https://doi.org/10.1016/j.ssresearch.2013.07.002 .
Marshman, E. M., Kalender, Z. Y., Nokes-Malach, T., Schunn, C., & Singh, C. (2018). Female students with A’s have similar physics self-efficacy as male students with C’s in introductory courses: A cause for alarm? Physical Review Physics Education Research , 14 (2), 020123. https://doi.org/10.1103/PhysRevPhysEducRes.14.020123 .
Morgan, S. L., Gelbgiser, D., & Weeden, K. A. (2013). Feeding the Pipeline: Gender, Occupational Plans, and College Major Selection. Social Science Research , 42 (4), 989–1005. https://doi.org/10.1016/j.ssresearch.2013.03.008 .
Neugebauer, M., Heublein, U., & Daniel, A. (2019). Studienabbruch in Deutschland: Ausmaß, Ursachen, Folgen, Präventionsmöglichkeiten. Zeitschrift für Erziehungswissenschaft, 22 (5), 1025–1046. https://doi.org/10.1007/s11618-019-00904-1
Nguyen, N. K. (2001). Theory & methods: Cutting experimental designs into blocks. Australian & New Zealand Journal of Statistics , 43 (3), 367–374. https://doi.org/10.1111/1467-842X.00183 .
Niederle, M., & Vesterlund, L. (2007). Do Women Shy Away From Competition? Do Men Compete Too Much? The Quarterly Journal of Economics, 122 (3), 1067–1101. https://doi.org/10.1162/qjec.122.3.1067
Niederle, M., & Vesterlund, L. (2011). Gender and competition. Annual Review of Economics, 3 (1), 601–630. https://doi.org/10.1146/annurev-economics-111809-125122
Ochsenfeld, F. (2016). Preferences, constraints, and the process of sex segregation in College Majors: A choice analysis. Social Science Research , 56 (March), 117–132. https://doi.org/10.1016/j.ssresearch.2015.12.008 .
Perez-Felkner, L., Nix, S., & Thomas, K. (2017). Gendered pathways: How mathematics ability beliefs shape secondary and postsecondary course and degree field choices. Frontiers in Psychology , 386. https://doi.org/10.3389/fpsyg.2017.00386 .
Pietrzyk, I., & Erdmann, M. (2020). Investigating the impact of interventions on educational disparities: Estimating average treatment effects (ATEs) is not sufficient. Research in Social Stratification and Mobility, 65 , 100471. https://doi.org/10.1016/j.rssm.2019.100471.
Reimer, D., & Steinmetz, S. (2009). Educational specialisation and labour market risks of men and women in Spain and Germany. European Societies, 11 (5), 723–746. https://doi.org/10.1080/14616690802326400
Ruder, A. I., & Van Noy, M. (2017). Knowledge of earnings risk and major choice: Evidence from an information experiment. Economics of Education Review, 57 , 80–90. https://doi.org/10.1016/j.econedurev.2017.02.001
Sanabria, T., & Penner, A. (2017). Weeded out? Gendered responses to failing Calculus. Social Sciences (Basel Switzerland), 6 (2), 47. https://doi.org/10.3390/socsci6020047
Sax, L. J., Kathleen, J., Lehman, J. A., Jacobs, M., Allison Kanny, G., Lim, L., Monje-Paulson, & Zimmerman, H. B. (2017). Anatomy of an Enduring gender gap: The evolution of women’s participation in Computer Science. The Journal of Higher Education , 88 (2), 258–293. https://doi.org/10.1080/00221546.2016.1257306 .
Scheeren, Lotte, Herman, G., van de Werfhorst, & Bol, T. (2018). The gender revolution in Context: How later tracking in education benefits girls. Social Forces , 97 (1), 193–220. https://doi.org/10.1093/sf/soy025 .
Schwerter, J., & Ilg, L. (2021). Gender differences in the labour market entry of STEM graduates. European Journal of Higher Education, 0 (0), 1–19. https://doi.org/10.1080/21568235.2021.2010226
Silander, C., Haake, U., Lindberg, L., & Riis, U. (2022). Nordic Research on gender Equality in Academic careers: A Literature Review. European Journal of Higher Education, 12 (1), 72–97. https://doi.org/10.1080/21568235.2021.1895858
Smyth, E. (2005). Gender differentiation and early labour market integration across Europe. European Societies , 7 (3), 451–479. https://doi.org/10.1080/14616690500194084 .
Sutter, M., & Glätzle-Rützler, D. (2014). Gender differences in the willingness to Compete Emerge Early in Life and Persist. Management Science, 61 (10), 2339–2354. https://doi.org/10.1287/mnsc.2014.1981
Sutter, M., Glätzle-Rützler, D., Balafoutas, L., & Czermak, S. (2016). Cancelling out early age gender differences in competition: An analysis of policy interventions. Experimental Economics, 19 (2), 412–432. https://doi.org/10.1007/s10683-015-9447-y
Tutić, A. (2017). Revisiting the Breen–Goldthorpe Model of Educational Stratification. Rationality and Society , 29 (4), 389–407. https://doi.org/10.1177/1043463117734177 .
Wolter, I., Ehrtmann, L., Seidel, T., & Drechsel, B. (2019). Social or Economic goals? The professional goal orientation of students enrolled in STEM and Non-STEM majors in University. Frontiers in Psychology , 10 . https://doi.org/10.3389/fpsyg.2019.02065 .
Xie, Y., Fang, M., & Shauman, K. (2015). STEM Education. Annual Review of Sociology, 41 (1), 331–357. https://doi.org/10.1146/annurev-soc-071312-145659
Download references
Open Access funding enabled and organized by Projekt DEAL. This research received funding from the BMBF (Bundesministerium für Bildung und Forschung) Funding Priority—“Academic success and dropout phenomena” (funding code (FKZ): 01PX16004).
Author information
Authors and affiliations.
German Institute for Adult Education (DIE), Heinemannstraße 12-14, Bonn, 53175, Germany
Lukas Fervers & Joachim G. Piepenburg
Institute of Sociology and Social Psychology (ISS), University of Cologne, Universitätsstraße 24, Cologne, 50931, Germany
Lukas Fervers & Marita Jacob
Bundesinstitut für Berufsbildung (BIBB), Robert-Schuman-Platz 3, Bonn, 53175, Germany
Janina Beckmann
GESIS Leibniz Institute for the Social Sciences, B6 4-5, Mannheim, 68159, Germany
Joachim G. Piepenburg
You can also search for this author in PubMed Google Scholar
Corresponding author
Correspondence to Lukas Fervers .
Ethics declarations
Conflict of interest.
The authors declare no competing interests.
Additional information
Publisher’s note.
Springer Nature remains neutral with regard to jurisdictional claims in published maps and institutional affiliations.
Supplementary Information
Below is the link to the electronic supplementary material.
Supplementary Material 1
Rights and permissions.
Open Access This article is licensed under a Creative Commons Attribution 4.0 International License, which permits use, sharing, adaptation, distribution and reproduction in any medium or format, as long as you give appropriate credit to the original author(s) and the source, provide a link to the Creative Commons licence, and indicate if changes were made. The images or other third party material in this article are included in the article's Creative Commons licence, unless indicated otherwise in a credit line to the material. If material is not included in the article's Creative Commons licence and your intended use is not permitted by statutory regulation or exceeds the permitted use, you will need to obtain permission directly from the copyright holder. To view a copy of this licence, visit http://creativecommons.org/licenses/by/4.0/ .
Reprints and permissions
About this article
Fervers, L., Jacob, M., Beckmann, J. et al. Risk–return preferences, gender inequalities and the moderating role of a counselling intervention on choice of major: evidence from a field and survey experiment. High Educ (2024). https://doi.org/10.1007/s10734-024-01237-7
Download citation
Accepted : 03 May 2024
Published : 14 May 2024
DOI : https://doi.org/10.1007/s10734-024-01237-7
Share this article
Anyone you share the following link with will be able to read this content:
Sorry, a shareable link is not currently available for this article.
Provided by the Springer Nature SharedIt content-sharing initiative
- Choice of major
- Gender inequality
- Intervention
- Factorial survey
- Find a journal
- Publish with us
- Track your research
Quality Signaling and Demand for Renewable Energy Technology: Evidence from a Randomized Field Experiment
Solar technologies have been associated with private and social returns, but their technological potential often remains unachieved because of persistently low demand for high-quality products. In a randomized field experiment in Senegal, we assess the potential of three types of quality signaling to increase demand for high-quality solar lamps. We find no effect on demand when consumers are offered a money-back guarantee but increased demand with a third-party certification or warranty, consistent with the notion that consumers are uncertain about product durability rather than their utility. However, despite the higher willingness to pay, the prices they would pay are still well below market prices for the average household, suggesting that reducing information asymmetries alone is insufficient to encourage wider adoption. Surprisingly, we also find that the effective quality signals in our setting stimulate demand for low-quality products by creating product-class effects among those least familiar with the product.
The team is grateful to the joint Lighting Africa program of the World Bank and International Finance Cooperation and to the World Bank Energy & Extractives Global Practice for financial support and feedback during the impact-evaluation design and implementation. In particular, we thank Raihan Elahi and Olivier Gallou for their review of the initial design and guidance on Lighting Africa and Lighting Global materials and objectives. We also thank Ousmane Sarr (ASER), Michele Laleye (Total Senegal), and the World Bank Senegal Country Office team—including Chris Trimble, Manuel Berlengiero, Eric Dacosta, Micheline Moreira, and Aminata Ndiaye Bob—for support and recommendations throughout the project. The work was made possible by the excellent field and research assistance led by Marco Valenza and supported by Amadou Racine Dia. We also thank Kevin Winseck and seminar participants at Leibniz University Hannover, University of Passau, KDI School-World Bank DIME Conference (online), German Development Economics Conference (Stuttgart), NOVAFRICA Conference on Economic Development (Lisbon), and London School of Economics for valuable comments and suggestions. The findings, interpretations, and conclusions expressed in this paper are entirely those of the authors. They do not necessarily represent the views of the World Bank and its affiliated organizations, nor those of the executive directors of the World Bank, nor the governments they represent, nor the National Bureau of Economic Research.
MARC RIS BibTeΧ
Download Citation Data
More from NBER
In addition to working papers , the NBER disseminates affiliates’ latest findings through a range of free periodicals — the NBER Reporter , the NBER Digest , the Bulletin on Retirement and Disability , the Bulletin on Health , and the Bulletin on Entrepreneurship — as well as online conference reports , video lectures , and interviews .
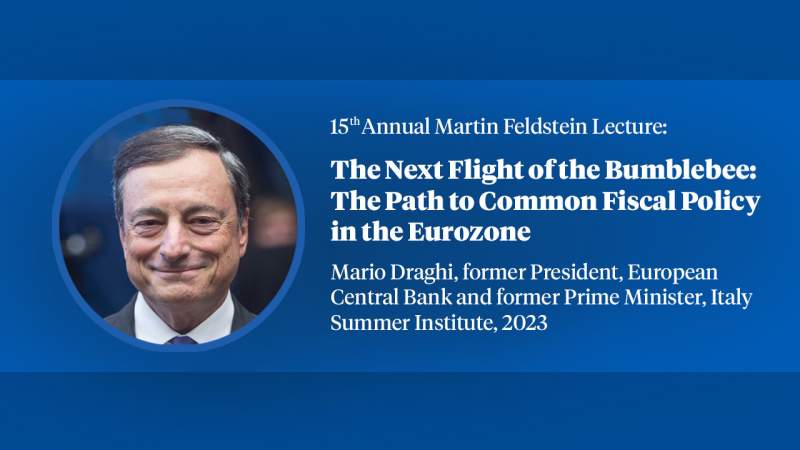

Alfalfa: Flexible amid drought and high in protein
Field day offers insight into latest research.
- by Trina Kleist
- May 15, 2024
As growers face continued reductions in water available to irrigate crops, and while the world needs more food produced and more protein in particular, alfalfa offers an attractive option. It yields remarkedly well under reduced irrigation, and its protein can be consumed by both animals and people.
Researchers are working to answer questions and solve obstacles that remain to its wider use, visitors learned at the UC Davis Small Grains/Alfalfa Field Day held May 9. A range of topics were covered:
Producing protein directly from alfalfa
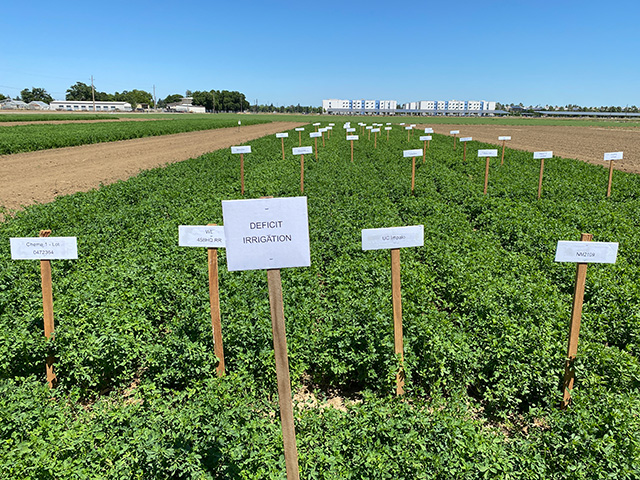
“Alfalfa is the highest-protein-producing crop in the United States on a per-acre basis,” said Dan Putnam, an alfalfa expert and professor emeritus of Cooperative Extension in the Department of Plant Sciences. “However, this protein is primarily leaf protein, compared with the protein produced in grain crops, which is easier to handle. Questions remain: Can we extract protein directly from alfalfa for human consumption as well as for animals other than cows?”
Many factors affect alfalfa’s yield and protein availability, including when it’s planted and harvested, the type of soil and when water is applied. The availability of the plant’s protein depends on who is eating it (cows have an easier time), how it’s harvested and handled and, for people, the protein extraction process.
In this research at UC Davis, researchers have planted six varieties of alfalfa to see how fall dormancy affects yield and the potential to extract protein. Samples were collected throughout 2023 and again in 2024. Scientists looked at different harvest times (from immature to more mature growth) and their effect on crude, true and soluble protein after immediate drying or liquid extraction.
Breeding addresses barriers to yield
Charlie Brummer and team explained the objectives of their plant breeding activities for alfalfa. They are experimenting with 10 varieties for potential release, with trials over several years in various locations. “The big issue has been lack of yield improvement… Why is that?” Brummer said.
Cree King discussed the on-going research to understand the basis of yield. Studies look at deficit irrigation, persistence, salinity tolerance, pest and disease resistance, plus genetic analysis to understand the basis of yield. Details are in this hand-out . King is a graduate student working with Brummer , a professor and alfalfa breeding expert, and Grey Monroe , an assistant professor with a focus on climate adaptation
Resilience to drought
While alfalfa is remarkedly resilient in times of drought, researchers are examining how different cultivars and breeding lines might respond when water is severely limited. Charles Janssen described his research to develop alfalfa with greater resilience amid highly variable water supplies. The goal is both to maximize yield under reduced water applications and to assure long-term root survival, so a drought-stricken plant can revive when water becomes available again. Details of the trials are in this hand-out . Janssen is a first-year graduate student working with Brummer and Putnam.
“We’re going have to continue to grow alfalfa under water-variable conditions,” Putnam added. He noted the severe to exceptional drought that has affected up to half of alfalfa-growing areas nationally in the past 12 years.
Yet, when partially irrigated, alfalfa still can produce good yields. That makes it a viable alternative to leaving the land fallow when water is scanty. Putnam discussed four key strategies for irrigating alfalfa amid drought, detailed in this hand-out .
Almond shells improve water infiltration
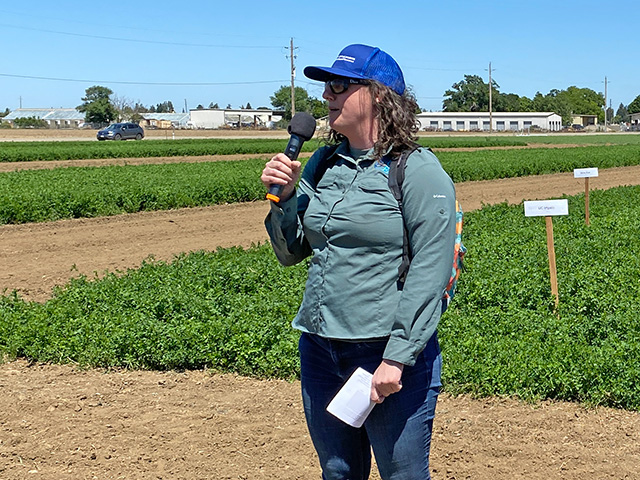
Sarah Light explained the benefits of putting almond shells on alfalfa fields: Unlike almond hulls, which are fed to dairy cows, almond shells are very high in carbon. They could improve soil carbon and soil health when applied to alfalfa fields, in addition to acting as mulch to conserve water.
In her research on farmer’s fields, Light found that yields did not differ between plots where the shells were applied and control areas with no shells. However, water infiltration improved in the plots with shells, and there was some indication of improved soil quality.
Light is a UC Cooperative Extension farm advisor in Sutter and Yuba counties. This field research was sponsored by UC Davis and UCCE Agriculture and Natural Resources; and funded by USDA-NIFA, the Alfalfa Checkoff program of the National Alfalfa and Forage Alliance, the California Alfalfa & Forage Association and donations by companies and individuals.
More resources
Get hand-outs from Small Grains/Alfalfa Field Day here .
Get more from the UC Alfalfa Research and Information Center here .
Media Resources
- Trina Kleist, UC Davis Department of Plant Sciences, [email protected], (530) 754-6148 or (530) 601-6846
Primary Category
Secondary categories.
Chapter 10 Experimental Research
Experimental research, often considered to be the “gold standard” in research designs, is one of the most rigorous of all research designs. In this design, one or more independent variables are manipulated by the researcher (as treatments), subjects are randomly assigned to different treatment levels (random assignment), and the results of the treatments on outcomes (dependent variables) are observed. The unique strength of experimental research is its internal validity (causality) due to its ability to link cause and effect through treatment manipulation, while controlling for the spurious effect of extraneous variable.
Experimental research is best suited for explanatory research (rather than for descriptive or exploratory research), where the goal of the study is to examine cause-effect relationships. It also works well for research that involves a relatively limited and well-defined set of independent variables that can either be manipulated or controlled. Experimental research can be conducted in laboratory or field settings. Laboratory experiments , conducted in laboratory (artificial) settings, tend to be high in internal validity, but this comes at the cost of low external validity (generalizability), because the artificial (laboratory) setting in which the study is conducted may not reflect the real world. Field experiments , conducted in field settings such as in a real organization, and high in both internal and external validity. But such experiments are relatively rare, because of the difficulties associated with manipulating treatments and controlling for extraneous effects in a field setting.
Experimental research can be grouped into two broad categories: true experimental designs and quasi-experimental designs. Both designs require treatment manipulation, but while true experiments also require random assignment, quasi-experiments do not. Sometimes, we also refer to non-experimental research, which is not really a research design, but an all-inclusive term that includes all types of research that do not employ treatment manipulation or random assignment, such as survey research, observational research, and correlational studies.
Basic Concepts
Treatment and control groups. In experimental research, some subjects are administered one or more experimental stimulus called a treatment (the treatment group ) while other subjects are not given such a stimulus (the control group ). The treatment may be considered successful if subjects in the treatment group rate more favorably on outcome variables than control group subjects. Multiple levels of experimental stimulus may be administered, in which case, there may be more than one treatment group. For example, in order to test the effects of a new drug intended to treat a certain medical condition like dementia, if a sample of dementia patients is randomly divided into three groups, with the first group receiving a high dosage of the drug, the second group receiving a low dosage, and the third group receives a placebo such as a sugar pill (control group), then the first two groups are experimental groups and the third group is a control group. After administering the drug for a period of time, if the condition of the experimental group subjects improved significantly more than the control group subjects, we can say that the drug is effective. We can also compare the conditions of the high and low dosage experimental groups to determine if the high dose is more effective than the low dose.
Treatment manipulation. Treatments are the unique feature of experimental research that sets this design apart from all other research methods. Treatment manipulation helps control for the “cause” in cause-effect relationships. Naturally, the validity of experimental research depends on how well the treatment was manipulated. Treatment manipulation must be checked using pretests and pilot tests prior to the experimental study. Any measurements conducted before the treatment is administered are called pretest measures , while those conducted after the treatment are posttest measures .
Random selection and assignment. Random selection is the process of randomly drawing a sample from a population or a sampling frame. This approach is typically employed in survey research, and assures that each unit in the population has a positive chance of being selected into the sample. Random assignment is however a process of randomly assigning subjects to experimental or control groups. This is a standard practice in true experimental research to ensure that treatment groups are similar (equivalent) to each other and to the control group, prior to treatment administration. Random selection is related to sampling, and is therefore, more closely related to the external validity (generalizability) of findings. However, random assignment is related to design, and is therefore most related to internal validity. It is possible to have both random selection and random assignment in well-designed experimental research, but quasi-experimental research involves neither random selection nor random assignment.
Threats to internal validity. Although experimental designs are considered more rigorous than other research methods in terms of the internal validity of their inferences (by virtue of their ability to control causes through treatment manipulation), they are not immune to internal validity threats. Some of these threats to internal validity are described below, within the context of a study of the impact of a special remedial math tutoring program for improving the math abilities of high school students.
- History threat is the possibility that the observed effects (dependent variables) are caused by extraneous or historical events rather than by the experimental treatment. For instance, students’ post-remedial math score improvement may have been caused by their preparation for a math exam at their school, rather than the remedial math program.
- Maturation threat refers to the possibility that observed effects are caused by natural maturation of subjects (e.g., a general improvement in their intellectual ability to understand complex concepts) rather than the experimental treatment.
- Testing threat is a threat in pre-post designs where subjects’ posttest responses are conditioned by their pretest responses. For instance, if students remember their answers from the pretest evaluation, they may tend to repeat them in the posttest exam. Not conducting a pretest can help avoid this threat.
- Instrumentation threat , which also occurs in pre-post designs, refers to the possibility that the difference between pretest and posttest scores is not due to the remedial math program, but due to changes in the administered test, such as the posttest having a higher or lower degree of difficulty than the pretest.
- Mortality threat refers to the possibility that subjects may be dropping out of the study at differential rates between the treatment and control groups due to a systematic reason, such that the dropouts were mostly students who scored low on the pretest. If the low-performing students drop out, the results of the posttest will be artificially inflated by the preponderance of high-performing students.
- Regression threat , also called a regression to the mean, refers to the statistical tendency of a group’s overall performance on a measure during a posttest to regress toward the mean of that measure rather than in the anticipated direction. For instance, if subjects scored high on a pretest, they will have a tendency to score lower on the posttest (closer to the mean) because their high scores (away from the mean) during the pretest was possibly a statistical aberration. This problem tends to be more prevalent in non-random samples and when the two measures are imperfectly correlated.
Two-Group Experimental Designs
The simplest true experimental designs are two group designs involving one treatment group and one control group, and are ideally suited for testing the effects of a single independent variable that can be manipulated as a treatment. The two basic two-group designs are the pretest-posttest control group design and the posttest-only control group design, while variations may include covariance designs. These designs are often depicted using a standardized design notation, where R represents random assignment of subjects to groups, X represents the treatment administered to the treatment group, and O represents pretest or posttest observations of the dependent variable (with different subscripts to distinguish between pretest and posttest observations of treatment and control groups).
Pretest-posttest control group design . In this design, subjects are randomly assigned to treatment and control groups, subjected to an initial (pretest) measurement of the dependent variables of interest, the treatment group is administered a treatment (representing the independent variable of interest), and the dependent variables measured again (posttest). The notation of this design is shown in Figure 10.1.

Figure 10.1. Pretest-posttest control group design
The effect E of the experimental treatment in the pretest posttest design is measured as the difference in the posttest and pretest scores between the treatment and control groups:
E = (O 2 – O 1 ) – (O 4 – O 3 )
Statistical analysis of this design involves a simple analysis of variance (ANOVA) between the treatment and control groups. The pretest posttest design handles several threats to internal validity, such as maturation, testing, and regression, since these threats can be expected to influence both treatment and control groups in a similar (random) manner. The selection threat is controlled via random assignment. However, additional threats to internal validity may exist. For instance, mortality can be a problem if there are differential dropout rates between the two groups, and the pretest measurement may bias the posttest measurement (especially if the pretest introduces unusual topics or content).
Posttest-only control group design . This design is a simpler version of the pretest-posttest design where pretest measurements are omitted. The design notation is shown in Figure 10.2.

Figure 10.2. Posttest only control group design.
The treatment effect is measured simply as the difference in the posttest scores between the two groups:
E = (O 1 – O 2 )
The appropriate statistical analysis of this design is also a two- group analysis of variance (ANOVA). The simplicity of this design makes it more attractive than the pretest-posttest design in terms of internal validity. This design controls for maturation, testing, regression, selection, and pretest-posttest interaction, though the mortality threat may continue to exist.
Covariance designs . Sometimes, measures of dependent variables may be influenced by extraneous variables called covariates . Covariates are those variables that are not of central interest to an experimental study, but should nevertheless be controlled in an experimental design in order to eliminate their potential effect on the dependent variable and therefore allow for a more accurate detection of the effects of the independent variables of interest. The experimental designs discussed earlier did not control for such covariates. A covariance design (also called a concomitant variable design) is a special type of pretest posttest control group design where the pretest measure is essentially a measurement of the covariates of interest rather than that of the dependent variables. The design notation is shown in Figure 10.3, where C represents the covariates:

Figure 10.3. Covariance design
Because the pretest measure is not a measurement of the dependent variable, but rather a covariate, the treatment effect is measured as the difference in the posttest scores between the treatment and control groups as:

Figure 10.4. 2 x 2 factorial design
Factorial designs can also be depicted using a design notation, such as that shown on the right panel of Figure 10.4. R represents random assignment of subjects to treatment groups, X represents the treatment groups themselves (the subscripts of X represents the level of each factor), and O represent observations of the dependent variable. Notice that the 2 x 2 factorial design will have four treatment groups, corresponding to the four combinations of the two levels of each factor. Correspondingly, the 2 x 3 design will have six treatment groups, and the 2 x 2 x 2 design will have eight treatment groups. As a rule of thumb, each cell in a factorial design should have a minimum sample size of 20 (this estimate is derived from Cohen’s power calculations based on medium effect sizes). So a 2 x 2 x 2 factorial design requires a minimum total sample size of 160 subjects, with at least 20 subjects in each cell. As you can see, the cost of data collection can increase substantially with more levels or factors in your factorial design. Sometimes, due to resource constraints, some cells in such factorial designs may not receive any treatment at all, which are called incomplete factorial designs . Such incomplete designs hurt our ability to draw inferences about the incomplete factors.
In a factorial design, a main effect is said to exist if the dependent variable shows a significant difference between multiple levels of one factor, at all levels of other factors. No change in the dependent variable across factor levels is the null case (baseline), from which main effects are evaluated. In the above example, you may see a main effect of instructional type, instructional time, or both on learning outcomes. An interaction effect exists when the effect of differences in one factor depends upon the level of a second factor. In our example, if the effect of instructional type on learning outcomes is greater for 3 hours/week of instructional time than for 1.5 hours/week, then we can say that there is an interaction effect between instructional type and instructional time on learning outcomes. Note that the presence of interaction effects dominate and make main effects irrelevant, and it is not meaningful to interpret main effects if interaction effects are significant.
Hybrid Experimental Designs
Hybrid designs are those that are formed by combining features of more established designs. Three such hybrid designs are randomized bocks design, Solomon four-group design, and switched replications design.
Randomized block design. This is a variation of the posttest-only or pretest-posttest control group design where the subject population can be grouped into relatively homogeneous subgroups (called blocks ) within which the experiment is replicated. For instance, if you want to replicate the same posttest-only design among university students and full -time working professionals (two homogeneous blocks), subjects in both blocks are randomly split between treatment group (receiving the same treatment) or control group (see Figure 10.5). The purpose of this design is to reduce the “noise” or variance in data that may be attributable to differences between the blocks so that the actual effect of interest can be detected more accurately.
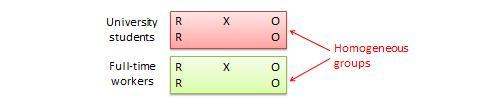
Figure 10.5. Randomized blocks design.
Solomon four-group design . In this design, the sample is divided into two treatment groups and two control groups. One treatment group and one control group receive the pretest, and the other two groups do not. This design represents a combination of posttest-only and pretest-posttest control group design, and is intended to test for the potential biasing effect of pretest measurement on posttest measures that tends to occur in pretest-posttest designs but not in posttest only designs. The design notation is shown in Figure 10.6.
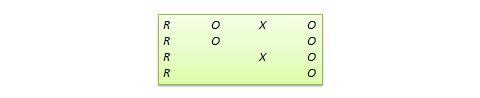
Figure 10.6. Solomon four-group design
Switched replication design . This is a two-group design implemented in two phases with three waves of measurement. The treatment group in the first phase serves as the control group in the second phase, and the control group in the first phase becomes the treatment group in the second phase, as illustrated in Figure 10.7. In other words, the original design is repeated or replicated temporally with treatment/control roles switched between the two groups. By the end of the study, all participants will have received the treatment either during the first or the second phase. This design is most feasible in organizational contexts where organizational programs (e.g., employee training) are implemented in a phased manner or are repeated at regular intervals.

Figure 10.7. Switched replication design.
Quasi-Experimental Designs
Quasi-experimental designs are almost identical to true experimental designs, but lacking one key ingredient: random assignment. For instance, one entire class section or one organization is used as the treatment group, while another section of the same class or a different organization in the same industry is used as the control group. This lack of random assignment potentially results in groups that are non-equivalent, such as one group possessing greater mastery of a certain content than the other group, say by virtue of having a better teacher in a previous semester, which introduces the possibility of selection bias . Quasi-experimental designs are therefore inferior to true experimental designs in interval validity due to the presence of a variety of selection related threats such as selection-maturation threat (the treatment and control groups maturing at different rates), selection-history threat (the treatment and control groups being differentially impact by extraneous or historical events), selection-regression threat (the treatment and control groups regressing toward the mean between pretest and posttest at different rates), selection-instrumentation threat (the treatment and control groups responding differently to the measurement), selection-testing (the treatment and control groups responding differently to the pretest), and selection-mortality (the treatment and control groups demonstrating differential dropout rates). Given these selection threats, it is generally preferable to avoid quasi-experimental designs to the greatest extent possible.
Many true experimental designs can be converted to quasi-experimental designs by omitting random assignment. For instance, the quasi-equivalent version of pretest-posttest control group design is called nonequivalent groups design (NEGD), as shown in Figure 10.8, with random assignment R replaced by non-equivalent (non-random) assignment N . Likewise, the quasi -experimental version of switched replication design is called non-equivalent switched replication design (see Figure 10.9).

Figure 10.8. NEGD design.

Figure 10.9. Non-equivalent switched replication design.
In addition, there are quite a few unique non -equivalent designs without corresponding true experimental design cousins. Some of the more useful of these designs are discussed next.
Regression-discontinuity (RD) design . This is a non-equivalent pretest-posttest design where subjects are assigned to treatment or control group based on a cutoff score on a preprogram measure. For instance, patients who are severely ill may be assigned to a treatment group to test the efficacy of a new drug or treatment protocol and those who are mildly ill are assigned to the control group. In another example, students who are lagging behind on standardized test scores may be selected for a remedial curriculum program intended to improve their performance, while those who score high on such tests are not selected from the remedial program. The design notation can be represented as follows, where C represents the cutoff score:

Figure 10.10. RD design.
Because of the use of a cutoff score, it is possible that the observed results may be a function of the cutoff score rather than the treatment, which introduces a new threat to internal validity. However, using the cutoff score also ensures that limited or costly resources are distributed to people who need them the most rather than randomly across a population, while simultaneously allowing a quasi-experimental treatment. The control group scores in the RD design does not serve as a benchmark for comparing treatment group scores, given the systematic non-equivalence between the two groups. Rather, if there is no discontinuity between pretest and posttest scores in the control group, but such a discontinuity persists in the treatment group, then this discontinuity is viewed as evidence of the treatment effect.
Proxy pretest design . This design, shown in Figure 10.11, looks very similar to the standard NEGD (pretest-posttest) design, with one critical difference: the pretest score is collected after the treatment is administered. A typical application of this design is when a researcher is brought in to test the efficacy of a program (e.g., an educational program) after the program has already started and pretest data is not available. Under such circumstances, the best option for the researcher is often to use a different prerecorded measure, such as students’ grade point average before the start of the program, as a proxy for pretest data. A variation of the proxy pretest design is to use subjects’ posttest recollection of pretest data, which may be subject to recall bias, but nevertheless may provide a measure of perceived gain or change in the dependent variable.

Figure 10.11. Proxy pretest design.
Separate pretest-posttest samples design . This design is useful if it is not possible to collect pretest and posttest data from the same subjects for some reason. As shown in Figure 10.12, there are four groups in this design, but two groups come from a single non-equivalent group, while the other two groups come from a different non-equivalent group. For instance, you want to test customer satisfaction with a new online service that is implemented in one city but not in another. In this case, customers in the first city serve as the treatment group and those in the second city constitute the control group. If it is not possible to obtain pretest and posttest measures from the same customers, you can measure customer satisfaction at one point in time, implement the new service program, and measure customer satisfaction (with a different set of customers) after the program is implemented. Customer satisfaction is also measured in the control group at the same times as in the treatment group, but without the new program implementation. The design is not particularly strong, because you cannot examine the changes in any specific customer’s satisfaction score before and after the implementation, but you can only examine average customer satisfaction scores. Despite the lower internal validity, this design may still be a useful way of collecting quasi-experimental data when pretest and posttest data are not available from the same subjects.
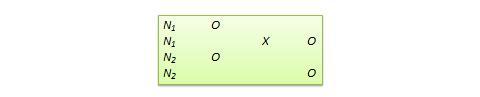
Figure 10.12. Separate pretest-posttest samples design.
Nonequivalent dependent variable (NEDV) design . This is a single-group pre-post quasi-experimental design with two outcome measures, where one measure is theoretically expected to be influenced by the treatment and the other measure is not. For instance, if you are designing a new calculus curriculum for high school students, this curriculum is likely to influence students’ posttest calculus scores but not algebra scores. However, the posttest algebra scores may still vary due to extraneous factors such as history or maturation. Hence, the pre-post algebra scores can be used as a control measure, while that of pre-post calculus can be treated as the treatment measure. The design notation, shown in Figure 10.13, indicates the single group by a single N , followed by pretest O 1 and posttest O 2 for calculus and algebra for the same group of students. This design is weak in internal validity, but its advantage lies in not having to use a separate control group.
An interesting variation of the NEDV design is a pattern matching NEDV design , which employs multiple outcome variables and a theory that explains how much each variable will be affected by the treatment. The researcher can then examine if the theoretical prediction is matched in actual observations. This pattern-matching technique, based on the degree of correspondence between theoretical and observed patterns is a powerful way of alleviating internal validity concerns in the original NEDV design.

Figure 10.13. NEDV design.
Perils of Experimental Research
Experimental research is one of the most difficult of research designs, and should not be taken lightly. This type of research is often best with a multitude of methodological problems. First, though experimental research requires theories for framing hypotheses for testing, much of current experimental research is atheoretical. Without theories, the hypotheses being tested tend to be ad hoc, possibly illogical, and meaningless. Second, many of the measurement instruments used in experimental research are not tested for reliability and validity, and are incomparable across studies. Consequently, results generated using such instruments are also incomparable. Third, many experimental research use inappropriate research designs, such as irrelevant dependent variables, no interaction effects, no experimental controls, and non-equivalent stimulus across treatment groups. Findings from such studies tend to lack internal validity and are highly suspect. Fourth, the treatments (tasks) used in experimental research may be diverse, incomparable, and inconsistent across studies and sometimes inappropriate for the subject population. For instance, undergraduate student subjects are often asked to pretend that they are marketing managers and asked to perform a complex budget allocation task in which they have no experience or expertise. The use of such inappropriate tasks, introduces new threats to internal validity (i.e., subject’s performance may be an artifact of the content or difficulty of the task setting), generates findings that are non-interpretable and meaningless, and makes integration of findings across studies impossible.
The design of proper experimental treatments is a very important task in experimental design, because the treatment is the raison d’etre of the experimental method, and must never be rushed or neglected. To design an adequate and appropriate task, researchers should use prevalidated tasks if available, conduct treatment manipulation checks to check for the adequacy of such tasks (by debriefing subjects after performing the assigned task), conduct pilot tests (repeatedly, if necessary), and if doubt, using tasks that are simpler and familiar for the respondent sample than tasks that are complex or unfamiliar.
In summary, this chapter introduced key concepts in the experimental design research method and introduced a variety of true experimental and quasi-experimental designs. Although these designs vary widely in internal validity, designs with less internal validity should not be overlooked and may sometimes be useful under specific circumstances and empirical contingencies.
- Social Science Research: Principles, Methods, and Practices. Authored by : Anol Bhattacherjee. Provided by : University of South Florida. Located at : http://scholarcommons.usf.edu/oa_textbooks/3/ . License : CC BY-NC-SA: Attribution-NonCommercial-ShareAlike
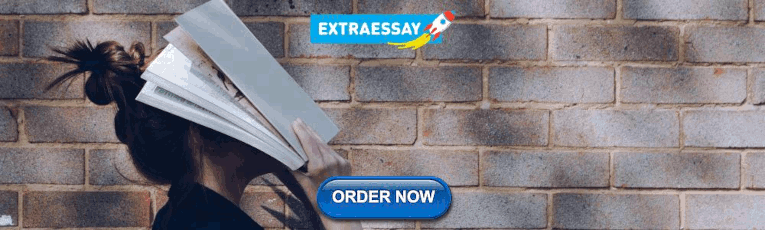
COMMENTS
Field experiments, explained. Editor's note: This is part of a series called "The Day Tomorrow Began," which explores the history of breakthroughs at UChicago. Learn more here. A field experiment is a research method that uses some controlled elements of traditional lab experiments, but takes place in natural, real-world settings.
Field Experiment. A field experiment is a research method in psychology that takes place in a natural, real-world setting. It is similar to a laboratory experiment in that the experimenter manipulates one or more independent variables and measures the effects on the dependent variable. ... Field experiments are often used to study social ...
There are limitations of and arguments against using field experiments in place of other research designs (e.g. lab experiments, survey experiments, observational studies, etc.). Given that field experiments necessarily take place in a specific geographic and political setting, there is a concern about extrapolating outcomes to formulate a ...
In this article, we offer an overview of field experimentation and its importance in discerning cause and effect relationships. We outline how randomized experiments represent an unbiased method for determining what works. Furthermore, we discuss key aspects of experiments, such as intervention, excludability, and non-interference.
In a controlled experiment, you must be able to: Systematically and precisely manipulate the independent variable(s). Precisely measure the dependent variable(s). Control any potential confounding variables. If your study system doesn't match these criteria, there are other types of research you can use to answer your research question.
Field experiments have grown significantly in prominence since the 1990s. In this article, we provide a summary of the major types of field experiments, explore their uses, and describe a few examples. We show how field experiments can be used for both positive and normative purposes within economics. We also discuss more generally why data ...
The study design used to answer a particular research question depends on the nature of the question and the availability of resources. In this article, which is the first part of a series on "study designs," we provide an overview of research study designs and their classification. The subsequent articles will focus on individual designs.
Field studies can be used to meet one of the central aims of social psychology: to develop theory and design interventions that tackle societal problems 1. By operating within the context that ...
In such research studies, the effects of multiple treatments may interact and show some effects contrary to our expectations. ... A field experiment is a true experimental study performed outside the laboratory, that is, in the real-world situations such as farms, forests, grasslands, ponds, polluting sources, rivers, watersheds, factories ...
Therefore, many political scientists turn their attention to conducting field experiments or lab-in-the-field experiments to reveal causality (Druckman et al. 2006; Beath, Christia, and Enikolopov 2013; Finseraas and Kotsadam 2017), or to leveraging in-depth insights or historical records gained through qualitative or archival research in ...
Field experiments require well-defined participant selection procedures. It is important to standardize experimental procedures to minimize extraneous variables, including experimenter expectancy effects. It is important to conduct one or more small-scale pilot tests of an experiment to be sure that the procedure works as planned.
10 Experimental research. 10. Experimental research. Experimental research—often considered to be the 'gold standard' in research designs—is one of the most rigorous of all research designs. In this design, one or more independent variables are manipulated by the researcher (as treatments), subjects are randomly assigned to different ...
The next major distinction between research methods is between laboratory and field studies. A laboratory study is a study that is conducted in the laboratory environment. In contrast, a field study is a study that is conducted in the real-world, in a natural environment. Laboratory experiments typically have high internal validity. Internal ...
An important difference between field experiments and other types of studies is that field experiments can entail substantially greater costs for the researchers. This is especially true when comparing field experiments with survey experiments, which typically are easy to conduct and entail low costs (Mutz 2011). The costs of field experiments ...
The experimental method involves manipulating one variable to determine if this causes changes in another variable. This method relies on controlled research methods and random assignment of study subjects to test a hypothesis. For example, researchers may want to learn how different visual patterns may impact our perception.
Field experiments aren't the most widely used research method in Sociology, but the examiners seem to love asking questions about them - below are seven examples of this research method.. Looked at collectively, the results of the field experiments below reveal punishingly depressing findings about human action - they suggest that people are racist, sexist, shallow, passive, and prepared ...
Olivia Guy-Evans, MSc. Research methods in psychology are systematic procedures used to observe, describe, predict, and explain behavior and mental processes. They include experiments, surveys, case studies, and naturalistic observations, ensuring data collection is objective and reliable to understand and explain psychological phenomena.
Experiments look for the effect that manipulated variables (independent variables) have on measured variables (dependent variables), i.e. causal effects. Field experiments are conducted in a natural setting (e.g. at a sports event or on public transport), as opposed to the artificial environment created in laboratory experiments. Some variables cannot be controlled due to the unpredictability ...
Field Experiments take place in real-life settings such as a classroom, the work place or even the high street. Field experiments are much more common in sociology than laboratory experiments. In fact sociologists hardly ever use lab experiments because the artificial environment of the laboratory is so far removed from real life that most sociologists believe that the results gained from such ...
(field experiments), (iii) field studies and (iv) survey research. In this unit you will learn about experiments, types of experimental research design etc. You will also learn about the criteria of a good experimental design. 3.1 OBJECTIVES After reading this unit, you will be able to: • Define experimental research and field experiments;
Even a Little Bit of Expertise Can Go a Long Way. My usual approach to helping students get past this floundering stage is to tell them to avoid thinking up a study altogether. Instead, I tell ...
Ignition with high-field, compact tokamaks* John A. Schmidt, in Fusion Technology 1990, 1991 Compact, high-field experiments offer the most direct path to study burning plasma behavior.Several designs for these experiments have been proposed. The IGNITOR device has been proposed for construction at Ispra in northern Italy, and the CIT device has been proposed for construction at Princeton, N.J ...
Essentially, our research design combines a survey and field experiment. The field experiment consists of a counselling workshop, with participants randomly assigned into treatment and control group (randomized controlled trial, RCT), while the survey experiment consists of a factorial survey conducted after the counselling workshops.
In a randomized field experiment in Senegal, we assess the potential of three types of quality signaling to increase demand for high-quality solar lamps. We find no effect on demand when consumers are offered a money-back guarantee but increased demand with a third-party certification or warranty, consistent with the notion that consumers are ...
Previous studies using litter-only reciprocal transplant experiments in the field have concluded that differences in the functional ability of the overall soil microbial community explain litter decomposition (Keiser et al., 2014; Keiser & Bradford, 2017). However, it seems likely that this captures the climate influence produced by the strong ...
Calibration and validation of a hybrid traffic flow model based on vehicle trajectory data from a field car-following experiment. Roberta Di Pace a Sustainable Transportation Systems Engineering and ... This study was carried out within the MOST - Sustainable Mobility National Research Centre and received funding from the European Union Next ...
This field research was sponsored by UC Davis and UCCE Agriculture and Natural Resources; and funded by USDA-NIFA, the Alfalfa Checkoff program of the National Alfalfa and Forage Alliance, the California Alfalfa & Forage Association and donations by companies and individuals. More resources. Get hand-outs from Small Grains/Alfalfa Field Day here.
A novel low-NOx burner was proposed in this study to achieve the stable and clean combustion of low- and medium-calorific-value gas and promote energy sustainability, and the influence of the gas pipe structure on the burner's characteristics was studied with coke oven gas as a fuel. A 40 kW burner test bench was established to conduct cold-state experiments to investigate the influences of ...
Research Article Effects of agricultural informatization on agricultural carbon emissions: a quasi natural experiment study in China Zhuang Zhang a School of Business Administration, Zhongnan University of Economics and Law, Wuhan, PR China Correspondence [email protected]
Experimental research can be conducted in laboratory or field settings. Laboratory experiments , conducted in laboratory (artificial) settings, tend to be high in internal validity, but this comes at the cost of low external validity (generalizability), because the artificial (laboratory) setting in which the study is conducted may not reflect ...