Global main menu
- School of Electronic Engineering and Computer Science
- PhD studentships
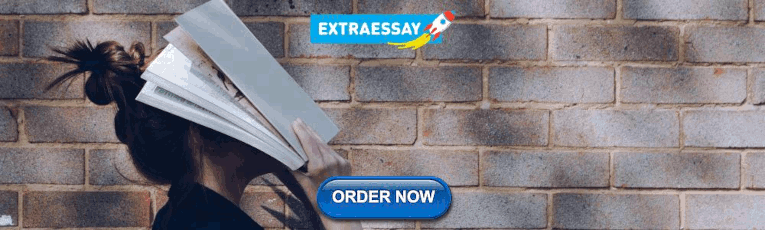
DeepMind PhD Studentship in AI or Machine Learning
About the Studentship
Queen Mary University of London is inviting applications for the DeepMind PhD Studentship for September 2023.
The DeepMind PhD Studentship programme is established at Queen Mary University of London in partnership with leading British AI company, DeepMind.
The PhD Studentship supports and encourages under-represented groups, namely female and Black researchers, to pursue postgraduate research in AI or Machine Learning.
The PhD DeepMind Studentship will cover tuition fees and offer a London weighted stipend of £19,668 per year minimum together with an annual £2,200 travel and conference allowance and a one-off equipment grant of £1,700.
- 3-year fully-funded PhD Studentship
- Access to cutting-edge facilities and expertise in AI
- Partnership and mentorship with DeepMind employees working at the cutting edge of AI research and technologies.
Who can apply Queen Mary is on the lookout for the best and brightest students in the fields of AI and Machine Learning.
Successful applicants will have the following profile:
- Identify as female and/or are of Black ethnicity, each being under-represented groups in the field of Artificial Intelligence and Computer Science
- Should hold, or is expected to obtain an MSc in Computer Science, Electronic Engineering, AI, Physics or Mathematics or a closely related discipline; or can demonstrate evidence of equivalent work experience
- Having obtained distinction or first-class level degree is highly desirable
- Programming skills are strongly desirable; however, we do not consider this to be an essential criterion if candidates have complementary strengths.
We actively encourage applications from candidates who are ordinarily resident in the UK. The studentship is also open to International applicants.
About the School of Electronic Engineering and Computer Science at Queen Mary
The PhD Studentship will be based in the School of Electronic Engineering and Computer Science (EECS) at Queen Mary University of London. As a multidisciplinary School, we are well known for our pioneering research and pride ourselves on our world-class projects. We are 8th in the UK for computer science research (REF 2021) and 7th in the UK for engineering research (REF 2021). The School is a dynamic community of approximately 350 PhD students and 80 research assistants working on research centred around a number of research groups in several areas, including Antennas and Electromagnetics, Computing and Data Science, Communication Systems, Computer Vision, Cognitive Science, Digital Music, Games and AI, Multimedia and Vision, Networks, Risk and Information Management, Robotics and Theory
For further information about research in the school of Electronic Engineering and Computer Science, please visit: http://eecs.qmul.ac.uk/research/ .
How to apply
Queen Mary is interested in developing the next generation of outstanding researchers - whether in academia, industry or government – therefore the project undertaken under this Studentship is expected to fit into the wider research programme of School. Applicants should select a supervisor (a first and second choice) from the School at application stage. Visit our website for information about our research groups and supervisors: eecs.qmul.ac.uk/phd/phd-opportunities/
Applicants should submit their interest by returning the following to [email protected] by 12pm (noon), 10 April 2023:
- Indicate first and second choice academic supervisor
- CV (max 2 pages)
- Cover letter (max 4,500 characters)
- Research proposal (max 500 words)
- 2 References
- Certificate of English Language (for students whose first language is not English)
- Other Certificates
Application deadline: 10 April 2023
Applications will be reviewed by a panel of academic staff: May 2023
Interviews: April/May 2023
Start date: September 2023
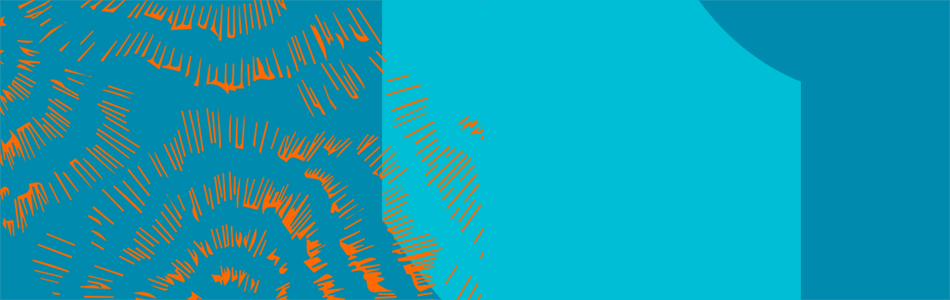
How we work in artificial intelligence
Ukri artificial intelligence centres for doctoral training.
The UK Research and Innovation (UKRI) artificial intelligence (AI) Centres for Doctoral Training (CDTs) are training a new generation of PhD students. They will develop novel AI methodology and use AI technology in areas such as:
- improving healthcare
- tackling climate change
- creating new commercial opportunities
Following the £100 million investment in 2018 in 16 UKRI AI CDTs, in 2023 UKRI invested a further £117 million in 12 new centres to continue training doctoral researchers in AI across the remit of UKRI. These new centres will train around 900 students over eight years. The first cohort will start in the 2024 to 2025 academic year.
The 2023 investment supported CDTs based at 16 universities and included partnerships with 370 different organisations from the private, public and third sector. These partnerships leveraged an additional £71 million from project partners and £40 million from the universities. EPSRC has also funded CDTs relevant to AI.
If you are interested in studying at one of the CDTs or partnering with them, contact the centre directly.
Ask a question about AI CDTs
Email: [email protected]
We aim to respond in two working days.
2023 centres
The centres are:
UKRI AI Centre for Doctoral Training in Lifelong Safety Assurance of AI-enabled Autonomous Systems (SAINTS) Led by Professor Simon Parsons, University of Lincoln, with universities of Aberdeen, Strathclyde and Queen’s University Belfast
UKRI AI Centre for Doctoral Training in Responsible and Trustworthy in-the-world Natural Language Processing Led by Professor John Vines, The University of Edinburgh
UKRI AI Centre for Doctoral Training in AI for Sustainability Led by Professor Enrico Gerding, University of Southampton
UKRI AI Centre for Doctoral Training in Citizen-Centred Artificial Intelligence Led by Professor Shaun Lawson, Northumbria University
UKRI AI Centre for Doctoral Training in Practice-Oriented Artificial Intelligence (PrO-AI) Led by Professor Peter Flach, University of Bristol
UKRI AI Centre for Doctoral Training in Decision Making for Complex Systems Led by Dr Mauricio Alvarez Lopez, The University of Manchester with University of Cambridge
UKRI AI Centre for Doctoral Training in Dependable and Deployable Artificial Intelligence for Robotics (D2AIR) Led by Professor Ronald Petrick, Heriot-Watt University with The University of Edinburgh
UKRI AI Centre for Doctoral Training in Biomedical Innovation Led by Professor Ian Simpson, The University of Edinburgh
UKRI AI Centre for Doctoral Training in AI for the Environment (Intelligent Earth) Led by Professor Philip Stier, University of Oxford
UKRI AI Centre for Doctoral Training in AI for Digital Media Inclusion Led by Professor Adrian Hilton, University of Surrey with Royal Holloway, University of London
UKRI AI Centre for Doctoral Training in Digital Healthcare Led by Professor Aldo Faisal, Imperial College London
2018 centres
UKRI AI Centre for Doctoral Training in Foundational Artificial Intelligence Led by Professor David Barber, University College London
UKRI AI Centre for Doctoral Training in AI Enabled Healthcare Systems Led by Professor Paul Taylor, University College London
UKRI AI Centre for Doctoral Training in Environmental Intelligence: Data Science and AI for Sustainable Futures Led by Professor Gavin Shaddick, University of Exeter
UKRI AI Centre for Doctoral Training in Natural Language Processing Led by Professor Mirella Lapata, The University of Edinburgh
UKRI AI Centre for Doctoral Training in Artificial Intelligence and Music Led by Professor Simon Dixon, Queen Mary University of London
UKRI AI Centre for Doctoral Training in Speech and Language Technologies and their Applications Led by Professor Thomas Hain, The University of Sheffield
UKRI AI Centre for Doctoral Training in AI for Healthcare Led by Professor Aldo Faisal, Imperial College London
UKRI AI Centre for Doctoral Training in Accountable, Responsible and Transparent AI Led by Professor Eamonn O’Neill, University of Bath
UKRI AI Centre for Doctoral Training in Artificial Intelligence, Machine Learning & Advanced Computing Led by Professor Gert Aarts, Swansea University with universities of Bangor, Cardiff, Aberystwyth and Bristol
UKRI AI Centre for Doctoral Training in Machine Intelligence for Nano- Electronic Devices and Systems Led by Professor Tim Norman, University of Southampton
UKRI AI Centre for Doctoral Training in Socially Intelligent Artificial Agents Led by Professor Alessandro Vinciarelli, University of Glasgow
UKRI AI Centre for Doctoral Training in Biomedical Artificial Intelligence Led by Professor Ian Simpson, The University of Edinburgh
UKRI AI Centre for Doctoral Training in Interactive Artificial Intelligence Led by Professor Peter Flach, University of Bristol
UKRI AI Centre for Doctoral Training in AI for the Study of Environmental Risks Led by Dr Emily Shuckburgh, University of Cambridge
UKRI AI Centre for Doctoral Training in Safe and Trusted Artificial Intelligence Led by Dr Elizabeth Black, King’s College London with Imperial College London
UKRI AI Centre for Doctoral Training in Artificial Intelligence for Medical Diagnosis and Care Led by Professor David Hogg, University of Leeds
Meeting reports
UKRI AI CDT meeting reports
Last updated: 15 May 2024
This is the website for UKRI: our seven research councils, Research England and Innovate UK. Let us know if you have feedback or would like to help improve our online products and services .
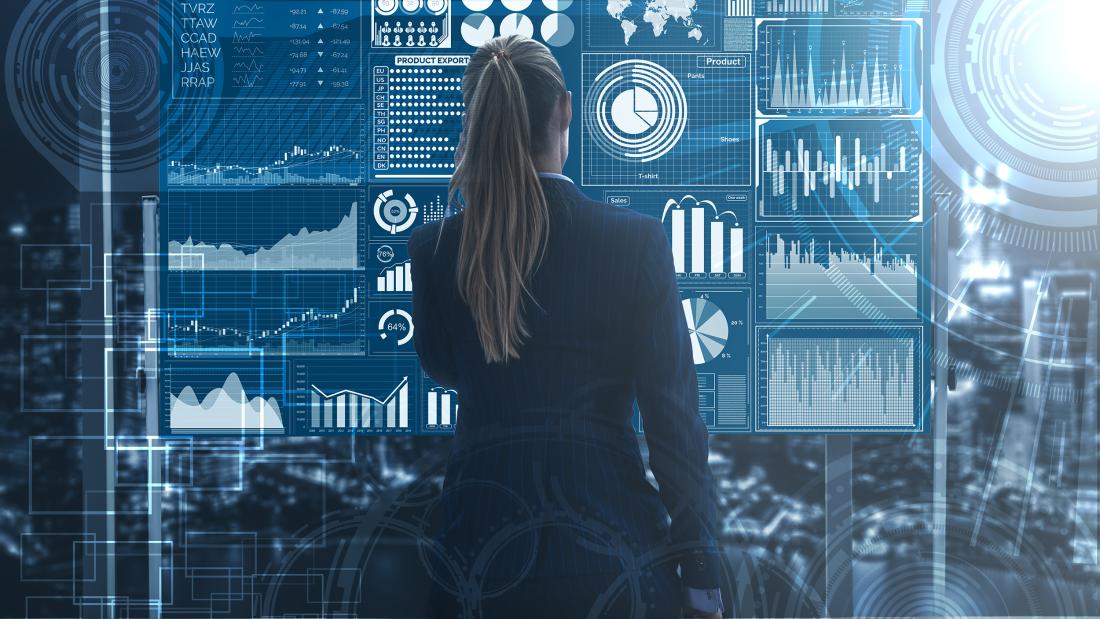
Artificial Intelligence Machine Learning and Advanced Computing Postgraduate Research - 2024 Entry
Course details.
- Qualification PhD
- Duration 4 years
About This Course
One fully-funded 4-year PhD scholarship is available to start in September 2023 in the area of Artificial Intelligence machine learning and advanced computing (AIMLAC). The PhD is suitable for a graduate with a keen interest in AI algorithms for big data, optimisation, 3D modelling, and visualisation. The exciting project will research Smart Optimisation of Big Data for Geometry Generation and 3D Models, with Applications in Optimisation and Machine Learning of Big Data from various sources such as LiDAR (3D point clouds) obtained from architectural buildings and historical heritage.
The 4-year PhD, scholarship will sit within the UKRI Centre for Doctoral Training in Artificial Intelligence, Machine Learning & Advanced Computing (CDT-AIMLAC). The students will be based at Bangor University, located within the School of Computer Science and Electronic Engineering (CSEE). Funding will cover the full cost of tuition fees and an annual stipend of approximately £15,900. Additional funding is available for research expenses.
Candidates must be resident in the UK without any immigration restriction. Applicants are required to submit a research proposal, on this topic, and written in their own words, when they submit their application. Candidates will be shortlisted, and then invited for interview.
Additional information of the project can be found here .
Project title: Smart Optimisation of Big Data for Geometry Generation and 3D Models
1st supervisor: Dr Mosab Bazargani (School of Computer Science and Engineering)
2nd supervisor: Prof Jonathan C. Roberts (School of Computer Science and Engineering)
The successful candidate will be required to attend the AIMLAC taught components in year 1 (such as foundations of AI, research methods, information visualisation), residential meetings at Aberystwyth, Bristol, Cardiff or Swansea Universities, deliver responsible innovation, and engage with placements with external partners throughout the four-year programme. Placements may be six-month, or shorter three-month or two-week blocks. Successful applicants will be registered at Bangor University, hosted by the School of Computer Science and Engineering throughout their period of study, with the delivery of the related training in the PhD programme being shared between the Universities of Aberystwyth, Bangor, Bristol, Cardiff and Swansea.
Entry Requirements
Applicants should have at least a 2:1 degree. Applicants must demonstrate excellent programming skills, and have followed a suitable degree programme, e.g., in computer science, mathematics or electronic engineering (with substantial programming), or closely related discipline. Applicants must have an interest in AI, machine learning and advanced computing and one of the topics, above. Applicants must have excellent written and spoken English. Applicants should have an aptitude and ability in computational thinking and methods (as evidenced by your degree and application information). Shortlisted candidates will be interviewed around the second half of July to the beginning of August.
To qualify as a UK applicant, prospective students must have been ordinarily resident in the UK for three years immediately prior to the start of the award, with no restrictions on how long they can remain in the UK. Overseas applicants are not eligible, as we have met our quota that is applied across the whole AIMLAC CDT cohort.
Application
To apply for the AIMLAC funded position at Bangor University, for the 2023 intake, applicants must complete Bangor’s PhD Direct Application process, and include the relevant and required information as below:
Select “Apply Now” from the menu. Applicants must include the following information.
- One research proposal , written in their own words, and based on the topic.
- An up-to-date CV , evidencing suitable experience for the PhD positions.
- An accompanying letter , including a statement of no longer than 1000 words that explains (a) why you want to join our Centre, and (b) your coding experience, with examples.
- certificates and transcripts (if you are still an undergraduate, provide a transcript of results known to date),
- Academic references - all scholarship applications require two supporting references to be submitted. Please ensure that your chosen referees are aware of the funding deadline (to be determined), as their references form a vital part of the evaluation process. Please include these with your scholarship application.
Applicants must also complete equality, diversity and inclusivity information. This is a requirement of the funders. Due to collaborative nature of the award, this detail must also be submitted to the AIMLAC central email, separately to the application. C omplete the Monitoring Equality, Diversity and Inclusivity form at time of your BU application.
Interviews (using video conferencing or in person) will occur during the second half of July to the beginning of August.
The deadline for applications is August 17th 2023; with interviews planned for the week starting 21 August, with a start date of 18th September. However applications will be accepted until all positions are filled.
For more information please contact Professor Jonathan Roberts
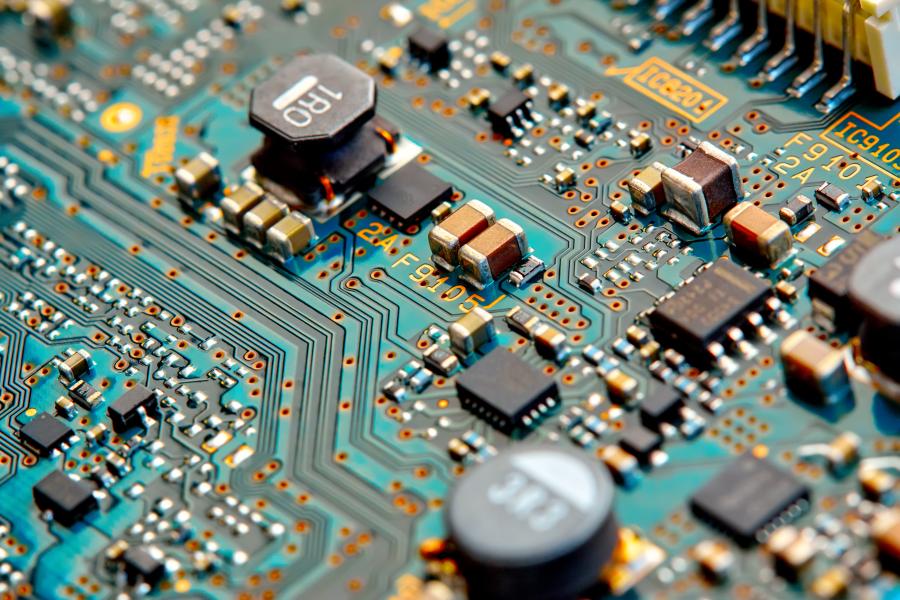
Explore More in Electronic Engineering
The advent of the digital era makes electronics and electronic devices more important than ever. Our world-leading experts expose our students to cutting-edge technologies and research. Our ambitions centre around employing micro and nanotechnology to exploit new materials and techniques.
It looks like you’re visiting from outside the UK, would you like to be redirected to the international page?
Our cookies
We use cookies for three reasons: to give you the best experience on PGS, to make sure the PGS ads you see on other sites are relevant , and to measure website usage. Some of these cookies are necessary to help the site work properly and can’t be switched off. Cookies also support us to provide our services for free, and by click on “Accept” below, you are agreeing to our use of cookies .You can manage your preferences now or at any time.
Privacy overview
We use cookies, which are small text files placed on your computer, to allow the site to work for you, improve your user experience, to provide us with information about how our site is used, and to deliver personalised ads which help fund our work and deliver our service to you for free.
The information does not usually directly identify you, but it can give you a more personalised web experience.
You can accept all, or else manage cookies individually. However, blocking some types of cookies may affect your experience of the site and the services we are able to offer.
You can change your cookies preference at any time by visiting our Cookies Notice page. Please remember to clear your browsing data and cookies when you change your cookies preferences. This will remove all cookies previously placed on your browser.
For more detailed information about the cookies we use, or how to clear your browser cookies data see our Cookies Notice
Manage consent preferences
Strictly necessary cookies
These cookies are necessary for the website to function and cannot be switched off in our systems.
They are essential for you to browse the website and use its features.
You can set your browser to block or alert you about these cookies, but some parts of the site will not then work. We can’t identify you from these cookies.
Functional cookies
These help us personalise our sites for you by remembering your preferences and settings. They may be set by us or by third party providers, whose services we have added to our pages. If you do not allow these cookies, then these services may not function properly.
Performance cookies
These cookies allow us to count visits and see where our traffic comes from, so we can measure and improve the performance of our site. They help us to know which pages are popular and see how visitors move around the site. The cookies cannot directly identify any individual users.
If you do not allow these cookies we will not know when you have visited our site and will not be able to improve its performance for you.
Marketing cookies
These cookies may be set through our site by social media services or our advertising partners. Social media cookies enable you to share our content with your friends and networks. They can track your browser across other sites and build up a profile of your interests. If you do not allow these cookies you may not be able to see or use the content sharing tools.
Advertising cookies may be used to build a profile of your interests and show you relevant adverts on other sites. They do not store directly personal information, but work by uniquely identifying your browser and internet device. If you do not allow these cookies, you will still see ads, but they won’t be tailored to your interests.
Course type
Qualification, university name, doctorate degrees in artificial intelligence (ai).
16 degrees at 13 universities in the UK.
Customise your search
Select the start date, qualification, and how you want to study

Related subjects:
- Doctorate Artificial Intelligence (AI)
- Doctorate Animation Software
- Doctorate Bioinformatics
- Doctorate Business Information Systems
- Doctorate Computer Animation
- Doctorate Computer Architectures
- Doctorate Computer Communications and Networking
- Doctorate Computer Cybernetics
- Doctorate Computer Games Design
- Doctorate Computer Graphics
- Doctorate Computer Network Components
- Doctorate Computer Science and Information Technology
- Doctorate Computer Security Systems
- Doctorate Computer Support Operations
- Doctorate Computer Systems
- Doctorate Computing Methodologies
- Doctorate Data Science
- Doctorate Database Management
- Doctorate Expert Systems
- Doctorate Geographical Information Systems Software
- Doctorate Graphics And Multimedia Software
- Doctorate Health Informatics
- Doctorate Human Computer Interface Development
- Doctorate Image Processing
- Doctorate Informatics
- Doctorate Information Management
- Doctorate Information Security
- Doctorate Information Systems
- Doctorate Information Technology
- Doctorate Information Work and Information Use
- Doctorate Internet Security Systems
- Doctorate Internet Systems
- Doctorate Knowledge Management Systems
- Doctorate Librarianship and Library Management
- Doctorate Libraries and Librarianship
- Doctorate Mobile Computing
- Doctorate Modelling and Simulation Systems
- Doctorate Multimedia
- Doctorate Network Systems Management
- Doctorate Network Systems Management Software
- Doctorate Pattern Recognition
- Doctorate Software Development
- Doctorate Software Engineering
- Doctorate Software Testing
- Doctorate Software for Specific Subjects and Industries
- Doctorate Systems Analysis and Design
- Doctorate Using Software

- Course title (A-Z)
- Course title (Z-A)
- Price: high - low
- Price: low - high
Artificial Intelligence and Intelligent Agents PhD
Bangor university.
Research topics include knowledge-based systems, logic, multi-agent systems, distributed systems, machine learning, data mining, Read more...
- 3 years Full time degree: £4,712 per year (UK)
Computer Science PhD, MPhil - Knowledge Discovery and Machine Learning
University of leicester.
Computing at Leicester offers supervision for the degrees of Doctor of Philosophy (PhD) - full-time and part-time Master of Philosophy Read more...
- 3 years Full time degree: £4,786 per year (UK)
- 6 years Part time degree: £2,393 per year (UK)
PhD Robotics and Systems Engineering
University of salford.
INTRODUCTION Automation for the Food Industry Research The food industry is very labour intensive and as a result is under threat from Read more...
- 5 years Online/Distance degree: £2,390 per year (UK)
- 3 years Online/Distance degree: £4,780 per year (UK)
Robotics and Autonomous Systems PhD
University of surrey.
Why choose this programme On our Robotics and Autonomous Systems PhD, you’ll study, design and build novel solutions and behaviours for Read more...
- 4 years Full time degree: £4,712 per year (UK)
- 8 years Part time degree: £2,356 per year (UK)
Artificial Intelligence Enabled Healthcare MRes and MPhil/PhD
Ucl (university college london).
The CDT programme consists of a 1 year MRes followed by a 3 year PhD. Throughout this period the CDT will continue to closely monitor the Read more...
- 1 year Full time degree: £6,035 per year (UK)
- 2 years Part time degree: £2,930 per year (UK)
PhD Artificial Intelligence and Music
Queen mary university of london.
The UKRI Centre for Doctoral Training in Artificial Intelligence and Music (AIM) is a leading PhD research programme aimed at the Read more...
PhD with Integrated Study Machine Intelligence for Nano-electronic Devices (MINDS)
University of southampton.
This four-year iPhD is designed to develop and nurture the next generation of technology pioneers who will have the skills, assets and Read more...
- 4 years Full time degree
PhD Robotics
Sheffield hallam university.
Course summary Undertake extensive, supervised studies in the Centre for Automation and Robotics Research Specialise in pertinent Read more...
- 7 years Part time degree: £2,356 per year (UK)
Artificial Intelligence Machine Learning and Advanced Computing PhD
Three fully-funded 4-year PhD scholarships are available to start in October 2021 in the area of Artificial Intelligence machine learning Read more...
DPhil in Autonomous Intelligent Machines and Systems (EPSRC Centre for Doctoral Training)
University of oxford.
The Autonomous Intelligent Machines and Systems (AIMS) Centre for Doctoral Training (CDT) provides graduates with the opportunity to Read more...
- 4 years Full time degree: £9,500 per year (UK)
- 8 years Part time degree: £4,750 per year (UK)
Text and Data Mining (PhD/MPhil)
Cardiff university.
Focus your studies on text and data mining through our Computer Science and Informatics research programmes (MPhil, PhD). Studying for a Read more...
- 3 years Full time degree
- 5 years Part time degree
Practice-Oriented Artificial Intelligence PhD
University of bristol.
Practice-oriented artificial intelligence is about bridging the gap between complex problem domains such as those found in science and Read more...
- 4 years Full time degree: £4,758 per year (UK)
Informatics: ANC: Machine Learning, Computational Neuroscience, Computational Biology PhD
The university of edinburgh.
The Institute for Adaptive and Neural Computation (IANC) is a world-leading institute dedicated to the theoretical and empirical study of Read more...
- 6 years Part time degree
PhD Intelligent Systems
Ulster university.
The vision is to develop a bio-inspired computational basis for Artificial Intelligence to power future cognitive technologies. Our mission Read more...
- 6 years Part time degree: £2,390 per year (UK)
Statistics and Machine Learning (DPhil)
The Modern Statistics and Statistical Machine Learning CDT is a four-year DPhil research programme (or eight years if studying Read more...
Informatics: AIAI: Foundations and Applications of Artificial Intelligence, Automated Reasoning, Agents, Data Intensive Research PhD
At the Artificial Intelligence and its Applications Institute, we enable computer systems to reproduce and complement human abilities, work Read more...
Course type:
- Full time Doctorate
- Part time Doctorate
Qualification:
Related subjects:.
- View all courses
- Taught postgraduate study
- Postgraduate taught degree courses
- Postgraduate taught tuition fees
- Pre-masters for international students
- Funding your postgraduate taught studies
- How to apply for a postgraduate taught degree
- Pre-sessional English courses
- PhDs and research degrees
- Create your own research project
- Find a PhD project
- Funding your research degree
- How to apply for a PhD or research degree
- How to make a PhD enquiry
- Support while studying your PhD or research degree
- Exchanges and studying abroad
- Undergraduate study
- Undergraduate degree courses
- Foundation year programmes
- Undergraduate tuition fees
- Customise your degree
- Funding undergraduate studies
- How to apply
- Tuition fees and funding
- Short courses
- Lunchtime evening and weekend courses
- Summer schools
- Get a prospectus
- Student life
- Accommodation
- Choose your halls of residence
- Apply for accommodation
- Guaranteed accommodation
- Your accommodation options
- Accommodation for those with additional requirements
- International and pre-sessional students
- Postgraduate accommodation
- Couples and students with children
- Renting privately
- Our accommodation areas
- Privacy notice
- Terms and conditions
- Fees and contracts
- Southampton
- Sports and gyms
- Sports facilities
- Sports clubs
- Watersports centres
- Our campuses
- Avenue Campus
- Boldrewood Innovation Campus
- City Centre Campus
- Highfield Campus
- University Hospital Southampton
- Waterfront Campus
- Winchester Campus
- Join our student community
- What's on
- Clubs and societies
- Sports teams
- SUSU places
- Representing you
- SUSU support and advice
- Support and money
- Living costs
- Academic and mental health support
- Support for disabled students
- Part-time work
- Health services
- Research projects
- Research areas
- Research facilities
- Collaborate with us
- Institutes, centres and groups
- Support for researchers
- Faculties, schools and departments
- Research jobs
- Find people and expertise
- Why work with us?
- Collaboration
- Consultancy
- Commercialisation
- Use our facilities
- Connect with our students
- How we operate
- Make a business enquiry
- International students
- International Office
- Partnerships and initiatives
- Visiting delegations
- Visiting fellowships
Postgraduate research project
Responsible and trustworthy ai, about the project.
The global artificial intelligence (AI) market is valued at over $387.4 billion and is projected to more than triple by 2029. For the world to realise the benefits brought by AI, it is important to ensure AI systems are responsibly developed, used throughout their entire life cycle, and trusted by the humans expected to rely on them.
This PhD studentship is in the broad area of responsible and trustworthy AI. Potential research topics include:
- multi-robot systems
- autonomous systems
- multi-agent systems
- goal-driven systems
- recognition, human-computer interaction
Modern AI technologies have great potential to advance our society. With this power comes great responsibility to ensure AI is used for good and in an equitable, transparent manner. AI has a significant impact, and ensuring that AI is designed, deployed, and used responsibly is critical to ensuring its impact is positive.
The PhD studentship is related to our flagship £31M Responsible AI UK programme (RAI UK) . This national centre, based at the University of Southampton, will create an international ecosystem for responsible AI research and innovation.
Potential supervisors
Lead supervisor.
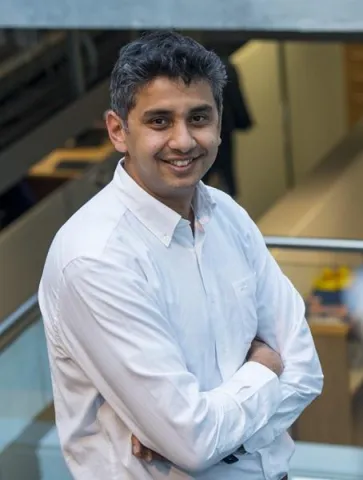
Professor Gopal Ramchurn
Supervisors.
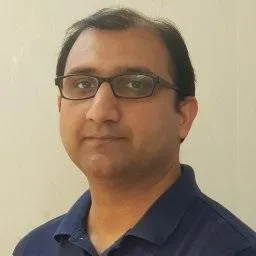
Dr Shoaib Ehsan
Research interests.
- Robotics (Localisation and Mapping)
- Embedded Systems
- Computer Vision
Entry requirements
A UK First class honours degree, or its international equivalent , in one of the following:
- computer science
- electronics
- mathematics
- a related discipline
The following are essential:
- attention to detail
- ability to work with diverse people and communities to understand their particular needs
- enthusiasm to work in the broader area of responsible and trustworthy AI to develop systems with beneficial human impact
This project is only open to UK applicants.
Fees and funding
For UK students, tuition fees will be paid and you'll receive a stipend (living allowance) of £18,622 tax-free per year for up to 3.5 years.
You need to:
- choose programme type (Research), 2023/24, Faculty of Engineering and Physical Sciences
- choose PhD in Computer science (Full time)
- add supervisor Professor Gopal Ramchurn in section 2
Applications should include:
- your CV (resumé)
- 2 reference letters
- degree transcripts to date
- a 2-page research proposal explaining what you're interested in, why it is exciting for you and what you have already read about it.
Your research idea could focus on the topics mentioned in the About the project section on the broader area of responsible and trustworthy AI.
In your research proposal, we will be looking for your:
- excitement for your chosen topic
- enthusiasm for the opportunity to do research aligned with the Responsible AI UK programme and the UKRI Trustworthy Autonomous Systems (TAS) Hub
- ability to communicate your ideas clearly
Faculty of Engineering and Physical Sciences
If you have a general question, email our doctoral college ([email protected]) .
- Course modules
- Acoustical engineering
- Biomedical and medical engineering
- Civil engineering
- Every day I’m completely immersed in an environment that’s creative in all aspects
- Everything I learn feels so relevant, even If it’s a subject rooted in the past
- Maritime engineering
- Photonics and optoelectronics
- Social statistics and demography
- A missing link between continental shelves and the deep sea: Have we underestimated the importance of land-detached canyons?
- A seismic study of the continent-ocean transition southwest of the UK
- A study of rolling contact fatigue in electric vehicles (EVs)
- Acoustic monitoring of forest exploitation to establish community perspectives of sustainable hunting
- Acoustic sensing and characterisation of soil organic matter
- Advancing intersectional geographies of diaspora-led development in times of multiple crises
- Aero engine fan wake turbulence – Simulation and wind tunnel experiments
- Against Climate Change (DACC): improving the estimates of forest fire smoke emissions
- All-in-one Mars in-situ resource utilisation (ISRU) system and life-supporting using non-thermal plasma
- An electromagnetic study of the continent-ocean transition southwest of the UK
- An investigation of the relationship between health, home and law in the context of poor and precarious housing, and complex and advanced illness
- Antibiotic resistance genes in chalk streams
- Being autistic in care: Understanding differences in care experiences including breakdowns in placements for autistic and non-autistic children
- Biogeochemical cycling in the critical coastal zone: Developing novel methods to make reliable measurements of geochemical fluxes in permeable sediments
- Bloom and bust: seasonal cycles of phytoplankton and carbon flux
- British Black Lives Matter: The emergence of a modern civil rights movement
- Building physics for low carbon comfort using artificial intelligence
- Building-resolved large-eddy simulations of wind and dispersion over a city scale urban area
- Business studies and management: accounting
- Business studies and management: banking and finance
- Business studies and management: decision analytics and risk
- Business studies and management: digital and data driven marketing
- Business studies and management: human resources (HR) management and organisational behaviour
- Business studies and management: strategy, innovation and entrepreneurship
- Carbon storage in reactive rock systems: determining the coupling of geo-chemo-mechanical processes in reactive transport
- Cascading hazards from the largest volcanic eruption in over a century: What happened when Hunga Tonga-Hunga Ha’apai erupted in January 2022?
- Characterisation of cast austenitic stainless steels using ultrasonic backscatter and artificial intelligence
- Climate Change effects on the developmental physiology of the small-spotted catshark
- Climate at the time of the Human settlement of the Eastern Pacific
- Collaborative privacy in data marketplaces
- Compatibility of climate and biodiversity targets under future land use change
- Cost of living in modern and fossil animals
- Creative clusters in rural, coastal and post-industrial towns
- Deep oceanic convection: the outsized role of small-scale processes
- Defect categories and their realisation in supersymmetric gauge theory
- Defining the Marine Fisheries-Energy-Environment Nexus: Learning from shocks to enhance natural resource resilience
- Design and fabrication of next generation optical fibres
- Developing a practical application of unmanned aerial vehicle technologies for conservation research and monitoring of endangered wildlife
- Development and evolution of animal biomineral skeletons
- Development of all-in-one in-situ resource utilisation system for crewed Mars exploration missions
- Ecological role of offshore artificial structures
- Effect of embankment and subgrade weathering on railway track performance
- Efficient ‘whole-life’ anchoring systems for offshore floating renewables
- Electrochemical sensing of the sea surface microlayer
- Engagement with nature among children from minority ethnic backgrounds
- Enhancing UAV manoeuvres and control using distributed sensor arrays
- Ensuring the Safety and Security of Autonomous Cyber-Physical Systems
- Environmental and genetic determinants of Brassica crop damage by the agricultural pest Diamondback moth
- Estimating marine mammal abundance and distribution from passive acoustic and biotelemetry data
- Evolution of symbiosis in a warmer world
- Examining evolutionary loss of calcification in coccolithophores
- Explainable AI (XAI) for health
- Explaining process, pattern and dynamics of marine predator hotspots in the Southern Ocean
- Exploring dynamics of natural capital in coastal barrier systems
- Exploring the mechanisms of microplastics incorporation and their influence on the functioning of coral holobionts
- Exploring the potential electrical activity of gut for healthcare and wellbeing
- Exploring the trans-local nature of cultural scene
- Facilitating forest restoration sustainability of tropical swidden agriculture
- Faulting, fluids and geohazards within subduction zone forearcs
- Faulting, magmatism and fluid flow during volcanic rifting in East Africa
- Fingerprinting environmental releases from nuclear facilities
- Flexible hybrid thermoelectric materials for wearable energy harvesting
- Floating hydrokinetic power converter
- Glacial sedimentology associated subglacial hydrology
- Green and sustainable Internet of Things
- How do antimicrobial peptides alter T cell cytokine production?
- How do calcifying marine organisms grow? Determining the role of non-classical precipitation processes in biogenic marine calcite formation
- How do neutrophils alter T cell metabolism?
- How well can we predict future changes in biodiversity using machine learning?
- Hydrant dynamics for acoustic leak detection in water pipes
- If ‘Black Lives Matter’, do ‘Asian Lives Matter’ too? Impact trajectories of organisation activism on wellbeing of ethnic minority communities
- Illuminating luciferin bioluminescence in dinoflagellates
- Imaging quantum materials with an XFEL
- Impact of neuromodulating drugs on gut microbiome homeostasis
- Impact of pharmaceuticals in the marine environment in a changing world
- Impacts of environmental change on coastal habitat restoration
- Improving subsea navigation using environment observations for long term autonomy
- Information theoretic methods for sensor management
- Installation effect on the noise of small high speed fans
- Integrated earth observation mapping change land sea
- Interconnections of past greenhouse climates
- Investigating IgG cell depletion mechanisms
- Is ocean mixing upside down? How mixing processes drive upwelling in a deep-ocean basin
- Landing gear aerodynamics and aeroacoustics
- Lightweight gas storage: real-world strategies for the hydrogen economy
- Long-term change in the benthos – creating robust data from varying camera systems
- Machine learning for multi-robot perception
- Marine ecosystem responses to past climate change and its oceanographic impacts
- Mechanical effects in the surf zone - in situ electrochemical sensing
- Microfluidic cell isolation systems for sepsis
- Migrant entrepreneurship, gender and generation: context and family dynamics in small town Britain
- Miniaturisation in fishes: evolutionary and ecological perspectives
- Modelling high-power fibre laser and amplifier stability
- Modelling soil dewatering and recharge for cost-effective and climate resilient infrastructure
- Modelling the evolution of adaptive responses to climate change across spatial landscapes
- Nanomaterials sensors for biomedicine and/or the environment
- New high-resolution observations of ocean surface current and winds from innovative airborne and satellite measurements
- New perspectives on ocean photosynthesis
- Novel methods of detecting carbon cycling pathways in lakes and their impact on ecosystem change
- Novel technologies for cyber-physical security
- Novel transparent conducting films with unusual optoelectronic properties
- Novel wavelength fibre lasers for industrial applications
- Ocean circulation and the Southern Ocean carbon sink
- Ocean influence on recent climate extremes
- Ocean methane sensing using novel surface plasmon resonance technology
- Ocean physics and ecology: can robots disentangle the mix?
- Ocean-based Carbon Dioxide Removal: Assessing the utility of coastal enhanced weathering
- Offshore renewable energy (ORE) foundations on rock seabeds: advancing design through analogue testing and modelling
- Optical fibre sensing for acoustic leak detection in buried pipelines
- Optimal energy transfer in nonlinear systems
- Optimizing machine learning for embedded systems
- Oxidation of fossil organic matter as a source of atmospheric CO2
- Partnership dissolution and re-formation in later life among individuals from minority ethnic communities in the UK
- Personalized multimodal human-robot interactions
- Preventing disease by enhancing the cleaning power of domestic water taps using sound
- Quantifying riparian vegetation dynamics and flow interactions for Nature Based Solutions using novel environmental sensing techniques
- Quantifying the response and sensitivity of tropical forest carbon sinks to various drivers
- Quantifying variability in phytoplankton electron requirements for carbon fixation
- Resilient and sustainable steel-framed building structures
- Resolving Antarctic meltwater events in Southern Ocean marine sediments and exploring their significance using climate models
- Robust acoustic leak detection in water pipes using contact sound guides
- Silicon synapses for artificial intelligence hardware
- Smart photon delivery via reconfigurable optical fibres
- The Gulf Stream control of the North Atlantic carbon sink
- The Mayflower Studentship: a prestigious fully funded PhD studentship in bioscience
- The calming effect of group living in social fishes
- The duration of ridge flank hydrothermal exchange and its role in global biogeochemical cycles
- The evolution of symmetry in echinoderms
- The impact of early life stress on neuronal enhancer function
- The oceanic fingerprints on changing monsoons over South and Southeast Asia
- The role of iron in nitrogen fixation and photosynthesis in changing polar oceans
- The role of singlet oxygen signaling in plant responses to heat and drought stress
- Time variability on turbulent mixing of heat around melting ice in the West Antarctic
- Triggers and Feedbacks of Climate Tipping Points
- Uncovering the drivers of non-alcoholic fatty liver disease progression using patient derived organoids
- Understanding recent land-use change in Snowdonia to plan a sustainable future for uplands: integrating palaeoecology and conservation practice
- Understanding the role of cell motility in resource acquisition by marine phytoplankton
- Understanding the structure and engagement of personal networks that support older people with complex care needs in marginalised communities and their ability to adapt to increasingly ‘digitalised’ health and social care
- Unpicking the Anthropocene in the Hawaiian Archipelago
- Unraveling oceanic multi-element cycles using single cell ionomics
- Unravelling southwest Indian Ocean biological productivity and physics: a machine learning approach
- Using acoustics to monitor how small cracks develop into bursts in pipelines
- Using machine learning to improve predictions of ocean carbon storage by marine life
- Vulnerability of low-lying coastal transportation networks to natural hazards
- Wideband fibre optical parametric amplifiers for Space Division Multiplexing technology
- Will it stick? Exploring the role of turbulence and biological glues on ocean carbon storage
- X-ray imaging and property characterisation of porous materials
- Postgraduate Taught Diversity Scholarship (Environmental and Life Sciences)
- Southampton Business School Postgraduate UK Scholarship
- Southampton Genomics Talent Scholarship
- Southampton History Patricia Mather and Helen Patterson Scholarship
- Southampton MA Holocaust scholarships
- Southampton Philosophy David Humphris-Norman Scholarship
- Southampton Physics and Astronomy Achievement Scholarship
- GREAT Scholarships 2024 – Greece
- Undergraduate scholarships for UK students
- Winchester School of Art Postgraduate Global Talent Scholarship
- Southampton University Corporate Civil Engineering Scholarship Scheme
- Merit scholarships for international postgraduates
- Merit scholarships for international undergraduates
- Scholarships, awards and funding opportunities
- Becas Chile Scholarship
- Chevening Scholarships
- China Scholarship Council Scholarships
- COLFUTURO Scholarships
- Commonwealth Distance Learning Scholarships
- Commonwealth Master's Scholarships
- Commonwealth PhD Scholarships
- Commonwealth PhD Scholarships for high income countries
- Commonwealth Shared Scholarships
- Commonwealth Split-Site Scholarships
- FIDERH Scholarships
- Fulbright Awards
- FUNED Scholarships
- Great Scholarships 2024 – India
- Great Scholarships 2024 – Bangladesh
- Great Scholarships 2024 – Mexico
- Great Scholarships 2024 – Nigeria
- Marshall Scholarship
- Saïd Foundation Scholarships
- British Council Scholarships for Women in STEM
- Xiamen University PhD Scholarships
- GREAT scholarships for justice and law 2024 – Indonesia
- Scholarship terms and conditions
- Southampton Canadian Prestige Scholarship for Law
- Southampton Presidential International Scholarship
- Continuing professional development
- Archers Road
- City Gateway
- Erasmus Park
- Highfield Hall
- Lucia Foster Welch
- Orion Point
- Wessex Lane
- Cancer Sciences Protein Facility
- Geotechnical Centrifuge
- Maritime Robotics and Instrumentation Laboratory (MRIL)
- Active Living
- Advanced Fibre Applications
- Advanced Laser Laboratory
- Advanced Project Management Research Centre
- Antibody and Vaccine Group
- Astronomy Group
- Autism Community Research Network @ Southampton (ACoRNS)
- Bioarchaeology and Osteoarchaeology at Southampton (BOS)
- Bladder and Bowel Management
- Cell and Developmental Biology
- Centre for Defence and Security Research
- Centre for Developmental Origins of Health and Disease
- Centre for Digital Finance
- Centre for Eastern European and Eurasian Studies (CEEES)
- Centre for Empirical Research in Finance and Banking (CERFIB)
- Centre for Geometry, Topology, and Applications
- Centre for Global Englishes
- Centre for Global Health and Policy (GHaP)
- Centre for Health Technologies
- Centre for Healthcare Analytics
- Centre for Human Development, Stem Cells and Regeneration
- Centre for Imperial and Postcolonial Studies
- Centre for Inclusive and Sustainable Entrepreneurship and Innovation (CISEI)
- Centre for International Film Research (CIFR)
- Centre for International Law and Globalisation
- Centre for Internet of Things and Pervasive Systems
- Centre for Justice Studies
- Centre for Linguistics, Language Education and Acquisition Research
- Centre for Machine Intelligence
- Centre for Maritime Archaeology
- Centre for Medieval and Renaissance Culture (CMRC)
- Centre for Modern and Contemporary Writing (CMCW)
- Centre for Music Education and Social Justice
- Centre for Political Ethnography (CPE)
- Centre for Research in Accounting, Accountability and Governance
- Centre for Research on Work and Organisations
- Centre for Resilient Socio-Technical Systems
- Centre for Transnational Studies
- Child and Adolescent Research Group
- Clinical Ethics, Law and Society (CELS)
- Computational Nonlinear Optics
- Cyber Security Academy
- Data Science Group
- Digital Oceans
- EPSRC and MOD Centre for Doctoral Training in Complex Integrated Systems for Defence and Security
- Economic Theory and Experimental Economics
- Economy, Society and Governance
- Electrical Power Engineering
- Environmental Hydraulics
- Gas Photonics in Hollow Core Fibres
- Geochemistry
- Global Health (Demography)
- Global Health Community of Practice
- Gravity group
- Healthy Oceans
- High Power Fibre Lasers
- Hollow Core Fibre
- Human Genetics and Genomic Medicine
- Infrastructure Group
- Institute of Maritime Law (IML)
- Integrated Photonic Devices
- Integrative Molecular Phenotyping Centre
- Interdisciplinary Musculoskeletal Health
- International Centre for Ecohydraulics Research (ICER)
- Language Assessment and Testing Unit (LATU)
- Laser-Direct-Write (LDW) Technologies for Biomedical Applications
- Law and Technology Centre
- Long Term Conditions
- Magnetic Resonance
- Mathematical Modelling
- Medicines Management
- Molecular and Precision Biosciences
- Multiwavelength Accretion and Astronomical Transients
- National Biofilms Innovation Centre (NBIC)
- National Centre for Research Methods
- National Infrastructure Laboratory
- Nature-Based Ocean Solutions
- Nonlinear Semiconductor Photonics
- Ocean Perception Group
- Operational Research
- Optical Engineering and Quantum Photonics Group
- Paediatrics and Child Health - Clinical and Experimental Sciences
- People, Property, Community
- Photonic Systems, Circuits and Sensors Group
- Physical Optics
- Primary Care Research Centre
- Product Returns Research Group (PRRG)
- Quantum, Light and Matter Group
- Silica Fibre Fabrication
- Silicon Photonics
- Skin Sensing Research Group
- Southampton Centre for Nineteenth-Century Research
- Southampton Ethics Centre
- Southampton Health Technology Assessments Centre (SHTAC)
- Southampton High Energy Physics group
- Southampton Imaging
- Southampton Theory Astrophysics and Gravity (STAG) Research Centre
- Stefan Cross Centre for Women, Equality and Law
- String theory and holography
- The India Centre for Inclusive Growth and Sustainable Development
- The Parkes Institute
- Tony Davies High Voltage Laboratory
- Ultrafast X-ray Group
- Vision Science
- Work Futures Research Centre (WFRC)
- Departments
Cookies on our website
We use some essential cookies to make this website work.
We'd like to set additional cookies to understand how you use our site. And we'd like to serve you some cookies set by other services to show you relevant content.
PhD/MSc programmes
The AI research group are heavily involved in multiple PhD and Masters programmes.
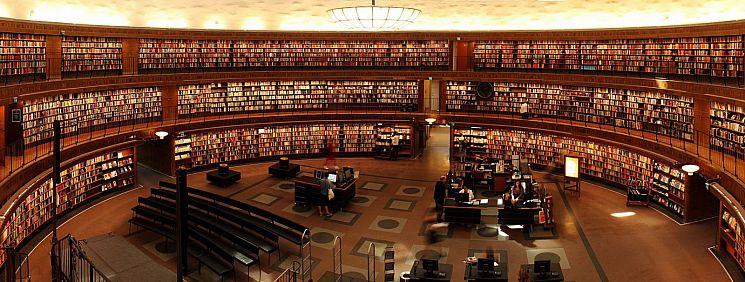
PhD Programmes
Sussex AI is offering an elite PhD programme as part of our newly established interdisciplinary centre of excellence, with 3 fully-funded (including internationals) studentships per year. Students on this programme will conduct innovative research into a range of theoretical, applied and interdisciplinary AI topics. To learn more and find out how to apply please see the Sussex AI PhD programme page .
The Sussex Neuroscience 4 year PhD programme is an elite 4 year programme with a taught first year including 3 lab rotations. It is run by Sussex Neuroscience, a university research programme, bringing together labs from across four schools. You can learn more , read about Sussex Neuroscience and find out how to apply .
Besides the specific programmes listed above, we are always open for applications of talented students with other sources of funding. Current funding opportunities can be found on the Sussex PhD funding pages .
Masters Programmes
The study of natural and artificial intelligent and adaptive systems is at the heart of rapidly developing areas. These range from artificial intelligence and autonomous robotics to neuroscience, consciousness and cognitive science. The AIAS MSc provides a solid grounding in the major themes of the area. This well established programme (formally the EASy MSc) is taught by leading experts and there are many opportunities to interact with the thriving local community of researchers in this field. More information is available in the AIAS course prospectus .
This programme provides advanced training in state-of-the-art AI research approaches. Modules will cover machine learning, bioinspired AI, AI research methods as well as applications and implications of AI technology. MRes students will perform an in-depth original research project, supervised by one of our world-leading researchers. This programme is suitable for students with a technical background who are already confident in the fundamentals of AI and want to learn the skills for high-quality AI research and development. More details will be made available in the next few months, for additional information please contact the convenor Dr Ivor Simpson .
This MSc programme covers the theory and practice of state-of-the-art data science with applications ranging from mathematics to physics, and from biology to computer science. While it is taught in four streams, Mathematics, Physics, Computer Science, Life Sciences, the overarching principles of how to tackle big data and the tools and techniques used to extract useful knowledge from it are surprisingly similar across the board. You will learn about these techniques, acquire the skills to apply them to the different application domains and so learn how take advantage of the explosion of data across science and society. The syllabus and other details are available in the Data Science course prospectus .
This MSc is aimed at understanding how and why data is used, and the societal and ethical implications of doing so. It relates closely to how data is collected, processed and stored throughout modern life, ranging from simple business interactions, our education, government, to our online presence on social media and otehr platforms. Taught content covers both, practical skills for getting involved with data science and reflections on the ethical implications and how to improve ethical use and interpretability. Nore details including the syllabus are in the HSDS course prospectus .
Recent PhD Programmes
Members of the AI group were heavily involved in running two Leverhulme Doctoral Scholarship Programmes (DSP) that are no longer accepting applicants. These were prestigious, inter-disciplinary doctoral training centers with generous provisions, including for student-led activities, summer schools and research equipment.
Directors: Prof Anil Seth and Prof Jamie Ward
The Leverhulme Doctoral Scholarship Programme (DSP) in ‘From sensation and perception to awareness’ is one of twelve DSPs in the UK that started in 2018, funded in a part by a generous £1M grant from the Leverhulme Trust. The programme bring together researchers from across neuroscience, robotics, psychology, and the arts, with the ultimate aim of advancing our understanding of interactions between sensing, perceiving, and awareness in humans, animal, and machines. Reflecting the interdisciplinary ethos at the heart of the programme, the 21 Leverhulme Scholars are working under the supervision of supervisors from across five of Sussex's Schools (Psychology, Informatics, Medicine, Life Sciences, and Media, Film and Music), and tackle important questions ranging from embodied music to the Neuroscience of visual perception. More details about the programme are on its web pages , including descriptions of the projects of the Leverhulme Scholars .
Directors: Prof Thomas Nowotny , Prof Andrew Philippides and Prof Paul Graham
The be.AI Doctoral Scholarship Programme is supported in a part by a generous £1M grant from the Leverhulme Trust awarded in late 2020. The first intake of 6 Leverhulme Scholars will commence work on their PhDs in September 2021. They will explore how intelligence arises from the interaction of a brain with the body and the environment and what we can learn for new approaches and applications of AI. This involves many disciplines ranging from Biology to Music, Computer Science to Philosophy. There will be three intakes of students with applications open from December for a start in September the following year. You can learn more , read about our research themes and find out how to apply .
Imperial College London Imperial College London
Latest news.

Food swaps could cut greenhouse gas emissions from groceries by a quarter

Cell division decisions and cancer recommendations: News from Imperial

Impact of Giving Event to commemorate the impact of philanthropy
Artificial intelligence
Take a deep dive into the cutting edge world of machine learning and artificial intelligence
Artificial intelligence and machine learning harness computation to simulate human intelligence and solve complex problems
What is artificial intelligence?
- Jobs for the future Demand for AI professionals is growing as it permeates everyday life, from driverless cars to disease detection
- Work in almost any industry With applications in wide ranging sectors and academic fields – from medicine to business
- 1st in the UK Computer Science and Informatics Research Excellence Framework (REF) 2021 – based on the proportion of world-leading research
Why Imperial ?
Access expertise spanning theoretical foundations and applications of AI, including machine learning, robotics, and augmented reality and cognition
Benefit first-hand from research from a network of 20+ AI-related research groups and centres
Accelerate you career with a world-class learning experience delivered by leading experts
The flexibility of AI is demonstrated in the fact that a number of Imperial departments offer courses in the field. Explore our courses below to see the different ways you can apply it.
Undergraduate courses Showing courses for 2025
- Undergraduate
Biomedical Technology Ventures
Learn how to improve human healthcare through the application of technology.
Computing (Artificial Intelligence and Machine Learning)
Focus your study of computing on artificial intelligence in this integrated Master’s degree.
Computing (Visual Computing and Robotics)
Focus on the implementation and evaluation of systems that depend on computer vision or computer generated imagery.
Economics, Finance and Data Science
A first-of-its-kind degree combining economics, finance, and data science and its applications
Postgraduate taught courses
- Postgraduate taught
Applied Computational Science and Engineering
Discover how numerical methods and computational science can help solve large scale problems.
Applied Machine Learning
Learn how to design, implement and evaluate machine learning systems.
Artificial Intelligence
Receive intensive training in programming and the fundamentals of artificial intelligence.
Artificial Intelligence and Machine Learning
Develop advanced artificial intelligence and machine learning skills and apply these to real-world problems.
Artificial Intelligence Applications and Innovation
Learn AI fundamentals, explore real-world AI applications, and acquire expertise needed to become a leader in AI technologies and business
Biomedical Research (Data Science)
Interdisciplinary training in 'big data' analysis, building skills towards a career in biomedical research.
Deepen your knowledge and understanding of artificial intelligence and machine learning to prepare for a career in the computing industry.
Specialise in the study of vision, graphics, intelligent behaviour and biomedical image computing.
Digital Chemistry with AI and Automation (MSc)
Discover how advances in automation, artificial intelligence, and big data are revolutionising the study of chemistry.
Study options
- Digital Chemistry with AI and Automation (PG Cert)
Which option would you like to add to compare? (Select up to 3)
- Digital Chemistry with AI and Automation Save to Course Compare
Environmental Data Science and Machine Learning
Apply computing techniques and machine learning to real-world environmental problems.
Environmental Engineering with Data Science
Explore environmental engineering practices and the use of computational methods and statistical modelling techniques.
Geo-Energy with Machine Learning and Data Science
Develop the essential data science and machine learning skills needed to embark on a career as a geoscientist or engineer.
Geotechnical Engineering with Data Science
Study key areas of geotechnical engineering and specialise in analysing large volumes of geotechnical data to provide efficient, sustainable, resilient geotechnical solutions.
Health Data Analytics and Machine Learning (MSc)
Develop skills in using cutting-edge quantitative methods to fully exploit complex data.
Human and Biological Robotics
Advance your knowledge on how bioengineers are leading the way in tackling modern healthcare challenges.
- Postgraduate taught, Online
Machine Learning and Data Science (Online)
Develop an in-depth understanding of machine learning models and learn to apply them to real-world problems.
Nanomedicine and Nanodiagnostics MRes
Investigate the unique properties of nanoscale materials for the treatment, detection, visualisation and prevention of diseases.
Statistics (Data Science)
Build familiarity with a wide range of data science techniques and their application in a variety of settings.
Transport with Data Science
Specialise in analysing large volumes of transport data and in developing models for efficient, sustainable, and resilient transport systems.
Online courses
View our 2024 entry undergraduate courses .
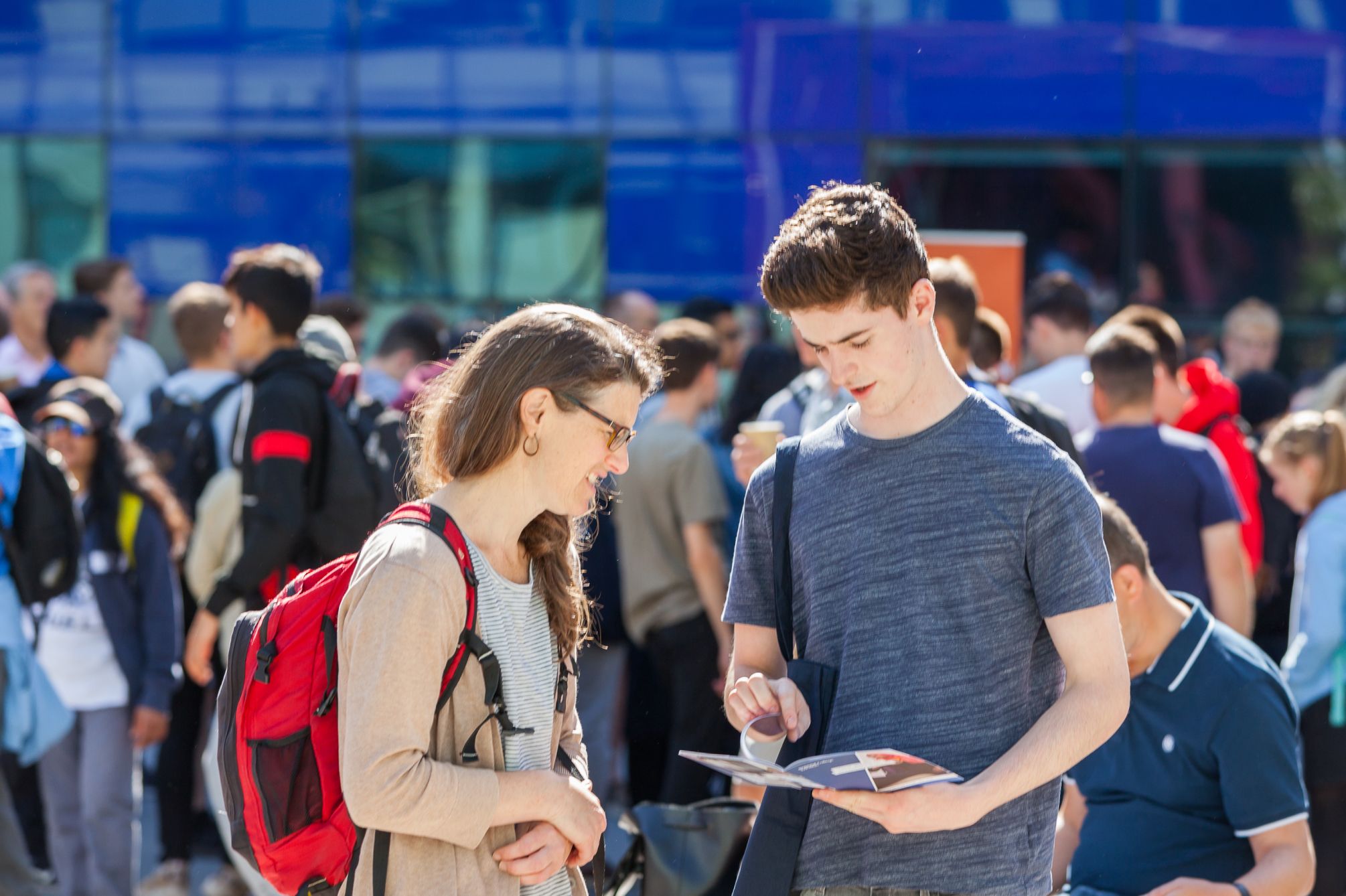
Tue, 4 June 2024 10.00 – 11.00
Undergraduate Study | Virtual Drop-in Session | Europe and International
- Online only
- Recruitment
Wed, 12 June 2024 11.00 – 17.00
Undergraduate Study | Year 12 Taster Course | Future Computing
- Huxley Building, South Kensington Campus
Fri, 31 May 2024 17.00 – 18.00
Postgraduate Study | PGT Webinar | Africa
Wed, 5 June 2024 17.00 – 18.00
Allergy | Postgraduate Information Session
- Internal wiki
PhD Programme in Advanced Machine Learning
The Cambridge Machine Learning Group (MLG) runs a PhD programme in Advanced Machine Learning. The supervisors are Jose Miguel Hernandez-Lobato , Carl Rasmussen , Richard E. Turner , Adrian Weller , Hong Ge and David Krueger . Zoubin Ghahramani is currently on academic leave and not accepting new students at this time.
We encourage applications from outstanding candidates with academic backgrounds in Mathematics, Physics, Computer Science, Engineering and related fields, and a keen interest in doing basic research in machine learning and its scientific applications. There are no additional restrictions on the topic of the PhD, but for further information on our current research areas, please consult our webpages at http://mlg.eng.cam.ac.uk .
The typical duration of the PhD will be four years.
Applicants must formally apply through the Applicant Portal at the University of Cambridge by the deadline, indicating “PhD in Engineering” as the course (supervisor Hernandez-Lobato, Rasmussen, Turner, Weller, Ge and/or Krueger). Applicants who want to apply for University funding need to reply ‘Yes’ to the question ‘Apply for Cambridge Scholarships’. See http://www.admin.cam.ac.uk/students/gradadmissions/prospec/apply/deadlines.html for details. Note that applications will not be complete until all the required material has been uploaded (including reference letters), and we will not be able to see any applications until that happens.
Gates funding applicants (US or other overseas) need to fill out the dedicated Gates Cambridge Scholarships section later on the form which is sent on to the administrators of Gates funding.
Deadline for PhD Application: noon 5 December, 2023
Applications from outstanding individuals may be considered after this time, but applying later may adversely impact your chances for both admission and funding.
FURTHER INFORMATION ABOUT COMPLETING THE ADMISSIONS FORMS:
The Machine Learning Group is based in the Department of Engineering, not Computer Science.
We will assess your application on three criteria:
1 Academic performance (ensure evidence for strong academic achievement, e.g. position in year, awards, etc.) 2 references (clearly your references will need to be strong; they should also mention evidence of excellence as quotes will be drawn from them) 3 research (detail your research experience, especially that which relates to machine learning)
You will also need to put together a research proposal. We do not offer individual support for this. It is part of the application assessment, i.e. ascertaining whether you can write about a research area in a sensible way and pose interesting questions. It is not a commitment to what you will work on during your PhD. Most often PhD topics crystallise over the first year. The research proposal should be about 2 pages long and can be attached to your application (you can indicate that your proposal is attached in the 1500 character count Research Summary box). This aspect of the application does not carry a huge amount of weight so do not spend a large amount of time on it. Please also attach a recent CV to your application too.
INFORMATION ABOUT THE CAMBRIDGE-TUEBINGEN PROGRAMME:
We also offer a small number of PhDs on the Cambridge-Tuebingen programme. This stream is for specific candidates whose research interests are well-matched to both the machine learning group in Cambridge and the MPI for Intelligent Systems in Tuebingen. For more information about the Cambridge-Tuebingen programme and how to apply see here . IMPORTANT: remember to download your application form before you submit so that you can send a copy to the administrators in Tuebingen directly . Note that the application deadline for the Cambridge-Tuebingen programme is noon, 5th December, 2023, CET.
What background do I need?
An ideal background is a top undergraduate or Masters degree in Mathematics, Physics, Computer Science, or Electrical Engineering. You should be both very strong mathematically and have an intuitive and practical grasp of computation. Successful applicants often have research experience in statistical machine learning. Shortlisted applicants are interviewed.
Do you have funding?
There are a number of funding sources at Cambridge University for PhD students, including for international students. All our students receive partial or full funding for the full three years of the PhD. We do not give preference to “self-funded” students. To be eligible for funding it is important to apply early (see https://www.graduate.study.cam.ac.uk/finance/funding – current deadlines are 10 October for US students, and 1 December for others). Also make sure you tick the box on the application saying you wish to be considered for funding!
If you are applying to the Cambridge-Tuebingen programme, note that this source of funding will not be listed as one of the official funding sources, but if you apply to this programme, please tick the other possible sources of funding if you want to maximise your chances of getting funding from Cambridge.
What is my likelihood of being admitted?
Because we receive so many applications, unfortunately we can’t admit many excellent candidates, even some who have funding. Successful applicants tend to be among the very top students at their institution, have very strong mathematics backgrounds, and references, and have some research experience in statistical machine learning.
Do I have to contact one of the faculty members first or can I apply formally directly?
It is not necessary, but if you have doubts about whether your background is suitable for the programme, or if you have questions about the group, you are welcome to contact one of the faculty members directly. Due to their high email volume you may not receive an immediate response but they will endeavour to get back to you as quickly as possible. It is important to make your official application to Graduate Admissions at Cambridge before the funding deadlines, even if you don’t hear back from us; otherwise we may not be able to consider you.
Do you take Masters students, or part-time PhD students?
We generally don’t admit students for a part-time PhD. We also don’t usually admit students just for a pure-research Masters in machine learning , except for specific programs such as the Churchill and Marshall scholarships. However, please do note that we run a one-year taught Master’s Programme: The MPhil in Machine Learning, and Machine Intelligence . You are welcome to apply directly to this.
What Department / course should I indicate on my application form?
This machine learning group is in the Department of Engineering. The degree you would be applying for is a PhD in Engineering (not Computer Science or Statistics).
How long does a PhD take?
A typical PhD from our group takes 3-4 years. The first year requires students to pass some courses and submit a first-year research report. Students must submit their PhD before the 4th year.
What research topics do you have projects on?
We don’t generally pre-specify projects for students. We prefer to find a research area that suits the student. For a sample of our research, you can check group members’ personal pages or our research publications page.
What are the career prospects for PhD students from your group?
Students and postdocs from the group have moved on to excellent positions both in academia and industry. Have a look at our list of recent alumni on the Machine Learning group webpage . Research expertise in machine learning is in very high demand these days.
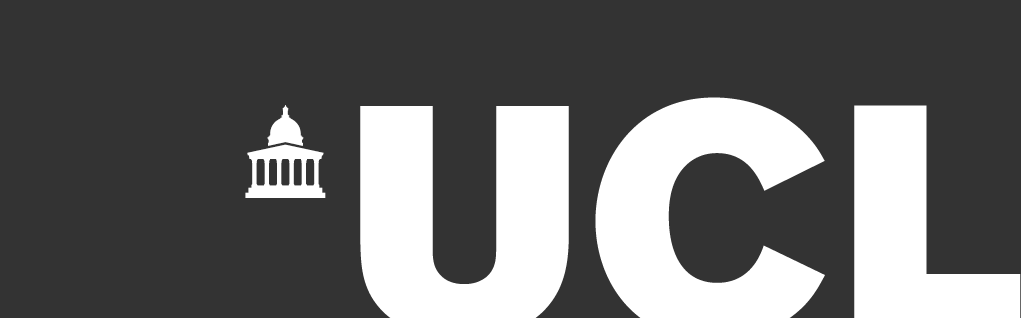
Artificial Intelligence Enabled Healthcare MRes + MPhil/PhD
London, Bloomsbury
Artificial Intelligence (AI) has the potential to transform health and healthcare systems globally, yet few individuals have the required skills and training. To address this challenge, our Centre For Doctoral Training (CDT) in AI-Enabled Healthcare Systems will create a unique interdisciplinary environment to train the brightest and best healthcare artificial intelligence scientists and innovators of the future.
UK tuition fees (2024/25)
Overseas tuition fees (2024/25), programme starts, applications accepted.
Applications closed
The Centre for Doctoral Training recruits in at least two rounds. Applicants are advised to apply early, priority will be given to those who have applied in round one.
- Entry requirements
A minimum of an upper second class honours undergraduate degree, or a Master's degree in a relevant discipline (or equivalent international qualifications or experience). Our preferred subject areas are Physical Sciences (Computer Science, Engineering, Mathematics and Physics) or Clinical / Biomedical Science. Applicants with a clinical background or degree in Biomedical Science must be able to demonstrate strong computational skills. You must be able to demonstrate an interest in creating, developing or evaluating AI-enabled Healthcare systems.
The English language level for this programme is: Level 2
UCL Pre-Master's and Pre-sessional English courses are for international students who are aiming to study for a postgraduate degree at UCL. The courses will develop your academic English and academic skills required to succeed at postgraduate level.
Further information can be found on our English language requirements page.
If you are intending to apply for a time-limited visa to complete your UCL studies (e.g., Student visa, Skilled worker visa, PBS dependant visa etc.) you may be required to obtain ATAS clearance . This will be confirmed to you if you obtain an offer of a place. Please note that ATAS processing times can take up to six months, so we recommend you consider these timelines when submitting your application to UCL.
Equivalent qualifications
Country-specific information, including details of when UCL representatives are visiting your part of the world, can be obtained from the International Students website .
International applicants can find out the equivalent qualification for their country by selecting from the list below. Please note that the equivalency will correspond to the broad UK degree classification stated on this page (e.g. upper second-class). Where a specific overall percentage is required in the UK qualification, the international equivalency will be higher than that stated below. Please contact Graduate Admissions should you require further advice.
About this degree
Every student who is accepted onto the AI-enabled Healthcare Systems Centre for Doctoral Training (CDT) must take the MRes Artificial Intelligence Enabled Healthcare in their first year. This will be followed by a 3 year PhD. Throughout this period the CDT will continue to closely monitor the need for continuing training and support, tailored to each student, and provide ongoing training in research skills. The MRes is not currently available as a stand-alone programme.
The MRes programme covers the core competencies of artificial intelligence and has a central emphasis on how healthcare organisations work. Ethical training for medical artificial intelligence will be explicitly emphasised alongside a broader approach to responsible research, innovation and entrepreneurship.
During the MRes year, students will learn the statistical underpinnings of machine learning theory, get a practical grounding in research software engineering and the principles of healthcare and medical research, as well as a thorough treatment of topics in machine learning, advanced statistics and principles of data science.
As part of the MRes, alongside the core and elective modules, you will complete a substantial Masters-level project of your choice, working with a supervisory team that will normally include a clinician and an academic. The project you work on during your MRes normally leads to the chosen PhD research topic.
The remaining years will be more like a traditional PhD, which leads to the presentation of a PhD thesis at the end of the fourth year. During your PhD you will remain involved in CDT activities and will continue to work closely with relevant health professionals and clinical teams through our NHS partners and leading academics at UCL.
As a cohort based PhD programme, students will also have the opportunity to participate in a range of seminars, training programmes, placements and other activities, including UCL's Doctoral Skills Development Programme.
Training Opportunities The CDT programme consists of a range of activities and events including:
- A Mini-MD programme where trainees undertake an immersive clinical experience within an NHS setting
- Annual CDT Conference
- Seminar series
- PPI Training
- Responsible Research & Innovation
- Communication Skills
- Entrepreneurship
- Ethical Training
- The opportunity to attend training programmes offered by the Alan Turing Institute
- Opportunities for internships and placements with industry partners
More information can be found on the CDT Website .
Who this course is for
The Centre for Doctoral Training programme is for students with an interest in creating and developing AI solutions aimed to transform and solve healthcare challenges. The CDT programme is embedded within a NHS setting, and should appeal to students keen to develop clinical knowledge and algorithmic/ programming expertise.
What this course will give you
- Benefit from UCL's excellence both in computational science and biomedical research innovating in AI;
- Be supervised by world-leading clinicians and AI researchers in areas related to your research;
- Work within a real-world setting, embedded within hospitals, allowing you to gain a practical understanding of the value and limitations of the datasets and the translational skills required to put systems into practice;
- Have the opportunity to not only apply AI to healthcare but to apply healthcare to AI, generating novel large-scale open datasets driving methodological innovation in AI;
- Become a future leader in solving the most pressing healthcare challenges with the most innovative AI solutions;
- Study at UCL, which is rated No.1 for research power and impact in medicine, health and life sciences (REF 2021) and 9th in the world as a university (QS World Rankings 2024).
The foundation of your career
We do not yet have any graduates from the four-year programme, our first cohort of students will be graduating over the next few months. We expect them to stay within the field of AI and healthcare, and much like previous graduates from our experienced CDT supervisors, they will go onto successful careers in academia and industry.
Employability
The distinctive characteristics of our programme allow us to produce graduates who are prepared to:
- engineer adaptive and responsive solutions that use AI to deal with complexity;
- innovate across all levels of care, from community services to specialist hospitals;
- be comfortable working with patients and professionals, and responding to their input;
- appreciate the importance of addressing health needs rather than creating new demand.
The Institute's research departments collaborate with third-sector and governmental organisations, as well as members of the media, both nationally and internationally to ensure the highest possible impact of their work beyond the academic community. Students are encouraged to do internships with relevant organisations where funding permits. Members of staff also collaborate closely with academics from leading institutions globally.
Teaching and learning
Various teaching and learning methods are employed to facilitate effective learning and cater to different learning styles. Below are some common types of teaching methods that may be used across the programme:
Interdisciplinary Teaching: Interdisciplinary teaching involves integrating knowledge and skills from multiple disciplines or subject areas to provide a comprehensive understanding of a topic, particularly AI and healthcare. This approach encourages students to make connections between different subjects and fosters critical thinking and problem-solving abilities.
Lecture-Based Teaching: Lecture-based teaching is a traditional method where the instructor presents information to students through spoken words. It involves the teacher sharing knowledge, concepts, and theories, while students take notes and listen actively. This method is effective for conveying large amounts of information and providing foundational knowledge.
Practical Coding Sessions: Practical coding sessions are hands-on learning experiences where students actively engage in coding exercises, programming tasks, and problem-solving activities. These sessions are essential for AI and programming-related subjects (machine learning, etc) as they allow students to apply theoretical knowledge to real-world scenarios.
Interactive Teaching: Interactive teaching methods encourage active participation and engagement from students. These methods can include discussions, debates, group activities, and case studies, in particular in several modules such as Journal Club. Interactive teaching fosters collaboration, communication skills, and a deeper understanding of the subject matter.
Project-Based Learning: Project-Based Learning involves assigning students long-term projects that require them to investigate and address real-world problems or challenges (such as AI & healthcare group project). It enhances critical thinking, research skills, and creativity while promoting independent learning and teamwork.
Collaborative Learning: Collaborative learning involves students working together in small groups or pairs to solve problems, discuss ideas, and complete tasks. This method promotes teamwork, communication, and the exchange of diverse perspectives.
The use of these teaching/learning methods can vary depending on the subject matter, the goals of the programme, and the preferences of the instructors in the MRes year. Our educational programme incorporates a mix of these methods to cater to the diverse needs of learners and create a well-rounded learning experience.
Compulsory Modules:
CHME0033 Dissertation in Artificial Intelligence Enabled Healthcare
CHME0032 Healthcare Artificial Intelligence Journal Club
Optional Modules
CHME0012 Principles of Health Data Science
CHME0013 Data Methods for Health Research
CHME0015 Advanced Statistics for Records Research
CHME0016 Machine Learning in Healthcare and Biomedicine
CHME0031 Programming with Python for Health Research
CHME0034 Computational Genetics of Healthcare
CHME0035 Advanced Machine Learning for Healthcare
CHME0039 Artificial Intelligence in Healthcare Group Project
COMP0084 Information Retrieval and Data Mining
Please note that the list of modules given here is indicative. This information is published a long time in advance of enrolment and module content and availability is subject to change.
Assessment methods are crucial components of an educational programme, as they evaluate students' understanding, knowledge, skills, and application of concepts. Here are various types of assessment methods that may be used across the programme:
Exams: Traditional exams are a common assessment method that tests students' knowledge and understanding of the course material. These exams typically involve a time-bound written assessment, where students respond to questions related to the subject matter.
Open-Book Exam: In an open-book exam, students are allowed to refer to their textbooks, notes, or other resources during the assessment. The questions in these exams are often designed to test higher-order thinking and problem-solving abilities, as students have access to reference materials.
Coursework: Coursework assessments involve various assignments, essays, reports, or projects that students complete throughout the course. These assessments may cover specific topics or practical applications and help to assess students' comprehension and critical thinking skills.
Coding Exam: A coding exam is specifically designed for courses related to computer science, software development, or programming. Students are given coding challenges or programming tasks that assess their coding proficiency and problem-solving abilities.
Collaborative Project: In a collaborative project assessment, students work in groups to tackle a complex problem or complete a substantial task. This assessment measures teamwork, communication, time management, and the ability to achieve shared goals.
Presentation and Q&A: Presentations require students to deliver a talk on a given topic or project. The presentation assesses their ability to communicate effectively, organize information, and present ideas coherently. Often, a question and answer (Q&A) session follows the presentation to delve deeper into the topic.
Research Proposal: A research proposal is a preliminary plan for a research project that students submit to demonstrate their research capabilities. It outlines the research question, objectives, methodology, and potential outcomes of the study.
Dissertation Writing: Dissertation writing is typically reserved for higher education levels, such as undergraduate and postgraduate studies. It involves an extended research project on a specific subject, allowing students to demonstrate research, analytical, and academic writing skills.
Online Quizzes and Tests: Online quizzes and tests are digital assessments that may be used for formative or summative purposes. They are often employed in blended or online learning environments.
The use of assessment methods will vary based on the nature of the programme, the subject matter throughout the MRes year. A well-balanced combination of assessment types ensures that students' diverse abilities and learning styles are appropriately evaluated while providing a comprehensive understanding of their progress and achievements.
During the MRes 4 hours of a student's time is spent in tutorials per week and/or, 6-8 hours in lectures per week, and a further 20-24 hours in independent study per week.
Research areas and structure
- AI-enabled diagnostics or prognostics
- AI-enabled operations
- AI-enabled therapeutics
- Public Health Data Science
- Machine Learning in Health Care
- Public Health informatics
- Learning health systems
- Electronic health records and clinical knowledge management
- e-health and m-health
- Clinical Decision Support Systems
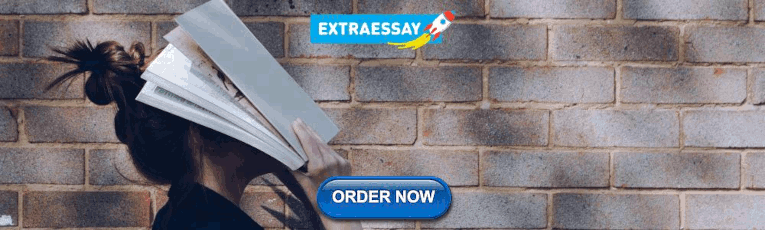
Research environment
Our research environment offers a unique degree programme that stands out among competitors. We provide students with the exceptional opportunity to explore the cutting-edge intersection of AI technology and healthcare applications. Our curriculum emphasizes research and innovation skills, empowering students to become independent researchers and adept problem solvers. A key difference is our close collaboration with clinicians and front-line practitioners. This interaction fosters a holistic understanding of healthcare challenges and real-world applications, ensuring that our graduates are equipped with practical knowledge and solutions. Our programme is inclusive, welcoming students from both computational and clinical backgrounds, creating a diverse and dynamic learning environment.
Students studying the programme full-time will be expected to complete 180 credits during the academic year.
Students studying the programme part-time will be expected to complete 180 credits across two academic years.
Accessibility
Details of the accessibility of UCL buildings can be obtained from AccessAble accessable.co.uk . Further information can also be obtained from the UCL Student Support and Wellbeing team .
Fees and funding
Fees for this course.
The tuition fees shown are for the year indicated above. Fees for subsequent years may increase or otherwise vary. Where the programme is offered on a flexible/modular basis, fees are charged pro-rata to the appropriate full-time Master's fee taken in an academic session. Further information on fee status, fee increases and the fee schedule can be viewed on the UCL Students website: ucl.ac.uk/students/fees .
Additional costs
All studentships include a research training support grant, which covers additional research costs throughout students' time on the programme.
For more information on additional costs for prospective students please go to our estimated cost of essential expenditure at Accommodation and living costs .
Funding your studies
Please visit the CDT website for funding information.
For a comprehensive list of the funding opportunities available at UCL, including funding relevant to your nationality, please visit the Scholarships and Funding website .
Note for applicants: When applying on UCL Select, please select MRes Artificial Intelligence enabled healthcare to apply for programme.
Please note that you may submit applications for a maximum of two graduate programmes (or one application for the Law LLM) in any application cycle.
Got questions? Get in touch
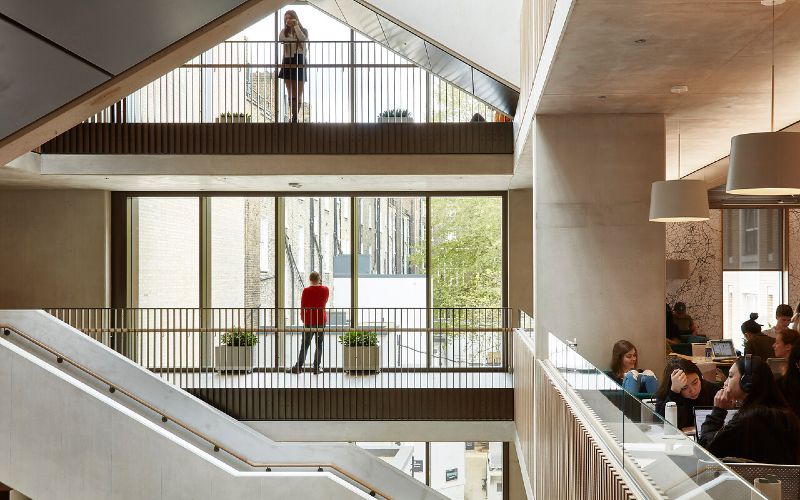
Institute of Health Informatics
UCL is regulated by the Office for Students .
Prospective Students Graduate
- Graduate degrees
- Taught degrees
- Taught Degrees
- Applying for Graduate Taught Study at UCL
- Research degrees
- Research Degrees
- Funded Research Opportunities
- Doctoral School
- Funded Doctoral Training Programmes
- Applying for Graduate Research Study at UCL
- Teacher training
- Teacher Training
- Early Years PGCE programmes
- Primary PGCE programmes
- Secondary PGCE programmes
- Further Education PGCE programme
- How to apply
- The IOE approach
- Teacher training in the heart of London
- Why choose UCL?
- Inspiring facilities and resources
- Careers and employability
- Your global alumni community
- Your wellbeing
- Postgraduate Students' Association
- Your life in London
- Accommodation
- Funding your Master's
Student News
Tuesday, 28 may, search for news, browse student news stories.
- All Student news
- All featured
Fully Funded PhDs in Data Science, AI and Machine Learning

The University of Liverpool’s Centre for Doctoral training in Distributed Algorithms (CDT) are currently looking for students to join their fully funded PhDs in Data Science, AI and Machine Learning.
The team aim to develop 60 PhD students to meet the world’s pressing need for highly-trained data scientists and work with industry and government to help solve real-world problems.
Applicants come from a range of subjects and backgrounds, including:
- Computer Science
- Department of Civil Engineering and Industrial Design
- Earth, Ocean and Ecological Sciences
- Electrical Engineering and Electronics
- Geography and Planning
- Mathematical Sciences
- Mechanical and Aerospace Engineering
The fully funded PhD studentships are open to home and international students. You’ll be working as part of a cohort in a collaborative environment alongside other PhD students, postdoc researchers and data scientists. Other benefits include:
- PhD projects co-defined and co-supervised with a project partner
- Monthly tax-free payment of £1,338.50
- Annual research grant
- Placements in year 3
- Long-term employment potential
- Inclusive and supportive cohort environment
- Technical, professional and personal training and development
- Access to state-of-the-art high-performance computers
Interested?
The team would love to hear from you. Please do get in touch to find out more.
Email Kelli or Sara ( [email protected] ) if you have any questions.
They will also be at the Careers Studio on Friday 22 July between 11am – 1pm – drop-in to speak to the team, no appointment necessary.
Click here to find out more and apply
Further reading
- Click here to discover what our current students are working on and who with.
- Email one of our Student Ambassadors here and arrange a call with them.
- View our applicant information and guidance.
- Simon Maskell, CDT Director Bio
- Student Featured 2
- Careers and Employability
- CDT in Distributed Algorithms.
- Centre for Doctoral Training in Distributed Algorithms
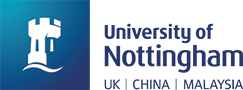
- About the School
- Computer Science Open Day Building Guide
- Buying a Computer
- Scholarships
- Alumni Scholarships
- Masters degrees
- Nottingham Doctoral Training Centre in AI
- Cohorts and Projects
- Study Abroad
- New and returning students
- Disability information
- Visiting us
- Work with us
- Equality Diversity and Inclusion
- Seminar series
- Email this Page
- Student Intranet
Faculty of Science Doctoral Training Centre in Artificial Intelligence

The Faculty of Science AI DTC is a new initiative by the University of Nottingham to train future researchers and leaders to address the most pressing challenges of the 21st Century through foundational and applied AI research on a cohort basis. The training and supervision will be delivered by a team of outstanding scholars from different disciplines cutting across Arts , Engineering , Medicine and Health Sciences , Science and Social Sciences .
The Faculty of Science will invite applications from Home students for fully-funded PhD studentships to carry out multidisciplinary research in the world-transforming field of artificial intelligence. The PhD students will have the opportunity to:
- Choose from a wide choice of AI-related multidisciplinary research projects available, working with world-class academic experts in their fields;
- Benefit from a fully-funded PhD with an attractive annual tax-free stipend;
- Join a multidisciplinary cohort to benefit from peer-to-peer learning and transferable skills development.
The deadline to have completed and submitted your application to NottinghamHub has now closed.
Research Topics
Rooted in the exceptional research environments of our Schools/Faculties at the University of Nottingham, the third cohort of the AI DTC will be organised around seventeen multidisciplinary research topics. It is important that you identify a research topic aligned with the expected skill set, your background and particular areas of interest. You will need to obtain support from the supervisors associated with your research topic choice before submitting your official application. You can do this by exploring the research projects below and contacting the main supervisor of the project that is of interest to you, directly, to discuss the further details and to arrange an interview as appropriate. In your PhD studentship application, you will be asked to provide your CV, and a personal statement including a research/project topic from the following list and explaining why you are interested in that research/project topic and your motivation for doing a PhD, and the names of the supervisors you have support from. We encourage applicants to complete the personal statement in their own words based on their background and experience. Please follow the instructions above on how to apply.

AI for additive manufacture of complex flow devices
AI based generative methods can allow us to generate designs of structures with optimal or new functionality. These structures are often complex, which means it is difficult to manufacture them, but with additive manufacturing and its design freedoms such constraints do not apply. Consequently, the combination of AI, numerical methods and additive manufacturing can lead to a new paradigm in functionality, with any device dependent on shape for its function being available for augmentation in behaviour.
Our approach is to use recent developments in generative design, such as AI diffusion models, to identify designs that match the requirements of the user, allowing us to ‘dial up’ or select function and have the design provided to us. The building and training of this model will be via numerical or computational models, validated by experiment, that will allow us to provide training data and indeed, augment that with data over the course of the PhD. As a final part of the jigsaw, additive manufacturing built parts will be used to validate both the numerical models and the AI model.
Our initial focus will be on the automatic generation of mixing devices, commonly used for process intensification and downstream processing in multiple high value manufacturing operations such as pharmaceutical and chemical synthesis, but a general methodology is sought that can be applied across multiple applications.
This project will in part be supported by a Programme Grant hosted by the University of Nottingham ‘Dialling up performance for on demand management’, with over 10 industrial partners supporting the projects. The studentship will have access to resources (physical, chemical and financial) to support the research, and also access to an exchange fund that enables extended research visits to collaborative institutions, including UC Berkeley, ETH Zurich and CSIRO.
Supervisors: Prof Ricky Wildman (Faculty of Engineering) [email protected] , Dr Mirco Magnini (Faculty of Engineering), Prof Ender Ozcan (School of Computer Science).
For further details and to arrange an interview please contact Prof Ricky Wildman (Faculty of Engineering) [email protected]
AI-based decoding of evoked neural activity to study bilingual language processing
There are more people in the world who can speak two or more languages fluently than people who speak only one language. How are the languages of these bilinguals represented and processed in their brain? Traditionally, neuroimaging and behavioural techniques have been used to develop theories about language storage and processing. Recent studies have shown that insight about how multiple languages are represented in the brain can be gained from decoding language from neural activity. A particular interesting approach is cross-language decoding, which involves decoding brain activity of words in one language and use that to decode words of another language. Whereas most studies in the literature have used functional magnetic resonance imaging (fMRI) data for decoding language, recent studies have shown that decoding language from evoked non-invasive electroencephalography (EEG) data is also possible. However, decoding accuracies obtained have generally been low.
This project aims to study the time course of the activation of within- and between-language representations in the bilingual brain by decoding evoked EEG activity across multiple modalities (visual and auditory). In particular, the project will focus on semantic representations. The project will involve designing and conducting EEG experiments with bilinguals to obtain data for decoding. Furthermore, the project will make use of state-of-the art machine learning techniques and advanced large language models to improve decoding accuracy.
Applicants are expected to have a degree in Psychology, Mathematics, Computer Science, Physics, or related areas, and have knowledge of programming and a strong interest in machine learning, cognitive neuroscience, and psycholinguistics. Experience with EEG and experimental techniques are desirable, but not essential.
Supervisors: Dr Walter van Heuven (School of Psychology), Dr Matias Ison (School of Psychology), Dr Ruediger Thul (School of Mathematical Sciences) [email protected] .
For further details and to arrange an interview please contact Dr Walter van Heuven (School of Psychology).
AI-based digital twins and active control in composites manufacturing
Applications are invited for a PhD studentship to conduct interdisciplinary research in the rapidly evolving area of AI for manufacturing of fibre-reinforced polymer composites. A student working on this project will develop and test novel AI algorithms for digital twins of manufactured composite parts and for active control of composites manufacturing processes. The project will include developing effective surrogate models (e.g., via physics-informed neural networks (PINNs)) and active learning of underlying physical processes. The algorithms will be used for on-the-fly estimation of properties of composites parts and for real-time active control to produce parts according to specification and avoiding defects. The project will include working with real data from experiments conducted in our Composites Research lab at the Faculty of Engineering and a range of our industrial partners.
Supervisors: Prof Michael Tretyakov (School of Mathematical Sciences) , Dr Mikhail Matveev (Faculty of Engineering), Dr Marco Iglesias (School of Mathematical Sciences).
For further details and to arrange an interview please contact Prof Michael Tretyakov (School of Mathematical Sciences).
Artificial-Intelligent-Aided Energy Management for Future Electric Aircraft
The COP26 has set a clear goal of securing global net zero by mid-century and keep 1.5oC within reach. To deliver this target , it is critical that the transportation sector speeds up the electrification process. For Aviation, the Intergovernmental Panel on Climate Change has set the target of at least 50% reduction of CO2 emissions by 2050 within which aviation is responsible for about 20% of overall emissions. Hybrid/Electric aircraft, which are powered by batteries, fuel cells and generators, are with great potential to reduce the CO2 emission in the Aviation Sector.
This PhD project aims to optimise energy management on board future electric aircraft using the recently advanced machine learning and artificial intelligent (AI) technologies. The student will be working within two world-leading research groups in the University of Nottingham. The applicant should have electrical engineering background with strong math skills. Basic AI knowledge would be expected from applicant.
Supervisors: Dr. Tao Yang (Electrical Engineering), Dr Grazziela Figueredo (Faculty of Medicine & Health Sciences) [email protected]
For further details and to arrange an interview please contact Dr. Tao Yang (Electrical Engineering).
Camera-based movement analysis to provide real-time optimisation of therapeutic non-invasive neuromodulation for neurological diseases: A Human-in-the-Loop and Machine Learning Approach
Involuntary movements, such as tremor, tics, sudden jerks (chorea) and muscle spasms (dystonia) occur in many neurological disorders. These involuntary movements are difficult to treat, requiring medications (which often have unpleasant side effects) or invasive deep brain stimulation (requiring electrodes to be implanted in the brain). Recent innovative research by Prof Stephen Jackson (UoN Psychology) found that non-invasive median nerve stimulation (MNS), which involves stimulating a nerve in the wrist using a wearable electronic device, suppresses involuntary movements in Tourette syndrome by entraining oscillations in relevant brain circuits when the device is active. Clinical trials are now underway, or being developed, to test the therapeutic effect in a number of neurological disorders, including Parkinson disease (PD) ataxia telangiectasia (A-T) and restless legs syndrome (RLS).
However, involuntary movements are intermittent and highly variable, meaning that continuous stimulation by the MNS device is not required or desirable. Real-time detection of involuntary movements could allow personally-tailored therapeutic stimulation strategies. optimised to detect different types of involuntary movements seen in diseases such as PD, AT and RLS, and used to optimise the stimulation regime on an individual basis.
In this project, we will analyse videos of trial participants to understand how MNS affects the patients' symptoms, and to optimise the device in real time to provide the maximum benefit for each individual. To achieve this, we will utilise state-of-the-art marker-less pose estimation in combination with multi-modal machine learning to better understand the complex movements of those with movement disorders. This will enable us to tailor the treatment to each individual's specific needs in real time and maximise its effectiveness by tuning the MNS device. Demonstrating the feasibility of the human-in-the-loop approach will directly enable clinical trials of the effectiveness of personalised home-administered MNS stimulation in reducing the unwanted movements, and allow exploration of the potential benefits of this technology in improving quality of life for individuals with movement disorders.
This PhD project will benefit from a strong multidisciplinary approach, combining computer science, psychology, and neuroscience. Applicants are expected to have a combination of programming experience (python) and an interest/background in neuroscience.
Supervisors: Dr Alexander Turner (School of Computer Science), Prof Stephen Jackson (School of Psychology), Prof Robert Dineen (Faculty of Medicine & Health Sciences)
For further details and to arrange an interview please contact Dr Alexander Turner (School of Computer Science).
Computer vision for dynamic materials analysis
Electronic materials development is reliant upon the use of computational chemistry tools for elucidating structure-property relationships on the atomic scale. Molecular dynamics simulations can capture macroscopic material changes involving over 100 million atoms, such as catastrophic electrical breakdown, whilst retaining information down to the level of individual atoms. As electronic device miniaturisation has reached the nanoscale, using dynamic simulations as a theoretical microscope will be vital for overcoming the materials bottleneck we are facing at the end of Moore’s Law.
One of the challenges in calculations of this scale is the analysis of structural changes including crystallinity, grain boundaries and nanodomain formation. These features are critical in determining properties from electronic and thermal conductivity to optical processes and response to electric fields. However, their complex dynamical behaviour involves thousands of atoms moving over hundreds of thousands of timesteps, making them unsuited to traditional modes of analysis developed for regular crystalline materials.
This project will take advantage of AI tools in computer vision as a new approach to analyse structural features in dynamical materials simulations. These have advanced to enable analysis of images of self-similar repeating patterns, which will be highly applicable to subtle changes in atomic configurations. Deep learning methods will be used to develop classification, segmentation, and regression models to identify structural transitions, anomaly detection to identify point defects, and edge detection for revealing grain boundaries. These will be applied to the most pressing materials chemistry problems hindering electronic device miniaturisation.
Potential applicants are expected to have some background in machine learning and/or computational materials/chemistry, in addition to some experience in (and desire to learn) scientific programming such as Python, scikit-learn, and relevant deep learning libraries, such as PyTorch.
Supervisors: Dr Katherine Inzani (School of Chemistry), Dr Valerio Giuffrida (School of Computer Science), Dr Julie Greensmith (School of Computer Science)
For further details and to arrange an interview please contact Dr Katherine Inzani (School of Chemistry).
Computer Vision-Based Monitoring of Equine Welfare and Wellness
We are seeking a dynamic and passionate PhD student to join our team and help drive enhancements to equine health and welfare through AI based monitoring. Through this PhD, you will have the unique opportunity to gain an exceptional skillset through interdisciplinary collaboration between the UoN School of Veterinary Medicine and Science, School of Computer Science, and industry partner Vet Vision AI; helping to shape the future of the animal health and welfare.
Numerous potential welfare issues impact equine welfare when stabled. However, many of these are preventable. Current processes to improve welfare are extremely limited, often relying on owner “know-how” rather than objective and continuous monitoring. The use of AI to automatically monitor welfare outcome will provide a step change in animal health and welfare monitoring, empowering vets and horse owners to revolutionise equine welfare monitoring.
This PhD aims to develop and deploy cutting-edge computer vision algorithms alongside veterinary insights to monitor and improve equine welfare outcomes accurately and automatically. Successful applicants will be based in the School of Veterinary Medicine, with a close-knit team environment within which mentoring, collaboration and idea sharing is strongly promoted, with input from world-leading computer scientists from the School of Computer Science. They will also work directly with experienced computer vision developers alongside partner company Vet Vision AI; a spinout from the School of Veterinary Medicine and Science on a mission to revolutionise animal health and welfare by combining veterinary insights and computer vision technology.
This PhD aims to combine world leading veterinary expertise and equine domain knowledge with advanced skills in computer vision and machine learning. Their aim is to translate this knowledge into cutting edge solutions that will help owners and veterinary surgeons improve the lives of millions of animals worldwide.
Supervisors: Supervisors: Dr Robert Hyde (School of Veterinary Medicine and Science), Prof Sarah Freeman (School of Veterinary Medicine and Science), Dr Katie Burrel (School of Veterinary Medicine and Science), Dr Zhun Zhong (School of Computer science), Prof Andrew French (School of Computer Science)
For further details and to arrange an interview please contact Dr Robert Hyde .
Energy requirements of neuromorphic learning systems
Large neural networks are rapidly invading many parts of science, and have yielded some very exciting results. However, particularly the training of large networks requires a lot of energy. This energy is needed to compute, but also to store information in the synaptic connections between neurons. Interestingly, also biological systems require substantial amounts of energy to learn. Under metabolically challenging conditions, these requirements can be so large that in small animals learning reduces the lifespan. Based on these findings we have started to design algorithms that reduce the energy needed to train neural networks.
This project will explore energy requirements for learning in neuromorphics system with memristors. Neuromorphic systems mimic the biological nervous system in their design principles and are currently being explored to create highly energy efficient neural networks. Memristors that are a key technology in such devices. Specifically, we will 1) develop models that describe the energy needs for learning in neuromorphic networks, 2) use inspiration from biology to design efficient algorithms that are more energy efficient and test them in simulations, and 3) contrast energy requirements to the energy needs in biology as well as conventional hardware.
The ideal applicant will have a strong background in physics, mathematics, computer science or engineering with both analytical and programming skills. Interest in biology and/or engineering will be beneficial.
Supervisors: Prof Mark van Rossum (School of Psychology), Dr Neil Kemp (School of Physics and Astronomy) [email protected] .
For further details and to arrange an interview please contact Prof Mark van Rossum (School of Psychology).
Imaging Molecules in Action: A Journey from Atoms to Energy Materials
Over 300 years scientists have devised many ways to illustrate atoms and molecules and their behaviour, using everything from elemental symbols to sophisticated 3D models. It’s easy to think these representations are reality, but how we picture molecules is usually based on bulk measurements where information is averaged over billions of billions of molecules (spectroscopy) or reciprocal space (diffractometry). Recently, electron microscopy began revolutionising the way we see matter and allowed us to glimpse at atomically resolved images of individual molecules. Early breakthroughs are exciting, but they raised three key questions:
• What does it mean to ‘see a molecule’?
• How can we reconstruct the 3D shape of a molecule from 2D micrographs?
• How can we achieve resolution in space and time for tracking the movement of individual molecules?
In this project, we aim to answer fundamental questions related to electron microscopy by advancing image analysis methods. We plan to make a significant step-change by applying advanced image analysis methods (Xin Chen) to problems related to single-molecule imaging (Andrei Khlobystov). While sub-Angstrom resolution can be achieved routinely, there's a pressing need for robust techniques to denoise electron microscopy images that allow comparing experimental and theoretically simulated images. For example, image denoising using a diffusion probabilistic model has the potential to identify atom positions while simultaneously enhancing the temporal resolution of electron microscopy. This breakthrough in spatiotemporal continuity of atomic imaging could allow us to watch molecules in action and film chemical reactions with atomic resolution in real-time.
The Nanoscale & Microscale Research Centre at Nottingham, along with leading European centres in Ulm, Germany and Diamond Light Source in the UK, will provide training for the student to image molecules with atomic resolution. In addition, the student will learn to develop modern artificial intelligence algorithms for image processing and analysis to enhance the data to the highest level of spatiotemporal resolution.
The project aims to revolutionize the imaging of molecules, turning it into a valuable tool for discovering new chemical processes. This will be particularly useful in catalysis and net-zero technologies, which are crucial for 70% of the UK chemical industry. The University of Nottingham holds the largest EPSRC grant in this area (EP/V000055/1).
Supervisors: Prof Andrei Khlobystov (School of Chemistry), Dr Xin Chen (School of Computer Science).
For further details and to arrange an interview please contact Prof Andrei Khlobystov (School of Chemistry).
Intelligent Modelling of RNA Nanomedicines using Cryo-OrbiSIMS, Machine Learning and Molecular Simulations
Motivation: Imagine a new virus emerges, causing a global disease outbreak. Scientists quickly sequence the virus's genome, but developing a traditional vaccine or treatment can take months to years, and a faster solution is needed to control the outbreak. Our project addresses this critical challenge by developing an innovative approach to aid rapid and intelligent design of RNA nanomedicines.
Challenges to be addressed: Designing RNA-based nanomedicines, such as mRNA vaccines, presents a unique challenge for computational scientists. RNA molecules adopt complex 3D folds that significantly impact their function within a cell. For instance, the way the mRNA folds within the vaccine can affect how well the body can read its instructions and elicit an effective immune protection. Thus, it is important to determine the 3D structure of the mRNA within the vaccine. However, current experimental and computational techniques struggle to resolve its 3D architecture and flexibility.
To address this technology gap, we have pioneered the integration of cryo-OrbiSIMS, a unique mass spectroscopic imaging capability of University of Nottingham, with molecular modelling and computer simulations to model the three-dimensional structures of RNAs at atomic resolution.
However, studying RNA nanomedicines, such as mRNA vaccines and viral vectors, using our method presents two specific challenges:
1. The cryo-OrbiSIMS data becomes significantly complex and hinders efficient data analysis using traditional computer programming.
2. The RNA molecules adopt increasingly complex folds and interactions, which are challenging to model using 3D structure prediction algorithms.
Proposed solution: Through this AI DTC project, we aim to use AI/ML techniques to learn the complex relationships between RNA structures and its corresponding cryo-OrbiSIMS data to enhance our data analysis and interpretation pipelines.
Skillsets, Training and Development: The project sits at an interdisciplinary interface of structural biophysics and computational sciences. Thus, a strong background in data handling, statistical analysis or computer programming would be essential in the DTC candidate. Alternatively, experience in computational structural biology would be desirable.
The core-supervisory team for this project comprises of experts from RNA biology, structural biology, mass spectroscopy and computer science. Thus, they are very well suited to provide a cross-institutional, dynamic, intellectually stimulating and highly resourceful research environment. The doctoral student will be based within the Wolfson Centre for Global Virus Research at University of Nottingham with supervisory links to the School of Pharmacy, School of Computer Science and Nanoscale and Microscale Research Centre, where a number of PhDs, postdocs and technical staff will provide day-to-day assistance in state-of-art, interdisciplinary techniques.
Supervisors: Dr Aditi Borkar , (School of Veterinary Medicine and Science), Dr Grazziela Figueredo (Faculty of Medicine & Health Sciences), Dr David Scurr (School of Pharmacy), Prof Morgan Alexander (School of Pharmacy), Dr Anna Kotowska (School of Pharmacy).
For further details and to arrange an interview please contact Dr Aditi Borkar , (School of Veterinary Medicine and Science).
"Learning by Doing": A Cross-Disciplinary Exploration of Human Motor Learning, AI, and Robot Learning
AI and Robotics technologies are becoming pervasive in everyday human life, e.g. telepresence robots, robot vacuums, humanoids in the factories, etc. To operate in and interact with humans and made-for-human environments, robot learning plays a vital role, allowing robots to be more easily extended and adapted to novel situations. An example of applying AI and machine learning to robotics is learning-by-demonstration, a supervised learning technique, where robots acquire new skills by learning to imitate an expert.
The research challenge lies in developing robot learning models by modelling the different stages of motor learning in humans, i.e., modelling humans as naïve learners, rather than experts. The fundamental research in human motor learning (HML), by which humans acquire and refine motor skills through practice, feedback, and adaptation, proves critical in this regard. This can allow the robot to mimic human motor control mechanisms, such as joint flexibility and compliance, more naturally, with the benefit of refining the acquired skill over time and input from humans. Example applications include: an assistive robotic arm assisting stroke patients guide their movements and providing corrective cues; therapy patients learning to adjust their motor patterns for better outcomes, based on the AI models, etc.
This research will address the challenge noted, focussing on three measurable outcomes – (i) novel research into AI to model HML in subjects, including adults and children, especially with the goal of mapping the HML model to robot motion; (ii) implementing the developed HML models in a robot learning architecture (in robotic manipulation or navigation) evaluated against novel benchmarking metrics (to be investigated) for the applicability and utility of developed research in real-world; and (iii) annotated and labelled dataset (videos, sensor data, etc.) of human as well as robot motions made publicly available, for the benefit of the scientific communities in psychology, AI, and robotics.
Prospective PhD applicants may have a degree in Computer Science or Robotics (or Psychology with an experimental focus), and with knowledge of Machine Learning, Deep Learning, AI, and Robotics (preferably). This project will require excellent programming skills with evidence of proficient working knowledge in one or more of the following: C++, Python, ROS.
Supervisors: Dr Nikhil Deshpande (School of Computer Science), Dr Deborah Serrien (School of Psychology) [email protected] .
For further details and to arrange an interview please contact Dr Nikhil Deshpande (School of Computer Science).
Learning Heuristics for Computer Algebra Software
Computer Algebra Systems (CASs) play an increasingly important role in pure mathematics research. These are immensely complicated pieces of software that allow the user to represent and handle abstract mathematical objects within a computer. Handing these objects requires expensive computations and involves heuristics that choose the most appropriate computational methods.
Each heuristic attempts to predict which of the available computational methods will be most effective in the current circumstances. The choice does not affect the correctness of the solution – it is essential that the output remains correct – but affects running times. Because of the exponential nature of many of the algorithms used by a CAS, a poor choice can make the difference between a computation finishing in under a second, to requiring years to complete.
Traditionally, such heuristics are designed by humans who study at most a few hundred examples and produce common-sense-based algorithms. They are strongly influenced by the use cases they are familiar with. It has been shown that computers usually outperform humans in such predictions, although it is often a non-trivial task to employ machine learning within computational software due to the complexity of the patterns and the huge variability in use cases.
This project will develop machine learning tools for algorithm selection, which can be embedded in an existing CAS. These tools will replace the existing hard-coded heuristics, and a life-long-learning mechanism will ensure that they develop over time in response to real-world use cases.
This pioneering project will place the student at the forefront of an exciting new area of research: the application of machine learning techniques to exact symbolic computations. This work has enormous potential for collaborative projects with CAS research groups worldwide: for example, the hugely successful SAGE open source project; the DFG-funded Singular and OSCAR groups in Germany; and the Magma group in Australia.
An ideal candidate will have computer science or engineering background, with interest in mathematics and artificial intelligence. Good programming skills are essential.
Supervisors: Dr Daniel Karapetyan (School of Computer Science), Dr Alexander Kasprzyk (School of Mathematical Sciences).
For further details and to arrange an interview please contact Dr Daniel Karapetyan (School of Computer Science).
Machine learning-assisted growth of atomically thin semiconductors
Development of quantum systems and understanding of their complex behaviour - from quantum tunnelling to entanglement - have led to revolutionary discoveries in science. Quantum science has great potential, but future progress requires a shift towards transformative materials and advanced fabrication methods. This project will use a bespoke cluster (EPI2SEM) for EPitaxial growth and In-situ analysis of two dimensional semiconductors (2DSEM) to create the high-purity materials and research tools required to advance the field beyond present state-of-the art. By using computational modelling and machine learning (ML), we aim to realise an artificial intelligence semiconductor-synthesis control system. The successful growth of 2DSEM demands strict control of many conditions, including temperature, pressure, atom fluxes and their ratio, growth rate, etc.
We will explore the thermodynamics and growth kinetics including reaction pathways, surface migration, and reaction rate of 2DSEM on specific substrate surfaces. The proposed approach of “cooking” (i.e. define recipes for the growth) and “tasting” (i.e. growth and measurement) will be applied to the fabrication of atomically thin-semiconductors with ultra-high electron mobilities for nanoelectronics. Advanced computational and ML simulations (with Prof. Elena Besley, School of Chemistry), combined with complementary experimental tests (with Prof. Amalia Patanè, School of Physics), will provide a powerful toolkit for unveiling the real-time growth mechanism.
In this project, machine learning (ML) methods will be utilised to predict thin-film growth of semiconductor materials. The ML predictions will be tested in a unique in the world, bespoke facility EPI2SEM (School of Physics) and by our industrial partner Paragraf (Greater Cambridge Area). Synthesis-by-design (with Prof. Amalia Patanè, School of Physics) guided by computation (with Prof. Elena Besley, School of Chemistry) will reduce the need for cost- and time- consuming trial-and-error experiments.
Applicants will be expected to have a numerate background from a first degree in Maths, Chemistry, Physics or similar, strong interest in applied machine learning and developing new coding and data science skills.
Supervisors: Prof Elena Besley (School of Chemistry), Prof Amalia Patanè (School of Physics).
For further details and to arrange an interview please contact Prof Amalia Patanè (School of Physics).
Multimodal machine learning of parent-child interactions as a predictor of child cognitive functions
The first five years of the child’s life play a critical role for cementing cognitive functions. Several studies have shown that the quantity and quality of parent-child interactions can affect children’s cognitive development later on. Periods of shared attention between caregivers and children have important implications for developing the child’s attention span and language skills. Recent neuroimaging research has also found that some parts of the brain may be activated in similar ways for both parents and children during these interactions, and interestingly, the extent of this analogous brain activation might be influenced by factors like how stressful the home environment is. Despite what is known about the association between child cognitive functions and the modalities (i.e., audio-visual and brain activity) involved in parent-child interactions, the extent to which these can be combined to inform better cognitive developmental outcomes for infants is unknown. If we can better understand these associations, we can help caregivers interact with young children in more effective ways that could potentially transform their development in the crucial early stages of life.
In this project you will work to better understand the interactions between young children and caregivers as they interact during exploratory play. You will apply state-of-the art machine learning techniques to analyse videos of interactions, detecting poses, activities and key events. You will explore novel deep learning methods for integrating multi-modal information sources, to combine video events, audio data and FNIRS data and extract perceptual, verbalization, affect, brain function information (to name a few) during parent-child interactions to predict cognitive functions in children. Video, questionnaire and neuroimaging data are already available from an ongoing, longitudinal project assessing neurocognition in children in the School of Psychology at the University of Nottingham. Separately, it might be possible to design and collect more data in the future.
Applicants will be expected to have a good working experience with current machine learning and image processing tools and techniques. Prior knowledge of biomedical signal processing and natural language processing is desirable but not essential.
Supervisors: Dr Joy Egede (School of Computer Science), Dr Sobana Wijeakumar , (School of Psychology), Dr Aly Magassouba (School of Computer Science) [email protected] .
For further details and to arrange an interview please contact Dr Joy Egede (School of Computer Science)
Physics-informed Machine Learning for Climate Wind Change
Machine learning simulation strategies for fluid flows have been extensively developed in recent years. Particular attention has been paid to physics-informed deep neural networks in a statistical learning context. Such models combine measurements with physical properties to improve the reconstruction quality, especially when there are not enough velocity measurements. In this project, we will develop novel methods to reconstruct and predict the velocity field of incompressible flows given a finite set of measurements. Specifically, using wind data from the Met Office, we aim at reconstructing the wind in the UK for the last 50 years and predict the main features of the wind in the UK in the upcoming decades and compare it against climate change models (CMIP6 and ERA5) based on classical data assimilation. For the spatiotemporal approximation, we will further develop the Physics-informed Spectral Learning (PiSL) framework [1,2,3] which has controllable accuracy. Our computational framework thus combines supervised (wind data) and unsupervised (physical conservation laws) learning techniques. From a mathematical standpoint, we will study the stability and robustness of the method whereas, from a computer science standpoint, we will develop efficient algorithms for the adaptive construction of the sparse spectral approximation.
We are looking for master's degree holders who are interested in interdisciplinary research projects that revolve around computational methods such as mathematical models, simulation methods, and data science techniques. Applicants are expected to have a numerate background from a first degree in Maths, Physics, Computer Science or Engineering; and an interest in developing novel physics-informed machine learning approaches and in developing their coding and data science skills. It is essential that the applicant is proficient in using either Python or similar high-level programming languages.
[1] Espath, L., Kabanov, D., Kiessling, J. and Tempone, R., 2021. Statistical learning for fluid flows: Sparse Fourier divergence-free approximations. Physics of Fluids, 33(9), p.097108.
[2] Kabanov, D.I., Espath, L., Kiessling, J. and Tempone, R.F., 2021. Estimating divergence‐free flows via neural networks. PAMM, 21(1), p.e202100173.
[3] Saidaoui, H., Espath, L. and Tempone, R., 2022. Deep NURBS–admissible neural networks. arXiv preprint arXiv:2210.13900.
Supervisors: Dr Luis Espath (School of Mathematical Sciences), Dr Xin Chen (School of Computer Science).
For further details and to arrange an interview please contact Dr Luis Espath (School of Mathematical Sciences).
Uncertainty quantification for machine learning models of chemical reactivity
In this PhD project, we will develop and implement approaches for estimating the uncertainty in AI predictions of chemical reactivity, to help strengthen the interaction between human chemists and machine learning algorithms and to assess when AI predictions are likely to be correct and when, for example, first principles quantum chemical calculations might be helpful.
Predicting chemical reactivity is, in general, a challenging problem and one for which there is relatively little data, because experimental chemistry takes time and is expensive. Within our research group, we have a highly automated workflow for high-level quantum chemical calculations and we have generated thousands of examples relating to the reactivity of molecules for a specific chemical reaction. This project will evaluate a variety of machine learning algorithms trained on these data and, most crucially, will develop and implement techniques for computing the uncertainty in the prediction.
The algorithms developed in the project will be implemented in our ai4green electronic lab notebook, which is available as a web-based application: http://ai4green.app and which is the focus of a major ongoing project supported by the Royal Academy of Engineering. The results of the project will help chemists to make molecules in a greener and more sustainable fashion, by identifying routes with fewer steps or routes involving more benign reagents.
Applicants should have, or expected to achieve, at least a 2:1 Honours degree (or equivalent if from other countries) in Chemistry or Mathematics or a related subject. A MChem/MSc-4-year integrated Masters, a BSc + MSc or a BSc with substantial research experience will be highly advantageous. Experience in computer programming will be essential.
Supervisors: Prof Jonathan Hirst (School of Chemistry), Prof Simon Preston (School of Mathematical Sciences).
For further details and to arrange an interview please contact Prof Jonathan Hirst (School of Chemistry).
Using AI to create ‘super-glasses’ to see cells deep inside living tissue for biomedicine
Imaging with cellular resolution (~1 micron) is a critically important tool for understanding biological processes that underpin diseases from Alzheimer’s to cancer. Images are usually captured with light (e.g. with microscopes), but this can penetrate less than 1mm into tissue. This limitation prevents imaging deep inside large organs of the body, such as the brain, without greatly reducing resolution. The main reasons for the limited penetration depth of light into tissue is aberrations and scattering, which cause blurry images, akin to what a glasses-wearer might see without corrective lenses, or diffuse images, akin to trying to see through fog.
Recent work has shown that special ‘glasses’, in the form of adaptive optical systems, can be created for microscopes that reverse the distortion caused by aberrations and scattering to produce clear images. Scattering and aberrations are a result of the light travelling through the inhomogeneous tissue, the properties of which vary in spatial but also in time for living samples. To become a truly transformative technology these glasses must in future be able to correct in real-time to account for the sample, and hence the image distortions, changing with time. To do this, they must incorporate real-time measurements that help with estimating changes to the sample. In other words, they must be upgraded to ‘super-glasses’. Such technology has only recently become feasible due to advances in generative image AI.
In this Ph.D. you will apply and extend one of the most successful generative image reconstruction techniques, Diffusion models, to correct for scattering and aberrations in two important real-world case studies: imaging deep in biological tissue models (e.g. for personalized medicine), and imaging through ultra-thin optical fibers to make medical endoscopes for disease diagnosis. You will implement an autoregressive learning model to incorporate recent measurement data into your diffusion models via a conditioning approach. Working with collaborators in the lab you will also explore options to control microscope wavefront shaping using reinforcement learning. The final system will maintain a real-time physical approximation of the sample state in a dynamically changing environment.
Applicants are expected to have a strong background in computing and programming, and some knowledge of machine learning and deep learning would be idea. Any knowledge of the relevant physics (optics/electromagnetism) would be useful but it is not essential and can be learned on the job. The candidate will be supported in the different areas of knowledge required by a multidisciplinary team from Computer Science and Engineering, and will work with datasets measured in-house.
Supervisors: Dr Michael Pound (School of Computer Science), Prof Amanda Wright (Faculty of Engineering), Dr George Gordon (Faculty of Engineering).
For further details and to arrange an interview please contact Dr Michael Pound (School of Computer Science).
Further information For further enquiries, please contact Professor Ender Özcan - School of Computer Science
School of Computer Science
University of Nottingham Jubilee Campus Wollaton Road Nottingham, NG8 1BB
For all enquires please visit: www.nottingham.ac.uk/enquire
Legal information
- Terms and conditions
- Posting rules
- Accessibility
- Freedom of information
- Charity gateway
- Cookie policy
Connect with the University of Nottingham through social media and our blogs .

Create profile
Like courses
Apply direct
Create your IDP profile
To continue your research, create your profile with IDP. Your profile allows you to:
- Apply direct to courses and receive a response within the same day
- Shortlist and save courses
- Get the AI course recomendations
- Access our cost of living calculator
Match with universities
Now create a profile
Create a profile and start liking courses. We’ll show you recommendations that match what you’re looking for.
Your password must include
- One upper case letter
- One lower case letter
- One special character
- At least 8 characters
- IDP Education
- Computing and IT Courses
- Artificial Intelligence Courses
- Doctorate Artificial Intelligence Courses
- Artificial Intelligence in United Kingdom

Doctorate Artificial Intelligence courses in United Kingdom
- IELTS score (low to high)
- IELTS score (high to low)
- Course name (A-Z)
- Course name (Z-A)
- THE World University Rankings
- Next starting
- Course fee (low to high)
- Course fee (high to low)
Filter courses (4) Start a new search
Subject area, specific subject area 1 selected.
- Artificial Intelligence
Study Level 1 selected
- Postgraduate
- Undergraduate
- Doctorate
- Foundation
Study destination 1 selected
Study mode any.
- On campus study
Course fee range
- Loughborugh
- Coventry
- Leeds
- Bangor
- Belfast
- Bristol
- Coleraine
- Dundee
- London
- Londonderry/Derry
- Newtownabbey
- Staffordshire
Institution Any
- Loughborough University
- Coventry University
- University of Leeds
- University of the Arts, London
- University of Bristol
- Bangor University
- Keele University
- University of Hull
- Ulster University
- Abertay University
Select subject area Selecting a new subject will reset your search and filters
- Agriculture Sciences
- All Accounting
- All Geography
- All Human Welfare Studies And Services
- All Pharmacy
- Art and Design
- Biological and life Sciences
- Building and Architecture
- Computing and IT
- Engineering
- Environmental Science
- Health and Medicine
- Hospitality and Tourism
- Information Science and Librarianship
- Language and Culture
- Law and Legal Studies
- Marketing, Media and Communication
- Performing Arts and Music
- Political and Social Sciences
- Teaching and Education
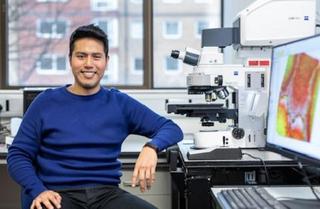
PhD in Ethics of Artificial Intelligence and Big Data
Leeds , United Kingdom
THE world university rank: 129
Course qualification
Entry score
Total course fee
AUD 128,944 ? GBP 69,000 Program fees are indicative only. Speak to your IDP study counsellor to get up-to-date course prices.
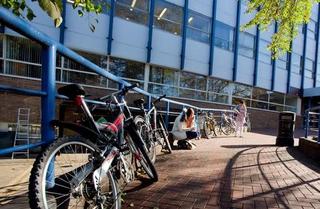
PhD in Fundamental Algorithms for Artificial Intelligence
Coventry , United Kingdom
THE world university rank: 601
AUD 83,326 ? GBP 44,589 Program fees are indicative only. Speak to your IDP study counsellor to get up-to-date course prices.
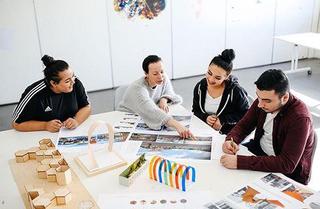
PhD Creativity, Machine Learning, AI and Robotics
London , United Kingdom
AUD 93,662 ? GBP 50,120 Program fees are indicative only. Speak to your IDP study counsellor to get up-to-date course prices.
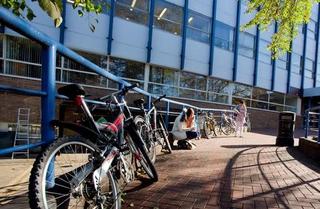
PhD in Artificial Intelligence for Cyberphysical Systems

PhD/MPhil in Artificial Intelligence and Intelligent Agents
Bangor , United Kingdom
THE world university rank: 401
AUD 105,118 ? GBP 56,250 Program fees are indicative only. Speak to your IDP study counsellor to get up-to-date course prices.
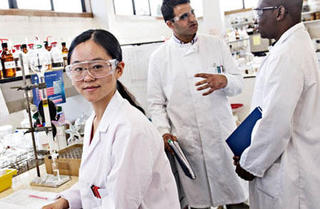
PhD Explainable AI
Loughborugh , United Kingdom
THE world university rank: 251
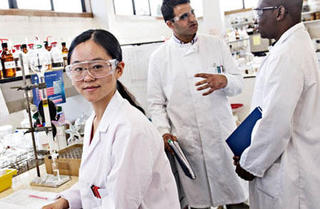
PhD Machine Learning and Deep Learning for Advanced Medical Imaging and Di...
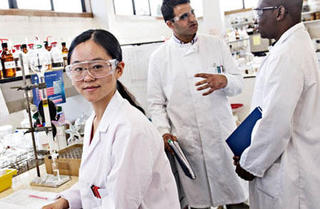
PhD Self-Supervised Learning for Complex Visual Understanding
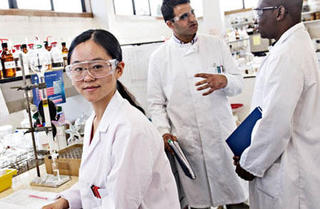
PhD AI-Enabled Acoustic Emission System for Industrial Condition Monitorin...
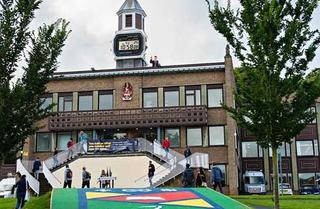
PhD/MPhil Computer Science - Theoretical computer science
Staffordshire , United Kingdom
THE world university rank: 501
Showing 1-10 of 17 courses
- 1 (current)
How does IDP FastLane work?
With the FastLane 'Offer in Principle', you'll know in minutes if you'll be accepted!
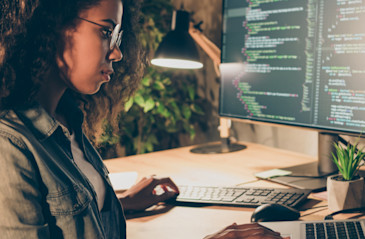
As technology continues to take up a bigger role in our lives, web Development presents a great pathway for technically-minded ...
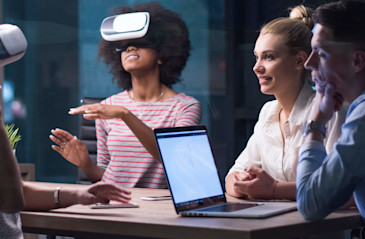
Artificial Intelligence (AI) continues reshaping the world. From improving people’s lives to optimising business outcomes, stud...
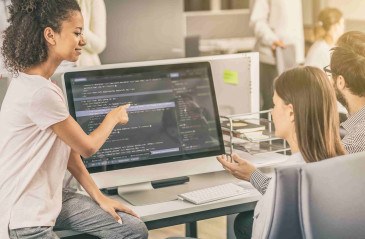
Love creating digital magic? Studying Software Engineering could be the pathway you’re searching for, allowing you to use the t...
To find out more about the information shown here – read about How we collect and display course information . IDP assumes no responsibility or liability for any errors or omissions in the content of this site. We always recommend that you speak to an IDP counsellor to get the latest and most accurate advice.

- Postgraduate Artificial Intelligence courses in United Kingdom
- Undergraduate Artificial Intelligence courses in United Kingdom
- Foundation Artificial Intelligence courses in United Kingdom

- Doctorate Artificial Intelligence courses in United States
- Doctorate Artificial Intelligence courses in Canada
- Doctorate Artificial Intelligence courses in Australia
- Search for courses
- Find a university
- Find a scholarship
Please select a level of study
Enter subject, choose from the list or hit search
Start typing, choose from the list or hit search
Enter subject, choose from the list or or hit search
Please type and select an institution
Type 3 characters of a university name and select from the list
Enter a university or school name and select from the list
Got any ideal countries in mind?
No Event Found.
Let’s get started
Sign up or login in with one click, sign up or login to save your courses, let's get started with "shortlist".
Your profile page will have the liked courses.
has been saved to your shortlist
View your shortlist or close this box to continue researching.
- Courses for you
Cookies on GOV.UK
We use some essential cookies to make this website work.
We’d like to set additional cookies to understand how you use GOV.UK, remember your settings and improve government services.
We also use cookies set by other sites to help us deliver content from their services.
You have accepted additional cookies. You can change your cookie settings at any time.
You have rejected additional cookies. You can change your cookie settings at any time.

- Business and industry
- Science and innovation
- Artificial intelligence
Tech Secretary unveils £8.5 million research funding set to break new grounds in AI safety testing
Up to £8.5 million of government research grants will be offered to improve society’s resilience to the risks arising from the development of new AI technologies.
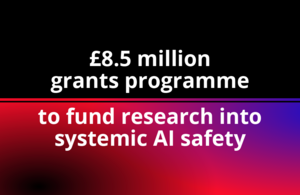
£8.5 million grants programme to fund research into systemic AI safety.
- New grants offered to researchers to push boundaries of AI safety research
- funding programme launched as UK government seeks to explore new methods to increase safe and trustworthy deployment of AI Funded research
- grants will aim to understand how society can adapt to the transformations generated by the advent of AI .
At the AI Seoul Summit today (22 May), which is co-hosted by the UK and Republic of Korea, Technology Secretary Michelle Donelan announced that the UK government will offer grants to researchers to study how to protect society from AI risks such as deepfakes and cyberattacks, as well as helping to harness its benefits, such as increased productivity.
The most promising proposals will be developed into longer-term projects and could receive further funding.
The programme (published on www.aisi.gov.uk) will be led within the UK government’s pioneering AI Safety Institute by Shahar Avin, an AI safety researcher who will be joining the UK’s Institute on secondment and Christopher Summerfield, UK AI Safety Institute Research Director. The research programme will be delivered in partnership with UK Research and Innovation and The Alan Turing Institute and the UK AI Safety Institute will aim to collaborate with other AI Safety Institutes internationally. Applicants will need to be based in the UK but will be encouraged to collaborate with other researchers from around the world.
The UK government’s pioneering AI Safety Institute is leading the world in the testing and evaluation of AI models, advancing the cause of safe and trustworthy AI . Earlier this week, the AI Safety Institute released its first set of public results from tests of AI models. It also announced a new office in the US and a partnership with the Canadian AI Safety Institute – building on a landmark agreement with the US earlier this year.
The new grants programme is designed to broaden the Institute’s remit to include the emerging field of ‘systemic AI safety’, which aims to understand how to mitigate the impacts of AI at a societal level and study how our institutions, systems and infrastructure can adapt to the transformations this technology has brought about.
Examples of proposals within scope would include ideas on how to curb the spread of fake images and misinformation by intervening on the platforms that spread them, rather than on the AI models that generate them.
Technology Secretary Michelle Donelan, said:
When the UK launched the world’s first AI Safety Institute last year, we committed to achieving an ambitious yet urgent mission to reap the positive benefits of AI by advancing the cause of AI safety. With evaluation systems for AI models now in place, Phase 2 of my plan to safely harness the opportunities of AI needs to be about making AI safe across the whole of society. This is exactly what we are making possible with this funding which will allow our Institute to partner with academia and industry to ensure we continue to be proactive in developing new approaches that can help us ensure AI continues to be a transformative force for good. I am acutely aware that we can only achieve this momentous challenge by tapping into a broad and diverse pool of talent and disciplines, and forging ahead with new approaches that push the limit of existing knowledge and methodologies.
The Honourable François-Philippe Champagne, Minister of Innovation, Science and Industry, said:
Canada continues to play a leading role on the global governance and responsible use of AI . From our role championing the creation of the Global Partnership on AI ( GPAI ), to pioneering a national AI strategy, to being among the first to propose a legislative framework to regulate AI , we will continue engaging with the global community to shape the international discourse to build trust around this transformational technology.
The AISI Systemic Safety programme aims to attract proposals from a broad range of researchers across both the public and private sectors, who will work closely with the UK government to ensure their ideas have maximum impact.
It will run alongside the Institute’s evaluation and testing of AI Models, where the Institute will continue to work with AI labs to set standards for development and help steer AI towards having positive impact.
Christopher Summerfield, UK AI Safety Institute Research Director, said:
This new programme of grants is a major step towards ensuring that AI is deployed safely into society. We need to think carefully about how to adapt our infrastructure and systems for a new world in which AI is embedded in everything we do. This programme is designed to generate a huge body of ideas for how to tackle this problem, and to help make sure great ideas can be put into practice.
The AI Seoul Summit builds on the inaugural AI Safety Summit hosted by the United Kingdom at Bletchley Park in November last year and is one the largest ever gathering of nations, companies and civil society on AI .
UKRI Chief Executive, Professor Dame Ottoline Leyser said:
The AI Safety Institute’s work is vital for understanding AI risks and creating solutions to maximise the societal and economic value of AI for all citizens. UKRI is delighted to be working closely with the Institute on this new programme to ensure that institutions, systems and infrastructures across the UK can benefit safely from AI . This programme leverages the UK’s world-leading AI expertise, and UKRI ’s AI investment portfolio encompassing skills, research, infrastructure and innovation, to ensure effective governance of AI deployment across society and the economy. The programme will bring safety research right into the heart of government, underpinning the pro-innovation regulation that will shape the UKs digital future.
Professor Helen Margetts, director of public policy at The Alan Turing Institute, said:
We’re delighted to be part of this important initiative which we hope will have a significant impact on the UK’s ability to tackle threats from AI technology and keep people safe. Rapidly advancing technology is bringing profound changes to the information environment, shaping our social, economic and democratic interactions. That is why funding AI safety is vital – to ensure we are all protected from the potential risks of misuse while maximising the benefits of AI for a positive impact on society.
Notes to editors
AI researcher Shahar Avin will lead the grants programme from within the UK AI Safety Institute, and bring a wealth of knowledge and experience to ensure proposals reach their fullest potential in protecting the public from risks of AI while harnessing its benefits. He is a senior researcher associate at the Centre for the Study of Existential Risk ( CSER ) and previously worked at Google.
The programme will be delivered in partnership with UK Research and Innovation and The Alan Turing Institute.
You can read more about the recent announcement on the Institute’s office opening in San Francisco, the AI models testing result, and UK AISI ’s partnership with the US and Canadian AI Safety Institutes .
Share this page
The following links open in a new tab
- Share on Facebook (opens in new tab)
- Share on Twitter (opens in new tab)
Is this page useful?
- Yes this page is useful
- No this page is not useful
Help us improve GOV.UK
Don’t include personal or financial information like your National Insurance number or credit card details.
To help us improve GOV.UK, we’d like to know more about your visit today. Please fill in this survey (opens in a new tab) .
- Work & Careers
- Life & Arts
Become an FT subscriber
Try unlimited access Only $1 for 4 weeks
Then $75 per month. Complete digital access to quality FT journalism on any device. Cancel anytime during your trial.
- Global news & analysis
- Expert opinion
- Special features
- FirstFT newsletter
- Videos & Podcasts
- Android & iOS app
- FT Edit app
- 10 gift articles per month
Explore more offers.
Standard digital.
- FT Digital Edition
Premium Digital
Print + premium digital, ft professional, weekend print + standard digital, weekend print + premium digital.
Essential digital access to quality FT journalism on any device. Pay a year upfront and save 20%.
- Global news & analysis
- Exclusive FT analysis
- FT App on Android & iOS
- FirstFT: the day's biggest stories
- 20+ curated newsletters
- Follow topics & set alerts with myFT
- FT Videos & Podcasts
- 20 monthly gift articles to share
- Lex: FT's flagship investment column
- 15+ Premium newsletters by leading experts
- FT Digital Edition: our digitised print edition
- Weekday Print Edition
- Videos & Podcasts
- Premium newsletters
- 10 additional gift articles per month
- FT Weekend Print delivery
- Everything in Standard Digital
- Everything in Premium Digital
Complete digital access to quality FT journalism with expert analysis from industry leaders. Pay a year upfront and save 20%.
- 10 monthly gift articles to share
- Everything in Print
- Make and share highlights
- FT Workspace
- Markets data widget
- Subscription Manager
- Workflow integrations
- Occasional readers go free
- Volume discount
Terms & Conditions apply
Explore our full range of subscriptions.
Why the ft.
See why over a million readers pay to read the Financial Times.
International Edition
UK opens office in San Francisco to tackle AI risk

Ahead of the AI safety summit kicking off in Seoul, South Korea later this week , its co-host, the United Kingdom, is expanding its own efforts in the field. The AI Safety Institute, a U.K. body set up in November 2023 with the ambitious goal of assessing and addressing risks in AI platforms, has said it will open a second location in San Francisco.
The idea is to get closer to the epicenter of AI development. The Bay Area is the home of companies like OpenAI, Anthropic, Google and Meta that are building foundational AI technology.
Foundational models are the building blocks of generative AI services and other applications, and it’s interesting that although the U.K. has signed an MOU with the U.S. to collaborate on AI safety initiatives, the U.K. is still choosing to set up in the U.S. to tackle the issue.
“By having people on the ground in San Francisco, it will give them access to the headquarters of many of these AI companies,” Michelle Donelan, the U.K. secretary of state for science, innovation and technology, said in an interview with TechCrunch. “A number of them have bases here in the United Kingdom, but we think that would be very useful to have a base there as well, and access to an additional pool of talent, and be able to work even more collaboratively and hand-in-glove with the United States.”
Part of the reason is that being closer to that epicenter is useful not just for understanding what is being built, it also gives the U.K. more visibility with these firms. That’s important, since AI and technology is seen by the U.K. as a huge opportunity for economic growth and investment.
And given the latest drama at OpenAI around its Superalignment team , it feels like an especially timely moment to establish a presence there.
The AI Safety Institute, launched in November 2023, is a relatively modest affair today. The organization has just 32 employees, a veritable David to the Goliath of AI tech, when you consider the billions of dollars of investment riding on the companies building AI models and their own economic motivations for getting their technologies into the hands of paying users.
One of the AI Safety Institute’s most notable developments was the release of Inspect , its first set of tools for testing the safety of foundational AI models, earlier this month.
Donelan today referred to that release as a “phase one” effort. Not only has it proven challenging to benchmark models, but for now, engagement is very much an opt-in and inconsistent arrangement. As one senior source at a U.K. regulator pointed out, companies are under no legal obligation to have their models vetted at this point; and not every company is willing to have their models vetted before release. That could mean, in cases where risk might be identified, the horse may have already bolted.
Donelan said the AI Safety Institute was still working on strategies to engage with AI companies to evaluate them. “Our evaluation process is an emerging science in itself,” she said. “So with every evaluation, we will develop the process and finesse it even more.”
Donelan said that one goal of the conference in Seoul is to present Inspect to regulators, aiming to get them to adopt it, too.
“Now we have an evaluation system. Phase two needs to also be about making AI safe across the whole of society,” she said.
Longer term, Donelan believes the U.K. will be building more AI legislation, although, repeating what Prime Minister Rishi Sunak has said on the topic, it will resist doing so until it better understands the scope of AI risks.
“We do not believe in legislating before we properly have a grip and full understanding,” she said, noting that the institute’s recent international AI safety report, focused primarily on trying to get a comprehensive picture of research to date, “highlighted that there are big gaps missing and that we need to incentivize and encourage more research globally.”
“Also, legislation takes about a year in the United Kingdom. If we had just started legislation when we started instead of [organizing] the AI Safety Summit [held in November last year], we’d still be legislating now, and we wouldn’t actually have anything to show for that,” Donelan said.
“Since day one of the Institute, we have been clear on the importance of taking an international approach to AI safety, share research, and work collaboratively with other countries to test models and anticipate risks of frontier AI,” said Ian Hogarth, chair of the AI Safety Institute, in a statement. “Today marks a pivotal moment that allows us to further advance this agenda, and we are proud to be scaling our operations in an area bursting with tech talent, adding to the incredible expertise that our staff in London has brought since the very beginning.”
More TechCrunch
Get the industry’s biggest tech news, techcrunch daily news.
Every weekday and Sunday, you can get the best of TechCrunch’s coverage.
Startups Weekly
Startups are the core of TechCrunch, so get our best coverage delivered weekly.
TechCrunch Fintech
The latest Fintech news and analysis, delivered every Sunday.
TechCrunch Mobility
TechCrunch Mobility is your destination for transportation news and insight.
Rock band’s hidden hacking-themed website gets hacked
On Friday, Pal Kovacs was listening to the long-awaited new album from rock and metal giants Bring Me The Horizon when he noticed a strange sound at the end of…

Anthropic hires former OpenAI safety lead to head up new team
Jan Leike, a leading AI researcher who earlier this month resigned from OpenAI before publicly criticizing the company’s approach to AI safety, has joined OpenAI rival Anthropic to lead a…

The demise of BaaS fintech Synapse could derail the funding prospects for other startups in the space
Welcome to TechCrunch Fintech! This week, we’re looking at the long-term implications of Synapse’s bankruptcy on the fintech sector, Majority’s impressive ARR milestone, and more! To get a roundup of…

YouTube’s free games catalog ‘Playables’ rolls out to all users
YouTube’s free Playables don’t directly challenge the app store model or break Apple’s rules. However, they do compete with the App Store’s free games.

Featured Article
A comprehensive list of 2024 tech layoffs
The tech layoff wave is still going strong in 2024. Following significant workforce reductions in 2022 and 2023, this year has already seen 60,000 job cuts across 254 companies, according to independent layoffs tracker Layoffs.fyi. Companies like Tesla, Amazon, Google, TikTok, Snap and Microsoft have conducted sizable layoffs in the first months of 2024. Smaller-sized…
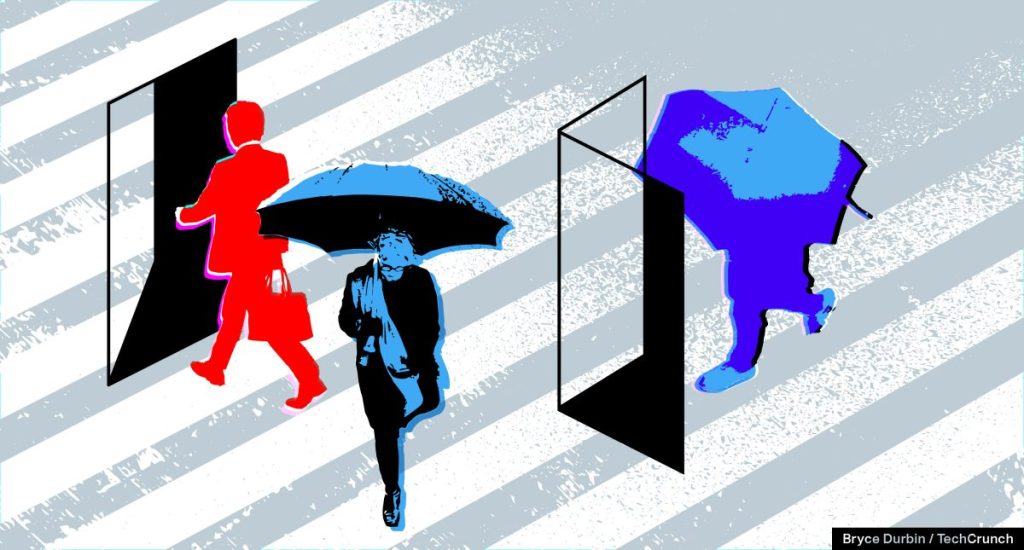
OpenAI’s new safety committee is made up of all insiders
OpenAI has formed a new committee to oversee “critical” safety and security decisions related to the company’s projects and operations. But, in a move that’s sure to raise the ire…

Early bird gets the savings — 4 days left for Disrupt sale
Time is running out for tech enthusiasts and entrepreneurs to secure their early-bird tickets for TechCrunch Disrupt 2024! With only four days left until the May 31 deadline, now is…

Skej’s AI meeting scheduling assistant works like adding an EA to your email
AI may not be up to the task of replacing Google Search just yet, but it can be useful in more specific contexts — including handling the drudgery that comes…

Faircado raises $3M to nudge people to buy pre-owned goods
Faircado has built a browser extension that suggests pre-owned alternatives for ecommerce listings.

Tumblr launches its semi-private Communities in open beta
Tumblr, the blogging site acquired twice, is launching its “Communities” feature in open beta, the Tumblr Labs division has announced. The feature offers a dedicated space for users to connect…
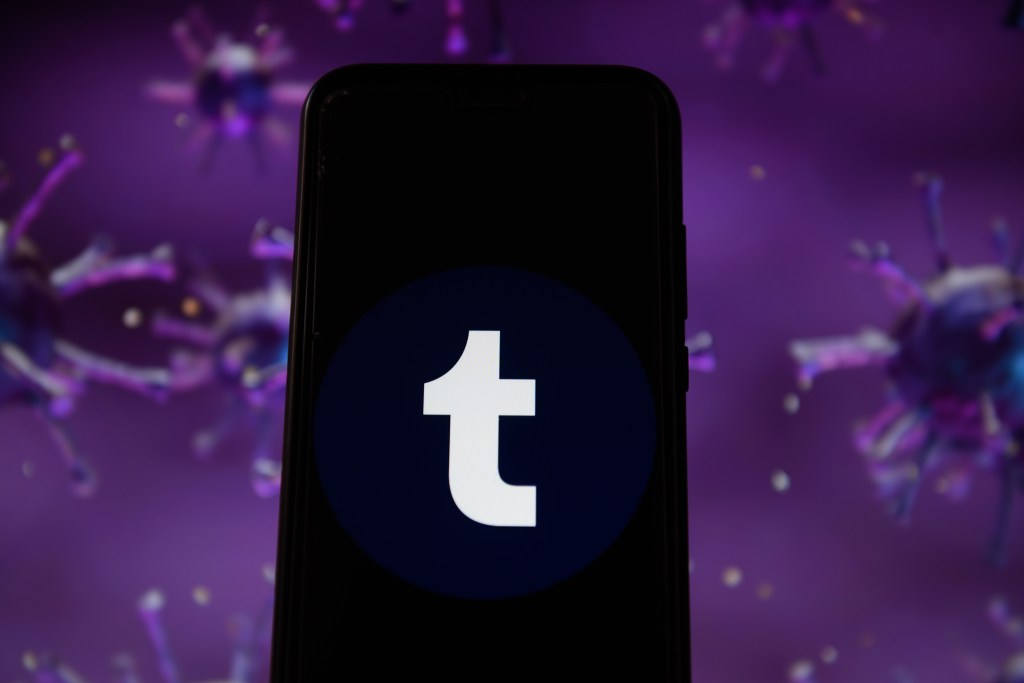
Félix Pago raises $15.5 million to help Latino workers send money home via WhatsApp
Remittances from workers in the U.S. to their families and friends in Latin America amounted to $155 billion in 2023. With such a huge opportunity, banks, money transfer companies, retailers,…

Google adds AI-powered features to Chromebook
Google said today it’s adding new AI-powered features such as a writing assistant and a wallpaper creator and providing easy access to Gemini chatbot to its Chromebook Plus line of…

The Chainsmokers light up Disrupt 2024
The dynamic duo behind the Grammy Award–winning music group the Chainsmokers, Alex Pall and Drew Taggart, are set to bring their entrepreneurial expertise to TechCrunch Disrupt 2024. Known for their…

LumApps, the French ‘intranet super app,’ sells majority stake to Bridgepoint in a $650M deal
The deal will give LumApps a big nest egg to make acquisitions and scale its business.

More neobanks are becoming mobile networks — and Nubank wants a piece of the action
Nubank is taking its first tentative steps into the mobile network realm, as the NYSE-traded Brazilian neobank rolls out an eSIM (embedded SIM) service for travelers. The service will give customers access to 10GB of free roaming internet in more than 40 countries without having to switch out their own existing physical SIM card or…

MARS doubles down on India’s Infra.Market with new $50M investment
Infra.Market, an Indian startup that helps construction and real estate firms procure materials, has raised $50M from MARS Unicorn Fund.

Cloover wants to speed solar adoption by helping installers finance new sales
Small operations can lose customers by not offering financing, something the Berlin-based startup wants to change.

Adani looks to battle Reliance, Walmart in India’s e-commerce, payments race, report says
India’s Adani Group is in discussions to venture into digital payments and e-commerce, according to a report.

Ledger starts shipping its high-end hardware crypto wallet
Ledger, a French startup mostly known for its secure crypto hardware wallets, has started shipping new wallets nearly 18 months after announcing the latest Ledger Stax devices. The updated wallet…

EU’s ChatGPT taskforce offers first look at detangling the AI chatbot’s privacy compliance
A data protection taskforce that’s spent over a year considering how the European Union’s data protection rulebook applies to OpenAI’s viral chatbot, ChatGPT, reported preliminary conclusions Friday. The top-line takeaway…

LatAm startups: Apply to Startup Battlefield 200
Here’s a shoutout to LatAm early-stage startup founders! We want YOU to apply for the Startup Battlefield 200 at TechCrunch Disrupt 2024. But you’d better hurry — time is running…

5 days left to get your early-bird Disrupt passes
The countdown to early-bird savings for TechCrunch Disrupt, taking place October 28–30 in San Francisco, continues. You have just five days left to save up to $800 on the price…

Spanish startups reached €100 billion in aggregate value last year
Venture investment into Spanish startups also held up quite well, with €2.2 billion raised across some 850 funding rounds.

Onyx Motorbikes was in trouble — and then its 37-year-old owner died
James Khatiblou, the owner and CEO of Onyx Motorbikes, was watching his e-bike startup fall apart. Onyx was being evicted from its warehouse in El Segundo, near Los Angeles. The company’s unpaid bills were stacking up. Its chief operating officer had abruptly resigned. A shipment of around 100 CTY2 dirt bikes from Chinese supplier Suzhou…

Iyo thinks its gen AI earbuds can succeed where Humane and Rabbit stumbled
Iyo represents a third form factor in the push to deliver standalone generative AI devices: Bluetooth earbuds.

Women in AI: Arati Prabhakar thinks it’s crucial to get AI ‘right’
Arati Prabhakar, profiled as part of TechCrunch’s Women in AI series, is director of the White House Office of Science and Technology Policy.

Doly lets you generate 3D product videos from your iPhone
AniML, the French startup behind a new 3D capture app called Doly, wants to create the PhotoRoom of product videos, sort of. If you’re selling sneakers on an online marketplace…

Elon Musk’s xAI raises $6B from Valor, a16z, and Sequoia
Elon Musk’s AI startup, xAI, has raised $6 billion in a new funding round, it said today, as Musk shores up capital to aggressively compete with rivals including OpenAI, Microsoft,…

Indian EV startup Zypp Electric secures backing to fund expansion to Southeast Asia
Indian startup Zypp Electric plans to use fresh investment from Japanese oil and energy conglomerate ENEOS to take its EV rental service into Southeast Asia early next year, TechCrunch has…

A venture capital firm looks back on changing norms, from board seats to backing rival startups
Last month, one of the Bay Area’s better-known early-stage venture capital firms, Uncork Capital, marked its 20th anniversary with a party in a renovated church in San Francisco’s SoMa neighborhood,…

UK Government in £8.5m Bid to Tackle AI Cyber-Threats

Phil Muncaster
UK / EMEA News Reporter , Infosecurity Magazine
- Follow @philmuncaster
The UK has promised £8.5m ($10.8m) to fund new AI safety research designed to tackle cyber-threats including deepfakes.
Announced by technology secretary Michelle Donelan at the AI Seoul Summit today, the research grants will focus on “systemic AI safety” – that is, understanding how better to protect society from AI risks and harness the technology’s benefits.
The research program will be led by researcher Shahar Avin at the government’s AI Safety Institute and delivered in partnership with UK Research and Innovation and The Alan Turing Institute. Although applicants will need to be based in the UK, they will be encouraged to collaborate with other researchers in AI safety institutes around the world.
AI represents a two-pronged threat to economic and social stability. On the one hand, AI systems could be targeted by techniques such as prompt injection and data poisoning, and on the other, the technology can be used by threat actors themselves to gain an advantage.
The UK’s National Cyber Security Centre (NCSC) warned in January that malicious AI use will “almost certainly” lead to an increase in the volume and impact of cyber-attacks over the next two years, particularly ransomware.
Read more on AI threats: UK Financial Regulator Urges Banks to Tackle AI-Based Fraud
In fact, new research from compliance specialist ISMS.online released this week revealed that 30% of information security professionals experienced a deepfake-related incident in the past 12 months, the second most popular answer after “malware infection.”
At the same time, three-quarters (76%) of respondents claimed that AI technology is improving information security and 64% said they are increasing their budgets accordingly over the coming year.
AI Safety Institute research director, Christopher Summerfield, claimed the new funding represents a “major step” to ensuring AI is deployed safely in society.
“We need to think carefully about how to adapt our infrastructure and systems for a new world in which AI is embedded in everything we do,” he added . “This program is designed to generate a huge body of ideas for how to tackle this problem, and to help make sure great ideas can be put into practice.”
The institute has already been conducting valuable research into AI threats. A May update published on Monday revealed that four of the most used generative AI chatbots are vulnerable to basic jailbreak attempts.
The UK and South Korea yesterday hailed a “historic first” as 16 major AI companies signed new commitments to safely develop AI models.
You may also like
Uk government: cyber-attacks could kill or maim thousands, mod receives funding boost and confirms increase in cyber-spending, #cyberuk18: nsa claims attack tactics rarely change, lawmakers slam uk government’s “ostrich strategy” for cybersecurity, south korean police develops deepfake detection tool ahead of april elections, what’s hot on infosecurity magazine.
- Editor's Choice
Fake Pegasus Spyware Strains Populate Clear and Dark Web
Health information published online after medisecure ransomware attack, indian election faces cyber-attacks, data leaks on dark web, how to backup and restore database in sql server, microsoft: gift card fraud rising, costing businesses up to $100,000 a day, how to open and view ost files without outlook, why culture is the bedrock of cybersecurity, russia’s doppelgänger campaign manipulates social media, 70% of cisos expect cyber-attacks in next year, report finds, national records of scotland data breached in nhs cyber-attack, nvd leaves exploited vulnerabilities unchecked, report reveals 341% rise in advanced phishing attacks, how to optimize third-party risk management programs through nist csf 2.0, why ddos simulation testing is critical for proactive network defense, learn from the nhs - proactive password security for improved cybersecurity, adapting to tomorrow's threat landscape: ai's role in cybersecurity and security operations in 2024, understand and combat the top healthcare cloud threats today, untangling the web: navigating third-party risk in a hyperconnected world, women in cybersecurity at infosecurity europe 2024, rsac: cisa launches vulnrichment program to address nvd challenges, lockbit leader aka lockbitsupp identity revealed, how to proactively remediate rising web application threats, live roundtable event: secure enterprise browsing, new ways to strengthen endpoint security.
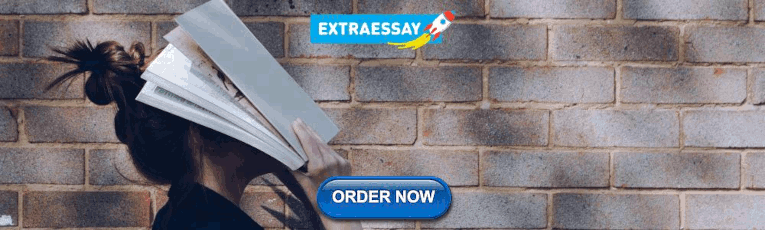
IMAGES
VIDEO
COMMENTS
The UKRI Centre for Doctoral Training in Artificial Intelligence and Music (AIM) is a leading PhD research programme aimed at the Read more... 3 years Full time degree: £4,786 per year (UK) 6 years Part time degree: £2,393 per year (UK) Apply now Visit website Request info Book event. View 8 additional courses.
22 Artificial Intelligence PhDs in United Kingdom. Robotics and Autonomous Systems. A robust and accurate facial 3D reconstruction method from images acquired by mobile devices at home for facial growth monitorin. Robust Deep Learning for Medical Image Reconstruction. Interactive Artificial Intelligence. Artificial Intelligence and Intelligent ...
We have 302 artificial intelligence PhD Projects, Programmes & Scholarships in the UK. PhD studentship in Machine Learning and Artificial Intelligence (AI) Systems: Distributed Deep Learning, Personalising Foundation Models and Building Efficient Autonomous Distributed AI. Sep 24 start.
The PhD Studentship supports and encourages under-represented groups, namely female and Black researchers, to pursue postgraduate research in AI or Machine Learning. The PhD DeepMind Studentship will cover tuition fees and offer a London weighted stipend of £19,668 per year minimum together with an annual £2,200 travel and conference ...
The UK Research and Innovation (UKRI) artificial intelligence (AI) Centres for Doctoral Training (CDTs) are training a new generation of PhD students. They will develop novel AI methodology and use AI technology in areas such as: improving healthcare. tackling climate change.
Find the best PhD programmes in the field of Artificial Intelligence from top universities in United Kingdom. Check all 22 programmes.
New MRes and PhD in AI-enabled Healthcare. The new Centre for Doctoral Training (CDT) in AI-enabled Healthcare, supported by UK Research and Innovation (UKRI), offers a unique programme consisting of a 1 year MRes followed by a 3 year PhD. ... The UCL Artificial Intelligence Society is the first society in the UK to focus on AI, and one of the ...
The Centre runs a range of programmes including PhD and Masters programmes in Machine Learning and related topics in AI, UCL has a proud history of excellence in AI education. The scientific and mathematical principles behind AI take priority over studying existing AI tools. We offer the capability to create new AI algorithms giving students a depth of understanding and the skills to lead ...
About This Course. One fully-funded 4-year PhD scholarship is available to start in September 2023 in the area of Artificial Intelligence machine learning and advanced computing (AIMLAC). The PhD is suitable for a graduate with a keen interest in AI algorithms for big data, optimisation, 3D modelling, and visualisation.
The UKRI Centre for Doctoral Training in Artificial Intelligence and Music (AIM) is a leading PhD research programme aimed at the Read more... 3 years Full time degree: £4,786 per year (UK) 6 years Part time degree: £2,393 per year (UK) Apply now Visit website Request info Book event. View 8 additional courses.
With this power comes great responsibility to ensure AI is used for good and in an equitable, transparent manner. AI has a significant impact, and ensuring that AI is designed, deployed, and used responsibly is critical to ensuring its impact is positive. The PhD studentship is related to our flagship £31M Responsible AI UK programme (RAI UK ...
PhD Programmes. Sussex AI PhD programme (New for 2024) Sussex Neuroscience 4-year PhD programme. Besides the specific programmes listed above, we are always open for applications of talented students with other sources of funding. Current funding opportunities can be found on the Sussex PhD funding pages.
PhD at the Data Science Institute. ... Hao Dong is an assistant professor at Peking University studying AI, robotics and vision. He has a broad interest in the generalisation problem using generative models and self-supervised learning. ... London SW7 2AZ, UK tel: +44 (0)20 7589 5111 Campuses & maps Follow Imperial. Facebook X, formerly known ...
1st in the UK Computer Science and Informatics Research Excellence Framework (REF) 2021 - based on the proportion of world-leading research Why Imperial ? Access expertise spanning theoretical foundations and applications of AI, including machine learning, robotics, and augmented reality and cognition
The Cambridge Machine Learning Group (MLG) runs a PhD programme in Advanced Machine Learning. The supervisors are Jose Miguel Hernandez-Lobato, Carl Rasmussen, Richard E. Turner, Adrian Weller, Hong Ge and David Krueger. Zoubin Ghahramani is currently on academic leave and not accepting new students at this time.. We encourage applications from outstanding candidates with academic backgrounds ...
Artificial Intelligence (AI) has the potential to transform health and healthcare systems globally, yet few individuals have the required skills and training. To address this challenge, our Centre For Doctoral Training (CDT) in AI-Enabled Healthcare Systems will create a unique interdisciplinary environment to train the brightest and best healthcare artificial intelligence
The fully funded PhD studentships are open to home and international students. You'll be working as part of a cohort in a collaborative environment alongside other PhD students, postdoc researchers and data scientists. Other benefits include: PhD projects co-defined and co-supervised with a project partner. Monthly tax-free payment of £1,338.50.
The Faculty of Science AI DTC is a new initiative by the University of Nottingham to train future researchers and leaders to address the most pressing challenges of the 21st Century through foundational and applied AI research on a cohort basis. The training and supervision will be delivered by a team of outstanding scholars from different disciplines cutting across Arts, Engineering, Medicine ...
Designing chocolate products with enhanced health, wellbeing and technical performance using artificial intelligence. University of Leeds Faculty of Environment. One full scholarship is available in the School of Food Science and Nutrition in 2024. This scholarship is open to UK and covers UK tuition fees plus UKRI maintenance per year (£ ...
Explore the AI UK 2024 stages. AI UK 2024 featured three main stages - Conversation, Future and Research - as well as hands-on workshops, and the Turing Hub for networking and idea exchange. Click here for the AI UK 2024 programme PDF and see the floorplan here. We'll be uploading videos of the sessions from AI UK 2024, subscribe to our ...
We always recommend that you speak to an IDP counsellor to get the latest and most accurate advice. 12 Doctorate Artificial Intelligence courses 📖 in United Kingdom. Course price ranging from AUD 83,326 - AUD 128,944 with a max.Hurry the courses start from 27 May 2024.
Funded PhD Studentships at the EPSRC Centre for Doctoral Training: NET2ZERO. Explore PhD opportunities within the Institute for Digital Technologies. Fully Funded PhD Scholarships in Engineering and Informatics. Apply now for 2024. Apply today to start your PhD in Quantum Technology at The University of Glasgow.
Up to £8.5 million of government research grants will be offered to improve society's resilience to the risks arising from the development of new AI technologies.
The company, which produces AI voice assistants for call centres, said it has closed a new $50mn funding round led by Hedosophia, a group run by investor Ian Osborne, and NVentures, the venture ...
Ahead of the AI safety summit kicking off in Seoul, South Korea later this week, its co-host, the United Kingdom, is expanding its own efforts in the field. The AI Safety Institute, a U.K. body ...
The UK has promised £8.5m ($10.8m) to fund new AI safety research designed to tackle cyber-threats including deepfakes. Announced by technology secretary Michelle Donelan at the AI Seoul Summit today, the research grants will focus on "systemic AI safety" - that is, understanding how better to protect society from AI risks and harness the technology's benefits.