- Thesis Action Plan New
- Academic Project Planner
- Literature Navigator
- Thesis Dialogue Blueprint
- Writing Wizard's Template
- Research Proposal Compass
- Why students love us
- Why professors love us
- Why we are different
- All Products
- Coming Soon

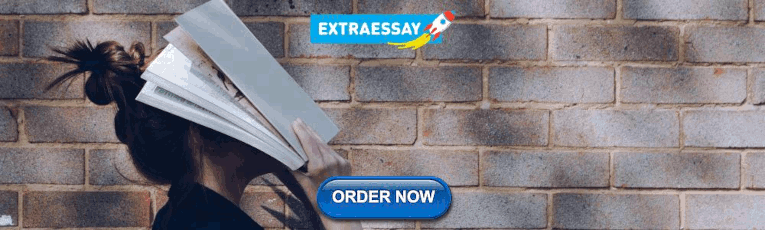
Navigating Thesis Writing Anxiety: Tips for a Smooth Process

Overcoming Dissertation Test Anxiety: Strategies for Success

A Comprehensive Guide on How a Thesis is Written

Determining the Ideal Word Count for a Bachelor Thesis
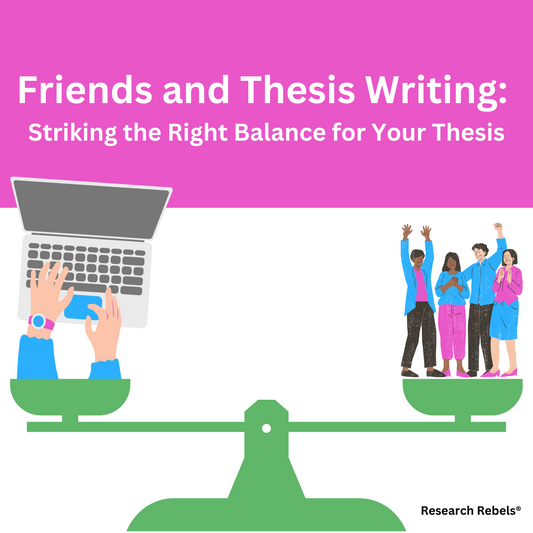
Friends and Thesis Writing: Striking the Right Balance for Your Thesis
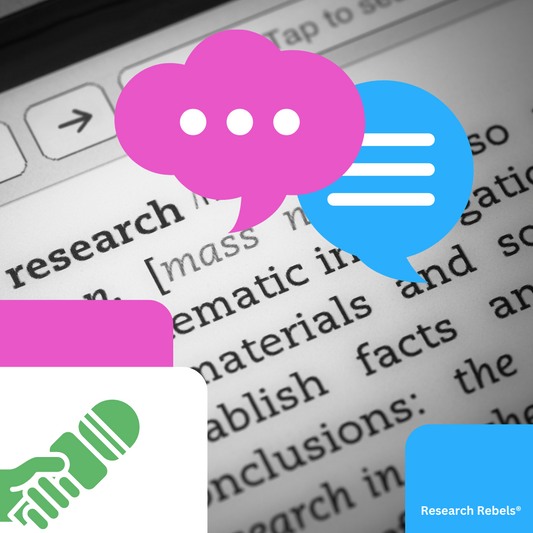
Mastering the Art of Communication: Effective Strategies for Voice Your Research in Interviews
Navigating ethical dilemmas: the morality of conducting interviews.
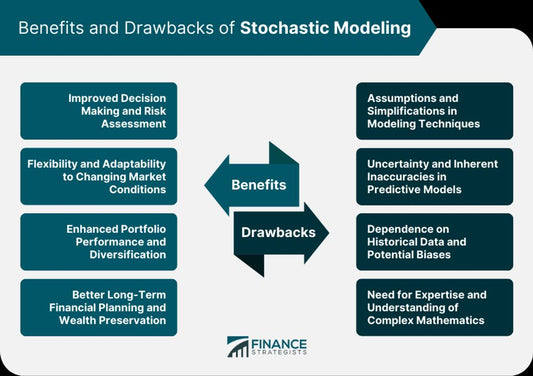
Data Anomalies: Strategies for Analyzing and Interpreting Outlier Data
Decoding the research articles: finding and understanding hypotheses.

Research articles are the cornerstones of scientific progress, presenting new findings and hypotheses that contribute to the collective understanding of various fields. Deciphering these articles is crucial for students, researchers, and professionals alike. Understanding how to find and interpret the hypotheses within these articles is essential for engaging with the research, critiquing its validity, and applying its conclusions to further study or practice. This article guides readers through the process of identifying and evaluating hypotheses in research articles, providing a structured approach to navigate the complexity of scholarly literature.
Key Takeaways
- The introduction of a research article is key to understanding the presented hypotheses, as it sets the stage for the research question and the gap the study aims to fill.
- A critical evaluation of hypotheses includes assessing their alignment with research goals, the rigor of their formulation, and the ethical considerations they entail.
- Understanding the null hypothesis and its role in research is crucial, as it forms the basis for statistical testing and interpretation of the study's results.
Navigating the Structure of Research Articles to Identify Hypotheses
Understanding the role of the introduction in presenting hypotheses.
When you embark on the journey of dissecting a research article, the introduction is your starting point. It is here that the authors lay the groundwork for their study, often weaving in the hypothesis amidst the context of their work. A thesis or hypothesis is not always clearly labeled ; you may need to read through the introductory paragraphs to determine what the authors are proposing.
The introduction should succinctly build off related empirical research, highlighting a gap that the current study aims to fill. It is essential to look for the research question(s) that the paper will address, as these are often directly tied to the hypothesis. Avoid the common pitfall of getting lost in an extensive literature review—focus on finding the statement of purpose that guides the research.
To aid in your understanding, consider the following points when reading an introduction:
- Identify the research question or problem the study addresses.
- Look for a clear statement of the hypothesis or thesis.
- Assess how the introduction frames the importance and novelty of the research.
- Determine if the introduction provides a logical path through the critical literature supporting the study's aims.
Remember, the introduction is not just a background summary; it is a strategic component that sets the stage for the hypothesis and the ensuing research.
Analyzing the Methods Section for Hypothesis Testing Procedures
When you delve into the Methods section of a research paper, you are essentially decoding the blueprint of the study's hypothesis testing. Begin with a summary that encapsulates the research design, which should align with the study's objectives. This summary often includes the type of study conducted, whether it be experimental, correlational, or observational.
Next, detail the participant information, which is crucial for understanding the context and scope of the hypothesis testing. This includes demographics, selection criteria, and sample size. For instance:
- Participant demographics: Age, gender, occupation
- Selection criteria: Inclusion and exclusion parameters
- Sample size: Number of participants
The subsequent step involves explaining the research procedures. This includes the specific actions taken to test the hypothesis, such as data collection methods, experimental manipulations, or survey administration. It's important to scrutinize the tools and materials used, as they must provide reliable and valid measurements relevant to the hypothesis.
Finally, key statistical concepts such as hypothesis testing, significance, and sampling underpin the credibility of the findings. Understanding the difference between statistical and practical significance is crucial for interpreting the results in a meaningful way. This section often culminates in statistical storytelling , which weaves the numerical data into a coherent narrative about the hypothesis.
Interpreting Results and Discussion in the Context of Hypotheses
Once you've navigated through the methodological labyrinth and arrived at the results section , you're ready to connect the dots between your findings and the original hypotheses. This section is where you report the findings of your study based on the methodology you applied to gather information. It's crucial to approach this part with a critical eye, ensuring that the interpretation of results aligns with the objectives and hypotheses set forth in the introduction.
In the discussion section, you'll delve deeper into the implications of your results. It's typically easier to read than the methods and results sections, as it helps you understand the broader significance of your findings. Here, you should check if the discussion clearly addresses the objectives and hypotheses, and whether it provides a mere repetition of the results or offers a thoughtful interpretation against them.
To effectively interpret the results and discussion, consider the following points:
- Compare your findings with previous research to contextualize your study.
- Assess whether the results support or refute your hypotheses.
- Discuss any unexpected outcomes and their possible explanations.
- Consider the limitations of your study and how they might affect the interpretation of the results.
- Suggest directions for future research based on your findings.
Critical Evaluation of Hypotheses in Scholarly Research
Assessing the alignment of hypotheses with research goals.
When you delve into a research article, it's crucial to assess whether the hypotheses are well-aligned with the overarching research goals. A well-formulated hypothesis acts as a guiding star , leading the inquiry in a clear direction. It should be a reflection of the research questions and objectives that stem from identifying knowledge gaps and reviewing existing literature.
Consider the following points to evaluate the alignment:
- Does the hypothesis address the core problem statement of the research?
- Is there a logical connection between the literature review and the hypothesis?
- Are the research questions, as outlined in the introduction, directly linked to the hypothesis?
Remember, a hypothesis that is misaligned with the research goals can lead to a convoluted study, where the results may not provide meaningful contributions to the field. It helps researchers to identify the research questions and objectives, to develop hypotheses, and to determine the appropriate methodology for the study. As you scrutinize the hypothesis, ensure that it is clear, specific, and concise, and that it articulates the problem being addressed with precision.
Examining the Rigor and Ethical Considerations in Hypothesis Formulation
When you delve into the world of research, the formulation of a hypothesis is not merely about posing a plausible explanation or prediction; it is about ensuring the rigor and integrity of your scientific inquiry. Rigor in hypothesis formulation involves a systematic approach that is both logical and empirical. It requires that your hypothesis is not only testable but also grounded in existing literature and theory. Ethical considerations, on the other hand, demand that the research question is significant enough to warrant investigation and that it does not pose harm or deception to participants.
To examine the rigor of your hypothesis, consider the following points:
- The hypothesis should be clear and concise, allowing for precise testing.
- It must be based on a logical rationale and tied to theory .
- The hypothesis should be falsifiable, meaning it can be proven wrong through evidence.
Ethical considerations in hypothesis formulation include:
- Ensuring the research question addresses a gap in knowledge that benefits society.
- Avoiding harm to research participants and respecting their autonomy.
- Transparency in the research process, including the potential limitations of your study.
Remember, a hypothesis that lacks rigor or overlooks ethical standards can compromise the entire research project. As you craft your hypothesis, keep in mind the importance of aligning it with the broader goals of your research and the ethical framework within which you operate.
The Importance of Null Hypothesis and Its Implications for Research
In the realm of research, the null hypothesis serves as a critical benchmark, positing that no significant difference or relationship exists between the variables under investigation. It is the foundation against which all new findings are tested. By default, the null hypothesis assumes that any observed effect is due to chance, and it is only rejected when there is sufficient evidence to support an alternative hypothesis .
The null hypothesis is not merely a formality but a pivotal component of the scientific method. It provides a structured approach to determine the validity of an effect or relationship. When you encounter a research article, scrutinize the null hypothesis to understand the baseline expectations of the study. This will allow you to better appreciate the significance of the research findings.
Remember, the inability to reject the null hypothesis is not an indication of failure but rather an important result in itself. It can reveal the absence of an effect or relationship, contributing valuable knowledge to the field. Here's a simple list to guide you through the implications of the null hypothesis in research:
- Establishes a default position for testing
- Facilitates the calculation of statistical significance
- Helps prevent false positives
- Informs the direction of future research
By grasping the role of the null hypothesis, you can critically evaluate the outcomes of a study and the robustness of its conclusions.
In the realm of scholarly research, the ability to critically evaluate hypotheses is paramount. It's a skill that can make the difference between a compelling study and one that fails to persuade. If you're grappling with the intricacies of thesis writing or simply seeking to refine your research methodology, our step-by-step Thesis Action Plan is designed to guide you through the process. Don't let anxiety and uncertainty hinder your academic progress. Visit our website now to learn more about our innovative approach and claim your special offer. Empower your research journey with the support and expertise of Research Rebels.
In conclusion, the ability to locate and comprehend hypotheses within research articles is a fundamental skill for students, academics, and professionals alike. This article has provided a structured approach to identifying hypotheses, understanding their significance, and evaluating their construction within the broader context of scholarly research. By emphasizing the importance of hypotheses as the backbone of empirical studies, we have underscored their role in guiding research design, methodology, and analysis. It is our hope that readers will emerge from this exploration with a heightened appreciation for the hypothesis as a tool for scientific inquiry and a clearer path to engaging with research literature. As we continue to bridge the gap between academic jargon and everyday language, we empower ourselves to contribute more effectively to the collective body of knowledge and to advance our understanding of the world around us.
Frequently Asked Questions
How can i identify the hypothesis in a research article.
To identify the hypothesis in a research article, focus on the introduction section where the authors present the research questions and hypotheses. Look for statements that propose a relationship or effect between variables. The hypothesis is often presented after a brief review of existing literature and is a clear, testable statement that the research aims to investigate.
Why is the null hypothesis important in research?
The null hypothesis is crucial because it provides a baseline assumption that there is no effect or relationship between variables. It is a starting point for statistical testing, allowing researchers to determine the likelihood that any observed effects in the data are due to chance. Rejecting or failing to reject the null hypothesis helps in drawing conclusions about the validity of the research hypothesis.
What should I look for in the methods section regarding hypotheses?
In the methods section, examine how the research hypothesis will be tested. Look for descriptions of the experimental design, procedures, variables, and data analysis techniques. This section should provide detailed information on how the study is structured to test the stated hypothesis and whether it includes appropriate controls and replication to ensure reliable results.

- Rebels Blog
- Blog Articles
- Terms and Conditions
- Payment and Shipping Terms
- Privacy Policy
- Return Policy
© 2024 Research Rebels, All rights reserved.
Your cart is currently empty.

Holman Library

Research Guide: Scholarly Journals
- Introduction: Hypothesis/Thesis
- Why Use Scholarly Journals?
- What does "Peer-Reviewed" mean?
- What is *NOT* a Scholarly Journal Article?
- Interlibrary Loan for Journal Articles
- Reading the Citation
- Authors' Credentials
- Literature Review
- Methodology
- Results/Data
- Discussion/Conclusions
- APA Citations for Scholarly Journal Articles
- MLA Citations for Scholarly Journal Articles
Hypothesis or Thesis
Looking for the author's thesis or hypothesis.
The image below shows the part of the scholarly article that shows where the authors are making their argument.
(click on image to enlarge)

- The first few paragraphs of a journal article serve to introduce the topic, to provide the author's hypothesis or thesis, and to indicate why the research was done.
- A thesis or hypothesis is not always clearly labeled; you may need to read through the introductory paragraphs to determine what the authors are proposing.
- << Previous: How to Read a Scholarly Article
- Next: Reading the Citation >>
- Last Updated: May 4, 2024 2:04 PM
- URL: https://libguides.greenriver.edu/scholarlyjournals
Have a language expert improve your writing
Run a free plagiarism check in 10 minutes, automatically generate references for free.
- Knowledge Base
- Methodology
- How to Write a Strong Hypothesis | Guide & Examples
How to Write a Strong Hypothesis | Guide & Examples
Published on 6 May 2022 by Shona McCombes .
A hypothesis is a statement that can be tested by scientific research. If you want to test a relationship between two or more variables, you need to write hypotheses before you start your experiment or data collection.
Table of contents
What is a hypothesis, developing a hypothesis (with example), hypothesis examples, frequently asked questions about writing hypotheses.
A hypothesis states your predictions about what your research will find. It is a tentative answer to your research question that has not yet been tested. For some research projects, you might have to write several hypotheses that address different aspects of your research question.
A hypothesis is not just a guess – it should be based on existing theories and knowledge. It also has to be testable, which means you can support or refute it through scientific research methods (such as experiments, observations, and statistical analysis of data).
Variables in hypotheses
Hypotheses propose a relationship between two or more variables . An independent variable is something the researcher changes or controls. A dependent variable is something the researcher observes and measures.
In this example, the independent variable is exposure to the sun – the assumed cause . The dependent variable is the level of happiness – the assumed effect .
Prevent plagiarism, run a free check.
Step 1: ask a question.
Writing a hypothesis begins with a research question that you want to answer. The question should be focused, specific, and researchable within the constraints of your project.
Step 2: Do some preliminary research
Your initial answer to the question should be based on what is already known about the topic. Look for theories and previous studies to help you form educated assumptions about what your research will find.
At this stage, you might construct a conceptual framework to identify which variables you will study and what you think the relationships are between them. Sometimes, you’ll have to operationalise more complex constructs.
Step 3: Formulate your hypothesis
Now you should have some idea of what you expect to find. Write your initial answer to the question in a clear, concise sentence.
Step 4: Refine your hypothesis
You need to make sure your hypothesis is specific and testable. There are various ways of phrasing a hypothesis, but all the terms you use should have clear definitions, and the hypothesis should contain:
- The relevant variables
- The specific group being studied
- The predicted outcome of the experiment or analysis
Step 5: Phrase your hypothesis in three ways
To identify the variables, you can write a simple prediction in if … then form. The first part of the sentence states the independent variable and the second part states the dependent variable.
In academic research, hypotheses are more commonly phrased in terms of correlations or effects, where you directly state the predicted relationship between variables.
If you are comparing two groups, the hypothesis can state what difference you expect to find between them.
Step 6. Write a null hypothesis
If your research involves statistical hypothesis testing , you will also have to write a null hypothesis. The null hypothesis is the default position that there is no association between the variables. The null hypothesis is written as H 0 , while the alternative hypothesis is H 1 or H a .
Hypothesis testing is a formal procedure for investigating our ideas about the world using statistics. It is used by scientists to test specific predictions, called hypotheses , by calculating how likely it is that a pattern or relationship between variables could have arisen by chance.
A hypothesis is not just a guess. It should be based on existing theories and knowledge. It also has to be testable, which means you can support or refute it through scientific research methods (such as experiments, observations, and statistical analysis of data).
A research hypothesis is your proposed answer to your research question. The research hypothesis usually includes an explanation (‘ x affects y because …’).
A statistical hypothesis, on the other hand, is a mathematical statement about a population parameter. Statistical hypotheses always come in pairs: the null and alternative hypotheses. In a well-designed study , the statistical hypotheses correspond logically to the research hypothesis.
Cite this Scribbr article
If you want to cite this source, you can copy and paste the citation or click the ‘Cite this Scribbr article’ button to automatically add the citation to our free Reference Generator.
McCombes, S. (2022, May 06). How to Write a Strong Hypothesis | Guide & Examples. Scribbr. Retrieved 14 May 2024, from https://www.scribbr.co.uk/research-methods/hypothesis-writing/
Is this article helpful?
Shona McCombes
Other students also liked, operationalisation | a guide with examples, pros & cons, what is a conceptual framework | tips & examples, a quick guide to experimental design | 5 steps & examples.

HOW TO: Use Articles for Research: Introduction: Hypothesis/Thesis
- What's a Scholarly Journal?
- Reading the Citation
- Authors' Credentials
- Introduction: Hypothesis/Thesis
- Literature Review
- Research Method
- Results/Data
- Discussion/Conclusions
Hypothesis or Thesis
The first few paragraphs of a journal article serve to introduce the topic, to provide the author's hypothesis or thesis, and to indicate why the research was done. A thesis or hypothesis is not always clearly labled; you may need to read through the introductory paragraphs to determine what the authors are proposing.
- << Previous: Abstract
- Next: Literature Review >>
- Last Updated: Jan 29, 2024 3:35 PM
- URL: https://libguides.cayuga-cc.edu/1ST-PRIORITY/articles
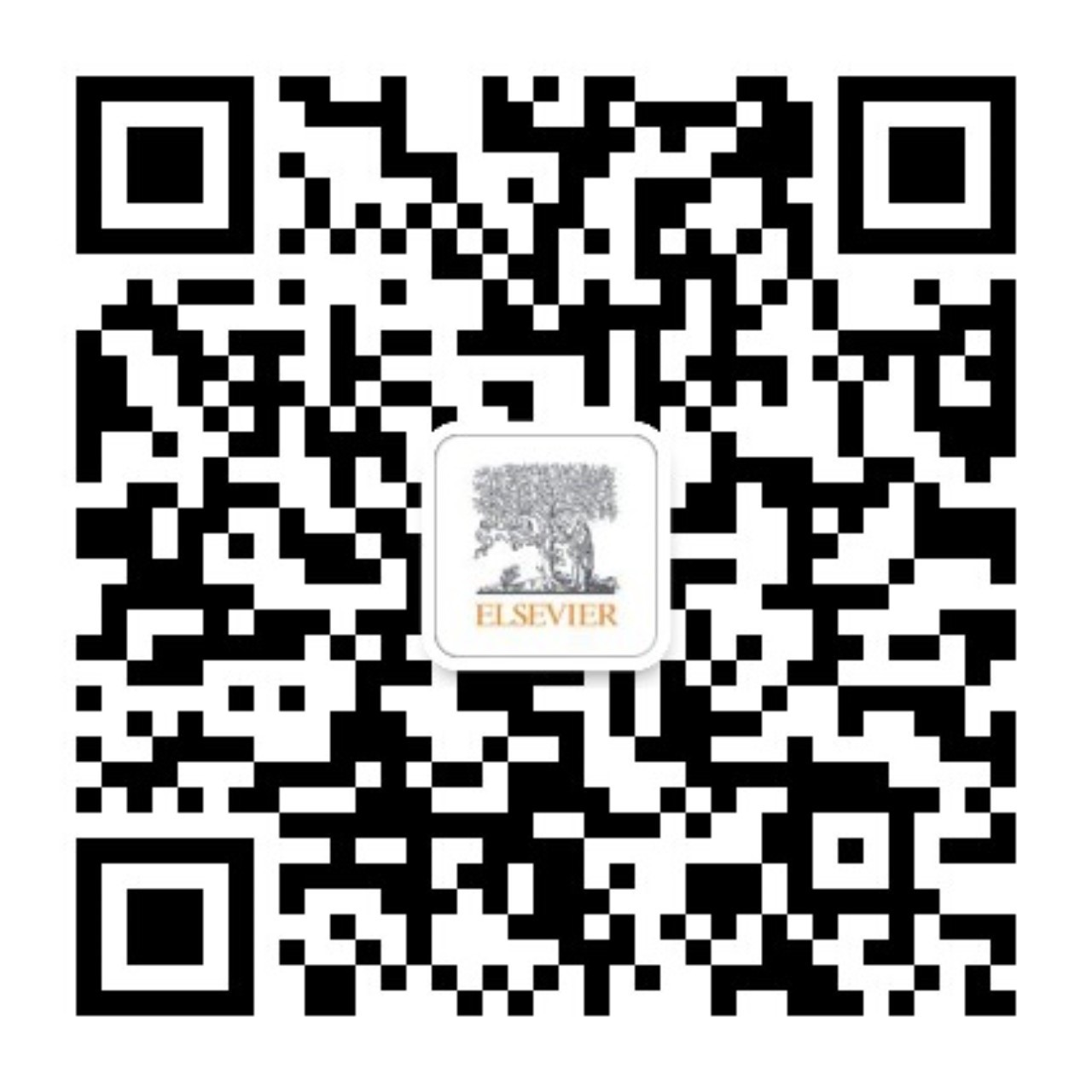
- Manuscript Preparation
What is and How to Write a Good Hypothesis in Research?
- 4 minute read
- 313.7K views
Table of Contents
One of the most important aspects of conducting research is constructing a strong hypothesis. But what makes a hypothesis in research effective? In this article, we’ll look at the difference between a hypothesis and a research question, as well as the elements of a good hypothesis in research. We’ll also include some examples of effective hypotheses, and what pitfalls to avoid.
What is a Hypothesis in Research?
Simply put, a hypothesis is a research question that also includes the predicted or expected result of the research. Without a hypothesis, there can be no basis for a scientific or research experiment. As such, it is critical that you carefully construct your hypothesis by being deliberate and thorough, even before you set pen to paper. Unless your hypothesis is clearly and carefully constructed, any flaw can have an adverse, and even grave, effect on the quality of your experiment and its subsequent results.
Research Question vs Hypothesis
It’s easy to confuse research questions with hypotheses, and vice versa. While they’re both critical to the Scientific Method, they have very specific differences. Primarily, a research question, just like a hypothesis, is focused and concise. But a hypothesis includes a prediction based on the proposed research, and is designed to forecast the relationship of and between two (or more) variables. Research questions are open-ended, and invite debate and discussion, while hypotheses are closed, e.g. “The relationship between A and B will be C.”
A hypothesis is generally used if your research topic is fairly well established, and you are relatively certain about the relationship between the variables that will be presented in your research. Since a hypothesis is ideally suited for experimental studies, it will, by its very existence, affect the design of your experiment. The research question is typically used for new topics that have not yet been researched extensively. Here, the relationship between different variables is less known. There is no prediction made, but there may be variables explored. The research question can be casual in nature, simply trying to understand if a relationship even exists, descriptive or comparative.
How to Write Hypothesis in Research
Writing an effective hypothesis starts before you even begin to type. Like any task, preparation is key, so you start first by conducting research yourself, and reading all you can about the topic that you plan to research. From there, you’ll gain the knowledge you need to understand where your focus within the topic will lie.
Remember that a hypothesis is a prediction of the relationship that exists between two or more variables. Your job is to write a hypothesis, and design the research, to “prove” whether or not your prediction is correct. A common pitfall is to use judgments that are subjective and inappropriate for the construction of a hypothesis. It’s important to keep the focus and language of your hypothesis objective.
An effective hypothesis in research is clearly and concisely written, and any terms or definitions clarified and defined. Specific language must also be used to avoid any generalities or assumptions.
Use the following points as a checklist to evaluate the effectiveness of your research hypothesis:
- Predicts the relationship and outcome
- Simple and concise – avoid wordiness
- Clear with no ambiguity or assumptions about the readers’ knowledge
- Observable and testable results
- Relevant and specific to the research question or problem
Research Hypothesis Example
Perhaps the best way to evaluate whether or not your hypothesis is effective is to compare it to those of your colleagues in the field. There is no need to reinvent the wheel when it comes to writing a powerful research hypothesis. As you’re reading and preparing your hypothesis, you’ll also read other hypotheses. These can help guide you on what works, and what doesn’t, when it comes to writing a strong research hypothesis.
Here are a few generic examples to get you started.
Eating an apple each day, after the age of 60, will result in a reduction of frequency of physician visits.
Budget airlines are more likely to receive more customer complaints. A budget airline is defined as an airline that offers lower fares and fewer amenities than a traditional full-service airline. (Note that the term “budget airline” is included in the hypothesis.
Workplaces that offer flexible working hours report higher levels of employee job satisfaction than workplaces with fixed hours.
Each of the above examples are specific, observable and measurable, and the statement of prediction can be verified or shown to be false by utilizing standard experimental practices. It should be noted, however, that often your hypothesis will change as your research progresses.
Language Editing Plus
Elsevier’s Language Editing Plus service can help ensure that your research hypothesis is well-designed, and articulates your research and conclusions. Our most comprehensive editing package, you can count on a thorough language review by native-English speakers who are PhDs or PhD candidates. We’ll check for effective logic and flow of your manuscript, as well as document formatting for your chosen journal, reference checks, and much more.
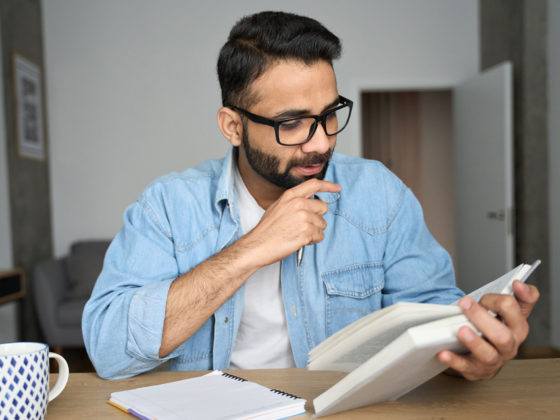
- Research Process
Systematic Literature Review or Literature Review?
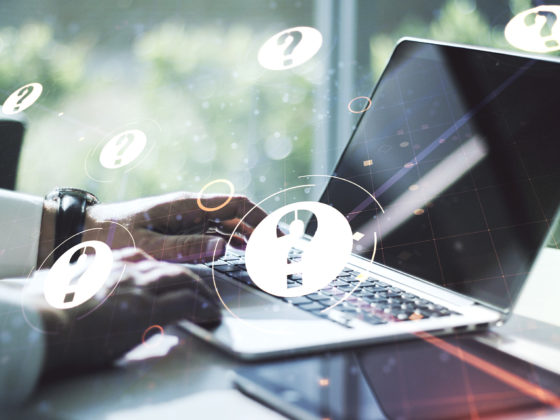
What is a Problem Statement? [with examples]
You may also like.
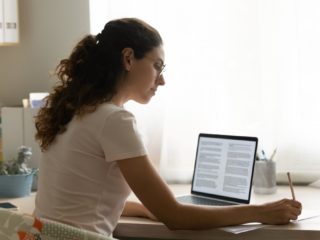
Make Hook, Line, and Sinker: The Art of Crafting Engaging Introductions
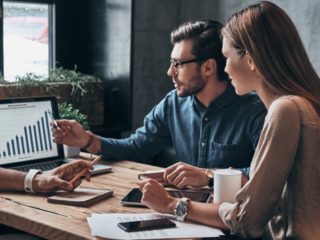
Can Describing Study Limitations Improve the Quality of Your Paper?
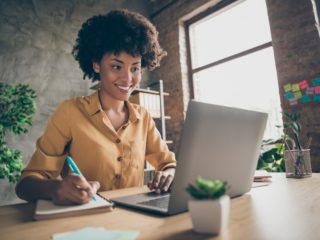
A Guide to Crafting Shorter, Impactful Sentences in Academic Writing
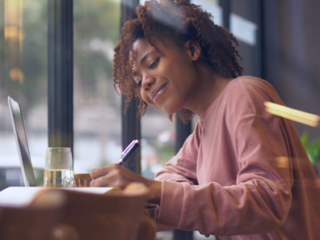
6 Steps to Write an Excellent Discussion in Your Manuscript
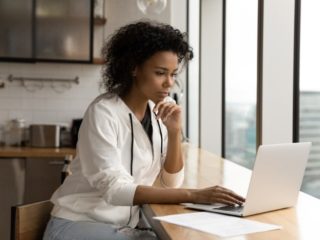
How to Write Clear and Crisp Civil Engineering Papers? Here are 5 Key Tips to Consider
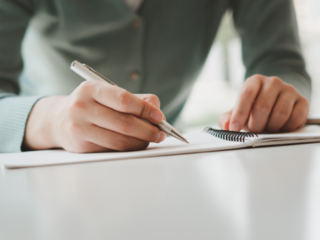
The Clear Path to An Impactful Paper: ②
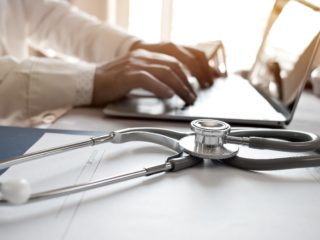
The Essentials of Writing to Communicate Research in Medicine
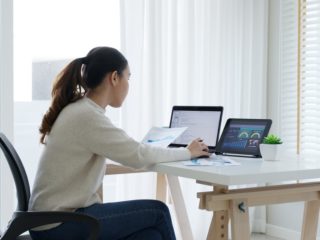
Changing Lines: Sentence Patterns in Academic Writing
Input your search keywords and press Enter.

What Is A Research (Scientific) Hypothesis? A plain-language explainer + examples
By: Derek Jansen (MBA) | Reviewed By: Dr Eunice Rautenbach | June 2020
If you’re new to the world of research, or it’s your first time writing a dissertation or thesis, you’re probably noticing that the words “research hypothesis” and “scientific hypothesis” are used quite a bit, and you’re wondering what they mean in a research context .
“Hypothesis” is one of those words that people use loosely, thinking they understand what it means. However, it has a very specific meaning within academic research. So, it’s important to understand the exact meaning before you start hypothesizing.
Research Hypothesis 101
- What is a hypothesis ?
- What is a research hypothesis (scientific hypothesis)?
- Requirements for a research hypothesis
- Definition of a research hypothesis
- The null hypothesis
What is a hypothesis?
Let’s start with the general definition of a hypothesis (not a research hypothesis or scientific hypothesis), according to the Cambridge Dictionary:
Hypothesis: an idea or explanation for something that is based on known facts but has not yet been proved.
In other words, it’s a statement that provides an explanation for why or how something works, based on facts (or some reasonable assumptions), but that has not yet been specifically tested . For example, a hypothesis might look something like this:
Hypothesis: sleep impacts academic performance.
This statement predicts that academic performance will be influenced by the amount and/or quality of sleep a student engages in – sounds reasonable, right? It’s based on reasonable assumptions , underpinned by what we currently know about sleep and health (from the existing literature). So, loosely speaking, we could call it a hypothesis, at least by the dictionary definition.
But that’s not good enough…
Unfortunately, that’s not quite sophisticated enough to describe a research hypothesis (also sometimes called a scientific hypothesis), and it wouldn’t be acceptable in a dissertation, thesis or research paper . In the world of academic research, a statement needs a few more criteria to constitute a true research hypothesis .
What is a research hypothesis?
A research hypothesis (also called a scientific hypothesis) is a statement about the expected outcome of a study (for example, a dissertation or thesis). To constitute a quality hypothesis, the statement needs to have three attributes – specificity , clarity and testability .
Let’s take a look at these more closely.
Need a helping hand?
Hypothesis Essential #1: Specificity & Clarity
A good research hypothesis needs to be extremely clear and articulate about both what’ s being assessed (who or what variables are involved ) and the expected outcome (for example, a difference between groups, a relationship between variables, etc.).
Let’s stick with our sleepy students example and look at how this statement could be more specific and clear.
Hypothesis: Students who sleep at least 8 hours per night will, on average, achieve higher grades in standardised tests than students who sleep less than 8 hours a night.
As you can see, the statement is very specific as it identifies the variables involved (sleep hours and test grades), the parties involved (two groups of students), as well as the predicted relationship type (a positive relationship). There’s no ambiguity or uncertainty about who or what is involved in the statement, and the expected outcome is clear.
Contrast that to the original hypothesis we looked at – “Sleep impacts academic performance” – and you can see the difference. “Sleep” and “academic performance” are both comparatively vague , and there’s no indication of what the expected relationship direction is (more sleep or less sleep). As you can see, specificity and clarity are key.
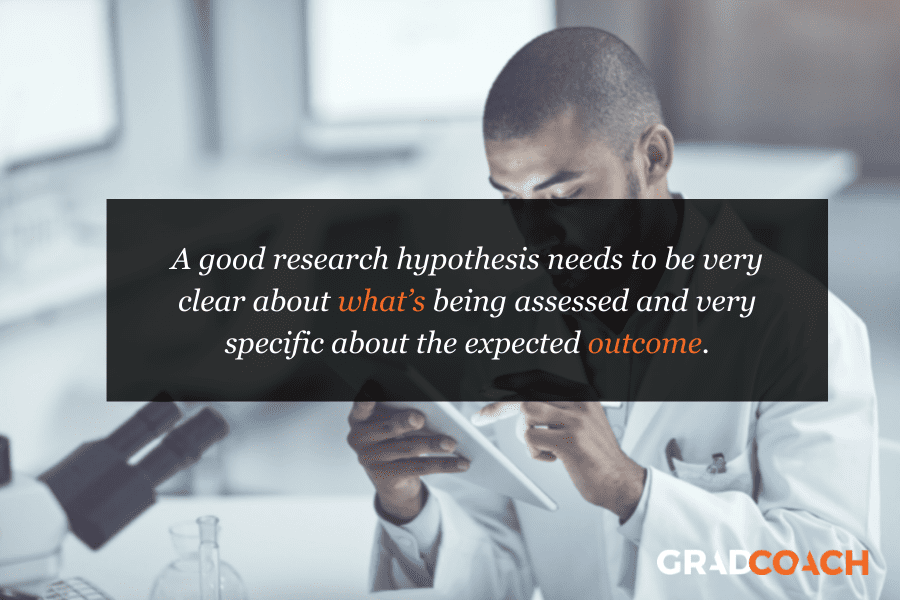
Hypothesis Essential #2: Testability (Provability)
A statement must be testable to qualify as a research hypothesis. In other words, there needs to be a way to prove (or disprove) the statement. If it’s not testable, it’s not a hypothesis – simple as that.
For example, consider the hypothesis we mentioned earlier:
Hypothesis: Students who sleep at least 8 hours per night will, on average, achieve higher grades in standardised tests than students who sleep less than 8 hours a night.
We could test this statement by undertaking a quantitative study involving two groups of students, one that gets 8 or more hours of sleep per night for a fixed period, and one that gets less. We could then compare the standardised test results for both groups to see if there’s a statistically significant difference.
Again, if you compare this to the original hypothesis we looked at – “Sleep impacts academic performance” – you can see that it would be quite difficult to test that statement, primarily because it isn’t specific enough. How much sleep? By who? What type of academic performance?
So, remember the mantra – if you can’t test it, it’s not a hypothesis 🙂
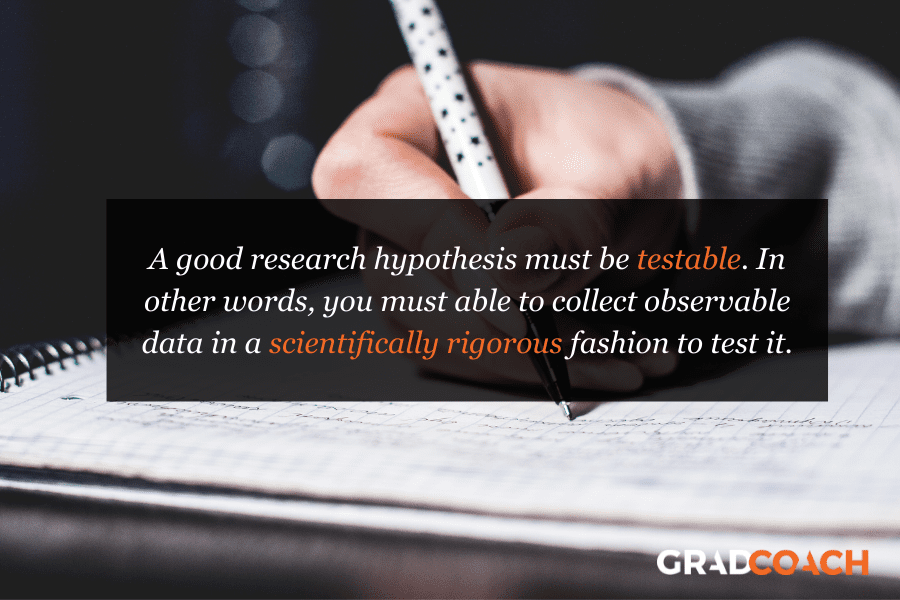
Defining A Research Hypothesis
You’re still with us? Great! Let’s recap and pin down a clear definition of a hypothesis.
A research hypothesis (or scientific hypothesis) is a statement about an expected relationship between variables, or explanation of an occurrence, that is clear, specific and testable.
So, when you write up hypotheses for your dissertation or thesis, make sure that they meet all these criteria. If you do, you’ll not only have rock-solid hypotheses but you’ll also ensure a clear focus for your entire research project.
What about the null hypothesis?
You may have also heard the terms null hypothesis , alternative hypothesis, or H-zero thrown around. At a simple level, the null hypothesis is the counter-proposal to the original hypothesis.
For example, if the hypothesis predicts that there is a relationship between two variables (for example, sleep and academic performance), the null hypothesis would predict that there is no relationship between those variables.
At a more technical level, the null hypothesis proposes that no statistical significance exists in a set of given observations and that any differences are due to chance alone.
And there you have it – hypotheses in a nutshell.
If you have any questions, be sure to leave a comment below and we’ll do our best to help you. If you need hands-on help developing and testing your hypotheses, consider our private coaching service , where we hold your hand through the research journey.
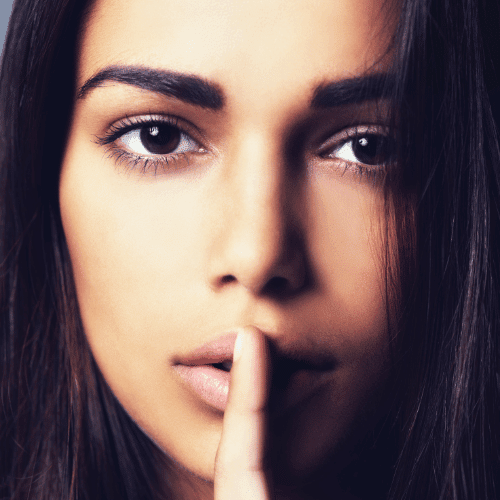
Psst... there’s more!
This post was based on one of our popular Research Bootcamps . If you're working on a research project, you'll definitely want to check this out ...
You Might Also Like:
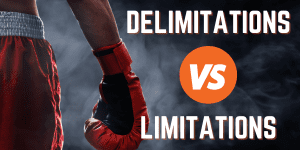
16 Comments
Very useful information. I benefit more from getting more information in this regard.
Very great insight,educative and informative. Please give meet deep critics on many research data of public international Law like human rights, environment, natural resources, law of the sea etc
In a book I read a distinction is made between null, research, and alternative hypothesis. As far as I understand, alternative and research hypotheses are the same. Can you please elaborate? Best Afshin
This is a self explanatory, easy going site. I will recommend this to my friends and colleagues.
Very good definition. How can I cite your definition in my thesis? Thank you. Is nul hypothesis compulsory in a research?
It’s a counter-proposal to be proven as a rejection
Please what is the difference between alternate hypothesis and research hypothesis?
It is a very good explanation. However, it limits hypotheses to statistically tasteable ideas. What about for qualitative researches or other researches that involve quantitative data that don’t need statistical tests?
In qualitative research, one typically uses propositions, not hypotheses.
could you please elaborate it more
I’ve benefited greatly from these notes, thank you.
This is very helpful
well articulated ideas are presented here, thank you for being reliable sources of information
Excellent. Thanks for being clear and sound about the research methodology and hypothesis (quantitative research)
I have only a simple question regarding the null hypothesis. – Is the null hypothesis (Ho) known as the reversible hypothesis of the alternative hypothesis (H1? – How to test it in academic research?
this is very important note help me much more
Trackbacks/Pingbacks
- What Is Research Methodology? Simple Definition (With Examples) - Grad Coach - […] Contrasted to this, a quantitative methodology is typically used when the research aims and objectives are confirmatory in nature. For example,…
Submit a Comment Cancel reply
Your email address will not be published. Required fields are marked *
Save my name, email, and website in this browser for the next time I comment.
- Print Friendly
- Bipolar Disorder
- Therapy Center
- When To See a Therapist
- Types of Therapy
- Best Online Therapy
- Best Couples Therapy
- Best Family Therapy
- Managing Stress
- Sleep and Dreaming
- Understanding Emotions
- Self-Improvement
- Healthy Relationships
- Student Resources
- Personality Types
- Guided Meditations
- Verywell Mind Insights
- 2024 Verywell Mind 25
- Mental Health in the Classroom
- Editorial Process
- Meet Our Review Board
- Crisis Support
How to Write a Great Hypothesis
Hypothesis Definition, Format, Examples, and Tips
Kendra Cherry, MS, is a psychosocial rehabilitation specialist, psychology educator, and author of the "Everything Psychology Book."
:max_bytes(150000):strip_icc():format(webp)/IMG_9791-89504ab694d54b66bbd72cb84ffb860e.jpg)
Amy Morin, LCSW, is a psychotherapist and international bestselling author. Her books, including "13 Things Mentally Strong People Don't Do," have been translated into more than 40 languages. Her TEDx talk, "The Secret of Becoming Mentally Strong," is one of the most viewed talks of all time.
:max_bytes(150000):strip_icc():format(webp)/VW-MIND-Amy-2b338105f1ee493f94d7e333e410fa76.jpg)
Verywell / Alex Dos Diaz
- The Scientific Method
Hypothesis Format
Falsifiability of a hypothesis.
- Operationalization
Hypothesis Types
Hypotheses examples.
- Collecting Data
A hypothesis is a tentative statement about the relationship between two or more variables. It is a specific, testable prediction about what you expect to happen in a study. It is a preliminary answer to your question that helps guide the research process.
Consider a study designed to examine the relationship between sleep deprivation and test performance. The hypothesis might be: "This study is designed to assess the hypothesis that sleep-deprived people will perform worse on a test than individuals who are not sleep-deprived."
At a Glance
A hypothesis is crucial to scientific research because it offers a clear direction for what the researchers are looking to find. This allows them to design experiments to test their predictions and add to our scientific knowledge about the world. This article explores how a hypothesis is used in psychology research, how to write a good hypothesis, and the different types of hypotheses you might use.
The Hypothesis in the Scientific Method
In the scientific method , whether it involves research in psychology, biology, or some other area, a hypothesis represents what the researchers think will happen in an experiment. The scientific method involves the following steps:
- Forming a question
- Performing background research
- Creating a hypothesis
- Designing an experiment
- Collecting data
- Analyzing the results
- Drawing conclusions
- Communicating the results
The hypothesis is a prediction, but it involves more than a guess. Most of the time, the hypothesis begins with a question which is then explored through background research. At this point, researchers then begin to develop a testable hypothesis.
Unless you are creating an exploratory study, your hypothesis should always explain what you expect to happen.
In a study exploring the effects of a particular drug, the hypothesis might be that researchers expect the drug to have some type of effect on the symptoms of a specific illness. In psychology, the hypothesis might focus on how a certain aspect of the environment might influence a particular behavior.
Remember, a hypothesis does not have to be correct. While the hypothesis predicts what the researchers expect to see, the goal of the research is to determine whether this guess is right or wrong. When conducting an experiment, researchers might explore numerous factors to determine which ones might contribute to the ultimate outcome.
In many cases, researchers may find that the results of an experiment do not support the original hypothesis. When writing up these results, the researchers might suggest other options that should be explored in future studies.
In many cases, researchers might draw a hypothesis from a specific theory or build on previous research. For example, prior research has shown that stress can impact the immune system. So a researcher might hypothesize: "People with high-stress levels will be more likely to contract a common cold after being exposed to the virus than people who have low-stress levels."
In other instances, researchers might look at commonly held beliefs or folk wisdom. "Birds of a feather flock together" is one example of folk adage that a psychologist might try to investigate. The researcher might pose a specific hypothesis that "People tend to select romantic partners who are similar to them in interests and educational level."
Elements of a Good Hypothesis
So how do you write a good hypothesis? When trying to come up with a hypothesis for your research or experiments, ask yourself the following questions:
- Is your hypothesis based on your research on a topic?
- Can your hypothesis be tested?
- Does your hypothesis include independent and dependent variables?
Before you come up with a specific hypothesis, spend some time doing background research. Once you have completed a literature review, start thinking about potential questions you still have. Pay attention to the discussion section in the journal articles you read . Many authors will suggest questions that still need to be explored.
How to Formulate a Good Hypothesis
To form a hypothesis, you should take these steps:
- Collect as many observations about a topic or problem as you can.
- Evaluate these observations and look for possible causes of the problem.
- Create a list of possible explanations that you might want to explore.
- After you have developed some possible hypotheses, think of ways that you could confirm or disprove each hypothesis through experimentation. This is known as falsifiability.
In the scientific method , falsifiability is an important part of any valid hypothesis. In order to test a claim scientifically, it must be possible that the claim could be proven false.
Students sometimes confuse the idea of falsifiability with the idea that it means that something is false, which is not the case. What falsifiability means is that if something was false, then it is possible to demonstrate that it is false.
One of the hallmarks of pseudoscience is that it makes claims that cannot be refuted or proven false.
The Importance of Operational Definitions
A variable is a factor or element that can be changed and manipulated in ways that are observable and measurable. However, the researcher must also define how the variable will be manipulated and measured in the study.
Operational definitions are specific definitions for all relevant factors in a study. This process helps make vague or ambiguous concepts detailed and measurable.
For example, a researcher might operationally define the variable " test anxiety " as the results of a self-report measure of anxiety experienced during an exam. A "study habits" variable might be defined by the amount of studying that actually occurs as measured by time.
These precise descriptions are important because many things can be measured in various ways. Clearly defining these variables and how they are measured helps ensure that other researchers can replicate your results.
Replicability
One of the basic principles of any type of scientific research is that the results must be replicable.
Replication means repeating an experiment in the same way to produce the same results. By clearly detailing the specifics of how the variables were measured and manipulated, other researchers can better understand the results and repeat the study if needed.
Some variables are more difficult than others to define. For example, how would you operationally define a variable such as aggression ? For obvious ethical reasons, researchers cannot create a situation in which a person behaves aggressively toward others.
To measure this variable, the researcher must devise a measurement that assesses aggressive behavior without harming others. The researcher might utilize a simulated task to measure aggressiveness in this situation.
Hypothesis Checklist
- Does your hypothesis focus on something that you can actually test?
- Does your hypothesis include both an independent and dependent variable?
- Can you manipulate the variables?
- Can your hypothesis be tested without violating ethical standards?
The hypothesis you use will depend on what you are investigating and hoping to find. Some of the main types of hypotheses that you might use include:
- Simple hypothesis : This type of hypothesis suggests there is a relationship between one independent variable and one dependent variable.
- Complex hypothesis : This type suggests a relationship between three or more variables, such as two independent and dependent variables.
- Null hypothesis : This hypothesis suggests no relationship exists between two or more variables.
- Alternative hypothesis : This hypothesis states the opposite of the null hypothesis.
- Statistical hypothesis : This hypothesis uses statistical analysis to evaluate a representative population sample and then generalizes the findings to the larger group.
- Logical hypothesis : This hypothesis assumes a relationship between variables without collecting data or evidence.
A hypothesis often follows a basic format of "If {this happens} then {this will happen}." One way to structure your hypothesis is to describe what will happen to the dependent variable if you change the independent variable .
The basic format might be: "If {these changes are made to a certain independent variable}, then we will observe {a change in a specific dependent variable}."
A few examples of simple hypotheses:
- "Students who eat breakfast will perform better on a math exam than students who do not eat breakfast."
- "Students who experience test anxiety before an English exam will get lower scores than students who do not experience test anxiety."
- "Motorists who talk on the phone while driving will be more likely to make errors on a driving course than those who do not talk on the phone."
- "Children who receive a new reading intervention will have higher reading scores than students who do not receive the intervention."
Examples of a complex hypothesis include:
- "People with high-sugar diets and sedentary activity levels are more likely to develop depression."
- "Younger people who are regularly exposed to green, outdoor areas have better subjective well-being than older adults who have limited exposure to green spaces."
Examples of a null hypothesis include:
- "There is no difference in anxiety levels between people who take St. John's wort supplements and those who do not."
- "There is no difference in scores on a memory recall task between children and adults."
- "There is no difference in aggression levels between children who play first-person shooter games and those who do not."
Examples of an alternative hypothesis:
- "People who take St. John's wort supplements will have less anxiety than those who do not."
- "Adults will perform better on a memory task than children."
- "Children who play first-person shooter games will show higher levels of aggression than children who do not."
Collecting Data on Your Hypothesis
Once a researcher has formed a testable hypothesis, the next step is to select a research design and start collecting data. The research method depends largely on exactly what they are studying. There are two basic types of research methods: descriptive research and experimental research.
Descriptive Research Methods
Descriptive research such as case studies , naturalistic observations , and surveys are often used when conducting an experiment is difficult or impossible. These methods are best used to describe different aspects of a behavior or psychological phenomenon.
Once a researcher has collected data using descriptive methods, a correlational study can examine how the variables are related. This research method might be used to investigate a hypothesis that is difficult to test experimentally.
Experimental Research Methods
Experimental methods are used to demonstrate causal relationships between variables. In an experiment, the researcher systematically manipulates a variable of interest (known as the independent variable) and measures the effect on another variable (known as the dependent variable).
Unlike correlational studies, which can only be used to determine if there is a relationship between two variables, experimental methods can be used to determine the actual nature of the relationship—whether changes in one variable actually cause another to change.
The hypothesis is a critical part of any scientific exploration. It represents what researchers expect to find in a study or experiment. In situations where the hypothesis is unsupported by the research, the research still has value. Such research helps us better understand how different aspects of the natural world relate to one another. It also helps us develop new hypotheses that can then be tested in the future.
Thompson WH, Skau S. On the scope of scientific hypotheses . R Soc Open Sci . 2023;10(8):230607. doi:10.1098/rsos.230607
Taran S, Adhikari NKJ, Fan E. Falsifiability in medicine: what clinicians can learn from Karl Popper [published correction appears in Intensive Care Med. 2021 Jun 17;:]. Intensive Care Med . 2021;47(9):1054-1056. doi:10.1007/s00134-021-06432-z
Eyler AA. Research Methods for Public Health . 1st ed. Springer Publishing Company; 2020. doi:10.1891/9780826182067.0004
Nosek BA, Errington TM. What is replication ? PLoS Biol . 2020;18(3):e3000691. doi:10.1371/journal.pbio.3000691
Aggarwal R, Ranganathan P. Study designs: Part 2 - Descriptive studies . Perspect Clin Res . 2019;10(1):34-36. doi:10.4103/picr.PICR_154_18
Nevid J. Psychology: Concepts and Applications. Wadworth, 2013.
By Kendra Cherry, MSEd Kendra Cherry, MS, is a psychosocial rehabilitation specialist, psychology educator, and author of the "Everything Psychology Book."
- Affiliate Program

- UNITED STATES
- 台灣 (TAIWAN)
- TÜRKIYE (TURKEY)
- Academic Editing Services
- - Research Paper
- - Journal Manuscript
- - Dissertation
- - College & University Assignments
- Admissions Editing Services
- - Application Essay
- - Personal Statement
- - Recommendation Letter
- - Cover Letter
- - CV/Resume
- Business Editing Services
- - Business Documents
- - Report & Brochure
- - Website & Blog
- Writer Editing Services
- - Script & Screenplay
- Our Editors
- Client Reviews
- Editing & Proofreading Prices
- Wordvice Points
- Partner Discount
- Plagiarism Checker
- APA Citation Generator
- MLA Citation Generator
- Chicago Citation Generator
- Vancouver Citation Generator
- - APA Style
- - MLA Style
- - Chicago Style
- - Vancouver Style
- Writing & Editing Guide
- Academic Resources
- Admissions Resources
How to Write a Research Hypothesis: Good & Bad Examples
What is a research hypothesis?
A research hypothesis is an attempt at explaining a phenomenon or the relationships between phenomena/variables in the real world. Hypotheses are sometimes called “educated guesses”, but they are in fact (or let’s say they should be) based on previous observations, existing theories, scientific evidence, and logic. A research hypothesis is also not a prediction—rather, predictions are ( should be) based on clearly formulated hypotheses. For example, “We tested the hypothesis that KLF2 knockout mice would show deficiencies in heart development” is an assumption or prediction, not a hypothesis.
The research hypothesis at the basis of this prediction is “the product of the KLF2 gene is involved in the development of the cardiovascular system in mice”—and this hypothesis is probably (hopefully) based on a clear observation, such as that mice with low levels of Kruppel-like factor 2 (which KLF2 codes for) seem to have heart problems. From this hypothesis, you can derive the idea that a mouse in which this particular gene does not function cannot develop a normal cardiovascular system, and then make the prediction that we started with.
What is the difference between a hypothesis and a prediction?
You might think that these are very subtle differences, and you will certainly come across many publications that do not contain an actual hypothesis or do not make these distinctions correctly. But considering that the formulation and testing of hypotheses is an integral part of the scientific method, it is good to be aware of the concepts underlying this approach. The two hallmarks of a scientific hypothesis are falsifiability (an evaluation standard that was introduced by the philosopher of science Karl Popper in 1934) and testability —if you cannot use experiments or data to decide whether an idea is true or false, then it is not a hypothesis (or at least a very bad one).
So, in a nutshell, you (1) look at existing evidence/theories, (2) come up with a hypothesis, (3) make a prediction that allows you to (4) design an experiment or data analysis to test it, and (5) come to a conclusion. Of course, not all studies have hypotheses (there is also exploratory or hypothesis-generating research), and you do not necessarily have to state your hypothesis as such in your paper.
But for the sake of understanding the principles of the scientific method, let’s first take a closer look at the different types of hypotheses that research articles refer to and then give you a step-by-step guide for how to formulate a strong hypothesis for your own paper.
Types of Research Hypotheses
Hypotheses can be simple , which means they describe the relationship between one single independent variable (the one you observe variations in or plan to manipulate) and one single dependent variable (the one you expect to be affected by the variations/manipulation). If there are more variables on either side, you are dealing with a complex hypothesis. You can also distinguish hypotheses according to the kind of relationship between the variables you are interested in (e.g., causal or associative ). But apart from these variations, we are usually interested in what is called the “alternative hypothesis” and, in contrast to that, the “null hypothesis”. If you think these two should be listed the other way round, then you are right, logically speaking—the alternative should surely come second. However, since this is the hypothesis we (as researchers) are usually interested in, let’s start from there.
Alternative Hypothesis
If you predict a relationship between two variables in your study, then the research hypothesis that you formulate to describe that relationship is your alternative hypothesis (usually H1 in statistical terms). The goal of your hypothesis testing is thus to demonstrate that there is sufficient evidence that supports the alternative hypothesis, rather than evidence for the possibility that there is no such relationship. The alternative hypothesis is usually the research hypothesis of a study and is based on the literature, previous observations, and widely known theories.
Null Hypothesis
The hypothesis that describes the other possible outcome, that is, that your variables are not related, is the null hypothesis ( H0 ). Based on your findings, you choose between the two hypotheses—usually that means that if your prediction was correct, you reject the null hypothesis and accept the alternative. Make sure, however, that you are not getting lost at this step of the thinking process: If your prediction is that there will be no difference or change, then you are trying to find support for the null hypothesis and reject H1.
Directional Hypothesis
While the null hypothesis is obviously “static”, the alternative hypothesis can specify a direction for the observed relationship between variables—for example, that mice with higher expression levels of a certain protein are more active than those with lower levels. This is then called a one-tailed hypothesis.
Another example for a directional one-tailed alternative hypothesis would be that
H1: Attending private classes before important exams has a positive effect on performance.
Your null hypothesis would then be that
H0: Attending private classes before important exams has no/a negative effect on performance.
Nondirectional Hypothesis
A nondirectional hypothesis does not specify the direction of the potentially observed effect, only that there is a relationship between the studied variables—this is called a two-tailed hypothesis. For instance, if you are studying a new drug that has shown some effects on pathways involved in a certain condition (e.g., anxiety) in vitro in the lab, but you can’t say for sure whether it will have the same effects in an animal model or maybe induce other/side effects that you can’t predict and potentially increase anxiety levels instead, you could state the two hypotheses like this:
H1: The only lab-tested drug (somehow) affects anxiety levels in an anxiety mouse model.
You then test this nondirectional alternative hypothesis against the null hypothesis:
H0: The only lab-tested drug has no effect on anxiety levels in an anxiety mouse model.
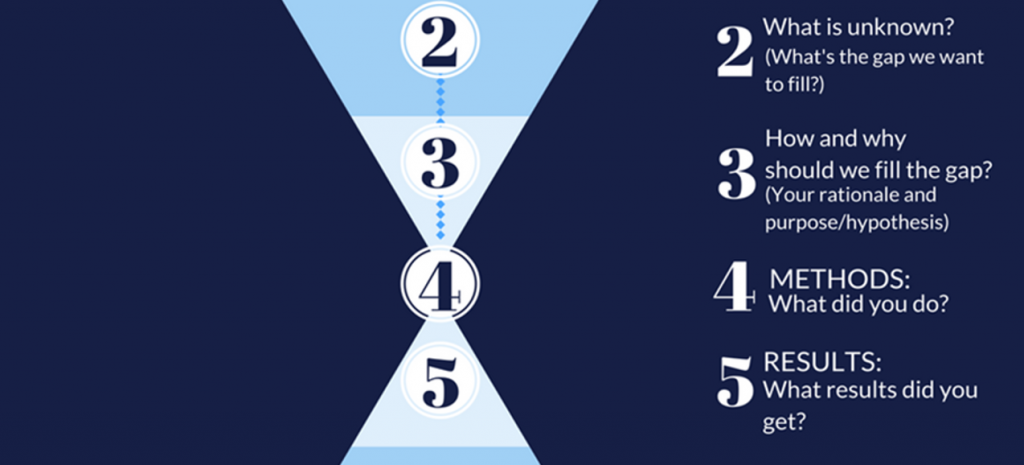
How to Write a Hypothesis for a Research Paper
Now that we understand the important distinctions between different kinds of research hypotheses, let’s look at a simple process of how to write a hypothesis.
Writing a Hypothesis Step:1
Ask a question, based on earlier research. Research always starts with a question, but one that takes into account what is already known about a topic or phenomenon. For example, if you are interested in whether people who have pets are happier than those who don’t, do a literature search and find out what has already been demonstrated. You will probably realize that yes, there is quite a bit of research that shows a relationship between happiness and owning a pet—and even studies that show that owning a dog is more beneficial than owning a cat ! Let’s say you are so intrigued by this finding that you wonder:
What is it that makes dog owners even happier than cat owners?
Let’s move on to Step 2 and find an answer to that question.
Writing a Hypothesis Step 2:
Formulate a strong hypothesis by answering your own question. Again, you don’t want to make things up, take unicorns into account, or repeat/ignore what has already been done. Looking at the dog-vs-cat papers your literature search returned, you see that most studies are based on self-report questionnaires on personality traits, mental health, and life satisfaction. What you don’t find is any data on actual (mental or physical) health measures, and no experiments. You therefore decide to make a bold claim come up with the carefully thought-through hypothesis that it’s maybe the lifestyle of the dog owners, which includes walking their dog several times per day, engaging in fun and healthy activities such as agility competitions, and taking them on trips, that gives them that extra boost in happiness. You could therefore answer your question in the following way:
Dog owners are happier than cat owners because of the dog-related activities they engage in.
Now you have to verify that your hypothesis fulfills the two requirements we introduced at the beginning of this resource article: falsifiability and testability . If it can’t be wrong and can’t be tested, it’s not a hypothesis. We are lucky, however, because yes, we can test whether owning a dog but not engaging in any of those activities leads to lower levels of happiness or well-being than owning a dog and playing and running around with them or taking them on trips.
Writing a Hypothesis Step 3:
Make your predictions and define your variables. We have verified that we can test our hypothesis, but now we have to define all the relevant variables, design our experiment or data analysis, and make precise predictions. You could, for example, decide to study dog owners (not surprising at this point), let them fill in questionnaires about their lifestyle as well as their life satisfaction (as other studies did), and then compare two groups of active and inactive dog owners. Alternatively, if you want to go beyond the data that earlier studies produced and analyzed and directly manipulate the activity level of your dog owners to study the effect of that manipulation, you could invite them to your lab, select groups of participants with similar lifestyles, make them change their lifestyle (e.g., couch potato dog owners start agility classes, very active ones have to refrain from any fun activities for a certain period of time) and assess their happiness levels before and after the intervention. In both cases, your independent variable would be “ level of engagement in fun activities with dog” and your dependent variable would be happiness or well-being .
Examples of a Good and Bad Hypothesis
Let’s look at a few examples of good and bad hypotheses to get you started.
Good Hypothesis Examples
Bad hypothesis examples, tips for writing a research hypothesis.
If you understood the distinction between a hypothesis and a prediction we made at the beginning of this article, then you will have no problem formulating your hypotheses and predictions correctly. To refresh your memory: We have to (1) look at existing evidence, (2) come up with a hypothesis, (3) make a prediction, and (4) design an experiment. For example, you could summarize your dog/happiness study like this:
(1) While research suggests that dog owners are happier than cat owners, there are no reports on what factors drive this difference. (2) We hypothesized that it is the fun activities that many dog owners (but very few cat owners) engage in with their pets that increases their happiness levels. (3) We thus predicted that preventing very active dog owners from engaging in such activities for some time and making very inactive dog owners take up such activities would lead to an increase and decrease in their overall self-ratings of happiness, respectively. (4) To test this, we invited dog owners into our lab, assessed their mental and emotional well-being through questionnaires, and then assigned them to an “active” and an “inactive” group, depending on…
Note that you use “we hypothesize” only for your hypothesis, not for your experimental prediction, and “would” or “if – then” only for your prediction, not your hypothesis. A hypothesis that states that something “would” affect something else sounds as if you don’t have enough confidence to make a clear statement—in which case you can’t expect your readers to believe in your research either. Write in the present tense, don’t use modal verbs that express varying degrees of certainty (such as may, might, or could ), and remember that you are not drawing a conclusion while trying not to exaggerate but making a clear statement that you then, in a way, try to disprove . And if that happens, that is not something to fear but an important part of the scientific process.
Similarly, don’t use “we hypothesize” when you explain the implications of your research or make predictions in the conclusion section of your manuscript, since these are clearly not hypotheses in the true sense of the word. As we said earlier, you will find that many authors of academic articles do not seem to care too much about these rather subtle distinctions, but thinking very clearly about your own research will not only help you write better but also ensure that even that infamous Reviewer 2 will find fewer reasons to nitpick about your manuscript.
Perfect Your Manuscript With Professional Editing
Now that you know how to write a strong research hypothesis for your research paper, you might be interested in our free AI proofreader , Wordvice AI, which finds and fixes errors in grammar, punctuation, and word choice in academic texts. Or if you are interested in human proofreading , check out our English editing services , including research paper editing and manuscript editing .
On the Wordvice academic resources website , you can also find many more articles and other resources that can help you with writing the other parts of your research paper , with making a research paper outline before you put everything together, or with writing an effective cover letter once you are ready to submit.
How to Write a Research Hypothesis
- Research Process
- Peer Review
Since grade school, we've all been familiar with hypotheses. The hypothesis is an essential step of the scientific method. But what makes an effective research hypothesis, how do you create one, and what types of hypotheses are there? We answer these questions and more.
Updated on April 27, 2022

What is a research hypothesis?
General hypothesis.
Since grade school, we've all been familiar with the term “hypothesis.” A hypothesis is a fact-based guess or prediction that has not been proven. It is an essential step of the scientific method. The hypothesis of a study is a drive for experimentation to either prove the hypothesis or dispute it.
Research Hypothesis
A research hypothesis is more specific than a general hypothesis. It is an educated, expected prediction of the outcome of a study that is testable.
What makes an effective research hypothesis?
A good research hypothesis is a clear statement of the relationship between a dependent variable(s) and independent variable(s) relevant to the study that can be disproven.
Research hypothesis checklist
Once you've written a possible hypothesis, make sure it checks the following boxes:
- It must be testable: You need a means to prove your hypothesis. If you can't test it, it's not a hypothesis.
- It must include a dependent and independent variable: At least one independent variable ( cause ) and one dependent variable ( effect ) must be included.
- The language must be easy to understand: Be as clear and concise as possible. Nothing should be left to interpretation.
- It must be relevant to your research topic: You probably shouldn't be talking about cats and dogs if your research topic is outer space. Stay relevant to your topic.
How to create an effective research hypothesis
Pose it as a question first.
Start your research hypothesis from a journalistic approach. Ask one of the five W's: Who, what, when, where, or why.
A possible initial question could be: Why is the sky blue?
Do the preliminary research
Once you have a question in mind, read research around your topic. Collect research from academic journals.
If you're looking for information about the sky and why it is blue, research information about the atmosphere, weather, space, the sun, etc.
Write a draft hypothesis
Once you're comfortable with your subject and have preliminary knowledge, create a working hypothesis. Don't stress much over this. Your first hypothesis is not permanent. Look at it as a draft.
Your first draft of a hypothesis could be: Certain molecules in the Earth's atmosphere are responsive to the sky being the color blue.
Make your working draft perfect
Take your working hypothesis and make it perfect. Narrow it down to include only the information listed in the “Research hypothesis checklist” above.
Now that you've written your working hypothesis, narrow it down. Your new hypothesis could be: Light from the sun hitting oxygen molecules in the sky makes the color of the sky appear blue.
Write a null hypothesis
Your null hypothesis should be the opposite of your research hypothesis. It should be able to be disproven by your research.
In this example, your null hypothesis would be: Light from the sun hitting oxygen molecules in the sky does not make the color of the sky appear blue.
Why is it important to have a clear, testable hypothesis?
One of the main reasons a manuscript can be rejected from a journal is because of a weak hypothesis. “Poor hypothesis, study design, methodology, and improper use of statistics are other reasons for rejection of a manuscript,” says Dr. Ish Kumar Dhammi and Dr. Rehan-Ul-Haq in Indian Journal of Orthopaedics.
According to Dr. James M. Provenzale in American Journal of Roentgenology , “The clear declaration of a research question (or hypothesis) in the Introduction is critical for reviewers to understand the intent of the research study. It is best to clearly state the study goal in plain language (for example, “We set out to determine whether condition x produces condition y.”) An insufficient problem statement is one of the more common reasons for manuscript rejection.”
Characteristics that make a hypothesis weak include:
- Unclear variables
- Unoriginality
- Too general
- Too specific
A weak hypothesis leads to weak research and methods . The goal of a paper is to prove or disprove a hypothesis - or to prove or disprove a null hypothesis. If the hypothesis is not a dependent variable of what is being studied, the paper's methods should come into question.
A strong hypothesis is essential to the scientific method. A hypothesis states an assumed relationship between at least two variables and the experiment then proves or disproves that relationship with statistical significance. Without a proven and reproducible relationship, the paper feeds into the reproducibility crisis. Learn more about writing for reproducibility .
In a study published in The Journal of Obstetrics and Gynecology of India by Dr. Suvarna Satish Khadilkar, she reviewed 400 rejected manuscripts to see why they were rejected. Her studies revealed that poor methodology was a top reason for the submission having a final disposition of rejection.
Aside from publication chances, Dr. Gareth Dyke believes a clear hypothesis helps efficiency.
“Developing a clear and testable hypothesis for your research project means that you will not waste time, energy, and money with your work,” said Dyke. “Refining a hypothesis that is both meaningful, interesting, attainable, and testable is the goal of all effective research.”
Types of research hypotheses
There can be overlap in these types of hypotheses.
Simple hypothesis
A simple hypothesis is a hypothesis at its most basic form. It shows the relationship of one independent and one independent variable.
Example: Drinking soda (independent variable) every day leads to obesity (dependent variable).
Complex hypothesis
A complex hypothesis shows the relationship of two or more independent and dependent variables.
Example: Drinking soda (independent variable) every day leads to obesity (dependent variable) and heart disease (dependent variable).
Directional hypothesis
A directional hypothesis guesses which way the results of an experiment will go. It uses words like increase, decrease, higher, lower, positive, negative, more, or less. It is also frequently used in statistics.
Example: Humans exposed to radiation have a higher risk of cancer than humans not exposed to radiation.
Non-directional hypothesis
A non-directional hypothesis says there will be an effect on the dependent variable, but it does not say which direction.
Associative hypothesis
An associative hypothesis says that when one variable changes, so does the other variable.
Alternative hypothesis
An alternative hypothesis states that the variables have a relationship.
- The opposite of a null hypothesis
Example: An apple a day keeps the doctor away.
Null hypothesis
A null hypothesis states that there is no relationship between the two variables. It is posed as the opposite of what the alternative hypothesis states.
Researchers use a null hypothesis to work to be able to reject it. A null hypothesis:
- Can never be proven
- Can only be rejected
- Is the opposite of an alternative hypothesis
Example: An apple a day does not keep the doctor away.
Logical hypothesis
A logical hypothesis is a suggested explanation while using limited evidence.
Example: Bats can navigate in the dark better than tigers.
In this hypothesis, the researcher knows that tigers cannot see in the dark, and bats mostly live in darkness.
Empirical hypothesis
An empirical hypothesis is also called a “working hypothesis.” It uses the trial and error method and changes around the independent variables.
- An apple a day keeps the doctor away.
- Two apples a day keep the doctor away.
- Three apples a day keep the doctor away.
In this case, the research changes the hypothesis as the researcher learns more about his/her research.
Statistical hypothesis
A statistical hypothesis is a look of a part of a population or statistical model. This type of hypothesis is especially useful if you are making a statement about a large population. Instead of having to test the entire population of Illinois, you could just use a smaller sample of people who live there.
Example: 70% of people who live in Illinois are iron deficient.
Causal hypothesis
A causal hypothesis states that the independent variable will have an effect on the dependent variable.
Example: Using tobacco products causes cancer.
Final thoughts
Make sure your research is error-free before you send it to your preferred journal . Check our our English Editing services to avoid your chances of desk rejection.

Jonny Rhein, BA
See our "Privacy Policy"

How to Develop a Good Research Hypothesis
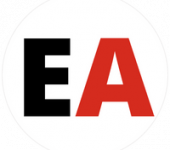
The story of a research study begins by asking a question. Researchers all around the globe are asking curious questions and formulating research hypothesis. However, whether the research study provides an effective conclusion depends on how well one develops a good research hypothesis. Research hypothesis examples could help researchers get an idea as to how to write a good research hypothesis.
This blog will help you understand what is a research hypothesis, its characteristics and, how to formulate a research hypothesis
Table of Contents
What is Hypothesis?
Hypothesis is an assumption or an idea proposed for the sake of argument so that it can be tested. It is a precise, testable statement of what the researchers predict will be outcome of the study. Hypothesis usually involves proposing a relationship between two variables: the independent variable (what the researchers change) and the dependent variable (what the research measures).
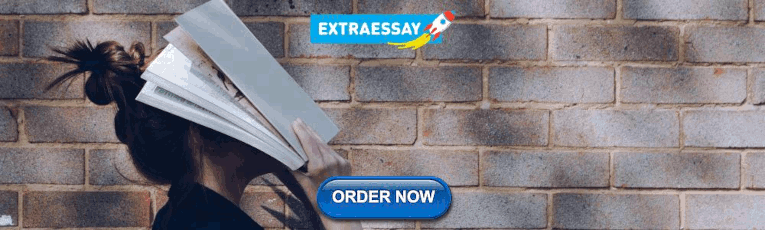
What is a Research Hypothesis?
Research hypothesis is a statement that introduces a research question and proposes an expected result. It is an integral part of the scientific method that forms the basis of scientific experiments. Therefore, you need to be careful and thorough when building your research hypothesis. A minor flaw in the construction of your hypothesis could have an adverse effect on your experiment. In research, there is a convention that the hypothesis is written in two forms, the null hypothesis, and the alternative hypothesis (called the experimental hypothesis when the method of investigation is an experiment).
Characteristics of a Good Research Hypothesis
As the hypothesis is specific, there is a testable prediction about what you expect to happen in a study. You may consider drawing hypothesis from previously published research based on the theory.
A good research hypothesis involves more effort than just a guess. In particular, your hypothesis may begin with a question that could be further explored through background research.
To help you formulate a promising research hypothesis, you should ask yourself the following questions:
- Is the language clear and focused?
- What is the relationship between your hypothesis and your research topic?
- Is your hypothesis testable? If yes, then how?
- What are the possible explanations that you might want to explore?
- Does your hypothesis include both an independent and dependent variable?
- Can you manipulate your variables without hampering the ethical standards?
- Does your research predict the relationship and outcome?
- Is your research simple and concise (avoids wordiness)?
- Is it clear with no ambiguity or assumptions about the readers’ knowledge
- Is your research observable and testable results?
- Is it relevant and specific to the research question or problem?
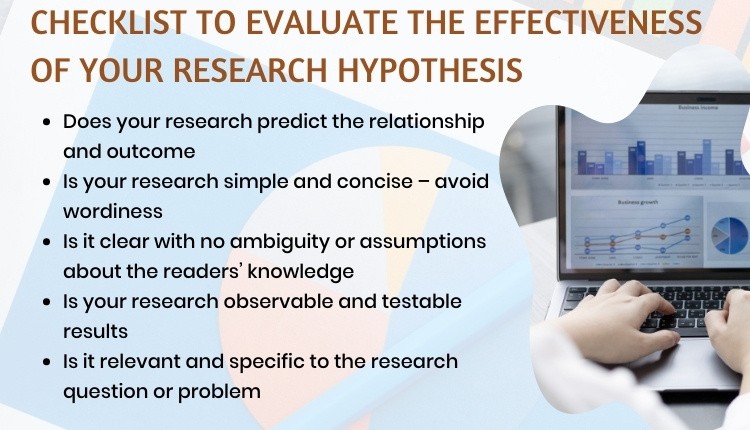
The questions listed above can be used as a checklist to make sure your hypothesis is based on a solid foundation. Furthermore, it can help you identify weaknesses in your hypothesis and revise it if necessary.
Source: Educational Hub
How to formulate a research hypothesis.
A testable hypothesis is not a simple statement. It is rather an intricate statement that needs to offer a clear introduction to a scientific experiment, its intentions, and the possible outcomes. However, there are some important things to consider when building a compelling hypothesis.
1. State the problem that you are trying to solve.
Make sure that the hypothesis clearly defines the topic and the focus of the experiment.
2. Try to write the hypothesis as an if-then statement.
Follow this template: If a specific action is taken, then a certain outcome is expected.
3. Define the variables
Independent variables are the ones that are manipulated, controlled, or changed. Independent variables are isolated from other factors of the study.
Dependent variables , as the name suggests are dependent on other factors of the study. They are influenced by the change in independent variable.
4. Scrutinize the hypothesis
Evaluate assumptions, predictions, and evidence rigorously to refine your understanding.
Types of Research Hypothesis
The types of research hypothesis are stated below:
1. Simple Hypothesis
It predicts the relationship between a single dependent variable and a single independent variable.
2. Complex Hypothesis
It predicts the relationship between two or more independent and dependent variables.
3. Directional Hypothesis
It specifies the expected direction to be followed to determine the relationship between variables and is derived from theory. Furthermore, it implies the researcher’s intellectual commitment to a particular outcome.
4. Non-directional Hypothesis
It does not predict the exact direction or nature of the relationship between the two variables. The non-directional hypothesis is used when there is no theory involved or when findings contradict previous research.
5. Associative and Causal Hypothesis
The associative hypothesis defines interdependency between variables. A change in one variable results in the change of the other variable. On the other hand, the causal hypothesis proposes an effect on the dependent due to manipulation of the independent variable.
6. Null Hypothesis
Null hypothesis states a negative statement to support the researcher’s findings that there is no relationship between two variables. There will be no changes in the dependent variable due the manipulation of the independent variable. Furthermore, it states results are due to chance and are not significant in terms of supporting the idea being investigated.
7. Alternative Hypothesis
It states that there is a relationship between the two variables of the study and that the results are significant to the research topic. An experimental hypothesis predicts what changes will take place in the dependent variable when the independent variable is manipulated. Also, it states that the results are not due to chance and that they are significant in terms of supporting the theory being investigated.
Research Hypothesis Examples of Independent and Dependent Variables
Research Hypothesis Example 1 The greater number of coal plants in a region (independent variable) increases water pollution (dependent variable). If you change the independent variable (building more coal factories), it will change the dependent variable (amount of water pollution).
Research Hypothesis Example 2 What is the effect of diet or regular soda (independent variable) on blood sugar levels (dependent variable)? If you change the independent variable (the type of soda you consume), it will change the dependent variable (blood sugar levels)
You should not ignore the importance of the above steps. The validity of your experiment and its results rely on a robust testable hypothesis. Developing a strong testable hypothesis has few advantages, it compels us to think intensely and specifically about the outcomes of a study. Consequently, it enables us to understand the implication of the question and the different variables involved in the study. Furthermore, it helps us to make precise predictions based on prior research. Hence, forming a hypothesis would be of great value to the research. Here are some good examples of testable hypotheses.
More importantly, you need to build a robust testable research hypothesis for your scientific experiments. A testable hypothesis is a hypothesis that can be proved or disproved as a result of experimentation.
Importance of a Testable Hypothesis
To devise and perform an experiment using scientific method, you need to make sure that your hypothesis is testable. To be considered testable, some essential criteria must be met:
- There must be a possibility to prove that the hypothesis is true.
- There must be a possibility to prove that the hypothesis is false.
- The results of the hypothesis must be reproducible.
Without these criteria, the hypothesis and the results will be vague. As a result, the experiment will not prove or disprove anything significant.
What are your experiences with building hypotheses for scientific experiments? What challenges did you face? How did you overcome these challenges? Please share your thoughts with us in the comments section.
Frequently Asked Questions
The steps to write a research hypothesis are: 1. Stating the problem: Ensure that the hypothesis defines the research problem 2. Writing a hypothesis as an 'if-then' statement: Include the action and the expected outcome of your study by following a ‘if-then’ structure. 3. Defining the variables: Define the variables as Dependent or Independent based on their dependency to other factors. 4. Scrutinizing the hypothesis: Identify the type of your hypothesis
Hypothesis testing is a statistical tool which is used to make inferences about a population data to draw conclusions for a particular hypothesis.
Hypothesis in statistics is a formal statement about the nature of a population within a structured framework of a statistical model. It is used to test an existing hypothesis by studying a population.
Research hypothesis is a statement that introduces a research question and proposes an expected result. It forms the basis of scientific experiments.
The different types of hypothesis in research are: • Null hypothesis: Null hypothesis is a negative statement to support the researcher’s findings that there is no relationship between two variables. • Alternate hypothesis: Alternate hypothesis predicts the relationship between the two variables of the study. • Directional hypothesis: Directional hypothesis specifies the expected direction to be followed to determine the relationship between variables. • Non-directional hypothesis: Non-directional hypothesis does not predict the exact direction or nature of the relationship between the two variables. • Simple hypothesis: Simple hypothesis predicts the relationship between a single dependent variable and a single independent variable. • Complex hypothesis: Complex hypothesis predicts the relationship between two or more independent and dependent variables. • Associative and casual hypothesis: Associative and casual hypothesis predicts the relationship between two or more independent and dependent variables. • Empirical hypothesis: Empirical hypothesis can be tested via experiments and observation. • Statistical hypothesis: A statistical hypothesis utilizes statistical models to draw conclusions about broader populations.

Wow! You really simplified your explanation that even dummies would find it easy to comprehend. Thank you so much.
Thanks a lot for your valuable guidance.
I enjoy reading the post. Hypotheses are actually an intrinsic part in a study. It bridges the research question and the methodology of the study.
Useful piece!
This is awesome.Wow.
It very interesting to read the topic, can you guide me any specific example of hypothesis process establish throw the Demand and supply of the specific product in market
Nicely explained
It is really a useful for me Kindly give some examples of hypothesis
It was a well explained content ,can you please give me an example with the null and alternative hypothesis illustrated
clear and concise. thanks.
So Good so Amazing
Good to learn
Thanks a lot for explaining to my level of understanding
Explained well and in simple terms. Quick read! Thank you
It awesome. It has really positioned me in my research project
Rate this article Cancel Reply
Your email address will not be published.
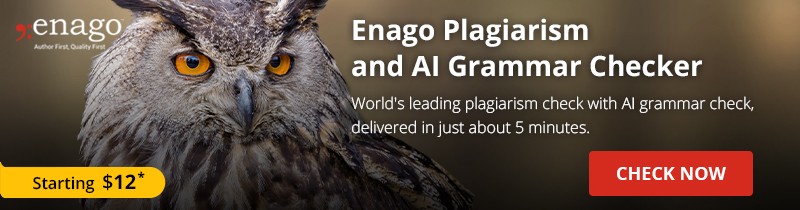
Enago Academy's Most Popular Articles
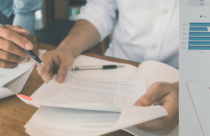
- Reporting Research
Choosing the Right Analytical Approach: Thematic analysis vs. content analysis for data interpretation
In research, choosing the right approach to understand data is crucial for deriving meaningful insights.…
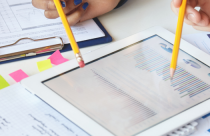
Comparing Cross Sectional and Longitudinal Studies: 5 steps for choosing the right approach
The process of choosing the right research design can put ourselves at the crossroads of…
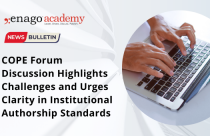
- Industry News
COPE Forum Discussion Highlights Challenges and Urges Clarity in Institutional Authorship Standards
The COPE forum discussion held in December 2023 initiated with a fundamental question — is…
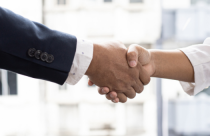
- Career Corner
Unlocking the Power of Networking in Academic Conferences
Embarking on your first academic conference experience? Fear not, we got you covered! Academic conferences…
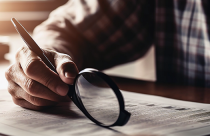
Research Recommendations – Guiding policy-makers for evidence-based decision making
Research recommendations play a crucial role in guiding scholars and researchers toward fruitful avenues of…
Choosing the Right Analytical Approach: Thematic analysis vs. content analysis for…
Comparing Cross Sectional and Longitudinal Studies: 5 steps for choosing the right…
How to Design Effective Research Questionnaires for Robust Findings

Sign-up to read more
Subscribe for free to get unrestricted access to all our resources on research writing and academic publishing including:
- 2000+ blog articles
- 50+ Webinars
- 10+ Expert podcasts
- 50+ Infographics
- 10+ Checklists
- Research Guides
We hate spam too. We promise to protect your privacy and never spam you.
I am looking for Editing/ Proofreading services for my manuscript Tentative date of next journal submission:
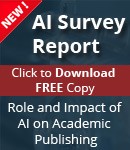
As a researcher, what do you consider most when choosing an image manipulation detector?

Where to Find The Hypothesis in a Research Article
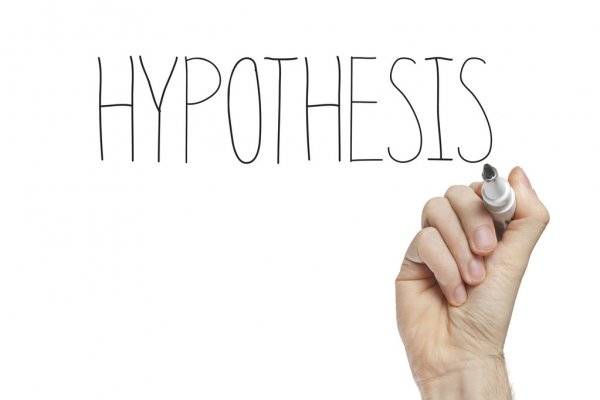
The question of “Where to Find The Hypothesis in a Research Article” can only be answered by exploring how research articles represent scientific methods.
Table of Contents
Introduction
A research article represents a compilation of information by a scientist concerning an original research idea. It is characterized by a wide range of information including, the purpose of the study, the thesis statement, hypothesis, literature review, methodology, results and conclusion.
The examination of a research article is an important process, and the ability to identify crucial elements of research is paramount for the effective analysis of a research article.
Research articles are usually arranged in specific ways. A hypothesis in a research article is usually located in a specific position in an article. The ability to quickly pinpoint where the hypothesis is located is crucial in becoming an expert in exploring research articles as well as formulating them.
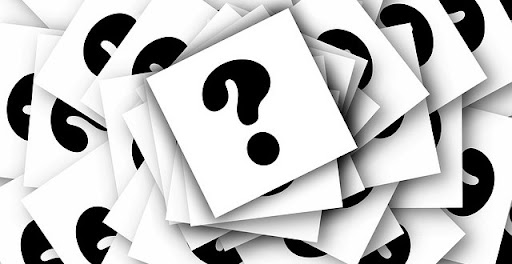
What is a hypothesis
A hypothesis represents a scientific guess that is stated in research. It is a speculative statement concerning the relationship between two or more variables in research.
Therefore, a good hypothesis is a prediction that is testable, specific, and explores what a researcher expects to find in the study.
Formulating a Hypothesis
The creation of a hypothesis represents a critical part of the scientific method. Formulating a hypothesis is important, especially when testing a theory. Most scientific research involves testing theories. Theories, in this case, refer to ideas about the way things relate to one another. For one to formulate a hypothesis to be used in research, they have to be to predict the outcome of the research.
If one cannot predict the outcome, then the research does not need the formulating of a hypothesis because it is either exploratory or descriptive. These forms of research cannot have a hypothesis, and the reason is that there is a limited base of knowledge concerning the subject matter for the prediction of the outcome to be possible.
A good Hypothesis
A good hypothesis has to have two or more variables. These variables have to be measurable or have the potential to be measured. The hypothesis also has to specify how the variables are related to one another.
Where to Find the Hypothesis in a Research Article
The scientific method is characterized by several steps. They include:
- Coming up with a question or the problem that needs to be solved.
- Conducting background research on the problem
- Formulating a hypothesis
- Establishing how the research will be conducted using a research design
- Collecting data
- Analyzing the data and coming up with results
- Provide conclusions
- Presenting the information through a research article.
Based on the above structure, it is evident that the hypothesis is located in the introduction section of a research article. One should look out for “if-then” statements. However, for such statements to be hypotheses, they need to:
- Demonstrates the relationship between variables,
- The relationship needs to be testable and
- The prediction needs to be measurable
A hypothesis is not always clearly labeled. This means that the statement can appear in different forms apart from when formulated using the “if-then” statements. One should, therefore, look out for a statement that offers a prediction of what readers need to expect from the research.
The ability to identify where to find a hypothesis is located in a research article is very important in several ways:
- One can quickly know what the researcher wants to prove using the research.
- It makes individuals effective in reading research articles.
- It enhances an individual’s ability to formulate their own hypothesis when conducting research
Entrust your assignments to our expert writers for excellent results!
Eagle Academics prides itself in providing quality custom papers for many students around the world. We guarantee exceptional standards, and this is based on the selection of highly professional and experienced tutors and writers in different fields. Our experts can handle any work regardless of the deadline.
You must choose what you do with your time. Let our experts help you with your schoolwork while you focus on other essential things in your life. Talk to us, and we will give you the help that you need.
Calculate the price of your paper
Free features.
- Formatting (APA, MLA, Harvard, Chicago/Turabian)
- Bibliography
- Upload custom grading criteria
Additional services
- Part-by-part payment
- Links to used sources
- Review your writer’s samples
- Charts and PowerPoint slides
Don’t look far for academic help. We have you covered
Money back guarantee.
Our goal is to give the best services to our clients. However, if for one reason or the other a client is not satisfied, we will refund your money. So the funds paid to us are safe.
Confidentiality
We value our clients’ privacy. No single information of our clients can be shared by third parties.
Our service is legit
Our services follow the laid down academic guidelines. We cannot blackmail our clients, but only strive to offer them the best academic assistance they need.
Get a plagiarism-free paper
Our writers work hand in hand with the quality assurance department to ensure whatever paper you get is totally plagiarism-free.
We can help with urgent tasks
We have standby writers who can handle papers even within 6 hours. Just get in touch and let us know.
Pay a fair price
We have competitive prices that are pocket friendly. In addition, we have plenty of discounts to offer.
Need a better grade? We've got you covered.
The conundrum of porter hypothesis, pollution haven hypothesis, and pollution halo hypothesis: evidence from the Indian manufacturing sector
- Original Paper
- Published: 16 May 2024
Cite this article
- Prantik Bagchi ORCID: orcid.org/0000-0002-9056-5835 1 &
- Santosh Kumar Sahu ORCID: orcid.org/0000-0003-3480-6507 2 , 3
1 Altmetric
In the globalized world, factors, such as environmental regulations and outcomes, are interlinked with foreign direct investment and technological innovation. However, firm-level theories mostly treat them independently. We have filled the gap by carrying out empirical research with an integrated approach at the firm level. The theoretical framework is based on Porter’s hypothesis and the pollution haven hypothesis/pollution halo hypothesis. We collect the data from the center for monitoring Indian Economy Prowess IQ and the Ministry of Environment, Forest, and Climate Change. Using the modified Krugman specialization index, we find that Indian manufacturing firms are neither converging nor specialized in terms of technical progress. Estimating a z -score for environmental stringency, we interact that with the pollution loads of the firms. Our findings suggest that environmental regulation does not ensure a “win–win” situation for the producers, refuting Porter’s hypothesis. Rather, factors such as profit margin and R&D produce robust results across different models to induce the productivity of the firm. One of the concerning facts is older firms using vintage capital are detrimental to productivity enhancement, and there is evidence of layoff at the cost of increasing profits to improve the firm performance. Also, more dependence for export-intensive firms on material increases the cost and, thereby, reduces productivity. In addition, we apply a panel threshold regression model and conclude that there is evidence of a single threshold, and irrespective of the choice of technology foreign firms induces the energy intensity, confirming a pollution Haven hypothesis.
Graphical abstract
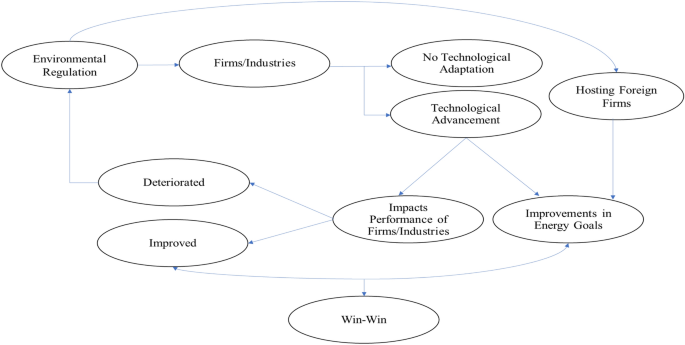
This is a preview of subscription content, log in via an institution to check access.
Access this article
Price includes VAT (Russian Federation)
Instant access to the full article PDF.
Rent this article via DeepDyve
Institutional subscriptions
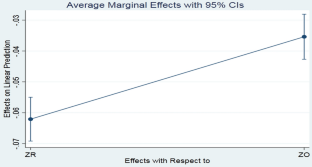
Similar content being viewed by others
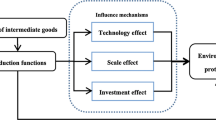
The role of imports in reducing emissions: evidence from Chinese listed companies
Racing to the bottom and racing to the top: the crucial role of firm characteristics in foreign direct investment choices, the impact of environmental policy stringency on industrial r&d conditional on pollution intensity and relocation costs, data availability.
Enquiries about data availability should be directed to the authors.
Environment is considered to be a factor of production.
One of the threat perceptions is that the resource-rich countries are vulnerable to PHH, known as the resource curse hypothesis, although the evidence is mixed (Liang et al. 2023 ).
India imports a large volume of non-renewable resources despite a large volume of production, and this has been increasing over the years (Energy Statistics 2023). A substantial part of it is exported as it does not meet the environmental standard. As there is an excess demand for the same, they import it from the developed countries. A part of this import might be the surplus that does not meet environmental standards in those countries, and the laxity of regulations may induce the threat of both PH and PHH/PHaH.
To the best of our knowledge, this is the first empirical paper to integrate PH and PHH for Indian manufacturing firms.
Since PH incentivizes the firms with a “win–win” situation, the decision of the firm will be taken based on the possibility of the improvement of their outcome. Often this is measured in terms of total factor productivity (TFP).
In this paper, we are not interested in focusing on the narrow version because: i. The interest of the paper does not lie in validating this; and ii. there is an acute shortage of the data.
The list of the indicators will be discussed later. Input intensities refer to labor intensity, capital intensity, material intensity, and energy intensity.
Z = (X it -X t )/σ it.
Intensities are not considered separately to omit the problem of correlation and multicollinearity problems as these variables are used while calculating the TFP.
We have not reported the green firms as it will be considered as base in the regression models.
There is a possibility of the existence of a nonlinearity; specifically, it may have an exponential effect. This may lead to the probabilistic measures to cross their bounds.
As a rule of thumb, we have tested for multicollinearity, stationarity, and cointegration. Since the independent variables have VIFs less than 10, they are found to be non-colinear. Besides, they are stationary in the first difference, and they are found to exhibit a long-term relationship due to the significant cointegration.
We have calculated the mean spending on R&D by domestic and foreign firms. It is seen that foreign firms are spending four to five times more on R&D than domestic firms.
We have tested the correlation of the dependent variable and the covariates. It exhibits that tax and profit are highly correlated (0.49), and hence, we keep both of them in separate regression and compare them. This was carried out for the unbalanced panel as well, but in that case, the correlation was less than 0.3.
Abid N, Ahmad F, Aftab J, Razzaq A (2023) A blessing or a burden? Assessing the impact of climate change mitigation efforts in Europe using quantile regression models. Energy Policy 178:113589
Article Google Scholar
Al-Mulali U, Tang CF (2013) Investigating the validity of pollution haven hypothesis in the gulf cooperation council (GCC) countries. Energy Policy 60:813–819
Ambec S, Cohen MA, Elgie S, Lanoie P (2013) The Porter hypothesis at 20: Can environmental regulation enhance innovation and competitiveness? Rev Environ Econ Policy 7(1):2–22. https://doi.org/10.1093/reep/res016
Ashford, N. A. (1993). Understanding technological responses of industrial firms to environmental problems: Implications for government policy (chapter).
Bagchi P, Sahu SK (2020) Energy intensity, productivity and pollution loads:empirical evidence from manufacturing sector of India. Stud Microecon 8(2):194–211
Bagchi P, Sahu SK, Kumar A, Tan KH (2022) Analysis of carbon productivity for firms in the manufacturing sector of India. Technol Forecast Soc Chang 178:121606
Bradford DF, Fender RA, Shore SH, Wagner M (2005) The environmental Kuznets curve: exploring a fresh specification. Contrib Econ Anal Policy 4(1):1–28
Bramber T, Clark WR, Golder M (2006) Understanding interaction models: improving empirical analysis. Polit Anal 14(3):63–82
Brunnermeier SB, Cohen MA (2003) Determinants of environmental innovation in US manufacturing industries. J Environ Econ Manag 45(2):278–293
Bu M, Qiao Z, Liu B (2020) Voluntary environmental regulation and firm innovation in China. Econ Model 89:10–18
Chandrika R, Mahesh R, Tripathy N (2022) Is India a pollution haven? Evidence from cross-border mergers and acquisitions. J Clean Prod 376:134355
Cole MA (2004) Trade, the pollution haven hypothesis and the environmental Kuznets curve: examining the linkages. Ecol Econ 48(1):71–81
D’Agostino LM (2015) How MNEs respond to environmental regulation: integrating the Porter hypothesis and the pollution haven hypothesis. Economia Politica 32:245–269
Falkowska A (2020) The impact of environmental policy on location patterns in the waste management industry. Economia Politica 37(1):167–195
Ghosh A, Kayal P, Bagchi P (2024) Climate change and tourism: Assessing the nexus and climate-related disasters in diverse economies. J Clean Prod 443:141097. https://doi.org/10.1016/j.jclepro.2024.141097
M Greenstone, J A List, C Syverson. (2012). The effects of environmental regulation on the competitiveness of US manufacturing (No. w18392). National Bureau of Economic Research
Grossman GM, Krueger AB (1991) Environmental impacts of a North American free trade agreement. NBER Working Paper No. 3914. National Bureau of Economic Research.
Hansen BE (1996) Inference when a nuisance parameter is not identified under the null hypothesis. Econometrica 64(2):413. https://doi.org/10.2307/2171789
Hansen BE (1999) Threshold effects in non-dynamic panels: Estimation, testing, and inference. J Econ 93(2):345–368
Jaffe AB, Palmer K (1997) Environmental regulation and innovation: a panel data study. Rev Econ Stat 79(4):610–619
Kearsley A, Riddel M (2010) A further inquiry into the pollution Haven hypothesis and the environmental Kuznets curve. Ecol Econ 69(4):905–919
Kheder SB, Zugravu N (2012) Environmental regulation and French firms location abroad: an economic geography model in an international comparative study. Ecol Econ 77:48–61
Liang H, Shi C, Abid N, Yu Y (2023) Are digitalization and human development discarding the resource curse in emerging economies? Resour Policy 85:103844
Liu J, Qu J, Zhao K (2019) Is China’s development conforms to the environmental Kuznets curve hypothesis and the pollution haven hypothesis? J Clean Prod 234:787–796
Ma R, Abid N, Yang S, Ahmad F (2023) From crisis to resilience: strengthening climate action in OECD countries through environmental policy and energy transition. Environ Sci Pollut Res 30(54):115480–115495
Markusen JR, Venables AJ (1999) Foreign direct investment as a catalyst for industrial development. Eur Econ Rev 43(2):335–356
Pethig R (1976) Pollution, welfare, and environmental policy in the theory of comparative advantage. J Environ Econ Manag 2(3):160–169
Porter ME, Linde CVD (1995) Toward a new conception of the environment-competitiveness relationship. J Econ Perspect 9(4):97–118
Rubashkina Y, Galeotti M, Verdolini E (2015) Environmental regulation and competitiveness: empirical evidence on the Porter hypothesis from European manufacturing sectors. Energy Policy 83:288–300
Sahu SK, Bagchi P (2023) Waste from production: an analysis at the firm level. Qual Quant 57(3):2641–2656
Sahu SK, Bagchi P, Kumar A, Tan KH (2022) Technology, price instruments and energy intensity: a study of firms in the manufacturing sector of the Indian economy. Ann Oper Res 313(1):319–339
Shen F, Liu B, Luo F, Wu C, Chen H, Wei W (2021) The effect of economic growth target constraints on green technology innovation. J Environ Manage 292:112765
Wang X, Luo Y (2020) Has technological innovation capability addressed environmental pollution from the dual perspective of FDI quantity and quality? Evidence from China. J Clean Prod 258:120941. https://doi.org/10.1016/j.jclepro.2020.120941
Wei D, Ahmad F, Abid N, Khan I (2023) The impact of digital inclusive finance on the growth of the renewable energy industry: theoretical and logical Chinese experience. J Clean Prod 428:139357
Yuan B, Zhang K (2017) Can environmental regulation promote industrial innovation and productivity? Based on the strong and weak Porter hypothesis. Chinese J Popul Resou Environ 15(4):322–336
Google Scholar
Zhang N, Deng J, Ahmad F, Draz MU, Abid N (2023a) The dynamic association between public environmental demands, government environmental governance, and green technology innovation in China: evidence from panel VAR model. Environ Dev Sustain 25(9):9851–9875
Zhang Y, Zeng S, Wu Q, Fu J, Li T (2023b) A study on the impact of the carbon emissions trading policy on the mining industry based on Porter hypothesis. Resour Policy 87:104349
Download references
Acknowledgement
The paper is presented in the SHADG 2024 Conference, CoE-SEA, IIT Kharagpur. The authors are highly benefitted with the valueable comments and suggestions received by the reviewers and the editor of the paper.
The authors have not disclosed any funding.
Author information
Authors and affiliations.
Department of Humanities and Social Sciences, Indian Institute of Technology Kharagpur, Kharagpur, West Bengal, 721302, India
Prantik Bagchi
Department of Humanities and Social Sciences, Indian Institute of Technology Madras, Chennai, Tamil Nadu, 600036, India
Santosh Kumar Sahu
School of Sustainability, Indian Institute of Technology Madras, Chennai, Tamil Nadu, 600036, India
You can also search for this author in PubMed Google Scholar
Contributions
The first author conceptualizes the paper, estimates, and writes the paper. The second author conceptualizes the paper, downloads and cleans the data, and edits the manuscript.
Corresponding author
Correspondence to Prantik Bagchi .
Ethics declarations
Conflict of interest.
The authors did not receive any funding from anywhere. Also, there is no direct or indirect financial or non-financial interest to disclose. There is no other conflict of interest.
Additional information
Publisher's note.
Springer Nature remains neutral with regard to jurisdictional claims in published maps and institutional affiliations.
Rights and permissions
Springer Nature or its licensor (e.g. a society or other partner) holds exclusive rights to this article under a publishing agreement with the author(s) or other rightsholder(s); author self-archiving of the accepted manuscript version of this article is solely governed by the terms of such publishing agreement and applicable law.
Reprints and permissions
About this article
Bagchi, P., Sahu, S.K. The conundrum of porter hypothesis, pollution haven hypothesis, and pollution halo hypothesis: evidence from the Indian manufacturing sector. Clean Techn Environ Policy (2024). https://doi.org/10.1007/s10098-024-02886-z
Download citation
Received : 14 January 2024
Accepted : 06 May 2024
Published : 16 May 2024
DOI : https://doi.org/10.1007/s10098-024-02886-z
Share this article
Anyone you share the following link with will be able to read this content:
Sorry, a shareable link is not currently available for this article.
Provided by the Springer Nature SharedIt content-sharing initiative
- Productivity
- Pollution haven hypothesis
- Pollution halo hypothesis
- Energy transition
- Indian manufacturing
- Find a journal
- Publish with us
- Track your research
Click through the PLOS taxonomy to find articles in your field.
For more information about PLOS Subject Areas, click here .
Loading metrics
Open Access
Peer-reviewed
Research Article
The impact of population aging on SME digital transformation: Evidence from China
Roles Conceptualization, Formal analysis, Methodology, Resources, Supervision, Visualization, Writing – review & editing
Affiliation Beijing Technology and Business University, China, Beijing
Roles Conceptualization, Data curation, Software, Writing – original draft
* E-mail: [email protected]

- Xueyan Xie,
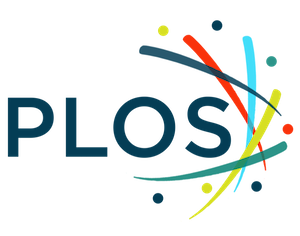
- Published: May 16, 2024
- https://doi.org/10.1371/journal.pone.0300660
- Reader Comments
Many societies around the world are rapidly aging and this has implications for social and economic development. We collect data on NEEQ-listed enterprises from 2010 to 2021 in China and empirically test the effect of population aging on the digital transformation of small and medium-sized enterprises (SMEs). The findings show that population aging has a significant positive impact on SME digital transformation, and private enterprises and enterprises in eastern regions of China tend to benefit more than other regions. The mechanism studies find that population aging positively impacts SME digital transformation by increasing labor costs, facilitating human capital accumulation, and raising savings rates. Furthermore, the threshold effect analyses find that the marginal promotion effect of population aging will weaken with greater aging and will strengthen with a higher level of marketization. Finally, we provide policy recommendations for promoting digital transformation in SMEs against the background of population aging.
Citation: Xie X, Xu X (2024) The impact of population aging on SME digital transformation: Evidence from China. PLoS ONE 19(5): e0300660. https://doi.org/10.1371/journal.pone.0300660
Editor: Laura Hannah Kelly, Public Library of Science, UNITED KINGDOM
Received: October 29, 2023; Accepted: February 28, 2024; Published: May 16, 2024
Copyright: © 2024 Xie, Xu. This is an open access article distributed under the terms of the Creative Commons Attribution License , which permits unrestricted use, distribution, and reproduction in any medium, provided the original author and source are credited.
Data Availability: All relevant data are within the Supporting information .
Funding: The author(s) received no specific funding for this work.
Competing interests: The authors have declared that no competing interests exist.
1. Introduction
Population aging refers to changes in the age structure of the population and an increase in the proportion of the elderly in the total population. It is a global social phenomenon and a reality that many countries must face. According to the data from the National Bureau of Statistics, the total population of China at the end of 2022 decreased by 850,000 compared with the end of the previous year, and the natural population growth rate was -0.60%, marking the first negative population growth rate in China in more than 60 years. Meanwhile, the elderly population aged 65 and above exceeded 210 million, accounting for 14.9% of the total population. According to international standards, China has entered the stage of moderate aging. Studies show that population aging has significant effects on social and economic development. At the macro level, population aging can promote the application of automation technology [ 1 ], enhance technological innovation [ 2 , 3 ], and drive industrial structure upgrading [ 4 ] and economic growth [ 5 ]. At the micro level, population aging can affect the allocation of household financial assets [ 6 ], enterprises’ debt financing decisions [ 7 ], and investment behaviors [ 8 ].
At the same time, SMEs have become an important part of the Chinese economy by playing an essential role in expanding employment, narrowing income gaps, promoting market competition, and driving innovation. With the development of the digital economy in recent years, SMEs are actively seeking digital transformation. Enterprise digital transformation is the deep integration of digital technology and enterprise strategies, which can trigger the digital evolution of resources and capabilities, business models, business processes, products and services, and business ecosystems [ 9 ]. Studies find that digital transformation is beneficial in reducing enterprise debt costs [ 10 ], alleviating financial constraints [ 11 ], improving internal and external governance environments [ 12 , 13 ], enhancing performance levels [ 14 , 15 ], reducing business risks [ 16 ], promoting technological innovation [ 17 – 19 ], and increasing total factor productivity [ 20 , 21 ]. Therefore, the digital transformation of SMEs is crucial to the development of the enterprises themselves as well as the national economy, and this topic has received wide attention from governments and researchers.
What kind of impact will population aging have on SME digital transformation? There are few studies that directly explore the relationship between the two. It is well known that enterprise digital transformation based on innovation in enterprise digital technology is an investment behavior of enterprises. Relevant studies explore the impact of population aging on technological innovation and investment. For example, Liu et al. [ 2 ] find that population aging could stimulate enterprises to increase R&D investment, introduce high-tech personnel, and improve the level of technological innovation. Similarly, Baldanzi et al. [ 3 ] show that aging leads to an increase in life expectancy, aggregate savings, and demand for innovation, thereby causing higher employment in the R&D sector and faster technological progress. Xian et al. [ 8 ] document that population aging affects the investment decisions of enterprises by increasing the labor cost of manufacturing enterprises and reducing the output efficiency of unit wages. Therefore, we use data on NEEQ-listed enterprises from 2010 to 2021 in China and find that population aging has a significant positive impact on SME digital transformation. Moreover, we explore three influence mechanisms. First, population aging leads to higher labor costs, forcing enterprises to replace labor with capital and technology, which drives SMEs’ digital transformation. Second, population aging means longer life expectancy and higher return on investment in education, promoting human capital accumulation, which provides intellectual support to SMEs’ digital transformation. Third, population aging raises savings rates, alleviating financial constraints, which provides financial support for SMEs’ digital transformation. Studies on heterogeneity find that the positive impact of population aging on enterprise digital transformation is more significant for private enterprises and enterprises in eastern regions of China. Finally, we find that the impact of population aging on SME digital transformation is nonlinear. The marginal effect of population aging weakens with greater aging but strengthens with a higher level of marketization.
The theoretical contributions of our paper are as follows. First, in terms of the research perspective, we examine the impact of population aging, an indispensable macro factor, on enterprise digital transformation, thus enriching the literature on population aging and the digital transformation of enterprises. Many studies focus on the economic consequences of population aging, such as the level of technological innovation [ 2 , 3 ], the upgrading of industrial structure [ 4 ], and economic growth [ 5 ], but what will be the impact on the digital transformation of enterprises, an important trend of enterprise development? Relevant studies are still lacking. Moreover, existing studies discuss the factors influencing enterprise digital transformation from multiple perspectives, such as organizational structure [ 22 ], corporate strategy [ 23 ], and policy support [ 24 ], but few studies pay attention to the impact of changes in demographics, which is an important macro factor. Second, in terms of research content, we analyze the influence paths of population aging on SME digital transformation based on the effects of labor costs, human capital, and savings rates. The literature has not yet focused on the effect of population aging on enterprise digital transformation and its mechanisms and our paper fills this gap. Third, in terms of research methodology, we adopt a textual analysis method to characterize SME digital transformation by constructing a more comprehensive keyword dictionary of digital transformation that includes 169 keywords and then counting the keyword frequency, hence providing a useful reference for measuring the degree of SMEs’ digital transformation. Compared to established studies, we not only include keywords in digital technology but also expand keywords in digital technology applications.
The remainder of this paper is organized as follows. Section 2 provides the literature review. Section 3 describes the theoretical analysis and hypothesis. Section 4 describes the variable constructions, data, and model. Section 5 presents the results for the baseline regression, robustness checks, and endogeneity tests. Section 6 explores the influence mechanisms. Section 7 tests the nonlinear impact. Section 8 draws conclusions and issues recommendations.
2. Literature review
With population aging becoming a global problem, scholars have conducted a series of studies on the economic impacts of population aging. At the macro level, studies find that population aging has both positive and negative impacts. On the positive side, Annabi et al. [ 25 ] construct an overlapping generations (OLG) model and find that the labor supply shortage caused by aging increases the average wage level and promotes the relative expansion of nonlabor-intensive industries. Siliverstovs et al. [ 26 ] use data from developing and developed countries from 1970–2004 and indicate that population aging has a significant positive effect on the employment share of the tertiary sector. Chu et al. [ 4 ] construct a spatial econometric SEM from the perspective of production and consumption and discover that population aging has a dynamic impact on industrial structure upgrading by promoting the substitution of capital or technology for labor and increasing per capita education years. On the negative side, Sanz and Velázquez [ 27 ] use government expenditure data from OECD countries during 1970–1997 and reveal that the increase in the proportion of elderly people would lead to an increase in public expenditure and undermine the sustainability of public finances, negatively impacting economic growth. Using state-level data from the US, Maestas et al. [ 28 ] estimate that slower labor force growth due to aging negatively affects national per capita output growth. Prettner et al. [ 29 ] propose a dynamic consumer optimization model and show that the economic decline caused by a slowdown in labor supply can be partially offset by increasing investment in higher education and health care. At the micro level, Bressan et al. [ 6 ], based on data from the Health, Aging and Retirement in Europe survey, find that people would reduce risk-taking behaviors and prefer safer investment portfolios as they become older and less healthy. Chen et al. [ 7 ] use data from 2007 to 2019 of Chinese A-share listed companies and discover that population aging reduces the level of corporate debt financing and aggravates the mismatch of financial resources. Xian et al. [ 8 ] use data from 2012 to 2020 of A-share listed manufacturing companies in China and document that population aging reduces the output efficiency of unit labor costs and prompts companies to increase foreign investment.
In recent years, with the development and application of digital technology worldwide, more studies on digital transformation have emerged. Some studies focus on the factors that influence digital transformation. At the micro level, these factors include manager characteristics [ 30 ], organizational structure [ 22 ], dynamic capabilities [ 31 ], profit expectations [ 32 ], digital strategies [ 23 ], financial asset allocation [ 33 ], and equity structure [ 34 ]. At the macro level, these factors include digital technology [ 35 ], financial technology [ 36 ], digital infrastructure construction [ 37 ], policy guarantees [ 24 ], and the business environment [ 38 ]. In addition, some studies focus on the economic effects of digital transformation. Cui et al. [ 16 ] use data from 2012 to 2021 of Chinese A-share listed companies and find that digital transformation alleviates financial distress by reducing operational risks and easing financial constraints. Manita et al. [ 13 ], based on qualitative data analysis, reveal that the application of digital technology reduces information asymmetry and improves the internal governance environment of enterprises. Zhai et al. [ 14 ] use data from 2009 to 2019 of A-share listed companies in China and conduct empirical studies which show that digital transformation reduces operating costs, improves operational efficiency, and thereby enhances performance levels. Blichfeldt and Faullant [ 18 ] use European Manufacturing Survey (EMS) data and find that companies with a high level of digital technology implementation (in both breadth and depth) could introduce more radical product and service innovations. Wen et al. [ 19 ], based on data from Chinese A-share listed manufacturing companies from 2007 to 2019, discover that digital transformation increases companies’ investment in innovation activities and promotes technological innovation. Yu et al. [ 21 ] select data from listed companies from 2015 to 2021 as a research sample and show that enterprises that use digital technology can accelerate internal knowledge spillovers and improve enterprise competitiveness, thereby increasing total factor productivity.
3. Theoretical analysis and hypothesis
3.1 the impact of population aging on sme digital transformation.
Digital transformation is characterized by long cycles and high uncertainty, requiring low-cost funds in the long term as well as talent and technology as complements. Because of this, SMEs face greater challenges in digital transformation than large enterprises. Population aging leads to higher labor costs, human capital, and savings rates, which creates favorable conditions for enterprises to utilize capital, talent, and technology, thus promoting the digital transformation of SMEs. First, the rise in the proportion of the elderly population causes a shortage of working-age laborers, leading to an increase in labor costs and forcing enterprises to shift their factor preference from labor to capital and technology [ 39 ], thus becoming a push for SMEs’ digital transformation. Second, population aging will extend life expectancy and provide a longer time window [ 40 ] and greater incentives [ 41 ] for people to pursue higher education. These factors promote human capital accumulation, and improve the quality of the workforce, which can meet the demand of enterprises for talent and become a pull for SMEs’ digital transformation. Third, population aging may lead to a rise in the savings rate [ 42 ], which alleviates the financial constraints faced by SMEs. This is conducive to improving the affordability of digital transformation to SMEs, becoming another pull for SMEs’ digital transformation. Based on the above analysis, the following hypothesis is proposed.
- Hypothesis 1: Population aging can positively impact the digital transformation of SMEs.
The mechanisms of population aging on the digital transformation of SMEs are displayed in Fig 1 .
- PPT PowerPoint slide
- PNG larger image
- TIFF original image
https://doi.org/10.1371/journal.pone.0300660.g001
3.2 The mediating role of labor costs
Population aging is accompanied by a decline in the birth rate, which leads to an increase in the average age and a decline in the labor participation rate of working-age laborers. Therefore, as population aging deepens, the labor supply becomes relatively scarce in the factor market, labor costs increase [ 25 ], and the relative prices of production factors change. Compared with large enterprises, SMEs are more inclined to labor-intensive industries. Meanwhile, labor is the most basic and important production factor for SMEs, and a reduction in its supply and cost increases will inevitably have a greater negative influence on SMEs. To offset these negative impacts, enterprises are forced to adjust production strategies, redistribute limited funds among different factors, and replace labor with capital and technology [ 39 ]. From the experience of developed countries, countries turn to high-tech industries as the population ages to create more value with less labor. In recent years, with the development of digital technologies such as big data, artificial intelligence, and automation, the trend of using machines to fill labor shortages has become even more apparent. Acemoglu and Restrepo [ 1 ] use panel data from 126 countries during 1990–2015 and find that countries with a higher degree of aging offset the negative effects of labor reduction through large-scale industrial automation, thus minimizing the risk of economic downturns. From this, the replacement of labor by capital and technology becomes an important component of strategic adjustments as the population ages, thus promoting the digital transformation of SMEs. Hence, the following hypothesis is postulated.
- Hypothesis 2: Population aging can affect SMEs by the push effect of increasing labor costs and thus positively impact the digital transformation of SMEs.
3.3 The mediating role of human capital accumulation
Enterprise digital transformation means the digitalization, automation, and intelligence of production, which requires highly skilled labor. For example, enterprises need to hire highly skilled engineers to install, debug, use, and maintain advanced production equipment after it has been purchased. In addition, technological innovation promotes enterprise digital transformation, which itself is a technological innovation activity [ 43 ]. Technological research requires advanced abilities in thinking, learning, cooperation, psychological qualities, and innovation in workers. Therefore, enterprise digital transformation requires high-level human capital. At the same time, population aging extends life expectancy. Since it increases the time that people can use to enrich themselves, more young people will choose to postpone entering the labor market to receive more education [ 40 ]. It also increases the expected rate of return on investment of education, which incentivizes people to improve the qualities of family members, thus increasing human capital investment [ 41 ]. In summary, population aging facilitates human capital accumulation and knowledge spillover, which provides the necessary intellectual support for SMEs to implement digital transformation. Consequently, the following hypothesis is proposed.
- Hypothesis 3: Population aging can affect SMEs by the pull effect of facilitating human capital accumulation and thus positively impact the digital transformation of SMEs.
3.4 The mediating role of savings rates
A lack of funds is one significant constraint for SMEs seeking digital transformation. Studies show that increases in the social savings rate can increase private sector investment and thus promote capital deepening [ 44 ]. As the savings rate rises, the scale of deposits that can be transformed into investment in society will increase accordingly, which is conducive to alleviating financial constraints, thereby promoting SMEs’ digital transformation. Then, how will population aging affect the savings rate? In theory, there are positive and negative aspects. On the one hand, the elderly population belongs to the “negative saving” group, and the increase in the proportion of the elderly population will reduce the social savings rate [ 45 ]. On the other hand, population aging is accompanied by longer lifespans and fewer children, and to prepare for retirement, people will save more when they are young, which may lead to a rise in the savings rate [ 46 ]. Additionally, the impact of population aging on savings rates will vary by the stage of aging and the policy environment. For example, in the early stages of aging, the proportion of the young population is much larger than that of the elderly population, which leads to a larger positive than negative effect of aging on the savings rate, thereby raising the savings rate. However, in the later stages of aging, the proportion of the elderly population significantly increases, which results in the negative effect outweighing the positive effect, thus decreasing the overall savings rate. Furthermore, establishing a mandatory pension system and providing tax incentives for individual contributions could also raise the savings rate. China is in the middle stage of aging where the proportion of the young population is still larger than that of the elderly population. In the meantime, China has established a basic social pension insurance system and is expanding coverage. Thus, in present China, it is expected that the positive effect of aging is greater than the negative effect and many studies support this view [ 42 , 47 ]. Therefore, the following can be hypothesized.
- Hypothesis 4: Population aging can affect SMEs by the pull effect of raising savings rates and thus positively impact the digital transformation of SMEs.
4. Research design
4.1 variable selection, 4.1.1 dependent variable..
The dependent variable is the degree of digital transformation of the SMEs. There is no unified measurement standard for such an index. Since the frequency of digital-related words in annual reports disclosed by SMEs can reflect the importance of digital strategy for enterprises, we choose keyword frequency as the measure of this index. First, we construct a more comprehensive keyword dictionary. Referring to the existing studies of Wu et al. [ 48 ] and Zhao et al. [ 49 ], the keywords measuring enterprise digital transformation are divided into two categories: “bottom-level digital technology” and “digital technology application”, totaling 169 keywords. The bottom-level digital technology dimension is divided into four subdimensions: big data, cloud computing, artificial intelligence, and blockchain. The digital technology application dimension is also divided into four subdimensions: internet business model, industrial digitalization and intelligence, digital financial services, and modern information systems (see S1 Appendix ). Second, Python is used to download the annual reports of NEEQ-listed enterprises and segment the text information. Third, we count the keyword frequency in annual report segments. Considering the differences in the text length of different annual reports, the sums of keyword frequencies are divided by the length of annual report segments to obtain the index. For ease of expression, this paper multiplies the index by 100. The larger the index is, the higher the degree of digital transformation of SMEs.
4.1.2 Explanatory variable.
The explanatory variable is the degree of population aging. The acceleration of population aging is reflected in two aspects: a rise in the proportion of the elderly population and an increase in the burden of dependency of the elderly population. Therefore, both the proportion of the population aged 65 and above in the total population and the proportion of the population aged 65 and above in the working-age population (15–64 years old) are used to measure it.
4.1.3 Control variable.
Given the existing studies, we select firm-level and regional-level control variables. The firm-level control variables include enterprise age ( Age ), enterprise size ( Size ), return on total assets ( Roa ), leverage ratio ( Lev ), fixed asset ratio ( Fix ), first largest shareholder holding ratio ( Shr ), revenue growth ( Growth ), operating cash flow ratio ( Cashflow ) and R&D investment ratio ( RD ). These variables may affect enterprise decision-making in the digital field, thus affecting enterprise digital transformation. The regional-level control variables include the urbanization level ( Urban ) and per capita GDP ( GDP ) of the city where the enterprise is registered. The definitions of the main variables are provided in Table 1 .
https://doi.org/10.1371/journal.pone.0300660.t001
4.2 Data sources
This paper selects NEEQ-listed enterprises from 2010 to 2021 in China as samples and obtains unbalanced panel data containing 7,700 enterprises after excluding financial enterprises, ST enterprises and enterprises with severe missing data. The main sources of the data are as follows: (1) the enterprise micro data are from the Wind database and the CSMAR database, (2) the enterprise digital transformation indices are derived from textual analytics, and (3) the macro data of 31 provinces in China are from the China Statistical Yearbook, China Population Statistics Yearbook, China Education Statistics Yearbook, and Provincial Statistical Yearbook. To alleviate the influence of extreme values, all of the continuous variables are winsorized at the 1% and 99% levels. The descriptive statistics of the main variables are shown in Table 2 , and the trends of population aging and SME digital transformation are presented in Fig 2 .
https://doi.org/10.1371/journal.pone.0300660.g002
https://doi.org/10.1371/journal.pone.0300660.t002
4.3 Model construction
5. Results and discussion
5.1 baseline regression analysis.
Table 3 reports the results of the baseline regression. The explanatory variable in columns (1) and (3) is the proportion of the elderly population, while the explanatory variable in columns (2) and (4) is the elderly dependency ratio. The results show that when no control variables are included (columns (1) and (2)), the coefficients of both the elderly population proportion and the elderly dependency ratio are significantly positive at the 1% level. After adding control variables in the regression (columns (3) and (4)), the regression coefficient of the proportion of the elderly population is 0.1568 and passes the 1% significance test, while that of the elderly dependency ratio is 0.0970 and passes the 1% significance test. The above results indicate that the current process of population aging in China has a positive effect on SME digital transformation, thus confirming Hypothesis 1.
https://doi.org/10.1371/journal.pone.0300660.t003
5.2 Robustness test
5.2.1 disaggregated explained variables..
For the robustness of the results, this paper disaggregates the total index of SMEs’ digital transformation degree ( Digital ) into two subindices: bottom-level digital technology ( Digital_tech ) and digital technology application ( Digital_apply ) for retesting. The results are reported in Table 4 . Both subindices pass the 10% significance test, indicating that after changing the measurement method of digital transformation, the conclusion that population aging positively impacts the digital transformation of SMEs still holds, thus verifying the robustness of the results.
https://doi.org/10.1371/journal.pone.0300660.t004
5.2.2 Alternative explained variable.
Referring to Li et al. [ 50 ], we use the Chinese pre-trained BERT model to construct the word vector for each initial digital transformation keyword. Subsequently, we extract words from the annual report vocabulary that have 80% similarity to the digital transformation word vectors and filter 42 additional keywords representing the digital transformation of SMEs. Finally, we use the expanded 118 keywords to construct Digital_2 and then replace Digital with Digital_2 for retesting. The results are shown in Table 5 , which are consistent with the baseline results, thus confirming the robustness of the results.
https://doi.org/10.1371/journal.pone.0300660.t005
5.2.3 Sample selection transformation.
To eliminate possible estimation bias caused by sample selection bias, we retest by replacing samples. Most studies use data at the provincial level, with insufficient analysis of data at the city level. Hence, this paper selects population aging data at the city level in Anhui, Gansu, Henan, and Jiangxi provinces, which have the most complete data among all of the provinces. The results are listed in Table 6 . The conclusion that population aging positively impacts SME digital transformation is significant, based on both provincial and city data, thus checking the robustness of the results.
https://doi.org/10.1371/journal.pone.0300660.t006
5.3 Endogeneity analysis
5.3.1 lagging explanatory variables..
To eliminate the endogeneity problem due to reverse causality, this paper lags the explanatory variables for one, two, and three periods. The results are provided in Table 7 . The coefficients of the explanatory variables lagged by one period and two periods are both significantly positive and pass the 1% significance test, while the coefficients of the explanatory variables lagged by three periods are also both significantly positive and pass the 10% significance test. This reveals that the impact of population aging on SME digital transformation has a lag effect and tests the robustness of the results.
https://doi.org/10.1371/journal.pone.0300660.t007
5.3.2 Instrumental variable approach.
In addition, this paper uses the instrumental variable method to alleviate the endogeneity problem. Referring to Cai et al. [ 51 ], we select the birth rate in 1980 of the province where the enterprise is located as the instrumental variable for the degree of population aging from 2010 to 2021. This instrumental variable was chosen because the historical birth rate has good predictive ability for the degree of population aging, and it is difficult for the birth rate 30 years ago to directly influence current decisions on enterprise digital transformation. The results are presented in Table 8 . There is still a significant positive relationship between population aging and SMEs’ digital transformation after retesting with the instrumental variable. We also report the results of a weak instrumental variable test at the end of Table 8 , and the statistical values are much larger than 100, which supports the rationality of the instrumental variable selection.
https://doi.org/10.1371/journal.pone.0300660.t008
5.4 Heterogeneity analysis
5.4.1 regional heterogeneity..
There are visible differences in economic development between the eastern and central-western regions in China. Therefore, according to the locations of enterprises, this paper divides the samples into eastern and central-western regions. The regression results in Table 9 show that the digital transformation coefficient is positively significant at the 5% level for enterprises in eastern regions but is not significant for those in central-western regions. One possible explanation is that compared with central-western regions, eastern regions have developed economies, advanced technologies, abundant financial resources, and higher population quality. Therefore, the labor substitution effect of capital and technology, the human capital accumulation effect, and the raising savings rate effect caused by population aging are greater. Thus, the promotion effects on SME digital transformation are also greater in the eastern regions of China.
https://doi.org/10.1371/journal.pone.0300660.t009
5.4.2 Corporate heterogeneity.
To explore the impact of population aging on SME digital transformation under different types of ownership, this paper divides the samples into state-owned enterprises and private enterprises. The estimation results in Table 10 show that the impact is significantly positive for enterprises with state-owned ownership but is not significant for private enterprises. This suggests that population aging has a stronger driving force for private enterprise digital transformation. The reason is that state-owned enterprises have advantages in acquiring resources such as capital, talent, and technology, as well as in market competitiveness. Consequently, the marginal impacts of higher labor costs, increased human capital, and higher savings rates caused by population aging are relatively small. In contrast, private enterprises that have poor financial environments and weak abilities to access resources such as talent and technology are more affected by the above effects, thus having a larger incentive to digitally transform.
https://doi.org/10.1371/journal.pone.0300660.t010
6. Influence mechanism analysis
6.1 test of the mediating role of labor costs.
To verify the mediating effect of labor costs, following Wang et al. [ 52 ], the relative wage of the province’s average wage level compared to that of the country is used as a measure of relative labor costs. The results are listed in Table 11 . Columns (1) and (3) show that population aging has a significant positive impact on the relative average wage level, which means that population aging increases labor costs. In columns (2) and (4), after adding the mediating variable of the relative average wage level, the impact of population aging on enterprise digital transformation is still positive, which means that population aging positively impacts SME digital transformation by increasing labor costs, thus proving Hypothesis 2. This is because population aging leads to higher labor costs. Under the burden of higher labor costs, SMEs are pushed to replace labor with capital and technology, leading to more digital transformation.
https://doi.org/10.1371/journal.pone.0300660.t011
6.2 Test of the mediating role of human capital accumulation
To verify the mediating effect of human capital, following Guo et al. [ 53 ], the proportion of students in higher education to the total population is used as a measure of human capital. The results are provided in Table 12 . Columns (1) and (3) show that population aging has a significant positive impact on human capital, which means that population aging can facilitate human capital accumulation. Columns (2) and (4) regress both human capital level and aging degree into the model, and the coefficient of enterprise digital transformation is still significantly positive, indicating that population aging positively impacts enterprise digital transformation by facilitating human capital accumulation, thus proving Hypothesis 3. The reason is that population aging means longer life expectancy and higher return on educational investment, thus promoting human capital accumulation. As the quality of the workforce improves, enterprise demand for highly skilled laborers can be better met, which makes SMEs obtain the pull from increasing human capital for digital transformation.
https://doi.org/10.1371/journal.pone.0300660.t012
6.3 Test of the mediating role of savings rates
To verify the mediating effect of savings rates, following Xu et al. [ 54 ], the proportion of total savings to regional GDP is used as a measure of the savings rate. In Table 13 , columns (1) and (3) show the impact of population aging on the savings rate. At the 5% level, the coefficient is significantly positive, indicating that population aging can raise savings rates. Columns (2) and (4) display the results of the regression after adding the savings rate. At the 10% level, the regression coefficient of population aging and enterprise digital transformation is significant, which means that population aging positively impacts enterprise digital transformation by raising savings rates, thus verifying Hypothesis 4. This result can be explained by the following reasons. First, China is in the middle stage of aging, and the proportion of the young population is still much larger than that of the elderly population. Second, China has an expanding basic social pension insurance system. Therefore, population aging has a positive effect on savings rates in China at this stage. As the savings rate rises, creditable funds in the financial market increase, which makes SMEs obtain the pull from alleviating financial constraints for digital transformation.
https://doi.org/10.1371/journal.pone.0300660.t013
7. Further analysis
7.1 threshold effect of aging degree.
To study the impact of differing degrees of population aging on SME digital transformation, this paper uses the threshold effect model. As reported in Table 14 , when the elderly population proportion is used as a threshold variable, there is a double threshold at the 5% significance level, and the threshold values are 12.5% and 15.01%. The test results in Table 15 show that when the proportion of the elderly population is lower than 12.5%, the coefficient of population aging on enterprise digital transformation is 0.5401. When the proportion of the elderly population is between 12.5% and 15.01%, the coefficient is 0.4105. When the proportion of the elderly population is higher than 15.01%, the coefficient is 0.2890. The above results reveal that although population aging has a positive impact on enterprise digital transformation, the marginal positive impact decreases as population aging progresses. One possible reason is that in the early stages of population aging, higher labor costs can be easily offset by technological progress, and the extension of life expectancy increases the savings rate, which results in a greater positive impact on enterprise digital transformation. However, in the later stages of population aging, severe labor shortages make the substitution effect of capital and technology negligible, and the upward trend of the social savings rate is slowed by increasing social pension payouts, which weakens the positive impact on digital transformation.
https://doi.org/10.1371/journal.pone.0300660.t014
https://doi.org/10.1371/journal.pone.0300660.t015
7.2 Threshold effect of marketization level
To study the impact of population aging on SME digital transformation under different marketization levels, this paper selects the marketization process index in China’s Provincial Marketization Index Report (2021) [ 55 ] as a measure of the level of marketization. Since this report only includes marketization indices from 2010 to 2019, we extend the data to the whole sample period according to the annual average growth rate of the marketization index.
As presented in Table 16 , when the marketization level is used as a threshold variable, two threshold values are obtained at 9.01 and 10.15. The test results in Table 17 show that when the marketization degree is at a low level ( Market <9.01), the impact coefficient of population aging on enterprise digital transformation is 0.1401. When the marketization degree is at a medium level (9.01≤ Market <10.15), the impact coefficient of population aging on enterprise digital transformation increases to 0.1924. When the marketization degree is at a high level ( Market ≥10.15), the impact coefficient of population aging on enterprise digital transformation increases to 0.2379. The above results suggest that with increasing marketization levels, the impact of population aging on enterprise digital transformation gradually increases. One possible reason is that enterprises become more sensitive to production factor supply and price changes as the marketization level increases. Therefore, changes in the supply and relative prices of production factors such as labor, human capital, and finance caused by population aging will have a greater impact on enterprises in regions with a higher level of marketization.
https://doi.org/10.1371/journal.pone.0300660.t016
https://doi.org/10.1371/journal.pone.0300660.t017
8. Conclusions and recommendations
8.1 conclusions.
This paper explores the impact and mechanisms of population aging on SME digital transformation. The study finds that, first, population aging has a significant positive impact on SME digital transformation and the result is robust to robustness checks and endogeneity tests. Second, the impact of population aging on SME digital transformation shows heterogeneity. For private enterprises and enterprises in the eastern regions of China, population aging can positively impact enterprise digital transformation; meanwhile, for state-owned enterprises and enterprises in the central-western regions of China, this impact is not significant. Third, regarding the influence mechanisms, population aging forces enterprises to replace labor with capital and technology and drives SMEs’ digital transformation; population aging facilitates the accumulation of human capital and provides intellectual support for SMEs’ digital transformation; population aging raises savings rates, alleviates financial constraints, and provides financial support for SMEs’ digital transformation. Fourth, the impact of population aging on SME digital transformation is dynamic and is influenced by both the degree of aging and the level of marketization. As the degree of population aging increases, the marginal positive impact of population aging on SME digital transformation shows a decreasing trend. As the marketization level improves, the impact of population aging on enterprise digital transformation gradually increases. This indicates that with the deepening of population aging, only by continuously improving the marketization level can the positive impact of population aging be attained.
8.2 Recommendations
Based on the research conclusions, this paper makes the following recommendations:
Improve the fertility security policy and plan a delayed retirement policy. Deepening population aging coupled with declines in the fertility rate will have a negative effect on labor in China. The state should support women’s reproductive rights at all levels of society. Tax incentives or subsidies to enterprises could reduce employment discrimination against women. The government should lower the threshold for maternity insurance, expand the coverage of maternity insurance, reduce fertility costs by rewarding multiple births, and increase women’s reproductive willingness. At the same time, the government should delay the legal retirement age to leverage the experience of elderly workers.
Improve public education quality and facilitate human capital accumulation. The state should continue to expand the coverage years and scope of compulsory education beyond the nine-year compulsory education. The government should support quality vocational education beyond guaranteeing a basic level of education for people. Highly skilled talent is necessary for leveraging innovative technologies after successful research and development. In addition, the government should increase investment in public education, reduce the cost of human capital accumulation, and enhance social education willingness.
Improve technological innovation ability and accelerate digital transformation. The state should increase investment in digital innovation and provide guaranteed technical support. The government should optimize relevant policies, guide resources toward enterprises with slower transformation speeds, and appeal to backward regions to raise their awareness of digital transformation. Enterprises should introduce digital technologies, reduce their reliance on low-end labor, and enhance their market competitiveness. Enterprises should also develop highly skilled employees, realize synergy between technology and capital factors, improve production efficiency, and enhance enterprise performance.
Strengthen marketization reform efforts and coordinate regional development levels. The state should prioritize speeding up marketization processes in the central-western regions of China, improving the allocation efficiency of production factors, enhancing market intermediary organizations, and optimizing the legal system environment. For eastern regions, the state should reduce unnecessary government intervention and allow market forces to freely operate to achieve equitable development among different regions.
Supporting information
S1 appendix. digital transformation keywords..
https://doi.org/10.1371/journal.pone.0300660.s001
S1 Data. Sharing data.
https://doi.org/10.1371/journal.pone.0300660.s002
- View Article
- Google Scholar
- PubMed/NCBI
- 55. Wang X, Hu L, Fan G. Marketization Index of China’s Provinces: NERI Report 2021.Beijing: Social Sciences Academic Press(China),2022.
Thank you for visiting nature.com. You are using a browser version with limited support for CSS. To obtain the best experience, we recommend you use a more up to date browser (or turn off compatibility mode in Internet Explorer). In the meantime, to ensure continued support, we are displaying the site without styles and JavaScript.
- View all journals
- Explore content
- About the journal
- Publish with us
- Sign up for alerts
- 08 May 2024
‘Milestone’ discovery as JWST confirms atmosphere on an Earth-like exoplanet
- Sumeet Kulkarni
You can also search for this author in PubMed Google Scholar
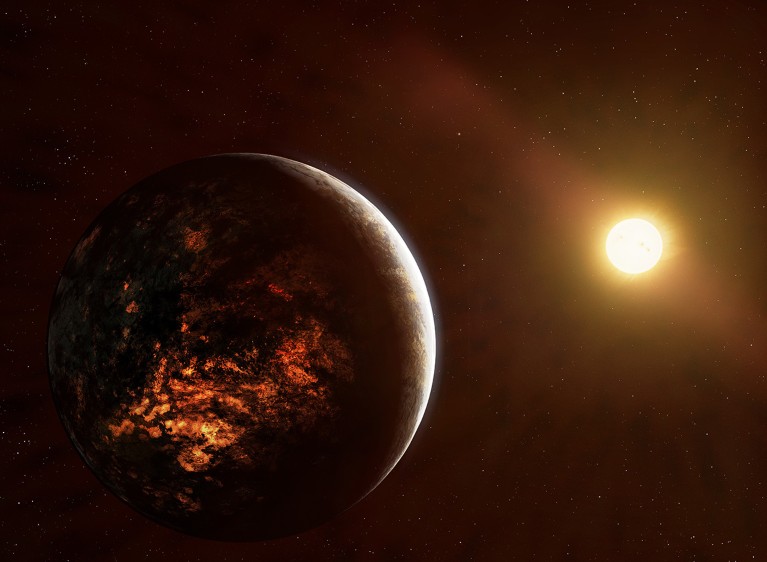
The planet 55 Cancri e (artist’s impression) orbits very close to its star. Credit: Mark Garlick/Science Photo Library
Astronomers say that they have used the James Webb Space Telescope (JWST) to detect for the first time an atmosphere surrounding a rocky planet outside the Solar System 1 . Although this planet cannot support life as we know it, in part because it is probably covered by a magma ocean, scientists might learn something from it about the early history of Earth — which is also a rocky planet and was once molten.
Finding a gaseous envelope around an Earth-like planet is a big milestone in exoplanet research, says Sara Seager, a planetary scientist at the Massachusetts Institute of Technology in Cambridge who was not involved with the research. Earth’s thin atmosphere is crucial for sustaining life, and being able to spot atmospheres on similar terrestrial planets is an important step in the search for life beyond the Solar System.
The planet investigated by JWST, called 55 Cancri e, orbits a Sun-like star 12.6 parsecs away and is considered a super-Earth, a terrestrial planet a little bigger than Earth — in this case, with about twice Earth’s radius and more than eight times as heavy. According to a paper published today in Nature 1 , its atmosphere is probably rich in carbon dioxide or carbon monoxide and has a thickness that is “up to a few per cent” of the planet’s radius.
A mysterious planet
Another reason that 55 Cancri e is uninhabitable is that it is very close to its star — around one sixty-fifth of the distance from Earth to the Sun. And yet, “it’s perhaps the most studied rocky planet”, says Aaron Bello-Arufe, an astrophysicist at the Jet Propulsion Laboratory (JPL) in Pasadena, California, and a co-author of the paper. Its host star is bright in our night sky, and because it is large for a rocky planet, it’s easier to study than other planets outside the Solar System. “Every telescope or instrument that you can think of in astronomy has pointed to this planet at some point,” Bello-Arufe says.
55 Cancri e was so well studied that after JWST launched in December 2021 , engineers pointed the observatory’s infrared spectrometers towards it for testing. These instruments can detect the chemical fingerprints of gases swirling around planets as they absorb infrared wavelengths from starlight. Bello-Arufe and his colleagues then decided to dig deeper to confirm whether the planet had an atmosphere.
Before the latest observations, astronomers had changed their minds about 55 Cancri e myriad times. The planet was discovered in 2004 2 . At first, researchers thought it was probably the core of a gas giant similar to Jupiter. But in 2011, the Spitzer Space Telescope observed the planet as it passed in front of its star 3 , and researchers found that 55 Cancri e is in fact a lot smaller and denser than a gas giant, making it a rocky super-Earth.
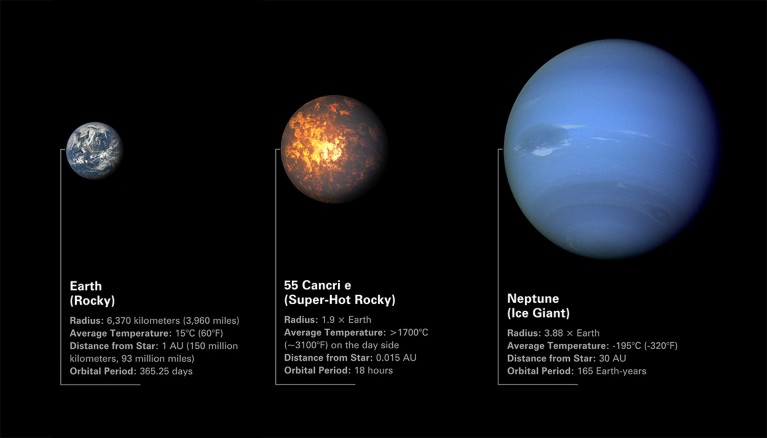
55 Cancri e is a little bigger than Earth, but much smaller than the Solar System’s giant planets, such as Neptune. Credit: NASA, ESA, CSA, Dani Player (STScI)
Some years later, researchers noticed that 55 Cancri e was cooler than it should have been for a planet so close to its star, indicating that it probably has an atmosphere 4 . One hypothesis was that the planet is a ‘water world’ enveloped by supercritical water molecules; another was that it is surrounded by an expansive, primordial atmosphere composed mainly of hydrogen and helium 5 . But these ideas were eventually disproved.
A planet so close to its star would be bombarded by stellar winds and have a hard time holding on to volatile molecules in its atmosphere, says Renyu Hu, a planetary scientist at JPL and a co-author of the latest study. Two possibilities remained, he says. The first was that the planet is completely dry, with an ultrathin atmosphere of vaporized rock. The second was that it has a thick atmosphere composed of heavier, volatile molecules that do not bleed away easily.
A clearer picture
The latest data indicate that 55 Cancri e’s atmosphere contains carbon-based gases, pointing to option two. The team collected bona fide evidence of an atmosphere, Seager says, but more observations are needed to determine its full composition, the relative quantities of gases present and its precise thickness.
Laura Schaefer, a planetary geologist at Stanford University in California, is interested in learning how 55 Cancri e’s atmosphere interacts with materials beneath the planet’s surface. It’s still possible that the atmosphere is being eroded by stellar winds, the study’s authors say, but the gases could be getting replenished by the melting and outgassing of rocks in the magma ocean.
“Earth probably went through at least one magma-ocean stage, maybe several,” Schaefer says. “Having actual present-day examples of magma oceans can help us understand the early history of our Solar System.”
doi: https://doi.org/10.1038/d41586-024-01332-w
Hu, R. et al. Nature https://doi.org/10.1038/s41586-024-07432-x (2024).
Article Google Scholar
McArthur, B. E. et al. Astrophys. J. 614 , L81 (2004).
Demory, B.-O. et al. Astron. Astrophys. 533 , A114 (2011).
Tsiaras, A. et al. Astrophys. J. 820 , 99 (2016).
Gillon, M. et al. Astron. Astrophys. 539 , A28 (2012).
Download references
Reprints and permissions
Related Articles
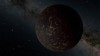
- Planetary science
- Astronomy and astrophysics
- Astronomical instrumentation
A secondary atmosphere on the rocky Exoplanet 55 Cancri e
Article 08 MAY 24
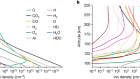
Venus water loss is dominated by HCO+ dissociative recombination
Article 06 MAY 24
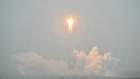
China’s Chang’e-6 launched successfully — what happens next?
News 06 MAY 24
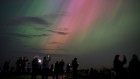
Dazzling auroras are just a warm-up as more solar storms are likely, scientists say
News Explainer 13 MAY 24
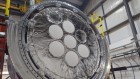
‘Best view ever’: observatory will map Big Bang’s afterglow in new detail
News 22 MAR 24
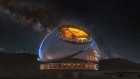
Two giant US telescopes threatened by funding cap
News 29 FEB 24
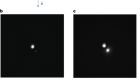
The Dimorphos ejecta plume properties revealed by LICIACube
Article 28 FEB 24
Postdoc in CRISPR Meta-Analytics and AI for Therapeutic Target Discovery and Priotisation (OT Grant)
APPLICATION CLOSING DATE: 14/06/2024 Human Technopole (HT) is a new interdisciplinary life science research institute created and supported by the...
Human Technopole
Research Associate - Metabolism
Houston, Texas (US)
Baylor College of Medicine (BCM)
Postdoc Fellowships
Train with world-renowned cancer researchers at NIH? Consider joining the Center for Cancer Research (CCR) at the National Cancer Institute
Bethesda, Maryland
NIH National Cancer Institute (NCI)
Faculty Recruitment, Westlake University School of Medicine
Faculty positions are open at four distinct ranks: Assistant Professor, Associate Professor, Full Professor, and Chair Professor.
Hangzhou, Zhejiang, China
Westlake University
PhD/master's Candidate
PhD/master's Candidate Graduate School of Frontier Science Initiative, Kanazawa University is seeking candidates for PhD and master's students i...
Kanazawa University
Sign up for the Nature Briefing newsletter — what matters in science, free to your inbox daily.
Quick links
- Explore articles by subject
- Guide to authors
- Editorial policies

- SUGGESTED TOPICS
- The Magazine
- Newsletters
- Managing Yourself
- Managing Teams
- Work-life Balance
- The Big Idea
- Data & Visuals
- Reading Lists
- Case Selections
- HBR Learning
- Topic Feeds
- Account Settings
- Email Preferences

How to Weigh the Risks of Disclosing a Disability
- Ludmila N. Praslova

Disclosing a challenging health condition at work can be risky. You may get the accommodations you need, but you may also be met with suspicion, resentment, and accusations of making it all up. Research shows that most disabled employees experience some form of workplace discrimination, including hiring biases, pay gaps, bullying, and mistreatment. In addition, when people with nonapparent conditions disclose them, they often get a “You look fine” response, adding to their reluctance to disclose. In this article, the author discusses why disclosure is challenging, how to decide whether the risk is worth taking, and how a network can support you.
A guide to help you decide — and find support.
Whether to disclose your long Covid, or any other nonapparent condition or disability, to your employer is a deeply personal and consequential decision. While disclosing may help you access accommodations, it carries risks stemming from stigma and ableism. You might get support, but you might also be met with suspicion, resentment, and accusations of making it all up.
And what their employers are — and aren’t — doing to support them.
- Ludmila N. Praslova , PhD, SHRM-SCP, uses her extensive experience with neurodiversity and global and cultural inclusion to help create talent-rich workplaces. The author of The Canary Code , she is a professor of graduate industrial-organizational psychology and the accreditation liaison officer at Vanguard University of Southern California. Follow Ludmila on LinkedIn .
Partner Center
- Open supplemental data
- Reference Manager
- Simple TEXT file
People also looked at
Original research article, the potential protective role of parkinson’s disease against hypothyroidism: co-localisation and bidirectional mendelian randomization study.
- 1 Department of Neurology, The First Affiliated Hospital of Guangxi Medical University, Nanning, China
- 2 Department of Neurology, The First People’s Hospital of Nanning, Nanning, China
- 3 Department of Rehabilitation Medicine, Jiangbin Hospital of Guangxi Zhuang Autonomous Region, Nanning, China
Background: The association between hypothyroidism and Parkinson’s disease (PD) has sparked intense debate in the medical community due to conflicting study results. A better understanding of this association is crucial because of its potential implications for both pathogenesis and treatment strategies.
Methods: To elucidate this complex relationship, we used Bayesian co-localisation (COLOC) and bidirectional Mendelian randomization (MR) analysis. COLOC was first used to determine whether hypothyroidism and PD share a common genetic basis. Subsequently, genetic variants served as instrumental variables in a bidirectional MR to explore causal interactions between these conditions.
Results: COLOC analysis revealed no shared genetic variants between hypothyroidism and PD, with a posteriori probability of hypothesis 4 (PPH4) = 0.025. Furthermore, MR analysis indicated that hypothyroidism does not have a substantial causal effect on PD (OR = 0.990, 95% CI = 0.925, 1.060, p = 0.774). Conversely, PD appears to have a negative causal effect on hypothyroidism (OR = 0.776, 95% CI = 0.649, 0.928, p = 0.005).
Conclusion: Our findings suggest the absence of shared genetic variants between hypothyroidism and PD. Interestingly, PD may inversely influence the risk of developing hypothyroidism, a finding that may inform future research and clinical approaches.
1 Introduction
Parkinson’s disease (PD) is the second most common neurodegenerative disease after Alzheimer’s disease, and its incidence continues to increase ( Pavlou and Outeiro, 2017 ). The dyskinesia symptoms of PD–bradykinesia, tremor, rigidity and postural balance deficits–are the result of dopaminergic neuronal deficits and the accumulation of α-synuclein ( Jakubowski and Labrie, 2017 ; Mohd Murshid et al., 2022 ). Dysregulation of dopamine metabolism, oxidative stress and neuroinflammation are key factors contributing to dopaminergic neuronal deficits ( Dexter and Jenner, 2013 ; Labbé et al., 2016 ; Chang and Chen, 2020 ). Hypothyroidism, characterised by low thyroid hormone levels, is associated with oxidative stress and inflammatory responses ( Mancini et al., 2016 ; de Carvalho et al., 2018 ). There is conflicting evidence about the relationship between hypothyroidism and the risk of PD. For example, a Danish study involving more than 80,000 people found no significant association between hypothyroidism and PD, whereas studies in Taiwan and South Korea have shown that patients with hypothyroidism have an increased risk of PD ( Rugbjerg et al., 2009 ; Chen et al., 2020 ; Kim et al., 2021 ). Hypothyroidism is known to increase the risk of anaemia, obesity and hyperlipidaemia, all of which are associated with an increased risk of developing PD ( Mohammadi et al., 2021 ). Given the mixed results of observational studies and potential confounding factors, it remains difficult to establish a causal relationship between hypothyroidism and PD.
Mendelian randomization (MR), which uses single-nucleotide polymorphisms (SNPs) as instrumental variables to estimate the causal effect of exposure on outcomes, can help researchers identify causal relationships between diseases and avoid the confounding problems often found in traditional studies ( Liu et al., 2021 ). MR has proven to be a powerful and convenient tool for investigating risk factors associated with PD ( Senkevich et al., 2023 ; Song et al., 2024 ). Bayesian co-localisation (COLOC) is a method that uses Bayesian statistical principles to assess whether two or more traits share the same genetic variation ( Chen et al., 2024 ). This is important for understanding how different traits are linked by common biological pathways or mechanisms. Some studies have used MR in conjunction with COLOC, an approach that has revealed a common genetic basis between psoriasis and multiple sclerosis and helped to identify potential therapeutic targets for multiple sclerosis ( Lin et al., 2023 ; Patrick et al., 2023 ).
Traditional observational studies often encounter difficulties in establishing a causal relationship between PD and hypothyroidism, the main obstacle being the exclusion of confounding factors. To overcome this limitation, MR offers a powerful alternative approach that is particularly effective in overcoming the limitations of traditional studies in making causal inferences. Our study uses a genome-wide association study (GWAS) dataset containing two diseases, which provides a good basis for using both COLOC and MR analysis methods. The combination of these two approaches could provide a solid basis for genetic commonality and causal inference to reveal genetic and causal links between hypothyroidism and PD, and provide new biological insights into these two potentially related disorders, thus opening up new avenues for clinical practice and future research directions.
2 Materials and methods
2.1 data sources and study populations.
We obtained data from published GWAS, 1 focusing on datasets that provide comprehensive insights into PD and hypothyroidism ( Table 1 ). As this study used publicly available data, ethical approval was not required as each dataset had already received ethical approval.

Table 1 . Source of exposure and outcome GWAS data.
2.2 Colocalization analysis
The COLOC analysis was based on the following assumptions ( Wallace, 2020 ). Independence assumption: associations between traits are caused directly by a common genetic variant and not by other indirect factors such as confounding variables. COLOC uses a Bayesian probability model to assess the strength of the evidence that two traits share the same SNPs. COLOC can derive five types of probability, including cases where the two traits are independent and have no common variant, each has its own variant, and one variant is shared. We used the hypothetical posterior probability 4 (PPH4) in the COLOC algorithm to assess whether the two share genetic variants, with PPH4 > 80% defined as the two sharing the same genetic variant as an influence ( Deng et al., 2022 ). This analysis was performed using the “coloc” software package. 2
2.3 MR study design
Our MR study design was based on three critical assumptions: (A) SNPs are significantly associated with exposure, serving as an instrumental variable; (B) these SNPs are not associated with known confounders; and (C) the effect of SNPs on outcome is entirely mediated by exposure factors.
2.4 Selection of instrumental variables
SNPs were used as instrumental variables according to the following four criteria: a. p < 5 × 10 8 ; b. removal of chain imbalances (kb = 10,000 and r 2 = 0.001) ( Hu et al., 2023 ); c. search for these SNPs in PhenoScanner 3 and removal of SNPs associated with confounders and outcomes ( Hu et al., 2022 ); d. calculation and selection of SNPs with F-statistics >10, which can mitigate the effects of potential bias ( Hu et al., 2023 ).
2.5 MR analysis
We chose inverse variance weighted (IVW), weighted median, MR-Egger and weighted mode for MR analyses, and considered p < 0.05 to be statistically significant ( Han et al., 2022 ). Heterogeneity was assessed using MR-Egger and IVW regressions, with p > 0.05 indicating no heterogeneity. The presence of horizontal pleiotropy was assessed using the Egger intercept, with p > 0.05 indicating the absence of horizontal pleiotropy ( Hu et al., 2022 , 2023 ). Visualization results are presented as rejection-by-exclusion tests, forest plots, scatter plots and funnel plots. All statistical analyses were performed using the “TwoSampleMR” package in R (version 4.2.3).
3.1 Co-localization between hypothyroidism and PD
In our paired GWAS analysis of hypothyroidism and PD, the COLOC PPH4 was below 80% (PPH4 = 0.024), suggesting that there is insufficient evidence to support that they share a common genetic locus ( Figure 1 ). This finding suggests that there may not be a direct genetic link between hypothyroidism and PD, and that they may affect patients through different biological pathways.
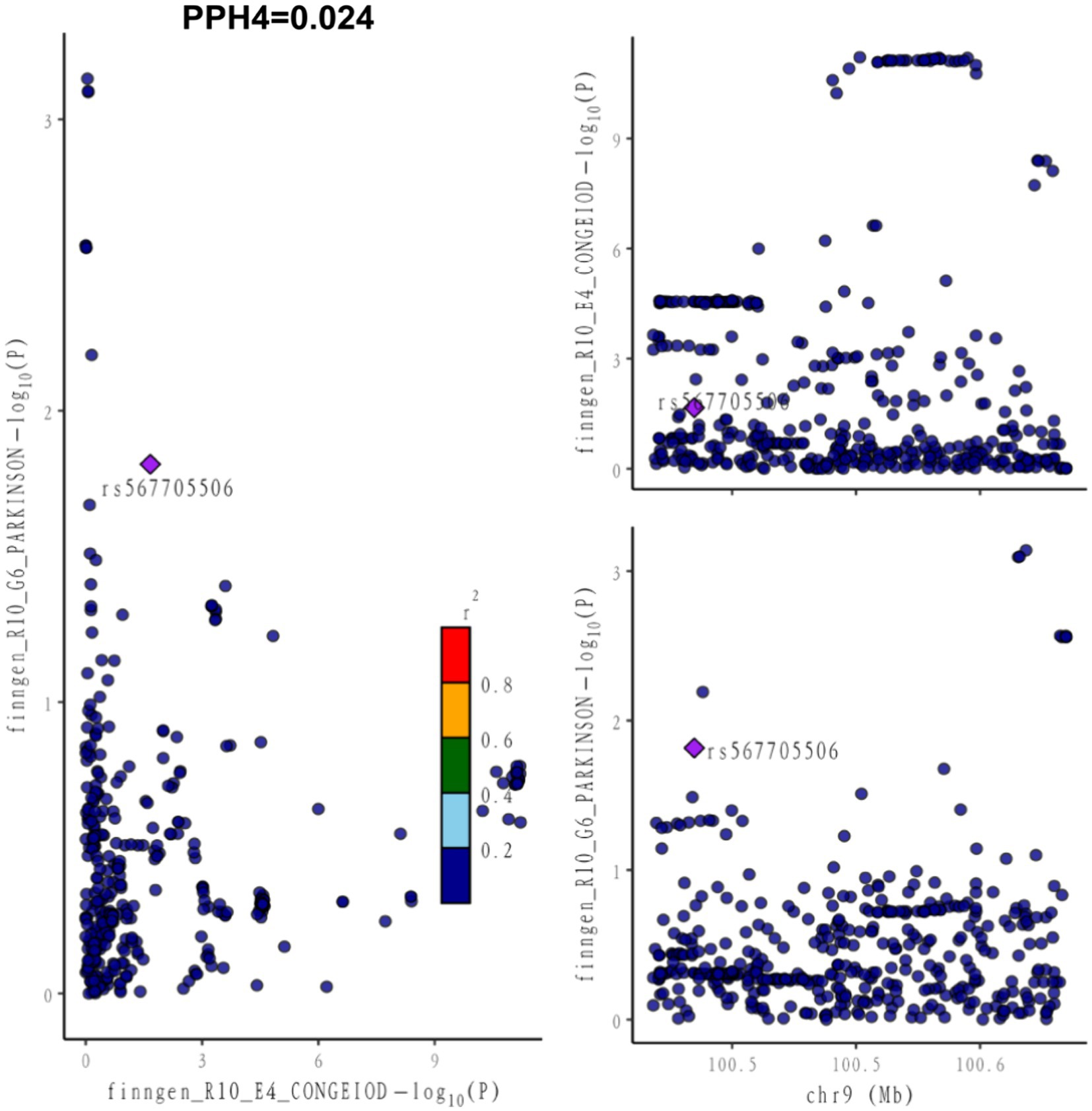
Figure 1 . Co-localization between hypothyroidism and PD. PPH4 stands for posterior probability of hypothesis 4. PPH4 > 80% defined as both sharing the same genetic variant as an influencing factor.
3.2 For performing MR analysis SNPs
When selecting SNPs as instrumental variables from the GWAS dataset, we used methodological mention criteria to ensure that these SNPs were strongly associated with the phenotype, as detailed in Supplementary Table S1 . This ensured the precision and reliability of our analysis.
3.3 MR analysis, sensitivity analysis and visualization
3.3.1 results of mr analysis of hypothyroidism on pd.
Using a MR analysis with validated instrumental variables, the MR-IVW results showed no causal effect of hypothyroidism on PD (OR = 0.990, 95% CI = 0.925, 1.060, p = 0.774). The MR Egger (OR = 1. 006, 95% CI = 0.824, 1.227, p = 0.953), weighted median (OR = 0.968, 95% CI = 0.899, 1.042, p = 0.389) and weighted mode (OR = 1.034, 95% CI = 0.902, 1.185, p = 0.641) analyses were consistent with the MR-IVW analysis ( Table 2 ). Although our analysis did not show a causal effect of hypothyroidism on PD. This result helps to rule out hypothyroidism as a potential risk factor or protective factor for PD. The Cochran’s Q statistic in the MR-Egger and IVW regressions showed heterogeneity in the Cochran’s Q test ( p < 0.05) ( Supplementary Table S2 ). Our horizontal multivariate test showed no horizontal multivariate p > 0.05 in the instrumental variables ( Supplementary Table S3 ). Sensitivity analyses using the leave-one-out method confirmed the stability of our results ( Supplementary Figure S1A ). Forest, scatter and funnel plots supported the results of our MR analyses ( Supplementary Figures S2A–C ).
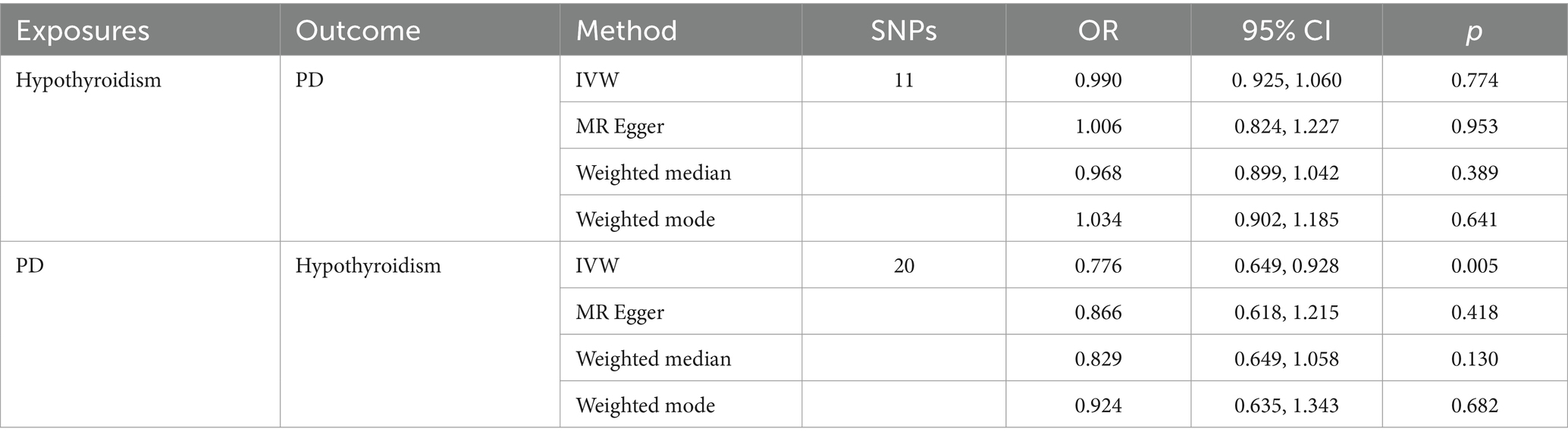
Table 2 . Bidirectional MR analysis of the relationship between hypothyroidism and PD.
3.3.2 Results of MR analysis of PD on hypothyroidism
The same MR analysis method was used to infer the causal effect of PD on hypothyroidism. Interestingly, although the MR Egger (OR = 0.886, 95% CI = 0.618, 1.215, p = 0.418), weighted median (OR = 0.829 95% CI = 0.649, 1.058, p = 0.130) and weighted mode (OR = 0.682 95% CI = 0.635, 1.343, p = 0.682) analyses did not agree with the results of the MR-IVW analyses, the MR-IVW results suggested that PD reduced the risk of developing hypothyroidism (OR = 0.776 95% CI = 0.649, 0.928, p = 0.005). The Cochran’s Q statistic for the MR-Egger and IVW regressions suggested that the Cochran’s Q test was not heterogeneous ( p > 0.05) ( Supplementary Table S2 ). Our multilevel pleiotropy test indicated no level pleiotropy ( p > 0.05) for the instrumental variables used ( Supplementary Table S3 ). This suggests that the instrumental variables were causally related to PD via hypothyroidism, without confounding by other factors. The robustness of our findings was further confirmed in the leave-one-out sensitivity analysis ( Supplementary Figure S2B ). In addition, forest plots, scatter plots and funnel plots also confirmed the consistency of our MR analysis results ( Supplementary Figures S3A–C ).
4 Discussion
Our study shows that there is no common genetic variant between hypothyroidism and PD. Hypothyroidism does not have a causal effect on PD, but PD has a negative causal effect on hypothyroidism. This finding is inconsistent with previous studies showing that hypothyroidism increases the risk of developing PD ( Charoenngam et al., 2022 ). A concurrent and prospective study using traditional statistics and novel machine learning models found that hypothyroidism is associated with an increased risk of PD ( Gialluisi et al., 2023 ). In vitro and in vivo experiments have shown that thyroid hormones can prevent apoptosis of dopamine neurons ( Kincaid, 2001 ; Lee et al., 2019 ; Menezes et al., 2019 ), suggesting a protective role of thyroid hormones on dopamine neurons in PD. However, hypothyroidism, characterised by insufficient thyroid hormone production, may exacerbate or promote the progression of PD. Recent bidirectional MR studies do not support a causal relationship between hypothyroidism and PD ( Zeng et al., 2024 ). Our results are reliable and we used the most recent Finnish database with the largest sample size available for the study. First, in the co-localisation analysis of the two diseases did not find any common genetic variants were found, suggesting that variants in one disease do not increase the risk of the other. Second, despite the heterogeneity in the MR analysis of hypothyroidism on PD, the MR analysis overcame the confounding factors in traditional studies and ensured the reliability of causal inferences.
PD and hypothyroidism share similar pathogenic mechanisms, such as oxidative stress and inflammation. Studies have shown that apo D expression is upregulated in both diseases, and its antioxidant and anti-inflammatory effects provide an interesting avenue for further research ( Fyfe-Desmarais et al., 2023 ). The mechanisms by which PD reduces the risk of hypothyroidism are unknown. We speculate that antioxidant mechanisms upregulated in PD may have a protective effect against the oxidative damage commonly seen in hypothyroidism. Future studies could investigate specific antioxidant levels and markers of oxidative stress in patients with PD compared to those with hypothyroidism. Altered immune-inflammatory pathways in PD may also play a protective role in the development of hypothyroidism. This hypothesis could be explored by examining cytokine levels and inflammatory markers in patients with PD versus those diagnosed with hypothyroidism to see if the reduced inflammatory response in PD is associated with a lower incidence of hypothyroidism. In addition, all current clinical pharmacological treatments can only alleviate the symptoms of PD, rather than slowing and curing the disease process. Investigating the role of oxidative stress and immune-inflammatory responses may provide new targets for PD treatment.
The motor dysfunctions associated with hypothyroidism may resemble those of PD, and given its treatability, accurate clinical diagnosis is important ( Schneider et al., 2023 ). The high prevalence of PD and hypothyroidism in the elderly and of subclinical hypothyroidism in PD patients suggests a complex interaction between the two diseases ( Munhoz et al., 2004 ). There is an association between thyroid hormones and PD, and most of the 12 SNPs may influence PD and thyroid function through immune mechanisms ( Xu et al., 2022 ). The cardiovascular effects of hypothyroidism in PD and the improvement of fluctuating symptoms with thyroxine therapy in some patients further emphasise the need to assess thyroid function in the management of PD ( García-Moreno and Chacón-Peña, 2003 ; Rodrigues et al., 2020 ). Although we did not find a common genetic variant between PD and hypothyroidism, the interaction between hypothyroidism and PD warrants further investigation.
Nevertheless, our study has limitations, including the heterogeneity of the instrumental variables in our MR analyses, which could be due to unobserved confounders. Also, as our GWAS data are predominantly from European populations, the applicability of our findings to other ethnic groups, such as Asians, remains uncertain. In addition, gender differences in the prevalence of hypothyroidism and PD ( Chaker et al., 2017 ; Cerri et al., 2019 ), which were not accounted for in our analysis, may influence our results and should be a focus of future research.
In conclusion, our findings suggest that there is no shared genetic variation between hypothyroidism and PD, and interestingly, PD may have an inverse effect on the risk of developing hypothyroidism, a finding that may inform future research and clinical approaches.
Data availability statement
Publicly available datasets were analyzed in this study. This data can be found at: https://www.finngen.fi/en/access_results .
Author contributions
JLe: Writing – original draft. WH: Writing – original draft. YaL: Formal analysis, Writing – original draft. QZ: Investigation, Writing – review & editing. YiL: Formal analysis, Writing – original draft. QO: Investigation, Writing – original draft. XW: Formal analysis, Writing – review & editing. FL: Formal analysis, Writing – original draft. JLi: Investigation, Writing – review & editing. YX: Writing – review & editing.
The author(s) declare financial support was received for the research, authorship, and/or publication of this article. This research received funding from the National Natural Science Foundation of China, under Grant/Award Numbers 81801268 and 82060248. Additionally, it was supported by the Clinical Research “Climbing” Program of the First Affiliated Hospital of Guangxi Medical University, Grant/Award Number YYZS2020017. This study was also funded by First-class discipline innovation-driven talentprogram of Guangxi Medical University.
Conflict of interest
The authors declare that the research was conducted in the absence of any commercial or financial relationships that could be construed as a potential conflict of interest.
Publisher’s note
All claims expressed in this article are solely those of the authors and do not necessarily represent those of their affiliated organizations, or those of the publisher, the editors and the reviewers. Any product that may be evaluated in this article, or claim that may be made by its manufacturer, is not guaranteed or endorsed by the publisher.
Supplementary material
The Supplementary material for this article can be found online at: https://www.frontiersin.org/articles/10.3389/fnagi.2024.1377719/full#supplementary-material
1. ^ https://www.finngen.fi/en/access_results
2. ^ https://github.com/chr1swallace/coloc
3. ^ www.phenoscanner.medschl.cam.ac.uk
Cerri, S., Mus, L., and Blandini, F. (2019). Parkinson’s disease in women and men: What’s the difference? J. Parkinsons Dis. 9, 501–515. doi: 10.3233/JPD-191683
PubMed Abstract | Crossref Full Text | Google Scholar
Chaker, L., Bianco, A. C., Jonklaas, J., and Peeters, R. P. (2017). Hypothyroidism. Lancet 390, 1550–1562. doi: 10.1016/S0140-6736(17)30703-1
Chang, K. H., and Chen, C. M. (2020). The role of oxidative stress in Parkinson’s disease. Antioxidants (Basel) 9:597. doi: 10.3390/antiox9070597
Charoenngam, N., Rittiphairoj, T., Ponvilawan, B., and Prasongdee, K. (2022). Thyroid dysfunction and risk of Parkinson’s disease: a systematic review and Meta-analysis. Front. Endocrinol. (Lausanne) 13:863281. doi: 10.3389/fendo.2022.863281
Chen, Y., Fan, C., and Liu, J. (2024). Investigating the shared genetic architecture between COVID-19 and obesity: a large-scale genome wide cross-trait analysis. Front. Endocrinol. (Lausanne) 15:1325939. doi: 10.3389/fendo.2024.1325939
Chen, S. F., Yang, Y. C., Hsu, C. Y., and Shen, Y. C. (2020). Risk of Parkinson’s disease in patients with hypothyroidism: a nationwide population-based cohort study. Parkinsonism Relat. Disord. 74, 28–32. doi: 10.1016/j.parkreldis.2020.04.001
de Carvalho, G. P.-F. G. A., Junior, C. M., and Graf, H. (2018). Management of endocrine disease: pitfalls on the replacement therapy for primary and central hypothyroidism in adults. Eur. J. Endocrinol. 178, R231–R244. doi: 10.1530/EJE-17-0947
Deng, Y.-T., Ou, Y.-N., Wu, B.-S., Yang, Y.-X., Jiang, Y., Huang, Y.-Y., et al. (2022). Identifying causal genes for depression via integration of the proteome and transcriptome from brain and blood. Mol. Psychiatry 27, 2849–2857. doi: 10.1038/s41380-022-01507-9
Crossref Full Text | Google Scholar
Dexter, D. T., and Jenner, P. (2013). Parkinson disease: from pathology to molecular disease mechanisms. Free Radic. Biol. Med. 62, 132–144. doi: 10.1016/j.freeradbiomed.2013.01.018
Fyfe-Desmarais, G., Desmarais, F., Rassart, É., and Mounier, C. (2023). Apolipoprotein D in oxidative stress and inflammation. Antioxidants 12:1027. doi: 10.3390/antiox12051027
García-Moreno, J. M., and Chacón-Peña, J. (2003). Hypothyroidism and Parkinson’s disease and the issue of diagnostic confusion. Mov. Disord. 18, 1058–1059. doi: 10.1002/mds.10475
Gialluisi, A., De Bartolo, M. I., Costanzo, S., Belvisi, D., Falciglia, S., Ricci, M., et al. (2023). Risk and protective factors in Parkinson’s disease: a simultaneous and prospective study with classical statistical and novel machine learning models. J. Neurol. 270, 4487–4497. doi: 10.1007/s00415-023-11803-1
Han, F., Zhang, C., Xuan, M., Xie, Z., Zhang, K., and Li, Y. (2022). Effects of hyperthyroidism on venous thromboembolism: a Mendelian randomization study. J Immunol Res 2022:2339678. doi: 10.1155/2022/2339678
Hu, H., Mei, J., Cai, Y., Ding, H., Niu, S., Zhang, W., et al. (2023). No genetic causal association between Alzheimer’s disease and osteoporosis: a bidirectional two-sample Mendelian randomization study. Front. Aging Neurosci. 15:1090223. doi: 10.3389/fnagi.2023.1090223
Hu, M. J., Tan, J. S., Gao, X. J., Yang, J. G., and Yang, Y. J. (2022). Effect of cheese intake on cardiovascular diseases and cardiovascular biomarkers. Nutrients 14:2936. doi: 10.3390/nu14142936
Jakubowski, J. L., and Labrie, V. (2017). Epigenetic biomarkers for Parkinson’s disease: from diagnostics to therapeutics. J. Parkinsons Dis. 7, 1–12. doi: 10.3233/JPD-160914
Kim, J. H., Lee, H. S., Ahn, J. H., Oh, J. K., Chang, I. B., Song, J. H., et al. (2021). Association between thyroid diseases and Parkinson’s disease: a nested case-control study using a National Health Screening Cohort. J. Parkinsons Dis. 11, 211–220. doi: 10.3233/JPD-202265
Kincaid, A. E. (2001). Spontaneous circling behavior and dopamine neuron loss in a genetically hypothyroid mouse. Neuroscience 105, 891–898. doi: 10.1016/S0306-4522(01)00229-9
Labbé, C., Lorenzo-Betancor, O., and Ross, O. A. (2016). Epigenetic regulation in Parkinson’s disease. Acta Neuropathol. 132, 515–530. doi: 10.1007/s00401-016-1590-9
Lee, E. H., Kim, S. M., Kim, C. H., Pagire, S. H., Pagire, H. S., Chung, H. Y., et al. (2019). Dopamine neuron induction and the neuroprotective effects of thyroid hormone derivatives. Sci. Rep. 9:13659. doi: 10.1038/s41598-019-49876-6
Lin, J., Zhou, J., and Xu, Y. (2023). Potential drug targets for multiple sclerosis identified through Mendelian randomization analysis. Brain 146, 3364–3372. doi: 10.1093/brain/awad070
Liu, H., Zhang, Y., Zhang, H., Wang, L., Wang, T., Han, Z., et al. (2021). Effect of plasma vitamin C levels on Parkinson’s disease and age at onset: a Mendelian randomization study. J. Transl. Med. 19:221. doi: 10.1186/s12967-021-02892-5
Mancini, A., Di Segni, C., Raimondo, S., Olivieri, G., Silvestrini, A., Meucci, E., et al. (2016). Thyroid hormones, oxidative stress, and inflammation. Mediat. Inflamm. 2016:6757154. doi: 10.1155/2016/6757154
Menezes, E. C., Santos, P. R., Goes, T. C., Carvalho, V. C. B., Teixeira-Silva, F., Stevens, H. E., et al. (2019). Effects of a rat model of gestational hypothyroidism on forebrain dopaminergic, GABAergic, and serotonergic systems and related behaviors. Behav. Brain Res. 366, 77–87. doi: 10.1016/j.bbr.2019.03.027
Mohammadi, S., Dolatshahi, M., and Rahmani, F. (2021). Shedding light on thyroid hormone disorders and Parkinson disease pathology: mechanisms and risk factors. J. Endocrinol. Investig. 44, 1–13. doi: 10.1007/s40618-020-01314-5
Mohd Murshid, N., Aminullah Lubis, F., and Makpol, S. (2022). Epigenetic changes and its intervention in age-related neurodegenerative diseases. Cell. Mol. Neurobiol. 42, 577–595. doi: 10.1007/s10571-020-00979-z
Munhoz, R. P., Teive, H. A., Troiano, A. R., Hauck, P. R., Herdoiza Leiva, M. H., Graff, H., et al. (2004). Parkinson’s disease and thyroid dysfunction. Parkinsonism Relat. Disord. 10, 381–383. doi: 10.1016/j.parkreldis.2004.03.008
Patrick, M. T., Nair, R. P., He, K., Stuart, P. E., Billi, A. C., Zhou, X., et al. (2023). Shared genetic risk factors for multiple sclerosis/psoriasis suggest involvement of Interleukin-17 and Janus kinase-signal transducers and activators of transcription signaling. Ann. Neurol. 94, 384–397. doi: 10.1002/ana.26672
Pavlou, M. A. S., and Outeiro, T. F. (2017). Epigenetics in Parkinson’s disease. Adv. Exp. Med. Biol. 978, 363–390. doi: 10.1007/978-3-319-53889-1_19
Rodrigues, L. D., Oliveira, L. F., Scorza, C. A., Scorza, F. A., Andersen, M. L., Tufik, S., et al. (2020). Sudden unexpected death in Parkinson’s disease: who would think of the thyroid gland? Parkinsonism Relat. Disord. 81, 54–55. doi: 10.1016/j.parkreldis.2020.10.010
Rugbjerg, K., Friis, S., Ritz, B., Schernhammer, E. S., Korbo, L., and Olsen, J. H. (2009). Autoimmune disease and risk for Parkinson disease: a population-based case-control study. Neurology 73, 1462–1468. doi: 10.1212/WNL.0b013.e3181c06635
Schneider, S. A., Tschaidse, L., and Reisch, N. (2023). Thyroid disorders and movement disorders-a systematic review. Mov. Disord. Clin. Pract. 10, 360–368. doi: 10.1002/mdc3.13656
Senkevich, K., Alipour, P., Chernyavskaya, E., Yu, E., Noyce, A. J., and Gan-Or, Z. (2023). Potential protective link between type I diabetes and Parkinson’s disease risk and progression. Mov. Disord. 38, 1350–1355. doi: 10.1002/mds.29424
Song, J., Qin, Y., Wang, L., Quan, W., Xu, J., Li, J., et al. (2024). Exploring the causal relationship between B lymphocytes and Parkinson’s disease: a bidirectional, two-sample Mendelian randomization study. Sci. Rep. 14:2783. doi: 10.1038/s41598-024-53287-7
Wallace, C. (2020). Eliciting priors and relaxing the single causal variant assumption in colocalisation analyses. PLoS Genet. 16:e1008720. doi: 10.1371/journal.pgen.1008720
Xu, J., Zhao, C., Liu, Y., Xu, C., Qin, B., and Liang, H. (2022). Genetic correlation between thyroid hormones and Parkinson’s disease. Clin. Exp. Immunol. 208, 372–379. doi: 10.1093/cei/uxac044
Zeng, Y., Cao, S., and Yang, H. (2024). No causal relationship between thyroid function and Parkinson’s disease: a bidirectional Mendelian randomization study. Neurol. Sci. 45, 1481–1487. doi: 10.1007/s10072-023-07176-y
Keywords: Parkinson’s disease, Mendelian randomization, single-nucleotide polymorphism, hypothyroidism, co-localization
Citation: Lei J, He W, Liu Y, Zhang Q, Liu Y, Ou Q, Wu X, Li F, Liao J and Xiao Y (2024) The potential protective role of Parkinson’s disease against hypothyroidism: co-localisation and bidirectional Mendelian randomization study. Front. Aging Neurosci . 16:1377719. doi: 10.3389/fnagi.2024.1377719
Received: 28 January 2024; Accepted: 29 April 2024; Published: 14 May 2024.
Reviewed by:
Copyright © 2024 Lei, He, Liu, Zhang, Liu, Ou, Wu, Li, Liao and Xiao. This is an open-access article distributed under the terms of the Creative Commons Attribution License (CC BY) . The use, distribution or reproduction in other forums is permitted, provided the original author(s) and the copyright owner(s) are credited and that the original publication in this journal is cited, in accordance with accepted academic practice. No use, distribution or reproduction is permitted which does not comply with these terms.
*Correspondence: Jiajia Liao, [email protected] ; Yousheng Xiao, [email protected]
† These authors share first authorship
This article is part of the Research Topic
Common pathophysiology underpinning Parkinson's disease and other neurodegenerative diseases

An official website of the United States government
The .gov means it’s official. Federal government websites often end in .gov or .mil. Before sharing sensitive information, make sure you’re on a federal government site.
The site is secure. The https:// ensures that you are connecting to the official website and that any information you provide is encrypted and transmitted securely.
- Publications
- Account settings
Preview improvements coming to the PMC website in October 2024. Learn More or Try it out now .
- Advanced Search
- Journal List
- v.53(4); 2010 Aug

Research questions, hypotheses and objectives
Patricia farrugia.
* Michael G. DeGroote School of Medicine, the
Bradley A. Petrisor
† Division of Orthopaedic Surgery and the
Forough Farrokhyar
‡ Departments of Surgery and
§ Clinical Epidemiology and Biostatistics, McMaster University, Hamilton, Ont
Mohit Bhandari
There is an increasing familiarity with the principles of evidence-based medicine in the surgical community. As surgeons become more aware of the hierarchy of evidence, grades of recommendations and the principles of critical appraisal, they develop an increasing familiarity with research design. Surgeons and clinicians are looking more and more to the literature and clinical trials to guide their practice; as such, it is becoming a responsibility of the clinical research community to attempt to answer questions that are not only well thought out but also clinically relevant. The development of the research question, including a supportive hypothesis and objectives, is a necessary key step in producing clinically relevant results to be used in evidence-based practice. A well-defined and specific research question is more likely to help guide us in making decisions about study design and population and subsequently what data will be collected and analyzed. 1
Objectives of this article
In this article, we discuss important considerations in the development of a research question and hypothesis and in defining objectives for research. By the end of this article, the reader will be able to appreciate the significance of constructing a good research question and developing hypotheses and research objectives for the successful design of a research study. The following article is divided into 3 sections: research question, research hypothesis and research objectives.
Research question
Interest in a particular topic usually begins the research process, but it is the familiarity with the subject that helps define an appropriate research question for a study. 1 Questions then arise out of a perceived knowledge deficit within a subject area or field of study. 2 Indeed, Haynes suggests that it is important to know “where the boundary between current knowledge and ignorance lies.” 1 The challenge in developing an appropriate research question is in determining which clinical uncertainties could or should be studied and also rationalizing the need for their investigation.
Increasing one’s knowledge about the subject of interest can be accomplished in many ways. Appropriate methods include systematically searching the literature, in-depth interviews and focus groups with patients (and proxies) and interviews with experts in the field. In addition, awareness of current trends and technological advances can assist with the development of research questions. 2 It is imperative to understand what has been studied about a topic to date in order to further the knowledge that has been previously gathered on a topic. Indeed, some granting institutions (e.g., Canadian Institute for Health Research) encourage applicants to conduct a systematic review of the available evidence if a recent review does not already exist and preferably a pilot or feasibility study before applying for a grant for a full trial.
In-depth knowledge about a subject may generate a number of questions. It then becomes necessary to ask whether these questions can be answered through one study or if more than one study needed. 1 Additional research questions can be developed, but several basic principles should be taken into consideration. 1 All questions, primary and secondary, should be developed at the beginning and planning stages of a study. Any additional questions should never compromise the primary question because it is the primary research question that forms the basis of the hypothesis and study objectives. It must be kept in mind that within the scope of one study, the presence of a number of research questions will affect and potentially increase the complexity of both the study design and subsequent statistical analyses, not to mention the actual feasibility of answering every question. 1 A sensible strategy is to establish a single primary research question around which to focus the study plan. 3 In a study, the primary research question should be clearly stated at the end of the introduction of the grant proposal, and it usually specifies the population to be studied, the intervention to be implemented and other circumstantial factors. 4
Hulley and colleagues 2 have suggested the use of the FINER criteria in the development of a good research question ( Box 1 ). The FINER criteria highlight useful points that may increase the chances of developing a successful research project. A good research question should specify the population of interest, be of interest to the scientific community and potentially to the public, have clinical relevance and further current knowledge in the field (and of course be compliant with the standards of ethical boards and national research standards).
FINER criteria for a good research question
Adapted with permission from Wolters Kluwer Health. 2
Whereas the FINER criteria outline the important aspects of the question in general, a useful format to use in the development of a specific research question is the PICO format — consider the population (P) of interest, the intervention (I) being studied, the comparison (C) group (or to what is the intervention being compared) and the outcome of interest (O). 3 , 5 , 6 Often timing (T) is added to PICO ( Box 2 ) — that is, “Over what time frame will the study take place?” 1 The PICOT approach helps generate a question that aids in constructing the framework of the study and subsequently in protocol development by alluding to the inclusion and exclusion criteria and identifying the groups of patients to be included. Knowing the specific population of interest, intervention (and comparator) and outcome of interest may also help the researcher identify an appropriate outcome measurement tool. 7 The more defined the population of interest, and thus the more stringent the inclusion and exclusion criteria, the greater the effect on the interpretation and subsequent applicability and generalizability of the research findings. 1 , 2 A restricted study population (and exclusion criteria) may limit bias and increase the internal validity of the study; however, this approach will limit external validity of the study and, thus, the generalizability of the findings to the practical clinical setting. Conversely, a broadly defined study population and inclusion criteria may be representative of practical clinical practice but may increase bias and reduce the internal validity of the study.
PICOT criteria 1
A poorly devised research question may affect the choice of study design, potentially lead to futile situations and, thus, hamper the chance of determining anything of clinical significance, which will then affect the potential for publication. Without devoting appropriate resources to developing the research question, the quality of the study and subsequent results may be compromised. During the initial stages of any research study, it is therefore imperative to formulate a research question that is both clinically relevant and answerable.
Research hypothesis
The primary research question should be driven by the hypothesis rather than the data. 1 , 2 That is, the research question and hypothesis should be developed before the start of the study. This sounds intuitive; however, if we take, for example, a database of information, it is potentially possible to perform multiple statistical comparisons of groups within the database to find a statistically significant association. This could then lead one to work backward from the data and develop the “question.” This is counterintuitive to the process because the question is asked specifically to then find the answer, thus collecting data along the way (i.e., in a prospective manner). Multiple statistical testing of associations from data previously collected could potentially lead to spuriously positive findings of association through chance alone. 2 Therefore, a good hypothesis must be based on a good research question at the start of a trial and, indeed, drive data collection for the study.
The research or clinical hypothesis is developed from the research question and then the main elements of the study — sampling strategy, intervention (if applicable), comparison and outcome variables — are summarized in a form that establishes the basis for testing, statistical and ultimately clinical significance. 3 For example, in a research study comparing computer-assisted acetabular component insertion versus freehand acetabular component placement in patients in need of total hip arthroplasty, the experimental group would be computer-assisted insertion and the control/conventional group would be free-hand placement. The investigative team would first state a research hypothesis. This could be expressed as a single outcome (e.g., computer-assisted acetabular component placement leads to improved functional outcome) or potentially as a complex/composite outcome; that is, more than one outcome (e.g., computer-assisted acetabular component placement leads to both improved radiographic cup placement and improved functional outcome).
However, when formally testing statistical significance, the hypothesis should be stated as a “null” hypothesis. 2 The purpose of hypothesis testing is to make an inference about the population of interest on the basis of a random sample taken from that population. The null hypothesis for the preceding research hypothesis then would be that there is no difference in mean functional outcome between the computer-assisted insertion and free-hand placement techniques. After forming the null hypothesis, the researchers would form an alternate hypothesis stating the nature of the difference, if it should appear. The alternate hypothesis would be that there is a difference in mean functional outcome between these techniques. At the end of the study, the null hypothesis is then tested statistically. If the findings of the study are not statistically significant (i.e., there is no difference in functional outcome between the groups in a statistical sense), we cannot reject the null hypothesis, whereas if the findings were significant, we can reject the null hypothesis and accept the alternate hypothesis (i.e., there is a difference in mean functional outcome between the study groups), errors in testing notwithstanding. In other words, hypothesis testing confirms or refutes the statement that the observed findings did not occur by chance alone but rather occurred because there was a true difference in outcomes between these surgical procedures. The concept of statistical hypothesis testing is complex, and the details are beyond the scope of this article.
Another important concept inherent in hypothesis testing is whether the hypotheses will be 1-sided or 2-sided. A 2-sided hypothesis states that there is a difference between the experimental group and the control group, but it does not specify in advance the expected direction of the difference. For example, we asked whether there is there an improvement in outcomes with computer-assisted surgery or whether the outcomes worse with computer-assisted surgery. We presented a 2-sided test in the above example because we did not specify the direction of the difference. A 1-sided hypothesis states a specific direction (e.g., there is an improvement in outcomes with computer-assisted surgery). A 2-sided hypothesis should be used unless there is a good justification for using a 1-sided hypothesis. As Bland and Atlman 8 stated, “One-sided hypothesis testing should never be used as a device to make a conventionally nonsignificant difference significant.”
The research hypothesis should be stated at the beginning of the study to guide the objectives for research. Whereas the investigators may state the hypothesis as being 1-sided (there is an improvement with treatment), the study and investigators must adhere to the concept of clinical equipoise. According to this principle, a clinical (or surgical) trial is ethical only if the expert community is uncertain about the relative therapeutic merits of the experimental and control groups being evaluated. 9 It means there must exist an honest and professional disagreement among expert clinicians about the preferred treatment. 9
Designing a research hypothesis is supported by a good research question and will influence the type of research design for the study. Acting on the principles of appropriate hypothesis development, the study can then confidently proceed to the development of the research objective.
Research objective
The primary objective should be coupled with the hypothesis of the study. Study objectives define the specific aims of the study and should be clearly stated in the introduction of the research protocol. 7 From our previous example and using the investigative hypothesis that there is a difference in functional outcomes between computer-assisted acetabular component placement and free-hand placement, the primary objective can be stated as follows: this study will compare the functional outcomes of computer-assisted acetabular component insertion versus free-hand placement in patients undergoing total hip arthroplasty. Note that the study objective is an active statement about how the study is going to answer the specific research question. Objectives can (and often do) state exactly which outcome measures are going to be used within their statements. They are important because they not only help guide the development of the protocol and design of study but also play a role in sample size calculations and determining the power of the study. 7 These concepts will be discussed in other articles in this series.
From the surgeon’s point of view, it is important for the study objectives to be focused on outcomes that are important to patients and clinically relevant. For example, the most methodologically sound randomized controlled trial comparing 2 techniques of distal radial fixation would have little or no clinical impact if the primary objective was to determine the effect of treatment A as compared to treatment B on intraoperative fluoroscopy time. However, if the objective was to determine the effect of treatment A as compared to treatment B on patient functional outcome at 1 year, this would have a much more significant impact on clinical decision-making. Second, more meaningful surgeon–patient discussions could ensue, incorporating patient values and preferences with the results from this study. 6 , 7 It is the precise objective and what the investigator is trying to measure that is of clinical relevance in the practical setting.
The following is an example from the literature about the relation between the research question, hypothesis and study objectives:
Study: Warden SJ, Metcalf BR, Kiss ZS, et al. Low-intensity pulsed ultrasound for chronic patellar tendinopathy: a randomized, double-blind, placebo-controlled trial. Rheumatology 2008;47:467–71.
Research question: How does low-intensity pulsed ultrasound (LIPUS) compare with a placebo device in managing the symptoms of skeletally mature patients with patellar tendinopathy?
Research hypothesis: Pain levels are reduced in patients who receive daily active-LIPUS (treatment) for 12 weeks compared with individuals who receive inactive-LIPUS (placebo).
Objective: To investigate the clinical efficacy of LIPUS in the management of patellar tendinopathy symptoms.
The development of the research question is the most important aspect of a research project. A research project can fail if the objectives and hypothesis are poorly focused and underdeveloped. Useful tips for surgical researchers are provided in Box 3 . Designing and developing an appropriate and relevant research question, hypothesis and objectives can be a difficult task. The critical appraisal of the research question used in a study is vital to the application of the findings to clinical practice. Focusing resources, time and dedication to these 3 very important tasks will help to guide a successful research project, influence interpretation of the results and affect future publication efforts.
Tips for developing research questions, hypotheses and objectives for research studies
- Perform a systematic literature review (if one has not been done) to increase knowledge and familiarity with the topic and to assist with research development.
- Learn about current trends and technological advances on the topic.
- Seek careful input from experts, mentors, colleagues and collaborators to refine your research question as this will aid in developing the research question and guide the research study.
- Use the FINER criteria in the development of the research question.
- Ensure that the research question follows PICOT format.
- Develop a research hypothesis from the research question.
- Develop clear and well-defined primary and secondary (if needed) objectives.
- Ensure that the research question and objectives are answerable, feasible and clinically relevant.
FINER = feasible, interesting, novel, ethical, relevant; PICOT = population (patients), intervention (for intervention studies only), comparison group, outcome of interest, time.
Competing interests: No funding was received in preparation of this paper. Dr. Bhandari was funded, in part, by a Canada Research Chair, McMaster University.
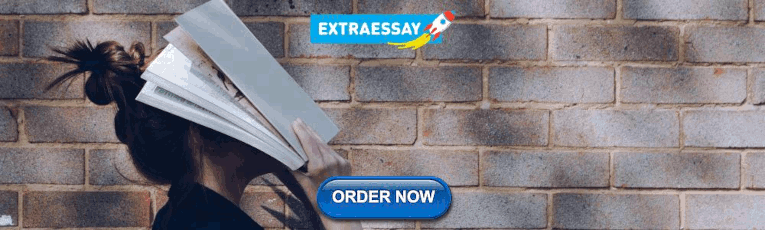
IMAGES
VIDEO
COMMENTS
Developing a hypothesis (with example) Step 1. Ask a question. Writing a hypothesis begins with a research question that you want to answer. The question should be focused, specific, and researchable within the constraints of your project. Example: Research question.
To identify the hypothesis in a research article, focus on the introduction section where the authors present the research questions and hypotheses. Look for statements that propose a relationship or effect between variables. The hypothesis is often presented after a brief review of existing literature and is a clear, testable statement that ...
The first few paragraphs of a journal article serve to introduce the topic, to provide the author's hypothesis or thesis, and to indicate why the research was done. A thesis or hypothesis is not always clearly labeled; you may need to read through the introductory paragraphs to determine what the authors are proposing.
Step 5: Phrase your hypothesis in three ways. To identify the variables, you can write a simple prediction in if … then form. The first part of the sentence states the independent variable and the second part states the dependent variable. If a first-year student starts attending more lectures, then their exam scores will improve.
The first few paragraphs of a journal article serve to introduce the topic, to provide the author's hypothesis or thesis, and to indicate why the research was done. A thesis or hypothesis is not always clearly labled; you may need to read through the introductory paragraphs to determine what the authors are proposing.
INTRODUCTION. Scientific research is usually initiated by posing evidenced-based research questions which are then explicitly restated as hypotheses.1,2 The hypotheses provide directions to guide the study, solutions, explanations, and expected results.3,4 Both research questions and hypotheses are essentially formulated based on conventional theories and real-world processes, which allow the ...
3. Simple hypothesis. A simple hypothesis is a statement made to reflect the relation between exactly two variables. One independent and one dependent. Consider the example, "Smoking is a prominent cause of lung cancer." The dependent variable, lung cancer, is dependent on the independent variable, smoking. 4.
Interestingly, a recent analysis of 111 publications related to Strachan's hygiene hypothesis, stating that the lack of exposure to infections in early life increases the risk of rhinitis, revealed a selection bias of 5,551 citations on Web of Science.37 The articles supportive of the hypothesis were cited more than nonsupportive ones (odds ...
It seeks to explore and understand a particular aspect of the research subject. In contrast, a research hypothesis is a specific statement or prediction that suggests an expected relationship between variables. It is formulated based on existing knowledge or theories and guides the research design and data analysis. 7.
An effective hypothesis in research is clearly and concisely written, and any terms or definitions clarified and defined. Specific language must also be used to avoid any generalities or assumptions. Use the following points as a checklist to evaluate the effectiveness of your research hypothesis: Predicts the relationship and outcome.
A research hypothesis (also called a scientific hypothesis) is a statement about the expected outcome of a study (for example, a dissertation or thesis). To constitute a quality hypothesis, the statement needs to have three attributes - specificity, clarity and testability. Let's take a look at these more closely.
Present the findings in your results and discussion section. Though the specific details might vary, the procedure you will use when testing a hypothesis will always follow some version of these steps. Table of contents. Step 1: State your null and alternate hypothesis. Step 2: Collect data. Step 3: Perform a statistical test.
A hypothesis is a tentative statement about the relationship between two or more variables. It is a specific, testable prediction about what you expect to happen in a study. It is a preliminary answer to your question that helps guide the research process. Consider a study designed to examine the relationship between sleep deprivation and test ...
Tips for Writing a Research Hypothesis. If you understood the distinction between a hypothesis and a prediction we made at the beginning of this article, then you will have no problem formulating your hypotheses and predictions correctly. To refresh your memory: We have to (1) look at existing evidence, (2) come up with a hypothesis, (3) make a ...
HYPOTHESIS TESTING. A clinical trial begins with an assumption or belief, and then proceeds to either prove or disprove this assumption. In statistical terms, this belief or assumption is known as a hypothesis. Counterintuitively, what the researcher believes in (or is trying to prove) is called the "alternate" hypothesis, and the opposite ...
Research hypothesis checklist. Once you've written a possible hypothesis, make sure it checks the following boxes: It must be testable: You need a means to prove your hypothesis. If you can't test it, it's not a hypothesis. It must include a dependent and independent variable: At least one independent variable ( cause) and one dependent ...
Section 1. displayed as a theoretical analysis or interpreta-tion of the research question. Finally, the research article will conclude with a discussion and summary of the study. In the discussion,you will find that the hypothesis is usually restated, and perhaps a small discussion of why this is the hypothesis.
The steps to write a research hypothesis are: 1. Stating the problem: Ensure that the hypothesis defines the research problem. 2. Writing a hypothesis as an 'if-then' statement: Include the action and the expected outcome of your study by following a 'if-then' structure. 3.
Introduction. A research article represents a compilation of information by a scientist concerning an original research idea. It is characterized by a wide range of information including, the purpose of the study, the thesis statement, hypothesis, literature review, methodology, results and conclusion.
Research problems and hypotheses are important means for attaining valuable knowledge. They are pointers or guides to such knowledge, or as formulated by Kerlinger ( 1986, p. 19): " … they direct investigation.". There are many kinds of problems and hypotheses, and they may play various roles in knowledge construction.
This is why I wrote this article: to explain hypothesis testing the way I wish it had been explained to me in the first place. And hopefully to get you to the same aha moment I had. The article will be structured in the following paragraphs: Step 0. Looking at the confusion matrix; Step 1. Defining the hypothesis; Step 2.
Precision in formulating your hypothesis cannot be overstated. A good hypothesis is specific, stating exactly what you expect to find. It should be simple, focused, and to the point, avoiding any ...
Identifying a hypothesis allows students to know what is being proven by a particular experiment or paper. Being able to determine the overall point not only makes you a more effective reader but also better at formulating your own theories when writing your own paper. By asking a few simple questions while you read, ...
The hypothesis is only one of the things that we will be extracting from the articles. At the moment we are focusing on atmospheric science papers (that's my major) and I've been able to extract the papers by sections and like you mentioned, we will be focusing on the last few paragraphs of the introduction.
In the globalized world, factors, such as environmental regulations and outcomes, are interlinked with foreign direct investment and technological innovation. However, firm-level theories mostly treat them independently. We have filled the gap by carrying out empirical research with an integrated approach at the firm level. The theoretical framework is based on Porter's hypothesis and the ...
Many societies around the world are rapidly aging and this has implications for social and economic development. We collect data on NEEQ-listed enterprises from 2010 to 2021 in China and empirically test the effect of population aging on the digital transformation of small and medium-sized enterprises (SMEs). The findings show that population aging has a significant positive impact on SME ...
One hypothesis was that the planet is a 'water world' enveloped by supercritical water molecules; another was that it is surrounded by an expansive, primordial atmosphere composed mainly of ...
Research shows that most disabled employees experience some form of workplace discrimination, including hiring biases, pay gaps, bullying, and mistreatment. In addition, when people with ...
BackgroundThe association between hypothyroidism and Parkinson's disease (PD) has sparked intense debate in the medical community due to conflicting study results. A better understanding of this association is crucial because of its potential implications for both pathogenesis and treatment strategies.MethodsTo elucidate this complex relationship, we used Bayesian co-localisation (COLOC) and ...
The development of the research question, including a supportive hypothesis and objectives, is a necessary key step in producing clinically relevant results to be used in evidence-based practice. A well-defined and specific research question is more likely to help guide us in making decisions about study design and population and subsequently ...