Animation Studies
The Peer-reviewed Open Access Online Journal for Animation History and Theory – ISSN 1930-1928
The journal invites original English-language essays (5,000-10,000 words, including endnotes and bibliography) addressing animated works from any part of the world.
Advertisement
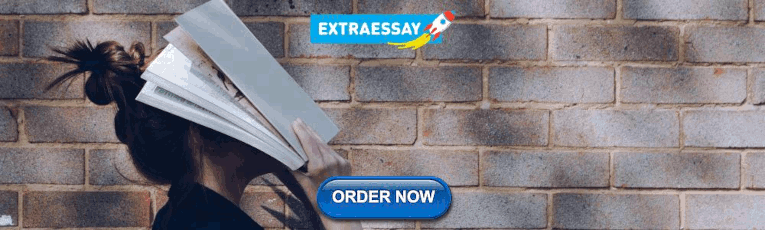
When learning from animations is more successful than learning from static pictures: learning the specifics of change
- Original Research
- Open access
- Published: 26 May 2021
- Volume 49 , pages 497–514, ( 2021 )
Cite this article
You have full access to this open access article
- Rolf Ploetzner ORCID: orcid.org/0000-0003-4894-6631 1 ,
- Sandra Berney ORCID: orcid.org/0000-0002-6643-9302 2 &
- Mireille Bétrancourt ORCID: orcid.org/0000-0001-7144-167X 2
7551 Accesses
12 Citations
2 Altmetric
Explore all metrics
The results of three meta-analyses show that the effectiveness of learning from animations, when compared to learning from static pictures, is rather limited. A recent re-analysis of one of these meta-analyses, however, supports that learning from animations is considerably more effective than learning from static pictures if the specifics of the displayed changes need to be learned. In order to further validate this finding as well as to clarify the educational strengths and weaknesses of animations and static pictures, an experimental study with three groups was conducted. Overall, 88 university students participated in the study. One group of learners ( n = 30) watched a single picture of a gear mechanism, one group of learners ( n = 28) watched four pictures, and one group of learners ( n = 30) watched an animation. All groups had to identify specific motions and spatial arrangements covered by the gear mechanism. While learners who watched the animation exhibited the best performance with respect to the identification of motions, learners who watched the pictures showed the best performance with respect to the identification of spatial arrangements. The effect sizes are large. The results of the study help to clarify when animations and when static pictures are most suitable for learning.
Similar content being viewed by others
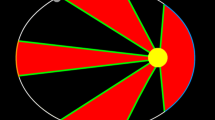
The moderating role of additional information when learning with animations compared to static pictures
Learning from Animations: From 2D to 3D?
Looking across instead of back and forth: how the simultaneous presentation of multiple animation episodes facilitates learning.
Avoid common mistakes on your manuscript.
Introduction
In education, animations are employed to support the perception, mental representation, and understanding of changes in space and time. However, the educational effectiveness of animations has been challenged by three meta-analyses which compared learning from animations with learning from static pictures. In the first meta-analysis, Höffler and Leutner ( 2007 ) analyzed 76 pair-wise comparisons. They observed a merely small overall effect size of d = 0.37 in favor of animations and videos. Almost 10 years later, the second analysis by Berney and Bétrancourt ( 2016 ) covered 140 pair-wise comparisons. It resulted in an even smaller overall effect size of g = 0.226 in favor of animations. The third analysis by Castro-Alonso et al. ( 2019 ) involved 82 pair-wise comparisons. It also yielded an overall effect size of merely g = 0.23 in favor of animations.
The disillusioning results of these meta-analyses resulted in an increasingly growing skepticism over the educational benefits of animations. For instance, Clark and Mayer ( 2016 , p. 84) suggest “… to use static illustrations unless there is a compelling instructional rationale for animation. In particular, when you have an explanative illustration, we recommend presenting a series of static frames to depict the various states of the system rather than a lock-step animation.” However, what denotes a compelling instructional rationale for the use of animation? One important reason for the educational use of animations is that learners need to grasp what animations can overtly present: how change in space and time occurs. Already 2002 Tversky, Morrison, and Bétrancourt assumed “… if there are benefits to animation, they should be evident especially for continuous rather than discrete changes, in particular, for manner of change and for microsteps, the subtle and intricate timing relations among parts of a complex system.” (Tversky et al., 2002 , p. 258). Until recently, however, the specific potential of animations has not received sufficient attention in empirical research comparing the educational effectiveness of animations and static pictures.
More recently, Ploetzner et al. ( 2020 ) re-analyzed the meta-analysis originally published by Berney and Bétrancourt ( 2016 ). They investigated a new moderator that encodes whether the specific features of the displayed changes were irrelevant or relevant to learning. They distinguished between simple and complex features with respect to the features of change. A frequent type of change is motion, for instance. If it merely had to be learned in which direction an object moves, this was coded as a simple feature of change relevant to learning. However, if it had to be learned whether an object moves slow or fast, or whether an object speeds up or slows down, this was coded as a complex feature of change relevant to learning.
Learning from animations was significantly more successful than learning from static pictures if either simple ( g = 0.340) or complex ( g = 0.647) features of the displayed changes had to be learned. If neither simple nor complex features of the presented changes had to be learned, it was irrelevant whether learning took place on the basis of animations or static pictures ( g = 0.043). These results suggest that when the learning domain includes only simple forms of change, many learners who are presented static pictures seem to be able to construct suitable mental animations. However, when challenging forms of change are to be learned, mental animation is more likely to be difficult and prone to error, thus learning from animated displays is more beneficial (cf. Hegarty et al., 2003 ).
The authors conclude that their re-analysis is of heuristic value and that future research on learning from animations needs to validate this finding by means of experimental studies. In this paper, we present an experimental study that compares learning from an animation with learning from static pictures. It aims to experimentally challenge the findings reported by Ploetzner et al. ( 2020 ) as well as to investigate the potential and limitations of animations for learning in relation to static pictures. The study is therefore not only based on the representational characteristics of animations and static pictures, but also on a model of how animations are perceptually and cognitively processed.
In the following sections, the theoretical background is described. Thereafter, the study that experimentally compares learning from an animation with learning from static pictures is presented. A discussion and conclusions complete the paper.
Theoretical background
Animations and static pictures have different representational characteristics. Both display visuospatial information (cf. Ploetzner & Lowe, 2012 ; Schnotz & Lowe, 2008 ). Visuospatial information refers to the set of graphic entities that make up the display as well as how these entities are arranged in space. Spatial arrangements can be specified by referring to the absolute positions of entities in the display (e.g. an entity is located in the upper left corner) or by referring to the relative positions of entities (e.g. an entity is located left of another entity). Furthermore, visual spatial information refers to how entities are spatially organized (e.g. an entity is made up of two other entities).
In contrast to static pictures, animations display not only visuospatial but also spatiotemporal information, i.e., changes in space over time (cf. Scaife & Rogers, 1996 ). Animations consist of a sequence of static pictures in which each picture differs slightly from the preceding picture. If the separate pictures are displayed at a sufficient rate (e.g. 24 pictures per second), humans experience the optical illusion of continuous change in the display. Spatiotemporal information refers to the set of events that constitute an animation. Events denote entities and how they change over time. During events, entities might change (e.g. an entity gets larger) and/or the spatial arrangements of entities might change (e.g. an entity rotates around another entity). Furthermore, spatiotemporal information refers to how events are temporarily organized (e.g. an event takes place before another event or two events take place simultaneously).
Whereas animations display changes explicitly, static pictures can merely indicate them by means of arrows or onion skins, for example (cf. Jenkinson, 2017 ). Hence, when learning from animations, the learners can directly perceive the changes. In contrast, learners have to infer the changes when learning from static pictures – a process that is often demanding and prone to error (cf. Hegarty et al., 2003 ). Thus, animations are more informative than static pictures with regard to the changes that occur in the displayed subject matter (see also Kühl et al., 2018 ). Therefore, it is frequently assumed that learning from animations is more effective than learning from static pictures if the displayed subject matter involves changes in space and time.
Even if an animation explicitly depicts visuospatial and spatiotemporal information, in order to be educationally effective, it needs to be sufficiently perceived and comprehended. The Animation Process Model (APM; Lowe & Boucheix, 2008 , 2011 ; Lowe & Schnotz, 2014 ) describes how learning from unnarrated animations progresses in order to construct a more and more complete mental model of the presented subject matter (see also Kriz & Hegarty, 2007 ). The model describes five phases in which perceptual bottom-up and cognitive top-down processes interact. However, it is not assumed that learners process these phases in linear order. Especially learners with only little prior knowledge will have to repeatedly apply the different processes before adequate understanding is reached.
According to Lowe and Boucheix ( 2008 , 2011 ), during Phase 1 the learners identify confined event units which may be presented at different spatial and temporal locations. Event units represent graphic entities and the behavior they exhibit. If the learners possess only little prior knowledge about the displayed subject matter, the separation of event units will mostly be a bottom-up process. That is, it relies mainly on the perceptual properties of the visual display, such as the colors and sizes of presented areas or the relative rates at which areas in the display change.
The event units identified in Phase 1 make up the basic components for the succeeding phase. In Phase 2, event units are gradually and iteratively merged into larger but still restricted structures. Essential to this activity is the construction of visuospatial and spatiotemporal relations that depend on the perceptual properties of the animated display. For instance, event units that are close to each other in space or time may be combined into spatiotemporal structures named dynamic micro-chunks. During Phase 3, spatially and temporarily distributed dynamic micro-chunks are combined to produce more extensive relational structures such as causal chains. This demands the use of domain-relevant general knowledge. The iterative combination of established relational structures can finally embrace the animation’s entire spatial and temporal extent, yielding a global characterization of the animation.
In Phase 4, by taking advantage of domain-specific prior knowledge, the learners associate functional tasks with the established structures. As a consequence, the structures are described as functional episodes which represent the functionality of the displayed subject matter. During Phase 5, the learners refine the established functionality to identify the conditions under which the animated system operates, for example. This may result in a mental model of the animated subject matter that is complete, coherent, and consistent. Subsequently, such a model can be applied to new but analogous situations.
In studies of learning from animation, the participants are mostly beginners with respect to the animated subject area. In order to compensate for the learners’ lack of domain-specific knowledge, the dynamic visualizations are often combined with spoken or written narrations. The narrations may guide the learners’ attention to specific events in the display, comment and describe events, or explain relationships between events such as causes and effects. If an animation involves both pictorial and verbal information, the learners need to sufficiently relate both sources of information. The cognitive theory of multimedia learning (Mayer, 2009 , 2014 ) and the integrated model of text and picture comprehension (Schnotz, 2014 ) both delineate the perceptual processes as well as the cognitive processes that are important to learning from pictorial and verbal information.
The distinction between perceptual and cognitive processes drawn in the APM emphasizes that learning at the perceptual level and learning at the cognitive level can make up educational objectives in their own rights. Although education very often strives for the acquisition of conceptual models, the meta-analyses by Höffler and Leutner ( 2007 ), Berney and Bétrancourt ( 2016 ), and Castro-Alonso et al. ( 2019 ), together with the meta-analysis by Ploetzner et al. ( 2020 ) indicate that the teaching of conceptual models is not a specific strength of animations. Instead, the meta-analysis by Ploetzner et al. ( 2020 ) suggests that animations can be more successfully employed if kinematic models need to be learned, i.e. if the learners need to construct mental representations of the displayed changes and how they unfold in time (cf. Hegarty & Just, 1993 ; Hegarty et al., 2003 ).
Experimental study
Research questions and hypotheses.
Due to the different representational characteristics of animations and static pictures, the educational effectiveness of animations and static pictures might depend on the learning tasks that have to be accomplished. The meta-analysis conducted by Ploetzner et al. ( 2020 ) gives raise to the assumption that learning from animations is more successful than learning from static pictures if the specifics of the displayed changes have to be learned. It is hypothesized that this result is due to the fact that animations display spatiotemporal information completely and explicitly. Static pictures, in contrast, depict spatiotemporal information merely incompletely and implicitly.
Both animations and static pictures display visuospatial information completely and explicitly. It can, therefore, be hypothesized that visuospatial arrangements can be learned equally well from animations and static pictures. It might even be that visuospatial information is more successfully learned from static pictures than from animations. Visuospatial arrangements are stationary in static pictures. As a consequence, the learners can attend to and identify them without being visually distracted. In contrast, visuospatial arrangements are permanently changing in animations. These changes might make it difficult for the learners to attend to and identify specific arrangements at specific points in time. That is, while animations might lead the learners to focus on spatiotemporal aspects, static pictures might lead the learners to focus on visuospatial aspects (cf. Ploetzner & Fillisch, 2017 ).
Thus, two hypotheses were tested in an experimental study:
If spatiotemporal information – especially the specifics of the displayed change – are relevant to the learning task, then learning from an animation is expected to be more successful than learning from static pictures.
If visuospatial information – such as spatial arrangements – has to be learned, then learning from static pictures is expected to be equally or more successful than learning from an animation.
Learning task
The learning task refers to a six-bar linkage, a gear mechanism that moves in a plane (cf. Fig. 1 ). Six-bar linkages are constructed from six links – including the rack – and seven joints (cf. Volmer, 1992 ). They very often transform continuous rotation into complex motion.
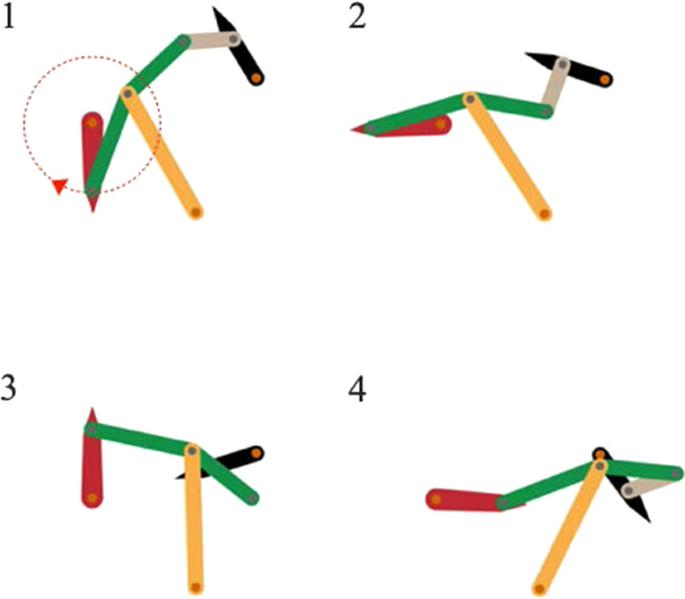
A sequence of four states of the six-bar linkage employed in the study (reconstructed with permission of the digital mechanism and gear library, www.dmg-lib.org )
The employed six-bar linkage Footnote 1 converts continuous clockwise rotation of the input, or drive gear (red link), into discontinuous counterclockwise rotation of the output gear (black link) with three halts of different durations. The transformation is realized by two nonuniformly moving couplers (green link and beige link) and an asymmetrically oscillating crank (yellow link). The links are connected by three joints fixed to the rack (orange joints) and four joints moving in a plane (grey joints).
Thus, with the exception of the input gear, the links move non-uniformly, asymmetrically, or even discontinuously. In machine engineering, gear atlases were traditionally used to describe the motion of linkages by static diagrams (e.g. Hain, 1972 ). These diagrams depict the paths of links as well as the relative gradients of links over time, for example. However, because even machine engineers may have difficulty in inferring the motion of linkages on the basis of static representations (Brix et al., 2005 ), digital gear libraries have been established in order to dynamically present the linkages (e.g. www.dmg-lib.org ).
The learning task consisted of watching either one picture of the six-bar linkage, four pictures, or an animation. Thereafter, the learners had to identify the correct motion of each link out of four motions. Furthermore, the leaners had to identify the correct arrangement of each pair of joined links out of four arrangements.
In the main study, the learners have to identify the motion of each link out of four different motions as well as the arrangement of each pair of joined links out of four different arrangements. It was therefore investigated in a pre-study, as to whether the learners could sufficiently distinguish between the different motions and arrangements they were shown.
Two groups of learners were investigated. While one group had to distinguish between different motions of each link, the other group had to distinguish between different arrangements of each pair of joined links.
Participants
A total of 33 students volunteered for the study and received financial compensation for their participation. All students were enrolled in undergraduate pre-service teacher programs in the STEM and other disciplines at a university in southwest Germany. The students were randomly assigned to the group that had to distinguish between motions (14 females and 3 males, mean age M = 21.29 years, SD = 3.33) and the group that had to distinguish between arrangements (13 females and 3 males, mean age M = 23.25 years, SD = 2.86).
The six-bar linkage consists of five moving links. With respect to each link four kinds of motion were animated: (1) continuous uniform motion (red, green, grey, and black link) or continuous symmetrical oscillation (yellow link), (2) continuous non-uniform motion (red, green, grey, and black link) or continuous asymmetrical oscillation (yellow link), (3) mirror-inverted continuous, nonuniform motion (red, green, grey, and black link) or mirror-inverted, continuous asymmetrical oscillation (yellow link), and (4) discontinuous motion (all links). All animations of a link moved in the same direction and covered the same path. This resulted in 5 × 4 = 20 animations covering five correct and 15 incorrect motions. Each animation lasted 6.2 s.
Because the six-bar linkage consists of n = 5 moving but joined links, n−1 = 4 pairs of joined links can be considered. With respect to each pair four kinds of arrangement were displayed: (1) correct joint and correct relative position, (2) correct joint and mirror-inverted relative position of one link, (3) incorrect joint and correct relative position, and (4) incorrect joint and mirror-inverted relative position of one link. This resulted in 4 × 4 = 16 pictures covering four correct and 12 incorrect arrangements.
All animations of links and pictures of arrangements were produced with Adobe Animate CC and Adobe Illustrator CC. The left-hand side of Fig. 2 shows snapshots of the four animations of the green link at three seconds. Because the animations exhibit different motions, they only display the same positions and orientations at the start. The right-hand side of Fig. 2 shows the four arrangements of the green and yellow links. Of interest is if the learners are presented any of the four animations or any of the four arrangements, would they be able to distinguish the animation or arrangement they had watched from the other three animations or arrangements?
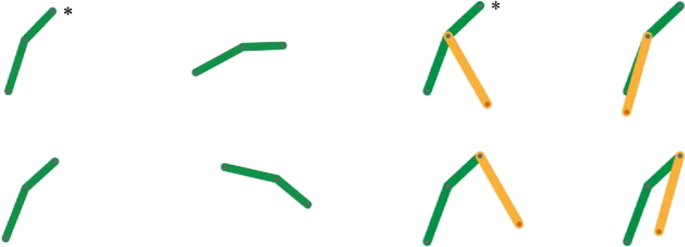
Snapshots of four animations at three seconds (left) and pictures of four arrangements (right). The correct animation and arrangement are marked with an asterisk
The learning tasks were presented to the learners by a computer program made with MatchWare Mediator 9. The size of the presentation area was 1200 × 1000 pixels. Initially, the use of the program and the learning task were described to the learners. Thereafter, an example of an animation or of an arrangement was shown for 31 s, i.e. the duration of five iterations of an animation. The examples relied on a four-bar linkage. Next, four animations or four arrangements were presented in quadrants labelled A, B, C, and D (cf. Fig. 3 ). In order to not confuse the leaners by presenting four animations simultaneously, none of the animations or arrangements were initially visible. When the mouse was moved over a quadrant, the looping animation or the stationary arrangement became immediately visible. Thus, the learners were able to watch one animation or arrangement at a time. Furthermore, the learners were able to move the mouse back and forth between quadrants as long as they wished. After the learners decided which animation or arrangement corresponded to the one they had seen before, they received feedback as to whether their decision was correct.
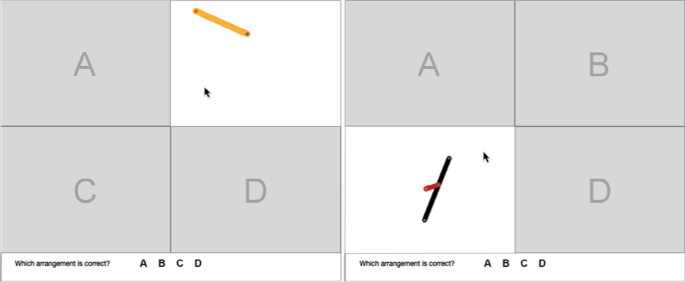
The four quadrants with an example animation (left) and an example arrangement (right) uncovered (translated by the authors)
After the example was finished, the 20 animations or the 16 arrangements described above were presented to the learners in random order. Each animation or arrangement was presented in the same manner as the animation or arrangement in the example. Only when all of the animations or arrangements were processed by the learners did they receive feedback as to how many of their decisions were correct. Every correct response was scored with one point. The maximum score with respect to the identification of motions was 20 points; the maximum score with respect to the identification of arrangements was 16 points.
Students participated in groups of up to 12 individuals. Each student was individually seated in front of a computer with a 21-inch screen. The computers were placed on separate tables. One group of students watched a randomized sequence of 20 moving links. After watching a moving link, the students had to identify the motion out of four motions. The other group of students watched a randomized sequence of 16 arrangements of pairs of joined links. After watching a pair of joined links, the students had to identify the arrangement out of four arrangements. The procedure took about 30 min.
On average, 18.53 moving links (92.65%, SD = 1.18) and 15.56 arrangements of pairs of joined links were identified correctly (97.25%, SD = 0.81). The results demonstrate that the learners were able to sufficiently distinguish between the motions as well as the arrangements they were shown.
Three groups of learners were investigated (cf. Fig. 4 ). In group ‘Picture’, the learners watched a single picture of the six-bar linkage. In group ‘Four Pictures’, the learners viewed a sequence of four pictures. In group ‘Animation’, the learners watched an animation.
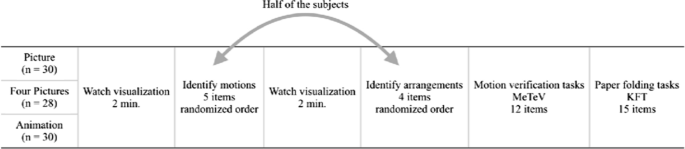
The design of the main study
To compare learning from an animation to learning from a single picture might be sound from a methodological point of view (cf. Castro-Alonso et al., 2016 ). However, it might be problematic from a psychological point of view. If an animation is replaced by pictures, information gets lost. The fewer pictures are presented, the more information gets lost. Novice learners are hardly able to infer motions from a single picture. Therefore, we also presented a sequence of four pictures of the six-bar linkage. This gave the learners – at least in principle – the opportunity to infer motions by comparing and contrasting the presented pictures (cf. Ploetzner & Lowe, 2014 ).
In each group, the learners had to accomplish two learning tasks and were therefore required to watch the static or dynamic visualization of the six-bar linkage twice. After one viewing, the learners had to identify the correct motion of each link. After the other viewing, the learners had to identify the correct arrangement of each pair of joined links. To counterbalance possible sequencing effects (e.g. Jhangiani et al., 2019 ), within each group, half of the learners received the learning tasks in inverse order than the other half. Furthermore, the learners’ mechanical ability and spatial ability were assessed as potential covariates.
A total of 88 students volunteered for the study and received financial compensation for their participation. All students were enrolled in undergraduate pre-service teacher programs in the STEM and other disciplines at a university in southwest Germany. The students were randomly assigned to the group ‘Picture’ (23 females and 7 males, mean age M = 21.47 years, SD = 2.54), the group ‘Four Pictures’ (21 females and 7 males, mean age M = 22.04 years, SD = 2.37), and the group ‘Animation’ (23 females and 7 males, mean age M = 21.63 years, SD = 3.01).
Learning tasks
The single picture, the sequence of four pictures, and the non-interactive animation of the six-bar linkage were produced with Adobe Illustrator CC and Adobe Animate CC. Figure 5 shows how the single picture (left-hand side), the sequence of four pictures (middle) and the animation (right-hand side) were presented to the learners. All visualizations of the six-bar linkage were of the same size. The single picture equals the first picture of the sequence of four pictures. The four pictures equal four frames shown in the animation. The single picture as well as the first picture of the sequence of pictures indicate the direction of rotation of the drive gear (red link) by a dashed circle with an arrow. The four pictures were numbered according to the succession of frames they depict. In all groups, the learners were additionally instructed in written form that the drive gear (red link) uniformly rotates in clockwise direction.
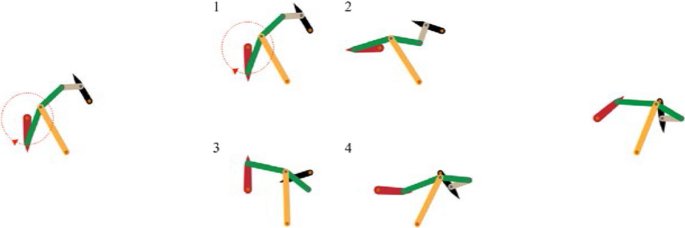
How the picture (left), the sequence of four pictures (middle), and the animation (right) of the six-bar linkage were presented to the learners
The five correct and 15 incorrect animations of individual links as well as the four correct and 12 incorrect pictures of arrangements of pairs of joined links were the same as those employed in the pre-study.
The learning tasks were presented to the learners by a computer program made with MatchWare Mediator 9. The size of the presentation area was 1200 × 1000 pixels. Initially, the use of the program and the learning tasks were described to the learners. Thereafter, a single picture, a sequence of four pictures, or an animation of an example four-bar linkage were presented to the learners for 90s. Next, the learners had to identify the motion of each link out of four motions or the arrangement of each pair of joined links out of four arrangements. All animations of individual links and all pictures of arrangements of pairs of joined links were presented in the same way as in the pre-study (cf. Fig. 3 ). After all animations or arrangements were processed by the learners, they received feedback as to how many of their decisions were correct.
After the example was finished, the single picture, the sequence of four pictures, or the animation of the six-bar linkage were shown to the learners for two minutes. Thereafter, the learners had to identify the motion of each link out of four motions or the arrangement of each pair of joined links out of four arrangements. Again, all animations of individual links and all pictures of arrangements of pairs of joined links were presented in the same way as in the pre-study (cf. Fig. 3 ). The correct and incorrect animations of links and the correct and incorrect pictures of arrangements were presented in random order. After all animations or arrangements were processed by the learners, they received feedback as to how many of their decisions were correct. Every correct response was scored with one point. The maximum score with respect to the animated links was 5 points, the maximum score with respect to the identification of arrangements was 4 points.
Mechanical ability
With respect to gear mechanisms, the learners’ mechanical ability makes up a domain-relevant competence. This competence might influence how the learners imagine and perceive the motion of the six-bar linkage. Thus, the learners’ mechanical ability was assessed by 12 motion verification tasks from the Test of Mechanical and Technical Understanding (MeTeV; Hartweg, 2010 ). Motion verification tasks can be solved on the basis of general mechanical principles (cf. Hegarty, 1992 ). Typically, they present a schematic picture of a mechanical system to the learners. The picture includes graphical or verbal information as to how certain components of the system move. The learners’ task is to infer from the picture how other components of the system move. Hegarty et al. ( 1988 ) termed the inference processes required by motion verification tasks “mechanical reasoning” (see also Hegarty, 1992 , 2004 ).
Figure 6 shows an example of a motion verification task taken from the Test of Mechanical and Technical Understanding. The format of all tasks was multiple-choice with four response options. Each task had to be processed within 90 s (cf. Hartweg, 2010 ). Every correct response was scored with one point. The maximum score was 12 points.
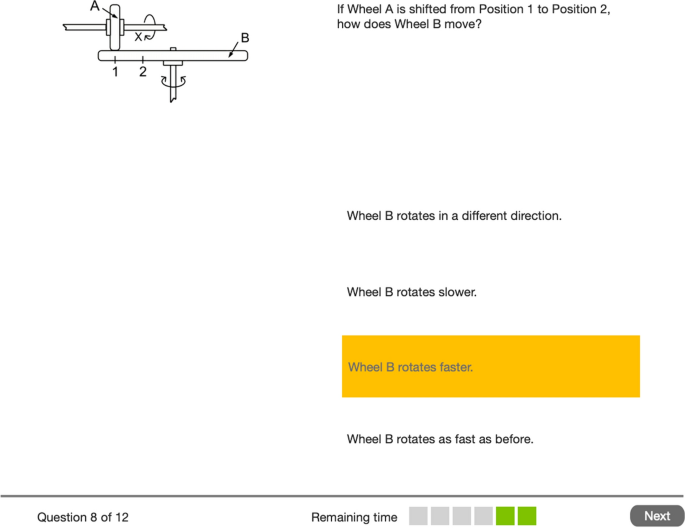
An example motion verification task from the Test of Mechanical and Technical Understanding (MeTeV; reconstructed with permission of Verena Hartweg; translated by the authors)
The Test of Mechanical and Technical Understanding has a reliability of 0.69 (Cronbach`s Alpha, cf. Hartweg, 2010 ). It correlates 0.56 with spatial-visual ability as measured by the Mannheimer Test for the Assessment of Physical-Technical Problem Solving (Conrad et al., 1980 ) and 0.67 with mechanical-technical understanding as measured by the Wilde Intelligence Test 2 (Kersting et al., 2008 ).
Spatial ability
Empirical research has demonstrated that learners with low or high spatial ability learn differently from static and dynamic visualizations (e.g. Höffler, 2010 ; Höffler & Leutner, 2011 ). Learners with high spatial ability are often more successful in constructing mental animations than learners with low spatial ability. As a consequence, the former learn more successfully from static pictures than the latter. In the present study, the learners’ spatial ability was assessed by the Subtest N3 of the Cognitive Ability Test (KFT; Heller & Perleth, 2000 ). It employs 15 paper folding tasks comparable to those originally proposed by Ekstrom et al. ( 1976 ). The format of all tasks was multiple-choice with five response options. Each task had to be processed within 40 s (cf. Heller & Perleth, 2000 ). Every correct response was scored with one point. The maximum score was 15 points.
The Subtest N3 of the Cognitive Ability Test has a reliability of 0.79 (Kuder-Richardson, cf. Heller & Perleth, 2000 ). Because the validation of the initial version of the Cognitive Ability Test took place before the Subtest N3 was included, the test manual does not report the construct validity for the Subtest N3 (cf. Heller & Perleth, 2000 ).
The motion verification tasks and the paper folding tasks were presented to the learners by the same computer program that displayed the learning tasks. After each set of tasks, the learners received feedback as to how many tasks they solved correctly.
Students participated in groups of up to 16 individuals. Each student was individually seated in front of a computer with a 21-inch screen. The computers were placed on separate tables. The computers presented the material to the students in the following order: (1) first presentation of the visualization (one picture, four pictures, or animation), (2) identification of motions (first half of the students) or arrangements (second half of the students), (3) second presentation of the same visualization, (4) identification of motions (second half of the students) or arrangements (first half of the students), (5) motion verification tasks, and (6) paper folding tasks. The procedure took about 50 min.
The means and standard deviations for each dependent variable are shown in Table 1 . The intercorrelations among the dependent variables are provided in Table 2 . While the group ‘Animation’ exhibited the best performance with respect to the identification of motions, the group ‘Picture’ showed the best performance with respect to the identification of arrangements.
The investigated groups did not significantly differ with respect to their mechanical ability ( F (2, 85) = 0.73, p = 0.484) or with respect to their spatial ability ( F (2, 85) = 1.16, p = 0.319). The correlations between the learners’ mechanical ability and their identifications of motions as well as between the learners’ mechanical ability and their identifications of arrangements were nonsignificant (cf. Table 2 ). Likewise, the correlations between the learners’ spatial ability and their identifications of motions as well as between the learners’ spatial ability and their identifications of arrangements were nonsignificant (cf. Table 2 ). Therefore, the learners’ mechanical and spatial ability were not considered as covariates in the analysis of variance.
The results of a multivariate analysis of variance are shown in Table 3 . On the multivariate level, the investigated groups differ significantly. The effect size is large (cf. Cohen, 1988 ; Rosenthal, 1994 ). On the univariate level, the groups differ significantly with respect to the identification of motions as well as with respect to the identification of arrangements. The effect sizes are large (cf. Cohen, 1988 ; Rosenthal, 1994 ). Concerning the identification of motions, post-hoc analyses further reveal that the group ‘Animation’ significantly differs from the group ‘Picture’ (Fisher’s Least Significant Difference LSD = 2.03, p < 0.01, d = 2.33) as well as from the group ‘Four Pictures’ ( LSD = 2.16, p < 0.01, d = 2.41). The group ‘Picture’ and the group ‘Four Pictures’ do not significantly differ from each other ( LSD = 0.12, p = 0.606).
With regard to the identification of arrangements, post-hoc analyses reveal that the group ‘Picture’ significantly differs from the group ‘Four Pictures’ ( LSD = 1.00, p < 0.01, d = 1.11) and the group ‘Animation’ ( LSD = 1.00, p < 0.01, d = 1.30). The group ‘Four Pictures’ and the group ‘Animation’ do not differ from each other ( LSD = 0.00, p = 0.985).
The research reported in this paper originated from the fact that three meta-analyses which compared learning from animation to learning from static pictures found only small overall effect sizes in favor of animations (Berney & Bétrancourt, 2016 ; Castro-Alonso et al., 2019 ; Höffler & Leutner, 2007 ). At the same time, the production of animations can be much more challenging, time-consuming, and costly than the production of static graphics. The potentially harmful consequences that researchers as well as practitioners may draw from the above findings is that the production of animations is not worth the extra effort (cf. Clark & Mayer, 2016 ).
In contrast, a recent meta-analysis by Ploetzner et al. ( 2020 ) found that learning from animation is considerably more effective than learning from static pictures if especially challenging features of change have to be learned. An experimental study was conducted in order to validate this finding. The learning task was made up of a mechanical device – a six-bar linkage – that produces accelerated, asymmetric, nonuniform, and discontinuous patterns of motion. Due to their irregularities, these patterns are difficult to predict by novices and even engineers (cf. Brix et al. 2005 ) on the basis of static pictures and everyday perceptual schemata (cf. Lowe & Schnotz, 2014 ). It was, therefore, hypothesized that watching an animation is more effective than watching static pictures for mentally representing the spatiotemporal information of the gear mechanism. However, because both animations and static pictures display visuospatial information completely and explicitly, it was hypothesized that watching an animation is not more effective than watching static pictures for mentally representing the visuospatial information of the gear mechanism. The results of the experimental study support both hypotheses and are in accord with the theoretical assumptions by Tversky et al. ( 2002 ) as well as with the results of the meta-analysis reported by Ploetzner et al. ( 2020 ).
While visuospatial information was more successfully learned from one picture than from the animation, learning from four pictures was not more successful than learning from the animation. This finding might indicate that both the four pictures and the animation provided abundant information to the learners. As a consequence, in both groups the learners might have had comparable difficulties in deciding on which information to focus and which information to extract.
The animation process model (APM, Lowe & Boucheix, 2008 , 2011 ; Lowe & Schnotz, 2014 ) distinguishes between perceptual and cognitive processes during learning from animation. Especially, the APM emphasizes that learning at the perceptual level and learning at the cognitive level each make up educational objectives in their own rights. To demonstrate the potential of animations for learning at the perceptual level, the focus of the experimental study was on perceptual processing during learning from animation. Correspondingly, the employed tasks for identifying motions and arrangements have a certain proximity to perceptual memory tasks (e.g. Castro-Alonso & Atit, 2019 ; Schurgin, 2018 ). Conceptual processing, in contrast, was not investigated. Whether the neglect of conceptual processing is considered a limitation of the present study depends on the educational objectives. In many domains, perceptual processing and learning is an educational objective in its own right: e.g. motion patterns of technical devices in science and engineering (cf. Lowe & Boucheix, 2011 ; Ploetzner & Fillisch, 2017 ) as well as motion patterns of body parts in biology, medicine, physiotherapy, and physical training (cf. Chen & Wu, 2016 ; Sukel et al., 2003 ).
In many learning contexts, however, both perceptual and conceptual processing is required (e.g. how a technical device needs to be constructed in order to produce a certain motion pattern). Although the animation processing model (Lowe & Boucheix, 2008 , 2011 ; Lowe & Schnotz, 2014 ) as well as the cognitive theory of multimedia learning (Mayer, 2009 , 2014 ) abstractly address the interplay between perceptual and conceptual processing, it still remains to be described in more detail as to precisely how perceptual processes during learning from animations can facilitate conceptual understanding and vice versa. Therefore, future studies could not only focus on perceptual processing during learning from animation but on both perceptual and conceptual processing. Such studies could help in better understanding of how perceptual processing contributes to conceptual understanding.
Unexpectedly, the learners’ spatial ability was not significantly related to learning performance. Especially with respect to the group ‘Picture’ and the group ‘Four Pictures’, it was expected that learners with higher spatial ability are more successful in mentally animating the relevant motions than learners with lower spatial ability (cf. Höffler, 2010 ; Höffler & Leutner, 2011 ). The descriptive data, together with the sporadic feedback of individual learners, give rise to the assumption that the learners in these groups were mostly overburdened with having to mentally animate the relevant motions. Even high spatial ability did not possibly help them in constructing suitable mental animations. Hence, this might indicate that even well-developed cognitive abilities cannot always compensate for learning material that is inadequate with respect to the learning task. Alternatively, because spatial ability constitutes a multidimensional construct (e.g. Buckley et al., 2018 ; Castro-Alonso & Atit, 2019 ), this might indicate that the employed assessment of spatial ability did not correspond well to the demands of the investigated learning situation.
The finding that instructional animations are especially beneficial when the specifics of change need to be learned certainly requires further validation. For instance, future studies could investigate learners with different characteristics (e.g. learners who possess more pre-knowledge about the investigated subject matter) or the learning of different subject matters. Furthermore, future studies could examine how learners construct mental representations of the observed movements. For instance, some learners might have tried to visually memorize the shown movements. Others might have tried to run a mental animation in order to mentally reconstruct the shown movements. Others might have produced internal verbalizations of the shown movements (e.g. the yellow link swings forth and back like a pendulum, fast from the right to the left and slow from the left to the right; cf. Lloyd-Jones et al., 2008 ). Still others might have used gestures (e.g. mimicking movements with their fingers) in order to the support the encoding of the shown movements (cf. Brucker et al., 2015 ; Lajevardi et al., 2017 ). Learners might have taken advantage of such strategies either in isolation or in combination. Understanding better how learners construct mental representations while they process an animation perceptually could help in developing measures that support learners during this phase of learning.
After years of growing skepticism with respect to the educational effectiveness of animations, the reported findings may help to cast instructional animations in a more positive light again. Reliable empirical evidence about the instructional strengths of animations as well as of static pictures would certainly help multimedia designers and educators to decide when to make use of animations and when to resort to static pictures.
Conclusions
In past research, animations have been frequently employed in a way that their potential for learning was not fully realized. In particular, the representational characteristics of the animated display were often not well aligned with the demands of the learning task. As a consequence, learning from animations was found to be only modestly more effective than learning from static pictures. In accord with a meta-analysis conducted by Ploetzner et al. ( 2020 ), the experimental study presented in this paper demonstrates that learning from animation is significantly more successful than learning from static pictures if the specific features of the displayed change are to be learned. Thus, animations can be highly effective tools to support dynamic perceptual learning and the acquisition of kinematic models. Static pictures, in contrast, are especially promising in teaching spatial configurations.
Data availability
The data set is available from the Open Science Foundation: https://doi.org/10.17605/OSF.IO/QP6UG .
Code availability
The text provides a link to the animation that underlies our reconstruction of the six-bar linkage. We have permission to reconstruct the linkage, to use the reconstruction in our research, and to include pictures of the reconstruction in publications. However, we have no permission to make the reconstruction itself available to the public. The employed mechanical ability and cognitive ability tests are available through the references provided in the text.
A video of the original linkage can be watched in the Digital Mechanism and Gear Library ( https://www.dmg-lib.org/dmglib/handler?manim=209022 ).
Berney, S., & Bétrancourt, M. (2016). Does animation enhance learning? A meta-analysis. Computers & Education, 101 , 150–167. https://doi.org/10.1016/j.compedu.2016.06.005
Article Google Scholar
Brix, T., Döring, U., & Trott, S. (2005). DMG-Lib: Ein moderner Wissensraum für die Getriebetechnik [DMG-Lib: A modern knowledge space for gear technology]. In Zentralbibliothek des Forschungszentrums Jülich (Hg.), Knowledge eXtended: Die Kooperation von Wissenschaftlern, Bibliothekaren und IT-Spezialisten [Knowledge eXtended: The cooperation of scientists, librarians, and specialists for information technology] (S. 251–262). Jülich: Forschungszentrum Jülich
Brucker, B., Ehlis, A.-C., Häußinger, F. B., Fallgatter, A. J., & Gerjets, P. (2015). Watching corresponding gestures facilitates learning with animations by activating human mirror-neurons: An fNIRS study. Learning and Instruction, 36 , 27–37. https://doi.org/10.1016/j.learninstruc.2014.11.003
Buckley, J., Seery, N., & Canty, D. (2018). A heuristic framework of spatial ability: A review and synthesis of spatial factor literature to support its translation into STEM education. Educational Psychology Review, 30 , 947–972. https://doi.org/10.1007/s10648-018-9432-z
Castro-Alonso, J. C., & Atit, K. (2019). Different abilities controlled by visuospatial processing. In J. C. Castro-Alonso (Ed.), Visuospatial processing for education in health and natural sciences. (pp. 23–51). Springer.
Chapter Google Scholar
Castro-Alonso, J. C., Ayres, P., & Paas, F. (2016). Comparing apples and oranges? A critical look at research on learning from statics versus animations. Computers & Education, 102 , 234–243. https://doi.org/10.1016/j.compedu.2016.09.004
Castro-Alonso, J. C., Wong, M., Adesope, O. O., Ayres, P., & Paas, F. (2019). Gender imbalance in instructional dynamic versus static visualizations: A meta-analysis. Educational Psychology Review, 31 , 361–387. https://doi.org/10.1007/s10648-019-09469-1
Chen, Y.-J., & Wu, J.-L. (2016). A computer-aided articulation learning system for subjects with articulation disorders. Engineering Computations, 33 , 2185–2197. https://doi.org/10.1108/EC-08-2015-0235
Clark, R. C., & Mayer, R. E. (2016). E-learning and the science of instruction . (4th ed.). Wiley.
Book Google Scholar
Cohen, J. (1988). Statistical power analysis for the behavioral sciences . (2nd ed.). Lawrence Erlbaum Associates.
Google Scholar
Conrad, W., Baumann, E., & Mohr, V. (1980). Mannheimer test zur erfassung des physikalisch-technischen problemlösens (MTP) [mannheimer test for the assessment of physical-technical problem solving] . Hogrefe Verlag.
Ekstrom, R. B., French, J. W., Harman, H. H., & Dermen, D. (1976). Kit of factor-referenced cognitive tests . Educational Testing Service.
Hain, K. (1973). Getriebebeispiel-atlas: Eine zusammenstellung ungleichförmig übersetzender getriebe für den konstrukteur [atlas of gear examples: a collection of nonuniformly transmitting gears for the constructing engineer] . VDI Verlag.
Hartweg, V. (2010). Mechanisch-technisches Verständnis als Konstrukt in der testbasierten Studienberatung [Mechanical-technical ability as a construct in the test-based student advisory service] (Doctoral Dissertation). Aachen: Rheinisch-Westfälische Technische Hochschule Aachen.
Hegarty, M. (1992). Mental animation: Inferring motion from static displays of mechanical systems. Journal of Experimental Psychology, 18 , 1084–1102. https://doi.org/10.1037/0278-7393.18.5.1084
Hegarty, M. (2004). Mechanical reasoning by mental simulation. Trends in Cognitive Sciences, 8 , 280–285. https://doi.org/10.1016/j.tics.2004.04.001
Hegarty, M., & Just, M. A. (1993). Constructing mental models of machines from text and diagrams. Journal of Memory and Language, 32 , 717–742. https://doi.org/10.1006/jmla.1993.1036
Hegarty, M., Just, M. A., & Morrison, J. (1988). Mental models of mechanical systems: Individual differences in qualitative and quantitative reasoning. Cognitive Psychology, 20 , 191–236. https://doi.org/10.1016/0010-0285(88)90019-9
Hegarty, M., Kriz, S., & Cate, C. (2003). The roles of mental animations and external animations in understanding mechanical systems. Cognition and Instruction, 21 , 325–360. https://doi.org/10.1207/s1532690xci2104_1
Heller, K. A., & Perleth, C. (2000). Kognitiver Fähigkeitstest für 4. bis 12. Klassen, Revision [Cognitive ability test for grades 4 to 12, revision]. Göttingen: Beltz Test GmbH
Höffler, T. N. (2010). Spatial ability: Its influence on learning with visualizations – A meta-analytic review. Educational Psychology Review, 22 , 245–269. https://doi.org/10.1007/s10648-010-9126-7
Höffler, T. N., & Leutner, D. (2007). Instructional animation versus static pictures: A meta-analysis. Learning and Instruction, 17 , 722–738. https://doi.org/10.1016/j.learninstruc.2007.09.013
Höffler, T. N., & Leutner, D. (2011). The role of spatial ability in learning from instructional animations – Evidence for an ability-as-compensator hypothesis. Computers in Human Behavior, 27 , 209–216. https://doi.org/10.1016/j.chb.2010.07.042
Jenkinson, J. (2017). The role of craft-based knowledge in the design of dynamic visualizations. In R. Lowe & R. Ploetzner (Eds.), Learning from dynamic visualization – Innovations in research and application. (pp. 93–117). Springer.
Jhangiani, R. S., Chiang, I.-C.A., Cuttler, C., & Leighton, D. C. (2019). Research methods in psychology . (4th ed.). Kwantlen Polytechnic University.
Kersting, M., Althoff, K., & Jäger, A. O. (2008). Wilde-intelligenz-test 2 (WIT-2) [wilde intelligence test 2] . Hogrefe Verlag.
Kriz, S., & Hegarty, M. (2007). Top-down and bottom-up influences on learning from animations. International Journal of Human Computer Studies, 65 , 911–930. https://doi.org/10.1016/j.ijhcs.2007.06.005
Kühl, T., Navratil, S. D., & Münzer, S. (2018). Animations and static pictures: The influence of prompting and time of testing. Learning and Instruction, 58 , 201–209. https://doi.org/10.1016/j.learninstruc.2018.07.006
Lajevardi, N., Narang, N. S., Marcus, N., & Ayres, P. (2017). Can mimicking gestures facilitate learning from instructional animations and static graphics? Computers & Education, 110 , 64–76. https://doi.org/10.1016/j.compedu.2017.03.010
Lloyd-Jones, T. J., Brandimonte, M. A., & Bäuml, K.-H. (2008). Verbalising visual memories. European Journal of Cognitive Psychology, 20 , 387–395. https://doi.org/10.1080/09541440701755543
Lowe, R., & Boucheix, J.-M. (2008). Learning from animated diagrams: How are mental models built? In G. Stapleton, J. Howse, & J. Lee (Eds.), Diagrammatic representations and inference – Proceedings of the fifth international conference, diagrams 2008. (pp. 266–281). Springer.
Lowe, R., & Boucheix, J.-M. (2011). Cueing complex animations: Does direction of attention foster learning processes? Learning and Instruction, 21 , 650–663. https://doi.org/10.1016/j.learninstruc.2011.02.002
Lowe, R. K., & Schnotz, W. (2014). Animation principles in multimedia learning. In R. E. Mayer (Ed.), The Cambridge handbook of multimedia learning. (2nd ed., pp. 513–546). Cambridge University Press.
Mayer, R. E. (2009). Multimedia learning . (2nd ed.). Cambridge University Press.
Mayer, R. E. (2014). Cognitive theory of multimedia learning. In R. E. Mayer (Ed.), The Cambridge handbook of multimedia learning. (2nd ed., pp. 43–71). Cambridge University Press.
Ploetzner, R., Berney, S., & Bétrancourt, M. (2020). A review of learning demands in instructional animations: The educational effectiveness of animations unfolds if the features of change need to be learned. Journal of Computer Assisted Learning, 36 , 838–860. https://doi.org/10.1111/jcal.12476
Ploetzner, R., & Fillisch, B. (2017). Not the silver bullet: Learner-generated drawings make it difficult to understand broader spatiotemporal structures in complex animations. Learning and Instruction, 47 , 13–24. https://doi.org/10.1016/j.learninstruc.2016.10.002
Ploetzner, R., & Lowe, R. (2012). A systematic characterisation of expository animations. Computers in Human Behavior, 28 , 781–794. https://doi.org/10.1016/j.chb.2011.12.001
Ploetzner, R., & Lowe, R. (2014). Simultaneously presented animations facilitate the learning of higher-order relationships. Computers in Human Behavior, 34 , 12–22. https://doi.org/10.1016/j.chb.2014.01.039
Rosenthal, R. (1994). Parametric measures of effect size. In H. Cooper & L. V. Hedges (Eds.), The handbook of research synthesis. (pp. 231–244). Russell Sage Foundation.
Scaife, M., & Rogers, Y. (1996). External cognition: How do graphical representations work? International Journal of Human-Computer Studies, 45 , 185–213. https://doi.org/10.1006/ijhc.1996.0048
Schnotz, W. (2014). Integrated model of text and picture comprehension. In R. E. Mayer (Ed.), The Cambridge handbook of multimedia learning. (2nd ed., pp. 72–103). Cambridge University Press.
Schnotz, W., & Lowe, R. K. (2008). A unified view of learning from animated and static graphics. In R. K. Lowe & W. Schnotz (Eds.), Learning with animation: Research implications for design. (pp. 304–356). Cambridge University Press.
Schurgin, M. W. (2018). Visual memory, the long and the short of it: A review of visual working memory and long-term memory. Attention, Perception, & Psychophysics, 80 , 1035–1056. https://doi.org/10.3758/s13414-018-1522-y
Sukel, K. E., Catrambone, R., Essa, I., & Brostow, G. (2003). Presenting movement in a computer-based dance tutor. International Journal of Human-Computer Interaction, 15 , 433–452
Tversky, B., Morrison, J., & Bétrancourt, M. (2002). Animation: Can it facilitate? International Journal of Human-Computer Studies, 57 , 247–262. https://doi.org/10.1006/ijhc.2002.1017
Volmer, J. (Ed.). (1992). Getriebetechnik – Grundlagen [gear technology – basic principles] . Verlag Technik.
Download references
Acknowledgements
We thank Olesya Lang for supporting the data collection.
Open Access funding enabled and organized by Projekt DEAL. This research did not receive any specific grant from funding agencies in the public, commercial, or not-for-profit sectors.
Author information
Authors and affiliations.
Department of Psychology, University of Education, Kunzenweg 21, 79117, Freiburg, Germany
Rolf Ploetzner
TECFA-FAPSE, University of Geneva, 40, Boulevard du Pont d’Arve, 1211, Geneva, Switzerland
Sandra Berney & Mireille Bétrancourt
You can also search for this author in PubMed Google Scholar
Contributions
All authors contributed to the conception of the study. The experimental design, the preparation of material, the data collection, the data analysis, and the writing of the first draft was performed by the first author. All authors contributed to the refinement of the draft and approved the final manuscript.
Corresponding author
Correspondence to Rolf Ploetzner .
Ethics declarations
Conflict of interest.
The authors have no conflicts of interest to declare that are relevant to the content of this article.
Additional information
Publisher's note.
Springer Nature remains neutral with regard to jurisdictional claims in published maps and institutional affiliations.
Rights and permissions
Open Access This article is licensed under a Creative Commons Attribution 4.0 International License, which permits use, sharing, adaptation, distribution and reproduction in any medium or format, as long as you give appropriate credit to the original author(s) and the source, provide a link to the Creative Commons licence, and indicate if changes were made. The images or other third party material in this article are included in the article's Creative Commons licence, unless indicated otherwise in a credit line to the material. If material is not included in the article's Creative Commons licence and your intended use is not permitted by statutory regulation or exceeds the permitted use, you will need to obtain permission directly from the copyright holder. To view a copy of this licence, visit http://creativecommons.org/licenses/by/4.0/ .
Reprints and permissions
About this article
Ploetzner, R., Berney, S. & Bétrancourt, M. When learning from animations is more successful than learning from static pictures: learning the specifics of change. Instr Sci 49 , 497–514 (2021). https://doi.org/10.1007/s11251-021-09541-w
Download citation
Received : 04 September 2020
Accepted : 04 May 2021
Published : 26 May 2021
Issue Date : August 2021
DOI : https://doi.org/10.1007/s11251-021-09541-w
Share this article
Anyone you share the following link with will be able to read this content:
Sorry, a shareable link is not currently available for this article.
Provided by the Springer Nature SharedIt content-sharing initiative
- Static pictures
- Specifics of change
- Educational effectiveness
- Find a journal
- Publish with us
- Track your research
Technology
Publications
Papers & talks.
We engage with the global research community through collaborations with universities around the world, publications in academic journals, and participation in top-tier conferences. Explore our publications below!

Talk The Versatile Rigging of Splat in Strange World SIGGRAPH 2023
Cameron Black, Christoffer Pedersen

Talk Swoop: Animating Characters Along a Path SIGGRAPH 2023
Andy Lin, Hannah Swan, Justin Walker, Cathy Lam, Ricky Arietta

Talk Splat: Developing a "Strange" Shader SIGGRAPH 2023
Kendall Litaker, Brent Burley, Dan Lipson, Mason Khoo

Talk Honing USD: Lessons Learned and Workflow Enhancements at Walt Disney Animation Studios SIGGRAPH 2023
Emily Vo, George "Ricky" Rieckenberg, Ernest Petti

Talk Diving Deeper into Volume Style Transfer SIGGRAPH 2023
Mike Navarro

Talk Creating the Art-Directed Groom for Legend in Disney’s Strange World SIGGRAPH 2023
Courtney Chun, Jose Velasquez, Haixiang Liu

Talk Creating Curve-Based Garments with Custom Weave Patterns SIGGRAPH 2023
Dan Lipson, Jose Velasquez

Talk Creating Creature Chaos: The Methods that Brought Crowds to the Forefront on Disney’s Strange World SIGGRAPH 2023
Nathan Devlin, Yasser Hamed, Alberto Luceño Ros, Jeff Sullivan, D’Lun Wong

Talk Visualizing the Production Process of Encanto with the Command Center SIGGRAPH 2022
Justin Tennant, Mitch Counsell, Far Jangtrakool, Salina Ortega, Rajesh Sharma, Tad Miller, Scott Kersavage

Talk Practical Multiple-Scattering Sheen Using Linearly Transformed Cosines SIGGRAPH 2022
Tizian Zeltner, Brent Burley, Matt Jen-Yuan Chiang

Talk Modeling Animated Jumbo Floral Display on Disney’s Encanto SIGGRAPH 2022
Andrew Finley, Jesse Erickson, Peter De Mund, Ying Liu

Talk Making Encanto with USD SIGGRAPH 2022
Tad Miller, Harmony M Li, Neelima Karanam, Nadim Sinno, Todd Scopio

Talk Gravity Preloading for Maintaining Hair Shape Using the Simulator as a Closed-Box Function SIGGRAPH 2022
Haixiang Liu

Talk Fracture-Aware Tessellation of Subdivision Surfaces SIGGRAPH 2022
Brent Burley, Francisco Rodriguez

Talk Encanto - Let’s Talk About Bruno’s Visions SIGGRAPH 2022
Corey Butler, Brent Burley, Wei-Feng Wayne Huang, Karl Li, Benjamin Huang

Talk Embroidery and Cloth Fiber Workflows on Disney’s Encanto SIGGRAPH 2022
Jose Velasquez, Alexander Alvarado, Ying Liu, Maryann Simmons

Talk Wrapped Clothing on Disney’s Raya and the Last Dragon SIGGRAPH 2021
Avneet Kaur, Johann Francois Coetzee

Talk Weaving The Druun’s Webbing SIGGRAPH 2021

Talk Unbiased Emission and Scattering Importance Sampling For Heterogeneous Volumes SIGGRAPH 2021
Wei-Feng Wayne Huang, Peter Kutz*, Yining Karl Li, Matt Jen-Yuan Chiang

Talk The Atmosphere of Raya and the Last Dragon SIGGRAPH 2021
Marc Bryant, Ryan DeYoung, Wei-Feng Wayne Huang, Joe Longson, Noel Villegas

Talk Stylizing Volumes with Neural Networks SIGGRAPH 2021
Mike Navarro, Jacob Rice

Talk Populating the World of Kumandra: Animation at Scale for Disney’s Raya and the Last Dragon SIGGRAPH 2021
Alberto Luceño Ros, Kristin Chow, Jack Geckler, Norman Moses Joseph, Nicolas Ngheim

Talk Mathematical Tricks for Scalable and Appealing Crowds in Walt Disney Animation Studios’ Raya and the Last Dragon SIGGRAPH 2021
Nicolas Nghiem

Talk Creating Diversity and Variety in the People of Kumandra for Disney’s Raya and The Last Dragon SIGGRAPH 2021
Avneet Kaur, Erik Eulen, Johann Francois Coetzee

Talk Cooking Southeast Asia-Inspired Soup in Animated Film SIGGRAPH 2021
Cong Wang, Dale Mayeda, Jacob Rice, Thom Wickes, Benjamin Huang

Paper Discretization-Error-Accurate Mixed-Precision Multigrid Solvers SIAM J. SCI. COMPUT.
Rasmus Tamstorf, Joseph Benzaken, Stephen F. McCormick

Paper Algebraic Error Analysis for Mixed-Precision Multigrid Solvers SIAM J. SCI. COMPUT.
Stephen F. McCormick, Joseph Benzaken, Rasmus Tamstorf

Talk The Look and Lighting of "Show Yourself" from Frozen 2 SIGGRAPH 2020
Amol Sathe, Lance Summers, Matt Chiang, James Newland

Talk The Collaboration between Art & Technology: Making Disney Animation's Myth: a Frozen Tale SIGGRAPH 2020
Jose Luis Gomez Diaz, Jeff Gipson, Michael R.W. Anderson, Edward Robbins, Brittney Lee, Nick Russell

Talk Myth: a Frozen Tale Stylized Effects for Real-Time VR SIGGRAPH 2020
Ian Coony, Dan Lund, Michael R.W. Andersen

Talk Making Beautiful Embroidery for Frozen 2 SIGGRAPH 2020
Ying Liu, Jared Wright, Alexander Alvarado

Talk How Short Circuit Experiments: Experimental Filmmaking at Walt Disney Animation Studios SIGGRAPH 2020
Jennifer Newfield, Josh Staub

Talk Frozen 2 : Effects Vegetation Pipeline SIGGRAPH 2020
Norman Joseph, Benjamin Fiske, Vijoy Gaddipati, Marie Tollec, Tad Miller

Talk Frozen 2 : Creating the Water Horse SIGGRAPH 2020
David Hutchins, Cameron Black, Marc Bryant, Richard Lehmann, Svetla Radivoeva

Talk Deconstructing Destruction: Making & Breaking Frozen 2 's Dam SIGGRAPH 2020
Marie Tollec, Charles Cunningham-Scott, Sean Jenkins, Lance Summers

Talk Creating the Wind Spirit SIGGRAPH 2020
Cameron Black, Benjamin Fiske, Trent Correy

Paper The Challenges of Releasing the Moana Island Scene EGSR 2019
Rasmus Tamstorf, Heather Pritchett

Talk Taming the Shadow Terminator SIGGRAPH 2019
Matt Jen-Yuan Chiang, Yining Karl Li, Brent Burley

Paper Subdivision-Based Nonlinear Multiscale Cloth Simulation SIAM J. SCI. COMPUT. 2019
Alena Kopaničáková, Rolf Krause, Rasmus Tamstorf

Talk Optimizing Rig Manipulation with GPU and Parallel Evaluation SIGGRAPH 2019
Andy Lin, John Kahwaty, Walter Yoder, Gene S. Lee, David Suroviec

Talk Optimizing Large Scale Crowds in Ralph Breaks the Internet SIGGRAPH 2019
Josh Richards, Le Joyce Tong, Moe El-Ali,Tuan Nguyen

Talk Machine-Learning Denoising in Feature Film Production SIGGRAPH 2019
Henrik D. Dahlberg (Industrial Light & Magic), David M. Adler (Walt Disney Animation Studios), Jeremy Newlin (Pixar Animation Studios)

Talk Creating Ralphzilla: Moshpit, Skeleton Library, and Automation Framework SIGGRAPH 2019
Dong Joo Byun, Alberto Luceno Ros, Alexander Moaveni, Marc Bryant, Joyce Le Tong, Moe El-Ali

Talk 2D Animation in VR Clouds: The Making of Disney’s a kite’s tale SIGGRAPH 2019
Bruce Wright, Michael Anderson, Angela McBride, Henrik Falt, Daniel Peixe, Tony DeRosa

Paper A Material Point Method for Thin Shells with Frictional Contact SIGGRAPH 2018
Qi Guo, Xuchen Han, Chuyuan Fu, Theodore Gast, Rasmus Tamstorf, Joseph Teran

Paper The Design and Evolution of Disney’s Hyperion Renderer ACM Transactions on Graphics (TOG) 2018
Brent Burley, David Adler, Matt Jen-Yuan Chiang, Hank Driskill, Ralf Habel, Patrick Kelly, Peter Kutz, Yining Karl Li, Daniel Teece

Paper Denoising with Kernel Prediction and Asymmetric Loss Functions SIGGRAPH 2018
Thijs Vogels, Fabrice Rousselle, Brian McWilliams, Gerhard Rothlin, Alex Harvill, David Adler, Mark Meyer, Jan Novak

Talk SimpleBullet: Collaborating on a Modular Destruction Toolkit SIGGRAPH 2018
Marie Tollec

Talk Hierarchical Workflow for Art-Directed Hair Animation SIGGRAPH 2018
Avneet Kaur, Maryann Simmons

Paper Denoising Deep Monte Carlo Renderings EG Computer Graphics Forum 2018
Delio Vicini, David Adler, Jan Novak, Fabrice Rousselle, Brent Burley

Talk The Role of Hand-Drawn Animation in Disney's Moana SIGGRAPH 2017
Kimberly Keech, Brett Achorn, Brian Whitted and Rachel Bibb

Talk The Ocean and Water Pipeline of Disney's Moana : The Line Where the Sky Meets the Sea SIGGRAPH 2017
Ralf Habel, Patrick Kelly, Peter Kutz, Sara Drakeley, Jonathan Garcia, Sean Palmer

Talk The Art and Technology of Simulating Hair in Disney's Moana SIGGRAPH 2017
Marc Thyng, Christopher Evart, Toby Jones, Alex McAdams

Talk Moana : Performing Water SIGGRAPH 2017
Ben Frost, Alexey Stomakhin, Hiroaki Narita

Talk Moana : Geometry Based Disco Ball Lighting for Tamatoa’s Lair SIGGRAPH 2017
DJ Byun, Shant Ergenian, Gregory Culp

Talk Moana : Foundation of a Lava Monster SIGGRAPH 2017
Marc Bryant, Ian Coony, Jonathan Garcia

Talk Moana : Crashing Waves SIGGRAPH 2017
Alexey Stomakhin, DJ Byun

Talk Handling Scene Constraints for Pose-Based Caching SIGGRAPH 2017
Gene Lee, Christian Eisenacher, Andy Lin & Noel Villegas

Talk Building Moana ’s Kakamora Barge: You’re Gonna Need a Bigger Boat SIGGRAPH 2017
Brett Achorn, Sean Palmer, Larry Wu

Paper Fluxed Animated Boundary Method SIGGRAPH 2017
Alexey Stomakhin, Andrew Selle

Paper Spectral and Decomposition Tracking for Rendering Heterogeneous Volumes SIGGRAPH 2017
Peter Kutz, Ralf Habel, Yining Karl Li, Jan Novák

Paper All’s Well That Ends Well: Guaranteed Resolution of Simultaneous Rigid Body Impact SIGGRAPH 2017
Etienne Vouga, Breannan Smith, Danny M. Kaufman, Rasmus Tamstorf, Eitan Grinspun

Paper Modeling and Data-Driven Parameter Estimation for Woven Fabrics Symposium on Computer Animation
David Clyde, Joseph Teran, Rasmus Tamstorf

Paper Efficient Elasticity for Character Flesh Simulation on Octrees Tech Report 2017
Andy Milne, Mark A. McLaughlin, Haixiang Liu, Alexey Stomakhin, Rasmus Tamstorf

Talk Practical and Controllable Subsurface Scattering for Production Path Tracing SIGGRAPH 2016
Matt Jen-Yuan Chiang, Peter Kutz, Brent Burley

Talk Zootopia Crowd Pipeline SIGGRAPH 2016
Alberto Luceno Ros, Le Tong, Josh Richards, Tuan Nguyen, Moe El-Ali, Norman Joseph

Paper Subdivision Next-Event Estimation for Path-Traced Subsurface Scattering EGSR 2016
David Koerner, Jan Novák, Peter Kutz, Ralf Habel, and Wojciech Jarosz

Talk From Armadillo to Zebra: Creating the Diverse Characters and the World of Zootopia SIGGRAPH 2016
Ernest Petti, Sean Palmer, Nick Burkard, Hans Keim, Brian Leach, Michelle Robinson

Talk Flesh, Flab, and Fascia Simulation on Zootopia SIGGRAPH 2016
Andy Milne, Mark McLaughlin, Rasmus Tamstorf, Alexey Stomakhin, Nicolas Burkhard, Mitch Counsell, Jesus Canal, David Komorowski, Evan Goldberg

Talk Delicious Looking Ice Cream Effects with Non-Simulation Approaches SIGGRAPH 2016
Dong Joo Byun, James Mansfield, Cesar Veazquez

Talk Coda: The Cultural Effects of Queueing at Disney Animation SIGGRAPH 2016
Marc Jordan, Kevin Constantine

Talk Artist-Friendly Level-of-Detail in a Fur-Filled World SIGGRAPH 2016
Kendall Litaker, Sean Palmer

Talk Art-Directable Procedural Vegetation in Disney's Zootopia SIGGRAPH 2016
Hans Keim, Maryann Simmons, Daniel Teece, Jared Reisweber, Sara Drekely

David Koerner, Jan Novak, Peter Kutz, Ralf Habel, Wojciech Jarosz

Paper A Practical and Controllable Hair and Fur Model for Production Path Tracing Eurographics 2016
Matt Jen-Yuan Chiang, Benedikt Bitterli, Chuck Tappan, Brent Burley

Paper Smoothed Aggregation Multigrid for Cloth Simulation ACM Transactions on Graphics, 34,6, 2015; SIGGRAPH Asia 2015
Rasmus Tamstorf, Toby Jones, Stephen F. McCormick

Paper Sackcloth or Silk? The Impact of Appearance vs Dynamics on the Perception of Animated Cloth Symposium on Applied Perception 2015
Carlos Aliaga, Carol O'Sullivan, Diego Gutierrez, Rasmus Tamstorf

Paper The Affine Particle-In-Cell Method ACM SIGGRAPH 2015
Chenfanfu Jiang, Craig Schroeder, Andrew Selle, Joseph Teran, Alexey Stomakhin

Talk Interactive Script Based Dynamics in Big Hero 6 SIGGRAPH 2015
Dong Joo Byun, Zubin Wadia, Michael Kaschalk

Talk Crowd Character Complexity on Big Hero 6 SIGGRAPH 2015
Yasser Hamed, John Kahwaty, Andy Lin, Evan Goldberg, Lawrence Chai

Talk Big Hero 6 : Into the Portal SIGGRAPH 2015
David Hutchins, Olun Riley, Jesse Erickson, Alexey Stomakhin, Ralf Habel, Michael Kaschalk

Talk A Practical and Controllable Hair and Fur Model for Production Path Tracing SIGGRAPH 2015

Talk Achieving Real-Time Playback with Production Rigs SIGGRAPH 2015
Andy Lin, Gene S. Lee, Joe Longson, Jay Steele, Evan Goldberg, Rastko Stefanovic

Paper Scalable Asynchronous Contact Mechanics Using Charm++ IEEE IPDPS 2015
Xiang Ni, Laxmikant Kale, Rasmus Tamstorf

Paper Adaptive Nonlinearity for Collisions in Complex Rod Assemblies ACM SIGGRAPH 2014, 33(4), pp. 123:1-123:12
Danny Kaufman, Rasmus Tamstorf, Breannan Smith, Jean-Marie Aubry, Eitan Grinspun

Paper Augmented MPM for Phase-Change and Varied Materials ACM SIGGRAPH 2014, 33(4), pp. 138:1-138:11
Alexey Stomakhin, Craig Schroeder, C. Jiang, Lawrence Chai, Joseph Teran, Andrew Selle

Talk Simulating Wind Effects on Cloth and Hair in Disney's Frozen Siggraph 2014
Keith Wilson, Aleka McAdams, Hubert Leo, and Maryann Simmons

Talk Modeling Tools at Disney Animation Symposium on Digital Production Digi Pro 2014
Dmitriy Pinskiy, Jose L. Gomez Diaz

Paper Ink-and-Ray: Bas-Relief Meshes for Adding Global Illumination Effects to Hand-Drawn Characters ACM Transactions on Graphics, 33,2, 2014 (SIGGRAPH 2014)
Daniel Sykora, Ladislav Kavan, Martin Cadik, Ondrej Jamriska, Alec Jacobson, Brian Whited, Maryann Simmons, Olga Sorkine-Hornung

Talk Disney's Hair Pipeline: Crafting Hair Styles From Design to Motion Eurographics Industrial Presentation 2014
Maryann Simmons, Brian Whited

Paper A Material Point Method For Snow Simulation Siggraph 2013
Alexey Stomakhin, Craig Schroeder, Lawrence Chai, Joseph Teran, Andrew Selle

Paper Modeling and Estimation of Internal Friction in Cloth Siggraph 2013
Eder Miguel, Rasmus Tamstorf, Derek Bradley, Sara C. Schvartzman, Bernhard Thomaszewski, Bernd Bickel, Wojciech Matusik, Steve Marschner, Miguel A. Otaduy

Talk Pixels to Parks: New Animation Techniques for Fantasyland SIGGRAPH 2013
Gene Lee, Aaron Adams, Evan Goldberg, Justin Walker

Paper Enhanced Dual Quaternion Skinning for Production Use SIGGRAPH 2013
Gene Lee, Andy Lin, Matt Schiller, Scott Peters, Mark McLaughlin, Frank Hanner

Paper Discrete Bending Forces and Their Jacobians GMOD 2013
Rasmus Tamstorf, Eitan Grinspun

Talk A Deformer-Based Approach to Facial Rigging SIGGRAPH 2013
John Kahwaty, Gene Lee, Greg Smith, Andy Lin, Matthew Schiller

Paper Stereo Compositing Accelerated by Quadtree Structures in Piecewise Linear and Curvilinear Spaces Symposium on Digital Production Digi Pro 2013
Dmitriy Pinskiy, Joseph Longson, Peter Kristof, Evan Goldberg, Robert Neuman

Paper Sorted Deferred Shading for Production Path Tracing EGSR 2013
Christian Eisenacher, Gregory Nichols, Andrew Selle, Brent Burley

Paper Speculative Parallel Asynchronous Contact Mechanics Siggraph Asia 2012
Samantha Ainsley, Etienne Vouga, Eitan Grinspun, Rasmus Tamstorf

Paper Smart Scribbles for Sketch Segmentation Computer Graphics Forum. 31:8, 2012
Gioacchino Noris, Daniel Sykora, Brian Whited, Alexander Hornung, Maryann Simmons, Stelian Coros, Ariel Shamir, Robert Sumner, Markus Gross

Paper Reflections on Simultaneous Impact Siggraph 2012
Breannan Smith, Danny M. Kaufman, Etienne Vouga, Rasmus Tamstorf, Eitan Grinspun

Talk Physically-Based Shading at Disney SIGGRAPH 2012
Brent Burley

Talk dRig: An Artist-Friendly, Object-Oriented Approach to Rig Building Siggraph 2012
Greg Smith, Mark McLaughlin, Andy Lin, Evan Goldberg, Frank Hanner

Talk Computer-Assisted Animation of Line and Paint in Disney's Paperman SIGGRAPH 2012
Brian Whited, Eric Daniels, Michael Kaschalk, Patrick Osborne, Kyle Odermatt

Paper Topology-Driven Vectorization of Clean Line Drawings ACM Transactions on Graphics 2012
Gioacchino Noris, Alexander Hornung, Robert W. Sumner, Maryann Simmons, Markus Gross

Paper Asynchronous Contact Mechanics Communications of the ACM Paper 2012
David Harmon, Etienne Vouga, Breannan Smith, Rasmus Tamstorf, Eitan Grinspun

Paper TexToons: Practical Texture Mapping for Hand-Drawn Cartoon Animations Symposium on Non-photorealistic Animation and Rendering 2011
Daniel Sykora, Mirela Ben-Chen, Martin Cadk, Brian Whited, Maryann Simmons

Paper Temporal Noise Control for Sketchy Animation Symposium on Non-photorealistic Animation and Rendering (NPAR'11), 2011
Gioacchino Noris, Daniel Sykora, Stelian Coros, Brian Whited, Maryann Simmons, Alexander Hornung, Markus Gross, Robert Sumner

Paper Perceptually-Based Compensation of Light Pollution in Display Systems APGV 2011
Jeroen van Baar, Steven Poulakos, Wojciech Jarosz, Derek Nowrouzezahrai, Rasmus Tamstorf, Markus Gross

Paper Ball-morph: Definition, Implementation and Comparative Evaluation IEEE TVCG, June 2011, 17:6, pp 757-769, © 2011 IEEE Paper
G. Noris, D. Sýkora, S. Coros, B. Whited, M. Simmons, A. Hornung, M. Gross, R. W. Sumner

Paper A Programmable System for Artistic Volumetric Lighting Siggraph 2011
Derek Nowrouzezahrai, Jared Johnson, Andrew Selle, Dylan Lacewell, Michael Kaschalk, Wojciech Jarosz

Talk Tangled Choreographing Destruction: Art Directing a Dam Break Siggraph 2011
Brett Boggs, Lawrence Chai, Michael Kaschalk, Andrew Selle

Talk Gaussian Quadrature for Photon Beams in Tangled Siggraph 2011
Jared M. Johnson, Dylan Lacewell, Andrew Selle, Wojciech Jarosz

Paper Efficient Elasticity for Character Skinning with Contact and Collisions Siggraph 2011
Aleka McAdams, Yongning Zhu, Andrew Selle, Mark Empey, Rasmus Tamstorf, Joseph Teran, Eftychios Sifakis

Talk Directing Hair Motion on Tangled Siggraph 2011
Maryann Simmons, Kelly Ward, Hidetaka Yosumi, Hubert Leo, Xinmin Zhao

Paper Collision-Driven Volumetric Deformation on the GPU Eurographics 2011
G. Aldrich, D. Pinskiy, B. Hamann

Talk Point-Based Hair Global Illumination Siggraph Asia 2010
Ramón Montoya-Vozmediano

Talk Automated Target Selection for DrivenShape Siggraph Asia 2010
Gene S. Lee

Talk A Hybrid Approach to Facial Rigging Siggraph Asia 2010
David Komorowski, Vinod Melapudi, Darren Mortillaro, Gene S. Lee

Paper BetweenIT: An Interactive Tool for Tight Inbetweening Computer Graphics Forum, 2010
Brian Whited, Gioacchino Noris, Maryann Simmons, Robert W. Sumner, Markus Gross, Jarek Rossignac

Talk Simulating Rapunzel’s Hair in Disney’s Tangled Siggraph 2010
Kelly Ward, Maryann Simmons, Andy Milne, Hidetaka Yosumi, Xinmin Zhao

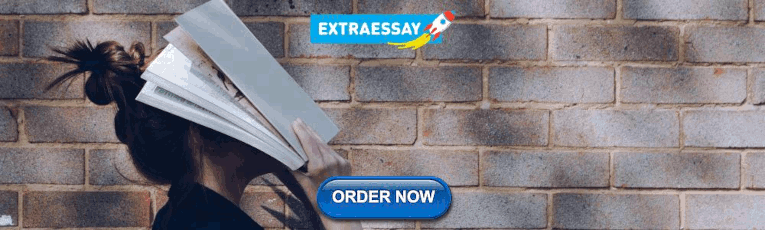
Talk Set’m and Forget’m: A Motion Graphics Pipeline for Effects Siggraph 2010
Ian J. Coony, Brian Silva, Bruce Wright, Andrew Kinney

Talk Example-Based Texture Synthesis on Disney’s Tangled Siggraph 2010
Christian Eisenacher, Chuck Tappan, Brent Burley, Daniel Teece, Arthur Shek

Talk Christmas in July: The Effects Snow Process Siggraph 2010
Ian J. Coony - David Hutchins - Kevin Lee - Mike Harris - Tim Molinder - Kee Suong

Talk Art-Directing Disney’s Tangled Procedural Trees Siggraph 2010
Arthur Shek, Dylan Lacewell, Andrew Selle, Daniel Teece, Tom Thompson

Paper An Artist Friendly Hair Shading System Siggraph 2010
Iman Sadeghi, Heather Pritchett, Henrik Wann Jensen, Rasmus Tamstorf

Paper Sliding Deformation: Shape Preserving Per-Vertex Displacement Eurographics 2010
Dmitriy Pinskiy

Paper CASE STUDY - Beauty and the Beast 3D Benefits of 3D Viewing for 2D to 3D Conversion SPIE Electronic Imaging 2010/Stereoscopic Displays and Applications XXI Paper
Tara Handy Turner

Paper Synthetic Turbulence Using Artificial Boundary Layers Siggraph Asia 2009
Tobias Pfaff, Nils Thuerey, Andrew Selle, Markus Gross

Talk Practical Experiences with Pose Space Deformation Siggraph Asia 2009
Gene S. Lee, Frank Hanner

Talk Evaluation of the Radial Basis Function Space Siggraph Asia 2009

Talk Rhino-Palooza: Procedural Animation and Mesh Smoothing Siggraph 2009
Evan Goldberg, Dmitriy Pinskiy

Talk Realistic Eye Motion Using Procedural Geometric Methods Siggraph 2009
Dmitriy Pinskiy, Erick Miller

Talk Medial Axis Techniques for Stereoscopic Extraction Siggraph 2009
Evan Goldberg

Talk Interactive Lighting of Effects Using Point Clouds in Bolt Siggraph 2009
Dale Mayeda

Talk iBind: Smooth Indirect Binding Using Segmented Thin-Layers Siggraph 2009
Chung-An Lin, Erick Miller, Gene S. Lee

Talk Geometric Fracture Modeling in BOLT Siggraph 2009
Jeffrey Hellrung, Andrew Selle, Arthur Shek, Eftychios Sifakis, Joseph Teran

Paper Detail Preserving Continuum Simulation of Straight Hair Siggraph 2009
Aleka McAdams, Andrew Selle, Kelly Ward, Eftychios Sifakis, Joseph Teran

Talk Composite Based Refraction for Fur and Other Complex Objects Siggraph 2009
Lewis Siegel, Sean Jenkins

Paper Asynchronous Contact Mechanics Siggraph 2009 and CMAME Journal Paper
David Harmon, Etienne Vouga, Brennan Smith, Rasmus Tamstorf, Eitan Grinspun

Talk Applying Painterly Concepts in a CG Film - BOLT Siggraph 2009
Adolph Lusinsky, Paul Felix, Ernie Petti, Sean Jenkins, Adrienne Othon, Patrick Dalton, Hank Driskill, John Murrah

Paper Implicit Contact Handling for Deformable Objects Eurographics 2009 Paper
Miguel A. Otaduy, Rasmus Tamstorf, Denis Steinemann, Markus Gross

Paper Dent Removal: Geodesic Curve-Based Mesh Fairing Eurographics 2009

Paper Robust Treatment of Simultaneous Collisions Siggraph 2008
David Harmon, Etienne Vouga, Rasmus Tamstorf, Eitan Grinspun

Paper Approximate Topological Matching of Quadrilateral Meshes The Visual Computer 2009 and Shape Modeling International 2008 Paper
David Eppstein, Michael T. Goodrich, Ethan Kim, Rasmus Tamstorf

Paper The Hybrid Theatre Stereoscopic Displays and Applications 2008

Paper Raytracing Prefiltered Occlusion for Aggregate Geometry IEEE Symposium on Interactive Raytracing 2008
Dylan Lacewell, Brent Burley, Solomon Boulos

Paper Motorcycle Graphs: Canonical Quad Mesh Partitioning Symposium on Geometry Processing 2008

Paper Ptex: Per-Face Texture Mapping for Production Rendering Eurographics Symposium on Rendering 2008
Brent Burley, Dylan Lacewell

Paper Fast Collision Detection for Deformable Models Using Representative-Triangles i3D 2008
Sean Curtis, Rasmus Tamstorf, Dinesh Manocha

Paper Exact Evaluation of Catmull-Clark Subdivision Surfaces Near B-Spline Boundaries Journal of Graphics Tools 2007 Paper
Dylan Lacewell, Brent Burley

Paper Interactive Collision Detection Between Deformable Models using Chromatic Decomposition Siggraph 2005
Naga K. Govindaraju, David Knott, Nitin Jain, Ilknur Kabul, Rasmus Tamstorf, Russell Gayle, Ming C. Lin, Dinesh Manocha

Paper Texture Mapping for Cel Animation Siggraph 1998
Wagner Toledo Correa, Robert J. Jensen, Craig E. Thayer, Adam Finkelstein

Paper Multiperspective Panoramas for Cel Animation Siggraph 1997
Daniel N. Wood, Adam Finkelstein, John F. Hughes, Craig E. Thayer, David H. Salesin

Paper Painterly Rendering for Animation Siggraph 1996
Barbara J. Meier
Collaboration through Sharing
We are committed to advancing technology through sharing of Data Sets and Open Source Software. We encourage you to explore and use the technologies.
Downloadable data sets from our animated films, for research purposes, to discover and inspire.
Open Source
Open Source software and technologies for your exploration and use.
Be Part of Our Story Careers
Each individual at our Studio contributes to the creation of our films. Be a part of our rich legacy of storytelling.
Teams that Collaborate Our Teams
Production, Technology, and Studio teams collaborate closely throughout the filmmaking process. Learn more about each area of the Studio and the role they play within Disney Animation.
Inside the Hat Life at Disney
Our culture inspires the creative and innovative spirit found throughout our Studio.
Thank you for visiting nature.com. You are using a browser version with limited support for CSS. To obtain the best experience, we recommend you use a more up to date browser (or turn off compatibility mode in Internet Explorer). In the meantime, to ensure continued support, we are displaying the site without styles and JavaScript.
- View all journals
- Explore content
- About the journal
- Publish with us
- Sign up for alerts
- CAREER COLUMN
- 07 January 2022
Broaden your scientific audience with video animation
- Alvina Lai 0
Alvina Lai is an associate professor at University College London. Her research involves genetics, statistics, modelling and informatics.
You can also search for this author in PubMed Google Scholar
Scientists often struggle to explain their research in lay terms — whether to funding agencies and tenure and promotion committees, or to friends and family.
Access options
Access Nature and 54 other Nature Portfolio journals
Get Nature+, our best-value online-access subscription
$29.99 / 30 days
cancel any time
Subscribe to this journal
Receive 51 print issues and online access
$199.00 per year
only $3.90 per issue
Rent or buy this article
Prices vary by article type
Prices may be subject to local taxes which are calculated during checkout
doi: https://doi.org/10.1038/d41586-022-00045-2
This is an article from the Nature Careers Community, a place for Nature readers to share their professional experiences and advice. Guest posts are encouraged .
Competing Interests
The author declares no competing interests.
Related Articles
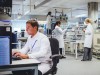
- Communication
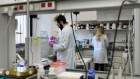
Brazil’s plummeting graduate enrolments hint at declining interest in academic science careers
Career News 21 MAY 24
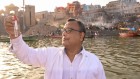
How religious scientists balance work and faith
Career Feature 20 MAY 24
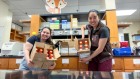
How to set up your new lab space
Career Column 20 MAY 24
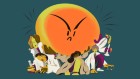
Harassment of scientists is surging — institutions aren’t sure how to help
News Feature 21 MAY 24
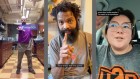
US TikTok ban: how the looming restriction is affecting scientists on the app
News 09 MAY 24
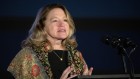
‘Shrugging off failure is hard’: the $400-million grant setback that shaped the Smithsonian lead scientist’s career
Career Q&A 15 APR 24
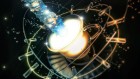
Time’s restless ocean
Futures 22 MAY 24
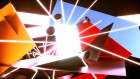
Explaining novel scientific concepts to people whose technical acumen does not extend to turning it off, then turning it on again
Futures 15 MAY 24
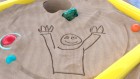
Tashni’s first tunnel
Futures 08 MAY 24
Editor (Structural biology, experimental and/or computational biophysics)
We are looking for an Editor to join Nature Communications, the leading multidisciplinary OA journal, publishing high-quality scientific research.
London or New York - hybrid working model.
Springer Nature Ltd
Wissenschaftliche/r Mitarbeiter/in - Quantencomputing mit gespeicherten Ionen
Wissenschaftliche/r Mitarbeiter/in - Quantencomputing mit gespeicherten Ionen Bereich: Fakultät IV - Naturwissenschaftlich-Technische Fakultät | St...
Siegen, Nordrhein-Westfalen (DE)
Universität Siegen
Wissenschaftliche/r Mitarbeiter/in (PostDoc) - Quantencomputing mit gespeicherten Ionen
Wissenschaftliche/r Mitarbeiter/in (PostDoc) - Quantencomputing mit gespeicherten Ionen Bereich: Fakultät IV - Naturwissenschaftlich-Technische Fak...
Professor Helminthology
Excellent track record on the biology and immunobiology of zoonotic helminths and co-infections, with a strong scientific network.
Antwerp, New York
Institute of Tropical Medicine
Assistant Professor in Plant Biology
The Plant Science Program in the Biological and Environmental Science and Engineering (BESE) Division at King Abdullah University of Science and Te...
Saudi Arabia (SA)
King Abdullah University of Science and Technology
Sign up for the Nature Briefing newsletter — what matters in science, free to your inbox daily.
Quick links
- Explore articles by subject
- Guide to authors
- Editorial policies
animation industry Recently Published Documents
Total documents.
- Latest Documents
- Most Cited Documents
- Contributed Authors
- Related Sources
- Related Keywords
Animation Character Detection Algorithm Based on Clustering and Cascaded SSD
With the evolution of the Internet and information technology, the era of big data is a new digital one. Accordingly, animation IP has been more and more widely welcomed and concerned with the continuous development of the domestic and international animation industry. Hence, animation video analysis will be a good landing application for computers. This paper proposes an algorithm based on clustering and cascaded SSD for object detection of animation characters in the big data environment. In the training process, the improved classification Loss function based on Focal Loss and Truncated Gradient was used to enhance the initial detection effect. In the detection phase, this algorithm designs a small target enhanced detection module cascaded with an SSD network. In this way, the high-level features corresponding to the small target region can be extracted separately to detect small targets, which can effectively enhance the detection effect of small targets. In order to further improve the effect of small target detection, the regional candidate box is reconstructed by a k-means clustering algorithm to improve the detection accuracy of the algorithm. Experimental results demonstrate that this method can effectively detect animation characters, and performance indicators are better than other existing algorithms.
Performance Measurement of Animation Design Pre-Production Artist During COVID-19 Pandemic in India
This research focuses on the development of the Indian animation industry. In Animation Pipeline pre-production is an important stage that determines the success of a film. Create and develop a story is the fast step and all other steps have to follow that storyline till final film realized. In this research paper present the survey base online questionnaire and the data has been collected of 300 artist who belong into Indian animation industry conducted in September 2020 by using google form. In general, Indian animation industry mostly run into production and post production (technical) base work so Indian animation industry has a smaller number of vacancies for pre-production (design and planning) artist and also having a very a smaller number of design and planning artist because of that they have highly demand. To evaluate and determine the factors that may affect the level of Indian animation pre-production industry. The study helpful to identify the animation industry current need and it focuses on planning stage to production of a movie. This research paper concludes 95% of artist working into the industry for production and post production if they are properly working into preproduction and then start into the movie work then there will be more vacancy for pre-production artist and end of the day production cost reduced up to 25 %.
Research on the Computer Case Design of 3D Human Animation Visual Experience
In the animation industry, with the development of computer software and hardware technology, a new technology began to emerge, that is, three-dimensional animation. Three-dimensional animation software first creates a virtual world in the computer. In this virtual three-dimensional world, the designer builds the model and scene according to the shape and size of the object to be represented and then sets the motion trajectory of the model, the motion of the virtual camera, and the scene according to the requirements. When setting other animation parameters, we need to assign specific materials to the model and turn on lights. When all this is completed, the computer can automatically calculate and generate the final picture. The software Maya can just help animators to complete this work. When using Maya, we can apply many professional courses such as action design, scene design, and storyboarding script design that we have learned. Maya is a 3D software with convenient operability. It can combine the rendered sequence frames with AE to show unique animations. Therefore, the three-dimensional production method is preferred in the production method. The production of animation based on the 3D software Maya brings infinite challenges. At the same time, it also helps everyone grow and has a good position for our employment direction.
History of Japanese Animation Industry and New Technology
Scotland’s history of animation: an exploratory account of the key figures and influential events.
Scotland’s history of animation is a forgotten past accomplishment in the animation/VFX sector, with key influential animation professionals having had an impact both at home and abroad. Yet, to date, this history has not been meaningfully documented and such documentation can help inform policy initiatives to help nurture and develop the industry. These developments could help ensure that the importance and accomplishments of its achievements will not be forgotten or remain undeveloped. Indeed, it is argued here that Scotland suffers from historical amnesia with regard to the country’s past accomplishments and missed opportunities, but that public funding and further investment in talent development and retention can help establish the industry as a key player in society and economy. This article presents the results from an investigative literature collection and consultation with central figures in the Scottish animation industry, providing for the first time a clearer picture of the importance of animation in Scotland both for the country and for the industry worldwide. Discussing the initiatives and funding models of other European countries such as France, the article concludes by suggesting ways in which future policy initiatives could help assist Scotland’s animation industry grow and establish itself both for the future development of animation in Scotland and worldwide.
Sustainable Development Strategy of Chinese Animation Industry
The animation industry is a crucial part of cultural and creative industries and has formed a huge consumer market all over the world and even become a pillar industry in developed countries. However, in China, the animation industry is still in its infancy, and its development is relatively backward. The main reason is that there is no sustainable development model in the industry. Thus, how to carry out sustainable development has become an important research topic for the Chinese animation industry. This study probed into the challenges and problems faced by China′s animation industry, and based on Porter′s Diamond Model, analyzed the current situation of China′s animation industry from six aspects: production factors, demand conditions, industrial chain, enterprise strategy, cultural factors, and government policies, clarifies the key direction for industrial development, and puts forward relatively comprehensive reference strategies to promote the formation of a sustainable development model for the animation industry. The research shows that the sustainable development of the animation industry must be completed by integrating resources within the industry, improving the chain, upgrading the enterprises, technological innovations, cultural embedding, external personnel training, government support, legal protection, and other aspects.
Design and Realization of Animation Composition and Tone Space Conversion Algorithm
In recent years, with the development of society and the rapid development of the animation industry, people are paying more and more attention to and requirements for animation production. As an indispensable part of animation production, picture composition plays a major role in animation production. It can give full play to the application of color matching and light and shadow design and enhance the depth and space of the animation screen. Tone space conversion refers to the conversion or representation of color data in one color space into corresponding data in another color space. Its purpose is to distinguish and process color components such as hue and saturation in an image. This article first introduces the domestic and foreign research status of digital image preprocessing and analyzes the basic principles of several color space conversions in detail. Then, several color space conversion algorithms are studied, and the performance of the algorithms is compared and analyzed. The paper focuses on the hardware implementation and optimization of the algorithm for converting RGB color space into HSI color space to meet the real-time requirements. This article focuses on the mutual conversion between the RGB tone space and the HSI tone space and describes in detail how each color component in the HSI tone space is converted from the three RGB color components from a geometric perspective, and then the conversion is derived, and several general conversion methods of RGB to HSI tone space are introduced; two conversion methods of geometric derivation method and standard modulus algorithm are implemented in the software, and the comparison verification is carried out, and the comparison is made from the perspective of hardware implementation. The pros and cons of the two methods are discussed. Finally, the paper summarizes the shortcomings in the design and proposes further research directions in the future.
Analisis dan Pengembangan Pipeline Cloth Simulation Pada Produksi Animasi 3D di MSV Studio
Pengaruh penerapan pipeline dalam sebuah industry animasi 3d sangat mempengaruhi produksi animasi agar maksimal. Dalam masing-masing divisi juga terdapat pipeline yang digunakan contohnya pipeline pada divisi cloth simulation. Pada MSV Studio pipeline simulasi yang diterapkan di divisi cloth simulation masih terdapat beberapa kendala pada saat produksi. Cara kerja pipeline yang sudah ada yaitu pada saat proses pengecekan data asset yang masih manual antara Sceneres dan Renderes yang diperlukan untuk simulasi cloth masih sering terjadi kesalahan seperti human error dan set up cloth masih dilakukan disetiap shot. Dari masalah tersebut diusulkan untuk dilakukan pengembangan pipeline cloth simulation agar bisa meminimalisir kesalahan dan menghemat waktu pengerjaan. Hasil dari produksi film animasi sangat dipengaruhi oleh pipeline yang digunakan dalam produksi tersebut. Studi ini memberikan gambaran bagaimana sebuah pipeline ikut andil besar dalam sebuah hasil dari produksi film animasi 3D terutama dalam masalah waktu. Pada akhirnya studi ini dapat menjadi acuan dalam pembuatan sebuah pipeline film animasi khususnya pada divisi cloth simulation.Kata Kunci — pipeline, cloth simulation, animasi 3DThe effect of pipeline application in a 3D animation industry greatly influences the production of animation for maximizing. In each division. there is also a pipeline that is used for example the pipeline in the cloth simulation division. In the MSV studio pipeline simulation applied in the cloth simulation division there were still some obstacles during production. The work of the existing pipeline during the process of checking asset data which is still manual between Sceneres and Renderes needed for cloth simulation is still often had an error such as human error and set up cloth still done in every shot. From this problem it is proposed to develop a pipeline cloth simulation in order to minimize errors and saving time processing. The results of the production of animated films are strongly influenced by the pipeline used in the production. This studyprovides an illustration of how a pipeline contributes greatly to the results of the production of 3D animated films, especially in the matter of time. In the end, this study can be a reference in making an animation film pipeline, especially in the cloth simulation division.Keywords — pipeline, cloth simulation, 3D animation
SEKOLAH MENENGAH KEJURUAN DESAIN ANIMASI DI BANJARBARU
Animation is a sub-sector of the creative industry that has promising business opportunities and is able to absorb a lot of creative labor. The increasing number of animation creators in Indonesia shows the increasing demand for animated films, unfortunately the quality of animators has not been able to compete internationally due to inadequate infrastructure and low education. The government also encourages the development of the animation industry by opening vocational education, where the Animation Vocational School can become a special forum for learning activities in the field of animation technology which aims to improve Human Resources (HR). The Interactive Space theme offers problem-solving for Animated Vocational Schools which have an interactive platform. The method used is to create a place to stimulate ideas. Like a waiting room which can be a collaborative space for discussion and relaxation.
The Destiny of a “Baby” Industry: Turkish Animation
The word “destiny” is rooted in the word “destination”, the place where someone is going. In order to draw a map for our journey we have to know where we are standing and we have to have a groundwork. Animation in Turkey dates back to the first animation experimentations of Turkish cartoonists and the first public screening of Disney’s “The Skeleton Dance” in 1932. The pioneering animations are either unfinished, lost or obscure. Just like the doomed faith of the first -unfinished- animated feature film project “Once Upon a Time” that has been carried out for almost 9 years or the questionable and -sued- authenticity of the first highly popular domestic cartoon character on Turkish TV, animation in Turkey have so many low points. Nevertheless there is a growing number of domestic feature films with record breaking number of audiences. Animation education is only 30 years old with little number of departments devoted to it, -yet- the numbers are growing. “Design Centers” are established by the encouragement of Turkish Ministry of Industry and Technology to support animation studios, professional associations are forming, intellectual property rights are the talk of the day, the academy and industry interaction is getting stronger, little festivals flourish... These are indeed turning points for the -baby- animation industry in Turkey. We need to study this map in order to get to our destination: a mature industry with established work ethics, high artistic standards and rich economic outcome and make a good destiny out of it.
Export Citation Format
Share document.
- Open access
- Published: 17 October 2022
Influence of 3D models and animations on students in natural subjects
- Milada Teplá 1 ,
- Pavel Teplý ORCID: orcid.org/0000-0003-2180-510X 1 &
- Petr Šmejkal 1
International Journal of STEM Education volume 9 , Article number: 65 ( 2022 ) Cite this article
15k Accesses
6 Citations
5 Altmetric
Metrics details
Studies comparing the effect of dynamic and static visualization suggest a predominantly positive effect of dynamic visualization. However, the results of individual comparisons are highly heterogeneous. In this study, we assess whether dynamic visualization (3D models and animations) used in the experimental group has a stronger positive influence on the intrinsic motivation and learning outcomes of science students (Biology, Chemistry and Geology) than static visualization used in the control group, and whether selected variables (students’ gender, age, educational level, learning domain, and teacher personality) significantly affect the results.
This study was conducted in 2019 with a sample of 565 students from Czech middle (aged 11–15 years) and high (aged 15–19 years) schools using the following research tools: Motivated Strategies for Learning Questionnaire, Intrinsic Motivation Inventory and knowledge tests. The results show that using 3D models and animations in the teaching process significantly increased the students’ intrinsic motivation for learning natural sciences (more specifically, its components (1) interest, (2) effort to actively participate in the educational process, (3) perceived competence and (4) understanding the usefulness of the subject matter), with a mean Hedges’ g = 0.38. In addition, students in the experimental group reached a significantly higher level of Chemistry knowledge than their peers in the control group. Furthermore, by moderator analysis, we identified three moderator variables, namely student age, instructional domain and teacher personality. These variables significantly affect intrinsic motivation in different ways. The strongest positive effect of dynamic visualizations was found among students aged 11–13, whereas the weakest positive effect was identified among students aged 14–16. Regarding instructional domain animations and 3D models, the strongest positive effect is found in Chemistry ( g = 0.74) and Biology ( g = 0.72), whereas the positive impact on Geology is significantly weaker ( g = 0.45). Teacher personality was found to be a major moderator in student motivation, with significant differences ( g = 0.40—1.24). Teachers’ attitude towards modern technology plays an important role concerning this effect.
Conclusions
Based on these findings, we conclude that 3D models and animations have a positive effect on students and that teachers should include these visual aids in their lessons. For this reason, teachers are encouraged to implement these dynamic visual aids in their lessons regardless of their beliefs, and to get an adequate support in the process of implementation if necessary.
Introduction
A lack of Science, Technology, Engineering, and Mathematics (STEM) graduates has long troubled the European Union in general, and the Czech Republic in particular (Gago et al., 2005 ). Despite the growing number of scientific publications in the STEM field (Li, 2021 ; Takeuchi et al., 2020 ) there is a current shortage of STEM graduates resulting from the relatively low number of high school students who enroll in science and technology degrees at universities (Eurostat, 2020 ), mostly due to the low popularity of some subjects. For example, Chemistry is an unpopular subject based on the results of student surveys (Beauchamp & Parkinson, 2008 ; Pavelková et al., 2010 ) because this subject is apparently too abstract for students who struggle to visualize some fundamental concepts, such as an atomic orbital (Chen et al., 2015 ), and to understand the particulate nature of matter (Williamson & Abraham, 1995 ). Even Biology, which is regarded as an easy subject (Hanzalová, 2019 ), covers numerous topics requiring a high level of abstraction, including anatomic structures (Mitsuhashi et al., 2009 ) and cellular biology (Jenkinson, 2018 ), as well as molecular genetics concepts and processes (Malacinski & Zell, 1996 ; Rotbain et al., 2006 ). It is therefore necessary that students are sufficiently motivated to study science subjects, which means increasing students ’ interest in the topics taught, along with overcoming challenging (mostly abstract) topics.
Visual representations have been developed to aid thinking and we generally use it to better understand various data (Mazza, 2009 ; Ware, 2004 ). The goal is to visually represent data in such a way that the most important patterns are clearly distinguishable from their surroundings (Mazza, 2009 ) to enable us capturing and incorporating a new piece of information into the long-term memory (Craik & Lockhart, 1972 ). This predetermines visualizations to be used in teaching these particular abstract topics.
Visualization can be divided into static visualizations (e.g., still illustrations, slides and photographs) and dynamic visualizations (e.g., animations, three-dimensional rotating models, simulations and videos). The latter have been gaining popularity as the use of graphics in computer-based educational environments has increasingly become commonplace (Lin & Atkinson, 2011 ).
Yet individual comparisons between the differential effects of static and dynamic visualization have yielded highly heterogeneous results (Kaushal & Panda, 2019 ).
Considering the above, this study aims to identify the best approach to increase students’ internal motivation for science subjects, because students who are more interested in natural sciences are also more motivated to study these subjects (Berg et al., 2003 ; Klahr & Nigam, 2004 ) and to understand them (Khishfe & Abd-El-Khalick, 2002 ). For this purpose, we assess the influence of dynamic visualization on primary and secondary school students in comparison with static visualization in science subjects (biology, chemistry and geology). More specifically, we examine the influence of static and dynamic visualization on students’ internal motivation (interest/enjoyment, effort, perceived competence, value/usefulness) and on the level of acquired knowledge on the subject matter.
One way to increase students’ interest in science subjects and to support their cognitive processes is to use visualization aids (Bilbokaitė, 2015 ; Nodzyńska, 2012 ; Popelka et al., 2019 ; Rotbain et al., 2006 ; Ryoo & Linn, 2012 ; Wu et al., 2001 ). Visual aids can help students understand particularly difficult and abstract topics (Bunce & Gabel, 2002 ; Harrison & Treagust, 2006 ) by stimulating their imagination and enhancing their ability to understand the subject matter, thereby improving the memorization of these concepts. Visualization can also enable students to adequately understand preconcepts (Tarmizi, 2010 ) while preventing the formation of misconcepts. Generally, visualization plays a key role in explaining the subject matter, focusing on features of microelements invisible to the naked eye (DiSpezio, 2010 ; Gomez-Zwiep, 2008 ; Herman et al., 2011 ). Some subjects, such as Biochemistry (Schönborn & Anderson, 2006) and closely related molecular biology (Jenkinson, 2018 ; Marbach-Ad et al., 2008 ), cannot be effectively taught without visualization. Therefore, visualization tools are crucial for understanding and research in the molecular and cellular biological sciences (Schönborn & Anderson, 2006).
The benefits of visualization tools lie in facilitating the understanding process best described by the so-called scaffolding theory (Eshach et al., 2011 ; Wood et al., 1976 ). “The scaffolding metaphor means that given appropriate assistance, a learner can perform a task otherwise outside his/her independent reach” (Eshach et al., 2011 , p. 552). The scaffolding theory, originally requiring an adult to assist and help students (Wood et al., 1976 ), has been subsequently extended by Puntambekar and Hübscher ( 2005 ) to teaching tools able to control and measure the amount of information given, thus reducing the number of acts needed to reach understanding (Puntambekar & Hübscher, 2005 ; Tabak, 2004 ; Wood et al., 1976 ). More recently, Chang and Linn ( 2013 ) showed that interactions with visualization tools are even more beneficial than visualization itself. Therefore, visualization aids that promote further interactions aim to be more effective.
Dynamic visualization
Dynamic visualization aids (e.g., animations, simulations, three-dimensional rotating models and videos) can be used in the teaching process for several purposes. First, animations can serve as a means of gaining attention. This category includes various animated arrows or highlights (Berney & Bétrancourt, 2016 ). Secondly, animation may be used to demonstrate concrete or abstract procedures required to be memorized and performed by the learner, such as tying nautical knots (Ayres et al., 2009 ; Schwan & Riempp, 2004 ). Thirdly, animation-based teaching is effective in describing processes that change over time and space (Ainsworth & VanLabeke, 2004 ; Rieber, 1990 ; Schnotz & Lowe, 2003 ). Dynamic visualization is especially suitable for dynamically visualizing abstract objects which students cannot easily imagine. Therefore, teaching through dynamic visualization is significantly more effective, especially in difficult scientific disciplines in which dynamic visualization can support the students’ cognitive processes (Bilbokaite, 2015 ; McElhaney et al., 2015 ).
This correlation is evident in processes that change over time (Ainsworth & VanLabeke, 2004 ; Rieber, 1990 ). For this reason, visualization is widely used in areas related to physical, chemical or biological disciplines. McElhaney et al. ( 2015 ) specifically mention that dynamic visualization can help pupils/students visualize unobservable dynamic phenomena, such as global climate change, tectonic plate motion, heat transfer, gene expression, cellular respiration and other cellular processes (e.g., cell division)—i.e., topics taught in science subjects such as geology, biology and chemistry.
Advantages and disadvantages of dynamic visualization
Both advantages and disadvantages of using dynamic visualization in teaching have been reported in comparison with static visualization. The benefits of dynamic visualization include enabling and facilitating effects (Kühl et al., 2011 ; Schnotz, 2005 ; Schnotz & Rasch, 2005 ) because the continuous representation of changes supports the perceptual and conceptual processing of dynamic information (Berney & Bétrancourt, 2016 ), in addition to preventing students from developing misconceptions and drawing erroneous conclusions from a mere static representation of the curriculum (e.g., misinterpreting a picture), which is related to an unnecessary cognitive load (Bétrancourt et al., 2001 ; Kühl et al., 2011 ). Dynamic visualization also reduces cognitive load associated with gradual steps (Berney & Bétrancourt, 2016 ), by helping students contextualize separate knowledge, for example relationships among pictures or schemes, which subsequently reduces working memory demands. Another benefit of dynamic visualization includes the ability to control its pace, such as pausing, rewinding or replaying (McElhaney et al., 2015 ).
Conversely, a disadvantage of dynamic visualization is the great amount of information given (Ainsworth & VanLabeke, 2004 ; Bétrancourt & Réalini, 2005 ), all of which (even transient) is processed and stored in the working memory, which could potentially lead to cognitive overload (Chandler, 2004 ; Chandler & Sweller, 1991 ; Jones & Scaife, 2000 ; Lowe, 1999 ; Mayer & Moreno, 2002 ). Dynamic visualization offers only temporary information, which (due to working memory overload) can be replaced by subsequent information (Bétrancourt & Tversky, 2000 ). By contrast, static images presenting different states or steps allow students to examine and compare these states, whereas dynamic visualization provides one step at a time. This stepwise presentation results in another disadvantage of dynamic visualization, which is the inability to compare individual steps (Bétrancourt et al., 2001 ). Another disadvantage of dynamic visualization is the split attention effect. When multiple events overlap in dynamic visualization (animation), attention fragmentation may occur, causing imperfect information acquisition (Löwe, 2003 ). Other disadvantages include oversimplifying a curriculum problem, which may give students a false impression that they understand the problem (Schnotz & Rasch, 2005 ).
Dynamic vs static visualization impact
Considering the widespread use of dynamic visualization, researchers have sought to study its impact on students. In 2000, a review conducted by Bétrancourt and Tversky ( 2000 ) compared 17 studies on the differences between common educational methods (extrapolation and analysis, among others) and the educational process supported by animations. Most studies (10 of 17) showed a positive impact of using animations, but the remaining 7 found no effect or only non-significant effects of incorporating animations into the educational process. In 2007, Höffler and Leutner conducted a meta-analysis of 26 studies published in 1973–2003 (Höffler & Leutner, 2007 ), including 76 pairwise comparisons of the effect between dynamic visualizations and static visualizations. This meta-analysis showed a positive effect of the animations compared with the static visualizations, with an average effect size d = 0.37, which indicates a small to medium effect. However, the authors also included video-based visualization in their study. Even studies comparing static to video-based visualization have shown a significantly higher effect on average ( d = 0.76; a total of 12 comparisons) than other comparisons based on computer graphics ( d = 0.36; a total of 64 comparisons). The total number of participants was not specified.
The research of Höffler and Leutner was closely followed by a similar review study by Berney and Bétrancourt ( 2016 ), who also analyzed research articles published up to 2013, and focused on comparing differences in the benefits of static and dynamic visualization. The authors included 61 published studies, totaling 140 pairwise comparisons of dynamic and static visualizations intended for teaching. In contrast to the previous study, which assessed effect size based on Cohen’s d (Cohen, 1988 ), the magnitude of the effect was expressed as Hedges’ g (Hedges, 1981 ) in this meta-analysis, but the results confirmed the positive effect of animations when compared with static visualizations, with a difference in effect magnitude g = 0.23, which represents a small effect. As the studies included more than 7000 subjects, the results can be considered reliable. The comparison between Cohen’s d of the previous meta-analysis and Hedges’ g of this analysis shows a decrease in effect size. The authors explain that the effect size is smaller because they included more total and pairwise comparisons in the analysis. The authors further highlight that, although the overall effect was positive, almost 60% of the studies did not show significant differences between dynamic and static visualization.
The results from the aforementioned meta-analyses suggest a predominant, albeit slight, positive effect of dynamic visualization (most often in the form of animation). Moreover, the results from individual comparisons are highly heterogeneous. Some studies show the positive influence of animations on the educational process (Lin & Atkinson, 2011 ; Marbach-Ad et al., 2008 ; Özmen, 2011 ), whereas others are less clear in their conclusions (Boucheix & Schneider, 2009 ; Bulman & Fairlie, 2016 ; Mayer et al., 2007 ; Tversky et al., 2002 ).
Moderator variables influencing the effect of dynamic visualization on students
As detailed in the section above, previous empirical studies exploring the influence of animations lack uniform results (Kaushal & Panda, 2019 ). These disparities have led researchers to search for potential moderators of the effect of using dynamic visual aids on student performance. These factors, which our study also addressed, include the instructional domain (subject), student gender and education level.
Instructional domain (subject)
The influence of the instructional domain, for which animation was created, was studied in the meta-analysis by Höffler and Leutner, ( 2007 ). The results showed that the instructional domain in which the analysis is performed is a determining factor of the effect size. The highest magnitude of the effect was measured in chemistry ( d = 0.75; a total of 7 comparisons), followed by mathematics ( d = 0.62; 5 comparisons) and physics ( d = 0.28; 39 comparisons). The smallest effect was found surprisingly in biology ( d = 0.13; 12 comparisons). However, due to the low number of comparisons, whose final effect size has been included in the overall result, the statistical power of this comparison was low (Höffler & Leutner, 2007 ).
The meta-analysis conducted by Berney and Bétrancourt ( 2016 ) also examined the influence of moderating variables that affect the effectiveness of animations in the teaching process. This meta-analysis among other things showed the subject in which the analysis is performed is a determinant of effect size as well. The highest effect was measured in “natural sciences” ( g = 1.26; 8 comparisons), with a relatively large effect in chemistry as well (g = 0.77; 8 comparisons), but with a low effect size in biology (g = 0.20; 33 comparisons). However, even in these results, only a few subjects were compared, which reduced the statistical power of the results.
In the meta-analysis by Castro-Alonso et al. ( 2019 ), the influence of the subject on the effectiveness of dynamic visualization (animation) in teaching was also investigated. The authors focused on STEM and found that the dynamic type of visualization is more effective in geology and other sciences ( g = 0.38; 11 comparisons) and subsequently in biology and medical sciences ( g = 0.27; 11 comparisons) than in technical or mathematical subjects ( g = 0.15; 15 comparisons) or even physics and chemistry ( g = 0.19; 23 comparisons). Nevertheless, the number of overall comparisons was relatively low, which reduces the statistical power of the results.
The division into the instructional domain also entails some difficulties, which may be, for example, the attractiveness of the discussed topic. The whole content of individual scientific disciplines is not homogeneous, and one chapter may be more attractive for students than another, which has a great influence on the overall results.
Student gender
In their meta-analysis, Castro-Alonso et al. ( 2019 ) found that student gender is a key factor because dynamic visualizations are less effective in a sample of participants with fewer females than males. In particular, studies involving fewer than 59% of females showed a moderately positive effect of dynamic visualization ( g = 0.36, 35 comparisons) and studies involving 60% or more females did not show any dynamic visualization effect (g = 0.07, 47 comparisons). The authors suggested that the unequal ratio of females to males, in some studies, may be a significant factor in explaining variations in effect size across studies.
Unfortunately, student gender factor has been overlooked in many studies (Garland & Sanchez, 2013 ; Schnotz et al., 1999 ; Wang et al., 2011 ), and most of which do not even provide gender ratios for the whole sample (Castro-Alonso et al., 2019 ). In addition, many studies are conducted with undergraduate pedagogy and psychology students, and males are markedly under-represented in these degrees (Castro-Alonso et al., 2019 ).
Gender has a strong influence on cognitive load (Bevilacqua, 2017 ). Thus, this factor must be analyzed. In their meta-analysis, Zell et al. ( 2015 ) concluded that gender has a significant effect on attention, memory and problem solving ( d = 0.22), especially among the participants with the best results. Gender can also affect the participants’ perceptions of spatial imagination (Höffler, 2010 ; Ikwuka & Samuel, 2017 ; Wong et al., 2018 ; Zell et al., 2015 ).
Education level
Level of education plays a huge role, mainly because cognitive ability correlates with age (within individual differences) (Damon et al., 2006 ). This is reflected not only in different subjects (instructional domain), but more specifically in individual topics. The level of education must also be taken into account when choosing teaching methods, because it is at the age of middle school students when abstract and scientific thinking gradually develops (Damon et al., 2006 ; Goswami, 2010 ).
The literature shows that dynamic visualizations and animations have a positive impact on school children (Bétrancourt & Chassot, 2008 ), university students (Jaffar, 2012 ) and adults (Türkay, 2016 ). McElhaney et al. ( 2015 ) assessed, whether the effect of dynamic visualization depended on education level, and found that the difference between the effects of dynamic and static visualizations is higher in primary and secondary school students ( g = 0.27; 10 comparisons) than in post-secondary level students ( g = 0.07; 37 comparisons), which showed almost no effect.
The variable education level was also examined by Castro-Alonso et al. ( 2019 ), who concluded that the use of dynamic visualization is most effective among primary school students ( g = 0.53), followed by secondary school students ( g = 0.44), and the least effective among university students ( g = 0.19) (Castro-Alonso et al., 2019 ).
Teacher personality
A substantial amount of variance in instructional quality can be explained by teacher characteristics such as cognitive ability, personality, professional knowledge, constructivist beliefs, enthusiasm and instructional quality (Baier et al., 2019 ). Teacher personality plays an important role in the educational process and should not be omitted. Kim et al. ( 2018 ) showed that even though domains of teacher personality do not predict academic achievement, they are able to predict subjective measures of teacher effectiveness as well as evaluation of teaching (Kim et al., 2019 ). Especially extraversion and enthusiasm have been identified as very strong predictors of instructional quality (Baier et al., 2019 ). Some of these domains are also crucial factors in the implementation and acceptance of technology in education (Tzima et al., 2019 ).
Objectives, hypothesis and research questions
The results from empirical studies are not uniform. Thus, further research is required to determine when animations are more effective than static visual aids (Kaushal & Panda, 2019 ) by continuously exploring dynamic visualizations and by defining potential moderators, which may significantly affect the potential impact of these aids on students.
Currently, many ongoing discussions (especially among teachers and politicians) address the use of dynamic visualizations (e.g., animations, simulations, three-dimensional rotating model, and videos) and their impact on the quality of the education process. Furthermore, the Strategy of Digital Education of the Czech Republic has already been approved since 2014 (MEYS, 2020 ). This strategy, aimed at the digitalization of education in middle and high schools, prioritized opening up the education system to new teaching methods through the use of digital technologies. Accordingly, new visualization equipment was purchased for 60 Czech schools. However, the effectiveness of these visual aids, their impact on the quality of educational process and the influence of potential moderator variables must be evaluated before expanding this strategy to the entire country.
Considering the above, we conducted a proof-of-concept study to assess whether using visual aids positively influenced students. The basic research method was a comparative study in the form of a pedagogical experiment, which investigated the impact of dynamic visualization as a teaching tool on chemistry students (and students of other science subjects) in comparison with those taught using static representations. A different representation of the curriculum was chosen as the independent variable, and it was investigated as to whether the difference could cause a change in both the intrinsic motivation of the students as well as the level of acquired knowledge (dependent variable). Thus, this is research in science didactics using ICT technology to serve as a teaching tool, delivering teaching content and motivating students in the process. Our study was designed and conducted at middle and high schools and mainly focused on the influence of using 3D models and animations in lessons of natural sciences (Biology, Chemistry and Geology)—more specifically on students’ intrinsic motivation and on their level of knowledge. Furthermore, the roles of potential moderator variables, such as gender, level of education, instructional domains and teacher personality, are discussed in our research.
The aim of our research was to assess the effect of 3D models and animations used in natural science classes on students. The size effect was measured on Hedges’ g scale.
The following research questions were developed:
How does the use of 3D models and animations affect students’ intrinsic motivation—more specifically students’: (1) interest; (2) effort to actively participate in the educational process; (3) perceived competence; (4) understanding of the usefulness of the subject matter?
How does this effect change after the intervention time (three months) of regular usage of 3D models and animations?
What is the effect of using 3D models and animations on acquired knowledge in Chemistry and Biology?
What roles do potential moderators (instructional domains, gender, level of education and teacher personality) play in the effectiveness of 3D models and animations?
Based on the results mentioned in the previous section, we set the following hypothesis:
3D models and animations have a positive influence on the intrinsic motivation of students in comparison with static visualization.
3D models and animations have a positive effect on learning outcomes in comparison with static visualization.
The variables of gender, age, educational level, learning domain, and teacher personality significantly affect the results.
The first and second hypotheses are based on the assumption that visualization can serve as a scaffolding tool for learners (Puntambekar & Hübscher, 2005 ). These hypotheses are supported by the benefits of dynamic visualization reported in Chapters 1.1 and 1.2, i.e., dynamic visualization helps students visualize abstract objects that they struggled to imagine (Bilbokaite, 2015 ; McElhaney et al., 2015 ) and unobservable dynamic phenomena (McElhaney et al., 2015 ), preventing misconceptions (Bétrancourt et al., 2001 ; Kühl et al., 2011 ) and reducing cognitive load (Berney & Bétrancourt, 2016 ). The hypotheses are contradicted by the findings of several meta-analyses (e.g., Berney & Bétrancourt, 2016 ; Castro-Alonso et al., 2019 ; Höffler & Leutner, 2007 ; McElhaney et al., 2015 ), as summarized in more detail in Chapters 1.3 and 1.4.
Participants
In total, 565 (middle and high school) students (321 females and 238 males; 6 students omitted this information), aged 11 to 20, were included in this study and divided into two groups (242 students in the control group and 323 students in the experimental group). Most of them were Biology students (350), in addition to Chemistry (124) and Geology (70) students. All students of both groups had similar educational and socioeconomic backgrounds.
In accordance with the precepts of a proof-of-concept study, the teachers and consequently their students who participated in this research were randomly selected. The teachers involved in this research were required to teach the same subject (Chemistry, Biology or Geology) in two classes of the same grade so that each teacher taught students in both the experimental class and control class, that is, to enable a direct comparison between the two classes. Of the 50 teachers who met the criteria for participation in this study, 11 were randomly selected to participate in this research. The teachers were employed at a middle or high school and had a master’s degree. The median years of experience in teaching science was 15.5 years, and all teachers agreed to use 3D models and visualizations in some of their classes.
As explained above, all teachers taught in two classes of the same grade, an experimental class and a control class. All students of the experimental classes formed the experimental group (EG), whereas all students of the control classes formed the control group (CG).
In this article, experimental teaching is teaching in which EG students were taught using dynamic visualization aids. The teachers incorporated dynamic visualizations (three-dimensional rotating models and animations) into the lessons in the experimental class for 3 months, without changing their teaching methods. Teachers were instructed to use dynamic visualization in almost every lesson depending on the topic under discussion.
In turn, control teaching herein is classical (traditional) teaching in which the traditional way of teaching (thus far) was followed, i.e., in the same way as in the experimental group, albeit without dynamic visualization aids. The teacher could use visual aids in the control class as well (pictures and schemes, among others), but not dynamic visualizations (three-dimensional rotating models or animations).
Each teacher taught the same topics in both control and experimental classes.
Topics from general chemistry (the state of substances, the formation of chemical bonds, ions and acid–base reactions) and organic chemistry (hybridization, stereochemistry, the structure of hydrocarbons and their derivatives) were taught in chemistry. In turn, Biology introduced mainly topics from human biology (human anatomy, muscles, blood circulation, the human skeleton and digestive system), zoology (differences in systems and body structure), general biology (prokaryotic and eukaryotic cell) and botany. Lastly, in Geology, mainly external and internal geological processes were taught.
Learning environment
The application software Corinth was used as the source of 3D models and animations. This app was developed by experts from several universities and is designed to support the digitalization of the educational process at middle and high schools (Corinth s.r.o., 2020). In addition, Charles University, primarily experts in didactics of natural sciences (including authors of this article), helped to develop this application. Corinth is mainly intended for lessons of natural sciences and offers various visual aids for the educational process. The software consists of a library with 1500 visual objects—mostly 3D models, microscope images, videos, photo galleries and animations (Fig. 1 ). The following topics are covered in this application: Biology, Geology, Chemistry, Physics, Astronomy, Geometry and a few cultural and historical topics. In contrast to common textbooks, online videos or presentations, students can manipulate the object as if they were holding the actual object in their own hands. Therefore, each student can focus on specific details overlooked in 2D images. Moreover, students can turn the 3D models around, zoom in or out on the picture, highlight the objects or look inside them and pause the animations. All models also provide a short description of individual parts, as well as other important comments and notes—for example visualization in augmented reality (AR). This function uses the camera of the equipment to project the chosen 3D model or animation on real time captured backgroud. Application Corinth is known in the US thanks to the educational platform Lifeliqe, which received the 2017 Best App for Teaching and Learning award from the American Association of School Librarians (ALA, 2017).
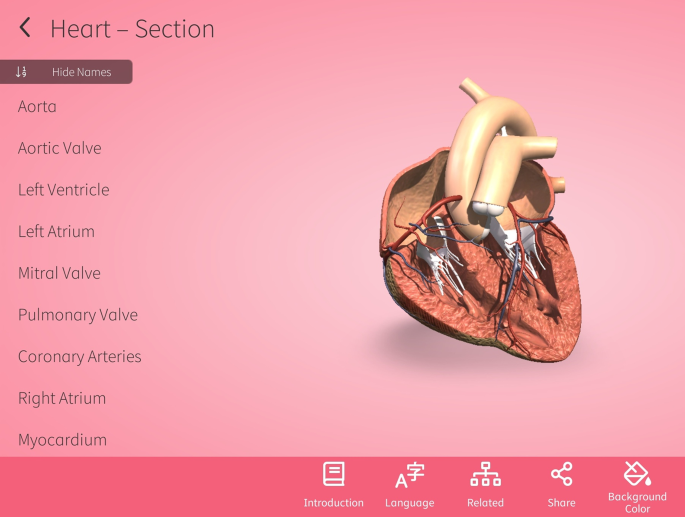
One of the models used in experimental teaching—a 3D model of the heart (Corinth s.r.o., 2020)
Measures, knowledge test and questionnaires
Several research tools were used in the preliminary study and subsequent research.
Two types of research tools were used to determine the effect on students’ motivational orientation:
Motivated Strategies for Learning Questionnaire (MSLQ) (Pintrich et al., 1991 ) Intrinsic Motivation Inventory (IMI) (McAuley et al., 1989 ; Ryan, 1982 ).
The level of knowledge was determined through knowledge pretests and posttests.
At the beginning of the research, each teacher was interviewed to assess their expectations and experience. At the end of the research, each teacher was interviewed, providing feedback on the lessons taught in this project.
Standardized questionnaires: MSLQ and IMI
The MSLQ (Motivated Strategies for Learning Questionnaire) is a tool for identifying students’ motivational strategies in the learning process, compiled by Pintrich, Smith, Garcia and McKeachie, and serves to identify and evaluate students’ motivational orientations and their use of different strategies for self-learning, i.e., in the process of self-regulation (Pintrich et al., 1991 ). Based on this questionnaire, a Pre-questionnaire was designed by selecting 16 statements from the four following scales:
intrinsic goal motivation (e.g., “in a class like this, I prefer course material that really challenges me so I can learn new things. The most satisfying thing for me in this course is trying to understand the content as thoroughly as possible.”);
self-efficacy for learning and performance (e.g., “I’m confident I can do an excellent job on the assignments and tests in this course. Considering the difficulty of this course, the teacher, and my skills, I think I will do well in this class.”);
extrinsic goal motivation (e.g., “Getting a good grade in this class is the most satisfying thing for me right now. If I can, I want to get better grades in this class than most of the other students.”);
control beliefs (e.g., “It is my own fault if I don’t learn the material in this course. If I don’t understand the course material, it is because I didn’t try hard enough.”).
This Pre-questionnaire was used in both the experimental and control classes at the beginning of the second lesson—before using the experimental teaching methods for the first time.
The IMI (Intrinsic Motivation Inventory) tool is an internal motivation questionnaire based on Ryan’s research ( 1982 ), but its final form was compiled by McAuley et al. ( 1989 ) and is used to assess the subjective experience related to the student’s internal motivation and personal self-reflection. Based on IMI, three questionnaires (Post-questionnaire 1, Post-questionnaire 2_1 and Post-questionnaire 2_2) were created, each of which consisted of 25 statements from the four following scales:
interest/enjoyment (e.g., “This activity was fun to do. I enjoyed doing this activity very much.”);
effort/importance (e.g., “I put a lot of effort into this. I tried very hard on this activity.”);
perceived competence (e.g., “I was pretty skilled at this activity. I am satisfied with my performance on this task.”);
value/usefulness (e.g., “I think this is an important activity. I believe doing this activity could be beneficial to me.”).
Both tools use a seven-item Likert’s scale for each statement (Likert, 1932 ) enabling participants to express their level of agreement with each statement from “strongly agree” = 1 to “strongly disagree” = 7 (Pintrich et al., 1991 ; Ryan, 1982 ). Both tools have been used in many earlier studies in the field of intrinsic motivation and self-regulation (Monetti, 2002 ; Niemi et al., 2003 ; Wolters, 2004 ). These tools were also used to measure intrinsic motivation for natural sciences (Šmejkal et al., 2016 ). An advantage of such research tools is their flexibility as modular aids adaptable to specific research needs. Therefore, they do not require using their full versions (Markland & Hardy, 1997 ; Pintrich et al., 1991 ; Rotgans & Schmidt, 2010 ).
Knowledge tests
The acquired knowledge was evaluated using knowledge tests. Due to the difficult process of developing these tests, this analysis was performed only at randomly selected schools and in randomly selected classes of those schools, totaling 4 tests (2 Chemistry tests and 2 Biology tests). The tests were created specifically for each school and class by a panel of experts, more specifically two experts in didactics and three teachers of the subject (Chemistry/ Biology). The tests were compiled based on the curriculum and on the goals set by the teacher, in line with the revised version of Bloom's taxonomy of cognitive goals (Airasian et al., 2001 ). In chemistry in particular, we were able to include a larger number of tasks focused on engaging of higher level thinking skills, such as conceptual and procedural knowledge in Knowledge Dimension and remembering, understanding and application in Cognitive Process Dimension. Each knowledge test was administered twice, once during the first lesson (Pretest) and then during the penultimate lesson (Posttest). The tests were identical for the experimental and control groups.
The research survey was performed in 2019. All teachers involved in the research completed a 2-day training course before the research survey to acquaint themselves with the educational aid, its content and technical aspects (e.g., how to install Corinth software on their mobile device or how to incorporate educational content in presentations and other educational materials). Throughout this study, the teachers were in contact with the researchers and with the Corinth technical support as well. All teachers were also familiarized in detail with the course of the research, all research tools and their purpose in the research, and with the way in which students were supposed to fill in the questionnaires. Students were informed about the pedagogical research, and the research questionnaires were filled in anonymously.
The pedagogical experiment proceeded as follows. Before the actual start of the experiment, an initial interview was conducted with all the teachers. The aim of the initial interview was to find out what the teachers’ expectations are in relation to the implementation of dynamic visualization, specifically the implementation of Corinth in the classroom. The interview included a total of 17 questions, which were thematically divided into four areas: teacher-oriented questions (5), student-oriented questions (4), questions oriented to the content of the Corinth application (5), questions oriented to the school’s attitude towards the implementation of the Corinth application in the classroom (3).
In the first to third lessons, both the experimental and control groups used the classical teaching style. All students filled the Pretest during the first lesson, the Pre-questionnaire at the beginning of the second lesson, and the Post-Questionnaire 1 at the end of the third lesson. From the fourth lesson onward, control group (CG) students were taught using a classical teaching style, whereas the experimental teaching style was implemented in the experimental group (EG). The EG students filled in Post-Questionnaire 2_1 after the first experimental lesson. The same questionnaire, Post-Questionnaire 2_2, was filled in by the EG students again in the last lesson, after three months of intensive learning using dynamic visualizations. In the penultimate lesson of the pedagogical experiment, the students filled in the posttests. After the pedagogical experiment, an output interview was conducted with the teachers.
Figure 2 schematically shows the time course of research and the sequence of research tools.
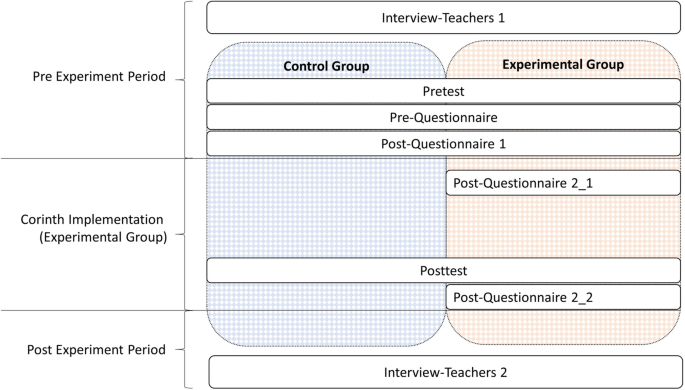
Diagram showing the timeline of the implementation of each research tool
Results and discussion
Data from 565 students were used in the statistical analysis. The anonymized data were processed in the statistical software IBM SPSS using appropriate statistical methods. Significance was assessed using both parametric and non-parametric tests, setting the significance level at α = 0.05. Initially, the effect size was calculated based on Hedges’ g (Hedges & Olkin, 1985 ).
Reliability of the data from the questionnaires and calculation of the study variables
In all scales, the reliability of each questionnaire mentioned above was assessed by calculating the Cronbach’s alpha coefficient (Cronbach, 1951 ).
Almost all values of reliability exceeded the generally accepted minimum of 0.70 (Nunnally, 1978 ), except for the Cronbach’s alpha of “control beliefs” of the Pre-Questionnaire, which was 0.60 (see Table 1 ). This value was nevertheless close to the required level and was therefore accepted. In conclusion, the data are internally consistent and reliable. Based on this model approved by confirmation analysis (Šmejkal et al., 2016 ), new variables were calculated as an average of each item from one of the scales described in Methodology.
The influence of 3D models and animations on students’ intrinsic motivation
To assess the influence of using 3D models and animations on students (RQ1), specifically: (1) interest in the subject matter; (2) effort during the educational process; (3) perceived competence; (4) usefulness of the subject matter, two statistical tests were performed.
First, the students’ motivation in the control lesson was evaluated using data from the Pre-Questionnaire and from the Post-Questionnaire 1. Based on the data, we assessed whether the CG and EG significantly differed. Because the data did not conclusively show a normal distribution, the Mann–Whitney U test was used as the appropriate statistical test, albeit showing no significant difference between the CG and the EG. The significance level of all scales exceeded 0.05, except for “self-efficacy for learning and performance”. Although a significant difference was found in this scale, the Hedges’ g demonstrated that the difference was very small (Table 2 ). Therefore, the students of the EG and CG reached similar values of intrinsic motivation in most scales.
Second, we assessed whether the perception of control and experimental lessons significantly differed among students who had experienced both teaching styles (only students in the EG). The corresponding data were retrieved from the Post-Questionnaire 1 and Post-Questionnaire 2_1 and analyzed statistically. For this purpose, the non-parametric Wilcoxon signed-rank test was used because some of the data did not show a normal distribution. The results from the test highlighted significant differences between the students’ evaluation of the control and the first experimental lessons in all scales ( p -values were significantly lower than 0.05 in all scales, see Table 3 ). The values of Hedges’ g also suggested that using 3D models and animations had a strong positive effect on the students’ intrinsic motivation, particularly in their interest/enjoyment of the teaching process ( g = 1.05) and perceived value/usefulness of the subject matter ( g = 1.02), in addition to a medium positive effect on perceived competence ( g = 0.41) and a low, albeit positive influence on effort/ importance ( g = 0.27). In short, after the first experimental lesson the students’ motivation (more specifically their interest in the subject matter, their effort to understand the subject matter and their perceived teacher competence and subject matter importance) significantly differed between the control and experimental lessons, with a large weighted mean effect size ( g = 0.69). It can be concluded that 3D models and animations have a significant, positive effect on all components of intrinsic motivation, thus corroborating the findings of Berney and Bétrancourt ( 2016 ). All components of intrinsic motivation are positively influenced by the use of 3D models and animations when comparing experimental and control lessons. Overall, owing to the incorporation of 3D models and animations into the educational process, students are more interested in the subject matter and therefore willing to put more effort into learning new skills, thereby improving their learning outcomes.
To assess whether the positive effect of the application decreases with the intervention time of its incorporation into the educational process over time (3 months) (RQ2), data from the Post-Questionnaire 2_1 were compared with data from the Post-Questionnaire 2_2. Based on the character of the data, the non-parametric Wilcoxon signed-rank test was used for this analysis, rejecting the null hypothesis in 3 of the 4 scales because significant differences were found between the answers of the two questionnaires (see Table 4 ). The comparison of the effect size showed that the decreases in the scales were low ( g = 0.32) in perceived competence, medium ( g = 0.41) in value/usefulness and medium/large ( g = 0.60) in interest/enjoyment. However, no significant decrease was found in effort/importance over time. As in similar cases it can be expected that after starting to use 3D dynamic animations, the so-called “Novelty Effect” (Clark & Sugrue, 1988 ) may be observed. Therefore, a study was carried out to monitor changes (in motivation, knowledge) depending on the intervention time of using dynamic 3D animations, for it has been shown that the intervention time of using the aid can lead to a decrease in students attention and motivation (Tsay et al., 2018 ).
The comparison between the traditional teaching style and the experimental method after 3 months of intensive use of 3D models and animations in the lessons showed a consistently significant difference in 3 of the 4 scales (based on the Wilcoxon signed-rank test on the data from the Post-Questionnaire 1 and Post-Questionnaire 2_2, Table 5 and Fig. 3 ), with a medium/large positive effect size in the value/usefulness scale ( g = 0.64), a medium effect size in the interest/enjoyment scale ( g = 0.49) and a small but positive effect size in the effort/importance scale ( g = 0.26). Based on the results, using 3D models and animations primarily affects the students’ interest and perceived value of the subject matter. The overall positive effect was evident, even after three months of using the 3D models and animations, as shown by the weighted mean effect size ( g = 0.38). In other words, the use of 3D models and animations enhances the perceived importance of the subject matter, most likely by lowering the level of cognitive processes and abstraction necessary for understanding the concepts of phenomena studied in natural sciences (Chandler & Sweller, 1991 ), which proves a scaffolding potential of used visualization. This experimental approach to teaching prevents the decrease (and in some cases even leads to an increase) in the students’ interest in the subject matter. Furthermore, the students are also willing to put more effort into understanding a given topic. From a long-term perspective, these two trends are crucial because effort/importance reach the same values over time. Accordingly, the occasional use of 3D models and animations helps students understand the importance of the subject, thereby increasing the long-term efforts that they put into the educational process (Ryan & Deci, 2000 ). Based on the above stated findings, it can be declared that the first hypothesis was confirmed.
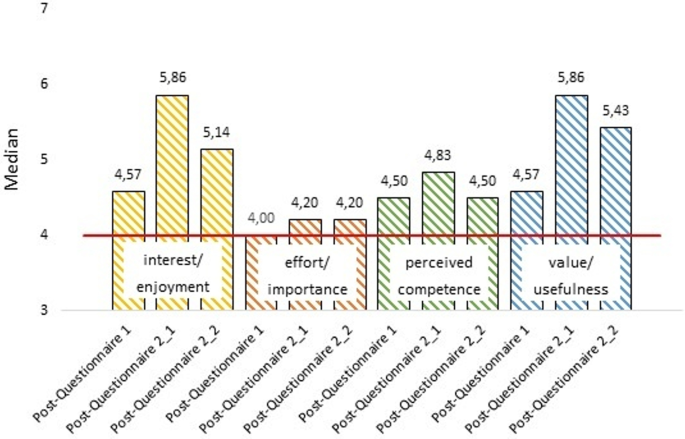
The bar chart illustrates the medians of the components of intrinsic motivation after the control lesson, after the first experimental lesson and after the last experimental lesson (seven-item Likert’s scale)
In comparison with the findings of previous studies, our positive effects of the use of 3D models and animations are significantly stronger than the results from the meta-analysis performed by Berney and Bétrancourt ( 2016 ) and by Castro-Alonso et al. ( 2019 ), with an average effect size of 0.23 (Hedges’ g ). In turn, the results from this study are similar to those of the meta-analysis by Höffler and Leutner ( 2007 ), who reported an average effect size of 0.37 (Cohen’s d ). The differences in results of the studies may be caused by the heterogeneity of the studies included in the analyses. Moreover, Castro-Alonso et al. ( 2019 ) also address this issue in their meta-analysis where they observed a significant heterogeneity between effect sizes. Therefore, they recommend focusing on different variables influencing these results.
The effect of using 3D models and animations on the level of acquired knowledge
The effect of using 3D models and animations on the level of acquired knowledge (RQ3) was assessed based on the results from knowledge tests.
The reliability of each knowledge test was determined by calculating the corresponding Cronbach’s alpha (see Table 6 for results). The required value of reliability of the test used for individual pedagogical diagnosis is 0.8 (Chráska, 1999 ). According to George and Mallery ( 2003 ), a Cronbach’s alpha value between 0.7 and 0.8 is also acceptable. Therefore, based on the Cronbach’s alpha values calculated in this study, the knowledge tests meet the required reliability standards.
The data were analyzed using the parametric, two-tailed t -test. The results showed no significant difference in the Pretest between the CG and EG at the beginning of the research (Pretest Chemistry: t = -0.192, df = 54, p = 0.848, M control = 4.29, SD = 2.532, M experimental = 4.44, SD = 3.292, g = 0.050; Pretest Biology: t = − 1.283, df = 54, p = 0.205, M control = 15.55; SD = 5.954; M experimental = 17.52; SD = 5.402, g = 0.342).
At the end of the research, the students were asked to complete the same knowledge tests (Posttests). The results from the two-tailed t -test conclusively demonstrate that Chemistry students in the EG performed better than their peers in the CG. The calculated difference was significant and deemed large (Posttest Chemistry: t = − 3.601, df = 58, p = 0.001, M control = 16.531, SD = 7.326, M experimental = 23.839, SD = 8.394, g = 0.916). Furthermore, in the Biology tests, students in the EG also performed better than the students in the CG (Posttest Biology: t = − 1.189, df = 50, p = 0.240, M control = 25.92, SD = 10.488, M experimental = 29.04, SD = 8.373, g = 0.322), albeit non-significantly. One of the possible explanations is the higher level of abstraction and visualization required in Chemistry. Considering the individual development of visual orientation and abstract thinking, 3D models require a lower level of visual orientation from students, and animations can support the understanding of abstract concepts. Thus, the combination of these tools improves the understanding of the subject matter and therefore the results from the evaluation phase of the educational process. The second hypothesis (H2) was as well as confirmed. The higher level of visual orientation and abstract thinking necessary for understanding Chemistry may account for the stronger impact of using visual aids in the educational process. The results from this analysis are in line with the Cognitive Load Theory (Chandler & Sweller, 1991 ), according to which visualization decreases the cognitive load and therefore lowers the total cognitive steps necessary for succeeding in a given task. The combination of this factor with the significant, positive influence on the students’ interest is one of the signs of scientific literacy, as defined by PISA (OECD, 2006), thus opening up new research opportunities.
The results from knowledge pretests and posttests are summarized in Fig. 4 . The chart shows box diagrams of the results of 4 tests (2 pretests and 2 posttests) separately for both subjects, that is, chemistry and natural sciences. The comparison of the box diagrams shows no significant differences between CG and EG in knowledge pretests (especially in chemistry), but the differences become more pronounced in knowledge posttests (again, mainly in chemistry).
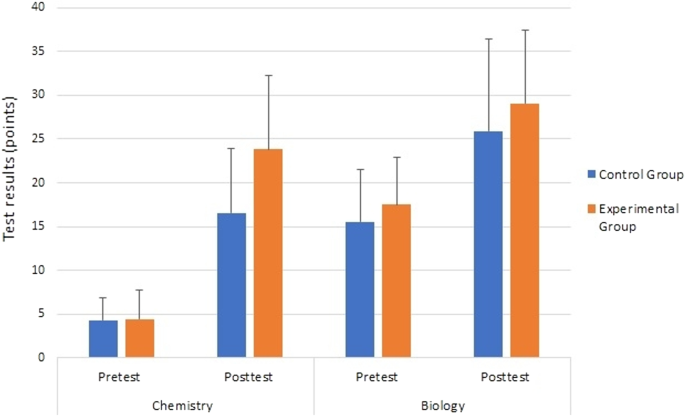
Results from the knowledge tests in CG and EG
The results shown above are in line with the outcomes of the teacher interviews. The teachers reported increased interest and motivation of the students during the lessons with 3D models and animations.
“The pupils ’ interest increased, they were drawn into the lessons, everyone was paying attention, they were enjoying it. The lessons were more interesting for the them. Thank you.”
Furthermore, the teachers also reported that passive students were more easily activated.
“The pupils were curious what new things they would see.”
Moreover, the improvements in illustration of the subject matter facilitated the explanation and understanding of abstract concepts for teachers and students, respectively.
“Teaching has become more interesting and students ’ imagination and understanding of the subject matter has improved.”
Educators also mentioned that incorporating 3D models and animations is helpful, especially for students with a lower level of visual orientation or abstract thinking.
The influence of potential moderators on dynamic visualizations
The next step of our research was the analysis of the impact of the following potential moderators of the effect of using animations and 3D models on the students’ motivation: student gender, level of education, student age, instructional domain and teacher personality (RQ4). However, the obtained conclusions did not fully confirm the third hypothesis (H3).
Based on the results from the Mann–Whitney U test, student gender played no role in the evaluation of the first experimental lesson ( g = 0.10, N female = 151, N male = 104), and all intrinsic motivation components were equal between male and female students in the first experimental lesson. Similar results were found when comparing the corresponding data from the first experimental lesson with the data from the control lesson (separately for each gender). The weighted mean effect size on female students ( g = 0.69, N = 129) and the weighted mean effect size on male students ( g = 0.68, N = 91) were virtually equal (see Table 7 ). In our study, student gender is not a moderator variable of intrinsic motivation. In contrast, other studies have shown that student gender is a strong moderator variable of the effect of dynamic visualizations on learning (Castro-Alonso et al., 2019 ). The variability in the findings of these studies may be caused by the differences in individual methodologies, learning environments and male:female ratios of participants. Therefore, this potential moderator must be further explored to find more evidence about its role.
Level of education
The results from the Mann–Whitney U test showed no level-of-education effect on the results of the students ( g = 0.13, N middle school = 128, N high school = 129) in the first experimental lesson. Similar results were found when comparing the corresponding data from the first experimental lessons with the data from the control lessons (separately for middle and high school students), as shown by the weighted mean effect sizes for middle ( g = 0.67, N = 108) and high school ( g = 0.70, N = 112) students (see Table 7 ). The level of education shows no effect on the results of our study, despite the findings of Castro-Alonso et al. ( 2019 ), who reported that dynamic visualizations were more effective among middle school students than among high school students, but the difference was quite small. This finding could be caused by the non-linear variation of the results with student age.
Student age
The first experimental lesson was attended by 257 students aged 11 to 20. Data analysis highlighted a significant, quadratic relationship between student age and all components of intrinsic motivation [interest: F (255) = 5.07, p = 0.007; effort: F (255) = 9.08, p = 0.000, competence F (255) = 4.60, p = 0.011: value: F (255) = 3.34, p = 0.037, see Fig. 5 ]. Based on the relationship between these pairs of variables, a few general trends can be described, for example: younger students (11–12 years of age) perceive the incorporation of dynamic visualizations into the teaching process highly positively. However, as the students become older, they gradually evaluate the use of animations and 3D models in the educational process less favorably—the students’ evaluation is the least positive among 15-year-old students (at the age when they graduate from middle school in the Czech Republic). However, student feedback becomes more positive among high school students aged 16–18. Unfortunately, the sample of students older than 18 years was too small to enable any prediction. In any case, the power of the models is low.
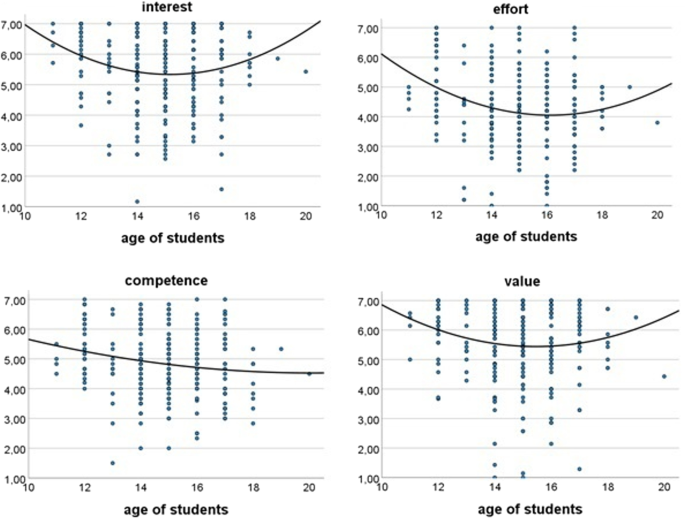
Fitted line plot of the quadratic model for the relationship between student age and interest, effort, competence or value
Instructional domain
The Kruskal–Wallis test showed a significant influence of the subject Biology ( N = 154), Chemistry ( N = 64) or Geology ( N = 36) on two components of intrinsic motivation, interest/ enjoyment ( η 2 = 0.079) and value/usefulness ( η 2 = 0.066) in the first experimental lesson. Biology students showed the highest interest in the subject, whereas the lowest interest was found among Geology students. Furthermore, the same trend was observed in the scale value/ usefulness. As for the other components of intrinsic motivation (effort/ importance and perceived competence), the students in the first experimental lesson reached similar values in each school subject.
Comparing the data from the first experimental lesson with those from the control lesson (separately for each subject), we can summarize the results as follows: animations and 3D models have the strongest positive effect on Chemistry ( g = 0.74, N = 56) and Biology ( g = 0.72, N Biology = 133), whereas the positive impact on Geology is significantly weaker ( g = 0.45, N Geology = 31) than on the other subjects (see Table 7 ). Considering these results, the instructional domain is a significant moderator variable. Given the limited number of questionnaires from the experimental lessons in Geology and Chemistry, and since only selected topics were taught, the results cannot be generalized. However, based on our findings, we assume that including dynamic visualization (3D models and animations) in biological, chemical and geological disciplines is beneficial, as evidenced by other authors (Jenkinson, 2018 ; McElhaney et al., 2015 ; Mitsuhashi et al., 2009 ).
In total, 11 teachers (9 females and 2 males) participated in this study. Using the Kruskal–Wallis test, we found a significant influence of teacher personality on all components of intrinsic motivation ( η 2 is between 0.070 and 0.132) in the first experimental lesson.
The overall effect of animations and 3D models on the students’ intrinsic motivation was evaluated based on the comparison between the data from the first experimental lesson and the data from the first control lesson. The calculated values of the weighted mean effect sizes (Hedges’ g ) ranged from 0.40 to 1.21 (see Table 7 ).
The largest differences in size effect were found between individual teachers. Therefore, teacher personality is a significant moderator variable. However, due to the limited number of questionnaires from the experimental lesson, the power of the test comparing subgroups is low. The analysis of structured interviews with teachers shows that students whose teachers worry about the failure of the educational application, and question the positive effect of visualization on learning, evaluate the experimental lesson less positively than students whose teachers are more confident about the experimental teaching process. In their interviews, all teachers also mentioned the time needed to adjust their lesson plans to incorporate visual aids appropriately.
“Initially, I spent more time and put more effort into lesson preparation process because I wanted the app to fit into my teaching plan.”
This concern was justified because many teachers had to learn how to work with the application software before they could use its visual aids in the teaching process. Based on these results, teachers must have a positive attitude towards modern technology, as innovators and early adopters, according to Aldunate and Nussbaum ( 2013 ), in addition to adequate technical support at schools in case of any technical difficulties. Furthermore, teachers should be familiar not only with the teaching content of the application software but also with all technical issues. However, teacher personality was not analyzed as a moderator variable of the effectiveness of dynamic visualizations in the teaching process, and therefore should be the subject of further studies.
Study limitations
The main limitation of this study is that all data reflect only the students’ attitude towards the teaching process and their own level of understanding of the subject matter. Therefore, the students’ level of self-confidence also interferes with the results. Nevertheless, this effect is partly compensated for by structured interviews with the teachers, who also evaluated the teaching process from their perspective. The data collected from students and teachers matched. During the interviews, teachers stated that the incorporation of 3D models and animations into the teaching process had a positive impact on their students, who found the models interesting, entertaining and attractive. Therefore, the students appeared more motivated to learn and interested in the subject matter. Furthermore, the teachers expressed a deeper interest in 3D models and animations of physiological processes in plants and humans from not only a biological but also a chemical standpoint (reaction mechanisms, for example).
The effect of teacher personality on the results of the experiment was also partly compensated for by the fact that all teachers taught both groups (experimental and control classes of the same grade). Moreover, the teachers included the same topics in the teaching process in both classes, further offsetting this factor. The generalizability of the results might be limited also by the small sample size. Especially small number of teachers with different teaching styles and personality characteristics may have influenced some of the research results. In this regard, the findings of the present study may provide a good starting point for the design of such studies in a larger scale aiming toward equal sample sizes and similar education level.
The use of 3D models and animations in lessons of natural sciences is positively perceived by students at both middle and high schools. These conclusions are supported by the positive impact of dynamic visualizations on intrinsic motivation in comparison with static images of 3 subject matters (Biology, Chemistry and Geology), as shown by the weighted mean effect size (Hedges’ g = 0.38). Accordingly, the Czech educational system must respond to the specific needs of the current generation, by updating education materials in lockstep with the most recent advances in technology, and by introducing subject matter topics in a more dynamic, realistic and effective way. Our research demonstrates that appropriately incorporating visual aids simplifies abstract processes and enhances understanding. As a result, students may be more interested in learning and may even consider studying the subject matter at a higher level (for example at university). For this reason, teachers should include these visual aids in their lessons regardless of their age or beliefs. Similarly, university educators should also train future teachers in working with digital technologies (Evagorou et al., 2015 ), so that they are more confident in using them without fear of potential technical failures.
Availability of data and materials
Not applicable.
Ainsworth, S., & VanLabeke, N. (2004). Multiple forms of dynamic representation. Learning and Instruction, 14 (3), 241–255. https://doi.org/10.1016/j.learninstruc.2004.06.002
Article Google Scholar
Airasian, P., Cruikshank, K., Mayer, R., Pintrich, P., Raths, J., & Wittrock, M. (2001). Taxonomy for learning, teaching, and assessing: A revision of Bloom’s taxonomy of educational objectives (L. W. Anderson & D. R. Krathwohl (eds.)).
Aldunate, R., & Nussbaum, M. (2013). Teacher adoption of technology. Computers in Human Behavior, 29 (3), 519–524. https://doi.org/10.1016/j.chb.2012.10.017
Ayres, P., Marcus, N., Chan, C., & Qian, N. (2009). Learning hand manipulative tasks: When instructional animations are superior to equivalent static representations. Computers in Human Behavior, 25 (2), 348–353. https://doi.org/10.1016/j.chb.2008.12.013
Baier, F., Decker, A. T., Voss, T., Kleickmann, T., Klusmann, U., & Kunter, M. (2019). What makes a good teacher? The relative importance of mathematics teachers’ cognitive ability, personality, knowledge, beliefs, and motivation for instructional quality. British Journal of Educational Psychology, 89 (4), 767–786. https://doi.org/10.1111/BJEP.12256
Beauchamp, G., & Parkinson, J. (2008). Pupils’ attitudes towards school science as they transfer from an ICT-rich primary school to a secondary school with fewer ICT resources: Does ICT matter? Education and Information Technologies, 13 (2), 103–118. https://doi.org/10.1007/s10639-007-9053-5
Berg, C. A. R., Bergendahl, V. C. B., Lundberg, B. K. S., & Tibell, L. A. E. (2003). Benefiting from an open-ended experiment? A comparison of attitudes to, and outcomes of, an expository versus an open-inquiry version of the same experiment. International Journal of Science Education, 25 (3), 351–372. https://doi.org/10.1080/09500690210145738
Berney, S., & Bétrancourt, M. (2016). Does animation enhance learning? A meta-analysis. Computers and Education, 101 , 150–167. https://doi.org/10.1016/j.compedu.2016.06.005
Bétrancourt, M., & Chassot, A. (2008). Making sense of animation. In R. Löwe & W. Schnotz (Eds.), Learning with animation: Research implications for design. Cambridge University Press.
Google Scholar
Bétrancourt, M., & Réalini, N. (2005). 11th Journe´es d’Etude sur le Traitement Cognitif des Syste`mes d’Information Complexes (JETCSIC). Le Contrôle Sur Le Déroulement de l’animation . https://telearn.archives-ouvertes.fr/hal-00016538/document
Bétrancourt, M., & Tversky, B. (2000). Effect of computer animation on users’ performance: a review/(Effet de l’animation sur les performances des utilisateurs: une sythèse). Le Travail Humain , 63 (4).
Bétrancourt, M., Tversky, B., & Bauer-Morrison, J. (2001). Les animations sont-elles vraiment plus efficaces. Revue d’intelligence Artificielle , 14 (1–2).
Bevilacqua, A. (2017). Commentary: Should gender differences be included in the evolutionary upgrade to cognitive load theory? Educational Psychology Review, 29 , 189–194. https://doi.org/10.1007/s10648-016-9362-6
Bilbokaitė, R. (2015). Effect of computer based visualization on students’ cognitive processes in education process. Society, Integration, Education., 4 , 349. https://doi.org/10.17770/sie2015vol4.417
Boucheix, J. M., & Schneider, E. (2009). Static and animated presentations in learning dynamic mechanical systems. Learning and Instruction, 19 (2), 112–127. https://doi.org/10.1016/j.learninstruc.2008.03.004
Bulman, G., & Fairlie, R. W. (2016). Technology and Education: Computers, Software, and the Internet. In Handbook of the Economics of Education (Vol. 5, pp. 239–280). Elsevier. https://doi.org/10.1016/B978-0-444-63459-7.00005-1
Bunce, D. M., & Gabel, D. (2002). Differential effects on the achievement of males and females of teaching the particulate nature of chemistry. Journal of Research in Science Teaching, 39 (10), 911–927. https://doi.org/10.1002/tea.10056
Castro-Alonso, J. C., Wong, M., Adesope, O. O., Ayres, P., & Paas, F. (2019). Gender imbalance in instructional dynamic versus static visualizations: A meta-analysis. Educational Psychology Review, 31 , 361–387. https://doi.org/10.1007/s10648-019-09469-1
Chandler, P. (2004). The crucial role of cognitive processes in the design of dynamic visualizations. Learning and Instruction, 14 , 353–357. https://doi.org/10.1016/j.learninstruc.2004.06.009
Chandler, P., & Sweller, J. (1991). Cognitive load theory and the format of instruction. Cognition and Instruction, 8 (4), 293–332. https://doi.org/10.1207/s1532690xci0804_2
Chang, H.-Y., & Linn, M. C. (2013). Scaffolding learning from molecular visualizations. Journal of Research in Science Teaching, 50 (7), 858–886. https://doi.org/10.1002/tea.21089
Chen, S. C., Hsiao, M. S., & She, H. C. (2015). The effects of static versus dynamic 3D representations on 10th grade students’ atomic orbital mental model construction: Evidence from eye movement behaviors. Computers in Human Behavior, 53 , 169–180. https://doi.org/10.1016/j.chb.2015.07.003
Chráska, M. (1999). Didaktické testy: příručka pro učitele a studenty učitelství . Paido.
Clark, R. E., & Sugrue, B. M. (1988). Research on instructional media 1978–88 . Libraries Unlimited, Inc.
Cohen, J. (1988). Statistical power analysis for the behavioural sciences (2nd ed.). L. Erlbaum Associates.
Craik, F. I. M., & Lockhart, R. S. (1972). Levels of processing: A framework for memory research. Journal of Verbal Learning and Verbal Behavior, 11 (6), 671–684. https://doi.org/10.1016/S0022-5371(72)80001-X
Cronbach, L. J. (1951). Coefficient alpha and the internal structure of tests. Psychometrika, 16 (3), 297–334. https://doi.org/10.1007/BF02310555
Damon, W., Lerner, R. M., Kuhn, D., & Siegler, R. S. (2006). Handbook of child psychology: Cognition, Perception, and Language (6th ed.). John Wiley Sons.
DiSpezio, M. (2010). Misconceptions in the science classroom. Science Scope, 34 (1), 16.
Eshach, H., Dor-Ziderman, Y., & Arbel, Y. (2011). Scaffolding the “Scaffolding” metaphor: From inspiration to a practical tool for kindergarten teachers. Journal of Science Education and Technology, 20 (5), 550–565. https://doi.org/10.1007/S10956-011-9323-2/TABLES/6
Eurostat. (2020). Distribution of tertiary education students by broad field and sex . https://ec.europa.eu/eurostat/statistics-explained/index.php?title=File:Distribution_of_tertiary_education_students_by_broad_field_and_sex,_EU-27,_2018_(%25)_ET2020.png
Evagorou, M., Erduran, S., & Mäntylä, T. (2015). The role of visual representations in scientific practices: From conceptual understanding and knowledge generation to ‘seeing’ how science works. International Journal of STEM Education, 2 (1), 1–13. https://doi.org/10.1186/S40594-015-0024-X/FIGURES/6
Gago, J., Ziman, J., Caro, P., Constantinou, C., Davies, G., Parchmann, I., Rannikmae, M., & Sjoberg, S. (2005). Europe needs more scientists: Report by the high level group on increasing human resources for science and technology . Office for Official Publications of the European Communities, Luxembourg. http://eprints.uni-kiel.de/id/eprint/38088
Garland, T. B., & Sanchez, C. A. (2013). Rotational perspective and learning procedural tasks from dynamic media. Computers and Education, 69 , 31–37. https://doi.org/10.1016/j.compedu.2013.06.014
George, D., & Mallery, P. (2003). SPSS for Windows step by step: A simple guide and reference .
Gomez-Zwiep, S. (2008). Elementary teachers’ understanding of students’ science misconceptions: Implications for practice and teacher education. Journal of Science Teacher Education, 19 (5), 437–454. https://doi.org/10.1007/s10972-008-9102-y
Goswami, U. C. (2010). The Wiley-Blackwell handbook of childhood cognitive development (2nd ed.). Wiley-Blackwell.
Book Google Scholar
Hanzalová, P. (2019). Oblíbenost témat výuky přírodopisu na 2. stupni základní školy [Univerzita Karlova, Pedagogická fakulta]. https://dspace.cuni.cz/handle/20.500.11956/106185
Harrison, A. G., & Treagust, D. F. (2006). The particulate nature of matter: Challenges in understanding the submicroscopic world. In Chemical Education: Towards Research-based Practice (pp. 189–212). Kluwer Academic Publishers. https://doi.org/10.1007/0-306-47977-x_9
Hedges, L. V. (1981). Distribution theory for glass’s estimator of effect size and related estimators. Journal of Educational Statistics, 6 (2), 107–128. https://doi.org/10.3102/10769986006002107
Hedges, L. V., & Olkin, I. (1985). Statistical methods for meta-analysis . Academic Press, Inc.
Herman, G. L., Loui, M. C., & Zilles, C. (2011). Students’ misconceptions about medium-scale integrated circuits. IEEE Transactions on Education, 54 (4), 637–645. https://doi.org/10.1109/TE.2011.2104361
Höffler, T. N. (2010). Spatial ability: Its influence on learning with visualizations-a meta-analytic review. Educational Psychology Review, 22 , 245–269. https://doi.org/10.1007/s10648-010-9126-7
Höffler, T. N., & Leutner, D. (2007). Instructional animation versus static pictures: A meta-analysis. Learning and Instruction, 17 (6), 722–738. https://doi.org/10.1016/j.learninstruc.2007.09.013
Ikwuka, O. I., & Samuel, N. N. C. (2017). Effect of computer animation on chemistry academic achievement of secondary school students in Anambra State, Nigeria. Journal of Emerging Trends in Educational Research and Policy Studies, 8 (2), 98–102. https://doi.org/10.10520/EJC-9b95fd597
Jaffar, A. A. (2012). YouTube: An emerging tool in anatomy education. Anatomical Sciences Education, 5 (3), 158–164. https://doi.org/10.1002/ase.1268
Jenkinson, J. (2018). Molecular biology meets the learning sciences: Visualizations in education and outreach. Journal of Molecular Biology, 430 (21), 4013–4027. https://doi.org/10.1016/j.jmb.2018.08.020
Jones, S., & Scaife, M. (2000). Animated diagrams: An investigation into the cognitive effects of using animation to illustrate dynamic processes. Lecture Notes in Computer Science (Including Subseries Lecture Notes in Artificial Intelligence and Lecture Notes in Bioinformatics), 1889 , 231–244. https://doi.org/10.1007/3-540-44590-0_22
Kaushal, R. K., & Panda, S. N. (2019). A meta analysis on effective conditions to offer animation based teaching style. Malaysian Journal of Learning and Instruction , 16 (1), 129–153. https://eric.ed.gov/?id=EJ1219792
Khishfe, R., & Abd-El-Khalick, F. (2002). Influence of explicit and reflective versus implicit inquiry-oriented instruction on sixth graders’ views of nature of science. Journal of Research in Science Teaching, 39 (7), 551–578. https://doi.org/10.1002/tea.10036
Kim, L. E., Dar-Nimrod, I., & MacCann, C. (2018). Teacher personality and teacher effectiveness in secondary school: Personality predicts teacher support and student self-efficacy but not academic achievement. Journal of Educational Psychology , 110 (3), 309–323. https://psycnet.apa.org/buy/2017-52843-001
Kim, L. E., Jörg, V., & Klassen, R. M. (2019). A meta-analysis of the effects of teacher personality on teacher effectiveness and burnout. Educational Psychology Review, 31 (1), 163–195. https://doi.org/10.1007/S10648-018-9458-2/TABLES/2
Klahr, D., & Nigam, M. (2004). The equivalence of learning paths in early science instruction: Effects of direct instruction and discovery learning. Psychological Science, 15 (10), 661–667. https://doi.org/10.1111/j.0956-7976.2004.00737.x
Kühl, T., Scheiter, K., Gerjets, P., & Gemballa, S. (2011). Can differences in learning strategies explain the benefits of learning from static and dynamic visualizations? Computers and Education, 56 (1), 176–187. https://doi.org/10.1016/j.compedu.2010.08.008
Li, Y. (2021). Seven years of development as building a foundation for the journal’s leadership in promoting STEM education internationally. International Journal of STEM Education, 8 (1), 1–6. https://doi.org/10.1186/S40594-021-00316-W/TABLES/5
Likert, R. (1932). A technique for the measurement of attitudes. Archives of Psychology, 140 , 44–53.
Lin, L., & Atkinson, R. K. (2011). Using animations and visual cueing to support learning of scientific concepts and processes. Computers and Education, 56 (3), 650–658. https://doi.org/10.1016/j.compedu.2010.10.007
Lowe, R. K. (1999). Extracting information from an animation during complex visual learning. European Journal of Psychology of Education, 14 (2), 225–244. https://doi.org/10.1007/BF03172967
Löwe, R. K. (2003). Animation and learning: Selective processing of information in dynamic graphics. Learning and Instruction, 13 (2), 157–176. https://doi.org/10.1016/S0959-4752(02)00018-X
Malacinski, G. M., & Zell, P. W. (1996). Manipulating the “Invisible”: Learning molecular biology using inexpensive models. American Biology Teacher , 58 (7). https://eric.ed.gov/?id=EJ531590
Marbach-Ad, G., Rotbain, Y., & Stavy, R. (2008). Using computer animation and illustration activities to improve high school students’ achievement in molecular genetics. Journal of Research in Science Teaching, 45 (3), 273–292. https://doi.org/10.1002/tea.20222
Markland, D., & Hardy, L. (1997). On the factorial and construct validity of the intrinsic motivation inventory: Conceptual and operational concerns. Research Quarterly for Exercise and Sport, 68 (1), 20–32. https://doi.org/10.1080/02701367.1997.10608863
Mayer, R. E., DeLeeuw, K. E., & Ayres, P. (2007). Creating retroactive and proactive interference in multimedia learning. Applied Cognitive Psychology, 21 (6), 795–809. https://doi.org/10.1002/acp.1350
Mayer, R. E., & Moreno, R. (2002). Aids to computer-based multimedia learning. Learning and Instruction, 12 (1), 107–119. https://doi.org/10.1016/S0959-4752(01)00018-4
Mazza, R. (2009). Introduction to information visualization. Introduction to Information Visualization . https://doi.org/10.1007/978-1-84800-219-7
McAuley, E. D., Duncan, T., & Tammen, V. V. (1989). Psychometric properties of the intrinsic motivation inventory in a competitive sport setting: A confirmatory factor analysis. Research Quarterly for Exercise and Sport, 60 (1), 48–58. https://doi.org/10.1080/02701367.1989.10607413
McElhaney, K. W., Chang, H. Y., Chiu, J. L., & Linn, M. C. (2015). Evidence for effective uses of dynamic visualisations in science curriculum materials. Studies in Science Education, 51 (1), 49–85. https://doi.org/10.1080/03057267.2014.984506
MEYS. (2020). Strategy for the Education Policy of the Czech Republic up to 2030+ . https://www.msmt.cz/uploads/brozura_S2030_en_fin_online.pdf
Mitsuhashi, N., Fujieda, K., Tamura, T., Kawamoto, S., Takagi, T., & Okubo, K. (2009). BodyParts3D: 3D structure database for anatomical concepts. Nucleic Acids Research, 37 (SUPPL. 1), D782–D785. https://doi.org/10.1093/nar/gkn613
Monetti, D. M. (2002). A multiple regression analysis of self-regulated learning, epistemology, and student achievement . 62 , 3294. http://search.epnet.com/login.aspx?direct=true&db=aph&authdb=epref&an=ABJGBGDGB
Niemi, H., Nevgi, A., & Virtanen, P. (2003). Towards self-regulation in web-based learning. Journal of Educational Media, 28 (1), 49–71. https://doi.org/10.1080/1358165032000156437
Nodzyńska, M. (2012). Vizualizace V Chemii a Ve Výuce Chemie. Chem Listy , 106 , 519–527. http://chemicke-listy.cz/docs/full/2012_06_519-527.pdf
Nunnally, J. C. (1978). An overview of psychological measurement. In Clinical Diagnosis of Mental Disorders (pp. 97–146). Springer US. https://doi.org/10.1007/978-1-4684-2490-4_4
Özmen, H. (2011). Effect of animation enhanced conceptual change texts on 6th grade students’ understanding of the particulate nature of matter and transformation during phase changes. Computers and Education, 57 (1), 1114–1126. https://doi.org/10.1016/j.compedu.2010.12.004
Pavelková, I., Škaloudová, A., & Hrabal, V. (2010). Analýza vyučovacích předmětu na základě výpovědí žáků. Pedagogika . https://doi.org/10.14712/23362189.2018.861
Pintrich, P., Smith, D. A. F., Garcia, T., & McKeachie, W. J. (1991). A Manual for the Use of the Motivated Strategies for Learning Questionnaire (MSLQ) . https://files.eric.ed.gov/fulltext/ED338122.pdf
Popelka, S., Vondrakova, A., & Hujnakova, P. (2019). Eye-tracking evaluation of weather web maps. ISPRS International Journal of Geo-Information, 8 (6), 256. https://doi.org/10.3390/ijgi8060256
Puntambekar, S., & Hübscher, R. (2005). Tools for scaffolding students in a complex learning environment: What have we gained and what have we missed? Educational Psychologist, 40 (1), 1–12. https://doi.org/10.1207/s15326985ep4001_1
Rieber, L. P. (1990). Using computer animated graphics in science instruction with children. Journal of Educational Psychology, 82 (1), 135–140. https://doi.org/10.1037/0022-0663.82.1.135
Rotbain, Y., Marbach-Ad, G., & Stavy, R. (2006). Effect of bead and illustrations models on high school students’ achievement in molecular genetics. Journal of Research in Science Teaching., 43 (5), 500–529. https://doi.org/10.1002/tea.20144
Rotgans, J. I., & Schmidt, H. (2010). The motivated strategies for learning questionnaire: A measure for students’ general motivational beliefs and want more papers like this? The Asia-Paciic Education Researcher, 19 (2), 357–369.
Ryan, R. M. (1982). Control and information in the intrapersonal sphere: An extension of cognitive evaluation theory. Journal of Personality and Social Psychology, 43 (3), 450–461. https://doi.org/10.1037/0022-3514.43.3.450
Ryan, R. M., & Deci, E. L. (2000). Self-determination theory and the facilitation of intrinsic motivation, social development, and well-being. American Psychologist, 55 (1), 68–78. https://doi.org/10.1037/0003-066X.55.1.68
Ryoo, K., & Linn, M. C. (2012). Can dynamic visualizations improve middle school students’ understanding of energy in photosynthesis? Journal of Research in Science Teaching, 49 (2), 218–243. https://doi.org/10.1002/tea.21003
Schnotz, W. (2005). An integrated model of text and picture comprehension. In R. E. Mayer (Ed.), The Cambridge Handbook of Multimedia Learning (1st ed.). Cambridge University Press. https://doi.org/10.1017/CBO9780511816819
Chapter Google Scholar
Schnotz, W., Böckheler, J., & Grzondziel, H. (1999). Individual and co-operative learning with interactive animated pictures. European Journal of Psychology of Education, 14 (2), 245–265. https://doi.org/10.1007/bf03172968
Schnotz, W., & Lowe, R. (2003). External and internal representations in multimedia learning. Learning and Instruction, 13 (2), 117–123. https://doi.org/10.1016/S0959-47520200015-4
Schnotz, W., & Rasch, T. (2005). Enabling, facilitating, and inhibiting effects of animations in multimedia learning: Why reduction of cognitive load can have negative results on learning. Educational Technology Research and Development, 53 (3), 47–58. https://doi.org/10.1007/BF02504797
Schwan, S., & Riempp, R. (2004). The cognitive benefits of interactive videos: Learning to tie nautical knots. Learning and Instruction, 14 (3), 293–305. https://doi.org/10.1016/j.learninstruc.2004.06.005
Šmejkal, P., Skoršepa, M., Stratilová Urválková, E., & Teplý, P. (2016). Chemické úlohy se školními měřicími systémy: motivační orientace žáků v badatelsky orientovaných úlohách. Scientia in Educatione, 7 (1), 29–48. https://doi.org/10.14712/18047106.280
Tabak, I. (2004). Synergy: A complement to emerging patterns of distributed scaffolding. Journal of the Learning Sciences, 13 (3), 305–335. https://doi.org/10.1207/s15327809jls1303_3
Takeuchi, M. A., Sengupta, P., Shanahan, M. C., Adams, J. D., & Hachem, M. (2020). Transdisciplinarity in STEM education: A critical review. Studies in Science Education, 56 (2), 213–253. https://doi.org/10.1080/03057267.2020.1755802
Tarmizi, R. A. (2010). Visualizing students’ difficulties in learning calculus. In A. Tarmizi & R. Ayub (Eds.), International on mathematics education research 2010—ICMER 2010 (Vol. 8, pp. 377–383). Elsevier Science BV.
Tsay, C.H.-H., Kofinas, A., & Trivedi, S. K. (2018). Novelty effect and student engagement in a technology-mediated gamified learning system. Academy of Management Annual Meeting Proceedings, 2018 (1), 13030. https://doi.org/10.5465/AMBPP.2018.13030ABSTRACT
Türkay, S. (2016). The effects of whiteboard animations on retention and subjective experiences when learning advanced physics topics. Computers and Education, 98 , 102–114. https://doi.org/10.1016/j.compedu.2016.03.004
Tversky, B., Morrison, J., & Betrancourt, M. (2002). Animation: Can it facilitate? International Journal of Human Computer Studies, 57 (4), 247–262. https://doi.org/10.1006/ijhc.2002.1017
Tzima, S., Styliaras, G., & Bassounas, A. (2019). Augmented reality applications in education: Teachers point of view. Education Sciences, 9 (2), 99. https://doi.org/10.3390/EDUCSCI9020099
Wang, P. Y., Vaughn, B. K., & Liu, M. (2011). The impact of animation interactivity on novices’ learning of introductory statistics. Computers and Education, 56 (1), 300–311. https://doi.org/10.1016/j.compedu.2010.07.011
Ware, C. (2004). Information Visualization: Perception for Design. In Information Visualization (2nd ed.). Morgan Kaufmann.
Williamson, V. M., & Abraham, M. R. (1995). The effects of computer animation on the particulate mental models of college chemistry students. Journal of Research in Science Teaching, 32 (5), 521–534. https://doi.org/10.1002/tea.3660320508
Wolters, C. A. (2004). Advancing achievement goal theory: Using goal structures and goal orientations to predict students’ motivation, cognition, and achievement. Journal of Educational Psychology, 96 (2), 236–250. https://doi.org/10.1037/0022-0663.96.2.236
Wong, M., Castro-Alonso, J. C., Ayres, P., & Paas, F. (2018). Investigating gender and spatial measurements in instructional animation research. Computers in Human Behavior, 89 , 446–456. https://doi.org/10.1016/j.chb.2018.02.017
Wood, D., Bruner, J. S., & Ross, G. (1976). The role of tutoring in problem solving. Journal of Child Psychology and Psychiatry, 17 (2), 89–100. https://doi.org/10.1111/j.1469-7610.1976.tb00381.x
Wu, H. K., Krajcik, J. S., & Soloway, E. (2001). Promoting understanding of chemical representations: Students’ use of a visualization tool in the classroom. Journal of Research in Science Teaching, 38 (7), 821–842. https://doi.org/10.1002/tea.1033
Zell, E., Krizan, Z., & Teeter, S. R. (2015). Evaluating gender similarities and differences using metasynthesis. American Psychologist, 70 (1), 10–20. https://doi.org/10.1037/a0038208
Download references
Acknowledgements
The authors thank all the collaborating teachers in this research for their enthusiasm and valuable feedback. The authors also thank Dr. Carlos V. Melo for editing the manuscript.
This work was supported by University research centres of Charles University: UNCE/HUM/024 and funding project Progres Q17 .
Author information
Authors and affiliations.
Department of Chemistry Education, Faculty of Science, Charles University, Hlavova 8, 128 43, Prague 2, Czech Republic
Milada Teplá, Pavel Teplý & Petr Šmejkal
You can also search for this author in PubMed Google Scholar
Contributions
All authors read and approved the final manuscript.
Corresponding author
Correspondence to Pavel Teplý .
Ethics declarations
Competing interests.
The authors declare that they have no competing interests.
Additional information
Publisher's note.
Springer Nature remains neutral with regard to jurisdictional claims in published maps and institutional affiliations.
Rights and permissions
Open Access This article is licensed under a Creative Commons Attribution 4.0 International License, which permits use, sharing, adaptation, distribution and reproduction in any medium or format, as long as you give appropriate credit to the original author(s) and the source, provide a link to the Creative Commons licence, and indicate if changes were made. The images or other third party material in this article are included in the article's Creative Commons licence, unless indicated otherwise in a credit line to the material. If material is not included in the article's Creative Commons licence and your intended use is not permitted by statutory regulation or exceeds the permitted use, you will need to obtain permission directly from the copyright holder. To view a copy of this licence, visit http://creativecommons.org/licenses/by/4.0/ .
Reprints and permissions
About this article
Cite this article.
Teplá, M., Teplý, P. & Šmejkal, P. Influence of 3D models and animations on students in natural subjects. IJ STEM Ed 9 , 65 (2022). https://doi.org/10.1186/s40594-022-00382-8
Download citation
Received : 17 February 2022
Accepted : 30 September 2022
Published : 17 October 2022
DOI : https://doi.org/10.1186/s40594-022-00382-8
Share this article
Anyone you share the following link with will be able to read this content:
Sorry, a shareable link is not currently available for this article.
Provided by the Springer Nature SharedIt content-sharing initiative
- Natural subjects
Help | Advanced Search
Computer Science > Computer Vision and Pattern Recognition
Title: avatarclip: zero-shot text-driven generation and animation of 3d avatars.
Abstract: 3D avatar creation plays a crucial role in the digital age. However, the whole production process is prohibitively time-consuming and labor-intensive. To democratize this technology to a larger audience, we propose AvatarCLIP, a zero-shot text-driven framework for 3D avatar generation and animation. Unlike professional software that requires expert knowledge, AvatarCLIP empowers layman users to customize a 3D avatar with the desired shape and texture, and drive the avatar with the described motions using solely natural languages. Our key insight is to take advantage of the powerful vision-language model CLIP for supervising neural human generation, in terms of 3D geometry, texture and animation. Specifically, driven by natural language descriptions, we initialize 3D human geometry generation with a shape VAE network. Based on the generated 3D human shapes, a volume rendering model is utilized to further facilitate geometry sculpting and texture generation. Moreover, by leveraging the priors learned in the motion VAE, a CLIP-guided reference-based motion synthesis method is proposed for the animation of the generated 3D avatar. Extensive qualitative and quantitative experiments validate the effectiveness and generalizability of AvatarCLIP on a wide range of avatars. Remarkably, AvatarCLIP can generate unseen 3D avatars with novel animations, achieving superior zero-shot capability.
Submission history
Access paper:.
- Other Formats

References & Citations
- Google Scholar
- Semantic Scholar
BibTeX formatted citation

Bibliographic and Citation Tools
Code, data and media associated with this article, recommenders and search tools.
- Institution
arXivLabs: experimental projects with community collaborators
arXivLabs is a framework that allows collaborators to develop and share new arXiv features directly on our website.
Both individuals and organizations that work with arXivLabs have embraced and accepted our values of openness, community, excellence, and user data privacy. arXiv is committed to these values and only works with partners that adhere to them.
Have an idea for a project that will add value for arXiv's community? Learn more about arXivLabs .
Navigation Menu
Search code, repositories, users, issues, pull requests..., provide feedback.
We read every piece of feedback, and take your input very seriously.
Saved searches
Use saved searches to filter your results more quickly.
To see all available qualifiers, see our documentation .
- Notifications
Papers, datasets, and resources related to 2D cartoon video research. Contributions welcome.
zhenglinpan/Awesome-Animation-Research
Folders and files, repository files navigation.
ENGLISH | 简体中文
Awesome Animation Research 🎥📚
This repository provides a curated collection of dataset, research, and resources related to 🎞️cel animation / 🎞️hand-drawn cartoons specifically.
💁♀️ What You'll Find Here: Papers/Dataset/Repo closely related to cel animation(cartoon video) that could potentially assist creating animation. e.g. Inbetweening, Genga Colorization.
🤷♀️ What's Not Included: General Anime Research. i.e. Anime Style Transfer, Anime Image Enhancement, Anime Image generation. If you are interested in general anime research, please refer to AwesomeAnimeResearch .
🙇♀️Creating animation is time-consuming and often involves manual work. AI tools are changing this landscape. Researchers are tackling animation-specific challenges like inbetweening and frame-to-frame color propagation.
You might notice the repo's list is currently short. Animation research is a relatively new and niche area, and we look forward to more researchers, including you, contributing to its growth.
The repo will keep track of the latest research. Feel free to follow and star ! 🌟
Colorization
Inbetweening & interpolation, tracking & matching, segmentation, 3d rotoscoping&assistance, how to contribute.
We encourage animation enthusiasts, researchers, and scholars to contribute to this repository by adding relevant papers, articles, and resources. Your contributions will help build a valuable reference for anyone interested in the art and science of animation.
To contribute, simply fork this repository, make your additions or improvements, and submit a pull request. Please follow the contribution guidelines outlined in the repository's README file.

icon by Twitter ©illufinch

An official website of the United States government
The .gov means it’s official. Federal government websites often end in .gov or .mil. Before sharing sensitive information, make sure you’re on a federal government site.
The site is secure. The https:// ensures that you are connecting to the official website and that any information you provide is encrypted and transmitted securely.
- Publications
- Account settings
Preview improvements coming to the PMC website in October 2024. Learn More or Try it out now .
- Advanced Search
- Journal List
- Comput Intell Neurosci
- v.2022; 2022

Research on the Application of Animation Design Based on Machine Learning and Dynamic Image Index
Yaodong yin.
Department of Fine Arts, Taiyuan Normal University, Jinzhong, China
Associated Data
The data used to support the findings of this study are currently under embargo while the research findings are commercialized. Requests for data, 6 months after publication of this article, will be considered by the corresponding author.
With the development of computer technology, animation is more and more used because of its simple, effective, and higher performance. Machine learning has become the core of artificial intelligence at present. Intelligent learning algorithms are widely used in practical problems such as evaluation. Knowledge-based automatic animation production system faces two challenges: (1) lack of learning ability and waste of data on the website; (2) the quality of animation produced that depends on the level of system designer and the inability of system users to participate in animation production.In order to solve these two problems, an active animation learning system enables the animation system to constantly learn experience and produce the most popular animation, for the first time, for animation production system design and implementation of applied research. Image retrieval technology is a research center in the field of image application. It is widely used in many fields, such as electronic commerce. Animation design will use dynamic image and machine learning to innovate.
1. Introduction
Animation design is based on all-weather computer-aided animation automatic production technology, which is based on computer-aided animation technology [ 1 ]. In the whole process from receiving SMS to output animation, there is no manual process. The animation design production system consists of four basic units: information extraction, plot qualitative planning (ADL), animation quantitative calculation (CAL), and network development. After receiving SMS from animation production system, the staff will process natural language text and semantic analysis text, and conduct qualitative analysis and quantitative calculation of text [ 2 ]. Finally, animation design system faces two challenges through animation demonstration and production: (1) there may be a lack of learning ability and waste of a large amount of animation short message accumulated data; (2) the quality of the animation produced depends on the design level of the system, originally designed for the animation production system, and a dynamic animation learning system has been established to enable the animation production system to continuously learn experience and generate more animation information [ 3 ].
Animation is a kind of digital learning resource, it is an important means to spread information content, and it is also an important online learning resource, including text, image, audio, video, interaction, dynamic effect, their powerful interactive ability in the field of distance education, excellent course website and simulation platform, wide use of this animation and multimedia performance, and accumulated network animation resources [ 4 ]. The dynamic learning system is a system that contains many system parameters, including the number of basic decision trees for random forest models and the setting of limit values in the analysis. It is a dynamic learning system [ 5 ]. With the necessity of data management of these parameters and the validity time of testing, a part of the dynamic animation learning system is currently semiautomatic, needs system administrators to participate in updating the animation model, ensures the stability and control of the system in the early stages of operation, and gradually reduces the participation manual as the active learning system matures [ 6 ]. Learning animation system adopts an active learning method to evaluate some user-driven information. At present, active learning animation system and user interaction mode, from e-mail to user, access automatic animation generation system, and the system administrator evaluates the user in the demonstration database of the animation learning system and studies the animation design application by machine learning and dynamic image index technology.
2. Related Work
Some research suggested designing and improving the learning ability of active animation learning system and designing a random forest model for automatic animation production so as to draw lessons from the accumulated animation data and guide animation production [ 7 ]. The content structure characteristics of online animation learning resources are comprehensively analyzed, the feature description model of content structure is developed, the description of animation content structure is improved, and the infrastructure of animation content structure is established [ 8 ]. The characteristic content of image structure is extracted from a theoretical basis. The animation image structure description model is built to combine color density with edge density to determine the boundaries of the visual scene. Using label data, logical images are extracted from learning resources [ 9 ]. The emphasis is on obtaining representative tables through the average master chromatography of each visual scene, the number of which is determined by the number and length of the scene. Based on animation creation principles and related animation techniques, static visual features, visual dynamic effects, and animation interactive processing genes are introduced and quantified through appropriate algorithms [ 10 ]. The literature recommends the inclusion of attribute values in the content structure attribute database in order to subsequently identify higher emotional semantics [ 11 ]. Based on the content, the literature animated flash semantics representative models, the quantification of abstract emotional semantics into space based on relevant model recognition theories and models, emotional computing, and emotional psychology, combined with the extraction of visual feature vectors using neural network BP, SVM, and the comparative analysis of neural analytic networks, such as learning low-level visual (static visual features and dynamic effects) expressive high-level semantics [ 12 ]. To realize the perceptual recognition of images, the literature is explained, mainly by establishing an image index based on K-Means and making a visual dictionary in classification, establishing an image base index, and studying user feedback algorithm [ 13 ]. The index results are improved on the basis of information, and the images received from users are searched quickly and effectively.
In this paper, for the first time, an “active” animation learning system using machine learning and dynamic image retrieval technology is designed and implemented [ 14 ]. The animation production system can receive the text after selecting the user's favorite background scene and improve the user satisfaction with the animation product. Animation active learning system is closely related to all links of animation system, and animation production and system influence are closely related to these links.
3. Design of Dynamic Target Detection Algorithm Based on Machine Learning
3.1. interframe difference method.
Differential method is the most commonly used method to detect dynamic targets in image sequences, also known as interframe difference method.
The change is detected in the image sequence of the two tables. The time interval between the adjacent image sequence tables is very small, and the constant position of only the dynamic target is changed. Therefore, when the value changes, it can be regarded as the destination. Finally, the moving area is removed, and a dynamic target is achieved by different size threshold, as shown in formula ( 1 ):
Determine dynamic target areas:
After subtracting every two adjacent frames, the difference image is obtained:
Then, the binary image is obtained by threshold judgment:
The dynamic target image of the intermediate frame is detected by logic and operation of the two difference results:
M ′ k can be converted to
When the pixel value is 1 k ( x , y ), M k is a dynamic target, otherwise it will be a background.
The frame difference principle (in Figure 1 ) is very simple. It uses the images in the above table as the current background model and does not accumulate in the calculation. Therefore, its advantages are real-time, fast, and simple. At the same time, because the interval between two adjacent tables is very short, the change in two consecutive tables is not particularly obvious, and the difference in the image is not sensitive to the change of light in the environment.

Frame difference method.
Background difference method (in Figure 2 ), also called the background subtraction method, is to model the background and calculate the current image and background in turn.

Background difference method.
Detection of moving targets is as follows:
Basically different, the VCR (video cassette recorder) is static, and it can detect a complete moving target, but the light is sensitive; therefore, providing a background model adapted to the changing environment is crucial for the effectiveness of the method of changing the environment. A number of general background modeling approaches are currently being studied:
- (1) Statistical averaging.
- Statistical average method is also called the gray level merging method. B x , y = 1 k ∑ k = 1 k f k x , y . (10)
- (2) Single Gaussian background modeling.
- The gray scale is represented by a Gaussian distribution as follows: P f = 1 2 π 1 / 2 c t exp − f − σ t 2 2 σ t 2 . (11)
- After the model is established, a confidence interval can be obtained. Formula ( 13 ) is used to determine the current pixel points: f x , y = 1 , Foreground f − μ t σ t > T h , 0 , Background other. (12)
- After judging whether the pixel is a background point, each gray image in the background image needs to be updated: μ t ∗ = 1 − α u t + α f σ t 2 ∗ = 1 − α σ t 2 + α f − σ t 2 . (13)
- α is a constant that controls the speed of updating.
- (3) Codebook background modeling method.
The codewords for a video frame can be expressed as
Condition 1 . —
For a given threshold,
When Coolordist ( k ) < the given threshold, the condition is satisfied.
Condition 2 . —
Determine a of adjustment coefficient according to visual characteristics μ :
When the brightness is within the confidence interval, the condition is satisfied.
When the two conditions are satisfied at the same time, the minimum codeword is selected as the current matching codeword. Update replacement:
The update rule of the maximum number of rejected lines in codewords is
When it is judged that it does not belong to the same class, the eigenvector of the point is calculated and added to the codebook.
Deletion of scene vector is as follows:
The basic principle of detecting dynamic targets by the optical flow method (in Figure 3 ) is to use gray values of dynamic image series to change time zone and stage.

Schematic illustration of the pyramid optical flow method.
The background and target motion images are determined and calculated, and the Taylor mode of obtaining the optical flow limit equation is amplified:
Assuming that the optical flow is smooth, the speed change rate is 0. At this point, the optical flow should be satisfied:
The optical flow estimation error is defined as
Among them, W 2 ( x ) represents a window weight function. Among the LK algorithms, some cases will lead to the irreversibility of the matrix, and the design error is very large when the object has a large range of motion.
3.2. Classic Machine Learning Algorithm
BP neural network model is a three-layer structure, including input layer, hidden layer, and output layer. When input data Xn is from the first input layer to the second hidden layer, the following mathematical model shall be converted:-
Among them, P ij , which is the weight between layers, is an important parameter affecting the accuracy of the model, from the second hidden layer to the third layer.
The following mathematical models should be transformed:
3.3. Improved Foreground Target Extraction for Five-Frame Difference by Fusion
Save the same information to the maximum extent, and dynamic targets such as background pixels are accurately preserved, but this also means that there will be more targets. The image operations N 1 ( X , Y ) and N 2 ( X , Y ) ensure the removal of the moving target area. N of results 1 ( X , Y ) N results 2 ( X , Y ) Postaction M to outcome 1 X , Y and M of results 2 ( X , Y ) “Same or”
Conduct “transactions” to obtain
where s 1 ( x , y ) and s 2 ( x , y ) perform “or” operations to obtain the final target area m ( x , y ); namely, m x , y = s 2 x , y ∩ s 2 x , y = 1 , m 2 x , y ∐ n 2 x , y = 1 , 0 , m 2 x , y ∐ n 2 x , y ≠ 1. (27)
The maximum interclass variance method is used to set the binarization threshold, which further improves the extraction speed, and the illumination information is also integrated into the threshold judgment:
Gamma value is the premise that the basic light does not change. When the light changes, the gamma value rises, and the judgment threshold rises, thus reducing light to a certain extent. These changes reduce the effect of light on the accuracy of test results.
3.4. Comparative Analysis of Experimental Results
The research on the above three kinds of target dynamic detection algorithms shows that each kind of target is in a specific environment. As shown in Table 1 , the three algorithms in the current table should be more clearly explained for each of the three algorithms applying different environmental conditions.
Comparison of three dynamic target detection methods.
In order to show the effect of the algorithm test more intuitively, two videos have been taken, the real animation of the same image has been taken, and the image contrast analysis is carried out directly on the interface. The difference between the two tables is most obvious in the internal extraction of dynamic targets. Animation and coverage are not allowed to extract target graphics and are not very effective. Three different tabular methods also allow to appear on the screen in the form of clear and nonrepeated borders, extracting graphics from dynamic targets, but with some flaws in the targets. Under variable light conditions, the overall defect of the measurement target cannot be extracted from the target and cannot be rehearsed. GMM animation can get the outline of the target animation icon, but it is affected by light; there will be some shortcomings, and the animation algorithm running speed is shown in Table 2 .
Comparison of four algorithms.
In principle, for a good dynamic target detection algorithm, the following conditions should be satisfied:
- Insensitive to slow changes in the environment.
- Can handle complex background and complex target situation.
- Can adapt to the interference in the environment.
- Can reduce the shadow effect of the algorithm results.
- Meet the accuracy requirements of subsequent processing such as identification.
The above dynamic target testing algorithm meets the requirements of accuracy and can be processed for a long time, and the operation time is very short. The algorithm cannot detect the whole target; everyone has a real disadvantage. ADEM in these algorithms: many can be used, and each algorithm of different applications can be used in different applications. K -Means clustering algorithm can process a large number of high-resolution impact data sets; these data sets are simple and effective and have been applied. Nevertheless, the average K itself has many defects, the first of which is the K value, which is determined in some complex environments. It should be a dynamic value for change, and sometimes manual estimation of experience and applied data sources in the PRA is required. Several attributes of the main data set are selected as cluster centers. However, if the difference between the data sets is not very clear and the density of the data sets has many noise points, the results of the data sets are often not very stable or effective. Given the initial group concentration, the algorithm is selected to minimize the value of the objective function. However, when the selection value is not appropriate, the result is often the best result locally, and the understanding of the destination function is not as good as possible. Image attributes should be minimized to speed up the retrieval and optimization of random starting points of the K-means group algorithm. In order to improve the application of visual dynamic image search help, animation designers can understand the current popular animation style and content and better design and production of animation.
In order to ensure that the results of the enhanced algorithm combination are better than those of the randomly determined initial central cluster, the distance between the classification centers should be judged according to the degree of separation of the classification. The difference between the grouped data and the central values of the aggregate level in the classification is used to judge. When calculating the higher value of the grouping result, the greater the distance between the classifications, the higher the level and the longer the edge description algorithm works for all levels.
First, as shown in Table 3 , the interval distance between K-Means classification results is larger than that between K-Means normal classification results, and its interval distance is less than that of K-Means. This indicates that if SITF point coordinates are used to group in different image groups, the effect of the enhancement algorithm group is the greatest. ADEM is the improvement of the K-Means cluster, which requires less repetition times and is more effective. The K-Means algorithm is shown in Table 4 .
Cluster results.
Improved K-Means algorithm.
4. Application of Machine Learning and Dynamic Image Index in Animation Design Generation
With the development of the times, information technology and our life are closely linked, and information technology affects all levels of our daily life; every angle of our daily life cannot be separated from information technology. Education is very important for the development of a country. Education embodies the future of a nation. The combination of education and information technology can open up a new development path for educational practice and accord with the development trend of the new era. With the development of the times, animation plays an increasingly important role in our daily life. It is no longer the exclusive film to educate children; animation can be closely linked with people's daily life through information technology for the development of the animation industry into a new development power. At present, the development of information technology promotes the development of artificial intelligence technology, and artificial intelligence technology is very popular in today's era. It is a machine learning chip. Artificial intelligence technology can combine animation creation, identify, classify, and process some animation data, organize some animation works, and search the images of animation works. In the past, the scope of image retrieval was very small, limited to the three aspects of article content, semantics, and text. With the popularization of artificial intelligence technology, the scope of image retrieval technology is gradually expanding. People can fully use machine learning to retrieve content but also add artificial intelligence recognition and data recognition and further improve the accuracy of image retrieval. Image retrieval and animation design can also be combined to improve the animation creation efficiency of creators.
4.1. Automatic Animation Generation Based on Machine Learning
The automatic animation production system is based on artificial intelligence technology, receives the text information after natural processing and semantic analysis, then takes the text as the starting point, carries on the qualitative and quantitative analysis of the animation environment, and finally carries on the production.
Acoustic animation and synthesis for output animation. The procedures are shown in Figure 4 .

Schematic diagram of interactive content.
As a whole, the automatic animation production system has successfully adopted a knowledge-based method. Therefore, this paper develops a dynamic animation learning system for automatic animation production system using a random forest model. Learn from a large amount of accumulated information, this information can guide animation production through the animation production system, and actively learn how to let users participate. Users and managers interact to achieve learning. The learning machine is divided into supervised learning and semisupervised active learning. As a means of semisupervised learning, it is a progressive sample prediction method. It can select some unlabeled samples according to some principles, actively communicate with the outside world to obtain the true marks of these samples, and then use these samples to complete the research marks. Compared with the general semimonitoring learning method, active learning can greatly reduce the demand for samples through similar correction rates, and the active learning process can be shown in Figure 5 . Experimental determination of optimal relative parameters of random forest model and comparative experiment of decision tree model show that random forest model automatically produces advantages through animation. At the same time, two sets of experiments are designed to verify the feasibility and effectiveness of the active animation learning system.

Flowchart of active learning.
The active learning animation system is based on the experimental results and uses the random forest model as the learning model, which includes 11 decision trees with an error rate of 0.21. In order to solve these two problems, we have been expanding, on the one hand, with the operation of animation production system; on the other hand, the parameters of the random forest algorithm are adjusted through new experiments.
Active learning animation system uses the historical data of user animation product evaluation and animation system as training samples and uses the machine learning algorithm to produce a classification model based on user evaluation in order to guide the animation production system, to produce the most satisfactory animation. However, the user's evaluation of animation is a subjective judgment; The user's understanding of the text, the user's psychological state, color, music, and the movement of the animation itself will affect the evaluation results. Therefore, an active learning system is needed to help the animation production system select a preferred background scene. The decision tree model is used to improve the satisfaction of animation product users and reduce the error rate.
4.2. Animation Design Based on Dynamic Image Retrieval
The overall features of an image are general image information, in which the most common features are color, texture, and graphics. Some search systems use the features of images to build image search indices because the advantages of these features include variability, simple computation, and visualization. Search of image index is shown in Figure 6 .

Establishment and search of image index.
Because of the benefits of related search, it is inevitable to apply it to image retrieval, especially in the aspect of the “semantic gap” in image-based image retrieval. This is mainly because the user's abstract interpretation of the image, the use of visual descriptions such as background color, shape in the CBIR, and the differences between high-level semantics and background visual features may lead to unsatisfactory search results. Therefore, the content-based image retrieval feedback process requires continuous capture of images. After multiple interactions between the user and the system, the user needs to fully incorporate the user's subjective views into the system.
5. Realization of Animation Design Based on Machine Learning and Dynamic Image Indexing Technology
5.1. completion of animation design.
The active animation learning system designed in this paper allows the continuous receiving of sample data from the animation system and the automatic expansion of the sample number through interaction with the animation production system, users, and managers to carry out the “initiative action.” The algorithm is used for active learning, selecting samples that need to interact with users, and solving problems. Because the KFF method does not take into account the diversity of samples to be marked, the active animation learning system uses statistical methods to learn the uniqueness of samples.
3D animation automatic production system faces two challenges: (1) there may be a lack of learning ability and waste of a large amount of animation information data accumulated since the system was launched in 2008; (2) the quality of animation produced depends on the level of system designers. Users of the system cannot participate in the production of the system. It was originally designed for animation production system and implemented a dynamic animation learning system so that the animation production system can continue to learn experience and produce the most popular animation users.
5.2. Learning Ability of Active Animation Learning System
First, the master designed a random forest model for automatic animation production to draw lessons from accumulated animation data, guide animation production, implement animation production systems, and abstractly develop the characteristics and categories of random forest models; standardize large amounts of historical data and receive training samples for random forest model development; and continuously test and optimize random forest model parameters so that the learning model can better guide animation production systems to form a complete system user: active learning animation system, “active” learning ability developed.
Secondly, the animation active learning system designed in this paper interacts with the animation production system, system users, and managers so that the sample data of the system can be continuously received, the number of training samples is automatically expanded, the status of the sample database is analyzed, and suggestions for updating the learning model are made to the system managers when necessary. We use KFF algorithms and K-Means statistics to actively learn and select samples that need to interact with users.
Through the subjective interpretation of the image, the image content is summed up into the key text, time, place, and image text information to determine the correlation of the image and ensure that the image becomes text. This method is relatively simple, but with the increase of image volume, the labor consumption of manual text identification is also increasing, the subjectivity of manual identification is also increasing, and the content of text summary image is not comprehensive. This may lead to differences in image understanding and defects in text description.
5.3. Shortcomings in Animation Design
At the same time, a number of experiments in active learning animation systems have identified potential problems and deficiencies requiring further research, including the following.
First, expand model learning. The animation active learning system designed in this paper is based on the choice of background animation scene. It is necessary to further study whether this learning model based on background scene can be extended to the model.
Secondly, optimize the parameters of dynamic animation learning system. Dynamic learning system is a system that contains many parameters of the system, including the number of basic decision trees of random forest models. Limit configuration in data management analysis, the necessity of further testing these parameters, and the validity of time testing.
Third, reduce the participation of system users and managers. The dynamic animation learning system is semiautomatic and needs system administrators to participate in updating the animation model. Manual participation ensures that, in the initial stage of system operation, the stability and control of the system and the maturity of the active learning animation system will need to gradually reduce the participation of manual operation. On the other hand, the active animation learning system adopts a positive learning method to obtain the user's evaluation of part of the animation text. Now, the active animation learning system interacts with the user by displaying the amount of text information. Users can use the 3D animation automatic generation system generated by automatic animation to find digital short messages that need assessment. System administrators evaluate users of the sample database of active animation learning system. In the next version of the active animation learning system, a more direct and faster interface can be established for users.
Based on the advantages of animation, students need to regain the learning resources of online animation. This study has begun to analyze and restore the structural features of the content of online learning resources. By analyzing the content of animation learning resource files, visual scenes and their visual features extraction, composition elements characteristics, internal animation graphics, emotional features, and so on, a database with animation content structure characteristics will be created, which will eventually be used in a network-based dynamic learning resource search system. This study is very important in theory for the educational application of learning animation resources on the Internet and confirms that animation content has a positive impact on students' interest in learning. It complements the model of applying online animation resources in teaching and enriches the content of online learning resources. The analysis of content structure features helps to improve the efficiency of finding learning resources. Animation is based on rich dynamic image content to introduce knowledge to students. In many cases, students express emotional content according to the characteristics of animation content, such as tone, texture, dynamic effect, and button. Through the content-based Internet developed by the Institute, the animation search system can improve the efficiency and accuracy of searching for learning resources on the Internet and increase the use of Internet animation resources. It strengthens the self-study contribution of many students and provides educational information services.
6. Conclusion
Information technology has been integrated into all aspects of work, life, and learning. Adopting the development concept of “Educational Informatization 2.0”, information technology and educational practice are deeply brought into the development trend, and animation is becoming more and more important in our life. It is very important to explore the role of new technology in animation design. Machine learning is the basic technology of artificial intelligence at present, which can be very helpful to determine the characteristics of animation. The research experience of image retrieval technology is based on three stages: text, content, and semantic search. These stages mainly rely on semantic image search, including machine learning knowledge, content retrieval-based artificial intelligence, model recognition, and data extraction, in order to improve resilience genes. Animation is also an important medium for transmitting information today. It not only is an entertainment media but also carries the function of learning. Animation is a kind of multimedia interactive ability and expressive ability. Animation is used not only in entertainment but also in teaching. In order to establish an animation active learning system, the animation resources can be summarized, the automation state of the animation learning system can be improved, the stability of the animation learning system can be guaranteed, and the animation active learning system can be matured as soon as possible. The application of image retrieval to the design and production of animation enables producers to understand the current trend of animation production. The role of these two techniques in animation image design enables animated images to better adapt to people's aesthetics and guide the future.
Acknowledgments
This work was supported by the Shanxi Educational Science Planning Project “Shanxi Shadow Puppet Art Applied Research in College Animation Practice Teaching” (no. ZJ-17044).
Data Availability
Conflicts of interest.
The author has declared no conflicts of interest.

Our PhD-trained science communicators and professional artists will UNLEASH YOUR IMPACT through our animation services and SciComm training programs.

ACADEMIA HAS A PROBLEM
14000 papers are published
every single day!
Many never get cited or even read.
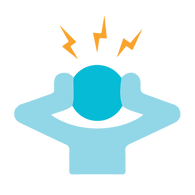
Your research could
get lost too!
WE HAVE A SOLUTION
Our animations, infographics and training programs make your research stand out
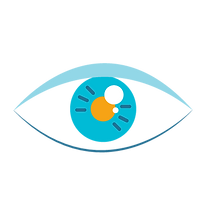
...and obtain the visibility it deserves
WITH MANY BENEFITS
more citations and
higher impact metrics

For society:
engaging and understandable science out there
Services Science Animations and Graphics
Animate Your Science has helped 332+ researchers and institutions in 32 countries tell their stories by producing animations and graphics that generate impact.
Animated Video Abstracts
To tell the story of your paper in a short, fun and engaging animation
Ready in 3-4 weeks when you order today
Custom Science Explainer Animations
A tailored animation or graphic for your unique story
Ready in 4-6 weeks when you order today
Custom Scientific Graphics & Posters
Make a splash with an eye-catching scientific poster
Graphical Abstracts
To tell the story of your paper with an eye-grabbing infographic
Ready in 1-2 weeks when you order today
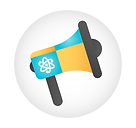
The Science Communication Training Academy
Join thousands of other researchers and learn the skills to captivate your audience and communicate your research in a compelling way. Animate Your Science has trained 4250+ researchers across 45 countries. Want to unleash your impact?
Online Courses
Learn at your own pace with
our pre-recorded courses.

Practical evidence-based science communication training.

In-person or online
Keynote Presentations
Captivating presentations tailored to your event.
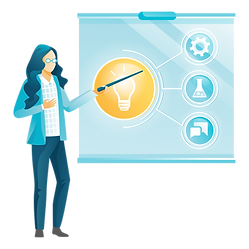
This company was founded by a scientist for scientists: so we understand your needs.
Our PhD-trained science communicators work side by side with professional designers, following a process that ensures scientific rigour while taking little more than an hour of your time.
With us, you will get head-turning videos and graphics that truly engage your audience. Hands-off.
What sets us apart
The Animate Your Science approach is
Key Benefits
Videos and graphics draw more eyes to your research.

Articles published with a video abstract benefit from:
more citations
Source: Zong et al. 2019

Articles promoted on Twitter with a graphical abstract benefit from a:
boost in article views
Source: Ibrahim et al. 2017

On average, people spend:
more time on pages with video
Source: Wistia Internal Study

Journalists need video content they can use for free
Therefore, videos boost your chances of being picked up by the media
Testimonials
What Clients Think of Us
"The experience of getting a video abstract from Animate Your Science has been flawless.
All we had to do was to fill a short questionnaire and the rest was pretty much hands-off.
We are very happy with the final result and can definitely recommend this service.”
Dr. Federico Gaiti
Cornell University
"We are happy with the result and have had a dynamic, patient and responsive working process with AYS.
Other partners recommended us AYS, and they have lived up to their reputation as professional, competent and engaged."
Henrik Oksfeldt Enevoldsen
"Animate Your Science have done a wonderful job translating our work for a lay audience; exactly as requested and with a really quick turnaround.
Thanks Tullio and team!"
Dr. Clare Bradley
"The way AYS extracted the information needed for our video made the whole process very clear and very easy.
Our whole team was rapt with the end result and feedback from the public has been excellent. Highly recommended!"
Prof. Gretta Pecl
University of Tasmania
“A seamless, effective, visually-appealing, and fun way to get the message of our paper out to the general public.
It is so important to translate scientific findings so they can be utilised beyond our immediate scientific community, and this is a great way to do it!”
Prof. Jodie Rummer
James Cook University

Some of the world's most respected institutions trust us
Interested in our services or training, just click the button below, hear what they say about us.

Launchpad Meetup

Tomorrow Maker

Department for Innovation and Skills
Small Business Award

Our satisfied clients
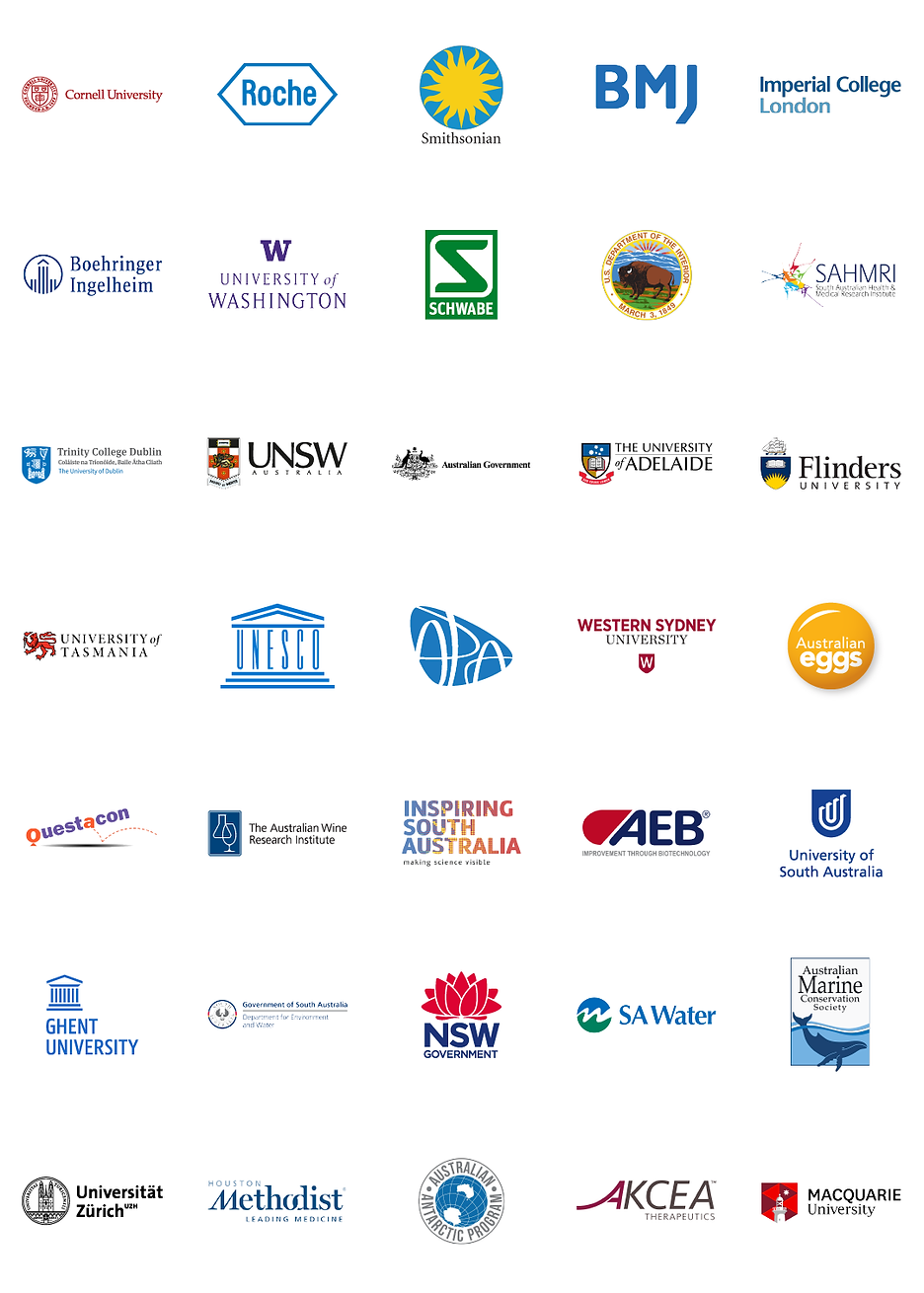
We use an easy-to-use client portal to efficiently communicate with you and share our progress.
You'll be in full control the entire time, with opportunities to provide feedback at each key stage of the process: so there will be no surprises.

Watch our animation process explainer
A proven process that lets us work together at a distance
- What is a science animation? Science animation refers to the use of animation to portray, communicate, and explain scientific concepts. They are often used for the purposes of academic teaching, awareness campaigning, as well as for entertainment. We provide two science animation services: 1. Video abstracts Video abstracts are short animated summaries about the main findings of an academic paper. They are most effective at promoting a paper's public visibility through media releases and on social media. We offer video abstracts in three artistic formats: Whiteboard 2D motion graphic Hybrid whiteboard/motion graphic 2. Custom explainer videos The scope of an explainer video can branch out infinitely. Whether you need a video to explain your grant application ideas, or simply want an animation to introduce your research team, we'll be able to craft a customised video to suit your needs. Custom explainer videos are offered in the same artistic formats as with video abstracts. However, higher levels of motion and artistic detail can also be achieved in our custom explainer video service. You can learn more about our product tiers here. Examples of animations we have created include: Government project explainers Recruitment campaigns in science and health Animations for research team websites Protocols in a scientific industry Promotional explainers for books with a research context Have an idea for your custom animation? Contact us to find out how we can bring your story to life.
- What is the process? Your research is well on its way to more citations, a healthy boost in metrics, and maybe even onto newsstands around the world. And, believe it or not, it’s as easy as 1 - 2 - 3. Move 1 - Questionnaire Effortlessly tell us the story of your paper with our guided questionnaire. This speeds up the process and ensures that you remain in control over the way your research is portrayed. Move 2 - Concept & storyboard Our team breathes life into your research by writing a script and sketching out your video or graphical abstract for your approval. Move 3 - Illustration/video production Sit back and relax as we work our magic in turning your complex science into a beautiful visual that you’ll be proud to share with the world. Customers that choose our custom animations will start with a strategy session instead of the questionnaire.
- What license covers the graphical and video abstracts? Don’t worry, you’re covered. All of our products are licensed under Creative Commons – Attribution, No Derivatives by default. This means you and anyone else are free to share, copy, and redistribute your video or graphical abstract in any medium, as long as the following conditions are met: 1. Attribution is given to the paper (title, authors, journal, year, DOI), and to www.animateyour.science 2. The product is not modified in any way If you need a whitelabeled product that's ok too, a small fee applies in that case.
- What payment methods do you take? We accept payment by Credit Card, PayPal and Bank transfers. While we do accept purchase orders, we highly encourage instant payment (usingthe options above) for a more streamlined process and much faster turnaround time.
- Will you keep my work confidential? Absolutely! Your project remains top secret until the embargo is lifted. And you choose the date and time you want to release your animated research to the world.
- Who designs the artwork and animates the video? While the concepts are created by a science communicator, the illustrations and animations are created by our professional designers. You can see our beautiful faces on the about page.
- Do you promote the video/graphical abstracts? We do! We offer social sharing through our media channels so that your research is positioned to make a splash from the start. For no extra cost!
- Can I show graphs in the visual abstract? Yes and no. Here’s the thing — we advise against the use of graphs as they can be a turnoff to the layperson. With that said, if your target audience is exclusively scientists, we can include one simple graph in your animation.
Interested in our services?
Or email us at
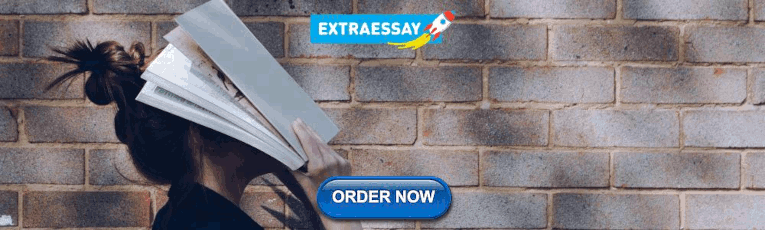
IMAGES
VIDEO
COMMENTS
Animation is increasingly pervasive and implemented in many ways in many disciplines. Animation: an interdisciplinary journal provides the first cohesive, international peer-reviewed publishing platform for animation that unites contributions from a wide range of research agendas and creative practice. View full journal description
This research paper aims to provide a comprehensive analysis of various animation techniques and their applications. This study examines hand-drawn animation, computer-generated imagery (CGI ...
Character Animation aims to generating character videos from still images through driving signals. Currently, diffusion models have become the mainstream in visual generation research, owing to their robust generative capabilities. However, challenges persist in the realm of image-to-video, especially in character animation, where temporally maintaining consistency with detailed information ...
This paper propose an animation for Yorùbá folktale in order to motivate the reading and create socio-cultural awareness among children and adults. The 3D animated base was created using Adobe ...
This paper presents a new methodology for studying anime visual effects and addresses the use of convolutional neural networks in this context. The established algorithm is incorporated into the new visual effects research model of animation film as a method for studying the development of these effects.
Animation Studies is the Society for Animation Studies ' double-blind peer-reviewed online journal. It is devoted to the history, theory, aesthetics and practice of animated media.. The journal invites original English-language essays (5,000-10,000 words, including endnotes and bibliography) addressing animated works from any part of the world.
The research reported in this paper originated from the fact that three meta-analyses which compared learning from animation to learning from static pictures found only small overall effect sizes in favor of animations (Berney & Bétrancourt, 2016; Castro-Alonso et al., 2019; Höffler & Leutner, 2007). At the same time, the production of ...
Papers & Talks We engage with the global research community through collaborations with universities around the world, publications in academic journals, and participation in top-tier conferences. ... Downloadable data sets from our animated films, for research purposes, to discover and inspire. All Data Sets. Open Source. Open Source software ...
Sakuga-42M Dataset: Scaling Up Cartoon Research. Zhenglin Pan, Yu Zhu, Yuxuan Mu. Hand-drawn cartoon animation employs sketches and flat-color segments to create the illusion of motion. While recent advancements like CLIP, SVD, and Sora show impressive results in understanding and generating natural video by scaling large models with extensive ...
Broaden your scientific audience with video animation. Academic writing can go only so far. Use video and animations in plain language to explain why your research matters, says Alvina Lai. Alvina ...
Animation - a study and comparison of concepts and software issues . Abstract . This paper examines animation from several different views - historical through the modern computer generated. It covers a short history of animation with a detailed look at some of the early examples with an emphasis on the techniques and tricks used in their ...
This research paper concludes 95% of artist working into the industry for production and post production if they are properly working into preproduction and then start into the movie work then there will be more vacancy for pre-production artist and end of the day production cost reduced up to 25 %. Download Full-text.
Several important animation research fields, such as gaming, medical, and entertainment, were not covered in this paper. Future research should include the recently published articles to enhance the quality and validate the findings in this study. In addition, the future study focuses on assessing the influence of animation motion and animated ...
Explore the latest full-text research PDFs, articles, conference papers, preprints and more on 3D-ANIMATION. Find methods information, sources, references or conduct a literature review on 3D ...
Studies comparing the effect of dynamic and static visualization suggest a predominantly positive effect of dynamic visualization. However, the results of individual comparisons are highly heterogeneous. In this study, we assess whether dynamic visualization (3D models and animations) used in the experimental group has a stronger positive influence on the intrinsic motivation and learning ...
3D avatar creation plays a crucial role in the digital age. However, the whole production process is prohibitively time-consuming and labor-intensive. To democratize this technology to a larger audience, we propose AvatarCLIP, a zero-shot text-driven framework for 3D avatar generation and animation. Unlike professional software that requires expert knowledge, AvatarCLIP empowers layman users ...
AI tools are changing this landscape. Researchers are tackling animation-specific challenges like inbetweening and frame-to-frame color propagation. You might notice the repo's list is currently short. Animation research is a relatively new and niche area, and we look forward to more researchers, including you, contributing to its growth.
Three Dimensional (3D) animation scene design, as a key element in digital entertainment, gaming, and advertising (Yu and Ma, 2022), has attracted widespread attention and research in recent years.
Secondly, the animation active learning system designed in this paper interacts with the animation production system, system users, and managers so that the sample data of the system can be continuously received, the number of training samples is automatically expanded, the status of the sample database is analyzed, and suggestions for updating ...
His research interests involve animation, film, design education and folk art. His animated shorts have already won many domestic and international awards, including the gold award of the 10th Xiamen International Animation Festival (2017).His research on these topics has been published in high-quality conferences such as HCI, and in journals ...
We provide two science animation services: 1. Video abstracts Video abstracts are short animated summaries about the main findings of an academic paper. They are most effective at promoting a paper's public visibility through media releases and on social media. We offer video abstracts in three artistic formats: Whiteboard 2D motion graphic ...