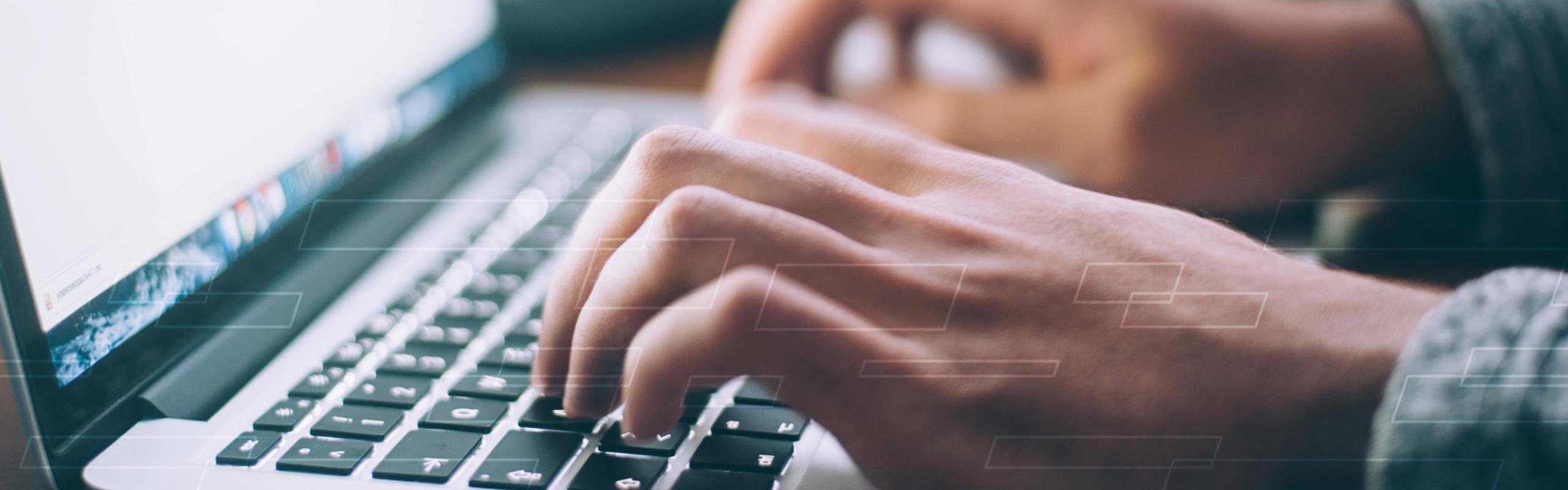
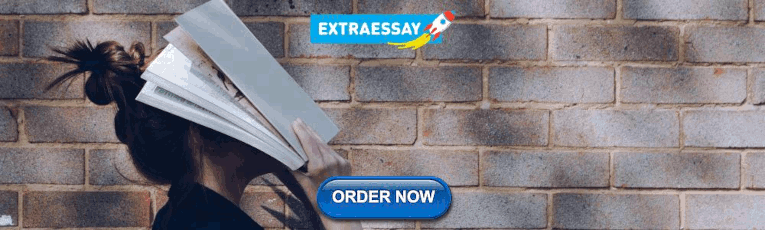
User Preferences
Content preview.
Arcu felis bibendum ut tristique et egestas quis:
- Ut enim ad minim veniam, quis nostrud exercitation ullamco laboris
- Duis aute irure dolor in reprehenderit in voluptate
- Excepteur sint occaecat cupidatat non proident
Keyboard Shortcuts
S.3.2 hypothesis testing (p-value approach).
The P -value approach involves determining "likely" or "unlikely" by determining the probability — assuming the null hypothesis was true — of observing a more extreme test statistic in the direction of the alternative hypothesis than the one observed. If the P -value is small, say less than (or equal to) \(\alpha\), then it is "unlikely." And, if the P -value is large, say more than \(\alpha\), then it is "likely."
If the P -value is less than (or equal to) \(\alpha\), then the null hypothesis is rejected in favor of the alternative hypothesis. And, if the P -value is greater than \(\alpha\), then the null hypothesis is not rejected.
Specifically, the four steps involved in using the P -value approach to conducting any hypothesis test are:
- Specify the null and alternative hypotheses.
- Using the sample data and assuming the null hypothesis is true, calculate the value of the test statistic. Again, to conduct the hypothesis test for the population mean μ , we use the t -statistic \(t^*=\frac{\bar{x}-\mu}{s/\sqrt{n}}\) which follows a t -distribution with n - 1 degrees of freedom.
- Using the known distribution of the test statistic, calculate the P -value : "If the null hypothesis is true, what is the probability that we'd observe a more extreme test statistic in the direction of the alternative hypothesis than we did?" (Note how this question is equivalent to the question answered in criminal trials: "If the defendant is innocent, what is the chance that we'd observe such extreme criminal evidence?")
- Set the significance level, \(\alpha\), the probability of making a Type I error to be small — 0.01, 0.05, or 0.10. Compare the P -value to \(\alpha\). If the P -value is less than (or equal to) \(\alpha\), reject the null hypothesis in favor of the alternative hypothesis. If the P -value is greater than \(\alpha\), do not reject the null hypothesis.
Example S.3.2.1
Mean gpa section .
In our example concerning the mean grade point average, suppose that our random sample of n = 15 students majoring in mathematics yields a test statistic t * equaling 2.5. Since n = 15, our test statistic t * has n - 1 = 14 degrees of freedom. Also, suppose we set our significance level α at 0.05 so that we have only a 5% chance of making a Type I error.
Right Tailed
The P -value for conducting the right-tailed test H 0 : μ = 3 versus H A : μ > 3 is the probability that we would observe a test statistic greater than t * = 2.5 if the population mean \(\mu\) really were 3. Recall that probability equals the area under the probability curve. The P -value is therefore the area under a t n - 1 = t 14 curve and to the right of the test statistic t * = 2.5. It can be shown using statistical software that the P -value is 0.0127. The graph depicts this visually.
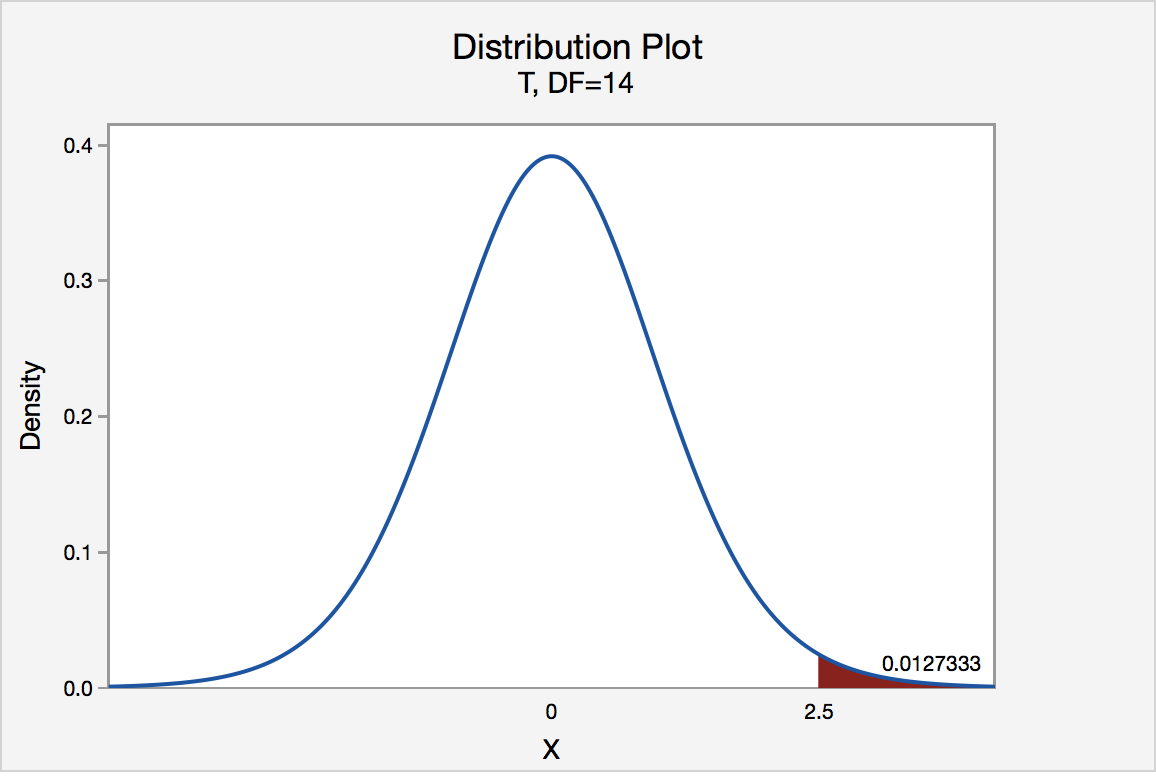
The P -value, 0.0127, tells us it is "unlikely" that we would observe such an extreme test statistic t * in the direction of H A if the null hypothesis were true. Therefore, our initial assumption that the null hypothesis is true must be incorrect. That is, since the P -value, 0.0127, is less than \(\alpha\) = 0.05, we reject the null hypothesis H 0 : μ = 3 in favor of the alternative hypothesis H A : μ > 3.
Note that we would not reject H 0 : μ = 3 in favor of H A : μ > 3 if we lowered our willingness to make a Type I error to \(\alpha\) = 0.01 instead, as the P -value, 0.0127, is then greater than \(\alpha\) = 0.01.
Left Tailed
In our example concerning the mean grade point average, suppose that our random sample of n = 15 students majoring in mathematics yields a test statistic t * instead of equaling -2.5. The P -value for conducting the left-tailed test H 0 : μ = 3 versus H A : μ < 3 is the probability that we would observe a test statistic less than t * = -2.5 if the population mean μ really were 3. The P -value is therefore the area under a t n - 1 = t 14 curve and to the left of the test statistic t* = -2.5. It can be shown using statistical software that the P -value is 0.0127. The graph depicts this visually.
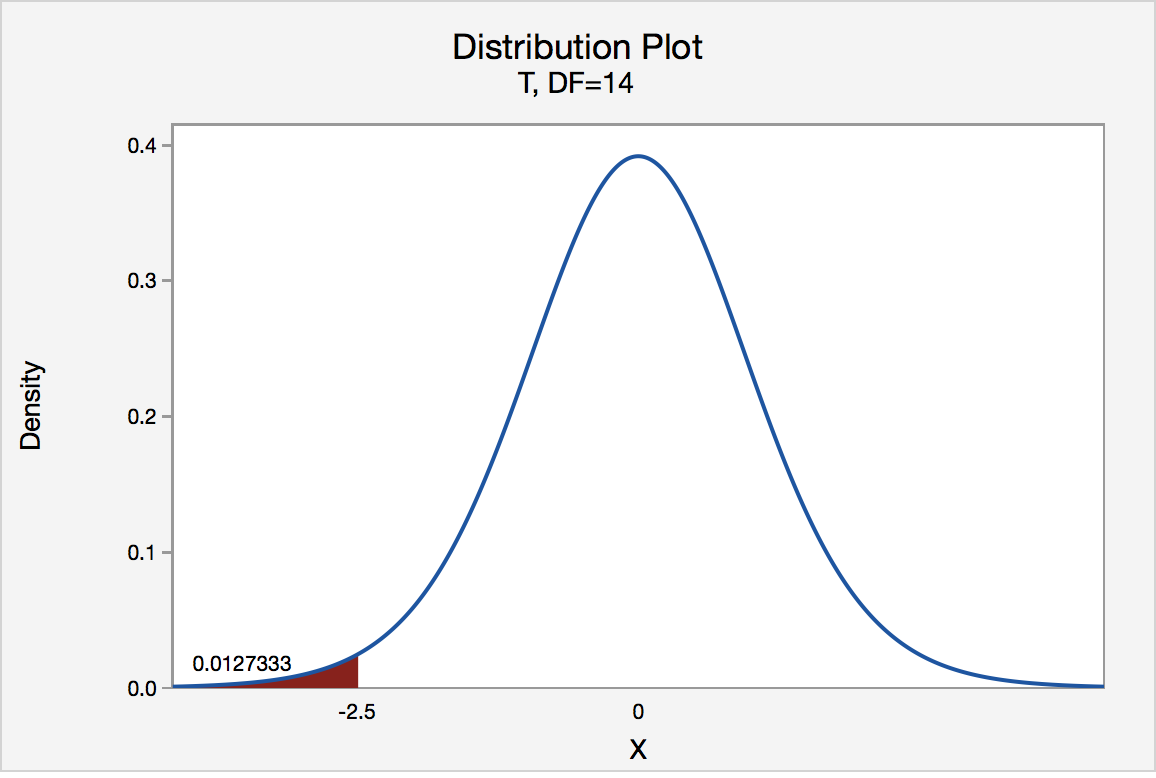
The P -value, 0.0127, tells us it is "unlikely" that we would observe such an extreme test statistic t * in the direction of H A if the null hypothesis were true. Therefore, our initial assumption that the null hypothesis is true must be incorrect. That is, since the P -value, 0.0127, is less than α = 0.05, we reject the null hypothesis H 0 : μ = 3 in favor of the alternative hypothesis H A : μ < 3.
Note that we would not reject H 0 : μ = 3 in favor of H A : μ < 3 if we lowered our willingness to make a Type I error to α = 0.01 instead, as the P -value, 0.0127, is then greater than \(\alpha\) = 0.01.
In our example concerning the mean grade point average, suppose again that our random sample of n = 15 students majoring in mathematics yields a test statistic t * instead of equaling -2.5. The P -value for conducting the two-tailed test H 0 : μ = 3 versus H A : μ ≠ 3 is the probability that we would observe a test statistic less than -2.5 or greater than 2.5 if the population mean μ really was 3. That is, the two-tailed test requires taking into account the possibility that the test statistic could fall into either tail (hence the name "two-tailed" test). The P -value is, therefore, the area under a t n - 1 = t 14 curve to the left of -2.5 and to the right of 2.5. It can be shown using statistical software that the P -value is 0.0127 + 0.0127, or 0.0254. The graph depicts this visually.
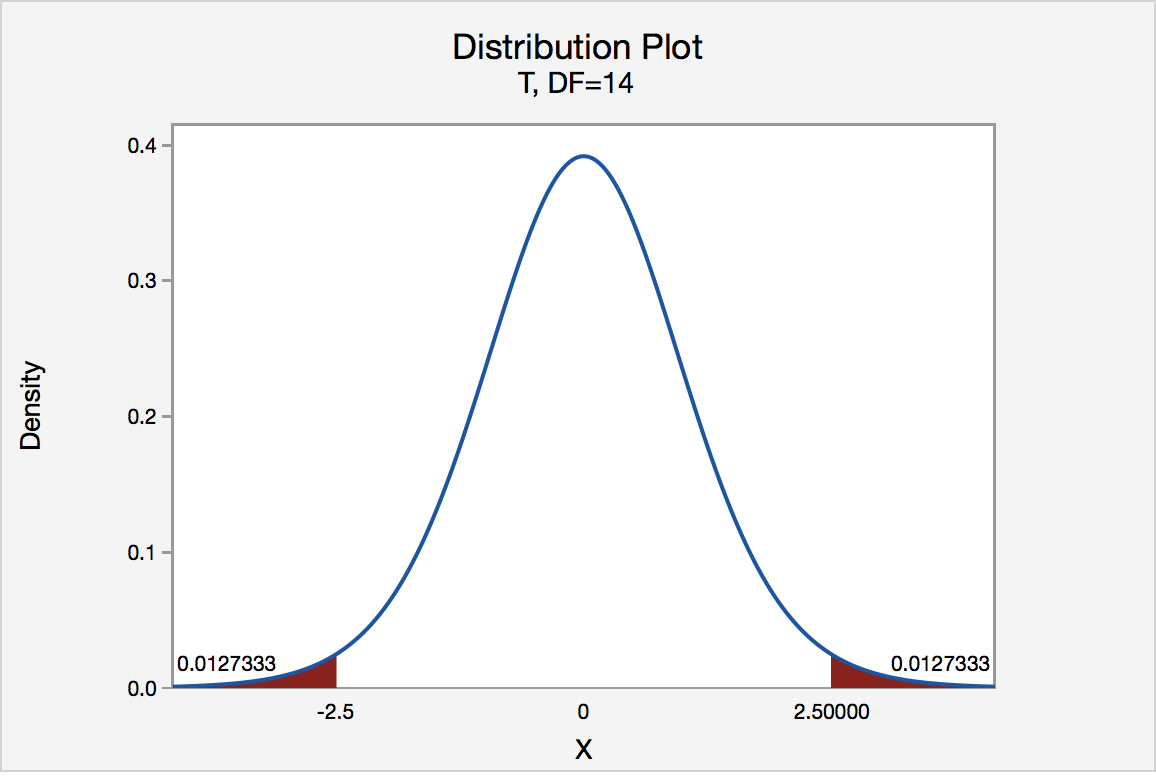
Note that the P -value for a two-tailed test is always two times the P -value for either of the one-tailed tests. The P -value, 0.0254, tells us it is "unlikely" that we would observe such an extreme test statistic t * in the direction of H A if the null hypothesis were true. Therefore, our initial assumption that the null hypothesis is true must be incorrect. That is, since the P -value, 0.0254, is less than α = 0.05, we reject the null hypothesis H 0 : μ = 3 in favor of the alternative hypothesis H A : μ ≠ 3.
Note that we would not reject H 0 : μ = 3 in favor of H A : μ ≠ 3 if we lowered our willingness to make a Type I error to α = 0.01 instead, as the P -value, 0.0254, is then greater than \(\alpha\) = 0.01.
Now that we have reviewed the critical value and P -value approach procedures for each of the three possible hypotheses, let's look at three new examples — one of a right-tailed test, one of a left-tailed test, and one of a two-tailed test.
The good news is that, whenever possible, we will take advantage of the test statistics and P -values reported in statistical software, such as Minitab, to conduct our hypothesis tests in this course.

An official website of the United States government
The .gov means it's official. Federal government websites often end in .gov or .mil. Before sharing sensitive information, make sure you're on a federal government site.
The site is secure. The https:// ensures that you are connecting to the official website and that any information you provide is encrypted and transmitted securely.
- Publications
- Account settings
- Browse Titles
NCBI Bookshelf. A service of the National Library of Medicine, National Institutes of Health.
StatPearls [Internet]. Treasure Island (FL): StatPearls Publishing; 2024 Jan-.

StatPearls [Internet].
Hypothesis testing, p values, confidence intervals, and significance.
Jacob Shreffler ; Martin R. Huecker .
Affiliations
Last Update: March 13, 2023 .
- Definition/Introduction
Medical providers often rely on evidence-based medicine to guide decision-making in practice. Often a research hypothesis is tested with results provided, typically with p values, confidence intervals, or both. Additionally, statistical or research significance is estimated or determined by the investigators. Unfortunately, healthcare providers may have different comfort levels in interpreting these findings, which may affect the adequate application of the data.
- Issues of Concern
Without a foundational understanding of hypothesis testing, p values, confidence intervals, and the difference between statistical and clinical significance, it may affect healthcare providers' ability to make clinical decisions without relying purely on the research investigators deemed level of significance. Therefore, an overview of these concepts is provided to allow medical professionals to use their expertise to determine if results are reported sufficiently and if the study outcomes are clinically appropriate to be applied in healthcare practice.
Hypothesis Testing
Investigators conducting studies need research questions and hypotheses to guide analyses. Starting with broad research questions (RQs), investigators then identify a gap in current clinical practice or research. Any research problem or statement is grounded in a better understanding of relationships between two or more variables. For this article, we will use the following research question example:
Research Question: Is Drug 23 an effective treatment for Disease A?
Research questions do not directly imply specific guesses or predictions; we must formulate research hypotheses. A hypothesis is a predetermined declaration regarding the research question in which the investigator(s) makes a precise, educated guess about a study outcome. This is sometimes called the alternative hypothesis and ultimately allows the researcher to take a stance based on experience or insight from medical literature. An example of a hypothesis is below.
Research Hypothesis: Drug 23 will significantly reduce symptoms associated with Disease A compared to Drug 22.
The null hypothesis states that there is no statistical difference between groups based on the stated research hypothesis.
Researchers should be aware of journal recommendations when considering how to report p values, and manuscripts should remain internally consistent.
Regarding p values, as the number of individuals enrolled in a study (the sample size) increases, the likelihood of finding a statistically significant effect increases. With very large sample sizes, the p-value can be very low significant differences in the reduction of symptoms for Disease A between Drug 23 and Drug 22. The null hypothesis is deemed true until a study presents significant data to support rejecting the null hypothesis. Based on the results, the investigators will either reject the null hypothesis (if they found significant differences or associations) or fail to reject the null hypothesis (they could not provide proof that there were significant differences or associations).
To test a hypothesis, researchers obtain data on a representative sample to determine whether to reject or fail to reject a null hypothesis. In most research studies, it is not feasible to obtain data for an entire population. Using a sampling procedure allows for statistical inference, though this involves a certain possibility of error. [1] When determining whether to reject or fail to reject the null hypothesis, mistakes can be made: Type I and Type II errors. Though it is impossible to ensure that these errors have not occurred, researchers should limit the possibilities of these faults. [2]
Significance
Significance is a term to describe the substantive importance of medical research. Statistical significance is the likelihood of results due to chance. [3] Healthcare providers should always delineate statistical significance from clinical significance, a common error when reviewing biomedical research. [4] When conceptualizing findings reported as either significant or not significant, healthcare providers should not simply accept researchers' results or conclusions without considering the clinical significance. Healthcare professionals should consider the clinical importance of findings and understand both p values and confidence intervals so they do not have to rely on the researchers to determine the level of significance. [5] One criterion often used to determine statistical significance is the utilization of p values.
P values are used in research to determine whether the sample estimate is significantly different from a hypothesized value. The p-value is the probability that the observed effect within the study would have occurred by chance if, in reality, there was no true effect. Conventionally, data yielding a p<0.05 or p<0.01 is considered statistically significant. While some have debated that the 0.05 level should be lowered, it is still universally practiced. [6] Hypothesis testing allows us to determine the size of the effect.
An example of findings reported with p values are below:
Statement: Drug 23 reduced patients' symptoms compared to Drug 22. Patients who received Drug 23 (n=100) were 2.1 times less likely than patients who received Drug 22 (n = 100) to experience symptoms of Disease A, p<0.05.
Statement:Individuals who were prescribed Drug 23 experienced fewer symptoms (M = 1.3, SD = 0.7) compared to individuals who were prescribed Drug 22 (M = 5.3, SD = 1.9). This finding was statistically significant, p= 0.02.
For either statement, if the threshold had been set at 0.05, the null hypothesis (that there was no relationship) should be rejected, and we should conclude significant differences. Noticeably, as can be seen in the two statements above, some researchers will report findings with < or > and others will provide an exact p-value (0.000001) but never zero [6] . When examining research, readers should understand how p values are reported. The best practice is to report all p values for all variables within a study design, rather than only providing p values for variables with significant findings. [7] The inclusion of all p values provides evidence for study validity and limits suspicion for selective reporting/data mining.
While researchers have historically used p values, experts who find p values problematic encourage the use of confidence intervals. [8] . P-values alone do not allow us to understand the size or the extent of the differences or associations. [3] In March 2016, the American Statistical Association (ASA) released a statement on p values, noting that scientific decision-making and conclusions should not be based on a fixed p-value threshold (e.g., 0.05). They recommend focusing on the significance of results in the context of study design, quality of measurements, and validity of data. Ultimately, the ASA statement noted that in isolation, a p-value does not provide strong evidence. [9]
When conceptualizing clinical work, healthcare professionals should consider p values with a concurrent appraisal study design validity. For example, a p-value from a double-blinded randomized clinical trial (designed to minimize bias) should be weighted higher than one from a retrospective observational study [7] . The p-value debate has smoldered since the 1950s [10] , and replacement with confidence intervals has been suggested since the 1980s. [11]
Confidence Intervals
A confidence interval provides a range of values within given confidence (e.g., 95%), including the accurate value of the statistical constraint within a targeted population. [12] Most research uses a 95% CI, but investigators can set any level (e.g., 90% CI, 99% CI). [13] A CI provides a range with the lower bound and upper bound limits of a difference or association that would be plausible for a population. [14] Therefore, a CI of 95% indicates that if a study were to be carried out 100 times, the range would contain the true value in 95, [15] confidence intervals provide more evidence regarding the precision of an estimate compared to p-values. [6]
In consideration of the similar research example provided above, one could make the following statement with 95% CI:
Statement: Individuals who were prescribed Drug 23 had no symptoms after three days, which was significantly faster than those prescribed Drug 22; there was a mean difference between the two groups of days to the recovery of 4.2 days (95% CI: 1.9 – 7.8).
It is important to note that the width of the CI is affected by the standard error and the sample size; reducing a study sample number will result in less precision of the CI (increase the width). [14] A larger width indicates a smaller sample size or a larger variability. [16] A researcher would want to increase the precision of the CI. For example, a 95% CI of 1.43 – 1.47 is much more precise than the one provided in the example above. In research and clinical practice, CIs provide valuable information on whether the interval includes or excludes any clinically significant values. [14]
Null values are sometimes used for differences with CI (zero for differential comparisons and 1 for ratios). However, CIs provide more information than that. [15] Consider this example: A hospital implements a new protocol that reduced wait time for patients in the emergency department by an average of 25 minutes (95% CI: -2.5 – 41 minutes). Because the range crosses zero, implementing this protocol in different populations could result in longer wait times; however, the range is much higher on the positive side. Thus, while the p-value used to detect statistical significance for this may result in "not significant" findings, individuals should examine this range, consider the study design, and weigh whether or not it is still worth piloting in their workplace.
Similarly to p-values, 95% CIs cannot control for researchers' errors (e.g., study bias or improper data analysis). [14] In consideration of whether to report p-values or CIs, researchers should examine journal preferences. When in doubt, reporting both may be beneficial. [13] An example is below:
Reporting both: Individuals who were prescribed Drug 23 had no symptoms after three days, which was significantly faster than those prescribed Drug 22, p = 0.009. There was a mean difference between the two groups of days to the recovery of 4.2 days (95% CI: 1.9 – 7.8).
- Clinical Significance
Recall that clinical significance and statistical significance are two different concepts. Healthcare providers should remember that a study with statistically significant differences and large sample size may be of no interest to clinicians, whereas a study with smaller sample size and statistically non-significant results could impact clinical practice. [14] Additionally, as previously mentioned, a non-significant finding may reflect the study design itself rather than relationships between variables.
Healthcare providers using evidence-based medicine to inform practice should use clinical judgment to determine the practical importance of studies through careful evaluation of the design, sample size, power, likelihood of type I and type II errors, data analysis, and reporting of statistical findings (p values, 95% CI or both). [4] Interestingly, some experts have called for "statistically significant" or "not significant" to be excluded from work as statistical significance never has and will never be equivalent to clinical significance. [17]
The decision on what is clinically significant can be challenging, depending on the providers' experience and especially the severity of the disease. Providers should use their knowledge and experiences to determine the meaningfulness of study results and make inferences based not only on significant or insignificant results by researchers but through their understanding of study limitations and practical implications.
- Nursing, Allied Health, and Interprofessional Team Interventions
All physicians, nurses, pharmacists, and other healthcare professionals should strive to understand the concepts in this chapter. These individuals should maintain the ability to review and incorporate new literature for evidence-based and safe care.
- Review Questions
- Access free multiple choice questions on this topic.
- Comment on this article.
Disclosure: Jacob Shreffler declares no relevant financial relationships with ineligible companies.
Disclosure: Martin Huecker declares no relevant financial relationships with ineligible companies.
This book is distributed under the terms of the Creative Commons Attribution-NonCommercial-NoDerivatives 4.0 International (CC BY-NC-ND 4.0) ( http://creativecommons.org/licenses/by-nc-nd/4.0/ ), which permits others to distribute the work, provided that the article is not altered or used commercially. You are not required to obtain permission to distribute this article, provided that you credit the author and journal.
- Cite this Page Shreffler J, Huecker MR. Hypothesis Testing, P Values, Confidence Intervals, and Significance. [Updated 2023 Mar 13]. In: StatPearls [Internet]. Treasure Island (FL): StatPearls Publishing; 2024 Jan-.
In this Page
Bulk download.
- Bulk download StatPearls data from FTP
Related information
- PMC PubMed Central citations
- PubMed Links to PubMed
Similar articles in PubMed
- The reporting of p values, confidence intervals and statistical significance in Preventive Veterinary Medicine (1997-2017). [PeerJ. 2021] The reporting of p values, confidence intervals and statistical significance in Preventive Veterinary Medicine (1997-2017). Messam LLM, Weng HY, Rosenberger NWY, Tan ZH, Payet SDM, Santbakshsing M. PeerJ. 2021; 9:e12453. Epub 2021 Nov 24.
- Review Clinical versus statistical significance: interpreting P values and confidence intervals related to measures of association to guide decision making. [J Pharm Pract. 2010] Review Clinical versus statistical significance: interpreting P values and confidence intervals related to measures of association to guide decision making. Ferrill MJ, Brown DA, Kyle JA. J Pharm Pract. 2010 Aug; 23(4):344-51. Epub 2010 Apr 13.
- Interpreting "statistical hypothesis testing" results in clinical research. [J Ayurveda Integr Med. 2012] Interpreting "statistical hypothesis testing" results in clinical research. Sarmukaddam SB. J Ayurveda Integr Med. 2012 Apr; 3(2):65-9.
- Confidence intervals in procedural dermatology: an intuitive approach to interpreting data. [Dermatol Surg. 2005] Confidence intervals in procedural dermatology: an intuitive approach to interpreting data. Alam M, Barzilai DA, Wrone DA. Dermatol Surg. 2005 Apr; 31(4):462-6.
- Review Is statistical significance testing useful in interpreting data? [Reprod Toxicol. 1993] Review Is statistical significance testing useful in interpreting data? Savitz DA. Reprod Toxicol. 1993; 7(2):95-100.
Recent Activity
- Hypothesis Testing, P Values, Confidence Intervals, and Significance - StatPearl... Hypothesis Testing, P Values, Confidence Intervals, and Significance - StatPearls
Your browsing activity is empty.
Activity recording is turned off.
Turn recording back on
Connect with NLM
National Library of Medicine 8600 Rockville Pike Bethesda, MD 20894
Web Policies FOIA HHS Vulnerability Disclosure
Help Accessibility Careers
P-Value And Statistical Significance: What It Is & Why It Matters
Saul Mcleod, PhD
Editor-in-Chief for Simply Psychology
BSc (Hons) Psychology, MRes, PhD, University of Manchester
Saul Mcleod, PhD., is a qualified psychology teacher with over 18 years of experience in further and higher education. He has been published in peer-reviewed journals, including the Journal of Clinical Psychology.
Learn about our Editorial Process
Olivia Guy-Evans, MSc
Associate Editor for Simply Psychology
BSc (Hons) Psychology, MSc Psychology of Education
Olivia Guy-Evans is a writer and associate editor for Simply Psychology. She has previously worked in healthcare and educational sectors.
On This Page:
The p-value in statistics quantifies the evidence against a null hypothesis. A low p-value suggests data is inconsistent with the null, potentially favoring an alternative hypothesis. Common significance thresholds are 0.05 or 0.01.
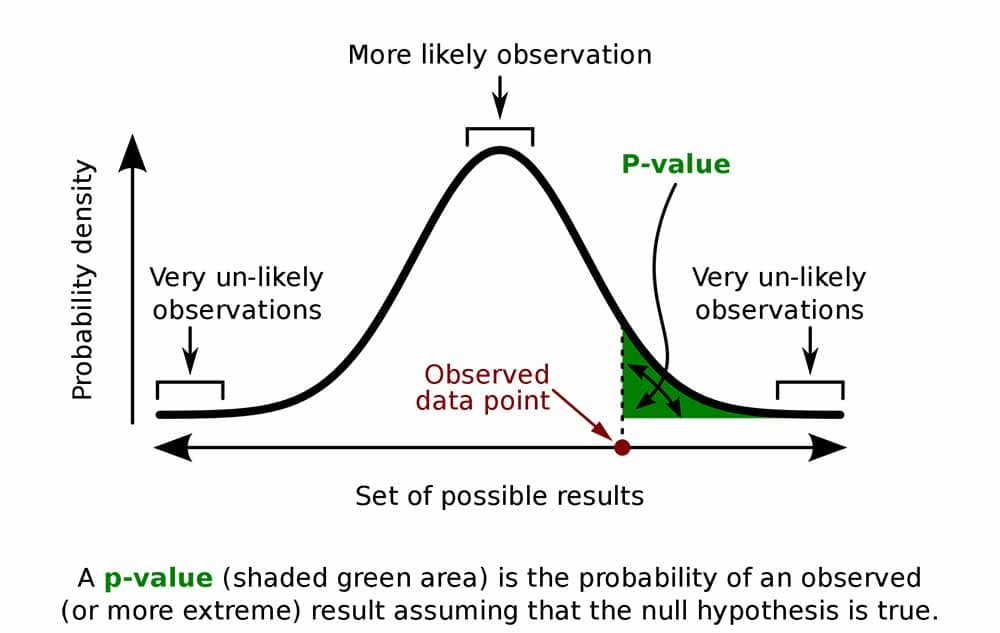
Hypothesis testing
When you perform a statistical test, a p-value helps you determine the significance of your results in relation to the null hypothesis.
The null hypothesis (H0) states no relationship exists between the two variables being studied (one variable does not affect the other). It states the results are due to chance and are not significant in supporting the idea being investigated. Thus, the null hypothesis assumes that whatever you try to prove did not happen.
The alternative hypothesis (Ha or H1) is the one you would believe if the null hypothesis is concluded to be untrue.
The alternative hypothesis states that the independent variable affected the dependent variable, and the results are significant in supporting the theory being investigated (i.e., the results are not due to random chance).
What a p-value tells you
A p-value, or probability value, is a number describing how likely it is that your data would have occurred by random chance (i.e., that the null hypothesis is true).
The level of statistical significance is often expressed as a p-value between 0 and 1.
The smaller the p -value, the less likely the results occurred by random chance, and the stronger the evidence that you should reject the null hypothesis.
Remember, a p-value doesn’t tell you if the null hypothesis is true or false. It just tells you how likely you’d see the data you observed (or more extreme data) if the null hypothesis was true. It’s a piece of evidence, not a definitive proof.
Example: Test Statistic and p-Value
Suppose you’re conducting a study to determine whether a new drug has an effect on pain relief compared to a placebo. If the new drug has no impact, your test statistic will be close to the one predicted by the null hypothesis (no difference between the drug and placebo groups), and the resulting p-value will be close to 1. It may not be precisely 1 because real-world variations may exist. Conversely, if the new drug indeed reduces pain significantly, your test statistic will diverge further from what’s expected under the null hypothesis, and the p-value will decrease. The p-value will never reach zero because there’s always a slim possibility, though highly improbable, that the observed results occurred by random chance.
P-value interpretation
The significance level (alpha) is a set probability threshold (often 0.05), while the p-value is the probability you calculate based on your study or analysis.
A p-value less than or equal to your significance level (typically ≤ 0.05) is statistically significant.
A p-value less than or equal to a predetermined significance level (often 0.05 or 0.01) indicates a statistically significant result, meaning the observed data provide strong evidence against the null hypothesis.
This suggests the effect under study likely represents a real relationship rather than just random chance.
For instance, if you set α = 0.05, you would reject the null hypothesis if your p -value ≤ 0.05.
It indicates strong evidence against the null hypothesis, as there is less than a 5% probability the null is correct (and the results are random).
Therefore, we reject the null hypothesis and accept the alternative hypothesis.
Example: Statistical Significance
Upon analyzing the pain relief effects of the new drug compared to the placebo, the computed p-value is less than 0.01, which falls well below the predetermined alpha value of 0.05. Consequently, you conclude that there is a statistically significant difference in pain relief between the new drug and the placebo.
What does a p-value of 0.001 mean?
A p-value of 0.001 is highly statistically significant beyond the commonly used 0.05 threshold. It indicates strong evidence of a real effect or difference, rather than just random variation.
Specifically, a p-value of 0.001 means there is only a 0.1% chance of obtaining a result at least as extreme as the one observed, assuming the null hypothesis is correct.
Such a small p-value provides strong evidence against the null hypothesis, leading to rejecting the null in favor of the alternative hypothesis.
A p-value more than the significance level (typically p > 0.05) is not statistically significant and indicates strong evidence for the null hypothesis.
This means we retain the null hypothesis and reject the alternative hypothesis. You should note that you cannot accept the null hypothesis; we can only reject it or fail to reject it.
Note : when the p-value is above your threshold of significance, it does not mean that there is a 95% probability that the alternative hypothesis is true.
One-Tailed Test
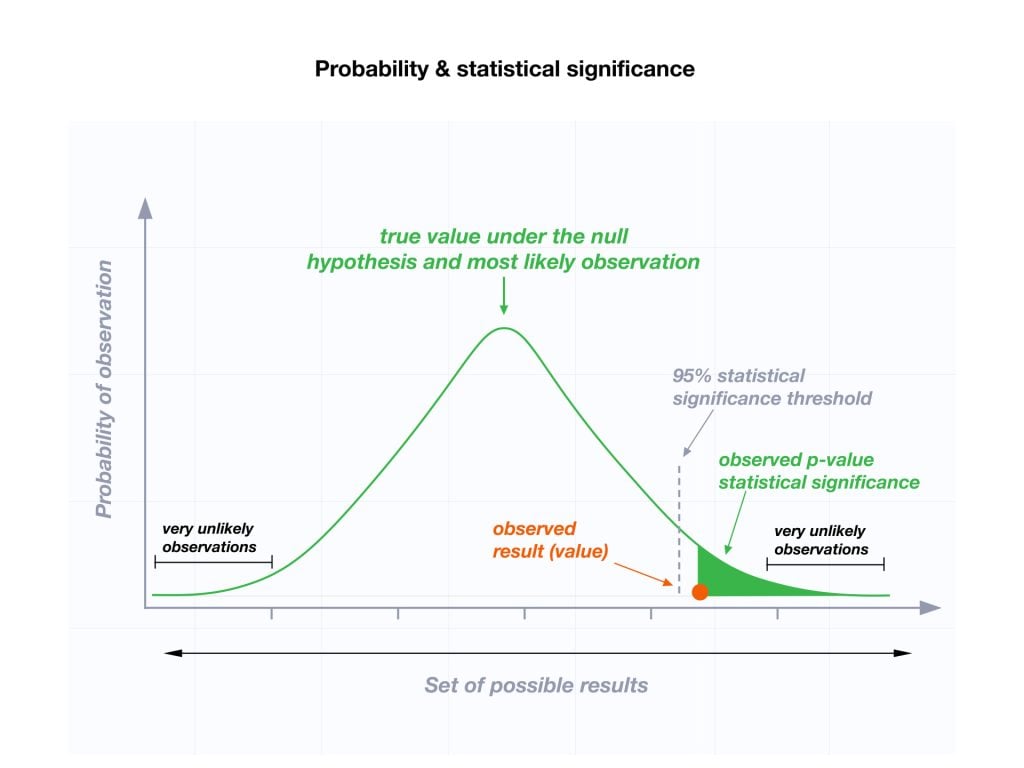
Two-Tailed Test
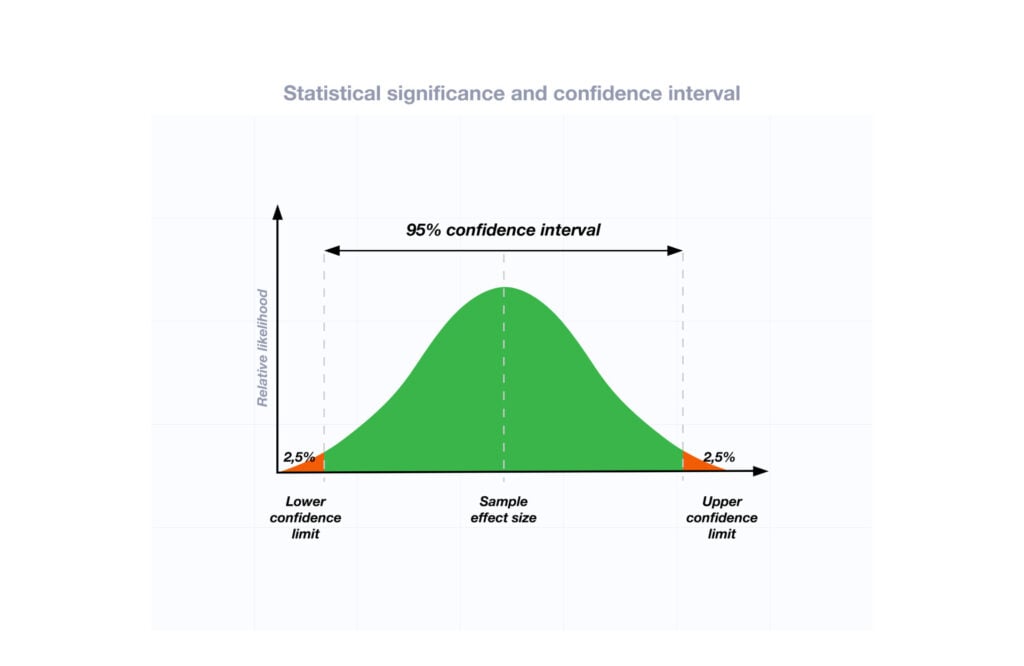
How do you calculate the p-value ?
Most statistical software packages like R, SPSS, and others automatically calculate your p-value. This is the easiest and most common way.
Online resources and tables are available to estimate the p-value based on your test statistic and degrees of freedom.
These tables help you understand how often you would expect to see your test statistic under the null hypothesis.
Understanding the Statistical Test:
Different statistical tests are designed to answer specific research questions or hypotheses. Each test has its own underlying assumptions and characteristics.
For example, you might use a t-test to compare means, a chi-squared test for categorical data, or a correlation test to measure the strength of a relationship between variables.
Be aware that the number of independent variables you include in your analysis can influence the magnitude of the test statistic needed to produce the same p-value.
This factor is particularly important to consider when comparing results across different analyses.
Example: Choosing a Statistical Test
If you’re comparing the effectiveness of just two different drugs in pain relief, a two-sample t-test is a suitable choice for comparing these two groups. However, when you’re examining the impact of three or more drugs, it’s more appropriate to employ an Analysis of Variance ( ANOVA) . Utilizing multiple pairwise comparisons in such cases can lead to artificially low p-values and an overestimation of the significance of differences between the drug groups.
How to report
A statistically significant result cannot prove that a research hypothesis is correct (which implies 100% certainty).
Instead, we may state our results “provide support for” or “give evidence for” our research hypothesis (as there is still a slight probability that the results occurred by chance and the null hypothesis was correct – e.g., less than 5%).
Example: Reporting the results
In our comparison of the pain relief effects of the new drug and the placebo, we observed that participants in the drug group experienced a significant reduction in pain ( M = 3.5; SD = 0.8) compared to those in the placebo group ( M = 5.2; SD = 0.7), resulting in an average difference of 1.7 points on the pain scale (t(98) = -9.36; p < 0.001).
The 6th edition of the APA style manual (American Psychological Association, 2010) states the following on the topic of reporting p-values:
“When reporting p values, report exact p values (e.g., p = .031) to two or three decimal places. However, report p values less than .001 as p < .001.
The tradition of reporting p values in the form p < .10, p < .05, p < .01, and so forth, was appropriate in a time when only limited tables of critical values were available.” (p. 114)
- Do not use 0 before the decimal point for the statistical value p as it cannot equal 1. In other words, write p = .001 instead of p = 0.001.
- Please pay attention to issues of italics ( p is always italicized) and spacing (either side of the = sign).
- p = .000 (as outputted by some statistical packages such as SPSS) is impossible and should be written as p < .001.
- The opposite of significant is “nonsignificant,” not “insignificant.”
Why is the p -value not enough?
A lower p-value is sometimes interpreted as meaning there is a stronger relationship between two variables.
However, statistical significance means that it is unlikely that the null hypothesis is true (less than 5%).
To understand the strength of the difference between the two groups (control vs. experimental) a researcher needs to calculate the effect size .
When do you reject the null hypothesis?
In statistical hypothesis testing, you reject the null hypothesis when the p-value is less than or equal to the significance level (α) you set before conducting your test. The significance level is the probability of rejecting the null hypothesis when it is true. Commonly used significance levels are 0.01, 0.05, and 0.10.
Remember, rejecting the null hypothesis doesn’t prove the alternative hypothesis; it just suggests that the alternative hypothesis may be plausible given the observed data.
The p -value is conditional upon the null hypothesis being true but is unrelated to the truth or falsity of the alternative hypothesis.
What does p-value of 0.05 mean?
If your p-value is less than or equal to 0.05 (the significance level), you would conclude that your result is statistically significant. This means the evidence is strong enough to reject the null hypothesis in favor of the alternative hypothesis.
Are all p-values below 0.05 considered statistically significant?
No, not all p-values below 0.05 are considered statistically significant. The threshold of 0.05 is commonly used, but it’s just a convention. Statistical significance depends on factors like the study design, sample size, and the magnitude of the observed effect.
A p-value below 0.05 means there is evidence against the null hypothesis, suggesting a real effect. However, it’s essential to consider the context and other factors when interpreting results.
Researchers also look at effect size and confidence intervals to determine the practical significance and reliability of findings.
How does sample size affect the interpretation of p-values?
Sample size can impact the interpretation of p-values. A larger sample size provides more reliable and precise estimates of the population, leading to narrower confidence intervals.
With a larger sample, even small differences between groups or effects can become statistically significant, yielding lower p-values. In contrast, smaller sample sizes may not have enough statistical power to detect smaller effects, resulting in higher p-values.
Therefore, a larger sample size increases the chances of finding statistically significant results when there is a genuine effect, making the findings more trustworthy and robust.
Can a non-significant p-value indicate that there is no effect or difference in the data?
No, a non-significant p-value does not necessarily indicate that there is no effect or difference in the data. It means that the observed data do not provide strong enough evidence to reject the null hypothesis.
There could still be a real effect or difference, but it might be smaller or more variable than the study was able to detect.
Other factors like sample size, study design, and measurement precision can influence the p-value. It’s important to consider the entire body of evidence and not rely solely on p-values when interpreting research findings.
Can P values be exactly zero?
While a p-value can be extremely small, it cannot technically be absolute zero. When a p-value is reported as p = 0.000, the actual p-value is too small for the software to display. This is often interpreted as strong evidence against the null hypothesis. For p values less than 0.001, report as p < .001
Further Information
- P-values and significance tests (Kahn Academy)
- Hypothesis testing and p-values (Kahn Academy)
- Wasserstein, R. L., Schirm, A. L., & Lazar, N. A. (2019). Moving to a world beyond “ p “< 0.05”.
- Criticism of using the “ p “< 0.05”.
- Publication manual of the American Psychological Association
- Statistics for Psychology Book Download
Bland, J. M., & Altman, D. G. (1994). One and two sided tests of significance: Authors’ reply. BMJ: British Medical Journal , 309 (6958), 874.
Goodman, S. N., & Royall, R. (1988). Evidence and scientific research. American Journal of Public Health , 78 (12), 1568-1574.
Goodman, S. (2008, July). A dirty dozen: twelve p-value misconceptions . In Seminars in hematology (Vol. 45, No. 3, pp. 135-140). WB Saunders.
Lang, J. M., Rothman, K. J., & Cann, C. I. (1998). That confounded P-value. Epidemiology (Cambridge, Mass.) , 9 (1), 7-8.

Related Articles
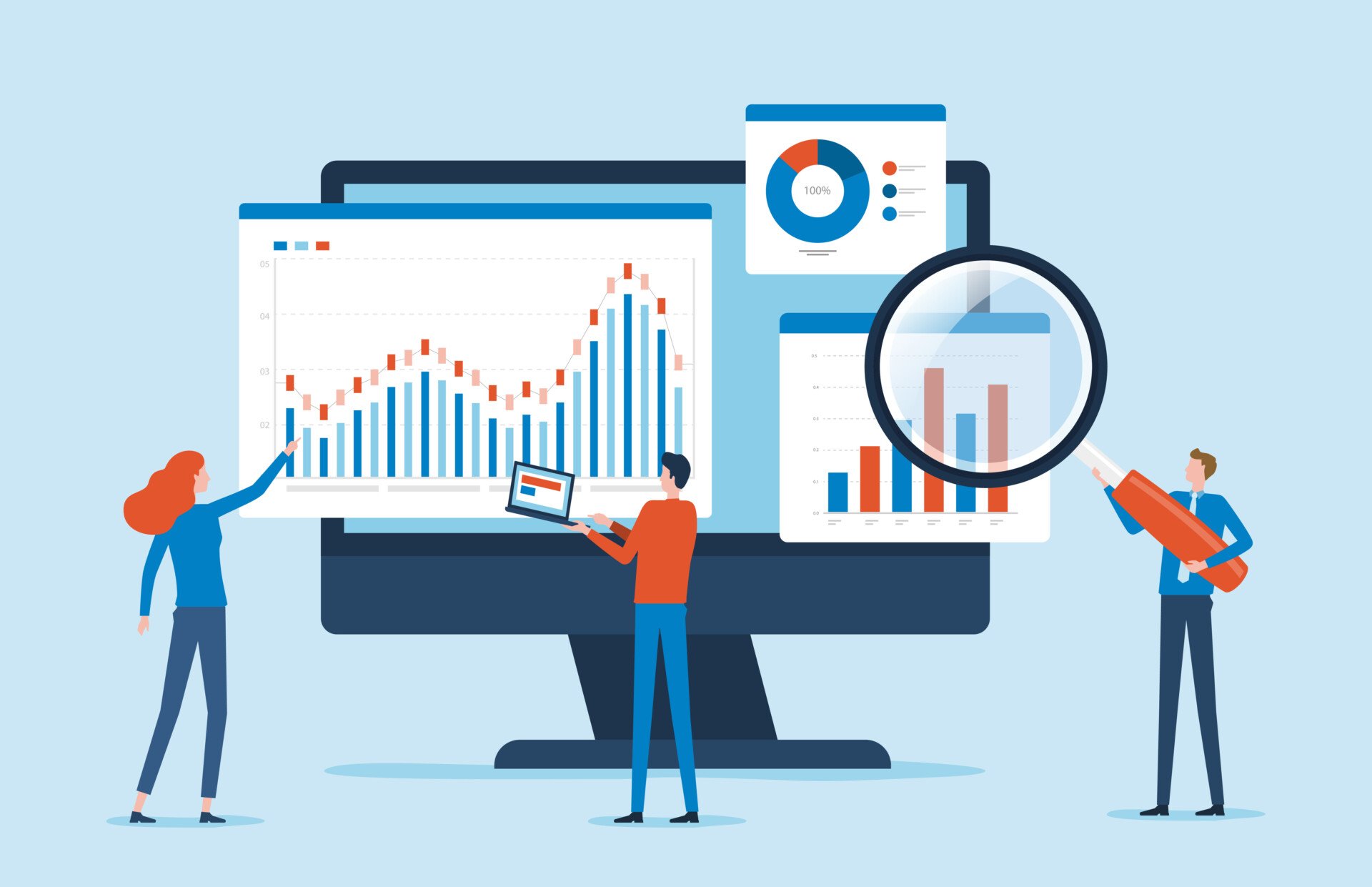
Exploratory Data Analysis
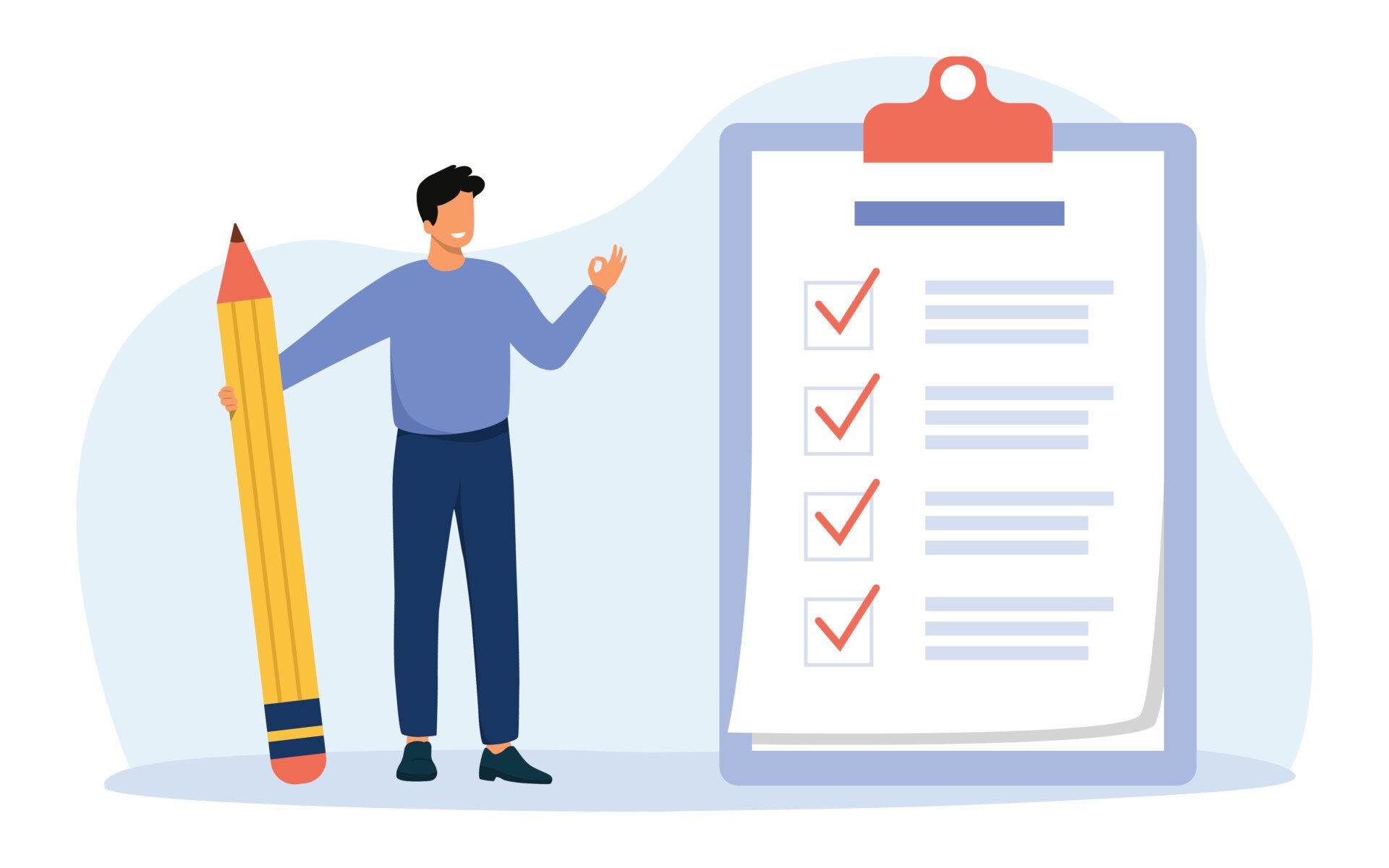
Research Methodology , Statistics
What Is Face Validity In Research? Importance & How To Measure
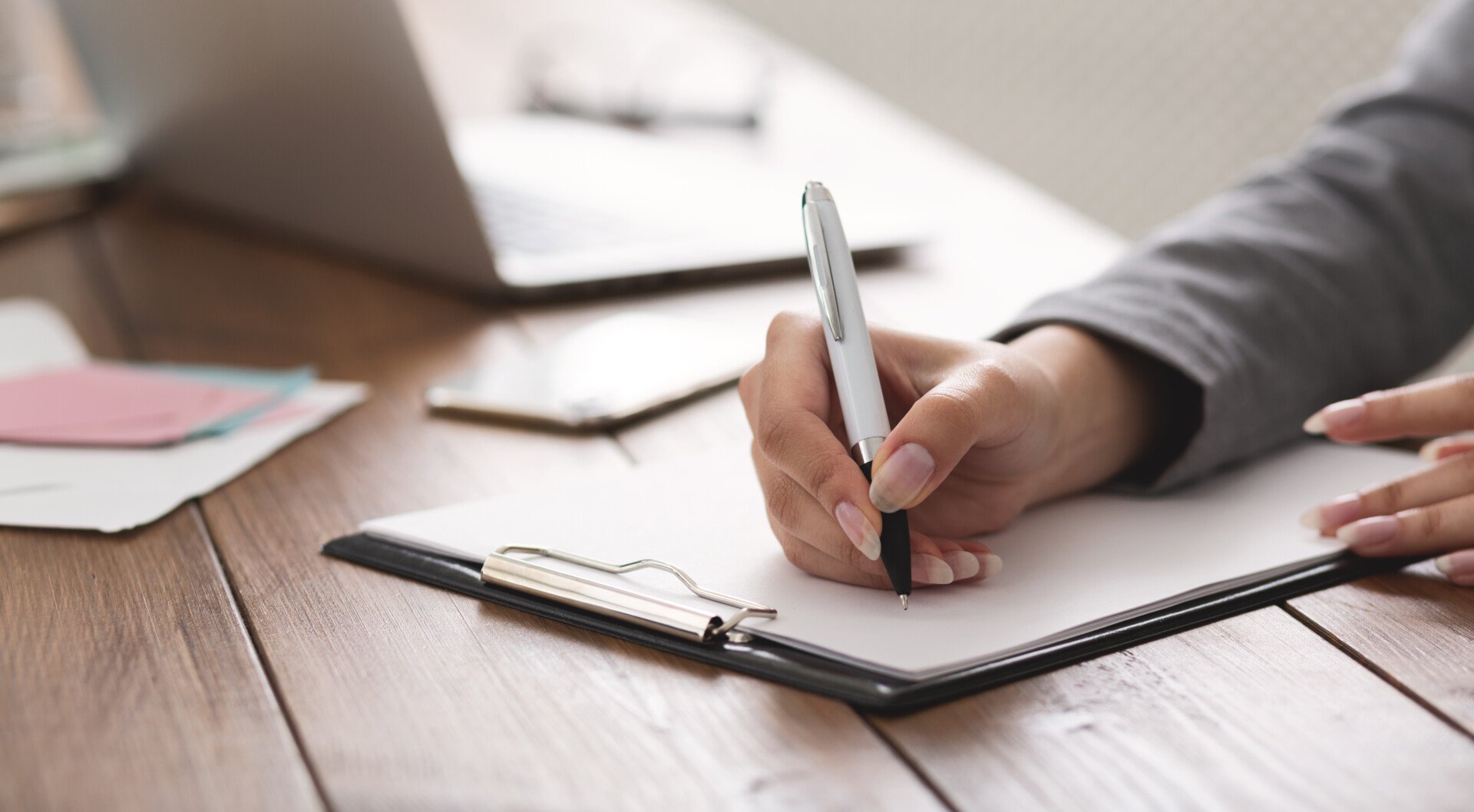
Criterion Validity: Definition & Examples
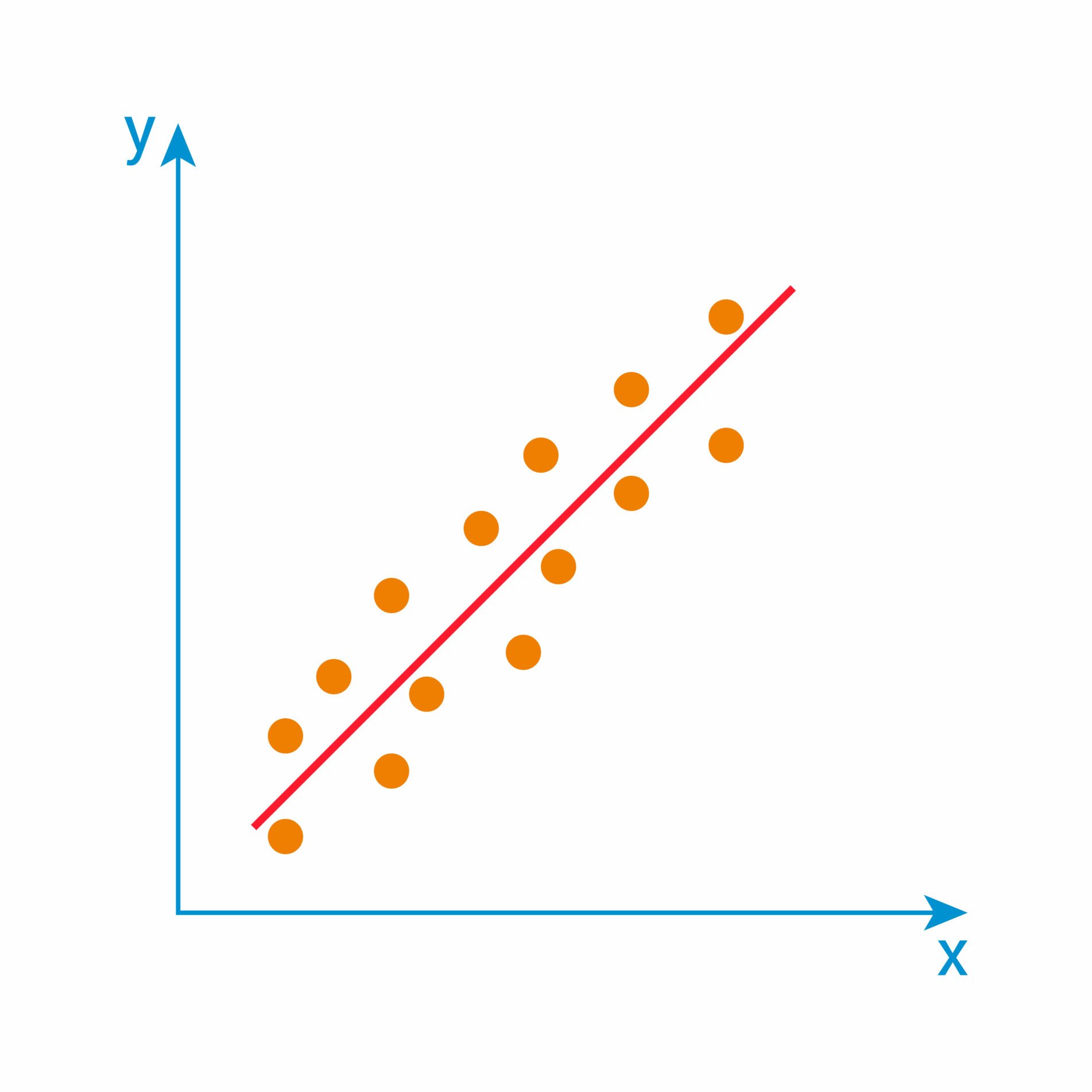
Convergent Validity: Definition and Examples
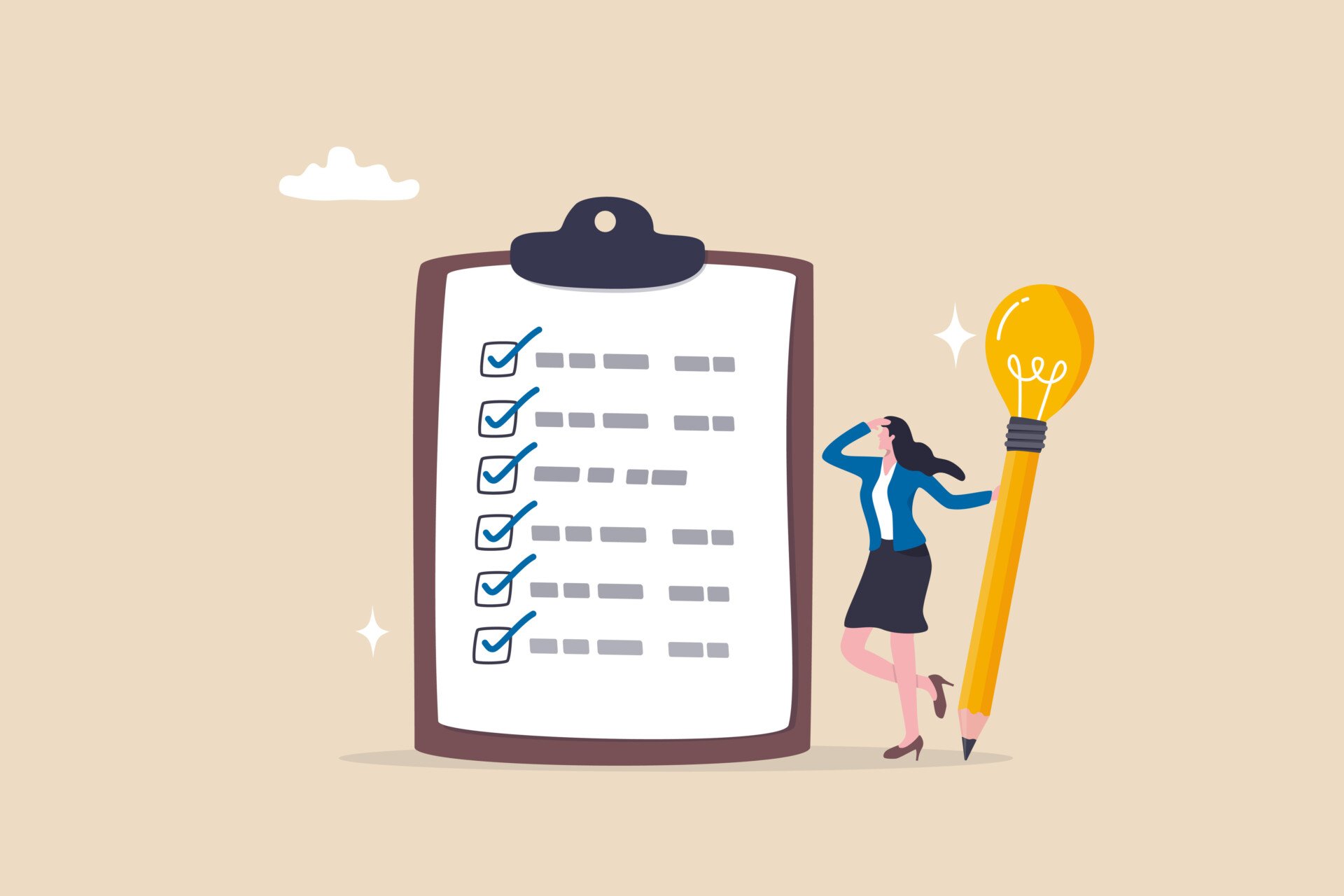
Content Validity in Research: Definition & Examples
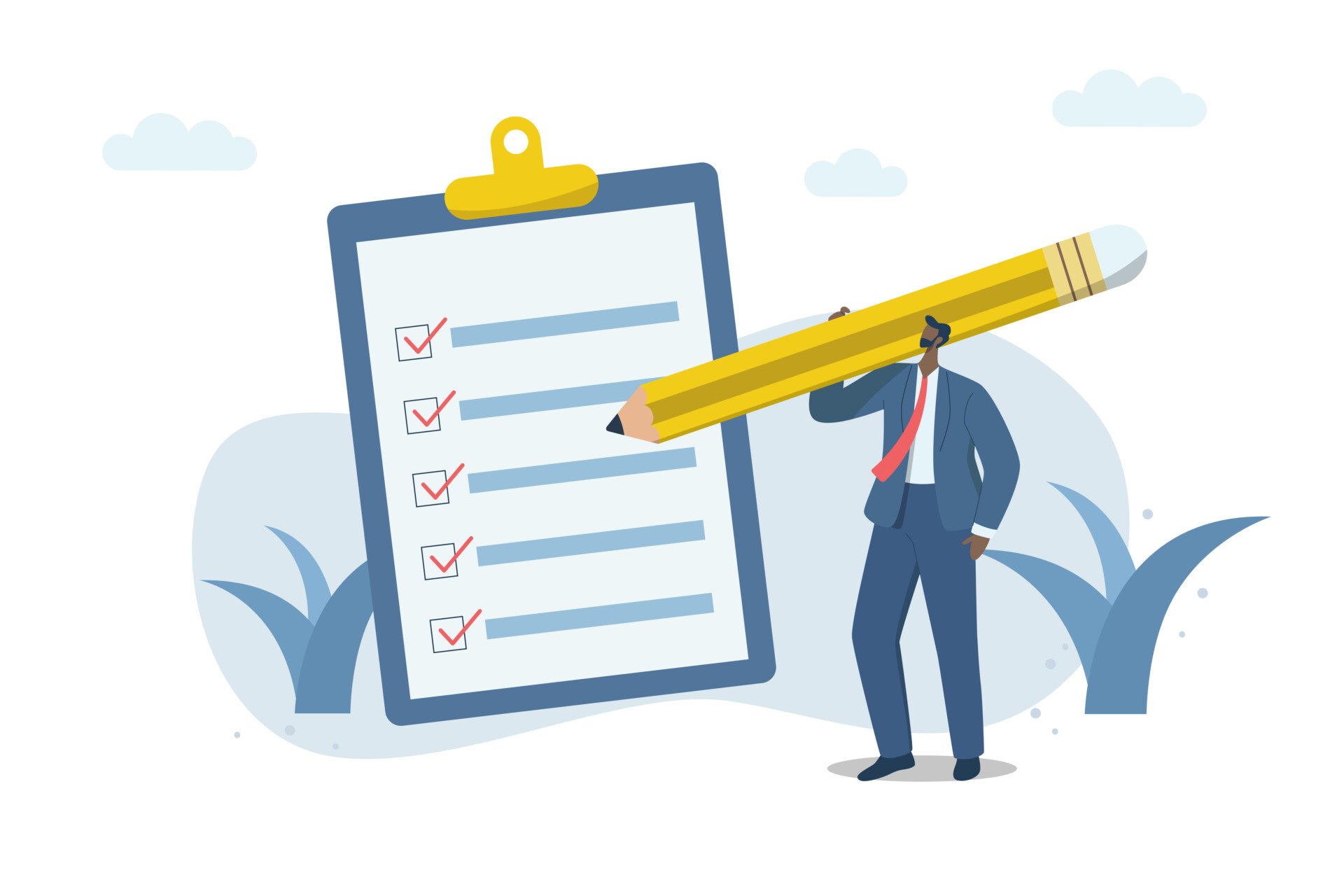
Construct Validity In Psychology Research

- Quality Improvement
- Talk To Minitab
How to Correctly Interpret P Values
Topics: Hypothesis Testing
The P value is used all over statistics, from t-tests to regression analysis . Everyone knows that you use P values to determine statistical significance in a hypothesis test . In fact, P values often determine what studies get published and what projects get funding.
Despite being so important, the P value is a slippery concept that people often interpret incorrectly. How do you interpret P values?
In this post, I'll help you to understand P values in a more intuitive way and to avoid a very common misinterpretation that can cost you money and credibility.
What Is the Null Hypothesis in Hypothesis Testing?
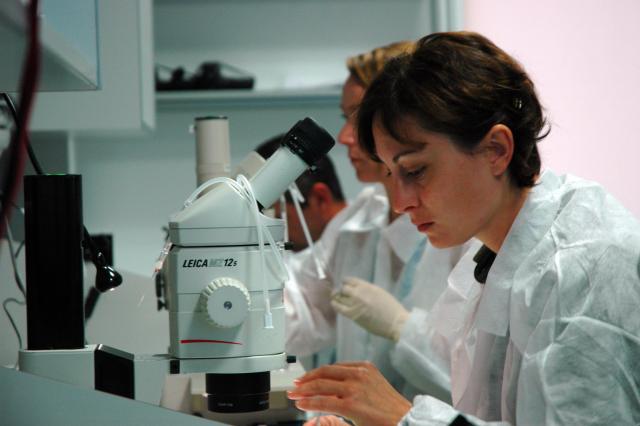
In every experiment, there is an effect or difference between groups that the researchers are testing. It could be the effectiveness of a new drug, building material, or other intervention that has benefits. Unfortunately for the researchers, there is always the possibility that there is no effect, that is, that there is no difference between the groups. This lack of a difference is called the null hypothesis , which is essentially the position a devil’s advocate would take when evaluating the results of an experiment.
To see why, let’s imagine an experiment for a drug that we know is totally ineffective. The null hypothesis is true: there is no difference between the experimental groups at the population level.
Despite the null being true, it’s entirely possible that there will be an effect in the sample data due to random sampling error. In fact, it is extremely unlikely that the sample groups will ever exactly equal the null hypothesis value. Consequently, the devil’s advocate position is that the observed difference in the sample does not reflect a true difference between populations .
What Are P Values?
- High P values: your data are likely with a true null.
- Low P values: your data are unlikely with a true null.
A low P value suggests that your sample provides enough evidence that you can reject the null hypothesis for the entire population.
How Do You Interpret P Values?
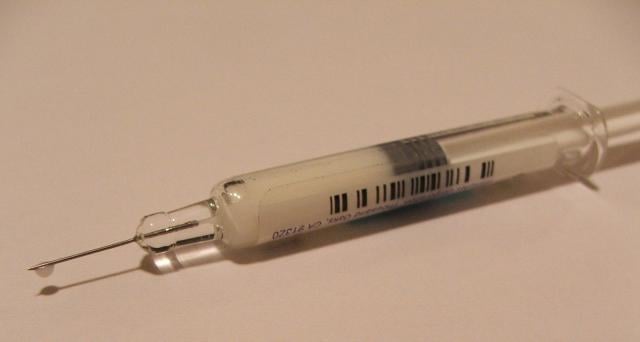
For example, suppose that a vaccine study produced a P value of 0.04. This P value indicates that if the vaccine had no effect, you’d obtain the observed difference or more in 4% of studies due to random sampling error.
P values address only one question: how likely are your data, assuming a true null hypothesis? It does not measure support for the alternative hypothesis. This limitation leads us into the next section to cover a very common misinterpretation of P values.
hbspt.cta._relativeUrls=true;hbspt.cta.load(3447555, '16128196-352b-4dd2-8356-f063c37c5b2a', {"useNewLoader":"true","region":"na1"});
P values are not the probability of making a mistake.
Incorrect interpretations of P values are very common. The most common mistake is to interpret a P value as the probability of making a mistake by rejecting a true null hypothesis (a Type I error ).
There are several reasons why P values can’t be the error rate.
First, P values are calculated based on the assumptions that the null is true for the population and that the difference in the sample is caused entirely by random chance. Consequently, P values can’t tell you the probability that the null is true or false because it is 100% true from the perspective of the calculations.
Second, while a low P value indicates that your data are unlikely assuming a true null, it can’t evaluate which of two competing cases is more likely:
- The null is true but your sample was unusual.
- The null is false.
Determining which case is more likely requires subject area knowledge and replicate studies.
Let’s go back to the vaccine study and compare the correct and incorrect way to interpret the P value of 0.04:
- Correct: Assuming that the vaccine had no effect, you’d obtain the observed difference or more in 4% of studies due to random sampling error.
- Incorrect: If you reject the null hypothesis, there’s a 4% chance that you’re making a mistake.
To see a graphical representation of how hypothesis tests work, see my post: Understanding Hypothesis Tests: Significance Levels and P Values .
What Is the True Error Rate?
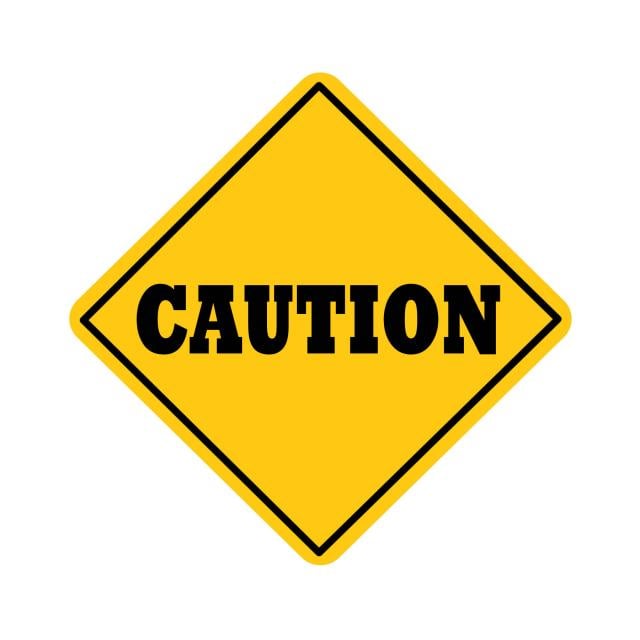
If a P value is not the error rate, what the heck is the error rate? (Can you guess which way this is heading now?)
Sellke et al.* have estimated the error rates associated with different P values. While the precise error rate depends on various assumptions (which I discuss here ), the table summarizes them for middle-of-the-road assumptions.
Do the higher error rates in this table surprise you? Unfortunately, the common misinterpretation of P values as the error rate creates the illusion of substantially more evidence against the null hypothesis than is justified. As you can see, if you base a decision on a single study with a P value near 0.05, the difference observed in the sample may not exist at the population level. That can be costly!
Now that you know how to interpret P values, read my five guidelines for how to use P values and avoid mistakes .
You can also read my rebuttal to an academic journal that actually banned P values !
An exciting study about the reproducibility of experimental results was published in August 2015. This study highlights the importance of understanding the true error rate. For more information, read my blog post: P Values and the Replication of Experiments .
The American Statistical Association speaks out on how to use p-values!
*Thomas SELLKE, M. J. BAYARRI, and James O. BERGER, Calibration of p Values for Testing Precise Null Hypotheses, The American Statistician, February 2001, Vol. 55, No. 1
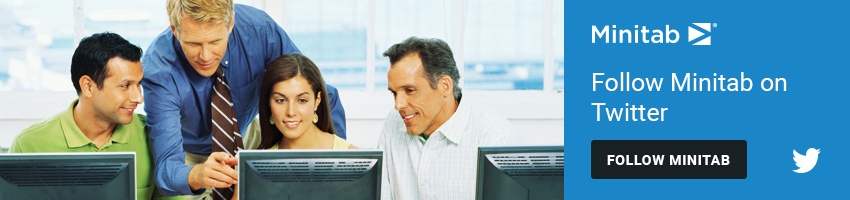
You Might Also Like
- Trust Center
© 2023 Minitab, LLC. All Rights Reserved.
- Terms of Use
- Privacy Policy
- Cookies Settings
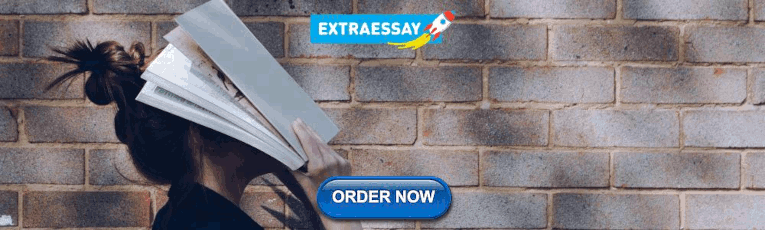
The p value – definition and interpretation of p-values in statistics
This article examines the most common statistic reported in scientific papers and used in applied statistical analyses – the p -value . The article goes through the definition illustrated with examples, discusses its utility, interpretation, and common misinterpretations of observed statistical significance and significance levels. It is structured as follows:
What does ‘ p ‘ in ‘ p -value’ stand for?
What does p measure and how to interpret it.
- A p-value only makes sense under a specified null hypothesis
How to calculate a p -value?
A practical example, p -values as convenient summary statistics.
- Quantifying the relative uncertainty of data
Easy comparison of different statistical tests
- p -value interpretation in outcomes of experiments (randomized controlled trials)
- p -value interpretation in regressions and correlations of observational data
Mistaking statistical significance with practical significance
Treating the significance level as likelihood for the observed effect, treating p -values as likelihoods attached to hypotheses, a high p -value means the null hypothesis is true, lack of statistical significance suggests a small effect size, p -value definition and meaning.
The technical definition of the p -value is (based on [4,5,6]):
A p -value is the probability of the data-generating mechanism corresponding to a specified null hypothesis to produce an outcome as extreme or more extreme than the one observed.
However, it is only straightforward to understand for those already familiar in detail with terms such as ‘probability’, ‘null hypothesis’, ‘data generating mechanism’, ‘extreme outcome’. These, in turn, require knowledge of what a ‘hypothesis’, a ‘statistical model’ and ‘statistic’ mean, and so on. While some of these will be explained on a cursory level in the following paragraphs, those looking for deeper understanding should consider consulting the following glossary definitions: statistical model , hypothesis , null hypothesis , statistic .
A slightly less technical and therefore more accessible definition is:
A p -value quantifies how likely it is to erroneously reject a specific statistical hypothesis, were it true, based on a given set of data.
Let us break these down and examine several examples to make both of these definitions make sense.
p stands for p robability where probability means the frequency with which an event occurs under certain assumptions. The most common example is the frequency with which a coin lands heads under the assumption that it is equally balanced (a fair coin toss ). That frequency is 0.5 (50%).
Capital ‘P’ stands for probability in general, whereas lowercase ‘ p ‘ refers to the probability of a particular data realization. To expand on the coin toss example: P would stand for the probability of heads in general, whereas p could refer to the probability of landing a series of five heads in a row, or the probability of landing less than or equal to 38 heads out of 100 coin flips.
Given that it was established that p stands for probability, it is easy to figure out it measures a sort of probability.
In everyday language the term ‘probability’ might be used as synonymous to ‘chance’, ‘likelihood’, ‘odds’, e.g. there is 90% probability that it will rain tomorrow. However, in statistics one cannot speak of ‘probability’ without specifying a mechanism which generates the observed data. A simple example of such a mechanism is a device which produces fair coin tosses. A statistical model based on this data-generating mechanism can be put forth and under that model the probability of 38 or less heads out of 100 tosses can be estimated to be 1.05%, for example by using a binomial calculator . The p -value against the model of a fair coin would be ~0.01 (rounding it to 0.01 from hereon for the purposes of the article).
The way to interpret that p -value is: observing 38 heads or less out of the 100 tosses could have happened in only 1% of infinitely many series of 100 fair coin tosses. The null hypothesis in this case is defined as the coin being fair, therefore having a 50% chance for heads and 50% chance for tails on each toss.
Assuming the null hypothesis is true allows the comparison of the observed data to what would have been expected under the null. It turns out the particular observation of 38/100 heads is a rather improbable and thus surprising outcome under the assumption of the null hypothesis. This is measured by the low p -value which also accounts for more extreme outcomes such as 37/100, 36/100, and so on all the way to 0/100.
If one had a predefined level of statistical significance at 0.05 then one would claim that the outcome is statistically significant since it’s p -value of 0.01 meets the 0.05 significance level (0.01 ≤ 0.05). A visual representation of the relationship between p -values, significance level ( p -value threshold), and statistical significance of an outcome is illustrated visually in this graph:
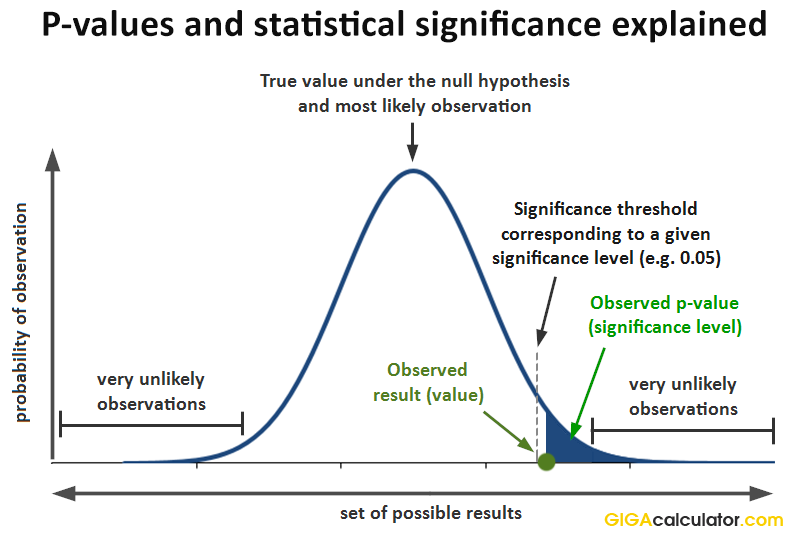
In fact, had the significance threshold been at any value above 0.01, the outcome would have been statistically significant, therefore it is usually said that with a p -value of 0.01, the outcome is statistically significant at any level above 0.01 .
Continuing with the interpretation: were one to reject the null hypothesis based on this p -value of 0.01, they would be acting as if a significance level of 0.01 or lower provides sufficient evidence against the hypothesis of the coin being fair. One could interpret this as a rule for a long-run series of experiments and inferences . In such a series, by using this p -value threshold one would incorrectly reject the fair coin hypothesis in at most 1 out of 100 cases, regardless of whether the coin is actually fair in any one of them. An incorrect rejection of the null is often called a type I error as opposed to a type II error which is to incorrectly fail to reject a null.
A more intuitive interpretation proceeds without reference to hypothetical long-runs. This second interpretation comes in the form of a strong argument from coincidence :
- there was a low probability (0.01 or 1%) that something would have happened assuming the null was true
- it did happen so it has to be an unusual (to the extent that the p -value is low) coincidence that it happened
- this warrants the conclusion to reject the null hypothesis
( source ). It stems from the concept of severe testing as developed by Prof. Deborah Mayo in her various works [1,2,3,4,5] and reflects an error-probabilistic approach to inference.
A p -value only makes sense under a specified null hypothesis
It is important to understand why a specified ‘null hypothesis’ should always accompany any reported p -value and why p-values are crucial in so-called Null Hypothesis Statistical Tests (NHST) . Statistical significance only makes sense when referring to a particular statistical model which in turn corresponds to a given null hypothesis. A p -value calculation has a statistical model and a statistical null hypothesis defined within it as prerequisites, and a statistical null is only interesting because of some tightly related substantive null such as ‘this treatment improves outcomes’. The relationship is shown in the chart below:

In the coin example, the substantive null that is interesting to (potentially) reject is the claim that the coin is fair. It translates to a statistical null hypothesis (model) with the following key properties:
- heads having 50% chance and tails having 50% chance, on each toss
- independence of each toss from any other toss. The outcome of any given coin toss does not depend on past or future coin tosses.
- homogeneity of the coin behavior over time (the true chance does not change across infinitely many tosses)
- a binomial error distribution
The resulting p -value of 0.01 from the coin toss experiment should be interpreted as the probability only under these particular assumptions.
What happens, however, if someone is interested in rejecting the claim that the coin is somewhat biased against heads? To be precise: the claim that it has a true frequency of heads of 40% or less (hence 60% for tails) is the one they are looking to deny with a certain evidential threshold.
The p -value needs to be recalculated under their null hypothesis so now the same 38 heads out of 100 tosses result in a p -value of ~0.38 ( calculation ). If they were interested in rejecting such a null hypothesis, then this data provide poor evidence against it since a 38/100 outcome would not be unusual at all if it were in fact true (p ≤ 0.38 would occur with probability 38%).
Similarly, the p -value needs to be recalculated for a claim of bias in the other direction, say that the coin produces heads with a frequency of 60% or more. The probability of observing 38 or fewer out of 100 under this null hypothesis is so extremely small ( p -value ~= 0.000007364 or 7.364 x 10 -6 in standard form , calculation ) that maintaining a claim for 60/40 bias in favor of heads becomes near-impossible for most practical purposes.
A p -value can be calculated for any frequentist statistical test. Common types of statistical tests include tests for:
- absolute difference in proportions;
- absolute difference in means;
- relative difference in means or proportions;
- goodness-of-fit;
- homogeneity
- independence
- analysis of variance (ANOVA)
and others. Different statistics would be computed depending on the error distribution of the parameter of interest in each case, e.g. a t value, z value, chi-square (Χ 2 ) value, f -value, and so on.
p -values can then be calculated based on the cumulative distribution functions (CDFs) of these statistics whereas pre-test significance thresholds (critical values) can be computed based on the inverses of these functions. You can try these by plugging different inputs in our critical value calculator , and also by consulting its documentation.
In its generic form, a p -value formula can be written down as:
p = P(d(X) ≥ d(x 0 ); H 0 )
where P stands for probability, d(X) is a test statistic (distance function) of a random variable X , x 0 is a typical realization of X and H 0 is the selected null hypothesis. The semi-colon means ‘assuming’. The distance function is the aforementioned cumulative distribution function for the relevant error distribution. In its generic form a distance function equation can be written as:
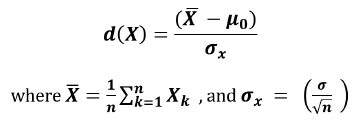
X -bar is the arithmetic mean of the observed values, μ 0 is a hypothetical or expected mean to which X is compared, and n is the sample size. The result of a distance function will often be expressed in a standardized form – the number of standard deviations between the observed value and the expected value.
The p -value calculation is different in each case and so a different formula will be applied depending on circumstances. You can see examples in the p -values reported in our statistical calculators, such as the statistical significance calculator for difference of means or proportions , the Chi-square calculator , the risk ratio calculator , odds ratio calculator , hazard ratio calculator , and the normality calculator .
A very fresh (as of late 2020) example of the application of p -values in scientific hypothesis testing can be found in the recently concluded COVID-19 clinical trials. Multiple vaccines for the virus which spread from China in late 2019 and early 2020 have been tested on tens of thousands of volunteers split randomly into two groups – one gets the vaccine and the other gets a placebo. This is called a randomized controlled trial (RCT). The main parameter of interest is the difference between the rates of infections in the two groups. An appropriate test is the t-test for difference of proportions, but the same data can be examined in terms of risk ratios or odds ratio.
The null hypothesis in many of these medical trials is that the vaccine is at least 30% efficient. A statistical model can be built about the expected difference in proportions if the vaccine’s efficiency is 30% or less, and then the actual observed data from a medical trial can be compared to that null hypothesis. Most trials set their significance level at the minimum required by the regulatory bodies (FDA, EMA, etc.), which is usually set at 0.05 . So, if the p -value from a vaccine trial is calculated to be below 0.05, the outcome would be statistically significant and the null hypothesis of the vaccine being less than or equal to 30% efficient would be rejected.
Let us say a vaccine trial results in a p -value of 0.0001 against that null hypothesis. As this is highly unlikely under the assumption of the null hypothesis being true, it provides very strong evidence against the hypothesis that the tested treatment has less than 30% efficiency.
However, many regulators stated that they require at least 50% proven efficiency. They posit a different null hypothesis and so the p -value presented before these bodies needs to be calculated against it. This p -value would be somewhat increased since 50% is a higher null value than 30%, but given that the observed effects of the first vaccines to finalize their trials are around 95% with 95% confidence interval bounds hovering around 90%, the p -value against a null hypothesis stating that the vaccine’s efficiency is 50% or less is likely to still be highly statistically significant, say at 0.001 . Such an outcome is to be interpreted as follows: had the efficiency been 50% or below, such an extreme outcome would have most likely not been observed, therefore one can proceed to reject the claim that the vaccine has efficiency of 50% or less with a significance level of 0.001 .
While this example is fictitious in that it doesn’t reference any particular experiment, it should serve as a good illustration of how null hypothesis statistical testing (NHST) operates based on p -values and significance thresholds.
The utility of p -values and statistical significance
It is not often appreciated how much utility p-values bring to the practice of performing statistical tests for scientific and business purposes.
Quantifying relative uncertainty of data
First and foremost, p -values are a convenient expression of the uncertainty in the data with respect to a given claim. They quantify how unexpected a given observation is, assuming some claim which is put to the test is true. If the p-value is low the probability that it would have been observed under the null hypothesis is low. This means the uncertainty the data introduce is high. Therefore, anyone defending the substantive claim which corresponds to the statistical null hypothesis would be pressed to concede that their position is untenable in the face of such data.
If the p-value is high, then the uncertainty with regard to the null hypothesis is low and we are not in a position to reject it, hence the corresponding claim can still be maintained.
As evident by the generic p -value formula and the equation for a distance function which is a part of it, a p -value incorporates information about:
- the observed effect size relative to the null effect size
- the sample size of the test
- the variance and error distribution of the statistic of interest
It would be much more complicated to communicate the outcomes of a statistical test if one had to communicate all three pieces of information. Instead, by way of a single value on the scale of 0 to 1 one can communicate how surprising an outcome is. This value is affected by any change in any of these variables.
This quality stems from the fact that assuming that a p -value from one statistical test can easily and directly be compared to another. The minimal assumptions behind significance tests mean that given that all of them are met, the strength of the statistical evidence offered by data relative to a null hypothesis of interest is the same in two tests if they have approximately equal p -values.
This is especially useful in conducting meta-analyses of various sorts, or for combining evidence from multiple tests.
p -value interpretation in outcomes of experiments
When a p -value is calculated for the outcome of a randomized controlled experiment, it is used to assess the strength of evidence against a null hypothesis of interest, such as that a given intervention does not have a positive effect. If H 0 : μ 0 ≤ 0% and the observed effect is μ 1 = 30% and the calculated p -value is 0.025, this can be used to reject the claim H 0 : μ 0 ≤ 0% at any significance level ≥ 0.025. This, in turn, allows us to claim that H 1 , a complementary hypothesis called the ‘alternative hypothesis’, is in fact true. In this case since H 0 : μ 0 ≤ 0% then H 1 : μ 1 > 0% in order to exhaust the parameter space, as illustrated below:
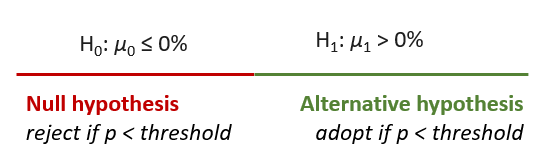
A claim as the above corresponds to what is called a one-sided null hypothesis . There could be a point null as well, for example the claim that an intervention has no effect whatsoever translates to H 0 : μ 0 = 0%. In such a case the corresponding p -value refers to that point null and hence should be interpreted as rejecting the claim of the effect being exactly zero. For those interested in the differences between point null hypotheses and one-sided hypotheses the articles on onesided.org should be an interesting read. TLDR: most of the time you’d want to reject a directional claim and hence a one-tailed p -value should be reported [8] .
These finer points aside, after observing a low enough p -value, one can claim the rejection of the null and hence the adoption of the complementary alternative hypothesis as true. The alternative hypothesis is simply a negation of the null and is therefore a composite claim such as ‘there is a positive effect’ or ‘there is some non-zero effect’. Note that any inference about a particular effect size within the alternative space has not been tested and hence claiming it has probability equal to p calculated against a zero effect null hypothesis (a.k.a. the nil hypothesis) does not make sense.
p – value interpretation in regressions and correlations of observational data
When performing statistical analyses of observational data p -values are often calculated for regressors in addition to regression coefficients and for the correlation in addition to correlation coefficients. A p -value falling below a specific statistical significance threshold measures how surprising the observed correlation or regression coefficient would be if the variable of interest is in fact orthogonal to the outcome variable. That is – how likely would it be to observe the apparent relationship, if there was no actual relationship between the variable and the outcome variable.
Our correlation calculator outputs both p -values and confidence intervals for the calculated coefficients and is an easy way to explore the concept in the case of correlations. Extrapolating to regressions is then straightforward.
Misinterpretations of statistically significant p -values
There are several common misinterpretations [7] of p -values and statistical significance and no calculator can save one from falling for them. The following errors are often committed when a result is seen as statistically significant.
A result may be highly statistically significant (e.g. p -value 0.0001) but it might still have no practical consequences due to a trivial effect size. This often happens with overpowered designs, but it can also happen in a properly designed statistical test. This error can be avoided by always reporting the effect size and confidence intervals around it.
Observing a highly significant result, say p -value 0.01 does not mean that the likelihood that the observed difference is the true difference. In fact, that likelihood is much, much smaller. Remember that statistical significance has a strict meaning in the NHST framework.
For example, if the observed effect size μ 1 from an intervention is 20% improvement in some outcome and a p -value against the null hypothesis of μ 0 ≤ 0% has been calculated to be 0.01, it does not mean that one can reject μ 0 ≤ 20% with a p -value of 0.01. In fact, the p -value against μ 0 ≤ 20% would be 0.5, which is not statistically significant by any measure.
To make claims about a particular effect size it is recommended to use confidence intervals or severity, or both.
For example, stating that a p -value of 0.02 means that there is 98% probability that the alternative hypothesis is true or that there is 2% probability that the null hypothesis is true . This is a logical error.
By design, even if the null hypothesis is true, p -values equal to or lower than 0.02 would be observed exactly 2% of the time, so one cannot use the fact that a low p -value has been observed to argue there is only 2% probability that the null hypothesis is true. Frequentist and error-statistical methods do not allow one to attach probabilities to hypotheses or claims, only to events [4] . Doing so requires an exhaustive list of hypotheses and prior probabilities attached to them which goes firmly into decision-making territory. Put in Bayesian terms, the p -value is not a posterior probability.
Misinterpretations of statistically non-significant outcomes
Statistically non-significant p-values – that is, p is greater than the specified significance threshold α (alpha), can lead to a different set of misinterpretations. Due to the ubiquitous use of p -values, these are committed often as well.
Treating a high p -value / low significance level as evidence, by itself, that the null hypothesis is true is a common mistake. For example, after observing p = 0.2 one may claim this is evidence that there is no effect, e.g. no difference between two means, is a common mistake.
However, it is trivial to demonstrate why it is wrong to interpret a high p -value as providing support for the null hypothesis. Take a simple experiment in which one measures only 2 (two) people or objects in the control and treatment groups. The p -value for this test of significance will surely not be statistically significant. Does that mean that the intervention is ineffective? Of course not, since that claim has not been tested severely enough. Using a statistic such as severity can completely eliminate this error [4,5] .
A more detailed response would say that failure to observe a statistically significant result, given that the test has enough statistical power, can be used to argue for accepting the null hypothesis to the extent warranted by the power and with reference to the minimum detectable effect for which it was calculated. For example, if the statistical test had 99% power to detect an effect of size μ 1 at level α and it failed, then it could be argued that it is quite unlikely that there exists and effect of size μ 1 or greater as in that case one would have most likely observed a significant p -value.
This is a softer version of the above mistake wherein instead of claiming support for the null hypothesis, a low p -value is taken, by itself, as indicating that the effect size must be small.
This is a mistake since the test might have simply lacked power to exclude many effects of meaningful size. Examining confidence intervals and performing severity calculations against particular hypothesized effect sizes would be a way to avoid this issue.
References:
[1] Mayo, D.G. 1983. “An Objective Theory of Statistical Testing.” Synthese 57 (3): 297–340. DOI:10.1007/BF01064701. [2] Mayo, D.G. 1996 “Error and the Growth of Experimental Knowledge.” Chicago, Illinois: University of Chicago Press. DOI:10.1080/106351599260247. [3] Mayo, D.G., and A. Spanos. 2006. “Severe Testing as a Basic Concept in a Neyman–Pearson Philosophy of Induction.” The British Journal for the Philosophy of Science 57 (2): 323–357. DOI:10.1093/bjps/axl003. [4] Mayo, D.G., and A. Spanos. 2011. “Error Statistics.” Vol. 7, in Handbook of Philosophy of Science Volume 7 – Philosophy of Statistics , by D.G., Spanos, A. et al. Mayo, 1-46. Elsevier. [5] Mayo, D.G. 2018 “Statistical Inference as Severe Testing.” Cambridge: Cambridge University Press. ISBN: 978-1107664647 [6] Georgiev, G.Z. (2019) “Statistical Methods in Online A/B Testing”, ISBN: 978-1694079725 [7] Greenland, S. et al. (2016) “Statistical tests, P values, confidence intervals, and power: a guide to misinterpretations”, European Journal of Epidemiology 31:337–350; DOI:10.1007/s10654-016-0149-3 [8] Georgiev, G.Z. (2018) “Directional claims require directional (statistical) hypotheses” [online, accessed on Dec 07, 2020, at https://www.onesided.org/articles/directional-claims-require-directional-hypotheses.php]
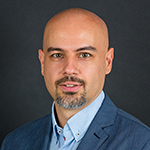
An applied statistician, data analyst, and optimizer by calling, Georgi has expertise in web analytics, statistics, design of experiments, and business risk management. He covers a variety of topics where mathematical models and statistics are useful. Georgi is also the author of “Statistical Methods in Online A/B Testing”.
Recent Articles
- Mastering Formulas in Baking: Baker’s Math, Kitchen Conversions, + More
- Margin vs. Markup: Decoding Profitability in Simple Terms
- How Much Do I Have to Earn Per Hour to Afford the Cost of Living?
- How to Calculate for VAT When Traveling Abroad
- Mathematics in the Kitchen
- Search GIGA Articles
- Cybersecurity
- Home & Garden
- Mathematics
If you're seeing this message, it means we're having trouble loading external resources on our website.
If you're behind a web filter, please make sure that the domains *.kastatic.org and *.kasandbox.org are unblocked.
To log in and use all the features of Khan Academy, please enable JavaScript in your browser.
AP®︎/College Statistics
Course: ap®︎/college statistics > unit 10.
- Idea behind hypothesis testing
Examples of null and alternative hypotheses
- Writing null and alternative hypotheses
- P-values and significance tests
- Comparing P-values to different significance levels
- Estimating a P-value from a simulation
- Estimating P-values from simulations
- Using P-values to make conclusions

Want to join the conversation?
- Upvote Button navigates to signup page
- Downvote Button navigates to signup page
- Flag Button navigates to signup page

Video transcript
9.1 Null and Alternative Hypotheses
The actual test begins by considering two hypotheses . They are called the null hypothesis and the alternative hypothesis . These hypotheses contain opposing viewpoints.
H 0 , the — null hypothesis: a statement of no difference between sample means or proportions or no difference between a sample mean or proportion and a population mean or proportion. In other words, the difference equals 0.
H a —, the alternative hypothesis: a claim about the population that is contradictory to H 0 and what we conclude when we reject H 0 .
Since the null and alternative hypotheses are contradictory, you must examine evidence to decide if you have enough evidence to reject the null hypothesis or not. The evidence is in the form of sample data.
After you have determined which hypothesis the sample supports, you make a decision. There are two options for a decision. They are reject H 0 if the sample information favors the alternative hypothesis or do not reject H 0 or decline to reject H 0 if the sample information is insufficient to reject the null hypothesis.
Mathematical Symbols Used in H 0 and H a :
H 0 always has a symbol with an equal in it. H a never has a symbol with an equal in it. The choice of symbol depends on the wording of the hypothesis test. However, be aware that many researchers use = in the null hypothesis, even with > or < as the symbol in the alternative hypothesis. This practice is acceptable because we only make the decision to reject or not reject the null hypothesis.
Example 9.1
H 0 : No more than 30 percent of the registered voters in Santa Clara County voted in the primary election. p ≤ 30 H a : More than 30 percent of the registered voters in Santa Clara County voted in the primary election. p > 30
A medical trial is conducted to test whether or not a new medicine reduces cholesterol by 25 percent. State the null and alternative hypotheses.
Example 9.2
We want to test whether the mean GPA of students in American colleges is different from 2.0 (out of 4.0). The null and alternative hypotheses are the following: H 0 : μ = 2.0 H a : μ ≠ 2.0
We want to test whether the mean height of eighth graders is 66 inches. State the null and alternative hypotheses. Fill in the correct symbol (=, ≠, ≥, <, ≤, >) for the null and alternative hypotheses.
- H 0 : μ __ 66
- H a : μ __ 66
Example 9.3
We want to test if college students take fewer than five years to graduate from college, on the average. The null and alternative hypotheses are the following: H 0 : μ ≥ 5 H a : μ < 5
We want to test if it takes fewer than 45 minutes to teach a lesson plan. State the null and alternative hypotheses. Fill in the correct symbol ( =, ≠, ≥, <, ≤, >) for the null and alternative hypotheses.
- H 0 : μ __ 45
- H a : μ __ 45
Example 9.4
An article on school standards stated that about half of all students in France, Germany, and Israel take advanced placement exams and a third of the students pass. The same article stated that 6.6 percent of U.S. students take advanced placement exams and 4.4 percent pass. Test if the percentage of U.S. students who take advanced placement exams is more than 6.6 percent. State the null and alternative hypotheses. H 0 : p ≤ 0.066 H a : p > 0.066
On a state driver’s test, about 40 percent pass the test on the first try. We want to test if more than 40 percent pass on the first try. Fill in the correct symbol (=, ≠, ≥, <, ≤, >) for the null and alternative hypotheses.
- H 0 : p __ 0.40
- H a : p __ 0.40
Collaborative Exercise
Bring to class a newspaper, some news magazines, and some internet articles. In groups, find articles from which your group can write null and alternative hypotheses. Discuss your hypotheses with the rest of the class.
As an Amazon Associate we earn from qualifying purchases.
This book may not be used in the training of large language models or otherwise be ingested into large language models or generative AI offerings without OpenStax's permission.
Want to cite, share, or modify this book? This book uses the Creative Commons Attribution License and you must attribute Texas Education Agency (TEA). The original material is available at: https://www.texasgateway.org/book/tea-statistics . Changes were made to the original material, including updates to art, structure, and other content updates.
Access for free at https://openstax.org/books/statistics/pages/1-introduction
- Authors: Barbara Illowsky, Susan Dean
- Publisher/website: OpenStax
- Book title: Statistics
- Publication date: Mar 27, 2020
- Location: Houston, Texas
- Book URL: https://openstax.org/books/statistics/pages/1-introduction
- Section URL: https://openstax.org/books/statistics/pages/9-1-null-and-alternative-hypotheses
© Jan 23, 2024 Texas Education Agency (TEA). The OpenStax name, OpenStax logo, OpenStax book covers, OpenStax CNX name, and OpenStax CNX logo are not subject to the Creative Commons license and may not be reproduced without the prior and express written consent of Rice University.

Statistics Made Easy
5 Tips for Interpreting P-Values Correctly in Hypothesis Testing

Hypothesis testing is a critical part of statistical analysis and is often the endpoint where conclusions are drawn about larger populations based on a sample or experimental dataset. Central to this process is the p-value. Broadly, the p-value quantifies the strength of evidence against the null hypothesis. Given the importance of the p-value, it is essential to ensure its interpretation is correct. Here are five essential tips for ensuring the p-value from a hypothesis test is understood correctly.
1. Know What the P-value Represents
First, it is essential to understand what a p-value is. In hypothesis testing, the p-value is defined as the probability of observing your data, or data more extreme, if the null hypothesis is true. As a reminder, the null hypothesis states no difference between your data and the expected population.
For example, in a hypothesis test to see if changing a company’s logo drives more traffic to the website, a null hypothesis would state that the new traffic numbers are equal to the old traffic numbers. In this context, the p-value would be the probability that the data you observed, or data more extreme, would occur if this null hypothesis were true.
Therefore, a smaller p-value indicates that what you observed is unlikely to have occurred if the null were true, offering evidence to reject the null hypothesis. Typically, a cut-off value of 0.05 is used where any p-value below this is considered significant evidence against the null.
2. Understand the Directionality of Your Hypothesis
Based on the research question under exploration, there are two types of hypotheses: one-sided and two-sided. A one-sided test specifies a particular direction of effect, such as traffic to a website increasing after a design change. On the other hand, a two-sided test allows the change to be in either direction and is effective when the researcher wants to see any effect of the change.
Either way, determining the statistical significance of a p-value is the same: if the p-value is below a threshold value, it is statistically significant. However, when calculating the p-value, it is important to ensure the correct sided calculations have been completed.
Additionally, the interpretation of the meaning of a p-value will differ based on the directionality of the hypothesis. If a one-sided test is significant, the researchers can use the p-value to support a statistically significant increase or decrease based on the direction of the test. If a two-sided test is significant, the p-value can only be used to say that the two groups are different, but not that one is necessarily greater.
3. Avoid Threshold Thinking
A common pitfall in interpreting p-values is falling into the threshold thinking trap. The most commonly used cut-off value for whether a calculated p-value is statistically significant is 0.05. Typically, a p-value of less than 0.05 is considered statistically significant evidence against the null hypothesis.
However, this is just an arbitrary value. Rigid adherence to this or any other predefined cut-off value can obscure business-relevant effect sizes. For example, a hypothesis test looking at changes in traffic after a website design may find that an increase of 10,000 views is not statistically significant with a p-value of 0.055 since that value is above 0.05. However, the actual increase of 10,000 may be important to the growth of the business.
Therefore, a p-value can be practically significant while not being statistically significant. Both types of significance and the broader context of the hypothesis test should be considered when making a final interpretation.
4. Consider the Power of Your Study
Similarly, some study conditions can result in a non-significant p-value even if practical significance exists. Statistical power is the ability of a study to detect an effect when it truly exists. In other words, it is the probability that the null hypothesis will be rejected when it is false.
Power is impacted by a lot of factors. These include sample size, the effect size you are looking for, and variability within the data. In the example of website traffic after a design change, if the number of visits overall is too small, there may not be enough views to have enough power to detect a difference.
Simple ways to increase the power of a hypothesis test and increase the chances of detecting an effect are increasing the sample size, looking for a smaller effect size, changing the experiment design to control for variables that can increase variability, or adjusting the type of statistical test being run.
5. Be Aware of Multiple Comparisons
Whenever multiple p-values are calculated in a single study due to multiple comparisons, there is an increased risk of false positives. This is because each individual comparison introduces random fluctuations, and each additional comparison compounds these fluctuations.
For example, in a hypothesis test looking at traffic before and after a website redesign, the team may be interested in making more than one comparison. This can include total visits, page views, and average time spent on the website. Since multiple comparisons are being made, there must be a correction made when interpreting the p-value.
The Bonferroni correction is one of the most commonly used methods to account for this increased probability of false positives. In this method, the significance cut-off value, typically 0.05, is divided by the number of comparisons made. The result is used as the new significance cut-off value. Applying this correction mitigates the risk of false positives and improves the reliability of findings from a hypothesis test.
In conclusion, interpreting p-values requires a nuanced understanding of many statistical concepts and careful consideration of the hypothesis test’s context. By following these five tips, the interpretation of the p-value from a hypothesis test can be more accurate and reliable, leading to better data-driven decision-making.
Featured Posts

Leave a Reply Cancel reply
Your email address will not be published. Required fields are marked *
Join the Statology Community
Sign up to receive Statology's exclusive study resource: 100 practice problems with step-by-step solutions. Plus, get our latest insights, tutorials, and data analysis tips straight to your inbox!
By subscribing you accept Statology's Privacy Policy.
- Math Article
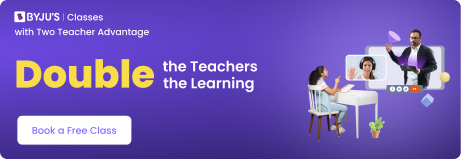
In Statistics, the researcher checks the significance of the observed result, which is known as test static . For this test, a hypothesis test is also utilized. The P-value or probability value concept is used everywhere in statistical analysis. It determines the statistical significance and the measure of significance testing. In this article, let us discuss its definition, formula, table, interpretation and how to use P-value to find the significance level etc. in detail.
Table of Contents:
P-value Definition
The P-value is known as the probability value. It is defined as the probability of getting a result that is either the same or more extreme than the actual observations. The P-value is known as the level of marginal significance within the hypothesis testing that represents the probability of occurrence of the given event. The P-value is used as an alternative to the rejection point to provide the least significance at which the null hypothesis would be rejected. If the P-value is small, then there is stronger evidence in favour of the alternative hypothesis.
P-value Table
The P-value table shows the hypothesis interpretations:
Generally, the level of statistical significance is often expressed in p-value and the range between 0 and 1. The smaller the p-value, the stronger the evidence and hence, the result should be statistically significant. Hence, the rejection of the null hypothesis is highly possible, as the p-value becomes smaller.
Let us look at an example to better comprehend the concept of P-value.
Let’s say a researcher flips a coin ten times with the null hypothesis that it is fair. The total number of heads is the test statistic, which is two-tailed. Assume the researcher notices alternating heads and tails on each flip (HTHTHTHTHT). As this is the predicted number of heads, the test statistic is 5 and the p-value is 1 (totally unexceptional).
Assume that the test statistic for this research was the “number of alternations” (i.e., the number of times H followed T or T followed H), which is two-tailed once again. This would result in a test statistic of 9, which is extremely high and has a p-value of 1/2 8 = 1/256, or roughly 0.0039. This would be regarded as extremely significant, much beyond the 0.05 level. These findings suggest that the data set is exceedingly improbable to have happened by random in terms of one test statistic, yet they do not imply that the coin is biased towards heads or tails.
The data have a high p-value according to the first test statistic, indicating that the number of heads observed is not impossible. The data have a low p-value according to the second test statistic, indicating that the pattern of flips observed is extremely unlikely. There is no “alternative hypothesis,” (therefore only the null hypothesis can be rejected), and such evidence could have a variety of explanations – the data could be falsified, or the coin could have been flipped by a magician who purposefully swapped outcomes.
This example shows that the p-value is entirely dependent on the test statistic used and that p-values can only be used to reject a null hypothesis, not to explore an alternate hypothesis.
P-value Formula
We Know that P-value is a statistical measure, that helps to determine whether the hypothesis is correct or not. P-value is a number that lies between 0 and 1. The level of significance(α) is a predefined threshold that should be set by the researcher. It is generally fixed as 0.05. The formula for the calculation for P-value is
Step 1: Find out the test static Z is
P0 = assumed population proportion in the null hypothesis
N = sample size
Step 2: Look at the Z-table to find the corresponding level of P from the z value obtained.
P-Value Example
An example to find the P-value is given here.
Question: A statistician wants to test the hypothesis H 0 : μ = 120 using the alternative hypothesis Hα: μ > 120 and assuming that α = 0.05. For that, he took the sample values as
n =40, σ = 32.17 and x̄ = 105.37. Determine the conclusion for this hypothesis?
We know that,
Now substitute the given values
Now, using the test static formula, we get
t = (105.37 – 120) / 5.0865
Therefore, t = -2.8762
Using the Z-Score table , we can find the value of P(t>-2.8762)
From the table, we get
P (t<-2.8762) = P(t>2.8762) = 0.003
If P(t>-2.8762) =1- 0.003 =0.997
P- value =0.997 > 0.05
Therefore, from the conclusion, if p>0.05, the null hypothesis is accepted or fails to reject.
Hence, the conclusion is “fails to reject H 0. ”
Frequently Asked Questions on P-Value
What is meant by p-value.
The p-value is defined as the probability of obtaining the result at least as extreme as the observed result of a statistical hypothesis test, assuming that the null hypothesis is true.
What does a smaller P-value represent?
The smaller the p-value, the greater the statistical significance of the observed difference, which results in the rejection of the null hypothesis in favour of alternative hypotheses.
What does the p-value greater than 0.05 represent?
If the p-value is greater than 0.05, then the result is not statistically significant.
Can the p-value be greater than 1?
P-value means probability value, which tells you the probability of achieving the result under a certain hypothesis. Since it is a probability, its value ranges between 0 and 1, and it cannot exceed 1.
What does the p-value less than 0.05 represent?
If the p-value is less than 0.05, then the result is statistically significant, and hence we can reject the null hypothesis in favour of the alternative hypothesis.

Register with BYJU'S & Download Free PDFs
Register with byju's & watch live videos.
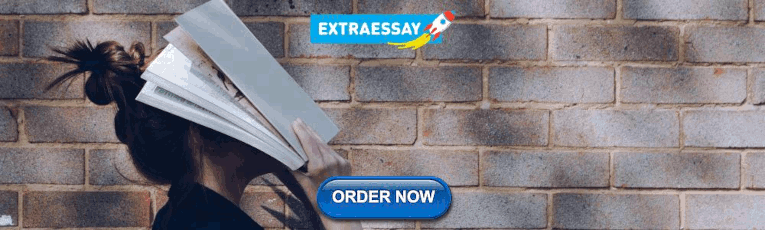
IMAGES
VIDEO
COMMENTS
The p value is a proportion: if your p value is 0.05, that means that 5% of the time you would see a test statistic at least as extreme as the one you found if the null hypothesis was true. Example: Test statistic and p value If the mice live equally long on either diet, then the test statistic from your t test will closely match the test ...
Two-Tailed. In our example concerning the mean grade point average, suppose again that our random sample of n = 15 students majoring in mathematics yields a test statistic t* instead of equaling -2.5.The P-value for conducting the two-tailed test H 0: μ = 3 versus H A: μ ≠ 3 is the probability that we would observe a test statistic less than -2.5 or greater than 2.5 if the population mean ...
One of the most commonly used p-value is 0.05. If the calculated p-value turns out to be less than 0.05, the null hypothesis is considered to be false, or nullified (hence the name null hypothesis). And if the value is greater than 0.05, the null hypothesis is considered to be true. Let me elaborate a bit on that.
Reject the null hypothesis when the p-value is less than or equal to your significance level. Your sample data favor the alternative hypothesis, which suggests that the effect exists in the population. ... Below are typical examples of writing a null hypothesis for various parameters and hypothesis tests. Related posts: Descriptive vs ...
The null hypothesis states that there is no statistical difference between groups based on the stated research hypothesis. ... For example, a p-value from a double-blinded randomized clinical trial (designed to minimize bias) should be weighted higher than one from a retrospective observational study .
A p-value, or probability value, is a number describing how likely it is that your data would have occurred by random chance (i.e., that the null hypothesis is true). The level of statistical significance is often expressed as a p-value between 0 and 1. The smaller the p -value, the less likely the results occurred by random chance, and the ...
In this case, our t-value of 2.289 produces a p value between 0.02 and 0.05 for a two-tailed test. Our results are statistically significant, and they are consistent with the calculator's more precise results. Displaying the P value in a Chart. In the example above, you saw how to calculate a p-value starting with the sample statistics.
The p-value represents the strength of your sample evidence against the null. Lower p-values represent stronger evidence. Like the significance level, the p-value is stated in terms of the likelihood of your sample evidence if the null is true. For example, a p-value of 0.03 indicates that the sample effect you observe, or more extreme, had a 3 ...
Onward! We use p -values to make conclusions in significance testing. More specifically, we compare the p -value to a significance level α to make conclusions about our hypotheses. If the p -value is lower than the significance level we chose, then we reject the null hypothesis H 0 in favor of the alternative hypothesis H a .
Then, if the null hypothesis is wrong, then the data will tend to group at a point that is not the value in the null hypothesis (1.2), and then our p-value will wind up being very small. If the null hypothesis is correct, or close to being correct, then the p-value will be larger, because the data values will group around the value we hypothesized.
The p value is the evidence against a null hypothesis. The smaller the p-value, the stronger the evidence that you should reject the null hypothesis. P values are expressed as decimals although it may be easier to understand what they are if you convert them to a percentage. For example, a p value of 0.0254 is 2.54%.
, the p-value = 0.03, then the probability of randomly selecting a sample of 100 users who spend, on average, 25 minutes on the yellow-background website is only 3%! In other words, the likelihood of getting a sample mean of 25 given that all sample means should be near 20 is only 3%. So, in a world where the null hypothesis is true--a hypothetical world where the yellow background has no ...
The textbook definition of a p-value is: A p-value is the probability of observing a sample statistic that is at least as extreme as your sample statistic, given that the null hypothesis is true. For example, suppose a factory claims that they produce tires that have a mean weight of 200 pounds. An auditor hypothesizes that the true mean weight ...
The p -value is used in the context of null hypothesis testing in order to quantify the statistical significance of a result, the result being the observed value of the chosen statistic . [note 2] The lower the p -value is, the lower the probability of getting that result if the null hypothesis were true. A result is said to be statistically ...
In technical terms, a P value is the probability of obtaining an effect at least as extreme as the one in your sample data, assuming the truth of the null hypothesis. For example, suppose that a vaccine study produced a P value of 0.04. This P value indicates that if the vaccine had no effect, you'd obtain the observed difference or more in 4 ...
The way to interpret that p-value is: observing 38 heads or less out of the 100 tosses could have happened in only 1% of infinitely many series of 100 fair coin tosses. The null hypothesis in this case is defined as the coin being fair, therefore having a 50% chance for heads and 50% chance for tails on each toss.. Assuming the null hypothesis is true allows the comparison of the observed data ...
It is the opposite of your research hypothesis. The alternative hypothesis--that is, the research hypothesis--is the idea, phenomenon, observation that you want to prove. If you suspect that girls take longer to get ready for school than boys, then: Alternative: girls time > boys time. Null: girls time <= boys time.
The actual test begins by considering two hypotheses.They are called the null hypothesis and the alternative hypothesis.These hypotheses contain opposing viewpoints. H 0, the —null hypothesis: a statement of no difference between sample means or proportions or no difference between a sample mean or proportion and a population mean or proportion. In other words, the difference equals 0.
The p-value (or the observed level of significance) is the smallest level of significance at which you can reject the null hypothesis, assuming the null hypothesis is true. You can also think about the p-value as the total area of the region of rejection. Remember that in a one-tailed test, the regi
Whenever we perform a hypothesis test, we always write a null hypothesis and an alternative hypothesis, which take the following forms: H0 (Null Hypothesis): Population parameter =, ≤, ≥ some value. HA (Alternative Hypothesis): Population parameter <, >, ≠ some value. Note that the null hypothesis always contains the equal sign.
Here are five essential tips for ensuring the p-value from a hypothesis test is understood correctly. 1. Know What the P-value Represents. First, it is essential to understand what a p-value is. In hypothesis testing, the p-value is defined as the probability of observing your data, or data more extreme, if the null hypothesis is true.
This example shows that the p-value is entirely dependent on the test statistic used and that p-values can only be used to reject a null hypothesis, not to explore an alternate hypothesis. P-value Formula. We Know that P-value is a statistical measure, that helps to determine whether the hypothesis is correct or not. P-value is a number that ...
P-values are the backbone of many statistical tests, providing a metric to gauge whether observed data deviates from what is expected under the null hypothesis. Essentially, a p-value is the ...